- Integrative Review
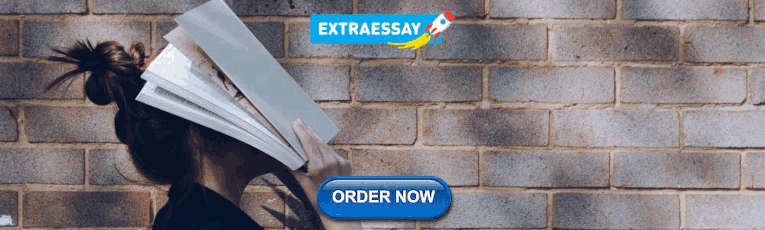
What is an Integrative Review?
An integrative review provides a broader summary of the literature and includes findings from a range of research designs. It gathers and synthesizes both empirical and theoretical evidence relevant to a clearly defined problem. It may include case studies, observational studies, and meta-analyses, but may also include practice applications, theory, and guidelines. It is the only approach that allows for the combination of diverse methodologies. Its aim is to develop a holistic understanding of the topic, present the state of the science and contribute to theory development. The integrative review has been advocated as important for evidence-based practice initiatives in nursing (Hopia et al., 2016).
Integrative reviews are popular in nursing because they use diverse data sources to investigate the complexity of nursing practice. An integrative review addresses the current state of the evidence, the quality of the available evidence, identifies gaps in the literature and suggests future directions for research and practice The clinical question(s) of an integrative review is broader than that of a systematic review, yet should be clearly stated and well-defined. As with a systematic review, an integrative review requires a transparent and rigorous systematic approach (Remington & Toronto, 2020).
Integrative reviews synthesize research data from various research designs to reach comprehensive and reliable conclusions. An integrative review helps to develop a comprehensive understanding of the topic by synthesizing all forms of available evidence (Dhollande et al., 2021). They allow healthcare professionals to use all available evidence from both qualitative and quantitative research to provide a more holistic understanding of the topic, which can then be applied to clinical practice. Sampling for an integrative review may include experimental and nonexperimental (empirical) and theoretical literature (Remington & Toronto, 2020).
From: Kutcher, & LeBaron, V. T. (2022). A simple guide for completing an integrative review using an example article. Journal of Professional Nursing, 40 , 13-19. https://doi.org/10.1016/j.profnurs.2022.02.004
See Table 2: Steps of the integrative review (IR) process with key points and lessons learned
Steps of the Integrative Review Process
1: Select a Topic: Formulate a purpose and/or review question(s). An integrative review can be used to answer research questions related to nursing and other disciplines. Clearly identify a problem from a gap in the literature. Perform a quick search for other literature reviews related to the topic of interest to avoid duplication. Integrative review questions should be broad in scope, but narrow enough that the search is manageable. It should be well-defined, and clearly stated . Provide background on the topic and justification for the integrative review. Do a quick literature search to determine if any recent integrative or other types of reviews on or related to the topic have been performed.
Quality Appraisal Tools for Integrative Reviews
Critical Appraisal Skills Programme (CASP) Checklists Appraisal checklists designed for use with Systematic Reviews, Randomized Controlled Trials, Cohort Studies, Case Control Studies, Economic Evaluations, Diagnostic Studies, Qualitative studies and Clinical Prediction Rule.
Mixed Methods Appraisal Tool (MMAT) The MMAT is a critical appraisal tool that is designed for the appraisal stage of systematic mixed studies reviews, i.e., reviews that include qualitative, quantitative and mixed methods studies. It permits to appraise the methodological quality of five categories to studies: qualitative research, randomized controlled trials, non-randomized studies, quantitative descriptive studies, and mixed methods studies. (Hong et al., 2018).
Hong, Q. N., Fàbregues, S., Bartlett, G., Boardman, F., Cargo, M., Dagenais, P., Gagnon, M.-P., Griffiths, F., Nicolau, B., O’Cathain, A., Rousseau, M.-C., Vedel, I., & Pluye, P. (2018). The Mixed Methods Appraisal Tool (MMAT) version 2018 for information professionals and researchers. Education for Information, 34 (4), 285–291. https://doi.org/10.3233/EFI-180221
More Information
For more information on integrative reviews:
Dhollande, S., Taylor, A., Meyer, S., & Scott, M. (2021). Conducting integrative reviews: A guide for novice nursing researchers. Journal of Research in Nursing, 26( 5), 427–438. https://doi.org/10.1177/1744987121997907
Evans, D. (2007). Integrative reviews: Overview of methods. In C. Webb, & B. Roe (Eds.), Reviewing research evidence for nursing practice: Systematic reviews (pp. 135 - 148). John Wiley & Sons, Incorporated.
Hopia, Latvala, E., & Liimatainen, L. (2016). Reviewing the methodology of an integrative review. Scandinavian Journal of Caring Sciences, 30 (4), 662–669. https://doi.org/10.1111/scs.12327
Kutcher, & LeBaron, V. T. (2022). A simple guide for completing an integrative review using an example article. Journal of Professional Nursing, 40 , 13-19. https://doi.org/10.1016/j.profnurs.2022.02.004
Oermann, M. H., & Knafl, K. A. (2021). Strategies for completing a successful integrative review. Nurse Author & Editor (Blackwell) , 31 (3/4), 65–68. https://doi-org.libproxy.adelphi.edu/10.1111/nae2.30
Toronto, C. E., & Remington, R. (Eds.). (2020). A step-by-step guide to conducting an integrative review . Springer.
Whittemore, R., & Knafl, K. (2005). The integrative review: updated methodology. Journal of Advanced Nursing , 52 (5), 546–553. https://doi.org/10.1111/j.1365-2648.2005.03621.x
Whittemore, R. (2007). Rigour in integrative reviews. In C. Webb, & B. Roe (Eds.), Reviewing research evidence for nursing practice: Systematic reviews (pp. 149 - 156). John Wiley & Sons, Incorporated.
- << Previous: Evidence Synthesis Review Types - Overview
- Next: Scoping Review >>
- Types of Questions
- Key Features and Limitations
- Is a Systematic Review Right for Your Research?
- Scoping Review
- Rapid Review
- Meta-Analysis/Meta-Synthesis
- Selecting a Review Type
- Reducing Bias
- Guidelines for Student Researchers
- Training Resources
- Register Your Protocol
- Handbooks & Manuals
- Reporting Guidelines
- PRESS 2015 Guidelines
- Search Strategies
- Selected Databases
- Grey Literature
- Handsearching
- Citation Searching
- Study Types & Terminology
- Quantitative vs. Qualitative Research
- Critical Appraisal of Studies
- Broad Functionality Programs & Tools
- Search Strategy Tools
- Deduplication Tools
- CItation Screening
- Critical Appraisal Tools
- Quality Assessment/Risk of Bias Tools
- Data Collection/Extraction
- Meta Analysis Tools
- Books on Systematic Reviews
- Finding Systematic Review Articles in the Databases
- Systematic Review Journals
- More Resources
- Evidence-Based Practice Research in Nursing
- Citation Management Programs
- Last Updated: Apr 5, 2024 2:54 PM
- URL: https://libguides.adelphi.edu/Systematic_Reviews
AACN Levels of Evidence
Add to Collection
Added to Collection
Level A — Meta-analysis of quantitative studies or metasynthesis of qualitative studies with results that consistently support a specific action, intervention, or treatment (including systematic review of randomized controlled trials).
Level B — Well-designed, controlled studies with results that consistently support a specific action, intervention, or treatment.
Level C — Qualitative studies, descriptive or correlational studies, integrative review, systematic review, or randomized controlled trials with inconsistent results.
Level D — Peer-reviewed professional and organizational standards with the support of clinical study recommendations.
Level E — Multiple case reports, theory-based evidence from expert opinions, or peer-reviewed professional organizational standards without clinical studies to support recommendations.
Level M — Manufacturer’s recommendations only.
(Excerpts from Peterson et al. Choosing the Best Evidence to Guide Clinical Practice: Application of AACN Levels of Evidence. Critical Care Nurse. 2014;34[2]:58-68.)
What is the purpose of levels of evidence (LOEs)?
“The amount and availability of research supporting evidence-based practice can be both useful and overwhelming for critical care clinicians. Therefore, clinicians must critically evaluate research before attempting to put the findings into practice. Evaluation of research generally occurs on 2 levels: rating or grading the evidence by using a formal level-of-evidence system and individually critiquing the quality of the study. Determining the level of evidence is a key component of appraising the evidence.1-3 Levels or hierarchies of evidence are used to evaluate and grade evidence. The purpose of determining the level of evidence and then critiquing the study is to ensure that the evidence is credible (eg, reliable and valid) and appropriate for inclusion into practice.3 Critique questions and checklists are available in most nursing research and evidence-based practice texts to use as a starting point in evaluation.”
How are LOEs determined?
“The most common method used to classify or determine the level of evidence is to rate the evidence according to the methodological rigor or design of the research study.3,4 The rigor of a study refers to the strict precision or exactness of the design. In general, findings from experimental research are considered stronger than findings from nonexperimental studies, and similar findings from more than 1 study are considered stronger than results of single studies. Systematic reviews of randomized controlled trials are considered the highest level of evidence, despite the inability to provide answers to all questions in clinical practice.”4,5
Who developed the AACN LOEs?
“As interest in promoting evidence-based practice has grown, many professional organizations have adopted criteria to evaluate evidence and develop evidence-based guidelines for their members.”1,5 Originally developed in 1995, AACN’s rating scale has been updated in 2008 and 2014 by the Evidence-Based Practice Resources Workgroup (EBPRWG). The 2011-2013 EBPRWG continued the tradition of previous workgroups to move research to the patient bedside.
What are the AACN LOEs and how are they used?
The AACN levels of evidence are structured in an alphabetical hierarchy in which the highest form of evidence is ranked as A and includes meta-analyses and meta-syntheses of the results of controlled trials. Evidence from controlled trials is rated B. Level C, the highest level for nonexperimental studies includes systematic reviews of qualitative, descriptive, or correlational studies. “Levels A, B, and C are all based on research (either experimental or nonexperimental designs) and are considered evidence. Levels D, E, and M are considered recommendations drawn from articles, theory, or manufacturers’ recommendations.”
“Clinicians must critically evaluate research before attempting to implement the findings into practice. The clinical relevance of any research must be evaluated as appropriate for inclusion into practice.”
- Polit DF, Beck CT. Resource Manual for Nursing Research: Generating and Assessing Evidence for Nursing Practice. 9th ed. Philadelphia, PA: Williams & Wilkins; 2012.
- Armola RR, Bourgault AM, Halm MA, et al; 2008-2009 Evidence-Based Practice Resource Work Group of the American Association of Critical-Care Nurses. Upgrading the American Association of Critical-Care Nurses’ evidence-leveling hierarchy. Am J Crit Care. 2009;18(5):405-409.
- Melnyk BM, Fineout-Overholt, E. Evidence-Based Practice in Nursing and Healthcare: A Guide to Best Practice. 2nd ed. Philadelphia, PA: Lippincott Williams & Wilkins; 2011.
- Gugiu PC, Gugiu MR. A critical appraisal of standard guidelines for grading levels of evidence. Eval Health Prof. 2010;33(3):233-255. doi:10.1177/0163278710373980.
- Evans D. Hierarchy of evidence: a framework for ranking evidence evaluating healthcare interventions. J Clin Nurs. 2003;12(1):77-84.

Gerstein Science Information Centre
Knowledge syntheses: systematic & scoping reviews, and other review types.
- Before you start
- Getting Started
- Different Types of Knowledge Syntheses
- Assemble a Team
- Develop your Protocol
- Eligibility Criteria
- Screening for articles
- Data Extraction
- Critical appraisal
- What are Systematic Reviews?
- What is a Meta-Analysis?
- What are Scoping Reviews?
- What are Rapid Reviews?
- What are Realist Reviews?
- What are Mapping Reviews?
When is an integrative review methodology appropiate?
Elements of an integrative review, methods and guidance.
- What are Umbrella Reviews?
- Standards and Guidelines
- Supplementary Resources for All Review Types
- Resources for Qualitative Synthesis
- Resources for Quantitative Synthesis
- Resources for Mixed Methods Synthesis
- Bibliography
- More Questions?
- Common Mistakes in Systematic Reviews, scoping reviews, and other review types
An integrative review is a specific review method that summarises past empirical or theoretical literature to provide a greater comprehensive understanding of a particular phenomenon or healthcare problem (Broome 1993). Thus, integrative reviews have the potential to build upon nursing science, informing research, practice, and policy initiatives.
An integrative review method is an approach that allows for the inclusion of diverse methodologies (i.e. experimental and non-experimental research) and has the potential to play a greater role in evidence-based practice for nursing (Whittemore et al., 2005) .
When to Use It: According to Toronto, C., & Remington, R.(2020) , Whitmore et al. (2005) , Broome (1993): an integrative review approach is best suited for:
A research scope focused more broadly at a phenomenon of interest rather than a systematic review and allows for diverse research, which may contain theoretical and methodological literature to address the aim of the review
Supporting a wide range of inquiry, such as defining concepts, reviewing theories, or analyzing methodological issues
Examining the complexity of nursing practice more broadly by using diverse data sources
The following characteristics, strengths, and challenges of integrative reviews are derived from Toronto, C., & Remington, R.(2020) , Whitmore et al. (2005) , Broome (1993):
Characteristics:
A review method that summarises past empirical or theoretical literature to provide a more comprehensive understanding of a particular phenomenon or healthcare problem
An integrative review is best designed for nursing practice
The problem must be clearly defined
The aim of the review is to analyze experimental and non-experimental research simultaneously in order to:
Define concepts
Review theories
Review evidence/point out gaps in the literature
Analyze methodological issues
Best designed for nursing research
Evidence produced from well-conducted integrative reviews contributes to nursing knowledge by clarifying phenomena, which in turn informs nursing practice and clinical practice guidelines
Challenges:
The combination and complexity of incorporating diverse methodologies can contribute to a lack of rigour, inaccuracy, and bias
Methods of analysis, synthesis, and conclusion-drawing remain poorly formulated
Combining empirical and theoretical reports can be difficult
There is no current guidance on reporting
The following resources are considered to be the best guidance for conduct in the field of integrative reviews.
METHODS & GUIDANCE
Hopia, H., Latvala, E., & Liimatainen, L. (2016). Reviewing the methodology of an integrative review. Scandinavian journal of caring sciences , 30 (4), 662–669. https://doi.org/10.1111/scs.12327
Russell C. L. (2005). An overview of the integrative research review. Progress in transplantation (Aliso Viejo, Calif.) , 15 (1), 8–13
Toronto, & Remington, R. (2020). A Step-By-Step Guide to Conducting an Integrative Review (1st ed.). Springer International Publishing AG.
Whittemore, R., & Knafl, K. (2005). The integrative review: updated methodology . Journal of advanced nursing , 52 (5), 546–553. https://doi.org/10.1111/j.1365-2648.2005.03621.x
REPORTING GUIDELINE
There is currently no reporting guideline for integrative reviews.
- << Previous: What are Mapping Reviews?
- Next: What are Umbrella Reviews? >>
- Last Updated: Apr 16, 2024 1:53 PM
- URL: https://guides.library.utoronto.ca/systematicreviews
Library links
- Gerstein Home
- U of T Libraries Home
- Renew items and pay fines
- Library hours
- Contact Gerstein
- University of Toronto Libraries
- UT Mississauga Library
- UT Scarborough Library
- Information Commons
- All libraries

© University of Toronto . All rights reserved.
Connect with us

- more social media
- Library databases
- Library website
Evidence-Based Research: Levels of Evidence Pyramid
Introduction.
One way to organize the different types of evidence involved in evidence-based practice research is the levels of evidence pyramid. The pyramid includes a variety of evidence types and levels.
- systematic reviews
- critically-appraised topics
- critically-appraised individual articles
- randomized controlled trials
- cohort studies
- case-controlled studies, case series, and case reports
- Background information, expert opinion
Levels of evidence pyramid
The levels of evidence pyramid provides a way to visualize both the quality of evidence and the amount of evidence available. For example, systematic reviews are at the top of the pyramid, meaning they are both the highest level of evidence and the least common. As you go down the pyramid, the amount of evidence will increase as the quality of the evidence decreases.

Text alternative for Levels of Evidence Pyramid diagram
EBM Pyramid and EBM Page Generator, copyright 2006 Trustees of Dartmouth College and Yale University. All Rights Reserved. Produced by Jan Glover, David Izzo, Karen Odato and Lei Wang.
Filtered Resources
Filtered resources appraise the quality of studies and often make recommendations for practice. The main types of filtered resources in evidence-based practice are:
Scroll down the page to the Systematic reviews , Critically-appraised topics , and Critically-appraised individual articles sections for links to resources where you can find each of these types of filtered information.
Systematic reviews
Authors of a systematic review ask a specific clinical question, perform a comprehensive literature review, eliminate the poorly done studies, and attempt to make practice recommendations based on the well-done studies. Systematic reviews include only experimental, or quantitative, studies, and often include only randomized controlled trials.
You can find systematic reviews in these filtered databases :
- Cochrane Database of Systematic Reviews Cochrane systematic reviews are considered the gold standard for systematic reviews. This database contains both systematic reviews and review protocols. To find only systematic reviews, select Cochrane Reviews in the Document Type box.
- JBI EBP Database (formerly Joanna Briggs Institute EBP Database) This database includes systematic reviews, evidence summaries, and best practice information sheets. To find only systematic reviews, click on Limits and then select Systematic Reviews in the Publication Types box. To see how to use the limit and find full text, please see our Joanna Briggs Institute Search Help page .

You can also find systematic reviews in this unfiltered database :

To learn more about finding systematic reviews, please see our guide:
- Filtered Resources: Systematic Reviews
Critically-appraised topics
Authors of critically-appraised topics evaluate and synthesize multiple research studies. Critically-appraised topics are like short systematic reviews focused on a particular topic.
You can find critically-appraised topics in these resources:
- Annual Reviews This collection offers comprehensive, timely collections of critical reviews written by leading scientists. To find reviews on your topic, use the search box in the upper-right corner.
- Guideline Central This free database offers quick-reference guideline summaries organized by a new non-profit initiative which will aim to fill the gap left by the sudden closure of AHRQ’s National Guideline Clearinghouse (NGC).
- JBI EBP Database (formerly Joanna Briggs Institute EBP Database) To find critically-appraised topics in JBI, click on Limits and then select Evidence Summaries from the Publication Types box. To see how to use the limit and find full text, please see our Joanna Briggs Institute Search Help page .
- National Institute for Health and Care Excellence (NICE) Evidence-based recommendations for health and care in England.
- Filtered Resources: Critically-Appraised Topics
Critically-appraised individual articles
Authors of critically-appraised individual articles evaluate and synopsize individual research studies.
You can find critically-appraised individual articles in these resources:
- EvidenceAlerts Quality articles from over 120 clinical journals are selected by research staff and then rated for clinical relevance and interest by an international group of physicians. Note: You must create a free account to search EvidenceAlerts.
- ACP Journal Club This journal publishes reviews of research on the care of adults and adolescents. You can either browse this journal or use the Search within this publication feature.
- Evidence-Based Nursing This journal reviews research studies that are relevant to best nursing practice. You can either browse individual issues or use the search box in the upper-right corner.
To learn more about finding critically-appraised individual articles, please see our guide:
- Filtered Resources: Critically-Appraised Individual Articles
Unfiltered resources
You may not always be able to find information on your topic in the filtered literature. When this happens, you'll need to search the primary or unfiltered literature. Keep in mind that with unfiltered resources, you take on the role of reviewing what you find to make sure it is valid and reliable.
Note: You can also find systematic reviews and other filtered resources in these unfiltered databases.
The Levels of Evidence Pyramid includes unfiltered study types in this order of evidence from higher to lower:
You can search for each of these types of evidence in the following databases:
TRIP database
Background information & expert opinion.
Background information and expert opinions are not necessarily backed by research studies. They include point-of-care resources, textbooks, conference proceedings, etc.
- Family Physicians Inquiries Network: Clinical Inquiries Provide the ideal answers to clinical questions using a structured search, critical appraisal, authoritative recommendations, clinical perspective, and rigorous peer review. Clinical Inquiries deliver best evidence for point-of-care use.
- Harrison, T. R., & Fauci, A. S. (2009). Harrison's Manual of Medicine . New York: McGraw-Hill Professional. Contains the clinical portions of Harrison's Principles of Internal Medicine .
- Lippincott manual of nursing practice (8th ed.). (2006). Philadelphia, PA: Lippincott Williams & Wilkins. Provides background information on clinical nursing practice.
- Medscape: Drugs & Diseases An open-access, point-of-care medical reference that includes clinical information from top physicians and pharmacists in the United States and worldwide.
- Virginia Henderson Global Nursing e-Repository An open-access repository that contains works by nurses and is sponsored by Sigma Theta Tau International, the Honor Society of Nursing. Note: This resource contains both expert opinion and evidence-based practice articles.
- Previous Page: Phrasing Research Questions
- Next Page: Evidence Types
- Office of Student Disability Services
Walden Resources
Departments.
- Academic Residencies
- Academic Skills
- Career Planning and Development
- Customer Care Team
- Field Experience
- Military Services
- Student Success Advising
- Writing Skills
Centers and Offices
- Center for Social Change
- Office of Academic Support and Instructional Services
- Office of Degree Acceleration
- Office of Research and Doctoral Services
- Office of Student Affairs
Student Resources
- Doctoral Writing Assessment
- Form & Style Review
- Quick Answers
- ScholarWorks
- SKIL Courses and Workshops
- Walden Bookstore
- Walden Catalog & Student Handbook
- Student Safety/Title IX
- Legal & Consumer Information
- Website Terms and Conditions
- Cookie Policy
- Accessibility
- Accreditation
- State Authorization
- Net Price Calculator
- Contact Walden
Walden University is a member of Adtalem Global Education, Inc. www.adtalem.com Walden University is certified to operate by SCHEV © 2024 Walden University LLC. All rights reserved.
University of Houston Libraries
- Literature Reviews in the Health Sciences
- Review Comparison Chart
- Decision Tools
- Systematic Review
- Meta-Analysis
- Scoping Review
- Mapping Review
- Integrative Review
- Rapid Review
- Realist Review
- Umbrella Review
- Review of Complex Interventions
- Diagnostic Test Accuracy Review
- Narrative Literature Reviews
- Standards and Guidelines
Navigate the links below to jump to a specific section of the page:
When is an Integrative Review methodology appropriate?
Outline of stages, methods and guidance, examples of integrative reviews, supplementary resources.
"An integrative review is a specific review method that summarizes past empirical or theoretical literature to provide a greater comprehensive understanding of a particular phenomenon or healthcare problem" (Broome, 1993). Thus, integrative reviews have the potential to build upon nursing science, informing research, practice, and policy initiatives.
An integrative review method is an approach that allows for the inclusion of diverse methodologies (i.e. experimental and non-experimental research) and have the potential to play a greater role in evidence-based practice for nursing ( Whittemore & Knafl, 2005 ).
Characteristics:
- An integrative review is best designed for nursing research
- The problem must be clearly defined
- define concepts
- review theories
- review evidence/point out gaps in the literature
- analyze methodological issues
When to Use It: According to Toronto & Remington (2020) , Whittmore & Knafl (2005) , and Broome (2000) an integrative review approach is best suited for:
- A research scope focused more broadly at a phenomenon of interest rather than a systematic review and allows for diverse research, which may contain theoretical and methodological literature to address the aim of the review.
- Supporting a wide range of inquiry, such as defining concepts, reviewing theories, or analyzing methodological issues.
- Examining the complexity of nursing practice more broadly by using diverse data sources.
The following stages of conducting an integrative review are derived from Whittemore & Knafl (2005) .
Timeframe: 12+ months
*Varies beyond the type of review. Depends on many factors such as but not limited to: resources available, the quantity and quality of the literature, and the expertise or experience of reviewers" ( Grant & Booth, 2009 ).
Question: Formulation of a problem, may be related to practice and/or policy especially in nursing.
Is your review question a complex intervention? Learn more about Reviews of Complex Interventions .
Sources and searches: Comprehensive but with a specific focus, integrated methodologies-experimental and non-experimental research. Purposive Sampling may be employed. Database searching is recommended along with grey literature searching. "Other recommended approaches to searching the literature include ancestry searching, journal hand searching, networking, and searching research registries." Search is transparent and reproducible.
Selection: Selected as related to problem identified or question, Inclusion of empirical and theoretical reports and diverse study methodologies.
Appraisal: "How quality is evaluated in an integrative review will vary depending on the sampling frame." Limited/varying methods of critical appraisal and can be complex. "In a review that encompasses theoretical and empirical sources, two quality criteria instruments could be developed for each type of source and scores could be used as criteria for inclusion/exclusion or as a variable in the data analysis stage."
Synthesis: Narrative synthesis for qualitative and quantitative studies. Data extracted for study characteristics and concept. Synthesis may be in the form of a table, diagram or model to portray results. "Extracted data are compared item by item so that similar data are categorized and grouped together."
The method consists of:
- data reduction
- data display
- data comparison
- conclusion drawing,
- verification
The following resources are considered to be the best guidance for conduct in the field of integrative reviews.
Methods & Guidance
- Hopia, H., Latvala, E., & Liimatainen, L. (2016). Reviewing the methodology of an integrative review . Scandinavian journal of caring sciences , 30 (4), 662–669. doi: 10.1111/scs.12327
- Russell C. L. (2005). An overview of the integrative research review . Progress in transplantation , 15 (1), 8–13. doi: 10.1177/152692480501500102
- Whittemore, R., & Knafl, K. (2005). The integrative review: updated methodology . Journal of advanced nursing , 52 (5), 546–553. doi: 10.1111/j.1365-2648.2005.03621.x
Reporting Guideline
There is currently no reporting guideline for integrative reviews.
- Collins, J. W., Zoucha, R., Lockhart, J. S., & Mixer, S. J. (2018). Cultural aspects of end-of-life care planning for African Americans: an integrative review of literature . Journal of transcultural nursing , 29 (6), 578–590. doi: 10.1177/1043659617753042
- Cowdell, F., Booth, A., & Appleby, B. (2017). Knowledge mobilization in bridging patient-practitioner-researcher boundaries: a systematic integrative review protocol . Journal of advanced nursing , 73 (11), 2757–2764. doi: 10.1111/jan.13378
- Frisch, N. C., & Rabinowitsch, D. (2019). What's in a definition? Holistic nursing, integrative health care, and integrative nursing: report of an integrated literature review . Journal of holistic nursing , 37 (3), 260–272. doi: 10.1177/0898010119860685
- Kim, J., Kim, Y. L., Jang, H., Cho, M., Lee, M., Kim, J., & Lee, H. (2020). Living labs for health: an integrative literature review . European journal of public health , 30 (1), 55–63. doi: 10.1093/eurpub/ckz105
- Luckett, T., Sellars, M., Tieman, J., Pollock, C. A., Silvester, W., Butow, P. N., Detering, K. M., Brennan, F., & Clayton, J. M. (2014). Advance care planning for adults with CKD: a systematic integrative review . American journal of kidney diseases , 63 (5), 761–770. doi: 10.1053/j.ajkd.2013.12.007
- Shinners, L., Aggar, C., Grace, S., & Smith, S. (2020). Exploring healthcare professionals' understanding and experiences of artificial intelligence technology use in the delivery of healthcare: an integrative review . Health informatics journal , 26 (2), 1225–1236. doi: 10.1177/1460458219874641
- Silva, D., Tavares, N. V., Alexandre, A. R., Freitas, D. A., Brêda, M. Z., Albuquerque, M. C., & Melo, V. L. (2015). Depressão e risco de suicídio entre profissionais de Enfermagem: revisão integrative [Depression and suicide risk among nursing professionals: an integrative review] . Revista da Escola de Enfermagem da U S P , 49 (6), 1027–1036. doi: 10.1590/S0080-623420150000600020
- Stormacq, C., Van den Broucke, S., & Wosinski, J. (2019). Does health literacy mediate the relationship between socioeconomic status and health disparities? integrative review . Health promotion international , 34 (5), e1–e17. doi: 10.1093/heapro/day062
- Broome M.E. (1993). Integrative literature reviews for the development of concepts. In Rodgers, B. L., & Knafl, K. A. (Eds.), Concept development in nursing (2nd ed., pp. 231-250). W.B. Saunders Company.
- da Silva, R. N., Brandão, M., & Ferreira, M. A. (2020). Integrative Review as a Method to Generate or to Test Nursing Theory . Nursing science quarterly , 33 (3), 258–263. doi: 10.1177/0894318420920602
- Garritty, C., Gartlehner, G., Nussbaumer-Streit, B., King, V. J., Hamel, C., Kamel, C., Affengruber, L., & Stevens, A. (2021). Cochrane Rapid Reviews Methods Group offers evidence-informed guidance to conduct rapid reviews . Journal of clinical epidemiology , 130 , 13–22. doi: 10.1016/j.jclinepi.2020.10.007
- Grant, M. J., & Booth, A. (2009). A typology of reviews: an analysis of 14 review types and associated methodologies . Health information and libraries journal , 26 (2), 91–108. doi: 10.1111/j.1471-1842.2009.00848.x
Toronto, C. E., & Remington, R. (2020). A Step-By-Step Guide to Conducting an Integrative Review. Springer International Publishing AG. doi: 10.1007/978-3-030-37504-1
- Torraco, R. J. (2005). Writing integrative literature reviews: guidelines and examples . Human Resource Development Review, 4 (3), 356–367. doi: 10.1177/1534484305278283
- Whittemore. (2007). Rigour in Integrative Reviews . In Webb, C., & Roe, B. (Eds.), Reviewing Research Evidence for Nursing Practice (pp. 149–156). Blackwell Publishing Ltd. https://doi.org/10.1002/9780470692127.ch11
- << Previous: Mapping Review
- Next: Rapid Review >>
Other Names for an Integrative Review
- Integrative Literature Review
- Systematic Integrative Review
- Integrative Research Review
Limitations of an Integrative Review
The following challenges of integrative reviews are derived from Toronto & Remington (2020) , Whitmore & Knafl (2005) , and Broome (2000) .
- The combination and complexity of incorporating diverse methodologies can contribute to lack of rigor, inaccuracy, and bias.
- Methods of analysis, synthesis, and conclusion-drawing remain poorly formulated.
- Combining empirical and theoretical reports can be difficult.
- There is no current guidance on reporting.
Medical Librarian

- Last Updated: Sep 5, 2023 11:14 AM
- URL: https://guides.lib.uh.edu/reviews
Integrative literature review of evidence-based patient-centred care guidelines
Affiliations.
- 1 Department of Nursing Science, Faculty of Health Sciences, Nelson Mandela University, Port Elizabeth, South Africa.
- 2 Faculty of Health Sciences, Nelson Mandela University, Port Elizabeth, South Africa.
- 3 Department of Nursing and Midwifery, Faculty of Medicine and Health Sciences, Stellenbosch University, Cape Town, South Africa.
- PMID: 33314226
- DOI: 10.1111/jan.14716
Aim: To summarize what facilitates patient-centred care for adult patients in acute healthcare settings from evidence-based patient-centred care guidelines.
Design: An integrative literature review.
Data sources: The following data sources were searched between 2002-2020: Citation databases: CINAHL, Medline, Biomed Central, Academic Search Complete, Health Source: Nursing/Academic Edition and Google Scholar. Guideline databases: US National Guideline Clearinghouse, Guidelines International Network, and National Institute for Health and Clinical Excellence (NICE). Websites of guideline developers: Scottish Intercollegiate Guidelines Network, Royal College of Nurses, Registered Nurses Association of Ontario, New Zealand Guidelines Group, National Health and Medical Research Council, and Canadian Medical Association.
Review methods: Whittemore and Knafl's five-step integrative literature review: (1) identification of research problem; (2) search of the literature; (3) evaluation of data; (4) analysis of data; and (5) presentation of results.
Results: Following critical appraisal, nine guidelines were included for data extraction and synthesis. The following three groups of factors were found to facilitate patient-centred care: 1) Patient care practices: embracing values foundational to patient-centred care, optimal communication in all aspects of care, rendering basic nursing care practices, and family involvement; 2) Educational factors: staff and patient education; and 3) Organizational and policy factors: organizational and managerial support, organizational champions, healthy work environment, and organizational structures promoting interdisciplinary partnership.
Conclusion: Evidence from included guidelines can be used by nurses, with the required support and buy-in from management, to promote patient-centred care.
Impact: Patient-centred care is essential for quality care. No other literature review has been conducted in the English language to summarize evidence-based patient-centred care guidelines. Patient care practices and educational, organizational, and policy factors promote patient-centred care to improve quality of care and raise levels of awareness of patient-centred care among nursing staff and patients.
Keywords: Evidence-based; best practice guideline; literature review; nurses; patient-centred care.
© 2020 John Wiley & Sons Ltd.
Publication types
- New Zealand
- Nursing Staff*
- Patient-Centered Care*
- Quality of Health Care
MSU Libraries
- Need help? Ask a Librarian
Nursing Literature and Other Types of Reviews
- Literature and Other Types of Reviews
- Starting Your Search
- Constructing Your Search
- Selecting Databases and Saving Your Search
Levels of Evidence
- Creating a PRISMA Table
- Literature Table and Synthesis
- Other Resources
Levels of evidence (sometimes called hierarchy of evidence) are assigned to studies based on the methodological quality of their design, validity, and applicability to patient care. These decisions gives the grade (or strength) of recommendation. Just because something is lower on the pyramid doesn't mean that the study itself is lower-quality, it just means that the methods used may not be as clinically rigorous as higher levels of the pyramid. In nursing, the system for assigning levels of evidence is often from Melnyk & Fineout-Overholt's 2011 book, Evidence-based Practice in Nursing and Healthcare: A Guide to Best Practice . The Levels of Evidence below are adapted from Melnyk & Fineout-Overholt's (2011) model.

Melnyk & Fineout-Overholt (2011)
- Meta-Analysis: A systematic review that uses quantitative methods to summarize the results. (Level 1)
- Systematic Review: A comprehensive review that authors have systematically searched for, appraised, and summarized all of the medical literature for a specific topic (Level 1)
- Randomized Controlled Trials: RCT's include a randomized group of patients in an experimental group and a control group. These groups are followed up for the variables/outcomes of interest. Examples of RCTs are clinical trials that compare the effects of drugs, surgical techniques, medical devices, diagnostic procedures, diets or other medical treatments. (can be Level 2 or Level 4, depending on how expansive the study)
- Non-Randomized Controlled Trials: A clinical trial in which the participants are not assigned by chance to different treatment groups. Participants may choose which group they want to be in, or they may be assigned to the groups by the researchers.
- Cohort Study: Identifies two groups (cohorts) of patients, one which did receive the exposure of interest, and one which did not, and following these cohorts forward for the outcome of interest. ( Level 5)
- Case-Control Study: Involves identifying patients who have the outcome of interest (cases) and control patients without the same outcome, and looking to see if they had the exposure of interest.
- Background Information/Expert Opinion: Handbooks, encyclopedias, and textbooks often provide a good foundation or introduction and often include generalized information about a condition. While background information presents a convenient summary, often it takes about three years for this type of literature to be published. (Level 7)
- << Previous: Selecting Databases and Saving Your Search
- Next: Creating a PRISMA Table >>
- Last Updated: Mar 27, 2024 3:56 PM
- URL: https://libguides.lib.msu.edu/nursinglitreview
Evaluating and Using Medical Evidence in Integrative Mental Health Care: Literature Review, Evidence Tables, Algorithms, and the Promise of Artificial Intelligence
- First Online: 29 May 2019
Cite this chapter
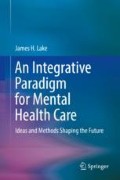
- James H. Lake 2
572 Accesses
1 Citations
The problem of evidence in medicine is discussed. Criteria are introduced for assigning levels of evidence to CAM modalities. In Western medicine findings from laboratory studies comprise the highest level of evidence for a putative mechanism of action and the relationship between “treatment” and “outcomes.” In contrast, in non-Western systems of medicine “evidence” reflects the values and beliefs of the parent culture. Important differences between quantitative and qualitative evidence are described. Special problems pertaining to literature research on CAM are discussed including how to formulate a question, identifying resources most likely to yield pertinent information on a particular subject, and using methods for optimizing and streamlining literature research. A clearly phrased question is the basis for any literature search. If the question is ambiguous or unfocused important resources will be overlooked, and relevant information will be missed. Valuable web-based resources are identified and practical tips are provided for obtaining current reliable information. Techniques for using prefiltered databases and evidence mapping are reviewed. The concepts of the evidence table and the algorithm are introduced. A methodology is proposed for using these tools when planning integrative mental health care. The accuracy and quality of information put into an algorithm will determine the effectiveness and relevance of clinical solutions generated by it for each unique patient. The optimal integrative care plan for a patient depends on history, symptoms, circumstances, preferences, and financial constraints in the context of locally available health care resources, and the professional judgment and clinical experience of the practitioner. The chapter concludes with a discussion of advances in artificial intelligence (AI) software and AI’s implications for the future of mental health care.
“Three things cannot be long hidden: the sun, the moon, and the truth.” —The Buddha
Links to all websites mentioned in this chapter are included in the book’s companion website http://integrativementalhealthplan.com
This is a preview of subscription content, log in via an institution to check access.
Access this chapter
- Available as PDF
- Read on any device
- Instant download
- Own it forever
- Available as EPUB and PDF
- Compact, lightweight edition
- Dispatched in 3 to 5 business days
- Free shipping worldwide - see info
- Durable hardcover edition
Tax calculation will be finalised at checkout
Purchases are for personal use only
Institutional subscriptions
ISPOR Connections. (2012). International Society for Pharmacoeconomics and Outcomes Research (ISPOR) (pp. 3–4). Retrieved from http://www.ispor.org
Acion, L., Kelmansky, D., van der Laan, M., Sahker, E., Jones, D., & Arndt, S. (2017). Use of a machine learning framework to predict substance use disorder treatment success. PLoS One, 12 (4), e0175383.
Article Google Scholar
Appelboom, G., LoPresti, M., Reginster, J. Y., Sander Connolly, E., & Dumont, E. P. (2014). The quantified patient: A patient participatory culture. Current Medical Research and Opinion, 30 (12), 2585–2587.
Banks, J. (1998). Handbook of simulation . New York: Wiley.
Book Google Scholar
Barry, C. A. (2006). The role of evidence in alternative medicine: Contrasting biomedical and anthropological approaches. Social Science & Medicine, 62 (11), 2646–2657.
Baumgartner Jr., W. A., Cohen, K. B., Fox, L., Acquaah-Mensah, G. K., & Hunter, L. (2007). Manual curation is not sufficient for annotation of genomic databases. Bioinformatics, 23 , i41–i48.
Beckner, W., & Berman, B. (2003). Complementary therapies on the Internet . St. Louis, MO: Churchill Livingstone.
Google Scholar
Bernardo, T. M., Rajic, A., Young, I., Robiadek, K., Pham, M. T., & Funk, J. A. (2013). Scoping review on search queries and social media for disease surveillance: A chronology of innovation. Journal of Medical Internet Research, 15 (7), e147.
Brailsford, S. C., Harper, P. R., Patel, B., & Pitt, M. (2009). An analysis of the academic literature on simulation and modelling in health care. Journal of Simulation, 3 (3), 130–140.
Browman, G. (2001). Development and aftercare of clinical guidelines: The balance between rigor and pragmatism. Journal of the American Medical Association, 286 , 1509–1511.
Dilsizian, S. E., & Siegel, E. L. (2014). Artificial intelligence in medicine and cardiac imaging: Harnessing big data and advanced computing to provide personalized medical diagnosis and treatment. Current Cardiology Reports, 16 , 441.
Doan, S., Conway, M., Phuong, T. M., & Ohno-Machado, L. (2014). Natural language processing in biomedicine: A unified system architecture overview. In R. J. A. Trent (Ed.), Clinical bioinformatics . New York: Springer.
Doshi, P., Kickersin, K., Healy, D., Vedula, S., & Jefferson, T. (2013, June). Restoring invisible and abandoned trials: A call for people to publish the findings, BMJ, 13 , 346.
Doshi, P., Shamseer, L., Jones, M., & Jefferson, T. (2018, April 26). Restoring biomedical literature with RIAT. BMJ, 361 , k1742.
Gantz, J., & Reinsel, D. (2011). Extracting value from chaos. IDC Iview, 1142 , 9–10. Good Practices Task Force. Value Health. 2015b;18(1):5–16.
Graham, J. (2016). Artificial intelligence, machine learning, and the FDA . Retrieved from https://www.forbes.com/sites/theapothecary/2016/08/19/artificial-intelligence-machine-learning-and-the-fda/#4aca26121aa1
Gray, G. (2004). Concise guide to evidence-based psychiatry . Washington, DC: American Psychiatric Publishing.
Guyatt, G., & Rennie, D. (Eds.). (2002). Users’ guide to the medical literature: Essentials of evidence-based clinical practice . Chicago: AMA Press.
Harrison, J. R., Lin, Z., Carroll, G. R., & Carley, K. M. (2007). Simulation modeling in organizational and management research. The Academy of Management Review, 32 (4), 1229–1245.
Hayes, M. J., & Prasad, V. (2018). Financial Conflicts of Interest at FDA Drug Advisory Committee Meetings. Cent Rep. 48(2):10–13.
Jadad, A., & Gagliardi, A. (1998). Rating health information on the Internet: Navigating to knowledge or to Babel? Journal of the American Medical Association, 279 , 611–614.
Jašović-Gašić, M., Dunjic-Kostić, B., Pantović, M., Cvetić, T., Marić, N. P., & Jovanović, A. A. (2013). Algorithms in psychiatry: State of the art. Psychiatria Danubina, 25 (3), 280–283.
PubMed Google Scholar
Katz, D. L., Williams, A. L., Girard, C., Goodman, J., Comerford, B., Behrman, A., et al. (2003). The evidence base for complementary and alternative medicine: Methods of evidence mapping with applications to CAM. Alternative Therapies in Health and Medicine, 9 (4), 22–30.
Kayyali, B., Knott, D., & Kuiken, S. V. (2013). The big-data revolution in US health care: Accelerating value and innovation . Retrieved from http://www.mckinsey.com/industries/healthcare-systems-and-services/ourinsights/the-big-data-revolution-in-us-health-care
Laney, D. (2012). The importance of ‘big data’: A definition . Gartner. Retrieved from https://www.scirp.org/(S(oyulxb452alnt1aej1nfow45))/reference/ReferencesPapers.aspx?ReferenceID=1287330
Leeper, N. J., Bauer-Mehren, A., Iyer, S. V., LePendu, P., Olson, C., & Shah, N. H. (2013). Practice-based evidence: Profiling the safety of cilostazol by text-mining of clinical notes. PLoS One, 8 (5), e63499.
LePendu, P., Iyer, S. V., Bauer-Mehren, A., Harpaz, R., Mortensen, J. M., Podchiyska, T., et al. (2013). Pharmacovigilance using clinical notes. Clinical Pharmacology & Therapeutics, 93 (6), 547–555.
Lohr, S. (2016). IBM is counting on its bet on Watson, and paying big money for it . Retrieved from https://www.nytimes.com/2016/10/17/technology/ibm-is-counting-on-its-bet-on-watson-and-paying-big-money-for-it.html
Lu, Z. (2011). PubMed and beyond: A survey of web tools for searching biomedical literature. Database, 2011 , baq036.
Lurie, P. (2018). Suggestions for improving conflict of interest processes in the US Food and Drug Administration Advisory Committees-Past Imperfect. JAMA Internal Medicine, 178 (7), 997–998. https://doi.org/10.1001/jamainternmed.2018.1324
Article PubMed Google Scholar
Mancano, M., & Bullano, M. (1998). Meta-analysis: Methodology, utility, and limitations. Journal of Pharmacy Practice, 11 (4), 239–250.
Manchikanti, L., Kaye, A. D., Boswell, M. V., & Hirsch, J. A. (2015, January–February). Medical journal peer review: Process and bias. Pain Physician, 18 (1), E1–E14.
Marshall, D. A. (2012). Getting connected: Systems solutions for generating maximal value from health care resources. In International Society for Pharmacoeconomics and Outcomes Research (ISPOR) Connections. 2012, International Society for Pharmacoeconomics and Outcomes Research (ISPOR) (pp. 3–4).
Marshall, D. A., Burgos-Liz, L., IJzerman, M. J., Crown, W., Padula, W. V., Wong, P. K., et al. (2015). Selecting a dynamic simulation modeling method for health care delivery research—part 2: Report of the ISPOR Dynamic Simulation Modeling Emerging Good Practices Task Force. Value in Health, 18 (2), 147–160.
Marshall, D. A., Burgos-Liz, L., IJzerman, M. J., Osgood, N. D., Padula, W. V., Higashi, M. K., et al. (2015). Applying dynamic simulation modeling methods in health care delivery research—The SIMULATE checklist: Report of the ISPOR Simulation Modeling Emerging Good Practices Task Force. Value in Health, 18 (1), 5–16.
Matthews, P. M., Edison, P., Geraghty, O. C., & Johnson, M. R. (2014). The emerging agenda of stratified medicine in neurology. Nature Reviews Neurology, 10 (1), 15–26.
Mayer-Schönberger, V., & Cukier, K. (2013). Big data: A revolution that will transform how we live, work, and think . New York: Houghton Mifflin Harcourt.
Mewes, H. W. (2013). Perspectives of a systems biology of the brain: The big data conundrum understanding psychiatric diseases. Pharmacopsychiatry, 46 (Suppl 1), S2–S9.
Miller, A. L., Crismon, M. L., Rush, A. J., Chiles, J., Kashner, M., Toprac, M., et al. (2004). The Texas medication algorithm project: Clinical results for schizophrenia. Schizophrenia Bulletin, 30 , 627–647.
Moore, M., & McQuay, H. (2006). Bandolier’s little book of making sense of the medical evidence . New York, NY: Oxford University Press.
Morley, J., Rosner, A. L., & Redwood, D. (2001). A case study of misrepresentation of the scientific literature: Recent reviews of chiropractic. Journal of Alternative and Complementary Medicine, 7 (1), 65–78.
Neill, D. B. (2013). Using artificial intelligence to improve hospital inpatient care. IEEE Intelligent Systems, 28 , 92–95.
Ong, J. B. S., Chen, M. I., Cook, A. R., Lee, H. C., Lee, V. J., Lin, R. T., et al. (2010). Real-time epidemic monitoring and forecasting of H1N1-2009 using influenza-like illness from general practice and family doctor clinics in Singapore. PLoS One, 5 (4), e10036.
Osgood, N., & Liu, J. (2014). Towards closed loop modeling: Evaluating the prospects for creating recurrently regrounded aggregate simulation models using particle filtering. In Proceedings of the 2014 Winter Simulation Conference . IEEE Press.
Patel, V. L., Shortliffe, E. H., Stefanelli, M., Szolovits, P., Berthold, M. R., Bellazzi, R., et al. (2009). The coming of age of artificial intelligence in medicine. Artificial Intelligence in Medicine, 46 , 5–17.
Pathak, J., Bailey, K. R., Beebe, C. E., Bethard, S., Carrell, D. S., Chen, P. J., et al. (2013). Normalization and standardization of electronic health records for high-throughput phenotyping: The SHARPn consortium. Journal of the American Medical Informatics Association, 20 (e2), e341–e348.
Pathak, J., Kho, A. N., & Denny, J. C. (2013). Electronic health records-driven phenotyping: Challenges, recent advances, and perspectives. Journal of the American Medical Informatics Association, 20 (e2), e206–e211.
Pearson, T. (2011). How to replicate Watson hardware and systems design for your own use in your basement . https://www.ibm.com/developerworks/community/blogs/insideSystemStorage/entry/ibm_watson_how_to_build_your_own_watson_jr_in_your_basement7?lang=en
Pham-Kanter, G. (2014, September). Revisiting financial conflicts of interest in FDA advisory committees. The Milbank Quarterly, 92 (3), 446–470.
Rankin-Box, D. (2006, May). Shaping medical knowledge II: Bias and balance. Complementary Therapies in Clinical Practice, 12 (2), 77–79.
Resch, K., Ernst, E., & Garrow, J. (2000). A randomized controlled study of reviewer bias against an unconventional therapy. Journal of the Royal Society of Medicine, 93 (4), 164–167.
Sokolowski, J. A., & Banks, C. M. (2009). Principles of modeling and simulation: A multidisciplinary approach . Hoboken: Wiley.
Vieiraa, S., Pinayab, W. H. L., & Mechellia, A. (2017). Using deep learning to investigate the neuroimaging correlates of psychiatric and neurological disorders: Methods and applications. Neuroscience and Biobehavioral Reviews, 74 , 58–75.
Whitaker, R., & Cosgrove, L. (2015). Psychiatry Under the Influence: Institutional corruption, social injury and prescriptions for reform . New York, NY: Palgrave Macmillan.
White, R. W., Tatonetti, N. P., Shah, N. H., Altman, R. B., & Horvitz, E. (2013). Web-scale pharmacovigilance: Listening to signals from the crowd. Journal of the American Medical Informatics Association, 20 (3), 404–408.
Download references
Author information
Authors and affiliations.
Center for Integrative Medicine, University of Arizona College of Medicine, Tucson, AZ, USA
James H. Lake
You can also search for this author in PubMed Google Scholar
Rights and permissions
Reprints and permissions
Copyright information
© 2019 Springer Nature Switzerland AG
About this chapter
Lake, J.H. (2019). Evaluating and Using Medical Evidence in Integrative Mental Health Care: Literature Review, Evidence Tables, Algorithms, and the Promise of Artificial Intelligence. In: An Integrative Paradigm for Mental Health Care. Springer, Cham. https://doi.org/10.1007/978-3-030-15285-7_6
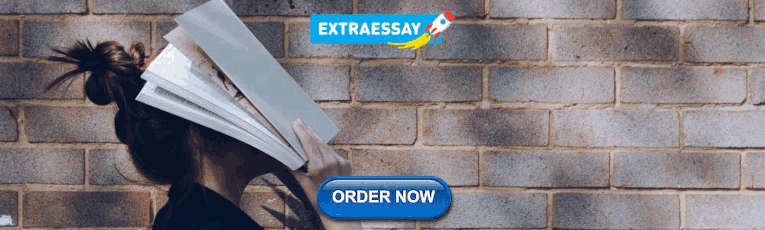
Download citation
DOI : https://doi.org/10.1007/978-3-030-15285-7_6
Published : 29 May 2019
Publisher Name : Springer, Cham
Print ISBN : 978-3-030-15284-0
Online ISBN : 978-3-030-15285-7
eBook Packages : Behavioral Science and Psychology Behavioral Science and Psychology (R0)
Share this chapter
Anyone you share the following link with will be able to read this content:
Sorry, a shareable link is not currently available for this article.
Provided by the Springer Nature SharedIt content-sharing initiative
- Publish with us
Policies and ethics
- Find a journal
- Track your research

Darrell W. Krueger Library Krueger Library
Evidence based practice toolkit.
- What is EBP?
- Asking Your Question
Levels of Evidence / Evidence Hierarchy
Evidence pyramid (levels of evidence), definitions, research designs in the hierarchy, clinical questions --- research designs.
- Evidence Appraisal
- Find Research
- Standards of Practice

Levels of evidence (sometimes called hierarchy of evidence) are assigned to studies based on the research design, quality of the study, and applicability to patient care. Higher levels of evidence have less risk of bias .
Levels of Evidence (Melnyk & Fineout-Overholt 2023)
*Adapted from: Melnyk, & Fineout-Overholt, E. (2023). Evidence-based practice in nursing & healthcare: A guide to best practice (Fifth edition.). Wolters Kluwer.
Levels of Evidence (LoBiondo-Wood & Haber 2022)
Adapted from LoBiondo-Wood, G. & Haber, J. (2022). Nursing research: Methods and critical appraisal for evidence-based practice (10th ed.). Elsevier.

" Evidence Pyramid " is a product of Tufts University and is licensed under BY-NC-SA license 4.0
Tufts' "Evidence Pyramid" is based in part on the Oxford Centre for Evidence-Based Medicine: Levels of Evidence (2009)
- Oxford Centre for Evidence Based Medicine Glossary
Different types of clinical questions are best answered by different types of research studies. You might not always find the highest level of evidence (i.e., systematic review or meta-analysis) to answer your question. When this happens, work your way down to the next highest level of evidence.
This table suggests study designs best suited to answer each type of clinical question.
- << Previous: Asking Your Question
- Next: Evidence Appraisal >>
- Last Updated: Apr 2, 2024 7:02 PM
- URL: https://libguides.winona.edu/ebptoolkit

Infection prevention and control studies for care of patients with suspected or confirmed filovirus disease in healthcare settings, with focus on Ebola and Marburg: an integrative review

Victoria Willet ,
Eugene T Richardson ,
George W Rutherford ,
April Baller ,
https://doi.org/ 10.1136/bmjph-2023-000556
Objective To review evidence pertaining to methods for preventing healthcare-associated filovirus infections (including the survivability of filoviruses in clinical environments and the chlorine concentration required for effective disinfection), and to assess protocols for determining the risk of health worker (HW) exposures to filoviruses.
Design Integrative review.
Data sources PubMed, Embase, Google Scholar, internet-based sources of international health organisations (eg, WHO, CDC), references of the included literature and grey literature.
Study selection Laboratory science, clinical research and real-world observational studies identified through comprehensive search strings that pertained to Ebola disease and Marburg disease and the three research objectives.
Methods Using the framework of population, intervention or exposure, outcomes, study types and report characteristics, reviewers extracted data and critically appraised the evidence using predefined data extraction forms and summary tables. The extraction forms, summary tables and critical appraisals varied based on the included literature; we used both the QUIPS Risk-of-Bias tool when possible and an internally developed instrument to systematically extract and review the evidence from observational and experimental studies. Evidence was then synthesised and summarised to create summary recommendations.
Results Thirty-six studies (including duplicates across research questions) were included in our reviews. All studies that related to the review questions were either (1) descriptive, real-world studies (ie, environmental audits of various surfaces in operational Ebola Treatment Units) or (2) controlled, laboratory studies (ie, experimental studies on the survivability of ebolaviruses in controlled conditions), presenting a range of concerns pertaining to bias and external validity. Our reviews of viral survivability evidence revealed significant disconnections between laboratory-based and real-world findings. However, there is greater viral persistence in liquid than dried body fluids, with the possible exception of blood, and ebolaviruses can survive for significant periods of time in dried substrate. Evidence suggests that 0.5% hypochlorite solution should be used for disinfection activity. Spills should be cleaned with covering and soaking for 15 min. Existing literature suggests that within a well-resourced clinical environment with trained, foreign HWs and established protocols, transmission of ebolaviruses as an occupational risk is a rare event. Despite the high rates of HW infections within public African healthcare settings, no evidence with low risk of bias exists to assess the risk of various occupational exposures given that all high-quality studies were conducted on foreign Ebola clinicians who had low overall rates of infection. This review underscores the critical need for better-quality evidence to inform best practices to ensure HW safety during filovirus disease epidemics.
- Notes about this article
What is already known on this topic
The strong evidence base related to filovirus disease transmission modes has led to the development of health worker (HW) infection prevention protocols (both disinfection and occupational risk assessments) within designated treatment units, but evaluations of algorithms and disinfection practices within these protocols are relatively few and have generated a weak evidence base.
What this study adds
Basic science (laboratory) evidence on safe disinfection protocols is reviewed and specific practices using hypochlorite solution are proposed. Gaps in evidence surrounding algorithms for HW risk assessment are reviewed.
How this study might affect research, practice or policy
Our results and proposed evidence-based protocols contribute to efforts to standardise practices within filovirus treatment units and can be implemented by outbreak response programs.
- Introduction
Filovirus disease (FVD) epidemics present a variety of challenges pertaining to health worker (HW) safety and exposure mitigation. The causative agents of these outbreaks—most notably, ebolaviruses—are known to be highly infectious and lead to clinical diseases with high mortality rates, even in the context of supportive care 1 and monoclonal antibody therapies, 2 though there is evidence that effective intensive care unit-level care can significantly improve outcomes. 3 Given that patients suffering from FVDs often produce copious amounts of infectious body fluids at the height of their illness each day, infection prevention and control (IPC) measures are important to adhere to while often being challenging to implement. 4 Compounding this, most haemorrhagic filoviruses are endemic to extremely impoverished regions of the world—particularly Western and Central Africa—and outbreaks emerge in the context of systemically underdeveloped public healthcare systems where frontline HWs often do not have the materials to protect themselves. 5 6 Health facilities have amplified viral transmission due to the absence of routine personal protective equipment (PPE) and safety protocols. 7 8
Novel Ebola disease (EBOD) vaccines offer promising approaches for epidemic control, while, at the same time, recent advances in antiviral and monoclonal antibody therapies open up the possibility for effective HW postexposure prophylaxis regimens. 9 10 However, there are not standardised, evidence-based criteria for how to stratify the risk of various forms of occupational exposure, which types of occupational exposures warrant postexposure prophylaxis, nor how these postexposure prophylaxis regimens might be tailored to individuals who have received pre-exposure vaccination. Given the high mortality rates and relative rarity and fast pace of filovirus outbreaks, exposure risk studies have been limited. 11 , 12 13 As a result, epidemic response institutions and organisations have developed their own protocols for HW safety and risk exposure assessment given the state of scientific knowledge about the infectiousness, transmission dynamics and survivability of filoviruses.
Due to these issues, guidelines issued by international agencies and non-governmental organizations (NGOs) for HW protection are often inconsistent and not always evidence-based. In 2022, the World Health Organization (WHO) established a guideline development group (GDG) to re-evaluate the current IPC recommendations and protocols for HW safety in hopes of disseminating best and evidence-based practices and aligning institutional protocols (the prior guidelines for PPE use were published in 2016 14 and for general interim IPC guidance were published in 2014 15 ). In response to a series of priority questions identified by the WHO GDG members, we conducted a review of the literature to provide evidence to support development of key recommendations. As an integrative review, we sought to critically appraise and synthesise findings systematically from multiple types of literature to generate frameworks and summary recommendations (thus our review would not be a purely ‘scoping’ review). 16 Given that this integrative review had no statistical analyses, it fell under the category of ‘Literature reviews that use a systematic search’, which precludes registration in the PROSPERO database. 17
Our integrative review addresses three priority questions. First, we sought to review the existing literature on established systems used by active epidemic response organisations to classify the level of risk of exposure of an HW to ebolaviruses and Marburg virus (MARV). (Throughout, we use the new WHO filovirus disease classification system, which stipulates that EBOD reference clinical syndromes caused by any of the ebolaviruses, MARD a clinical syndrome caused by marburgvirus infection, EVD a clinical syndrome caused by the Zaire ebolavirus , EBOV as the Zaire ebolavirus, and MARV as the Marburg marburgvirus.) 18 Our reviews focused on the evidence surrounding particular risk-assessment algorithms that can be implemented within high-risk settings rather than particular pathways for Ebola transmission, as the modes of Ebola and Marburg transmission have been well-defined. 19 20 Then, as a secondary aim, we reviewed literature on two more fundamental questions with relevance for HW safety protocols: What is the survivability of ebolaviruses and MARV in the environment (eg, water, septic systems, dirt) and on surfaces? And what is the chlorine concentration and contact time required to disinfect materials or surfaces contaminated with ebolaviruses and MARV, respectively? These latter two more laboratory science questions have also been explored as part of a series of recent systematic reviews on viral survivability and disinfection practices, 21 22 however, in our review, we sought to address particular gaps in the evidence-base that can readily inform HW protection protocols during (specifically) FVD outbreaks. Together, our reviews on both the basic science and implementation of HW safety in filovirus epidemics can be used to formulate questions for further study and inform the ongoing revision and study of IPC guidelines.
We devised a systematic search strategy for each question. To create eligible criteria, we used the framework of population, intervention or exposure, outcomes, study types and report characteristics. To assess the eligible literature, we created comprehensive search strings with terms that included combinations of Medical Subject Headings (MeSH) and text words for Ebola disease (EBOD) and Marburg disease (MARD) (see online supplemental materials ). This search strategy was applied against the following information sources to identify and screen for the eligible literature: PubMed, Embase, Google Scholar, internet-based sources of international health organisations (eg, WHO, CDC), references of the included literature and grey literature. Other systematic reviews were not included in our review. The flow from articles identified and screened to articles included is described in the Preferred Reporting Items for Systematic Reviews and Meta-Analyses (PRISMA) diagrams.
Two reviewers (RGF, JDK) extracted data and critically appraised the evidence using predefined data extraction forms and summary tables (see online supplemental materials ), and in turn verified each other’s work. The extraction forms and summary tables for questions 1–3 varied based on the included literature and are also included in online supplemental materials , as are the PICO frameworks for each question and a complete list of studies included in our review for each question. No grey literature manuscripts ended up meeting inclusion criteria and being included in our review. Notably, all studies included in the review that related to the background questions were either (1) descriptive, real-world studies (ie, environmental audits of various surfaces in operational Ebola Treatment Units (ETUs)) or (2) controlled, laboratory studies (ie, experimental studies on the survivability of ebolaviruses in controlled conditions). Since there are no validated risk-of-bias assessment tools for descriptive or controlled, experimental studies, our approach drew both on the QUIPS Risk-of-Bias tool when possible 23 ( online supplemental table 1 ) and an instrument we developed to systematically extract and review the evidence from observational and experimental studies. Thus, while we were not able to consistently perform bias assessments due to the nature of the study designs, we recognise that experimental laboratory studies and descriptive studies are inherently biased by a high likelihood of selection and measurement biases, as well as other issues.
Existing systems to classify level of risk exposure of an HW to filoviruses
The search returned 410 unique results which were screened to assess if they met inclusion or exclusion criteria. Of these screened records, 36 full-text articles were assessed for eligibility, and 6 studies were included in these results. A flow diagram describing the process can be found in figure 1 and the screening eligibility in figure 2 . In this search, we found two types of research studies: high-quality studies of international HWs and one low-quality study of local HWs. Box 1 describes the key findings of this review.
Key findings
There are multiple avenues to develop algorithms to classify level of HW exposure risk. 9 24 25
Multiple studies of exposed HWs from Global North countries did not show PCR or antibody evidence of infection with ebolaviruses. 9 24 25
Studies of local HWs are difficult to interpret because of community-based exposures without stratifying based on isolated exposure events. 29
HW, health worker.
Question 1 Preferred Reporting Items for Systematic Reviews and Meta-Analyses diagram.
Question 1 inclusion criteria. EBOD, Ebola disease; MARD, Marburg virus disease
The 2013–2016 Ebola epidemic in West Africa appears to be the first EBOD outbreak during which researchers attempted to develop risk assessment algorithms specifically for HWs involved in the response. These algorithms were largely adapted from existing occupational health literature. The need for risk assessment algorithms emerged from the availability of potential EBOD therapeutics for postexposure prophylaxis, as well as the need to identify the types of exposure that should warrant rapid evacuation to well-equipped intensive care centres. (Of note: it is no coincidence that this effort to define exposure algorithms overlapped with a major influx of European and American HWs to affected regions of West Africa; local HWs who worked on the frontlines in this and other filovirus epidemics often bore the risk of exposure without assurance of evacuation to higher levels of care.) 8
In a study by Jacobs and colleagues, 9 an initial risk assessment algorithm was proposed based on interviews from eight HWs who were potentially exposed to EBOV. This risk assessment algorithm was further developed and modified in a study by Houlihan and colleagues and used to assess risk among 268 HWs who travelled to West Africa in response to the Ebola epidemic. 24 Key additions to the algorithm this latter study included the addition of PPE and breeches. Both studies were conducted in the UK and considered HWs who responded to the outbreak in Sierra Leone. At the same time, however, a prospective study of French military providers in Guinea sought to classify exposures and present a parallel risk assessment algorithm. 25 Key additions from this study were consideration of HWs’ indirect contact with infectious individuals by more than 1 m, and cumulative incidents and exposures to ebolavirus, particularly during the removal of PPE.
All of these studies advanced algorithms for use within ETUs that attempt to stratify risk based off of particular breaches, exposures and pathways. However, none of these studies actually identified any infections by using these risk algorithms, thus rendering the risk assessment algorithms not evidenced-based. Together, these studies suggest that HW infection rates are exceedingly low in the settings in which these algorithms were implemented; with the relatively small cohorts of responding HWs there is no existing, high-quality evidence to link HW infection to particular types of exposure.
In contrast to these research studies, there have been a series of case reports among international laboratory scientists and high-profile media reports of international HWs who have been exposed and subsequently infected with ebolaviruses (with variable clinical outcomes). 26–28 These reports are low quality given the risk of bias and represent a very weak evidence base; however, given that HWs have been infected via occupational exposure, the available risk algorithms should be further evaluated with cohorts that include HWs who do develop EBOD from occupational exposure.
In contrast to what is suggested by the literature available from international HWs and the relative paucity of HW infections in this population, a considerable number of local healthcare providers from West Africa were infected with the disease and thus beckon further consideration of the highest profile risks. A retrospective descriptive study based in Sierra Leone by Olu et al attempted to associate mode and type of exposures with healthcare provider infections. 29 This study is difficult to interpret because of the widespread possibility that national HWs acquired EVD from community sources (ie, through contact within their homes or families) among this study population. This study thus has a high risk of bias and represents weak evidence for consideration in the current development of a risk assessment algorithm. However, this study points to the critical need to pursue high-quality research among national healthcare providers that focus on isolated health facility-based events without potential community-based exposures in future outbreaks, and that the overall risk of HW infections across healthcare settings and with varying resources and safety protocols in place remains unclear. It also reaffirms the fact that local HWs often operate on the front lines of FVD outbreaks at great peril given inadequate supplies and that the safety of future HWs in countries impacted by FVD epidemics depends on further exploration of occupational hazards. 30 Of note, a review of FVD risk factors and HW infection rates by Selvaraj and colleagues likewise found widely variable rates of HW infections depending on context, and affirmed that over multiple outbreaks inadequate PPE supplies were commonly linked to national HW infections. 8 This lends credence to the idea that while there is a critical need for evidence based guidelines to assess occupational exposure risk, many HW infections in frontline clinics may simply be caused by scarce supplies of basic PPE.
- Survivability of ebolaviruses and MARV in the environment (eg, water, septic systems, dirt) and on surfaces
The second search focused on the survivability of ebolaviruses and MARV and returned 142 unique results, which were screened to assess if they met inclusion or exclusion criteria. Of these screened records, 81 full-text articles were assessed for eligibility, and 20 studies were included in these results. A flow diagram describing the process can be found in figure 3 and the screening eligibility in figure 4 . See box 2 for key findings.
Ebolaviruses and Marburg virus have similar survivability, and non-pourous surfaces have similar survivability times for ebolaviruses. 31 32
Ebolaviruses maintain viability for longer in liquid rather than dried substrates, with the possible exception of blood. 32 39
In real-world ETU environmental audit studies, ebolavirus RNA has only been found on visibly soiled surfaces and in immediate vicinity of patients. 42–47
ETU, Ebola treatment unit.
Question 2 Preferred Reporting Items for Systematic Reviews and Meta-Analyses diagram.
Question 2 inclusion criteria.
Evidence pertaining to the survivability of ebolaviruses and MARV on various relevant surfaces derives from two sources: experimental studies that have assessed the survivability of the viruses in a variety of controlled conditions, and real-world environmental audits in which various surfaces in actively used ETUs were swabbed and then assessed for the presence of viral RNA.
There are fewer studies pertaining to the survivability of MARV than that of ebolaviruses. However, Piercy and colleagues showed that these filoviruses have similar decay rates on both liquid and dried substrates at 4°C. 31 While there is limited data in more real-world conditions of MARV, this study suggests that the general principles pertaining to the survivability of ebolaviruses from other investigations can likely be applied to MARV as well.
Schuit and colleagues found that when multiple strains of ebolaviruses were spiked onto three different dried human body fluid matrices and then inoculated on a variety of clinically relevant non-porous test surfaces in multiple temperatures and humidity levels, the decay rates of ebolaviruses were dependent on the fluid matrix (eg, vomitus, faeces, blood) and the environment (eg, temperature, humidity), not the surface itself. 32 Cook and colleagues found that ebolaviruses on porous surfaces, such as a cotton gown, survives for much less time than on nonporous clinical surfaces. 33 Sagripanti and colleagues also found a decay rate of nearly 6 days for ebolaviruses inoculated on a non-porous surfaces in darkness. 34
The Schuit study further showed that ebolaviruses did not survive for significant time periods in dried, simulated vomitus or faeces, but did survive for up to 240 hours in dried blood 32 ; multiple other studies also found that ebolaviruses or surrogate viruses may survive within blood and plasma samples for several days or more. 35 , 36 37 , 38 One concerning finding from the Schuit study is that ebolaviruses persisted longest in dried blood at higher relative humidity, (conditions most similar to real-world, African ETU settings). Fischer and colleagues revealed similar findings pertaining to surfaces, but showed that ebolaviruses maintained an even longer duration of viability in liquid blood than in dried blood. 39
Wastewater has been another fluid matrix under consideration in terms of ebolavirus survivability. 40 Bibby and colleagues showed that ebolaviruses may survive in wastewater for up to 8 days; however, the viral titre rapidly declined (approximately 99%) within the first day of the experiment, suggesting diminished risk of transmission via wastewater after 1 day of contamination. 41 Cook and colleagues used viral decay rates to calculate an estimated upper range of survivability. These investigators found that on stainless steel in an organic soil load, in a low-humidity, 22°C setting, ebolaviruses may survive for up to 365 hours. 33
These findings about the long survivability of filoviruses on relevant fluids and surfaces are, however, belied by real-world descriptive studies. Six such real-world studies report the results of environmental audits, during which high-risk and low-risk surfaces from in-use ETUs were (often unsystematically) swabbed and tested for the presence of viral RNA and, in some cases, live viral particles. Of note: these studies were all carried out in relatively well-resourced clinical contexts that may not resemble the national clinics and hospitals in which FVD epidemics are first managed. In Youkee et al (Sierra Leone), 42 Bausch et al (Uganda), 43 Poliquin et al (Sierra Leone), 44 Puro et al (Italy), 45 Palich et al (Guinea) 46 and Wu et al (Sierra Leone), 47 ebolaviruses were only recovered in areas in the immediate vicinity to patients or on visibly soiled surfaces . While Youkee and colleagues suggested that some viral RNA may have been displaced in the process of cleaning (as, for instance, a patients’ bedframe was only found to harbour viral RNA after a routine cleaning, not before), these studies suggest that while ebolaviruses may survive for an extended period on surfaces (as also evidenced by the experimental studies), current disinfection procedures as used in a variety of well-resourced contexts are generally effective.
All these studies are descriptive and thus may be affected by a high likelihood of selection/measurement bias and other issues. The findings from the experimental studies included are subject to the limitations of the assays and the controlled conditions in which they were carried out. The fact that there are such discrepancies in the implications of the findings from the experimental studies (which suggest potentially weeks-long survivability of ebolaviruses) versus the real-world studies (which suggest that disinfection protocols are effective and ebolaviruses is unlikely to be found outside of direct patient care areas) suggest an urgent need for further high-quality research. More environmental audits that systematically test for viable viral particles on ETU surfaces would be helpful to elucidate the survivability of these viruses in real-world settings.
- Chlorine concentration and contact time required to disinfect materials or surfaces soiled with bolaviruses and MARV
The third search focused on the concentration and contact time of chlorine as an environmental disinfectant and returned 36 unique results which were screened to assess if they met inclusion or exclusion criteria. Of these screened records, 26 full-text articles were assessed for eligibility, and 10 studies were included in these results. A flow diagram describing the process can be found in figure 5 and the screening eligibility in figure 6 . See box 3 for key findings.
0.5% hypochlorite solution is effective for surface disinfection activity, though may not be completely effective at disinfecting dried blood. 33 48 50 56
On non-porous, contaminated surfaces without visible spills, 10 min of contact time is consistently effective. 33 48 49
For visible spills, covering and pouring for 15 min is the most conservative recommendation. 48
Question 3 Preferred Reporting Items for Systematic Reviews and Meta-Analyses diagram.
Question 3 inclusion criteria.
There are at least three different institutional protocols (MSF, WHO and CDC) pertaining to chlorine disinfection recommendations for Ebola response efforts, 48 none of which are evidence based. All these protocols, however, call for 0.5% chlorine to be used as the primary surface cleaning solution in ETUs.
In our review of the literature, we again found a range of experimental studies which are subject to the same limitations as stated above: low external validity, limitations with the assays used and more. Cook and colleagues found that solutions of sodium hypochlorite (NaOCl) greater than or equal to 0.5% chlorine inactivated all strains of ebolaviruses in organic soil load after 5 min of contact. 33 Weaker concentrations (for instance 0.05% and 0.1%) were not able to fully inactivate ebolaviruses. Gallandat and colleagues corroborated this finding about the effectiveness of 0.5% chlorine with a viral surrogate inoculated in an organic soil load. They also found that covering spills with a cloth soaked in 0.5% chlorine and leaving it for 15 min was an effective method of disinfection, while avoiding the risk of splash from spraying. 48 Smither and colleagues found that a 0.75% NaOCl exposure for 10 min led to no recoverable ebolavirus on all surface types tested, however this investigation did not assess the effectiveness of 0.5% chlorine. 49
In another study by Smither and colleagues, neither 0.5% nor 1% hypochlorite was able to completely reduce ebolavirus viability when the virus was inoculated on surfaces in dried blood. 50 More research is needed on whether higher concentrations of chlorine—or chlorine applied during the cover-and-wipe method of disinfection—are effective at disinfecting ebolaviruses in dried blood.
Finally, Cook and colleagues found that high amounts of viral RNA could be recovered in the absence of infectious virus after chlorine disinfection, suggesting that PCR alone likely overestimates the survivability (and thus ineffectiveness) of a disinfection method. 33
In the context of the real-world studies reviewed for question 2 (above) that have shown low risk of recoverable infectious virus after routine disinfection protocols, and given the several studies that have corroborated the efficacy of 0.5% chlorine, these studies offer ample evidence for 0.5% chlorine being an effective disinfectant for surfaces, with the caveat that the issue of viral persistence in dried blood needs further investigation.
In this review, we have surveyed the existing literature on FVD HW exposure risk algorithms, and the survivability and effective disinfection of filoviruses in healthcare settings. Throughout, we found inconsistencies between experimental and real-world findings, but the lack of systematic, high-quality research has likely contributed to conservative IPC practices and other implications for both EBOD and MARD care.
Broadly, the existing literature suggests that within a well-resourced ETU environment with trained HWs, the transmission of ebolaviruses from occupational risks is a rare event (although exposures that result in asymptomatic infection are overlooked). 51 52 Systematic studies that include a larger number of these internationally staffed ETUs would increase the probability of capturing infected HWs who were evacuated to home countries and add power to descriptive and analytical estimates. Given that most transmission of ebolaviruses has been occurring within local HW populations, high-quality studies may be able to identify HWs with isolated occupational exposures and decrease potential biases. We wish to emphasise that these conclusions pertain to the delivery of care within designated treatment units during known FVD epidemics, when paradigms of FVD HW protection have been enacted; ‘mistakes’ in FVD identification, diagnosis and HW protection protocols—not to mention scarcities in crucial PPE resources—have historically been drivers of FVD emergence and transmission in both African and, in the case of the small 2014 outbreak in Dallas county, the USA as well. 53 54 Of course, in real-world settings, as HWs must meet the demands of routine care delivery even as ebolaviruses and MARV may be circulating in communities, this distinction is less clear-cut; there is a crucial need for more research to guide the protocols that should be enacted within non-ETU hospitals and clinics in FVD-endemic or high-risk areas, both during and in-between FVD outbreaks. There is also a need for more data on the rates of particular procedural failures and their contribution to disease transmission during care within ETUs and general health facilities.
The studies that have assessed HW exposure algorithms are potentially subject to a range of biases and methodological limitations, including: (1) the lack of a control group (ie, no study randomised ETUs to use vs not use aspects of risk algorithms or algorithms in their entirety); (2) low overall numbers of ebolavirus infections which limit the power of these studies to evaluate algorithms; and (3) publication biases: given that Ebola care is often delivered by humanitarian organisations and public clinical facilities, there may have been other ETUs or response organisations who have used algorithms included in these studies but did not report findings. It is unclear how this lack of publication may have influenced our findings.
Pertaining to viral survivability, we found a significant disconnection between laboratory-based and real-world findings. Still, general principles hold: there is greater viral persistence in liquid than dried body fluids, though ebolaviruses can survive for significant periods of time in dried substrates, particularly dried blood. There is a need for concerted effort to coordinate further environmental contamination studies to learn more about real-world survivability of infectious virus using culture in BSL-4 facilities.
Finally, evidence suggests that 0.5% hypochlorite solution should be used for disinfection activity. Spills should be cleaned with covering and soaking for 15 min. There is a need for further evaluation of decontamination techniques in real-world settings (ie, surfaces for which sustained contact with chlorine is not easy to maintain) and regarding the disinfection of dried spills.
These findings on viral survivability and disinfection practices were also possibly subject to biases including (1) the lack of external validity from laboratory-based experiments to real-world settings; (2) the lack of validated instruments to evaluate risk of biases in such experimental, laboratory studies; and (3) publication bias: given the biosecurity threat of viral haemorrhagic fevers, there are likely other experiments on viral survivability that have been conducted but which are not published due to government restrictions. Finally, in all three of our reviews, our search terms were carefully selected by the study team, but it is possible that we have missed related studies that could contribute to the evidence base presented here.
There continue to be grave disparities in HW FVD infection and mortality rates across contexts, with large numbers of HWs contracting these diseases in under-resourced settings, and very low infection rates and deaths occurring among ‘expatriate’ HWs. 55 These disparities evince the fact that FVD epidemics should be studied as historically-situated phenomena, shaped by legacies of colonialism and ongoing systems of structural violence. 5 6 As a more proximal intervention to address these disparities, research protocols on HW safety during FVD epidemics could be developed in pre-outbreak periods, so that when the next filovirus outbreak occurs, high-quality research studies can be rapidly enacted and higher-quality evidence produced to inform HW safety protocols. This review underscores the need for ongoing efforts to protect frontline workers from filoviruses with know-how, supplies and other components of comprehensive health systems during future outbreaks.
- Supplementary files

An official website of the United States government
The .gov means it’s official. Federal government websites often end in .gov or .mil. Before sharing sensitive information, make sure you’re on a federal government site.
The site is secure. The https:// ensures that you are connecting to the official website and that any information you provide is encrypted and transmitted securely.
- Publications
- Account settings
Preview improvements coming to the PMC website in October 2024. Learn More or Try it out now .
- Advanced Search
- Journal List
- J Med Internet Res
- PMC10646672

Data Quality in Health Research: Integrative Literature Review
Filipe andrade bernardi.
1 Ribeirão Preto School of Medicine, University of Sao Paulo, Ribeirão Preto, Brazil
Domingos Alves
Nathalia crepaldi, diego bettiol yamada, vinícius costa lima.
2 Polytechnic Institute of Leiria, Leiria, Portugal
3 Institute for Systems and Computers Engineering, Coimbra, Portugal
4 Center for Research in Health Technologies and Services, Porto, Portugal
Associated Data
Preferred Reporting Items for Systematic Reviews and Meta-Analyses (PRISMA) checklist.
Individual studies.
The data sets generated and analyzed during this study are available as Multimedia Appendix 2 or can be obtained from the corresponding author upon reasonable request.
Decision-making and strategies to improve service delivery must be supported by reliable health data to generate consistent evidence on health status. The data quality management process must ensure the reliability of collected data. Consequently, various methodologies to improve the quality of services are applied in the health field. At the same time, scientific research is constantly evolving to improve data quality through better reproducibility and empowerment of researchers and offers patient groups tools for secured data sharing and privacy compliance.
Through an integrative literature review, the aim of this work was to identify and evaluate digital health technology interventions designed to support the conducting of health research based on data quality.
A search was conducted in 6 electronic scientific databases in January 2022: PubMed, SCOPUS, Web of Science, Institute of Electrical and Electronics Engineers Digital Library, Cumulative Index of Nursing and Allied Health Literature, and Latin American and Caribbean Health Sciences Literature. The Preferred Reporting Items for Systematic Reviews and Meta-Analyses checklist and flowchart were used to visualize the search strategy results in the databases.
After analyzing and extracting the outcomes of interest, 33 papers were included in the review. The studies covered the period of 2017-2021 and were conducted in 22 countries. Key findings revealed variability and a lack of consensus in assessing data quality domains and metrics. Data quality factors included the research environment, application time, and development steps. Strategies for improving data quality involved using business intelligence models, statistical analyses, data mining techniques, and qualitative approaches.
Conclusions
The main barriers to health data quality are technical, motivational, economical, political, legal, ethical, organizational, human resources, and methodological. The data quality process and techniques, from precollection to gathering, postcollection, and analysis, are critical for the final result of a study or the quality of processes and decision-making in a health care organization. The findings highlight the need for standardized practices and collaborative efforts to enhance data quality in health research. Finally, context guides decisions regarding data quality strategies and techniques.
International Registered Report Identifier (IRRID)
RR2-10.1101/2022.05.31.22275804
Introduction
In health care settings, the priceless value of data must be emphasized, and the relevance and performance of digital media are evidenced by the efforts of governments worldwide to develop infrastructure and technology, aiming to expand their ability to take advantage of generated data. It is important to emphasize that technology, by itself, cannot transform data into information, and the participation of health care professionals is essential for knowledge production from a set of data. Through research that optimizes health interventions and contributes to aligning more effective policies, knowledge combines concrete experiences, values, contexts, and insights, which may enable a framework for evaluation and decision-making [ 1 ].
The low quality, nonavailability, and lack of integration (fragmentation) of health data can be highlighted among the main factors that negatively influence research and health decision-making. In addition, it is worth noting the existence of a large number of remote databases accessible only in a particular context. Such factors cause data quality problems and, consequently, information loss. Despite the intense volume, information remains decentralized, but it needs to help the decision-making process [ 2 ], making its coordination and evaluation challenging.
The crucial role of data spans a wide range of areas and sectors, ranging from health care data to financial data, social media, transportation, scientific research, and e-commerce. Each data type presents its own challenges and requirements regarding quality, standardization, and privacy. Ensuring the quality and reliability of these data is essential to support the combination of different sources and types of data that can lead to even more powerful discoveries [ 3 ].
For example, using poor-quality data in developing artificial intelligence (AI) models can lead to decision-making processes with erroneous conclusions. AI systems, which are increasingly used to aid decision-making, have used labeled big data sets to build their models. Data are often collected and marked by poorly trained algorithms, and research often demonstrates this method’s problems. Algorithms can present biases in judgments about a person’s profession, nationality, or character and basic errors hidden in the data used to train and test their models. Consequently, prediction can be masked, making it difficult to distinguish between right and wrong models [ 4 ].
Principles are also established in the semantic web domain to ensure adequate data quality for use in linked data environments. Such recommendations are divided into 4 dimensions: quality of data sources, quality of raw data, quality of the semantic conversion, and quality of the linking process. The first principle is related to the availability, accessibility, and reliability of the data source, as well as technical issues, such as performance and verifiability [ 5 ]. The second dimension refers to the absence of noise, inconsistencies, and duplicates in the raw data from these data sources. In addition, it also addresses issues regarding the completeness, accuracy, cleanness, and formatting of the data to be helpful and easily converted into other models, if necessary. The last 2 dimensions refer to the use of high-quality validated vocabularies, flexible for semantic conversion, and the ability of these data to be combined with other semantic data, thus generating sophisticated informational intelligence. Such factors depend on correctness, granularity, consistency, connectedness, isomorphism, and directionality [ 6 ].
The heterogeneity of data in this area is intrinsically connected to the type of information generated by health services and research, which are considered diverse and complex. The highly heterogeneous and sometimes ambiguous nature of medical language and its constant evolution, the enormous amount of data constantly generated by process automation and the emergence of new technologies, and the need to process and analyze data for decision-making constitute the foundation for the inevitable computerization of health systems and research and to promote the production and management of knowledge [ 7 ].
There are different concepts of data quality [ 8 ]. According to the World Health Organization, quality data portray what was determined by their official source and must encompass the following characteristics: accuracy and validity, reliability, completeness, readability, timeliness and punctuality, accessibility, meaning or usefulness, confidentiality, and security [ 9 ]. Data quality can be affected at different stages, such as the collection process, coding, and nonstandardization of terms. It can be interfered with by technical, organizational, behavioral, and environmental aspects [ 10 ].
Even when data exist, some aspects make their use unfeasible by researchers, managers, and health care professionals, such as the noncomputerization of processes, heterogeneity, duplicity, and errors in collecting and processing data in health information systems [ 11 ]. Reliable health data must support decision-making and strategies to improve service delivery to generate consistent evidence on health status, so the data quality management process must ensure the reliability of the data collected [ 12 ].
Some health institutions have action protocols that require their departments to adopt quality improvement and resource-saving initiatives. Consequently, various methodologies to improve the quality of services have been applied in the health field. Mulgund et al [ 13 ] demonstrated, for example, how data quality from physician-rating sites can empower patients’ voices and increase the transparency of health care processes.
Research in scientific communities about new strategies constantly evolves to improve research quality through better reproducibility and empowerment of researchers and provides patient groups with tools for secure data sharing and privacy compliance [ 14 ]. Raising a hypothesis and defining a methodology are a standard scientific approach in health research, which will lead to the acquisition of specific data. In contrast, data production in the big data era is often completely independent of the possible use of the data. One of the hallmarks of the big data era is that the data are often used for a purpose other than the one for which they were acquired. In this sense, influencing the modification of acquisition processes in clinical contexts requires more structured approaches [ 13 ].
The health sector is increasingly using advanced technologies, such as sophisticated information systems, knowledge-based platforms, machine learning algorithms, semantic web applications, and AI software [ 15 ]. These mechanisms use structured data sets to identify patterns, resolve complex problems, assist with managerial and strategic decision-making, and predict future events. However, it is crucial to ensure that the data used for these analyses adhere to the best practices and metrics for evaluating data quality to avoid biases in the conclusions generated by these technologies. Failure to do so can make it challenging to elucidate previously unknown health phenomena and events [ 16 ].
To use the best practices, institutions use the results of literature reviews due to the significant time savings and high reliability of their studies. Thus, through an integrative literature review, the main objective of this work is to identify and evaluate digital health technology interventions designed to support the conduct of health research based on data quality.
Study Design
The Population, Concept, and Context (PCC) strategy was applied to define the research question. The PCC strategy guides the question of the study and its elaboration, helping in the process of bibliographic search for evidence. The adequate definition of the research question indicates the information necessary to answer it and avoids the error of unnecessary searches [ 17 ].
“Population” refers to the population or problem to be investigated in the study. “Content” refers to all the detailed elements relevant to what would be considered in a formal integrative review, such as interventions and phenomena of interest and outcomes. “Context” is defined according to the objective and the review question. It can be determined by cultural factors, such as geographic location, gender, or ethnicity [ 18 ]. For this study, the following were defined: P=digital technology, C=data accuracy, and C=health research.
In this sense, the following research questions were defined:
- What is the definition of health research data quality?
- What are the health research data quality techniques and tools?
- What are the indicators of the data confidence level in health research?
Health Research
Numerous classifications characterize scientific research, depending on its objective, type of approach, and nature. Regardless of the purpose of how surveys can be classified, levels of confidence in data quality must be ubiquitous at all stages of the survey. Detailed cost-effectiveness analysis may inform decisions to adopt technology methods and tools that support electronic data collection of such interventions as an alternative to traditional methods.
Health research systems have invested heavily in research and development to support sound decisions. In this sense, all types of studies were observed that presented results of recent opportunities to apply the value of digital technology to the quality of the information in the direct or indirect evaluation of the promotion of health research. Therefore, in a transversal way, we considered all types of studies dealing with such aspects.
Types of Approaches
Various methods for setting priorities in health technology research and development have been proposed, and some have been used to identify priority areas for research. They include surveys and measurements of epidemiological estimates, clinical research, and cost-effectiveness assessments of devices and drugs. The technical challenges and estimation of losses due to variations in clinical practice and deviations from protocols have been supported by recommendation manuals and good practice guidelines. However, each of these proposed methods has specific severe methodological problems.
First, all these approaches see research simply as a method of changing clinical practice. However, there are many ways to change clinical practice, and conducting research may not be the most effective or cost-effective way. Research’s real value is generating information about what clinical practice should be. The question of how to implement survey results is a separate but related issue. Therefore, these methods implicitly assume no uncertainty surrounding the decision that the proposed research should inform.
Types of Interventions and Evaluated Results
Technology-based interventions that affect and aggregate concepts, designs, methods, processes, and outcomes promote data quality from all health research.
Measures demonstrate how results can address political, ethical, and legal issues, including the need to support and use technological mechanisms that bring added value regardless of the type and stage at which they are applied to research. We looked at how the results can be evaluated to address other questions, such as which subgroups of domains should be prioritized, which comparators and outcomes should be included, and which follow-up duration and moments would be most valuable for improving interventions on the reliability of health research data.
Eligibility Criteria
Research carried out in English and Portuguese, with quantitative and qualitative approaches, primary studies, systematic reviews, meta-analyses, meta-synthesis, books, and guidelines, published from 2016 onward was included. This choice is justified because we sought scientific indications that were minimally evaluated by our community. In this sense, websites, white papers, reports, abstracts only, letters, and commentaries were not considered. The year limitation is justified because knowledge is considered an adequate degree of being up to date.
In addition to the methodological design, we included any studies that described the definition, techniques, or tools that have the essential functions of synthesis, integration, and verification of existing data from different research sources to guarantee acceptable levels of data quality. In this way, we expected to monitor trends in health research, highlight areas for action on this topic, and, finally, identify gaps in health data arising from quality control applications.
Although the primary objective of this review was to seek evidence of data quality from health research, we also independently included studies on health data quality and research data quality. The exclusion criteria were applied to studies with a lack of information (eg, the paper was not found), studies whose primary focus was not health and research, and papers not relevant to the objective of the research, papers not available as full text in the final search, and papers not written in English or Portuguese. In addition, the titles and respective authors were checked to verify possible database repetitions. All criteria are presented in Table 1 .
Inclusion and exclusion criteria for eligibility of studies.
a Not applicable.
Databases and Search Strategies
A search was carried out in 6 electronic scientific databases in January 2022 because of their quality parameters and broad scope: PubMed, SCOPUS, Web of Science, Institute of Electrical and Electronics Engineers (IEEE) Digital Library, Cumulative Index of Nursing and Allied Health Literature (CINAHL), and Latin American and Caribbean Health Sciences Literature (LILACS). For the search, descriptors and their synonyms were combined according to the Health Sciences Descriptors (DeCS) [ 19 ] and Medical Subject Headings (MeSH) [ 20 ]. The following descriptors and keywords were selected, combined with the Boolean connectors AND and OR: “Data Accuracy,” “Data Gathering,” and “Health Research.” These descriptors and keywords come from an iterative and tuning process after an exploratory phase. The same search strategy was used in all databases.
Google Scholar was used for manual searching, searching for other references, and searching for dissertations. These documents are considered gray literature because they are not published in commercial media. However, they may thus reduce publication bias, increase reviews’ comprehensiveness and timeliness, and foster a balanced picture of available evidence [ 21 ].
We created a list of all the studies we found and removed duplicates. A manual search was performed for possible studies/reports not found in the databases. The references of each analyzed study were also reviewed for inclusion in the search. The search was carried out in January 2022, and based on the inclusion and exclusion criteria described, the final number of papers included in the proposed integrative review was reached. The search procedure in the databases and data platforms is described in Table 2 , according to the combination of descriptors.
Search procedure on databases.
a IEEE: Institute of Electrical and Electronics Engineers.
b LILACS: Latin American and Caribbean Health Sciences Literature.
c CINAHL: Cumulative Index of Nursing and Allied Health Literature.
Data Collection
First, 2 independent reviewers with expertise in information and data science performed a careful reading of the title of each paper. The selected papers were filtered after reading the abstract and selected according to the presence of keywords and descriptors of interest. The reviewers were not blinded to the journal’s title, study authors, or associated institutions. The established inclusion and exclusion criteria adequacy was verified for all screened publications. Any disagreements between the 2 reviewers were resolved by a senior third independent evaluator. The Mendeley reference manager [ 22 ] was used to organize the papers. Subsequently, the extracted findings were shared and discussed with the other team members.
Data synthesis aims to gather findings into themes/topics that represent, describe, and explain the phenomena under study. The extracted data were analyzed to identify themes arising from the data and facilitate the integration and development of the theory. Two reviewers performed data analysis and shared it with other team members to ensure the synthesis adequately reflected the original data.
Data Extraction
Data extraction involved first-order (participants’ citations) or second-order (researchers’ interpretation, statements, assumptions, and ideas) concepts in qualitative research. Second-order concepts were extracted to answer the questions of this study [ 17 ].
We looked at data quality characteristics in the studies examined, the assessment methods used, and basic descriptive information, including the type of data under study. Before starting this analysis, we looked for preexisting data quality and governance models specific to health research but needed help finding them. Thus, 2 reviewers were responsible for extracting the following data from each paper:
- Bibliographic information (title, publication date and journal, and authors)
- Study objectives
- Methods (study design, data collection, and analysis)
- Results (researchers’ interpretation, statements, assumptions, and ideas)
Result Presentation
The PRISMA (Preferred Reporting Items for Systematic Reviews and Meta-Analyses) checklist ( Multimedia Appendix 1 ) and flowchart were used to visualize the search strategy results in the databases. PRISMA follows a minimum set of items to improve reviews and meta-analyses [ 23 ]. Based on the PRISMA flowchart, a narrative synthesis was prepared, in which we described the objectives and purposes of the selected and reviewed papers, the concepts adopted, and the results related to the theme of this review.
Data Synthesis
The data synthesis process involved several steps to ensure a systematic and comprehensive analysis of the findings. After a rigorous study selection process, the extracted data were analyzed using a coding and categorization approach.
Initially, a coding framework was developed based on the research objectives and key themes identified in the literature. This framework served as a guide for organizing and categorizing the extracted data. At least 2 independent reviewers performed this coding process to ensure consistency and minimize bias. Any discrepancies or disagreements were resolved through consensus discussions. Relevant data points from each study were coded and assigned to specific categories or themes ( Multimedia Appendix 2 ), capturing the main aspects related to data quality in health research, as shown in Table 3 .
Search procedure used on databases.
Once the data were coded and categorized, a thorough analysis was conducted to identify patterns, trends, and commonalities across the studies. Quantitative data, such as frequencies or percentages of reported data quality issues, were analyzed using descriptive statistics. Qualitative data, such as themes or explanations provided by the authors, were analyzed using thematic analysis techniques to identify recurring concepts or narratives related to data quality.
The synthesized findings were then summarized and organized into coherent themes or subtopics. This involved integrating the coded data from different studies to identify overarching patterns and relationships. Similar results were grouped, and relationships between different themes or categories were explored to derive meaningful insights and generate a comprehensive picture of data quality in health research.
As part of the data synthesis process, the quality of the included studies was also assessed. This involved evaluating the studies’ methodological rigor, reliability, and validity using established quality assessment tools or frameworks. The quality assessment results were considered when interpreting and discussing the synthesized findings, providing a context for understanding the strength and limitations of the evidence.
Study Characteristics
In this review, 27,709 occurrences were returned from the search procedure, with 789 (2.84%) records from the SCOPUS database, 2 (0.01%) from LILACS, 1989 (7.18%) from the IEEE Digital Library, 5589 (20.17%) from the Web of Science, and 19,340 (69.80%) from PubMed. Searches were also performed in the World Health Organization Library and Information Networks for Knowledge (WHOLIS) and CINAHL databases, but no results were found. Of these, 25,202 (90.95%) records were flagged as ineligible by the automation tools and filters available in the databases, because they were mainly reports, editorial papers, letters or comments, book chapters, dissertations, and theses or because they did not specifically address the topic of interest according to the use of descriptors. Furthermore, 204 (0.74%) records were duplicated between databases and were removed.
After carefully evaluating the titles and abstracts (first screening step), 1221 (80.22%) of 1522 search results were excluded. For inclusion of papers after reading the abstracts, 81 () of 301 (26.9%) papers were listed for a full reading. After analyzing and extracting the desired results, 33 (40.7%) papers were included in the review because they answered the research questions. The entire selection, sorting, extraction, and synthesis process is described through the PRISMA flowchart [ 23 ], represented in Figure 1 .

PRISMA flowchart with the results of study selection. PRISMA: Preferred Reporting Items for Systematic Reviews and Meta-Analyses.
The 33 studies covered the period of 2017-2021 and were conducted in 22 countries. Most studies were concentrated in Europe (n=11, 33.3%) and North America (the United States and Canada; n=10, 30.3%). Others were carried out in Oceania (Australia; n=4, 12.1%), Asia (China and Taiwan; n=3, 9.1%), and the Middle East (Iran and Saudi Arabia; n=2, 6.1%). In addition, studies were carried out collaboratively or in a network (the United States and India; the United States and African countries; the US Consortium, the United Kingdom, South Africa, Costa Rica, Canada, Sweden, Switzerland, and Bahrain; n=3, 9.1%).
In their entirety, the studies were carried out in high-income countries, and most of the assessments were based on the evidence available in English. The United States (n=11, 33.3%) and Australia (n=4, 12.1%) led in studies involving the investigated topic. No studies conducted or coordinated by middle-income countries were reported. In addition to the low economic diversity of countries where the research was conducted, all papers were evaluated in a single language. The involvement and collaboration of emerging countries took place exclusively through partnerships and participation in consortia.
Regarding the domains described in the studies, there was tremendous variability and inconsistency between the terms presented (n=38 terms). Note that no consensus existed between critical and noncritical variables for data quality assessment. The lack of consensus reflected that the definitions of concepts vary and their relationships are not homogeneous across studies. The discrepancy between domains and evaluated concepts did not allow an evaluation of parity between metrics and was present during all phases of the studies found. The subtopic distribution into the defined categories also evidenced the high-variability factors and strategies in the literature to lead with data quality. The distribution of the improvement strategies for data quality is shown in Table 4 and that of the related influencing factors for data quality in Table 5 .
Improvement strategies for data quality.
Influencing factors for data quality.
Data Quality Issues and Challenges
The metrics extracted from the studies comprised domains related to the methodology adopted by them, that is, concepts that supported the definition of data quality and their respective individual or combined categorizations regarding the adjusted use for the purpose (n=8, 24.2%) of frameworks (n=6, 18.2%), ontologies (n=2, 6.1%), good practice guides (n=15, 45.5%), or combinations of methodologies (n=2, 6.1%).
Among the studies that used the concept of purpose-adjusted use, terms such as “gold standard according to experts” [ 24 ], “intrinsic quality” [ 25 ], “ideal record” [ 26 ], “data fitness” [ 27 , 28 ], and “data culture” [ 29 , 30 ] were addressed. In general, the use of frameworks and ontologies was based on previously published studies and available in development libraries as modules for mapping-adapted entities, proprietary or embedded systems, and data-based strategies for process improvement [ 31 - 34 ].
The central guides and guidelines adopted in data quality studies refer to the adoption of national protocols and policies, agreements signed between research networks and consortia, guides to good clinical practices (International Conference on Harmonization—Good Clinical Practice, ICHGCP [ 35 - 38 ]; Food and Drug Administration, FDA [ 35 , 38 ]; Health Insurance Portability and Accountability Act, HIPPA [ 39 ]), or information governance principles, models, and strategies (International Organization for Standardization, ISO [ 40 , 41 ]; Joint Action Cross-Border Patient Registries Initiative, PARENT [ 41 ]; Findability, Accessibility, Interoperability, and Reuse, FAIR [ 25 , 40 , 42 ]).
Regarding data quality, dimensions were interposed in all research stages, thus being a fundamental factor in being incorporated with good practices and recommendations, giving light to health research, regardless of their methodological designs. The distribution of dimensions evaluated in our findings showed significant heterogeneity, as shown in Table 6 .
Distribution of quality dimensions in health research.
Factors Affecting Data Quality
The study considered factors such as the environment, application time, and development steps, all influencing data quality. Controlled environments were reported in research-only scenarios with planning and proof-of-concept development [ 34 , 35 , 37 , 38 , 43 - 45 ]. Transition and validation environments were identified where research and service were combined [ 25 , 27 , 31 , 40 , 46 - 49 ]. Most studies were conducted in restricted environments specific to health services. Most studies also used their own research repositories, while others relied on external sources, such as preexisting data models [ 25 , 26 , 33 , 40 ] or public databases [ 38 , 50 ]. The research applications spanned diverse health areas, including electronic health records, cancer, intensive care units, rare diseases, maternal health, and more. However, the research areas were more concentrated in specialties such as clinical research [ 27 , 31 , 35 , 37 , 48 ], health informatics [ 43 , 45 ], and research networks [ 25 , 34 , 40 , 44 , 49 ]. Collaborative research networks and clinical trials played a prominent role in the application areas.
Data sources used in the research included literature papers, institutional records, clinical documents, expert perceptions, data models, simulation models, and government databases. Technical limitations were related to performance concerns, infrastructure differences, security measures, visualization methods, and access to data sources.
Other aspects mentioned included the disparity in professionals’ knowledge, the inability to process large volumes of information, and the lack of human and material resources. Legal limitations were attributed to organizational policies that restricted extensive analysis.
The main challenge reported in the studies was related to methodological approaches, particularly the inability to evaluate solutions across multiple scopes, inadequate sample sizes, limited evaluation periods, the lack of a gold standard, and the need for validation and evaluation in different study designs.
Overall, the integrated findings highlight the importance of considering the environment, application time, and methodological approaches in ensuring data quality in health research. The identified challenges and limitations provide valuable insights for future research and the development of strategies to enhance data quality assurance in various health domains.
Strategies for Improving Data Quality
In the analyzed studies, various strategies and interventions were used to plan, manage, and analyze the impact of implementing procedures on data quality assurance. Business intelligence models guided some studies, using extraction, transform, and load (ETL) [ 32 , 40 , 41 , 47 , 51 ]; preprocessing [ 28 , 45 , 52 - 54 ]; Six Sigma practices [ 32 , 48 ]; and the business process management (BPM) model [ 33 ]. Data monitoring strategies included risk-based approaches [ 36 , 37 ], data source verification [ 35 , 37 , 38 ], central monitoring [ 37 , 38 ], remote monitoring (eg, telephone contact) [ 31 , 38 ], and training [ 29 ]. Benchmarking strategies were applied across systems or projects in some cases [ 26 , 50 , 51 ].
Quantitative analyses primarily involved combined strategies, with data triangulation often paired with statistical analyses. Data mining techniques [ 24 ], deep learning, and natural language processing [ 45 ] were also used in combination or individually in different studies. Statistics alone was the most commonly used quantitative technique. The qualitative analysis encompassed diverse approaches, with consultation with specialists [ 30 , 34 , 43 , 44 , 54 , 55 ], structured instruments [ 29 , 38 , 44 , 46 ], data set validation [ 41 , 42 , 56 ], and visual analysis [ 33 , 40 , 48 ] being prominent. Various qualitative techniques, such as interviews [ 27 ], the Delphi technique [ 24 ], feedback audit [ 35 ], grammatical rules [ 39 ], and compliance enforcement [ 49 ], were reported.
Different computational resources were used for analysis and processes. The R language (R Core Team and the R Foundation for Statistical Computing) was commonly used for planning and defining data sets, while Python and Java were mentioned in specific cases for auditing databases and error detection. Clinical and administrative software, web portals, and electronic data capture platforms (eg, Research Electronic Data Capture [REDCap], CommonCarecom, MalariaCare, Assistance Publique–Hôpitaux de Paris–Clinical Data Repository [AP-HP-CDR], Intensive Care Unit DaMa–Clinical information System [ICU-DaMa-CIS]) were used for support, decision-making, data set planning, collection, and auditing. Additional tools, such as dictionaries, data plans, quality indicators, data monitoring plans, electronic measurements (e-measures), and Microsoft Excel spreadsheets, were also used.
It is evident that a range of strategies, interventions, and computational resources were used to ensure data quality in the studies. Business intelligence models, statistical analyses, data mining techniques, and qualitative approaches played significant roles in analyzing and managing data quality. Various programming languages and software tools were used for different tasks, while electronic data capture platforms facilitated data collection and auditing. The integration of these findings highlights the diverse approaches and resources used to address data quality in the analyzed studies.
Synthesis of Findings
The main barriers reported related to the theme of research in the area of health data quality cite circumstances regarding use, systems, and health services. Such barriers are influenced by technical, organizational, behavioral, and environmental factors that cover significant contexts of information systems, specific knowledge, and multidisciplinary techniques [ 43 ]. The quality of each data element in the 9 categories can be assessed by checking its adherence to institutional norms or by comparing and validating it with external sources [ 41 ]. Table 7 summarizes the main types of obstacles reported in the studies.
Barriers to health data quality.
Although many electronic records provide a dictionary of data from their sources, units of measurement were often neglected and adopted outside of established standards. Such “human errors” are inevitable, reinforcing the need for continuous quality assessment from the beginning of collection. However, some studies have tried to develop ontologies to allow the automated and reproducible calculation of data quality measures, although this strategy did not have great acceptance. For Feder [ 55 ], “The harmonized data quality assessment terminology, although not comprehensive, covers common and important aspects of the quality assessment practice.” Therefore, generating a data dictionary with its determined types and creating a data management plan are fundamental in the planning of research [ 28 ].
Both the way of collecting and the way of inputting data impact the expected result from a data set. Therefore, with a focus on minimizing data entry errors as an essential control strategy for clinical research studies, implementing intervention modes of technical barriers was presented as pre- and postanalysis [ 56 ]. The problems were caused by errors in the data source, extraction, transform, and load process or by limitations of the data entry tool. Extracting information to identify actionable insights by mining clinical documents can help answer quantitative questions derived from structured health quality research data sources [ 39 ].
Given the time and effort involved in the iterative error detection process, typical manual curation was considered insufficient. The primary sources of error included human and technological errors [ 35 ]. However, outliers identified by automated algorithms should be considered potential outliers, leaving the field specialists in charge [ 51 ]. In contrast, different and ambiguous definitions of data quality and related characteristics in emergency medical services were presented [ 55 ]. Such divergences were based on intuition, previous experiences, and evaluation purposes. Using definitions based on ontology or standardization is suggested to compare research methods and their results. The definitions and relationships between the different data quality dimensions were unclear, making the quality of comparative assessment difficult [ 52 ].
In terms of evaluation methods, similar definitions overlapped. The difference lay in the distribution comparison and validity verification, where the definition of distribution comparison was based on comparing a data element with an official external reference [ 54 ]. Meanwhile, the validity check was concerned with whether a particular value wass an outlier, a value outside the normal range. The reasons for the existence of multiple evaluation practices were the heterogeneity of data sources about syntax (file format), schema (data structure models), and semantics (meaning and varied interpretations) [ 50 ]. There should be a standard set of data to deal with such inconsistencies and allow data transformation into a structure capable of interoperating with its electronic records [ 40 ].
Data standardization transforms databases from disparate sources into a standard format with shared specifications and structures. It also allows users from different institutions to share digital resources and can facilitate the merging of multicenter data and the development of federated research networks [ 34 ]. For this, 2 processes are necessary: (1) standardization of individual data elements, adhering to terminology specifications [ 49 ], and (2) standardization of the database structure through a minimum data set, which specifies where data values are located and stored in the database [ 50 ]. Improvements in electronic collection software functionality and its coding structures have also been reported to result in lower error rates [ 36 ].
In addition, it is recommended to know the study platform and access secondary data sources that can be used. In this way, transparency in the systemic dissemination of data quality with clear communication, well-defined processes, and instruments can improve the multidisciplinary cooperation that the area requires [ 44 ].
Awareness campaigns on the topic at the organizational level contributed to improving aspects of data governance. The most reported error prevention activities were the continuing education of professionals with regular training of data collectors during their studies [ 50 ]. In this sense, in-service education should promote the correct use of names formulated by structured systems to improve the consistency and accuracy of records and favor their regular auditing. Health systems that received financial incentives for their research obtained more satisfactory results regarding the degree of reliability of their data [ 53 ].
Figure 2 depicts the great diversity of elements involved in the data quality process in health research, representing the planning (precollection), development (data acquisition and monitoring), and analysis (postcollection) stages. In our findings, each phase presented a set of strategies and tools implemented to provide resources that helped the interaction between phases.

Elements involved in the research data quality process. Elements involved in the data quality process. FAIR: Findability, Accessibility, Interoperability, and Reuse; ICHGCP: International Conference on Harmonization—Good Clinical Practice; ISO: International Organization for Standardization.
For the success of research, the processes and techniques must be fluid and applied in a direction based on good guides and recommendations. The research must go through phases, with well-established bases and tools suitable for its purpose, using sources and instruments available through digital strategies and systems, models, guides and feedback, and audit mechanisms.
In addition, every beginning of a new phase must be supported by well-defined pillars that encompass the exhaustive use of validations and pretests; plans for monitoring, management, and data analysis; precautions for ethical and legal issues; training of the team; and channels for effective communication.
In the broadest sense, incorporating data quality techniques and tools is analogous to going on a trip, that is, going from point A to point B. The starting point refers to good planning of issues, such as the year’s season, the quantity and type of items that will be transported, the most appropriate means of transport, the budget available, and tips and guidance available in the different means of communication. Even if the path is already known, an important step that precedes the beginning of its execution is always the definition of the best route. Consulting maps and updated conditions are always recommended since they can change over time.
However, the execution phase of a trip is not limited to reaching the final destination. During the journey, we should always be attentive to signs and directions, without obviously failing to enjoy the landscape and all its opportunities. Finally, when we arrive at our destination, we must bear in mind that to obtain the best results, it is necessary to know the best guides and tourist attractions. A wrong choice or decision can provide us with a low-quality photograph, an unexpected experience, and, as an effect, an epilogue of bad memories.
Principal Findings
This study presented contributions to aid the ultimate goal of good data quality focused on findings that used some digital technology (ie, to develop a disciplined process of identifying data sources, preparing the data for use, and evaluating the value of those sources for their intended use). Key findings revealed variability and a lack of consensus in assessing data quality domains and metrics. Data quality factors included the research environment, application time, and development steps. Strategies for improving data quality involved using business intelligence models, statistical analyses, data mining techniques, and qualitative approaches. The findings highlight the need for standardized practices and collaborative efforts to enhance data quality in health research.
The routine of health services that deal with demands for collecting and consuming data and information can benefit from the set of evidence on tools, processes, and evaluation techniques presented here. Increasingly ubiquitous in the daily lives of professionals, managers, and patients, technology should not be adopted without a specific purpose, as doing so can generate misinterpreted information obtained from unreliable digital health devices and systems. The resources presented can help guide medical decisions that not only involve medical professionals but also indirectly contribute to avoiding decisions based on low-quality information that can put patients’ lives at risk.
With the promotion of the data culture increasingly present in a transversal way, research and researchers can offer increasingly more reliable evidence and, in this way, benefit the promotion and approach to the health area. This mutual cycle must be transparent so that there is awareness that adherence to such a practice can favor the potential strengthening of a collaborative network based on results and promote fluidity and methodological transparency. In addition, it encourages data sharing and, consequently, the reuse of data into reliable information silos, enhancing the development and credibility of health research. At the international level, platforms with a centralized structure of reliable data repositories of patient records that offer data sharing have reduced duplication of efforts and costs. This collaboration can further decrease disparate inequities between middle- and high-income, giving celerity and minimizing risks in the development and integrity of studies.
Reliable data can play a crucial role in enlightening health institutions that prioritize cultivating a data-centric culture and are well equipped to deliver high-quality information. This, in turn, facilitates improved conditions for patient care. In addition to mapping concepts between different sources and application scenarios, it is essential to understand how initial data quality approaches are anchored in previous concepts and domains, with significant attention to suitability for use, following guidelines or using frameworks in a given context [ 41 ]. Since the concept in the same data source can change over time, it is still necessary to carry out mapping with an emphasis on its dimensions in a sensible way and on how the evolution of concepts, processes, and tools impacts the quality assessment of research and health services [ 47 ].
The realization of mapping with emphasis on domains or concepts must coexist in health information systems. The outcome favors maximizing processes, increasing productivity, reducing costs, and meeting research needs [ 26 ]. Consequently, within legal and ethical limits, it is increasingly necessary to use data comprehensively and efficiently to benefit patients [ 57 ]. For example, recent clinical and health service research has adopted the “fit for use” concept proposed in the information science literature. This concept implies that data quality dimensions do not have objective definitions but depend on tasks characterized by research methods and processes [ 48 ]. Increasingly, data quality research has borrowed concepts from various referencing disciplines. More importantly, with many different referencing disciplines using data quality as a context within their discipline, the identity of the field of research has become increasingly less distinct [ 33 ].
Comparison With Prior Work
The large dissonance between domain definitions has increasingly motivated the search for a gold standard to be followed [ 30 ]. The area has received particular attention, especially after the term “big data” gained increasing strength [ 58 ]. The human inability to act with a large volume of information in research and the need to control this high data volume are increasingly driving the emergence of digital solutions. Although the definition of these digital data quality tools occurs from the end user’s perspective, their implementation occurs from the researcher’s perspective; a data set is highly context specific [ 33 ]. So, a generic assessment framework is unlikely to provide a comprehensive data quality analysis for a specific study, making its selection dependent on the study’s analysis plan [ 40 ].
The use of ontologies, for example, can help quantify the impact of likely problems, promote the validity of an effective electronic measure, and allow a generalization of the assessment approach to other data analysis tasks in more specific domains [ 55 ]. This benefit allows the decision-making process and planning of corrective actions and resource allocation faster [ 47 ]. However, the complex coding process can generate inconsistencies and incompleteness due to the characterization of clinically significant conditions, insufficient clinical documentation, and variability in interpretation [ 30 ]. Therefore, it is critical to use specific rules that capture relevant associations in their corresponding information groups. Administrative health data can also capture valuable information about such difficulties using standardized terminologies and monitor and compare coded data between institutions [ 24 ].
Nevertheless, as a consequence of this lack of standard, the use of integrated quality assurance methods combined with standard operating procedures (SOPs) [ 58 ], the use of rapid data feedback [ 38 ], and supportive supervision during the implementation of surveys are feasible, effective, and necessary to ensure high-quality data [ 31 ]. Adopting such well-defined interventions still plays an essential role in data quality management. It is possible to perform these activities through process control and monitoring methods, data manipulation and visualization tools, techniques, and analysis to discover patterns and perspectives on the target information subset [ 27 ]. Regardless of the model adopted, these tools should aim to discover abnormalities and provide the ability to stop and correct them in an acceptable time, also allowing for the investigation of the cause of the problem [ 56 ].
Technology is an excellent ally in these processes, and in parallel with the tools of the Lean Six Sigma philosophy, it can partially replace human work [ 31 ]. To maximize the potential of this combination, the value derived from using analytics must dictate data quality requirements. Computer vision/deep learning, a technology to visualize multidimensional data, has demonstrated data quality checks with a systematic approach to guarantee a reliable and viable developed asset for health care organizations for the holistic implementation of machine learning processes [ 53 ]. However, most of these analytical tools still assume that the analyzed data have high intrinsic quality, which can thus allow possible failures in the process, in addition to the final experiments’ lack of optimization, safety, and reliability [ 37 ].
In this way, the reuse of information has a tremendous negative impact [ 48 ]. The centralized storage of variables without excellent mapping to changes in system paradigms (metadata) and with a mechanism to trace the effects of changes in concepts that are frequent in the health area can also affect the reliability of research [ 37 ]. For example, the severity classification of a given condition can change over time and, consequently, mitigate the comparability power of a study or even prevent it from being used as a basis for planning or evaluating a new one [ 52 ]. In addition, the cultural background and experience of researchers can influence the interpretation of data [ 44 ]. Therefore, a combination of integrated tools located centrally and at each partner site for decentralized research networks can increase the quality of research data [ 40 ].
A central metadata repository contains common data elements and value definitions used to validate the content of data warehouses operated at each location [ 34 ]. So, the consortium can work with standardized reports on data quality, preserving the autonomy of each partner site and allowing individual centers to improve data in their locally sourced systems [ 29 ]. It is, therefore, essential to consider the quality of a record’s content, the data quality usability, and what mechanisms can make data available for broader use [ 41 ]. As outlined by Kodra et al [ 42 ], managing data at the source and applying the FAIR guiding principles for data management are recognized as fundamental strategies in interdisciplinary research network collaboration.
Data production and quality information dissemination depend on establishing a record governance model; identifying the correct data sources; specifying data elements, case report forms, and standardization; and building an IT infrastructure per agreed principles [ 29 ]. Developing adequate documentation, training staff, and providing audit data quality are also essential and can serve as a reference for teaching material for health service education [ 25 ]. This can facilitate more quality studies in low- and middle-income countries.
The lack of such studies implies that health systems and research performance in these countries still face significant challenges at strategic stages, such as planning and managing complete data, leading to errors in population health management and clinical care [ 43 ]. In turn, the low use of health information and poor management of health information systems in these countries make evidence-based decisions and planning at the community level difficult [ 2 ]. The results also demonstrate that, despite existing, such individual training efforts focus mainly on transmitting data analysis skills [ 33 ].
Identifying systematic and persistent defects in advance and correctly directing human, technical, and financial resources are essential to promote better management and increase the quality of information and results achieved in research [ 42 ]. This step can provide improvements and benefits to health managers, allowing greater efficiency in services and better allocation of resources. Promoting such benefits to society through relevant data impacts the performance and effectiveness of public health services [ 39 ] and boosts areas of research, innovation, and enterprise development [ 59 ].
Creative approaches to decision-making in data quality and usability require good use of transdisciplinary collaboration among experts from various fields regardless of study design planning or application area [ 59 ]. This use may be reaching the threshold of significant growth and thus forcing the need for a metamorphosis from the measurement and evaluation of data quality, today focused on content, to a direction focused on use and context [ 57 ].
Without a standard definition, the use of the “fit for purpose” concept for performance monitoring, program management, and data quality decision-making is growing. As a large part of this quality depends on the collection stage, interventions must target the local level where it occurs and must encompass professionals at the operational level and forms at the technical level. Identifying and addressing behavioral and organizational challenges and building technical capacity are critical [ 60 ], increasingly fostering a data-driven culture [ 29 , 30 ].
Limitations
Among the limitations of our review, we first highlight the search for works written in English and Portuguese, since the interpretation of concepts and even the literal translations of terms referring to the dimensions and adaptations to different cultural realities can vary, and thus influenced part of our evaluation [ 31 ]. The limitation may impact the results by excluding relevant research published in other languages and overlooking diverse cultural perspectives. To mitigate this, we suggest expanding collaboration with multilingual experts and including studies in various languages to ensure a comprehensive and unbiased evaluation of data quality.
Second, the absence of evidence in middle-income countries prevented the authors from conducting an adequate synthesis regarding the performance and application of the evidence found in these countries [ 2 ]. Limited representation from middle-income countries hinders the generalizability and applicability of findings, risking a biased understanding of intervention effectiveness. Inclusion of more studies from middle-income countries is vital for comprehensive evidence synthesis, enabling better comprehension of intervention performance in worldwide contexts and avoiding oversight of critical perspectives and outcome variations.
Third, due to the rapid growth of technologies applied to the area, we conducted a search focused on the past 5 years, which may draw attention away from other fundamentals and relevant procedures. The limited time span may lead to incomplete findings and conclusions, hindering a comprehensive understanding of the field’s knowledge and advancements. To address this limitation, future research should consider a broader time frame to include older studies, allowing for a more thorough examination of fundamentals and relevant procedures impacted by the rapid evolution of technologies in the area.
Future Directions
Once the technical and organizational barriers have been overcome, with data managed, reused, stored, extracted, and appropriately distributed [ 46 ], health care must also pay attention to behavior focused on interactions between human, artificial, and hybrid actors. This interaction reflects the importance of adhering to social, ethical, and professional norms, including demands related to justice, responsibility, and transparency [ 60 ]. In short, increasing dependence on quality information increases its possibilities [ 61 ], but it also presents regulators and policy makers with considerable challenges related to their governance in health.
For future work, developing a toolkit based on process indicators is desirable to verify the quality of existing records and provide a score and feedback on the aspects of the registry that require improvements. There is a need for coordination between undergoing initiatives at national and international levels. At the national level, we recommend developing a centralized, public, national “registration as a service” platform, which will guarantee access to highly trained personnel on all topics mentioned in this paper, promoting the standardization of registries. In addition to allowing cost and time savings in creating new registries, the strategy should allow for linking essential data sources on different diseases and increase the capacity to develop cooperation at the regional level.
We also suggest using the data models found in this study to serve as a structured information base for decision support information system development and health observatories, which are increasingly relevant to public health. Furthermore, concerning the health context, it may allow the execution of implementation research projects and the combination with frameworks that relate to health behavior interventions, for example, the Reach, Effectiveness, Adoption, Implementation, and Maintenance (RE-AIM) framework [ 62 ], among others.
This study will help researchers, data managers, auditors, and systems engineers think about the conception, monitoring, tools, and methodologies used to design, execute, and evaluate their research and proposals concerned with data quality. With a well-established and validated data quality workflow for health care, it is expected to assist in mapping the management processes of health care research and promote the identification of gaps in the collection flow where any necessary data quality intervention can be accordingly evaluated with the best tools described here. In conclusion, the results provide evidence of the best practices using data quality approaches involving many other stakeholders, not just researchers and research networks. Although there are some well-known data quality guidelines, they are context specific and not found in the identified scientific publications. So, the information collected in this study can support better decision-making in the area and provide insights that are distinct from the context-specific information typically found in scientific publications.
Abbreviations
Multimedia appendix 1, multimedia appendix 2, data availability.
Conflicts of Interest: None declared.
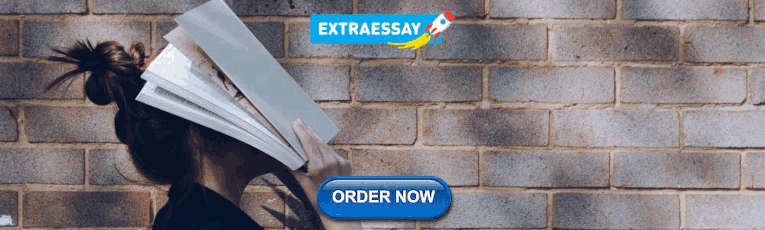
IMAGES
VIDEO
COMMENTS
Findings are evaluated based on hierarchies of evidence from levels 1-6, with meta-analyses of RCT's as the highest level of evidence (1), and opinion-based articles constitute the lowest (6). ... An integrative review of the literature. Canadian Oncology Nursing Journal, 31 (4) (2021), pp. 412-450, 10.5737/23688076314412429.
An integrative review, similar to other reviews, begins with a description of the problem and content of interest: the concepts, target population, and healthcare problem to be addressed in the review. For an integrative review, these variables indicate the need to examine a broad range of study types and literature. Literature search
A literature review is a systematic way of collecting and synthesizing previous research (Snyder, 2019).An integrative literature review provides an integration of the current state of knowledge as a way of generating new knowledge (Holton, 2002).HRDR is labeling Integrative Literature Review as one of the journal's four non-empirical research article types as in theory and conceptual ...
Step 1: Write the review question. The review question acts as a foundation for an integrative study (Riva et al. 2012).Yet, a review question may be difficult to articulate for the novice nursing researcher as it needs to consider multiple factors specifically, the population or sample, the interventions or area under investigation, the research design and outcomes and any benefit to the ...
An integrative review provides a broader summary of the literature and includes findings from a range of research designs. It gathers and synthesizes both empirical and theoretical evidence relevant to a clearly defined problem. It may include case studies, observational studies, and meta-analyses, but may also include practice applications ...
The purpose of a review is to summarize what is known about a topic and communicate the synthesis of literature to a targeted community. Before the advent of evidence-based practice, reviews were unsystematic, and there was no formal guidance on how to produce quality-synthesized evidence (Grant and Booth 2009).Conducting a review should parallel the steps a researcher undertakes when ...
The integrative literature review has many benefits to the scholarly reviewer, including evaluating the strength of the scientific evidence, identifying gaps in current research, identifying the need for future research, bridging between related areas of work, identifying central issues in an area, generating a research question, identifying a theoretical or conceptual framework, and exploring ...
Please consider conducting an integrative or systematic literature review. Be sure to review the Manuscript Submission Guidelines and use established guidelines, such as the Preferred Reporting Items for Systematic Reviews and Meta-Analyses (PRISMA), along with a description of the hierarchy of research evidence (levels of evidence).
Evaluation of research generally occurs on 2 levels: rating or grading the evidence by using a formal level-of-evidence system and individually critiquing the quality of the study. Determining the level of evidence is a key component of appraising the evidence.1-3 Levels or hierarchies of evidence are used to evaluate and grade evidence.
Levels of Evidence for Integrative, Alternative, and Complementary Therapies is about how to weigh the strength of the evidence obtained in cited research studies. ... The summary reflects an independent review of the literature and does not represent a policy statement of NCI or the National Institutes of Health (NIH). Board members review ...
The term integrative review has been used in different ways by knowledge synthesis scholars. Some have considered it broadly, as an overarching term for various types of knowledge syntheses, including scoping, critical, and meta-narrative reviews. ... She decides that conducting a literature review would be a savvy way to examine the evidence ...
An integrative review is a specific review method that summarises past empirical or theoretical literature to provide a greater comprehensive understanding of a particular phenomenon or healthcare problem (Broome 1993). Thus, integrative reviews have the potential to build upon nursing science, informing research, practice, and policy initiatives.
An integrative literature review is a non-experimental design in which the researchers objectively ... level of evidence of articles involved. European Journal of Research in Medical Sciences Vol. 5 No. 1, 2017 ISSN 2056-600X Progressive Academic Publishing, UK Page 12 www.idpublications.org
One way to organize the different types of evidence involved in evidence-based practice research is the levels of evidence pyramid. The pyramid includes a variety of evidence types and levels. Filtered resources: pre-evaluated in some way. systematic reviews. critically-appraised topics. critically-appraised individual articles.
This article presents the integrative review of literature as a distinctive form of research that uses existing literature to create new knowledge. ... Salas E., Mathieu J. E., Rayne S. R. (2012). Task types and team-level attributes: Synthesis of team classification literature. Human Resource ... Integrative literature review of evidence ...
An integrative review is best designed for nursing research. The problem must be clearly defined. The aim of an integrative review is to analyze experimental and non-experimental research simultaneously in order to: define concepts. review theories. review evidence/point out gaps in the literature.
Most reviews fall into the following types: literature review, narrative review, integrative review, evidenced based review, meta-analysis and systematic review. This LibGuide will provide you a general overview of the specific review, offer starting points, and outline the reporting process. ... Presented below are three pyramids that show the ...
An Integrative Literature Review (ILR) allows researchers to go beyond an analysis and synthesis of primary research findings and provides new insights and summarised knowledge about a specific topic. Although an ILR aims to follow similar approaches to that of a systematic review, it allows for the inclusion of both primary research studies, along with other documents (including opinions ...
Conclusion: Evidence from included guidelines can be used by nurses, with the required support and buy-in from management, to promote patient-centred care. Impact: Patient-centred care is essential for quality care. No other literature review has been conducted in the English language to summarize evidence-based patient-centred care guidelines.
Meta-Analysis: A systematic review that uses quantitative methods to summarize the results.(Level 1) Systematic Review: A comprehensive review that authors have systematically searched for, appraised, and summarized all of the medical literature for a specific topic (Level 1) Randomized Controlled Trials: RCT's include a randomized group of patients in an experimental group and a control group.
In cases where the evidence for a specific Western medical or CAM modality is ambiguous and does not clearly meet criteria for one of the above three levels, the modality is assigned to the lower of two levels pending additional literature review or publication of additional studies or systematic reviews.
Evidence from well-designed case-control or cohort studies. Level 5. Evidence from systematic reviews of descriptive and qualitative studies (meta-synthesis) Level 6. Evidence from a single descriptive or qualitative study, EBP, EBQI and QI projects. Level 7. Evidence from the opinion of authorities and/or reports of expert committees, reports ...
Objective To review evidence pertaining to methods for preventing healthcare-associated filovirus infections (including the survivability of filoviruses in clinical environments and the chlorine concentration required for effective disinfection), and to assess protocols for determining the risk of health worker (HW) exposures to filoviruses.Design Integrative review.Data sources PubMed, Embase ...
Background. Decision-making and strategies to improve service delivery must be supported by reliable health data to generate consistent evidence on health status. The data quality management process must ensure the reliability of collected data. Consequently, various methodologies to improve the quality of services are applied in the health field.