Click through the PLOS taxonomy to find articles in your field.
For more information about PLOS Subject Areas, click here .
Loading metrics
Open Access
Peer-reviewed
Research Article
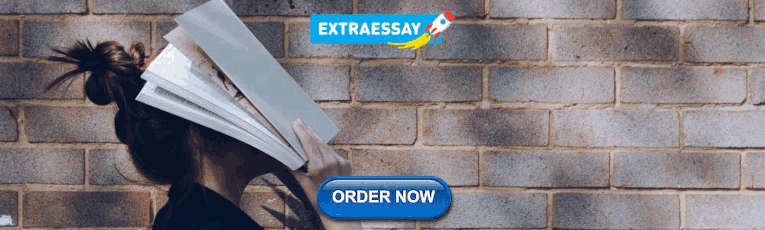
Perceptions of health risks of cigarette smoking: A new measure reveals widespread misunderstanding
Affiliation Department of Communication, Stanford University, Stanford, California, United States of America
Affiliation Graduate School of Business, Stanford University, Stanford, California, United States of America
* E-mail: [email protected]
Affiliations Department of Political Science, Vanderbilt University, Nashville, Tennessee, United States of America, Hoover Institution, Stanford University, Stanford, California, United States of America

Affiliation U.S. Department of Treasury, Washington, D.C., United States of America
Affiliation LinChiat Chang Consulting, LLC, San Francisco, California, United States of America
Affiliation Department of Communication Studies, University of Michigan, Ann Arbor, Michigan, United States of America
Affiliation GfK Custom Research North America, New York City, New York, United States of America
- Jon A. Krosnick,
- Neil Malhotra,
- Cecilia Hyunjung Mo,
- Eduardo F. Bruera,
- LinChiat Chang,
- Josh Pasek,
- Randall K. Thomas
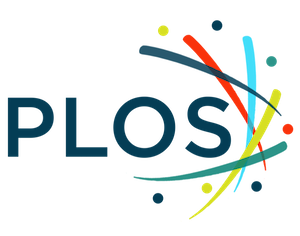
- Published: August 14, 2017
- https://doi.org/10.1371/journal.pone.0182063
- Reader Comments
15 Feb 2019: Krosnick JA, Malhotra N, Mo CH, Bruera EF, Chang L, et al. (2019) Correction: Perceptions of health risks of cigarette smoking: A new measure reveals widespread misunderstanding. PLOS ONE 14(2): e0212705. https://doi.org/10.1371/journal.pone.0212705 View correction
Most Americans recognize that smoking causes serious diseases, yet many Americans continue to smoke. One possible explanation for this paradox is that perhaps Americans do not accurately perceive the extent to which smoking increases the probability of adverse health outcomes. This paper examines the accuracy of Americans’ perceptions of the absolute risk, attributable risk, and relative risk of lung cancer, and assesses which of these beliefs drive Americans’ smoking behavior. Using data from three national surveys, statistical analyses were performed by comparing means, medians, and distributions, and by employing Generalized Additive Models. Perceptions of relative risk were associated as expected with smoking onset and smoking cessation, whereas perceptions of absolute risk and attributable risk were not. Additionally, the relation of relative risk with smoking status was stronger among people who held their risk perceptions with more certainty. Most current smokers, former smokers, and never-smokers considerably underestimated the relative risk of smoking. If, as this paper suggests, people naturally think about the health consequences of smoking in terms of relative risk, smoking rates might be reduced if public understanding of the relative risks of smoking were more accurate and people held those beliefs with more confidence.
Citation: Krosnick JA, Malhotra N, Mo CH, Bruera EF, Chang L, Pasek J, et al. (2017) Perceptions of health risks of cigarette smoking: A new measure reveals widespread misunderstanding. PLoS ONE 12(8): e0182063. https://doi.org/10.1371/journal.pone.0182063
Editor: Raymond Niaura, Legacy, Schroeder Institute for Tobacco Research and Policy Studies, UNITED STATES
Received: May 7, 2016; Accepted: June 20, 2017; Published: August 14, 2017
This is an open access article, free of all copyright, and may be freely reproduced, distributed, transmitted, modified, built upon, or otherwise used by anyone for any lawful purpose. The work is made available under the Creative Commons CC0 public domain dedication.
Data Availability: Data are available at: https://dataverse.harvard.edu/dataset.xhtml?persistentId=doi:10.7910/DVN/JP2JHH , doi: 10.7910/DVN/JP2JHH .
Funding: LC and RKT have commercial affiliations with LinChiat Chang Consulting and GfK Custom Research North America, respectively. These companies provided support in the form of salaries for authors LC and RKT, but did not have any additional role in the study design, data collection and analysis, decision to publish, or preparation of the manuscript. The specific roles of these authors are articulated in the ‘author contributions’ section.
Competing interests: LC and RKT have commercial affiliations with LinChiat Chang Consulting and GfK Custom Research North America, respectively. This does not alter our adherence to PLOS ONE policies on sharing data and materials.
Introduction
Despite a constant flow of messages reminding Americans of the health risks of cigarette smoking, and despite a steady decline in the proportion of Americans who smoke during the last 50 years, more than 20% of Americans continue to smoke regularly today [ 1 ]. This paper explores whether the continued prevalence of smoking may, in part, stem from a failure to acknowledge these risks. At first blush, this assertion may seem patently implausible; much research indicates that increasingly large proportions of Americans recognize the various dangers of smoking, and some studies even suggest that most Americans overestimate the proportion of smokers who suffer from certain smoking-related ailments [ 2 ]. Nonetheless, it is possible that people underestimate the magnitude of some of the health risks caused by smoking. Because individuals seem to base their decisions about whether to smoke on how they believe the act of smoking changes the risk of contracting specific diseases, correcting any underestimation of risk may yield future reductions in smoking onset and increases in cessation [ 3 ]. To explore these possibilities, we conducted three studies of national samples of American adults documenting risk perceptions and their relations to smoking behavior.
Challenges in the study of risk perception
One way to gauge the accuracy of people’s perceptions of the health dangers of smoking is to focus simply on the list of maladies that become more likely as a result of smoking. This list includes various cancers, heart diseases, respiratory diseases, premature death, and more [ 4 , 5 ]. By asking representative national samples of American adults to identify which diseases and medical conditions on a provided list are linked with smoking, researchers have illuminated three interesting patterns. First, since the 1950s, the proportion of Americans who failed to identify any health risks of smoking dropped consistently [ 6 ]. Second, according to Gallup [ 7 ], a sizable proportion of Americans still fails to recognize a link between smoking and some related ailments (see S1 Fig ). Other contemporary surveys support these same conclusions [ 8 – 10 ]. The proportion of American adults who associate smoking with a particular ailment varies considerably across ailments, from a high of 81% who report a link between smoking and cancer to single-digit proportions who identify links with asthma, hypertension, bronchitis, and stroke [ 11 ]. Thus, even today, Americans apparently underestimate the breadth of the danger.
A more refined way to gauge the accuracy of perceptions is to focus on the amount of increased risk of each malady that results from smoking. According to epidemiological studies, each of these increases is a function of many attributes, including age of smoking onset, number of years of regular smoking, number of cigarettes consumed per day, and more [ 4 , 5 ]. Therefore, actual risks must be expressed as variables that are functions of such factors, and perceptions of these risks must be ascertained specifying such factors.
Furthermore, even holding constant age of onset, length of smoking, and dosage, a smoking-related risk can be perceived in three different ways: (1) absolute risk (i.e., “what is the chance that a person will get lung cancer if he/she smokes?”), (2) attributable risk (i.e., “how much does smoking raise the chances that a person will get lung cancer compared to not smoking?”), and (3) relative risk (i.e., “how much more likely is a person to get lung cancer if he/she smokes?”) [ 12 , 13 ]. Mausner and Bahn [ 14 ] provide a thorough review of how epidemiologists calculate and use each of these different measures of risk. Assessing Americans’ perceptions of all three seems most sensible in order to determine whether people tend to perceive all types of risk accurately, overestimate all types of risk, underestimate all types of risk, or overestimate some while underestimating others. Attributable fraction is another measure of risk perceptions, but we do not investigate this measure in this study [ 15 ].
One way to think about the goal of such an investigation is to identify any ways in which people underestimate risk, so that public health education campaigns can correct this misunderstanding. But it could turn out that people underestimate one particular type of risk (e.g., absolute risk) and yet do not use that particular perception of risk in their decision-making about whether to start or stop smoking. Therefore, efforts to correct the public’s misunderstanding would not translate into changes in smoking behavior. So to draw out implications of measurements of perceived risk, we need evidence indicating which perceptions may be behaviorally consequential.
The research described in this paper set out to do so by gauging perceptions of absolute risk, attributable risk, and relative risk with a focus specifically on lung cancer. And we explored which of these risk perceptions might drive smoking onset and cessation. We focus on lung cancer specifically rather than all health risks associated with smoking following Viscusi’s seminal work on smoking-related risks [ 2 ]. While the share of American adults who associate smoking with a particular health malady varies across maladies [ 11 ], an assessment of which type of risk perception—absolute risk, attributable risk, and relative risk—impacts Americans’ smoking behavior the most should not be sensitive to the health malady of interest. In other words, if perceptions of relative risk of lung cancer affects smoking behavior more than perceptions of absolute and attributable risk of lung cancer, then perceptions of relative risk of another disease should similarly be most effective at driving smoking behavior.
Prior studies of perceptions of the magnitude of risk
A number of past studies have attempted to measure perceptions of the magnitude of the risk of smoking in representative samples of American adults, but their methodologies entailed a series of limitations, as we outline next. It is worth noting that this paper focuses on the U.S. and therefore does not discuss the many interesting studies of smoking-related risk perceptions that have been done in countries other than the U.S [ 16 – 18 ].
We also do not discuss studies that examined people’s perceptions of their own personal smoking-related risks (e.g., Boney-McCoy et al. [ 19 ]; Strecher et al. [ 20 ]) because our focus is on Americans’ perceptions of the risk of smoking to people in general. Many studies have produced interesting results involving people’s perceptions of their own personal risks of smoking-related health problems (e.g., [ 19 , 21 – 27 ]). However, according to Gigerenzer [ 28 ], people naturally think about the population rather than personal chance, and perceptions of personal risk likely mediate the relationship between general risk and behavior.
Because this paper is focused on the beliefs of adults, we also do not discuss the findings of many interesting studies of youth. For example, Romer and Jamieson [ 29 ] asked questions similar to Viscusi’s [ 2 ] of a national sample of 14- and 15-year-olds: “Out of every 100 cigarette smokers, how many do you think will: (a) get lung cancer because they smoke? (b) have heart problems, like a heart attack, because they smoke? (c) die from a smoking-related illness?” Their results mirror Viscusi’s [ 2 ]: on average; respondents said 61.4% of smokers would develop lung cancer, much higher than the true rate. Likewise, a representative sample of 20–22 year olds said 52.6% on average. Many other studies have explored the beliefs of children and adolescents as well [ 21 , 30 – 37 ].
Some past studies have asked people to describe their perceptions of the magnitude of a smoking-related risk of some malady by asking people to select a point on a rating scale with a small number of verbally labeled response options. For example, Weinstein et al. [ 27 ] asked “How likely do you think it is that (the average male cigarette smoker/the average female cigarette smoker/you) will develop lung cancer in the future?” and offered a 5-point scale ranging from “very low” to “very high.” Similarly, Romer and Jamieson [ 29 ] asked respondents “In your opinion, is smoking very risky for a person’s health, somewhat risky, only a little risky, or not risky at all?” It is not clear whether “somewhat risky” or “very risky” is an overestimate or underestimate of risk. In other words, measures that assess perceptions of smoking’s dangers on these non-numeric subjective probability scales do not permit assessing the degree to which magnitudes of perceived risk reflect true numeric risk levels.
Other studies have measured perceptions of risks quantitatively but did not specify the population of people being described or the dosage of smoking being addressed. For example, in a survey conducted by Audits & Surveys Worldwide, respondents were asked, “Among 100 cigarette smokers, how many of them do you think will get lung cancer because they smoke?” [ 2 ]. The characteristics of a smoker are important contextual considerations with regards to actual health risks a given smoker faces. The probabilities of various smoking-related ailments differ for occasional and daily smokers and depend on the age of a smoker as well as the duration of smoking. Because this type of question does not specify what population is to be described or how much smoking was done for how long, it is impossible to gauge the accuracy of responses by comparing them with the results of epidemiological studies, which show risk to vary across populations and age, smoking duration, and dosage. Some scholarly work has begun to remedy this issue, specifying the exact quantity of cigarettes smoked per day [ 38 ].
Another potential limitation of the Audits & Surveys question is the phrase “because they smoke.” This phrase was presumably meant to lead respondents to estimate the number of lung cancer cases completely attributable to smoking. As Slovic [ 36 ] observed, this phrase can be interpreted in various different ways. Specifically, people may believe that smoking, along with other factors, enhances the chances of contracting lung cancer, leading them to respond that smoking is partially responsible for some lung cancer cases. This, too, makes it difficult to identify the appropriate true rate of smoking-induced lung cancer cases to which to compare risk perceptions.
Finally, the notions of “subadditivity” and “the focus of judgment effect” point to another potential problem with the Audits & Surveys question [ 39 – 41 ]. The question, “Among 100 cigarette smokers, how many of them do you think will get lung cancer because they smoke?” focuses respondents’ attention on just one possible outcome of smoking: getting lung cancer. This approach typically leads to overestimation of the probability of the event in question. Asking respondents instead to report the number of smokers who will not get lung cancer would focus attention on that outcome instead, probably leading to overstatement of that probability. So the sum of the average answers to these two forms of the question would most likely total more than 100. A more desirable measurement approach would overcome the bias induced by arbitrarily asking about only one outcome (e.g., either getting lung cancer or not getting lung cancer).
The present research
To overcome the limitations of past studies, we conducted three surveys measuring Americans’ beliefs about smoking-related health risks in different ways. To gauge perceived risk, we asked two questions: one about the risk to nonsmokers, and the other about the risk to smokers. This approach is advantageous if a researcher wants to measure perceptions of attributable risk or relative risk, because (1) subadditivity is likely to bias both reports upward, so subtracting or dividing one judgment from or by the other will minimize the impact of overestimation, (2) answers to these questions can be used to generate assessments of perceived absolute risk, attributable risk, and relative risk, and (3) this approach employs the principle of decomposition, which enhances the accuracy of measures of people’s beliefs [ 15 ]. It is worth noting one limitation of our research is the fact that we only ask about lung cancer, and do not consider other health risks linked with smoking. However, most likely people’s perceptions of risk across multiple disease categories would be positively correlated. Consequently, our general conclusions about lung cancer would likely be similar if respondents were forced to consider multiple disease categories.
In decomposition, a single, global judgment is broken down into a series of sub-judgments, each of which a respondent must make in the process of generating the global judgment. Here, in order to gauge people’s perceptions of relative risk, we could ask, “how many more times likely is a smoker to get lung cancer than a nonsmoker?” To answer the global question, a respondent must estimate both the likelihood a nonsmoker will get lung cancer and estimate the likelihood that a smoker will get lung cancer, and then mentally compute the ratio of the probabilities. Because respondents can accidentally make a computational error when executing that last step, surveyors can more accurately measure people’s beliefs by asking directly about the sub-judgments, leaving the researcher to compute the ratio. The same logic applies to the measurement of perceived attributable risk (see S1 Appendix for a discussion of measuring probabilities and numeracy).
When measuring perceptions of the lung cancer risks of nonsmokers and smokers, we expressed specifically a volume of smoking and at what age it began, so we could more accurately gauge the extent to which people overestimated or under-estimated the health risks of smoking. And rather than asking survey respondents to report probabilities, we asked them to report frequencies, since a variety of studies suggest that people think more naturally in terms of frequencies [ 42 , 43 ].
We compared the three risk perception measures (absolute, attributable, and relative risk) in terms of their associations with cessation among a sample of current and former smokers. We also compared the risk perception measures in terms of their associations with the desire to quit among current smokers. Although previous studies have found positive and significant correlations between risk perceptions and the desire to quit, none of these studies compared different risk perception measures to one another or analyzed numerical risk estimates [ 27 , 44 , 45 ].
Such associations can occur for at least two reasons. First, beliefs about the health risks of smoking may be instigators of smoking cessation (for a review of this literature, see S2 Appendix ). Second, people may adjust their beliefs about smoking’s health risks in order to rationalize their status as a smoker or a non-smoker [ 46 – 48 ]. If perceptions of health risks are motivators of smoking cessation and/or if quitting smoking induces people to inflate their risk perceptions, then perceived risk should be lower among people who currently smoke than among people who have quit. That is, the higher a person’s perceived risk, the more likely he or she is to have quit. Likewise, the higher a current smoker’s perception of risk, the more motivated he or she should be to quit smoking. Therefore, the more strongly a risk perception measure is associated with whether a person has quit smoking and a smoker’s desire to quit, the more likely that risk perception is to capture the way people naturally think about risk in this arena.
Many possible patterns of risk perception types could be found in a population. The most heterogeneous pattern would be one in which one-third of people think in terms of absolute risk, while another one-third of people think in terms of attributable risk, and the remaining people think in terms of relative risk. The most homogeneous case would be one in which everyone thinks in terms of just one of these risk perceptions to make behavioral choices regarding smoking. Our analyses explored the extent of use of each of the three risk perception measures.
We also explored whether people who felt more certain about risk perceptions manifested stronger relations of those perceptions with cessation and desire to quit. Psychological research on attitude strength suggests that people hold beliefs and attitudes with varying degrees of certainty, and beliefs held with more certainty are more likely to shape thinking and action [ 49 ]. Therefore, we explored whether any of the risk perceptions were more strongly related to cessation among people who held their risk perceptions with more certainty.
Three studies
Our three studies explored five main questions: (1) How many people overestimate and underestimate absolute risk, attributable risk, and relative risk of lung cancer due to smoking? (2) How strongly are perceived absolute risk, attributable risk, and relative risk related to quitting? (3) How strongly are perceived absolute risk, attributable risk, and relative risk related to desire to quit among current smokers? (4) Are the relations between risk perceptions and quitting strongest among respondents who are most certain about their risk perceptions? (5) How strongly are perceived absolute risk, attributable risk, and relative risk related to having initiated smoking?
Study 1 was a random digit dial telephone survey of a nationally representative sample of American adults who were current or former smokers, conducted in 2000 by Schulman, Ronca, and Bucuvalas, Inc. (hereafter SRBI). Study 2 was a 2006 survey of a national non-representative sample of current and former smokers who volunteered to complete Internet surveys for Harris Interactive in exchange for points that could be redeemed for gifts. Study 3 was a 2009 survey of a nationally representative sample of all Americans, including people who had never smoked, via the Face-to-Face Recruited Internet Survey Platform (the FFRISP; see S3 Appendix for descriptions of the methodologies of the three studies, and see S4 Appendix for the demographic characteristics of the three samples).
The telephone survey respondents who were current or former smokers were asked:
(1) “Next, I'd like to turn to a different topic: what you personally think about the effect of cigarette smoking on people's health. I'm going to read these next two questions very slowly to let you think about each part of them, and I can repeat each question as many times as you like before you answer, so you can be sure they are clear to you. First, if we were to randomly choose one thousand American adults who never smoked cigarettes at all during their lives, how many of those one thousand people do you think would get lung cancer sometime during their lives?” (2) “And if we were to randomly choose one thousand American adults who each smoked one pack of cigarettes a day every day for 20 years starting when they were 20 years old, how many of those one thousand people do you think would get lung cancer sometime during their lives?” (3) “You said that smokers are [more likely/as likely/less likely] to get lung cancer than nonsmokers. How certain are you about this? Extremely certain, very certain, moderately certain, slightly certain, or not certain at all?”
We ask respondents to assess the prospect of lung cancer incidence generally like Viscusi [ 2 ]. We emphasized “personally” so that people would feel comfortable providing their own best guess of a fact, specifically general population risk of contracting lung cancer. This wording is designed to avoid the question seeming like a “quiz” (or their guess of what a public health authority might say), but rather their personal assessment of risk. For the two Internet surveys, the wording was adapted for self-administration. In all three studies, the response choices for the last question were presented in descending order for a randomly chosen half of the respondents and in ascending order for the other half. By implementing the same internally valid research design three separate times, it is possible to assess whether our findings are replicable.
Each of the three studies discussed above were deemed as suitable for exempt IRB review status by Stanford University’s review board, as no identifying information on the respondents was retained, and disclosure of answers to the survey questions would not place the respondents at risk. Informed consent for Study 1 was provided verbally given that Study 1 was a telephone survey. Written informed consent was provided for both Study 2 and Study 3, and Stanford’s IRB approved use of oral consent in Study 1 and written consent in Study 2 and 3.
Actual risk
We used data reported by Peto et al. [ 50 ] to compute the actual absolute risk, attributable risk, and relative risk of contracting lung cancer for one-pack-a-day smokers who started smoking at age 20 and smoked for 20 years. To do so, we divided the absolute risk of mortality due to lung cancer among these smokers (about 3%) by the absolute risk of mortality due to lung cancer among non-smokers (about 0.4%, yielding a relative risk of about 7). Although Peto et al. [ 50 ] examined mortality instead of incidence, the probability of dying from lung cancer conditional on developing lung cancer is 74.4% within a thirteen-year period according to Marcus et al. [ 51 ], and even higher among smokers [ 52 ]. If relative risk is higher, then our results understate the proportion of Americans who underestimate this relative risk. According to these figures, the attributable risk of lung cancer due to smoking is then about 3% (3% minus 0.4%, rounds to 3%). It is worth noting that although one might imagine that it is difficult to estimate risk rates because of complex functional forms, interactions of smoking with other risk factors, cohort effects, and other complications, research suggests that in fact, risk rates are largely robust to some potential complexities [ 53 – 55 ].
Perceived risk
In Study 1, the mean of current and former smokers’ perceptions of absolute risk of lung cancer among smokers was 48% (i.e., 480.1 smokers out of 1,000 smokers would get lung cancer); the median was 50% (see columns 1 and 2 of Table 1 ). 10.3% of respondents perceived absolute risks between 0% and 5.0%, and the remaining respondents gave answers above 5.0%. 99.5% of respondents overestimated absolute risk, only about 0.3% estimated it correctly (by giving an answer of 30), and 0.2% underestimated it (by giving an answer less than 30).
- PPT PowerPoint slide
- PNG larger image
- TIFF original image
https://doi.org/10.1371/journal.pone.0182063.t001
As expected, the mean and median perceived absolute risk of nonsmokers getting lung cancer were less: 22% and 10%, respectively. Thirty-six percent of respondents gave answers between 0% and 5.0%. Thus, most people vastly overestimated this absolute risk.
Only 5.2% of respondents thought smokers were less likely to get lung cancer than nonsmokers (a belief revealed by attributable risks less than 0; see columns 1 and 2 of Table 2 ). Attributable risk was calculated by subtracting each respondent’s answer to the question about nonsmokers from his or her answer to the question about smokers. 9.6% of respondents thought smokers and nonsmokers were equally likely to contract lung cancer, reporting an attributable risk of 0. A large majority, 85.2% of respondents, reported that smokers were more likely than nonsmokers to contract lung cancer. 76.1% overestimated attributable risk by reporting figures greater than 4%. The mean perceived attributable risk was about 27%, and the median was 20%.
https://doi.org/10.1371/journal.pone.0182063.t002
In contrast, a large majority of respondents (74.6%) underestimated relative risk, because they reported perceptions that implied a relative risk less than 7 (see columns 1 and 2 of Table 3 ). Relative risk was computed by dividing each respondent's answer to the question about 1,000 smokers by his or her answer to the question about 1,000 nonsmokers. Because this quantity is undefined for respondents who said none of the 1,000 nonsmokers would get lung cancer (because the denominator would be zero), 1 was added to these respondents’ answers to the questions about smokers and nonsmokers to allow the relative risk quantity to be defined for all respondents. Note that re-computing all analyses reported below treating these people as having missing data on the relative risk measure had negligible impact on the reported results. 54.6% of the respondents could be said to have vastly underestimated relative risk, because their reports implied a value less than 3. Only about 1.5% of respondents perceived relative risk approximately correctly (e.g., 7), and only 23.9% of respondents overestimated relative risk. 5.2% of respondents perceived a relative risk of less than 1, meaning they thought smokers developed lung cancer less often than nonsmokers, and 9.6% of the sample perceived a relative risk of 1.0, meaning they thought smokers and nonsmokers were equally likely to develop lung cancer. Mean perceived relative risk was 26.7, much higher than the true value, and the median was 2.5, lower than the true value. Thus, relative risk tells a very different story about the prevalent errors in risk perceptions than does attributable risk: most people overestimated the latter, whereas most people underestimated the former.
https://doi.org/10.1371/journal.pone.0182063.t003
Compared to the representative sample of current and formers smokers interviewed in Study 1, Study 2’s non-probability sample of current and former smokers reported: (1) lower perceived absolute risk of lung cancer among nonsmokers and smokers (e.g., 49.5% and 25.7%, respectively, gave answers between 0 and 50 out of 1,000 who would get lung cancer, compared to 36.0% and 10.3% in Study 1; see seventh and eighth columns in Table 1 ); (2) lower perceived attributable risk (e.g., 50.9% had a value of 99 or less, compared to 30.7% of the Study 1 respondents; see the eighth column of Table 2 ); and (3) lower perceived relative risk (e.g., 59.5% had values of 2.99 or less, as compared with 54.6% of the Study 1 respondents; see the eighth column of Table 3 ).
Using all three risk measures, Study 3’s representative sample of current and former smokers perceived less risk than the Study 1’s respondents did 9 years earlier. Study 3’s current and former smokers reported lower absolute risk among nonsmokers (mean = 11.9%, median = 5%) than did the Study 1 respondents (mean = 21.5%, median = 10%; see columns nine and one, respectively, of Table 1 ). Study 3’s current and former smokers perceived lower absolute risk for smokers than did the Study 1 respondents (means = 33.1% vs. 48.0%; medians = 30.0% vs. 50.0%; see columns ten and two, respectively, of Table 1 ). And Study 3’s current and former smokers perceived lower attributable risk of smoking than did the Study 1 respondents (means = 21.1% vs. 26.7%; medians = 11.5% vs. 20.0%; see columns nine and one, respectively, of Table 2 ) and lower relative risk than did the Study 1 respondents (means = 12.9 vs. 26.7; medians = 2.5 vs. 2.5; see columns 9 and 1, respectively, of Table 3 ).
Study 3 suggests that the perceived risk of lung cancer may have declined among current and former smokers between 2000 and 2009. That is, the two representative sample surveys indicated that respondents’ assessments of the absolute risk of lung cancer for both smokers and non-smokers became notably more accurate during this period.
Comparing risk measures
Which of these measures is an appropriate focus for claims about public risk perceptions and their accuracy? One way to answer this question is to determine which of these risk perceptions drives people’s decisions about whether or not to smoke. Many possible patterns of risk perception use are possible in any population. The most heterogeneous pattern would be one in which some people decide whether to smoke or quit based upon their perceptions of the attributable risk, while others make this decision with reference to perceptions of relative risk, and still others make their decisions based on perceptions of absolute risk, with the three groups being of roughly equal size. The most homogeneous case is that in which everyone uses just one of these risk perceptions to make their behavioral choices regarding smoking. By gauging which risk perceptions have how much impact for how many people, we can begin to understand whether smoking behavior overall in a population is driven mostly by perceptions that overestimate risk, mostly by perceptions that underestimate risk, or by a mixture of perceptions that sometimes overestimate and other times underestimate.
The data of all three studies allowed us to explore whether perceptions of attributable risk, relative risk, and absolute risk inspire people to quit smoking by comparing current and former smokers. If perceptions of health risks are indeed a principal motivator of smoking cessation, then perceived risk should be lower among people who currently smoke than among people who used to smoke but have quit. In other words, the higher a person’s perceived risk, the more likely he or she should be to have quit smoking. Based upon this assumption, the better a risk perception measure predicts whether a person has quit smoking, the more likely that risk perception is to have driven quitting decisions.
To adjudicate whether absolute risk, attributable risk, or relative risk drove people’s decisions to quit, we estimated the parameters of generalized additive models (GAMs) comparing current smokers to former smokers by using a Gaussian link function predicting a binary variable representing whether a respondent was a current or former smoker using the various measures of perceived risk and the weights for unequal probability of selection and demographic post-stratification (see S5 Appendix for more details on GAMs). GAMs are especially useful for estimating models containing two highly correlated predictors (as we have here) because relaxing the assumption of linearity prevents model misspecification, allowing for better isolation of the unique relations of different risk perceptions with other variables.
Using this flexible approach, we first estimated a model in which relative and attributable risk predicted quitting (more precisely, having quit). It might seem appealing to estimate GAMs predicting quitting using all three measures, but non-independence among the three measures of perceived risk makes that impossible. When examining Study 1’s data, we see that perceptions of relative risk were sensibly correlated with diminished chances of remaining a smoker (see the top-left panel of S2 Fig ). The dark line in the figure represents the estimated relation, and the two light lines demark the bounds of the 95% confidence interval around the estimates. The small vertical lines at the bottom of the figure (called “rugmarks”) indicate whether one or more respondents provided a data point at each point along the x-axis. Increasing perceived relative risk was associated with decreased log-odds of remaining a smoker. Movement from the 25 th percentile to the 75 th percentile (weighted) of relative risk increased the probability of quitting by 13.8 percentage points (see the first row of the first column of Table 4 ).
https://doi.org/10.1371/journal.pone.0182063.t004
In contrast, over the range of the bulk of the data (where the majority of the rugmarks on the x-axis are located), the relation between attributable risk and quitting was fairly flat (see bottom-left panel of S2 Fig ). Movement across the interquartile range of attributable risk increased the probability of quitting negligibly, by only 0.3% (see second row of the first column of Table 4 ).
To more formally gauge and compare these relations, we estimated a set of nested GAMs. First, we estimated a model predicting quitting using only attributable risk and then observed the improvement in goodness of fit of the model when we added relative risk as a predictor. A likelihood ratio (hereafter LR) test comparing the log likelihood of the two-variable model to the nested one-variable model indicated that the addition of the extra variable resulted in a significantly better fit (p=.03), meaning that relative risk was a reliable unique predictor of quitting (see third row of the first column of Table 4 ). Next, we estimated a model predicting quitting using only relative risk and then estimated the improvement in goodness of fit when attributable risk was added as a predictor. This addition did not improve the model’s fit significantly (p=.64; see fourth row of the first column of Table 4 ). Thus, relative risk perceptions appear to have been related to decisions to quit smoking, whereas perceptions of attributable risk were not.
To explore whether absolute risk outperforms relative risk, we estimated a GAM in which quitting was predicted by both measures. As shown in the right panels of S2 Fig , relative risk was again sensibly related to quitting (with probability of remaining a smoker declining smoothly as perceived risk increased), whereas absolute risk was not. Again, adding relative risk to a model fitted with only absolute risk improved the fit significantly (p=.002), whereas adding absolute risk to a model with relative risk did not yield a significant improvement in fit (p=.15; see rows seven and eight of the first column of Table 4 ). Movement across the interquartile range of absolute risk was associated with a 10.5% decrease in the chances of quitting, whereas movement across the interquartile range of relative risk was associated with a sizable and more reasonable 15.2% increase in the likelihood of quitting (see rows five and six of the first column of Table 4 ). As shown in columns two and three of Table 4 (as well as S3 and S4 Figs), these same results were replicated in Studies 2 and 3.
There may be an illusion hidden in these results. When people are asked to report a probability but do not know the answer, they sometimes answer “50,” meaning “fifty-fifty” or “I don’t know,” rather than meaning a 50% chance [ 56 ]. To explore the impact of this potential source of measurement error on our conclusions, we re-estimated the logistic GAM by: (1) dropping the respondents who answered “500” to the question about nonsmokers or to the question about smokers; (2) replacing the 500s with values generated by multiple imputation; and (3) replacing the 500s with answers obtained by a follow-up probe. The results supported the above conclusions even more strongly (for details of these approaches and results, see S6 Appendix ).
Next, we explored whether certainty moderated the associations of risk perceptions with quitting behavior. In Study 1, as expected, the correlation of relative risk with quitting was significantly stronger among high certainty respondents (people who were extremely certain, 27% of the sample) than among lower certainty respondents. Among the high certainty respondents, the probability of quitting increased over the interquartile range of relative risk by 23.7 percentage points (p=.008), a much larger increase than among the low certainty respondents, whose positive change was just 10.5 percentage points (p=.054). Accounting for certainty significantly improved the goodness of fit of the model (p=.03).
Likewise, in Study 2, the positive relation between perceived relative risk and quitting was significantly stronger among high certainty respondents than among low certainty respondents (p=.009). Among the high certainty respondents (18% of the sample), movement across the interquartile range of relative risk increased the probability of quitting by 44.1% (p<.001), whereas movement across this interquartile range in the low certainty group was associated with an increase in quitting probability of only 13.6% (p<.001). Accounting for certainty significantly improved the goodness of fit of the model (p=.009).
In Study 3, among high certainty individuals (30.5% of the sample), movement across the interquartile range of relative risk was associated with an increased probability of quitting smoking of 15.8% (p=.06), whereas movement across this interquartile range in the low certainty group was associated with an increase in quitting probability of 11.1% (p=.03). Accounting for certainty again significantly improved the goodness of fit of the model (p=.03).
Desire to quit.
Next, we examined whether current smokers’ risk perceptions were associated with their desire to quit. While a desire to quit does not automatically translate to smoking cessation, a strong desire to quit is predictive of subsequent quitting behavior, and is a necessary condition for quitting [ 57 ]. In Study 1, adding relative risk to a GAM model predicting desire to quit among current smokers with attributable risk caused a marginally non-significant improvement in fit (p=.09; see the third row of column four in Table 4 ). Movement from the 25 th to the 75 th percentile of relative risk raised the probability of wanting to quit by 17.0% (see the first row of column four in Table 4 ). But adding attributable risk to a model predicting desire to quit with relative risk did not improve fit significantly (p=.27; see row four of column four in Table 4 ). Movement across the interquartile range of attributable risk slightly lowered desire to quit by 1.1% (see row two of column four in Table 4 ). Likewise, adding relative risk to a model including absolute risk yielded a significant improvement in fit (p=.046; see row seven of column four in Table 4 ). Movement across the interquartile range of relative risk increased desire to quit by 13.9% (see row five in Table 4 ). But adding absolute risk to a model including relative risk marginally significantly decreased desire to quit (interquartile range movement = 15.6%, p=.06; see rows six and eight of column four in Table 4 ). The data from Studies 2 and 3 yielded similar results (see columns five and six of Table 4 ). This further supports the contention that people think in terms of relative risk perceptions.
Smoking onset.
We observed the expected results when we used the three measures in Study 3 to explore whether perceived risk was greater among people who ever smoked than among people who never smoked. Comparing the distributions in the ninth and tenth columns in Table 1 with the distributions in the last two columns of the table, we see that: (1) both groups had similar expectations for the proportion of nonsmokers who would get lung cancer (mean = 11% for people who never smoked vs. 12% for people who ever smoked), but (2) the expected proportion of smokers who would get lung cancer was higher among people who had never smoked (mean = 43.3%) than among people who ever smoked (mean = 33.1%).
Also as expected, people who never smoked perceived higher attributable risk of smoking than did people who ever smoked (see the last two columns in Table 2 ): (1) 3.9% thought that smokers were less likely to contract lung cancer than nonsmokers (attributable risk of less than 0); (2) 6.3% thought that smokers and nonsmokers were equally likely to get lung cancer (attributable risk of 0); and (3) 89.7% thought that smokers were more likely to contract lung cancer than nonsmokers. Respondents who never smoked thought smokers were 32 percentage points more likely than nonsmokers to get lung cancer, on average (see columns 11 and 12 of Table 2 ). Thus, these individuals perceived a higher attributable risk than did current and former smokers (21.1 percentage points; see column nine of Table 2 ). Likewise, respondents who never smoked also perceived higher relative risk than did current and former smokers (compare the last two columns of Table 3 with the ninth and tenth columns of that table).
As expected, perceptions of relative risk were strongly associated with status as a never smoker vs. a current smoker in GAMs (see the left panels of S5 Fig ). Adding relative risk to a model predicting current smoking with attributable risk considerably improved fit (p<.001), whereas adding attributable risk to a model with relative risk did not significantly improve fit (p=.57). Movement across the interquartile range of relative risk yielded a 22.7 percentage point decrease in the likelihood that respondents were smokers. Movement across the interquartile range of attributable risk yielded a decrease in the probability of being a smoker of only 0.7 percentage points.
Likewise, adding relative risk to a model with only absolute risk improved fit significantly (p<.001), whereas adding absolute risk to a model including relative risk was associated with only a marginally significant improvement in fit (p=.07). Movement across the interquartile range of relative risk (when controlling for absolute risk) was associated with a 22.3 percentage point decrease in the probability of ever having smoked (see the right panels of S5 Fig ). In contrast, movement across the interquartile range of absolute risk (when controlling for relative risk) produced only an 8.5 percentage point decrease in the likelihood of ever having smoked.
Summary and implications
Taken together, this evidence suggests that while Americans have overestimated the absolute risk and risk difference of lung cancer associated with cigarette smoking, Americans have generally underestimated the relative risk. Furthermore, this evidence suggests that people may think more about smoking health risks in terms of relative risk than in terms of absolute risk or risk difference. The relations we saw here may result from the influence of health risk beliefs on decisions to quit smoking, decisions to start smoking, and regret about smoking, or these relations may occur because people rationalize their smoking status by adjusting their risk perceptions, or from some other process. Having seen here that these are possibilities, we look forward to future research exploring them to characterize the basis for the relations we observed.
Communication of risk has been a difficult task for medical professionals, and our findings encourage consideration of a different approach to communicating health risks than has been typical on American cigarette packages and in other prominent health communications [ 58 , 59 ]. There are a large number of studies that show that the design of and warnings on cigarette packs can influence perceptions of the risks of smoking [ 60 – 68 ]. However, much constructive work can perhaps still be done by informing individuals about how much smoking increases their health risks. If the findings reported here are correct in suggesting that people use perceptions of relative risk when deciding whether to quit smoking, and if relative risk is indeed underestimated by most current and former smokers, corrective steps in this regard might be consequential. More specifically, if public health efforts are initiated in the future to encourage Americans to more accurately recognize the magnitudes of relative risks for various undesirable health outcomes of cigarette consumption, this may well lead to a reduction in the nation’s smoking rate and a consequent reduction in smoking-related morbidity and mortality. This may be why quantitative information about relative risk on cigarette packages in Australia (e.g., “Tobacco smoking causes more than four times the number of deaths caused by car accidents.”) appears to have been effective in encouraging smoking cessation [ 69 ].
Future research could explore these possibilities with experiments gauging the effects of different ways of describing risks on cigarette packages and other health communication mediums like television advertisements, poster campaigns, and doctor-patient communication [ 70 ]. Our findings suggest that when conducting such experiments, it may be desirable to attempt to alter people’s perceptions of relative risk in order to most directly address people’s natural approach to thinking about health risks in this arena. Perceptions of relative risk might be changed best by making such direct statements. But it may also be that such perceptions can be changed even more effectively by inducing affective reactions or in other non-quantitative ways, while simultaneously maximizing trust in the source of the information [ 71 , 72 ]. It is important to bear in mind that even successful efforts to change risk perceptions may not produce changes in behavior, so it will be important for future investigations to assess whether risk perception changes are translated into action [ 73 ].
In addition to their applied value, the findings reported here are interesting in basic psychological terms. By distinguishing between absolute, attributable, and relative risk, the present findings encourage future study with such measures to understand how people make many types of risky decisions and, more generally, how people trade off probabilities when making choices. And many important questions remain regarding risk perceptions involving smoking, such as how people arrive at their perceptions of relative, attributable, and absolute risk, and when and why some people use one measure rather than another to make behavioral decisions. Future studies of these sorts of issues seem merited, both in the smoking and other domains.
Resonance with other findings
Various findings reported here resonate with findings of some past studies. For example, Viscusi [ 2 ] and Borland [ 69 ] found that people overestimated the absolute risk of smoking. Khwaja et al. [ 74 ] found that both smokers and non-smokers overestimated their risks of dying from all sorts of causes [ 69 ]. When Weinstein et al. [ 27 ] asked respondents to assess the relative risk of smoking (“Would you say the average smoker has about the same lung cancer risk as a nonsmoker, a little higher lung cancer risk than a nonsmoker, twice the nonsmoker’s risk, five times the nonsmoker’s risk, or ten times the nonsmoker’s risk?”), smokers offered underestimates.
Boney-McCoy et al. [ 19 ] found that current smokers perceived the absolute risk of smoking to be significantly lower than that perceived by former smokers. This is consistent with the evidence reported here that when considered alone, absolute risk perceptions are related to quitting in the same way. However, when controlling for relative risk, the relation of quitting to absolute risk perceptions was close to zero in the present data.
Antoñanzas et al. [ 75 ] found distributions of Spaniards’ perceptions of attributable and relative risk (regarding the impact of cigarette smoking on lung cancer and heart disease) very similar to those reported here. Viscusi et al. [ 76 ] found that each of these risk perceptions predicted Spaniards’ status as a smoker or nonsmoker when considered alone, and relative risk was a considerably stronger predictor than attributable risk, though Viscusi et al. [ 76 ] did not assess the predictive abilities of perceived attributable risk and relative risk in a single regression equation.
The present evidence that people seem to think in terms of relative risk rather than attributable or absolute risk resonates with research on effective ways to communicate risks to patients [ 77 , 78 ]. For example, Malenka et al. [ 13 ] asked respondents to imagine they had a disease and could choose to take one of two medications—one described in terms of its impact on relative risk (“reduces risk of dying by 80%”) and the other (statistically equivalent) described in terms of impact on attributable risk (“can prevent 8 deaths per 100 people”). Most respondents preferred the medication described in terms of relative risk, perhaps because this portrayal resonated with people’s natural way of thinking about medication benefits found that relative risk information had more impact than did attributable risk information [ 79 – 83 ]. These findings contrast with Saitz’s [ 84 ] and Gigerenzer et al.’s [ 85 ] speculations that people will respond as well or better to attributable risk information (presented as two absolute risks) than to relative risk information, a finding challenged by our data as well.
A preference for thinking about health risks in terms of relative risk is also apparent in news media stories. In one study, 83% of such stories reported benefits of medications in terms of relative risk only, 2% reported benefits in terms of attributable risk only, and 15% reported benefits in terms of both indicators [ 86 ]. Similarly, medical journal articles tend to focus on reports of relative risk rather than attributable risk [ 87 ].
Other directions for further research
Future research might gain more insight into people’s natural ways of thinking about health risks by asking people to describe the health risks of smoking with whatever language they wish. With enough probing, open-ended data gathering might reveal whether people naturally use language evoking absolute risk, attributable risks, or relative risk levels, or a non-numeric representation, and such evidence is worthwhile to collect in future research [ 37 , 88 ]. Future work should also incorporate how much life is lost when calculating risk (see Viscusi [ 38 ] for a discussion of how this might affect an understanding of these results).
Generalizing beyond lung cancer
The focus of the analyses reported here has been people’s perceptions of the risk of getting lung cancer due to smoking. Because lung cancer is one of the best-known health risks of smoking [ 11 ], Americans may be less likely to underestimate the relative risk of lung cancer than of other diseases that are known to be caused by smoking. If we had asked survey questions about heart disease, oral cancers, or stroke instead of lung cancer, the prevalence of underestimation of relative risk may have been even greater than was observed for lung cancer. Correcting these misunderstandings may decrease the expected smoking rate even more. Future studies can explore these possibilities.
Implications regarding other domains of risk perception.
Differentiating perceived relative risk from perceived attributable risk may be useful in other health domains as well. For example, Meltzer and Egleston [ 89 ] reported that patients with diabetes vastly overestimated their own absolute risk of experiencing various complications. But perhaps their perceptions of relative risk are more accurate.
Implications for health education.
Psychological research on health counseling communication has revealed errors in people’s understanding of risk information [ 90 – 92 ]. However, educational efforts can present risk rates in various different ways, and some presentation approaches can cause misunderstandings [ 93 , 92 ]. The present evidence bolsters the conclusions of some past studies suggesting that future research may be most successful when presenting relative risk information to yield better quality decisions [ 94 – 99 ].
Supporting information
S1 fig. proportions of americans who failed to assert that smoking is dangerous to human health: gallup organization surveys..
https://doi.org/10.1371/journal.pone.0182063.s001
S2 Fig. Generalized Additive Models predicting the probability of being a current smoker: SRBI Survey (n = 456).
https://doi.org/10.1371/journal.pone.0182063.s002
S3 Fig. Generalized Additive Models predicting the probability of being a current smoker: Harris Interactive Survey (n = 795).
https://doi.org/10.1371/journal.pone.0182063.s003
S4 Fig. Generalized Additive Models predicting the probability of being a current smoker vs. former smoker: FFRISP (n = 471).
https://doi.org/10.1371/journal.pone.0182063.s004
S5 Fig. Generalized Additive Models predicting the probability of being a current smoker vs. never smoker: FFRISP (n = 714).
https://doi.org/10.1371/journal.pone.0182063.s005
S1 Appendix. Measuring risk.
https://doi.org/10.1371/journal.pone.0182063.s006
S2 Appendix. Literature on the relation of health risk perceptions with quitting smoking.
https://doi.org/10.1371/journal.pone.0182063.s007
S3 Appendix. Survey methodology.
https://doi.org/10.1371/journal.pone.0182063.s008
S4 Appendix. Demographics of current and former smokers in the SRBI Survey, current and former smokers in the Harris Interactive Survey, all individuals in the FFRISP Survey, and the nation’s population.
https://doi.org/10.1371/journal.pone.0182063.s009
S5 Appendix. GAMs.
https://doi.org/10.1371/journal.pone.0182063.s010
S6 Appendix. Exploring responses of 500.
https://doi.org/10.1371/journal.pone.0182063.s011
S7 Appendix. References for supporting information.
https://doi.org/10.1371/journal.pone.0182063.s012
Acknowledgments
The first survey described in this paper was funded by Empire Blue Cross/Blue Shield of New York. The third data set described was collected via the Face-to-Face Recruited Internet Survey Platform (FFRISP), funded by NSF Grant 0619956, Jon A. Krosnick, Principal Investigator. The authors thank Geoffrey Fong and Paul Slovic for very helpful suggestions. The authors acknowledge the excellent research assistance of Virginia Lovison. Jon Krosnick is University Fellow at Resources for the Future.
Author Contributions
- Conceptualization: JAK LC.
- Data curation: JAK NM CHM LC JP RKT.
- Formal analysis: NM CHM LC JP.
- Funding acquisition: JAK RKT.
- Investigation: JAK LC RKT.
- Methodology: NM LC JP.
- Project administration: JAK NM CHM.
- Resources: JAK RKT.
- Software: NM CHM LC JP RKT.
- Supervision: JAK.
- Validation: NM CHM JP.
- Visualization: NM CHM LC JP.
- Writing – original draft: JAK NM CHM EFB JP.
- Writing – review & editing: JAK NM CHM EFB JP.
- 1. Centers for Disease Control and Prevention. Cigarette smoking among adults—United States, 2007.
- 2. Viscusi WK. Smoking: Making the risky decision. New York: Oxford University Press; 1992.
- View Article
- Google Scholar
- PubMed/NCBI
- 6. Newport F, Moore DW, Saad L (Gallup O. Long term Gallup Poll trends: A portrait of American public opinion through the century [Internet]. http://www.gallup.com/poll/3400/longterm-gallup-poll-trends-portrait-american-public-opinion.aspx
- 7. Gallup Organization. National survey. Retrieved from iPOLL Databank, The Roper Center for Public Opinion Research, University of Connecticut. http://www.ropercenter.uconn.edu/ipoll.html . Accessed August 1, 2014.
- 8. Department of Health and Human Services, Public Health Service, Office of the Assistant Secretary for Health, Office on Smoking and Health. Use of Tobacco Survey (ARC Identifier 607143),1986.
- 9. American Lung Association and Gallup Organization. National survey, June 1987. Retrieved from iPOLL Databank, The Roper Center for Public Opinion Research, University of Connecticut. http://www.ropercenter.uconn.edu/ipoll.html . Accessed.
- 12. Manski CF. Identification problems in the social sciences. Cambridge, MA: Harvard University Press; 1995.
- 14. Mausner JS, Bahn JK. Epidemiology: An introductory text. Philadelphia, PA: Saunders; 1974.
- 47. Festinger L. A theory of cognitive dissonance. Stanford, CA: Stanford University Press; 1957.
- 49. Petty RE, Krosnick JA. Attitude strength: Antecedents and consequences. Hillsdale, NJ: Erlbaum; 1995.
- Open access
- Published: 30 July 2021
Impact of tobacco and/or nicotine products on health and functioning: a scoping review and findings from the preparatory phase of the development of a new self-report measure
- Esther F. Afolalu ORCID: orcid.org/0000-0001-8866-4765 1 ,
- Erica Spies 1 ,
- Agnes Bacso 1 ,
- Emilie Clerc 1 ,
- Linda Abetz-Webb 2 ,
- Sophie Gallot 1 &
- Christelle Chrea 1
Harm Reduction Journal volume 18 , Article number: 79 ( 2021 ) Cite this article
15k Accesses
4 Citations
10 Altmetric
Metrics details
Measuring self-reported experience of health and functioning is important for understanding the changes in the health status of individuals switching from cigarettes to less harmful tobacco and/or nicotine products (TNP) or reduced-risk products (RRP) and for supporting tobacco harm reduction strategies.
This paper presents insights from three research activities from the preparatory phase of the development of a new self-report health and functioning measure. A scoping literature review was conducted to identify the positive and negative impact of TNP use on health and functioning. Focus groups ( n = 29) on risk perception and individual interviews ( n = 40) on perceived dependence in people who use TNPs were reanalyzed in the context of health and functioning, and expert opinion was gathered from five key opinion leaders and five technical consultants.
Triangulating the findings of the review of 97 articles, qualitative input from people who use TNPs, and expert feedback helped generate a preliminary conceptual framework including health and functioning and conceptually-related domains impacted by TNP use. Domains related to the future health and functioning measurement model include physical health signs and symptoms, general physical appearance, functioning (physical, sexual, cognitive, emotional, and social), and general health perceptions.
Conclusions
This preliminary conceptual framework can inform future research on development and validation of new measures for assessment of overall health and functioning impact of TNPs from the consumers’ perspective.
As a leading cause of preventable morbidity and mortality worldwide, smoking remains a major public health problem. Compared with those who do not smoke, people who smoke are significantly more likely to develop heart diseases, lung cancer, chronic obstructive pulmonary disease (COPD), and other diseases [ 1 , 2 ]. It is well established that the best way to avoid the health risks associated with smoking is for people to never start and for those who smoke to quit [ 1 , 3 ]. Tobacco harm reduction is one way to alleviate the health risk for individuals who choose not to quit smoking [ 4 ], by providing less harmful tobacco and/or nicotine products (TNP), such as reduced-risk products (RRP) (used here to refer to products that present, are likely to present, or have the potential to present, less risk of harm to people who smoke and switch to these products versus continued smoking) or modified risk tobacco products (MRTP).
Several smokeless tobacco products and a heated tobacco product were recently authorized for marketing with modified risk claims through the United States (US) Food and Drug Administration (FDA) MRTP pathway [ 5 ]. The guidance on MRTP applications [ 6 ] specifies that health outcomes should be assessed during premarket evaluation and postmarket surveillance of modified risk TNPs such as these. These health outcomes comprise not only objective clinical and biological measures but also self-reported outcomes [ 6 , 7 ]. Studies and reports have recently started providing evidence on the health impact of new TNPs [ 8 ]. For instance, recent papers have investigated the effects of e-cigarettes and heated tobacco products on cardiopulmonary outcomes [ 9 , 10 , 11 , 12 , 13 , 14 ]. However, the papers have mainly focused on clinical measurements, such as spirometry and other lung function tests; consumer perception is rarely explored or the focus of the research. Measuring self-reported experience is important for understanding the changes in the health status of individuals switching from cigarettes to RRPs and is a key component of tobacco harm reduction strategies [ 7 ]. Self-reported ratings of RRP effectiveness or adverse events might differ from clinical measures and provide another perspective as useful as the clinicians. In addition, consumer perception of positive changes in health status, functioning and other behavioral outcomes will also subsequently influence use behaviors and switching to RRPs rather than continuing smoking.
Self-perceived health status is a complex concept to define and measure, particularly within the context of TNP use [ 15 ]. While generic health status measures, such as the Medical Outcomes Study 36-item Short-Form Health Survey (SF-36), have been used to evaluate the health status of people who smoke [ 16 , 17 ], comparisons have mainly been made between those who currently smoke, those who used to smoke, and those who never smoked [ 18 , 19 ]. Results from these studies strongly suggest that, in healthy populations, existing generic measures are not sensitive enough to detect change over time when stopping or switching from cigarettes to other TNPs, owing to high ceiling effects [ 20 ]. While a few smoking-specific quality of life measures have been developed, these measures have not been widely implemented or standardized [ 15 , 17 , 21 , 22 ], and the application of these smoking-specific measures to different TNP use across the risk continuum is scarce [ 20 ].
As part of the A ssessment of B ehavioral OU tcomes related to T obacco and Nicotine Products (ABOUT™) Toolbox initiative [ 23 ], the present project aims at developing a new self-report measure (ABOUT™— Health and Functioning ) to address the current gap and assess the impact of TNPs on health and functioning (including health status, functional status and other health-related quality of life constructs). This paper presents insights from three research activities [ 24 , 25 ] from the preparatory phase of development of the measure—that is, a scoping literature review, reanalysis of consumer focus groups/interviews, and expert opinion. These three activities serve as background research to support the development of a preliminary conceptual framework of health and functioning associated with the use of TNPs.
Scoping literature review
The purpose of the review was to address two main questions among individuals who use TNPs:
What are the positive and negative health and functioning impacts of TNP use?
What concepts are evaluated by measures used to assess the positive and negative impacts of TNP use?
Given the nature and breadth of the research questions and the number of potentially relevant publications, a scoping literature review was used as it provides a means of identifying the literature and mapping the concepts and evidence on a topic by using an informative and iterative research process [ 26 ]. The scoping review involved a PubMed search (August 2018) and application of Sciome’s rapid Evidence Mapping (rEM) [ 27 ], followed by additional manual screening and review. rEM is a proprietary methodology developed by Sciome ( https://www.sciome.com/ ) to rapidly summarize and produce a quantitative representation of the available body of scientific evidence in a particular area. The study by Lam et al. demonstrated a proof-of-concept application of the rEM methodology [ 27 ]. The PubMed search terms targeted qualitative and quantitative research among people who use TNPs (Table 1 ). This was supplemented by a second, parallel step of manually identifying relevant literature through other known sources. Table 2 describes the general inclusion and exclusion criteria that were applied to the scoping literature review.
After the initial rEM exercise, two reviewers (EC, SG) further manually screened the titles and abstracts of the articles identified through the automated rEM exercise against the inclusion and exclusion criteria. Finally, the selected publications underwent a full screening by two reviewers (VL and DF) for determining their relevance to the research questions for data extraction and one of the co-authors (LA-W) cross-checked the screening and resolved differences in opinion among the reviewers.
The World Health Organization (WHO) International Classification of Functioning, Disability and Health (ICF) [ 28 ] framework and the revised Wilson and Cleary [ 29 , 30 ] model were used as a guide to broadly inform categories for data extraction from the literature on TNP use and health and functioning. These established models enable the conceptualization and description of health status and functioning (the combination of which is often referred to as health-related quality of life) [ 31 , 32 ], and related outcomes and determinants. To complement and refine this and to ensure relevance to those who use TNPs, the data extracted from the literature was also grouped and labeled based on the contents of the literature reviewed.
The elements extracted from the selected papers were as follows:
Author, citation details, and publication type
Objectives and/or research questions
Sample type, size, and principle demographics
Type(s) of TNP and definitions of levels of consumption
Methodology, questionnaires, and statistical methods used
Main results
Results grouped in broad categories: Health Signs and Symptoms; General Health Perceptions; Quality of Life, Health-Related Quality of Life, and Functional Status; Individual Characteristics; Environmental and Social Characteristics; Biomarkers and Biological Endpoints.
Reanalysis of focus groups/in-depth interviews
The objective of the secondary analyses of existing qualitative data in people who use TNPs was to inform the drafting of the initial conceptual framework, as well as interview guides for planned concept elicitation qualitative studies to identify concepts and develop items to detect what is relevant to measure in this context. Two sets of qualitative data containing information related to health and functioning were reanalyzed and participants had consented for their data to be used in future studies. The first was from 29 focus groups (total number of participants n = 229) that were originally designed to discuss perceived risk, appeal, and intent to use TNPs [ 33 , 34 ]. The focus groups—stratified by smoking status—were conducted in the United States (US; n = 12), Japan ( n = 4), Italy ( n = 4), and the United Kingdom (UK; n = 9) between December 2012 and August 2013. The second dataset included 40 in-depth interviews conducted in North Carolina, USA, with people who use TNPs, to discuss issues centered on perceived dependence on TNPs [ 35 ]. While 21 interviewees were people who were poly-TNPs users, 19 were people who were exclusive users of one of the following types of TNPs: cigarettes ( n = 5), smokeless tobacco ( n = 5), e-cigarettes ( n = 5), or another type of TNP (pipes, waterpipes, or nicotine replacement therapy [NRT] products; n = 4). These interviews were conducted in August 2017. The demographics of both data sets are presented in Table 3 . For reanalyzing the data, an initial codebook guided by the literature review data extraction categories was developed; however, new codes were created to complement these categories based on the thematic content analysis of the transcripts. The qualitative analysis software Quirkos [ 36 ] was used for the reanalysis.
Expert panel review
An expert panel consisting of five key opinion leaders (KOL) and five technical consultants was convened in August 28, 2018, in Neuchâtel, Switzerland. The KOLs were subject matter experts in the fields of nicotine and smoking cessation ( n = 1), Patients Reported Outcomes (PRO) evaluation and scale development ( n = 3), and health economics ( n = 1). The consultants were experts on nicotine dependence ( n = 1), psychometric validation ( n = 2), market research ( n = 1), and PRO development and validation ( n = 1). The meeting followed an agenda and semi-structured discussion guide to facilitate conversations. First, the panel was presented with the principles underlying the tobacco harm reduction assessment strategy [ 4 ]. This session was followed by an open elicitation phase, during which two experienced moderators asked the panel to identify and discuss concepts related to health and functioning in people who use TNPs that different stakeholders might find important. Then, the panel was asked to review and respond to the concepts identified in the literature review and in the qualitative research reanalysis. These findings were discussed in depth to arrive at a consolidated preliminary conceptual framework. Each concept was presented, and the experts were asked to rank and agree on concepts to be included and how the concepts should be grouped by domains in the framework. In generating the framework, the project team and expert panel considered the themes and concepts identified under each of the categories from the scoping literature review, specific concepts from the secondary analyses of the qualitative data, and the expert panel meeting. The authors then synthesized and re-organized concepts emerging from the different preparatory phase activities under main health and functioning and conceptually-related domains. The participants also provided their input on the best strategies for planned qualitative studies to inform and support the development and validity of the proposed health and functioning measure.
The literature search identified 4761 articles. Figure 1 (flow diagram) depicts the results of the search and screening process. Titles and abstracts were screened by the rEM exercise until the machine learning algorithms predicted 97.7% relevant references; thus, 707 abstracts were not screened. After applying the inclusion/exclusion criteria to the remaining 4,054 abstracts, 281 were identified as part of the rEM exercise. After additional manual screening and review of the abstracts and articles against the inclusion/exclusion criteria, 90 full-text articles were included for data extraction [ 20 , 37 – 125 ]. Seven additional full-text articles were also included on the basis of a manual search [ 126 , 127 , 128 , 129 , 130 , 131 , 132 ]. Findings are summarized in Table 4 and a detailed description and data extracted from all the articles from the literature review is presented in Additional File 1 .
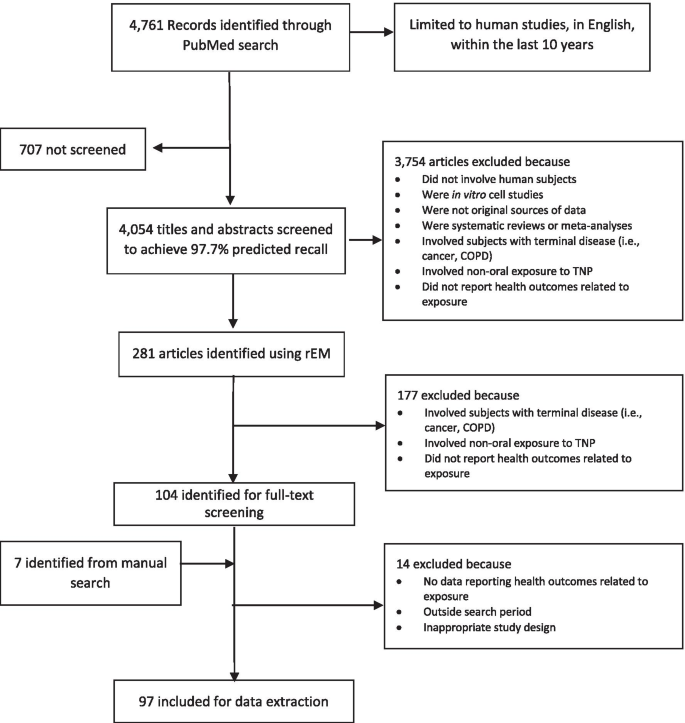
Flow diagram Sciome’s rapid Evidence Mapping (rEM) and manual screening processes of the scoping literature review
Fifty-six publications (56/97; 58%) presented data related to health signs and symptoms . These are grouped under five core areas: mental health and cognitive functioning (28/97; 29%); pain and physical trauma (6/97; 6%); respiratory, cardiovascular and inflammatory conditions (5/97; 5%); “other” health conditions , which included insomnia, liver disease, eye health, and hearing loss (5/97; 5%); and oral health (4/97; 4%). There were also eight publications related to the effects of smoking cessation on health signs and symptoms, mostly benefits of cessation but also including perceived dependence, addiction, and withdrawal symptoms (8/97; 8%). Overall, the burden and impact of cigarette smoking on both physical and mental health symptoms was negative and generally worse among people who smoke relative to those who do not smoke. On the other hand, quitting smoking was accompanied by improvements in general physical health and psychological wellbeing. However, in spite of the positive impact of quitting smoking, loss of moments of pleasure, struggle to manage stress, the social aspects of smoking, and withdrawal symptoms were seen as barriers to quitting.
The general health perceptions of various adults who use TNPs were reported in 18 of the 97 articles (18%), with 9 of them detailing the general health perceptions related to cigarettes and 9 being related to e-cigarettes and other TNPs. Perceptions were determined by questionnaires and focus groups for evaluating the health impacts, fear of diseases, harm to others and self, social impacts (both positive [e.g., inclusion and looking “cool”] and negative [e.g., stigma and exclusion]), and other reasons for taking up or considering/attempting smoking cessation.
Quality of life, health-related quality of life, and functional status was studied in 9 of the 97 included articles (9%). These studies mostly demonstrated with generic and specific QoL, HRQoL, or functional status questionnaires that cigarette smoking was associated with a worse quality of life and that smoking cessation often resulted in an improved quality of life. However, in some cases, the use of TNPs also reportedly enabled individuals to manage their levels of anxiety and improve some aspects of social engagement and functional status.
Individual, environmental and social characteristics were found to influence the decision to smoke and/or consider or attempt to quit smoking or switching to other TNPs, as reported in 8 (8%) and 11 (11%) of 97 publications, respectively. Some key characteristics and determinants of smoking behavior included low socioeconomic status, male sex, living alone, family, and close social environment, societal stigma, and local regulations.
Finally, 12 of the 97 publications (12%) were related to studies on biomarkers and biological endpoints in people who use TNPs and showed that smoking cigarettes negatively influenced cardiovascular, respiratory, oral, renal, stress, metabolic, and inflammatory-related biomarkers and physiological assessments.
The themes from this reanalysis are summarized below and organized on the basis of the narrative of the participants of their experiences.
Perceived negative impact of smoking
Other than health, the biggest and most salient reported negative impact of smoking was the perceived lack of control related to addiction and emotional health and wellbeing. Participants reported feeling that cigarette smoking was running their lives or “holding them hostage.” They reported that this perceived lack of a sense of control or willpower often led to feelings of weakness or a feeling that they were a “slave” to cigarettes. Many respondents reported smoking even when they did not necessarily want to and experiencing feelings of obsession and craving.
Perceived lack of control and addiction were also related to the activities of the participants throughout the day. People who smoke often reported altering their activities to smoke because of patterns of behavior or routine and the experienced need for a smoke. They reported that the “need for a smoke” sensation would cause them to leave work or social events early, not attend events if smoking was not allowed, interrupt what they were doing to smoke, and get up in the middle of the night.
Fear of withdrawal symptoms, with a strong emphasis on mental/emotional health, was also prominent among reported negative impacts of smoking. This fear was often reported as limiting the willingness of individuals to try to quit smoking or facilitating a return to prior smoking behavior. Individuals reported fearing the following symptoms they associated with withdrawal: mood swings and irritability, violent or aggressive behavior, inability to concentrate, anxiety, anger, and weight gain.
Perceived benefits of smoking
Several perceived benefits were identified that keep individuals smoking or using cigarettes. These included perceptions of enhanced cognitive functioning, relaxation, a way to take a break, use as a coping strategy, a social function, a weight management tool, the perception that it feels good, and being part of one’s identity. It is also important to note that the perceived benefits of smoking often outweighed the risks and the feeling of lack of control in the participant discussions. Even people who used to smoke noted they missed the relaxation and breaks they associated with smoking.
Recognition of symptoms/diseases related to smoking
Table 5 summarizes the negative symptoms and diseases related to smoking recognized by participants in both the focus groups and interviews. These were mostly related to six main body systems (cardiovascular, digestive, oral, neurological, reproductive, and respiratory).
Impacts on physical functioning
The participants noted how smoking impacts their physical functioning. In particular, they noted how their exercise capacity during running, playing sports, walking upstairs, and general physical activity was diminished. They also reported reduced stamina and endurance, decreased physical strength, and feeling tired more easily.
Effects on emotional health
The participants also described how smoking impacts their emotional health and wellbeing. People who smoke reported feelings of shame, guilt, weakness, and a lack of control or powerlessness. They also reported feelings of depression and anxiety associated with worry about health risks. Furthermore, the participants indicated that they experienced a fear of going to places where they could not smoke, being a bad role model for their children, and (in case of people who used to smoke) going back to smoking.
Positive and negative social impacts
Smoking was perceived to have both negative and positive impacts on the social lives of participants. Smoking impacted life negatively when it was not allowed in certain environments, such as in homes, at work, and in cars and airplanes. Stigma was also associated with smoking in an environment where peers and family members do not smoke, but it was also seen as a source of group identity within social networks that had a higher prevalence of smoking behaviors. Participants reported that smoking had some positive impacts on their social interaction, because it facilitated work breaks and increased communication with peers.
Reasons people decided to try to quit
Throughout the focus groups and interviews, individuals identified several reasons why they tried to quit smoking. These included: health, diagnosis of cancer (self, family, or friend), gum disease, pregnancy, hospital stay, worry that it will “kill me,” dislike of taste or odor, social reasons, change in surroundings (fewer smoking spaces), and price.
Reasons people do not like alternatives to cigarettes
The participants’ reasons for not liking alternatives to cigarettes (i.e., less harmful TNPs/RRPs) included perceptions that the alternatives did not work (i.e., the participants still had cravings and experienced withdrawal symptoms), made them feel or get ill (nausea and vomiting), were not “the same” as cigarettes in terms of the ritual, taste, or “feeling,” or were inconvenient/too big to carry.
The conclusions of the expert panel widely supported the findings of the literature review and the input from the reanalyzed focus groups and interviews. Some of the experts working in field of tobacco and nicotine provided additional insights based on their extensive experience with people who use TNPs; they highlighted the importance of the enjoyment of smoking for people who find it difficult to quit, the positive immediate benefits of quitting, and the smoking-related biomarkers that might be on a causal pathway between switching and changes in health and functioning status.
The following main areas were discussed and agreed during the meeting: (1) utility of use, referring to the perceived satisfaction and enjoyment of smoking (e.g., craving relief, weight control, and social affiliation); (2) signs and symptoms of withdrawal (e.g., anxiety, depression, and anger) and the positive immediate physical health effects of quitting smoking (e.g., better general and oral hygiene, less coughing, and improved exercise capacity); (3) functioning, including cognitive, physical, sexual, social, emotional, and role functioning; (4) worry associated with smoking and smoking-related diseases; (5) general health perceptions and quality of life; (6) association with smoking-related biomarkers that could be on the causal pathway between switching and changes in health and functioning; and (7) TNP use patterns and maintenance of switching to RRPs.
Generation of the preliminary conceptual framework
Triangulation of the findings from the literature review, qualitative input from people who use TNPs, and expert panel feedback helped generate a preliminary descriptive conceptual framework that includes the health and functioning and conceptually-related domains impacted by TNP use (Fig. 2 ).

Health and functioning conceptual framework related to tobacco and/or nicotine product use from the preparatory phase research findings
Four domains related to the future health and functioning measurement model for TNP use are indicated in grey rectangular boxes and include (moving down from proximal to distal parameters) physical health symptoms (e.g., oral and respiratory symptoms), general physical condition (e.g., appearance and hygiene), functioning (physical, sexual, cognitive, emotional, and social functioning), and general health perceptions, which will be the most distal measure of health and functioning. The preparatory phase research also identified six conceptually-related domains (in dashed rectangular boxes), which are not direct indicators of health status but might influence the impact of TNP use on health and functioning. These include attitudinal variables (worry about the health risks of using TNPs and perceived dependence/fear of withdrawal symptoms associated with quitting smoking), and utilitarian ones (perceived appeal, satisfaction, and benefits of TNP use). In addition, personal factors (e.g., sociodemographic) and environmental factors (e.g., peer/family influence, policies and regulations and sociocultural context) are also reflected in the conceptual framework as indirect indicators of health and functioning.
The framework further indicates that specific behavioral indicators (i.e., TNP use patterns over time) might influence any impact of TNP use on health and functioning. Whilst other causal and reciprocal relationships and hierarchies might exist within the domains, these are not explicitly characterized in this initial draft of the framework and will have to be tested with further empirical data. Finally, identified biomarkers of potential harm (in italics and dashed box) are also integrated in this conceptual framework as part of the conceptually-related domains, because they are on a causal pathway between TNP use and changes in health and functioning [ 133 , 134 ]. Biomarkers are not part of the measurement model that will be considered for a new self-report measure; however, because they are the most proximal parameters to health and functioning, they will be assessed independently as appropriate endpoints by objective clinical or biological analyses.
Triangulation of published literature, reanalysis of qualitative data, and expert opinion helped develop the presented preliminary conceptual framework as the foundation for a new measure to assess the impact of TNPs on self-reported health and functioning. This is essential for identifying relevant concepts and understanding what is important to measure in people who use TNPs. The findings reveal the importance of not only the perceived impacts of TNP use on physical health and physical functioning, but also on aspects of mental health and social interactions and functioning, and general perceptions of health and health-related quality of life.
For the literature review, the WHO ICF [ 28 ] and Wilson and Cleary model [ 29 , 30 ] served as useful guides to develop categories for data abstraction. The scoping literature review yielded 97 articles on TNP use and the relationship to health, perceptions of health, social and individual functioning, and quality of life. Overall, most studies had focused on the known negative effects of cigarette smoking (e.g., mental, respiratory, and oral health) and the rationale and motivation to quit smoking. The WHO ICF and Wilson and Clearly models were not always sufficient for identifying the breadth of relevant concepts, especially from the perspective of TNP use. Development of new codes for the reanalysis of existing qualitative data allowed for the development, extension, and exploration of the topic and provided valuable insights reported in the qualitative data reanalysis, such as the perceived benefits/satisfaction from cigarette smoking, and the rationale for quitting smoking or switching to an RRP. The findings show how this manner of secondary analysis can be valuable in health-related fields where the topic is broad and an existing body of knowledge can contribute by offering a different perspective [ 135 ].
The presentation of the preliminary conceptual framework from this preparatory phase is specific to TNP use and marks a slight departure from the established norms and characterization of the variables typically observed in existing generic health and functioning and health-related quality of life models, such as the WHO ICF and Wilson and Clearly models. Notably, specific hypothesized relationships and the hierarchy between domains are not explicitly characterized in the current draft of the framework. The framework provided an exploratory representation of the current findings to reflect a measurement instrument in people who use TNPs that would ideally be able to assess and demonstrate improvements in self-reported health and functioning status, stability of perceived positive aspects of using TNPs, and no worsening in key areas of physical and emotional health and functioning upon switching to RRPs. Nevertheless, the framework could still undergo further refinement to support the development and validation of a new measure and to further characterize and test the relationships and hierarchies between domains.
This work is not without limitations. For the scoping literature review, among the reviewed articles, not many reported on the use of e-cigarettes and other alternative tobacco or nicotine-delivery devices, because most studies had focused exclusively on cigarettes. It is possible that concepts associated with health and functioning that are relevant to other TNPs were not identified. This is most likely the consequence of the large number of publications related to cigarette use. Some concepts might also have been missed, given the large evidence base on health and functioning-related themes and concepts. However, this was also not a systematic literature search; a scoping review is generally broader than a systematic review in terms of the former having a less-defined research question, broader inclusion and exclusion criteria, and no systematic appraisal of study quality [ 26 ]. Nevertheless, the present scoping review methodology provides a lens on the overall evidence base, and regular updates on the search—specifically related to RRPs and novel TNPs and their health and functioning impacts—could be considered for fully understanding the evolving state of the art in this context. The reanalysis of existing qualitative data also has limitations related to data fit and completeness of preexisting data [ 136 ]. The insights collected from these reanalyzed studies were originally for a different purpose several years prior to the present research, and this might not completely and accurately reflect the objectives of the new project.
Considering the findings of the current research, the development of a health and functioning measure can continue to follow the FDA’s Guidance on PRO measures. As specified within the guideline, gaining input directly from the intended use populations through concept elicitation is a critical activity for ensuring content validity during the development of any new self-reported measure [ 137 ]. Continuous engagement with an expert panel can also support the refinement of the conceptual framework as well as the development of the draft and final measure.
The goal of this research was to identify from varied research activities key concepts and aspects of health and functioning and related changes associated with the use of TNPs. The resulting preliminary conceptual framework provides the basis for informing future research to further understand health and functioning concepts important to measure in individual who switch to RRPs and to develop a new self-report measure to assess this from the consumers’ perspective.
Availability of data and materials
All data generated or analyzed during this study are included in this published article and its supplementary information files.
Abbreviations
Assessment of Behavioral OUtcomes related to Tobacco and Nicotine Products Toolbox
Chronic obstructive pulmonary disease
Food and Drug Administration
Health-related quality of life
International Classification of Functioning, Disability and Health
- Modified risk tobacco products
Nicotine replacement therapy
Patient-Reported Outcomes
Quality of life
Reduced-risk products
Rapid Evidence Mapping
- Tobacco and/or nicotine products
United Kingdom
United States
36-Item Short-Form Health Survey
World Health Organization
U.S. Department of Health and Human Services. The health consequences of smoking: 50 years of progress. A report of the surgeon general. Atlanta, GA: U.S. Department of Health and Human Services, Centers for Disease Control and Prevention, National Center for Chronic Disease Prevention and Health Promotion, Office on Smoking and Health; 2014.
Google Scholar
Danaei G, Ding EL, Mozaffarian D, Taylor B, Rehm J, Murray CJL, et al. The preventable causes of death in the United States: comparative risk assessment of dietary, lifestyle, and metabolic risk factors. PLoS Med. 2009;6(4):e1000058.
Article PubMed PubMed Central Google Scholar
Bilano V, Gilmour S, Moffiet T, d’Espaignet ET, Stevens GA, Commar A, et al. Global trends and projections for tobacco use, 1990–2025: an analysis of smoking indicators from the WHO Comprehensive Information Systems for Tobacco Control. Lancet. 2015;385(9972):966–76.
Article PubMed Google Scholar
Zeller M, Hatsukami D. The strategic dialogue on tobacco harm reduction: a vision and blueprint for action in the US. Tob Control. 2009;18(4):324–32.
Food and Drug Administration. Modified risk orders 2020. https://www.fda.gov/tobacco-products/advertising-and-promotion/modified-risk-orders .
Food and Drug Administration. Modified risk tobacco product applications: draft guidance for industry 2012. https://www.fda.gov/regulatory-information/search-fda-guidance-documents/modified-risk-tobacco-product-applications .
Institute of Medicine. Scientific standards for studies on modified risk tobacco products. Washington, DC: The National Academies Press; 2012. p. 370.
Stratton K, Kwan LY, Eaton DL, editors. National Academies of Sciences Engineering and Medicine. Public health consequences of e-cigarettes. Washington, DC: The National Academies Press; 2018. p. 774.
Lappas AS, Tzortzi AS, Konstantinidi EM, Teloniatis SI, Tzavara CK, Gennimata SA, et al. Short-term respiratory effects of e-cigarettes in healthy individuals and smokers with asthma. Respirology. 2018;23(3):291–7.
Meo SA, Ansary MA, Barayan FR, Almusallam AS, Almehaid AM, Alarifi NS, et al. Electronic cigarettes: impact on lung function and fractional exhaled nitric oxide among healthy adults. Am J Men’s Health. 2019;13(1):1557988318806073.
Article Google Scholar
Polosa R, Cibella F, Caponnetto P, Maglia M, Prosperini U, Russo C, et al. Health impact of E-cigarettes: a prospective 3.5-year study of regular daily users who have never smoked. Sci Rep. 2017;7(1):13825.
Article PubMed PubMed Central CAS Google Scholar
Sharman A, Zhussupov B, Sharman D, Kim I, Yerenchina E. Lung function in users of a smoke-free electronic device with HeatSticks (iQOS) versus smokers of conventional cigarettes: protocol for a longitudinal cohort observational study. JMIR Res Protoc. 2018;7(11):e10006.
Skotsimara G, Antonopoulos AS, Oikonomou E, Siasos G, Ioakeimidis N, Tsalamandris S, et al. Cardiovascular effects of electronic cigarettes: a systematic review and meta-analysis. Eur J Prev Cardiol. 2019;26(11):1219–28.
Wang JB, Olgin JE, Nah G, Vittinghoff E, Cataldo JK, Pletcher MJ, et al. Cigarette and e-cigarette dual use and risk of cardiopulmonary symptoms in the Health eHeart Study. PLoS ONE. 2018;13(7):e0198681.
Ware JE Jr, Gandek B, Kulasekaran A, Guyer R. Evaluation of smoking-specific and generic quality of life measures in current and former smokers in Germany and the United States. Health Qual Life Outcomes. 2015;13:128.
Frendl DM, Ware JE Jr. Patient-reported functional health and well-being outcomes with drug therapy: a systematic review of randomized trials using the SF-36 health survey. Med Care. 2014;52(5):439–45.
Goldenberg M, Danovitch I, IsHak WW. Quality of life and smoking. Am J Addict. 2014;23(6):540–62.
Olufade AO, Shaw JW, Foster SA, Leischow SJ, Hays RD, Coons SJ. Development of the smoking cessation quality of life questionnaire. Clin Ther. 1999;21(12):2113–30.
Article CAS PubMed Google Scholar
Sarna L, Bialous SA, Cooley ME, Jun HJ, Feskanich D. Impact of smoking and smoking cessation on health-related quality of life in women in the Nurses’ Health Study. Qual Life Res. 2008;17(10):1217–27.
Kulasekaran A, Proctor C, Papadopoulou E, Shepperd CJ, Guyer R, Gandek B, et al. Preliminary evaluation of a new german translated tobacco quality of life impact tool to discriminate between healthy current and former smokers and to explore the effect of switching smokers to a reduced toxicant prototype cigarette. Nicotine Tob Res. 2015;17(12):1456–64.
Edelen MO. The PROMIS smoking assessment toolkit–background and introduction to supplement. Nicotine Tob Res. 2014;16(Suppl 3):S170–4.
Shaw JW, Coons SJ, Foster SA, Leischow SJ, Hays RD. Responsiveness of the Smoking Cessation Quality of Life (SCQoL) questionnaire. Clin Ther. 2001;23(6):957–69.
Chrea C, Acquadro C, Afolalu EF, Spies E, Salzberger T, Abetz-Webb L, et al. Developing fit-for-purpose self-report instruments for assessing consumer responses to tobacco and nicotine products: the ABOUT Toolbox initiative. F1000Res. 2018;7:1878.
Food and Drug Administration. Patient-reported outcome measures: use in medical product development to support labeling claims 2009. https://www.fda.gov/regulatory-information/search-fda-guidance-documents/patient-reported-outcome-measures-use-medical-product-development-support-labeling-claims .
Food and Drug Administration. Patient-focused drug development: methods to identify what is important to patients guidance for industry, Food and Drug Administration Staff, and Other Stakeholders 2019. https://www.fda.gov/regulatory-information/search-fda-guidance-documents/patient-focused-drug-development-methods-identify-what-important-patients-guidance-industry-food-and .
Munn Z, Peters MDJ, Stern C, Tufanaru C, McArthur A, Aromataris E. Systematic review or scoping review? Guidance for authors when choosing between a systematic or scoping review approach. BMC Med Res Methodol. 2018;18(1):143.
Lam J, Howard BE, Thayer K, Shah RR. Low-calorie sweeteners and health outcomes: a demonstration of rapid evidence mapping (rEM). Environ Int. 2019;123:451–8.
World Health Organisation. International classification of functioning, disability and health (ICF) 2001. https://www.who.int/classifications/icf/en/ .
Ferrans CE, Zerwic JJ, Wilbur JE, Larson JL. Conceptual model of health-related quality of life. J Nurs Scholarsh. 2005;37(4):336–42.
Wilson IB, Cleary PD. Linking clinical variables with health-related quality of life. A conceptual model of patient outcomes. JAMA. 1995;273(1):59–65.
Karimi M, Brazier J. Health, health-related quality of life, and quality of life: what is the difference? Pharmacoeconomics. 2016;34(7):645–9.
Moons P. Why call it health-related quality of life when you mean perceived health status? Eur J Cardiovasc Nurs. 2004;3(4):275–7.
Cano S, Chrea C, Salzberger T, Alfieri T, Emilien G, Mainy N, et al. Development and validation of a new instrument to measure perceived risks associated with the use of tobacco and nicotine-containing products. Health Qual Life Outcomes. 2018;16(1):192.
Salzberger T, Chrea C, Cano SJ, Martin M, Atkison M, Emilien G, et al. Perceived risks associated with the use of tobacco and nicotine-containing products: findings from qualitative research. Tobacco Sci Technol. 2017;50:32–42.
Chrea C, Salzberger T, Abetz-Webb L, Afolalu EF, Cano S, Rose J, et al. PRM183—development of a fit-for-purpose tobacco and nicotine products dependence instrument. Barcelona: ISPOR Europe; 2018.
Book Google Scholar
Quirkos 2.3.1 [Computer Software] 2020. https://www.quirkos.com .
Abu-Helalah MA, Alshraideh HA, Al-Serhan AA, Nesheiwat AI, Da’na M, Al-Nawafleh A. Epidemiology, attitudes and perceptions toward cigarettes and hookah smoking amongst adults in Jordan. Environ Health Prev Med. 2015;20(6):422–33.
Akturk E, Yağmur J, Açıkgöz N, Ermi N, Cansel M, Karaku Y, et al. Assessment of atrial conduction time by tissue Doppler echocardiography and P-wave dispersion in smokers. J Interv Card Electrophysiol. 2012;34(2):247–53.
Aubin HJ, Peiffer G, Stoebner-Delbarre A, Vicaut E, Jeanpetit Y, Solesse A, et al. The French Observational Cohort of Usual Smokers (FOCUS) cohort: French smokers perceptions and attitudes towards smoking cessation. BMC Public Health. 2010;10(100):1–8.
Becoña E, Vázquez MI, Míguez MC, Fernández del Río E, López-Durán A, Martínez Ú, et al. Smoking habit profile and health-related quality of life. Psicothema. 2013;25(4):421–6.
PubMed Google Scholar
Bennasar Veny M, Pericas Beltrán J, González Torrente S, Segui González P, Aguiló Pons A, Tauler RP. Self-perceived factors associated with smoking cessation among primary health care nurses: a qualitative study. Rev Latino-Am Enfermagem. 2011;19(6):1437–44.
Bommelé J, Schoenmakers TM, Kleinjan M, van Straaten B, Wits E, Snelleman M, et al. Perceived pros and cons of smoking and quitting in hard-core smokers: a focus group study. BMC Public Health. 2014;14:175–85.
Borland R, Yong HH, O’Connor RJ, Hyland A, Thompson ME. The reliability and predictive validity of the Heaviness of Smoking Index and its two components: findings from the International Tobacco Control Four Country study. Nicotine Tob Res. 2010;12(Suppl 1):S45-50.
Bot M, Vink J, Milaneschi Y, Smit JH, Kluft C, Neuteboom J, et al. Plasma cotinine levels in cigarette smokers: impact of mental health and other correlates. Eur Addict Res. 2014;20(4):183–91.
Brody AL, Olmstead RE, Abrams AL, Costello MR, Khan A, Kozman D, et al. Effect of a history of major depressive disorder on smoking-induced dopamine release. Biol Psychiatry. 2009;66(9):898–901.
Article CAS PubMed PubMed Central Google Scholar
Brook DW, Rubenstone E, Zhang C, Brook JS. Trajectories of cigarette smoking in adulthood predict insomnia among women in late mid-life. Sleep Med. 2012;13(9):1130–7.
Brotman RM, He X, Gajer P, Fadrosh D, Sharma E, Mongodin EF, et al. Association between cigarette smoking and the vaginal microbiota: a pilot study. BMC Infect Dis. 2014;14:471–82.
Brown-Johnson CG, Cataldo JK, Orozco N, Lisha NE, Hickman NJ 3rd, Prochaska JJ. Validity and reliability of the Internalized Stigma of Smoking Inventory: an exploration of shame, isolation, and discrimination in smokers with mental health diagnoses. Am J Addict. 2015;24(5):410–8.
Bush T, Hsu C, Levine MD, Magnusson B, Miles L. Weight gain and smoking: perceptions and experiences of obese quitline participants. BMC Public Health. 2014;14:1229.
Caldirola D, Cavedini P, Riva A, Di Chiaro NV, Perna G. Cigarette smoking has no pro-cognitive effect in subjects with obsessive-compulsive disorder: a preliminary study. Psychiatr Danub. 2016;28(1):86–90.
Caldirola D, Daccò S, Grassi M, Citterio A, Menotti R, Cavedini P, et al. Effects of cigarette smoking on neuropsychological performance in mood disorders: a comparison between smoking and nonsmoking inpatients. J Clin Psychiatry. 2013;74(2):e130–6.
Carpenter MJ, Gray KM. A pilot randomized study of smokeless tobacco use among smokers not interested in quitting: changes in smoking behavior and readiness to quit. Nicotine Tob Res. 2010;12(2):136–43.
Croucher R, Haque MF, Kassim S. Oral pain before and after smokeless tobacco cessation in U.K.-resident Bangladeshi women: cross-sectional analyses. Nicotine Tobacco Res. 2013;15(5):896–903.
Article CAS Google Scholar
Depp CA, Bowie CR, Mausbach BT, Wolyniec P, Thornquist MH, Luke JR, et al. Current smoking is associated with worse cognitive and adaptive functioning in serious mental illness. Acta Psychiatr Scand. 2015;131(5):333–41.
Ditre JW, Zale EL, Heckman BW, Hendricks PS. A measure of perceived pain and tobacco smoking interrelations: pilot validation of the pain and smoking inventory. Cogn Behav Ther. 2017;46(6):339–51.
Doiron M, Dupré N, Langlois M, Provencher P, Simard M. Smoking history is associated to cognitive impairment in Parkinson’s disease. Aging Ment Health. 2017;21(3):322–6.
Gonzalez A, Zvolensky MJ, Vujanovic AA, Leyro TM, Marshall EC. An evaluation of anxiety sensitivity, emotional dysregulation, and negative affectivity among daily cigarette smokers: relation to smoking motives and barriers to quitting. J Psychiatr Res. 2008;43(2):138–47.
Grogan S, Fry G, Gough B, Conner M. Smoking to stay thin or giving up to save face? Young men and women talk about appearance concerns and smoking. Br J Health Psychol. 2009;14(1):175–86.
Hawari FI, Obeidat NA, Ghonimat IM, Ayub HS, Dawahreh SS. The effect of habitual waterpipe tobacco smoking on pulmonary function and exercise capacity in young healthy males: A pilot study. Respir Med. 2017;122:71–5.
Heffernan TM, O’Neill TS, Moss M. Smoking-related prospective memory deficits in a real-world task. Drug Alcohol Depend. 2012;120(1–3):1–6.
Highland KB, McChargue DE. Stress-induced cardiovascular reactivity among African American smokers. Am J Health Behav. 2011;35(1):51–9.
Holley AL, Law EF, Tham SW, Myaing M, Noonan C, Strachan E, et al. Current smoking as a predictor of chronic musculoskeletal pain in young adult twins. J Pain. 2013;14(10):1131–9.
Ichikawa Y, Kitagawa K, Kato S, Dohi K, Hirano T, Ito M, et al. Altered coronary endothelial function in young smokers detected by magnetic resonance assessment of myocardial blood flow during the cold pressor test. Int J Cardiovasc Imaging. 2014;30:73–80.
Javed F, Abduljabbar T, Vohra F, Malmstrom H, Rahman I, Romanos GE. Comparison of periodontal parameters and self-perceived oral symptoms among cigarette smokers, individuals vaping electronic cigarettes, and never-smokers. J Periodontol. 2017;88(10):1059–65.
Johnson AL, McLeish AC. Differences in panic psychopathology between smokers with and without asthma. Psychol Health Med. 2017;22(1):110–20.
Kahler CW, Daughters SB, Leventhal AM, Rogers ML, Clark MA, Colby SM, et al. Personality, psychiatric disorders, and smoking in middle-aged adults. Nicotine Tob Res. 2009;11(7):833–41.
Karadoğan D, Önal Ö, Şahin D, Yazıcı S, Kanbay Y. Evaluation of school teachers’ sociodemographic characteristics and quality of life according to their cigarette smoking status: a cross-sectional study from eastern Black Sea region of Turkey. Tuberkuloz ve toraks. 2017;65(1):18–24.
Kim J, Gall SL, Dewey HM, Macdonell RA, Sturm JW, Thrift AG. Baseline smoking status and the long-term risk of death or nonfatal vascular event in people with stroke: a 10-year survival analysis. Stroke. 2012;43(12):3173–8.
Kim J, Lee S. Using focus group interviews to analyze the behavior of users of new types of tobacco products. J Prev Med Public Health. 2017;50(5):336–46.
Kralikova E, Novak J, West O, Kmetova A, Hajek P. Do e-cigarettes have the potential to compete with conventional cigarettes?: a survey of conventional cigarette smokers’ experiences with e-cigarettes. Chest. 2013;144(5):1609–14.
Lao XQ, Jiang CQ, Zhang WS, Adab P, Lam TH, Cheng KK, et al. Smoking, smoking cessation and inflammatory markers in older Chinese men: The Guangzhou Biobank Cohort Study. Atherosclerosis. 2009;203(1):304–10.
Lappan S, Thorne CB, Long D, Hendricks PS. Longitudinal and reciprocal relationships between psychological well-being and smoking. Nicotine Tob Res. 2018;22(1):18–23.
Article PubMed Central Google Scholar
Lynch ME, Johnson KC, Kable JA, Carroll J, Coles CD. Smoking in pregnancy and parenting stress: maternal psychological symptoms and socioeconomic status as potential mediating variables. Nicotine Tob Res. 2011;13(7):532–9.
Lyvers M, Carlopio C, Honours VB, Edwards MS. Mood, mood regulation, and frontal systems functioning in current smokers, long-term abstinent ex-smokers, and never-smokers. J Psychoactive Drugs. 2014;46(2):133–9.
Martin LM, Sayette MA. A review of the effects of nicotine on social functioning. Exp Clin Psychopharmacol. 2018;26(5):425–39.
Martinasek MP, McDermott RJ, Bryant CA. Antecedents of university students’ hookah smoking intention. Am J Health Behav. 2013;37(5):599–609.
Mattey DL, Dawson SR, Healey EL, Packham JC. Relationship between smoking and patient-reported measures of disease outcome in ankylosing spondylitis. J Rheumatol. 2011;38(12):2608–15.
McCann SJ. Subjective well-being, personality, demographic variables, and American state differences in smoking prevalence. Nicotine Tob Res. 2010;12(9):895–904.
McDonald EA, Ling PM. One of several “toys” for smoking: young adult experiences with electronic cigarettes in New York City. Tob Control. 2015;24(6):588–93.
McLeish AC, Zvolensky MJ, Del Ben KS, Burke RS. Anxiety sensitivity as a moderator of the association between smoking rate and panic-relevant symptoms among a community sample of middle-aged adult daily smokers. Am J Addict. 2009;18(1):93–9.
Melis M, Lobo SL, Ceneviz C, Ruparelia UN, Zawawi KH, Chandwani BP, et al. Effect of cigarette smoking on pain intensity of TMD patients: a pilot study. Cranio. 2010;28(3):187–92.
Memon A, Barber J, Rumsby E, Parker S, Mohebati L, de Visser RO, et al. What factors are important in smoking cessation and relapse in women from deprived communities? A qualitative study in Southeast England. Public Health. 2016;134:39–45.
Mendiondo MS, Alexander LA, Crawford T. Health profile differences for menthol and non-menthol smokers: findings from the National Health Interview Survey. Addiction. 2010;105(Suppl 1):124–40.
Miyatake N, Numata T, Nishii K, Sakano N, Suzue T, Hirao T, et al. Influence of cigarette smoking on estimated glomerular filtration rate (eGFR) in Japanese male workers. Acta Med Okayama. 2010;64:385–90.
CAS PubMed Google Scholar
Mohammadi S, Mazhari MM, Mehrparvar AH, Attarchi MS. Cigarette smoking and occupational noise-induced hearing loss. Eur J Public Health. 2009;20(4):452–5.
Mohammadnezhad M, Tsourtos G, Wilson C, Ratcliffe J, Ward P. “I have never experienced any problem with my health. So far, it hasn’t been harmful”: older Greek-Australian smokers’ views on smoking: a qualitative study. BMC Public Health. 2015;15:304–15.
Morris MC, Mielock AS, Rao U. Salivary stress biomarkers of recent nicotine use and dependence. Am J Drug Alcohol Abuse. 2016;42(6):640–8.
Muir S, Marshall B. Changes in health perceptions of male prisoners following a smoking cessation program. J Correct Health Care. 2016;22(3):247–56.
Nelson JP, Pederson LL, Lewis J. Tobacco use in the Army: illuminating patterns, practices, and options for treatment. Mil Med. 2009;174(2):162–9.
Nemeth JM, Liu ST, Klein EG, Ferketich AK, Kwan MP, Wewers ME. Factors influencing smokeless tobacco use in rural Ohio Appalachia. J Community Health. 2012;37(6):1208–17.
Nikcevic AV, Spada MM. Metacognitions about smoking: a preliminary investigation. Clin Psychol Psychother. 2010;17(6):536–42.
Nunes SO, Vargas HO, Brum J, Prado E, Vargas MM, de Castro MR, et al. A comparison of inflammatory markers in depressed and nondepressed smokers. Nord J Psychiatry. 2012;14:540–6.
CAS Google Scholar
Oh DL, Heck JE, Dresler C, Allwright S, Haglund M, Del Mazo SS, et al. Determinants of smoking initiation among women in five European countries: a cross-sectional survey. BMC Public Health. 2010;10(74):1–11.
Pasco JA, Williams LJ, Jacka FN, Ng F, Henry MJ, Nicholson GC, et al. Tobacco smoking as a risk factor for major depressive disorder: population-based study. Br J Psychiatry. 2008;193(4):322–6.
Pina JA, Namba MD, Leyrer-Jackson JM, Cabrera-Brown G, Gipson CD. Social influences on nicotine-related behaviors. Int Rev Neurobiol. 2018;140:1–32.
Poole-Di Salvo E, Liu YH, Brenner S, Weitzman M. Adult household smoking is associated with increased child emotional and behavioral problems. J Dev Behav Pediatr. 2010;31(2):107–15.
Rahman MA, Mahmood MA, Spurrier N, Rahman M, Choudhury SR, Leeder S. Why do Bangladeshi people use smokeless tobacco products? Asia-Pacific J Public Health. 2015;27(2):NP2197–209.
Rollini F, Franchi F, Cho JR, Degroat C, Bhatti M, Ferrante E, et al. Cigarette smoking and antiplatelet effects of aspirin monotherapy versus clopidogrel monotherapy in patients with atherosclerotic disease: results of a prospective pharmacodynamic study. J Cardiovasc Transl Res. 2014;7(1):53–63.
Romijnders K, van Osch L, de Vries H, Talhout R. Perceptions and reasons regarding e-cigarette use among users and non-users: a narrative literature review. Int J Environ Res Public Health. 2018;15(6):1190.
Rooke C, Cunningham-Burley S, Amos A. Smokers’ and ex-smokers’ understanding of electronic cigarettes: a qualitative study. Tob Control. 2016;25:e60–6.
Roth B, Bengtsson M, Ohlsson B. Diarrhoea is not the only symptom that needs to be treated in patients with microscopic colitis. Eur J Intern Med. 2013;24(6):573–8.
Sales MP, Oliveira MI, Mattos IM, Viana CM, Pereira ED. The impact of smoking cessation on patient quality of life. J Bras Pneumol. 2009;35(5):436–41.
Schane RE, Prochaska JJ, Glantz SA. Counseling nondaily smokers about secondhand smoke as a cessation message: a pilot randomized trial. Nicotine Tob Res. 2013;15(2):334–42.
Schnoll RA, Goren A, Annunziata K, Suaya JA. The prevalence, predictors and associated health outcomes of high nicotine dependence using three measures among US smokers. Addiction. 2013;108(11):1989–2000.
Segarra R, Zabala A, Eguíluz JI, Ojeda N, Elizagarate E, Sánchez P, et al. Cognitive performance and smoking in first-episode psychosis: the self-medication hypothesis. Eur Arch Psychiatry Clin Neurosci. 2011;261(4):241–50.
Sherratt FC, Newson L, Marcus MW, Field JK, Robinson J. Perceptions towards electronic cigarettes for smoking cessation among Stop Smoking Service users. Br J Health Psychol. 2015;21(2):421–33.
Soneji SS, Sung HY, Primack BA, Pierce JP, Sargent JD. Quantifying population-level health benefits and harms of e-cigarette use in the United States. PLoS ONE. 2018;13(3):e0193328.
Talati A, Wickramaratne PJ, Keyes KM, Hasin DS, Levin FR, Weissman MM. Smoking and psychopathology increasingly associated in recent birth cohorts. Drug Alcohol Depend. 2013;133(2):724–32.
Tan QH. Smoking spaces as enabling spaces of wellbeing. Health Place. 2013;24:173–82.
Tatullo M, Gentile S, Paduano F, Santacroce L, Marrelli M. Crosstalk between oral and general health status in e-smokers. Medicine. 2016;95(49):e5589.
Taylor G, McNeill A, Girling A, Farley A, Lindson-Hawley N, Aveyard P. Change in mental health after smoking cessation: systematic review and meta-analysis. BMJ. 2014;348:g1151-g.
Thompson TP, Greaves CJ, Ayres R, Aveyard P, Warren FC, Byng R, et al. An exploratory analysis of the smoking and physical activity outcomes from a pilot randomized controlled trial of an exercise assisted reduction to stop smoking intervention in disadvantaged groups. Nicotine Tob Res. 2016;18(3):289–97.
Torigian DA, Green-McKenzie J, Liu X, Shofer FS, Werner T, Smith CE, et al. A study of the feasibility of FDG-PET/CT to systematically detect and quantify differential metabolic effects of chronic tobacco use in organs of the whole body—a prospective pilot study. Acad Radiol. 2017;24:930–40.
Ulvik A, Ebbing M, Hustad S, Midttun Ø, Nygård O, Vollset SE, et al. Long- and short-term effects of tobacco smoking on circulating concentrations of B vitamins. Clin Chem. 2010;56(5):755–63.
Vidrine JI, Businelle MS, Cinciripini P, Li Y, Marcus MT, Waters AJ, et al. Associations of mindfulness with nicotine dependence, withdrawal, and agency. Subst Abus. 2009;30(4):318–27.
Volkman JE, DeRycke EC, Driscoll MA, Becker WC, Brandt CA, Mattocks KM, et al. Smoking status and pain intensity among OEF/OIF/OND veterans. Pain Med. 2015;16(9):1690–6.
Vujanovic AA, Marshall-Berenz EC, Beckham JC, Bernstein A, Zvolensky MJ. Posttraumatic stress symptoms and cigarette deprivation in the prediction of anxious responding among trauma-exposed smokers: a laboratory test. Nicotine Tob Res. 2010;12(11):1080–8.
Wadsworth E, Neale J, McNeill A, Hitchman SC. How and why do smokers start using e-cigarettes? Qualitative study of Vapers in London, UK. Int J Environ Res Public Health. 2016;13(7):661–74.
Article PubMed Central CAS Google Scholar
Xie X, Dijkstra AE, Vonk JM, Oudkerk M, Vliegenthart R, Groen HJ. Chronic respiratory symptoms associated with airway wall thickening measured by thin-slice low-dose CT. Am J Roentgenol. 2014;203(4):W383–90.
Yang T, Shiffman S, Rockett IR, Cui X, Cao R. Nicotine dependence among Chinese city dwellers: a population-based cross-sectional study. Nicotine Tob Res. 2011;13(7):556–64.
Yu A, Cai X, Zhang Z, Shi H, Liu D, Zhang P, et al. Effect of nicotine dependence on opioid requirements of patients after thoracic surgery. Acta Anaesthesiol Scand. 2015;59(1):115–22.
Zhang X, Kahende J, Fan AZ, Barker L, Thompson TJ, Mokdad AH, et al. Smoking and visual impairment among older adults with age-related eye diseases. Prev Chronic Dis. 2011;8(4):A84.
PubMed PubMed Central Google Scholar
Zhao L, Xu L, Lai Y, Che C, Zhou Y. Temporal changes of smoking status and motivation in Chinese patients with hepatitis B: relationship with anxiety and depression. J Clin Nurs. 2012;21(15–16):2193–201.
Zorlu N, Cropley VL, Zorlu PK, Delibas DH, Adibelli ZH, Baskin EP, et al. Effects of cigarette smoking on cortical thickness in major depressive disorder. J Psychiatr Res. 2017;84:1–8.
Miyatake N, Numata T, Nishii K, Sakano N, Suzue T, Hirao T, et al. Relation between cigarette smoking and ventilatory threshold in the Japanese. Environ Health Prev Med. 2011;16(3):185–90.
Bjørngaard JH, Gunnell D, Elvestad MB, Davey Smith G, Skorpen F, Krokan H, et al. The causal role of smoking in anxiety and depression: a Mendelian randomization analysis of the HUNT study. Psychol Med. 2012;43(4):711–9.
Chen SC, Chen HF, Peng HL, Lee LY, Chiang TY, Chiu HC. Psychometric testing of the Chinese-Version Glover-Nilsson Smoking Behavioral Questionnaire (GN-SBQ-C) for the identification of nicotine dependence in adult smokers in Taiwan. Int J Behav Med. 2017;24(2):272–9.
Doran N, Spring B, McChargue D, Pergadia M, Richmond M. Impulsivity and smoking relapse. Nicotine Tob Res. 2004;6:641–7.
Haller CS, Etter JF, Courvoisier DS. Trajectories in cigarette dependence as a function of anxiety: a multilevel analysis. Drug Alcohol Depend. 2014;139:115–20.
Jamal M, Van der Does AJW, Cuijpers P, Penninx BW. Association of smoking and nicotine dependence with severity and course of symptoms in patients with depressive or anxiety disorder. Drug Alcohol Depend. 2012;126(1–2):138–46.
Krishnan-Sarin S, Reynolds B, Duhig A, Smith A, Liss T, McFetridge A, et al. Behavioral impulsivity predicts treatment outcome in a smoking cessation program for adolescent smokers. Drug Alcohol Depend. 2007;88:79–82.
Tidey JW, Pacek LR, Koopmeiners JS, Vandrey R, Nardone N, Drobes DJ, et al. Effects of 6-week use of reduced-nicotine content cigarettes in smokers with and without elevated depressive symptoms. Nicotine Tob Res. 2017;19(Suppl 1):59–67.
Chang CM, Cheng YC, Cho TM, Mishina EV, Del Valle-Pinero AY, van Bemmel DM, et al. Biomarkers of potential harm: summary of an FDA-sponsored public workshop. Nicotine Tob Res. 2019;21(1):3–13.
Haziza C, de La Bourdonnaye G, Donelli A, Skiada D, Poux V, Weitkunat R, et al. Favorable changes in biomarkers of potential harm to reduce the adverse health effects of smoking in smokers switching to the menthol tobacco heating system 2.2 for three months (part 2). Nicotine Tob Res. 2019;22(4):549–59.
Bishop L, Kuula-Luumi A. Revisiting qualitative data reuse: a decade on. SAGE Open. 2017;7(1):1–15.
Sherif V. Evaluating preexisting qualitative research data for secondary analysis. In: Forum: qualitative social research, vol. 19, no. 2. 2018.
Patrick D, Burke L, Gwaltney C, Leidy N, Martin M, Molsen E, et al. Content validity—establishing and reporting the evidence in newly developed patient-reported outcomes (PRO) instruments for medical product evaluation: ISPOR PRO good research practices task force report: part 1—eliciting concepts for a new PRO instrument. Value Health. 2011;14(8):967–77.
Download references
Acknowledgements
We thank the team at Sciome LLC for their assistance and contribution to the literature review. We thank Vivienne Law and David Floyd for their contributions to the literature review, reanalysis of qualitative data, and assistance with review of the draft manuscript. We thank Catherine Acquadro for her review of the draft manuscript. We also thank John Ware, Jed Rose, Ashley Slagle, Donald Patrick, Karl Fagerström, Stefan Cano, and Thomas Salzberger for their input and review.
Philip Morris International is the sole source of funding and sponsor of this research.
Author information
Authors and affiliations.
PMI R&D, Philip Morris Product S.A., Quai Jeanrenaud 5, 2000, Neuchâtel, Switzerland
Esther F. Afolalu, Erica Spies, Agnes Bacso, Emilie Clerc, Sophie Gallot & Christelle Chrea
Patient-Centered Outcomes Assessments Ltd., 1 Springbank, Bollington, Macclesfield, Cheshire, SK10 5LQ, UK
Linda Abetz-Webb
You can also search for this author in PubMed Google Scholar
Contributions
EA, ES and CC performed conceptualization. EA, ES and LA-W performed methodology. EA, ES, SG, EC and LA-W were involved in the investigation. EA and ES were involved in writing—original draft. EA, EC, LA-W and CC were involved in writing—review & editing. EA performed visualization. ES and CC performed supervision. AB, EC and SG were involved in data curation. AB and EC were involved in project administration. LA-W performed formal analysis. CC was involved in funding acquisition. All authors read and approved the final manuscript.
Corresponding author
Correspondence to Esther F. Afolalu .
Ethics declarations
Ethics approval and consent to participate.
Not applicable.
Consent for publication
Competing interests.
Esther F. Afolalu, Emilie Clerc, and Christelle Chrea are employees of Philip Morris International. Agnes Bacso, Erica Spies, and Sophie Gallot completed the work during prior employment with Philip Morris International. Linda Abetz-Webb is a consultant for Philip Morris International.
Additional information
Publisher's note.
Springer Nature remains neutral with regard to jurisdictional claims in published maps and institutional affiliations.
Work completed during prior affiliation with PMI R&D: Erica Spies, Agnes Bacso, Sophie Gallot.
Supplementary Information
Additional file 1..
Summary tables of results of scoping literature review
Rights and permissions
Open Access This article is licensed under a Creative Commons Attribution 4.0 International License, which permits use, sharing, adaptation, distribution and reproduction in any medium or format, as long as you give appropriate credit to the original author(s) and the source, provide a link to the Creative Commons licence, and indicate if changes were made. The images or other third party material in this article are included in the article's Creative Commons licence, unless indicated otherwise in a credit line to the material. If material is not included in the article's Creative Commons licence and your intended use is not permitted by statutory regulation or exceeds the permitted use, you will need to obtain permission directly from the copyright holder. To view a copy of this licence, visit http://creativecommons.org/licenses/by/4.0/ . The Creative Commons Public Domain Dedication waiver ( http://creativecommons.org/publicdomain/zero/1.0/ ) applies to the data made available in this article, unless otherwise stated in a credit line to the data.
Reprints and permissions
About this article
Cite this article.
Afolalu, E.F., Spies, E., Bacso, A. et al. Impact of tobacco and/or nicotine products on health and functioning: a scoping review and findings from the preparatory phase of the development of a new self-report measure. Harm Reduct J 18 , 79 (2021). https://doi.org/10.1186/s12954-021-00526-z
Download citation
Received : 14 October 2020
Accepted : 21 July 2021
Published : 30 July 2021
DOI : https://doi.org/10.1186/s12954-021-00526-z
Share this article
Anyone you share the following link with will be able to read this content:
Sorry, a shareable link is not currently available for this article.
Provided by the Springer Nature SharedIt content-sharing initiative
- Health and functioning
- Scoping review
- Qualitative research
- Conceptual framework
Harm Reduction Journal
ISSN: 1477-7517
- Submission enquiries: [email protected]
- Research article
- Open access
- Published: 30 June 2021
The negative impact of chronic tobacco smoking on adult neuropsychological function: a cross-sectional study
- Mohammed Sh. Nadar ORCID: orcid.org/0000-0003-4281-5630 1 ,
- Abdullah M. Hasan 2 &
- Mohammed Alsaleh 2
BMC Public Health volume 21 , Article number: 1278 ( 2021 ) Cite this article
5056 Accesses
13 Citations
2 Altmetric
Metrics details
The evidence on the effects of chronic tobacco smoking on neuropsychological functions is conflicting. The literature remains limited by inconsistent accounting for potentially confounding biomedical and psychiatric conditions. This study aimed to assess the neuropsychological functions of adult chronic tobacco smokers in comparison to group-matched non-smokers.
The study included 73 smokers and 84 group-matched non-smokers. The data was collected during the year 2019. After an initial interview to collect demographics and smoking profile, the subjects undertook neuropsychological assessments that targeted a wide range of cognitive domains.
The performance of smokers was poorer on almost all neuropsychological domains, namely selective attention ( p ≤ .001, p = .044), alternating attention ( p = .002) working memory ( p ≤ .001), Short-term memory ( p = .006 and .003), Long-term memory ( p ≤ .001), processing accuracy ( p ≤ .001), and executive function ( p = .011 and .026). Smokers were intact on processing speed. Smoking accumulation and lower age onset of regular smoking were correlated with lower neuropsychological function.
Our findings add to the growing body of evidence suggesting that chronic tobacco smoking impacts cognition negatively.
Peer Review reports
In 2015, around a quarter (24.9%) of the global population were current users of some form of tobacco [ 1 ]. Smoking is one form of tobacco exposure that is prevalent across the world.
The harmful impact of chronic tobacco smoking on physical health is well documented and includes cardiovascular diseases, respiratory diseases, and various forms of cancer [ 2 ]. Additionally, chronic smoking is implicated in the pathogenesis of neuropsychological dysfunction and has been directly linked to increased risk of depression and cognitive impairment [ 1 , 3 , 4 ].
A large number of studies have examined the effects of smoking on neuropsychological function across multiple variables. Compared to non-smokers, chronic smoking was cumulatively reported to have detrimental effects on various neuropsychological domains, including general intellectual abilities, processing speed, attention, memory, cognitive flexibility and executive functions [ 2 , 3 , 5 ]. The effects of smoking on cognition are believed to vary based on the dose and onset of regular use. Although the majority of studies provide evidence on the effect of chronic tobacco smoking on neuropsychological impairments, conflicting evidence also exists. For example, a 10-year longitudinal study of 1436 older adults found that smokers were less likely to develop cognitive impairment than those who had never smoked [ 6 ]. Another study consisting of 2553 adults found smokers to have lower rates of cognitive impairment compared to non-smokers [ 7 ], and both studies concluded that smoking may be protective of cognitive function. Several other studies also support the notion that cigarette smoking seems not to affect cognition or to have a positive effect on some aspects of cognitive function of smokers [ 8 , 9 , 10 , 11 ].
Based on the current literature, it is difficult to draw solid conclusions on the impact of smoking on neuropsychological function [ 2 ]. The influence of tobacco smoking on specific domains of cognition is complex and much remains to be known about its impact on neuropsychology and cognition. The literature remains limited by inconsistent accounting for potentially confounding biomedical and psychiatric conditions. For example, many of the studies did not account for confounding variables such as psychiatric disorders and comorbid substance abuse (i.e., alcohol, cannabis, and other drugs). In other studies, the duration of tobacco use was not taken into account [ 12 ]. Other studies varied in terms of which subcategories of specific neuropsychological domains were tested. Some controversy also remains regarding smoking effect on specific cognitive functions, and individual differences in smoking cognitive effects. Consequently, it is essential to continue to investigate the association between chronic tobacco smoking and potential neuropsychological impairments while controlling for possible confounding variables. This cross-sectional study aimed to assess the neuropsychological functions of chronic tobacco smokers in comparison to group-matched non-smokers. It was hypothesized that chronic tobacco smokers would have significantly poorer global neuropsychological functions compared to non-smokers.
Participants and procedure
We recruited the participants via social medial and local adverts during the year 2019. The participants were interviewed regarding cigarette smoking and use of other tobacco products. We excluded participants who reported current use of other substances that are known to affect cognition (i.e., alcohol, cannabis, and psychotropic medications, except for caffeine) up to 3 months prior to study enrollment. Since psychiatric illness is strongly correlated to cognitive impairment, we excluded subjects with any known psychiatric problems or mental diseases. Participants with any medical condition or history of serious head injury that are known to influence cognition were also excluded. To be included in the smoking group, the participant must have been a smoker for at least 10 years and smoked a minimum of one pack per day. The smokers and non-smokers were matched as a group for age, sex, ethnicity, educational, and socioeconomic status. Past smokers and second-hand smokers were excluded from the non-smokers’ group.
The study was approved by the Institutional Review Board, and all participants signed a written consent form to participate in the study before data collection. An initial interview collected information related to socio-demographic, health, and smoking profiles. After the interview session, all participants completed a comprehensive battery of outcome measures assessing neuropsychological functions that target a wide range of cognitive domains. All the measures used in this study have well-established and comprehensive psychometric properties, and were used in other studies on neuropsychological function (a brief description of each measure is provided in Table 1 ). In addition to the neuropsychological measures, we used the Grooved Pegboard (Lafayette Instrument, Lafayette, IN) as a measure of fine motor dexterity, which requires visual-spatial and motor coordination. The test battery was administered by a trained researcher, and the entire battery required about 60–70 min to complete. The participants were allowed short breaks between tests, and smokers were free to smoke during the breaks if desired. The sequence of outcome measures was administered consistently across all participants.
After completion of all measures, the participants were presented with two questions which they were required to answer by “Yes” or “No”. 1) “Do you believe that smoking increases the risk of physical health problems, such as getting heart disease, lung disease, stroke and cancer?”, and 2) “Do you believe that smoking increases the risk of cognitive health problems, such as reduced memory, attention, and concentration?”.
Statistical analysis
All statistical analysis was performed with Statistical Package for the Social Sciences (SPSS – Windows version 25; SPSS Inc., Chicago, IL). Comparison between groups of participants in terms of demographic characteristics and quantitative outcome measures were performed using t-tests for normally distributed data. Where homogeneity of variance was violated in a given model (Levine’s test), we used the Mann-Whitney U test for skewed data. Two-tailed statistics were used, and statistical significance was set at P < 0.05. Cohen’s D effect sizes were reported when significant effects of group on a cognitive variable were identified. Pearson correlations were used to assess the influences of the smoking variables of accumulation (number of years smoking), Cigarettes/day, and Pack-Years of smoking and decline in cognitive function.
The final sample included 73 smokers (M = 52.1 years, SD = 7.2) and 84 non-smokers (M = 51.7 years, SD = 8.1). Independent t-tests showed that both groups were comparable across participant socio-demographic and health characteristics. Table 2 shows the general characteristics of the study population.
Data for all neuropsychological tasks are summarized in Table 3 . In the Montreal Cognitive Assessment (MoCA), both groups performed above the cutoff score of 26/30, indicating global cognition scores within the “normal” range. Nonetheless, the smokers’ group scored significantly lower than the non-smokers’ group ( p = .042) with a moderate effect size of .49.
The Word condition (W) and Color condition (C) component of the Stroop Color and Word Test revealed no significant differences between both groups, demonstrating comparable processing speed abilities. The Color-word condition (CW) yielded a significant difference between groups ( p ≤ .001, d = .62) demonstrating better selective attention of non-smokers. The Comprehensive Trail making test (CTMT) Trial 1 subtest, which measures processing speed, revealed no significant differences between groups, but the Trial 5 subtest revealed a significant difference ( p = .002, d = .47) representing healthier alternating attention function of non-smokers. For the Wisconsin Card Sorting Test-64 (WCST-64), a small but significant effect size was detected in favor of the non-smokers’ group, showing a slightly better executive function capacity for non-smokers as evident by fewer Perseveration errors ( p < .011, d = .34) and Non-perseveration errors ( p < .026, d = 0.23).
In the Contextual Memory Test, the non-smokers’ group performed significantly better with small effect size for short-term memory ( p = .006, d = .38), and with moderate effect size for long-term memory ( p ≤ .001, d = .66). The Digit Span Task revealed comparable short-term memory results to the Contextual Memory Test, where the non-smokers’ group performed better than the smoking group ( p = .003, d = .40). For working memory, a significant and large effect size of .75 was detected in favor of the non-smokers’ group ( p ≤ .001).
The total number of items processed in the d2 Test of Attention was similar in both groups, indicating parallel processing speed. However, when factoring in the errors in performance, the smoking group had significantly poorer processing accuracy than their non-smokers’ counterpart in both measures of error (OE-Omission errors: p ≤ .001, d = .55, CE-Commission errors: p ≤ .001, d = .54, and TE-Total errors: p ≤ .001, d = .67). The overall TP-Total performance of the d2 Test of Attention was significant with a small effect size ( p = .044, d = .19) reflecting superior selective attention ability of the non-smokers’ group. As per the psychomotor domain, the non-smokers’ group outperformed the smoking group in the Grooved Pegboard test of fine motor dexterity ( p = .007, d = .37).
A set of Pearson correlations were conducted to explore the relationship between smoking variables with scores of the neuropsychological measures. Higher smoking accumulation (total lifetime years of smoking) was significantly correlated with the lower neuropsychological performance of selective attention of the Stroop Color and Word Test ( r = − 0.58; p = .009), alternating attention of the Comprehensive Trail making test (CTMT) ( r = − 0.32; p = .027), and working memory of the Digit Span task ( r = − 0.57; p ≤ .001). There was an association between lifetime years of smoking and poorer performance on the Grooved Pegboard psychomotor measure of fine motor dexterity ( r = −.41; p = .047). The age onset of regular smoking was also correlated with the lower performance in the same measures above; namely selective attention ( r = − 0.41; p = .037), alternating attention ( r = − 0.29; p = .048), working memory ( r = − 0.62; p ≤ .001), and also processing accuracy of the d2 Test of Attention ( r = − 0.46; p = .039). There were no other significant correlations between the number of cigarettes smoked per day or Pack-Years and neuropsychological performance.
When asking the participants about the effects of smoking on health, 100% of non-smokers and 92% of smokers believe that smoking has negative effects on physical health, while 37% of non-smokers and 19% of smokers believed that smoking has negative effects on cognitive health.
The current study aimed to assess and compare the neuropsychological functions of chronic tobacco smokers in comparison to non-smokers. As hypothesized, the results indicated that chronic tobacco smokers had significantly poorer neuropsychological functions compared to their group-matched non-smokers. The poorer performance was apparent in almost all cognitive domains, namely attention, memory, processing accuracy, and executive function, but not processing speed.
With respect to global neuropsychological function, the performance of smokers in the Montreal Cognitive Assessment (MoCA) was not impaired since they scored within the “normal” range. Nonetheless, their performance was significantly weaker compared to their matched non-smokers. Despite the “normal” results of the MoCA, the more dedicated measures in this study clearly revealed a sub-optimal neuropsychological performance of our smokers’ group.
The effects of smoking on memory performance is inconsistent in the literature, with studies reporting significant differences between smokers and non-smokers in some memory measures [ 4 , 21 , 22 , 23 , 24 , 25 ] while others reporting insignificant differences [ 10 , 21 , 22 , 26 , 27 , 28 ]. Our results showed the neuropsychological domain of memory to be affected in our smoking sample and with group differences of moderate to strong effect sizes, as our non-smokers’ group outperformed the smokers in all components of memory measures. The non-smokers’ group had better short-term and long-term memory as indicated by their superior capacity to recall information in the Contextual Memory Test, which involves visual presentation of pictures, as well as the Digit Span Task, which involves auditory and verbal presentation of numbers. Working memory was particularly compromised in our chronic tobacco smokers, as evident by the largest effect size (d = .75) amongst the neuropsychological measures employed in this study. Similar results for working memory impairments were found in middle-aged adults [ 22 , 29 ], young adults [ 30 , 31 , 32 , 33 ] and even adolescent smokers [ 34 ]. Based on the compromised overall memory performance of smokers, it is plausible to state that chronic tobacco smoking may predispose the development of dementia.
While the effects of tobacco smoking on memory have been widely studied, their effects on attention have been less investigated. Attention is central for learning and memory since encoding information requires attention in the first place. The smokers in our study were less able to block irrelevant information and focus their selective attention on the task at hand. This was strongly demonstrated in the Stroop Color and Word Test and less significantly demonstrated in the d2 Test of Attention. Alternating attention was also affected in our smokers’ CTMT test, indicating more difficulty to disengage and reengage the focus of attention in response to environmental stimuli, in comparison to the non-smokers’ group. Our findings on the domain of attention are consistent with the literature where attention was found to be affected in smokers [ 28 , 31 ], but contrasted several other studies where attention was not affected [ 10 , 26 , 33 , 34 ]. Given the intertwined relationship between attention and memory, it is reasonable to suggest that smoking may diminish memory as a result of decreasing attentional capacities, reflected in reduced ability to resist distraction and blocking irrelevant stimuli.
Executive function involves the simultaneous use of a set of cognitive abilities to allow the individual to perform higher-level complex tasks. The findings in our study detected a significant executive function difference in favor of the non-smokers’ group, who performed better in both subtests of the WCST-64. These results are consistent with previous literature findings of executive function limitation among smokers [ 9 , 10 , 22 , 23 , 25 , 26 , 31 , 35 ], indicating that chronic tobacco smokers have inferior mental flexibility and abstract thinking compared to non-smokers.
Interestingly, processing speed was not significantly affected in the smoking group across all the four subtests we used in this study, albeit two of the subtests were statistically borderline ( p = .052, .055). These findings agree with few studies [ 10 , 26 , 31 , 33 ], but contrast with most other studies that assessed processing speed for their smoking participants [ 4 , 21 , 22 , 36 , 37 , 38 ]. Despite this finding, the processing accuracy of our smokers’ sample was significantly lower than non-smokers ( p ≤ .001). That is, the smokers’ processing speed matched that of non-smokers, but with a significantly higher number of errors. The substandard performance of smokers on measures of processing accuracy, as evident by a significantly higher number of cognitive errors, should be added to a growing list of neuropsychological sequelae associated with persistent smoking.
Within the smoker group, smoking onset was correlated with lower performance on working memory, selective attention, alternating attention, and the number of errors made. This means that individuals who start smoking at a younger age are at a greater risk of developing neuropsychological dysfunction. The impairments appear to manifest very early in smokers, as demonstrated in the inferior working memory of adolescents with a mean of only 4 years of smoking [ 34 ]. Additional support on the effects of smoking on the young brain was objectively shown in functional magnetic resonance imaging where young adult smokers had reduced prefrontal cortex activation during attentional tasks when compared with non-smokers [ 39 ]. This pronounced negative effects of smoking on the young brain might be explained by the fact that the prefrontal cortex has not completed its maturation, as the prefrontal cortex is one of the last brain areas to mature and is still developing during adolescence and early adulthood [ 40 ]. This stage of ongoing development makes the brain more susceptible to the influence of tobacco smoking and other psychoactive substances [ 40 ].
Correspondingly, smoking accumulation in this study similarly affected neuropsychological function, meaning the longer an individual smoked during their lifetime, the more prone they become to cognitive dysfunction. These results are consistent with other studies that reported total lifetime years of smoking to be correlated with inferior neuropsychological efficiency [ 22 ] and executive function [ 27 ]. Interestingly, the number of cigarettes per day or pack-years were not associated with neuropsychological performance in this study, contrasting the main findings of a recent review conducted by Conti et al. (2019), in which several studies included in their review reported a negative link between the number of pack-years and neuropsychological function [ 2 ]. This discrepancy in results may be confounded by the considerable variations in the number of pack-years reported across studies (ranging from 4.26 to 73.73) [ 2 ].
The magnitude of differences between smokers and non-smokers in this study extend beyond neuropsychological function to include the psychomotor domain as well. Our non-smokers’ group outperformed the smoking group in the Grooved Pegboard test of fine motor dexterity, albeit with a relatively small effect size of .37. Durazzo et al. [ 22 ] also reported significantly poorer fine motor dexterity performance in their smoking sample, but with much larger effect sizes (i.e., .72). The psychomotor function was also negatively correlated with smoking accumulation, where longer lifetime smoking was associated with reduced fine motor dexterity.
The question arises related to the reasons for heterogeneity in study outcomes between studies of comparable designs. For example, processing speed was reported in some studies to be reduced among smokers [ 4 , 21 , 22 , 36 , 37 , 38 ] but not in other studies [ 10 , 26 , 31 , 33 ]. The same point can be raised for memory and attention. Several reasons could explain such variations in the performance; 1) The outcome measures used to assess the target variable (i.e., processing speed) are different across studies, which may vary in their sensitivity and other psychometric properties. 2) Some studies did not report which version of the tests they used (e.g., original version, modified version, pen and paper, or electronic version). Depending on the assessment used, it could involve a visual task, an auditory task, or even a motor task. An assessment that involves the use of pen and paper will recruit a variety of motor and cognitive pathways in the brain to facilitate writing or tracing, while an electronic version of the same assessment will involve different brain pathways. Such variations in assessment components could yield different results. These factors make it less accurate to perform cross-study comparisons or to compare the study scores with normative data. A possible solution to help makes the results more comparable across studies, and thus more clinically meaningful, is to use complete reporting of the specific testing procedures (e.g., test version, subtests used, and scoring methods) and to use consistent and systematic data collection and analysis procedures.
The participants awareness about the negative effects of smoking on physical health were very high. However, their awareness about the negative effects of smoking on neuropsychological health was low, particularly among smokes (37% of non-smokers and 19% of smokers). This very low awareness of the negative consequences of smoking on neuropsychological function among smokers and non-smokers is a major public health issue that should be properly addressed.
Since tobacco smoking remains highly prevalent across the globe and is known to be influenced by public perception of risk and associations with negative outcomes [ 31 ], it is imperative to invest further in policy initiatives to control smoking. The emphasis of public health campaigns primarily focuses on physical health, and less commonly address neuropsychological health. Increasing public awareness should go beyond the already established physical health consequences to include the negative impact of smoking on neuropsychological function. We hope that raising awareness about the wider effects of tobacco smoking on cognition could help encourage people to stop smoking.
Limitations and future research
There are several limitations and areas for improvement in future research. Although we attempted group matching for demographics and health variables, the self-reported health & medical profile can be problematic due to matters of inaccuracy of some participants. Future research should adopt standardized screening measures to provide accurate objective measures. The scope of neuropsychological impairment associated with chronic cigarette smoking has yet to be fully delineated. Large-scale prospective studies with more consistent, highly sensitive, and robust cognitive outcome measures are required to determine the true links between smoking and neuropsychological dysfunction.
Chronic tobacco smoking seems to be a prospective risk factor for neuropsychological impairment, as expressed in our data by reduced attention, memory, executive function, and processing accuracy of smokers compared to their group-matched non-smokers. The cognitive performance of participants decreased as the number of years smoking increased. Similarly, the younger the age when regular smoking started, the lower was the cognitive performance. The consequences of smoking go beyond neuropsychological performance to encompass fine motor dexterity tasks as well.
Availability of data and materials
The datasets used during the current study are available from the corresponding author on reasonable request.
Abbreviations
Montreal Cognitive Assessment
Comprehensive Trail making test
Wisconsin Card Sorting Test-64
World Health Organization, “WHO global report on trends in prevalence of tobacco use 2000–2025,” 2019.
Google Scholar
Conti AA, Lauren M, Serenella T, Steele JD, Baldacchino A. Chronic tobacco smoking and neuropsychological impairments: a systematic review and meta-analysis. Neurosci Biobehav Rev. 2019;96:143–54.
Article CAS PubMed Google Scholar
Waisman Campos M, Serebrisky D, Castaldelli-Maia JM. Smoking and cognition. Curr Drug Abuse Rev. 2016;9(76):1–4.
Richards M, Jarvis MJ, Thompson N, Wadsworth ME. Cigarette smoking and cognitive decline in midlife: evidence from a prospective birth cohort study. Am J Public Health. 2003;93(6):994–8. https://doi.org/10.2105/AJPH.93.6.994 .
Article PubMed PubMed Central Google Scholar
Durazzo TC, Meyerhoff DJ, Nixon SJ. Chronic cigarette smoking: implications for neurocognition and brain neurobiology. Int J Environ Res Public Health. 2010;7(10):3760–91. https://doi.org/10.3390/ijerph7103760 .
Wang C-C, Lu T-H, Liao W-C, Yuan S-C, Kuo P-C, Chuang H-L, et al. Cigarette smoking and cognitive impairment: a 10-year cohort study in Taiwan. Arch Gerontol Geriatr. 2010;51(2):143–8.
Article PubMed Google Scholar
Momtaz YA, Ibrahim R, Hamid TA, Chai ST. Smoking and cognitive impairment among older persons in Malaysia. Am J Alzheimers Dis Other Dement. 2015;30(4):405–11. https://doi.org/10.1177/1533317514552318 .
Article Google Scholar
Pandey K, Panday D, Sapkota N, Dhami A, Sarraf A, Shrestha S, et al. Effect of smoking in cognition. J Pulm Respir Med. 2017;3(399):2.
Razani J, Boone K, Lesser I, Weiss D. Effects of cigarette smoking history on cognitive functioning in healthy older adults. Am J Geriatr Psychiatry. 2004;12(4):404–11. https://doi.org/10.1097/00019442-200407000-00008 .
Caspers K, Arndt S, Yucuis R, McKirgan L, Spinks R. Effects of alcohol- and cigaretteuse disorders on global and specific measures of cognition in middle-age adults. J Stud Alcohol Drugs. 2010;71(2):192–200. https://doi.org/10.15288/jsad.2010.71.192 .
Heishman S, Kleykamp B, Singleton E. Meta-analysis of the acute effects of nicotine and smoking on human performance. Psychopharmacology (Berl.). 2010;210(4):453–69. https://doi.org/10.1007/s00213-010-1848-1 .
Wagner M, Schulze-Rauschenbach S, Petrovsky N, Brinkmeyer J, von der Goltz C, Gründer G, et al. Neurocognitive impairments in non-deprived smokers-results from a population-based multi-center study on smoking-related behavior. Addict Biol. 2013;18(4):752–61. https://doi.org/10.1111/j.1369-1600.2011.00429.x .
Nasreddine ZS, Phillips NA, Bédirian V, Charbonneau S, Whitehead V, Collin I, et al. The Montreal Cognitive Assessment, MoCA: a brief screening tool for mild cognitive impairment. J Am Geriatr Soc. 2005;53(4):695–9. https://doi.org/10.1111/j.1532-5415.2005.53221.x .
Golden CJ, Freshwater SM. The Stroop color word test: a manual for clinical and experimental uses. Los Angeles: Western Psychological Services; 2002.
Moses JA. Test review-Comprehensive Trail Making Test (CTMT). Arch Clin Neuropsychol. 2004;19(5):703–8. https://doi.org/10.1016/j.acn.2004.02.004 .
Kongs SK, Thompson LL, Iverson GL, Heaton RK. Wisconsin card sorting test-, 64 card version: WCST-64. Lutz: PAR; 2000.
Toglia JP. Contextual memory test. San Antonio: Therapy Skill Builders; 1993.
Wechsler D. Wechsler adult intelligence scale: administration and scoring manual. 3rd ed. San Antonio: The Psychological Corporation; 1997.
Bates ME, Lemay EP Jr. The d2 test of attention: construct validity and extensions in scoring techniques. J Int Neuropsychol Soc. 2004;10(3):392–400. https://doi.org/10.1017/S135561770410307X .
Ruff RM, Parker SB. Gender-and age-specific changes in motor speed and eye-hand coordination in adults: normative values for the Finger Tapping and Grooved Pegboard Tests. Perceptual Motor Skills. 1993;76(3_suppl):1219–30.
Nooyens AC, Gelder BM v, Verschuren WM. Smoking and cognitive decline among middle-aged men and women: the Doetinchem cohort study. Am J Public Health. 2008;98(12):2244–50. https://doi.org/10.2105/AJPH.2007.130294 .
Durazzo T, Meyerhoff D, Nixon S. A comprehensive assessment of neurocognition in middle-aged chronic cigarette smokers. Drug Alcohol Depend. 2012;122(1):105–11. https://doi.org/10.1016/j.drugalcdep.2011.09.019 .
Sabia S, Marmot M, Dufouil C, Singh-Manoux A. Smoking history and cognitive function in middle age from the Whitehall II study. Arch Intern Med. 2008;168(11):1165–73. https://doi.org/10.1001/archinte.168.11.1165 .
Reitz C, Luchsinger J, Tang M-X, Mayeux R. Effect of smoking and time on cognitive function in the elderly without dementia. Neurology. 2005;65(6):870–5. https://doi.org/10.1212/01.wnl.0000176057.22827.b7 .
Sabia S, Elbaz A, Dugravot A, Head J, Shipley M, Hagger-Johnson G, et al. Impact of smoking on cognitive decline in early old age: the Whitehall II cohort study. Arch Gen Psychiatry. 2012;69(6):627–35. https://doi.org/10.1001/archgenpsychiatry.2011.2016 .
Paul RH, Brickman AM, Cohen RA, Williams LM, Niaura R, Pogun S, et al. Cognitive status of young and older cigarette smokers: data from the international brain database. J Clin Neurosci. 2006;13(4):457–65. https://doi.org/10.1016/j.jocn.2005.04.012 .
Durazzo TC, Rothlind JC, Gazdzinski S, Banys P, Meyerhoff DJ. A comparison of neurocognitive function in nonsmoking and chronically smoking short-term abstinent alcoholics. Alcohol. 2006;39(1):1–11. https://doi.org/10.1016/j.alcohol.2006.06.006 .
Bashir S, Alghamdi F, Alhussien A, Alohali M, Alatawi A, Almusned T, et al. Effect of smoking on cognitive functioning in Young Saudi Adults. Med Sci Monitor Basic Res. 2017;23:31.
George TP, Vessicchio JC, Termine A, Sahady DM, Head CA, Pepper WT, et al. Effects of smoking abstinence on visuospatial working memory function in schizophrenia. Neuropsychopharmacology. 2002;26(1):75–85. https://doi.org/10.1016/S0893-133X(01)00296-2 .
Sutherland MT, Ross TJ, Shakleya DM, Huestis MA, Stein EA. Chronic smoking, but not acute nicotine administration, modulates neural correlates of working memory. Psychopharmacology. 2011;213(1):29–42.
Chamberlain SR, Odlaug BL, Schreiber LR, Grant JE. Association between tobacco smoking and cognitive functioning in young adults. Am J Addict. 2012;21:S14–9.
Spilich GJ, June L, Renner J. Cigarette smoking and cognitive performance. Br J Addict. 1992;87(9):1313–26. https://doi.org/10.1111/j.1360-0443.1992.tb02740.x .
Fried PA, Watkinson B, Gray R. Neurocognitive consequences of cigarette smoking in young adults – a comparison with pre-drug performance. Neurotoxicology. 2006;28(4):517–25. https://doi.org/10.1016/j.ntt.2006.06.003 .
Article CAS Google Scholar
Jacobsen LK, Krystal JH, Mencl WE, Westerveld M, Frost SJ, Pugh KR. Effects of smoking and smoking abstinence on cognition in adolescent tobacco smokers. Biol Psychiatry. 2005;57(1):56–66. https://doi.org/10.1016/j.biopsych.2004.10.022 .
Whalley LJ, Fox HC, Deary IJ, Starr JM. Childhood IQ, smoking, and cognitive change from age 11 to 64 years. Addict Behav. 2005;30(1):77–88. https://doi.org/10.1016/j.addbeh.2004.04.014 .
Valentine G, Sofuoglu M. Cognitive effects of nicotine: recent Progress. Curr Neuropharmacol. 2018;16(4):403–14. https://doi.org/10.2174/1570159X15666171103152136 .
Article CAS PubMed PubMed Central Google Scholar
Starr JM, Deary IJ, Fox HC, Whalley LJ. Smoking and cognitive change from age 11 to 66 years: a confirmatory investigation. Addict Behav. 2007;32(1):63–8. https://doi.org/10.1016/j.addbeh.2006.03.020 .
Corley J, Gow AJ, Starr JM, Deary IJ. Smoking, childhood IQ, and cognitive function in old age. J Psychosom Res. 2012;73(2):132–8. https://doi.org/10.1016/j.jpsychores.2012.03.006 .
Musso F, Bettermann F, Vucurevic G, Stoeter P, Konrad A, Winterer G. Smoking impacts on prefrontal attentional network function in young adult brains. Psychopharmacology. 2007;191(1):159–69.
Goriounova NA, Mansvelder HD. Short- and long-term consequences of nicotine exposure during adoles¬cence for prefrontal cortex neuronal network function. Cold Spring Harbor Perspect Med. 2012;2(12):a012120.
Download references
Acknowledgements
Not applicable.
Author information
Authors and affiliations.
Occupational Therapy Department, Faculty of Allied Health Sciences, Kuwait University, Jabriah, Kuwait
Mohammed Sh. Nadar
School of Pharmacy and Pharmaceutical Sciences, Cardiff University, Cardiff, UK
Abdullah M. Hasan & Mohammed Alsaleh
You can also search for this author in PubMed Google Scholar
Contributions
MN designed the study, coordinated the implementation of the study and data collection, and drafted the manuscript. AH and MA managed the literature review and references cited. AH and MA reviewed and revised the manuscript. All authors have read and approved the final manuscript.
Corresponding author
Correspondence to Mohammed Sh. Nadar .
Ethics declarations
Ethics approval and consent to participate.
The study was approved by Kuwait University Health Sciences Center Ethical Committee. All participants signed a written consent form to participate in the study before data collection.
Consent for publication
Competing interests.
The authors declare that they have no competing interests.
Additional information
Publisher’s note.
Springer Nature remains neutral with regard to jurisdictional claims in published maps and institutional affiliations.
Rights and permissions
Open Access This article is licensed under a Creative Commons Attribution 4.0 International License, which permits use, sharing, adaptation, distribution and reproduction in any medium or format, as long as you give appropriate credit to the original author(s) and the source, provide a link to the Creative Commons licence, and indicate if changes were made. The images or other third party material in this article are included in the article's Creative Commons licence, unless indicated otherwise in a credit line to the material. If material is not included in the article's Creative Commons licence and your intended use is not permitted by statutory regulation or exceeds the permitted use, you will need to obtain permission directly from the copyright holder. To view a copy of this licence, visit http://creativecommons.org/licenses/by/4.0/ . The Creative Commons Public Domain Dedication waiver ( http://creativecommons.org/publicdomain/zero/1.0/ ) applies to the data made available in this article, unless otherwise stated in a credit line to the data.
Reprints and permissions
About this article
Cite this article.
Nadar, M.S., Hasan, A.M. & Alsaleh, M. The negative impact of chronic tobacco smoking on adult neuropsychological function: a cross-sectional study. BMC Public Health 21 , 1278 (2021). https://doi.org/10.1186/s12889-021-11287-6
Download citation
Received : 12 August 2020
Accepted : 15 June 2021
Published : 30 June 2021
DOI : https://doi.org/10.1186/s12889-021-11287-6
Share this article
Anyone you share the following link with will be able to read this content:
Sorry, a shareable link is not currently available for this article.
Provided by the Springer Nature SharedIt content-sharing initiative
- Working memory
- Cognitive impairment
- Chronic smoking
BMC Public Health
ISSN: 1471-2458
- Submission enquiries: [email protected]
- General enquiries: [email protected]
Harmful health effects of cigarette smoking
- Published: November 2003
- Volume 253 , pages 159–165, ( 2003 )
Cite this article
- Salil K. Das 1
3932 Accesses
85 Citations
2 Altmetric
Explore all metrics
This is a comprehensive review on the harmful health effects of cigarette smoking. Tobacco smoking is a reprehensible habit that has spread all over the world as an epidemic. It reduces the life expectancy among smokers. It increases overall medical costs and contributes to the loss of productivity during the life span. Smoking has been shown to be linked with various neurological, cardiovascular, and pulmonary diseases. Cigarette smoke not only affects the smokers but also contributes to the health problems of the non-smokers. Exposure to environmental tobacco smoke contributes to health problems in children and is a significant risk factor for asthma. Cigarette smoke contains several carcinogens that alter biochemical defense systems leading to lung cancer.
This is a preview of subscription content, log in via an institution to check access.
Access this article
Price includes VAT (Russian Federation)
Instant access to the full article PDF.
Rent this article via DeepDyve
Institutional subscriptions
Similar content being viewed by others
Heavy Metal Toxicity and the Environment
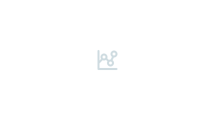
E-Cigarette Use Among High School Students—a Cross-Sectional Study of Associated Risk Factors for the Use of Flavour-Only and Nicotine Vapes
Janni Leung, Calvert Tisdale, … Leanne Hides
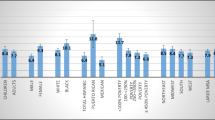
Asthma epidemiology and risk factors
Jessica Stern, Jennifer Pier & Augusto A. Litonjua
Cigarette smoking among adults — United States, 1999. MMWR Morb Mortal Wkly Rep Oct 12, 50: 869-873, 2001
Google Scholar
Bang KM, Kim JH: Prevalence of cigarette smoking by occupation and industry in the United States. Am J Int Med 40: 233-239, 2001
Bronnum-Hansen H, Juel K: Abstention from smoking extends life and compresses morbidity: A population based study of health expectancy among smokers and never smokers in Denmark. Tob Control 10: 273-278, 2001
Max W: The financial impact of smoking on health-related costs: A review of the literature. Am J Health Promot 15: 321-331, 2001
Adams EK, Miller VP, Ernst C, Nishimura BK, Melvin C, Merritt R: Neonatal health care costs related to smoking during pregnancy. Health Econ 11: 193-206, 2002
Bratzler DW, Oehlert WH, Austelle A: Smoking in the elderly — it's never too late to quit. J Okla State Med Assoc 95: 185-191, 2002
Hesketh T, Ding QJ, Tomkins A: Smoking among youths in China. Am J Public Health 91: 1653-1655, 2001
Maziak W: Smoking in Syria: Profile of a developing Arab country. Int J Tuberc Lung Dis 6: 183-191, 2002
Kirby JB: The influence of parental separation on smoking initiation in adolescents. J Health Soc Behav 43: 56-71, 2002
Trends in cigarette smoking among high school students — United States, 1991-1999. MMWR Morb Mortal Wkly Rep 49: 755-758, 2000
John U, Hanke M: Tobacco smoking attributable mortality in Germany. Gesundheitsaesen 63: 363-369, 2001
Criado-Alvarez JJ, Morant Ginestar C, de Lucas Veguillas A: Mortality attributable to tobacco consumption in the years 1987 and 1997 in Castilla la Mancha, Spain. Rev Esp Salud Publica 76: 27-36, 2002
Lam TH, Jiang CQ, Ho SY, Zhang WS, Liu WW, He JM: Smoking and mortality in 81,344 drivers in Guangzhou, China. Occup Environ Med 59: 135-138, 2002
Marable S, Crim C, Dennis GC, Epps RP, Freeman H, Mills S, Coolchan ET, Robinson L, Robinson R, Cole L, Payne PH: Tobacco control: Consensus report of the National Medical Association. J Natl Med Assoc 94: 78-87, 2002
Turner J, Page-Shafer K, Chin DP, Osmond D, Mossar M, Markstein L, Huitsing J, Barnes S, Clemente V, Chesney M: Pulmonary Complications of HIV Iinfection Study Group: Adverse impact of cigarette smoking on dimensions of health-related quality of life in persons with HIV infection. AIDS Patient Care STDS 15: 615-624, 2001
Vogt MT, Hanscom B, Lauerman WC, Kang JD: Influence of smoking on the health status of spinal patients: The National Spine Network database. Spine 27: 313-319, 2002
Hamalainen J, Kaprio J, Isometsa E, Heikkinen M, Poikolainen K, Lindeman S, Aro H: Cigarette smoking, alcohol intoxication and major depressive episode in a representative population sample. J Epidemiol Community Health 55: 573-576, 2001
Berard RM, Lockhart IA, Boermeester F, Tredoux C: Cigarette smoking in an adolescent psychiatric population. S Afr Med J 92: 58-61, 2002
Fratiglioni L, Wang HX: Smoking and Parkinson's and Alzheimer's disease: Review of the epidemiological studies. Behav Brain Res 113: 117-120, 2000
Hernan MA, Zhang SM, Rueda-deCastro AM, Colditz GA, Speizer FE, Ascherio A: Cigarette smoking and the incidence of Parkinson's disease in two prospective studies. Ann Neurol 50: 780-786, 2001
Checkoway H, Powers K, Smith-Weller T, Franklin GM, Longstreth WT Jr, Swanson PD: Parkinson's disease risks associated with cigarette smoking, alcohol consumption, and caffeine intake. Am J Epidemiol 155: 732-738, 2002
Almeida OP, Hulse GK, Lawrence D, Flickler L: Smoking as a risk factor for Alzheimer's disease: Contrasting evidence from a systematic review of case-control and cohort studies. Addiction 97: 15-28, 2002
Frank CW, Weinblatt E, Shapiro S, Sager RV: Myocardial infarction in men. Role of physical activity and smoking in incidence and mortality. JAMA 198: 1241-1245, 1966
Hay DR, Turbott S: Changes in smoking habits in men under 65 years after myocardial infarction and coronary insufficiency. Br Heart J 32: 738-740, 1970
Aronow WS: Smoking, carbon monoxide and coronary heart disease. Circulation 48: 1169-1172, 1973
Al-Delaimy WK, Manson JE, Solomon CG, Kawachi I, Stampfer MJ, Willett WC, Hu FB: Smoking and risk of coronary heart disease among women with type 2 diabetes mellitus. Arch Intern Med 162: 273-279, 2002
Al-Delaimy WK, Willett WC, Manson JE, Speizer FE, Hu FB: Smoking and mortality among women with type 2 diabetes: The Nurses' Health Study Cohort. Diabetes Care 24: 2043-2048, 2001
Johnson KH, Bazargan M, Cherpitel CJ: Alcohol, tobacco, and drug use and the onset of type 2 diabetes among inner-city minority patients. J Am Board Fam Pract 14: 430-436, 2001
Halimi JM, Giraudeau B, Vol S, Caces E, Nivet H, Tichet J: The risk of hypertension in men: Direct and indirect effects of chronic smoking. J Hypertens 20: 171-172, 2002
Louie D: The effects of cigarette smoking on cardiopulmonary function and exercise tolerance in teenagers. Can Respir J 8: 289-291, 2001
Gladston M, Feldman JG, Levytska V, Magnusson B: Antioxidant activity of serum ceruloplasmin and transferring available iron-binding capacity in smokers and non-smokers. Am Rev Respir Dis 135: 783-787, 1987
Strain JJ, Carville DGM, Barker ME, Thompson KA, Welch RW, Young P, Rice DA: Smoking and blood antioxidant enzyme activities. Biochem Soc Trans 17: 497-498, 1989
McGowan SE, Henley SA: Iron and ferritin contents and distribution in human alveolar macrophages. J Lab Clin Med 111: 611-617, 1988
Mukherjee S, Woods L, Weston Z, Williams AB, Das SK: The effect of mainstream and sidestream cigarette smoke exposure on oxygen defense mechanisms of guinea pig erythrocytes. J Biochem Toxicology 8: 119-125, 1993
Van Hoydonck PG, Temme EH, Schouten EG: Serum bilirubin concentration in a Belgium population: The association with smoking status and type of cigarettes. Int J Epidemiol 30: 1465-1472, 2001
Haustein KO: Health consequences of passive smoking. Z Arztl Fortbild Qualitatssich 95: 377-386, 2001
Auerbach O, Hammond EL, Garfinker L, Benante C: Relation of smoking and age to emphysema. Whole-lung section study. N Eng J Med 286: 853-857, 1972
U.S. Public Health Service (USPHS). Smoking and health: A report of the Surgeon General, DHEW Publication (PHS) 79-50066. Washington DC: US. Department of Health, Education, and Welfare, Public Health Service; 10-35, 1979
Hunninghake GW, Crystal RG: Cigarette smoking and lung destruction. Am Rev Respir Dis 128: 833-838, 1983
Bartal M: Health effects of tobacco use and exposure. Monaldi Arch Chest Dis 56: 545-554, 2001
Janson C, Chinn S, Jarvis D, Zock JP, Toren K, Burney P, European Community Respiratory Health Survey: Effect of passive smoking on respiratory symptoms, bronchial responsiveness, lung function, and total serum IgE in the European Community Respiratory Health Survey: A cross-sectional study. Lancet 358: 2103-2109, 2001. Erratum in Lancet 359: 360, 2002
Wang H, Liu X, Umino T, Skold CM, Zhu Y, Kohyama T, Spurzem JR, Romberger DJ, Rennard SI: Cigarette smoke inhibits human bronchial epithelial cell repair processes. Am J Respir Cell Mol Biol 25: 772-779, 2001
American Thoracic Society Task Force Report: Future directions for research on diseases of the lung. Am J Respir Crit Care Med 152: pp 1713-1735, 1995
Balint JA, Bondurant S, Kynakides EC: Lecithin biosynthesis in cigarette smoking dogs. Arch Intern Med 127: 740-747, 1971
Subramaniam S, Bummer P, Gairola CG: Biochemical and biophysical characterization of pulmonary surfactant in rats exposed chronically to cigarette smoke. Fundam Appl Toxicol 27: 63-69, 1995
Le Mesurier SM, Stewart BW, Lykke AW: Injury to type-2 pneumocytes in rats exposed to cigarette smoke. Environ Res 24: 207-217, 1981
Zetterberg G, Curstedt T, Eklund A: A possible alteration of surfactant in broncho-alveolar lavage fluid from healthy smokers compared to non-smokers and patients with sarcoidosis. Sarcoidosis 12: 46-50, 1995
Mancini NM, Bene MC, Gerard H, Chabot F, Faure G, Polu JM, Lesur O: Early effects of short-time cigarette smoking on the human lung: A study of bronchoalveolar lavage fluids. Lung 171: 277-291, 1993
Haagsman HP, Van Golde LM: Lung surfactant and pulmonary toxicology. Lung 163: 275-303, 1985
Mukherjee S, Nayyar T, Chytil F, Das SK: Mainstream and sidestream cigarette smoke exposure increases retinol in guinea pig lungs. Free Radic Biol Med 18: 507-514, 1995
Mukherjee S, Das SK: Effects of cigarette smoke exposure on the binding capacity of β-adrenergic receptors in guinea pig alveolar type II cells. FASEB J 6: 259, 1992
Whitsett JA, Manton MA, Darovec-Beckerman C, Adams K: Beta adrenergic receptors and catecholamine sensitive adenylate cyclase in the developing rat lung. Life Sci 28: 339-365, 1981
Das SK, Chakrabarti P, Tsao FHC, Nayyar T, Mukherjee S: Identification of calcium-dependent phospholipid-binding proteins (annexins) from guinea pig alveolar type II cells. Mol Cell Biochem 115: 79-84, 1992
Das SK, Tsao FHC, Mukherjee S: Mainstream and sidestream cigarette smoke exposure increases Ca 2+ -dependent phospholipid binding proteins in guinea pig alveolar type II cells. Mol Cell Biochem 231: 37-42, 2002
Ulrik CS, Lange P: Cigarette smoking and asthma. Monaldi Arch Chest Dis 56: 349-353, 2001
Larsson ML, Frisk M, Hallstrom J, Kiviloog J, Lundback B: Environmental tobacco smoke exposure during childhood is associated with increased prevalence of asthma in adults. Chest 120: 711-717, 2001
Weitzman M, Gortmaker S, Walker DK, Sobol A: Maternal smoking and childhood asthma. Pediatrics 85: 505-511, 1990
Buczko GB, Day A, Vanderdoelen JL, Boucher R, Zamel N: Effects of cigarette smoking and short-term smoking cessation on airway responsiveness to inhaled methacholine. Am Rev Respir Dis 129: 12-14, 1984
Murray AB, Morrison BJ: The effect of cigarette smoke from the mother on bronchial responsiveness and severity of symptoms in children with asthma. J Allergy Clin Immunol 77: 575-581, 1986
Meijer GG, Postma DS, Van der Heide S, de Reus DM et al. : Exogenous stimuli and circadian peak expiratory flow variation in allergic asthmatic children. Am J Respir Crit Care Med 153: 237-242, 1996
Evans D, Levison MJ, Feldman C et al. : The impact of passive smoking on emergency room visits of urban children with asthma. Am Rev Respir Dis 135: 567-572, 1987
Pitman JD, Snapper JR: Non-specific airway hyperresponsiveness: Mechanisms and Meaning. In: D.H. Simmons, D.F. Tierney (eds). Current Pulmonology, Vol. 13. Mosby-Yearbook, Chicago, IL, 1992, pp 143-192
Chapman KR: Therapeutic approaches to chronic obstructive pulmonary disease: An emerging consensus. Am J Med 100: 5S-10S, 1996
Menton P, Rando RJ, Stankus RP, Salvaggio JE, Lehrer SB: Passive cigarette smoke-challenge studies: Increase in bronchial hyperreactivity. J Allergy Clin Immunol 77: 575-581, 1986
Murray AB, Morrison BJ: Passive smoking by asthmatics: Its greater effect on boys than on girls and on older than on younger children. Pediatrics 84: 451-459, 1989
Prischer T, Kuehr J, Meinert R et al. : Maternal smoking in early childhood: A risk factor for bronchial responsiveness to exercise in primary-school children. J Pediatr 121: 17-22, 1992
Chilmonczyk BA, Salmun LM, Megathlin KN, Neveux LM, Palomaki GE, Knight GJ, Pulkkinen AJ, Haddow JE: Association between exposure to environmental tobacco smoke and exacerbations of asthma in children. New Eng J Med 328: 1665-1669, 1993
Martinez FD, Antognoni G, Macri F, Bonci E, Midulla F, De Castro G, Ronchetti R: Parental smoking enhances bronchial responsiveness in nine-year-old children. Am Rev Respir Dis 138: 518-523, 1988
Tager IB, Weiss ST, Munoz A, Rosner B, Spizer FE: Longitudinal study of the effects of maternal smoking on pulmonary function in children. New Engl J Med 309: 699-703, 1983
Willers S, Attewell R, Bensryd, I, Schultz A, Skarping A, Vahter M: Exposure to environmental tobacco smoke in the household and urinary cotinine excretion, heavy metals retention and lung function. Arch Environ Health 47: 357-363, 1992
Baughman RP, Corser BC, Strohofer S, Hendricks D: Spontaneous hydrogen peroxide release from alveolar macrophages of some cigarette smokers. J Lab Clin Med 107: 233-237, 1986
Higgins M: Risk factors associated with chronic obstructive lung disease. In: G. Weinbaun, R.E. Giles, R.D. Krell (eds). Pulmonary Emphysema. The Rationale for Therapeutic Intervention. Annals NY Acad Sci 624: 7-17, 1991
U.S. Department of Health and Human Services. The health consequences of involuntary smoking. A Report of the Surgeon General. U.S. Department of Health and Human Services, Public Health Services, Centers for Disease Control, Center for Chronic Disease Prevention and Health Promotion, Office on Smoking and Health. DHHS Publication, 1986
Hendrick D: Asthma: Epidemics and epidemiology. Thorax 44: 609-613, 1989
Platts-Mills TAE, De Weck AL: House dust mites: A world wide problem. J Allergy Clin Immunol 83: 416-427, 1989
Sears MR, Herbison GP, Holdaway MD, Hewitt CJ, Flannery EM, Silva PA: The relative risks of sensitivity to grass pollen, house dust mite and cat dader in the development of childhood asthma. Clin Exp Allergy 19: 419-424, 1989
Fleming DM, Cromble DL: Prevalence of asthma and hay fever in England and Wales. Br Med J 294: 279-283, 1987
Charpin D, Kleisbauer JP, Lanteaume A, Razzouk H, Vervloet D, Toumi M, Faraj F, Charpin J: Asthma and allergy to house-dust mites in populations living in high altitude. Chest 93: 758-761, 1988
Andrae SO, Axelson O, Bjorksten B, Fredriksson M, Kjellman NIM: Symptoms of bronchial hyperreactivity and asthma in relation to environmental factors. Arch Dis Child 63: 473-478, 1988
Tager IB: Passive smoking-bronchial responsiveness and atopy. Am Rev Respir Dis 138: 507-579, 1988
Langhammaer A, Johnsen R, Holmen J, Gulsvik A, Bjermer L: Cigarette smoking gives more respiratory symptoms among women than among men. The Nord-Trondelag Health Study (HUNT). J Epidemiol Community Health 54: 917-922, 2000
Wang Z, Chen C, Niu T, Wu D, Yang J, Wang B, Fang Z, Yandava CN, Drazen JM, Weiss ST, Xu X: Association of asthma with beta(2)-adrenergic receptor gene polymorphism and cigarette smoking. Am J Respir Crit Care Med 163: 1404-1409, 2001
Nair CR, Davis MM, Das SK: Effect of vitamin A deficiency on pulmonary defense systems of guinea pig lung. Internat J Vit Nutr Res 58: 375-380, 1988
Mascio PD, Devassagayam TPA, Kaiser S, Sies H: Carotenoids, tocopherols and thiols as biological singlet molecular oxygen quenchers. Biochem Soc Trans 18: 1054-1056, 1990
Jenkinson SG, Lawrence RD, Burk RF, Gregory PE: Non-selenium-dependent glutathione peroxidase activity in rat lung: Association with lung glutathione S-transferase activity and the effects of hyperoxia. Toxicol Appl Pharmacol 68: 399-404, 1983
Cresanta JL: Epidemiology of cancer in the United States. Prim Care 19: 419-441, 1992
Goodman GE, Omenn GS: Carotene and retinol efficacy trail in heavy cigarette smokers and asbestos-exposed smokers. CARET coinvestigators and staff. Adv Exp Med Biol 320: 137-140, 1992
Pastorino U, Infante M, Maioli M, Chiesa G, Buyse M, Firket P, Rosmentz N, Clerici M, Soresi E, Valente M et al. : Adjuvant treatment of stage 1 lung cancer with high-dose vitamin A. J Clin Oncol 11: 1216-1222, 1993
Burns DM: Cigarette smoking among the elderly: Disease consequences and the benefits of cessation.
Rachtan J: Smoking, passive smoking and lung cancer cell types among women in Polland. Lung Cancer 35: 129-136, 2002
Taylor R, Cumming R, Woodward A, Black M: Passive smoking and lung cancer: A cumulative meta-analysis. Aust NZ J Public Health 25: 203-211, 2001
Cooley ME, Kaiser LR, Abrahm JL, Giarelli E: The silent epidemic: Tobacco and the evolution of lung cancer and its treatment. Cancer Invest 19: 739-751, 2001
Stellman SD, Takezaki T, Wang L, Chen Y, Citron ML, Djordjevic MV, Harlap S, Muscat JE, Neugut AI, Wynder EL, Ogawa H, Tajima K, Aoki K: Smoking and lung cancer risk in American and Japanese men: An international case-control study. Cancer Epidemiol Biomarkers Prev 10: 1193-1199, 2001
Shields PG: Epidemiology of tobacco carcinogenesis. Curr Oncol Rep 2: 257-262, 2000
Download references
Author information
Authors and affiliations.
Department of Biochemistry, Meharry Medical College, 1005 D.B. Todd Boulevard, Nashville, TN, 37208, USA
Salil K. Das
You can also search for this author in PubMed Google Scholar
Rights and permissions
Reprints and permissions
About this article
Das, S.K. Harmful health effects of cigarette smoking. Mol Cell Biochem 253 , 159–165 (2003). https://doi.org/10.1023/A:1026024829294
Download citation
Issue Date : November 2003
DOI : https://doi.org/10.1023/A:1026024829294
Share this article
Anyone you share the following link with will be able to read this content:
Sorry, a shareable link is not currently available for this article.
Provided by the Springer Nature SharedIt content-sharing initiative
- health effects
- Find a journal
- Publish with us
- Track your research
- Open access
- Published: 18 May 2021
An updated overview of e-cigarette impact on human health
- Patrice Marques ORCID: orcid.org/0000-0003-0465-1727 1 , 2 ,
- Laura Piqueras ORCID: orcid.org/0000-0001-8010-5168 1 , 2 , 3 &
- Maria-Jesus Sanz ORCID: orcid.org/0000-0002-8885-294X 1 , 2 , 3
Respiratory Research volume 22 , Article number: 151 ( 2021 ) Cite this article
385k Accesses
127 Citations
350 Altmetric
Metrics details
The electronic cigarette ( e-cigarette ), for many considered as a safe alternative to conventional cigarettes, has revolutionised the tobacco industry in the last decades. In e-cigarettes , tobacco combustion is replaced by e-liquid heating, leading some manufacturers to propose that e-cigarettes have less harmful respiratory effects than tobacco consumption. Other innovative features such as the adjustment of nicotine content and the choice of pleasant flavours have won over many users. Nevertheless, the safety of e-cigarette consumption and its potential as a smoking cessation method remain controversial due to limited evidence. Moreover, it has been reported that the heating process itself can lead to the formation of new decomposition compounds of questionable toxicity. Numerous in vivo and in vitro studies have been performed to better understand the impact of these new inhalable compounds on human health. Results of toxicological analyses suggest that e-cigarettes can be safer than conventional cigarettes, although harmful effects from short-term e-cigarette use have been described. Worryingly, the potential long-term effects of e-cigarette consumption have been scarcely investigated. In this review, we take stock of the main findings in this field and their consequences for human health including coronavirus disease 2019 (COVID-19).
Electronic nicotine dispensing systems (ENDS), commonly known as electronic cigarettes or e-cigarettes , have been popularly considered a less harmful alternative to conventional cigarette smoking since they first appeared on the market more than a decade ago. E-cigarettes are electronic devices, essentially consisting of a cartridge, filled with an e-liquid, a heating element/atomiser necessary to heat the e-liquid to create a vapour that can be inhaled through a mouthpiece, and a rechargeable battery (Fig. 1 ) [ 1 , 2 ]. Both the electronic devices and the different e-liquids are easily available in shops or online stores.
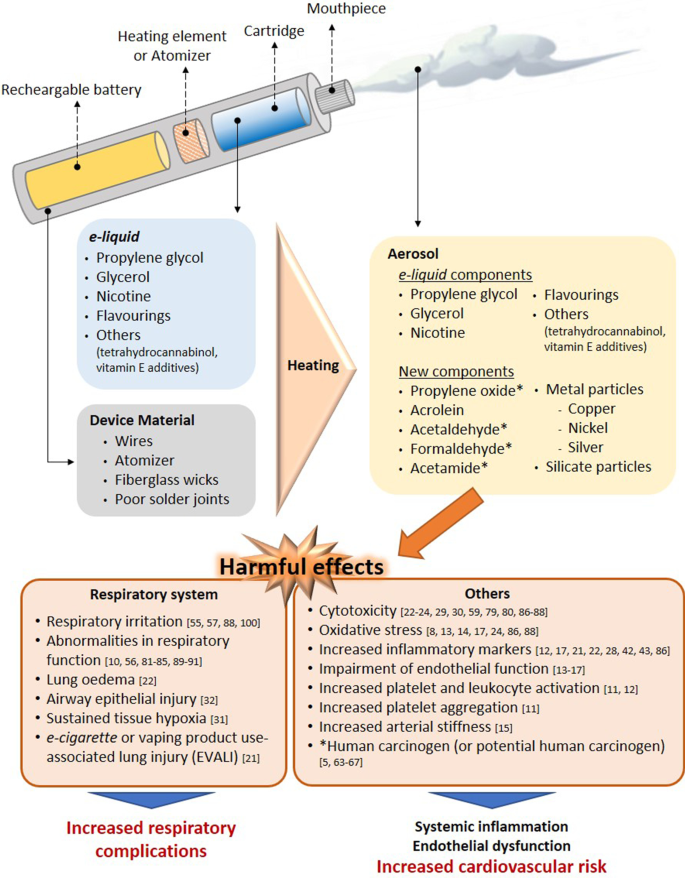
Effect of the heating process on aerosol composition. Main harmful effects documented. Several compounds detected in e-cigarette aerosols are not present in e-liquid s and the device material also seems to contribute to the presence of metal and silicate particles in the aerosols. The heating conditions especially on humectants, flavourings and the low-quality material used have been identified as the generator of the new compounds in aerosols. Some compounds generated from humectants (propylene glycol and glycerol) and flavourings, have been associated with clear airways impact, inflammation, impairment of cardiovascular function and toxicity. In addition, some of them are carcinogens or potential carcinogens
The e-liquid typically contains humectants and flavourings, with or without nicotine; once vapourised by the atomiser, the aerosol (vapour) provides a sensation similar to tobacco smoking, but purportedly without harmful effects [ 3 ]. However, it has been reported that the heating process can lead to the generation of new decomposition compounds that may be hazardous [ 4 , 5 ]. The levels of nicotine, which is the key addictive component of tobacco, can also vary between the commercially available e-liquids, and even nicotine-free options are available. For this particular reason, e-cigarettes are often viewed as a smoking cessation tool, given that those with nicotine can prevent smoking craving, yet this idea has not been fully demonstrated [ 2 , 6 , 7 ].
Because e-cigarettes are combustion-free, and because most of the damaging and well-known effects of tobacco are derived from this reaction, there is a common and widely spread assumption that e-cigarette consumption or “vaping” is safer than conventional cigarette smoking. However, are they risk-free? Is there sufficient toxicological data on all the components employed in e-liquids ? Do we really know the composition of the inhaled vapour during the heating process and its impact on health? Can e-cigarettes be used to curb tobacco use? Do their consumption impact on coronavirus disease 2019 (COVID-19)? In the present review, we have attempted to clarify these questions based on the existing scientific literature, and we have compiled new insights related with the toxicity derived from the use of these devices.
Effect of e-cigarette vapour versus conventional cigarette exposure: in vivo and in vitro effects
Numerous studies have been performed to evaluate the safety/toxicity of e-cigarette use both in vivo and in in vitro cell culture.
One of the first studies in humans involved the analysis of 9 volunteers that consumed e-cigarettes , with or without nicotine, in a ventilated room for 2 h [ 8 ]. Pollutants in indoor air, exhaled nitric oxide (NO) and urinary metabolite profiles were analysed. The results of this acute experiment revealed that e-cigarettes are not emission-free, and ultrafine particles formed from propylene glycol (PG) could be detected in the lungs. The study also suggested that the presence of nicotine in e-cigarettes increased the levels of NO exhaled from consumers and provoked marked airway inflammation; however, no differences were found in the levels of exhaled carbon monoxide (CO), an oxidative stress marker, before and after e-cigarette consumption [ 8 ]. A more recent human study detected significantly higher levels of metabolites of hazardous compounds including benzene, ethylene oxide, acrylonitrile, acrolein and acrylamide in the urine of adolescent dual users ( e-cigarettes and conventional tobacco consumers) than in adolescent e-cigarette -only users (Table 1 ) [ 9 ]. Moreover, the urine levels of metabolites of acrylonitrile, acrolein, propylene oxide, acrylamide and crotonaldehyde, all of which are detrimental for human health, were significantly higher in e-cigarette -only users than in non-smoker controls, reaching up to twice the registered values of those from non-smoker subjects (Table 1 ) [ 9 ]. In line with these observations, dysregulation of lung homeostasis has been documented in non-smokers subjected to acute inhalation of e-cigarette aerosols [ 10 ].
Little is known about the effect of vaping on the immune system. Interestingly, both traditional and e-cigarette consumption by non-smokers was found to provoke short-term effects on platelet function, increasing platelet activation (levels of soluble CD40 ligand and the adhesion molecule P-selectin) and platelet aggregation, although to a lesser extent with e-cigarettes [ 11 ]. As found with platelets, the exposure of neutrophils to e-cigarette aerosol resulted in increased CD11b and CD66b expression being both markers of neutrophil activation [ 12 ]. Additionally, increased oxidative stress, vascular endothelial damage, impaired endothelial function, and changes in vascular tone have all been reported in different human studies on vaping [ 13 , 14 , 15 , 16 , 17 ]. In this context, it is widely accepted that platelet and leukocyte activation as well as endothelial dysfunction are associated with atherogenesis and cardiovascular morbidity [ 18 , 19 ]. In line with these observations the potential association of daily e-cigarettes consumption and the increased risk of myocardial infarction remains controversial but benefits may occur when switching from tobacco to chronic e-cigarette use in blood pressure regulation, endothelial function and vascular stiffness (reviewed in [ 20 ]). Nevertheless, whether or not e-cigarette vaping has cardiovascular consequences requires further investigation.
More recently, in August 2019, the US Centers for Disease Control and Prevention (CDC) declared an outbreak of the e-cigarette or vaping product use-associated lung injury (EVALI) which caused several deaths in young population (reviewed in [ 20 ]). Indeed, computed tomography (CT scan) revealed local inflammation that impaired gas exchange caused by aerosolised oils from e-cigarettes [ 21 ]. However, most of the reported cases of lung injury were associated with use of e-cigarettes for tetrahydrocannabinol (THC) consumption as well as vitamin E additives [ 20 ] and not necessarily attributable to other e-cigarette components.
On the other hand, in a comparative study of mice subjected to either lab air, e-cigarette aerosol or cigarette smoke (CS) for 3 days (6 h-exposure per day), those exposed to e-cigarette aerosols showed significant increases in interleukin (IL)-6 but normal lung parenchyma with no evidence of apoptotic activity or elevations in IL-1β or tumour necrosis factor-α (TNFα) [ 22 ]. By contrast, animals exposed to CS showed lung inflammatory cell infiltration and elevations in inflammatory marker expression such as IL-6, IL-1β and TNFα [ 22 ]. Beyond airway disease, exposure to aerosols from e-liquids with or without nicotine has also been also associated with neurotoxicity in an early-life murine model [ 23 ].
Results from in vitro studies are in general agreement with the limited number of in vivo studies. For example, in an analysis using primary human umbilical vein endothelial cells (HUVEC) exposed to 11 commercially-available vapours, 5 were found to be acutely cytotoxic, and only 3 of those contained nicotine [ 24 ]. In addition, 5 of the 11 vapours tested (including 4 that were cytotoxic) reduced HUVEC proliferation and one of them increased the production of intracellular reactive oxygen species (ROS) [ 24 ]. Three of the most cytotoxic vapours—with effects similar to those of conventional high-nicotine CS extracts—also caused comparable morphological changes [ 24 ]. Endothelial cell migration is an important mechanism of vascular repair than can be disrupted in smokers due to endothelial dysfunction [ 25 , 26 ]. In a comparative study of CS and e-cigarette aerosols, Taylor et al . found that exposure of HUVEC to e-cigarette aqueous extracts for 20 h did not affect migration in a scratch wound assay [ 27 ], whereas equivalent cells exposed to CS extract showed a significant inhibition in migration that was concentration dependent [ 27 ].
In cultured human airway epithelial cells, both e-cigarette aerosol and CS extract induced IL-8/CXCL8 (neutrophil chemoattractant) release [ 28 ]. In contrast, while CS extract reduced epithelial barrier integrity (determined by the translocation of dextran from the apical to the basolateral side of the cell layer), e-cigarette aerosol did not, suggesting that only CS extract negatively affected host defence [ 28 ]. Moreover, Higham et al . also found that e-cigarette aerosol caused IL-8/CXCL8 and matrix metallopeptidase 9 (MMP-9) release together with enhanced activity of elastase from neutrophils [ 12 ] which might facilitate neutrophil migration to the site of inflammation [ 12 ].
In a comparative study, repeated exposure of human gingival fibroblasts to CS condensate or to nicotine-rich or nicotine-free e-vapour condensates led to alterations in morphology, suppression of proliferation and induction of apoptosis, with changes in all three parameters greater in cells exposed to CS condensate [ 29 ]. Likewise, both e-cigarette aerosol and CS extract increased cell death in adenocarcinomic human alveolar basal epithelial cells (A549 cells), and again the effect was more damaging with CS extract than with e-cigarette aerosol (detrimental effects found at 2 mg/mL of CS extract vs. 64 mg/mL of e-cigarette extract) [ 22 ], which is in agreement with another study examining battery output voltage and cytotoxicity [ 30 ].
All this evidence would suggest that e-cigarettes are potentially less harmful than conventional cigarettes (Fig. 2 ) [ 11 , 14 , 22 , 24 , 27 , 28 , 29 ]. Importantly, however, most of these studies have investigated only short-term effects [ 10 , 14 , 15 , 22 , 27 , 28 , 29 , 31 , 32 ], and the long-term effects of e-cigarette consumption on human health are still unclear and require further study.
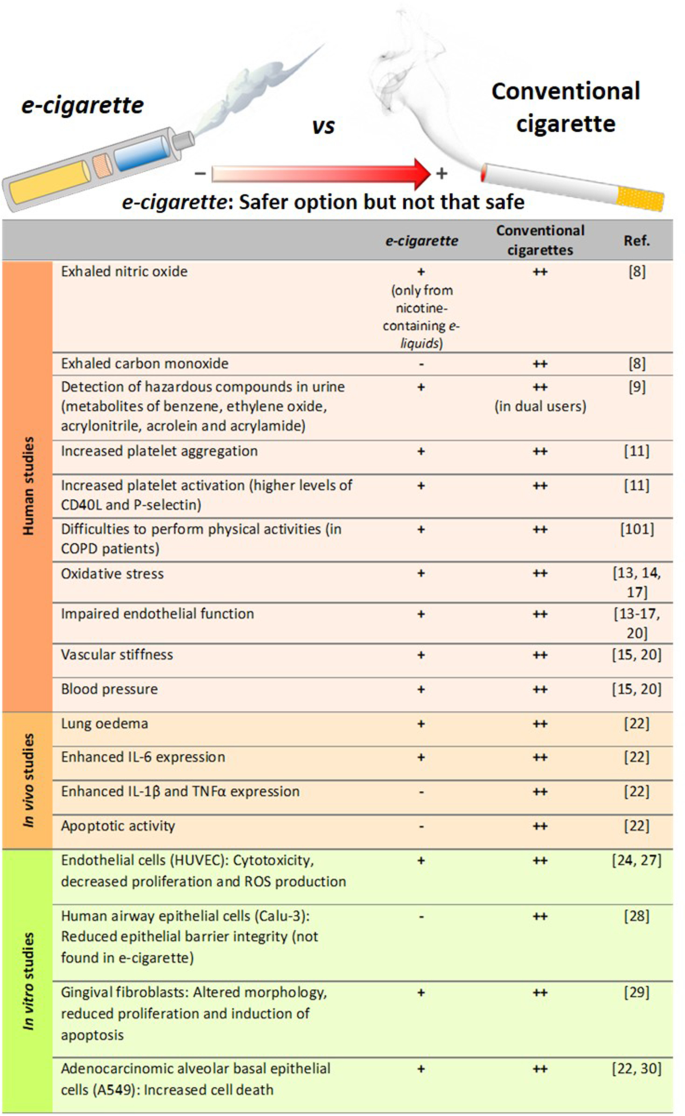
Comparison of the degree of harmful effects documented from e-cigarette and conventional cigarette consumption. Human studies, in vivo mice exposure and in vitro studies. All of these effects from e-cigarettes were documented to be lower than those exerted by conventional cigarettes, which may suggest that e-cigarette consumption could be a safer option than conventional tobacco smoking but not a clear safe choice
Consequences of nicotine content
Beyond flavour, one of the major issues in the e-liquid market is the range of nicotine content available. Depending on the manufacturer, the concentration of this alkaloid can be presented as low , medium or high , or expressed as mg/mL or as a percentage (% v/v). The concentrations range from 0 (0%, nicotine-free option) to 20 mg/mL (2.0%)—the maximum nicotine threshold according to directive 2014/40/EU of the European Parliament and the European Union Council [ 33 , 34 ]. Despite this normative, however, some commercial e-liquids have nicotine concentrations close to 54 mg/mL [ 35 ], much higher than the limits established by the European Union.
The mislabelling of nicotine content in e-liquids has been previously addressed [ 8 , 34 ]. For instance, gas chromatography with a flame ionisation detector (GC-FID) revealed inconsistencies in the nicotine content with respect to the manufacturer´s declaration (average of 22 ± 0.8 mg/mL vs. 18 mg/mL) [ 8 ], which equates to a content ~ 22% higher than that indicated in the product label. Of note, several studies have detected nicotine in those e-liquids labelled as nicotine-free [ 5 , 35 , 36 ]. One study detected the presence of nicotine (0.11–6.90 mg/mL) in 5 of 23 nicotine-free labelled e-liquids by nuclear magnetic resonance spectroscopy [ 35 ], and another study found nicotine (average 8.9 mg/mL) in 13.6% (17/125) of the nicotine-free e-liquids as analysed by high performance liquid chromatography (HPLC) [ 36 ]. Among the 17 samples tested in this latter study 14 were identified to be counterfeit or suspected counterfeit. A third study detected nicotine in 7 of 10 nicotine-free refills, although the concentrations were lower than those identified in the previous analyses (0.1–15 µg/mL) [ 5 ]. Not only is there evidence of mislabelling of nicotine content among refills labelled as nicotine-free, but there also seems to be a history of poor labelling accuracy in nicotine-containing e-liquids [ 37 , 38 ].
A comparison of the serum levels of nicotine from e-cigarette or conventional cigarette consumption has been recently reported [ 39 ]. Participants took one vape from an e-cigarette , with at least 12 mg/mL of nicotine, or inhaled a conventional cigarette, every 20 s for 10 min. Blood samples were collected 1, 2, 4, 6, 8, 10, 12 and 15 min after the first puff, and nicotine serum levels were measured by liquid chromatography-mass spectrometry (LC–MS). The results revealed higher serum levels of nicotine in the conventional CS group than in the e-cigarette group (25.9 ± 16.7 ng/mL vs. 11.5 ± 9.8 ng/mL). However, e-cigarettes containing 20 mg/mL of nicotine are more equivalent to normal cigarettes, based on the delivery of approximately 1 mg of nicotine every 5 min [ 40 ].
In this line, a study compared the acute impact of CS vs. e-cigarette vaping with equivalent nicotine content in healthy smokers and non-smokers. Both increased markers of oxidative stress and decreased NO bioavailability, flow-mediated dilation, and vitamin E levels showing no significant differences between tobacco and e-cigarette exposure (reviewed in [ 20 ]). Inasmuch, short-term e-cigarette use in healthy smokers resulted in marked impairment of endothelial function and an increase in arterial stiffness (reviewed in [ 20 ]). Similar effects on endothelial dysfunction and arterial stiffness were found in animals when they were exposed to e-cigarette vapor either for several days or chronically (reviewed in [ 20 ]). In contrast, other studies found acute microvascular endothelial dysfunction, increased oxidative stress and arterial stiffness in smokers after exposure to e-cigarettes with nicotine, but not after e-cigarettes without nicotine (reviewed in [ 20 ]). In women smokers, a study found a significant difference in stiffness after smoking just one tobacco cigarette, but not after use of e-cigarettes (reviewed in [ 20 ]).
It is well known that nicotine is extremely addictive and has a multitude of harmful effects. Nicotine has significant biologic activity and adversely affects several physiological systems including the cardiovascular, respiratory, immunological and reproductive systems, and can also compromise lung and kidney function [ 41 ]. Recently, a sub-chronic whole-body exposure of e-liquid (2 h/day, 5 days/week, 30 days) containing PG alone or PG with nicotine (25 mg/mL) to wild type (WT) animals or knockout (KO) mice in α7 nicotinic acetylcholine receptor (nAChRα7-KO) revealed a partly nAChRα7-dependent lung inflammation [ 42 ]. While sub-chronic exposure to PG/nicotine promote nAChRα7-dependent increased levels of different cytokines and chemokines in the bronchoalveolar lavage fluid (BALF) such as IL-1α, IL-2, IL-9, interferon γ (IFNγ), granulocyte-macrophage colony-stimulating factor (GM-CSF), monocyte chemoattractant protein-1 (MCP-1/CCL2) and regulated on activation, normal T cell expressed and secreted (RANTES/CCL5), the enhanced levels of IL-1β, IL-5 and TNFα were nAChRα7 independent. In general, most of the cytokines detected in BALF were significantly increased in WT mice exposed to PG with nicotine compared to PG alone or air control [ 42 ]. Some of these effects were found to be through nicotine activation of NF-κB signalling albeit in females but not in males. In addition, PG with nicotine caused increased macrophage and CD4 + /CD8 + T-lymphocytes cell counts in BALF compared to air control, but these effects were ameliorated when animals were sub-chronically exposed to PG alone [ 42 ].
Of note, another study indicated that although RANTES/CCL5 and CCR1 mRNA were upregulated in flavour/nicotine-containing e-cigarette users, vaping flavour and nicotine-less e-cigarettes did not significantly dysregulate cytokine and inflammasome activation [ 43 ].
In addition to its toxicological effects on foetus development, nicotine can disrupt brain development in adolescents and young adults [ 44 , 45 , 46 ]. Several studies have also suggested that nicotine is potentially carcinogenic (reviewed in [ 41 ]), but more work is needed to prove its carcinogenicity independently of the combustion products of tobacco [ 47 ]. In this latter regard, no differences were encountered in the frequency of tumour appearance in rats subjected to long-term (2 years) inhalation of nicotine when compared with control rats [ 48 ]. Despite the lack of carcinogenicity evidence, it has been reported that nicotine promotes tumour cell survival by decreasing apoptosis and increasing proliferation [ 49 ], indicating that it may work as a “tumour enhancer”. In a very recent study, chronic administration of nicotine to mice (1 mg/kg every 3 days for a 60-day period) enhanced brain metastasis by skewing the polarity of M2 microglia, which increases metastatic tumour growth [ 50 ]. Assuming that a conventional cigarette contains 0.172–1.702 mg of nicotine [ 51 ], the daily nicotine dose administered to these animals corresponds to 40–400 cigarettes for a 70 kg-adult, which is a dose of an extremely heavy smoker. We would argue that further studies with chronic administration of low doses of nicotine are required to clearly evaluate its impact on carcinogenicity.
In the aforementioned study exposing human gingival fibroblasts to CS condensate or to nicotine-rich or nicotine-free e-vapour condensates [ 29 ], the detrimental effects were greater in cells exposed to nicotine-rich condensate than to nicotine-free condensate, suggesting that the possible injurious effects of nicotine should be considered when purchasing e-refills . It is also noteworthy that among the 3 most cytotoxic vapours for HUVEC evaluated in the Putzhammer et al . study, 2 were nicotine-free, which suggests that nicotine is not the only hazardous component in e-cigarettes [ 24 ] .
The lethal dose of nicotine for an adult is estimated at 30–60 mg [ 52 ]. Given that nicotine easily diffuses from the dermis to the bloodstream, acute nicotine exposure by e-liquid spilling (5 mL of a 20 mg/mL nicotine-containing refill is equivalent to 100 mg of nicotine) can easily be toxic or even deadly [ 8 ]. Thus, devices with rechargeable refills are another issue of concern with e-cigarettes , especially when e-liquids are not sold in child-safe containers, increasing the risk of spilling, swallowing or breathing.
These data overall indicate that the harmful effects of nicotine should not be underestimated. Despite the established regulations, some inaccuracies in nicotine content labelling remain in different brands of e-liquids . Consequently, stricter regulation and a higher quality control in the e-liquid industry are required.
Effect of humectants and their heating-related products
In this particular aspect, again the composition of the e-liquid varies significantly among different commercial brands [ 4 , 35 ]. The most common and major components of e-liquids are PG or 1,2-propanediol, and glycerol or glycerine (propane-1,2,3-triol). Both types of compounds are used as humectants to prevent the e-liquid from drying out [ 2 , 53 ] and are classified by the Food and Drug Administration (FDA) as “Generally Recognised as Safe” [ 54 ]. In fact, they are widely used as alimentary and pharmaceutical products [ 2 ]. In an analysis of 54 commercially available e-liquids , PG and glycerol were detected in almost all samples at concentrations ranging from 0.4% to 98% (average 57%) and from 0.3% to 95% (average 37%), respectively [ 35 ].
With regards to toxicity, little is known about the effects of humectants when they are heated and chronically inhaled. Studies have indicated that PG can induce respiratory irritation and increase the probability of asthma development [ 55 , 56 ], and both PG and glycerol from e-cigarettes might reach concentrations sufficiently high to potentially cause irritation of the airways [ 57 ]. Indeed, the latter study established that one e-cigarette puff results in a PG exposure of 430–603 mg/m 3 , which is higher than the levels reported to cause airway irritation (average 309 mg/m 3 ) based on a human study [ 55 ]. The same study established that one e-cigarette puff results in a glycerol exposure of 348–495 mg/m 3 [ 57 ], which is close to the levels reported to cause airway irritation in rats (662 mg/m 3 ) [ 58 ].
Airway epithelial injury induced by acute vaping of PG and glycerol aerosols (50:50 vol/vol), with or without nicotine, has been reported in two randomised clinical trials in young tobacco smokers [ 32 ]. In vitro, aerosols from glycerol only-containing refills showed cytotoxicity in A549 and human embryonic stem cells, even at a low battery output voltage [ 59 ]. PG was also found to affect early neurodevelopment in a zebrafish model [ 60 ]. Another important issue is that, under heating conditions PG can produce acetaldehyde or formaldehyde (119.2 or 143.7 ng/puff at 20 W, respectively, on average), while glycerol can also generate acrolein (53.0, 1000.0 or 5.9 ng/puff at 20 W, respectively, on average), all carbonyls with a well-documented toxicity [ 61 ]. Although, assuming 15 puffs per e-cigarette unit, carbonyls produced by PG or glycerol heating would be below the maximum levels found in a conventional cigarette combustion (Table 2 ) [ 51 , 62 ]. Nevertheless, further studies are required to properly test the deleterious effects of all these compounds at physiological doses resembling those to which individuals are chronically exposed.
Although PG and glycerol are the major components of e-liquids other components have been detected. When the aerosols of 4 commercially available e-liquids chosen from a top 10 list of “ Best E-Cigarettes of 2014” , were analysed by gas chromatography-mass spectrometry (GC–MS) after heating, numerous compounds were detected, with nearly half of them not previously identified [ 4 ], thus suggesting that the heating process per se generates new compounds of unknown consequence. Of note, the analysis identified formaldehyde, acetaldehyde and acrolein [ 4 ], 3 carbonyl compounds with known high toxicity [ 63 , 64 , 65 , 66 , 67 ]. While no information was given regarding formaldehyde and acetaldehyde concentrations, the authors calculated that one puff could result in an acrolein exposure of 0.003–0.015 μg/mL [ 4 ]. Assuming 40 mL per puff and 15 puffs per e-cigarette unit (according to several manufacturers) [ 4 ], each e-cigarette unit would generate approximately 1.8–9 μg of acrolein, which is less than the levels of acrolein emitted by a conventional tobacco cigarette (18.3–98.2 μg) [ 51 ]. However, given that e-cigarette units of vaping are not well established, users may puff intermittently throughout the whole day. Thus, assuming 400 to 500 puffs per cartridge, users could be exposed to up to 300 μg of acrolein.
In a similar study, acrolein was found in 11 of 12 aerosols tested, with a similar content range (approximately 0.07–4.19 μg per e-cigarette unit) [ 68 ]. In the same study, both formaldehyde and acetaldehyde were detected in all of the aerosols tested, with contents of 0.2–5.61 μg and 0.11–1.36 μg, respectively, per e-cigarette unit [ 68 ]. It is important to point out that the levels of these toxic products in e-cigarette aerosols are significantly lower than those found in CS: 9 times lower for formaldehyde, 450 times lower for acetaldehyde and 15 times lower for acrolein (Table 2 ) [ 62 , 68 ].
Other compounds that have been detected in aerosols include acetamide, a potential human carcinogen [ 5 ], and some aldehydes [ 69 ], although their levels were minimal. Interestingly, the existence of harmful concentrations of diethylene glycol, a known cytotoxic agent, in e-liquid aerosols is contentious with some studies detecting its presence [ 4 , 68 , 70 , 71 , 72 ], and others finding low subtoxic concentrations [ 73 , 74 ]. Similar observations were reported for the content ethylene glycol. In this regard, either it was detected at concentrations that did not exceed the authorised limit [ 73 ], or it was absent from the aerosols produced [ 4 , 71 , 72 ]. Only one study revealed its presence at high concentration in a very low number of samples [ 5 ]. Nevertheless, its presence above 1 mg/g is not allowed by the FDA [ 73 ]. Figure 1 lists the main compounds detected in aerosols derived from humectant heating and their potential damaging effects. It would seem that future studies should analyse the possible toxic effects of humectants and related products at concentrations similar to those that e-cigarette vapers are exposed to reach conclusive results.
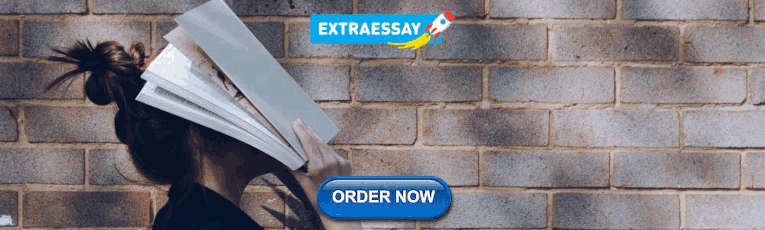
Impact of flavouring compounds
The range of e-liquid flavours available to consumers is extensive and is used to attract both current smokers and new e-cigarette users, which is a growing public health concern [ 6 ]. In fact, over 5 million middle- and high-school students were current users of e-cigarettes in 2019 [ 75 ], and appealing flavours have been identified as the primary reason for e-cigarette consumption in 81% of young users [ 76 ]. Since 2016, the FDA regulates the flavours used in the e-cigarette market and has recently published an enforcement policy on unauthorised flavours, including fruit and mint flavours, which are more appealing to young users [ 77 ]. However, the long-term effects of all flavour chemicals used by this industry (which are more than 15,000) remain unknown and they are not usually included in the product label [ 78 ]. Furthermore, there is no safety guarantee since they may harbour potential toxic or irritating properties [ 5 ].
With regards to the multitude of available flavours, some have demonstrated cytotoxicity [ 59 , 79 ]. Bahl et al. evaluated the toxicity of 36 different e-liquids and 29 different flavours on human embryonic stem cells, mouse neural stem cells and human pulmonary fibroblasts using a metabolic activity assay. In general, those e-liquids that were bubblegum-, butterscotch- and caramel-flavoured did not show any overt cytotoxicity even at the highest dose tested. By contrast, those e-liquids with Freedom Smoke Menthol Arctic and Global Smoke Caramel flavours had marked cytotoxic effects on pulmonary fibroblasts and those with Cinnamon Ceylon flavour were the most cytotoxic in all cell lines [ 79 ]. A further study from the same group [ 80 ] revealed that high cytotoxicity is a recurrent feature of cinnamon-flavoured e-liquids. In this line, results from GC–MS and HPLC analyses indicated that cinnamaldehyde (CAD) and 2-methoxycinnamaldehyde, but not dipropylene glycol or vanillin, were mainly responsible for the high cytotoxicity of cinnamon-flavoured e-liquids [ 80 ]. Other flavouring-related compounds that are associated with respiratory complications [ 81 , 82 , 83 ], such as diacetyl, 2,3-pentanedione or acetoin, were found in 47 out of 51 aerosols of flavoured e-liquids tested [ 84 ] . Allen et al . calculated an average of 239 μg of diacetyl per cartridge [ 84 ]. Assuming again 400 puffs per cartridge and 40 mL per puff, is it is possible to estimate an average of 0.015 ppm of diacetyl per puff, which could compromise normal lung function in the long-term [ 85 ].
The cytotoxic and pro-inflammatory effects of different e-cigarette flavouring chemicals were also tested on two human monocytic cell lines—mono mac 6 (MM6) and U937 [ 86 ]. Among the flavouring chemicals tested, CAD was found to be the most toxic and O-vanillin and pentanedione also showed significant cytotoxicity; by contrast, acetoin, diacetyl, maltol, and coumarin did not show any toxicity at the concentrations assayed (10–1000 µM). Of interest, a higher toxicity was evident when combinations of different flavours or mixed equal proportions of e-liquids from 10 differently flavoured e-liquids were tested, suggesting that vaping a single flavour is less toxic than inhaling mixed flavours [ 86 ]. Also, all the tested flavours produced significant levels of ROS in a cell-free ROS production assay. Finally, diacetyl, pentanedione, O-vanillin, maltol, coumarin, and CAD induced significant IL-8 secretion from MM6 and U937 monocytes [ 86 ]. It should be borne in mind, however, that the concentrations assayed were in the supra-physiological range and it is likely that, once inhaled, these concentrations are not reached in the airway space. Indeed, one of the limitations of the study was that human cells are not exposed to e-liquids per se, but rather to the aerosols where the concentrations are lower [ 86 ]. In this line, the maximum concentration tested (1000 µM) would correspond to approximately 80 to 150 ppm, which is far higher than the levels found in aerosols of some of these compounds [ 84 ]. Moreover, on a day-to-day basis, lungs of e-cigarette users are not constantly exposed to these chemicals for 24 h at these concentrations. Similar limitations were found when five of seven flavourings were found to cause cytotoxicity in human bronchial epithelial cells [ 87 ].
Recently, a commonly commercialized crème brûlée -flavoured aerosol was found to contain high concentrations of benzoic acid (86.9 μg/puff), a well-established respiratory irritant [ 88 ]. When human lung epithelial cells (BEAS-2B and H292) were exposed to this aerosol for 1 h, a marked cytotoxicity was observed in BEAS-2B but not in H292 cells, 24 h later. However, increased ROS production was registered in H292 cells [ 88 ].
Therefore, to fully understand the effects of these compounds, it is relevant the cell cultures selected for performing these assays, as well as the use of in vivo models that mimic the real-life situation of chronic e-cigarette vapers to clarify their impact on human health.
The e-cigarette device
While the bulk of studies related to the impact of e-cigarette use on human health has focused on the e-liquid components and the resulting aerosols produced after heating, a few studies have addressed the material of the electronic device and its potential consequences—specifically, the potential presence of metals such as copper, nickel or silver particles in e-liquids and aerosols originating from the filaments and wires and the atomiser [ 89 , 90 , 91 ].
Other important components in the aerosols include silicate particles from the fiberglass wicks or silicone [ 89 , 90 , 91 ]. Many of these products are known to cause abnormalities in respiratory function and respiratory diseases [ 89 , 90 , 91 ], but more in-depth studies are required. Interestingly, the battery output voltage also seems to have an impact on the cytotoxicity of the aerosol vapours, with e-liquids from a higher battery output voltage showing more toxicity to A549 cells [ 30 ].
A recent study compared the acute effects of e-cigarette vapor (with PG/vegetable glycerine plus tobacco flavouring but without nicotine) generated from stainless‐steel atomizer (SS) heating element or from a nickel‐chromium alloy (NC) [ 92 ]. Some rats received a single e-cigarette exposure for 2 h from a NC heating element (60 or 70 W); other rats received a similar exposure of e-cigarette vapor using a SS heating element for the same period of time (60 or 70 W) and, a final group of animals were exposed for 2 h to air. Neither the air‐exposed rats nor those exposed to e-cigarette vapor using SS heating elements developed respiratory distress. In contrast, 80% of the rats exposed to e-cigarette vapor using NC heating units developed clinical acute respiratory distress when a 70‐W power setting was employed. Thus, suggesting that operating units at higher than recommended settings can cause adverse effects. Nevertheless, there is no doubt that the deleterious effects of battery output voltage are not comparable to those exerted by CS extracts [ 30 ] (Figs. 1 and 2 ).
E-cigarettes as a smoking cessation tool
CS contains a large number of substances—about 7000 different constituents in total, with sizes ranging from atoms to particulate matter, and with many hundreds likely responsible for the harmful effects of this habit [ 93 ]. Given that tobacco is being substituted in great part by e-cigarettes with different chemical compositions, manufacturers claim that e -cigarette will not cause lung diseases such as lung cancer, chronic obstructive pulmonary disease, or cardiovascular disorders often associated with conventional cigarette consumption [ 3 , 94 ]. However, the World Health Organisation suggests that e-cigarettes cannot be considered as a viable method to quit smoking, due to a lack of evidence [ 7 , 95 ]. Indeed, the results of studies addressing the use of e-cigarettes as a smoking cessation tool remain controversial [ 96 , 97 , 98 , 99 , 100 ]. Moreover, both FDA and CDC are actively investigating the incidence of severe respiratory symptoms associated with the use of vaping products [ 77 ]. Because many e-liquids contain nicotine, which is well known for its powerful addictive properties [ 41 ], e-cigarette users can easily switch to conventional cigarette smoking, avoiding smoking cessation. Nevertheless, the possibility of vaping nicotine-free e-cigarettes has led to the branding of these devices as smoking cessation tools [ 2 , 6 , 7 ].
In a recently published randomised trial of 886 subjects who were willing to quit smoking [ 100 ], the abstinence rate was found to be twice as high in the e-cigarette group than in the nicotine-replacement group (18.0% vs. 9.9%) after 1 year. Of note, the abstinence rate found in the nicotine-replacement group was lower than what is usually expected with this therapy. Nevertheless, the incidence of throat and mouth irritation was higher in the e-cigarette group than in the nicotine-replacement group (65.3% vs. 51.2%, respectively). Also, the participant adherence to the treatment after 1-year abstinence was significantly higher in the e-cigarette group (80%) than in nicotine-replacement products group (9%) [ 100 ].
On the other hand, it is estimated that COPD could become the third leading cause of death in 2030 [ 101 ]. Given that COPD is generally associated with smoking habits (approximately 15 to 20% of smokers develop COPD) [ 101 ], smoking cessation is imperative among COPD smokers. Published data revealed a clear reduction of conventional cigarette consumption in COPD smokers that switched to e-cigarettes [ 101 ]. Indeed, a significant reduction in exacerbations was observed and, consequently, the ability to perform physical activities was improved when data was compared with those non-vapers COPD smokers. Nevertheless, a longer follow-up of these COPD patients is required to find out whether they have quitted conventional smoking or even vaping, since the final goal under these circumstances is to quit both habits.
Based on the current literature, it seems that several factors have led to the success of e-cigarette use as a smoking cessation tool. First, some e-cigarette flavours positively affect smoking cessation outcomes among smokers [ 102 ]. Second, e-cigarettes have been described to improve smoking cessation rate only among highly-dependent smokers and not among conventional smokers, suggesting that the individual degree of nicotine dependence plays an important role in this process [ 97 ]. Third, the general belief of their relative harmfulness to consumers' health compared with conventional combustible tobacco [ 103 ]. And finally, the exposure to point-of-sale marketing of e-cigarette has also been identified to affect the smoking cessation success [ 96 ].
Implication of e-cigarette consumption in COVID-19 time
Different reports have pointed out that smokers and vapers are more vulnerable to SARS-CoV-2 (Severe Acute Respiratory Syndrome Coronavirus 2) infections or more prone to adverse outcomes if they suffer COVID-19 [ 104 ]. However, while a systematic review indicated that cigarette smoking is probably associated with enhanced damage from COVID-19, a meta-analysis did not, yet the latter had several limitations due to the small sample sizes [ 105 ].
Interestingly, most of these reports linking COVID-19 harmful effects with smoking or vaping, are based on their capability of increasing the expression of angiotensin-converting enzyme 2 (ACE2) in the lung. It is well known that ACE2 is the gate for SARS-CoV-2 entrance to the airways [ 106 ] and it is mainly expressed in type 2 alveolar epithelial cells and alveolar macrophages [ 107 ]. To date, most of the studies in this field indicate that current smokers have higher expression of ACE2 in the airways (reviewed by [ 108 ]) than healthy non-smokers [ 109 , 110 ]. However, while a recent report indicated that e-cigarette vaping also caused nicotine-dependent ACE2 up-regulation [ 42 ], others have revealed that neither acute inhalation of e-cigarette vapour nor e-cigarette users had increased lung ACE2 expression regardless nicotine presence in the e-liquid [ 43 , 110 ].
In regard to these contentions, current knowledge suggests that increased ACE2 expression is not necessarily linked to enhanced susceptibility to SARS-CoV-2 infection and adverse outcome. Indeed, elderly population express lower levels of ACE2 than young people and SARS-CoV-2/ACE2 interaction further decreases ACE2 expression. In fact, most of the deaths provoked by COVID-19 took place in people over 60 years old of age [ 111 ]. Therefore, it is plausible that the increased susceptibility to disease progression and the subsequent fatal outcome in this population is related to poor angiotensin 1-7 (Ang-1-7) generation, the main peptide generated by ACE2, and probably to their inaccessibility to its anti-inflammatory effects. Furthermore, it seems that all the efforts towards increasing ACE2 expression may result in a better resolution of the pneumonic process associated to this pandemic disease.
Nevertheless, additional complications associated to COVID-19 are increased thrombotic events and cytokine storm. In the lungs, e-cigarette consumption has been correlated to toxicity, oxidative stress, and inflammatory response [ 32 , 112 ]. More recently, a study revealed that while the use of nicotine/flavour-containing e-cigarettes led to significant cytokine dysregulation and potential inflammasome activation, none of these effects were detected in non-flavoured and non-nicotine-containing e-cigarettes [ 43 ]. Therefore, taken together these observations, e-cigarette use may still be a potent risk factor for severe COVID-19 development depending on the flavour and nicotine content.
In summary, it seems that either smoking or nicotine vaping may adversely impact on COVID-19 outcome. However, additional follow up studies are required in COVID-19 pandemic to clarify the effect of e-cigarette use on lung and cardiovascular complications derived from SARS-CoV-2 infection.
Conclusions
The harmful effects of CS and their deleterious consequences are both well recognised and widely investigated. However, and based on the studies carried out so far, it seems that e-cigarette consumption is less toxic than tobacco smoking. This does not necessarily mean, however, that e-cigarettes are free from hazardous effects. Indeed, studies investigating their long-term effects on human health are urgently required. In this regard, the main additional studies needed in this field are summarized in Table 3 .
The composition of e-liquids requires stricter regulation, as they can be easily bought online and many incidences of mislabelling have been detected, which can seriously affect consumers’ health. Beyond their unknown long-term effects on human health, the extended list of appealing flavours available seems to attract new “never-smokers”, which is especially worrying among young users. Additionally, there is still a lack of evidence of e-cigarette consumption as a smoking cessation method. Indeed, e-cigarettes containing nicotine may relieve the craving for smoking, but not the conventional cigarette smoking habit.
Interestingly, there is a strong difference of opinion on e-cigarettes between countries. Whereas countries such as Brazil, Uruguay and India have banned the sale of e-cigarettes , others such as the United Kingdom support this device to quit smoking. The increasing number of adolescent users and reported deaths in the United States prompted the government to ban the sale of flavoured e-cigarettes in 2020. The difference in opinion worldwide may be due to different restrictions imposed. For example, while no more than 20 ng/mL of nicotine is allowed in the EU, e-liquids with 59 mg/dL are currently available in the United States. Nevertheless, despite the national restrictions, users can easily access foreign or even counterfeit products online.
In regard to COVID-19 pandemic, the actual literature suggests that nicotine vaping may display adverse outcomes. Therefore, follow up studies are necessary to clarify the impact of e-cigarette consumption on human health in SARS-CoV-2 infection.
In conclusion, e-cigarettes could be a good alternative to conventional tobacco cigarettes, with less side effects; however, a stricter sale control, a proper regulation of the industry including flavour restriction, as well as further toxicological studies, including their chronic effects, are warranted.
Availability of data and materials
Not applicable.
Abbreviations
Angiotensin-converting enzyme 2
Angiotensin 1-7
Bronchoalveolar lavage fluid
Cinnamaldehyde
US Centers for Disease Control and Prevention
Carbon monoxide
Chronic obstructive pulmonary disease
Coronavirus disease 2019
Cigarette smoke
Electronic nicotine dispensing systems
e-cigarette or vaping product use-associated lung injury
Food and Drug Administration
Gas chromatography with a flame ionisation detector
Gas chromatography-mass spectrometry
Granulocyte–macrophage colony-stimulating factor
High performance liquid chromatography
Human umbilical vein endothelial cells
Interleukin
Interferon γ
Liquid chromatography-mass spectrometry
Monocyte chemoattractant protein-1
Matrix metallopeptidase 9
α7 Nicotinic acetylcholine receptor
Nickel‐chromium alloy
Nitric oxide
Propylene glycol
Regulated on activation, normal T cell expressed and secreted
Reactive oxygen species
Severe acute respiratory syndrome coronavirus 2
Stainless‐steel atomizer
Tetrahydrocannabinol
Tumour necrosis factor-α
Hiemstra PS, Bals R. Basic science of electronic cigarettes: assessment in cell culture and in vivo models. Respir Res. 2016;17(1):127.
Article PubMed PubMed Central CAS Google Scholar
Bertholon JF, Becquemin MH, Annesi-Maesano I, Dautzenberg B. Electronic cigarettes: a short review. Respiration. 2013;86(5):433–8.
Article CAS PubMed Google Scholar
Rowell TR, Tarran R. Will chronic e-cigarette use cause lung disease? Am J Physiol Lung Cell Mol Physiol. 2015;309(12):L1398–409.
Article CAS PubMed PubMed Central Google Scholar
Herrington JS, Myers C. Electronic cigarette solutions and resultant aerosol profiles. J Chromatogr A. 2015;1418:192–9.
Hutzler C, Paschke M, Kruschinski S, Henkler F, Hahn J, Luch A. Chemical hazards present in liquids and vapors of electronic cigarettes. Arch Toxicol. 2014;88(7):1295–308.
Pokhrel P, Herzog TA, Muranaka N, Fagan P. Young adult e-cigarette users’ reasons for liking and not liking e-cigarettes: a qualitative study. Psychol Health. 2015;30(12):1450–69.
Article PubMed PubMed Central Google Scholar
Harrell PT, Simmons VN, Correa JB, Padhya TA, Brandon TH. Electronic nicotine delivery systems (“e-cigarettes”): review of safety and smoking cessation efficacy. Otolaryngol Head Neck Surg. 2014;151(3):381–93.
Schober W, Szendrei K, Matzen W, Osiander-Fuchs H, Heitmann D, Schettgen T, et al. Use of electronic cigarettes (e-cigarettes) impairs indoor air quality and increases FeNO levels of e-cigarette consumers. Int J Hyg Environ Health. 2014;217(6):628–37.
Rubinstein ML, Delucchi K, Benowitz NL, Ramo DE. Adolescent exposure to toxic volatile organic chemicals from E-cigarettes. Pediatrics. 2018;141(4):e20173557.
Article PubMed Google Scholar
Staudt MR, Salit J, Kaner RJ, Hollmann C, Crystal RG. Altered lung biology of healthy never smokers following acute inhalation of E-cigarettes. Respir Res. 2018;19(1):78.
Nocella C, Biondi-Zoccai G, Sciarretta S, Peruzzi M, Pagano F, Loffredo L, et al. Impact of tobacco versus electronic cigarette smoking on platelet function. Am J Cardiol. 2018;122(9):1477–81.
Higham A, Rattray NJW, Dewhurst JA, Trivedi DK, Fowler SJ, Goodacre R, et al. Electronic cigarette exposure triggers neutrophil inflammatory responses. Respir Res. 2016;17(1):56.
Antoniewicz L, Bosson JA, Kuhl J, Abdel-Halim SM, Kiessling A, Mobarrez F, et al. Electronic cigarettes increase endothelial progenitor cells in the blood of healthy volunteers. Atherosclerosis. 2016;255:179–85.
Carnevale R, Sciarretta S, Violi F, Nocella C, Loffredo L, Perri L, et al. Acute impact of tobacco vs electronic cigarette smoking on oxidative stress and vascular function. Chest. 2016;150(3):606–12.
Vlachopoulos C, Ioakeimidis N, Abdelrasoul M, Terentes-Printzios D, Georgakopoulos C, Pietri P, et al. Electronic cigarette smoking increases aortic stiffness and blood pressure in young smokers. J Am Coll Cardiol. 2016;67(23):2802–3.
Franzen KF, Willig J, Cayo Talavera S, Meusel M, Sayk F, Reppel M, et al. E-cigarettes and cigarettes worsen peripheral and central hemodynamics as well as arterial stiffness: a randomized, double-blinded pilot study. Vasc Med. 2018;23(5):419–25.
Caporale A, Langham MC, Guo W, Johncola A, Chatterjee S, Wehrli FW. Acute effects of electronic cigarette aerosol inhalation on vascular function detected at quantitative MRI. Radiology. 2019;293(1):97–106.
von Hundelshausen P, Schmitt MM. Platelets and their chemokines in atherosclerosis-clinical applications. Front Physiol. 2014;5:294.
Google Scholar
Landmesser U, Hornig B, Drexler H. Endothelial function: a critical determinant in atherosclerosis? Circulation. 2004;109(21 Suppl 1):Ii27-33.
PubMed Google Scholar
Münzel T, Hahad O, Kuntic M, Keaney JF, Deanfield JE, Daiber A. Effects of tobacco cigarettes, e-cigarettes, and waterpipe smoking on endothelial function and clinical outcomes. Eur Heart J. 2020;41:4057–70.
Javelle E. Electronic cigarette and vaping should be discouraged during the new coronavirus SARS-CoV-2 pandemic. Arch Toxicol. 2020;94(6):2261–2.
Husari A, Shihadeh A, Talih S, Hashem Y, El Sabban M, Zaatari G. Acute exposure to electronic and combustible cigarette aerosols: effects in an animal model and in human alveolar cells. Nicotine Tob Res. 2016;18(5):613–9.
Zelikoff JT, Parmalee NL, Corbett K, Gordon T, Klein CB, Aschner M. Microglia activation and gene expression alteration of neurotrophins in the hippocampus following early-life exposure to E-cigarette aerosols in a murine model. Toxicol Sci. 2018;162(1):276–86.
Putzhammer R, Doppler C, Jakschitz T, Heinz K, Forste J, Danzl K, et al. Vapours of US and EU market leader electronic cigarette brands and liquids are cytotoxic for human vascular endothelial cells. PLoS One. 2016;11(6):e0157337.
Bernhard D, Pfister G, Huck CW, Kind M, Salvenmoser W, Bonn GK, et al. Disruption of vascular endothelial homeostasis by tobacco smoke: impact on atherosclerosis. Faseb J. 2003;17(15):2302–4.
Newby DE, Wright RA, Labinjoh C, Ludlam CA, Fox KA, Boon NA, et al. Endothelial dysfunction, impaired endogenous fibrinolysis, and cigarette smoking: a mechanism for arterial thrombosis and myocardial infarction. Circulation. 1999;99(11):1411–5.
Taylor M, Jaunky T, Hewitt K, Breheny D, Lowe F, Fearon IM, et al. A comparative assessment of e-cigarette aerosols and cigarette smoke on in vitro endothelial cell migration. Toxicol Lett. 2017;277:123–8.
Herr C, Tsitouras K, Niederstraßer J, Backes C, Beisswenger C, Dong L, et al. Cigarette smoke and electronic cigarettes differentially activate bronchial epithelial cells. Respir Res. 2020;21(1):67.
Alanazi H, Park HJ, Chakir J, Semlali A, Rouabhia M. Comparative study of the effects of cigarette smoke and electronic cigarettes on human gingival fibroblast proliferation, migration and apoptosis. Food Chem Toxicol. 2018;118:390–8.
Otreba M, Kosmider L. E-cigarettes: voltage- and concentration-dependent loss in human lung adenocarcinoma viability. J Appl Toxicol. 2018;38(8):1135–43.
Chaumont M, Bernard A, Pochet S, Melot C, El Khattabi C, Reye F, et al. High-wattage E-cigarettes induce tissue hypoxia and lower airway injury: a randomized clinical trial. Am J Respir Crit Care Med. 2018;198(1):123–6.
Chaumont M, van de Borne P, Bernard A, Van Muylem A, Deprez G, Ullmo J, et al. Fourth generation e-cigarette vaping induces transient lung inflammation and gas exchange disturbances: results from two randomized clinical trials. Am J Physiol Lung Cell Mol Physiol. 2019;316(5):L705–19.
European Parliament and the council of the European Union. Directive 2014/40/EU. 2014 (updated April 29, 2014). https://ec.europa.eu/health//sites/health/files/tobacco/docs/dir_201440_en.pdf . Accessed 17 April 2020.
Cameron JM, Howell DN, White JR, Andrenyak DM, Layton ME, Roll JM. Variable and potentially fatal amounts of nicotine in e-cigarette nicotine solutions. Tob Control. 2014;23(1):77–8.
Hahn J, Monakhova YB, Hengen J, Kohl-Himmelseher M, Schussler J, Hahn H, et al. Electronic cigarettes: overview of chemical composition and exposure estimation. Tob Induc Dis. 2014;12(1):23.
Omaiye EE, Cordova I, Davis B, Talbot P. Counterfeit electronic cigarette products with mislabeled nicotine concentrations. Tob Regul Sci. 2017;3(3):347–57.
Buettner-Schmidt K, Miller DR, Balasubramanian N. Electronic cigarette refill liquids: child-resistant packaging, nicotine content, and sales to minors. J Pediatr Nurs. 2016;31(4):373–9.
Jackson R, Huskey M, Brown S. Labelling accuracy in low nicotine e-cigarette liquids from a sampling of US manufacturers. Int J Pharm Pract. 2019;28(3):290–4.
Yingst JM, Foulds J, Veldheer S, Hrabovsky S, Trushin N, Eissenberg TT, et al. Nicotine absorption during electronic cigarette use among regular users. PLoS One. 2019;14(7):e0220300.
Farsalinos KE, Romagna G, Tsiapras D, Kyrzopoulos S, Voudris V. Evaluation of electronic cigarette use (vaping) topography and estimation of liquid consumption: implications for research protocol standards definition and for public health authorities’ regulation. Int J Environ Res Public Health. 2013;10(6):2500–14.
Mishra A, Chaturvedi P, Datta S, Sinukumar S, Joshi P, Garg A. Harmful effects of nicotine. Indian J Med Paediatr Oncol. 2015;36(1):24–31.
Wang Q, Sundar IK, Li D, Lucas JH, Muthumalage T, McDonough SR, et al. E-cigarette-induced pulmonary inflammation and dysregulated repair are mediated by nAChR α7 receptor: role of nAChR α7 in SARS-CoV-2 Covid-19 ACE2 receptor regulation. Respir Res. 2020;21(1):154.
Lee AC, Chakladar J, Li WT, Chen C, Chang EY, Wang-Rodriguez J, et al. Tobacco, but not nicotine and flavor-less electronic cigarettes, induces ACE2 and immune dysregulation. Int J Mol Sci. 2020;21(15):5513.
Article CAS PubMed Central Google Scholar
England LJ, Bunnell RE, Pechacek TF, Tong VT, McAfee TA. Nicotine and the developing human: a neglected element in the electronic cigarette debate. Am J Prev Med. 2015;49(2):286–93.
Yuan M, Cross SJ, Loughlin SE, Leslie FM. Nicotine and the adolescent brain. J Physiol. 2015;593(16):3397–412.
Holbrook BD. The effects of nicotine on human fetal development. Birth Defects Res C Embryo Today. 2016;108(2):181–92.
Sanner T, Grimsrud TK. Nicotine: carcinogenicity and effects on response to cancer treatment—a review. Front Oncol. 2015;5:196.
Waldum HL, Nilsen OG, Nilsen T, Rørvik H, Syversen V, Sanvik AK, et al. Long-term effects of inhaled nicotine. Life Sci. 1996;58(16):1339–46.
Cucina A, Dinicola S, Coluccia P, Proietti S, D’Anselmi F, Pasqualato A, et al. Nicotine stimulates proliferation and inhibits apoptosis in colon cancer cell lines through activation of survival pathways. J Surg Res. 2012;178(1):233–41.
Wu SY, Xing F, Sharma S, Wu K, Tyagi A, Liu Y, et al. Nicotine promotes brain metastasis by polarizing microglia and suppressing innate immune function. J Exp Med. 2020;217(8):e20191131.
Roemer E, Stabbert R, Rustemeier K, Veltel DJ, Meisgen TJ, Reininghaus W, et al. Chemical composition, cytotoxicity and mutagenicity of smoke from US commercial and reference cigarettes smoked under two sets of machine smoking conditions. Toxicology. 2004;195(1):31–52.
Mayer B. How much nicotine kills a human? Tracing back the generally accepted lethal dose to dubious self-experiments in the nineteenth century. Arch Toxicol. 2014;88(1):5–7.
Brown CJ, Cheng JM. Electronic cigarettes: product characterisation and design considerations. Tob Control. 2014;23(Suppl 2):ii4-10.
Food and Drug Administration. SCOGS (Select Committee on GRAS Substances). 2019 (updated April 29, 2019). https://www.accessdata.fda.gov/scripts/fdcc/index.cfm?set=SCOGS&sort=Sortsubstance&order=ASC&startrow=251&type=basic&search= . Accessed 14 April 2020.
Wieslander G, Norback D, Lindgren T. Experimental exposure to propylene glycol mist in aviation emergency training: acute ocular and respiratory effects. Occup Environ Med. 2001;58(10):649–55.
Choi H, Schmidbauer N, Sundell J, Hasselgren M, Spengler J, Bornehag CG. Common household chemicals and the allergy risks in pre-school age children. PLoS One. 2010;5(10):e13423.
Kienhuis AS, Soeteman-Hernandez LG, Bos PMJ, Cremers HWJM, Klerx WN, Talhout R. Potential harmful health effects of inhaling nicotine-free shisha-pen vapor: a chemical risk assessment of the main components propylene glycol and glycerol. Tob Induc Dis. 2015;13(1):15.
Renne RA, Wehner AP, Greenspan BJ, Deford HS, Ragan HA, Westerberg RB, et al. 2-Week and 13-week inhalation studies of aerosolized glycerol in rats. Inhal Toxicol. 1992;4(2):95–111.
Article CAS Google Scholar
Behar R, Wang Y, Talbot P. Comparing the cytotoxicity of electronic cigarette fluids, aerosols and solvents. Tob Control. 2018;27(3):325.
Massarsky A, Abdel A, Glazer L, Levin ED, Di Giulio RT. Neurobehavioral effects of 1,2-propanediol in zebrafish (Danio rerio). Neurotoxicology. 2018;65:111–24.
Geiss O, Bianchi I, Barrero-Moreno J. Correlation of volatile carbonyl yields emitted by e-cigarettes with the temperature of the heating coil and the perceived sensorial quality of the generated vapours. Int J Hyg Environ Health. 2016;219(3):268–77.
Counts ME, Morton MJ, Laffoon SW, Cox RH, Lipowicz PJ. Smoke composition and predicting relationships for international commercial cigarettes smoked with three machine-smoking conditions. Regul Toxicol Pharmacol. 2005;41(3):185–227.
Agency for Toxic Substances & Disease Registry. Toxicological Profile for Formaldehyde. 2019 (updated September 26, 2019). https://www.atsdr.cdc.gov/ToxProfiles/tp.asp?id=220&tid=39 . Accessed 9 April 2020.
Agency for Toxic Substances & Disease Registry. Toxicological Profile for Acrolein. 2019 (updated September 26, 2019). https://www.atsdr.cdc.gov/toxprofiles/TP.asp?id=557&tid=102 . Accessed 9 April 2020
Moghe A, Ghare S, Lamoreau B, Mohammad M, Barve S, McClain C, et al. Molecular mechanisms of acrolein toxicity: relevance to human disease. Toxicol Sci. 2015;143(2):242–55.
Seitz HK, Stickel F. Acetaldehyde as an underestimated risk factor for cancer development: role of genetics in ethanol metabolism. Genes Nutr. 2010;5(2):121–8.
Faroon O, Roney N, Taylor J, Ashizawa A, Lumpkin MH, Plewak DJ. Acrolein health effects. Toxicol Ind Health. 2008;24(7):447–90.
Goniewicz ML, Knysak J, Gawron M, Kosmider L, Sobczak A, Kurek J, et al. Levels of selected carcinogens and toxicants in vapour from electronic cigarettes. Tob Control. 2014;23(2):133–9.
Farsalinos KE, Voudris V. Do flavouring compounds contribute to aldehyde emissions in e-cigarettes? Food Chem Toxicol. 2018;115:212–7.
Kavvalakis MP, Stivaktakis PD, Tzatzarakis MN, Kouretas D, Liesivuori J, Alegakis AK, et al. Multicomponent analysis of replacement liquids of electronic cigarettes using chromatographic techniques. J Anal Toxicol. 2015;39(4):262–9.
Etter JF, Zather E, Svensson S. Analysis of refill liquids for electronic cigarettes. Addiction. 2013;108(9):1671–9.
Etter JF, Bugey A. E-cigarette liquids: constancy of content across batches and accuracy of labeling. Addict Behav. 2017;73:137–43.
Varlet V, Farsalinos K, Augsburger M, Thomas A, Etter JF. Toxicity assessment of refill liquids for electronic cigarettes. Int J Environ Res Public Health. 2015;12(5):4796–815.
McAuley TR, Hopke PK, Zhao J, Babaian S. Comparison of the effects of e-cigarette vapor and cigarette smoke on indoor air quality. Inhal Toxicol. 2012;24(12):850–7.
Cullen KA, Gentzke AS, Sawdey MD, Chang JT, Anic GM, Wang TW, et al. e-Cigarette use among youth in the United States, 2019. JAMA. 2019;322(21):2095–103.
Villanti AC, Johnson AL, Ambrose BK, Cummings KM, Stanton CA, Rose SW, et al. Flavored tobacco product use in youth and adults: findings from the first wave of the PATH Study (2013–2014). Am J Prev Med. 2017;53(2):139–51.
Food and Drug Administration. Vaporizers, E-Cigarettes, and other Electronic Nicotine Delivery Systems (ENDS) 2020 (updated April 13, 2020). https://www.fda.gov/tobacco-products/products-ingredients-components/vaporizers-e-cigarettes-and-other-electronic-nicotine-delivery-systems-ends . Accessed 15 April 2020
Omaiye EE, McWhirter KJ, Luo W, Tierney PA, Pankow JF, Talbot P. High concentrations of flavor chemicals are present in electronic cigarette refill fluids. Sci Rep. 2019;9(1):2468.
Bahl V, Lin S, Xu N, Davis B, Wang YH, Talbot P. Comparison of electronic cigarette refill fluid cytotoxicity using embryonic and adult models. Reprod Toxicol. 2012;34(4):529–37.
Behar R, Davis B, Wang Y, Bahl V, Lin S, Talbot P. Identification of toxicants in cinnamon-flavored electronic cigarette refill fluids. Toxicol In Vitro. 2014;28(2):198–208.
Morgan DL, Flake GP, Kirby PJ, Palmer SM. Respiratory toxicity of diacetyl in C57BL/6 mice. Toxicol Sci. 2008;103(1):169–80.
Hubbs AF, Cumpston AM, Goldsmith WT, Battelli LA, Kashon ML, Jackson MC, et al. Respiratory and olfactory cytotoxicity of inhaled 2,3-pentanedione in Sprague-Dawley rats. Am J Pathol. 2012;181(3):829–44.
Vas CA, Porter A, Mcadam K. Acetoin is a precursor to diacetyl in e-cigarette liquids. Food Chem Toxicol. 2019;133:110727.
Allen JG, Flanigan SS, LeBlanc M, Vallarino J, MacNaughton P, Stewart JH, et al. Flavoring chemicals in E-cigarettes: diacetyl, 2,3-pentanedione, and acetoin in a sample of 51 products, including fruit-, candy-, and cocktail-flavored E-cigarettes. Environ Health Perspect. 2016;124(6):733–9.
Park RM, Gilbert SJ. Pulmonary impairment and risk assessment in a diacetyl-exposed population: microwave popcorn workers. J Occup Environ Med. 2018;60(6):496–506.
Muthumalage T, Prinz M, Ansah KO, Gerloff J, Sundar IK, Rahman I. Inflammatory and oxidative responses induced by exposure to commonly used e-cigarette flavoring chemicals and flavored e-liquids without nicotine. Front Physiol. 2017;8:1130.
Sherwood CL, Boitano S. Airway epithelial cell exposure to distinct e-cigarette liquid flavorings reveals toxicity thresholds and activation of CFTR by the chocolate flavoring 2,5-dimethypyrazine. Respir Res. 2016;17(1):57.
Pinkston R, Zaman H, Hossain E, Penn AL, Noël A. Cell-specific toxicity of short-term JUUL aerosol exposure to human bronchial epithelial cells and murine macrophages exposed at the air–liquid interface. Respir Res. 2020;21(1):269.
Williams M, Villarreal A, Bozhilov K, Lin S, Talbot P. Metal and silicate particles including nanoparticles are present in electronic cigarette cartomizer fluid and aerosol. PLoS One. 2013;8(3):e57987.
Mikheev VB, Brinkman MC, Granville CA, Gordon SM, Clark PI. Real-time measurement of electronic cigarette aerosol size distribution and metals content analysis. Nicotine Tob Res. 2016;18(9):1895–902.
Williams M, Bozhilov K, Ghai S, Talbot P. Elements including metals in the atomizer and aerosol of disposable electronic cigarettes and electronic hookahs. PLoS One. 2017;12(4):e0175430.
Kleinman MT, Arechavala RJ, Herman D, Shi J, Hasen I, Ting A, et al. E-cigarette or vaping product use-associated lung injury produced in an animal model from electronic cigarette vapor exposure without tetrahydrocannabinol or vitamin E oil. J Am Heart Assoc. 2020;9(18):e017368.
Patnode CD, Henderson JT, Thompson JH, Senger CA, Fortmann SP, Whitlock EP. Behavioral counseling and pharmacotherapy interventions for tobacco cessation in adults, including pregnant women: a review of reviews for the U.S. preventive services task force. Ann Intern Med. 2015;163(8):608–21.
Messner B, Bernhard D. Smoking and cardiovascular disease: mechanisms of endothelial dysfunction and early atherogenesis. Arterioscler Thromb Vasc Biol. 2014;34(3):509–15.
Bansal V, Kim K-H. Review on quantitation methods for hazardous pollutants released by e-cigarette (EC) smoking. Trends Analyt Chem. 2016;78:120–33.
Mantey DS, Pasch KE, Loukas A, Perry CL. Exposure to point-of-sale marketing of cigarettes and E-cigarettes as predictors of smoking cessation behaviors. Nicotine Tob Res. 2019;21(2):212–9.
Selya AS, Dierker L, Rose JS, Hedeker D, Mermelstein RJ. The role of nicotine dependence in E-cigarettes’ potential for smoking reduction. Nicotine Tob Res. 2018;20(10):1272–7.
Kalkhoran S, Glantz SA. E-cigarettes and smoking cessation in real-world and clinical settings: a systematic review and meta-analysis. Lancet Respir Med. 2016;4(2):116–28.
Levy DT, Yuan Z, Luo Y, Abrams DB. The relationship of e-cigarette use to cigarette quit attempts and cessation: insights from a large, nationally representative U.S. survey. Nicotine Tob Res. 2017;20(8):931–9.
Article PubMed Central Google Scholar
Hajek P, Phillips-Waller A, Przulj D, Pesola F, Myers Smith K, Bisal N, et al. A randomized trial of E-cigarettes versus nicotine-replacement therapy. N Engl J Med. 2019;380(7):629–37.
Polosa R, Morjaria JB, Caponnetto P, Prosperini U, Russo C, Pennisi A, et al. Evidence for harm reduction in COPD smokers who switch to electronic cigarettes. Respir Res. 2016;17(1):166.
Litt MD, Duffy V, Oncken C. Cigarette smoking and electronic cigarette vaping patterns as a function of e-cigarette flavourings. Tob Control. 2016;25(Suppl 2):ii67–72.
Palmer AM, Brandon TH. How do electronic cigarettes affect cravings to smoke or vape? Parsing the influences of nicotine and expectancies using the balanced-placebo design. J Consult Clin Psychol. 2018;86(5):486–91.
Majmundar A, Allem JP, Cruz TB, Unger JB. Public health concerns and unsubstantiated claims at the intersection of vaping and COVID-19. Nicotine Tob Res. 2020;22(9):1667–8.
Berlin I, Thomas D, Le Faou A-L, Cornuz J. COVID-19 and smoking. Nicotine Tob Res. 2020;22(9):1650–2.
Wrapp D, Wang N, Corbett KS, Goldsmith JA, Hsieh C-L, Abiona O, et al. Cryo-EM structure of the 2019-nCoV spike in the prefusion conformation. Science. 2020;367(6483):1260–3.
Wang K, Gheblawi M, Oudit GY. Angiotensin Converting Enzyme 2: A Double-Edged Sword. Circulation. 2020;142(5):426–8.
Sharma P, Zeki AA. Does vaping increase susceptibility to COVID-19? Am J Respir Crit Care Med. 2020;202(7):1055–6.
Brake SJ, Barnsley K, Lu W, McAlinden KD, Eapen MS, Sohal SS. Smoking upregulates angiotensin-converting enzyme-2 receptor: a potential adhesion site for novel coronavirus SARS-CoV-2 (Covid-19). J Clin Med. 2020;9(3):841.
Zhang H, Rostamim MR, Leopold PL, Mezey JG, O’Beirne SL, Strulovici-Barel Y, et al. Reply to sharma and zeki: does vaping increase susceptibility to COVID-19? Am J Respir Crit Care Med. 2020;202(7):1056–7.
Cheng H, Wang Y, Wang GQ. Organ-protective effect of angiotensin-converting enzyme 2 and its effect on the prognosis of COVID-19. J Med Virol. 2020;92(7):726–30.
Lerner CA, Sundar IK, Yao H, Gerloff J, Ossip DJ, McIntosh S, et al. Vapors produced by electronic cigarettes and e-juices with flavorings induce toxicity, oxidative stress, and inflammatory response in lung epithelial cells and in mouse lung. PLoS ONE. 2015;10(2):e0116732.
Download references
Acknowledgements
The authors gratefully acknowledge Dr. Cruz González, Pulmonologist at University Clinic Hospital of Valencia (Valencia, Spain) for her thoughtful suggestions and support.
This work was supported by the Spanish Ministry of Science and Innovation [Grant Number SAF2017-89714-R]; Carlos III Health Institute [Grant Numbers PIE15/00013, PI18/00209]; Generalitat Valenciana [Grant Number PROMETEO/2019/032, Gent T CDEI-04/20-A and AICO/2019/250], and the European Regional Development Fund.
Author information
Authors and affiliations.
Department of Pharmacology, Faculty of Medicine, University of Valencia, Avda. Blasco Ibañez 15, 46010, Valencia, Spain
Patrice Marques, Laura Piqueras & Maria-Jesus Sanz
Institute of Health Research INCLIVA, University Clinic Hospital of Valencia, Valencia, Spain
CIBERDEM-Spanish Biomedical Research Centre in Diabetes and Associated Metabolic Disorders, ISCIII, Av. Monforte de Lemos 3-5, 28029, Madrid, Spain
Laura Piqueras & Maria-Jesus Sanz
You can also search for this author in PubMed Google Scholar
Contributions
All authors discussed and agreed to the scope of the manuscript and contributed to the development of the manuscript at all stages. All authors read and approved the final manuscript.
Corresponding author
Correspondence to Maria-Jesus Sanz .
Ethics declarations
Ethics approval and consent to participate, consent for publication, competing interests.
The authors of the manuscript declare no conflicts of interest and take sole responsibility for the writing and content of the manuscript. None of the authors have been involved in legal or regulatory matters related to the contents of this paper.
Additional information
Publisher's note.
Springer Nature remains neutral with regard to jurisdictional claims in published maps and institutional affiliations.
Rights and permissions
Open Access This article is licensed under a Creative Commons Attribution 4.0 International License, which permits use, sharing, adaptation, distribution and reproduction in any medium or format, as long as you give appropriate credit to the original author(s) and the source, provide a link to the Creative Commons licence, and indicate if changes were made. The images or other third party material in this article are included in the article's Creative Commons licence, unless indicated otherwise in a credit line to the material. If material is not included in the article's Creative Commons licence and your intended use is not permitted by statutory regulation or exceeds the permitted use, you will need to obtain permission directly from the copyright holder. To view a copy of this licence, visit http://creativecommons.org/licenses/by/4.0/ . The Creative Commons Public Domain Dedication waiver ( http://creativecommons.org/publicdomain/zero/1.0/ ) applies to the data made available in this article, unless otherwise stated in a credit line to the data.
Reprints and permissions
About this article
Cite this article.
Marques, P., Piqueras, L. & Sanz, MJ. An updated overview of e-cigarette impact on human health. Respir Res 22 , 151 (2021). https://doi.org/10.1186/s12931-021-01737-5
Download citation
Received : 22 October 2020
Accepted : 03 May 2021
Published : 18 May 2021
DOI : https://doi.org/10.1186/s12931-021-01737-5
Share this article
Anyone you share the following link with will be able to read this content:
Sorry, a shareable link is not currently available for this article.
Provided by the Springer Nature SharedIt content-sharing initiative
- Electronic cigarette
- E-cigarette
- Flavourings
- Smoking cessation tool
Respiratory Research
ISSN: 1465-993X
- General enquiries: [email protected]
Health Effects of Cigarette Smoking
Smoking and death, smoking and increased health risks, smoking and cardiovascular disease, smoking and respiratory disease, smoking and cancer, smoking and other health risks, quitting and reduced risks.
Cigarette smoking harms nearly every organ of the body, causes many diseases, and reduces the health of smokers in general. 1,2
Quitting smoking lowers your risk for smoking-related diseases and can add years to your life. 1,2
Cigarette smoking is the leading cause of preventable death in the United States. 1
- Cigarette smoking causes more than 480,000 deaths each year in the United States. This is nearly one in five deaths. 1,2,3
- Human immunodeficiency virus (HIV)
- Illegal drug use
- Alcohol use
- Motor vehicle injuries
- Firearm-related incidents
- More than 10 times as many U.S. citizens have died prematurely from cigarette smoking than have died in all the wars fought by the United States. 1
- Smoking causes about 90% (or 9 out of 10) of all lung cancer deaths. 1,2 More women die from lung cancer each year than from breast cancer. 5
- Smoking causes about 80% (or 8 out of 10) of all deaths from chronic obstructive pulmonary disease (COPD). 1
- Cigarette smoking increases risk for death from all causes in men and women. 1
- The risk of dying from cigarette smoking has increased over the last 50 years in the U.S. 1
Smokers are more likely than nonsmokers to develop heart disease, stroke, and lung cancer. 1
- For coronary heart disease by 2 to 4 times 1,6
- For stroke by 2 to 4 times 1
- Of men developing lung cancer by 25 times 1
- Of women developing lung cancer by 25.7 times 1
- Smoking causes diminished overall health, increased absenteeism from work, and increased health care utilization and cost. 1
Smokers are at greater risk for diseases that affect the heart and blood vessels (cardiovascular disease). 1,2
- Smoking causes stroke and coronary heart disease, which are among the leading causes of death in the United States. 1,3
- Even people who smoke fewer than five cigarettes a day can have early signs of cardiovascular disease. 1
- Smoking damages blood vessels and can make them thicken and grow narrower. This makes your heart beat faster and your blood pressure go up. Clots can also form. 1,2
- A clot blocks the blood flow to part of your brain;
- A blood vessel in or around your brain bursts. 1,2
- Blockages caused by smoking can also reduce blood flow to your legs and skin. 1,2
Smoking can cause lung disease by damaging your airways and the small air sacs (alveoli) found in your lungs. 1,2
- Lung diseases caused by smoking include COPD, which includes emphysema and chronic bronchitis. 1,2
- Cigarette smoking causes most cases of lung cancer. 1,2
- If you have asthma, tobacco smoke can trigger an attack or make an attack worse. 1,2
- Smokers are 12 to 13 times more likely to die from COPD than nonsmokers. 1
Smoking can cause cancer almost anywhere in your body: 1,2
- Blood (acute myeloid leukemia)
- Colon and rectum (colorectal)
- Kidney and ureter
- Oropharynx (includes parts of the throat, tongue, soft palate, and the tonsils)
- Trachea, bronchus, and lung
Smoking also increases the risk of dying from cancer and other diseases in cancer patients and survivors. 1
If nobody smoked, one of every three cancer deaths in the United States would not happen. 1,2
Smoking harms nearly every organ of the body and affects a person’s overall health. 1,2
- Preterm (early) delivery
- Stillbirth (death of the baby before birth)
- Low birth weight
- Sudden infant death syndrome (known as SIDS or crib death)
- Ectopic pregnancy
- Orofacial clefts in infants
- Smoking can also affect men’s sperm, which can reduce fertility and also increase risks for birth defects and miscarriage. 2
- Women past childbearing years who smoke have weaker bones than women who never smoked. They are also at greater risk for broken bones.
- Smoking affects the health of your teeth and gums and can cause tooth loss. 1
- Smoking can increase your risk for cataracts (clouding of the eye’s lens that makes it hard for you to see). It can also cause age-related macular degeneration (AMD). AMD is damage to a small spot near the center of the retina, the part of the eye needed for central vision. 1
- Smoking is a cause of type 2 diabetes mellitus and can make it harder to control. The risk of developing diabetes is 30–40% higher for active smokers than nonsmokers. 1,2
- Smoking causes general adverse effects on the body, including inflammation and decreased immune function. 1
- Smoking is a cause of rheumatoid arthritis. 1
- Quitting smoking is one of the most important actions people can take to improve their health. This is true regardless of their age or how long they have been smoking. Visit the Benefits of Quitting page for more information about how quitting smoking can improve your health.
- U.S. Department of Health and Human Services. The Health Consequences of Smoking—50 Years of Progress: A Report of the Surgeon General . Atlanta: U.S. Department of Health and Human Services, Centers for Disease Control and Prevention, National Center for Chronic Disease Prevention and Health Promotion, Office on Smoking and Health, 2014 [accessed 2017 Apr 20].
- U.S. Department of Health and Human Services. How Tobacco Smoke Causes Disease: What It Means to You . Atlanta: U.S. Department of Health and Human Services, Centers for Disease Control and Prevention, National Center for Chronic Disease Prevention and Health Promotion, Office on Smoking and Health, 2010 [accessed 2017 Apr 20].
- Centers for Disease Control and Prevention. QuickStats: Number of Deaths from 10 Leading Causes—National Vital Statistics System, United States, 2010 . Morbidity and Mortality Weekly Report 2013:62(08);155. [accessed 2017 Apr 20].
- Mokdad AH, Marks JS, Stroup DF, Gerberding JL. Actual Causes of Death in the United States . JAMA: Journal of the American Medical Association 2004;291(10):1238–45 [cited 2017 Apr 20].
- U.S. Department of Health and Human Services. Women and Smoking: A Report of the Surgeon General . Rockville (MD): U.S. Department of Health and Human Services, Public Health Service, Office of the Surgeon General, 2001 [accessed 2017 Apr 20].
- U.S. Department of Health and Human Services. Reducing the Health Consequences of Smoking: 25 Years of Progress. A Report of the Surgeon General . Rockville (MD): U.S. Department of Health and Human Services, Public Health Service, Centers for Disease Control, National Center for Chronic Disease Prevention and Health Promotion, Office on Smoking and Health, 1989 [accessed 2017 Apr 20].
To receive email updates about Smoking & Tobacco Use, enter your email address:
- Tips From Former Smokers ®
- Division of Cancer Prevention and Control
- Lung Cancer
- National Comprehensive Cancer Control Program
- Division of Reproductive Health

Exit Notification / Disclaimer Policy
- The Centers for Disease Control and Prevention (CDC) cannot attest to the accuracy of a non-federal website.
- Linking to a non-federal website does not constitute an endorsement by CDC or any of its employees of the sponsors or the information and products presented on the website.
- You will be subject to the destination website's privacy policy when you follow the link.
- CDC is not responsible for Section 508 compliance (accessibility) on other federal or private website.
Smoking and Its Negative Effects on Human Beings Research Paper
Smoking is one of the most common negative habits that people indulge in. Many health experts have warned that smoking is unhealthy and dangerous to the human health. This essay will discuss the negative effects of smoking on human beings.
Smoking cigarette is addictive that is why many smokers have difficulties in giving up the habit. Cigarettes are produced of tobacco with a large percent of other additives, which account for the largest number of preventable deaths in the world. People who smoke commonly face different health problems, which are caused by tobacco consumption. Therefore, smoking has negative health consequences for smokers and people who live with them and become passive smokers as a result.
The WHO and other health organisations have sensitised people on the dangers of smoking. There are many health conditions which smokers are likely to suffer from (Pampel 61). Their bodies absorb harmful toxins which cigarettes contain which are dangerous to their health.
Smoking is a major health risk which results in heart attacks, strokes, bronchitis, and other respiratory diseases. The accumulation of tobacco and other toxins in the respiratory tract of a smoker makes a person suffer from respiratory health conditions.
Smokers, therefore, are likely to incur huge medical bills when they seek for treatment for these diseases. Many governments spend a lot of money on treating smoking related diseases, which increases the cost of healthcare. Pampel argues that smokers can succumb to such illnesses unless they stop smoking (64).
Tobacco consumption causes dental problems which are difficult to reverse. Smokers are likely to have bad breath, stained teeth and smelly gums. Toxic elements, which cigarettes contain, for instance, tar, have dangerous impacts on human health. These substances cause smokers to have poor dents and even lose their teeth (Peate 362).
Smokers are likely to suffer emotionally and psychologically because poor health and unattractive appearance, caused, for example, by stained or broken teeth, make a person lose his/her own self-esteem. Smokers are likely to be shunned by people close to them because of fetid breath, bad body odour and poor outward appearance. Therefore, people need to be made aware of dental and other health problems they are likely to experience as a result of smoking.
Tobacco consumption causes a lot of deaths in developing countries. These countries have weak laws which do not effectively regulate cigarette selling and consumption. Advertisement implicit messages encourage the young to become smokers. Tobacco advertising in many developed countries has been prohibited. However, some third world countries still allow tobacco advertising, which encourages more people to acquire this bad habit.
The images of sophistication, bravery and glamour which are carried by tobacco adverts easily persuade the young to become smokers. Peate reveals that tobacco companies target adolescents and women to increase their sales (363). These people are easily influenced by what they see in the media. People who begin smoking at early age are likely to be addicted for a longer period than those who develop the habit at mature age (Cox).
Smokers are exposed to various carcinogens in cigarettes. These carcinogens cause cancer and negatively affect human health. Lung, throat, brain, bladder, cervical cancer as well as other forms are caused by smoking. The symptoms are often detected at the time when the smoker’s health condition is already chronic.
Cancer is one of the leading causes of death world wide. A significant number of cancer patients have a history of smoking and tobacco consumption (Peate 365). If people get exposed to exhaled smoke, they are likely to be affected by it. They breathe in toxic components of the exhaled smoke that deposit in their lungs and other respiratory organs. These people can suffer from respiratory illnesses as well.
Women, who smoke during pregnancy, are likely to expose their unborn babies to toxic substances contained in cigarettes. The tar that is present in cigarettes is likely to be embedded in the DNA of a mother, who may pass it on to the child in her womb. These toxic components inhibit the normal growth of a baby in the fetus, which results in death and still births. Cox reveals that if the pregnancy proceeds to full term, the delivered child can have severe brain disorders.
Such children are very slow at learning because their cognitive functions are impaired. Female smokers are likely to become infertile or their reproductive abilities are limited. Nicotine restricts the ability of the female reproductive system to generate estrogen. Many physiological and reproductive functions in women depend on estrogen.
Nicotine is a substance found in cigarettes which is very addictive. People who try to give up smoking experience severe withdrawal symptoms, which restrict their ability to function effectively. They are likely to experience several episodes of depression.
This is because their bodies are used to the intake of nicotine and have difficulties in performing its functions without it (Cox). Nicotine stimulates the human mind just like any other drug, which increases the risk of high blood pressure in a smoker. From the above mentioned, it is easy to conclude that smoking has negative effects on people’s health.
Works Cited
Cox, Jack. “ The Lesser Known Harmful Effects of Smoking .” The Register . 2012. Orange Country Register News . Web.
Pampel, Fred C. Tobacco Industry and Smoking . New York: Infobase Publishing, 2009. Print.
Peate, Ian. “The Effects of Smoking on the Reproductive Health of Men”. British Journal of Nursing 14.7 (2005): 362–366. Print.
- Chicago (A-D)
- Chicago (N-B)
IvyPanda. (2024, March 28). Smoking and Its Negative Effects on Human Beings. https://ivypanda.com/essays/smoking-effects/
"Smoking and Its Negative Effects on Human Beings." IvyPanda , 28 Mar. 2024, ivypanda.com/essays/smoking-effects/.
IvyPanda . (2024) 'Smoking and Its Negative Effects on Human Beings'. 28 March.
IvyPanda . 2024. "Smoking and Its Negative Effects on Human Beings." March 28, 2024. https://ivypanda.com/essays/smoking-effects/.
1. IvyPanda . "Smoking and Its Negative Effects on Human Beings." March 28, 2024. https://ivypanda.com/essays/smoking-effects/.
Bibliography
IvyPanda . "Smoking and Its Negative Effects on Human Beings." March 28, 2024. https://ivypanda.com/essays/smoking-effects/.
- Importance of Discovery of Occupational Carcinogens
- Dr. Paul Demers Presentations: Asbestos and Occupational Cancers and Carcinogens
- Detoxification: The Use of Hydrotherapy
- Discussion on Alberta Tar Sands
- Causes and Effects of Smoking in Public
- Use of Household Disinfectants
- Health Effects of Tobacco Smoking in Hispanic Men
- Nicotine: E-Cigarettes and Tobacco Use
- Pharmacology of Nicotine: Absorption and Distribution
- How Smoking Cigarettes Effects Your Health
- Langley Park: Public Health Services
- Importance of Nutrition and Exercise in the Society
- A Major Health Issue in our Society
- Fatigue in workplace
- Problems of Everyday Addictions in Society
Numbers, Facts and Trends Shaping Your World
Read our research on:
Full Topic List
Regions & Countries
- Publications
- Our Methods
- Short Reads
- Tools & Resources
Read Our Research On:
How Pew Research Center will report on generations moving forward
Journalists, researchers and the public often look at society through the lens of generation, using terms like Millennial or Gen Z to describe groups of similarly aged people. This approach can help readers see themselves in the data and assess where we are and where we’re headed as a country.
Pew Research Center has been at the forefront of generational research over the years, telling the story of Millennials as they came of age politically and as they moved more firmly into adult life . In recent years, we’ve also been eager to learn about Gen Z as the leading edge of this generation moves into adulthood.
But generational research has become a crowded arena. The field has been flooded with content that’s often sold as research but is more like clickbait or marketing mythology. There’s also been a growing chorus of criticism about generational research and generational labels in particular.
Recently, as we were preparing to embark on a major research project related to Gen Z, we decided to take a step back and consider how we can study generations in a way that aligns with our values of accuracy, rigor and providing a foundation of facts that enriches the public dialogue.
A typical generation spans 15 to 18 years. As many critics of generational research point out, there is great diversity of thought, experience and behavior within generations.
We set out on a yearlong process of assessing the landscape of generational research. We spoke with experts from outside Pew Research Center, including those who have been publicly critical of our generational analysis, to get their take on the pros and cons of this type of work. We invested in methodological testing to determine whether we could compare findings from our earlier telephone surveys to the online ones we’re conducting now. And we experimented with higher-level statistical analyses that would allow us to isolate the effect of generation.
What emerged from this process was a set of clear guidelines that will help frame our approach going forward. Many of these are principles we’ve always adhered to , but others will require us to change the way we’ve been doing things in recent years.
Here’s a short overview of how we’ll approach generational research in the future:
We’ll only do generational analysis when we have historical data that allows us to compare generations at similar stages of life. When comparing generations, it’s crucial to control for age. In other words, researchers need to look at each generation or age cohort at a similar point in the life cycle. (“Age cohort” is a fancy way of referring to a group of people who were born around the same time.)
When doing this kind of research, the question isn’t whether young adults today are different from middle-aged or older adults today. The question is whether young adults today are different from young adults at some specific point in the past.
To answer this question, it’s necessary to have data that’s been collected over a considerable amount of time – think decades. Standard surveys don’t allow for this type of analysis. We can look at differences across age groups, but we can’t compare age groups over time.
Another complication is that the surveys we conducted 20 or 30 years ago aren’t usually comparable enough to the surveys we’re doing today. Our earlier surveys were done over the phone, and we’ve since transitioned to our nationally representative online survey panel , the American Trends Panel . Our internal testing showed that on many topics, respondents answer questions differently depending on the way they’re being interviewed. So we can’t use most of our surveys from the late 1980s and early 2000s to compare Gen Z with Millennials and Gen Xers at a similar stage of life.
This means that most generational analysis we do will use datasets that have employed similar methodologies over a long period of time, such as surveys from the U.S. Census Bureau. A good example is our 2020 report on Millennial families , which used census data going back to the late 1960s. The report showed that Millennials are marrying and forming families at a much different pace than the generations that came before them.
Even when we have historical data, we will attempt to control for other factors beyond age in making generational comparisons. If we accept that there are real differences across generations, we’re basically saying that people who were born around the same time share certain attitudes or beliefs – and that their views have been influenced by external forces that uniquely shaped them during their formative years. Those forces may have been social changes, economic circumstances, technological advances or political movements.
When we see that younger adults have different views than their older counterparts, it may be driven by their demographic traits rather than the fact that they belong to a particular generation.
The tricky part is isolating those forces from events or circumstances that have affected all age groups, not just one generation. These are often called “period effects.” An example of a period effect is the Watergate scandal, which drove down trust in government among all age groups. Differences in trust across age groups in the wake of Watergate shouldn’t be attributed to the outsize impact that event had on one age group or another, because the change occurred across the board.
Changing demographics also may play a role in patterns that might at first seem like generational differences. We know that the United States has become more racially and ethnically diverse in recent decades, and that race and ethnicity are linked with certain key social and political views. When we see that younger adults have different views than their older counterparts, it may be driven by their demographic traits rather than the fact that they belong to a particular generation.
Controlling for these factors can involve complicated statistical analysis that helps determine whether the differences we see across age groups are indeed due to generation or not. This additional step adds rigor to the process. Unfortunately, it’s often absent from current discussions about Gen Z, Millennials and other generations.
When we can’t do generational analysis, we still see value in looking at differences by age and will do so where it makes sense. Age is one of the most common predictors of differences in attitudes and behaviors. And even if age gaps aren’t rooted in generational differences, they can still be illuminating. They help us understand how people across the age spectrum are responding to key trends, technological breakthroughs and historical events.
Each stage of life comes with a unique set of experiences. Young adults are often at the leading edge of changing attitudes on emerging social trends. Take views on same-sex marriage , for example, or attitudes about gender identity .
Many middle-aged adults, in turn, face the challenge of raising children while also providing care and support to their aging parents. And older adults have their own obstacles and opportunities. All of these stories – rooted in the life cycle, not in generations – are important and compelling, and we can tell them by analyzing our surveys at any given point in time.
When we do have the data to study groups of similarly aged people over time, we won’t always default to using the standard generational definitions and labels. While generational labels are simple and catchy, there are other ways to analyze age cohorts. For example, some observers have suggested grouping people by the decade in which they were born. This would create narrower cohorts in which the members may share more in common. People could also be grouped relative to their age during key historical events (such as the Great Recession or the COVID-19 pandemic) or technological innovations (like the invention of the iPhone).
By choosing not to use the standard generational labels when they’re not appropriate, we can avoid reinforcing harmful stereotypes or oversimplifying people’s complex lived experiences.
Existing generational definitions also may be too broad and arbitrary to capture differences that exist among narrower cohorts. A typical generation spans 15 to 18 years. As many critics of generational research point out, there is great diversity of thought, experience and behavior within generations. The key is to pick a lens that’s most appropriate for the research question that’s being studied. If we’re looking at political views and how they’ve shifted over time, for example, we might group people together according to the first presidential election in which they were eligible to vote.
With these considerations in mind, our audiences should not expect to see a lot of new research coming out of Pew Research Center that uses the generational lens. We’ll only talk about generations when it adds value, advances important national debates and highlights meaningful societal trends.
- Age & Generations
- Demographic Research
- Generation X
- Generation Z
- Generations
- Greatest Generation
- Methodological Research
- Millennials
- Silent Generation
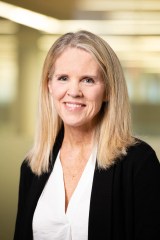
Kim Parker is director of social trends research at Pew Research Center
How Teens and Parents Approach Screen Time
Who are you the art and science of measuring identity, u.s. centenarian population is projected to quadruple over the next 30 years, older workers are growing in number and earning higher wages, teens, social media and technology 2023, most popular.
1615 L St. NW, Suite 800 Washington, DC 20036 USA (+1) 202-419-4300 | Main (+1) 202-857-8562 | Fax (+1) 202-419-4372 | Media Inquiries
Research Topics
- Coronavirus (COVID-19)
- Economy & Work
- Family & Relationships
- Gender & LGBTQ
- Immigration & Migration
- International Affairs
- Internet & Technology
- News Habits & Media
- Non-U.S. Governments
- Other Topics
- Politics & Policy
- Race & Ethnicity
- Email Newsletters
ABOUT PEW RESEARCH CENTER Pew Research Center is a nonpartisan fact tank that informs the public about the issues, attitudes and trends shaping the world. It conducts public opinion polling, demographic research, media content analysis and other empirical social science research. Pew Research Center does not take policy positions. It is a subsidiary of The Pew Charitable Trusts .
Copyright 2024 Pew Research Center
Terms & Conditions
Privacy Policy
Cookie Settings
Reprints, Permissions & Use Policy

An official website of the United States government
The .gov means it’s official. Federal government websites often end in .gov or .mil. Before sharing sensitive information, make sure you’re on a federal government site.
The site is secure. The https:// ensures that you are connecting to the official website and that any information you provide is encrypted and transmitted securely.
- Publications
- Account settings
Preview improvements coming to the PMC website in October 2024. Learn More or Try it out now .
- Advanced Search
- Journal List
- Indian J Med Paediatr Oncol
- v.36(1); Jan-Mar 2015
Harmful effects of nicotine
Aseem mishra.
Department of Surgical Oncology, Head and Neck Services, Tata Memorial Hospital, Parel, Mumbai, Maharashtra, India
Pankaj Chaturvedi
Sourav datta, snita sinukumar, poonam joshi, apurva garg.
With the advent of nicotine replacement therapy, the consumption of the nicotine is on the rise. Nicotine is considered to be a safer alternative of tobacco. The IARC monograph has not included nicotine as a carcinogen. However there are various studies which show otherwise. We undertook this review to specifically evaluate the effects of nicotine on the various organ systems. A computer aided search of the Medline and PubMed database was done using a combination of the keywords. All the animal and human studies investigating only the role of nicotine were included. Nicotine poses several health hazards. There is an increased risk of cardiovascular, respiratory, gastrointestinal disorders. There is decreased immune response and it also poses ill impacts on the reproductive health. It affects the cell proliferation, oxidative stress, apoptosis, DNA mutation by various mechanisms which leads to cancer. It also affects the tumor proliferation and metastasis and causes resistance to chemo and radio therapeutic agents. The use of nicotine needs regulation. The sale of nicotine should be under supervision of trained medical personnel.
INTRODUCTION
Tobacco is the leading cause of preventable cancers. WHO estimated around 1.27 billion tobacco users world-wide. Tobacco consumption alone accounts for nearly 5.4 million deaths per year and one billion people may die in this century if global tobacco consumption remained at the current levels.[ 1 ] An international treaty spearheaded by WHO in 2003 and signed by 170 countries, aims to encourage governments to reduce the production, sales, distribution advertisement and promotion of tobacco products. Despite strong opposition from the Industry, the treaty has been making steady progress in achieving its goal of comprehensive tobacco control around the world.[ 2 ] As tobacco consumption is being curbed, there is a growing demand for cessation. Pharmacological treatment of nicotine addiction remains an active area of research. There are many nicotine preparations (nicotine gums, patches, e cigarettes and inhalational agents) that are freely available in most parts of the world. These products are being heavily promoted and marketed as magical remedies. Nicotine gums are available in 2 mg and 4 mg preparation that deliver around 1 mg and 3 mg nicotine to the blood stream respectively. E-cigarette, a sophisticated nicotine delivery device, delivers nicotine in a vapor form and it closely mimics the act of smoking. Currently, these products constitute approximately 1% of total nicotine consumption and are showing an increasing trend in most countries.[ 3 ]
Nicotine is well known to have serious systemic side effects in addition to being highly addictive. It adversely affects the heart, reproductive system, lung, kidney etc. Many studies have consistently demonstrated its carcinogenic potential. [ Table 1 ] The only other known use of nicotine has been as an insecticide since 17 th century.[ 4 ] After World War II, its use has declined owing to the availability of cheaper, more potent pesticides that are less harmful to mammals. The environment Protection Agency of United States has banned use of nicotine as a pesticide from 1 st January 2014.[ 4 ] India, one of the largest producer and exporter of nicotine sulphate, has progressively banned its use as agricultural pesticide.[ 5 ] We undertook this review to evaluate the systemic adverse effects of nicotine.
Studies showing nicotine as a carcinogen

MATERIALS AND METHODS
A computer aided search of the Medline and PubMed databases was done using different combination of the keywords “nicotine,” “chemical composition,” “history,” “metabolism,” “addiction,” “cancer,” “toxic,” “endocrine system,” “cardiovascular system,” “respiratory system,” “lung carcinogenesis, “gastrointestinal system,” “immune system,” “ocular,” “cataract,” “central nervous system,” “renal system,” “reproductive system,” “menstrual cycle,” “oocytes,” “foetus,”. Initial search buildup was done using “Nicotine/adverse effects” [Mesh], which showed 3436 articles. Articles were analyzed and 90 relevant articles were included in the review. All the animal and human studies that investigated the role of nicotine on organ systems were analyzed. Studies that evaluated tobacco use and smoking were excluded. All possible physiological effects were considered for this review. We did not exclude studies that reported beneficial effects of nicotine. The objective was to look at the effects of nicotine without confounding effects of other toxins and carcinogens present in tobacco or tobacco smoke.
CHEMICAL PROPERTIES AND METABOLISM
Nicotine was first extracted from tobacco by German physicians Wilhelm Heinrich Posselt and Karl Ludwig Reimann. Nicotine, a strong alkaloid, in its pure form is a clear liquid with a characteristic odour. It turns brown on exposure to air. It is water soluble and separates preferentially from organic solvents. It is an amine composed of pyridine and pyrrolidine rings.
Nicotine is a dibasic compound and the availability and absorption in human body depends upon the pH of the solution.[ 7 ] The absorption can occur through oral mucosa, lungs, skin or gut.[ 6 ] The increase in pH of a solution causes an increase in concentrations of uncharged lipophilic nicotine, in this form it can actively pass through all biological membranes.[ 7 ] The addition of slaked lime and catechu to tobacco increases the absorption of nicotine from the oral cavity.
Nicotine once ingested, is absorbed and metabolized by the liver. The metabolic process can be categorized into two phases. In phase I there is microsomal oxidation of the nicotine via multiple pathways.[ 8 ] This leads to formation of various metabolites like cotinine and nornicotine, demethyl cotinine, trans-3-hydroxy-cotinine and d-(3-pyridyl)-g-methylaminobutyric acid.[ 9 , 10 ] Thereafter in phase II there is N’-and O’-glucuronidation of the metabolites and excretion via urine, feces, bile, saliva, sweat etc.[ 11 , 12 ]5-10% of elimination is by renal excretion of unchanged nicotine, however there is reabsorption from the bladder when the urinary pH is high.[ 14 ] There is evidence that nitrosation of nicotine in vivo could lead to formation of N-nitrosonornicotine (NNN) and 4-(methylnitrosamino)-1-(3-pyridyl)-1-butanone (NNK).[ 13 ] which are known to be highly carcinogenic. Inflammation in the oral cavity increases risk of endogenous nitrosation.
MECHANISM OF ACTION
Nicotine acts via 3 major mechanisms, producing physiological and pathological effects on a variety of organ systems.[ 15 , 16 ]
- Ganglionic transmission.
- Nicotinic acetylcholine receptors (nAChRs) on chromaffin cells via catecholamines.
- Central nervous system (CNS) stimulation of nAChRs.
Brain imaging studies demonstrate that nicotine acutely increases activity in the prefrontal cortex and visual systems. There is release of a variety of neurotransmitters important in drug-induced reward. Nicotine also causes an increased oxidative stress and neuronal apoptosis, DNA damage, reactive oxygen species and lipid peroxide increase. nAChRs were originally thought to be limited to neuronal cells, however, studies have identified functional nAChRs in tissues outside the nervous system. Actions on nicotinic receptors produce a wide variety of acute and long-term effects on organ systems, cell multiplication and apoptosis, throughout the body.
IMMEDIATE EFFECTS AND TOXICITY
Nicotine on direct application in humans causes irritation and burning sensation in the mouth and throat, increased salivation, nausea, abdominal pain, vomiting and diarrhea.[ 17 ] Gastrointestinal effects are less severe but can occur even after cutaneous and respiratory exposure.[ 18 ] Predominant immediate effects as seen in animal studies and in humans consist of increase in pulse rate and blood pressure. Nicotine also causes an increase in plasma free fatty acids, hyperglycemia, and an increase in the level of catecholamines in the blood.[ 19 , 20 ] There is reduced coronary blood flow but an increased skeletal muscle blood flow.[ 20 , 22 ] The increased rate of respiration causes hypothermia, a hypercoagulable state, decreases skin temperature, and increases the blood viscosity.
Nicotine is one of the most toxic of all poisons and has a rapid onset of action. Apart from local actions, the target organs are the peripheral and central nervous systems. In severe poisoning, there are tremors, prostration, cyanosis, dypnoea, convulsion, progression to collapse and coma. Even death may occur from paralysis of respiratory muscles and/or central respiratory failure with a LD50 in adults of around 30-60 mg of nicotine. In children the LD50 is around 10 mg.[ 23 ]
GREEN TOBACCO SICKNESS
This is an acute form of nicotine toxicity that is known to occur due to handling of green tobacco leaves, with symptoms lasting from 12 to 24 h. The acute symptoms include headache, nausea, vomiting, giddiness, loss of appetite, fatigue and tachyarrythmias.[ 24 ] No significant mortality has been reported due to green tobacco sickness (GTS) but it significantly affects the health of workers in the tobacco industry.[ 25 ]
NICOTINE ADDICTION
Nicotine is one of the most addicting agent. The US surgeon general (2010) has concluded nicotine to be as addictive as cocaine or heroin. Nicotine interacts with the nicotinic acetyl choline receptors and stimulates the dopaminergic transmission.[ 26 ] This in turn stimulates the reward centre and is responsible for the mood elevation and apparent improvement in cognitive function.[ 27 ] With chronic stimulation by nicotine the GABAergic neurons are desensitized and thus lose their inhibitory effect on dopamine.[ 28 ] This in turn reinforces the addiction by inducing craving. This effect has been shown to affect the CYP2A6 gene and leads to heritable dependence to nicotine. Studies have shown the nicotine dependence to be transmitted maternally and grand maternally by epigenetic mechanism.[ 29 ]
EFFECTS ON METABOLISM
Nicotine causes catecholamine release and stimulates the autonomic system. There is increased glycogen synthesis due to α-adrenoceptor stimulation. This leads to reduction in the fasting blood glucose levels. It also causes lipolysis thus decreasing body weight. Nicotine affects insulin resistance and predisposes to metabolic syndrome. In an animal study prenatal exposure was toxic to pancreatic β-cell and leads to decreased B cell population, thus increasing the risk of diabetes.[ 30 , 31 ]
NICOTINE AND CANCER
The stimulation of nAChRs by nicotine has biologic effects on cells important for initiation and progression of cancer.[ 26 ] It activates signal transduction pathways directly through receptor-mediated events, allowing the survival of damaged epithelial cells. In addition, nicotine is a precursor of tobacco specific nitrosamines (TSNAs), through nitrosation in the oral cavity.[ 32 , 33 ] It is shown that nitrosation of nicotine could lead to formation of NNN and NNK. This effect of nicotine may be important because of its high concentration in tobacco and nicotine replacement products.[ 13 ] NNN and NNK are strongly carcinogenic.[ 34 ]
Nicotine forms arachidonic acid metabolites which cause increased cell division. Binding to Bcl-2 and action on vascular endothelial growth factor and cyclooxygenase-2 (COX-2) causes increased cancer proliferation and survival.[ 35 , 36 ] Promotion of tumor angiogenesis accelerates tumor growth which is mediated by β-adrenergic activation and stimulation of nAChRs.[ 35 , 37 , 28 , 39 ] Nicotine also suppresses apoptosis by phosphorylation mediated extracellular signal regulated kinases of Bcl-2.[ 40 , 41 ] Recent studies show that nicotine, activates nuclear factor kappa B (NF-kB)-dependent survival of cancer cell and proliferation.[ 42 ]
In normal cells, nicotine can stimulate properties consistent with cell transformation and the early stages of cancer formation, such as increased cell proliferation, decreased cellular dependence on the extracellular matrix for survival, and decreased contact inhibition. Thus, the induced activation of nAChRs in lung and other tissues by nicotine can promote carcinogenesis by causing DNA mutations[ 26 ] Through its tumor promoter effects, it acts synergistically with other carcinogens from automobile exhausts or wood burning and potentially shorten the induction period of cancers[ 43 ] [ Table 2 ].
Studies showing the role of nicotine as tumor promoter

LUNG CARCINOGENESIS
A study relates lung carcinogenesis by nicotine due to genetic variation in CYP2B6.[ 44 ] Its simultaneous exposure with hyperoxia has been found to induce cancer in hamsters.[ 45 ] Cotinine has been found to promote lung tumorigenesis by inhibiting anti-apoptotic pathway.[ 46 ] Nuclear translocation of ARB1 gene by nicotine has found in proliferation and progression of nonsmall-cell lung cancer. Several Studies have shown that nicotine has significant role in tumor progression and metastasis via CXCR4 and increased angiogenesis.[ 36 , 47 ] Carriers of the lung-cancer-susceptibility loci in their DNA extract more nicotine. Smokers carrying the gene CHRNA3 and CHRNA5 were found to extract more nicotine and cells were thus exposed to a higher internal dose of carcinogenic nicotine-derived nitrosamines.[ 48 ] Additionally modulation of the mitochondrial signaling pathway leads to resistance to the chemotherapeutic agents.[ 49 ]
GASTRO INTESTINAL CARCINOGENESIS
The carcinogenic role may be mediated by the MAPK/COX-2 pathways, α-7 nAchR and β-adrenergic receptor expression, and mi RNAs α-BTX anatagonist.[ 50 ] Nicotine forms adducts with liver DNA which enhances its mutagenic potential.[ 49 , 51 , 52 ] activation of cell-surface receptors by nicotine stimulates downstream kinases that can mediate resistance to chemotherapy. It has been shown by the finding that smokers who continue to smoke during chemotherapy have a worse prognosis. Moreover they also have increased toxicity and lower efficacy of chemo therapeutic drugs.[ 53 ] Nicotine affects the periostin gene, α-7-nAChR and e-cadherin suppression which explains the mechanism of gastric cancer growth, invasion and metastasis.[ 54 , 55 ] Nicotine negatively impacts tumor biology by promoting angiogenesis, tumor invasion and increased risk of metastasis.[ 53 ]
PANCREATIC CANCER
Nicotine has been found to induce pancreatic adenocarcinoma in mice model, by stimulating the stress neurotransmitters.[ 56 , 57 ] In another study nicotine promoted the growth of nonsmall cell lung cancer and pancreatic cancer in a receptor dependent fashion. It also increased tumor metastasis, and resistance to gemcitabine induced apoptosis, causing chemoresistance.[ 58 ] The MUC-4 upregulation, NF-kB and GRP78 activation and Id1 expression by Src dependent manner are the probable mechanism leading to tumor growth, metastasis and chemotherapeutic drug resistance.[ 57 , 58 ]
BREAST CANCER
Nicotine causes α9-nAChR-mediated cyclin D3 overexpression which might cause transformation of normal breast epithelial cells and induce cancer. Nicotine and cotinine has been found to be present in the breast fluid of lactating women.[ 59 ] Several studies have found that α9-nAChR mediated mechanism leads to increased tumor growth, metastasis and tumor cells resistant to chemotherapeutic drugs in breast cancer.[ 59 , 60 ]
CARDIOVASCULAR SYSTEM
The acute hemodynamic effects of cigarette smoking or smokeless tobacco are mediated primarily by the sympathomimetic action. The intensity of its hemodynamic effect is greater with rapid nicotine delivery.[ 61 ] Nicotine causes catecholamine release both locally and systemically leading to an increase in heart rate, blood pressure and cardiac contractility. It reduces blood flow in cutaneous and coronary vessels; and increases blood flow in the skeletal muscles. Due to restricted myocardial oxygen delivery there is reduced cardiac work. In a study, chewing a low dose (4 mg) of nicotine gum by healthy nonsmokers blunted the increase in coronary blood flow that occurs with increased heart rate produced by cardiac pacing.[ 21 ] Thus, persistent stimulation by nicotine can contribute to Coronary Vascular Disease by producing acute myocardial ischemia. In the presence of coronary disease, myocardial dysfunction can be worsened. In a placebo-controlled experiment that produced transient ischemia in anesthetized dogs myocardial dysfunction was produced at doses, that did not alter heart rate, blood pressure, or blood flow or myocyte necrosis.[ 62 ]
Nicotine alters the structural and functional characteristics of vascular smooth muscle and endothelial cells.[ 63 ] It enhances release of the basic fibroblast growth factor and inhibits production of transforming growth factor-β1.[ 64 ] These effects lead to increased DNA synthesis, mitogenic activity, endothelial proliferation and increases atherosclerotic plaque formation.[ 65 ] Neovascularization stimulated by nicotine can help progression of atherosclerotic plaques.[ 66 ] These effects lead to myointimal thickening and atherogenic and ischemic changes, increasing the incidence of hypertension and cardiovascular disorders. A study on dogs demonstrated the deleterious effects of nicotine on the heart.[ 67 ]
Nicotinic acetylcholine receptor's actions on vascular smooth muscle proliferation and plaque neovascularization increases the risk of peripheral arterial disorders. In a murine model of hind limb ischemia, short-term exposure to nicotine paradoxically increased capillary density and improved regional blood flow in the ischemic hind limb.[ 35 ] However, long-term exposure to nicotine for 16 weeks (about one-third of the life span of a mouse) before induction of ischemia obliterated angiogenic response to nicotine.[ 68 ]
RESPIRATORY SYSTEM
The effects of nicotine on respiratory system are twofold. One, directly by a local exposure of lungs to nicotine through smoking or inhaled nicotine, and second via a central nervous system mechanism. Nicotine plays a role in the development of emphysema in smokers, by decreasing elastin in the lung parenchyma and increasing the alveolar volume. Nicotine stimulates vagal reflex and parasympathetic ganglia and causes an increased airway resistance by causing bronchoconstriction.[ 69 ] Nicotine alters respiration through its effects on the CNS. The simultaneous effect of bronchoconstriction and apnea increases the tracheal tension and causes several respiratory disorders. In a study microinjection of nicotine were administered to the prebotzinger complex and adjacent nuclei in the brain. The firing pattern of the brain signals and breathing pattern were monitored. There was an increased frequency of bursts and decreased amplitude and a shallow and rapid rhythm of respiration.[ 70 ]
GASTROINTESTINAL SYSTEM
Nicotine use has been associated with Gastro Esophageal Reflux Disorder (GERD) and peptic ulcer disease (PUD).[ 36 , 71 ] This effect is mediated by increased gastric acid, pepsinogen secretion and stimulatory effects on vasopressin. The action on the cyclo-oxygenase pathway also increases the risk of GERD and PUD.[ 72 ] Nicotine causes smooth muscle relaxation by action of endogenous nitric oxide as a nonadrenergic noncholinergic neurotransmitter.[ 73 ] The decrease in tone of the colon and gastric motility and reduced lower esophageal sphincteric pressure might be the reason of increased incidence of GERD.[ 74 ]
There is an increased incidence of treatment resistant Helicobacter pylori infection in smokers. It potentiates the effects of toxins of H. pylori by its action on the gastric parietal cells.[ 75 ] This effect could be due to histamine mediated response of nicotine.
IMMUNOLOGICAL SYSTEM
Nicotine has been known to be immunosuppressive through central and peripheral mechanisms. It impairs antigen and receptor mediated signal transduction in the lymphoid system leading to decreased immunological response. The T-cell population is reduced due to arrest of cell cycle. Even the macrophage response, which forms the first line defense against tuberculosis becomes dysfunctional and causes increased incidence of tuberculosis.[ 76 ] The migration of fibroblasts and inflammatory cells to the inflamed site is reduced. There is decreased epithelialization and cell adhesion and thus there is a delayed wound healing as well as increased risk of infection in nicotine exposed individuals.
The action on the hypothalamo-pituitary adrenal axis and autonomic nervous system stimulation via sympathetic and parasympathetic pathways affects the immune system. The adrenocorticotropic hormone (ACTH) secretion pathway and corticotrophin release is affected and this causes immunosuppression.[ 77 ]
OCULAR SYSTEM
Nicotine promotes pathologic angiogenesis and retinal neovascularization in murine models. It causes age-related macular degeneration in mice.[ 78 ] In a clinical study, the most virulent form of age-related maculopathy was associated with retinal neovascularization that contributed to visual deterioration. Tobacco smokers are known to be at greater risk of age-related macular degeneration than are nonsmokers.[ 79 ] In animal model, spraguely Dawley rats with type 1 diabetes treated with nicotine, developed cataract.[ 80 ] Thus the syngergistic relationship between nicotine and glucose metabolism exaggerating diabetes might cause accelerated cataract formation. There is synergistic relationship between nicotine and glucose metabolism which increases the risk of diabetes mellitus. This might cause accelerated cataract formation.
RENAL SYSTEM
Risk of chronic kidney disease in smokers is high. Cigarette smoking has been found to increase albumin excretion in urine, decrease glomerular filtration rate, causes increased incidence of renal artery stenosis and is associated with an increased mortality in patients with end-stage renal disease. The pathogenesis of renal effects is due to the action of nicotine via COX-2 isoform induction. The COX-2 isoforms causes increased glomerular inflammation, acute glomerulonephritis and ureteral obstruction.[ 81 ] There is impaired response of kidneys to the increased systemic blood pressure in smokers. This loss of renoprotective mechanism in smokers also leads to pathogenetic effects of nicotine on the renal system.[ 82 ]
REPRODUCTIVE SYSTEM – MALES
Nitrous oxide liberated from parasympathetico-nergic nerves plays a pivotal role in generating immediate penile vasodilatation and corpus cavernosum relaxation, and NO derived from endothelial cells contributes to maintaining penile erection. Nicotine causes impairment of NO synthesis. This may lead to loss of penile erections and erectile dysfunction.[ 83 ]
Various animal studies suggest that nicotine causes seminiferous tubules degeneration, disrupts the spermatogenesis and at cellular level, affect germ cell structure and function in males.[ 84 ] It decreases testosterone levels which is secondary to decreased production of StAR.[ 85 ] StAR is the protein which plays an important role in testosterone biosynthesis.
REPRODUCTIVE SYSTEM – FEMALE
Menstrual cycle.
Nicotine by inhibiting the 21 hydoxylase causes hypoestrogenic state. It shunts the metabolites to formation of androgen. This leads to chronic anovulation and irregular menstrual cycles. Nicotine can predispose the endometrium to inappropriate cytokine production and irregular bleeding.[ 86 ] There is consistent evidence that increase in follicle-stimulating hormone levels and decreases in estrogen and progesterone that are associated with cigarette smoking in women, is atleast in part due to effects of nicotine on the endocrine system.[ 26 ]
Effect on oocytes
Nicotine affects the ovaries and alters the production of oocytes in various animal studies. Nicotine-treated oocytes appeared nonspherical with rough surface and torn and irregular zona-pellucida. Nicotine also caused disturbed oocyte maturation. There is a decreased blood flow to the oviducts and thus impaired fertilization.[ 87 ]
Peri-natal effects
Maternal smoking has always been known to have deleterious effects on the fetal outcome. There is an increased incidence of intrauterine growth restriction, still birth, miscarriages and mental retardation.[ 88 ] Various animal studies show retarded fetal growth and lower birth weight when treated perinatally with nicotine. The lower levels of ACTH and cortisol due to nicotine are probable reasons for the incidence of lower birth weight in the newborns.[ 89 ]
Maternal as well as grand maternal smoking has been found to increase risk of pediatric asthma. Another serious and important effect is the transgenic transmission of the addictive pattern.[ 29 ]
Nicotine is the fundamental cause of addiction among tobacco users. Nicotine adversely affects many organs as shown in human and animal studies. Its biological effects are widespread and extend to all systems of the body including cardiovascular, respiratory, renal and reproductive systems. Nicotine has also been found to be carcinogenic in several studies. It promotes tumorigenesis by affecting cell proliferation, angiogenesis and apoptotic pathways. It causes resistance to the chemotherapeutic agents. Nicotine replacement therapy (NRT) is an effective adjunct in management of withdrawal symptoms and improves the success of cessation programs. Any substantive beneficial effect of nicotine on human body is yet to be proven. Nicotine should be used only under supervision of trained cessation personnel therefore its sale needs to be strictly regulated. Needless to say, that research for safer alternative to nicotine must be taken on priority.
Source of Support: Nil
Conflict of Interest: None declared.
Thank you for visiting nature.com. You are using a browser version with limited support for CSS. To obtain the best experience, we recommend you use a more up to date browser (or turn off compatibility mode in Internet Explorer). In the meantime, to ensure continued support, we are displaying the site without styles and JavaScript.
- View all journals
- My Account Login
- Explore content
- About the journal
- Publish with us
- Sign up for alerts
- Open access
- Published: 17 April 2024
The economic commitment of climate change
- Maximilian Kotz ORCID: orcid.org/0000-0003-2564-5043 1 , 2 ,
- Anders Levermann ORCID: orcid.org/0000-0003-4432-4704 1 , 2 &
- Leonie Wenz ORCID: orcid.org/0000-0002-8500-1568 1 , 3
Nature volume 628 , pages 551–557 ( 2024 ) Cite this article
70k Accesses
3527 Altmetric
Metrics details
- Environmental economics
- Environmental health
- Interdisciplinary studies
- Projection and prediction
Global projections of macroeconomic climate-change damages typically consider impacts from average annual and national temperatures over long time horizons 1 , 2 , 3 , 4 , 5 , 6 . Here we use recent empirical findings from more than 1,600 regions worldwide over the past 40 years to project sub-national damages from temperature and precipitation, including daily variability and extremes 7 , 8 . Using an empirical approach that provides a robust lower bound on the persistence of impacts on economic growth, we find that the world economy is committed to an income reduction of 19% within the next 26 years independent of future emission choices (relative to a baseline without climate impacts, likely range of 11–29% accounting for physical climate and empirical uncertainty). These damages already outweigh the mitigation costs required to limit global warming to 2 °C by sixfold over this near-term time frame and thereafter diverge strongly dependent on emission choices. Committed damages arise predominantly through changes in average temperature, but accounting for further climatic components raises estimates by approximately 50% and leads to stronger regional heterogeneity. Committed losses are projected for all regions except those at very high latitudes, at which reductions in temperature variability bring benefits. The largest losses are committed at lower latitudes in regions with lower cumulative historical emissions and lower present-day income.
Similar content being viewed by others
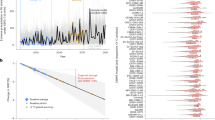
Climate damage projections beyond annual temperature
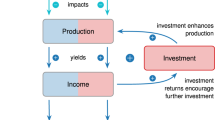
Investment incentive reduced by climate damages can be restored by optimal policy
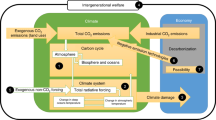
Climate economics support for the UN climate targets
Projections of the macroeconomic damage caused by future climate change are crucial to informing public and policy debates about adaptation, mitigation and climate justice. On the one hand, adaptation against climate impacts must be justified and planned on the basis of an understanding of their future magnitude and spatial distribution 9 . This is also of importance in the context of climate justice 10 , as well as to key societal actors, including governments, central banks and private businesses, which increasingly require the inclusion of climate risks in their macroeconomic forecasts to aid adaptive decision-making 11 , 12 . On the other hand, climate mitigation policy such as the Paris Climate Agreement is often evaluated by balancing the costs of its implementation against the benefits of avoiding projected physical damages. This evaluation occurs both formally through cost–benefit analyses 1 , 4 , 5 , 6 , as well as informally through public perception of mitigation and damage costs 13 .
Projections of future damages meet challenges when informing these debates, in particular the human biases relating to uncertainty and remoteness that are raised by long-term perspectives 14 . Here we aim to overcome such challenges by assessing the extent of economic damages from climate change to which the world is already committed by historical emissions and socio-economic inertia (the range of future emission scenarios that are considered socio-economically plausible 15 ). Such a focus on the near term limits the large uncertainties about diverging future emission trajectories, the resulting long-term climate response and the validity of applying historically observed climate–economic relations over long timescales during which socio-technical conditions may change considerably. As such, this focus aims to simplify the communication and maximize the credibility of projected economic damages from future climate change.
In projecting the future economic damages from climate change, we make use of recent advances in climate econometrics that provide evidence for impacts on sub-national economic growth from numerous components of the distribution of daily temperature and precipitation 3 , 7 , 8 . Using fixed-effects panel regression models to control for potential confounders, these studies exploit within-region variation in local temperature and precipitation in a panel of more than 1,600 regions worldwide, comprising climate and income data over the past 40 years, to identify the plausibly causal effects of changes in several climate variables on economic productivity 16 , 17 . Specifically, macroeconomic impacts have been identified from changing daily temperature variability, total annual precipitation, the annual number of wet days and extreme daily rainfall that occur in addition to those already identified from changing average temperature 2 , 3 , 18 . Moreover, regional heterogeneity in these effects based on the prevailing local climatic conditions has been found using interactions terms. The selection of these climate variables follows micro-level evidence for mechanisms related to the impacts of average temperatures on labour and agricultural productivity 2 , of temperature variability on agricultural productivity and health 7 , as well as of precipitation on agricultural productivity, labour outcomes and flood damages 8 (see Extended Data Table 1 for an overview, including more detailed references). References 7 , 8 contain a more detailed motivation for the use of these particular climate variables and provide extensive empirical tests about the robustness and nature of their effects on economic output, which are summarized in Methods . By accounting for these extra climatic variables at the sub-national level, we aim for a more comprehensive description of climate impacts with greater detail across both time and space.
Constraining the persistence of impacts
A key determinant and source of discrepancy in estimates of the magnitude of future climate damages is the extent to which the impact of a climate variable on economic growth rates persists. The two extreme cases in which these impacts persist indefinitely or only instantaneously are commonly referred to as growth or level effects 19 , 20 (see Methods section ‘Empirical model specification: fixed-effects distributed lag models’ for mathematical definitions). Recent work shows that future damages from climate change depend strongly on whether growth or level effects are assumed 20 . Following refs. 2 , 18 , we provide constraints on this persistence by using distributed lag models to test the significance of delayed effects separately for each climate variable. Notably, and in contrast to refs. 2 , 18 , we use climate variables in their first-differenced form following ref. 3 , implying a dependence of the growth rate on a change in climate variables. This choice means that a baseline specification without any lags constitutes a model prior of purely level effects, in which a permanent change in the climate has only an instantaneous effect on the growth rate 3 , 19 , 21 . By including lags, one can then test whether any effects may persist further. This is in contrast to the specification used by refs. 2 , 18 , in which climate variables are used without taking the first difference, implying a dependence of the growth rate on the level of climate variables. In this alternative case, the baseline specification without any lags constitutes a model prior of pure growth effects, in which a change in climate has an infinitely persistent effect on the growth rate. Consequently, including further lags in this alternative case tests whether the initial growth impact is recovered 18 , 19 , 21 . Both of these specifications suffer from the limiting possibility that, if too few lags are included, one might falsely accept the model prior. The limitations of including a very large number of lags, including loss of data and increasing statistical uncertainty with an increasing number of parameters, mean that such a possibility is likely. By choosing a specification in which the model prior is one of level effects, our approach is therefore conservative by design, avoiding assumptions of infinite persistence of climate impacts on growth and instead providing a lower bound on this persistence based on what is observable empirically (see Methods section ‘Empirical model specification: fixed-effects distributed lag models’ for further exposition of this framework). The conservative nature of such a choice is probably the reason that ref. 19 finds much greater consistency between the impacts projected by models that use the first difference of climate variables, as opposed to their levels.
We begin our empirical analysis of the persistence of climate impacts on growth using ten lags of the first-differenced climate variables in fixed-effects distributed lag models. We detect substantial effects on economic growth at time lags of up to approximately 8–10 years for the temperature terms and up to approximately 4 years for the precipitation terms (Extended Data Fig. 1 and Extended Data Table 2 ). Furthermore, evaluation by means of information criteria indicates that the inclusion of all five climate variables and the use of these numbers of lags provide a preferable trade-off between best-fitting the data and including further terms that could cause overfitting, in comparison with model specifications excluding climate variables or including more or fewer lags (Extended Data Fig. 3 , Supplementary Methods Section 1 and Supplementary Table 1 ). We therefore remove statistically insignificant terms at later lags (Supplementary Figs. 1 – 3 and Supplementary Tables 2 – 4 ). Further tests using Monte Carlo simulations demonstrate that the empirical models are robust to autocorrelation in the lagged climate variables (Supplementary Methods Section 2 and Supplementary Figs. 4 and 5 ), that information criteria provide an effective indicator for lag selection (Supplementary Methods Section 2 and Supplementary Fig. 6 ), that the results are robust to concerns of imperfect multicollinearity between climate variables and that including several climate variables is actually necessary to isolate their separate effects (Supplementary Methods Section 3 and Supplementary Fig. 7 ). We provide a further robustness check using a restricted distributed lag model to limit oscillations in the lagged parameter estimates that may result from autocorrelation, finding that it provides similar estimates of cumulative marginal effects to the unrestricted model (Supplementary Methods Section 4 and Supplementary Figs. 8 and 9 ). Finally, to explicitly account for any outstanding uncertainty arising from the precise choice of the number of lags, we include empirical models with marginally different numbers of lags in the error-sampling procedure of our projection of future damages. On the basis of the lag-selection procedure (the significance of lagged terms in Extended Data Fig. 1 and Extended Data Table 2 , as well as information criteria in Extended Data Fig. 3 ), we sample from models with eight to ten lags for temperature and four for precipitation (models shown in Supplementary Figs. 1 – 3 and Supplementary Tables 2 – 4 ). In summary, this empirical approach to constrain the persistence of climate impacts on economic growth rates is conservative by design in avoiding assumptions of infinite persistence, but nevertheless provides a lower bound on the extent of impact persistence that is robust to the numerous tests outlined above.
Committed damages until mid-century
We combine these empirical economic response functions (Supplementary Figs. 1 – 3 and Supplementary Tables 2 – 4 ) with an ensemble of 21 climate models (see Supplementary Table 5 ) from the Coupled Model Intercomparison Project Phase 6 (CMIP-6) 22 to project the macroeconomic damages from these components of physical climate change (see Methods for further details). Bias-adjusted climate models that provide a highly accurate reproduction of observed climatological patterns with limited uncertainty (Supplementary Table 6 ) are used to avoid introducing biases in the projections. Following a well-developed literature 2 , 3 , 19 , these projections do not aim to provide a prediction of future economic growth. Instead, they are a projection of the exogenous impact of future climate conditions on the economy relative to the baselines specified by socio-economic projections, based on the plausibly causal relationships inferred by the empirical models and assuming ceteris paribus. Other exogenous factors relevant for the prediction of economic output are purposefully assumed constant.
A Monte Carlo procedure that samples from climate model projections, empirical models with different numbers of lags and model parameter estimates (obtained by 1,000 block-bootstrap resamples of each of the regressions in Supplementary Figs. 1 – 3 and Supplementary Tables 2 – 4 ) is used to estimate the combined uncertainty from these sources. Given these uncertainty distributions, we find that projected global damages are statistically indistinguishable across the two most extreme emission scenarios until 2049 (at the 5% significance level; Fig. 1 ). As such, the climate damages occurring before this time constitute those to which the world is already committed owing to the combination of past emissions and the range of future emission scenarios that are considered socio-economically plausible 15 . These committed damages comprise a permanent income reduction of 19% on average globally (population-weighted average) in comparison with a baseline without climate-change impacts (with a likely range of 11–29%, following the likelihood classification adopted by the Intergovernmental Panel on Climate Change (IPCC); see caption of Fig. 1 ). Even though levels of income per capita generally still increase relative to those of today, this constitutes a permanent income reduction for most regions, including North America and Europe (each with median income reductions of approximately 11%) and with South Asia and Africa being the most strongly affected (each with median income reductions of approximately 22%; Fig. 1 ). Under a middle-of-the road scenario of future income development (SSP2, in which SSP stands for Shared Socio-economic Pathway), this corresponds to global annual damages in 2049 of 38 trillion in 2005 international dollars (likely range of 19–59 trillion 2005 international dollars). Compared with empirical specifications that assume pure growth or pure level effects, our preferred specification that provides a robust lower bound on the extent of climate impact persistence produces damages between these two extreme assumptions (Extended Data Fig. 3 ).
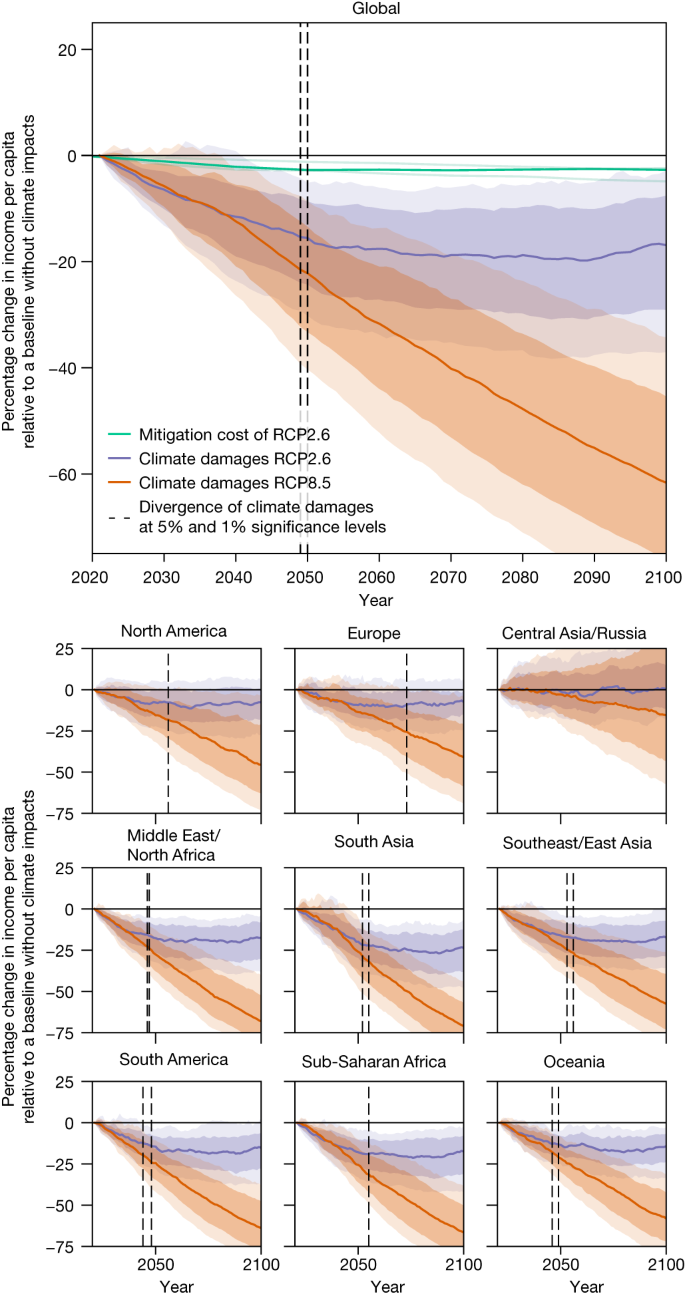
Estimates of the projected reduction in income per capita from changes in all climate variables based on empirical models of climate impacts on economic output with a robust lower bound on their persistence (Extended Data Fig. 1 ) under a low-emission scenario compatible with the 2 °C warming target and a high-emission scenario (SSP2-RCP2.6 and SSP5-RCP8.5, respectively) are shown in purple and orange, respectively. Shading represents the 34% and 10% confidence intervals reflecting the likely and very likely ranges, respectively (following the likelihood classification adopted by the IPCC), having estimated uncertainty from a Monte Carlo procedure, which samples the uncertainty from the choice of physical climate models, empirical models with different numbers of lags and bootstrapped estimates of the regression parameters shown in Supplementary Figs. 1 – 3 . Vertical dashed lines show the time at which the climate damages of the two emission scenarios diverge at the 5% and 1% significance levels based on the distribution of differences between emission scenarios arising from the uncertainty sampling discussed above. Note that uncertainty in the difference of the two scenarios is smaller than the combined uncertainty of the two respective scenarios because samples of the uncertainty (climate model and empirical model choice, as well as model parameter bootstrap) are consistent across the two emission scenarios, hence the divergence of damages occurs while the uncertainty bounds of the two separate damage scenarios still overlap. Estimates of global mitigation costs from the three IAMs that provide results for the SSP2 baseline and SSP2-RCP2.6 scenario are shown in light green in the top panel, with the median of these estimates shown in bold.
Damages already outweigh mitigation costs
We compare the damages to which the world is committed over the next 25 years to estimates of the mitigation costs required to achieve the Paris Climate Agreement. Taking estimates of mitigation costs from the three integrated assessment models (IAMs) in the IPCC AR6 database 23 that provide results under comparable scenarios (SSP2 baseline and SSP2-RCP2.6, in which RCP stands for Representative Concentration Pathway), we find that the median committed climate damages are larger than the median mitigation costs in 2050 (six trillion in 2005 international dollars) by a factor of approximately six (note that estimates of mitigation costs are only provided every 10 years by the IAMs and so a comparison in 2049 is not possible). This comparison simply aims to compare the magnitude of future damages against mitigation costs, rather than to conduct a formal cost–benefit analysis of transitioning from one emission path to another. Formal cost–benefit analyses typically find that the net benefits of mitigation only emerge after 2050 (ref. 5 ), which may lead some to conclude that physical damages from climate change are simply not large enough to outweigh mitigation costs until the second half of the century. Our simple comparison of their magnitudes makes clear that damages are actually already considerably larger than mitigation costs and the delayed emergence of net mitigation benefits results primarily from the fact that damages across different emission paths are indistinguishable until mid-century (Fig. 1 ).
Although these near-term damages constitute those to which the world is already committed, we note that damage estimates diverge strongly across emission scenarios after 2049, conveying the clear benefits of mitigation from a purely economic point of view that have been emphasized in previous studies 4 , 24 . As well as the uncertainties assessed in Fig. 1 , these conclusions are robust to structural choices, such as the timescale with which changes in the moderating variables of the empirical models are estimated (Supplementary Figs. 10 and 11 ), as well as the order in which one accounts for the intertemporal and international components of currency comparison (Supplementary Fig. 12 ; see Methods for further details).
Damages from variability and extremes
Committed damages primarily arise through changes in average temperature (Fig. 2 ). This reflects the fact that projected changes in average temperature are larger than those in other climate variables when expressed as a function of their historical interannual variability (Extended Data Fig. 4 ). Because the historical variability is that on which the empirical models are estimated, larger projected changes in comparison with this variability probably lead to larger future impacts in a purely statistical sense. From a mechanistic perspective, one may plausibly interpret this result as implying that future changes in average temperature are the most unprecedented from the perspective of the historical fluctuations to which the economy is accustomed and therefore will cause the most damage. This insight may prove useful in terms of guiding adaptation measures to the sources of greatest damage.
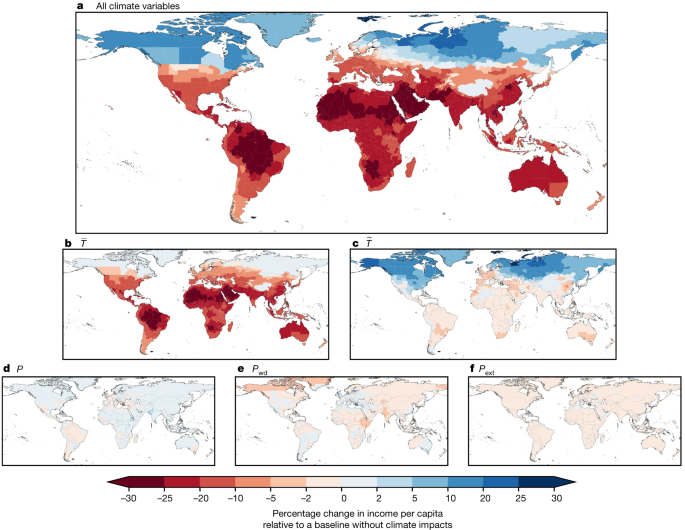
Estimates of the median projected reduction in sub-national income per capita across emission scenarios (SSP2-RCP2.6 and SSP2-RCP8.5) as well as climate model, empirical model and model parameter uncertainty in the year in which climate damages diverge at the 5% level (2049, as identified in Fig. 1 ). a , Impacts arising from all climate variables. b – f , Impacts arising separately from changes in annual mean temperature ( b ), daily temperature variability ( c ), total annual precipitation ( d ), the annual number of wet days (>1 mm) ( e ) and extreme daily rainfall ( f ) (see Methods for further definitions). Data on national administrative boundaries are obtained from the GADM database version 3.6 and are freely available for academic use ( https://gadm.org/ ).
Nevertheless, future damages based on empirical models that consider changes in annual average temperature only and exclude the other climate variables constitute income reductions of only 13% in 2049 (Extended Data Fig. 5a , likely range 5–21%). This suggests that accounting for the other components of the distribution of temperature and precipitation raises net damages by nearly 50%. This increase arises through the further damages that these climatic components cause, but also because their inclusion reveals a stronger negative economic response to average temperatures (Extended Data Fig. 5b ). The latter finding is consistent with our Monte Carlo simulations, which suggest that the magnitude of the effect of average temperature on economic growth is underestimated unless accounting for the impacts of other correlated climate variables (Supplementary Fig. 7 ).
In terms of the relative contributions of the different climatic components to overall damages, we find that accounting for daily temperature variability causes the largest increase in overall damages relative to empirical frameworks that only consider changes in annual average temperature (4.9 percentage points, likely range 2.4–8.7 percentage points, equivalent to approximately 10 trillion international dollars). Accounting for precipitation causes smaller increases in overall damages, which are—nevertheless—equivalent to approximately 1.2 trillion international dollars: 0.01 percentage points (−0.37–0.33 percentage points), 0.34 percentage points (0.07–0.90 percentage points) and 0.36 percentage points (0.13–0.65 percentage points) from total annual precipitation, the number of wet days and extreme daily precipitation, respectively. Moreover, climate models seem to underestimate future changes in temperature variability 25 and extreme precipitation 26 , 27 in response to anthropogenic forcing as compared with that observed historically, suggesting that the true impacts from these variables may be larger.
The distribution of committed damages
The spatial distribution of committed damages (Fig. 2a ) reflects a complex interplay between the patterns of future change in several climatic components and those of historical economic vulnerability to changes in those variables. Damages resulting from increasing annual mean temperature (Fig. 2b ) are negative almost everywhere globally, and larger at lower latitudes in regions in which temperatures are already higher and economic vulnerability to temperature increases is greatest (see the response heterogeneity to mean temperature embodied in Extended Data Fig. 1a ). This occurs despite the amplified warming projected at higher latitudes 28 , suggesting that regional heterogeneity in economic vulnerability to temperature changes outweighs heterogeneity in the magnitude of future warming (Supplementary Fig. 13a ). Economic damages owing to daily temperature variability (Fig. 2c ) exhibit a strong latitudinal polarisation, primarily reflecting the physical response of daily variability to greenhouse forcing in which increases in variability across lower latitudes (and Europe) contrast decreases at high latitudes 25 (Supplementary Fig. 13b ). These two temperature terms are the dominant determinants of the pattern of overall damages (Fig. 2a ), which exhibits a strong polarity with damages across most of the globe except at the highest northern latitudes. Future changes in total annual precipitation mainly bring economic benefits except in regions of drying, such as the Mediterranean and central South America (Fig. 2d and Supplementary Fig. 13c ), but these benefits are opposed by changes in the number of wet days, which produce damages with a similar pattern of opposite sign (Fig. 2e and Supplementary Fig. 13d ). By contrast, changes in extreme daily rainfall produce damages in all regions, reflecting the intensification of daily rainfall extremes over global land areas 29 , 30 (Fig. 2f and Supplementary Fig. 13e ).
The spatial distribution of committed damages implies considerable injustice along two dimensions: culpability for the historical emissions that have caused climate change and pre-existing levels of socio-economic welfare. Spearman’s rank correlations indicate that committed damages are significantly larger in countries with smaller historical cumulative emissions, as well as in regions with lower current income per capita (Fig. 3 ). This implies that those countries that will suffer the most from the damages already committed are those that are least responsible for climate change and which also have the least resources to adapt to it.
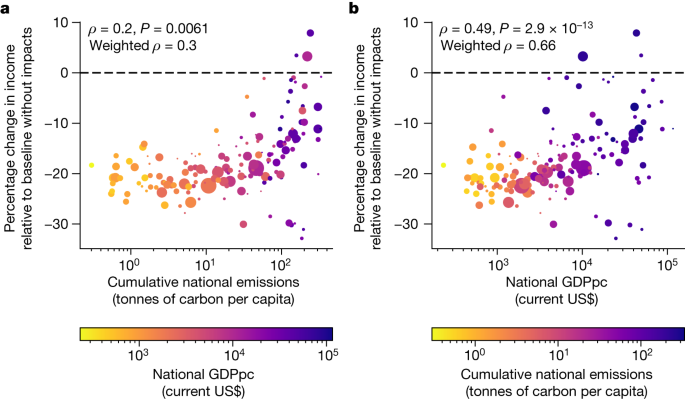
Estimates of the median projected change in national income per capita across emission scenarios (RCP2.6 and RCP8.5) as well as climate model, empirical model and model parameter uncertainty in the year in which climate damages diverge at the 5% level (2049, as identified in Fig. 1 ) are plotted against cumulative national emissions per capita in 2020 (from the Global Carbon Project) and coloured by national income per capita in 2020 (from the World Bank) in a and vice versa in b . In each panel, the size of each scatter point is weighted by the national population in 2020 (from the World Bank). Inset numbers indicate the Spearman’s rank correlation ρ and P -values for a hypothesis test whose null hypothesis is of no correlation, as well as the Spearman’s rank correlation weighted by national population.
To further quantify this heterogeneity, we assess the difference in committed damages between the upper and lower quartiles of regions when ranked by present income levels and historical cumulative emissions (using a population weighting to both define the quartiles and estimate the group averages). On average, the quartile of countries with lower income are committed to an income loss that is 8.9 percentage points (or 61%) greater than the upper quartile (Extended Data Fig. 6 ), with a likely range of 3.8–14.7 percentage points across the uncertainty sampling of our damage projections (following the likelihood classification adopted by the IPCC). Similarly, the quartile of countries with lower historical cumulative emissions are committed to an income loss that is 6.9 percentage points (or 40%) greater than the upper quartile, with a likely range of 0.27–12 percentage points. These patterns reemphasize the prevalence of injustice in climate impacts 31 , 32 , 33 in the context of the damages to which the world is already committed by historical emissions and socio-economic inertia.
Contextualizing the magnitude of damages
The magnitude of projected economic damages exceeds previous literature estimates 2 , 3 , arising from several developments made on previous approaches. Our estimates are larger than those of ref. 2 (see first row of Extended Data Table 3 ), primarily because of the facts that sub-national estimates typically show a steeper temperature response (see also refs. 3 , 34 ) and that accounting for other climatic components raises damage estimates (Extended Data Fig. 5 ). However, we note that our empirical approach using first-differenced climate variables is conservative compared with that of ref. 2 in regard to the persistence of climate impacts on growth (see introduction and Methods section ‘Empirical model specification: fixed-effects distributed lag models’), an important determinant of the magnitude of long-term damages 19 , 21 . Using a similar empirical specification to ref. 2 , which assumes infinite persistence while maintaining the rest of our approach (sub-national data and further climate variables), produces considerably larger damages (purple curve of Extended Data Fig. 3 ). Compared with studies that do take the first difference of climate variables 3 , 35 , our estimates are also larger (see second and third rows of Extended Data Table 3 ). The inclusion of further climate variables (Extended Data Fig. 5 ) and a sufficient number of lags to more adequately capture the extent of impact persistence (Extended Data Figs. 1 and 2 ) are the main sources of this difference, as is the use of specifications that capture nonlinearities in the temperature response when compared with ref. 35 . In summary, our estimates develop on previous studies by incorporating the latest data and empirical insights 7 , 8 , as well as in providing a robust empirical lower bound on the persistence of impacts on economic growth, which constitutes a middle ground between the extremes of the growth-versus-levels debate 19 , 21 (Extended Data Fig. 3 ).
Compared with the fraction of variance explained by the empirical models historically (<5%), the projection of reductions in income of 19% may seem large. This arises owing to the fact that projected changes in climatic conditions are much larger than those that were experienced historically, particularly for changes in average temperature (Extended Data Fig. 4 ). As such, any assessment of future climate-change impacts necessarily requires an extrapolation outside the range of the historical data on which the empirical impact models were evaluated. Nevertheless, these models constitute the most state-of-the-art methods for inference of plausibly causal climate impacts based on observed data. Moreover, we take explicit steps to limit out-of-sample extrapolation by capping the moderating variables of the interaction terms at the 95th percentile of the historical distribution (see Methods ). This avoids extrapolating the marginal effects outside what was observed historically. Given the nonlinear response of economic output to annual mean temperature (Extended Data Fig. 1 and Extended Data Table 2 ), this is a conservative choice that limits the magnitude of damages that we project. Furthermore, back-of-the-envelope calculations indicate that the projected damages are consistent with the magnitude and patterns of historical economic development (see Supplementary Discussion Section 5 ).
Missing impacts and spatial spillovers
Despite assessing several climatic components from which economic impacts have recently been identified 3 , 7 , 8 , this assessment of aggregate climate damages should not be considered comprehensive. Important channels such as impacts from heatwaves 31 , sea-level rise 36 , tropical cyclones 37 and tipping points 38 , 39 , as well as non-market damages such as those to ecosystems 40 and human health 41 , are not considered in these estimates. Sea-level rise is unlikely to be feasibly incorporated into empirical assessments such as this because historical sea-level variability is mostly small. Non-market damages are inherently intractable within our estimates of impacts on aggregate monetary output and estimates of these impacts could arguably be considered as extra to those identified here. Recent empirical work suggests that accounting for these channels would probably raise estimates of these committed damages, with larger damages continuing to arise in the global south 31 , 36 , 37 , 38 , 39 , 40 , 41 , 42 .
Moreover, our main empirical analysis does not explicitly evaluate the potential for impacts in local regions to produce effects that ‘spill over’ into other regions. Such effects may further mitigate or amplify the impacts we estimate, for example, if companies relocate production from one affected region to another or if impacts propagate along supply chains. The current literature indicates that trade plays a substantial role in propagating spillover effects 43 , 44 , making their assessment at the sub-national level challenging without available data on sub-national trade dependencies. Studies accounting for only spatially adjacent neighbours indicate that negative impacts in one region induce further negative impacts in neighbouring regions 45 , 46 , 47 , 48 , suggesting that our projected damages are probably conservative by excluding these effects. In Supplementary Fig. 14 , we assess spillovers from neighbouring regions using a spatial-lag model. For simplicity, this analysis excludes temporal lags, focusing only on contemporaneous effects. The results show that accounting for spatial spillovers can amplify the overall magnitude, and also the heterogeneity, of impacts. Consistent with previous literature, this indicates that the overall magnitude (Fig. 1 ) and heterogeneity (Fig. 3 ) of damages that we project in our main specification may be conservative without explicitly accounting for spillovers. We note that further analysis that addresses both spatially and trade-connected spillovers, while also accounting for delayed impacts using temporal lags, would be necessary to adequately address this question fully. These approaches offer fruitful avenues for further research but are beyond the scope of this manuscript, which primarily aims to explore the impacts of different climate conditions and their persistence.
Policy implications
We find that the economic damages resulting from climate change until 2049 are those to which the world economy is already committed and that these greatly outweigh the costs required to mitigate emissions in line with the 2 °C target of the Paris Climate Agreement (Fig. 1 ). This assessment is complementary to formal analyses of the net costs and benefits associated with moving from one emission path to another, which typically find that net benefits of mitigation only emerge in the second half of the century 5 . Our simple comparison of the magnitude of damages and mitigation costs makes clear that this is primarily because damages are indistinguishable across emissions scenarios—that is, committed—until mid-century (Fig. 1 ) and that they are actually already much larger than mitigation costs. For simplicity, and owing to the availability of data, we compare damages to mitigation costs at the global level. Regional estimates of mitigation costs may shed further light on the national incentives for mitigation to which our results already hint, of relevance for international climate policy. Although these damages are committed from a mitigation perspective, adaptation may provide an opportunity to reduce them. Moreover, the strong divergence of damages after mid-century reemphasizes the clear benefits of mitigation from a purely economic perspective, as highlighted in previous studies 1 , 4 , 6 , 24 .
Historical climate data
Historical daily 2-m temperature and precipitation totals (in mm) are obtained for the period 1979–2019 from the W5E5 database. The W5E5 dataset comes from ERA-5, a state-of-the-art reanalysis of historical observations, but has been bias-adjusted by applying version 2.0 of the WATCH Forcing Data to ERA-5 reanalysis data and precipitation data from version 2.3 of the Global Precipitation Climatology Project to better reflect ground-based measurements 49 , 50 , 51 . We obtain these data on a 0.5° × 0.5° grid from the Inter-Sectoral Impact Model Intercomparison Project (ISIMIP) database. Notably, these historical data have been used to bias-adjust future climate projections from CMIP-6 (see the following section), ensuring consistency between the distribution of historical daily weather on which our empirical models were estimated and the climate projections used to estimate future damages. These data are publicly available from the ISIMIP database. See refs. 7 , 8 for robustness tests of the empirical models to the choice of climate data reanalysis products.
Future climate data
Daily 2-m temperature and precipitation totals (in mm) are taken from 21 climate models participating in CMIP-6 under a high (RCP8.5) and a low (RCP2.6) greenhouse gas emission scenario from 2015 to 2100. The data have been bias-adjusted and statistically downscaled to a common half-degree grid to reflect the historical distribution of daily temperature and precipitation of the W5E5 dataset using the trend-preserving method developed by the ISIMIP 50 , 52 . As such, the climate model data reproduce observed climatological patterns exceptionally well (Supplementary Table 5 ). Gridded data are publicly available from the ISIMIP database.
Historical economic data
Historical economic data come from the DOSE database of sub-national economic output 53 . We use a recent revision to the DOSE dataset that provides data across 83 countries, 1,660 sub-national regions with varying temporal coverage from 1960 to 2019. Sub-national units constitute the first administrative division below national, for example, states for the USA and provinces for China. Data come from measures of gross regional product per capita (GRPpc) or income per capita in local currencies, reflecting the values reported in national statistical agencies, yearbooks and, in some cases, academic literature. We follow previous literature 3 , 7 , 8 , 54 and assess real sub-national output per capita by first converting values from local currencies to US dollars to account for diverging national inflationary tendencies and then account for US inflation using a US deflator. Alternatively, one might first account for national inflation and then convert between currencies. Supplementary Fig. 12 demonstrates that our conclusions are consistent when accounting for price changes in the reversed order, although the magnitude of estimated damages varies. See the documentation of the DOSE dataset for further discussion of these choices. Conversions between currencies are conducted using exchange rates from the FRED database of the Federal Reserve Bank of St. Louis 55 and the national deflators from the World Bank 56 .
Future socio-economic data
Baseline gridded gross domestic product (GDP) and population data for the period 2015–2100 are taken from the middle-of-the-road scenario SSP2 (ref. 15 ). Population data have been downscaled to a half-degree grid by the ISIMIP following the methodologies of refs. 57 , 58 , which we then aggregate to the sub-national level of our economic data using the spatial aggregation procedure described below. Because current methodologies for downscaling the GDP of the SSPs use downscaled population to do so, per-capita estimates of GDP with a realistic distribution at the sub-national level are not readily available for the SSPs. We therefore use national-level GDP per capita (GDPpc) projections for all sub-national regions of a given country, assuming homogeneity within countries in terms of baseline GDPpc. Here we use projections that have been updated to account for the impact of the COVID-19 pandemic on the trajectory of future income, while remaining consistent with the long-term development of the SSPs 59 . The choice of baseline SSP alters the magnitude of projected climate damages in monetary terms, but when assessed in terms of percentage change from the baseline, the choice of socio-economic scenario is inconsequential. Gridded SSP population data and national-level GDPpc data are publicly available from the ISIMIP database. Sub-national estimates as used in this study are available in the code and data replication files.
Climate variables
Following recent literature 3 , 7 , 8 , we calculate an array of climate variables for which substantial impacts on macroeconomic output have been identified empirically, supported by further evidence at the micro level for plausible underlying mechanisms. See refs. 7 , 8 for an extensive motivation for the use of these particular climate variables and for detailed empirical tests on the nature and robustness of their effects on economic output. To summarize, these studies have found evidence for independent impacts on economic growth rates from annual average temperature, daily temperature variability, total annual precipitation, the annual number of wet days and extreme daily rainfall. Assessments of daily temperature variability were motivated by evidence of impacts on agricultural output and human health, as well as macroeconomic literature on the impacts of volatility on growth when manifest in different dimensions, such as government spending, exchange rates and even output itself 7 . Assessments of precipitation impacts were motivated by evidence of impacts on agricultural productivity, metropolitan labour outcomes and conflict, as well as damages caused by flash flooding 8 . See Extended Data Table 1 for detailed references to empirical studies of these physical mechanisms. Marked impacts of daily temperature variability, total annual precipitation, the number of wet days and extreme daily rainfall on macroeconomic output were identified robustly across different climate datasets, spatial aggregation schemes, specifications of regional time trends and error-clustering approaches. They were also found to be robust to the consideration of temperature extremes 7 , 8 . Furthermore, these climate variables were identified as having independent effects on economic output 7 , 8 , which we further explain here using Monte Carlo simulations to demonstrate the robustness of the results to concerns of imperfect multicollinearity between climate variables (Supplementary Methods Section 2 ), as well as by using information criteria (Supplementary Table 1 ) to demonstrate that including several lagged climate variables provides a preferable trade-off between optimally describing the data and limiting the possibility of overfitting.
We calculate these variables from the distribution of daily, d , temperature, T x , d , and precipitation, P x , d , at the grid-cell, x , level for both the historical and future climate data. As well as annual mean temperature, \({\bar{T}}_{x,y}\) , and annual total precipitation, P x , y , we calculate annual, y , measures of daily temperature variability, \({\widetilde{T}}_{x,y}\) :
the number of wet days, Pwd x , y :
and extreme daily rainfall:
in which T x , d , m , y is the grid-cell-specific daily temperature in month m and year y , \({\bar{T}}_{x,m,{y}}\) is the year and grid-cell-specific monthly, m , mean temperature, D m and D y the number of days in a given month m or year y , respectively, H the Heaviside step function, 1 mm the threshold used to define wet days and P 99.9 x is the 99.9th percentile of historical (1979–2019) daily precipitation at the grid-cell level. Units of the climate measures are degrees Celsius for annual mean temperature and daily temperature variability, millimetres for total annual precipitation and extreme daily precipitation, and simply the number of days for the annual number of wet days.
We also calculated weighted standard deviations of monthly rainfall totals as also used in ref. 8 but do not include them in our projections as we find that, when accounting for delayed effects, their effect becomes statistically indistinct and is better captured by changes in total annual rainfall.
Spatial aggregation
We aggregate grid-cell-level historical and future climate measures, as well as grid-cell-level future GDPpc and population, to the level of the first administrative unit below national level of the GADM database, using an area-weighting algorithm that estimates the portion of each grid cell falling within an administrative boundary. We use this as our baseline specification following previous findings that the effect of area or population weighting at the sub-national level is negligible 7 , 8 .
Empirical model specification: fixed-effects distributed lag models
Following a wide range of climate econometric literature 16 , 60 , we use panel regression models with a selection of fixed effects and time trends to isolate plausibly exogenous variation with which to maximize confidence in a causal interpretation of the effects of climate on economic growth rates. The use of region fixed effects, μ r , accounts for unobserved time-invariant differences between regions, such as prevailing climatic norms and growth rates owing to historical and geopolitical factors. The use of yearly fixed effects, η y , accounts for regionally invariant annual shocks to the global climate or economy such as the El Niño–Southern Oscillation or global recessions. In our baseline specification, we also include region-specific linear time trends, k r y , to exclude the possibility of spurious correlations resulting from common slow-moving trends in climate and growth.
The persistence of climate impacts on economic growth rates is a key determinant of the long-term magnitude of damages. Methods for inferring the extent of persistence in impacts on growth rates have typically used lagged climate variables to evaluate the presence of delayed effects or catch-up dynamics 2 , 18 . For example, consider starting from a model in which a climate condition, C r , y , (for example, annual mean temperature) affects the growth rate, Δlgrp r , y (the first difference of the logarithm of gross regional product) of region r in year y :
which we refer to as a ‘pure growth effects’ model in the main text. Typically, further lags are included,
and the cumulative effect of all lagged terms is evaluated to assess the extent to which climate impacts on growth rates persist. Following ref. 18 , in the case that,
the implication is that impacts on the growth rate persist up to NL years after the initial shock (possibly to a weaker or a stronger extent), whereas if
then the initial impact on the growth rate is recovered after NL years and the effect is only one on the level of output. However, we note that such approaches are limited by the fact that, when including an insufficient number of lags to detect a recovery of the growth rates, one may find equation ( 6 ) to be satisfied and incorrectly assume that a change in climatic conditions affects the growth rate indefinitely. In practice, given a limited record of historical data, including too few lags to confidently conclude in an infinitely persistent impact on the growth rate is likely, particularly over the long timescales over which future climate damages are often projected 2 , 24 . To avoid this issue, we instead begin our analysis with a model for which the level of output, lgrp r , y , depends on the level of a climate variable, C r , y :
Given the non-stationarity of the level of output, we follow the literature 19 and estimate such an equation in first-differenced form as,
which we refer to as a model of ‘pure level effects’ in the main text. This model constitutes a baseline specification in which a permanent change in the climate variable produces an instantaneous impact on the growth rate and a permanent effect only on the level of output. By including lagged variables in this specification,
we are able to test whether the impacts on the growth rate persist any further than instantaneously by evaluating whether α L > 0 are statistically significantly different from zero. Even though this framework is also limited by the possibility of including too few lags, the choice of a baseline model specification in which impacts on the growth rate do not persist means that, in the case of including too few lags, the framework reverts to the baseline specification of level effects. As such, this framework is conservative with respect to the persistence of impacts and the magnitude of future damages. It naturally avoids assumptions of infinite persistence and we are able to interpret any persistence that we identify with equation ( 9 ) as a lower bound on the extent of climate impact persistence on growth rates. See the main text for further discussion of this specification choice, in particular about its conservative nature compared with previous literature estimates, such as refs. 2 , 18 .
We allow the response to climatic changes to vary across regions, using interactions of the climate variables with historical average (1979–2019) climatic conditions reflecting heterogenous effects identified in previous work 7 , 8 . Following this previous work, the moderating variables of these interaction terms constitute the historical average of either the variable itself or of the seasonal temperature difference, \({\hat{T}}_{r}\) , or annual mean temperature, \({\bar{T}}_{r}\) , in the case of daily temperature variability 7 and extreme daily rainfall, respectively 8 .
The resulting regression equation with N and M lagged variables, respectively, reads:
in which Δlgrp r , y is the annual, regional GRPpc growth rate, measured as the first difference of the logarithm of real GRPpc, following previous work 2 , 3 , 7 , 8 , 18 , 19 . Fixed-effects regressions were run using the fixest package in R (ref. 61 ).
Estimates of the coefficients of interest α i , L are shown in Extended Data Fig. 1 for N = M = 10 lags and for our preferred choice of the number of lags in Supplementary Figs. 1 – 3 . In Extended Data Fig. 1 , errors are shown clustered at the regional level, but for the construction of damage projections, we block-bootstrap the regressions by region 1,000 times to provide a range of parameter estimates with which to sample the projection uncertainty (following refs. 2 , 31 ).
Spatial-lag model
In Supplementary Fig. 14 , we present the results from a spatial-lag model that explores the potential for climate impacts to ‘spill over’ into spatially neighbouring regions. We measure the distance between centroids of each pair of sub-national regions and construct spatial lags that take the average of the first-differenced climate variables and their interaction terms over neighbouring regions that are at distances of 0–500, 500–1,000, 1,000–1,500 and 1,500–2000 km (spatial lags, ‘SL’, 1 to 4). For simplicity, we then assess a spatial-lag model without temporal lags to assess spatial spillovers of contemporaneous climate impacts. This model takes the form:
in which SL indicates the spatial lag of each climate variable and interaction term. In Supplementary Fig. 14 , we plot the cumulative marginal effect of each climate variable at different baseline climate conditions by summing the coefficients for each climate variable and interaction term, for example, for average temperature impacts as:
These cumulative marginal effects can be regarded as the overall spatially dependent impact to an individual region given a one-unit shock to a climate variable in that region and all neighbouring regions at a given value of the moderating variable of the interaction term.
Constructing projections of economic damage from future climate change
We construct projections of future climate damages by applying the coefficients estimated in equation ( 10 ) and shown in Supplementary Tables 2 – 4 (when including only lags with statistically significant effects in specifications that limit overfitting; see Supplementary Methods Section 1 ) to projections of future climate change from the CMIP-6 models. Year-on-year changes in each primary climate variable of interest are calculated to reflect the year-to-year variations used in the empirical models. 30-year moving averages of the moderating variables of the interaction terms are calculated to reflect the long-term average of climatic conditions that were used for the moderating variables in the empirical models. By using moving averages in the projections, we account for the changing vulnerability to climate shocks based on the evolving long-term conditions (Supplementary Figs. 10 and 11 show that the results are robust to the precise choice of the window of this moving average). Although these climate variables are not differenced, the fact that the bias-adjusted climate models reproduce observed climatological patterns across regions for these moderating variables very accurately (Supplementary Table 6 ) with limited spread across models (<3%) precludes the possibility that any considerable bias or uncertainty is introduced by this methodological choice. However, we impose caps on these moderating variables at the 95th percentile at which they were observed in the historical data to prevent extrapolation of the marginal effects outside the range in which the regressions were estimated. This is a conservative choice that limits the magnitude of our damage projections.
Time series of primary climate variables and moderating climate variables are then combined with estimates of the empirical model parameters to evaluate the regression coefficients in equation ( 10 ), producing a time series of annual GRPpc growth-rate reductions for a given emission scenario, climate model and set of empirical model parameters. The resulting time series of growth-rate impacts reflects those occurring owing to future climate change. By contrast, a future scenario with no climate change would be one in which climate variables do not change (other than with random year-to-year fluctuations) and hence the time-averaged evaluation of equation ( 10 ) would be zero. Our approach therefore implicitly compares the future climate-change scenario to this no-climate-change baseline scenario.
The time series of growth-rate impacts owing to future climate change in region r and year y , δ r , y , are then added to the future baseline growth rates, π r , y (in log-diff form), obtained from the SSP2 scenario to yield trajectories of damaged GRPpc growth rates, ρ r , y . These trajectories are aggregated over time to estimate the future trajectory of GRPpc with future climate impacts:
in which GRPpc r , y =2020 is the initial log level of GRPpc. We begin damage estimates in 2020 to reflect the damages occurring since the end of the period for which we estimate the empirical models (1979–2019) and to match the timing of mitigation-cost estimates from most IAMs (see below).
For each emission scenario, this procedure is repeated 1,000 times while randomly sampling from the selection of climate models, the selection of empirical models with different numbers of lags (shown in Supplementary Figs. 1 – 3 and Supplementary Tables 2 – 4 ) and bootstrapped estimates of the regression parameters. The result is an ensemble of future GRPpc trajectories that reflect uncertainty from both physical climate change and the structural and sampling uncertainty of the empirical models.
Estimates of mitigation costs
We obtain IPCC estimates of the aggregate costs of emission mitigation from the AR6 Scenario Explorer and Database hosted by IIASA 23 . Specifically, we search the AR6 Scenarios Database World v1.1 for IAMs that provided estimates of global GDP and population under both a SSP2 baseline and a SSP2-RCP2.6 scenario to maintain consistency with the socio-economic and emission scenarios of the climate damage projections. We find five IAMs that provide data for these scenarios, namely, MESSAGE-GLOBIOM 1.0, REMIND-MAgPIE 1.5, AIM/GCE 2.0, GCAM 4.2 and WITCH-GLOBIOM 3.1. Of these five IAMs, we use the results only from the first three that passed the IPCC vetting procedure for reproducing historical emission and climate trajectories. We then estimate global mitigation costs as the percentage difference in global per capita GDP between the SSP2 baseline and the SSP2-RCP2.6 emission scenario. In the case of one of these IAMs, estimates of mitigation costs begin in 2020, whereas in the case of two others, mitigation costs begin in 2010. The mitigation cost estimates before 2020 in these two IAMs are mostly negligible, and our choice to begin comparison with damage estimates in 2020 is conservative with respect to the relative weight of climate damages compared with mitigation costs for these two IAMs.
Data availability
Data on economic production and ERA-5 climate data are publicly available at https://doi.org/10.5281/zenodo.4681306 (ref. 62 ) and https://www.ecmwf.int/en/forecasts/datasets/reanalysis-datasets/era5 , respectively. Data on mitigation costs are publicly available at https://data.ene.iiasa.ac.at/ar6/#/downloads . Processed climate and economic data, as well as all other necessary data for reproduction of the results, are available at the public repository https://doi.org/10.5281/zenodo.10562951 (ref. 63 ).
Code availability
All code necessary for reproduction of the results is available at the public repository https://doi.org/10.5281/zenodo.10562951 (ref. 63 ).
Glanemann, N., Willner, S. N. & Levermann, A. Paris Climate Agreement passes the cost-benefit test. Nat. Commun. 11 , 110 (2020).
Article ADS CAS PubMed PubMed Central Google Scholar
Burke, M., Hsiang, S. M. & Miguel, E. Global non-linear effect of temperature on economic production. Nature 527 , 235–239 (2015).
Article ADS CAS PubMed Google Scholar
Kalkuhl, M. & Wenz, L. The impact of climate conditions on economic production. Evidence from a global panel of regions. J. Environ. Econ. Manag. 103 , 102360 (2020).
Article Google Scholar
Moore, F. C. & Diaz, D. B. Temperature impacts on economic growth warrant stringent mitigation policy. Nat. Clim. Change 5 , 127–131 (2015).
Article ADS Google Scholar
Drouet, L., Bosetti, V. & Tavoni, M. Net economic benefits of well-below 2°C scenarios and associated uncertainties. Oxf. Open Clim. Change 2 , kgac003 (2022).
Ueckerdt, F. et al. The economically optimal warming limit of the planet. Earth Syst. Dyn. 10 , 741–763 (2019).
Kotz, M., Wenz, L., Stechemesser, A., Kalkuhl, M. & Levermann, A. Day-to-day temperature variability reduces economic growth. Nat. Clim. Change 11 , 319–325 (2021).
Kotz, M., Levermann, A. & Wenz, L. The effect of rainfall changes on economic production. Nature 601 , 223–227 (2022).
Kousky, C. Informing climate adaptation: a review of the economic costs of natural disasters. Energy Econ. 46 , 576–592 (2014).
Harlan, S. L. et al. in Climate Change and Society: Sociological Perspectives (eds Dunlap, R. E. & Brulle, R. J.) 127–163 (Oxford Univ. Press, 2015).
Bolton, P. et al. The Green Swan (BIS Books, 2020).
Alogoskoufis, S. et al. ECB Economy-wide Climate Stress Test: Methodology and Results European Central Bank, 2021).
Weber, E. U. What shapes perceptions of climate change? Wiley Interdiscip. Rev. Clim. Change 1 , 332–342 (2010).
Markowitz, E. M. & Shariff, A. F. Climate change and moral judgement. Nat. Clim. Change 2 , 243–247 (2012).
Riahi, K. et al. The shared socioeconomic pathways and their energy, land use, and greenhouse gas emissions implications: an overview. Glob. Environ. Change 42 , 153–168 (2017).
Auffhammer, M., Hsiang, S. M., Schlenker, W. & Sobel, A. Using weather data and climate model output in economic analyses of climate change. Rev. Environ. Econ. Policy 7 , 181–198 (2013).
Kolstad, C. D. & Moore, F. C. Estimating the economic impacts of climate change using weather observations. Rev. Environ. Econ. Policy 14 , 1–24 (2020).
Dell, M., Jones, B. F. & Olken, B. A. Temperature shocks and economic growth: evidence from the last half century. Am. Econ. J. Macroecon. 4 , 66–95 (2012).
Newell, R. G., Prest, B. C. & Sexton, S. E. The GDP-temperature relationship: implications for climate change damages. J. Environ. Econ. Manag. 108 , 102445 (2021).
Kikstra, J. S. et al. The social cost of carbon dioxide under climate-economy feedbacks and temperature variability. Environ. Res. Lett. 16 , 094037 (2021).
Article ADS CAS Google Scholar
Bastien-Olvera, B. & Moore, F. Persistent effect of temperature on GDP identified from lower frequency temperature variability. Environ. Res. Lett. 17 , 084038 (2022).
Eyring, V. et al. Overview of the Coupled Model Intercomparison Project Phase 6 (CMIP6) experimental design and organization. Geosci. Model Dev. 9 , 1937–1958 (2016).
Byers, E. et al. AR6 scenarios database. Zenodo https://zenodo.org/records/7197970 (2022).
Burke, M., Davis, W. M. & Diffenbaugh, N. S. Large potential reduction in economic damages under UN mitigation targets. Nature 557 , 549–553 (2018).
Kotz, M., Wenz, L. & Levermann, A. Footprint of greenhouse forcing in daily temperature variability. Proc. Natl Acad. Sci. 118 , e2103294118 (2021).
Article CAS PubMed PubMed Central Google Scholar
Myhre, G. et al. Frequency of extreme precipitation increases extensively with event rareness under global warming. Sci. Rep. 9 , 16063 (2019).
Min, S.-K., Zhang, X., Zwiers, F. W. & Hegerl, G. C. Human contribution to more-intense precipitation extremes. Nature 470 , 378–381 (2011).
England, M. R., Eisenman, I., Lutsko, N. J. & Wagner, T. J. The recent emergence of Arctic Amplification. Geophys. Res. Lett. 48 , e2021GL094086 (2021).
Fischer, E. M. & Knutti, R. Anthropogenic contribution to global occurrence of heavy-precipitation and high-temperature extremes. Nat. Clim. Change 5 , 560–564 (2015).
Pfahl, S., O’Gorman, P. A. & Fischer, E. M. Understanding the regional pattern of projected future changes in extreme precipitation. Nat. Clim. Change 7 , 423–427 (2017).
Callahan, C. W. & Mankin, J. S. Globally unequal effect of extreme heat on economic growth. Sci. Adv. 8 , eadd3726 (2022).
Diffenbaugh, N. S. & Burke, M. Global warming has increased global economic inequality. Proc. Natl Acad. Sci. 116 , 9808–9813 (2019).
Callahan, C. W. & Mankin, J. S. National attribution of historical climate damages. Clim. Change 172 , 40 (2022).
Burke, M. & Tanutama, V. Climatic constraints on aggregate economic output. National Bureau of Economic Research, Working Paper 25779. https://doi.org/10.3386/w25779 (2019).
Kahn, M. E. et al. Long-term macroeconomic effects of climate change: a cross-country analysis. Energy Econ. 104 , 105624 (2021).
Desmet, K. et al. Evaluating the economic cost of coastal flooding. National Bureau of Economic Research, Working Paper 24918. https://doi.org/10.3386/w24918 (2018).
Hsiang, S. M. & Jina, A. S. The causal effect of environmental catastrophe on long-run economic growth: evidence from 6,700 cyclones. National Bureau of Economic Research, Working Paper 20352. https://doi.org/10.3386/w2035 (2014).
Ritchie, P. D. et al. Shifts in national land use and food production in Great Britain after a climate tipping point. Nat. Food 1 , 76–83 (2020).
Dietz, S., Rising, J., Stoerk, T. & Wagner, G. Economic impacts of tipping points in the climate system. Proc. Natl Acad. Sci. 118 , e2103081118 (2021).
Bastien-Olvera, B. A. & Moore, F. C. Use and non-use value of nature and the social cost of carbon. Nat. Sustain. 4 , 101–108 (2021).
Carleton, T. et al. Valuing the global mortality consequences of climate change accounting for adaptation costs and benefits. Q. J. Econ. 137 , 2037–2105 (2022).
Bastien-Olvera, B. A. et al. Unequal climate impacts on global values of natural capital. Nature 625 , 722–727 (2024).
Malik, A. et al. Impacts of climate change and extreme weather on food supply chains cascade across sectors and regions in Australia. Nat. Food 3 , 631–643 (2022).
Article ADS PubMed Google Scholar
Kuhla, K., Willner, S. N., Otto, C., Geiger, T. & Levermann, A. Ripple resonance amplifies economic welfare loss from weather extremes. Environ. Res. Lett. 16 , 114010 (2021).
Schleypen, J. R., Mistry, M. N., Saeed, F. & Dasgupta, S. Sharing the burden: quantifying climate change spillovers in the European Union under the Paris Agreement. Spat. Econ. Anal. 17 , 67–82 (2022).
Dasgupta, S., Bosello, F., De Cian, E. & Mistry, M. Global temperature effects on economic activity and equity: a spatial analysis. European Institute on Economics and the Environment, Working Paper 22-1 (2022).
Neal, T. The importance of external weather effects in projecting the macroeconomic impacts of climate change. UNSW Economics Working Paper 2023-09 (2023).
Deryugina, T. & Hsiang, S. M. Does the environment still matter? Daily temperature and income in the United States. National Bureau of Economic Research, Working Paper 20750. https://doi.org/10.3386/w20750 (2014).
Hersbach, H. et al. The ERA5 global reanalysis. Q. J. R. Meteorol. Soc. 146 , 1999–2049 (2020).
Cucchi, M. et al. WFDE5: bias-adjusted ERA5 reanalysis data for impact studies. Earth Syst. Sci. Data 12 , 2097–2120 (2020).
Adler, R. et al. The New Version 2.3 of the Global Precipitation Climatology Project (GPCP) Monthly Analysis Product 1072–1084 (University of Maryland, 2016).
Lange, S. Trend-preserving bias adjustment and statistical downscaling with ISIMIP3BASD (v1.0). Geosci. Model Dev. 12 , 3055–3070 (2019).
Wenz, L., Carr, R. D., Kögel, N., Kotz, M. & Kalkuhl, M. DOSE – global data set of reported sub-national economic output. Sci. Data 10 , 425 (2023).
Article PubMed PubMed Central Google Scholar
Gennaioli, N., La Porta, R., Lopez De Silanes, F. & Shleifer, A. Growth in regions. J. Econ. Growth 19 , 259–309 (2014).
Board of Governors of the Federal Reserve System (US). U.S. dollars to euro spot exchange rate. https://fred.stlouisfed.org/series/AEXUSEU (2022).
World Bank. GDP deflator. https://data.worldbank.org/indicator/NY.GDP.DEFL.ZS (2022).
Jones, B. & O’Neill, B. C. Spatially explicit global population scenarios consistent with the Shared Socioeconomic Pathways. Environ. Res. Lett. 11 , 084003 (2016).
Murakami, D. & Yamagata, Y. Estimation of gridded population and GDP scenarios with spatially explicit statistical downscaling. Sustainability 11 , 2106 (2019).
Koch, J. & Leimbach, M. Update of SSP GDP projections: capturing recent changes in national accounting, PPP conversion and Covid 19 impacts. Ecol. Econ. 206 (2023).
Carleton, T. A. & Hsiang, S. M. Social and economic impacts of climate. Science 353 , aad9837 (2016).
Article PubMed Google Scholar
Bergé, L. Efficient estimation of maximum likelihood models with multiple fixed-effects: the R package FENmlm. DEM Discussion Paper Series 18-13 (2018).
Kalkuhl, M., Kotz, M. & Wenz, L. DOSE - The MCC-PIK Database Of Subnational Economic output. Zenodo https://zenodo.org/doi/10.5281/zenodo.4681305 (2021).
Kotz, M., Wenz, L. & Levermann, A. Data and code for “The economic commitment of climate change”. Zenodo https://zenodo.org/doi/10.5281/zenodo.10562951 (2024).
Dasgupta, S. et al. Effects of climate change on combined labour productivity and supply: an empirical, multi-model study. Lancet Planet. Health 5 , e455–e465 (2021).
Lobell, D. B. et al. The critical role of extreme heat for maize production in the United States. Nat. Clim. Change 3 , 497–501 (2013).
Zhao, C. et al. Temperature increase reduces global yields of major crops in four independent estimates. Proc. Natl Acad. Sci. 114 , 9326–9331 (2017).
Wheeler, T. R., Craufurd, P. Q., Ellis, R. H., Porter, J. R. & Prasad, P. V. Temperature variability and the yield of annual crops. Agric. Ecosyst. Environ. 82 , 159–167 (2000).
Rowhani, P., Lobell, D. B., Linderman, M. & Ramankutty, N. Climate variability and crop production in Tanzania. Agric. For. Meteorol. 151 , 449–460 (2011).
Ceglar, A., Toreti, A., Lecerf, R., Van der Velde, M. & Dentener, F. Impact of meteorological drivers on regional inter-annual crop yield variability in France. Agric. For. Meteorol. 216 , 58–67 (2016).
Shi, L., Kloog, I., Zanobetti, A., Liu, P. & Schwartz, J. D. Impacts of temperature and its variability on mortality in New England. Nat. Clim. Change 5 , 988–991 (2015).
Xue, T., Zhu, T., Zheng, Y. & Zhang, Q. Declines in mental health associated with air pollution and temperature variability in China. Nat. Commun. 10 , 2165 (2019).
Article ADS PubMed PubMed Central Google Scholar
Liang, X.-Z. et al. Determining climate effects on US total agricultural productivity. Proc. Natl Acad. Sci. 114 , E2285–E2292 (2017).
Desbureaux, S. & Rodella, A.-S. Drought in the city: the economic impact of water scarcity in Latin American metropolitan areas. World Dev. 114 , 13–27 (2019).
Damania, R. The economics of water scarcity and variability. Oxf. Rev. Econ. Policy 36 , 24–44 (2020).
Davenport, F. V., Burke, M. & Diffenbaugh, N. S. Contribution of historical precipitation change to US flood damages. Proc. Natl Acad. Sci. 118 , e2017524118 (2021).
Dave, R., Subramanian, S. S. & Bhatia, U. Extreme precipitation induced concurrent events trigger prolonged disruptions in regional road networks. Environ. Res. Lett. 16 , 104050 (2021).
Download references
Acknowledgements
We gratefully acknowledge financing from the Volkswagen Foundation and the Deutsche Gesellschaft für Internationale Zusammenarbeit (GIZ) GmbH on behalf of the Government of the Federal Republic of Germany and Federal Ministry for Economic Cooperation and Development (BMZ).
Open access funding provided by Potsdam-Institut für Klimafolgenforschung (PIK) e.V.
Author information
Authors and affiliations.
Research Domain IV, Research Domain IV, Potsdam Institute for Climate Impact Research, Potsdam, Germany
Maximilian Kotz, Anders Levermann & Leonie Wenz
Institute of Physics, Potsdam University, Potsdam, Germany
Maximilian Kotz & Anders Levermann
Mercator Research Institute on Global Commons and Climate Change, Berlin, Germany
Leonie Wenz
You can also search for this author in PubMed Google Scholar
Contributions
All authors contributed to the design of the analysis. M.K. conducted the analysis and produced the figures. All authors contributed to the interpretation and presentation of the results. M.K. and L.W. wrote the manuscript.
Corresponding author
Correspondence to Leonie Wenz .
Ethics declarations
Competing interests.
The authors declare no competing interests.
Peer review
Peer review information.
Nature thanks Xin-Zhong Liang, Chad Thackeray and the other, anonymous, reviewer(s) for their contribution to the peer review of this work. Peer reviewer reports are available.
Additional information
Publisher’s note Springer Nature remains neutral with regard to jurisdictional claims in published maps and institutional affiliations.
Extended data figures and tables
Extended data fig. 1 constraining the persistence of historical climate impacts on economic growth rates..
The results of a panel-based fixed-effects distributed lag model for the effects of annual mean temperature ( a ), daily temperature variability ( b ), total annual precipitation ( c ), the number of wet days ( d ) and extreme daily precipitation ( e ) on sub-national economic growth rates. Point estimates show the effects of a 1 °C or one standard deviation increase (for temperature and precipitation variables, respectively) at the lower quartile, median and upper quartile of the relevant moderating variable (green, orange and purple, respectively) at different lagged periods after the initial shock (note that these are not cumulative effects). Climate variables are used in their first-differenced form (see main text for discussion) and the moderating climate variables are the annual mean temperature, seasonal temperature difference, total annual precipitation, number of wet days and annual mean temperature, respectively, in panels a – e (see Methods for further discussion). Error bars show the 95% confidence intervals having clustered standard errors by region. The within-region R 2 , Bayesian and Akaike information criteria for the model are shown at the top of the figure. This figure shows results with ten lags for each variable to demonstrate the observed levels of persistence, but our preferred specifications remove later lags based on the statistical significance of terms shown above and the information criteria shown in Extended Data Fig. 2 . The resulting models without later lags are shown in Supplementary Figs. 1 – 3 .
Extended Data Fig. 2 Incremental lag-selection procedure using information criteria and within-region R 2 .
Starting from a panel-based fixed-effects distributed lag model estimating the effects of climate on economic growth using the real historical data (as in equation ( 4 )) with ten lags for all climate variables (as shown in Extended Data Fig. 1 ), lags are incrementally removed for one climate variable at a time. The resulting Bayesian and Akaike information criteria are shown in a – e and f – j , respectively, and the within-region R 2 and number of observations in k – o and p – t , respectively. Different rows show the results when removing lags from different climate variables, ordered from top to bottom as annual mean temperature, daily temperature variability, total annual precipitation, the number of wet days and extreme annual precipitation. Information criteria show minima at approximately four lags for precipitation variables and ten to eight for temperature variables, indicating that including these numbers of lags does not lead to overfitting. See Supplementary Table 1 for an assessment using information criteria to determine whether including further climate variables causes overfitting.
Extended Data Fig. 3 Damages in our preferred specification that provides a robust lower bound on the persistence of climate impacts on economic growth versus damages in specifications of pure growth or pure level effects.
Estimates of future damages as shown in Fig. 1 but under the emission scenario RCP8.5 for three separate empirical specifications: in orange our preferred specification, which provides an empirical lower bound on the persistence of climate impacts on economic growth rates while avoiding assumptions of infinite persistence (see main text for further discussion); in purple a specification of ‘pure growth effects’ in which the first difference of climate variables is not taken and no lagged climate variables are included (the baseline specification of ref. 2 ); and in pink a specification of ‘pure level effects’ in which the first difference of climate variables is taken but no lagged terms are included.
Extended Data Fig. 4 Climate changes in different variables as a function of historical interannual variability.
Changes in each climate variable of interest from 1979–2019 to 2035–2065 under the high-emission scenario SSP5-RCP8.5, expressed as a percentage of the historical variability of each measure. Historical variability is estimated as the standard deviation of each detrended climate variable over the period 1979–2019 during which the empirical models were identified (detrending is appropriate because of the inclusion of region-specific linear time trends in the empirical models). See Supplementary Fig. 13 for changes expressed in standard units. Data on national administrative boundaries are obtained from the GADM database version 3.6 and are freely available for academic use ( https://gadm.org/ ).
Extended Data Fig. 5 Contribution of different climate variables to overall committed damages.
a , Climate damages in 2049 when using empirical models that account for all climate variables, changes in annual mean temperature only or changes in both annual mean temperature and one other climate variable (daily temperature variability, total annual precipitation, the number of wet days and extreme daily precipitation, respectively). b , The cumulative marginal effects of an increase in annual mean temperature of 1 °C, at different baseline temperatures, estimated from empirical models including all climate variables or annual mean temperature only. Estimates and uncertainty bars represent the median and 95% confidence intervals obtained from 1,000 block-bootstrap resamples from each of three different empirical models using eight, nine or ten lags of temperature terms.
Extended Data Fig. 6 The difference in committed damages between the upper and lower quartiles of countries when ranked by GDP and cumulative historical emissions.
Quartiles are defined using a population weighting, as are the average committed damages across each quartile group. The violin plots indicate the distribution of differences between quartiles across the two extreme emission scenarios (RCP2.6 and RCP8.5) and the uncertainty sampling procedure outlined in Methods , which accounts for uncertainty arising from the choice of lags in the empirical models, uncertainty in the empirical model parameter estimates, as well as the climate model projections. Bars indicate the median, as well as the 10th and 90th percentiles and upper and lower sixths of the distribution reflecting the very likely and likely ranges following the likelihood classification adopted by the IPCC.
Supplementary information
Supplementary information, peer review file, rights and permissions.
Open Access This article is licensed under a Creative Commons Attribution 4.0 International License, which permits use, sharing, adaptation, distribution and reproduction in any medium or format, as long as you give appropriate credit to the original author(s) and the source, provide a link to the Creative Commons licence, and indicate if changes were made. The images or other third party material in this article are included in the article’s Creative Commons licence, unless indicated otherwise in a credit line to the material. If material is not included in the article’s Creative Commons licence and your intended use is not permitted by statutory regulation or exceeds the permitted use, you will need to obtain permission directly from the copyright holder. To view a copy of this licence, visit http://creativecommons.org/licenses/by/4.0/ .
Reprints and permissions
About this article
Cite this article.
Kotz, M., Levermann, A. & Wenz, L. The economic commitment of climate change. Nature 628 , 551–557 (2024). https://doi.org/10.1038/s41586-024-07219-0
Download citation
Received : 25 January 2023
Accepted : 21 February 2024
Published : 17 April 2024
Issue Date : 18 April 2024
DOI : https://doi.org/10.1038/s41586-024-07219-0
Share this article
Anyone you share the following link with will be able to read this content:
Sorry, a shareable link is not currently available for this article.
Provided by the Springer Nature SharedIt content-sharing initiative
By submitting a comment you agree to abide by our Terms and Community Guidelines . If you find something abusive or that does not comply with our terms or guidelines please flag it as inappropriate.
Quick links
- Explore articles by subject
- Guide to authors
- Editorial policies
Sign up for the Nature Briefing newsletter — what matters in science, free to your inbox daily.

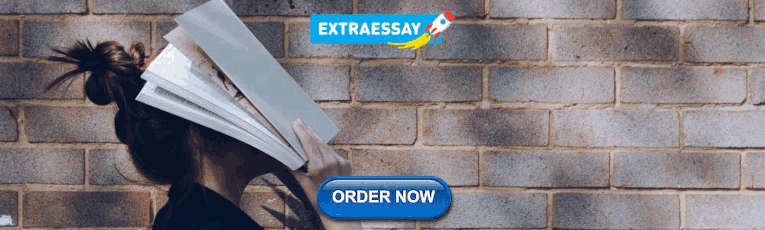
IMAGES
VIDEO
COMMENTS
Background and objectives: Despite reductions in prevalence in recent years, tobacco smoking remains one of the main preventable causes of ill-health and premature death worldwide.This paper reviews the extent and nature of harms caused by smoking, the benefits of stopping, patterns of smoking, psychological, pharmacological and social factors that contribute to uptake and maintenance of ...
We found a very strong and significant harmful relationship between pack-years of current smoking and the RR of lung cancer (Fig. 1b).The mean RR of lung cancer at 20 pack-years of smoking was 5. ...
This paper provides a broad overview of smoking in terms of: the health effects, ... has been the subject of by far the largest volume of research and is the most harmful form of tobacco use. Stopping smoking usually involves an intention not to smoke any more cigarettes ... Stopping smoking has different effects on different smoking-related ...
Abstract. Tobacco smoking is a major determinant of preventable morbidity and mortality worldwide. More than a billion people smoke, and without major increases in cessation, at least half will ...
We all know that smoking is bad for us, but now new research has revealed that it also has long lasting effects on our immune system even years after quitting. Darragh Duffy. Smoking has a strong ...
In their Article in The Lancet Public Health, Marissa Reitsma and colleagues1 report their comprehensive analysis of smoking tobacco use in young people from more than 3000 tobacco surveys from 204 countries and territories around the world. The result is an invaluable overview of an epidemic that causes millions of deaths every year. Their detailed mapping of the prevalence of smoking tobacco ...
Introduction. Despite a constant flow of messages reminding Americans of the health risks of cigarette smoking, and despite a steady decline in the proportion of Americans who smoke during the last 50 years, more than 20% of Americans continue to smoke regularly today [].This paper explores whether the continued prevalence of smoking may, in part, stem from a failure to acknowledge these risks.
Measuring self-reported experience of health and functioning is important for understanding the changes in the health status of individuals switching from cigarettes to less harmful tobacco and/or nicotine products (TNP) or reduced-risk products (RRP) and for supporting tobacco harm reduction strategies. This paper presents insights from three research activities from the preparatory phase of ...
Background The evidence on the effects of chronic tobacco smoking on neuropsychological functions is conflicting. The literature remains limited by inconsistent accounting for potentially confounding biomedical and psychiatric conditions. This study aimed to assess the neuropsychological functions of adult chronic tobacco smokers in comparison to group-matched non-smokers. Method The study ...
This is a comprehensive review on the harmful health effects of cigarette smoking. Tobacco smoking is a reprehensible habit that has spread all over the world as an epidemic. It reduces the life expectancy among smokers. It increases overall medical costs and contributes to the loss of productivity during the life span. Smoking has been shown to be linked with various neurological ...
estimated that smoking increases the risk of. coronary heart disease about 2-4 times, stroke 2-4 times, lung cancer 25 times in. men, and 25.7 times in women. Be sides, smoking can lead to an ...
Research on medicine from mangroves for the treatment of cancer has not only been shown to have an effect on cancer, but also provided important methods for the study of cancer therapy and mechanism. ... Other harmful effects of cigarette smoking. Cigarette smoking contributes to remarkable risk factors of noncommunicable diseases, including ...
Decades after its ill effects on human health were first documented, tobacco smoking remains one of the major global drivers of premature death and disability. In 2017, smoking was responsible for ...
Comparison of the degree of harmful effects documented from e-cigarette and conventional cigarette consumption. Human studies, in vivo mice exposure and in vitro studies. All of these effects from e-cigarettes were documented to be lower than those exerted by conventional cigarettes, which may suggest that e-cigarette consumption could be a safer option than conventional tobacco smoking but ...
Smoking causes stroke and coronary heart disease, which are among the leading causes of death in the United States. 1,3. Even people who smoke fewer than five cigarettes a day can have early signs of cardiovascular disease. 1. Smoking damages blood vessels and can make them thicken and grow narrower.
These carcinogens cause cancer and negatively affect human health. Lung, throat, brain, bladder, cervical cancer as well as other forms are caused by smoking. The symptoms are often detected at the time when the smoker's health condition is already chronic. Cancer is one of the leading causes of death world wide.
Despite a gradual decline in smoking rates over time, exposure to secondhand smoke (SHS) continues to cause harm to nonsmokers, who are disproportionately children and women living in low- and ...
Tobacco use is a global epidemic among young people. As with adults, it poses a serious health threat to youth and young adults in the United States and has significant implications for this nation's public and economic health in the future (Perry et al. 1994; Kessler 1995). The impact of cigarette smoking and other tobacco use on chronic disease, which accounts for 75% of American spending ...
How Pew Research Center will report on generations moving forward. Journalists, researchers and the public often look at society through the lens of generation, using terms like Millennial or Gen Z to describe groups of similarly aged people. This approach can help readers see themselves in the data and assess where we are and where we're ...
The acute hemodynamic effects of cigarette smoking or smokeless tobacco are mediated primarily by the sympathomimetic action. The intensity of its hemodynamic effect is greater with rapid nicotine delivery. Nicotine causes catecholamine release both locally and systemically leading to an increase in heart rate, blood pressure and cardiac ...
Starting from a panel-based fixed-effects distributed lag model estimating the effects of climate on economic growth using the real historical data (as in equation ) with ten lags for all climate ...