G-STA-PHD - Statistical Science - PhD
Degree designation.
The Department of Statistical Science at Duke University offers graduate study leading to PhD and MS degrees in statistical science. The PhD program offers thorough preparation in the theory and methods of statistics, with major emphases on modern, model-based statistical science, Bayesian and classical approaches to inference, computational statistics, and machine learning. A hallmark of the program is the integration of interdisciplinary applications into teaching and research, reflecting the department’s broad and deep engagements in leadership and innovation in statistical science and its intersections with many other areas, including the biomedical sciences, computational sciences, data and information sciences, economic and policy sciences, environmental sciences, engineering, machine learning, physical sciences, and social sciences. The rich opportunities for students in interdisciplinary statistical research at Duke are complemented by opportunities for engagement in research in summer projects with nonprofit agencies, industry, and academia.
For an up-to-date faculty list and description of graduate programs in statistical science visit the website at stat.duke.edu .
Traditional features of the curriculum include parallel development of theory and applications as well as coverage of specific biostatistical topic areas and ethical issues in the conduct of statistical and medical research. The core curriculum covers the principles of epidemiologic studies in detail. Embedded throughout the curriculum are examples of conflict of interest situations faced by biostatisticians, along with principles of reproducible research and strategies for implementation.
The PhD program follows the Duke Graduate School Academic Calendar .
View the timeline for students with and without an Applicable Quantitative Master's Degree.
For students with a Master's degree in Biostatistics, some of the required 700 level courses listed below may be waived if they have taken those courses or their equivalents previously.
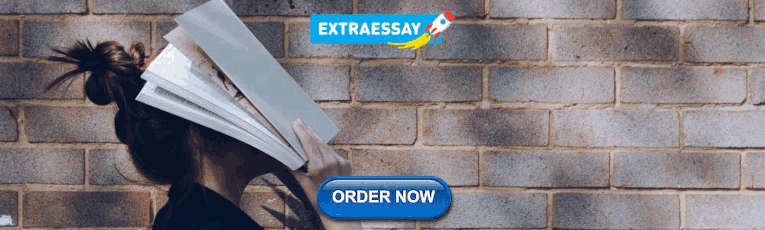
Required Knowledge in the Following Core Courses
This course provides a formal introduction to the basic theory and methods of probability and statistics. It covers topics in probability theory with an emphasis on those needed in statistics, including probability and sample spaces, independence, conditional probability, random variables, parametric families of distributions, and sampling distributions. Core concepts are mastered through mathematical exploration and linkage with the applied concepts studied in BIOSTAT 704. Prerequisite(s): 2 semesters of calculus or its equivalent (multivariate calculus preferred). Familiarity with linear algebras is helpful. Corequisite(s): BIOSTAT 702, BIOSTAT 703. Credits: 3
This course provides an introduction to study design, descriptive statistics, and analysis of statistical models with one or two predictor variables. Topics include principles of study design, basic study designs, descriptive statistics, sampling, contingency tables, one- and two-way analysis of variance, simple linear regression, and analysis of covariance. Both parametric and non-parametric techniques are explored. Core concepts are mastered through team-based case studies and analysis of authentic research problems encountered by program faculty and demonstrated in practicum experiences in concert with BIOSTAT 703. Computational exercises will use the R and SAS packages. Prerequisite(s): 2 semesters of calculus or its equivalent (multivariate calculus preferred). Familiarity with linear algebras is helpful. Corequisites(s): BIOSTAT 701, BIOSTAT 703, BIOSTAT 721. Credits: 3
This course provides an introduction to biology at a level suitable for practicing biostatisticians and directed practice in techniques of statistical collaboration and communication. With an emphasis on the connection between biomedical content and statistical approach, this course helps unify the statistical concepts and applications learned in BIOSTAT 701 and BIOSTAT 702. In addition to didactic sessions on biomedical issues, students are introduced to different areas of biostatistical practice at Duke University Medical Center. Biomedical topics are organized around the fundamental mechanisms of disease from both evolutionary and mechanistic perspectives, illustrated using examples from infectious disease, cancer and chronic /degenerative disease. In addition, students learn how to read and interpret research and clinical trial papers. Core concepts and skills are mastered through individual reading and class discussion of selected biomedical papers, team-based case studies and practical sessions introducing the art of collaborative statistics. Corequisite(s): BIOSTAT 701, BIOSTAT 702. Credits: 3
The lab will be an extension of the course. The lab will be run like a journal club. The lab will instruct students how to dissect a research article from a statistical and scientific perspective. The lab will also give students the opportunity to present on material covered in the co-requisite course and to practice the communication skills that are a core tenant of the program. Corequisite(s): BIOSTAT 703 or permission of the Director of Graduate Studies. Credits: 0
This course provides formal introduction to the basic theory and methods of probability and statistics. It covers topics in statistical inference, including classical and Bayesian methods, and statistical models for discrete, continuous and categorical outcomes. Core concepts are mastered through mathematical exploration, simulations, and linkage with the applied concepts studied in BIOSTAT 705. Prerequisite(s): BIOSTAT 701 or its equivalent. Corequisite(s): BIOSTAT 705, BIOSTAT 706. Credits: 3
This course provides an introduction to general linear models and the concept of experimental designs. Topics include linear regression models, analysis of variance, mixed-effects models, generalized linear models (GLM) including binary, multinomial responses and log-linear models, basic models for survival analysis and regression models for censored survival data, and model assessment, validation and prediction. Core concepts are mastered through statistical methods application and analysis of practical research problems encountered by program faculty and demonstrated in practicum experiences in concert with BIOSTAT 706. Computational examples and exercises will use the SAS and R packages. Prerequisite(s): BIOSTAT 702 or its equivalent. Corequisite(s): BIOSTAT 704, BIOSTAT 706, BIOSTAT 722. Credits: 3
This course revisits the topics covered in BIOSTAT 703 in the context of high-throughput, high-dimensional studies such as genomics and transcriptomics. The course will be based on reading of both the textbook and research papers. Students will learn the biology and technology underlying the generation of “big data,” and the computational and statistical challenges associated with the analysis of such data sets. As with BIOSTAT 703, there will be strong emphasis on the development of communication skills via written and oral presentations. Prerequisite(s): BIOSTAT 703. Corequisite(s): BIOSTAT 704, BIOSTAT 705. Credits: 3
Introduction to concepts and techniques used in the analysis of time to event data, including censoring, hazard rates, estimation of survival curves, regression techniques, applications to clinical trials. Interval censoring, informative censoring, competing risks, multiple events and multiple endpoints, time dependent covariates; nonparametric and semi-parametric methods. Prerequisite(s): BIOSTAT 701, 702, 704, 705, and 721 or 722 or their equivalents, or permission of the Director of Graduate Studies. Credits: 3
Topics include linear and nonlinear mixed models; generalized estimating equations; subject specific versus population average interpretation; and hierarchical model. Prerequisite(s): BIOSTAT 701, 702, 704, 705, and 721 or 722 or their equivalents, or permission of the Director of Graduate Studies. Credits: 3
The class introduces the concept of exponential family of distributions and link function, and their use in generalizing the standard linear regression to accommodate various outcome types. Theoretical framework will be presented but detailed practical analyses will be performed as well, including logistic regression and Poisson regression with extensions. Majority of the course will deal with the independent observations framework. However, there will be substantial discussion of longitudinal/clustered data where correlations within clusters are expected. To deal with such data the Generalized Estimating Equations and the Generalized Linear Mixed models will be introduced. An introduction to a Bayesian analysis approach will be presented, time permitting.Prerequisite(s): BIOSTAT 701, 702, 704, 705, and 721 or 722 or their equivalents, or permission of the Director of Graduate Studies. Credits: 3
Advanced seminar on topics at the research frontiers in biostatistics. Readings of current biostatistical research and presentations by faculty and advanced students of current research in their area of specialization. Instructor: O’Brien. 1 unit.
Introduction to linear models and linear inference from the coordinate-free viewpoint. Topics: identifiability and estimability, key properties of and results for finite-dimensional vector spaces, linear transformations, self-adjoint transformations, spectral theorem, properties and geometry of orthogonal projectors, Cochran's theorem, estimation and inference for normal models, distributional properties of quadratic forms, minimum variance linear unbiased estimation, Gauss-Markov theorem and estimation, calculus of differentials, analysis of variance and covariance. Prerequisite: Biostatistics 906. Instructor: Owzar. 3 units.
Introduce decision theory and optimality criteria, sufficiency, methods for point estimation, confidence interval and hypothesis testing methods and theory. Prerequisite: Biostatistics 704 or equivalent. Instructor consent required. Instructor: Xie. 3 units.
Student gains a holistic view of career choices and individual development plans including tools they will need to succeed as professionals in the world of work. The curriculum focuses on the unique challenges of PhD candidates and tools needed for successful careers in academia or in industry. May be repeated with consent of the advisor and the Director of Graduate Studies. Instructor: Baker. 1 unit.
The theory for M- and Z- estimators and applications. Semiparametric models, geometry of efficient score functions and efficient influence functions, construction of semiparametric efficient estimators. Introduction to the bootstrap: consistency, inconsistency and remedy, correction for bias, and double bootstrap. U statistics and rank and permutation tests. Prerequisites: Statistical Sciences 711 and Biostatistics 906. Instructor: Li. 3 units.
The goal of this course is to provide motivated Ph.D. and master’s students with background knowledge of high-dimensional statistics/machine learning for their research, especially in their methodology and theory development. Discussions cover theory, methodology, and applications. Selected topics in this course include the basics of high-dimensional statistics, matrix and tensor modeling, concentration inequity, nonconvex optimization, applications in genomics, and biomedical informatics. Prerequisite: Knowledge in probability, inference, and basic algebra are required. Credits: 3
Introduction to probability spaces, the theory of measure and integration, random variables, and limit theorems. Distribution functions, densities, and characteristic functions; convergence of random variables and of their distributions; uniform integrability and the Lebesgue convergence theorems. Weak and strong laws of large numbers, central limit theorem. Prerequisite: elementary real analysis and elementary probability theory. Instructor: Staff. 3 units.
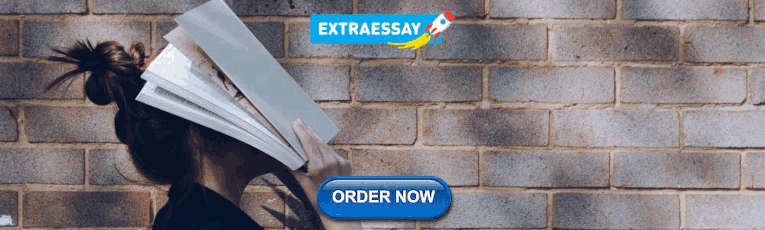
Elective Courses
This course surveys a number of techniques for high dimensional data analysis useful for data mining, machine learning and genomic applications, among others. Topics include principal and independent component analysis, multidimensional scaling, tree-based classifiers, clustering techniques, support vector machines and networks, and techniques for model validation. Core concepts are mastered through the analysis and interpretation of several actual high dimensional genomics datasets. Prerequisite(s): BIOSTAT 701, 702, 704, 705, and 721 or 722 or their equivalents, or permission of the Director of Graduate Studies. Credits: 3
Topics include: history/background and process for clinical trial, key concepts for good statistics practice (GSP)/good clinical practice (GCP), regulatory requirement for pharmaceutical/clinical development, basic considerations for clinical trials, designs for clinical trials, classification of clinical trials, power analysis for sample size calculation, statistical analysis for efficacy evaluation, statistical analysis for safety assessment, implementation of a clinical protocol, statistical analysis plan, data safety monitoring, adaptive design methods in clinical trials (general concepts, group sequential design, dose finding design, and phase I/II or phase II/III seamless design) and controversial issues in clinical trials. Prerequisite(s): BIOSTAT 701, 702, 704, 705, and 721 or 722 or their equivalents, or permission of the Director of Graduate Studies. Credits: 3
Methods for causal inference, including confounding and selection bias in observational or quasi-experimental research designs, propensity score methodology, instrumental variables, and methods for non-compliance in randomized clinical trials. Prerequisite(s): BIOSTAT 701, 702, 704, 705, and 721 or 722 or their equivalents, or permission of the Director of Graduate Studies. Credits: 3
Topics from current and classical methods for assessing familiality and heritability, linkage analysis of Mendelian and complex traits, family-based and population-based association studies, genetic heterogeneity, epistasis, and gene-environmental interactions. Computational methods and applications in current research areas. The course will include a simple overview of genetic data, terminology, and essential population genetic results. Topics will include sampling designs in human genetics, gene frequency estimation, segregation analysis, linkage analysis, tests of association, and detection of errors in genetic data. Prerequisite(s): BIOSTAT 701, 702, 704, 705, and 721 or 722 or their equivalents, or permission of the Director of Graduate Studies. Credits: 3
Theory and application of missing data methodology, ad hoc methods, missing data mechanism, selection models, pattern mixture models, likelihood-based methods, multiple imputation, inverse probability weighting, sensitivity analysis. Prerequisites: Statistical Science 711, 721, and 732, or consent of instructor. Instructor: Allen. 3 units.
Designed for PhD students in Biostatistics or DSS departments who may be interested in conducting methodological research in the area of Survival Data Analysis. Applications of counting process and martingale theory to right censored survival data. Applications of empirical process theory to more general and possibly more complex statistical models using nonparametric analysis of interval-censored data as illustrating examples. After completion, students are anticipated to understand the statistical method papers on survival analysis appearing in top tier statistical journals. Prerequisites: BIOSTAT 701, 704, and 713, or equivalent, or consent of instructor. Instructor: Wu. 3 units.
Introduction to diverse statistical design and analytical methods for randomized phase II clinical trials. Topics: Minimax, optimal, and admissible clinical trials Inference methods for phase II clinical trials; clinical trials with a survival endpoint; clinical trials with heterogeneous patient populations; and randomized phase II clinical trials. Instructor consent required. Instructor: Jung. 3 units.
Faculty directed statistical methodology research. Instructor consent required. Instructor: O’Brien. 1 unit.
Student gains practical experience by taking an internship in industry/government and writes a report about this experience. Requires prior consent from the student's advisor and from the Director of Graduate Studies. May be repeated with consent of the advisor and the Director of Graduate Studies. Credit/no credit grading only. Instructor: O’Brien. 1 unit.
This course provides an introduction to the basic theory and application of empirical processes. Topics include: concepts of stochastic processes, Brownian motion and Brownian bridge process, stochastic integrals, weak convergence of sequences of random elements, convergence of empirical distribution functions, general Glivenko-Cantelli theorems and Donsker theorems, functional Delta method. An emphasis is put on applications in various biostatistical problems. Pre-requisites: Stat 711. Instructor: Li. Units: 3
Introduction to probabilistic graphical models and structured prediction, with applications in genetics and genomics. Hidden Markov Models, conditional random fields, stochastic grammars, Bayesian hierarchical models, neural networks, and approaches to integrative modeling. Algorithms for exact and approximate inference. Applications in DNA/RNA analysis, phylogenetics, sequence alignment, gene expression, allelic phasing and imputation, genome/epigenome annotation, and gene regulation. Department consent required. Instructor: Majoros. 3 units. C-L: Computational Biology and Bioinformatics 914.
Introduction to concepts in robabilistic machine learning with a focus on discriminative and hierarchical generative models. Topics include directed and undirected graphical models, kernel methods, exact and approximate parameter estimation methods, and structure learning. Prerequisites: Linear algebra, Statistical Science 250 or Statistical Science 611. Instructor: Heller, Mukherjee, or Reeves. 3 units.
Principles of data analysis and modern statistical modeling. Exploratory data analysis. Introduction to Bayesian inference, prior and posterior distributions, predictive distributions, hierarchical models, model checking and selection, missing data, introduction to stochastic simulation by Markov Chain Monte Carlo using a higher level statistical language such as R or Matlab. Applications drawn from various disciplines. Not open to students with credit for Statistical Science 360. Prerequisite: Statistical Science 210, 230 and 250, or close equivalents. Instructor: Clyde, Dunson, Reiter, or Volfovsky. 3 units.
Statistical issues in causality and methods for estimating causal effects. Randomized designs and alternative designs and methods for when randomization is infeasible: matching methods, propensity scores, longitudinal treatments, regression discontinuity, instrumental variables, and principal stratification. Methods are motivated by examples from social sciences, policy and health sciences. Instructor: Li or VolfovskyStatistical issues in causality and methods for estimating causal effects. Randomized designs and alternative designs and methods for when randomization is infeasible: matching methods, propensity scores, longitudinal treatments, regression discontinuity, instrumental variables, and principal stratification. Methods are motivated by examples from social sciences, policy and health sciences. Instructor: Li or Volfovsky
Statistical modeling and machine learning involving large data sets and challenging computation. Data pipelines and data bases, big data tools, sequential algorithms and subsampling methods for massive data sets, efficient programming for multi-core and cluster machines, including topics drawn from GPU programming, cloud computing, Map/Reduce and general tools of distributed computing environments. Intense use of statistical and data manipulation software will be required. Data from areas such as astronomy, genomics, finance, social media, networks, neuroscience. Instructor consent required. Prerequisites: Statistics 521L, 523L; Statistics 531, 532 (or co-registration). (3 units)
Ph.D. Programs
Biological and biomedical sciences, physical sciences and engineering, social sciences.
* – Denotes Ph.D. admitting programs. Students may apply and be admitted directly to these departments or programs, but the Ph.D. is offered only through one of the participating departments identified in the program description. After their second year of study at Duke, students must select a participating department in which they plan to earn the Ph.D.
Biochemistry Biology Biostatistics Cell and Molecular Biology Cell Biology Cognitive Neuroscience* Computational Biology and Bioinformatics Developmental and Stem Cell Biology* Ecology Evolutionary Anthropology Genetics and Genomics
Immunology Integrated Toxicology and Environmental Health* Medical Physics Medical Scientist Training Molecular Cancer Biology Molecular Genetics and Microbiology Neurobiology Pathology Pharmacology Population Health Sciences
Art, Art History and Visual Studies Classical Studies Computational Media, Arts & Cultures English German Studies (Carolina-Duke German Program)
Literature Music Philosophy Religious Studies Romance Studies
Biomedical Engineering Chemistry Civil and Environmental Engineering Computer Science Earth and Climate Sciences Electrical and Computer Engineering Environment
Marine Science and Conservation Materials Science and Engineering Mathematics Mechanical Engineering and Materials Science Physics Statistical Science
Business Administration Cultural Anthropology Economics Environmental Policy History
Nursing Political Science Psychology and Neuroscience Public Policy Sociology
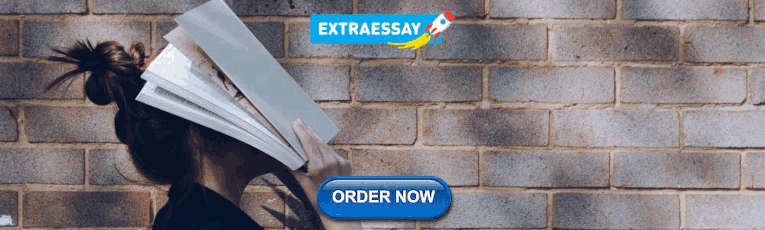
IMAGES
VIDEO
COMMENTS
Statistical Science at Duke is the world's leading graduate research and educational environment for Bayesian statistics, emphasizing the major themes of 21st century statistical science: foundational concepts of statistics, theory and methods of complex stochastic modeling, interdisciplinary applications of statistics, computational statistics, big data analytics, and machine learning.
Biostatistics PhD candidate at Duke University, School of Medicine interested in graphical models and machine learning. Durham, North Carolina, United States 209 followers 207 connections
PhD Student at Duke University · *Incoming Decision Science Graduate Intern @ Disney*<br><br>I have always loved working with numbers. As a young child, I just couldn't get enough ...
Duke University School of Medicine. Aug 2021 - Present 2 years 7 months. Durham, North Carolina, United States. Advisors: Professors Cynthia Rudin (Duke Computer Science), David Page (Duke ...
Merlise Clyde Director of Graduate Studies Department of Statistical Science Duke University Box 90251 Durham, NC 27708-0251 Phone: (919) 684-8029. Contact: Director of Graduate Studies (for PhD) [email protected]. PhD Program: https://stat.duke.edu/phd
Ph.D. Candidate, Statistics @ Duke | Ex-Biostatistics Intern @ Merck Durham, NC. Connect ... Statistics PhD Candidate at Stanford University Stanford, CA. Connect ...
The PhD program offers thorough preparation in the theory and methods of statistics, with major emphases on modern, model-based statistical science, Bayesian and classical approaches to inference, computational statistics, and machine learning. A hallmark of the program is the integration of interdisciplinary applications into teaching and ...
About Us. The Department of Statistical Science is helping lead the data and computational revolution through its research, teaching, and service. Our faculty and students produce groundbreaking research in theory, methods, and applications that ultimately advances science and positively impacts society. We offer undergraduate, master's, and Ph ...
Director of Graduate Studies Department of Statistical Science Duke University Box 90251 Durham, NC 27708-0251 Phone: (919) 684-8029. Contact: Director of Graduate Studies (for PhD) [email protected]. PhD Program: https://stat.duke.edu/phd
The Master's in Statistical Science (MSS) is a 2-year graduate degree program that provides a modern, comprehensive education in statistical theory, methods and computation. The MSS is attractive ...
The Department of Biostatistics and Bioinformatics offers a Ph.D. degree in Biostatistics through the Duke University Graduate School. A distinguishing feature of the program is its integration within the world-class biomedical research enterprise at Duke University and the Duke School of Medicine. The goal of the program is to train students ...
Are you interested in pursuing a PhD in Biostatistics at Duke University? Find out how long it takes to complete the program, what are the graduation requirements, and how many students have earned their degrees in the past years. Learn more about the Biostatistics PhD Time to Degree Statistics at The Graduate School.
Applications to the Ph.D. in Biostatistics is through the Duke University Graduate School application website. There you will find instructions and the needed information to apply. The online application for the 2023 - 2024 program is open. Please note: Application materials emailed or mailed to individual faculty members will not be reviewed ...
James B. Duke Distinguished Professor. [email protected]. Fan Li. Professor of Statistical Science. ... Arts and Sciences Distinguished Professor of Statistics and Decision Sciences. Personal site. Jason Xu. Assistant Professor of Statistical Science ... Admission Statistics; Graduate Placements; Living in Durham; Course Help & Tutoring; MSS ...
These affiliations provide a wide range of experiences and opportunities for graduate study. Statistics. Biostatistics: Ph.D. Admissions and Enrollment Statistics; Biostatistics: PhD Time to Degree Statistics; Biostatistics: PhD Career Outcomes Statistics Application Information. Application Terms Available: Fall. Application Deadline: November 30
M.S. Program. Master's in Statistical Science (MSS) program is a rigorous two-year graduate experience, where you'll delve into the very core of statistical theory, methods, computation, and their real-world applications. This is the pathway to unlock a world of professional opportunities in industry, business, and government.
Postdoctoral Researcher, Stanford University, Sep 2022. PhD Dissertation - Tree-Based Methods for Learning Probability Distribution. Li Ma. 2022. Caprio, Michele. Postdoctoral Researcher, University of Pennsylvania, Department of Computer & Information Science, June 2022. Advances in Choquet Theories. Sayan Mukherjee. 2022.
The PhD program follows the Duke Graduate School Academic Calendar. View the timeline for students with and without an Applicable Quantitative Master's Degree. For students with a Master's degree in Biostatistics, some of the required 700 level courses listed below may be waived if they have taken those courses or their equivalents previously.
Statistics. In an effort to provide comprehensive information for all interested individuals, The Duke University Graduate School posts summary data on its Ph.D. and master's programs. These data include information such as total applications, admissions, matriculations, demographics, median GRE and GPA scores, and career outcomes.
Associate Professor of Economics, Department of Statistics, Graduate School of Economics, The University of Tokyo, Nov 2022 MSS En-Route 2014: Kyzyurova, Ksenia N MSS En-Route 2014: Larson, Gary J: Statistical Analyst, Social & Scientific Systems, Oct. 2018 MSS En-Route 2014: Liang, Jiawei Wesley: Product Science Lead, Indeed.com, Jul 2019
Ph.D. Programs. * - Denotes Ph.D. admitting programs. Students may apply and be admitted directly to these departments or programs, but the Ph.D. is offered only through one of the participating departments identified in the program description. After their second year of study at Duke, students must select a participating department in which ...
Statistical Science undergraduate student Ryan Mitchell was a winner of the 2023 Fall Undergraduate Statistics Research Project Competition, earning 3rd place among all submissions. Mitchell — whose advisor was Assistant Professor of the Practice Yue Jiang — will graduate this Spring with a double major in Computer Science and Statistical Science with a concentration in Data Science.