- Privacy Policy
Buy Me a Coffee

Home » What is a Hypothesis – Types, Examples and Writing Guide
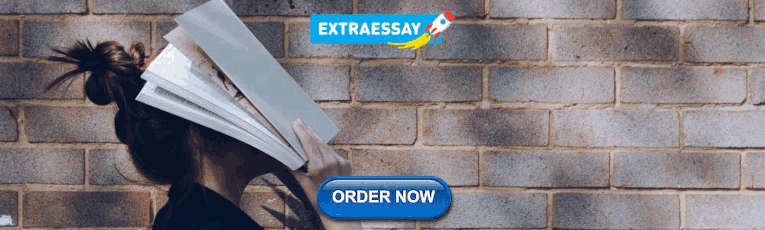
What is a Hypothesis – Types, Examples and Writing Guide
Table of Contents

Definition:
Hypothesis is an educated guess or proposed explanation for a phenomenon, based on some initial observations or data. It is a tentative statement that can be tested and potentially proven or disproven through further investigation and experimentation.
Hypothesis is often used in scientific research to guide the design of experiments and the collection and analysis of data. It is an essential element of the scientific method, as it allows researchers to make predictions about the outcome of their experiments and to test those predictions to determine their accuracy.
Types of Hypothesis
Types of Hypothesis are as follows:
Research Hypothesis
A research hypothesis is a statement that predicts a relationship between variables. It is usually formulated as a specific statement that can be tested through research, and it is often used in scientific research to guide the design of experiments.
Null Hypothesis
The null hypothesis is a statement that assumes there is no significant difference or relationship between variables. It is often used as a starting point for testing the research hypothesis, and if the results of the study reject the null hypothesis, it suggests that there is a significant difference or relationship between variables.
Alternative Hypothesis
An alternative hypothesis is a statement that assumes there is a significant difference or relationship between variables. It is often used as an alternative to the null hypothesis and is tested against the null hypothesis to determine which statement is more accurate.
Directional Hypothesis
A directional hypothesis is a statement that predicts the direction of the relationship between variables. For example, a researcher might predict that increasing the amount of exercise will result in a decrease in body weight.
Non-directional Hypothesis
A non-directional hypothesis is a statement that predicts the relationship between variables but does not specify the direction. For example, a researcher might predict that there is a relationship between the amount of exercise and body weight, but they do not specify whether increasing or decreasing exercise will affect body weight.
Statistical Hypothesis
A statistical hypothesis is a statement that assumes a particular statistical model or distribution for the data. It is often used in statistical analysis to test the significance of a particular result.
Composite Hypothesis
A composite hypothesis is a statement that assumes more than one condition or outcome. It can be divided into several sub-hypotheses, each of which represents a different possible outcome.
Empirical Hypothesis
An empirical hypothesis is a statement that is based on observed phenomena or data. It is often used in scientific research to develop theories or models that explain the observed phenomena.
Simple Hypothesis
A simple hypothesis is a statement that assumes only one outcome or condition. It is often used in scientific research to test a single variable or factor.
Complex Hypothesis
A complex hypothesis is a statement that assumes multiple outcomes or conditions. It is often used in scientific research to test the effects of multiple variables or factors on a particular outcome.
Applications of Hypothesis
Hypotheses are used in various fields to guide research and make predictions about the outcomes of experiments or observations. Here are some examples of how hypotheses are applied in different fields:
- Science : In scientific research, hypotheses are used to test the validity of theories and models that explain natural phenomena. For example, a hypothesis might be formulated to test the effects of a particular variable on a natural system, such as the effects of climate change on an ecosystem.
- Medicine : In medical research, hypotheses are used to test the effectiveness of treatments and therapies for specific conditions. For example, a hypothesis might be formulated to test the effects of a new drug on a particular disease.
- Psychology : In psychology, hypotheses are used to test theories and models of human behavior and cognition. For example, a hypothesis might be formulated to test the effects of a particular stimulus on the brain or behavior.
- Sociology : In sociology, hypotheses are used to test theories and models of social phenomena, such as the effects of social structures or institutions on human behavior. For example, a hypothesis might be formulated to test the effects of income inequality on crime rates.
- Business : In business research, hypotheses are used to test the validity of theories and models that explain business phenomena, such as consumer behavior or market trends. For example, a hypothesis might be formulated to test the effects of a new marketing campaign on consumer buying behavior.
- Engineering : In engineering, hypotheses are used to test the effectiveness of new technologies or designs. For example, a hypothesis might be formulated to test the efficiency of a new solar panel design.
How to write a Hypothesis
Here are the steps to follow when writing a hypothesis:
Identify the Research Question
The first step is to identify the research question that you want to answer through your study. This question should be clear, specific, and focused. It should be something that can be investigated empirically and that has some relevance or significance in the field.
Conduct a Literature Review
Before writing your hypothesis, it’s essential to conduct a thorough literature review to understand what is already known about the topic. This will help you to identify the research gap and formulate a hypothesis that builds on existing knowledge.
Determine the Variables
The next step is to identify the variables involved in the research question. A variable is any characteristic or factor that can vary or change. There are two types of variables: independent and dependent. The independent variable is the one that is manipulated or changed by the researcher, while the dependent variable is the one that is measured or observed as a result of the independent variable.
Formulate the Hypothesis
Based on the research question and the variables involved, you can now formulate your hypothesis. A hypothesis should be a clear and concise statement that predicts the relationship between the variables. It should be testable through empirical research and based on existing theory or evidence.
Write the Null Hypothesis
The null hypothesis is the opposite of the alternative hypothesis, which is the hypothesis that you are testing. The null hypothesis states that there is no significant difference or relationship between the variables. It is important to write the null hypothesis because it allows you to compare your results with what would be expected by chance.
Refine the Hypothesis
After formulating the hypothesis, it’s important to refine it and make it more precise. This may involve clarifying the variables, specifying the direction of the relationship, or making the hypothesis more testable.
Examples of Hypothesis
Here are a few examples of hypotheses in different fields:
- Psychology : “Increased exposure to violent video games leads to increased aggressive behavior in adolescents.”
- Biology : “Higher levels of carbon dioxide in the atmosphere will lead to increased plant growth.”
- Sociology : “Individuals who grow up in households with higher socioeconomic status will have higher levels of education and income as adults.”
- Education : “Implementing a new teaching method will result in higher student achievement scores.”
- Marketing : “Customers who receive a personalized email will be more likely to make a purchase than those who receive a generic email.”
- Physics : “An increase in temperature will cause an increase in the volume of a gas, assuming all other variables remain constant.”
- Medicine : “Consuming a diet high in saturated fats will increase the risk of developing heart disease.”
Purpose of Hypothesis
The purpose of a hypothesis is to provide a testable explanation for an observed phenomenon or a prediction of a future outcome based on existing knowledge or theories. A hypothesis is an essential part of the scientific method and helps to guide the research process by providing a clear focus for investigation. It enables scientists to design experiments or studies to gather evidence and data that can support or refute the proposed explanation or prediction.
The formulation of a hypothesis is based on existing knowledge, observations, and theories, and it should be specific, testable, and falsifiable. A specific hypothesis helps to define the research question, which is important in the research process as it guides the selection of an appropriate research design and methodology. Testability of the hypothesis means that it can be proven or disproven through empirical data collection and analysis. Falsifiability means that the hypothesis should be formulated in such a way that it can be proven wrong if it is incorrect.
In addition to guiding the research process, the testing of hypotheses can lead to new discoveries and advancements in scientific knowledge. When a hypothesis is supported by the data, it can be used to develop new theories or models to explain the observed phenomenon. When a hypothesis is not supported by the data, it can help to refine existing theories or prompt the development of new hypotheses to explain the phenomenon.
When to use Hypothesis
Here are some common situations in which hypotheses are used:
- In scientific research , hypotheses are used to guide the design of experiments and to help researchers make predictions about the outcomes of those experiments.
- In social science research , hypotheses are used to test theories about human behavior, social relationships, and other phenomena.
- I n business , hypotheses can be used to guide decisions about marketing, product development, and other areas. For example, a hypothesis might be that a new product will sell well in a particular market, and this hypothesis can be tested through market research.
Characteristics of Hypothesis
Here are some common characteristics of a hypothesis:
- Testable : A hypothesis must be able to be tested through observation or experimentation. This means that it must be possible to collect data that will either support or refute the hypothesis.
- Falsifiable : A hypothesis must be able to be proven false if it is not supported by the data. If a hypothesis cannot be falsified, then it is not a scientific hypothesis.
- Clear and concise : A hypothesis should be stated in a clear and concise manner so that it can be easily understood and tested.
- Based on existing knowledge : A hypothesis should be based on existing knowledge and research in the field. It should not be based on personal beliefs or opinions.
- Specific : A hypothesis should be specific in terms of the variables being tested and the predicted outcome. This will help to ensure that the research is focused and well-designed.
- Tentative: A hypothesis is a tentative statement or assumption that requires further testing and evidence to be confirmed or refuted. It is not a final conclusion or assertion.
- Relevant : A hypothesis should be relevant to the research question or problem being studied. It should address a gap in knowledge or provide a new perspective on the issue.
Advantages of Hypothesis
Hypotheses have several advantages in scientific research and experimentation:
- Guides research: A hypothesis provides a clear and specific direction for research. It helps to focus the research question, select appropriate methods and variables, and interpret the results.
- Predictive powe r: A hypothesis makes predictions about the outcome of research, which can be tested through experimentation. This allows researchers to evaluate the validity of the hypothesis and make new discoveries.
- Facilitates communication: A hypothesis provides a common language and framework for scientists to communicate with one another about their research. This helps to facilitate the exchange of ideas and promotes collaboration.
- Efficient use of resources: A hypothesis helps researchers to use their time, resources, and funding efficiently by directing them towards specific research questions and methods that are most likely to yield results.
- Provides a basis for further research: A hypothesis that is supported by data provides a basis for further research and exploration. It can lead to new hypotheses, theories, and discoveries.
- Increases objectivity: A hypothesis can help to increase objectivity in research by providing a clear and specific framework for testing and interpreting results. This can reduce bias and increase the reliability of research findings.
Limitations of Hypothesis
Some Limitations of the Hypothesis are as follows:
- Limited to observable phenomena: Hypotheses are limited to observable phenomena and cannot account for unobservable or intangible factors. This means that some research questions may not be amenable to hypothesis testing.
- May be inaccurate or incomplete: Hypotheses are based on existing knowledge and research, which may be incomplete or inaccurate. This can lead to flawed hypotheses and erroneous conclusions.
- May be biased: Hypotheses may be biased by the researcher’s own beliefs, values, or assumptions. This can lead to selective interpretation of data and a lack of objectivity in research.
- Cannot prove causation: A hypothesis can only show a correlation between variables, but it cannot prove causation. This requires further experimentation and analysis.
- Limited to specific contexts: Hypotheses are limited to specific contexts and may not be generalizable to other situations or populations. This means that results may not be applicable in other contexts or may require further testing.
- May be affected by chance : Hypotheses may be affected by chance or random variation, which can obscure or distort the true relationship between variables.
About the author
Muhammad Hassan
Researcher, Academic Writer, Web developer
You may also like

Data Collection – Methods Types and Examples

Delimitations in Research – Types, Examples and...

Research Process – Steps, Examples and Tips

Research Design – Types, Methods and Examples

Institutional Review Board – Application Sample...

Evaluating Research – Process, Examples and...
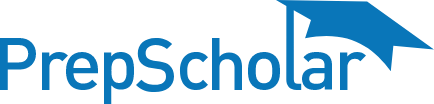
Choose Your Test
Sat / act prep online guides and tips, what is a hypothesis and how do i write one.
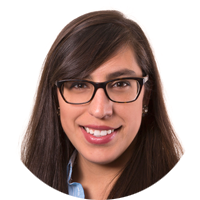
General Education
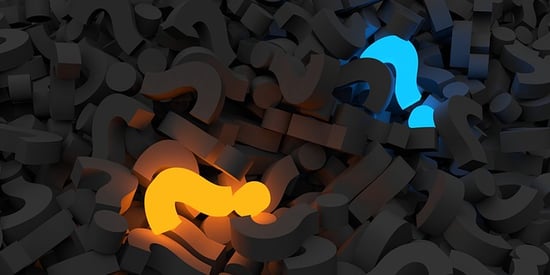
Think about something strange and unexplainable in your life. Maybe you get a headache right before it rains, or maybe you think your favorite sports team wins when you wear a certain color. If you wanted to see whether these are just coincidences or scientific fact, you would form a hypothesis, then create an experiment to see whether that hypothesis is true or not.
But what is a hypothesis, anyway? If you’re not sure about what a hypothesis is--or how to test for one!--you’re in the right place. This article will teach you everything you need to know about hypotheses, including:
- Defining the term “hypothesis”
- Providing hypothesis examples
- Giving you tips for how to write your own hypothesis
So let’s get started!
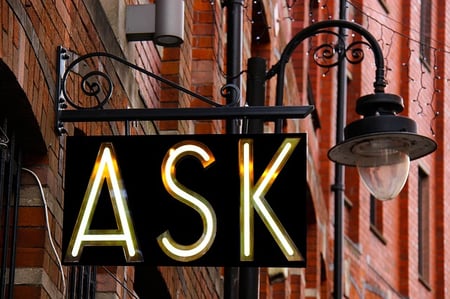
What Is a Hypothesis?
Merriam Webster defines a hypothesis as “an assumption or concession made for the sake of argument.” In other words, a hypothesis is an educated guess . Scientists make a reasonable assumption--or a hypothesis--then design an experiment to test whether it’s true or not. Keep in mind that in science, a hypothesis should be testable. You have to be able to design an experiment that tests your hypothesis in order for it to be valid.
As you could assume from that statement, it’s easy to make a bad hypothesis. But when you’re holding an experiment, it’s even more important that your guesses be good...after all, you’re spending time (and maybe money!) to figure out more about your observation. That’s why we refer to a hypothesis as an educated guess--good hypotheses are based on existing data and research to make them as sound as possible.
Hypotheses are one part of what’s called the scientific method . Every (good) experiment or study is based in the scientific method. The scientific method gives order and structure to experiments and ensures that interference from scientists or outside influences does not skew the results. It’s important that you understand the concepts of the scientific method before holding your own experiment. Though it may vary among scientists, the scientific method is generally made up of six steps (in order):
- Observation
- Asking questions
- Forming a hypothesis
- Analyze the data
- Communicate your results
You’ll notice that the hypothesis comes pretty early on when conducting an experiment. That’s because experiments work best when they’re trying to answer one specific question. And you can’t conduct an experiment until you know what you’re trying to prove!
Independent and Dependent Variables
After doing your research, you’re ready for another important step in forming your hypothesis: identifying variables. Variables are basically any factor that could influence the outcome of your experiment . Variables have to be measurable and related to the topic being studied.
There are two types of variables: independent variables and dependent variables. I ndependent variables remain constant . For example, age is an independent variable; it will stay the same, and researchers can look at different ages to see if it has an effect on the dependent variable.
Speaking of dependent variables... dependent variables are subject to the influence of the independent variable , meaning that they are not constant. Let’s say you want to test whether a person’s age affects how much sleep they need. In that case, the independent variable is age (like we mentioned above), and the dependent variable is how much sleep a person gets.
Variables will be crucial in writing your hypothesis. You need to be able to identify which variable is which, as both the independent and dependent variables will be written into your hypothesis. For instance, in a study about exercise, the independent variable might be the speed at which the respondents walk for thirty minutes, and the dependent variable would be their heart rate. In your study and in your hypothesis, you’re trying to understand the relationship between the two variables.
Elements of a Good Hypothesis
The best hypotheses start by asking the right questions . For instance, if you’ve observed that the grass is greener when it rains twice a week, you could ask what kind of grass it is, what elevation it’s at, and if the grass across the street responds to rain in the same way. Any of these questions could become the backbone of experiments to test why the grass gets greener when it rains fairly frequently.
As you’re asking more questions about your first observation, make sure you’re also making more observations . If it doesn’t rain for two weeks and the grass still looks green, that’s an important observation that could influence your hypothesis. You'll continue observing all throughout your experiment, but until the hypothesis is finalized, every observation should be noted.
Finally, you should consult secondary research before writing your hypothesis . Secondary research is comprised of results found and published by other people. You can usually find this information online or at your library. Additionally, m ake sure the research you find is credible and related to your topic. If you’re studying the correlation between rain and grass growth, it would help you to research rain patterns over the past twenty years for your county, published by a local agricultural association. You should also research the types of grass common in your area, the type of grass in your lawn, and whether anyone else has conducted experiments about your hypothesis. Also be sure you’re checking the quality of your research . Research done by a middle school student about what minerals can be found in rainwater would be less useful than an article published by a local university.
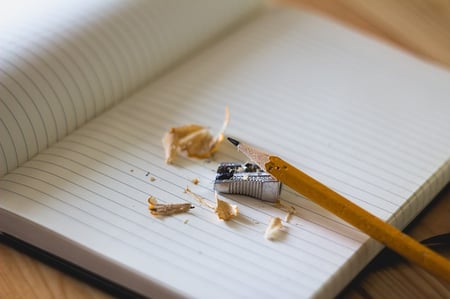
Writing Your Hypothesis
Once you’ve considered all of the factors above, you’re ready to start writing your hypothesis. Hypotheses usually take a certain form when they’re written out in a research report.
When you boil down your hypothesis statement, you are writing down your best guess and not the question at hand . This means that your statement should be written as if it is fact already, even though you are simply testing it.
The reason for this is that, after you have completed your study, you'll either accept or reject your if-then or your null hypothesis. All hypothesis testing examples should be measurable and able to be confirmed or denied. You cannot confirm a question, only a statement!
In fact, you come up with hypothesis examples all the time! For instance, when you guess on the outcome of a basketball game, you don’t say, “Will the Miami Heat beat the Boston Celtics?” but instead, “I think the Miami Heat will beat the Boston Celtics.” You state it as if it is already true, even if it turns out you’re wrong. You do the same thing when writing your hypothesis.
Additionally, keep in mind that hypotheses can range from very specific to very broad. These hypotheses can be specific, but if your hypothesis testing examples involve a broad range of causes and effects, your hypothesis can also be broad.
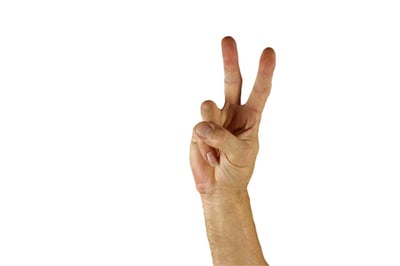
The Two Types of Hypotheses
Now that you understand what goes into a hypothesis, it’s time to look more closely at the two most common types of hypothesis: the if-then hypothesis and the null hypothesis.
#1: If-Then Hypotheses
First of all, if-then hypotheses typically follow this formula:
If ____ happens, then ____ will happen.
The goal of this type of hypothesis is to test the causal relationship between the independent and dependent variable. It’s fairly simple, and each hypothesis can vary in how detailed it can be. We create if-then hypotheses all the time with our daily predictions. Here are some examples of hypotheses that use an if-then structure from daily life:
- If I get enough sleep, I’ll be able to get more work done tomorrow.
- If the bus is on time, I can make it to my friend’s birthday party.
- If I study every night this week, I’ll get a better grade on my exam.
In each of these situations, you’re making a guess on how an independent variable (sleep, time, or studying) will affect a dependent variable (the amount of work you can do, making it to a party on time, or getting better grades).
You may still be asking, “What is an example of a hypothesis used in scientific research?” Take one of the hypothesis examples from a real-world study on whether using technology before bed affects children’s sleep patterns. The hypothesis read s:
“We hypothesized that increased hours of tablet- and phone-based screen time at bedtime would be inversely correlated with sleep quality and child attention.”
It might not look like it, but this is an if-then statement. The researchers basically said, “If children have more screen usage at bedtime, then their quality of sleep and attention will be worse.” The sleep quality and attention are the dependent variables and the screen usage is the independent variable. (Usually, the independent variable comes after the “if” and the dependent variable comes after the “then,” as it is the independent variable that affects the dependent variable.) This is an excellent example of how flexible hypothesis statements can be, as long as the general idea of “if-then” and the independent and dependent variables are present.
#2: Null Hypotheses
Your if-then hypothesis is not the only one needed to complete a successful experiment, however. You also need a null hypothesis to test it against. In its most basic form, the null hypothesis is the opposite of your if-then hypothesis . When you write your null hypothesis, you are writing a hypothesis that suggests that your guess is not true, and that the independent and dependent variables have no relationship .
One null hypothesis for the cell phone and sleep study from the last section might say:
“If children have more screen usage at bedtime, their quality of sleep and attention will not be worse.”
In this case, this is a null hypothesis because it’s asking the opposite of the original thesis!
Conversely, if your if-then hypothesis suggests that your two variables have no relationship, then your null hypothesis would suggest that there is one. So, pretend that there is a study that is asking the question, “Does the amount of followers on Instagram influence how long people spend on the app?” The independent variable is the amount of followers, and the dependent variable is the time spent. But if you, as the researcher, don’t think there is a relationship between the number of followers and time spent, you might write an if-then hypothesis that reads:
“If people have many followers on Instagram, they will not spend more time on the app than people who have less.”
In this case, the if-then suggests there isn’t a relationship between the variables. In that case, one of the null hypothesis examples might say:
“If people have many followers on Instagram, they will spend more time on the app than people who have less.”
You then test both the if-then and the null hypothesis to gauge if there is a relationship between the variables, and if so, how much of a relationship.
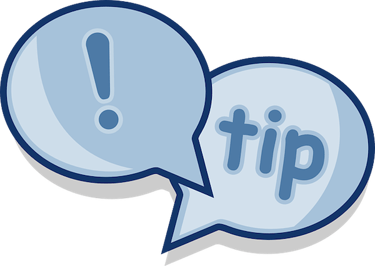
4 Tips to Write the Best Hypothesis
If you’re going to take the time to hold an experiment, whether in school or by yourself, you’re also going to want to take the time to make sure your hypothesis is a good one. The best hypotheses have four major elements in common: plausibility, defined concepts, observability, and general explanation.
#1: Plausibility
At first glance, this quality of a hypothesis might seem obvious. When your hypothesis is plausible, that means it’s possible given what we know about science and general common sense. However, improbable hypotheses are more common than you might think.
Imagine you’re studying weight gain and television watching habits. If you hypothesize that people who watch more than twenty hours of television a week will gain two hundred pounds or more over the course of a year, this might be improbable (though it’s potentially possible). Consequently, c ommon sense can tell us the results of the study before the study even begins.
Improbable hypotheses generally go against science, as well. Take this hypothesis example:
“If a person smokes one cigarette a day, then they will have lungs just as healthy as the average person’s.”
This hypothesis is obviously untrue, as studies have shown again and again that cigarettes negatively affect lung health. You must be careful that your hypotheses do not reflect your own personal opinion more than they do scientifically-supported findings. This plausibility points to the necessity of research before the hypothesis is written to make sure that your hypothesis has not already been disproven.
#2: Defined Concepts
The more advanced you are in your studies, the more likely that the terms you’re using in your hypothesis are specific to a limited set of knowledge. One of the hypothesis testing examples might include the readability of printed text in newspapers, where you might use words like “kerning” and “x-height.” Unless your readers have a background in graphic design, it’s likely that they won’t know what you mean by these terms. Thus, it’s important to either write what they mean in the hypothesis itself or in the report before the hypothesis.
Here’s what we mean. Which of the following sentences makes more sense to the common person?
If the kerning is greater than average, more words will be read per minute.
If the space between letters is greater than average, more words will be read per minute.
For people reading your report that are not experts in typography, simply adding a few more words will be helpful in clarifying exactly what the experiment is all about. It’s always a good idea to make your research and findings as accessible as possible.
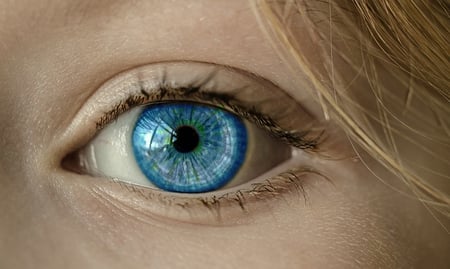
Good hypotheses ensure that you can observe the results.
#3: Observability
In order to measure the truth or falsity of your hypothesis, you must be able to see your variables and the way they interact. For instance, if your hypothesis is that the flight patterns of satellites affect the strength of certain television signals, yet you don’t have a telescope to view the satellites or a television to monitor the signal strength, you cannot properly observe your hypothesis and thus cannot continue your study.
Some variables may seem easy to observe, but if you do not have a system of measurement in place, you cannot observe your hypothesis properly. Here’s an example: if you’re experimenting on the effect of healthy food on overall happiness, but you don’t have a way to monitor and measure what “overall happiness” means, your results will not reflect the truth. Monitoring how often someone smiles for a whole day is not reasonably observable, but having the participants state how happy they feel on a scale of one to ten is more observable.
In writing your hypothesis, always keep in mind how you'll execute the experiment.
#4: Generalizability
Perhaps you’d like to study what color your best friend wears the most often by observing and documenting the colors she wears each day of the week. This might be fun information for her and you to know, but beyond you two, there aren’t many people who could benefit from this experiment. When you start an experiment, you should note how generalizable your findings may be if they are confirmed. Generalizability is basically how common a particular phenomenon is to other people’s everyday life.
Let’s say you’re asking a question about the health benefits of eating an apple for one day only, you need to realize that the experiment may be too specific to be helpful. It does not help to explain a phenomenon that many people experience. If you find yourself with too specific of a hypothesis, go back to asking the big question: what is it that you want to know, and what do you think will happen between your two variables?
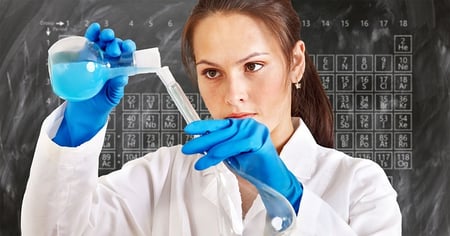
Hypothesis Testing Examples
We know it can be hard to write a good hypothesis unless you’ve seen some good hypothesis examples. We’ve included four hypothesis examples based on some made-up experiments. Use these as templates or launch pads for coming up with your own hypotheses.
Experiment #1: Students Studying Outside (Writing a Hypothesis)
You are a student at PrepScholar University. When you walk around campus, you notice that, when the temperature is above 60 degrees, more students study in the quad. You want to know when your fellow students are more likely to study outside. With this information, how do you make the best hypothesis possible?
You must remember to make additional observations and do secondary research before writing your hypothesis. In doing so, you notice that no one studies outside when it’s 75 degrees and raining, so this should be included in your experiment. Also, studies done on the topic beforehand suggested that students are more likely to study in temperatures less than 85 degrees. With this in mind, you feel confident that you can identify your variables and write your hypotheses:
If-then: “If the temperature in Fahrenheit is less than 60 degrees, significantly fewer students will study outside.”
Null: “If the temperature in Fahrenheit is less than 60 degrees, the same number of students will study outside as when it is more than 60 degrees.”
These hypotheses are plausible, as the temperatures are reasonably within the bounds of what is possible. The number of people in the quad is also easily observable. It is also not a phenomenon specific to only one person or at one time, but instead can explain a phenomenon for a broader group of people.
To complete this experiment, you pick the month of October to observe the quad. Every day (except on the days where it’s raining)from 3 to 4 PM, when most classes have released for the day, you observe how many people are on the quad. You measure how many people come and how many leave. You also write down the temperature on the hour.
After writing down all of your observations and putting them on a graph, you find that the most students study on the quad when it is 70 degrees outside, and that the number of students drops a lot once the temperature reaches 60 degrees or below. In this case, your research report would state that you accept or “failed to reject” your first hypothesis with your findings.
Experiment #2: The Cupcake Store (Forming a Simple Experiment)
Let’s say that you work at a bakery. You specialize in cupcakes, and you make only two colors of frosting: yellow and purple. You want to know what kind of customers are more likely to buy what kind of cupcake, so you set up an experiment. Your independent variable is the customer’s gender, and the dependent variable is the color of the frosting. What is an example of a hypothesis that might answer the question of this study?
Here’s what your hypotheses might look like:
If-then: “If customers’ gender is female, then they will buy more yellow cupcakes than purple cupcakes.”
Null: “If customers’ gender is female, then they will be just as likely to buy purple cupcakes as yellow cupcakes.”
This is a pretty simple experiment! It passes the test of plausibility (there could easily be a difference), defined concepts (there’s nothing complicated about cupcakes!), observability (both color and gender can be easily observed), and general explanation ( this would potentially help you make better business decisions ).
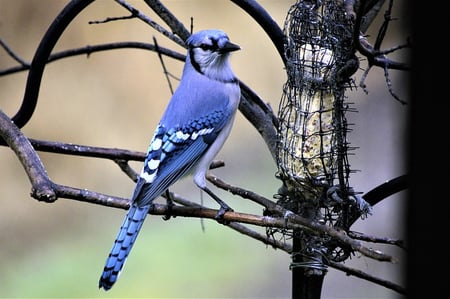
Experiment #3: Backyard Bird Feeders (Integrating Multiple Variables and Rejecting the If-Then Hypothesis)
While watching your backyard bird feeder, you realized that different birds come on the days when you change the types of seeds. You decide that you want to see more cardinals in your backyard, so you decide to see what type of food they like the best and set up an experiment.
However, one morning, you notice that, while some cardinals are present, blue jays are eating out of your backyard feeder filled with millet. You decide that, of all of the other birds, you would like to see the blue jays the least. This means you'll have more than one variable in your hypothesis. Your new hypotheses might look like this:
If-then: “If sunflower seeds are placed in the bird feeders, then more cardinals will come than blue jays. If millet is placed in the bird feeders, then more blue jays will come than cardinals.”
Null: “If either sunflower seeds or millet are placed in the bird, equal numbers of cardinals and blue jays will come.”
Through simple observation, you actually find that cardinals come as often as blue jays when sunflower seeds or millet is in the bird feeder. In this case, you would reject your “if-then” hypothesis and “fail to reject” your null hypothesis . You cannot accept your first hypothesis, because it’s clearly not true. Instead you found that there was actually no relation between your different variables. Consequently, you would need to run more experiments with different variables to see if the new variables impact the results.
Experiment #4: In-Class Survey (Including an Alternative Hypothesis)
You’re about to give a speech in one of your classes about the importance of paying attention. You want to take this opportunity to test a hypothesis you’ve had for a while:
If-then: If students sit in the first two rows of the classroom, then they will listen better than students who do not.
Null: If students sit in the first two rows of the classroom, then they will not listen better or worse than students who do not.
You give your speech and then ask your teacher if you can hand out a short survey to the class. On the survey, you’ve included questions about some of the topics you talked about. When you get back the results, you’re surprised to see that not only do the students in the first two rows not pay better attention, but they also scored worse than students in other parts of the classroom! Here, both your if-then and your null hypotheses are not representative of your findings. What do you do?
This is when you reject both your if-then and null hypotheses and instead create an alternative hypothesis . This type of hypothesis is used in the rare circumstance that neither of your hypotheses is able to capture your findings . Now you can use what you’ve learned to draft new hypotheses and test again!
Key Takeaways: Hypothesis Writing
The more comfortable you become with writing hypotheses, the better they will become. The structure of hypotheses is flexible and may need to be changed depending on what topic you are studying. The most important thing to remember is the purpose of your hypothesis and the difference between the if-then and the null . From there, in forming your hypothesis, you should constantly be asking questions, making observations, doing secondary research, and considering your variables. After you have written your hypothesis, be sure to edit it so that it is plausible, clearly defined, observable, and helpful in explaining a general phenomenon.
Writing a hypothesis is something that everyone, from elementary school children competing in a science fair to professional scientists in a lab, needs to know how to do. Hypotheses are vital in experiments and in properly executing the scientific method . When done correctly, hypotheses will set up your studies for success and help you to understand the world a little better, one experiment at a time.
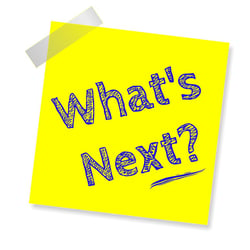
What’s Next?
If you’re studying for the science portion of the ACT, there’s definitely a lot you need to know. We’ve got the tools to help, though! Start by checking out our ultimate study guide for the ACT Science subject test. Once you read through that, be sure to download our recommended ACT Science practice tests , since they’re one of the most foolproof ways to improve your score. (And don’t forget to check out our expert guide book , too.)
If you love science and want to major in a scientific field, you should start preparing in high school . Here are the science classes you should take to set yourself up for success.
If you’re trying to think of science experiments you can do for class (or for a science fair!), here’s a list of 37 awesome science experiments you can do at home
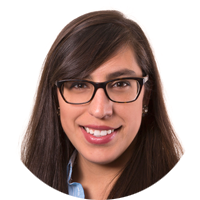
Ashley Sufflé Robinson has a Ph.D. in 19th Century English Literature. As a content writer for PrepScholar, Ashley is passionate about giving college-bound students the in-depth information they need to get into the school of their dreams.
Student and Parent Forum
Our new student and parent forum, at ExpertHub.PrepScholar.com , allow you to interact with your peers and the PrepScholar staff. See how other students and parents are navigating high school, college, and the college admissions process. Ask questions; get answers.

Ask a Question Below
Have any questions about this article or other topics? Ask below and we'll reply!
Improve With Our Famous Guides
- For All Students
The 5 Strategies You Must Be Using to Improve 160+ SAT Points
How to Get a Perfect 1600, by a Perfect Scorer
Series: How to Get 800 on Each SAT Section:
Score 800 on SAT Math
Score 800 on SAT Reading
Score 800 on SAT Writing
Series: How to Get to 600 on Each SAT Section:
Score 600 on SAT Math
Score 600 on SAT Reading
Score 600 on SAT Writing
Free Complete Official SAT Practice Tests
What SAT Target Score Should You Be Aiming For?
15 Strategies to Improve Your SAT Essay
The 5 Strategies You Must Be Using to Improve 4+ ACT Points
How to Get a Perfect 36 ACT, by a Perfect Scorer
Series: How to Get 36 on Each ACT Section:
36 on ACT English
36 on ACT Math
36 on ACT Reading
36 on ACT Science
Series: How to Get to 24 on Each ACT Section:
24 on ACT English
24 on ACT Math
24 on ACT Reading
24 on ACT Science
What ACT target score should you be aiming for?
ACT Vocabulary You Must Know
ACT Writing: 15 Tips to Raise Your Essay Score
How to Get Into Harvard and the Ivy League
How to Get a Perfect 4.0 GPA
How to Write an Amazing College Essay
What Exactly Are Colleges Looking For?
Is the ACT easier than the SAT? A Comprehensive Guide
Should you retake your SAT or ACT?
When should you take the SAT or ACT?
Stay Informed

Get the latest articles and test prep tips!
Looking for Graduate School Test Prep?
Check out our top-rated graduate blogs here:
GRE Online Prep Blog
GMAT Online Prep Blog
TOEFL Online Prep Blog
Holly R. "I am absolutely overjoyed and cannot thank you enough for helping me!”
- Bipolar Disorder
- Therapy Center
- When To See a Therapist
- Types of Therapy
- Best Online Therapy
- Best Couples Therapy
- Best Family Therapy
- Managing Stress
- Sleep and Dreaming
- Understanding Emotions
- Self-Improvement
- Healthy Relationships
- Student Resources
- Personality Types
- Guided Meditations
- Verywell Mind Insights
- 2023 Verywell Mind 25
- Mental Health in the Classroom
- Editorial Process
- Meet Our Review Board
- Crisis Support
How to Write a Great Hypothesis
Hypothesis Format, Examples, and Tips
Kendra Cherry, MS, is a psychosocial rehabilitation specialist, psychology educator, and author of the "Everything Psychology Book."
:max_bytes(150000):strip_icc():format(webp)/IMG_9791-89504ab694d54b66bbd72cb84ffb860e.jpg)
Amy Morin, LCSW, is a psychotherapist and international bestselling author. Her books, including "13 Things Mentally Strong People Don't Do," have been translated into more than 40 languages. Her TEDx talk, "The Secret of Becoming Mentally Strong," is one of the most viewed talks of all time.
:max_bytes(150000):strip_icc():format(webp)/VW-MIND-Amy-2b338105f1ee493f94d7e333e410fa76.jpg)
Verywell / Alex Dos Diaz
- The Scientific Method
Hypothesis Format
Falsifiability of a hypothesis, operational definitions, types of hypotheses, hypotheses examples.
- Collecting Data
Frequently Asked Questions
A hypothesis is a tentative statement about the relationship between two or more variables. It is a specific, testable prediction about what you expect to happen in a study.
One hypothesis example would be a study designed to look at the relationship between sleep deprivation and test performance might have a hypothesis that states: "This study is designed to assess the hypothesis that sleep-deprived people will perform worse on a test than individuals who are not sleep-deprived."
This article explores how a hypothesis is used in psychology research, how to write a good hypothesis, and the different types of hypotheses you might use.
The Hypothesis in the Scientific Method
In the scientific method , whether it involves research in psychology, biology, or some other area, a hypothesis represents what the researchers think will happen in an experiment. The scientific method involves the following steps:
- Forming a question
- Performing background research
- Creating a hypothesis
- Designing an experiment
- Collecting data
- Analyzing the results
- Drawing conclusions
- Communicating the results
The hypothesis is a prediction, but it involves more than a guess. Most of the time, the hypothesis begins with a question which is then explored through background research. It is only at this point that researchers begin to develop a testable hypothesis. Unless you are creating an exploratory study, your hypothesis should always explain what you expect to happen.
In a study exploring the effects of a particular drug, the hypothesis might be that researchers expect the drug to have some type of effect on the symptoms of a specific illness. In psychology, the hypothesis might focus on how a certain aspect of the environment might influence a particular behavior.
Remember, a hypothesis does not have to be correct. While the hypothesis predicts what the researchers expect to see, the goal of the research is to determine whether this guess is right or wrong. When conducting an experiment, researchers might explore a number of factors to determine which ones might contribute to the ultimate outcome.
In many cases, researchers may find that the results of an experiment do not support the original hypothesis. When writing up these results, the researchers might suggest other options that should be explored in future studies.
In many cases, researchers might draw a hypothesis from a specific theory or build on previous research. For example, prior research has shown that stress can impact the immune system. So a researcher might hypothesize: "People with high-stress levels will be more likely to contract a common cold after being exposed to the virus than people who have low-stress levels."
In other instances, researchers might look at commonly held beliefs or folk wisdom. "Birds of a feather flock together" is one example of folk wisdom that a psychologist might try to investigate. The researcher might pose a specific hypothesis that "People tend to select romantic partners who are similar to them in interests and educational level."
Elements of a Good Hypothesis
So how do you write a good hypothesis? When trying to come up with a hypothesis for your research or experiments, ask yourself the following questions:
- Is your hypothesis based on your research on a topic?
- Can your hypothesis be tested?
- Does your hypothesis include independent and dependent variables?
Before you come up with a specific hypothesis, spend some time doing background research. Once you have completed a literature review, start thinking about potential questions you still have. Pay attention to the discussion section in the journal articles you read . Many authors will suggest questions that still need to be explored.
To form a hypothesis, you should take these steps:
- Collect as many observations about a topic or problem as you can.
- Evaluate these observations and look for possible causes of the problem.
- Create a list of possible explanations that you might want to explore.
- After you have developed some possible hypotheses, think of ways that you could confirm or disprove each hypothesis through experimentation. This is known as falsifiability.
In the scientific method , falsifiability is an important part of any valid hypothesis. In order to test a claim scientifically, it must be possible that the claim could be proven false.
Students sometimes confuse the idea of falsifiability with the idea that it means that something is false, which is not the case. What falsifiability means is that if something was false, then it is possible to demonstrate that it is false.
One of the hallmarks of pseudoscience is that it makes claims that cannot be refuted or proven false.
A variable is a factor or element that can be changed and manipulated in ways that are observable and measurable. However, the researcher must also define how the variable will be manipulated and measured in the study.
For example, a researcher might operationally define the variable " test anxiety " as the results of a self-report measure of anxiety experienced during an exam. A "study habits" variable might be defined by the amount of studying that actually occurs as measured by time.
These precise descriptions are important because many things can be measured in a number of different ways. One of the basic principles of any type of scientific research is that the results must be replicable. By clearly detailing the specifics of how the variables were measured and manipulated, other researchers can better understand the results and repeat the study if needed.
Some variables are more difficult than others to define. How would you operationally define a variable such as aggression ? For obvious ethical reasons, researchers cannot create a situation in which a person behaves aggressively toward others.
In order to measure this variable, the researcher must devise a measurement that assesses aggressive behavior without harming other people. In this situation, the researcher might utilize a simulated task to measure aggressiveness.
Hypothesis Checklist
- Does your hypothesis focus on something that you can actually test?
- Does your hypothesis include both an independent and dependent variable?
- Can you manipulate the variables?
- Can your hypothesis be tested without violating ethical standards?
The hypothesis you use will depend on what you are investigating and hoping to find. Some of the main types of hypotheses that you might use include:
- Simple hypothesis : This type of hypothesis suggests that there is a relationship between one independent variable and one dependent variable.
- Complex hypothesis : This type of hypothesis suggests a relationship between three or more variables, such as two independent variables and a dependent variable.
- Null hypothesis : This hypothesis suggests no relationship exists between two or more variables.
- Alternative hypothesis : This hypothesis states the opposite of the null hypothesis.
- Statistical hypothesis : This hypothesis uses statistical analysis to evaluate a representative sample of the population and then generalizes the findings to the larger group.
- Logical hypothesis : This hypothesis assumes a relationship between variables without collecting data or evidence.
A hypothesis often follows a basic format of "If {this happens} then {this will happen}." One way to structure your hypothesis is to describe what will happen to the dependent variable if you change the independent variable .
The basic format might be: "If {these changes are made to a certain independent variable}, then we will observe {a change in a specific dependent variable}."
A few examples of simple hypotheses:
- "Students who eat breakfast will perform better on a math exam than students who do not eat breakfast."
- Complex hypothesis: "Students who experience test anxiety before an English exam will get lower scores than students who do not experience test anxiety."
- "Motorists who talk on the phone while driving will be more likely to make errors on a driving course than those who do not talk on the phone."
Examples of a complex hypothesis include:
- "People with high-sugar diets and sedentary activity levels are more likely to develop depression."
- "Younger people who are regularly exposed to green, outdoor areas have better subjective well-being than older adults who have limited exposure to green spaces."
Examples of a null hypothesis include:
- "Children who receive a new reading intervention will have scores different than students who do not receive the intervention."
- "There will be no difference in scores on a memory recall task between children and adults."
Examples of an alternative hypothesis:
- "Children who receive a new reading intervention will perform better than students who did not receive the intervention."
- "Adults will perform better on a memory task than children."
Collecting Data on Your Hypothesis
Once a researcher has formed a testable hypothesis, the next step is to select a research design and start collecting data. The research method depends largely on exactly what they are studying. There are two basic types of research methods: descriptive research and experimental research.
Descriptive Research Methods
Descriptive research such as case studies , naturalistic observations , and surveys are often used when it would be impossible or difficult to conduct an experiment . These methods are best used to describe different aspects of a behavior or psychological phenomenon.
Once a researcher has collected data using descriptive methods, a correlational study can then be used to look at how the variables are related. This type of research method might be used to investigate a hypothesis that is difficult to test experimentally.
Experimental Research Methods
Experimental methods are used to demonstrate causal relationships between variables. In an experiment, the researcher systematically manipulates a variable of interest (known as the independent variable) and measures the effect on another variable (known as the dependent variable).
Unlike correlational studies, which can only be used to determine if there is a relationship between two variables, experimental methods can be used to determine the actual nature of the relationship—whether changes in one variable actually cause another to change.
A Word From Verywell
The hypothesis is a critical part of any scientific exploration. It represents what researchers expect to find in a study or experiment. In situations where the hypothesis is unsupported by the research, the research still has value. Such research helps us better understand how different aspects of the natural world relate to one another. It also helps us develop new hypotheses that can then be tested in the future.
Some examples of how to write a hypothesis include:
- "Staying up late will lead to worse test performance the next day."
- "People who consume one apple each day will visit the doctor fewer times each year."
- "Breaking study sessions up into three 20-minute sessions will lead to better test results than a single 60-minute study session."
The four parts of a hypothesis are:
- The research question
- The independent variable (IV)
- The dependent variable (DV)
- The proposed relationship between the IV and DV
Castillo M. The scientific method: a need for something better? . AJNR Am J Neuroradiol. 2013;34(9):1669-71. doi:10.3174/ajnr.A3401
Nevid J. Psychology: Concepts and Applications. Wadworth, 2013.
By Kendra Cherry, MSEd Kendra Cherry, MS, is a psychosocial rehabilitation specialist, psychology educator, and author of the "Everything Psychology Book."
- Resources Home 🏠
- Try SciSpace Copilot
- Search research papers
- Add Copilot Extension
- Try AI Detector
- Try Paraphraser
- Try Citation Generator
- April Papers
- June Papers
- July Papers

The Craft of Writing a Strong Hypothesis

Table of Contents
Writing a hypothesis is one of the essential elements of a scientific research paper. It needs to be to the point, clearly communicating what your research is trying to accomplish. A blurry, drawn-out, or complexly-structured hypothesis can confuse your readers. Or worse, the editor and peer reviewers.
A captivating hypothesis is not too intricate. This blog will take you through the process so that, by the end of it, you have a better idea of how to convey your research paper's intent in just one sentence.
What is a Hypothesis?
The first step in your scientific endeavor, a hypothesis, is a strong, concise statement that forms the basis of your research. It is not the same as a thesis statement , which is a brief summary of your research paper .
The sole purpose of a hypothesis is to predict your paper's findings, data, and conclusion. It comes from a place of curiosity and intuition . When you write a hypothesis, you're essentially making an educated guess based on scientific prejudices and evidence, which is further proven or disproven through the scientific method.
The reason for undertaking research is to observe a specific phenomenon. A hypothesis, therefore, lays out what the said phenomenon is. And it does so through two variables, an independent and dependent variable.
The independent variable is the cause behind the observation, while the dependent variable is the effect of the cause. A good example of this is “mixing red and blue forms purple.” In this hypothesis, mixing red and blue is the independent variable as you're combining the two colors at your own will. The formation of purple is the dependent variable as, in this case, it is conditional to the independent variable.
Different Types of Hypotheses

Types of hypotheses
Some would stand by the notion that there are only two types of hypotheses: a Null hypothesis and an Alternative hypothesis. While that may have some truth to it, it would be better to fully distinguish the most common forms as these terms come up so often, which might leave you out of context.
Apart from Null and Alternative, there are Complex, Simple, Directional, Non-Directional, Statistical, and Associative and casual hypotheses. They don't necessarily have to be exclusive, as one hypothesis can tick many boxes, but knowing the distinctions between them will make it easier for you to construct your own.
1. Null hypothesis
A null hypothesis proposes no relationship between two variables. Denoted by H 0 , it is a negative statement like “Attending physiotherapy sessions does not affect athletes' on-field performance.” Here, the author claims physiotherapy sessions have no effect on on-field performances. Even if there is, it's only a coincidence.
2. Alternative hypothesis
Considered to be the opposite of a null hypothesis, an alternative hypothesis is donated as H1 or Ha. It explicitly states that the dependent variable affects the independent variable. A good alternative hypothesis example is “Attending physiotherapy sessions improves athletes' on-field performance.” or “Water evaporates at 100 °C. ” The alternative hypothesis further branches into directional and non-directional.
- Directional hypothesis: A hypothesis that states the result would be either positive or negative is called directional hypothesis. It accompanies H1 with either the ‘<' or ‘>' sign.
- Non-directional hypothesis: A non-directional hypothesis only claims an effect on the dependent variable. It does not clarify whether the result would be positive or negative. The sign for a non-directional hypothesis is ‘≠.'
3. Simple hypothesis
A simple hypothesis is a statement made to reflect the relation between exactly two variables. One independent and one dependent. Consider the example, “Smoking is a prominent cause of lung cancer." The dependent variable, lung cancer, is dependent on the independent variable, smoking.
4. Complex hypothesis
In contrast to a simple hypothesis, a complex hypothesis implies the relationship between multiple independent and dependent variables. For instance, “Individuals who eat more fruits tend to have higher immunity, lesser cholesterol, and high metabolism.” The independent variable is eating more fruits, while the dependent variables are higher immunity, lesser cholesterol, and high metabolism.
5. Associative and casual hypothesis
Associative and casual hypotheses don't exhibit how many variables there will be. They define the relationship between the variables. In an associative hypothesis, changing any one variable, dependent or independent, affects others. In a casual hypothesis, the independent variable directly affects the dependent.
6. Empirical hypothesis
Also referred to as the working hypothesis, an empirical hypothesis claims a theory's validation via experiments and observation. This way, the statement appears justifiable and different from a wild guess.
Say, the hypothesis is “Women who take iron tablets face a lesser risk of anemia than those who take vitamin B12.” This is an example of an empirical hypothesis where the researcher the statement after assessing a group of women who take iron tablets and charting the findings.
7. Statistical hypothesis
The point of a statistical hypothesis is to test an already existing hypothesis by studying a population sample. Hypothesis like “44% of the Indian population belong in the age group of 22-27.” leverage evidence to prove or disprove a particular statement.
Characteristics of a Good Hypothesis
Writing a hypothesis is essential as it can make or break your research for you. That includes your chances of getting published in a journal. So when you're designing one, keep an eye out for these pointers:
- A research hypothesis has to be simple yet clear to look justifiable enough.
- It has to be testable — your research would be rendered pointless if too far-fetched into reality or limited by technology.
- It has to be precise about the results —what you are trying to do and achieve through it should come out in your hypothesis.
- A research hypothesis should be self-explanatory, leaving no doubt in the reader's mind.
- If you are developing a relational hypothesis, you need to include the variables and establish an appropriate relationship among them.
- A hypothesis must keep and reflect the scope for further investigations and experiments.
Separating a Hypothesis from a Prediction
Outside of academia, hypothesis and prediction are often used interchangeably. In research writing, this is not only confusing but also incorrect. And although a hypothesis and prediction are guesses at their core, there are many differences between them.
A hypothesis is an educated guess or even a testable prediction validated through research. It aims to analyze the gathered evidence and facts to define a relationship between variables and put forth a logical explanation behind the nature of events.
Predictions are assumptions or expected outcomes made without any backing evidence. They are more fictionally inclined regardless of where they originate from.
For this reason, a hypothesis holds much more weight than a prediction. It sticks to the scientific method rather than pure guesswork. "Planets revolve around the Sun." is an example of a hypothesis as it is previous knowledge and observed trends. Additionally, we can test it through the scientific method.
Whereas "COVID-19 will be eradicated by 2030." is a prediction. Even though it results from past trends, we can't prove or disprove it. So, the only way this gets validated is to wait and watch if COVID-19 cases end by 2030.
Finally, How to Write a Hypothesis
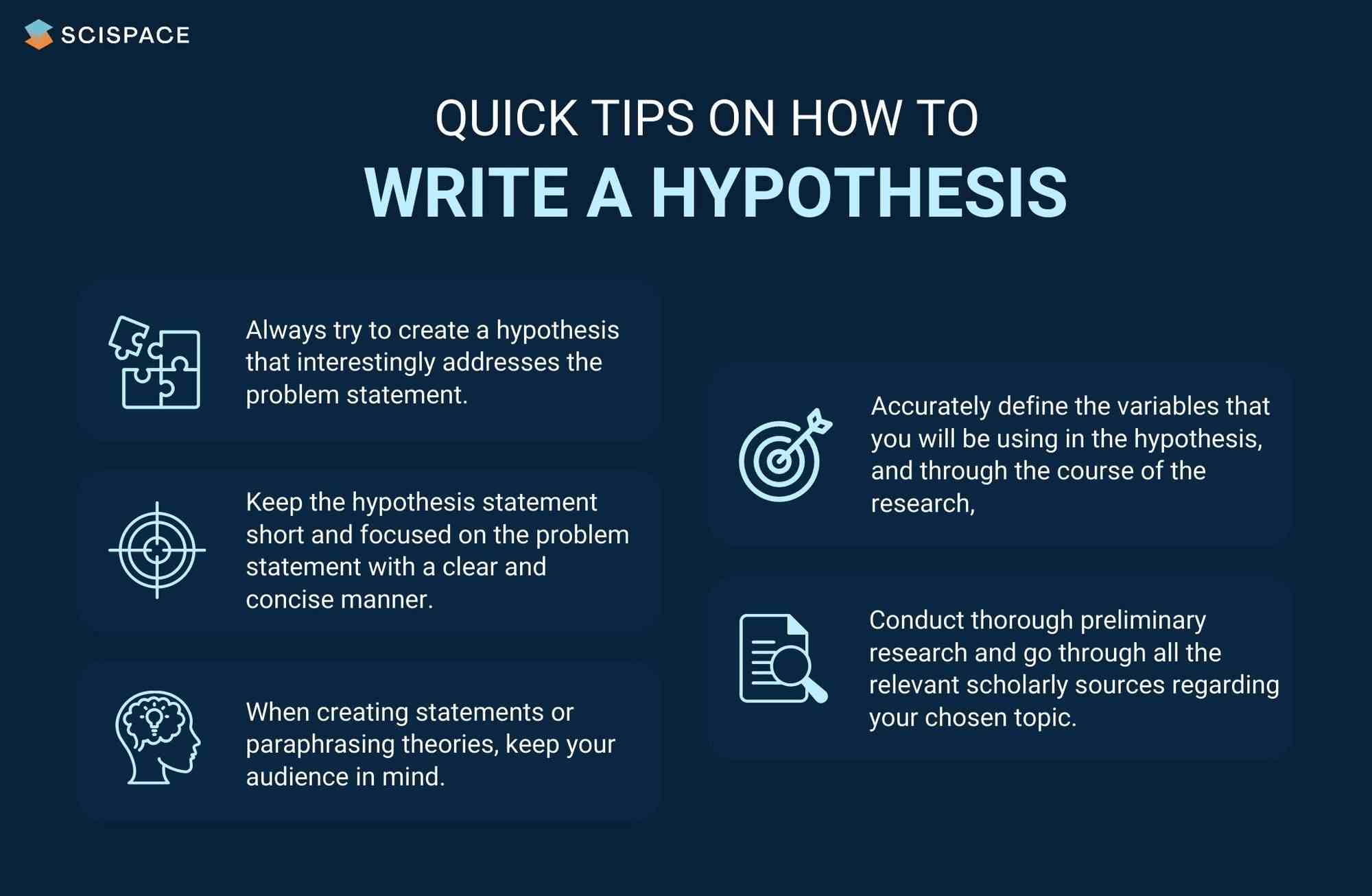
Quick tips on writing a hypothesis
1. Be clear about your research question
A hypothesis should instantly address the research question or the problem statement. To do so, you need to ask a question. Understand the constraints of your undertaken research topic and then formulate a simple and topic-centric problem. Only after that can you develop a hypothesis and further test for evidence.
2. Carry out a recce
Once you have your research's foundation laid out, it would be best to conduct preliminary research. Go through previous theories, academic papers, data, and experiments before you start curating your research hypothesis. It will give you an idea of your hypothesis's viability or originality.
Making use of references from relevant research papers helps draft a good research hypothesis. SciSpace Discover offers a repository of over 270 million research papers to browse through and gain a deeper understanding of related studies on a particular topic. Additionally, you can use SciSpace Copilot , your AI research assistant, for reading any lengthy research paper and getting a more summarized context of it. A hypothesis can be formed after evaluating many such summarized research papers. Copilot also offers explanations for theories and equations, explains paper in simplified version, allows you to highlight any text in the paper or clip math equations and tables and provides a deeper, clear understanding of what is being said. This can improve the hypothesis by helping you identify potential research gaps.
3. Create a 3-dimensional hypothesis
Variables are an essential part of any reasonable hypothesis. So, identify your independent and dependent variable(s) and form a correlation between them. The ideal way to do this is to write the hypothetical assumption in the ‘if-then' form. If you use this form, make sure that you state the predefined relationship between the variables.
In another way, you can choose to present your hypothesis as a comparison between two variables. Here, you must specify the difference you expect to observe in the results.
4. Write the first draft
Now that everything is in place, it's time to write your hypothesis. For starters, create the first draft. In this version, write what you expect to find from your research.
Clearly separate your independent and dependent variables and the link between them. Don't fixate on syntax at this stage. The goal is to ensure your hypothesis addresses the issue.
5. Proof your hypothesis
After preparing the first draft of your hypothesis, you need to inspect it thoroughly. It should tick all the boxes, like being concise, straightforward, relevant, and accurate. Your final hypothesis has to be well-structured as well.
Research projects are an exciting and crucial part of being a scholar. And once you have your research question, you need a great hypothesis to begin conducting research. Thus, knowing how to write a hypothesis is very important.
Now that you have a firmer grasp on what a good hypothesis constitutes, the different kinds there are, and what process to follow, you will find it much easier to write your hypothesis, which ultimately helps your research.
Now it's easier than ever to streamline your research workflow with SciSpace Discover . Its integrated, comprehensive end-to-end platform for research allows scholars to easily discover, write and publish their research and fosters collaboration.
It includes everything you need, including a repository of over 270 million research papers across disciplines, SEO-optimized summaries and public profiles to show your expertise and experience.
If you found these tips on writing a research hypothesis useful, head over to our blog on Statistical Hypothesis Testing to learn about the top researchers, papers, and institutions in this domain.
Frequently Asked Questions (FAQs)
1. what is the definition of hypothesis.
According to the Oxford dictionary, a hypothesis is defined as “An idea or explanation of something that is based on a few known facts, but that has not yet been proved to be true or correct”.
2. What is an example of hypothesis?
The hypothesis is a statement that proposes a relationship between two or more variables. An example: "If we increase the number of new users who join our platform by 25%, then we will see an increase in revenue."
3. What is an example of null hypothesis?
A null hypothesis is a statement that there is no relationship between two variables. The null hypothesis is written as H0. The null hypothesis states that there is no effect. For example, if you're studying whether or not a particular type of exercise increases strength, your null hypothesis will be "there is no difference in strength between people who exercise and people who don't."
4. What are the types of research?
• Fundamental research
• Applied research
• Qualitative research
• Quantitative research
• Mixed research
• Exploratory research
• Longitudinal research
• Cross-sectional research
• Field research
• Laboratory research
• Fixed research
• Flexible research
• Action research
• Policy research
• Classification research
• Comparative research
• Causal research
• Inductive research
• Deductive research
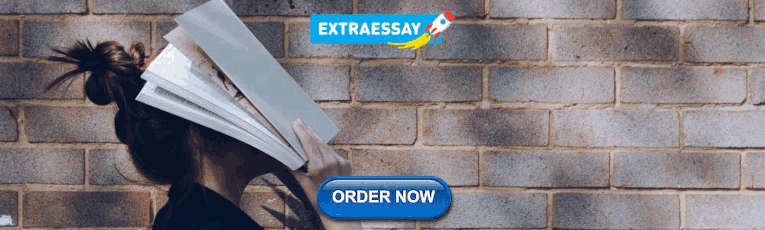
5. How to write a hypothesis?
• Your hypothesis should be able to predict the relationship and outcome.
• Avoid wordiness by keeping it simple and brief.
• Your hypothesis should contain observable and testable outcomes.
• Your hypothesis should be relevant to the research question.
6. What are the 2 types of hypothesis?
• Null hypotheses are used to test the claim that "there is no difference between two groups of data".
• Alternative hypotheses test the claim that "there is a difference between two data groups".
7. Difference between research question and research hypothesis?
A research question is a broad, open-ended question you will try to answer through your research. A hypothesis is a statement based on prior research or theory that you expect to be true due to your study. Example - Research question: What are the factors that influence the adoption of the new technology? Research hypothesis: There is a positive relationship between age, education and income level with the adoption of the new technology.
8. What is plural for hypothesis?
The plural of hypothesis is hypotheses. Here's an example of how it would be used in a statement, "Numerous well-considered hypotheses are presented in this part, and they are supported by tables and figures that are well-illustrated."
9. What is the red queen hypothesis?
The red queen hypothesis in evolutionary biology states that species must constantly evolve to avoid extinction because if they don't, they will be outcompeted by other species that are evolving. Leigh Van Valen first proposed it in 1973; since then, it has been tested and substantiated many times.
10. Who is known as the father of null hypothesis?
The father of the null hypothesis is Sir Ronald Fisher. He published a paper in 1925 that introduced the concept of null hypothesis testing, and he was also the first to use the term itself.
11. When to reject null hypothesis?
You need to find a significant difference between your two populations to reject the null hypothesis. You can determine that by running statistical tests such as an independent sample t-test or a dependent sample t-test. You should reject the null hypothesis if the p-value is less than 0.05.

You might also like

Consensus GPT vs. SciSpace GPT: Choose the Best GPT for Research
Literature Review and Theoretical Framework: Understanding the Differences

Types of Essays in Academic Writing - Quick Guide (2024)
Frequently asked questions
What is a hypothesis.
A hypothesis states your predictions about what your research will find. It is a tentative answer to your research question that has not yet been tested. For some research projects, you might have to write several hypotheses that address different aspects of your research question.
A hypothesis is not just a guess — it should be based on existing theories and knowledge. It also has to be testable, which means you can support or refute it through scientific research methods (such as experiments, observations and statistical analysis of data).
Frequently asked questions: Methodology
Attrition refers to participants leaving a study. It always happens to some extent—for example, in randomized controlled trials for medical research.
Differential attrition occurs when attrition or dropout rates differ systematically between the intervention and the control group . As a result, the characteristics of the participants who drop out differ from the characteristics of those who stay in the study. Because of this, study results may be biased .
Action research is conducted in order to solve a particular issue immediately, while case studies are often conducted over a longer period of time and focus more on observing and analyzing a particular ongoing phenomenon.
Action research is focused on solving a problem or informing individual and community-based knowledge in a way that impacts teaching, learning, and other related processes. It is less focused on contributing theoretical input, instead producing actionable input.
Action research is particularly popular with educators as a form of systematic inquiry because it prioritizes reflection and bridges the gap between theory and practice. Educators are able to simultaneously investigate an issue as they solve it, and the method is very iterative and flexible.
A cycle of inquiry is another name for action research . It is usually visualized in a spiral shape following a series of steps, such as “planning → acting → observing → reflecting.”
To make quantitative observations , you need to use instruments that are capable of measuring the quantity you want to observe. For example, you might use a ruler to measure the length of an object or a thermometer to measure its temperature.
Criterion validity and construct validity are both types of measurement validity . In other words, they both show you how accurately a method measures something.
While construct validity is the degree to which a test or other measurement method measures what it claims to measure, criterion validity is the degree to which a test can predictively (in the future) or concurrently (in the present) measure something.
Construct validity is often considered the overarching type of measurement validity . You need to have face validity , content validity , and criterion validity in order to achieve construct validity.
Convergent validity and discriminant validity are both subtypes of construct validity . Together, they help you evaluate whether a test measures the concept it was designed to measure.
- Convergent validity indicates whether a test that is designed to measure a particular construct correlates with other tests that assess the same or similar construct.
- Discriminant validity indicates whether two tests that should not be highly related to each other are indeed not related. This type of validity is also called divergent validity .
You need to assess both in order to demonstrate construct validity. Neither one alone is sufficient for establishing construct validity.
- Discriminant validity indicates whether two tests that should not be highly related to each other are indeed not related
Content validity shows you how accurately a test or other measurement method taps into the various aspects of the specific construct you are researching.
In other words, it helps you answer the question: “does the test measure all aspects of the construct I want to measure?” If it does, then the test has high content validity.
The higher the content validity, the more accurate the measurement of the construct.
If the test fails to include parts of the construct, or irrelevant parts are included, the validity of the instrument is threatened, which brings your results into question.
Face validity and content validity are similar in that they both evaluate how suitable the content of a test is. The difference is that face validity is subjective, and assesses content at surface level.
When a test has strong face validity, anyone would agree that the test’s questions appear to measure what they are intended to measure.
For example, looking at a 4th grade math test consisting of problems in which students have to add and multiply, most people would agree that it has strong face validity (i.e., it looks like a math test).
On the other hand, content validity evaluates how well a test represents all the aspects of a topic. Assessing content validity is more systematic and relies on expert evaluation. of each question, analyzing whether each one covers the aspects that the test was designed to cover.
A 4th grade math test would have high content validity if it covered all the skills taught in that grade. Experts(in this case, math teachers), would have to evaluate the content validity by comparing the test to the learning objectives.
Snowball sampling is a non-probability sampling method . Unlike probability sampling (which involves some form of random selection ), the initial individuals selected to be studied are the ones who recruit new participants.
Because not every member of the target population has an equal chance of being recruited into the sample, selection in snowball sampling is non-random.
Snowball sampling is a non-probability sampling method , where there is not an equal chance for every member of the population to be included in the sample .
This means that you cannot use inferential statistics and make generalizations —often the goal of quantitative research . As such, a snowball sample is not representative of the target population and is usually a better fit for qualitative research .
Snowball sampling relies on the use of referrals. Here, the researcher recruits one or more initial participants, who then recruit the next ones.
Participants share similar characteristics and/or know each other. Because of this, not every member of the population has an equal chance of being included in the sample, giving rise to sampling bias .
Snowball sampling is best used in the following cases:
- If there is no sampling frame available (e.g., people with a rare disease)
- If the population of interest is hard to access or locate (e.g., people experiencing homelessness)
- If the research focuses on a sensitive topic (e.g., extramarital affairs)
The reproducibility and replicability of a study can be ensured by writing a transparent, detailed method section and using clear, unambiguous language.
Reproducibility and replicability are related terms.
- Reproducing research entails reanalyzing the existing data in the same manner.
- Replicating (or repeating ) the research entails reconducting the entire analysis, including the collection of new data .
- A successful reproduction shows that the data analyses were conducted in a fair and honest manner.
- A successful replication shows that the reliability of the results is high.
Stratified sampling and quota sampling both involve dividing the population into subgroups and selecting units from each subgroup. The purpose in both cases is to select a representative sample and/or to allow comparisons between subgroups.
The main difference is that in stratified sampling, you draw a random sample from each subgroup ( probability sampling ). In quota sampling you select a predetermined number or proportion of units, in a non-random manner ( non-probability sampling ).
Purposive and convenience sampling are both sampling methods that are typically used in qualitative data collection.
A convenience sample is drawn from a source that is conveniently accessible to the researcher. Convenience sampling does not distinguish characteristics among the participants. On the other hand, purposive sampling focuses on selecting participants possessing characteristics associated with the research study.
The findings of studies based on either convenience or purposive sampling can only be generalized to the (sub)population from which the sample is drawn, and not to the entire population.
Random sampling or probability sampling is based on random selection. This means that each unit has an equal chance (i.e., equal probability) of being included in the sample.
On the other hand, convenience sampling involves stopping people at random, which means that not everyone has an equal chance of being selected depending on the place, time, or day you are collecting your data.
Convenience sampling and quota sampling are both non-probability sampling methods. They both use non-random criteria like availability, geographical proximity, or expert knowledge to recruit study participants.
However, in convenience sampling, you continue to sample units or cases until you reach the required sample size.
In quota sampling, you first need to divide your population of interest into subgroups (strata) and estimate their proportions (quota) in the population. Then you can start your data collection, using convenience sampling to recruit participants, until the proportions in each subgroup coincide with the estimated proportions in the population.
A sampling frame is a list of every member in the entire population . It is important that the sampling frame is as complete as possible, so that your sample accurately reflects your population.
Stratified and cluster sampling may look similar, but bear in mind that groups created in cluster sampling are heterogeneous , so the individual characteristics in the cluster vary. In contrast, groups created in stratified sampling are homogeneous , as units share characteristics.
Relatedly, in cluster sampling you randomly select entire groups and include all units of each group in your sample. However, in stratified sampling, you select some units of all groups and include them in your sample. In this way, both methods can ensure that your sample is representative of the target population .
A systematic review is secondary research because it uses existing research. You don’t collect new data yourself.
The key difference between observational studies and experimental designs is that a well-done observational study does not influence the responses of participants, while experiments do have some sort of treatment condition applied to at least some participants by random assignment .
An observational study is a great choice for you if your research question is based purely on observations. If there are ethical, logistical, or practical concerns that prevent you from conducting a traditional experiment , an observational study may be a good choice. In an observational study, there is no interference or manipulation of the research subjects, as well as no control or treatment groups .
It’s often best to ask a variety of people to review your measurements. You can ask experts, such as other researchers, or laypeople, such as potential participants, to judge the face validity of tests.
While experts have a deep understanding of research methods , the people you’re studying can provide you with valuable insights you may have missed otherwise.
Face validity is important because it’s a simple first step to measuring the overall validity of a test or technique. It’s a relatively intuitive, quick, and easy way to start checking whether a new measure seems useful at first glance.
Good face validity means that anyone who reviews your measure says that it seems to be measuring what it’s supposed to. With poor face validity, someone reviewing your measure may be left confused about what you’re measuring and why you’re using this method.
Face validity is about whether a test appears to measure what it’s supposed to measure. This type of validity is concerned with whether a measure seems relevant and appropriate for what it’s assessing only on the surface.
Statistical analyses are often applied to test validity with data from your measures. You test convergent validity and discriminant validity with correlations to see if results from your test are positively or negatively related to those of other established tests.
You can also use regression analyses to assess whether your measure is actually predictive of outcomes that you expect it to predict theoretically. A regression analysis that supports your expectations strengthens your claim of construct validity .
When designing or evaluating a measure, construct validity helps you ensure you’re actually measuring the construct you’re interested in. If you don’t have construct validity, you may inadvertently measure unrelated or distinct constructs and lose precision in your research.
Construct validity is often considered the overarching type of measurement validity , because it covers all of the other types. You need to have face validity , content validity , and criterion validity to achieve construct validity.
Construct validity is about how well a test measures the concept it was designed to evaluate. It’s one of four types of measurement validity , which includes construct validity, face validity , and criterion validity.
There are two subtypes of construct validity.
- Convergent validity : The extent to which your measure corresponds to measures of related constructs
- Discriminant validity : The extent to which your measure is unrelated or negatively related to measures of distinct constructs
Naturalistic observation is a valuable tool because of its flexibility, external validity , and suitability for topics that can’t be studied in a lab setting.
The downsides of naturalistic observation include its lack of scientific control , ethical considerations , and potential for bias from observers and subjects.
Naturalistic observation is a qualitative research method where you record the behaviors of your research subjects in real world settings. You avoid interfering or influencing anything in a naturalistic observation.
You can think of naturalistic observation as “people watching” with a purpose.
A dependent variable is what changes as a result of the independent variable manipulation in experiments . It’s what you’re interested in measuring, and it “depends” on your independent variable.
In statistics, dependent variables are also called:
- Response variables (they respond to a change in another variable)
- Outcome variables (they represent the outcome you want to measure)
- Left-hand-side variables (they appear on the left-hand side of a regression equation)
An independent variable is the variable you manipulate, control, or vary in an experimental study to explore its effects. It’s called “independent” because it’s not influenced by any other variables in the study.
Independent variables are also called:
- Explanatory variables (they explain an event or outcome)
- Predictor variables (they can be used to predict the value of a dependent variable)
- Right-hand-side variables (they appear on the right-hand side of a regression equation).
As a rule of thumb, questions related to thoughts, beliefs, and feelings work well in focus groups. Take your time formulating strong questions, paying special attention to phrasing. Be careful to avoid leading questions , which can bias your responses.
Overall, your focus group questions should be:
- Open-ended and flexible
- Impossible to answer with “yes” or “no” (questions that start with “why” or “how” are often best)
- Unambiguous, getting straight to the point while still stimulating discussion
- Unbiased and neutral
A structured interview is a data collection method that relies on asking questions in a set order to collect data on a topic. They are often quantitative in nature. Structured interviews are best used when:
- You already have a very clear understanding of your topic. Perhaps significant research has already been conducted, or you have done some prior research yourself, but you already possess a baseline for designing strong structured questions.
- You are constrained in terms of time or resources and need to analyze your data quickly and efficiently.
- Your research question depends on strong parity between participants, with environmental conditions held constant.
More flexible interview options include semi-structured interviews , unstructured interviews , and focus groups .
Social desirability bias is the tendency for interview participants to give responses that will be viewed favorably by the interviewer or other participants. It occurs in all types of interviews and surveys , but is most common in semi-structured interviews , unstructured interviews , and focus groups .
Social desirability bias can be mitigated by ensuring participants feel at ease and comfortable sharing their views. Make sure to pay attention to your own body language and any physical or verbal cues, such as nodding or widening your eyes.
This type of bias can also occur in observations if the participants know they’re being observed. They might alter their behavior accordingly.
The interviewer effect is a type of bias that emerges when a characteristic of an interviewer (race, age, gender identity, etc.) influences the responses given by the interviewee.
There is a risk of an interviewer effect in all types of interviews , but it can be mitigated by writing really high-quality interview questions.
A semi-structured interview is a blend of structured and unstructured types of interviews. Semi-structured interviews are best used when:
- You have prior interview experience. Spontaneous questions are deceptively challenging, and it’s easy to accidentally ask a leading question or make a participant uncomfortable.
- Your research question is exploratory in nature. Participant answers can guide future research questions and help you develop a more robust knowledge base for future research.
An unstructured interview is the most flexible type of interview, but it is not always the best fit for your research topic.
Unstructured interviews are best used when:
- You are an experienced interviewer and have a very strong background in your research topic, since it is challenging to ask spontaneous, colloquial questions.
- Your research question is exploratory in nature. While you may have developed hypotheses, you are open to discovering new or shifting viewpoints through the interview process.
- You are seeking descriptive data, and are ready to ask questions that will deepen and contextualize your initial thoughts and hypotheses.
- Your research depends on forming connections with your participants and making them feel comfortable revealing deeper emotions, lived experiences, or thoughts.
The four most common types of interviews are:
- Structured interviews : The questions are predetermined in both topic and order.
- Semi-structured interviews : A few questions are predetermined, but other questions aren’t planned.
- Unstructured interviews : None of the questions are predetermined.
- Focus group interviews : The questions are presented to a group instead of one individual.
Deductive reasoning is commonly used in scientific research, and it’s especially associated with quantitative research .
In research, you might have come across something called the hypothetico-deductive method . It’s the scientific method of testing hypotheses to check whether your predictions are substantiated by real-world data.
Deductive reasoning is a logical approach where you progress from general ideas to specific conclusions. It’s often contrasted with inductive reasoning , where you start with specific observations and form general conclusions.
Deductive reasoning is also called deductive logic.
There are many different types of inductive reasoning that people use formally or informally.
Here are a few common types:
- Inductive generalization : You use observations about a sample to come to a conclusion about the population it came from.
- Statistical generalization: You use specific numbers about samples to make statements about populations.
- Causal reasoning: You make cause-and-effect links between different things.
- Sign reasoning: You make a conclusion about a correlational relationship between different things.
- Analogical reasoning: You make a conclusion about something based on its similarities to something else.
Inductive reasoning is a bottom-up approach, while deductive reasoning is top-down.
Inductive reasoning takes you from the specific to the general, while in deductive reasoning, you make inferences by going from general premises to specific conclusions.
In inductive research , you start by making observations or gathering data. Then, you take a broad scan of your data and search for patterns. Finally, you make general conclusions that you might incorporate into theories.
Inductive reasoning is a method of drawing conclusions by going from the specific to the general. It’s usually contrasted with deductive reasoning, where you proceed from general information to specific conclusions.
Inductive reasoning is also called inductive logic or bottom-up reasoning.
Triangulation can help:
- Reduce research bias that comes from using a single method, theory, or investigator
- Enhance validity by approaching the same topic with different tools
- Establish credibility by giving you a complete picture of the research problem
But triangulation can also pose problems:
- It’s time-consuming and labor-intensive, often involving an interdisciplinary team.
- Your results may be inconsistent or even contradictory.
There are four main types of triangulation :
- Data triangulation : Using data from different times, spaces, and people
- Investigator triangulation : Involving multiple researchers in collecting or analyzing data
- Theory triangulation : Using varying theoretical perspectives in your research
- Methodological triangulation : Using different methodologies to approach the same topic
Many academic fields use peer review , largely to determine whether a manuscript is suitable for publication. Peer review enhances the credibility of the published manuscript.
However, peer review is also common in non-academic settings. The United Nations, the European Union, and many individual nations use peer review to evaluate grant applications. It is also widely used in medical and health-related fields as a teaching or quality-of-care measure.
Peer assessment is often used in the classroom as a pedagogical tool. Both receiving feedback and providing it are thought to enhance the learning process, helping students think critically and collaboratively.
Peer review can stop obviously problematic, falsified, or otherwise untrustworthy research from being published. It also represents an excellent opportunity to get feedback from renowned experts in your field. It acts as a first defense, helping you ensure your argument is clear and that there are no gaps, vague terms, or unanswered questions for readers who weren’t involved in the research process.
Peer-reviewed articles are considered a highly credible source due to this stringent process they go through before publication.
In general, the peer review process follows the following steps:
- First, the author submits the manuscript to the editor.
- Reject the manuscript and send it back to author, or
- Send it onward to the selected peer reviewer(s)
- Next, the peer review process occurs. The reviewer provides feedback, addressing any major or minor issues with the manuscript, and gives their advice regarding what edits should be made.
- Lastly, the edited manuscript is sent back to the author. They input the edits, and resubmit it to the editor for publication.
Exploratory research is often used when the issue you’re studying is new or when the data collection process is challenging for some reason.
You can use exploratory research if you have a general idea or a specific question that you want to study but there is no preexisting knowledge or paradigm with which to study it.
Exploratory research is a methodology approach that explores research questions that have not previously been studied in depth. It is often used when the issue you’re studying is new, or the data collection process is challenging in some way.
Explanatory research is used to investigate how or why a phenomenon occurs. Therefore, this type of research is often one of the first stages in the research process , serving as a jumping-off point for future research.
Exploratory research aims to explore the main aspects of an under-researched problem, while explanatory research aims to explain the causes and consequences of a well-defined problem.
Explanatory research is a research method used to investigate how or why something occurs when only a small amount of information is available pertaining to that topic. It can help you increase your understanding of a given topic.
Clean data are valid, accurate, complete, consistent, unique, and uniform. Dirty data include inconsistencies and errors.
Dirty data can come from any part of the research process, including poor research design , inappropriate measurement materials, or flawed data entry.
Data cleaning takes place between data collection and data analyses. But you can use some methods even before collecting data.
For clean data, you should start by designing measures that collect valid data. Data validation at the time of data entry or collection helps you minimize the amount of data cleaning you’ll need to do.
After data collection, you can use data standardization and data transformation to clean your data. You’ll also deal with any missing values, outliers, and duplicate values.
Every dataset requires different techniques to clean dirty data , but you need to address these issues in a systematic way. You focus on finding and resolving data points that don’t agree or fit with the rest of your dataset.
These data might be missing values, outliers, duplicate values, incorrectly formatted, or irrelevant. You’ll start with screening and diagnosing your data. Then, you’ll often standardize and accept or remove data to make your dataset consistent and valid.
Data cleaning is necessary for valid and appropriate analyses. Dirty data contain inconsistencies or errors , but cleaning your data helps you minimize or resolve these.
Without data cleaning, you could end up with a Type I or II error in your conclusion. These types of erroneous conclusions can be practically significant with important consequences, because they lead to misplaced investments or missed opportunities.
Data cleaning involves spotting and resolving potential data inconsistencies or errors to improve your data quality. An error is any value (e.g., recorded weight) that doesn’t reflect the true value (e.g., actual weight) of something that’s being measured.
In this process, you review, analyze, detect, modify, or remove “dirty” data to make your dataset “clean.” Data cleaning is also called data cleansing or data scrubbing.
Research misconduct means making up or falsifying data, manipulating data analyses, or misrepresenting results in research reports. It’s a form of academic fraud.
These actions are committed intentionally and can have serious consequences; research misconduct is not a simple mistake or a point of disagreement but a serious ethical failure.
Anonymity means you don’t know who the participants are, while confidentiality means you know who they are but remove identifying information from your research report. Both are important ethical considerations .
You can only guarantee anonymity by not collecting any personally identifying information—for example, names, phone numbers, email addresses, IP addresses, physical characteristics, photos, or videos.
You can keep data confidential by using aggregate information in your research report, so that you only refer to groups of participants rather than individuals.
Research ethics matter for scientific integrity, human rights and dignity, and collaboration between science and society. These principles make sure that participation in studies is voluntary, informed, and safe.
Ethical considerations in research are a set of principles that guide your research designs and practices. These principles include voluntary participation, informed consent, anonymity, confidentiality, potential for harm, and results communication.
Scientists and researchers must always adhere to a certain code of conduct when collecting data from others .
These considerations protect the rights of research participants, enhance research validity , and maintain scientific integrity.
In multistage sampling , you can use probability or non-probability sampling methods .
For a probability sample, you have to conduct probability sampling at every stage.
You can mix it up by using simple random sampling , systematic sampling , or stratified sampling to select units at different stages, depending on what is applicable and relevant to your study.
Multistage sampling can simplify data collection when you have large, geographically spread samples, and you can obtain a probability sample without a complete sampling frame.
But multistage sampling may not lead to a representative sample, and larger samples are needed for multistage samples to achieve the statistical properties of simple random samples .
These are four of the most common mixed methods designs :
- Convergent parallel: Quantitative and qualitative data are collected at the same time and analyzed separately. After both analyses are complete, compare your results to draw overall conclusions.
- Embedded: Quantitative and qualitative data are collected at the same time, but within a larger quantitative or qualitative design. One type of data is secondary to the other.
- Explanatory sequential: Quantitative data is collected and analyzed first, followed by qualitative data. You can use this design if you think your qualitative data will explain and contextualize your quantitative findings.
- Exploratory sequential: Qualitative data is collected and analyzed first, followed by quantitative data. You can use this design if you think the quantitative data will confirm or validate your qualitative findings.
Triangulation in research means using multiple datasets, methods, theories and/or investigators to address a research question. It’s a research strategy that can help you enhance the validity and credibility of your findings.
Triangulation is mainly used in qualitative research , but it’s also commonly applied in quantitative research . Mixed methods research always uses triangulation.
In multistage sampling , or multistage cluster sampling, you draw a sample from a population using smaller and smaller groups at each stage.
This method is often used to collect data from a large, geographically spread group of people in national surveys, for example. You take advantage of hierarchical groupings (e.g., from state to city to neighborhood) to create a sample that’s less expensive and time-consuming to collect data from.
No, the steepness or slope of the line isn’t related to the correlation coefficient value. The correlation coefficient only tells you how closely your data fit on a line, so two datasets with the same correlation coefficient can have very different slopes.
To find the slope of the line, you’ll need to perform a regression analysis .
Correlation coefficients always range between -1 and 1.
The sign of the coefficient tells you the direction of the relationship: a positive value means the variables change together in the same direction, while a negative value means they change together in opposite directions.
The absolute value of a number is equal to the number without its sign. The absolute value of a correlation coefficient tells you the magnitude of the correlation: the greater the absolute value, the stronger the correlation.
These are the assumptions your data must meet if you want to use Pearson’s r :
- Both variables are on an interval or ratio level of measurement
- Data from both variables follow normal distributions
- Your data have no outliers
- Your data is from a random or representative sample
- You expect a linear relationship between the two variables
Quantitative research designs can be divided into two main categories:
- Correlational and descriptive designs are used to investigate characteristics, averages, trends, and associations between variables.
- Experimental and quasi-experimental designs are used to test causal relationships .
Qualitative research designs tend to be more flexible. Common types of qualitative design include case study , ethnography , and grounded theory designs.
A well-planned research design helps ensure that your methods match your research aims, that you collect high-quality data, and that you use the right kind of analysis to answer your questions, utilizing credible sources . This allows you to draw valid , trustworthy conclusions.
The priorities of a research design can vary depending on the field, but you usually have to specify:
- Your research questions and/or hypotheses
- Your overall approach (e.g., qualitative or quantitative )
- The type of design you’re using (e.g., a survey , experiment , or case study )
- Your sampling methods or criteria for selecting subjects
- Your data collection methods (e.g., questionnaires , observations)
- Your data collection procedures (e.g., operationalization , timing and data management)
- Your data analysis methods (e.g., statistical tests or thematic analysis )
A research design is a strategy for answering your research question . It defines your overall approach and determines how you will collect and analyze data.
Questionnaires can be self-administered or researcher-administered.
Self-administered questionnaires can be delivered online or in paper-and-pen formats, in person or through mail. All questions are standardized so that all respondents receive the same questions with identical wording.
Researcher-administered questionnaires are interviews that take place by phone, in-person, or online between researchers and respondents. You can gain deeper insights by clarifying questions for respondents or asking follow-up questions.
You can organize the questions logically, with a clear progression from simple to complex, or randomly between respondents. A logical flow helps respondents process the questionnaire easier and quicker, but it may lead to bias. Randomization can minimize the bias from order effects.
Closed-ended, or restricted-choice, questions offer respondents a fixed set of choices to select from. These questions are easier to answer quickly.
Open-ended or long-form questions allow respondents to answer in their own words. Because there are no restrictions on their choices, respondents can answer in ways that researchers may not have otherwise considered.
A questionnaire is a data collection tool or instrument, while a survey is an overarching research method that involves collecting and analyzing data from people using questionnaires.
The third variable and directionality problems are two main reasons why correlation isn’t causation .
The third variable problem means that a confounding variable affects both variables to make them seem causally related when they are not.
The directionality problem is when two variables correlate and might actually have a causal relationship, but it’s impossible to conclude which variable causes changes in the other.
Correlation describes an association between variables : when one variable changes, so does the other. A correlation is a statistical indicator of the relationship between variables.
Causation means that changes in one variable brings about changes in the other (i.e., there is a cause-and-effect relationship between variables). The two variables are correlated with each other, and there’s also a causal link between them.
While causation and correlation can exist simultaneously, correlation does not imply causation. In other words, correlation is simply a relationship where A relates to B—but A doesn’t necessarily cause B to happen (or vice versa). Mistaking correlation for causation is a common error and can lead to false cause fallacy .
Controlled experiments establish causality, whereas correlational studies only show associations between variables.
- In an experimental design , you manipulate an independent variable and measure its effect on a dependent variable. Other variables are controlled so they can’t impact the results.
- In a correlational design , you measure variables without manipulating any of them. You can test whether your variables change together, but you can’t be sure that one variable caused a change in another.
In general, correlational research is high in external validity while experimental research is high in internal validity .
A correlation is usually tested for two variables at a time, but you can test correlations between three or more variables.
A correlation coefficient is a single number that describes the strength and direction of the relationship between your variables.
Different types of correlation coefficients might be appropriate for your data based on their levels of measurement and distributions . The Pearson product-moment correlation coefficient (Pearson’s r ) is commonly used to assess a linear relationship between two quantitative variables.
A correlational research design investigates relationships between two variables (or more) without the researcher controlling or manipulating any of them. It’s a non-experimental type of quantitative research .
A correlation reflects the strength and/or direction of the association between two or more variables.
- A positive correlation means that both variables change in the same direction.
- A negative correlation means that the variables change in opposite directions.
- A zero correlation means there’s no relationship between the variables.
Random error is almost always present in scientific studies, even in highly controlled settings. While you can’t eradicate it completely, you can reduce random error by taking repeated measurements, using a large sample, and controlling extraneous variables .
You can avoid systematic error through careful design of your sampling , data collection , and analysis procedures. For example, use triangulation to measure your variables using multiple methods; regularly calibrate instruments or procedures; use random sampling and random assignment ; and apply masking (blinding) where possible.
Systematic error is generally a bigger problem in research.
With random error, multiple measurements will tend to cluster around the true value. When you’re collecting data from a large sample , the errors in different directions will cancel each other out.
Systematic errors are much more problematic because they can skew your data away from the true value. This can lead you to false conclusions ( Type I and II errors ) about the relationship between the variables you’re studying.
Random and systematic error are two types of measurement error.
Random error is a chance difference between the observed and true values of something (e.g., a researcher misreading a weighing scale records an incorrect measurement).
Systematic error is a consistent or proportional difference between the observed and true values of something (e.g., a miscalibrated scale consistently records weights as higher than they actually are).
On graphs, the explanatory variable is conventionally placed on the x-axis, while the response variable is placed on the y-axis.
- If you have quantitative variables , use a scatterplot or a line graph.
- If your response variable is categorical, use a scatterplot or a line graph.
- If your explanatory variable is categorical, use a bar graph.
The term “ explanatory variable ” is sometimes preferred over “ independent variable ” because, in real world contexts, independent variables are often influenced by other variables. This means they aren’t totally independent.
Multiple independent variables may also be correlated with each other, so “explanatory variables” is a more appropriate term.
The difference between explanatory and response variables is simple:
- An explanatory variable is the expected cause, and it explains the results.
- A response variable is the expected effect, and it responds to other variables.
In a controlled experiment , all extraneous variables are held constant so that they can’t influence the results. Controlled experiments require:
- A control group that receives a standard treatment, a fake treatment, or no treatment.
- Random assignment of participants to ensure the groups are equivalent.
Depending on your study topic, there are various other methods of controlling variables .
There are 4 main types of extraneous variables :
- Demand characteristics : environmental cues that encourage participants to conform to researchers’ expectations.
- Experimenter effects : unintentional actions by researchers that influence study outcomes.
- Situational variables : environmental variables that alter participants’ behaviors.
- Participant variables : any characteristic or aspect of a participant’s background that could affect study results.
An extraneous variable is any variable that you’re not investigating that can potentially affect the dependent variable of your research study.
A confounding variable is a type of extraneous variable that not only affects the dependent variable, but is also related to the independent variable.
In a factorial design, multiple independent variables are tested.
If you test two variables, each level of one independent variable is combined with each level of the other independent variable to create different conditions.
Within-subjects designs have many potential threats to internal validity , but they are also very statistically powerful .
Advantages:
- Only requires small samples
- Statistically powerful
- Removes the effects of individual differences on the outcomes
Disadvantages:
- Internal validity threats reduce the likelihood of establishing a direct relationship between variables
- Time-related effects, such as growth, can influence the outcomes
- Carryover effects mean that the specific order of different treatments affect the outcomes
While a between-subjects design has fewer threats to internal validity , it also requires more participants for high statistical power than a within-subjects design .
- Prevents carryover effects of learning and fatigue.
- Shorter study duration.
- Needs larger samples for high power.
- Uses more resources to recruit participants, administer sessions, cover costs, etc.
- Individual differences may be an alternative explanation for results.
Yes. Between-subjects and within-subjects designs can be combined in a single study when you have two or more independent variables (a factorial design). In a mixed factorial design, one variable is altered between subjects and another is altered within subjects.
In a between-subjects design , every participant experiences only one condition, and researchers assess group differences between participants in various conditions.
In a within-subjects design , each participant experiences all conditions, and researchers test the same participants repeatedly for differences between conditions.
The word “between” means that you’re comparing different conditions between groups, while the word “within” means you’re comparing different conditions within the same group.
Random assignment is used in experiments with a between-groups or independent measures design. In this research design, there’s usually a control group and one or more experimental groups. Random assignment helps ensure that the groups are comparable.
In general, you should always use random assignment in this type of experimental design when it is ethically possible and makes sense for your study topic.
To implement random assignment , assign a unique number to every member of your study’s sample .
Then, you can use a random number generator or a lottery method to randomly assign each number to a control or experimental group. You can also do so manually, by flipping a coin or rolling a dice to randomly assign participants to groups.
Random selection, or random sampling , is a way of selecting members of a population for your study’s sample.
In contrast, random assignment is a way of sorting the sample into control and experimental groups.
Random sampling enhances the external validity or generalizability of your results, while random assignment improves the internal validity of your study.
In experimental research, random assignment is a way of placing participants from your sample into different groups using randomization. With this method, every member of the sample has a known or equal chance of being placed in a control group or an experimental group.
“Controlling for a variable” means measuring extraneous variables and accounting for them statistically to remove their effects on other variables.
Researchers often model control variable data along with independent and dependent variable data in regression analyses and ANCOVAs . That way, you can isolate the control variable’s effects from the relationship between the variables of interest.
Control variables help you establish a correlational or causal relationship between variables by enhancing internal validity .
If you don’t control relevant extraneous variables , they may influence the outcomes of your study, and you may not be able to demonstrate that your results are really an effect of your independent variable .
A control variable is any variable that’s held constant in a research study. It’s not a variable of interest in the study, but it’s controlled because it could influence the outcomes.
Including mediators and moderators in your research helps you go beyond studying a simple relationship between two variables for a fuller picture of the real world. They are important to consider when studying complex correlational or causal relationships.
Mediators are part of the causal pathway of an effect, and they tell you how or why an effect takes place. Moderators usually help you judge the external validity of your study by identifying the limitations of when the relationship between variables holds.
If something is a mediating variable :
- It’s caused by the independent variable .
- It influences the dependent variable
- When it’s taken into account, the statistical correlation between the independent and dependent variables is higher than when it isn’t considered.
A confounder is a third variable that affects variables of interest and makes them seem related when they are not. In contrast, a mediator is the mechanism of a relationship between two variables: it explains the process by which they are related.
A mediator variable explains the process through which two variables are related, while a moderator variable affects the strength and direction of that relationship.
There are three key steps in systematic sampling :
- Define and list your population , ensuring that it is not ordered in a cyclical or periodic order.
- Decide on your sample size and calculate your interval, k , by dividing your population by your target sample size.
- Choose every k th member of the population as your sample.
Systematic sampling is a probability sampling method where researchers select members of the population at a regular interval – for example, by selecting every 15th person on a list of the population. If the population is in a random order, this can imitate the benefits of simple random sampling .
Yes, you can create a stratified sample using multiple characteristics, but you must ensure that every participant in your study belongs to one and only one subgroup. In this case, you multiply the numbers of subgroups for each characteristic to get the total number of groups.
For example, if you were stratifying by location with three subgroups (urban, rural, or suburban) and marital status with five subgroups (single, divorced, widowed, married, or partnered), you would have 3 x 5 = 15 subgroups.
You should use stratified sampling when your sample can be divided into mutually exclusive and exhaustive subgroups that you believe will take on different mean values for the variable that you’re studying.
Using stratified sampling will allow you to obtain more precise (with lower variance ) statistical estimates of whatever you are trying to measure.
For example, say you want to investigate how income differs based on educational attainment, but you know that this relationship can vary based on race. Using stratified sampling, you can ensure you obtain a large enough sample from each racial group, allowing you to draw more precise conclusions.
In stratified sampling , researchers divide subjects into subgroups called strata based on characteristics that they share (e.g., race, gender, educational attainment).
Once divided, each subgroup is randomly sampled using another probability sampling method.
Cluster sampling is more time- and cost-efficient than other probability sampling methods , particularly when it comes to large samples spread across a wide geographical area.
However, it provides less statistical certainty than other methods, such as simple random sampling , because it is difficult to ensure that your clusters properly represent the population as a whole.
There are three types of cluster sampling : single-stage, double-stage and multi-stage clustering. In all three types, you first divide the population into clusters, then randomly select clusters for use in your sample.
- In single-stage sampling , you collect data from every unit within the selected clusters.
- In double-stage sampling , you select a random sample of units from within the clusters.
- In multi-stage sampling , you repeat the procedure of randomly sampling elements from within the clusters until you have reached a manageable sample.
Cluster sampling is a probability sampling method in which you divide a population into clusters, such as districts or schools, and then randomly select some of these clusters as your sample.
The clusters should ideally each be mini-representations of the population as a whole.
If properly implemented, simple random sampling is usually the best sampling method for ensuring both internal and external validity . However, it can sometimes be impractical and expensive to implement, depending on the size of the population to be studied,
If you have a list of every member of the population and the ability to reach whichever members are selected, you can use simple random sampling.
The American Community Survey is an example of simple random sampling . In order to collect detailed data on the population of the US, the Census Bureau officials randomly select 3.5 million households per year and use a variety of methods to convince them to fill out the survey.
Simple random sampling is a type of probability sampling in which the researcher randomly selects a subset of participants from a population . Each member of the population has an equal chance of being selected. Data is then collected from as large a percentage as possible of this random subset.
Quasi-experimental design is most useful in situations where it would be unethical or impractical to run a true experiment .
Quasi-experiments have lower internal validity than true experiments, but they often have higher external validity as they can use real-world interventions instead of artificial laboratory settings.
A quasi-experiment is a type of research design that attempts to establish a cause-and-effect relationship. The main difference with a true experiment is that the groups are not randomly assigned.
Blinding is important to reduce research bias (e.g., observer bias , demand characteristics ) and ensure a study’s internal validity .
If participants know whether they are in a control or treatment group , they may adjust their behavior in ways that affect the outcome that researchers are trying to measure. If the people administering the treatment are aware of group assignment, they may treat participants differently and thus directly or indirectly influence the final results.
- In a single-blind study , only the participants are blinded.
- In a double-blind study , both participants and experimenters are blinded.
- In a triple-blind study , the assignment is hidden not only from participants and experimenters, but also from the researchers analyzing the data.
Blinding means hiding who is assigned to the treatment group and who is assigned to the control group in an experiment .
A true experiment (a.k.a. a controlled experiment) always includes at least one control group that doesn’t receive the experimental treatment.
However, some experiments use a within-subjects design to test treatments without a control group. In these designs, you usually compare one group’s outcomes before and after a treatment (instead of comparing outcomes between different groups).
For strong internal validity , it’s usually best to include a control group if possible. Without a control group, it’s harder to be certain that the outcome was caused by the experimental treatment and not by other variables.
An experimental group, also known as a treatment group, receives the treatment whose effect researchers wish to study, whereas a control group does not. They should be identical in all other ways.
Individual Likert-type questions are generally considered ordinal data , because the items have clear rank order, but don’t have an even distribution.
Overall Likert scale scores are sometimes treated as interval data. These scores are considered to have directionality and even spacing between them.
The type of data determines what statistical tests you should use to analyze your data.
A Likert scale is a rating scale that quantitatively assesses opinions, attitudes, or behaviors. It is made up of 4 or more questions that measure a single attitude or trait when response scores are combined.
To use a Likert scale in a survey , you present participants with Likert-type questions or statements, and a continuum of items, usually with 5 or 7 possible responses, to capture their degree of agreement.
In scientific research, concepts are the abstract ideas or phenomena that are being studied (e.g., educational achievement). Variables are properties or characteristics of the concept (e.g., performance at school), while indicators are ways of measuring or quantifying variables (e.g., yearly grade reports).
The process of turning abstract concepts into measurable variables and indicators is called operationalization .
There are various approaches to qualitative data analysis , but they all share five steps in common:
- Prepare and organize your data.
- Review and explore your data.
- Develop a data coding system.
- Assign codes to the data.
- Identify recurring themes.
The specifics of each step depend on the focus of the analysis. Some common approaches include textual analysis , thematic analysis , and discourse analysis .
There are five common approaches to qualitative research :
- Grounded theory involves collecting data in order to develop new theories.
- Ethnography involves immersing yourself in a group or organization to understand its culture.
- Narrative research involves interpreting stories to understand how people make sense of their experiences and perceptions.
- Phenomenological research involves investigating phenomena through people’s lived experiences.
- Action research links theory and practice in several cycles to drive innovative changes.
Hypothesis testing is a formal procedure for investigating our ideas about the world using statistics. It is used by scientists to test specific predictions, called hypotheses , by calculating how likely it is that a pattern or relationship between variables could have arisen by chance.
Operationalization means turning abstract conceptual ideas into measurable observations.
For example, the concept of social anxiety isn’t directly observable, but it can be operationally defined in terms of self-rating scores, behavioral avoidance of crowded places, or physical anxiety symptoms in social situations.
Before collecting data , it’s important to consider how you will operationalize the variables that you want to measure.
When conducting research, collecting original data has significant advantages:
- You can tailor data collection to your specific research aims (e.g. understanding the needs of your consumers or user testing your website)
- You can control and standardize the process for high reliability and validity (e.g. choosing appropriate measurements and sampling methods )
However, there are also some drawbacks: data collection can be time-consuming, labor-intensive and expensive. In some cases, it’s more efficient to use secondary data that has already been collected by someone else, but the data might be less reliable.
Data collection is the systematic process by which observations or measurements are gathered in research. It is used in many different contexts by academics, governments, businesses, and other organizations.
There are several methods you can use to decrease the impact of confounding variables on your research: restriction, matching, statistical control and randomization.
In restriction , you restrict your sample by only including certain subjects that have the same values of potential confounding variables.
In matching , you match each of the subjects in your treatment group with a counterpart in the comparison group. The matched subjects have the same values on any potential confounding variables, and only differ in the independent variable .
In statistical control , you include potential confounders as variables in your regression .
In randomization , you randomly assign the treatment (or independent variable) in your study to a sufficiently large number of subjects, which allows you to control for all potential confounding variables.
A confounding variable is closely related to both the independent and dependent variables in a study. An independent variable represents the supposed cause , while the dependent variable is the supposed effect . A confounding variable is a third variable that influences both the independent and dependent variables.
Failing to account for confounding variables can cause you to wrongly estimate the relationship between your independent and dependent variables.
To ensure the internal validity of your research, you must consider the impact of confounding variables. If you fail to account for them, you might over- or underestimate the causal relationship between your independent and dependent variables , or even find a causal relationship where none exists.
Yes, but including more than one of either type requires multiple research questions .
For example, if you are interested in the effect of a diet on health, you can use multiple measures of health: blood sugar, blood pressure, weight, pulse, and many more. Each of these is its own dependent variable with its own research question.
You could also choose to look at the effect of exercise levels as well as diet, or even the additional effect of the two combined. Each of these is a separate independent variable .
To ensure the internal validity of an experiment , you should only change one independent variable at a time.
No. The value of a dependent variable depends on an independent variable, so a variable cannot be both independent and dependent at the same time. It must be either the cause or the effect, not both!
You want to find out how blood sugar levels are affected by drinking diet soda and regular soda, so you conduct an experiment .
- The type of soda – diet or regular – is the independent variable .
- The level of blood sugar that you measure is the dependent variable – it changes depending on the type of soda.
Determining cause and effect is one of the most important parts of scientific research. It’s essential to know which is the cause – the independent variable – and which is the effect – the dependent variable.
In non-probability sampling , the sample is selected based on non-random criteria, and not every member of the population has a chance of being included.
Common non-probability sampling methods include convenience sampling , voluntary response sampling, purposive sampling , snowball sampling, and quota sampling .
Probability sampling means that every member of the target population has a known chance of being included in the sample.
Probability sampling methods include simple random sampling , systematic sampling , stratified sampling , and cluster sampling .
Using careful research design and sampling procedures can help you avoid sampling bias . Oversampling can be used to correct undercoverage bias .
Some common types of sampling bias include self-selection bias , nonresponse bias , undercoverage bias , survivorship bias , pre-screening or advertising bias, and healthy user bias.
Sampling bias is a threat to external validity – it limits the generalizability of your findings to a broader group of people.
A sampling error is the difference between a population parameter and a sample statistic .
A statistic refers to measures about the sample , while a parameter refers to measures about the population .
Populations are used when a research question requires data from every member of the population. This is usually only feasible when the population is small and easily accessible.
Samples are used to make inferences about populations . Samples are easier to collect data from because they are practical, cost-effective, convenient, and manageable.
There are seven threats to external validity : selection bias , history, experimenter effect, Hawthorne effect , testing effect, aptitude-treatment and situation effect.
The two types of external validity are population validity (whether you can generalize to other groups of people) and ecological validity (whether you can generalize to other situations and settings).
The external validity of a study is the extent to which you can generalize your findings to different groups of people, situations, and measures.
Cross-sectional studies cannot establish a cause-and-effect relationship or analyze behavior over a period of time. To investigate cause and effect, you need to do a longitudinal study or an experimental study .
Cross-sectional studies are less expensive and time-consuming than many other types of study. They can provide useful insights into a population’s characteristics and identify correlations for further research.
Sometimes only cross-sectional data is available for analysis; other times your research question may only require a cross-sectional study to answer it.
Longitudinal studies can last anywhere from weeks to decades, although they tend to be at least a year long.
The 1970 British Cohort Study , which has collected data on the lives of 17,000 Brits since their births in 1970, is one well-known example of a longitudinal study .
Longitudinal studies are better to establish the correct sequence of events, identify changes over time, and provide insight into cause-and-effect relationships, but they also tend to be more expensive and time-consuming than other types of studies.
Longitudinal studies and cross-sectional studies are two different types of research design . In a cross-sectional study you collect data from a population at a specific point in time; in a longitudinal study you repeatedly collect data from the same sample over an extended period of time.
There are eight threats to internal validity : history, maturation, instrumentation, testing, selection bias , regression to the mean, social interaction and attrition .
Internal validity is the extent to which you can be confident that a cause-and-effect relationship established in a study cannot be explained by other factors.
In mixed methods research , you use both qualitative and quantitative data collection and analysis methods to answer your research question .
The research methods you use depend on the type of data you need to answer your research question .
- If you want to measure something or test a hypothesis , use quantitative methods . If you want to explore ideas, thoughts and meanings, use qualitative methods .
- If you want to analyze a large amount of readily-available data, use secondary data. If you want data specific to your purposes with control over how it is generated, collect primary data.
- If you want to establish cause-and-effect relationships between variables , use experimental methods. If you want to understand the characteristics of a research subject, use descriptive methods.
A confounding variable , also called a confounder or confounding factor, is a third variable in a study examining a potential cause-and-effect relationship.
A confounding variable is related to both the supposed cause and the supposed effect of the study. It can be difficult to separate the true effect of the independent variable from the effect of the confounding variable.
In your research design , it’s important to identify potential confounding variables and plan how you will reduce their impact.
Discrete and continuous variables are two types of quantitative variables :
- Discrete variables represent counts (e.g. the number of objects in a collection).
- Continuous variables represent measurable amounts (e.g. water volume or weight).
Quantitative variables are any variables where the data represent amounts (e.g. height, weight, or age).
Categorical variables are any variables where the data represent groups. This includes rankings (e.g. finishing places in a race), classifications (e.g. brands of cereal), and binary outcomes (e.g. coin flips).
You need to know what type of variables you are working with to choose the right statistical test for your data and interpret your results .
You can think of independent and dependent variables in terms of cause and effect: an independent variable is the variable you think is the cause , while a dependent variable is the effect .
In an experiment, you manipulate the independent variable and measure the outcome in the dependent variable. For example, in an experiment about the effect of nutrients on crop growth:
- The independent variable is the amount of nutrients added to the crop field.
- The dependent variable is the biomass of the crops at harvest time.
Defining your variables, and deciding how you will manipulate and measure them, is an important part of experimental design .
Experimental design means planning a set of procedures to investigate a relationship between variables . To design a controlled experiment, you need:
- A testable hypothesis
- At least one independent variable that can be precisely manipulated
- At least one dependent variable that can be precisely measured
When designing the experiment, you decide:
- How you will manipulate the variable(s)
- How you will control for any potential confounding variables
- How many subjects or samples will be included in the study
- How subjects will be assigned to treatment levels
Experimental design is essential to the internal and external validity of your experiment.
I nternal validity is the degree of confidence that the causal relationship you are testing is not influenced by other factors or variables .
External validity is the extent to which your results can be generalized to other contexts.
The validity of your experiment depends on your experimental design .
Reliability and validity are both about how well a method measures something:
- Reliability refers to the consistency of a measure (whether the results can be reproduced under the same conditions).
- Validity refers to the accuracy of a measure (whether the results really do represent what they are supposed to measure).
If you are doing experimental research, you also have to consider the internal and external validity of your experiment.
A sample is a subset of individuals from a larger population . Sampling means selecting the group that you will actually collect data from in your research. For example, if you are researching the opinions of students in your university, you could survey a sample of 100 students.
In statistics, sampling allows you to test a hypothesis about the characteristics of a population.
Quantitative research deals with numbers and statistics, while qualitative research deals with words and meanings.
Quantitative methods allow you to systematically measure variables and test hypotheses . Qualitative methods allow you to explore concepts and experiences in more detail.
Methodology refers to the overarching strategy and rationale of your research project . It involves studying the methods used in your field and the theories or principles behind them, in order to develop an approach that matches your objectives.
Methods are the specific tools and procedures you use to collect and analyze data (for example, experiments, surveys , and statistical tests ).
In shorter scientific papers, where the aim is to report the findings of a specific study, you might simply describe what you did in a methods section .
In a longer or more complex research project, such as a thesis or dissertation , you will probably include a methodology section , where you explain your approach to answering the research questions and cite relevant sources to support your choice of methods.
Ask our team
Want to contact us directly? No problem. We are always here for you.
- Email [email protected]
- Start live chat
- Call +1 (510) 822-8066
- WhatsApp +31 20 261 6040
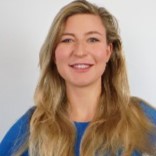
Our team helps students graduate by offering:
- A world-class citation generator
- Plagiarism Checker software powered by Turnitin
- Innovative Citation Checker software
- Professional proofreading services
- Over 300 helpful articles about academic writing, citing sources, plagiarism, and more
Scribbr specializes in editing study-related documents . We proofread:
- PhD dissertations
- Research proposals
- Personal statements
- Admission essays
- Motivation letters
- Reflection papers
- Journal articles
- Capstone projects
Scribbr’s Plagiarism Checker is powered by elements of Turnitin’s Similarity Checker , namely the plagiarism detection software and the Internet Archive and Premium Scholarly Publications content databases .
The add-on AI detector is powered by Scribbr’s proprietary software.
The Scribbr Citation Generator is developed using the open-source Citation Style Language (CSL) project and Frank Bennett’s citeproc-js . It’s the same technology used by dozens of other popular citation tools, including Mendeley and Zotero.
You can find all the citation styles and locales used in the Scribbr Citation Generator in our publicly accessible repository on Github .

What Is A Research (Scientific) Hypothesis? A plain-language explainer + examples
By: Derek Jansen (MBA) | Reviewed By: Dr Eunice Rautenbach | June 2020
If you’re new to the world of research, or it’s your first time writing a dissertation or thesis, you’re probably noticing that the words “research hypothesis” and “scientific hypothesis” are used quite a bit, and you’re wondering what they mean in a research context .
“Hypothesis” is one of those words that people use loosely, thinking they understand what it means. However, it has a very specific meaning within academic research. So, it’s important to understand the exact meaning before you start hypothesizing.
Research Hypothesis 101
- What is a hypothesis ?
- What is a research hypothesis (scientific hypothesis)?
- Requirements for a research hypothesis
- Definition of a research hypothesis
- The null hypothesis
What is a hypothesis?
Let’s start with the general definition of a hypothesis (not a research hypothesis or scientific hypothesis), according to the Cambridge Dictionary:
Hypothesis: an idea or explanation for something that is based on known facts but has not yet been proved.
In other words, it’s a statement that provides an explanation for why or how something works, based on facts (or some reasonable assumptions), but that has not yet been specifically tested . For example, a hypothesis might look something like this:
Hypothesis: sleep impacts academic performance.
This statement predicts that academic performance will be influenced by the amount and/or quality of sleep a student engages in – sounds reasonable, right? It’s based on reasonable assumptions , underpinned by what we currently know about sleep and health (from the existing literature). So, loosely speaking, we could call it a hypothesis, at least by the dictionary definition.
But that’s not good enough…
Unfortunately, that’s not quite sophisticated enough to describe a research hypothesis (also sometimes called a scientific hypothesis), and it wouldn’t be acceptable in a dissertation, thesis or research paper . In the world of academic research, a statement needs a few more criteria to constitute a true research hypothesis .
What is a research hypothesis?
A research hypothesis (also called a scientific hypothesis) is a statement about the expected outcome of a study (for example, a dissertation or thesis). To constitute a quality hypothesis, the statement needs to have three attributes – specificity , clarity and testability .
Let’s take a look at these more closely.
Need a helping hand?
Hypothesis Essential #1: Specificity & Clarity
A good research hypothesis needs to be extremely clear and articulate about both what’ s being assessed (who or what variables are involved ) and the expected outcome (for example, a difference between groups, a relationship between variables, etc.).
Let’s stick with our sleepy students example and look at how this statement could be more specific and clear.
Hypothesis: Students who sleep at least 8 hours per night will, on average, achieve higher grades in standardised tests than students who sleep less than 8 hours a night.
As you can see, the statement is very specific as it identifies the variables involved (sleep hours and test grades), the parties involved (two groups of students), as well as the predicted relationship type (a positive relationship). There’s no ambiguity or uncertainty about who or what is involved in the statement, and the expected outcome is clear.
Contrast that to the original hypothesis we looked at – “Sleep impacts academic performance” – and you can see the difference. “Sleep” and “academic performance” are both comparatively vague , and there’s no indication of what the expected relationship direction is (more sleep or less sleep). As you can see, specificity and clarity are key.
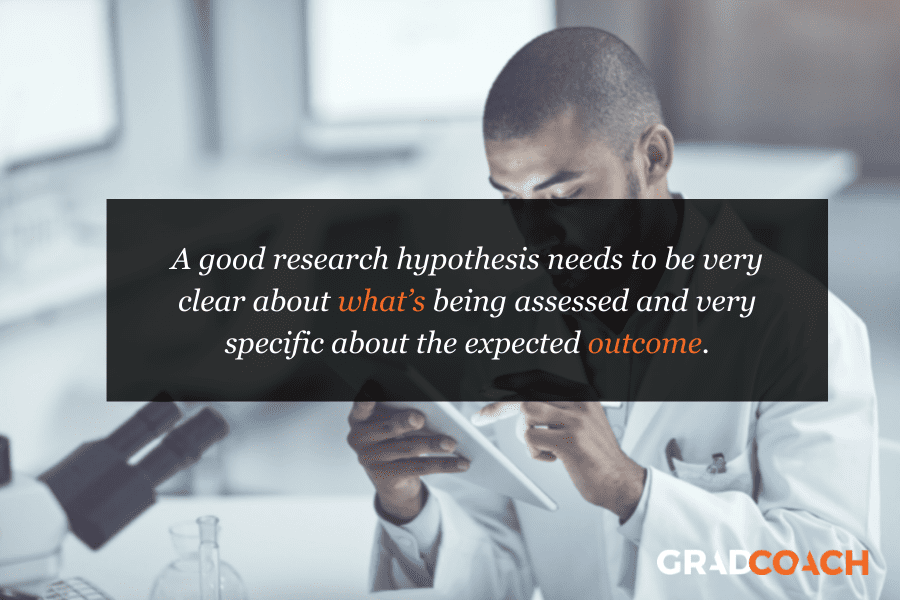
Hypothesis Essential #2: Testability (Provability)
A statement must be testable to qualify as a research hypothesis. In other words, there needs to be a way to prove (or disprove) the statement. If it’s not testable, it’s not a hypothesis – simple as that.
For example, consider the hypothesis we mentioned earlier:
Hypothesis: Students who sleep at least 8 hours per night will, on average, achieve higher grades in standardised tests than students who sleep less than 8 hours a night.
We could test this statement by undertaking a quantitative study involving two groups of students, one that gets 8 or more hours of sleep per night for a fixed period, and one that gets less. We could then compare the standardised test results for both groups to see if there’s a statistically significant difference.
Again, if you compare this to the original hypothesis we looked at – “Sleep impacts academic performance” – you can see that it would be quite difficult to test that statement, primarily because it isn’t specific enough. How much sleep? By who? What type of academic performance?
So, remember the mantra – if you can’t test it, it’s not a hypothesis 🙂
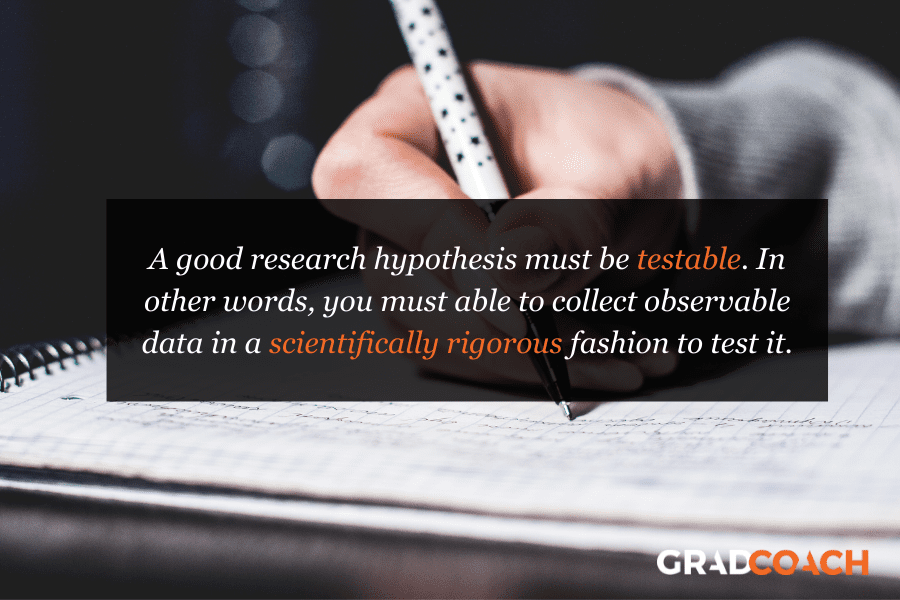
Defining A Research Hypothesis
You’re still with us? Great! Let’s recap and pin down a clear definition of a hypothesis.
A research hypothesis (or scientific hypothesis) is a statement about an expected relationship between variables, or explanation of an occurrence, that is clear, specific and testable.
So, when you write up hypotheses for your dissertation or thesis, make sure that they meet all these criteria. If you do, you’ll not only have rock-solid hypotheses but you’ll also ensure a clear focus for your entire research project.
What about the null hypothesis?
You may have also heard the terms null hypothesis , alternative hypothesis, or H-zero thrown around. At a simple level, the null hypothesis is the counter-proposal to the original hypothesis.
For example, if the hypothesis predicts that there is a relationship between two variables (for example, sleep and academic performance), the null hypothesis would predict that there is no relationship between those variables.
At a more technical level, the null hypothesis proposes that no statistical significance exists in a set of given observations and that any differences are due to chance alone.
And there you have it – hypotheses in a nutshell.
If you have any questions, be sure to leave a comment below and we’ll do our best to help you. If you need hands-on help developing and testing your hypotheses, consider our private coaching service , where we hold your hand through the research journey.
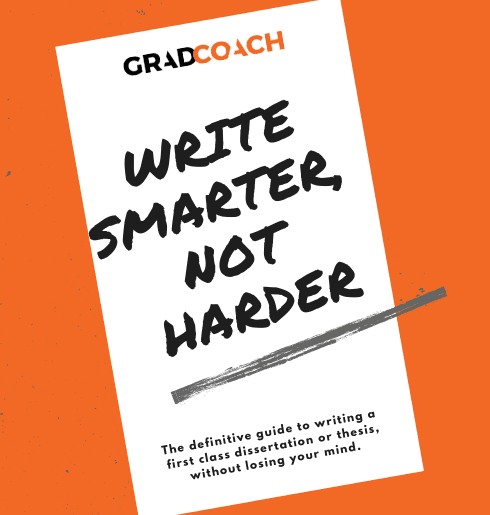
Psst… there’s more (for free)
This post is part of our dissertation mini-course, which covers everything you need to get started with your dissertation, thesis or research project.
You Might Also Like:
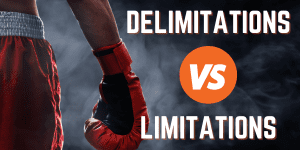
16 Comments
Very useful information. I benefit more from getting more information in this regard.
Very great insight,educative and informative. Please give meet deep critics on many research data of public international Law like human rights, environment, natural resources, law of the sea etc
In a book I read a distinction is made between null, research, and alternative hypothesis. As far as I understand, alternative and research hypotheses are the same. Can you please elaborate? Best Afshin
This is a self explanatory, easy going site. I will recommend this to my friends and colleagues.
Very good definition. How can I cite your definition in my thesis? Thank you. Is nul hypothesis compulsory in a research?
It’s a counter-proposal to be proven as a rejection
Please what is the difference between alternate hypothesis and research hypothesis?
It is a very good explanation. However, it limits hypotheses to statistically tasteable ideas. What about for qualitative researches or other researches that involve quantitative data that don’t need statistical tests?
In qualitative research, one typically uses propositions, not hypotheses.
could you please elaborate it more
I’ve benefited greatly from these notes, thank you.
This is very helpful
well articulated ideas are presented here, thank you for being reliable sources of information
Excellent. Thanks for being clear and sound about the research methodology and hypothesis (quantitative research)
I have only a simple question regarding the null hypothesis. – Is the null hypothesis (Ho) known as the reversible hypothesis of the alternative hypothesis (H1? – How to test it in academic research?
this is very important note help me much more
Trackbacks/Pingbacks
- What Is Research Methodology? Simple Definition (With Examples) - Grad Coach - […] Contrasted to this, a quantitative methodology is typically used when the research aims and objectives are confirmatory in nature. For example,…
Submit a Comment Cancel reply
Your email address will not be published. Required fields are marked *
Save my name, email, and website in this browser for the next time I comment.
- Print Friendly
Frequently asked questions
What is the definition of a hypothesis.
A hypothesis states your predictions about what your research will find. It is a tentative answer to your research question that has not yet been tested. For some research projects, you might have to write several hypotheses that address different aspects of your research question.
A hypothesis is not just a guess. It should be based on existing theories and knowledge. It also has to be testable, which means you can support or refute it through scientific research methods (such as experiments, observations, and statistical analysis of data).
Frequently asked questions: Methodology
Quantitative observations involve measuring or counting something and expressing the result in numerical form, while qualitative observations involve describing something in non-numerical terms, such as its appearance, texture, or color.
To make quantitative observations , you need to use instruments that are capable of measuring the quantity you want to observe. For example, you might use a ruler to measure the length of an object or a thermometer to measure its temperature.
Scope of research is determined at the beginning of your research process , prior to the data collection stage. Sometimes called “scope of study,” your scope delineates what will and will not be covered in your project. It helps you focus your work and your time, ensuring that you’ll be able to achieve your goals and outcomes.
Defining a scope can be very useful in any research project, from a research proposal to a thesis or dissertation . A scope is needed for all types of research: quantitative , qualitative , and mixed methods .
To define your scope of research, consider the following:
- Budget constraints or any specifics of grant funding
- Your proposed timeline and duration
- Specifics about your population of study, your proposed sample size , and the research methodology you’ll pursue
- Any inclusion and exclusion criteria
- Any anticipated control , extraneous , or confounding variables that could bias your research if not accounted for properly.
Inclusion and exclusion criteria are predominantly used in non-probability sampling . In purposive sampling and snowball sampling , restrictions apply as to who can be included in the sample .
Inclusion and exclusion criteria are typically presented and discussed in the methodology section of your thesis or dissertation .
The purpose of theory-testing mode is to find evidence in order to disprove, refine, or support a theory. As such, generalisability is not the aim of theory-testing mode.
Due to this, the priority of researchers in theory-testing mode is to eliminate alternative causes for relationships between variables . In other words, they prioritise internal validity over external validity , including ecological validity .
Convergent validity shows how much a measure of one construct aligns with other measures of the same or related constructs .
On the other hand, concurrent validity is about how a measure matches up to some known criterion or gold standard, which can be another measure.
Although both types of validity are established by calculating the association or correlation between a test score and another variable , they represent distinct validation methods.
Validity tells you how accurately a method measures what it was designed to measure. There are 4 main types of validity :
- Construct validity : Does the test measure the construct it was designed to measure?
- Face validity : Does the test appear to be suitable for its objectives ?
- Content validity : Does the test cover all relevant parts of the construct it aims to measure.
- Criterion validity : Do the results accurately measure the concrete outcome they are designed to measure?
Criterion validity evaluates how well a test measures the outcome it was designed to measure. An outcome can be, for example, the onset of a disease.
Criterion validity consists of two subtypes depending on the time at which the two measures (the criterion and your test) are obtained:
- Concurrent validity is a validation strategy where the the scores of a test and the criterion are obtained at the same time
- Predictive validity is a validation strategy where the criterion variables are measured after the scores of the test
Attrition refers to participants leaving a study. It always happens to some extent – for example, in randomised control trials for medical research.
Differential attrition occurs when attrition or dropout rates differ systematically between the intervention and the control group . As a result, the characteristics of the participants who drop out differ from the characteristics of those who stay in the study. Because of this, study results may be biased .
Criterion validity and construct validity are both types of measurement validity . In other words, they both show you how accurately a method measures something.
While construct validity is the degree to which a test or other measurement method measures what it claims to measure, criterion validity is the degree to which a test can predictively (in the future) or concurrently (in the present) measure something.
Construct validity is often considered the overarching type of measurement validity . You need to have face validity , content validity , and criterion validity in order to achieve construct validity.
Convergent validity and discriminant validity are both subtypes of construct validity . Together, they help you evaluate whether a test measures the concept it was designed to measure.
- Convergent validity indicates whether a test that is designed to measure a particular construct correlates with other tests that assess the same or similar construct.
- Discriminant validity indicates whether two tests that should not be highly related to each other are indeed not related. This type of validity is also called divergent validity .
You need to assess both in order to demonstrate construct validity. Neither one alone is sufficient for establishing construct validity.
Face validity and content validity are similar in that they both evaluate how suitable the content of a test is. The difference is that face validity is subjective, and assesses content at surface level.
When a test has strong face validity, anyone would agree that the test’s questions appear to measure what they are intended to measure.
For example, looking at a 4th grade math test consisting of problems in which students have to add and multiply, most people would agree that it has strong face validity (i.e., it looks like a math test).
On the other hand, content validity evaluates how well a test represents all the aspects of a topic. Assessing content validity is more systematic and relies on expert evaluation. of each question, analysing whether each one covers the aspects that the test was designed to cover.
A 4th grade math test would have high content validity if it covered all the skills taught in that grade. Experts(in this case, math teachers), would have to evaluate the content validity by comparing the test to the learning objectives.
Content validity shows you how accurately a test or other measurement method taps into the various aspects of the specific construct you are researching.
In other words, it helps you answer the question: “does the test measure all aspects of the construct I want to measure?” If it does, then the test has high content validity.
The higher the content validity, the more accurate the measurement of the construct.
If the test fails to include parts of the construct, or irrelevant parts are included, the validity of the instrument is threatened, which brings your results into question.
Construct validity refers to how well a test measures the concept (or construct) it was designed to measure. Assessing construct validity is especially important when you’re researching concepts that can’t be quantified and/or are intangible, like introversion. To ensure construct validity your test should be based on known indicators of introversion ( operationalisation ).
On the other hand, content validity assesses how well the test represents all aspects of the construct. If some aspects are missing or irrelevant parts are included, the test has low content validity.
- Discriminant validity indicates whether two tests that should not be highly related to each other are indeed not related
Construct validity has convergent and discriminant subtypes. They assist determine if a test measures the intended notion.
The reproducibility and replicability of a study can be ensured by writing a transparent, detailed method section and using clear, unambiguous language.
Reproducibility and replicability are related terms.
- A successful reproduction shows that the data analyses were conducted in a fair and honest manner.
- A successful replication shows that the reliability of the results is high.
- Reproducing research entails reanalysing the existing data in the same manner.
- Replicating (or repeating ) the research entails reconducting the entire analysis, including the collection of new data .
Snowball sampling is a non-probability sampling method . Unlike probability sampling (which involves some form of random selection ), the initial individuals selected to be studied are the ones who recruit new participants.
Because not every member of the target population has an equal chance of being recruited into the sample, selection in snowball sampling is non-random.
Snowball sampling is a non-probability sampling method , where there is not an equal chance for every member of the population to be included in the sample .
This means that you cannot use inferential statistics and make generalisations – often the goal of quantitative research . As such, a snowball sample is not representative of the target population, and is usually a better fit for qualitative research .
Snowball sampling relies on the use of referrals. Here, the researcher recruits one or more initial participants, who then recruit the next ones.
Participants share similar characteristics and/or know each other. Because of this, not every member of the population has an equal chance of being included in the sample, giving rise to sampling bias .
Snowball sampling is best used in the following cases:
- If there is no sampling frame available (e.g., people with a rare disease)
- If the population of interest is hard to access or locate (e.g., people experiencing homelessness)
- If the research focuses on a sensitive topic (e.g., extra-marital affairs)
Stratified sampling and quota sampling both involve dividing the population into subgroups and selecting units from each subgroup. The purpose in both cases is to select a representative sample and/or to allow comparisons between subgroups.
The main difference is that in stratified sampling, you draw a random sample from each subgroup ( probability sampling ). In quota sampling you select a predetermined number or proportion of units, in a non-random manner ( non-probability sampling ).
Random sampling or probability sampling is based on random selection. This means that each unit has an equal chance (i.e., equal probability) of being included in the sample.
On the other hand, convenience sampling involves stopping people at random, which means that not everyone has an equal chance of being selected depending on the place, time, or day you are collecting your data.
Convenience sampling and quota sampling are both non-probability sampling methods. They both use non-random criteria like availability, geographical proximity, or expert knowledge to recruit study participants.
However, in convenience sampling, you continue to sample units or cases until you reach the required sample size.
In quota sampling, you first need to divide your population of interest into subgroups (strata) and estimate their proportions (quota) in the population. Then you can start your data collection , using convenience sampling to recruit participants, until the proportions in each subgroup coincide with the estimated proportions in the population.
A sampling frame is a list of every member in the entire population . It is important that the sampling frame is as complete as possible, so that your sample accurately reflects your population.
Stratified and cluster sampling may look similar, but bear in mind that groups created in cluster sampling are heterogeneous , so the individual characteristics in the cluster vary. In contrast, groups created in stratified sampling are homogeneous , as units share characteristics.
Relatedly, in cluster sampling you randomly select entire groups and include all units of each group in your sample. However, in stratified sampling, you select some units of all groups and include them in your sample. In this way, both methods can ensure that your sample is representative of the target population .
When your population is large in size, geographically dispersed, or difficult to contact, it’s necessary to use a sampling method .
This allows you to gather information from a smaller part of the population, i.e. the sample, and make accurate statements by using statistical analysis. A few sampling methods include simple random sampling , convenience sampling , and snowball sampling .
The two main types of social desirability bias are:
- Self-deceptive enhancement (self-deception): The tendency to see oneself in a favorable light without realizing it.
- Impression managemen t (other-deception): The tendency to inflate one’s abilities or achievement in order to make a good impression on other people.
Response bias refers to conditions or factors that take place during the process of responding to surveys, affecting the responses. One type of response bias is social desirability bias .
Demand characteristics are aspects of experiments that may give away the research objective to participants. Social desirability bias occurs when participants automatically try to respond in ways that make them seem likeable in a study, even if it means misrepresenting how they truly feel.
Participants may use demand characteristics to infer social norms or experimenter expectancies and act in socially desirable ways, so you should try to control for demand characteristics wherever possible.
A systematic review is secondary research because it uses existing research. You don’t collect new data yourself.
Ethical considerations in research are a set of principles that guide your research designs and practices. These principles include voluntary participation, informed consent, anonymity, confidentiality, potential for harm, and results communication.
Scientists and researchers must always adhere to a certain code of conduct when collecting data from others .
These considerations protect the rights of research participants, enhance research validity , and maintain scientific integrity.
Research ethics matter for scientific integrity, human rights and dignity, and collaboration between science and society. These principles make sure that participation in studies is voluntary, informed, and safe.
Research misconduct means making up or falsifying data, manipulating data analyses, or misrepresenting results in research reports. It’s a form of academic fraud.
These actions are committed intentionally and can have serious consequences; research misconduct is not a simple mistake or a point of disagreement but a serious ethical failure.
Anonymity means you don’t know who the participants are, while confidentiality means you know who they are but remove identifying information from your research report. Both are important ethical considerations .
You can only guarantee anonymity by not collecting any personally identifying information – for example, names, phone numbers, email addresses, IP addresses, physical characteristics, photos, or videos.
You can keep data confidential by using aggregate information in your research report, so that you only refer to groups of participants rather than individuals.
Peer review is a process of evaluating submissions to an academic journal. Utilising rigorous criteria, a panel of reviewers in the same subject area decide whether to accept each submission for publication.
For this reason, academic journals are often considered among the most credible sources you can use in a research project – provided that the journal itself is trustworthy and well regarded.
In general, the peer review process follows the following steps:
- First, the author submits the manuscript to the editor.
- Reject the manuscript and send it back to author, or
- Send it onward to the selected peer reviewer(s)
- Next, the peer review process occurs. The reviewer provides feedback, addressing any major or minor issues with the manuscript, and gives their advice regarding what edits should be made.
- Lastly, the edited manuscript is sent back to the author. They input the edits, and resubmit it to the editor for publication.
Peer review can stop obviously problematic, falsified, or otherwise untrustworthy research from being published. It also represents an excellent opportunity to get feedback from renowned experts in your field.
It acts as a first defence, helping you ensure your argument is clear and that there are no gaps, vague terms, or unanswered questions for readers who weren’t involved in the research process.
Peer-reviewed articles are considered a highly credible source due to this stringent process they go through before publication.
Many academic fields use peer review , largely to determine whether a manuscript is suitable for publication. Peer review enhances the credibility of the published manuscript.
However, peer review is also common in non-academic settings. The United Nations, the European Union, and many individual nations use peer review to evaluate grant applications. It is also widely used in medical and health-related fields as a teaching or quality-of-care measure.
Peer assessment is often used in the classroom as a pedagogical tool. Both receiving feedback and providing it are thought to enhance the learning process, helping students think critically and collaboratively.
- In a single-blind study , only the participants are blinded.
- In a double-blind study , both participants and experimenters are blinded.
- In a triple-blind study , the assignment is hidden not only from participants and experimenters, but also from the researchers analysing the data.
Blinding is important to reduce bias (e.g., observer bias , demand characteristics ) and ensure a study’s internal validity .
If participants know whether they are in a control or treatment group , they may adjust their behaviour in ways that affect the outcome that researchers are trying to measure. If the people administering the treatment are aware of group assignment, they may treat participants differently and thus directly or indirectly influence the final results.
Blinding means hiding who is assigned to the treatment group and who is assigned to the control group in an experiment .
Explanatory research is a research method used to investigate how or why something occurs when only a small amount of information is available pertaining to that topic. It can help you increase your understanding of a given topic.
Explanatory research is used to investigate how or why a phenomenon occurs. Therefore, this type of research is often one of the first stages in the research process , serving as a jumping-off point for future research.
Exploratory research is a methodology approach that explores research questions that have not previously been studied in depth. It is often used when the issue you’re studying is new, or the data collection process is challenging in some way.
Exploratory research is often used when the issue you’re studying is new or when the data collection process is challenging for some reason.
You can use exploratory research if you have a general idea or a specific question that you want to study but there is no preexisting knowledge or paradigm with which to study it.
To implement random assignment , assign a unique number to every member of your study’s sample .
Then, you can use a random number generator or a lottery method to randomly assign each number to a control or experimental group. You can also do so manually, by flipping a coin or rolling a die to randomly assign participants to groups.
Random selection, or random sampling , is a way of selecting members of a population for your study’s sample.
In contrast, random assignment is a way of sorting the sample into control and experimental groups.
Random sampling enhances the external validity or generalisability of your results, while random assignment improves the internal validity of your study.
Random assignment is used in experiments with a between-groups or independent measures design. In this research design, there’s usually a control group and one or more experimental groups. Random assignment helps ensure that the groups are comparable.
In general, you should always use random assignment in this type of experimental design when it is ethically possible and makes sense for your study topic.
Clean data are valid, accurate, complete, consistent, unique, and uniform. Dirty data include inconsistencies and errors.
Dirty data can come from any part of the research process, including poor research design , inappropriate measurement materials, or flawed data entry.
Data cleaning takes place between data collection and data analyses. But you can use some methods even before collecting data.
For clean data, you should start by designing measures that collect valid data. Data validation at the time of data entry or collection helps you minimize the amount of data cleaning you’ll need to do.
After data collection, you can use data standardisation and data transformation to clean your data. You’ll also deal with any missing values, outliers, and duplicate values.
Data cleaning involves spotting and resolving potential data inconsistencies or errors to improve your data quality. An error is any value (e.g., recorded weight) that doesn’t reflect the true value (e.g., actual weight) of something that’s being measured.
In this process, you review, analyse, detect, modify, or remove ‘dirty’ data to make your dataset ‘clean’. Data cleaning is also called data cleansing or data scrubbing.
Data cleaning is necessary for valid and appropriate analyses. Dirty data contain inconsistencies or errors , but cleaning your data helps you minimise or resolve these.
Without data cleaning, you could end up with a Type I or II error in your conclusion. These types of erroneous conclusions can be practically significant with important consequences, because they lead to misplaced investments or missed opportunities.
Observer bias occurs when a researcher’s expectations, opinions, or prejudices influence what they perceive or record in a study. It usually affects studies when observers are aware of the research aims or hypotheses. This type of research bias is also called detection bias or ascertainment bias .
The observer-expectancy effect occurs when researchers influence the results of their own study through interactions with participants.
Researchers’ own beliefs and expectations about the study results may unintentionally influence participants through demand characteristics .
You can use several tactics to minimise observer bias .
- Use masking (blinding) to hide the purpose of your study from all observers.
- Triangulate your data with different data collection methods or sources.
- Use multiple observers and ensure inter-rater reliability.
- Train your observers to make sure data is consistently recorded between them.
- Standardise your observation procedures to make sure they are structured and clear.
Naturalistic observation is a valuable tool because of its flexibility, external validity , and suitability for topics that can’t be studied in a lab setting.
The downsides of naturalistic observation include its lack of scientific control , ethical considerations , and potential for bias from observers and subjects.
Naturalistic observation is a qualitative research method where you record the behaviours of your research subjects in real-world settings. You avoid interfering or influencing anything in a naturalistic observation.
You can think of naturalistic observation as ‘people watching’ with a purpose.
Closed-ended, or restricted-choice, questions offer respondents a fixed set of choices to select from. These questions are easier to answer quickly.
Open-ended or long-form questions allow respondents to answer in their own words. Because there are no restrictions on their choices, respondents can answer in ways that researchers may not have otherwise considered.
You can organise the questions logically, with a clear progression from simple to complex, or randomly between respondents. A logical flow helps respondents process the questionnaire easier and quicker, but it may lead to bias. Randomisation can minimise the bias from order effects.
Questionnaires can be self-administered or researcher-administered.
Self-administered questionnaires can be delivered online or in paper-and-pen formats, in person or by post. All questions are standardised so that all respondents receive the same questions with identical wording.
Researcher-administered questionnaires are interviews that take place by phone, in person, or online between researchers and respondents. You can gain deeper insights by clarifying questions for respondents or asking follow-up questions.
In a controlled experiment , all extraneous variables are held constant so that they can’t influence the results. Controlled experiments require:
- A control group that receives a standard treatment, a fake treatment, or no treatment
- Random assignment of participants to ensure the groups are equivalent
Depending on your study topic, there are various other methods of controlling variables .
An experimental group, also known as a treatment group, receives the treatment whose effect researchers wish to study, whereas a control group does not. They should be identical in all other ways.
A true experiment (aka a controlled experiment) always includes at least one control group that doesn’t receive the experimental treatment.
However, some experiments use a within-subjects design to test treatments without a control group. In these designs, you usually compare one group’s outcomes before and after a treatment (instead of comparing outcomes between different groups).
For strong internal validity , it’s usually best to include a control group if possible. Without a control group, it’s harder to be certain that the outcome was caused by the experimental treatment and not by other variables.
A questionnaire is a data collection tool or instrument, while a survey is an overarching research method that involves collecting and analysing data from people using questionnaires.
A Likert scale is a rating scale that quantitatively assesses opinions, attitudes, or behaviours. It is made up of four or more questions that measure a single attitude or trait when response scores are combined.
To use a Likert scale in a survey , you present participants with Likert-type questions or statements, and a continuum of items, usually with five or seven possible responses, to capture their degree of agreement.
Individual Likert-type questions are generally considered ordinal data , because the items have clear rank order, but don’t have an even distribution.
Overall Likert scale scores are sometimes treated as interval data. These scores are considered to have directionality and even spacing between them.
The type of data determines what statistical tests you should use to analyse your data.
A research hypothesis is your proposed answer to your research question. The research hypothesis usually includes an explanation (‘ x affects y because …’).
A statistical hypothesis, on the other hand, is a mathematical statement about a population parameter. Statistical hypotheses always come in pairs: the null and alternative hypotheses. In a well-designed study , the statistical hypotheses correspond logically to the research hypothesis.
Cross-sectional studies are less expensive and time-consuming than many other types of study. They can provide useful insights into a population’s characteristics and identify correlations for further research.
Sometimes only cross-sectional data are available for analysis; other times your research question may only require a cross-sectional study to answer it.
Cross-sectional studies cannot establish a cause-and-effect relationship or analyse behaviour over a period of time. To investigate cause and effect, you need to do a longitudinal study or an experimental study .
Longitudinal studies and cross-sectional studies are two different types of research design . In a cross-sectional study you collect data from a population at a specific point in time; in a longitudinal study you repeatedly collect data from the same sample over an extended period of time.
Longitudinal studies are better to establish the correct sequence of events, identify changes over time, and provide insight into cause-and-effect relationships, but they also tend to be more expensive and time-consuming than other types of studies.
The 1970 British Cohort Study , which has collected data on the lives of 17,000 Brits since their births in 1970, is one well-known example of a longitudinal study .
Longitudinal studies can last anywhere from weeks to decades, although they tend to be at least a year long.
A correlation reflects the strength and/or direction of the association between two or more variables.
- A positive correlation means that both variables change in the same direction.
- A negative correlation means that the variables change in opposite directions.
- A zero correlation means there’s no relationship between the variables.
A correlational research design investigates relationships between two variables (or more) without the researcher controlling or manipulating any of them. It’s a non-experimental type of quantitative research .
A correlation coefficient is a single number that describes the strength and direction of the relationship between your variables.
Different types of correlation coefficients might be appropriate for your data based on their levels of measurement and distributions . The Pearson product-moment correlation coefficient (Pearson’s r ) is commonly used to assess a linear relationship between two quantitative variables.
Controlled experiments establish causality, whereas correlational studies only show associations between variables.
- In an experimental design , you manipulate an independent variable and measure its effect on a dependent variable. Other variables are controlled so they can’t impact the results.
- In a correlational design , you measure variables without manipulating any of them. You can test whether your variables change together, but you can’t be sure that one variable caused a change in another.
In general, correlational research is high in external validity while experimental research is high in internal validity .
The third variable and directionality problems are two main reasons why correlation isn’t causation .
The third variable problem means that a confounding variable affects both variables to make them seem causally related when they are not.
The directionality problem is when two variables correlate and might actually have a causal relationship, but it’s impossible to conclude which variable causes changes in the other.
As a rule of thumb, questions related to thoughts, beliefs, and feelings work well in focus groups . Take your time formulating strong questions, paying special attention to phrasing. Be careful to avoid leading questions , which can bias your responses.
Overall, your focus group questions should be:
- Open-ended and flexible
- Impossible to answer with ‘yes’ or ‘no’ (questions that start with ‘why’ or ‘how’ are often best)
- Unambiguous, getting straight to the point while still stimulating discussion
- Unbiased and neutral
Social desirability bias is the tendency for interview participants to give responses that will be viewed favourably by the interviewer or other participants. It occurs in all types of interviews and surveys , but is most common in semi-structured interviews , unstructured interviews , and focus groups .
Social desirability bias can be mitigated by ensuring participants feel at ease and comfortable sharing their views. Make sure to pay attention to your own body language and any physical or verbal cues, such as nodding or widening your eyes.
This type of bias in research can also occur in observations if the participants know they’re being observed. They might alter their behaviour accordingly.
A focus group is a research method that brings together a small group of people to answer questions in a moderated setting. The group is chosen due to predefined demographic traits, and the questions are designed to shed light on a topic of interest. It is one of four types of interviews .
The four most common types of interviews are:
- Structured interviews : The questions are predetermined in both topic and order.
- Semi-structured interviews : A few questions are predetermined, but other questions aren’t planned.
- Unstructured interviews : None of the questions are predetermined.
- Focus group interviews : The questions are presented to a group instead of one individual.
An unstructured interview is the most flexible type of interview, but it is not always the best fit for your research topic.
Unstructured interviews are best used when:
- You are an experienced interviewer and have a very strong background in your research topic, since it is challenging to ask spontaneous, colloquial questions
- Your research question is exploratory in nature. While you may have developed hypotheses, you are open to discovering new or shifting viewpoints through the interview process.
- You are seeking descriptive data, and are ready to ask questions that will deepen and contextualise your initial thoughts and hypotheses
- Your research depends on forming connections with your participants and making them feel comfortable revealing deeper emotions, lived experiences, or thoughts
A semi-structured interview is a blend of structured and unstructured types of interviews. Semi-structured interviews are best used when:
- You have prior interview experience. Spontaneous questions are deceptively challenging, and it’s easy to accidentally ask a leading question or make a participant uncomfortable.
- Your research question is exploratory in nature. Participant answers can guide future research questions and help you develop a more robust knowledge base for future research.
The interviewer effect is a type of bias that emerges when a characteristic of an interviewer (race, age, gender identity, etc.) influences the responses given by the interviewee.
There is a risk of an interviewer effect in all types of interviews , but it can be mitigated by writing really high-quality interview questions.
A structured interview is a data collection method that relies on asking questions in a set order to collect data on a topic. They are often quantitative in nature. Structured interviews are best used when:
- You already have a very clear understanding of your topic. Perhaps significant research has already been conducted, or you have done some prior research yourself, but you already possess a baseline for designing strong structured questions.
- You are constrained in terms of time or resources and need to analyse your data quickly and efficiently
- Your research question depends on strong parity between participants, with environmental conditions held constant
More flexible interview options include semi-structured interviews , unstructured interviews , and focus groups .
When conducting research, collecting original data has significant advantages:
- You can tailor data collection to your specific research aims (e.g., understanding the needs of your consumers or user testing your website).
- You can control and standardise the process for high reliability and validity (e.g., choosing appropriate measurements and sampling methods ).
However, there are also some drawbacks: data collection can be time-consuming, labour-intensive, and expensive. In some cases, it’s more efficient to use secondary data that has already been collected by someone else, but the data might be less reliable.
Data collection is the systematic process by which observations or measurements are gathered in research. It is used in many different contexts by academics, governments, businesses, and other organisations.
A mediator variable explains the process through which two variables are related, while a moderator variable affects the strength and direction of that relationship.
A confounder is a third variable that affects variables of interest and makes them seem related when they are not. In contrast, a mediator is the mechanism of a relationship between two variables: it explains the process by which they are related.
If something is a mediating variable :
- It’s caused by the independent variable
- It influences the dependent variable
- When it’s taken into account, the statistical correlation between the independent and dependent variables is higher than when it isn’t considered
Including mediators and moderators in your research helps you go beyond studying a simple relationship between two variables for a fuller picture of the real world. They are important to consider when studying complex correlational or causal relationships.
Mediators are part of the causal pathway of an effect, and they tell you how or why an effect takes place. Moderators usually help you judge the external validity of your study by identifying the limitations of when the relationship between variables holds.
You can think of independent and dependent variables in terms of cause and effect: an independent variable is the variable you think is the cause , while a dependent variable is the effect .
In an experiment, you manipulate the independent variable and measure the outcome in the dependent variable. For example, in an experiment about the effect of nutrients on crop growth:
- The independent variable is the amount of nutrients added to the crop field.
- The dependent variable is the biomass of the crops at harvest time.
Defining your variables, and deciding how you will manipulate and measure them, is an important part of experimental design .
Discrete and continuous variables are two types of quantitative variables :
- Discrete variables represent counts (e.g., the number of objects in a collection).
- Continuous variables represent measurable amounts (e.g., water volume or weight).
Quantitative variables are any variables where the data represent amounts (e.g. height, weight, or age).
Categorical variables are any variables where the data represent groups. This includes rankings (e.g. finishing places in a race), classifications (e.g. brands of cereal), and binary outcomes (e.g. coin flips).
You need to know what type of variables you are working with to choose the right statistical test for your data and interpret your results .
Determining cause and effect is one of the most important parts of scientific research. It’s essential to know which is the cause – the independent variable – and which is the effect – the dependent variable.
You want to find out how blood sugar levels are affected by drinking diet cola and regular cola, so you conduct an experiment .
- The type of cola – diet or regular – is the independent variable .
- The level of blood sugar that you measure is the dependent variable – it changes depending on the type of cola.
No. The value of a dependent variable depends on an independent variable, so a variable cannot be both independent and dependent at the same time. It must be either the cause or the effect, not both.
Yes, but including more than one of either type requires multiple research questions .
For example, if you are interested in the effect of a diet on health, you can use multiple measures of health: blood sugar, blood pressure, weight, pulse, and many more. Each of these is its own dependent variable with its own research question.
You could also choose to look at the effect of exercise levels as well as diet, or even the additional effect of the two combined. Each of these is a separate independent variable .
To ensure the internal validity of an experiment , you should only change one independent variable at a time.
To ensure the internal validity of your research, you must consider the impact of confounding variables. If you fail to account for them, you might over- or underestimate the causal relationship between your independent and dependent variables , or even find a causal relationship where none exists.
A confounding variable is closely related to both the independent and dependent variables in a study. An independent variable represents the supposed cause , while the dependent variable is the supposed effect . A confounding variable is a third variable that influences both the independent and dependent variables.
Failing to account for confounding variables can cause you to wrongly estimate the relationship between your independent and dependent variables.
There are several methods you can use to decrease the impact of confounding variables on your research: restriction, matching, statistical control, and randomisation.
In restriction , you restrict your sample by only including certain subjects that have the same values of potential confounding variables.
In matching , you match each of the subjects in your treatment group with a counterpart in the comparison group. The matched subjects have the same values on any potential confounding variables, and only differ in the independent variable .
In statistical control , you include potential confounders as variables in your regression .
In randomisation , you randomly assign the treatment (or independent variable) in your study to a sufficiently large number of subjects, which allows you to control for all potential confounding variables.
In scientific research, concepts are the abstract ideas or phenomena that are being studied (e.g., educational achievement). Variables are properties or characteristics of the concept (e.g., performance at school), while indicators are ways of measuring or quantifying variables (e.g., yearly grade reports).
The process of turning abstract concepts into measurable variables and indicators is called operationalisation .
In statistics, ordinal and nominal variables are both considered categorical variables .
Even though ordinal data can sometimes be numerical, not all mathematical operations can be performed on them.
A control variable is any variable that’s held constant in a research study. It’s not a variable of interest in the study, but it’s controlled because it could influence the outcomes.
Control variables help you establish a correlational or causal relationship between variables by enhancing internal validity .
If you don’t control relevant extraneous variables , they may influence the outcomes of your study, and you may not be able to demonstrate that your results are really an effect of your independent variable .
‘Controlling for a variable’ means measuring extraneous variables and accounting for them statistically to remove their effects on other variables.
Researchers often model control variable data along with independent and dependent variable data in regression analyses and ANCOVAs . That way, you can isolate the control variable’s effects from the relationship between the variables of interest.
An extraneous variable is any variable that you’re not investigating that can potentially affect the dependent variable of your research study.
A confounding variable is a type of extraneous variable that not only affects the dependent variable, but is also related to the independent variable.
There are 4 main types of extraneous variables :
- Demand characteristics : Environmental cues that encourage participants to conform to researchers’ expectations
- Experimenter effects : Unintentional actions by researchers that influence study outcomes
- Situational variables : Eenvironmental variables that alter participants’ behaviours
- Participant variables : Any characteristic or aspect of a participant’s background that could affect study results
The difference between explanatory and response variables is simple:
- An explanatory variable is the expected cause, and it explains the results.
- A response variable is the expected effect, and it responds to other variables.
The term ‘ explanatory variable ‘ is sometimes preferred over ‘ independent variable ‘ because, in real-world contexts, independent variables are often influenced by other variables. This means they aren’t totally independent.
Multiple independent variables may also be correlated with each other, so ‘explanatory variables’ is a more appropriate term.
On graphs, the explanatory variable is conventionally placed on the x -axis, while the response variable is placed on the y -axis.
- If you have quantitative variables , use a scatterplot or a line graph.
- If your response variable is categorical, use a scatterplot or a line graph.
- If your explanatory variable is categorical, use a bar graph.
A correlation is usually tested for two variables at a time, but you can test correlations between three or more variables.
An independent variable is the variable you manipulate, control, or vary in an experimental study to explore its effects. It’s called ‘independent’ because it’s not influenced by any other variables in the study.
Independent variables are also called:
- Explanatory variables (they explain an event or outcome)
- Predictor variables (they can be used to predict the value of a dependent variable)
- Right-hand-side variables (they appear on the right-hand side of a regression equation)
A dependent variable is what changes as a result of the independent variable manipulation in experiments . It’s what you’re interested in measuring, and it ‘depends’ on your independent variable.
In statistics, dependent variables are also called:
- Response variables (they respond to a change in another variable)
- Outcome variables (they represent the outcome you want to measure)
- Left-hand-side variables (they appear on the left-hand side of a regression equation)
Deductive reasoning is commonly used in scientific research, and it’s especially associated with quantitative research .
In research, you might have come across something called the hypothetico-deductive method . It’s the scientific method of testing hypotheses to check whether your predictions are substantiated by real-world data.
Deductive reasoning is a logical approach where you progress from general ideas to specific conclusions. It’s often contrasted with inductive reasoning , where you start with specific observations and form general conclusions.
Deductive reasoning is also called deductive logic.
Inductive reasoning is a method of drawing conclusions by going from the specific to the general. It’s usually contrasted with deductive reasoning, where you proceed from general information to specific conclusions.
Inductive reasoning is also called inductive logic or bottom-up reasoning.
In inductive research , you start by making observations or gathering data. Then, you take a broad scan of your data and search for patterns. Finally, you make general conclusions that you might incorporate into theories.
Inductive reasoning is a bottom-up approach, while deductive reasoning is top-down.
Inductive reasoning takes you from the specific to the general, while in deductive reasoning, you make inferences by going from general premises to specific conclusions.
There are many different types of inductive reasoning that people use formally or informally.
Here are a few common types:
- Inductive generalisation : You use observations about a sample to come to a conclusion about the population it came from.
- Statistical generalisation: You use specific numbers about samples to make statements about populations.
- Causal reasoning: You make cause-and-effect links between different things.
- Sign reasoning: You make a conclusion about a correlational relationship between different things.
- Analogical reasoning: You make a conclusion about something based on its similarities to something else.
It’s often best to ask a variety of people to review your measurements. You can ask experts, such as other researchers, or laypeople, such as potential participants, to judge the face validity of tests.
While experts have a deep understanding of research methods , the people you’re studying can provide you with valuable insights you may have missed otherwise.
Face validity is important because it’s a simple first step to measuring the overall validity of a test or technique. It’s a relatively intuitive, quick, and easy way to start checking whether a new measure seems useful at first glance.
Good face validity means that anyone who reviews your measure says that it seems to be measuring what it’s supposed to. With poor face validity, someone reviewing your measure may be left confused about what you’re measuring and why you’re using this method.
Face validity is about whether a test appears to measure what it’s supposed to measure. This type of validity is concerned with whether a measure seems relevant and appropriate for what it’s assessing only on the surface.
Statistical analyses are often applied to test validity with data from your measures. You test convergent validity and discriminant validity with correlations to see if results from your test are positively or negatively related to those of other established tests.
You can also use regression analyses to assess whether your measure is actually predictive of outcomes that you expect it to predict theoretically. A regression analysis that supports your expectations strengthens your claim of construct validity .
When designing or evaluating a measure, construct validity helps you ensure you’re actually measuring the construct you’re interested in. If you don’t have construct validity, you may inadvertently measure unrelated or distinct constructs and lose precision in your research.
Construct validity is often considered the overarching type of measurement validity , because it covers all of the other types. You need to have face validity , content validity, and criterion validity to achieve construct validity.
Construct validity is about how well a test measures the concept it was designed to evaluate. It’s one of four types of measurement validity , which includes construct validity, face validity , and criterion validity.
There are two subtypes of construct validity.
- Convergent validity : The extent to which your measure corresponds to measures of related constructs
- Discriminant validity: The extent to which your measure is unrelated or negatively related to measures of distinct constructs
Attrition bias can skew your sample so that your final sample differs significantly from your original sample. Your sample is biased because some groups from your population are underrepresented.
With a biased final sample, you may not be able to generalise your findings to the original population that you sampled from, so your external validity is compromised.
There are seven threats to external validity : selection bias , history, experimenter effect, Hawthorne effect , testing effect, aptitude-treatment, and situation effect.
The two types of external validity are population validity (whether you can generalise to other groups of people) and ecological validity (whether you can generalise to other situations and settings).
The external validity of a study is the extent to which you can generalise your findings to different groups of people, situations, and measures.
Attrition bias is a threat to internal validity . In experiments, differential rates of attrition between treatment and control groups can skew results.
This bias can affect the relationship between your independent and dependent variables . It can make variables appear to be correlated when they are not, or vice versa.
Internal validity is the extent to which you can be confident that a cause-and-effect relationship established in a study cannot be explained by other factors.
There are eight threats to internal validity : history, maturation, instrumentation, testing, selection bias , regression to the mean, social interaction, and attrition .
A sampling error is the difference between a population parameter and a sample statistic .
A statistic refers to measures about the sample , while a parameter refers to measures about the population .
Populations are used when a research question requires data from every member of the population. This is usually only feasible when the population is small and easily accessible.
Systematic sampling is a probability sampling method where researchers select members of the population at a regular interval – for example, by selecting every 15th person on a list of the population. If the population is in a random order, this can imitate the benefits of simple random sampling .
There are three key steps in systematic sampling :
- Define and list your population , ensuring that it is not ordered in a cyclical or periodic order.
- Decide on your sample size and calculate your interval, k , by dividing your population by your target sample size.
- Choose every k th member of the population as your sample.
Yes, you can create a stratified sample using multiple characteristics, but you must ensure that every participant in your study belongs to one and only one subgroup. In this case, you multiply the numbers of subgroups for each characteristic to get the total number of groups.
For example, if you were stratifying by location with three subgroups (urban, rural, or suburban) and marital status with five subgroups (single, divorced, widowed, married, or partnered), you would have 3 × 5 = 15 subgroups.
You should use stratified sampling when your sample can be divided into mutually exclusive and exhaustive subgroups that you believe will take on different mean values for the variable that you’re studying.
Using stratified sampling will allow you to obtain more precise (with lower variance ) statistical estimates of whatever you are trying to measure.
For example, say you want to investigate how income differs based on educational attainment, but you know that this relationship can vary based on race. Using stratified sampling, you can ensure you obtain a large enough sample from each racial group, allowing you to draw more precise conclusions.
In stratified sampling , researchers divide subjects into subgroups called strata based on characteristics that they share (e.g., race, gender, educational attainment).
Once divided, each subgroup is randomly sampled using another probability sampling method .
Multistage sampling can simplify data collection when you have large, geographically spread samples, and you can obtain a probability sample without a complete sampling frame.
But multistage sampling may not lead to a representative sample, and larger samples are needed for multistage samples to achieve the statistical properties of simple random samples .
In multistage sampling , you can use probability or non-probability sampling methods.
For a probability sample, you have to probability sampling at every stage. You can mix it up by using simple random sampling , systematic sampling , or stratified sampling to select units at different stages, depending on what is applicable and relevant to your study.
Cluster sampling is a probability sampling method in which you divide a population into clusters, such as districts or schools, and then randomly select some of these clusters as your sample.
The clusters should ideally each be mini-representations of the population as a whole.
There are three types of cluster sampling : single-stage, double-stage and multi-stage clustering. In all three types, you first divide the population into clusters, then randomly select clusters for use in your sample.
- In single-stage sampling , you collect data from every unit within the selected clusters.
- In double-stage sampling , you select a random sample of units from within the clusters.
- In multi-stage sampling , you repeat the procedure of randomly sampling elements from within the clusters until you have reached a manageable sample.
Cluster sampling is more time- and cost-efficient than other probability sampling methods , particularly when it comes to large samples spread across a wide geographical area.
However, it provides less statistical certainty than other methods, such as simple random sampling , because it is difficult to ensure that your clusters properly represent the population as a whole.
If properly implemented, simple random sampling is usually the best sampling method for ensuring both internal and external validity . However, it can sometimes be impractical and expensive to implement, depending on the size of the population to be studied,
If you have a list of every member of the population and the ability to reach whichever members are selected, you can use simple random sampling.
The American Community Survey is an example of simple random sampling . In order to collect detailed data on the population of the US, the Census Bureau officials randomly select 3.5 million households per year and use a variety of methods to convince them to fill out the survey.
Simple random sampling is a type of probability sampling in which the researcher randomly selects a subset of participants from a population . Each member of the population has an equal chance of being selected. Data are then collected from as large a percentage as possible of this random subset.
Sampling bias occurs when some members of a population are systematically more likely to be selected in a sample than others.
In multistage sampling , or multistage cluster sampling, you draw a sample from a population using smaller and smaller groups at each stage.
This method is often used to collect data from a large, geographically spread group of people in national surveys, for example. You take advantage of hierarchical groupings (e.g., from county to city to neighbourhood) to create a sample that’s less expensive and time-consuming to collect data from.
In non-probability sampling , the sample is selected based on non-random criteria, and not every member of the population has a chance of being included.
Common non-probability sampling methods include convenience sampling , voluntary response sampling, purposive sampling , snowball sampling , and quota sampling .
Probability sampling means that every member of the target population has a known chance of being included in the sample.
Probability sampling methods include simple random sampling , systematic sampling , stratified sampling , and cluster sampling .
Samples are used to make inferences about populations . Samples are easier to collect data from because they are practical, cost-effective, convenient, and manageable.
While a between-subjects design has fewer threats to internal validity , it also requires more participants for high statistical power than a within-subjects design .
Advantages:
- Prevents carryover effects of learning and fatigue.
- Shorter study duration.
Disadvantages:
- Needs larger samples for high power.
- Uses more resources to recruit participants, administer sessions, cover costs, etc.
- Individual differences may be an alternative explanation for results.
In a factorial design, multiple independent variables are tested.
If you test two variables, each level of one independent variable is combined with each level of the other independent variable to create different conditions.
Yes. Between-subjects and within-subjects designs can be combined in a single study when you have two or more independent variables (a factorial design). In a mixed factorial design, one variable is altered between subjects and another is altered within subjects.
Within-subjects designs have many potential threats to internal validity , but they are also very statistically powerful .
- Only requires small samples
- Statistically powerful
- Removes the effects of individual differences on the outcomes
- Internal validity threats reduce the likelihood of establishing a direct relationship between variables
- Time-related effects, such as growth, can influence the outcomes
- Carryover effects mean that the specific order of different treatments affect the outcomes
Quasi-experimental design is most useful in situations where it would be unethical or impractical to run a true experiment .
Quasi-experiments have lower internal validity than true experiments, but they often have higher external validity as they can use real-world interventions instead of artificial laboratory settings.
In experimental research, random assignment is a way of placing participants from your sample into different groups using randomisation. With this method, every member of the sample has a known or equal chance of being placed in a control group or an experimental group.
A quasi-experiment is a type of research design that attempts to establish a cause-and-effect relationship. The main difference between this and a true experiment is that the groups are not randomly assigned.
In a between-subjects design , every participant experiences only one condition, and researchers assess group differences between participants in various conditions.
In a within-subjects design , each participant experiences all conditions, and researchers test the same participants repeatedly for differences between conditions.
The word ‘between’ means that you’re comparing different conditions between groups, while the word ‘within’ means you’re comparing different conditions within the same group.
A confounding variable , also called a confounder or confounding factor, is a third variable in a study examining a potential cause-and-effect relationship.
A confounding variable is related to both the supposed cause and the supposed effect of the study. It can be difficult to separate the true effect of the independent variable from the effect of the confounding variable.
In your research design , it’s important to identify potential confounding variables and plan how you will reduce their impact.
Triangulation can help:
- Reduce bias that comes from using a single method, theory, or investigator
- Enhance validity by approaching the same topic with different tools
- Establish credibility by giving you a complete picture of the research problem
But triangulation can also pose problems:
- It’s time-consuming and labour-intensive, often involving an interdisciplinary team.
- Your results may be inconsistent or even contradictory.
There are four main types of triangulation :
- Data triangulation : Using data from different times, spaces, and people
- Investigator triangulation : Involving multiple researchers in collecting or analysing data
- Theory triangulation : Using varying theoretical perspectives in your research
- Methodological triangulation : Using different methodologies to approach the same topic
Experimental designs are a set of procedures that you plan in order to examine the relationship between variables that interest you.
To design a successful experiment, first identify:
- A testable hypothesis
- One or more independent variables that you will manipulate
- One or more dependent variables that you will measure
When designing the experiment, first decide:
- How your variable(s) will be manipulated
- How you will control for any potential confounding or lurking variables
- How many subjects you will include
- How you will assign treatments to your subjects
Exploratory research explores the main aspects of a new or barely researched question.
Explanatory research explains the causes and effects of an already widely researched question.
The key difference between observational studies and experiments is that, done correctly, an observational study will never influence the responses or behaviours of participants. Experimental designs will have a treatment condition applied to at least a portion of participants.
An observational study could be a good fit for your research if your research question is based on things you observe. If you have ethical, logistical, or practical concerns that make an experimental design challenging, consider an observational study. Remember that in an observational study, it is critical that there be no interference or manipulation of the research subjects. Since it’s not an experiment, there are no control or treatment groups either.
These are four of the most common mixed methods designs :
- Convergent parallel: Quantitative and qualitative data are collected at the same time and analysed separately. After both analyses are complete, compare your results to draw overall conclusions.
- Embedded: Quantitative and qualitative data are collected at the same time, but within a larger quantitative or qualitative design. One type of data is secondary to the other.
- Explanatory sequential: Quantitative data is collected and analysed first, followed by qualitative data. You can use this design if you think your qualitative data will explain and contextualise your quantitative findings.
- Exploratory sequential: Qualitative data is collected and analysed first, followed by quantitative data. You can use this design if you think the quantitative data will confirm or validate your qualitative findings.
Triangulation in research means using multiple datasets, methods, theories and/or investigators to address a research question. It’s a research strategy that can help you enhance the validity and credibility of your findings.
Triangulation is mainly used in qualitative research , but it’s also commonly applied in quantitative research . Mixed methods research always uses triangulation.
Operationalisation means turning abstract conceptual ideas into measurable observations.
For example, the concept of social anxiety isn’t directly observable, but it can be operationally defined in terms of self-rating scores, behavioural avoidance of crowded places, or physical anxiety symptoms in social situations.
Before collecting data , it’s important to consider how you will operationalise the variables that you want to measure.
Hypothesis testing is a formal procedure for investigating our ideas about the world using statistics. It is used by scientists to test specific predictions, called hypotheses , by calculating how likely it is that a pattern or relationship between variables could have arisen by chance.
There are five common approaches to qualitative research :
- Grounded theory involves collecting data in order to develop new theories.
- Ethnography involves immersing yourself in a group or organisation to understand its culture.
- Narrative research involves interpreting stories to understand how people make sense of their experiences and perceptions.
- Phenomenological research involves investigating phenomena through people’s lived experiences.
- Action research links theory and practice in several cycles to drive innovative changes.
There are various approaches to qualitative data analysis , but they all share five steps in common:
- Prepare and organise your data.
- Review and explore your data.
- Develop a data coding system.
- Assign codes to the data.
- Identify recurring themes.
The specifics of each step depend on the focus of the analysis. Some common approaches include textual analysis , thematic analysis , and discourse analysis .
In mixed methods research , you use both qualitative and quantitative data collection and analysis methods to answer your research question .
Methodology refers to the overarching strategy and rationale of your research project . It involves studying the methods used in your field and the theories or principles behind them, in order to develop an approach that matches your objectives.
Methods are the specific tools and procedures you use to collect and analyse data (e.g. experiments, surveys , and statistical tests ).
In shorter scientific papers, where the aim is to report the findings of a specific study, you might simply describe what you did in a methods section .
In a longer or more complex research project, such as a thesis or dissertation , you will probably include a methodology section , where you explain your approach to answering the research questions and cite relevant sources to support your choice of methods.
The research methods you use depend on the type of data you need to answer your research question .
- If you want to measure something or test a hypothesis , use quantitative methods . If you want to explore ideas, thoughts, and meanings, use qualitative methods .
- If you want to analyse a large amount of readily available data, use secondary data. If you want data specific to your purposes with control over how they are generated, collect primary data.
- If you want to establish cause-and-effect relationships between variables , use experimental methods. If you want to understand the characteristics of a research subject, use descriptive methods.
Ask our team
Want to contact us directly? No problem. We are always here for you.
- Chat with us
- Email [email protected]
- Call +44 (0)20 3917 4242
- WhatsApp +31 20 261 6040
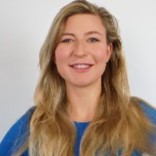
Our support team is here to help you daily via chat, WhatsApp, email, or phone between 9:00 a.m. to 11:00 p.m. CET.
Our APA experts default to APA 7 for editing and formatting. For the Citation Editing Service you are able to choose between APA 6 and 7.
Yes, if your document is longer than 20,000 words, you will get a sample of approximately 2,000 words. This sample edit gives you a first impression of the editor’s editing style and a chance to ask questions and give feedback.
How does the sample edit work?
You will receive the sample edit within 24 hours after placing your order. You then have 24 hours to let us know if you’re happy with the sample or if there’s something you would like the editor to do differently.
Read more about how the sample edit works
Yes, you can upload your document in sections.
We try our best to ensure that the same editor checks all the different sections of your document. When you upload a new file, our system recognizes you as a returning customer, and we immediately contact the editor who helped you before.
However, we cannot guarantee that the same editor will be available. Your chances are higher if
- You send us your text as soon as possible and
- You can be flexible about the deadline.
Please note that the shorter your deadline is, the lower the chance that your previous editor is not available.
If your previous editor isn’t available, then we will inform you immediately and look for another qualified editor. Fear not! Every Scribbr editor follows the Scribbr Improvement Model and will deliver high-quality work.
Yes, our editors also work during the weekends and holidays.
Because we have many editors available, we can check your document 24 hours per day and 7 days per week, all year round.
If you choose a 72 hour deadline and upload your document on a Thursday evening, you’ll have your thesis back by Sunday evening!
Yes! Our editors are all native speakers, and they have lots of experience editing texts written by ESL students. They will make sure your grammar is perfect and point out any sentences that are difficult to understand. They’ll also notice your most common mistakes, and give you personal feedback to improve your writing in English.
Every Scribbr order comes with our award-winning Proofreading & Editing service , which combines two important stages of the revision process.
For a more comprehensive edit, you can add a Structure Check or Clarity Check to your order. With these building blocks, you can customize the kind of feedback you receive.
You might be familiar with a different set of editing terms. To help you understand what you can expect at Scribbr, we created this table:
View an example
When you place an order, you can specify your field of study and we’ll match you with an editor who has familiarity with this area.
However, our editors are language specialists, not academic experts in your field. Your editor’s job is not to comment on the content of your dissertation, but to improve your language and help you express your ideas as clearly and fluently as possible.
This means that your editor will understand your text well enough to give feedback on its clarity, logic and structure, but not on the accuracy or originality of its content.
Good academic writing should be understandable to a non-expert reader, and we believe that academic editing is a discipline in itself. The research, ideas and arguments are all yours – we’re here to make sure they shine!
After your document has been edited, you will receive an email with a link to download the document.
The editor has made changes to your document using ‘Track Changes’ in Word. This means that you only have to accept or ignore the changes that are made in the text one by one.
It is also possible to accept all changes at once. However, we strongly advise you not to do so for the following reasons:
- You can learn a lot by looking at the mistakes you made.
- The editors don’t only change the text – they also place comments when sentences or sometimes even entire paragraphs are unclear. You should read through these comments and take into account your editor’s tips and suggestions.
- With a final read-through, you can make sure you’re 100% happy with your text before you submit!
You choose the turnaround time when ordering. We can return your dissertation within 24 hours , 3 days or 1 week . These timescales include weekends and holidays. As soon as you’ve paid, the deadline is set, and we guarantee to meet it! We’ll notify you by text and email when your editor has completed the job.
Very large orders might not be possible to complete in 24 hours. On average, our editors can complete around 13,000 words in a day while maintaining our high quality standards. If your order is longer than this and urgent, contact us to discuss possibilities.
Always leave yourself enough time to check through the document and accept the changes before your submission deadline.
Scribbr is specialised in editing study related documents. We check:
- Graduation projects
- Dissertations
- Admissions essays
- College essays
- Application essays
- Personal statements
- Process reports
- Reflections
- Internship reports
- Academic papers
- Research proposals
- Prospectuses
Calculate the costs
The fastest turnaround time is 24 hours.
You can upload your document at any time and choose between three deadlines:
At Scribbr, we promise to make every customer 100% happy with the service we offer. Our philosophy: Your complaint is always justified – no denial, no doubts.
Our customer support team is here to find the solution that helps you the most, whether that’s a free new edit or a refund for the service.
Yes, in the order process you can indicate your preference for American, British, or Australian English .
If you don’t choose one, your editor will follow the style of English you currently use. If your editor has any questions about this, we will contact you.
- More from M-W
- To save this word, you'll need to log in. Log In
Definition of hypothesis
Did you know.
The Difference Between Hypothesis and Theory
A hypothesis is an assumption, an idea that is proposed for the sake of argument so that it can be tested to see if it might be true.
In the scientific method, the hypothesis is constructed before any applicable research has been done, apart from a basic background review. You ask a question, read up on what has been studied before, and then form a hypothesis.
A hypothesis is usually tentative; it's an assumption or suggestion made strictly for the objective of being tested.
A theory , in contrast, is a principle that has been formed as an attempt to explain things that have already been substantiated by data. It is used in the names of a number of principles accepted in the scientific community, such as the Big Bang Theory . Because of the rigors of experimentation and control, it is understood to be more likely to be true than a hypothesis is.
In non-scientific use, however, hypothesis and theory are often used interchangeably to mean simply an idea, speculation, or hunch, with theory being the more common choice.
Since this casual use does away with the distinctions upheld by the scientific community, hypothesis and theory are prone to being wrongly interpreted even when they are encountered in scientific contexts—or at least, contexts that allude to scientific study without making the critical distinction that scientists employ when weighing hypotheses and theories.
The most common occurrence is when theory is interpreted—and sometimes even gleefully seized upon—to mean something having less truth value than other scientific principles. (The word law applies to principles so firmly established that they are almost never questioned, such as the law of gravity.)
This mistake is one of projection: since we use theory in general to mean something lightly speculated, then it's implied that scientists must be talking about the same level of uncertainty when they use theory to refer to their well-tested and reasoned principles.
The distinction has come to the forefront particularly on occasions when the content of science curricula in schools has been challenged—notably, when a school board in Georgia put stickers on textbooks stating that evolution was "a theory, not a fact, regarding the origin of living things." As Kenneth R. Miller, a cell biologist at Brown University, has said , a theory "doesn’t mean a hunch or a guess. A theory is a system of explanations that ties together a whole bunch of facts. It not only explains those facts, but predicts what you ought to find from other observations and experiments.”
While theories are never completely infallible, they form the basis of scientific reasoning because, as Miller said "to the best of our ability, we’ve tested them, and they’ve held up."
- proposition
- supposition
hypothesis , theory , law mean a formula derived by inference from scientific data that explains a principle operating in nature.
hypothesis implies insufficient evidence to provide more than a tentative explanation.
theory implies a greater range of evidence and greater likelihood of truth.
law implies a statement of order and relation in nature that has been found to be invariable under the same conditions.
Examples of hypothesis in a Sentence
These examples are programmatically compiled from various online sources to illustrate current usage of the word 'hypothesis.' Any opinions expressed in the examples do not represent those of Merriam-Webster or its editors. Send us feedback about these examples.
Word History
Greek, from hypotithenai to put under, suppose, from hypo- + tithenai to put — more at do
1641, in the meaning defined at sense 1a
Phrases Containing hypothesis
- null hypothesis
- planetesimal hypothesis
- counter - hypothesis
- Whorfian hypothesis
- nebular hypothesis
Articles Related to hypothesis
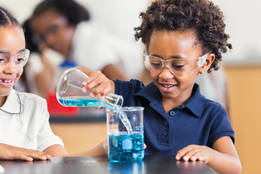
This is the Difference Between a...
This is the Difference Between a Hypothesis and a Theory
In scientific reasoning, they're two completely different things
Dictionary Entries Near hypothesis
hypothermia
hypothesize
Cite this Entry
“Hypothesis.” Merriam-Webster.com Dictionary , Merriam-Webster, https://www.merriam-webster.com/dictionary/hypothesis. Accessed 10 Apr. 2024.
Kids Definition
Kids definition of hypothesis, medical definition, medical definition of hypothesis, more from merriam-webster on hypothesis.
Nglish: Translation of hypothesis for Spanish Speakers
Britannica English: Translation of hypothesis for Arabic Speakers
Britannica.com: Encyclopedia article about hypothesis
Subscribe to America's largest dictionary and get thousands more definitions and advanced search—ad free!
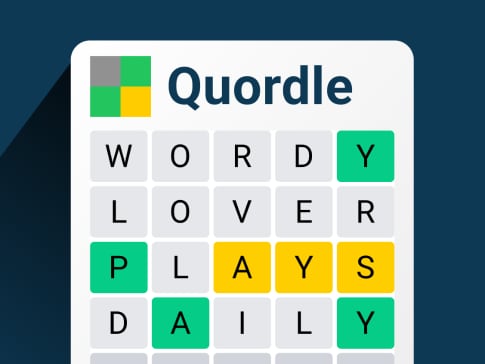
Can you solve 4 words at once?
Word of the day.
See Definitions and Examples »
Get Word of the Day daily email!
Popular in Grammar & Usage
Your vs. you're: how to use them correctly, every letter is silent, sometimes: a-z list of examples, more commonly mispronounced words, how to use em dashes (—), en dashes (–) , and hyphens (-), absent letters that are heard anyway, popular in wordplay, the words of the week - apr. 5, 12 bird names that sound like compliments, 10 scrabble words without any vowels, 12 more bird names that sound like insults (and sometimes are), 8 uncommon words related to love, games & quizzes.
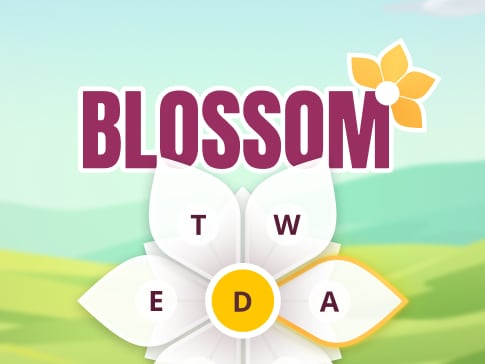
Definition of a Hypothesis
What it is and how it's used in sociology
- Key Concepts
- Major Sociologists
- News & Issues
- Research, Samples, and Statistics
- Recommended Reading
- Archaeology
A hypothesis is a prediction of what will be found at the outcome of a research project and is typically focused on the relationship between two different variables studied in the research. It is usually based on both theoretical expectations about how things work and already existing scientific evidence.
Within social science, a hypothesis can take two forms. It can predict that there is no relationship between two variables, in which case it is a null hypothesis . Or, it can predict the existence of a relationship between variables, which is known as an alternative hypothesis.
In either case, the variable that is thought to either affect or not affect the outcome is known as the independent variable, and the variable that is thought to either be affected or not is the dependent variable.
Researchers seek to determine whether or not their hypothesis, or hypotheses if they have more than one, will prove true. Sometimes they do, and sometimes they do not. Either way, the research is considered successful if one can conclude whether or not a hypothesis is true.
Null Hypothesis
A researcher has a null hypothesis when she or he believes, based on theory and existing scientific evidence, that there will not be a relationship between two variables. For example, when examining what factors influence a person's highest level of education within the U.S., a researcher might expect that place of birth, number of siblings, and religion would not have an impact on the level of education. This would mean the researcher has stated three null hypotheses.
Alternative Hypothesis
Taking the same example, a researcher might expect that the economic class and educational attainment of one's parents, and the race of the person in question are likely to have an effect on one's educational attainment. Existing evidence and social theories that recognize the connections between wealth and cultural resources , and how race affects access to rights and resources in the U.S. , would suggest that both economic class and educational attainment of the one's parents would have a positive effect on educational attainment. In this case, economic class and educational attainment of one's parents are independent variables, and one's educational attainment is the dependent variable—it is hypothesized to be dependent on the other two.
Conversely, an informed researcher would expect that being a race other than white in the U.S. is likely to have a negative impact on a person's educational attainment. This would be characterized as a negative relationship, wherein being a person of color has a negative effect on one's educational attainment. In reality, this hypothesis proves true, with the exception of Asian Americans , who go to college at a higher rate than whites do. However, Blacks and Hispanics and Latinos are far less likely than whites and Asian Americans to go to college.
Formulating a Hypothesis
Formulating a hypothesis can take place at the very beginning of a research project , or after a bit of research has already been done. Sometimes a researcher knows right from the start which variables she is interested in studying, and she may already have a hunch about their relationships. Other times, a researcher may have an interest in a particular topic, trend, or phenomenon, but he may not know enough about it to identify variables or formulate a hypothesis.
Whenever a hypothesis is formulated, the most important thing is to be precise about what one's variables are, what the nature of the relationship between them might be, and how one can go about conducting a study of them.
Updated by Nicki Lisa Cole, Ph.D
- What Is a Hypothesis? (Science)
- Understanding Path Analysis
- Null Hypothesis Examples
- What Are the Elements of a Good Hypothesis?
- What 'Fail to Reject' Means in a Hypothesis Test
- How Intervening Variables Work in Sociology
- Null Hypothesis Definition and Examples
- Understanding Simple vs Controlled Experiments
- Scientific Method Vocabulary Terms
- Null Hypothesis and Alternative Hypothesis
- Six Steps of the Scientific Method
- What Are Examples of a Hypothesis?
- Structural Equation Modeling
- Scientific Method Flow Chart
- How To Design a Science Fair Experiment
- Hypothesis Test for the Difference of Two Population Proportions

Understanding a Hypothesis (Definition, Null, and Examples)
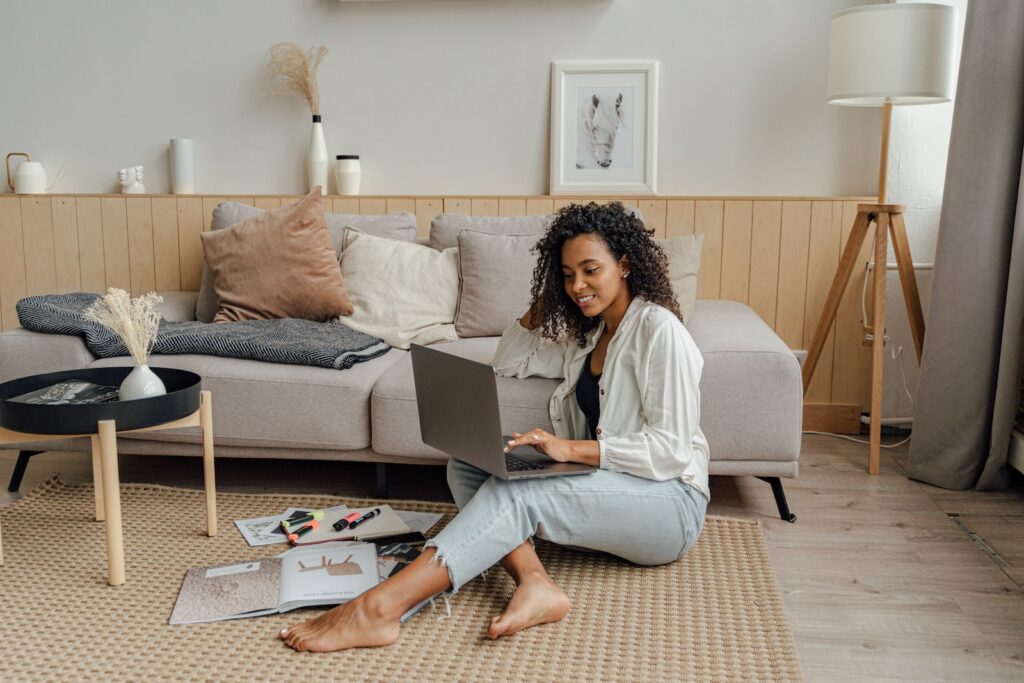
You come home exhausted and plop down on the couch. You don’t know why you are feeling so weary. You think about several possible reasons. Is it because you stayed up late last night? Is it because you skipped breakfast? Or is it because you had to take the stairs due to a power outage? Or is it because of all the above reasons?
What you are doing is hypothesizing about why you are feeling tired.
If you enjoy reading detective stories, you would have already come across a hypothesis. A good whodunit mystery confounds the reader with multiple hypotheses about who committed the crime.
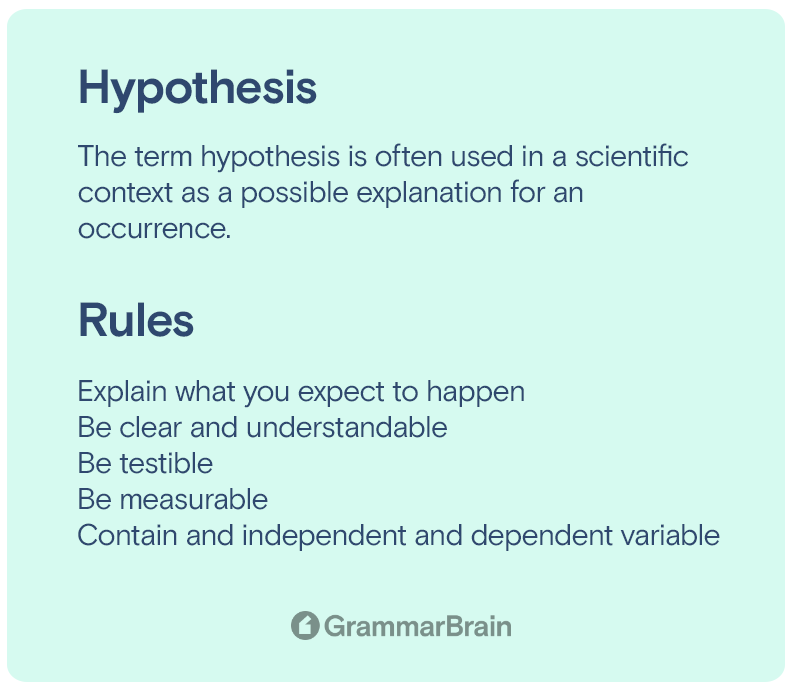
- What is a Hypothesis?
The term hypothesis is often used in a scientific context as a possible explanation for an occurrence.
The word originated from ancient Greek and means “putting under” indicating its early association with experimentation.
A hypothesis is:
- An assumption that serves as a starting point for further research
- A supposition made on the basis of insufficient evidence
- A tentative and logical statement that can be tested for its authenticity
- An idea that seeks to explain why a phenomenon takes place
- A prediction about the outcome of a study according to known facts
- A proposal about the possible relationship between two or more variables
A scientist testing a hypothesis is no different from a detective investigating a crime scene. Famous detectives such as Sherlock Holmes combine the evidence with their powers of prediction to identify the criminal from several potential suspects.
The scientist examines each hypothesis rigorously for any inconsistencies through experiments before it can receive the stamp of approval.
Scientists accept a hypothesis as a theory only after it has been validated several times in different conditions. This includes use of scientific methods and protocols involving observation and analysis of results.
A good hypothesis seeks to establish a causal relationship between two or more variables, primarily between the independent and the dependent variable.
Brushing your teeth at least twice in a day reduces the incidence of dental caries.
The independent variable or cause in the above example is the number of times you brush in a day. The dependent variable or effect is the incidence of dental caries or cavities.
A scientist or researcher tests a hypothesis by changing the independent variable and measuring its effect on the dependent variable.
A relationship between a single independent and dependent variable is known as a simple hypothesis.
The mathematical expression of this relationship is:
- where x is the independent variable and Y is the dependent variable and
- where x is the input and Y is the output or a function of x
So, brushing your teeth at least twice daily is an input and the reduction of dental caries is an output or a function of the action of brushing your teeth.
If there are multiple independent variables or in some cases more than a single dependent variable, the statement is a complex hypothesis.
Brushing your teeth at least twice a day and using dental floss reduces the incidence of cavities and periodontitis.
In the above example the two independent variables are brushing teeth and using dental floss. The dependent variables are reduction in cavities and periodontitis or gum infection. In this example the two independent variables are common for the two dependent variables.
The equation of a complex hypothesis can be written as:
Y = f(x 1 +x 2 +x 3 …)
Y 1 = f(z 1 +z 2 +z 3 …)
where z is a different set of independent variables for Y1 as the dependent variable
- Developing a Hypothesis
A hypothesis is a frame of reference or a window through which you observe a phenomenon. The phenomenon is the dependent variable. Your job is to determine the independent variables that are causing the event.
Cultivate the habit of looking for patterns in anything that happens. Train your mind to think in terms of stimulus and reaction or cause and effect.
This will enable you to glean insights from the knowledge you gather. You will then be able to write a strong hypothesis that focuses on the variables that matter over the noise.
The six steps to developing a hypothesis are:
- Ask a question
- Preliminary research
- Formulate the hypothesis
- Refine the hypothesis
- Phrase your hypothesis in three ways
- Write a null hypothesis
Ask a Question
The first step is to write a research question.
To write an effective research question be as curious as possible. Start with asking yourself a ton of questions.
Begin with broad and open-ended questions before narrowing it down to more specific ones.
You can use the 5W1H method to get into the mode of writing a research question.
- What took place?
- When did it happen?
- Where did it occur?
- Why did it take place?
- Who did it affect ?
- How did it happen?
The research question needs to be clear, objective , well-defined and measurable.
Do people who take health supplements log in fewer sick days at work in a year than those who don’t?
After you have framed the right question you can make an educated guess to answer it. This answer will be your preliminary hypothesis. Your hypothesis will attempt to answer the research question with observable facts through various experiments.
Preliminary Research
You don’t have to start from scratch. You can draw from preexisting knowledge and well-established theories to discount fallacious premises at the outset.
Resources that you can refer to include case studies, research papers and theses published in academic or scientific journals. A thorough background research will help you to look at the research question from several angles.
Do keep an open mind or a blank slate to avoid falling in the trap of preconceived notions and prejudices. Your initial research should help you focus on the areas where you are most likely to find the answers.
You can come up with a blueprint or outline highlighting the variables that you think are most relevant to your research question.
Think how changing the attributes of a single variable potentially affects others. You may need to operationalize or define how you are going to measure the variables and their effects.
Formulate the Hypothesis
It’s time now to put together your hypothesis into words.
A sound hypothesis states:
- Who or what is being studied?
- The relationship between the variables
- A measurable and reproducible outcome
- The possibility to prove it as true or false
Teenagers in the 14-16 age group who eat a high-protein diet are taller by two inches than the average height for that age group.
The next step is to ensure your statement ticks all the boxes for a strong hypothesis.
Is the hypothesis:
- Precise and quantifiable without any ambiguity
- Lucid and focused on the results described in the research question
Does the hypothesis include:
- An independent and dependent variable
- Variables that can be changed or controlled
- Terms that even a layman can understand
- A well-defined outcome
Phrase your Hypothesis in Three Ways
A hypothesis is often written in an If-then format. This format describes the cause and effect relationship between an independent variable and a dependent variable.
Phrase your hypothesis as “If {you make changes to an independent variable} then {you will observe this change in the dependent variable}.”
If employees are given more autonomy to take work-related decisions then their overall performance improves.
Another way to write a hypothesis is by directly stating the outcome between the two variables.
More autonomy in terms of taking work-related decisions helps to improve an employee’s overall performance.
You can also state a hypothesis as a comparison between two groups.
Employees who are offered more autonomy to take work-related decisions show better overall performance than those who work in a micro-managed environment.
Write a Null Hypothesis
The next step is to frame a null hypothesis, especially if your study requires you to analyze the data statistically. A null hypothesis by default takes a converse position to the researcher’s hypothesis.
Your statement is known as the alternative hypothesis while its opposite outcome is referred to as the null hypothesis.
If you expect a change according to a relationship between the variables the null hypothesis denies the possibility of any change or association between the variables. If you expect the conditions to remain constant the null hypothesis states that change will take place.
The null hypothesis is referred to as H 0. Your hypothesis which is the alternative is written as H 1 or H a .
H 1 : A player who is more than two meters tall has a better chance of winning the National Basketball Association Most Valuable Player Award.
H 0 : The height of a player does not affect his prospects of winning the National Basketball Association Most Valuable Player Award.
Hypothesis Examples
Examples of research questions.
- Which loop diuretic drug is more effective for treating heart failure?
- Does attending online learning sessions help students to improve their exam scores?
- Does talking on the phone while driving cause more accidents?
- Does increasing the pressure affect the rate of reaction between gases?
- Is a person more likely to be obese if she or he eats unhealthy foods at least four times in a week?
Examples of a Hypothesis
- The clinical trial of the new drug Furosemide proved that it is better at treating heart failure than other loop diuretic drugs such as Bumetanide.
- The students who attended online learning sessions had better exam scores than those who skipped the sessions.
- Drivers who talk on the phone are likely to have an accident than those who don’t.
- Increasing the pressure affects the concentration of gases and it acts as a catalyst in speeding up the rate of reaction.
- People who eat processed foods frequently are more likely to be obese than people who limit their intake of such foods.
Examples of a Null Hypothesis
- The clinical trial proved that there is no difference between the effectiveness of Furosemide and other loop diuretic drugs, such as Bumetanide, for treating heart failure.
- There is no difference in the exam scores of students who attended online learning sessions and those who did not attend.
- There is no difference in the rate of accidents experienced by drivers who talk on the phone compared with those who don’t talk on the phone while driving.
- The elevation of pressure has no effect on the rate of reaction between gases.
- The food consumed and its frequency of consumption do not affect the probability of a person becoming obese.
What are Null Hypotheses?
The null hypothesis states the opposite outcome to the researcher’s hypothesis.
In most cases, the null hypothesis’s default position is a prediction that no relationship exists between any two or more variables. The null hypothesis denies the possibility of a causal relationship existing between an assumed independent and dependent variable.
The symbol of the null hypothesis is H 0 .
The notion of a null hypothesis fulfills the requirement of the falsifiability of a hypothesis before it can be accepted as valid.
A null hypothesis is often written as a negative statement that posits that the original hypothesis is false. It either claims that the results obtained are due to chance or there is no evidence to prove any change.
Original Hypothesis: Use of nitrogen fertilizers helps plants grow faster as compared to use of phosphorus or potassium fertilizers.
Null Hypothesis (H 0 ): The fertilizer used has no bearing on the rate of plant growth
What are Alternative Hypotheses?
An alternative hypothesis states the researcher’s supposition of a causal relationship between any two or more variables. Alternative hypotheses are based upon an observable effect and seek to predict how changing an independent variable will affect the dependent variable.
An alternative hypothesis is symbolized as H 1 or H a . It’s often written together with a null hypothesis with the two statements existing as a dual pair of opposite assumptions. Only a single statement among two can be true.
Alternative hypotheses try to determine that the results are obtained due to significant changes related to the variables and not due to chance.
Research Question: Does washing hands thoroughly with soap before eating a meal reduce the rate of recurrence of respiratory ailments?
Alternative Hypothesis (H 1 ): Washing hands with soap before eating reduces the rate of recurrence of respiratory ailments by 30% compared with those who neglect hand hygiene.
Null Hypothesis (H 0 ): Washing hands with soap before eating has no effect on the rate of recurrence of respiratory ailments.
What is Hypothesis Testing?
After you have formulated a hypothesis, you need to choose a research and testing method.
Use a descriptive approach when experiments are difficult to conduct. A descriptive method incorporates case studies and surveys to collect data.
You can employ statistical tools such as a correlational study to measure the relationship between variables.
A correlational study calculates the probability of whether a linkage between two variables can be determined or do the changes occur purely due to chance. Do note that correlation is not equivalent to causality.
This method lets you arrive at a conclusion by generalizing the data obtained without performing any actual experiments. A hypothesis proved using this approach is known as a statistical hypothesis.
The other approach is the experimental method in which causal relationships are established between different variables through demonstrations. A working or empirical hypothesis often makes use of the experimental method to determine the relationships between the variables.
The steps for testing a hypothesis experimentally are:
- Design of experiments
- Collating data
- Analysis of observable facts
- Summarizing the conclusions
- Validating the hypothesis as a theory
How to Write a Good Hypothesis
To find ideas for a hypothesis, you can look through discussion sections in academic and scientific journals or browse online publications. You will come across questions that can be investigated further.
Simple Steps
The steps to write a strong hypothesis are:
- Choose your frame of reference or direction for determining the cause
- Such an approach is known as a directional hypothesis
- If you are unable to determine a starting point or the current theories are ambiguous and contradictory, you can choose a non-directional approach
- This method involves stating the facts and observations randomly and then seeking to find a pattern
- Identify the key variables
- A variable is any attribute that can have measurable values such as temperature, time, or length
- Tentatively label some variables as independent and some as dependent
- State the relationship between the variables using clear and objective language
- Operationalize or define how you will measure the variables for testability
- Write the statement in the If-then format. You can also write it as a declarative sentence
- Avoid jargon and use simple words that can be understood by a layman
- Write a null hypothesis to satisfy the condition of falsifiability
If you watch television for more than three hours a day, then your ability to concentrate diminishes.
How to Write a Scientific Hypothesis
A good scientific hypothesis is:
- Consistent: Use preexisting knowledge as a springboard for further research
- Testable: Include words that are quantifiable or measurable
- Concise: Cut down on verbose phrases and use precise words
- Scalable: Formulate the statement in a universal context based on the variables
- Promising: State unexplained occurrences as loose ends that can be investigated further
Simple steps
- Record your observations and facts about the topic
- Evaluate your statements for possible links to determine the cause and effect
- Document all potential explanations to analyze further
- Write the null hypothesis along with your own hypothesis
- This satisfies the requisite condition for a valid hypothesis. It can either be confirmed or disproved
If you plant cotton in black soil, then the production is boosted by 20% as compared to the output from red soil.
How to write a Psychology Hypothesis
A psychology hypothesis often begins with how the environment or certain parameters within it influence or cause a specific behavior.
To write a sound psychology hypothesis:
- Choose a topic that you are genuinely interested in
- Do not ramble. Keep it short and simple
- Use previous research and your own study to direct your vision
- Ascertain and define the variables
- You can write the hypothesis either as an If-then statement
- Other alternatives are to write the hypothesis as a direct sentence or a comparative supposition
Use the following questions to guide your understanding of the topic.
- Is your hypothesis based on a preexisting theory or your own research?
- Can your hypothesis be tested for falsifiability?
- What are the independent and dependent variables?
People who exercise regularly are less at risk from depression than people who lead a sedentary life.
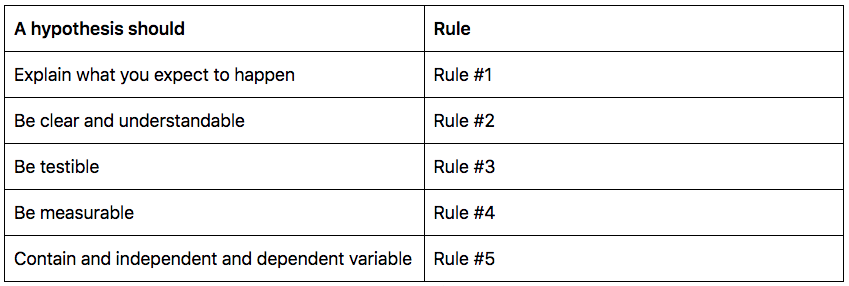
- What is and How to Write a Good Hypothesis in Research?
- How to Write a Hypothesis in 6 Steps
- Developing Hypothesis and Research Questions
- Forming a Good Hypothesis for Scientific Research
- 6 Hypothesis Examples in Psychology
- Correlational Research | When & How to Use
- How to Write a Strong Hypothesis in 6 Simple Steps
- How to Develop a Good Research Hypothesis
- How To Develop a Hypothesis (With Elements, Types and Examples)
- Definition of Hypothesis
Inside this article
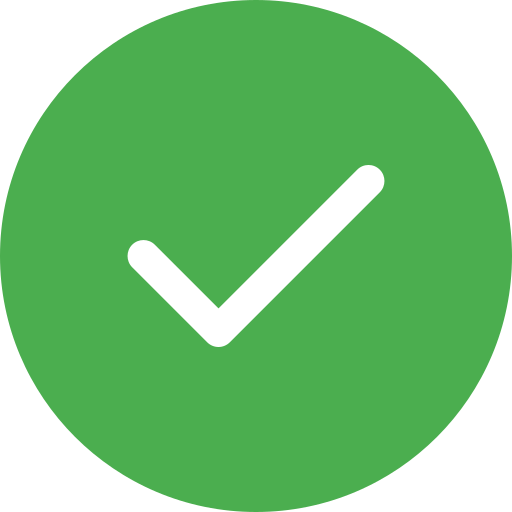
Fact checked: Content is rigorously reviewed by a team of qualified and experienced fact checkers. Fact checkers review articles for factual accuracy, relevance, and timeliness. Learn more.
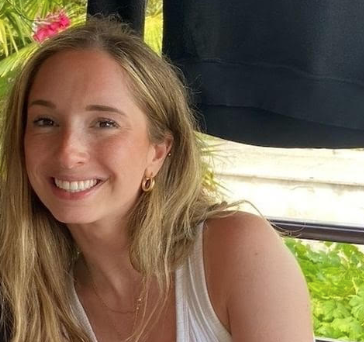
About the author
Dalia Y.: Dalia is an English Major and linguistics expert with an additional degree in Psychology. Dalia has featured articles on Forbes, Inc, Fast Company, Grammarly, and many more. She covers English, ESL, and all things grammar on GrammarBrain.
Core lessons
- Abstract Noun
- Accusative Case
- Active Sentence
- Alliteration
- Adjective Clause
- Adjective Phrase
- Adverbial Clause
- Appositive Phrase
- Body Paragraph
- Compound Adjective
- Complex Sentence
- Compound Words
- Compound Predicate
- Common Noun
- Comparative Adjective
- Comparative and Superlative
- Compound Noun
- Compound Subject
- Compound Sentence
- Copular Verb
- Collective Noun
- Colloquialism
- Conciseness
- Conditional
- Concrete Noun
- Conjunction
- Conjugation
- Conditional Sentence
- Comma Splice
- Correlative Conjunction
- Coordinating Conjunction
- Coordinate Adjective
- Cumulative Adjective
- Dative Case
- Declarative Statement
- Direct Object Pronoun
- Direct Object
- Dangling Modifier
- Demonstrative Pronoun
- Demonstrative Adjective
- Direct Characterization
- Definite Article
- Doublespeak
- Equivocation Fallacy
- Future Perfect Progressive
- Future Simple
- Future Perfect Continuous
- Future Perfect
- First Conditional
- Gerund Phrase
- Genitive Case
- Helping Verb
- Irregular Adjective
- Irregular Verb
- Imperative Sentence
- Indefinite Article
- Intransitive Verb
- Introductory Phrase
- Indefinite Pronoun
- Indirect Characterization
- Interrogative Sentence
- Intensive Pronoun
- Inanimate Object
- Indefinite Tense
- Infinitive Phrase
- Interjection
- Intensifier
- Indicative Mood
- Juxtaposition
- Linking Verb
- Misplaced Modifier
- Nominative Case
- Noun Adjective
- Object Pronoun
- Object Complement
- Order of Adjectives
- Parallelism
- Prepositional Phrase
- Past Simple Tense
- Past Continuous Tense
- Past Perfect Tense
- Past Progressive Tense
- Present Simple Tense
- Present Perfect Tense
- Personal Pronoun
- Personification
- Persuasive Writing
- Parallel Structure
- Phrasal Verb
- Predicate Adjective
- Predicate Nominative
- Phonetic Language
- Plural Noun
- Punctuation
- Punctuation Marks
- Preposition
- Preposition of Place
- Parts of Speech
- Possessive Adjective
- Possessive Determiner
- Possessive Case
- Possessive Noun
- Proper Adjective
- Proper Noun
- Present Participle
- Quotation Marks
- Relative Pronoun
- Reflexive Pronoun
- Reciprocal Pronoun
- Subordinating Conjunction
- Simple Future Tense
- Stative Verb
- Subjunctive
- Subject Complement
- Subject of a Sentence
- Sentence Variety
- Second Conditional
- Superlative Adjective
- Slash Symbol
- Topic Sentence
- Types of Nouns
- Types of Sentences
- Uncountable Noun
- Vowels and Consonants
Popular lessons
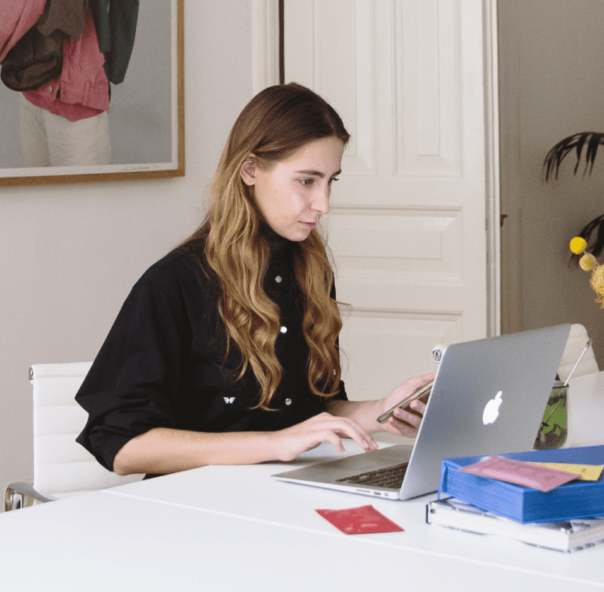
Stay awhile. Your weekly dose of grammar and English fun.

The world's best online resource for learning English. Understand words, phrases, slang terms, and all other variations of the English language.
- Abbreviations
- Editorial Policy
Sapir–Whorf hypothesis (Linguistic Relativity Hypothesis)
Mia Belle Frothingham
Author, Researcher, Science Communicator
BA with minors in Psychology and Biology, MRes University of Edinburgh
Mia Belle Frothingham is a Harvard University graduate with a Bachelor of Arts in Sciences with minors in biology and psychology
Learn about our Editorial Process
Saul Mcleod, PhD
Editor-in-Chief for Simply Psychology
BSc (Hons) Psychology, MRes, PhD, University of Manchester
Saul Mcleod, PhD., is a qualified psychology teacher with over 18 years of experience in further and higher education. He has been published in peer-reviewed journals, including the Journal of Clinical Psychology.
Olivia Guy-Evans, MSc
Associate Editor for Simply Psychology
BSc (Hons) Psychology, MSc Psychology of Education
Olivia Guy-Evans is a writer and associate editor for Simply Psychology. She has previously worked in healthcare and educational sectors.
On This Page:
There are about seven thousand languages heard around the world – they all have different sounds, vocabularies, and structures. As you know, language plays a significant role in our lives.
But one intriguing question is – can it actually affect how we think?
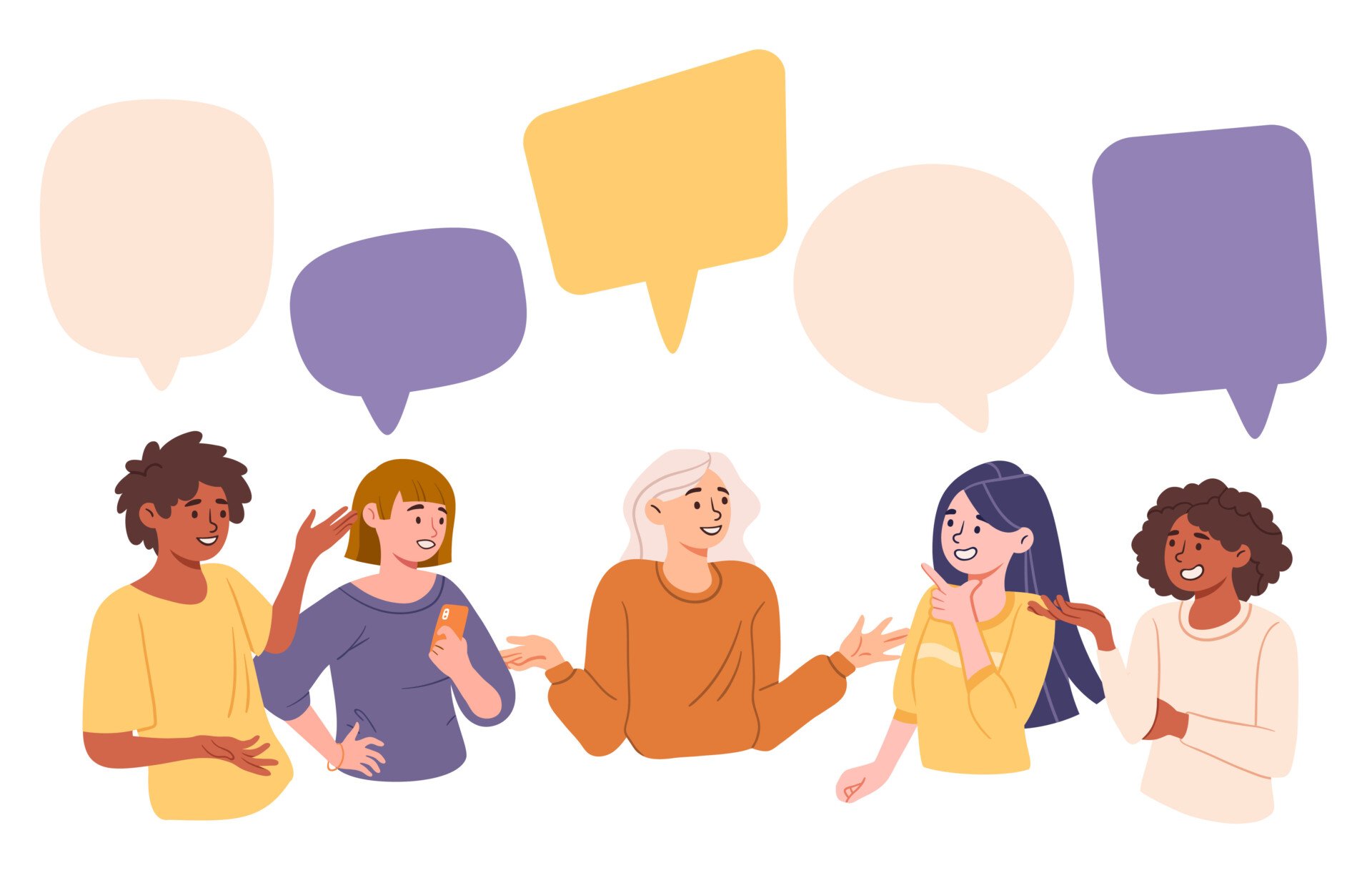
It is widely thought that reality and how one perceives the world is expressed in spoken words and are precisely the same as reality.
That is, perception and expression are understood to be synonymous, and it is assumed that speech is based on thoughts. This idea believes that what one says depends on how the world is encoded and decoded in the mind.
However, many believe the opposite.
In that, what one perceives is dependent on the spoken word. Basically, that thought depends on language, not the other way around.
What Is The Sapir-Whorf Hypothesis?
Twentieth-century linguists Edward Sapir and Benjamin Lee Whorf are known for this very principle and its popularization. Their joint theory, known as the Sapir-Whorf Hypothesis or, more commonly, the Theory of Linguistic Relativity, holds great significance in all scopes of communication theories.
The Sapir-Whorf hypothesis states that the grammatical and verbal structure of a person’s language influences how they perceive the world. It emphasizes that language either determines or influences one’s thoughts.
The Sapir-Whorf hypothesis states that people experience the world based on the structure of their language, and that linguistic categories shape and limit cognitive processes. It proposes that differences in language affect thought, perception, and behavior, so speakers of different languages think and act differently.
For example, different words mean various things in other languages. Not every word in all languages has an exact one-to-one translation in a foreign language.
Because of these small but crucial differences, using the wrong word within a particular language can have significant consequences.
The Sapir-Whorf hypothesis is sometimes called “linguistic relativity” or the “principle of linguistic relativity.” So while they have slightly different names, they refer to the same basic proposal about the relationship between language and thought.
How Language Influences Culture
Culture is defined by the values, norms, and beliefs of a society. Our culture can be considered a lens through which we undergo the world and develop a shared meaning of what occurs around us.
The language that we create and use is in response to the cultural and societal needs that arose. In other words, there is an apparent relationship between how we talk and how we perceive the world.
One crucial question that many intellectuals have asked is how our society’s language influences its culture.
Linguist and anthropologist Edward Sapir and his then-student Benjamin Whorf were interested in answering this question.
Together, they created the Sapir-Whorf hypothesis, which states that our thought processes predominantly determine how we look at the world.
Our language restricts our thought processes – our language shapes our reality. Simply, the language that we use shapes the way we think and how we see the world.
Since the Sapir-Whorf hypothesis theorizes that our language use shapes our perspective of the world, people who speak different languages have different views of the world.
In the 1920s, Benjamin Whorf was a Yale University graduate student studying with linguist Edward Sapir, who was considered the father of American linguistic anthropology.
Sapir was responsible for documenting and recording the cultures and languages of many Native American tribes disappearing at an alarming rate. He and his predecessors were well aware of the close relationship between language and culture.
Anthropologists like Sapir need to learn the language of the culture they are studying to understand the worldview of its speakers truly. Whorf believed that the opposite is also true, that language affects culture by influencing how its speakers think.
His hypothesis proposed that the words and structures of a language influence how its speaker behaves and feels about the world and, ultimately, the culture itself.
Simply put, Whorf believed that you see the world differently from another person who speaks another language due to the specific language you speak.
Human beings do not live in the matter-of-fact world alone, nor solitary in the world of social action as traditionally understood, but are very much at the pardon of the certain language which has become the medium of communication and expression for their society.
To a large extent, the real world is unconsciously built on habits in regard to the language of the group. We hear and see and otherwise experience broadly as we do because the language habits of our community predispose choices of interpretation.
Studies & Examples
The lexicon, or vocabulary, is the inventory of the articles a culture speaks about and has classified to understand the world around them and deal with it effectively.
For example, our modern life is dictated for many by the need to travel by some vehicle – cars, buses, trucks, SUVs, trains, etc. We, therefore, have thousands of words to talk about and mention, including types of models, vehicles, parts, or brands.
The most influential aspects of each culture are similarly reflected in the dictionary of its language. Among the societies living on the islands in the Pacific, fish have significant economic and cultural importance.
Therefore, this is reflected in the rich vocabulary that describes all aspects of the fish and the environments that islanders depend on for survival.
For example, there are over 1,000 fish species in Palau, and Palauan fishers knew, even long before biologists existed, details about the anatomy, behavior, growth patterns, and habitat of most of them – far more than modern biologists know today.
Whorf’s studies at Yale involved working with many Native American languages, including Hopi. He discovered that the Hopi language is quite different from English in many ways, especially regarding time.
Western cultures and languages view times as a flowing river that carries us continuously through the present, away from the past, and to the future.
Our grammar and system of verbs reflect this concept with particular tenses for past, present, and future.
We perceive this concept of time as universal in that all humans see it in the same way.
Although a speaker of Hopi has very different ideas, their language’s structure both reflects and shapes the way they think about time. Seemingly, the Hopi language has no present, past, or future tense; instead, they divide the world into manifested and unmanifest domains.
The manifested domain consists of the physical universe, including the present, the immediate past, and the future; the unmanifest domain consists of the remote past and the future and the world of dreams, thoughts, desires, and life forces.
Also, there are no words for minutes, minutes, or days of the week. Native Hopi speakers often had great difficulty adapting to life in the English-speaking world when it came to being on time for their job or other affairs.
It is due to the simple fact that this was not how they had been conditioned to behave concerning time in their Hopi world, which followed the phases of the moon and the movements of the sun.
Today, it is widely believed that some aspects of perception are affected by language.
One big problem with the original Sapir-Whorf hypothesis derives from the idea that if a person’s language has no word for a specific concept, then that person would not understand that concept.
Honestly, the idea that a mother tongue can restrict one’s understanding has been largely unaccepted. For example, in German, there is a term that means to take pleasure in another person’s unhappiness.
While there is no translatable equivalent in English, it just would not be accurate to say that English speakers have never experienced or would not be able to comprehend this emotion.
Just because there is no word for this in the English language does not mean English speakers are less equipped to feel or experience the meaning of the word.
Not to mention a “chicken and egg” problem with the theory.
Of course, languages are human creations, very much tools we invented and honed to suit our needs. Merely showing that speakers of diverse languages think differently does not tell us whether it is the language that shapes belief or the other way around.
Supporting Evidence
On the other hand, there is hard evidence that the language-associated habits we acquire play a role in how we view the world. And indeed, this is especially true for languages that attach genders to inanimate objects.
There was a study done that looked at how German and Spanish speakers view different things based on their given gender association in each respective language.
The results demonstrated that in describing things that are referred to as masculine in Spanish, speakers of the language marked them as having more male characteristics like “strong” and “long.” Similarly, these same items, which use feminine phrasings in German, were noted by German speakers as effeminate, like “beautiful” and “elegant.”
The findings imply that speakers of each language have developed preconceived notions of something being feminine or masculine, not due to the objects” characteristics or appearances but because of how they are categorized in their native language.
It is important to remember that the Theory of Linguistic Relativity (Sapir-Whorf Hypothesis) also successfully achieves openness. The theory is shown as a window where we view the cognitive process, not as an absolute.
It is set forth to look at a phenomenon differently than one usually would. Furthermore, the Sapir-Whorf Hypothesis is very simple and logically sound. Understandably, one’s atmosphere and culture will affect decoding.
Likewise, in studies done by the authors of the theory, many Native American tribes do not have a word for particular things because they do not exist in their lives. The logical simplism of this idea of relativism provides parsimony.
Truly, the Sapir-Whorf Hypothesis makes sense. It can be utilized in describing great numerous misunderstandings in everyday life. When a Pennsylvanian says “yuns,” it does not make any sense to a Californian, but when examined, it is just another word for “you all.”
The Linguistic Relativity Theory addresses this and suggests that it is all relative. This concept of relativity passes outside dialect boundaries and delves into the world of language – from different countries and, consequently, from mind to mind.
Is language reality honestly because of thought, or is it thought which occurs because of language? The Sapir-Whorf Hypothesis very transparently presents a view of reality being expressed in language and thus forming in thought.
The principles rehashed in it show a reasonable and even simple idea of how one perceives the world, but the question is still arguable: thought then language or language then thought?
Modern Relevance
Regardless of its age, the Sapir-Whorf hypothesis, or the Linguistic Relativity Theory, has continued to force itself into linguistic conversations, even including pop culture.
The idea was just recently revisited in the movie “Arrival,” – a science fiction film that engagingly explores the ways in which an alien language can affect and alter human thinking.
And even if some of the most drastic claims of the theory have been debunked or argued against, the idea has continued its relevance, and that does say something about its importance.
Hypotheses, thoughts, and intellectual musings do not need to be totally accurate to remain in the public eye as long as they make us think and question the world – and the Sapir-Whorf Hypothesis does precisely that.
The theory does not only make us question linguistic theory and our own language but also our very existence and how our perceptions might shape what exists in this world.
There are generalities that we can expect every person to encounter in their day-to-day life – in relationships, love, work, sadness, and so on. But thinking about the more granular disparities experienced by those in diverse circumstances, linguistic or otherwise, helps us realize that there is more to the story than ours.
And beautifully, at the same time, the Sapir-Whorf Hypothesis reiterates the fact that we are more alike than we are different, regardless of the language we speak.
Isn’t it just amazing that linguistic diversity just reveals to us how ingenious and flexible the human mind is – human minds have invented not one cognitive universe but, indeed, seven thousand!
Kay, P., & Kempton, W. (1984). What is the Sapir‐Whorf hypothesis?. American anthropologist, 86(1), 65-79.
Whorf, B. L. (1952). Language, mind, and reality. ETC: A review of general semantics, 167-188.
Whorf, B. L. (1997). The relation of habitual thought and behavior to language. In Sociolinguistics (pp. 443-463). Palgrave, London.
Whorf, B. L. (2012). Language, thought, and reality: Selected writings of Benjamin Lee Whorf. MIT press.

- Dictionaries home
- American English
- Collocations
- German-English
- Grammar home
- Practical English Usage
- Learn & Practise Grammar (Beta)
- Word Lists home
- My Word Lists
- Recent additions
- Resources home
- Text Checker
Definition of hypothesis noun from the Oxford Advanced American Dictionary
- formulate/advance a theory/hypothesis
- build/construct/create/develop a simple/theoretical/mathematical model
- develop/establish/provide/use a theoretical/conceptual framework/an algorithm
- advance/argue/develop the thesis that…
- explore an idea/a concept/a hypothesis
- make a prediction/an inference
- base a prediction/your calculations on something
- investigate/evaluate/accept/challenge/reject a theory/hypothesis/model
- design an experiment/a questionnaire/a study/a test
- do research/an experiment/an analysis
- make observations/calculations
- take/record measurements
- carry out/conduct/perform an experiment/a test/a longitudinal study/observations/clinical trials
- run an experiment/a simulation/clinical trials
- repeat an experiment/a test/an analysis
- replicate a study/the results/the findings
- observe/study/examine/investigate/assess a pattern/a process/a behavior
- fund/support the research/project/study
- seek/provide/get/secure funding for research
- collect/gather/extract data/information
- yield data/evidence/similar findings/the same results
- analyze/examine the data/soil samples/a specimen
- consider/compare/interpret the results/findings
- fit the data/model
- confirm/support/verify a prediction/a hypothesis/the results/the findings
- prove a conjecture/hypothesis/theorem
- draw/make/reach the same conclusions
- read/review the records/literature
- describe/report an experiment/a study
- present/publish/summarize the results/findings
- present/publish/read/review/cite a paper in a scientific journal
Want to learn more?
Find out which words work together and produce more natural-sounding English with the Oxford Collocations Dictionary app. Try it for free as part of the Oxford Advanced Learner’s Dictionary app.

An official website of the United States government
The .gov means it’s official. Federal government websites often end in .gov or .mil. Before sharing sensitive information, make sure you’re on a federal government site.
The site is secure. The https:// ensures that you are connecting to the official website and that any information you provide is encrypted and transmitted securely.
- Publications
- Account settings
Preview improvements coming to the PMC website in October 2024. Learn More or Try it out now .
- Advanced Search
- Journal List
- HHS Author Manuscripts
- PMC10312134

From Social Contingency to Verbal Reference: A Constructivist Hypothesis
Elena luchkina.
1 Department of Psychology, Northwestern University
2 Department of Psychology, University of California, Berkeley
In the first year of life, infants’ word learning is slow, laborious, and requires repeated exposure to word-referent co-occurrences. In contrast, by 14–18 months, infants learn words from just a few labeling events, use joint attention and eye gaze to decipher word meaning, and begin to use speech to communicate about absent things. We propose that this remarkable advancement in word learning results from attaining a referential understanding of words —that words are linked to mental representations and used intentionally to communicate about real-world entities. We suggest that verbal reference is supported by codeveloping conceptual, social, representational, and statistical learning capacities. We also propose that infants’ recognition of this tri-directional link between words, referents, and mental representations is enabled by their experience participating in and observing socially contingent interactions . Understanding verbal reference signals a qualitative shift in infants’ word learning. This shift enables infants to bootstrap word meanings from syntax and semantics, learn novel words and facts from nonostensive communication, and make inferences about speakers’ epistemic competence based on their language production. In this paper, we review empirical findings across multiple facets of infant cognition and propose a novel developmental theory of verbal reference. Finally, we suggest new directions of empirical research that may provide stronger and more direct evidence for our theory and contribute to our understanding of the development of verbal reference and language-mediated learning in infancy and beyond.
Human language permits us to call to mind objects, events, and ideas that we cannot witness directly ( Deacon, 1997 ). We learn about people we have never met, about time that has not passed (“tomorrow”), and about concepts as abstract as weak subatomic force. We propose that this communicative power of words is enabled by verbal reference —a link between words, real-world phenomena, and their mental representations that enables words to refer to those phenomena even when they are perceptually unavailable. How do infants establish this tridirectional link and begin to engage in language-mediated learning by extracting information encoded in language to establish, retrieve, and modify mental representations of absent or abstract referents?
With the rapid accumulation of empirical evidence on infants’ vocabulary development in the recent decades ( Bloom, 2002 ; Carey, 2010 ; Frank et al., 2009 ; Hidaka & Smith, 2010 ; Markman, 1989 ; Perszyk & Waxman, 2018 ; Swingley, 2010 ; Waxman & Lidz, 2007 ; Yurovsky, 2018 ), when and how young children understand the referential nature of words has become an apple of discord in language acquisition ( Golinkoff et al., 2000 ; Sloutsky, 2009 ; Waxman & Gelman, 2009 ). The proponents of perception-driven early word learning theories tend to explain the emergence of verbal reference through domain-general cognitive mechanisms, such as associative learning and cross-situational statistics (e.g., Howard, et al., 2005 ; Samuelson & Smith, 1999 ; Smith & Yu, 2013 ; see Smith et al., 2014 , for a review). In contrast, some have argued that verbal reference may be inherent in human communication, a conceptual primitive hard-wired in infants’ minds, present in preverbal communication in infancy, and pertaining to words from the outset of verbal communication (e.g., Burge, 2010 ; Csibra, 2003 , 2010 ; Macnamara, 1982 ). Still others have proposed that the interplay between conceptual knowledge, social cognition, and language enables the development of verbal reference in infancy, in the spirit of constructivist theories of cognitive development ( Karmiloff-Smith, 1992 ; Piaget, 1954 ; Tomasello, 2010 ; Waxman, 2003 ; Xu, 2019 ).
To address this divergence of views, we integrate insight from multiple accounts of word learning, draw on findings across several lines of empirical research on cognitive and language development, and propose a novel constructivist theory of the development of verbal reference in the first 2 years of life. We aim to better understand the processes governing this development by considering its precursor capacities (see Appendix ) and the mechanisms facilitating its emergence.
Following Bloom (1993) , we define verbal reference as a property of words (or other signals) that are linked to mental representations and are used intentionally by people to communicate about real-world or abstract entities 1 . This tridirectional link ( Figure 1 ) allows us to establish, retrieve, and modify mental representations based on language alone. An important property of this link is referential specificity , which entails that words do more than just indexing parts of a scene, like objects, events, or locations (“there is something in this location”). Instead, they allow us to communicate about specific aspects, states, or changes of state of those entities (“the car is damaged,” “the car is red,” “there is a Prius in the garage”). This property distinguishes verbal reference from its nonverbal precursors, like pointing or gaze direction. In this article, we will make the following three claims and discuss the supporting empirical evidence to date.

Note . See the online article for the color version of this figure.
We propose that (1) the precursor capacities of verbal reference include the ability to represent absent entities and the understanding that those entities can be communicated about and that words are the means of such communication. At the same time, infants employ powerful statistical learning mechanisms that segment speech into words, track their co-occurrences with objects or events, conduct cross-situational comparisons, and propose and revise word-referent links as more distributional information becomes available. These capacities must operate in concert to get word learning off the ground.
We also propose that (2) infants’ experience with socially contingent communicative exchange enables them to employ these precursor capacities and attain a referential understanding of words. As infants accumulate observations of people interacting in a contingent manner via language and as they accumulate first-hand experiences participating in such interactions (e.g., by vocalizing and being responded to with actions accompanied by language), they infer that language is the means of such communication and recognize the referential status of words. Thus, attaining a referential understanding of words is a gradual process scaffolded by a combination of precursor capacities and experience with socially contingent interactions.
Finally, we propose that (3) attaining a referential understanding of words leads to a qualitative shift in word learning. Understanding the referential nature of words enables infants to engage in language-mediated learning, such as learning new word meanings and novel facts from language alone (directed to them or overheard), integrating multiple cues to meaning from syntax, semantics, and pragmatics, and making inferences about speakers’ epistemic competence from their language use.
Development of the Referential Understanding of Words in Infancy
We begin by summarizing the findings in the literature on the development of verbal reference from the last two decades and tracing its trajectory over the first 2 years of life. Infants’ comprehension of verbal reference has been researched using multiple methods, such as pitting referential cues (e.g., eye gaze) in a labeling situation against visual salience of an object ( Pruden et al., 2006 ) or against infants’ intrinsic preference for an object ( Baldwin, 1993b ). However, when the referent object is present at the time its label is introduced, there is always the possibility that infants’ success in learning the label is driven by perceptual association.
We, therefore, focus here on studies that leveraged infants’ ability to comprehend speech about absent objects 2 . In such studies, infants either first observe a labeling event (usually a count noun that refers to an object), after which the referent is hidden, and an experimenter subsequently enquires about the absent object (“How about the wug? Where is the wug?”), or they first hear utterances containing object labels (“I have a bike”) and are subsequently presented with objects corresponding to those labels and distracter objects, displayed in silence. Infants’ looking direction (suggesting their search for the object in a particular location) and pointing are typically measured to evaluate their comprehension of the target words. If infants can map a label to its referent, even if it is perceptually unavailable, it constitutes strong evidence that they understand that words refer.
Developmental Timeline of Absent Verbal Reference
By 12 months, infants show the first signs of understanding “anchored” absent verbal reference —reference to recently removed objects primed by perceptually available entities associated with it (“anchors”), such as their former locations ( Gallerani et al., 2009 ; Ganea, 2005 ; Osina et al., 2013 , 2014 ). In Osina et al. (2013) , for example, infants are first presented with a toy dog, which is subsequently placed inside an ottoman, so that infants can no longer see the toy. After a delay, during which the experimenter sang “Twinkle, Twinkle, Little Star” and pointed to decals on the ceiling, the experimenter then asked infants about the hidden toy, first in a hint-like manner (“What about the dog? Have you seen the dog?”) and then directly (“Where is the dog? Could you find the dog?”). After being prompted 3–5 times, infants looked, or pointed to, or approached the ottoman, suggesting that they understood reference to the hidden object.
At 14 months, infants interpret verbal requests for hidden objects using social information, such as individuals’ history of interacting with an object (e.g., which of the two balls the experimenter played with; Saylor & Ganea, 2007 ). At this age, infants track properties of recently removed objects, such as their color or former location, whose labels are mentioned in utterances ( Saylor, 2004 ) and produce communicative behaviors toward these anchors (e.g., pointing to the panel that occludes the absent referent, or searching for an absent person; Ganea & Saylor, 2013 ; Saylor & Baldwin, 2004 ). Around 14 months infants also begin to comprehend reference to objects hidden from view without “anchoring,” albeit provided with visual exposure to those objects before they are removed from view ( Hendrickson & Sundara, 2017 ).
Infants begin to retrieve mental representations of objects based on language input alone, without pre-exposure to those objects within the experiment by 16 months. For example, Luchkina et al. (2020) showed that 16- but not 13-month-olds look more to familiar objects previously mentioned in speech unaccompanied by any images when those images become available. Sixteen-month-olds also use language to update their representations of the locations of recently shown objects (e.g., “Now the dog goes on the table”) and look longer if the revealed outcomes mismatch the language input (e.g., a cat is on the table; Ganea et al., 2016 ).
By 18–22 months, infants not only retrieve detailed mental representations of familiar objects based on language alone, but also can form representations 3 of novel word-referent mappings even if those referents are perceptually unavailable ( Baldwin, 1993a ; Ferguson et al., 2014 ). Baldwin (1993a) , for example, showed that 19-month-olds (but not 15-month-olds) successfully learn labels for novel objects that are first demonstrated but subsequently removed from infants’ view and labeled while being sealed in an opaque container (e.g., the experimenter looks into the container and says, “Look, a fep!”). At this age, infants also update mental representations of states of the absent objects that are being referred to in speech (“Lucy is wet now!” ; see Galazka & Ganea, 2014 ; Ganea et al., 2007 ).
Notably, younger infants may have a nascent ability to form a representation of a perceptually unavailable object based on language input. Xu et al. (2005) , for example, showed that 12-month-olds search longer in an opaque box after retrieving one object, if they previously saw an experimenter peek into the box and utter two distinct labels than if the experimenter uttered the same label twice. This finding suggests that these infants appreciate the link between the number of words and the number of referents (a rudimentary form of mutual exclusivity; Markman, 1989 ; see also Pomiechowska et al., 2021 ). However, it is not until several months later that infants show the signs of moving beyond this link and establish a specific representation of a previously unseen object based on language input alone.
Taken together, this evidence suggests that the ability to extract information encoded in language to establish, retrieve, and update mental representations based on language develops between 12 and 18 months. This ability allows infants to comprehend speech about absent things without “anchors” and ultimately engage in language-mediated learning. What are the learning mechanisms that drive this development?
Existing Developmental Accounts of Verbal Reference
From the perception-driven bottom-up view , the explanation lies in the accumulation of linguistic data via domain-general associative learning mechanisms. The second year of life is marked by substantial advances in syntactic learning and a rapidly growing cache of associative information about word-referent co-occurrences (see Smith et al., 2014 , for a review). With this growing body of linguistic data, young learners become better positioned to take advantage of their semantic and syntactic knowledge to make inferences about the meanings of words that are uttered in the absence of any referents (e.g., Sloutsky, 2009 ). These capacities, however, are insufficient to form or modify mental representations on the basis of language alone: Without having previously experienced a referent of a novel word, one cannot form a new perceptual association or use that word to access the representation of its referent.
Alternatively, some have argued that reference is a primitive inherent in our conceptual and linguistic system (e.g., Burge, 2010 ; Csibra, 2003 , 2010 ; Macnamara, 1982 ). Six-month-old infants, for example, exhibit a nascent understanding of the referential nature of gaze by following an actor’s gaze toward a present object if cues signaling communicative intent—eye contact or infant-directed speech—were present ( Hernik & Broesch, 2019 ; Senju & Csibra, 2008 ). By 12 months, infants reliably recognize the referential nature of gaze to a hidden object and are surprised if the location indicated by an actor’s gaze is revealed to be empty ( Csibra & Volein, 2008 ). At 12 months, infants also reliably comprehend and produce pointing gestures to refer to not only present but also absent entities. They begin to point at an object’s empty location to request that object from an adult or to draw the adult’s attention to it ( Behne et al., 2012 ; Bohn et al., 2018 ; Gräfenhain et al., 2009 ; Liszkowski et al., 2009 ).
This comprehension of nonverbal reference may serve as a steppingstone to understanding verbal reference to absent entities. However, nonverbal reference is limited in two significant ways. First, nonverbal reference, such as pointing or gaze direction, lacks referential specificity —a fundamental feature of verbal reference that enables us to communicate about various aspects of objects or events. 4 For example, pointing can direct attention (e.g., there is something there; or even “there is a car” if a car had been seen previously ), but only words can refer to specific aspects and properties of an object and communicative about it with precision (e.g., “there is a car, not a cup”; “the red car” if there are more than one car; “the car is broken”). Referential specificity entails that infants not only realize that words can index nonpresent entities, but also discover that different kinds of words (e.g., nouns, adjectives, verbs) take on different kinds of meaning, even if these words reference the same object. Further, while pointing or gaze can reference objects, it is significantly harder to use these referential tools for events or changes of state. For example, pointing to one’s eye is unlikely to communicate that one is seeing ; pointing to the direction of the wind is unlikely to communicate that it became windy . Second and relatedly, only verbal reference enables one to create, maintain, and update detailed mental representations based on language alone , thereby enabling effective language-mediated learning and communication. Verbal reference, but not nonverbal referential actions, allows one to learn about objects, events, or ideas that one has not experienced directly or that do not have stable perceptual forms and thus cannot be pointed to or looked at (e.g., atoms, viruses, yesterday, tomorrow, or the 23rd century).
New Proposal: A Constructivist Account of Verbal Reference Development
In our view, infants’ vocabulary in the first year of life and early, nonverbal, reference are marked by the lack of (or fragile and unstructured) connection between words and reference. 5 That is, 6–12-month-old infants possess some word knowledge—likely acquired via associative mechanisms—and possess an understanding that reference is possible, but they have not yet made the connection between the two. Indeed, although 9–12-month-olds show some understanding of nonverbal reference, their word knowledge appears to be chiefly driven by perceptual salience and associative mechanisms (e.g., Hollich et al., 2000 ; Pruden et al., 2006 ). For example, Pruden et al. (2006) found that 10-month-olds learn novel words by relying on the perceptual salience of an object instead of social cues provided by a speaker 6 .
By 12–14 months, infants begin to treat concurrently present words and deictic gestures alike, realizing that both can index hidden objects ( Gliga & Csibra, 2009 ; see also Graham & Kilbreath, 2007 ). They also use gesture to disambiguate between possible referents of a novel word ( Pomiechowska & Csibra, 2020 ; c.f. Lucca & Wilbourn, 2018 ). Furthermore, infants begin to establish links between different types of words and different types of meaning during this developmental window, for example, count nouns map onto object categories, adjectives map onto properties or attributes, and verbs map onto actions or events (see Perszyk & Waxman, 2018 , for a review). This signals the beginning of referential specificity. Thus, by the beginning of the second year, infants start to realize that words refer, and this realization qualitatively changes their word learning strategies, enabling referentially specific use of words to communicate about both present and absent entities.
Our key question remains: What mechanisms underlie this realization and enable the development of verbal reference? As Hollich et al. (2000 , p. 5) put it: “The development of reference is a mystery.” We propose that first, the codevelopment of conceptual, social, representational, and statistical learning precursor capacities is necessary to enable the emergence of verbal reference, and reference to perceptually unavailable objects or events in particular, and second, an understanding of socially contingent interactions drives the establishment of the tridirectional link that is the core of verbal reference ( Figure 1 ). We outline the respective contributions of these precursor capacities and the effects of their concurrent development in the next section (for a more concise discussion, see Luchkina & Waxman, in press ) before turning our attention to discussing the key role of social contingency.
Development of Precursor Capacities
Representing absent entities.
The ability to store representations of objects or events and to access those representations through their names entails that infants form representations of real-world phenomena, link them with words, and possess sufficient memory capacity to store such links. In some cases, verbal reference can be built on representations of concrete objects or events (e.g., persons, their manner of moving or speaking). However, to enable reference to previously unencountered instances that belong to the same object or event categories, these representations need to abstract away from concrete instances. Moreover, such abstraction allows for a more efficient use of the learner’s memory capacity: By focusing on category-defining features, infants can use fewer resources to store information about objects or events they encounter and extend relevant properties to those entities by recognizing their category membership. Evidence from the developmental literature suggests that infants begin to form abstract representations from an early age.
Infants begin to form visual categories based on perceptual features by about 3–4 months of age. For example, 3–4-month-olds readily categorize dot patterns and create prototypes by abstracting away from concrete exemplars ( Bomba & Siqueland, 1983 ; Quinn, 1985 ). At this age, infants also categorize and distinguish pictures of different animal kinds (e.g., Eimas & Quinn, 1994 ; Quinn et al., 1993 ). By 9 months, infants form both perceptual and conceptual categories based on visual features (e.g., they can generalize category information from static images to dynamic point-light displays; see Arterberry & Bornstein, 2002 ). In addition to categorizing visual stimuli, 3–4-month-olds create auditory categories, such as the time of voice onset in consonant-vowel syllables ( Eimas et al., 1971 ). By 6 months, they also categorize speech sounds ( Kuhl, 1979 , 1980 , 1983 ) and intonation patterns ( Morse, 1972 ). Between 6 and 12 months, infants fine-tune their speech sound categories and hone in on the relevant categories for their native language ( Werker, 2018 ; Werker & Tees, 1984 ).
This parallel development of visual and auditory categorization enables infants to create and recognize word-object links and use them to retrieve object representations. At 6 months, infants preferentially look to familiar object images upon hearing their names (e.g., looking to an image of a banana when hearing “banana” vs. an image of a sock; Bergelson & Swingley, 2012 ; see also Tincoff & Jusczyk, 1999 ). By 9 months, they begin to treat words as “invitations” to create visual categories: Upon observing several instances of a novel category (e.g., dinosaurs) consistently paired with the same word (e.g., “blicket”), infants establish a corresponding category and subsequently recognize its novel instances 7 (e.g., Balaban & Waxman, 1997 ). At this age, words also guide infants’ flexible category formation: The number of labels influences the number of discrete categories infants create along a continuum of object stimuli (e.g., Althaus & Westermann, 2016 ; Havy & Waxman, 2016 ). Further, research on infants’ object individuation indicates that by 9 months, infants also rely on words to make judgments about internal properties of object kinds ( Dewar & Xu, 2007 , 2009 ) and to keep track of individual objects that have been recently displaced ( Xu, 2002 ). At least by 12 months, infants not only expect words to be linked with categories but also use nonverbal category knowledge to extend the meanings of newly learned words ( Pomiechowska & Gliga, 2019 ; see also Graham et al., 2004 ). This bidirectional link between words and categories positions infants well to begin accessing mental representations of objects or events when hearing their names.
Finally, infants need accessible and sufficiently durable memory to enable effective access to representations and thus support the development of verbal reference. Although the earliest evidence of infants’ accessing their declarative (vs. procedural) memory has been reported in 3.5–4-month-olds (see Mandler, 1988 , for a reivew), this memory is short-lived (3–4 min; see Baillargeon et al., 1989 ; Baillargeon & Graber, 1988 ; Luo et al., 2003 ) and depends on perceptual availability of features or events that cooccurred with the represented object or event. By 6 months, however, infants can retain memory of an event for at least 24 hr, represent the existence and location of recently hidden objects ( Baillargeon, 1986 ), and imitate demonstrated actions upon encountering the object used in the demonstration ( Hayne et al., 2000 ). By 9–10 months, infants can remember such sequences of events over the span of months ( Bauer, 2002 ; Bauer et al., 2000 ; Carver et al., 2000 ; Mandler & McDonough, 1995 ).
Thus, investigations of infants’ categorization and memory development suggest that at least by 9 months, infants are capable of forming sufficiently abstract representations of objects or events, store them over extended periods of time, and access them by hearing words linked to those representations. Albeit crucial for the development of verbal reference, however, these capacities do not guarantee reference. A link between a representation and a corresponding word does not automatically confer the understanding that the word encodes that representation for communication purposes. How and when do infants gain such an understanding?
Using Speech to Communicate About Absent Entities
Infants’ ability to understand that speech can communicate about perceptually unavailable entities emerges around 6 months and is reliably present by the first birthday ( Cheung et al., 2012 ; Martin et al., 2012 ; Vouloumanos, 2018 ; Vouloumanos et al., 2012 , 2014 ). Vouloumanos et al. (2012) , for example, showed that 6-month-olds expect speech to communicate unobservable intentions. Infants first observed an actor (A1) who looked at two objects and indicated her preference for one of the objects by grasping it. Subsequently, A1 no longer had access to the objects and turned to another actor (A2), who had access to the objects but had not seen A1’s preference demonstration, uttering a nonsensical word. Infants looked significantly longer when A2 handed A1 the object she did not prefer, suggesting that they understood that A1’s utterance must have transferred information about her preference to A2. By 12 months, infants can link their inferences about the intended outcome of an attempted but failed action (by A1; e.g., attempting to reach for an object) with the communicative function of speech. They expect A2 to achieve A1’s intended outcome upon hearing speech. Crucially, such an understanding requires that infants (1) appreciate others’ beliefs and intentions and (2) recognize that language can be used to communicate about such beliefs and intentions.
Consistent with Vouloumanos et al. (2012) results, research on infants’ understanding of intentions suggests that by 6 months, they attribute goal-directedness to others’ actions (e.g., Woodward, 1998 ), appreciate the causal role of intentions in such actions ( Southgate & Vernetti, 2014 ), and recognize the role of access to information in interpreting those actions (e.g., Luo & Johnson, 2009 ). At 7 months, infants keep track of others’ beliefs and show surprisal, as they would expect others to show, when an outcome of an occlusion event is inconsistent with others’ beliefs ( Kovacs et al., 2010 ). By 10–15 months, they recognize others’ false beliefs and how those beliefs guide actions ( Luo, 2011 ; Onishi & Baillargeon, 2005 ; see Heyes, 2014 , for a review).
Recall that around their first birthdays, in addition to recognizing that others have beliefs and intentions, infants begin to recognize that these beliefs or intentions can be about absent objects, which can be referred to, at least in an indexical way. Combined with the advances in infants’ ability to form abstract representations and access them via associated words, the understanding that speech can communicate about perceptually unavailable entities prepares them to make the leap toward verbal reference. Indeed, the first signs of understanding reference to absent objects appear around 12–14 months (see section I). By 14 months, infants reliably use verbal cues to retrieve memories of absent objects ( Ganea, 2005 ; Moore & Meltzoff, 2004 ; Osina et al., 2013 , 2014 ) and past events ( Bauer et al., 2000 ).
Proposing and Revising Links Between Words and Their Meanings
Finally, effective command of verbal reference entails that infants not only recognize that reference to absent or abstract entities via words is possible in principle, but are also able to propose, or hypothesize, and revise the meanings of words that refer to those entities. To succeed on this task, infants need to resolve at least two computational problems. First, they need to extract structure from the language input: Because most of the time, infants hear continuous streams of speech, they need to determine where word boundaries are and what governs word-word co-occurrences. Second, infants need to resolve instances of referential ambiguity when learning novel word meanings. Does a word refer to an object, its parts, its properties, or its super-ordinate category? In order to learn correct word meanings, infants need to consider different mapping hypotheses and determine the most likely word-referent mappings.
The first problem is addressed by infants’ capacity to discover structure in language input and segment it based on statistical regularities. This capacity enables 8-month-olds ( Aslin et al., 1998 ; Jusczyk & Hohne, 1997 ; Saffran et al., 1996 ; see Saffran, 2020 , for a review on word segmentation) and even 6-month-olds ( Shukla et al., 2011 ), to extract words from a continuous stream of speech and visual input ( Kirkham et al., 2002 ). At this age, infants can generalize their acoustic representations of words to novel speakers and can also recognize familiar words, even when they were mispronounced ( Bergelson & Swingley, 2018 ; interestingly, 11–14-month-olds perform poorly with mispronounced words, suggesting a shift in infants’ understanding of linguistically relevant sources of variation). At least by 7 months, infants are able to recruit their statistical learning capacity to distill abstract rules from auditory ( Ferguson & Lew-Williams, 2016 ) and visual input (e.g., Ferguson, Franconeri, et al., 2018 ; Rabagliati et al., 2012 , 2019 ). By 9–10 months, infants integrate distributional cues both within and between words and with a combination of prosodic and phonotactic cues to determine the location of word boundaries ( Mattys et al., 1999 ; see Jusczyk, 1999 , for a review). By 12 months, infants use distributional cues not only to determine word boundaries but also to infer the rules of grammar ( Gomez & Gerken, 1999 ; Morgan & Demuth, 1996 ). In this way, infants can identify unfamiliar words in a stream of speech and register their pattern of occurrence within utterances.
The second problem may be addressed by cross-situational comparison. By 14 months, infants become competent statistical learners and use a combination for co-occurrence statistics and cross-situational comparison to strip away noise in labeling data and learn correct word-referent associations ( Smith et al., 2014 ; Smith & Yu, 2008 ). In considering their hypotheses about the meanings of novel words, infants may rely on a variety of strategies ( Yurovsky & Frank, 2015 ). Some accounts claim that infants simultaneously acquire information about multiple candidate referents for the same word (e.g., McMurray et al., 2012 ; Yu & Smith, 2007 ; Yurovsky et al., 2014 ). Others have argued that word learners maintain a single hypothesis about the referent of any given word (e.g., Medina et al., 2011 ; Trueswell et al., 2013 ; these accounts however mainly draw on evidence from adults and preschoolers).
In addition, infants need to recognize that when different words regularly co-occur with the same object, they may pick out different aspects of that object. For example, when someone mentions a neighbor’s car, they can be talking about its category membership (“car”), its color (“red”), or its speed (“fast”). By leveraging their ability to register commonalities and differences among the different situations and utterances in which they encounter a given word, infants may infer which aspect of an object or an event is the intended referent of that word. Indeed, both infants and adults rely, at least in part, on cross-situational statistics to establish mappings between syntactic categories (e.g., nouns, verbs) and kinds of meaning (e.g., objects, actions) ( Monaghan & Mattock, 2009 ; Rebuschat et al., 2021 ). This capacity for cross-situational comparison, combined with the capacity to extract syntactic rules from word co-occurrence patterns, enables 14-month-olds to map nouns to kinds and adjectives to kind properties (e.g., Booth & Waxman, 2009 ). Infants’ appropriate extensions of novel adjectives to object properties, and not kinds, illustrate that they recognize that a given entity can be described by different words depending on communicative intention and context.
Taken together, the reviewed evidence illustrates that by the time the first signs of verbal reference are observed, infants (1) possess representational capacities to maintain memories of absent entities and retrieve them upon hearing their names, (2) appreciate others’ mental states and recognize the communicative function of speech, and (3) are equipped with powerful statistical learning mechanisms—distributional analysis and cross-situational comparison—that enable them to detect word boundaries, propose a set of possible word-referent mappings, and revise their hypotheses about those mappings as they accumulate more linguistic data. A question that remains open is what brings these precursor capacities together to enable verbal reference.
The Role of Social Contingency in Recognizing That Words Refer
As our review suggests, most precursor capacities of verbal reference are in place between 6 and 12 months of age. Yet infants’ early word learning is slow and laborious ( Carey, 1978 ; Fenson et al., 1994 ; Hollich et al., 2000 ; Nelson, 1973 ; Woodward, 2000 ) and there has been little evidence of referential understanding of words before age 1 (e.g., Pruden et al., 2006 ). In contrast, at 12–18 months, infants demonstrate substantial advances in their rate of word learning ( Goldfield & Reznick, 1990 ; Nazzi & Bertoncini, 2003 ; Werker et al., 1998 ; but see Ganger & Brent, 2004 , for an alternative view), begin integrating multiple cues to meaning ( Beier & Carey, 2014 ; Pruden et al., 2006 ), and comprehend reference to absent entities. What changes in infants’ word learning in this developmental window and what drives this qualitative shift (see Bergelson & Swingley, 2013 , for a discussion)? We propose that infants gain insight into the words’ referential nature and realize the tridirectional link between words, real-world referents, and mental representations ( Figure 1 ) through their experience observing the power of words to trigger behaviors and other changes in their surroundings.
This key phenomenon has been described as socially contingent responsivity —a property of human interactions, in which an action or an utterance of one interlocutor is contingent 8 on actions or utterances of the other ( Csibra, 2010 ). We will refer to this construct as “social contingency,” for brevity. As infants experience and accumulate observations of language-mediated socially contingent interactions, they learn that others’speech and their own vocalizations and behaviors can elicit targeted responses from other people. Social contingency plays a critical role in establishing the tridirectional link: (a) By observing specific words (or vocalizations, if their own) used in socially contingent interactions resulting in fulfilling specific needs (e.g., getting food or a toy), infants realize that words are connected to real-world entities in a principled, lawful way, (b) By observing others produce specific intentional behaviors in response to specific words uttered in socially contingent interactions, infants realize that words are also linked to mental states and mental representations in a principled, lawful way, and (c) Finally, by observing others’ intentional behaviors that are not ostensibly motivated by anything in their environment other than speech (e.g., wanting a cup of tea and asking for it) resulting in changes in that environment (e.g., an interlocutor brings a tea), infants may infer that mental representations are linked to representations of real-world entities , and words may be used to elicit specific mental representations in others that lead to corresponding changes in the world. Thus, social contingency may serve as the “glue” that enables an understanding of verbal reference that includes all three key components: Words, mental representations, and the real world. Moreover, infants’sensitivity to social contingency undergoes significant development throughout the first year (see below). This development, along with the development of the precursor capacities of verbal reference, may explain why the first signs of infants’ comprehension of verbal reference to absent entities are observed by 12–14 months of age. Notably, this developmental window is marked by a nonlinear 9 qualitative shift in infants’ word comprehension ( Bergelson, 2020 ). Infant’s word comprehension becomes substantially more robust, as measured by the proportion of looking to the target object in looking-while-listening procedures ( Fernald et al., 2008 ). Bergelson shows that this shift is not explained by changes in infants’ word input and instead must be driven by changes in infants’ ability to take advantage of their word input. Our proposal is consistent with this argument and offers a mechanism that enables infants to take better advantage of word input.
If our hypotheses are correct and the shift toward appreciating the referential nature of words is indeed driven by social contingency, then several assertions must be supported by empirical evidence in developmental research. First, infants must attend to social contingency and show a preference to observe and participate in socially contingent interactions. Second, we should be able to show that increased exposure to socially contingent interactions predicts the quality of infants’ language development, and word learning in particular. And third, infants’ processing and perception of language should be enhanced by social contingency.
Infants’ Sensitivity to Social Contingency
Investigations of parent–child interactions suggest that infants are indeed sensitive to social contingency and that parents’ responsiveness to infants’ vocalizations shapes infants’ expectations about the power of those vocalizations to trigger others’ behaviors. Among the first socially contingent interactions experienced by an infant is breast feeding, which is sometimes described as a proto-conversation between an infant and a mother (see Csibra, 2010 ). As an infant ceases to suck, a mother encourages sucking by jiggling him or her. The infant then resumes sucking after jiggling stops ( Kaye, 1977 ). Soon after, at 3–5 months, when infants begin to engage in contingent face-to-face interactions with others, they show a preference for more contingent partners, associate the level of contingency with their personal identities, and retain this association over time ( Bigelow, 1996 ; Rochat et al., 1998 ). By 5 months, infants appear to infer that their own actions evoke responses from others. For example, using a still-face procedure, 10 Goldstein et al. (2009) showed that 5-month-olds significantly increased noncry vocalizations in response to the experimenter’s ceased contingent responsiveness. These vocalizations may signal attempts to initiate contingent interactions with the experimenter and indicate that infants are not only capable of engaging in contingent interactions but also actively seek them.
Further, by setting expectations about the level of social contingency and selectively responding more to some behaviors than others, caregivers help infants clarify the link between their own behaviors and expected responses. For example, in Bigelow and Rochat (2006) , infants were less responsive to strangers whose level of contingency was either significantly higher or lower than that of their mothers. Evidence from still-face experiments also shows a strong predictive effect of caregivers’ responsiveness on infants’ own level of contingency. Mcquaid et al. (2009) found that maternal contingent smiling predicted infants’ individual differences in smiling during the still-face phase of the procedure, which indicated that infants had expectations about social interactions and their own ability to initiate an interaction. Bornstein et al. (2015) investigated the development of infant–parent contingent interactions in naturalistic settings and found a strong tendency among mothers of 5.5-month-olds to speak to their infants following nondistress (not related to hunger or discomfort) vocalizations. Moreover, as parents shift communicative actions directed at their infants from mood regulation to behavior influence over time (e.g., from 7 months to 15 months; see Kochanska & Aksan, 2004 ), infants’ positive responsiveness to those actions increases. In particular, infants’social overtures and influence attempts increased and distress reactions decreased in response to parents’ communicative bids.
In sum, studies of infant–adult interactions strongly suggest that social contingency plays a significant role in infants’ communicative development, guiding both their social expectations and communicative behaviors directed at others. What about the effects of social contingency on spoken language development? Do infants’sensitivity to social contingency and their strong association between social contingency, agency, and communicative signals influence their language acquisition, and word learning in particular?
Looking to establish a link between social contingency and language outcomes, Gros-Louis et al. (2014) observed 8–14-month-olds’ interactions with their mothers in naturalistic settings over the course of 6 months. Mothers’ responses and imitation of infants’ vocalization correlated with the increase in infants’ mother-directed vocalizations in the following month and predicted more mature consonant–vowel vocalizations. Further, infants of more responsive mothers had larger productive vocabularies at 15 months. These results suggest that social contingency may indeed facilitate language development, and that this facilitative effect permeates multiple facets of language acquisition.
A number of correlational and longitudinal studies also found a predictive effect of parents’ contingent responsiveness on verbal and social competence later in development. For example, Bornstein and Tamis-LeMonda (1989) observed mother–infant dyads when infants were 2–5-months and assessed their cognitive development at 13 months and 4 years. Maternal responsiveness predicted language comprehension and pretend play at 13 months and higher scores on Wechsler Preschool and Primary Scale of Intelligence at age 4. Similarly, Olson et al. (1984) reported a significant relation between maternal responsiveness 11 when infants are 6 and 13 months and their receptive vocabulary at 24 months (measured by Bayley Mental Developmental Index [Bayley MDI] and Peabody Picture Vocabulary Test [PPVT]). Moreover, when maternal responsiveness was measured specifically in terms of mothers’ verbal activity in response to infants’ vocal or exploratory behaviors 12 , the analyses revealed that such responsiveness at 9 months predicted infants’ language comprehension at 13 months ( Baumwell et al., 1997 ). Similarly, Rollins (2003) observed parent–infant dyads engaged in naturalistic interactions and found that words uttered within socially contingent interactions at 9 months, where the mother talked about an object of joint focus of attention or narrated an ongoing activity, predicted language comprehension at 12 months.
These studies lend support to our hypothesis that increased exposure to and participation in socially contingent interactions benefits infants’ word learning and broader language development at later ages. Notably, the reviewed longitudinal studies report language learning benefits of exposure to social contingency at 12–14 months—the developmental window associated with the emerging ability to comprehend verbal reference to absent objects and marked by a nonlinear increase in infants’ word comprehension (see Bergelson, 2020 ). Thus, the observed effects may indeed reflect the hypothesized facilitative influence of social contingency on infants’ understanding of the referential nature of words.
It is possible, however, that infants who experience more socially contingent exchanges simply hear more language from their parents and benefit from the increased exposure to more types and tokens of words compared to infants who experience fewer such exchanges. To our knowledge, there has been no direct empirical investigation of the relation between social contingency and the development of verbal reference. However, converging evidence from several lines of work suggests that the effect of social contingency on the development of verbal reference cannot be explained by the mere increase in exposure to word input.
First, findings from the investigations linking children’s exposure to vocabulary and their language learning outcomes provide evidence that rich vocabulary input alone is not sufficient to facilitate qualitative shifts in one’s understanding of the referential nature of words. Although some findings suggest that children who hear a lot of child-directed speech (CDS) and children whose CDS input is relatively scarce are exposed to comparable total word input, after ambient speech has been taken into consideration (e.g., Sperry et al., 2019 ), only child-directed speech has been consistently linked to vocabulary growth (e.g., Hoff, 2003 ; Shneidman et al., 2013 ; Shneidman & Goldin-Meadow, 2012 ). Importantly, child-directed speech is more likely to be socially contingent as caregivers respond to infants’ and children’s vocalizations and word productions (e.g., Gratier et al., 2015 ; Tomasello & Farrar, 1986 ; Veneziano & Parisse, 2010 ), suggesting that the effect of social contingency on language development goes above and beyond mere exposure to words. Indeed, Donnelly and Kidd (2021) examined infants’ natural language environment (using LENA recordings) between 9 and 24 months, measuring their language input and participation in socially contingent verbal exchange, operationalized as conversational turns. They found that conversational turns predicted change in infants’ vocabulary even after word input variables (i.e., types and tokens) were controlled for.
Second, Tamis-LeMonda et al. (1996) showed that maternal verbal responsiveness to infants’ verbal bids (vocalizations, word production), but not their verbal responsiveness to play behavior, predicted 13- and 20-month-olds’ language comprehension and production (see also Tamis-LeMonda et al., 2001 ). This finding suggests that the rate of mothers’ verbal responses specifically to verbal behaviors and not mothers’ overall verbal responsiveness predicted infants’success with language. Further, a study with older slow-to-talk children revealed that maternal expansions, imitations, interpretations, and responsive questions positively correlated with 24-month-olds’ language comprehension and production (evaluated on Preschool Language Scale, Zimmerman et al., 2002 ), while labeling responses negatively correlated with these measures ( Levickis et al., 2014 ). This finding provides additional support to the idea that increased exposure to word input alone is unlikely to explain the effects of social contingency on the development of verbal reference in infancy.
Third, young children appear to ascribe communicative properties to signals that are embedded in socially contingent interactions, even if those signals are not typically associated with human behaviors. Six-month-old infants privilege speech sounds over sine-waves ( Vouloumanos & Werker, 2004 ) and preferentially treat speech but non sine-waves as invitations to form categories ( Fulkerson & Waxman, 2007 ), which suggests that at this age they have a strong bias to treat speech as a communicative signal. Ferguson and Waxman (2016) , however, showed that despite not normally treating sine-wave tones as language, after a period of exposure to those tones used in a rich communicative exchange between two actors, 6-month-olds extended word-like properties to them. Infants formed object categories based on a series of tone-image presentations—an effect normally observed for words but not sine-wave tones at this age (see Fulkerson & Waxman, 2007 ). Similarly, hearing 26-month-olds, who have accumulated substantial experience using speech, and not sign language, to communicate, accepted signs as object labels after observing sign language embedded in a socially contingent exchange between two interlocutors ( Namy & Waxman, 1998 ). These results provide a powerful demonstration of the effects of social contingency on the perceived referential status of new stimuli, which can be achieved even within a short experimental exposure to those stimuli. In contrast, infants and children with autism spectrum disorders, who have difficulties detecting and responding to social contingency, tend to have difficulties attributing communicative properties even to natural languages (see Northrup, 2017 , for a review).
Finally, corroborating evidence comes from investigations comparing infants’ and young children’s speech processing and word learning in contingent and noncontingent settings. Kuhl et al. (2003) , for example, evaluated 9-month-olds’sensitivity to a foreign language phonetic contrast after 5 hr of exposure, split into 12 laboratory sessions. One group of English-acquiring infants interacted with a native speaker of Mandarin Chinese. The speaker played with the infants and read books to them. The other two groups were exposed to a video- or an audio-recording of the same speaker. In the video, the speaker looked to the camera to emulate eye contact. Only infants in the live interaction condition performed above chance on the phonetic discrimination test after 12 weeks, at the level comparable to that of Mandarin-acquiring infants. Consistent with our hypothesis, these results suggest that phonetic learning is facilitated by social contingency, which may be due to infants’ enhanced processing of linguistic information: Socially contingent interactions may have boosted infants’ understanding of Mandarin Chinese as a form of communicative signal. Further, although the same word-referent co-occurrence cues are present in both live and video-recorded speech, infants often fail to learn new word-referent links from watching video-recordings—a phenomenon known as the “video deficit” ( Anderson & Pempek, 2005 ). Infants and young children exhibit such a deficit even when watching television programs that emphasize repetition and emulate infant-directed speech in labeling events ( DeLoache et al., 2010 ; O’Doherty et al., 2011 ; Richert et al., 2011 ; Robb et al., 2009 ). In contrast, when offered an opportunity to learn novel words via socially contingent video chat, 24–30-month-old children succeed and show word-learning outcomes comparable to that in a live in-person interaction ( Roseberry et al., 2014 ). A recent finding also demonstrates that 12-month-olds infants’ word learning from a digital screen is enhanced by the presence of a contingent virtual agent who uses referential cues ( Tsuji et al., 2020 ). Critically, a mere co-presence of a socially contingent interaction does not enhance infants’ word learning outcomes. DeLoache et al. (2010) found that 12–18-month-olds did not learn words presented on video by an experimenter , if parents contingently interacted with their infants during the video exposure. They only learned the new words presented by the parents during socially contingent interactions, suggesting that social contingency enhances infants’ word learning if those words are taught within a socially contingent interaction, rather than merely co-occurring with it.
Together, cross-sectional and longitudinal investigations suggest that increased exposure to social contingency early in infancy predicts larger vocabularies in the long term and enhances phonetic and word learning in the short term. These studies provide highly suggestive evidence for our hypothesis and illustrate that accumulated exposure to social contingency and infants’ growing sensitivity to socially contingent displays indeed facilitate infants’ realization that words are used for communication and they refer to mental representations that correspond to the real world.
Importantly, infants’ attention to social contingency increases with age and becomes dissociated from morphological properties of interlocutors, which may be the reason why we begin to observe its effects on the development of verbal reference by 12–14 months and not earlier. For example (as reviewed in Thiele et al., 2021 ), at 6 months, infants shift visual attention to a reciprocal social interaction of two people facing each other ( Augusti, 2010 ). By 12 months, infants look longer at socially contingent over nonsocially contingent events ( Bakker et al., 2011 ) and engage in gaze following (or orientation) with entities that act in a socially contingent way, even if they do not have faces ( Johnson et al., 1998 ). Shimizu and Johnson (2004) also found that 12-month-olds will attribute goal-directedness to morphologically unfamiliar but socially contingent agents who engage in reciprocal communication. Finally, at 13 months, infants’ preference for socially contingent displays manifests even in associative learning scenarios: Infants are better at learning a predictive relation between a visual cue and a socially contingent display than a visual cue and a non-contingent display ( Thiele et al., 2021 ).
These findings suggest that at this age infants may not only prefer socially contingent displays but also recognize socially contingent entities as agents and privilege the value of information coming from those agents (see Rochat, 2007 , for a review). Thus, between 6 and 13 months infants’ developing sensitivity to socially contingent interactions begins to influence their interpretation of the information presented in the context of such interactions (see Rochat, 2001 , for a review). Infants’ growing social competence factors into this development by informing their inferences about social and nonsocial type of contingency and selectively privileging information presented in the context of socially contingent interactions.
In sum, extant research suggests that participating in and observing socially contingent interactions, and especially language-mediated interactions, affects infants’ communicative development by providing insight into the referential status of words . Infants are more likely to learn novel words and phonetic contrasts in socially contingent contexts. They are also willing to extend word-like communicative properties to non-linguistic signals used in socially contingent interactions. Infants who participate in and observe socially contingent interactions more often tend to have larger vocabularies. Although socially contingent interactions are present early, infants’ gradually increasing attention to such interactions, combined with the development of other precursor capacities, likely explains why the first signs of understanding that words refer (measured as absent reference comprehension) emerge at around 12–14 months of age.
Developmental Advances of Attaining a Referential Understanding of Words
Attaining an understanding of the referential nature of words allows infants to establish strong bidirectional links between words and mental representations, as well as the links between mental representations and their real-world counterparts, and to realize how words can affect the world via mental representations. This implies an appreciation that words are used intentionally and are meant to cause change in others’ actions or mental states. It also entails the capacity to propose hypotheses for word meanings and revise these hypotheses as more linguistic (e.g., syntactic contexts) and word-referent co-occurrence data are accumulated. In concert, these capacities constitute verbal reference and enable infants to integrate multiple cues to word meanings (by 12–14 months; see Luchkina & Waxman, in press ) and acquire vocabulary with high efficiency (see Hollich et al., 2000 , for a review).
Gaining the appreciation of the tridirectional link between words, mental representations, and real-world referents enables infants to recognize that words likely encode and transmit relevant information and are worth their attention, without needing a socially contingent context any longer. Knowing that words are linked to the world in a principled, lawful way, they realize that even speech not addressed to them has that property. This realization enables them to engage in increasingly decontextualized forms of language-mediated learning and expand their social knowledge and factual knowledge, rapidly acquire new vocabulary and syntax. We elaborate on several key advantages of attaining a referential understanding of words below.
One such advantage is the ability to learn new information in nonostensive contexts, such as overhearing a conversation. Floor and Akhtar (2006) compared 18-month-olds’ ability to learn novel object labels in two contexts—while being directly addressed and while observing an interaction as a third party. In the Addressed condition, an experimenter interacted with the infant, made eye contact, and ostensibly labeled a novel object (additional three novel objects were ostensibly demonstrated but not labeled). In the overhearing condition, the experimenter interacted with an adult confederate while the infant was present and had an opportunity to observe their interaction. The same objects were demonstrated and one labeled, as in the Addressed condition, in which the interaction was between the experimenter and the infant. The results revealed that 18-month-olds learned the novel object-labels equivalently well in both conditions and their test performance was not driven by an intrinsic preference for a specific object. These findings illustrate that 18-month-olds have the ability to learn word meanings in nonostensive scenarios, without being directly addressed.
At this age, infants also begin to make sophisticated inferences about word meanings in nonostensive contexts based on social cues. For example, Tomasello et al. (1996) found that 18-month-olds learn novel words equivalently well in a situation in which an experimenter introduces a novel word and retrieves its referent from a container immediately after (“Now let’s find the gazzler”; ostensive context), and in a situation, in which she first retrieved an incorrect object, frowned at it, and replaced it with the correct one (non-ostensive context). Despite having seen a distractor object demonstrated right after the novel word was introduced, infants used social cues to infer the intended meaning of the word. By 24–30 months, children are able to learn novel word meanings in non-ostensive contexts from a third-party interaction, even when they are distracted by an engaging activity ( Akhtar, 2005 ). Finally, at least by age 3, children can learn object names and facts about those objects from overhearing a seemingly irrelevant cell-phone conversation, or “halfalogue” ( Emberson et al., 2010 ; Foushee et al., 2021 ). Critically, this form of learning cannot be explained from a strictly associationist perspective. Not only is the linguistic information presented in a nonostensive manner, but the naming events often happen in the absence of word referents, which necessarily entails that children map words onto mental representations and recognize that these word-representation links extend to real-world objects or events. These advances in learning from overhearing substantially expand the reach of children’s learning beyond the here-and-now and allow them to benefit from the information exchanged in their social environments but not directed at them. Such information can include diverse vocabularies (e.g., used in adult-directed speech), social routines (e.g., greeting, expressions of sympathy), and facts about the world (“The neighbor’s dog is dangerous”).
Another significant advantage of attaining a referential understanding of words is the ability to create and update mental representations of objects based on language alone. For example, by 18–19 months, infants can establish a representation of non-visible novel object, learn its name, and subsequently use this name to identify the correct referent when it becomes perceptually available, based on referential cues and mutual exclusivity inferences ( Baldwin, 1993b ; Tomasello et al., 1996 ). By 22 months, children update mental representations of previously seen objects based on language alone. In Ganea et al. (2007) , for example, 22-month-olds were familiarized with a toy frog “Lucy” that was subsequently hidden, and infants heard a story in which Lucy became wet. When presented with a wet toy frog and an identical-looking dry toy frog and asked to identify “Lucy,” 22-month-olds selected the wet toy, demonstrating having successfully extracted novel information and updated existing representations based on language alone. By 30 months, children can learn names of novel objects and facts about them from verbal descriptions and subsequently identify the correct objects without having previously seen them ( Saylor et al., 2016 ). Similar to learning words from overhearing, learning facts from language alone necessarily requires that a child’s understanding of word-referent link surpasses the level of perceptual associations. Without being able to link language input with representations of word referents, a learner should have no basis for identifying a referent of a word or an utterance they had heard in the absence of any objects or events that can be associated with it. Thus, these studies illustrate the far-reaching impact of verbal reference on children’s capacity to extract novel information from language, create and modify mental representations on its basis, and use this knowledge to identify their real-world counterparts. This capacity is central to formal and much of informal instruction (as both convey information via language) and has profound implications for knowledge acquisition and school readiness.
In addition to gaining access to information encoded in language and learning in non-ostensive contexts, the understanding that words refer may boost children’s ability to take advantage of linguistic cues to infer the meaning of novel words from language alone. Before realizing that words are linked to mental representations, infants may notice a pattern in which an unfamiliar word co-occurs with known words within utterances. However, without anything to map that word onto, they may not be able to leverage this information. The referential understanding of words may benefit vocabulary acquisition by allowing infants to create a representation of a novel referent based on semantic and syntactic bootstrapping. For example, at 19 but not at 15 months, infants can establish a mental representation of an object, paired with a novel label, without seeing that object and just relying on semantic properties of the carrier phrase ( Ferguson, Graf, et al., 2018 ). Specifically, 19-month-olds inferred the animacy of an object corresponding to a novel name, presented in the absence of any conceivable referents, based on the predicate that modifies it, “the dax is crying” versus “the dax is right here.” When shown a pair of images and asked to identify the “dax,” infants who encountered “dax” in an utterance that implied its animacy, were significantly more likely to select the animate referent than infants who heard a neutral utterance. By age 2, children can use syntactic information, such as verb transitivity, to create a representation of a novel action ( Arunachalam et al., 2013 ; Yuan & Fisher, 2009 ). Arunachalam and Waxman (2010) demonstrated that children who observed two speakers converse and mention a novel verb in a transitive sentence (“The lady mooped my brother”) subsequently were significantly more likely to choose a causative video scene than toddlers who heard that verb in an intransitive sentence (“The lady and my brother mooped”). At this age, children also generalize animacy-selecting restrictions of novel verbs, learned in the absence of any visual information, to other nouns within the animate and the inanimate categories (e.g., first hearing “I stiped the pig” and subsequently selecting an animate object when asked to identify an object that can be “stiped”; Yuan et al., 2011 ). Finally, by 27 months, children can make these inferences even when they hear the novel words in socially -impoverished contexts: Mentioned in adult-directed speech, with no discourse, and with linguistic information unaccompanied with any visual information ( Arunachalam, 2013 ). Combined, these studies suggest that from the onset of the referential understanding of words, infants leverage syntactic and semantic bootstrapping to infer the meanings of novel words in increasingly decontextualized situations. Being able to infer the properties of a novel referent from the word’s surrounding linguistic context helps infants narrow the range of possible candidate meanings of the novel word and increase the likelihood of identifying the correct referent when it becomes perceptually available.
Yet another advantage of attaining a referential understanding of words is that it changes infants’sensitivity to distributional information, such as word-referent co-occurrence statistics. By realizing that words refer, infants gain additional appreciation of the relevance of this information: Knowing that words refer to mental representations and their real-world counterparts and encode information intentionally communicated by others, infants may pay more attention to word input and referential cues in their learning. Combined with targeted consideration of the most likely word-referent hypotheses, this enables infants to transition from slow and laborious word learning that results from repeated exposure to co-occurrence statistics, to learning novel word meanings from witnessing only a few word-referent co-occurrences. For example, Woodward et al. (1994) show that by 18 months, infants successfully learn novel word meanings after just nine mentions of the target word and retain them after 24 hr. Notably, 18-month-olds in this study responded correctly on test trials 76% of the time, while 13-month-olds’ performance averaged around 62%. In a recent report, LaTourrette and Waxman (2019) show that by age 2, children learn novel word meanings after observing only two members of a category labeled as well as when all six members of that category are labeled. By age 4, children learn novel word meanings from a single labeled example as well as from three examples and successfully generalize those meanings to other members of the same subordinate, basic-level, or superordinate category ( Xu & Tenenbaum, 2007b ). One may argue that this developmental progression is driven by children’s increasing memory capacity. However, investigations of infants’ memory development indicate that by 9–10 months, infants successfully remember sequences of events over the span of months ( Bauer, 2002 ; Carver et al., 2000 ; Mandler & McDonough, 1995 ). Thus, it is unlikely that this rapidly increasing ability to learn novel word meanings from only a few examples can be entirely explained by the growing memory capacity. Instead, we suggest that these effects may be a consequence of children’s encoding of labeling information being enhanced by attaining a referential understanding of words.
Other developmental benefits of attaining a referential understanding of words include advances in social reasoning, such as the ability to make inferences about others’ knowledge based on their language production. Recall that we define verbal reference as a tridirectional link between words, real-world referents, and their mental representations used for intentional communication between people. This implies that a naming event reflects both their intention to communicate information about a referent, their knowledge of the word-referent mapping, and their ability to recognize a given object or event as an instance of a known referent category paired with a word. Thus, upon attaining a referential understanding of words and aided by recognizing the conventional nature of words (by at least 14 months; Buresh & Woodward, 2007 ; Colomer & Sebastian-Galles, 2020 ; Henderson & Woodward, 2012 ; Scott & Henderson, 2013 ), infants begin to make inferences about such states based on the quality of information they communicate via language. For example, by 18 months, infants track whether others use correct words to describe familiar objects and only learn novel word meanings from speakers who do not make mistakes in naming (e.g., Brooker & Poulin-Dubois, 2013 ; Luchkina et al., 2018 ). Luchkina et al. (2018) showed infants a video of two actors, one of which demonstrated familiar to the infant objects and labeled them accurately and the other demonstrated a different set of familiar objects and labeled them inaccurately (e.g., “Look, a shoe,” while showing a book). The infants subsequently saw a video, in which either the accurate or the inaccurate speaker (between-subject) introduced two novel objects and labeled them. Infants’ word knowledge was probed in an intermodal preferential looking procedure. While infants in both groups performed well on control trials and consistently looked to the correct familiar objects, only infants who learned the novel labels from the accurate speaker looked significantly more to the target novel object. These findings suggest that 18-month-olds track others’ accuracy in object naming, use this information to make inferences about others’ knowledge states and selectively learn new words from speakers they deem epistemically competent. This developing capacity allows infants to leverage their word knowledge to monitor the quality of the language input they receive and selectively learn and retain information from reliable sources. In preschoolers, studies also found that learners can take into account the epistemic state of the speaker and decide accordingly how best to integrate the language input in learning new words ( Harris & Corriveau, 2011 ; Koenig & Harris, 2005 ; Sobel & Kushnir, 2013 ; Xu & Tenenbaum, 2007a ; see Harris, 2012 , for a review).
The empirical evidence reviewed above illustrates the profound and far-reaching effects of understanding the referential nature of words. We propose that this understanding fundamentally changes the way infants attend to, process, and retain linguistic information and powers multiple learning capacities that are essential for cognitive development. These proposed effects invite new empirical investigations, some of which we outline in the final section.
Conclusions and Future Directions
In this paper, our goal was to explain the development of verbal reference through aggregating insights from multiple facets of research on language and cognitive development. We reviewed empirical evidence on infants’ emerging understanding of the referential nature of words; argued that conceptual, social, representational, and statistical learning capacities must operate in concert to enable such an understanding; and hypothesized that infants arrive at the realization that words refer via engaging in and observing socially contingent interactions. We also argued that by serving as “cement” that brings together multiple cues to meaning, verbal reference facilitates a qualitative shift in infants’ word learning, enabling them to acquire novel words with remarkable efficiency. Finally, we proposed that this shift ultimately enables infants to engage in language-mediated learning and acquire knowledge about the world and about their communicative partners via language.
In sum, we reviewed multiple literatures on early cognitive development and proposed a constructivist account of the origins and mechanisms of the development of verbal reference, having discussed cross-sectional and longitudinal evidence corroborating our arguments. Our account represents the constructivist approach to language and cognitive development because it offers a set of primitives (or building blocks) that in combination drives conceptual advances in infants’ understanding of word-referent relations, which in turn, lead to shifts and new constraints in infants’ interpretation of linguistic and social input. These properties are characteristic of constructivist views of human development and contrast sharply with pure nativist (which emphasizes innate cognitive architectures) and pure empiricist (which emphasizes the role of associative mechanisms operating on primitives) approaches ( Cohen et al., 2002 ; Xu, 2019 ; Xu & Kushnir, 2013 ; see also Hirsh-Pasek et al., 2004 ; Hollich et al., 2000 ).
In addition to the existing evidence, more direct empirical tests will be necessary to provide strong evidence for our proposed account. We, therefore, invite such investigations and suggest several directions of research that will contribute to our understanding of the development of verbal reference and its role in transforming lexical acquisition and enabling language-mediated learning.
One direction of empirical investigations is to test whether social contingency indeed facilitates the referential understanding of words. For example, longitudinal studies can test whether infants of more socially contingent parents begin to show signs of comprehending speech about absent entities earlier and whether changes in infants’ own socially contingent vocalizations predict the onset of this comprehension. Cross-sectional laboratory studies can investigate caregiver–infant interactions and examine whether and how caregivers’ contingent responsiveness helps infants recognize that words, referents, and others’ behaviors are linked in a principled, lawful way. Similarly, observations of such interactions can be instrumental in determining whether infants who observe and participate in socially contingent interactions more often are more likely to recognize the communicative nature of speech and link it to mental states. Further, laboratory and longitudinal investigations measuring the properties of parents’ language production will distinguish between the effects of social contingency and the effects of the quality and quantity of verbal input. The combination of cross-sectional and longitudinal studies will provide a more complete picture the mechanisms driving the development of verbal reference. It will also help address the outstanding questions about infants’ ability to learn novel words without seeing their referents and their ability to retain those mappings.
Another direction of empirical research is to test whether having attained a referential understanding of words indeed predicts qualitative shifts in infants’ word learning and whether it marks the onset of language-mediated learning. For example, are infants who display the comprehension of speech about absent entities more likely to learn novel words from just a few labeling events than their peers who do not yet display such a comprehension? Similarly, are infants who demonstrate an ability to learn novel word meanings in the absence of their referents earlier also more likely to learn novel facts from language alone at earlier ages, and be able to update their mental representations of objects and events via language alone? To conduct these investigations, it is critical to establish an experimental procedure that can effectively measure whether infants have attained a referential understanding of words. One such procedure, which leverages infants’ ability to learn the meanings of words in the absence of their referents using infants semantic networks, has been proposed by Luchkina and Waxman (2021) . A longitudinal follow-up that investigates whether differences in this ability at 15 months predict differences in language-mediated learning at 22 months, is underway.
These and other empirical investigations will help us gain a better understanding of the mechanisms underlying word learning and the development of verbal reference in the first 2 years of life. Combined, these insights will advance our understanding of the development of the fundamental human capacity to establish, retrieve, and modify knowledge based on information encoded in and transmitted via language.
Developmental Timelines of Verbal Reference and its Precursor Capacities
We thank James Morgan, David Sobel, Sandra Waxman, Athena Vouloumanos, Bertram Malle, Dima Amso, Mahesh Srinivasan, Kali Woodruff Carr, Alexander LaTourrette, and Miriam Novack for helpful discussion about this research.
1 Abbott (2010) points out a distinction between pragmatic reference and semantic reference. In the case of pragmatic reference, words cannot refer on their own, they are tools used for speakers to communicate about something (see Clark & Bangerter, 2004 ; Clark & Wilkes-Gibbs, 1986 ; see also Michaelson & Reimer, 2019 , for. a discussion of the “intentionalist” model). Semantic reference, which, as Abbot remarks, has arisen within formal logic, relates expressions to things out in the world (e.g., Wittgenstein, 2017 ). In this latter sense, any expression reliably linked to a phenomenon or a class of phenomena can be considered referential, so long as it signifies those phenomena. Our definition emphasizes the pragmatic aspect of reference, while embracing the semantic aspect as well, that is, words are linked both to representations and to things in the world.
2 For brevity, we refer to both objects that have been recently seen but hidden and objects that exist but have not been displayed before infants’ word comprehension is probed as “absent.” Importantly, some objects can be absent and not retrievable (e.g., ones that ceased to exist or are imaginary) or do not have stable physical manifestations (“democracy”). The latter kind of permanently absent word referents is beyond the scope of our discussion (but see Bergelson & Swingley, 2013 , for a discussion on infants’ acquisition of abstract words).
3 It is unclear, however, how lasting these representations are outside of the context of the experiment (see Horst & Samuelson, 2008 ). To elucidate this matter, an investigation involving a delay (or a longitudinal investigation) is necessary.
4 Great apes, similar to human 12-month-olds, request absent entities (e.g., treats) by pointing to their former locations (e.g., Bohn et al., 2015 ). However, they do not seem to move beyond indexical reference and develop referentially specific links between words (or other symbols) and their present and absent referents. Even great apes trained to use sign language to request objects do not respond to messages about states or locations of those objects and appear to be using signs by pairing them with perceptual categories of the corresponding objects ( Savage-Rumbaugh et al., 1993 ), suggesting that referential specificity does not characterize apes’ verbal communication.
5 An electrophysiological investigation found that even 9-month-olds exhibit mismatch negativity if they hear a word (e.g., a “duck”) in the absence of an object and then see an object with a different name (e.g., a cookie; Parise & Csibra, 2012 ), suggesting a possibility that hearing words activates visual representations of objects. This finding may reflect 9-montholds’ referential understanding of words, or alternatively demonstrate a stable link between the word “duck” and a mental picture of a duck. However, because this evidence is limited, future research is needed to further test these possibilities.
6 Bergelson and Swingley (2012) showed that 6–9-month-olds can recognize a handful of concrete object referents of highly familiar nouns (e.g., “banana”; see also Jusczyk & Aslin, 1995 ; Mandel et al., 1995 ). Yet, infants of the same age fail to recognize the referents of common verbs (e.g., kiss, drink; Bergelson & Swingley, 2015 ). This is likely because verbs take on arguments (events often also contain multiple objects; see Gentner, 2006 ), which means that perceptual salience is a poor cue to meaning in the case of verbs. In contrast, by 12–14 months, when infants’ word knowledge is increasingly driven by conceptual representations ( Pomiechowska & Gliga, 2019 ; Waxman & Leddon, 2011 ; Yin & Csibra, 2015 ), their verb comprehension also becomes more robust.
7 Some evidence suggests that this effect might be present as early as 3–4 months of age (e.g., Ferry et al., 2010 ). This evidence, however, should be taken with caution, as this facilitative effects appears to be sensitive to the acoustic properties of the stimuli ( Lau et al., in press ) and not necessarily represent word-referent links. For example 3- and 4-month-olds show a categorization effect driven by lemur vocalizations and English utterances, but not Cantonese utterances or birdsongs (see Woodruff Carr et al., 2021 ).
8 While contingency does not guarantee causality, it is likely that contingent behaviors are perceived as causal. From 3 months, infants appreciate causal relations between events ( White, 1988 ) and may understand causal effects of human actions ( Leslie, 1982 ).
9 Some have proposed that this shift, described geometrically as an inflection point on the graph of infants’ receptive vocabulary as a function of their age, is best modelled by a logistic function (e.g., Ganger & Brent, 2004 ).
10 An infant first interacts with an adult who responds contingently to infant’s actions and vocalizations. The adult then turns away and turns back, showing a “still face,” or a lack of responsiveness to the infant. The adult later resumes a contingent interaction with the infant (see Cohn & Tronick, 1983 ).
11 Maternal responsiveness was measured as (a) contact and proximity behaviors (look at infant for at least 2 s, approach infant, pick up infant, put down infant, affectionate touch, bounce, jiggle, or rock, put to shoulder, leave infant’s view), (b) facial expression (smile, grin at infant, frown/grimace at infant), (c) verbal or vocal stimulation(distal vocalization to infant, proximal vocalization, “sweet, musical quality”; proximal vocalization, normal quality; negative vocalization), (d) play stimulation (give toy or object, give toy or object and work it for infant, return dropped toy, tickle or poke infant), and (e) physical needs caregiving.
12 Responsiveness was defined as a positive and meaningful change in mother’s verbal behavior subsequent to and dependent on a child exhibiting a vocal or exploratory act. If a child looked at the brush and mother said “brush,” mother was credited with responsiveness. In order for a maternal verbal activity to be credited as responsive, mother had to change her ongoing behavior within 5 s of a change in a child visual or vocal activity.
- Abbott BK (2010). Reference (Oxford surveys in semantics & pragmatics; 2) . Oxford University Press. [ Google Scholar ]
- Akhtar N (2005). The robustness of learning through overhearing . Developmental Science , 8 ( 2 ), 199–209. 10.1111/j.1467-7687.2005.00406.x [ PubMed ] [ CrossRef ] [ Google Scholar ]
- Althaus N, & Westermann G (2016). Labels constructively shape object categories in 10-month-old infants . Journal of Experimental Child Psychology , 151 , 5–17. 10.1016/j.jecp.2015.11.013 [ PubMed ] [ CrossRef ] [ Google Scholar ]
- Anderson DR, & Pempek TA (2005). Television and very young children . American Behavioral Scientist , 48 ( 5 ), 505–522. 10.1177/0002764204271506 [ CrossRef ] [ Google Scholar ]
- Arterberry ME, & Bornstein MH (2002). Infant perceptual and conceptual categorization: The roles of static and dynamic stimulus attributes . Cognition , 86 ( 1 ), 1–24. 10.1016/S0010-0277(02)00108-7 [ PubMed ] [ CrossRef ] [ Google Scholar ]
- Arunachalam S (2013). Two-year-olds can begin to acquire verb meanings in socially impoverished contexts . Cognition , 129 ( 3 ), 569–573. 10.1016/j.cognition.2013.08.021 [ PubMed ] [ CrossRef ] [ Google Scholar ]
- Arunachalam S, Escovar E, Hansen MA, & Waxman SR (2013). Out of sight, but not out of mind: 21-month-olds use syntactic information to learn verbs even in the absence of a corresponding event . Language and Cognitive Processes , 28 ( 4 ), 417–425. 10.1080/01690965.2011.641744 [ PMC free article ] [ PubMed ] [ CrossRef ] [ Google Scholar ]
- Arunachalam S, & Waxman SR (2010). Meaning from syntax: Evidence from 2-year-olds . Cognition , 114 ( 3 ), 442–446. 10.1016/j.cognition.2009.10.015 [ PMC free article ] [ PubMed ] [ CrossRef ] [ Google Scholar ]
- Aslin RN, Saffran JR, & Newport EL (1998). Computation of conditional probability statistics by 8-month-old infants . Psychological Science , 9 ( 4 ), 321–324. 10.1111/1467-9280.00063 [ CrossRef ] [ Google Scholar ]
- Augusti E-M (2010). Look who’s talking: Pre-verbal infants’ perception of face-to-face and back-to-back social interactions . Frontiers in Psychology , 1 , Article 161. 10.3389/fpsyg.2010.00161 [ PMC free article ] [ PubMed ] [ CrossRef ] [ Google Scholar ]
- Baillargeon R (1986). Representing the existence and the location of hidden objects: Object permanence in 6-and 8-month-old infants . Cognition , 23 ( 1 ), 21–41. 10.1016/0010-0277(86)90052-1 [ PubMed ] [ CrossRef ] [ Google Scholar ]
- Baillargeon R, Devos J, & Graber M (1989). Location memory in 8-month-old infants in a non-search AB task: Further evidence . Cognitive Development , 4 ( 4 ), 345–367. 10.1016/S0885-2014(89)90040-3 [ CrossRef ] [ Google Scholar ]
- Baillargeon R, & Graber M (1988). Evidence of location memory in 8-month-old infants in a nonsearch AB task . Developmental Psychology , 24 ( 4 ), 502–511. 10.1037/0012-1649.24.4.502 [ CrossRef ] [ Google Scholar ]
- Bakker M, Kochukhova O, & von Hofsten C (2011). Development of social perception: A conversation study of 6-, 12- and 36-month-old children . Infant Behavior and Development , 34 ( 2 ), 363–370. 10.1016/j.infbeh.2011.03.001 [ PubMed ] [ CrossRef ] [ Google Scholar ]
- Balaban MT, & Waxman SR (1997). Do words facilitate object categorization in 9-month-old infants? Journal of Experimental Child Psychology , 64 ( 1 ), 3–26. 10.1006/jecp.1996.2332 [ PubMed ] [ CrossRef ] [ Google Scholar ]
- Baldwin DA (1993a). Early referential understanding: Infants’ ability to recognize referential acts for what they are . Developmental Psychology , 29 ( 5 ), 832–843. 10.1037/0012-1649.29.5.832 [ CrossRef ] [ Google Scholar ]
- Baldwin DA (1993b). Infants’ ability to consult the speaker for clues to word reference . Journal of Child Language , 20 ( 2 ), 395–418. 10.1017/S0305000900008345 [ PubMed ] [ CrossRef ] [ Google Scholar ]
- Bauer PJ (2002). Long-term recall memory: Behavioral and neuro–developmental changes in the first 2 years of life . Current Directions in Psychological Science , 11 ( 4 ), 137–141. 10.1111/1467-8721.00186 [ CrossRef ] [ Google Scholar ]
- Bauer PJ, Wenner JA, Dropik PL, & Werwerka SS (2000). Parameters of remembering and forgetting in the transition from infancy to early childhood . Monographs of the Society for Research in Child Development , 65 ( 4 ), i–vi+1–213. [ PubMed ] [ Google Scholar ]
- Baumwell L, Tamis-LeMonda CS, & Bornstein MH (1997). Maternal verbal sensitivity and child language comprehension . Infant Behavior and Development , 20 ( 2 ), 247–258. 10.1016/S0163-6383(97)90026-6 [ CrossRef ] [ Google Scholar ]
- Behne T, Liszkowski U, Carpenter M, & Tomasello M (2012). Twelve-month-olds’ comprehension and production of pointing: Twelve-month-olds comprehend pointing . British Journal of Developmental Psychology , 30 ( 3 ), 359–375. 10.1111/j.2044-835X.2011.02043.x [ PubMed ] [ CrossRef ] [ Google Scholar ]
- Beier JS, & Carey S (2014). Contingency is not enough: Social context guides third-party attributions of intentional agency . Developmental Psychology , 50 ( 3 ), 889–902. 10.1037/a0034171 [ PubMed ] [ CrossRef ] [ Google Scholar ]
- Bergelson E (2020). The comprehension boost in early word learning: Older infants are better learners . Child Development Perspectives , 14 ( 3 ), 142–149. 10.1111/cdep.12373 [ PMC free article ] [ PubMed ] [ CrossRef ] [ Google Scholar ]
- Bergelson E, & Swingley D (2012). At 6–9 months, human infants know the meanings of many common nouns . Proceedings of the National Academy of Sciences , 109 ( 9 ), 3253–3258. 10.1073/pnas.1113380109 [ PMC free article ] [ PubMed ] [ CrossRef ] [ Google Scholar ]
- Bergelson E, & Swingley D (2013). The acquisition of abstract words by young infants . Cognition , 127 ( 3 ), 391–397. 10.1016/j.cognition.2013.02.011 [ PMC free article ] [ PubMed ] [ CrossRef ] [ Google Scholar ]
- Bergelson E, & Swingley D (2015). Early word comprehension in infants: Replication and extension . Language Learning and Development , 11 ( 4 ), 369–380. 10.1080/15475441.2014.979387 [ PMC free article ] [ PubMed ] [ CrossRef ] [ Google Scholar ]
- Bergelson E, & Swingley D (2018). Young infants’ word comprehension given an unfamiliar talker or altered pronunciations . Child Development , 89 ( 5 ), 1567–1576. 10.1111/cdev.12888 [ PMC free article ] [ PubMed ] [ CrossRef ] [ Google Scholar ]
- Bigelow AE (1996). Infants’ memory for contingently responding persons . Infant Behavior and Development , 19 , 334. 10.1016/S0163-6383(96)90388-4 [ CrossRef ] [ Google Scholar ]
- Bigelow AE, & Rochat P (2006). Two-month-old infants’sensitivity to social contingency in mother-infant and stranger-infant interaction . Infancy , 9 ( 3 ), 313–325. 10.1207/s15327078in0903_3 [ PubMed ] [ CrossRef ] [ Google Scholar ]
- Bloom L (1993). The transition from infancy to language: Acquiring the power of expression (1st ed.). Cambridge University Press. 10.1017/CBO9780511752797 [ CrossRef ] [ Google Scholar ]
- Bloom P (2002). How children learn the meanings of words . MIT Press. [ Google Scholar ]
- Bohn M, Call J, & Tomasello M (2015). Communication about absent entities in great apes and human infants . Cognition , 145 , 63–72. 10.1016/j.cognition.2015.08.009 [ PubMed ] [ CrossRef ] [ Google Scholar ]
- Bohn M, Zimmermann L, Call J, & Tomasello M (2018). The social-cognitive basis of infants’ reference to absent entities . Cognition , 177 , 41–48. 10.1016/j.cognition.2018.03.024 [ PubMed ] [ CrossRef ] [ Google Scholar ]
- Bomba PC, & Siqueland ER (1983). The nature and structure of infant form categories . Journal of Experimental Child Psychology , 35 ( 2 ), 294–328. 10.1016/0022-0965(83)90085-1 [ CrossRef ] [ Google Scholar ]
- Booth AE, & Waxman SR (2009). A horse of a different color: Specifying with precision infants’ mappings of novel nouns and adjectives . Child Development , 80 ( 1 ), 15–22. 10.1111/j.1467-8624.2008.01242.x [ PMC free article ] [ PubMed ] [ CrossRef ] [ Google Scholar ]
- Bornstein MH, Putnick DL, Cote LR, Haynes OM, & Suwalsky JTD (2015). Mother-infant contingent vocalizations in 11 countries . Psychological Science , 26 ( 8 ), 1272–1284. 10.1177/0956797615586796 [ PMC free article ] [ PubMed ] [ CrossRef ] [ Google Scholar ]
- Bornstein MH, & Tamis-LeMonda CS (1989). Maternal responsiveness and cognitive development in children . New Directions for Child and Adolescent Development , 1989 ( 43 ), 49–61. 10.1002/cd.23219894306 [ PubMed ] [ CrossRef ] [ Google Scholar ]
- Brooker I, & Poulin-Dubois D (2013). Is a bird an apple? The Effect of speaker labeling accuracy on infants’ word learning, imitation, and helping behaviors . Infancy , 18 , E46–E68. 10.1111/infa.12027 [ PMC free article ] [ PubMed ] [ CrossRef ] [ Google Scholar ]
- Buresh JS, & Woodward AL (2007). Infants track action goals within and across agents . Cognition , 104 ( 2 ), 287–314. 10.1016/j.cognition.2006.07.001 [ PubMed ] [ CrossRef ] [ Google Scholar ]
- Burge T (2010). Origins of objectivity . Oxford University Press. [ Google Scholar ]
- Carey S (1978). The child as word learner. Linguistic theory and psychological reality. In Halle M, Bresnan J, & Miller GA (Eds.), Linguistic theory and psychological reality (pp. 264–293). MIT Press. [ Google Scholar ]
- Carey S (2010). Beyond fast mapping . Language Learning and Development , 6 ( 3 ), 184–205. 10.1080/15475441.2010.484379 [ PMC free article ] [ PubMed ] [ CrossRef ] [ Google Scholar ]
- Carver LJ, Bauer PJ, & Nelson CA (2000). Associations between infant brain activity and recall memory . Developmental Science , 3 ( 2 ), 234–246. 10.1111/1467-7687.00116 [ CrossRef ] [ Google Scholar ]
- Cheung H, Xiao W, & Lai CM (2012). Twelve-month-olds’ understanding of intention transfer through communication . PLoS ONE , 7 ( 9 ), Article e46168. 10.1371/journal.pone.0046168 [ PMC free article ] [ PubMed ] [ CrossRef ] [ Google Scholar ]
- Clark HH, & Bangerter A (2004). Changing ideas about reference. In Noveck IA & Sperber D (Eds.), Experimental pragmatics (pp. 25–49). Palgrave Macmillan. 10.1057/9780230524125_2 [ CrossRef ] [ Google Scholar ]
- Clark HH, & Wilkes-Gibbs D (1986). Referring as a collaborative process . Cognition , 22 ( 1 ), 1–39. 10.1016/0010-0277(86)90010-7 [ PubMed ] [ CrossRef ] [ Google Scholar ]
- Cohen LB, Chaput HH, & Cashon CH (2002). A constructivist model of infant cognition . Cognitive Development , 17 ( 3–4 ), 1323–1343. 10.1016/S0885-2014(02)00124-7 [ CrossRef ] [ Google Scholar ]
- Cohn JF, & Tronick EZ (1983). Three-month-old infants’ reaction to simulated maternal depression . Child Development , 54 ( 1 ), 185–193. 10.2307/1129876 [ PubMed ] [ CrossRef ] [ Google Scholar ]
- Colomer M, & Sebastian-Galles N (2020). Language background shapes third-party communication expectations in 14-month-old infants . Cognition , 202 , Article 104292. 10.1016/j.cognition.2020.104292 [ PubMed ] [ CrossRef ] [ Google Scholar ]
- Csibra G (2003). Teleological and referential understanding of action in infancy . Philosophical Transactions of the Royal Society of London. Series B: Biological Sciences , 358 ( 1431 ), 447–458. 10.1098/rstb.2002.1235 [ PMC free article ] [ PubMed ] [ CrossRef ] [ Google Scholar ]
- Csibra G (2010). Recognizing communicative intentions in infancy . Mind & Language , 25 ( 2 ), 141–168. 10.1111/j.1468-0017.2009.01384.x [ CrossRef ] [ Google Scholar ]
- Csibra Gergely., & Volein Ágnes. (2008). Infants can infer the presence of hidden objects from referential gaze information . British Journal of Developmental Psychology , 26 ( 1 ), 1–11. 10.1348/026151007X185987 [ CrossRef ] [ Google Scholar ]
- Deacon T (1997). The symbolic species . W. W. Norton & Company. [ Google Scholar ]
- DeLoache JS, Chiong C, Sherman K, Islam N, Vanderborght M, Troseth GL, Strouse GA, & O’Doherty K (2010). Do babies learn from baby media? Psychological Science , 21 ( 11 ), 1570–1574. 10.1177/0956797610384145 [ PubMed ] [ CrossRef ] [ Google Scholar ]
- Dewar K, & Xu F (2007). Do 9-month-old infants expect distinct words to refer to kinds? Developmental Psychology , 43 ( 5 ), 1227–1238. 10.1037/0012-1649.43.5.1227 [ PubMed ] [ CrossRef ] [ Google Scholar ]
- Dewar K, & Xu F (2009). Do early nouns refer to kinds or distinct shapes?: Evidence from 10-month-old infants . Psychological Science , 20 ( 2 ), 252–257. 10.1111/j.1467-9280.2009.02278.x [ PubMed ] [ CrossRef ] [ Google Scholar ]
- Donnelly S, & Kidd E (2021). The longitudinal relationship between conversational turn-taking and vocabulary growth in early language development . Child Development , 92 ( 2 ), 609–625. 10.1111/cdev.13511 [ PubMed ] [ CrossRef ] [ Google Scholar ]
- Eimas PD, & Quinn PC (1994). Studies on the formation of perceptually based basic-level categories in young infants . Child Development , 65 ( 3 ), 903–917. 10.2307/1131427 [ PubMed ] [ CrossRef ] [ Google Scholar ]
- Eimas PD, Siqueland ER, Jusczyk P, & Vigorito J (1971). Speech perception in infants . Science , 171 ( 3968 ), 303–306. 10.1126/science.171.3968.303 [ PubMed ] [ CrossRef ] [ Google Scholar ]
- Emberson LL, Lupyan G, Goldstein MH, & Spivey MJ (2010). Overheard cell-phone conversations: When less speech is more distracting . Psychological Science , 21 ( 10 ), 1383–1388. 10.1177/0956797610382126 [ PubMed ] [ CrossRef ] [ Google Scholar ]
- Fenson L, Dale PS, Reznick JS, Bates E, Thal DJ, Pethick SJ, Tomasello M, Mervis CB, & Stiles J (1994). Variability in early communicative development . Monographs of the Society for Research in Child Development , 59 ( 5 ), i+iii–v+1–185. 10.2307/1166093 [ PubMed ] [ CrossRef ] [ Google Scholar ]
- Ferguson B, Franconeri SL, & Waxman SR (2018). Very young infants learn abstract rules in the visual modality . PLOS ONE , 13 ( 1 ), Article e0190185. 10.1371/journal.pone.0190185 [ PMC free article ] [ PubMed ] [ CrossRef ] [ Google Scholar ]
- Ferguson B, Graf E, & Waxman SR (2014). Infants use known verbs to learn novel nouns: Evidence from 15- and 19-month-olds . Cognition , 131 ( 1 ), 139–146. 10.1016/j.cognition.2013.12.014 [ PubMed ] [ CrossRef ] [ Google Scholar ]
- Ferguson B, Graf E, & Waxman SR (2018). When veps cry: Two-year-olds efficiently learn novel words from linguistic contexts alone . Language Learning and Development , 14 ( 1 ), 1–12. 10.1080/15475441.2017.1311260 [ PMC free article ] [ PubMed ] [ CrossRef ] [ Google Scholar ]
- Ferguson B, & Lew-Williams C (2016). Communicative signals support abstract rule learning by 7-month-old infants . Scientific Reports , 6 ( 1 ), Article 25434. 10.1038/srep25434 [ PMC free article ] [ PubMed ] [ CrossRef ] [ Google Scholar ]
- Ferguson B, & Waxman SR (2016). What the [beep]? Six-month-olds link novel communicative signals to meaning . Cognition , 146 , 185–189. 10.1016/j.cognition.2015.09.020 [ PMC free article ] [ PubMed ] [ CrossRef ] [ Google Scholar ]
- Fernald AE, Zangl R, Portillo AL, & Marchman VA (2008). Looking while listening: Using eye movements to monitor spoken language comprehension by infants and young children. In Sekerina IA, Fernández EM, & Clahsen H (Eds.), Language acquisition and language disorders (Vol. 44 , pp. 97–135). John Benjamins Publishing Company. 10.1075/lald.44.06fer [ CrossRef ] [ Google Scholar ]
- Ferry AL, Hespos SJ, & Waxman SR (2010). Categorization in 3- and 4-month-old infants: An advantage of words over tones . Child Development , 81 ( 2 ), 472–479. 10.1111/j.1467-8624.2009.01408.x [ PMC free article ] [ PubMed ] [ CrossRef ] [ Google Scholar ]
- Floor P, & Akhtar N (2006). Can 18-month-old infants learn words by listening in on conversations? Infancy , 9 ( 3 ), 327–339. 10.1207/s15327078in0903_4 [ PubMed ] [ CrossRef ] [ Google Scholar ]
- Foushee R, Srinivasan M, & Xu F (2021). Self-directed learning by preschoolers in a naturalistic overhearing context . Cognition , 206 , Article 104415. 10.1016/j.cognition.2020.104415 [ PubMed ] [ CrossRef ] [ Google Scholar ]
- Frank MC, Goodman ND, & Tenenbaum JB (2009). Using speakers’ referential intentions to model early cross-situational word learning . Psychological Science , 20 ( 5 ), 578–585. 10.1111/j.1467-9280.2009.02335.x [ PubMed ] [ CrossRef ] [ Google Scholar ]
- Fulkerson AL, & Waxman SR (2007). Words (but not tones) facilitate object categorization: Evidence from 6- and 12-month-olds . Cognition , 105 ( 1 ), 218–228. 10.1016/j.cognition.2006.09.005 [ PMC free article ] [ PubMed ] [ CrossRef ] [ Google Scholar ]
- Galazka MA, & Ganea PA (2014). The role of representational strength in verbal updating: Evidence from 19- and 24-month-olds . Journal of Experimental Child Psychology , 121 , 156–168. 10.1016/j.jecp.2013.12.002 [ PubMed ] [ CrossRef ] [ Google Scholar ]
- Gallerani CM, Saylor MM, & Adwar S (2009). Mother–infant conversation about absent things . Language Learning and Development , 5 ( 4 ), 282–293. 10.1080/15475440902897604 [ CrossRef ] [ Google Scholar ]
- Ganea PA (2005). Contextual factors affect absent reference comprehension in 14-month-olds . Child Development , 76 ( 5 ), 989–998. 10.1111/j.1467-8624.2005.00892.x [ PubMed ] [ CrossRef ] [ Google Scholar ]
- Ganea PA, Fitch A, Harris PL, & Kaldy Z (2016). Sixteen-month-olds can use language to update their expectations about the visual world . Journal of Experimental Child Psychology , 151 , 65–76. 10.1016/j.jecp.2015.12.005 [ PubMed ] [ CrossRef ] [ Google Scholar ]
- Ganea PA, & Saylor MM (2013). Talking about the near and dear: Infants’ comprehension of displaced speech . Developmental Psychology , 49 ( 7 ), 1299–1307. 10.1037/a0030086 [ PubMed ] [ CrossRef ] [ Google Scholar ]
- Ganea PA, Shutts K, Spelke ES, & DeLoache JS (2007). Thinking of things unseen: Infants’ use of language to update mental representations . Psychological Science , 18 ( 8 ), 734–739. [ PubMed ] [ Google Scholar ]
- Ganger J, & Brent MR (2004). Reexamining the vocabulary spurt . Developmental Psychology , 40 ( 4 ), 621–632. 10.1037/0012-1649.40.4.621 [ PubMed ] [ CrossRef ] [ Google Scholar ]
- Gentner D (2006). Why verbs are hard to learn. In Golinkoff RM & Hirsh-Pasek K (Eds.), Action meets word: How children learn verbs (pp. 544–564). Oxford University Press. http://site.ebrary.com/id/10160546 [ Google Scholar ]
- Gliga T, & Csibra G (2009). One-year-old infants appreciate the referential nature of deictic gestures and words . Psychological Science , 20 ( 3 ), 347–353. 10.1111/j.1467-9280.2009.02295.x [ PubMed ] [ CrossRef ] [ Google Scholar ]
- Goldfield BA, & Reznick JS (1990). Early lexical acquisition: Rate, content, and the vocabulary spurt . Journal of Child Language , 17 ( 1 ), 171–183. 10.1017/S0305000900013167 [ PubMed ] [ CrossRef ] [ Google Scholar ]
- Goldstein MH, Schwade JA, & Bornstein MH (2009). The value of vocalizing: Five-month-old infants associate their own noncry vocalizations with responses from caregivers . Child Development , 80 ( 3 ), 636–644. 10.1111/j.1467-8624.2009.01287.x [ PMC free article ] [ PubMed ] [ CrossRef ] [ Google Scholar ]
- Golinkoff RM, Hirsch-Pasek K, Bloom L, Smith LB, Woodward AL, Akhtar N, Tomasello M, & Hollich GJ (2000). Becoming a word learner: A debate on lexical acquisition . Oxford University Press. [ Google Scholar ]
- Gomez RL, & Gerken L (1999). Artificial grammar learning by 1-year-olds leads to specific and abstract knowledge . Cognition , 70 ( 2 ), 109–135. 10.1016/S0010-0277(99)00003-7 [ PubMed ] [ CrossRef ] [ Google Scholar ]
- Gräfenhain M, Behne T, Carpenter M, & Tomasello M (2009). Young children’s understanding of joint commitments . Developmental Psychology , 45 ( 5 ), 1430–1443. 10.1037/a0016122 [ PubMed ] [ CrossRef ] [ Google Scholar ]
- Graham SA, & Kilbreath CS (2007). It’s a sign of the kind: Gestures and words guide infants’ inductive inferences . Developmental Psychology , 43 ( 5 ), 1111–1123. 10.1037/0012-1649.43.5.1111 [ PubMed ] [ CrossRef ] [ Google Scholar ]
- Graham SA, Kilbreath CS, & Welder AN (2004). Thirteen-month-olds rely on shared labels and shape similarity for inductive inferences . Child Development , 75 ( 2 ), 409–427. 10.1111/j.1467-8624.2004.00683.x [ PubMed ] [ CrossRef ] [ Google Scholar ]
- Gratier M, Devouche E, Guellai B, Infanti R, Yilmaz E, & Parlato-Oliveira E (2015). Early development of turn-taking in vocal interaction between mothers and infants . Frontiers in Psychology , 6 , Article 1167. 10.3389/fpsyg.2015.01167 [ PMC free article ] [ PubMed ] [ CrossRef ] [ Google Scholar ]
- Gros-Louis J, West MJ, & King AP (2014). Maternal responsiveness and the development of directed vocalizing in social interactions . Infancy , 19 ( 4 ), 385–408. 10.1111/infa.12054 [ CrossRef ] [ Google Scholar ]
- Harris PL (2012). Trusting what you’re told: How children learn from others . Harvard University Press. [ Google Scholar ]
- Harris PL, & Corriveau KH (2011). Young children’s selective trust in informants . Philosophical Transactions of the Royal Society B: Biological Sciences , 366 ( 1567 ), 1179–1187. 10.1098/rstb.2010.0321 [ PMC free article ] [ PubMed ] [ CrossRef ] [ Google Scholar ]
- Havy M, & Waxman SR (2016). Naming influences 9-month-olds’ identification of discrete categories along a perceptual continuum . Cognition , 156 , 41–51. 10.1016/j.cognition.2016.07.011 [ PMC free article ] [ PubMed ] [ CrossRef ] [ Google Scholar ]
- Hayne H, Boniface J, & Barr R (2000). The development of declarative memory in human infants: Age-related changes in deffered imitation . Behavioral Neuroscience , 114 ( 1 ), 77–83. 10.1037/0735-7044.114.1.77 [ PubMed ] [ CrossRef ] [ Google Scholar ]
- Henderson AME, & Woodward AL (2012). Nine-month-old infants generalize object labels, but not object preferences across individuals: Nine-month-olds generalize object labels . Developmental Science , 15 ( 5 ), 641–652. 10.1111/j.1467-7687.2012.01157.x [ PMC free article ] [ PubMed ] [ CrossRef ] [ Google Scholar ]
- Hendrickson K, & Sundara M (2017). Fourteen-month-olds’ decontextualized understanding of words for absent objects . Journal of Child Language , 44 ( 1 ), 239–254. 10.1017/S0305000915000756 [ PubMed ] [ CrossRef ] [ Google Scholar ]
- Hernik M, & Broesch T (2019). Infant gaze following depends on communicative signals: An eye-tracking study of 5- to 7-month-olds in Vanuatu . Developmental Science , 22 ( 4 ), Article e12779. 10.1111/desc.12779 [ PMC free article ] [ PubMed ] [ CrossRef ] [ Google Scholar ]
- Heyes C (2014). False belief in infancy: A fresh look . Developmental Science , 17 ( 5 ), 647–659. 10.1111/desc.12148 [ PubMed ] [ CrossRef ] [ Google Scholar ]
- Hidaka S, & Smith LB (2010). A single word in a population of words . Language Learning and Development , 6 ( 3 ), 206–222. 10.1080/15475441.2010.484380 [ PMC free article ] [ PubMed ] [ CrossRef ] [ Google Scholar ]
- Hirsh-Pasek K, Golinkoff RM, Hennon EA, & Maguire MJ (2004). Hybrid theories at the frontier of developmental psychology: The emergentist coalition model of word learning as a case in point. In Geoffrey Hall D & Waxman SR (Eds.), Weaving a lexicon (pp. 173–204). MIT Press. [ Google Scholar ]
- Hoff E (2003). The specificity of environmental influence: Socioeconomic status affects early vocabulary development via maternal speech . Child Development , 74 ( 5 ), 1368–1378. 10.1111/1467-8624.00612 [ PubMed ] [ CrossRef ] [ Google Scholar ]
- Hollich GJ, Hirsh-Pasek K, Michnick Golinkoff R, Brand RJ, Brown E, Chung HL, Hennon E, Rocroi C, & Bloom L (2000). Breaking the language barrier: An emergentist coalition model for the origins of word learning. In Liben LS (Ed.), Monographs of the society for research in child development (Vol. 65 , No. 3, pp. i–vi 1–123). Wiley on behalf of the Society for Research in Child Development. https://www.jstor.org/stable/3181533 [ PubMed ] [ Google Scholar ]
- Horst JS, & Samuelson LK (2008). Fast mapping but poor retention by 24-month-old infants . Infancy , 13 ( 2 ), 128–157. 10.1080/15250000701795598 [ PubMed ] [ CrossRef ] [ Google Scholar ]
- Howard EM, Robinson CW, & Sloutsky VM (2005). The nature of early word comprehension: Symbols or associations? [Conference session]. Proceedings of the 27th Annual Meeting of the Cognitive Science Society. Stresa, Italy. [ Google Scholar ]
- Johnson S, Slaughter V, & Carey S (1998). Whose gaze will infants follow? The elicitation of gaze-following in 12-month-olds . Developmental Science , 1 ( 2 ), 233–238. 10.1111/1467-7687.00036 [ CrossRef ] [ Google Scholar ]
- Jusczyk PW (1999). How infants begin to extract words from speech . Trends in Cognitive Sciences , 3 ( 9 ), 323–328. 10.1016/S1364-6613(99)01363-7 [ PubMed ] [ CrossRef ] [ Google Scholar ]
- Jusczyk PW, & Aslin RN (1995). Infants′ detection of the sound patterns of words in fluent speech . Cognitive Psychology , 29 ( 1 ), 1–23. 10.1006/cogp.1995.1010 [ PubMed ] [ CrossRef ] [ Google Scholar ]
- Jusczyk PW, & Hohne EA (1997). Infants’ memory for spoken words . Science , 277 ( 5334 ), 1984–1986. 10.1126/science.277.5334.1984 [ PubMed ] [ CrossRef ] [ Google Scholar ]
- Karmiloff-Smith A (1992). Nature, nurture and PDP: Preposterous developmental postulates? Connection Science , 4 ( 3–4 ), 253–269. 10.1080/09540099208946618 [ CrossRef ] [ Google Scholar ]
- Kaye K (1977). Toward the origin of dialogue. In Schaffer HR (Ed.), Studies in mother-infant interaction (pp. 89–117). Academic Press. [ Google Scholar ]
- Kirkham NZ, Slemmer JA, & Johnson SP (2002). Visual statistical learning in infancy: Evidence for a domain general learning mechanism . Cognition , 83 ( 2 ), B35–B42. 10.1016/S0010-0277(02)00004-5 [ PubMed ] [ CrossRef ] [ Google Scholar ]
- Kochanska G, & Aksan N (2004). Development of Mutual responsiveness between parents and their young children . Child Development , 75 ( 6 ), 1657–1676. 10.1111/j.1467-8624.2004.00808.x [ PubMed ] [ CrossRef ] [ Google Scholar ]
- Koenig MA, & Harris PL (2005). The role of social cognition in early trust . Trends in Cognitive Sciences , 9 ( 10 ), 457–459. 10.1016/j.tics.2005.08.006 [ PubMed ] [ CrossRef ] [ Google Scholar ]
- Kovacs AM, Teglas E, & Endress AD (2010). The social sense: Susceptibility to others’ beliefs in human infants and adults . Science , 330 ( 6012 ), 1830–1834. 10.1126/science.1190792 [ PubMed ] [ CrossRef ] [ Google Scholar ]
- Kuhl PK (1979). Speech perception in early infancy: Perceptual constancy for spectrally dissimilar vowel categories . The Journal of the Acoustical Society of America , 66 ( 6 ), 1668–1679. 10.1121/1.383639 [ PubMed ] [ CrossRef ] [ Google Scholar ]
- Kuhl PK (1980). Perceptual constancy for speech-sound categories in early infancy. In Yeni-Komshian GH, Kavanaugh JF, & Ferguson CA (Eds.), Child phonology: Vol. 2. Perception (pp. 41–66). Academic Press. [ Google Scholar ]
- Kuhl PK (1983). Perception of auditory equivalence classes for speech in early infancy . Infant Behavior and Development , 6 ( 2–3 ), 263–285. 10.1016/S0163-6383(83)80036-8 [ CrossRef ] [ Google Scholar ]
- Kuhl PK, Tsao F-M, & Liu H-M (2003). Foreign-language experience in infancy: Effects of short-term exposure and social interaction on phonetic learning . Proceedings of the National Academy of Sciences , 100 ( 15 ), 9096–9101. 10.1073/pnas.1532872100 [ PMC free article ] [ PubMed ] [ CrossRef ] [ Google Scholar ]
- LaTourrette A, & Waxman SR (2019). A little labeling goes a long way: Semi-supervised learning in infancy . Developmental Science , 22 ( 1 ), Article e12736. 10.1111/desc.12736 [ PMC free article ] [ PubMed ] [ CrossRef ] [ Google Scholar ]
- Lau JCY, Fyshe A, & Waxman SR (in press). Which acoustic features support the language-cognition link in infancy: A machine-learning approach [Conference session]. Proceedings of the 43rd Annual Meeting of the Cognitive Science Society, Austin, Texas, United States. [ Google Scholar ]
- Leslie AM (1982). The perception of causality in infants . Perception , 11 ( 2 ), 173–186. 10.1068/p110173 [ PubMed ] [ CrossRef ] [ Google Scholar ]
- Levickis P, Reilly S, Girolametto L, Ukoumunne OC, & Wake M (2014). Maternal behaviors promoting language acquisition in slow-to-talk toddlers: Prospective community-based study . Journal of Developmental & Behavioral Pediatrics , 35 ( 4 ), 274–281. 10.1097/DBP.0000000000000056 [ PubMed ] [ CrossRef ] [ Google Scholar ]
- Liszkowski U, Schäfer M, Carpenter M, & Tomasello M (2009). Prelinguistic infants, but not chimpanzees, communicate about absent entities . Psychological Science , 20 ( 5 ), 654–660. [ PubMed ] [ Google Scholar ]
- Lucca K, & Wilbourn MP (2018). Communicating to learn: Infants’ pointing gestures result in optimal learning . Child Development , 89 ( 3 ), 941–960. 10.1111/cdev.12707 [ PubMed ] [ CrossRef ] [ Google Scholar ]
- Luchkina E, Sobel DM, & Morgan JL (2018). Eighteen-month-olds selectively generalize words from accurate speakers to novel contexts . Developmental Science , 21 ( 6 ), Article e12663. 10.1111/desc.12663 [ PMC free article ] [ PubMed ] [ CrossRef ] [ Google Scholar ]
- Luchkina E, & Waxman SR (2021). Semantic priming supports infants’ ability to learn names of unseen objects . PLOS ONE , 16 ( 1 ), Article e0244968. 10.1371/journal.pone.0244968 [ PMC free article ] [ PubMed ] [ CrossRef ] [ Google Scholar ]
- Luchkina E, & Waxman SR (in press). Acquiring verbal reference: The interplay of cognitive, linguistic, and general learning capacities . Infant Behavior and Development . [ PMC free article ] [ PubMed ] [ Google Scholar ]
- Luchkina E, Xu F, Sobel D, & Morgan J (2020). Developing a Mind: Learning in Humans, Animals, and Machines [Conference session]. Proceedings of the 42nd annual meeting of the cognitive science society. 10.31219/osf.io/5tc6d [ CrossRef ] [ Google Scholar ]
- Luo Y (2011). Do 10-month-old infants understand others’ false beliefs? Cognition , 121 ( 3 ), 289–298. 10.1016/j.cognition.2011.07.011 [ PubMed ] [ CrossRef ] [ Google Scholar ]
- Luo Y, Baillargeon R, Brueckner L, & Munakata Y (2003). Reasoning about a hidden object after a delay: Evidence for robust representations in 5-month-old infants . Cognition , 88 ( 3 ), B23–B32. 10.1016/S0010-0277(03)00045-3 [ PMC free article ] [ PubMed ] [ CrossRef ] [ Google Scholar ]
- Luo Y, & Johnson SC (2009). Recognizing the role of perception in action at 6 months . Developmental Science , 12 ( 1 ), 142–149. 10.1111/j.1467-7687.2008.00741.x [ PubMed ] [ CrossRef ] [ Google Scholar ]
- Macnamara J (1982). Names for things: A study of human learning . MIT Press. [ Google Scholar ]
- Mandel DR, Jusczyk PW, & Pisoni DB (1995). Infants’ recognition of the sound patterns of their own names . Psychological Science , 6 ( 5 ), 314–317. 10.1111/j.1467-9280.1995.tb00517.x [ PMC free article ] [ PubMed ] [ CrossRef ] [ Google Scholar ]
- Mandler JM (1988). How to build a baby: On the development of an accessible representational system . Cognitive Development , 3 ( 2 ), 113–136. 10.1016/0885-2014(88)90015-9 [ CrossRef ] [ Google Scholar ]
- Mandler JM, & McDonough L (1995). Long-term recall of event sequences in infancy . Journal of Experimental Child Psychology , 59 ( 3 ), 457–474. 10.1006/jecp.1995.1021 [ PubMed ] [ CrossRef ] [ Google Scholar ]
- Markman EM (1989). Categorization and naming in children: Problems of induction . MIT Press. [ Google Scholar ]
- Martin A, Onishi KH, & Vouloumanos A (2012). Understanding the abstract role of speech in communication at 12months . Cognition , 123 ( 1 ), 50–60. 10.1016/j.cognition.2011.12.003 [ PubMed ] [ CrossRef ] [ Google Scholar ]
- Mattys S, Jusczyk PW, Luce PA, & Morgan JL (1999). Phonotactic and prosodic effects on word segmentation in infants . Cognitive Psychology , 38 ( 4 ), 465–494. [ PubMed ] [ Google Scholar ]
- McMurray B, Horst JS, & Samuelson LK (2012). Word learning emerges from the interaction of online referent selection and slow associative learning . Psychological Review , 119 ( 4 ), 831–877. 10.1037/a0029872 [ PMC free article ] [ PubMed ] [ CrossRef ] [ Google Scholar ]
- Mcquaid NE, Bibok MB, & Carpendale JIM (2009). Relation between maternal contingent responsiveness and infant social expectations . Infancy , 14 ( 3 ), 390–401. 10.1080/15250000902839955 [ PubMed ] [ CrossRef ] [ Google Scholar ]
- Medina TN, Snedeker J, Trueswell JC, & Gleitman LR (2011). How words can and cannot be learned by observation . Proceedings of the National Academy of Sciences , 108 ( 22 ), 9014–9019. 10.1073/pnas.1105040108 [ PMC free article ] [ PubMed ] [ CrossRef ] [ Google Scholar ]
- Michaelson E, & Reimer M (2019). Reference. In Zalta EN (Ed.), The Stanford encyclopedia of philosophy (Spring 2019 ed.). Metaphysics Research Laboratory, Stanford University. https://plato.stanford.edu/archives/spr2019/entries/reference/ [ Google Scholar ]
- Monaghan P, & Mattock K (2009). Cross-situational language learning: The effects of grammatical categories as constraints on referential labeling . Proceedings of the thirty first annual cognitive science society conference (pp. 2226–2231). Cognitive Science Society. [ Google Scholar ]
- Moore MK, & Meltzoff AN (2004). Object permanence after a 24-hr delay and leaving the locale of disappearance: The role of memory, space, and identity . Developmental Psychology , 40 ( 4 ), 606–620. 10.1037/0012-1649.40.4.606 [ PMC free article ] [ PubMed ] [ CrossRef ] [ Google Scholar ]
- Morgan JL, & Demuth K (Eds.). (1996). Signal to syntax: Bootstrapping from speech to grammar in early acquisition . Lawrence Erlbaum. [ Google Scholar ]
- Morse PA (1972). The discrimination of speech and nonspeech stimuli in early infancy . Journal of Experimental Child Psychology , 14 ( 3 ), 477–492. 10.1016/0022-0965(72)90066-5 [ PubMed ] [ CrossRef ] [ Google Scholar ]
- Namy LL, & Waxman SR (1998). Words and gestures: Infants’ interpretations of different forms of symbolic reference . Child Development , 69 ( 2 ), 295–308. 10.1111/j.1467-8624.1998.tb06189.x [ PubMed ] [ CrossRef ] [ Google Scholar ]
- Nazzi T, & Bertoncini J (2003). Before and after the vocabulary spurt: Two modes of word acquisition? Developmental Science , 6 ( 2 ), 136–142. 10.1111/1467-7687.00263 [ CrossRef ] [ Google Scholar ]
- Nelson K (1973). Structure and strategy in learning to talk . Monographs of the Society for Research in Child Development , 38 ( 1/2 ), 1–135. 10.2307/1165788 [ CrossRef ] [ Google Scholar ]
- Northrup JB (2017). Contingency detection in a complex world: A developmental model and implications for atypical development . International Journal of Behavioral Development , 41 ( 6 ), 723–734. 10.1177/0165025416668582 [ CrossRef ] [ Google Scholar ]
- O’Doherty K, Troseth GL, Shimpi PM, Goldenberg E, Akhtar N, & Saylor MM (2011). Third-party social interaction and word learning from video: Social interaction and video . Child Development , 82 ( 3 ), 902–915. 10.1111/j.1467-8624.2011.01579.x [ PMC free article ] [ PubMed ] [ CrossRef ] [ Google Scholar ]
- Olson SL, Bates JE, & Bayles K (1984). Mother–infant interaction and the development of individual differences in children’s cognitive competence . Developmental Psychology , 20 ( 1 ), 166–179. 10.1037/0012-1649.20.1.166 [ CrossRef ] [ Google Scholar ]
- Onishi KH, & Baillargeon R (2005). Do 15-month-old infants understand false beliefs? Science , 308 ( 5719 ), 255–258. 10.1126/science.1107621 [ PMC free article ] [ PubMed ] [ CrossRef ] [ Google Scholar ]
- Osina MA, Saylor MM, & Ganea PA (2013). When familiar is not better: 12-month-old infants respond to talk about absent objects . Developmental Psychology , 49 ( 1 ), 138–145. 10.1037/a0027903 [ PubMed ] [ CrossRef ] [ Google Scholar ]
- Osina MA, Saylor MM, & Ganea PA (2014). Object locations, identity and absent reference understanding at 12 months . Infancy , 19 ( 1 ), 65–81. 10.1111/infa.12031 [ CrossRef ] [ Google Scholar ]
- Parise E, & Csibra G (2012). Electrophysiological evidence for the understanding of maternal speech by 9-month-old infants . Psychological Science , 23 ( 7 ), 728–733. 10.1177/0956797612438734 [ PMC free article ] [ PubMed ] [ CrossRef ] [ Google Scholar ]
- Perszyk DR, & Waxman SR (2018). Linking language and cognition in infancy . Annual Review of Psychology , 69 ( 1 ), 231–250. 10.1146/annurev-psych-122216-011701 [ PMC free article ] [ PubMed ] [ CrossRef ] [ Google Scholar ]
- Piaget J (1954). The construction of reality in the child . Routledge. [ Google Scholar ]
- Pomiechowska B, Bródy G, Csibra G, & Gliga T (2021). Twelve-month-olds disambiguate new words using mutual-exclusivity inferences . Cognition . [ PubMed ] [ Google Scholar ]
- Pomiechowska B, & Csibra G (2020). Nonverbal action interpretation guides novel word disambiguation in 12-month-olds . PsyArXiv . 10.31234/osf.io/tkhsp [ PMC free article ] [ PubMed ] [ CrossRef ] [ Google Scholar ]
- Pomiechowska B, & Gliga T (2019). Lexical acquisition through category matching: 12-month-old infants associate words to visual categories . Psychological Science , 30 ( 2 ), 288–299. 10.1177/0956797618817506 [ PubMed ] [ CrossRef ] [ Google Scholar ]
- Pruden SM, Hirsh-Pasek K, Golinkoff RM, & Hennon EA (2006). The birth of words: Ten-month-olds learn words through perceptual salience . Child Development , 77 ( 2 ), 266–280. 10.1111/j.1467-8624.2006.00869.x [ PMC free article ] [ PubMed ] [ CrossRef ] [ Google Scholar ]
- Quinn PC (1985). Form categorization in early infancy [Doctoral dissertation] . Brown University. [ Google Scholar ]
- Quinn PC, Eimas PD, & Rosenkrantz SL (1993). Evidence for representations of perceptually similar natural categories by 3-month-old and 4-month-old infants . Perception , 22 ( 4 ), 463–475. 10.1068/p220463 [ PubMed ] [ CrossRef ] [ Google Scholar ]
- Rabagliati H, Ferguson B, & Lew-Williams C (2019). The profile of abstract rule learning in infancy: Meta-analytic and experimental evidence . Developmental Science , 22 ( 1 ), Article e12704. 10.1111/desc.12704 [ PMC free article ] [ PubMed ] [ CrossRef ] [ Google Scholar ]
- Rabagliati H, Senghas A, Johnson S, & Marcus GF (2012). Infant rule learning: Advantage language, or advantage speech? PLoS ONE , 7 ( 7 ), Article e40517. 10.1371/journal.pone.0040517 [ PMC free article ] [ PubMed ] [ CrossRef ] [ Google Scholar ]
- Rebuschat P, Monaghan P, & Schoetensack C (2021). Learning vocabulary and grammar from cross-situational statistics . Cognition , 206 , Article 104475. 10.1016/j.cognition.2020.104475 [ PubMed ] [ CrossRef ] [ Google Scholar ]
- Richert RA, Robb MB, & Smith EI (2011). Media as social partners: The social nature of young children’s learning from screen media: Media as social partners . Child Development , 82 ( 1 ), 82–95. 10.1111/j.1467-8624.2010.01542.x [ PubMed ] [ CrossRef ] [ Google Scholar ]
- Robb MB, Richert RA, & Wartella EA (2009). Just a talking book? Word learning from watching baby videos . British Journal of Developmental Psychology , 27 ( 1 ), 27–45. 10.1348/026151008X320156 [ PubMed ] [ CrossRef ] [ Google Scholar ]
- Rochat P (2007). Intentional action arises from early reciprocal exchanges . Acta Psychologica , 124 ( 1 ), 8–25. 10.1016/j.actpsy.2006.09.004 [ PubMed ] [ CrossRef ] [ Google Scholar ]
- Rochat P, Neisser U, & Marian V (1998). Are young infants sensitive to interpersonal contingency? Infant Behavior and Development , 21 ( 2 ), 355–366. 10.1016/S0163-6383(98)90012-1 [ CrossRef ] [ Google Scholar ]
- Rochat PR (2001). Social contingency detection and infant development . Bulletin of the Menninger Clinic , 65 ( 3 ), 347–360. 10.1521/bumc.65.3.347.19847 [ PubMed ] [ CrossRef ] [ Google Scholar ]
- Rollins PR (2003). Caregivers’ contingent comments to 9-month-old infants: Relationships with later language . Applied Psycholinguistics , 24 ( 2 ), 221–234. 10.1017/S0142716403000110 [ CrossRef ] [ Google Scholar ]
- Roseberry S, Hirsh-Pasek K, & Golinkoff RM (2014). Skype me! Socially contingent interactions help toddlers learn language . Child Development , 85 ( 3 ), 956–970. 10.1111/cdev.12166 [ PMC free article ] [ PubMed ] [ CrossRef ] [ Google Scholar ]
- Saffran JR (2020). Statistical language learning in infancy . Child Developmental Perspectives , 14 ( 1 ), 49–54. 10.1111/cdep.12355 [ PMC free article ] [ PubMed ] [ CrossRef ] [ Google Scholar ]
- Saffran JR, Aslin RN, & Newport EL (1996). Statistical learning by 8-month-old infants . Science , 274 ( 5294 ), 1926–1928. 10.1126/science.274.5294.1926 [ PubMed ] [ CrossRef ] [ Google Scholar ]
- Samuelson LK, & Smith LB (1999). Early noun vocabularies: Do ontology, category structure and syntax correspond? Cognition , 73 ( 1 ), 1–33. 10.1016/S0010-0277(99)00034-7 [ PubMed ] [ CrossRef ] [ Google Scholar ]
- Savage-Rumbaugh ES, Murphy J, Sevcik RA, Brakke KE, Williams SL, Rumbaugh DM, & Bates E (1993). Language comprehension in ape and child . Monographs of the Society for Research in Child Development , 58 ( 3/4 ), i+iii+v–vi+1–252. 10.2307/1166068 [ PubMed ] [ CrossRef ] [ Google Scholar ]
- Saylor MM (2004). Twelve- and 16-month-old infants recognize properties of mentioned absent things . Developmental Science , 7 ( 5 ), 599–611. 10.1111/j.1467-7687.2004.00383.x [ PubMed ] [ CrossRef ] [ Google Scholar ]
- Saylor MM, & Baldwin DA (2004). Discussing those not present: Comprehension of references to absent caregivers . Journal of Child Language , 31 ( 3 ), 537–560. 10.1017/S0305000904006282 [ PubMed ] [ CrossRef ] [ Google Scholar ]
- Saylor MM, & Ganea P (2007). Infants interpret ambiguous requests for absent objects . Developmental Psychology , 43 ( 3 ), 696–704. 10.1037/0012-1649.43.3.696 [ PubMed ] [ CrossRef ] [ Google Scholar ]
- Saylor MM, Osina M, Tassin T, Rose R, & Ganea P (2016). Creature feature: Preschoolers use verbal descriptions to identify referents . Journal of Experimental Child Psychology , 152 , 205–220. 10.1016/j.jecp.2016.07.005 [ PubMed ] [ CrossRef ] [ Google Scholar ]
- Scott JC, & Henderson AME (2013). Language matters: Thirteen-month-olds understand that the language a speaker uses constrains conventionality . Developmental Psychology , 49 ( 11 ), 2102–2111. 10.1037/a0031981 [ PubMed ] [ CrossRef ] [ Google Scholar ]
- Senju A, & Csibra G (2008). Gaze following in human infants depends on communicative signals . Current Biology , 18 ( 9 ), 668–671. 10.1016/j.cub.2008.03.059 [ PubMed ] [ CrossRef ] [ Google Scholar ]
- Shimizu YA, & Johnson SC (2004). Infants’ attribution of a goal to a morphologically unfamiliar agent . Developmental Science , 7 ( 4 ), 425–430. 10.1111/j.1467-7687.2004.00362.x [ PubMed ] [ CrossRef ] [ Google Scholar ]
- Shneidman LA, Arroyo ME, Levine SC, & Goldin-Meadow S (2013). What counts as effective input for word learning? Journal of Child Language , 40 ( 3 ), 672–686. 10.1017/S0305000912000141 [ PMC free article ] [ PubMed ] [ CrossRef ] [ Google Scholar ]
- Shneidman LA, & Goldin-Meadow S (2012). Language input and acquisition in a Mayan village: How important is directed speech?: Mayan village . Developmental Science , 15 ( 5 ), 659–673. 10.1111/j.1467-7687.2012.01168.x [ PMC free article ] [ PubMed ] [ CrossRef ] [ Google Scholar ]
- Shukla M, White KS, & Aslin RN (2011). Prosody guides the rapid mapping of auditory word forms onto visual objects in 6-mo-old infants . Proceedings of the National Academy of Sciences , 108 ( 15 ), 6038–6043. 10.1073/pnas.1017617108 [ PMC free article ] [ PubMed ] [ CrossRef ] [ Google Scholar ]
- Sloutsky VM (2009). Theories about “theories”: Where is the explanation? Comment on Waxman and Gelman . Trends in Cognitive Sciences , 13 ( 8 ), 331–332. 10.1016/j.tics.2009.05.003 [ PubMed ] [ CrossRef ] [ Google Scholar ]
- Smith L, & Yu C (2008). Infants rapidly learn word–referent mappings via cross-situational statistics . Cognition , 106 ( 3 ), 1558–1568. 10.1016/j.cognition.2007.06.010 [ PMC free article ] [ PubMed ] [ CrossRef ] [ Google Scholar ]
- Smith LB, Suanda SH, & Yu C (2014). The unrealized promise of infant statistical word–referent learning . Trends in Cognitive Sciences , 18 ( 5 ), 251–258. 10.1016/j.tics.2014.02.007 [ PMC free article ] [ PubMed ] [ CrossRef ] [ Google Scholar ]
- Smith LB, & Yu C (2013). Visual attention is not enough: Individual differences in statistical word-referent learning in infants . Language Learning and Development , 9 ( 1 ), 25–49. 10.1080/15475441.2012.707104 [ PMC free article ] [ PubMed ] [ CrossRef ] [ Google Scholar ]
- Sobel DM, & Kushnir T (2013). Knowledge matters: How children evaluate the reliability of testimony as a process of rational inference . Psychological Review , 120 ( 4 ), 779–797. 10.1037/a0034191 [ PubMed ] [ CrossRef ] [ Google Scholar ]
- Southgate V, & Vernetti A (2014). Belief-based action prediction in preverbal infants . Cognition , 130 ( 1 ), 1–10. 10.1016/j.cognition.2013.08.008 [ PMC free article ] [ PubMed ] [ CrossRef ] [ Google Scholar ]
- Sperry DE, Sperry LL, & Miller PJ (2019). Reexamining the verbal environments of children from different socioeconomic back-grounds . Child Development , 90 ( 4 ), 1303–1318. 10.1111/cdev.13072 [ PubMed ] [ CrossRef ] [ Google Scholar ]
- Swingley D (2010). Fast mapping and slow mapping in children’s word learning . Language Learning and Development , 6 ( 3 ), 179–183. 10.1080/15475441.2010.484412 [ CrossRef ] [ Google Scholar ]
- Tamis-LeMonda CS, Bornstein MH, & Baumwell L (2001). Maternal responsiveness and children’s achievement of language milestones . Child Development , 72 ( 3 ), 748–767. 10.1111/1467-8624.00313 [ PubMed ] [ CrossRef ] [ Google Scholar ]
- Tamis-LeMonda CS, Bornstein MH, Baumwell L, & Melstein Damast A (1996). Responsive parenting in the second year: Specific influences on children’s language and play . Early Development and Parenting: An International Journal of Research and Practice , 5 ( 4 ), 173–183. [ Google Scholar ]
- Thiele M, Hepach R, Michel C, Gredebäck G, & Haun DBM (2021). Social interaction targets enhance 13-month-old infants’ associative learning . Infancy , Article 12393. 10.1111/infa.12393 [ PubMed ] [ CrossRef ] [ Google Scholar ]
- Tincoff R, & Jusczyk PW (1999). Some beginnings of word comprehension in 6-month-olds . Psychological Science , 10 ( 2 ), 172–175. 10.1111/1467-9280.00127 [ CrossRef ] [ Google Scholar ]
- Tomasello M (2010). Origins of human communication . MIT Press. [ Google Scholar ]
- Tomasello M, & Farrar MJ (1986). Joint attention and early language . Child Development , 57 ( 6 ), 1454–1463. 10.2307/1130423 [ PubMed ] [ CrossRef ] [ Google Scholar ]
- Tomasello M, Strosberg R, & Akhtar N (1996). Eighteen-month-old children learn words in non-ostensive contexts . Journal of Child Language , 23 ( 1 ), 157–176. 10.1017/S0305000900010138 [ PubMed ] [ CrossRef ] [ Google Scholar ]
- Trueswell JC,Medina TN, Hafri A, & Gleitman LR (2013). Propose but verify: Fast mapping meets cross-situational word learning . Cognitive Psychology , 66 ( 1 ), 126–156. 10.1016/j.cogpsych.2012.10.001 [ PMC free article ] [ PubMed ] [ CrossRef ] [ Google Scholar ]
- Tsuji S, Jincho N, Mazuka R, & Cristia A (2020). Communicative cues in the absence of a human interaction partner enhance 12-month-old infants’ word learning . Journal of Experimental Child Psychology , 191 , Article 104740. 10.1016/j.jecp.2019.104740 [ PubMed ] [ CrossRef ] [ Google Scholar ]
- Veneziano E, & Parisse C (2010). The acquisition of early verbs in French: Assessing the role of conversation and of child-directed speech . First Language , 30 ( 3–4 ), 287–311. 10.1177/0142723710379785 [ CrossRef ] [ Google Scholar ]
- Vouloumanos A, Onishi KH, & Pogue A (2012). Twelve-month-old infants recognize that speech can communicate unobservable intentions . Proceedings of the National Academy of Sciences , 109 ( 32 ), 12933–12937. 10.1073/pnas.1121057109 [ PMC free article ] [ PubMed ] [ CrossRef ] [ Google Scholar ]
- Vouloumanos A (2018). Voulez-vous jouer avec moi? Twelve-month-olds understand that foreign languages can communicate . Cognition , 173 , 87–92. 10.1016/j.cognition.2018.01.002 [ PMC free article ] [ PubMed ] [ CrossRef ] [ Google Scholar ]
- Vouloumanos A, Martin A, & Onishi KH (2014). Do 6-month-olds understand that speech can communicate? Developmental Science , 17 ( 6 ), 872–879. 10.1111/desc.12170 [ PubMed ] [ CrossRef ] [ Google Scholar ]
- Vouloumanos A, & Werker JF (2004). Tuned to the signal: The privileged status of speech for young infants . Developmental Science , 7 ( 3 ), 270–276. 10.1111/j.1467-7687.2004.00345.x [ PubMed ] [ CrossRef ] [ Google Scholar ]
- Waxman SR (2003). Links between object categorization and naming. In Rakison DH & Oakes LM (Eds.), Early category and concept development: Making sense of the blooming, buzzing confusion (pp. 213–241). Oxford University Press. [ Google Scholar ]
- Waxman SR, & Gelman SA (2009). Early word-learning entails reference, not merely associations . Trends in Cognitive Sciences , 13 ( 6 ), 258–263. 10.1016/j.tics.2009.03.006 [ PMC free article ] [ PubMed ] [ CrossRef ] [ Google Scholar ]
- Waxman SR, & Leddon EM (2011). Early word-learning and conceptual development: Everything had a name, and each name gave birth to a new thought. In Goswami U (Ed.), Blackwell handbook of childhood cognitive development (pp. 180–208). Blackwell Publishing. [ Google Scholar ]
- Waxman SR, & Lidz JL (2007). Early world learning. In Damon W & Lerner RM (Eds.), Handbook of child psychology . Wiley. 10.1002/9780470147658.chpsy0207 [ CrossRef ] [ Google Scholar ]
- Werker JF (2018). Perceptual beginnings to language acquisition . Applied Psycholinguistics , 39 ( 4 ), 703–728. 10.1017/S0142716418000152 [ CrossRef ] [ Google Scholar ]
- Werker JF, Cohen LB, Lloyd VL, Casasola M, & Stager CL (1998). Acquisition of word–object associations by 14-month-old infants . Developmental Psychology , 34 ( 6 ), 1289–1309. 10.1037/0012-1649.34.6.1289 [ PubMed ] [ CrossRef ] [ Google Scholar ]
- Werker JF, & Tees RC (1984). Cross-language speech perception: Evidence for perceptual reorganization during the first year of life . Infant Behavior and Development , 7 ( 1 ), 49–63. 10.1016/S0163-6383(84)80022-3 [ CrossRef ] [ Google Scholar ]
- White PA (1988). Causal processing: Origins and development . Psychological Bulletin , 104 ( 1 ), 36–52. 10.1037/0033-2909.104.1.36 [ CrossRef ] [ Google Scholar ]
- Wittgenstein L (2017). Tractatus logico-philosophicus: The original 1922 edition with an introduction by Bertram Russell . e-artnow. [ Google Scholar ]
- Woodruff Carr K, Perszyk DR, & Waxman SR (2021). Birdsong fails to support object categorization in human infants . PLOS ONE , 16 ( 3 ), Article e0247430. 10.1371/journal.pone.0247430 [ PMC free article ] [ PubMed ] [ CrossRef ] [ Google Scholar ]
- Woodward A (1998). Infants selectively encode the goal object of an actor’s reach . Cognition , 69 ( 1 ), 1–34. 10.1016/S0010-0277(98)00058-4 [ PubMed ] [ CrossRef ] [ Google Scholar ]
- Woodward AL (2000). Constraining the problem space in early word learning. In Michnick Golinkoff R, Hirsch-Pasek K, Smith LB, Woodward AL, Akhtar N, Tomasello M, & Hollich GJ (Eds.), Becoming a word learner: A debate on lexical acquisition (pp. 81–114). Oxford University Press. [ Google Scholar ]
- Woodward AL, Markman EM, & Fitzsimmons CM (1994). Rapid word learning in 13- and 18-month-olds . Developmental Psychology , 30 ( 4 ), 553–566. 10.1037/0012-1649.30.4.553 [ CrossRef ] [ Google Scholar ]
- Xu F (2002). The role of language in acquiring object kind concepts in infancy . Cognition , 85 ( 3 ), 223–250. 10.1016/S0010-0277(02)00109-9 [ PubMed ] [ CrossRef ] [ Google Scholar ]
- Xu F (2019). Towards a rational constructivist theory of cognitive development . Psychological Review , 126 ( 6 ), 841–864. 10.1037/rev0000153 [ PubMed ] [ CrossRef ] [ Google Scholar ]
- Xu F, Cote M, & Baker A (2005). Labeling guides object individuation in 12-month-old infants . Psychological Science , 16 ( 5 ), 372–377. 10.1111/j.0956-7976.2005.01543.x [ PubMed ] [ CrossRef ] [ Google Scholar ]
- Xu F, & Kushnir T (2013). Infants are rational constructivist learners . Current Directions in Psychological Science , 22 ( 1 ), 28–32. 10.1177/0963721412469396 [ CrossRef ] [ Google Scholar ]
- Xu F, & Tenenbaum JB (2007a). Sensitivity to sampling in Bayesian word learning . Developmental Science , 10 ( 3 ), 288–297. 10.1111/j.1467-7687.2007.00590.x [ PubMed ] [ CrossRef ] [ Google Scholar ]
- Xu F, & Tenenbaum JB (2007b). Word learning as Bayesian inference . Psychological Review , 114 ( 2 ), 245–272. 10.1037/0033-295X.114.2.245 [ PubMed ] [ CrossRef ] [ Google Scholar ]
- Yin J, & Csibra G (2015). Concept-based word learning in human infants . Psychological Science , 26 ( 8 ), 1316–1324. 10.1177/0956797615588753 [ PMC free article ] [ PubMed ] [ CrossRef ] [ Google Scholar ]
- Yu C, & Smith LB (2007). Rapid word learning under uncertainty via cross-situational statistics . Psychological Science , 18 ( 5 ), 414–420. 10.1111/j.1467-9280.2007.01915.x [ PubMed ] [ CrossRef ] [ Google Scholar ]
- Yuan S, & Fisher C (2009). “Really? She blicked the baby?”: Two-year-olds learn combinatorial facts about verbs by listening . Psychological Science , 20 ( 5 ), 619–626. 10.1111/j.1467-9280.2009.02341.x [ PMC free article ] [ PubMed ] [ CrossRef ] [ Google Scholar ]
- Yuan S, Fisher C, Kandhadai P, & Fernald A (2011). Cooperative Minds: Social Interaction and Group Dynamics [Conference session]. Proceedings of the 35th Annual Meeting of the Cognitive Science Society, Berlin, Germany. [ Google Scholar ]
- Yurovsky D (2018). A communicative approach to early word learning . New Ideas in Psychology , 50 , 73–79. 10.1016/j.newideapsych.2017.09.001 [ CrossRef ] [ Google Scholar ]
- Yurovsky D, & Frank MC (2015). An integrative account of constraints on cross-situational learning . Cognition , 145 , 53–62. 10.1016/j.cognition.2015.07.013 [ PMC free article ] [ PubMed ] [ CrossRef ] [ Google Scholar ]
- Yurovsky D, Fricker DC, Yu C, & Smith LB (2014). The role of partial knowledge in statistical word learning . Psychonomic Bulletin & Review , 21 ( 1 ), 1–22. 10.3758/s13423-013-0443-y [ PMC free article ] [ PubMed ] [ CrossRef ] [ Google Scholar ]
- Zimmerman IL, Steiner VG, & Pond RE (2002). Preschool language scales, fourth edition (PLSTM-4) is an interactive assessment of developmental language skills . The Psychological Corporation. [ Google Scholar ]
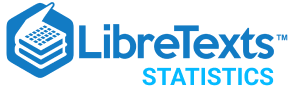
- school Campus Bookshelves
- menu_book Bookshelves
- perm_media Learning Objects
- login Login
- how_to_reg Request Instructor Account
- hub Instructor Commons
- Download Page (PDF)
- Download Full Book (PDF)
- Periodic Table
- Physics Constants
- Scientific Calculator
- Reference & Cite
- Tools expand_more
- Readability
selected template will load here
This action is not available.
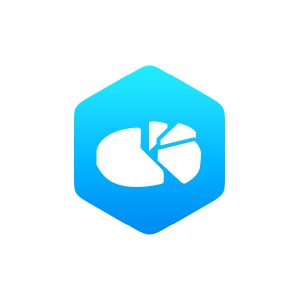
8.4: The Alternative Hypothesis
- Last updated
- Save as PDF
- Page ID 14493
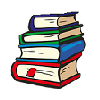
- Foster et al.
- University of Missouri-St. Louis, Rice University, & University of Houston, Downtown Campus via University of Missouri’s Affordable and Open Access Educational Resources Initiative
If the null hypothesis is rejected, then we will need some other explanation, which we call the alternative hypothesis, \(H_A\) or \(H_1\). The alternative hypothesis is simply the reverse of the null hypothesis, and there are three options, depending on where we expect the difference to lie. Thus, our alternative hypothesis is the mathematical way of stating our research question. If we expect our obtained sample mean to be above or below the null hypothesis value, which we call a directional hypothesis, then our alternative hypothesis takes the form:
\[\mathrm{H}_{\mathrm{A}}: \mu>7.47 \quad \text { or } \quad \mathrm{H}_{\mathrm{A}}: \mu<7.47 \nonumber \]
based on the research question itself. We should only use a directional hypothesis if we have good reason, based on prior observations or research, to suspect a particular direction. When we do not know the direction, such as when we are entering a new area of research, we use a non-directional alternative:
\[\mathrm{H}_{\mathrm{A}}: \mu \neq 7.47 \nonumber \]
We will set different criteria for rejecting the null hypothesis based on the directionality (greater than, less than, or not equal to) of the alternative. To understand why, we need to see where our criteria come from and how they relate to \(z\)-scores and distributions.
Writing hypotheses in words
As we alluded to in the null hypothesis section, we can write our hypotheses in word statements (in addition to the statements with symbols). These statements should be specific enough to the particular experiment or situation being referred to. That is, don't make them generic enough so that they would apply to any hypothesis test that you would conduct.
Examples for how to write null and alternate hypotheses in words for directional and non-directional situations are given throughout the chapters.
Contributors and Attributions
Foster et al. (University of Missouri-St. Louis, Rice University, & University of Houston, Downtown Campus)

- Cambridge Dictionary +Plus
Definition of hypothesis – Learner’s Dictionary
Your browser doesn't support HTML5 audio
(Definition of hypothesis from the Cambridge Learner's Dictionary © Cambridge University Press)
Translations of hypothesis
Get a quick, free translation!
Word of the Day
a type of singing in which four, usually male, voices in close combination perform popular romantic songs, especially from the 1920s and 1930s

Alike and analogous (Talking about similarities, Part 1)

Learn more with +Plus
- Recent and Recommended {{#preferredDictionaries}} {{name}} {{/preferredDictionaries}}
- Definitions Clear explanations of natural written and spoken English English Learner’s Dictionary Essential British English Essential American English
- Grammar and thesaurus Usage explanations of natural written and spoken English Grammar Thesaurus
- Pronunciation British and American pronunciations with audio English Pronunciation
- English–Chinese (Simplified) Chinese (Simplified)–English
- English–Chinese (Traditional) Chinese (Traditional)–English
- English–Dutch Dutch–English
- English–French French–English
- English–German German–English
- English–Indonesian Indonesian–English
- English–Italian Italian–English
- English–Japanese Japanese–English
- English–Norwegian Norwegian–English
- English–Polish Polish–English
- English–Portuguese Portuguese–English
- English–Spanish Spanish–English
- English–Swedish Swedish–English
- Dictionary +Plus Word Lists
- Learner’s Dictionary Noun
- Translations
- All translations
Add hypothesis to one of your lists below, or create a new one.
{{message}}
Something went wrong.
There was a problem sending your report.
- Skip to main content
- Keyboard shortcuts for audio player
Muslim politicians in the U.K. have faced Islamophobia through Ramadan
Fatima Al-Kassab
In the U.K., Muslim politicians are getting verbal abuse. Many worry the government's new "extremism" definition targets them. Watching Gaza, it's an especially difficult Ramadan.
AILSA CHANG, HOST:
This week, a huge chunk of humanity was looking skyward for a glimpse of the solar eclipse. And tonight, many in the Muslim world will be looking up, searching for a crescent moon signifying the end of a month of fasting and the start of the Eid holiday. But in the United Kingdom, tensions over the war in Gaza and rising Islamophobia mean that it's been a difficult month for Muslims, as NPR's Fatima Al-Kassab reports from London.
(SOUNDBITE OF ARCHIVED RECORDING)
UNIDENTIFIED MUSICAL ARTIST #1: (Singing in non-English language).
FATIMA AL-KASSAB, BYLINE: This ornate hotel banquet hall in the posh area of Mayfair is decorated with lanterns and golden crescent moons. Some of the biggest names in British politics, entertainment and the arts are breaking their fast with an iftar meal. But the guests say that the mood is somber this year.
SAYEEDA WARSI: Every Ramadan iftar event that I've been to, there's no celebrations. I always say that when you wake up in the morning, you think about Gaza. When you do iftar, you think about Gaza.
AL-KASSAB: Sayeeda Warsi is the former chairwoman of the U.K.'s ruling Conservative Party. She says that, as a Muslim, she feels the pain of Palestinian suffering in Gaza.
WARSI: There is a collective sense of trauma that is happening because of what we are watching unfold in front - on our screens.
AL-KASSAB: She's worrying about the situation at home, too. In the U.K., Islamophobic incidents have more than tripled since October 7, when Hamas-led militants attacked Israel. Antisemitism has spiked, too. Death threats have been directed at Muslim politicians, especially the mayor of London, Sadiq Khan. In between speeches at this iftar, he told NPR how all of this abuse leaves him questioning whether the job is worth it.
SADIQ KHAN: Why would you, as somebody, you know, who is of Islamic faith, want to be a politician knowing what politicians of our background go through? But also, if you're a parent, if you're an uncle, if you're an auntie, why would you encourage your children, your nephews and nieces to become politicians?
AL-KASSAB: Khan is the first London mayor to get the same level of round-the-clock security as the King and the Prime Minister. He's also the first Muslim to hold the job.
KHAN: You know, when my father first came to this country in the 1960s, there were signs on guesthouses and public buildings saying no Blacks, no Irish, no dogs. And by Blacks, they meant anybody who was a person of color. Within one generation, one of his children became the mayor of London, so we should be incredibly proud of the progress we've made.
AL-KASSAB: He's a member of the Labour Party. The U.K. government is run by the conservatives, and they've recently issued a new legal definition of extremism amid ongoing demonstrations over the war in Gaza. Khan and other critics say it is vague and could be used to cut off government funding to some of Britain's biggest Muslim charities.
KHAN: This conservative government can't even define Islamophobia or anti-Muslim hatred or racism. What the government's doing is using this to try and divide communities against each other.
UNIDENTIFIED PERSON: Salaam aleikum. Ticketholders this way. Salaam aleikum. Peace be upon you.
UNIDENTIFIED MUSICAL ARTIST #2: (Singing in non-English language).
AL-KASSAB: Here in London's Trafalgar Square, the clouds have broken, and it's pouring with rain. I'm looking at a dozen rows of people who are lining up the steps down onto Trafalgar Square. They're huddled under umbrellas, and the rain has not deterred them. And they're waiting to break their fast here. It's one of the last nights of Ramadan.
FAIZA JAVAID: Ever since everything in Gaza started, it's made me feel closer to the religion - definitely felt, you know, more connected.
AL-KASSAB: Faiza Javaid (ph) is one of those breaking her fast in the square. She says that, growing up, she never felt out of place as a Muslim in London.
JAVAID: I felt quite - you know, I'm from here. I belong here. There's no issue at all. For the first time, I feel like I need to hold my identity a bit closer and, you know, let it shine a bit. Yeah. It's made me want to hold onto the faith that people in Gaza are holding onto.
AL-KASSAB: At a time when many Muslims are feeling despondent, Faiza says it is her Muslim identity that gives her hope.
Fatima Al-Kassab, NPR News, London.
Copyright © 2024 NPR. All rights reserved. Visit our website terms of use and permissions pages at www.npr.org for further information.
NPR transcripts are created on a rush deadline by an NPR contractor. This text may not be in its final form and may be updated or revised in the future. Accuracy and availability may vary. The authoritative record of NPR’s programming is the audio record.
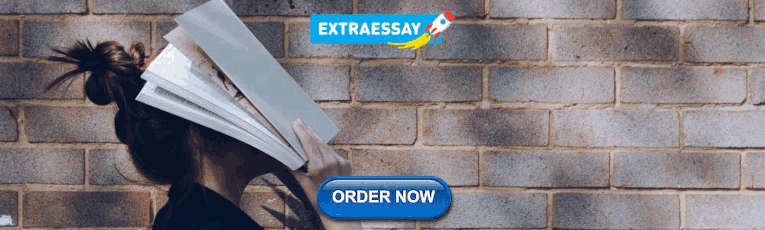
IMAGES
VIDEO
COMMENTS
5. Phrase your hypothesis in three ways. To identify the variables, you can write a simple prediction in if…then form. The first part of the sentence states the independent variable and the second part states the dependent variable. If a first-year student starts attending more lectures, then their exam scores will improve.
Definition: Hypothesis is an educated guess or proposed explanation for a phenomenon, based on some initial observations or data. It is a tentative statement that can be tested and potentially proven or disproven through further investigation and experimentation. Hypothesis is often used in scientific research to guide the design of experiments ...
Merriam Webster defines a hypothesis as "an assumption or concession made for the sake of argument.". In other words, a hypothesis is an educated guess. Scientists make a reasonable assumption--or a hypothesis--then design an experiment to test whether it's true or not.
What is a hypothesis and how can you write a great one for your research? A hypothesis is a tentative statement about the relationship between two or more variables that can be tested empirically. Find out how to formulate a clear, specific, and testable hypothesis with examples and tips from Verywell Mind, a trusted source of psychology and mental health information.
3. Simple hypothesis. A simple hypothesis is a statement made to reflect the relation between exactly two variables. One independent and one dependent. Consider the example, "Smoking is a prominent cause of lung cancer." The dependent variable, lung cancer, is dependent on the independent variable, smoking. 4.
A hypothesis states your predictions about what your research will find. It is a tentative answer to your research question that has not yet been tested. For some research projects, you might have to write several hypotheses that address different aspects of your research question. A hypothesis is not just a guess — it should be based on ...
A hypothesis (plural hypotheses) is a proposed explanation for an observation. The definition depends on the subject. In science, a hypothesis is part of the scientific method. It is a prediction or explanation that is tested by an experiment. Observations and experiments may disprove a scientific hypothesis, but can never entirely prove one.
A research hypothesis (also called a scientific hypothesis) is a statement about the expected outcome of a study (for example, a dissertation or thesis). To constitute a quality hypothesis, the statement needs to have three attributes - specificity, clarity and testability. Let's take a look at these more closely.
A hypothesis states your predictions about what your research will find. It is a tentative answer to your research question that has not yet been tested. For some research projects, you might have to write several hypotheses that address different aspects of your research question. A hypothesis is not just a guess. It should be based on ...
hypothesis: [noun] an assumption or concession made for the sake of argument. an interpretation of a practical situation or condition taken as the ground for action.
A hypothesis is a prediction of what will be found at the outcome of a research project and is typically focused on the relationship between two different variables studied in the research. It is usually based on both theoretical expectations about how things work and already existing scientific evidence. Within social science, a hypothesis can ...
A tentative and logical statement that can be tested for its authenticity. An idea that seeks to explain why a phenomenon takes place. A prediction about the outcome of a study according to known facts. A proposal about the possible relationship between two or more variables.
The Sapir-Whorf hypothesis states that people experience the world based on the structure of their language, and that linguistic categories shape and limit cognitive processes. It proposes that differences in language affect thought, perception, and behavior, so speakers of different languages think and act differently.
The hypothesis predicts that children will perform better on task A than on task B. The results confirmed his hypothesis on the use of modal verbs. These observations appear to support our working hypothesis. a speculative hypothesis concerning the nature of matter; an interesting hypothesis about the development of language
In hypothesis testing, the goal is to see if there is sufficient statistical evidence to reject a presumed null hypothesis in favor of a conjectured alternative hypothesis.The null hypothesis is usually denoted \(H_0\) while the alternative hypothesis is usually denoted \(H_1\). An hypothesis test is a statistical decision; the conclusion will either be to reject the null hypothesis in favor ...
1 [countable] an idea or explanation of something that is based on a few known facts but that has not yet been proved to be true or correct synonym theory to formulate/confirm a hypothesis a hypothesis about the function of dreams There is little evidence to support these hypotheses. Topic Collocations Scientific Research theory. formulate/advance a theory/hypothesis
Others have argued that word learners maintain a single hypothesis about the referent of any given word (e.g., Medina et al., 2011; Trueswell et al., ... Recall that we define verbal reference as a tridirectional link between words, real-world referents, and their mental representations used for intentional communication between people. ...
HYPOTHESIS definition: 1. an idea or explanation for something that is based on known facts but has not yet been proved…. Learn more.
HYPOTHESIS meaning: 1. an idea or explanation for something that is based on known facts but has not yet been proved…. Learn more.
Thus, our alternative hypothesis is the mathematical way of stating our research question. If we expect our obtained sample mean to be above or below the null hypothesis value, which we call a directional hypothesis, then our alternative hypothesis takes the form: HA: μ > 7.47 or HA: μ < 7.47 H A: μ > 7.47 or H A: μ < 7.47.
Hypothesis definition: a proposition, or set of propositions, set forth as an explanation for the occurrence of some specified group of phenomena, either asserted merely as a provisional conjecture to guide investigation (working hypothesis ) or accepted as highly probable in the light of established facts. See examples of HYPOTHESIS used in a sentence.
HYPOTHESIS meaning: a suggested explanation for something that has not yet been proved to be true. Learn more.
In the U.K., Muslim politicians are getting verbal abuse. Many worry the government's new "extremism" definition targets them. Watching Gaza, it's an especially difficult Ramadan. AILSA CHANG ...
Hypothesis definition, a proposition, or set of propositions, set forth as an explanation for the occurrence of some specified group of phenomena, either asserted merely as a provisional conjecture to guide investigation (working hypothesis ) or accepted as highly probable in the light of established facts. See more.