Synonyms of assignment
- as in lesson
- as in appointment
- More from M-W
- To save this word, you'll need to log in. Log In
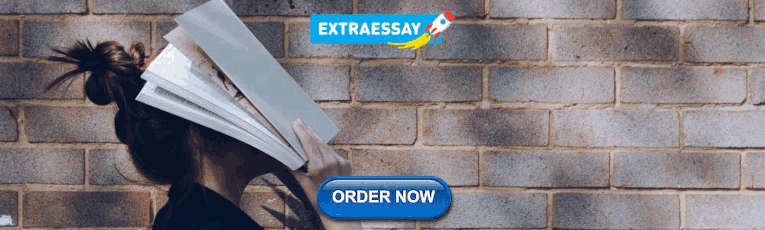
Thesaurus Definition of assignment
Synonyms & Similar Words
- responsibility
- undertaking
- requirement
- designation
- appointment
- authorization
- installment
- installation
- destination
- emplacement
- investiture
- singling (out)
Antonyms & Near Antonyms
- dethronement
Synonym Chooser
How does the noun assignment contrast with its synonyms?
Some common synonyms of assignment are chore , duty , job , stint , and task . While all these words mean "a piece of work to be done," assignment implies a definite limited task assigned by one in authority.
When is it sensible to use chore instead of assignment ?
While the synonyms chore and assignment are close in meaning, chore implies a minor routine activity necessary for maintaining a household or farm.
When is duty a more appropriate choice than assignment ?
Although the words duty and assignment have much in common, duty implies an obligation to perform or responsibility for performance.
When might job be a better fit than assignment ?
The synonyms job and assignment are sometimes interchangeable, but job applies to a piece of work voluntarily performed; it may sometimes suggest difficulty or importance.
When could stint be used to replace assignment ?
In some situations, the words stint and assignment are roughly equivalent. However, stint implies a carefully allotted or measured quantity of assigned work or service.
When can task be used instead of assignment ?
The meanings of task and assignment largely overlap; however, task implies work imposed by a person in authority or an employer or by circumstance.
Thesaurus Entries Near assignment
assignments
Cite this Entry
“Assignment.” Merriam-Webster.com Thesaurus , Merriam-Webster, https://www.merriam-webster.com/thesaurus/assignment. Accessed 12 Apr. 2024.
More from Merriam-Webster on assignment
Nglish: Translation of assignment for Spanish Speakers
Britannica English: Translation of assignment for Arabic Speakers
Subscribe to America's largest dictionary and get thousands more definitions and advanced search—ad free!
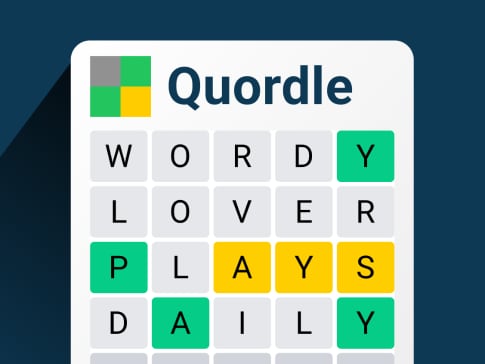
Can you solve 4 words at once?
Word of the day.
See Definitions and Examples »
Get Word of the Day daily email!
Popular in Grammar & Usage
Your vs. you're: how to use them correctly, every letter is silent, sometimes: a-z list of examples, more commonly mispronounced words, how to use em dashes (—), en dashes (–) , and hyphens (-), absent letters that are heard anyway, popular in wordplay, the words of the week - apr. 12, 10 scrabble words without any vowels, 12 more bird names that sound like insults (and sometimes are), 8 uncommon words related to love, 9 superb owl words, games & quizzes.
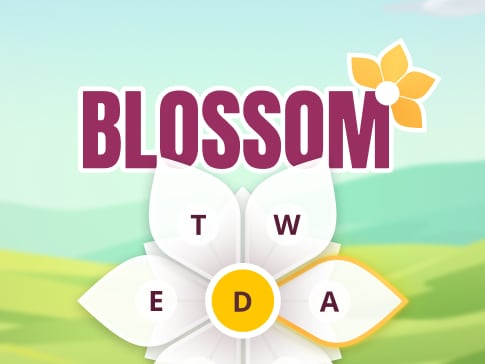
- Dictionaries home
- American English
- Collocations
- German-English
- Grammar home
- Practical English Usage
- Learn & Practise Grammar (Beta)
- Word Lists home
- My Word Lists
- Recent additions
- Resources home
- Text Checker
Definition of assign verb from the Oxford Advanced American Dictionary
Want to learn more?
Find out which words work together and produce more natural-sounding English with the Oxford Collocations Dictionary app. Try it for free as part of the Oxford Advanced Learner’s Dictionary app.

Definition of 'assignment'

Video: pronunciation of assignment
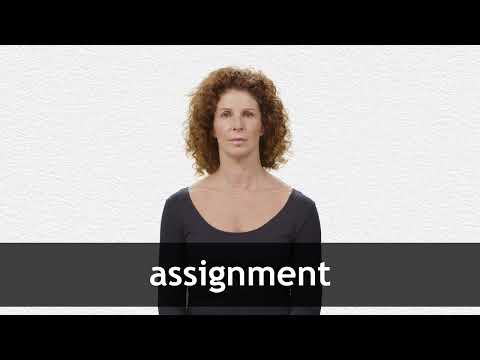
assignment in American English
Assignment in british english, examples of 'assignment' in a sentence assignment, related word partners assignment, trends of assignment.
View usage over: Since Exist Last 10 years Last 50 years Last 100 years Last 300 years
Browse alphabetically assignment
- assigned randomly
- assigned risk
- assimilability
- assimilable
- All ENGLISH words that begin with 'A'
Related terms of assignment
- seat assignment
- tough assignment
- writing assignment
- challenging assignment
- difficult assignment
- View more related words
Quick word challenge
Quiz Review
Score: 0 / 5

Wordle Helper

Scrabble Tools


Understanding Assignments
What this handout is about.
The first step in any successful college writing venture is reading the assignment. While this sounds like a simple task, it can be a tough one. This handout will help you unravel your assignment and begin to craft an effective response. Much of the following advice will involve translating typical assignment terms and practices into meaningful clues to the type of writing your instructor expects. See our short video for more tips.
Basic beginnings
Regardless of the assignment, department, or instructor, adopting these two habits will serve you well :
- Read the assignment carefully as soon as you receive it. Do not put this task off—reading the assignment at the beginning will save you time, stress, and problems later. An assignment can look pretty straightforward at first, particularly if the instructor has provided lots of information. That does not mean it will not take time and effort to complete; you may even have to learn a new skill to complete the assignment.
- Ask the instructor about anything you do not understand. Do not hesitate to approach your instructor. Instructors would prefer to set you straight before you hand the paper in. That’s also when you will find their feedback most useful.
Assignment formats
Many assignments follow a basic format. Assignments often begin with an overview of the topic, include a central verb or verbs that describe the task, and offer some additional suggestions, questions, or prompts to get you started.
An Overview of Some Kind
The instructor might set the stage with some general discussion of the subject of the assignment, introduce the topic, or remind you of something pertinent that you have discussed in class. For example:
“Throughout history, gerbils have played a key role in politics,” or “In the last few weeks of class, we have focused on the evening wear of the housefly …”
The Task of the Assignment
Pay attention; this part tells you what to do when you write the paper. Look for the key verb or verbs in the sentence. Words like analyze, summarize, or compare direct you to think about your topic in a certain way. Also pay attention to words such as how, what, when, where, and why; these words guide your attention toward specific information. (See the section in this handout titled “Key Terms” for more information.)
“Analyze the effect that gerbils had on the Russian Revolution”, or “Suggest an interpretation of housefly undergarments that differs from Darwin’s.”
Additional Material to Think about
Here you will find some questions to use as springboards as you begin to think about the topic. Instructors usually include these questions as suggestions rather than requirements. Do not feel compelled to answer every question unless the instructor asks you to do so. Pay attention to the order of the questions. Sometimes they suggest the thinking process your instructor imagines you will need to follow to begin thinking about the topic.
“You may wish to consider the differing views held by Communist gerbils vs. Monarchist gerbils, or Can there be such a thing as ‘the housefly garment industry’ or is it just a home-based craft?”
These are the instructor’s comments about writing expectations:
“Be concise”, “Write effectively”, or “Argue furiously.”
Technical Details
These instructions usually indicate format rules or guidelines.
“Your paper must be typed in Palatino font on gray paper and must not exceed 600 pages. It is due on the anniversary of Mao Tse-tung’s death.”
The assignment’s parts may not appear in exactly this order, and each part may be very long or really short. Nonetheless, being aware of this standard pattern can help you understand what your instructor wants you to do.
Interpreting the assignment
Ask yourself a few basic questions as you read and jot down the answers on the assignment sheet:
Why did your instructor ask you to do this particular task?
Who is your audience.
- What kind of evidence do you need to support your ideas?
What kind of writing style is acceptable?
- What are the absolute rules of the paper?
Try to look at the question from the point of view of the instructor. Recognize that your instructor has a reason for giving you this assignment and for giving it to you at a particular point in the semester. In every assignment, the instructor has a challenge for you. This challenge could be anything from demonstrating an ability to think clearly to demonstrating an ability to use the library. See the assignment not as a vague suggestion of what to do but as an opportunity to show that you can handle the course material as directed. Paper assignments give you more than a topic to discuss—they ask you to do something with the topic. Keep reminding yourself of that. Be careful to avoid the other extreme as well: do not read more into the assignment than what is there.
Of course, your instructor has given you an assignment so that he or she will be able to assess your understanding of the course material and give you an appropriate grade. But there is more to it than that. Your instructor has tried to design a learning experience of some kind. Your instructor wants you to think about something in a particular way for a particular reason. If you read the course description at the beginning of your syllabus, review the assigned readings, and consider the assignment itself, you may begin to see the plan, purpose, or approach to the subject matter that your instructor has created for you. If you still aren’t sure of the assignment’s goals, try asking the instructor. For help with this, see our handout on getting feedback .
Given your instructor’s efforts, it helps to answer the question: What is my purpose in completing this assignment? Is it to gather research from a variety of outside sources and present a coherent picture? Is it to take material I have been learning in class and apply it to a new situation? Is it to prove a point one way or another? Key words from the assignment can help you figure this out. Look for key terms in the form of active verbs that tell you what to do.
Key Terms: Finding Those Active Verbs
Here are some common key words and definitions to help you think about assignment terms:
Information words Ask you to demonstrate what you know about the subject, such as who, what, when, where, how, and why.
- define —give the subject’s meaning (according to someone or something). Sometimes you have to give more than one view on the subject’s meaning
- describe —provide details about the subject by answering question words (such as who, what, when, where, how, and why); you might also give details related to the five senses (what you see, hear, feel, taste, and smell)
- explain —give reasons why or examples of how something happened
- illustrate —give descriptive examples of the subject and show how each is connected with the subject
- summarize —briefly list the important ideas you learned about the subject
- trace —outline how something has changed or developed from an earlier time to its current form
- research —gather material from outside sources about the subject, often with the implication or requirement that you will analyze what you have found
Relation words Ask you to demonstrate how things are connected.
- compare —show how two or more things are similar (and, sometimes, different)
- contrast —show how two or more things are dissimilar
- apply—use details that you’ve been given to demonstrate how an idea, theory, or concept works in a particular situation
- cause —show how one event or series of events made something else happen
- relate —show or describe the connections between things
Interpretation words Ask you to defend ideas of your own about the subject. Do not see these words as requesting opinion alone (unless the assignment specifically says so), but as requiring opinion that is supported by concrete evidence. Remember examples, principles, definitions, or concepts from class or research and use them in your interpretation.
- assess —summarize your opinion of the subject and measure it against something
- prove, justify —give reasons or examples to demonstrate how or why something is the truth
- evaluate, respond —state your opinion of the subject as good, bad, or some combination of the two, with examples and reasons
- support —give reasons or evidence for something you believe (be sure to state clearly what it is that you believe)
- synthesize —put two or more things together that have not been put together in class or in your readings before; do not just summarize one and then the other and say that they are similar or different—you must provide a reason for putting them together that runs all the way through the paper
- analyze —determine how individual parts create or relate to the whole, figure out how something works, what it might mean, or why it is important
- argue —take a side and defend it with evidence against the other side
More Clues to Your Purpose As you read the assignment, think about what the teacher does in class:
- What kinds of textbooks or coursepack did your instructor choose for the course—ones that provide background information, explain theories or perspectives, or argue a point of view?
- In lecture, does your instructor ask your opinion, try to prove her point of view, or use keywords that show up again in the assignment?
- What kinds of assignments are typical in this discipline? Social science classes often expect more research. Humanities classes thrive on interpretation and analysis.
- How do the assignments, readings, and lectures work together in the course? Instructors spend time designing courses, sometimes even arguing with their peers about the most effective course materials. Figuring out the overall design to the course will help you understand what each assignment is meant to achieve.
Now, what about your reader? Most undergraduates think of their audience as the instructor. True, your instructor is a good person to keep in mind as you write. But for the purposes of a good paper, think of your audience as someone like your roommate: smart enough to understand a clear, logical argument, but not someone who already knows exactly what is going on in your particular paper. Remember, even if the instructor knows everything there is to know about your paper topic, he or she still has to read your paper and assess your understanding. In other words, teach the material to your reader.
Aiming a paper at your audience happens in two ways: you make decisions about the tone and the level of information you want to convey.
- Tone means the “voice” of your paper. Should you be chatty, formal, or objective? Usually you will find some happy medium—you do not want to alienate your reader by sounding condescending or superior, but you do not want to, um, like, totally wig on the man, you know? Eschew ostentatious erudition: some students think the way to sound academic is to use big words. Be careful—you can sound ridiculous, especially if you use the wrong big words.
- The level of information you use depends on who you think your audience is. If you imagine your audience as your instructor and she already knows everything you have to say, you may find yourself leaving out key information that can cause your argument to be unconvincing and illogical. But you do not have to explain every single word or issue. If you are telling your roommate what happened on your favorite science fiction TV show last night, you do not say, “First a dark-haired white man of average height, wearing a suit and carrying a flashlight, walked into the room. Then a purple alien with fifteen arms and at least three eyes turned around. Then the man smiled slightly. In the background, you could hear a clock ticking. The room was fairly dark and had at least two windows that I saw.” You also do not say, “This guy found some aliens. The end.” Find some balance of useful details that support your main point.
You’ll find a much more detailed discussion of these concepts in our handout on audience .
The Grim Truth
With a few exceptions (including some lab and ethnography reports), you are probably being asked to make an argument. You must convince your audience. It is easy to forget this aim when you are researching and writing; as you become involved in your subject matter, you may become enmeshed in the details and focus on learning or simply telling the information you have found. You need to do more than just repeat what you have read. Your writing should have a point, and you should be able to say it in a sentence. Sometimes instructors call this sentence a “thesis” or a “claim.”
So, if your instructor tells you to write about some aspect of oral hygiene, you do not want to just list: “First, you brush your teeth with a soft brush and some peanut butter. Then, you floss with unwaxed, bologna-flavored string. Finally, gargle with bourbon.” Instead, you could say, “Of all the oral cleaning methods, sandblasting removes the most plaque. Therefore it should be recommended by the American Dental Association.” Or, “From an aesthetic perspective, moldy teeth can be quite charming. However, their joys are short-lived.”
Convincing the reader of your argument is the goal of academic writing. It doesn’t have to say “argument” anywhere in the assignment for you to need one. Look at the assignment and think about what kind of argument you could make about it instead of just seeing it as a checklist of information you have to present. For help with understanding the role of argument in academic writing, see our handout on argument .
What kind of evidence do you need?
There are many kinds of evidence, and what type of evidence will work for your assignment can depend on several factors–the discipline, the parameters of the assignment, and your instructor’s preference. Should you use statistics? Historical examples? Do you need to conduct your own experiment? Can you rely on personal experience? See our handout on evidence for suggestions on how to use evidence appropriately.
Make sure you are clear about this part of the assignment, because your use of evidence will be crucial in writing a successful paper. You are not just learning how to argue; you are learning how to argue with specific types of materials and ideas. Ask your instructor what counts as acceptable evidence. You can also ask a librarian for help. No matter what kind of evidence you use, be sure to cite it correctly—see the UNC Libraries citation tutorial .
You cannot always tell from the assignment just what sort of writing style your instructor expects. The instructor may be really laid back in class but still expect you to sound formal in writing. Or the instructor may be fairly formal in class and ask you to write a reflection paper where you need to use “I” and speak from your own experience.
Try to avoid false associations of a particular field with a style (“art historians like wacky creativity,” or “political scientists are boring and just give facts”) and look instead to the types of readings you have been given in class. No one expects you to write like Plato—just use the readings as a guide for what is standard or preferable to your instructor. When in doubt, ask your instructor about the level of formality she or he expects.
No matter what field you are writing for or what facts you are including, if you do not write so that your reader can understand your main idea, you have wasted your time. So make clarity your main goal. For specific help with style, see our handout on style .
Technical details about the assignment
The technical information you are given in an assignment always seems like the easy part. This section can actually give you lots of little hints about approaching the task. Find out if elements such as page length and citation format (see the UNC Libraries citation tutorial ) are negotiable. Some professors do not have strong preferences as long as you are consistent and fully answer the assignment. Some professors are very specific and will deduct big points for deviations.
Usually, the page length tells you something important: The instructor thinks the size of the paper is appropriate to the assignment’s parameters. In plain English, your instructor is telling you how many pages it should take for you to answer the question as fully as you are expected to. So if an assignment is two pages long, you cannot pad your paper with examples or reword your main idea several times. Hit your one point early, defend it with the clearest example, and finish quickly. If an assignment is ten pages long, you can be more complex in your main points and examples—and if you can only produce five pages for that assignment, you need to see someone for help—as soon as possible.
Tricks that don’t work
Your instructors are not fooled when you:
- spend more time on the cover page than the essay —graphics, cool binders, and cute titles are no replacement for a well-written paper.
- use huge fonts, wide margins, or extra spacing to pad the page length —these tricks are immediately obvious to the eye. Most instructors use the same word processor you do. They know what’s possible. Such tactics are especially damning when the instructor has a stack of 60 papers to grade and yours is the only one that low-flying airplane pilots could read.
- use a paper from another class that covered “sort of similar” material . Again, the instructor has a particular task for you to fulfill in the assignment that usually relates to course material and lectures. Your other paper may not cover this material, and turning in the same paper for more than one course may constitute an Honor Code violation . Ask the instructor—it can’t hurt.
- get all wacky and “creative” before you answer the question . Showing that you are able to think beyond the boundaries of a simple assignment can be good, but you must do what the assignment calls for first. Again, check with your instructor. A humorous tone can be refreshing for someone grading a stack of papers, but it will not get you a good grade if you have not fulfilled the task.
Critical reading of assignments leads to skills in other types of reading and writing. If you get good at figuring out what the real goals of assignments are, you are going to be better at understanding the goals of all of your classes and fields of study.
You may reproduce it for non-commercial use if you use the entire handout and attribute the source: The Writing Center, University of North Carolina at Chapel Hill
Make a Gift
- Privacy Policy
Buy Me a Coffee

Home » Assignment – Types, Examples and Writing Guide
Assignment – Types, Examples and Writing Guide
Table of Contents

Definition:
Assignment is a task given to students by a teacher or professor, usually as a means of assessing their understanding and application of course material. Assignments can take various forms, including essays, research papers, presentations, problem sets, lab reports, and more.
Assignments are typically designed to be completed outside of class time and may require independent research, critical thinking, and analysis. They are often graded and used as a significant component of a student’s overall course grade. The instructions for an assignment usually specify the goals, requirements, and deadlines for completion, and students are expected to meet these criteria to earn a good grade.
History of Assignment
The use of assignments as a tool for teaching and learning has been a part of education for centuries. Following is a brief history of the Assignment.
- Ancient Times: Assignments such as writing exercises, recitations, and memorization tasks were used to reinforce learning.
- Medieval Period : Universities began to develop the concept of the assignment, with students completing essays, commentaries, and translations to demonstrate their knowledge and understanding of the subject matter.
- 19th Century : With the growth of schools and universities, assignments became more widespread and were used to assess student progress and achievement.
- 20th Century: The rise of distance education and online learning led to the further development of assignments as an integral part of the educational process.
- Present Day: Assignments continue to be used in a variety of educational settings and are seen as an effective way to promote student learning and assess student achievement. The nature and format of assignments continue to evolve in response to changing educational needs and technological innovations.
Types of Assignment
Here are some of the most common types of assignments:
An essay is a piece of writing that presents an argument, analysis, or interpretation of a topic or question. It usually consists of an introduction, body paragraphs, and a conclusion.
Essay structure:
- Introduction : introduces the topic and thesis statement
- Body paragraphs : each paragraph presents a different argument or idea, with evidence and analysis to support it
- Conclusion : summarizes the key points and reiterates the thesis statement
Research paper
A research paper involves gathering and analyzing information on a particular topic, and presenting the findings in a well-structured, documented paper. It usually involves conducting original research, collecting data, and presenting it in a clear, organized manner.
Research paper structure:
- Title page : includes the title of the paper, author’s name, date, and institution
- Abstract : summarizes the paper’s main points and conclusions
- Introduction : provides background information on the topic and research question
- Literature review: summarizes previous research on the topic
- Methodology : explains how the research was conducted
- Results : presents the findings of the research
- Discussion : interprets the results and draws conclusions
- Conclusion : summarizes the key findings and implications
A case study involves analyzing a real-life situation, problem or issue, and presenting a solution or recommendations based on the analysis. It often involves extensive research, data analysis, and critical thinking.
Case study structure:
- Introduction : introduces the case study and its purpose
- Background : provides context and background information on the case
- Analysis : examines the key issues and problems in the case
- Solution/recommendations: proposes solutions or recommendations based on the analysis
- Conclusion: Summarize the key points and implications
A lab report is a scientific document that summarizes the results of a laboratory experiment or research project. It typically includes an introduction, methodology, results, discussion, and conclusion.
Lab report structure:
- Title page : includes the title of the experiment, author’s name, date, and institution
- Abstract : summarizes the purpose, methodology, and results of the experiment
- Methods : explains how the experiment was conducted
- Results : presents the findings of the experiment
Presentation
A presentation involves delivering information, data or findings to an audience, often with the use of visual aids such as slides, charts, or diagrams. It requires clear communication skills, good organization, and effective use of technology.
Presentation structure:
- Introduction : introduces the topic and purpose of the presentation
- Body : presents the main points, findings, or data, with the help of visual aids
- Conclusion : summarizes the key points and provides a closing statement
Creative Project
A creative project is an assignment that requires students to produce something original, such as a painting, sculpture, video, or creative writing piece. It allows students to demonstrate their creativity and artistic skills.
Creative project structure:
- Introduction : introduces the project and its purpose
- Body : presents the creative work, with explanations or descriptions as needed
- Conclusion : summarizes the key elements and reflects on the creative process.
Examples of Assignments
Following are Examples of Assignment templates samples:
Essay template:
I. Introduction
- Hook: Grab the reader’s attention with a catchy opening sentence.
- Background: Provide some context or background information on the topic.
- Thesis statement: State the main argument or point of your essay.
II. Body paragraphs
- Topic sentence: Introduce the main idea or argument of the paragraph.
- Evidence: Provide evidence or examples to support your point.
- Analysis: Explain how the evidence supports your argument.
- Transition: Use a transition sentence to lead into the next paragraph.
III. Conclusion
- Restate thesis: Summarize your main argument or point.
- Review key points: Summarize the main points you made in your essay.
- Concluding thoughts: End with a final thought or call to action.
Research paper template:
I. Title page
- Title: Give your paper a descriptive title.
- Author: Include your name and institutional affiliation.
- Date: Provide the date the paper was submitted.
II. Abstract
- Background: Summarize the background and purpose of your research.
- Methodology: Describe the methods you used to conduct your research.
- Results: Summarize the main findings of your research.
- Conclusion: Provide a brief summary of the implications and conclusions of your research.
III. Introduction
- Background: Provide some background information on the topic.
- Research question: State your research question or hypothesis.
- Purpose: Explain the purpose of your research.
IV. Literature review
- Background: Summarize previous research on the topic.
- Gaps in research: Identify gaps or areas that need further research.
V. Methodology
- Participants: Describe the participants in your study.
- Procedure: Explain the procedure you used to conduct your research.
- Measures: Describe the measures you used to collect data.
VI. Results
- Quantitative results: Summarize the quantitative data you collected.
- Qualitative results: Summarize the qualitative data you collected.
VII. Discussion
- Interpretation: Interpret the results and explain what they mean.
- Implications: Discuss the implications of your research.
- Limitations: Identify any limitations or weaknesses of your research.
VIII. Conclusion
- Review key points: Summarize the main points you made in your paper.
Case study template:
- Background: Provide background information on the case.
- Research question: State the research question or problem you are examining.
- Purpose: Explain the purpose of the case study.
II. Analysis
- Problem: Identify the main problem or issue in the case.
- Factors: Describe the factors that contributed to the problem.
- Alternative solutions: Describe potential solutions to the problem.
III. Solution/recommendations
- Proposed solution: Describe the solution you are proposing.
- Rationale: Explain why this solution is the best one.
- Implementation: Describe how the solution can be implemented.
IV. Conclusion
- Summary: Summarize the main points of your case study.
Lab report template:
- Title: Give your report a descriptive title.
- Date: Provide the date the report was submitted.
- Background: Summarize the background and purpose of the experiment.
- Methodology: Describe the methods you used to conduct the experiment.
- Results: Summarize the main findings of the experiment.
- Conclusion: Provide a brief summary of the implications and conclusions
- Background: Provide some background information on the experiment.
- Hypothesis: State your hypothesis or research question.
- Purpose: Explain the purpose of the experiment.
IV. Materials and methods
- Materials: List the materials and equipment used in the experiment.
- Procedure: Describe the procedure you followed to conduct the experiment.
- Data: Present the data you collected in tables or graphs.
- Analysis: Analyze the data and describe the patterns or trends you observed.
VI. Discussion
- Implications: Discuss the implications of your findings.
- Limitations: Identify any limitations or weaknesses of the experiment.
VII. Conclusion
- Restate hypothesis: Summarize your hypothesis or research question.
- Review key points: Summarize the main points you made in your report.
Presentation template:
- Attention grabber: Grab the audience’s attention with a catchy opening.
- Purpose: Explain the purpose of your presentation.
- Overview: Provide an overview of what you will cover in your presentation.
II. Main points
- Main point 1: Present the first main point of your presentation.
- Supporting details: Provide supporting details or evidence to support your point.
- Main point 2: Present the second main point of your presentation.
- Main point 3: Present the third main point of your presentation.
- Summary: Summarize the main points of your presentation.
- Call to action: End with a final thought or call to action.
Creative writing template:
- Setting: Describe the setting of your story.
- Characters: Introduce the main characters of your story.
- Rising action: Introduce the conflict or problem in your story.
- Climax: Present the most intense moment of the story.
- Falling action: Resolve the conflict or problem in your story.
- Resolution: Describe how the conflict or problem was resolved.
- Final thoughts: End with a final thought or reflection on the story.
How to Write Assignment
Here is a general guide on how to write an assignment:
- Understand the assignment prompt: Before you begin writing, make sure you understand what the assignment requires. Read the prompt carefully and make note of any specific requirements or guidelines.
- Research and gather information: Depending on the type of assignment, you may need to do research to gather information to support your argument or points. Use credible sources such as academic journals, books, and reputable websites.
- Organize your ideas : Once you have gathered all the necessary information, organize your ideas into a clear and logical structure. Consider creating an outline or diagram to help you visualize your ideas.
- Write a draft: Begin writing your assignment using your organized ideas and research. Don’t worry too much about grammar or sentence structure at this point; the goal is to get your thoughts down on paper.
- Revise and edit: After you have written a draft, revise and edit your work. Make sure your ideas are presented in a clear and concise manner, and that your sentences and paragraphs flow smoothly.
- Proofread: Finally, proofread your work for spelling, grammar, and punctuation errors. It’s a good idea to have someone else read over your assignment as well to catch any mistakes you may have missed.
- Submit your assignment : Once you are satisfied with your work, submit your assignment according to the instructions provided by your instructor or professor.
Applications of Assignment
Assignments have many applications across different fields and industries. Here are a few examples:
- Education : Assignments are a common tool used in education to help students learn and demonstrate their knowledge. They can be used to assess a student’s understanding of a particular topic, to develop critical thinking skills, and to improve writing and research abilities.
- Business : Assignments can be used in the business world to assess employee skills, to evaluate job performance, and to provide training opportunities. They can also be used to develop business plans, marketing strategies, and financial projections.
- Journalism : Assignments are often used in journalism to produce news articles, features, and investigative reports. Journalists may be assigned to cover a particular event or topic, or to research and write a story on a specific subject.
- Research : Assignments can be used in research to collect and analyze data, to conduct experiments, and to present findings in written or oral form. Researchers may be assigned to conduct research on a specific topic, to write a research paper, or to present their findings at a conference or seminar.
- Government : Assignments can be used in government to develop policy proposals, to conduct research, and to analyze data. Government officials may be assigned to work on a specific project or to conduct research on a particular topic.
- Non-profit organizations: Assignments can be used in non-profit organizations to develop fundraising strategies, to plan events, and to conduct research. Volunteers may be assigned to work on a specific project or to help with a particular task.
Purpose of Assignment
The purpose of an assignment varies depending on the context in which it is given. However, some common purposes of assignments include:
- Assessing learning: Assignments are often used to assess a student’s understanding of a particular topic or concept. This allows educators to determine if a student has mastered the material or if they need additional support.
- Developing skills: Assignments can be used to develop a wide range of skills, such as critical thinking, problem-solving, research, and communication. Assignments that require students to analyze and synthesize information can help to build these skills.
- Encouraging creativity: Assignments can be designed to encourage students to be creative and think outside the box. This can help to foster innovation and original thinking.
- Providing feedback : Assignments provide an opportunity for teachers to provide feedback to students on their progress and performance. Feedback can help students to understand where they need to improve and to develop a growth mindset.
- Meeting learning objectives : Assignments can be designed to help students meet specific learning objectives or outcomes. For example, a writing assignment may be designed to help students improve their writing skills, while a research assignment may be designed to help students develop their research skills.
When to write Assignment
Assignments are typically given by instructors or professors as part of a course or academic program. The timing of when to write an assignment will depend on the specific requirements of the course or program, but in general, assignments should be completed within the timeframe specified by the instructor or program guidelines.
It is important to begin working on assignments as soon as possible to ensure enough time for research, writing, and revisions. Waiting until the last minute can result in rushed work and lower quality output.
It is also important to prioritize assignments based on their due dates and the amount of work required. This will help to manage time effectively and ensure that all assignments are completed on time.
In addition to assignments given by instructors or professors, there may be other situations where writing an assignment is necessary. For example, in the workplace, assignments may be given to complete a specific project or task. In these situations, it is important to establish clear deadlines and expectations to ensure that the assignment is completed on time and to a high standard.
Characteristics of Assignment
Here are some common characteristics of assignments:
- Purpose : Assignments have a specific purpose, such as assessing knowledge or developing skills. They are designed to help students learn and achieve specific learning objectives.
- Requirements: Assignments have specific requirements that must be met, such as a word count, format, or specific content. These requirements are usually provided by the instructor or professor.
- Deadline: Assignments have a specific deadline for completion, which is usually set by the instructor or professor. It is important to meet the deadline to avoid penalties or lower grades.
- Individual or group work: Assignments can be completed individually or as part of a group. Group assignments may require collaboration and communication with other group members.
- Feedback : Assignments provide an opportunity for feedback from the instructor or professor. This feedback can help students to identify areas of improvement and to develop their skills.
- Academic integrity: Assignments require academic integrity, which means that students must submit original work and avoid plagiarism. This includes citing sources properly and following ethical guidelines.
- Learning outcomes : Assignments are designed to help students achieve specific learning outcomes. These outcomes are usually related to the course objectives and may include developing critical thinking skills, writing abilities, or subject-specific knowledge.
Advantages of Assignment
There are several advantages of assignment, including:
- Helps in learning: Assignments help students to reinforce their learning and understanding of a particular topic. By completing assignments, students get to apply the concepts learned in class, which helps them to better understand and retain the information.
- Develops critical thinking skills: Assignments often require students to think critically and analyze information in order to come up with a solution or answer. This helps to develop their critical thinking skills, which are important for success in many areas of life.
- Encourages creativity: Assignments that require students to create something, such as a piece of writing or a project, can encourage creativity and innovation. This can help students to develop new ideas and perspectives, which can be beneficial in many areas of life.
- Builds time-management skills: Assignments often come with deadlines, which can help students to develop time-management skills. Learning how to manage time effectively is an important skill that can help students to succeed in many areas of life.
- Provides feedback: Assignments provide an opportunity for students to receive feedback on their work. This feedback can help students to identify areas where they need to improve and can help them to grow and develop.
Limitations of Assignment
There are also some limitations of assignments that should be considered, including:
- Limited scope: Assignments are often limited in scope, and may not provide a comprehensive understanding of a particular topic. They may only cover a specific aspect of a topic, and may not provide a full picture of the subject matter.
- Lack of engagement: Some assignments may not engage students in the learning process, particularly if they are repetitive or not challenging enough. This can lead to a lack of motivation and interest in the subject matter.
- Time-consuming: Assignments can be time-consuming, particularly if they require a lot of research or writing. This can be a disadvantage for students who have other commitments, such as work or extracurricular activities.
- Unreliable assessment: The assessment of assignments can be subjective and may not always accurately reflect a student’s understanding or abilities. The grading may be influenced by factors such as the instructor’s personal biases or the student’s writing style.
- Lack of feedback : Although assignments can provide feedback, this feedback may not always be detailed or useful. Instructors may not have the time or resources to provide detailed feedback on every assignment, which can limit the value of the feedback that students receive.
About the author
Muhammad Hassan
Researcher, Academic Writer, Web developer
You may also like

Data Collection – Methods Types and Examples

Delimitations in Research – Types, Examples and...

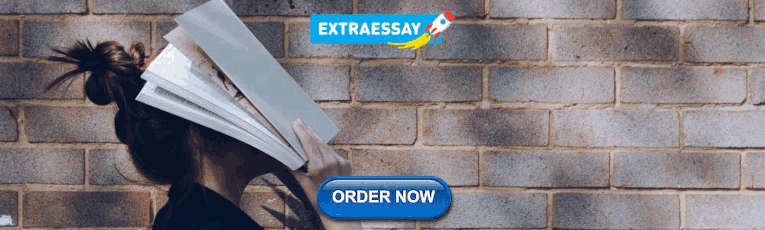
Research Process – Steps, Examples and Tips

Research Design – Types, Methods and Examples

Institutional Review Board – Application Sample...

Evaluating Research – Process, Examples and...
Primary tabs
Assignment is a legal term whereby an individual, the “assignor,” transfers rights, property, or other benefits to another known as the “ assignee .” This concept is used in both contract and property law. The term can refer to either the act of transfer or the rights /property/benefits being transferred.
Contract Law
Under contract law, assignment of a contract is both: (1) an assignment of rights; and (2) a delegation of duties , in the absence of evidence otherwise. For example, if A contracts with B to teach B guitar for $50, A can assign this contract to C. That is, this assignment is both: (1) an assignment of A’s rights under the contract to the $50; and (2) a delegation of A’s duty to teach guitar to C. In this example, A is both the “assignor” and the “delegee” who d elegates the duties to another (C), C is known as the “ obligor ” who must perform the obligations to the assignee , and B is the “ assignee ” who is owed duties and is liable to the “ obligor ”.
(1) Assignment of Rights/Duties Under Contract Law
There are a few notable rules regarding assignments under contract law. First, if an individual has not yet secured the contract to perform duties to another, he/she cannot assign his/her future right to an assignee . That is, if A has not yet contracted with B to teach B guitar, A cannot assign his/her rights to C. Second, rights cannot be assigned when they materially change the obligor ’s duty and rights. Third, the obligor can sue the assignee directly if the assignee does not pay him/her. Following the previous example, this means that C ( obligor ) can sue B ( assignee ) if C teaches guitar to B, but B does not pay C $50 in return.
(2) Delegation of Duties
If the promised performance requires a rare genius or skill, then the delegee cannot delegate it to the obligor. It can only be delegated if the promised performance is more commonplace. Further, an obligee can sue if the assignee does not perform. However, the delegee is secondarily liable unless there has been an express release of the delegee. That is, if B does want C to teach guitar but C refuses to, then B can sue C. If C still refuses to perform, then B can compel A to fulfill the duties under secondary liability.
Lastly, a related concept is novation , which is when a new obligor substitutes and releases an old obligor. If novation occurs, then the original obligor’s duties are wiped out. However, novation requires an original obligee’s consent .
Property Law
Under property law, assignment typically arises in landlord-tenant situations. For example, A might be renting from landlord B but wants to another party (C) to take over the property. In this scenario, A might be able to choose between assigning and subleasing the property to C. If assigning , A would be giving C the entire balance of the term, with no reversion to anyone whereas if subleasing , A would be giving C for a limited period of the remaining term. Significantly, under assignment C would have privity of estate with the landlord while under a sublease, C would not.
[Last updated in May of 2020 by the Wex Definitions Team ]
- business law
- landlord & tenant
- property & real estate law
- trusts, inheritances & estates
- wex definitions
HTML conversions sometimes display errors due to content that did not convert correctly from the source. This paper uses the following packages that are not yet supported by the HTML conversion tool. Feedback on these issues are not necessary; they are known and are being worked on.
- failed: optidef
- failed: complexity
- failed: crossreftools
Authors: achieve the best HTML results from your LaTeX submissions by following these best practices .
Approximation Algorithms for School Assignment: Group Fairness and Multi-criteria Optimization
We consider the problem of assigning students to schools, when students have different utilities for schools and schools have capacity. There are additional group fairness considerations over students that can be captured either by concave objectives, or additional constraints on the groups. We present approximation algorithms for this problem via convex program rounding that achieve various trade-offs between utility violation, capacity violation, and running time. We also show that our techniques easily extend to the setting where there are arbitrary covering constraints on the feasible assignment, capturing multi-criteria and ranking optimization.
1 Introduction
In this paper, we consider a general model of assignment with a group fairness objective or constraints, motivated by school choice. In the school assignment problem, we are required to assign students to schools, subject to: (1) matching every student, (2) respecting the capacities of schools, and (3) being fair on the utilities to a pre-defined set of g 𝑔 g italic_g groups of students, which can potentially overlap. These groups could capture demographic features like income, race, etc. Each student has arbitrary cardinal utilities bounded in [ 0 , 1 ] 0 1 [0,1] [ 0 , 1 ] over schools, and the group fairness could either be captured in the objective defined over the utilities obtained by each group, or as a set of constraints capturing the same.
Our main contribution is a set of approximation algorithms for this problem. For arbitrary fairness objectives, we present two algorithms based on rounding a natural convex programming relaxation, which yield somewhat different guarantees:
1 subscript 𝛿 𝑣 1+\delta_{v} 1 + italic_δ start_POSTSUBSCRIPT italic_v end_POSTSUBSCRIPT , where ∑ v δ v ≤ 2 g subscript 𝑣 subscript 𝛿 𝑣 2 𝑔 \sum_{v}\delta_{v}\leq 2g ∑ start_POSTSUBSCRIPT italic_v end_POSTSUBSCRIPT italic_δ start_POSTSUBSCRIPT italic_v end_POSTSUBSCRIPT ≤ 2 italic_g . This algorithm is a careful adaptation of the rounding algorithm in [ 7 ] .
A n O ( g ) superscript 𝑛 𝑂 𝑔 n^{O(g)} italic_n start_POSTSUPERSCRIPT italic_O ( italic_g ) end_POSTSUPERSCRIPT time algorithm where each group receives at least its utility in the relaxation, while each school v 𝑣 v italic_v ’s capacity is violated by δ v subscript 𝛿 𝑣 \delta_{v} italic_δ start_POSTSUBSCRIPT italic_v end_POSTSUBSCRIPT , where ∑ v δ v = O ( g 2 ) subscript 𝑣 subscript 𝛿 𝑣 𝑂 superscript 𝑔 2 \sum_{v}\delta_{v}=O(g^{2}) ∑ start_POSTSUBSCRIPT italic_v end_POSTSUBSCRIPT italic_δ start_POSTSUBSCRIPT italic_v end_POSTSUBSCRIPT = italic_O ( italic_g start_POSTSUPERSCRIPT 2 end_POSTSUPERSCRIPT ) .
For practical school choice scenarios [ 1 ] , the number of students significantly outweigh the number of available schools, while the number of groups g 𝑔 g italic_g is typically small, even constant. The above violations are therefore quite mild.
General Covering Constraints.
We subsequently present an extension of our framework to handle assignments with more general covering constraints. As one application, suppose the utility of a student for a school is multi-dimensional, capturing aspects like academic excellence, or location, or diversity of student body. The goal is to achieve at least a certain total utility value in each dimension. Such multi-objective optimization can be modeled using covering constraints.
1 subscript 𝛿 𝑣 1+\delta_{v} 1 + italic_δ start_POSTSUBSCRIPT italic_v end_POSTSUBSCRIPT , where ∑ v δ v ≤ 2 r subscript 𝑣 subscript 𝛿 𝑣 2 𝑟 \sum_{v}\delta_{v}\leq 2r ∑ start_POSTSUBSCRIPT italic_v end_POSTSUBSCRIPT italic_δ start_POSTSUBSCRIPT italic_v end_POSTSUBSCRIPT ≤ 2 italic_r . We also give a O ( n O ( r ) ) 𝑂 superscript 𝑛 𝑂 𝑟 O(n^{O(r)}) italic_O ( italic_n start_POSTSUPERSCRIPT italic_O ( italic_r ) end_POSTSUPERSCRIPT ) time algorithm that finds such a matching, while violating the capacity of school v 𝑣 v italic_v by δ v subscript 𝛿 𝑣 \delta_{v} italic_δ start_POSTSUBSCRIPT italic_v end_POSTSUBSCRIPT , where ∑ v δ v = O ( r 2 ) subscript 𝑣 subscript 𝛿 𝑣 𝑂 superscript 𝑟 2 \sum_{v}\delta_{v}=O(r^{2}) ∑ start_POSTSUBSCRIPT italic_v end_POSTSUBSCRIPT italic_δ start_POSTSUBSCRIPT italic_v end_POSTSUBSCRIPT = italic_O ( italic_r start_POSTSUPERSCRIPT 2 end_POSTSUPERSCRIPT ) . In comparison to the algorithm in [ 6 ] that runs in time n O ( r 2 ) superscript 𝑛 𝑂 superscript 𝑟 2 n^{O(r^{2})} italic_n start_POSTSUPERSCRIPT italic_O ( italic_r start_POSTSUPERSCRIPT 2 end_POSTSUPERSCRIPT ) end_POSTSUPERSCRIPT and uses multivariate polynomial interpolation, our algorithms present substantial improvements in both runtime and ease of implementation at the cost of a small capacity violation, when students are given a small choice r 𝑟 r italic_r of ranks.
1.1 Related Work and Techniques
Gap rounding..
Our algorithm uses LP rounding, and borrows ideas from the semimal Generalized Assignment Problem (GAP) rounding technique of Lenstra, Shmoys, and Tardos [ 7 , 11 ] . Their iterative rounding procedure involves the observation that the number of fractional variables in a vertex solution to a linear programming relaxation is bounded. We build on this idea and apply it to a linear program written on paths and cycles instead of assignments, enabling us to combine it with recent methods for finding proportional allocations mentioned below.
In addition to GAP rounding, closely related rounding techniques include that for the bounded degree minimum spanning tree problem [ 4 , 12 ] , which outputs a spanning tree whose cost is at most the optimal solution and whose degree constraints are violated by small additive constants.
School Assignment and Matching.
The school assignment problem as formulated above was first considered recently in Procaccia, Robinson, and Tucker-Foltz [ 10 ] . This work only considers the objective of proportionality — in the assignment, each of the g 𝑔 g italic_g groups is required to achieve at least 1 / g 1 𝑔 1/g 1 / italic_g fraction of the utility it could have achieved had it been the only group in the system. They present an algorithm (not based on rounding) tailored to proportionality by applying a theorem of Stromquist and Woodall [ 14 ] , which they call “cake frosting”. The theorem in [ 14 ] is a consequence of the celebrated ham-sandwich theorem [ 13 ] , and is hence non-constructive. Using this technique, the algorithm for proportional school choice in [ 10 ] loses an additive O ( g log g ) 𝑂 𝑔 𝑔 O(g\log g) italic_O ( italic_g roman_log italic_g ) on utilities (hence achieving approximate proportionality), in addition to violating the total capacity by O ( g log g ) 𝑂 𝑔 𝑔 O(g\log g) italic_O ( italic_g roman_log italic_g ) . In contrast, we use convex programming relaxation to handle arbitrary fairness objectives, for instance, proportional fairness, Pareto-optimality, and leximin fairness, hence vastly generalizing the space of objectives. Our method only loses O ( g 2 ) 𝑂 superscript 𝑔 2 O(g^{2}) italic_O ( italic_g start_POSTSUPERSCRIPT 2 end_POSTSUPERSCRIPT ) on the total capacity, while preserving utilities from the fractional relaxation. For instance, our method would achieve exact proportionality.
At a high level, our main technical contribution is to show how the cake frosting method can be applied to certain types of fractional solutions, in particular, a vertex solution constructed via GAP rounding of the convex programming relaxation. We hence showcase the full power of the technique in [ 14 ] . In addition, as discussed above, our techniques extend smoothly to handle arbitrary covering constraints on the allocations, which is motivated by multi-objective optimization and rank optimization.
We note that the idea of using cake frosting to round fractional solutions has appeared before for packing problems in Grandoni et al. [ 5 ] , where the authors develop a PTAS for matchings in general graphs with O ( 1 ) 𝑂 1 O(1) italic_O ( 1 ) budget constraints on the set of chosen edges. At a high level, all these approaches – the ones in [ 10 , 5 ] and our work – apply cake frosting to decompose paths and cycles to approximately preserve constraints, but differ in the details of how the paths and cycles are constructed from the integer or fractional solutions. For instance, in contrast to [ 10 ] that defines the frosting function based on schools, we define it based on students, hence avoiding an additive violation on the utility. Further, since [ 5 ] consider packing problems, their reduction to cake frosting is entirely different in the technical details.
Matching with Violations.
Recently, several papers have considered assignment problems with small capacity violations. These papers mostly fall into two categories - those that try to directly optimize the capacity violations in some form while achieving a set goal like stability or perfectness [ 2 , 3 , 6 ] and those that optimize some other objective like fairness with provably small capacity violations [ 5 , 9 , 10 ] . Our model falls in the latter category – we want to find a matching that satisfies some notion of fairness, while violating capacities by as little as possible.
1.2 Roadmap
We present the group fairness problem in school choice, along with the statement of our main result ( Theorem 1 ) in Section 2 . We present the two approximation algorithms that prove Theorem 1 in Section 3 . We generalize the model to covering constraints in Section 4 , specializing it to the ranking problem mentioned above in Section 4.3 .
2 Preliminaries and Main Result
An assignment of students to schools is a feasible solution y → ∈ 𝒫 → 𝑦 𝒫 \vec{y}\in\mathcal{P} over→ start_ARG italic_y end_ARG ∈ caligraphic_P , where 𝒫 𝒫 \mathcal{P} caligraphic_P is defined by:
Utility and Objective.
The utility of assigning student i 𝑖 i italic_i to school j 𝑗 j italic_j is u i j ∈ [ 0 , 1 ] subscript 𝑢 𝑖 𝑗 0 1 u_{ij}\in[0,1] italic_u start_POSTSUBSCRIPT italic_i italic_j end_POSTSUBSCRIPT ∈ [ 0 , 1 ] . Given an assignment y → ∈ 𝒫 → 𝑦 𝒫 \vec{y}\in\mathcal{P} over→ start_ARG italic_y end_ARG ∈ caligraphic_P , we define the utility of each group S k subscript 𝑆 𝑘 S_{k} italic_S start_POSTSUBSCRIPT italic_k end_POSTSUBSCRIPT as
As an example, the celebrated proportional fairness objective sets f = log 𝑓 f=\log italic_f = roman_log , and the optimal solution U * → → superscript 𝑈 \vec{U^{*}} over→ start_ARG italic_U start_POSTSUPERSCRIPT * end_POSTSUPERSCRIPT end_ARG has the following property: For any other feasible utility vector U ′ → → superscript 𝑈 ′ \vec{U^{\prime}} over→ start_ARG italic_U start_POSTSUPERSCRIPT ′ end_POSTSUPERSCRIPT end_ARG :
Such an allocation is not only proportional to the groups: U k ′ ≤ g ⋅ U k * subscript superscript 𝑈 ′ 𝑘 ⋅ 𝑔 subscript superscript 𝑈 𝑘 U^{\prime}_{k}\leq g\cdot U^{*}_{k} italic_U start_POSTSUPERSCRIPT ′ end_POSTSUPERSCRIPT start_POSTSUBSCRIPT italic_k end_POSTSUBSCRIPT ≤ italic_g ⋅ italic_U start_POSTSUPERSCRIPT * end_POSTSUPERSCRIPT start_POSTSUBSCRIPT italic_k end_POSTSUBSCRIPT , but also proportional to various subsets of groups.
2.1 Main Result
Our main result is the following:
Theorem 1 .
Let U * → normal-→ superscript 𝑈 \vec{U^{*}} over→ start_ARG italic_U start_POSTSUPERSCRIPT * end_POSTSUPERSCRIPT end_ARG be the optimal solution for any concave fairness function. Then, we can compute an assignment y → ∈ 𝒫 normal-→ 𝑦 𝒫 \vec{y}\in\mathcal{P} over→ start_ARG italic_y end_ARG ∈ caligraphic_P that satisfies relaxed school capacities C ′ → normal-→ superscript 𝐶 normal-′ \vec{C^{\prime}} over→ start_ARG italic_C start_POSTSUPERSCRIPT ′ end_POSTSUPERSCRIPT end_ARG and yields utilities U ′ → normal-→ superscript 𝑈 normal-′ \vec{U^{\prime}} over→ start_ARG italic_U start_POSTSUPERSCRIPT ′ end_POSTSUPERSCRIPT end_ARG with U k ′ ≥ U k * subscript superscript 𝑈 normal-′ 𝑘 subscript superscript 𝑈 𝑘 U^{\prime}_{k}\geq U^{*}_{k} italic_U start_POSTSUPERSCRIPT ′ end_POSTSUPERSCRIPT start_POSTSUBSCRIPT italic_k end_POSTSUBSCRIPT ≥ italic_U start_POSTSUPERSCRIPT * end_POSTSUPERSCRIPT start_POSTSUBSCRIPT italic_k end_POSTSUBSCRIPT for all groups k 𝑘 k italic_k , with one of the following guarantees on capacity:
subscript 𝐶 𝑗 1 subscript 𝛿 𝑗 C^{\prime}_{j}\leq C_{j}+1+\delta_{j} italic_C start_POSTSUPERSCRIPT ′ end_POSTSUPERSCRIPT start_POSTSUBSCRIPT italic_j end_POSTSUBSCRIPT ≤ italic_C start_POSTSUBSCRIPT italic_j end_POSTSUBSCRIPT + 1 + italic_δ start_POSTSUBSCRIPT italic_j end_POSTSUBSCRIPT , where ∑ j δ j ≤ 2 g subscript 𝑗 subscript 𝛿 𝑗 2 𝑔 \sum_{j}\delta_{j}\leq 2g ∑ start_POSTSUBSCRIPT italic_j end_POSTSUBSCRIPT italic_δ start_POSTSUBSCRIPT italic_j end_POSTSUBSCRIPT ≤ 2 italic_g .
subscript 𝐶 𝑗 subscript 𝛿 𝑗 C^{\prime}_{j}\leq C_{j}+\delta_{j} italic_C start_POSTSUPERSCRIPT ′ end_POSTSUPERSCRIPT start_POSTSUBSCRIPT italic_j end_POSTSUBSCRIPT ≤ italic_C start_POSTSUBSCRIPT italic_j end_POSTSUBSCRIPT + italic_δ start_POSTSUBSCRIPT italic_j end_POSTSUBSCRIPT with ∑ j δ j = O ( g 2 ) subscript 𝑗 subscript 𝛿 𝑗 𝑂 superscript 𝑔 2 \sum_{j}\delta_{j}=O(g^{2}) ∑ start_POSTSUBSCRIPT italic_j end_POSTSUBSCRIPT italic_δ start_POSTSUBSCRIPT italic_j end_POSTSUBSCRIPT = italic_O ( italic_g start_POSTSUPERSCRIPT 2 end_POSTSUPERSCRIPT ) .
Note that the latter algorithm is slower, but yields better violation of capacities if g ≪ | T | much-less-than 𝑔 𝑇 g\ll|T| italic_g ≪ | italic_T | .
2.2 Hardness Results
The hardness results below motivate the need to violate capacities in Theorem 1 if we want to preserve utilities exactly. We first show that the problem under the max-min fairness objective is strongly NP-Hard when the number of groups g 𝑔 g italic_g is part of the input.
Theorem 2 .
Suppose the number of groups g 𝑔 g italic_g is part of the input, and the objective is to decide if the minimum utility received by any group is at least one. Then the school redistricting problem is NP-Complete even when there are only two schools.
We reduce from Set Cover with a collection 𝒞 𝒞 \mathcal{C} caligraphic_C of sets, and a universe U 𝑈 U italic_U of elements. Suppose the goal is to decide if a set cover instance has k 𝑘 k italic_k sets that cover U 𝑈 U italic_U . Then each element becomes a group, and each set a student. A student belongs to a group if the corresponding set covers the corresponding element. There are two schools s 1 subscript 𝑠 1 s_{1} italic_s start_POSTSUBSCRIPT 1 end_POSTSUBSCRIPT and s 2 subscript 𝑠 2 s_{2} italic_s start_POSTSUBSCRIPT 2 end_POSTSUBSCRIPT . The former school has capacity k 𝑘 k italic_k and the latter has capacity ∞ \infty ∞ . Each student has utility 1 1 1 1 for s 1 subscript 𝑠 1 s_{1} italic_s start_POSTSUBSCRIPT 1 end_POSTSUBSCRIPT and 0 0 for s 2 subscript 𝑠 2 s_{2} italic_s start_POSTSUBSCRIPT 2 end_POSTSUBSCRIPT . Then the goal of matching k 𝑘 k italic_k students to s 1 subscript 𝑠 1 s_{1} italic_s start_POSTSUBSCRIPT 1 end_POSTSUBSCRIPT to give each group utility at least one is exactly the same as finding a set cover of size k 𝑘 k italic_k , completing the proof. ∎
We next show that the school redistricting problem under the max-min fairness (or proportionality) objective is weakly NP-Hard even when the number of groups g = 2 𝑔 2 g=2 italic_g = 2 .
Theorem 3 .
Suppose the number of groups g = 2 𝑔 2 g=2 italic_g = 2 , and the objective is to decide if an exactly proportional allocation exists. Then the school redistricting problem is weakly NP-Complete .
We reduce from Partition . Given a set of numbers x 1 , . . , x n x_{1},..,x_{n} italic_x start_POSTSUBSCRIPT 1 end_POSTSUBSCRIPT , . . , italic_x start_POSTSUBSCRIPT italic_n end_POSTSUBSCRIPT , the goal is to decide if there is a subset of sum exactly X / 2 𝑋 2 X/2 italic_X / 2 where X = ∑ i x i 𝑋 subscript 𝑖 subscript 𝑥 𝑖 X=\sum_{i}x_{i} italic_X = ∑ start_POSTSUBSCRIPT italic_i end_POSTSUBSCRIPT italic_x start_POSTSUBSCRIPT italic_i end_POSTSUBSCRIPT .
For every number x i subscript 𝑥 𝑖 x_{i} italic_x start_POSTSUBSCRIPT italic_i end_POSTSUBSCRIPT , create two students p i subscript 𝑝 𝑖 p_{i} italic_p start_POSTSUBSCRIPT italic_i end_POSTSUBSCRIPT and q i subscript 𝑞 𝑖 q_{i} italic_q start_POSTSUBSCRIPT italic_i end_POSTSUBSCRIPT , and one school S i subscript 𝑆 𝑖 S_{i} italic_S start_POSTSUBSCRIPT italic_i end_POSTSUBSCRIPT of capacity 1. There is also a dummy school S 0 subscript 𝑆 0 S_{0} italic_S start_POSTSUBSCRIPT 0 end_POSTSUBSCRIPT of capacity n 𝑛 n italic_n . The students p i subscript 𝑝 𝑖 p_{i} italic_p start_POSTSUBSCRIPT italic_i end_POSTSUBSCRIPT and q i subscript 𝑞 𝑖 q_{i} italic_q start_POSTSUBSCRIPT italic_i end_POSTSUBSCRIPT have edges only to S i subscript 𝑆 𝑖 S_{i} italic_S start_POSTSUBSCRIPT italic_i end_POSTSUBSCRIPT and S 0 subscript 𝑆 0 S_{0} italic_S start_POSTSUBSCRIPT 0 end_POSTSUBSCRIPT , where p i subscript 𝑝 𝑖 p_{i} italic_p start_POSTSUBSCRIPT italic_i end_POSTSUBSCRIPT and q i subscript 𝑞 𝑖 q_{i} italic_q start_POSTSUBSCRIPT italic_i end_POSTSUBSCRIPT have utility x i subscript 𝑥 𝑖 x_{i} italic_x start_POSTSUBSCRIPT italic_i end_POSTSUBSCRIPT for S i subscript 𝑆 𝑖 S_{i} italic_S start_POSTSUBSCRIPT italic_i end_POSTSUBSCRIPT and 0 for S 0 subscript 𝑆 0 S_{0} italic_S start_POSTSUBSCRIPT 0 end_POSTSUBSCRIPT .
All the p i subscript 𝑝 𝑖 p_{i} italic_p start_POSTSUBSCRIPT italic_i end_POSTSUBSCRIPT students belong to group 1 and all the q i subscript 𝑞 𝑖 q_{i} italic_q start_POSTSUBSCRIPT italic_i end_POSTSUBSCRIPT students belong to group 2. We want to find a matching that gives utility X / 2 𝑋 2 X/2 italic_X / 2 to both groups, which is the proportional share.
Suppose there is a subset T 𝑇 T italic_T of the numbers that sums to exactly X / 2 𝑋 2 X/2 italic_X / 2 . Then for every x i ∈ T subscript 𝑥 𝑖 𝑇 x_{i}\in T italic_x start_POSTSUBSCRIPT italic_i end_POSTSUBSCRIPT ∈ italic_T , we assign p i subscript 𝑝 𝑖 p_{i} italic_p start_POSTSUBSCRIPT italic_i end_POSTSUBSCRIPT to S i subscript 𝑆 𝑖 S_{i} italic_S start_POSTSUBSCRIPT italic_i end_POSTSUBSCRIPT and q i subscript 𝑞 𝑖 q_{i} italic_q start_POSTSUBSCRIPT italic_i end_POSTSUBSCRIPT to S 0 subscript 𝑆 0 S_{0} italic_S start_POSTSUBSCRIPT 0 end_POSTSUBSCRIPT . For every x i ∉ T subscript 𝑥 𝑖 𝑇 x_{i}\notin T italic_x start_POSTSUBSCRIPT italic_i end_POSTSUBSCRIPT ∉ italic_T , we assign q i subscript 𝑞 𝑖 q_{i} italic_q start_POSTSUBSCRIPT italic_i end_POSTSUBSCRIPT to S i subscript 𝑆 𝑖 S_{i} italic_S start_POSTSUBSCRIPT italic_i end_POSTSUBSCRIPT and p i subscript 𝑝 𝑖 p_{i} italic_p start_POSTSUBSCRIPT italic_i end_POSTSUBSCRIPT to S 0 subscript 𝑆 0 S_{0} italic_S start_POSTSUBSCRIPT 0 end_POSTSUBSCRIPT . Both groups get utility X / 2 𝑋 2 X/2 italic_X / 2 each. The reverse direction is similar, completing the proof. ∎
3 Proof of Theorem 1
In this section, we prove Theorem 1 . We begin with a convex programming relaxation to the problem, and then present two rounding schemes that yield the two guarantees in the theorem.
3.1 Convex Program Relaxation
The first step is to write the following convex programming relaxation:
This can clearly be solved in polynomial time. Let the optimal solution to the convex program yield utility vector U * → → superscript 𝑈 \vec{U^{*}} over→ start_ARG italic_U start_POSTSUPERSCRIPT * end_POSTSUPERSCRIPT end_ARG . We now need to round the following set of constraints, so that the { y i j } subscript 𝑦 𝑖 𝑗 \{y_{ij}\} { italic_y start_POSTSUBSCRIPT italic_i italic_j end_POSTSUBSCRIPT } values are integer. Denote this formulation as (LP1).
We now present two rounding algorithms that yields the corresponding guarantees in Theorem 1 .
3.2 Generalized Assignment Rounding
The first rounding algorithm is similar to rounding for generalized assignment (GAP) [ 7 , 11 ] , and is presented in Algorithm 1 . We remark that the iterative procedure from Step 1 - 6 is not necessary; the same can be achieved with a single LP solution. We present it this way for ease of exposition. We show that it achieves the following guarantee, yielding the first part of Theorem 1 .
Theorem 4 .
Algorithm 1 runs in polynomial time and finds an integer solution y → ∈ 𝒫 normal-→ 𝑦 𝒫 \vec{y}\in\mathcal{P} over→ start_ARG italic_y end_ARG ∈ caligraphic_P that satisfies relaxed school capacities C ′ → normal-→ superscript 𝐶 normal-′ \vec{C^{\prime}} over→ start_ARG italic_C start_POSTSUPERSCRIPT ′ end_POSTSUPERSCRIPT end_ARG and yields utilities U ′ → normal-→ superscript 𝑈 normal-′ \vec{U^{\prime}} over→ start_ARG italic_U start_POSTSUPERSCRIPT ′ end_POSTSUPERSCRIPT end_ARG , where
U k ′ ≥ U k * subscript superscript 𝑈 ′ 𝑘 subscript superscript 𝑈 𝑘 U^{\prime}_{k}\geq U^{*}_{k} italic_U start_POSTSUPERSCRIPT ′ end_POSTSUPERSCRIPT start_POSTSUBSCRIPT italic_k end_POSTSUBSCRIPT ≥ italic_U start_POSTSUPERSCRIPT * end_POSTSUPERSCRIPT start_POSTSUBSCRIPT italic_k end_POSTSUBSCRIPT for all groups k 𝑘 k italic_k , and
Proof of Theorem 4 ..
We denote the number of incident edges with y i j > 0 subscript 𝑦 𝑖 𝑗 0 y_{ij}>0 italic_y start_POSTSUBSCRIPT italic_i italic_j end_POSTSUBSCRIPT > 0 as the “degree” of a vertex. First note that since each student i 𝑖 i italic_i is assigned to argmax j { u i j | y i j > 0 } subscript argmax 𝑗 conditional-set subscript 𝑢 𝑖 𝑗 subscript 𝑦 𝑖 𝑗 0 \mbox{argmax}_{j}\{u_{ij}|y_{ij}>0\} argmax start_POSTSUBSCRIPT italic_j end_POSTSUBSCRIPT { italic_u start_POSTSUBSCRIPT italic_i italic_j end_POSTSUBSCRIPT | italic_y start_POSTSUBSCRIPT italic_i italic_j end_POSTSUBSCRIPT > 0 } , the utility in the integer solution is at least that in (LP1).
We now argue the capacity violation. We note that Step 4 cannot violate any capacities, since y → → 𝑦 \vec{y} over→ start_ARG italic_y end_ARG was feasible for (LP1). Thus, it only remains to argue that Step 7 does not incur too many capacity violations. Let E 𝐸 E italic_E be the set of remaining edges with y i j ∈ ( 0 , 1 ) subscript 𝑦 𝑖 𝑗 0 1 y_{ij}\in(0,1) italic_y start_POSTSUBSCRIPT italic_i italic_j end_POSTSUBSCRIPT ∈ ( 0 , 1 ) . Since y → → 𝑦 \vec{y} over→ start_ARG italic_y end_ARG was an extreme point solution to (LP1), | E | 𝐸 |E| | italic_E | constraints of (LP1) must be tight.
At the beginning of Step 7 , let T ′ superscript 𝑇 ′ T^{\prime} italic_T start_POSTSUPERSCRIPT ′ end_POSTSUPERSCRIPT be the set of remaining schools and among these, let T ^ ^ 𝑇 \hat{T} over^ start_ARG italic_T end_ARG be those whose capacity constraints are tight. Let S ′ superscript 𝑆 ′ S^{\prime} italic_S start_POSTSUPERSCRIPT ′ end_POSTSUPERSCRIPT be the set of remaining students. Note that each student has a tight constraint associated with it. Suppose g ′ superscript 𝑔 ′ g^{\prime} italic_g start_POSTSUPERSCRIPT ′ end_POSTSUPERSCRIPT of the g 𝑔 g italic_g constraints corresponding to the groups are tight. Since we have a vertex solution,
From the Handshaking lemma, we also have
2 subscript 𝛿 𝑣 2+\delta_{v} 2 + italic_δ start_POSTSUBSCRIPT italic_v end_POSTSUBSCRIPT , while school v ∈ T ′ ∖ T ^ 𝑣 superscript 𝑇 ′ ^ 𝑇 v\in T^{\prime}\setminus\hat{T} italic_v ∈ italic_T start_POSTSUPERSCRIPT ′ end_POSTSUPERSCRIPT ∖ over^ start_ARG italic_T end_ARG have degree δ v subscript 𝛿 𝑣 \delta_{v} italic_δ start_POSTSUBSCRIPT italic_v end_POSTSUBSCRIPT . We will refer to these δ v subscript 𝛿 𝑣 \delta_{v} italic_δ start_POSTSUBSCRIPT italic_v end_POSTSUBSCRIPT terms as the excess degrees. Then the above implies
To bound the capacity violation in 7 , we just observe that
If a school in T ^ ^ 𝑇 \hat{T} over^ start_ARG italic_T end_ARG had degree 2, then it must have had capacity at least 1, and in the worst case, both students with edges to it will match to it. This leads to a violation of 1 in this school’s capacity.
1 subscript 𝛿 𝑣 1+\delta_{v} 1 + italic_δ start_POSTSUBSCRIPT italic_v end_POSTSUBSCRIPT .
For schools v ∈ T ′ ∖ T ^ 𝑣 superscript 𝑇 ′ ^ 𝑇 v\in T^{\prime}\setminus\hat{T} italic_v ∈ italic_T start_POSTSUPERSCRIPT ′ end_POSTSUPERSCRIPT ∖ over^ start_ARG italic_T end_ARG , since the degree is δ v subscript 𝛿 𝑣 \delta_{v} italic_δ start_POSTSUBSCRIPT italic_v end_POSTSUBSCRIPT , this leads to a violation of at most δ v subscript 𝛿 𝑣 \delta_{v} italic_δ start_POSTSUBSCRIPT italic_v end_POSTSUBSCRIPT .
In total, this leads to a violation of 1 per school and the excess degrees ∑ v δ v subscript 𝑣 subscript 𝛿 𝑣 \sum_{v}\delta_{v} ∑ start_POSTSUBSCRIPT italic_v end_POSTSUBSCRIPT italic_δ start_POSTSUBSCRIPT italic_v end_POSTSUBSCRIPT lead to an additional 2 g 2 𝑔 2g 2 italic_g violation overall. This completes the proof. ∎
3.3 Improved Capacity Violation via Cake Cutting
In this part, we show the following theorem, corresponding to the second part of Theorem 1 . The main advantage is that we don’t need extra capacity at each school, albeit at the expense of a worse running time.
Theorem 5 .
There is a n O ( g ) superscript 𝑛 𝑂 𝑔 n^{O(g)} italic_n start_POSTSUPERSCRIPT italic_O ( italic_g ) end_POSTSUPERSCRIPT time algorithm that computes an integer assignment y → ∈ 𝒫 normal-→ 𝑦 𝒫 \vec{y}\in\mathcal{P} over→ start_ARG italic_y end_ARG ∈ caligraphic_P that satisfies relaxed school capacities C ′ → normal-→ superscript 𝐶 normal-′ \vec{C^{\prime}} over→ start_ARG italic_C start_POSTSUPERSCRIPT ′ end_POSTSUPERSCRIPT end_ARG and yields utilities U ′ → normal-→ superscript 𝑈 normal-′ \vec{U^{\prime}} over→ start_ARG italic_U start_POSTSUPERSCRIPT ′ end_POSTSUPERSCRIPT end_ARG , such that:
U k ′ ≥ U k * subscript superscript 𝑈 ′ 𝑘 subscript superscript 𝑈 𝑘 U^{\prime}_{k}\geq U^{*}_{k} italic_U start_POSTSUPERSCRIPT ′ end_POSTSUPERSCRIPT start_POSTSUBSCRIPT italic_k end_POSTSUBSCRIPT ≥ italic_U start_POSTSUPERSCRIPT * end_POSTSUPERSCRIPT start_POSTSUBSCRIPT italic_k end_POSTSUBSCRIPT for all groups k 𝑘 k italic_k ; and
We prove the above theorem by replace Step 7 in Algorithm 1 with a more involved cake cutting process, building on the work of [ 10 ] .
Paths and Cycles.
Let G 𝐺 G italic_G be the graph at the beginning of Step 7 in Algorithm 1 . Recall that the maximum degree in G 𝐺 G italic_G was 2, save for some vertices with excess degrees in Eq. 2 . We will process the graph into a graph of maximum degree 2, with some additional properties, in Algorithm 2 .
At the end of the process, let G ( V , E ) 𝐺 𝑉 𝐸 G(V,E) italic_G ( italic_V , italic_E ) be the resulting graph on fractional edges. Any vertex has degree at most two, and hence we get a graph with the following structure:
Every connected component is a path or a cycle.
Every student has degree exactly two, and is an internal node of a path or cycle.
Every school j ∈ T ′ 𝑗 superscript 𝑇 ′ j\in T^{\prime} italic_j ∈ italic_T start_POSTSUPERSCRIPT ′ end_POSTSUPERSCRIPT has capacity one and degree at most two.
School j ∈ T ′ ∖ T ^ 𝑗 superscript 𝑇 ′ ^ 𝑇 j\in T^{\prime}\setminus\hat{T} italic_j ∈ italic_T start_POSTSUPERSCRIPT ′ end_POSTSUPERSCRIPT ∖ over^ start_ARG italic_T end_ARG has degree one and capacity one, and is therefore a leaf of a path.
Algorithm 2 violates the total capacity by an additional 4 g 4 𝑔 4g 4 italic_g .
1 subscript 𝛿 𝑗 2 subscript 𝛿 𝑗 1+\delta_{j}\leq 2\delta_{j} 1 + italic_δ start_POSTSUBSCRIPT italic_j end_POSTSUBSCRIPT ≤ 2 italic_δ start_POSTSUBSCRIPT italic_j end_POSTSUBSCRIPT . For j ∈ S 2 𝑗 subscript 𝑆 2 j\in S_{2} italic_j ∈ italic_S start_POSTSUBSCRIPT 2 end_POSTSUBSCRIPT , the new capacity is the same as the original capacity. For j ∈ T ′ ∖ T ^ 𝑗 superscript 𝑇 ′ ^ 𝑇 j\in T^{\prime}\setminus\hat{T} italic_j ∈ italic_T start_POSTSUPERSCRIPT ′ end_POSTSUPERSCRIPT ∖ over^ start_ARG italic_T end_ARG , suppose degree ( j ) = δ j degree 𝑗 subscript 𝛿 𝑗 \mbox{degree}(j)=\delta_{j} degree ( italic_j ) = italic_δ start_POSTSUBSCRIPT italic_j end_POSTSUBSCRIPT , then we increase the capacity by δ j subscript 𝛿 𝑗 \delta_{j} italic_δ start_POSTSUBSCRIPT italic_j end_POSTSUBSCRIPT . By Eq. 2 , the total increase is therefore at most 4 g 4 𝑔 4g 4 italic_g . ∎
subscript 𝑥 subscript 𝑒 1 subscript 𝑥 subscript 𝑒 2 1 x_{e_{1}}+x_{e_{2}}=1 italic_x start_POSTSUBSCRIPT italic_e start_POSTSUBSCRIPT 1 end_POSTSUBSCRIPT end_POSTSUBSCRIPT + italic_x start_POSTSUBSCRIPT italic_e start_POSTSUBSCRIPT 2 end_POSTSUBSCRIPT end_POSTSUBSCRIPT = 1 if the two edges incident on v 𝑣 v italic_v are e 1 subscript 𝑒 1 e_{1} italic_e start_POSTSUBSCRIPT 1 end_POSTSUBSCRIPT and e 2 subscript 𝑒 2 e_{2} italic_e start_POSTSUBSCRIPT 2 end_POSTSUBSCRIPT . This follows because a degree-two school must belong to T ^ ^ 𝑇 \hat{T} over^ start_ARG italic_T end_ARG and corresponds to a tight constraint, and any student is associated with a tight constraint. This implies the following claim:
For every component (path or cycle) C 𝐶 C italic_C of G 𝐺 G italic_G , there is some α ∈ ( 0 , 1 ) 𝛼 0 1 \alpha\in(0,1) italic_α ∈ ( 0 , 1 ) such that every even edge e 𝑒 e italic_e in the component has x e = α subscript 𝑥 𝑒 𝛼 x_{e}=\alpha italic_x start_POSTSUBSCRIPT italic_e end_POSTSUBSCRIPT = italic_α and every odd edge has x e = 1 − α subscript 𝑥 𝑒 1 𝛼 x_{e}=1-\alpha italic_x start_POSTSUBSCRIPT italic_e end_POSTSUBSCRIPT = 1 - italic_α .
Bounding the number of fractional components.
We view this fractional solution in the following way. For a component C 𝐶 C italic_C , set z C = α subscript 𝑧 𝐶 𝛼 z_{C}=\alpha italic_z start_POSTSUBSCRIPT italic_C end_POSTSUBSCRIPT = italic_α if x e = α subscript 𝑥 𝑒 𝛼 x_{e}=\alpha italic_x start_POSTSUBSCRIPT italic_e end_POSTSUBSCRIPT = italic_α for every even edge. Let u C e v e n ( i ) superscript subscript 𝑢 𝐶 𝑒 𝑣 𝑒 𝑛 𝑖 u_{C}^{even}(i) italic_u start_POSTSUBSCRIPT italic_C end_POSTSUBSCRIPT start_POSTSUPERSCRIPT italic_e italic_v italic_e italic_n end_POSTSUPERSCRIPT ( italic_i ) be the utility that group i 𝑖 i italic_i gets in the assignment that selects all even edges (and none of the odd edges) of component C 𝐶 C italic_C . Let u C o d d ( ℓ ) superscript subscript 𝑢 𝐶 𝑜 𝑑 𝑑 ℓ u_{C}^{odd}(\ell) italic_u start_POSTSUBSCRIPT italic_C end_POSTSUBSCRIPT start_POSTSUPERSCRIPT italic_o italic_d italic_d end_POSTSUPERSCRIPT ( roman_ℓ ) be the utility that group ℓ ℓ \ell roman_ℓ gets in the assignment that selects all odd edges of component C 𝐶 C italic_C . We modify (LP1) to the following, where U ^ ℓ * subscript superscript ^ 𝑈 ℓ \hat{U}^{*}_{\ell} over^ start_ARG italic_U end_ARG start_POSTSUPERSCRIPT * end_POSTSUPERSCRIPT start_POSTSUBSCRIPT roman_ℓ end_POSTSUBSCRIPT is the modified utility after removing all the integral variables.
Let s 𝑠 s italic_s denote the number of variables. In any extreme point solution, at least s − g 𝑠 𝑔 s-g italic_s - italic_g of the constraints z C ∈ [ 0 , 1 ] subscript 𝑧 𝐶 0 1 z_{C}\in[0,1] italic_z start_POSTSUBSCRIPT italic_C end_POSTSUBSCRIPT ∈ [ 0 , 1 ] are tight, which means at most g 𝑔 g italic_g of the z C subscript 𝑧 𝐶 z_{C} italic_z start_POSTSUBSCRIPT italic_C end_POSTSUBSCRIPT variables can be fractional, in ( 0 , 1 ) 0 1 (0,1) ( 0 , 1 ) . For all integral z C subscript 𝑧 𝐶 z_{C} italic_z start_POSTSUBSCRIPT italic_C end_POSTSUBSCRIPT , we select the even matching if z C = 1 subscript 𝑧 𝐶 1 z_{C}=1 italic_z start_POSTSUBSCRIPT italic_C end_POSTSUBSCRIPT = 1 or the odd matching if z C = 0 subscript 𝑧 𝐶 0 z_{C}=0 italic_z start_POSTSUBSCRIPT italic_C end_POSTSUBSCRIPT = 0 . Remove these variables and rewrite the above LP just on the fractional variables.
Reduction to Cake Frosting.
For the g 𝑔 g italic_g components with fractional z C subscript 𝑧 𝐶 z_{C} italic_z start_POSTSUBSCRIPT italic_C end_POSTSUBSCRIPT , we need to find an integral solution that approximately preserves the utilities. This would be achieved if we could ‘interpolate’ z C subscript 𝑧 𝐶 z_{C} italic_z start_POSTSUBSCRIPT italic_C end_POSTSUBSCRIPT fraction of the way from the odd matching to the even matching. We can view this as a cake-frosting problem as in [ 10 ] where the g 𝑔 g italic_g groups are the players. First, we convert each cycle into a path as follows: Pick some student i 𝑖 i italic_i on this cycle, assign i 𝑖 i italic_i to argmax j { u i j | y i j > 0 } subscript argmax 𝑗 conditional-set subscript 𝑢 𝑖 𝑗 subscript 𝑦 𝑖 𝑗 0 \mbox{argmax}_{j}\{u_{ij}|y_{ij}>0\} argmax start_POSTSUBSCRIPT italic_j end_POSTSUBSCRIPT { italic_u start_POSTSUBSCRIPT italic_i italic_j end_POSTSUBSCRIPT | italic_y start_POSTSUBSCRIPT italic_i italic_j end_POSTSUBSCRIPT > 0 } , and delete this student. This step increases the capacity of at most g 𝑔 g italic_g schools by one, and reduces each cycle to a path that begins and ends at a school.
Before proceeding, we recall the “cake frosting” lemma first presented in [ 14 ] and generalized in [ 5 , 10 ] .
Lemma 8 (Cake Frosting Lemma) .
We now show how to apply the above lemma in a fashion similar to [ 10 , 5 ] . Fix a path C 𝐶 C italic_C . Let z C = α subscript 𝑧 𝐶 𝛼 z_{C}=\alpha italic_z start_POSTSUBSCRIPT italic_C end_POSTSUBSCRIPT = italic_α . Let there be r 𝑟 r italic_r students in C 𝐶 C italic_C , indexed from 1 1 1 1 to r 𝑟 r italic_r . We divide the interval [ 0 , 1 ] 0 1 [0,1] [ 0 , 1 ] into r 𝑟 r italic_r parts where [ i − 1 r , i r ) 𝑖 1 𝑟 𝑖 𝑟 [\frac{i-1}{r},\frac{i}{r}) [ divide start_ARG italic_i - 1 end_ARG start_ARG italic_r end_ARG , divide start_ARG italic_i end_ARG start_ARG italic_r end_ARG ) belongs to the i t h superscript 𝑖 𝑡 ℎ i^{th} italic_i start_POSTSUPERSCRIPT italic_t italic_h end_POSTSUPERSCRIPT student. 2 2 2 This is in contrast to the method in [ 10 ] , which defines intervals based on schools. Define for every group ℓ ℓ \ell roman_ℓ ,
For x ∈ [ i − 1 r , i r ) 𝑥 𝑖 1 𝑟 𝑖 𝑟 x\in[\frac{i-1}{r},\frac{i}{r}) italic_x ∈ [ divide start_ARG italic_i - 1 end_ARG start_ARG italic_r end_ARG , divide start_ARG italic_i end_ARG start_ARG italic_r end_ARG ) , define f ℓ ( x ) subscript 𝑓 ℓ 𝑥 f_{\ell}(x) italic_f start_POSTSUBSCRIPT roman_ℓ end_POSTSUBSCRIPT ( italic_x ) as
Rounding Procedure.
For path C 𝐶 C italic_C , we now apply the cake frosting lemma to the function f 𝑓 f italic_f as defined above, with α = z C 𝛼 subscript 𝑧 𝐶 \alpha=z_{C} italic_α = italic_z start_POSTSUBSCRIPT italic_C end_POSTSUBSCRIPT to find the perfect frosting X 𝑋 X italic_X that is a union of at most 2 g − 1 2 𝑔 1 2g-1 2 italic_g - 1 intervals. Given X 𝑋 X italic_X , we construct the assignment as in Algorithm 3 .
The final algorithm applies this procedure separately to each of the g 𝑔 g italic_g fractional paths. Note that the α 𝛼 \alpha italic_α value depends on the path.
We first bound the utility of each group ℓ ℓ \ell roman_ℓ in path C 𝐶 C italic_C . Define T 3 := [ r ] ∖ ( T 1 ∪ T 2 ) assign subscript 𝑇 3 delimited-[] 𝑟 subscript 𝑇 1 subscript 𝑇 2 T_{3}:=[r]\setminus(T_{1}\cup T_{2}) italic_T start_POSTSUBSCRIPT 3 end_POSTSUBSCRIPT := [ italic_r ] ∖ ( italic_T start_POSTSUBSCRIPT 1 end_POSTSUBSCRIPT ∪ italic_T start_POSTSUBSCRIPT 2 end_POSTSUBSCRIPT ) , i.e. the set of students in C 𝐶 C italic_C not in T 1 subscript 𝑇 1 T_{1} italic_T start_POSTSUBSCRIPT 1 end_POSTSUBSCRIPT or T 2 subscript 𝑇 2 T_{2} italic_T start_POSTSUBSCRIPT 2 end_POSTSUBSCRIPT . The utility of group ℓ ℓ \ell roman_ℓ in the integer solution is:
To bound the total capacity violation, note that Algorithm 3 violates the capacity by one at every interval boundary. By the Cake Frosting lemma, this is an additional violation of O ( g ) 𝑂 𝑔 O(g) italic_O ( italic_g ) per path, and hence O ( g 2 ) 𝑂 superscript 𝑔 2 O(g^{2}) italic_O ( italic_g start_POSTSUPERSCRIPT 2 end_POSTSUPERSCRIPT ) overall. This completes the proof of Theorem 5 , and hence Theorem 1 .
4 Generalization to Covering Constraints
We now generalize the setting in Theorems 4 and 5 . As before, we are given a set T 𝑇 T italic_T of schools, where school j 𝑗 j italic_j has capacity C j subscript 𝐶 𝑗 C_{j} italic_C start_POSTSUBSCRIPT italic_j end_POSTSUBSCRIPT , a set S 𝑆 S italic_S of students, and a bipartite graph G = ( S ∪ T , E ) 𝐺 𝑆 𝑇 𝐸 G=(S\cup T,E) italic_G = ( italic_S ∪ italic_T , italic_E ) between the students and schools. The objective is to find an integral assignment y → → 𝑦 \vec{y} over→ start_ARG italic_y end_ARG of all the students that satisfies an additional set of r 𝑟 r italic_r covering constraints with non-negative coefficients.
In other words, we wish solve the following integer program:
As motivation, each q i j ℓ superscript subscript 𝑞 𝑖 𝑗 ℓ q_{ij}^{\ell} italic_q start_POSTSUBSCRIPT italic_i italic_j end_POSTSUBSCRIPT start_POSTSUPERSCRIPT roman_ℓ end_POSTSUPERSCRIPT could capture a different type of utility student i 𝑖 i italic_i has for school j 𝑗 j italic_j , for instance, academic excellence, closeness, diversity of student body, etc, and we want a solution that achieves high average utility in all dimensions.
4.1 Generalizing Theorem 4
Our algorithm runs in the following steps, which build on Algorithm 1 .
Solve the linear programming relaxation, fix and remove the integral variables, and find a vertex solution. Let E 𝐸 E italic_E be the set of fractional variables, and S ′ superscript 𝑆 ′ S^{\prime} italic_S start_POSTSUPERSCRIPT ′ end_POSTSUPERSCRIPT be the remaining students.
Rewrite LP on the variables E 𝐸 E italic_E and without the capacity constraints Eq. 4 .
Keep eliminating integer variables, stopping at a vertex solution where all variables are fractional. Let E ′ superscript 𝐸 ′ E^{\prime} italic_E start_POSTSUPERSCRIPT ′ end_POSTSUPERSCRIPT be the remaining variables and S ′′ superscript 𝑆 ′′ S^{\prime\prime} italic_S start_POSTSUPERSCRIPT ′ ′ end_POSTSUPERSCRIPT be the remaining students.
Set an arbitrary y i j > 0 subscript 𝑦 𝑖 𝑗 0 y_{ij}>0 italic_y start_POSTSUBSCRIPT italic_i italic_j end_POSTSUBSCRIPT > 0 to 1 1 1 1 for each i ∈ S ′′ 𝑖 superscript 𝑆 ′′ i\in S^{\prime\prime} italic_i ∈ italic_S start_POSTSUPERSCRIPT ′ ′ end_POSTSUPERSCRIPT .
Theorem 9 .
For arbitrary covering constraints, when the linear programming relaxation has a feasible solution, there is a polynomial time algorithm that outputs an integer matching and that achieves the following guarantee:
The constraints Eq. 5 are preserved up to an additive r ⋅ Q max ⋅ 𝑟 subscript 𝑄 max r\cdot Q_{\mathrm{max}} italic_r ⋅ italic_Q start_POSTSUBSCRIPT roman_max end_POSTSUBSCRIPT ; and
If each school is given one unit extra capacity, the total violation in Eq. 4 over this is 2 r 2 𝑟 2r 2 italic_r .
First, the proof of Theorem 4 shows that regardless of how the students in S ′ superscript 𝑆 ′ S^{\prime} italic_S start_POSTSUPERSCRIPT ′ end_POSTSUPERSCRIPT are assigned, if each school is given one extra unit of capacity, then the total violation in capacity is at most 2 r 2 𝑟 2r 2 italic_r .
superscript 𝑆 ′′ 𝑟 |S^{\prime\prime}|+r | italic_S start_POSTSUPERSCRIPT ′ ′ end_POSTSUPERSCRIPT | + italic_r , we obtain | S ′′ | ≤ r superscript 𝑆 ′′ 𝑟 |S^{\prime\prime}|\leq r | italic_S start_POSTSUPERSCRIPT ′ ′ end_POSTSUPERSCRIPT | ≤ italic_r . Therefore Step (4) violates each constraint by an additive r ⋅ Q max ⋅ 𝑟 subscript 𝑄 max r\cdot Q_{\mathrm{max}} italic_r ⋅ italic_Q start_POSTSUBSCRIPT roman_max end_POSTSUBSCRIPT , completing the proof. ∎
4.2 Generalizing Theorem 5
We next generalize Theorem 5 . We first apply Algorithm 1 to the linear programming relaxation. We then follow the procedure in Section 3.3 and sequentially apply Algorithms 2 and 3 to the fractional solution. To set up the cake frosting game to apply Algorithm 3 , we view each of the r 𝑟 r italic_r Eq. 5 constraints as a player of the cake frosting instance, and the function u e v e n ( ℓ , i ) := q i j ℓ assign subscript 𝑢 𝑒 𝑣 𝑒 𝑛 ℓ 𝑖 superscript subscript 𝑞 𝑖 𝑗 ℓ u_{even}(\ell,i):=q_{ij}^{\ell} italic_u start_POSTSUBSCRIPT italic_e italic_v italic_e italic_n end_POSTSUBSCRIPT ( roman_ℓ , italic_i ) := italic_q start_POSTSUBSCRIPT italic_i italic_j end_POSTSUBSCRIPT start_POSTSUPERSCRIPT roman_ℓ end_POSTSUPERSCRIPT where ( i , j ) 𝑖 𝑗 (i,j) ( italic_i , italic_j ) is the even matching edge adjacent to j 𝑗 j italic_j and u o d d ( ℓ , i ) subscript 𝑢 𝑜 𝑑 𝑑 ℓ 𝑖 u_{odd}(\ell,i) italic_u start_POSTSUBSCRIPT italic_o italic_d italic_d end_POSTSUBSCRIPT ( roman_ℓ , italic_i ) is defined similarly. The only steps that are different are the assignment steps – Step 3 in Algorithm 2 and Step 7 in Algorithm 3 . Here, we perform an arbitrary assignment of the students to the schools. We present the complete algorithm in Algorithm 4 for completeness.
Theorem 10 .
When the linear programming relaxation has a feasible solution, if r 𝑟 r italic_r is the number of constraints Eq. 5 , there is a n O ( r ) superscript 𝑛 𝑂 𝑟 n^{O(r)} italic_n start_POSTSUPERSCRIPT italic_O ( italic_r ) end_POSTSUPERSCRIPT time algorithm that outputs an integer matching and that achieves the following guarantee:
The constraints Eq. 5 are preserved up to an additive O ( r 2 ⋅ Q max ) 𝑂 ⋅ superscript 𝑟 2 subscript 𝑄 max O(r^{2}\cdot Q_{\mathrm{max}}) italic_O ( italic_r start_POSTSUPERSCRIPT 2 end_POSTSUPERSCRIPT ⋅ italic_Q start_POSTSUBSCRIPT roman_max end_POSTSUBSCRIPT ) ; and
The total violation in Eq. 4 is O ( r 2 ) 𝑂 superscript 𝑟 2 O(r^{2}) italic_O ( italic_r start_POSTSUPERSCRIPT 2 end_POSTSUPERSCRIPT ) .
We first argue about the violation in Eq. 5 . The only steps that affect the constraints are the assignment steps – Steps 9 and 27 in Algorithm 4 . In Step 9 , the number of students assigned is O ( r ) 𝑂 𝑟 O(r) italic_O ( italic_r ) from Eq. 2 , while that in Step 27 is O ( r 2 ) 𝑂 superscript 𝑟 2 O(r^{2}) italic_O ( italic_r start_POSTSUPERSCRIPT 2 end_POSTSUPERSCRIPT ) . If these students are arbitrarily assigned, each assignment loses an additive Q max subscript 𝑄 max Q_{\mathrm{max}} italic_Q start_POSTSUBSCRIPT roman_max end_POSTSUBSCRIPT in the constraint. Therefore, the overall additive loss is O ( r 2 ⋅ Q max ) 𝑂 ⋅ superscript 𝑟 2 subscript 𝑄 max O(r^{2}\cdot Q_{\mathrm{max}}) italic_O ( italic_r start_POSTSUPERSCRIPT 2 end_POSTSUPERSCRIPT ⋅ italic_Q start_POSTSUBSCRIPT roman_max end_POSTSUBSCRIPT ) . Note that the capacity bound violation follows from the proof of Theorem 5 and holds even when these students are arbitrarily assigned. ∎
Using Theorem 4.12 in [ 5 ] , we can improve Theorem 10 to the following corollary. It follows by guessing 8 r 2 / ϵ 8 superscript 𝑟 2 italic-ϵ 8r^{2}/\epsilon 8 italic_r start_POSTSUPERSCRIPT 2 end_POSTSUPERSCRIPT / italic_ϵ chosen edges with highest utility for each group, and applying Algorithms 2 and 3 subsequently. This yields the following corollary, and we omit the standard details.
Corollary 11 .
When the linear programming relaxation has a feasible solution, if r 𝑟 r italic_r is the number of constraints Eq. 5 , then for any constant ϵ > 0 italic-ϵ 0 \epsilon>0 italic_ϵ > 0 , there is a n O ( r 3 / ϵ ) superscript 𝑛 𝑂 superscript 𝑟 3 italic-ϵ n^{O(r^{3}/\epsilon)} italic_n start_POSTSUPERSCRIPT italic_O ( italic_r start_POSTSUPERSCRIPT 3 end_POSTSUPERSCRIPT / italic_ϵ ) end_POSTSUPERSCRIPT time algorithm that outputs an integer matching and that achieves the following guarantee:
The constraints Eq. 5 are preserved up to a multiplicative factor of ( 1 − ϵ ) 1 italic-ϵ (1-\epsilon) ( 1 - italic_ϵ ) ; and
4.3 Better Bounds for Monotonic Constraints
𝑘 1 ℓ q_{ij_{k}}^{\ell}\geq q_{ij_{k+1}}^{\ell} italic_q start_POSTSUBSCRIPT italic_i italic_j start_POSTSUBSCRIPT italic_k end_POSTSUBSCRIPT end_POSTSUBSCRIPT start_POSTSUPERSCRIPT roman_ℓ end_POSTSUPERSCRIPT ≥ italic_q start_POSTSUBSCRIPT italic_i italic_j start_POSTSUBSCRIPT italic_k + 1 end_POSTSUBSCRIPT end_POSTSUBSCRIPT start_POSTSUPERSCRIPT roman_ℓ end_POSTSUPERSCRIPT .
Theorem 12 .
If the constraints Q 𝑄 Q italic_Q are monotone, when the linear programming relaxation has a feasible solution, there is a polynomial time algorithm that outputs an integer matching and that achieves the following guarantee:
The constraints Eq. 5 are preserved; and
Theorem 13 .
If the constraints Q 𝑄 Q italic_Q are monotone, when the linear programming relaxation has a feasible solution, there is a n O ( r ) superscript 𝑛 𝑂 𝑟 n^{O(r)} italic_n start_POSTSUPERSCRIPT italic_O ( italic_r ) end_POSTSUPERSCRIPT time algorithm that outputs an integer matching and that achieves the following guarantee:
The total violation in Eq. 4 over this is O ( r 2 ) 𝑂 superscript 𝑟 2 O(r^{2}) italic_O ( italic_r start_POSTSUPERSCRIPT 2 end_POSTSUPERSCRIPT ) .
Proof of Theorems 12 and 13 .
We proceed as in Theorems 4 and 5 . At each point where the algorithm assigns a student to j * = arg max j ∈ X u i j superscript 𝑗 subscript 𝑗 𝑋 subscript 𝑢 𝑖 𝑗 j^{*}=\arg\max_{j\in X}u_{ij} italic_j start_POSTSUPERSCRIPT * end_POSTSUPERSCRIPT = roman_arg roman_max start_POSTSUBSCRIPT italic_j ∈ italic_X end_POSTSUBSCRIPT italic_u start_POSTSUBSCRIPT italic_i italic_j end_POSTSUBSCRIPT for some set X 𝑋 X italic_X , we simply assign it to min k { j k | j k ∈ X } subscript 𝑘 conditional subscript 𝑗 𝑘 subscript 𝑗 𝑘 𝑋 \min_{k}\{j_{k}\ |j_{k}\in X\} roman_min start_POSTSUBSCRIPT italic_k end_POSTSUBSCRIPT { italic_j start_POSTSUBSCRIPT italic_k end_POSTSUBSCRIPT | italic_j start_POSTSUBSCRIPT italic_k end_POSTSUBSCRIPT ∈ italic_X } . That is, assign it to the most preferred school (according to ⪰ i subscript succeeds-or-equals 𝑖 \succeq_{i} ⪰ start_POSTSUBSCRIPT italic_i end_POSTSUBSCRIPT ). This also preserves the r 𝑟 r italic_r constraints because of monotonicity. ∎
Application: Weak Dominance of Ranks.
As a special case, we consider the setting in [ 6 ] . Here, every student ranks the schools it has an edge to, and this ranking may have ties. Let r 𝑟 r italic_r be the largest rank any student has, which can be much smaller than the number of schools. Given a matching, the rank of edge ( p , q ) 𝑝 𝑞 (p,q) ( italic_p , italic_q ) is the rank of school q 𝑞 q italic_q in student p 𝑝 p italic_p ’s ranking. A matching M 𝑀 M italic_M has signature σ = ( σ 1 , σ 2 , … , σ r ) 𝜎 subscript 𝜎 1 subscript 𝜎 2 … subscript 𝜎 𝑟 \sigma=(\sigma_{1},\sigma_{2},\ldots,\sigma_{r}) italic_σ = ( italic_σ start_POSTSUBSCRIPT 1 end_POSTSUBSCRIPT , italic_σ start_POSTSUBSCRIPT 2 end_POSTSUBSCRIPT , … , italic_σ start_POSTSUBSCRIPT italic_r end_POSTSUBSCRIPT ) if it has σ t subscript 𝜎 𝑡 \sigma_{t} italic_σ start_POSTSUBSCRIPT italic_t end_POSTSUBSCRIPT rank t 𝑡 t italic_t edges for every t ∈ [ r ] 𝑡 delimited-[] 𝑟 t\in[r] italic_t ∈ [ italic_r ] . We say that signature σ 𝜎 \sigma italic_σ weakly dominates 3 3 3 The authors of [ 6 ] use ‘cumulatively better than’. signature ρ 𝜌 \rho italic_ρ , or σ ≻ ρ succeeds 𝜎 𝜌 \sigma\succ\rho italic_σ ≻ italic_ρ if
Given an input signature ρ 𝜌 \rho italic_ρ , the goal is to find a matching whose signature weakly dominates ρ 𝜌 \rho italic_ρ . We term this the matching with ranking problem. We have the following theorems, which directly follow from the observation that the constraints satisfy the monotonicity assumption. At each step where, for some set X 𝑋 X italic_X , we assign i 𝑖 i italic_i to arg max j ∈ X u i j subscript 𝑗 𝑋 subscript 𝑢 𝑖 𝑗 \arg\max_{j\in X}{u_{ij}} roman_arg roman_max start_POSTSUBSCRIPT italic_j ∈ italic_X end_POSTSUBSCRIPT italic_u start_POSTSUBSCRIPT italic_i italic_j end_POSTSUBSCRIPT , we instead assign it to its most preferred school from X 𝑋 X italic_X . This preserves the signature of any fractional assignment. Our approach yields faster n O ( r ) superscript 𝑛 𝑂 𝑟 n^{O(r)} italic_n start_POSTSUPERSCRIPT italic_O ( italic_r ) end_POSTSUPERSCRIPT time deterministic algorithms at the cost of small violations in capacities whereas the algorithm in [ 6 ] is randomized and takes n O ( r 2 ) superscript 𝑛 𝑂 superscript 𝑟 2 n^{O(r^{2})} italic_n start_POSTSUPERSCRIPT italic_O ( italic_r start_POSTSUPERSCRIPT 2 end_POSTSUPERSCRIPT ) end_POSTSUPERSCRIPT time. Their approach essentially uses the algorithm for Exact Matchings [ 8 ] . It has been a longstanding open question whether it is possible to derandomize it.
Theorem 14 .
subscript 𝐶 𝑗 1 subscript 𝛿 𝑗 C^{\prime}_{j}\leq C_{j}+1+\delta_{j} italic_C start_POSTSUPERSCRIPT ′ end_POSTSUPERSCRIPT start_POSTSUBSCRIPT italic_j end_POSTSUBSCRIPT ≤ italic_C start_POSTSUBSCRIPT italic_j end_POSTSUBSCRIPT + 1 + italic_δ start_POSTSUBSCRIPT italic_j end_POSTSUBSCRIPT , and ∑ j δ j ≤ 2 r subscript 𝑗 subscript 𝛿 𝑗 2 𝑟 \sum_{j}\delta_{j}\leq 2r ∑ start_POSTSUBSCRIPT italic_j end_POSTSUBSCRIPT italic_δ start_POSTSUBSCRIPT italic_j end_POSTSUBSCRIPT ≤ 2 italic_r .
Theorem 15 .
subscript 𝐶 𝑗 subscript 𝛿 𝑗 C^{\prime}_{j}\leq C_{j}+\delta_{j} italic_C start_POSTSUPERSCRIPT ′ end_POSTSUPERSCRIPT start_POSTSUBSCRIPT italic_j end_POSTSUBSCRIPT ≤ italic_C start_POSTSUBSCRIPT italic_j end_POSTSUBSCRIPT + italic_δ start_POSTSUBSCRIPT italic_j end_POSTSUBSCRIPT , and ∑ j δ j = O ( r 2 ) subscript 𝑗 subscript 𝛿 𝑗 𝑂 superscript 𝑟 2 \sum_{j}\delta_{j}=O(r^{2}) ∑ start_POSTSUBSCRIPT italic_j end_POSTSUBSCRIPT italic_δ start_POSTSUBSCRIPT italic_j end_POSTSUBSCRIPT = italic_O ( italic_r start_POSTSUPERSCRIPT 2 end_POSTSUPERSCRIPT ) .
- [1] Atila Abdulkadiroğlu and Tayfun Sönmez. School choice: A mechanism design approach. American economic review , 93(3):729–747, 2003.
- [2] Federico Bobbio, Margarida Carvalho, Andrea Lodi, and Alfredo Torrico. Capacity variation in the many-to-one stable matching, 2022.
- [3] Jiehua Chen and Gergely Csáji. Optimal capacity modification for many-to-one matching problems. In Proceedings of the 2023 International Conference on Autonomous Agents and Multiagent Systems , AAMAS ’23, page 2880–2882, Richland, SC, 2023. International Foundation for Autonomous Agents and Multiagent Systems.
- [4] Michel X Goemans. Minimum bounded degree spanning trees. In 2006 47th Annual IEEE Symposium on Foundations of Computer Science (FOCS’06) , pages 273–282. IEEE, 2006.
- [5] Fabrizio Grandoni, R. Ravi, Mohit Singh, and Rico Zenklusen. New approaches to multi-objective optimization. Math. Program. , 146(1-2):525–554, 2014.
- [6] Santhini K. A., Govind S. Sankar, and Meghana Nasre. Optimal matchings with one-sided preferences: Fixed and cost-based quotas. In Proceedings of the 21st International Conference on Autonomous Agents and Multiagent Systems , AAMAS ’22, page 696–704, Richland, SC, 2022. International Foundation for Autonomous Agents and Multiagent Systems.
- [7] Jan Karel Lenstra, David B. Shmoys, and Eva Tardos. Approximation algorithms for scheduling unrelated parallel machines. In 28th Annual Symposium on Foundations of Computer Science (sfcs 1987) , pages 217–224, 1987.
- [8] Ketan Mulmuley, Umesh V Vazirani, and Vijay V Vazirani. Matching is as easy as matrix inversion. In Proceedings of the nineteenth annual ACM symposium on Theory of computing , pages 345–354, 1987.
- [9] Thanh Nguyen and Rakesh Vohra. Near-feasible stable matchings with couples. American Economic Review , 108(11):3154–3169, 2018.
- [10] Ariel D. Procaccia, Isaac Robinson, and Jamie Tucker-Foltz. School redistricting: Wiping unfairness off the map. In Proc. ACM-SIAM SODA , 2024.
- [11] David B. Shmoys and Éva Tardos. An approximation algorithm for the generalized assignment problem. Math. Program. , 62:461–474, 1993.
- [12] Mohit Singh and Lap Chi Lau. Approximating minimum bounded degree spanning trees to within one of optimal. In Proceedings of the Thirty-Ninth Annual ACM Symposium on Theory of Computing , STOC ’07, page 661–670, New York, NY, USA, 2007. Association for Computing Machinery.
- [13] A. H. Stone and J. W. Tukey. Generalized “sandwich” theorems. Duke Mathematical Journal , 9(2):356 – 359, 1942.
- [14] Walter Stromquist and D.R Woodall. Sets on which several measures agree. Journal of Mathematical Analysis and Applications , 108(1):241–248, 1985.

- Cambridge Dictionary +Plus
Meaning of on assignment in English
On assignment, on assignment | intermediate english, translations of on assignment.
Get a quick, free translation!
Word of the Day
Your browser doesn't support HTML5 audio
acting or speaking together, or at the same time

Alike and analogous (Talking about similarities, Part 1)

Learn more with +Plus
- Recent and Recommended {{#preferredDictionaries}} {{name}} {{/preferredDictionaries}}
- Definitions Clear explanations of natural written and spoken English English Learner’s Dictionary Essential British English Essential American English
- Grammar and thesaurus Usage explanations of natural written and spoken English Grammar Thesaurus
- Pronunciation British and American pronunciations with audio English Pronunciation
- English–Chinese (Simplified) Chinese (Simplified)–English
- English–Chinese (Traditional) Chinese (Traditional)–English
- English–Dutch Dutch–English
- English–French French–English
- English–German German–English
- English–Indonesian Indonesian–English
- English–Italian Italian–English
- English–Japanese Japanese–English
- English–Norwegian Norwegian–English
- English–Polish Polish–English
- English–Portuguese Portuguese–English
- English–Spanish Spanish–English
- English–Swedish Swedish–English
- Dictionary +Plus Word Lists
- English Phrase
- Intermediate Idiom
- Translations
- All translations
Add on assignment to one of your lists below, or create a new one.
{{message}}
Something went wrong.
There was a problem sending your report.
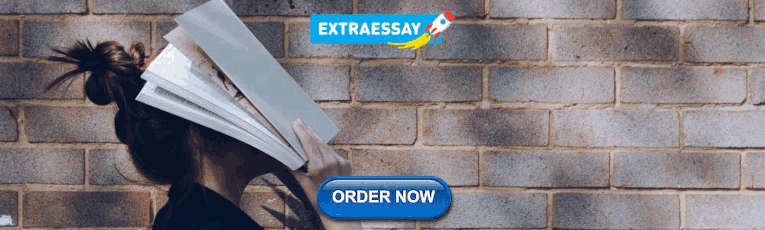
IMAGES
VIDEO
COMMENTS
The meaning of ASSIGNMENT is the act of assigning something. How to use assignment in a sentence. Synonym Discussion of Assignment.
ASSIGNMENT definition: 1. a piece of work given to someone, typically as part of their studies or job: 2. a job that…. Learn more.
Assignment definition: something assigned, as a particular task or duty. See examples of ASSIGNMENT used in a sentence.
assignment: 1 n an undertaking that you have been assigned to do (as by an instructor) Types: show 6 types... hide 6 types... school assignment , schoolwork a school task performed by a student to satisfy the teacher writing assignment , written assignment an assignment to write something classroom project a school task requiring considerable ...
7 meanings: 1. something that has been assigned, such as a mission or task 2. a position or post to which a person is assigned.... Click for more definitions.
Definition of assignment noun in Oxford Advanced Learner's Dictionary. Meaning, pronunciation, picture, example sentences, grammar, usage notes, synonyms and more. ... on assignment one of our reporters on assignment in China; Extra Examples. The students handed in their assignments.
ASSIGNMENT definition: a piece of work or job that you are given to do: . Learn more.
Definition of assignment noun in Oxford Advanced American Dictionary. Meaning, pronunciation, picture, example sentences, grammar, usage notes, synonyms and more. ... She is in Greece on an assignment for one of the Sunday newspapers. one of our reporters on assignment in China I had given myself a tough assignment. a business/special assignment.
assign: [verb] to transfer (property) to another especially in trust or for the benefit of creditors.
1. : a job or duty that is given to someone : a task someone is required to do. [count] My assignment was to clean the equipment. = They gave me the assignment of cleaning the equipment. The students were given a homework assignment. The reporter's assignment is to interview the candidate. The reporter is here on an assignment.
ASSIGNMENT meaning: 1. a piece of work given to someone, typically as part of their studies or job: 2. a job that…. Learn more.
assignment - WordReference English dictionary, questions, discussion and forums. All Free.
Synonyms for ASSIGNMENT: task, job, duty, project, mission, chore, responsibility, function; Antonyms of ASSIGNMENT: dismissal, discharge, firing, expulsion ...
1 to give someone something that they can use, or some work or responsibility assign something (to somebody) The two large classrooms have been assigned to us. The teacher assigned a different task to each of the children. assign somebody something We have been assigned the two large classrooms. The teacher assigned each of the children a different task.
assignment meaning: a piece of work or job that you are given to do: . Learn more.
An assignment is a task given to a specific person or group to complete.It can also mean the act of assigning.In some legal fields it can refer to the transferring of ownership of property. An assignation is the act of assigning or the actual assignment.But it also means a secret rendezvous for lovers, most especially for affairs or illicit relationships.
assignment in American English. (əˈsainmənt) noun. 1. something assigned, as a particular task or duty. She completed the assignment and went on to other jobs. 2. a position of responsibility, post of duty, or the like, to which one is appointed. He left for his assignment in the Middle East.
The assignment's parts may not appear in exactly this order, and each part may be very long or really short. Nonetheless, being aware of this standard pattern can help you understand what your instructor wants you to do. Interpreting the assignment. Ask yourself a few basic questions as you read and jot down the answers on the assignment sheet:
Assignment. Definition: Assignment is a task given to students by a teacher or professor, usually as a means of assessing their understanding and application of course material. Assignments can take various forms, including essays, research papers, presentations, problem sets, lab reports, and more.
Assignment definition: something assigned, as a particular task or duty. See examples of ASSIGNMENT used in a sentence.
ASSIGN meaning: 1. to give a particular job or piece of work to someone: 2. If you assign a time for a job or…. Learn more.
Assignment is a legal term whereby an individual, the "assignor," transfers rights, property, or other benefits to another known as the " assignee .". This concept is used in both contract and property law. The term can refer to either the act of transfer or the rights /property/benefits being transferred.
In this paper, we consider a general model of assignment with a group fairness objective or constraints, motivated by school choice. In the school assignment problem, we are required to assign students to schools, subject to: (1) matching every student, (2) respecting the capacities of schools, and (3) being fair on the utilities to a pre-defined set of g 𝑔 g italic_g groups of students ...
ON ASSIGNMENT meaning: 1. Someone who is on assignment is doing a particular job or piece of work, usually in a particular…. Learn more.