
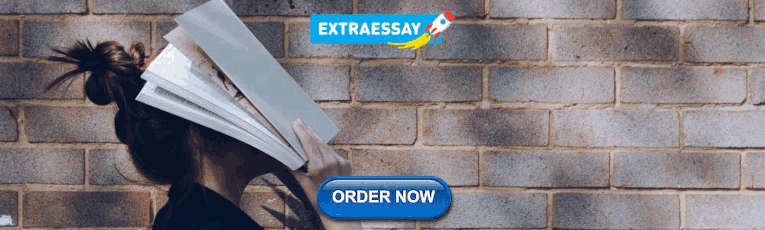
How To Write The Results/Findings Chapter
For quantitative studies (dissertations & theses).
By: Derek Jansen (MBA). Expert Reviewed By: Kerryn Warren (PhD) | July 2021
So, you’ve completed your quantitative data analysis and it’s time to report on your findings. But where do you start? In this post, we’ll walk you through the results chapter (also called the findings or analysis chapter), step by step, so that you can craft this section of your dissertation or thesis with confidence. If you’re looking for information regarding the results chapter for qualitative studies, you can find that here .
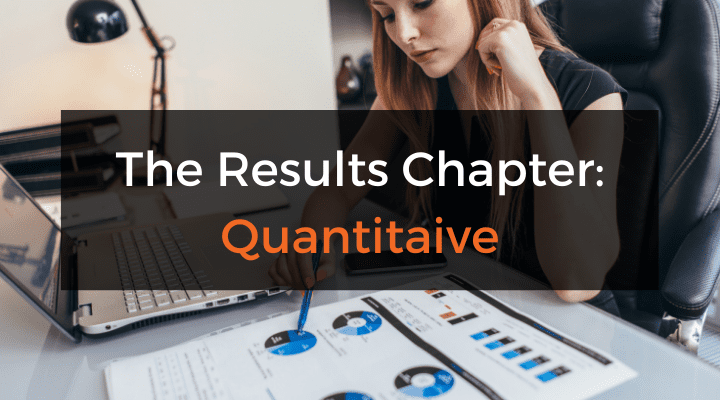
Overview: Quantitative Results Chapter
- What exactly the results/findings/analysis chapter is
- What you need to include in your results chapter
- How to structure your results chapter
- A few tips and tricks for writing top-notch chapter
What exactly is the results chapter?
The results chapter (also referred to as the findings or analysis chapter) is one of the most important chapters of your dissertation or thesis because it shows the reader what you’ve found in terms of the quantitative data you’ve collected. It presents the data using a clear text narrative, supported by tables, graphs and charts. In doing so, it also highlights any potential issues (such as outliers or unusual findings) you’ve come across.
But how’s that different from the discussion chapter?
Well, in the results chapter, you only present your statistical findings. Only the numbers, so to speak – no more, no less. Contrasted to this, in the discussion chapter , you interpret your findings and link them to prior research (i.e. your literature review), as well as your research objectives and research questions . In other words, the results chapter presents and describes the data, while the discussion chapter interprets the data.
Let’s look at an example.
In your results chapter, you may have a plot that shows how respondents to a survey responded: the numbers of respondents per category, for instance. You may also state whether this supports a hypothesis by using a p-value from a statistical test. But it is only in the discussion chapter where you will say why this is relevant or how it compares with the literature or the broader picture. So, in your results chapter, make sure that you don’t present anything other than the hard facts – this is not the place for subjectivity.
It’s worth mentioning that some universities prefer you to combine the results and discussion chapters. Even so, it is good practice to separate the results and discussion elements within the chapter, as this ensures your findings are fully described. Typically, though, the results and discussion chapters are split up in quantitative studies. If you’re unsure, chat with your research supervisor or chair to find out what their preference is.
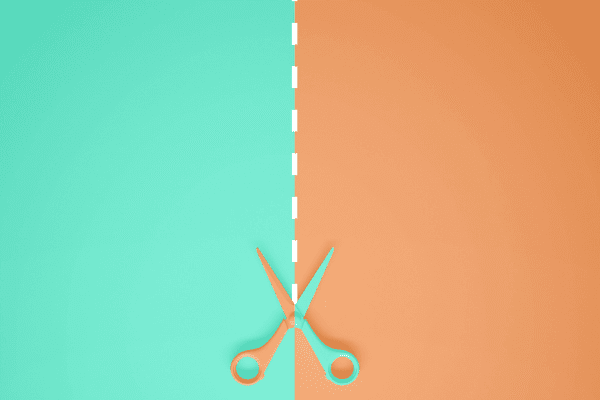
What should you include in the results chapter?
Following your analysis, it’s likely you’ll have far more data than are necessary to include in your chapter. In all likelihood, you’ll have a mountain of SPSS or R output data, and it’s your job to decide what’s most relevant. You’ll need to cut through the noise and focus on the data that matters.
This doesn’t mean that those analyses were a waste of time – on the contrary, those analyses ensure that you have a good understanding of your dataset and how to interpret it. However, that doesn’t mean your reader or examiner needs to see the 165 histograms you created! Relevance is key.
How do I decide what’s relevant?
At this point, it can be difficult to strike a balance between what is and isn’t important. But the most important thing is to ensure your results reflect and align with the purpose of your study . So, you need to revisit your research aims, objectives and research questions and use these as a litmus test for relevance. Make sure that you refer back to these constantly when writing up your chapter so that you stay on track.
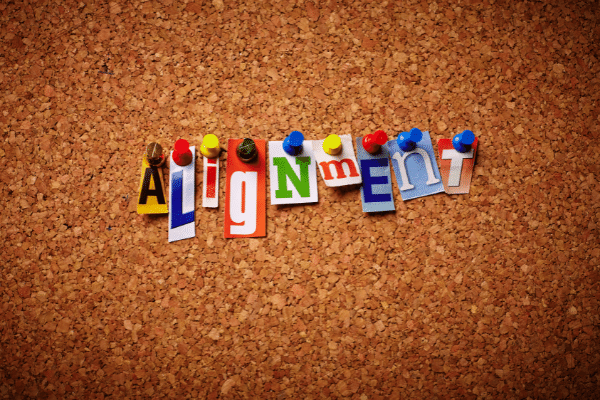
As a general guide, your results chapter will typically include the following:
- Some demographic data about your sample
- Reliability tests (if you used measurement scales)
- Descriptive statistics
- Inferential statistics (if your research objectives and questions require these)
- Hypothesis tests (again, if your research objectives and questions require these)
We’ll discuss each of these points in more detail in the next section.
Importantly, your results chapter needs to lay the foundation for your discussion chapter . This means that, in your results chapter, you need to include all the data that you will use as the basis for your interpretation in the discussion chapter.
For example, if you plan to highlight the strong relationship between Variable X and Variable Y in your discussion chapter, you need to present the respective analysis in your results chapter – perhaps a correlation or regression analysis.
Need a helping hand?
How do I write the results chapter?
There are multiple steps involved in writing up the results chapter for your quantitative research. The exact number of steps applicable to you will vary from study to study and will depend on the nature of the research aims, objectives and research questions . However, we’ll outline the generic steps below.
Step 1 – Revisit your research questions
The first step in writing your results chapter is to revisit your research objectives and research questions . These will be (or at least, should be!) the driving force behind your results and discussion chapters, so you need to review them and then ask yourself which statistical analyses and tests (from your mountain of data) would specifically help you address these . For each research objective and research question, list the specific piece (or pieces) of analysis that address it.
At this stage, it’s also useful to think about the key points that you want to raise in your discussion chapter and note these down so that you have a clear reminder of which data points and analyses you want to highlight in the results chapter. Again, list your points and then list the specific piece of analysis that addresses each point.
Next, you should draw up a rough outline of how you plan to structure your chapter . Which analyses and statistical tests will you present and in what order? We’ll discuss the “standard structure” in more detail later, but it’s worth mentioning now that it’s always useful to draw up a rough outline before you start writing (this advice applies to any chapter).
Step 2 – Craft an overview introduction
As with all chapters in your dissertation or thesis, you should start your quantitative results chapter by providing a brief overview of what you’ll do in the chapter and why . For example, you’d explain that you will start by presenting demographic data to understand the representativeness of the sample, before moving onto X, Y and Z.
This section shouldn’t be lengthy – a paragraph or two maximum. Also, it’s a good idea to weave the research questions into this section so that there’s a golden thread that runs through the document.
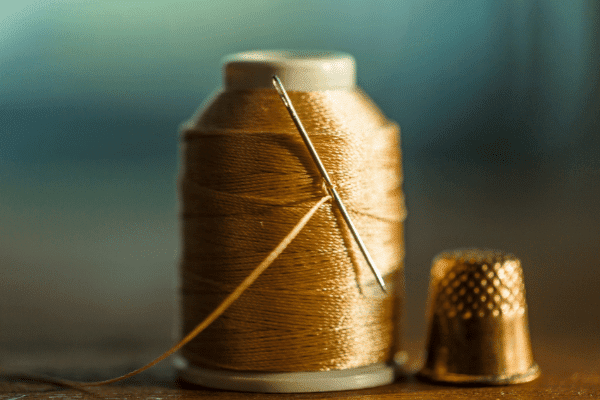
Step 3 – Present the sample demographic data
The first set of data that you’ll present is an overview of the sample demographics – in other words, the demographics of your respondents.
For example:
- What age range are they?
- How is gender distributed?
- How is ethnicity distributed?
- What areas do the participants live in?
The purpose of this is to assess how representative the sample is of the broader population. This is important for the sake of the generalisability of the results. If your sample is not representative of the population, you will not be able to generalise your findings. This is not necessarily the end of the world, but it is a limitation you’ll need to acknowledge.
Of course, to make this representativeness assessment, you’ll need to have a clear view of the demographics of the population. So, make sure that you design your survey to capture the correct demographic information that you will compare your sample to.
But what if I’m not interested in generalisability?
Well, even if your purpose is not necessarily to extrapolate your findings to the broader population, understanding your sample will allow you to interpret your findings appropriately, considering who responded. In other words, it will help you contextualise your findings . For example, if 80% of your sample was aged over 65, this may be a significant contextual factor to consider when interpreting the data. Therefore, it’s important to understand and present the demographic data.
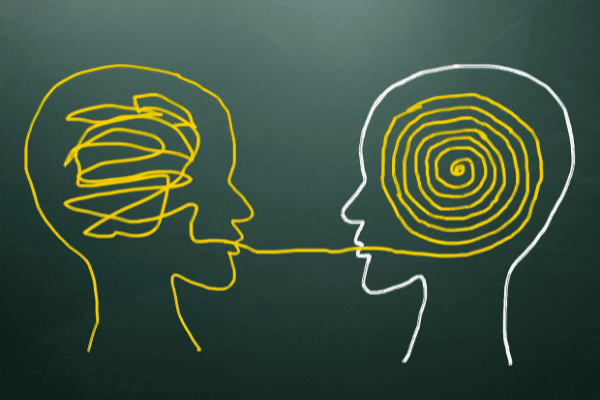
Step 4 – Review composite measures and the data “shape”.
Before you undertake any statistical analysis, you’ll need to do some checks to ensure that your data are suitable for the analysis methods and techniques you plan to use. If you try to analyse data that doesn’t meet the assumptions of a specific statistical technique, your results will be largely meaningless. Therefore, you may need to show that the methods and techniques you’ll use are “allowed”.
Most commonly, there are two areas you need to pay attention to:
#1: Composite measures
The first is when you have multiple scale-based measures that combine to capture one construct – this is called a composite measure . For example, you may have four Likert scale-based measures that (should) all measure the same thing, but in different ways. In other words, in a survey, these four scales should all receive similar ratings. This is called “ internal consistency ”.
Internal consistency is not guaranteed though (especially if you developed the measures yourself), so you need to assess the reliability of each composite measure using a test. Typically, Cronbach’s Alpha is a common test used to assess internal consistency – i.e., to show that the items you’re combining are more or less saying the same thing. A high alpha score means that your measure is internally consistent. A low alpha score means you may need to consider scrapping one or more of the measures.
#2: Data shape
The second matter that you should address early on in your results chapter is data shape. In other words, you need to assess whether the data in your set are symmetrical (i.e. normally distributed) or not, as this will directly impact what type of analyses you can use. For many common inferential tests such as T-tests or ANOVAs (we’ll discuss these a bit later), your data needs to be normally distributed. If it’s not, you’ll need to adjust your strategy and use alternative tests.
To assess the shape of the data, you’ll usually assess a variety of descriptive statistics (such as the mean, median and skewness), which is what we’ll look at next.
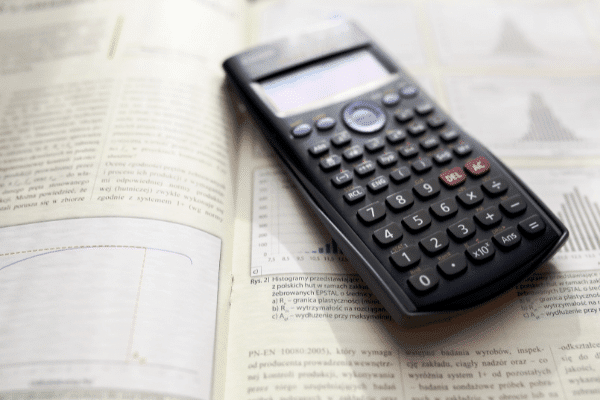
Step 5 – Present the descriptive statistics
Now that you’ve laid the foundation by discussing the representativeness of your sample, as well as the reliability of your measures and the shape of your data, you can get started with the actual statistical analysis. The first step is to present the descriptive statistics for your variables.
For scaled data, this usually includes statistics such as:
- The mean – this is simply the mathematical average of a range of numbers.
- The median – this is the midpoint in a range of numbers when the numbers are arranged in order.
- The mode – this is the most commonly repeated number in the data set.
- Standard deviation – this metric indicates how dispersed a range of numbers is. In other words, how close all the numbers are to the mean (the average).
- Skewness – this indicates how symmetrical a range of numbers is. In other words, do they tend to cluster into a smooth bell curve shape in the middle of the graph (this is called a normal or parametric distribution), or do they lean to the left or right (this is called a non-normal or non-parametric distribution).
- Kurtosis – this metric indicates whether the data are heavily or lightly-tailed, relative to the normal distribution. In other words, how peaked or flat the distribution is.
A large table that indicates all the above for multiple variables can be a very effective way to present your data economically. You can also use colour coding to help make the data more easily digestible.
For categorical data, where you show the percentage of people who chose or fit into a category, for instance, you can either just plain describe the percentages or numbers of people who responded to something or use graphs and charts (such as bar graphs and pie charts) to present your data in this section of the chapter.
When using figures, make sure that you label them simply and clearly , so that your reader can easily understand them. There’s nothing more frustrating than a graph that’s missing axis labels! Keep in mind that although you’ll be presenting charts and graphs, your text content needs to present a clear narrative that can stand on its own. In other words, don’t rely purely on your figures and tables to convey your key points: highlight the crucial trends and values in the text. Figures and tables should complement the writing, not carry it .
Depending on your research aims, objectives and research questions, you may stop your analysis at this point (i.e. descriptive statistics). However, if your study requires inferential statistics, then it’s time to deep dive into those .
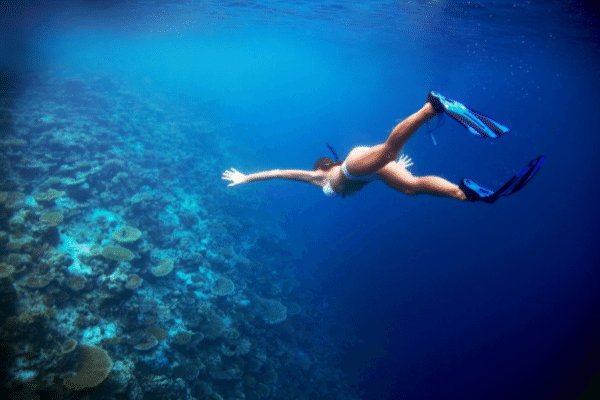
Step 6 – Present the inferential statistics
Inferential statistics are used to make generalisations about a population , whereas descriptive statistics focus purely on the sample . Inferential statistical techniques, broadly speaking, can be broken down into two groups .
First, there are those that compare measurements between groups , such as t-tests (which measure differences between two groups) and ANOVAs (which measure differences between multiple groups). Second, there are techniques that assess the relationships between variables , such as correlation analysis and regression analysis. Within each of these, some tests can be used for normally distributed (parametric) data and some tests are designed specifically for use on non-parametric data.
There are a seemingly endless number of tests that you can use to crunch your data, so it’s easy to run down a rabbit hole and end up with piles of test data. Ultimately, the most important thing is to make sure that you adopt the tests and techniques that allow you to achieve your research objectives and answer your research questions .
In this section of the results chapter, you should try to make use of figures and visual components as effectively as possible. For example, if you present a correlation table, use colour coding to highlight the significance of the correlation values, or scatterplots to visually demonstrate what the trend is. The easier you make it for your reader to digest your findings, the more effectively you’ll be able to make your arguments in the next chapter.
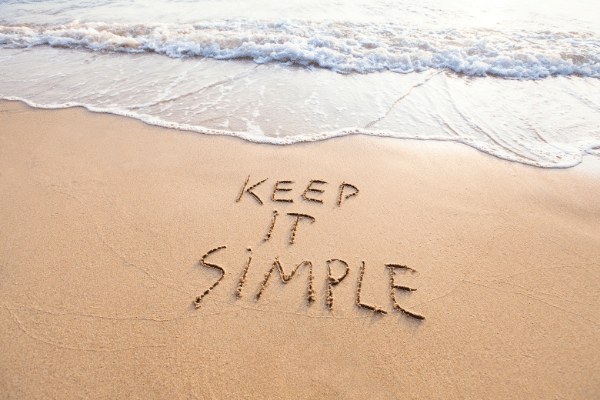
Step 7 – Test your hypotheses
If your study requires it, the next stage is hypothesis testing. A hypothesis is a statement , often indicating a difference between groups or relationship between variables, that can be supported or rejected by a statistical test. However, not all studies will involve hypotheses (again, it depends on the research objectives), so don’t feel like you “must” present and test hypotheses just because you’re undertaking quantitative research.
The basic process for hypothesis testing is as follows:
- Specify your null hypothesis (for example, “The chemical psilocybin has no effect on time perception).
- Specify your alternative hypothesis (e.g., “The chemical psilocybin has an effect on time perception)
- Set your significance level (this is usually 0.05)
- Calculate your statistics and find your p-value (e.g., p=0.01)
- Draw your conclusions (e.g., “The chemical psilocybin does have an effect on time perception”)
Finally, if the aim of your study is to develop and test a conceptual framework , this is the time to present it, following the testing of your hypotheses. While you don’t need to develop or discuss these findings further in the results chapter, indicating whether the tests (and their p-values) support or reject the hypotheses is crucial.
Step 8 – Provide a chapter summary
To wrap up your results chapter and transition to the discussion chapter, you should provide a brief summary of the key findings . “Brief” is the keyword here – much like the chapter introduction, this shouldn’t be lengthy – a paragraph or two maximum. Highlight the findings most relevant to your research objectives and research questions, and wrap it up.
Some final thoughts, tips and tricks
Now that you’ve got the essentials down, here are a few tips and tricks to make your quantitative results chapter shine:
- When writing your results chapter, report your findings in the past tense . You’re talking about what you’ve found in your data, not what you are currently looking for or trying to find.
- Structure your results chapter systematically and sequentially . If you had two experiments where findings from the one generated inputs into the other, report on them in order.
- Make your own tables and graphs rather than copying and pasting them from statistical analysis programmes like SPSS. Check out the DataIsBeautiful reddit for some inspiration.
- Once you’re done writing, review your work to make sure that you have provided enough information to answer your research questions , but also that you didn’t include superfluous information.
If you’ve got any questions about writing up the quantitative results chapter, please leave a comment below. If you’d like 1-on-1 assistance with your quantitative analysis and discussion, check out our hands-on coaching service , or book a free consultation with a friendly coach.
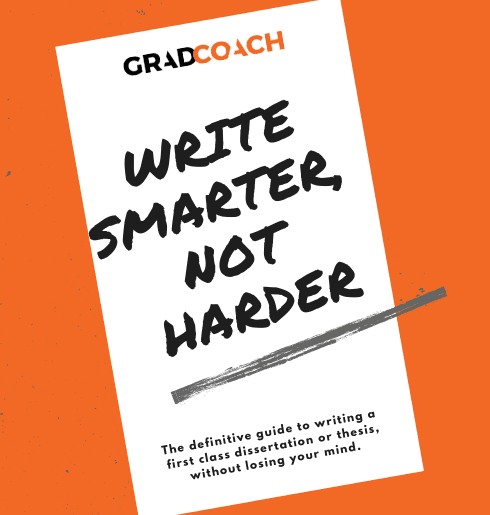
Psst… there’s more (for free)
This post is part of our dissertation mini-course, which covers everything you need to get started with your dissertation, thesis or research project.
You Might Also Like:
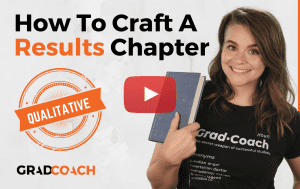
Thank you. I will try my best to write my results.
Awesome content 👏🏾
this was great explaination
Submit a Comment Cancel reply
Your email address will not be published. Required fields are marked *
Save my name, email, and website in this browser for the next time I comment.
- Print Friendly
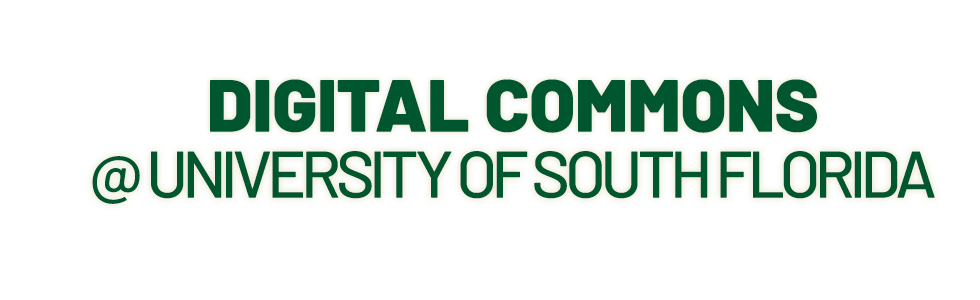
- USF Research
- USF Libraries
Digital Commons @ USF > College of Arts and Sciences > Mathematics and Statistics > Theses and Dissertations
Mathematics and Statistics Theses and Dissertations
Theses/dissertations from 2023 2023.
Classification of Finite Topological Quandles and Shelves via Posets , Hitakshi Lahrani
Applied Analysis for Learning Architectures , Himanshu Singh
Rational Functions of Degree Five That Permute the Projective Line Over a Finite Field , Christopher Sze
Theses/Dissertations from 2022 2022
New Developments in Statistical Optimal Designs for Physical and Computer Experiments , Damola M. Akinlana
Advances and Applications of Optimal Polynomial Approximants , Raymond Centner
Data-Driven Analytical Predictive Modeling for Pancreatic Cancer, Financial & Social Systems , Aditya Chakraborty
On Simultaneous Similarity of d-tuples of Commuting Square Matrices , Corey Connelly
Symbolic Computation of Lump Solutions to a Combined (2+1)-dimensional Nonlinear Evolution Equation , Jingwei He
Boundary behavior of analytic functions and Approximation Theory , Spyros Pasias
Stability Analysis of Delay-Driven Coupled Cantilevers Using the Lambert W-Function , Daniel Siebel-Cortopassi
A Functional Optimization Approach to Stochastic Process Sampling , Ryan Matthew Thurman
Theses/Dissertations from 2021 2021
Riemann-Hilbert Problems for Nonlocal Reverse-Time Nonlinear Second-order and Fourth-order AKNS Systems of Multiple Components and Exact Soliton Solutions , Alle Adjiri
Zeros of Harmonic Polynomials and Related Applications , Azizah Alrajhi
Combination of Time Series Analysis and Sentiment Analysis for Stock Market Forecasting , Hsiao-Chuan Chou
Uncertainty Quantification in Deep and Statistical Learning with applications in Bio-Medical Image Analysis , K. Ruwani M. Fernando
Data-Driven Analytical Modeling of Multiple Myeloma Cancer, U.S. Crop Production and Monitoring Process , Lohuwa Mamudu
Long-time Asymptotics for mKdV Type Reduced Equations of the AKNS Hierarchy in Weighted L 2 Sobolev Spaces , Fudong Wang
Online and Adjusted Human Activities Recognition with Statistical Learning , Yanjia Zhang
Theses/Dissertations from 2020 2020
Bayesian Reliability Analysis of The Power Law Process and Statistical Modeling of Computer and Network Vulnerabilities with Cybersecurity Application , Freeh N. Alenezi
Discrete Models and Algorithms for Analyzing DNA Rearrangements , Jasper Braun
Bayesian Reliability Analysis for Optical Media Using Accelerated Degradation Test Data , Kun Bu
On the p(x)-Laplace equation in Carnot groups , Robert D. Freeman
Clustering methods for gene expression data of Oxytricha trifallax , Kyle Houfek
Gradient Boosting for Survival Analysis with Applications in Oncology , Nam Phuong Nguyen
Global and Stochastic Dynamics of Diffusive Hindmarsh-Rose Equations in Neurodynamics , Chi Phan
Restricted Isometric Projections for Differentiable Manifolds and Applications , Vasile Pop
On Some Problems on Polynomial Interpolation in Several Variables , Brian Jon Tuesink
Numerical Study of Gap Distributions in Determinantal Point Process on Low Dimensional Spheres: L -Ensemble of O ( n ) Model Type for n = 2 and n = 3 , Xiankui Yang
Non-Associative Algebraic Structures in Knot Theory , Emanuele Zappala
Theses/Dissertations from 2019 2019
Field Quantization for Radiative Decay of Plasmons in Finite and Infinite Geometries , Maryam Bagherian
Probabilistic Modeling of Democracy, Corruption, Hemophilia A and Prediabetes Data , A. K. M. Raquibul Bashar
Generalized Derivations of Ternary Lie Algebras and n-BiHom-Lie Algebras , Amine Ben Abdeljelil
Fractional Random Weighted Bootstrapping for Classification on Imbalanced Data with Ensemble Decision Tree Methods , Sean Charles Carter
Hierarchical Self-Assembly and Substitution Rules , Daniel Alejandro Cruz
Statistical Learning of Biomedical Non-Stationary Signals and Quality of Life Modeling , Mahdi Goudarzi
Probabilistic and Statistical Prediction Models for Alzheimer’s Disease and Statistical Analysis of Global Warming , Maryam Ibrahim Habadi
Essays on Time Series and Machine Learning Techniques for Risk Management , Michael Kotarinos
The Systems of Post and Post Algebras: A Demonstration of an Obvious Fact , Daviel Leyva
Reconstruction of Radar Images by Using Spherical Mean and Regular Radon Transforms , Ozan Pirbudak
Analyses of Unorthodox Overlapping Gene Segments in Oxytricha Trifallax , Shannon Stich
An Optimal Medium-Strength Regularity Algorithm for 3-uniform Hypergraphs , John Theado
Power Graphs of Quasigroups , DayVon L. Walker
Theses/Dissertations from 2018 2018
Groups Generated by Automata Arising from Transformations of the Boundaries of Rooted Trees , Elsayed Ahmed
Non-equilibrium Phase Transitions in Interacting Diffusions , Wael Al-Sawai
A Hybrid Dynamic Modeling of Time-to-event Processes and Applications , Emmanuel A. Appiah
Lump Solutions and Riemann-Hilbert Approach to Soliton Equations , Sumayah A. Batwa
Developing a Model to Predict Prevalence of Compulsive Behavior in Individuals with OCD , Lindsay D. Fields
Generalizations of Quandles and their cohomologies , Matthew J. Green
Hamiltonian structures and Riemann-Hilbert problems of integrable systems , Xiang Gu
Optimal Latin Hypercube Designs for Computer Experiments Based on Multiple Objectives , Ruizhe Hou
Human Activity Recognition Based on Transfer Learning , Jinyong Pang
Signal Detection of Adverse Drug Reaction using the Adverse Event Reporting System: Literature Review and Novel Methods , Minh H. Pham
Statistical Analysis and Modeling of Cyber Security and Health Sciences , Nawa Raj Pokhrel
Machine Learning Methods for Network Intrusion Detection and Intrusion Prevention Systems , Zheni Svetoslavova Stefanova
Orthogonal Polynomials With Respect to the Measure Supported Over the Whole Complex Plane , Meng Yang
Theses/Dissertations from 2017 2017
Modeling in Finance and Insurance With Levy-It'o Driven Dynamic Processes under Semi Markov-type Switching Regimes and Time Domains , Patrick Armand Assonken Tonfack
Prevalence of Typical Images in High School Geometry Textbooks , Megan N. Cannon
On Extending Hansel's Theorem to Hypergraphs , Gregory Sutton Churchill
Contributions to Quandle Theory: A Study of f-Quandles, Extensions, and Cohomology , Indu Rasika U. Churchill
Linear Extremal Problems in the Hardy Space H p for 0 p , Robert Christopher Connelly
Statistical Analysis and Modeling of Ovarian and Breast Cancer , Muditha V. Devamitta Perera
Statistical Analysis and Modeling of Stomach Cancer Data , Chao Gao
Structural Analysis of Poloidal and Toroidal Plasmons and Fields of Multilayer Nanorings , Kumar Vijay Garapati
Dynamics of Multicultural Social Networks , Kristina B. Hilton
Cybersecurity: Stochastic Analysis and Modelling of Vulnerabilities to Determine the Network Security and Attackers Behavior , Pubudu Kalpani Kaluarachchi
Generalized D-Kaup-Newell integrable systems and their integrable couplings and Darboux transformations , Morgan Ashley McAnally
Patterns in Words Related to DNA Rearrangements , Lukas Nabergall
Time Series Online Empirical Bayesian Kernel Density Segmentation: Applications in Real Time Activity Recognition Using Smartphone Accelerometer , Shuang Na
Schreier Graphs of Thompson's Group T , Allen Pennington
Cybersecurity: Probabilistic Behavior of Vulnerability and Life Cycle , Sasith Maduranga Rajasooriya
Bayesian Artificial Neural Networks in Health and Cybersecurity , Hansapani Sarasepa Rodrigo
Real-time Classification of Biomedical Signals, Parkinson’s Analytical Model , Abolfazl Saghafi
Lump, complexiton and algebro-geometric solutions to soliton equations , Yuan Zhou
Theses/Dissertations from 2016 2016
A Statistical Analysis of Hurricanes in the Atlantic Basin and Sinkholes in Florida , Joy Marie D'andrea
Statistical Analysis of a Risk Factor in Finance and Environmental Models for Belize , Sherlene Enriquez-Savery
Putnam's Inequality and Analytic Content in the Bergman Space , Matthew Fleeman
On the Number of Colors in Quandle Knot Colorings , Jeremy William Kerr
Statistical Modeling of Carbon Dioxide and Cluster Analysis of Time Dependent Information: Lag Target Time Series Clustering, Multi-Factor Time Series Clustering, and Multi-Level Time Series Clustering , Doo Young Kim
Some Results Concerning Permutation Polynomials over Finite Fields , Stephen Lappano
Hamiltonian Formulations and Symmetry Constraints of Soliton Hierarchies of (1+1)-Dimensional Nonlinear Evolution Equations , Solomon Manukure
Modeling and Survival Analysis of Breast Cancer: A Statistical, Artificial Neural Network, and Decision Tree Approach , Venkateswara Rao Mudunuru
Generalized Phase Retrieval: Isometries in Vector Spaces , Josiah Park
Leonard Systems and their Friends , Jonathan Spiewak
Resonant Solutions to (3+1)-dimensional Bilinear Differential Equations , Yue Sun
Statistical Analysis and Modeling Health Data: A Longitudinal Study , Bhikhari Prasad Tharu
Global Attractors and Random Attractors of Reaction-Diffusion Systems , Junyi Tu
Time Dependent Kernel Density Estimation: A New Parameter Estimation Algorithm, Applications in Time Series Classification and Clustering , Xing Wang
On Spectral Properties of Single Layer Potentials , Seyed Zoalroshd
Theses/Dissertations from 2015 2015
Analysis of Rheumatoid Arthritis Data using Logistic Regression and Penalized Approach , Wei Chen
Active Tile Self-assembly and Simulations of Computational Systems , Daria Karpenko
Nearest Neighbor Foreign Exchange Rate Forecasting with Mahalanobis Distance , Vindya Kumari Pathirana
Statistical Learning with Artificial Neural Network Applied to Health and Environmental Data , Taysseer Sharaf
Radial Versus Othogonal and Minimal Projections onto Hyperplanes in l_4^3 , Richard Alan Warner
Ensemble Learning Method on Machine Maintenance Data , Xiaochuang Zhao
Theses/Dissertations from 2014 2014
Properties of Graphs Used to Model DNA Recombination , Ryan Arredondo
Recursive Methods in Number Theory, Combinatorial Graph Theory, and Probability , Jonathan Burns
On the Classification of Groups Generated by Automata with 4 States over a 2-Letter Alphabet , Louis Caponi
Statistical Analysis, Modeling, and Algorithms for Pharmaceutical and Cancer Systems , Bong-Jin Choi
Topological Data Analysis of Properties of Four-Regular Rigid Vertex Graphs , Grant Mcneil Conine
Trend Analysis and Modeling of Health and Environmental Data: Joinpoint and Functional Approach , Ram C. Kafle
Advanced Search
- Email Notifications and RSS
- All Collections
- USF Faculty Publications
- Open Access Journals
- Conferences and Events
- Theses and Dissertations
- Textbooks Collection
Useful Links
- Mathematics and Statistics Department
- Rights Information
- SelectedWorks
- Submit Research
Home | About | Help | My Account | Accessibility Statement | Language and Diversity Statements
Privacy Copyright
The Data Deep Dive: Statistical Analysis Guide

Table of contents
- 1 What Is Statistical Analysis and Its Role?
- 2 Preparing for Statistical Analysis
- 3 Data Collection and Management
- 4 Performing Descriptive Statistical Analysis
- 5 Performing Inferential Statistics Analysis
- 6 Writing the Statistical Research Paper
- 7 Common Mistakes in Statistical Analysis
- 8 Ethical Considerations
- 9 Concluding the Research Paper
- 10 Examples of Good and Poor Statistical Analysis in Research Paper
- 11 Key Insights: Navigating Statistical Analysis
Statistical analysis is fundamental if you need to reveal patterns or identify trends in datasets, employing numerical data analysis to eliminate bias and extract meaningful vision. Accordingly, it is crucial in research explanation, model evolution, and survey planning.
Statistical analysts make valuable results from the raw data, facilitating informed decision-making and predictive statistical analytics based on historical information.
Do you need to make a statistical analysis for your university studies? In this statistical study article, you will find instructions on how to write statistical analysis, as well as types of statistical analysis, statistical tools, and common mistakes students face.
What Is Statistical Analysis and Its Role?
Statistical analysis is the systematic process of collecting, organizing, and interpreting numbers to reveal patterns and identify trends and relationships. It plays a crucial role in research by providing tools to analyze data objectively, remove bias, and draw conclusions. Moreover, statistical analysis aids in identifying correlations, testing hypotheses, and making predictions, thereby informing decision-making in various fields such as computer science, medicine, economics, and social sciences. Thus, it enables quantitative data and statistical analytics researchers to assess results.
Struggling with how to analyze data in research? Feel free to address our specialists to get skilled and qualified help with research paper data analysis.
Preparing for Statistical Analysis
Preparing for statistical analysis requires some essential steps to ensure the validity and reliability of results.
- Firstly, formulating understandable and measurable questions is critical for valid statistics in a research paper. Questions lead the entire data analysis process and help define the scope of the study. Accordingly, scientists should develop specific, relevant, and capable issues that can be answered through statistical methods.
- Secondly, identifying appropriate data is vital. Picking an accordant data set that aligns with the investigations guarantees the analysis and business intelligence are focused and meaningful. For this purpose, researchers should consider data origin, quality, and responsibility when selecting data for analysis.
By scrupulously formulating problems and selecting appropriate statistical analytics data, researchers can lay a solid foundation for statistical analysis, guiding to strong and prudent results.
Data Collection and Management
Information collection and management are integral components of the statistical analysis process, ensuring the accuracy and reliability of results. Firstly, considering the techniques of data collection is essential. Here, researchers may employ primary approaches, such as examinations, interviews, or experiments, to gather direct information. Secondary methods involve utilizing existing data sources like databases, statistical analysis software, literature reviews, or archival records.
To collect data, specialists need to analyze:
- dependent variable;
- categorical variables;
- outcome variable;
- patterns and trends;
- alternative hypothesis states.
Once data is collected, organizing it is crucial for efficient analysis. As a rule, researchers utilize statistical analysis software tools or spreadsheets to manage data systematically, ensuring clarity and accessibility. Besides, proper organization includes labeling variables, formatting data consistently, and documenting any transformations or cleaning statistical procedures undertaken.
Effective data management also facilitates coherent analysis, empowering scientists to get meaningful insights and draw valid conclusions. By using suitable data collection approaches and organizing data systematically, researchers can unlock the full potential of statistical analysis, advancing knowledge and driving proof-based replies.
Performing Descriptive Statistical Analysis
Performing descriptive statistics is essential in knowing and summarizing data sets for statistics in research. Usually, it involves exploring the crucial characteristics of the data to gain insights into its normal allotting and changeability.
The basics of descriptive statistics encompass measures of central tendency, dispersion, and graphical representations.
- Measures of main bias , such as mean, median, and mode, summarize a dataset’s typical or main value.
- Dispersion repeated measures , including range, variance, and standard deviation, quantify the spread or variability of the data points.
- Graphical representations , such as histograms, box plots, and scatter plots, offer visual insights into the distribution and patterns within the data based on statistical observations.
Explaining descriptive statistical analysis results involves understanding and presenting the findings effectively. Accordingly, researchers should show understandable explanations of the descriptive statistics in the research paper calculated, highlighting key insights and trends within the data. Indeed, visual representations can enhance understanding by illustrating the distribution and relationships in the data. Hence, it’s essential to consider the context of the analysis and the questions when interpreting the results, ensuring that the conclusions drawn are suggestive and relevant.
Overall, performing descriptive statistical data analysis enables researchers to summarize and derive the crucial characteristics after collecting data. It is vital to provide a foundation for further research study and interpretation. By mastering the basics of different types of statistical analysis and correctly explaining the results, experimentals can uncover valuable insights and communicate their findings clearly and precisely.
If you struggle on this step, ask for help from our writers. They can’t write an essay or paper for you but are eager to assist you with each step.
Performing Inferential Statistics Analysis
When students perform statistical analysis, it involves making statistical inference and drawing conclusions about a population based on sample data. For this reason, the inferential statistical tool in research revolves around hypothesis testing, confidence intervals, and significance levels.
On the one side, hypothesis testing allows researchers to assess the validity of assumptions underlying entire population parameters by comparing one sample data to theoretical expectations. On the other side, sample data, null hypothesis, and confidence intervals provide a range of normal and extreme values within which the proper population parameter will likely fall. Lastly, significance levels indicate the probability of obtaining the observed results by chance alone, helping researchers determine the reliability of their findings.
Choosing the proper approach is crucial for conducting meaningful inferential statistics analysis. Accordingly, researchers must select appropriate parametric tests based on the research design, collect data type, null hypothesis, and hypothesis being tested. For example, standard parametric tests and non parametric tests include:
- T-tests: a parametric statistical test used to determine if there is a significant difference between the means of two groups. It is commonly used when the sample size is small, and the population standard deviation is unknown.
- Z test: similar to the t-test, the z-test is a parametric test used to compare means, but it is typically employed when the sample size is large and/or the population standard deviation is known.
- ANOVA: this parametric statistical test compares the means of three or more groups simultaneously. It assesses whether there are any statistically significant differences between the means of the groups.
- Regression: a statistical method used to examine the relationship between one dependent variable (often denoted as Y) and one or more independent variables (often denoted as X) within one case study analysis . Thus, it helps in understanding how the value of the dependent variable changes when one or more independent variables are varied. Here, case study analysis refers to applying regression analysis in specific scenarios or case studies to explore relationships between quantitative variables.
Importantly to note, interpreting results from inferential studies requires a nuanced understanding of statistical concepts and diligent consideration of the context. Here, investigators should assess the strength of evidence supporting their conclusions, considering factors such as effect size, statistical power, and potential biases. Besides, communicating inferential statistics results involves presenting findings and standard deviation to highlight the implications for the research question or troublesome under investigation.
Writing the Statistical Research Paper
Writing a research paper involves integrating and presenting your findings coherently. You need to know the answers to the questions: “What is statistical analysis?” and “How do you do a statistical analysis?”. As a rule, the typical structure includes several essential sections:
- Introduction : This section provides backdrop information on the research theme, states the research questions or null hypothesis, patterns, and trends, and outlines the study’s objectives and statistical attention.
- Methodology : Here, researchers detail the methods and procedures for analyzing and collecting data. This section should be thorough enough for other researchers to replicate the study.
- Results : This section presents the study’s findings, often through descriptive and inferential statistical data analysis. It’s essential to present results objectively and accurately, using appropriate statistical study measures and techniques.
- Discussion : In this segment, investigators interpret statistics and the results, discuss their implications, and compare them to existing literature. It’s an opportunity to critically evaluate the findings and address any limitations or potential biases.
- Conclusion : The conclusion summarizes the study’s key findings, discusses their significance, and suggests avenues for future research.
When you present or write a statistical report in each section, it’s crucial to clearly and concisely explain the methods, results, and research design. Therefore, students usually need to test it in the sample group. In the methodology section, describe the statistical techniques used and justify their appropriateness for the research question. Otherwise, use descriptive statistics to summarize data and inferential statistics to test hypotheses or explore relationships between variables.
Whereas, graphics and tables are potent statistical instruments for presenting data effectively. Choose the most appropriate format for your data, whether it’s a bar graph, scatter plot, or table of descriptive statistics for research.
As a result, writing your research essay must involve such steps:
- Arranging your decisions analytically;
- Integrating statistical analysis throughout;
- Using visuals and tables to enhance clarity and understanding.
Common Mistakes in Statistical Analysis
Common mistakes in statistical analysis can undermine the validity and reliability of research findings. Here are some key pitfalls to avoid:
- Confusing terms like ‘mean’ and ‘median’ or misinterpreting p value and confidence intervals can lead to incorrect conclusions.
- Selecting the wrong test for the research question or ignoring test assumptions can compromise the accuracy of the results.
- Ignoring missing data and outliers or failing to preprocess data properly can introduce bias and skew results.
- Focusing solely on statistical significance without considering practical significance or engaging in p-hacking practices can lead to misleading conclusions.
- Failing to share facts or selectively report results can hinder research reproducibility and transparency.
- Both small and large sample sizes can impact the reliability and generalizability of findings.
- Repeatedly testing hypotheses on the same data set or creating overly complicated models can result in spurious decisions.
- Failing to interpret statistical results within the broader context or generalize findings appropriately can limit the practical relevance of research.
- Misrepresenting graphics or neglecting to label and interval scale graphs correctly can distort the statistical analysis of data.
- Managing redundant analyses or ignoring existing knowledge in the field can hinder the promotion of research.
Avoiding common mistakes in statistical analysis requires diligence and attention to detail. Consequently, researchers should prioritize understanding statistical concepts systematically and using appropriate methods for exploratory data analysis. Thus, it’s essential to double-check calculations, verify assumptions, and seek guidance from statistical analysts if needed.
Furthermore, maintaining transparency and reproducibility in research practices is leading. It includes sharing data, code, and methodology details to facilitate equivalent surveys and replication of findings.
Continuous data learning and staying updated on best practices in statistical analysis are also vital for avoiding pitfalls and improving the quality of research. By addressing these common mistakes and adopting robust practices, researchers can ensure the morality and reliability of their findings, contributing to advancing knowledge in their respective fields.
Ethical Considerations
Ethical considerations in statistical analysis encompass safeguarding data privacy and integrity. That being said, researchers must uphold ethical practices in handling data, ensuring confidentiality, and respecting participants’ rights. Indeed, transparent reporting of results is vital, as is disclosing potential conflicts of passion and holding to moral guidelines data dedicated to relevant institutions and controlling bodies. By prioritizing ethical principles, researchers can maintain trust and integrity in their work, fostering a culture of responsible data analysis in research and decision-making.
Concluding the Research Paper
Concluding a research paper involves summarizing key findings and suggesting future research directions. Here, reiterating the paper’s main points and highlighting the significance of the results is essential. Statistical analysts can also discuss limitations and areas for further investigation, providing context for future studies. By showing insightful outcomes and figuring out avenues for future research, scientists can contribute to the ongoing discourse in their field and inspire further inquiry and exploration.
Examples of Good and Poor Statistical Analysis in Research Paper
Good statistical analysis examples in research:
- A study on the effectiveness of a new drug uses appropriate parametric tests, presents results clearly with confidence intervals, and discusses both statistical and practical significance.
- A survey-based research project employs stratified random sampling, ensuring a representative sample, and utilizes advanced regression analysis to explore complex relationships between variables.
- An experiment investigating the impact of a teaching method on student performance controls for potential confounding variables and conducts power statistical analysis basics to determine sample size, ensuring adequate statistical power.
Examples of poor stat analysis in research:
- A study fails to report key details about information collection and statistical methods, making it impossible to evaluate the validity of the findings.
- A research paper relies solely on p value to conclude without considering effect sizes or practical significance, leading to misleading interpretations.
- An analysis uses an inappropriate statistical test for the research question, resulting in flawed conclusions and misinterpretation of the data.
Here are two good examples.
Example 1: The Effect of Regular Exercise on Anxiety Levels among College Students
Introduction: In recent years, mental health issues among college students have become a growing concern. Anxiety, in particular, is prevalent among this demographic. This study aims to investigate the potential impact of regular exercise on anxiety levels among college students. Understanding this relationship could inform interventions aimed at improving mental well-being in this population.
Methodology: Participants (N = 100) were recruited from a local university and randomly assigned to either an exercise or control group. The exercise group engaged in a supervised 30-minute aerobic exercise session three times a week for eight weeks, while the control group maintained regular activities. Anxiety levels were assessed using the State-Trait Anxiety Inventory (STAI) before and after the intervention period.
Results: The results revealed a significant decrease in anxiety levels among participants in the exercise group compared to the control group (t(98) = -2.45, p < 0.05). Specifically, the mean anxiety score decreased from 45.2 (SD = 7.8) to 38.6 (SD = 6.4) in the exercise group, while it remained relatively stable in the control group (mean = 44.5, SD = 8.2).
Discussion: These findings suggest that regular aerobic exercise may have a beneficial effect on reducing anxiety levels among college students. Engaging in physical activity could serve as a potential non-pharmacological intervention for managing anxiety symptoms in this population. Further research is warranted to explore this relationship’s underlying mechanisms and determine optimal exercise duration and intensity for maximum mental health benefits.
Example 2: The Relationship between Service Quality, Customer Satisfaction, and Loyalty in Retail Settings
Introduction: Maintaining high levels of customer satisfaction and loyalty is essential for the success of retail businesses. This study investigates the relationship between service quality, customer satisfaction, and loyalty in a local retail chain context. Understanding these dynamics can help businesses identify areas for improvement and develop strategies to enhance customer retention.
Methodology: A survey was conducted among the retail chain’s customers (N = 300) to assess their perceptions of service quality, satisfaction with their shopping experience, and intention to repurchase from the store. Service quality was measured using the SERVQUAL scale, while customer satisfaction and loyalty were assessed using Likert-type scales.
Results: The results indicated a strong positive correlation between service quality, customer satisfaction, and loyalty (r = 0.75, p < 0.001). Furthermore, regression analysis revealed that service quality significantly predicted both customer satisfaction (β = 0.60, p < 0.001) and loyalty (β = 0.45, p < 0.001). Additionally, customer satisfaction emerged as a significant predictor of loyalty (β = 0.50, p < 0.001), indicating its mediating role in the relationship between service quality and loyalty.
Discussion: These findings underscore the importance of high-quality service in enhancing customer satisfaction and fostering loyalty in retail settings. Businesses should prioritize investments in service training, infrastructure, and customer relationship management to ensure positive shopping experiences and promote repeat patronage. Future research could explore additional factors influencing customer loyalty and examine the effectiveness of specific loyalty programs and incentives in driving repeat business.
Key Insights: Navigating Statistical Analysis
To sum up, mastering a statistical analysis system is essential for researchers to derive meaningful insights from data. Understanding statistical concepts, choosing appropriate methods, and adhering to ethical guidelines are paramount.
Additionally, transparent reporting, rigorous methodology, and careful interpretation ensure the integrity and reliability of research findings. By avoiding common pitfalls and embracing best practices, researchers can contribute to advancing knowledge and making informed decisions across various fields.
Ultimately, statistical analysis is a powerful tool for unlocking the mysteries hidden within data, guiding us toward more profound understanding and innovation.
Readers also enjoyed

WHY WAIT? PLACE AN ORDER RIGHT NOW!
Just fill out the form, press the button, and have no worries!
We use cookies to give you the best experience possible. By continuing we’ll assume you board with our cookie policy.
Have a language expert improve your writing
Run a free plagiarism check in 10 minutes, generate accurate citations for free.
- Knowledge Base
- Choosing the Right Statistical Test | Types & Examples
Choosing the Right Statistical Test | Types & Examples
Published on January 28, 2020 by Rebecca Bevans . Revised on June 22, 2023.
Statistical tests are used in hypothesis testing . They can be used to:
- determine whether a predictor variable has a statistically significant relationship with an outcome variable.
- estimate the difference between two or more groups.
Statistical tests assume a null hypothesis of no relationship or no difference between groups. Then they determine whether the observed data fall outside of the range of values predicted by the null hypothesis.
If you already know what types of variables you’re dealing with, you can use the flowchart to choose the right statistical test for your data.
Statistical tests flowchart
Table of contents
What does a statistical test do, when to perform a statistical test, choosing a parametric test: regression, comparison, or correlation, choosing a nonparametric test, flowchart: choosing a statistical test, other interesting articles, frequently asked questions about statistical tests.
Statistical tests work by calculating a test statistic – a number that describes how much the relationship between variables in your test differs from the null hypothesis of no relationship.
It then calculates a p value (probability value). The p -value estimates how likely it is that you would see the difference described by the test statistic if the null hypothesis of no relationship were true.
If the value of the test statistic is more extreme than the statistic calculated from the null hypothesis, then you can infer a statistically significant relationship between the predictor and outcome variables.
If the value of the test statistic is less extreme than the one calculated from the null hypothesis, then you can infer no statistically significant relationship between the predictor and outcome variables.
Here's why students love Scribbr's proofreading services
Discover proofreading & editing
You can perform statistical tests on data that have been collected in a statistically valid manner – either through an experiment , or through observations made using probability sampling methods .
For a statistical test to be valid , your sample size needs to be large enough to approximate the true distribution of the population being studied.
To determine which statistical test to use, you need to know:
- whether your data meets certain assumptions.
- the types of variables that you’re dealing with.
Statistical assumptions
Statistical tests make some common assumptions about the data they are testing:
- Independence of observations (a.k.a. no autocorrelation): The observations/variables you include in your test are not related (for example, multiple measurements of a single test subject are not independent, while measurements of multiple different test subjects are independent).
- Homogeneity of variance : the variance within each group being compared is similar among all groups. If one group has much more variation than others, it will limit the test’s effectiveness.
- Normality of data : the data follows a normal distribution (a.k.a. a bell curve). This assumption applies only to quantitative data .
If your data do not meet the assumptions of normality or homogeneity of variance, you may be able to perform a nonparametric statistical test , which allows you to make comparisons without any assumptions about the data distribution.
If your data do not meet the assumption of independence of observations, you may be able to use a test that accounts for structure in your data (repeated-measures tests or tests that include blocking variables).
Types of variables
The types of variables you have usually determine what type of statistical test you can use.
Quantitative variables represent amounts of things (e.g. the number of trees in a forest). Types of quantitative variables include:
- Continuous (aka ratio variables): represent measures and can usually be divided into units smaller than one (e.g. 0.75 grams).
- Discrete (aka integer variables): represent counts and usually can’t be divided into units smaller than one (e.g. 1 tree).
Categorical variables represent groupings of things (e.g. the different tree species in a forest). Types of categorical variables include:
- Ordinal : represent data with an order (e.g. rankings).
- Nominal : represent group names (e.g. brands or species names).
- Binary : represent data with a yes/no or 1/0 outcome (e.g. win or lose).
Choose the test that fits the types of predictor and outcome variables you have collected (if you are doing an experiment , these are the independent and dependent variables ). Consult the tables below to see which test best matches your variables.
Parametric tests usually have stricter requirements than nonparametric tests, and are able to make stronger inferences from the data. They can only be conducted with data that adheres to the common assumptions of statistical tests.
The most common types of parametric test include regression tests, comparison tests, and correlation tests.
Regression tests
Regression tests look for cause-and-effect relationships . They can be used to estimate the effect of one or more continuous variables on another variable.
Comparison tests
Comparison tests look for differences among group means . They can be used to test the effect of a categorical variable on the mean value of some other characteristic.
T-tests are used when comparing the means of precisely two groups (e.g., the average heights of men and women). ANOVA and MANOVA tests are used when comparing the means of more than two groups (e.g., the average heights of children, teenagers, and adults).
Correlation tests
Correlation tests check whether variables are related without hypothesizing a cause-and-effect relationship.
These can be used to test whether two variables you want to use in (for example) a multiple regression test are autocorrelated.
Non-parametric tests don’t make as many assumptions about the data, and are useful when one or more of the common statistical assumptions are violated. However, the inferences they make aren’t as strong as with parametric tests.
Prevent plagiarism. Run a free check.
This flowchart helps you choose among parametric tests. For nonparametric alternatives, check the table above.
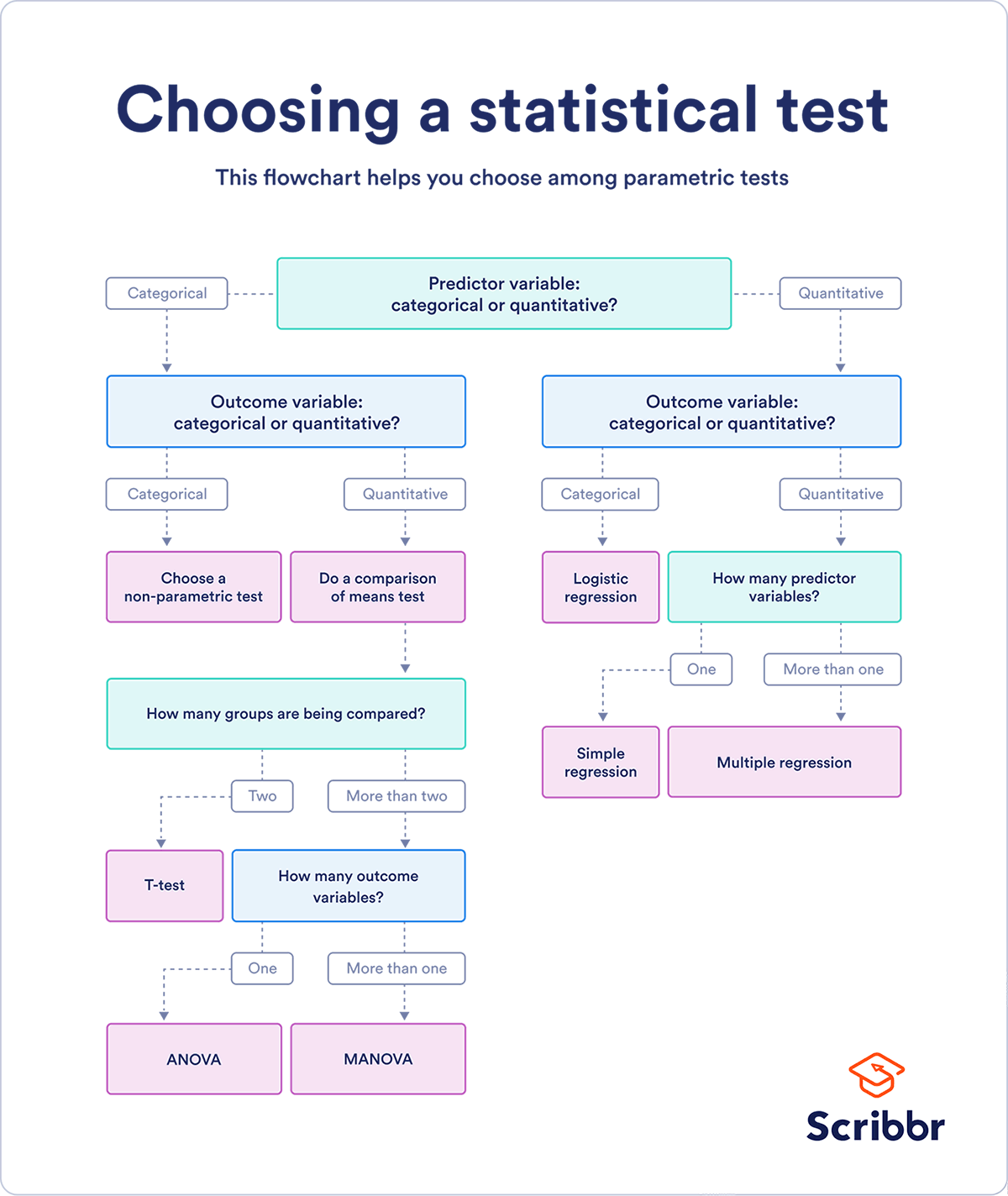
If you want to know more about statistics , methodology , or research bias , make sure to check out some of our other articles with explanations and examples.
- Normal distribution
- Descriptive statistics
- Measures of central tendency
- Correlation coefficient
- Null hypothesis
Methodology
- Cluster sampling
- Stratified sampling
- Types of interviews
- Cohort study
- Thematic analysis
Research bias
- Implicit bias
- Cognitive bias
- Survivorship bias
- Availability heuristic
- Nonresponse bias
- Regression to the mean
Statistical tests commonly assume that:
- the data are normally distributed
- the groups that are being compared have similar variance
- the data are independent
If your data does not meet these assumptions you might still be able to use a nonparametric statistical test , which have fewer requirements but also make weaker inferences.
A test statistic is a number calculated by a statistical test . It describes how far your observed data is from the null hypothesis of no relationship between variables or no difference among sample groups.
The test statistic tells you how different two or more groups are from the overall population mean , or how different a linear slope is from the slope predicted by a null hypothesis . Different test statistics are used in different statistical tests.
Statistical significance is a term used by researchers to state that it is unlikely their observations could have occurred under the null hypothesis of a statistical test . Significance is usually denoted by a p -value , or probability value.
Statistical significance is arbitrary – it depends on the threshold, or alpha value, chosen by the researcher. The most common threshold is p < 0.05, which means that the data is likely to occur less than 5% of the time under the null hypothesis .
When the p -value falls below the chosen alpha value, then we say the result of the test is statistically significant.
Quantitative variables are any variables where the data represent amounts (e.g. height, weight, or age).
Categorical variables are any variables where the data represent groups. This includes rankings (e.g. finishing places in a race), classifications (e.g. brands of cereal), and binary outcomes (e.g. coin flips).
You need to know what type of variables you are working with to choose the right statistical test for your data and interpret your results .
Discrete and continuous variables are two types of quantitative variables :
- Discrete variables represent counts (e.g. the number of objects in a collection).
- Continuous variables represent measurable amounts (e.g. water volume or weight).
Cite this Scribbr article
If you want to cite this source, you can copy and paste the citation or click the “Cite this Scribbr article” button to automatically add the citation to our free Citation Generator.
Bevans, R. (2023, June 22). Choosing the Right Statistical Test | Types & Examples. Scribbr. Retrieved April 16, 2024, from https://www.scribbr.com/statistics/statistical-tests/
Is this article helpful?
Rebecca Bevans
Other students also liked, hypothesis testing | a step-by-step guide with easy examples, test statistics | definition, interpretation, and examples, normal distribution | examples, formulas, & uses, what is your plagiarism score.
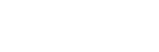
Statistical Science
- Undergraduate theses
- Navigating the library and website
- Off-campus access
- Finding information @ Duke
- Data sets & collections
- Data & visualization services This link opens in a new window
- Statistics consulting This link opens in a new window
- Citing sources
- Excel This link opens in a new window
- Bayesian statistics
- Actuarial science
- Sports analytics
Science Librarian

Ask a Librarian
Submit thesis to dukespace.
If you are an undergraduate honors student interested in submitting your thesis to DukeSpace , Duke University's online repository for publications and other archival materials in digital format, please contact Joan Durso to get this process started.
DukeSpace Electronic Theses and Dissertations (ETD) Submission Tutorial
- DukeSpace Electronic Theses and Dissertation Self-Submission Guide
Need help submitting your thesis? Contact [email protected] .
- << Previous: Sports analytics
- Last Updated: Apr 10, 2024 11:17 AM
- URL: https://guides.library.duke.edu/stats

Services for...
- Faculty & Instructors
- Graduate Students
- Undergraduate Students
- International Students
- Patrons with Disabilities

- Harmful Language Statement
- Re-use & Attribution / Privacy
- Support the Libraries

- Weblog home
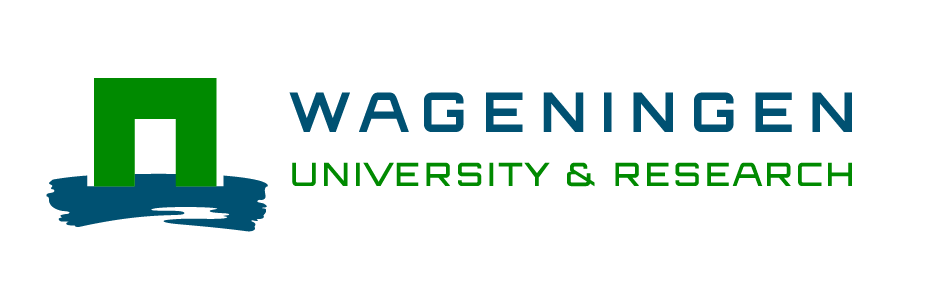
International Students blog
Thesis life: 7 ways to tackle statistics in your thesis.
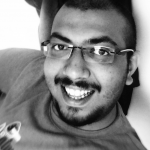
By Pranav Kulkarni
Thesis is an integral part of your Masters’ study in Wageningen University and Research. It is the most exciting, independent and technical part of the study. More often than not, most departments in WU expect students to complete a short term independent project or a part of big on-going project for their thesis assignment.
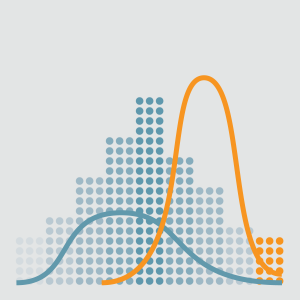
Source : www.coursera.org
This assignment involves proposing a research question, tackling it with help of some observations or experiments, analyzing these observations or results and then stating them by drawing some conclusions.
Since it is an immitigable part of your thesis, you can neither run from statistics nor cry for help.
The penultimate part of this process involves analysis of results which is very crucial for coherence of your thesis assignment.This analysis usually involve use of statistical tools to help draw inferences. Most students who don’t pursue statistics in their curriculum are scared by this prospect. Since it is an immitigable part of your thesis, you can neither run from statistics nor cry for help. But in order to not get intimidated by statistics and its “greco-latin” language, there are a few ways in which you can make your journey through thesis life a pleasant experience.
Make statistics your friend
The best way to end your fear of statistics and all its paraphernalia is to befriend it. Try to learn all that you can about the techniques that you will be using, why they were invented, how they were invented and who did this deed. Personifying the story of statistical techniques makes them digestible and easy to use. Each new method in statistics comes with a unique story and loads of nerdy anecdotes.
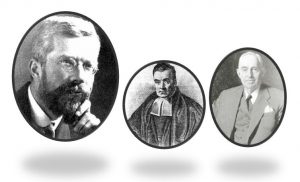
If you cannot make friends with statistics, at least make a truce
If you cannot still bring yourself about to be interested in the life and times of statistics, the best way to not hate statistics is to make an agreement with yourself. You must realise that although important, this is only part of your thesis. The better part of your thesis is something you trained for and learned. So, don’t bother to fuss about statistics and make you all nervous. Do your job, enjoy thesis to the fullest and complete the statistical section as soon as possible. At the end, you would have forgotten all about your worries and fears of statistics.
Visualize your data
The best way to understand the results and observations from your study/ experiments, is to visualize your data. See different trends, patterns, or lack thereof to understand what you are supposed to do. Moreover, graphics and illustrations can be used directly in your report. These techniques will also help you decide on which statistical analyses you must perform to answer your research question. Blind decisions about statistics can often influence your study and make it very confusing or worse, make it completely wrong!
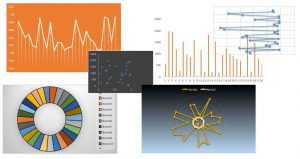
Simplify with flowcharts and planning
Similar to graphical visualizations, making flowcharts and planning various steps of your study can prove beneficial to make statistical decisions. Human brain can analyse pictorial information faster than literal information. So, it is always easier to understand your exact goal when you can make decisions based on flowchart or any logical flow-plans.
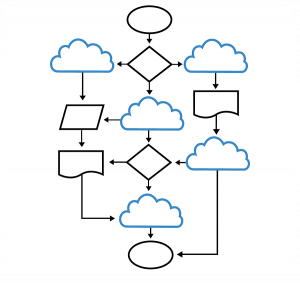
Source: www.imindq.com
Find examples on internet
Although statistics is a giant maze of complicated terminologies, the internet holds the key to this particular maze. You can find tons of examples on the web. These may be similar to what you intend to do or be different applications of the similar tools that you wish to engage. Especially, in case of Statistical programming languages like R, SAS, Python, PERL, VBA, etc. there is a vast database of example codes, clarifications and direct training examples available on the internet. Various forums are also available for specialized statistical methodologies where different experts and students discuss the issues regarding their own projects.
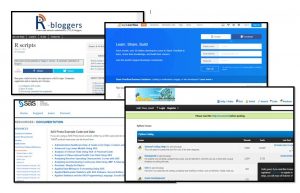
Comparative studies
Much unlike blindly searching the internet for examples and taking word of advice from online faceless people, you can systematically learn which quantitative tests to perform by rigorously studying literature of relevant research. Since you came up with a certain problem to tackle in your field of study, chances are, someone else also came up with this issue or something quite similar. You can find solutions to many such problems by scouring the internet for research papers which address the issue. Nevertheless, you should be cautious. It is easy to get lost and disheartened when you find many heavy statistical studies with lots of maths and derivations with huge cryptic symbolical text.
When all else fails, talk to an expert
All the steps above are meant to help you independently tackle whatever hurdles you encounter over the course of your thesis. But, when you cannot tackle them yourself it is always prudent and most efficient to ask for help. Talking to students from your thesis ring who have done something similar is one way of help. Another is to make an appointment with your supervisor and take specific questions to him/ her. If that is not possible, you can contact some other teaching staff or researchers from your research group. Try not to waste their as well as you time by making a list of specific problems that you will like to discuss. I think most are happy to help in any way possible.
Talking to students from your thesis ring who have done something similar is one way of help.
Sometimes, with the help of your supervisor, you can make an appointment with someone from the “Biometris” which is the WU’s statistics department. These people are the real deal; chances are, these people can solve all your problems without any difficulty. Always remember, you are in the process of learning, nobody expects you to be an expert in everything. Ask for help when there seems to be no hope.
Apart from these seven ways to make your statistical journey pleasant, you should always engage in reading, watching, listening to stuff relevant to your thesis topic and talking about it to those who are interested. Most questions have solutions in the ether realm of communication. So, best of luck and break a leg!!!
Related posts:
No related posts.
MSc Animal Science
View articles
There are 4 comments.
A perfect approach in a very crisp and clear manner! The sequence suggested is absolutely perfect and will help the students very much. I particularly liked the idea of visualisation!
You are write! I get totally stuck with learning and understanding statistics for my Dissertation!
Statistics is a technical subject that requires extra effort. With the highlighted tips you already highlighted i expect it will offer the much needed help with statistics analysis in my course.
this is so much relevant to me! Don’t forget one more point: try to enrol specific online statistics course (in my case, I’m too late to join any statistic course). The hardest part for me actually to choose what type of statistical test to choose among many options
Leave a reply Cancel reply
Your email address will not be published. Required fields are marked *

An official website of the United States government
The .gov means it’s official. Federal government websites often end in .gov or .mil. Before sharing sensitive information, make sure you’re on a federal government site.
The site is secure. The https:// ensures that you are connecting to the official website and that any information you provide is encrypted and transmitted securely.
- Publications
- Account settings
Preview improvements coming to the PMC website in October 2024. Learn More or Try it out now .
- Advanced Search
- Journal List
- Indian J Anaesth
- v.60(9); 2016 Sep
Basic statistical tools in research and data analysis
Zulfiqar ali.
Department of Anaesthesiology, Division of Neuroanaesthesiology, Sheri Kashmir Institute of Medical Sciences, Soura, Srinagar, Jammu and Kashmir, India
S Bala Bhaskar
1 Department of Anaesthesiology and Critical Care, Vijayanagar Institute of Medical Sciences, Bellary, Karnataka, India
Statistical methods involved in carrying out a study include planning, designing, collecting data, analysing, drawing meaningful interpretation and reporting of the research findings. The statistical analysis gives meaning to the meaningless numbers, thereby breathing life into a lifeless data. The results and inferences are precise only if proper statistical tests are used. This article will try to acquaint the reader with the basic research tools that are utilised while conducting various studies. The article covers a brief outline of the variables, an understanding of quantitative and qualitative variables and the measures of central tendency. An idea of the sample size estimation, power analysis and the statistical errors is given. Finally, there is a summary of parametric and non-parametric tests used for data analysis.
INTRODUCTION
Statistics is a branch of science that deals with the collection, organisation, analysis of data and drawing of inferences from the samples to the whole population.[ 1 ] This requires a proper design of the study, an appropriate selection of the study sample and choice of a suitable statistical test. An adequate knowledge of statistics is necessary for proper designing of an epidemiological study or a clinical trial. Improper statistical methods may result in erroneous conclusions which may lead to unethical practice.[ 2 ]
Variable is a characteristic that varies from one individual member of population to another individual.[ 3 ] Variables such as height and weight are measured by some type of scale, convey quantitative information and are called as quantitative variables. Sex and eye colour give qualitative information and are called as qualitative variables[ 3 ] [ Figure 1 ].

Classification of variables
Quantitative variables
Quantitative or numerical data are subdivided into discrete and continuous measurements. Discrete numerical data are recorded as a whole number such as 0, 1, 2, 3,… (integer), whereas continuous data can assume any value. Observations that can be counted constitute the discrete data and observations that can be measured constitute the continuous data. Examples of discrete data are number of episodes of respiratory arrests or the number of re-intubations in an intensive care unit. Similarly, examples of continuous data are the serial serum glucose levels, partial pressure of oxygen in arterial blood and the oesophageal temperature.
A hierarchical scale of increasing precision can be used for observing and recording the data which is based on categorical, ordinal, interval and ratio scales [ Figure 1 ].
Categorical or nominal variables are unordered. The data are merely classified into categories and cannot be arranged in any particular order. If only two categories exist (as in gender male and female), it is called as a dichotomous (or binary) data. The various causes of re-intubation in an intensive care unit due to upper airway obstruction, impaired clearance of secretions, hypoxemia, hypercapnia, pulmonary oedema and neurological impairment are examples of categorical variables.
Ordinal variables have a clear ordering between the variables. However, the ordered data may not have equal intervals. Examples are the American Society of Anesthesiologists status or Richmond agitation-sedation scale.
Interval variables are similar to an ordinal variable, except that the intervals between the values of the interval variable are equally spaced. A good example of an interval scale is the Fahrenheit degree scale used to measure temperature. With the Fahrenheit scale, the difference between 70° and 75° is equal to the difference between 80° and 85°: The units of measurement are equal throughout the full range of the scale.
Ratio scales are similar to interval scales, in that equal differences between scale values have equal quantitative meaning. However, ratio scales also have a true zero point, which gives them an additional property. For example, the system of centimetres is an example of a ratio scale. There is a true zero point and the value of 0 cm means a complete absence of length. The thyromental distance of 6 cm in an adult may be twice that of a child in whom it may be 3 cm.
STATISTICS: DESCRIPTIVE AND INFERENTIAL STATISTICS
Descriptive statistics[ 4 ] try to describe the relationship between variables in a sample or population. Descriptive statistics provide a summary of data in the form of mean, median and mode. Inferential statistics[ 4 ] use a random sample of data taken from a population to describe and make inferences about the whole population. It is valuable when it is not possible to examine each member of an entire population. The examples if descriptive and inferential statistics are illustrated in Table 1 .
Example of descriptive and inferential statistics

Descriptive statistics
The extent to which the observations cluster around a central location is described by the central tendency and the spread towards the extremes is described by the degree of dispersion.
Measures of central tendency
The measures of central tendency are mean, median and mode.[ 6 ] Mean (or the arithmetic average) is the sum of all the scores divided by the number of scores. Mean may be influenced profoundly by the extreme variables. For example, the average stay of organophosphorus poisoning patients in ICU may be influenced by a single patient who stays in ICU for around 5 months because of septicaemia. The extreme values are called outliers. The formula for the mean is

where x = each observation and n = number of observations. Median[ 6 ] is defined as the middle of a distribution in a ranked data (with half of the variables in the sample above and half below the median value) while mode is the most frequently occurring variable in a distribution. Range defines the spread, or variability, of a sample.[ 7 ] It is described by the minimum and maximum values of the variables. If we rank the data and after ranking, group the observations into percentiles, we can get better information of the pattern of spread of the variables. In percentiles, we rank the observations into 100 equal parts. We can then describe 25%, 50%, 75% or any other percentile amount. The median is the 50 th percentile. The interquartile range will be the observations in the middle 50% of the observations about the median (25 th -75 th percentile). Variance[ 7 ] is a measure of how spread out is the distribution. It gives an indication of how close an individual observation clusters about the mean value. The variance of a population is defined by the following formula:

where σ 2 is the population variance, X is the population mean, X i is the i th element from the population and N is the number of elements in the population. The variance of a sample is defined by slightly different formula:

where s 2 is the sample variance, x is the sample mean, x i is the i th element from the sample and n is the number of elements in the sample. The formula for the variance of a population has the value ‘ n ’ as the denominator. The expression ‘ n −1’ is known as the degrees of freedom and is one less than the number of parameters. Each observation is free to vary, except the last one which must be a defined value. The variance is measured in squared units. To make the interpretation of the data simple and to retain the basic unit of observation, the square root of variance is used. The square root of the variance is the standard deviation (SD).[ 8 ] The SD of a population is defined by the following formula:

where σ is the population SD, X is the population mean, X i is the i th element from the population and N is the number of elements in the population. The SD of a sample is defined by slightly different formula:

where s is the sample SD, x is the sample mean, x i is the i th element from the sample and n is the number of elements in the sample. An example for calculation of variation and SD is illustrated in Table 2 .
Example of mean, variance, standard deviation

Normal distribution or Gaussian distribution
Most of the biological variables usually cluster around a central value, with symmetrical positive and negative deviations about this point.[ 1 ] The standard normal distribution curve is a symmetrical bell-shaped. In a normal distribution curve, about 68% of the scores are within 1 SD of the mean. Around 95% of the scores are within 2 SDs of the mean and 99% within 3 SDs of the mean [ Figure 2 ].

Normal distribution curve
Skewed distribution
It is a distribution with an asymmetry of the variables about its mean. In a negatively skewed distribution [ Figure 3 ], the mass of the distribution is concentrated on the right of Figure 1 . In a positively skewed distribution [ Figure 3 ], the mass of the distribution is concentrated on the left of the figure leading to a longer right tail.

Curves showing negatively skewed and positively skewed distribution
Inferential statistics
In inferential statistics, data are analysed from a sample to make inferences in the larger collection of the population. The purpose is to answer or test the hypotheses. A hypothesis (plural hypotheses) is a proposed explanation for a phenomenon. Hypothesis tests are thus procedures for making rational decisions about the reality of observed effects.
Probability is the measure of the likelihood that an event will occur. Probability is quantified as a number between 0 and 1 (where 0 indicates impossibility and 1 indicates certainty).
In inferential statistics, the term ‘null hypothesis’ ( H 0 ‘ H-naught ,’ ‘ H-null ’) denotes that there is no relationship (difference) between the population variables in question.[ 9 ]
Alternative hypothesis ( H 1 and H a ) denotes that a statement between the variables is expected to be true.[ 9 ]
The P value (or the calculated probability) is the probability of the event occurring by chance if the null hypothesis is true. The P value is a numerical between 0 and 1 and is interpreted by researchers in deciding whether to reject or retain the null hypothesis [ Table 3 ].
P values with interpretation

If P value is less than the arbitrarily chosen value (known as α or the significance level), the null hypothesis (H0) is rejected [ Table 4 ]. However, if null hypotheses (H0) is incorrectly rejected, this is known as a Type I error.[ 11 ] Further details regarding alpha error, beta error and sample size calculation and factors influencing them are dealt with in another section of this issue by Das S et al .[ 12 ]
Illustration for null hypothesis

PARAMETRIC AND NON-PARAMETRIC TESTS
Numerical data (quantitative variables) that are normally distributed are analysed with parametric tests.[ 13 ]
Two most basic prerequisites for parametric statistical analysis are:
- The assumption of normality which specifies that the means of the sample group are normally distributed
- The assumption of equal variance which specifies that the variances of the samples and of their corresponding population are equal.
However, if the distribution of the sample is skewed towards one side or the distribution is unknown due to the small sample size, non-parametric[ 14 ] statistical techniques are used. Non-parametric tests are used to analyse ordinal and categorical data.
Parametric tests
The parametric tests assume that the data are on a quantitative (numerical) scale, with a normal distribution of the underlying population. The samples have the same variance (homogeneity of variances). The samples are randomly drawn from the population, and the observations within a group are independent of each other. The commonly used parametric tests are the Student's t -test, analysis of variance (ANOVA) and repeated measures ANOVA.
Student's t -test
Student's t -test is used to test the null hypothesis that there is no difference between the means of the two groups. It is used in three circumstances:

where X = sample mean, u = population mean and SE = standard error of mean

where X 1 − X 2 is the difference between the means of the two groups and SE denotes the standard error of the difference.
- To test if the population means estimated by two dependent samples differ significantly (the paired t -test). A usual setting for paired t -test is when measurements are made on the same subjects before and after a treatment.
The formula for paired t -test is:

where d is the mean difference and SE denotes the standard error of this difference.
The group variances can be compared using the F -test. The F -test is the ratio of variances (var l/var 2). If F differs significantly from 1.0, then it is concluded that the group variances differ significantly.
Analysis of variance
The Student's t -test cannot be used for comparison of three or more groups. The purpose of ANOVA is to test if there is any significant difference between the means of two or more groups.
In ANOVA, we study two variances – (a) between-group variability and (b) within-group variability. The within-group variability (error variance) is the variation that cannot be accounted for in the study design. It is based on random differences present in our samples.
However, the between-group (or effect variance) is the result of our treatment. These two estimates of variances are compared using the F-test.
A simplified formula for the F statistic is:

where MS b is the mean squares between the groups and MS w is the mean squares within groups.
Repeated measures analysis of variance
As with ANOVA, repeated measures ANOVA analyses the equality of means of three or more groups. However, a repeated measure ANOVA is used when all variables of a sample are measured under different conditions or at different points in time.
As the variables are measured from a sample at different points of time, the measurement of the dependent variable is repeated. Using a standard ANOVA in this case is not appropriate because it fails to model the correlation between the repeated measures: The data violate the ANOVA assumption of independence. Hence, in the measurement of repeated dependent variables, repeated measures ANOVA should be used.
Non-parametric tests
When the assumptions of normality are not met, and the sample means are not normally, distributed parametric tests can lead to erroneous results. Non-parametric tests (distribution-free test) are used in such situation as they do not require the normality assumption.[ 15 ] Non-parametric tests may fail to detect a significant difference when compared with a parametric test. That is, they usually have less power.
As is done for the parametric tests, the test statistic is compared with known values for the sampling distribution of that statistic and the null hypothesis is accepted or rejected. The types of non-parametric analysis techniques and the corresponding parametric analysis techniques are delineated in Table 5 .
Analogue of parametric and non-parametric tests

Median test for one sample: The sign test and Wilcoxon's signed rank test
The sign test and Wilcoxon's signed rank test are used for median tests of one sample. These tests examine whether one instance of sample data is greater or smaller than the median reference value.
This test examines the hypothesis about the median θ0 of a population. It tests the null hypothesis H0 = θ0. When the observed value (Xi) is greater than the reference value (θ0), it is marked as+. If the observed value is smaller than the reference value, it is marked as − sign. If the observed value is equal to the reference value (θ0), it is eliminated from the sample.
If the null hypothesis is true, there will be an equal number of + signs and − signs.
The sign test ignores the actual values of the data and only uses + or − signs. Therefore, it is useful when it is difficult to measure the values.
Wilcoxon's signed rank test
There is a major limitation of sign test as we lose the quantitative information of the given data and merely use the + or – signs. Wilcoxon's signed rank test not only examines the observed values in comparison with θ0 but also takes into consideration the relative sizes, adding more statistical power to the test. As in the sign test, if there is an observed value that is equal to the reference value θ0, this observed value is eliminated from the sample.
Wilcoxon's rank sum test ranks all data points in order, calculates the rank sum of each sample and compares the difference in the rank sums.
Mann-Whitney test
It is used to test the null hypothesis that two samples have the same median or, alternatively, whether observations in one sample tend to be larger than observations in the other.
Mann–Whitney test compares all data (xi) belonging to the X group and all data (yi) belonging to the Y group and calculates the probability of xi being greater than yi: P (xi > yi). The null hypothesis states that P (xi > yi) = P (xi < yi) =1/2 while the alternative hypothesis states that P (xi > yi) ≠1/2.
Kolmogorov-Smirnov test
The two-sample Kolmogorov-Smirnov (KS) test was designed as a generic method to test whether two random samples are drawn from the same distribution. The null hypothesis of the KS test is that both distributions are identical. The statistic of the KS test is a distance between the two empirical distributions, computed as the maximum absolute difference between their cumulative curves.
Kruskal-Wallis test
The Kruskal–Wallis test is a non-parametric test to analyse the variance.[ 14 ] It analyses if there is any difference in the median values of three or more independent samples. The data values are ranked in an increasing order, and the rank sums calculated followed by calculation of the test statistic.
Jonckheere test
In contrast to Kruskal–Wallis test, in Jonckheere test, there is an a priori ordering that gives it a more statistical power than the Kruskal–Wallis test.[ 14 ]
Friedman test
The Friedman test is a non-parametric test for testing the difference between several related samples. The Friedman test is an alternative for repeated measures ANOVAs which is used when the same parameter has been measured under different conditions on the same subjects.[ 13 ]
Tests to analyse the categorical data
Chi-square test, Fischer's exact test and McNemar's test are used to analyse the categorical or nominal variables. The Chi-square test compares the frequencies and tests whether the observed data differ significantly from that of the expected data if there were no differences between groups (i.e., the null hypothesis). It is calculated by the sum of the squared difference between observed ( O ) and the expected ( E ) data (or the deviation, d ) divided by the expected data by the following formula:

A Yates correction factor is used when the sample size is small. Fischer's exact test is used to determine if there are non-random associations between two categorical variables. It does not assume random sampling, and instead of referring a calculated statistic to a sampling distribution, it calculates an exact probability. McNemar's test is used for paired nominal data. It is applied to 2 × 2 table with paired-dependent samples. It is used to determine whether the row and column frequencies are equal (that is, whether there is ‘marginal homogeneity’). The null hypothesis is that the paired proportions are equal. The Mantel-Haenszel Chi-square test is a multivariate test as it analyses multiple grouping variables. It stratifies according to the nominated confounding variables and identifies any that affects the primary outcome variable. If the outcome variable is dichotomous, then logistic regression is used.
SOFTWARES AVAILABLE FOR STATISTICS, SAMPLE SIZE CALCULATION AND POWER ANALYSIS
Numerous statistical software systems are available currently. The commonly used software systems are Statistical Package for the Social Sciences (SPSS – manufactured by IBM corporation), Statistical Analysis System ((SAS – developed by SAS Institute North Carolina, United States of America), R (designed by Ross Ihaka and Robert Gentleman from R core team), Minitab (developed by Minitab Inc), Stata (developed by StataCorp) and the MS Excel (developed by Microsoft).
There are a number of web resources which are related to statistical power analyses. A few are:
- StatPages.net – provides links to a number of online power calculators
- G-Power – provides a downloadable power analysis program that runs under DOS
- Power analysis for ANOVA designs an interactive site that calculates power or sample size needed to attain a given power for one effect in a factorial ANOVA design
- SPSS makes a program called SamplePower. It gives an output of a complete report on the computer screen which can be cut and paste into another document.
It is important that a researcher knows the concepts of the basic statistical methods used for conduct of a research study. This will help to conduct an appropriately well-designed study leading to valid and reliable results. Inappropriate use of statistical techniques may lead to faulty conclusions, inducing errors and undermining the significance of the article. Bad statistics may lead to bad research, and bad research may lead to unethical practice. Hence, an adequate knowledge of statistics and the appropriate use of statistical tests are important. An appropriate knowledge about the basic statistical methods will go a long way in improving the research designs and producing quality medical research which can be utilised for formulating the evidence-based guidelines.
Financial support and sponsorship
Conflicts of interest.
There are no conflicts of interest.
Secondary Menu
- Master's Thesis
As an integral component of the Master of Science in Statistical Science program, you can submit and defend a Master's Thesis. Your Master's Committee administers this oral examination. If you choose to defend a thesis, it is advisable to commence your research early, ideally during your second semester or the summer following your first year in the program. It's essential to allocate sufficient time for the thesis writing process. Your thesis advisor, who also serves as the committee chair, must approve both your thesis title and proposal. The final thesis work necessitates approval from all committee members and must adhere to the Master's thesis requirements set forth by the Duke University Graduate School.
Master’s BEST Award
Each second-year Duke Master’s of Statistical Science (MSS) student defending their MSS thesis may be eligible for the Master’s BEST Award . The Statistical Science faculty BEST Award Committee selects the awardee based on the submitted thesis of MSS thesis students, and the award is presented at the departmental graduation ceremony.
Thesis Proposal
All second-year students choosing to do a thesis must submit a proposal (not more than two pages) approved by their thesis advisor to the Master's Director via Qualtrics by November 10th. The thesis proposal should include a title, the thesis advisor, committee members, and a description of your work. The description must introduce the research topic, outline its main objectives, and emphasize the significance of the research and its implications while identifying gaps in existing statistical literature. In addition, it can include some of the preliminary results.
Committee members
MSS Students will have a thesis committee, which includes three faculty members - two must be departmental primary faculty, and the third could be from an external department in an applied area of the student’s interest, which must be a Term Graduate Faculty through the Graduate School or have a secondary appointment with the Department of Statistical Science. All Committee members must be familiar with the Student’s work. The department coordinates Committee approval. The thesis defense committee must be approved at least 30 days before the defense date.
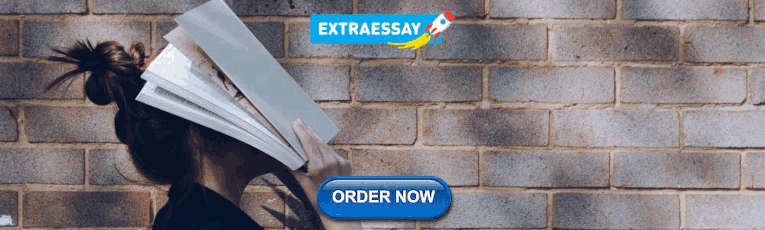
Thesis Timeline and Departmental Process:
Before defense:.
Intent to Graduate: Students must file an Intent to Graduate in ACES, specifying "Thesis Defense" during the application. For graduation deadlines, please refer to https://gradschool.duke.edu/academics/preparing-graduate .
Scheduling Thesis Defense: The student collaborates with the committee to set the date and time for the defense and communicates this information to the department, along with the thesis title. The defense must be scheduled during regular class sessions. Be sure to review the thesis defense and submission deadlines at https://gradschool.duke.edu/academics/theses-and-dissertations/
Room Reservations: The department arranges room reservations and sends confirmation details to the student, who informs committee members of the location.
Defense Announcement: The department prepares a defense announcement, providing a copy to the student and chair. After approval, it is signed by the Master's Director and submitted to the Graduate School. Copies are also posted on department bulletin boards.
Initial Thesis Submission: Two weeks before the defense, the student submits the initial thesis to the committee and the Graduate School. Detailed thesis formatting guidelines can be found at https://gradschool.duke.edu/academics/theses-and-dissertations.
Advisor Notification: The student requests that the advisor email [email protected] , confirming the candidate's readiness for defense. This step should be completed before the exam card appointment.
Format Check Appointment: One week before the defense, the Graduate School contacts the student to schedule a format check appointment. Upon approval, the Graduate School provides the Student Master’s Exam Card, which enables the student to send a revised thesis copy to committee members.
MSS Annual Report Form: The department provides the student with the MSS Annual Report Form to be presented at the defense.
Post Defense:
Communication of Defense Outcome: The committee chair conveys the defense results to the student, including any necessary follow-up actions in case of an unsuccessful defense.
In Case of Failure: If a student does not pass the thesis defense, the committee's decision to fail the student must be accompanied by explicit and clear comments from the chair, specifying deficiencies and areas that require attention for improvement.
Documentation: The student should ensure that the committee signs the Title Page, Abstract Page, and Exam Card.
Annual Report Form: The committee chair completes the Annual Report Form.
Master's Director Approval: The Master's director must provide their approval by signing the Exam Card.
Form Submission: Lastly, the committee chair is responsible for returning all completed and signed forms to the Department.
Final Thesis Submission: The student must meet the Graduate School requirement by submitting the final version of their Thesis to the Graduate School via ProQuest before the specified deadline. For detailed information, visit https://gradschool.duke.edu/academics/preparinggraduate .
- The Stochastic Proximal Distance Algorithm
- Logistic-tree Normal Mixture for Clustering Microbiome Compositions
- Inference for Dynamic Treatment Regimes using Overlapping Sampling Splitting
- Bayesian Modeling for Identifying Selection in B Cell Maturation
- Differentially Private Verification with Survey Weights
- Stable Variable Selection for Sparse Linear Regression in a Non-uniqueness Regime
- A Cost-Sensitive, Semi-Supervised, and Active Learning Approach for Priority Outlier Investigation
- Bayesian Decoupling: A Decision Theory-Based Approach to Bayesian Variable Selection
- A Differentially Private Bayesian Approach to Replication Analysis
- Numerical Approximation of Gaussian-Smoothed Optimal Transport
- Computational Challenges to Bayesian Density Discontinuity Regression
- Hierarchical Signal Propagation for Household Level Sales in Bayesian Dynamic Models
- Logistic Tree Gaussian Processes (LoTgGaP) for Microbiome Dynamics and Treatment Effects
- Bayesian Inference on Ratios Subject to Differentially Private Noise
- Multiple Imputation Inferences for Count Data
- An Euler Characteristic Curve Based Representation of 3D Shapes in Statistical Analysis
- An Investigation Into the Bias & Variance of Almost Matching Exactly Methods
- Comparison of Bayesian Inference Methods for Probit Network Models
- Differentially Private Counts with Additive Constraints
- Multi-Scale Graph Principal Component Analysis for Connectomics
- MCMC Sampling Geospatial Partitions for Linear Models
- Bayesian Dynamic Network Modeling with Censored Flow Data
- An Application of Graph Diffusion for Gesture Classification
- Easy and Efficient Bayesian Infinite Factor Analysis
- Analyzing Amazon CD Reviews with Bayesian Monitoring and Machine Learning Methods
- Missing Data Imputation for Voter Turnout Using Auxiliary Margins
- Generalized and Scalable Optimal Sparse Decision Trees
- Construction of Objective Bayesian Prior from Bertrand’s Paradox and the Principle of Indifference
- Rethinking Non-Linear Instrumental Variables
- Clustering-Enhanced Stochastic Gradient MCMC for Hidden Markov Models
- Optimal Sparse Decision Trees
- Bayesian Density Regression with a Jump Discontinuity at a Given Threshold
- Forecasting the Term Structure of Interest Rates: A Bayesian Dynamic Graphical Modeling Approach
- Testing Between Different Types of Poisson Mixtures with Applications to Neuroscience
- Multiple Imputation of Missing Covariates in Randomized Controlled Trials
- A Bayesian Strategy to the 20 Question Game with Applications to Recommender Systems
- Applied Factor Dynamic Analysis for Macroeconomic Forecasting
- A Theory of Statistical Inference for Ensuring the Robustness of Scientific Results
- Bayesian Inference Via Partitioning Under Differential Privacy
- A Bayesian Forward Simulation Approach to Establishing a Realistic Prior Model for Complex Geometrical Objects
- Two Applications of Summary Statistics: Integrating Information Across Genes and Confidence Intervals with Missing Data
- Our Mission
- Diversity, Equity, and Inclusion
- International Recognition
- Department History
- Past Recipients
- Considering a Statistical Science major at Duke?
- Careers for Statisticians
- Typical Pathways
- Applied Electives for BS
- Interdepartmental Majors
- Minor in Statistical Science
- Getting Started with Statistics
- Student Learning Outcomes
- Study Abroad
- Course Help & Tutoring
- Past Theses
- Research Teams
- Independent Study
- Transfer Credit
- Conference Funding for Research
- Statistical Science Majors Union
- Duke Actuarial Society
- Duke Sports Analytics Club
- Trinity Ambassadors
- Frequently Asked Questions
- Summer Session Courses
- How to Apply
- Financial Support
- Graduate Placements
- Living in Durham
- Preliminary Examination
- Dissertation
- English Language Requirement
- TA Guidelines
- Progress Toward Completion
- Ph.D. Committees
- Terminal MS Degree
- Student Governance
- Program Requirements
- PhD / Research
- Data Science & Analytics
- Health Data Science
- Finance & Economics
- Marketing Research & Business Analytics
- Social Science & Policy
- Admission Statistics
- Portfolio of Work
- Capstone Project
- Statistical Science Proseminar
- Primary Faculty
- Secondary Faculty
- Visiting Faculty
- Postdoctoral Fellows
- Ph.D. Students
- M.S. Students
- Theory, Methods, and Computation
- Interdisciplinary Collaborations
- Statistical Consulting Center
- Alumni Profiles
- For Current Students
- Assisting Duke Students
- StatSci Alumni Network
- Ph.D. Student - Alumni Fund
- Our Ph.D. Alums
- Our M.S. Alums
- Our Undergrad Alums
- Our Postdoc Alums
- How it works
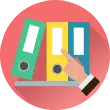
Dissertation Statistical Analysis Samples and Examples
Are you done with the rest of your dissertation but unable to perform the statistical analysis? To help you overcome this issue, our professionals have gathered a list of samples of dissertation statistical analysis for you to take inspiration from and start working on your dissertation. These high-quality dissertation statistical analysis samples have been specially prepared to provide students with a path to follow. Our writers can work with all statistical analysis softwares, including SPSS, Stata, SAS, R, MATLAB, JMP, Python, and Excel.
Dissertation Statistical Analysis Sample
Discipline: Linguistic
Quality: 2:1 / 69%
Discipline: Public Health
Based on the graphical representation, at least 49% of the total participants have a household..
Statistical Analysis
Geography Statistical
The graphs below show the distribution companies’ operating margins..
Our dissertation statistical analysis service features, subject specialists.
We have writers specialising in their respective subjects to ensure high quality.
Thoroughly Researched
We make sure that the content is thoroughly researched and referenced.
Analysis Software
We work in all statistical softwares and can use the one according to your requirements.
Free Revisions
We offer free unlimited revisions until the client is satisfied with the results.
Our focus is on providing services that are affordable to everyone to help the majority of people.
On-Time Delivery
We deliver work timely, and if for some reason we fail, our refund policy is smooth.
Loved by over 100,000 students
Thousands of students have used ResearchProspect academic support services to improve their grades. Why are you waiting?

“I couldn’t figure out how to do statistical analysis for my literature dissertation. I saw their dissertation statistical analysis sample and placed my order. Very happy with the results!"
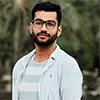
Law Student
“I want to thank ResearchProspect for my excellent grades in my dissertation. Their statistical analysis was very helpful."
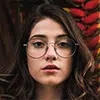
Economics Student
Frequently Ask Questions?
How our statistical analysis samples can help.
Statistical analysis is a critical aspect of a dissertation and makes up the fourth chapter of a thesis, i.e., results and findings. Statistical analysis is the collection and interpretation of data to reveal trends and patterns or test a hypothesis.
SPSS, STATA, reviews, R, Nvivo, SAS, and others are some of the most commonly used statistical analysis software in colleges and universities worldwide.
When conducting statistical analysis for your dissertation, you can expect to work with numbers, descriptions, and themes. Accurately identify your research variables in the preceding chapters. The data interpretation stems from establishing the aim and objectives.
Choosing the correct variables will help you group data according to your research objectives and conduct statistical analysis accurately.
Your topic, academic level, and academic subjective determine what type of statistical test you should conduct. Based on your research aim and objective, you will have to decide which tests should be conducted.
We suggest that you start your data analysis off by considering the following seven statistical techniques before moving to more complex techniques for quantitative data.
- Arithmetic Mean Statistical Analysis Technique
- Standard Deviation Statistical Analysis Technique
- Skewness Statistical Analysis Technique
- Hypothesis Testing Statistical Analysis Technique
- Regression Statistical Analysis Technique
- Correlation Statistical Analysis Technique
- Monte Carlo Simulation Statistical Analysis Technique
Interpret the results after statistical analysis. Include all relevant tables, graphs, and charts to present your data and results explicitly. You must provide a brief explanation justifying the relevance and signification of each table, graph, and chart.
Your data interpretation and results explanation should be simple and easy to understand so that readers can comprehend all results.
The dissertation statistical analysis is the meat of your study. After you have presented and interpreted the results, it is recommended that you crosscheck the data and the results. Errors in data handling can lead to incorrect analysis and interpretation.
Recheck the identified variables to make sure they are appropriate. Validate whether the data input process was error-free and the results obtained are reliable.
Finally, make sure to move any less relevant graphics and visuals to the appendices section.
Do not be hasty when conducting statistical analysis because readers are particularly interested in your research findings. Presenting incorrect data and misleading patterns can undermine your academic integrity. Make sure that you verify your data and results immediately after running tests.
How ResearchProspect can help?
Are you stuck with the statistical analysis of your dissertation? Unsure about the accuracy of the results? Whatever your situation is, get in touch with our team now if you have hit the dead end.
Our statistical analysis experts and highly qualified writers are here to help! We will handle your data collection and testing woes. The statistical analysis will be exactly in line with your research question or hypothesis and make your dissertation stand out.
What is the dissertation statistical analysis process?
On receiving your order, our customer services team will get in touch. We request prompt payment to avoid delays. We’ll find a suitable writer to get into your statistical analysis. It will require input from you so we can conduct appropriate analysis on your complete and uncontaminated data. This is how we can produce the cleanest most concise results.
What are the features of our dissertation statistical analysis service?
- Using our dissertation statistics service means you can be sure your data analysis will be completed by a writer with the relevant skills and experience.
- Every statistical analysis order we fulfil is measured against our strict quality control process. This ensures it’s in line with the necessary academic standards.
- You can specify the software you prefer the writer to use to carry out the statistical analysis: SPSS, Excel, STATA, eViews… we are familiar with them all.
- The dissertation statistical analysis service allows you free unlimited revisions until you are fully satisfied with the work. This is a standard part of the services we offer.
- When you receive your analysis, you will also get a plagiarism report, produced by our in-house plagiarism-checking software. It will confirm that your work is totally original and free of plagiarism.
Explore More Samples
View our professional samples to be certain that we have the portofilio and capabilities to deliver what you need.
USEFUL LINKS
LEARNING RESOURCES
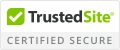
COMPANY DETAILS
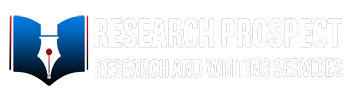
- How It Works
Have a thesis expert improve your writing
Check your thesis for plagiarism in 10 minutes, generate your apa citations for free.
- Knowledge Base
The Beginner's Guide to Statistical Analysis | 5 Steps & Examples
Statistical analysis means investigating trends, patterns, and relationships using quantitative data . It is an important research tool used by scientists, governments, businesses, and other organisations.
To draw valid conclusions, statistical analysis requires careful planning from the very start of the research process . You need to specify your hypotheses and make decisions about your research design, sample size, and sampling procedure.
After collecting data from your sample, you can organise and summarise the data using descriptive statistics . Then, you can use inferential statistics to formally test hypotheses and make estimates about the population. Finally, you can interpret and generalise your findings.
This article is a practical introduction to statistical analysis for students and researchers. We’ll walk you through the steps using two research examples. The first investigates a potential cause-and-effect relationship, while the second investigates a potential correlation between variables.
Table of contents
Step 1: write your hypotheses and plan your research design, step 2: collect data from a sample, step 3: summarise your data with descriptive statistics, step 4: test hypotheses or make estimates with inferential statistics, step 5: interpret your results, frequently asked questions about statistics.
To collect valid data for statistical analysis, you first need to specify your hypotheses and plan out your research design.
Writing statistical hypotheses
The goal of research is often to investigate a relationship between variables within a population . You start with a prediction, and use statistical analysis to test that prediction.
A statistical hypothesis is a formal way of writing a prediction about a population. Every research prediction is rephrased into null and alternative hypotheses that can be tested using sample data.
While the null hypothesis always predicts no effect or no relationship between variables, the alternative hypothesis states your research prediction of an effect or relationship.
- Null hypothesis: A 5-minute meditation exercise will have no effect on math test scores in teenagers.
- Alternative hypothesis: A 5-minute meditation exercise will improve math test scores in teenagers.
- Null hypothesis: Parental income and GPA have no relationship with each other in college students.
- Alternative hypothesis: Parental income and GPA are positively correlated in college students.
Planning your research design
A research design is your overall strategy for data collection and analysis. It determines the statistical tests you can use to test your hypothesis later on.
First, decide whether your research will use a descriptive, correlational, or experimental design. Experiments directly influence variables, whereas descriptive and correlational studies only measure variables.
- In an experimental design , you can assess a cause-and-effect relationship (e.g., the effect of meditation on test scores) using statistical tests of comparison or regression.
- In a correlational design , you can explore relationships between variables (e.g., parental income and GPA) without any assumption of causality using correlation coefficients and significance tests.
- In a descriptive design , you can study the characteristics of a population or phenomenon (e.g., the prevalence of anxiety in U.S. college students) using statistical tests to draw inferences from sample data.
Your research design also concerns whether you’ll compare participants at the group level or individual level, or both.
- In a between-subjects design , you compare the group-level outcomes of participants who have been exposed to different treatments (e.g., those who performed a meditation exercise vs those who didn’t).
- In a within-subjects design , you compare repeated measures from participants who have participated in all treatments of a study (e.g., scores from before and after performing a meditation exercise).
- In a mixed (factorial) design , one variable is altered between subjects and another is altered within subjects (e.g., pretest and posttest scores from participants who either did or didn’t do a meditation exercise).
- Experimental
- Correlational
First, you’ll take baseline test scores from participants. Then, your participants will undergo a 5-minute meditation exercise. Finally, you’ll record participants’ scores from a second math test.
In this experiment, the independent variable is the 5-minute meditation exercise, and the dependent variable is the math test score from before and after the intervention. Example: Correlational research design In a correlational study, you test whether there is a relationship between parental income and GPA in graduating college students. To collect your data, you will ask participants to fill in a survey and self-report their parents’ incomes and their own GPA.
Measuring variables
When planning a research design, you should operationalise your variables and decide exactly how you will measure them.
For statistical analysis, it’s important to consider the level of measurement of your variables, which tells you what kind of data they contain:
- Categorical data represents groupings. These may be nominal (e.g., gender) or ordinal (e.g. level of language ability).
- Quantitative data represents amounts. These may be on an interval scale (e.g. test score) or a ratio scale (e.g. age).
Many variables can be measured at different levels of precision. For example, age data can be quantitative (8 years old) or categorical (young). If a variable is coded numerically (e.g., level of agreement from 1–5), it doesn’t automatically mean that it’s quantitative instead of categorical.
Identifying the measurement level is important for choosing appropriate statistics and hypothesis tests. For example, you can calculate a mean score with quantitative data, but not with categorical data.
In a research study, along with measures of your variables of interest, you’ll often collect data on relevant participant characteristics.
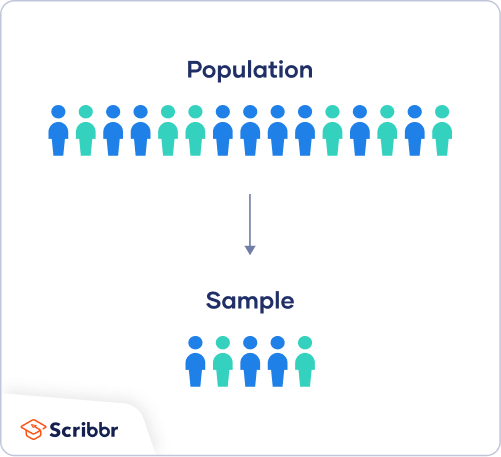
In most cases, it’s too difficult or expensive to collect data from every member of the population you’re interested in studying. Instead, you’ll collect data from a sample.
Statistical analysis allows you to apply your findings beyond your own sample as long as you use appropriate sampling procedures . You should aim for a sample that is representative of the population.
Sampling for statistical analysis
There are two main approaches to selecting a sample.
- Probability sampling: every member of the population has a chance of being selected for the study through random selection.
- Non-probability sampling: some members of the population are more likely than others to be selected for the study because of criteria such as convenience or voluntary self-selection.
In theory, for highly generalisable findings, you should use a probability sampling method. Random selection reduces sampling bias and ensures that data from your sample is actually typical of the population. Parametric tests can be used to make strong statistical inferences when data are collected using probability sampling.
But in practice, it’s rarely possible to gather the ideal sample. While non-probability samples are more likely to be biased, they are much easier to recruit and collect data from. Non-parametric tests are more appropriate for non-probability samples, but they result in weaker inferences about the population.
If you want to use parametric tests for non-probability samples, you have to make the case that:
- your sample is representative of the population you’re generalising your findings to.
- your sample lacks systematic bias.
Keep in mind that external validity means that you can only generalise your conclusions to others who share the characteristics of your sample. For instance, results from Western, Educated, Industrialised, Rich and Democratic samples (e.g., college students in the US) aren’t automatically applicable to all non-WEIRD populations.
If you apply parametric tests to data from non-probability samples, be sure to elaborate on the limitations of how far your results can be generalised in your discussion section .
Create an appropriate sampling procedure
Based on the resources available for your research, decide on how you’ll recruit participants.
- Will you have resources to advertise your study widely, including outside of your university setting?
- Will you have the means to recruit a diverse sample that represents a broad population?
- Do you have time to contact and follow up with members of hard-to-reach groups?
Your participants are self-selected by their schools. Although you’re using a non-probability sample, you aim for a diverse and representative sample. Example: Sampling (correlational study) Your main population of interest is male college students in the US. Using social media advertising, you recruit senior-year male college students from a smaller subpopulation: seven universities in the Boston area.
Calculate sufficient sample size
Before recruiting participants, decide on your sample size either by looking at other studies in your field or using statistics. A sample that’s too small may be unrepresentative of the sample, while a sample that’s too large will be more costly than necessary.
There are many sample size calculators online. Different formulas are used depending on whether you have subgroups or how rigorous your study should be (e.g., in clinical research). As a rule of thumb, a minimum of 30 units or more per subgroup is necessary.
To use these calculators, you have to understand and input these key components:
- Significance level (alpha): the risk of rejecting a true null hypothesis that you are willing to take, usually set at 5%.
- Statistical power : the probability of your study detecting an effect of a certain size if there is one, usually 80% or higher.
- Expected effect size : a standardised indication of how large the expected result of your study will be, usually based on other similar studies.
- Population standard deviation: an estimate of the population parameter based on a previous study or a pilot study of your own.
Once you’ve collected all of your data, you can inspect them and calculate descriptive statistics that summarise them.
Inspect your data
There are various ways to inspect your data, including the following:
- Organising data from each variable in frequency distribution tables .
- Displaying data from a key variable in a bar chart to view the distribution of responses.
- Visualising the relationship between two variables using a scatter plot .
By visualising your data in tables and graphs, you can assess whether your data follow a skewed or normal distribution and whether there are any outliers or missing data.
A normal distribution means that your data are symmetrically distributed around a center where most values lie, with the values tapering off at the tail ends.
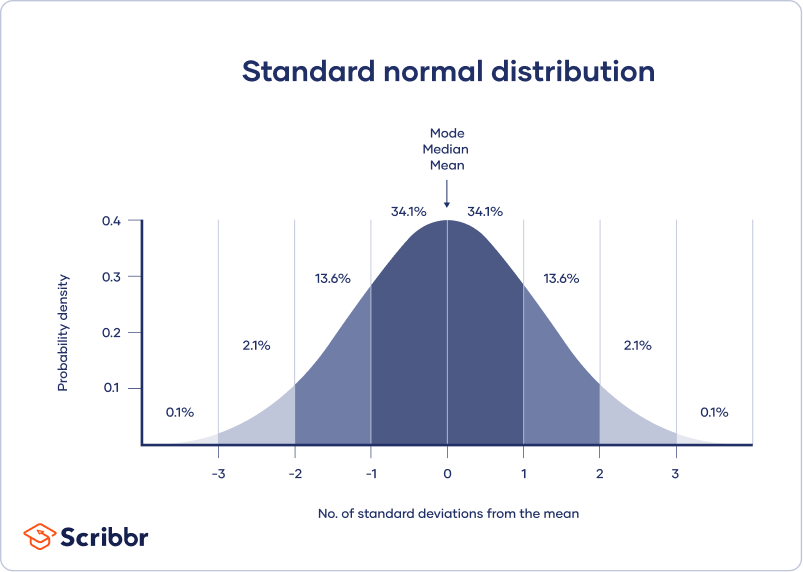
In contrast, a skewed distribution is asymmetric and has more values on one end than the other. The shape of the distribution is important to keep in mind because only some descriptive statistics should be used with skewed distributions.
Extreme outliers can also produce misleading statistics, so you may need a systematic approach to dealing with these values.
Calculate measures of central tendency
Measures of central tendency describe where most of the values in a data set lie. Three main measures of central tendency are often reported:
- Mode : the most popular response or value in the data set.
- Median : the value in the exact middle of the data set when ordered from low to high.
- Mean : the sum of all values divided by the number of values.
However, depending on the shape of the distribution and level of measurement, only one or two of these measures may be appropriate. For example, many demographic characteristics can only be described using the mode or proportions, while a variable like reaction time may not have a mode at all.
Calculate measures of variability
Measures of variability tell you how spread out the values in a data set are. Four main measures of variability are often reported:
- Range : the highest value minus the lowest value of the data set.
- Interquartile range : the range of the middle half of the data set.
- Standard deviation : the average distance between each value in your data set and the mean.
- Variance : the square of the standard deviation.
Once again, the shape of the distribution and level of measurement should guide your choice of variability statistics. The interquartile range is the best measure for skewed distributions, while standard deviation and variance provide the best information for normal distributions.
Using your table, you should check whether the units of the descriptive statistics are comparable for pretest and posttest scores. For example, are the variance levels similar across the groups? Are there any extreme values? If there are, you may need to identify and remove extreme outliers in your data set or transform your data before performing a statistical test.
From this table, we can see that the mean score increased after the meditation exercise, and the variances of the two scores are comparable. Next, we can perform a statistical test to find out if this improvement in test scores is statistically significant in the population. Example: Descriptive statistics (correlational study) After collecting data from 653 students, you tabulate descriptive statistics for annual parental income and GPA.
It’s important to check whether you have a broad range of data points. If you don’t, your data may be skewed towards some groups more than others (e.g., high academic achievers), and only limited inferences can be made about a relationship.
A number that describes a sample is called a statistic , while a number describing a population is called a parameter . Using inferential statistics , you can make conclusions about population parameters based on sample statistics.
Researchers often use two main methods (simultaneously) to make inferences in statistics.
- Estimation: calculating population parameters based on sample statistics.
- Hypothesis testing: a formal process for testing research predictions about the population using samples.
You can make two types of estimates of population parameters from sample statistics:
- A point estimate : a value that represents your best guess of the exact parameter.
- An interval estimate : a range of values that represent your best guess of where the parameter lies.
If your aim is to infer and report population characteristics from sample data, it’s best to use both point and interval estimates in your paper.
You can consider a sample statistic a point estimate for the population parameter when you have a representative sample (e.g., in a wide public opinion poll, the proportion of a sample that supports the current government is taken as the population proportion of government supporters).
There’s always error involved in estimation, so you should also provide a confidence interval as an interval estimate to show the variability around a point estimate.
A confidence interval uses the standard error and the z score from the standard normal distribution to convey where you’d generally expect to find the population parameter most of the time.
Hypothesis testing
Using data from a sample, you can test hypotheses about relationships between variables in the population. Hypothesis testing starts with the assumption that the null hypothesis is true in the population, and you use statistical tests to assess whether the null hypothesis can be rejected or not.
Statistical tests determine where your sample data would lie on an expected distribution of sample data if the null hypothesis were true. These tests give two main outputs:
- A test statistic tells you how much your data differs from the null hypothesis of the test.
- A p value tells you the likelihood of obtaining your results if the null hypothesis is actually true in the population.
Statistical tests come in three main varieties:
- Comparison tests assess group differences in outcomes.
- Regression tests assess cause-and-effect relationships between variables.
- Correlation tests assess relationships between variables without assuming causation.
Your choice of statistical test depends on your research questions, research design, sampling method, and data characteristics.
Parametric tests
Parametric tests make powerful inferences about the population based on sample data. But to use them, some assumptions must be met, and only some types of variables can be used. If your data violate these assumptions, you can perform appropriate data transformations or use alternative non-parametric tests instead.
A regression models the extent to which changes in a predictor variable results in changes in outcome variable(s).
- A simple linear regression includes one predictor variable and one outcome variable.
- A multiple linear regression includes two or more predictor variables and one outcome variable.
Comparison tests usually compare the means of groups. These may be the means of different groups within a sample (e.g., a treatment and control group), the means of one sample group taken at different times (e.g., pretest and posttest scores), or a sample mean and a population mean.
- A t test is for exactly 1 or 2 groups when the sample is small (30 or less).
- A z test is for exactly 1 or 2 groups when the sample is large.
- An ANOVA is for 3 or more groups.
The z and t tests have subtypes based on the number and types of samples and the hypotheses:
- If you have only one sample that you want to compare to a population mean, use a one-sample test .
- If you have paired measurements (within-subjects design), use a dependent (paired) samples test .
- If you have completely separate measurements from two unmatched groups (between-subjects design), use an independent (unpaired) samples test .
- If you expect a difference between groups in a specific direction, use a one-tailed test .
- If you don’t have any expectations for the direction of a difference between groups, use a two-tailed test .
The only parametric correlation test is Pearson’s r . The correlation coefficient ( r ) tells you the strength of a linear relationship between two quantitative variables.
However, to test whether the correlation in the sample is strong enough to be important in the population, you also need to perform a significance test of the correlation coefficient, usually a t test, to obtain a p value. This test uses your sample size to calculate how much the correlation coefficient differs from zero in the population.
You use a dependent-samples, one-tailed t test to assess whether the meditation exercise significantly improved math test scores. The test gives you:
- a t value (test statistic) of 3.00
- a p value of 0.0028
Although Pearson’s r is a test statistic, it doesn’t tell you anything about how significant the correlation is in the population. You also need to test whether this sample correlation coefficient is large enough to demonstrate a correlation in the population.
A t test can also determine how significantly a correlation coefficient differs from zero based on sample size. Since you expect a positive correlation between parental income and GPA, you use a one-sample, one-tailed t test. The t test gives you:
- a t value of 3.08
- a p value of 0.001
The final step of statistical analysis is interpreting your results.
Statistical significance
In hypothesis testing, statistical significance is the main criterion for forming conclusions. You compare your p value to a set significance level (usually 0.05) to decide whether your results are statistically significant or non-significant.
Statistically significant results are considered unlikely to have arisen solely due to chance. There is only a very low chance of such a result occurring if the null hypothesis is true in the population.
This means that you believe the meditation intervention, rather than random factors, directly caused the increase in test scores. Example: Interpret your results (correlational study) You compare your p value of 0.001 to your significance threshold of 0.05. With a p value under this threshold, you can reject the null hypothesis. This indicates a statistically significant correlation between parental income and GPA in male college students.
Note that correlation doesn’t always mean causation, because there are often many underlying factors contributing to a complex variable like GPA. Even if one variable is related to another, this may be because of a third variable influencing both of them, or indirect links between the two variables.
Effect size
A statistically significant result doesn’t necessarily mean that there are important real life applications or clinical outcomes for a finding.
In contrast, the effect size indicates the practical significance of your results. It’s important to report effect sizes along with your inferential statistics for a complete picture of your results. You should also report interval estimates of effect sizes if you’re writing an APA style paper .
With a Cohen’s d of 0.72, there’s medium to high practical significance to your finding that the meditation exercise improved test scores. Example: Effect size (correlational study) To determine the effect size of the correlation coefficient, you compare your Pearson’s r value to Cohen’s effect size criteria.
Decision errors
Type I and Type II errors are mistakes made in research conclusions. A Type I error means rejecting the null hypothesis when it’s actually true, while a Type II error means failing to reject the null hypothesis when it’s false.
You can aim to minimise the risk of these errors by selecting an optimal significance level and ensuring high power . However, there’s a trade-off between the two errors, so a fine balance is necessary.
Frequentist versus Bayesian statistics
Traditionally, frequentist statistics emphasises null hypothesis significance testing and always starts with the assumption of a true null hypothesis.
However, Bayesian statistics has grown in popularity as an alternative approach in the last few decades. In this approach, you use previous research to continually update your hypotheses based on your expectations and observations.
Bayes factor compares the relative strength of evidence for the null versus the alternative hypothesis rather than making a conclusion about rejecting the null hypothesis or not.
Hypothesis testing is a formal procedure for investigating our ideas about the world using statistics. It is used by scientists to test specific predictions, called hypotheses , by calculating how likely it is that a pattern or relationship between variables could have arisen by chance.
The research methods you use depend on the type of data you need to answer your research question .
- If you want to measure something or test a hypothesis , use quantitative methods . If you want to explore ideas, thoughts, and meanings, use qualitative methods .
- If you want to analyse a large amount of readily available data, use secondary data. If you want data specific to your purposes with control over how they are generated, collect primary data.
- If you want to establish cause-and-effect relationships between variables , use experimental methods. If you want to understand the characteristics of a research subject, use descriptive methods.
Statistical analysis is the main method for analyzing quantitative research data . It uses probabilities and models to test predictions about a population from sample data.
Is this article helpful?
Other students also liked, a quick guide to experimental design | 5 steps & examples, controlled experiments | methods & examples of control, between-subjects design | examples, pros & cons, more interesting articles.
- Central Limit Theorem | Formula, Definition & Examples
- Central Tendency | Understanding the Mean, Median & Mode
- Correlation Coefficient | Types, Formulas & Examples
- Descriptive Statistics | Definitions, Types, Examples
- How to Calculate Standard Deviation (Guide) | Calculator & Examples
- How to Calculate Variance | Calculator, Analysis & Examples
- How to Find Degrees of Freedom | Definition & Formula
- How to Find Interquartile Range (IQR) | Calculator & Examples
- How to Find Outliers | Meaning, Formula & Examples
- How to Find the Geometric Mean | Calculator & Formula
- How to Find the Mean | Definition, Examples & Calculator
- How to Find the Median | Definition, Examples & Calculator
- How to Find the Range of a Data Set | Calculator & Formula
- Inferential Statistics | An Easy Introduction & Examples
- Levels of measurement: Nominal, ordinal, interval, ratio
- Missing Data | Types, Explanation, & Imputation
- Normal Distribution | Examples, Formulas, & Uses
- Null and Alternative Hypotheses | Definitions & Examples
- Poisson Distributions | Definition, Formula & Examples
- Skewness | Definition, Examples & Formula
- T-Distribution | What It Is and How To Use It (With Examples)
- The Standard Normal Distribution | Calculator, Examples & Uses
- Type I & Type II Errors | Differences, Examples, Visualizations
- Understanding Confidence Intervals | Easy Examples & Formulas
- Variability | Calculating Range, IQR, Variance, Standard Deviation
- What is Effect Size and Why Does It Matter? (Examples)
- What Is Interval Data? | Examples & Definition
- What Is Nominal Data? | Examples & Definition
- What Is Ordinal Data? | Examples & Definition
- What Is Ratio Data? | Examples & Definition
- What Is the Mode in Statistics? | Definition, Examples & Calculator
Table of Contents
Types of statistical analysis, importance of statistical analysis, benefits of statistical analysis, statistical analysis process, statistical analysis methods, statistical analysis software, statistical analysis examples, career in statistical analysis, choose the right program, become proficient in statistics today, what is statistical analysis types, methods and examples.
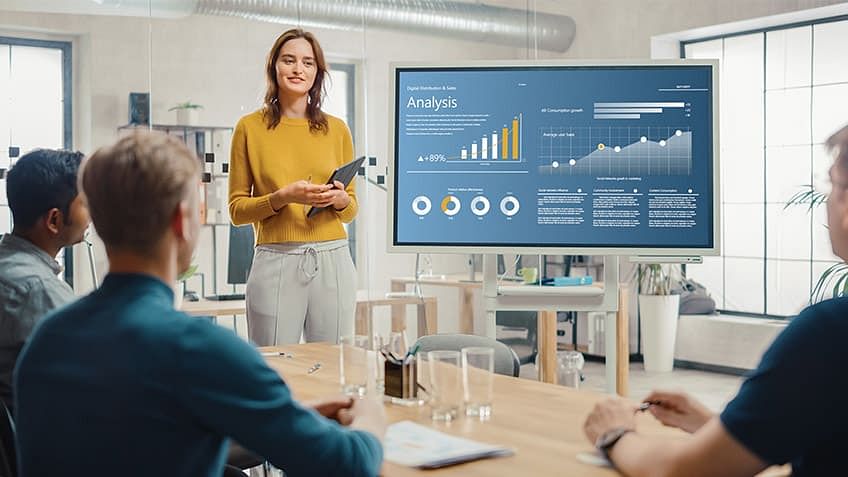
Statistical analysis is the process of collecting and analyzing data in order to discern patterns and trends. It is a method for removing bias from evaluating data by employing numerical analysis. This technique is useful for collecting the interpretations of research, developing statistical models, and planning surveys and studies.
Statistical analysis is a scientific tool in AI and ML that helps collect and analyze large amounts of data to identify common patterns and trends to convert them into meaningful information. In simple words, statistical analysis is a data analysis tool that helps draw meaningful conclusions from raw and unstructured data.
The conclusions are drawn using statistical analysis facilitating decision-making and helping businesses make future predictions on the basis of past trends. It can be defined as a science of collecting and analyzing data to identify trends and patterns and presenting them. Statistical analysis involves working with numbers and is used by businesses and other institutions to make use of data to derive meaningful information.
Given below are the 6 types of statistical analysis:
Descriptive Analysis
Descriptive statistical analysis involves collecting, interpreting, analyzing, and summarizing data to present them in the form of charts, graphs, and tables. Rather than drawing conclusions, it simply makes the complex data easy to read and understand.
Inferential Analysis
The inferential statistical analysis focuses on drawing meaningful conclusions on the basis of the data analyzed. It studies the relationship between different variables or makes predictions for the whole population.
Predictive Analysis
Predictive statistical analysis is a type of statistical analysis that analyzes data to derive past trends and predict future events on the basis of them. It uses machine learning algorithms, data mining , data modelling , and artificial intelligence to conduct the statistical analysis of data.
Prescriptive Analysis
The prescriptive analysis conducts the analysis of data and prescribes the best course of action based on the results. It is a type of statistical analysis that helps you make an informed decision.
Exploratory Data Analysis
Exploratory analysis is similar to inferential analysis, but the difference is that it involves exploring the unknown data associations. It analyzes the potential relationships within the data.
Causal Analysis
The causal statistical analysis focuses on determining the cause and effect relationship between different variables within the raw data. In simple words, it determines why something happens and its effect on other variables. This methodology can be used by businesses to determine the reason for failure.
Statistical analysis eliminates unnecessary information and catalogs important data in an uncomplicated manner, making the monumental work of organizing inputs appear so serene. Once the data has been collected, statistical analysis may be utilized for a variety of purposes. Some of them are listed below:
- The statistical analysis aids in summarizing enormous amounts of data into clearly digestible chunks.
- The statistical analysis aids in the effective design of laboratory, field, and survey investigations.
- Statistical analysis may help with solid and efficient planning in any subject of study.
- Statistical analysis aid in establishing broad generalizations and forecasting how much of something will occur under particular conditions.
- Statistical methods, which are effective tools for interpreting numerical data, are applied in practically every field of study. Statistical approaches have been created and are increasingly applied in physical and biological sciences, such as genetics.
- Statistical approaches are used in the job of a businessman, a manufacturer, and a researcher. Statistics departments can be found in banks, insurance businesses, and government agencies.
- A modern administrator, whether in the public or commercial sector, relies on statistical data to make correct decisions.
- Politicians can utilize statistics to support and validate their claims while also explaining the issues they address.
Become a Data Science & Business Analytics Professional
- 28% Annual Job Growth By 2026
- 11.5 M Expected New Jobs For Data Science By 2026
Data Analyst
- Industry-recognized Data Analyst Master’s certificate from Simplilearn
- Dedicated live sessions by faculty of industry experts
Data Scientist
- Add the IBM Advantage to your Learning
- 25 Industry-relevant Projects and Integrated labs
Here's what learners are saying regarding our programs:

Gayathri Ramesh
Associate data engineer , publicis sapient.
The course was well structured and curated. The live classes were extremely helpful. They made learning more productive and interactive. The program helped me change my domain from a data analyst to an Associate Data Engineer.
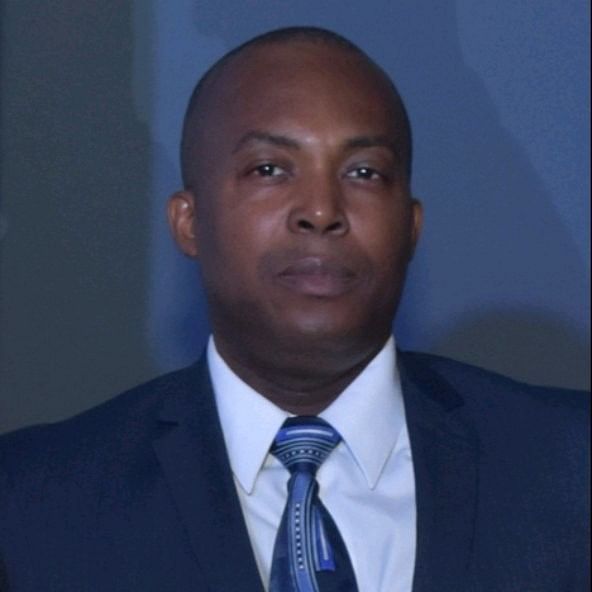
A.Anthony Davis
Simplilearn has one of the best programs available online to earn real-world skills that are in demand worldwide. I just completed the Machine Learning Advanced course, and the LMS was excellent.
Statistical analysis can be called a boon to mankind and has many benefits for both individuals and organizations. Given below are some of the reasons why you should consider investing in statistical analysis:
- It can help you determine the monthly, quarterly, yearly figures of sales profits, and costs making it easier to make your decisions.
- It can help you make informed and correct decisions.
- It can help you identify the problem or cause of the failure and make corrections. For example, it can identify the reason for an increase in total costs and help you cut the wasteful expenses.
- It can help you conduct market analysis and make an effective marketing and sales strategy.
- It helps improve the efficiency of different processes.
Given below are the 5 steps to conduct a statistical analysis that you should follow:
- Step 1: Identify and describe the nature of the data that you are supposed to analyze.
- Step 2: The next step is to establish a relation between the data analyzed and the sample population to which the data belongs.
- Step 3: The third step is to create a model that clearly presents and summarizes the relationship between the population and the data.
- Step 4: Prove if the model is valid or not.
- Step 5: Use predictive analysis to predict future trends and events likely to happen.
Although there are various methods used to perform data analysis, given below are the 5 most used and popular methods of statistical analysis:
Mean or average mean is one of the most popular methods of statistical analysis. Mean determines the overall trend of the data and is very simple to calculate. Mean is calculated by summing the numbers in the data set together and then dividing it by the number of data points. Despite the ease of calculation and its benefits, it is not advisable to resort to mean as the only statistical indicator as it can result in inaccurate decision making.
Standard Deviation
Standard deviation is another very widely used statistical tool or method. It analyzes the deviation of different data points from the mean of the entire data set. It determines how data of the data set is spread around the mean. You can use it to decide whether the research outcomes can be generalized or not.
Regression is a statistical tool that helps determine the cause and effect relationship between the variables. It determines the relationship between a dependent and an independent variable. It is generally used to predict future trends and events.
Hypothesis Testing
Hypothesis testing can be used to test the validity or trueness of a conclusion or argument against a data set. The hypothesis is an assumption made at the beginning of the research and can hold or be false based on the analysis results.
Sample Size Determination
Sample size determination or data sampling is a technique used to derive a sample from the entire population, which is representative of the population. This method is used when the size of the population is very large. You can choose from among the various data sampling techniques such as snowball sampling, convenience sampling, and random sampling.
Everyone can't perform very complex statistical calculations with accuracy making statistical analysis a time-consuming and costly process. Statistical software has become a very important tool for companies to perform their data analysis. The software uses Artificial Intelligence and Machine Learning to perform complex calculations, identify trends and patterns, and create charts, graphs, and tables accurately within minutes.
Look at the standard deviation sample calculation given below to understand more about statistical analysis.
The weights of 5 pizza bases in cms are as follows:
Calculation of Mean = (9+2+5+4+12)/5 = 32/5 = 6.4
Calculation of mean of squared mean deviation = (6.76+19.36+1.96+5.76+31.36)/5 = 13.04
Sample Variance = 13.04
Standard deviation = √13.04 = 3.611
A Statistical Analyst's career path is determined by the industry in which they work. Anyone interested in becoming a Data Analyst may usually enter the profession and qualify for entry-level Data Analyst positions right out of high school or a certificate program — potentially with a Bachelor's degree in statistics, computer science, or mathematics. Some people go into data analysis from a similar sector such as business, economics, or even the social sciences, usually by updating their skills mid-career with a statistical analytics course.
Statistical Analyst is also a great way to get started in the normally more complex area of data science. A Data Scientist is generally a more senior role than a Data Analyst since it is more strategic in nature and necessitates a more highly developed set of technical abilities, such as knowledge of multiple statistical tools, programming languages, and predictive analytics models.
Aspiring Data Scientists and Statistical Analysts generally begin their careers by learning a programming language such as R or SQL. Following that, they must learn how to create databases, do basic analysis, and make visuals using applications such as Tableau. However, not every Statistical Analyst will need to know how to do all of these things, but if you want to advance in your profession, you should be able to do them all.
Based on your industry and the sort of work you do, you may opt to study Python or R, become an expert at data cleaning, or focus on developing complicated statistical models.
You could also learn a little bit of everything, which might help you take on a leadership role and advance to the position of Senior Data Analyst. A Senior Statistical Analyst with vast and deep knowledge might take on a leadership role leading a team of other Statistical Analysts. Statistical Analysts with extra skill training may be able to advance to Data Scientists or other more senior data analytics positions.
Supercharge your career in AI and ML with Simplilearn's comprehensive courses. Gain the skills and knowledge to transform industries and unleash your true potential. Enroll now and unlock limitless possibilities!
Program Name AI Engineer Post Graduate Program In Artificial Intelligence Post Graduate Program In Artificial Intelligence Geo All Geos All Geos IN/ROW University Simplilearn Purdue Caltech Course Duration 11 Months 11 Months 11 Months Coding Experience Required Basic Basic No Skills You Will Learn 10+ skills including data structure, data manipulation, NumPy, Scikit-Learn, Tableau and more. 16+ skills including chatbots, NLP, Python, Keras and more. 8+ skills including Supervised & Unsupervised Learning Deep Learning Data Visualization, and more. Additional Benefits Get access to exclusive Hackathons, Masterclasses and Ask-Me-Anything sessions by IBM Applied learning via 3 Capstone and 12 Industry-relevant Projects Purdue Alumni Association Membership Free IIMJobs Pro-Membership of 6 months Resume Building Assistance Upto 14 CEU Credits Caltech CTME Circle Membership Cost $$ $$$$ $$$$ Explore Program Explore Program Explore Program
Hope this article assisted you in understanding the importance of statistical analysis in every sphere of life. Artificial Intelligence (AI) can help you perform statistical analysis and data analysis very effectively and efficiently.
If you are a science wizard and fascinated by the role of AI in statistical analysis, check out this amazing Caltech Post Graduate Program in AI & ML course in collaboration with Caltech. With a comprehensive syllabus and real-life projects, this course is one of the most popular courses and will help you with all that you need to know about Artificial Intelligence.
Our AI & Machine Learning Courses Duration And Fees
AI & Machine Learning Courses typically range from a few weeks to several months, with fees varying based on program and institution.
Get Free Certifications with free video courses
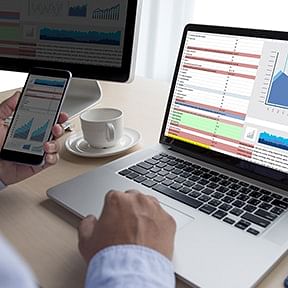
Data Science & Business Analytics
Introduction to Data Analytics Course
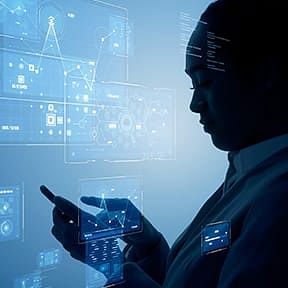
Introduction to Data Science
Learn from Industry Experts with free Masterclasses
Ai & machine learning.
Gain Gen AI expertise in Purdue's Applied Gen AI Specialization
Unlock Your Career Potential: Land Your Dream Job with Gen AI Tools
Make Your Gen AI & ML Career Shift in 2024 a Success with iHUB DivyaSampark, IIT Roorkee
Recommended Reads
Free eBook: Guide To The CCBA And CBAP Certifications
Understanding Statistical Process Control (SPC) and Top Applications
A Complete Guide on the Types of Statistical Studies
Digital Marketing Salary Guide 2021
What Is Data Analysis: A Comprehensive Guide
A Complete Guide to Get a Grasp of Time Series Analysis
Get Affiliated Certifications with Live Class programs
- PMP, PMI, PMBOK, CAPM, PgMP, PfMP, ACP, PBA, RMP, SP, and OPM3 are registered marks of the Project Management Institute, Inc.
- How It Works
Statistical Analysis for Thesis
Statistical Analysis for Thesis: Elevate Your Research with Our Customized Statistics, Methodology, and Results Writing Services. Specializing in SPSS, R, STATA, JASP, Nvivo, and More for Comprehensive Assistance.
Top-Tier Statisticians 👩🔬 | Free Unlimited Revisions 🔄 | NDA-Protected Service 🛡️ | Plagiarism-Free Work 🎓 | On-Time Delivery 🎯 | 24/7 Support 🕒 | Strict Privacy Assured 🔒 | Satisfaction with Every Data Analysis ✅

Our Expertise Recognized by Students at Leading Universities:

Comprehensive Statistical Services for Academic Research
Statistical data analysis help for thesis is a comprehensive service tailored to meet the specific needs of your academic research, ensuring that you receive expert support in the following key areas:
- Methodology Writing : We develop a detailed plan for your research approach, outlining the statistical methods to be used in your study. This foundational step ensures that your research is built on a robust methodological framework.
- Data Management : Our services include importing your data into the preferred statistical software, recoding variables to suit analysis requirements, and data cleaning to ensure accuracy and reliability. This process prepares your data for meaningful analysis, setting the stage for insightful findings.
- Data Analysis & Hypothesis Testing: Whether your research calls for quantitative data analysis or qualitative data analysis , our experts are equipped to handle it. We apply appropriate statistical tests for your hypothesis and techniques to analyse your data, uncovering the patterns and insights that support your research objectives.
- Results Writing: We present your findings in a clear and academically rigorous format, adhering to APA, Harvard , or other academic styles as required. This includes preparing tables and graphs that effectively communicate your results , making them accessible to your intended audience.
Let us help you transform raw data into insightful, coherent findings that propel your research forward. Get a Free Quote Now!
Why Choose Us Choosing SPSSanalysis.com for your dissertation statistical analysis comes with a set of clear promises and benefits, designed to ensure your absolute satisfaction and confidence in our services
Experienced statisticians.
Our team comprises PhD-holding statisticians with a minimum of 7+ years of experience in a variety of fields
Privacy Guarantee
We pledge never to share your details or data with any third party, maintaining the anonymity of your project.
Plagiarism-Free Work
We provide work that is completely free from plagiarism, adhering to the highest standards of academic integrity.
On-Time Delivery
We commit to meeting your deadlines, ensuring that your project is completed within the agreed timeframe.
Free Unlimited Revisions
Your satisfaction is our priority. We offer unlimited revisions until your expectations are fully met.
24/7 Support Service
Our support team is available around the clock to answer your questions and provide assistance whenever you need it.
Thesis Stats Service: How It Works

1. Submit Your Data Task
Start by clicking on GET a FREE QUOTE button. Indicate the instructions, the requirements, and the deadline, and upload supporting files for your Thesis Statistics Task .
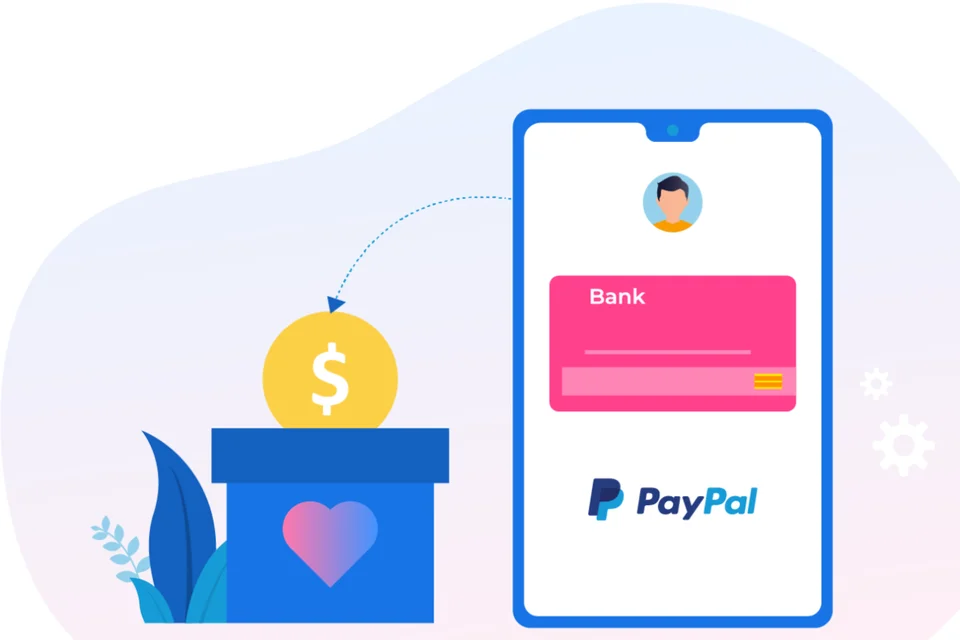
2. Make the Payment
Our experts will review and update the quote for your qualitative or quantitative dissertation task. Once you agree, make a secure payment via PayPal, which secures a safe transaction

3. Get the Results
Once your thesis statistics results is ready, we’ll email you the original solution attachment. You will receive a high-quality result that is 100% plagiarism-free within the promised deadline.
Introduction
At SPSSanalysis.com , we empower PhD students, researchers, and academics by offering customised services in statistical data analysis and consulting . Our platform simplifies the complex process of statistical analysis, making it accessible for projects of any scale. From Data Processing to Reporting, our expertise spans across leading statistical software such as SPSS , R, STATA, and JASP. By connecting you with seasoned statisticians, we ensure your project is not just completed, but comprehensively understood and expertly presented.
Embarking on your statistical journey with us involves three straightforward steps, designed to integrate seamlessly into your research workflow. This simplicity, coupled with our deep commitment to accuracy and insight, makes SPSSanalysis.com the go-to destination for those seeking Thesis Statistics Help . Dive into a world where data becomes decisions, and analysis reveals insights, all tailored to propel your academic and research projects forward.

Discover how our expert statistical services can transform your project by visiting our Get a Free Quote page, where detailing your data analysis needs allows us to provide tailored assistance.
1. Understanding Statistics Help
Thesis Statistics Help is a crucial support system for students grappling with the statistical elements of their research. This service streamlines the process of data analysis , from identifying the correct statistical tests to interpreting the results accurately. It’s not just about crunching numbers; it’s about leveraging statistical insight to bolster your research’s validity and reliability. With the right support, students can transform their data into compelling evidence that supports their thesis argument .
Navigating the complexities of statistical analysis can be daunting, especially for those without a strong background in statistics . This is where Thesis Statistics Help becomes invaluable, providing expert guidance to demystify the process. By tapping into this resource, students can ensure their thesis stands on a solid foundation of accurately analyzed data, enhancing their research’s overall quality and impact.
Navigate the complexities of your thesis with confidence by seeking our expert advice; simply submit your requirements for thesis statistics help on our Get a Free Quote page for personalized support.
How to Get Thesis Support
Our process for thesis statistics help is straightforward and efficient, encapsulated in three simple steps :
- Get a Free Quote : First, Fill out the form on our website detailing your project requirements. This step helps us understand your needs and provide a precise quote.
- Make a Payment : If you’re satisfied with the quote, proceed with payment through our secure PayPal system to initiate your project.
- Receive Your Results: Our experts will then conduct the statistical analysis, ensuring high-quality results directly to your email by the set deadline.

2. Statistical Analysis Services
Our Thesis Statistics Help services cater to a diverse clientele, ensuring that anyone in need of statistical assistance can benefit. This includes:
- PhD Students: Enhancing their research with advanced statistical analysis.
- Academicians: Supporting their scholarly work with precise data interpretation.
- Researchers: Empowering their investigations with robust statistical tools.
- Master Students: Assisting in the completion of their thesis with accurate data analysis.
- Individuals: Offering personal projects the benefit of statistical expertise.
- Companies: Improving business decisions through data-driven insights.

Regardless of your academic or professional background, our services are designed to meet your statistical analysis needs . We cater to projects of all sizes and complexities, ensuring that every client receives the support necessary to elevate their research. Thesis Statistics Help for Get a Free Quote Now!
3. PhD Data Analysis Help: For Master’s and PhD Candidates
PhD Thesis Statistics Help is an essential service tailored specifically for Master’s and PhD candidates who are embarking on the rigorous journey of thesis writing. This specialized support goes beyond basic statistical analysis, addressing the unique challenges and expectations faced at the doctoral level. Expert statisticians can provide guidance on advanced statistical methods, help in interpreting complex data sets, and offer advice on presenting findings clearly and compellingly. This level of support is invaluable for candidates looking to make a significant contribution to their field of study.

Elevate your PhD or Master’s thesis with our advanced statistical support. Visit our Get a Free Quote page, where our experts are ready to address your complex data analysis challenges.
4. Exploring Quantitative Theses
Quantitative theses are characterized by their use of statistical methods to analyze numerical data, offering a clear, objective lens through which research questions can be explored. This approach is integral to many fields, particularly the sciences and social sciences, where quantifiable evidence is paramount. Students embarking on quantitative dissertations must develop a strong understanding of statistical principles to apply the correct methodologies and interpret their data effectively.
The backbone of a quantitative thesis is its reliance on empirical evidence derived from statistical analysis . This requires a meticulous approach to data collection, from designing surveys to conducting experiments. Each step must be carefully planned to ensure the integrity of the data, which in turn, supports the validity of the thesis’s conclusions. Mastery of statistical tools and techniques is essential, turning raw data into meaningful insights that drive research forward.
Utilizing Statistics in Quantitative Theses
In quantitative theses, statistics are not just tools but critical components that shape the research narrative. The proper application of statistical methods enables researchers to test hypotheses, identify patterns, and draw conclusions with confidence. This process begins with selecting the right statistical tests, which must align with the research design and objectives. It’s a step that demands not only technical skill but also a strategic understanding of the thesis’s goals.
For precise and insightful quantitative analysis, visit our Get a Free Quote page. Tell us about your data, and we’ll craft a statistical approach that illuminates your research.
5. The Nature of Qualitative Theses
Qualitative theses explore the depth of human experiences, beliefs, and interactions, offering a nuanced understanding of research questions. This approach values the complexity of social phenomena, seeking to uncover the meanings and motivations behind human behavior. Through interviews, observations, and textual analysis, the qualitative dissertation provides a rich tapestry of insights that numerical data alone cannot capture. It challenges researchers to look beyond the surface, engaging with the subjective experiences of their subjects.
The strength of a qualitative thesis lies in its ability to provide detailed, context-rich insights that illuminate the intricacies of its subject matter. This requires a delicate balance between data collection and interpretation, where the researcher’s skill in analyzing and presenting data becomes paramount. Qualitative research demands not just technical proficiency but also empathy and an open mind, allowing for a deep connection with the research topic and participants. It’s a journey into the heart of the subject matter, where statistics complement narratives to build a compelling argument.
Applying Statistics in Qualitative Theses
While qualitative theses primarily focus on narrative and thematic analysis, integrating statistical elements can significantly enhance their depth and validity. Statistics in qualitative research are not about reducing experiences to numbers but about supporting and validating the emerging themes with quantifiable evidence. This complementary approach can illuminate patterns and trends within the data, providing a firmer ground for conclusions and recommendations.
Enhance your qualitative research with thesis statistics help. Outline your project needs on our Get a Free Quote page to explore how our expertise can elevate your thesis.
7. Key Thesis Sections Concerning Statistics: Methodology, Methods, Results
The methodology , methods, and results sections of a thesis are crucial for showcasing the statistical underpinnings of the research. The methodology outlines the overarching approach, detailing how the research was conducted and why certain statistical methods were chosen. This section sets the stage, explaining the framework within which the data was analyzed and interpreted. It’s here that the researcher justifies their methodological choices, highlighting the rigor and reliability of their approach.

Following the methodology, the methods section dives deeper into the specifics of data collection and analysis. It describes the statistical tests used, the rationale behind their selection, and how they were applied to the research data. This section is key for demonstrating the technical competence of the researcher and the validity of the research design. Finally, the results section presents the findings in a clear, logical manner, supported by statistical evidence. This triad of sections forms the backbone of the thesis, underpinning the research with solid statistical foundations.
Subject Areas
SPSSanalysis.com offers expert statistical support across a wide range of subject areas for your dissertation, including but not limited to:
- Psychology : We assist with statistical analysis for studies in behaviours, cognition, and emotion, among other topics.
- Medical Research : Our services cover clinical trials, epidemiology, and other health-related research.
- Nursing : We provide a wide range of PhD-level statistical consultation and data analysis services for DNP students.
- Education: We support research in teaching methods, learning outcomes, and educational policy analysis.
- Sociology : Our team helps with analyses of social behaviour, community studies, and demographic research.
- Business and Marketing: We provide statistical insights for market research, consumer behaviour, and business strategy studies.
- Economics: Our expertise extends to economic models, financial analysis, and policy impact assessments.
- Sports : Statistical support for research on athletic performance, sports psychology, and physical education.
- Nutrition : Analysis for dietary studies, nutritional epidemiology, and health outcome research related to nutrition
This list represents the core areas where we offer specialized statistical support, ensuring that your dissertation benefits from precise and insightful analysis tailored to your specific field of study.
8. Conducting Statistical Analysis for Your Thesis
Conducting statistical analysis for your thesis requires a careful blend of technical skill and critical thinking. It begins with a thorough understanding of your research questions and a strategic approach to data collection. This foundation ensures that the data you gather is both relevant and robust, suitable for the statistical tests you plan to apply. The next step involves selecting the appropriate statistical methods, a decision that hinges on the nature of your data and the objectives of your research. This process is critical for generating valid, reliable results that can support your thesis claims.
Dive deeper into your data with our statistical analysis services. Describe your thesis project on our Get a Free Quote page for insights that can redefine your research.
9. Support for Interpreting Thesis Results
Interpreting the results of your thesis requires a keen understanding of both your research context and the statistical methods employed. It’s a critical phase where data speaks to your hypotheses, guiding the conclusions you draw. Expert support in this stage can illuminate the nuances of your findings, helping you to articulate the implications of your research with clarity and precision. This support is particularly crucial for complex analyses, where the interpretation of results can determine the impact of your research.

At SPSSanalysis.com , our statisticians are not just experts in numbers; they are skilled in translating statistical outcomes into meaningful insights. This support extends beyond mere interpretation, offering guidance on how to present your findings in a way that is accessible to your audience. Whether it’s discussing the significance of your results in the broader context of your field or identifying areas for future research, expert interpretation can elevate the quality of your thesis, making your contributions stand out.
10. Assistance with Writing the Methodology Section
Writing the methodology section of your thesis is about more than just listing the steps you took in your research; it’s about justifying your choices and demonstrating the rigor of your approach. This section is foundational, setting the stage for the credibility of your entire thesis. Expert assistance in crafting this section can ensure that your methodology is clearly articulated, from the selection of your sample to the choice of statistical tests. This clarity is essential for readers and reviewers, who must understand and trust your research process.
Strengthen your methodology section with our expert advice. Fill in your project details on our Get a Free Quote page for statistical support that ensures your research stands out.
11. Statistical Analyses Employed in Theses
The range of statistical analyses employed in theses is vast and varied, tailored to suit the specific demands of each research question. From basic descriptive statistics that summarize data to complex inferential tests that explore relationships and causality, the choice of analysis is critical. This decision should be informed by the research design, the nature of the data, and the hypotheses under investigation. Each statistical method offers unique insights, and selecting the most appropriate one is key to unlocking the full potential of your data.
Understanding the various statistical analyses available and their applications is essential for any researcher. Whether it’s a simple t-test to compare two groups or a multivariate regression analysis to explore multiple predictors of an outcome , the right statistical tools can illuminate the path to significant, meaningful findings. Familiarity with these methods not only enhances the robustness of your thesis but also equips you with the skills to contribute valuable knowledge to your field.
Whether it’s regression analysis or ANOVA, get the statistical guidance your thesis deserves by visiting our Get a Free Quote page and sharing your analytical requirements.
Statistical Tests for Dissertation Statistics
Choosing the right statistical tests is pivotal for analyzing dissertation data effectively. The main tests include:
- Descriptive Statistics : This involves summarizing and organizing data to understand its central tendencies and variability.
- Comparative Statistics : Utilizes tests such as T-tests, ANOVA (Analysis of Variance), and Mann-Whitney tests to evaluate differences between groups.
- Inferential Statistics : Employs statistical methods to infer properties about a population based on a sample.
- Correlation Analysis : Measures the degree and direction of association between two variables. For example Pearson Correlation , Spearman’s Rho rank order , Kendall’s Tau , Partial Correlation , and Canonical Correlation .
- Regression Analysis : This analysis is key for predicting outcomes and understanding the strength and character of the relationship between variables. For Example Simple Linear Regression , Binary Logistic Regression , and Hierarchical Regression . Probit Regression
- Univariate Analysis : Focuses on analyzing a single variable to describe its characteristics and distribution. This includes measures of central tendency, dispersion, and skewness, providing insights into the pattern of data for that variable.
- Multivariate Analysis : Involves examining multiple variables simultaneously to understand relationships and influences among them.
12. Selecting the Appropriate Statistical Test for Your Thesis
Selecting the appropriate statistical test is a pivotal step in the research process, one that requires careful consideration of your data and research questions. This choice is guided by several factors, including the type of data you have collected, the distribution of that data, and the specific hypotheses you aim to test. The correct test will provide the most accurate and relevant insights, helping you to draw meaningful conclusions from your research.
Make informed decisions on statistical tests by consulting our experts. Submit your project details on our Get a Free Quote page for a strategy that strengthens your thesis.
13. The Importance of Seeking Help with Thesis Statistics
Engaging with Thesis Statistics Help is not merely a convenience; it’s a strategic decision that can significantly elevate the quality of your thesis. Statistical analysis, with its inherent complexity, can be a formidable challenge for many students. Expert guidance can simplify these complexities, providing clarity and confidence in your statistical choices. This support is invaluable for ensuring your research methodologies are sound and your interpretations of data are accurate, lending credibility and authority to your findings.

Moreover, seeking help with thesis statistics can save invaluable time and resources, allowing you to focus more on the substantive aspects of your research. Expert statisticians bring a level of proficiency and insight that can transform your data analysis from a daunting task into a clear, manageable process. This collaboration not only enriches your research experience but also enhances the overall integrity and impact of your thesis. By investing in professional statistical support, you’re ensuring your thesis stands as a testament to high-quality, rigorous research.
14. The Cost of Hiring a Statistician for Your Dissertation
The cost of hiring a statistician for your thesis starts from £250, however, Investing in a statistician for your dissertation represents a significant step towards ensuring the quality and integrity of your research. The cost of such services can vary, reflecting the complexity of the statistical analysis required and the level of expertise of the statistician. At SPSSanalysis.com , we understand the financial constraints faced by students and researchers, which is why we strive to offer competitive rates without compromising on the quality of our services. Our pricing structure is transparent and tailored to meet the needs of a diverse client base, ensuring you receive value for your investment.

Understanding the cost and value of expert statistical analysis is just a step away. Share your thesis statneeds on our Get a Free Quote page, and we’ll outline how our services can fit your budget.
15. What Statistical Software is Used in Theses?
Statistical software plays a pivotal role in theses, offering the tools needed to conduct sophisticated analyses with efficiency and accuracy. The choice of software often depends on the specific needs of the research, including the complexity of the data and the preferred statistical methods. Commonly used software includes:
- SPSS: Renowned for its user-friendly interface, SPSS is widely used across social sciences for a variety of statistical tests.
- R: A powerful and flexible open-source software, R is favored for its extensive range of packages and capabilities, suitable for advanced statistical modeling.
- STATA: Popular in economics and health sciences, STATA offers robust data management and statistical analysis features.
- JASP: An open-source alternative known for its ease of use, JASP is gaining popularity for standard statistical tests and Bayesian analyses.

Selecting the right software is a crucial decision that can influence the efficiency and effectiveness of your statistical analysis. Each program has its strengths, and the best choice for your thesis will depend on your specific research needs and familiarity with the software. Engaging with statistical experts can provide valuable insights into the most appropriate software for your project, ensuring your analysis is conducted with the utmost precision.
16. SPSS Data Analysis Help for Academic Research
Our SPSS data analysis help extends across various fields, assisting students to excel in their respective domains:
- Medical : Applying statistical analysis to medical research for groundbreaking findings.
- Nursing : Enhancing nursing studies with accurate data interpretation.
- Healthcare : Supporting healthcare research with comprehensive statistical insights.
- Education : Analyzing educational data to improve teaching and learning outcomes.
- Sociology : Examining social phenomena through detailed statistical analysis.
- Psychology : Interpreting psychological data to understand human behavior better.
- Marketing : Interpreting marketing data to understand human behavior better.
Our services are designed to meet the unique needs of each field, providing tailored support that enhances your research. With our expert guidance, you can harness the power of SPSS to uncover insights that make a difference. Get a FREE Quote Now!
Stay connected with SPSSanalysis.com on LinkedIn for the latest updates and insights!
Looking for a Statistician to Do Your Data Analysis?
- Bangalore, India
- +91-9731988227
- [email protected]

- Vision & Mission
- Dr. Ganasoundari
- PhD thesis consultation
- DBA thesis consultation
- Thesis support for PG Students
- Thesis writing
- Topic Selection
- PhD Proposal Service
- Research Article Writing
- Systematic Review
- Paraphrasing
- Questionnaire Preparation
- Reference Editing
- Thesis Formatting
- Plagiarism Checking
Statistical Analysis
- Completed & Future Workshops
- Thesis Writing workshop
- Literature Review Writing Workshop
- Research Methodology Workshop
- Research Paper Writing Workshop
- Proposal Writing workshop
- Increasing Citation Score
- Systematic Review Workshop
- Academic Integrity Workshop
- Formatting workshop
- SPSS Foundation Training
- SPSS Advanced Training
- SEM Statistical Workshop
- Copyediting Training
- Reference Management Workshop
PhD Statistical Analysis for Thesis, projects & Reports
Most of the types of data collected for research require statistical analysis. Not all are experts in statistically analysing the data. You can count on us to analyse the data. At Rehoboth, our statistical consultants strive to present you with accurate results through our data analysis services. You can tell us to use specific statistical tools to use to arrive at the outcome. If you are not sure of which statistical tools to be used, we will select the appropriate tools. However, it should be borne in mind that we do not modify the data to get the desired outcome.
We can also interpret the results and write it as a chapter if required.
After we submit the report, we also ensure that you understand the analytic techniques used for obtaining the results.
Statistical tools that we use
· Descriptive statistics
· Chi-square test
· Student t-test
· ANOVA
· Correlation
· Regression
· Multivariate test (MANOVA, MANCOVA)
· Validity Test (Factor analysis)
· Reliability Test (Cronbach’s alpha)
· Friedman Rank test
· Structure Equation Modelling (SEM), etc.
Deliverables
· Analysed data presented in the form of tables and figures.
· Interpretation written in the form of a chapter with relevant and adequate references (optional).
· Revision based on your inputs (up to 1 month).
What does Rehoboth need from you?
· Objectives, hypothesis and purpose of the research.
· Data collected.
· Research instruments used in the research.
“Rehoboth Academic Services” is a premium institute supporting PhD & Master’s Thesis since 2013. We offer editing , proofreading, Paper Preparation, statistical analysis , formatting and plagiarism checking services. We have helped more than 1000+ research scholars in most of the subjects and universities across the globe in the last nine years. We also conduct Copyediting & Proofreading, Art of thesis writing, Research Paper writing , Statistical Analysis with SPSS Foundation & Advanced workshops. If you need our assistance please call 9731988227, 9741871657 or mail to jo****@re***************.com
- Disclaimer Policy
- Terms and Conditions
- Cancellation & Refund Policy
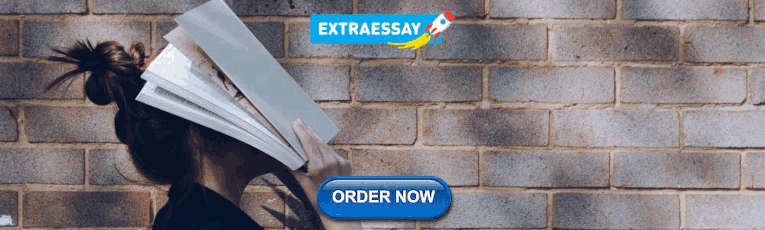
IMAGES
VIDEO
COMMENTS
Table of contents. Step 1: Write your hypotheses and plan your research design. Step 2: Collect data from a sample. Step 3: Summarize your data with descriptive statistics. Step 4: Test hypotheses or make estimates with inferential statistics.
The results chapter (also referred to as the findings or analysis chapter) is one of the most important chapters of your dissertation or thesis because it shows the reader what you've found in terms of the quantitative data you've collected. It presents the data using a clear text narrative, supported by tables, graphs and charts.
Statistical Learning of Biomedical Non-Stationary Signals and Quality of Life Modeling, Mahdi Goudarzi. PDF. Probabilistic and Statistical Prediction Models for Alzheimer's Disease and Statistical Analysis of Global Warming, Maryam Ibrahim Habadi. PDF. Essays on Time Series and Machine Learning Techniques for Risk Management, Michael ...
Introduction. Statistical analysis is necessary for any research project seeking to make quantitative conclusions. The following is a primer for research-based statistical analysis. It is intended to be a high-level overview of appropriate statistical testing, while not diving too deep into any specific methodology.
A master's thesis is an independent scientific work and is meant to prepare students for future professional or academic work. Largely, the thesis is expected to be similar to papers published in statistical journals. It is not set in stone exactly how the thesis should be organized. The following outline should however be followed. Title Page
Misrepresenting graphics or neglecting to label and interval scale graphs correctly can distort the statistical analysis of data. Managing redundant analyses or ignoring existing knowledge in the field can hinder the promotion of research. Avoiding common mistakes in statistical analysis requires diligence and attention to detail.
Categorical variables represent groupings of things (e.g. the different tree species in a forest). Types of categorical variables include: Ordinal: represent data with an order (e.g. rankings). Nominal: represent group names (e.g. brands or species names). Binary: represent data with a yes/no or 1/0 outcome (e.g. win or lose).
A beginner's guide to statistical analysis and review. Statistical analysis—the backbone of scientific research studies—uses statistical methods to obtain usable information from raw data. It gives meaning to data and the study, determines if the proposed method is more efficient than the existing or conventional methods, and indicates ...
Submit thesis to DukeSpace. If you are an undergraduate honors student interested in submitting your thesis to DukeSpace, Duke University's online repository for publications and other archival materials in digital format, please contact Joan Durso to get this process started. DukeSpace Electronic Theses and Dissertations (ETD) Submission Tutorial.
Research Design and Methodology. Chapter 3 consists of three parts: (1) Purpose of the. study and research design, (2) Methods, and (3) Statistical. Data analysis procedure. Part one, Purpose of ...
1 Introduction. Statistics is a set of methods used to analyze data. The statistic is present in all areas of science involving the. collection, handling and sorting of data, given the insight of ...
Study Design and Statistical Analysis A Practical Guide for Clinicians This book takes the reader through the entire research process: choosing a question, designing a study, collecting the data, using univariate, bivariate and multivariable analysis, and publishing the results. It does so by using plain language rather than complex
Since it is an immitigable part of your thesis, you can neither run from statistics nor cry for help. The penultimate part of this process involves analysis of results which is very crucial for coherence of your thesis assignment.This analysis usually involve use of statistical tools to help draw inferences. Most students who don't pursue ...
Statistical methods involved in carrying out a study include planning, designing, collecting data, analysing, drawing meaningful interpretation and reporting of the research findings. The statistical analysis gives meaning to the meaningless numbers, thereby breathing life into a lifeless data. The results and inferences are precise only if ...
Master's Thesis. As an integral component of the Master of Science in Statistical Science program, you can submit and defend a Master's Thesis. Your Master's Committee administers this oral examination. If you choose to defend a thesis, it is advisable to commence your research early, ideally during your second semester or the summer following ...
PhD Theses. 2023. Title. Author. Supervisor. Statistical Methods for the Analysis and Prediction of Hierarchical Time Series Data with Applications to Demography. Daphne Liu. Adrian E Raftery. Statistical methods for genomic sequencing data.
The statistical analysis for your thesis or dissertation should be appropriate for what you are researching and should fit with your needs and capabilities. I know, that's not saying much, but it's important that you're comfortable with the statistical analysis you will be conducting. An experienced dissertation consultant will help you ...
Statistical analysis is a critical aspect of a dissertation and makes up the fourth chapter of a thesis, i.e., results and findings. Statistical analysis is the collection and interpretation of data to reveal trends and patterns or test a hypothesis. SPSS, STATA, reviews, R, Nvivo, SAS, and others are some of the most commonly used statistical ...
Table of contents. Step 1: Write your hypotheses and plan your research design. Step 2: Collect data from a sample. Step 3: Summarise your data with descriptive statistics. Step 4: Test hypotheses or make estimates with inferential statistics.
Statistical analysis is the process of collecting and analyzing data in order to discern patterns and trends. It is a method for removing bias from evaluating data by employing numerical analysis. This technique is useful for collecting the interpretations of research, developing statistical models, and planning surveys and studies.
Statistical data analysis help for thesis is a comprehensive service tailored to meet the specific needs of your academic research, ensuring that you receive expert support in the following key areas: Methodology Writing: We develop a detailed plan for your research approach, outlining the statistical methods to be used in your study. This ...
Statistical Analysis on Students' Perf ormance. Elepo, Tayo Afusat 1 & Balogun, Oluwafemi Samson *2. 1 Department of Statistics, Kwara State Polytechnics, Ilorin, Kwara State, Nigeria. 1 ...
We also conduct Copyediting & Proofreading, Art of thesis writing, Research Paper writing, Statistical Analysis with SPSS Foundation & Advanced workshops. If you need our assistance please call 9731988227, 9741871657 or mail to jo****@re***************.com. Sample size calculation, reliability of your Pilot Study, Expert Statistical SEM ...