6.2 Correlational Research
Learning objectives.
- Define correlational research and give several examples.
- Explain why a researcher might choose to conduct correlational research rather than experimental research or another type of non-experimental research.
- Interpret the strength and direction of different correlation coefficients.
- Explain why correlation does not imply causation.
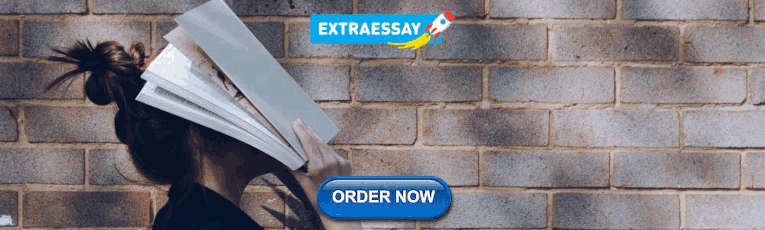
What Is Correlational Research?
Correlational research is a type of non-experimental research in which the researcher measures two variables and assesses the statistical relationship (i.e., the correlation) between them with little or no effort to control extraneous variables. There are many reasons that researchers interested in statistical relationships between variables would choose to conduct a correlational study rather than an experiment. The first is that they do not believe that the statistical relationship is a causal one or are not interested in causal relationships. Recall two goals of science are to describe and to predict and the correlational research strategy allows researchers to achieve both of these goals. Specifically, this strategy can be used to describe the strength and direction of the relationship between two variables and if there is a relationship between the variables then the researchers can use scores on one variable to predict scores on the other (using a statistical technique called regression).
Another reason that researchers would choose to use a correlational study rather than an experiment is that the statistical relationship of interest is thought to be causal, but the researcher cannot manipulate the independent variable because it is impossible, impractical, or unethical. For example, while I might be interested in the relationship between the frequency people use cannabis and their memory abilities I cannot ethically manipulate the frequency that people use cannabis. As such, I must rely on the correlational research strategy; I must simply measure the frequency that people use cannabis and measure their memory abilities using a standardized test of memory and then determine whether the frequency people use cannabis use is statistically related to memory test performance.
Correlation is also used to establish the reliability and validity of measurements. For example, a researcher might evaluate the validity of a brief extraversion test by administering it to a large group of participants along with a longer extraversion test that has already been shown to be valid. This researcher might then check to see whether participants’ scores on the brief test are strongly correlated with their scores on the longer one. Neither test score is thought to cause the other, so there is no independent variable to manipulate. In fact, the terms independent variable and dependent variabl e do not apply to this kind of research.
Another strength of correlational research is that it is often higher in external validity than experimental research. Recall there is typically a trade-off between internal validity and external validity. As greater controls are added to experiments, internal validity is increased but often at the expense of external validity. In contrast, correlational studies typically have low internal validity because nothing is manipulated or control but they often have high external validity. Since nothing is manipulated or controlled by the experimenter the results are more likely to reflect relationships that exist in the real world.
Finally, extending upon this trade-off between internal and external validity, correlational research can help to provide converging evidence for a theory. If a theory is supported by a true experiment that is high in internal validity as well as by a correlational study that is high in external validity then the researchers can have more confidence in the validity of their theory. As a concrete example, correlational studies establishing that there is a relationship between watching violent television and aggressive behavior have been complemented by experimental studies confirming that the relationship is a causal one (Bushman & Huesmann, 2001) [1] . These converging results provide strong evidence that there is a real relationship (indeed a causal relationship) between watching violent television and aggressive behavior.
Data Collection in Correlational Research
Again, the defining feature of correlational research is that neither variable is manipulated. It does not matter how or where the variables are measured. A researcher could have participants come to a laboratory to complete a computerized backward digit span task and a computerized risky decision-making task and then assess the relationship between participants’ scores on the two tasks. Or a researcher could go to a shopping mall to ask people about their attitudes toward the environment and their shopping habits and then assess the relationship between these two variables. Both of these studies would be correlational because no independent variable is manipulated.
Correlations Between Quantitative Variables
Correlations between quantitative variables are often presented using scatterplots . Figure 6.3 shows some hypothetical data on the relationship between the amount of stress people are under and the number of physical symptoms they have. Each point in the scatterplot represents one person’s score on both variables. For example, the circled point in Figure 6.3 represents a person whose stress score was 10 and who had three physical symptoms. Taking all the points into account, one can see that people under more stress tend to have more physical symptoms. This is a good example of a positive relationship , in which higher scores on one variable tend to be associated with higher scores on the other. A negative relationship is one in which higher scores on one variable tend to be associated with lower scores on the other. There is a negative relationship between stress and immune system functioning, for example, because higher stress is associated with lower immune system functioning.

Figure 6.3 Scatterplot Showing a Hypothetical Positive Relationship Between Stress and Number of Physical Symptoms. The circled point represents a person whose stress score was 10 and who had three physical symptoms. Pearson’s r for these data is +.51.
The strength of a correlation between quantitative variables is typically measured using a statistic called Pearson’s Correlation Coefficient (or Pearson’s r ) . As Figure 6.4 shows, Pearson’s r ranges from −1.00 (the strongest possible negative relationship) to +1.00 (the strongest possible positive relationship). A value of 0 means there is no relationship between the two variables. When Pearson’s r is 0, the points on a scatterplot form a shapeless “cloud.” As its value moves toward −1.00 or +1.00, the points come closer and closer to falling on a single straight line. Correlation coefficients near ±.10 are considered small, values near ± .30 are considered medium, and values near ±.50 are considered large. Notice that the sign of Pearson’s r is unrelated to its strength. Pearson’s r values of +.30 and −.30, for example, are equally strong; it is just that one represents a moderate positive relationship and the other a moderate negative relationship. With the exception of reliability coefficients, most correlations that we find in Psychology are small or moderate in size. The website http://rpsychologist.com/d3/correlation/ , created by Kristoffer Magnusson, provides an excellent interactive visualization of correlations that permits you to adjust the strength and direction of a correlation while witnessing the corresponding changes to the scatterplot.

Figure 6.4 Range of Pearson’s r, From −1.00 (Strongest Possible Negative Relationship), Through 0 (No Relationship), to +1.00 (Strongest Possible Positive Relationship)
There are two common situations in which the value of Pearson’s r can be misleading. Pearson’s r is a good measure only for linear relationships, in which the points are best approximated by a straight line. It is not a good measure for nonlinear relationships, in which the points are better approximated by a curved line. Figure 6.5, for example, shows a hypothetical relationship between the amount of sleep people get per night and their level of depression. In this example, the line that best approximates the points is a curve—a kind of upside-down “U”—because people who get about eight hours of sleep tend to be the least depressed. Those who get too little sleep and those who get too much sleep tend to be more depressed. Even though Figure 6.5 shows a fairly strong relationship between depression and sleep, Pearson’s r would be close to zero because the points in the scatterplot are not well fit by a single straight line. This means that it is important to make a scatterplot and confirm that a relationship is approximately linear before using Pearson’s r . Nonlinear relationships are fairly common in psychology, but measuring their strength is beyond the scope of this book.

Figure 6.5 Hypothetical Nonlinear Relationship Between Sleep and Depression
The other common situations in which the value of Pearson’s r can be misleading is when one or both of the variables have a limited range in the sample relative to the population. This problem is referred to as restriction of range . Assume, for example, that there is a strong negative correlation between people’s age and their enjoyment of hip hop music as shown by the scatterplot in Figure 6.6. Pearson’s r here is −.77. However, if we were to collect data only from 18- to 24-year-olds—represented by the shaded area of Figure 6.6—then the relationship would seem to be quite weak. In fact, Pearson’s r for this restricted range of ages is 0. It is a good idea, therefore, to design studies to avoid restriction of range. For example, if age is one of your primary variables, then you can plan to collect data from people of a wide range of ages. Because restriction of range is not always anticipated or easily avoidable, however, it is good practice to examine your data for possible restriction of range and to interpret Pearson’s r in light of it. (There are also statistical methods to correct Pearson’s r for restriction of range, but they are beyond the scope of this book).

Figure 6.6 Hypothetical Data Showing How a Strong Overall Correlation Can Appear to Be Weak When One Variable Has a Restricted Range.The overall correlation here is −.77, but the correlation for the 18- to 24-year-olds (in the blue box) is 0.
Correlation Does Not Imply Causation
You have probably heard repeatedly that “Correlation does not imply causation.” An amusing example of this comes from a 2012 study that showed a positive correlation (Pearson’s r = 0.79) between the per capita chocolate consumption of a nation and the number of Nobel prizes awarded to citizens of that nation [2] . It seems clear, however, that this does not mean that eating chocolate causes people to win Nobel prizes, and it would not make sense to try to increase the number of Nobel prizes won by recommending that parents feed their children more chocolate.
There are two reasons that correlation does not imply causation. The first is called the directionality problem . Two variables, X and Y , can be statistically related because X causes Y or because Y causes X . Consider, for example, a study showing that whether or not people exercise is statistically related to how happy they are—such that people who exercise are happier on average than people who do not. This statistical relationship is consistent with the idea that exercising causes happiness, but it is also consistent with the idea that happiness causes exercise. Perhaps being happy gives people more energy or leads them to seek opportunities to socialize with others by going to the gym. The second reason that correlation does not imply causation is called the third-variable problem . Two variables, X and Y , can be statistically related not because X causes Y , or because Y causes X , but because some third variable, Z , causes both X and Y . For example, the fact that nations that have won more Nobel prizes tend to have higher chocolate consumption probably reflects geography in that European countries tend to have higher rates of per capita chocolate consumption and invest more in education and technology (once again, per capita) than many other countries in the world. Similarly, the statistical relationship between exercise and happiness could mean that some third variable, such as physical health, causes both of the others. Being physically healthy could cause people to exercise and cause them to be happier. Correlations that are a result of a third-variable are often referred to as spurious correlations.
Some excellent and funny examples of spurious correlations can be found at http://www.tylervigen.com (Figure 6.7 provides one such example).

“Lots of Candy Could Lead to Violence”
Although researchers in psychology know that correlation does not imply causation, many journalists do not. One website about correlation and causation, http://jonathan.mueller.faculty.noctrl.edu/100/correlation_or_causation.htm , links to dozens of media reports about real biomedical and psychological research. Many of the headlines suggest that a causal relationship has been demonstrated when a careful reading of the articles shows that it has not because of the directionality and third-variable problems.
One such article is about a study showing that children who ate candy every day were more likely than other children to be arrested for a violent offense later in life. But could candy really “lead to” violence, as the headline suggests? What alternative explanations can you think of for this statistical relationship? How could the headline be rewritten so that it is not misleading?
As you have learned by reading this book, there are various ways that researchers address the directionality and third-variable problems. The most effective is to conduct an experiment. For example, instead of simply measuring how much people exercise, a researcher could bring people into a laboratory and randomly assign half of them to run on a treadmill for 15 minutes and the rest to sit on a couch for 15 minutes. Although this seems like a minor change to the research design, it is extremely important. Now if the exercisers end up in more positive moods than those who did not exercise, it cannot be because their moods affected how much they exercised (because it was the researcher who determined how much they exercised). Likewise, it cannot be because some third variable (e.g., physical health) affected both how much they exercised and what mood they were in (because, again, it was the researcher who determined how much they exercised). Thus experiments eliminate the directionality and third-variable problems and allow researchers to draw firm conclusions about causal relationships.
Key Takeaways
- Correlational research involves measuring two variables and assessing the relationship between them, with no manipulation of an independent variable.
- Correlation does not imply causation. A statistical relationship between two variables, X and Y , does not necessarily mean that X causes Y . It is also possible that Y causes X , or that a third variable, Z , causes both X and Y .
- While correlational research cannot be used to establish causal relationships between variables, correlational research does allow researchers to achieve many other important objectives (establishing reliability and validity, providing converging evidence, describing relationships and making predictions)
- Correlation coefficients can range from -1 to +1. The sign indicates the direction of the relationship between the variables and the numerical value indicates the strength of the relationship.
- A cognitive psychologist compares the ability of people to recall words that they were instructed to “read” with their ability to recall words that they were instructed to “imagine.”
- A manager studies the correlation between new employees’ college grade point averages and their first-year performance reports.
- An automotive engineer installs different stick shifts in a new car prototype, each time asking several people to rate how comfortable the stick shift feels.
- A food scientist studies the relationship between the temperature inside people’s refrigerators and the amount of bacteria on their food.
- A social psychologist tells some research participants that they need to hurry over to the next building to complete a study. She tells others that they can take their time. Then she observes whether they stop to help a research assistant who is pretending to be hurt.
2. Practice: For each of the following statistical relationships, decide whether the directionality problem is present and think of at least one plausible third variable.
- People who eat more lobster tend to live longer.
- People who exercise more tend to weigh less.
- College students who drink more alcohol tend to have poorer grades.
- Bushman, B. J., & Huesmann, L. R. (2001). Effects of televised violence on aggression. In D. Singer & J. Singer (Eds.), Handbook of children and the media (pp. 223–254). Thousand Oaks, CA: Sage. ↵
- Messerli, F. H. (2012). Chocolate consumption, cognitive function, and Nobel laureates. New England Journal of Medicine, 367 , 1562-1564. ↵

Share This Book
- Increase Font Size

Want to create or adapt books like this? Learn more about how Pressbooks supports open publishing practices.
7.2 Correlational Research
Learning objectives.
- Define correlational research and give several examples.
- Explain why a researcher might choose to conduct correlational research rather than experimental research or another type of nonexperimental research.
What Is Correlational Research?
Correlational research is a type of nonexperimental research in which the researcher measures two variables and assesses the statistical relationship (i.e., the correlation) between them with little or no effort to control extraneous variables. There are essentially two reasons that researchers interested in statistical relationships between variables would choose to conduct a correlational study rather than an experiment. The first is that they do not believe that the statistical relationship is a causal one. For example, a researcher might evaluate the validity of a brief extraversion test by administering it to a large group of participants along with a longer extraversion test that has already been shown to be valid. This researcher might then check to see whether participants’ scores on the brief test are strongly correlated with their scores on the longer one. Neither test score is thought to cause the other, so there is no independent variable to manipulate. In fact, the terms independent variable and dependent variable do not apply to this kind of research.
The other reason that researchers would choose to use a correlational study rather than an experiment is that the statistical relationship of interest is thought to be causal, but the researcher cannot manipulate the independent variable because it is impossible, impractical, or unethical. For example, Allen Kanner and his colleagues thought that the number of “daily hassles” (e.g., rude salespeople, heavy traffic) that people experience affects the number of physical and psychological symptoms they have (Kanner, Coyne, Schaefer, & Lazarus, 1981). But because they could not manipulate the number of daily hassles their participants experienced, they had to settle for measuring the number of daily hassles—along with the number of symptoms—using self-report questionnaires. Although the strong positive relationship they found between these two variables is consistent with their idea that hassles cause symptoms, it is also consistent with the idea that symptoms cause hassles or that some third variable (e.g., neuroticism) causes both.
A common misconception among beginning researchers is that correlational research must involve two quantitative variables, such as scores on two extraversion tests or the number of hassles and number of symptoms people have experienced. However, the defining feature of correlational research is that the two variables are measured—neither one is manipulated—and this is true regardless of whether the variables are quantitative or categorical. Imagine, for example, that a researcher administers the Rosenberg Self-Esteem Scale to 50 American college students and 50 Japanese college students. Although this “feels” like a between-subjects experiment, it is a correlational study because the researcher did not manipulate the students’ nationalities. The same is true of the study by Cacioppo and Petty comparing college faculty and factory workers in terms of their need for cognition. It is a correlational study because the researchers did not manipulate the participants’ occupations.
Figure 7.2 “Results of a Hypothetical Study on Whether People Who Make Daily To-Do Lists Experience Less Stress Than People Who Do Not Make Such Lists” shows data from a hypothetical study on the relationship between whether people make a daily list of things to do (a “to-do list”) and stress. Notice that it is unclear whether this is an experiment or a correlational study because it is unclear whether the independent variable was manipulated. If the researcher randomly assigned some participants to make daily to-do lists and others not to, then it is an experiment. If the researcher simply asked participants whether they made daily to-do lists, then it is a correlational study. The distinction is important because if the study was an experiment, then it could be concluded that making the daily to-do lists reduced participants’ stress. But if it was a correlational study, it could only be concluded that these variables are statistically related. Perhaps being stressed has a negative effect on people’s ability to plan ahead (the directionality problem). Or perhaps people who are more conscientious are more likely to make to-do lists and less likely to be stressed (the third-variable problem). The crucial point is that what defines a study as experimental or correlational is not the variables being studied, nor whether the variables are quantitative or categorical, nor the type of graph or statistics used to analyze the data. It is how the study is conducted.
Figure 7.2 Results of a Hypothetical Study on Whether People Who Make Daily To-Do Lists Experience Less Stress Than People Who Do Not Make Such Lists

Data Collection in Correlational Research
Again, the defining feature of correlational research is that neither variable is manipulated. It does not matter how or where the variables are measured. A researcher could have participants come to a laboratory to complete a computerized backward digit span task and a computerized risky decision-making task and then assess the relationship between participants’ scores on the two tasks. Or a researcher could go to a shopping mall to ask people about their attitudes toward the environment and their shopping habits and then assess the relationship between these two variables. Both of these studies would be correlational because no independent variable is manipulated. However, because some approaches to data collection are strongly associated with correlational research, it makes sense to discuss them here. The two we will focus on are naturalistic observation and archival data. A third, survey research, is discussed in its own chapter.
Naturalistic Observation
Naturalistic observation is an approach to data collection that involves observing people’s behavior in the environment in which it typically occurs. Thus naturalistic observation is a type of field research (as opposed to a type of laboratory research). It could involve observing shoppers in a grocery store, children on a school playground, or psychiatric inpatients in their wards. Researchers engaged in naturalistic observation usually make their observations as unobtrusively as possible so that participants are often not aware that they are being studied. Ethically, this is considered to be acceptable if the participants remain anonymous and the behavior occurs in a public setting where people would not normally have an expectation of privacy. Grocery shoppers putting items into their shopping carts, for example, are engaged in public behavior that is easily observable by store employees and other shoppers. For this reason, most researchers would consider it ethically acceptable to observe them for a study. On the other hand, one of the arguments against the ethicality of the naturalistic observation of “bathroom behavior” discussed earlier in the book is that people have a reasonable expectation of privacy even in a public restroom and that this expectation was violated.
Researchers Robert Levine and Ara Norenzayan used naturalistic observation to study differences in the “pace of life” across countries (Levine & Norenzayan, 1999). One of their measures involved observing pedestrians in a large city to see how long it took them to walk 60 feet. They found that people in some countries walked reliably faster than people in other countries. For example, people in the United States and Japan covered 60 feet in about 12 seconds on average, while people in Brazil and Romania took close to 17 seconds.
Because naturalistic observation takes place in the complex and even chaotic “real world,” there are two closely related issues that researchers must deal with before collecting data. The first is sampling. When, where, and under what conditions will the observations be made, and who exactly will be observed? Levine and Norenzayan described their sampling process as follows:
Male and female walking speed over a distance of 60 feet was measured in at least two locations in main downtown areas in each city. Measurements were taken during main business hours on clear summer days. All locations were flat, unobstructed, had broad sidewalks, and were sufficiently uncrowded to allow pedestrians to move at potentially maximum speeds. To control for the effects of socializing, only pedestrians walking alone were used. Children, individuals with obvious physical handicaps, and window-shoppers were not timed. Thirty-five men and 35 women were timed in most cities. (p. 186)
Precise specification of the sampling process in this way makes data collection manageable for the observers, and it also provides some control over important extraneous variables. For example, by making their observations on clear summer days in all countries, Levine and Norenzayan controlled for effects of the weather on people’s walking speeds.
The second issue is measurement. What specific behaviors will be observed? In Levine and Norenzayan’s study, measurement was relatively straightforward. They simply measured out a 60-foot distance along a city sidewalk and then used a stopwatch to time participants as they walked over that distance. Often, however, the behaviors of interest are not so obvious or objective. For example, researchers Robert Kraut and Robert Johnston wanted to study bowlers’ reactions to their shots, both when they were facing the pins and then when they turned toward their companions (Kraut & Johnston, 1979). But what “reactions” should they observe? Based on previous research and their own pilot testing, Kraut and Johnston created a list of reactions that included “closed smile,” “open smile,” “laugh,” “neutral face,” “look down,” “look away,” and “face cover” (covering one’s face with one’s hands). The observers committed this list to memory and then practiced by coding the reactions of bowlers who had been videotaped. During the actual study, the observers spoke into an audio recorder, describing the reactions they observed. Among the most interesting results of this study was that bowlers rarely smiled while they still faced the pins. They were much more likely to smile after they turned toward their companions, suggesting that smiling is not purely an expression of happiness but also a form of social communication.
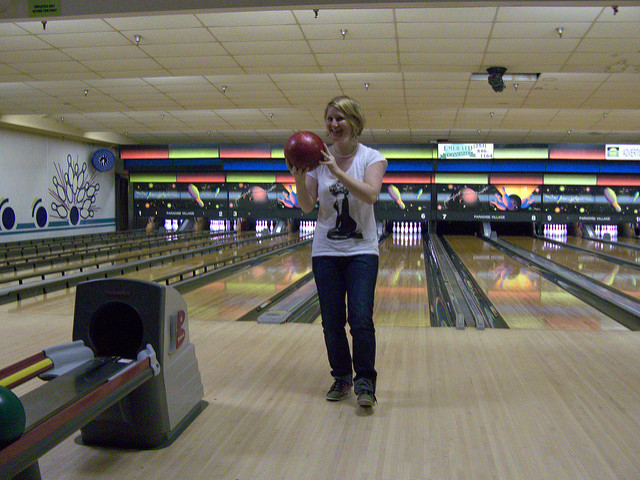
Naturalistic observation has revealed that bowlers tend to smile when they turn away from the pins and toward their companions, suggesting that smiling is not purely an expression of happiness but also a form of social communication.
sieneke toering – bowling big lebowski style – CC BY-NC-ND 2.0.
When the observations require a judgment on the part of the observers—as in Kraut and Johnston’s study—this process is often described as coding . Coding generally requires clearly defining a set of target behaviors. The observers then categorize participants individually in terms of which behavior they have engaged in and the number of times they engaged in each behavior. The observers might even record the duration of each behavior. The target behaviors must be defined in such a way that different observers code them in the same way. This is the issue of interrater reliability. Researchers are expected to demonstrate the interrater reliability of their coding procedure by having multiple raters code the same behaviors independently and then showing that the different observers are in close agreement. Kraut and Johnston, for example, video recorded a subset of their participants’ reactions and had two observers independently code them. The two observers showed that they agreed on the reactions that were exhibited 97% of the time, indicating good interrater reliability.
Archival Data
Another approach to correlational research is the use of archival data , which are data that have already been collected for some other purpose. An example is a study by Brett Pelham and his colleagues on “implicit egotism”—the tendency for people to prefer people, places, and things that are similar to themselves (Pelham, Carvallo, & Jones, 2005). In one study, they examined Social Security records to show that women with the names Virginia, Georgia, Louise, and Florence were especially likely to have moved to the states of Virginia, Georgia, Louisiana, and Florida, respectively.
As with naturalistic observation, measurement can be more or less straightforward when working with archival data. For example, counting the number of people named Virginia who live in various states based on Social Security records is relatively straightforward. But consider a study by Christopher Peterson and his colleagues on the relationship between optimism and health using data that had been collected many years before for a study on adult development (Peterson, Seligman, & Vaillant, 1988). In the 1940s, healthy male college students had completed an open-ended questionnaire about difficult wartime experiences. In the late 1980s, Peterson and his colleagues reviewed the men’s questionnaire responses to obtain a measure of explanatory style—their habitual ways of explaining bad events that happen to them. More pessimistic people tend to blame themselves and expect long-term negative consequences that affect many aspects of their lives, while more optimistic people tend to blame outside forces and expect limited negative consequences. To obtain a measure of explanatory style for each participant, the researchers used a procedure in which all negative events mentioned in the questionnaire responses, and any causal explanations for them, were identified and written on index cards. These were given to a separate group of raters who rated each explanation in terms of three separate dimensions of optimism-pessimism. These ratings were then averaged to produce an explanatory style score for each participant. The researchers then assessed the statistical relationship between the men’s explanatory style as college students and archival measures of their health at approximately 60 years of age. The primary result was that the more optimistic the men were as college students, the healthier they were as older men. Pearson’s r was +.25.
This is an example of content analysis —a family of systematic approaches to measurement using complex archival data. Just as naturalistic observation requires specifying the behaviors of interest and then noting them as they occur, content analysis requires specifying keywords, phrases, or ideas and then finding all occurrences of them in the data. These occurrences can then be counted, timed (e.g., the amount of time devoted to entertainment topics on the nightly news show), or analyzed in a variety of other ways.
Key Takeaways
- Correlational research involves measuring two variables and assessing the relationship between them, with no manipulation of an independent variable.
- Correlational research is not defined by where or how the data are collected. However, some approaches to data collection are strongly associated with correlational research. These include naturalistic observation (in which researchers observe people’s behavior in the context in which it normally occurs) and the use of archival data that were already collected for some other purpose.
Discussion: For each of the following, decide whether it is most likely that the study described is experimental or correlational and explain why.
- An educational researcher compares the academic performance of students from the “rich” side of town with that of students from the “poor” side of town.
- A cognitive psychologist compares the ability of people to recall words that they were instructed to “read” with their ability to recall words that they were instructed to “imagine.”
- A manager studies the correlation between new employees’ college grade point averages and their first-year performance reports.
- An automotive engineer installs different stick shifts in a new car prototype, each time asking several people to rate how comfortable the stick shift feels.
- A food scientist studies the relationship between the temperature inside people’s refrigerators and the amount of bacteria on their food.
- A social psychologist tells some research participants that they need to hurry over to the next building to complete a study. She tells others that they can take their time. Then she observes whether they stop to help a research assistant who is pretending to be hurt.
Kanner, A. D., Coyne, J. C., Schaefer, C., & Lazarus, R. S. (1981). Comparison of two modes of stress measurement: Daily hassles and uplifts versus major life events. Journal of Behavioral Medicine, 4 , 1–39.
Kraut, R. E., & Johnston, R. E. (1979). Social and emotional messages of smiling: An ethological approach. Journal of Personality and Social Psychology, 37 , 1539–1553.
Levine, R. V., & Norenzayan, A. (1999). The pace of life in 31 countries. Journal of Cross-Cultural Psychology, 30 , 178–205.
Pelham, B. W., Carvallo, M., & Jones, J. T. (2005). Implicit egotism. Current Directions in Psychological Science, 14 , 106–110.
Peterson, C., Seligman, M. E. P., & Vaillant, G. E. (1988). Pessimistic explanatory style is a risk factor for physical illness: A thirty-five year longitudinal study. Journal of Personality and Social Psychology, 55 , 23–27.
Research Methods in Psychology Copyright © 2016 by University of Minnesota is licensed under a Creative Commons Attribution-NonCommercial-ShareAlike 4.0 International License , except where otherwise noted.
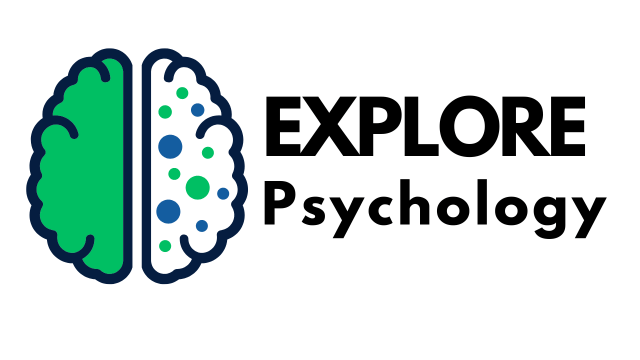
Correlational Research in Psychology: Definition and How It Works
Categories Research Methods
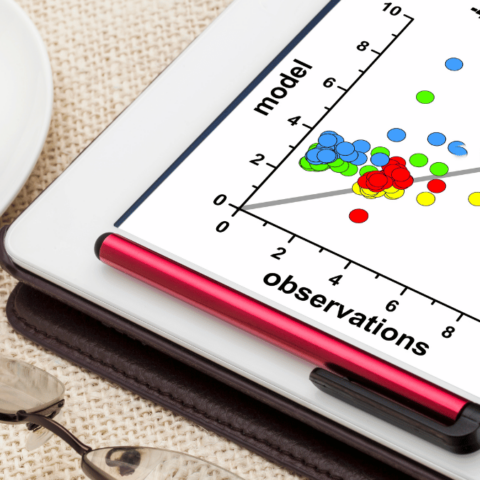
Sharing is caring!
Correlational research is a type of scientific investigation in which a researcher looks at the relationships between variables but does not vary, manipulate, or control them. It can be a useful research method for evaluating the direction and strength of the relationship between two or more different variables.
When examining how variables are related to one another, researchers may find that the relationship is positive or negative. Or they may also find that there is no relationship at all.
Table of Contents
How Does Correlational Research Work?
In correlational research, the researcher measures the values of the variables of interest and calculates a correlation coefficient, which quantifies the strength and direction of the relationship between the variables.
The correlation coefficient ranges from -1.0 to +1.0, where -1.0 represents a perfect negative correlation, 0 represents no correlation, and +1.0 represents a perfect positive correlation.
A negative correlation indicates that as the value of one variable increases, the value of the other variable decreases, while a positive correlation indicates that as the value of one variable increases, the value of the other variable also increases. A zero correlation indicates that there is no relationship between the variables.
Correlational Research vs. Experimental Research
Correlational research differs from experimental research in that it does not involve manipulating variables. Instead, it focuses on analyzing the relationship between two or more variables.
In other words, correlational research seeks to determine whether there is a relationship between two variables and, if so, the nature of that relationship.
Experimental research, on the other hand, involves manipulating one or more variables to determine the effect on another variable. Because of this manipulation and control of variables, experimental research allows for causal conclusions to be drawn, while correlational research does not.
Both types of research are important in understanding the world around us, but they serve different purposes and are used in different situations.
Types of Correlational Research
There are three main types of correlational studies:
Cohort Correlational Study
This type of study involves following a cohort of participants over a period of time. This type of research can be useful for understanding how certain events might influence outcomes.
For example, researchers might study how exposure to a traumatic natural disaster influences the mental health of a group of people over time.
By examining the data collected from these individuals, researchers can determine whether there is a correlation between the two variables under investigation. This information can be used to develop strategies for preventing or treating certain conditions or illnesses.
Cross-Sectional Correlational Study
A cross-sectional design is a research method that examines a group of individuals at a single time. This type of study collects information from a diverse group of people, usually from different backgrounds and age groups, to gain insight into a particular phenomenon or issue.
The data collected from this type of study is used to analyze relationships between variables and identify patterns and trends within the group.
Cross-sectional studies can help identify potential risk factors for certain conditions or illnesses, and can also be used to evaluate the prevalence of certain behaviors, attitudes, or beliefs within a population.
Case-Control Correlational Study
A case-control correlational study is a type of research design that investigates the relationship between exposure and health outcomes. In this study, researchers identify a group of individuals with the health outcome of interest (cases) and another group of individuals without the health outcome (controls).
The researchers then compare the exposure history of the cases and controls to determine whether the exposure and health outcome correlate.
This type of study design is often used in epidemiology and can provide valuable information about potential risk factors for a particular disease or condition.
When to Use Correlational Research
There are a number of situations where researchers might opt to use a correlational study instead of some other research design.
Correlational research can be used to investigate a wide range of psychological phenomena, including the relationship between personality traits and academic performance, the association between sleep duration and mental health, and the correlation between parental involvement and child outcomes.
To Generate Hypotheses
Correlational research can also be used to generate hypotheses for further research by identifying variables that are associated with each other.
To Investigate Variables Without Manipulating Them
Researchers should use correlational research when they want to investigate the relationship between two variables without manipulating them. This type of research is useful when the researcher cannot or should not manipulate one of the variables or when it is impossible to conduct an experiment due to ethical or practical concerns.
To Identify Patterns
Correlational research allows researchers to identify patterns and relationships between variables, which can inform future research and help to develop theories. However, it is important to note that correlational research does not prove that one variable causes changes in the other.
While correlational research has its limitations, it is still a valuable tool for researchers in many fields, including psychology, sociology, and education.
How to Collect Data in Correlational Research
Researchers can collect data for correlational research in a few different ways. To conduct correlational research, data can be collected using the following:
- Surveys : One method is through surveys, where participants are asked to self-report their behaviors or attitudes. This approach allows researchers to gather large amounts of data quickly and affordably.
- Naturalistic observation : Another method is through observation, where researchers observe and record behaviors in a natural or controlled setting. This method allows researchers to learn more about the behavior in question and better generalize the results to real-world settings.
- Archival, retrospective data : Additionally, researchers can collect data from archival sources, such as medical, school records, official records, or past polls.
The key is to collect data from a large and representative sample to measure the relationship between two variables accurately.
Pros and Cons of Correlational Research
There are some advantages of using correlational research, but there are also some downsides to consider.
- One of the strengths of correlational research is its ability to identify patterns and relationships between variables that may be difficult or unethical to manipulate in an experimental study.
- Correlational research can also be used to examine variables that are not under the control of the researcher , such as age, gender, or socioeconomic status.
- Correlational research can be used to make predictions about future behavior or outcomes, which can be valuable in a variety of fields.
- Correlational research can be conducted quickly and inexpensively , making it a practical option for researchers with limited resources.
- Correlational research is limited by its inability to establish causality between variables. Correlation does not imply causation, and it is possible that a third variable may be influencing both variables of interest, creating a spurious correlation. Therefore, it is important for researchers to use multiple methods of data collection and to be cautious when interpreting correlational findings.
- Correlational research relies heavily on self-reported data , which can be biased or inaccurate.
- Correlational research is limited in its ability to generalize findings to larger populations, as it only measures the relationship between two variables in a specific sample.
Frequently Asked Questions About Correlational Research
What are the main problems with correlational research.
Some of the main problems that can occur in correlational research include selection bias, confounding variables. and misclassification.
- Selecting participants based on their exposure to an event means that the sample might be biased since the selection was not randomized.
- Correlational studies may also be impacted by extraneous factors that researchers cannot control.
- Finally, there may be problems with how accurately data is recorded and classified, which can be particularly problematic in retrospective studies.
What are the variables in a correlational study?
In a correlational study, variables refer to any measurable factors being examined for their potential relationship or association with each other. These variables can be continuous (meaning they can take on a range of values) or categorical (meaning they fall into distinct categories or groups).
For example, in a study examining the correlation between exercise and mental health, the independent variable would be exercise frequency (measured in times per week), while the dependent variable would be mental health (measured using a standardized questionnaire).
What is the goal of correlational research?
The goal of correlational research is to examine the relationship between two or more variables. It involves analyzing data to determine if there is a statistically significant connection between the variables being studied.
Correlational research is useful for identifying patterns and making predictions but cannot establish causation. Instead, it helps researchers to better understand the nature of the relationship between variables and to generate hypotheses for further investigation.
How do you identify correlational research?
To identify correlational research, look for studies that measure two or more variables and analyze their relationship using statistical techniques. The results of correlational studies are typically presented in the form of correlation coefficients or scatterplots, which visually represent the degree of association between the variables being studied.
Correlational research can be useful for identifying potential causal relationships between variables but cannot establish causation on its own.
Curtis EA, Comiskey C, Dempsey O. Importance and use of correlational research . Nurse Researcher . 2016;23(6):20-25. doi10.7748/nr.2016.e1382
Lau F. Chapter 12 Methods for Correlational Studies . University of Victoria; 2017.
Mitchell TR. An evaluation of the validity of correlational research conducted in organizations . The Academy of Management Review . 1985;10(2):192. doi:10.5465/amr.1985.4277939
Seeram E. An overview of correlational research . Radiol Technol . 2019;91(2):176-179.
- Bipolar Disorder
- Therapy Center
- When To See a Therapist
- Types of Therapy
- Best Online Therapy
- Best Couples Therapy
- Best Family Therapy
- Managing Stress
- Sleep and Dreaming
- Understanding Emotions
- Self-Improvement
- Healthy Relationships
- Student Resources
- Personality Types
- Guided Meditations
- Verywell Mind Insights
- 2023 Verywell Mind 25
- Mental Health in the Classroom
- Editorial Process
- Meet Our Review Board
- Crisis Support
Correlation Studies in Psychology Research
Determining the relationship between two or more variables.
Kendra Cherry, MS, is a psychosocial rehabilitation specialist, psychology educator, and author of the "Everything Psychology Book."
:max_bytes(150000):strip_icc():format(webp)/IMG_9791-89504ab694d54b66bbd72cb84ffb860e.jpg)
Emily is a board-certified science editor who has worked with top digital publishing brands like Voices for Biodiversity, Study.com, GoodTherapy, Vox, and Verywell.
:max_bytes(150000):strip_icc():format(webp)/Emily-Swaim-1000-0f3197de18f74329aeffb690a177160c.jpg)
Verywell / Brianna Gilmartin
- Characteristics
Potential Pitfalls
Frequently asked questions.
A correlational study is a type of research design that looks at the relationships between two or more variables. Correlational studies are non-experimental, which means that the experimenter does not manipulate or control any of the variables.
A correlation refers to a relationship between two variables. Correlations can be strong or weak and positive or negative. Sometimes, there is no correlation.
There are three possible outcomes of a correlation study: a positive correlation, a negative correlation, or no correlation. Researchers can present the results using a numerical value called the correlation coefficient, a measure of the correlation strength. It can range from –1.00 (negative) to +1.00 (positive). A correlation coefficient of 0 indicates no correlation.
- Positive correlations : Both variables increase or decrease at the same time. A correlation coefficient close to +1.00 indicates a strong positive correlation.
- Negative correlations : As the amount of one variable increases, the other decreases (and vice versa). A correlation coefficient close to -1.00 indicates a strong negative correlation.
- No correlation : There is no relationship between the two variables. A correlation coefficient of 0 indicates no correlation.
Characteristics of a Correlational Study
Correlational studies are often used in psychology, as well as other fields like medicine. Correlational research is a preliminary way to gather information about a topic. The method is also useful if researchers are unable to perform an experiment.
Researchers use correlations to see if a relationship between two or more variables exists, but the variables themselves are not under the control of the researchers.
While correlational research can demonstrate a relationship between variables, it cannot prove that changing one variable will change another. In other words, correlational studies cannot prove cause-and-effect relationships.
When you encounter research that refers to a "link" or an "association" between two things, they are most likely talking about a correlational study.
Types of Correlational Research
There are three types of correlational research: naturalistic observation, the survey method, and archival research. Each type has its own purpose, as well as its pros and cons.
Naturalistic Observation
The naturalistic observation method involves observing and recording variables of interest in a natural setting without interference or manipulation.
Can inspire ideas for further research
Option if lab experiment not available
Variables are viewed in natural setting
Can be time-consuming and expensive
Extraneous variables can't be controlled
No scientific control of variables
Subjects might behave differently if aware of being observed
This method is well-suited to studies where researchers want to see how variables behave in their natural setting or state. Inspiration can then be drawn from the observations to inform future avenues of research.
In some cases, it might be the only method available to researchers; for example, if lab experimentation would be precluded by access, resources, or ethics. It might be preferable to not being able to conduct research at all, but the method can be costly and usually takes a lot of time.
Naturalistic observation presents several challenges for researchers. For one, it does not allow them to control or influence the variables in any way nor can they change any possible external variables.
However, this does not mean that researchers will get reliable data from watching the variables, or that the information they gather will be free from bias.
For example, study subjects might act differently if they know that they are being watched. The researchers might not be aware that the behavior that they are observing is not necessarily the subject's natural state (i.e., how they would act if they did not know they were being watched).
Researchers also need to be aware of their biases, which can affect the observation and interpretation of a subject's behavior.
Surveys and questionnaires are some of the most common methods used for psychological research. The survey method involves having a random sample of participants complete a survey, test, or questionnaire related to the variables of interest. Random sampling is vital to the generalizability of a survey's results.
Cheap, easy, and fast
Can collect large amounts of data in a short amount of time
Results can be affected by poor survey questions
Results can be affected by unrepresentative sample
Outcomes can be affected by participants
If researchers need to gather a large amount of data in a short period of time, a survey is likely to be the fastest, easiest, and cheapest option.
It's also a flexible method because it lets researchers create data-gathering tools that will help ensure they get the information they need (survey responses) from all the sources they want to use (a random sample of participants taking the survey).
Survey data might be cost-efficient and easy to get, but it has its downsides. For one, the data is not always reliable—particularly if the survey questions are poorly written or the overall design or delivery is weak. Data is also affected by specific faults, such as unrepresented or underrepresented samples .
The use of surveys relies on participants to provide useful data. Researchers need to be aware of the specific factors related to the people taking the survey that will affect its outcome.
For example, some people might struggle to understand the questions. A person might answer a particular way to try to please the researchers or to try to control how the researchers perceive them (such as trying to make themselves "look better").
Sometimes, respondents might not even realize that their answers are incorrect or misleading because of mistaken memories .
Archival Research
Many areas of psychological research benefit from analyzing studies that were conducted long ago by other researchers, as well as reviewing historical records and case studies.
For example, in an experiment known as "The Irritable Heart ," researchers used digitalized records containing information on American Civil War veterans to learn more about post-traumatic stress disorder (PTSD).
Large amount of data
Can be less expensive
Researchers cannot change participant behavior
Can be unreliable
Information might be missing
No control over data collection methods
Using records, databases, and libraries that are publicly accessible or accessible through their institution can help researchers who might not have a lot of money to support their research efforts.
Free and low-cost resources are available to researchers at all levels through academic institutions, museums, and data repositories around the world.
Another potential benefit is that these sources often provide an enormous amount of data that was collected over a very long period of time, which can give researchers a way to view trends, relationships, and outcomes related to their research.
While the inability to change variables can be a disadvantage of some methods, it can be a benefit of archival research. That said, using historical records or information that was collected a long time ago also presents challenges. For one, important information might be missing or incomplete and some aspects of older studies might not be useful to researchers in a modern context.
A primary issue with archival research is reliability. When reviewing old research, little information might be available about who conducted the research, how a study was designed, who participated in the research, as well as how data was collected and interpreted.
Researchers can also be presented with ethical quandaries—for example, should modern researchers use data from studies that were conducted unethically or with questionable ethics?
You've probably heard the phrase, "correlation does not equal causation." This means that while correlational research can suggest that there is a relationship between two variables, it cannot prove that one variable will change another.
For example, researchers might perform a correlational study that suggests there is a relationship between academic success and a person's self-esteem. However, the study cannot show that academic success changes a person's self-esteem.
To determine why the relationship exists, researchers would need to consider and experiment with other variables, such as the subject's social relationships, cognitive abilities, personality, and socioeconomic status.
The difference between a correlational study and an experimental study involves the manipulation of variables. Researchers do not manipulate variables in a correlational study, but they do control and systematically vary the independent variables in an experimental study. Correlational studies allow researchers to detect the presence and strength of a relationship between variables, while experimental studies allow researchers to look for cause and effect relationships.
If the study involves the systematic manipulation of the levels of a variable, it is an experimental study. If researchers are measuring what is already present without actually changing the variables, then is a correlational study.
The variables in a correlational study are what the researcher measures. Once measured, researchers can then use statistical analysis to determine the existence, strength, and direction of the relationship. However, while correlational studies can say that variable X and variable Y have a relationship, it does not mean that X causes Y.
The goal of correlational research is often to look for relationships, describe these relationships, and then make predictions. Such research can also often serve as a jumping off point for future experimental research.
Heath W. Psychology Research Methods . Cambridge University Press; 2018:134-156.
Schneider FW. Applied Social Psychology . 2nd ed. SAGE; 2012:50-53.
Curtis EA, Comiskey C, Dempsey O. Importance and use of correlational research . Nurse Researcher . 2016;23(6):20-25. doi:10.7748/nr.2016.e1382
Carpenter S. Visualizing Psychology . 3rd ed. John Wiley & Sons; 2012:14-30.
Pizarro J, Silver RC, Prause J. Physical and mental health costs of traumatic war experiences among civil war veterans . Arch Gen Psychiatry . 2006;63(2):193. doi:10.1001/archpsyc.63.2.193
Post SG. The echo of Nuremberg: Nazi data and ethics . J Med Ethics . 1991;17(1):42-44. doi:10.1136/jme.17.1.42
Lau F. Chapter 12 Methods for Correlational Studies . In: Lau F, Kuziemsky C, eds. Handbook of eHealth Evaluation: An Evidence-based Approach . University of Victoria.
Akoglu H. User's guide to correlation coefficients . Turk J Emerg Med . 2018;18(3):91-93. doi:10.1016/j.tjem.2018.08.001
Price PC. Research Methods in Psychology . California State University.
By Kendra Cherry, MSEd Kendra Cherry, MS, is a psychosocial rehabilitation specialist, psychology educator, and author of the "Everything Psychology Book."
Have a language expert improve your writing
Run a free plagiarism check in 10 minutes, automatically generate references for free.
- Knowledge Base
- Methodology
- Correlational Research | Guide, Design & Examples
Correlational Research | Guide, Design & Examples
Published on 5 May 2022 by Pritha Bhandari . Revised on 5 December 2022.
A correlational research design investigates relationships between variables without the researcher controlling or manipulating any of them.
A correlation reflects the strength and/or direction of the relationship between two (or more) variables. The direction of a correlation can be either positive or negative.
Table of contents
Correlational vs experimental research, when to use correlational research, how to collect correlational data, how to analyse correlational data, correlation and causation, frequently asked questions about correlational research.
Correlational and experimental research both use quantitative methods to investigate relationships between variables. But there are important differences in how data is collected and the types of conclusions you can draw.
Prevent plagiarism, run a free check.
Correlational research is ideal for gathering data quickly from natural settings. That helps you generalise your findings to real-life situations in an externally valid way.
There are a few situations where correlational research is an appropriate choice.
To investigate non-causal relationships
You want to find out if there is an association between two variables, but you don’t expect to find a causal relationship between them.
Correlational research can provide insights into complex real-world relationships, helping researchers develop theories and make predictions.
To explore causal relationships between variables
You think there is a causal relationship between two variables, but it is impractical, unethical, or too costly to conduct experimental research that manipulates one of the variables.
Correlational research can provide initial indications or additional support for theories about causal relationships.
To test new measurement tools
You have developed a new instrument for measuring your variable, and you need to test its reliability or validity .
Correlational research can be used to assess whether a tool consistently or accurately captures the concept it aims to measure.
There are many different methods you can use in correlational research. In the social and behavioural sciences, the most common data collection methods for this type of research include surveys, observations, and secondary data.
It’s important to carefully choose and plan your methods to ensure the reliability and validity of your results. You should carefully select a representative sample so that your data reflects the population you’re interested in without bias .
In survey research , you can use questionnaires to measure your variables of interest. You can conduct surveys online, by post, by phone, or in person.
Surveys are a quick, flexible way to collect standardised data from many participants, but it’s important to ensure that your questions are worded in an unbiased way and capture relevant insights.
Naturalistic observation
Naturalistic observation is a type of field research where you gather data about a behaviour or phenomenon in its natural environment.
This method often involves recording, counting, describing, and categorising actions and events. Naturalistic observation can include both qualitative and quantitative elements, but to assess correlation, you collect data that can be analysed quantitatively (e.g., frequencies, durations, scales, and amounts).
Naturalistic observation lets you easily generalise your results to real-world contexts, and you can study experiences that aren’t replicable in lab settings. But data analysis can be time-consuming and unpredictable, and researcher bias may skew the interpretations.
Secondary data
Instead of collecting original data, you can also use data that has already been collected for a different purpose, such as official records, polls, or previous studies.
Using secondary data is inexpensive and fast, because data collection is complete. However, the data may be unreliable, incomplete, or not entirely relevant, and you have no control over the reliability or validity of the data collection procedures.
After collecting data, you can statistically analyse the relationship between variables using correlation or regression analyses, or both. You can also visualise the relationships between variables with a scatterplot.
Different types of correlation coefficients and regression analyses are appropriate for your data based on their levels of measurement and distributions .
Correlation analysis
Using a correlation analysis, you can summarise the relationship between variables into a correlation coefficient : a single number that describes the strength and direction of the relationship between variables. With this number, you’ll quantify the degree of the relationship between variables.
The Pearson product-moment correlation coefficient, also known as Pearson’s r , is commonly used for assessing a linear relationship between two quantitative variables.
Correlation coefficients are usually found for two variables at a time, but you can use a multiple correlation coefficient for three or more variables.
Regression analysis
With a regression analysis , you can predict how much a change in one variable will be associated with a change in the other variable. The result is a regression equation that describes the line on a graph of your variables.
You can use this equation to predict the value of one variable based on the given value(s) of the other variable(s). It’s best to perform a regression analysis after testing for a correlation between your variables.
It’s important to remember that correlation does not imply causation . Just because you find a correlation between two things doesn’t mean you can conclude one of them causes the other, for a few reasons.
Directionality problem
If two variables are correlated, it could be because one of them is a cause and the other is an effect. But the correlational research design doesn’t allow you to infer which is which. To err on the side of caution, researchers don’t conclude causality from correlational studies.
Third variable problem
A confounding variable is a third variable that influences other variables to make them seem causally related even though they are not. Instead, there are separate causal links between the confounder and each variable.
In correlational research, there’s limited or no researcher control over extraneous variables . Even if you statistically control for some potential confounders, there may still be other hidden variables that disguise the relationship between your study variables.
Although a correlational study can’t demonstrate causation on its own, it can help you develop a causal hypothesis that’s tested in controlled experiments.
A correlation reflects the strength and/or direction of the association between two or more variables.
- A positive correlation means that both variables change in the same direction.
- A negative correlation means that the variables change in opposite directions.
- A zero correlation means there’s no relationship between the variables.
A correlational research design investigates relationships between two variables (or more) without the researcher controlling or manipulating any of them. It’s a non-experimental type of quantitative research .
Controlled experiments establish causality, whereas correlational studies only show associations between variables.
- In an experimental design , you manipulate an independent variable and measure its effect on a dependent variable. Other variables are controlled so they can’t impact the results.
- In a correlational design , you measure variables without manipulating any of them. You can test whether your variables change together, but you can’t be sure that one variable caused a change in another.
In general, correlational research is high in external validity while experimental research is high in internal validity .
A correlation is usually tested for two variables at a time, but you can test correlations between three or more variables.
A correlation coefficient is a single number that describes the strength and direction of the relationship between your variables.
Different types of correlation coefficients might be appropriate for your data based on their levels of measurement and distributions . The Pearson product-moment correlation coefficient (Pearson’s r ) is commonly used to assess a linear relationship between two quantitative variables.
Cite this Scribbr article
If you want to cite this source, you can copy and paste the citation or click the ‘Cite this Scribbr article’ button to automatically add the citation to our free Reference Generator.
Bhandari, P. (2022, December 05). Correlational Research | Guide, Design & Examples. Scribbr. Retrieved 22 April 2024, from https://www.scribbr.co.uk/research-methods/correlational-research-design/
Is this article helpful?
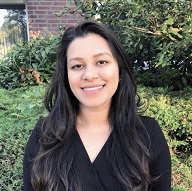
Pritha Bhandari
Other students also liked, a quick guide to experimental design | 5 steps & examples, quasi-experimental design | definition, types & examples, qualitative vs quantitative research | examples & methods.
- Privacy Policy

Home » Correlational Research – Methods, Types and Examples
Correlational Research – Methods, Types and Examples
Table of Contents

Correlational Research
Correlational Research is a type of research that examines the statistical relationship between two or more variables without manipulating them. It is a non-experimental research design that seeks to establish the degree of association or correlation between two or more variables.
Types of Correlational Research
There are three types of correlational research:
Positive Correlation
A positive correlation occurs when two variables increase or decrease together. This means that as one variable increases, the other variable also tends to increase. Similarly, as one variable decreases, the other variable also tends to decrease. For example, there is a positive correlation between the amount of time spent studying and academic performance. The more time a student spends studying, the higher their academic performance is likely to be. Similarly, there is a positive correlation between a person’s age and their income level. As a person gets older, they tend to earn more money.
Negative Correlation
A negative correlation occurs when one variable increases while the other decreases. This means that as one variable increases, the other variable tends to decrease. Similarly, as one variable decreases, the other variable tends to increase. For example, there is a negative correlation between the number of hours spent watching TV and physical activity level. The more time a person spends watching TV, the less physically active they are likely to be. Similarly, there is a negative correlation between the amount of stress a person experiences and their overall happiness. As stress levels increase, happiness levels tend to decrease.
Zero Correlation
A zero correlation occurs when there is no relationship between two variables. This means that the variables are unrelated and do not affect each other. For example, there is zero correlation between a person’s shoe size and their IQ score. The size of a person’s feet has no relationship to their level of intelligence. Similarly, there is zero correlation between a person’s height and their favorite color. The two variables are unrelated to each other.
Correlational Research Methods
Correlational research can be conducted using different methods, including:
Surveys are a common method used in correlational research. Researchers collect data by asking participants to complete questionnaires or surveys that measure different variables of interest. Surveys are useful for exploring the relationships between variables such as personality traits, attitudes, and behaviors.
Observational Studies
Observational studies involve observing and recording the behavior of participants in natural settings. Researchers can use observational studies to examine the relationships between variables such as social interactions, group dynamics, and communication patterns.
Archival Data
Archival data involves using existing data sources such as historical records, census data, or medical records to explore the relationships between variables. Archival data is useful for investigating the relationships between variables that cannot be manipulated or controlled.
Experimental Design
While correlational research does not involve manipulating variables, researchers can use experimental design to establish cause-and-effect relationships between variables. Experimental design involves manipulating one variable while holding other variables constant to determine the effect on the dependent variable.
Meta-Analysis
Meta-analysis involves combining and analyzing the results of multiple studies to explore the relationships between variables across different contexts and populations. Meta-analysis is useful for identifying patterns and inconsistencies in the literature and can provide insights into the strength and direction of relationships between variables.
Data Analysis Methods
Correlational research data analysis methods depend on the type of data collected and the research questions being investigated. Here are some common data analysis methods used in correlational research:
Correlation Coefficient
A correlation coefficient is a statistical measure that quantifies the strength and direction of the relationship between two variables. The correlation coefficient ranges from -1 to +1, with -1 indicating a perfect negative correlation, +1 indicating a perfect positive correlation, and 0 indicating no correlation. Researchers use correlation coefficients to determine the degree to which two variables are related.
Scatterplots
A scatterplot is a graphical representation of the relationship between two variables. Each data point on the plot represents a single observation. The x-axis represents one variable, and the y-axis represents the other variable. The pattern of data points on the plot can provide insights into the strength and direction of the relationship between the two variables.
Regression Analysis
Regression analysis is a statistical method used to model the relationship between two or more variables. Researchers use regression analysis to predict the value of one variable based on the value of another variable. Regression analysis can help identify the strength and direction of the relationship between variables, as well as the degree to which one variable can be used to predict the other.
Factor Analysis
Factor analysis is a statistical method used to identify patterns among variables. Researchers use factor analysis to group variables into factors that are related to each other. Factor analysis can help identify underlying factors that influence the relationship between two variables.
Path Analysis
Path analysis is a statistical method used to model the relationship between multiple variables. Researchers use path analysis to test causal models and identify direct and indirect effects between variables.
Applications of Correlational Research
Correlational research has many practical applications in various fields, including:
- Psychology : Correlational research is commonly used in psychology to explore the relationships between variables such as personality traits, behaviors, and mental health outcomes. For example, researchers may use correlational research to examine the relationship between anxiety and depression, or the relationship between self-esteem and academic achievement.
- Education : Correlational research is useful in educational research to explore the relationships between variables such as teaching methods, student motivation, and academic performance. For example, researchers may use correlational research to examine the relationship between student engagement and academic success, or the relationship between teacher feedback and student learning outcomes.
- Business : Correlational research can be used in business to explore the relationships between variables such as consumer behavior, marketing strategies, and sales outcomes. For example, marketers may use correlational research to examine the relationship between advertising spending and sales revenue, or the relationship between customer satisfaction and brand loyalty.
- Medicine : Correlational research is useful in medical research to explore the relationships between variables such as risk factors, disease outcomes, and treatment effectiveness. For example, researchers may use correlational research to examine the relationship between smoking and lung cancer, or the relationship between exercise and heart health.
- Social Science : Correlational research is commonly used in social science research to explore the relationships between variables such as socioeconomic status, cultural factors, and social behavior. For example, researchers may use correlational research to examine the relationship between income and voting behavior, or the relationship between cultural values and attitudes towards immigration.
Examples of Correlational Research
- Psychology : Researchers might be interested in exploring the relationship between two variables, such as parental attachment and anxiety levels in young adults. The study could involve measuring levels of attachment and anxiety using established scales or questionnaires, and then analyzing the data to determine if there is a correlation between the two variables. This information could be useful in identifying potential risk factors for anxiety in young adults, and in developing interventions that could help improve attachment and reduce anxiety.
- Education : In a correlational study in education, researchers might investigate the relationship between two variables, such as teacher engagement and student motivation in a classroom setting. The study could involve measuring levels of teacher engagement and student motivation using established scales or questionnaires, and then analyzing the data to determine if there is a correlation between the two variables. This information could be useful in identifying strategies that teachers could use to improve student motivation and engagement in the classroom.
- Business : Researchers might explore the relationship between two variables, such as employee satisfaction and productivity levels in a company. The study could involve measuring levels of employee satisfaction and productivity using established scales or questionnaires, and then analyzing the data to determine if there is a correlation between the two variables. This information could be useful in identifying factors that could help increase productivity and improve job satisfaction among employees.
- Medicine : Researchers might examine the relationship between two variables, such as smoking and the risk of developing lung cancer. The study could involve collecting data on smoking habits and lung cancer diagnoses, and then analyzing the data to determine if there is a correlation between the two variables. This information could be useful in identifying risk factors for lung cancer and in developing interventions that could help reduce smoking rates.
- Sociology : Researchers might investigate the relationship between two variables, such as income levels and political attitudes. The study could involve measuring income levels and political attitudes using established scales or questionnaires, and then analyzing the data to determine if there is a correlation between the two variables. This information could be useful in understanding how socioeconomic factors can influence political beliefs and attitudes.
How to Conduct Correlational Research
Here are the general steps to conduct correlational research:
- Identify the Research Question : Start by identifying the research question that you want to explore. It should involve two or more variables that you want to investigate for a correlation.
- Choose the research method: Decide on the research method that will be most appropriate for your research question. The most common methods for correlational research are surveys, archival research, and naturalistic observation.
- Choose the Sample: Select the participants or data sources that you will use in your study. Your sample should be representative of the population you want to generalize the results to.
- Measure the variables: Choose the measures that will be used to assess the variables of interest. Ensure that the measures are reliable and valid.
- Collect the Data: Collect the data from your sample using the chosen research method. Be sure to maintain ethical standards and obtain informed consent from your participants.
- Analyze the data: Use statistical software to analyze the data and compute the correlation coefficient. This will help you determine the strength and direction of the correlation between the variables.
- Interpret the results: Interpret the results and draw conclusions based on the findings. Consider any limitations or alternative explanations for the results.
- Report the findings: Report the findings of your study in a research report or manuscript. Be sure to include the research question, methods, results, and conclusions.
Purpose of Correlational Research
The purpose of correlational research is to examine the relationship between two or more variables. Correlational research allows researchers to identify whether there is a relationship between variables, and if so, the strength and direction of that relationship. This information can be useful for predicting and explaining behavior, and for identifying potential risk factors or areas for intervention.
Correlational research can be used in a variety of fields, including psychology, education, medicine, business, and sociology. For example, in psychology, correlational research can be used to explore the relationship between personality traits and behavior, or between early life experiences and later mental health outcomes. In education, correlational research can be used to examine the relationship between teaching practices and student achievement. In medicine, correlational research can be used to investigate the relationship between lifestyle factors and disease outcomes.
Overall, the purpose of correlational research is to provide insight into the relationship between variables, which can be used to inform further research, interventions, or policy decisions.
When to use Correlational Research
Here are some situations when correlational research can be particularly useful:
- When experimental research is not possible or ethical: In some situations, it may not be possible or ethical to manipulate variables in an experimental design. In these cases, correlational research can be used to explore the relationship between variables without manipulating them.
- When exploring new areas of research: Correlational research can be useful when exploring new areas of research or when researchers are unsure of the direction of the relationship between variables. Correlational research can help identify potential areas for further investigation.
- When testing theories: Correlational research can be useful for testing theories about the relationship between variables. Researchers can use correlational research to examine the relationship between variables predicted by a theory, and to determine whether the theory is supported by the data.
- When making predictions: Correlational research can be used to make predictions about future behavior or outcomes. For example, if there is a strong positive correlation between education level and income, one could predict that individuals with higher levels of education will have higher incomes.
- When identifying risk factors: Correlational research can be useful for identifying potential risk factors for negative outcomes. For example, a study might find a positive correlation between drug use and depression, indicating that drug use could be a risk factor for depression.
Characteristics of Correlational Research
Here are some common characteristics of correlational research:
- Examines the relationship between two or more variables: Correlational research is designed to examine the relationship between two or more variables. It seeks to determine if there is a relationship between the variables, and if so, the strength and direction of that relationship.
- Non-experimental design: Correlational research is typically non-experimental in design, meaning that the researcher does not manipulate any variables. Instead, the researcher observes and measures the variables as they naturally occur.
- Cannot establish causation : Correlational research cannot establish causation, meaning that it cannot determine whether one variable causes changes in another variable. Instead, it only provides information about the relationship between the variables.
- Uses statistical analysis: Correlational research relies on statistical analysis to determine the strength and direction of the relationship between variables. This may include calculating correlation coefficients, regression analysis, or other statistical tests.
- Observes real-world phenomena : Correlational research is often used to observe real-world phenomena, such as the relationship between education and income or the relationship between stress and physical health.
- Can be conducted in a variety of fields : Correlational research can be conducted in a variety of fields, including psychology, sociology, education, and medicine.
- Can be conducted using different methods: Correlational research can be conducted using a variety of methods, including surveys, observational studies, and archival studies.
Advantages of Correlational Research
There are several advantages of using correlational research in a study:
- Allows for the exploration of relationships: Correlational research allows researchers to explore the relationships between variables in a natural setting without manipulating any variables. This can help identify possible relationships between variables that may not have been previously considered.
- Useful for predicting behavior: Correlational research can be useful for predicting future behavior. If a strong correlation is found between two variables, researchers can use this information to predict how changes in one variable may affect the other.
- Can be conducted in real-world settings: Correlational research can be conducted in real-world settings, which allows for the collection of data that is representative of real-world phenomena.
- Can be less expensive and time-consuming than experimental research: Correlational research is often less expensive and time-consuming than experimental research, as it does not involve manipulating variables or creating controlled conditions.
- Useful in identifying risk factors: Correlational research can be used to identify potential risk factors for negative outcomes. By identifying variables that are correlated with negative outcomes, researchers can develop interventions or policies to reduce the risk of negative outcomes.
- Useful in exploring new areas of research: Correlational research can be useful in exploring new areas of research, particularly when researchers are unsure of the direction of the relationship between variables. By conducting correlational research, researchers can identify potential areas for further investigation.
Limitation of Correlational Research
Correlational research also has several limitations that should be taken into account:
- Cannot establish causation: Correlational research cannot establish causation, meaning that it cannot determine whether one variable causes changes in another variable. This is because it is not possible to control all possible confounding variables that could affect the relationship between the variables being studied.
- Directionality problem: The directionality problem refers to the difficulty of determining which variable is influencing the other. For example, a correlation may exist between happiness and social support, but it is not clear whether social support causes happiness, or whether happy people are more likely to have social support.
- Third variable problem: The third variable problem refers to the possibility that a third variable, not included in the study, is responsible for the observed relationship between the two variables being studied.
- Limited generalizability: Correlational research is often limited in terms of its generalizability to other populations or settings. This is because the sample studied may not be representative of the larger population, or because the variables studied may behave differently in different contexts.
- Relies on self-reported data: Correlational research often relies on self-reported data, which can be subject to social desirability bias or other forms of response bias.
- Limited in explaining complex behaviors: Correlational research is limited in explaining complex behaviors that are influenced by multiple factors, such as personality traits, situational factors, and social context.
About the author
Muhammad Hassan
Researcher, Academic Writer, Web developer
You may also like

Questionnaire – Definition, Types, and Examples

Case Study – Methods, Examples and Guide

Observational Research – Methods and Guide

Quantitative Research – Methods, Types and...

Qualitative Research Methods

Explanatory Research – Types, Methods, Guide
Using Science to Inform Educational Practices
Correlational Research
Correlation means that there is a relationship between two or more variables (such as ice cream consumption and crime), but this relationship does not necessarily imply cause and effect. When two variables are correlated, it simply means that as one variable changes, so does the other. We can measure correlation by calculating a statistic known as a correlation coefficient. A correlation coefficient is a number from -1 to +1 that indicates the strength and direction of the relationship between variables. The correlation coefficient is usually represented by the letter r .
The number portion of the correlation coefficient indicates the strength of the relationship. The closer the number is to 1 (be it negative or positive), the more strongly related the variables are, and the more predictable changes in one variable will be as the other variable changes. The closer the number is to zero, the weaker the relationship and the less predictable the relationship between the variables becomes. For instance, a correlation coefficient of 0.9 indicates a far stronger relationship than a correlation coefficient of 0.3. If the variables are not related to one another at all, the correlation coefficient is 0. The example above about ice cream and crime is an example of two variables that we might expect to have no relationship to each other.
The sign—positive or negative—of the correlation coefficient indicates the direction of the relationship (Figure 2.2). A positive correlation means that the variables move in the same direction. Put another way, it means that as one variable increases so does the other, and conversely, when one variable decreases so does the other. A negative correlation means that the variables move in opposite directions. If two variables are negatively correlated, a decrease in one variable is associated with an increase in the other and vice versa.
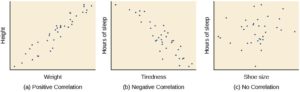
Figure 2.7.1. Scatterplots are a graphical view of the strength and direction of correlations. The stronger the correlation, the closer the data points are to a straight line. In these examples, we see that there is (a) a positive correlation between weight and height, (b) a negative correlation between tiredness and hours of sleep, and (c) no correlation between shoe size and hours of sleep.
The example of ice cream and crime rates is a positive correlation because both variables increase when temperatures are warmer. Other examples of positive correlations are the relationship between an individual’s height and weight or the relationship between a person’s age and number of wrinkles. One might expect a negative correlation to exist between someone’s tiredness during the day and the number of hours they slept the previous night: the amount of sleep decreases as the feelings of tiredness increase. In a real-world example of negative correlation, student researchers at the University of Minnesota found a weak negative correlation ( r = -0.29) between the average number of days per week that students got fewer than 5 hours of sleep and their GPA (Lowry, Dean, & Manders, 2010). Keep in mind that a negative correlation is not the same as no correlation. For example, we would probably find no correlation between hours of sleep and shoe size.
Video 2.7.1. Correlational Research Design provides explanation and examples for correlational research. A closed-captioned version of this video is available here .
Exercise 2.1. Manipulating Scatterplots
Manipulate this interactive scatterplot to practice your understanding of positive and negative correlations.
As mentioned earlier, correlations have predictive value. Imagine that you are on the admissions committee of a major university. You are faced with a massive number of applications, but you are able to accommodate only a small percentage of the applicant pool. How might you decide who should be admitted? You might try to correlate your current students’ college GPA with their scores on standardized tests like the SAT or ACT. By observing which correlations were strongest for your current students, you could use this information to predict the relative success of those students who have applied for admission into the university.
Correlation Does Not Indicate Causation
Correlational research is useful because it allows us to discover the strength and direction of relationships that exist between two variables. However, correlation is limited because establishing the existence of a relationship tells us little about cause and effect. While variables are sometimes correlated because one does cause the other, it could also be that some other factor, a confounding variable, is actually causing the systematic movement in our variables of interest. In the ice cream/crime rate example mentioned earlier, temperature is a confounding variable that could account for the relationship between the two variables.
Even when we cannot point to clear confounding variables, we should not assume that a correlation between two variables implies that one variable causes changes in another. This can be frustrating when a cause-and-effect relationship seems clear and intuitive. Think back to our discussion of the research done by the American Cancer Society and how their research projects were some of the first demonstrations of the link between smoking and cancer. It seems reasonable to assume that smoking causes cancer, but if we were limited to correlational research, we would be overstepping our bounds by making this assumption.
Unfortunately, people mistakenly make claims of causation as a function of correlations all the time. Such claims are especially common in advertisements and news stories. For example, recent research found that people who eat cereal on a regular basis achieve healthier weights than those who rarely eat cereal (Frantzen, Treviño, Echon, Garcia-Dominic, & DiMarco, 2013; Barton et al., 2005). Guess how the cereal companies report this finding. Does eating cereal really cause an individual to maintain a healthy weight, or are there other possible explanations, such as, someone at a healthy weight is more likely to regularly eat a healthy breakfast than someone who is obese or someone who avoids meals in an attempt to diet? While correlational research is invaluable in identifying relationships among variables, a significant limitation is the inability to establish causality. Psychologists want to make statements about cause and effect, but the only way to do that is to conduct an experiment to answer a research question. The next section describes how scientific experiments incorporate methods that eliminate or control for alternative explanations, which allow researchers to explore how changes in one variable cause changes in another variable.
Video 2.7.2. Correlation and Causality provides explanationfor why correlation does not imply causality.
Illusory Correlations
The temptation to make erroneous cause-and-effect statements based on correlational research is not the only way we tend to misinterpret data. We also tend to make the mistake of illusory correlations, especially with unsystematic observations. Illusory correlations , or false correlations, occur when people believe that relationships exist between two things when no such relationship exists. One well-known illusory correlation is the supposed effect that the moon’s phases have on human behavior. Many people passionately assert that human behavior is affected by the phase of the moon, and specifically, that people act strangely when the moon is full (Figure 2).
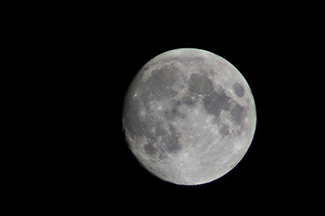
There is no denying that the moon exerts a powerful influence on our planet. The ebb and flow of the ocean’s tides are tightly tied to the gravitational forces of the moon. Many people believe, therefore, that it is logical that we are affected by the moon as well. After all, our bodies are largely made up of water. A meta-analysis of nearly 40 studies consistently demonstrated, however, that the relationship between the moon and our behavior does not exist (Rotton & Kelly, 1985). While we may pay more attention to odd behavior during the full phase of the moon, the rates of odd behavior remain constant throughout the lunar cycle. Why are we so apt to believe in illusory correlations like this? Often we read or hear about them and simply accept the information as valid. Or, we have a hunch about how something works and then look for evidence to support that hunch, ignoring evidence that would tell us our hunch is false; this is known as confirmation bias . Other times, we find illusory correlations based on the information that comes most easily to mind, even if that information is severely limited. And while we may feel confident that we can use these relationships to better understand and predict the world around us, illusory correlations can have significant drawbacks. For example, research suggests that illusory correlations—in which certain behaviors are inaccurately attributed to certain groups—are involved in the formation of prejudicial attitudes that can ultimately lead to discriminatory behavior (Fiedler, 2004).
Candela Citations
- Correlational Research. Authored by : Nicole Arduini-Van Hoose. Provided by : Hudson Valley Community College. Retrieved from : https://courses.lumenlearning.com/edpsy/chapter/correlational-research/. License : CC BY-NC-SA: Attribution-NonCommercial-ShareAlike
- Correlational Research. Authored by : Nicole Arduini-Van Hoose. Provided by : Hudson Valley Community College. Retrieved from : https://courses.lumenlearning.com/adolescent/chapter/correlational-research/. License : CC BY-NC-SA: Attribution-NonCommercial-ShareAlike
Educational Psychology Copyright © 2020 by Nicole Arduini-Van Hoose is licensed under a Creative Commons Attribution-NonCommercial-ShareAlike 4.0 International License , except where otherwise noted.
Share This Book
Correlation in Psychology: Meaning, Types, Examples & coefficient
Saul Mcleod, PhD
Editor-in-Chief for Simply Psychology
BSc (Hons) Psychology, MRes, PhD, University of Manchester
Saul Mcleod, PhD., is a qualified psychology teacher with over 18 years of experience in further and higher education. He has been published in peer-reviewed journals, including the Journal of Clinical Psychology.
Learn about our Editorial Process
Olivia Guy-Evans, MSc
Associate Editor for Simply Psychology
BSc (Hons) Psychology, MSc Psychology of Education
Olivia Guy-Evans is a writer and associate editor for Simply Psychology. She has previously worked in healthcare and educational sectors.
On This Page:
Correlation means association – more precisely, it measures the extent to which two variables are related. There are three possible results of a correlational study: a positive correlation, a negative correlation, and no correlation.
- A positive correlation is a relationship between two variables in which both variables move in the same direction. Therefore, one variable increases as the other variable increases, or one variable decreases while the other decreases. An example of a positive correlation would be height and weight. Taller people tend to be heavier.
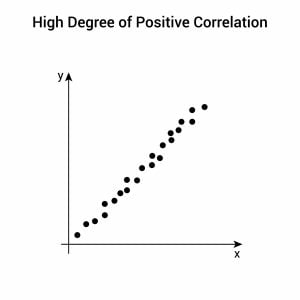
- A negative correlation is a relationship between two variables in which an increase in one variable is associated with a decrease in the other. An example of a negative correlation would be the height above sea level and temperature. As you climb the mountain (increase in height), it gets colder (decrease in temperature).
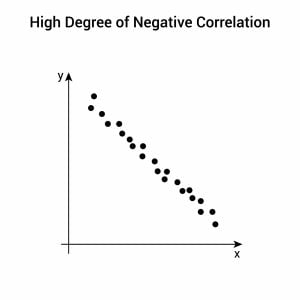
- A zero correlation exists when there is no relationship between two variables. For example, there is no relationship between the amount of tea drunk and the level of intelligence.
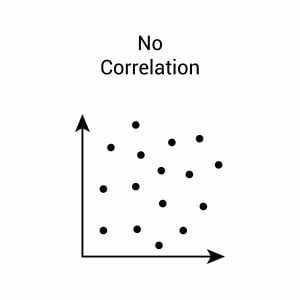
Scatter Plots
A correlation can be expressed visually. This is done by drawing a scatter plot (also known as a scattergram, scatter graph, scatter chart, or scatter diagram).
A scatter plot is a graphical display that shows the relationships or associations between two numerical variables (or co-variables), which are represented as points (or dots) for each pair of scores.
A scatter plot indicates the strength and direction of the correlation between the co-variables.
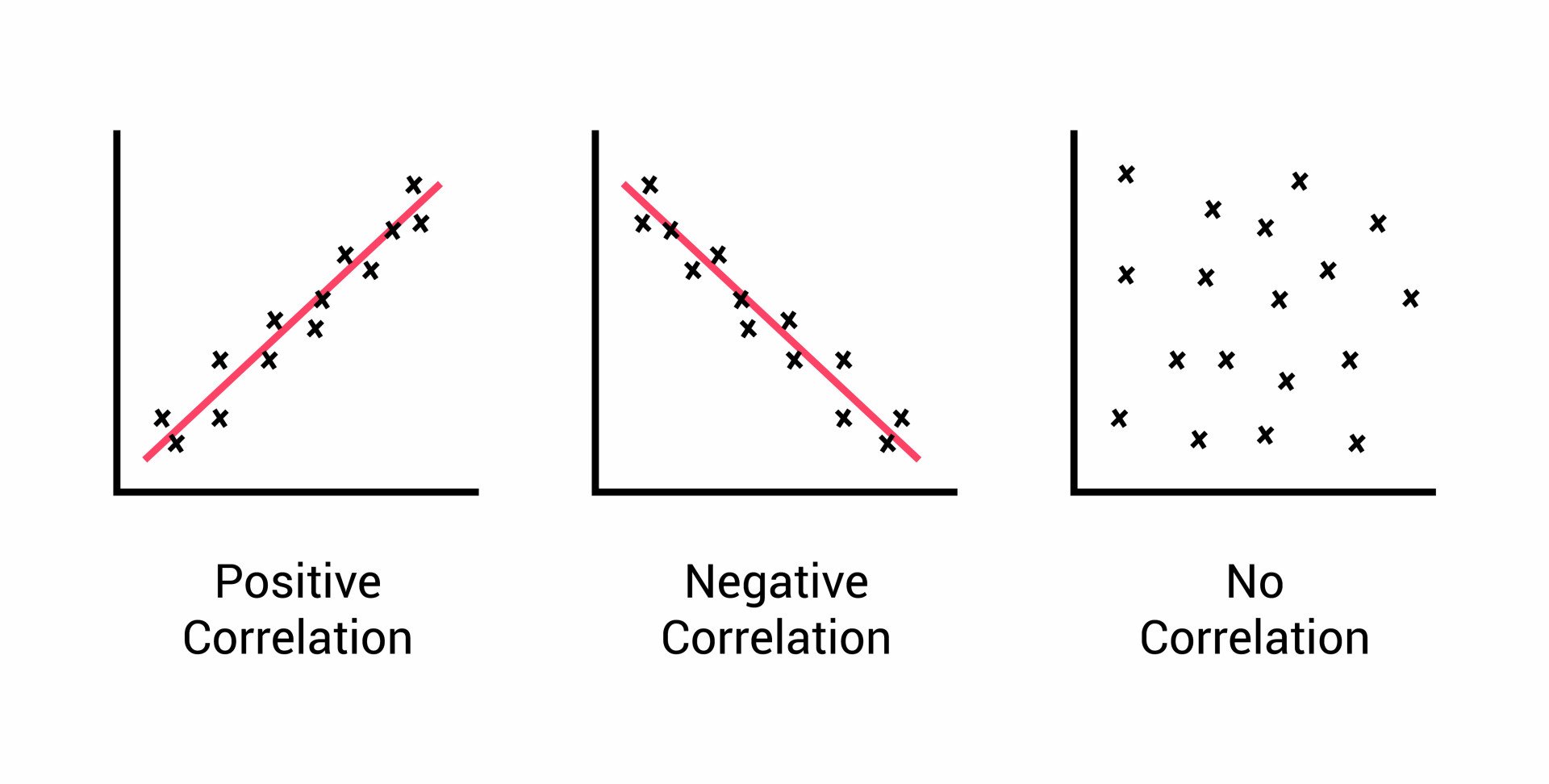
When you draw a scatter plot, it doesn’t matter which variable goes on the x-axis and which goes on the y-axis.
Remember, in correlations, we always deal with paired scores, so the values of the two variables taken together will be used to make the diagram.
Decide which variable goes on each axis and then simply put a cross at the point where the two values coincide.
Uses of Correlations
- If there is a relationship between two variables, we can make predictions about one from another.
- Concurrent validity (correlation between a new measure and an established measure).
Reliability
- Test-retest reliability (are measures consistent?).
- Inter-rater reliability (are observers consistent?).
Theory verification
- Predictive validity.
Correlation Coefficients
Instead of drawing a scatter plot, a correlation can be expressed numerically as a coefficient, ranging from -1 to +1. When working with continuous variables, the correlation coefficient to use is Pearson’s r.
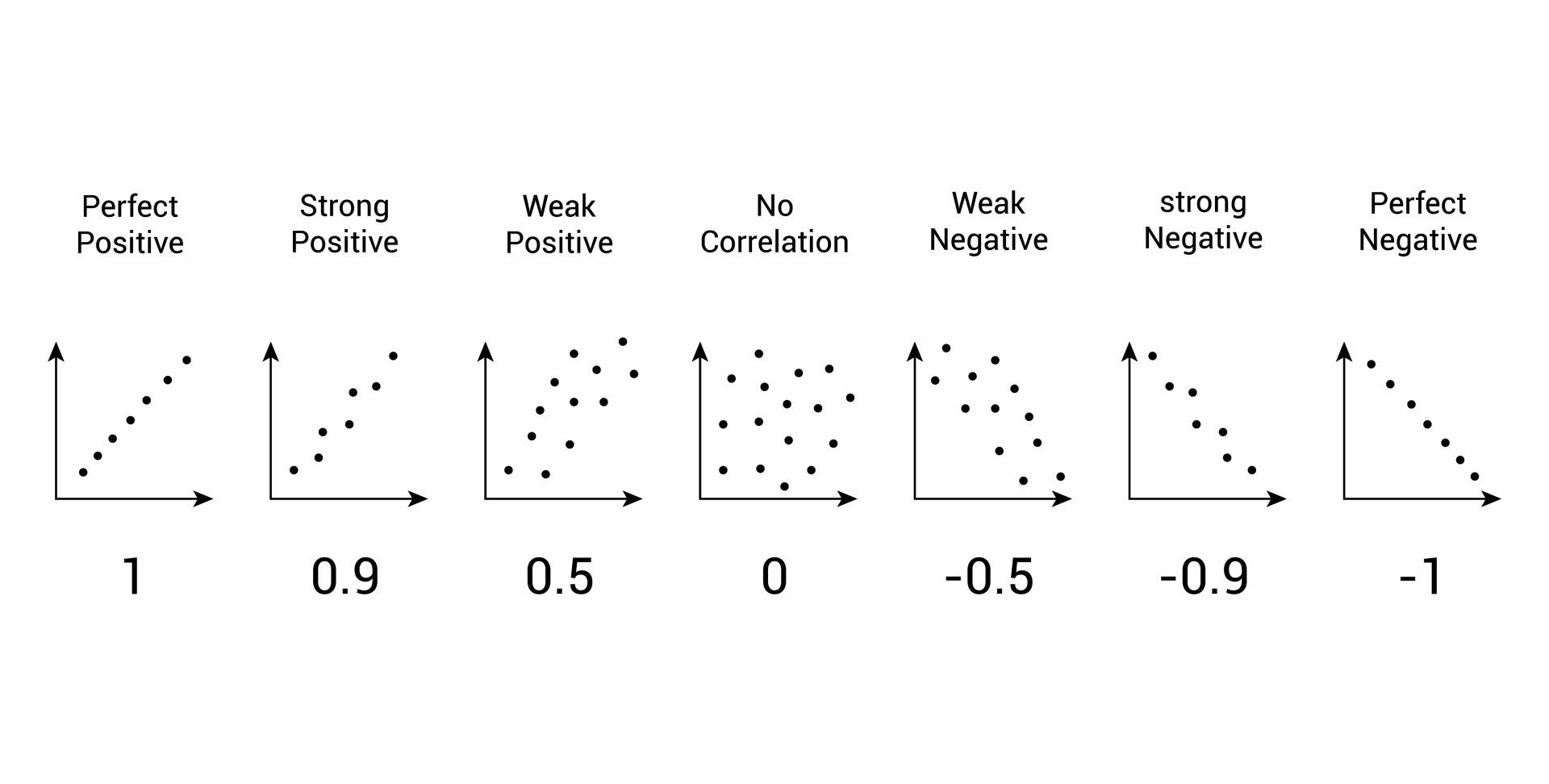
The correlation coefficient ( r ) indicates the extent to which the pairs of numbers for these two variables lie on a straight line. Values over zero indicate a positive correlation, while values under zero indicate a negative correlation.
A correlation of –1 indicates a perfect negative correlation, meaning that as one variable goes up, the other goes down. A correlation of +1 indicates a perfect positive correlation, meaning that as one variable goes up, the other goes up.
There is no rule for determining what correlation size is considered strong, moderate, or weak. The interpretation of the coefficient depends on the topic of study.
When studying things that are difficult to measure, we should expect the correlation coefficients to be lower (e.g., above 0.4 to be relatively strong). When we are studying things that are easier to measure, such as socioeconomic status, we expect higher correlations (e.g., above 0.75 to be relatively strong).)
In these kinds of studies, we rarely see correlations above 0.6. For this kind of data, we generally consider correlations above 0.4 to be relatively strong; correlations between 0.2 and 0.4 are moderate, and those below 0.2 are considered weak.
When we are studying things that are more easily countable, we expect higher correlations. For example, with demographic data, we generally consider correlations above 0.75 to be relatively strong; correlations between 0.45 and 0.75 are moderate, and those below 0.45 are considered weak.
Correlation vs. Causation
Causation means that one variable (often called the predictor variable or independent variable) causes the other (often called the outcome variable or dependent variable).
Experiments can be conducted to establish causation. An experiment isolates and manipulates the independent variable to observe its effect on the dependent variable and controls the environment in order that extraneous variables may be eliminated.
A correlation between variables, however, does not automatically mean that the change in one variable is the cause of the change in the values of the other variable. A correlation only shows if there is a relationship between variables.
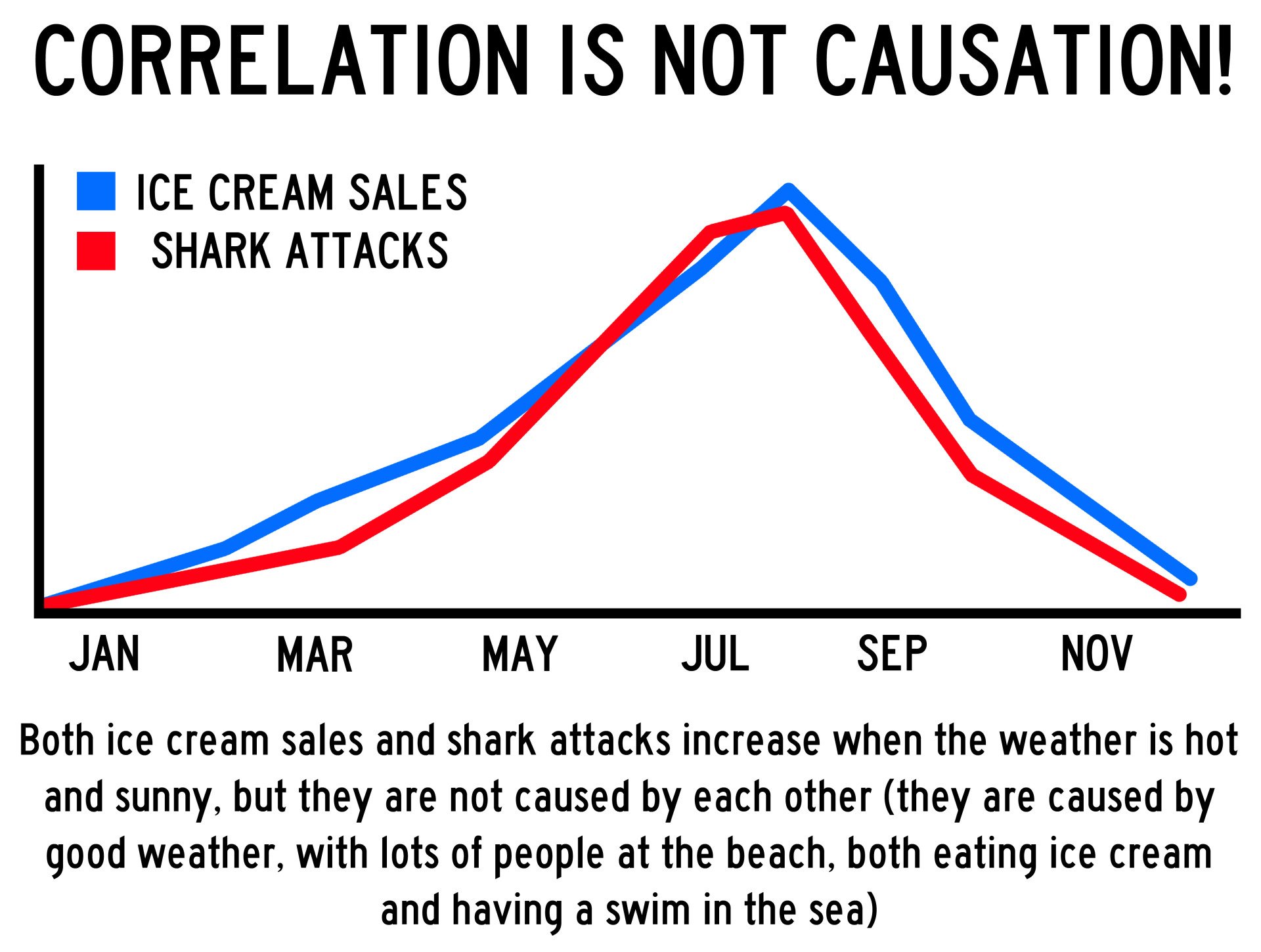
While variables are sometimes correlated because one does cause the other, it could also be that some other factor, a confounding variable , is actually causing the systematic movement in our variables of interest.
Correlation does not always prove causation, as a third variable may be involved. For example, being a patient in a hospital is correlated with dying, but this does not mean that one event causes the other, as another third variable might be involved (such as diet and level of exercise).
“Correlation is not causation” means that just because two variables are related it does not necessarily mean that one causes the other.
A correlation identifies variables and looks for a relationship between them. An experiment tests the effect that an independent variable has upon a dependent variable but a correlation looks for a relationship between two variables.
This means that the experiment can predict cause and effect (causation) but a correlation can only predict a relationship, as another extraneous variable may be involved that it not known about.
1. Correlation allows the researcher to investigate naturally occurring variables that may be unethical or impractical to test experimentally. For example, it would be unethical to conduct an experiment on whether smoking causes lung cancer.
2 . Correlation allows the researcher to clearly and easily see if there is a relationship between variables. This can then be displayed in a graphical form.
Limitations
1 . Correlation is not and cannot be taken to imply causation. Even if there is a very strong association between two variables, we cannot assume that one causes the other.
For example, suppose we found a positive correlation between watching violence on T.V. and violent behavior in adolescence.
It could be that the cause of both these is a third (extraneous) variable – for example, growing up in a violent home – and that both the watching of T.V. and the violent behavior is the outcome of this.
2 . Correlation does not allow us to go beyond the given data. For example, suppose it was found that there was an association between time spent on homework (1/2 hour to 3 hours) and the number of G.C.S.E. passes (1 to 6).
It would not be legitimate to infer from this that spending 6 hours on homework would likely generate 12 G.C.S.E. passes.
How do you know if a study is correlational?
A study is considered correlational if it examines the relationship between two or more variables without manipulating them. In other words, the study does not involve the manipulation of an independent variable to see how it affects a dependent variable.
One way to identify a correlational study is to look for language that suggests a relationship between variables rather than cause and effect.
For example, the study may use phrases like “associated with,” “related to,” or “predicts” when describing the variables being studied.
Another way to identify a correlational study is to look for information about how the variables were measured. Correlational studies typically involve measuring variables using self-report surveys, questionnaires, or other measures of naturally occurring behavior.
Finally, a correlational study may include statistical analyses such as correlation coefficients or regression analyses to examine the strength and direction of the relationship between variables.
Why is a correlational study used?
Correlational studies are particularly useful when it is not possible or ethical to manipulate one of the variables.
For example, it would not be ethical to manipulate someone’s age or gender. However, researchers may still want to understand how these variables relate to outcomes such as health or behavior.
Additionally, correlational studies can be used to generate hypotheses and guide further research.
If a correlational study finds a significant relationship between two variables, this can suggest a possible causal relationship that can be further explored in future research.
What is the goal of correlational research?
The ultimate goal of correlational research is to increase our understanding of how different variables are related and to identify patterns in those relationships.
This information can then be used to generate hypotheses and guide further research aimed at establishing causality.

What is Correlational Research? (+ Design, Examples)
Appinio Research · 04.03.2024 · 30min read
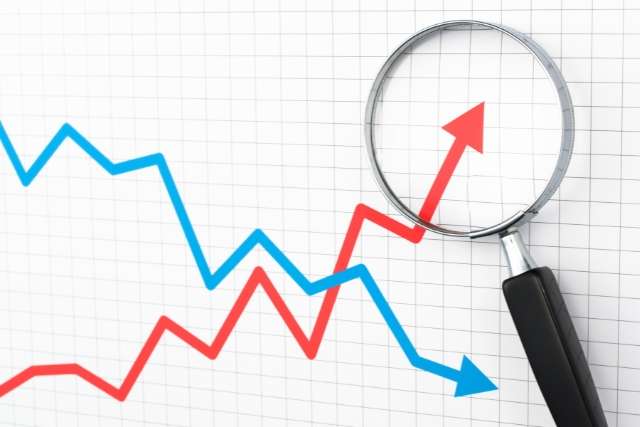
Ever wondered how researchers explore connections between different factors without manipulating them? Correlational research offers a window into understanding the relationships between variables in the world around us. From examining the link between exercise habits and mental well-being to exploring patterns in consumer behavior, correlational studies help us uncover insights that shape our understanding of human behavior, inform decision-making, and drive innovation. In this guide, we'll dive into the fundamentals of correlational research, exploring its definition, importance, ethical considerations, and practical applications across various fields. Whether you're a student delving into research methods or a seasoned researcher seeking to expand your methodological toolkit, this guide will equip you with the knowledge and skills to conduct and interpret correlational studies effectively.
What is Correlational Research?
Correlational research is a methodological approach used in scientific inquiry to examine the relationship between two or more variables. Unlike experimental research, which seeks to establish cause-and-effect relationships through manipulation and control of variables, correlational research focuses on identifying and quantifying the degree to which variables are related to one another. This method allows researchers to investigate associations, patterns, and trends in naturalistic settings without imposing experimental manipulations.
Importance of Correlational Research
Correlational research plays a crucial role in advancing scientific knowledge across various disciplines. Its importance stems from several key factors:
- Exploratory Analysis : Correlational studies provide a starting point for exploring potential relationships between variables. By identifying correlations, researchers can generate hypotheses and guide further investigation into causal mechanisms and underlying processes.
- Predictive Modeling: Correlation coefficients can be used to predict the behavior or outcomes of one variable based on the values of another variable. This predictive ability has practical applications in fields such as economics, psychology, and epidemiology, where forecasting future trends or outcomes is essential.
- Diagnostic Purposes: Correlational analyses can help identify patterns or associations that may indicate the presence of underlying conditions or risk factors. For example, correlations between certain biomarkers and disease outcomes can inform diagnostic criteria and screening protocols in healthcare.
- Theory Development: Correlational research contributes to theory development by providing empirical evidence for proposed relationships between variables. Researchers can refine and validate theoretical models in their respective fields by systematically examining correlations across different contexts and populations.
- Ethical Considerations: In situations where experimental manipulation is not feasible or ethical, correlational research offers an alternative approach to studying naturally occurring phenomena. This allows researchers to address research questions that may otherwise be inaccessible or impractical to investigate.
Correlational vs. Causation in Research
It's important to distinguish between correlation and causation in research. While correlational studies can identify relationships between variables, they cannot establish causal relationships on their own. Several factors contribute to this distinction:
- Directionality: Correlation does not imply the direction of causation. A correlation between two variables does not indicate which variable is causing the other; it merely suggests that they are related in some way. Additional evidence, such as experimental manipulation or longitudinal studies , is needed to establish causality.
- Third Variables: Correlations may be influenced by third variables, also known as confounding variables, that are not directly measured or controlled in the study. These third variables can create spurious correlations or obscure true causal relationships between the variables of interest.
- Temporal Sequence: Causation requires a temporal sequence, with the cause preceding the effect in time. Correlational studies alone cannot establish the temporal order of events, making it difficult to determine whether one variable causes changes in another or vice versa.
Understanding the distinction between correlation and causation is critical for interpreting research findings accurately and drawing valid conclusions about the relationships between variables. While correlational research provides valuable insights into associations and patterns, establishing causation typically requires additional evidence from experimental studies or other research designs.
Key Concepts in Correlation
Understanding key concepts in correlation is essential for conducting meaningful research and interpreting results accurately.
Correlation Coefficient
The correlation coefficient is a statistical measure that quantifies the strength and direction of the relationship between two variables. It's denoted by the symbol r and ranges from -1 to +1.
- A correlation coefficient of -1 indicates a perfect negative correlation, meaning that as one variable increases, the other decreases in a perfectly predictable manner.
- A coefficient of +1 signifies a perfect positive correlation, where both variables increase or decrease together in perfect sync.
- A coefficient of 0 implies no correlation, indicating no systematic relationship between the variables.
Strength and Direction of Correlation
The strength of correlation refers to how closely the data points cluster around a straight line on the scatterplot. A correlation coefficient close to -1 or +1 indicates a strong relationship between the variables, while a coefficient close to 0 suggests a weak relationship.
- Strong correlation: When the correlation coefficient approaches -1 or +1, it indicates a strong relationship between the variables. For example, a correlation coefficient of -0.9 suggests a strong negative relationship, while a coefficient of +0.8 indicates a strong positive relationship.
- Weak correlation: A correlation coefficient close to 0 indicates a weak or negligible relationship between the variables. For instance, a coefficient of -0.1 or +0.1 suggests a weak correlation where the variables are minimally related.
The direction of correlation determines how the variables change relative to each other.
- Positive correlation: When one variable increases, the other variable also tends to increase. Conversely, when one variable decreases, the other variable tends to decrease. This is represented by a positive correlation coefficient.
- Negative correlation: In a negative correlation, as one variable increases, the other variable tends to decrease. Similarly, when one variable decreases, the other variable tends to increase. This relationship is indicated by a negative correlation coefficient.
Scatterplots
A scatterplot is a graphical representation of the relationship between two variables. Each data point on the plot represents the values of both variables for a single observation. By plotting the data points on a Cartesian plane, you can visualize patterns and trends in the relationship between the variables.
- Interpretation: When examining a scatterplot, observe the pattern of data points. If the points cluster around a straight line, it indicates a strong correlation. However, if the points are scattered randomly, it suggests a weak or no correlation.
- Outliers: Identify any outliers or data points that deviate significantly from the overall pattern. Outliers can influence the correlation coefficient and may warrant further investigation to determine their impact on the relationship between variables.
- Line of Best Fit: In some cases, you may draw a line of best fit through the data points to visually represent the overall trend in the relationship. This line can help illustrate the direction and strength of the correlation between the variables.
Understanding these key concepts will enable you to interpret correlation coefficients accurately and draw meaningful conclusions from your data.
How to Design a Correlational Study?
When embarking on a correlational study, careful planning and consideration are crucial to ensure the validity and reliability of your research findings.
Research Question Formulation
Formulating clear and focused research questions is the cornerstone of any successful correlational study. Your research questions should articulate the variables you intend to investigate and the nature of the relationship you seek to explore. When formulating your research questions:
- Be Specific: Clearly define the variables you are interested in studying and the population to which your findings will apply.
- Be Testable: Ensure that your research questions are empirically testable using correlational methods. Avoid vague or overly broad questions that are difficult to operationalize.
- Consider Prior Research: Review existing literature to identify gaps or unanswered questions in your area of interest. Your research questions should build upon prior knowledge and contribute to advancing the field.
For example, if you're interested in examining the relationship between sleep duration and academic performance among college students, your research question might be: "Is there a significant correlation between the number of hours of sleep per night and GPA among undergraduate students?"
Participant Selection
Selecting an appropriate sample of participants is critical to ensuring the generalizability and validity of your findings. Consider the following factors when selecting participants for your correlational study:
- Population Characteristics: Identify the population of interest for your study and ensure that your sample reflects the demographics and characteristics of this population.
- Sampling Method: Choose a sampling method that is appropriate for your research question and accessible, given your resources and constraints. Standard sampling methods include random sampling, stratified sampling, and convenience sampling.
- Sample Size: Determine the appropriate sample size based on factors such as the effect size you expect to detect, the desired level of statistical power, and practical considerations such as time and budget constraints.
For example, suppose you're studying the relationship between exercise habits and mental health outcomes in adults aged 18-65. In that case, you might use stratified random sampling to ensure representation from different age groups within the population.
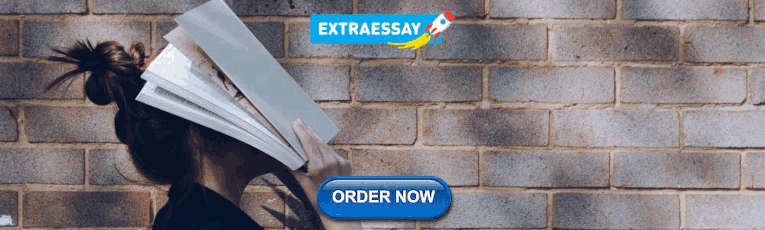
Variables Identification
Identifying and operationalizing the variables of interest is essential for conducting a rigorous correlational study. When identifying variables for your research:
- Independent and Dependent Variables: Clearly distinguish between independent variables (factors that are hypothesized to influence the outcome) and dependent variables (the outcomes or behaviors of interest).
- Control Variables: Identify any potential confounding variables or extraneous factors that may influence the relationship between your independent and dependent variables. These variables should be controlled for in your analysis.
- Measurement Scales: Determine the appropriate measurement scales for your variables (e.g., nominal, ordinal, interval, or ratio) and select valid and reliable measures for assessing each construct.
For instance, if you're investigating the relationship between socioeconomic status (SES) and academic achievement, SES would be your independent variable, while academic achievement would be your dependent variable. You might measure SES using a composite index based on factors such as income, education level, and occupation.
Data Collection Methods
Selecting appropriate data collection methods is essential for obtaining reliable and valid data for your correlational study. When choosing data collection methods:
- Quantitative vs. Qualitative : Determine whether quantitative or qualitative methods are best suited to your research question and objectives. Correlational studies typically involve quantitative data collection methods like surveys, questionnaires, or archival data analysis.
- Instrument Selection: Choose measurement instruments that are valid, reliable, and appropriate for your variables of interest. Pilot test your instruments to ensure clarity and comprehension among your target population.
- Data Collection Procedures : Develop clear and standardized procedures for data collection to minimize bias and ensure consistency across participants and time points.
For example, if you're examining the relationship between smartphone use and sleep quality among adolescents, you might administer a self-report questionnaire assessing smartphone usage patterns and sleep quality indicators such as sleep duration and sleep disturbances.
Crafting a well-designed correlational study is essential for yielding meaningful insights into the relationships between variables. By meticulously formulating research questions , selecting appropriate participants, identifying relevant variables, and employing effective data collection methods, researchers can ensure the validity and reliability of their findings.
With Appinio , conducting correlational research becomes even more seamless and efficient. Our intuitive platform empowers researchers to gather real-time consumer insights in minutes, enabling them to make informed decisions with confidence.
Experience the power of Appinio and unlock valuable insights for your research endeavors. Schedule a demo today and revolutionize the way you conduct correlational studies!
Book a Demo
How to Analyze Correlational Data?
Once you have collected your data in a correlational study, the next crucial step is to analyze it effectively to draw meaningful conclusions about the relationship between variables.
How to Calculate Correlation Coefficients?
The correlation coefficient is a numerical measure that quantifies the strength and direction of the relationship between two variables. There are different types of correlation coefficients, including Pearson's correlation coefficient (for linear relationships), Spearman's rank correlation coefficient (for ordinal data ), and Kendall's tau (for non-parametric data). Here, we'll focus on calculating Pearson's correlation coefficient (r), which is commonly used for interval or ratio-level data.
To calculate Pearson's correlation coefficient (r), you can use statistical software such as SPSS, R, or Excel. However, if you prefer to calculate it manually, you can use the following formula:
r = Σ((X - X̄)(Y - Ȳ)) / ((n - 1) * (s_X * s_Y))
- X and Y are the scores of the two variables,
- X̄ and Ȳ are the means of X and Y, respectively,
- n is the number of data points,
- s_X and s_Y are the standard deviations of X and Y, respectively.
Interpreting Correlation Results
Once you have calculated the correlation coefficient (r), it's essential to interpret the results correctly. When interpreting correlation results:
- Magnitude: The absolute value of the correlation coefficient (r) indicates the strength of the relationship between the variables. A coefficient close to 1 or -1 suggests a strong correlation, while a coefficient close to 0 indicates a weak or no correlation.
- Direction: The sign of the correlation coefficient (positive or negative) indicates the direction of the relationship between the variables. A positive correlation coefficient indicates a positive relationship (as one variable increases, the other tends to increase), while a negative correlation coefficient indicates a negative relationship (as one variable increases, the other tends to decrease).
- Statistical Significance : Assess the statistical significance of the correlation coefficient to determine whether the observed relationship is likely to be due to chance. This is typically done using hypothesis testing, where you compare the calculated correlation coefficient to a critical value based on the sample size and desired level of significance (e.g., α =0.05).
Statistical Significance
Determining the statistical significance of the correlation coefficient involves conducting hypothesis testing to assess whether the observed correlation is likely to occur by chance. The most common approach is to use a significance level (alpha, α ) of 0.05, which corresponds to a 5% chance of obtaining the observed correlation coefficient if there is no true relationship between the variables.
To test the null hypothesis that the correlation coefficient is zero (i.e., no correlation), you can use inferential statistics such as the t-test or z-test. If the calculated p-value is less than the chosen significance level (e.g., p <0.05), you can reject the null hypothesis and conclude that the correlation coefficient is statistically significant.
Remember that statistical significance does not necessarily imply practical significance or the strength of the relationship. Even a statistically significant correlation with a small effect size may not be meaningful in practical terms.
By understanding how to calculate correlation coefficients, interpret correlation results, and assess statistical significance, you can effectively analyze correlational data and draw accurate conclusions about the relationships between variables in your study.
Correlational Research Limitations
As with any research methodology, correlational studies have inherent considerations and limitations that researchers must acknowledge and address to ensure the validity and reliability of their findings.
Third Variables
One of the primary considerations in correlational research is the presence of third variables, also known as confounding variables. These are extraneous factors that may influence or confound the observed relationship between the variables under study. Failing to account for third variables can lead to spurious correlations or erroneous conclusions about causality.
For example, consider a correlational study examining the relationship between ice cream consumption and drowning incidents. While these variables may exhibit a positive correlation during the summer months, the true causal factor is likely to be a third variable—such as hot weather—that influences both ice cream consumption and swimming activities, thereby increasing the risk of drowning.
To address the influence of third variables, researchers can employ various strategies, such as statistical control techniques, experimental designs (when feasible), and careful operationalization of variables.
Causal Inferences
Correlation does not imply causation—a fundamental principle in correlational research. While correlational studies can identify relationships between variables, they cannot determine causality. This is because correlation merely describes the degree to which two variables co-vary; it does not establish a cause-and-effect relationship between them.
For example, consider a correlational study that finds a positive relationship between the frequency of exercise and self-reported happiness. While it may be tempting to conclude that exercise causes happiness, it's equally plausible that happier individuals are more likely to exercise regularly. Without experimental manipulation and control over potential confounding variables, causal inferences cannot be made.
To strengthen causal inferences in correlational research, researchers can employ longitudinal designs, experimental methods (when ethical and feasible), and theoretical frameworks to guide their interpretations.
Sample Size and Representativeness
The size and representativeness of the sample are critical considerations in correlational research. A small or non-representative sample may limit the generalizability of findings and increase the risk of sampling bias .
For example, if a correlational study examines the relationship between socioeconomic status (SES) and educational attainment using a sample composed primarily of high-income individuals, the findings may not accurately reflect the broader population's experiences. Similarly, an undersized sample may lack the statistical power to detect meaningful correlations or relationships.
To mitigate these issues, researchers should aim for adequate sample sizes based on power analyses, employ random or stratified sampling techniques to enhance representativeness and consider the demographic characteristics of the target population when interpreting findings.
Ensure your survey delivers accurate insights by using our Sample Size Calculator . With customizable options for margin of error, confidence level, and standard deviation, you can determine the optimal sample size to ensure representative results. Make confident decisions backed by robust data.
Reliability and Validity
Ensuring the reliability and validity of measures is paramount in correlational research. Reliability refers to the consistency and stability of measurement over time, whereas validity pertains to the accuracy and appropriateness of measurement in capturing the intended constructs.
For example, suppose a correlational study utilizes self-report measures of depression and anxiety. In that case, it's essential to assess the measures' reliability (e.g., internal consistency, test-retest reliability) and validity (e.g., content validity, criterion validity) to ensure that they accurately reflect participants' mental health status.
To enhance reliability and validity in correlational research, researchers can employ established measurement scales, pilot-test instruments, use multiple measures of the same construct, and assess convergent and discriminant validity.
By addressing these considerations and limitations, researchers can enhance the robustness and credibility of their correlational studies and make more informed interpretations of their findings.
Correlational Research Examples and Applications
Correlational research is widely used across various disciplines to explore relationships between variables and gain insights into complex phenomena. We'll examine examples and applications of correlational studies, highlighting their practical significance and impact on understanding human behavior and societal trends across various industries and use cases.
Psychological Correlational Studies
In psychology, correlational studies play a crucial role in understanding various aspects of human behavior, cognition, and mental health. Researchers use correlational methods to investigate relationships between psychological variables and identify factors that may contribute to or predict specific outcomes.
For example, a psychological correlational study might examine the relationship between self-esteem and depression symptoms among adolescents. By administering self-report measures of self-esteem and depression to a sample of teenagers and calculating the correlation coefficient between the two variables, researchers can assess whether lower self-esteem is associated with higher levels of depression symptoms.
Other examples of psychological correlational studies include investigating the relationship between:
- Parenting styles and academic achievement in children
- Personality traits and job performance in the workplace
- Stress levels and coping strategies among college students
These studies provide valuable insights into the factors influencing human behavior and mental well-being, informing interventions and treatment approaches in clinical and counseling settings.
Business Correlational Studies
Correlational research is also widely utilized in the business and management fields to explore relationships between organizational variables and outcomes. By examining correlations between different factors within an organization, researchers can identify patterns and trends that may impact performance, productivity, and profitability.
For example, a business correlational study might investigate the relationship between employee satisfaction and customer loyalty in a retail setting. By surveying employees to assess their job satisfaction levels and analyzing customer feedback and purchase behavior, researchers can determine whether higher employee satisfaction is correlated with increased customer loyalty and retention.
Other examples of business correlational studies include examining the relationship between:
- Leadership styles and employee motivation
- Organizational culture and innovation
- Marketing strategies and brand perception
These studies provide valuable insights for organizations seeking to optimize their operations, improve employee engagement, and enhance customer satisfaction.
Marketing Correlational Studies
In marketing, correlational studies are instrumental in understanding consumer behavior, identifying market trends, and optimizing marketing strategies. By examining correlations between various marketing variables, researchers can uncover insights that drive effective advertising campaigns, product development, and brand management.
For example, a marketing correlational study might explore the relationship between social media engagement and brand loyalty among millennials. By collecting data on millennials' social media usage, brand interactions, and purchase behaviors, researchers can analyze whether higher levels of social media engagement correlate with increased brand loyalty and advocacy.
Another example of a marketing correlational study could focus on investigating the relationship between pricing strategies and customer satisfaction in the retail sector. By analyzing data on pricing fluctuations, customer feedback , and sales performance, researchers can assess whether pricing strategies such as discounts or promotions impact customer satisfaction and repeat purchase behavior.
Other potential areas of inquiry in marketing correlational studies include examining the relationship between:
- Product features and consumer preferences
- Advertising expenditures and brand awareness
- Online reviews and purchase intent
These studies provide valuable insights for marketers seeking to optimize their strategies, allocate resources effectively, and build strong relationships with consumers in an increasingly competitive marketplace. By leveraging correlational methods, marketers can make data-driven decisions that drive business growth and enhance customer satisfaction.
Correlational Research Ethical Considerations
Ethical considerations are paramount in all stages of the research process, including correlational studies. Researchers must adhere to ethical guidelines to ensure the rights, well-being, and privacy of participants are protected. Key ethical considerations to keep in mind include:
- Informed Consent: Obtain informed consent from participants before collecting any data. Clearly explain the purpose of the study, the procedures involved, and any potential risks or benefits. Participants should have the right to withdraw from the study at any time without consequence.
- Confidentiality: Safeguard the confidentiality of participants' data. Ensure that any personal or sensitive information collected during the study is kept confidential and is only accessible to authorized individuals. Use anonymization techniques when reporting findings to protect participants' privacy.
- Voluntary Participation: Ensure that participation in the study is voluntary and not coerced. Participants should not feel pressured to take part in the study or feel that they will suffer negative consequences for declining to participate.
- Avoiding Harm: Take measures to minimize any potential physical, psychological, or emotional harm to participants. This includes avoiding deceptive practices, providing appropriate debriefing procedures (if necessary), and offering access to support services if participants experience distress.
- Deception: If deception is necessary for the study, it must be justified and minimized. Deception should be disclosed to participants as soon as possible after data collection, and any potential risks associated with the deception should be mitigated.
- Researcher Integrity: Maintain integrity and honesty throughout the research process. Avoid falsifying data, manipulating results, or engaging in any other unethical practices that could compromise the integrity of the study.
- Respect for Diversity: Respect participants' cultural, social, and individual differences. Ensure that research protocols are culturally sensitive and inclusive, and that participants from diverse backgrounds are represented and treated with respect.
- Institutional Review: Obtain ethical approval from institutional review boards or ethics committees before commencing the study. Adhere to the guidelines and regulations set forth by the relevant governing bodies and professional organizations.
Adhering to these ethical considerations ensures that correlational research is conducted responsibly and ethically, promoting trust and integrity in the scientific community.
Correlational Research Best Practices and Tips
Conducting a successful correlational study requires careful planning, attention to detail, and adherence to best practices in research methodology. Here are some tips and best practices to help you conduct your correlational research effectively:
- Clearly Define Variables: Clearly define the variables you are studying and operationalize them into measurable constructs. Ensure that your variables are accurately and consistently measured to avoid ambiguity and ensure reliability.
- Use Valid and Reliable Measures: Select measurement instruments that are valid and reliable for assessing your variables of interest. Pilot test your measures to ensure clarity, comprehension, and appropriateness for your target population.
- Consider Potential Confounding Variables: Identify and control for potential confounding variables that could influence the relationship between your variables of interest. Consider including control variables in your analysis to isolate the effects of interest.
- Ensure Adequate Sample Size: Determine the appropriate sample size based on power analyses and considerations of statistical power. Larger sample sizes increase the reliability and generalizability of your findings.
- Random Sampling: Whenever possible, use random sampling techniques to ensure that your sample is representative of the population you are studying. If random sampling is not feasible, carefully consider the characteristics of your sample and the extent to which findings can be generalized.
- Statistical Analysis : Choose appropriate statistical techniques for analyzing your data, taking into account the nature of your variables and research questions. Consult with a statistician if necessary to ensure the validity and accuracy of your analyses.
- Transparent Reporting: Transparently report your methods, procedures, and findings in accordance with best practices in research reporting. Clearly articulate your research questions, methods, results, and interpretations to facilitate reproducibility and transparency.
- Peer Review: Seek feedback from colleagues, mentors, or peer reviewers throughout the research process. Peer review helps identify potential flaws or biases in your study design, analysis, and interpretation, improving your research's overall quality and credibility.
By following these best practices and tips, you can conduct your correlational research with rigor, integrity, and confidence, leading to valuable insights and contributions to your field.
Conclusion for Correlational Research
Correlational research serves as a powerful tool for uncovering connections between variables in the world around us. By examining the relationships between different factors, researchers can gain valuable insights into human behavior, health outcomes, market trends, and more. While correlational studies cannot establish causation on their own, they provide a crucial foundation for generating hypotheses, predicting outcomes, and informing decision-making in various fields. Understanding the principles and practices of correlational research empowers researchers to explore complex phenomena, advance scientific knowledge, and address real-world challenges. Moreover, embracing ethical considerations and best practices in correlational research ensures the integrity, validity, and reliability of study findings. By prioritizing informed consent, confidentiality, and participant well-being, researchers can conduct studies that uphold ethical standards and contribute meaningfully to the body of knowledge. Incorporating transparent reporting, peer review, and continuous learning further enhances the quality and credibility of correlational research. Ultimately, by leveraging correlational methods responsibly and ethically, researchers can unlock new insights, drive innovation, and make a positive impact on society.
How to Collect Data for Correlational Research in Minutes?
Discover the revolutionary power of Appinio , the real-time market research platform. With Appinio, conducting your own correlational research has never been easier or more exciting. Gain access to real-time consumer insights, empowering you to make data-driven decisions in minutes. Here's why Appinio stands out:
- From questions to insights in minutes: Say goodbye to lengthy research processes. With Appinio, you can gather valuable insights swiftly, allowing you to act on them immediately.
- Intuitive platform for everyone: No need for a PhD in research. Appinio's user-friendly interface makes it accessible to anyone, empowering you to conduct professional-grade research effortlessly.
- Extensive reach, global impact: Define your target group from over 1200 characteristics and survey consumers in over 90 countries. With Appinio, the world is your research playground.
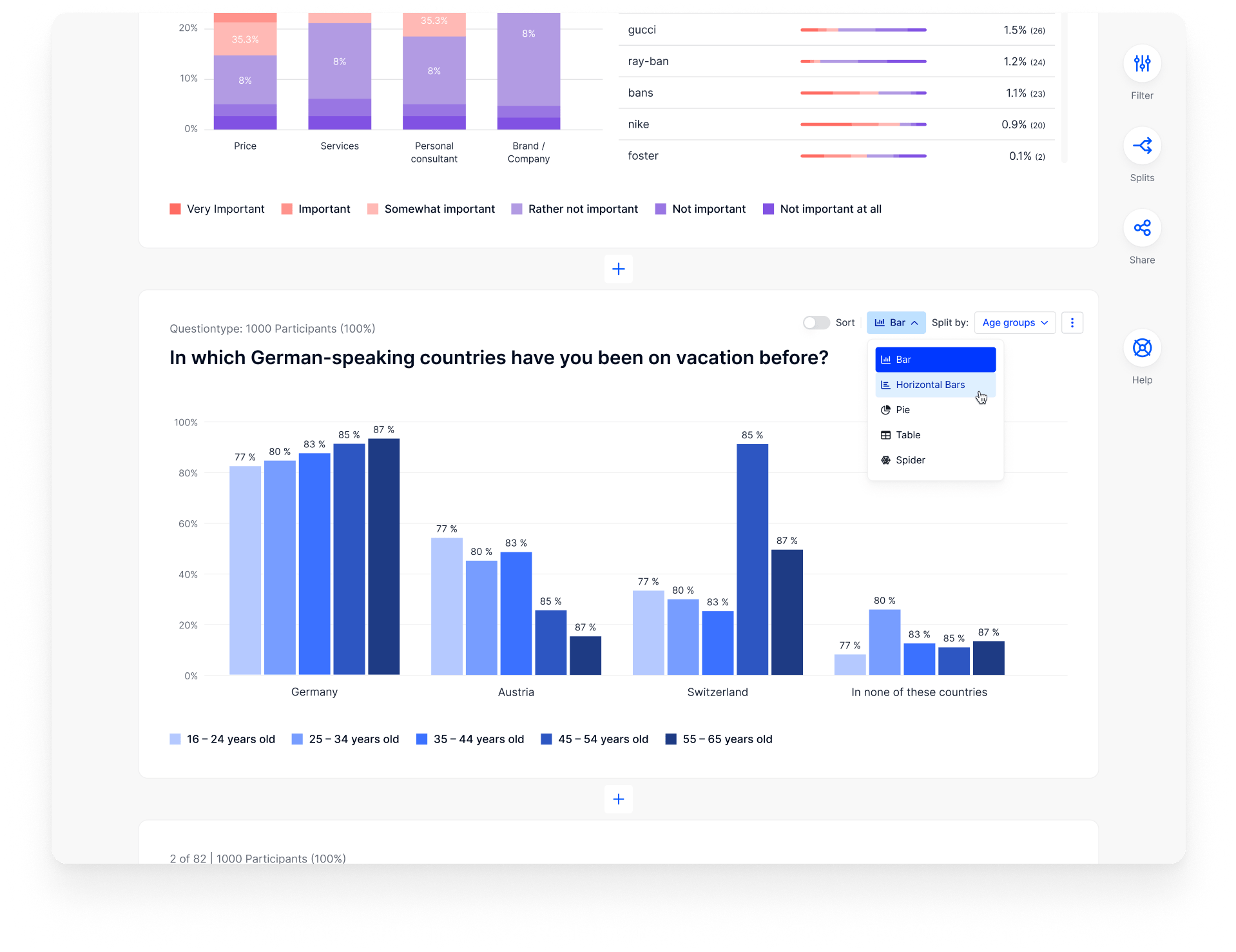
Get free access to the platform!
Join the loop 💌
Be the first to hear about new updates, product news, and data insights. We'll send it all straight to your inbox.
Get the latest market research news straight to your inbox! 💌
Wait, there's more
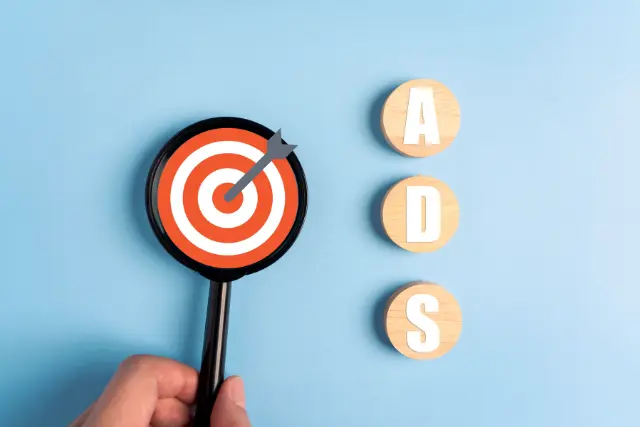
25.04.2024 | 37min read
Targeted Advertising: Definition, Benefits, Examples
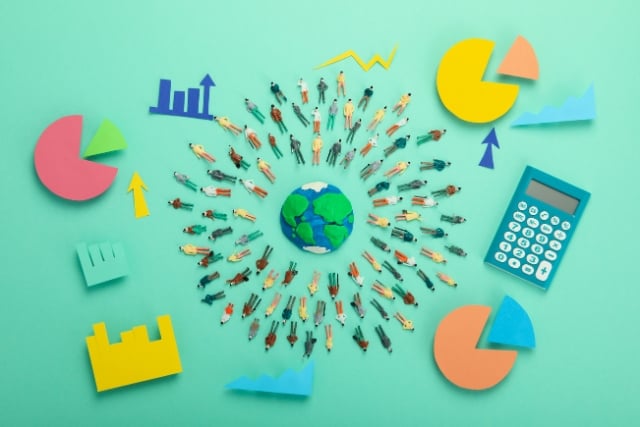
17.04.2024 | 25min read
Quota Sampling: Definition, Types, Methods, Examples
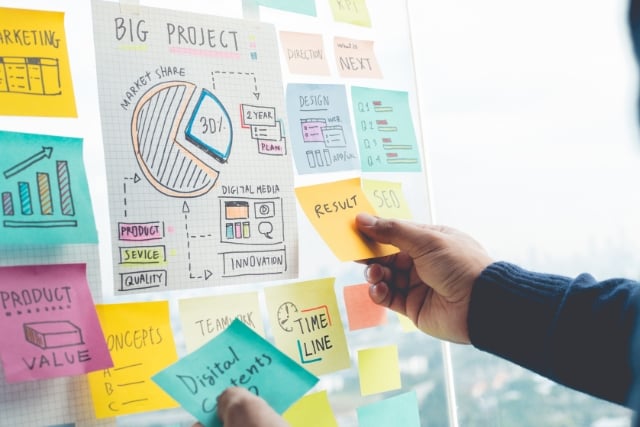
15.04.2024 | 34min read
What is Market Share? Definition, Formula, Examples

- > Statistics
What is Correlational Research? Types and Characteristics
- Hrithik Saini
- Jun 13, 2022
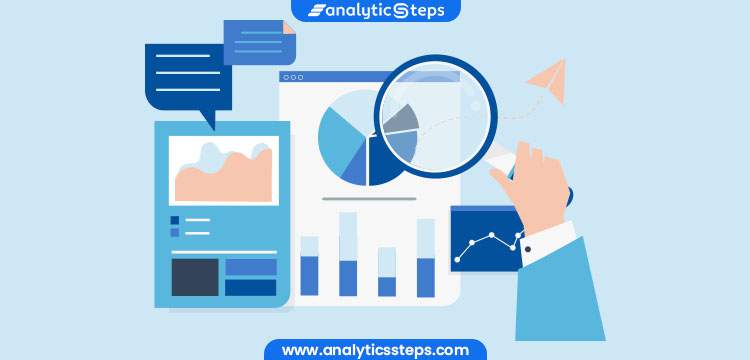
The human psyche is a remarkable instrument for sifting through unconnected elements and establishing a link with a certain matter at hand. If we discuss correlational research, this competence emerges.
We do correlational study on a daily basis; consider how you develop a link between phone ringing at a precise moment and the appearance of the delivery driver. As it's crucial to grasp the many forms of correlation which are accessible, but also how to do them.
What is Correlational Research ?
Correlational analysis is a way of study that includes studying 2 factors in order to obtain a statistically relevant link amongst them. The goal of correlational research is to find factors that are related to each other to the point that a change in one causes a difference in the other.
In its most basic form, correlational research aims to determine if two factors are connected and, if so, how. Of course, knowing what a factor is would be beneficial, right? Variables may be thought of as areas of focus which can take on various forms. A natural source variable itself has not been made by the researchers in any way.
It's crucial to keep in mind that correlation does not indicate causality. Only because two factors have a correlation will not really indicate one of them will be the cause of the other for a myriad of purposes.
The Issue of Directionality
It's possible that two variables are connected because one is a causation and the other is a consequence. However, the correlational study design prevents you from determining which is which. To be safe, academics don't draw conclusions about causality from correlational studies.
Problem with the Third Variable
A mitigating factor is a third variable that has an effect on other variables, making them appear causally connected when they aren't. Instead, each variable and the confounder have their own causal linkages.
Extraneous factors are controlled to a limited extent or not at all in correlational research. Even if certain possible confounding variables are statistically controlled for, there may still be additional hidden factors that obscure the link between your research variables.
Types of Correlational Research
High co - relational research, low correlational research, and no correlational research are the three forms of correlational study. All of these categories have their own combination of traits.
Positive Correlational Analysis (PCA)
Positive correlational research is an important strategy that uses two significantly correlated variables to see if an adjustment in one causes a similar transformation in the other. For instance, a rise in employee wages can lead to a rise in the cost of the product, and likewise.
Negative Correlational Analysis (NCRA)
Negative correlational research is a study strategy that involves two numerically opposing characteristics, at which an increase in one variable has an opposite reaction or a drop from the other. If the price of products or services rises, prices plummet, and inversely, this is an example of a negative correlation.
Zero Correlational Analysis (ZCA)
Zero Correlational Analysis is a method of analysis in which there is no connection between. A form of similar experiment known as zero correlational research combines multiple parameters which were not mathematically related.
A movement from one of the factors might not even cause an equal or opposite modification in the other variable in this scenario. Reasons for the difference in ambiguous causal links are accommodated by zero correlational research. Even though money and endurance are linearly separable, these can be factors in zero correlational study.
Also Read | Hypothesis Testing
When Must Correlational Research be Used ?
Correlational research is a great way to quickly collect data from natural situations. This allows you to apply your results to real-life problems in a way that is externally legitimate.
There are a few instances where correlational research is the best option.
To look into non-causal connections.
You want to see if there's a link between two parameters, but you don't expect to uncover a cause-and-effect relationship. Correlational research can help academics construct hypotheses and make predictions by providing insights into complicated real-world interactions.
To look into the causal links between variables.
You believe there is still a causative link between two factors, but conducting experimental study that tries to influence one of several variables is impracticable, immoral, or too expensive. Correlational research can give preliminary evidence or more support for causal connection ideas.
To put new measuring instruments to the test
You've created a new tool for assessing your variable and want to see if it's reliable or valid. Correlational research can be done to see if an instrument consistently and properly measures the notion it's supposed to.
Best Ways to Examine Correlational Data
After gathering data, you can use correlation or statistical modeling, or both, to statistically assess the relationship among variables. A scatter plot could also be used to depict the relation between variables.
Depending on the degrees of quantification and patterns of your data, several forms of statistical parameters and multiple regression are applicable.
Analyzing Correlations
You may summarize the link between variables using a correlation analysis by calculating a regression equation, which is a specific number that indicates the degree and strength of the association between factors. You'll become capable of determining the strength of the association between variables using this quantity.
For analyzing relationships between the latent quantitative variables, the Pearson ’s product moment coefficient of correlation, generally known as Pearson's r, is widely employed.
Correlation coefficients are typically calculated for two variables . in addition, but a multivariate relationship between two variables can be calculated for three or more factors.
Analysis of Regression
You can anticipate how often a single independent variable will be connected with a movement in another factor using regression analysis. As a consequence, you'll get a linear relationship that explains the curve on your graphing of variables.
This equation can be used to estimate the value of the dependent variable given the value(s) of all the other parameter (s). After you've checked for a correlation amongst your factors, you should do a regression analysis .
Characteristics of Correlational Research
There are three important tenets of correlational research. They are as follows:
Non-Experimental
Correlational research is a non-experimental method. It indicates that investigators do not have to use formal technique to modify factors in agreeing or dispute with such a concept. The investigator just analyzes and examines the relationship among variables, not changing or modifying them in any way.
Backward-Looking
Correlational study is solely willing to look backwards at historical information and observe the past. It is used by scientists to assess and identify long term trends among 2 factors. A correlational analysis may reveal an advantageous association between variables, but that link might shift in the upcoming years.
Correlational study results involving 2 factors are never static and are continually evolving. Based on a variety of causes, two parameters with a negative correlation in the prior may well have a positive correlation connection in the future.
Also Read | Types of Sampling Methods
Examples of Correlational Research
Correlational research examples abound, highlighting a variety of scenarios in which a correlational study may be used to discover a statistical behavioral trend for the variables examined. Here are three correlational research examples :
You want to know if those who are rich are much less tolerant. You feel that affluent individuals are impatient based on your personal experience.
However, you want to find a statistical tendency that supports or refutes your hypothesis. In this scenario, correlational research can be used to find a trend that connects both parameters.
You want to know if there's a link between how much money individuals make and how many children they have. You don't think that people who have more money have more offspring than individuals who have less money.
Domestic abuse, you suppose, produces a brain hemorrhage. You can't do an experiment since it's unacceptable to subject individuals to domestic abuse on purpose.
You believe that a person's income has little bearing on the number of children they have. However, doing correlational study on both variables might disclose whether or not there is a correlational link between them. You can, nevertheless, do correlational study to see if victims of crime experience greater brain bleeding than non-victims.
What is the Correlation Coefficient ?
In correlational research, a coefficient value reveals if there is a favorable, unfavorable, or non-existent network of connected variables. It is commonly denoted by the letter [r] and falls within a spectrum of -1.0 to +1.0 factor loadings.
Pearson's Link Factor (or Pearson's r ) is a metric that is used to test the stability of a relationship amongst variables. A result of 1.0 indicates a positive correlation, a value of -1.0 indicates a negative correlation, and a result of 0.0 indicates zero similarity.
It's necessary to keep in mind that a coefficient of correlation simply represents the linear relationship between the dependent variables; it can't distinguish between dependent and independent variables .
Advantages and Disadvantages of Correlational Research
Advantages of correlational research :.
Correlational research can be conducted to identify the link between two variables when conducting exploratory study is inappropriate. When researching humans, for example, doing an experiment might be considered as risky or immoral; so, correlational research is the ideal alternative.
You can quickly identify the statistical link between two variables using research methodology .
Correlational research takes shorter time and costs less money to conduct than experimental investigation. When dealing with a small number of researchers and limited funds, or when the amount of variables used in this study is kept to a minimum, this becomes a significant benefit.
Relationship between two variables allows researchers to collect data quickly utilizing a variety of approaches, such as a brief survey. Because a brief survey does not need the researcher to conduct it directly, it allows the researcher to deal with a small group of people.
Disadvantages of Correlational Research :
Because correlational research could only be used to discover the statistical link between two parameters, it is limited. It can't be used to find a connection among more than dependent parameters.
It doesn't accommodate for action and reaction between two variables because it doesn't specify which of the two factors is to blame for the observer and record pattern. Finding a favorable correlation between education and vegetarians, for example, does not explain why being informed contributes to becoming a vegetarian or meat consumption leads to greater education.
Although there are plausible explanations for both, causality cannot be established until additional study is conducted. A third, unidentified variable might also be to blame for both. Living in Detroit, for example, can lead to both knowledge and vegetarians.
To discover the connection between variables, correlational research relies on prior statistical trends. As a result, the data cannot be completely trusted for future study.
The researcher has no influence over the variables in correlational study. Correlational study, unlike experimental research, merely enables the researchers to monitor the factors for the purpose of correlating patterns in data without the use of a catalyst.
Correlational study yields a limited amount of data. Correlational study just demonstrates the association between variables; it does not imply causality.
Also Read | What is Statistics?
Correlational research allows researchers to find a quantitative pattern connecting two apparently unrelated variables, and it serves as the foundation for all types of research. It helps you to connect two variables by monitoring their natural behavior.
Correlational research, with exception of experimental investigation, does not focus on the causal factor impacting two variables, making the data generated by correlational research prone to continual change. Experimental research, on the other hand, is faster, simpler, less costly, and more convenient.
Share Blog :

Be a part of our Instagram community
Trending blogs
5 Factors Influencing Consumer Behavior
Elasticity of Demand and its Types
What is PESTLE Analysis? Everything you need to know about it
An Overview of Descriptive Analysis
What is Managerial Economics? Definition, Types, Nature, Principles, and Scope
5 Factors Affecting the Price Elasticity of Demand (PED)
6 Major Branches of Artificial Intelligence (AI)
Dijkstra’s Algorithm: The Shortest Path Algorithm
Scope of Managerial Economics
Different Types of Research Methods
Latest Comments
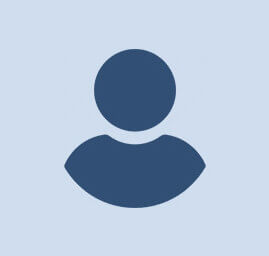
umeshchandradhasmana01
Hi Dear Correlational research is a method used to examine the relationship between variables without manipulating them. It aims to identify if changes in one variable are associated with changes in another variable. There are three types of correlations: positive (both variables increase or decrease together), negative (one variable increases while the other decreases), and zero (no relationship). Characteristics of correlational research include the use of statistical analysis, data collection from existing sources, and the ability to identify patterns and trends in data. Best regards, Mobiloitte


An official website of the United States government
The .gov means it's official. Federal government websites often end in .gov or .mil. Before sharing sensitive information, make sure you're on a federal government site.
The site is secure. The https:// ensures that you are connecting to the official website and that any information you provide is encrypted and transmitted securely.
- Publications
- Account settings
- Browse Titles
NCBI Bookshelf. A service of the National Library of Medicine, National Institutes of Health.
Lau F, Kuziemsky C, editors. Handbook of eHealth Evaluation: An Evidence-based Approach [Internet]. Victoria (BC): University of Victoria; 2017 Feb 27.

Handbook of eHealth Evaluation: An Evidence-based Approach [Internet].
Chapter 12 methods for correlational studies.
Francis Lau .
12.1. Introduction
Correlational studies aim to find out if there are differences in the characteristics of a population depending on whether or not its subjects have been exposed to an event of interest in the naturalistic setting. In eHealth, correlational studies are often used to determine whether the use of an eHealth system is associated with a particular set of user characteristics and/or quality of care patterns ( Friedman & Wyatt, 2006 ). An example is a computerized provider order entry ( cpoe ) study to differentiate the background, usage and performance between clinical users and non-users of the cpoe system after its implementation in a hospital.
Correlational studies are different from comparative studies in that the evaluator does not control the allocation of subjects into comparison groups or assignment of the intervention to specific groups. Instead, the evaluator defines a set of variables including an outcome of interest then tests for hypothesized relations among these variables. The outcome is known as the dependent variable and the variables being tested for association are the independent variables. Correlational studies are similar to comparative studies in that they take on an objectivist view where the variables can be defined, measured and analyzed for the presence of hypothesized relations. As such, correlational studies face the same challenges as comparative studies in terms of their internal and external validity. Of particular importance are the issues of design choices, selection bias, confounders, and reporting consistency.
In this chapter we describe the basic types of correlational studies seen in the eHealth literature and their methodological considerations. Also included are three case examples to show how these studies are done.
12.2. Types of Correlational Studies
Correlational studies, better known as observational studies in epidemiology, are used to examine event exposure, disease prevalence and risk factors in a population ( Elwood, 2007 ). In eHealth, the exposure typically refers to the use of an eHealth system by a population of subjects in a given setting. These subjects may be patients, providers or organizations identified through a set of variables that are thought to differ in their measured values depending on whether or not the subjects were “exposed” to the eHealth system.
There are three basic types of correlational studies that are used in eHealth evaluation: cohort, cross-sectional, and case-control studies ( Vandenbroucke et al., 2014 ). These are described below.
- Cohort studies – A sample of subjects is observed over time where those exposed and not exposed to the eHealth system are compared for differences in one or more predefined outcomes, such as adverse event rates. Cohort studies may be prospective in nature where subjects are followed for a time period into the future or retrospective for a period into the past. The comparisons are typically made at the beginning of the study as baseline measures, then repeated over time at predefined intervals for differences and trends. Some cohort studies involve only a single group of subjects. Their focus is to describe the characteristics of subjects based on a set of variables, such as the pattern of ehr use by providers and their quality of care in an organization over a given time period.
- Cross-sectional studies – These are considered a type of cohort study where only one comparison is made between exposed and unexposed subjects. They provide a snapshot of the outcome and the associated characteristics of the cohort at a specific point in time.
- Case-control studies – Subjects in a sample that are exposed to the eHealth system are matched with those not exposed but otherwise similar in composition, then compared for differences in some predefined outcomes. Case-control studies are retrospective in nature where subjects already exposed to the event are selected then matched with unexposed subjects, using historical cases to ensure they have similar characteristics.
A cross-sectional survey is a type of cross-sectional study where the data source is drawn from postal questionnaires and interviews. This topic will be covered in the chapter on methods for survey studies.
12.3. Methodological Considerations
While correlational studies are considered less rigorous than rct s, they are the preferred designs when it is neither feasible nor ethical to conduct experimental trials. Key methodological issues arise in terms of: (a) design options, (b) biases and confounders, (c) controlling for confounding effects, (d) adherence to good practices, and (e) reporting consistency. These issues are discussed below.
12.3.1. Design Options
There are growing populations with multiple chronic conditions and healthcare interventions. They have made it difficult to design rct s with sufficient sample size and long-term follow-up to account for all the variability this phenomenon entails. Also rct s are intended to test the efficacy of an intervention in a restricted sample of subjects under ideal settings. They have limited generalizability to the population at large in routine settings ( Fleurence, Naci, & Jansen, 2010 ). As such, correlational studies, especially those involving the use of routinely collected ehr data from the general population, have become viable alternatives to rct s. There are advantages and disadvantages to each of the three design options presented above. They are listed below.
- Cohort studies – These studies typically follow the cohorts over time, which allow one to examine causal relationships between exposure and one or more outcomes. They also allow one to measure change in exposure and outcomes over time. However, these studies can be costly and time-consuming to conduct if the outcomes are rare or occur in the future. With prospective cohorts they can be prone to dropout. With retrospective cohorts accurate historical records are required which may not be available or complete ( Levin, 2003a ).
- Case-control studies – These studies are suited to examine infrequent or rare outcomes since they are selected at the outset to ensure sufficient cases. Yet the selection of exposed and matching cases can be problematic, as not all relevant characteristics are known. Moreover, the cases may not be representative of the population of interest. The focus on exposed cases that occur infrequently may overestimate their risks ( Levin, 2003b ).
- Cross-sectional studies – These studies are easier and quicker to conduct than others as they involve a one-time effort over a short period using a sample from the population of interest. They can be used to generate hypotheses and examine multiple outcomes and characteristics at the same time with no loss to follow-up. On the other hand, these studies only give a snapshot of the situation at one time point, making it difficult for causal inference of the exposure and outcomes. The results might be different had another time period been chosen ( Levin, 2006 ).
12.3.2. Biases and Confounders
Shamliyan, Kane, and Dickinson (2010) conducted a systematic review on tools used to assess the quality of observational studies. Despite the large number of quality scales and checklists found in the literature, they concluded that the universal concerns are in the areas of selection bias, confounding, and misclassification. These concerns, also mentioned by Vandenbroucke and colleagues (2014) in their reporting guidelines for observational studies, are summarized below.
- Selection bias – When subjects are selected through their exposure to the event rather than by random or concealed allocation, there is a risk that the subjects are not comparable due to the presence of systematic differences in their baseline characteristics. For example, a correlational study that examines the association between ehr use and quality of care may have younger providers with more computer savvy in the exposed group because they use ehr more and with more facility than those in the unexposed group. It is also possible to have sicker patients in the exposed group since they require more frequent ehr use than unexposed patients who may be healthier and have less need for the ehr . This is sometimes referred to as response bias, where the characteristics of subjects agreed to be in the study are different from those who declined to take part.
- Confounding – Extraneous factors that influence the outcome but are also associated with the exposure are said to have a confounding effect. One such type is confounding by indication where sicker patients are both more likely to receive treatments and also more likely to have adverse outcomes. For example, a study of cds alerts and adverse drug events may find a positive but spurious association due to the inclusion of sicker patients with multiple conditions and medications, which increases their chance of adverse events regardless of cds alerts.
- Misclassification – When there are systematic differences in the completeness or accuracy of the data recorded on the subjects, there is a risk of misclassification in their exposures or outcomes. This is also known as information or detection bias. An example is where sicker patients may have more complete ehr data because they received more tests, treatments and outcome tracking than those who are healthier and require less attention. As such, the exposure and outcomes of sicker patients may be overestimated.
It is important to note that bias and confounding are not synonymous. Bias is caused by finding the wrong association from flawed information or subject selection. Confounding is factually correct with respect to the relationship found, but is incorrect in its interpretation due to an extraneous factor that is associated with both the exposure and outcome.
12.3.3. Controlling for Confounding Effects
There are three common methods to control for confounding effects. These are by matching, stratification, and modelling. They are described below ( Higgins & Green, 2011 ).
- Matching – The selection of subjects with similar characteristics so that they are comparable; the matching can be done at the individual subject level where each exposed subject is matched with one or more unexposed subjects as controls. It can also be done at the group level with equal numbers of exposed and unexposed subjects. Another way to match subjects is by propensity score, that is, a measure derived from a set of characteristics in the subjects. An example is the retrospective cohort study by Zhou, Leith, Li, and Tom (2015) to examine the association between caregiver phr use and healthcare utilization by pediatric patients. In that study, a propensity score-matching algorithm was used to match phr -registered children to non-registered children. The matching model used registration as the outcome variable and all child and caregiver characteristics as the independent variables.
- Stratification – Subjects are categorized into subgroups based on a set of characteristics such as age and sex then analyzed for the effect within each subgroup. An example is the retrospective cohort study by Staes et al. (2008) , examining the impact of computerized alerts on the quality of outpatient lab monitoring for transplant patients. In that study, the before/after comparison of the timeliness of reporting and clinician responses was stratified by the type of test (creatinine, cyclosporine A, and tacrolimus) and report source (hospital laboratory or other labs).
- Modelling – The use of statistical models to compute adjusted effects while accounting for relevant characteristics such as age and sex differences among subjects. An example is the retrospective cohort study by Beck and colleagues (2012) to compare documentation consistency and care plan improvement before and after the implementation of an electronic asthma-specific history and physical template. In that study, before/after group characteristics were compared for differences using t -tests for continuous variables and χ 2 statistics for categorical variables. Logistic regression was used to adjust for group differences in age, gender, insurance, albuterol use at admission, and previous hospitalization.
12.3.4. Adherence to Good Practices in Prospective Observational Studies
The ispor Good Research Practices Task Force published a set of recommendations in designing, conducting and reporting prospective observational studies for comparative effectiveness research ( Berger et al., 2012 ) that are relevant to eHealth evaluation. Their key recommendations are listed below.
- Key policy questions should be defined to allow inferences to be drawn.
- Hypothesis testing protocol design to include the hypothesis/questions, treatment groups and outcomes, measured and unmeasured confounders, primary analyses, and required sample size.
- Rationale for prospective observational study design over others (e.g., rct ) is based on question, feasibility, intervention characteristics and ability to answer the question versus cost and timeliness.
- Study design choice is able to address potential biases and confounders through the use of inception cohorts, multiple comparator groups, matching designs and unaffected outcomes.
- Explanation of study design and analytic choices is transparent.
- Study execution is carried out in ways that ensure relevance and reasonable follow-up is not different from the usual practice.
- Study registration takes place on publicly available sites prior to its initiation.
12.3.5. The Need for Reporting Consistency
Vandenbroucke et al. (2014) published an expanded version of the Strengthening the Reporting of Observational Studies in Epidemiology ( strobe ) statement to improve the reporting of observational studies that can be applied in eHealth evaluation. It is made up of 22 items, of which 18 are common to cohort, case-control and cross-sectional studies, with four being specific to each of the three designs. The 22 reporting items are listed below (for details refer to the cited reference).
- Title and abstract – one item that covers the type of design used, and a summary of what was done and found.
- Introduction – two items on study background/rationale, objectives and/or hypotheses.
- Methods – nine items on design, setting, participants, variables, data sources/measurement, bias, study size, quantitative variables and statistical methods used.
- Results – five items on participants, descriptive, outcome data, main results and other analyses.
- Discussion – four items on key results, limitations, interpretation and generalizability.
- Other information – one item on funding source.
The four items specific to study design relate to the reporting of participants, statistical methods, descriptive results and outcome data. They are briefly described below for the three types of designs.
- Cohort studies – Participant eligibility criteria and sources, methods of selection, follow-up and handling dropouts, description of follow-up time and duration, and number of outcome events or summary measures over time. For matched studies include matching criteria and number of exposed and unexposed subjects.
- Cross-sectional studies – Participant eligibility criteria, sources and methods of selection, analytical methods accounting for sampling strategy as needed, and number of outcome events or summary measures.
- Case-control studies – Participant eligibility criteria, sources and methods of case/control selection with rationale for choices, methods of matching cases/controls, and number of exposures by category or summary measures of exposures. For matched studies include matching criteria and number of controls per case.
12.4. Case Examples
12.4.1. cohort study of automated immunosuppressive care.
Park and colleagues (2010) conducted a retrospective cohort study to examine the association between the use of a cds (clinical decision support) system in post-liver transplant immunosuppressive care and the rates of rejection episode and drug toxicity. The study is summarized below.
- Setting – A liver transplant program in the United States that had implemented an automated cds system to manage immunosuppressive therapy for its post-liver transplant recipients after discharge. The system consolidated all clinical information to expedite immunosuppressive review, ordering, and follow-up with recipients. Prior to automation, a paper charting system was used that involved manually tracking lab tests, transcribing results into a paper spreadsheet, finding physicians to review results and orders, and contacting recipients to notify them of changes.
- Subjects – The study population included recipients of liver transplants between 2004 and 2008 who received outpatient immunosuppressive therapy that included tacrolimus medications.
- Design – A retrospective cohort study with a before/after design to compare recipients managed by the paper charting system against those managed by the cds system for up to one year after discharge.
- Measures – The outcome variables were the percentages of recipients with at least one rejection and/or tacrolimus toxicity episode during the one-year follow-up period. The independent variables included recipient, intraoperative, donor and postoperative characteristics, and use of paper charting or cds . Examples of recipient variables were age, gender, body mass index, presence of diabetes and hypertension, and pre-transplant lab results. Examples of intraoperative data were blood type match, type of transplant and volume of blood transfused. Examples of donor data included percentage of fat in the liver. Examples of post-transplantation data included the type of immunosuppressive induction therapy and the management method.
- Analysis – Mean, standard deviation and t -tests were computed for continuous variables after checking for normal distribution. Percentages and Fisher’s exact test were computed for categorical variables. Autoregressive integrated moving average analysis was done to determine change in outcomes over time. Logistic regression with variables thought to be clinically relevant was used to identify significant univariable and multivariable factors associated with the outcomes. P values of less than 0.05 were considered significant.
- Findings – Overall, the cds system was associated with significantly fewer episodes of rejection and tacrolimus toxicity. The integrated moving average analysis showed a significant decrease in outcome rates after the cds system was implemented compared with paper charting. Multivariable analysis showed the cds system had lower odds of a rejection episode than paper charting ( or 0.20; p < 0.01) and lower odds of tacrolimus toxicity ( or 0.5; p < 0.01). Other significant non-system related factors included the use of specific drugs, the percentage of fat in the donor liver and the volume of packed red cells transfused.
12.4.2. Cross-sectional Analysis of EHR Documentation and Care Quality
Linder, Schnipper, and Middleton (2012) conducted a cross-sectional study to examine the association between the type of ehr documentation used by physicians and the quality of care provided. The study is summarized below.
- Setting – An integrated primary care practice-based research network affiliated with an academic centre in the United States. The network uses an in-house ehr system with decision support for preventive services, chronic care management, and medication monitoring and alerts. The ehr data include problem and medication lists, coded allergies and lab tests.
- Subjects – Physicians and patients from 10 primary care practices that were part of an rct to examine the use of a decision support tool to manage patients with coronary artery disease and diabetes ( cad/DM ). Eligible patients were those with cad/DM in their ehr problem list prior to the rct start date.
- Design – A nine-month retrospective cross-sectional analysis of ehr data collected from the rct . Three physician documentation styles were defined based on 188,554 visit notes in the ehr : (a) dictation, (b) structured documentation, and (c) free text note. Physicians were divided into three groups based on their predominant style defined as more than 25% of their notes composed by a given method.
- Measures – The outcome variables were 15 ehr -based cad/DM quality measures assessed 30 days after primary care visits. They covered quality of documentation, medication use, lab testing, physiologic measures, and vaccinations. Measures collected prior to the day of visit were eligible and considered fulfilled with the presence of coded ehr data on vital signs, medications, allergies, problem lists, lab tests, and vaccinations. Independent variables on physicians and patients were included as covariates. For physicians, they included age, gender, training level, proportion of cad/DM patients in their panel, total patient visits, and self-reported experience with the ehr . For patients, they included socio-demographic factors, the number of clinic visits and hospitalizations, the number of problems and medications in the ehr , and whether their physician was in the intervention group.
- Analysis – Baseline characteristics of physicians and patients were compared using descriptive statistics. Continuous variables were compared using anova . For categorical variables, Fisher’s exact test was used for physician variables and χ 2 test for patient variables. Multivariate logistic regression models were used for each quality measure to adjust for patient and physician clustering and potential confounders. Bonferroni procedure was used to account for multiple comparisons for the 15 quality measures.
- Findings – During the study period, 234 physicians documented 18,569 visits from 7,000 cad/DM patients. Of these physicians, 146 (62%) typed free-text notes, 68 (25%) used structured documentation, and 20 (9%) dictated notes. After adjusting for cluster effect, physicians who dictated their notes had the worst quality of care in all 15 measures. In particular, physicians who dictated notes were significantly worse in three of 15 measures (antiplatelet medication, tobacco use, diabetic eye exam); physicians who used structured documentation were better in three measures (blood pressure, body mass, diabetic foot exam); and those who used free-text were better in one measure (influenza vaccination). In summary, physicians who dictated notes had worse quality of care than those with structured documentation.
12.4.3. Case-control Comparison of Internet Portal Use
Nielsen, Halamka, and Kinkel (2012) conducted a case-control study to evaluate whether there was an association between active Internet patient portal use by Multiple Sclerosis ( ms ) patients and medical resource utilization. Patient predictors and barriers to portal use were also identified. The study is summarized below.
- Setting – An academic ms centre in the United States with an in-house Internet patient portal site that was accessed by ms patients to schedule clinic appointments, request prescription refills and referrals, view test results, upload personal health information, and communicate with providers via secure e-mails.
- Subjects – 240 adult ms patients actively followed during 2008 and 2009 were randomly selected from the ehr ; 120 of these patients had submitted at least one message during that period and were defined as portal users. Another 120 patients who did not enrol in the portal or send any message were selected as non-users for comparison.
- Design – A retrospective case-control study facilitated through a chart review comparing portal users against non-users from the same period. Patient demographic and clinical information was extracted from the ehr , while portal usage, including feature access type and frequency and e-mail message content, were provided by it staff.
- Measures – Patient variables included age, gender, race, insurance type, employment status, number of medical problems, disease duration, psychiatric history, number of medications, and physical disability scores. Provider variables included prescription type and frequency. Portal usage variables included feature access type and frequency for test results, appointments, prescription requests and logins, and categorized messaging contents.
- Analysis – Comparison of patient demographic, clinical and medical resource utilization data from users and non-users were made using descriptive statistics, Wilcoxon rank sum test, Fisher’s exact test and χ 2 test. Multivariate logistic regression was used to identify patient predictors and barriers to portal use. Provider prescribing habits against patient’s psychiatric history and portal use were examined by two-way analysis of variance. All statistical tests used p value of 0.05 with no adjustment made for multiple comparisons. A logistic multivariate regression model was created to predict portal use based on patient demographics, clinical condition, socio-economic status, and physical disability metrics.
- Findings – Portal users were mostly young professionals with little physical disability. The most frequently used feature was secure patient-provider messaging, often for medication requests or refills, and self-reported side effects. Predictors and barriers of portal use were the number of medications prescribed ( or 1.69, p < 0.0001), Caucasian ethnicity ( or 5.04, p = 0.007), arm and hand disability ( or 0.23, p = 0.01), and impaired vision ( or 0.31, p = 0.01). For medical resource utilization, portal users had more frequent clinic visits, medication use and prescriptions from centre staff providers. Patients with a history of psychiatric disease were prescribed more ms medications than those without any history ( p < 0.0001). In summary, ms patients used the Internet more than the general population, but physical disability limited their access and need to be addressed.
12.4.4. Limitations
A general limitation of a correlational study is that it can determine association between exposure and outcomes but cannot predict causation. The more specific limitations of the three case examples cited by the authors are listed below.
- Automated immunosuppressive care – Baseline differences existed between groups with unknown effects; possible other unmeasured confounders; possible Hawthorne effects from focus on immunosuppressive care.
- ehr documentation and care quality – Small sample size; only three documentation styles were considered (e.g., scribe and voice recognition software were excluded) and unsure if they were stable during study period; quality measures specific to cad/DM conditions only; complex methods of adjusting for clustering and confounding that did not account for unmeasured confounders; the level of physician training (e.g., attending versus residents) not adjusted.
- Internet portal use – Small sample size not representative of the study population; referral centre site could over-represent complex patients requiring advanced care; all patients had health insurance.
12.5. Summary
In this chapter we described cohort, case-control and cross-sectional studies as three types of correlational studies used in eHealth evaluation. The methodological issues addressed include bias and confounding, controlling for confounders, adherence to good practices and consistency in reporting. Three case examples were included to show how eHealth correlational studies are done.
1 ISPOR – International Society for Pharmacoeconomics and Outcomes Research
- Beck A. F., Sauers H. S., Kahn R. S., Yau C., Weiser J., Simmons J.M. Improved documentation and care planning with an asthma-specific history and physical. Hospital Pediatrics. 2012; 2 (4):194–201. [ PubMed : 24313025 ]
- Berger M. L., Dreyer N., Anderson F., Towse A., Sedrakyan A., Normand S.L. Prospective observational studies to address comparative effectiveness: The ispor good research practices task force report. Value in Health. 2012; 15 (2):217–230. Retrieved from http://www .sciencedirect .com/science/article /pii/S1098301512000071 . [ PubMed : 22433752 ]
- Elwood M. Critical appraisal of epidemiological studies and clinical studies. 3rd ed. Oxford: Oxford University Press; 2007.
- Fleurence R. L., Naci H., Jansen J.P. The critical role of observational evidence in comparative effectiveness research. Health Affairs. 2010; 29 (10):1826–1833. [ PubMed : 20921482 ]
- Friedman C. P., Wyatt J.C. Evaluation methods in biomedical informatics. 2nd ed. New York: Springer Science + Business Media, Inc; 2006.
- Higgins J. P. T., Green S., editors. Cochrane handbook for systematic reviews of interventions. London: The Cochrane Collaboration; 2011. (Version 5.1.0, updated March 2011) Retrieved from http://handbook .cochrane.org/
- Levin K. A. Study design iv : Cohort studies. Evidence-based Dentistry. 2003a; 7 :51–52. [ PubMed : 16858385 ]
- Levin K. A. Study design v : Case-control studies. Evidence-based Dentistry. 2003b; 7 :83–84. [ PubMed : 17003803 ]
- Levin K. A. Study design iii : Cross-sectional studies. Evidence-based Dentistry. 2006; 7 :24–25. [ PubMed : 16557257 ]
- Linder J. A., Schnipper J. L., Middleton B. Method of electronic health record documentation and quality of primary care. Journal of the American Medical Informatics Association. 2012; 19 (6):1019–1024. [ PMC free article : PMC3534457 ] [ PubMed : 22610494 ]
- Nielsen A. S., Halamka J. D., Kinkel R.P. Internet portal use in an academic multiple sclerosis center. Journal of the American Medical Informatics Association. 2012; 19 (1):128–133. [ PMC free article : PMC3240754 ] [ PubMed : 21571744 ]
- Park E. S., Peccoud M. R., Wicks K. A., Halldorson J. B., Carithers R. L. Jr., Reyes J. D., Perkins J.D. Use of an automated clinical management system improves outpatient immunosuppressive care following liver transplantation. Journal of the American Medical Informatics Association. 2010; 17 (4):396–402. [ PMC free article : PMC2995663 ] [ PubMed : 20595306 ]
- Shamliyan T., Kane R. L., Dickinson S. A systematic review of tools used to assess the quality of observational studies that examine incidence or prevalence and risk factors for diseases. Journal of Clinical Epidemiology. 2010; 63 (10):1061–1070. [ PubMed : 20728045 ]
- Staes C. J., Evans R. S., Rocha B. H. S. C., Sorensen J. B., Huff S. M., Arata J., Narus S.P. Computerized alerts improve outpatient laboratory monitoring of transplant patients. Journal of the American Medical Informatics Association. 2008; 15 (3):324–332. [ PMC free article : PMC2410008 ] [ PubMed : 18308982 ]
- Vandenbroucke J. P., von Elm E., Altman D. G., Gotzsche P. C., Mulrow C. D., Pocock S. J., Egger M. for the strobe Initiative. Strengthening the reporting of observational studies in epidemiology ( strobe ): explanation and elaboration. International Journal of Surgery. 2014; 12 (12):1500–1524. Retrieved from http://www.sciencedirect.com/science/article/pii/ s174391911400212x . [ PubMed : 25046751 ]
- Zhou Y. Y., Leith W. M., Li H., Tom J.O. Personal health record use for children and health care utilization: propensity score-matched cohort analysis. Journal of the American Medical Informatics Association. 2015; 22 (4):748–754. [ PubMed : 25656517 ]
This publication is licensed under a Creative Commons License, Attribution-Noncommercial 4.0 International License (CC BY-NC 4.0): see https://creativecommons.org/licenses/by-nc/4.0/
- Cite this Page Lau F. Chapter 12 Methods for Correlational Studies. In: Lau F, Kuziemsky C, editors. Handbook of eHealth Evaluation: An Evidence-based Approach [Internet]. Victoria (BC): University of Victoria; 2017 Feb 27.
- PDF version of this title (4.5M)
- Disable Glossary Links
In this Page
- Introduction
- Types of Correlational Studies
- Methodological Considerations
- Case Examples
Related information
- PMC PubMed Central citations
- PubMed Links to PubMed
Recent Activity
- Chapter 12 Methods for Correlational Studies - Handbook of eHealth Evaluation: A... Chapter 12 Methods for Correlational Studies - Handbook of eHealth Evaluation: An Evidence-based Approach
Your browsing activity is empty.
Activity recording is turned off.
Turn recording back on
Connect with NLM
National Library of Medicine 8600 Rockville Pike Bethesda, MD 20894
Web Policies FOIA HHS Vulnerability Disclosure
Help Accessibility Careers

Want to create or adapt books like this? Learn more about how Pressbooks supports open publishing practices.
Correlational Research
Rajiv S. Jhangiani; I-Chant A. Chiang; Carrie Cuttler; and Dana C. Leighton
Learning Objectives
- Define correlational research and give several examples.
- Explain why a researcher might choose to conduct correlational research rather than experimental research or another type of non-experimental research.
- Interpret the strength and direction of different correlation coefficients.
- Explain why correlation does not imply causation.
What Is Correlational Research?
Correlational research is a type of non-experimental research in which the researcher measures two variables (binary or continuous) and assesses the statistical relationship (i.e., the correlation) between them with little or no effort to control extraneous variables. There are many reasons that researchers interested in statistical relationships between variables would choose to conduct a correlational study rather than an experiment. The first is that they do not believe that the statistical relationship is a causal one or are not interested in causal relationships. Recall two goals of science are to describe and to predict and the correlational research strategy allows researchers to achieve both of these goals. Specifically, this strategy can be used to describe the strength and direction of the relationship between two variables and if there is a relationship between the variables then the researchers can use scores on one variable to predict scores on the other (using a statistical technique called regression, which is discussed further in the section on Complex Correlation in this chapter).
Another reason that researchers would choose to use a correlational study rather than an experiment is that the statistical relationship of interest is thought to be causal, but the researcher cannot manipulate the independent variable because it is impossible, impractical, or unethical. For example, while a researcher might be interested in the relationship between the frequency people use cannabis and their memory abilities they cannot ethically manipulate the frequency that people use cannabis. As such, they must rely on the correlational research strategy; they must simply measure the frequency that people use cannabis and measure their memory abilities using a standardized test of memory and then determine whether the frequency people use cannabis is statistically related to memory test performance.
Correlation is also used to establish the reliability and validity of measurements. For example, a researcher might evaluate the validity of a brief extraversion test by administering it to a large group of participants along with a longer extraversion test that has already been shown to be valid. This researcher might then check to see whether participants’ scores on the brief test are strongly correlated with their scores on the longer one. Neither test score is thought to cause the other, so there is no independent variable to manipulate. In fact, the terms independent variable and dependent variabl e do not apply to this kind of research.
Another strength of correlational research is that it is often higher in external validity than experimental research. Recall there is typically a trade-off between internal validity and external validity. As greater controls are added to experiments, internal validity is increased but often at the expense of external validity as artificial conditions are introduced that do not exist in reality. In contrast, correlational studies typically have low internal validity because nothing is manipulated or controlled but they often have high external validity. Since nothing is manipulated or controlled by the experimenter the results are more likely to reflect relationships that exist in the real world.
Finally, extending upon this trade-off between internal and external validity, correlational research can help to provide converging evidence for a theory. If a theory is supported by a true experiment that is high in internal validity as well as by a correlational study that is high in external validity then the researchers can have more confidence in the validity of their theory. As a concrete example, correlational studies establishing that there is a relationship between watching violent television and aggressive behavior have been complemented by experimental studies confirming that the relationship is a causal one (Bushman & Huesmann, 2001) [1] .
Does Correlational Research Always Involve Quantitative Variables?
A common misconception among beginning researchers is that correlational research must involve two quantitative variables, such as scores on two extraversion tests or the number of daily hassles and number of symptoms people have experienced. However, the defining feature of correlational research is that the two variables are measured—neither one is manipulated—and this is true regardless of whether the variables are quantitative or categorical. Imagine, for example, that a researcher administers the Rosenberg Self-Esteem Scale to 50 American college students and 50 Japanese college students. Although this “feels” like a between-subjects experiment, it is a correlational study because the researcher did not manipulate the students’ nationalities. The same is true of the study by Cacioppo and Petty comparing college faculty and factory workers in terms of their need for cognition. It is a correlational study because the researchers did not manipulate the participants’ occupations.
Figure 6.2 shows data from a hypothetical study on the relationship between whether people make a daily list of things to do (a “to-do list”) and stress. Notice that it is unclear whether this is an experiment or a correlational study because it is unclear whether the independent variable was manipulated. If the researcher randomly assigned some participants to make daily to-do lists and others not to, then it is an experiment. If the researcher simply asked participants whether they made daily to-do lists, then it is a correlational study. The distinction is important because if the study was an experiment, then it could be concluded that making the daily to-do lists reduced participants’ stress. But if it was a correlational study, it could only be concluded that these variables are statistically related. Perhaps being stressed has a negative effect on people’s ability to plan ahead (the directionality problem). Or perhaps people who are more conscientious are more likely to make to-do lists and less likely to be stressed (the third-variable problem). The crucial point is that what defines a study as experimental or correlational is not the variables being studied, nor whether the variables are quantitative or categorical, nor the type of graph or statistics used to analyze the data. What defines a study is how the study is conducted.
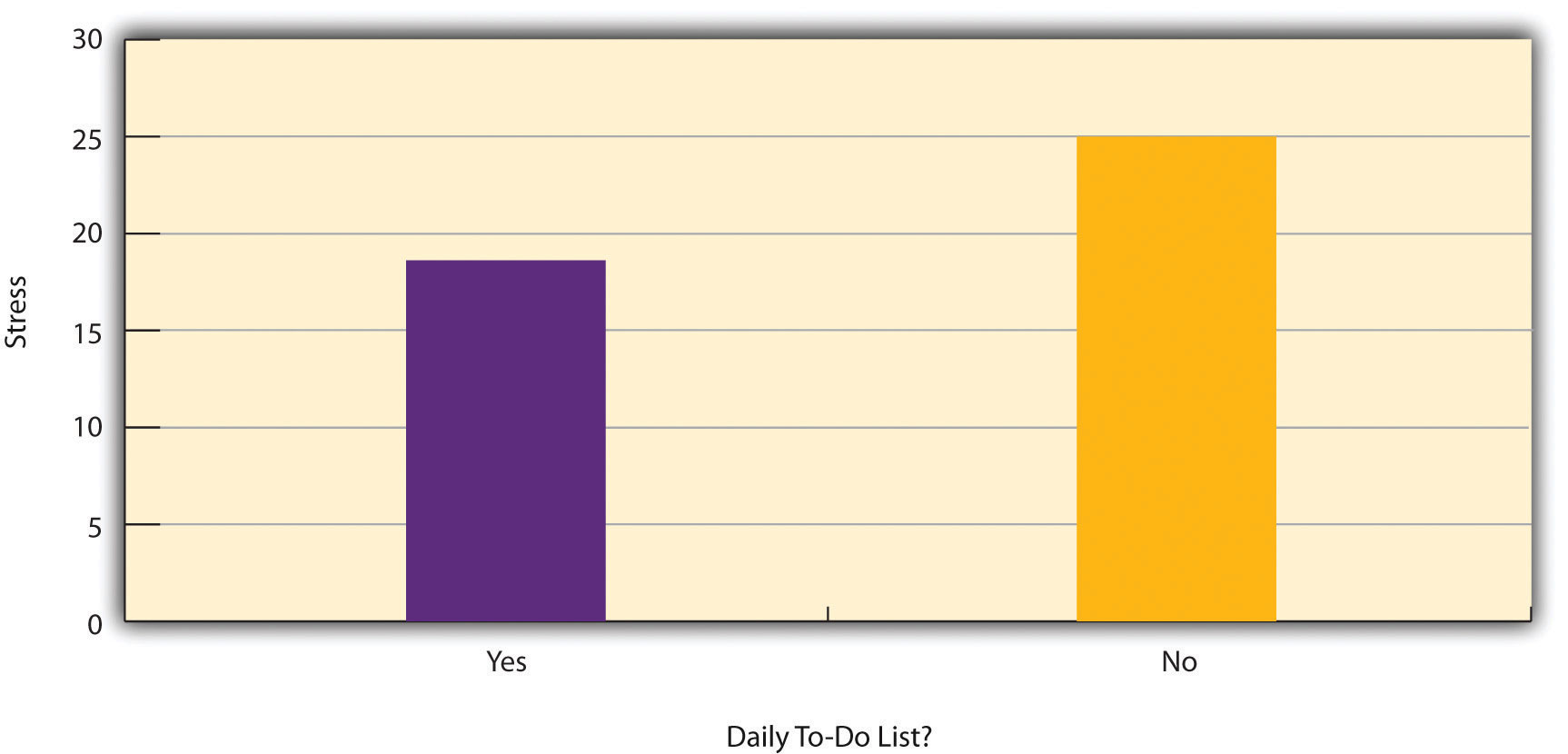
Data Collection in Correlational Research
Again, the defining feature of correlational research is that neither variable is manipulated. It does not matter how or where the variables are measured. A researcher could have participants come to a laboratory to complete a computerized backward digit span task and a computerized risky decision-making task and then assess the relationship between participants’ scores on the two tasks. Or a researcher could go to a shopping mall to ask people about their attitudes toward the environment and their shopping habits and then assess the relationship between these two variables. Both of these studies would be correlational because no independent variable is manipulated.
Correlations Between Quantitative Variables
Correlations between quantitative variables are often presented using scatterplots . Figure 6.3 shows some hypothetical data on the relationship between the amount of stress people are under and the number of physical symptoms they have. Each point in the scatterplot represents one person’s score on both variables. For example, the circled point in Figure 6.3 represents a person whose stress score was 10 and who had three physical symptoms. Taking all the points into account, one can see that people under more stress tend to have more physical symptoms. This is a good example of a positive relationship , in which higher scores on one variable tend to be associated with higher scores on the other. In other words, they move in the same direction, either both up or both down. A negative relationship is one in which higher scores on one variable tend to be associated with lower scores on the other. In other words, they move in opposite directions. There is a negative relationship between stress and immune system functioning, for example, because higher stress is associated with lower immune system functioning.
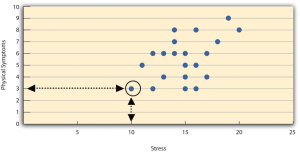
The strength of a correlation between quantitative variables is typically measured using a statistic called Pearson’s Correlation Coefficient (or Pearson's r ) . As Figure 6.4 shows, Pearson’s r ranges from −1.00 (the strongest possible negative relationship) to +1.00 (the strongest possible positive relationship). A value of 0 means there is no relationship between the two variables. When Pearson’s r is 0, the points on a scatterplot form a shapeless “cloud.” As its value moves toward −1.00 or +1.00, the points come closer and closer to falling on a single straight line. Correlation coefficients near ±.10 are considered small, values near ± .30 are considered medium, and values near ±.50 are considered large. Notice that the sign of Pearson’s r is unrelated to its strength. Pearson’s r values of +.30 and −.30, for example, are equally strong; it is just that one represents a moderate positive relationship and the other a moderate negative relationship. With the exception of reliability coefficients, most correlations that we find in Psychology are small or moderate in size. The website http://rpsychologist.com/d3/correlation/ , created by Kristoffer Magnusson, provides an excellent interactive visualization of correlations that permits you to adjust the strength and direction of a correlation while witnessing the corresponding changes to the scatterplot.
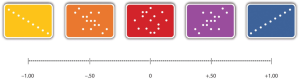
There are two common situations in which the value of Pearson’s r can be misleading. Pearson’s r is a good measure only for linear relationships, in which the points are best approximated by a straight line. It is not a good measure for nonlinear relationships, in which the points are better approximated by a curved line. Figure 6.5, for example, shows a hypothetical relationship between the amount of sleep people get per night and their level of depression. In this example, the line that best approximates the points is a curve—a kind of upside-down “U”—because people who get about eight hours of sleep tend to be the least depressed. Those who get too little sleep and those who get too much sleep tend to be more depressed. Even though Figure 6.5 shows a fairly strong relationship between depression and sleep, Pearson’s r would be close to zero because the points in the scatterplot are not well fit by a single straight line. This means that it is important to make a scatterplot and confirm that a relationship is approximately linear before using Pearson’s r . Nonlinear relationships are fairly common in psychology, but measuring their strength is beyond the scope of this book.
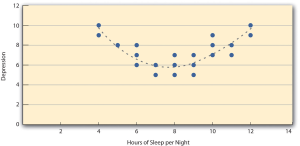
The other common situations in which the value of Pearson’s r can be misleading is when one or both of the variables have a limited range in the sample relative to the population. This problem is referred to as restriction of range . Assume, for example, that there is a strong negative correlation between people’s age and their enjoyment of hip hop music as shown by the scatterplot in Figure 6.6. Pearson’s r here is −.77. However, if we were to collect data only from 18- to 24-year-olds—represented by the shaded area of Figure 6.6—then the relationship would seem to be quite weak. In fact, Pearson’s r for this restricted range of ages is 0. It is a good idea, therefore, to design studies to avoid restriction of range. For example, if age is one of your primary variables, then you can plan to collect data from people of a wide range of ages. Because restriction of range is not always anticipated or easily avoidable, however, it is good practice to examine your data for possible restriction of range and to interpret Pearson’s r in light of it. (There are also statistical methods to correct Pearson’s r for restriction of range, but they are beyond the scope of this book).
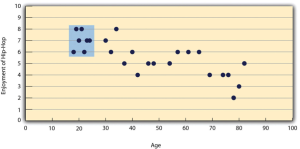
Correlation Does Not Imply Causation
You have probably heard repeatedly that “Correlation does not imply causation.” An amusing example of this comes from a 2012 study that showed a positive correlation (Pearson’s r = 0.79) between the per capita chocolate consumption of a nation and the number of Nobel prizes awarded to citizens of that nation [2] . It seems clear, however, that this does not mean that eating chocolate causes people to win Nobel prizes, and it would not make sense to try to increase the number of Nobel prizes won by recommending that parents feed their children more chocolate.
There are two reasons that correlation does not imply causation. The first is called the directionality problem . Two variables, X and Y , can be statistically related because X causes Y or because Y causes X . Consider, for example, a study showing that whether or not people exercise is statistically related to how happy they are—such that people who exercise are happier on average than people who do not. This statistical relationship is consistent with the idea that exercising causes happiness, but it is also consistent with the idea that happiness causes exercise. Perhaps being happy gives people more energy or leads them to seek opportunities to socialize with others by going to the gym. The second reason that correlation does not imply causation is called the third-variable problem . Two variables, X and Y , can be statistically related not because X causes Y , or because Y causes X , but because some third variable, Z , causes both X and Y . For example, the fact that nations that have won more Nobel prizes tend to have higher chocolate consumption probably reflects geography in that European countries tend to have higher rates of per capita chocolate consumption and invest more in education and technology (once again, per capita) than many other countries in the world. Similarly, the statistical relationship between exercise and happiness could mean that some third variable, such as physical health, causes both of the others. Being physically healthy could cause people to exercise and cause them to be happier. Correlations that are a result of a third-variable are often referred to as spurious correlations .
Some excellent and amusing examples of spurious correlations can be found at http://www.tylervigen.com (Figure 6.7 provides one such example).
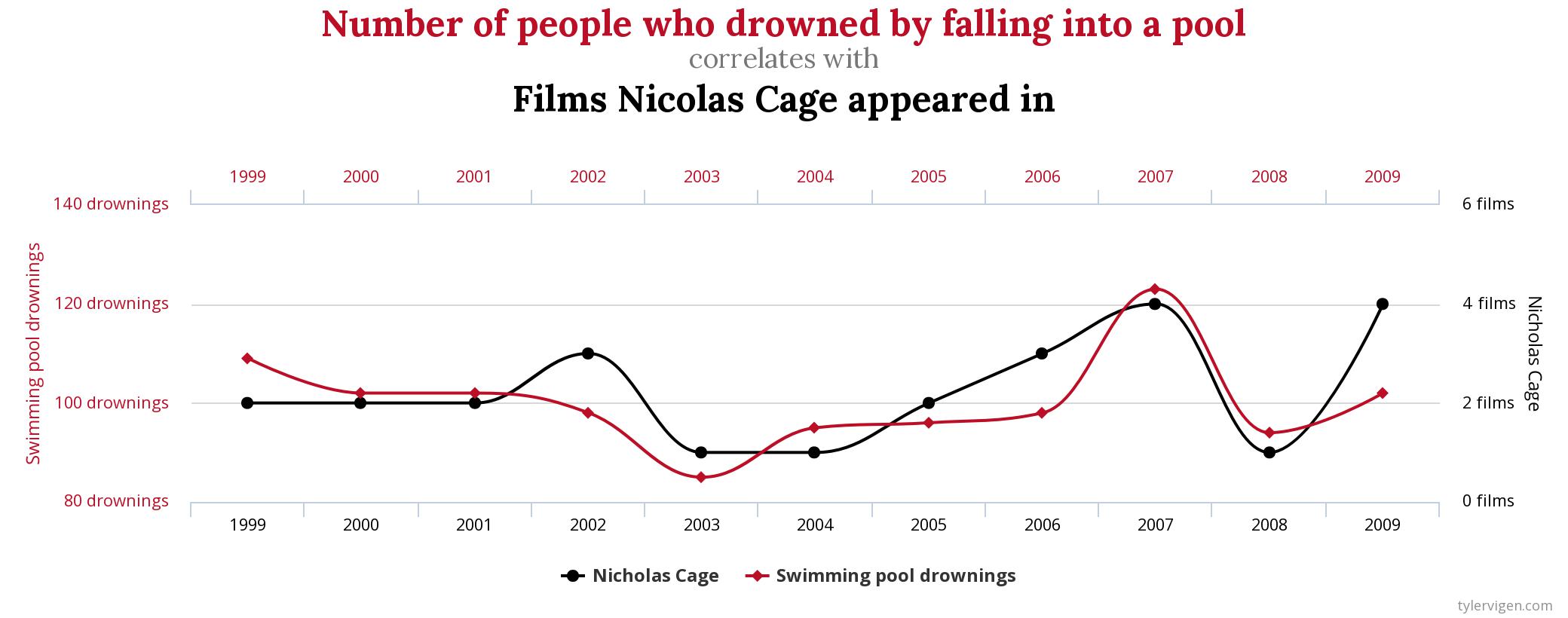
“Lots of Candy Could Lead to Violence”
Although researchers in psychology know that correlation does not imply causation, many journalists do not. One website about correlation and causation, http://jonathan.mueller.faculty.noctrl.edu/100/correlation_or_causation.htm , links to dozens of media reports about real biomedical and psychological research. Many of the headlines suggest that a causal relationship has been demonstrated when a careful reading of the articles shows that it has not because of the directionality and third-variable problems.
One such article is about a study showing that children who ate candy every day were more likely than other children to be arrested for a violent offense later in life. But could candy really “lead to” violence, as the headline suggests? What alternative explanations can you think of for this statistical relationship? How could the headline be rewritten so that it is not misleading?
As you have learned by reading this book, there are various ways that researchers address the directionality and third-variable problems. The most effective is to conduct an experiment. For example, instead of simply measuring how much people exercise, a researcher could bring people into a laboratory and randomly assign half of them to run on a treadmill for 15 minutes and the rest to sit on a couch for 15 minutes. Although this seems like a minor change to the research design, it is extremely important. Now if the exercisers end up in more positive moods than those who did not exercise, it cannot be because their moods affected how much they exercised (because it was the researcher who used random assignment to determine how much they exercised). Likewise, it cannot be because some third variable (e.g., physical health) affected both how much they exercised and what mood they were in. Thus experiments eliminate the directionality and third-variable problems and allow researchers to draw firm conclusions about causal relationships.
Media Attributions
- Nicholas Cage and Pool Drownings © Tyler Viegen is licensed under a CC BY (Attribution) license
- Bushman, B. J., & Huesmann, L. R. (2001). Effects of televised violence on aggression. In D. Singer & J. Singer (Eds.), Handbook of children and the media (pp. 223–254). Thousand Oaks, CA: Sage. ↵
- Messerli, F. H. (2012). Chocolate consumption, cognitive function, and Nobel laureates. New England Journal of Medicine, 367 , 1562-1564. ↵
A graph that presents correlations between two quantitative variables, one on the x-axis and one on the y-axis. Scores are plotted at the intersection of the values on each axis.
A relationship in which higher scores on one variable tend to be associated with higher scores on the other.
A relationship in which higher scores on one variable tend to be associated with lower scores on the other.
A statistic that measures the strength of a correlation between quantitative variables.
When one or both variables have a limited range in the sample relative to the population, making the value of the correlation coefficient misleading.
The problem where two variables, X and Y , are statistically related either because X causes Y, or because Y causes X , and thus the causal direction of the effect cannot be known.
Two variables, X and Y, can be statistically related not because X causes Y, or because Y causes X, but because some third variable, Z, causes both X and Y.
Correlations that are a result not of the two variables being measured, but rather because of a third, unmeasured, variable that affects both of the measured variables.
Correlational Research Copyright © 2022 by Rajiv S. Jhangiani; I-Chant A. Chiang; Carrie Cuttler; and Dana C. Leighton is licensed under a Creative Commons Attribution-NonCommercial-ShareAlike 4.0 International License , except where otherwise noted.
Share This Book
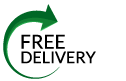
The Plagiarism Checker Online For Your Academic Work
Start Plagiarism Check
Editing & Proofreading for Your Research Paper
Get it proofread now
Online Printing & Binding with Free Express Delivery
Configure binding now
- Academic essay overview
- The writing process
- Structuring academic essays
- Types of academic essays
- Academic writing overview
- Sentence structure
- Academic writing process
- Improving your academic writing
- Titles and headings
- APA style overview
- APA citation & referencing
- APA structure & sections
- Citation & referencing
- Structure and sections
- APA examples overview
- Commonly used citations
- Other examples
- British English vs. American English
- Chicago style overview
- Chicago citation & referencing
- Chicago structure & sections
- Chicago style examples
- Citing sources overview
- Citation format
- Citation examples
- College essay overview
- Application
- How to write a college essay
- Types of college essays
- Commonly confused words
- Definitions
- Dissertation overview
- Dissertation structure & sections
- Dissertation writing process
- Graduate school overview
- Application & admission
- Study abroad
- Master degree
- Harvard referencing overview
- Language rules overview
- Grammatical rules & structures
- Parts of speech
- Punctuation
- Methodology overview
- Analyzing data
- Experiments
- Observations
- Inductive vs. Deductive
- Qualitative vs. Quantitative
- Types of validity
- Types of reliability
- Sampling methods
- Theories & Concepts
- Types of research studies
- Types of variables
- MLA style overview
- MLA examples
- MLA citation & referencing
- MLA structure & sections
- Plagiarism overview
- Plagiarism checker
- Types of plagiarism
- Printing production overview
- Research bias overview
- Types of research bias
- Example sections
- Types of research papers
- Research process overview
- Problem statement
- Research proposal
- Research topic
- Statistics overview
- Levels of measurment
- Frequency distribution
- Measures of central tendency
- Measures of variability
- Hypothesis testing
- Parameters & test statistics
- Types of distributions
- Correlation
- Effect size
- Hypothesis testing assumptions
- Types of ANOVAs
- Types of chi-square
- Statistical data
- Statistical models
- Spelling mistakes
- Tips overview
- Academic writing tips
- Dissertation tips
- Sources tips
- Working with sources overview
- Evaluating sources
- Finding sources
- Including sources
- Types of sources
Your Step to Success
Plagiarism Check within 10min
Printing & Binding with 3D Live Preview
Correlational Research – How and When to Use It
How do you like this article cancel reply.
Save my name, email, and website in this browser for the next time I comment.
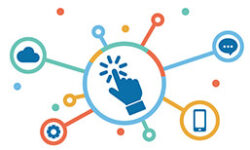
Correlational research, a commonly used methodology in academic writing, aids in determining the degree of relationship between two variables. Understanding its proper execution can empower you to uncover intriguing patterns and relationships, strengthening the depth and breadth of your study’s conclusions. Learn about correlational research and how to use it in your paper properly below. Harness its potential to elevate your research impact.
Inhaltsverzeichnis
- 1 Correlational Research – In a Nutshell
- 2 Definition: Correlational research
- 3 Correlational research vs. experimental research
- 4 When correlational research is used
- 5 Data collection methods in correlational research
- 6 How to analyze correlational data
Correlational Research – In a Nutshell
- Correlational research is used to measure the statistical relationship of two (or more) variables.
- Researchers tend to conduct correlational research when they don’t expect a causal relationship or when manipulation of variables is not possible.
- You can apply many data-gathering methods, including in-person methods and secondary, archival data collection , to correlational research.
Definition: Correlational research
Correlational research examines the relationship between variables. It is done without manipulating any of the variables themselves and is therefore used to measure the strength or direction of a relationship if one is found.
The direction of this relationship can be positive, negative , or non-existent (zero).

Correlational research vs. experimental research
Correlational research and experimental research are both used to study relationships with quantitative methods. However, there are some crucial differences:
When correlational research is used
Correlational research should be utilized for data gathering when looking for general results in real-world studies.
Correlational research can help determine whether variables are related within a group, which can then be applied to other situations.
Investigating non-causal relationships
If you want to discover whether a relationship between two variables exists, but you’re not expecting it to be causal in nature, correlational research can be valuable.
Correlational research is particularly useful for recognizing general patterns, that can then help inform decisions.
You may investigate the political voting patterns of families that sit down for dinner together.
It’s unlikely that family dinners cause people to vote a particular way (non-causal), but it could be indicative of other ideological or religious issues that can be applied to voting predictions.
Exploring causal relationships between variables
Correlational research can be used to identify causation when experimental research is too costly or unethical.
It may provide the impetus for further research as a base dataset to help develop causal theories.
You’d like to study the relationship between sewage treatment and gastroenteritis.
While a strong causal relationship can be found, you cannot ethically control the effects of sewage treatment on a population.
Testing new measurement tools
If you’ve developed a new tool for measuring a variable and its effects, you can use correlational research to test its reliability.
You’ve created a new scale for measuring life satisfaction.
To test the scale, you collect data on life satisfaction using existing tools and your new test scale.
Correlation between the different measurements indicates reliability in the new scale.
Data collection methods in correlational research
There are many data collection methods to choose from in the social sciences. These include everything from surveys and observation methods to historical statistical data.
Choosing the correct method is crucial to creating a solid piece of research. Begin by choosing a representational sample that’s free from personal bias.
Surveys are a common method for generating strong data and results. Commonly gathered through questionnaires, you can conduct this research in person or on the street, or with postal, phone, or online surveys where you can’t guide the subject(s).
Surveys are useful because they’re quick and flexible. Still, you must avoid bias when wording surveys and keep data relevant without guiding the answer(s).
Naturalistic observation
Naturalistic observation is a form of field research that involves studying real effects as they happen in real life. It can involve recording events like counting visitors to an exhibit or describing actions as they happen.
While naturalistic observation can be both qualitative and quantitative, the correlation should be analyzed through quantitative data.
Naturalistic observation is crucial for recording real-world events and context, but it can be extremely time-consuming. As it’s done personally, bias is also possible and must be addressed in the methodology outline.
You may want to study attention spans in college lectures based on gender.
You’d have to record all observations of students checking smartphones, for instance, as an indicator of engagement.
Secondary data
Secondary data is any data that you haven’t conducted yourself. This can be an extremely valuable and effective source of historical correlational research. The more comprehensive and official the data, the more reliable the research.
Census studies, historic polls, and other records are all potential data sources.
While inexpensive and ready-made, secondary data does lack reliability if you cannot check how data is gathered.
You could study the effects of alcohol consumption on the health of a population.
For this, you’d rely on historic consumption studies and historic hospital data.
When comparing this data across countries, you do risk comparing unequal data gathering methods, as not all countries record data similarly. However, you can still propose a general correlation.
How to analyze correlational data
Data alone isn’t capable of indicating correlation. You need to analyze it to elaborate on the relationship. You can do this through correlation or regression analysis.
Regression analysis
Regression analysis predicts how much change in one variable affects change in another.
This is visualized with a regression equation that describes the relationship on a graph. This equation can then be used to predict the value of a certain variable based on the value of another.
Only use regression analysis after correlation is confirmed
Correlation analysis
Correlation analysis is used to create a correlation coefficient, where the strength of a relationship and its direction are described by a number. This number expresses the degree or intensity of this correlation.
Most social studies utilize the Pearson product-moment coefficient, where an r is used to assess linear relationships.
What is the importance of correlational research?
Correlational research is important because it identifies and provides insight into real-life relationships. This can be used to develop effective policies and theories, or conduct more thorough experimental studies.
What is a correlation?
Correlation is a mutual connection between variables that isn’t always causal.
How many variables are involved in correlational research?
At least two variables are traditionally studied in correlational research – but it’s also possible to study more than three.
We use cookies on our website. Some of them are essential, while others help us to improve this website and your experience.
- External Media
Individual Privacy Preferences
Cookie Details Privacy Policy Imprint
Here you will find an overview of all cookies used. You can give your consent to whole categories or display further information and select certain cookies.
Accept all Save
Essential cookies enable basic functions and are necessary for the proper function of the website.
Show Cookie Information Hide Cookie Information
Statistics cookies collect information anonymously. This information helps us to understand how our visitors use our website.
Content from video platforms and social media platforms is blocked by default. If External Media cookies are accepted, access to those contents no longer requires manual consent.
Privacy Policy Imprint
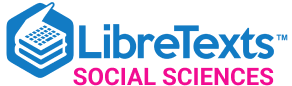
- school Campus Bookshelves
- menu_book Bookshelves
- perm_media Learning Objects
- login Login
- how_to_reg Request Instructor Account
- hub Instructor Commons
- Download Page (PDF)
- Download Full Book (PDF)
- Periodic Table
- Physics Constants
- Scientific Calculator
- Reference & Cite
- Tools expand_more
- Readability
selected template will load here
This action is not available.
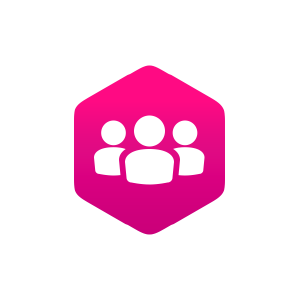
5.3: Correlational Research
- Last updated
- Save as PDF
- Page ID 20165
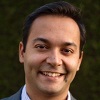
- Rajiv S. Jhangiani, I-Chant A. Chiang, Carrie Cuttler, & Dana C. Leighton
- Kwantlen Polytechnic U., Washington State U., & Texas A&M U.—Texarkana
- Define correlational research and give several examples.
- Explain why a researcher might choose to conduct correlational research rather than experimental research or another type of non-experimental research.
- Interpret the strength and direction of different correlation coefficients.
- Explain why correlation does not imply causation.
What Is Correlational Research?
Correlational research is a type of non-experimental research in which the researcher measures two variables (binary or continuous) and assesses the statistical relationship (i.e., the correlation) between them with little or no effort to control extraneous variables. There are many reasons that researchers interested in statistical relationships between variables would choose to conduct a correlational study rather than an experiment. The first is that they do not believe that the statistical relationship is a causal one or are not interested in causal relationships. Recall two goals of science are to describe and to predict and the correlational research strategy allows researchers to achieve both of these goals. Specifically, this strategy can be used to describe the strength and direction of the relationship between two variables and if there is a relationship between the variables then the researchers can use scores on one variable to predict scores on the other (using a statistical technique called regression, which is discussed further in the section on Complex Correlation in this chapter).
Another reason that researchers would choose to use a correlational study rather than an experiment is that the statistical relationship of interest is thought to be causal, but the researcher cannot manipulate the independent variable because it is impossible, impractical, or unethical. For example, while a researcher might be interested in the relationship between the frequency people use cannabis and their memory abilities they cannot ethically manipulate the frequency that people use cannabis. As such, they must rely on the correlational research strategy; they must simply measure the frequency that people use cannabis and measure their memory abilities using a standardized test of memory and then determine whether the frequency people use cannabis is statistically related to memory test performance.
Correlation is also used to establish the reliability and validity of measurements. For example, a researcher might evaluate the validity of a brief extraversion test by administering it to a large group of participants along with a longer extraversion test that has already been shown to be valid. This researcher might then check to see whether participants’ scores on the brief test are strongly correlated with their scores on the longer one. Neither test score is thought to cause the other, so there is no independent variable to manipulate. In fact, the terms independent variable and dependent variabl e do not apply to this kind of research.
Another strength of correlational research is that it is often higher in external validity than experimental research. Recall there is typically a trade-off between internal validity and external validity. As greater controls are added to experiments, internal validity is increased but often at the expense of external validity as artificial conditions are introduced that do not exist in reality. In contrast, correlational studies typically have low internal validity because nothing is manipulated or controlled but they often have high external validity. Since nothing is manipulated or controlled by the experimenter the results are more likely to reflect relationships that exist in the real world.
Finally, extending upon this trade-off between internal and external validity, correlational research can help to provide converging evidence for a theory. If a theory is supported by a true experiment that is high in internal validity as well as by a correlational study that is high in external validity then the researchers can have more confidence in the validity of their theory. As a concrete example, correlational studies establishing that there is a relationship between watching violent television and aggressive behavior have been complemented by experimental studies confirming that the relationship is a causal one (Bushman & Huesmann, 2001) [1] .
A common misconception among beginning researchers is that correlational research must involve two quantitative variables, such as scores on two extraversion tests or the number of daily hassles and number of symptoms people have experienced. However, the defining feature of correlational research is that the two variables are measured—neither one is manipulated—and this is true regardless of whether the variables are quantitative or categorical. Imagine, for example, that a researcher administers the Rosenberg Self-Esteem Scale to 50 American college students and 50 Japanese college students. Although this “feels” like a between-subjects experiment, it is a correlational study because the researcher did not manipulate the students’ nationalities. The same is true of the study by Cacioppo and Petty comparing college faculty and factory workers in terms of their need for cognition. It is a correlational study because the researchers did not manipulate the participants’ occupations.
Figure \(\PageIndex{1}\) shows data from a hypothetical study on the relationship between whether people make a daily list of things to do (a “to-do list”) and stress. Notice that it is unclear whether this is an experiment or a correlational study because it is unclear whether the independent variable was manipulated. If the researcher randomly assigned some participants to make daily to-do lists and others not to, then it is an experiment. If the researcher simply asked participants whether they made daily to-do lists, then it is a correlational study. The distinction is important because if the study was an experiment, then it could be concluded that making the daily to-do lists reduced participants’ stress. But if it was a correlational study, it could only be concluded that these variables are statistically related. Perhaps being stressed has a negative effect on people’s ability to plan ahead (the directionality problem). Or perhaps people who are more conscientious are more likely to make to-do lists and less likely to be stressed (the third-variable problem). The crucial point is that what defines a study as experimental or correlational is not the variables being studied, nor whether the variables are quantitative or categorical, nor the type of graph or statistics used to analyze the data. What defines a study is how the study is conducted.
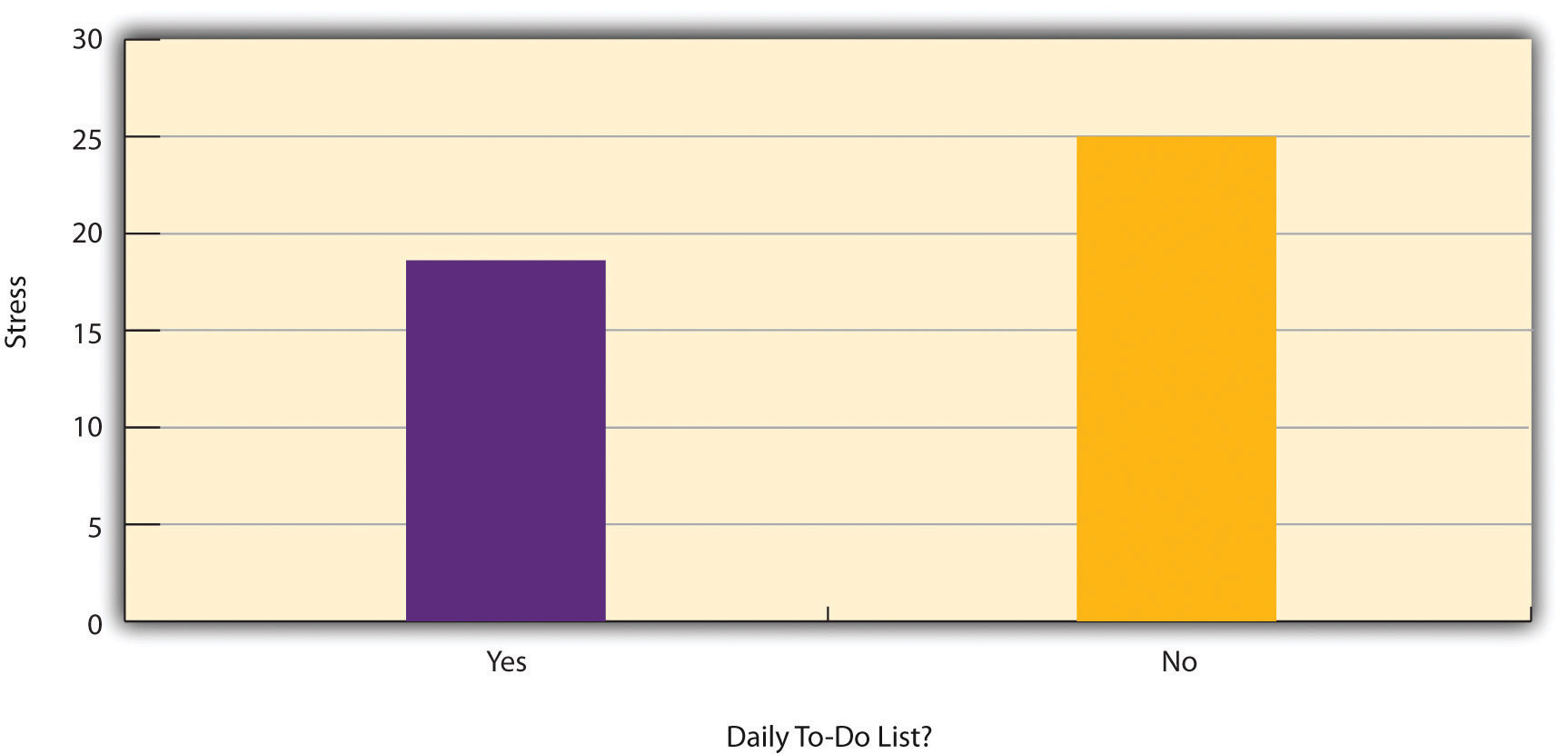
Data Collection in Correlational Research
Again, the defining feature of correlational research is that neither variable is manipulated. It does not matter how or where the variables are measured. A researcher could have participants come to a laboratory to complete a computerized backward digit span task and a computerized risky decision-making task and then assess the relationship between participants’ scores on the two tasks. Or a researcher could go to a shopping mall to ask people about their attitudes toward the environment and their shopping habits and then assess the relationship between these two variables. Both of these studies would be correlational because no independent variable is manipulated.
scatterplots. Figure \(\PageIndex{2}\) shows some hypothetical data on the relationship between the amount of stress people are under and the number of physical symptoms they have. Each point in the scatterplot represents one person’s score on both variables. For example, the circled point in Figure \(\PageIndex{2}\) represents a person whose stress score was 10 and who had three physical symptoms. Taking all the points into account, one can see that people under more stress tend to have more physical symptoms. This is a good example of a positive relationship , in which higher scores on one variable tend to be associated with higher scores on the other. In other words, they move in the same direction, either both up or both down. A negative relationship is one in which higher scores on one variable tend to be associated with lower scores on the other. In other words, they move in opposite directions. There is a negative relationship between stress and immune system functioning, for example, because higher stress is associated with lower immune system functioning.
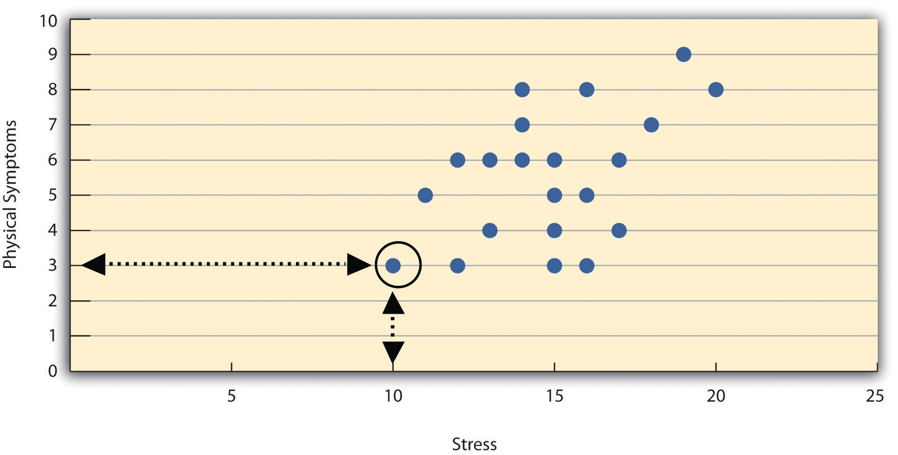
The strength of a correlation between quantitative variables is typically measured using a statistic called Pearson’s Correlation Coefficient (or Pearson's r ) . As Figure \(\PageIndex{3}\) shows, Pearson’s r ranges from −1.00 (the strongest possible negative relationship) to +1.00 (the strongest possible positive relationship). A value of 0 means there is no relationship between the two variables. When Pearson’s r is 0, the points on a scatterplot form a shapeless “cloud.” As its value moves toward −1.00 or +1.00, the points come closer and closer to falling on a single straight line. Correlation coefficients near ±.10 are considered small, values near ± .30 are considered medium, and values near ±.50 are considered large. Notice that the sign of Pearson’s r is unrelated to its strength. Pearson’s r values of +.30 and −.30, for example, are equally strong; it is just that one represents a moderate positive relationship and the other a moderate negative relationship. With the exception of reliability coefficients, most correlations that we find in Psychology are small or moderate in size. The website http://rpsychologist.com/d3/correlation/ , created by Kristoffer Magnusson, provides an excellent interactive visualization of correlations that permits you to adjust the strength and direction of a correlation while witnessing the corresponding changes to the scatterplot.
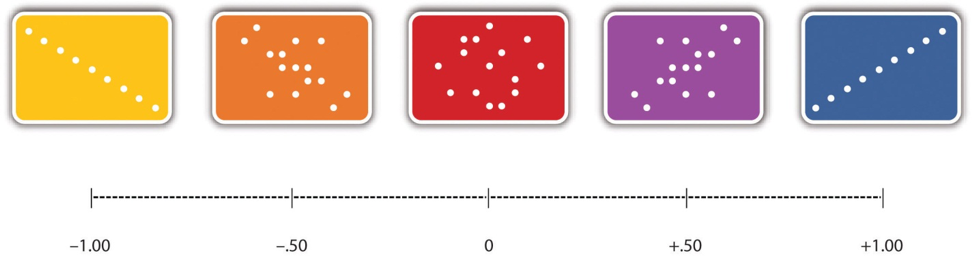
There are two common situations in which the value of Pearson’s r can be misleading. Pearson’s r is a good measure only for linear relationships, in which the points are best approximated by a straight line. It is not a good measure for nonlinear relationships, in which the points are better approximated by a curved line. Figure \(\PageIndex{4}\), for example, shows a hypothetical relationship between the amount of sleep people get per night and their level of depression. In this example, the line that best approximates the points is a curve—a kind of upside-down “U”—because people who get about eight hours of sleep tend to be the least depressed. Those who get too little sleep and those who get too much sleep tend to be more depressed. Even though Figure \(\PageIndex{4}\) shows a fairly strong relationship between depression and sleep, Pearson’s r would be close to zero because the points in the scatterplot are not well fit by a single straight line. This means that it is important to make a scatterplot and confirm that a relationship is approximately linear before using Pearson’s r . Nonlinear relationships are fairly common in psychology, but measuring their strength is beyond the scope of this book.
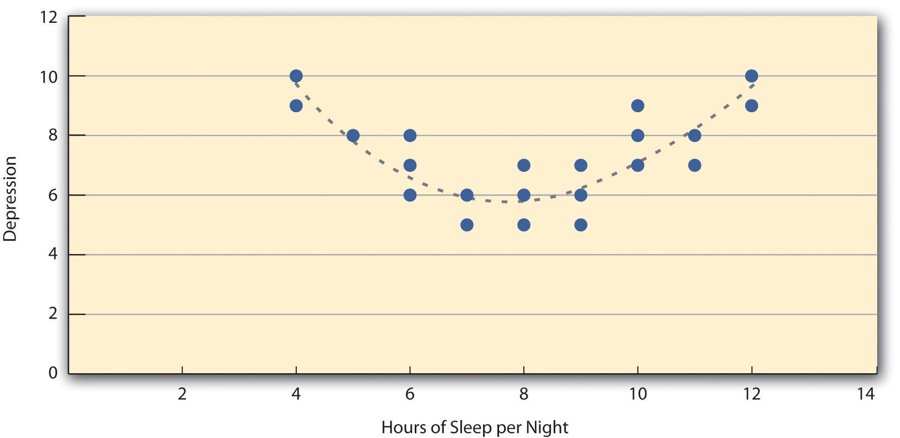
The other common situations in which the value of Pearson’s r can be misleading is when one or both of the variables have a limited range in the sample relative to the population. This problem is referred to as restriction of range . Assume, for example, that there is a strong negative correlation between people’s age and their enjoyment of hip hop music as shown by the scatterplot in Figure \(\PageIndex{5}\). Pearson’s r here is −.77. However, if we were to collect data only from 18- to 24-year-olds—represented by the shaded area of Figure \(\PageIndex{5}\)—then the relationship would seem to be quite weak. In fact, Pearson’s r for this restricted range of ages is 0. It is a good idea, therefore, to design studies to avoid restriction of range. For example, if age is one of your primary variables, then you can plan to collect data from people of a wide range of ages. Because restriction of range is not always anticipated or easily avoidable, however, it is good practice to examine your data for possible restriction of range and to interpret Pearson’s r in light of it. (There are also statistical methods to correct Pearson’s r for restriction of range, but they are beyond the scope of this book).
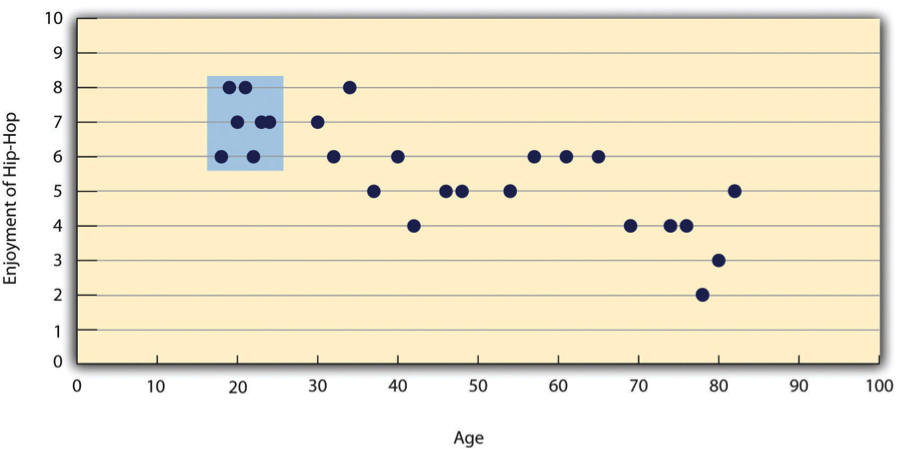
Correlation Does Not Imply Causation
You have probably heard repeatedly that “Correlation does not imply causation.” An amusing example of this comes from a 2012 study that showed a positive correlation (Pearson’s r = 0.79) between the per capita chocolate consumption of a nation and the number of Nobel prizes awarded to citizens of that nation [2] . It seems clear, however, that this does not mean that eating chocolate causes people to win Nobel prizes, and it would not make sense to try to increase the number of Nobel prizes won by recommending that parents feed their children more chocolate.
There are two reasons that correlation does not imply causation. The first is called the directionality problem . Two variables, X and Y , can be statistically related because X causes Y or because Y causes X . Consider, for example, a study showing that whether or not people exercise is statistically related to how happy they are—such that people who exercise are happier on average than people who do not. This statistical relationship is consistent with the idea that exercising causes happiness, but it is also consistent with the idea that happiness causes exercise. Perhaps being happy gives people more energy or leads them to seek opportunities to socialize with others by going to the gym. The second reason that correlation does not imply causation is called the third-variable problem . Two variables, X and Y , can be statistically related not because X causes Y , or because Y causes X , but because some third variable, Z , causes both X and Y . For example, the fact that nations that have won more Nobel prizes tend to have higher chocolate consumption probably reflects geography in that European countries tend to have higher rates of per capita chocolate consumption and invest more in education and technology (once again, per capita) than many other countries in the world. Similarly, the statistical relationship between exercise and happiness could mean that some third variable, such as physical health, causes both of the others. Being physically healthy could cause people to exercise and cause them to be happier. Correlations that are a result of a third-variable are often referred to as spurious correlations.
Some excellent and amusing examples of spurious correlations can be found at http://www.tylervigen.com (Figure \(\PageIndex{6}\) provides one such example).
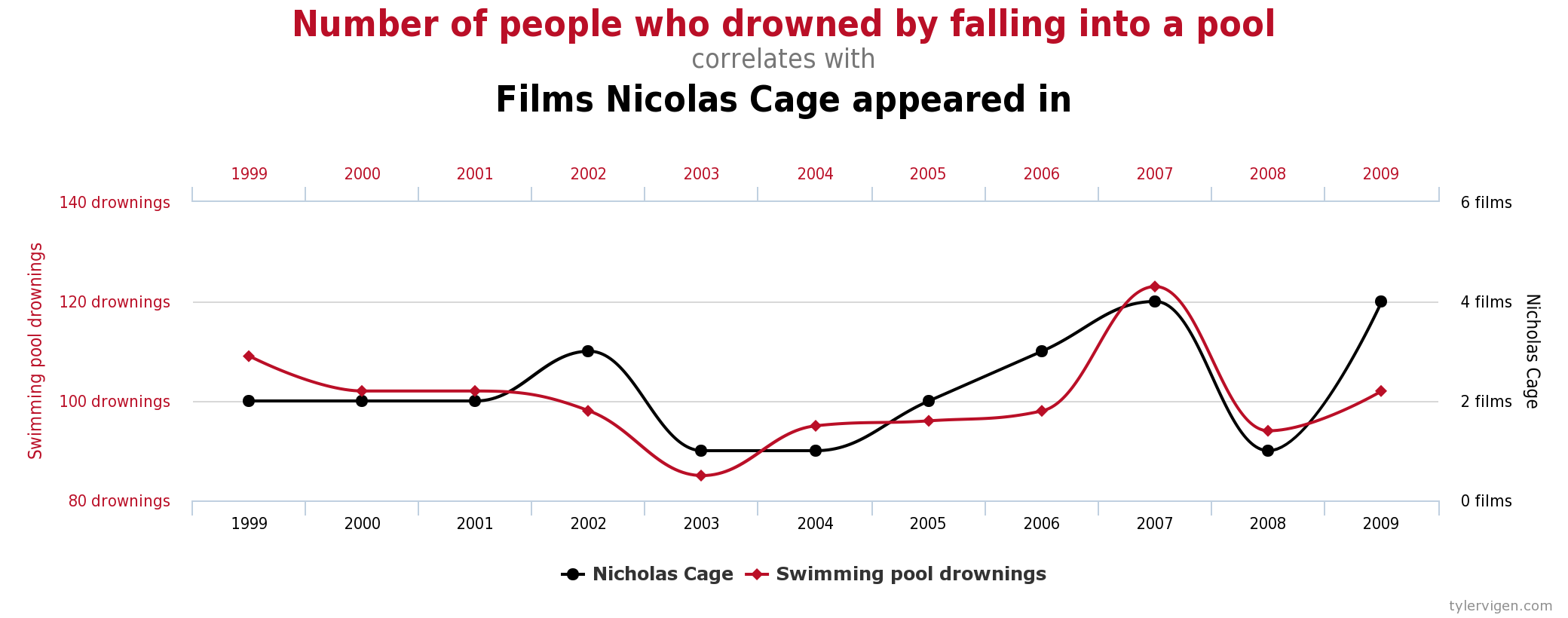
Although researchers in psychology know that correlation does not imply causation, many journalists do not. One website about correlation and causation, http://jonathan.mueller.faculty.noctrl.edu/100/correlation_or_causation.htm , links to dozens of media reports about real biomedical and psychological research. Many of the headlines suggest that a causal relationship has been demonstrated when a careful reading of the articles shows that it has not because of the directionality and third-variable problems.
One such article is about a study showing that children who ate candy every day were more likely than other children to be arrested for a violent offense later in life. But could candy really “lead to” violence, as the headline suggests? What alternative explanations can you think of for this statistical relationship? How could the headline be rewritten so that it is not misleading?
As you have learned by reading this book, there are various ways that researchers address the directionality and third-variable problems. The most effective is to conduct an experiment. For example, instead of simply measuring how much people exercise, a researcher could bring people into a laboratory and randomly assign half of them to run on a treadmill for 15 minutes and the rest to sit on a couch for 15 minutes. Although this seems like a minor change to the research design, it is extremely important. Now if the exercisers end up in more positive moods than those who did not exercise, it cannot be because their moods affected how much they exercised (because it was the researcher who used random assignment to determine how much they exercised). Likewise, it cannot be because some third variable (e.g., physical health) affected both how much they exercised and what mood they were in. Thus experiments eliminate the directionality and third-variable problems and allow researchers to draw firm conclusions about causal relationships.
- Bushman, B. J., & Huesmann, L. R. (2001). Effects of televised violence on aggression. In D. Singer & J. Singer (Eds.), Handbook of children and the media (pp. 223–254). Thousand Oaks, CA: Sage. ↵
- Messerli, F. H. (2012). Chocolate consumption, cognitive function, and Nobel laureates. New England Journal of Medicine, 367 , 1562-1564. ↵

- News Releases
New research confirms plastic production is directly linked to plastic pollution
udy finds that more than half of global branded plastic pollution can be tracked to just 56 companies
Break Free From Plastic
Volunteers with Greenpeace Indonesia record data on branded plastic waste during a brand audit in Jakarta, Indonesia on January 20, 2024.
Credit: Ezra Acayan, Break Free From Plastic, 2024
APRIL 24, 2024 – A research paper published today in Science Advances reveals a direct correlation between plastic production and plastic pollution, such that every 1% increase in plastic production is associated with a 1% increase in plastic pollution in the environment. The study finds that fast-moving consumer goods companies disproportionately contribute to the problem more than household and retail companies. The study marks the first robust quantification of the global relationship between plastic production and pollution.
The research, led by scientists from a dozen different universities in the United States of America, Australia, the Philippines, New Zealand, Estonia, Chile, Sweden, Canada, and the United Kingdom, found that 56 global companies are responsible for more than half of all branded plastic pollution. The Coca-Cola Company was responsible for 11% of branded waste, followed by PepsiCo (5%), Nestlé (3%), Danone (3%), and Altria/Philip Morris International (2%). The top companies identified produce food, beverage, or tobacco products.
The five-year analysis used #BreakFreeFromPlastic brand audit data from 1,576 audit events across 84 countries. Brand audits are citizen science initiatives in which volunteers conduct waste clean-ups and document the brands found on the pollution collected. Over five years, more than 200,000 volunteers submitted data through Break Free From Plastic or 5 Gyres’ TrashBlitz app.
The strong relationship between plastic production and pollution, across geographies and widely varying waste management systems, suggests that reducing plastic production in the fast-moving consumer goods sector is a viable solution to curb global plastic pollution. As world leaders negotiate a Global Plastics Treaty at INC-4 this month in Ottawa, Canada, this research serves as a tool to support a high-ambition legally binding treaty that includes provisions on corporate accountability, prioritizing plastic production reduction measures, and promoting reuse and refill systems.
Read the paper here .
Co-Author Quotes:
“When I first saw the relationship between production and pollution, I was shocked. I wanted to throw up, it was the reality of my worst nightmare. It means that producers big and small are toeing the line, despite all the things big brands say they are doing, we see no positive impact from their efforts. But on the other hand, it gives me hope that fast-moving consumer goods companies reducing their plastic production and shifting towards more durable and reusable products would have a strong positive impact on the environment.”
- Win Cowger, Research Director, The Moore Institute for Plastic Pollution Research
“Our study underscores the critical role of corporate accountability in tackling plastic pollution. We, as individuals, are not responsible for the plastics crisis; the onus lies on these 56 global companies to take decisive action. I urge world leaders at INC-4 to listen to the science, and to consider the clear link between plastic production and pollution during negotiations for a Global Plastics Treaty.”
- Dr. Lisa Erdle, Director of Science & Innovation, The 5 Gyres Institute
“This scientific study affirms what activists and communities impacted by plastic pollution have been saying for years: the more plastic is produced, the more plastic is found in the environment. It’s that simple. Yet again, plastic polluters like The Coca-Cola Company, PepsiCo, and Nestlé continue to fail on their voluntary commitment to reduce their plastic footprint. We need a legally binding Global Plastics Treaty that mandates significant cuts in plastic production and stops corporations from flooding the planet with single-use plastic.”
- Sybil Bullock, Associate Campaign Manager, Break Free From Plastic
“The research identifies the top 56 multinational companies contributing to global branded plastic litter. Past studies have ranked countries like the Philippines, Indonesia, Sri Lanka, Bangladesh, Nigeria, etc. among the top sources of plastic waste into the ocean. This has led to a narrative in social media that blames poor countries for global plastic pollution, ignoring the fact that around the 1960s global companies flooded developing countries with cheap, single-use plastics displacing traditional biodegradable materials and sustainable reuse-refill systems which, in the case of the Philippines, dated back to the 16th century. The current study focuses instead on the role of corporations and global plastic production.”
- Dr. Jorge Emmanuel, Adjunct Professor and Research Faculty Fellow, Institute of Environmental & Marine Sciences, and College of Engineering & Design, Silliman University
“This research provides the first quantification of global producer contribution to branded plastic pollution. The findings suggest that single-use packaging significantly contributes to branded plastic pollution. This data can help inform ways to address plastic production and reduce plastic waste ending up in the environment.”
- Dr. Kathy Willis, Postdoctoral Fellow from CSIRO, Australia’s national science agency
About Break Free From Plastic (BFFP)
#BreakFreeFromPlastic is a global movement envisioning a future free from plastic pollution. Since its launch in 2016, more than 2,000 organizations and 11,000 individual supporters from across the world have joined the movement to demand massive reductions in single-use plastics and push for lasting solutions to the plastic pollution crisis. BFFP member organizations and individuals share the values of environmental protection and social justice and work together through a holistic approach to bring about systemic change. This means tackling plastic pollution across the whole plastics value chain—from extraction to disposal—focusing on prevention rather than cure and providing effective solutions. www.breakfreefromplastic.org .
About The 5 Gyres Institute
The 5 Gyres Institute (5 Gyres) is a leader in the global movement against plastic pollution with more than 10 years of expertise in scientific research, engagement, and education. With the original goal of answering a few key scientific questions about ocean plastics, co- founders Marcus Eriksen and Anna Cummins led 19 research expeditions in all five subtropical gyres, as well as many of the world’s lakes and rivers. 5 Gyres continues to lead with scientific research to drive upstream solutions through education, advocacy, and community building. Learn more at 5gyres.org and @5gyres .
Special Note to Reporters
More information, including a copy of the paper, can be found online at the Science Advances press package at https://www.eurekalert.org/press/vancepak/ . Several scientists who contributed to this paper will be present at INC-4 and available for interviews upon request.
Science Advances
10.1126/sciadv.adj8275
Method of Research
Data/statistical analysis
Subject of Research
Not applicable
Article Title
Global Producer Responsibility for Plastic Pollution
Article Publication Date
24-Apr-2024
COI Statement
Lead coauthor Win Cowger received funding from Break Free From Plastic to conduct the research resulting in this manuscript.
Disclaimer: AAAS and EurekAlert! are not responsible for the accuracy of news releases posted to EurekAlert! by contributing institutions or for the use of any information through the EurekAlert system.
Original Source
Translations.
An official website of the United States government
Here's how you know
Official websites use .gov A .gov website belongs to an official government organization in the United States.
Secure .gov websites use HTTPS. A lock ( Lock Locked padlock ) or https:// means you've safely connected to the .gov website. Share sensitive information only on official, secure websites.
Most NSF systems and services, including NSF.gov, Research.gov and FastLane, will be unavailable from Friday, April 26, 11 p.m. EDT – Saturday, April 27, 8:00 a.m. EDT due to extended maintenance. We apologize for any inconvenience.
Dear Colleague Letter: Using Long-Term Research Associated Data (ULTRA-Data)
April 23, 2024
Dear Colleague:
With this Dear Colleague Letter (DCL), the U.S. National Science Foundation (NSF) seeks to stimulate and encourage the use and reuse of data from environmental time series research to improve generalizable understanding in fields including (but not limited to) ecology, organismal evolution/adaptation, geoscience, and oceanography.
The collection and comparison of long-term environmental measurements are critical to generate an integrated understanding of how ecosystem components interact, test ecological and evolutionary theories, and support the development and testing of ecological models. To advance the understanding of long-term dynamics of populations, communities, and ecosystems, NSF has made substantial investments in the collection and archiving of long-term data. Projects like Hawaii Ocean Time-series (HOT), Arctic Observing Network (AON), Bermuda Atlantic Time-series Study (BATS), Centers for Transformative Environmental Monitoring Programs (CTEMPs), Critical Zone research (CZ), National Ecological Observatory Network (NEON), Long-Term Research in Environmental Biology (LTREB), and Long-term Ecological Research (LTER) sites collect environmental data, make observations, test hypotheses, and in some cases conduct experiments, but few resources are dedicated to accomplishing larger-scale synthesis.
Data collected by long-term projects are often multidisciplinary (including biology, chemistry, geology, and other fields of study) and may cover broad spatial scales in addition to an extended temporal aspect. These data are valuable because they can be used to explore regional, continental, and global scale questions regarding environmental and ecological processes. While all resulting data are publicly accessible, differences in how they are recorded, reported, and accessed, mean significant time and training may need to be invested to harmonize the data for use.
GOALS OF THE DCL
- Synthesize, compare, and/or combine long- and short-term datasets to advance understanding of ecosystem and environmental dynamics, ecology, and evolution;
- Conduct new modeling activities, including ecological or environmental forecasting;
- Increase the interoperability of data sets that are available from public repositories/databases such as the Community Surface Dynamics Modeling System (CSDMS), the Consortium of Universities for the Advancement of Hydrologic Science (CUAHSI), National Ecological Observatory Network (NEON), Biological & Chemical Oceanography Data Management Office (BCO-DMO), United States Antarctic Program Data Center (USAP-DC), Arctic Data Center, Environmental Data Initiative (EDI), EPA Environmental Dataset Gateway (EDG), Ocean Observing Initiative (OOI), and DataONE, Paleobiology Database;
- Propose workshops for both researchers and data scientists on accessing and using long-term data sets, with dissemination of the products to the scientific community (e.g., ESIIL).
NSF seeks to support diverse teams of investigators and institutions in the scientific activities that it funds. Submissions that benefit and involve the full breadth of the research community, including undergraduates, graduate students, postgraduates, and faculty at all institutions of higher education are encouraged.
Programs within the following NSF divisions/offices welcome submission of proposals responsive to this DCL. See the "How to Respond to this DCL" section for additional guidance on identifying a program.
Directorate for Biological Sciences
- Division of Environmental Biology
- Division of Integrative and Organismal Systems
Directorate for Geosciences
- Division of Earth Sciences
- Division of Ocean Sciences
- Office of Polar Programs
HOW TO RESPOND TO THIS DCL
Principal Investigators should contact program officers in the participating areas of NSF listed above about the suitability of submission to an individual program in response to this DCL, and to discuss the scope and size of potential proposals.
Proposals should follow the guidelines, deadlines (if any), budget limitations (if any), and solicitation-specific criteria of the relevant NSF program(s), once identified. Awards for projects responsive to this DCL will be funded through the relevant NSF program(s).
The proposal title should begin with "ULTRA-Data:" after any NSF Proposal & Award Policies & Procedures Guide (PAPPG) and/or solicitation-specific title requirements, if applicable. At the end of the Overview section of the Project Summary, include a sentence indicating that the proposal is being submitted in response to this DCL. Proposals that fail to address the objectives and guidance described in this DCL and in the relevant funding opportunity will be returned without review.
NSF is broadly interested in enabling discovery through the use and reuse of existing resources with untapped potential. Proposals responsive to this DCL should be primarily focused on utilizing data from environmental time series. Proposals primarily focused on innovative use of physical specimens and of metadata tracing back to physical specimens may be appropriate for the Innovative Use of Scientific Collections DCL ( NSF 24-069 ), and we encourage PIs to consider that document.
Questions should be directed to program directors in the relevant NSF research program(s); not the signatories to this DCL.
Susan Marqusee, Assistant Director Directorate for Biological Sciences
Alexandra Isern, Assistant Director Directorate for Geosciences

An official website of the United States government
Here’s how you know
Official websites use .gov A .gov website belongs to an official government organization in the United States.
Secure .gov websites use HTTPS A lock ( Lock A locked padlock ) or https:// means you’ve safely connected to the .gov website. Share sensitive information only on official, secure websites.
JavaScript appears to be disabled on this computer. Please click here to see any active alerts .
ORD Strategic Goals and Objectives
ORD has identified four overarching goals—each with a set of clearly articulated and achievable objectives—to guide its work.
Goal 1: Advance Science and Technology
Goal 2: support decision making, goal 3: build a healthy workforce and organization, goal 4: be a trusted scientific source.
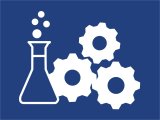
Improve protection of human health and the environment through scientific advancements, innovative research, and technology development using systems-level approaches.
Objective 1.1 Develop holistic scientific understanding using systems approaches
Today’s environmental challenges are increasingly complex, far reaching, and interconnected. As recommended by the National Academies of Sciences, Engineering, and Medicine (see Transforming EPA Science to Meet Today’s and Tomorrow’s Challenges ) ORD’s research will embrace a One Environment-One Health approach that emphasizes the dynamic connectivity between people; animals, plants, and other life; and our environment. In this overarching systems framework, our intramural and extramural research will encompass social, economic, and environmental systems; integrate across multiple levels from molecules to communities and ecosystems; address different geographic areas and timelines; and break down traditional boundaries between scientific disciplines. Applying systems approaches will enable more complete understanding of environmental challenges, such as climate change and the cumulative impacts of multiple stressors, increase our ability to identify connections between systems, and improve our understanding of the impact of potential solutions.
Objective 1.2 Innovate at the leading edge of environmental research
Innovation includes conceptualizing, developing, and implementing novel research, technology, or scientific research processes that add value for meeting the mission of ORD. ORD must have an innovative, creative, and nimble culture that pioneers better approaches to address existing challenges while also enabling us to anticipate and effectively respond to new and emerging challenges. As a leader in research that informs decisions to protect human health and the environment, we will embrace and support cross-disciplinary and diverse ideas at all levels of the organization, explore high-risk/high-reward research opportunities, and advance near-term and next generation scientific, technological, and process solutions. We will expand and strengthen our internal culture of innovation and seek new ideas and solutions from a diversity of external sources.
Objective 1.3 Expand and strengthen partnerships and collaboration
Tackling increasingly complex health and environmental challenges requires integration of knowledge and resources across organizations with complementary expertise, experience, perspectives, facilities, technologies, and reach. We will strategically enhance and expand external research collaborations to leverage the diverse talents and resources of existing and new partners, including federal agencies, states, Tribes, local governments, communities, academic institutions, non-governmental organizations, industry, international organizations, and others. We will work with our partners to integrate diverse bodies of knowledge and information, including Indigenous Knowledge, into our research efforts. Collaborative efforts will enable EPA to address complex challenges more effectively.
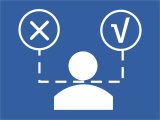
Deliver the scientific and technical information our partners need to inform their decisions to protect human health and the environment.
Objective 2.1 Deliver science to inform EPA’s actions
ORD’s science, technology, and research inform EPA’s actions to protect human health and the environment. We will engage extensively with EPA program and regional offices to identify and address current, emerging, and longer-term research needs and integrate research efforts across EPA programs. We will provide timely, relevant, and high-quality scientific information and technology, and reliable and accessible data, to our EPA partners to help support the Agency’s actions, develop solutions, and inform decisions.
Objective 2.2 Translate science into solutions with our external partners
We are committed to developing and translating research, consistent with EPA’s mission, that meets the needs of our external partners, including states, Tribes, local governments, and communities. We will emphasize stakeholder engagement throughout the research process, including problem formulation, research planning, implementation, dissemination, and evaluation. We will integrate and enhance community-engaged, solutions-driven research and participatory science in our research portfolio, including research aimed at helping those communities facing health inequities or environmental justice concerns.
Objective 2.3 Provide technical assistance and support
Internal and external partners rely on ORD’s expertise for making scientifically defensible decisions. Our technical support activities include direct assistance, training, outreach activities, and site-specific support to internal and external partners. We will continue to provide technical assistance and support to help our partners tackle existing, emerging, and future environmental challenges, including at Superfund and other contaminated sites, in response to and in preparation for emergencies and disasters, and in other situations as needed. We will also continue integrating technical support into our research programs to assist our partners with both proactive and reactive needs.
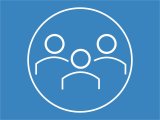
Strengthen and sustain a workforce and organization that have the skills and expertise necessary to anticipate and respond to current and future challenges to human health and the environment and that value inclusivity.
Objective 3.1 Recruit, develop, and retain a resilient workforce
ORD is committed to harnessing the power of a diverse workforce to drive innovation and creativity and to anticipate and respond to emerging human health and environmental challenges. Our workforce needs breadth, depth, and diversity of expertise, experience, and perspectives to enable us to be effective and innovative in conducting our work. We will continue to build a diverse, multi-talented, highly capable, and highly effective workforce that embraces ORD’s Principles, shares a common sense of purpose, and is empowered to contribute to the impactful environmental research needs of the Agency and the nation.
Objective 3.2 Strengthen our organization through inclusivity and equity
ORD must have an inclusive and equitable work environment where every employee feels valued, respected, and empowered to contribute their unique perspectives and talents. We are committed to strengthening our programs and activities to be inclusive, to provide opportunities for all our workforce, and to result in equitable impacts to our workforce. We strive to build a culture of physical, mental, and social well-being; collegiality; mutual respect; mutual support and mentoring; psychological safety; opportunity; recognition; and development. By creating an environment where every employee is valued and empowered, we enhance our ability to address complex environmental, administrative, managerial, and operational challenges and deliver impactful and beneficial outcomes for ORD, EPA, and our partners.
Objective 3.3 Use resources strategically
ORD’s efficient management of limited resources is critical to achieve our mission. We will comprehensively assess resource needs and allocation, explore innovative operations and continuous improvement opportunities, and leverage resource-sharing collaborations to meet modern scientific expectations. To maintain the scope, rigor, and quality of our scientific enterprise, we need state-of-the-art research equipment, specialized research assets, and resources for the administrative, managerial, and operational functions that support our scientific enterprise. We will be a leader in adoption of sustainable practices and promote a culture of resource stewardship amongst our workforce and at our facilities.
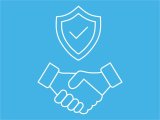
Serve as a trusted source of scientific information for the Agency, the nation, and the world.
Objective 4.1 Ensure science of the highest quality
With the advent of digital, social media, and artificial intelligence technologies that rapidly generate and circulate information for public consumption, it is essential that research organizations uphold the highest standards of scientific quality and credibility. ORD will ensure that our research and development activities continually meet the most rigorous scientific standards by following robust processes and procedures for data gathering, generation, management, and verification; quality control; and independent peer review. We will lead efforts with EPA programs and regions to develop and coordinate consistent and quality science practices and standards across the Agency. By upholding scientific rigor, we maintain our long-standing reputation as a leading trusted source of environmental expertise nationally and globally.
Objective 4.2 Be leaders for EPA’s scientific integrity
Scientific integrity is essential to ensure the public can trust the quality and validity of EPA’s work. We will continue to uphold scientific and ethical standards, communicate effectively with the public, enhance transparency within scientific processes, and safeguard EPA’s scientists and science from political interference or personal motivations. By upholding these principles and practices, we reinforce the integrity, quality, rigor, and objectivity of the scientific information and processes relied upon in policymaking, further strengthening public confidence in EPA’s work. We will maintain our leadership role in EPA’s commitment to sustain and grow a culture of scientific integrity.
Objective 4.3 Communicate science effectively
ORD is committed to transparency and open communication. We will continue to implement communications and outreach activities at all levels of ORD that engage the American people, the scientific community, elected officials, and other stakeholders in our research. Our effective communication practices will help translate ORD research to be understandable and useful. We will continue to make our data, results, and tools publicly available and explore ways to improve accessibility. We will seek opportunities to enhance our communications through new technologies and platforms, collaborations, and educational partners.
Measuring Our Success
The information below conveys the Key Performance Indicators (KPIs) that have been developed to ensure we meet our strategic goals and objectives. KPIs provide a measurable way to assess the effectiveness of the strategies outlined in the strategic plan and determine whether it is producing the desired results. We will regularly monitor each of its KPIs to identify areas of success and those that require additional attention, allowing for adjustments to be made to the strategy as needed. These actions will not only assist to ensure we stay on track to achieve its goals, but also provide valuable insights into the effectiveness of operations, allowing for continuous improvement.
The Office of Research and Development additionally contributes to broader Agency-wide metrics under the EPA FY 2022-2026 Long-Term Performance Goals, which can be found in EPA’s FY 2022-2026 Strategic Plan, available at: https://www.epa.gov/planandbudget/strategicplan .
To learn more about ORD’s Strategic Plan, including specific performance measures of success and how the plan’s goals and objectives map to the EPA Cross-Agency Strategies and Strategic Goals outlined above and in EPA’s FY 2022-2026 Strategic Plan, please download Office of Research and Development Strategic Plan (EPA Publication Number 600/R-24/001, March 2024, US EPA).
- EPA Research Home
- Research Topics
- Publications
- Funding & Career Opportunities
- Stay Connected
- EPA Research Events
- About Our Research
- Science Models and Research Tools (SMaRT) Search
- Strategic Research Action Plans Fiscal Years 2023-2026
We've detected unusual activity from your computer network
To continue, please click the box below to let us know you're not a robot.
Why did this happen?
Please make sure your browser supports JavaScript and cookies and that you are not blocking them from loading. For more information you can review our Terms of Service and Cookie Policy .
For inquiries related to this message please contact our support team and provide the reference ID below.
Numbers, Facts and Trends Shaping Your World
Read our research on:
Full Topic List
Regions & Countries
- Publications
- Our Methods
- Short Reads
- Tools & Resources
Read Our Research On:
What Are Americans’ Top Foreign Policy Priorities?
Protecting the u.s. from terrorism and reducing the flow of illegal drugs are top issues overall, but democrats and republicans have very different priorities, table of contents.
- Differences by partisanship
- Differences by age
- Acknowledgments
- The American Trends Panel survey methodology
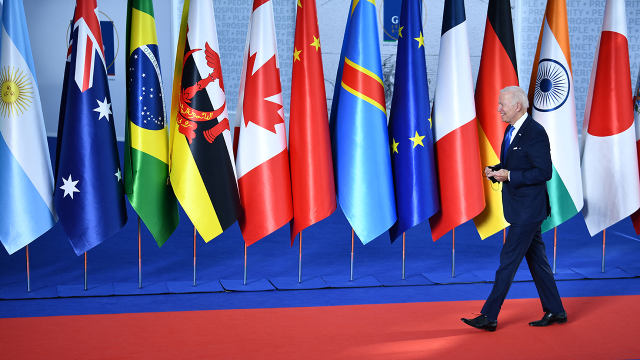
Pew Research Center conducted this analysis to better understand Americans’ long-range foreign policy priorities. For this analysis, we surveyed 3,600 U.S. adults from April 1 to April 7, 2024. Everyone who took part in this survey is a member of the Center’s American Trends Panel (ATP), an online survey panel that is recruited through national, random sampling of residential addresses. This way nearly all U.S. adults have a chance of selection. The survey is weighted to be representative of the U.S. adult population by gender, race, ethnicity, partisan affiliation, education and other categories. Read more about the ATP’s methodology .
Here are the questions used for this analysis, along with responses, and its methodology .
Americans have a lot on their plates in 2024, including an important election to determine who will remain or become again president. But the world does not stop for a U.S. election, and multiple conflicts around the world as well as other issues of global prominence continue to concern Americans.

When asked to prioritize the long-range foreign policy goals of the United States, the majority of Americans say preventing terrorist attacks (73%), keeping illegal drugs out of the country (64%) and preventing the spread of weapons of mass destruction (63%) are top priorities. Over half of Americans also see maintaining the U.S. military advantage over other countries (53%) and preventing the spread of infectious diseases (52%) as primary foreign policy responsibilities.
About half of Americans say limiting the power and influence of Russia and China are top priorities. A recent annual threat assessment from the U.S. intelligence community focused heavily on those countries’ strengthening military relationship and their ability to shape the global narrative against U.S. interests.
Fewer than half of Americans say dealing with global climate change (44%) and getting other countries to assume more of the costs of maintaining world order (42%) are top priorities. The partisan gaps on these two issues are quite large:
- 70% of Democrats and Democratic-leaning independents say climate change should be a top priority, while 15% of Republicans and Republican leaners say this.
- 54% of Republicans say getting other countries to assume more of the costs of maintaining world order should be a top priority, compared with 33% of Democrats.
About four-in-ten Americans see limiting the power and influence of North Korea and Iran as top priorities. (The survey was conducted before Iran’s large-scale missile attack on Israel on April 13.) And about a third say the same about the U.S. being a leader in artificial intelligence, a technology that governments around the world are increasingly concerned about .
When it comes to goals that focus on international engagement, like strengthening the United Nations and NATO or finding a solution to the Israeli-Palestinian conflict, fewer than a third of Americans mark these as top foreign policy priorities.
Related: Fewer Americans view the United Nations favorably than in 2023
Only about a quarter of Americans prioritize promoting human rights in other countries, leading other countries in space exploration and reducing military commitments overseas. And similar shares say supporting Ukraine (23%) and Israel (22%) are top issues.
At the bottom of this list of foreign policy priorities are promoting global democracy ( a major policy push from the Biden administration ) and aiding refugees fleeing violence around the world – about two-in-ten Americans describe these as top concerns. These assessments come amid a recent global surge in asylum claims . Still, in Center surveys, democracy promotion has typically been at the bottom of Americans’ list of foreign policy priorities, even dating back to George W. Bush’s and Barack Obama’s administrations .
Overall, a majority of Americans say that all 22 long-range foreign policy goals we asked about should be given at least some priority. Still, about three-in-ten Americans say supporting Israel (31%), promoting democracy (28%) and supporting Ukraine (27%) should be given no priority.

The long-range foreign policy priority questions were also asked in 2018 and 2021, and since then there have been some significant shifts in responses:
- Since 2018, the public has become significantly more likely to say limiting the power and influence of China (+17 percentage points) and finding a solution to the Israeli-Palestinian conflict (+11) are top foreign policy priorities.
- Americans have also increased the emphasis they place on limiting the power and influence of Russia, particularly in the wake of the Russian invasion of Ukraine (+8 points since 2021).
- On the decline since 2018 are strengthening the UN and aiding refugees (-8 points each), reducing foreign military commitments (-6), and promoting and defending human rights in other countries (-5).
- Preventing the spread of infectious diseases is down 19 percentage points since 2021 – during the height of the COVID-19 pandemic – and about back to where it was in 2018.
These are among the findings from a Pew Research Center survey conducted April 1-7, 2024.
The survey of 3,600 U.S. adults shows that foreign policy remains a partisan issue. Republicans prioritize the prevention of terrorism, reducing the flow of illegal drugs into the country, and maintaining a military advantage over other nations. Meanwhile, Democrats prioritize dealing with climate change and preventing the spread of weapons of mass destruction (WMDs), but also preventing terrorist attacks.

There are also stark age differences on many of the policy goals mentioned, but for the most part, young adults are less likely than older Americans to say the issues we asked about are top priorities. The exceptions are dealing with climate change, reducing military commitments overseas, and promoting and defending human rights abroad – on these issues, 18- to 29-year-olds are significantly more likely than older Americans to assign top priority.
Even with these priorities, foreign policy generally takes the backset to domestic policy for most Americans: 83% say it is more important for President Joe Biden to focus on domestic policy, compared with 14% who say he should focus on foreign policy.
Americans are even less likely to prioritize international affairs than they were in 2019, when 74% wanted then-President Donald Trump to focus on domestic policy and 23% said he should focus on foreign policy.
Americans’ foreign policy priorities differ greatly by party. The largest divide, by a significant margin, is the 55 percentage point gap between Democrats and Republicans on dealing with global climate change (70% vs. 15%, respectively, see it as a top priority).

Supporting Ukraine, aiding refugees, reducing the spread of diseases, protecting human rights, and strengthening the UN are also issues on which Democrats are at least 20 points more likely than Republicans to prioritize. For example, 63% of Democrats say reducing the spread of infectious diseases is a top priority, compared with 41% of Republicans.
Republicans prioritize supporting Israel, reducing the flow of illegal drugs and maintaining a military advantage over other countries – among other security and hard power issues – significantly more than Democrats do. For example, more than half of Republicans (54%) say getting other countries to assume more of the costs of maintaining world order should be a top focus in foreign policy. Only a third of Democrats say the same.
The priority assigned to several issues is divided even further by ideology within parties. Take support for Israel and Ukraine as examples. Supporting Israel is generally a higher priority for Republicans than Democrats, but within the Republican Party, 48% of conservatives say it’s a top concern, while 18% of moderates and liberals agree. Previous Center research shows that conservative Republicans are especially likely to favor military aid to Israel .
Supporting Ukraine, something Democrats emphasize more than Republicans, is a top priority particularly for liberal Democrats (47%), while about three-in-ten moderate and conservative Democrats agree (29%). Democrats have also shown more willingness than Republicans to provide aid to Ukraine in its conflict with Russia.
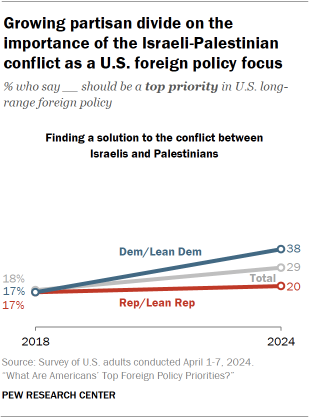
Generally, the partisan differences on the importance of several foreign policy issues have gotten smaller since 2021 , when most of these questions were last fielded. This is especially true for items related to the relative power of major countries, like the U.S. maintaining a military advantage and limiting the power and influence of both Russia and China.
However, finding a solution to the conflict between Israelis and Palestinians – a priority that saw no partisan difference at all when it was last asked about in 2018 – has an emerging partisan gap today. The share of Democrats who call this a top priority has more than doubled, while the share of Republicans has changed little.
Age differences persist on foreign policy issues. Older Americans prioritize most of the issues we asked about at higher rates than those ages 18 t0 29.
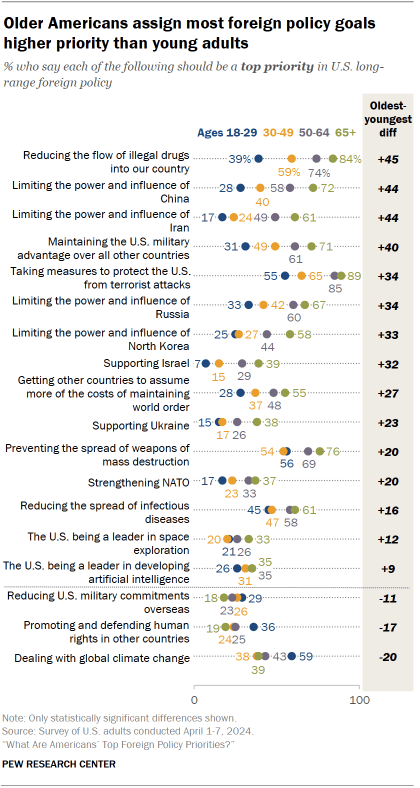
On four issues, there is at least a 40 percentage point gap between Americans ages 65 and older and young adults ages 18 to 29. The oldest Americans are more likely to prioritize reducing the flow of illegal drugs, limiting the power and influence of China and Iran, and maintaining a U.S. military advantage.
Those in the oldest age group are also more concerned than their younger counterparts on an additional 11 issues, ranging from support for Israel to U.S. leadership in space exploration.
For their part, young adults are more likely to say dealing with global climate change, reducing U.S. military commitments overseas, and promoting and defending human rights in other countries should be top foreign policy priorities.
Even starker patterns appear when looking at partisanship within two age groups – adults ages 18 to 49 and those 50 and older.
Among Democrats, older adults place particularly high priority on supporting Ukraine, strengthening NATO, and limiting the power and influence of Russia amid its war with Ukraine. Older Democrats are also more likely than younger ones to prioritize preventing the development of WMDs, curbing the spread of diseases, strengthening the UN and promoting democracy around the world, among other issues.
Among Republicans, those ages 50 and older are more likely than those ages 18 to 49 to prioritize supporting Israel, limiting the power and influence of Iran and China, getting other countries to assume more foreign policy costs, reducing the amount of illegal drugs entering the U.S., preventing terrorism, and maintaining a military advantage.
Sign up for our weekly newsletter
Fresh data delivery Saturday mornings
Sign up for The Briefing
Weekly updates on the world of news & information
- Environment & Climate
- Global Health
- Human Rights
- International Affairs
- United Nations
- War & International Conflict
A growing share of Americans have little or no confidence in Netanyahu
Fewer americans view the united nations favorably than in 2023, rising numbers of americans say jews and muslims face a lot of discrimination, younger americans stand out in their views of the israel-hamas war, how u.s. muslims are experiencing the israel-hamas war, most popular, report materials.
1615 L St. NW, Suite 800 Washington, DC 20036 USA (+1) 202-419-4300 | Main (+1) 202-857-8562 | Fax (+1) 202-419-4372 | Media Inquiries
Research Topics
- Age & Generations
- Coronavirus (COVID-19)
- Economy & Work
- Family & Relationships
- Gender & LGBTQ
- Immigration & Migration
- Internet & Technology
- Methodological Research
- News Habits & Media
- Non-U.S. Governments
- Other Topics
- Politics & Policy
- Race & Ethnicity
- Email Newsletters
ABOUT PEW RESEARCH CENTER Pew Research Center is a nonpartisan fact tank that informs the public about the issues, attitudes and trends shaping the world. It conducts public opinion polling, demographic research, media content analysis and other empirical social science research. Pew Research Center does not take policy positions. It is a subsidiary of The Pew Charitable Trusts .
Copyright 2024 Pew Research Center
Terms & Conditions
Privacy Policy
Cookie Settings
Reprints, Permissions & Use Policy
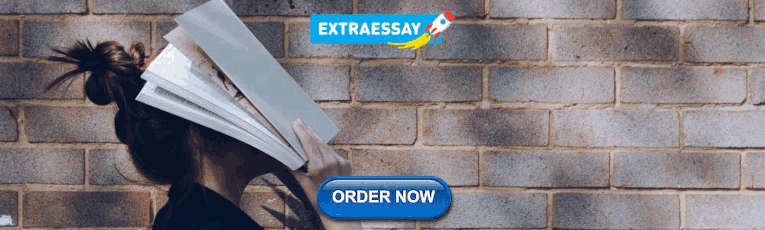
IMAGES
VIDEO
COMMENTS
Correlational research is a type of study that explores how variables are related to each other. It can help you identify patterns, trends, and predictions in your data. In this guide, you will learn when and how to use correlational research, and what its advantages and limitations are. You will also find examples of correlational research questions and designs. If you want to know the ...
Recall two goals of science are to describe and to predict and the correlational research strategy allows researchers to achieve both of these goals. Specifically, this strategy can be used to describe the strength and direction of the relationship between two variables and if there is a relationship between the variables then the researchers ...
This page titled 6.2: Correlational Research is shared under a CC BY-NC-SA 3.0 license and was authored, remixed, and/or curated by Anonymous via source content that was edited to the style and standards of the LibreTexts platform; a detailed edit history is available upon request. Correlational research is a type of non-experimental research ...
What Is Correlational Research? Correlational research is a type of nonexperimental research in which the researcher measures two variables and assesses the statistical relationship (i.e., the correlation) between them with little or no effort to control extraneous variables. There are essentially two reasons that researchers interested in ...
What is the goal of correlational research? The goal of correlational research is to examine the relationship between two or more variables. It involves analyzing data to determine if there is a statistically significant connection between the variables being studied. Correlational research is useful for identifying patterns and making ...
A correlational study is a type of research design that looks at the relationships between two or more variables. Correlational studies are non-experimental, which means that the experimenter does not manipulate or control any of the variables. A correlation refers to a relationship between two variables. Correlations can be strong or weak and ...
A correlational research design investigates relationships between variables without the researcher controlling or manipulating any of them. A correlation reflects the strength and/or direction of the relationship between two (or more) variables. The direction of a correlation can be either positive or negative. Positive correlation.
Correlational Research. Correlation means that there is a relationship between two or more variables (such as ice cream consumption and crime), but this relationship does not necessarily imply cause and effect. When two variables are correlated, it simply means that as one variable changes, so does the other. We can measure correlation by calculating a statistic known as a correlation coefficient.
Correlational research allows researchers to identify whether there is a relationship between variables, and if so, the strength and direction of that relationship. This information can be useful for predicting and explaining behavior, and for identifying potential risk factors or areas for intervention.
A correlational study is an experimental design that evaluates only the correlation between variables. The researchers record measurements but do not control or manipulate the variables. Correlational research is a form of observational study. A correlation indicates that as the value of one variable increases, the other tends to change in a ...
Correlational Research. Correlation means that there is a relationship between two or more variables (such as ice cream consumption and crime), but this relationship does not necessarily imply cause and effect. When two variables are correlated, it simply means that as one variable changes, so does the other.
The ultimate goal of correlational research is to increase our understanding of how different variables are related and to identify patterns in those relationships. This information can then be used to generate hypotheses and guide further research aimed at establishing causality.
Conclusion for Correlational Research. Correlational research serves as a powerful tool for uncovering connections between variables in the world around us. By examining the relationships between different factors, researchers can gain valuable insights into human behavior, health outcomes, market trends, and more.
The goal of correlational research is to identify variables that have a relationship in which a change in one creates a change in the other—without influence from any extraneous variable. Correlational research has, for example, identified a relationship between watching violent television and aggressive behaviors. But we must remember that ...
The goal of correlational research is to find factors that are related to each other to the point that a change in one causes a difference in the other. In its most basic form, correlational research aims to determine if two factors are connected and, if so, how.
Correlational studies aim to find out if there are differences in the characteristics of a population depending on whether or not its subjects have been exposed to an event of interest in the naturalistic setting. In eHealth, correlational studies are often used to determine whether the use of an eHealth system is associated with a particular set of user characteristics and/or quality of care ...
Correlational research is a type of non-experimental research in which the researcher measures two variables (binary or continuous) and assesses the statistical relationship (i.e., the correlation) between them with little or no effort to control extraneous variables. ... Recall two goals of science are to describe and to predict and the ...
Definition: Correlational research. Correlational research examines the relationship between variables. It is done without manipulating any of the variables themselves and is therefore used to measure the strength or direction of a relationship if one is found. The direction of this relationship can be positive, negative, or non-existent (zero).
Terms in this set (14) The goal of correlational research is to: Describe relationships between variables. To accomplish its goal, a correlational study involves the: Measurement of two or more variables. The primary difference between a differential study and a correlational study is that:
The goal of correlational research is to: Describe relationships between variables. To accomplish its goal, a correlational study involves the: Measurement of two or more variables. The primary difference between a differential study and a correlational study is that:
Study with Quizlet and memorize flashcards containing terms like The goal of the correlational research strategy is to _____. a. describe mean differences between groups b. describe mean differences over time c. examine and describe the relationship between variables d. describe an individual nonhuman subject in great detail, In a correlational study, commonly, _____. a. one variable is ...
Correlational research is a type of non-experimental research in which the researcher measures two variables and assesses the statistical relationship ... Recall two goals of science are to describe and to predict and the correlational research strategy allows researchers to achieve both of these goals. Specifically, this strategy can be used ...
APRIL 24, 2024 - A research paper published today in Science Advances reveals a direct correlation between plastic production and plastic pollution, such that every 1% increase in plastic ...
goals of the dcl To stimulate research that makes use of long-term datasets, the participating NSF divisions/offices listed below encourage the submission of proposals that: Synthesize, compare, and/or combine long- and short-term datasets to advance understanding of ecosystem and environmental dynamics, ecology, and evolution;
Goal 3: Build a Healthy Workforce and Organization. Strengthen and sustain a workforce and organization that have the skills and expertise necessary to anticipate and respond to current and future challenges to human health and the environment and that value inclusivity.. Objective 3.1 Recruit, develop, and retain a resilient workforce. ORD is committed to harnessing the power of a diverse ...
Recall two goals of science are to describe and to predict and the correlational research strategy allows researchers to achieve both of these goals. Specifically, this strategy can be used to describe the strength and direction of the relationship between two variables and if there is a relationship between the variables then the researchers ...
Alphabet Inc. Chief Executive Officer Sundar Pichai announced changes to Google's workplace teams structure, saying the moves will help the company develop artificial intelligence products and ...
Americans have a lot on their plates in 2024, including an important election to determine who will remain or become again president. But the world does not stop for a U.S. election, and multiple conflicts around the world as well as other issues of global prominence continue to concern Americans.. When asked to prioritize the long-range foreign policy goals of the United States, the majority ...