- How it works
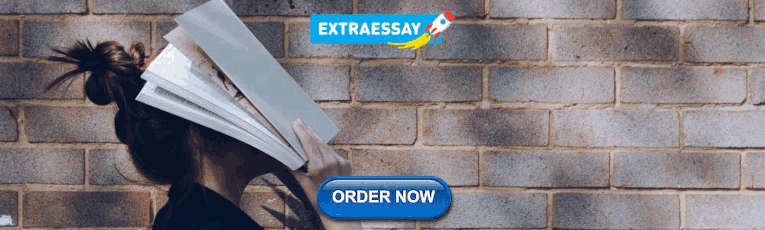
Meta-Analysis – Guide with Definition, Steps & Examples
Published by Owen Ingram at April 26th, 2023 , Revised On April 26, 2023
“A meta-analysis is a formal, epidemiological, quantitative study design that uses statistical methods to generalise the findings of the selected independent studies. “
Meta-analysis and systematic review are the two most authentic strategies in research. When researchers start looking for the best available evidence concerning their research work, they are advised to begin from the top of the evidence pyramid. The evidence available in the form of meta-analysis or systematic reviews addressing important questions is significant in academics because it informs decision-making.
What is Meta-Analysis
Meta-analysis estimates the absolute effect of individual independent research studies by systematically synthesising or merging the results. Meta-analysis isn’t only about achieving a wider population by combining several smaller studies. It involves systematic methods to evaluate the inconsistencies in participants, variability (also known as heterogeneity), and findings to check how sensitive their findings are to the selected systematic review protocol.
When Should you Conduct a Meta-Analysis?
Meta-analysis has become a widely-used research method in medical sciences and other fields of work for several reasons. The technique involves summarising the results of independent systematic review studies.
The Cochrane Handbook explains that “an important step in a systematic review is the thoughtful consideration of whether it is appropriate to combine the numerical results of all, or perhaps some, of the studies. Such a meta-analysis yields an overall statistic (together with its confidence interval) that summarizes the effectiveness of an experimental intervention compared with a comparator intervention” (section 10.2).
A researcher or a practitioner should choose meta-analysis when the following outcomes are desirable.
For generating new hypotheses or ending controversies resulting from different research studies. Quantifying and evaluating the variable results and identifying the extent of conflict in literature through meta-analysis is possible.
To find research gaps left unfilled and address questions not posed by individual studies. Primary research studies involve specific types of participants and interventions. A review of these studies with variable characteristics and methodologies can allow the researcher to gauge the consistency of findings across a wider range of participants and interventions. With the help of meta-analysis, the reasons for differences in the effect can also be explored.
To provide convincing evidence. Estimating the effects with a larger sample size and interventions can provide convincing evidence. Many academic studies are based on a very small dataset, so the estimated intervention effects in isolation are not fully reliable.
Elements of a Meta-Analysis
Deeks et al. (2019), Haidilch (2010), and Grant & Booth (2009) explored the characteristics, strengths, and weaknesses of conducting the meta-analysis. They are briefly explained below.
Characteristics:
- A systematic review must be completed before conducting the meta-analysis because it provides a summary of the findings of the individual studies synthesised.
- You can only conduct a meta-analysis by synthesising studies in a systematic review.
- The studies selected for statistical analysis for the purpose of meta-analysis should be similar in terms of comparison, intervention, and population.
Strengths:
- A meta-analysis takes place after the systematic review. The end product is a comprehensive quantitative analysis that is complicated but reliable.
- It gives more value and weightage to existing studies that do not hold practical value on their own.
- Policy-makers and academicians cannot base their decisions on individual research studies. Meta-analysis provides them with a complex and solid analysis of evidence to make informed decisions.
Criticisms:
- The meta-analysis uses studies exploring similar topics. Finding similar studies for the meta-analysis can be challenging.
- When and if biases in the individual studies or those related to reporting and specific research methodologies are involved, the meta-analysis results could be misleading.
Steps of Conducting the Meta-Analysis
The process of conducting the meta-analysis has remained a topic of debate among researchers and scientists. However, the following 5-step process is widely accepted.
Step 1: Research Question
The first step in conducting clinical research involves identifying a research question and proposing a hypothesis . The potential clinical significance of the research question is then explained, and the study design and analytical plan are justified.
Step 2: Systematic Review
The purpose of a systematic review (SR) is to address a research question by identifying all relevant studies that meet the required quality standards for inclusion. While established journals typically serve as the primary source for identified studies, it is important to also consider unpublished data to avoid publication bias or the exclusion of studies with negative results.
While some meta-analyses may limit their focus to randomized controlled trials (RCTs) for the sake of obtaining the highest quality evidence, other experimental and quasi-experimental studies may be included if they meet the specific inclusion/exclusion criteria established for the review.
Step 3: Data Extraction
After selecting studies for the meta-analysis, researchers extract summary data or outcomes, as well as sample sizes and measures of data variability for both intervention and control groups. The choice of outcome measures depends on the research question and the type of study, and may include numerical or categorical measures.
For instance, numerical means may be used to report differences in scores on a questionnaire or changes in a measurement, such as blood pressure. In contrast, risk measures like odds ratios (OR) or relative risks (RR) are typically used to report differences in the probability of belonging to one category or another, such as vaginal birth versus cesarean birth.
Step 4: Standardisation and Weighting Studies
After gathering all the required data, the fourth step involves computing suitable summary measures from each study for further examination. These measures are typically referred to as Effect Sizes and indicate the difference in average scores between the control and intervention groups. For instance, it could be the variation in blood pressure changes between study participants who used drug X and those who used a placebo.
Since the units of measurement often differ across the included studies, standardization is necessary to create comparable effect size estimates. Standardization is accomplished by determining, for each study, the average score for the intervention group, subtracting the average score for the control group, and dividing the result by the relevant measure of variability in that dataset.
In some cases, the results of certain studies must carry more significance than others. Larger studies, as measured by their sample sizes, are deemed to produce more precise estimates of effect size than smaller studies. Additionally, studies with less variability in data, such as smaller standard deviation or narrower confidence intervals, are typically regarded as higher quality in study design. A weighting statistic that aims to incorporate both of these factors, known as inverse variance, is commonly employed.
Step 5: Absolute Effect Estimation
The ultimate step in conducting a meta-analysis is to choose and utilize an appropriate model for comparing Effect Sizes among diverse studies. Two popular models for this purpose are the Fixed Effects and Random Effects models. The Fixed Effects model relies on the premise that each study is evaluating a common treatment effect, implying that all studies would have estimated the same Effect Size if sample variability were equal across all studies.
Conversely, the Random Effects model posits that the true treatment effects in individual studies may vary from each other, and endeavors to consider this additional source of interstudy variation in Effect Sizes. The existence and magnitude of this latter variability is usually evaluated within the meta-analysis through a test for ‘heterogeneity.’
Forest Plot
The results of a meta-analysis are often visually presented using a “Forest Plot”. This type of plot displays, for each study, included in the analysis, a horizontal line that indicates the standardized Effect Size estimate and 95% confidence interval for the risk ratio used. Figure A provides an example of a hypothetical Forest Plot in which drug X reduces the risk of death in all three studies.
However, the first study was larger than the other two, and as a result, the estimates for the smaller studies were not statistically significant. This is indicated by the lines emanating from their boxes, including the value of 1. The size of the boxes represents the relative weights assigned to each study by the meta-analysis. The combined estimate of the drug’s effect, represented by the diamond, provides a more precise estimate of the drug’s effect, with the diamond indicating both the combined risk ratio estimate and the 95% confidence interval limits.
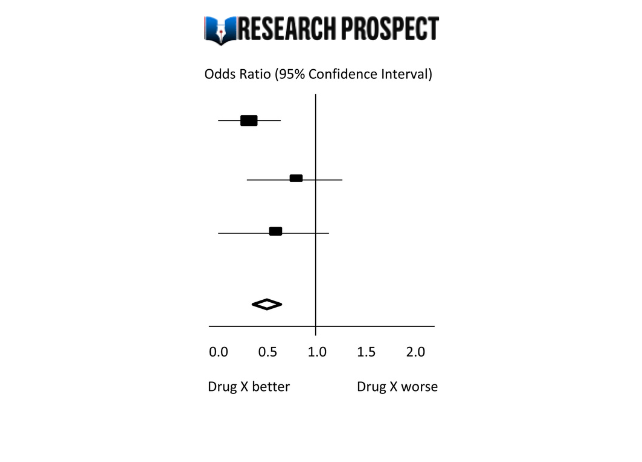
Figure-A: Hypothetical Forest Plot
Relevance to Practice and Research
Evidence Based Nursing commentaries often include recently published systematic reviews and meta-analyses, as they can provide new insights and strengthen recommendations for effective healthcare practices. Additionally, they can identify gaps or limitations in current evidence and guide future research directions.
The quality of the data available for synthesis is a critical factor in the strength of conclusions drawn from meta-analyses, and this is influenced by the quality of individual studies and the systematic review itself. However, meta-analysis cannot overcome issues related to underpowered or poorly designed studies.
Therefore, clinicians may still encounter situations where the evidence is weak or uncertain, and where higher-quality research is required to improve clinical decision-making. While such findings can be frustrating, they remain important for informing practice and highlighting the need for further research to fill gaps in the evidence base.
Methods and Assumptions in Meta-Analysis
Ensuring the credibility of findings is imperative in all types of research, including meta-analyses. To validate the outcomes of a meta-analysis, the researcher must confirm that the research techniques used were accurate in measuring the intended variables. Typically, researchers establish the validity of a meta-analysis by testing the outcomes for homogeneity or the degree of similarity between the results of the combined studies.
Homogeneity is preferred in meta-analyses as it allows the data to be combined without needing adjustments to suit the study’s requirements. To determine homogeneity, researchers assess heterogeneity, the opposite of homogeneity. Two widely used statistical methods for evaluating heterogeneity in research results are Cochran’s-Q and I-Square, also known as I-2 Index.
Difference Between Meta-Analysis and Systematic Reviews
Meta-analysis and systematic reviews are both research methods used to synthesise evidence from multiple studies on a particular topic. However, there are some key differences between the two.
Systematic reviews involve a comprehensive and structured approach to identifying, selecting, and critically appraising all available evidence relevant to a specific research question. This process involves searching multiple databases, screening the identified studies for relevance and quality, and summarizing the findings in a narrative report.
Meta-analysis, on the other hand, involves using statistical methods to combine and analyze the data from multiple studies, with the aim of producing a quantitative summary of the overall effect size. Meta-analysis requires the studies to be similar enough in terms of their design, methodology, and outcome measures to allow for meaningful comparison and analysis.
Therefore, systematic reviews are broader in scope and summarize the findings of all studies on a topic, while meta-analyses are more focused on producing a quantitative estimate of the effect size of an intervention across multiple studies that meet certain criteria. In some cases, a systematic review may be conducted without a meta-analysis if the studies are too diverse or the quality of the data is not sufficient to allow for statistical pooling.
Software Packages For Meta-Analysis
Meta-analysis can be done through software packages, including free and paid options. One of the most commonly used software packages for meta-analysis is RevMan by the Cochrane Collaboration.
Assessing the Quality of Meta-Analysis
Assessing the quality of a meta-analysis involves evaluating the methods used to conduct the analysis and the quality of the studies included. Here are some key factors to consider:
- Study selection: The studies included in the meta-analysis should be relevant to the research question and meet predetermined criteria for quality.
- Search strategy: The search strategy should be comprehensive and transparent, including databases and search terms used to identify relevant studies.
- Study quality assessment: The quality of included studies should be assessed using appropriate tools, and this assessment should be reported in the meta-analysis.
- Data extraction: The data extraction process should be systematic and clearly reported, including any discrepancies that arose.
- Analysis methods: The meta-analysis should use appropriate statistical methods to combine the results of the included studies, and these methods should be transparently reported.
- Publication bias: The potential for publication bias should be assessed and reported in the meta-analysis, including any efforts to identify and include unpublished studies.
- Interpretation of results: The results should be interpreted in the context of the study limitations and the overall quality of the evidence.
- Sensitivity analysis: Sensitivity analysis should be conducted to evaluate the impact of study quality, inclusion criteria, and other factors on the overall results.
Overall, a high-quality meta-analysis should be transparent in its methods and clearly report the included studies’ limitations and the evidence’s overall quality.
Hire an Expert Writer
Orders completed by our expert writers are
- Formally drafted in an academic style
- Free Amendments and 100% Plagiarism Free – or your money back!
- 100% Confidential and Timely Delivery!
- Free anti-plagiarism report
- Appreciated by thousands of clients. Check client reviews
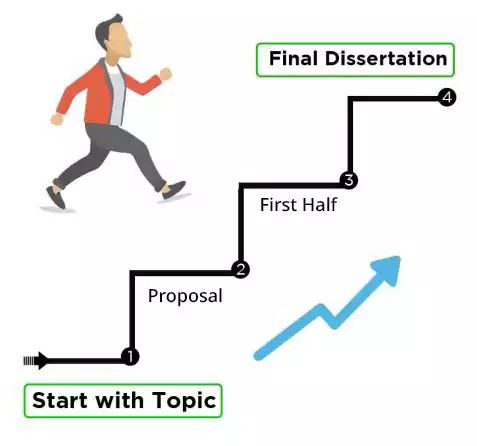
Examples of Meta-Analysis
- STANLEY T.D. et JARRELL S.B. (1989), « Meta-regression analysis : a quantitative method of literature surveys », Journal of Economics Surveys, vol. 3, n°2, pp. 161-170.
- DATTA D.K., PINCHES G.E. et NARAYANAN V.K. (1992), « Factors influencing wealth creation from mergers and acquisitions : a meta-analysis », Strategic Management Journal, Vol. 13, pp. 67-84.
- GLASS G. (1983), « Synthesising empirical research : Meta-analysis » in S.A. Ward and L.J. Reed (Eds), Knowledge structure and use : Implications for synthesis and interpretation, Philadelphia : Temple University Press.
- WOLF F.M. (1986), Meta-analysis : Quantitative methods for research synthesis, Sage University Paper n°59.
- HUNTER J.E., SCHMIDT F.L. et JACKSON G.B. (1982), « Meta-analysis : cumulating research findings across studies », Beverly Hills, CA : Sage.
Frequently Asked Questions
What is a meta-analysis in research.
Meta-analysis is a statistical method used to combine results from multiple studies on a specific topic. By pooling data from various sources, meta-analysis can provide a more precise estimate of the effect size of a treatment or intervention and identify areas for future research.
Why is meta-analysis important?
Meta-analysis is important because it combines and summarizes results from multiple studies to provide a more precise and reliable estimate of the effect of a treatment or intervention. This helps clinicians and policymakers make evidence-based decisions and identify areas for further research.
What is an example of a meta-analysis?
A meta-analysis of studies evaluating physical exercise’s effect on depression in adults is an example. Researchers gathered data from 49 studies involving a total of 2669 participants. The studies used different types of exercise and measures of depression, which made it difficult to compare the results.
Through meta-analysis, the researchers calculated an overall effect size and determined that exercise was associated with a statistically significant reduction in depression symptoms. The study also identified that moderate-intensity aerobic exercise, performed three to five times per week, was the most effective. The meta-analysis provided a more comprehensive understanding of the impact of exercise on depression than any single study could provide.
What is the definition of meta-analysis in clinical research?
Meta-analysis in clinical research is a statistical technique that combines data from multiple independent studies on a particular topic to generate a summary or “meta” estimate of the effect of a particular intervention or exposure.
This type of analysis allows researchers to synthesise the results of multiple studies, potentially increasing the statistical power and providing more precise estimates of treatment effects. Meta-analyses are commonly used in clinical research to evaluate the effectiveness and safety of medical interventions and to inform clinical practice guidelines.
Is meta-analysis qualitative or quantitative?
Meta-analysis is a quantitative method used to combine and analyze data from multiple studies. It involves the statistical synthesis of results from individual studies to obtain a pooled estimate of the effect size of a particular intervention or treatment. Therefore, meta-analysis is considered a quantitative approach to research synthesis.
You May Also Like
Struggling to figure out “whether I should choose primary research or secondary research in my dissertation?” Here are some tips to help you decide.
In correlational research, a researcher measures the relationship between two or more variables or sets of scores without having control over the variables.
This article provides the key advantages of primary research over secondary research so you can make an informed decision.
USEFUL LINKS
LEARNING RESOURCES
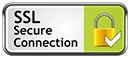
COMPANY DETAILS
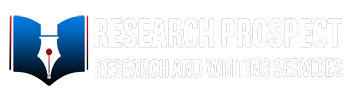
- How It Works

Meta-analysis
Reviewed by Psychology Today Staff
Meta-analysis is an objective examination of published data from many studies of the same research topic identified through a literature search. Through the use of rigorous statistical methods, it can reveal patterns hidden in individual studies and can yield conclusions that have a high degree of reliability. It is a method of analysis that is especially useful for gaining an understanding of complex phenomena when independent studies have produced conflicting findings.
Meta-analysis provides much of the underpinning for evidence-based medicine. It is particularly helpful in identifying risk factors for a disorder, diagnostic criteria, and the effects of treatments on specific populations of people, as well as quantifying the size of the effects. Meta-analysis is well-suited to understanding the complexities of human behavior.
- How Does It Differ From Other Studies?
- When Is It Used?
- What Are Some Important Things Revealed by Meta-analysis?
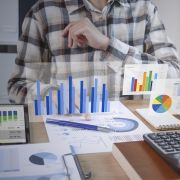
There are well-established scientific criteria for selecting studies for meta-analysis. Usually, meta-analysis is conducted on the gold standard of scientific research—randomized, controlled, double-blind trials. In addition, published guidelines not only describe standards for the inclusion of studies to be analyzed but also rank the quality of different types of studies. For example, cohort studies are likely to provide more reliable information than case reports.
Through statistical methods applied to the original data collected in the included studies, meta-analysis can account for and overcome many differences in the way the studies were conducted, such as the populations studied, how interventions were administered, and what outcomes were assessed and how. Meta-analyses, and the questions they are attempting to answer, are typically specified and registered with a scientific organization, and, with the protocols and methods openly described and reviewed independently by outside investigators, the research process is highly transparent.
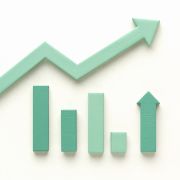
Meta-analysis is often used to validate observed phenomena, determine the conditions under which effects occur, and get enough clarity in clinical decision-making to indicate a course of therapeutic action when individual studies have produced disparate findings. In reviewing the aggregate results of well-controlled studies meeting criteria for inclusion, meta-analysis can also reveal which research questions, test conditions, and research methods yield the most reliable results, not only providing findings of immediate clinical utility but furthering science.
The technique can be used to answer social and behavioral questions large and small. For example, to clarify whether or not having more options makes it harder for people to settle on any one item, a meta-analysis of over 53 conflicting studies on the phenomenon was conducted. The meta-analysis revealed that choice overload exists—but only under certain conditions. You will have difficulty selecting a TV show to watch from the massive array of possibilities, for example, if the shows differ from each other in multiple ways or if you don’t have any strong preferences when you finally get to sit down in front of the TV.
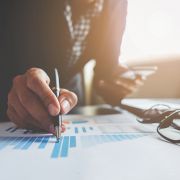
A meta-analysis conducted in 2000, for example, answered the question of whether physically attractive people have “better” personalities . Among other traits, they prove to be more extroverted and have more social skills than others. Another meta-analysis, in 2014, showed strong ties between physical attractiveness as rated by others and having good mental and physical health. The effects on such personality factors as extraversion are too small to reliably show up in individual studies but real enough to be detected in the aggregate number of study participants. Together, the studies validate hypotheses put forth by evolutionary psychologists that physical attractiveness is important in mate selection because it is a reliable cue of health and, likely, fertility.
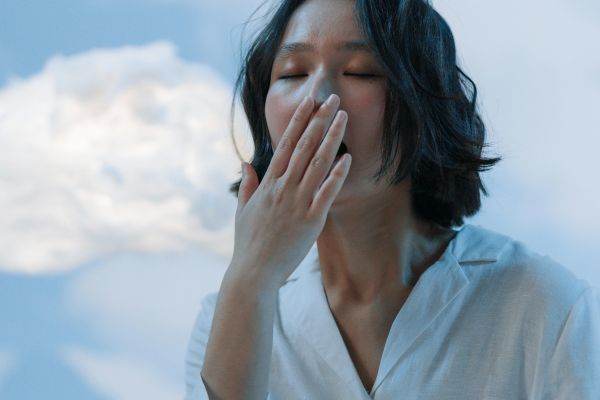
Investing in building a positive classroom climate holds benefits for students and teachers alike.
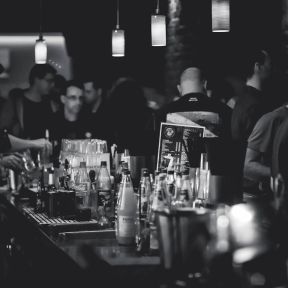
Mistakenly blaming cancer-causing chemicals and radiation for most cancers lets us avoid the simple lifestyle changes that could protect us from cancer far more.
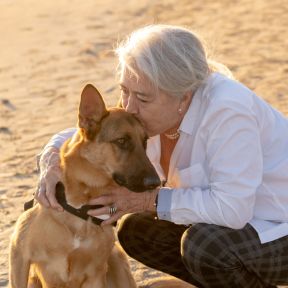
According to astronomer Carl Sagan, "Extraordinary claims require extraordinary evidence." Does the claim that pet owners live longer pass the extraordinary evidence requirement?
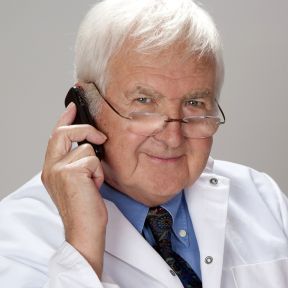
People, including leading politicians, are working later in life than ever before. Luckily, social science suggests that aging does not get in the way of job performance.
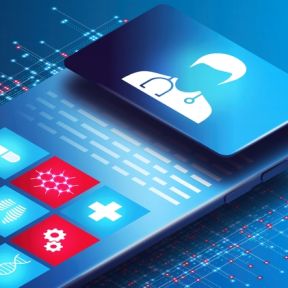
The healthcare industry is regulated to ensure patient safety, efficacy of treatments, and ethical practices. Why aren't these standards applied to mental health apps?
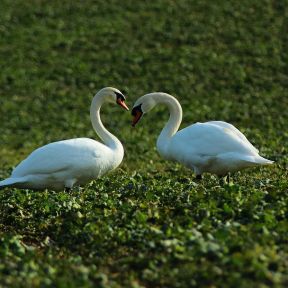
Being able to forgive others makes you more resilient. You can learn to let go of anger and bitterness.
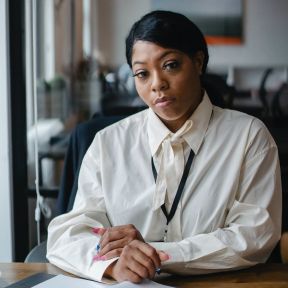
Discover how recent findings reveal a more promising outlook on the accuracy of performance appraisals.
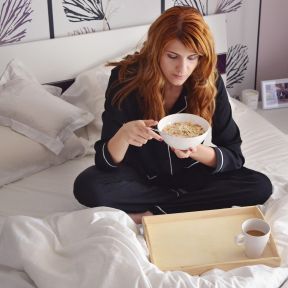
We all love the thrill of experiencing something new. However, recent research can help us understand the powerful appeal of the familiar.
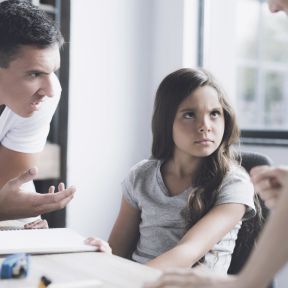
Amid growing calls to make verbal abuse a defined category of child maltreatment, a new meta-analysis identifies at least 22 types of verbally abusive behavior.
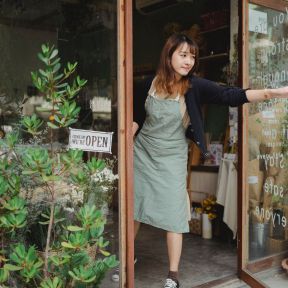
Incorporating science-based tools like cognitive restructuring, behavioral activation, and social skills training into your life can be profoundly empowering.
- Find a Therapist
- Find a Treatment Center
- Find a Psychiatrist
- Find a Support Group
- Find Teletherapy
- United States
- Brooklyn, NY
- Chicago, IL
- Houston, TX
- Los Angeles, CA
- New York, NY
- Portland, OR
- San Diego, CA
- San Francisco, CA
- Seattle, WA
- Washington, DC
- Asperger's
- Bipolar Disorder
- Chronic Pain
- Eating Disorders
- Passive Aggression
- Personality
- Goal Setting
- Positive Psychology
- Stopping Smoking
- Low Sexual Desire
- Relationships
- Child Development
- Therapy Center NEW
- Diagnosis Dictionary
- Types of Therapy
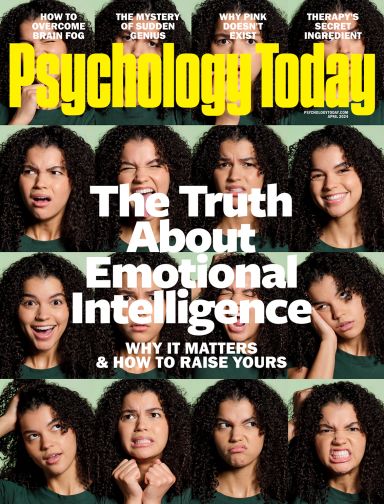
Understanding what emotional intelligence looks like and the steps needed to improve it could light a path to a more emotionally adept world.
- Coronavirus Disease 2019
- Affective Forecasting
- Neuroscience
What Is Meta-Analysis? Definition, Research & Examples
Appinio Research · 01.02.2024 · 39min read
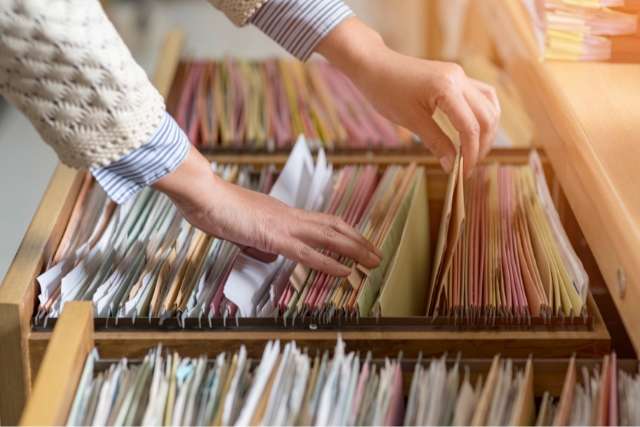
Are you looking to harness the power of data and uncover meaningful insights from a multitude of research studies? In a world overflowing with information, meta-analysis emerges as a guiding light, offering a systematic and quantitative approach to distilling knowledge from a sea of research.
This guide will demystify the art and science of meta-analysis, walking you through the process, from defining your research question to interpreting the results. Whether you're an academic researcher, a policymaker, or a curious mind eager to explore the depths of data, this guide will equip you with the tools and understanding needed to undertake robust and impactful meta-analyses.
What is a Meta Analysis?
Meta-analysis is a quantitative research method that involves the systematic synthesis and statistical analysis of data from multiple individual studies on a particular topic or research question. It aims to provide a comprehensive and robust summary of existing evidence by pooling the results of these studies, often leading to more precise and generalizable conclusions.
The primary purpose of meta-analysis is to:
- Quantify Effect Sizes: Determine the magnitude and direction of an effect or relationship across studies.
- Evaluate Consistency: Assess the consistency of findings among studies and identify sources of heterogeneity.
- Enhance Statistical Power: Increase the statistical power to detect significant effects by combining data from multiple studies.
- Generalize Results: Provide more generalizable results by analyzing a more extensive and diverse sample of participants or contexts.
- Examine Subgroup Effects: Explore whether the effect varies across different subgroups or study characteristics.
Importance of Meta-Analysis
Meta-analysis plays a crucial role in scientific research and evidence-based decision-making. Here are key reasons why meta-analysis is highly valuable:
- Enhanced Precision: By pooling data from multiple studies, meta-analysis provides a more precise estimate of the effect size, reducing the impact of random variation.
- Increased Statistical Power: The combination of numerous studies enhances statistical power, making it easier to detect small but meaningful effects.
- Resolution of Inconsistencies: Meta-analysis can help resolve conflicting findings in the literature by systematically analyzing and synthesizing evidence.
- Identification of Moderators: It allows for the identification of factors that may moderate the effect, helping to understand when and for whom interventions or treatments are most effective.
- Evidence-Based Decision-Making: Policymakers, clinicians, and researchers use meta-analysis to inform evidence-based decision-making, leading to more informed choices in healthcare , education, and other fields.
- Efficient Use of Resources: Meta-analysis can guide future research by identifying gaps in knowledge, reducing duplication of efforts, and directing resources to areas with the most significant potential impact.
Types of Research Questions Addressed
Meta-analysis can address a wide range of research questions across various disciplines. Some common types of research questions that meta-analysis can tackle include:
- Treatment Efficacy: Does a specific medical treatment, therapy, or intervention have a significant impact on patient outcomes or symptoms?
- Intervention Effectiveness: How effective are educational programs, training methods, or interventions in improving learning outcomes or skills?
- Risk Factors and Associations: What are the associations between specific risk factors, such as smoking or diet, and the likelihood of developing certain diseases or conditions?
- Impact of Policies: What is the effect of government policies, regulations, or interventions on social, economic, or environmental outcomes?
- Psychological Constructs: How do psychological constructs, such as self-esteem, anxiety, or motivation, influence behavior or mental health outcomes?
- Comparative Effectiveness: Which of two or more competing interventions or treatments is more effective for a particular condition or population?
- Dose-Response Relationships: Is there a dose-response relationship between exposure to a substance or treatment and the likelihood or severity of an outcome?
Meta-analysis is a versatile tool that can provide valuable insights into a wide array of research questions, making it an indispensable method in evidence synthesis and knowledge advancement.
Meta-Analysis vs. Systematic Review
In evidence synthesis and research aggregation, meta-analysis and systematic reviews are two commonly used methods, each serving distinct purposes while sharing some similarities. Let's explore the differences and similarities between these two approaches.
Meta-Analysis
- Purpose: Meta-analysis is a statistical technique used to combine and analyze quantitative data from multiple individual studies that address the same research question. The primary aim of meta-analysis is to provide a single summary effect size that quantifies the magnitude and direction of an effect or relationship across studies.
- Data Synthesis: In meta-analysis, researchers extract and analyze numerical data, such as means, standard deviations, correlation coefficients, or odds ratios, from each study. These effect size estimates are then combined using statistical methods to generate an overall effect size and associated confidence interval.
- Quantitative: Meta-analysis is inherently quantitative, focusing on numerical data and statistical analyses to derive a single effect size estimate.
- Main Outcome: The main outcome of a meta-analysis is the summary effect size, which provides a quantitative estimate of the research question's answer.
Systematic Review
- Purpose: A systematic review is a comprehensive and structured overview of the available evidence on a specific research question. While systematic reviews may include meta-analysis, their primary goal is to provide a thorough and unbiased summary of the existing literature.
- Data Synthesis: Systematic reviews involve a meticulous process of literature search, study selection, data extraction, and quality assessment. Researchers may narratively synthesize the findings, providing a qualitative summary of the evidence.
- Qualitative: Systematic reviews are often qualitative in nature, summarizing and synthesizing findings in a narrative format. They do not always involve statistical analysis .
- Main Outcome: The primary outcome of a systematic review is a comprehensive narrative summary of the existing evidence. While some systematic reviews include meta-analyses, not all do so.
Key Differences
- Nature of Data: Meta-analysis primarily deals with quantitative data and statistical analysis , while systematic reviews encompass both quantitative and qualitative data, often presenting findings in a narrative format.
- Focus on Effect Size: Meta-analysis focuses on deriving a single, quantitative effect size estimate, whereas systematic reviews emphasize providing a comprehensive overview of the literature, including study characteristics, methodologies, and key findings.
- Synthesis Approach: Meta-analysis is a quantitative synthesis method, while systematic reviews may use both quantitative and qualitative synthesis approaches.
Commonalities
- Structured Process: Both meta-analyses and systematic reviews follow a structured and systematic process for literature search, study selection, data extraction, and quality assessment.
- Evidence-Based: Both approaches aim to provide evidence-based answers to specific research questions, offering valuable insights for decision-making in various fields.
- Transparency: Both meta-analyses and systematic reviews prioritize transparency and rigor in their methodologies to minimize bias and enhance the reliability of their findings.
While meta-analysis and systematic reviews share the overarching goal of synthesizing research evidence, they differ in their approach and main outcomes. Meta-analysis is quantitative, focusing on effect sizes, while systematic reviews provide comprehensive overviews, utilizing both quantitative and qualitative data to summarize the literature. Depending on the research question and available data, one or both of these methods may be employed to provide valuable insights for evidence-based decision-making.
How to Conduct a Meta-Analysis?
Planning a meta-analysis is a critical phase that lays the groundwork for a successful and meaningful study. We will explore each component of the planning process in more detail, ensuring you have a solid foundation before diving into data analysis.
How to Formulate Research Questions?
Your research questions are the guiding compass of your meta-analysis. They should be precise and tailored to the topic you're investigating. To craft effective research questions:
- Clearly Define the Problem: Start by identifying the specific problem or topic you want to address through meta-analysis.
- Specify Key Variables: Determine the essential variables or factors you'll examine in the included studies.
- Frame Hypotheses: If applicable, create clear hypotheses that your meta-analysis will test.
For example, if you're studying the impact of a specific intervention on patient outcomes, your research question might be: "What is the effect of Intervention X on Patient Outcome Y in published clinical trials?"
Eligibility Criteria
Eligibility criteria define the boundaries of your meta-analysis. By establishing clear criteria, you ensure that the studies you include are relevant and contribute to your research objectives. Key considerations for eligibility criteria include:
- Study Types: Decide which types of studies will be considered (e.g., randomized controlled trials, cohort studies, case-control studies).
- Publication Time Frame: Specify the publication date range for included studies.
- Language: Determine whether studies in languages other than your primary language will be included.
- Geographic Region: If relevant, define any geographic restrictions.
Your eligibility criteria should strike a balance between inclusivity and relevance. Excluding certain studies based on valid criteria ensures the quality and relevance of the data you analyze.
Search Strategy
A robust search strategy is fundamental to identifying all relevant studies. To create an effective search strategy:
- Select Databases: Choose appropriate databases that cover your research area (e.g., PubMed, Scopus, Web of Science).
- Keywords and Search Terms: Develop a comprehensive list of relevant keywords and search terms related to your research questions.
- Search Filters: Utilize search filters and Boolean operators (AND, OR) to refine your search queries.
- Manual Searches: Consider conducting hand-searches of key journals and reviewing the reference lists of relevant studies for additional sources.
Remember that the goal is to cast a wide net while maintaining precision to capture all relevant studies.
Data Extraction
Data extraction is the process of systematically collecting information from each selected study. It involves retrieving key data points, including:
- Study Characteristics: Author(s), publication year, study design, sample size, duration, and location.
- Outcome Data: Effect sizes, standard errors, confidence intervals, p-values, and any other relevant statistics.
- Methodological Details: Information on study quality, risk of bias, and potential sources of heterogeneity.
Creating a standardized data extraction form is essential to ensure consistency and accuracy throughout this phase. Spreadsheet software, such as Microsoft Excel, is commonly used for data extraction.
Quality Assessment
Assessing the quality of included studies is crucial to determine their reliability and potential impact on your meta-analysis. Various quality assessment tools and checklists are available, depending on the study design. Some commonly used tools include:
- Newcastle-Ottawa Scale: Used for assessing the quality of non-randomized studies (e.g., cohort, case-control studies).
- Cochrane Risk of Bias Tool: Designed for evaluating randomized controlled trials.
Quality assessment typically involves evaluating aspects such as study design, sample size, data collection methods, and potential biases. This step helps you weigh the contribution of each study to the overall analysis.
How to Conduct a Literature Review?
Conducting a thorough literature review is a critical step in the meta-analysis process. We will explore the essential components of a literature review, from designing a comprehensive search strategy to establishing clear inclusion and exclusion criteria and, finally, the study selection process.
Comprehensive Search
To ensure the success of your meta-analysis, it's imperative to cast a wide net when searching for relevant studies. A comprehensive search strategy involves:
- Selecting Relevant Databases: Identify databases that cover your research area comprehensively, such as PubMed, Scopus, Web of Science, or specialized databases specific to your field.
- Creating a Keyword List: Develop a list of relevant keywords and search terms related to your research questions. Think broadly and consider synonyms, acronyms, and variations.
- Using Boolean Operators: Utilize Boolean operators (AND, OR) to combine keywords effectively and refine your search.
- Applying Filters: Employ search filters (e.g., publication date range, study type) to narrow down results based on your eligibility criteria.
Remember that the goal is to leave no relevant stone unturned, as missing key studies can introduce bias into your meta-analysis.
Inclusion and Exclusion Criteria
Clearly defined inclusion and exclusion criteria are the gatekeepers of your meta-analysis. These criteria ensure that the studies you include meet your research objectives and maintain the quality of your analysis. Consider the following factors when establishing criteria:
- Study Types: Determine which types of studies are eligible for inclusion (e.g., randomized controlled trials, observational studies, case reports).
- Publication Time Frame: Specify the time frame within which studies must have been published.
- Language: Decide whether studies in languages other than your primary language will be included or excluded.
- Geographic Region: If applicable, define any geographic restrictions.
- Relevance to Research Questions: Ensure that selected studies align with your research questions and objectives.
Your inclusion and exclusion criteria should strike a balance between inclusivity and relevance. Rigorous criteria help maintain the quality and applicability of the studies included in your meta-analysis.
Study Selection Process
The study selection process involves systematically screening and evaluating each potential study to determine whether it meets your predefined inclusion criteria. Here's a step-by-step guide:
- Screen Titles and Abstracts: Begin by reviewing the titles and abstracts of the retrieved studies. Exclude studies that clearly do not meet your inclusion criteria.
- Full-Text Assessment: Assess the full text of potentially relevant studies to confirm their eligibility. Pay attention to study design, sample size, and other specific criteria.
- Data Extraction: For studies that meet your criteria, extract the necessary data, including study characteristics, effect sizes, and other relevant information.
- Record Exclusions: Keep a record of the reasons for excluding studies. This transparency is crucial for the reproducibility of your meta-analysis.
- Resolve Discrepancies: If multiple reviewers are involved, resolve any disagreements through discussion or a third-party arbitrator.
Maintaining a clear and organized record of your study selection process is essential for transparency and reproducibility. Software tools like EndNote or Covidence can facilitate the screening and data extraction process.
By following these systematic steps in conducting a literature review, you ensure that your meta-analysis is built on a solid foundation of relevant and high-quality studies.
Data Extraction and Management
As you progress in your meta-analysis journey, the data extraction and management phase becomes paramount. We will delve deeper into the critical aspects of this phase, including the data collection process, data coding and transformation, and how to handle missing data effectively.
Data Collection Process
The data collection process is the heart of your meta-analysis, where you systematically extract essential information from each selected study. To ensure accuracy and consistency:
- Create a Data Extraction Form: Develop a standardized data extraction form that includes all the necessary fields for collecting relevant data. This form should align with your research questions and inclusion criteria.
- Data Extractors: Assign one or more reviewers to extract data from the selected studies. Ensure they are familiar with the form and the specific data points to collect.
- Double-Check Accuracy: Implement a verification process where a second reviewer cross-checks a random sample of data extractions to identify discrepancies or errors.
- Extract All Relevant Information: Collect data on study characteristics, participant demographics, outcome measures, effect sizes, confidence intervals, and any additional information required for your analysis.
- Maintain Consistency: Use clear guidelines and definitions for data extraction to ensure uniformity across studies.
To optimize your data collection process and streamline the extraction and management of crucial information, consider leveraging innovative solutions like Appinio . With Appinio, you can effortlessly collect real-time consumer insights, ensuring your meta-analysis benefits from the latest data trends and user perspectives. Ready to learn more? Book a demo today and unlock a world of data-driven possibilities!

Get a free demo and see the Appinio platform in action!
Data Coding and Transformation
After data collection, you may need to code and transform the extracted data to ensure uniformity and compatibility across studies. This process involves:
- Coding Categorical Variables: If studies report data differently, code categorical variables consistently . For example, ensure that categories like "male" and "female" are coded consistently across studies.
- Standardizing Units of Measurement: Convert all measurements to a common unit if studies use different measurement units. For instance, if one study reports height in inches and another in centimeters, standardize to one unit for comparability.
- Calculating Effect Sizes: Calculate effect sizes and their standard errors or variances if they are not directly reported in the studies. Common effect size measures include Cohen's d, odds ratio (OR), and hazard ratio (HR).
- Data Transformation: Transform data if necessary to meet assumptions of statistical tests. Common transformations include log transformation for skewed data or arcsine transformation for proportions.
- Heterogeneity Adjustment: Consider using transformation methods to address heterogeneity among studies, such as applying the Freeman-Tukey double arcsine transformation for proportions.
The goal of data coding and transformation is to make sure that data from different studies are compatible and can be effectively synthesized during the analysis phase. Spreadsheet software like Excel or statistical software like R can be used for these tasks.
Handling Missing Data
Missing data is a common challenge in meta-analysis, and how you handle it can impact the validity and precision of your results. Strategies for handling missing data include:
- Contact Authors: If feasible, contact the authors of the original studies to request missing data or clarifications.
- Imputation: Consider using appropriate imputation methods to estimate missing values, but exercise caution and report the imputation methods used.
- Sensitivity Analysis: Conduct sensitivity analyses to assess the impact of missing data on your results by comparing the main analysis to alternative scenarios.
Remember that transparency in reporting how you handled missing data is crucial for the credibility of your meta-analysis.
By following these steps in data extraction and management, you will ensure the integrity and reliability of your meta-analysis dataset.
Meta-Analysis Example
Meta-analysis is a versatile research method that can be applied to various fields and disciplines, providing valuable insights by synthesizing existing evidence.
Example 1: Analyzing the Impact of Advertising Campaigns on Sales
Background: A market research agency is tasked with assessing the effectiveness of advertising campaigns on sales outcomes for a range of consumer products. They have access to multiple studies and reports conducted by different companies, each analyzing the impact of advertising on sales revenue.
Meta-Analysis Approach:
- Study Selection: Identify relevant studies that meet specific inclusion criteria, such as the type of advertising campaign (e.g., TV commercials, social media ads), the products examined, and the sales metrics assessed.
- Data Extraction: Collect data from each study, including details about the advertising campaign (e.g., budget, duration), sales data (e.g., revenue, units sold), and any reported effect sizes or correlations.
- Effect Size Calculation: Calculate effect sizes (e.g., correlation coefficients) based on the data provided in each study, quantifying the strength and direction of the relationship between advertising and sales.
- Data Synthesis: Employ meta-analysis techniques to combine the effect sizes from the selected studies. Compute a summary effect size and its confidence interval to estimate the overall impact of advertising on sales.
- Publication Bias Assessment: Use funnel plots and statistical tests to assess the potential presence of publication bias, ensuring that the meta-analysis results are not unduly influenced by selective reporting.
Findings: Through meta-analysis, the market research agency discovers that advertising campaigns have a statistically significant and positive impact on sales across various product categories. The findings provide evidence for the effectiveness of advertising efforts and assist companies in making data-driven decisions regarding their marketing strategies.
These examples illustrate how meta-analysis can be applied in diverse domains, from tech startups seeking to optimize user engagement to market research agencies evaluating the impact of advertising campaigns. By systematically synthesizing existing evidence, meta-analysis empowers decision-makers with valuable insights for informed choices and evidence-based strategies.
How to Assess Study Quality and Bias?
Ensuring the quality and reliability of the studies included in your meta-analysis is essential for drawing accurate conclusions. We'll show you how you can assess study quality using specific tools, evaluate potential bias, and address publication bias.
Quality Assessment Tools
Quality assessment tools provide structured frameworks for evaluating the methodological rigor of each included study. The choice of tool depends on the study design. Here are some commonly used quality assessment tools:
For Randomized Controlled Trials (RCTs):
- Cochrane Risk of Bias Tool: This tool assesses the risk of bias in RCTs based on six domains: random sequence generation, allocation concealment, blinding of participants and personnel, blinding of outcome assessment, incomplete outcome data, and selective reporting.
- Jadad Scale: A simpler tool specifically for RCTs, the Jadad Scale focuses on randomization, blinding, and the handling of withdrawals and dropouts.
For Observational Studies:
- Newcastle-Ottawa Scale (NOS): The NOS assesses the quality of cohort and case-control studies based on three categories: selection, comparability, and outcome.
- ROBINS-I: Designed for non-randomized studies of interventions, the Risk of Bias in Non-randomized Studies of Interventions tool evaluates bias in domains such as confounding, selection bias, and measurement bias.
- MINORS: The Methodological Index for Non-Randomized Studies (MINORS) assesses non-comparative studies and includes items related to study design, reporting, and statistical analysis.
Bias Assessment
Evaluating potential sources of bias is crucial to understanding the limitations of the included studies. Common sources of bias include:
- Selection Bias: Occurs when the selection of participants is not random or representative of the target population.
- Performance Bias: Arises when participants or researchers are aware of the treatment or intervention status, potentially influencing outcomes.
- Detection Bias: Occurs when outcome assessors are not blinded to the treatment groups.
- Attrition Bias: Results from incomplete data or differential loss to follow-up between treatment groups.
- Reporting Bias: Involves selective reporting of outcomes, where only positive or statistically significant results are published.
To assess bias, reviewers often use the quality assessment tools mentioned earlier, which include domains related to bias, or they may specifically address bias concerns in the narrative synthesis.
We'll move on to the core of meta-analysis: data synthesis. We'll explore different effect size measures, fixed-effect versus random-effects models, and techniques for assessing and addressing heterogeneity among studies.
Data Synthesis
Now that you've gathered data from multiple studies and assessed their quality, it's time to synthesize this information effectively.
Effect Size Measures
Effect size measures quantify the magnitude of the relationship or difference you're investigating in your meta-analysis. The choice of effect size measure depends on your research question and the type of data provided by the included studies. Here are some commonly used effect size measures:
Continuous Outcome Data:
- Cohen's d: Measures the standardized mean difference between two groups. It's suitable for continuous outcome variables.
- Hedges' g: Similar to Cohen's d but incorporates a correction factor for small sample sizes.
Binary Outcome Data:
- Odds Ratio (OR): Used for dichotomous outcomes, such as success/failure or presence/absence.
- Risk Ratio (RR): Similar to OR but used when the outcome is relatively common.
Time-to-Event Data:
- Hazard Ratio (HR): Used in survival analysis to assess the risk of an event occurring over time.
- Risk Difference (RD): Measures the absolute difference in event rates between two groups.
Selecting the appropriate effect size measure depends on the nature of your data and the research question. When effect sizes are not directly reported in the studies, you may need to calculate them using available data, such as means, standard deviations, and sample sizes.
Formula for Cohen's d:
d = (Mean of Group A - Mean of Group B) / Pooled Standard Deviation
Fixed-Effect vs. Random-Effects Models
In meta-analysis, you can choose between fixed-effect and random-effects models to combine the results of individual studies:
Fixed-Effect Model:
- Assumes that all included studies share a common true effect size.
- Accounts for only within-study variability (sampling error).
- Appropriate when studies are very similar or when there's minimal heterogeneity.
Random-Effects Model:
- Acknowledges that there may be variability in effect sizes across studies.
- Accounts for both within-study variability (sampling error) and between-study variability (real differences between studies).
- More conservative and applicable when there's substantial heterogeneity.
The choice between these models should be guided by the degree of heterogeneity observed among the included studies. If heterogeneity is significant, the random-effects model is often preferred, as it provides a more robust estimate of the overall effect.
Forest Plots
Forest plots are graphical representations commonly used in meta-analysis to display the results of individual studies along with the combined summary estimate. Key components of a forest plot include:
- Vertical Line: Represents the null effect (e.g., no difference or no effect).
- Horizontal Lines: Represent the confidence intervals for each study's effect size estimate.
- Diamond or Square: Represents the summary effect size estimate, with its width indicating the confidence interval around the summary estimate.
- Study Names: Listed on the left side of the plot, identifying each study.
Forest plots help visualize the distribution of effect sizes across studies and provide insights into the consistency and direction of the findings.
Heterogeneity Assessment
Heterogeneity refers to the variability in effect sizes among the included studies. It's important to assess and understand heterogeneity as it can impact the interpretation of your meta-analysis results. Standard methods for assessing heterogeneity include:
- Cochran's Q Test: A statistical test that assesses whether there is significant heterogeneity among the effect sizes of the included studies.
- I² Statistic: A measure that quantifies the proportion of total variation in effect sizes that is due to heterogeneity. I² values range from 0% to 100%, with higher values indicating greater heterogeneity.
Assessing heterogeneity is crucial because it informs your choice of meta-analysis model (fixed-effect vs. random-effects) and whether subgroup analyses or sensitivity analyses are warranted to explore potential sources of heterogeneity.
How to Interpret Meta-Analysis Results?
With the data synthesis complete, it's time to make sense of the results of your meta-analysis.
Meta-Analytic Summary
The meta-analytic summary is the culmination of your efforts in data synthesis. It provides a consolidated estimate of the effect size and its confidence interval, combining the results of all included studies. To interpret the meta-analytic summary effectively:
- Effect Size Estimate: Understand the primary effect size estimate, such as Cohen's d, odds ratio, or hazard ratio, and its associated confidence interval.
- Significance: Determine whether the summary effect size is statistically significant. This is indicated when the confidence interval does not include the null value (e.g., 0 for Cohen's d or 1 for odds ratio).
- Magnitude: Assess the magnitude of the effect size. Is it large, moderate, or small, and what are the practical implications of this magnitude?
- Direction: Consider the direction of the effect. Is it in the hypothesized direction, or does it contradict the expected outcome?
- Clinical or Practical Significance: Reflect on the clinical or practical significance of the findings. Does the effect size have real-world implications?
- Consistency: Evaluate the consistency of the findings across studies. Are most studies in agreement with the summary effect size estimate, or are there outliers?
Subgroup Analyses
Subgroup analyses allow you to explore whether the effect size varies across different subgroups of studies or participants. This can help identify potential sources of heterogeneity or assess whether the intervention's effect differs based on specific characteristics. Steps for conducting subgroup analyses:
- Define Subgroups: Clearly define the subgroups you want to investigate based on relevant study characteristics (e.g., age groups, study design , intervention type).
- Analyze Subgroups: Calculate separate summary effect sizes for each subgroup and compare them to the overall summary effect.
- Assess Heterogeneity: Evaluate whether subgroup differences are statistically significant. If so, this suggests that the effect size varies significantly among subgroups.
- Interpretation: Interpret the subgroup findings in the context of your research question. Are there meaningful differences in the effect across subgroups? What might explain these differences?
Subgroup analyses can provide valuable insights into the factors influencing the overall effect size and help tailor recommendations for specific populations or conditions.
Sensitivity Analyses
Sensitivity analyses are conducted to assess the robustness of your meta-analysis results by exploring how different choices or assumptions might affect the findings. Common sensitivity analyses include:
- Exclusion of Low-Quality Studies: Repeating the meta-analysis after excluding studies with low quality or a high risk of bias.
- Changing Effect Size Measure: Re-running the analysis using a different effect size measure to assess whether the choice of measure significantly impacts the results.
- Publication Bias Adjustment: Applying methods like the trim-and-fill procedure to adjust for potential publication bias.
- Subsample Analysis: Analyzing a subset of studies based on specific criteria or characteristics to investigate their impact on the summary effect.
Sensitivity analyses help assess the robustness and reliability of your meta-analysis results, providing a more comprehensive understanding of the potential influence of various factors.
Reporting and Publication
The final stages of your meta-analysis involve preparing your findings for publication.
Manuscript Preparation
When preparing your meta-analysis manuscript, consider the following:
- Structured Format: Organize your manuscript following a structured format, including sections such as introduction, methods, results, discussion, and conclusions.
- Clarity and Conciseness: Write your findings clearly and concisely, avoiding jargon or overly technical language. Use tables and figures to enhance clarity.
- Transparent Methods: Provide detailed descriptions of your methods, including eligibility criteria, search strategy, data extraction, and statistical analysis.
- Incorporate Tables and Figures: Present your meta-analysis results using tables and forest plots to visually convey key findings.
- Interpretation: Interpret the implications of your findings, discussing the clinical or practical significance and limitations.
Transparent Reporting Guidelines
Adhering to transparent reporting guidelines ensures that your meta-analysis is transparent, reproducible, and credible. Some widely recognized guidelines include:
- PRISMA (Preferred Reporting Items for Systematic Reviews and Meta-Analyses): PRISMA provides a checklist and flow diagram for reporting systematic reviews and meta-analyses, enhancing transparency and rigor.
- MOOSE (Meta-analysis of Observational Studies in Epidemiology): MOOSE guidelines are designed for meta-analyses of observational studies and provide a framework for transparent reporting.
- ROBINS-I: If your meta-analysis involves non-randomized studies, follow the Risk Of Bias In Non-randomized Studies of Interventions guidelines for reporting.
Adhering to these guidelines ensures that your meta-analysis is transparent, reproducible, and credible. It enhances the quality of your research and aids readers and reviewers in assessing the rigor of your study.
PRISMA Statement
The PRISMA statement is a valuable resource for conducting and reporting systematic reviews and meta-analyses. Key elements of PRISMA include:
- Title: Clearly indicate that your paper is a systematic review or meta-analysis.
- Structured Abstract: Provide a structured summary of your study, including objectives, methods, results, and conclusions.
- Transparent Reporting: Follow the PRISMA checklist, which covers items such as the rationale, eligibility criteria, search strategy, data extraction, and risk of bias assessment.
- Flow Diagram: Include a flow diagram illustrating the study selection process.
By adhering to the PRISMA statement, you enhance the transparency and credibility of your meta-analysis, facilitating its acceptance for publication and aiding readers in evaluating the quality of your research.
Conclusion for Meta-Analysis
Meta-analysis is a powerful tool that allows you to combine and analyze data from multiple studies to find meaningful patterns and make informed decisions. It helps you see the bigger picture and draw more accurate conclusions than individual studies alone. Whether you're in healthcare, education, business, or any other field, the principles of meta-analysis can be applied to enhance your research and decision-making processes. Remember that conducting a successful meta-analysis requires careful planning, attention to detail, and transparency in reporting. By following the steps outlined in this guide, you can embark on your own meta-analysis journey with confidence, contributing to the advancement of knowledge and evidence-based practices in your area of interest.
How to Elevate Your Meta-Analysis With Real-Time Insights?
Introducing Appinio , the real-time market research platform that brings a new level of excitement to your meta-analysis journey. With Appinio, you can seamlessly collect your own market research data in minutes, empowering your meta-analysis with fresh, real-time consumer insights.
Here's why Appinio is your ideal partner for efficient data collection:
- From Questions to Insights in Minutes: Appinio's lightning-fast platform ensures you get the answers you need when you need them, accelerating your meta-analysis process.
- No Research PhD Required: Our intuitive platform is designed for everyone, eliminating the need for specialized research skills and putting the power of data collection in your hands.
- Global Reach, Minimal Time: With an average field time of less than 23 minutes for 1,000 respondents and access to over 90 countries, you can define precise target groups and gather data swiftly.
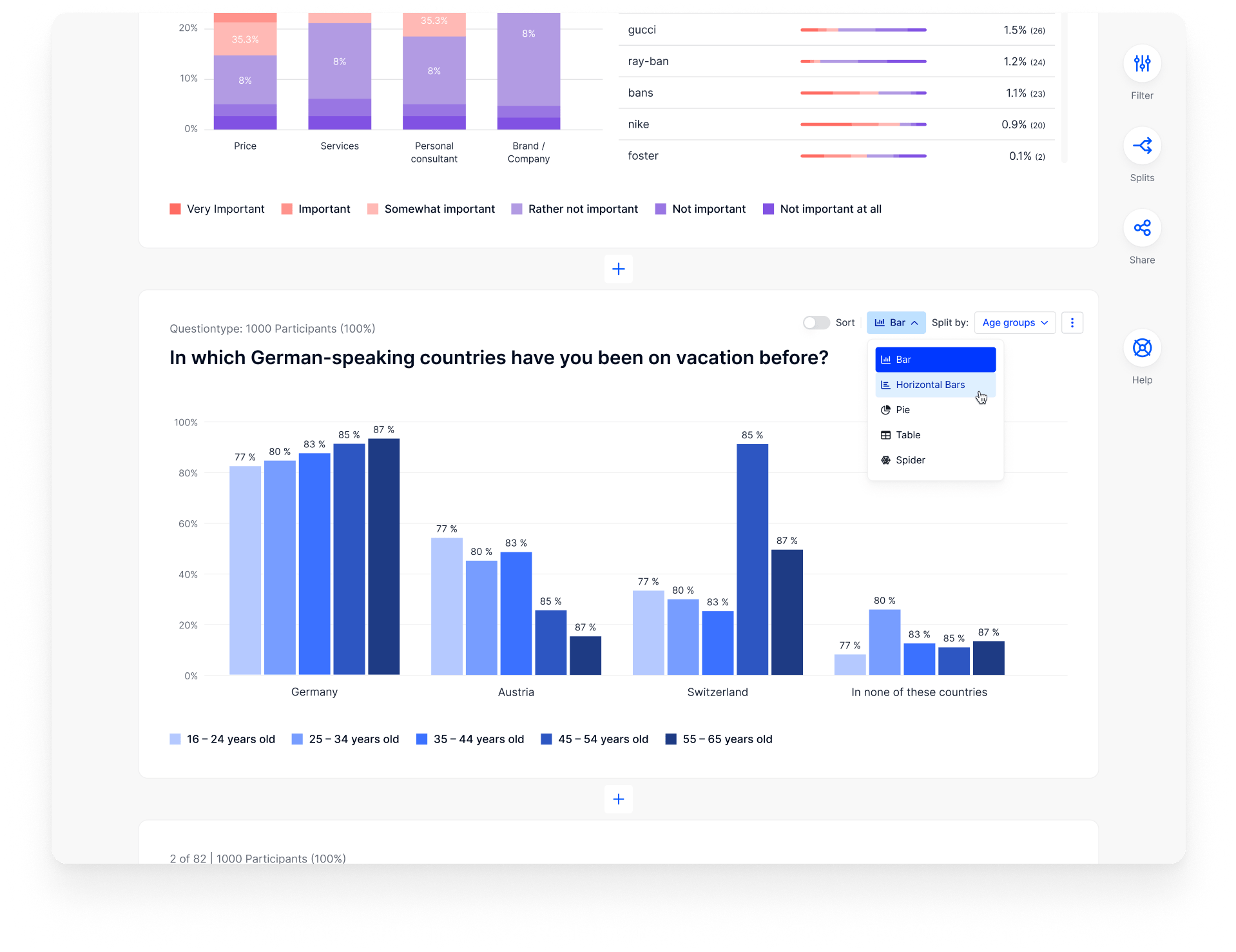
Get free access to the platform!
Join the loop 💌
Be the first to hear about new updates, product news, and data insights. We'll send it all straight to your inbox.
Get the latest market research news straight to your inbox! 💌
Wait, there's more
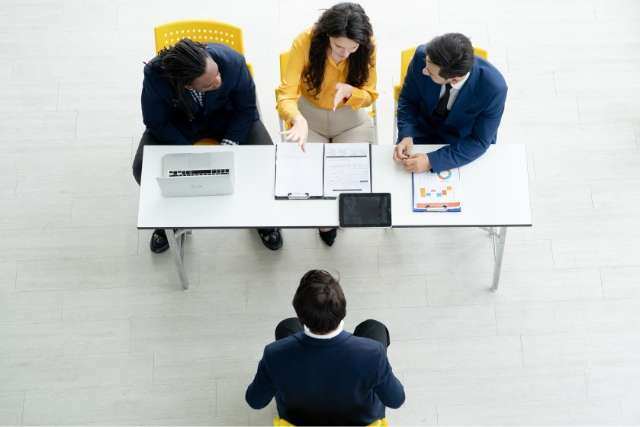
05.04.2024 | 27min read
What is Field Research? Definition, Types, Methods, Examples
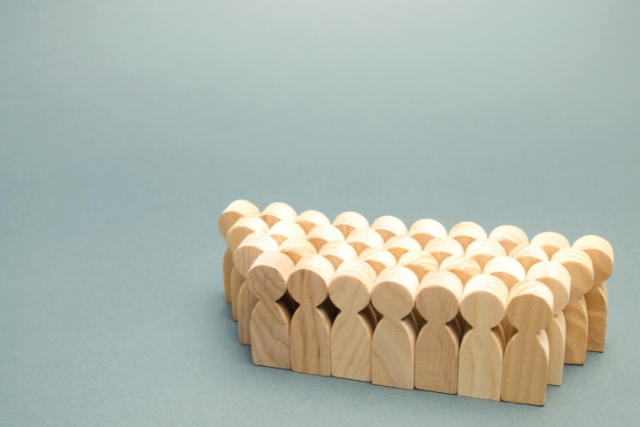
03.04.2024 | 29min read
What is Cluster Sampling? Definition, Methods, Examples
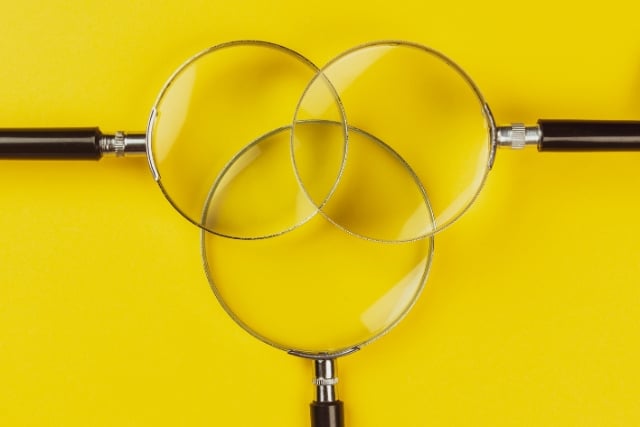
01.04.2024 | 26min read
Cross-Tabulation Analysis: A Full Guide (+ Examples)
Thank you for visiting nature.com. You are using a browser version with limited support for CSS. To obtain the best experience, we recommend you use a more up to date browser (or turn off compatibility mode in Internet Explorer). In the meantime, to ensure continued support, we are displaying the site without styles and JavaScript.
- View all journals
- Explore content
- About the journal
- Publish with us
- Sign up for alerts
- Published: 05 January 2022
The 5 min meta-analysis: understanding how to read and interpret a forest plot
- Yaping Chang ORCID: orcid.org/0000-0002-0549-5087 1 , 2 ,
- Mark R. Phillips ORCID: orcid.org/0000-0003-0923-261X 1 , 3 ,
- Robyn H. Guymer ORCID: orcid.org/0000-0002-9441-4356 4 , 5 ,
- Lehana Thabane ORCID: orcid.org/0000-0003-0355-9734 1 , 6 ,
- Mohit Bhandari ORCID: orcid.org/0000-0001-9608-4808 1 , 2 , 3 &
- Varun Chaudhary ORCID: orcid.org/0000-0002-9988-4146 1 , 3
on behalf of the R.E.T.I.N.A. study group
Eye volume 36 , pages 673–675 ( 2022 ) Cite this article
78k Accesses
21 Citations
249 Altmetric
Metrics details
- Outcomes research
A Correction to this article was published on 08 May 2023
This article has been updated
Introduction
In the evidence-based practice of ophthalmology, we often read systematic reviews. Why do we bother about systematic reviews? In science, new findings are built cumulatively on multiple and repeatable experiments [ 1 ]. In clinical research, rarely is one study definitive. Using a comprehensive and cumulative approach, systematic reviews synthesize results of individual studies to address a focused question that can guide important decisions, when well-conducted and current [ 2 , 3 , 4 , 5 ].
A systematic review may or may not include a meta-analysis, which provides a statistical approach to quantitatively combine results of studies eligible for a systematic review topic [ 2 , 3 , 4 , 5 ]. Such pooling also improves precision [ 2 , 4 , 5 ]. A “forest plot” is a form of graphical result presentation [ 2 , 4 ]. In this editorial, we start with introducing the anatomy of a forest plot and present 5 tips for understanding the results of a meta-analysis.
Anatomy of a forest plot
We demonstrate the components of a typical forest plot in Fig. 1 , using a topic from a recently published systematic review [ 6 ] but replaced with mockup numbers in analysis. In this example, four randomized trials (Studies #1 to #4) are included to compare a new surgical approach with the conventional surgery for patients with pseudoexfoliation glaucoma. Outcomes of intraocular pressure (IOP) and incidence of minor zonulolysis are evaluated at 1-year follow-up after surgery.
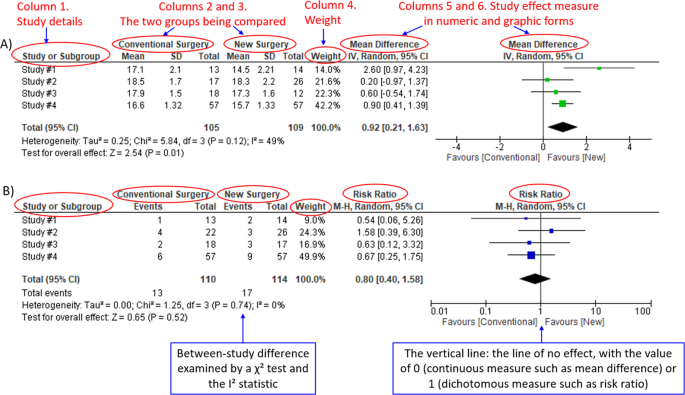
A Example of a continuous outcome measure: Intraocular pressure assessed with mean difference; B Example of a dichotomous outcome measure: Incidence of minor zonulolysis, at 1 year after surgery. Tau, the estimated standard deviation of underlying effects across studies (Tau 2 is only displayed in the random model). Chi 2 , the value of Chi-square test for heterogeneity. Random, random model (an analysis model in meta-analysis).
In a forest plot, the box in the middle of each horizontal line (confidence interval, CI) represents the point estimate of the effect for a single study. The size of the box is proportional to the weight of the study in relation to the pooled estimate. The diamond represents the overall effect estimate of the meta-analysis. The placement of the center of the diamond on the x-axis represents the point estimate, and the width of the diamond represents the 95% CI around the point estimate of the pooled effect.
Tip 1: Know the type of outcome than
There are differences in a forest plot depending on the type of outcomes. For a continuous outcome, the mean, standard deviation and number of patients are provided in Columns 2 and 3. A mean difference (MD, the absolute difference between the mean scores in the two groups) with its 95% CI is presented in Column 5 (Fig. 1A ). Some examples of continuous outcomes include IOP (mmHg), visual acuity in rank values, subfoveal choroidal thickness (μm) and cost.
For a dichotomous outcome, the number of events and number of patients, and a risk ratio (RR), also called relative risk, along with its 95% CI are presented in Columns 2,3 and 5 (Fig. 1B ). Examples of dichotomous outcomes include incidence of any adverse events, zonulolysis, capsulotomy and patients’ needing of medication (yes or no).
Tip 2: Understand the weight in a forest plot
Weights (Column 4) are assigned to individual studies according to their contributions to the pooled estimate, by calculating the inverse of the variance of the treatment effect, i.e., one over the square of the standard error. The weight is closely related to a study’s sample size [ 2 ]. In our example, Study #4 consisting of the largest sample size of 114 patients (57 in each group) has the greatest weight, 42.2% in IOP result (Figs. 1A ) and 49.9% in zonulolysis result (Fig. 1B ).
Tip 3: Pay attention to heterogeneity
Heterogeneity represents variation in results that might relate to population, intervention, comparator, outcome measure, risk of bias, study method, healthcare systems and other factors of the individual studies in a meta-analysis [ 2 , 7 ]. If no important heterogeneity is observed, we can trust the pooled estimate more because most or all the individual studies are telling the same answer [ 7 ].
We can identify heterogeneity by visual inspection of similarity of point estimates, overlapping of confidence intervals, and looking at the results of statistical heterogeneity tests outlined at near the bottom of a forest plot [ 2 , 7 ]. When more similarity of point estimates and more overlapping of confidence intervals are observed, it means less heterogeneity [ 2 , 7 ]. The P value generated by the Chi-squared test is the probability of the null hypothesis that there is no heterogeneity between studies. When P < 0.10 is shown, we reject this null hypothesis and consider that there is heterogeneity across the studies [ 2 ]. P value of 0.10 is typically used for the test of heterogeneity because of the lack of power for the test [ 2 ]. The I 2 statistic ranging from 0 to 100%, indicates the magnitude of heterogeneity. Greater I 2 indicates more heterogeneity. The I 2 below 40% may suggest not important heterogeneity; while the I 2 over 75% may suggest considerable heterogeneity [ 2 ].
For example in Fig. 1A , the point estimate of Study #1 (i.e., the between-group difference of mean IOP, 2.60 mmHg) is different from the point estimates of Studies #2 to #4 (0.20, 0.60 and 0.90 mmHg, respectively). By virtual observation of 95% CI (the horizontal lines), the 95% of Study #1 just partly overlaps with the other studies’. P -value for heterogeneity of 0.12 is relatively small but still >0.05. The I 2 of 49% indicates that a moderate heterogeneity may present [ 2 ]. In Fig. 1B , the 95% CIs of all the four studies largely overlap. The large P value for heterogeneity of 0.74 and the I 2 of 0% both indicate that no important heterogeneity is detected.
Tip 4: Understand subgroups
When heterogeneity is detected, which may indicate the unexplained differences between study estimates, using a subgroup analysis is one of the approaches to explain heterogeneity [ 2 ]. In our example, Study #3 only studied patients who were equal and below 65 years; Studies #1, 2, and 4 also reported IOP for patients of the two different age groups separately (Fig. 2 ). We can find the pooled effects of the two subgroups respectively in the forest plot: 1.1.1 over 65 years, the overall effect favours the new surgery (Section A in Fig. 2 , subtotal MD and 95% CI does not include the line of no effect, P value for overall effect <0.00001, I 2 = 0); and 1.1.2 equal and below 65 years, there is no difference between the conventional and new surgeries (Section B in Fig. 2 , subtotal MD and 95% CI includes the line of no effect, P value for overall effect is 0.10, I 2 = 0%).
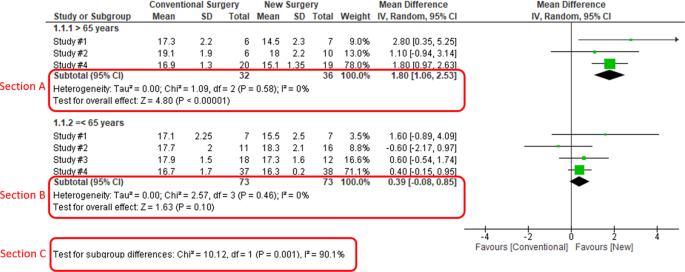
Subgroup results of IOP by age groups.
There is a subgroup effect by patients' age groups. We can find the result of test for subgroup difference in the last row of the forest plot (Section C in Fig. 2 ): P value of 0.001 and I 2 of 90.1% indicate a significant difference in treatment effects between the subgroups of patients of older or younger age.
Tip 5: Interpret the results in plain language
In our example, lower IOP and fewer zonulolysis are favoured outcomes. The statistical significance of a pooled estimate can be detected by visual inspection of the diamond (if the diamond width includes the line of no effect, there is no statistical difference between the two groups) or checking the p-value in the last row of a forest plot, “Test for overall effect” ( P < 0.05 indicates a significant difference).
In plain language, for patients with pseudoexfoliation glaucoma, the overall effect for IOP is in favour of the new surgery. More specifically, the new surgery is associated with the lower IOP compared to the conventional surgery 1 year after surgery (mean difference, 0.92 mmHg; 95% CI, 0.21 to 1.63 mmHg) with some concerns of heterogeneity and risk of bias. There is no difference in the incidence of minor zonulolysis between new and conventional surgeries.
In summary, knowing the structure of a forest plot, types of outcome measures, heterogeneity and risk of bias assessments will help us to understand the results of a systematic review. With more practice, the readers will gain more confidence in interpreting a forest plot and making application of systematic reviews’ results in your clinical practice.
Change history
08 may 2023.
A Correction to this paper has been published: https://doi.org/10.1038/s41433-023-02493-0
Zeigler D. Evolution and the cumulative nature of science. Evolution: Education Outreach. 2012;5:585–8. https://doi.org/10.1007/s12052-012-0454-6 .
Article Google Scholar
Higgins JP, Thomas J, Chandler J, Cumpston M, Li T, Page MJ, et al. Cochrane handbook for systematic reviews of interventions. John Wiley & Sons; 2019.
Haynes RB. Clinical epidemiology: how to do clinical practice research. Lippincott williams & wilkins; 2012.
Murad MH, Montori VM, Ioannidis JP, Neumann I, Hatala R, Meade MO, et al. Understanding and applying the results of a systematic review and meta-analysis. User’s guides to the medical literature: a manual for evidence-based clinical practice. 3rd edn. New York: JAMA/McGraw-Hill Global. 2015.
Guyatt GH, Oxman AD, Kunz R, Brozek J, Alonso-Coello P, Rind D, et al. GRADE guidelines 6. Rating the quality of evidence—imprecision. J Clin Epidemiol. 2011;64:1283–93. https://doi.org/10.1016/j.jclinepi.2011.01.012 .
Article PubMed Google Scholar
Pose-Bazarra S, López-Valladares MJ, López-de-Ullibarri I, Azuara-Blanco A. Surgical and laser interventions for pseudoexfoliation glaucoma systematic review of randomized controlled trials. Eye. 2021;35:1551–61. https://doi.org/10.1038/s41433-021-01424-1 .
Article PubMed PubMed Central Google Scholar
Guyatt GH, Oxman AD, Kunz R, Woodcock J, Brozek J, Helfand M, et al. GRADE guidelines: 7. Rating the quality of evidence—inconsistency. J Clinl Epidemiol. 2011;64:1294–302. https://doi.org/10.1016/j.jclinepi.2011.03.017 .
Download references
Author information
Authors and affiliations.
Department of Health Research Methods, Evidence & Impact, McMaster University, Hamilton, ON, Canada
Yaping Chang, Mark R. Phillips, Lehana Thabane, Mohit Bhandari & Varun Chaudhary
OrthoEvidence Inc., Burlington, ON, Canada
Yaping Chang & Mohit Bhandari
Department of Surgery, McMaster University, Hamilton, ON, Canada
Mark R. Phillips, Mohit Bhandari & Varun Chaudhary
Centre for Eye Research Australia, Royal Victorian Eye and Ear Hospital, East Melbourne, Australia
Robyn H. Guymer
Department of Surgery, (Ophthalmology), The University of Melbourne, Melbourne, Australia
Biostatistics Unit, St. Joseph’s Healthcare Hamilton, Hamilton, ON, Canada
Lehana Thabane
Retina Consultants of Texas (Retina Consultants of America), Houston, TX, USA
Charles C. Wykoff
Blanton Eye Institute, Houston Methodist Hospital, Houston, TX, USA
NIHR Moorfields Biomedical Research Centre, Moorfields Eye Hospital, London, UK
Sobha Sivaprasad
Cole Eye Institute, Cleveland Clinic, Cleveland, OH, USA
Peter Kaiser
Retinal Disorders and Ophthalmic Genetics, Stein Eye Institute, University of California, Los Angeles, CA, USA
David Sarraf
Department of Ophthalmology, Mayo Clinic, Rochester, MN, USA
Sophie Bakri
The Retina Service at Wills Eye Hospital, Philadelphia, PA, USA
Sunir J. Garg
Center for Ophthalmic Bioinformatics, Cole Eye Institute, Cleveland Clinic, Cleveland, OH, USA
Rishi P. Singh
Cleveland Clinic Lerner College of Medicine, Cleveland, OH, USA
Department of Ophthalmology, University of Bonn, Boon, Germany
Frank G. Holz
Singapore Eye Research Institute, Singapore, Singapore
Tien Y. Wong
Singapore National Eye Centre, Duke-NUD Medical School, Singapore, Singapore
You can also search for this author in PubMed Google Scholar
- Varun Chaudhary
- , Mohit Bhandari
- , Charles C. Wykoff
- , Sobha Sivaprasad
- , Lehana Thabane
- , Peter Kaiser
- , David Sarraf
- , Sophie Bakri
- , Sunir J. Garg
- , Rishi P. Singh
- , Frank G. Holz
- , Tien Y. Wong
- & Robyn H. Guymer
Contributions
YC was responsible for the conception of idea, writing of manuscript and review of manuscript. MRP was responsible for the conception of idea, and review of the manuscript. VC was responsible for conception of idea, and review of manuscript. MB was responsible for conception of idea, and review of manuscript. RHG was responsible for critical review and feedback on manuscript. LT was responsible for critical review and feedback on manuscript.
Corresponding author
Correspondence to Varun Chaudhary .
Ethics declarations
Competing interests.
YC: Nothing to disclose. MRP: Nothing to disclose. RHG: Advisory boards: Bayer, Novartis, Apellis, Roche, Genentech Inc. LT: Nothing to disclose. MB: Research funds: Pendopharm, Bioventus, Acumed – unrelated to this study. VC: Advisory Board Member: Alcon, Roche, Bayer, Novartis; Grants: Bayer, Novartis – unrelated to this study.
Additional information
Publisher’s note Springer Nature remains neutral with regard to jurisdictional claims in published maps and institutional affiliations.
The original online version of this article was revised: in part 'TIP 4: UNDERSTAND SUBGROUPS', the phrase "In our example, Study #3 only studied patients over 65 years" was corrected to read "In our example, Study #3 only studied patients who were equal and below 65 years".
Rights and permissions
Reprints and permissions
About this article
Cite this article.
Chang, Y., Phillips, M.R., Guymer, R.H. et al. The 5 min meta-analysis: understanding how to read and interpret a forest plot. Eye 36 , 673–675 (2022). https://doi.org/10.1038/s41433-021-01867-6
Download citation
Received : 11 November 2021
Revised : 12 November 2021
Accepted : 16 November 2021
Published : 05 January 2022
Issue Date : April 2022
DOI : https://doi.org/10.1038/s41433-021-01867-6
Share this article
Anyone you share the following link with will be able to read this content:
Sorry, a shareable link is not currently available for this article.
Provided by the Springer Nature SharedIt content-sharing initiative
This article is cited by
Estimate the burden of malnutrition among children with cerebral palsy in sub-saharan africa: a systematic review with meta-analysis.
- Ermias Sisay Chanie
- Natnael Moges
- Sewunt Sisay Chanie
Scientific Reports (2024)
Surrogate markers of metabolic syndrome and insulin resistance in children and young adults with type 1 diabetes: a systematic review & meta-analysis (MetS and IR in T1DM)
- Sukeshini B. Khandagale
- Vinesh S. Kamble
- Satyajeet P. Khare
International Journal of Diabetes in Developing Countries (2023)
Quick links
- Explore articles by subject
- Guide to authors
- Editorial policies

Log in using your username and password
- Search More Search for this keyword Advanced search
- Latest content
- Current issue
- Write for Us
- BMJ Journals More You are viewing from: Google Indexer
You are here
- Volume 16, Issue 1
What is meta-analysis?
- Article Text
- Article info
- Citation Tools
- Rapid Responses
- Article metrics

- Allison Shorten 1 ,
- Brett Shorten 2
- 1 School of Nursing , Yale University , New Haven, Connecticut , USA
- 2 Informed Health Choices Trust, Wollongong, New South Wales, Australia
- Correspondence to : Dr Allison Shorten Yale University School of Nursing, 100 Church Street South, PO Box 9740, New Haven, CT 06536, USA; allison.shorten{at}yale.edu
https://doi.org/10.1136/eb-2012-101118
Statistics from Altmetric.com
Request permissions.
If you wish to reuse any or all of this article please use the link below which will take you to the Copyright Clearance Center’s RightsLink service. You will be able to get a quick price and instant permission to reuse the content in many different ways.
When clinicians begin their search for the best available evidence to inform decision-making, they are usually directed to the top of the ‘evidence pyramid’ to find out whether a systematic review and meta-analysis have been conducted. The Cochrane Library 1 is fast filling with systematic reviews and meta-analyses that aim to answer important clinical questions and provide the most reliable evidence to inform practice and research. So what is meta-analysis and how can it contribute to practice?
The Five-step process
There is debate about the best practice for meta-analysis, however there are five common steps.
Step 1: the research question
A clinical research question is identified and a hypothesis proposed. The likely clinical significance is explained and the study design and analytical plan are justified.
Step 2: systematic review
A systematic review (SR) is specifically designed to address the research question and conducted to identify all studies considered to be both relevant and of sufficiently good quality to warrant inclusion. Often, only studies published in established journals are identified, but identification of ‘unpublished’ data is important to avoid ‘publication bias’ or exclusion of studies with negative findings. 4 Some meta-analyses only consider randomised control trials (RCTs) in the quest for highest quality evidence. Other types of ‘experimental’ and ‘quasi-experimental’ studies may be included if they satisfy the defined inclusion/exclusion criteria.
Step 3: data extraction
Once studies are selected for inclusion in the meta-analysis, summary data or outcomes are extracted from each study. In addition, sample sizes and measures of data variability for both intervention and control groups are required. Depending on the study and the research question, outcome measures could include numerical measures or categorical measures. For example, differences in scores on a questionnaire or differences in a measurement level such as blood pressure would be reported as a numerical mean. However, differences in the likelihood of being in one category versus another (eg, vaginal birth versus cesarean birth) are usually reported in terms of risk measures such as OR or relative risk (RR).
Step 4: standardisation and weighting studies
Having assembled all the necessary data, the fourth step is to calculate appropriate summary measures from each study for further analysis. These measures are usually called Effect Sizes and represent the difference in average scores between intervention and control groups. For example, the difference in change in blood pressure between study participants who used drug X compared with participants who used a placebo. Since units of measurement typically vary across included studies, they usually need to be ‘standardised’ in order to produce comparable estimates of this effect. When different outcome measures are used, such as when researchers use different tests, standardisation is imperative. Standardisation is achieved by taking, for each study, the mean score for the intervention group, subtracting the mean for the control group and dividing this result by the appropriate measure of variability in that data set.
The results of some studies need to carry more weight than others. Larger studies (as measured by sample sizes) are thought to produce more precise effect size estimates than smaller studies. Second, studies with less data variability, for example, smaller SD or narrower CIs are often regarded as ‘better quality’ in study design. A weighting statistic that seeks to incorporate both these factors, known as inverse variance , is commonly used.
Step 5: final estimates of effect
The final stage is to select and apply an appropriate model to compare Effect Sizes across different studies. The most common models used are Fixed Effects and Random Effects models. Fixed Effects models are based on the ‘assumption that every study is evaluating a common treatment effect’. 5 This means that the assumption is that all studies would estimate the same Effect Size were it not for different levels of sample variability across different studies. In contrast, the Random Effects model ‘assumes that the true treatment effects in the individual studies may be different from each other’. 5 and attempts to allow for this additional source of interstudy variation in Effect Sizes . Whether this latter source of variability is likely to be important is often assessed within the meta-analysis by testing for ‘heterogeneity’.
Forest plot
The final estimates from a meta-analysis are often graphically reported in the form of a ‘Forest Plot’.
In the hypothetical Forest Plot shown in figure 1 , for each study, a horizontal line indicates the standardised Effect Size estimate (the rectangular box in the centre of each line) and 95% CI for the risk ratio used. For each of the studies, drug X reduced the risk of death (the risk ratio is less than 1.0). However, the first study was larger than the other two (the size of the boxes represents the relative weights calculated by the meta-analysis). Perhaps, because of this, the estimates for the two smaller studies were not statistically significant (the lines emanating from their boxes include the value of 1). When all the three studies were combined in the meta-analysis, as represented by the diamond, we get a more precise estimate of the effect of the drug, where the diamond represents both the combined risk ratio estimate and the limits of the 95% CI.
- Download figure
- Open in new tab
- Download powerpoint
Hypothetical Forest Plot
Relevance to practice and research
Many Evidence Based Nursing commentaries feature recently published systematic review and meta-analysis because they not only bring new insight or strength to recommendations about the most effective healthcare practices but they also identify where future research should be directed to bridge the gaps or limitations in current evidence. The strength of conclusions from meta-analysis largely depends on the quality of the data available for synthesis. This reflects the quality of individual studies and the systematic review. Meta-analysis does not magically resolve the problem of underpowered or poorly designed studies and clinicians can be frustrated to find that even when a meta-analysis has been conducted, all that the researchers can conclude is that the evidence is weak, there is uncertainty about the effects of treatment and that higher quality research is needed to better inform practice. This is still an important finding and can inform our practice and challenge us to fill the evidence gaps with better quality research in the future.
- ↵ The Cochrane Library . http://www.thecochranelibrary.com/view/0/index.html (accessed 23 Oct 2012).
- Davey Smith G
- Davey Smoth G
- Higgins JPT ,
Competing interests None.
Read the full text or download the PDF:
Study Design 101: Meta-Analysis
- Case Report
- Case Control Study
- Cohort Study
- Randomized Controlled Trial
- Practice Guideline
- Systematic Review
Meta-Analysis
- Helpful Formulas
- Finding Specific Study Types
A subset of systematic reviews; a method for systematically combining pertinent qualitative and quantitative study data from several selected studies to develop a single conclusion that has greater statistical power. This conclusion is statistically stronger than the analysis of any single study, due to increased numbers of subjects, greater diversity among subjects, or accumulated effects and results.
Meta-analysis would be used for the following purposes:
- To establish statistical significance with studies that have conflicting results
- To develop a more correct estimate of effect magnitude
- To provide a more complex analysis of harms, safety data, and benefits
- To examine subgroups with individual numbers that are not statistically significant
If the individual studies utilized randomized controlled trials (RCT), combining several selected RCT results would be the highest-level of evidence on the evidence hierarchy, followed by systematic reviews, which analyze all available studies on a topic.
- Greater statistical power
- Confirmatory data analysis
- Greater ability to extrapolate to general population affected
- Considered an evidence-based resource
Disadvantages
- Difficult and time consuming to identify appropriate studies
- Not all studies provide adequate data for inclusion and analysis
- Requires advanced statistical techniques
- Heterogeneity of study populations
Design pitfalls to look out for
The studies pooled for review should be similar in type (i.e. all randomized controlled trials).
Are the studies being reviewed all the same type of study or are they a mixture of different types?
The analysis should include published and unpublished results to avoid publication bias.
Does the meta-analysis include any appropriate relevant studies that may have had negative outcomes?
Fictitious Example
Do individuals who wear sunscreen have fewer cases of melanoma than those who do not wear sunscreen? A MEDLINE search was conducted using the terms melanoma, sunscreening agents, and zinc oxide, resulting in 8 randomized controlled studies, each with between 100 and 120 subjects. All of the studies showed a positive effect between wearing sunscreen and reducing the likelihood of melanoma. The subjects from all eight studies (total: 860 subjects) were pooled and statistically analyzed to determine the effect of the relationship between wearing sunscreen and melanoma. This meta-analysis showed a 50% reduction in melanoma diagnosis among sunscreen-wearers.
Real-life Examples
Goyal, A., Elminawy, M., Kerezoudis, P., Lu, V., Yolcu, Y., Alvi, M., & Bydon, M. (2019). Impact of obesity on outcomes following lumbar spine surgery: A systematic review and meta-analysis. Clinical Neurology and Neurosurgery, 177 , 27-36. https://doi.org/10.1016/j.clineuro.2018.12.012
This meta-analysis was interested in determining whether obesity affects the outcome of spinal surgery. Some previous studies have shown higher perioperative morbidity in patients with obesity while other studies have not shown this effect. This study looked at surgical outcomes including "blood loss, operative time, length of stay, complication and reoperation rates and functional outcomes" between patients with and without obesity. A meta-analysis of 32 studies (23,415 patients) was conducted. There were no significant differences for patients undergoing minimally invasive surgery, but patients with obesity who had open surgery had experienced higher blood loss and longer operative times (not clinically meaningful) as well as higher complication and reoperation rates. Further research is needed to explore this issue in patients with morbid obesity.
Nakamura, A., van Der Waerden, J., Melchior, M., Bolze, C., El-Khoury, F., & Pryor, L. (2019). Physical activity during pregnancy and postpartum depression: Systematic review and meta-analysis. Journal of Affective Disorders, 246 , 29-41. https://doi.org/10.1016/j.jad.2018.12.009
This meta-analysis explored whether physical activity during pregnancy prevents postpartum depression. Seventeen studies were included (93,676 women) and analysis showed a "significant reduction in postpartum depression scores in women who were physically active during their pregnancies when compared with inactive women." Possible limitations or moderators of this effect include intensity and frequency of physical activity, type of physical activity, and timepoint in pregnancy (e.g. trimester).
Related Terms
A document often written by a panel that provides a comprehensive review of all relevant studies on a particular clinical or health-related topic/question.
Publication Bias
A phenomenon in which studies with positive results have a better chance of being published, are published earlier, and are published in journals with higher impact factors. Therefore, conclusions based exclusively on published studies can be misleading.
Now test yourself!
1. A Meta-Analysis pools together the sample populations from different studies, such as Randomized Controlled Trials, into one statistical analysis and treats them as one large sample population with one conclusion.
a) True b) False
2. One potential design pitfall of Meta-Analyses that is important to pay attention to is:
a) Whether it is evidence-based. b) If the authors combined studies with conflicting results. c) If the authors appropriately combined studies so they did not compare apples and oranges. d) If the authors used only quantitative data.
Evidence Pyramid - Navigation
- Meta- Analysis
- Case Reports
- << Previous: Systematic Review
- Next: Helpful Formulas >>

- Last Updated: Sep 25, 2023 10:59 AM
- URL: https://guides.himmelfarb.gwu.edu/studydesign101

- Himmelfarb Intranet
- Privacy Notice
- Terms of Use
- GW is committed to digital accessibility. If you experience a barrier that affects your ability to access content on this page, let us know via the Accessibility Feedback Form .
- Himmelfarb Health Sciences Library
- 2300 Eye St., NW, Washington, DC 20037
- Phone: (202) 994-2850
- [email protected]
- https://himmelfarb.gwu.edu
- - Google Chrome
Intended for healthcare professionals
- Access provided by Google Indexer
- My email alerts
- BMA member login
- Username * Password * Forgot your log in details? Need to activate BMA Member Log In Log in via OpenAthens Log in via your institution

Search form
- Advanced search
- Search responses
- Search blogs
- Meta-analysis:...
Meta-analysis: Principles and procedures
- Related content
- Peer review
- Matthias Egger , reader in social medicine and epidemiology ( egger{at}bristol.ac.uk ) a ,
- George Davey Smith , professor of clinical epidemiology a ,
This is the second in a series of seven articles examining the procedures in conducting reliable meta-analysis in medical research
- a Department of Social Medicine, University of Bristol, Bristol BS8 2PR
- b Department of Primary Care and Population Sciences, Royal Free Hospital School of Medicine, London NW3 2PF
- Correspondence to: Dr Eggerm
Introduction
Meta-analysis is a statistical procedure that integrates the results of several independent studies considered to be “combinable.” 1 Well conducted meta-analyses allow a more objective appraisal of the evidence than traditional narrative reviews, provide a more precise estimate of a treatment effect, and may explain heterogeneity between the results of individual studies. 2 Ill conducted meta-analyses, on the other hand, may be biased owing to exclusion of relevant studies or inclusion of inadequate studies. 3 Misleading analyses can generally be avoided if a few basic principles are observed. In this article we discuss these principles, along with the practical steps in performing meta-analysis.
Observational study of evidence
Meta-analysis should be viewed as an observational study of the evidence. The steps involved are similar to any other research undertaking: formulation of the problem to be addressed, collection and analysis of the data, and reporting of the results. Researchers should write in advance a detailed research protocol that clearly states the objectives, the hypotheses to be tested, the subgroups of interest, and the proposed methods and criteria for identifying and selecting relevant studies and extracting and analysing information.
As with criteria for including and excluding patients in clinical studies, eligibility criteria have to be defined for the data to be included. Criteria relate to the quality of trials and to the combinability of treatments, patients, outcomes, and lengths of follow up. Quality and design features of a study can influence the results. 4 5 Ideally, researchers should consider including only controlled trials with proper randomisation of patients that report on all initially included patients according to the intention to treat principle and with an objective, preferably blinded, outcome assessment. 6 Assessing the quality of a study can be a subjective process, however, especially since the information reported is often inadequate for this purpose. 7 It is therefore preferable to define only basic inclusion criteria and to perform a thorough sensitivity analysis (see below).
The strategy for identifying the relevant studies should be clearly delineated. In particular, it has to be decided whether the search will be extended to include unpublished studies, as their results may systematically differ from published trials. As will be discussed in later articles, a meta-analysis that is restricted to published evidence may produce distorted results owing to such publication bias. For locating published studies, electronic databases are useful, 8 but, used alone, they may miss a substantial proportion of relevant studies. 9 10 In an attempt to identify all published controlled trials, the Cochrane Collaboration has embarked on an extensive manual search of medical journals published in English and many other languages. 11 The Cochrane Controlled Trials Register 12 is probably the best single electronic source of trials; however, citation indices and the bibliographies of review articles, monographs, and the located studies should also be scrutinised.
Summary points
Meta-analysis should be as carefully planned as any other research project, with a detailed written protocol being prepared in advance
The a priori definition of eligibility criteria for studies to be included and a comprehensive search for such studies are central to high quality meta-analysis
The graphical display of results from individual studies on a common scale is an important intermediate step, which allows a visual examination of the degree of heterogeneity between studies
Different statistical methods exist for combining the data, but there is no single “correct” method
A thorough sensitivity analysis is essential to assess the robustness of combined estimates to different assumptions and inclusion criteria
A standardised record form is needed for data collection. It is useful if two independent observers extract the data, to avoid errors. At this stage the quality of the studies may be rated, with one of several specially designed scales. 13 14 Blinding observers to the names of the authors and their institutions, the names of the journals, sources of funding, and acknowledgments leads to more consistent scores. 14 This entails either photocopying papers, removing the title page, and concealing journal identifications and other characteristics with a black marker, or scanning the text of papers into a computer and preparing standardised formats. 15 16
Standardised outcome measure
Individual results have to be expressed in a standardised format to allow for comparison between studies. If the end point is continuous—for example, blood pressure—the mean difference between the treatment and control groups is used. The size of a difference, however, is influenced by the underlying population value. An antihypertensive drug, for example, is likely to have a greater absolute effect on blood pressure in overtly hypertensive patients than in borderline hypertensive patients. Differences are therefore often presented in units of standard deviation. If the end point is binary—for example, disease versus no disease, or dead versus alive) then odds ratios or relative risks are often calculated (box). The odds ratio has convenient mathematical properties, which allow for ease in combining data and testing the overall effect for significance. Absolute measures, such as the absolute risk reduction or the number of patients needed to be treated to prevent one event, 17 are more helpful when applying results in clinical practice (see below).
MARK HUDSON
- Download figure
- Open in new tab
- Download powerpoint
Odds ratio or relative risk?
Odds and odds ratio
The odds is the number of patients who fulfil the criteria for a given endpoint divided by the number of patients who do not. For example, the odds of diarrhoea during treatment with an antibiotic in a group of 10 patients may be 4 to 6 (4 with diarrhoea divided by 6 without, 0.66); in a control group the odds may be 1 to 9 (0.11) (a bookmaker would refer to this as 9 to 1). The odds ratio of treatment to control group would be 6 (0.66÷0.11).
Risk and relative risk
The risk is the number of patients who fulfil the criteria for a given end point divided by the total number of patients. In the example above the risks would be 4 in 10 in the treatment group and 1 in 10 in the control group, giving a risk ratio, or relative risk, of 4 (0.4÷0.1).
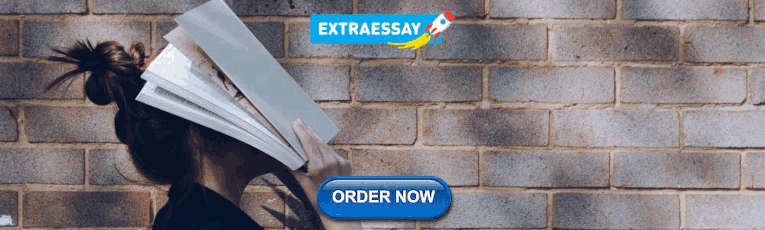
Statistical methods for calculating overall effect
The last step consists in calculating the overall effect by combining the data. A simple arithmetic average of the results from all the trials would give misleading results. The results from small studies are more subject to the play of chance and should therefore be given less weight. Methods used for meta-analysis use a weighted average of the results, in which the larger trials have more influence than the smaller ones. The statistical techniques to do this can be broadly classified into two models, 18 the difference consisting in the way the variability of the results between the studies is treated. The “fixed effects” model considers, often unreasonably, that this variability is exclusively due to random variation. 19 Therefore, if all the studies were infinitely large they would give identical results. The “random effects” model 20 assumes a different underlying effect for each study and takes this into consideration as an additional source of variation, which leads to somewhat wider confidence intervals than the fixed effects model. Effects are assumed to be randomly distributed, and the central point of this distribution is the focus of the combined effect estimate. Although neither of two models can be said to be “correct,” a substantial difference in the combined effect calculated by the fixed and random effects models will be seen only if studies are markedly heterogeneous. 18
Bayesian meta-analysis
Some statisticians feel that other statistical approaches are more appropriate than either of the above. One approach uses Bayes's theorem, named after an 18th century English clergyman. 21 Bayesian statisticians express their belief about the size of an effect by specifying some prior probability distribution before seeing the data, and then they update that belief by deriving a posterior probability distribution, taking the data into account. 22 Bayesian models are available under both the fixed and random effects assumption. 23 The confidence interval (or more correctly in bayesian terminology, the 95% credible interval, which covers 95% of the posterior probability distribution) will often be wider than that derived from using the conventional models because another component of variability, the prior distribution, is introduced. Bayesian approaches are controversial because the definition of prior probability will often be based on subjective assessments and opinion.
Heterogeneity between study results
If the results of the studies differ greatly then it may not be appropriate to combine the results. How to ascertain whether it is appropriate, however, is unclear. One approach is to examine statistically the degree of similarity in the studies' outcomes—in other words, to test for heterogeneity across studies. In such procedures, whether the results of a study reflect a single underlying effect, rather than a distribution of effects, is assessed. If this test shows homogeneous results then the differences between studies are assumed to be a consequence of sampling variation, and a fixed effects model is appropriate. If, however, the test shows that significant heterogeneity exists between study results then a random effects model is advocated. A major limitation with this approach is that the statistical tests lack power—they often fail to reject the null hypothesis of homogeneous results even if substantial differences between studies exist. Although there is no statistical solution to this issue, heterogeneity between study results should not be seen as purely a problem for meta-analysis—it also provides an opportunity for examining why treatment effects differ in different circumstances. Heterogeneity should not simply be ignored after a statistical test is applied; rather, it should be scrutinised, with an attempt to explain it. 24
Total mortality from trials of ß blockers in secondary prevention after myocardial infarction. The black square and horizontal line correspond to odds ratio and 95% confidence interval for each trial. The size of the black square reflects the weight of each trial. The diamond represents the combined odds ratio and 95% confidence interval, showing 22% a reduction in the odds of death (references are available from the authors)
Graphic display
Results from each trial are usefully graphically displayed, together with their confidence intervals. Figure 3 represents a meta-analysis of 17 trials of ß blockers in secondary prevention after myocardial infarction. Each study is represented by a black square and a horizontal line, which correspond to the point estimate and the 95% confidence intervals of the odds ratio. The 95% confidence intervals would contain the true underlying effect in 95% of the occasions if the study was repeated again and again. The solid vertical line corresponds to no effect of treatment (odds ratio 1.0). If the confidence interval includes 1, then the difference in the effect of experimental and control treatment is not significant at conventional levels (P>0.05). The area of the black squares reflects the weight of the study in the meta-analysis. The confidence interval of all but two studies cross this line, indicating that the effect estimates were non-significant (P>0.05).
The diamond represents the combined odds ratio, calculated using a fixed effects model, with its 95% confidence interval. The combined odds ratio shows that oral ß blockade starting a few days to a few weeks after the acute phase reduces subsequent mortality by an estimated 22% (odds ratio 0.78; 95% confidence interval 0.71 to 0.87). A dashed line is plotted vertically through the combined odds ratio. This line crosses the horizontal lines of all individual studies except one (N). This indicates a fairly homogenous set of studies. Indeed, the test for heterogeneity gives a non-significant P value of 0.2.
A logarithmic scale was used for plotting the odds ratios in figure 3 . There are several reasons that ratio measures are best plotted on logarithmic scales. 25 Most importantly, the value of an odds ratio and its reciprocal—for example, 0.5 and 2—which represent odds ratios of the same magnitude but opposite directions, will be equidistant from 1.0. Studies with odds ratios below and above 1.0 will take up equal space on the graph and thus look equally important. Also, confidence intervals will be symmetrical around the point estimate.
Relative and absolute measures of effect
Repeating the analysis by using relative risk instead of the odds ratio gives an overall relative risk of 0.80 (95% confidence interval 0.73 to 0.88). The odds ratio is thus close to the relative risk, as expected when the outcome is relatively uncommon (see box). The relative risk reduction, obtained by subtracting the relative risk from 1 and expressing the result as a percentage, is 20% (12% to 27%). The relative measures used in this analysis ignore the absolute underlying risk. The risk of death among patients who have survived the acute phase of myocardial infarction, however, varies widely. 26 For example, among patients with three or more cardiac risk factors the probability of death at two years after discharge ranged from 24% to 60%. 26 Conversely, two year mortality among patients with no risk factors was less than 3%. The absolute risk reduction or risk difference reflects both the underlying risk without treatment and the risk reduction associated with treatment. Taking the reciprocal of the risk difference gives the “number needed to treat” (the number of patients needed to be treated to prevent one event). 17
For a baseline risk of 1% a year, the absolute risk difference shows that two deaths are prevented per 1000 patients treated ( 1 ). This corresponds to 500 patients (1÷0.002) treated for one year to prevent one death. Conversely, if the risk is above 10%, less than 50 patients have to be treated to prevent one death. Many clinicians would probably decide not to treat patients at very low risk, given the large number of patients that have to be exposed to the adverse effects of ß blockade to prevent one death. Appraising the number needed to treat from a patient's estimated risk without treatment and the relative risk reduction with treatment is a helpful aid when making a decision in an individual patient. A nomogram that facilitates calculation of the number needed to treat at the bedside has recently been published. 27
β Blockade in secondary prevention after myocardial infarction-absolute risk reductions and numbers needed to treat for one year to prevent one death for different levels of mortality in control group
- View inline
Meta-analysis using absolute effect measures such as the risk difference may be useful to illustrate the range of absolute effects across studies. The combined risk difference (and the number needed to treat calculated from it) will, however, be essentially determined by the number and size of trials in patients at low, intermediate, or high risk. Combined results will thus be applicable only to patients at levels of risk corresponding to the average risk of the trials included. It is therefore generally more meaningful to use relative effect measures for summarising the evidence and absolute measures for applying it to a concrete clinical or public health situation.
Sensitivity analysis
Opinions will often diverge on the correct method for performing a particular meta-analysis. The robustness of the findings to different assumptions should therefore always be examined in a thorough sensitivity analysis. This is illustrated in figure 4 for the meta-analysis of ß blockade after myocardial infarction. Firstly, the overall effect was calculated by different statistical methods, by using both a fixed and a random effects model. The 4 shows that the overall estimates are virtually identical and that confidence intervals are only slightly wider with the random effects model. This is explained by the relatively small amount of variation between trials in this meta-analysis.
Sensitivity analysis of meta-analysis of ß blockers in secondary prevention after myocardial infarction (see text for explanation)
Secondly, methodological quality was assessed in terms of how patients were allocated to active treatment or control groups, how outcome was assessed, and how the data were analysed. 6 The maximum credit of nine points was given if patient allocation was truly random, if assessment of vital status was independent of treatment group, and if data from all patients initially included were analysed according to the intention to treat principle. Figure 4 shows that the three low quality studies (≤7 points) showed more benefit than the high quality trials. Exclusion of these three studies, however, leaves the overall effect and the confidence intervals practically unchanged.
Thirdly, significant results are more likely to get published than non-significant findings, 28 and this can distort the findings of meta-analyses. The presence of such publication bias can be identified by stratifying the analysis by study size—smaller effects can be significant in larger studies. If publication bias is present, it is expected that, of published studies, the largest ones will report the smallest effects. Figure 4 shows that this is indeed the case, with the smallest trials (50 or fewer deaths) showing the largest effect. However, exclusion of the smallest studies has little effect on the overall estimate.
Finally, two studies (J and N; see 3 ) were stopped earlier than anticipated on the grounds of the results from interim analyses. Estimates of treatment effects from trials that were stopped early are liable to be biased away from the null value. Bias may thus be introduced in a meta-analysis that includes such trials. 29 Exclusion of these trials, however, affects the overall estimate only marginally.
The sensitivity analysis thus shows that the results from this meta-analysis are robust to the choice of the statistical method and to the exclusion of trials of poorer quality or of studies stopped early. It also suggests that publication bias is unlikely to have distorted its findings.
Conclusions
Meta-analysis should be seen as structuring the processes through which a thorough review of previous research is carried out. The issues of completeness and combinability of evidence, which need to be considered in any review, 30 are made explicit. Was it sensible to have combined the individual trials that comprise the meta-analysis? How robust is the result to changes in assumptions? Does the conclusion reached make clinical and pathophysiological sense? Finally, has the analysis contributed to the process of making rational decisions about the management of patients? It is these issues that we explore further in later articles in this series.
Acknowledgments
Funding: ME was supported by the Swiss National Science Foundation.
The department of social medicine at the University of Bristol and the department of primary care and population sciences at the Royal Free Hospital School of Medicine, London, are part of the Medical Research Council's health services research collaboration.
- Davey Smith G
- Davey Smith G ,
- Schneider M ,
- Chalmers TC ,
- Schulz KF ,
- Chalmers I ,
- Prendiville W ,
- Elbourne D ,
- Eastwood S ,
- Greenhalgh T
- Dickersin K ,
- Chalmers TC
- Scherer R ,
- 12. ↵ The Cochrane Controlled Trials Register . In: Cochrane Library. CD ROM and online. Cochrane Collaboration (issue 1) . Oxford : Update Software , 1997 .
- Tugwell P ,
- Jenkinson C ,
- Reynolds DJM ,
- Gavaghan DJ ,
- Klassen T ,
- Le Lorier J ,
- Laupacis A ,
- Sackett DL ,
- Berlin JA ,
- Collins R ,
- DerSimonian R ,
- Lilford RJ ,
- Braunholtz D
- Hasselblad V ,
- Galbraith R
- Multicenter Postinfarction Research Group
- Chatellier G ,
- Zapletal E ,
- Lemaitre D ,
- Easterbrook PJ ,
- Gopalan R ,
- Matthews DR
- Fleming TR ,
The anatomy of an award-winning meta-analysis: Recommendations for authors, reviewers, and readers of meta-analytic reviews
- Open access
- Published: 05 January 2021
- Volume 52 , pages 23–44, ( 2021 )
Cite this article
You have full access to this open access article
- Piers Steel 1 ,
- Sjoerd Beugelsdijk 2 &
- Herman Aguinis 3
19k Accesses
66 Citations
17 Altmetric
Explore all metrics
Meta-analyses summarize a field’s research base and are therefore highly influential. Despite their value, the standards for an excellent meta-analysis, one that is potentially award-winning, have changed in the last decade. Each step of a meta-analysis is now more formalized, from the identification of relevant articles to coding, moderator analysis, and reporting of results. What was exemplary a decade ago can be somewhat dated today. Using the award-winning meta-analysis by Stahl et al. (Unraveling the effects of cultural diversity in teams: A meta-analysis of research on multicultural work groups. Journal of International Business Studies, 41(4):690–709, 2010) as an exemplar, we adopted a multi-disciplinary approach (e.g., management, psychology, health sciences) to summarize the anatomy (i.e., fundamental components) of a modern meta-analysis, focusing on: (1) data collection (i.e., literature search and screening, coding), (2) data preparation (i.e., treatment of multiple effect sizes, outlier identification and management, publication bias), (3) data analysis (i.e., average effect sizes, heterogeneity of effect sizes, moderator search), and (4) reporting (i.e., transparency and reproducibility, future research directions). In addition, we provide guidelines and a decision-making tree for when even foundational and highly cited meta-analyses should be updated. Based on the latest evidence, we summarize what journal editors and reviewers should expect, authors should provide, and readers (i.e., other researchers, practitioners, and policymakers) should consider about meta-analytic reviews.
Les méta-analyses résument la base d’un domaine de recherche et sont donc très influentes. Malgré leur valeur, les normes d’une excellente méta-analyse, celle qui peut être récompensée, ont changé au cours de la dernière décennie. Chaque étape d’une méta-analyse est désormais plus formalisée, de l’identification des articles pertinents au codage, à l’analyse des modérateurs et à la communication des résultats. Ce qui était exemplaire il y a dix ans peut être quelque peu daté aujourd’hui. En prenant comme exemple la méta-analyse primée de Stahl, Maznevski, Voigt et Jonsen (2010), nous avons adopté une approche pluridisciplinaire (par exemple, management, psychologie, sciences de la santé) pour résumer l’anatomie (c’est-à-dire les composantes fondamentales) d’une méta-analyse moderne, en nous concentrant sur : (i) la collecte de données (c’est-à-dire la recherche et le tri de la littérature, le codage), (ii) la préparation des données (c’est-à-dire le traitement des tailles d’effet multiples, l’identification et la gestion des valeurs aberrantes, le biais de publication), (iii) l’analyse des données (c’est-à-dire les tailles d’effet moyennes, l’hétérogénéité des tailles d’effet, la recherche de modérateurs) et (iv) la production de rapports (c’est-à-dire la transparence et la reproductibilité, les futures orientations de recherche). En outre, nous fournissons des lignes directrices et un arbre décisionnel pour les cas où même les méta-analyses fondamentales et fortement citées devraient être mises à jour. Sur la base des dernières données, nous résumons ce à quoi les rédacteurs et les réviseurs de revues doivent s’attendre, ce que les auteurs doivent fournir et ce que les lecteurs (c’est-à-dire les autres chercheurs, les praticiens et les décideurs politiques) doivent envisager concernant les études méta-analytiques.
Los meta-análisis resumen la base de investigación de un campo y, por esto, son altamente influyentes. A pesar de su valor, los estándares para un meta-análisis excelente, uno que sea potencialmente galardonado, han cambiado en la última década. Cada paso de un meta-análisis está ahora más formalizado, desde la identificación de los artículos relevantes para codificar, el análisis del moderador, y el reporte de los resultados. Lo que era ejemplar hace una década atrás puede estar obsoleto hoy. Usando el análisis galardonado de Stahl, Maznevski, Voigt, y Jonsen (2010) como ejemplar, adoptamos un enfoque multidisciplinario (por ejemplo, administración, psicología, ciencias de la salud) para resumir la anatomía (es decir, los componentes fundamentales) de un meta-análisis moderno, enfocándonos en: (i) recolección de datos (es decir, la búsqueda de literatura y examinación, codificación), (ii) preparación de los datos (es decir, los tratamientos de efectos de los tamaños de efecto múltiples, identificación y gestión de atípicos, sesgo de publicación), (iii) análisis de datos (es decir, tamaños del efecto promedio, la heterogeneidad de los tamaños del efecto, y la búsqueda de moderador), y (iv) reporte (es decir, transparencia y reproducibilidad, direcciones de investigación futura). Asimismo, proporcionamos lineamientos y un árbol de toma de decisiones para cuando se deben actualizar los meta-análisis de base y altamente citados. Sobre la base de las últimas evidencias, resumimos lo que los editores y revisores de revistas deben esperar, los autores deben proporcionar, y lectores (es decir, otros investigadores, profesionales, y formuladores de políticas) deben considerar acerca de las revisiones meta-analíticas.
Meta-análises resumem a base de pesquisa de um campo e, portanto, são altamente influentes. Apesar de seu valor, os padrões para uma excelente meta-análise, uma que seja potencialmente digna de ser premiada, mudaram na última década. Cada etapa de uma meta-análise é agora mais formalizada, desde a identificação de artigos relevantes até a codificação, análise do moderador e relato dos resultados. O que era exemplar há uma década pode ser um pouco ultrapassado hoje. Usando a premiada meta-análise de Stahl, Maznevski, Voigt e Jonsen (2010) como um exemplo, adotamos uma abordagem multidisciplinar (por exemplo, gestão, psicologia, ciências da saúde) para resumir a anatomia (ou seja, componentes fundamentais) de uma meta-análise moderna, com foco em: (i) coleta de dados (ou seja, pesquisa da literatura e triagem, codificação), (ii) preparação de dados (ou seja, tratamento de múltiplas magnitudes de efeito, identificação e gestão de outliers, viés de publicação), (iii) análise de dados (isto é, médias magnitudes de efeito, heterogeneidade de magnitudes de efeito, pesquisa de moderador) e (iv) relatórios (isto é, transparência e reprodutibilidade, direções de pesquisas futuras). Além disso, fornecemos diretrizes e uma árvore de tomada de decisão para quando até mesmo meta-análises fundamentais e altamente citadas devem ser atualizadas. Com base em evidências mais recentes, resumimos o que editores de periódicos e revisores devem esperar, autores devem fornecer e os leitores (ou seja, outros pesquisadores, praticantes e formuladores de políticas) devem considerar a respeito de revisões meta-analíticas.
荟萃分析总结一个领域的研究基础, 因而具有很大的影响。尽管有其价值, 有潜能获奖的出色的荟萃分析标准在过去十年中有了变化。荟萃分析的每个步骤, 从相关文章的识别到编码、调节变数分析、以及结果报告, 现在都更加正式化。十年前的样板在今天可能是过时的。我们以Stahl、Maznevski、Voigt和Jonsen(2010)获奖的荟萃分析为例, 采用多学科方法(例如管理学、心理学、健康科学)来总结现代荟萃分析的解剖结构(即基本成分) , 重点关注:(i)数据收集(即文献搜索与筛选, 编码), (ii) 数据准备(即多效应大小的处理, 异常值的识别与管理, 发布偏差), (iii)数据分析(即平均效应大小, 效果大小的异质性, 调节变数的搜索)和(iv)报告(即透明度和可重复性, 未来研究方向)。此外, 我们还提供了何时应更新甚至是基础的和高引用率的荟萃分析的指南和决策树。根据最新证据, 我们针对荟萃分析, 综述总结了期刊编辑和审阅者应有的期望, 作者应提供的内容, 以及读者(即其他研究人员、从业人员和政策制定者)应有的考虑。
Similar content being viewed by others
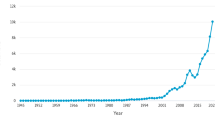
Literature reviews as independent studies: guidelines for academic practice
Sascha Kraus, Matthias Breier, … João J. Ferreira
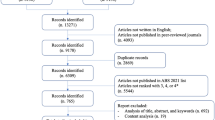
Authoritarian leadership styles and performance: a systematic literature review and research agenda
Elia Pizzolitto, Ida Verna & Michelina Venditti
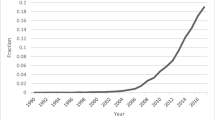
Mixed methods research: what it is and what it could be
Rob Timans, Paul Wouters & Johan Heilbron
Avoid common mistakes on your manuscript.
Introduction
Scientific knowledge is the result of a multi-generational collaboration where we cumulatively generate and connect findings gleaned from individual studies (Beugelsdijk, van Witteloostuijn, & Meyer, 2020 ). Meta-analysis is critical to this process, being the methodology of choice to quantitatively synthesize existing empirical evidence and draw evidence-based recommendations for practice and policymaking (Aguinis, Pierce, Bosco, Dalton, & Dalton, 2011 ; Davies, Nutley, & Smith, 1999 ). Although meta-analyses were first formally conducted in the 1970s, it was not until the following decade that they began to be promoted (e.g., Hedges, 1982 ; Hedges & Olkin, 1985 ; Hunter, Schmidt, & Jackson, 1982 ; Rosenthal & Rubin, 1982 ), which subsequently spread across almost all quantitative fields, including business and management (Cortina, Aguinis, & DeShon, 2017 ). Aguinis, Pierce, et al. ( 2011 ) reported a staggering increase from 55 business and management-related articles using meta-analysis for the 1970–1985 period to 6918 articles for the 1994–2009 period.
Although there are several notable examples of meta-analysis, there are many more that are of suspect quality (Ionnadis, 2016 ). Consequently, we take the opportunity to discuss components of a modern meta-analysis, noting how the methodology has continued to advance considerably (e.g., Havránek et al., 2020 ). To illustrate the evolution of meta-analysis, we use the award-winning contribution by Stahl, Maznevski, Voigt and Jonsen ( 2010 ) who effectively summarized and made sense of the voluminous correlational literature on team diversity and cultural differences.
It is difficult to overstate how relevant Stahl et al.’s ( 2010 ) topic of diversity has become. Having a diverse workforce that reflects the larger society has only grown as a social justice issue over the last decade (Fujimoto, Härtel, & Azmat, 2013 ; Tasheva & Hillman, 2019 ). Furthermore, team diversity also has potential organizational benefits, the “value-in-diversity” thesis (Fine, Sojo, & Lawford-Smith, 2020 ). Consequently, their meta-analysis speaks to the innumerable institutional efforts to increase diversity as well as those who question these efforts’ effectiveness (e.g., the “Google’s Ideological Echo Chamber” memo that challenged whether increasing gender diversity in the programming field would increase performance; Fortune, 2017 ).
The focus of our article is on meta-analytic methodology. Stahl et al. make a useful contrast as, although its methodology was advanced for its time, the field has evolved rapidly. We draw upon recently established developments to contrast traditional versus modern meta-analytic methodology, summarizing our recommendations in Table 1 . Our goal is to assist authors planning to carry out a meta-analytical study, journal editors and reviewers asked to evaluate their resulting work, and consumers of the knowledge produced (i.e., other researchers, practitioners, and policymakers) highlighting common areas of concern. Accordingly, we offer recommendations and, perhaps more importantly, specific implementation guidelines that make our recommendations concrete, tangible, and realistic.
Modern methodology
Using Stahl et al. as an exemplar, we summarize the anatomy (i.e., fundamental components) of a modern meta-analysis, focusing on: (1) data collection (i.e., literature search and screening, coding), (2) data preparation (i.e., treatment of multiple effect sizes, outlier identification and management, publication bias), (3) data analysis (i.e., average effect sizes, heterogeneity of effect sizes, moderator search), and (4) reporting (i.e., transparency and reproducibility, future research directions). Stahl et al. graciously shared their database with us, which we re-analyzed using more recently developed procedures.
Stage 1: Data Collection
Data collection is the creation of the database that enables a meta-analysis. Inherently, there is tension between making a meta-analysis manageable, that is small enough that it can be finished, and making it comprehensive and broad to make a meaningful contribution. With the research base growing exponentially but research time and efficiency remaining relatively constant, the temptation is to limit the topic arbitrarily by journals, by language, by publication year, or by the way constructs are measured (e.g., specific measure of cultural distance). The risk is that the meta-analysis is so narrowly conceived that, as Bem ( 1995 : 172) puts it, “Nobody will give a damn.” One solution is to acknowledge that meta-analysis is increasingly becoming a “Big Science” project, requiring larger groups of collaborators. Although well-funded meta-analytic laboratories do exist, they are almost exclusively in the medical field. In business, it is likely that influential reviews will increasingly become the purview of well-managed academic crowdsourcing projects (i.e., Massive Peer Production) whose leaders can tackle larger topics (i.e., community augmented meta-analyses; Tsuji, Bergmann, & Cristia, 2014 ), such as exemplified by Many Labs (e.g., Klein et al., 2018 ).
With a large team or a smaller but more dedicated group, researchers have a freer hand in determining how to define the topic and the edges that define the literature. To this end, Tranfield, Denyer and Smart ( 2003 ) discussed that the identification of a topic, described as Phase 0, “may be an iterative process of definition, clarification, and refinement” (Tranfield et al., 2003 : 214). Relatedly, Siddaway, Wood and Hedges ( 2019 ) highlighted scoping and planning as key stages that precede the literature search and screening procedures. Indeed, it is useful to conduct a pre-meta-analysis scoping study, ensuring that the research question is small enough to be manageable, large enough to be meaningful, there is sufficient research base for analysis, and that other recent or carried out reviews have not already addressed the same topic. Denyer and Tranfield ( 2008 ) stressed how an author’s prior and prolonged interest in the topic is immensely helpful, exemplified by a history of publishing in a particular domain. In fact, deep familiarity with the nuances of a field assists in every step of a meta-analytic review. Consistent with this point, Stahl et al.’s References section shows this familiarity, containing multiple publications by the first two authors. Gunter Stahl has emphasized cultural values while Martha Maznevski has focused on team development, with enough overlap between the two that Maznevski published in a handbook edited by Stahl (Maznevski, Davison, & Jonsen, 2006 ).
Once a worthy topic within one’s capabilities has been established, the most arduous part of meta-analysis begins. First is the literature search and screening (i.e., locating and obtaining relevant studies) and second is coding (i.e., extracting the data contained within the primary studies).
Literature search and screening
Bosco, Steel, Oswald, Uggerslev and Field ( 2015 ) alluded to academia’s “Tower of Babel” or what Larsen and Bong ( 2016 ) more formally labeled as the construct identity fallacy. These terms convey the idea that there can be dozens of terms and scores of measures for the same construct (i.e., jingle) and different constructs can go by the same name (i.e., jangle), such as cultural distance versus the Kogut and Singh index (Beugelsdijk, Ambos, & Nell, 2018 ; Maseland, Dow, & Steel, 2018 ). Furthermore, many research fields have exploded in size, almost exponentially (Bornmann & Mutz, 2015 ), making a literature search massively harder. Then there are the numerous databases within which the targeted articles may be hidden due to their often flawed or archaic organization (Gusenbauer & Haddaway, 2020 ), especially their keyword search functions. As per Spellman’s ( 2015 ) appraisal, “Our keyword system has become worthless, and we now rely too much on literal word searches that do not find similar (or analogous) research if the same terms are not used to describe it” (Spellman, 2015 : 894).
Given this difficulty and that literature searches often occur in an iterative manner, where researchers are learning the parameters of the search as they conduct them (i.e., “Realist Search”; Booth, Briscoe, & Wright, 2020 ), there is an incentive to filter or simplify the procedure and to not properly document such a fundamentally flawed process so as to not leave it open to critique from reviewers’ potentially idealistic standards (Aguinis, Ramani, & Alabduljader, 2018 ). The result can be an implicit selection bias, where the body of articles is a subset of what is of interest (Lee, Bosco, Steel, & Uggerslev, 2017 ). Rothstein, Sutton and Borenstein ( 2005 ) described four types of bias: availability bias (selective inclusion of studies that are easily accessible to the researcher), cost bias (selective inclusion of studies that are available free or at low costs), familiarity bias (selective inclusion of studies only from one’s own field or discipline), and language bias (selective inclusion of studies published in English). The last of these is particularly common as well as particularly ironic in international business (IB) research. To this list, we would like to add citation bias due to The Matthew Effect (Merton, 1968 ). With increased public information on citation structures thanks to software such as Google Scholar, there is the risk of a selective inclusion of those studies that are heavily cited, at the expense of studies that have not been picked up (yet). Each of these biases can be addressed, respectively, by searching the grey literature, finding access to pay-walled scientific journals, including databases outside one’s discipline, engaging in translation (at least those languages used in multiple sources), and not using a low citation rate as an exclusion criterion.
How was Stahl et al.’s literature search process? Adept for its time. They drew from multiple databases, which is recommended (Harari, Parola, Hartwell, & Riegelman, 2020 ), and they supplemented with a variety of other techniques, including manual searches. They provided a sensible set of keywords but also contacted researchers operating in the team field to acquire the “grey literature” of obscure or unpublished works. Some other techniques could be added, such Ones, Viswesvaran and Schmidt’s ( 2017 ) suggestion that “snowballing” (aka “ancestry searching” or “pearl growing”; Booth, 2008 ) should be de rigueur . In other words, “by working from the more contemporary references for meta-analysis, tracking these references for the prior meta-analytic work on which they relied, and iteratively continuing this process, it is possible to identify a set of common early references with no published predecessors” (Aguinis, Dalton, Bosco, Pierce, & Dalton, 2011 : 9). At present, however, some of Stahl et al.’s efforts would likely be critiqued in terms of replicability or reproducibility and transparency (Aguinis et al., 2018 ; Beugelsdijk et al., 2020 ). For example, if the keywords “team” and “diversity” are entered as search terms, Google Scholar alone yields close to two million hits. Other screening processes must have occurred, though are not reported, reflected in that Stahl et al. provided a sampling of techniques designed to reassure reviewers that they made a concerted effort (e.g., “searches were performed on several different databases, including…. search strategies included…”, Stahl et al., 2010 : 697).
Presently, in efforts to increase transparency and replicability, the PRISMA (Preferred Reporting Items for Systematic reviews and Meta-Analyses) method is often recommended, which requires being extremely explicit about the exact databases, the exact search terms, and the exact results, including duplicates and filtering criteria (Moher, Liberati, Tetzlaff, & Altman, 2009 ). Although more onerous, the PRISMA-P version goes even further in terms of transparency, advocating pre-registering of the entire systematic review protocol encapsulated in a 17-item checklist (Moher et al., 2015 ). And, at present, the 2020 version of PRISMA recommends a 27-item checklist, not including numerous sub-items, again with the goal of improving the trustworthiness of systematic reviews (Page et al., 2020 ). Given the attempt to minimize decisions in situ, proper adherence to the PRISMA protocols can be difficult when searches occur in an iterative manner, as researchers find new terms or measures as promising leads for relevant papers. When this happens, especially during the later stages of data preparation, researchers face the dilemma of either re-conducting the entire search process with the added criteria (substantively increasing the workload) or ignoring the new terms or measures (leading to a less than exhaustive search). New software has been developed to help address that search processes can be informed simultaneously with implementation, such as www.covidence.org , www.hubmeta.com , or https://revtools.net/ (with many more options curated at http://systematicreviewtools.com/ , The Systematic Review Tool Box). They provide a computer-assisted walk-through of the search as well as a screening process, which starts with deduplication, and filtering on abstract or title, followed by full text filtering (with annotated decisions). Reviewers should expect that this information be reported in a supplemental file, along with the final list of all articles coded and details regarding effect sizes, sample sizes, measures, moderators, and other specific details that would enable readers to readily reproduce the creation of the meta-analytic database.
It is a challenge to determine that a search approach has been thorough and exhaustive, given that reviewers may have an incomplete understanding of the search criteria or of how many articles can be expected. In other words, although the authors may have reported detailed inclusion and exclusion criteria, as per MARS (Kepes, McDaniel, Brannick, & Banks, 2013 ), how can reviewers evaluate their adequacy? We anticipate that in the future this need for construct intimacy may be emphasized and a meta-analysis would require first drawing upon or even publishing a deep review of the construct. For example, prior to publishing their own award-winning monograph on Hofstede’s cultural value dimensions (Taras, Kirkman, & Steel, 2010 ), two of the authors published a review of how culture itself was assessed (Taras, Rowney, & Steel, 2009 ), as well as a critique of the strengths and challenges of Hofstede’s measure (Taras & Steel, 2009 ). Another example is a pre-meta-analytic review of institutional distance (Kostova, Beugelsdijk, Scott, Kunst, Chua, & van Essen, 2020 ), where several of the authors previously published on the topic (e.g., Beugelsdijk, Kostova, Kunst, Spadafora, & van Essen, 2018 ; Kostova, Roth, & Dacin, 2008 ; Scott, 2014 ). Once authors have demonstrated prolonged and even affectionate familiarity with the topic (“immersion in the literature”; DeSimone, Köhler, & Schoen, 2019 : 883), reviewers may be further reassured that the technical aspects of the search were adequately carried out if a librarian (i.e., an information specialist) was reported to be involved (Johnson & Hennessy, 2019 ).
Coding of the primary studies
Extracting all the information from a primary study can be a lengthy procedure, as a myriad of material is typically needed beyond the basics of sample size and the estimated size of a relationship between variables (i.e., correlation coefficient). This includes details required for psychometric corrections, conversion from different statistical outputs to a common effect size (e.g., r or d ), and study conditions and context that permit later moderator analysis (i.e., conditions under which a relationship between variables is weaker or stronger). Properly implementing procedures such as applying psychometric corrections for measurement error and range restriction is not always straightforward (Aguinis, Hill, & Bailey, 2021 ; Hunter, Schmidt, & Le, 2006 ; Schmidt & Hunter, 2015 ; Yuan, Morgeson, & LeBreton, 2020 ). However, while this used to be a manual process requiring intimate statistical knowledge (e.g., including knowledge of how to correct for various methodological and statistical artifacts), fortunately, this process is increasingly semi-automated. For example, the meta-analytic program psychmeta (the psychometric meta-analysis toolkit) provides conversion to correlations for “Cohen’s d, independent samples t values (or their p values), two-group one-way ANOVA F values (or their p values), 1 df χ 2 values (or their p values), odds ratios, log odds ratios, Fisher z , and the common language effect size (CLES, A, AUC)” (Dahlke & Wiernik, 2019 ).
However, a pernicious coding challenge is related to the literature search and screening process described earlier. For initial forays into a topic, a certain degree of conceptual “clumping” is necessary to permit sufficient studies for meta-analytic summary, in which we trade increased measurement variance for a larger database. As more studies become available, it is possible to make more refined choices and to tease apart broad constructs into component dimensions or adeptly merge selected measures to minimize mono-method bias (Podsakoff, MacKenzie, & Podsakoff, 2012 ). For example, Richard, Devinney, Yip and Johnson’s ( 2009 ) study on organizational performance found not all measures to be commensurable, such as return on total assets often being radically different from return on sales. As a result, only a subset of the obtained literature actually represents the target construct, and this subset can be difficult to determine.
Stahl et al. methodically reported how they coded cultural diversity as well as each of their dependent variables. This is an essential start, but, reflecting the previous problem of construct proliferation, more information regarding how each dependent variable was operationalized in each study would be a welcome addition. Although some information regarding the exact measures used is available directly from the authors, which was readily provided upon request, today many journals require these data to be perpetually archived and available through an Open Science repository. The issue of commensurability applies here, as one of Stahl et al.’s dependent variables was creativity. Ma’s ( 2009 ) meta-analysis on creativity divided the concept into three groups, with many separating problem-solving from artistic creativity. With only five studies on creativity available, mingling of different varieties of creativity is necessary. Still, it is important to note that Stahl et al. chose to treat studies that focused on the quality of ideas generated (e.g., Cady & Valentine, 1999 ) as an indicator of creativity along with more explicit measures, such as creativity of story endings (Paletz, Peng, Erez, & Maslach, 2004 ), leaving room for this to be re-explored as the corpus of results expanded.
To help alleviate concerns of commensurability, it is commendable that Stahl et al. used two independent raters to code the articles, documenting agreement using Cohen’s kappa. Notably, kappa is used to quantify interrater reliability for qualitative decisions, where there is a lack of an irrefutable gold standard or “the ‘correctness’ of ratings cannot be determined in a typical situation” (Sun, 2011 : 147). Too often, kappa is used indiscriminately to include what should be indisputable decisions, such as sample size, and when there is disagreement, coders can simply reference the original document. Qualitative judgements, where there are no factual sources to adjudicate, reflect kappa’s intended purpose. Consequently, kappa can be inflated simply by including prosaic data entry decisions that reflect transcription (where it may suffice to mention double-coding with errors rectified by referencing the original document), and, with Stahl et al. reporting kappa “between .81 and .95” (Stahl et al., 2010 : 699), it is unclear how it was used in this case.
Consequently, reviewers should expect authors to provide additional reassurance beyond kappa that they grouped measures appropriately. This is not simply a case of using different indices of interrater agreement (LeBreton & Senter, 2008 ), which often prove interchangeable themselves, but using a battery of options to show measurement equivalence and that these measures are tapping into approximately the same construct. Although few measures will be completely identical (i.e., parallel forms), there are the traditional choices of showing different types of validity evidence (Wasserman & Bracken, 2003 ). For example, Taras et al. ( 2010 ) were faced with over 100 different measures of culture in their meta-analysis of Hofstede’s Values Survey Module. Their solution, which they document over several pages, was to begin with the available convergent validity evidence , that is factor or correlational studies. Given that the available associations were incomplete, they then proceeded to content validity evidence , examining not just the definitions but also the survey items for consistency with the target constructs. Finally, for more contentious decisions, they drew on 14 raters to gather further evidence regarding content validity.
As can be seen, demonstrating that different measures tap into the same construct can be laborious, and preferably future meta-analyses should be able to draw on previously established ontologies or taxonomic structures. As mentioned, there are some sources to rely on, such as Richard et al.’s ( 2009 ) work on organizational performance, Versteeg and Ginsburg’s ( 2017 ) assessment of rule of law indices, or Stanek and Ones’ ( 2018 ) taxonomy of personality and cognitive ability. Unfortunately, this work is still insufficient for many meta-analyses, and such a void is proving a major obstacle to the advancement of science. The multiplicity of overlapping terms and measures creates a knowledge management problem that is increasingly intractable for the individual researcher to solve. Larsen, Hekler, Paul and Gibson ( 2020 ) argued that a solution is manageable, but we need a sustained “collaborative research program between information systems, information science, and computer science researchers and social and behavioral science researchers to develop information system artifacts to address the problem” (Larsen et al., 2020 : 1). Once we have an organized system of knowledge, they concluded: “it would enable scholars to more easily conduct (possibly in a fully automated manner) literature reviews, meta-analyses, and syntheses across studies and scientific domains to advance our understanding about complex systems in the social and behavioral sciences” (Larsen et al., 2020 : 9).
Stage 2: Data Preparation
Literature search, screening, and coding provide the sample of primary studies and the preliminary meta-analytic database. Next, there are three aspects of the data preparation stage that leave quite a bit of discretionary room for the researcher, thus requiring explicit discussion useful not only for meta-analysts but also for reviewers as well as research consumers. First, there is the treatment of multiple effect sizes reported in a given primary-level study. Second, there is the identification and treatment of outliers. And third, the issue of publication bias.
Treatment of multiple effects sizes
A single study may choose to measure a construct in a variety of ways, each producing its own effect size estimate. In other words, effect sizes are calculated using the same sample and reported separately for each measure. Separately counting each result violates the principle of statistical independence, as all are based on the same sample. Stahl et al. chose to average effect sizes within articles, which addresses this issue; however, more effective options are now available (López‐López, Page, Lipsey, & Higgins, 2018 ).
Typically, the goal is to focus on the key construct, and so Schmidt and Hunter ( 2015 ) recommended the calculation of composite scores, drawing on the correlations between the different measures. Unless the measures are unrelated (which suggests that they assess different constructs and therefore should not be grouped), the resulting composite score will have better coverage of the underlying construct as well as higher reliability. Other techniques include the Robust Error Variance (RVE) approach (Tanner-Smith & Tipton, 2014 ), which considers the dependencies (i.e., covariation) between correlated effect sizes (i.e., from the same sample). Another option is adopting a multilevel meta-analytic approach, where Level 1 includes the effects sizes, Level 2 is the within-study variation, and Level 3 is the between-study variation (Pastor & Lazowski, 2018 ; Weisz et al., 2017 ). A potential practical limitation is that these alternatives to composite scores pose large data demands, as they typically require 40–80 studies per analysis to provide acceptable estimates (Viechtbauer, López-López, Sánchez-Meca, & Marín-Martínez, 2015 ).
Outlier identification and management
Although rarely carried out (Aguinis, Dalton, et al., 2011 ), outlier analysis is strongly recommended for meta-analysis. Some choices include doing nothing, reducing the weight given to the outlier, or eliminating the outlier altogether (Tabachnik & Fidell, 2014 ). However, whatever the choice, it should be transparent, with the option of reporting results both with and without outliers. To detect outliers, the statistical package metafor provides a variety of influential case diagnostics, ranging from externally standardized residuals to leave-one-out estimates (Viechtbauer, 2010 ). There are multiple outliers in Stahl et al.’s dataset, such as Polzer, Crisp, Jarvenpaa and Kim ( 2006 ) for Relationship Conflict, Maznevski ( 1995 ) for Process Conflict, and Gibson and Gibbs ( 2006 ) for Communication. In particular, Cady and Valentine ( 1999 ), which is the largest study for the outcome measure of Creativity and reports the sole negative correlation of − 0.14, almost triples the residual heterogeneity (Tau 2 ), increasing it from 0.025 to 0.065. As is the nature of outliers, and as will be shown later, their undue influence can substantially tilt results by their inclusion or exclusion.
Like the Black Swan effect, an outlier may be a legitimate effect size drawn by chance from the ends of a distribution, which would relinquish its outlier status as more effects reduce or balance its impact. Aguinis, Gottfredson and Joo ( 2013 ) offered a decision tree involving a sequence of steps to first identify outliers (i.e., whether a particular observation is far from the rest) and then decide whether specific outliers are errors, interesting, or influential. Based on the answer, a researcher can decide to eliminate it (i.e., if it is an error), retain it as is or decrease its influence, and then, regardless of the choices, it is recommended to report results with and without the outliers. Stahl et al. retained outliers, which is certainly preferable to using arbitrary cutoffs such as two standard deviations below or above the mean to omit observations from the analysis (a regrettable practice that artificially creates homogeneity; Aguinis et al., 2013 ). However, we do not have information on whether these outliers could have been errors.
Publication bias
Publication bias refers to a focus on statistically significant or strong effect sizes rather than a representative sample of results. This can happen for a wide of variety of reasons, including underpowered studies and questionable research practices such as p -hacking (Meyer, van Witteloostuijn & Beugelsdijk, 2017 ; Munafò et al., 2017 ), and it occurs frequently in a variety of fields (Ferguson & Brannick, 2012 ; Ioannidis, Munafò, Fusar-Poli, Nosek, & David, 2014 ), although not all (Dalton, Aguinis, Dalton, Bosco, & Pierce, 2012 ). When it does occur, it has the potential to severely distort findings (Friese & Frankenbach, 2020 ). It is notable that Stahl et al. tested for publication bias, while only 3–30% of meta-analyses include this step (Aguinis, Dalton, et al., 2011 ; Kepes, Banks, McDaniel, & Whetzel, 2012 ). To test for publication bias, Stahl et al. used the fail-safe N , devised by Rosenthal ( 1979 ) for experimental research. Although Rosenthal focused on the common “file drawer” problem, his statistic is more of a general indicator of the stability of meta-analytic results (Carson, Schriesheim, & Kinicki, 1990 ; Dalton et al., 2012 ). In particular, the fail-safe N estimates the number of null studies that would be needed to change the average effect size a group of studies to a specified statistical significance level, especially non-significance (e.g., p > .05).
While at one time the fail-safe N was a recommended component of a state-of-the-science meta-analysis, this time has now passed. It has a variety of problems. For example if the published literature indicates a lack of relationship, that is the null itself, the equation becomes unworkable. For example, Stahl et al. were unable to give a fail-safe N precisely for the variables which were not significant in the first place. Consequently, for decades, researchers have recommended its disuse (Begg, 1994 ; Johnson & Hennessy, 2019 ; Scargle, 2000 ). Sutton ( 2009 : 442) described it as “nothing more than a crude guide”, and Becker ( 2005 : 111) recommended that “the fail-safe N should be abandoned in favor of other more informative analyses.” At the very least, the fail-safe N should be supplemented.
Although there are no perfect methods to detect or correct for publication bias, there are a wide variety of better options (Kepes et al., 2012 ). We can use selection-based methods and compare study sources, typically published versus unpublished, with the expectation there should be little difference between the two (Dalton et al., 2012 ). Also, there are a variety of symmetry-based methods, essentially where the expectation is that sample sizes or standard errors should be unrelated to effect sizes. One of most popular of these symmetry techniques is Egger’s regression test, which we applied to Stahl et al. Confirming Stahl et al.’s findings, there was no detectable publication bias.
Henmi and Compas ( 2010 ) developed a simple method for reducing the effect of publication bias, which uses fixed-effect model weighting to reduce the impact of errant heterogeneity. Alternatively, the classic Trim-and-Fill technique (Duval, 2005 ) can also be employed, which will impute the “missing” correlations. For a more sophisticated option, there is the precision-effect test and a precision-effect estimate with standard errors (PET-PEESE), which can detect as well as correct for publication bias (see Stanley and Doucouliagos 2014 for illustrative examples and code for Stata and SPSS). Stanley ( 2017 ) identified when PET-PEESE becomes unreliable, typically when there are few studies, excessive heterogeneity, or small sample sizes, which are often the same conditions that weaken the effectiveness of meta-analytic techniques in general.
Stage 3: Data Analysis
Meta-analyses are overwhelmingly used to understand what is the overall (i.e., average) size of the relationship between variables across primary-level studies (DeSimone et al., 2019 ; Carlson & Ji, 2011 ). However, meta-analysis is just as useful, if not more so, to understand when and where a relationship is likely to be stronger or weaker (Aguinis, Pierce, et al., 2011 ). Consequently, we discuss the three basic elements of the data analysis stage – average effect sizes, heterogeneity, and moderators – and we emphasize theory implications.
Reflecting that many meta-analytic methodologies were under debate at that time, Stahl et al. used a combination of techniques, including psychometric meta-analysis, both a fixed-effect and random-effect approach, as well as converting correlations to Fisher’s z after psychometric adjustments. The motivation for this blend of techniques is clear: each has its advantages (Wiernik & Dahlke, 2020 ). However, procedures have been refined and, consequently, we contrast Stahl et al.’s results with a modern technique that better accomplishes their aim: Morris estimators (Brannick, Potter, Benitez, & Morris, 2019 ).
Average effect sizes
During the early years of meta-analysis, the main question of interest was: “Is there a consistent relationship between two variables when examined across a number of primary-level studies that seemingly report contradictory results?” As Gonzalez-Mulé and Aguinis ( 2018 ) reviewed, for many meta-analyses, this is all they provided. Showing association and connection represents the initial stages of theory testing, and most meta-analyses have some hypotheses attached to these estimates. Given that this is the lower-hanging empirical and theoretical fruit, much of it has already been plucked and, today, unlikely by itself to satisfy demands for a novel contribution. An improved test of theory at this stage is not just positing that a relationship exists, and that it is unlikely to be zero, but how big it is (Meehl, 1990 ); in other words, “Instead of treating meta-analytic results similarly to NHST (i.e., limiting the focus to the presence or absence of an overall relationship), reference and interpret the MAES (meta-analytic effect sizes) alongside any relevant qualifying information” (DeSimone et al., 2019 : 884). To this end, researchers have typically drawn on Cohen ( 1962 ), who made very rough benchmark estimates based on his review of articles published in the 1960 volume of Journal of Abnormal and Social Psychology . Contemporary effect-size estimates have been compiled by Bosco, Aguinis, Singh, Field and Pierce (2015) , who drew on 147,328 correlations reported in 1660 articles, and by Paterson, Harms, Steel and Credé ( 2016 ), who summarized results from more than 250 meta-analyses. Both ascertained that Cohen’s categorizations of small, medium, and large effects do not accurately reflect today’s research in management and related fields. Averaging Bosco et al.’s Table 2 and Paterson et al.’s Table 3, a better generic distribution remains as per Cohen 0.10 for small (i.e., 25th percentile), but 0.18 for medium (i.e., 50th percentile) and 0.32 for large (i.e., 75th percentile). Using these distributions of effect sizes, or those compiled from other analogous meta-analyses, meta-analysts can go beyond the simple conclusion that a relationship is different from zero and, instead, critically evaluate the size of the effect within the context of a specific domain.
Stahl et al. adopted a hybrid approach to calculate average effect sizes. Initially, they reported estimates using Schmidt and Hunter’s ( 2015 ) psychometric meta-analysis, correcting for dichotomization (i.e., uneven splits) and attenuation due to measurement error. They then departed from Schmidt’s and Hunter’s approach by transforming correlations to Fisher’s z s (Borenstein, Hedges, Higgins, & Rothstein, 2009 ) and weighting by N − 3, the inverse of sampling error after Fisher’s transformation. As Stahl et al. clearly acknowledged, this is a fixed-effects approach that assumes the existence of a single population effect. In contrast, a random-effects model assumes that there are multiple population effects that motivate the search for moderator (i.e., factors that account for substantive variability of observed effects).
Where does this leave Stahl et al., who corrected for attenuation but used a Fisher’s z transformation with an underlying fixed-effect approach? If correlations are between ± 0.30, Fisher’s z transformed versus untransformed correlations are almost identical. For Stahl et al.’s data, 81% of their effect sizes fell within this special case of near equivalence, making the matter almost moot. Similarly, Schmidt and Hunter ( 2015 ) used an attenuation factor, which can change weights drastically, but here the average absolute difference between raw and corrected correlation is less than 0.02, minimizing this concern. Consequently, although we do not recommend Stahl et al.’s fixed-effects approach, results should be close to equivalent to other methods, as noted by Aguinis, Gottfredson and Wright ( 2011 ).
As mentioned earlier, we re-analyzed Stahl et al.’s data using Morris weights. To calculate variance of effect sizes across primary-level studies, we used N − 1 in the formula rather than N , as the effect sizes are estimates and not population values. To calculate residual heterogeneity (i.e., whether variation of effect sizes is due to substantive rather than artifactual reasons), Morris estimators rely on restricted maximum likelihood. We conducted all analyses using the metafor (2.0-0) statistical package (Viechtbauer, 2010 ) in R (version 3.5.3). We found that the average effect size for creativity, for example, increased from Stahl et al.’s 0.16 to 0.18, although it was non-significant ( p = .20). Moreover, using the random-effects model, which increased the size of confidence intervals due the inclusion of the random-effects variance component (REVC), none of the effects were significant, with a caveat due to the consideration of outliers. If we exclude Cady and Valentine ( 1999 ), the effect size of creativity increases to 0.29 and became significant ( p = 0.02). In sum, Stahl et al. provided an excellent example that methodological choices, here regarding outliers and the model, are influential enough that a meta-analysis’ major conclusions can hinge upon them.
Stahl et al. presented a single column of effect sizes, which is now insufficient for modern meta-analyses. What is preferred is a grid of them. For example, meta-analytic structural equation modeling (MASEM) is based on expanding the scope of a meta-analysis from bivariate correlations to creating a full meta-analytic correlation matrix (Bergh et al., 2016 ; Cheung, 2018 ; Oh, 2020 ). Given that this allows for additional theory testing options enabled by standard structural equation modeling, the publication of a meta-analysis can pivot on its use of MASEM. Options range from factor analysis to path analysis, such as determining the total variance provided by predictors or if a predictor is particularly important (e.g., dominance or relative weights analysis). It also allows for mediation tests, that is, the “how” of theory or “reasons for connections.” It is even possible to use MASEM to test for interaction effects. Traditionally, the correlation between the interaction term and other variables is not reported and often must be requested directly from the original authors. Doing so is a high-risk endeavor given researchers’ traditionally low response rate (Aguinis, Beaty, Boik, & Pierce, 2005 ; Polanin et al., 2020a ), but the rise of Open Science and the concomitant Individual Participant Data (IPD) means that this information is increasingly available. Amalgamating IPD across multiple studies is usually referred to as a mega-analysis, and, as suggested here, can be used to supplement a standard meta-analysis (Boedhoe et al., 2019 ; Kaufmann, Reips, & Merki, 2016 ).
Reviewers will note that, as researchers move from simply an average of bivariate relationships towards MASEM, they can encounter incomplete and nonsensical matrices. For incomplete matrices, Landis ( 2013 ) and Bergh et al. ( 2016 ) provided sensible recommendations for filling blank cells in a matrix, such as drawing on previously published meta-analytic values or expanding the meta-analysis to target missing correlations. Nonsensical matrices (that occur increasingly as correlation matrices expand) create a non-positive definite “Frankenstein” matrix, stitched together from incompatible moderator patches. Landis ( 2013 ), as well as Sheng, Kong, Cortina and Hou ( 2016 ), provided remedies, such as excluding problematic cells or collapsing highly correlated variables into factors to avoid multicollinearity. In addition, we can employ more advanced methods that incorporate random effects and dovetail meta-regression with MASEM (e.g., Jak & Cheung, 2020 ). The benefit is a mature science that can adjust a matrix so that the resulting regression equations represent specific contexts. For example, synthetic validity is a MASEM application in which validity coefficients are predicted based on a meta-regression of job characteristics, meaning that we can create customized personnel selection platforms orders of magnitude less costly, faster, and more accurately (Steel, Johnson, Jeanneret, Scherbaum, Hoffman, & Foster, 2010 ).
Heterogeneity of effect sizes
A supplement to our previous discussion of average effect sizes is the degree of dispersion around the average effect. As noted by Borenstein et al. ( 2009 ), “the goal of a meta-analysis should be to synthesize the effect sizes, and not simply (or necessarily) to report a summary effect. If the effects are consistent, then the analysis shows that the effect is robust across the range of included studies. If there is modest dispersion, then this dispersion should serve to place the mean effect in context. If there is substantial dispersion, then the focus should shift from the summary effect to the dispersion itself. Researchers who report a summary effect are indeed missing the point of the synthesis” (Borenstein et al., 2009 : 378).
Stahl et al. examined whether the homogeneity Q statistic was significant, meaning that sufficient variability of effects around the mean exists, as a precursor to moderator examination. A modern meta-analysis should complement the Q statistic with other ways of assessing heterogeneity, because Q often leads to Type II errors (i.e., incorrect conclusions that heterogeneity is not present; Gonzalez-Mulé & Aguinis, 2018 ), especially when there is publication bias (Augusteijn, van Aert, & van Assen, 2019 ). Further reporting of heterogeneity by Stahl et al. is somewhat unclear. They provided in Table 2 “Variance explained by S.E. (%)” and “Range of effect sizes,” which were not otherwise explained. This oversight is, as Gonzalez-Mulé and Aguinis ( 2018 ) documented, regrettably common. In fact, they found that 16% of meta-analyses from major management journals fail to report heterogeneity at all. Stahl et al. reported the range of effect sizes for creativity was − .14 to .48. However, the actual credibility intervals, after removing the outlier, was .03 to .55, indicating that the result typically generalizes and can be strong. As per Gonzalez-Mulé and Aguinis, we recommend providing at a minimum: credibility intervals, T 2 (i.e., SD r or the REVC), and I 2 (i.e., percentage of total variance attributable to T 2 ). The ability to further assess heterogeneity is facilitated by recent methodological advances, such as the use of a Bayesian approach that corrects for artificial homogeneity created by small samples (Steel, Kammeyer-Mueller, & Paterson, 2015 ), and by the use of asymmetric distributions in cases of skewed credibility intervals (Baker & Jackson, 2016 ; Jackson, Turner, Rhodes, & Viechtbauer, 2014 ; Possolo, Merkatas, & Bodnar, 2019 ).
Moderator search
Moderating effects, which account for substantive heterogeneity, can be organized around Cattell’s Data Cube or the Data Box (Revelle & Wilt, 2019 ): (1) sample (e.g., firm or people characteristics), (2) variables (e.g., measurements), and (3) occasions (e.g., administration or setting). Typical moderator variables include country (e.g., developing vs developed), time period (e.g., decade) and published vs unpublished status (where comparison between the two can indicate the presence of publication bias). Particularly important from an IB perspective is the language and culture of survey administration, which has been shown to influence response styles (Harzing, 2006 ; Smith & Fischer, 2008 ) and response rates (Lyness & Brumit Kropf, 2007 ). Theory is often addressed as part of the moderator search, as per Cortina’s ( 2016 ) review, “a theory is a set of clearly identified variables and their connections, the reasons for those connections, and the primary boundary conditions for those connections” (Cortina, 2016 : 1142). Moderator search usually establishes the last of these – boundary conditions – although not exclusively. For example, Bowen, Rostami and Steel ( 2010 ) used the temporal sequence as a moderator to clarify the causal relationship between innovation and firm performance. Of note, untheorized moderators (e.g., control variables) are still a staple of meta-analyses but should be clearly delineated as robustness tests or sensitivity analyses (Bernerth & Aguinis, 2016 ).
After establishing average effect sizes (i.e., connections), Stahl et al. grappled deeply with the type of diversity, a boundary condition inquiry that determines how specific contexts affect these connections or effect sizes. Stahl et al. differentiated between the role of surface level (e.g., racio-ethnicity) vs deep level (e.g., cultural values) diversity and noted trade-offs. They expected diversity to be associated with higher levels of creativity, but at the potential cost of lower satisfaction and greater conflict, negative outcomes that likely diminish as team tenure increases. Note how well these moderators match up to core theoretical elements. Page’s ( 2008 ) book on diversity, The Difference , covers in detail the four conditions that lead to diversity creating superior performance. This includes that the task should be difficult enough that it needs more than a single brilliant problem solver (i.e., task complexity), that those in the group should have skills relevant to the problem (i.e., type of diversity), that there is synergy and sharing among the group members (i.e., team dispersion), and that the group should be large and genuinely diverse (i.e., team size). A clear connection between theory, data, and analysis is a hallmark of a great paper, reflected in that the more a meta-analysis attempts to test an existing theory, the larger the number of citations it receives (Aguinis, Dalton, et al., 2011 ).
However, the techniques that Stahl et al. used to assess moderators have evolved considerably. Stahl et al. used subgrouping methodology, which comes in two different forms: comparison of mean effect sizes and analysis of variance (Borenstein et al., 2009 ). The use of such subgrouping approach has come into debate. To begin with, subgrouping should be reserved for categorical variables as otherwise it requires dichotomizing continuous moderators, usually using a median split, which reduces statistical power (Cohen, 1983 ; Steel & Kammeyer-Mueller, 2002 ). Also, it appears that Stahl et al. used a fixed-effects model although meta-analytic comparisons are typically based on a random-effects model (Aguinis, Sturman, & Pierce, 2008 ), with some exceptions, such as when the subgroups are considered exhaustive (e.g., before and after a publication year; Borenstein & Higgins, 2013 ) or whether the research question focuses on dependent correlates differing within the same situation (Cheung & Chan, 2004 ). Furthermore, standard Wald-type comparisons result in massive increases in Type I errors (Gonzalez-Mulé & Aguinis, 2018 ), and, although useful to contrast two sets of correlations to determine whether they differ, they have limited application in determining moderators’ explanatory power (Lubinski & Humphreys, 1996 ). A superior alternative to subgrouping is meta-regression analysis or MARA (Aguinis, Gottfredson, & Wright, 2011 ; Gonzalez-Mulé & Aguinis, 2018 ; Viechtbauer et al., 2015 ). Essentially, MARA is a regression model in which effect sizes are the dependent variable and the moderators are the predictors (e.g., Steel & Kammeyer-Mueller, 2002 ). MARA tests whether the size of the effects can be predicted by fluctuations in the values of the hypothesized moderators, which therefore are conceptualized as boundary conditions for the size of the effect. If there are enough studies, MARA enables simultaneous testing of several moderators. Evaluating the weighting options for the predictors, Viechtbauer et al. settled on the Hartung–Knapp as the best alternative. Other recommendations for MARA are given by Gonzalez-Mulé and Aguinis ( 2018 ), such as making the sensible observation that we should use \(R^{2}_{\text{Meta}}\) , which adjusts R 2 to reflect I 2 , the known variance after excluding sampling error. Gonzalez-Mulé and Aguinis ( 2018 ) also included the R code to conduct all analyses as well as an illustrative study. Some analysis programs, such as metafor , provide \(R^{2}_{\text{Meta}}\) by default.
Stage 4: Reporting
A modern meta-analysis must be transparent and reproducible – meaning that all steps and procedures need to be described in such a way that a different team of researchers would obtain similar results with the same data. At present, this is among our greatest challenges. In psychology, half of 500 effect sizes sampled from 33 meta-analyses were not reproducible based on the available information (Maassen, van Assen, Nuijten, Olsson-Collentine, & Wicherts, 2020 ). Also, a modern meta-analysis not only provides more than a summary of past findings but also points towards the next steps. Consequently, it should consider future research directions, not just in terms of what studies should be conducted but when subsequent meta-analyses could be beneficial and what they should address.
Transparency and reproducibility
As Hohn, Slaney and Tafreshi ( 2020 : 207) concluded: “It is vitally important that meta-analytic work be reproducible, transparent, and able to be subjected to rigorous scrutiny so as to ensure that the validity of conclusions of any given question may be corroborated when necessary.” Stahl et al. provided their database to assist with our review, allowing the assessment of reproducibility because both of our analyses relied on the same meta-analytic data (Jasny, Chin, Chong, & Vignieri, 2011 ). Such responsiveness is commendable but also highlights the problem of using researchers’ personal computers as archives. The data are often difficult to obtain, lost, or incomplete, and even authors of recent meta-analyses, who claim that the references or data are available upon request, and such availability is an explicit requirement for many journals, are sporadically responsive (Wood, Müller, & Brown, 2018 ). Hence the call for Open Science, Open Data, Open Access, and Open Archive, and the increasing number of journals that have adopted this standard of transparency (Aguinis, Banks, Rogelberg, & Cascio, 2020 ; Vicente-Sáez & Martínez-Fuentes, 2018 ). Along with the complete database, if the statistical process deviates from standard practice, ideally a copy of the analysis script should be made available in an Open Science archive. The advantages of such heightened transparency and reproducibility are several (Aguinis et al., 2018 ; Polanin, Hennessy, & Tsuji, 2020b ), but it does introduce considerable challenges (Beugelsdijk et al., 2020 ).
To begin with, journal articles are an abridged version of the available data and the analysis process. By themselves, they can hide a multitude of virtues and vices. As per Stahl et al., we were unable to completely recreate some steps (though we did approximate them) as they were not sufficiently specified. Adopting an Open Science framework, choices can be examined and updated, improving the research quality, as it encourages increased vigilance by the source authors.
As Marshall and Wallace ( 2019 : 1) concluded, “Clearly, existing processes are not sustainable: reviews of current evidence cannot be produced efficiently and, in any case, often go out of date quickly once they are published. The fundamental problem is that current EBM [evidence-based medicine] methods, while rigorous, simply do not scale to meet the demands imposed by the voluminous scale of the (unstructured) evidence base.” Although originating from the medical field, this critique equally applies to management and IB (Rousseau, 2020 ). Our traditional methods of reporting, which Stahl et al. adopted, are flagging the extracted studies with an asterisk in the reference section or upon request from the authors. This is at present insufficient. Science is a social endeavor, and we need to be able to build on past meta-analyses to enable future ones; by making meta-analyses reproducible, that is, in having access to the coding database we are also making the process cumulative (Polanin et al., 2020b ). In fact, Open Science can be considered as a stepping stone towards living systematic reviews (LSRs; Elliot et al., 2017 ), essentially reviews that are continuously updated in real time. Having found traction in medicine, LSRs are based around critical topics that can enable broad collaborations (along with advances in technological innovations, such as online platforms and machine learning), although not without their own challenges (Millard, Synnot, Elliott, Green, McDonald, & Turner, 2019 ).
Such data sharing is not without its perils, exacerbating the moral hazards associated with a common pool resource, that is, the publication base (Alter & Gonzalez, 2018 ; Hess & Ostrom, 2003 ). Traditionally, in a meta-analysis, the information becomes “consumed” once published or “extracted” in a meta-analysis, and the research base needs time to “regenerate,” that is grow sufficiently that a new summary is justified. Since there is no definitive point when regeneration occurs, we encounter a tragedy of the commons, where one instrumental strategy is to rush marginal meta-analyses to the academic market, shopping them to multiple venues in search of acceptance (i.e., science’s first mover advantage; Newman 2009 ). Open Science is likely to exacerbate this practice, as the cost of updating meta-analyses would be substantially reduced and, as Beugelsdijk et al. ( 2020 : 897) discussed, “There would be nothing to stop others from using the fruits of their labor to write a competing article”. For example, in the field of ecology, the authors of a meta-analysis on marine habitats admirably provided their complete database, which was rapidly re-analyzed by a subsequent group with a slightly different taxonomy (Kinlock et al., 2019 ). In a charitable reply, they viewed this as an endorsement of Open Science, concluding “Without transparent methods, explicitly defined models, and fully transparent data and code, this advancement in scientific knowledge would have been delayed if not unobtainable” (Kinlock et al., 2019 : 1533). However, as they noted, it took a team of ten authors over two years to create the original database, and posting it allowed others to supersede them with relatively minimal effort. If the original authors adopt an Open Science philosophy for their meta-analytic database (which we strongly recommend), subsequent free-riding or predatory authors could take advantage and, by adding marginal updates, publish. Reviewers should be sensitive to whether a new meta-analysis provides a substantive threshold of contribution, preferably with the involvement of the previous lead authors upon whose work they are building (especially if recent). To help guide decisions, we further address this issue in our subsequent section, “ The next generation of meta-analyses .” In addition, journals can help to mitigate the moral hazard associated with meta-analysis’ common pool resource by allowing pre-registration and conditional pre-approval of large meta-analyses.
Future research directions
A good section on future research directions,” based on a close study of the entire field’s findings, although perhaps sporadically used (Carlson & Ji, 2011 ), can be as invaluable as the core results themselves. This information allows meta-analysts to steer the field itself. We can expect meta-analysts to expound on the gap between what is already known and what is required to move forward. The components of a good Future Research section touch on many of the very stages we previously emphasized here, especially Data Collection, Data Analysis, and Reporting.
During Data Collection, researchers have had to be sensitive to inclusion and exclusion criteria and how constructs were defined and measured. This provides several insights. To begin with, the development of inclusion and exclusion criteria, along with addressing issues of commensurability, allow researchers to consider construct definition and its measurement. Was the construct well defined? Often, there are as many definitions as there are researchers, so this is an opportunity to provide some clarity. With an enhanced understanding, an evaluation of the measures can proceed, especially where they could be improved. How well do they assess the construct? Should some be favored and others abandoned?
During Data Analysis, researchers likely attempted to assemble a correlation matrix to conduct meta-analytic structural equation modeling and meta-regression. One of the more frustrating aspects of this endeavor is when the matrix is almost complete, but some cells are missing. Here is where the researcher can direct future projects towards understudied elements, as well as highlight that other relationships have been overly emphasized, perhaps to the point of recommending a moratorium. Similarly, the issues of heterogeneity and moderators come up. The results may generalize, but this may be due to overly homogenous samples or settings. Also, there was likely a need by some moderators to address theory, but the field simply did not report or contain them. Additionally, informing reviewers that the field is not yet able to address such ambitions often helps curtail a critique of their absence. This is where Stahl et al. primarily dedicated their own Future Research Agenda: process moderators should be considered (alone and in combination) and different cultural settings should be explored. In short, the researcher should stress how every future study should contextualize or describe itself (i.e., based on the likely major moderators).
Finally, we emphasized during Reporting the need for an Open Science framework. For a meta-analyst, often the greatest challenge is not the choice of statistical technique but getting enough foundational studies, especially those that fully report and are of high quality. The methodological techniques tend to converge at higher k , and statistical legerdemain can mitigate but not overcome an inherent lack of data. Fortunately, the Open Science movement and the increased availability of a study’s underlying data (i.e., IPD) opens possibilities. Contextual and other detailed information may not be reported in a study, often due to journal space limitation, but are needed for meta-analytic moderator analyses. With Open Science, this information will be increasingly available, allowing for the improved application of many sophisticated techniques. For example, Jak and Cheung’s ( 2020 ) one-stage MASEM incorporates continuous moderators for MARA but requires a minimum of 30 studies. Consequently, researchers should consider what new findings would be possible with a growing research base. In short, journal editors and reviewers should expect a synopsis of when a follow up meta-analysis would be appropriate and what the next update could accomplish with a greater and more varied database to rely on.
The next generation of meta-analyses
Is Stahl et al. the last word on diversity? Of course not. The entire point of Stahl et al.’s future research direction section was that it should be acted upon. Since Stahl et al., there have been a variety of advances in diversity research, such as the greater adoption of Blau’s index used to calculate the actual proportion of diversity (Blau, 1977 ; Harrison & Klein, 2007 ), and Shemla et al.’s ( 2016 ) conclusions that perceived levels of diversity can be more revealing than the objective measures on which Stahl et al. focused. Furthermore, not only do research bases refine and grow, at times exponentially, but meta-analytical methodology continues to evolve. With the increased popularity of meta-analysis, we can expect continued technical refinements and advances, some of which we touched upon in our article. We have shown that some of the newer techniques affected Stahl et al. findings, which proved sensitive to outliers and whether a fixed- or random-effects model was used. As for the near future, Marshall and Wallace ( 2019 ), as well as Johnson, Bauer and Niederman ( 2017 ), argued that we will see increased adoption of machine-learning systems in literature search and screening, which already exist but tend to be in the domain of well-funded health topics such as immunization (Begert, Granek, Irwin, & Brogly, 2020 ). Machine learning is a response to the “torrential volume of unstructured published evidence has rendered existing (rigorous, but manual) approaches to evidence synthesis increasingly costly and impractical” (Johnson et al., 2017 : 8). The typical machine-learning strategy is to constantly sort the remaining articles based on researchers’ previous choices, until these researchers reject (screen out) a substantive number of articles in a row, whereupon screening stops. Since the system cannot predict perfectly, there is a tradeoff between false negatives and positives, meaning that adopters will sacrifice missing approximately 4–5% of relevant articles to reduce screening time by 30–78% (Créquit, Boutron, Meerpohl, Williams, Craig, & Ravaud, 2020 ). Complementing these efforts, meta-analyses may draw on a variant of the “Mark–Recapture” method commonly used in ecology to determine a population’s size. Essentially, such as determining the number of fish in a pond, some are captured, marked, and released. The number of these marked fish re-captured during a subsequent effort provides the total population through the Lincoln–Petersen method. As this applies to meta-analysis, when one has a variety of terms and databases to search for a construct, subsequent searches showing an ever-increasing number of duplicate articles (i.e., articles previously “marked” and “recaptured”) provides a strong indicator of thoroughness. This combination of research base growth and improved search and analysis means that meta-analyses should have a half-life and perhaps a short one (Shojania, Sampson, Ansari Ji, Doucette, & Moher, 2007 ).
Despite these ongoing advances, it is not uncommon for IB, management, and related fields to rely on meta-analyses not just one decade old but two, three or four, which can be contrasted with the Cochrane Database of Systematic Reviews where the median time for an update is approximately 3 years (Bashir, Surian, & Dunn, 2018 ; Bastian, Doust, Clarke, & Glasziou, 2019 ). For example, the classic meta-analysis on job satisfaction by Judge, Heller and Mount ( 2002 ) is still considered foundational and cited hundreds of times each year, although it relies on an unpublished personality matrix from the early 1980s, a choice of matrix that, as Park et al. ( 2020 : 25) noted, “can substantively alter their conclusions”. Because of this reliance on very early and very rough estimates, newer meta-analyses indicate that many of Judge et al.’s core findings do not replicate (Steel, Schmidt, Bosco, & Uggerslev, 2019 ). Exactly because techniques evolve and research bases continue to grow, it is critical to update meta-analyses, even those, or perhaps especially those, that have become classics in a field.
This issue of meta-analytic currency has been intensely debated, culminating in a two-day international workshop by the Cochrane Collaboration’s Panel for Updating Guidance for Systematic Review (Garner et al., 2016 ). Drawing on this panel’s work, as well as similar recommendations by Mendes, Wohlin, Felizardo and Kalinowski ( 2020 ), we provide a revised set of guidelines, summarized in Figure 1 . Next, we apply this sequence of steps to Stahl et al. Step one is the consideration of currency. Does the review still address a relevant question? In the case of Stahl et al., its topic has increased in relevance, as reflected by its frequent citations and the widespread concern with diversity. Step two is to the consideration of methodology and/or the research base. Have any new relevant methods been developed? Did Stahl et al. miss any appropriate applications? Meta-analysis has indeed rapidly developed, and, as we review here, there are numerous refinements that could be applied, from outlier analysis to MASEM. Alternatively, have any new relevant studies been published, or new information? This is related to currency, Step one , as, within the thousand studies alone that cited Stahl et al., the meta-analytic database would likely double or triple. Also, an expanded research base enables the application of more sophisticated analysis techniques. Step three is the probable impact of the new methodology and/or studies. Can they be expected to change the findings or reduce uncertainty? This has already been shown here, that taking a random-effects approach has changed statistical significance. Of note, there merely needs to be a likelihood of impact, not an inevitability. For example, narrowing extremely wide confidence intervals without changing the average effect size is still a valuable contribution simply because it reduces uncertainty. Similarly, providing a previously unavailable complete meta-analytic database in an Open Science archive (enabling cumulative growth) can still be considered impactful (especially as it motivates all researchers to data-share or risk their meta-analysis being rapidly superseded).
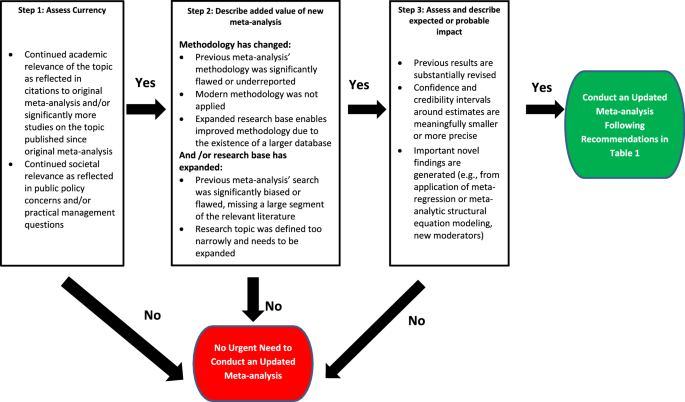
Decision framework to determine the need to update a meta-analysis.
By all standards, Stahl et al.’s meta-analysis is now worthy of updating, but, as mentioned, it is certainly not alone. Given that our Table 1 focuses on modern meta-analytic practices, it makes a useful litmus test in conjunction with Figure 1 ’s decision framework for determining whether newer meta-analyses should be pursued or whether existing ones provide a sufficiently novel contribution. The more of the elements expounded in Table 1 that the more recent meta-analysis has compared to its predecessor, the more it deserves favorable treatment.
Conclusions
We have discussed key methodological junctures in the design and execution of a modern meta-analytic study. We have shown that each stage in a meta-analytical study requires a series of critical decisions. These decisions are critical because they have an impact on the results obtained and substantive conclusions for theory as well as implications for practice and policymaking. We have discussed Stahl et al.’s meta-analysis as an exemplar to explain why their article was selected as the 2020 JIBS decade award, but also to show how the field of meta-analysis has progressed since. Table 1 summarizes recommendations and their implementation guidelines for a modern meta-analysis. By following the different steps described in Table 1 , we make explicit the anatomy of a successful meta-analysis, and we summarize what authors can be expected to do, what reviewers can be expected to ask for, and what consumers of meta-analytic reviews (i.e., other researchers, practitioners, and policymakers) can be expected to look for. Like any research method, meta-analysis is nuanced, and this is not an exhaustive list of all technical aspects or possible contributions or permutations. We can, though, summarize its spirit. When a phenomenon has been researched from a wide variety of perspectives, pulling these studies together and effectively exploring and explaining the shifting effect sizes and signs is invariably enriching.
Aguinis, H., Banks, G. C., Rogelberg, S., & Cascio, W. F. 2020. Actionable recommendations for narrowing the science-practice gap in open science. Organizational Behavior and Human Decision Processes, 158: 27–35.
Google Scholar
Aguinis, H., Beaty, J. C., Boik, R. J., & Pierce, C. A. 2005. Effect size and power in assessing moderating effects of categorical variables using multiple regression: A 30-year review. Journal of Applied Psychology, 90(1): 94–107.
Aguinis, H., Dalton, D. R., Bosco, F. A., Pierce, C. A., & Dalton, C. M. 2011a. Meta-analytic choices and judgment calls: Implications for theory building and testing, obtained effect sizes, and scholarly impact. Journal of Management, 37(1): 5–38.
Aguinis, H., Gottfredson, R. K., & Joo, H. 2013. Best-practice recommendations for defining, identifying, and handling outliers. Organizational Research Methods, 16(2): 270–301.
Aguinis, H., Gottfredson, R. K., & Wright, T. A. 2011b. Best-practice recommendations for estimating interaction effects using meta-analysis. Journal of Organizational Behavior, 32(8): 1033–1043.
Aguinis, H., Hill, N. S., & Bailey, J. R. 2021. Best practices in data collection and preparation: Recommendations for reviewers, editors, and authors. Organizational Research Methods . https://doi.org/10.1177/1094428119836485 .
Article Google Scholar
Aguinis, H., Pierce, C. A., Bosco, F. A., Dalton, D. R., & Dalton, C. M. 2011c. Debunking myths and urban legends about meta-analysis. Organizational Research Methods, 14(2): 306–331.
Aguinis, H., Ramani, R. S., & Alabduljader, N. 2018. What you see is what you get? Enhancing methodological transparency in management research. Academy of Management Annals, 12: 83–110.
Aguinis, H., Sturman, M. C., & Pierce, C. A. 2008. Comparison of three meta-analytic procedures for estimating moderating effects of categorical variables. Organizational Research Methods, 11(1): 9–34.
Alter, G., & Gonzalez, R. 2018. Responsible practices for data sharing. American Psychologist, 73(2): 146–156.
Augusteijn, H. E. M., van Aert, R. C. M., & van Assen, M. A. L. M. 2019. The effect of publication bias on the Q test and assessment of heterogeneity. Psychological Methods, 24(1): 116–134.
Baker, R., & Jackson, D. 2016. New models for describing outliers in meta-analysis. Research Synthesis Methods, 7(3): 314–328.
Bashir, R., Surian, D., & Dunn, A. G. 2018. Time-to-update of systematic reviews relative to the availability of new evidence. Systematic Reviews, 7(1): 195.
Bastian, H., Doust, J., Clarke, M., & Glasziou, P. 2019. The epidemiology of systematic review updates: A longitudinal study of updating of Cochrane reviews, 2003 to 2018 . medRxiv: 19014134.
Becker, B. J. 2005. Failsafe N or file-drawer number. In H. R. Rothstein, A. J. Sutton, & M. Borenstein (Eds.), Publication bias in meta-analysis: Prevention, assessment and adjustments : 111–125. West Sussex: Wiley.
Begert, D., Granek, J., Irwin, B., Brogly, C., & Xtract, A. I. 2020. Using automation for repetitive work involved in a systematic review. CCDR, 46(6): 174–179.
Begg, C. B. 1994. Publication bias. In H. M. Cooper & L. V. Hedges (Eds.), The handbook of research synthesis : 399–409. New York: Russell Sage.
Bem, D. J. 1995. Writing a review article for psychological bulletin. Psychological Bulletin, 118(2): 172–177.
Bergh, D. D., Aguinis, H., Heavey, C., Ketchen, D. J., Boyd, B. K., Su, P., et al. 2016. Using meta-analytic structural equation modeling to advance strategic management research: Guidelines and an empirical illustration via the strategic leadership-performance relationship. Strategic Management Journal, 37(3): 477–497.
Bernerth, J., & Aguinis, H. 2016. A critical review and best-practice recommendations for control variable usage. Personnel Psychology, 69(1): 229–283.
Beugelsdijk, S., Ambos, B., & Nell, P. 2018a. Conceptualizing and measuring distance in international business research: Recurring questions and best practice guidelines. Journal of International Business Studies, 49(9): 1113–1137.
Beugelsdijk, S., Kostova, T., Kunst, V. E., Spadafora, E., & van Essen, M. 2018b. Cultural distance and firm internationalization: A meta-analytical review and theoretical implications. Journal of Management, 44(1): 89–130.
Beugelsdijk, S., van Witteloostuijn, A., & Meyer, K. 2020. A new approach to data access and research transparency (DART). Journal of International Business Studies, 51(6): 887–905.
Blau, P. 1977. Inequality and heterogeneity. A primitive theory of social structure . New York: Free Press.
Boedhoe, P. S., Heymans, M. W., Schmaal, L., Abe, Y., Alonso, P., Ameis, S. H., et al. 2019. An empirical comparison of meta and mega-analysis with data from the ENIGMA obsessive-compulsive disorder working group. Frontiers in Neuroinformatics, 12: 102.
Booth, A. 2008. Unpacking your literature search toolbox: On search styles and tactics. Health Information and Libraries Journal, 25(4): 313–317.
Booth, A., Briscoe, S., & Wright, J. M. 2020. The “realist search”: A systematic scoping review of current practice and reporting. Research Synthesis Methods, 11(1): 14–35.
Borenstein, M., Hedges, L. V., Higgins, J. P., & Rothstein, H. R. 2009. Introduction to meta-analysis . Chichester: Wiley.
Borenstein, M., & Higgins, J. P. T. 2013. Meta-analysis and subgroups. Prevention Science, 14(2): 134–143.
Bornmann, L., & Mutz, R. 2015. Growth rates of modern science: A bibliometric analysis based on the number of publications and cited references. Journal of the Association for Information Science and Technology, 66(11): 2215–2222.
Bosco, F. A., Aguinis, H., Singh, K., Field, J. G., & Pierce, C. A. 2015a. Correlational effect size benchmarks. Journal of Applied Psychology, 100(2): 431–449.
Bosco, F. A., Steel, P., Oswald, F. L., Uggerslev, K., & Field, J. G. 2015b. Cloud-based meta-analysis to bridge science and practice: Welcome to metaBUS. Personnel Assessment and Decisions, 1(1): 3–17.
Bowen, F. E., Rostami, M., & Steel, P. 2010. Timing is everything: A meta-analysis of the relationships between organizational performance and innovation. Journal of Business Research, 63(11): 1179–1185.
Brannick, M. T., Potter, S. M., Benitez, B., & Morris, S. B. 2019. Bias and precision of alternate estimators in meta-analysis: Benefits of blending Schmidt-Hunter and Hedges approaches. Organizational Research Methods, 22(2): 490–514.
Cady, S. H., & Valentine, J. 1999. Team innovation and perceptions of consideration: What difference does diversity make? Small Group Research, 30(6): 730–750.
Carlson, K. D., & Ji, F. X. 2011. Citing and building on meta-analytic findings: A review and recommendations. Organizational Research Methods, 14(4): 696–717.
Carson, K. P., Schriesheim, C. A., & Kinicki, A. J. 1990. The usefulness of the “fail-safe” statistic in meta-analysis. Educational and Psychological Measurement, 50(2): 233–243.
Cheung, M. W.-L. 2018. Issues in solving the problem of effect size heterogeneity in meta-analytic structural equation modeling: A commentary and simulation study on Yu, Downes, Carter, and O’Boyle (2016). Journal of Applied Psychology, 103(7): 787–803.
Cheung, M. W. L., & Chan, W. 2004. Testing dependent correlation coefficients via structural equation modeling. Organizational Research Methods, 7(2): 206–223.
Cohen, J. 1962. The statistical power of abnormal-social psychological research: A review. Journal of Abnormal and Social Psychology, 65: 145–153.
Cohen, J. 1983. The cost of dichotomization. Applied Psychological Measurement, 7(3): 249–253.
Cortina, J. M. 2016. Defining and operationalizing theory. Journal of Organizational Behavior, 37(8): 1142–1149.
Cortina, J. M., Aguinis, H., & DeShon, R. P. 2017. Twilight of dawn or of evening? A century of research methods in the Journal of Applied Psychology. Journal of Applied Psychology, 102(3): 274–290.
Créquit, P., Boutron, I., Meerpohl, J., Williams, H., Craig, J., & Ravaud, P. 2020. Future of evidence ecosystem series: 2. Current opportunities and need for better tools and methods. Journal of Clinical Epidemiology, 123: 143–152.
Dahlke, J. A., & Wiernik, B. M. 2019. psychmeta: An R package for psychometric meta-analysis. Applied Psychological Measurement, 43(5): 415–416.
Dalton, D. R., Aguinis, H., Dalton, C. M., Bosco, F. A., & Pierce, C. A. 2012. Revisiting the file drawer problem in meta-analysis: An assessment of published and nonpublished correlation matrices. Personnel Psychology, 65(2): 221–249.
Davies, H. T. O., Nutley, S. M., & Smith, P. C. 1999. Editorial: What works? The role of evidence in public sector policy and practice. Public Money and Management, 19(1): 3–5.
Denyer, D., & Tranfield, D. 2008. Producing a systematic review. In D. Buchanan (Ed.), The Sage handbook of organizational research methods : 671–689. London: Sage.
DeSimone, J. A., Köhler, T., & Schoen, J. L. 2019. If it were only that easy: The use of meta-analytic research by organizational scholars. Organizational Research Methods, 22(4): 867–891.
Duval, S. J. 2005. The trim and fill method. In H. R. Rothstein, A. J. Sutton, & M. Borenstein (Eds.), Publication bias in meta-analysis: Prevention, assessment, and adjustments : 127–144. Chichester: Wiley.
Elliott, J. H., Synnot, A., Turner, T., Simmonds, M., Akl, E. A., McDonald, S., et al. 2017. Living systematic review: 1. Introduction – The why, what, when, and how. Journal of Clinical Epidemiology, 91: 23–30.
Ferguson, C. J., & Brannick, M. T. 2012. Publication bias in psychological science: Prevalence, methods for identifying and controlling, and implications for the use of meta-analyses. Psychological Methods, 17(1): 120–128.
Fine, C., Sojo, V., & Lawford-Smith, H. 2020. Why does workplace gender diversity matter? Justice, organizational benefits, and policy. Social Issues and Policy Review, 14(1): 36–72.
Fortune 2017 (August 8). Google’s gender problem is actually a tech problem . Retrieved from http://fortune.com/2017/08/08/google-gender-struggle-tech/ . Retrieved from June 15, 2020.
Friese, M., & Frankenbach, J. 2020. p-Hacking and publication bias interact to distort meta-analytic effect size estimates. Psychological Methods, 25(4): 456–471.
Fujimoto, Y., Härtel, C. E., & Azmat, F. 2013. Towards a diversity justice management model: Integrating organizational justice and diversity management. Social Responsibility Journal, 9(1): 148–166.
Garner, P., Hopewell, S., Chandler, J., MacLehose, H., Akl, E. A., Beyene, J., et al. 2016. When and how to update systematic reviews: Consensus and checklist. British Medical Journal, 354: i3507.
Gibson, C. B., & Gibbs, J. L. 2006. Unpacking the concept of virtuality: The effects of geographic dispersion, electronic dependence, dynamic structure, and national diversity on team innovation. Administrative Science Quarterly, 51(3): 451–495.
Gonzalez-Mulé, E., & Aguinis, H. 2018. Advancing theory by assessing boundary conditions with meta-regression: A critical review and best-practice recommendations. Journal of Management, 44: 2246–2273.
Gusenbauer, M., & Haddaway, N. R. 2020. Which academic search systems are suitable for systematic reviews or meta-analyses? Evaluating retrieval qualities of Google Scholar, PubMed, and 26 other resources. Research Synthesis Methods, 11(2): 181–217.
Harari, M. B., Parola, H. R., Hartwell, C. J., & Riegelman, A. 2020. Literature searches in systematic reviews and meta-analyses: A review, evaluation, and recommendations. Journal of Vocational Behavior, 118: 103377.
Harrison, D., & Klein, K. 2007. What’s the difference? Diversity constructs as separation, variety, or disparity in organizations. Academy of Management Review, 32(4): 1199–1228.
Harzing, A.-W. 2006. Response styles in cross-national survey research: A 26-country study. International Journal of Cross Cultural Management, 6(2): 243–264.
Havránek, T., Stanley, T. D., Doucouliagos, H., Bom, P., Geyer-Klingeberg, J., Iwasaki, I., et al. 2020. Reporting guidelines for meta-analysis in economics. Journal of Economic Surveys, 34: 469–475.
Hedges, L. V. 1982. Estimation of effect sizes from a series of experiments. Psychological Bulletin, 92: 490–499.
Hedges, L. V., & Olkin, I. 1985. Statistical methods for meta-analysis . Orlando: Academic.
Henmi, M., & Copas, J. B. 2010. Confidence intervals for random effects meta-analysis and robustness to publication bias. Statistics in Medicine, 29(29): 2969–2983.
Hess, C., & Ostrom, E. 2003. Ideas, artifacts, and facilities: Information as a common-pool resource. Law and Contemporary Problems, 66(1/2): 111–145.
Hohn, R. E., Slaney, K. L., & Tafreshi, D. 2020. An empirical review of research and reporting practices in psychological meta-analyses. Review of General Psychology, 24(3): 195–209.
Hunter, J. E., Schmidt, F. L., & Jackson, G. B. 1982. Meta-analysis: Cumulative research findings across studies . Beverly Hills: Sage.
Hunter, J. E., Schmidt, F. L., & Le, H. 2006. Implications of direct and indirect range restriction for meta-analysis methods and findings. Journal of Applied Psychology, 91(3): 594–612.
Ioannidis, J. P. 2016. The mass production of redundant, misleading, and conflicted systematic reviews and meta-analyses. The Milbank Quarterly, 94(3): 485–514.
Ioannidis, J. P., Munafò, M. R., Fusar-Poli, P., Nosek, B. A., & David, S. P. 2014. Publication and other reporting biases in cognitive sciences: Detection, prevalence, and prevention. Trends in Cognitive Sciences, 18(5): 235–241.
Jackson, D., Turner, R., Rhodes, K., & Viechtbauer, W. 2014. Methods for calculating confidence and credible intervals for the residual between-study variance in random effects meta-regression models. BMC Medical Research Methodology, 14(1): 103.
Jak, S., & Cheung, M. W. L. 2020. Meta-analytic structural equation modeling with moderating effects on SEM parameters. Psychological Methods, 25(4): 430–455.
Jasny, B. R., Chin, G., Chong, L., & Vignieri, S. 2011. Again, and again, and again…. Science, 334: 1225.
Johnson, B. T., & Hennessy, E. A. 2019. Systematic reviews and meta-analyses in the health sciences: Best practice methods for research syntheses. Social Science and Medicine, 233: 237–251.
Johnson, C. D., Bauer, B. C., & Niederman, F. 2017. The automation of management and business science. Academy of Management Perspectives . https://doi.org/10.5465/amp.2017.0159 .
Judge, T. A., Heller, D., & Mount, M. K. 2002. Five-factor model of personality and job satisfaction: A meta-analysis. Journal of Applied Psychology, 87(3): 530–541.
Kaufmann, E., Reips, U. D., & Maag Merki, K. 2016. Avoiding methodological biases in meta-analysis: Use of online versus offline individual participant data (IPD) in educational psychology. Zeitschrift für Psychologie, 224(3): 157–167.
Kepes, S., Banks, G. C., McDaniel, M., & Whetzel, D. L. 2012. Publication bias in the organizational sciences. Organizational Research Methods, 15(4): 624–662.
Kepes, S., McDaniel, M. A., Brannick, M. T., & Banks, G. C. 2013. Meta-analytic reviews in the organizational sciences: Two meta-analytic schools on the way to MARS (the Meta-analytic Reporting Standards). Journal of Business and Psychology, 28(2): 123–143.
Kinlock, N. L., Prowant, L., Herstoff, E. M., Foley, C. M., Akin-Fajiye, M., Bender, N., et al. 2019. Open science and meta‐analysis allow for rapid advances in ecology: A response to Menegotto et al. (2019). Global Ecology and Biogeography, 28(10): 1533–1534.
Klein, R. A., Vianello, M., Hasselman, F., Adams, B. G., Adams, R. B., Jr., Alper, S., et al. 2018. Many Labs 2: Investigating variation in replicability across samples and settings. Advances in Methods and Practices in Psychological Science, 1(4): 443–490.
Kostova, T., Beugelsdijk, S., Scott, W. R., Kunst, V., Chua, C. H., & van Essen, M. 2020. The construct of institutional distance through the lens of different institutional perspectives: Review, analysis and recommendations. Journal of International Business Studies, 51(4): 467–497.
Kostova, T., Roth, K., & Dacin, T. 2008. Institutional theory in the study of multinational corporations: A critique and new directions. Academy of Management Review, 33(4): 994–1006.
Landis, R. S. 2013. Successfully combining meta-analysis and structural equation modeling: Recommendations and strategies. Journal of Business and Psychology, 28(3): 251–261.
Larsen, K. R., & Bong, C. H. 2016. A tool for addressing construct identity in literature reviews and meta-analyses. MIS Quarterly, 40(3): 529–551.
Larsen, K. R., Hekler, E. B., Paul, M. J., & Gibson, B. S. 2020. Improving usability of social and behavioral sciences’ evidence: A call to action for a national infrastructure project for mining our knowledge. Communications of the Association for Information Systems, 46(1): 1.
LeBreton, J. M., & Senter, J. L. 2008. Answers to 20 questions about interrater reliability and interrater agreement. Organizational Research Methods, 11(4): 815–852.
Lee, C. I., Bosco, F. A., Steel, P., & Uggerslev, K. L. 2017. A metaBUS-enabled meta-analysis of career satisfaction. Career Development International, 22(5): 565–582.
López-López, J. A., Page, M. J., Lipsey, M. W., & Higgins, J. P. 2018. Dealing with effect size multiplicity in systematic reviews and meta-analyses. Research Synthesis Methods, 9(3): 336–351.
Lubinski, D., & Humphreys, L. 1996. Seeing the forest from the trees: When predicting the behavior or status of groups, correlate means. Psychology, Public Policy, and Law, 2: 363–376.
Lyness, K. S., & Brumit Kropf, M. 2007. Cultural values and potential nonresponse bias. Organizational Research Methods, 10(2): 210–224.
Ma, H. H. 2009. The effect size of variables associated with creativity: A meta-analysis. Creativity Research Journal, 21(1): 30–42.
Maassen, E., van Assen, M. A., Nuijten, M. B., Olsson-Collentine, A., & Wicherts, J. M. 2020. Reproducibility of individual effect sizes in meta-analyses in psychology. PLoS ONE, 15(5): e0233107.
Marshall, I. J., & Wallace, B. C. 2019. Toward systematic review automation: A practical guide to using machine learning tools in research synthesis. Systematic Reviews, 8(1): 163.
Maseland, R., Dow, D., & Steel, P. 2018. The Kogut and Singh national cultural distance index: Time to start using it as a springboard rather than a crutch. Journal of International Business Studies, 49(9): 1154–1166.
Maznevski, M. L. 1995. Process and performance in multicultural teams , Working Paper, University of Virginia, Charlottesville, VA.
Maznevski, M. L., Davison, S. C., & Jonsen, K. 2006. Global virtual team dynamics and effectiveness. In G. K. Stahl & I. Bjorkman (Eds.), Handbook of research in international human resource management : 364–384. Cheltenham: Edward Elgar.
Meehl, P. E. 1990. Appraising and amending theories: The strategy of Lakatosian defense and two principles that warrant it. Psychological Inquiry, 1(2): 108–141.
Mendes, E., Wohlin, C., Felizardo, K., & Kalinowski, M. 2020. When to update systematic literature reviews in software engineering. Journal of Systems and Software, 167: 110607.
Merton, R. K. 1968. The Matthew effect in science: The reward and communication systems of science are considered. Science, 159(3810): 56–63.
Meyer, K., van Witteloostuijn, A., & Beugelsdijk, S. 2017. What’s in a p? Reassessing best practices for conducting and reporting hypothesis-testing research. Journal of International Business Studies, 48(5): 535–551.
Millard, T., Synnot, A., Elliott, J., Green, S., McDonald, S., & Turner, T. 2019. Feasibility and acceptability of living systematic reviews: Results from a mixed-methods evaluation. Systematic Reviews, 8(1): 325.
Moher, D., Liberati, A., Tetzlaff, J., & Altman, D. G. 2009. Preferred reporting items for systematic reviews and meta-analyses: The PRISMA statement. Annals of Internal Medicine, 151(4): 264–269.
Moher, D., Shamseer, L., Clarke, M., Ghersi, D., Liberati, A., Petticrew, M., et al. 2015. Preferred reporting items for systematic review and meta-analysis protocols (PRISMA-P) 2015 statement. Systematic Reviews, 4(1): 1.
Munafò, M. R., Nosek, B. A., Bishop, D. V., Button, K. S., Chambers, C. D., Du Sert, N. P., et al. 2017. A manifesto for reproducible science. Nature Human Behaviour, 1(1): 1–9.
Newman, M. E. 2009. The first-mover advantage in scientific publication. Europhysics Letters, 86(6): 68001.
Oh, I. S. 2020. Beyond meta-analysis: Secondary uses of meta-analytic data. Annual Review of Organizational Psychology and Organizational Behavior, 7: 125–153.
Ones, D. S., Viswesvaran, C., & Schmidt, F. L. 2017. Realizing the full potential of psychometric meta-analysis for a cumulative science and practice of human resource management. Human Resource Management Review, 27(1): 201–215.
Page, S. E. 2008. The difference: How the power of diversity creates better groups, firms, schools, and societies . Princeton: Princeton University Press.
Page, M. J., et al. 2020. The PRISMA 2020 statement: an updated guideline for reporting systematic reviews . https://doi.org/10.31222/osf.io/v7gm2 .
Paletz, S. B., Peng, K., Erez, M., & Maslach, C. 2004. Ethnic composition and its differential impact on group processes in diverse teams. Small Group Research, 35(2): 128–157.
Park, H. H., Wiernik, B. M., Oh, I.-S., Gonzalez-Mulé, E., Ones, D. S., & Lee, Y. 2020. Meta-analytic five-factor model personality intercorrelations: Eeny, meeny, miney, moe, how, which, why, and where to go. Journal of Applied Psychology . https://doi.org/10.1037/apl0000476 .
Pastor, D. A., & Lazowski, R. A. 2018. On the multilevel nature of meta-analysis: A tutorial, comparison of software programs, and discussion of analytic choices. Multivariate Behavioral Research, 53(1): 74–89.
Paterson, T. A., Harms, P. D., Steel, P., & Credé, M. 2016. An assessment of the magnitude of effect sizes: Evidence from 30 years of meta-analysis in management. Journal of Leadership and Organizational Studies, 23(1): 66–81.
Podsakoff, P. M., MacKenzie, S. B., & Podsakoff, N. P. 2012. Sources of method bias in social science research and recommendations on how to control it. Annual Review of Psychology, 63: 539–569.
Polanin, J. R., Espelage, D. L., Grotpeter, J. K., Valido, A., Ingram, K. M., Torgal, C., et al. 2020a. Locating unregistered and unreported data for use in a social science systematic review and meta-analysis. Systematic Reviews, 9: 1–9.
Polanin, J. R., Hennessy, E. A., & Tsuji, S. 2020b. Transparency and reproducibility of meta-analysis in psychology: A meta-review. Perspectives on Psychological Science, 15(4): 1026–1041.
Polzer, J. T., Crisp, C. B., Jarvenpaa, S. L., & Kim, J. W. 2006. Extending the faultline model to geographically dispersed teams: How colocated subgroups can impair group functioning. Academy of Management Journal, 49(4): 679–692.
Possolo, A., Merkatas, C., & Bodnar, O. 2019. Asymmetrical uncertainties. Metrologia, 56(4): 045009.
Revelle, W., & Wilt, J. 2019. Analyzing dynamic data: A tutorial. Personality and Individual Differences, 136: 38–51.
Richard, P. J., Devinney, T. M., Yip, G. S., & Johnson, G. 2009. Measuring organizational performance: Towards methodological best practice. Journal of Management, 35(3): 718–804.
Rosenthal, R. 1979. The ‘‘file drawer problem’’ and tolerance for null results. Psychological Bulletin, 86: 638–641.
Rosenthal, R., & Rubin, D. B. 1982. Comparing effect sizes of independent studies. Psychological Bulletin, 92: 500–504.
Rothstein, H. R., Sutton, A. J., & Borenstein, M. 2005. Publication bias in meta-analysis: Prevention, assessment and adjustments . Chichester: Wiley.
Rousseau, D. 2020. The realist rationality of evidence-based management. Academy of Management Learning and Education, 19(3): 415–423.
Scargle, J. D. 2000. Publication bias: The “File Drawer” problem in scientific inference. Journal of Scientific Exploration, 14: 91–106.
Schmidt, F. L., & Hunter, J. E. 2015. Methods of meta-analysis (3rd ed.). Thousand Oaks: Sage.
Scott, R. W. 2014. Institutions and organizations (4th ed.). Thousand Oaks: Sage.
Shemla, M., Meyer, B., Greer, L., & Jehn, K. A. 2016. A review of perceived diversity in teams: Does how members perceive their team’s composition affect team processes and outcomes? Journal of Organizational Behavior, 37: 89–106.
Sheng, Z., Kong, W., Cortina, J. M., & Hou, S. 2016. Analyzing matrices of meta-analytic correlations: Current practices and recommendations. Research Synthesis Methods, 7(2): 187–208.
Shojania, K. G., Sampson, M., Ansari, M. T., Ji, J., Doucette, S., & Moher, D. 2007. How quickly do systematic reviews go out of date? A survival analysis. Annals of Internal Medicine, 147: 224–233.
Siddaway, A. P., Wood, A. M., & Hedges, L. V. 2019. How to do a systematic review: A best practice guide for conducting and reporting narrative reviews, meta-analyses, and meta-syntheses. Annual Review of Psychology, 70: 747–770.
Smith, P. B., & Fischer, R. 2008. Acquiescence, extreme response bias and culture: A multilevel analysis. In F. J. R. V. de Vijver, D. A. van Hemert, & Y. H. Poortinga (Eds.), Multilevel analysis of individuals and cultures : 285–314. New York: Taylor & Francis/Lawrence Erlbaum.
Spellman, B. A. 2015. A short (personal) future history of revolution 2.0. Perspectives on Psychological Science, 10(6): 886–889.
Stahl, G. K., Maznevski, M. L., Voigt, A., & Jonsen, K. 2010. Unraveling the effects of cultural diversity in teams: A meta-analysis of research on multicultural work groups. Journal of International Business Studies, 41(4): 690–709.
Stanek, K. C., & Ones, D. S. 2018. Taxonomies and compendia of cognitive ability and personality constructs and measures relevant to industrial, work and organizational psychology. In D. S. Ones, N. Anderson, C. Viswesvaran, & H. K. Sinangil (Eds.), The SAGE handbook of industrial, work and organizational psychology (2nd ed., Vol. 1): 366–407. Thousand Oaks: Sage.
Stanley, T. D. 2017. Limitations of PET-PEESE and other meta-analysis methods. Social Psychological and Personality Science, 8(5): 581–591.
Stanley, T. D., & Doucouliagos, H. 2014. Meta-regression approximations to reduce publication selection bias. Research Synthesis Methods, 5(1): 60–78.
Steel, P., Johnson, J. W., Jeanneret, P. R., Scherbaum, C. A., Hoffman, C. C., & Foster, J. 2010. At sea with synthetic validity. Industrial and Organizational Psychology, 3(3): 371–383.
Steel, P. D., & Kammeyer-Mueller, J. D. 2002. Comparing meta-analytic moderator estimation techniques under realistic conditions. Journal of Applied Psychology, 87(1): 96–111.
Steel, P., Kammeyer-Mueller, J., & Paterson, T. A. 2015. Improving the meta-analytic assessment of effect size variance with an informed Bayesian prior. Journal of Management, 41(2): 718–743.
Steel, P., Schmidt, J., Bosco, F., & Uggerslev, K. 2019. The effects of personality on job satisfaction and life satisfaction: A meta-analytic investigation accounting for bandwidth–fidelity and commensurability. Human Relations, 72(2): 217–247.
Sun, S. 2011. Meta-analysis of Cohen’s kappa. Health Services and Outcomes Research Methodology, 11(3–4): 145–163.
Sutton, A. J. 2009. Publication bias. In H. Cooper, L. V. Hedges, & J. C. Valentine (Eds.), The handbook of research synthesis and meta-analysis : 435–452. New York: Russell Sage.
Tabachnick, B. G., & Fidell, L. S. 2014. Using multivariate statistics . Harlow: Pearson.
Tanner-Smith, E. E., & Tipton, E. 2014. Robust variance estimation with dependent effect sizes: Practical considerations including a software tutorial in Stata and SPSS. Research Synthesis Methods, 5(1): 13–30.
Taras, V., Kirkman, B. L., & Steel, P. 2010. Examining the impact of culture’s consequences: A three-decade, multilevel, meta-analytic review of Hofstede’s cultural value dimensions. Journal of Applied Psychology, 95(3): 405–439.
Taras, V., Rowney, J., & Steel, P. 2009. Half a century of measuring culture: Review of approaches, challenges, and limitations based on the analysis of 121 instruments for quantifying culture. Journal of International Management, 15(4): 357–373.
Taras, V., & Steel, P. 2009. Beyond Hofstede: Challenging the ten commandments of cross-cultural research. In C. Nakata (Ed.), Beyond Hofstede: Culture frameworks for global marketing and management : 40–60. Chicago: Palgrave Macmillan.
Tasheva, S., & Hillman, A. J. 2019. Integrating diversity at different levels: Multilevel human capital, social capital, and demographic diversity and their implications for team effectiveness. Academy of Management Review, 44(4): 746–765.
Tranfield, D., Denyer, D., & Smart, P. 2003. Towards a methodology for developing evidence-informed management knowledge by means of systematic review. British Journal of Management, 14(3): 207–222.
Tsuji, S., Bergmann, C., & Cristia, A. 2014. Community-augmented meta-analyses: Toward cumulative data assessment. Perspectives on Psychological Science, 9(6): 661–665.
Versteeg, M., & Ginsburg, T. 2017. Measuring the rule of law: A comparison of indicators. Law and Social Inquiry, 42(1): 100–137.
Vicente-Sáez, R., & Martínez-Fuentes, C. 2018. Open Science now: A systematic literature review for an integrated definition. Journal of Business Research, 88: 428–436.
Viechtbauer, W. 2010. Conducting meta-analyses in R with the metafor package. Journal of Statistical Software, 36(3): 1–48.
Viechtbauer, W., Lopez-Lopez, J. A., Sanchez-Meca, J., & Marin-Martinez, F. 2015. A comparison of procedures to test for moderators in mixed-effects meta-regression models. Psychological Methods, 20: 360–374.
Wasserman, J. D., & Bracken, B. A. 2003. Psychometric characteristics of assessment procedures. In J. R. Graham & J. A. Naglieri (Eds.), Handbook of psychology : 43–66. New Jersey: Wiley.
Weisz, J. R., Kuppens, S., Ng, M. Y., Eckshtain, D., Ugueto, A. M., Vaughn-Coaxum, R., et al. 2017. What five decades of research tells us about the effects of youth psychological therapy: A multilevel meta-analysis and implications for science and practice. American Psychologist, 72(2): 79–117.
Wiernik, B. M., & Dahlke, J. A. 2020. Obtaining unbiased results in meta-analysis: The importance of correcting for statistical artifacts. Advances in Methods and Practices in Psychological Science, 3(1): 94–123.
Wood, B. D., Müller, R., & Brown, A. N. 2018. Push button replication: Is impact evaluation evidence for international development verifiable? PLoS ONE, 13(12): e0209416.
Yuan, Z., Morgeson, F. P., & LeBreton, J. M. 2020. Maybe not so independent after all: The possibility, prevalence, and consequences of violating the independence assumptions in psychometric meta-analysis. Personnel Psychology, 73(3): 491–516.
Download references
Acknowledgement
We thank Alain Verbeke and two Journal of International Business Studies anonymous reviewers for highly constructive and useful feedback that allowed us to improve our article.
Author information
Authors and affiliations.
Haskayne Business School, University of Calgary, 2500 University Dr NW, Calgary, AB, T2N 1N4, Canada
Piers Steel
Faculty of Economics and Business, University of Groningen, Nettelbosje 2, 9700 AV, Groningen, Netherlands
Sjoerd Beugelsdijk
Department of Management, School of Business, The George Washington University, 2201 G St. NW, Washington, DC, 20052, USA
Herman Aguinis
You can also search for this author in PubMed Google Scholar
Corresponding author
Correspondence to Piers Steel .
Additional information
Publisher's note.
Springer Nature remains neutral with regard to jurisdictional claims in published maps and institutional affiliations.
Accepted by Alain Verbeke, Editor-in-Chief, 20 October 2020. This article was single-blind reviewed.
Rights and permissions
Open Access This article is licensed under a Creative Commons Attribution 4.0 International License, which permits use, sharing, adaptation, distribution and reproduction in any medium or format, as long as you give appropriate credit to the original author(s) and the source, provide a link to the Creative Commons licence, and indicate if changes were made. The images or other third party material in this article are included in the article's Creative Commons licence, unless indicated otherwise in a credit line to the material. If material is not included in the article's Creative Commons licence and your intended use is not permitted by statutory regulation or exceeds the permitted use, you will need to obtain permission directly from the copyright holder. To view a copy of this licence, visit http://creativecommons.org/licenses/by/4.0/ .
Reprints and permissions
About this article
Steel, P., Beugelsdijk, S. & Aguinis, H. The anatomy of an award-winning meta-analysis: Recommendations for authors, reviewers, and readers of meta-analytic reviews. J Int Bus Stud 52 , 23–44 (2021). https://doi.org/10.1057/s41267-020-00385-z
Download citation
Received : 28 July 2020
Accepted : 20 October 2020
Published : 05 January 2021
Issue Date : February 2021
DOI : https://doi.org/10.1057/s41267-020-00385-z
Share this article
Anyone you share the following link with will be able to read this content:
Sorry, a shareable link is not currently available for this article.
Provided by the Springer Nature SharedIt content-sharing initiative
- meta-analysis
- literature review
- quantitative review
- research methodology
- Find a journal
- Publish with us
- Track your research
Cochrane UK is now closed
Due to changes in NIHR funding in evidence synthesis, Cochrane UK is now closed. We are so grateful to all our contributors and supporters.
If you would like to get involved with Cochrane, please visit the Join Cochrane pages
To learn more about Cochrane, visit Cochrane's homepage
If you would like to propose a topic for a Cochrane Review, please see our instructions for authors
If you have any queries, please contact Cochrane Support
Cochrane UK
Meta-analysis: what, why, and how.
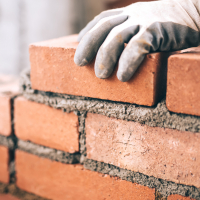
This is an excerpt from a blog originally published on Students 4 Best Evidence
What is a meta-analysis?
Meta-analysis is a statistical technique for combining data from multiple studies on a particular topic.
Meta-analyses play a fundamental role in evidence-based healthcare. Compared to other study designs (such as randomized controlled trials or cohort studies), the meta-analysis comes in at the top of the evidence-based medicine pyramid. This is a pyramid which enables us to weigh up the different levels of evidence available to us. As we go up the pyramid, each level of evidence is less subject to bias than the level below it. Therefore, meta-analyses can be seen as the pinnacle of evidence-based medicine (1).
Meta-analyses began to appear as a leading part of research in the late 70s. Since then, they have become a common way for synthesizing evidence and summarizing the results of individual studies (2).
Read the full article here
SYSTEMATIC REVIEW article
Social identity and social integration: a meta-analysis exploring the relationship between social identity and social integration.
- 1 School of Humanities, Jinan University, Guangdong, China
- 2 Department of Social and Behavioral Sciences, City University of Hong Kong, Hong Kong SAR, China
Social identity formation is crucial for psychosocial development, particularly in the case of migrating adults. A body of research exploring how social identity influences social integration among migrants shows that social identity affects social integration through a range of moderators and procedures. This study reports on a meta-analysis of 33 studies with 47 cases (total N = 33,777; Fisher’s z = 0.33, moderate effects) examining the relationship between social identity and social integration in research conducted from 2005–2020. The research findings suggest that social identity can affect social integration directly without any moderators, indicating that most of the identified moderators in the previous studies are sample-specific variables. More importantly, the effects of various aspects of identities exert similar degrees of impact (moderate effect) on social integration; in other words, the usefulness of analyzing different aspects of social identity on social integration is challenged.
In the last decade, immigration, a visible reflection of global integration related to well-being, freedom, life chances, etc., has become a significant facet of globalization, which has generated renewed interest in the rise of diverse identities. Social integration is recognized as the process of newcomers or minorities being incorporated into the host society ( Alba and Nee, 1997 ) and is particularly significant within ethnic minority groups as they are trying hard to adapt to the host culture to function adequately daily and increase their well-being, which is the main benefit of social integration. Social integration refers to the extent of social relations or social ties binding people together in the sense of social belonging and inclusion ( Wray et al., 2011 ). The effects of social integration have been well documented in the literature, including well-being ( Scottham and Dias, 2010 ), self-esteem ( Berry and Sabatier, 2010 ), health ( Curran, 2003 ), social identity conflict ( Ward et al., 2011 ), prosocial behaviors ( Schwartz et al., 2007 ), inter-group relations ( Zagefka and Brown, 2002 ), sociocultural adaptation problems ( Neto et al., 2005 ), and objective integration parameters, such as income and occupation ( Borjas, 1994 ). Individuals might also focus on the identity-affirming and acculturation strategies used in the host society in the face of demands linked to the context of immigration ( Berry and Sam, 1997 ). Immigrants are more likely to be accepted if they come from cities or countries considered identically and culturally compatible with the host society ( Wimmer and Soehl, 2014 ). At an individual level, the degree of identity assimilation into the host society results in social integration into the host culture ( Helbling and Traunmüller, 2016 ). Social identity changes to adapt to the local environment as we embrace and occupy various spaces and form new ties under the conditions that identities are not fixed or given, which is exactly the process of social integration.
Scholars often postulate that social identity is linked to the process of social integration ( Scott and Marshall, 2009 ). Social identity illustrates individual, interpersonal, and social processes to embed into the social structures ( Davis et al., 2019 ). Social psychologists from both sociology and psychology have developed robust theories of social identity and social integration, which is notably represented by social identity theory. Social identity theory was proposed by Tajfel and Turner (1979) making a new interpretation of group behavior. An individual’s choice of behavior is largely affected by the perceived inter-group relationship, in that individual recognition of groups is the basis of group behavior ( Tajfel and Turner, 1979 ). McCrone and Bechhofer (2008) and Reeskens and Wright (2013) suggested that social identity enacts identity meanings in social situations, which positively influenced social integration. Identities are internalized roles attached to the self as a unique person in a certain group ( Burke and Stets, 2009 ; Stets and Serpe, 2013 ; Stets and Burke, 2014 ). Individuals tend to enact prominent identities when the social situation allows ( Owens et al., 2010 ; Brenner et al., 2014 ). However, social identity may also hinder the process of social integration because of the strong ties those concerned have with their own countries ( Aalberg et al., 2011 ). Previous literature has discussed diverse identities, such as ethnic social identity ( Giles et al., 1979 ; Garcia, 1982 ; Moha, 2005 ), religious social identity ( Walters et al., 2007 ), regional social identity ( Moha, 2005 ) and cultural social identity ( Ghosh, 2000 ). Given above, social identity is a multi-dimensional construct; thus, existing literature has examined the relationship between various aspects of social identity and social integration, while multiple moderators have been identified to influence the relationship between different identities and social integration. To illustrate, life satisfaction ( Schotte et al., 2018 ), gender ( Litchmore and Safdar, 2015 ), self-esteem ( Vedder, 2005 ) and community involvement ( Ramirez-Valles et al., 2010 ) tend to affect the relationship between social identity and social integration. Given such diverse results, the strength of relationship between different types of identities and social integration remains unknown. More importantly, it also remains unclear whether there is a need to separately examine how each of our identities influences social integration and identify those moderators for such a relationship. There is a huge variation in data collection within the existing literature, with data generated from different areas, ethnicities, and nationalities. Hence, a meta-analysis on the relationship of social identity and social integration is crucial and timely.
Social psychologists from both sociology and psychology also have developed acculturation theory besides social identity theory to explain social identity and social integration. Immigrant identities form through immigrants’ cultural and social positions in the host majority group, which performs integration or exclusion behaviors. The transition of migrants from one culture to another culture and/or from one society to another society may induce social identity challenges, which is analyzable by Berry’s (1990) acculturation model. The model focuses on the process of cultural exchange with another culture with general acculturative changes in the original culture ( Berry, 1990 ), which can be summarized by four acculturation strategies (assimilation, separation, integration and marginalization). Furthermore, each acculturation strategy, effectively explaining the relationship between social identity and social integration, may lead to different psychological effects. For example, integration is the main characteristic of being a bicultural person and is the most commonly adopted acculturation strategy. Higher level of social identity increases immigrants’ social integration in the host countries through strong psychological linkage ( Aalberg et al., 2011 ). Individuals organize identity meanings, religious affiliation, community connection, to respond to identity-relevant feedback, such as social integration ( Walters et al., 2007 ) Existing literature on social identity offered two perspectives. One focused on the individual’s social identity and its relation to different social groups ( Tajfel, 1982 ), while the second focused on how an individual cope with multiple identities and the results of this ( Stryker and Burke, 2000 ). In addition, cross-cultural similarities are attributable to the integration tendency and the motivation of social integration and distinctiveness ( Gaertner and Dovidio, 2000 ). Immigrants are expected to assimilate well into the local culture and quickly adopt a new social identity ( Amit, 2012 ). The rapid development of technology and globalization effects has expanded the opportunities for people to acculturate ( Chen et al., 2008 ; Wang and Abosag, 2019 ). In other words, people can acculturate because of immigration-or technology-mediated globalization. Both acculturation types may require people to integrate different cultural identities. Helbling and Traunmüller (2016) found that immigrants who shared the same race, ethnicity, culture or religion as the ethnic majority group were more likely to be accepted. This however, refutes the reality, given the large cultural distance particularly between East Asian culture and Western culture that migrants face in terms of social identity challenges. Existing psychology literature suggests that some ethnic minorities have developed integrated cultural identities whereas others have developed separated cultural identities ( Haritatos and Benet-Martı́nez, 2002 ). The integrated cultural identities are more likely to lead to positive psychological well-being ( Haritatos and Benet-Martı́nez, 2002 ).
The aim of our paper is to examine the relationship between social identity and social integration based on social identity theory and acculturation theory. The effects of moderators, life satisfaction ( Schotte et al., 2018 ), gender ( Litchmore and Safdar, 2015 ), self-esteem ( Vedder, 2005 ) and community involvement ( Ramirez-Valles et al., 2010 ), remained uncertain in the relationship between diverse identities and social integration. Meta-analysis is already widely used in psychology research; however, it is still relatively new in sociological studies, especially in social psychology research. To fill the research gap, our research presents meta-analysis endeavors to address two questions. First, we examine the average effect size of the relationship of social identity and social integration. Second, this paper examines the potential moderators between social identity and social integration.
Literature review
Social identity and social integration.
“Social identity” firstly comes from psychology literature. As Freud (1921) suggested, social identity is the initial form of emotional connection with an objective object and it also implies an alternative to sexual instincts, as if it were that the object is injected into the self. One’s social identity includes a series of constituent multiple identities, and each sub-social identity differs from the others.
The impact of social norms on individual attitudes and behaviors is through one’s social identity ( Brown, 2000 ). Multiple identities may lead to social identity conflict. There are four identities which are widely recognized and examined in both psychology and social science literature—these are ethnic social identity ( Giles et al., 1979 ; Garcia, 1982 ; Moha, 2005 ), religious social identity ( Walters et al., 2007 ), regional social identity ( Moha, 2005 ) and cultural social identity ( Ghosh, 2000 ). Ethnic social identity, like other identities, serves as a multidimensional construct, involving ethnic attitudes, knowledge, and social behaviors ( Giles et al., 1979 ; Garcia, 1982 ). One’s religious social identity seems to influence immigrants’ adaptation to the host society; for example, Walters et al. (2007) found that the black community in Canada felt less Canadian because of their religious affiliation (Catholic or non-Catholic). Regional social identity includes national social identity and community social identity, among others. Investigated the case of Canada related to the measurement of national social identity, which could be deemed as the measurement of regional social identity. Cultural social identity is the social identity or belonging to the culture of a certain group, which is a part of self-perception relating to other types of social identity; for example, ethnic social identity and national social identity ( Moha, 2005 ). Ghosh (2000) postulated that our experiences of the past and present social, cultural and economic relations seemed to influence how we define ourselves. Although these four identities have been the most extensively studied, they nevertheless differ significantly from one another. For example, regional social identity is mainly developed from regional classification. As different ethnicity relate to different religious identity, ethnic social identity and religious social identity share some characteristics. Additionally, ethnicity also relates to region; that is, region is sometimes divided based on ethnic groups.
Social integration may be affected by social identity in that the strong ties those concerned have with their own countries ( Aalberg et al., 2011 ). Social integration is a result of allowing every member, particularly migrants, to feel part of the community and connected to the host society. Social integration takes place when minority groups from other regions are incorporated into mainstream society; however, such integration is a long-term process of adopting a shared system of meaning, language, culture and the like ( Sherman et al., 2006 ). Social integration is also a multi-dimensional construct which may include economic integration, cultural integration and psychological integration. Social identity integration is different from social integration and only captures the compatibility between one’s multiple identities which are strongly associated with social roles. Individuals may perceive their cultural identities conflicted and separated and have difficulties in integrating them in a unified sense ( Benet-Martínez et al., 2002 ; Benet-Martínez and Haritatos, 2005 ). However, social integration is a more comprehensive term which includes various aspects of integration into the host society. Therefore, social identity integration is only a dimension of social integration and cannot be perceived as equivalent to social integration.
There are no consistent terminologies that researchers use to examine the phenomenon of social integration. Social inclusion and social cohesion have been used as the proxy to measure social integration (e.g., Atkinson, 2002 ; Marlier, 2003 ; Atkinson et al., 2004 ; Cordier et al., 2017 ). Social integration embodies the implication of social inclusion and social cohesion at society, group or individual levels. To be more specific, social inclusion indicates a user-friendly term referring to individuals accessing limited resources to their benefit and returns ( Oxoby, 2009 ) in terms of improving the ability, opportunity, and dignity of those disadvantaged based on their social identity ( World Bank, 2013 ). Dayton-Johnson (2003) suggested that social cohesion can be a feature of society depending on social capital accumulation from the perspective of economics. Thus, social inclusion indicates the process of attaining certain rights, such as employment, adequate housing, medical care and education when people try to adapt to the host culture. A sense of belonging to a society or the opposite feelings of exclusion are often perceived as two important features of social cohesion. Social cohesion infers the desirable feature a society generating a sense of belonging within the person concerned; a sense of belonging to the community and society. The opposite, however, can also be the case when such feelings/features of social cohesion deteriorate ( Schmeets and te Riele, 2014 ). As Bernard (1999) suggested, social cohesion is a hybrid mental construction to achieve a consensus in a social entity. There are six common dimensions of social cohesion; these are social relations ( Friedkin, 2004 ), identification ( Jenson, 2010 ), equality ( Novy et al., 2012 ), orientation towards the common good ( Green and Janmaat, 2011 ), objective and subjective quality of life ( KfW Bankengruppe, 2010 ), and shared values ( Botterman et al., 2012 ).
Several empirical studies have examined the topics related to social integration ( Zhou et al., 2014 ; Mariyam and Jose, 2017 ; Negru-Subtirica et al., 2017 ). Social integration is generally measured by objective indicators and subjective indicators. Objective indicators refer to the situation of social security (e.g., medical insurance, etc.), housing, vocational training, working hours per day, and personal income. Subjective indicators refer to social identity, social status, willingness to relocate, acceptance of local cultural values and social satisfaction. In a similar vein, Giambona and Vassallo (2014) proposed that social inclusion (or social integration) could be estimated by four specific indicators, which are (i) the percentage of people at risk-of-poverty after migration, (ii) the proportion of severely materially deprived people, (iii) the percentage of people living with very low work intensity, and (iv) school dropout rates ( Finn and Rock, 1997 ).
The relationship between social identity and social integration
People encapsulate multiple identities. Existing literature has therefore examined the relationship between different aspects of social identity and social integration. Most scholars suggested that the perceived social identity, like national social identity, cultural social identity, and ethnic social identity, can greatly influence social integration. For example, national identity, a kind of social identity, is in the presence of other countries in the context of people to build a “country” belonging to the “sense of identity.” McCrone and Bechhofer (2008) argued that national social identity can be captured by social identity; which might eventually lead to social inclusion or social exclusion. Specifically, all individuals claim particular identities given their roles and the groups they belong to in the social structure, which implies that they search for positive distinctiveness in dealings with other groups, so as to increase permeability of group boundaries ( McCrone and Bechhofer, 2008 ; Hogg, 2018 ). Social identity is passed down in the form of fixed repertoire by power systems, under which people negotiate and ultimately transform identities ( McCrone, 2001 ). The degree of negotiated process influences the level of social integration. Bourdieu (1987) and Corneo and Jeanne (2010) argued that the relationship between career and people’s character traits (i.e., social identity) contributes to how individuals perceive themselves and influences how they are perceived by the rest of the society. The components of social integration come from various elements such as language, race, culture symbols, habits, geography and behaviors ( Orgad, 2015 ). Reeskens and Wright (2013) demonstrated that social integration for immigrants was influenced by the different types of identities and different conditions in a community. Social identity can influence life chances insofar as being “one of us” in the society, while interest or other factors in inclusion and exclusion is an issue of how can people integrate in a society ( McCrone and Bechhofer, 2008 ).
Nevertheless, the negative effect of social identity on social integration also exists ( Reitz et al., 2009 ). Reitz et al. (2009) contended that social identity sometimes hindered the process of social integration—for example, the ethnic social identity showed a clear negative effect on citizenship acquisition and the willingness to integrate into the Canadian society for immigrants in Canada ( Reitz et al., 2009 ). To be specific, according to social identity theory, individuals with negative or insecure identity cope with “jump ship” (social mobility) strategy, especially if group boundaries are sufficiently permeable to permit this ( Brown, 2019 ). Once a relevant social identity is thus engaged, the adaptation is occurred. However, if migrants hold a very strong social identity with their own society, it will be very hard for them to accept the new culture and values in the host society ( Aalberg et al., 2011 ). The two opposing opinions represent the diverse influence of social identity on social integration; however, the claim of positive effect occupies the dominant position.
Social identity theory and acculturation theory are widely used to explain the relationship of social identity and social integration in most of the literature. Based on social identity theory, identities have three bases: person, role, and group/social ( Stets and Serpe, 2013 ) denoting one’s position within the broader social structure ( Burke and Stets, 2009 ). Forging ties among these bases in social identity theory is a key step in constructing a general theory of the self ( Stets and Burke, 2000 ; Stets and Serpe, 2013 ). Herein, social identities influence both immigrants and host societies, particularly those that are related to social system and cultural development such as ethnic social identity ( Duroy, 2011 ). Moreover, the theoretical framework of social identity takes account of the heterogeneities which cause differences in social integration outcomes ( Merve, 2017 ). For example, the cultural social identity assimilation process requires people to accept and integrate into the host culture practices. Immigrants identify and classify themselves into social categories in the host society; namely, social identity (e.g., religious social identity and regional social identity) based on the sense of belonging. The process of forming social relationships is also a process of social integration.
Acculturation theory is also used to explain the mechanism of how social identity influences social integration. Acculturation is a process that occurs when minority groups in a society meet another culture ( Berry and Kim, 1988 ). The acculturation process may require ethnic minorities to adapt to different values, beliefs, and behaviors (e.g., language) of the dominant society ( Ramirez, 2007 ). Berry (2005) presented four ways—assimilation, separation, integration and marginalization—to manage social identity in a multicultural environment, particularly for immigrants. Goodman (2012) , Joppke (2007) and Sniderman and Hagendoorn (2007) claimed that the ethnic majority may perceive international immigrants as lacking commitment to assimilation. The marginalized feeling among international immigrants results in a vicious cycle of failed integration which is hard to break ( Adida et al., 2014 ). Even among integrated individuals, people may have developed different levels of integration. Social integration may be improved through educational classes (e.g., language class, literacy class and employment training programs), cultural assimilation, and social norms acceptance ( Billeke et al., 2015 ). Some migrants have developed integrated cultural identities, and others have not ( Benet-Martínez et al., 2002 ). Personality traits, accent, appearance and dress may contribute to these differences.
This study examines the relationship between social identity and social integration with the extension of social identity theory and acculturation model, since, to the best of the authors’ knowledge, no comprehensive study including various samples in terms of area, ethnicity, nationality and others has been undertaken. We are specifically interested in seeing whether this association differs according to which aspect of social identity is considered. We are also interested in whether this moderation effect would differ across social identity dimensions.
Search strategies
Because target participants are not limited to one nationality, the current study includes papers from databases, such as PsycINFO, Pro-Quest, CNKI. Relevant studies with key words social identity (identification, belonging, acculturation, multiculturalism) and to integration (inclusion, cohesion, adaption, transformation, exclusion) were identified when searching these databases. We followed Mol and Bus’s (2011) analytic procedures to search relevant studies in social science research. We selected all possible materials from the past 15 years from 2005 to 2020.
Inclusion criteria
The selected articles had to meet the following inclusion criteria: (a) not a single case study; (b) no opinion and non-empirical articles; (c) internal migration or international migration; and (d) reports social identity and social integration. The rest of the materials were closely reviewed using specific criteria described below.
First, the correlations, or means, or the percentage of variance ( R 2 ) in social integration accounted for by social identity, or standard deviations provided presented the association between social identity and social integration. These association could be transformed into a Fisher’s z effect size. All materials for controlling the potential impact of different writing systems in the relationship between social identity and social integration ( García and Cain, 2014 ). We retrieved all (published or unpublished) articles, dissertations, and conference papers before the year of 2021.
Second, we treated multiple, independent samples within one article as separate studies ( Mol and Bus, 2011 ). For each study, we tried to find a match on the following sample size, age, vocations, life satisfaction, community involvement, self-categorization, and cultural conformity. If data were absent from the original materials, we emailed the authors concerned for the required information.
Third, studies had to assess both social identity and social integration with objective and quantitative tasks. Meta-analysis incorporates the synthesized effect of related empirical studies into the analysis. This study is the aggregation of information leading to a higher statistical power on the relationship of social identity and social integration.
Fourth, if a composite measure of social integration (e.g., police effectiveness plus social integration; social system plus social inclusion; or cognitive of discrimination plus social cohesion) was used, the resulting correlation was not included because we wanted to examine how the relationship between social identity and social integration is affected by the methods used to measure the variables.
Fifth, the measures of social identity and social integration used to calculate the correlations had to be taken at the same time point and with a group of people who shared commonalities (i.e., all the people are migrants, internal migrants or international migrants at the beginning of their migration lives).
Coding procedures
Studies were coded according to participants characteristics and the characteristics of the assessments were used to measure social identity and social integration. Two independent coders completed a standard coding scheme per study, comprising (a) year of publication, (b) sample size (c) participant’s location or nationality, (d) first three authors, (e) type of publication, (f) type of social identity, (g) type of migration, and (h) where the data came from. Two coders coded 75% of all studies included. The inter-coder agreement for both study characteristics and outcome variables ranged between 77 and 100% across meta-analyses, all discrepancies between coders were settled in discussion, and consensus scores were used.
For each study included in our analysis, we coded for the key moderators of the relationship of social identity and social integration. With respect to type of measurement, we divided the independent variable ‘social identity’ into four kinds of social identity—ethnic, cultural, regional, and religious—which four kinds most used in research.
Overview of studies
The current study includes 33 articles with 47 cases which involved 33,777 participants in total. Most studies used a correlational design. All indicators come from the correlation index which includes regression analysis, correlation analysis and factor analysis (i.e., factor loading). In the current meta-analysis, 11 studies followed longitudinal research design and two studies applied experimental research design. Table 1 lists all details of the important features from the selected studies.
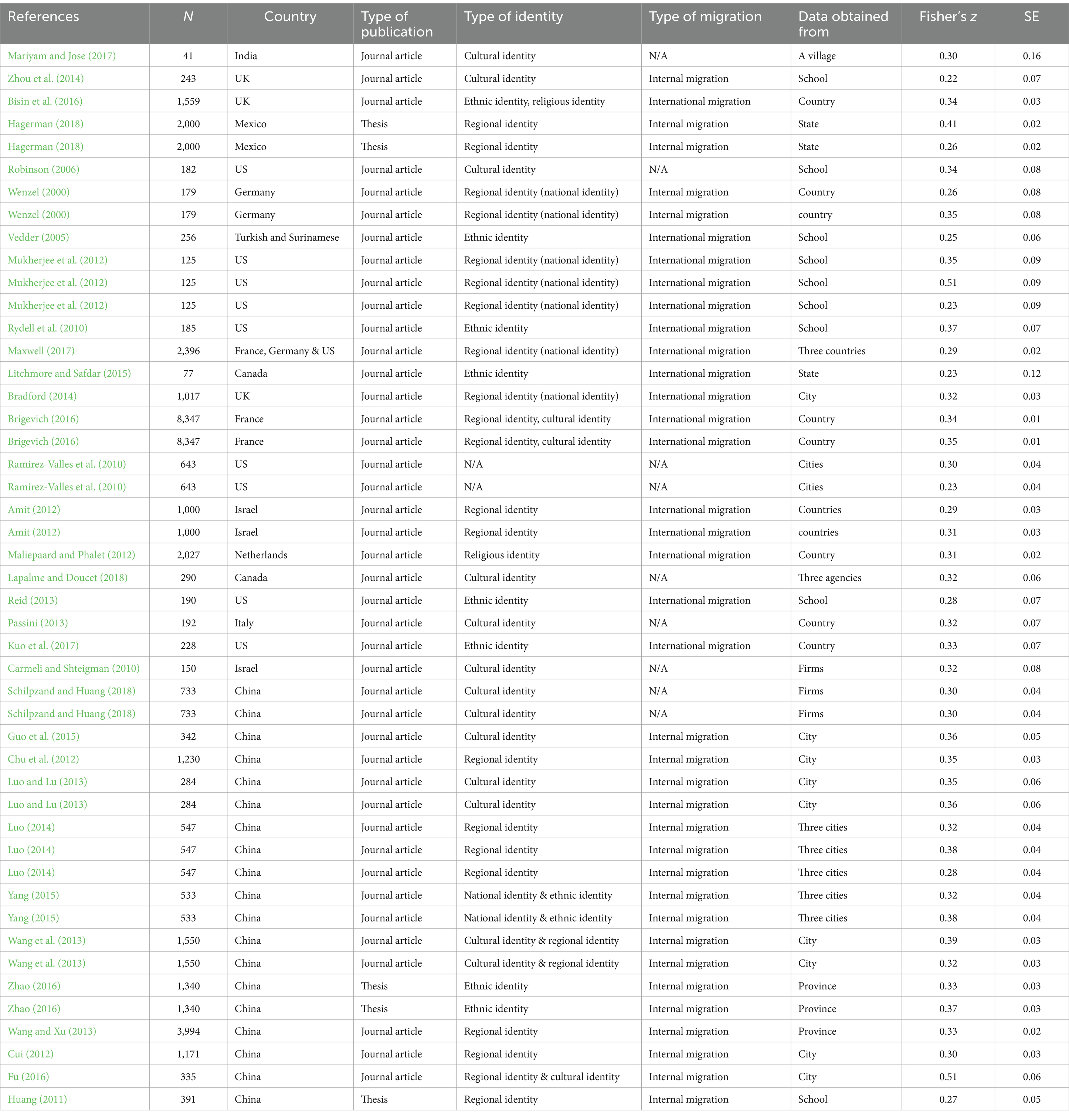
Table 1 . Summary of included studies.
Meta-analytic procedures
The Comprehensive Meta-Analysis 3.0 software was applied to Fisher’s z- effect sizes calculation. PRISMA was applied to the current method, which provided the detailed information for a meta-analysis ( Moher et al., 2010 ). All correlations between the social identity and any outcome variable were inserted into the computer program Comprehensive Meta-Analysis and transformed into Fisher’s z- effect sizes for further analyses, because the variance of Fisher’s z was nearly constant, whereas the variance of the correlation followed an asymmetrical distribution ( Borenstein et al., 2009 ). To ease interpretation of the Results section, Fisher’s z summary estimates were transformed back into a correlation with the formula r = tanh( z ’) ( Lipsey and Wilson, 2001 ). In general, a Fisher’s z value of 0.10 ( r = 0.10) counted as a small-effect size, 0.31 ( r = 0.30) as moderate, and 0.55 ( r = 0.50) as large ( Cohen, 1988 ).
To interpret the ES for the correlation between social integration and its domains (economics, psychological belonging, cultural acceptance, etc.), we regraded each outcome domain as an independent correlate ( Mol and Bus, 2011 ). If an article applied multiple tests to measure the correlation through different measurements, we reported the average ES to ensure each study only offers one ES in the analysis.
We coded samples as “ethnic” when the article offered the relevant information (i.e., language, history and common social norms). In the current study, we treat participants in the same way as those who live in one area with local people because they share similar social norms of the host society.
The current study applied a random-effect model for conservative consideration on ES estimation ( Borenstein et al., 2009 ). We also presented 95% confidence interval (CI) and ensured the interval did not cross the zero zone ( Pigott, 2012 ). Meanwhile, we presented the chi-square test for materials’ heterogeneity report; we selected a significant Q between the df value for moderator analyses (meta-regression would apply if the minimum number of cases exceeds four) ( Mol and Bus, 2011 ).
Because the impact of social identity and its domains (ethnic social identity, cultural social identity, regional social identity and religious social identity), studies in each domains showed different correlations. Therefore, we examined whether the results were moderated by the age, vocation, life satisfaction, community involvement, self-categorization, and cultural conformity, among other factors. As another indicator, we checked the publication bias. We applied funnel plot ( Duval and Tweedie, 2000 ), p -curve analysis ( Simonsohn et al., 2014 ) and PET-PEESE6 ( Stanley and Doucouliagos, 2014 ). Last, we presented Rosenthal’s fail-safe number which reveals the number of missing studies with null effects that would have to be retrieved and included in the analyses before the p value becomes non-significant ( Borenstein et al., 2009 ).
Overall analyses
Table 2 presents the overall effect of the relationship of social identity and social integration. From the table, the overall effect between different kinds of social identity and social integration is at a moderate level (Fisher’s z = 0.33, p < 0.001). The chi-square test shows that the differences in the indicators of the empirical studies involved in this research were not significant ( p > 0.05), which means that the heterogeneity had not reached a level of significance. The results show that the correlation between all kinds of social identity and social integration were at the moderate level, which also indicates that almost all potential moderators are not significant in this correlation. As for the heterogeneity analysis, we test all kinds of moderators. The results show that these moderators (e.g., ethnic group, age group) only explain 8% of the variance of the heterogeneity; 92% of the variances of the heterogeneity are not explainable by the current moderators.

Table 2 . Meta-analysis of the correlation between social identity and social integration.
The forest plot in Figure 1 shows the aggregate raw proportions and the 95% CIs for all the studies included in the meta-analysis. Figure 1 presents the ES and 95% CIs from all studies included in this research. The figure shows the overall ES at the bottom from the selected studies, and the dotted line at the 0.50 level reflects that the selected materials are randomly distributed with no researcher preferences.
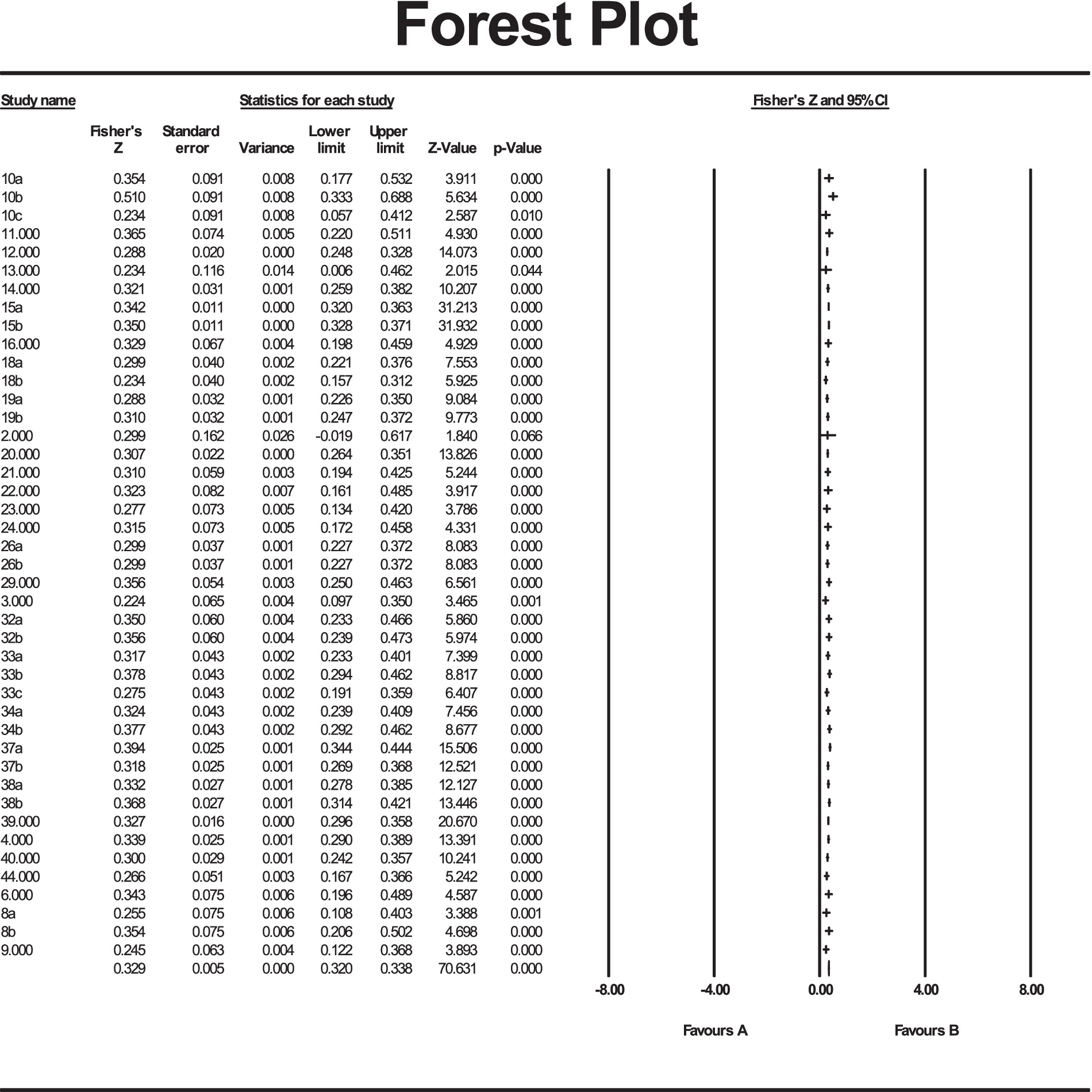
Figure 1 . Forest plot of precision by Fisher’s z of the effect sizes relating to social identity and social integration.
In the absence of publication bias we would expect the studies to be distributed symmetrically about the combined effect size. By contrast, in the presence of bias, we would expect that the bottom of the plot would show a higher concentration of studies on one side of the mean than on the other. This would reflect the fact that smaller studies (which appear toward the bottom) are more likely to be published if they have larger-than-average effects, which makes them more likely to meet the criterion for statistical significance.
Various statistical procedures can be accessed from the View menu to quantify or augment this display. The classic fail-Safe N and the Orwin fail-safe N ask if we need to be concerned that the entire observed effect may be an artifact of bias. Rank correlation and regression procedures can test for the presence of bias. Trim and fill analysis offers a more nuanced perspective, and asks how the effect size would shift if the apparent bias is to be removed.
An important caveat is confirmed by Sterne and Egger (2001) , which notes that while the plot and these procedures may detect a relationship between sample size and effect size, they cannot assign a causal mechanism to it.
Prior to exploring the effect of moderators found in the literature, however, we examined to what extent the overall effect may be influenced by publication bias.
Publication bias check
The funnel plot distributes the large SE studies across the top of the plot which shows the selected studies are of a high quality. Regarding the current study, most studies are dotted across the top of the funnel plot, which means the selected materials are of a higher quality. Also, from the plot (see Figure 2 ), we see that almost all studies are distributed randomly axisymmetric, which reflects that publication bias was not significant.
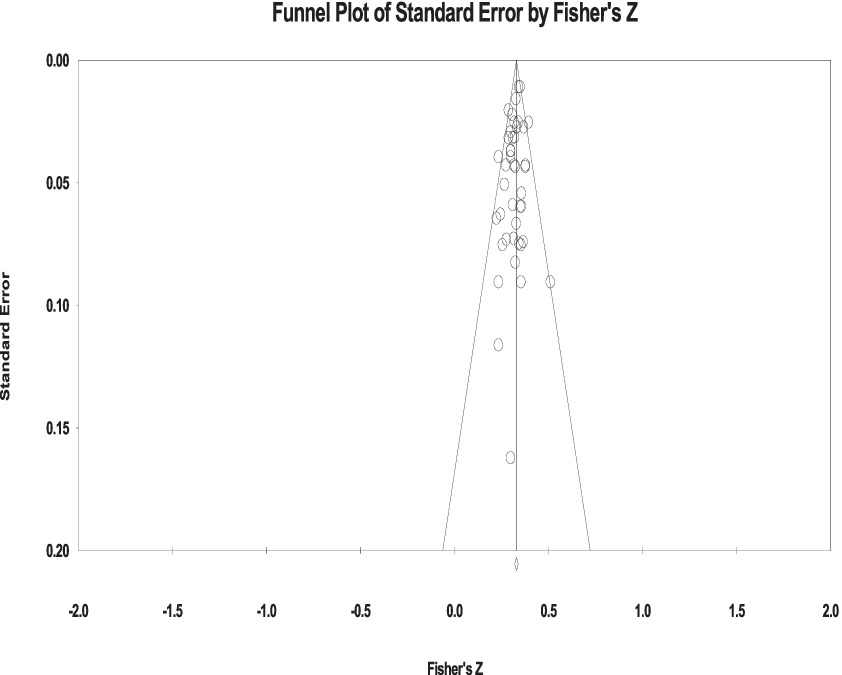
Figure 2 . Funnel plot of the effect sizes relating to social identity and social integration.
Then we use Egger’s regression intercept test which was suggested by Sterne and Egger (2001) to offer statistical cues to test the publication bias. From the conservative perspective view, we focus on the random effects model and test the asymmetry of the funnel plot distribution. The correlation rank test from Begg and Mazumdar (1994) showed that two indicators did not reach a level of significance ( p > 0.05), which means that from the statistical perspective, the publication bias was not significant.
We test the estimate indicator credibility through p -curve analysis ( Simonsohn et al., 2014 ). Both “file drawer effect” and “just significant effects” evinced that the obtained effect is strong and the sensitivity analysis is not significant ( p > 0.50). It also shows that the “right-skewed curve” is not significant ( z = 0.03, p > 0.05), and that the “left-skewed curve” also do not reach a level of significance ( p > 0.05). In sum, the results of p -curve analysis demonstrate the current study do not have significant publication bias.
Based on PET-PEESE method checking, because our results are significant ( r = 0.32, SE < 0.01, p < 0.001), either the fixed model or the random model shows similar results (i.e., r = 0.32, 95% CI [0.31, 0.33], p < 0.001). According to Stanley and Doucouliagos’s (2014) findings, the current study do not find significant publication bias.
Regarding the safe-N number, Table 1 shows that 16,214 samples are still needed to reverse the conclusion reached that the correlation is not significant. Also, the result is much more stable and the researcher preference factor is not significant. In terms of checking the unpublished studies, r = 0.31, 95% CI [0.30, 0.32]. This reveals a marginal difference in favor of published studies ( Q = 48.34; df = 41, p = 0.23). Since they share a similar ES with published studies, the overlapping CI achieves the level of legitimacy. In sum, the safe-N number analysis show that the current study do not have significant publication bias.
Moderation analyses
Unlike other meta-analysis research ( Lee and Ahn, 2013 ; Thielmann et al., 2020 ), this meta-analysis on the relationship of social identity and social integration (see Table 3 ) suggests that all the moderators in previous literature show weak influences on the relationship between social identity and social integration. Possibly, there is no need to find moderators for the relationship. The effect of social identity on social integration is robust to moderation. Community involvement ( Ramirez-Valles et al., 2010 ), life satisfaction ( Schotte et al., 2018 ), self-esteem ( Vedder, 2005 ) and gender ( Litchmore and Safdar, 2015 ) do not seem to demonstrate moderation effects (see Table 3 ).
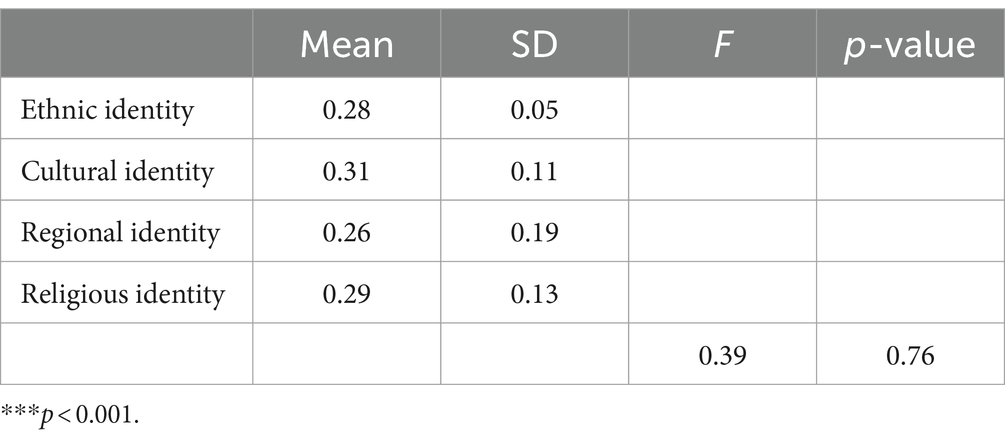
Table 3 . Moderation effect between social identity and social integration.
The research findings (shown in Table 2 ) suggests that various social identity types, such as ethnic social identity, religion social identity, regional social identity and cultural social identity classified by existing literature, exert similar degrees of impact on social integration; namely, around the moderate effect—Fisher’s z = 0.33. In other words, the influences of social identity on social integration is the same regardless of social identity types. This also suggests that the classification of social identity is not necessary since any classifications have a similar impact on social integration. There is no strong evidence to support the research which examines the influences of each type of social identity on social integration separately. Different types of social identity correlate with each other; in other words, it is hard to distinguish which one influences social integration without affecting other identities. For example, cultural social identity is a sense of belonging which in turn affects other identities such as ethnic social identity and national social identity ( Moha, 2005 ). Litchmore and Safdar (2015) attempted to distinguish religious social identity and ethnic social identity, but religious social identity usually affects ethnicity. More importantly, none of the moderators significantly influences the relationship between social identity and social integration, including community involvement ( Ramirez-Valles et al., 2010 ), life satisfaction ( Schotte et al., 2018 ) and self-esteem ( Vedder, 2005 ) that exists in the selected studies. The findings suggest that social identity and social integration have a stronger relationship without any moderators, which may indicate that moderators are context-specific and only suitable for a certain sample. The relationship between social identity and social integration cannot follow an exclusionary pattern ( Tatar, 2010 ). This meta-analysis provided convincing evidence for the direct relationship between social identity and social integration rather than occurring through moderators; therefore, the need for future research to identify the potential moderators between social identity and social integration should be cautious.
The research findings generate some practical implications. First, the governments of those multicultural societies (e.g., the US and the UK) should try to create opportunities for migrants to integrate into the mainstream culture in general without identifying which specific social identity the governments should focus on. For instance, the host government could offer language classes for migrants to learn the local language, which is generally deemed as increasing cultural social identity in the previous literature. The increasing cultural social identity of migrants will probably enhance their national social identity and other identities as well. Eventually, social integration among migrants will be improved; this is also the process to help migrants to adapt to the host environment. For the migrants, participating in and interacting with the host country could be a process that may lead to social integration. During this process, their levels of social identity increase when they participate in the local activities.
Limitations
This meta-analysis is not without limitations. First, the number of studies involved is limited, which may affect the results of the meta-analysis. Although we have searched almost all the literature between 2005 and 2020, literature that meet our selection requirement is limited. Eventually, 33 studies with a total number of 33,777 cases were selected. Similarly, this meta-analysis has involved 15 years of studies from 2005 to 2020. Future researchers may consider expanding the time frame and analyzing studies published before 2005 or after 2020. Second, only 8% of the variance on heterogeneity was explained, so other moderators which may explain the relationship between social identity and social integration need further exploration. Finally, improvements could not be made to the identified effects due to the lack of psychometric data. Some psychological factors may affect social identity, and then further influence the relationship between social identity and social integration. This limitation offers suggestions for future researchers that relevant psychometric data should be considered and collected.
This meta-analysis serves as the first study to provide a stable estimation of the relationship between social identity and social integration based on previous research (around from 2005 to 2020) with territorial (studies from all over the world), and numerical (34 independent studies, moderate effect, and the total sample number of 33,777) factors. This meta-analysis creatively focuses on social identity and social integration, which is one of only a few meta-analysis studies in psychosocial literature. Indeed, multilevel models provide more robust estimations for the observed effects and the effects of moderators. Although social identity has a strong connection with social integration, this study illustrates different types of social identity exert similar influence on social integration. Herein, there is not necessary to classify social identity. Moreover, this study also suggests that the moderators of the relationship between social identity and social integration are only suitable for particular samples. Findings on potential moderators between social identity and social integration are not yet generalizable.
Data availability statement
The original contributions presented in the study are included in the article/supplementary material, further inquiries can be directed to the corresponding author.
Author contributions
JH: Writing – original draft. CC: Writing – review & editing.
The author(s) declare financial support was received for the research, authorship, and/or publication of this article. This work was supported by the Fundamental Research Funds for the Central Universities (23JNQMX35), and the Fellowship of China Postdoctoral Science Foundation (2021M701429).
Conflict of interest
The authors declare that the research was conducted in the absence of any commercial or financial relationships that could be construed as a potential conflict of interest.
Publisher’s note
All claims expressed in this article are solely those of the authors and do not necessarily represent those of their affiliated organizations, or those of the publisher, the editors and the reviewers. Any product that may be evaluated in this article, or claim that may be made by its manufacturer, is not guaranteed or endorsed by the publisher.
*contain data used in the meta-analysis.
Google Scholar
Aalberg, T., Iyengar, S., and Messing, S. (2011). Who is a “deserving” immigrant? An experimental study of Norwegian attitudes. Scand. Polit. Stud. 35, 97–116. doi: 10.1111/j.1467-9477.2011.00280.x
Crossref Full Text | Google Scholar
Adida, C., Laitin, D., and Valfort, M. A. (2014). Muslims in France: identifying a discriminatory equilibrium. J. Popul. Econ. 27, 1039–1086. doi: 10.1007/s00148-014-0512-1
Alba, R., and Nee, V. (1997). Rethinking assimilation theory for a new era of immigration. Int. Migr. Rev 31, 826–874. doi: 10.1177/019791839703100403
PubMed Abstract | Crossref Full Text | Google Scholar
*Amit, K. (2012). Social integration and social identity of immigrants from western countries, the FSU and Ethiopia in Israel. Ethn. Racial Stud. , 35, 1287–1310, doi: 10.1080/01419870.2011.602091
Atkinson, A. B. (2002). Social inclusion and the European Union. J. Common Mark. Stud. 40, 625–643. doi: 10.1111/1468-5965.00391
Atkinson, A. B., Marlier, E., and Nolan, B. (2004). Indicators and targets for social inclusion in the European Union. J. Common Mark. Stud. 42, 47–75. doi: 10.1111/j.0021-9886.2004.00476.x
Begg, C. B., and Mazumdar, M. (1994). Operating characteristics of a rank correlation test for publication bias. Biometrics 50, 1088–1101. doi: 10.2307/2533446
Benet-Martínez, V., and Haritatos, J. (2005). Bicultural social identity integration (BII): components and psychosocial antecedents. J. Pers. 73, 1015–1050. doi: 10.1111/j.1467-6494.2005.00337.x
Benet-Martínez, V., Leu, J., Lee, F., and Morris, M. (2002). Negotiating biculturalism: cultural frame-switching in biculturals with “oppositional” vs. “compatible” cultural identities. J. Cross-Cult. Psychol. 33, 492–516. doi: 10.1177/0022022102033005005
Bernard, P. (1999). La cohésion sociale: critique dialectique d’un quasi-concept. Lien social et Politiques 41, 47–59. doi: 10.7202/005057ar
Berry, J. W. (1990). “Psychology of acculturation” in Cross-cultural perspectives: Nebraska symposium on motivation . ed. J. Berman (Lincoln, NE: University of Nebraska Press), 201–235.
Berry, J. W. (2005). Acculturation: living successfully in two cultures. Int. J. Intercult. Relat. 29, 697–712. doi: 10.1016/j.ijintrel.2005.07.013
Berry, J. W., and Kim, U. (1988). “Acculturation and mental health” in Health and cross-cultural psychology: Towards application . eds. J. W. Berry and N. Sartorius (London: Sage), 207–236.
Berry, J. W., and Sabatier, C. (2010). Acculturation, discrimination and adaptation among second generation immigrant youth in Montreal and Paris. Int. J. Intercult. Relat. 34, 191–207. doi: 10.1016/j.ijintrel.2009.11.007
Berry, J. W., and Sam, D. (1997). “Acculturation and adaptation” in Handbook of cross-cultural psychology . eds. J. W. Berry, M. H. Segall, and C. Kagitcibasi, vol. 3 (Boston, MA: Allyn & Bacon), 291–326.
Billeke, P., Armijo, A., Castillo, D., López, T., Zamorano, F., Cosmelli, D., et al. (2015). Paradoxical expectation: oscillatory brain activity reveals social interaction impairment in schizophrenia. Biol. Psychiatry 78, 421–431. doi: 10.1016/j.biopsych.2015.02.012
*Bisin, A., Patacchini, E., Verdier, T., and Zenou, Y. (2016). Bend it like Beckham: ethnic social identity and integration. Eur. Econ. Rev. , 90, 146–164, doi: 10.1016/j.euroecorev.2016.01.006
Borenstein, M., Hedges, L. V., Higgins, J. P. T., and Rothstein, H. R. (2009). Introduction to meta-analysis . Chichester, UK: John Wiley & Sons. Ltd
Borjas, G. J. (1994). Long-run convergence of ethnic skill differentials: the children and grandchildren of the great migration. Ind. Labor Relat. Rev. 47, 553–573. doi: 10.1177/001979399404700403
Botterman, S., Hooghe, M., and Reeskens, T. (2012). “One size fits all”? An empirical study into the multidimensionality of social cohesion indicators in Belgian local communities. Urban Stud. 49, 185–202. doi: 10.1177/0042098010397397
Bourdieu, P. (1987). What makes a social class? On the theoretical and practical existence of groups. Berkeley J. Sociol. 32, 1–17.
*Bradford, B. (2014). Policing and social identity: procedural justice, inclusion and cooperation between police and public. Polic. Soc. , 24, 22–43, doi: 10.1080/10439463.2012.724068
Brenner, P. S., Serpe, R. T., and Stryker, S. (2014). The causal ordering of prominence and salience in identity theory: an empirical examination. Soc. Psychol. Q. 77, 231–252. doi: 10.1177/0190272513518337
*Brigevich, A. (2016). Regional social identity and support for Europe: distinguishing between cultural and political social identity in France. Reg. Fed. Stud. , 26, 475–507, doi: 10.1080/13597566.2016.1223057
Brown, R. (2000). Social identity theory: past achievements, current problems and future challenges. Eur. J. Soc. Psychol. 30, 745–778. doi: 10.1002/1099-0992(200011/12)30:6<745::AID-EJSP24>3.0.CO;2-O
Brown, R. (2019). The social identity approach: appraising the Tajfellian legacy. Br. J. Soc. Psychol. 59, 5–25. doi: 10.1111/bjso.12349
Burke, P. J., and Stets, J. E. (2009). Identity theory . New York, NY: Oxford University Press
*Carmeli, A., and Shteigman, A. (2010). Top management team behavioral integration in small-sized firms: a social identity perspective. Group Dyn. Theory Res. Pract. , 14, 318–331, doi: 10.1037/a0018254
Chen, S. X., Benet-Martínez, V., and Harris Bond, M. (2008). Bicultural social identity, bilingualism, and psychological adjustment in multicultural societies: immigration-based and globalization-based acculturation. J. Pers. 76, 803–838. doi: 10.1111/j.1467-6494.2008.00505.x
Chu, R., Xiao, Z., and Zhang, X. (2012). Concept of urban integration of Chinese migrant workers and its effect on perceived relationship: a survey in Shanghai. J. Public Manag. 9, 44–51.
Cohen, J. (1988). Statistical power analysis for the behavioral sciences . In: N. J. Hillsdale, editor. L. Erlbaum Associates. 2nd Edn.
Cordier, R., Milbourn, B., Martin, R., Buchanan, A., Chung, D., and Speyer, R. (2017). A systematic review evaluating the psychometric properties of measures of social inclusion. PLoS One 12:e0179109. doi: 10.1371/journal.pone.0179109
Corneo, G., and Jeanne, O. (2010). Symbolic values, occupational choice, and economic development. Eur. Econ. Rev. 54, 237–251. doi: 10.1016/j.euroecorev.2009.04.006
*Cui, Y. (2012). A study on migrants' psychological integration and self-social identity. Sociol. Res. , 5, 141–160.
Curran, M. (2003). Across the water – The acculturation and health of Irish people in London . Dublin: Trinity College and Allen Library.
Davis, J. L., Love, T. P., and Fares, P. (2019). Collective social identity: synthesizing identity theory and social identity theory using digital data. Soc. Psychol. Q. 82, 254–273. doi: 10.1177/0190272519851025
Dayton-Johnson, J. (2003). The economic implications of social cohesion, vol. 16 . Toronto: University of Toronto Press.
Duroy, Q. (2011). North African social identity and racial discrimination in France: a social economic analysis of capability deprivation. Rev. Soc. Econ. 69, 307–332. doi: 10.1080/00346764.2010.502834
Duval, S., and Tweedie, R. (2000). Trim and fill: a simple funnel-plot–based method of testing and adjusting for publication bias in meta-analysis. Biometrics 56, 455–463. doi: 10.1111/j.0006-341X.2000.00455.x
Finn, J. D., and Rock, D. A. (1997). Academic success among students at risk for school failure. J. Appl. Psychol. 82, 221–234. doi: 10.1037/0021-9010.82.2.221
Freud, S. (1921). “Group psychology and the analysis of the ego” in The standard edition of the complete psychological works of Sigmund Freud . ed. J. Strachey (London: Hogarth Press)
Friedkin, N. E. (2004). Social cohesion. Annu. Rev. Sociol. 30, 409–425. doi: 10.1146/annurev.soc.30.012703.110625
*Fu, G. (2016). The construction of the social integration indicator system for migrant children: based on the perspective of social identity. Gungxi Soc. Sci. , 11, 155–159.
Gaertner, S. L., and Dovidio, J. (2000). Reducing intergroup bias . Hove, England: Psychology Press.
Garcia, J. (1982). Ethnicity and Chicanos: measurement of ethnic identification, social identity, and consciousness. Hisp. J. Behav. Sci. 4, 295–314. doi: 10.1177/07399863820043001
García, J. R., and Cain, K. (2014). Decoding and reading comprehension: a meta-analysis to identify which reader and assessment characteristics influence the strength of the relationship in English. Rev. Educ. Res. 84, 74–111. doi: 10.3102/0034654313499616
Ghosh, R. (2000). Social identity and social integration: girls from a minority ethno-cultural group in Canada. McGill J. Educ. 35, 279–296.
Giambona, F., and Vassallo, E. (2014). Composite indicator of social inclusion for European countries. Soc. Indic. Res. 116, 269–293. doi: 10.1007/s11205-013-0274-2
Giles, H., Llado, N., McKirnan, D., and Taylor, D. (1979). Social identity in Puerto Rico. Int. J. Psychol. 14, 185–201. doi: 10.1080/00207597908246730
Goodman, S. (2012). Fortifying citizenship: policy strategies for civic integration in Western Europe. World Polit. 64, 659–698. doi: 10.1017/S0043887112000184
Green, A., and Janmaat, J. G. (2011). Regimes of social cohesion: Societies and the crisis of globalization . Basingstoke, UK: Palgrave Macmillan
*Guo, Q., Guan, Y., and Zhao, Y. (2015). An empirical study of relationship between migrant workers’ awareness and behavior of sports participation and their integration into city. J. Chengdu Sport Univ. , 46, 22–27.
*Hagerman, K. L. (2018). Domestic ritual and social identity in the Teotihuacan state: Exploring regional processes of social integration through ceramic figurines . San Diego: UC.
Haritatos, J., and Benet-Martínez, V. (2002). Bicultural identities: The interface of cultural, personality, and socio-cognitive processes. J. Res. Pers. 36, 598–606.
Helbling, M., and Traunmüller, R. (2016). How state support of religion shapes attitudes toward Muslim immigrants. New evidence from a subnational comparison. Comp. Pol. Stud. 49, 391–424. doi: 10.1177/0010414015612388
Hogg, M. A. (2018). “Social identity theory” in Social psychological theories . ed. P. J. Burke (Stanford: Stanford University Press), 112–138.
*Huang, M. (2011). Influence of socio-structural characteristics of intergroup relations on the group identification and adaptation of migrant children . East China: Normal University.
Jenson, J. (2010). Defining and measuring social cohesion . London: UNRISD & Commonwealth Secretariat
Joppke, C. (2007). Transformation of immigrant integration: civic integration and antidiscrimination in the Netherlands, France, and Germany. World Polit. 59, 243–273. doi: 10.1353/wp.2007.0022
KfW Bankengruppe. (2010). Konzeptpapier des KfW-Nachhaltigkeitsindikators . Frankfurt am Main: KfW Bankengruppe.
*Kuo, A., Malhotra, N., and Mo, C. H. (2017). Social exclusion and political social identity: the case of Asian American partisanship. J. Polit. , 79, 17–32, doi: 10.1086/687570
*Lapalme, M.-È., and Doucet, O. (2018). The social integration of healthcare agency workers in long-term care facilities: a cross-sectional study. Int. J. Nurs. Stud. , 82, 106–112, doi: 10.1016/j.ijnurstu.2018.03.011
Lee, D. L., and Ahn, S. (2013). The relation of racial identity, ethnic identity, and racial socialization to discrimination-distress: a meta-analysis of black Americans. J. Couns. Psychol. 60, 1–14. doi: 10.1037/a0031275
Lipsey, M. W., and Wilson, D. B. (2001). Practical meta-analysis . Thousand Oaks, Calif: Sage Publications Inc.
*Litchmore, R., and Safdar, S. (2015). Perceptions of discrimination as a marker of integration among Muslim-Canadians: the role of religiosity, ethnic social identity, and gender. J. Int. Migr. Integr. , 16, 187–204, doi: 10.1007/s12134-014-0337-5
*Luo, S. (2014). Structure and status of migrant workers’ children to integrate into the city: an empirical analysis based on survey data in Xiamen, Changsha, Guiyang. Jianghan Acad. , 33, 20–25.
*Luo, M., and Lu, Y. (2013). An empirical analysis of the influence of migrant workers' professional social identity on their urban integration. China Rural Surv. , 5, 10–23.
*Maliepaard, M., and Phalet, K. (2012). Social integration and religious social identity expression among Dutch Muslims: the role of minority and majority group contact. Soc. Psychol. Q. , 75, 131–148, doi: 10.1177/0190272511436353
*Mariyam, S., and Jose, J. (2017). Age social identity and social exclusion of elderly persons: a cross sectional study. Int. J. Soc. Sci. , 6, 191–198.
Marlier, E. (2003). Setting targets: the use of indicators. EAPN Network News 98, 4–6.
*Maxwell, R. (2017). Occupations, national social identity, and immigrant integration. Comp. Pol. Stud. , 50, 232–263, doi: 10.1177/0010414016655535
McCrone, D. (2001). Understanding Scotland: The sociology of a nation . London: Routledge.
McCrone, D., and Bechhofer, F. (2008). National social identity and social inclusion. Ethn. Racial Stud. 31, 1245–1266. doi: 10.1080/01419870701704677
Merve, B. (2017). An social identity-based matching theory approach to integration. Forum Soc. Econ. 25 , 1–16.
Moha, E. (2005). Multilingualism, cultural social identity, and education in Morocco . New York: Springer Science & Business Media.
Moher, D., Liberati, A., Tetzlaff, J., and Altman, D. G.Group, P (2010). Preferred reporting items for systematic reviews and meta-analyses: the PRISMA statement. Int. J. Surg. 8, 336–341. doi: 10.1016/j.ijsu.2010.02.007
Mol, S. E., and Bus, A. G. (2011). To read or not to read: a meta-analysis of print exposure from infancy to early adulthood. Psychol. Bull. 137, 267–296. doi: 10.1037/a0021890
*Mukherjee, S., Molina, Ludwin E., and Adams, G. (2012). National social identity and immigration policy: concern for legality or ethnocentric exclusion? Anal. Soc. Issues Public Policy , 12, 21–32, doi: 10.1111/j.1530-2415.2011.01257.x
Negru-Subtirica, O., Pop, E. I., and Crocetti, E. (2017). A longitudinal integration of social identity styles and educational social identity processes in adolescence. Dev. Psychol. 53, 2127–2138. doi: 10.1037/dev0000325
Neto, F., Barros, J., and Schmitz, P. G. (2005). Acculturation attitudes and adaptation of Portuguese immigrants in Germany: integration or separation? Psychol. Dev. Soc. 17, 19–32. doi: 10.1177/097133360501700102
Novy, A., Swiatek, D. C., and Moulaert, F. (2012). Social cohesion: a conceptual and political elucidation. Urban Stud. 49, 1873–1889. doi: 10.1177/0042098012444878
Orgad, L. (2015). The cultural defense of nations: A Liberal theory of majority rights . Oxford, UK: Oxford University Press
Owens, T. J., Robinson, D. T., and Smith-Lovin, L. (2010). Three faces of identity. Annu. Rev. Sociol. 36, 477–499. doi: 10.1146/annurev.soc.34.040507.134725
Oxoby, R. (2009). Understanding social inclusion, social cohesion, and social capital. Int. J. Soc. Econ. 36, 1133–1152. doi: 10.1108/03068290910996963
*Passini, S. (2013). What do I think of others in relation to myself? Moral social identity and moral inclusion in explaining prejudice. J. Community Appl. Soc. Psychol. , 23, 261–269, doi: 10.1002/casp.2117
Pigott, T. (2012). Advances in meta-analysis . New York: Springer Science & Business Media.
Ramirez, I. (2007). The relationship of acculturation and social integration to assaults on intimate partners among Mexican American and non-Mexican white students. J. Fam. Violence 22, 533–542. doi: 10.1007/s10896-007-9102-2
*Ramirez-Valles, J., Kuhns, L. M., Campbell, R. T., and Diaz, R. M. (2010). Social integration and health: community involvement, stigmatized identities, and sexual risk in Latino sexual minorities. J. Health Soc. Behav. , 51, 30–47, doi: 10.1177/0022146509361176
Reeskens, T., and Wright, M. (2013). Nationalism and the cohesive society: a multi-level analysis of the interplay among diversity, national social identity, and social capital across 27 European societies. Comp. Pol. Stud. 46, 153–181. doi: 10.1177/0010414012453033
*Reid, K. W. (2013). Understanding the relationships among racial social identity, self-efficacy, institutional integration and academic achievement of black males attending research universities. J. Negro Educ. , 82, 75–93, doi: 10.7709/jnegroeducation.82.1.0075
Reitz, J. G., Breton, R., and Dion, K. K. (2009). Multiculturalism and social cohesion: Potentials and challenges of diversity . Dordrecht: Springer Netherlands.
*Robinson, T. N. (2006). Social identity as a mediator of institutional integration variables in the prediction of undergraduate persistence intentions. J. Adolesc. Res. , 18, 3–24, doi: 10.1177/0743558402238274
*Rydell, R. J., Hamilton, D. L., and Devos, T. (2010). Now they are American, now they are not: valence as a determinant of the inclusion of African Americans in the American social identity. Soc. Cogn. , 28, 161–179, doi: 10.1521/soco.2010.28.2.161
*Schilpzand, P., and Huang, L. (2018). When and how experienced incivility dissuades proactive performance: an integration of sociometer and self-social identity orientation perspectives. J. Appl. Psychol. , 103, 828–841, doi: 10.1037/apl0000303
Schmeets, H., and te Riele, S. (2014). Declining social cohesion in the Netherlands? Soc. Indic. Res. 115, 791–812. doi: 10.1007/s11205-013-0234-x
Schotte, K., Stanat, P., and Edele, A. (2018). Is integration always most adaptive? The role of cultural social identity in academic achievement and in psychological adaptation of immigrant students in Germany. J. Youth Adolesc. 47, 16–37. doi: 10.1007/s10964-017-0737-x
Schwartz, S., Zamboanga, B., and Jarvis, L. H. (2007). Rethinking the concept of acculturation: implications for theory and research. Am. Psychol. 65, 237–251. doi: 10.1037/a0019330
Scott, J., and Marshall, G. (2009). A dictionary of sociology . 3 rev. Oxford: Oxford University Press
Scottham, K. M., and Dias, R. H. (2010). Acculturative strategies and the psychological adaptation of Brazilian migrants to Japan. Social Identity Int. J. Theory Res. 10, 284–303. doi: 10.1080/15283488.2010.523587
Sherman, A. M., Shumaker, S. A., Jack, R. W., Morgan, T., Applegate, W. B., and Ettinger, W. (2006). Social support, social integration, and health-related quality of life over time: results from the fitness and arthritis in seniors trial (FAST). Psychol. Health 21, 463–480. doi: 10.1080/14768320500380881
Simonsohn, U., Nelson, L. D., and Simmons, J. P. (2014). P-curve: a key to the file-drawer. J. Exp. Psychol. Gen. 143, 534–547. doi: 10.1037/a0033242
Sniderman, P., and Hagendoorn, L. (2007). When ways of life collide: Multiculturalism and its discontents in the Netherlands . Princeton, NJ: Princeton University Press.
Stanley, T. D., and Doucouliagos, H. (2014). Meta-regression approximations to reduce publication selection bias. Res. Synth. Methods 5, 60–78. doi: 10.1002/jrsm.1095
Sterne, J. A. C., and Egger, M. (2001). Funnel plots for detecting bias in meta-analysis: guidelines on choice of axis. J. Clin. Epidemiol. 54, 1046–1055. doi: 10.1016/S0895-4356(01)00377-8
Stets, J. E., and Burke, P. J. (2000). Identity theory and social identity theory. Soc. Psychol. Q. 63, 224–237. doi: 10.2307/2695870
Stets, J. E., and Burke, P. J. (2014). “The development of identity theory” in Advances in group processes, vol. 31 . eds. S. R. Thye and E. J. Lawler (Bingley, UK: Emerald Group Publishing Limited), 57–97.
Stets, J. E., and Serpe, R. T. (2013). “Identity theory” in Handbook of social psychology . eds. J. DeLamater and A. Ward (New York: Springer), 31–60.
Stryker, S., and Burke, P. J. (2000). The past, present, and future of social identity theory. Soc. Psychol. Q. 63, 284–297. doi: 10.2307/2695840
Tajfel, H. (1982). Social identity and intergroup relations . Cambridge, UK: Cambridge University Press.
Tajfel, H., and Turner, J. C. (1979). “An integrative theory of intergroup conflict” in The social psychology of intergroup relations . eds. W. G. Austin and S. Worchel (Monterey, CA: Brooks/Cole), 33–47.
Tatar, M. (2010). Integration, social identity and participation in a changing Europe. J. Soc. Res. Policy 1, 47–62.
Thielmann, I., Spadaro, G., and Balliet, D. (2020). Personality and prosocial behavior: a theoretical framework and meta-analysis. Psychol. Bull. 146, 30–90. doi: 10.1037/bul0000217
*Vedder, P. (2005). Language, ethnic social identity, and the adaptation of immigrant youth in the Netherlands. J. Adolesc. Res. , 20, 396–416, doi: 10.1177/0743558405274853
Walters, D., Phythian, K., and Anisef, P. (2007). The acculturation of Canadian immigrants: determinants of ethnic identification. Can. Rev. Sociol. Anthropol. 44, 37–64. doi: 10.1111/j.1755-618X.2007.tb01147.x
Wang, W., and Abosag, I. (2019). Do immigration and social media facilitate or inhibit cognitive acculturation? The role of individual dialecticism in dual-focused cultural stimuli evaluation. Technol. Forecast. Soc. Change. 145, 523–531.
*Wang, L., Wang, L., and Du, Q. (2013). Cultural social identity, community integration and citizenization: an effective path for the new generation of migrant workers' urban integration. Acad. J. , 2, 101–107.
*Wang, S., and Xu, S. (2013). The structure and factors of social integration feelings of the floating population. Popul. J. , 35, 05–14.
Ward, C., Stuart, J., and Kus, L. (2011). The construction and validation of a measure of ethno-cultural social identity conflict. J. Pers. Assess. 93, 462–473. doi: 10.1080/00223891.2011.558872
*Wenzel, M. (2000). Justice and social identity: the significance of inclusion for perceptions of entitlement and the justice motive. Personal. Soc. Psychol. Bull. , 26, 157–176, doi: 10.1177/0146167200264004
Wimmer, A., and Soehl, T. (2014). Blocked acculturation: cultural heterodoxy among Europe’s immigrants. Am. J. Sociol. 120, 146–186. doi: 10.1086/677207
World Bank. (2013). Inclusion matters: The Foundation for Shared Prosperity. New Frontiers of social policy . Washington, DC. World Bank.
Wray, M., Colen, C., and Pescosolido, B. (2011). The sociology of suicide. Annu. Rev. Sociol. 37, 505–528. doi: 10.1146/annurev-soc-081309-150058
*Yang, K. (2015). National social identity and ethnic social identity: an empirical study about the change of ethnic social identity in Northwest China. Guangxi Ethnic Stud. , 4, 20–33.
Zagefka, H., and Brown, R. (2002). The relation between acculturation strategies, relative fit and intergroup relations: immigrant-majority relations in Germany. Eur. J. Soc. Psychol. 32, 171–188. doi: 10.1002/ejsp.73
*Zhao, J. (2016). Ethnic social identity and the research on Kwangsi minority migrant Children's social integration . Guangxi University for Nationalities
*Zhou, J., O’Brien, K. S., and Heim, D. P. (2014). Alcohol consumption in sportspeople: the role of social cohesion, social identity and happiness. Int. Rev. Sociol. Sport , 49, 278–293, doi: 10.1177/1012690213493105
Keywords: social identity, social integration, meta-analysis, quantitative research, social psychology
Citation: Hu J and Cheung CKJ (2024) Social identity and social integration: a meta-analysis exploring the relationship between social identity and social integration. Front. Psychol . 15:1361163. doi: 10.3389/fpsyg.2024.1361163
Received: 26 January 2024; Accepted: 19 March 2024; Published: 04 April 2024.
Reviewed by:
Copyright © 2024 Hu and Cheung. This is an open-access article distributed under the terms of the Creative Commons Attribution License (CC BY) . The use, distribution or reproduction in other forums is permitted, provided the original author(s) and the copyright owner(s) are credited and that the original publication in this journal is cited, in accordance with accepted academic practice. No use, distribution or reproduction is permitted which does not comply with these terms.
*Correspondence: Jieyi Hu, [email protected]
Disclaimer: All claims expressed in this article are solely those of the authors and do not necessarily represent those of their affiliated organizations, or those of the publisher, the editors and the reviewers. Any product that may be evaluated in this article or claim that may be made by its manufacturer is not guaranteed or endorsed by the publisher.
- Skip to primary navigation
- Skip to main content
- Skip to primary sidebar
information for practice
news, new scholarship & more from around the world
- gary.holden@nyu.edu
- @ Info4Practice
Therapist-guided remote versus in-person cognitive behavioural therapy: a systematic review and meta-analysis of randomized controlled trials [Research]
Background:
Cognitive behavioural therapy (CBT) has been shown to be effective for several psychiatric and somatic conditions; however, most randomized controlled trials (RCTs) have administered treatment in person and whether remote delivery is similarly effective remains uncertain. We sought to compare the effectiveness of therapist-guided remote CBT and in-person CBT.
We systematically searched MEDLINE, Embase, PsycINFO, CINAHL, and the Cochrane Central Register of Controlled Trials from inception to July 4, 2023, for RCTs that enrolled adults (aged ≥ 18 yr) presenting with any clinical condition and that randomized participants to either therapist-guided remote CBT (e.g., teleconference, videoconference) or in-person CBT. Paired reviewers assessed risk of bias and extracted data independently and in duplicate. We performed random-effects model meta-analyses to pool patient-important primary outcomes across eligible RCTs as standardized mean differences (SMDs). We used Grading of Recommendations Assessment, Development and Evaluation (GRADE) guidance to assess the certainty of evidence and used the Instrument to Assess the Credibility of Effect Modification Analyses (ICEMAN) to rate the credibility of subgroup effects.
We included 54 RCTs that enrolled a total of 5463 patients. Seventeen studies focused on treatment of anxiety and related disorders, 14 on depressive symptoms, 7 on insomnia, 6 on chronic pain or fatigue syndromes, 5 on body image or eating disorders, 3 on tinnitus, 1 on alcohol use disorder, and 1 on mood and anxiety disorders. Moderate-certainty evidence showed little to no difference in the effectiveness of therapist-guided remote and in-person CBT on primary outcomes (SMD –0.02, 95% confidence interval –0.12 to 0.07).
Interpretation:
Moderate-certainty evidence showed little to no difference in the effectiveness of in-person and therapist-guided remote CBT across a range of mental health and somatic disorders, suggesting potential for the use of therapist-guided remote CBT to facilitate greater access to evidence-based care. Systematic review registration: Open Science Framework https://osf.io/7asrc/)
Read the full article ›

Intensive Versus Conservative Blood Pressure Lowering after Endovascular Therapy in Stroke: a meta-analysis of randomized controlled trials
- Find this author on Google Scholar
- Find this author on PubMed
- Search for this author on this site
- For correspondence: [email protected]
- Info/History
- Supplementary material
- Preview PDF
Background The optimum systolic blood pressure after endovascular thrombectomy for acute ischemic stroke is uncertain. We aimed to perform an updated meta-analysis of randomized controlled trials to evaluate the safety and efficacy of more intensive blood pressure management as compared to less intensive blood pressure management. Methods We searched various electronic databases including Embase, MEDLINE (via PubMed), and CENTRAL to retrieve relevant randomized controlled trials (RCTs) on the clinical effects of more intensive blood pressure management after endovascular thrombectomy as compared to the less intensive management. We assessed the risk of bias using the revised Cochrane Risk of bias tool for randomized trials (RoB 2.0), calculated risk ratio (RR) with 95% confidence intervals (CI) for dichotomous outcomes, and mean difference (MD) with 95% CI for continuous outcomes. Results Our meta-analysis included 4 RCTs with a total of 1560 patients. According to our analysis, more intensive blood pressure management was associated with a statistically significant decrease in the number of patients showing functional independence (modified Rankin scale mRS score=0-2) at 90 days (RR 0.81; CI = 0.72-0.91; I2 = 12%). Regarding 90-day mortality, our pooled results from three RCTs showed no statistically significant difference between the more intensive blood pressure management group and the less intensive blood pressure management group (RR 1.17; CI = 0.90-1.52; I2 = 0%). There was no statistically significant difference between the two groups regarding the incidence of intracerebral hemorrhage (ICH) (RR 1.05; CI = 0.90-1.23; I2 = 0%) and the incidence of symptomatic intracerebral hemorrhage (sICH) (RR 1.10; CI = 0.76-1.60; I2 = 0%). Conclusion According to our meta-analysis, no benefit of intensive lowering of blood pressure was observed in terms of functional independence at 90 days, mortality rates, and incidence of intracerebral hemorrhage. Future large-scale trials should focus on other interventions to improve prognosis in these patients.
Competing Interest Statement
The authors have declared no competing interest.
Funding Statement
This study did not receive any funding.
Author Declarations
I confirm all relevant ethical guidelines have been followed, and any necessary IRB and/or ethics committee approvals have been obtained.
The details of the IRB/oversight body that provided approval or exemption for the research described are given below:
The study used only openly available human data and all the data has been published in different journals and referenced in the manuscript.
I confirm that all necessary patient/participant consent has been obtained and the appropriate institutional forms have been archived, and that any patient/participant/sample identifiers included were not known to anyone (e.g., hospital staff, patients or participants themselves) outside the research group so cannot be used to identify individuals.
I understand that all clinical trials and any other prospective interventional studies must be registered with an ICMJE-approved registry, such as ClinicalTrials.gov. I confirm that any such study reported in the manuscript has been registered and the trial registration ID is provided (note: if posting a prospective study registered retrospectively, please provide a statement in the trial ID field explaining why the study was not registered in advance).
I have followed all appropriate research reporting guidelines, such as any relevant EQUATOR Network research reporting checklist(s) and other pertinent material, if applicable.
Data Availability
All data produced in the present work are contained in the manuscript.
View the discussion thread.
Supplementary Material
Thank you for your interest in spreading the word about medRxiv.
NOTE: Your email address is requested solely to identify you as the sender of this article.

Citation Manager Formats
- EndNote (tagged)
- EndNote 8 (xml)
- RefWorks Tagged
- Ref Manager
- Tweet Widget
- Facebook Like
- Google Plus One
- Addiction Medicine (316)
- Allergy and Immunology (618)
- Anesthesia (159)
- Cardiovascular Medicine (2277)
- Dentistry and Oral Medicine (279)
- Dermatology (201)
- Emergency Medicine (370)
- Endocrinology (including Diabetes Mellitus and Metabolic Disease) (798)
- Epidemiology (11579)
- Forensic Medicine (10)
- Gastroenterology (678)
- Genetic and Genomic Medicine (3580)
- Geriatric Medicine (336)
- Health Economics (617)
- Health Informatics (2305)
- Health Policy (913)
- Health Systems and Quality Improvement (864)
- Hematology (335)
- HIV/AIDS (752)
- Infectious Diseases (except HIV/AIDS) (13154)
- Intensive Care and Critical Care Medicine (757)
- Medical Education (359)
- Medical Ethics (100)
- Nephrology (389)
- Neurology (3351)
- Nursing (191)
- Nutrition (507)
- Obstetrics and Gynecology (651)
- Occupational and Environmental Health (646)
- Oncology (1758)
- Ophthalmology (524)
- Orthopedics (209)
- Otolaryngology (284)
- Pain Medicine (223)
- Palliative Medicine (66)
- Pathology (438)
- Pediatrics (1004)
- Pharmacology and Therapeutics (422)
- Primary Care Research (406)
- Psychiatry and Clinical Psychology (3062)
- Public and Global Health (5992)
- Radiology and Imaging (1222)
- Rehabilitation Medicine and Physical Therapy (714)
- Respiratory Medicine (811)
- Rheumatology (367)
- Sexual and Reproductive Health (353)
- Sports Medicine (316)
- Surgery (386)
- Toxicology (50)
- Transplantation (170)
- Urology (142)
- Open access
- Published: 02 April 2024
The association of atherogenic index of plasma with cardiovascular outcomes in patients with coronary artery disease: A systematic review and meta-analysis
- Mehrdad Rabiee Rad 1 ,
- Ghazal Ghasempour Dabaghi 1 , 3 ,
- Bahar Darouei 2 &
- Reza Amani-Beni 2
Cardiovascular Diabetology volume 23 , Article number: 119 ( 2024 ) Cite this article
130 Accesses
Metrics details
Atherogenic index of plasma (AIP) represents a novel marker in the current era of cardiovascular diseases. In this meta-analysis, we aimed to evaluate the association of AIP with cardiovascular prognosis in patients with coronary artery disease (CAD).
PubMed, Scopus, and Web of Science databases were searched from inception through 2024. The primary outcome was major cardiovascular events (MACE). The secondary outcomes included all-causes death, cardiovascular death, myocardial infarction (MI), stroke, revascularization, and no-reflow phenomenon. AIP was determined by taking the logarithm of the ratio of triglyceride (TG) to high-density lipoprotein cholesterol (HDL-C). The data analysis was represented using the risk ratio (RR) along with a 95% confidence interval (CI).
Sixteen studies with a total number of 20,833 patients met the eligible criteria. The pooled-analysis showed a significant increased risk of MACE in the highest AIP group compared with the lowest AIP group (RR = 1.63; 95% CI, 1.44–1.85; P < 0.001). A similar result was observed when AIP was regarded as a continuous variable (RR = 1.54; 95% CI, 1.30–1.83; P < 0.001). Besides, elevated AIP was associated with increased risk of cardiovascular death (RR = 1.79; 95% CI, 1.09–2.78; P = 0.02), MI (RR = 2.21; 95% CI, 1.55–3.13; P < 0.001), revascularization (RR = 1.62; 95% CI, 1.34–1.97; P < 0.001), no-reflow phenomenon (RR = 3.12 95% CI, 1.09–8.96; P = 0.034), and stent thrombosis (RR = 13.46; 95%CI, 1.39-129.02; P = 0.025). However, AIP was not significantly associated with the risk of all-causes death and stroke among patients with CAD.
Conclusions
The results of this study demonstrated that increased AIP is an independent prognostic factors in patients with CAD. Further research is warranted to elucidate the potential development of targeted interventions to modify AIP levels and improve patient outcomes.
Introduction
Coronary artery diseases (CAD) are accountable for a high morbidity and mortality rate worldwide, with 17.8 million deaths annually [ 1 ]. Many studies have been conducted on the role of risk factors in predicting the risk of CAD; however, fewer studies have addressed the role of various factors in the short and long-term prognosis of patients with CAD. The short-term prognosis is mainly related to percutaneous coronary intervention (PCI) and in-hospital events such as the no-reflow phenomenon and in-hospital death, while the long-term prognosis mainly includes major adverse cardiovascular events (MACE) [ 2 ].
The prognosis of patients with CAD is dependent upon multiple factors. Traditional and modifiable risk factors for CAD include hypertension, diabetes mellitus, smoking, obesity, and dyslipidemia, which have been identified to play a role in the prognosis of CAD and, therefore, MACE [ 3 ]. However, clinicians frequently come across patients with novel CADs that have been misclassified due to these traditional cardiovascular risk factors in a way that necessitates establishing accurate predictors for CAD [ 4 ].
Atherogenic index of plasma (AIP) is calculated by Logarithm [triglyceride (TG) / high-denisity lipoprotein cholesterol (HDL-C)] and can be an independent cardiovascular risk factor by correlating with lipoprotein particle size [ 5 ]. A recent meta-analysis concluded that higher values of AIP can significantly increase the risk of CAD after adjusting for other risk factors [ 6 ]. Moreover, other studies have revealed the prognostic role of AIP in arterial stiffness, atherosclerotic disease, the risk of AMI, ischemic stroke, and MACE [ 7 , 8 , 9 ]. Fu et al. demonstrated that diabetic patients with MACE had higher values of AIP, introducing a novel MACE predictor for high-risk patients [ 10 ]. Similar results were observed in another study, including non-diabetic older adults with hypertension [ 11 ]. Nevertheless, no meta-analysis has been performed to reveal AIP’s prognostic effect in patients with CAD; therefore, we sought to determine the association between the levels of AIP and prognosis in patients with CAD.
Materials and methods
Data sources and searches.
This systematic review and meta-analysis is performed according to the guideline of the Preferred Reporting Items for Systematic Review and Meta-analyses statement (PRISMA) [ 12 ]. A systematic search of the electronic databases including PubMed, Scopus, and Web of Science was undertaken to identify relevant papers published before January 2024. Search strategy used the terms for AIP (“Atherogenic index of plasma”, “atherogenic index”, AIP) and CAD (“coronary disease”, “coronary diseases”, “disease coronary”, “coronary heart disease”, “coronary heart diseases”, “heart disease coronary”, “heart diseases coronary”, “left main”, “left main coronary disease”, “percutaneous coronary intervention”, “coronary artery disease”, “coronary artery diseases”, “coronary artery bypass”, “coronary artery bypass graft”, “coronary syndrome”, “acute coronary syndrome”, “chronic coronary syndrome”). We also conducted a manual search of reference lists and potential related articles. Two independent reviewers completed the electronic search in databases.
Eligible criteria
Two reviewers independently screened the eligible studies based on the following inclusion criteria: (1) Adult patients who were diagnosed with CAD including myocardial infarction (MI), and acute or chronic CAD; (2) Measured AIP and reported the odds ratios (ORs) or hazard ratios (HRs) were with 95% confidence interval (CI) for association of AIP with the outcomes; and (3) The full text was available and written in the English language. Abstracts, reviews, case reports and case series, nonhuman studies, and letters to editors were excluded. Any disagreement was resolved by consensus.
Data extraction and quality assessment
Major adverse cardiovascular events (MACE) was the primary outcome of interest. The secondary outcomes included all-causes mortality, cardiovascular mortality, MI, stroke, revascularization, and no-reflow phenomenon. The following information was abstracted by two independent investigators: the first author’s last name, publication date, sample size, country, study design, mean age, percent of female participants, type of CAD, length of follow-up, adjusted RRs with their 95% CI for the outcomes. Disagreements were resolved by a third reviewer.
Two reviewers independently conducted a quality assessment of each included study using the Newcastle–Ottawa Quality Assessment Scale (NOS), with scores of ≥ 7 considered as high-quality studies [ 13 ]. Any discrepancies were resolved through discussion.
Statistical analyses
Risk ratios (RRs) and 95% CIs from the fully adjusted models were pooled to obtain the association of AIP with the outcomes. In studies where the AIP was examined as categorized variable, the RR of the outcomes for patients with the highest AIP level compared to those with the lowest level were collected. In studies where the AIP was analyzed as a continuous variable, the RR of the outcomes per 1-unit increase in the AIP were extracted.
The I 2 statistic and Cochran’s Q test were utilized to assess heterogeneity. In cases where significant heterogeneity was observed (I 2 > 50%, p < 0.1) among the studies, a random-effects model was applied. A fixed-effects model was used in case of no significant heterogeneity. Visual inspection of Funnel plot and Egger test were used to evaluate possible publication bias. We performed subgroup analysis to identify the potential sources of heterogeneities. All data were analyzed with STATA (Version14). P value < 0.05 was considered as significant.
Following the abovementioned systematic search, we identified 1067 papers through databases. After duplicates removing and title/abstract screening, 118 studies eligible for full-text evaluation. After full-text screening, 16 studies [ 14 , 15 , 16 , 17 , 18 , 19 , 20 , 21 , 22 , 23 , 24 , 25 , 26 , 27 , 28 , 29 ] were included (Fig. 1 ).
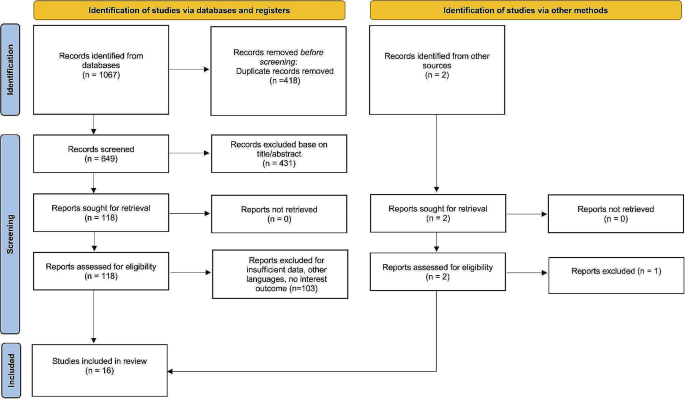
The flowchart of study selection
Characteristics of included studies
The basic characteristics of included studies are summarized in Table 1 . Sixteen studies with a total of 20,883 participants were published from 2020 to 2024. Twelve studies were retrospective cohort, three studies were prospective cohort, and one study was cross-sectional. The mean age and female proportion ranged from 55 to 63 years and 14.7–41.1%, respectively. The duration of follow-up ranged from three day to four years. All included studies evaluated the AIP under fasting condition. According to NOS score, all included studies had high quality (score ≥ 7).
Primary outcome
A total of eight studies investigated the association of AIP as a continues variable and MACE in patients with CAD. Overall, AIP level was found to increase the risk of MACE (RR = 1.54; 95% CI, 1.30–1.83; P < 0.001) with a significant heterogeneity (I 2 = 61.9%, P = 0.010) (Fig. 2 ). Six studies compared the highest vs. lowest category of AIP, and the pooled analysis showed an increased risk of MACE in those with higher AIP (RR = 1.63; 95% CI, 1.44–1.85; P < 0.001) with no significant heterogeneity (I 2 = 40.0%, P = 0.134) (Fig. 2 ).
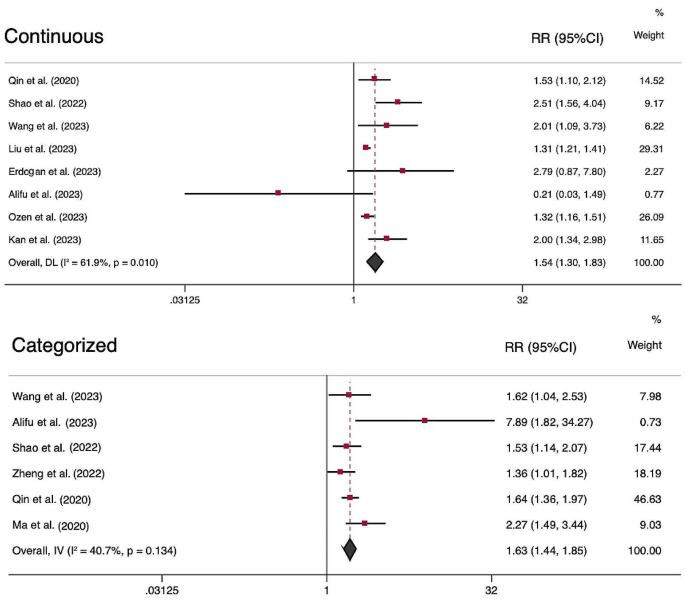
Forest plots showing the meta-analysis of major cardiovascular events using the AIP as categorial and continues variable
Secondary outcomes
Ten studies reported the RRs for the secondary outcomes. The pooled analysis indicated that higher AIP increase the risk of cardiovascular death (RR = 1.79; 95% CI, 1.09–2.78; P = 0.02), MI (RR = 2.21; 95% CI, 1.55–3.13; P < 0.001), revascularization (RR = 1.62; 95% CI, 1.34–1.97; P < 0.001), and no-reflow phenomenon (RR = 3.12 95% CI, 1.09–8.96; P = 0.034). However, AIP was not significantly associated with risk of all-causes death (RR = 1.15; 95% CI, 0.56–2.36; P = 0.699) and stroke (RR = 1.03; 95% CI, 0.69–1.52; P = 0.892) (Fig. 3 ). A significant heterogeneity was found for the no-reflow phenomenon (I 2 = 89.7%, P < 0.001). Three studies analyzed AIP as a continuous variable, which reported an of HR 1.21 (95% CI, 0.72–2.02, P = 0.460), 1.61 (95% CI, 1.12–2.32, P = 0.009), 3.77 (95% CI, 1.34–10.60, P = 0.012), and 13.46 (95%CI, 1.39-129.02; P = 0.025) for cardiovascular death [ 17 ], MI [ 17 ], all-causes death [ 21 ], and stent thrombosis [ 24 ], respectively.
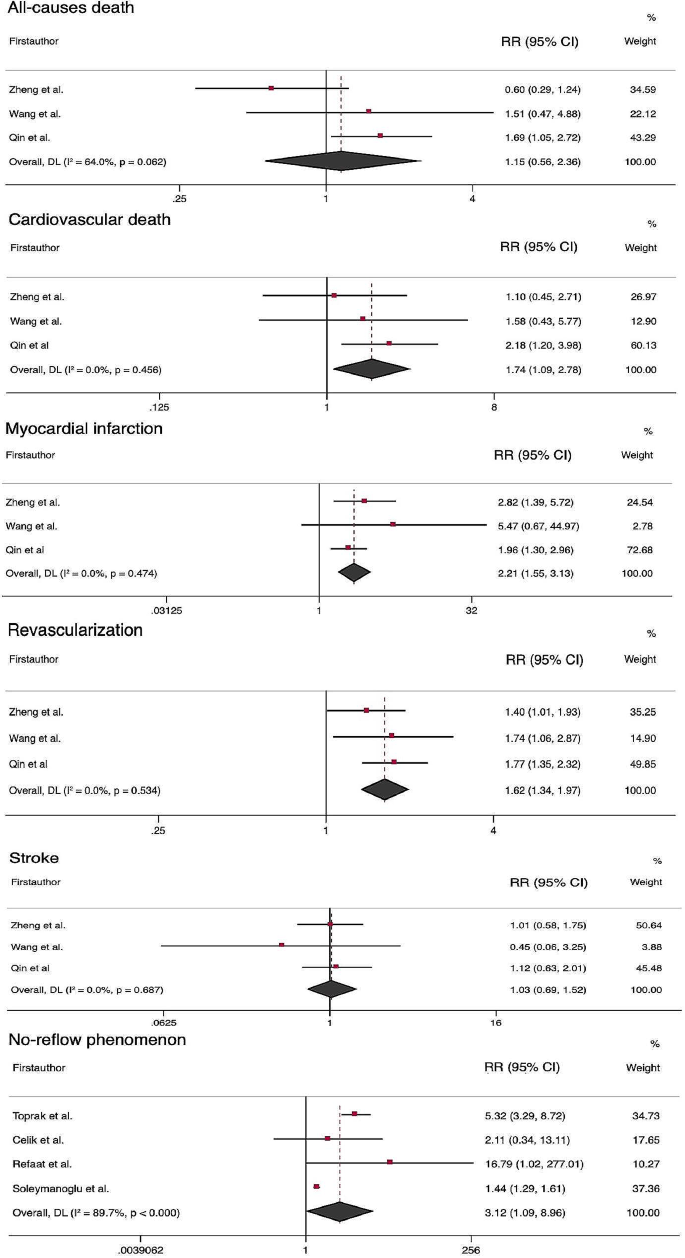
Forest plots showing the meta-analysis of secondary outcomes in comparison of highest AIP vs. lowest AIP group
Subgroup and sensitivity analysis
A subgroup analysis was performed for primary outcome according to the age (< 60 or ≥ 60 years), study design (retrospective or prospective), sample size (< 1,000 or ≥ 1,000), duration of follow-up (< 24 or ≥ 24 months), and LDL-C (< 1.8 or ≥ 1.8 mmol/L) to identify the sources of heterogeneity. A remarkable reduction in heterogeneity was found in prospective studies (I 2 = 0.0%) and LCL-C below 1.8 mmol/L (I 2 = 0.0%), suggesting that study design and LDL-C level might be factors contributing to heterogeneity. Besides, the analysis revealed no significant association between AIP and MACE in studies with a duration of follow-up below 24 months (RR = 1.56; 95% CI, 0.85, 2.87; P = 0.150) and mean age of over 60 years (RR = 1.43; 95% CI, 0.72, 2.82; P = 0.305) (Table 2 ).
A sensitivity analyses was performed including studies with a ≥ 2 years of follow-up. Consistent with our primary analysis, we revealed a significant association of AIP with MACE (RR = 1.66; 95% CI, 1.25, 2.20, P = 0.001). The results for other outcomes remained unchanged except for no-reflow phenomenon, which all studies reported a short duration of follow-up, and hence, the further analysis could not perform.
Publication bias
The funnel plots in Fig. 4 demonstrate the relationship between the AIP and the incidence of MACEs in CAD patients. Upon visual examination, the plots seem to be asymmetrical, suggesting a possible risk of publication bias. However, Egger test found no significant publication bias for categorial ( P = 0.052) and continues ( P = 0.178) analysis.
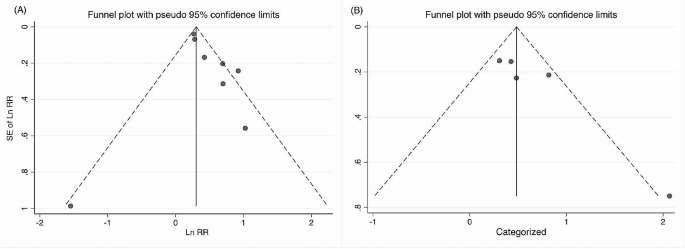
The publication bias assessment with funnel plot for the primary outcome
This meta-analysis showed that a higher AIP is associated with an increased risk of MACE, cardiovascular mortality, MI, revascularization, and the no-reflow phenomenon in patients with CAD. Subgroup analysis revealed that AIP may not be an indicator of MACE among patients aged ≥ 60 years and short follow-up times. Besides, AIP was not associated with all-causes mortality and stroke risk.
In this study, CAD patients with a higher AIP level had a < 1.5-fold higher risk for MACE compared with subjects with lower AIP. In line with our results, a fifteen-year cohort study conducted on 6323 healthy adults demonstrated a 1.2-fold greater risk for cardiovascular events among participants with higher AIP [ 30 ]. Moreover, a cross-sectional study compromising 7,362 adults showed that the third tertile of AIP had a 1.3-fold higher risk for cardiovascular disease compared to the first tertile [ 31 ]. These collective findings underscore the potential of AIP as a valuable biomarker for identifying individuals at higher risk for cardiovascular disease.
Our subgroup analysis did not detect a significant association between AIP and MACE in older patients. In this context, Nansseu et al. [ 32 ] enrolled 108 postmenopausal women, and found no significant correlation between AIP and cardiovascular risk evaluated with Framingham risk score. Similarly, there was no significant association between AIP and CAD in elderly females aged ≥ 65 years [ 33 ]. Moreover, AIP could not predict the presence of CAD in elderly males who underwent coronary angiography [ 34 ]. A possible explanation to address this finding is that AIP level is increased in elderly population [ 35 ]. Several studies showed a positive correlation between AIP and age among different populations [ 36 , 37 , 38 ], which might impact the likelihood of detecting a significant association between AIP level and cardiovascular events in this group. Further studies are warranted to better understand the impact of age on AIP levels and its implications for assessing cardiovascular risk in the elderly.
This meta-analysis included three studies evaluating the association of AIP as a categorial variable with all-causes death, and the results showed that AIP could not predict all-causes death in patients with CAD. However, Refs. [ 16 , 26 , 29 ]) considered AIP as a continuous variable, and found a significant positive correlation between AIP level and risk of all-causes mortality [ 21 ]. This discrepancy may be due to low number of studies and the differences in follow-up duration between the studies. The endpoint of et al. was the in-hospital mortality, and hence, their results showed the predictive value of AIP for all-causes mortality in a median follow-up duration three days. To evaluate the association of AIP with risk of short-term mortality, further studies are needed.
The disbalance of these plasma lipids leads to dyslipidemia, which is characterized by high levels of LDL-C, TG, and total cholesterol and low levels of HDL-C [ 39 ]. Although reducing LDL-C levels is a treatment goal in CAD, even after attaining this target, a notable residual cardiovascular risk remains present, encouraging the exploration of more accurate risk factors in these patients [ 40 ]. Regarding a practical predictor, the AIP strongly predicts cardiovascular events by reflecting the atherogenic lipid profile and providing valuable insights into the residual cardiovascular risk.
Despite the strengths of this study, including a comprehensive search strategy and evaluating several outcomes, there are limitations that should be considered. First, the majority of studies included in this meta-analysis were retrospective, and hence, further studies with prospective design are needed. Second, the presence of significant heterogeneity in some of the analyses suggests potential variations in methodologies and outcome definitions such as MACE, which may have influenced the results. Third, although this study found a significant association of AIP with MI and cardiovascular death, there was considerable variability across studies. As such, additional validation is required to confirm this tenuous relationship. Finally, the included studies were observational, which limits the ability to establish causal relationships between AIP and cardiovascular outcomes.
In conclusion, the findings of this meta-analysis support the notion that AIP is a potential prognostic marker for adverse cardiovascular events in patients with CAD. A higher AIP was consistently associated with an increased risk of MACE, cardiovascular death, MI, revascularization, and the no-reflow phenomenon. Notably, no association was found between AIP and all-causes death or stroke. These results have important implications for risk stratification and management strategies in CAD patients. Further research is needed to validate these findings.
Data availability
No datasets were generated or analysed during the current study.
Abbreviations
- Atherogenic index of plasma
- Coronary artery disease
High-density lipoprotein cholesterol
Hazard ratio
Low-density lipoprotein cholesterol
Percutaneous coronary intervention
Preferred Reporting Items for Systematic Review and Meta-analyses statement
Small dense low-density lipoprotein
Triglyceride
Roth GA, Abate D, Abate KH, Abay SM, Abbafati C, Abbasi N, et al. Global, regional, and national age-sex-specific mortality for 282 causes of death in 195 countries and territories, 1980–2017: a systematic analysis for the global burden of Disease Study 2017. Lancet. 2018;392(10159):1736–88.
Article Google Scholar
Bosco E, Hsueh L, McConeghy KW, Gravenstein S, Saade E. Major adverse cardiovascular event definitions used in observational analysis of administrative databases: a systematic review. BMC Med Res Methodol. 2021;21(1):1–18.
Pepine CJ, Kowey PR, Kupfer S, Kolloch RE, Benetos A, Mancia G, et al. Predictors of adverse outcome among patients with hypertension and coronary artery disease. J Am Coll Cardiol. 2006;47(3):547–51.
Article PubMed Google Scholar
Kim SH, Cho YK, Kim YJ, Jung CH, Lee WJ, Park JY, et al. Association of the atherogenic index of plasma with cardiovascular risk beyond the traditional risk factors: a nationwide population-based cohort study. Cardiovasc Diabetol. 2022;21(1):81.
Article CAS PubMed PubMed Central Google Scholar
Dobiasova M, Frohlich J. The new atherogenic plasma index reflects the triglyceride and HDL-cholesterol ratio, the lipoprotein particle size and the cholesterol esterification rate: changes during lipanor therapy. Vnitr Lek. 2000;46(3):152–6.
CAS PubMed Google Scholar
Wu J, Zhou Q, Wei Z, Wei J, Cui M. Atherogenic index of plasma and coronary artery disease in the adult population: a meta-analysis. Front Cardiovasc Med. 2021;8:817441.
Zhang Y, Chen S, Tian X, Wang P, Xu Q, Xia X, et al. Association between cumulative atherogenic index of plasma exposure and risk of myocardial infarction in the general population. Cardiovasc Diabetol. 2023;22(1):210.
Article PubMed PubMed Central Google Scholar
Fernández-Macías JC, Ochoa-Martínez AC, Varela-Silva JA, Pérez-Maldonado IN. Atherogenic index of plasma: novel predictive biomarker for cardiovascular illnesses. Arch Med Res. 2019;50(5):285–94.
Zheng H, Wu K, Wu W, Chen G, Chen Z, Cai Z, et al. Relationship between the cumulative exposure to atherogenic index of plasma and ischemic stroke: a retrospective cohort study. Cardiovasc Diabetol. 2023;22(1):313.
Fu L, Zhou Y, Sun J, Zhu Z, Xing Z, Zhou S, et al. Atherogenic index of plasma is associated with major adverse cardiovascular events in patients with type 2 diabetes mellitus. Cardiovasc Diabetol. 2021;20(1):1–11.
Hang F, Chen J, Wang Z, Zheng K, Wu Y. Association between the atherogenic index of plasma and major adverse cardiovascular events among non-diabetic hypertensive older adults. Lipids Health Dis. 2022;21(1):1–7.
Moher D, Liberati A, Tetzlaff J, Altman DG, PRISMA Group*. Preferred reporting items for systematic reviews and meta-analyses: the PRISMA statement. Ann Intern Med. 2009;151(4):264–9.
Peterson J, Welch V, Losos M, Tugwell P. The Newcastle-Ottawa scale (NOS) for assessing the quality of nonrandomised studies in meta-analyses. Ottawa: Ottawa Hospital Research Institute. 2011;2(1):1–12.
Ma X, Sun Y, Cheng Y, Shen H, Gao F, Qi J, et al. Prognostic impact of the atherogenic index of plasma in type 2 diabetes mellitus patients with acute coronary syndrome undergoing percutaneous coronary intervention. Lipids Health Dis. 2020;19(1):1–13.
Toprak K, Kaplangoray M, Akyol S, İnanır M, Memioğlu T, Taşcanov MB et al. The non-HDL-C/HDL-C ratio is a strong and independent predictor of the no-reflow phenomenon in patients with ST-elevation myocardial infarction. Acta Cardiol. 2023;1–12.
Wang Y, Wang S, Sun S, Li F, Zhao W, Yang H, et al. The predictive value of atherogenic index of plasma for cardiovascular outcomes in patients with acute coronary syndrome undergoing percutaneous coronary intervention with LDL-C below 1.8 mmol/L. Cardiovasc Diabetol. 2023;22(1):1–10.
Google Scholar
Liu Y, Feng X, Yang J, Zhai G, Zhang B, Guo Q, et al. The relation between atherogenic index of plasma and cardiovascular outcomes in prediabetic individuals with unstable angina pectoris. BMC Endocr Disorders. 2023;23(1):187.
Article CAS Google Scholar
Erdoğan A, İnan D, Genç Ö, Yıldız U, Demirtola Aİ, Çetin İ, et al. The triglyceride–glucose index might be a Better Indicator for Predicting Poor Cardiovascular Outcomes in Chronic Coronary Syndrome. J Clin Med. 2023;12(19):6201.
Çelik İE, Öztürk S, Yarlıoğlu M, Barutçu O, Akgün O, Duran M, et al. The Association between Atherogenic Index of plasma and No-Reflow Phenomenon in Acute Coronary Syndrome. Anatol J Cardiol. 2023;27(12):712.
Alifu J, Xiang L, Zhang W, Qi P, Chen H, Liu L, et al. Association between the atherogenic index of plasma and adverse long-term prognosis in patients diagnosed with chronic coronary syndrome. Cardiovasc Diabetol. 2023;22(1):255.
Kasapkara HA, Erdoğan M. Association between atherogenic index of plasma and in-hospital mortality in patients with STEMI undergoing primary percutaneous coronary intervention. J Health Sci Med. 2023;6(1):158–64.
Özen Y, BILAL ÖZBAY M, Yakut İ, Kanal Y, Abdelmottaleb W, Nriagu B et al. Atherogenic index of plasma and triglycerideglucose index to predict more advanced coronary artery diseases in patients with the first diagnosis of acute coronary syndrome. Eur Rev Med Pharmacol Sci. 2023;27(9).
Kan Y, Sun Y, Shen H, Liu X, Liu Y, Shi D, et al. Effect of body Mass Index on the Prognostic Value of Atherogenic Index of Plasma in patients with Acute Coronary Syndrome undergoing percutaneous coronary intervention. J Clin Med. 2023;12(20):6543.
Abacıoğlu ÖÖ, Yıldırım A, Koyunsever NY, Karadeniz M, Kılıç S. Relationship between atherogenic index of plasma and stent thrombosis in patients with acute coronary syndrome. Anatol J Cardiol. 2022;26(2):112.
Article PubMed Central Google Scholar
Qiao-Yu S, Xiao-Teng M, Zhi-Qiang Y, Qiu-Xuan L, Yu-Fei W, Liang J, et al. Prognostic significance of multiple triglycerides-derived metabolic indices in patients with acute coronary syndrome. J Geriatric Cardiology: JGC. 2022;19(6):456.
Zheng Y, Li C, Yang J, Seery S, Qi Y, Wang W, et al. Atherogenic index of plasma for non-diabetic, coronary artery disease patients after percutaneous coronary intervention: a prospective study of the long-term outcomes in China. Cardiovasc Diabetol. 2022;21(1):1–14.
Refaat H, Tantawy A, Gamal AS, Radwan H. Novel predictors and adverse long-term outcomes of No-reflow phenomenon in patients with acute ST elevation myocardial infarction undergoing primary percutaneous coronary intervention. Indian Heart J. 2021;73(1):35–43.
Süleymanoğlu M, Rencüzoğulları İ, Karabağ Y, Çağdaş M, Yesin M, Gümüşdağ A, et al. The relationship between atherogenic index of plasma and no-reflow in patients with acute ST-segment elevation myocardial infarction who underwent primary percutaneous coronary intervention. Int J Cardiovasc Imaging. 2020;36:789–96.
Qin Z, Zhou K, Li Y, Cheng W, Wang Z, Wang J, et al. The atherogenic index of plasma plays an important role in predicting the prognosis of type 2 diabetic subjects undergoing percutaneous coronary intervention: results from an observational cohort study in China. Cardiovasc Diabetol. 2020;19:1–11.
Sadeghi M, Heshmat-Ghahdarijani K, Talaei M, Safaei A, Sarrafzadegan N, Roohafza H. The predictive value of atherogenic index of plasma in the prediction of cardiovascular events; a fifteen-year cohort study. Adv Med Sci. 2021;66(2):418–23.
Hamzeh B, Pasdar Y, Mirzaei N, Faramani RS, Najafi F, Shakiba E, et al. Visceral adiposity index and atherogenic index of plasma as useful predictors of risk of cardiovascular diseases: evidence from a cohort study in Iran. Lipids Health Dis. 2021;20(1):82.
Nansseu JRN, Ama Moor VJ, Nouaga MED, Zing-Awona B, Tchanana G, Ketcha A. Atherogenic index of plasma and risk of cardiovascular disease among Cameroonian postmenopausal women. Lipids Health Dis. 2016;15(1):49.
Huang H, Yu X, Li L, Shi G, Li F, Xiao J, et al. Atherogenic index of plasma is related to coronary atherosclerotic disease in elderly individuals: a cross-sectional study. Lipids Health Dis. 2021;20(1):68.
Hong L, Han Y, Deng C, Chen A. Correlation between atherogenic index of plasma and coronary artery disease in males of different ages: a retrospective study. BMC Cardiovasc Disord. 2022;22(1):440.
Khakurel G, Kayastha R, Chalise S, Karki PK. Atherogenic index of plasma in postmenopausal women. 2018.
Tagoe EA, Dwamena-Akoto E, Nsaful J, Aikins AR, Clegg-Lamptey JN, Quaye O. High atherogenic index of plasma and cardiovascular risk factors among Ghanaian breast cancer patients. Exp Biol Med (Maywood). 2020;245(18):1648–55.
Article CAS PubMed Google Scholar
Nwagha U, Ikekpeazu E, Ejezie F, Neboh E, Maduka I. Atherogenic index of plasma as useful predictor of cardiovascular risk among postmenopausal women in Enugu. Nigeria Afr Health Sci. 2010;10(3).
Hegde SN. Low-density lipoprotein cholesterol, non-high-density lipoprotein cholesterol, and apolipoprotein b for cardiovascular risk assessment–what is the right choice? APIK J Intern Med. 2020;8(2):48–50.
Hoogeveen RC, Ballantyne CM. Residual cardiovascular risk at low LDL: remnants, lipoprotein (a), and inflammation. Clin Chem. 2021;67(1):143–53.
Ikezaki H, Lim E, Cupples LA, Liu C, Asztalos BF, Schaefer EJ. Small dense low-density lipoprotein cholesterol is the most atherogenic lipoprotein parameter in the prospective Framingham offspring study. J Am Heart Association. 2021;10(5):e019140.
Download references
Acknowledgements
Author information, authors and affiliations.
Interventional Cardiology Research Center, Cardiovascular Research Institute, Isfahan University of Medical Sciences, Isfahan, Iran
Mehrdad Rabiee Rad & Ghazal Ghasempour Dabaghi
School of Medicine, Isfahan University of Medical Science, Isfahan, Iran
Bahar Darouei & Reza Amani-Beni
Isfahan Cardiovascular Research Center, Cardiovascular Research Institute, Isfahan University of Medical Sciences, Isfahan, Iran
Ghazal Ghasempour Dabaghi
You can also search for this author in PubMed Google Scholar
Contributions
MRR and GGD designed the research. MRR, RAB, and BD collected data in electronic database. MRR performed statistical analysis. All authors contributed to drafting of the manuscript, had full access to all the data in the study, approved the final version of the manuscript and had final decision to submit for publication. All authors read and approved the final manuscript.
Corresponding author
Correspondence to Ghazal Ghasempour Dabaghi .
Ethics declarations
Competing interests.
The authors declare no competing interests.
Ethical approval and consent to participate
Ethical approval was not applicable for this systematic review and meta-analysis.
Consent for publication
Additional information, publisher’s note.
Springer Nature remains neutral with regard to jurisdictional claims in published maps and institutional affiliations.
Rights and permissions
Open Access This article is licensed under a Creative Commons Attribution 4.0 International License, which permits use, sharing, adaptation, distribution and reproduction in any medium or format, as long as you give appropriate credit to the original author(s) and the source, provide a link to the Creative Commons licence, and indicate if changes were made. The images or other third party material in this article are included in the article’s Creative Commons licence, unless indicated otherwise in a credit line to the material. If material is not included in the article’s Creative Commons licence and your intended use is not permitted by statutory regulation or exceeds the permitted use, you will need to obtain permission directly from the copyright holder. To view a copy of this licence, visit http://creativecommons.org/licenses/by/4.0/ . The Creative Commons Public Domain Dedication waiver ( http://creativecommons.org/publicdomain/zero/1.0/ ) applies to the data made available in this article, unless otherwise stated in a credit line to the data.
Reprints and permissions
About this article
Cite this article.
Rabiee Rad, M., Ghasempour Dabaghi, G., Darouei, B. et al. The association of atherogenic index of plasma with cardiovascular outcomes in patients with coronary artery disease: A systematic review and meta-analysis. Cardiovasc Diabetol 23 , 119 (2024). https://doi.org/10.1186/s12933-024-02198-y
Download citation
Received : 03 February 2024
Accepted : 12 March 2024
Published : 02 April 2024
DOI : https://doi.org/10.1186/s12933-024-02198-y
Share this article
Anyone you share the following link with will be able to read this content:
Sorry, a shareable link is not currently available for this article.
Provided by the Springer Nature SharedIt content-sharing initiative
Cardiovascular Diabetology
ISSN: 1475-2840
- Submission enquiries: [email protected]
- Open access
- Published: 31 March 2024
MicroRNA expression as a prognostic biomarker of tongue squamous cell carcinoma (TSCC): a systematic review and meta-analysis
- Yiwei Sun 1 na1 ,
- Yuxiao Li 2 na1 ,
- Wenjuan Zhou 3 , 4 , 5 &
- Zhonghao Liu 3 , 4 , 5
BMC Oral Health volume 24 , Article number: 406 ( 2024 ) Cite this article
166 Accesses
Metrics details
Recent studies have indicated that microRNA (miRNA) expression in tumour tissues has prognostic significance in Tongue squamous cell carcinoma (TSCC) patients. This study explored the possible prognostic value of miRNAs for TSCC based on published research.
A comprehensive literature search of multiple databases was conducted according to predefined eligibility criteria. Data were extracted from the included studies by two researchers, and HR results were determined based on Kaplan‒Meier curves according to the Tierney method. The Newcastle‒Ottawa Scale (NOS) and GRADE (Grading of Recommendations Assessment, Development, and Evaluation) pro-GDT were applied to assess the quality of all studies. Publication bias was estimated by funnel plot, Egger’s rank correlation test and sensitivity analysis.
Eleven studies (891patients) were included, of which 6 reported up-regulated miRNAs and 7 mentioned down-regulated miRNAs. The pooled hazard ratio (HR) from the prognostic indicator overall survival (OS) was 1.34 (1.25–1.44), p < 0.00001, indicating a significant difference in miRNA expression between TSCC patients with better or worse prognosis.
MiRNAs may have high prognostic value and could be used as prognostic biomarkers of TSCC.
Peer Review reports
Introduction
Oral squamous cell carcinoma (OSCC) accounts for approximately 90% of all oral malignancies, resulting in the highest morbidity rates in the head and neck region worldwide [ 1 , 2 , 3 ]. When compared with OSCC at other sites, tongue squamous cell carcinoma (TSCC), a principal subtype of oral cancer, represents 17.8% of occurrences and shares similarities in etiopathogenesis related to early regional lymph node metastasis [ 4 , 5 , 6 , 7 ] and malignant proliferation [ 8 , 9 ]. Notably, despite the application of advanced therapies, the 5-year survival rate and quality of life for TSCC patients are not promising [ 1 , 9 ]. Compared with the overt symptoms of advanced stage TSCC, assessments such as tissue biopsy are advantageous for identifying patients with early-stage disease and, as a result, increase the chances for better treatment and recovery [ 10 , 11 , 12 ]. Thus, in accordance with the World Health Organization (WHO) recommendations for the primacy of early diagnosis and prevention, measures should be taken to achieve early discovery and diagnosis of TSCC with the purpose of prolonging and improving the quality of patients’ lives [ 10 , 13 ].
According to current clinical practice, the present detection methods for TSCC include but not limited to brush biopsy, CT and MRI scanning, and tissue autofluorescence. Even though some of these applications are widely performed in routine clinical practice, there exist certain limitations, such as labour intensiveness, invasiveness and low sensitivity, that should not be ignored. Recent researches indicate that part of the genetic alterations for TSCC contain progressive change in DNA methylation, overexpression of carcinoembryonic antigen, histone modification and expression level alteration of miRNAs, etc. As a result, the epigenetic alterations, including DNA fragments in saliva [ 14 , 15 ], immune-related gene transcript [ 16 ], the neutrophil-to‐lymphocyte ratio [ 17 ], platelet-to-lymphocyte ratio [ 18 ] and miRNA expression [ 19 ], could be used as the promising biomarkers for early-stage detection for prognosis, which bridge the defect of traditional Tumor-Node-Metastasis (TNM) staging system [ 20 ]. In recent years, with the purpose of finding more reliable diagnostic techniques, research based on microRNA molecular mechanisms has become an important academic focus [ 10 ]. Studies have reported connections between miRNAs and predicting the prognosis of TSCC, as changes in miRNA expression can reflect the stage of a tumour to some extent.
MicroRNAs (miRNAs) are sophisticated epigenetic molecular markers linked to TSCC patient prognosis. Intrinsically, miRNAs are endogenous, noncoding, single-stranded RNA molecules that are approximately 20–24 nucleotides long and renowned for their conserved gene sequence and distinct expression patterns [ 21 , 22 , 23 , 24 ]. Generally, miRNAs are characterized by high gene sequence conservation, temporal expression specificity and tissue expression specificity [ 25 ]. By mediating dysregulation and translational repression, miRNAs regulate 30% of gene expression in the posttranscriptional stage [ 24 , 25 ]. During the biological process of TSCC deterioration, miRNAs partly act as oncogenes or suppressor genes in carcinogenesis by dysregulation, as well as having an indirect impact on the expression of proto-oncogenes and cancer suppressor gene [ 26 ]. Followed by that, miRNA deteriorate tumour cell proliferation by escaping normal suppressing signals, expediting malignant cell migration and stimulating angiogenesis in tumours [ 21 , 22 , 24 , 25 ].
Experiments based on miRNA as a biomarker of malignancy prognosis have made great progress in recent years. However, due to the comparatively high cost and complexity of the process, this technique has not been put into clinical use on a large scale. Distinct from existing meta-analyses of TSCC, this manuscript offers a fresh perspective, bridging the gap between the academic value of miRNAs and their usefulness in clinical applications. This meta-analysis aimed to consolidate and elucidate the prognostic significance of miRNAs in TSCC, paving the way for innovative clinical interventions to enhance patient longevity and quality of life.
Materials and methods
Protocol and registration.
Following the Preferred Reporting Items for Systematic Review and Meta-analyses (PRISMA) guidelines [ 27 ], researchers executed a systematic review encompassing aspects such as protocol, inclusion criteria, search strategy, and outcomes. This systematic review has been catalogued on PROSPERO under the registration number CRD 42,023,391,953.
Eligibility criteria
Researchers focused on all retrospective cohort studies evaluating the association between variations in miRNA expression and prognostic survival metrics of TSCC. The criteria were based on the PICO elements: participants (patients diagnosed with TSCC), intervention (deregulated miRNA levels in TSCC patients), control (TSCC patients with normal miRNA expression levels), and outcome (prognosis differences in TSCC patients based on miRNA expression variance). Consequently, the PICO question was as follows: is there a difference in prognostic survival indexes between TSCC patients with dysregulation of miRNA expression and those with normal miRNA expression?
The inclusion criteria were studies that reported interrelated prognostic survival indexes, including hazard ratios (HRs), Kaplan–Meier curves and univariate or multivariate Cox regression between TSCC patients with low and high miRNA expression levels.
Regarding the exclusion criteria, non-English studies and those without prognostic survival indexes from which HRs could be extracted or inferred were excluded. Case reports, meta-analyses, systematic reviews, historical reviews and studies at high risk of bias were excluded. Studies that did not elaborate clinical outcomes or that reported content that was not relevant to clinical patients were likewise excluded.
Sources of information, research and selection
For reference inclusion, relevant articles were identified through literature retrieval from online databases. Specifically, non-English articles were excluded. Databases, including PubMed, Web of Science, Scopus, EMBASE, Cochrane Library and Google Scholar, were searched to retrieve relevant articles focusing on miRNA dysregulation in the prognosis of TSCC.
A search strategy combining the terms (Tongue Squamous Cell Cancer OR TSCC OR neoplasm OR cancer OR malignancy OR malignant neoplasm OR malignant neoplasms OR neoplasia) AND (prognosis OR prognostic factor OR prognostic factors) AND (MicroRNAs OR Micro RNA OR MicroRNA OR Primary MicroRNA OR Primary miRNA OR RNA, Small Temporal OR Small Temporal RNA OR miRNA OR miRNAs OR pre-miRNA OR pri-miRNA OR stRNA) was developed. Moreover, identified articles were scrutinized separately by reviewing the content of abstracts and full texts for eligible articles meeting the inclusion criteria for this meta-analysis.
Data collection process and data characteristics
Investigators extracted data from studies that met the inclusion criteria and subsequently contrasted, collated and adopted the data mentioned above. If distinctions in data existed, one researcher expressed opinions that played a critical part in data selection. Data extracted from studies that met the inclusion criteria included Kaplan–Meier curves, first author and date, country, study design, number of patients, cut-off between low and high expression, miRNA types, miRNA expression levels and HRs of deregulated miRNA expression (OS, DSS, DFS, RFS, PFS). According to the Tierney method, prognostic index HR data, comprising overall survival (OS), disease-specific survival (DSS), disease-free survival (DFS), recurrence-free survival (RFS) and progression-free survival (PFS), were collected and extrapolated from Kaplan–Meier curves [ 28 ].
Risk of bias in individual studies, summary measures, summary of results, risk of bias between studies, and additional measures
Investigators evaluated the risk of bias in the studies respectively while ensuring the accuracy of the assessments. Under the guidance of reference factors derived from the Newcastle‒Ottawa Scale (NOS), each study was graded from 0 to 9. Those scoring above or equal to 7 were considered high-quality articles, while those scoring below 7 were eliminated from this meta-analysis. Forest plots were used to graphically illustrate the results mentioned above, and inconsistency indexes involving the Higgins index I² were used for evaluation. Software STATA 15.1 and Reviewer Manager 5.4 were used for statistical analysis. Collected data were aggregated to obtain a pooled sensitivity and specificity of OS, DSS, DFS, RFS and PFS. The risk of bias between the studies was assessed graphically through analysis of overlaps of the confidence intervals through the I² inconsistency index (an I² value greater than 50% was statistically significant, and a fixed-effect model was applied in specific cases). By using a funnel plot and Egger’s rank correlation test, publication bias was comprehensively estimated [ 29 ]. If the p value of Egger’s test was N > 0.05 and the funnel plot was symmetrical, there was no obvious publication bias in this meta-analysis. Subsequently, we carried out sensitivity analysis to accurately judge the heterogeneity of the selected studies.
Study characteristics
A total of 403 bibliographic citations were initially identified, 240 were excluded due to repetition, and 140 others were excluded according to the eligibility criteria. Fourteen studies reported prognostic data for OSCC only and thus were excluded. Moreover, another 3 articles from Google Scholar that met the inclusion criteria were additionally included in the meta-analysis. In the end, 12 articles were included in our study and 11 of these articles were included in this meta-analysis (Fig. 1 ).
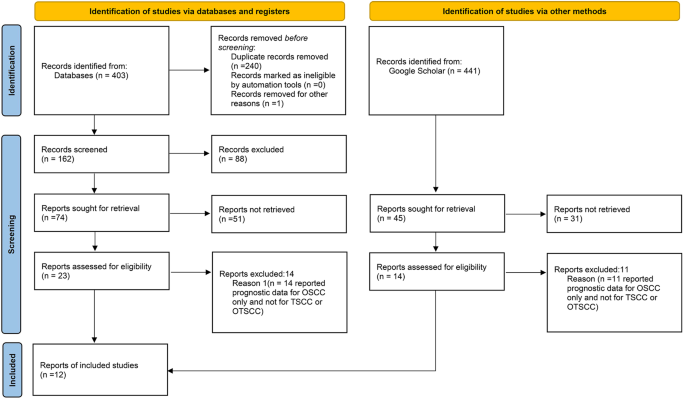
Flow diagram of studies selection process according to PRISMA guidelines
Data characteristics
According to the characteristics of the extracted data mentioned in the Data Collection Process and Data Characteristics section, all the extracted data are shown in Table 1 . All 12 included retrospective clinical studies with a follow-up period of 3 to 15 years and a total of 970 TSCC patients were published from 2009 to 2020. The studies by Jia et al. (2013) [ 30 ], Supic et al. (2018) [ 31 ], Zheng et al. (2016) [ 32 ], Jia et al. (2014) [ 33 ], Li et al. (2009) [ 34 ], Maruyama et al. (2018) [ 35 ], Xie et al. (2016) [ 36 ], Berania et al. (2017) [ 37 ], Jia et al. (2015) [ 38 ], W. Chen et al. (2019) [ 39 ], and S. Chen et al. (2019) [ 40 ] reported OS as a prognostic parameter; Kawakita et al. (2014) [ 41 ]used DSS; PFS was selected as another prognostic indicator by Berania et al. (2017) [ 37 ], and Maruyama et al. (2018) [ 35 ] regarded DFS as a prognostic index, while RFS was indicated by Supic et al. (2018) [ 31 ].
Risk of bias within studies
The risk of bias was assessed through factors derived from the NOS. According to the NOS guidelines, each study was graded from 0 to 9, and those scoring above or equal to 7 were considered high-quality studies (Table 2 ).
Additionally, GRADE pro-GDT was used to assess the quality of OS prognostic outcome (Table 3 ). The results indicated that the quality of the evidence is critical for the outcome.
Correlation of miRNA expression with survival outcomes
HR extracted from the included studies was the primary index for assessing TSCC patients with up-regulated and down-regulated miRNA expression. A fixed model was chosen to calculate the pooled HR value (95% CI).
The prognostic outcome OS from different miRNA expression levels was represented by a forest plot (Fig. 2 ), showing a pooled HR of 1.34 (1.25–1.44); heterogeneity of Chi 2 = 13.90; df = 12 ( p = 0.31); I 2 = 14%; and test for the overall effect of Z = 8.01 ( p < 0.00001).
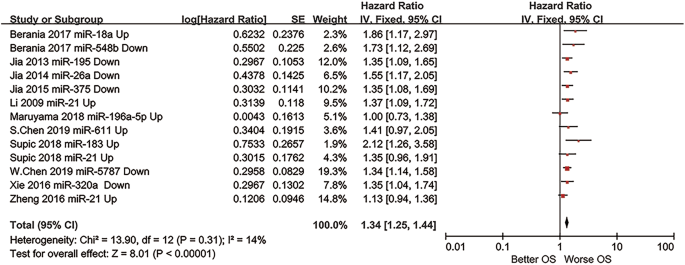
Forest plot of the OS prognostic outcome of the meta-analysis
Moreover, even if the prognostic outcomes of DFS PFS RFS DSS were excluded from our meta-analysis in reason of only one study was included in each group, these outcomes still indicated great statistical significance in demonstrating miRNA as a plausible prognostic biomarker for TSCC patients.
DFS was regarded as a prognostic indicator, the HR was 3.06 (1.32–7.12) with a test for the overall effect of Z = 0.19 ( p = 0.009) (Fig. 3 ).

Forest plot of DFS outcome
PFS was considered as a prognostic index, whose data were collected from the study, the HR was 2.31 (1.28,4.17), and the test for the overall effect was Z = 2.78 ( p = 0.005) (Fig. 4 ).

Forest plot of PFS outcome
The HR of another prognostic indicator RFS was 1.31 (0.97,1.78), and the test for the overall effect was Z = 1.74 ( p = 0.08) (Fig. 5 ).

Forest plot of RFS outcome
The HR of DSS prognostic indicator was 1.08 (0.87–1.34) and a test for the overall effect was Z = 0.69 ( p = 0.49) (Fig. 6 ).

Forest plot of DSS outcome
Heterogeneity and sensitivity analysis
There was no significant heterogeneity in this meta-analysis. To ascertain the effect of each included study on the pooled HR, we conducted a sensitivity analysis. With each study excluded successively, the remaining studies were meta-analysed, and the results of pooled HRs were observed. Eventually, the results suggested that no study had an influence on the pooled HR, which means that the results of the meta-analysis were statistically stable (Fig. 7 ).
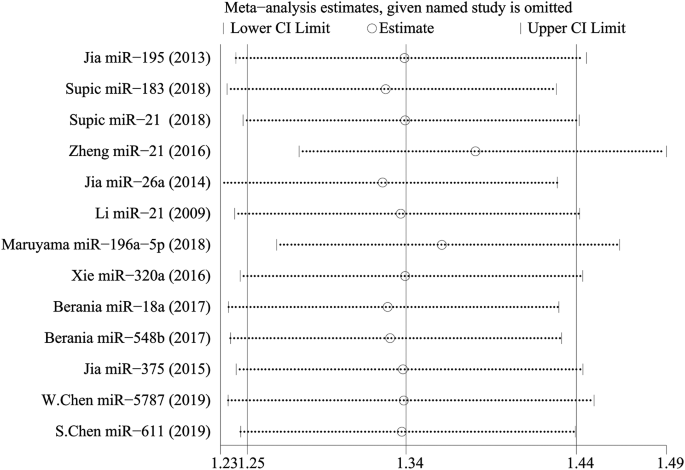
Outcome of sensitivity analysis to assess the heterogeneity in this meta-analysis
Publication Bias
A funnel plot and Egger’s test were conducted to assess publication bias. According to the figure displayed, there was no visible asymmetry shown by the funnel plot (Fig. 8 ). To be more accurate, Egger’s test was performed to assess publication bias, and the results showed p = 0.063 (≥ 0.05). Thus, there was no statistically significant indication of publication bias, which verified that our results were relatively stable (Fig. 9 ).
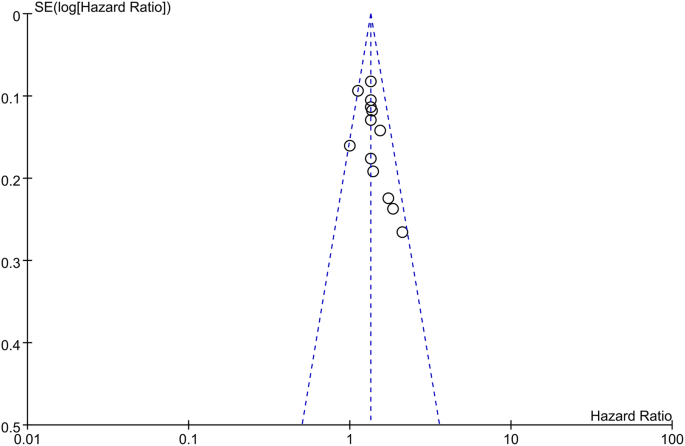
Funnel plot for the main outcome in the fixed-effects model
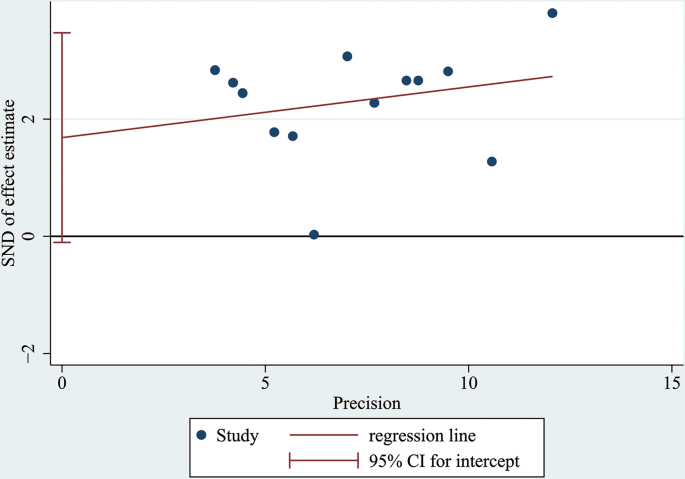
Egger’s test conducted to estimate publication bias; p = 0.063 (≥ 0.05)
Of the different OSCC subtypes, TSCC has the highest morbidity rate [ 1 ], with a low 5-year survival rate despite advancements in treatment during recent years [ 2 , 4 ]. To extend and improve the quality of patients’ lives, studies have investigated novel indicators targeting tumorigenesis and tumour metastasis, including but not limited to centromeric probes, gene expression profiling and standard karyotyping [ 5 ]. Among these possible methods, miRNAs providing regulatory mechanisms based on their expression level have become a hotspot among academic research. Previous studies confirmed down-regulated miRNAs (miR-26a, miR-137, miR-139-5p, miR-143-3p, miR-184, miR-375, miR-32, miR-20a, miR-16 and miR-125b) and up-regulated miRNAs (miR-21, miR-31, miR-134, miR-155, miR-196a, miR-196b, miR-218, miR-455-5p, miR-372 and miR-373) in TSCC prognosis [ 42 , 43 , 44 , 45 ]. In general, miRNAs are expected to be advanced epigenetic biomarkers in the prognosis and optimized therapy of TSCC [ 46 , 47 , 48 ].
In this meta-analysis, the researchers included 11 articles and 891 patients. The investigators further summarized and proposed miRNA expression as a prognostic biomarker for TSCC survival. From the researchers’ review, the pooled HR of the prognostic outcome overall survival (OS) was 1.34 (1.25–1.44), elucidating the potential prognostic value of miRNA expression in TSCC patients. This meta-analysis demonstrated the high feasibility of applying miRNA as a prognostic biomarker in TSCC. Nevertheless, further investigation and improvement are needed.
Based on the included studies and forest plots mentioned above, the expression levels of miRNA-18a, miRNA-611, miRNA-21, miRNA-183, and miRNA-196a-5p significantly increased, whereas those of miRNA-548b, miRNA-5787, miRNA-195, miRNA-26a, miRNA-357, and miRNA-320a apparently decreased, revealing that abnormal expression of miRNAs could be considered a signal for poor prognosis. In addition, miRNA-21, miRNA-183 and miRNA-18a have already been suggested in multiple studies as potential prognostic indicators of head and neck squamous cell carcinoma or oral squamous cell carcinoma [ 44 , 45 ]. Furthermore, recent studies have made new progress in considering miRNA-5787 and miRNA-357 as TSCC prognostic biomarkers [ 38 , 39 ]. Altogether, this review offers a new perspective on addressing refractory TSCC prognosis.
With respect to the statistical process, this meta-analysis applied the NOS to assess the quality of selected retrospective cohort studies. According to the criteria of this protocol, 11 included studies scored more than 7 points, which means that they had a low risk of bias and high quality. Pooled HRs (95% CI) of prognostic factors extracted from the forest plot was OS-1.34 (1.25–1.44), which implied low heterogeneity. Additionally, researchers determined that the data collected were credible and accurate enough to demonstrate miRNA as a plausible prognostic biomarker for TSCC patients via sensitivity analysis and Egger’s test.
Nevertheless, the limitations of this meta-analysis should not be ignored. First, it is inevitable that the quantity of patients included is relatively insufficient since supplementary cases could enhance credibility. Second, due to the intrinsic deficiency of the Kaplan‒Meier curve, it is difficult for researchers to verify part of the accuracy of the initial results since extraction based on image capture could not be perfected. Third, due to limited resources in online databases, the cases contained were entirely extracted from only East Asia and American countries, which obviously led to regional limitations. Hence, patients from other nations could not completely benefit from the current results. Therefore, these problems must be scientifically addressed in future studies.
During the information retrieval process, the investigators noted that exosome research, especially involving miRNAs, has drawn much attention from academic communities and has even been experimentally used as diagnostic and prognostic tools in several types of malignant tumors [ 49 , 50 ]. In comparison with other advanced techniques for prognosing TSCC, miRNA expression levels provide an original method for preventing recurrence and evaluating therapeutic effects. Not only could miRNA expression prospectively be used as a biomarker of residual or recrudescent TSCC malignant tissue, but it could also be utilized to make more accurate prognoses prior to clinical manifestations, which has significance for the timely initiation of adequate treatment. In summary, this meta-analysis aimed to contribute to the development of novel treatments and provide persuasive references for practical clinical applications and therapeutic guidance to some extent.
In conclusion, in this meta-analysis, the data extracted from the OS prognostic outcome suggest that alterations, including up-regulation and down-regulation of miRNA expression levels, could be used as promising prognostic factors of TSCC. According to the figures and tables presented above, the down-regulated miRNAs correlated with poor prognosis are miR-195, miR-26a, miR-320a, miR-548b, miR-375 and miR-5787, while the up-regulated miRNAs are miR-183, miR-21, miR-196a-5p, miR-18a, and miR-611. Given the limitations of the existing studies, it is necessary to conduct repetitive studies with more statistical tests and large-scale experiments that include patients from various regions and nations as well as various age groups. Consequently, this meta-analysis could provide references and prerequisites for further clinical trials and therapeutic applications.
Data availability
All data generated or analysed during this study are included in this published article.
Abbreviations
hazard ratio
Oral squamous cell carcinoma
Newcastle‒Ottawa Scale
World Health Organization
overall survival
disease-specific survival
disease-free survival
recurrence-free survival
progression-free survival
Ding L, Fu Y, Zhu N, Zhao M, Ding Z, Zhang X, Song Y, Jing Y, Zhang Q, Chen S, et al. OXTR(High) stroma fibroblasts control the invasion pattern of oral squamous cell carcinoma via ERK5 signaling. Nat Commun. 2022;13(1):5124.
Article CAS PubMed PubMed Central Google Scholar
Goldberg M, Manzi A, Birdi A, Laporte B, Conway P, Cantin S, Mishra V, Singh A, Pearson AT, Goldberg ER, et al. A nanoengineered topical transmucosal cisplatin delivery system induces anti-tumor response in animal models and patients with oral cancer. Nat Commun. 2022;13(1):4829.
Li Z, Fu R, Wen X, Zhang L. Network analysis reveals miRNA crosstalk between periodontitis and oral squamous cell carcinoma. BMC Oral Health. 2023;23(1):19.
Huang L, Luo EL, Xie J, Gan RH, Ding LC, Su BH, Zhao Y, Lin LS, Zheng DL, Lu YG. FZD2 regulates cell proliferation and invasion in tongue squamous cell carcinoma. Int J Biol Sci. 2019;15(11):2330–9.
Weatherspoon DJ, Chattopadhyay A, Boroumand S, Garcia I. Oral cavity and oropharyngeal cancer incidence trends and disparities in the United States: 2000–2010. Cancer Epidemiol. 2015;39(4):497–504.
Article PubMed PubMed Central Google Scholar
Xie N, Wang C, Liu X, Li R, Hou J, Chen X, Huang H. Tumor budding correlates with occult cervical lymph node metastasis and poor prognosis in clinical early-stage tongue squamous cell carcinoma. J Oral Pathol Med. 2015;44(4):266–72.
Article PubMed Google Scholar
Zhao W, Cui Y, Liu L, Qi X, Liu J, Ma S, Hu X, Zhang Z, Wang Y, Li H, et al. Splicing factor derived circular RNA circUHRF1 accelerates oral squamous cell carcinoma tumorigenesis via feedback loop. Cell Death Differ. 2020;27(3):919–33.
Article CAS PubMed Google Scholar
Li HF, Liu YQ, Shen ZJ, Gan XF, Han JJ, Liu YY, Li HG, Huang ZQ. Downregulation of MACC1 inhibits invasion, migration and proliferation, attenuates cisplatin resistance and induces apoptosis in tongue squamous cell carcinoma. Oncol Rep. 2015;33(2):651–60.
Xi L, Yang Y, Xu Y, Zhang F, Li J, Liu X, Zhang Z, Du Q. The enhanced genomic 6 mA metabolism contributes to the proliferation and migration of TSCC cells. Int J Oral Sci. 2022;14(1):11.
Abu-Ghanem S, Yehuda M, Carmel NN, Leshno M, Abergel A, Gutfeld O, Fliss DM. Elective neck dissection vs observation in early-stage squamous cell carcinoma of the oral tongue with no clinically apparent Lymph node metastasis in the neck: a systematic review and Meta-analysis. JAMA Otolaryngol Head Neck Surg. 2016;142(9):857–65.
Pich O, Bailey C, Watkins TBK, Zaccaria S, Jamal-Hanjani M, Swanton C. The translational challenges of precision oncology. Cancer Cell. 2022;40(5):458–78.
Sun L, Kang X, Wang C, Wang R, Yang G, Jiang W, Wu Q, Wang Y, Wu Y, Gao J, et al. Single-cell and spatial dissection of precancerous lesions underlying the initiation process of oral squamous cell carcinoma. Cell Discov. 2023;9(1):28.
Hussein AA, Forouzanfar T, Bloemena E, de Visscher J, Brakenhoff RH, Leemans CR, Helder MN. A review of the most promising biomarkers for early diagnosis and prognosis prediction of tongue squamous cell carcinoma. Br J Cancer. 2018;119(6):724–36.
Rapado-González Ó, López-Cedrún JL, Lago-Lestón RM, Abalo A, Rubin-Roger G, Salgado-Barreira Á, López-López R, Muinelo-Romay L, Suárez-Cunqueiro MM. Integrity and quantity of salivary cell-free DNA as a potential molecular biomarker in oral cancer: a preliminary study. J Oral Pathol Med. 2022;51(5):429–35.
Zhou Y, Liu Z. Saliva biomarkers in oral disease. Clin Chim Acta. 2023;548:117503.
Chen Y, Feng Y, Yan F, Zhao Y, Zhao H, Guo Y. A Novel Immune-related gene signature to identify the Tumor Microenvironment and Prognose Disease among patients with oral squamous cell carcinoma patients using ssGSEA: a Bioinformatics and Biological Validation Study. Front Immunol. 2022;13:922195.
Mariani P, Russo D, Maisto M, Troiano G, Caponio VCA, Annunziata M, Laino L. Pre-treatment neutrophil-to-lymphocyte ratio is an independent prognostic factor in head and neck squamous cell carcinoma: Meta-analysis and trial sequential analysis. J Oral Pathol Med. 2022;51(1):39–51.
Diana R, Pierluigi M, Dardo M, Claudia A, Rosario R, Luigi L. The prognostic role of pre-treatment platelet-to-lymphocyte ratio in head and neck squamous cell carcinoma. Meta-analysis and trial sequential analysis. J Evid Based Dent Pract. 2023;23(4):101898.
Bigagli E, Locatello LG, Di Stadio A, Maggiore G, Valdarnini F, Bambi F, Gallo O, Luceri C. Extracellular vesicles miR-210 as a potential biomarker for diagnosis and survival prediction of oral squamous cell carcinoma patients. J Oral Pathol Med. 2022;51(4):350–7.
Russo D, Mariani P, Caponio VCA, Lo Russo L, Fiorillo L, Zhurakivska K, Lo Muzio L, Laino L, Troiano G. Development and validation of prognostic models for oral squamous cell carcinoma: a systematic review and appraisal of the literature. Cancers (Basel). 2021;13(22).
Jadhav KB, Nagraj SK, Arora S. miRNA for the assessment of lymph node metastasis in patients with oral squamous cell carcinoma: systematic review and metanalysis. J Oral Pathol Med. 2021;50(4):345–52.
Lee YS, Dutta A. MicroRNAs in cancer. Annu Rev Pathol. 2009;4:199–227.
Vishnoi A, Rani S. miRNA biogenesis and regulation of diseases: an updated overview. Methods Mol Biol. 2023;2595:1–12.
Yete S, Saranath D. MicroRNAs in oral cancer: biomarkers with clinical potential. Oral Oncol. 2020;110:105002.
Budakoti M, Panwar AS, Molpa D, Singh RK, Büsselberg D, Mishra AP, Coutinho HDM, Nigam M. Micro-RNA: the darkhorse of cancer. Cell Signal. 2021;83:109995.
Di Stasio D, Romano A, Boschetti CE, Montella M, Mosca L, Lucchese A. Salivary miRNAs expression in potentially malignant disorders of the oral mucosa and oral squamous cell carcinoma: a pilot study on miR-21, miR-27b, and miR-181b. Cancers (Basel) 2022, 15(1).
Page MJ, McKenzie JE, Bossuyt PM, Boutron I, Hoffmann TC, Mulrow CD, Shamseer L, Tetzlaff JM, Akl EA, Brennan SE, et al. The PRISMA 2020 statement: an updated guideline for reporting systematic reviews. BMJ. 2021;372:n71.
Tierney JF, Stewart LA, Ghersi D, Burdett S, Sydes MR. Practical methods for incorporating summary time-to-event data into meta-analysis. Trials. 2007;8:16.
Bowden J, Davey Smith G, Burgess S. Mendelian randomization with invalid instruments: effect estimation and bias detection through Egger regression. Int J Epidemiol. 2015;44(2):512–25.
Jia LF, Wei SB, Gong K, Gan YH, Yu GY. Prognostic implications of micoRNA miR-195 expression in human tongue squamous cell carcinoma. PLoS ONE. 2013;8(2):e56634.
Supic G, Zeljic K, Rankov AD, Kozomara R, Nikolic A, Radojkovic D, Magic Z. miR-183 and miR-21 expression as biomarkers of progression and survival in tongue carcinoma patients. Clin Oral Investig. 2018;22(1):401–9.
Zheng G, Li N, Jia X, Peng C, Luo L, Deng Y, Yin J, Song Y, Liu H, Lu M, et al. MYCN-mediated miR-21 overexpression enhances chemo-resistance via targeting CADM1 in tongue cancer. J Mol Med (Berl). 2016;94(10):1129–41.
Jia LF, Wei SB, Gan YH, Guo Y, Gong K, Mitchelson K, Cheng J, Yu GY. Expression, regulation and roles of miR-26a and MEG3 in tongue squamous cell carcinoma. Int J Cancer. 2014;135(10):2282–93.
Li J, Huang H, Sun L, Yang M, Pan C, Chen W, Wu D, Lin Z, Zeng C, Yao Y, et al. MiR-21 indicates poor prognosis in tongue squamous cell carcinomas as an apoptosis inhibitor. Clin Cancer Res. 2009;15(12):3998–4008.
Maruyama T, Nishihara K, Umikawa M, Arasaki A, Nakasone T, Nimura F, Matayoshi A, Takei K, Nakachi S, Kariya KI, et al. MicroRNA-196a-5p is a potential prognostic marker of delayed lymph node metastasis in early-stage tongue squamous cell carcinoma. Oncol Lett. 2018;15(2):2349–63.
PubMed Google Scholar
Xie N, Wang C, Zhuang Z, Hou J, Liu X, Wu Y, Liu H, Huang H. Decreased miR-320a promotes invasion and metastasis of tumor budding cells in tongue squamous cell carcinoma. Oncotarget. 2016;7(40):65744–57.
Berania I, Cardin GB, Clément I, Guertin L, Ayad T, Bissada E, Nguyen-Tan PF, Filion E, Guilmette J, Gologan O, et al. Four PTEN-targeting co-expressed miRNAs and ACTN4- targeting miR-548b are independent prognostic biomarkers in human squamous cell carcinoma of the oral tongue. Int J Cancer. 2017;141(11):2318–28.
Jia L, Huang Y, Zheng Y, Lyu M, Zhang C, Meng Z, Gan Y, Yu G. miR-375 inhibits cell growth and correlates with clinical outcomes in tongue squamous cell carcinoma. Oncol Rep. 2015;33(4):2061–71.
Chen W, Wang P, Lu Y, Jin T, Lei X, Liu M, Zhuang P, Liao J, Lin Z, Li B, et al. Decreased expression of mitochondrial miR-5787 contributes to chemoresistance by reprogramming glucose metabolism and inhibiting MT-CO3 translation. Theranostics. 2019;9(20):5739–54.
Chen S, Zhang J, Sun L, Li X, Bai J, Zhang H, Li T. miR-611 promotes the proliferation, migration and invasion of tongue squamous cell carcinoma cells by targeting FOXN3. Oral Dis. 2019;25(8):1906–18.
Kawakita A, Yanamoto S, Yamada S, Naruse T, Takahashi H, Kawasaki G, Umeda M. MicroRNA-21 promotes oral cancer invasion via the Wnt/β-catenin pathway by targeting DKK2. Pathol Oncol Res. 2014;20(2):253–61.
Al Rawi N, Elmabrouk N, Abu Kou R, Mkadmi S, Rizvi Z, Hamdoon Z. The role of differentially expressed salivary microRNA in oral squamous cell carcinoma. A systematic review. Arch Oral Biol. 2021;125:105108.
Dioguardi M, Caloro GA, Laino L, Alovisi M, Sovereto D, Crincoli V, Aiuto R, Coccia E, Troiano G, Lo Muzio L. Circulating miR-21 as a potential biomarker for the diagnosis of oral Cancer: a systematic review with Meta-analysis. Cancers (Basel) 2020, 12(4).
Jamali Z, Asl Aminabadi N, Attaran R, Pournagiazar F, Ghertasi Oskouei S, Ahmadpour F. MicroRNAs as prognostic molecular signatures in human head and neck squamous cell carcinoma: a systematic review and meta-analysis. Oral Oncol. 2015;51(4):321–31.
Troiano G, Mastrangelo F, Caponio VCA, Laino L, Cirillo N, Lo Muzio L. Predictive prognostic value of tissue-based MicroRNA expression in oral squamous cell carcinoma: a systematic review and Meta-analysis. J Dent Res. 2018;97(7):759–66.
He Q, Chen Z, Cabay RJ, Zhang L, Luan X, Chen D, Yu T, Wang A, Zhou X. microRNA-21 and microRNA-375 from oral cytology as biomarkers for oral tongue cancer detection. Oral Oncol. 2016;57:15–20.
Moratin J, Hartmann S, Brands R, Brisam M, Mutzbauer G, Scholz C, Seher A, Müller-Richter U, Kübler AC, Linz C. Evaluation of miRNA-expression and clinical tumour parameters in oral squamous cell carcinoma (OSCC). J Craniomaxillofac Surg. 2016;44(7):876–81.
Yu X, Li Z. MicroRNA expression and its implications for diagnosis and therapy of tongue squamous cell carcinoma. J Cell Mol Med. 2016;20(1):10–6.
Sano D, Myers JN. Metastasis of squamous cell carcinoma of the oral tongue. Cancer Metastasis Rev. 2007;26(3–4):645–62.
Silverman S Jr. Demographics and occurrence of oral and pharyngeal cancers. The outcomes, the trends, the challenge. J Am Dent Assoc. 2001;132(Suppl):s7–11.
Article Google Scholar
Download references
Acknowledgements
This work is funded by the National Natural Science Foundation of China (Grant No. 82201110) and Yantai School-City. Integration Development Project (2023XDRHXMPT11). The authors are grateful to Prof. Chunlei Han for the advice on data analysis, Dr. Zhengrui Li for thesis writing instructions and AJE institution for language editing support.
The National Natural Science Foundation of China (Grant No. 82201110) and Yantai School-City; Integration Development Project (2023XDRHXMPT11).
Author information
Yiwei Sun and Yuxiao Li contributed equally to this work.
Authors and Affiliations
School of Stomatology, Binzhou Medical University, No. 346 The Guanhai Road Yantai, Yantai, Shandong Province, 264003, China
The Second School of Clinical Medicine, Binzhou Medical University, No. 346 The Guanhai Road Yantai, Yantai, Shandong Province, 264003, China
The affiliated Yantai Stomatological Hospital, Binzhou Medical University, Yantai, 264000, China
Wenjuan Zhou & Zhonghao Liu
Yantai Engineering Research Center for Digital Technology of Stomatology, Yantai, 264000, China
Characteristic Laboratories of Colleges and Universities in Shandong Province for Digital Stomatology, Yantai, 264003, China
You can also search for this author in PubMed Google Scholar
Contributions
Yuxiao Li and Yiwei Sun designed the study and completed the registration. Yuxiao Li conducted data extraction and performed the statistical analysis. Yuxiao Li and Yiwei Sun evaluated the risk of bias assessment and validated the result. Yuxiao Li and Yiwei Sun wrote the manuscript. Wenjuan Zhou and Zhonghao Liu supervised and offered support in language editing. All authors read and approved the final manuscript.
Corresponding authors
Correspondence to Wenjuan Zhou or Zhonghao Liu .
Ethics declarations
Ethics approval and consent to participate.
Not applicable.
Consent for publication
Competing interests.
The authors declare no conflict of interests.
Additional information
Publisher’s note.
Springer Nature remains neutral with regard to jurisdictional claims in published maps and institutional affiliations.
Rights and permissions
Open Access This article is licensed under a Creative Commons Attribution 4.0 International License, which permits use, sharing, adaptation, distribution and reproduction in any medium or format, as long as you give appropriate credit to the original author(s) and the source, provide a link to the Creative Commons licence, and indicate if changes were made. The images or other third party material in this article are included in the article’s Creative Commons licence, unless indicated otherwise in a credit line to the material. If material is not included in the article’s Creative Commons licence and your intended use is not permitted by statutory regulation or exceeds the permitted use, you will need to obtain permission directly from the copyright holder. To view a copy of this licence, visit http://creativecommons.org/licenses/by/4.0/ . The Creative Commons Public Domain Dedication waiver ( http://creativecommons.org/publicdomain/zero/1.0/ ) applies to the data made available in this article, unless otherwise stated in a credit line to the data.
Reprints and permissions
About this article
Cite this article.
Sun, Y., Li, Y., Zhou, W. et al. MicroRNA expression as a prognostic biomarker of tongue squamous cell carcinoma (TSCC): a systematic review and meta-analysis. BMC Oral Health 24 , 406 (2024). https://doi.org/10.1186/s12903-024-04182-0
Download citation
Received : 03 November 2023
Accepted : 26 March 2024
Published : 31 March 2024
DOI : https://doi.org/10.1186/s12903-024-04182-0
Share this article
Anyone you share the following link with will be able to read this content:
Sorry, a shareable link is not currently available for this article.
Provided by the Springer Nature SharedIt content-sharing initiative
- Tongue squamous cell carcinoma
BMC Oral Health
ISSN: 1472-6831
- Submission enquiries: [email protected]
- General enquiries: [email protected]
Automated Plot Analysis Intern (Summer 2024) – Illinois Applied Research Institute
- Student Employment & Internships
Illinois Applied Research Institute
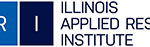
The University of Illinois’ Applied Research Institute is pleased to announce a Summer Internship Opportunity in developing Artificial Intelligence tools to automatically extract information from scientific plots.
This project aims to automate the extraction of information from scientific plots. Much of the information disseminated in scientific papers is embedded in graphs and cannot be readily extracted without manual intervention. We seek to automate this process using AI. The intern will develop code to generate training data and begin to develop the tools and models necessary to extract the relevant information.
Skill Base required:
Graduate, Junior / Senior undergraduate students in Computer Science, Data Science, Physics, or related programs with a strong academic record.
- Excited to learn new skills and capabilities
- Proficient in Python
- Familiar with numpy, matplotlib, scipy
- Should be a self-starter and work effectively in self-directed and proactive manner
- Preferred: background in image analysis, familiar with git
To apply contact: Dr. Heather Filippini, [email protected]
To apply for this job email your details to hrf@illinois.edu

An official website of the United States government
The .gov means it’s official. Federal government websites often end in .gov or .mil. Before sharing sensitive information, make sure you’re on a federal government site.
The site is secure. The https:// ensures that you are connecting to the official website and that any information you provide is encrypted and transmitted securely.
- Publications
- Account settings
Preview improvements coming to the PMC website in October 2024. Learn More or Try it out now .
- Advanced Search
- Journal List
- Hippokratia
- v.14(Suppl 1); 2010 Dec
Meta-analysis in medical research
The objectives of this paper are to provide an introduction to meta-analysis and to discuss the rationale for this type of research and other general considerations. Methods used to produce a rigorous meta-analysis are highlighted and some aspects of presentation and interpretation of meta-analysis are discussed.
Meta-analysis is a quantitative, formal, epidemiological study design used to systematically assess previous research studies to derive conclusions about that body of research. Outcomes from a meta-analysis may include a more precise estimate of the effect of treatment or risk factor for disease, or other outcomes, than any individual study contributing to the pooled analysis. The examination of variability or heterogeneity in study results is also a critical outcome. The benefits of meta-analysis include a consolidated and quantitative review of a large, and often complex, sometimes apparently conflicting, body of literature. The specification of the outcome and hypotheses that are tested is critical to the conduct of meta-analyses, as is a sensitive literature search. A failure to identify the majority of existing studies can lead to erroneous conclusions; however, there are methods of examining data to identify the potential for studies to be missing; for example, by the use of funnel plots. Rigorously conducted meta-analyses are useful tools in evidence-based medicine. The need to integrate findings from many studies ensures that meta-analytic research is desirable and the large body of research now generated makes the conduct of this research feasible.
Important medical questions are typically studied more than once, often by different research teams in different locations. In many instances, the results of these multiple small studies of an issue are diverse and conflicting, which makes the clinical decision-making difficult. The need to arrive at decisions affecting clinical practise fostered the momentum toward "evidence-based medicine" 1 – 2 . Evidence-based medicine may be defined as the systematic, quantitative, preferentially experimental approach to obtaining and using medical information. Therefore, meta-analysis, a statistical procedure that integrates the results of several independent studies, plays a central role in evidence-based medicine. In fact, in the hierarchy of evidence ( Figure 1 ), where clinical evidence is ranked according to the strength of the freedom from various biases that beset medical research, meta-analyses are in the top. In contrast, animal research, laboratory studies, case series and case reports have little clinical value as proof, hence being in the bottom.

Meta-analysis did not begin to appear regularly in the medical literature until the late 1970s but since then a plethora of meta-analyses have emerged and the growth is exponential over time ( Figure 2 ) 3 . Moreover, it has been shown that meta-analyses are the most frequently cited form of clinical research 4 . The merits and perils of the somewhat mysterious procedure of meta-analysis, however, continue to be debated in the medical community 5 – 8 . The objectives of this paper are to introduce meta-analysis and to discuss the rationale for this type of research and other general considerations.

Meta-Analysis and Systematic Review
Glass first defined meta-analysis in the social science literature as "The statistical analysis of a large collection of analysis results from individual studies for the purpose of integrating the findings" 9 . Meta-analysis is a quantitative, formal, epidemiological study design used to systematically assess the results of previous research to derive conclusions about that body of research. Typically, but not necessarily, the study is based on randomized, controlled clinical trials. Outcomes from a meta-analysis may include a more precise estimate of the effect of treatment or risk factor for disease, or other outcomes, than any individual study contributing to the pooled analysis. Identifying sources of variation in responses; that is, examining heterogeneity of a group of studies, and generalizability of responses can lead to more effective treatments or modifications of management. Examination of heterogeneity is perhaps the most important task in meta-analysis. The Cochrane collaboration has been a long-standing, rigorous, and innovative leader in developing methods in the field 10 . Major contributions include the development of protocols that provide structure for literature search methods, and new and extended analytic and diagnostic methods for evaluating the output of meta-analyses. Use of the methods outlined in the handbook should provide a consistent approach to the conduct of meta-analysis. Moreover, a useful guide to improve reporting of systematic reviews and meta-analyses is the PRISMA (Preferred Reporting Items for Systematic reviews and Meta-analyses) statement that replaced the QUOROM (QUality Of Reporting of Meta-analyses) statement 11 – 13 .
Meta-analyses are a subset of systematic review. A systematic review attempts to collate empirical evidence that fits prespecified eligibility criteria to answer a specific research question. The key characteristics of a systematic review are a clearly stated set of objectives with predefined eligibility criteria for studies; an explicit, reproducible methodology; a systematic search that attempts to identify all studies that meet the eligibility criteria; an assessment of the validity of the findings of the included studies (e.g., through the assessment of risk of bias); and a systematic presentation and synthesis of the attributes and findings from the studies used. Systematic methods are used to minimize bias, thus providing more reliable findings from which conclusions can be drawn and decisions made than traditional review methods 14 , 15 . Systematic reviews need not contain a meta-analysisthere are times when it is not appropriate or possible; however, many systematic reviews contain meta-analyses 16 .
The inclusion of observational medical studies in meta-analyses led to considerable debate over the validity of meta-analytical approaches, as there was necessarily a concern that the observational studies were likely to be subject to unidentified sources of confounding and risk modification 17 . Pooling such findings may not lead to more certain outcomes. Moreover, an empirical study showed that in meta-analyses were both randomized and non-randomized was included, nonrandomized studies tended to show larger treatment effects 18 .
Meta-analyses are conducted to assess the strength of evidence present on a disease and treatment. One aim is to determine whether an effect exists; another aim is to determine whether the effect is positive or negative and, ideally, to obtain a single summary estimate of the effect. The results of a meta-analysis can improve precision of estimates of effect, answer questions not posed by the individual studies, settle controversies arising from apparently conflicting studies, and generate new hypotheses. In particular, the examination of heterogeneity is vital to the development of new hypotheses.
Individual or Aggregated Data
The majority of meta-analyses are based on a series of studies to produce a point estimate of an effect and measures of the precision of that estimate. However, methods have been developed for the meta-analyses to be conducted on data obtained from original trials 19 , 20 . This approach may be considered the "gold standard" in metaanalysis because it offers advantages over analyses using aggregated data, including a greater ability to validate the quality of data and to conduct appropriate statistical analysis. Further, it is easier to explore differences in effect across subgroups within the study population than with aggregated data. The use of standardized individual-level information may help to avoid the problems encountered in meta-analyses of prognostic factors 21 , 22 . It is the best way to obtain a more global picture of the natural history and predictors of risk for major outcomes, such as in scleroderma 23 – 26 .This approach relies on cooperation between researchers who conducted the relevant studies. Researchers who are aware of the potential to contribute or conduct these studies will provide and obtain additional benefits by careful maintenance of original databases and making these available for future studies.
Literature Search
A sound meta-analysis is characterized by a thorough and disciplined literature search. A clear definition of hypotheses to be investigated provides the framework for such an investigation. According to the PRISMA statement, an explicit statement of questions being addressed with reference to participants, interventions, comparisons, outcomes and study design (PICOS) should be provided 11 , 12 . It is important to obtain all relevant studies, because loss of studies can lead to bias in the study. Typically, published papers and abstracts are identified by a computerized literature search of electronic databases that can include PubMed ( www.ncbi.nlm.nih.gov./entrez/query.fcgi ), ScienceDirect ( www.sciencedirect.com ), Scirus ( www.scirus.com/srsapp ), ISI Web of Knowledge ( http://www.isiwebofknowledge.com ), Google Scholar ( http://scholar.google.com ) and CENTRAL (Cochrane Central Register of Controlled Trials, http://www.mrw.interscience.wiley.com/cochrane/cochrane_clcentral_articles_fs.htm ). PRISMA statement recommends that a full electronic search strategy for at least one major database to be presented 12 . Database searches should be augmented with hand searches of library resources for relevant papers, books, abstracts, and conference proceedings. Crosschecking of references, citations in review papers, and communication with scientists who have been working in the relevant field are important methods used to provide a comprehensive search. Communication with pharmaceutical companies manufacturing and distributing test products can be appropriate for studies examining the use of pharmaceutical interventions.
It is not feasible to find absolutely every relevant study on a subject. Some or even many studies may not be published, and those that are might not be indexed in computer-searchable databases. Useful sources for unpublished trials are the clinical trials registers, such as the National Library of Medicine's ClinicalTrials.gov Website. The reviews should attempt to be sensitive; that is, find as many studies as possible, to minimize bias and be efficient. It may be appropriate to frame a hypothesis that considers the time over which a study is conducted or to target a particular subpopulation. The decision whether to include unpublished studies is difficult. Although language of publication can provide a difficulty, it is important to overcome this difficulty, provided that the populations studied are relevant to the hypothesis being tested.
Inclusion or Exclusion Criteria and Potential for Bias
Studies are chosen for meta-analysis based on inclusion criteria. If there is more than one hypothesis to be tested, separate selection criteria should be defined for each hypothesis. Inclusion criteria are ideally defined at the stage of initial development of the study protocol. The rationale for the criteria for study selection used should be clearly stated.
One important potential source of bias in meta-analysis is the loss of trials and subjects. Ideally, all randomized subjects in all studies satisfy all of the trial selection criteria, comply with all the trial procedures, and provide complete data. Under these conditions, an "intention-totreat" analysis is straightforward to implement; that is, statistical analysis is conducted on all subjects that are enrolled in a study rather than those that complete all stages of study considered desirable. Some empirical studies had shown that certain methodological characteristics, such as poor concealment of treatment allocation or no blinding in studies exaggerate treatment effects 27 . Therefore, it is important to critically appraise the quality of studies in order to assess the risk of bias.
The study design, including details of the method of randomization of subjects to treatment groups, criteria for eligibility in the study, blinding, method of assessing the outcome, and handling of protocol deviations are important features defining study quality. When studies are excluded from a meta-analysis, reasons for exclusion should be provided for each excluded study. Usually, more than one assessor decides independently which studies to include or exclude, together with a well-defined checklist and a procedure that is followed when the assessors disagree. Two people familiar with the study topic perform the quality assessment for each study, independently. This is followed by a consensus meeting to discuss the studies excluded or included. Practically, the blinding of reviewers from details of a study such as authorship and journal source is difficult.
Before assessing study quality, a quality assessment protocol and data forms should be developed. The goal of this process is to reduce the risk of bias in the estimate of effect. Quality scores that summarize multiple components into a single number exist but are misleading and unhelpful 28 . Rather, investigators should use individual components of quality assessment and describe trials that do not meet the specified quality standards and probably assess the effect on the overall results by excluding them, as part of the sensitivity analyses.
Further, not all studies are completed, because of protocol failure, treatment failure, or other factors. Nonetheless, missing subjects and studies can provide important evidence. It is desirable to obtain data from all relevant randomized trials, so that the most appropriate analysis can be undertaken. Previous studies have discussed the significance of missing trials to the interpretation of intervention studies in medicine 29 , 30 . Journal editors and reviewers need to be aware of the existing bias toward publishing positive findings and ensure that papers that publish negative or even failed trials be published, as long as these meet the quality guidelines for publication.
There are occasions when authors of the selected papers have chosen different outcome criteria for their main analysis. In practice, it may be necessary to revise the inclusion criteria for a meta-analysis after reviewing all of the studies found through the search strategy. Variation in studies reflects the type of study design used, type and application of experimental and control therapies, whether or not the study was published, and, if published, subjected to peer review, and the definition used for the outcome of interest. There are no standardized criteria for inclusion of studies in meta-analysis. Universal criteria are not appropriate, however, because meta-analysis can be applied to a broad spectrum of topics. Published data in journal papers should also be cross-checked with conference papers to avoid repetition in presented data.
Clearly, unpublished studies are not found by searching the literature. It is possible that published studies are systemically different from unpublished studies; for example, positive trial findings may be more likely to be published. Therefore, a meta-analysis based on literature search results alone may lead to publication bias.
Efforts to minimize this potential bias include working from the references in published studies, searching computerized databases of unpublished material, and investigating other sources of information including conference proceedings, graduate dissertations and clinical trial registers.
Statistical analysis
The most common measures of effect used for dichotomous data are the risk ratio (also called relative risk) and the odds ratio. The dominant method used for continuous data are standardized mean difference (SMD) estimation. Methods used in meta-analysis for post hoc analysis of findings are relatively specific to meta-analysis and include heterogeneity analysis, sensitivity analysis, and evaluation of publication bias.
All methods used should allow for the weighting of studies. The concept of weighting reflects the value of the evidence of any particular study. Usually, studies are weighted according to the inverse of their variance 31 . It is important to recognize that smaller studies, therefore, usually contribute less to the estimates of overall effect. However, well-conducted studies with tight control of measurement variation and sources of confounding contribute more to estimates of overall effect than a study of identical size less well conducted.
One of the foremost decisions to be made when conducting a meta-analysis is whether to use a fixed-effects or a random-effects model. A fixed-effects model is based on the assumption that the sole source of variation in observed outcomes is that occurring within the study; that is, the effect expected from each study is the same. Consequently, it is assumed that the models are homogeneous; there are no differences in the underlying study population, no differences in subject selection criteria, and treatments are applied the same way 32 . Fixed-effect methods used for dichotomous data include most often the Mantel-Haenzel method 33 and the Peto method 34 (only for odds ratios).
Random-effects models have an underlying assumption that a distribution of effects exists, resulting in heterogeneity among study results, known as τ2. Consequently, as software has improved, random-effects models that require greater computing power have become more frequently conducted. This is desirable because the strong assumption that the effect of interest is the same in all studies is frequently untenable. Moreover, the fixed effects model is not appropriate when statistical heterogeneity (τ2) is present in the results of studies in the meta-analysis. In the random-effects model, studies are weighted with the inverse of their variance and the heterogeneity parameter. Therefore, it is usually a more conservative approach with wider confidence intervals than the fixed-effects model where the studies are weighted only with the inverse of their variance. The most commonly used random-effects method is the DerSimonian and Laird method 35 . Furthermore, it is suggested that comparing the fixed-effects and random-effect models developed as this process can yield insights to the data 36 .
Heterogeneity
Arguably, the greatest benefit of conducting metaanalysis is to examine sources of heterogeneity, if present, among studies. If heterogeneity is present, the summary measure must be interpreted with caution 37 . When heterogeneity is present, one should question whether and how to generalize the results. Understanding sources of heterogeneity will lead to more effective targeting of prevention and treatment strategies and will result in new research topics being identified. Part of the strategy in conducting a meta-analysis is to identify factors that may be significant determinants of subpopulation analysis or covariates that may be appropriate to explore in all studies.
To understand the nature of variability in studies, it is important to distinguish between different sources of heterogeneity. Variability in the participants, interventions, and outcomes studied has been described as clinical diversity, and variability in study design and risk of bias has been described as methodological diversity 10 . Variability in the intervention effects being evaluated among the different studies is known as statistical heterogeneity and is a consequence of clinical or methodological diversity, or both, among the studies. Statistical heterogeneity manifests itself in the observed intervention effects varying by more than the differences expected among studies that would be attributable to random error alone. Usually, in the literature, statistical heterogeneity is simply referred to as heterogeneity.
Clinical variation will cause heterogeneity if the intervention effect is modified by the factors that vary across studies; most obviously, the specific interventions or participant characteristics that are often reflected in different levels of risk in the control group when the outcome is dichotomous. In other words, the true intervention effect will differ for different studies. Differences between studies in terms of methods used, such as use of blinding or differences between studies in the definition or measurement of outcomes, may lead to differences in observed effects. Significant statistical heterogeneity arising from differences in methods used or differences in outcome assessments suggests that the studies are not all estimating the same effect, but does not necessarily suggest that the true intervention effect varies. In particular, heterogeneity associated solely with methodological diversity indicates that studies suffer from different degrees of bias. Empirical evidence suggests that some aspects of design can affect the result of clinical trials, although this may not always be the case.
The scope of a meta-analysis will largely determine the extent to which studies included in a review are diverse. Meta-analysis should be conducted when a group of studies is sufficiently homogeneous in terms of subjects involved, interventions, and outcomes to provide a meaningful summary. However, it is often appropriate to take a broader perspective in a meta-analysis than in a single clinical trial. Combining studies that differ substantially in design and other factors can yield a meaningless summary result, but the evaluation of reasons for the heterogeneity among studies can be very insightful. It may be argued that these studies are of intrinsic interest on their own, even though it is not appropriate to produce a single summary estimate of effect.
Variation among k trials is usually assessed using Cochran's Q statistic, a chi-squared (χ 2 ) test of heterogeneity with k-1 degrees of freedom. This test has relatively poor power to detect heterogeneity among small numbers of trials; consequently, an α-level of 0.10 is used to test hypotheses 38 , 39 .
Heterogeneity of results among trials is better quantified using the inconsistency index I 2 , which describes the percentage of total variation across studies 40 . Uncertainty intervals for I 2 (dependent on Q and k) are calculated using the method described by Higgins and Thompson 41 . Negative values of I 2 are put equal to zero, consequently I 2 lies between 0 and 100%. A value >75% may be considered substantial heterogeneity 41 . This statistic is less influenced by the number of trials compared with other methods used to estimate the heterogeneity and provides a logical and readily interpretable metric but it still can be unstable when only a few studies are combined 42 .
Given that there are several potential sources of heterogeneity in the data, several steps should be considered in the investigation of the causes. Although random-effects models are appropriate, it may be still very desirable to examine the data to identify sources of heterogeneity and to take steps to produce models that have a lower level of heterogeneity, if appropriate. Further, if the studies examined are highly heterogeneous, it may be not appropriate to present an overall summary estimate, even when random effects models are used. As Petiti notes 43 , statistical analysis alone will not make contradictory studies agree; critically, however, one should use common sense in decision-making. Despite heterogeneity in responses, if all studies had a positive point direction and the pooled confidence interval did not include zero, it would not be logical to conclude that there was not a positive effect, provided that sufficient studies and subject numbers were present. The appropriateness of the point estimate of the effect is much more in question.
Some of the ways to investigate the reasons for heterogeneity; are subgroup analysis and meta-regression. The subgroup analysis approach, a variation on those described above, groups categories of subjects (e.g., by age, sex) to compare effect sizes. The meta-regression approach uses regression analysis to determine the influence of selected variables (the independent variables) on the effect size (the dependent variable). In a meta-regresregression, studies are regarded as if they were individual patients, but their effects are properly weighted to account for their different variances 44 .
Sensitivity analyses have also been used to examine the effects of studies identified as being aberrant concerning conduct or result, or being highly influential in the analysis. Recently, another method has been proposed that reduces the weight of studies that are outliers in meta-analyses 45 . All of these methods for examining heterogeneity have merit, and the variety of methods available reflects the importance of this activity.
Presentation of results
A useful graph, presented in the PRISMA statement 11 , is the four-phase flow diagram ( Figure 3 ).

This flow-diagram depicts the flow of information through the different phases of a systematic review or meta-analysis. It maps out the number of records identified, included and excluded, and the reasons for exclusions. The results of meta-analyses are often presented in a forest plot, where each study is shown with its effect size and the corresponding 95% confidence interval ( Figure 4 ).

The pooled effect and 95% confidence interval is shown in the bottom in the same line with "Overall". In the right panel of Figure 4 , the cumulative meta-analysis is graphically displayed, where data are entered successively, typically in the order of their chronological appearance 46 , 47 . Such cumulative meta-analysis can retrospectively identify the point in time when a treatment effect first reached conventional levels of significance. Cumulative meta-analysis is a compelling way to examine trends in the evolution of the summary-effect size, and to assess the impact of a specific study on the overall conclusions 46 . The figure shows that many studies were performed long after cumulative meta-analysis would have shown a significant beneficial effect of antibiotic prophylaxis in colon surgery.
Biases in meta-analysis
Although the intent of a meta-analysis is to find and assess all studies meeting the inclusion criteria, it is not always possible to obtain these. A critical concern is the papers that may have been missed. There is good reason to be concerned about this potential loss because studies with significant, positive results (positive studies) are more likely to be published and, in the case of interventions with a commercial value, to be promoted, than studies with non-significant or "negative" results (negative studies). Studies that produce a positive result, especially large studies, are more likely to have been published and, conversely, there has been a reluctance to publish small studies that have non-significant results. Further, publication bias is not solely the responsibility of editorial policy as there is reluctance among researchers to publish results that were either uninteresting or are not randomized 48 . There are, however, problems with simply including all studies that have failed to meet peer-review standards. All methods of retrospectively dealing with bias in studies are imperfect.
It is important to examine the results of each meta-analysis for evidence of publication bias. An estimation of likely size of the publication bias in the review and an approach to dealing with the bias is inherent to the conduct of many meta-analyses. Several methods have been developed to provide an assessment of publication bias; the most commonly used is the funnel plot. The funnel plot provides a graphical evaluation of the potential for bias and was developed by Light and Pillemer 49 and discussed in detail by Egger and colleagues 50 , 51 . A funnel plot is a scatterplot of treatment effect against a measure of study size. If publication bias is not present, the plot is expected to have a symmetric inverted funnel shape, as shown in Figure 5A .

In a study in which there is no publication bias, larger studies (i.e., have lower standard error) tend to cluster closely to the point estimate. As studies become less precise, such as in smaller trials (i.e., have a higher standard error), the results of the studies can be expected to be more variable and are scattered to both sides of the more precise larger studies. Figure 5A shows that the smaller, less precise studies are, indeed, scattered to both sides of the point estimate of effect and that these seem to be symmetrical, as an inverted funnel-plot, showing no evidence of publication bias. In contrast to Figure 5A , Figure 5B shows evidence of publication bias. There is evidence of the possibility that studies using smaller numbers of subjects and showing an decrease in effect size (lower odds ratio) were not published.
Asymmetry of funnel plots is not solely attributable to publication bias, but may also result from clinical heterogeneity among studies. Sources of clinical heterogeneity include differences in control or exposure of subjects to confounders or effect modifiers, or methodological heterogeneity between studies; for example, a failure to conceal treatment allocation. There are several statistical tests for detecting funnel plot asymmetry; for example, Eggers linear regression test 50 , and Begg's rank correlation test 52 but these do not have considerable power and are rarely used. However, the funnel plot is not without problems. If high precision studies really are different than low precision studies with respect to effect size (e.g., due different populations examined) a funnel plot may give a wrong impression of publication bias 53 . The appearance of the funnel plot plot can change quite dramatically depending on the scale on the y-axis - whether it is the inverse square error or the trial size 54 .
Other types of biases in meta-analysis include the time lag bias, selective reporting bias and the language bias. The time lag bias arises from the published studies, when those with striking results are published earlier than those with non-significant findings 55 . Moreover, it has been shown that positive studies with high early accrual of patients are published sooner than negative trials with low early accrual 56 . However, missing studies, either due to publication bias or time-lag bias may increasingly be identified from trials registries.
The selective reporting bias exists when published articles have incomplete or inadequate reporting. Empirical studies have shown that this bias is widespread and of considerable importance when published studies were compared with their study protocols 29 , 30 . Furthermore, recent evidence suggests that selective reporting might be an issue in safety outcomes and the reporting of harms in clinical trials is still suboptimal 57 . Therefore, it might not be possible to use quantitative objective evidence for harms in performing meta-analyses and making therapeutic decisions.
Excluding clinical trials reported in languages other than English from meta-analyses may introduce the language bias and reduce the precision of combined estimates of treatment effects. Trials with statistically significant results have been shown to be published in English 58 . In contrast, a later more extensive investigation showed that trials published in languages other than English tend to be of lower quality and produce more favourable treatment effects than trials published in English and concluded that excluding non-English language trials has generally only modest effects on summary treatment effect estimates but the effect is difficult to predict for individual meta-analyses 59 .
Evolution of meta-analyses
The classical meta-analysis compares two treatments while network meta-analysis (or multiple treatment metaanalysis) can provide estimates of treatment efficacy of multiple treatment regimens, even when direct comparisons are unavailable by indirect comparisons 60 . An example of a network analysis would be the following. An initial trial compares drug A to drug B. A different trial studying the same patient population compares drug B to drug C. Assume that drug A is found to be superior to drug B in the first trial. Assume drug B is found to be equivalent to drug C in a second trial. Network analysis then, allows one to potentially say statistically that drug A is also superior to drug C for this particular patient population. (Since drug A is better than drug B, and drug B is equivalent to drug C, then drug A is also better to drug C even though it was not directly tested against drug C.)
Meta-analysis can also be used to summarize the performance of diagnostic and prognostic tests. However, studies that evaluate the accuracy of tests have a unique design requiring different criteria to appropriately assess the quality of studies and the potential for bias. Additionally, each study reports a pair of related summary statistics (for example, sensitivity and specificity) rather than a single statistic (such as a risk ratio) and hence requires different statistical methods to pool the results of the studies 61 . Various techniques to summarize results from diagnostic and prognostic test results have been proposed 62 – 64 . Furthermore, there are many methodologies for advanced meta-analysis that have been developed to address specific concerns, such as multivariate meta-analysis 65 – 67 , and special types of meta-analysis in genetics 68 but will not be discussed here.
Meta-analysis is no longer a novelty in medicine. Numerous meta-analyses have been conducted for the same medical topic by different researchers. Recently, there is a trend to combine the results of different meta-analyses, known as a meta-epidemiological study, to assess the risk of bias 79 , 70 .
Conclusions
The traditional basis of medical practice has been changed by the use of randomized, blinded, multicenter clinical trials and meta-analysis, leading to the widely used term "evidence-based medicine". Leaders in initiating this change have been the Cochrane Collaboration who have produced guidelines for conducting systematic reviews and meta-analyses 10 and recently the PRISMA statement, a helpful resource to improve reporting of systematic reviews and meta-analyses has been released 11 . Moreover, standards by which to conduct and report meta-analyses of observational studies have been published to improve the quality of reporting 71 .
Meta-analysis of randomized clinical trials is not an infallible tool, however, and several examples exist of meta-analyses which were later contradicted by single large randomized controlled trials, and of meta-analyses addressing the same issue which have reached opposite conclusions 72 . A recent example, was the controversy between a meta-analysis of 42 studies 73 and the subsequent publication of the large-scale trial (RECORD trial) that did not support the cardiovascular risk of rosiglitazone 74 . However, the reason for this controversy was explained by the numerous methodological flaws found both in the meta-analysis and the large clinical trial 75 .
No single study, whether meta-analytic or not, will provide the definitive understanding of responses to treatment, diagnostic tests, or risk factors influencing disease. Despite this limitation, meta-analytic approaches have demonstrable benefits in addressing the limitations of study size, can include diverse populations, provide the opportunity to evaluate new hypotheses, and are more valuable than any single study contributing to the analysis. The conduct of the studies is critical to the value of a meta-analysis and the methods used need to be as rigorous as any other study conducted.
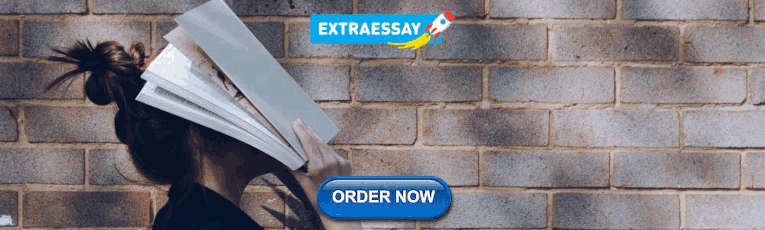
IMAGES
VIDEO
COMMENTS
2.1 Step 1: defining the research question. The first step in conducting a meta-analysis, as with any other empirical study, is the definition of the research question. Most importantly, the research question determines the realm of constructs to be considered or the type of interventions whose effects shall be analyzed.
It is easy to confuse systematic reviews and meta-analyses. A systematic review is an objective, reproducible method to find answers to a certain research question, by collecting all available studies related to that question and reviewing and analyzing their results. A meta-analysis differs from a systematic review in that it uses statistical ...
Meta-analysis is the statistical combination of the results of multiple studies addressing a similar research question. An important part of this method involves computing an effect size across all of the studies; this involves extracting effect sizes and variance measures from various studies.
Definition. "A meta-analysis is a formal, epidemiological, quantitative study design that uses statistical methods to generalise the findings of the selected independent studies. Meta-analysis and systematic review are the two most authentic strategies in research. When researchers start looking for the best available evidence concerning ...
Meta-analysis is an objective examination of published data from many studies of the same research topic identified through a literature search. Through the use of rigorous statistical methods, it ...
Basic research meta-analytic datasets are expected to be heterogeneous because (i) basic research literature searches tend to retrieve more studies than clinical literature searches and (ii) experimental methodologies used in basic research are more diverse and less standardized compared to clinical research. The presence of heterogeneity may ...
Meta-analysis is a quantitative research method that involves the systematic synthesis and statistical analysis of data from multiple individual studies on a particular topic or research question. It aims to provide a comprehensive and robust summary of existing evidence by pooling the results of these studies, often leading to more precise and ...
Meta-analysis is the quantitative, scientific synthesis of research results. Since the term and modern approaches to research synthesis were first introduced in the 1970s, meta-analysis has had a ...
First, the search scope of meta‐analysis should be expanded as much as possible to contain all relevant research, and it is important to remove language restrictions and actively search for non‐English bibliographic databases. Second, any meta‐analysis should include studies selected based on strict criteria established in advance.
A large part of conducting a meta-analysis involves quantifying and accounting for sources of heterogeneity that may compromise the validity of meta-analysis. Basic research meta-analytic datasets are expected to be heterogeneous because (i) basic research literature searches tend to retrieve more studies than clinical literature searches and ...
Meta-analysis is defined (p. vii) as, " … the statistical combination of results from multiple studies in order to yield results which make the best use of all available evidence." ... and frequency of meta-analytic research and systematic reviews provided by statisticians, practitioners, educators, and students. The Handbook of Meta ...
Tip 1: Know the type of outcome than. There are differences in a forest plot depending on the type of outcomes. For a continuous outcome, the mean, standard deviation and number of patients are ...
What is meta-analysis? Meta-analysis is a research process used to systematically synthesise or merge the findings of single, independent studies, using statistical methods to calculate an overall or 'absolute' effect.2 Meta-analysis does not simply pool data from smaller studies to achieve a larger sample size. Analysts use well recognised, systematic methods to account for differences in ...
Meta-analysis is a well-established approach to integrating research findings, with a long history in the sciences and in psychology in particular. Its use in summarizing research findings has special significance given increasing concerns about scientific replicability, but it has other important uses as well, such as integrating information ...
Meta-analysis would be used for the following purposes: To establish statistical significance with studies that have conflicting results. To develop a more correct estimate of effect magnitude. To provide a more complex analysis of harms, safety data, and benefits. To examine subgroups with individual numbers that are not statistically significant.
Meta-analysis is a statistical procedure that integrates the results of several independent studies considered to be "combinable."1 Well conducted meta-analyses allow a more objective appraisal of the evidence than traditional narrative reviews, provide a more precise estimate of a treatment effect, and may explain heterogeneity between the results of individual studies.2 Ill conducted ...
"Meta" comes from the Greek for "after" or "beyond"; a meta-analysis is an "analysis of analyses" . In other words, it is a statistical technique for combining the results from different studies on the same topic [ 7 ], and is becoming popular for resolving discrepancies in clinical research.
Meta-analyses summarize a field's research base and are therefore highly influential. Despite their value, the standards for an excellent meta-analysis, one that is potentially award-winning, have changed in the last decade. Each step of a meta-analysis is now more formalized, from the identification of relevant articles to coding, moderator analysis, and reporting of results. What was ...
Meta-analyses play a fundamental role in evidence-based healthcare. Compared to other study designs (such as randomized controlled trials or cohort studies), the meta-analysis comes in at the top of the evidence-based medicine pyramid. This is a pyramid which enables us to weigh up the different levels of evidence available to us.
Meta-analytic procedures. The Comprehensive Meta-Analysis 3.0 software was applied to Fisher's z-effect sizes calculation.PRISMA was applied to the current method, which provided the detailed information for a meta-analysis (Moher et al., 2010).All correlations between the social identity and any outcome variable were inserted into the computer program Comprehensive Meta-Analysis and ...
Therapist-guided remote versus in-person cognitive behavioural therapy: a systematic review and meta-analysis of randomized controlled trials [Research] Background: Cognitive behavioural therapy (CBT) has been shown to be effective for several psychiatric and somatic conditions; however, most randomized controlled trials (RCTs) have ...
Background The optimum systolic blood pressure after endovascular thrombectomy for acute ischemic stroke is uncertain. We aimed to perform an updated meta-analysis of randomized controlled trials to evaluate the safety and efficacy of more intensive blood pressure management as compared to less intensive blood pressure management. Methods We searched various electronic databases including ...
Sometimes the results of all of the studies found and included in a systematic review can be summarized and expressed as an overall result. This is known as a meta-analysis. The overall outcome of the studies is often more conclusive than the results of individual studies. But it only makes sense to do a meta-analysis if the results of the ...
Data sources and searches. This systematic review and meta-analysis is performed according to the guideline of the Preferred Reporting Items for Systematic Review and Meta-analyses statement (PRISMA) [].A systematic search of the electronic databases including PubMed, Scopus, and Web of Science was undertaken to identify relevant papers published before January 2024.
Meta-analysis would be used for the following purposes: To establish statistical significance with studies that have conflicting results. To develop a more correct estimate of effect magnitude. To provide a more complex analysis of harms, safety data, and benefits. To examine subgroups with individual numbers that are not statistically significant.
Background Recent studies have indicated that microRNA (miRNA) expression in tumour tissues has prognostic significance in Tongue squamous cell carcinoma (TSCC) patients. This study explored the possible prognostic value of miRNAs for TSCC based on published research. Methods A comprehensive literature search of multiple databases was conducted according to predefined eligibility criteria ...
Additional statistical research should include study of the impact of outliers on the meta-analysis and the potential insight that they could provide into a research question . Statistically valid methods to combine data across studies of varying quality and design, including data from case-control studies, will enable metaanalysts to maximize ...
Familiar with numpy, matplotlib, scipy. Should be a self-starter and work effectively in self-directed and proactive. manner. Preferred: background in image analysis, familiar with git. To apply contact: Dr. Heather Filippini, [email protected]. Previous Communications and Social Media Intern Summer 2024 - Research Park.
Meta-analysis is a quantitative, formal, epidemiological study design used to systematically assess previous research studies to derive conclusions about that body of research. Outcomes from a meta-analysis may include a more precise estimate of the effect of treatment or risk factor for disease, or other outcomes, than any individual study ...