Research vs. Study
What's the difference.
Research and study are two essential components of the learning process, but they differ in their approach and purpose. Research involves a systematic investigation of a particular topic or issue, aiming to discover new knowledge or validate existing theories. It often involves collecting and analyzing data, conducting experiments, and drawing conclusions. On the other hand, study refers to the process of acquiring knowledge or understanding through reading, memorizing, and reviewing information. It is typically focused on a specific subject or discipline and aims to deepen one's understanding or mastery of that subject. While research is more exploratory and investigative, study is more focused on acquiring and retaining information. Both research and study are crucial for intellectual growth and expanding our knowledge base.
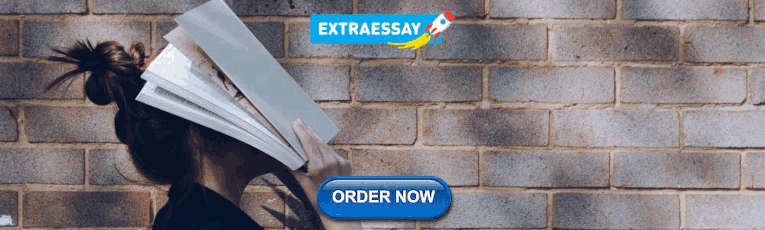
Further Detail
Introduction.
Research and study are two fundamental activities that play a crucial role in acquiring knowledge and understanding. While they share similarities, they also have distinct attributes that set them apart. In this article, we will explore the characteristics of research and study, highlighting their differences and similarities.
Definition and Purpose
Research is a systematic investigation aimed at discovering new knowledge, expanding existing knowledge, or solving specific problems. It involves gathering and analyzing data, formulating hypotheses, and drawing conclusions based on evidence. Research is often conducted in a structured and scientific manner, employing various methodologies and techniques.
On the other hand, study refers to the process of acquiring knowledge through reading, memorizing, and understanding information. It involves examining and learning from existing materials, such as textbooks, articles, or lectures. The purpose of study is to gain a comprehensive understanding of a particular subject or topic.
Approach and Methodology
Research typically follows a systematic approach, involving the formulation of research questions or hypotheses, designing experiments or surveys, collecting and analyzing data, and drawing conclusions. It often requires a rigorous methodology, including literature review, data collection, statistical analysis, and peer review. Research can be qualitative or quantitative, depending on the nature of the investigation.
Study, on the other hand, does not necessarily follow a specific methodology. It can be more flexible and personalized, allowing individuals to choose their own approach to learning. Study often involves reading and analyzing existing materials, taking notes, summarizing information, and engaging in discussions or self-reflection. While study can be structured, it is generally less formalized compared to research.
Scope and Depth
Research tends to have a broader scope and aims to contribute to the overall body of knowledge in a particular field. It often involves exploring new areas, pushing boundaries, and generating original insights. Research can be interdisciplinary, involving multiple disciplines and perspectives. The depth of research is often extensive, requiring in-depth analysis, critical thinking, and the ability to synthesize complex information.
Study, on the other hand, is usually more focused and specific. It aims to gain a comprehensive understanding of a particular subject or topic within an existing body of knowledge. Study can be deep and detailed, but it is often limited to the available resources and materials. While study may not contribute directly to the advancement of knowledge, it plays a crucial role in building a solid foundation of understanding.
Application and Output
Research is often driven by the desire to solve real-world problems or contribute to practical applications. The output of research can take various forms, including scientific papers, patents, policy recommendations, or technological advancements. Research findings are typically shared with the academic community and the public, aiming to advance knowledge and improve society.
Study, on the other hand, focuses more on personal development and learning. The application of study is often seen in academic settings, where individuals acquire knowledge to excel in their studies or careers. The output of study is usually reflected in improved understanding, enhanced critical thinking skills, and the ability to apply knowledge in practical situations.
Limitations and Challenges
Research faces several challenges, including limited resources, time constraints, ethical considerations, and the potential for bias. Conducting research requires careful planning, data collection, and analysis, which can be time-consuming and costly. Researchers must also navigate ethical guidelines and ensure the validity and reliability of their findings.
Study, on the other hand, may face challenges such as information overload, lack of motivation, or difficulty in finding reliable sources. It requires self-discipline, time management, and the ability to filter and prioritize information. Without proper guidance or structure, study can sometimes lead to superficial understanding or misconceptions.
In conclusion, research and study are both essential activities in the pursuit of knowledge and understanding. While research focuses on generating new knowledge and solving problems through a systematic approach, study aims to acquire and comprehend existing information. Research tends to be more formalized, rigorous, and contributes to the advancement of knowledge, while study is often more flexible, personalized, and focused on individual learning. Both research and study have their unique attributes and challenges, but together they form the foundation for intellectual growth and development.
Comparisons may contain inaccurate information about people, places, or facts. Please report any issues.
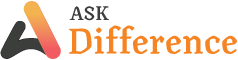
Study vs. Research — What's the Difference?

Difference Between Study and Research
Table of contents, key differences, comparison chart, methodology, compare with definitions, common curiosities, what is the main difference between study and research, is study less rigorous than research, can you research without studying, what's the goal of research, is research always scientific, can you study and research at the same time, what's the goal of study, is study always academic, who typically conducts research, can you study by yourself, who typically studies, is research always formal, do research findings affect general populations, is research always published, can study be informal, share your discovery.
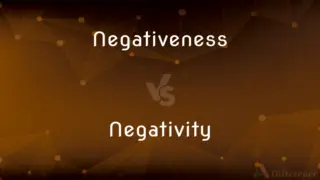
Author Spotlight

Popular Comparisons
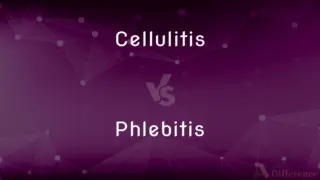
Trending Comparisons
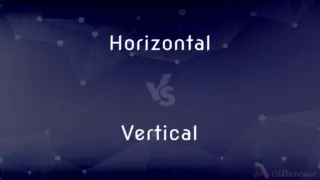
New Comparisons
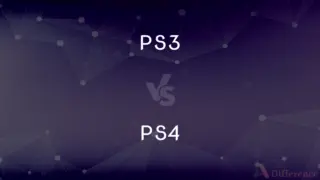
Trending Terms
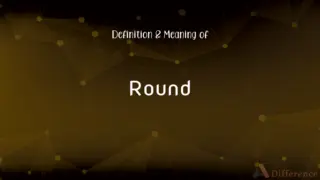
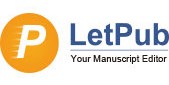
- Language Editing     For Manuscripts    For Response Letter new    For LaTeX    For Annual Review and Tenure    For Books new
- Scientific Editing     For Manuscripts    For Response Letter new
- Grant Editing 
- Translation 
- Publication Support  Journal Recommendation  Manuscript Formatting  Figure Formatting  Data Analysis new  Plagiarism Check  Conference Poster  Plain Language Summary
- Scientific Illustration  Journal Cover Design  Graphical Abstract  Infographic  Custom Illustration
- Scientific Videos  Video Abstract  Explainer Video  Scientific Animation
- Ethics and Confidentiality
- Editorial Certificate
- Testimonials
- Design Gallery
- Institutional Provider
- Publisher Portal
- Brand Localization
- Journal Selector Tool
- Learning Nexus
Research vs. study
The confusion about these words is that they can both be either nouns or verbs. If you ask someone, "Does 'studies' mean the same as 'researches'?" you may hear "Yes," but it is only true if they are used as verbs. As nouns, they have subtly different meanings.
"This team has done a lot of good research. I just read their latest study, which they wrote about calcium in germinating soybeans. It described several interesting experiments."
research 1. to perform a systematic investigation
1. "What kind of scientist is he? He's a botanist. He researches plants."
study 1. to perform a systematic investigation; 2. to actively learn or memorize academic material
1. "What kind of scientist is he? He's a botanist. He studies plants."
2. "Mindy studies every day. That is why she gets such excellent grades. She wants to go to college to study math."
Some authors say "research" when they mean "study." "Research," as a verb, means "to perform a study or studies," but "research" as a noun refers to the sum of many studies. "Chemical research" means the sum of all chemical studies. If you find yourself writing "a research" or "in this research," change it to "a study" or "in this study."
research The act of performing research. Also, the results of research. Note that "research" is a mass noun. It is already plural in meaning but grammatically singular. If you want to indicate more than one type, say "bodies of research" or "pieces of research," not "researches."
"Dr. Lee was a prolific scientist. She performed a great deal of research over her long career."
study A single research project or paper.
"Dr. Lee was a prolific scientist. She performed a great many studies over her long career."
The noun "study" refers to a single paper or project. You can replace "paper" with "study" in almost all cases (but not always the other way around), to the point where you can say "I wrote a study." The noun "research" means more like a whole body of research including many individual studies: The research of a field. The lifetime achievements of a scientist or research team.
Quick Links
- Language Editing Service
- Expert Scientific Editing Service
- Professional Translation Service
- Formatting Service
- Figure Preparation Service
Intentional Space Tag
Contact us
Your name *
Your email *
Your message *
Please fill in all fields and provide a valid email.
© 2010-2024 ACCDON LLC 400 5 th Ave, Suite 530, Waltham, MA 02451, USA Privacy • Terms of Service
© 2010-2024 United States: ACCDON LLC Tel: 1-781-202-9968 Email: [email protected]
Address: 400 5 th Ave, Suite 530, Waltham, Massachusetts 02451, United States
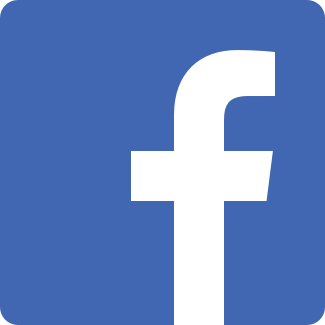

Difference Between Research and Study
Research and study are terms often used interchangeably but have distinct meanings. Understanding the difference between research and study is important, as it can help you approach your academic work more effectively.
The study refers to learning and acquiring knowledge through reading, lectures, and other forms of instruction. It involves a systematic approach to learning and often requires a significant amount of time and effort. Students engage in study to gain a better understanding of a particular subject or topic and to prepare for exams or other assessments.
On the other hand, research involves systematically investigating a particular topic or problem. It involves collecting and analyzing data and drawing conclusions based on the results. Research is often conducted to answer specific questions or to test hypotheses, and it can be used to inform policy decisions or to advance scientific knowledge.
Definition of Research
Research is a systematic and scientific investigation of a particular subject matter or problem. It collects, analyzes, and interprets data to answer a research question or hypothesis. Research can be conducted in various fields, such as science, social science, business, and technology.
Research differs from studying because it involves a more rigorous and structured approach. While studying involves learning and understanding a particular topic, research requires a more in-depth and critical analysis. Research also involves various methods and techniques, such as surveys, experiments, case studies, and observations.
One of the primary objectives of the research is to contribute to the existing knowledge in a particular field. Research findings can be used to inform policy decisions, improve products and services, and advance scientific knowledge. Research can also identify gaps in knowledge and inform future research directions.
Definition of Study
Regarding academia, “study” is often used interchangeably with “research.” However, there is a subtle difference between the two. A study systematically examines and analyzes a particular subject or topic. It involves gathering and interpreting data in order to draw conclusions or make recommendations.

Studies can take many forms, depending on the discipline and the research question being addressed. Some common studies include case studies, observational studies, and experimental studies. In a case study, a single subject or group is examined in detail, often over an extended period. Researchers observe and record behavior or phenomena without intervening in an observational study. In an experimental study, researchers manipulate one or more variables to observe the effect on an outcome.
Regardless of the type of study, the goal is always to gain a deeper understanding of the subject being examined. This may involve developing new theories, refining existing ones, or identifying practical solutions to real-world problems. A study is a rigorous and systematic process that requires careful planning, execution, and analysis.
Purpose of Research
Research is a systematic investigation of a particular topic or issue to discover new knowledge or verify existing knowledge. The research aims to answer questions, solve problems, or improve understanding of a particular phenomenon. Research can be conducted in various fields, including science, social sciences, humanities, and business.
The main purpose of research is to contribute to the advancement of knowledge in a particular field. Research can help to identify new phenomena, explain existing phenomena, or develop new theories. It can also help identify knowledge gaps and suggest areas for further investigation.
Another important purpose of research is to provide evidence-based information for decision-making. Research can inform policy decisions, guide the development of new products or services, or support clinical practice. By providing reliable, valid, and objective data, research can reduce uncertainty and improve the quality of decision-making.
Research can also help to identify and solve practical problems. By identifying the causes of a problem and testing potential solutions, research can help to improve processes, products, or services. For example, research can be used to develop new drugs, improve educational programs, or design more efficient transportation systems.
Purpose of Study
One of the main differences between research and study is the purpose for which they are conducted. While both involve acquiring knowledge, they differ in terms of their objectives and goals.
Studies are typically conducted to understand a specific topic or phenomenon better. They are often exploratory and seek to answer questions such as “what is happening?” or “what are the characteristics of this phenomenon?” Studies may also be conducted to test hypotheses or theories, but their primary goal is to understand a particular subject better.
Studies can be qualitative or quantitative in nature. Qualitative studies are typically used to explore complex phenomena and gain a deeper understanding of human behavior, attitudes, and experiences. Quantitative studies, on the other hand, are used to measure and quantify data and test hypotheses.
Overall, the purpose of a study is to gain a deeper understanding of a specific phenomenon or topic. This can be achieved through various methods, including surveys, interviews, observations, and experiments. Studies can be conducted in various fields, including psychology, sociology, education, and business.
Research Methodology
Research methodology is the systematic process of collecting and analyzing data to answer research questions or test hypotheses. It involves a series of steps researchers follow to ensure their study is valid and reliable. The following are some of the common research methodologies used in academic research:
- Experimental research involves manipulating one or more variables to observe the effect on another variable. It is often used to test cause-and-effect relationships.
- Survey research involves collecting data from a sample of individuals using questionnaires or interviews. It is often used to gather information about attitudes, opinions, and behaviors.
- Case study research involves an in-depth analysis of a single case or a small group of cases. It is often used to gain a detailed understanding of a complex phenomenon.
- Observational research: This involves observing and recording the behavior of individuals or groups in their natural environment. It is often used to gather data on behaviors that cannot be manipulated in an experimental setting.
Each research methodology has its own strengths and weaknesses, and researchers must carefully choose the most appropriate methodology for their research question. They must also ensure that their study design is rigorous and that their data collection and analysis procedures are reliable and valid.
Study Methodology
When conducting a study, the methodology used can vary depending on the study’s type. Here are a few common study methodologies:
- Observational studies: In this study, researchers observe and record participants’ behavior without intervening or manipulating any variables.
- Experimental studies: In an experimental study, researchers manipulate one or more variables to see how they affect the outcome. Participants are randomly assigned to different groups, each receiving a different treatment or intervention.
- Survey studies: This study involves gathering data from a large group through questionnaires or interviews. Researchers analyze the responses to conclude the population being studied.
When designing a study, researchers must also consider the sample size or the number of participants included. A larger sample size generally yields more reliable results but can be more costly and time-consuming to recruit and analyze.
Additionally, researchers must consider potential biases that could affect the study results. For example, selection bias can occur if participants are not randomly selected, while response bias can occur if participants provide inaccurate or incomplete information.
Overall, the methodology used in a study is crucial for ensuring the validity and reliability of the results. By carefully designing and conducting a study, researchers can draw meaningful conclusions and contribute to the body of knowledge in their field.
Types of Research
Research is a systematic and scientific approach to collecting and analyzing data to answer a specific research question. There are several types of research, each with its purpose and methodology. Here are some of the most common types of research:
Descriptive research
This type of research describes the characteristics of a particular phenomenon or group of people. It is often used to generate hypotheses or to identify patterns and trends.
Exploratory research
This type of research is conducted when the researcher needs to gain more knowledge about the subject of study. It is used to gain a better understanding of the research problem and to identify potential research questions.
Experimental research
This type of research involves manipulating one or more variables to observe the effect on another variable. It is used to establish cause-and-effect relationships between variables.
Correlational research
This type of research examines the relationship between two or more variables without manipulating them. It is used to identify the strength and direction of the relationship between variables.
Qualitative research
This type of research is used to explore and understand the meaning and experiences of individuals or groups. It is often conducted using interviews, observations, and focus groups.
Quantitative research
This type of research involves collecting and analyzing numerical data. It is often conducted using surveys, experiments, and statistical analysis.
Each type of research has its strengths and weaknesses, and the choice of research methodology depends on the research question, the nature of the research problem, and the available resources. Researchers must carefully consider the type of research that best suits their research question and design their study accordingly.
Types of Study
Studies can be classified into different types based on their objectives, design, and methodology. Here are some of the most common types of study:
Observational study
This type of study involves observing and recording the behavior of subjects in their natural environment without any intervention or manipulation. Observational studies can be classified into cross-sectional, case-control, and cohort studies.
Experimental study
This study involves manipulating one or more variables to observe the effect on the outcome of interest. Experimental studies can be classified into randomized controlled trials (RCTs), quasi-experimental studies, and single-subject designs.

Descriptive study
This type of study aims to describe the characteristics of a population or phenomenon. Descriptive studies can be further classified into case reports, case series, and surveys.
Exploratory study
This study aims to explore a new or under-researched topic or phenomenon. Exploratory studies can be further classified into qualitative, pilot, and feasibility studies.
Diagnostic study
This type of study aims to evaluate the accuracy of a diagnostic test or procedure. Diagnostic studies can be further classified into sensitivity and specificity studies, receiver operating characteristic (ROC) curve analysis, and likelihood ratio studies.
Each study type has its strengths and weaknesses, and the choice of study type depends on the research question, available resources, and ethical considerations. Researchers must carefully consider the study design and methodology to ensure the study is valid, reliable, and ethical.
Data Collection in Research
Data collection is a crucial aspect of research involving gathering information to answer research questions or test hypotheses. Data collection methods may vary depending on the research design, questions, and required data type. The most commonly used data collection methods in research include:
- Surveys/questionnaires
- Observations
- Experiments
Surveys or questionnaires are commonly used in research to collect data from many participants. Survey questions are usually closed-ended, and the responses are quantifiable. Surveys can be conducted online, by phone, or in person.
Conversely, interviews are more in-depth and can be conducted face-to-face or over the phone. Interviews are useful in collecting qualitative data, which is non-numerical data that provides insights into participants’ experiences, opinions, and attitudes.
Observations involve watching and recording the behavior of individuals or groups in their natural settings. Observations can be structured or unstructured and conducted in person or through video recordings.
Experiments are designed to test hypotheses and involve manipulating one or more variables to observe the effect on the outcome variable. Experiments can be conducted in a laboratory or the field.
Regardless of the data collection method used, it is essential to ensure that the data collected is valid and reliable. Validity refers to the extent to which the data collected measures what it is supposed to measure, while reliability refers to the consistency of the data collected.
Data Collection in Study
One of the most important aspects of a study is data collection. In a study, data is collected through various methods such as surveys, questionnaires, interviews, and observations. The collected data is then analyzed to draw conclusions and make recommendations.
Surveys and questionnaires are popular methods of data collection in studies. Surveys are used to gather information from a large group, while questionnaires are used to collect data from a smaller group. Both methods involve asking participants questions to gather information on a particular topic.
Interviews, on the other hand, are conducted on an individual basis. They are useful when in-depth information is required on a specific topic. The interviewer asks open-ended questions to gather information from the interviewee.
Observations are another method of data collection in studies. Observations involve watching and recording the behavior of individuals or groups. This method is useful when studying behavior or interactions between individuals or groups.
It is important to note that the quality of the data collected in a study depends on the accuracy of the data collection methods. Therefore, ensuring that the data collection methods used in a study are appropriate and reliable is crucial.
Data Analysis in Research
After collecting data through various methods, researchers analyze the data to derive meaningful conclusions. Data analysis is a crucial step in the research process, as it helps to identify patterns, relationships, and trends in the data.
Researchers can use different methods of data analysis, depending on the type of data collected and the research questions. Some common methods of data analysis in research include:
Descriptive statistics
This method involves summarizing the data using measures such as mean, median, mode, and standard deviation. Descriptive statistics help to provide a general overview of the data and identify any outliers or anomalies.
Inferential statistics
This method involves using statistical tests to make inferences about the population based on the sample data. Inferential statistics help determine the findings’ significance and draw conclusions about the research questions.
Content analysis
This method involves analyzing the content of written or verbal communication to identify themes, patterns, and meanings. Content analysis is commonly used in qualitative research to analyze data from interviews, focus groups, and open-ended survey questions.
Thematic analysis
This method involves identifying and analyzing patterns and themes in qualitative data. Thematic analysis is commonly used in research to explore participants’ experiences, perspectives, and attitudes.
Regardless of the method used, data analysis in research is a systematic process that involves organizing, coding, and interpreting the data. Researchers must use appropriate data analysis methods to ensure the findings are valid, reliable, and meaningful.
Data Analysis in Study
Data analysis is a crucial part of any study, as it helps to draw conclusions and make sense of the data collected. The process of data analysis involves cleaning, transforming, and modeling data to extract useful information and insights. The following are some common methods of data analysis used in studies:
This method involves summarizing and describing the main features of the data collected, such as mean, median, mode, and standard deviation.
This method involves making inferences or predictions about a population based on a sample of the data collected. It uses techniques such as hypothesis testing and confidence intervals.
Qualitative analysis
This method involves analyzing data that is not numerical, such as text or images. It is often used in social science research to understand the experiences and perspectives of participants.
Once the data has been analyzed, the researcher can conclude and make recommendations based on the findings. It is important to note that data analysis is not a one-size-fits-all process and may vary depending on the type of study being conducted.
Data analysis is critical to any study, as it helps ensure the findings are accurate and reliable. Using appropriate data analysis methods, researchers can draw meaningful conclusions and make informed decisions based on the results.
Research and study are two terms often used interchangeably, but they are not the same. While both involve acquiring knowledge, research is a more systematic and structured approach to gathering information. At the same time, study is a more general term that can refer to any learning or investigation.
Research involves using specific methods and techniques to collect and analyze data to answer a specific research question or hypothesis. The study, conversely, can refer to any type of learning or investigation, whether formal or informal, structured or unstructured.
Both research and study are important for advancing knowledge and understanding in a particular field, but they serve different purposes and require different approaches. Researchers must be skilled in designing research studies, collecting and analyzing data, and drawing valid conclusions. On the other hand, students must be able to absorb and retain information and apply it to their studies and future careers.
While research and study are different, they are both important for advancing knowledge and understanding in a particular field. By understanding the differences between these two terms, researchers and students can better appreciate the unique contributions that each makes to the pursuit of knowledge and understanding.
Related Posts

What is Research?
Characteristics of good research, leave a comment cancel reply.
Your email address will not be published. Required fields are marked *
Save my name, email, and website in this browser for the next time I comment.
- En español – ExME
- Em português – EME
An introduction to different types of study design
Posted on 6th April 2021 by Hadi Abbas
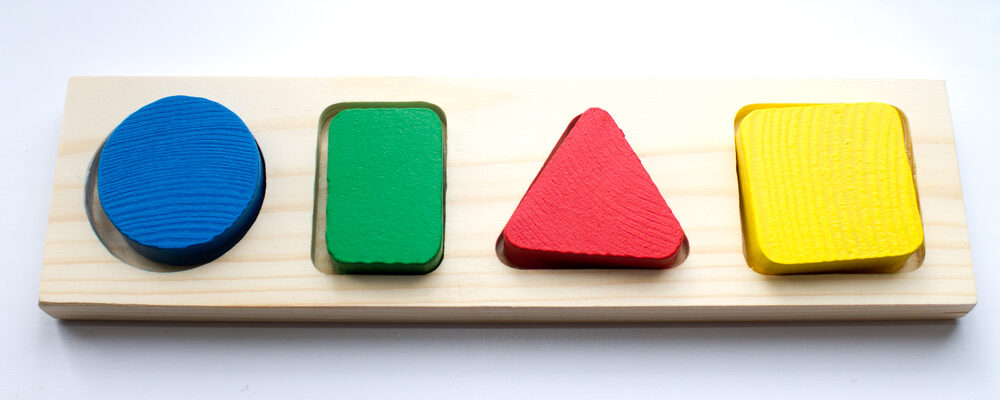
Study designs are the set of methods and procedures used to collect and analyze data in a study.
Broadly speaking, there are 2 types of study designs: descriptive studies and analytical studies.
Descriptive studies
- Describes specific characteristics in a population of interest
- The most common forms are case reports and case series
- In a case report, we discuss our experience with the patient’s symptoms, signs, diagnosis, and treatment
- In a case series, several patients with similar experiences are grouped.
Analytical Studies
Analytical studies are of 2 types: observational and experimental.
Observational studies are studies that we conduct without any intervention or experiment. In those studies, we purely observe the outcomes. On the other hand, in experimental studies, we conduct experiments and interventions.
Observational studies
Observational studies include many subtypes. Below, I will discuss the most common designs.
Cross-sectional study:
- This design is transverse where we take a specific sample at a specific time without any follow-up
- It allows us to calculate the frequency of disease ( p revalence ) or the frequency of a risk factor
- This design is easy to conduct
- For example – if we want to know the prevalence of migraine in a population, we can conduct a cross-sectional study whereby we take a sample from the population and calculate the number of patients with migraine headaches.
Cohort study:
- We conduct this study by comparing two samples from the population: one sample with a risk factor while the other lacks this risk factor
- It shows us the risk of developing the disease in individuals with the risk factor compared to those without the risk factor ( RR = relative risk )
- Prospective : we follow the individuals in the future to know who will develop the disease
- Retrospective : we look to the past to know who developed the disease (e.g. using medical records)
- This design is the strongest among the observational studies
- For example – to find out the relative risk of developing chronic obstructive pulmonary disease (COPD) among smokers, we take a sample including smokers and non-smokers. Then, we calculate the number of individuals with COPD among both.
Case-Control Study:
- We conduct this study by comparing 2 groups: one group with the disease (cases) and another group without the disease (controls)
- This design is always retrospective
- We aim to find out the odds of having a risk factor or an exposure if an individual has a specific disease (Odds ratio)
- Relatively easy to conduct
- For example – we want to study the odds of being a smoker among hypertensive patients compared to normotensive ones. To do so, we choose a group of patients diagnosed with hypertension and another group that serves as the control (normal blood pressure). Then we study their smoking history to find out if there is a correlation.
Experimental Studies
- Also known as interventional studies
- Can involve animals and humans
- Pre-clinical trials involve animals
- Clinical trials are experimental studies involving humans
- In clinical trials, we study the effect of an intervention compared to another intervention or placebo. As an example, I have listed the four phases of a drug trial:
I: We aim to assess the safety of the drug ( is it safe ? )
II: We aim to assess the efficacy of the drug ( does it work ? )
III: We want to know if this drug is better than the old treatment ( is it better ? )
IV: We follow-up to detect long-term side effects ( can it stay in the market ? )
- In randomized controlled trials, one group of participants receives the control, while the other receives the tested drug/intervention. Those studies are the best way to evaluate the efficacy of a treatment.
Finally, the figure below will help you with your understanding of different types of study designs.
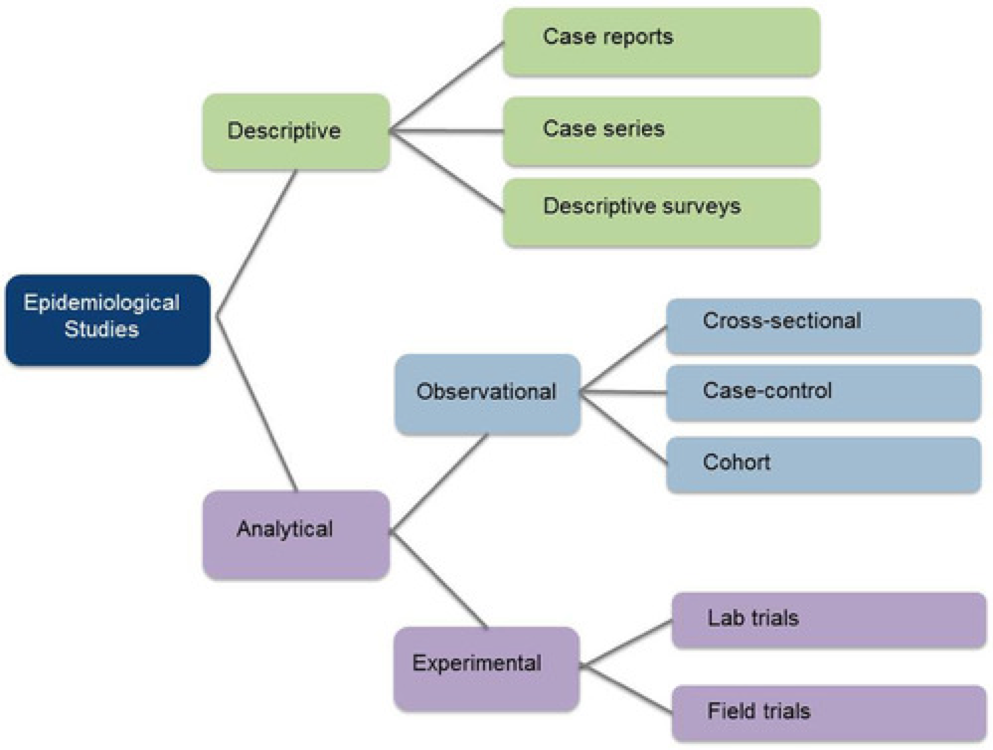
References (pdf)
You may also be interested in the following blogs for further reading:
An introduction to randomized controlled trials
Case-control and cohort studies: a brief overview
Cohort studies: prospective and retrospective designs
Prevalence vs Incidence: what is the difference?
Leave a Reply Cancel reply
Your email address will not be published. Required fields are marked *
Save my name, email, and website in this browser for the next time I comment.
No Comments on An introduction to different types of study design
you are amazing one!! if I get you I’m working with you! I’m student from Ethiopian higher education. health sciences student
Very informative and easy understandable
You are my kind of doctor. Do not lose sight of your objective.
Wow very erll explained and easy to understand
I’m Khamisu Habibu community health officer student from Abubakar Tafawa Balewa university teaching hospital Bauchi, Nigeria, I really appreciate your write up and you have make it clear for the learner. thank you
well understood,thank you so much
Well understood…thanks
Simply explained. Thank You.
Thanks a lot for this nice informative article which help me to understand different study designs that I felt difficult before
That’s lovely to hear, Mona, thank you for letting the author know how useful this was. If there are any other particular topics you think would be useful to you, and are not already on the website, please do let us know.
it is very informative and useful.
thank you statistician
Fabulous to hear, thank you John.
Thanks for this information
Thanks so much for this information….I have clearly known the types of study design Thanks
That’s so good to hear, Mirembe, thank you for letting the author know.
Very helpful article!! U have simplified everything for easy understanding
I’m a health science major currently taking statistics for health care workers…this is a challenging class…thanks for the simified feedback.
That’s good to hear this has helped you. Hopefully you will find some of the other blogs useful too. If you see any topics that are missing from the website, please do let us know!
Hello. I liked your presentation, the fact that you ranked them clearly is very helpful to understand for people like me who is a novelist researcher. However, I was expecting to read much more about the Experimental studies. So please direct me if you already have or will one day. Thank you
Dear Ay. My sincere apologies for not responding to your comment sooner. You may find it useful to filter the blogs by the topic of ‘Study design and research methods’ – here is a link to that filter: https://s4be.cochrane.org/blog/topic/study-design/ This will cover more detail about experimental studies. Or have a look on our library page for further resources there – you’ll find that on the ‘Resources’ drop down from the home page.
However, if there are specific things you feel you would like to learn about experimental studies, that are missing from the website, it would be great if you could let me know too. Thank you, and best of luck. Emma
Great job Mr Hadi. I advise you to prepare and study for the Australian Medical Board Exams as soon as you finish your undergrad study in Lebanon. Good luck and hope we can meet sometime in the future. Regards ;)
You have give a good explaination of what am looking for. However, references am not sure of where to get them from.
Subscribe to our newsletter
You will receive our monthly newsletter and free access to Trip Premium.
Related Articles
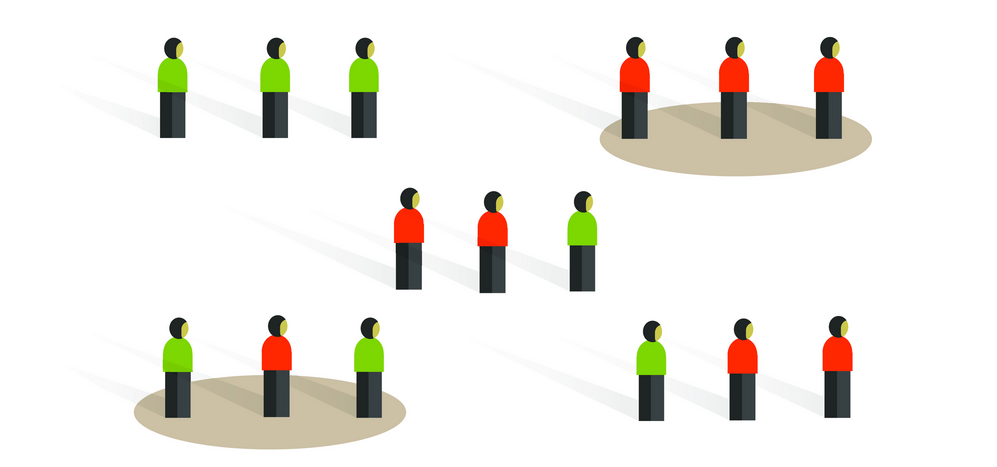
Cluster Randomized Trials: Concepts
This blog summarizes the concepts of cluster randomization, and the logistical and statistical considerations while designing a cluster randomized controlled trial.
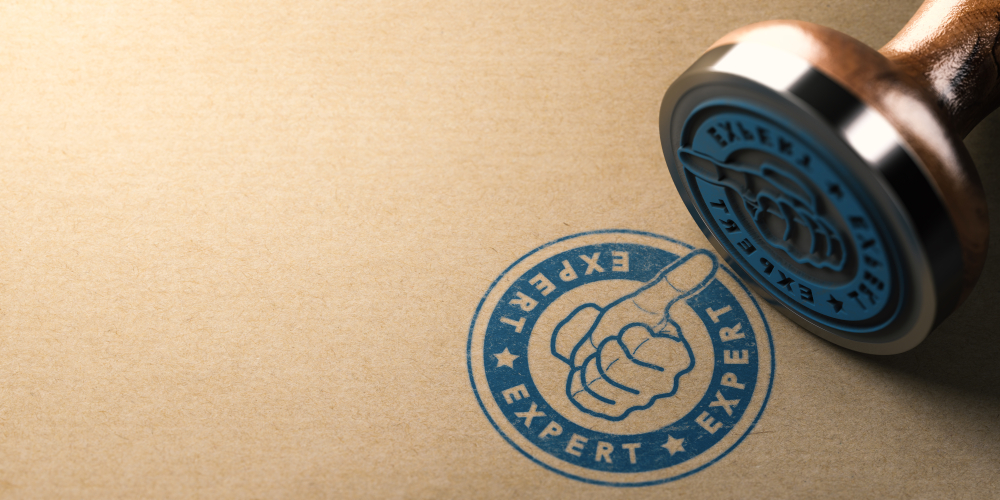
Expertise-based Randomized Controlled Trials
This blog summarizes the concepts of Expertise-based randomized controlled trials with a focus on the advantages and challenges associated with this type of study.
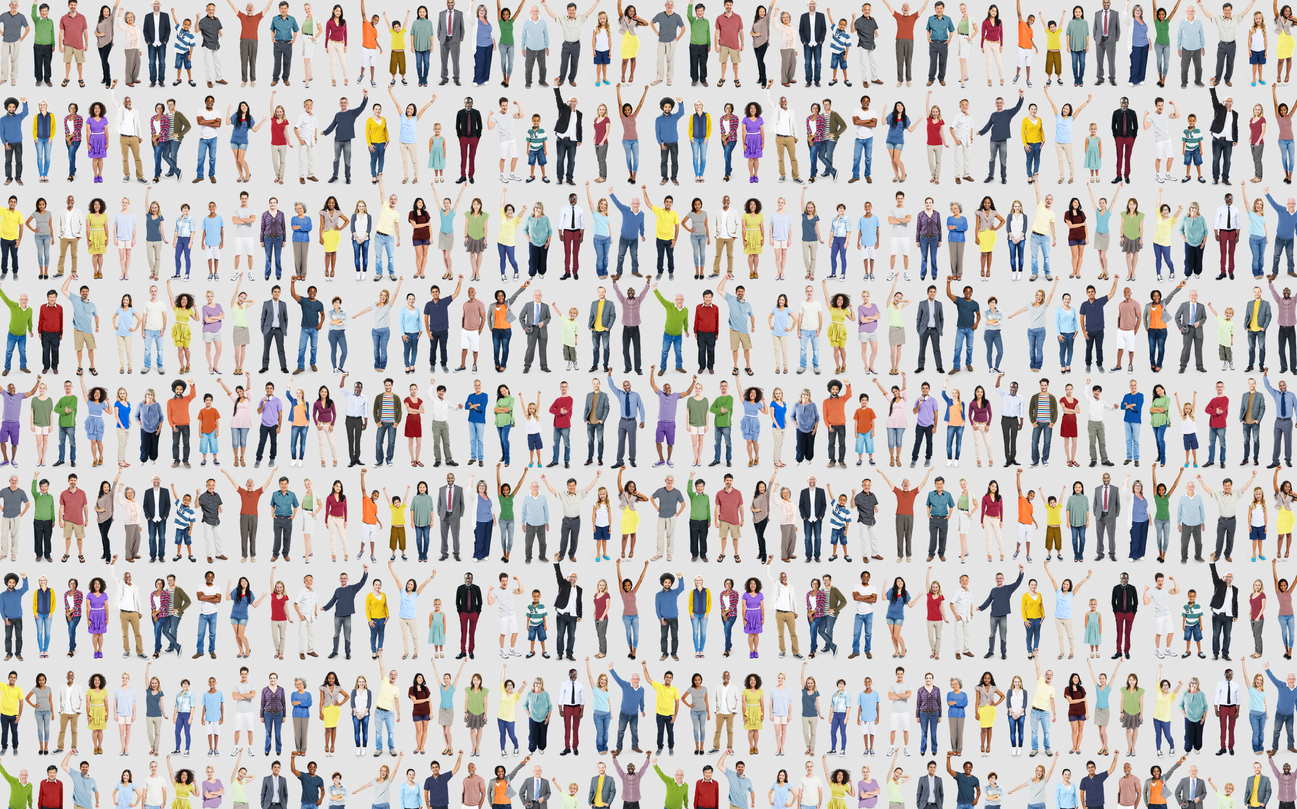
A well-designed cohort study can provide powerful results. This blog introduces prospective and retrospective cohort studies, discussing the advantages, disadvantages and use of these type of study designs.

Difference between Study and Research
What is the difference between study and research.
Study as a verb is to acquire knowledge on a subject through concentration on prepared learning materials. while Research as a verb is to search or examine with continued care; to seek diligently.
Part of speech: verb
Definition: To acquire knowledge on a subject through concentration on prepared learning materials.
Part of speech: noun
Definition: A state of mental perplexity or worried thought. Thought, as directed to a specific purpose; one's concern. Mental effort to acquire knowledge or learning. The act of studying; examination. A room in a house intended for reading and writing. An artwork made in order to practise or demonstrate a subject or technique.
Example sentence: Study strategy over the years and achieve the spirit of the warrior. Today is victory over yourself of yesterday; tomorrow is your victory over lesser men.
Definition: Diligent inquiry or examination to seek or revise facts, principles, theories, applications, et cetera; laborious or continued search after truth. A particular instance or piece of research.
Definition: to search or examine with continued care; to seek diligently. to make an extensive investigation into. to search again.
We hope you now know whether to use Study or Research in your sentence.
Difference between Lease and Mortgage
Autofluorescent and light - what's the difference, difference between undergrowth and underwood, obovate vs simple, labor and travail - what's the difference, peeing and pissing - what's the difference, blackberry vs boysenberry, difference between payload and load, popular articles, navic vs. gps vs. glonass vs. galileo: differences between navigation systems, vaccination vs. immunization: what's the difference, difference between level 1, level 2 and level 3 electric car chargers, difference between disney+ hotstar(indian version) and disney+ international version.
People often get confused between similar sounding words or synonyms. Most of the time these words have slightly different meanings, and some time entirely different meanings. We help people discover the difference between these words.

Research Philosophy & Paradigms
Positivism, Interpretivism & Pragmatism, Explained Simply
By: Derek Jansen (MBA) | Reviewer: Eunice Rautenbach (DTech) | June 2023
Research philosophy is one of those things that students tend to either gloss over or become utterly confused by when undertaking formal academic research for the first time. And understandably so – it’s all rather fluffy and conceptual. However, understanding the philosophical underpinnings of your research is genuinely important as it directly impacts how you develop your research methodology.
In this post, we’ll explain what research philosophy is , what the main research paradigms are and how these play out in the real world, using loads of practical examples . To keep this all as digestible as possible, we are admittedly going to simplify things somewhat and we’re not going to dive into the finer details such as ontology, epistemology and axiology (we’ll save those brain benders for another post!). Nevertheless, this post should set you up with a solid foundational understanding of what research philosophy and research paradigms are, and what they mean for your project.
Overview: Research Philosophy
- What is a research philosophy or paradigm ?
- Positivism 101
- Interpretivism 101
- Pragmatism 101
- Choosing your research philosophy
What is a research philosophy or paradigm?
Research philosophy and research paradigm are terms that tend to be used pretty loosely, even interchangeably. Broadly speaking, they both refer to the set of beliefs, assumptions, and principles that underlie the way you approach your study (whether that’s a dissertation, thesis or any other sort of academic research project).
For example, one philosophical assumption could be that there is an external reality that exists independent of our perceptions (i.e., an objective reality), whereas an alternative assumption could be that reality is constructed by the observer (i.e., a subjective reality). Naturally, these assumptions have quite an impact on how you approach your study (more on this later…).
The research philosophy and research paradigm also encapsulate the nature of the knowledge that you seek to obtain by undertaking your study. In other words, your philosophy reflects what sort of knowledge and insight you believe you can realistically gain by undertaking your research project. For example, you might expect to find a concrete, absolute type of answer to your research question , or you might anticipate that things will turn out to be more nuanced and less directly calculable and measurable . Put another way, it’s about whether you expect “hard”, clean answers or softer, more opaque ones.
So, what’s the difference between research philosophy and paradigm?
Well, it depends on who you ask. Different textbooks will present slightly different definitions, with some saying that philosophy is about the researcher themselves while the paradigm is about the approach to the study . Others will use the two terms interchangeably. And others will say that the research philosophy is the top-level category and paradigms are the pre-packaged combinations of philosophical assumptions and expectations.
To keep things simple in this video, we’ll avoid getting tangled up in the terminology and rather focus on the shared focus of both these terms – that is that they both describe (or at least involve) the set of beliefs, assumptions, and principles that underlie the way you approach your study .
Importantly, your research philosophy and/or paradigm form the foundation of your study . More specifically, they will have a direct influence on your research methodology , including your research design , the data collection and analysis techniques you adopt, and of course, how you interpret your results. So, it’s important to understand the philosophy that underlies your research to ensure that the rest of your methodological decisions are well-aligned .
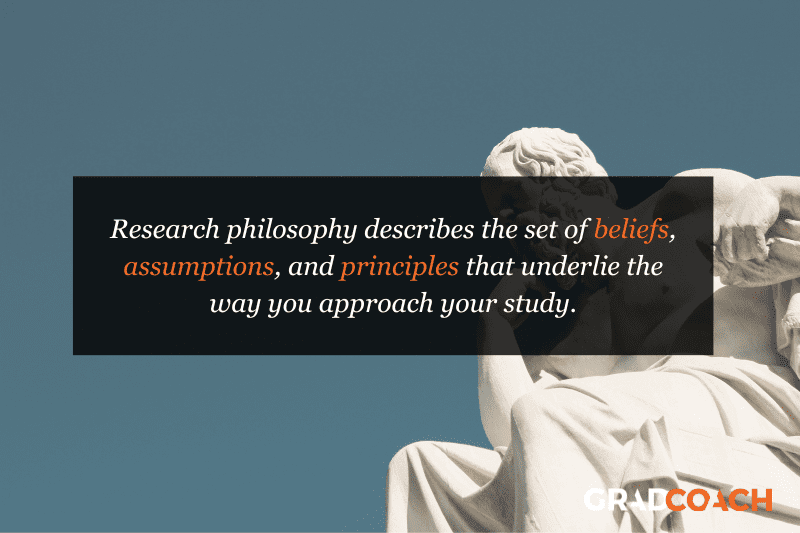
So, what are the options?
We’ll be straight with you – research philosophy is a rabbit hole (as with anything philosophy-related) and, as a result, there are many different approaches (or paradigms) you can take, each with its own perspective on the nature of reality and knowledge . To keep things simple though, we’ll focus on the “big three”, namely positivism , interpretivism and pragmatism . Understanding these three is a solid starting point and, in many cases, will be all you need.
Paradigm 1: Positivism
When you think positivism, think hard sciences – physics, biology, astronomy, etc. Simply put, positivism is rooted in the belief that knowledge can be obtained through objective observations and measurements . In other words, the positivist philosophy assumes that answers can be found by carefully measuring and analysing data, particularly numerical data .
As a research paradigm, positivism typically manifests in methodologies that make use of quantitative data , and oftentimes (but not always) adopt experimental or quasi-experimental research designs. Quite often, the focus is on causal relationships – in other words, understanding which variables affect other variables, in what way and to what extent. As a result, studies with a positivist research philosophy typically aim for objectivity, generalisability and replicability of findings.
Let’s look at an example of positivism to make things a little more tangible.
Assume you wanted to investigate the relationship between a particular dietary supplement and weight loss. In this case, you could design a randomised controlled trial (RCT) where you assign participants to either a control group (who do not receive the supplement) or an intervention group (who do receive the supplement). With this design in place, you could measure each participant’s weight before and after the study and then use various quantitative analysis methods to assess whether there’s a statistically significant difference in weight loss between the two groups. By doing so, you could infer a causal relationship between the dietary supplement and weight loss, based on objective measurements and rigorous experimental design.
As you can see in this example, the underlying assumptions and beliefs revolve around the viewpoint that knowledge and insight can be obtained through carefully controlling the environment, manipulating variables and analysing the resulting numerical data . Therefore, this sort of study would adopt a positivistic research philosophy. This is quite common for studies within the hard sciences – so much so that research philosophy is often just assumed to be positivistic and there’s no discussion of it within the methodology section of a dissertation or thesis.
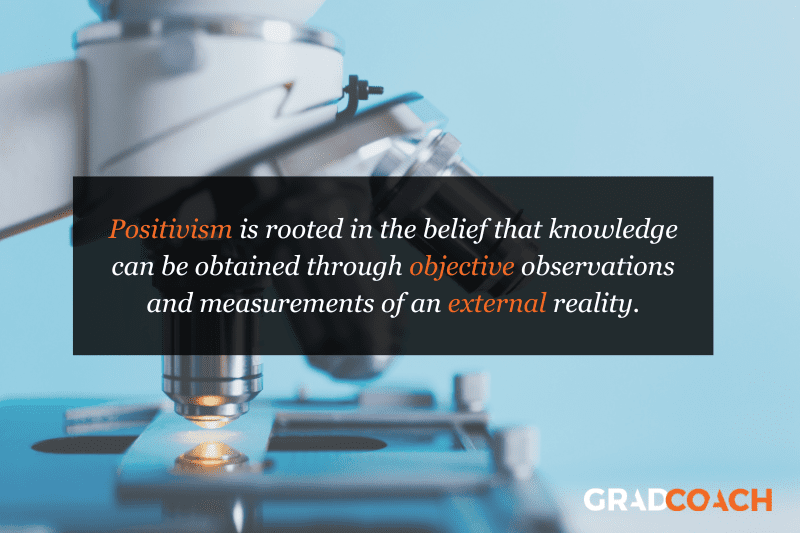
Paradigm 2: Interpretivism
If you can imagine a spectrum of research paradigms, interpretivism would sit more or less on the opposite side of the spectrum from positivism. Essentially, interpretivism takes the position that reality is socially constructed . In other words, that reality is subjective , and is constructed by the observer through their experience of it , rather than being independent of the observer (which, if you recall, is what positivism assumes).
The interpretivist paradigm typically underlies studies where the research aims involve attempting to understand the meanings and interpretations that people assign to their experiences. An interpretivistic philosophy also typically manifests in the adoption of a qualitative methodology , relying on data collection methods such as interviews , observations , and textual analysis . These types of studies commonly explore complex social phenomena and individual perspectives, which are naturally more subjective and nuanced.
Let’s look at an example of the interpretivist approach in action:
Assume that you’re interested in understanding the experiences of individuals suffering from chronic pain. In this case, you might conduct in-depth interviews with a group of participants and ask open-ended questions about their pain, its impact on their lives, coping strategies, and their overall experience and perceptions of living with pain. You would then transcribe those interviews and analyse the transcripts, using thematic analysis to identify recurring themes and patterns. Based on that analysis, you’d be able to better understand the experiences of these individuals, thereby satisfying your original research aim.
As you can see in this example, the underlying assumptions and beliefs revolve around the viewpoint that insight can be obtained through engaging in conversation with and exploring the subjective experiences of people (as opposed to collecting numerical data and trying to measure and calculate it). Therefore, this sort of study would adopt an interpretivistic research philosophy. Ultimately, if you’re looking to understand people’s lived experiences , you have to operate on the assumption that knowledge can be generated by exploring people’s viewpoints, as subjective as they may be.
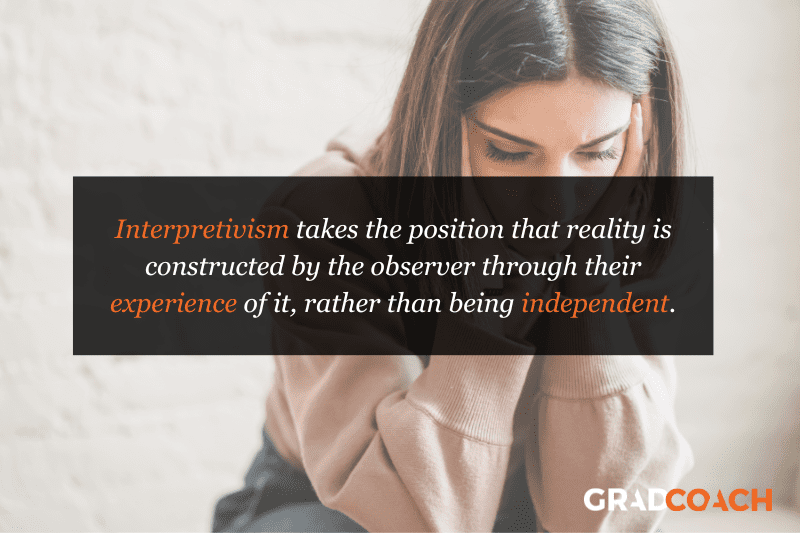
Paradigm 3: Pragmatism
Now that we’ve looked at the two opposing ends of the research philosophy spectrum – positivism and interpretivism, you can probably see that both of the positions have their merits , and that they both function as tools for different jobs . More specifically, they lend themselves to different types of research aims, objectives and research questions . But what happens when your study doesn’t fall into a clear-cut category and involves exploring both “hard” and “soft” phenomena? Enter pragmatism…
As the name suggests, pragmatism takes a more practical and flexible approach, focusing on the usefulness and applicability of research findings , rather than an all-or-nothing, mutually exclusive philosophical position. This allows you, as the researcher, to explore research aims that cross philosophical boundaries, using different perspectives for different aspects of the study .
With a pragmatic research paradigm, both quantitative and qualitative methods can play a part, depending on the research questions and the context of the study. This often manifests in studies that adopt a mixed-method approach , utilising a combination of different data types and analysis methods. Ultimately, the pragmatist adopts a problem-solving mindset , seeking practical ways to achieve diverse research aims.
Let’s look at an example of pragmatism in action:
Imagine that you want to investigate the effectiveness of a new teaching method in improving student learning outcomes. In this case, you might adopt a mixed-methods approach, which makes use of both quantitative and qualitative data collection and analysis techniques. One part of your project could involve comparing standardised test results from an intervention group (students that received the new teaching method) and a control group (students that received the traditional teaching method). Additionally, you might conduct in-person interviews with a smaller group of students from both groups, to gather qualitative data on their perceptions and preferences regarding the respective teaching methods.
As you can see in this example, the pragmatist’s approach can incorporate both quantitative and qualitative data . This allows the researcher to develop a more holistic, comprehensive understanding of the teaching method’s efficacy and practical implications, with a synthesis of both types of data . Naturally, this type of insight is incredibly valuable in this case, as it’s essential to understand not just the impact of the teaching method on test results, but also on the students themselves!
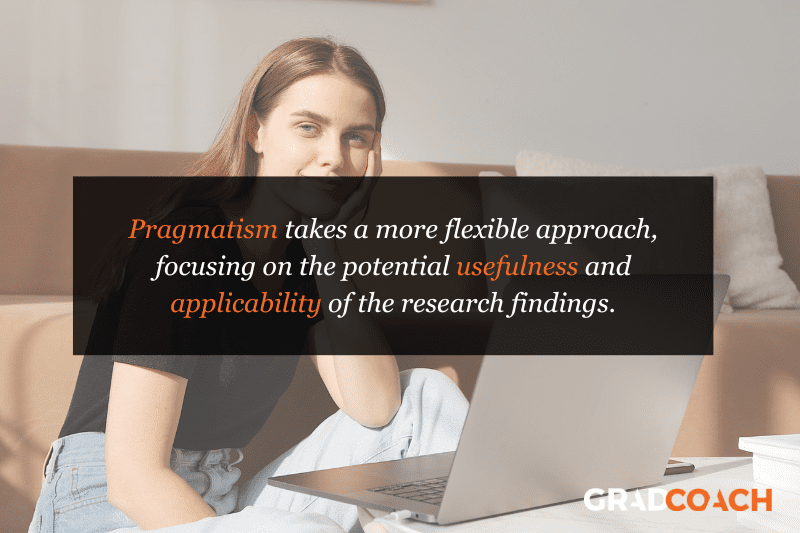
Wrapping Up: Philosophies & Paradigms
Now that we’ve unpacked the “big three” research philosophies or paradigms – positivism, interpretivism and pragmatism, hopefully, you can see that research philosophy underlies all of the methodological decisions you’ll make in your study. In many ways, it’s less a case of you choosing your research philosophy and more a case of it choosing you (or at least, being revealed to you), based on the nature of your research aims and research questions .
- Research philosophies and paradigms encapsulate the set of beliefs, assumptions, and principles that guide the way you, as the researcher, approach your study and develop your methodology.
- Positivism is rooted in the belief that reality is independent of the observer, and consequently, that knowledge can be obtained through objective observations and measurements.
- Interpretivism takes the (opposing) position that reality is subjectively constructed by the observer through their experience of it, rather than being an independent thing.
- Pragmatism attempts to find a middle ground, focusing on the usefulness and applicability of research findings, rather than an all-or-nothing, mutually exclusive philosophical position.
If you’d like to learn more about research philosophy, research paradigms and research methodology more generally, be sure to check out the rest of the Grad Coach blog . Alternatively, if you’d like hands-on help with your research, consider our private coaching service , where we guide you through each stage of the research journey, step by step.
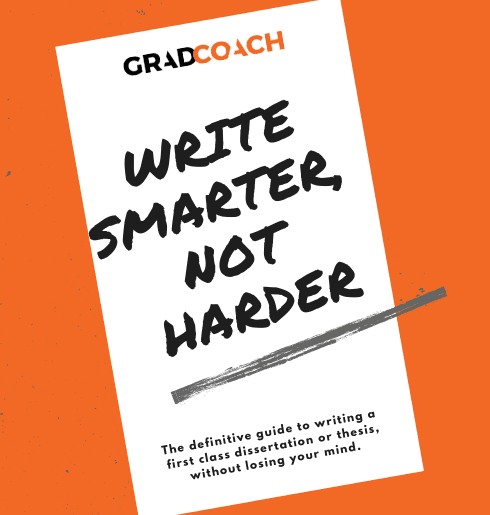
Psst… there’s more (for free)
This post is part of our dissertation mini-course, which covers everything you need to get started with your dissertation, thesis or research project.
You Might Also Like:
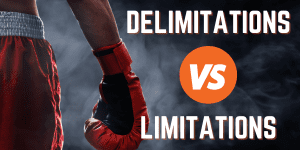
13 Comments
was very useful for me, I had no idea what a philosophy is, and what type of philosophy of my study. thank you
Thanks for this explanation, is so good for me
You contributed much to my master thesis development and I wish to have again your support for PhD program through research.
the way of you explanation very good keep it up/continuous just like this
Very precise stuff. It has been of great use to me. It has greatly helped me to sharpen my PhD research project!
Very clear and very helpful explanation above. I have clearly understand the explanation.
Very clear and useful. Thanks
Thanks so much for your insightful explanations of the research philosophies that confuse me
I would like to thank Grad Coach TV or Youtube organizers and presenters. Since then, I have been able to learn a lot by finding very informative posts from them.
thank you so much for this valuable and explicit explanation,cheers
Hey, at last i have gained insight on which philosophy to use as i had little understanding on their applicability to my current research. Thanks
Tremendously useful
thank you and God bless you. This was very helpful, I had no understanding before this.
Submit a Comment Cancel reply
Your email address will not be published. Required fields are marked *
Save my name, email, and website in this browser for the next time I comment.
- Print Friendly
Frequently asked questions
What’s the difference between research aims and objectives.
A research aim is a broad statement indicating the general purpose of your research project. It should appear in your introduction at the end of your problem statement , before your research objectives.
Research objectives are more specific than your research aim. They indicate the specific ways you’ll address the overarching aim.
Frequently asked questions: Writing a research paper
A research project is an academic, scientific, or professional undertaking to answer a research question . Research projects can take many forms, such as qualitative or quantitative , descriptive , longitudinal , experimental , or correlational . What kind of research approach you choose will depend on your topic.
The best way to remember the difference between a research plan and a research proposal is that they have fundamentally different audiences. A research plan helps you, the researcher, organize your thoughts. On the other hand, a dissertation proposal or research proposal aims to convince others (e.g., a supervisor, a funding body, or a dissertation committee) that your research topic is relevant and worthy of being conducted.
Formulating a main research question can be a difficult task. Overall, your question should contribute to solving the problem that you have defined in your problem statement .
However, it should also fulfill criteria in three main areas:
- Researchability
- Feasibility and specificity
- Relevance and originality
Research questions anchor your whole project, so it’s important to spend some time refining them.
In general, they should be:
- Focused and researchable
- Answerable using credible sources
- Complex and arguable
- Feasible and specific
- Relevant and original
All research questions should be:
- Focused on a single problem or issue
- Researchable using primary and/or secondary sources
- Feasible to answer within the timeframe and practical constraints
- Specific enough to answer thoroughly
- Complex enough to develop the answer over the space of a paper or thesis
- Relevant to your field of study and/or society more broadly
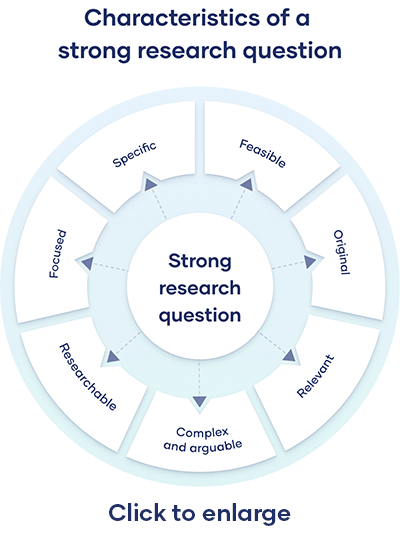
Once you’ve decided on your research objectives , you need to explain them in your paper, at the end of your problem statement .
Keep your research objectives clear and concise, and use appropriate verbs to accurately convey the work that you will carry out for each one.
I will compare …
Your research objectives indicate how you’ll try to address your research problem and should be specific:
Research objectives describe what you intend your research project to accomplish.
They summarize the approach and purpose of the project and help to focus your research.
Your objectives should appear in the introduction of your research paper , at the end of your problem statement .
The main guidelines for formatting a paper in Chicago style are to:
- Use a standard font like 12 pt Times New Roman
- Use 1 inch margins or larger
- Apply double line spacing
- Indent every new paragraph ½ inch
- Include a title page
- Place page numbers in the top right or bottom center
- Cite your sources with author-date citations or Chicago footnotes
- Include a bibliography or reference list
To automatically generate accurate Chicago references, you can use Scribbr’s free Chicago reference generator .
The main guidelines for formatting a paper in MLA style are as follows:
- Use an easily readable font like 12 pt Times New Roman
- Set 1 inch page margins
- Include a four-line MLA heading on the first page
- Center the paper’s title
- Use title case capitalization for headings
- Cite your sources with MLA in-text citations
- List all sources cited on a Works Cited page at the end
To format a paper in APA Style , follow these guidelines:
- Use a standard font like 12 pt Times New Roman or 11 pt Arial
- If submitting for publication, insert a running head on every page
- Apply APA heading styles
- Cite your sources with APA in-text citations
- List all sources cited on a reference page at the end
No, it’s not appropriate to present new arguments or evidence in the conclusion . While you might be tempted to save a striking argument for last, research papers follow a more formal structure than this.
All your findings and arguments should be presented in the body of the text (more specifically in the results and discussion sections if you are following a scientific structure). The conclusion is meant to summarize and reflect on the evidence and arguments you have already presented, not introduce new ones.
The conclusion of a research paper has several key elements you should make sure to include:
- A restatement of the research problem
- A summary of your key arguments and/or findings
- A short discussion of the implications of your research
Don’t feel that you have to write the introduction first. The introduction is often one of the last parts of the research paper you’ll write, along with the conclusion.
This is because it can be easier to introduce your paper once you’ve already written the body ; you may not have the clearest idea of your arguments until you’ve written them, and things can change during the writing process .
The way you present your research problem in your introduction varies depending on the nature of your research paper . A research paper that presents a sustained argument will usually encapsulate this argument in a thesis statement .
A research paper designed to present the results of empirical research tends to present a research question that it seeks to answer. It may also include a hypothesis —a prediction that will be confirmed or disproved by your research.
The introduction of a research paper includes several key elements:
- A hook to catch the reader’s interest
- Relevant background on the topic
- Details of your research problem
and your problem statement
- A thesis statement or research question
- Sometimes an overview of the paper
Ask our team
Want to contact us directly? No problem. We are always here for you.
- Email [email protected]
- Start live chat
- Call +1 (510) 822-8066
- WhatsApp +31 20 261 6040
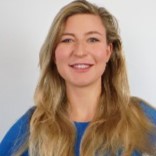
Our team helps students graduate by offering:
- A world-class citation generator
- Plagiarism Checker software powered by Turnitin
- Innovative Citation Checker software
- Professional proofreading services
- Over 300 helpful articles about academic writing, citing sources, plagiarism, and more
Scribbr specializes in editing study-related documents . We proofread:
- PhD dissertations
- Research proposals
- Personal statements
- Admission essays
- Motivation letters
- Reflection papers
- Journal articles
- Capstone projects
Scribbr’s Plagiarism Checker is powered by elements of Turnitin’s Similarity Checker , namely the plagiarism detection software and the Internet Archive and Premium Scholarly Publications content databases .
The add-on AI detector is powered by Scribbr’s proprietary software.
The Scribbr Citation Generator is developed using the open-source Citation Style Language (CSL) project and Frank Bennett’s citeproc-js . It’s the same technology used by dozens of other popular citation tools, including Mendeley and Zotero.
You can find all the citation styles and locales used in the Scribbr Citation Generator in our publicly accessible repository on Github .

- Richard G. Trefry Library
Q. What's the difference between a research article (or research study) and a review article?

- Course-Specific
- Textbooks & Course Materials
- Tutoring & Classroom Help
- Writing & Citing
- 44 Articles & Journals
- 11 Capstone/Thesis/Dissertation Research
- 37 Databases
- 56 Information Literacy
- 9 Interlibrary Loan
- 9 Need help getting started?
- 22 Technical Help
Answered By: Priscilla Coulter Last Updated: Jul 29, 2022 Views: 230750
A research paper is a primary source ...that is, it reports the methods and results of an original study performed by the authors . The kind of study may vary (it could have been an experiment, survey, interview, etc.), but in all cases, raw data have been collected and analyzed by the authors , and conclusions drawn from the results of that analysis.
Research papers follow a particular format. Look for:
- A brief introduction will often include a review of the existing literature on the topic studied, and explain the rationale of the author's study. This is important because it demonstrates that the authors are aware of existing studies, and are planning to contribute to this existing body of research in a meaningful way (that is, they're not just doing what others have already done).
- A methods section, where authors describe how they collected and analyzed data. Statistical analyses are included. This section is quite detailed, as it's important that other researchers be able to verify and/or replicate these methods.
- A results section describes the outcomes of the data analysis. Charts and graphs illustrating the results are typically included.
- In the discussion , authors will explain their interpretation of their results and theorize on their importance to existing and future research.
- References or works cited are always included. These are the articles and books that the authors drew upon to plan their study and to support their discussion.
You can use the library's article databases to search for research articles:
- A research article will nearly always be published in a peer-reviewed journal; click here for instructions on limiting your searches to peer-reviewed articles.
- If you have a particular type of study in mind, you can include keywords to describe it in your search . For instance, if you would like to see studies that used surveys to collect data, you can add "survey" to your topic in the database's search box. See this example search in our EBSCO databases: " bullying and survey ".
- Several of our databases have special limiting options that allow you to select specific methodologies. See, for instance, the " Methodology " box in ProQuest's PsycARTICLES Advanced Search (scroll down a bit to see it). It includes options like "Empirical Study" and "Qualitative Study", among many others.
A review article is a secondary source ...it is written about other articles, and does not report original research of its own. Review articles are very important, as they draw upon the articles that they review to suggest new research directions, to strengthen support for existing theories and/or identify patterns among exising research studies. For student researchers, review articles provide a great overview of the existing literature on a topic. If you find a literature review that fits your topic, take a look at its references/works cited list for leads on other relevant articles and books!
You can use the library's article databases to find literature reviews as well! Click here for tips.
- Share on Facebook
Was this helpful? Yes 7 No 0
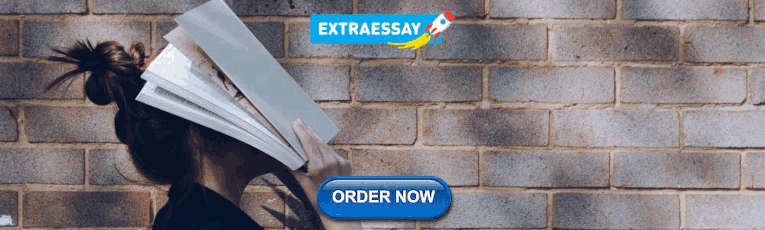
Related Topics
- Articles & Journals
- Information Literacy
Need personalized help? Librarians are available 365 days/nights per year! See our schedule.

Learn more about how librarians can help you succeed.
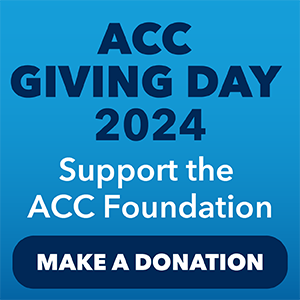
Create Free Account or

- Acute Coronary Syndromes
- Anticoagulation Management
- Arrhythmias and Clinical EP
- Cardiac Surgery
- Cardio-Oncology
- Cardiovascular Care Team
- Congenital Heart Disease and Pediatric Cardiology
- COVID-19 Hub
- Diabetes and Cardiometabolic Disease
- Dyslipidemia
- Geriatric Cardiology
- Heart Failure and Cardiomyopathies
- Invasive Cardiovascular Angiography and Intervention
- Noninvasive Imaging
- Pericardial Disease
- Pulmonary Hypertension and Venous Thromboembolism
- Sports and Exercise Cardiology
- Stable Ischemic Heart Disease
- Valvular Heart Disease
- Vascular Medicine
- Clinical Updates & Discoveries
- Advocacy & Policy
- Perspectives & Analysis
- Meeting Coverage
- ACC Member Publications
- ACC Podcasts
- View All Cardiology Updates
- Earn Credit
- View the Education Catalog
- ACC Anywhere: The Cardiology Video Library
- CardioSource Plus for Institutions and Practices
- ECG Drill and Practice
- Heart Songs
- Nuclear Cardiology
- Online Courses
- Collaborative Maintenance Pathway (CMP)
- Understanding MOC
- Image and Slide Gallery
- Annual Scientific Session and Related Events
- Chapter Meetings
- Live Meetings
- Live Meetings - International
- Webinars - Live
- Webinars - OnDemand
- Certificates and Certifications
- ACC Accreditation Services
- ACC Quality Improvement for Institutions Program
- CardioSmart
- National Cardiovascular Data Registry (NCDR)
- Advocacy at the ACC
- Cardiology as a Career Path
- Cardiology Careers
- Cardiovascular Buyers Guide
- Clinical Solutions
- Clinician Well-Being Portal
- Diversity and Inclusion
- Infographics
- Innovation Program
- Mobile and Web Apps
Study Links E-Cigarette Use with Higher Risk of Heart Failure
Large study adds to growing body of evidence that vaping may harm the heart.
Apr 02, 2024
Contact: Nicole Napoli , [email protected], 202-669-1465
WASHINGTON (Apr 02, 2024) -
People who use e-cigarettes are significantly more likely to develop heart failure compared with those who have never used them, according to one of the largest prospective studies to date investigating possible links between vaping and heart failure. The findings are being presented at the American College of Cardiology’s Annual Scientific Session.
Heart failure is a condition affecting more than 6 million U.S. adults in which the heart becomes too stiff or too weak to pump blood as effectively as it should. It can often lead to debilitating symptoms and frequent hospitalizations as people age. Electronic nicotine products, which include e-cigarettes, vape pens, hookah pens, personal vaporizers and mods, e-cigars, e-pipes and e-hookahs, deliver nicotine in aerosol form without combustion. Since they were first introduced in the U.S. in the late 2000s, electronic nicotine products have often been portrayed as a safer alternative to smoking, but a growing body of research has led to increased concern about potential negative health effects.
“More and more studies are linking e-cigarettes to harmful effects and finding that it might not be as safe as previously thought,” said Yakubu Bene-Alhasan, MD, a resident physician at MedStar Health in Baltimore and the study’s lead author. “The difference we saw was substantial. It’s worth considering the consequences to your health, especially with regard to heart health.”
For the study, researchers used data from surveys and electronic health records in All of Us, a large national study of U.S. adults run by the National Institutes of Health, to analyze associations between e-cigarette use and new diagnoses of heart failure in 175,667 study participants (an average age of 52 years and 60.5% female). Of this sample, 3,242 participants developed heart failure within a median follow-up time of 45 months.
The results showed that people who used e-cigarettes at any point were 19% more likely to develop heart failure compared with people who had never used e-cigarettes. In calculating this difference, researchers accounted for a variety of demographic and socioeconomic factors, other heart disease risk factors and participants’ past and current use of other substances, including alcohol and tobacco products. The researchers also found no evidence that participants’ age, sex or smoking status modified the relationship between e-cigarettes and heart failure.
Breaking the data down by type of heart failure, the increased risk associated with e-cigarette use was statistically significant for heart failure with preserved ejection fraction (HFpEF)—in which the heart muscle becomes stiff and does not properly fill with blood between contractions. However, this association was not significant for heart failure with reduced ejection fraction (HFrEF)—in which the heart muscle becomes weak and the left ventricle does not squeeze as hard as it should during contractions. Rates of HFpEF have risen in recent decades, which has led to an increased focus on determining risk factors and improving treatment options for this type of heart failure.
The findings align with previous studies conducted in animals, which signaled e-cigarette use can affect the heart in ways that are relevant to the heart changes involved in heart failure. Other studies in humans have also shown links between e-cigarette use and some risk factors associated with developing heart failure. However, previous studies attempting to assess the direct connection between e-cigarette use and heart failure have been inconclusive, which Bene-Alhasan said is due to the inherent limitations of the cross-sectional study designs, smaller sample sizes and the smaller number of heart failure events seen in previous research.
Researchers said the new study findings point to a need for additional investigations of the potential impacts of vaping on heart health, especially considering the prevalence of e-cigarette use among younger people. Surveys indicate that about 5% to 10% of U.S. teens and adults use e-cigarettes. In 2018, the U.S. Surgeon General called youth e-cigarette use an epidemic and warned about the health risks associated with nicotine addiction.
“I think this research is long overdue, especially considering how much e-cigarettes have gained traction,” Bene-Alhasan said. “We don’t want to wait too long to find out eventually that it might be harmful, and by that time a lot of harm might already have been done. With more research, we will get to uncover a lot more about the potential health consequences and improve the information out to the public.”
Bene-Alhasan also said e-cigarettes are not recommended as a tool to quit smoking, since many people may continue vaping long after they quit smoking. The U.S. Centers for Disease Control and Prevention recommends a combination of counseling and medications as the best strategy for quitting smoking.
Researchers said that the study’s prospective observational design allows them to infer, but not conclusively determine, a causal relationship between e-cigarette use and heart failure. However, with its large sample size and detailed data on substance use and health information, Bene-Alhasan said the study is one of the most comprehensive studies to assess this relationship to date.
For more information about the health effects of e-cigarettes, visit CardioSmart.org/StopSmoking .
Bene-Alhasan will present the study, “Electronic Nicotine Product Use Is Associated with Incident Heart Failure - The All of Us Research Program,” on Sunday, April 7, 2024, at 3:15 p.m. ET / 19:15 UTC in Hall B4-5.
ACC.24 will take place April 6-8, 2024, in Atlanta, bringing together cardiologists and cardiovascular specialists from around the world to share the newest discoveries in treatment and prevention. Follow @ACCinTouch , @ACCMediaCenter and #ACC24 for the latest news from the meeting.
The American College of Cardiology (ACC) is the global leader in transforming cardiovascular care and improving heart health for all. As the preeminent source of professional medical education for the entire cardiovascular care team since 1949, ACC credentials cardiovascular professionals in over 140 countries who meet stringent qualifications and leads in the formation of health policy, standards and guidelines. Through its world-renowned family of JACC Journals, NCDR registries, ACC Accreditation Services, global network of Member Sections, CardioSmart patient resources and more, the College is committed to ensuring a world where science, knowledge and innovation optimize patient care and outcomes. Learn more at ACC.org .
< Back to Listings
You must be logged in to save to your library.
Jacc journals on acc.org.
- JACC: Advances
- JACC: Basic to Translational Science
- JACC: CardioOncology
- JACC: Cardiovascular Imaging
- JACC: Cardiovascular Interventions
- JACC: Case Reports
- JACC: Clinical Electrophysiology
- JACC: Heart Failure
- Current Members
- Campaign for the Future
- Become a Member
- Renew Your Membership
- Member Benefits and Resources
- Member Sections
- ACC Member Directory
- ACC Innovation Program
- Our Strategic Direction
- Our History
- Our Bylaws and Code of Ethics
- Leadership and Governance
- Annual Report
- Industry Relations
- Support the ACC
- Jobs at the ACC
- Press Releases
- Social Media
- Book Our Conference Center
Clinical Topics
- Chronic Angina
- Congenital Heart Disease and Pediatric Cardiology
- Diabetes and Cardiometabolic Disease
- Hypertriglyceridemia
- Invasive Cardiovascular Angiography and Intervention
- Pulmonary Hypertension and Venous Thromboembolism
Latest in Cardiology
Education and meetings.
- Online Learning Catalog
- Products and Resources
- Annual Scientific Session
Tools and Practice Support
- Quality Improvement for Institutions
- Accreditation Services
- Practice Solutions

Heart House
- 2400 N St. NW
- Washington , DC 20037
- Email: [email protected]
- Phone: 1-202-375-6000
- Toll Free: 1-800-253-4636
- Fax: 1-202-375-6842
- Media Center
- ACC.org Quick Start Guide
- Advertising & Sponsorship Policy
- Clinical Content Disclaimer
- Editorial Board
- Privacy Policy
- Registered User Agreement
- Terms of Service
- Cookie Policy
© 2024 American College of Cardiology Foundation. All rights reserved.

An official website of the United States government
The .gov means it’s official. Federal government websites often end in .gov or .mil. Before sharing sensitive information, make sure you’re on a federal government site.
The site is secure. The https:// ensures that you are connecting to the official website and that any information you provide is encrypted and transmitted securely.
- Publications
- Account settings
Preview improvements coming to the PMC website in October 2024. Learn More or Try it out now .
- Advanced Search
- Journal List
- Indian J Anaesth
- v.60(9); 2016 Sep
Types of studies and research design
Mukul chandra kapoor.
Department of Anesthesiology, Max Smart Super Specialty Hospital, New Delhi, India
Medical research has evolved, from individual expert described opinions and techniques, to scientifically designed methodology-based studies. Evidence-based medicine (EBM) was established to re-evaluate medical facts and remove various myths in clinical practice. Research methodology is now protocol based with predefined steps. Studies were classified based on the method of collection and evaluation of data. Clinical study methodology now needs to comply to strict ethical, moral, truth, and transparency standards, ensuring that no conflict of interest is involved. A medical research pyramid has been designed to grade the quality of evidence and help physicians determine the value of the research. Randomised controlled trials (RCTs) have become gold standards for quality research. EBM now scales systemic reviews and meta-analyses at a level higher than RCTs to overcome deficiencies in the randomised trials due to errors in methodology and analyses.
INTRODUCTION
Expert opinion, experience, and authoritarian judgement were the norm in clinical medical practice. At scientific meetings, one often heard senior professionals emphatically expressing ‘In my experience,…… what I have said is correct!’ In 1981, articles published by Sackett et al . introduced ‘critical appraisal’ as they felt a need to teach methods of understanding scientific literature and its application at the bedside.[ 1 ] To improve clinical outcomes, clinical expertise must be complemented by the best external evidence.[ 2 ] Conversely, without clinical expertise, good external evidence may be used inappropriately [ Figure 1 ]. Practice gets outdated, if not updated with current evidence, depriving the clientele of the best available therapy.

Triad of evidence-based medicine
EVIDENCE-BASED MEDICINE
In 1971, in his book ‘Effectiveness and Efficiency’, Archibald Cochrane highlighted the lack of reliable evidence behind many accepted health-care interventions.[ 3 ] This triggered re-evaluation of many established ‘supposed’ scientific facts and awakened physicians to the need for evidence in medicine. Evidence-based medicine (EBM) thus evolved, which was defined as ‘the conscientious, explicit and judicious use of the current best evidence in making decisions about the care of individual patients.’[ 2 ]
The goal of EBM was scientific endowment to achieve consistency, efficiency, effectiveness, quality, safety, reduction in dilemma and limitation of idiosyncrasies in clinical practice.[ 4 ] EBM required the physician to diligently assess the therapy, make clinical adjustments using the best available external evidence, ensure awareness of current research and discover clinical pathways to ensure best patient outcomes.[ 5 ]
With widespread internet use, phenomenally large number of publications, training and media resources are available but determining the quality of this literature is difficult for a busy physician. Abstracts are available freely on the internet, but full-text articles require a subscription. To complicate issues, contradictory studies are published making decision-making difficult.[ 6 ] Publication bias, especially against negative studies, makes matters worse.
In 1993, the Cochrane Collaboration was founded by Ian Chalmers and others to create and disseminate up-to-date review of randomised controlled trials (RCTs) to help health-care professionals make informed decisions.[ 7 ] In 1995, the American College of Physicians and the British Medical Journal Publishing Group collaborated to publish the journal ‘Evidence-based medicine’, leading to the evolution of EBM in all spheres of medicine.
MEDICAL RESEARCH
Medical research needs to be conducted to increase knowledge about the human species, its social/natural environment and to combat disease/infirmity in humans. Research should be conducted in a manner conducive to and consistent with dignity and well-being of the participant; in a professional and transparent manner; and ensuring minimal risk.[ 8 ] Research thus must be subjected to careful evaluation at all stages, i.e., research design/experimentation; results and their implications; the objective of the research sought; anticipated benefits/dangers; potential uses/abuses of the experiment and its results; and on ensuring the safety of human life. Table 1 lists the principles any research should follow.[ 8 ]
General principles of medical research

Types of study design
Medical research is classified into primary and secondary research. Clinical/experimental studies are performed in primary research, whereas secondary research consolidates available studies as reviews, systematic reviews and meta-analyses. Three main areas in primary research are basic medical research, clinical research and epidemiological research [ Figure 2 ]. Basic research includes fundamental research in fields shown in Figure 2 . In almost all studies, at least one independent variable is varied, whereas the effects on the dependent variables are investigated. Clinical studies include observational studies and interventional studies and are subclassified as in Figure 2 .

Classification of types of medical research
Interventional clinical study is performed with the purpose of studying or demonstrating clinical or pharmacological properties of drugs/devices, their side effects and to establish their efficacy or safety. They also include studies in which surgical, physical or psychotherapeutic procedures are examined.[ 9 ] Studies on drugs/devices are subject to legal and ethical requirements including the Drug Controller General India (DCGI) directives. They require the approval of DCGI recognized Ethics Committee and must be performed in accordance with the rules of ‘Good Clinical Practice’.[ 10 ] Further details are available under ‘Methodology for research II’ section in this issue of IJA. In 2004, the World Health Organization advised registration of all clinical trials in a public registry. In India, the Clinical Trials Registry of India was launched in 2007 ( www.ctri.nic.in ). The International Committee of Medical Journal Editors (ICMJE) mandates its member journals to publish only registered trials.[ 11 ]
Observational clinical study is a study in which knowledge from treatment of persons with drugs is analysed using epidemiological methods. In these studies, the diagnosis, treatment and monitoring are performed exclusively according to medical practice and not according to a specified study protocol.[ 9 ] They are subclassified as per Figure 2 .
Epidemiological studies have two basic approaches, the interventional and observational. Clinicians are more familiar with interventional research, whereas epidemiologists usually perform observational research.
Interventional studies are experimental in character and are subdivided into field and group studies, for example, iodine supplementation of cooking salt to prevent hypothyroidism. Many interventions are unsuitable for RCTs, as the exposure may be harmful to the subjects.
Observational studies can be subdivided into cohort, case–control, cross-sectional and ecological studies.
- Cohort studies are suited to detect connections between exposure and development of disease. They are normally prospective studies of two healthy groups of subjects observed over time, in which one group is exposed to a specific substance, whereas the other is not. The occurrence of the disease can be determined in the two groups. Cohort studies can also be retrospective
- Case–control studies are retrospective analyses performed to establish the prevalence of a disease in two groups exposed to a factor or disease. The incidence rate cannot be calculated, and there is also a risk of selection bias and faulty recall.
Secondary research
Narrative review.
An expert senior author writes about a particular field, condition or treatment, including an overview, and this information is fortified by his experience. The article is in a narrative format. Its limitation is that one cannot tell whether recommendations are based on author's clinical experience, available literature and why some studies were given more emphasis. It can be biased, with selective citation of reports that reinforce the authors' views of a topic.[ 12 ]
Systematic review
Systematic reviews methodically and comprehensively identify studies focused on a specified topic, appraise their methodology, summate the results, identify key findings and reasons for differences across studies, and cite limitations of current knowledge.[ 13 ] They adhere to reproducible methods and recommended guidelines.[ 14 ] The methods used to compile data are explicit and transparent, allowing the reader to gauge the quality of the review and the potential for bias.[ 15 ]
A systematic review can be presented in text or graphic form. In graphic form, data of different trials can be plotted with the point estimate and 95% confidence interval for each study, presented on an individual line. A properly conducted systematic review presents the best available research evidence for a focused clinical question. The review team may obtain information, not available in the original reports, from the primary authors. This ensures that findings are consistent and generalisable across populations, environment, therapies and groups.[ 12 ] A systematic review attempts to reduce bias identification and studies selection for review, using a comprehensive search strategy and specifying inclusion criteria. The strength of a systematic review lies in the transparency of each phase and highlighting the merits of each decision made, while compiling information.
Meta-analysis
A review team compiles aggregate-level data in each primary study, and in some cases, data are solicited from each of the primary studies.[ 16 , 17 ] Although difficult to perform, individual patient meta-analyses offer advantages over aggregate-level analyses.[ 18 ] These mathematically pooled results are referred to as meta-analysis. Combining data from well-conducted primary studies provide a precise estimate of the “true effect.”[ 19 ] Pooling the samples of individual studies increases overall sample size, enhances statistical analysis power, reduces confidence interval and thereby improves statistical value.
The structured process of Cochrane Collaboration systematic reviews has contributed to the improvement of their quality. For the meta-analysis to be definitive, the primary RCTs should have been conducted methodically. When the existing studies have important scientific and methodological limitations, such as smaller sized samples, the systematic review may identify where gaps exist in the available literature.[ 20 ] RCTs and systematic review of several randomised trials are less likely to mislead us, and thereby help judge whether an intervention is better.[ 2 ] Practice guidelines supported by large RCTs and meta-analyses are considered as ‘gold standard’ in EBM. This issue of IJA is accompanied by an editorial on Importance of EBM on research and practice (Guyat and Sriganesh 471_16).[ 21 ] The EBM pyramid grading the value of different types of research studies is shown in Figure 3 .

The evidence-based medicine pyramid
In the last decade, a number of studies and guidelines brought about path-breaking changes in anaesthesiology and critical care. Some guidelines such as the ‘Surviving Sepsis Guidelines-2004’[ 22 ] were later found to be flawed and biased. A number of large RCTs were rejected as their findings were erroneous. Another classic example is that of ENIGMA-I (Evaluation of Nitrous oxide In the Gas Mixture for Anaesthesia)[ 23 ] which implicated nitrous oxide for poor outcomes, but ENIGMA-II[ 24 , 25 ] conducted later, by the same investigators, declared it as safe. The rise and fall of the ‘tight glucose control’ regimen was similar.[ 26 ]
Although RCTs are considered ‘gold standard’ in research, their status is at crossroads today. RCTs have conflicting interests and thus must be evaluated with careful scrutiny. EBM can promote evidence reflected in RCTs and meta-analyses. However, it cannot promulgate evidence not reflected in RCTs. Flawed RCTs and meta-analyses may bring forth erroneous recommendations. EBM thus should not be restricted to RCTs and meta-analyses but must involve tracking down the best external evidence to answer our clinical questions.
Financial support and sponsorship
Conflicts of interest.
There are no conflicts of interest.
Thank you for visiting nature.com. You are using a browser version with limited support for CSS. To obtain the best experience, we recommend you use a more up to date browser (or turn off compatibility mode in Internet Explorer). In the meantime, to ensure continued support, we are displaying the site without styles and JavaScript.
- View all journals
- My Account Login
- Explore content
- About the journal
- Publish with us
- Sign up for alerts
- Open access
- Published: 26 March 2024
Predicting and improving complex beer flavor through machine learning
- Michiel Schreurs ORCID: orcid.org/0000-0002-9449-5619 1 , 2 , 3 na1 ,
- Supinya Piampongsant 1 , 2 , 3 na1 ,
- Miguel Roncoroni ORCID: orcid.org/0000-0001-7461-1427 1 , 2 , 3 na1 ,
- Lloyd Cool ORCID: orcid.org/0000-0001-9936-3124 1 , 2 , 3 , 4 ,
- Beatriz Herrera-Malaver ORCID: orcid.org/0000-0002-5096-9974 1 , 2 , 3 ,
- Christophe Vanderaa ORCID: orcid.org/0000-0001-7443-5427 4 ,
- Florian A. Theßeling 1 , 2 , 3 ,
- Łukasz Kreft ORCID: orcid.org/0000-0001-7620-4657 5 ,
- Alexander Botzki ORCID: orcid.org/0000-0001-6691-4233 5 ,
- Philippe Malcorps 6 ,
- Luk Daenen 6 ,
- Tom Wenseleers ORCID: orcid.org/0000-0002-1434-861X 4 &
- Kevin J. Verstrepen ORCID: orcid.org/0000-0002-3077-6219 1 , 2 , 3
Nature Communications volume 15 , Article number: 2368 ( 2024 ) Cite this article
50k Accesses
850 Altmetric
Metrics details
- Chemical engineering
- Gas chromatography
- Machine learning
- Metabolomics
- Taste receptors
The perception and appreciation of food flavor depends on many interacting chemical compounds and external factors, and therefore proves challenging to understand and predict. Here, we combine extensive chemical and sensory analyses of 250 different beers to train machine learning models that allow predicting flavor and consumer appreciation. For each beer, we measure over 200 chemical properties, perform quantitative descriptive sensory analysis with a trained tasting panel and map data from over 180,000 consumer reviews to train 10 different machine learning models. The best-performing algorithm, Gradient Boosting, yields models that significantly outperform predictions based on conventional statistics and accurately predict complex food features and consumer appreciation from chemical profiles. Model dissection allows identifying specific and unexpected compounds as drivers of beer flavor and appreciation. Adding these compounds results in variants of commercial alcoholic and non-alcoholic beers with improved consumer appreciation. Together, our study reveals how big data and machine learning uncover complex links between food chemistry, flavor and consumer perception, and lays the foundation to develop novel, tailored foods with superior flavors.
Similar content being viewed by others
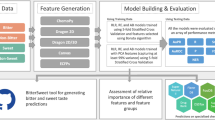
BitterSweet: Building machine learning models for predicting the bitter and sweet taste of small molecules
Rudraksh Tuwani, Somin Wadhwa & Ganesh Bagler
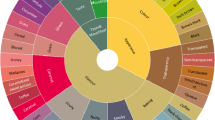
Sensory lexicon and aroma volatiles analysis of brewing malt
Xiaoxia Su, Miao Yu, … Tianyi Du
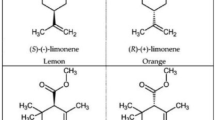
Predicting odor from molecular structure: a multi-label classification approach
Kushagra Saini & Venkatnarayan Ramanathan
Introduction
Predicting and understanding food perception and appreciation is one of the major challenges in food science. Accurate modeling of food flavor and appreciation could yield important opportunities for both producers and consumers, including quality control, product fingerprinting, counterfeit detection, spoilage detection, and the development of new products and product combinations (food pairing) 1 , 2 , 3 , 4 , 5 , 6 . Accurate models for flavor and consumer appreciation would contribute greatly to our scientific understanding of how humans perceive and appreciate flavor. Moreover, accurate predictive models would also facilitate and standardize existing food assessment methods and could supplement or replace assessments by trained and consumer tasting panels, which are variable, expensive and time-consuming 7 , 8 , 9 . Lastly, apart from providing objective, quantitative, accurate and contextual information that can help producers, models can also guide consumers in understanding their personal preferences 10 .
Despite the myriad of applications, predicting food flavor and appreciation from its chemical properties remains a largely elusive goal in sensory science, especially for complex food and beverages 11 , 12 . A key obstacle is the immense number of flavor-active chemicals underlying food flavor. Flavor compounds can vary widely in chemical structure and concentration, making them technically challenging and labor-intensive to quantify, even in the face of innovations in metabolomics, such as non-targeted metabolic fingerprinting 13 , 14 . Moreover, sensory analysis is perhaps even more complicated. Flavor perception is highly complex, resulting from hundreds of different molecules interacting at the physiochemical and sensorial level. Sensory perception is often non-linear, characterized by complex and concentration-dependent synergistic and antagonistic effects 15 , 16 , 17 , 18 , 19 , 20 , 21 that are further convoluted by the genetics, environment, culture and psychology of consumers 22 , 23 , 24 . Perceived flavor is therefore difficult to measure, with problems of sensitivity, accuracy, and reproducibility that can only be resolved by gathering sufficiently large datasets 25 . Trained tasting panels are considered the prime source of quality sensory data, but require meticulous training, are low throughput and high cost. Public databases containing consumer reviews of food products could provide a valuable alternative, especially for studying appreciation scores, which do not require formal training 25 . Public databases offer the advantage of amassing large amounts of data, increasing the statistical power to identify potential drivers of appreciation. However, public datasets suffer from biases, including a bias in the volunteers that contribute to the database, as well as confounding factors such as price, cult status and psychological conformity towards previous ratings of the product.
Classical multivariate statistics and machine learning methods have been used to predict flavor of specific compounds by, for example, linking structural properties of a compound to its potential biological activities or linking concentrations of specific compounds to sensory profiles 1 , 26 . Importantly, most previous studies focused on predicting organoleptic properties of single compounds (often based on their chemical structure) 27 , 28 , 29 , 30 , 31 , 32 , 33 , thus ignoring the fact that these compounds are present in a complex matrix in food or beverages and excluding complex interactions between compounds. Moreover, the classical statistics commonly used in sensory science 34 , 35 , 36 , 37 , 38 , 39 require a large sample size and sufficient variance amongst predictors to create accurate models. They are not fit for studying an extensive set of hundreds of interacting flavor compounds, since they are sensitive to outliers, have a high tendency to overfit and are less suited for non-linear and discontinuous relationships 40 .
In this study, we combine extensive chemical analyses and sensory data of a set of different commercial beers with machine learning approaches to develop models that predict taste, smell, mouthfeel and appreciation from compound concentrations. Beer is particularly suited to model the relationship between chemistry, flavor and appreciation. First, beer is a complex product, consisting of thousands of flavor compounds that partake in complex sensory interactions 41 , 42 , 43 . This chemical diversity arises from the raw materials (malt, yeast, hops, water and spices) and biochemical conversions during the brewing process (kilning, mashing, boiling, fermentation, maturation and aging) 44 , 45 . Second, the advent of the internet saw beer consumers embrace online review platforms, such as RateBeer (ZX Ventures, Anheuser-Busch InBev SA/NV) and BeerAdvocate (Next Glass, inc.). In this way, the beer community provides massive data sets of beer flavor and appreciation scores, creating extraordinarily large sensory databases to complement the analyses of our professional sensory panel. Specifically, we characterize over 200 chemical properties of 250 commercial beers, spread across 22 beer styles, and link these to the descriptive sensory profiling data of a 16-person in-house trained tasting panel and data acquired from over 180,000 public consumer reviews. These unique and extensive datasets enable us to train a suite of machine learning models to predict flavor and appreciation from a beer’s chemical profile. Dissection of the best-performing models allows us to pinpoint specific compounds as potential drivers of beer flavor and appreciation. Follow-up experiments confirm the importance of these compounds and ultimately allow us to significantly improve the flavor and appreciation of selected commercial beers. Together, our study represents a significant step towards understanding complex flavors and reinforces the value of machine learning to develop and refine complex foods. In this way, it represents a stepping stone for further computer-aided food engineering applications 46 .
To generate a comprehensive dataset on beer flavor, we selected 250 commercial Belgian beers across 22 different beer styles (Supplementary Fig. S1 ). Beers with ≤ 4.2% alcohol by volume (ABV) were classified as non-alcoholic and low-alcoholic. Blonds and Tripels constitute a significant portion of the dataset (12.4% and 11.2%, respectively) reflecting their presence on the Belgian beer market and the heterogeneity of beers within these styles. By contrast, lager beers are less diverse and dominated by a handful of brands. Rare styles such as Brut or Faro make up only a small fraction of the dataset (2% and 1%, respectively) because fewer of these beers are produced and because they are dominated by distinct characteristics in terms of flavor and chemical composition.
Extensive analysis identifies relationships between chemical compounds in beer
For each beer, we measured 226 different chemical properties, including common brewing parameters such as alcohol content, iso-alpha acids, pH, sugar concentration 47 , and over 200 flavor compounds (Methods, Supplementary Table S1 ). A large portion (37.2%) are terpenoids arising from hopping, responsible for herbal and fruity flavors 16 , 48 . A second major category are yeast metabolites, such as esters and alcohols, that result in fruity and solvent notes 48 , 49 , 50 . Other measured compounds are primarily derived from malt, or other microbes such as non- Saccharomyces yeasts and bacteria (‘wild flora’). Compounds that arise from spices or staling are labeled under ‘Others’. Five attributes (caloric value, total acids and total ester, hop aroma and sulfur compounds) are calculated from multiple individually measured compounds.
As a first step in identifying relationships between chemical properties, we determined correlations between the concentrations of the compounds (Fig. 1 , upper panel, Supplementary Data 1 and 2 , and Supplementary Fig. S2 . For the sake of clarity, only a subset of the measured compounds is shown in Fig. 1 ). Compounds of the same origin typically show a positive correlation, while absence of correlation hints at parameters varying independently. For example, the hop aroma compounds citronellol, and alpha-terpineol show moderate correlations with each other (Spearman’s rho=0.39 and 0.57), but not with the bittering hop component iso-alpha acids (Spearman’s rho=0.16 and −0.07). This illustrates how brewers can independently modify hop aroma and bitterness by selecting hop varieties and dosage time. If hops are added early in the boiling phase, chemical conversions increase bitterness while aromas evaporate, conversely, late addition of hops preserves aroma but limits bitterness 51 . Similarly, hop-derived iso-alpha acids show a strong anti-correlation with lactic acid and acetic acid, likely reflecting growth inhibition of lactic acid and acetic acid bacteria, or the consequent use of fewer hops in sour beer styles, such as West Flanders ales and Fruit beers, that rely on these bacteria for their distinct flavors 52 . Finally, yeast-derived esters (ethyl acetate, ethyl decanoate, ethyl hexanoate, ethyl octanoate) and alcohols (ethanol, isoamyl alcohol, isobutanol, and glycerol), correlate with Spearman coefficients above 0.5, suggesting that these secondary metabolites are correlated with the yeast genetic background and/or fermentation parameters and may be difficult to influence individually, although the choice of yeast strain may offer some control 53 .
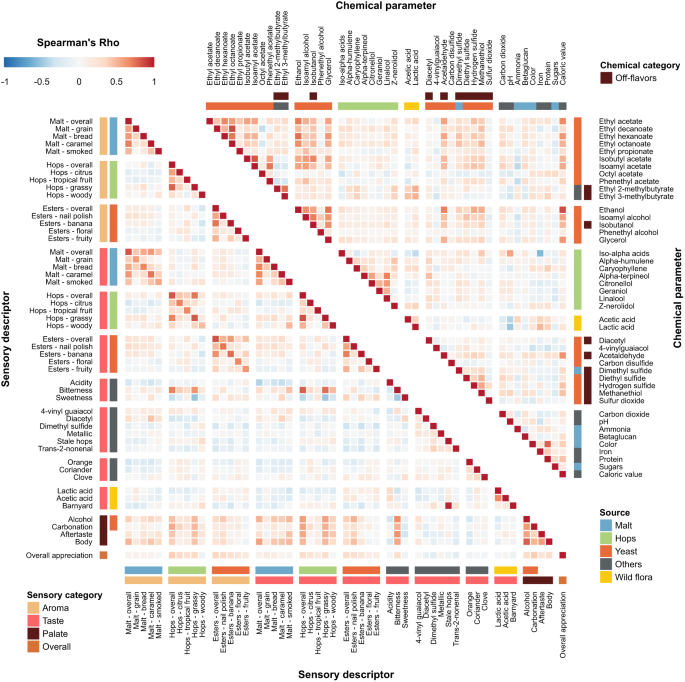
Spearman rank correlations are shown. Descriptors are grouped according to their origin (malt (blue), hops (green), yeast (red), wild flora (yellow), Others (black)), and sensory aspect (aroma, taste, palate, and overall appreciation). Please note that for the chemical compounds, for the sake of clarity, only a subset of the total number of measured compounds is shown, with an emphasis on the key compounds for each source. For more details, see the main text and Methods section. Chemical data can be found in Supplementary Data 1 , correlations between all chemical compounds are depicted in Supplementary Fig. S2 and correlation values can be found in Supplementary Data 2 . See Supplementary Data 4 for sensory panel assessments and Supplementary Data 5 for correlation values between all sensory descriptors.
Interestingly, different beer styles show distinct patterns for some flavor compounds (Supplementary Fig. S3 ). These observations agree with expectations for key beer styles, and serve as a control for our measurements. For instance, Stouts generally show high values for color (darker), while hoppy beers contain elevated levels of iso-alpha acids, compounds associated with bitter hop taste. Acetic and lactic acid are not prevalent in most beers, with notable exceptions such as Kriek, Lambic, Faro, West Flanders ales and Flanders Old Brown, which use acid-producing bacteria ( Lactobacillus and Pediococcus ) or unconventional yeast ( Brettanomyces ) 54 , 55 . Glycerol, ethanol and esters show similar distributions across all beer styles, reflecting their common origin as products of yeast metabolism during fermentation 45 , 53 . Finally, low/no-alcohol beers contain low concentrations of glycerol and esters. This is in line with the production process for most of the low/no-alcohol beers in our dataset, which are produced through limiting fermentation or by stripping away alcohol via evaporation or dialysis, with both methods having the unintended side-effect of reducing the amount of flavor compounds in the final beer 56 , 57 .
Besides expected associations, our data also reveals less trivial associations between beer styles and specific parameters. For example, geraniol and citronellol, two monoterpenoids responsible for citrus, floral and rose flavors and characteristic of Citra hops, are found in relatively high amounts in Christmas, Saison, and Brett/co-fermented beers, where they may originate from terpenoid-rich spices such as coriander seeds instead of hops 58 .
Tasting panel assessments reveal sensorial relationships in beer
To assess the sensory profile of each beer, a trained tasting panel evaluated each of the 250 beers for 50 sensory attributes, including different hop, malt and yeast flavors, off-flavors and spices. Panelists used a tasting sheet (Supplementary Data 3 ) to score the different attributes. Panel consistency was evaluated by repeating 12 samples across different sessions and performing ANOVA. In 95% of cases no significant difference was found across sessions ( p > 0.05), indicating good panel consistency (Supplementary Table S2 ).
Aroma and taste perception reported by the trained panel are often linked (Fig. 1 , bottom left panel and Supplementary Data 4 and 5 ), with high correlations between hops aroma and taste (Spearman’s rho=0.83). Bitter taste was found to correlate with hop aroma and taste in general (Spearman’s rho=0.80 and 0.69), and particularly with “grassy” noble hops (Spearman’s rho=0.75). Barnyard flavor, most often associated with sour beers, is identified together with stale hops (Spearman’s rho=0.97) that are used in these beers. Lactic and acetic acid, which often co-occur, are correlated (Spearman’s rho=0.66). Interestingly, sweetness and bitterness are anti-correlated (Spearman’s rho = −0.48), confirming the hypothesis that they mask each other 59 , 60 . Beer body is highly correlated with alcohol (Spearman’s rho = 0.79), and overall appreciation is found to correlate with multiple aspects that describe beer mouthfeel (alcohol, carbonation; Spearman’s rho= 0.32, 0.39), as well as with hop and ester aroma intensity (Spearman’s rho=0.39 and 0.35).
Similar to the chemical analyses, sensorial analyses confirmed typical features of specific beer styles (Supplementary Fig. S4 ). For example, sour beers (Faro, Flanders Old Brown, Fruit beer, Kriek, Lambic, West Flanders ale) were rated acidic, with flavors of both acetic and lactic acid. Hoppy beers were found to be bitter and showed hop-associated aromas like citrus and tropical fruit. Malt taste is most detected among scotch, stout/porters, and strong ales, while low/no-alcohol beers, which often have a reputation for being ‘worty’ (reminiscent of unfermented, sweet malt extract) appear in the middle. Unsurprisingly, hop aromas are most strongly detected among hoppy beers. Like its chemical counterpart (Supplementary Fig. S3 ), acidity shows a right-skewed distribution, with the most acidic beers being Krieks, Lambics, and West Flanders ales.
Tasting panel assessments of specific flavors correlate with chemical composition
We find that the concentrations of several chemical compounds strongly correlate with specific aroma or taste, as evaluated by the tasting panel (Fig. 2 , Supplementary Fig. S5 , Supplementary Data 6 ). In some cases, these correlations confirm expectations and serve as a useful control for data quality. For example, iso-alpha acids, the bittering compounds in hops, strongly correlate with bitterness (Spearman’s rho=0.68), while ethanol and glycerol correlate with tasters’ perceptions of alcohol and body, the mouthfeel sensation of fullness (Spearman’s rho=0.82/0.62 and 0.72/0.57 respectively) and darker color from roasted malts is a good indication of malt perception (Spearman’s rho=0.54).
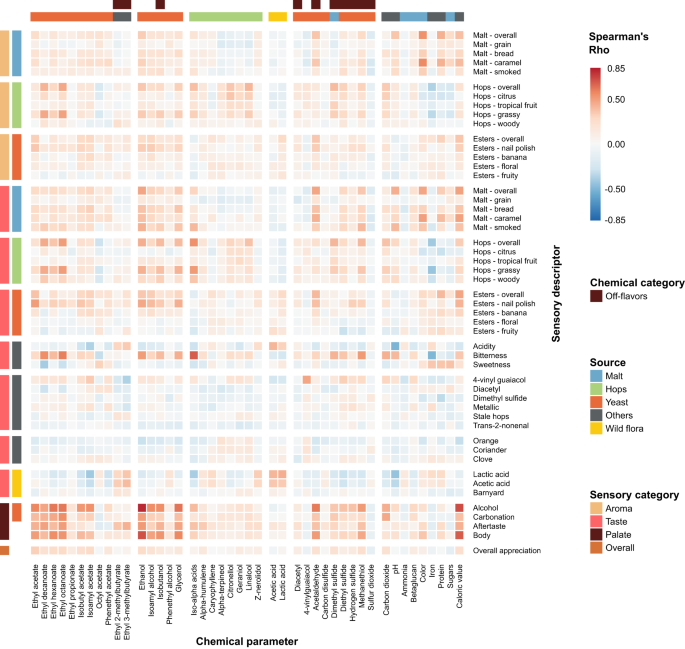
Heatmap colors indicate Spearman’s Rho. Axes are organized according to sensory categories (aroma, taste, mouthfeel, overall), chemical categories and chemical sources in beer (malt (blue), hops (green), yeast (red), wild flora (yellow), Others (black)). See Supplementary Data 6 for all correlation values.
Interestingly, for some relationships between chemical compounds and perceived flavor, correlations are weaker than expected. For example, the rose-smelling phenethyl acetate only weakly correlates with floral aroma. This hints at more complex relationships and interactions between compounds and suggests a need for a more complex model than simple correlations. Lastly, we uncovered unexpected correlations. For instance, the esters ethyl decanoate and ethyl octanoate appear to correlate slightly with hop perception and bitterness, possibly due to their fruity flavor. Iron is anti-correlated with hop aromas and bitterness, most likely because it is also anti-correlated with iso-alpha acids. This could be a sign of metal chelation of hop acids 61 , given that our analyses measure unbound hop acids and total iron content, or could result from the higher iron content in dark and Fruit beers, which typically have less hoppy and bitter flavors 62 .
Public consumer reviews complement expert panel data
To complement and expand the sensory data of our trained tasting panel, we collected 180,000 reviews of our 250 beers from the online consumer review platform RateBeer. This provided numerical scores for beer appearance, aroma, taste, palate, overall quality as well as the average overall score.
Public datasets are known to suffer from biases, such as price, cult status and psychological conformity towards previous ratings of a product. For example, prices correlate with appreciation scores for these online consumer reviews (rho=0.49, Supplementary Fig. S6 ), but not for our trained tasting panel (rho=0.19). This suggests that prices affect consumer appreciation, which has been reported in wine 63 , while blind tastings are unaffected. Moreover, we observe that some beer styles, like lagers and non-alcoholic beers, generally receive lower scores, reflecting that online reviewers are mostly beer aficionados with a preference for specialty beers over lager beers. In general, we find a modest correlation between our trained panel’s overall appreciation score and the online consumer appreciation scores (Fig. 3 , rho=0.29). Apart from the aforementioned biases in the online datasets, serving temperature, sample freshness and surroundings, which are all tightly controlled during the tasting panel sessions, can vary tremendously across online consumers and can further contribute to (among others, appreciation) differences between the two categories of tasters. Importantly, in contrast to the overall appreciation scores, for many sensory aspects the results from the professional panel correlated well with results obtained from RateBeer reviews. Correlations were highest for features that are relatively easy to recognize even for untrained tasters, like bitterness, sweetness, alcohol and malt aroma (Fig. 3 and below).
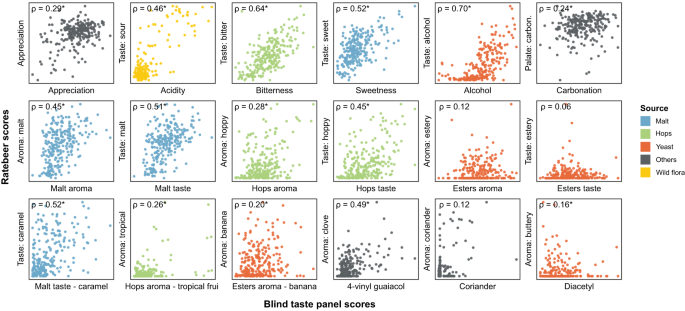
RateBeer text mining results can be found in Supplementary Data 7 . Rho values shown are Spearman correlation values, with asterisks indicating significant correlations ( p < 0.05, two-sided). All p values were smaller than 0.001, except for Esters aroma (0.0553), Esters taste (0.3275), Esters aroma—banana (0.0019), Coriander (0.0508) and Diacetyl (0.0134).
Besides collecting consumer appreciation from these online reviews, we developed automated text analysis tools to gather additional data from review texts (Supplementary Data 7 ). Processing review texts on the RateBeer database yielded comparable results to the scores given by the trained panel for many common sensory aspects, including acidity, bitterness, sweetness, alcohol, malt, and hop tastes (Fig. 3 ). This is in line with what would be expected, since these attributes require less training for accurate assessment and are less influenced by environmental factors such as temperature, serving glass and odors in the environment. Consumer reviews also correlate well with our trained panel for 4-vinyl guaiacol, a compound associated with a very characteristic aroma. By contrast, correlations for more specific aromas like ester, coriander or diacetyl are underrepresented in the online reviews, underscoring the importance of using a trained tasting panel and standardized tasting sheets with explicit factors to be scored for evaluating specific aspects of a beer. Taken together, our results suggest that public reviews are trustworthy for some, but not all, flavor features and can complement or substitute taste panel data for these sensory aspects.
Models can predict beer sensory profiles from chemical data
The rich datasets of chemical analyses, tasting panel assessments and public reviews gathered in the first part of this study provided us with a unique opportunity to develop predictive models that link chemical data to sensorial features. Given the complexity of beer flavor, basic statistical tools such as correlations or linear regression may not always be the most suitable for making accurate predictions. Instead, we applied different machine learning models that can model both simple linear and complex interactive relationships. Specifically, we constructed a set of regression models to predict (a) trained panel scores for beer flavor and quality and (b) public reviews’ appreciation scores from beer chemical profiles. We trained and tested 10 different models (Methods), 3 linear regression-based models (simple linear regression with first-order interactions (LR), lasso regression with first-order interactions (Lasso), partial least squares regressor (PLSR)), 5 decision tree models (AdaBoost regressor (ABR), extra trees (ET), gradient boosting regressor (GBR), random forest (RF) and XGBoost regressor (XGBR)), 1 support vector regression (SVR), and 1 artificial neural network (ANN) model.
To compare the performance of our machine learning models, the dataset was randomly split into a training and test set, stratified by beer style. After a model was trained on data in the training set, its performance was evaluated on its ability to predict the test dataset obtained from multi-output models (based on the coefficient of determination, see Methods). Additionally, individual-attribute models were ranked per descriptor and the average rank was calculated, as proposed by Korneva et al. 64 . Importantly, both ways of evaluating the models’ performance agreed in general. Performance of the different models varied (Table 1 ). It should be noted that all models perform better at predicting RateBeer results than results from our trained tasting panel. One reason could be that sensory data is inherently variable, and this variability is averaged out with the large number of public reviews from RateBeer. Additionally, all tree-based models perform better at predicting taste than aroma. Linear models (LR) performed particularly poorly, with negative R 2 values, due to severe overfitting (training set R 2 = 1). Overfitting is a common issue in linear models with many parameters and limited samples, especially with interaction terms further amplifying the number of parameters. L1 regularization (Lasso) successfully overcomes this overfitting, out-competing multiple tree-based models on the RateBeer dataset. Similarly, the dimensionality reduction of PLSR avoids overfitting and improves performance, to some extent. Still, tree-based models (ABR, ET, GBR, RF and XGBR) show the best performance, out-competing the linear models (LR, Lasso, PLSR) commonly used in sensory science 65 .
GBR models showed the best overall performance in predicting sensory responses from chemical information, with R 2 values up to 0.75 depending on the predicted sensory feature (Supplementary Table S4 ). The GBR models predict consumer appreciation (RateBeer) better than our trained panel’s appreciation (R 2 value of 0.67 compared to R 2 value of 0.09) (Supplementary Table S3 and Supplementary Table S4 ). ANN models showed intermediate performance, likely because neural networks typically perform best with larger datasets 66 . The SVR shows intermediate performance, mostly due to the weak predictions of specific attributes that lower the overall performance (Supplementary Table S4 ).
Model dissection identifies specific, unexpected compounds as drivers of consumer appreciation
Next, we leveraged our models to infer important contributors to sensory perception and consumer appreciation. Consumer preference is a crucial sensory aspects, because a product that shows low consumer appreciation scores often does not succeed commercially 25 . Additionally, the requirement for a large number of representative evaluators makes consumer trials one of the more costly and time-consuming aspects of product development. Hence, a model for predicting chemical drivers of overall appreciation would be a welcome addition to the available toolbox for food development and optimization.
Since GBR models on our RateBeer dataset showed the best overall performance, we focused on these models. Specifically, we used two approaches to identify important contributors. First, rankings of the most important predictors for each sensorial trait in the GBR models were obtained based on impurity-based feature importance (mean decrease in impurity). High-ranked parameters were hypothesized to be either the true causal chemical properties underlying the trait, to correlate with the actual causal properties, or to take part in sensory interactions affecting the trait 67 (Fig. 4A ). In a second approach, we used SHAP 68 to determine which parameters contributed most to the model for making predictions of consumer appreciation (Fig. 4B ). SHAP calculates parameter contributions to model predictions on a per-sample basis, which can be aggregated into an importance score.
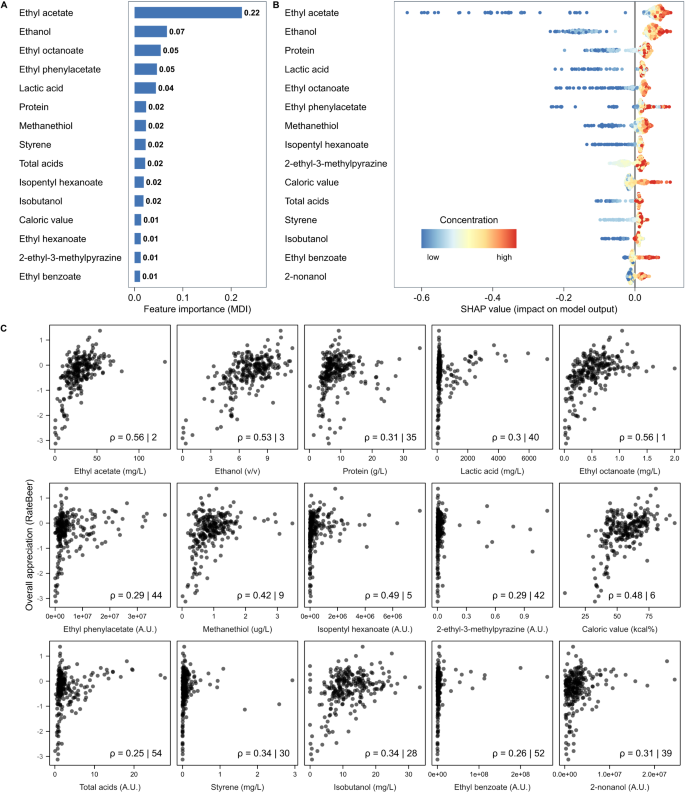
A The impurity-based feature importance (mean deviance in impurity, MDI) calculated from the Gradient Boosting Regression (GBR) model predicting RateBeer appreciation scores. The top 15 highest ranked chemical properties are shown. B SHAP summary plot for the top 15 parameters contributing to our GBR model. Each point on the graph represents a sample from our dataset. The color represents the concentration of that parameter, with bluer colors representing low values and redder colors representing higher values. Greater absolute values on the horizontal axis indicate a higher impact of the parameter on the prediction of the model. C Spearman correlations between the 15 most important chemical properties and consumer overall appreciation. Numbers indicate the Spearman Rho correlation coefficient, and the rank of this correlation compared to all other correlations. The top 15 important compounds were determined using SHAP (panel B).
Both approaches identified ethyl acetate as the most predictive parameter for beer appreciation (Fig. 4 ). Ethyl acetate is the most abundant ester in beer with a typical ‘fruity’, ‘solvent’ and ‘alcoholic’ flavor, but is often considered less important than other esters like isoamyl acetate. The second most important parameter identified by SHAP is ethanol, the most abundant beer compound after water. Apart from directly contributing to beer flavor and mouthfeel, ethanol drastically influences the physical properties of beer, dictating how easily volatile compounds escape the beer matrix to contribute to beer aroma 69 . Importantly, it should also be noted that the importance of ethanol for appreciation is likely inflated by the very low appreciation scores of non-alcoholic beers (Supplementary Fig. S4 ). Despite not often being considered a driver of beer appreciation, protein level also ranks highly in both approaches, possibly due to its effect on mouthfeel and body 70 . Lactic acid, which contributes to the tart taste of sour beers, is the fourth most important parameter identified by SHAP, possibly due to the generally high appreciation of sour beers in our dataset.
Interestingly, some of the most important predictive parameters for our model are not well-established as beer flavors or are even commonly regarded as being negative for beer quality. For example, our models identify methanethiol and ethyl phenyl acetate, an ester commonly linked to beer staling 71 , as a key factor contributing to beer appreciation. Although there is no doubt that high concentrations of these compounds are considered unpleasant, the positive effects of modest concentrations are not yet known 72 , 73 .
To compare our approach to conventional statistics, we evaluated how well the 15 most important SHAP-derived parameters correlate with consumer appreciation (Fig. 4C ). Interestingly, only 6 of the properties derived by SHAP rank amongst the top 15 most correlated parameters. For some chemical compounds, the correlations are so low that they would have likely been considered unimportant. For example, lactic acid, the fourth most important parameter, shows a bimodal distribution for appreciation, with sour beers forming a separate cluster, that is missed entirely by the Spearman correlation. Additionally, the correlation plots reveal outliers, emphasizing the need for robust analysis tools. Together, this highlights the need for alternative models, like the Gradient Boosting model, that better grasp the complexity of (beer) flavor.
Finally, to observe the relationships between these chemical properties and their predicted targets, partial dependence plots were constructed for the six most important predictors of consumer appreciation 74 , 75 , 76 (Supplementary Fig. S7 ). One-way partial dependence plots show how a change in concentration affects the predicted appreciation. These plots reveal an important limitation of our models: appreciation predictions remain constant at ever-increasing concentrations. This implies that once a threshold concentration is reached, further increasing the concentration does not affect appreciation. This is false, as it is well-documented that certain compounds become unpleasant at high concentrations, including ethyl acetate (‘nail polish’) 77 and methanethiol (‘sulfury’ and ‘rotten cabbage’) 78 . The inability of our models to grasp that flavor compounds have optimal levels, above which they become negative, is a consequence of working with commercial beer brands where (off-)flavors are rarely too high to negatively impact the product. The two-way partial dependence plots show how changing the concentration of two compounds influences predicted appreciation, visualizing their interactions (Supplementary Fig. S7 ). In our case, the top 5 parameters are dominated by additive or synergistic interactions, with high concentrations for both compounds resulting in the highest predicted appreciation.
To assess the robustness of our best-performing models and model predictions, we performed 100 iterations of the GBR, RF and ET models. In general, all iterations of the models yielded similar performance (Supplementary Fig. S8 ). Moreover, the main predictors (including the top predictors ethanol and ethyl acetate) remained virtually the same, especially for GBR and RF. For the iterations of the ET model, we did observe more variation in the top predictors, which is likely a consequence of the model’s inherent random architecture in combination with co-correlations between certain predictors. However, even in this case, several of the top predictors (ethanol and ethyl acetate) remain unchanged, although their rank in importance changes (Supplementary Fig. S8 ).
Next, we investigated if a combination of RateBeer and trained panel data into one consolidated dataset would lead to stronger models, under the hypothesis that such a model would suffer less from bias in the datasets. A GBR model was trained to predict appreciation on the combined dataset. This model underperformed compared to the RateBeer model, both in the native case and when including a dataset identifier (R 2 = 0.67, 0.26 and 0.42 respectively). For the latter, the dataset identifier is the most important feature (Supplementary Fig. S9 ), while most of the feature importance remains unchanged, with ethyl acetate and ethanol ranking highest, like in the original model trained only on RateBeer data. It seems that the large variation in the panel dataset introduces noise, weakening the models’ performances and reliability. In addition, it seems reasonable to assume that both datasets are fundamentally different, with the panel dataset obtained by blind tastings by a trained professional panel.
Lastly, we evaluated whether beer style identifiers would further enhance the model’s performance. A GBR model was trained with parameters that explicitly encoded the styles of the samples. This did not improve model performance (R2 = 0.66 with style information vs R2 = 0.67). The most important chemical features are consistent with the model trained without style information (eg. ethanol and ethyl acetate), and with the exception of the most preferred (strong ale) and least preferred (low/no-alcohol) styles, none of the styles were among the most important features (Supplementary Fig. S9 , Supplementary Table S5 and S6 ). This is likely due to a combination of style-specific chemical signatures, such as iso-alpha acids and lactic acid, that implicitly convey style information to the original models, as well as the low number of samples belonging to some styles, making it difficult for the model to learn style-specific patterns. Moreover, beer styles are not rigorously defined, with some styles overlapping in features and some beers being misattributed to a specific style, all of which leads to more noise in models that use style parameters.
Model validation
To test if our predictive models give insight into beer appreciation, we set up experiments aimed at improving existing commercial beers. We specifically selected overall appreciation as the trait to be examined because of its complexity and commercial relevance. Beer flavor comprises a complex bouquet rather than single aromas and tastes 53 . Hence, adding a single compound to the extent that a difference is noticeable may lead to an unbalanced, artificial flavor. Therefore, we evaluated the effect of combinations of compounds. Because Blond beers represent the most extensive style in our dataset, we selected a beer from this style as the starting material for these experiments (Beer 64 in Supplementary Data 1 ).
In the first set of experiments, we adjusted the concentrations of compounds that made up the most important predictors of overall appreciation (ethyl acetate, ethanol, lactic acid, ethyl phenyl acetate) together with correlated compounds (ethyl hexanoate, isoamyl acetate, glycerol), bringing them up to 95 th percentile ethanol-normalized concentrations (Methods) within the Blond group (‘Spiked’ concentration in Fig. 5A ). Compared to controls, the spiked beers were found to have significantly improved overall appreciation among trained panelists, with panelist noting increased intensity of ester flavors, sweetness, alcohol, and body fullness (Fig. 5B ). To disentangle the contribution of ethanol to these results, a second experiment was performed without the addition of ethanol. This resulted in a similar outcome, including increased perception of alcohol and overall appreciation.
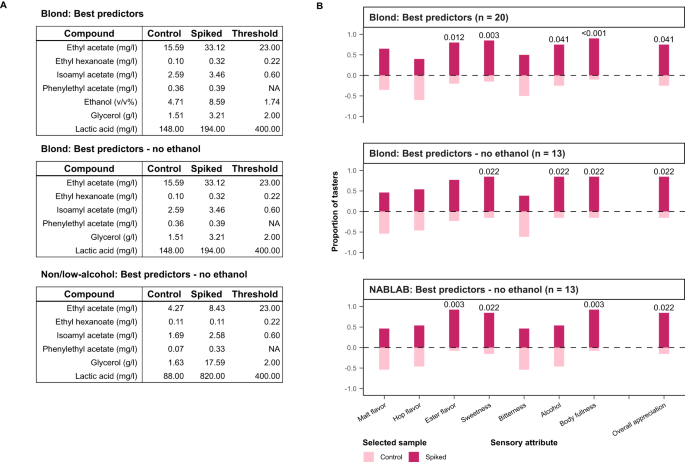
Adding the top chemical compounds, identified as best predictors of appreciation by our model, into poorly appreciated beers results in increased appreciation from our trained panel. Results of sensory tests between base beers and those spiked with compounds identified as the best predictors by the model. A Blond and Non/Low-alcohol (0.0% ABV) base beers were brought up to 95th-percentile ethanol-normalized concentrations within each style. B For each sensory attribute, tasters indicated the more intense sample and selected the sample they preferred. The numbers above the bars correspond to the p values that indicate significant changes in perceived flavor (two-sided binomial test: alpha 0.05, n = 20 or 13).
In a last experiment, we tested whether using the model’s predictions can boost the appreciation of a non-alcoholic beer (beer 223 in Supplementary Data 1 ). Again, the addition of a mixture of predicted compounds (omitting ethanol, in this case) resulted in a significant increase in appreciation, body, ester flavor and sweetness.
Predicting flavor and consumer appreciation from chemical composition is one of the ultimate goals of sensory science. A reliable, systematic and unbiased way to link chemical profiles to flavor and food appreciation would be a significant asset to the food and beverage industry. Such tools would substantially aid in quality control and recipe development, offer an efficient and cost-effective alternative to pilot studies and consumer trials and would ultimately allow food manufacturers to produce superior, tailor-made products that better meet the demands of specific consumer groups more efficiently.
A limited set of studies have previously tried, to varying degrees of success, to predict beer flavor and beer popularity based on (a limited set of) chemical compounds and flavors 79 , 80 . Current sensitive, high-throughput technologies allow measuring an unprecedented number of chemical compounds and properties in a large set of samples, yielding a dataset that can train models that help close the gaps between chemistry and flavor, even for a complex natural product like beer. To our knowledge, no previous research gathered data at this scale (250 samples, 226 chemical parameters, 50 sensory attributes and 5 consumer scores) to disentangle and validate the chemical aspects driving beer preference using various machine-learning techniques. We find that modern machine learning models outperform conventional statistical tools, such as correlations and linear models, and can successfully predict flavor appreciation from chemical composition. This could be attributed to the natural incorporation of interactions and non-linear or discontinuous effects in machine learning models, which are not easily grasped by the linear model architecture. While linear models and partial least squares regression represent the most widespread statistical approaches in sensory science, in part because they allow interpretation 65 , 81 , 82 , modern machine learning methods allow for building better predictive models while preserving the possibility to dissect and exploit the underlying patterns. Of the 10 different models we trained, tree-based models, such as our best performing GBR, showed the best overall performance in predicting sensory responses from chemical information, outcompeting artificial neural networks. This agrees with previous reports for models trained on tabular data 83 . Our results are in line with the findings of Colantonio et al. who also identified the gradient boosting architecture as performing best at predicting appreciation and flavor (of tomatoes and blueberries, in their specific study) 26 . Importantly, besides our larger experimental scale, we were able to directly confirm our models’ predictions in vivo.
Our study confirms that flavor compound concentration does not always correlate with perception, suggesting complex interactions that are often missed by more conventional statistics and simple models. Specifically, we find that tree-based algorithms may perform best in developing models that link complex food chemistry with aroma. Furthermore, we show that massive datasets of untrained consumer reviews provide a valuable source of data, that can complement or even replace trained tasting panels, especially for appreciation and basic flavors, such as sweetness and bitterness. This holds despite biases that are known to occur in such datasets, such as price or conformity bias. Moreover, GBR models predict taste better than aroma. This is likely because taste (e.g. bitterness) often directly relates to the corresponding chemical measurements (e.g., iso-alpha acids), whereas such a link is less clear for aromas, which often result from the interplay between multiple volatile compounds. We also find that our models are best at predicting acidity and alcohol, likely because there is a direct relation between the measured chemical compounds (acids and ethanol) and the corresponding perceived sensorial attribute (acidity and alcohol), and because even untrained consumers are generally able to recognize these flavors and aromas.
The predictions of our final models, trained on review data, hold even for blind tastings with small groups of trained tasters, as demonstrated by our ability to validate specific compounds as drivers of beer flavor and appreciation. Since adding a single compound to the extent of a noticeable difference may result in an unbalanced flavor profile, we specifically tested our identified key drivers as a combination of compounds. While this approach does not allow us to validate if a particular single compound would affect flavor and/or appreciation, our experiments do show that this combination of compounds increases consumer appreciation.
It is important to stress that, while it represents an important step forward, our approach still has several major limitations. A key weakness of the GBR model architecture is that amongst co-correlating variables, the largest main effect is consistently preferred for model building. As a result, co-correlating variables often have artificially low importance scores, both for impurity and SHAP-based methods, like we observed in the comparison to the more randomized Extra Trees models. This implies that chemicals identified as key drivers of a specific sensory feature by GBR might not be the true causative compounds, but rather co-correlate with the actual causative chemical. For example, the high importance of ethyl acetate could be (partially) attributed to the total ester content, ethanol or ethyl hexanoate (rho=0.77, rho=0.72 and rho=0.68), while ethyl phenylacetate could hide the importance of prenyl isobutyrate and ethyl benzoate (rho=0.77 and rho=0.76). Expanding our GBR model to include beer style as a parameter did not yield additional power or insight. This is likely due to style-specific chemical signatures, such as iso-alpha acids and lactic acid, that implicitly convey style information to the original model, as well as the smaller sample size per style, limiting the power to uncover style-specific patterns. This can be partly attributed to the curse of dimensionality, where the high number of parameters results in the models mainly incorporating single parameter effects, rather than complex interactions such as style-dependent effects 67 . A larger number of samples may overcome some of these limitations and offer more insight into style-specific effects. On the other hand, beer style is not a rigid scientific classification, and beers within one style often differ a lot, which further complicates the analysis of style as a model factor.
Our study is limited to beers from Belgian breweries. Although these beers cover a large portion of the beer styles available globally, some beer styles and consumer patterns may be missing, while other features might be overrepresented. For example, many Belgian ales exhibit yeast-driven flavor profiles, which is reflected in the chemical drivers of appreciation discovered by this study. In future work, expanding the scope to include diverse markets and beer styles could lead to the identification of even more drivers of appreciation and better models for special niche products that were not present in our beer set.
In addition to inherent limitations of GBR models, there are also some limitations associated with studying food aroma. Even if our chemical analyses measured most of the known aroma compounds, the total number of flavor compounds in complex foods like beer is still larger than the subset we were able to measure in this study. For example, hop-derived thiols, that influence flavor at very low concentrations, are notoriously difficult to measure in a high-throughput experiment. Moreover, consumer perception remains subjective and prone to biases that are difficult to avoid. It is also important to stress that the models are still immature and that more extensive datasets will be crucial for developing more complete models in the future. Besides more samples and parameters, our dataset does not include any demographic information about the tasters. Including such data could lead to better models that grasp external factors like age and culture. Another limitation is that our set of beers consists of high-quality end-products and lacks beers that are unfit for sale, which limits the current model in accurately predicting products that are appreciated very badly. Finally, while models could be readily applied in quality control, their use in sensory science and product development is restrained by their inability to discern causal relationships. Given that the models cannot distinguish compounds that genuinely drive consumer perception from those that merely correlate, validation experiments are essential to identify true causative compounds.
Despite the inherent limitations, dissection of our models enabled us to pinpoint specific molecules as potential drivers of beer aroma and consumer appreciation, including compounds that were unexpected and would not have been identified using standard approaches. Important drivers of beer appreciation uncovered by our models include protein levels, ethyl acetate, ethyl phenyl acetate and lactic acid. Currently, many brewers already use lactic acid to acidify their brewing water and ensure optimal pH for enzymatic activity during the mashing process. Our results suggest that adding lactic acid can also improve beer appreciation, although its individual effect remains to be tested. Interestingly, ethanol appears to be unnecessary to improve beer appreciation, both for blond beer and alcohol-free beer. Given the growing consumer interest in alcohol-free beer, with a predicted annual market growth of >7% 84 , it is relevant for brewers to know what compounds can further increase consumer appreciation of these beers. Hence, our model may readily provide avenues to further improve the flavor and consumer appreciation of both alcoholic and non-alcoholic beers, which is generally considered one of the key challenges for future beer production.
Whereas we see a direct implementation of our results for the development of superior alcohol-free beverages and other food products, our study can also serve as a stepping stone for the development of novel alcohol-containing beverages. We want to echo the growing body of scientific evidence for the negative effects of alcohol consumption, both on the individual level by the mutagenic, teratogenic and carcinogenic effects of ethanol 85 , 86 , as well as the burden on society caused by alcohol abuse and addiction. We encourage the use of our results for the production of healthier, tastier products, including novel and improved beverages with lower alcohol contents. Furthermore, we strongly discourage the use of these technologies to improve the appreciation or addictive properties of harmful substances.
The present work demonstrates that despite some important remaining hurdles, combining the latest developments in chemical analyses, sensory analysis and modern machine learning methods offers exciting avenues for food chemistry and engineering. Soon, these tools may provide solutions in quality control and recipe development, as well as new approaches to sensory science and flavor research.
Beer selection
250 commercial Belgian beers were selected to cover the broad diversity of beer styles and corresponding diversity in chemical composition and aroma. See Supplementary Fig. S1 .
Chemical dataset
Sample preparation.
Beers within their expiration date were purchased from commercial retailers. Samples were prepared in biological duplicates at room temperature, unless explicitly stated otherwise. Bottle pressure was measured with a manual pressure device (Steinfurth Mess-Systeme GmbH) and used to calculate CO 2 concentration. The beer was poured through two filter papers (Macherey-Nagel, 500713032 MN 713 ¼) to remove carbon dioxide and prevent spontaneous foaming. Samples were then prepared for measurements by targeted Headspace-Gas Chromatography-Flame Ionization Detector/Flame Photometric Detector (HS-GC-FID/FPD), Headspace-Solid Phase Microextraction-Gas Chromatography-Mass Spectrometry (HS-SPME-GC-MS), colorimetric analysis, enzymatic analysis, Near-Infrared (NIR) analysis, as described in the sections below. The mean values of biological duplicates are reported for each compound.
HS-GC-FID/FPD
HS-GC-FID/FPD (Shimadzu GC 2010 Plus) was used to measure higher alcohols, acetaldehyde, esters, 4-vinyl guaicol, and sulfur compounds. Each measurement comprised 5 ml of sample pipetted into a 20 ml glass vial containing 1.75 g NaCl (VWR, 27810.295). 100 µl of 2-heptanol (Sigma-Aldrich, H3003) (internal standard) solution in ethanol (Fisher Chemical, E/0650DF/C17) was added for a final concentration of 2.44 mg/L. Samples were flushed with nitrogen for 10 s, sealed with a silicone septum, stored at −80 °C and analyzed in batches of 20.
The GC was equipped with a DB-WAXetr column (length, 30 m; internal diameter, 0.32 mm; layer thickness, 0.50 µm; Agilent Technologies, Santa Clara, CA, USA) to the FID and an HP-5 column (length, 30 m; internal diameter, 0.25 mm; layer thickness, 0.25 µm; Agilent Technologies, Santa Clara, CA, USA) to the FPD. N 2 was used as the carrier gas. Samples were incubated for 20 min at 70 °C in the headspace autosampler (Flow rate, 35 cm/s; Injection volume, 1000 µL; Injection mode, split; Combi PAL autosampler, CTC analytics, Switzerland). The injector, FID and FPD temperatures were kept at 250 °C. The GC oven temperature was first held at 50 °C for 5 min and then allowed to rise to 80 °C at a rate of 5 °C/min, followed by a second ramp of 4 °C/min until 200 °C kept for 3 min and a final ramp of (4 °C/min) until 230 °C for 1 min. Results were analyzed with the GCSolution software version 2.4 (Shimadzu, Kyoto, Japan). The GC was calibrated with a 5% EtOH solution (VWR International) containing the volatiles under study (Supplementary Table S7 ).
HS-SPME-GC-MS
HS-SPME-GC-MS (Shimadzu GCMS-QP-2010 Ultra) was used to measure additional volatile compounds, mainly comprising terpenoids and esters. Samples were analyzed by HS-SPME using a triphase DVB/Carboxen/PDMS 50/30 μm SPME fiber (Supelco Co., Bellefonte, PA, USA) followed by gas chromatography (Thermo Fisher Scientific Trace 1300 series, USA) coupled to a mass spectrometer (Thermo Fisher Scientific ISQ series MS) equipped with a TriPlus RSH autosampler. 5 ml of degassed beer sample was placed in 20 ml vials containing 1.75 g NaCl (VWR, 27810.295). 5 µl internal standard mix was added, containing 2-heptanol (1 g/L) (Sigma-Aldrich, H3003), 4-fluorobenzaldehyde (1 g/L) (Sigma-Aldrich, 128376), 2,3-hexanedione (1 g/L) (Sigma-Aldrich, 144169) and guaiacol (1 g/L) (Sigma-Aldrich, W253200) in ethanol (Fisher Chemical, E/0650DF/C17). Each sample was incubated at 60 °C in the autosampler oven with constant agitation. After 5 min equilibration, the SPME fiber was exposed to the sample headspace for 30 min. The compounds trapped on the fiber were thermally desorbed in the injection port of the chromatograph by heating the fiber for 15 min at 270 °C.
The GC-MS was equipped with a low polarity RXi-5Sil MS column (length, 20 m; internal diameter, 0.18 mm; layer thickness, 0.18 µm; Restek, Bellefonte, PA, USA). Injection was performed in splitless mode at 320 °C, a split flow of 9 ml/min, a purge flow of 5 ml/min and an open valve time of 3 min. To obtain a pulsed injection, a programmed gas flow was used whereby the helium gas flow was set at 2.7 mL/min for 0.1 min, followed by a decrease in flow of 20 ml/min to the normal 0.9 mL/min. The temperature was first held at 30 °C for 3 min and then allowed to rise to 80 °C at a rate of 7 °C/min, followed by a second ramp of 2 °C/min till 125 °C and a final ramp of 8 °C/min with a final temperature of 270 °C.
Mass acquisition range was 33 to 550 amu at a scan rate of 5 scans/s. Electron impact ionization energy was 70 eV. The interface and ion source were kept at 275 °C and 250 °C, respectively. A mix of linear n-alkanes (from C7 to C40, Supelco Co.) was injected into the GC-MS under identical conditions to serve as external retention index markers. Identification and quantification of the compounds were performed using an in-house developed R script as described in Goelen et al. and Reher et al. 87 , 88 (for package information, see Supplementary Table S8 ). Briefly, chromatograms were analyzed using AMDIS (v2.71) 89 to separate overlapping peaks and obtain pure compound spectra. The NIST MS Search software (v2.0 g) in combination with the NIST2017, FFNSC3 and Adams4 libraries were used to manually identify the empirical spectra, taking into account the expected retention time. After background subtraction and correcting for retention time shifts between samples run on different days based on alkane ladders, compound elution profiles were extracted and integrated using a file with 284 target compounds of interest, which were either recovered in our identified AMDIS list of spectra or were known to occur in beer. Compound elution profiles were estimated for every peak in every chromatogram over a time-restricted window using weighted non-negative least square analysis after which peak areas were integrated 87 , 88 . Batch effect correction was performed by normalizing against the most stable internal standard compound, 4-fluorobenzaldehyde. Out of all 284 target compounds that were analyzed, 167 were visually judged to have reliable elution profiles and were used for final analysis.
Discrete photometric and enzymatic analysis
Discrete photometric and enzymatic analysis (Thermo Scientific TM Gallery TM Plus Beermaster Discrete Analyzer) was used to measure acetic acid, ammonia, beta-glucan, iso-alpha acids, color, sugars, glycerol, iron, pH, protein, and sulfite. 2 ml of sample volume was used for the analyses. Information regarding the reagents and standard solutions used for analyses and calibrations is included in Supplementary Table S7 and Supplementary Table S9 .
NIR analyses
NIR analysis (Anton Paar Alcolyzer Beer ME System) was used to measure ethanol. Measurements comprised 50 ml of sample, and a 10% EtOH solution was used for calibration.
Correlation calculations
Pairwise Spearman Rank correlations were calculated between all chemical properties.
Sensory dataset
Trained panel.
Our trained tasting panel consisted of volunteers who gave prior verbal informed consent. All compounds used for the validation experiment were of food-grade quality. The tasting sessions were approved by the Social and Societal Ethics Committee of the KU Leuven (G-2022-5677-R2(MAR)). All online reviewers agreed to the Terms and Conditions of the RateBeer website.
Sensory analysis was performed according to the American Society of Brewing Chemists (ASBC) Sensory Analysis Methods 90 . 30 volunteers were screened through a series of triangle tests. The sixteen most sensitive and consistent tasters were retained as taste panel members. The resulting panel was diverse in age [22–42, mean: 29], sex [56% male] and nationality [7 different countries]. The panel developed a consensus vocabulary to describe beer aroma, taste and mouthfeel. Panelists were trained to identify and score 50 different attributes, using a 7-point scale to rate attributes’ intensity. The scoring sheet is included as Supplementary Data 3 . Sensory assessments took place between 10–12 a.m. The beers were served in black-colored glasses. Per session, between 5 and 12 beers of the same style were tasted at 12 °C to 16 °C. Two reference beers were added to each set and indicated as ‘Reference 1 & 2’, allowing panel members to calibrate their ratings. Not all panelists were present at every tasting. Scores were scaled by standard deviation and mean-centered per taster. Values are represented as z-scores and clustered by Euclidean distance. Pairwise Spearman correlations were calculated between taste and aroma sensory attributes. Panel consistency was evaluated by repeating samples on different sessions and performing ANOVA to identify differences, using the ‘stats’ package (v4.2.2) in R (for package information, see Supplementary Table S8 ).
Online reviews from a public database
The ‘scrapy’ package in Python (v3.6) (for package information, see Supplementary Table S8 ). was used to collect 232,288 online reviews (mean=922, min=6, max=5343) from RateBeer, an online beer review database. Each review entry comprised 5 numerical scores (appearance, aroma, taste, palate and overall quality) and an optional review text. The total number of reviews per reviewer was collected separately. Numerical scores were scaled and centered per rater, and mean scores were calculated per beer.
For the review texts, the language was estimated using the packages ‘langdetect’ and ‘langid’ in Python. Reviews that were classified as English by both packages were kept. Reviewers with fewer than 100 entries overall were discarded. 181,025 reviews from >6000 reviewers from >40 countries remained. Text processing was done using the ‘nltk’ package in Python. Texts were corrected for slang and misspellings; proper nouns and rare words that are relevant to the beer context were specified and kept as-is (‘Chimay’,’Lambic’, etc.). A dictionary of semantically similar sensorial terms, for example ‘floral’ and ‘flower’, was created and collapsed together into one term. Words were stemmed and lemmatized to avoid identifying words such as ‘acid’ and ‘acidity’ as separate terms. Numbers and punctuation were removed.
Sentences from up to 50 randomly chosen reviews per beer were manually categorized according to the aspect of beer they describe (appearance, aroma, taste, palate, overall quality—not to be confused with the 5 numerical scores described above) or flagged as irrelevant if they contained no useful information. If a beer contained fewer than 50 reviews, all reviews were manually classified. This labeled data set was used to train a model that classified the rest of the sentences for all beers 91 . Sentences describing taste and aroma were extracted, and term frequency–inverse document frequency (TFIDF) was implemented to calculate enrichment scores for sensorial words per beer.
The sex of the tasting subject was not considered when building our sensory database. Instead, results from different panelists were averaged, both for our trained panel (56% male, 44% female) and the RateBeer reviews (70% male, 30% female for RateBeer as a whole).
Beer price collection and processing
Beer prices were collected from the following stores: Colruyt, Delhaize, Total Wine, BeerHawk, The Belgian Beer Shop, The Belgian Shop, and Beer of Belgium. Where applicable, prices were converted to Euros and normalized per liter. Spearman correlations were calculated between these prices and mean overall appreciation scores from RateBeer and the taste panel, respectively.
Pairwise Spearman Rank correlations were calculated between all sensory properties.
Machine learning models
Predictive modeling of sensory profiles from chemical data.
Regression models were constructed to predict (a) trained panel scores for beer flavors and quality from beer chemical profiles and (b) public reviews’ appreciation scores from beer chemical profiles. Z-scores were used to represent sensory attributes in both data sets. Chemical properties with log-normal distributions (Shapiro-Wilk test, p < 0.05 ) were log-transformed. Missing chemical measurements (0.1% of all data) were replaced with mean values per attribute. Observations from 250 beers were randomly separated into a training set (70%, 175 beers) and a test set (30%, 75 beers), stratified per beer style. Chemical measurements (p = 231) were normalized based on the training set average and standard deviation. In total, three linear regression-based models: linear regression with first-order interaction terms (LR), lasso regression with first-order interaction terms (Lasso) and partial least squares regression (PLSR); five decision tree models, Adaboost regressor (ABR), Extra Trees (ET), Gradient Boosting regressor (GBR), Random Forest (RF) and XGBoost regressor (XGBR); one support vector machine model (SVR) and one artificial neural network model (ANN) were trained. The models were implemented using the ‘scikit-learn’ package (v1.2.2) and ‘xgboost’ package (v1.7.3) in Python (v3.9.16). Models were trained, and hyperparameters optimized, using five-fold cross-validated grid search with the coefficient of determination (R 2 ) as the evaluation metric. The ANN (scikit-learn’s MLPRegressor) was optimized using Bayesian Tree-Structured Parzen Estimator optimization with the ‘Optuna’ Python package (v3.2.0). Individual models were trained per attribute, and a multi-output model was trained on all attributes simultaneously.
Model dissection
GBR was found to outperform other methods, resulting in models with the highest average R 2 values in both trained panel and public review data sets. Impurity-based rankings of the most important predictors for each predicted sensorial trait were obtained using the ‘scikit-learn’ package. To observe the relationships between these chemical properties and their predicted targets, partial dependence plots (PDP) were constructed for the six most important predictors of consumer appreciation 74 , 75 .
The ‘SHAP’ package in Python (v0.41.0) was implemented to provide an alternative ranking of predictor importance and to visualize the predictors’ effects as a function of their concentration 68 .
Validation of causal chemical properties
To validate the effects of the most important model features on predicted sensory attributes, beers were spiked with the chemical compounds identified by the models and descriptive sensory analyses were carried out according to the American Society of Brewing Chemists (ASBC) protocol 90 .
Compound spiking was done 30 min before tasting. Compounds were spiked into fresh beer bottles, that were immediately resealed and inverted three times. Fresh bottles of beer were opened for the same duration, resealed, and inverted thrice, to serve as controls. Pairs of spiked samples and controls were served simultaneously, chilled and in dark glasses as outlined in the Trained panel section above. Tasters were instructed to select the glass with the higher flavor intensity for each attribute (directional difference test 92 ) and to select the glass they prefer.
The final concentration after spiking was equal to the within-style average, after normalizing by ethanol concentration. This was done to ensure balanced flavor profiles in the final spiked beer. The same methods were applied to improve a non-alcoholic beer. Compounds were the following: ethyl acetate (Merck KGaA, W241415), ethyl hexanoate (Merck KGaA, W243906), isoamyl acetate (Merck KGaA, W205508), phenethyl acetate (Merck KGaA, W285706), ethanol (96%, Colruyt), glycerol (Merck KGaA, W252506), lactic acid (Merck KGaA, 261106).
Significant differences in preference or perceived intensity were determined by performing the two-sided binomial test on each attribute.
Reporting summary
Further information on research design is available in the Nature Portfolio Reporting Summary linked to this article.
Data availability
The data that support the findings of this work are available in the Supplementary Data files and have been deposited to Zenodo under accession code 10653704 93 . The RateBeer scores data are under restricted access, they are not publicly available as they are property of RateBeer (ZX Ventures, USA). Access can be obtained from the authors upon reasonable request and with permission of RateBeer (ZX Ventures, USA). Source data are provided with this paper.
Code availability
The code for training the machine learning models, analyzing the models, and generating the figures has been deposited to Zenodo under accession code 10653704 93 .
Tieman, D. et al. A chemical genetic roadmap to improved tomato flavor. Science 355 , 391–394 (2017).
Article ADS CAS PubMed Google Scholar
Plutowska, B. & Wardencki, W. Application of gas chromatography–olfactometry (GC–O) in analysis and quality assessment of alcoholic beverages – A review. Food Chem. 107 , 449–463 (2008).
Article CAS Google Scholar
Legin, A., Rudnitskaya, A., Seleznev, B. & Vlasov, Y. Electronic tongue for quality assessment of ethanol, vodka and eau-de-vie. Anal. Chim. Acta 534 , 129–135 (2005).
Loutfi, A., Coradeschi, S., Mani, G. K., Shankar, P. & Rayappan, J. B. B. Electronic noses for food quality: A review. J. Food Eng. 144 , 103–111 (2015).
Ahn, Y.-Y., Ahnert, S. E., Bagrow, J. P. & Barabási, A.-L. Flavor network and the principles of food pairing. Sci. Rep. 1 , 196 (2011).
Article CAS PubMed PubMed Central Google Scholar
Bartoshuk, L. M. & Klee, H. J. Better fruits and vegetables through sensory analysis. Curr. Biol. 23 , R374–R378 (2013).
Article CAS PubMed Google Scholar
Piggott, J. R. Design questions in sensory and consumer science. Food Qual. Prefer. 3293 , 217–220 (1995).
Article Google Scholar
Kermit, M. & Lengard, V. Assessing the performance of a sensory panel-panellist monitoring and tracking. J. Chemom. 19 , 154–161 (2005).
Cook, D. J., Hollowood, T. A., Linforth, R. S. T. & Taylor, A. J. Correlating instrumental measurements of texture and flavour release with human perception. Int. J. Food Sci. Technol. 40 , 631–641 (2005).
Chinchanachokchai, S., Thontirawong, P. & Chinchanachokchai, P. A tale of two recommender systems: The moderating role of consumer expertise on artificial intelligence based product recommendations. J. Retail. Consum. Serv. 61 , 1–12 (2021).
Ross, C. F. Sensory science at the human-machine interface. Trends Food Sci. Technol. 20 , 63–72 (2009).
Chambers, E. IV & Koppel, K. Associations of volatile compounds with sensory aroma and flavor: The complex nature of flavor. Molecules 18 , 4887–4905 (2013).
Pinu, F. R. Metabolomics—The new frontier in food safety and quality research. Food Res. Int. 72 , 80–81 (2015).
Danezis, G. P., Tsagkaris, A. S., Brusic, V. & Georgiou, C. A. Food authentication: state of the art and prospects. Curr. Opin. Food Sci. 10 , 22–31 (2016).
Shepherd, G. M. Smell images and the flavour system in the human brain. Nature 444 , 316–321 (2006).
Meilgaard, M. C. Prediction of flavor differences between beers from their chemical composition. J. Agric. Food Chem. 30 , 1009–1017 (1982).
Xu, L. et al. Widespread receptor-driven modulation in peripheral olfactory coding. Science 368 , eaaz5390 (2020).
Kupferschmidt, K. Following the flavor. Science 340 , 808–809 (2013).
Billesbølle, C. B. et al. Structural basis of odorant recognition by a human odorant receptor. Nature 615 , 742–749 (2023).
Article ADS PubMed PubMed Central Google Scholar
Smith, B. Perspective: Complexities of flavour. Nature 486 , S6–S6 (2012).
Pfister, P. et al. Odorant receptor inhibition is fundamental to odor encoding. Curr. Biol. 30 , 2574–2587 (2020).
Moskowitz, H. W., Kumaraiah, V., Sharma, K. N., Jacobs, H. L. & Sharma, S. D. Cross-cultural differences in simple taste preferences. Science 190 , 1217–1218 (1975).
Eriksson, N. et al. A genetic variant near olfactory receptor genes influences cilantro preference. Flavour 1 , 22 (2012).
Ferdenzi, C. et al. Variability of affective responses to odors: Culture, gender, and olfactory knowledge. Chem. Senses 38 , 175–186 (2013).
Article PubMed Google Scholar
Lawless, H. T. & Heymann, H. Sensory evaluation of food: Principles and practices. (Springer, New York, NY). https://doi.org/10.1007/978-1-4419-6488-5 (2010).
Colantonio, V. et al. Metabolomic selection for enhanced fruit flavor. Proc. Natl. Acad. Sci. 119 , e2115865119 (2022).
Fritz, F., Preissner, R. & Banerjee, P. VirtualTaste: a web server for the prediction of organoleptic properties of chemical compounds. Nucleic Acids Res 49 , W679–W684 (2021).
Tuwani, R., Wadhwa, S. & Bagler, G. BitterSweet: Building machine learning models for predicting the bitter and sweet taste of small molecules. Sci. Rep. 9 , 1–13 (2019).
Dagan-Wiener, A. et al. Bitter or not? BitterPredict, a tool for predicting taste from chemical structure. Sci. Rep. 7 , 1–13 (2017).
Pallante, L. et al. Toward a general and interpretable umami taste predictor using a multi-objective machine learning approach. Sci. Rep. 12 , 1–11 (2022).
Malavolta, M. et al. A survey on computational taste predictors. Eur. Food Res. Technol. 248 , 2215–2235 (2022).
Lee, B. K. et al. A principal odor map unifies diverse tasks in olfactory perception. Science 381 , 999–1006 (2023).
Mayhew, E. J. et al. Transport features predict if a molecule is odorous. Proc. Natl. Acad. Sci. 119 , e2116576119 (2022).
Niu, Y. et al. Sensory evaluation of the synergism among ester odorants in light aroma-type liquor by odor threshold, aroma intensity and flash GC electronic nose. Food Res. Int. 113 , 102–114 (2018).
Yu, P., Low, M. Y. & Zhou, W. Design of experiments and regression modelling in food flavour and sensory analysis: A review. Trends Food Sci. Technol. 71 , 202–215 (2018).
Oladokun, O. et al. The impact of hop bitter acid and polyphenol profiles on the perceived bitterness of beer. Food Chem. 205 , 212–220 (2016).
Linforth, R., Cabannes, M., Hewson, L., Yang, N. & Taylor, A. Effect of fat content on flavor delivery during consumption: An in vivo model. J. Agric. Food Chem. 58 , 6905–6911 (2010).
Guo, S., Na Jom, K. & Ge, Y. Influence of roasting condition on flavor profile of sunflower seeds: A flavoromics approach. Sci. Rep. 9 , 11295 (2019).
Ren, Q. et al. The changes of microbial community and flavor compound in the fermentation process of Chinese rice wine using Fagopyrum tataricum grain as feedstock. Sci. Rep. 9 , 3365 (2019).
Hastie, T., Friedman, J. & Tibshirani, R. The Elements of Statistical Learning. (Springer, New York, NY). https://doi.org/10.1007/978-0-387-21606-5 (2001).
Dietz, C., Cook, D., Huismann, M., Wilson, C. & Ford, R. The multisensory perception of hop essential oil: a review. J. Inst. Brew. 126 , 320–342 (2020).
CAS Google Scholar
Roncoroni, Miguel & Verstrepen, Kevin Joan. Belgian Beer: Tested and Tasted. (Lannoo, 2018).
Meilgaard, M. Flavor chemistry of beer: Part II: Flavor and threshold of 239 aroma volatiles. in (1975).
Bokulich, N. A. & Bamforth, C. W. The microbiology of malting and brewing. Microbiol. Mol. Biol. Rev. MMBR 77 , 157–172 (2013).
Dzialo, M. C., Park, R., Steensels, J., Lievens, B. & Verstrepen, K. J. Physiology, ecology and industrial applications of aroma formation in yeast. FEMS Microbiol. Rev. 41 , S95–S128 (2017).
Article PubMed PubMed Central Google Scholar
Datta, A. et al. Computer-aided food engineering. Nat. Food 3 , 894–904 (2022).
American Society of Brewing Chemists. Beer Methods. (American Society of Brewing Chemists, St. Paul, MN, U.S.A.).
Olaniran, A. O., Hiralal, L., Mokoena, M. P. & Pillay, B. Flavour-active volatile compounds in beer: production, regulation and control. J. Inst. Brew. 123 , 13–23 (2017).
Verstrepen, K. J. et al. Flavor-active esters: Adding fruitiness to beer. J. Biosci. Bioeng. 96 , 110–118 (2003).
Meilgaard, M. C. Flavour chemistry of beer. part I: flavour interaction between principal volatiles. Master Brew. Assoc. Am. Tech. Q 12 , 107–117 (1975).
Briggs, D. E., Boulton, C. A., Brookes, P. A. & Stevens, R. Brewing 227–254. (Woodhead Publishing). https://doi.org/10.1533/9781855739062.227 (2004).
Bossaert, S., Crauwels, S., De Rouck, G. & Lievens, B. The power of sour - A review: Old traditions, new opportunities. BrewingScience 72 , 78–88 (2019).
Google Scholar
Verstrepen, K. J. et al. Flavor active esters: Adding fruitiness to beer. J. Biosci. Bioeng. 96 , 110–118 (2003).
Snauwaert, I. et al. Microbial diversity and metabolite composition of Belgian red-brown acidic ales. Int. J. Food Microbiol. 221 , 1–11 (2016).
Spitaels, F. et al. The microbial diversity of traditional spontaneously fermented lambic beer. PLoS ONE 9 , e95384 (2014).
Blanco, C. A., Andrés-Iglesias, C. & Montero, O. Low-alcohol Beers: Flavor Compounds, Defects, and Improvement Strategies. Crit. Rev. Food Sci. Nutr. 56 , 1379–1388 (2016).
Jackowski, M. & Trusek, A. Non-Alcohol. beer Prod. – Overv. 20 , 32–38 (2018).
Takoi, K. et al. The contribution of geraniol metabolism to the citrus flavour of beer: Synergy of geraniol and β-citronellol under coexistence with excess linalool. J. Inst. Brew. 116 , 251–260 (2010).
Kroeze, J. H. & Bartoshuk, L. M. Bitterness suppression as revealed by split-tongue taste stimulation in humans. Physiol. Behav. 35 , 779–783 (1985).
Mennella, J. A. et al. A spoonful of sugar helps the medicine go down”: Bitter masking bysucrose among children and adults. Chem. Senses 40 , 17–25 (2015).
Wietstock, P., Kunz, T., Perreira, F. & Methner, F.-J. Metal chelation behavior of hop acids in buffered model systems. BrewingScience 69 , 56–63 (2016).
Sancho, D., Blanco, C. A., Caballero, I. & Pascual, A. Free iron in pale, dark and alcohol-free commercial lager beers. J. Sci. Food Agric. 91 , 1142–1147 (2011).
Rodrigues, H. & Parr, W. V. Contribution of cross-cultural studies to understanding wine appreciation: A review. Food Res. Int. 115 , 251–258 (2019).
Korneva, E. & Blockeel, H. Towards better evaluation of multi-target regression models. in ECML PKDD 2020 Workshops (eds. Koprinska, I. et al.) 353–362 (Springer International Publishing, Cham, 2020). https://doi.org/10.1007/978-3-030-65965-3_23 .
Gastón Ares. Mathematical and Statistical Methods in Food Science and Technology. (Wiley, 2013).
Grinsztajn, L., Oyallon, E. & Varoquaux, G. Why do tree-based models still outperform deep learning on tabular data? Preprint at http://arxiv.org/abs/2207.08815 (2022).
Gries, S. T. Statistics for Linguistics with R: A Practical Introduction. in Statistics for Linguistics with R (De Gruyter Mouton, 2021). https://doi.org/10.1515/9783110718256 .
Lundberg, S. M. et al. From local explanations to global understanding with explainable AI for trees. Nat. Mach. Intell. 2 , 56–67 (2020).
Ickes, C. M. & Cadwallader, K. R. Effects of ethanol on flavor perception in alcoholic beverages. Chemosens. Percept. 10 , 119–134 (2017).
Kato, M. et al. Influence of high molecular weight polypeptides on the mouthfeel of commercial beer. J. Inst. Brew. 127 , 27–40 (2021).
Wauters, R. et al. Novel Saccharomyces cerevisiae variants slow down the accumulation of staling aldehydes and improve beer shelf-life. Food Chem. 398 , 1–11 (2023).
Li, H., Jia, S. & Zhang, W. Rapid determination of low-level sulfur compounds in beer by headspace gas chromatography with a pulsed flame photometric detector. J. Am. Soc. Brew. Chem. 66 , 188–191 (2008).
Dercksen, A., Laurens, J., Torline, P., Axcell, B. C. & Rohwer, E. Quantitative analysis of volatile sulfur compounds in beer using a membrane extraction interface. J. Am. Soc. Brew. Chem. 54 , 228–233 (1996).
Molnar, C. Interpretable Machine Learning: A Guide for Making Black-Box Models Interpretable. (2020).
Zhao, Q. & Hastie, T. Causal interpretations of black-box models. J. Bus. Econ. Stat. Publ. Am. Stat. Assoc. 39 , 272–281 (2019).
Article MathSciNet Google Scholar
Hastie, T., Tibshirani, R. & Friedman, J. The Elements of Statistical Learning. (Springer, 2019).
Labrado, D. et al. Identification by NMR of key compounds present in beer distillates and residual phases after dealcoholization by vacuum distillation. J. Sci. Food Agric. 100 , 3971–3978 (2020).
Lusk, L. T., Kay, S. B., Porubcan, A. & Ryder, D. S. Key olfactory cues for beer oxidation. J. Am. Soc. Brew. Chem. 70 , 257–261 (2012).
Gonzalez Viejo, C., Torrico, D. D., Dunshea, F. R. & Fuentes, S. Development of artificial neural network models to assess beer acceptability based on sensory properties using a robotic pourer: A comparative model approach to achieve an artificial intelligence system. Beverages 5 , 33 (2019).
Gonzalez Viejo, C., Fuentes, S., Torrico, D. D., Godbole, A. & Dunshea, F. R. Chemical characterization of aromas in beer and their effect on consumers liking. Food Chem. 293 , 479–485 (2019).
Gilbert, J. L. et al. Identifying breeding priorities for blueberry flavor using biochemical, sensory, and genotype by environment analyses. PLOS ONE 10 , 1–21 (2015).
Goulet, C. et al. Role of an esterase in flavor volatile variation within the tomato clade. Proc. Natl. Acad. Sci. 109 , 19009–19014 (2012).
Article ADS CAS PubMed PubMed Central Google Scholar
Borisov, V. et al. Deep Neural Networks and Tabular Data: A Survey. IEEE Trans. Neural Netw. Learn. Syst. 1–21 https://doi.org/10.1109/TNNLS.2022.3229161 (2022).
Statista. Statista Consumer Market Outlook: Beer - Worldwide.
Seitz, H. K. & Stickel, F. Molecular mechanisms of alcoholmediated carcinogenesis. Nat. Rev. Cancer 7 , 599–612 (2007).
Voordeckers, K. et al. Ethanol exposure increases mutation rate through error-prone polymerases. Nat. Commun. 11 , 3664 (2020).
Goelen, T. et al. Bacterial phylogeny predicts volatile organic compound composition and olfactory response of an aphid parasitoid. Oikos 129 , 1415–1428 (2020).
Article ADS Google Scholar
Reher, T. et al. Evaluation of hop (Humulus lupulus) as a repellent for the management of Drosophila suzukii. Crop Prot. 124 , 104839 (2019).
Stein, S. E. An integrated method for spectrum extraction and compound identification from gas chromatography/mass spectrometry data. J. Am. Soc. Mass Spectrom. 10 , 770–781 (1999).
American Society of Brewing Chemists. Sensory Analysis Methods. (American Society of Brewing Chemists, St. Paul, MN, U.S.A., 1992).
McAuley, J., Leskovec, J. & Jurafsky, D. Learning Attitudes and Attributes from Multi-Aspect Reviews. Preprint at https://doi.org/10.48550/arXiv.1210.3926 (2012).
Meilgaard, M. C., Carr, B. T. & Carr, B. T. Sensory Evaluation Techniques. (CRC Press, Boca Raton). https://doi.org/10.1201/b16452 (2014).
Schreurs, M. et al. Data from: Predicting and improving complex beer flavor through machine learning. Zenodo https://doi.org/10.5281/zenodo.10653704 (2024).
Download references
Acknowledgements
We thank all lab members for their discussions and thank all tasting panel members for their contributions. Special thanks go out to Dr. Karin Voordeckers for her tremendous help in proofreading and improving the manuscript. M.S. was supported by a Baillet-Latour fellowship, L.C. acknowledges financial support from KU Leuven (C16/17/006), F.A.T. was supported by a PhD fellowship from FWO (1S08821N). Research in the lab of K.J.V. is supported by KU Leuven, FWO, VIB, VLAIO and the Brewing Science Serves Health Fund. Research in the lab of T.W. is supported by FWO (G.0A51.15) and KU Leuven (C16/17/006).
Author information
These authors contributed equally: Michiel Schreurs, Supinya Piampongsant, Miguel Roncoroni.
Authors and Affiliations
VIB—KU Leuven Center for Microbiology, Gaston Geenslaan 1, B-3001, Leuven, Belgium
Michiel Schreurs, Supinya Piampongsant, Miguel Roncoroni, Lloyd Cool, Beatriz Herrera-Malaver, Florian A. Theßeling & Kevin J. Verstrepen
CMPG Laboratory of Genetics and Genomics, KU Leuven, Gaston Geenslaan 1, B-3001, Leuven, Belgium
Leuven Institute for Beer Research (LIBR), Gaston Geenslaan 1, B-3001, Leuven, Belgium
Laboratory of Socioecology and Social Evolution, KU Leuven, Naamsestraat 59, B-3000, Leuven, Belgium
Lloyd Cool, Christophe Vanderaa & Tom Wenseleers
VIB Bioinformatics Core, VIB, Rijvisschestraat 120, B-9052, Ghent, Belgium
Łukasz Kreft & Alexander Botzki
AB InBev SA/NV, Brouwerijplein 1, B-3000, Leuven, Belgium
Philippe Malcorps & Luk Daenen
You can also search for this author in PubMed Google Scholar
Contributions
S.P., M.S. and K.J.V. conceived the experiments. S.P., M.S. and K.J.V. designed the experiments. S.P., M.S., M.R., B.H. and F.A.T. performed the experiments. S.P., M.S., L.C., C.V., L.K., A.B., P.M., L.D., T.W. and K.J.V. contributed analysis ideas. S.P., M.S., L.C., C.V., T.W. and K.J.V. analyzed the data. All authors contributed to writing the manuscript.
Corresponding author
Correspondence to Kevin J. Verstrepen .
Ethics declarations
Competing interests.
K.J.V. is affiliated with bar.on. The other authors declare no competing interests.
Peer review
Peer review information.
Nature Communications thanks Florian Bauer, Andrew John Macintosh and the other, anonymous, reviewer(s) for their contribution to the peer review of this work. A peer review file is available.
Additional information
Publisher’s note Springer Nature remains neutral with regard to jurisdictional claims in published maps and institutional affiliations.
Supplementary information
Supplementary information, peer review file, description of additional supplementary files, supplementary data 1, supplementary data 2, supplementary data 3, supplementary data 4, supplementary data 5, supplementary data 6, supplementary data 7, reporting summary, source data, source data, rights and permissions.
Open Access This article is licensed under a Creative Commons Attribution 4.0 International License, which permits use, sharing, adaptation, distribution and reproduction in any medium or format, as long as you give appropriate credit to the original author(s) and the source, provide a link to the Creative Commons licence, and indicate if changes were made. The images or other third party material in this article are included in the article’s Creative Commons licence, unless indicated otherwise in a credit line to the material. If material is not included in the article’s Creative Commons licence and your intended use is not permitted by statutory regulation or exceeds the permitted use, you will need to obtain permission directly from the copyright holder. To view a copy of this licence, visit http://creativecommons.org/licenses/by/4.0/ .
Reprints and permissions
About this article
Cite this article.
Schreurs, M., Piampongsant, S., Roncoroni, M. et al. Predicting and improving complex beer flavor through machine learning. Nat Commun 15 , 2368 (2024). https://doi.org/10.1038/s41467-024-46346-0
Download citation
Received : 30 October 2023
Accepted : 21 February 2024
Published : 26 March 2024
DOI : https://doi.org/10.1038/s41467-024-46346-0
Share this article
Anyone you share the following link with will be able to read this content:
Sorry, a shareable link is not currently available for this article.
Provided by the Springer Nature SharedIt content-sharing initiative
By submitting a comment you agree to abide by our Terms and Community Guidelines . If you find something abusive or that does not comply with our terms or guidelines please flag it as inappropriate.
Quick links
- Explore articles by subject
- Guide to authors
- Editorial policies
Sign up for the Nature Briefing: Translational Research newsletter — top stories in biotechnology, drug discovery and pharma.

- Share full article
Advertisement
Supported by
More Young People Than Ever Will Get Colorectal Cancer This Year
Colon and rectal cancers are increasing among people younger than 50. Experts have a few ideas about why.

By Knvul Sheikh
Marisa Peters had been experiencing symptoms for years: blood on her toilet paper after going to the bathroom, changes in her stool and difficulty controlling the urge to poop. But she was in her 30s, healthy and physically active. She did not have any abdominal pain, and doctors dismissed the symptoms as hemorrhoids, or normal postpartum changes after the birth of her first son. When Ms. Peters finally visited a gastroenterologist in 2021, after having her third child and experiencing worsening bleeding from her rectum along with changes in her stool consistency, an urgent colonoscopy confirmed that she had colorectal cancer.
It had been four or five years since her symptoms had first emerged. Yet “I did not expect that cancer was going to be what they found,” Ms. Peters said.
A report published by the American Cancer Society in January suggests that rates of colorectal cancer are rising rapidly among people in their 20s, 30s and 40s — even as incidence is declining in people over the age of 65.
“It’s unfortunately becoming a bigger problem every year,” said Dr. Michael Cecchini, a co-director of the colorectal program in the Center for Gastrointestinal Cancers and a medical oncologist at Yale Cancer Center. He added that early-onset colorectal cancers have been increasing by about 2 percent per year since the mid-1990s. This increase has moved colorectal cancer up to being the top cause of cancer deaths in men under the age of 50 and the second-leading cause of cancer deaths in women under 50 in the United States.
In fact, experts are noticing a rise in early-onset colorectal cancers around the world — a trend that they are racing to explain.
Why is colorectal cancer increasing among young people?
Colon and rectal cancers share many similarities and are typically lumped into one category, called colorectal cancer. Studies, however, show that the increase in diagnoses is mainly driven by a rise in rectal cancers and cancers found in the left, or distal, side of the colon, near the rectum. “That maybe provides an important clue for understanding what might be going on,” said Caitlin Murphy, an associate professor and cancer researcher at UTHealth Houston.
Colorectal cancers in younger people also tend to be more aggressive, and they are often found at a more advanced stage, Dr. Murphy said. But most people affected by early-onset colorectal cancer are too young to be recommended for routine cancer screenings, which have helped decrease rates in adults over 50. In 2021, the U.S. Preventive Services Task Force reduced the recommended age for starting colorectal cancer screening by just five years — from 50 to 45 .
A vast majority of colorectal cancer diagnoses are still made in people 50 and older. The American Cancer Society predicted last year that roughly 153,000 new diagnoses would be made in the U.S. in 2023, of which 19,550 would be in people younger than 50. But millennials born around 1990 now have twice the risk of colon cancer compared with people born around the 1950s, while millennials’ risk for rectal cancer is about four times higher than that of older age groups, according to a study published in the Journal of the National Cancer Institute . That means diagnoses are likely to “continue going up as these higher-risk generations age,” Dr. Murphy said.
When cancer is found at a younger-than-usual age, doctors usually suspect that genetic mutations may be to blame. And some molecular studies suggest that tumors in early-onset colorectal cancers do have different mutations driving the cancer compared with tumors in older adults. Another piece of evidence that there is a genetic component: It is clear that having a first-degree relative who had colorectal cancer — or even a precancerous polyp — can increase your risk, Dr. Cecchini said. But genetic changes do not explain the full picture, he said.
Some research has linked lifestyle and dietary changes to increased rates of colorectal cancer in both young people and older adults. Recent generations have consumed more red meat , ultraprocessed foods and sugary beverages , and have been known to binge drink more frequently; between 1992 and 1998, cigarette smoking also increased before declining again, while physical activity has continuously declined for decades. All of these factors — along with the rise in obesity rates since the 1980s — are associated with cancer risk. But once again, none of them fully account for the increase in early-onset colorectal cancer.
“For a lot of these risk factors, like smoking, you have to be exposed for long periods of time before the cancer develops,” said Dr. Andrea Cercek, a co-director of the Center for Young Onset Colorectal and Gastrointestinal Cancers at Memorial Sloan Kettering Cancer Center. And many patients in their 20s and 30s do not even fit in these risk groups, she said. “Many of our patients are athletes,” she said. “Many of them were never heavy, not even in childhood.”
Experts are beginning to investigate if there are other environmental drivers of early-onset cancer. For instance, some small studies have hinted at the idea that people who develop colorectal cancer at an early age have an imbalance of “good” and “bad” bacteria in their gut. Researchers are not only looking at antibiotic use, which can alter the gut microbiome, but also nonsteroidal anti-inflammatory drugs that are used as painkillers, proton pump inhibitors that are used to counter stomach acid issues and several psychiatric medications that may be absorbed through the intestinal lining and have increased in use in recent decades, Dr. Cercek said.
Some experts believe exposure to toxic chemicals in the environment may also be to blame. “There’s patterns of environmental exposures by geography, by race, by sex, by all the things that we know colorectal cancer rates also differ by,” Dr. Murphy said.
For instance, for many years, the rates of colorectal cancer diagnoses were highest among non-Hispanic Black people, but research shows that these cancers increased more among non-Hispanic white people in the 1990s and early 2000s, Dr. Murphy said. Now, both groups have fairly similar rates of cancer. “Does this mean that white people are now being exposed to something that Black people have been exposed to for many, many years? We just don’t know yet,” Dr. Murphy said.
There are also geographic disparities in the increase in cancer, with experts seeing more cases emerge in cities and towns along the Mississippi River, in Southeastern states and in Appalachia, which may be explained by occupational exposures to trace elements like arsenic, chromium, and nickel , which are often used in coal production, chemical plants and other industries in those regions. So-called forever chemicals like per- and polyfluoroalkyl substances, better known as PFAS , have been linked to other cancers and could also be driving some of the increase in early-onset colorectal cancer.
“I don’t think there’s going to be one smoking gun that explains everything,” Dr. Murphy said. “It’s a whole bunch of things.”
What can you do to identify and reduce your risk?
After Ms. Peters was diagnosed and started chemotherapy, radiation, and reconstructive surgery, she encouraged her younger sister and brother to get screened immediately. “Because now they had a family history of the disease,” she said.
The Colon Cancer Coalition has developed a script you can use to bring up colon and rectal cancer questions in conversations with relatives, which may help you determine whether you should be screened 10 to 15 years earlier than the current recommended age.
If you are not in contact with your immediate family or are unaware of their medical history, it is important to know the symptoms of colorectal cancer, such as unexplained abdominal pain, changes in your stool and rectal bleeding. If you have any of these symptoms, talk to a doctor and get tested to rule out cancer.
After her experience being dismissed by doctors, Ms. Peters founded an organization called Be Seen to raise awareness of symptoms and encourage people to pledge to be screened .
Colonoscopies remain the gold standard for screening because they allow medical experts to not only see where tumors are, but also to remove them in the same procedure. There are now several different ways patients can prepare their bowels — including liquid laxatives, pills and powders — that are not as uncomfortable as options that were available to previous generations. “I can promise you that doing a one-day cleanse to prep for a colonoscopy is far better than having poop coming out of your stomach into a bag,” Ms. Peters said. “Thankfully, it was temporary for me, but it’s not for many people.”
There is also a home test that can detect 92 percent of colorectal cancers through DNA in your stool, though it is less sensitive at picking up precancerous polyps and cannot be used to remove any tissue, Dr. Cercek said. A blood test that is on the horizon may further increase the number of people willing to get screened.
Even though the trend in early-onset colorectal cancers is concerning, “what I take away from it is that the time to intervene is even earlier,” Dr. Murphy said. “And certainly what is happening now is going to affect the health of generations many, many years from now.”
Knvul Sheikh is a Times reporter covering chronic and infectious diseases and other aspects of personal health. More about Knvul Sheikh
The Fight Against Cancer
Colon and rectal cancers are increasing among people younger than 50. Experts have a few ideas about why .
Risk calculators can offer a more personalized picture of an individual patient’s breast cancer risk. But experts warn that the results need to be interpreted with the help of a doctor .
Early detection is a powerful weapon in preventing deaths from colon cancer, but many patients are reluctant to undergo colonoscopies or conduct at-home fecal tests. Doctors see potential in another screening method .
The human papillomavirus vaccine provides powerful protection against the leading cause of cervical cancer and against a strong risk factor for anal cancer. Here’s what to know about the shot.
A recent study adds to growing evidence that exercise is an important part of preventing prostate cancer , the second most common and second most fatal cancer in the United States for men.
No single food can prevent cancer on its own, but experts say that there are some that may help you build the best defense .
This paper is in the following e-collection/theme issue:
Published on 8.4.2024 in Vol 26 (2024)
Longitudinal Monitoring of Clinician-Patient Video Visits During the Peak of the COVID-19 Pandemic: Adoption and Sustained Challenges in an Integrated Health Care Delivery System
Authors of this article:

Original Paper
- Jessica A Palakshappa 1, 2 , MD, MS ;
- Erica R Hale 1, 2 , MS ;
- Joshua D Brown 1 , PhD ;
- Carol A Kittel 2 , MA ;
- Emily Dressler 2 , PhD ;
- Gary E Rosenthal 1, 2 , MD ;
- Sarah L Cutrona 3, 4 , MD, MPH ;
- Kristie L Foley 2 , MS, PhD ;
- Emily R Haines 2 , PhD ;
- Thomas K Houston II 1, 2 , MD, MPH
1 Atrium Health Wake Forest Baptist, Winston Salem, NC, United States
2 Wake Forest University School of Medicine, Winston Salem, NC, United States
3 Department of Population and Quantitative Health Sciences, University of Massachusetts Chan Medical School, Worcester, MA, United States
4 Center for Healthcare Organization and Implementation Research, Veterans Affairs Bedford Healthcare System, Bedford, MA, United States
Corresponding Author:
Jessica A Palakshappa, MD, MS
Wake Forest University School of Medicine
1 Medical Center Blvd
Winston Salem, NC, 27157
United States
Phone: 1 336 716 8465
Email: [email protected]
Background: Numerous prior opinion papers, administrative electronic health record data studies, and cross-sectional surveys of telehealth during the pandemic have been published, but none have combined assessments of video visit success monitoring with longitudinal assessments of perceived challenges to the rapid adoption of video visits during the pandemic.
Objective: This study aims to quantify (1) the use of video visits (compared with in-person and telephone visits) over time during the pandemic, (2) video visit successful connection rates, and (3) changes in perceived video visit challenges.
Methods: A web-based survey was developed for the dual purpose of monitoring and improving video visit implementation in our health care system during the COVID-19 pandemic. The survey included questions regarding rates of in-person, telephone, and video visits for clinician-patient encounters; the rate of successful connection for video visits; and perceived challenges to video visits (eg, software, hardware, bandwidth, and technology literacy). The survey was distributed via email to physicians, advanced practice professionals, and clinicians in May 2020. The survey was repeated in March 2021. Differences between the 2020 and 2021 responses were adjusted for within-respondent correlation across surveys and tested using generalized estimating equations.
Results: A total of 1126 surveys were completed (511 surveys in 2020 and 615 surveys in 2021). In 2020, only 21.7% (73/336) of clinicians reported no difficulty connecting with patients during video visits and 28.6% (93/325) of clinicians reported no difficulty in 2021. The distribution of the percentage of successfully connected video visits (“Over the past two weeks of scheduled visits, what percentage did you successfully connect with patients by video?”) was not significantly different between 2020 and 2021 ( P =.74). Challenges in conducting video visits persisted over time. Poor connectivity was the most common challenge reported by clinicians. This response increased over time, with 30.5% (156/511) selecting it as a challenge in 2020 and 37.1% (228/615) in 2021 ( P =.01). Patients not having access to their electronic health record portals was also a commonly reported challenge (109/511, 21.3% in 2020 and 137/615, 22.3% in 2021, P =.73).
Conclusions: During the pandemic, our health care delivery system rapidly adopted synchronous patient-clinician communication using video visits. As experience with video visits increased, the reported failure rate did not significantly decline, and clinicians continued to report challenges related to general network connectivity and patient access to technology.
Introduction
Interest in telehealth from policy makers, health care providers, patients, and families continues to grow [ 1 ], including newer modalities such as video visits [ 2 ]. Video visits refer to clinician-patient communication that includes real time video and audio assessment of the patient when the clinician is in a different location. Video visits have the potential to improve efficiency for clinicians and to improve access for patients, particularly those who reside in rural areas or with transportation barriers [ 3 ]. The technology to support video visits has existed for decades; however, only a small minority of clinicians used this form of telehealth in their practices [ 4 ] prior to the COVID-19 pandemic. There are multiple reasons why telehealth adoption was slow despite its potential benefits including changing cost and reimbursement policies, federal and state licensing laws, incompatible electronic health records, and gaps in internet access in certain areas.
The COVID-19 pandemic and subsequent public health emergency led to fundamental shifts in how health care was delivered in the United States, including the rapid adoption of telehealth services. Before the public health emergency, approximately 13,000 fee-for-service Medicare beneficiaries received telehealth services in a week and that number increased to nearly 1.7 million beneficiaries by the last week of April 2020 [ 5 ]. While the need to avoid in-person contact fueled the initial rapid rise, regulations and restrictions were temporarily lifted during this time facilitating its use. Clinicians were also paid for telehealth services at the same rate as in-person medical services. Several studies have reported on the rapid uptake of telehealth, including video visits, in this context [ 6 - 8 ]. However, few reports have explored rates of success and failure of video visits over time. The challenges clinicians face in conducting video visits have also not been explored. Understanding these challenges will be important for improving and expanding the reach of telehealth services after the pandemic has ended.
In the context of the rapidly increasing use of telehealth to conduct video visits, and consistent with the sociotechnical model’s [ 9 ] emphasis on monitoring the implementation of health information technology in complex adaptive health care systems, our health care delivery system initiated a series of brief assessments of video visit adoption. The research objective of this report is to summarize the findings of the video visit monitoring including (1) the use of video visits (compared with in-person and telephone visits) over time during the pandemic, (2) video visit successful connection rates, and (3) changes in perceived video visit challenges. With patient and clinician skills and experience with video visits increasing over time, our primary hypothesis was that clinicians’ perceived challenges to completing video visits (eg, software, hardware, bandwidth, and technology literacy) would decline over time.
Study Design
The design was a longitudinal series of 2 cross-sectional assessments (2020 and 2021). In summary, for the dual purpose of monitoring and improving telehealth implementation in our health care delivery system, institutional leaders developed a brief web-based survey regarding the use of video visits and challenges. The survey was initially distributed in 2020. Given the ongoing public health emergency and the need to re-evaluate telehealth use, the survey was repeated in 2021. Institutional leaders encouraged clinicians to complete the survey, communicating encouragement via emails and announcements at in-person faculty and departmental meetings.
Ethical Considerations
As the brief assessments were distributed by the clinical system as part of ongoing quality improvement, the project was approved as an research protocol as exempt from human participants approval by the Wake Forest University School of Medicine Institutional Review Board (IRB00077473). The survey did not collect identifying information from the participants.
Survey Development
Published in 2010 by Sittig and Singh [ 9 ], the sociotechnical model of health information technology was the first to fully emphasize the importance of system monitoring in implementation frameworks. Key aspects of monitoring, including measuring how the technology is being used by clinicians and whether implementation outcomes are being achieved, were considered when developing the survey. The survey was developed with a literature search, expert review, and iterative pilot-testing (see Multimedia Appendix 1 ). The final survey included 12 questions related to rates of in-person, telephone, and video for clinician-patient encounters; the rate of successful connection for video visits; and perceived challenges to video visits (eg, software, hardware, bandwidth, and technology literacy).
Study Population
The study population included all outpatient clinicians practicing across the health care delivery system; we excluded clinicians without direct patient care responsibilities. The system includes 5 hospitals and over 350 primary care and specialty clinics that provide care to over 2 million persons annually. The brief assessment was distributed to clinicians in 2020 and 2021 (1937 clinicians and 2843 clinicians, respectively).
Survey Distribution and Data Collection
As we are an integrated health care delivery system, we had access to the contact details of all providers. Our group practice clinical operations executive committee facilitated the survey distribution by requesting that each department chair and clinical service line director send an email to their team of providers to notify them of the survey and encourage completion. Surveys were collected and managed using REDCap (Research Electronic Data Capture), a secure, web-based app designed to support data capture [ 10 , 11 ]. A unique survey link was distributed via email to each clinician in May 2020 and March 2021. The system sent up to 2 reminder emails if the recipient had not yet completed the survey.
Statistical Analysis
To take full advantage of the data collected, we first analyzed the results as 2 cross-sectional surveys. In this primary analysis, we included all respondents in each year. We recognize that a subset of clinicians also responded in both years. Thus, as a secondary analysis, we analyzed the data limited to the longitudinal cohort who participated in both years. First, summary statistics are presented as count (frequency) for categorical variables and mean (SD) or median (IQR) for continuous variables as appropriate. Generalized estimating equations were then used to model frequency distributions of in-person, telephone, and video visits, and patient and clinician challenges. These logit models were adjusted for within-respondent correlation across surveys via an exchangeable correlation structure. P values of .05 were considered statistically significant. P values for multiple comparisons in frequency distributions of in-person, telephone, and video visits between physicians, advanced practice professionals (APPs), and other clinicians were adjusted via the Tukey-Kramer method to control for type I errors with a corrected P value <.05 deemed statistically significant [ 12 ]. All statistical analyses were performed with R (version 4.2.1; R Core Team) [ 13 ].
We recognize that a subset of clinicians responded in both years. Thus, as a secondary analysis, we analyzed the data limited to the longitudinal cohort who participated in both surveys. For the secondary analysis, matched pairs analyses were performed as were performed in the entire sample with only those responses from clinicians that completed both surveys.
Surveillance Participation and Participant Characteristics
In 2020, 1937 surveys were sent and 511 responses were received (response rate 26.4%). In 2021, 2843 surveys were sent and 615 responses were received (response rate 21.6%). In both years, over 55% of the respondents were physicians from a wide range of clinical specialties. About half of the clinicians who completed the survey in 2020 also completed it in 2021 ( Table 1 ).
a n=511 responses in 2020 and n=615 responses in 2021.
b n=300 responses in 2020 and n=353 responses in 2021.
Health Care Delivery by In-Person and Telephone
To place the volume of telehealth in context, we first asked about the number of in-person encounters completed over the past 2 weeks ( Table 2 ). The distribution of responses differed between 2020 and 2021 ( P <.001). Modeled probabilities show fewer respondents reported zero (22.2% vs 4.9%) or 1 to 10 (33.5% vs 11.5%) in-person visits in 2021 as compared with 2020. The volume of in-person visits increased over time ( Table 2 ). Further, the majority of respondents (399/509, 78.4%) reported at least 1 telephone visit in 2020 and 65.7% (369/562) in 2021 although the distribution of responses differed from 2020 to 2021 ( P <.001).
a Differences in frequency distributions between 2020 and 2021 tested via generalized estimating equation modeling; P value adjusted using Tukey-Kramer method to control for type I errors.
Health Care Delivery by Video Visits
Many health care providers were engaged in virtual care, with 65.9% (336/510) health care providers reporting video visit encounters in 2020 and 57.6% (325/564) health care providers reporting video visit encounters in 2021 ( Table 2 ) although the distribution of responses again changed from 2020 to 2021 ( P <.001). Compared with 2020, fewer 2021 respondents reported 11-25 (18.6% vs 6.7%), 26-50 (5.9% vs 2.8%), or over 50 (2.4% vs 2%) visits.
Secondary analyses were robust to missing data and showed that the results (distributions of in-person, phone, and video visits) did not change when limiting the data to only respondents who participated in both surveys.
Comparing Health Care Delivery by Physicians, APPs, and Others
We also compared health care delivery modality by type of clinician (physicians, APPs, or others). Patterns of health care delivery reported in the overall sample were similar in the physician, APP, and other subgroups. There were no significant differences between physicians and APPs in the number of patient encounters that were completed as in-person, telephone visits, or video visits in 2020 or 2021.
Perceived Challenges to Patient-Clinician Connection Using Video Visits
The use of video visits came with challenges. In 2020, only 21.7% (73/336) of clinicians reported no difficulty connecting with patients during video visits and 28.6% (93/325) of clinicians reported no difficulty in 2021 ( Figure 1 ). The distribution of the percentage of successfully connected video visits (“Over the past two weeks of scheduled visits, what percentage did you successfully connect with patients by video?”) was not significantly different between 2020 and 2021 ( P =.74, Figure 1 ). There was also no significant difference between physicians and APPs in the rate of successful video connection with patients in either year.
Clinicians were asked about the challenges in successfully completing video visits (see Table 3 ). The most commonly reported challenge was poor connectivity. This response increased over time with 30.5% (156/511) selecting it as a challenge in 2020 and 37.1% (228/615) selecting it as a challenge in 2021 ( P =.01). Patients not having access to their electronic health record portals was also a commonly reported challenge (109/511, 21.3% in 2020 and 137/615, 22.3% in 2021; P =.73).

a P value adjusted using the Tukey-Kramer method to control for type I errors.
b For some clinical video visits, a prerequisite was that patients needed to have registered with the patient portal.
Principal Findings
Although telehealth technology was available in our health care delivery system prior to the COVID-19 pandemic, it saw only limited use for providing synchronous care to patients prior to the pandemic. Inconsistent reimbursement for services, restrictions on the physical location of patients and clinicians during telehealth, and rules about types of visits that were acceptable for telehealth services all contributed to its limited use [ 14 , 15 ]. With the pandemic, and consistent with other reports, our health care delivery system rapidly expanded the provision of clinical care by way of video visits. Later in the pandemic, in-person visits did increase, but the use of video visits remained well above prepandemic levels.
Overall, there has been a shift toward a more positive sentiment about telehealth and telemedicine since the start of the pandemic. A scoping review by Doraiswamy et al [ 16 ] reported 543 telehealth-related papers (mostly opinions, commentaries, and perspectives; 61%) published across 331 different journals from January to June 2020. Most of these new reports had a “celebratory” or favorable sentiment about the use of telehealth. The scope of the increase in telehealth during the public health emergency likely contributed to this sentiment though concerns about patient and clinician connection, the lack of physical examinations, and cost-effectiveness were still noted by some. Although our providers reported benefits for clinical video telehealth beyond audio-only calls for patient-provider visits, failure to connect using clinical video visits was common.
While reports have documented challenges with clinical video telehealth [ 17 ], few have monitored these challenges over time. During the pandemic, as our health care delivery system’s experience with video visits grew, clinicians did not report a meaningful reduction in connection failure rate. The most frequently reported challenges were general network connectivity and those related to the digital divide (eg, patient lack of internet access, needed software, or internet-connected cameras). Gaps in access to technology and the internet for telehealth may impact some patient groups more than others. For example, older age, rural residence, dual Medicare and Medicaid enrollment, and non-Hispanic Black or Hispanic race or ethnicity have been shown to be associated with a lower probability of technology ownership, access to the internet, and use of the internet for communication in cancer survivors [ 18 ]. Further, over 10% of clinicians also reported that they experienced software or hardware challenges (eg, limited availability of internet cameras at a clinical location). Expanding telehealth will require ongoing investments in technology for clinicians. New workflows to support successful connection during video visits and follow-up processes may also be needed.
Our video visit monitoring results were shared with clinical operations leadership. In response to the sustained challenges noted, we initiated a new video visit program to provide patient support prior to scheduled video visits. Our technology navigators are a specially trained, centralized team and are directed to reach out to vulnerable patients and families to facilitate video visit access. A new electronic health record dashboard identified patients with (1) a scheduled video visit and (2) 1 or more risk factors (eg, lack of a prior successful video visit and lack of patient portal access). We further prioritized patients older than 65 years and those living in rural areas. Technology navigators reached out by telephone to contact these at-risk patients to assess their technology access (eg, internet, software, webcam, or smartphone), technology literacy and perceived competence, and availability of at-home support from family and friends. The technology navigators then troubleshoot any challenges noted by the patients and offer to conduct a “practice” video visit. Evaluation of this program is ongoing. In 2022-2023, the navigators contacted 1266 patients at high risk for video visit failure. Among those contacted, 515 requested and were provided assistance. With previsit support from the navigators, the patient-provider scheduled video visit completion rate was 84% as compared with a 60% completion rate among those patients who did not receive support.
Limitations of our video visit surveillance analysis include that the data were collected across 1 health care delivery system with an integrated electronic health care record system. The perceptions and challenges may be different in a smaller health care system and in those with different health care record systems. The survey measured only clinician-reported telehealth use and success rates which may be limited by recall. As with all surveys, our results may be biased as only about one-quarter of the sample responded. It is possible that respondents experienced more challenges conducting video visits than those who did not respond. Further, not all clinicians longitudinally completed both the 2020 and 2021 surveys—due both to response rates and providers leaving and entering the health care system. Thus, changes over time may represent differences in the underlying sample. For example, new clinicians may have been more or less familiar with conducting video visits.
Conclusions
Recent reviews have noted the need for more evidence related to telehealth’s implementation, effectiveness, and health equity in telehealth access [ 16 , 19 , 20 ]. Although internet and smartphone access has increased over the last decade (with older adults being one of the fastest-growing subgroups of new adoption), our longitudinal video visit surveillance reveals that the digital divide is still a significant barrier to video visit access.
Although Healthy People 2030 (a set of national objectives to improve health and well-being) includes developmental and research objectives related to patient portals and increasing the use of telehealth to improve access to health services [ 21 ], some social determinants of health taxonomies do not include technology access. If telehealth is increasingly an important component of health care access, then technology access (eg, internet, smartphone, patient portal, and connected hardware, such as internet-connected video) should be considered a social determinant of health [ 22 ]. A comprehensive solution to overcoming the digital divide has not yet been achieved. However, some partial solutions include directly providing technology to patients, providing detailed instructions, and support services (eg, our technology navigator program), and engaging trusted caregivers (family and friends) who may be able to assist patients [ 23 - 27 ].
Acknowledgments
The project was supported through the National Cancer Institute Cancer Moonshot initiative—iDAPT: Implementation and Informatics–Developing Adaptable Processes and Technologies for Cancer Control (P50 CA244693); the Wake Forest Clinical and Translational Science Award (5UL1TR001420); and also a career development award to JAP (1K23AG073529).
Conflicts of Interest
None declared.
Brief Provider Telehealth Assessment.
- World Health Organization. Telemedicine: Opportunities and Developments in Member States: Report on the Second Global Survey on eHealth 2009. Geneva, Switzerland. World Health Organization; 2010.
- Moulaei K, Shanbehzadeh M, Bahaadinbeigy K, Kazemi-Arpanahi H. Survey of the patients' perspectives and preferences in adopting telepharmacy versus in-person visits to the pharmacy: a feasibility study during the COVID-19 pandemic. BMC Med Inform Decis Mak. 2022;22(1):99. [ FREE Full text ] [ CrossRef ] [ Medline ]
- Nesbitt TS. The evolution of telehealth: where have we been and where are we going? In: Lustig TA, editor. The Role of Telehealth in an Evolving Health Care Environment: Workshop Summary. Washington, DC. National Academies Press; 2012;11-16.
- Kane CK, Gillis K. The use of telemedicine by physicians: still the exception rather than the rule. Health Aff (Millwood). 2018;37(12):1923-1930. [ FREE Full text ] [ CrossRef ] [ Medline ]
- Kane CK, Gillis K. The use of telemedicine by physicians: still the exception rather than the rule. Health Aff (Millwood). Dec 2018;37(12):1923-1930. [ CrossRef ] [ Medline ]
- Weiner JP, Bandeian S, Hatef E, Lans D, Liu A, Lemke KW. In-person and telehealth ambulatory contacts and costs in a large US insured cohort before and during the COVID-19 pandemic. JAMA Netw Open. 2021;4(3):e212618. [ FREE Full text ] [ CrossRef ] [ Medline ]
- Gilson SF, Umscheid CA, Laiteerapong N, Ossey G, Nunes KJ, Shah SD. Growth of ambulatory virtual visits and differential use by patient sociodemographics at one urban academic medical center during the COVID-19 pandemic: retrospective analysis. JMIR Med Inform. 2020;8(12):e24544. [ FREE Full text ] [ CrossRef ] [ Medline ]
- Hatef E, Wilson RF, Hannum SM, Zhang A, Kharrazi H, Weiner JP, et al. Use of Telehealth During the COVID-19 Era. Rockville, MD. Agency for Healthcare Research and Quality; 2023.
- Sittig DF, Singh H. A new sociotechnical model for studying health information technology in complex adaptive healthcare systems. Qual Saf Health Care. 2010;19(Suppl 3):i68-i74. [ FREE Full text ] [ CrossRef ] [ Medline ]
- Harris PA, Taylor R, Thielke R, Payne J, Gonzalez N, Conde JG. Research electronic data capture (REDCap): a metadata-driven methodology and workflow process for providing translational research informatics support. J Biomed Inform. 2009;42(2):377-381. [ FREE Full text ] [ CrossRef ] [ Medline ]
- Harris PA, Taylor R, Minor BL, Elliott V, Fernandez M, O'Neal L, et al. The REDCap consortium: building an international community of software platform partners. J Biomed Inform. 2019;95:103208. [ FREE Full text ] [ CrossRef ] [ Medline ]
- Midway S, Robertson M, Flinn S, Kaller M. Comparing multiple comparisons: practical guidance for choosing the best multiple comparisons test. PeerJ. 2020;8:e10387. [ FREE Full text ] [ CrossRef ] [ Medline ]
- R Core Team. R: A Language and Environment for Statistical Computing. Vienna, Austria. R Foundation for Statistical Computing; 2023.
- Shaver J. The state of telehealth before and after the COVID-19 pandemic. Prim Care. 2022;49(4):517-530. [ FREE Full text ] [ CrossRef ] [ Medline ]
- Hyder MA, Razzak J. Telemedicine in the United States: an introduction for students and residents. J Med Internet Res. 2020;22(11):e20839. [ FREE Full text ] [ CrossRef ] [ Medline ]
- Doraiswamy S, Abraham A, Mamtani R, Cheema S. Use of telehealth during the COVID-19 pandemic: scoping review. J Med Internet Res. 2020;22(12):e24087. [ FREE Full text ] [ CrossRef ] [ Medline ]
- Garfan S, Alamoodi AH, Zaidan BB, Al-Zobbi M, Hamid RA, Alwan JK, et al. Telehealth utilization during the Covid-19 pandemic: a systematic review. Comput Biol Med. 2021;138:104878. [ FREE Full text ] [ CrossRef ] [ Medline ]
- Lama Y, Davidoff AJ, Vanderpool RC, Jensen RE. Telehealth availability and use of related technologies among medicare-enrolled cancer survivors: cross-sectional findings from the onset of the COVID-19 pandemic. J Med Internet Res. 2022;24(1):e34616. [ FREE Full text ] [ CrossRef ] [ Medline ]
- Moulaei K, Moulaei R, Bahaadinbeigy K. Barriers and facilitators of using health information technologies by women: a scoping review. BMC Med Inform Decis Mak. 2023;23(1):176. [ FREE Full text ] [ CrossRef ] [ Medline ]
- Rodriguez JA, Shachar C, Bates DW. Digital inclusion as health care: supporting health care equity with digital-infrastructure initiatives. N Engl J Med. 2022;386(12):1101-1103. [ FREE Full text ] [ CrossRef ] [ Medline ]
- Healthy people 2030: health IT. U.S. Department of Health and Human Services. URL: https://health.gov/healthypeople/objectives-and-data/browse-objectives/health-it [accessed 2024-03-14]
- Benda NC, Veinot TC, Sieck CJ, Ancker JS. Broadband internet access is a social determinant of health!. Am J Public Health. 2020;110(8):1123-1125. [ CrossRef ] [ Medline ]
- Francis J, Kadylak T, Makki TW, Rikard RV, Cotten SR. Catalyst to connection: when technical difficulties lead to social support for older adults. Am Behav Sci. 2018;62(9):1167-1185. [ CrossRef ]
- Cotten SR, Yost EA, Berkowsky RW, Winstead V, Anderson WA. Designing Technology Training for Older Adults in Continuing Care Retirement Communities, 1st Edition. Boca Raton, FL. CRC Press; 2016.
- Sadasivam RS, Kinney RL, Lemon SC, Shimada SL, Allison JJ, Houston TK. Internet health information seeking is a team sport: analysis of the Pew internet survey. Int J Med Inform. 2013;82(3):193-200. [ CrossRef ] [ Medline ]
- Luger TM, Hogan TP, Richardson LM, Cioffari-Bailiff L, Harvey K, Houston TK. Older veteran digital disparities: examining the potential for solutions within social networks. J Med Internet Res. 2016;18(11):e296. [ FREE Full text ] [ CrossRef ] [ Medline ]
- Houston TK, Richardson LM, Cotten SR. Patient-directed digital health technologies: is implementation outpacing evidence? Med Care. 2019;57(2):95-97. [ CrossRef ] [ Medline ]
Abbreviations
Edited by G Eysenbach, T Leung; submitted 26.10.23; peer-reviewed by K Moulaei; comments to author 19.12.23; revised version received 24.01.24; accepted 09.03.24; published 08.04.24.
©Jessica A Palakshappa, Erica R Hale, Joshua D Brown, Carol A Kittel, Emily Dressler, Gary E Rosenthal, Sarah L Cutrona, Kristie L Foley, Emily R Haines, Thomas K Houston II. Originally published in the Journal of Medical Internet Research (https://www.jmir.org), 08.04.2024.
This is an open-access article distributed under the terms of the Creative Commons Attribution License (https://creativecommons.org/licenses/by/4.0/), which permits unrestricted use, distribution, and reproduction in any medium, provided the original work, first published in the Journal of Medical Internet Research, is properly cited. The complete bibliographic information, a link to the original publication on https://www.jmir.org/, as well as this copyright and license information must be included.

Study finds link between quality of sibling relationships and loneliness, depression
Research shows that good relationships with siblings can help bolster against mental health concerns as life progresses.
Copyright 2024 NPR

Stand up for civility
This news story is funded in large part by Connecticut Public’s Members — listeners, viewers, and readers like you who value fact-based journalism and trustworthy information.
We hope their support inspires you to donate so that we can continue telling stories that inform, educate, and inspire you and your neighbors. As a community-supported public media service, Connecticut Public has relied on donor support for more than 50 years.
Your donation today will allow us to continue this work on your behalf. Give today at any amount and join the 50,000 members who are building a better—and more civil—Connecticut to live, work, and play.
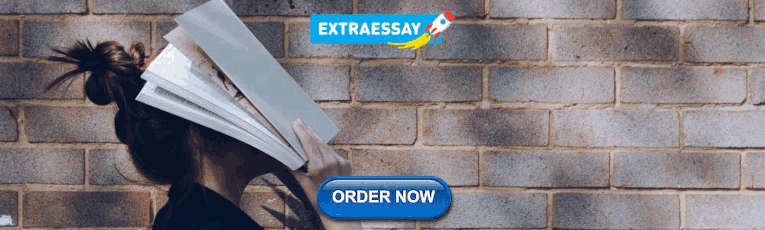
COMMENTS
Research and study are two fundamental activities that play a crucial role in acquiring knowledge and understanding. While they share similarities, they also have distinct attributes that set them apart. In this article, we will explore the characteristics of research and study, highlighting their differences and similarities. Definition and ...
If you're talking about learning or acquiring knowledge about a subject, then study is the appropriate term. If you're conducting a formal investigation or inquiry into a topic, then research is the correct word to use. Now that we've established the difference between study and research, let's dive deeper into each one.
Research is a synonym of study. As verbs the difference between study and research is that study is to revise materials already learned in order to make sure one does not forget them, usually in preparation for an examination while research is to search or examine with continued care; to seek diligently. As nouns the difference between study and research is that study is a state of mental ...
12. In summary, study and research are both means of acquiring knowledge. However, study is often a more flexible, learner-centric activity, whereas research is a structured, systematic process that seeks to add new information or perspectives to an academic or professional field. 15. ADVERTISEMENT.
"Research," as a verb, means "to perform a study or studies," but "research" as a noun refers to the sum of many studies. "Chemical research" means the sum of all chemical studies. If you find yourself writing "a research" or "in this research," change it to "a study" or "in this study." research The act of performing research.
There are various types of scientific studies such as experiments and comparative analyses, observational studies, surveys, or interviews. The choice of study type will mainly depend on the research question being asked. When making decisions, patients and doctors need reliable answers to a number of questions.
Difference Between Research and Study Definition of Research. Research is a systematic and scientific investigation of a particular subject matter or problem. It collects, analyzes, and interprets data to answer a research question or hypothesis. Research can be conducted in various fields, such as science, social science, business, and ...
Types of Research Designs Compared | Guide & Examples. Published on June 20, 2019 by Shona McCombes.Revised on June 22, 2023. When you start planning a research project, developing research questions and creating a research design, you will have to make various decisions about the type of research you want to do.. There are many ways to categorize different types of research.
INTRODUCTION. Scientific research is usually initiated by posing evidenced-based research questions which are then explicitly restated as hypotheses.1,2 The hypotheses provide directions to guide the study, solutions, explanations, and expected results.3,4 Both research questions and hypotheses are essentially formulated based on conventional theories and real-world processes, which allow the ...
The study design used to answer a particular research question depends on the nature of the question and the availability of resources. In this article, which is the first part of a series on "study designs," we provide an overview of research study designs and their classification. The subsequent articles will focus on individual designs.
Study designs are the set of methods and procedures used to collect and analyze data in a study. Broadly speaking, there are 2 types of study designs: descriptive studies and analytical studies. Descriptive studies. Describes specific characteristics in a population of interest; The most common forms are case reports and case series
Participants mentioned that differences in these areas are often due to differences between evaluation and research prior to the study. For instance, the purpose of the study, questions guiding the study, or amount of funding and time line can all affect the design and methodology of the study.
Definition: A state of mental perplexity or worried thought. Thought, as directed to a specific purpose; one's concern. Mental effort to acquire knowledge or learning. The act of studying; examination. A room in a house intended for reading and writing. An artwork made in order to practise or demonstrate a subject or technique.
A research design is a strategy for answering your research question using empirical data. Creating a research design means making decisions about: Your overall research objectives and approach. Whether you'll rely on primary research or secondary research. Your sampling methods or criteria for selecting subjects. Your data collection methods.
Research philosophy is one of those things that students tend to either gloss over or become utterly confused by when undertaking formal academic research for the first time. And understandably so - it's all rather fluffy and conceptual. However, understanding the philosophical underpinnings of your research is genuinely important as it directly impacts how you develop your research ...
The best way to remember the difference between a research plan and a research proposal is that they have fundamentally different audiences. A research plan helps you, the researcher, organize your thoughts. On the other hand, a dissertation proposal or research proposal aims to convince others (e.g., a supervisor, a funding body, or a dissertation committee) that your research topic is ...
A research paper is a primary source...that is, it reports the methods and results of an original study performed by the authors. The kind of study may vary (it could have been an experiment, survey, interview, etc.), but in all cases, raw data have been collected and analyzed by the authors, and conclusions drawn from the results of that analysis. ...
Research studies have a research question, research hypothesis, and one or more research objectives. A research question is what a study aims to answer, and a research hypothesis is a predictive statement about the relationship between two or more variables, which the study sets out to prove or disprove.
In short, PhD candidates present their research findings in the form of a dissertation, while professional doctorate candidates present their findings in a doctoral study. Let's look at the distinctions between these graduate-level degree programs and their capstone projects, the PhD dissertation and the doctoral study.
Define Research. Research is a systematic investigation or study of a particular subject or problem. The purpose of research is to discover new knowledge, validate existing theories, or develop new theories. Research can be conducted in various fields, such as science, medicine, social science, and humanities.
The study wasn't designed to answer questions about why these cancer types seemed to have the strongest ties to accelerated aging, but Ruiyi Tian, the graduate student who led the research, has ...
For the study, researchers used data from surveys and electronic health records in All of Us, a large national study of U.S. adults run by the National Institutes of Health, to analyze associations between e-cigarette use and new diagnoses of heart failure in 175,667 study participants (an average age of 52 years and 60.5% female).
Types of study design. Medical research is classified into primary and secondary research. Clinical/experimental studies are performed in primary research, whereas secondary research consolidates available studies as reviews, systematic reviews and meta-analyses. Three main areas in primary research are basic medical research, clinical research ...
Together, our study reveals how big data and machine learning uncover complex links between food chemistry, flavor and consumer perception, and lays the foundation to develop novel, tailored foods ...
Colon and rectal cancers are increasing among people younger than 50. Experts have a few ideas about why. Marisa Peters had been experiencing symptoms for years: blood on her toilet paper after ...
Objective: This study aims to quantify (1) the use of video visits (compared with in-person and telephone visits) over time during the pandemic, (2) video visit successful connection rates, and (3) changes in perceived video visit challenges. ... Differences between the 2020 and 2021 responses were adjusted for within-respondent correlation ...
Hagler Fellow to study connection between pig reproduction, cancer resistance. shutterstock/CHIRATH PHOTO. For the next three to five years, the Texas A&M School of Veterinary Medicine & Biomedical Sciences (VMBS) will partner with one of the top evolutionary biologists in the world who is researching whether swine reproduction holds the key to ...
Stand up for civility. This news story is funded in large part by Connecticut Public's Members — listeners, viewers, and readers like you who value fact-based journalism and trustworthy information. We hope their support inspires you to donate so that we can continue telling stories that inform, educate, and inspire you and your neighbors.