
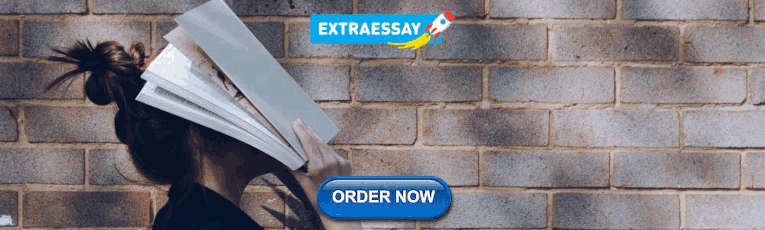
The Future of AI Research: 20 Thesis Ideas for Undergraduate Students in Machine Learning and Deep Learning for 2023!
A comprehensive guide for crafting an original and innovative thesis in the field of ai..
By Aarafat Islam on 2023-01-11
“The beauty of machine learning is that it can be applied to any problem you want to solve, as long as you can provide the computer with enough examples.” — Andrew Ng
This article provides a list of 20 potential thesis ideas for an undergraduate program in machine learning and deep learning in 2023. Each thesis idea includes an introduction , which presents a brief overview of the topic and the research objectives . The ideas provided are related to different areas of machine learning and deep learning, such as computer vision, natural language processing, robotics, finance, drug discovery, and more. The article also includes explanations, examples, and conclusions for each thesis idea, which can help guide the research and provide a clear understanding of the potential contributions and outcomes of the proposed research. The article also emphasized the importance of originality and the need for proper citation in order to avoid plagiarism.
1. Investigating the use of Generative Adversarial Networks (GANs) in medical imaging: A deep learning approach to improve the accuracy of medical diagnoses.
Introduction: Medical imaging is an important tool in the diagnosis and treatment of various medical conditions. However, accurately interpreting medical images can be challenging, especially for less experienced doctors. This thesis aims to explore the use of GANs in medical imaging, in order to improve the accuracy of medical diagnoses.
2. Exploring the use of deep learning in natural language generation (NLG): An analysis of the current state-of-the-art and future potential.
Introduction: Natural language generation is an important field in natural language processing (NLP) that deals with creating human-like text automatically. Deep learning has shown promising results in NLP tasks such as machine translation, sentiment analysis, and question-answering. This thesis aims to explore the use of deep learning in NLG and analyze the current state-of-the-art models, as well as potential future developments.
3. Development and evaluation of deep reinforcement learning (RL) for robotic navigation and control.
Introduction: Robotic navigation and control are challenging tasks, which require a high degree of intelligence and adaptability. Deep RL has shown promising results in various robotics tasks, such as robotic arm control, autonomous navigation, and manipulation. This thesis aims to develop and evaluate a deep RL-based approach for robotic navigation and control and evaluate its performance in various environments and tasks.
4. Investigating the use of deep learning for drug discovery and development.
Introduction: Drug discovery and development is a time-consuming and expensive process, which often involves high failure rates. Deep learning has been used to improve various tasks in bioinformatics and biotechnology, such as protein structure prediction and gene expression analysis. This thesis aims to investigate the use of deep learning for drug discovery and development and examine its potential to improve the efficiency and accuracy of the drug development process.
5. Comparison of deep learning and traditional machine learning methods for anomaly detection in time series data.
Introduction: Anomaly detection in time series data is a challenging task, which is important in various fields such as finance, healthcare, and manufacturing. Deep learning methods have been used to improve anomaly detection in time series data, while traditional machine learning methods have been widely used as well. This thesis aims to compare deep learning and traditional machine learning methods for anomaly detection in time series data and examine their respective strengths and weaknesses.
Photo by Joanna Kosinska on Unsplash
6. Use of deep transfer learning in speech recognition and synthesis.
Introduction: Speech recognition and synthesis are areas of natural language processing that focus on converting spoken language to text and vice versa. Transfer learning has been widely used in deep learning-based speech recognition and synthesis systems to improve their performance by reusing the features learned from other tasks. This thesis aims to investigate the use of transfer learning in speech recognition and synthesis and how it improves the performance of the system in comparison to traditional methods.
7. The use of deep learning for financial prediction.
Introduction: Financial prediction is a challenging task that requires a high degree of intelligence and adaptability, especially in the field of stock market prediction. Deep learning has shown promising results in various financial prediction tasks, such as stock price prediction and credit risk analysis. This thesis aims to investigate the use of deep learning for financial prediction and examine its potential to improve the accuracy of financial forecasting.
8. Investigating the use of deep learning for computer vision in agriculture.
Introduction: Computer vision has the potential to revolutionize the field of agriculture by improving crop monitoring, precision farming, and yield prediction. Deep learning has been used to improve various computer vision tasks, such as object detection, semantic segmentation, and image classification. This thesis aims to investigate the use of deep learning for computer vision in agriculture and examine its potential to improve the efficiency and accuracy of crop monitoring and precision farming.
9. Development and evaluation of deep learning models for generative design in engineering and architecture.
Introduction: Generative design is a powerful tool in engineering and architecture that can help optimize designs and reduce human error. Deep learning has been used to improve various generative design tasks, such as design optimization and form generation. This thesis aims to develop and evaluate deep learning models for generative design in engineering and architecture and examine their potential to improve the efficiency and accuracy of the design process.
10. Investigating the use of deep learning for natural language understanding.
Introduction: Natural language understanding is a complex task of natural language processing that involves extracting meaning from text. Deep learning has been used to improve various NLP tasks, such as machine translation, sentiment analysis, and question-answering. This thesis aims to investigate the use of deep learning for natural language understanding and examine its potential to improve the efficiency and accuracy of natural language understanding systems.
Photo by UX Indonesia on Unsplash
11. Comparing deep learning and traditional machine learning methods for image compression.
Introduction: Image compression is an important task in image processing and computer vision. It enables faster data transmission and storage of image files. Deep learning methods have been used to improve image compression, while traditional machine learning methods have been widely used as well. This thesis aims to compare deep learning and traditional machine learning methods for image compression and examine their respective strengths and weaknesses.
12. Using deep learning for sentiment analysis in social media.
Introduction: Sentiment analysis in social media is an important task that can help businesses and organizations understand their customers’ opinions and feedback. Deep learning has been used to improve sentiment analysis in social media, by training models on large datasets of social media text. This thesis aims to use deep learning for sentiment analysis in social media, and evaluate its performance against traditional machine learning methods.
13. Investigating the use of deep learning for image generation.
Introduction: Image generation is a task in computer vision that involves creating new images from scratch or modifying existing images. Deep learning has been used to improve various image generation tasks, such as super-resolution, style transfer, and face generation. This thesis aims to investigate the use of deep learning for image generation and examine its potential to improve the quality and diversity of generated images.
14. Development and evaluation of deep learning models for anomaly detection in cybersecurity.
Introduction: Anomaly detection in cybersecurity is an important task that can help detect and prevent cyber-attacks. Deep learning has been used to improve various anomaly detection tasks, such as intrusion detection and malware detection. This thesis aims to develop and evaluate deep learning models for anomaly detection in cybersecurity and examine their potential to improve the efficiency and accuracy of cybersecurity systems.
15. Investigating the use of deep learning for natural language summarization.
Introduction: Natural language summarization is an important task in natural language processing that involves creating a condensed version of a text that preserves its main meaning. Deep learning has been used to improve various natural language summarization tasks, such as document summarization and headline generation. This thesis aims to investigate the use of deep learning for natural language summarization and examine its potential to improve the efficiency and accuracy of natural language summarization systems.
Photo by Windows on Unsplash
16. Development and evaluation of deep learning models for facial expression recognition.
Introduction: Facial expression recognition is an important task in computer vision and has many practical applications, such as human-computer interaction, emotion recognition, and psychological studies. Deep learning has been used to improve facial expression recognition, by training models on large datasets of images. This thesis aims to develop and evaluate deep learning models for facial expression recognition and examine their performance against traditional machine learning methods.
17. Investigating the use of deep learning for generative models in music and audio.
Introduction: Music and audio synthesis is an important task in audio processing, which has many practical applications, such as music generation and speech synthesis. Deep learning has been used to improve generative models for music and audio, by training models on large datasets of audio data. This thesis aims to investigate the use of deep learning for generative models in music and audio and examine its potential to improve the quality and diversity of generated audio.
18. Study the comparison of deep learning models with traditional algorithms for anomaly detection in network traffic.
Introduction: Anomaly detection in network traffic is an important task that can help detect and prevent cyber-attacks. Deep learning models have been used for this task, and traditional methods such as clustering and rule-based systems are widely used as well. This thesis aims to compare deep learning models with traditional algorithms for anomaly detection in network traffic and analyze the trade-offs between the models in terms of accuracy and scalability.
19. Investigating the use of deep learning for improving recommender systems.
Introduction: Recommender systems are widely used in many applications such as online shopping, music streaming, and movie streaming. Deep learning has been used to improve the performance of recommender systems, by training models on large datasets of user-item interactions. This thesis aims to investigate the use of deep learning for improving recommender systems and compare its performance with traditional content-based and collaborative filtering approaches.
20. Development and evaluation of deep learning models for multi-modal data analysis.
Introduction: Multi-modal data analysis is the task of analyzing and understanding data from multiple sources such as text, images, and audio. Deep learning has been used to improve multi-modal data analysis, by training models on large datasets of multi-modal data. This thesis aims to develop and evaluate deep learning models for multi-modal data analysis and analyze their potential to improve performance in comparison to single-modal models.
I hope that this article has provided you with a useful guide for your thesis research in machine learning and deep learning. Remember to conduct a thorough literature review and to include proper citations in your work, as well as to be original in your research to avoid plagiarism. I wish you all the best of luck with your thesis and your research endeavors!
Continue Learning
100 valuable chatgpt prompts to boost startups and businesses.
Spark your startups and businesses with these invaluable ChatGPT prompts.
Mastering the GPT-3 Temperature Parameter with Ruby
Pooling layer — short and simple.
Here's all the information you should know about Pooling Layer in CNN
Prompt Engineering: How To Turn Your Words Into Works Of Art
Beginner’s guide to openai’s gpt-3.5-turbo model.
From GPT-3 to GPT-3.5-Turbo: Understanding the Latest Upgrades in OpenAI’s Language Model API.
The Role of AI in Enhancing Website Content Security and Clarity
A list of completed theses and new thesis topics from the Computer Vision Group.
Are you about to start a BSc or MSc thesis? Please read our instructions for preparing and delivering your work.
Below we list possible thesis topics for Bachelor and Master students in the areas of Computer Vision, Machine Learning, Deep Learning and Pattern Recognition. The project descriptions leave plenty of room for your own ideas. If you would like to discuss a topic in detail, please contact the supervisor listed below and Prof. Paolo Favaro to schedule a meeting. Note that for MSc students in Computer Science it is required that the official advisor is a professor in CS.
AI deconvolution of light microscopy images
Level: master.
Background Light microscopy became an indispensable tool in life sciences research. Deconvolution is an important image processing step in improving the quality of microscopy images for removing out-of-focus light, higher resolution, and beter signal to noise ratio. Currently classical deconvolution methods, such as regularisation or blind deconvolution, are implemented in numerous commercial software packages and widely used in research. Recently AI deconvolution algorithms have been introduced and being currently actively developed, as they showed a high application potential.
Aim Adaptation of available AI algorithms for deconvolution of microscopy images. Validation of these methods against state-of-the -art commercially available deconvolution software.
Material and Methods Student will implement and further develop available AI deconvolution methods and acquire test microscopy images of different modalities. Performance of developed AI algorithms will be validated against available commercial deconvolution software.

- Al algorithm development and implementation: 50%.
- Data acquisition: 10%.
- Comparison of performance: 40 %.
Requirements
- Interest in imaging.
- Solid knowledge of AI.
- Good programming skills.
Supervisors Paolo Favaro, Guillaume Witz, Yury Belyaev.
Institutes Computer Vison Group, Digital Science Lab, Microscopy imaging Center.
Contact Yury Belyaev, Microscopy imaging Center, [email protected] , + 41 78 899 0110.
Instance segmentation of cryo-ET images
Level: bachelor/master.
In the 1600s, a pioneering Dutch scientist named Antonie van Leeuwenhoek embarked on a remarkable journey that would forever transform our understanding of the natural world. Armed with a simple yet ingenious invention, the light microscope, he delved into uncharted territory, peering through its lens to reveal the hidden wonders of microscopic structures. Fast forward to today, where cryo-electron tomography (cryo-ET) has emerged as a groundbreaking technique, allowing researchers to study proteins within their natural cellular environments. Proteins, functioning as vital nano-machines, play crucial roles in life and understanding their localization and interactions is key to both basic research and disease comprehension. However, cryo-ET images pose challenges due to inherent noise and a scarcity of annotated data for training deep learning models.

Credit: S. Albert et al./PNAS (CC BY 4.0)
To address these challenges, this project aims to develop a self-supervised pipeline utilizing diffusion models for instance segmentation in cryo-ET images. By leveraging the power of diffusion models, which iteratively diffuse information to capture underlying patterns, the pipeline aims to refine and accurately segment cryo-ET images. Self-supervised learning, which relies on unlabeled data, reduces the dependence on extensive manual annotations. Successful implementation of this pipeline could revolutionize the field of structural biology, facilitating the analysis of protein distribution and organization within cellular contexts. Moreover, it has the potential to alleviate the limitations posed by limited annotated data, enabling more efficient extraction of valuable information from cryo-ET images and advancing biomedical applications by enhancing our understanding of protein behavior.
Methods The segmentation pipeline for cryo-electron tomography (cryo-ET) images consists of two stages: training a diffusion model for image generation and training an instance segmentation U-Net using synthetic and real segmentation masks.
1. Diffusion Model Training: a. Data Collection: Collect and curate cryo-ET image datasets from the EMPIAR database (https://www.ebi.ac.uk/empiar/). b. Architecture Design: Select an appropriate architecture for the diffusion model. c. Model Evaluation: Cryo-ET experts will help assess image quality and fidelity through visual inspection and quantitative measures 2. Building the Segmentation dataset: a. Synthetic and real mask generation: Use the trained diffusion model to generate synthetic cryo-ET images. The diffusion process will be seeded from either a real or a synthetic segmentation mask. This will yield to pairs of cryo-ET images and segmentation masks. 3. Instance Segmentation U-Net Training: a. Architecture Design: Choose an appropriate instance segmentation U-Net architecture. b. Model Evaluation: Evaluate the trained U-Net using precision, recall, and F1 score metrics.
By combining the diffusion model for cryo-ET image generation and the instance segmentation U-Net, this pipeline provides an efficient and accurate approach to segment structures in cryo-ET images, facilitating further analysis and interpretation.
References 1. Kwon, Diana. "The secret lives of cells-as never seen before." Nature 598.7882 (2021): 558-560. 2. Moebel, Emmanuel, et al. "Deep learning improves macromolecule identification in 3D cellular cryo-electron tomograms." Nature methods 18.11 (2021): 1386-1394. 3. Rice, Gavin, et al. "TomoTwin: generalized 3D localization of macromolecules in cryo-electron tomograms with structural data mining." Nature Methods (2023): 1-10.
Contacts Prof. Thomas Lemmin Institute of Biochemistry and Molecular Medicine Bühlstrasse 28, 3012 Bern ( [email protected] )
Prof. Paolo Favaro Institute of Computer Science Neubrückstrasse 10 3012 Bern ( [email protected] )
Adding and removing multiple sclerosis lesions with to imaging with diffusion networks
Background multiple sclerosis lesions are the result of demyelination: they appear as dark spots on t1 weighted mri imaging and as bright spots on flair mri imaging. image analysis for ms patients requires both the accurate detection of new and enhancing lesions, and the assessment of atrophy via local thickness and/or volume changes in the cortex. detection of new and growing lesions is possible using deep learning, but made difficult by the relative lack of training data: meanwhile cortical morphometry can be affected by the presence of lesions, meaning that removing lesions prior to morphometry may be more robust. existing ‘lesion filling’ methods are rather crude, yielding unrealistic-appearing brains where the borders of the removed lesions are clearly visible., aim: denoising diffusion networks are the current gold standard in mri image generation [1]: we aim to leverage this technology to remove and add lesions to existing mri images. this will allow us to create realistic synthetic mri images for training and validating ms lesion segmentation algorithms, and for investigating the sensitivity of morphometry software to the presence of ms lesions at a variety of lesion load levels., materials and methods: a large, annotated, heterogeneous dataset of mri data from ms patients, as well as images of healthy controls without white matter lesions, will be available for developing the method. the student will work in a research group with a long track record in applying deep learning methods to neuroimaging data, as well as experience training denoising diffusion networks..
Nature of the Thesis:
Literature review: 10%
Replication of Blob Loss paper: 10%
Implementation of the sliding window metrics:10%
Training on MS lesion segmentation task: 30%
Extension to other datasets: 20%
Results analysis: 20%
Fig. Results of an existing lesion filling algorithm, showing inadequate performance
Requirements:
Interest/Experience with image processing
Python programming knowledge (Pytorch bonus)
Interest in neuroimaging
Supervisor(s):
PD. Dr. Richard McKinley
Institutes: Diagnostic and Interventional Neuroradiology
Center for Artificial Intelligence in Medicine (CAIM), University of Bern
References: [1] Brain Imaging Generation with Latent Diffusion Models , Pinaya et al, Accepted in the Deep Generative Models workshop @ MICCAI 2022 , https://arxiv.org/abs/2209.07162
Contact : PD Dr Richard McKinley, Support Centre for Advanced Neuroimaging ( [email protected] )
Improving metrics and loss functions for targets with imbalanced size: sliding window Dice coefficient and loss.
Background The Dice coefficient is the most commonly used metric for segmentation quality in medical imaging, and a differentiable version of the coefficient is often used as a loss function, in particular for small target classes such as multiple sclerosis lesions. Dice coefficient has the benefit that it is applicable in instances where the target class is in the minority (for example, in case of segmenting small lesions). However, if lesion sizes are mixed, the loss and metric is biased towards performance on large lesions, leading smaller lesions to be missed and harming overall lesion detection. A recently proposed loss function (blob loss[1]) aims to combat this by treating each connected component of a lesion mask separately, and claims improvements over Dice loss on lesion detection scores in a variety of tasks.
Aim: The aim of this thesisis twofold. First, to benchmark blob loss against a simple, potentially superior loss for instance detection: sliding window Dice loss, in which the Dice loss is calculated over a sliding window across the area/volume of the medical image. Second, we will investigate whether a sliding window Dice coefficient is better corellated with lesion-wise detection metrics than Dice coefficient and may serve as an alternative metric capturing both global and instance-wise detection.
Materials and Methods: A large, annotated, heterogeneous dataset of MRI data from MS patients will be available for benchmarking the method, as well as our existing codebases for MS lesion segmentation. Extension of the method to other diseases and datasets (such as covered in the blob loss paper) will make the method more plausible for publication. The student will work alongside clinicians and engineers carrying out research in multiple sclerosis lesion segmentation, in particular in the context of our running project supported by the CAIM grant.

Fig. An annotated MS lesion case, showing the variety of lesion sizes
References: [1] blob loss: instance imbalance aware loss functions for semantic segmentation, Kofler et al, https://arxiv.org/abs/2205.08209
Idempotent and partial skull-stripping in multispectral MRI imaging
Background Skull stripping (or brain extraction) refers to the masking of non-brain tissue from structural MRI imaging. Since 3D MRI sequences allow reconstruction of facial features, many data providers supply data only after skull-stripping, making this a vital tool in data sharing. Furthermore, skull-stripping is an important pre-processing step in many neuroimaging pipelines, even in the deep-learning era: while many methods could now operate on data with skull present, they have been trained only on skull-stripped data and therefore produce spurious results on data with the skull present.
High-quality skull-stripping algorithms based on deep learning are now widely available: the most prominent example is HD-BET [1]. A major downside of HD-BET is its behaviour on datasets to which skull-stripping has already been applied: in this case the algorithm falsely identifies brain tissue as skull and masks it. A skull-stripping algorithm F not exhibiting this behaviour would be idempotent: F(F(x)) = F(x) for any image x. Furthermore, legacy datasets from before the availability of high-quality skull-stripping algorithms may still contain images which have been inadequately skull-stripped: currently the only solution to improve the skull-stripping on this data is to go back to the original datasource or to manually correct the skull-stripping, which is time-consuming and prone to error.
Aim: In this project, the student will develop an idempotent skull-stripping network which can also handle partially skull-stripped inputs. In the best case, the network will operate well on a large subset of the data we work with (e.g. structural MRI, diffusion-weighted MRI, Perfusion-weighted MRI, susceptibility-weighted MRI, at a variety of field strengths) to maximize the future applicability of the network across the teams in our group.
Materials and Methods: Multiple datasets, both publicly available and internal (encompassing thousands of 3D volumes) will be available. Silver standard reference data for standard sequences at 1.5T and 3T can be generated using existing tools such as HD-BET: for other sequences and field strengths semi-supervised learning or methods improving robustness to domain shift may be employed. Robustness to partial skull-stripping may be induced by a combination of learning theory and model-based approaches.

Dataset curation: 10%
Idempotent skull-stripping model building: 30%
Modelling of partial skull-stripping:10%
Extension of model to handle partial skull: 30%
Results analysis: 10%
Fig. An example of failed skull-stripping requiring manual correction
References: [1] Isensee, F, Schell, M, Pflueger, I, et al. Automated brain extraction of multisequence MRI using artificial neural networks. Hum Brain Mapp . 2019; 40: 4952– 4964. https://doi.org/10.1002/hbm.24750
Automated leaf detection and leaf area estimation (for Arabidopsis thaliana)
Correlating plant phenotypes such as leaf area or number of leaves to the genotype (i.e. changes in DNA) is a common goal for plant breeders and molecular biologists. Such data can not only help to understand fundamental processes in nature, but also can help to improve ecotypes, e.g., to perform better under climate change, or reduce fertiliser input. However, collecting data for many plants is very time consuming and automated data acquisition is necessary.
The project aims at building a machine learning model to automatically detect plants in top-view images (see examples below), segment their leaves (see Fig C) and to estimate the leaf area. This information will then be used to determine the leaf area of different Arabidopsis ecotypes. The project will be carried out in collaboration with researchers of the Institute of Plant Sciences at the University of Bern. It will also involve the design and creation of a dataset of plant top-views with the corresponding annotation (provided by experts at the Institute of Plant Sciences).

Contact: Prof. Dr. Paolo Favaro ( [email protected] )
Master Projects at the ARTORG Center
The Gerontechnology and Rehabilitation group at the ARTORG Center for Biomedical Engineering is offering multiple MSc thesis projects to students, which are interested in working with real patient data, artificial intelligence and machine learning algorithms. The goal of these projects is to transfer the findings to the clinic in order to solve today’s healthcare problems and thus to improve the quality of life of patients. Assessment of Digital Biomarkers at Home by Radar. [PDF] Comparison of Radar, Seismograph and Ballistocardiography and to Monitor Sleep at Home. [PDF] Sentimental Analysis in Speech. [PDF] Contact: Dr. Stephan Gerber ( [email protected] )
Internship in Computational Imaging at Prophesee
A 6 month intership at Prophesee, Grenoble is offered to a talented Master Student.
The topic of the internship is working on burst imaging following the work of Sam Hasinoff , and exploring ways to improve it using event-based vision.
A compensation to cover the expenses of living in Grenoble is offered. Only students that have legal rights to work in France can apply.
Anyone interested can send an email with the CV to Daniele Perrone ( [email protected] ).
Using machine learning applied to wearables to predict mental health
This Master’s project lies at the intersection of psychiatry and computer science and aims to use machine learning techniques to improve health. Using sensors to detect sleep and waking behavior has as of yet unexplored potential to reveal insights into health. In this study, we make use of a watch-like device, called an actigraph, which tracks motion to quantify sleep behavior and waking activity. Participants in the study consist of healthy and depressed adolescents and wear actigraphs for a year during which time we query their mental health status monthly using online questionnaires. For this masters thesis we aim to make use of machine learning methods to predict mental health based on the data from the actigraph. The ability to predict mental health crises based on sleep and wake behavior would provide an opportunity for intervention, significantly impacting the lives of patients and their families. This Masters thesis is a collaboration between Professor Paolo Favaro at the Institute of Computer Science ( [email protected] ) and Dr Leila Tarokh at the Universitäre Psychiatrische Dienste (UPD) ( [email protected] ). We are looking for a highly motivated individual interested in bridging disciplines.
Bachelor or Master Projects at the ARTORG Center
The Gerontechnology and Rehabilitation group at the ARTORG Center for Biomedical Engineering is offering multiple BSc- and MSc thesis projects to students, which are interested in working with real patient data, artificial intelligence and machine learning algorithms. The goal of these projects is to transfer the findings to the clinic in order to solve today’s healthcare problems and thus to improve the quality of life of patients. Machine Learning Based Gait-Parameter Extraction by Using Simple Rangefinder Technology. [PDF] Detection of Motion in Video Recordings [PDF] Home-Monitoring of Elderly by Radar [PDF] Gait feature detection in Parkinson's Disease [PDF] Development of an arthroscopic training device using virtual reality [PDF] Contact: Dr. Stephan Gerber ( [email protected] ), Michael Single ( [email protected]. ch )
Dynamic Transformer
Level: bachelor.
Visual Transformers have obtained state of the art classification accuracies [ViT, DeiT, T2T, BoTNet]. Mixture of experts could be used to increase the capacity of a neural network by learning instance dependent execution pathways in a network [MoE]. In this research project we aim to push the transformers to their limit and combine their dynamic attention with MoEs, compared to Switch Transformer [Switch], we will use a much more efficient formulation of mixing [CondConv, DynamicConv] and we will use this idea in the attention part of the transformer, not the fully connected layer.
- Input dependent attention kernel generation for better transformer layers.
Publication Opportunity: Dynamic Neural Networks Meets Computer Vision (a CVPR 2021 Workshop)
Extensions:
- The same idea could be extended to other ViT/Transformer based models [DETR, SETR, LSTR, TrackFormer, BERT]
Related Papers:
- Visual Transformers: Token-based Image Representation and Processing for Computer Vision [ViT]
- DeiT: Data-efficient Image Transformers [DeiT]
- Bottleneck Transformers for Visual Recognition [BoTNet]
- Tokens-to-Token ViT: Training Vision Transformers from Scratch on ImageNet [T2TViT]
- Outrageously Large Neural Networks: The Sparsely-Gated Mixture-of-Experts Layer [MoE]
- Switch Transformers: Scaling to Trillion Parameter Models with Simple and Efficient Sparsity [Switch]
- CondConv: Conditionally Parameterized Convolutions for Efficient Inference [CondConv]
- Dynamic Convolution: Attention over Convolution Kernels [DynamicConv]
- End-to-End Object Detection with Transformers [DETR]
- Rethinking Semantic Segmentation from a Sequence-to-Sequence Perspective with Transformers [SETR]
- End-to-end Lane Shape Prediction with Transformers [LSTR]
- TrackFormer: Multi-Object Tracking with Transformers [TrackFormer]
- BERT: Pre-training of Deep Bidirectional Transformers for Language Understanding [BERT]
Contact: Sepehr Sameni
Visual Transformers have obtained state of the art classification accuracies for 2d images[ViT, DeiT, T2T, BoTNet]. In this project, we aim to extend the same ideas to 3d data (videos), which requires a more efficient attention mechanism [Performer, Axial, Linformer]. In order to accelerate the training process, we could use [Multigrid] technique.
- Better video understanding by attention blocks.
Publication Opportunity: LOVEU (a CVPR workshop) , Holistic Video Understanding (a CVPR workshop) , ActivityNet (a CVPR workshop)
- Rethinking Attention with Performers [Performer]
- Axial Attention in Multidimensional Transformers [Axial]
- Linformer: Self-Attention with Linear Complexity [Linformer]
- A Multigrid Method for Efficiently Training Video Models [Multigrid]
GIRAFFE is a newly introduced GAN that can generate scenes via composition with minimal supervision [GIRAFFE]. Generative methods can implicitly learn interpretable representation as can be seen in GAN image interpretations [GANSpace, GanLatentDiscovery]. Decoding GIRAFFE could give us per-object interpretable representations that could be used for scene manipulation, data augmentation, scene understanding, semantic segmentation, pose estimation [iNeRF], and more.
In order to invert a GIRAFFE model, we will first train the generative model on Clevr and CompCars datasets, then we add a decoder to the pipeline and train this autoencoder. We can make the task easier by knowing the number of objects in the scene and/or knowing their positions.
Goals:
Scene Manipulation and Decomposition by Inverting the GIRAFFE
Publication Opportunity: DynaVis 2021 (a CVPR workshop on Dynamic Scene Reconstruction)
Related Papers:
- GIRAFFE: Representing Scenes as Compositional Generative Neural Feature Fields [GIRAFFE]
- Neural Scene Graphs for Dynamic Scenes
- pixelNeRF: Neural Radiance Fields from One or Few Images [pixelNeRF]
- NeRF: Representing Scenes as Neural Radiance Fields for View Synthesis [NeRF]
- Neural Volume Rendering: NeRF And Beyond
- GANSpace: Discovering Interpretable GAN Controls [GANSpace]
- Unsupervised Discovery of Interpretable Directions in the GAN Latent Space [GanLatentDiscovery]
- Inverting Neural Radiance Fields for Pose Estimation [iNeRF]
Quantized ViT
Visual Transformers have obtained state of the art classification accuracies [ViT, CLIP, DeiT], but the best ViT models are extremely compute heavy and running them even only for inference (not doing backpropagation) is expensive. Running transformers cheaply by quantization is not a new problem and it has been tackled before for BERT [BERT] in NLP [Q-BERT, Q8BERT, TernaryBERT, BinaryBERT]. In this project we will be trying to quantize pretrained ViT models.
Quantizing ViT models for faster inference and smaller models without losing accuracy
Publication Opportunity: Binary Networks for Computer Vision 2021 (a CVPR workshop)
Extensions:
- Having a fast pipeline for image inference with ViT will allow us to dig deep into the attention of ViT and analyze it, we might be able to prune some attention heads or replace them with static patterns (like local convolution or dilated patterns), We might be even able to replace the transformer with performer and increase the throughput even more [Performer].
- The same idea could be extended to other ViT based models [DETR, SETR, LSTR, TrackFormer, CPTR, BoTNet, T2TViT]
- Learning Transferable Visual Models From Natural Language Supervision [CLIP]
- Visual Transformers: Token-based Image Representation and Processing for Computer Vision [ViT]
- DeiT: Data-efficient Image Transformers [DeiT]
- BERT: Pre-training of Deep Bidirectional Transformers for Language Understanding [BERT]
- Q-BERT: Hessian Based Ultra Low Precision Quantization of BERT [Q-BERT]
- Q8BERT: Quantized 8Bit BERT [Q8BERT]
- TernaryBERT: Distillation-aware Ultra-low Bit BERT [TernaryBERT]
- BinaryBERT: Pushing the Limit of BERT Quantization [BinaryBERT]
- Rethinking Attention with Performers [Performer]
- End-to-End Object Detection with Transformers [DETR]
- Rethinking Semantic Segmentation from a Sequence-to-Sequence Perspective with Transformers [SETR]
- End-to-end Lane Shape Prediction with Transformers [LSTR]
- TrackFormer: Multi-Object Tracking with Transformers [TrackFormer]
- CPTR: Full Transformer Network for Image Captioning [CPTR]
- Bottleneck Transformers for Visual Recognition [BoTNet]
- Tokens-to-Token ViT: Training Vision Transformers from Scratch on ImageNet [T2TViT]
Multimodal Contrastive Learning
Recently contrastive learning has gained a lot of attention for self-supervised image representation learning [SimCLR, MoCo]. Contrastive learning could be extended to multimodal data, like videos (images and audio) [CMC, CoCLR]. Most contrastive methods require large batch sizes (or large memory pools) which makes them expensive for training. In this project we are going to use non batch size dependent contrastive methods [SwAV, BYOL, SimSiam] to train multimodal representation extractors.
Our main goal is to compare the proposed method with the CMC baseline, so we will be working with STL10, ImageNet, UCF101, HMDB51, and NYU Depth-V2 datasets.
Inspired by the recent works on smaller datasets [ConVIRT, CPD], to accelerate the training speed, we could start with two pretrained single-modal models and finetune them with the proposed method.
- Extending SwAV to multimodal datasets
- Grasping a better understanding of the BYOL
Publication Opportunity: MULA 2021 (a CVPR workshop on Multimodal Learning and Applications)
- Most knowledge distillation methods for contrastive learners also use large batch sizes (or memory pools) [CRD, SEED], the proposed method could be extended for knowledge distillation.
- One could easily extend this idea to multiview learning, for example one could have two different networks working on the same input and train them with contrastive learning, this may lead to better models [DeiT] by cross-model inductive biases communications.
- Self-supervised Co-training for Video Representation Learning [CoCLR]
- Learning Spatiotemporal Features via Video and Text Pair Discrimination [CPD]
- Audio-Visual Instance Discrimination with Cross-Modal Agreement [AVID-CMA]
- Self-Supervised Learning by Cross-Modal Audio-Video Clustering [XDC]
- Contrastive Multiview Coding [CPC]
- Contrastive Learning of Medical Visual Representations from Paired Images and Text [ConVIRT]
- A Simple Framework for Contrastive Learning of Visual Representations [SimCLR]
- Momentum Contrast for Unsupervised Visual Representation Learning [MoCo]
- Bootstrap your own latent: A new approach to self-supervised Learning [BYOL]
- Exploring Simple Siamese Representation Learning [SimSiam]
- Unsupervised Learning of Visual Features by Contrasting Cluster Assignments [SwAV]
- Contrastive Representation Distillation [CRD]
- SEED: Self-supervised Distillation For Visual Representation [SEED]
Robustness of Neural Networks
Neural Networks have been found to achieve surprising performance in several tasks such as classification, detection and segmentation. However, they are also very sensitive to small (controlled) changes to the input. It has been shown that some changes to an image that are not visible to the naked eye may lead the network to output an incorrect label. This thesis will focus on studying recent progress in this area and aim to build a procedure for a trained network to self-assess its reliability in classification or one of the popular computer vision tasks.
Contact: Paolo Favaro
Masters projects at sitem center
The Personalised Medicine Research Group at the sitem Center for Translational Medicine and Biomedical Entrepreneurship is offering multiple MSc thesis projects to the biomed eng MSc students that may also be of interest to the computer science students. Automated quantification of cartilage quality for hip treatment decision support. PDF Automated quantification of massive rotator cuff tears from MRI. PDF Deep learning-based segmentation and fat fraction analysis of the shoulder muscles using quantitative MRI. PDF Unsupervised Domain Adaption for Cross-Modality Hip Joint Segmentation. PDF Contact: Dr. Kate Gerber
Internships/Master thesis @ Chronocam
3-6 months internships on event-based computer vision. Chronocam is a rapidly growing startup developing event-based technology, with more than 15 PhDs working on problems like tracking, detection, classification, SLAM, etc. Event-based computer vision has the potential to solve many long-standing problems in traditional computer vision, and this is a super exciting time as this potential is becoming more and more tangible in many real-world applications. For next year we are looking for motivated Master and PhD students with good software engineering skills (C++ and/or python), and preferable good computer vision and deep learning background. PhD internships will be more research focused and possibly lead to a publication. For each intern we offer a compensation to cover the expenses of living in Paris. List of some of the topics we want to explore:
- Photo-realistic image synthesis and super-resolution from event-based data (PhD)
- Self-supervised representation learning (PhD)
- End-to-end Feature Learning for Event-based Data
- Bio-inspired Filtering using Spiking Networks
- On-the fly Compression of Event-based Streams for Low-Power IoT Cameras
- Tracking of Multiple Objects with a Dual-Frequency Tracker
- Event-based Autofocus
- Stabilizing an Event-based Stream using an IMU
- Crowd Monitoring for Low-power IoT Cameras
- Road Extraction from an Event-based Camera Mounted in a Car for Autonomous Driving
- Sign detection from an Event-based Camera Mounted in a Car for Autonomous Driving
- High-frequency Eye Tracking
Email with attached CV to Daniele Perrone at [email protected] .
Contact: Daniele Perrone
Object Detection in 3D Point Clouds
Today we have many 3D scanning techniques that allow us to capture the shape and appearance of objects. It is easier than ever to scan real 3D objects and transform them into a digital model for further processing, such as modeling, rendering or animation. However, the output of a 3D scanner is often a raw point cloud with little to no annotations. The unstructured nature of the point cloud representation makes it difficult for processing, e.g. surface reconstruction. One application is the detection and segmentation of an object of interest. In this project, the student is challenged to design a system that takes a point cloud (a 3D scan) as input and outputs the names of objects contained in the scan. This output can then be used to eliminate outliers or points that belong to the background. The approach involves collecting a large dataset of 3D scans and training a neural network on it.
Contact: Adrian Wälchli
Shape Reconstruction from a Single RGB Image or Depth Map
A photograph accurately captures the world in a moment of time and from a specific perspective. Since it is a projection of the 3D space to a 2D image plane, the depth information is lost. Is it possible to restore it, given only a single photograph? In general, the answer is no. This problem is ill-posed, meaning that many different plausible depth maps exist, and there is no way of telling which one is the correct one. However, if we cover one of our eyes, we are still able to recognize objects and estimate how far away they are. This motivates the exploration of an approach where prior knowledge can be leveraged to reduce the ill-posedness of the problem. Such a prior could be learned by a deep neural network, trained with many images and depth maps.
CNN Based Deblurring on Mobile
Deblurring finds many applications in our everyday life. It is particularly useful when taking pictures on handheld devices (e.g. smartphones) where camera shake can degrade important details. Therefore, it is desired to have a good deblurring algorithm implemented directly in the device. In this project, the student will implement and optimize a state-of-the-art deblurring method based on a deep neural network for deployment on mobile phones (Android). The goal is to reduce the number of network weights in order to reduce the memory footprint while preserving the quality of the deblurred images. The result will be a camera app that automatically deblurs the pictures, giving the user a choice of keeping the original or the deblurred image.
Depth from Blur
If an object in front of the camera or the camera itself moves while the aperture is open, the region of motion becomes blurred because the incoming light is accumulated in different positions across the sensor. If there is camera motion, there is also parallax. Thus, a motion blurred image contains depth information. In this project, the student will tackle the problem of recovering a depth-map from a motion-blurred image. This includes the collection of a large dataset of blurred- and sharp images or videos using a pair or triplet of GoPro action cameras. Two cameras will be used in stereo to estimate the depth map, and the third captures the blurred frames. This data is then used to train a convolutional neural network that will predict the depth map from the blurry image.
Unsupervised Clustering Based on Pretext Tasks
The idea of this project is that we have two types of neural networks that work together: There is one network A that assigns images to k clusters and k (simple) networks of type B perform a self-supervised task on those clusters. The goal of all the networks is to make the k networks of type B perform well on the task. The assumption is that clustering in semantically similar groups will help the networks of type B to perform well. This could be done on the MNIST dataset with B being linear classifiers and the task being rotation prediction.
Adversarial Data-Augmentation
The student designs a data augmentation network that transforms training images in such a way that image realism is preserved (e.g. with a constrained spatial transformer network) and the transformed images are more difficult to classify (trained via adversarial loss against an image classifier). The model will be evaluated for different data settings (especially in the low data regime), for example on the MNIST and CIFAR datasets.
Unsupervised Learning of Lip-reading from Videos
People with sensory impairment (hearing, speech, vision) depend heavily on assistive technologies to communicate and navigate in everyday life. The mass production of media content today makes it impossible to manually translate everything into a common language for assistive technologies, e.g. captions or sign language. In this project, the student employs a neural network to learn a representation for lip-movement in videos in an unsupervised fashion, possibly with an encoder-decoder structure where the decoder reconstructs the audio signal. This requires collecting a large dataset of videos (e.g. from YouTube) of speakers or conversations where lip movement is visible. The outcome will be a neural network that learns an audio-visual representation of lip movement in videos, which can then be leveraged to generate captions for hearing impaired persons.
Learning to Generate Topographic Maps from Satellite Images
Satellite images have many applications, e.g. in meteorology, geography, education, cartography and warfare. They are an accurate and detailed depiction of the surface of the earth from above. Although it is relatively simple to collect many satellite images in an automated way, challenges arise when processing them for use in navigation and cartography. The idea of this project is to automatically convert an arbitrary satellite image, of e.g. a city, to a map of simple 2D shapes (streets, houses, forests) and label them with colors (semantic segmentation). The student will collect a dataset of satellite image and topological maps and train a deep neural network that learns to map from one domain to the other. The data could be obtained from a Google Maps database or similar.
New Variables of Brain Morphometry: the Potential and Limitations of CNN Regression
Timo blattner · sept. 2022.
The calculation of variables of brain morphology is computationally very expensive and time-consuming. A previous work showed the feasibility of ex- tracting the variables directly from T1-weighted brain MRI images using a con- volutional neural network. We used significantly more data and extended their model to a new set of neuromorphological variables, which could become inter- esting biomarkers in the future for the diagnosis of brain diseases. The model shows for nearly all subjects a less than 5% mean relative absolute error. This high relative accuracy can be attributed to the low morphological variance be- tween subjects and the ability of the model to predict the cortical atrophy age trend. The model however fails to capture all the variance in the data and shows large regional differences. We attribute these limitations in part to the moderate to poor reliability of the ground truth generated by FreeSurfer. We further investigated the effects of training data size and model complexity on this regression task and found that the size of the dataset had a significant impact on performance, while deeper models did not perform better. Lack of interpretability and dependence on a silver ground truth are the main drawbacks of this direct regression approach.
Home Monitoring by Radar
Lars ziegler · sept. 2022.
Detection and tracking of humans via UWB radars is a promising and continuously evolving field with great potential for medical technology. This contactless method of acquiring data of a patients movement patterns is ideal for in home application. As irregularities in a patients movement patterns are an indicator for various health problems including neurodegenerative diseases, the insight this data could provide may enable earlier detection of such problems. In this thesis a signal processing pipeline is presented with which a persons movement is modeled. During an experiment 142 measurements were recorded by two separate radar systems and one lidar system which each consisted of multiple sensors. The models that were calculated on these measurements by the signal processing pipeline were used to predict the times when a person stood up or sat down. The predictions showed an accuracy of 72.2%.
Revisiting non-learning based 3D reconstruction from multiple images
Aaron sägesser · oct. 2021.
Arthroscopy consists of challenging tasks and requires skills that even today, young surgeons still train directly throughout the surgery. Existing simulators are expensive and rarely available. Through the growing potential of virtual reality(VR) (head-mounted) devices for simulation and their applicability in the medical context, these devices have become a promising alternative that would be orders of magnitude cheaper and could be made widely available. To build a VR-based training device for arthroscopy is the overall aim of our project, as this would be of great benefit and might even be applicable in other minimally invasive surgery (MIS). This thesis marks a first step of the project with its focus to explore and compare well-known algorithms in a multi-view stereo (MVS) based 3D reconstruction with respect to imagery acquired by an arthroscopic camera. Simultaneously with this reconstruction, we aim to gain essential measures to compare the VR environment to the real world, as validation of the realism of future VR tasks. We evaluate 3 different feature extraction algorithms with 3 different matching techniques and 2 different algorithms for the estimation of the fundamental (F) matrix. The evaluation of these 18 different setups is made with a reconstruction pipeline embedded in a jupyter notebook implemented in python based on common computer vision libraries and compared with imagery generated with a mobile phone as well as with the reconstruction results of state-of-the-art (SOTA) structure-from-motion (SfM) software COLMAP and Multi-View Environment (MVE). Our comparative analysis manifests the challenges of heavy distortion, the fish-eye shape and weak image quality of arthroscopic imagery, as all results are substantially worse using this data. However, there are huge differences regarding the different setups. Scale Invariant Feature Transform (SIFT) and Oriented FAST Rotated BRIEF (ORB) in combination with k-Nearest Neighbour (kNN) matching and Least Median of Squares (LMedS) present the most promising results. Overall, the 3D reconstruction pipeline is a useful tool to foster the process of gaining measurements from the arthroscopic exploration device and to complement the comparative research in this context.
Examination of Unsupervised Representation Learning by Predicting Image Rotations
Eric lagger · sept. 2020.
In recent years deep convolutional neural networks achieved a lot of progress. To train such a network a lot of data is required and in supervised learning algorithms it is necessary that the data is labeled. To label data there is a lot of human work needed and this takes a lot of time and money to be done. To avoid the inconveniences that come with this we would like to find systems that don’t need labeled data and therefore are unsupervised learning algorithms. This is the importance of unsupervised algorithms, even though their outcome is not yet on the same qualitative level as supervised algorithms. In this thesis we will discuss an approach of such a system and compare the results to other papers. A deep convolutional neural network is trained to learn the rotations that have been applied to a picture. So we take a large amount of images and apply some simple rotations and the task of the network is to discover in which direction the image has been rotated. The data doesn’t need to be labeled to any category or anything else. As long as all the pictures are upside down we hope to find some high dimensional patterns for the network to learn.
StitchNet: Image Stitching using Autoencoders and Deep Convolutional Neural Networks
Maurice rupp · sept. 2019.
This thesis explores the prospect of artificial neural networks for image processing tasks. More specifically, it aims to achieve the goal of stitching multiple overlapping images to form a bigger, panoramic picture. Until now, this task is solely approached with ”classical”, hardcoded algorithms while deep learning is at most used for specific subtasks. This thesis introduces a novel end-to-end neural network approach to image stitching called StitchNet, which uses a pre-trained autoencoder and deep convolutional networks. Additionally to presenting several new datasets for the task of supervised image stitching with each 120’000 training and 5’000 validation samples, this thesis also conducts various experiments with different kinds of existing networks designed for image superresolution and image segmentation adapted to the task of image stitching. StitchNet outperforms most of the adapted networks in both quantitative as well as qualitative results.
Facial Expression Recognition in the Wild
Luca rolshoven · sept. 2019.
The idea of inferring the emotional state of a subject by looking at their face is nothing new. Neither is the idea of automating this process using computers. Researchers used to computationally extract handcrafted features from face images that had proven themselves to be effective and then used machine learning techniques to classify the facial expressions using these features. Recently, there has been a trend towards using deeplearning and especially Convolutional Neural Networks (CNNs) for the classification of these facial expressions. Researchers were able to achieve good results on images that were taken in laboratories under the same or at least similar conditions. However, these models do not perform very well on more arbitrary face images with different head poses and illumination. This thesis aims to show the challenges of Facial Expression Recognition (FER) in this wild setting. It presents the currently used datasets and the present state-of-the-art results on one of the biggest facial expression datasets currently available. The contributions of this thesis are twofold. Firstly, I analyze three famous neural network architectures and their effectiveness on the classification of facial expressions. Secondly, I present two modifications of one of these networks that lead to the proposed STN-COV model. While this model does not outperform all of the current state-of-the-art models, it does beat several ones of them.
A Study of 3D Reconstruction of Varying Objects with Deformable Parts Models
Raoul grossenbacher · july 2019.
This work covers a new approach to 3D reconstruction. In traditional 3D reconstruction one uses multiple images of the same object to calculate a 3D model by taking information gained from the differences between the images, like camera position, illumination of the images, rotation of the object and so on, to compute a point cloud representing the object. The characteristic trait shared by all these approaches is that one can almost change everything about the image, but it is not possible to change the object itself, because one needs to find correspondences between the images. To be able to use different instances of the same object, we used a 3D DPM model that can find different parts of an object in an image, thereby detecting the correspondences between the different pictures, which we then can use to calculate the 3D model. To take this theory to practise, we gave a 3D DPM model, which was trained to detect cars, pictures of different car brands, where no pair of images showed the same vehicle and used the detected correspondences and the Factorization Method to compute the 3D point cloud. This technique leads to a completely new approach in 3D reconstruction, because changing the object itself was never done before.
Motion deblurring in the wild replication and improvements
Alvaro juan lahiguera · jan. 2019, coma outcome prediction with convolutional neural networks, stefan jonas · oct. 2018, automatic correction of self-introduced errors in source code, sven kellenberger · aug. 2018, neural face transfer: training a deep neural network to face-swap, till nikolaus schnabel · july 2018.
This thesis explores the field of artificial neural networks with realistic looking visual outputs. It aims at morphing face pictures of a specific identity to look like another individual by only modifying key features, such as eye color, while leaving identity-independent features unchanged. Prior works have covered the topic of symmetric translation between two specific domains but failed to optimize it on faces where only parts of the image may be changed. This work applies a face masking operation to the output at training time, which forces the image generator to preserve colors while altering the face, fitting it naturally inside the unmorphed surroundings. Various experiments are conducted including an ablation study on the final setting, decreasing the baseline identity switching performance from 81.7% to 75.8 % whilst improving the average χ2 color distance from 0.551 to 0.434. The provided code-based software gives users easy access to apply this neural face swap to images and videos of arbitrary crop and brings Computer Vision one step closer to replacing Computer Graphics in this specific area.
A Study of the Importance of Parts in the Deformable Parts Model
Sammer puran · june 2017, self-similarity as a meta feature, lucas husi · april 2017, a study of 3d deformable parts models for detection and pose-estimation, simon jenni · march 2015, amodal leaf segmentation, nicolas maier · nov. 2023.
Plant phenotyping is the process of measuring and analyzing various traits of plants. It provides essential information on how genetic and environmental factors affect plant growth and development. Manual phenotyping is highly time-consuming; therefore, many computer vision and machine learning based methods have been proposed in the past years to perform this task automatically based on images of the plants. However, the publicly available datasets (in particular, of Arabidopsis thaliana) are limited in size and diversity, making them unsuitable to generalize to new unseen environments. In this work, we propose a complete pipeline able to automatically extract traits of interest from an image of Arabidopsis thaliana. Our method uses a minimal amount of existing annotated data from a source domain to generate a large synthetic dataset adapted to a different target domain (e.g., different backgrounds, lighting conditions, and plant layouts). In addition, unlike the source dataset, the synthetic one provides ground-truth annotations for the occluded parts of the leaves, which are relevant when measuring some characteristics of the plant, e.g., its total area. This synthetic dataset is then used to train a model to perform amodal instance segmentation of the leaves to obtain the total area, leaf count, and color of each plant. To validate our approach, we create a small dataset composed of manually annotated real images of Arabidopsis thaliana, which is used to assess the performance of the models.
Assessment of movement and pose in a hospital bed by ambient and wearable sensor technology in healthy subjects
Tony licata · sept. 2022.
The use of automated systems describing the human motion has become possible in various domains. Most of the proposed systems are designed to work with people moving around in a standing position. Because such system could be interesting in a medical environment, we propose in this work a pipeline that can effectively predict human motion from people lying on beds. The proposed pipeline is tested with a data set composed of 41 participants executing 7 predefined tasks in a bed. The motion of the participants is measured with video cameras, accelerometers and pressure mat. Various experiments are carried with the information retrieved from the data set. Two approaches combining the data from the different measure technologies are explored. The performance of the different carried experiments is measured, and the proposed pipeline is composed with components providing the best results. Later on, we show that the proposed pipeline only needs to use the video cameras, which make the proposed environment easier to implement in real life situations.
Machine Learning Based Prediction of Mental Health Using Wearable-measured Time Series
Seyedeh sharareh mirzargar · sept. 2022.
Depression is the second major cause for years spent in disability and has a growing prevalence in adolescents. The recent Covid-19 pandemic has intensified the situation and limited in-person patient monitoring due to distancing measures. Recent advances in wearable devices have made it possible to record the rest/activity cycle remotely with high precision and in real-world contexts. We aim to use machine learning methods to predict an individual's mental health based on wearable-measured sleep and physical activity. Predicting an impending mental health crisis of an adolescent allows for prompt intervention, detection of depression onset or its recursion, and remote monitoring. To achieve this goal, we train three primary forecasting models; linear regression, random forest, and light gradient boosted machine (LightGBM); and two deep learning models; block recurrent neural network (block RNN) and temporal convolutional network (TCN); on Actigraph measurements to forecast mental health in terms of depression, anxiety, sleepiness, stress, sleep quality, and behavioral problems. Our models achieve a high forecasting performance, the random forest being the winner to reach an accuracy of 98% for forecasting the trait anxiety. We perform extensive experiments to evaluate the models' performance in accuracy, generalization, and feature utilization, using a naive forecaster as the baseline. Our analysis shows minimal mental health changes over two months, making the prediction task easily achievable. Due to these minimal changes in mental health, the models tend to primarily use the historical values of mental health evaluation instead of Actigraph features. At the time of this master thesis, the data acquisition step is still in progress. In future work, we plan to train the models on the complete dataset using a longer forecasting horizon to increase the level of mental health changes and perform transfer learning to compensate for the small dataset size. This interdisciplinary project demonstrates the opportunities and challenges in machine learning based prediction of mental health, paving the way toward using the same techniques to forecast other mental disorders such as internalizing disorder, Parkinson's disease, Alzheimer's disease, etc. and improving the quality of life for individuals who have some mental disorder.
CNN Spike Detector: Detection of Spikes in Intracranial EEG using Convolutional Neural Networks
Stefan jonas · oct. 2021.
The detection of interictal epileptiform discharges in the visual analysis of electroencephalography (EEG) is an important but very difficult, tedious, and time-consuming task. There have been decades of research on computer-assisted detection algorithms, most recently focused on using Convolutional Neural Networks (CNNs). In this thesis, we present the CNN Spike Detector, a convolutional neural network to detect spikes in intracranial EEG. Our dataset of 70 intracranial EEG recordings from 26 subjects with epilepsy introduces new challenges in this research field. We report cross-validation results with a mean AUC of 0.926 (+- 0.04), an area under the precision-recall curve (AUPRC) of 0.652 (+- 0.10) and 12.3 (+- 7.47) false positive epochs per minute for a sensitivity of 80%. A visual examination of false positive segments is performed to understand the model behavior leading to a relatively high false detection rate. We notice issues with the evaluation measures and highlight a major limitation of the common approach of detecting spikes using short segments, namely that the network is not capable to consider the greater context of the segment with regards to its origination. For this reason, we present the Context Model, an extension in which the CNN Spike Detector is supplied with additional information about the channel. Results show promising but limited performance improvements. This thesis provides important findings about the spike detection task for intracranial EEG and lays out promising future research directions to develop a network capable of assisting experts in real-world clinical applications.
PolitBERT - Deepfake Detection of American Politicians using Natural Language Processing
Maurice rupp · april 2021.
This thesis explores the application of modern Natural Language Processing techniques to the detection of artificially generated videos of popular American politicians. Instead of focusing on detecting anomalies and artifacts in images and sounds, this thesis focuses on detecting irregularities and inconsistencies in the words themselves, opening up a new possibility to detect fake content. A novel, domain-adapted, pre-trained version of the language model BERT combined with several mechanisms to overcome severe dataset imbalances yielded the best quantitative as well as qualitative results. Additionally to the creation of the biggest publicly available dataset of English-speaking politicians consisting of 1.5 M sentences from over 1000 persons, this thesis conducts various experiments with different kinds of text classification and sequence processing algorithms applied to the political domain. Furthermore, multiple ablations to manage severe data imbalance are presented and evaluated.
A Study on the Inversion of Generative Adversarial Networks
Ramona beck · march 2021.
The desire to use generative adversarial networks (GANs) for real-world tasks such as object segmentation or image manipulation is increasing as synthesis quality improves, which has given rise to an emerging research area called GAN inversion that focuses on exploring methods for embedding real images into the latent space of a GAN. In this work, we investigate different GAN inversion approaches using an existing generative model architecture that takes a completely unsupervised approach to object segmentation and is based on StyleGAN2. In particular, we propose and analyze algorithms for embedding real images into the different latent spaces Z, W, and W+ of StyleGAN following an optimization-based inversion approach, while also investigating a novel approach that allows fine-tuning of the generator during the inversion process. Furthermore, we investigate a hybrid and a learning-based inversion approach, where in the former we train an encoder with embeddings optimized by our best optimization-based inversion approach, and in the latter we define an autoencoder, consisting of an encoder and the generator of our generative model as a decoder, and train it to map an image into the latent space. We demonstrate the effectiveness of our methods as well as their limitations through a quantitative comparison with existing inversion methods and by conducting extensive qualitative and quantitative experiments with synthetic data as well as real images from a complex image dataset. We show that we achieve qualitatively satisfying embeddings in the W and W+ spaces with our optimization-based algorithms, that fine-tuning the generator during the inversion process leads to qualitatively better embeddings in all latent spaces studied, and that the learning-based approach also benefits from a variable generator as well as a pre-training with our hybrid approach. Furthermore, we evaluate our approaches on the object segmentation task and show that both our optimization-based and our hybrid and learning-based methods are able to generate meaningful embeddings that achieve reasonable object segmentations. Overall, our proposed methods illustrate the potential that lies in the GAN inversion and its application to real-world tasks, especially in the relaxed version of the GAN inversion where the weights of the generator are allowed to vary.
Multi-scale Momentum Contrast for Self-supervised Image Classification
Zhao xueqi · dec. 2020.
With the maturity of supervised learning technology, people gradually shift the research focus to the field of self-supervised learning. ”Momentum Contrast” (MoCo) proposes a new self-supervised learning method and raises the correct rate of self-supervised learning to a new level. Inspired by another article ”Representation Learning by Learning to Count”, if a picture is divided into four parts and passed through a neural network, it is possible to further improve the accuracy of MoCo. Different from the original MoCo, this MoCo variant (Multi-scale MoCo) does not directly pass the image through the encoder after the augmented images. Multi-scale MoCo crops and resizes the augmented images, and the obtained four parts are respectively passed through the encoder and then summed (upsampled version do not do resize to input but resize the contrastive samples). This method of images crop is not only used for queue q but also used for comparison queue k, otherwise the weights of queue k might be damaged during the moment update. This will further discussed in the experiments chapter between downsampled Multi-scale version and downsampled both Multi-scale version. Human beings also have the same principle of object recognition: when human beings see something they are familiar with, even if the object is not fully displayed, people can still guess the object itself with a high probability. Because of this, Multi-scale MoCo applies this concept to the pretext part of MoCo, hoping to obtain better feature extraction. In this thesis, there are three versions of Multi-scale MoCo, downsampled input samples version, downsampled input samples and contrast samples version and upsampled input samples version. The differences between these versions will be described in more detail later. The neural network architecture comparison includes ResNet50 , and the tested data set is STL-10. The weights obtained in pretext will be transferred to self-supervised learning, and in the process of self-supervised learning, the weights of other layers except the final linear layer are frozen without changing (these weights come from pretext).
Self-Supervised Learning Using Siamese Networks and Binary Classifier
Dušan mihajlov · march 2020.
In this thesis, we present several approaches for training a convolutional neural network using only unlabeled data. Our autonomously supervised learning algorithms are based on connections between image patch i. e. zoomed image and its original. Using the siamese architecture neural network we aim to recognize, if the image patch, which is input to the first neural network part, comes from the same image presented to the second neural network part. By applying transformations to both images, and different zoom sizes at different positions, we force the network to extract high level features using its convolutional layers. At the top of our siamese architecture, we have a simple binary classifier that measures the difference between feature maps that we extract and makes a decision. Thus, the only way that the classifier will solve the task correctly is when our convolutional layers are extracting useful representations. Those representations we can than use to solve many different tasks that are related to the data used for unsupervised training. As the main benchmark for all of our models, we used STL10 dataset, where we train a linear classifier on the top of our convolutional layers with a small amount of manually labeled images, which is a widely used benchmark for unsupervised learning tasks. We also combine our idea with recent work on the same topic, and the network called RotNet, which makes use of image rotations and therefore forces the network to learn rotation dependent features from the dataset. As a result of this combination we create a new procedure that outperforms original RotNet.
Learning Object Representations by Mixing Scenes
Lukas zbinden · may 2019.
In the digital age of ever increasing data amassment and accessibility, the demand for scalable machine learning models effective at refining the new oil is unprecedented. Unsupervised representation learning methods present a promising approach to exploit this invaluable yet unlabeled digital resource at scale. However, a majority of these approaches focuses on synthetic or simplified datasets of images. What if a method could learn directly from natural Internet-scale image data? In this thesis, we propose a novel approach for unsupervised learning of object representations by mixing natural image scenes. Without any human help, our method mixes visually similar images to synthesize new realistic scenes using adversarial training. In this process the model learns to represent and understand the objects prevalent in natural image data and makes them available for downstream applications. For example, it enables the transfer of objects from one scene to another. Through qualitative experiments on complex image data we show the effectiveness of our method along with its limitations. Moreover, we benchmark our approach quantitatively against state-of-the-art works on the STL-10 dataset. Our proposed method demonstrates the potential that lies in learning representations directly from natural image data and reinforces it as a promising avenue for future research.
Representation Learning using Semantic Distances
Markus roth · may 2019, zero-shot learning using generative adversarial networks, hamed hemati · dec. 2018, dimensionality reduction via cnns - learning the distance between images, ioannis glampedakis · sept. 2018, learning to play othello using deep reinforcement learning and self play, thomas simon steinmann · sept. 2018, aba-j interactive multi-modality tissue sectionto-volume alignment: a brain atlasing toolkit for imagej, felix meyenhofer · march 2018, learning visual odometry with recurrent neural networks, adrian wälchli · feb. 2018.
In computer vision, Visual Odometry is the problem of recovering the camera motion from a video. It is related to Structure from Motion, the problem of reconstructing the 3D geometry from a collection of images. Decades of research in these areas have brought successful algorithms that are used in applications like autonomous navigation, motion capture, augmented reality and others. Despite the success of these prior works in real-world environments, their robustness is highly dependent on manual calibration and the magnitude of noise present in the images in form of, e.g., non-Lambertian surfaces, dynamic motion and other forms of ambiguity. This thesis explores an alternative approach to the Visual Odometry problem via Deep Learning, that is, a specific form of machine learning with artificial neural networks. It describes and focuses on the implementation of a recent work that proposes the use of Recurrent Neural Networks to learn dependencies over time due to the sequential nature of the input. Together with a convolutional neural network that extracts motion features from the input stream, the recurrent part accumulates knowledge from the past to make camera pose estimations at each point in time. An analysis on the performance of this system is carried out on real and synthetic data. The evaluation covers several ways of training the network as well as the impact and limitations of the recurrent connection for Visual Odometry.
Crime location and timing prediction
Bernard swart · jan. 2018, from cartoons to real images: an approach to unsupervised visual representation learning, simon jenni · feb. 2017, automatic and large-scale assessment of fluid in retinal oct volume, nina mujkanovic · dec. 2016, segmentation in 3d using eye-tracking technology, michele wyss · july 2016, accurate scale thresholding via logarithmic total variation prior, remo diethelm · aug. 2014, novel techniques for robust and generalizable machine learning, abdelhak lemkhenter · sept. 2023.
Neural networks have transcended their status of powerful proof-of-concept machine learning into the realm of a highly disruptive technology that has revolutionized many quantitative fields such as drug discovery, autonomous vehicles, and machine translation. Today, it is nearly impossible to go a single day without interacting with a neural network-powered application. From search engines to on-device photo-processing, neural networks have become the go-to solution thanks to recent advances in computational hardware and an unprecedented scale of training data. Larger and less curated datasets, typically obtained through web crawling, have greatly propelled the capabilities of neural networks forward. However, this increase in scale amplifies certain challenges associated with training such models. Beyond toy or carefully curated datasets, data in the wild is plagued with biases, imbalances, and various noisy components. Given the larger size of modern neural networks, such models run the risk of learning spurious correlations that fail to generalize beyond their training data. This thesis addresses the problem of training more robust and generalizable machine learning models across a wide range of learning paradigms for medical time series and computer vision tasks. The former is a typical example of a low signal-to-noise ratio data modality with a high degree of variability between subjects and datasets. There, we tailor the training scheme to focus on robust patterns that generalize to new subjects and ignore the noisier and subject-specific patterns. To achieve this, we first introduce a physiologically inspired unsupervised training task and then extend it by explicitly optimizing for cross-dataset generalization using meta-learning. In the context of image classification, we address the challenge of training semi-supervised models under class imbalance by designing a novel label refinement strategy with higher local sensitivity to minority class samples while preserving the global data distribution. Lastly, we introduce a new Generative Adversarial Networks training loss. Such generative models could be applied to improve the training of subsequent models in the low data regime by augmenting the dataset using generated samples. Unfortunately, GAN training relies on a delicate balance between its components, making it prone mode collapse. Our contribution consists of defining a more principled GAN loss whose gradients incentivize the generator model to seek out missing modes in its distribution. All in all, this thesis tackles the challenge of training more robust machine learning models that can generalize beyond their training data. This necessitates the development of methods specifically tailored to handle the diverse biases and spurious correlations inherent in the data. It is important to note that achieving greater generalizability in models goes beyond simply increasing the volume of data; it requires meticulous consideration of training objectives and model architecture. By tackling these challenges, this research contributes to advancing the field of machine learning and underscores the significance of thoughtful design in obtaining more resilient and versatile models.
Automated Sleep Scoring, Deep Learning and Physician Supervision
Luigi fiorillo · oct. 2022.
Sleep plays a crucial role in human well-being. Polysomnography is used in sleep medicine as a diagnostic tool, so as to objectively analyze the quality of sleep. Sleep scoring is the procedure of extracting sleep cycle information from the wholenight electrophysiological signals. The scoring is done worldwide by the sleep physicians according to the official American Academy of Sleep Medicine (AASM) scoring manual. In the last decades, a wide variety of deep learning based algorithms have been proposed to automatise the sleep scoring task. In this thesis we study the reasons why these algorithms fail to be introduced in the daily clinical routine, with the perspective of bridging the existing gap between the automatic sleep scoring models and the sleep physicians. In this light, the primary step is the design of a simplified sleep scoring architecture, also providing an estimate of the model uncertainty. Beside achieving results on par with most up-to-date scoring systems, we demonstrate the efficiency of ensemble learning based algorithms, together with label smoothing techniques, in both enhancing the performance and calibrating the simplified scoring model. We introduced an uncertainty estimate procedure, so as to identify the most challenging sleep stage predictions, and to quantify the disagreement between the predictions given by the model and the annotation given by the physicians. In this thesis we also propose a novel method to integrate the inter-scorer variability into the training procedure of a sleep scoring model. We clearly show that a deep learning model is able to encode this variability, so as to better adapt to the consensus of a group of scorers-physicians. We finally address the generalization ability of a deep learning based sleep scoring system, further studying its resilience to the sleep complexity and to the AASM scoring rules. We can state that there is no need to train the algorithm strictly following the AASM guidelines. Most importantly, using data from multiple data centers results in a better performing model compared with training on a single data cohort. The variability among different scorers and data centers needs to be taken into account, more than the variability among sleep disorders.
Learning Representations for Controllable Image Restoration
Givi meishvili · march 2022.
Deep Convolutional Neural Networks have sparked a renaissance in all the sub-fields of computer vision. Tremendous progress has been made in the area of image restoration. The research community has pushed the boundaries of image deblurring, super-resolution, and denoising. However, given a distorted image, most existing methods typically produce a single restored output. The tasks mentioned above are inherently ill-posed, leading to an infinite number of plausible solutions. This thesis focuses on designing image restoration techniques capable of producing multiple restored results and granting users more control over the restoration process. Towards this goal, we demonstrate how one could leverage the power of unsupervised representation learning. Image restoration is vital when applied to distorted images of human faces due to their social significance. Generative Adversarial Networks enable an unprecedented level of generated facial details combined with smooth latent space. We leverage the power of GANs towards the goal of learning controllable neural face representations. We demonstrate how to learn an inverse mapping from image space to these latent representations, tuning these representations towards a specific task, and finally manipulating latent codes in these spaces. For example, we show how GANs and their inverse mappings enable the restoration and editing of faces in the context of extreme face super-resolution and the generation of novel view sharp videos from a single motion-blurred image of a face. This thesis also addresses more general blind super-resolution, denoising, and scratch removal problems, where blur kernels and noise levels are unknown. We resort to contrastive representation learning and first learn the latent space of degradations. We demonstrate that the learned representation allows inference of ground-truth degradation parameters and can guide the restoration process. Moreover, it enables control over the amount of deblurring and denoising in the restoration via manipulation of latent degradation features.
Learning Generalizable Visual Patterns Without Human Supervision
Simon jenni · oct. 2021.
Owing to the existence of large labeled datasets, Deep Convolutional Neural Networks have ushered in a renaissance in computer vision. However, almost all of the visual data we generate daily - several human lives worth of it - remains unlabeled and thus out of reach of today’s dominant supervised learning paradigm. This thesis focuses on techniques that steer deep models towards learning generalizable visual patterns without human supervision. Our primary tool in this endeavor is the design of Self-Supervised Learning tasks, i.e., pretext-tasks for which labels do not involve human labor. Besides enabling the learning from large amounts of unlabeled data, we demonstrate how self-supervision can capture relevant patterns that supervised learning largely misses. For example, we design learning tasks that learn deep representations capturing shape from images, motion from video, and 3D pose features from multi-view data. Notably, these tasks’ design follows a common principle: The recognition of data transformations. The strong performance of the learned representations on downstream vision tasks such as classification, segmentation, action recognition, or pose estimation validate this pretext-task design. This thesis also explores the use of Generative Adversarial Networks (GANs) for unsupervised representation learning. Besides leveraging generative adversarial learning to define image transformation for self-supervised learning tasks, we also address training instabilities of GANs through the use of noise. While unsupervised techniques can significantly reduce the burden of supervision, in the end, we still rely on some annotated examples to fine-tune learned representations towards a target task. To improve the learning from scarce or noisy labels, we describe a supervised learning algorithm with improved generalization in these challenging settings.
Learning Interpretable Representations of Images
Attila szabó · june 2019.
Computers represent images with pixels and each pixel contains three numbers for red, green and blue colour values. These numbers are meaningless for humans and they are mostly useless when used directly with classical machine learning techniques like linear classifiers. Interpretable representations are the attributes that humans understand: the colour of the hair, viewpoint of a car or the 3D shape of the object in the scene. Many computer vision tasks can be viewed as learning interpretable representations, for example a supervised classification algorithm directly learns to represent images with their class labels. In this work we aim to learn interpretable representations (or features) indirectly with lower levels of supervision. This approach has the advantage of cost savings on dataset annotations and the flexibility of using the features for multiple follow-up tasks. We made contributions in three main areas: weakly supervised learning, unsupervised learning and 3D reconstruction. In the weakly supervised case we use image pairs as supervision. Each pair shares a common attribute and differs in a varying attribute. We propose a training method that learns to separate the attributes into separate feature vectors. These features then are used for attribute transfer and classification. We also show theoretical results on the ambiguities of the learning task and the ways to avoid degenerate solutions. We show a method for unsupervised representation learning, that separates semantically meaningful concepts. We explain and show ablation studies how the components of our proposed method work: a mixing autoencoder, a generative adversarial net and a classifier. We propose a method for learning single image 3D reconstruction. It is done using only the images, no human annotation, stereo, synthetic renderings or ground truth depth map is needed. We train a generative model that learns the 3D shape distribution and an encoder to reconstruct the 3D shape. For that we exploit the notion of image realism. It means that the 3D reconstruction of the object has to look realistic when it is rendered from different random angles. We prove the efficacy of our method from first principles.
Learning Controllable Representations for Image Synthesis
Qiyang hu · june 2019.
In this thesis, our focus is learning a controllable representation and applying the learned controllable feature representation on images synthesis, video generation, and even 3D reconstruction. We propose different methods to disentangle the feature representation in neural network and analyze the challenges in disentanglement such as reference ambiguity and shortcut problem when using the weak label. We use the disentangled feature representation to transfer attributes between images such as exchanging hairstyle between two face images. Furthermore, we study the problem of how another type of feature, sketch, works in a neural network. The sketch can provide shape and contour of an object such as the silhouette of the side-view face. We leverage the silhouette constraint to improve the 3D face reconstruction from 2D images. The sketch can also provide the moving directions of one object, thus we investigate how one can manipulate the object to follow the trajectory provided by a user sketch. We propose a method to automatically generate video clips from a single image input using the sketch as motion and trajectory guidance to animate the object in that image. We demonstrate the efficiency of our approaches on several synthetic and real datasets.
Beyond Supervised Representation Learning
Mehdi noroozi · jan. 2019.
The complexity of any information processing task is highly dependent on the space where data is represented. Unfortunately, pixel space is not appropriate for the computer vision tasks such as object classification. The traditional computer vision approaches involve a multi-stage pipeline where at first images are transformed to a feature space through a handcrafted function and then consequenced by the solution in the feature space. The challenge with this approach is the complexity of designing handcrafted functions that extract robust features. The deep learning based approaches address this issue by end-to-end training of a neural network for some tasks that lets the network to discover the appropriate representation for the training tasks automatically. It turns out that image classification task on large scale annotated datasets yields a representation transferable to other computer vision tasks. However, supervised representation learning is limited to annotations. In this thesis we study self-supervised representation learning where the goal is to alleviate these limitations by substituting the classification task with pseudo tasks where the labels come for free. We discuss self-supervised learning by solving jigsaw puzzles that uses context as supervisory signal. The rational behind this task is that the network requires to extract features about object parts and their spatial configurations to solve the jigsaw puzzles. We also discuss a method for representation learning that uses an artificial supervisory signal based on counting visual primitives. This supervisory signal is obtained from an equivariance relation. We use two image transformations in the context of counting: scaling and tiling. The first transformation exploits the fact that the number of visual primitives should be invariant to scale. The second transformation allows us to equate the total number of visual primitives in each tile to that in the whole image. The most effective transfer strategy is fine-tuning, which restricts one to use the same model or parts thereof for both pretext and target tasks. We discuss a novel framework for self-supervised learning that overcomes limitations in designing and comparing different tasks, models, and data domains. In particular, our framework decouples the structure of the self-supervised model from the final task-specific finetuned model. Finally, we study the problem of multi-task representation learning. A naive approach to enhance the representation learned by a task is to train the task jointly with other tasks that capture orthogonal attributes. Having a diverse set of auxiliary tasks, imposes challenges on multi-task training from scratch. We propose a framework that allows us to combine arbitrarily different feature spaces into a single deep neural network. We reduce the auxiliary tasks to classification tasks and the multi-task learning to multi-label classification task consequently. Nevertheless, combining multiple representation space without being aware of the target task might be suboptimal. As our second contribution, we show empirically that this is indeed the case and propose to combine multiple tasks after the fine-tuning on the target task.
Motion Deblurring from a Single Image
Meiguang jin · dec. 2018.
With the information explosion, a tremendous amount photos is captured and shared via social media everyday. Technically, a photo requires a finite exposure to accumulate light from the scene. Thus, objects moving during the exposure generate motion blur in a photo. Motion blur is an image degradation that makes visual content less interpretable and is therefore often seen as a nuisance. Although motion blur can be reduced by setting a short exposure time, an insufficient amount of light has to be compensated through increasing the sensor’s sensitivity, which will inevitably bring large amount of sensor noise. Thus this motivates the necessity of removing motion blur computationally. Motion deblurring is an important problem in computer vision and it is challenging due to its ill-posed nature, which means the solution is not well defined. Mathematically, a blurry image caused by uniform motion is formed by the convolution operation between a blur kernel and a latent sharp image. Potentially there are infinite pairs of blur kernel and latent sharp image that can result in the same blurry image. Hence, some prior knowledge or regularization is required to address this problem. Even if the blur kernel is known, restoring the latent sharp image is still difficult as the high frequency information has been removed. Although we can model the uniform motion deblurring problem mathematically, it can only address the camera in-plane translational motion. Practically, motion is more complicated and can be non-uniform. Non-uniform motion blur can come from many sources, camera out-of-plane rotation, scene depth change, object motion and so on. Thus, it is more challenging to remove non-uniform motion blur. In this thesis, our focus is motion blur removal. We aim to address four challenging motion deblurring problems. We start from the noise blind image deblurring scenario where blur kernel is known but the noise level is unknown. We introduce an efficient and robust solution based on a Bayesian framework using a smooth generalization of the 0−1 loss to address this problem. Then we study the blind uniform motion deblurring scenario where both the blur kernel and the latent sharp image are unknown. We explore the relative scale ambiguity between the latent sharp image and blur kernel to address this issue. Moreover, we study the face deblurring problem and introduce a novel deep learning network architecture to solve it. We also address the general motion deblurring problem and particularly we aim at recovering a sequence of 7 frames each depicting some instantaneous motion of the objects in the scene.
Towards a Novel Paradigm in Blind Deconvolution: From Natural to Cartooned Image Statistics
Daniele perrone · july 2015.
In this thesis we study the blind deconvolution problem. Blind deconvolution consists in the estimation of a sharp image and a blur kernel from an observed blurry image. Because the blur model admits several solutions it is necessary to devise an image prior that favors the true blur kernel and sharp image. Recently it has been shown that a class of blind deconvolution formulations and image priors has the no-blur solution as global minimum. Despite this shortcoming, algorithms based on these formulations and priors can successfully solve blind deconvolution. In this thesis we show that a suitable initialization can exploit the non-convexity of the problem and yield the desired solution. Based on these conclusions, we propose a novel “vanilla” algorithm stripped of any enhancement typically used in the literature. Our algorithm, despite its simplicity, is able to compete with the top performers on several datasets. We have also investigated a remarkable behavior of a 1998 algorithm, whose formulation has the no-blur solution as global minimum: even when initialized at the no-blur solution, it converges to the correct solution. We show that this behavior is caused by an apparently insignificant implementation strategy that makes the algorithm no longer minimize the original cost functional. We also demonstrate that this strategy improves the results of our “vanilla” algorithm. Finally, we present a study of image priors for blind deconvolution. We provide experimental evidence supporting the recent belief that a good image prior is one that leads to a good blur estimate rather than being a good natural image statistical model. By focusing the attention on the blur estimation alone, we show that good blur estimates can be obtained even when using images quite different from the true sharp image. This allows using image priors, such as those leading to “cartooned” images, that avoid the no-blur solution. By using an image prior that produces “cartooned” images we achieve state-of-the-art results on different publicly available datasets. We therefore suggests a shift of paradigm in blind deconvolution: from modeling natural image statistics to modeling cartooned image statistics.
New Perspectives on Uncalibrated Photometric Stereo
Thoma papadhimitri · june 2014.
This thesis investigates the problem of 3D reconstruction of a scene from 2D images. In particular, we focus on photometric stereo which is a technique that computes the 3D geometry from at least three images taken from the same viewpoint and under different illumination conditions. When the illumination is unknown (uncalibrated photometric stereo) the problem is ambiguous: different combinations of geometry and illumination can generate the same images. First, we solve the ambiguity by exploiting the Lambertian reflectance maxima. These are points defined on curved surfaces where the normals are parallel to the light direction. Then, we propose a solution that can be computed in closed-form and thus very efficiently. Our algorithm is also very robust and yields always the same estimate regardless of the initial ambiguity. We validate our method on real world experiments and achieve state-of-art results. In this thesis we also solve for the first time the uncalibrated photometric stereo problem under the perspective projection model. We show that unlike in the orthographic case, one can uniquely reconstruct the normals of the object and the lights given only the input images and the camera calibration (focal length and image center). We also propose a very efficient algorithm which we validate on synthetic and real world experiments and show that the proposed technique is a generalization of the orthographic case. Finally, we investigate the uncalibrated photometric stereo problem in the case where the lights are distributed near the scene. In this case we propose an alternating minimization technique which converges quickly and overcomes the limitations of prior work that assumes distant illumination. We show experimentally that adopting a near-light model for real world scenes yields very accurate reconstructions.

Analytics Insight
Top 10 Research and Thesis Topics for ML Projects in 2022

This article features the top 10 research and thesis topics for ML projects for students to try in 2022
Text mining and text classification, image-based applications, machine vision, optimization, voice classification, sentiment analysis, recommendation framework project, mall customers’ project, object detection with deep learning.

Disclaimer: Any financial and crypto market information given on Analytics Insight are sponsored articles, written for informational purpose only and is not an investment advice. The readers are further advised that Crypto products and NFTs are unregulated and can be highly risky. There may be no regulatory recourse for any loss from such transactions. Conduct your own research by contacting financial experts before making any investment decisions. The decision to read hereinafter is purely a matter of choice and shall be construed as an express undertaking/guarantee in favour of Analytics Insight of being absolved from any/ all potential legal action, or enforceable claims. We do not represent nor own any cryptocurrency, any complaints, abuse or concerns with regards to the information provided shall be immediately informed here .
You May Also Like

Top 5 Trends Dominating the Blockchain Industry in 2023

Exclusive Interview with Piyush Mehta, CEO of Data Dynamics

IPOs Under 100Rs for High Return in 2024

XRP Price Prediction: Is the Price Strong Enough to Reach $1?

Analytics Insight® is an influential platform dedicated to insights, trends, and opinion from the world of data-driven technologies. It monitors developments, recognition, and achievements made by Artificial Intelligence, Big Data and Analytics companies across the globe.

- Select Language:
- Privacy Policy
- Content Licensing
- Terms & Conditions
- Submit an Interview
Special Editions
- Dec – Crypto Weekly Vol-1
- 40 Under 40 Innovators
- Women In Technology
- Market Reports
- AI Glossary
- Infographics
Latest Issue

Disclaimer: Any financial and crypto market information given on Analytics Insight is written for informational purpose only and is not an investment advice. Conduct your own research by contacting financial experts before making any investment decisions, more information here .
Second Menu


- Advisers & Contacts
- Bachelor of Arts & Bachelor of Science in Engineering
- Prerequisites
- Declaring Computer Science for AB Students
- Declaring Computer Science for BSE Students
- Class of '25, '26 & '27 - Departmental Requirements
- Class of 2024 - Departmental Requirements
- COS126 Information
- Important Steps and Deadlines
- Independent Work Seminars
- Guidelines and Useful Information
Undergraduate Research Topics
- AB Junior Research Workshops
- Undergraduate Program FAQ
- How to Enroll
- Requirements
- Certificate Program FAQ
- Interdepartmental Committee
- Minor Program
- Funding for Student Group Activities
- Mailing Lists and Policies
- Study Abroad
- Jobs & Careers
- Admissions Requirements
- Breadth Requirements
- Pre-FPO Checklist
- FPO Checklist
- M.S.E. Track
- M.Eng. Track
- Departmental Internship Policy (for Master's students)
- General Examination
- Fellowship Opportunities
- Travel Reimbursement Policy
- Communication Skills
- Course Schedule
- Course Catalog
- Research Areas
- Interdisciplinary Programs
- Technical Reports
- Computing Facilities
- Researchers
- Technical Staff
- Administrative Staff
- Graduate Students
- Undergraduate Students
- Graduate Alumni
- Climate and Inclusion Committee
- Resources for Undergraduate & Graduate Students
- Outreach Initiatives
- Resources for Faculty & Staff
- Spotlight Stories
- Job Openings
- Undergraduate Program
- Independent Work & Theses
Suggested Undergraduate Research Topics
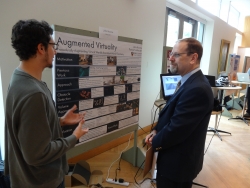
How to Contact Faculty for IW/Thesis Advising
Send the professor an e-mail. When you write a professor, be clear that you want a meeting regarding a senior thesis or one-on-one IW project, and briefly describe the topic or idea that you want to work on. Check the faculty listing for email addresses.
Parastoo Abtahi, Room 419
Available for single-semester IW and senior thesis advising, 2024-2025
- Research Areas: Human-Computer Interaction (HCI), Augmented Reality (AR), and Spatial Computing
- Input techniques for on-the-go interaction (e.g., eye-gaze, microgestures, voice) with a focus on uncertainty, disambiguation, and privacy.
- Minimal and timely multisensory output (e.g., spatial audio, haptics) that enables users to attend to their physical environment and the people around them, instead of a 2D screen.
- Interaction with intelligent systems (e.g., IoT, robots) situated in physical spaces with a focus on updating users’ mental model despite the complexity and dynamicity of these systems.
Ryan Adams, Room 411
Research areas:
- Machine learning driven design
- Generative models for structured discrete objects
- Approximate inference in probabilistic models
- Accelerating solutions to partial differential equations
- Innovative uses of automatic differentiation
- Modeling and optimizing 3d printing and CNC machining
Andrew Appel, Room 209
Available for Fall 2024 IW advising, only
- Research Areas: Formal methods, programming languages, compilers, computer security.
- Software verification (for which taking COS 326 / COS 510 is helpful preparation)
- Game theory of poker or other games (for which COS 217 / 226 are helpful)
- Computer game-playing programs (for which COS 217 / 226)
- Risk-limiting audits of elections (for which ORF 245 or other knowledge of probability is useful)
Sanjeev Arora, Room 407
- Theoretical machine learning, deep learning and its analysis, natural language processing. My advisees would typically have taken a course in algorithms (COS423 or COS 521 or equivalent) and a course in machine learning.
- Show that finding approximate solutions to NP-complete problems is also NP-complete (i.e., come up with NP-completeness reductions a la COS 487).
- Experimental Algorithms: Implementing and Evaluating Algorithms using existing software packages.
- Studying/designing provable algorithms for machine learning and implementions using packages like scipy and MATLAB, including applications in Natural language processing and deep learning.
- Any topic in theoretical computer science.
David August, Room 221
Not available for IW or thesis advising, 2024-2025
- Research Areas: Computer Architecture, Compilers, Parallelism
- Containment-based approaches to security: We have designed and tested a simple hardware+software containment mechanism that stops incorrect communication resulting from faults, bugs, or exploits from leaving the system. Let's explore ways to use containment to solve real problems. Expect to work with corporate security and technology decision-makers.
- Parallelism: Studies show much more parallelism than is currently realized in compilers and architectures. Let's find ways to realize this parallelism.
- Any other interesting topic in computer architecture or compilers.
Mark Braverman, 194 Nassau St., Room 231
- Research Areas: computational complexity, algorithms, applied probability, computability over the real numbers, game theory and mechanism design, information theory.
- Topics in computational and communication complexity.
- Applications of information theory in complexity theory.
- Algorithms for problems under real-life assumptions.
- Game theory, network effects
- Mechanism design (could be on a problem proposed by the student)
Sebastian Caldas, 221 Nassau Street, Room 105
- Research Areas: collaborative learning, machine learning for healthcare. Typically, I will work with students that have taken COS324.
- Methods for collaborative and continual learning.
- Machine learning for healthcare applications.
Bernard Chazelle, 194 Nassau St., Room 301
- Research Areas: Natural Algorithms, Computational Geometry, Sublinear Algorithms.
- Natural algorithms (flocking, swarming, social networks, etc).
- Sublinear algorithms
- Self-improving algorithms
- Markov data structures
Danqi Chen, Room 412
- My advisees would be expected to have taken a course in machine learning and ideally have taken COS484 or an NLP graduate seminar.
- Representation learning for text and knowledge bases
- Pre-training and transfer learning
- Question answering and reading comprehension
- Information extraction
- Text summarization
- Any other interesting topics related to natural language understanding/generation
Marcel Dall'Agnol, Corwin 034
- Research Areas: Theoretical computer science. (Specifically, quantum computation, sublinear algorithms, complexity theory, interactive proofs and cryptography)
- Research Areas: Machine learning
Jia Deng, Room 423
- Research Areas: Computer Vision, Machine Learning.
- Object recognition and action recognition
- Deep Learning, autoML, meta-learning
- Geometric reasoning, logical reasoning
Adji Bousso Dieng, Room 406
- Research areas: Vertaix is a research lab at Princeton University led by Professor Adji Bousso Dieng. We work at the intersection of artificial intelligence (AI) and the natural sciences. The models and algorithms we develop are motivated by problems in those domains and contribute to advancing methodological research in AI. We leverage tools in statistical machine learning and deep learning in developing methods for learning with the data, of various modalities, arising from the natural sciences.
Robert Dondero, Corwin Hall, Room 038
- Research Areas: Software engineering; software engineering education.
- Develop or evaluate tools to facilitate student learning in undergraduate computer science courses at Princeton, and beyond.
- In particular, can code critiquing tools help students learn about software quality?
Zeev Dvir, 194 Nassau St., Room 250
- Research Areas: computational complexity, pseudo-randomness, coding theory and discrete mathematics.
- Independent Research: I have various research problems related to Pseudorandomness, Coding theory, Complexity and Discrete mathematics - all of which require strong mathematical background. A project could also be based on writing a survey paper describing results from a few theory papers revolving around some particular subject.
Benjamin Eysenbach, Room 416
- Research areas: reinforcement learning, machine learning. My advisees would typically have taken COS324.
- Using RL algorithms to applications in science and engineering.
- Emergent behavior of RL algorithms on high-fidelity robotic simulators.
- Studying how architectures and representations can facilitate generalization.
Christiane Fellbaum, 1-S-14 Green
- Research Areas: theoretical and computational linguistics, word sense disambiguation, lexical resource construction, English and multilingual WordNet(s), ontology
- Anything having to do with natural language--come and see me with/for ideas suitable to your background and interests. Some topics students have worked on in the past:
- Developing parsers, part-of-speech taggers, morphological analyzers for underrepresented languages (you don't have to know the language to develop such tools!)
- Quantitative approaches to theoretical linguistics questions
- Extensions and interfaces for WordNet (English and WN in other languages),
- Applications of WordNet(s), including:
- Foreign language tutoring systems,
- Spelling correction software,
- Word-finding/suggestion software for ordinary users and people with memory problems,
- Machine Translation
- Sentiment and Opinion detection
- Automatic reasoning and inferencing
- Collaboration with professors in the social sciences and humanities ("Digital Humanities")
Adam Finkelstein, Room 424
- Research Areas: computer graphics, audio.
Robert S. Fish, Corwin Hall, Room 037
- Networking and telecommunications
- Learning, perception, and intelligence, artificial and otherwise;
- Human-computer interaction and computer-supported cooperative work
- Online education, especially in Computer Science Education
- Topics in research and development innovation methodologies including standards, open-source, and entrepreneurship
- Distributed autonomous organizations and related blockchain technologies
Michael Freedman, Room 308
- Research Areas: Distributed systems, security, networking
- Projects related to streaming data analysis, datacenter systems and networks, untrusted cloud storage and applications. Please see my group website at http://sns.cs.princeton.edu/ for current research projects.
Ruth Fong, Room 032
- Research Areas: computer vision, machine learning, deep learning, interpretability, explainable AI, fairness and bias in AI
- Develop a technique for understanding AI models
- Design a AI model that is interpretable by design
- Build a paradigm for detecting and/or correcting failure points in an AI model
- Analyze an existing AI model and/or dataset to better understand its failure points
- Build a computer vision system for another domain (e.g., medical imaging, satellite data, etc.)
- Develop a software package for explainable AI
- Adapt explainable AI research to a consumer-facing problem
Note: I am happy to advise any project if there's a sufficient overlap in interest and/or expertise; please reach out via email to chat about project ideas.
Tom Griffiths, Room 405
Available for Fall 2024 single-semester IW advising, only
Research areas: computational cognitive science, computational social science, machine learning and artificial intelligence
Note: I am open to projects that apply ideas from computer science to understanding aspects of human cognition in a wide range of areas, from decision-making to cultural evolution and everything in between. For example, we have current projects analyzing chess game data and magic tricks, both of which give us clues about how human minds work. Students who have expertise or access to data related to games, magic, strategic sports like fencing, or other quantifiable domains of human behavior feel free to get in touch.
Aarti Gupta, Room 220
- Research Areas: Formal methods, program analysis, logic decision procedures
- Finding bugs in open source software using automatic verification tools
- Software verification (program analysis, model checking, test generation)
- Decision procedures for logical reasoning (SAT solvers, SMT solvers)
Elad Hazan, Room 409
- Research interests: machine learning methods and algorithms, efficient methods for mathematical optimization, regret minimization in games, reinforcement learning, control theory and practice
- Machine learning, efficient methods for mathematical optimization, statistical and computational learning theory, regret minimization in games.
- Implementation and algorithm engineering for control, reinforcement learning and robotics
- Implementation and algorithm engineering for time series prediction
Felix Heide, Room 410
- Research Areas: Computational Imaging, Computer Vision, Machine Learning (focus on Optimization and Approximate Inference).
- Optical Neural Networks
- Hardware-in-the-loop Holography
- Zero-shot and Simulation-only Learning
- Object recognition in extreme conditions
- 3D Scene Representations for View Generation and Inverse Problems
- Long-range Imaging in Scattering Media
- Hardware-in-the-loop Illumination and Sensor Optimization
- Inverse Lidar Design
- Phase Retrieval Algorithms
- Proximal Algorithms for Learning and Inference
- Domain-Specific Language for Optics Design
Peter Henderson , 302 Sherrerd Hall
- Research Areas: Machine learning, law, and policy
Kyle Jamieson, Room 306
- Research areas: Wireless and mobile networking; indoor radar and indoor localization; Internet of Things
- See other topics on my independent work ideas page (campus IP and CS dept. login req'd)
Alan Kaplan, 221 Nassau Street, Room 105
Research Areas:
- Random apps of kindness - mobile application/technology frameworks used to help individuals or communities; topic areas include, but are not limited to: first response, accessibility, environment, sustainability, social activism, civic computing, tele-health, remote learning, crowdsourcing, etc.
- Tools automating programming language interoperability - Java/C++, React Native/Java, etc.
- Software visualization tools for education
- Connected consumer devices, applications and protocols
Brian Kernighan, Room 311
- Research Areas: application-specific languages, document preparation, user interfaces, software tools, programming methodology
- Application-oriented languages, scripting languages.
- Tools; user interfaces
- Digital humanities
Zachary Kincaid, Room 219
- Research areas: programming languages, program analysis, program verification, automated reasoning
- Independent Research Topics:
- Develop a practical algorithm for an intractable problem (e.g., by developing practical search heuristics, or by reducing to, or by identifying a tractable sub-problem, ...).
- Design a domain-specific programming language, or prototype a new feature for an existing language.
- Any interesting project related to programming languages or logic.
Gillat Kol, Room 316
Aleksandra korolova, 309 sherrerd hall.
- Research areas: Societal impacts of algorithms and AI; privacy; fair and privacy-preserving machine learning; algorithm auditing.
Advisees typically have taken one or more of COS 226, COS 324, COS 423, COS 424 or COS 445.
Pravesh Kothari, Room 320
- Research areas: Theory
Amit Levy, Room 307
- Research Areas: Operating Systems, Distributed Systems, Embedded Systems, Internet of Things
- Distributed hardware testing infrastructure
- Second factor security tokens
- Low-power wireless network protocol implementation
- USB device driver implementation
Kai Li, Room 321
- Research Areas: Distributed systems; storage systems; content-based search and data analysis of large datasets.
- Fast communication mechanisms for heterogeneous clusters.
- Approximate nearest-neighbor search for high dimensional data.
- Data analysis and prediction of in-patient medical data.
- Optimized implementation of classification algorithms on manycore processors.
Xiaoyan Li, 221 Nassau Street, Room 104
- Research areas: Information retrieval, novelty detection, question answering, AI, machine learning and data analysis.
- Explore new statistical retrieval models for document retrieval and question answering.
- Apply AI in various fields.
- Apply supervised or unsupervised learning in health, education, finance, and social networks, etc.
- Any interesting project related to AI, machine learning, and data analysis.
Lydia Liu, Room 414
- Research Areas: algorithmic decision making, machine learning and society
- Theoretical foundations for algorithmic decision making (e.g. mathematical modeling of data-driven decision processes, societal level dynamics)
- Societal impacts of algorithms and AI through a socio-technical lens (e.g. normative implications of worst case ML metrics, prediction and model arbitrariness)
- Machine learning for social impact domains, especially education (e.g. responsible development and use of LLMs for education equity and access)
- Evaluation of human-AI decision making using statistical methods (e.g. causal inference of long term impact)
Wyatt Lloyd, Room 323
- Research areas: Distributed Systems
- Caching algorithms and implementations
- Storage systems
- Distributed transaction algorithms and implementations
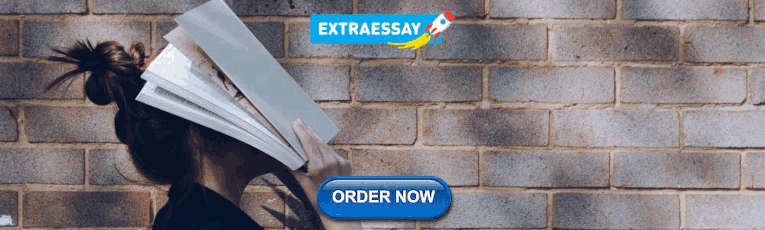
Alex Lombardi , Room 312
- Research Areas: Theory
Margaret Martonosi, Room 208
- Quantum Computing research, particularly related to architecture and compiler issues for QC.
- Computer architectures specialized for modern workloads (e.g., graph analytics, machine learning algorithms, mobile applications
- Investigating security and privacy vulnerabilities in computer systems, particularly IoT devices.
- Other topics in computer architecture or mobile / IoT systems also possible.
Jonathan Mayer, Sherrerd Hall, Room 307
Available for Spring 2025 single-semester IW, only
- Research areas: Technology law and policy, with emphasis on national security, criminal procedure, consumer privacy, network management, and online speech.
- Assessing the effects of government policies, both in the public and private sectors.
- Collecting new data that relates to government decision making, including surveying current business practices and studying user behavior.
- Developing new tools to improve government processes and offer policy alternatives.
Mae Milano, Room 307
- Local-first / peer-to-peer systems
- Wide-ares storage systems
- Consistency and protocol design
- Type-safe concurrency
- Language design
- Gradual typing
- Domain-specific languages
- Languages for distributed systems
Andrés Monroy-Hernández, Room 405
- Research Areas: Human-Computer Interaction, Social Computing, Public-Interest Technology, Augmented Reality, Urban Computing
- Research interests:developing public-interest socio-technical systems. We are currently creating alternatives to gig work platforms that are more equitable for all stakeholders. For instance, we are investigating the socio-technical affordances necessary to support a co-op food delivery network owned and managed by workers and restaurants. We are exploring novel system designs that support self-governance, decentralized/federated models, community-centered data ownership, and portable reputation systems. We have opportunities for students interested in human-centered computing, UI/UX design, full-stack software development, and qualitative/quantitative user research.
- Beyond our core projects, we are open to working on research projects that explore the use of emerging technologies, such as AR, wearables, NFTs, and DAOs, for creative and out-of-the-box applications.
Christopher Moretti, Corwin Hall, Room 036
- Research areas: Distributed systems, high-throughput computing, computer science/engineering education
- Expansion, improvement, and evaluation of open-source distributed computing software.
- Applications of distributed computing for "big science" (e.g. biometrics, data mining, bioinformatics)
- Software and best practices for computer science education and study, especially Princeton's 126/217/226 sequence or MOOCs development
- Sports analytics and/or crowd-sourced computing
Radhika Nagpal, F316 Engineering Quadrangle
- Research areas: control, robotics and dynamical systems
Karthik Narasimhan, Room 422
- Research areas: Natural Language Processing, Reinforcement Learning
- Autonomous agents for text-based games ( https://www.microsoft.com/en-us/research/project/textworld/ )
- Transfer learning/generalization in NLP
- Techniques for generating natural language
- Model-based reinforcement learning
Arvind Narayanan, 308 Sherrerd Hall
Research Areas: fair machine learning (and AI ethics more broadly), the social impact of algorithmic systems, tech policy
Pedro Paredes, Corwin Hall, Room 041
My primary research work is in Theoretical Computer Science.
* Research Interest: Spectral Graph theory, Pseudorandomness, Complexity theory, Coding Theory, Quantum Information Theory, Combinatorics.
The IW projects I am interested in advising can be divided into three categories:
1. Theoretical research
I am open to advise work on research projects in any topic in one of my research areas of interest. A project could also be based on writing a survey given results from a few papers. Students should have a solid background in math (e.g., elementary combinatorics, graph theory, discrete probability, basic algebra/calculus) and theoretical computer science (226 and 240 material, like big-O/Omega/Theta, basic complexity theory, basic fundamental algorithms). Mathematical maturity is a must.
A (non exhaustive) list of topics of projects I'm interested in: * Explicit constructions of better vertex expanders and/or unique neighbor expanders. * Construction deterministic or random high dimensional expanders. * Pseudorandom generators for different problems. * Topics around the quantum PCP conjecture. * Topics around quantum error correcting codes and locally testable codes, including constructions, encoding and decoding algorithms.
2. Theory informed practical implementations of algorithms Very often the great advances in theoretical research are either not tested in practice or not even feasible to be implemented in practice. Thus, I am interested in any project that consists in trying to make theoretical ideas applicable in practice. This includes coming up with new algorithms that trade some theoretical guarantees for feasible implementation yet trying to retain the soul of the original idea; implementing new algorithms in a suitable programming language; and empirically testing practical implementations and comparing them with benchmarks / theoretical expectations. A project in this area doesn't have to be in my main areas of research, any theoretical result could be suitable for such a project.
Some examples of areas of interest: * Streaming algorithms. * Numeric linear algebra. * Property testing. * Parallel / Distributed algorithms. * Online algorithms. 3. Machine learning with a theoretical foundation
I am interested in projects in machine learning that have some mathematical/theoretical, even if most of the project is applied. This includes topics like mathematical optimization, statistical learning, fairness and privacy.
One particular area I have been recently interested in is in the area of rating systems (e.g., Chess elo) and applications of this to experts problems.
Final Note: I am also willing to advise any project with any mathematical/theoretical component, even if it's not the main one; please reach out via email to chat about project ideas.
Iasonas Petras, Corwin Hall, Room 033
- Research Areas: Information Based Complexity, Numerical Analysis, Quantum Computation.
- Prerequisites: Reasonable mathematical maturity. In case of a project related to Quantum Computation a certain familiarity with quantum mechanics is required (related courses: ELE 396/PHY 208).
- Possible research topics include:
1. Quantum algorithms and circuits:
- i. Design or simulation quantum circuits implementing quantum algorithms.
- ii. Design of quantum algorithms solving/approximating continuous problems (such as Eigenvalue problems for Partial Differential Equations).
2. Information Based Complexity:
- i. Necessary and sufficient conditions for tractability of Linear and Linear Tensor Product Problems in various settings (for example worst case or average case).
- ii. Necessary and sufficient conditions for tractability of Linear and Linear Tensor Product Problems under new tractability and error criteria.
- iii. Necessary and sufficient conditions for tractability of Weighted problems.
- iv. Necessary and sufficient conditions for tractability of Weighted Problems under new tractability and error criteria.
3. Topics in Scientific Computation:
- i. Randomness, Pseudorandomness, MC and QMC methods and their applications (Finance, etc)
Yuri Pritykin, 245 Carl Icahn Lab
- Research interests: Computational biology; Cancer immunology; Regulation of gene expression; Functional genomics; Single-cell technologies.
- Potential research projects: Development, implementation, assessment and/or application of algorithms for analysis, integration, interpretation and visualization of multi-dimensional data in molecular biology, particularly single-cell and spatial genomics data.
Benjamin Raphael, Room 309
- Research interests: Computational biology and bioinformatics; Cancer genomics; Algorithms and machine learning approaches for analysis of large-scale datasets
- Implementation and application of algorithms to infer evolutionary processes in cancer
- Identifying correlations between combinations of genomic mutations in human and cancer genomes
- Design and implementation of algorithms for genome sequencing from new DNA sequencing technologies
- Graph clustering and network anomaly detection, particularly using diffusion processes and methods from spectral graph theory
Vikram Ramaswamy, 035 Corwin Hall
- Research areas: Interpretability of AI systems, Fairness in AI systems, Computer vision.
- Constructing a new method to explain a model / create an interpretable by design model
- Analyzing a current model / dataset to understand bias within the model/dataset
- Proposing new fairness evaluations
- Proposing new methods to train to improve fairness
- Developing synthetic datasets for fairness / interpretability benchmarks
- Understanding robustness of models
Ran Raz, Room 240
- Research Area: Computational Complexity
- Independent Research Topics: Computational Complexity, Information Theory, Quantum Computation, Theoretical Computer Science
Szymon Rusinkiewicz, Room 406
- Research Areas: computer graphics; computer vision; 3D scanning; 3D printing; robotics; documentation and visualization of cultural heritage artifacts
- Research ways of incorporating rotation invariance into computer visiontasks such as feature matching and classification
- Investigate approaches to robust 3D scan matching
- Model and compensate for imperfections in 3D printing
- Given a collection of small mobile robots, apply control policies learned in simulation to the real robots.
Olga Russakovsky, Room 408
- Research Areas: computer vision, machine learning, deep learning, crowdsourcing, fairness&bias in AI
- Design a semantic segmentation deep learning model that can operate in a zero-shot setting (i.e., recognize and segment objects not seen during training)
- Develop a deep learning classifier that is impervious to protected attributes (such as gender or race) that may be erroneously correlated with target classes
- Build a computer vision system for the novel task of inferring what object (or part of an object) a human is referring to when pointing to a single pixel in the image. This includes both collecting an appropriate dataset using crowdsourcing on Amazon Mechanical Turk, creating a new deep learning formulation for this task, and running extensive analysis of both the data and the model
Sebastian Seung, Princeton Neuroscience Institute, Room 153
- Research Areas: computational neuroscience, connectomics, "deep learning" neural networks, social computing, crowdsourcing, citizen science
- Gamification of neuroscience (EyeWire 2.0)
- Semantic segmentation and object detection in brain images from microscopy
- Computational analysis of brain structure and function
- Neural network theories of brain function
Jaswinder Pal Singh, Room 324
- Research Areas: Boundary of technology and business/applications; building and scaling technology companies with special focus at that boundary; parallel computing systems and applications: parallel and distributed applications and their implications for software and architectural design; system software and programming environments for multiprocessors.
- Develop a startup company idea, and build a plan/prototype for it.
- Explore tradeoffs at the boundary of technology/product and business/applications in a chosen area.
- Study and develop methods to infer insights from data in different application areas, from science to search to finance to others.
- Design and implement a parallel application. Possible areas include graphics, compression, biology, among many others. Analyze performance bottlenecks using existing tools, and compare programming models/languages.
- Design and implement a scalable distributed algorithm.
Mona Singh, Room 420
- Research Areas: computational molecular biology, as well as its interface with machine learning and algorithms.
- Whole and cross-genome methods for predicting protein function and protein-protein interactions.
- Analysis and prediction of biological networks.
- Computational methods for inferring specific aspects of protein structure from protein sequence data.
- Any other interesting project in computational molecular biology.
Robert Tarjan, 194 Nassau St., Room 308
- Research Areas: Data structures; graph algorithms; combinatorial optimization; computational complexity; computational geometry; parallel algorithms.
- Implement one or more data structures or combinatorial algorithms to provide insight into their empirical behavior.
- Design and/or analyze various data structures and combinatorial algorithms.
Olga Troyanskaya, Room 320
- Research Areas: Bioinformatics; analysis of large-scale biological data sets (genomics, gene expression, proteomics, biological networks); algorithms for integration of data from multiple data sources; visualization of biological data; machine learning methods in bioinformatics.
- Implement and evaluate one or more gene expression analysis algorithm.
- Develop algorithms for assessment of performance of genomic analysis methods.
- Develop, implement, and evaluate visualization tools for heterogeneous biological data.
David Walker, Room 211
- Research Areas: Programming languages, type systems, compilers, domain-specific languages, software-defined networking and security
- Independent Research Topics: Any other interesting project that involves humanitarian hacking, functional programming, domain-specific programming languages, type systems, compilers, software-defined networking, fault tolerance, language-based security, theorem proving, logic or logical frameworks.
Shengyi Wang, Postdoctoral Research Associate, Room 216
Available for Fall 2024 single-semester IW, only
- Independent Research topics: Explore Escher-style tilings using (introductory) group theory and automata theory to produce beautiful pictures.
Kevin Wayne, Corwin Hall, Room 040
- Research Areas: design, analysis, and implementation of algorithms; data structures; combinatorial optimization; graphs and networks.
- Design and implement computer visualizations of algorithms or data structures.
- Develop pedagogical tools or programming assignments for the computer science curriculum at Princeton and beyond.
- Develop assessment infrastructure and assessments for MOOCs.
Matt Weinberg, 194 Nassau St., Room 222
- Research Areas: algorithms, algorithmic game theory, mechanism design, game theoretical problems in {Bitcoin, networking, healthcare}.
- Theoretical questions related to COS 445 topics such as matching theory, voting theory, auction design, etc.
- Theoretical questions related to incentives in applications like Bitcoin, the Internet, health care, etc. In a little bit more detail: protocols for these systems are often designed assuming that users will follow them. But often, users will actually be strictly happier to deviate from the intended protocol. How should we reason about user behavior in these protocols? How should we design protocols in these settings?
Huacheng Yu, Room 310
- data structures
- streaming algorithms
- design and analyze data structures / streaming algorithms
- prove impossibility results (lower bounds)
- implement and evaluate data structures / streaming algorithms
Ellen Zhong, Room 314
Opportunities outside the department.
We encourage students to look in to doing interdisciplinary computer science research and to work with professors in departments other than computer science. However, every CS independent work project must have a strong computer science element (even if it has other scientific or artistic elements as well.) To do a project with an adviser outside of computer science you must have permission of the department. This can be accomplished by having a second co-adviser within the computer science department or by contacting the independent work supervisor about the project and having he or she sign the independent work proposal form.
Here is a list of professors outside the computer science department who are eager to work with computer science undergraduates.
Maria Apostolaki, Engineering Quadrangle, C330
- Research areas: Computing & Networking, Data & Information Science, Security & Privacy
Branko Glisic, Engineering Quadrangle, Room E330
- Documentation of historic structures
- Cyber physical systems for structural health monitoring
- Developing virtual and augmented reality applications for documenting structures
- Applying machine learning techniques to generate 3D models from 2D plans of buildings
- Contact : Rebecca Napolitano, rkn2 (@princeton.edu)
Mihir Kshirsagar, Sherrerd Hall, Room 315
Center for Information Technology Policy.
- Consumer protection
- Content regulation
- Competition law
- Economic development
- Surveillance and discrimination
Sharad Malik, Engineering Quadrangle, Room B224
Select a Senior Thesis Adviser for the 2020-21 Academic Year.
- Design of reliable hardware systems
- Verifying complex software and hardware systems
Prateek Mittal, Engineering Quadrangle, Room B236
- Internet security and privacy
- Social Networks
- Privacy technologies, anonymous communication
- Network Science
- Internet security and privacy: The insecurity of Internet protocols and services threatens the safety of our critical network infrastructure and billions of end users. How can we defend end users as well as our critical network infrastructure from attacks?
- Trustworthy social systems: Online social networks (OSNs) such as Facebook, Google+, and Twitter have revolutionized the way our society communicates. How can we leverage social connections between users to design the next generation of communication systems?
- Privacy Technologies: Privacy on the Internet is eroding rapidly, with businesses and governments mining sensitive user information. How can we protect the privacy of our online communications? The Tor project (https://www.torproject.org/) is a potential application of interest.
Ken Norman, Psychology Dept, PNI 137
- Research Areas: Memory, the brain and computation
- Lab: Princeton Computational Memory Lab
Potential research topics
- Methods for decoding cognitive state information from neuroimaging data (fMRI and EEG)
- Neural network simulations of learning and memory
Caroline Savage
Office of Sustainability, Phone:(609)258-7513, Email: cs35 (@princeton.edu)
The Campus as Lab program supports students using the Princeton campus as a living laboratory to solve sustainability challenges. The Office of Sustainability has created a list of campus as lab research questions, filterable by discipline and topic, on its website .
An example from Computer Science could include using TigerEnergy , a platform which provides real-time data on campus energy generation and consumption, to study one of the many energy systems or buildings on campus. Three CS students used TigerEnergy to create a live energy heatmap of campus .
Other potential projects include:
- Apply game theory to sustainability challenges
- Develop a tool to help visualize interactions between complex campus systems, e.g. energy and water use, transportation and storm water runoff, purchasing and waste, etc.
- How can we learn (in aggregate) about individuals’ waste, energy, transportation, and other behaviors without impinging on privacy?
Janet Vertesi, Sociology Dept, Wallace Hall, Room 122
- Research areas: Sociology of technology; Human-computer interaction; Ubiquitous computing.
- Possible projects: At the intersection of computer science and social science, my students have built mixed reality games, produced artistic and interactive installations, and studied mixed human-robot teams, among other projects.
David Wentzlaff, Engineering Quadrangle, Room 228
Computing, Operating Systems, Sustainable Computing.
- Instrument Princeton's Green (HPCRC) data center
- Investigate power utilization on an processor core implemented in an FPGA
- Dismantle and document all of the components in modern electronics. Invent new ways to build computers that can be recycled easier.
- Other topics in parallel computer architecture or operating systems

Bachelor and Master theses
If you are interested in working on a research project with us that results in a Bachelor or Master thesis we would like to hear about it. We usually have a couple of open Bachelor and Master thesis projects available here . Our websites should give you an impression about possible thesis topics, we can propose a project based on your preferences. If you already have an idea about a project we are happy to discuss that as well. In either case please send an email to [email protected] . For us to get to know you, it would be helpful to also include the following documents. If you are interested in working with a specific researcher in our group, you should state that in your email.
- transcripts of master/bachelor program (if applicable)
- high school documents (Abitur)

Computer Vision Group TUM School of Computation, Information and Technology Technical University of Munich
Technical university of munich.
- Our University
- Coronavirus
- Publications
- Departments
- Awards and Honors
- University Hospitals
- Teaching and QMS
- Working at TUM
- Contact & Directions
- Research Centers
- Excellence Strategy
- Research projects
- Research Partners
- Research promotion
- Doctorate (Ph.D.)
- Career openings
- Entrepreneurship
- Technology transfer
- Industry Liaison Office
- Lifelong learning
- Degree programs
- International Students
- Application
- Fees and Financial Aid
- During your Studies
- Completing your Studies
- Student Life
- Accommodation
- Music and Arts
- Alumni Services
- Career Service
- TUM for schools
- International Locations
- International Alliances
- Language Center

Javascript is disabled in your browser. Please enable it for full functionality and experience.
- Direkt zur Navigation springen
- Direkt zur Suche springen
- Direkt zum Inhalt springen
- Fraunhofer HHI
- Departments
- Start page >
- Departments >
- Vision and Imaging Technologies >
- Research Groups >
- Computer Vision & Graphics >
- CVG Research Overview
- AI-Based Building Digitalization
- Portrait Relighting
- Neural Speech-Driven Face Animation
- Video-driven Facial Animation
- Publications
- Student Opportunities
- IMC Research Overview
- Research Topics
- Pose and gesture analysis
- Behaviour analysis for human-computer interaction
- Contact-free Human-Computer Interaction
- Image Quality Estimation
- Subjective Tests
- Birgit Nierula
Research Topics of the Computer Vision & Graphics Group
Seeing, modelling and animating humans.
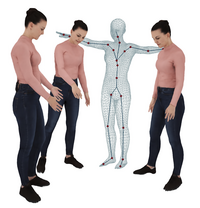
Realistic human modelling is a challenging task in Computer Vision and Graphics. We investigate new methods for capturing and analyzing human bodies and faces in images and videos as well as new compact models for the representation of facial expressions as well as human bodies and their motion. We combine model-based and image-and video based representations with generative AI models as well as neural rendering.
Read more about current research projects in this field.
Scenes, Structure and Motion
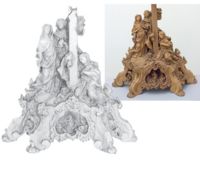
We have a long tradition in 3D scene analysis and continuously perform innovative research in 3D capturing as well as 3D reconstruction, ranging from highly detailed stereo as well as multi-view images of static objects and scenes, addressing even complex surface and shape properties, over monocular shape-from-X methods, to analyzing deforming objects in monocular video.
Computational Imaging and Video
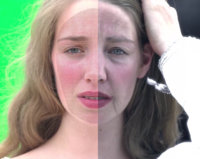
We perform innovative research in the field of video processing and computational video opening up new opportunities for how dynamic scenes can be analyzed and video footage can be represented, edited and seamlessly augmented with new content.
Learning and Inference
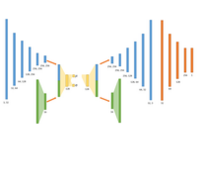
Our research combines computer vision, computer graphics, and machine learning to understand images and video data. In our research, we focus on the combination of deep learning with strong models or physical constraints in order to combine the advantages of model-based and data-driven methods.
Augmented and Mixed Reality
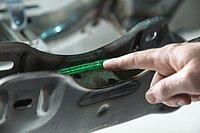
Our experience in tracking dynamic scenes and objects as well as photorealistic rendering enables new augmented reality solutions where virtual content is seamlessly blended into real video footage with applications e.g. multi-media, industry or medicine.
Previous Research Projects

We have performed various research projects in the above fields over the years.
Read more about older research projects here.
- Warning : Invalid argument supplied for foreach() in /home/customer/www/opendatascience.com/public_html/wp-includes/nav-menu.php on line 95 Warning : array_merge(): Expected parameter 2 to be an array, null given in /home/customer/www/opendatascience.com/public_html/wp-includes/nav-menu.php on line 102
- ODSC EUROPE
- AI+ Training
- Speak at ODSC

- Data Analytics
- Data Engineering
- Data Visualization
- Deep Learning
- Generative AI
- Machine Learning
- NLP and LLMs
- Business & Use Cases
- Career Advice
- Write for us
- ODSC Community Slack Channel
- Upcoming Webinars

10 Compelling Machine Learning Ph.D. Dissertations for 2020
Machine Learning Modeling Research posted by Daniel Gutierrez, ODSC August 19, 2020 Daniel Gutierrez, ODSC
As a data scientist, an integral part of my work in the field revolves around keeping current with research coming out of academia. I frequently scour arXiv.org for late-breaking papers that show trends and reveal fertile areas of research. Other sources of valuable research developments are in the form of Ph.D. dissertations, the culmination of a doctoral candidate’s work to confer his/her degree. Ph.D. candidates are highly motivated to choose research topics that establish new and creative paths toward discovery in their field of study. Their dissertations are highly focused on a specific problem. If you can find a dissertation that aligns with your areas of interest, consuming the research is an excellent way to do a deep dive into the technology. After reviewing hundreds of recent theses from universities all over the country, I present 10 machine learning dissertations that I found compelling in terms of my own areas of interest.
[Related article: Introduction to Bayesian Deep Learning ]
I hope you’ll find several that match your own fields of inquiry. Each thesis may take a while to consume but will result in hours of satisfying summer reading. Enjoy!
1. Bayesian Modeling and Variable Selection for Complex Data
As we routinely encounter high-throughput data sets in complex biological and environmental research, developing novel models and methods for variable selection has received widespread attention. This dissertation addresses a few key challenges in Bayesian modeling and variable selection for high-dimensional data with complex spatial structures.
2. Topics in Statistical Learning with a Focus on Large Scale Data
Big data vary in shape and call for different approaches. One type of big data is the tall data, i.e., a very large number of samples but not too many features. This dissertation describes a general communication-efficient algorithm for distributed statistical learning on this type of big data. The algorithm distributes the samples uniformly to multiple machines, and uses a common reference data to improve the performance of local estimates. The algorithm enables potentially much faster analysis, at a small cost to statistical performance.
Another type of big data is the wide data, i.e., too many features but a limited number of samples. It is also called high-dimensional data, to which many classical statistical methods are not applicable.
This dissertation discusses a method of dimensionality reduction for high-dimensional classification. The method partitions features into independent communities and splits the original classification problem into separate smaller ones. It enables parallel computing and produces more interpretable results.
3. Sets as Measures: Optimization and Machine Learning
The purpose of this machine learning dissertation is to address the following simple question:
How do we design efficient algorithms to solve optimization or machine learning problems where the decision variable (or target label) is a set of unknown cardinality?
Optimization and machine learning have proved remarkably successful in applications requiring the choice of single vectors. Some tasks, in particular many inverse problems, call for the design, or estimation, of sets of objects. When the size of these sets is a priori unknown, directly applying optimization or machine learning techniques designed for single vectors appears difficult. The work in this dissertation shows that a very old idea for transforming sets into elements of a vector space (namely, a space of measures), a common trick in theoretical analysis, generates effective practical algorithms.
4. A Geometric Perspective on Some Topics in Statistical Learning
Modern science and engineering often generate data sets with a large sample size and a comparably large dimension which puts classic asymptotic theory into question in many ways. Therefore, the main focus of this dissertation is to develop a fundamental understanding of statistical procedures for estimation and hypothesis testing from a non-asymptotic point of view, where both the sample size and problem dimension grow hand in hand. A range of different problems are explored in this thesis, including work on the geometry of hypothesis testing, adaptivity to local structure in estimation, effective methods for shape-constrained problems, and early stopping with boosting algorithms. The treatment of these different problems shares the common theme of emphasizing the underlying geometric structure.
5. Essays on Random Forest Ensembles
A random forest is a popular machine learning ensemble method that has proven successful in solving a wide range of classification problems. While other successful classifiers, such as boosting algorithms or neural networks, admit natural interpretations as maximum likelihood, a suitable statistical interpretation is much more elusive for a random forest. The first part of this dissertation demonstrates that a random forest is a fruitful framework in which to study AdaBoost and deep neural networks. The work explores the concept and utility of interpolation, the ability of a classifier to perfectly fit its training data. The second part of this dissertation places a random forest on more sound statistical footing by framing it as kernel regression with the proximity kernel. The work then analyzes the parameters that control the bandwidth of this kernel and discuss useful generalizations.
6. Marginally Interpretable Generalized Linear Mixed Models
A popular approach for relating correlated measurements of a non-Gaussian response variable to a set of predictors is to introduce latent random variables and fit a generalized linear mixed model. The conventional strategy for specifying such a model leads to parameter estimates that must be interpreted conditional on the latent variables. In many cases, interest lies not in these conditional parameters, but rather in marginal parameters that summarize the average effect of the predictors across the entire population. Due to the structure of the generalized linear mixed model, the average effect across all individuals in a population is generally not the same as the effect for an average individual. Further complicating matters, obtaining marginal summaries from a generalized linear mixed model often requires evaluation of an analytically intractable integral or use of an approximation. Another popular approach in this setting is to fit a marginal model using generalized estimating equations. This strategy is effective for estimating marginal parameters, but leaves one without a formal model for the data with which to assess quality of fit or make predictions for future observations. Thus, there exists a need for a better approach.
This dissertation defines a class of marginally interpretable generalized linear mixed models that leads to parameter estimates with a marginal interpretation while maintaining the desirable statistical properties of a conditionally specified model. The distinguishing feature of these models is an additive adjustment that accounts for the curvature of the link function and thereby preserves a specific form for the marginal mean after integrating out the latent random variables.
7. On the Detection of Hate Speech, Hate Speakers and Polarized Groups in Online Social Media
The objective of this dissertation is to explore the use of machine learning algorithms in understanding and detecting hate speech, hate speakers and polarized groups in online social media. Beginning with a unique typology for detecting abusive language, the work outlines the distinctions and similarities of different abusive language subtasks (offensive language, hate speech, cyberbullying and trolling) and how we might benefit from the progress made in each area. Specifically, the work suggests that each subtask can be categorized based on whether or not the abusive language being studied 1) is directed at a specific individual, or targets a generalized “Other” and 2) the extent to which the language is explicit versus implicit. The work then uses knowledge gained from this typology to tackle the “problem of offensive language” in hate speech detection.
8. Lasso Guarantees for Dependent Data
Serially correlated high dimensional data are prevalent in the big data era. In order to predict and learn the complex relationship among the multiple time series, high dimensional modeling has gained importance in various fields such as control theory, statistics, economics, finance, genetics and neuroscience. This dissertation studies a number of high dimensional statistical problems involving different classes of mixing processes.
9. Random forest robustness, variable importance, and tree aggregation
Random forest methodology is a nonparametric, machine learning approach capable of strong performance in regression and classification problems involving complex data sets. In addition to making predictions, random forests can be used to assess the relative importance of feature variables. This dissertation explores three topics related to random forests: tree aggregation, variable importance, and robustness.
10. Climate Data Computing: Optimal Interpolation, Averaging, Visualization and Delivery
This dissertation solves two important problems in the modern analysis of big climate data. The first is the efficient visualization and fast delivery of big climate data, and the second is a computationally extensive principal component analysis (PCA) using spherical harmonics on the Earth’s surface. The second problem creates a way to supply the data for the technology developed in the first. These two problems are computationally difficult, such as the representation of higher order spherical harmonics Y400, which is critical for upscaling weather data to almost infinitely fine spatial resolution.
I hope you enjoyed learning about these compelling machine learning dissertations.
Editor’s note: Interested in more data science research? Check out the Research Frontiers track at ODSC Europe this September 17-19 or the ODSC West Research Frontiers track this October 27-30.

Daniel Gutierrez, ODSC
Daniel D. Gutierrez is a practicing data scientist who’s been working with data long before the field came in vogue. As a technology journalist, he enjoys keeping a pulse on this fast-paced industry. Daniel is also an educator having taught data science, machine learning and R classes at the university level. He has authored four computer industry books on database and data science technology, including his most recent title, “Machine Learning and Data Science: An Introduction to Statistical Learning Methods with R.” Daniel holds a BS in Mathematics and Computer Science from UCLA.

Microsoft Invests $1.5B in Abu Dhabi-Based AI Company G42
AI and Data Science News posted by ODSC Team Apr 17, 2024 As reported by Reuters, Microsoft has committed $1.5 billion to Abu Dhabi-based artificial intelligence company G42....

What is AI Washing and Why is it a Concern?
Responsible AI Modeling posted by ODSC Team Apr 16, 2024 A new term has emerged, capturing the attention of industry insiders and regulators alike: AI washing....

The AI Expo Hall and Other Ways to Attend ODSC East 2024 for Free
East 2024 Conferences posted by ODSC Team Apr 16, 2024 Hoping to attend ODSC East 2024, but a bit short on cash? Don’t worry, the team...

- Skip to main content
- Skip to primary sidebar
- Skip to footer

The Best of Applied Artificial Intelligence, Machine Learning, Automation, Bots, Chatbots
10 Cutting Edge Research Papers In Computer Vision & Image Generation
January 24, 2019 by Mariya Yao

UPDATE: We’ve also summarized the top 2019 and top 2020 Computer Vision research papers.
Ever since convolutional neural networks began outperforming humans in specific image recognition tasks, research in the field of computer vision has proceeded at breakneck pace.
The basic architecture of CNNs (or ConvNets) was developed in the 1980s . Yann LeCun improved upon the original design in 1989 by using backpropagation to train models to recognize handwritten digits.
We’ve come a long way since then.
In 2018, we saw novel architecture designs that improve upon performance benchmarks and also expand the range of media that machine learning models can analyze. We also saw a number of breakthroughs with media generation which enable photorealistic style transfer, high-resolution image generation, and video-to-video synthesis.
Due to the importance and prevalence of computer vision and image generation for applied and enterprise AI, we did feature some of the papers below in our previous article summarizing the top overall machine learning papers of 2018 . Since you might not have read that previous piece, we chose to highlight the vision-related research ones again here.
We’ve done our best to summarize these papers correctly, but if we’ve made any mistakes, please contact us to request a fix . Special thanks also goes to computer vision specialist Rebecca BurWei for generously offering her expertise in editing and revising drafts of this article.
If these summaries of scientific AI research papers are useful for you, you can subscribe to our AI Research mailing list at the bottom of this article to be alerted when we release new summaries. We’re planning to release summaries of important papers in computer vision, reinforcement learning, and conversational AI in the next few weeks.
If you’d like to skip around, here are the papers we featured:
- Spherical CNNs
- Adversarial Examples that Fool both Computer Vision and Time-Limited Humans
- A Closed-form Solution to Photorealistic Image Stylization
- Group Normalization
- Taskonomy: Disentangling Task Transfer Learning
- Self-Attention Generative Adversarial Networks
- GANimation: Anatomically-aware Facial Animation from a Single Image
- Video-to-Video Synthesis
- Everybody Dance Now
- Large Scale GAN Training for High Fidelity Natural Image Synthesis
Important Computer Vision Research Papers of 2018
1. spherical cnns , by taco s. cohen, mario geiger, jonas koehler, and max welling, original abstract.
Convolutional Neural Networks (CNNs) have become the method of choice for learning problems involving 2D planar images. However, a number of problems of recent interest have created a demand for models that can analyze spherical images. Examples include omnidirectional vision for drones, robots, and autonomous cars, molecular regression problems, and global weather and climate modelling. A naive application of convolutional networks to a planar projection of the spherical signal is destined to fail, because the space-varying distortions introduced by such a projection will make translational weight sharing ineffective.
In this paper we introduce the building blocks for constructing spherical CNNs. We propose a definition for the spherical cross-correlation that is both expressive and rotation-equivariant. The spherical correlation satisfies a generalized Fourier theorem, which allows us to compute it efficiently using a generalized (non-commutative) Fast Fourier Transform (FFT) algorithm. We demonstrate the computational efficiency, numerical accuracy, and effectiveness of spherical CNNs applied to 3D model recognition and atomization energy regression.
Our Summary
Omnidirectional cameras that are already used by cars, drones, and other robots capture a spherical image of their entire surroundings. We could analyze such spherical signals by projecting them to the plane and using CNNs. However, any planar projection of a spherical signal results in distortions. To overcome this problem, the group of researchers from the University of Amsterdam introduces the theory of spherical CNNs, the networks that can analyze spherical images without being fooled by distortions. The approach demonstrates its effectiveness for classifying 3D shapes and Spherical MNIST images as well as for molecular energy regression, an important problem in computational chemistry.
What’s the core idea of this paper?
- Planar projections of spherical signals result in significant distortions as some areas look larger or smaller than they really are.
- Traditional CNNs are ineffective for spherical images because as objects move around the sphere, they also appear to shrink and stretch (think maps where Greenland looks much bigger than it actually is).
- The solution is to use a spherical CNN which is robust to spherical rotations in the input data. By preserving the original shape of the input data, spherical CNNs treat all objects on the sphere equally without distortion.
What’s the key achievement?
- Introducing a mathematical framework for building spherical CNNs.
- Providing easy to use, fast and memory efficient PyTorch code for implementation of these CNNs.
- classification of Spherical MNIST images
- classification of 3D shapes,
- molecular energy regression.
What does the AI community think?
- The paper won the Best Paper Award at ICLR 2018, one of the leading machine learning conferences.
What are future research areas?
- Development of a Steerable CNN for the sphere to analyze sections of vector bundles over the sphere (e.g., wind directions).
- Expanding the mathematical theory from 2D spheres to 3D point clouds for classification tasks that are invariant under reflections as well as rotations.
What are possible business applications?
- the omnidirectional vision for drones, robots, and autonomous cars;
- molecular regression problems in computational chemistry;
- global weather and climate modeling.
Where can you get implementation code?
- The authors provide the original implementation for this research paper on GitHub .

2. Adversarial Examples that Fool both Computer Vision and Time-Limited Humans , by Gamaleldin F. Elsayed, Shreya Shankar, Brian Cheung, Nicolas Papernot, Alex Kurakin, Ian Goodfellow, Jascha Sohl-Dickstein
Machine learning models are vulnerable to adversarial examples: small changes to images can cause computer vision models to make mistakes such as identifying a school bus as an ostrich. However, it is still an open question whether humans are prone to similar mistakes. Here, we address this question by leveraging recent techniques that transfer adversarial examples from computer vision models with known parameters and architecture to other models with unknown parameters and architecture, and by matching the initial processing of the human visual system. We find that adversarial examples that strongly transfer across computer vision models influence the classifications made by time-limited human observers.
Google Brain researchers seek an answer to the question: do adversarial examples that are not model-specific and can fool different computer vision models without access to their parameters and architectures, can also fool time-limited humans? They leverage key ideas from machine learning, neuroscience, and psychophysics to create adversarial examples that do in fact impact human perception in a time-limited setting. Thus, the paper introduces a new class of illusions that are shared between machines and humans.

- As the first step, the researchers use the black box adversarial example construction techniques that create adversarial examples without access to the model’s architecture or parameters.
- prepending each model with a retinal layer that pre-processes the input to incorporate some of the transformations performed by the human eye;
- performing an eccentricity-dependent blurring of the image to approximate the input which is received by the visual cortex of human subjects through their retinal lattice.
- Classification decisions of humans are evaluated in a time-limited setting to detect even subtle effects in human perception.
- Showing that adversarial examples that transfer across computer vision models do also successfully influence the perception of humans.
- Demonstrating the similarity between convolutional neural networks and the human visual system.
- The paper is widely discussed by the AI community. While most of the researchers are stunned by the results , some argue that we need a stricter definition of adversarial image because if humans classify the perturbated picture of a cat as a dog than it’s probably already a dog, not a cat.
- Researching which techniques are crucial for the transfer of adversarial examples to humans (i.e., retinal preprocessing, model ensembling).
- Practitioners should consider the risk that imagery could be manipulated to cause human observers to have unusual reactions because adversarial images can affect us below the horizon of awareness .
3. A Closed-form Solution to Photorealistic Image Stylization , by Yijun Li, Ming-Yu Liu, Xueting Li, Ming-Hsuan Yang, Jan Kautz
Photorealistic image stylization concerns transferring style of a reference photo to a content photo with the constraint that the stylized photo should remain photorealistic. While several photorealistic image stylization methods exist, they tend to generate spatially inconsistent stylizations with noticeable artifacts. In this paper, we propose a method to address these issues. The proposed method consists of a stylization step and a smoothing step. While the stylization step transfers the style of the reference photo to the content photo, the smoothing step ensures spatially consistent stylizations. Each of the steps has a closed-form solution and can be computed efficiently. We conduct extensive experimental validations. The results show that the proposed method generates photorealistic stylization outputs that are more preferred by human subjects as compared to those by the competing methods while running much faster. Source code and additional results are available at https://github.com/NVIDIA/FastPhotoStyle .
The team of scientists at NVIDIA and the University of California, Merced propose a new solution to photorealistic image stylization, FastPhotoStyle. The method consists of two steps: stylization and smoothing. Extensive experiments show that the suggested approach generates more realistic and compelling images than previous state-of-the-art. Even more, thanks to the closed-form solution, FastPhotoStyle can produce the stylized image 49 times faster than traditional methods.

- The goal of photorealistic image stylization is to transfer style of a reference photo to a content photo while keeping the stylized image photorealistic.
- The stylization step is based on the whitening and coloring transform (WCT), which processes images via feature projections. However, WCT was developed for artistic image stylizations, and thus, often generates structural artifacts for photorealistic image stylization. To overcome this problem, the paper introduces PhotoWCT method, which replaces the upsampling layers in the WCT with unpooling layers, and so, preserves more spatial information.
- The smoothing step is required to solve spatially inconsistent stylizations that could arise after the first step. Smoothing is based on a manifold ranking algorithm.
- Both steps have a closed-form solution, which means that the solution can be obtained in a fixed number of operations (i.e., convolutions, max-pooling, whitening, etc.). Thus, computations are much more efficient compared to the traditional methods.
- outperforms artistic stylization algorithms by rendering much fewer structural artifacts and inconsistent stylizations, and
- outperforms photorealistic stylization algorithms by synthesizing not only colors but also patterns in the style photos.
- The experiments demonstrate that users prefer FastPhotoStyle results over the previous state-of-the-art in terms of both stylization effects (63.1%) and photorealism (73.5%).
- FastPhotoSyle can synthesize an image of 1024 x 512 resolution in only 13 seconds, while the previous state-of-the-art method needs 650 seconds for the same task.
- The paper was presented at ECCV 2018, leading European Conference on Computer Vision.
- Finding the way to transfer small patterns from the style photo as they are smoothed away by the suggested method.
- Exploring the possibilities to further reduce the number of structural artifacts in the stylized photos.
- Content creators in the business settings can largely benefit from photorealistic image stylization as the tool basically allows you to automatically change the style of any photo based on what fits the narrative.
- The photographers also discuss the tremendous impact that this technology can have in real estate photography.
- NVIDIA team provides the original implementation for this research paper on GitHub .
4. Group Normalization , by Yuxin Wu and Kaiming He
Batch Normalization (BN) is a milestone technique in the development of deep learning, enabling various networks to train. However, normalizing along the batch dimension introduces problems – BN’s error increases rapidly when the batch size becomes smaller, caused by inaccurate batch statistics estimation. This limits BN’s usage for training larger models and transferring features to computer vision tasks including detection, segmentation, and video, which require small batches constrained by memory consumption. In this paper, we present Group Normalization (GN) as a simple alternative to BN. GN divides the channels into groups and computes within each group the mean and variance for normalization. GN’s computation is independent of batch sizes, and its accuracy is stable in a wide range of batch sizes. On ResNet-50 trained in ImageNet, GN has 10.6% lower error than its BN counterpart when using a batch size of 2; when using typical batch sizes, GN is comparably good with BN and outperforms other normalization variants. Moreover, GN can be naturally transferred from pre-training to fine-tuning. GN can outperform its BN-based counterparts for object detection and segmentation in COCO, and for video classification in Kinetics, showing that GN can effectively replace the powerful BN in a variety of tasks. GN can be easily implemented by a few lines of code in modern libraries.
Facebook AI research team suggest Group Normalization (GN) as an alternative to Batch Normalization (BN). They argue that BN’s error increases dramatically for small batch sizes. This limits the usage of BN when working with large models to solve computer vision tasks that require small batches due to memory constraints. On the contrary, Group Normalization is independent of batch sizes as it divides the channels into groups and computes the mean and variance for normalization within each group. The experiments confirm that GN outperforms BN in a variety of tasks, including object detection, segmentation, and video classification.

- Group Normalization is a simple alternative to Batch Normalization, especially in the scenarios where batch size tends to be small, for example, computer vision tasks, requiring high-resolution input.
- GN explores only the layer dimensions, and thus, its computation is independent of batch size. Specifically, GN divides channels, or feature maps, into groups and normalizes the features within each group.
- Group Normalization can be easily implemented by a few lines of code in PyTorch and TensorFlow.
- Introducing Group Normalization, new effective normalization method.
- GN’s accuracy is stable in a wide range of batch sizes as its computation is independent of batch size. For example, GN demonstrated a 10.6% lower error rate than its BN-based counterpart for ResNet-50 in ImageNet with a batch size of 2.
- GN can be also transferred to fine-tuning. The experiments show that GN can outperform BN counterparts for object detection and segmentation in COCO dataset and video classification in Kinetics dataset.
- The paper received an honorable mention at ECCV 2018, leading European Conference on Computer Vision.
- It is also the second most popular paper in 2018 based on the people’s libraries at Arxiv Sanity Preserver.
- Applying group normalization to sequential or generative models.
- Investigating GN’s performance on learning representations for reinforcement learning.
- Exploring if GN combined with a suitable regularizer will improve results.
- Business applications that rely on BN-based models for object detection, segmentation, video classification and other computer vision tasks that require high-resolution input may benefit from moving to GN-based models as they are more accurate in these settings.
- Facebook AI research team provides Mask R-CNN baseline results and models trained with Group Normalization .
- PyTorch implementation of group normalization is also available on GitHub.
5. Taskonomy: Disentangling Task Transfer Learning , by Amir R. Zamir, Alexander Sax, William Shen, Leonidas J. Guibas, Jitendra Malik, and Silvio Savarese
Do visual tasks have a relationship, or are they unrelated? For instance, could having surface normals simplify estimating the depth of an image? Intuition answers these questions positively, implying existence of a structure among visual tasks. Knowing this structure has notable values; it is the concept underlying transfer learning and provides a principled way for identifying redundancies across tasks, e.g., to seamlessly reuse supervision among related tasks or solve many tasks in one system without piling up the complexity.
We proposes a fully computational approach for modeling the structure of space of visual tasks. This is done via finding (first and higher-order) transfer learning dependencies across a dictionary of twenty six 2D, 2.5D, 3D, and semantic tasks in a latent space. The product is a computational taxonomic map for task transfer learning. We study the consequences of this structure, e.g. nontrivial emerged relationships, and exploit them to reduce the demand for labeled data. For example, we show that the total number of labeled datapoints needed for solving a set of 10 tasks can be reduced by roughly 2/3 (compared to training independently) while keeping the performance nearly the same. We provide a set of tools for computing and probing this taxonomical structure including a solver that users can employ to devise efficient supervision policies for their use cases.
Assertions of the existence of a structure among visual tasks have been made by many researchers since the early years of modern computer science. And now Amir Zamir and his team make an attempt to actually find this structure. They model it using a fully computational approach and discover lots of useful relationships between different visual tasks, including the nontrivial ones. They also show that by taking advantage of these interdependencies, it is possible to achieve the same model performance with the labeled data requirements reduced by roughly ⅔.

- A model aware of the relationships among different visual tasks demands less supervision, uses less computation, and behaves in more predictable ways.
- A fully computational approach to discovering the relationships between visual tasks is preferable because it avoids imposing prior, and possibly incorrect, assumptions: the priors are derived from either human intuition or analytical knowledge, while neural networks might operate on different principles.
- Identifying relationships between 26 common visual tasks.
- Showing how this structure helps in discovering types of transfer learning that will be most effective for each visual task.
- Creating a new dataset of 4 million images of indoor scenes including 600 buildings annotated with 26 tasks.
- The paper won the Best Paper Award at CVPR 2018, the key conference on computer vision and pattern recognition.
- The results are very important as for the most real-world tasks large-scale labeled datasets are not available .
- To move from a model where common visual tasks are entirely defined by humans and try an approach where human-defined visual tasks are viewed as observed samples which are composed of computationally found latent subtasks.
- Exploring the possibility to transfer the findings to not entirely visual tasks, e.g. robotic manipulation.
- Relationships discovered in this paper can be used to build more effective visual systems that will require less labeled data and lower computational costs.
6. Self-Attention Generative Adversarial Networks , by Han Zhang, Ian Goodfellow, Dimitris Metaxas, Augustus Odena
In this paper, we propose the Self-Attention Generative Adversarial Network (SAGAN) which allows attention-driven, long-range dependency modeling for image generation tasks. Traditional convolutional GANs generate high-resolution details as a function of only spatially local points in lower-resolution feature maps. In SAGAN, details can be generated using cues from all feature locations. Moreover, the discriminator can check that highly detailed features in distant portions of the image are consistent with each other. Furthermore, recent work has shown that generator conditioning affects GAN performance. Leveraging this insight, we apply spectral normalization to the GAN generator and find that this improves training dynamics. The proposed SAGAN achieves the state-of-the-art results, boosting the best published Inception score from 36.8 to 52.52 and reducing Frechet Inception distance from 27.62 to 18.65 on the challenging ImageNet dataset. Visualization of the attention layers shows that the generator leverages neighborhoods that correspond to object shapes rather than local regions of fixed shape.
Traditional convolutional GANs demonstrated some very promising results with respect to image synthesis. However, they have at least one important weakness – convolutional layers alone fail to capture geometrical and structural patterns in the images. Since convolution is a local operation, it is hardly possible for an output on the top-left position to have any relation to the output at bottom-right . The paper introduces a simple solution to this problem – incorporating the self-attention mechanism into the GAN framework. This solution combined with several stabilization techniques helps the Senf-Attention Generative Adversarial Networks (SAGANs) achieve the state-of-the-art results in image synthesis.

- Convolutional layers alone are computationally inefficient for modeling long-range dependencies in images. On the contrary, a self-attention mechanism incorporated into the GAN framework will enable both the generator and the discriminator to efficiently model relationships between widely separated spatial regions.
- The self-attention module calculates response at a position as a weighted sum of the features at all positions.
- Applying spectral normalization for both generator and discriminator – the researchers argue that not only the discriminator but also the generator can benefit from spectral normalization, as it can prevent the escalation of parameter magnitudes and avoid unusual gradients.
- Using separate learning rates for the generator and the discriminator to compensate for the problem of slow learning in a regularized discriminator and make it possible to use fewer generator steps per discriminator step.
- Showing that self-attention module incorporated into the GAN framework is, in fact, effective in modeling long-range dependencies.
- spectral normalization applied to the generator stabilizes GAN training;
- utilizing imbalanced learning rates speeds up training of regularized discriminators.
- Achieving state-of-the-art results in image synthesis by boosting the Inception Score from 36.8 to 52.52 and reducing Fréchet Inception Distance from 27.62 to 18.65.
- “The idea is simple and intuitive yet very effective, plus easy to implement.” – Sebastian Raschka , assistant professor of Statistics at the University of Wisconsin-Madison.
- Exploring the possibilities to reduce the number of weird samples generated by GANs.
- Image synthesis with GANs can replace expensive manual media creation for advertising and e-commerce purposes.
- PyTorch and TensorFlow implementations of Self-Attention GANs are available on GitHub.
7. GANimation: Anatomically-aware Facial Animation from a Single Image , by Albert Pumarola, Antonio Agudo, Aleix M. Martinez, Alberto Sanfeliu, Francesc Moreno-Noguer
Recent advances in Generative Adversarial Networks (GANs) have shown impressive results for task of facial expression synthesis. The most successful architecture is StarGAN, that conditions GANs generation process with images of a specific domain, namely a set of images of persons sharing the same expression. While effective, this approach can only generate a discrete number of expressions, determined by the content of the dataset. To address this limitation, in this paper, we introduce a novel GAN conditioning scheme based on Action Units (AU) annotations, which describes in a continuous manifold the anatomical facial movements defining a human expression. Our approach allows controlling the magnitude of activation of each AU and combine several of them. Additionally, we propose a fully unsupervised strategy to train the model, that only requires images annotated with their activated AUs, and exploit attention mechanisms that make our network robust to changing backgrounds and lighting conditions. Extensive evaluation show that our approach goes beyond competing conditional generators both in the capability to synthesize a much wider range of expressions ruled by anatomically feasible muscle movements, as in the capacity of dealing with images in the wild.
The paper introduces a novel GAN model that is able to generate anatomically-aware facial animations from a single image under changing backgrounds and illumination conditions. It advances current works, which had only addressed the problem for discrete emotions category editing and portrait images. The approach renders a wide range of emotions by encoding facial deformations as Action Units. The resulting animations demonstrate a remarkably smooth and consistent transformation across frames even with challenging light conditions and backgrounds.

- Facial expressions can be described in terms of Action Units (AUs), which anatomically describe the contractions of specific facial muscles. For example, the facial expression for ‘fear’ is generally produced with the following activations: Inner Brow Raiser (AU1), Outer Brow Raiser (AU2), Brow Lowerer (AU4), Upper Lid Raiser (AU5), Lid Tightener (AU7), Lip Stretcher (AU20) and Jaw Drop (AU26). The magnitude of each AU defines the extent of emotion.
- A model for synthetic facial animation is based on the GAN architecture, which is conditioned on a one-dimensional vector indicating the presence/absence and the magnitude of each Action Unit.
- To circumvent the need for pairs of training images of the same person under different expressions, a bidirectional generator is used to both transform an image into a desired expression and transform the synthesized image back into the original pose.
- To handle images under changing backgrounds and illumination conditions, the model includes an attention layer that focuses the action of the network only in those regions of the image that are relevant to convey the novel expression.
- Introducing a novel GAN model for face animation in the wild that can be trained in a fully unsupervised manner and generate visually compelling images with remarkably smooth and consistent transformation across frames even with challenging light conditions and non-real world data.
- Demonstrating how a wider range of emotions can be generated by interpolating between emotions the GAN has already seen.
- Applying the introduced approach to video sequences.
- The technology that automatically animates the facial expression from a single image can be applied in several areas including the fashion and e-commerce business, the movie industry, photography technologies.
- The authors provide the original implementation of this research paper on GitHub .
8. Video-to-Video Synthesis , by Ting-Chun Wang, Ming-Yu Liu, Jun-Yan Zhu, Guilin Liu, Andrew Tao, Jan Kautz, Bryan Catanzaro
We study the problem of video-to-video synthesis, whose goal is to learn a mapping function from an input source video (e.g., a sequence of semantic segmentation masks) to an output photorealistic video that precisely depicts the content of the source video. While its image counterpart, the image-to-image synthesis problem, is a popular topic, the video-to-video synthesis problem is less explored in the literature. Without understanding temporal dynamics, directly applying existing image synthesis approaches to an input video often results in temporally incoherent videos of low visual quality. In this paper, we propose a novel video-to-video synthesis approach under the generative adversarial learning framework. Through carefully-designed generator and discriminator architectures, coupled with a spatio-temporal adversarial objective, we achieve high-resolution, photorealistic, temporally coherent video results on a diverse set of input formats including segmentation masks, sketches, and poses. Experiments on multiple benchmarks show the advantage of our method compared to strong baselines. In particular, our model is capable of synthesizing 2K resolution videos of street scenes up to 30 seconds long, which significantly advances the state-of-the-art of video synthesis. Finally, we apply our approach to future video prediction, outperforming several state-of-the-art competing systems.
Researchers from NVIDIA have introduced a novel video-to-video synthesis approach. The framework is based on conditional GANs. Specifically, the method couples carefully-designed generator and discriminator with a spatio-temporal adversarial objective. The experiments demonstrate that the suggested vid2vid approach can synthesize high-resolution, photorealistic, temporally coherent videos on a diverse set of input formats including segmentation masks, sketches, and poses. It can also predict the next frames with far superior results than the baseline models.

- current source frame;
- past two source frames;
- past two generated frames.
- Conditional image discriminator ensures that each output frame resembles a real image given the same source image.
- Conditional video discriminator ensures that consecutive output frames resemble the temporal dynamics of a real video given the same optical flow.
- Foreground-background prior in the generator design further improves the synthesis performance of the proposed model.
- Using a soft occlusion mask instead of binary allows to better handle the “zoom in” scenario: we can add details by gradually blending the warped pixels and the newly synthesized pixels.
- Generating high-resolution (2048х2048), photorealistic, temporally coherent videos up to 30 seconds long.
- Outputting several videos with different visual appearances depending on sampling different feature vectors.
- Outperforming the baseline models in future video prediction.
- Converting semantic labels into realistic real-world videos.
- Generating multiple outputs of talking people from edge maps.
- Generating an entire human body given a pose.
- “NVIDIA’s new vid2vid is the first open-source code that lets you fake anybody’s face convincingly from one source video. […] interesting times ahead…”, Gene Kogan , an artist and a programmer.
- The paper has also received some criticism over the concern that it can be used to create deepfakes or tampered videos which can deceive people.
- Using object tracking information to make sure that each object has a consistent appearance across the whole video.
- Researching if training the model with coarser semantic labels will help reduce the visible artifacts that appear after semantic manipulations (e.g., turning trees into buildings).
- Adding additional 3D cues, such as depth maps, to enable synthesis of turning cars.
- Marketing and advertising can benefit from the opportunities created by the vid2vid method (e.g., replacing the face or even the entire body in the video). However, this should be used with caution, keeping in mind the ethical considerations.
- NVIDIA team provides the original implementation of this research paper on GitHub .
9. Everybody Dance Now , by Caroline Chan, Shiry Ginosar, Tinghui Zhou, Alexei A. Efros
This paper presents a simple method for “do as I do” motion transfer: given a source video of a person dancing we can transfer that performance to a novel (amateur) target after only a few minutes of the target subject performing standard moves. We pose this problem as a per-frame image-to-image translation with spatio-temporal smoothing. Using pose detections as an intermediate representation between source and target, we learn a mapping from pose images to a target subject’s appearance. We adapt this setup for temporally coherent video generation including realistic face synthesis. Our video demo can be found at https://youtu.be/PCBTZh41Ris .
UC Berkeley researchers present a simple method for generating videos with amateur dancers performing like professional dancers. If you want to take part in the experiment, all you need to do is to record a few minutes of yourself performing some standard moves and then pick up the video with the dance you want to repeat. The neural network will do the main job: it solves the problem as a per-frame image-to-image translation with spatio-temporal smoothing. By conditioning the prediction at each frame on that of the previous time step for temporal smoothness and applying a specialized GAN for realistic face synthesis, the method achieves really amazing results.

- A pre-trained state-of-the-art pose detector creates pose stick figures from the source video.
- Global pose normalization is applied to account for differences between the source and target subjects in body shapes and locations within the frame.
- Normalized pose stick figures are mapped to the target subject.
- To make videos smooth, the researchers suggest conditioning the generator on the previously generated frame and then giving both images to the discriminator. Gaussian smoothing on the pose keypoints allows to further reduce jitter.
- To generate more realistic faces, the method includes an additional face-specific GAN that brushes up the face after the main generation is finished.
- Suggesting a novel approach to motion transfer that outperforms a strong baseline (pix2pixHD), according to both qualitative and quantitative assessments.
- Demonstrating that face-specific GAN adds considerable detail to the output video.
- “Overall I thought this was really fun and well executed. Looking forward to the code release so that I can start training my dance moves.”, Tom Brown , member of technical staff at Google Brain.
- “’Everybody Dance Now’ from Caroline Chan, Alyosha Efros and team transfers dance moves from one subject to another. The only way I’ll ever dance well. Amazing work!!!”, Soumith Chintala, AI Research Engineer at Facebook.
- Replacing pose stick figures with temporally coherent inputs and representation specifically optimized for motion transfer.
- “Do as I do” motion transfer might be applied to replace subjects when creating marketing and promotional videos.
- PyTorch implementation of this research paper is available on GitHub .
10. Large Scale GAN Training for High Fidelity Natural Image Synthesis , by Andrew Brock, Jeff Donahue, and Karen Simonyan
Despite recent progress in generative image modeling, successfully generating high-resolution, diverse samples from complex datasets such as ImageNet remains an elusive goal. To this end, we train Generative Adversarial Networks at the largest scale yet attempted, and study the instabilities specific to such scale. We find that applying orthogonal regularization to the generator renders it amenable to a simple “truncation trick”, allowing fine control over the trade-off between sample fidelity and variety by truncating the latent space. Our modifications lead to models which set the new state of the art in class-conditional image synthesis. When trained on ImageNet at 128×128 resolution, our models (BigGANs) achieve an Inception Score (IS) of 166.3 and Frechet Inception Distance (FID) of 9.6, improving over the previous best IS of 52.52 and FID of 18.65.
DeepMind team finds that current techniques are sufficient for synthesizing high-resolution, diverse images from available datasets such as ImageNet and JFT-300M. In particular, they show that Generative Adversarial Networks (GANs) can generate images that look very realistic if they are trained at the very large scale, i.e. using two to four times as many parameters and eight times the batch size compared to prior art. These large-scale GANs, or BigGANs, are the new state-of-the-art in class-conditional image synthesis.

- GANs perform much better with the increased batch size and number of parameters.
- Applying orthogonal regularization to the generator makes the model responsive to a specific technique (“truncation trick”), which provides control over the trade-off between sample fidelity and variety.
- Demonstrating that GANs can benefit significantly from scaling.
- Building models that allow explicit, fine-grained control of the trade-off between sample variety and fidelity.
- Discovering instabilities of large-scale GANs and characterizing them empirically.
- an Inception Score (IS) of 166.3 with the previous best IS of 52.52;
- Frechet Inception Distance (FID) of 9.6 with the previous best FID of 18.65.
- The paper is under review for next ICLR 2019.
- After BigGAN generators become available on TF Hub, AI researchers from all over the world are playing with BigGANs to generate dogs, watches, bikini images, Mona Lisa, seashores and many more.
- Moving to larger datasets to mitigate GAN stability issues.
- Replacing expensive manual media creation for advertising and e-commerce purposes.
- A BigGAN demo implemented in TensorFlow is available to use on Google’s Colab tool.
- Aaron Leong has a Github repository for BigGAN implemented in PyTorch .
Want Deeper Dives Into Specific AI Research Topics?
Due to popular demand, we’ve released several of these easy-to-read summaries and syntheses of major research papers for different subtopics within AI and machine learning.
- Top 10 machine learning & AI research papers of 2018
- Top 10 AI fairness, accountability, transparency, and ethics (FATE) papers of 2018
- Top 14 natural language processing (NLP) research papers of 2018
- Top 10 computer vision and image generation research papers of 2018
- Top 10 conversational AI and dialog systems research papers of 2018
- Top 10 deep reinforcement learning research papers of 2018
Update: 2019 Research Summaries Are Released
- Top 10 AI & machine learning research papers from 2019
- Top 11 NLP achievements & papers from 2019
- Top 10 research papers in conversational AI from 2019
- Top 10 computer vision research papers from 2019
- Top 12 AI ethics research papers introduced in 2019
- Top 10 reinforcement learning research papers from 2019
Enjoy this article? Sign up for more AI research updates.
We’ll let you know when we release more summary articles like this one.
- Email Address *
- Name * First Last
- Natural Language Processing (NLP)
- Chatbots & Conversational AI
- Computer Vision
- Ethics & Safety
- Machine Learning
- Deep Learning
- Reinforcement Learning
- Generative Models
- Other (Please Describe Below)
- What is your biggest challenge with AI research? *
Reader Interactions
About Mariya Yao
Mariya is the co-author of Applied AI: A Handbook For Business Leaders and former CTO at Metamaven. She "translates" arcane technical concepts into actionable business advice for executives and designs lovable products people actually want to use. Follow her on Twitter at @thinkmariya to raise your AI IQ.
March 13, 2024 at 4:32 pm
If you have a patio, deck or pool and are looking for some fun ways to resurface it, you may be wondering how to do stamped concrete over existing patio surfaces. https://www.google.com/maps/place/?cid=10866013157741552281
March 21, 2024 at 6:18 am
Yes! Finally someone writes about tote bags.
March 27, 2024 at 7:39 am
A coloured concrete driveway can be a great option if you want to add character to a plain concrete driveway. It is durable, weatherproof, and offers many different design options. https://search.google.com/local/reviews?placeid=ChIJLRrbgctL4okRbNmXXl3Lpkk
Leave a Reply
You must be logged in to post a comment.
About TOPBOTS
- Expert Contributors
- Terms of Service & Privacy Policy
- Contact TOPBOTS

Exploring 250+ Machine Learning Research Topics

In recent years, machine learning has become super popular and grown very quickly. This happened because technology got better, and there’s a lot more data available. Because of this, we’ve seen lots of new and amazing things happen in different areas. Machine learning research is what makes all these cool things possible. In this blog, we’ll talk about machine learning research topics, why they’re important, how you can pick one, what areas are popular to study, what’s new and exciting, the tough problems, and where you can find help if you want to be a researcher.
Why Does Machine Learning Research Matter?
Table of Contents
Machine learning research is at the heart of the AI revolution. It underpins the development of intelligent systems capable of making predictions, automating tasks, and improving decision-making across industries. The importance of this research can be summarized as follows:
Advancements in Technology
The growth of machine learning research has led to the development of powerful algorithms, tools, and frameworks. Numerous industries, including healthcare, banking, autonomous cars, and natural language processing, have found use for these technology.
As researchers continue to push the boundaries of what’s possible, we can expect even more transformative technologies to emerge.
Real-world Applications
Machine learning research has brought about tangible changes in our daily lives. Voice assistants like Siri and Alexa, recommendation systems on streaming platforms, and personalized healthcare diagnostics are just a few examples of how this research impacts our world.
By working on new research topics, scientists can further refine these applications and create new ones.
Economic and Industrial Impacts
The economic implications of machine learning research are substantial. Companies that harness the power of machine learning gain a competitive edge in the market.
This creates a demand for skilled machine learning researchers, driving job opportunities and contributing to economic growth.
How to Choose the Machine Learning Research Topics?
Selecting the right machine learning research topics is crucial for your success as a machine learning researcher. Here’s a guide to help you make an informed decision:
- Understanding Your Interests
Start by considering your personal interests. Machine learning is a broad field with applications in virtually every sector. By choosing a topic that aligns with your passions, you’ll stay motivated and engaged throughout your research journey.
- Reviewing Current Trends
Stay updated on the latest trends in machine learning. Attend conferences, read research papers, and engage with the community to identify emerging research topics. Current trends often lead to exciting breakthroughs.
- Identifying Gaps in Existing Research
Sometimes, the most promising research topics involve addressing gaps in existing knowledge. These gaps may become evident through your own experiences, discussions with peers, or in the course of your studies.
- Collaborating with Experts
Collaboration is key in research. Working with experts in the field can help you refine your research topic and gain valuable insights. Seek mentors and collaborators who can guide you.
250+ Machine Learning Research Topics: Category-wise
Supervised learning.
- Explainable AI for Decision Support
- Few-shot Learning Methods
- Time Series Forecasting with Deep Learning
- Handling Imbalanced Datasets in Classification
- Regression Techniques for Non-linear Data
- Transfer Learning in Supervised Settings
- Multi-label Classification Strategies
- Semi-Supervised Learning Approaches
- Novel Feature Selection Methods
- Anomaly Detection in Supervised Scenarios
- Federated Learning for Distributed Supervised Models
- Ensemble Learning for Improved Accuracy
- Automated Hyperparameter Tuning
- Ethical Implications in Supervised Models
- Interpretability of Deep Neural Networks.
Unsupervised Learning
- Unsupervised Clustering of High-dimensional Data
- Semi-Supervised Clustering Approaches
- Density Estimation in Unsupervised Learning
- Anomaly Detection in Unsupervised Settings
- Transfer Learning for Unsupervised Tasks
- Representation Learning in Unsupervised Learning
- Outlier Detection Techniques
- Generative Models for Data Synthesis
- Manifold Learning in High-dimensional Spaces
- Unsupervised Feature Selection
- Privacy-Preserving Unsupervised Learning
- Community Detection in Complex Networks
- Clustering Interpretability and Visualization
- Unsupervised Learning for Image Segmentation
- Autoencoders for Dimensionality Reduction.
Reinforcement Learning
- Deep Reinforcement Learning in Real-world Applications
- Safe Reinforcement Learning for Autonomous Systems
- Transfer Learning in Reinforcement Learning
- Imitation Learning and Apprenticeship Learning
- Multi-agent Reinforcement Learning
- Explainable Reinforcement Learning Policies
- Hierarchical Reinforcement Learning
- Model-based Reinforcement Learning
- Curriculum Learning in Reinforcement Learning
- Reinforcement Learning in Robotics
- Exploration vs. Exploitation Strategies
- Reward Function Design and Ethical Considerations
- Reinforcement Learning in Healthcare
- Continuous Action Spaces in RL
- Reinforcement Learning for Resource Management.
Natural Language Processing (NLP)
- Multilingual and Cross-lingual NLP
- Contextualized Word Embeddings
- Bias Detection and Mitigation in NLP
- Named Entity Recognition for Low-resource Languages
- Sentiment Analysis in Social Media Text
- Dialogue Systems for Improved Customer Service
- Text Summarization for News Articles
- Low-resource Machine Translation
- Explainable NLP Models
- Coreference Resolution in NLP
- Question Answering in Specific Domains
- Detecting Fake News and Misinformation
- NLP for Healthcare: Clinical Document Understanding
- Emotion Analysis in Text
- Text Generation with Controlled Attributes.
Computer Vision
- Video Action Recognition and Event Detection
- Object Detection in Challenging Conditions (e.g., low light)
- Explainable Computer Vision Models
- Image Captioning for Accessibility
- Large-scale Image Retrieval
- Domain Adaptation in Computer Vision
- Fine-grained Image Classification
- Facial Expression Recognition
- Visual Question Answering
- Self-supervised Learning for Visual Representations
- Weakly Supervised Object Localization
- Human Pose Estimation in 3D
- Scene Understanding in Autonomous Vehicles
- Image Super-resolution
- Gaze Estimation for Human-Computer Interaction.
Deep Learning
- Neural Architecture Search for Efficient Models
- Self-attention Mechanisms and Transformers
- Interpretability in Deep Learning Models
- Robustness of Deep Neural Networks
- Generative Adversarial Networks (GANs) for Data Augmentation
- Neural Style Transfer in Art and Design
- Adversarial Attacks and Defenses
- Neural Networks for Audio and Speech Processing
- Explainable AI for Healthcare Diagnosis
- Automated Machine Learning (AutoML)
- Reinforcement Learning with Deep Neural Networks
- Model Compression and Quantization
- Lifelong Learning with Deep Learning Models
- Multimodal Learning with Vision and Language
- Federated Learning for Privacy-preserving Deep Learning.
Explainable AI
- Visualizing Model Decision Boundaries
- Saliency Maps and Feature Attribution
- Rule-based Explanations for Black-box Models
- Contrastive Explanations for Model Interpretability
- Counterfactual Explanations and What-if Analysis
- Human-centered AI for Explainable Healthcare
- Ethics and Fairness in Explainable AI
- Explanation Generation for Natural Language Processing
- Explainable AI in Financial Risk Assessment
- User-friendly Interfaces for Model Interpretability
- Scalability and Efficiency in Explainable Models
- Hybrid Models for Combined Accuracy and Explainability
- Post-hoc vs. Intrinsic Explanations
- Evaluation Metrics for Explanation Quality
- Explainable AI for Autonomous Vehicles.
Transfer Learning
- Zero-shot Learning and Few-shot Learning
- Cross-domain Transfer Learning
- Domain Adaptation for Improved Generalization
- Multilingual Transfer Learning in NLP
- Pretraining and Fine-tuning Techniques
- Lifelong Learning and Continual Learning
- Domain-specific Transfer Learning Applications
- Model Distillation for Knowledge Transfer
- Contrastive Learning for Transfer Learning
- Self-training and Pseudo-labeling
- Dynamic Adaption of Pretrained Models
- Privacy-Preserving Transfer Learning
- Unsupervised Domain Adaptation
- Negative Transfer Avoidance in Transfer Learning.
Federated Learning
- Secure Aggregation in Federated Learning
- Communication-efficient Federated Learning
- Privacy-preserving Techniques in Federated Learning
- Federated Transfer Learning
- Heterogeneous Federated Learning
- Real-world Applications of Federated Learning
- Federated Learning for Edge Devices
- Federated Learning for Healthcare Data
- Differential Privacy in Federated Learning
- Byzantine-robust Federated Learning
- Federated Learning with Non-IID Data
- Model Selection in Federated Learning
- Scalable Federated Learning for Large Datasets
- Client Selection and Sampling Strategies
- Global Model Update Synchronization in Federated Learning.
Quantum Machine Learning
- Quantum Neural Networks and Quantum Circuit Learning
- Quantum-enhanced Optimization for Machine Learning
- Quantum Data Compression and Quantum Principal Component Analysis
- Quantum Kernels and Quantum Feature Maps
- Quantum Variational Autoencoders
- Quantum Transfer Learning
- Quantum-inspired Classical Algorithms for ML
- Hybrid Quantum-Classical Models
- Quantum Machine Learning on Near-term Quantum Devices
- Quantum-inspired Reinforcement Learning
- Quantum Computing for Quantum Chemistry and Drug Discovery
- Quantum Machine Learning for Finance
- Quantum Data Structures and Quantum Databases
- Quantum-enhanced Cryptography in Machine Learning
- Quantum Generative Models and Quantum GANs.
Ethical AI and Bias Mitigation
- Fairness-aware Machine Learning Algorithms
- Bias Detection and Mitigation in Real-world Data
- Explainable AI for Ethical Decision Support
- Algorithmic Accountability and Transparency
- Privacy-preserving AI and Data Governance
- Ethical Considerations in AI for Healthcare
- Fairness in Recommender Systems
- Bias and Fairness in NLP Models
- Auditing AI Systems for Bias
- Societal Implications of AI in Criminal Justice
- Ethical AI Education and Training
- Bias Mitigation in Autonomous Vehicles
- Fair AI in Financial and Hiring Decisions
- Case Studies in Ethical AI Failures
- Legal and Policy Frameworks for Ethical AI.
Meta-Learning and AutoML
- Neural Architecture Search (NAS) for Efficient Models
- Transfer Learning in NAS
- Reinforcement Learning for NAS
- Multi-objective NAS
- Automated Data Augmentation
- Neural Architecture Optimization for Edge Devices
- Bayesian Optimization for AutoML
- Model Compression and Quantization in AutoML
- AutoML for Federated Learning
- AutoML in Healthcare Diagnostics
- Explainable AutoML
- Cost-sensitive Learning in AutoML
- AutoML for Small Data
- Human-in-the-Loop AutoML.
AI for Healthcare and Medicine
- Disease Prediction and Early Diagnosis
- Medical Image Analysis with Deep Learning
- Drug Discovery and Molecular Modeling
- Electronic Health Record Analysis
- Predictive Analytics in Healthcare
- Personalized Treatment Planning
- Healthcare Fraud Detection
- Telemedicine and Remote Patient Monitoring
- AI in Radiology and Pathology
- AI in Drug Repurposing
- AI for Medical Robotics and Surgery
- Genomic Data Analysis
- AI-powered Mental Health Assessment
- Explainable AI in Healthcare Decision Support
- AI in Epidemiology and Outbreak Prediction.
AI in Finance and Investment
- Algorithmic Trading and High-frequency Trading
- Credit Scoring and Risk Assessment
- Fraud Detection and Anti-money Laundering
- Portfolio Optimization with AI
- Financial Market Prediction
- Sentiment Analysis in Financial News
- Explainable AI in Financial Decision-making
- Algorithmic Pricing and Dynamic Pricing Strategies
- AI in Cryptocurrency and Blockchain
- Customer Behavior Analysis in Banking
- Explainable AI in Credit Decisioning
- AI in Regulatory Compliance
- Ethical AI in Financial Services
- AI for Real Estate Investment
- Automated Financial Reporting.
AI in Climate Change and Sustainability
- Climate Modeling and Prediction
- Renewable Energy Forecasting
- Smart Grid Optimization
- Energy Consumption Forecasting
- Carbon Emission Reduction with AI
- Ecosystem Monitoring and Preservation
- Precision Agriculture with AI
- AI for Wildlife Conservation
- Natural Disaster Prediction and Management
- Water Resource Management with AI
- Sustainable Transportation and Urban Planning
- Climate Change Mitigation Strategies with AI
- Environmental Impact Assessment with Machine Learning
- Eco-friendly Supply Chain Optimization
- Ethical AI in Climate-related Decision Support.
Data Privacy and Security
- Differential Privacy Mechanisms
- Federated Learning for Privacy-preserving AI
- Secure Multi-Party Computation
- Privacy-enhancing Technologies in Machine Learning
- Homomorphic Encryption for Machine Learning
- Ethical Considerations in Data Privacy
- Privacy-preserving AI in Healthcare
- AI for Secure Authentication and Access Control
- Blockchain and AI for Data Security
- Explainable Privacy in Machine Learning
- Privacy-preserving AI in Government and Public Services
- Privacy-compliant AI for IoT and Edge Devices
- Secure AI Models Sharing and Deployment
- Privacy-preserving AI in Financial Transactions
- AI in the Legal Frameworks of Data Privacy.
Global Collaboration in Research
- International Research Partnerships and Collaboration Models
- Multilingual and Cross-cultural AI Research
- Addressing Global Healthcare Challenges with AI
- Ethical Considerations in International AI Collaborations
- Interdisciplinary AI Research in Global Challenges
- AI Ethics and Human Rights in Global Research
- Data Sharing and Data Access in Global AI Research
- Cross-border Research Regulations and Compliance
- AI Innovation Hubs and International Research Centers
- AI Education and Training for Global Communities
- Humanitarian AI and AI for Sustainable Development Goals
- AI for Cultural Preservation and Heritage Protection
- Collaboration in AI-related Global Crises
- AI in Cross-cultural Communication and Understanding
- Global AI for Environmental Sustainability and Conservation.
Emerging Trends and Hot Topics in Machine Learning Research
The landscape of machine learning research topics is constantly evolving. Here are some of the emerging trends and hot topics that are shaping the field:
As AI systems become more prevalent, addressing ethical concerns and mitigating bias in algorithms are critical research areas.
Interpretable and Explainable Models
Understanding why machine learning models make specific decisions is crucial for their adoption in sensitive areas, such as healthcare and finance.
Meta-learning algorithms are designed to enable machines to learn how to learn, while AutoML aims to automate the machine learning process itself.
Machine learning is revolutionizing the healthcare sector, from diagnostic tools to drug discovery and patient care.
Algorithmic trading, risk assessment, and fraud detection are just a few applications of AI in finance, creating a wealth of research opportunities.
Machine learning research is crucial in analyzing and mitigating the impacts of climate change and promoting sustainable practices.
Challenges and Future Directions
While machine learning research has made tremendous strides, it also faces several challenges:
- Data Privacy and Security: As machine learning models require vast amounts of data, protecting individual privacy and data security are paramount concerns.
- Scalability and Efficiency: Developing efficient algorithms that can handle increasingly large datasets and complex computations remains a challenge.
- Ensuring Fairness and Transparency: Addressing bias in machine learning models and making their decisions transparent is essential for equitable AI systems.
- Quantum Computing and Machine Learning: The integration of quantum computing and machine learning has the potential to revolutionize the field, but it also presents unique challenges.
- Global Collaboration in Research: Machine learning research benefits from collaboration on a global scale. Ensuring that researchers from diverse backgrounds work together is vital for progress.
Resources for Machine Learning Researchers
If you’re looking to embark on a journey in machine learning research topics, there are various resources at your disposal:
- Journals and Conferences
Journals such as the “Journal of Machine Learning Research” and conferences like NeurIPS and ICML provide a platform for publishing and discussing research findings.
- Online Communities and Forums
Platforms like Stack Overflow, GitHub, and dedicated forums for machine learning provide spaces for collaboration and problem-solving.
- Datasets and Tools
Open-source datasets and tools like TensorFlow and PyTorch simplify the research process by providing access to data and pre-built models.
- Research Grants and Funding Opportunities
Many organizations and government agencies offer research grants and funding for machine learning projects. Seek out these opportunities to support your research.
Machine learning research is like a superhero in the world of technology. To be a part of this exciting journey, it’s important to choose the right machine learning research topics and keep up with the latest trends.
Machine learning research makes our lives better. It powers things like smart assistants and life-saving medical tools. It’s like the force driving the future of technology and society.
But, there are challenges too. We need to work together and be ethical in our research. Everyone should benefit from this technology. The future of machine learning research is incredibly bright. If you want to be a part of it, get ready for an exciting adventure. You can help create new solutions and make a big impact on the world.
Related Posts

Tips on How To Tackle A Machine Learning Project As A Beginner
Here in this blog, CodeAvail experts will explain to you tips on how to tackle a machine learning project as a beginner step by step…

Artificial Intelligence and Machine Learning Basics for Beginners
Here in this blog, CodeAvail experts will explain to you Artificial Intelligence and Machine Learning basics for beginners in detail step by step. What is…

Kindson The Genius
Providing the best learning experience for professionals
10 Machine Learning Project (Thesis) Topics for 2020
Are you looking for some interesting project ideas for your thesis, project or dissertation? Then be sure that a machine learning topic would be a very good topic to write on. I have outlined 10 different topics. These topics are really good because you can easily obtain the dataset (i will provide the link to the dataset) and you can as well get some support from me. Let me know if you need any support in preparing your thesis.
You can leave a comment below in the comment area.

1. Machine Learning Model for Classification and Detection of Breast Cancer (Classification)
The data is provided by the Oncology department and details instances and related attributes which are nine in all.
You can obtain the dataset from here
2. Intelligent Internet Ads Generation (Classification)
This is one of the most interesting topics for me. The reason is because the revenue generated or expended by ads campaign depends not just on the volume of the ads, but also on the relevance of the ads. Therefore it is possible to increase revenue and reduce spending by developing a Machine Learning model that select relevants ads with a high level of accuracy. The dataset provides a collection of ads as well as the structure and geometry of the ads.
Get the ads dataset from here
3. Feature Extraction for National Census Data (Clustering)
This looks like big data stuff. But no! It’s simply dataset you can use for analysis. It is the actual data obtained from the US census in 1990. There are 68 attributes for each of the records and clustering would be performed to identify trends in the data.
You can obtain census the dataset from here
4. Movie Outcome Prediction (Classification)
This is quite a tasking project but its quite interesting. Before now, there exists models to predict the ratings of movies on a scale of 0 to 10 or 1 to 5. But this takes it a step further. You actually need to determine the outcome of the movie. The data set is a large multivariate dataset of movie director, cast, individual roles of the actor, remarks, studio and relevant documents.
You can get the movies dataset from here
5. Forest Fire Area Coverage Prediction (Regression)
This project have been classified as difficult but I don’t think so. The objective to predict the the area affected by forest fires. Dataset include relevant meteological information and other parameters taken from a region of Portugal.
You can get the fire dataset from here
6. Atmospheric Ozone Level Analysis and Detection (Clustering)
Two ground ozone datasets are provided for this. Data includes temperatures at various times of the day as well as wind speed. The data included in the dataset was collected in a span of 6 years from 1998 to 2004.
You can get the Ozone dataset from here
7. Crime Prediction in New York City (Regression)
If you have watched the movie, ‘Person of Interest’ directed by Jonathan Nolan, then you will appreciate the fact that there is a possibility of predicting violent criminal activities before they actually occur. Dataset would contain historical data on crime rate, types of crimes occurrence per region.
You can get the crime dataset from here
8. Sentiment Analysis on Amazon ECommerce User Reviews (Classification)
The dataset for this project is derived from user review comments from Amazon users. The model should be able to perform analysis on the training dataset and come up with a model that classifies the reviews based on sentiments. Granularity can be improved by generating predictions based on location and other factors.
You can get the reviews dataset from here
9. Home Eletrical Power Consumption Analysis (Regression)
Everyone uses electricity at home. Or rather, almost everyone! Would is not be great to have a system that helps to predict electricity consumption. Training dataset provided for this project includes feature set such as the size of the home, duration and more
You can get the dataset from here
10. Predictive Modelling of Individual Human Knowledge (Classification and Clustering)
Here the available dataset provide a collection of data about an individual on a subject matter. You are required to create a model that would try to quantify the amount of knowledge the individual have on the given subject. You can be creating by trying to also infer the performance of the user on certain exams.
I hope these 10 Machine Learning Project topic would be helpful to you.
Thanks for reading and do leave a comment below if you need some support
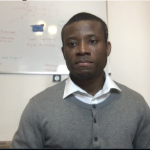
kindsonthegenius
Kindson Munonye is currently completing his doctoral program in Software Engineering in Budapest University of Technology and Economics
You might also like
Machine learning 101 – equation for a line and regression line, simple linear regression in machine learning (a simple tutorial), pca tutorial 1 – introduction to pca and dimensionality reduction, 2 thoughts on “ 10 machine learning project (thesis) topics for 2020 ”.
Is there any suggestion related to educational data mining?
I’m working on this. You can subscribe to my channel so when I make the update, you can get notified https://www.youtube.com/channel/UCvHgEAcw6VpcOA3864pSr5A
Leave a Reply Cancel reply
Your email address will not be published. Required fields are marked *
Save my name, email, and website in this browser for the next time I comment.
- Bibliography
- More Referencing guides Blog Automated transliteration Relevant bibliographies by topics
- Automated transliteration
- Relevant bibliographies by topics
- Referencing guides
Dissertations / Theses on the topic 'Machine vision; Computer'
Create a spot-on reference in apa, mla, chicago, harvard, and other styles.
Consult the top 50 dissertations / theses for your research on the topic 'Machine vision; Computer.'
Next to every source in the list of references, there is an 'Add to bibliography' button. Press on it, and we will generate automatically the bibliographic reference to the chosen work in the citation style you need: APA, MLA, Harvard, Chicago, Vancouver, etc.
You can also download the full text of the academic publication as pdf and read online its abstract whenever available in the metadata.
Browse dissertations / theses on a wide variety of disciplines and organise your bibliography correctly.
Luckman, Adrian John. "Active perception in machine vision." Thesis, University of York, 1991. http://ethos.bl.uk/OrderDetails.do?uin=uk.bl.ethos.280521.
Tsitiridis, Aristeidis. "Biologically-inspired machine vision." Thesis, Cranfield University, 2013. http://dspace.lib.cranfield.ac.uk/handle/1826/8029.
Park, Allen S. M. (Allen S. ). Massachusetts Institute of Technology. "Machine-vision assisted 3D printing." Thesis, Massachusetts Institute of Technology, 2016. http://hdl.handle.net/1721.1/113162.
Chen, Zhilu. "Computer Vision and Machine Learning for Autonomous Vehicles." Digital WPI, 2017. https://digitalcommons.wpi.edu/etd-dissertations/488.
Öberg, Filip. "Football analysis using machine learning and computer vision." Thesis, Luleå tekniska universitet, Institutionen för system- och rymdteknik, 2021. http://urn.kb.se/resolve?urn=urn:nbn:se:ltu:diva-85276.
Yang, Chen. "Machine Learning and Computer Vision for PCB Verification." Thesis, KTH, Skolan för elektroteknik och datavetenskap (EECS), 2020. http://urn.kb.se/resolve?urn=urn:nbn:se:kth:diva-290370.
Burns, James Ian. "Agricultural Crop Monitoring with Computer Vision." Thesis, Virginia Tech, 2014. http://hdl.handle.net/10919/52563.
Arteta, Carlos Federico. "Computer vision and machine learning for microscopy image analysis." Thesis, University of Oxford, 2015. https://ora.ox.ac.uk/objects/uuid:62a03c45-2616-49a4-8976-fb1ff481915f.
Landecker, Will. "Interpretable Machine Learning and Sparse Coding for Computer Vision." PDXScholar, 2014. https://pdxscholar.library.pdx.edu/open_access_etds/1937.
Tock, David. "FindFace : finding facial features by computer." Thesis, University of Aberdeen, 1992. http://ethos.bl.uk/OrderDetails.do?uin=uk.bl.ethos.314649.
Lim, Choon Kee. "Hypercube machine implementation of low-level vision algorithms." Ohio : Ohio University, 1989. http://www.ohiolink.edu/etd/view.cgi?ohiou1182864143.
Berry, David T. "A knowledge-based framework for machine vision." Thesis, Heriot-Watt University, 1987. http://hdl.handle.net/10399/1022.
Priestnall, Gary. "Machine recognition of engineering drawings." Thesis, University of Nottingham, 1994. http://ethos.bl.uk/OrderDetails.do?uin=uk.bl.ethos.283606.
Brown, Gary. "An object oriented model of machine vision." Thesis, Kingston University, 1997. http://eprints.kingston.ac.uk/20614/.
Mairal, Julien. "Sparse coding for machine learning, image processing and computer vision." Phd thesis, École normale supérieure de Cachan - ENS Cachan, 2010. http://tel.archives-ouvertes.fr/tel-00595312.
Jonsson, Erik. "Channel-Coded Feature Maps for Computer Vision and Machine Learning." Doctoral thesis, Linköping : Department of Electrical Engineering, Linköpings universitet, 2008. http://urn.kb.se/resolve?urn=urn:nbn:se:liu:diva-11040.
Rognvaldsson, Magnus Haukur. "Machine vision approach for visual servo controlled robotics." Thesis, Georgia Institute of Technology, 1996. http://hdl.handle.net/1853/25111.
Forsyth, D. A. "Colour constancy and its applications in machine vision." Thesis, University of Oxford, 1988. http://ethos.bl.uk/OrderDetails.do?uin=uk.bl.ethos.670357.
Lai, Bing-Chang. "Combining generic programming with vector processing for machine vision." Access electronically, 2005. http://www.library.uow.edu.au/adt-NWU/public/adt-NWU20060221.095043/index.html.
Cho, Tai-Hoon. "A knowledge-based machine vision system for automated industrial web inspection." Diss., This resource online, 1991. http://scholar.lib.vt.edu/theses/available/etd-07282008-134615/.
Folsom, Tyler C. "Neural networks modeling cortical cells for machine vision /." Thesis, Connect to this title online; UW restricted, 1994. http://hdl.handle.net/1773/6135.
Stoddart, Evan. "Computer Vision Techniques for Automotive Perception Systems." The Ohio State University, 2019. http://rave.ohiolink.edu/etdc/view?acc_num=osu1555357244145006.
Zarifi, Assad Allah. "Integrated inpection of sculptured surface products using machine vision and a coordinate measuring machine." Thesis, Loughborough University, 1996. https://dspace.lboro.ac.uk/2134/22084.
Kendall, Alex Guy. "Geometry and uncertainty in deep learning for computer vision." Thesis, University of Cambridge, 2019. https://www.repository.cam.ac.uk/handle/1810/287944.
Lyons, Laura Christine. "An investigation of systematic errors in machine vision hardware." Thesis, Georgia Institute of Technology, 1989. http://hdl.handle.net/1853/16759.
Billings, Rachel Mae. "On Efficient Computer Vision Applications for Neural Networks." Thesis, Virginia Tech, 2021. http://hdl.handle.net/10919/102957.
Borngrund, Carl. "Machine vision for automation of earth-moving machines : Transfer learning experiments with YOLOv3." Thesis, Luleå tekniska universitet, Institutionen för system- och rymdteknik, 2019. http://urn.kb.se/resolve?urn=urn:nbn:se:ltu:diva-75169.
Cheng, Kelvin. "0Direct interaction with large displays through monocular computer vision." Connect to full text, 2008. http://ses.library.usyd.edu.au/handle/2123/5331.
Volcy, Jerry. "Optimum illumination for machine vision using optical scatter data." Diss., Georgia Institute of Technology, 1996. http://hdl.handle.net/1853/17548.
Lorusso, Anthony Nicholas. "Construction of a machine vision system for autonomous applications." Thesis, Massachusetts Institute of Technology, 1996. http://hdl.handle.net/1721.1/40174.
Fang, Yajun Ph D. Massachusetts Institute of Technology. "Fusion-layer-based machine vision for intelligent transportation systems/." Thesis, Massachusetts Institute of Technology, 2010. http://hdl.handle.net/1721.1/60143.
La, Alex W. "Eigenblades: Application of Computer Vision and Machine Learning for Mode Shape Identification." BYU ScholarsArchive, 2017. https://scholarsarchive.byu.edu/etd/7228.
Yu, Haiyue. "Quantitative analysis of TMA images using computer vision and machine learning approaches." Thesis, University of Oxford, 2016. https://ora.ox.ac.uk/objects/uuid:e4cc472e-8c01-4121-b044-f3b4b19a8742.
Kulkarni, Amruta Kiran. "Classification of Faults in Railway Ties Using Computer Vision and Machine Learning." Thesis, Virginia Tech, 2017. http://hdl.handle.net/10919/86522.
Edwards, John M. "A reference architecture for flexibly integrating machine vision within manufacturing." Thesis, Loughborough University, 1993. https://dspace.lboro.ac.uk/2134/10322.
Andrews, Michael J. "An Information Theoretic Hierarchical Classifier for Machine Vision." Digital WPI, 1999. https://digitalcommons.wpi.edu/etd-theses/807.
Luwes, Nicolaas Johannes. "Artificial intelligence machine vision grading system." Thesis, Bloemfontein : Central University of Technology, Free State, 2014. http://hdl.handle.net/11462/35.
Beriat, Pelin. "Non-destructive Testing Of Textured Foods By Machine Vision." Master's thesis, METU, 2009. http://etd.lib.metu.edu.tr/upload/12610405/index.pdf.
Arthur, Richard B. "Vision-Based Human Directed Robot Guidance." Diss., CLICK HERE for online access, 2004. http://contentdm.lib.byu.edu/ETD/image/etd564.pdf.
MacLaren, Ian J. H. (Ian James Henry) Carleton University Dissertation Information and Systems Science. "Machine identification of facial images." Ottawa, 1989.
Alnestig, Henrik. "On the Feasibility of Low Cost Computer Vision : Building and Testing SimpleEye." Thesis, Mälardalens högskola, Akademin för innovation, design och teknik, 2014. http://urn.kb.se/resolve?urn=urn:nbn:se:mdh:diva-29363.
Shelley, Anthony N. "MONITORING DAIRY COW FEED INTAKE USING MACHINE VISION." UKnowledge, 2013. http://uknowledge.uky.edu/ece_etds/24.
Harekoppa, Pooja Puttaswamygowda. "Application of Computer Vision Techniques for Railroad Inspection using UAVs." Thesis, Virginia Tech, 2016. http://hdl.handle.net/10919/72273.
Bari, Farooq. "A machine vision system for classifying rectangular cabinet frames." Thesis, This resource online, 1994. http://scholar.lib.vt.edu/theses/available/etd-12042009-020159/.
Papaioannou, Athanasios. "Component analysis of complex-valued data for machine learning and computer vision tasks." Thesis, Imperial College London, 2017. http://hdl.handle.net/10044/1/49235.
Vendra, Soujanya. "Addressing corner detection issues for machine vision based UAV aerial refueling." Morgantown, W. Va. : [West Virginia University Libraries], 2006. https://eidr.wvu.edu/etd/documentdata.eTD?documentid=4551.
Miller, Erik G. (Erik Gundersen). "Learning from one example in machine vision by sharing probability densities." Thesis, Massachusetts Institute of Technology, 2002. http://hdl.handle.net/1721.1/29902.
Kulkarni, Sanjeev R. (Sanjeev Ramesh). "Problems of computational and informational complexity in machine vision and learning." Thesis, Massachusetts Institute of Technology, 1991. http://hdl.handle.net/1721.1/13878.
Netherwood, Paul. "Parallel machine vision for the inspection of surface mount electronic assemblies." Thesis, Kingston University, 1993. http://eprints.kingston.ac.uk/20569/.
Annavarjula, Vaishnavi. "Computer-Vision Based Retinal Image Analysis for Diagnosis and Treatment." Thesis, Blekinge Tekniska Högskola, Institutionen för datalogi och datorsystemteknik, 2017. http://urn.kb.se/resolve?urn=urn:nbn:se:bth-14979.

"The impact of cultural..."
Rebecca Geach

How It Works
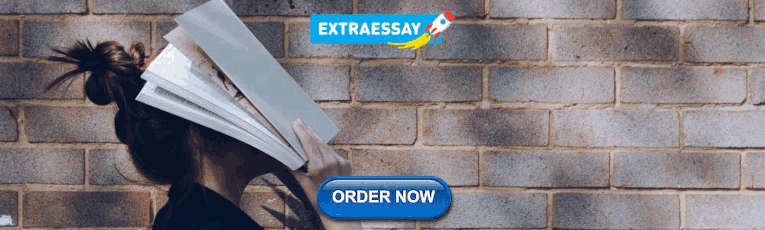
IMAGES
VIDEO
COMMENTS
Each thesis idea includes an introduction, which presents a brief overview of the topic and the research objectives. The ideas provided are related to different areas of machine learning and deep learning, such as computer vision, natural language processing, robotics, finance, drug discovery, and more.
A list of completed theses and new thesis topics from the Computer Vision Group. Are you about to start a BSc or MSc thesis? Please read our instructions for preparing and delivering your work. PhD Theses Master Theses Bachelor Theses Thesis Topics. Novel Techniques for Robust and Generalizable Machine Learning. PDF Abstract.
Selecting and working on a thesis topic in machine learning is not an easy task as machine learning uses statistical algorithms to make computers work in a certain way without being explicitly programmed. Achieving mastery over machine learning (ML) is becoming increasingly crucial for all the students in this field. ... Machine Vision Using ...
The thesis discusses a system named Myriad, a distributed computing framework for Machine Vision applications. Myriad is composed components, such as image processing engines and equipment controllers, which behave as enhanced web servers and communicate using simple HTTP requests.
widely used in machine vision problems. Today, the use of deep machine learning is a priority in the problems of classification and tracking, which is confirmed by the results of competitions at Kaggle (www.kaggle.com) and Image.net. The most popular neural network used in classification tasks is the convolutional neural network (CNN).
Machine Vision system, having to process images in real-time, while maintaining accuracy and precision. The objectives of this thesis are as follows: (1) Explore the variety of options when building a Machine Vision system, and (2) Detail how a Machine Vision system can be built and integrated into an existing quality control process using LabView.
How to Contact Faculty for IW/Thesis Advising. Send the professor an e-mail. When you write a professor, be clear that you want a meeting regarding a senior thesis or one-on-one IW project, and briefly describe the topic or idea that you want to work on. ... Computer Vision, Machine Learning. Independent Research Topics: 3D Vision; Object ...
The research topics can also be categorized by the equipment or techniques used, for example, image processing, computer vision, machine learning, and localization. This dissertation primarily reports on computer vision and machine learning algorithms and their implementations for autonomous vehicles.
That is, this thesis is about a different way of implementing machine vision systems. The work could be applied to prototype and in some cases implement machine vision systems in industrial ...
We usually have a couple of open Bachelor and Master thesis projects available here. Our websites should give you an impression about possible thesis topics, we can propose a project based on your preferences. If you already have an idea about a project we are happy to discuss that as well. In either case please send an email to d2-application ...
Research Areas Research Areas Our research group is working on a range of topics in Computer Vision and Image Processing, many of which are using Artifical Intelligence. Computer Vision is about interpreting images. More specifically the goal is to infer properties of the observed world from an image or a collection of images. Our work combines a range of mathematical domains including ...
Our research combines computer vision, computer graphics, and machine learning to understand images and video data. In our research, we focus on the combination of deep learning with strong models or physical constraints in order to combine the advantages of model-based and data-driven methods.
Machine learning is the part of artificial intelligence that provides solutions to the various medical imaging applications such as computer-aided diagnosis, lesion segmentation, medical image analysis, image-guided therapy, annotation and retrieval with 2D, 3D, and 4D data. Hence various machine learning techniques used in medical imaging is ...
This dissertation explores three topics related to random forests: tree aggregation, variable importance, and robustness. 10. Climate Data Computing: Optimal Interpolation, Averaging, Visualization and Delivery. This dissertation solves two important problems in the modern analysis of big climate data.
Machine Vision Thesis Topics - Free download as PDF File (.pdf), Text File (.txt) or read online for free. Scribd is the world's largest social reading and publishing site.
2. Adversarial Examples that Fool both Computer Vision and Time-Limited Humans, by Gamaleldin F. Elsayed, Shreya Shankar, Brian Cheung, Nicolas Papernot, Alex Kurakin, Ian Goodfellow, Jascha Sohl-Dickstein Original Abstract. Machine learning models are vulnerable to adversarial examples: small changes to images can cause computer vision models to make mistakes such as identifying a school bus ...
List of dissertations / theses on the topic 'Machine vision; Industrial inspection'. Scholarly publications with full text pdf download. Related research topic ideas.
Your thesis could be based on UI and computer vision as they really are changing the land scape and help an open source project in the process. We also want to add image homography and feature tracking to the next release (1.3). We have quick release cycles as well (about every 3 months).
Machine learning research is at the heart of the AI revolution. It underpins the development of intelligent systems capable of making predictions, automating tasks, and improving decision-making across industries. The importance of this research can be summarized as follows: Advancements in Technology.
2. Intelligent Internet Ads Generation (Classification) This is one of the most interesting topics for me. The reason is because the revenue generated or expended by ads campaign depends not just on the volume of the ads, but also on the relevance of the ads. Therefore it is possible to increase revenue and reduce spending by developing a ...
Computer Vision is the scientific subfield of AI concerned with developing algorithms to extract meaningful information from raw images, videos, and sensor data. This community is home to the academics and engineers both advancing and applying this interdisciplinary field, with backgrounds in computer science, machine learning, robotics ...
Dissertations / Theses on the topic 'Machine vision; Computer' To see the other types of publications on this topic, follow the link: Machine vision; Computer. Author: Grafiati. Published: 4 June 2021 Last updated: 2 February 2022 Create a spot-on reference in APA, MLA, Chicago, Harvard, and other styles ...
Machine Vision Thesis Topics: 100% Success rate Show More. 385 . Customer Reviews. Ask the experts to write an essay for me! Our writers will be by your side throughout the entire process of essay writing. After you have made the payment, the essay writer for me will take over 'my assignment' and start working on it, with commitment.