Do Your Students Know How to Analyze a Case—Really?
Explore more.
- Case Teaching
- Student Engagement
J ust as actors, athletes, and musicians spend thousands of hours practicing their craft, business students benefit from practicing their critical-thinking and decision-making skills. Students, however, often have limited exposure to real-world problem-solving scenarios; they need more opportunities to practice tackling tough business problems and deciding on—and executing—the best solutions.
To ensure students have ample opportunity to develop these critical-thinking and decision-making skills, we believe business faculty should shift from teaching mostly principles and ideas to mostly applications and practices. And in doing so, they should emphasize the case method, which simulates real-world management challenges and opportunities for students.
To help educators facilitate this shift and help students get the most out of case-based learning, we have developed a framework for analyzing cases. We call it PACADI (Problem, Alternatives, Criteria, Analysis, Decision, Implementation); it can improve learning outcomes by helping students better solve and analyze business problems, make decisions, and develop and implement strategy. Here, we’ll explain why we developed this framework, how it works, and what makes it an effective learning tool.
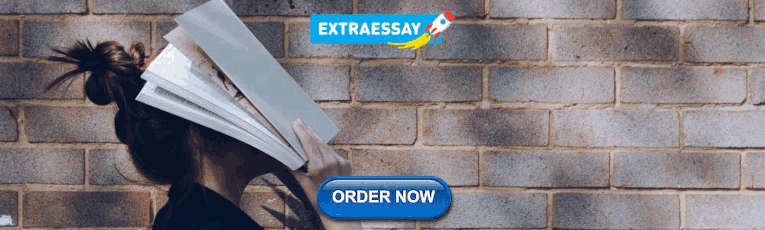
The Case for Cases: Helping Students Think Critically
Business students must develop critical-thinking and analytical skills, which are essential to their ability to make good decisions in functional areas such as marketing, finance, operations, and information technology, as well as to understand the relationships among these functions. For example, the decisions a marketing manager must make include strategic planning (segments, products, and channels); execution (digital messaging, media, branding, budgets, and pricing); and operations (integrated communications and technologies), as well as how to implement decisions across functional areas.
Faculty can use many types of cases to help students develop these skills. These include the prototypical “paper cases”; live cases , which feature guest lecturers such as entrepreneurs or corporate leaders and on-site visits; and multimedia cases , which immerse students into real situations. Most cases feature an explicit or implicit decision that a protagonist—whether it is an individual, a group, or an organization—must make.
For students new to learning by the case method—and even for those with case experience—some common issues can emerge; these issues can sometimes be a barrier for educators looking to ensure the best possible outcomes in their case classrooms. Unsure of how to dig into case analysis on their own, students may turn to the internet or rely on former students for “answers” to assigned cases. Or, when assigned to provide answers to assignment questions in teams, students might take a divide-and-conquer approach but not take the time to regroup and provide answers that are consistent with one other.
To help address these issues, which we commonly experienced in our classes, we wanted to provide our students with a more structured approach for how they analyze cases—and to really think about making decisions from the protagonists’ point of view. We developed the PACADI framework to address this need.
PACADI: A Six-Step Decision-Making Approach
The PACADI framework is a six-step decision-making approach that can be used in lieu of traditional end-of-case questions. It offers a structured, integrated, and iterative process that requires students to analyze case information, apply business concepts to derive valuable insights, and develop recommendations based on these insights.
Prior to beginning a PACADI assessment, which we’ll outline here, students should first prepare a two-paragraph summary—a situation analysis—that highlights the key case facts. Then, we task students with providing a five-page PACADI case analysis (excluding appendices) based on the following six steps.
Step 1: Problem definition. What is the major challenge, problem, opportunity, or decision that has to be made? If there is more than one problem, choose the most important one. Often when solving the key problem, other issues will surface and be addressed. The problem statement may be framed as a question; for example, How can brand X improve market share among millennials in Canada? Usually the problem statement has to be re-written several times during the analysis of a case as students peel back the layers of symptoms or causation.
Step 2: Alternatives. Identify in detail the strategic alternatives to address the problem; three to five options generally work best. Alternatives should be mutually exclusive, realistic, creative, and feasible given the constraints of the situation. Doing nothing or delaying the decision to a later date are not considered acceptable alternatives.
Step 3: Criteria. What are the key decision criteria that will guide decision-making? In a marketing course, for example, these may include relevant marketing criteria such as segmentation, positioning, advertising and sales, distribution, and pricing. Financial criteria useful in evaluating the alternatives should be included—for example, income statement variables, customer lifetime value, payback, etc. Students must discuss their rationale for selecting the decision criteria and the weights and importance for each factor.
Step 4: Analysis. Provide an in-depth analysis of each alternative based on the criteria chosen in step three. Decision tables using criteria as columns and alternatives as rows can be helpful. The pros and cons of the various choices as well as the short- and long-term implications of each may be evaluated. Best, worst, and most likely scenarios can also be insightful.
Step 5: Decision. Students propose their solution to the problem. This decision is justified based on an in-depth analysis. Explain why the recommendation made is the best fit for the criteria.
Step 6: Implementation plan. Sound business decisions may fail due to poor execution. To enhance the likeliness of a successful project outcome, students describe the key steps (activities) to implement the recommendation, timetable, projected costs, expected competitive reaction, success metrics, and risks in the plan.
“Students note that using the PACADI framework yields ‘aha moments’—they learned something surprising in the case that led them to think differently about the problem and their proposed solution.”
PACADI’s Benefits: Meaningfully and Thoughtfully Applying Business Concepts
The PACADI framework covers all of the major elements of business decision-making, including implementation, which is often overlooked. By stepping through the whole framework, students apply relevant business concepts and solve management problems via a systematic, comprehensive approach; they’re far less likely to surface piecemeal responses.
As students explore each part of the framework, they may realize that they need to make changes to a previous step. For instance, when working on implementation, students may realize that the alternative they selected cannot be executed or will not be profitable, and thus need to rethink their decision. Or, they may discover that the criteria need to be revised since the list of decision factors they identified is incomplete (for example, the factors may explain key marketing concerns but fail to address relevant financial considerations) or is unrealistic (for example, they suggest a 25 percent increase in revenues without proposing an increased promotional budget).
In addition, the PACADI framework can be used alongside quantitative assignments, in-class exercises, and business and management simulations. The structured, multi-step decision framework encourages careful and sequential analysis to solve business problems. Incorporating PACADI as an overarching decision-making method across different projects will ultimately help students achieve desired learning outcomes. As a practical “beyond-the-classroom” tool, the PACADI framework is not a contrived course assignment; it reflects the decision-making approach that managers, executives, and entrepreneurs exercise daily. Case analysis introduces students to the real-world process of making business decisions quickly and correctly, often with limited information. This framework supplies an organized and disciplined process that students can readily defend in writing and in class discussions.
PACADI in Action: An Example
Here’s an example of how students used the PACADI framework for a recent case analysis on CVS, a large North American drugstore chain.
The CVS Prescription for Customer Value*
PACADI Stage
Summary Response
How should CVS Health evolve from the “drugstore of your neighborhood” to the “drugstore of your future”?
Alternatives
A1. Kaizen (continuous improvement)
A2. Product development
A3. Market development
A4. Personalization (micro-targeting)
Criteria (include weights)
C1. Customer value: service, quality, image, and price (40%)
C2. Customer obsession (20%)
C3. Growth through related businesses (20%)
C4. Customer retention and customer lifetime value (20%)
Each alternative was analyzed by each criterion using a Customer Value Assessment Tool
Alternative 4 (A4): Personalization was selected. This is operationalized via: segmentation—move toward segment-of-1 marketing; geodemographics and lifestyle emphasis; predictive data analysis; relationship marketing; people, principles, and supply chain management; and exceptional customer service.
Implementation
Partner with leading medical school
Curbside pick-up
Pet pharmacy
E-newsletter for customers and employees
Employee incentive program
CVS beauty days
Expand to Latin America and Caribbean
Healthier/happier corner
Holiday toy drives/community outreach
*Source: A. Weinstein, Y. Rodriguez, K. Sims, R. Vergara, “The CVS Prescription for Superior Customer Value—A Case Study,” Back to the Future: Revisiting the Foundations of Marketing from Society for Marketing Advances, West Palm Beach, FL (November 2, 2018).
Results of Using the PACADI Framework
When faculty members at our respective institutions at Nova Southeastern University (NSU) and the University of North Carolina Wilmington have used the PACADI framework, our classes have been more structured and engaging. Students vigorously debate each element of their decision and note that this framework yields an “aha moment”—they learned something surprising in the case that led them to think differently about the problem and their proposed solution.
These lively discussions enhance individual and collective learning. As one external metric of this improvement, we have observed a 2.5 percent increase in student case grade performance at NSU since this framework was introduced.
Tips to Get Started
The PACADI approach works well in in-person, online, and hybrid courses. This is particularly important as more universities have moved to remote learning options. Because students have varied educational and cultural backgrounds, work experience, and familiarity with case analysis, we recommend that faculty members have students work on their first case using this new framework in small teams (two or three students). Additional analyses should then be solo efforts.
To use PACADI effectively in your classroom, we suggest the following:
Advise your students that your course will stress critical thinking and decision-making skills, not just course concepts and theory.
Use a varied mix of case studies. As marketing professors, we often address consumer and business markets; goods, services, and digital commerce; domestic and global business; and small and large companies in a single MBA course.
As a starting point, provide a short explanation (about 20 to 30 minutes) of the PACADI framework with a focus on the conceptual elements. You can deliver this face to face or through videoconferencing.
Give students an opportunity to practice the case analysis methodology via an ungraded sample case study. Designate groups of five to seven students to discuss the case and the six steps in breakout sessions (in class or via Zoom).
Ensure case analyses are weighted heavily as a grading component. We suggest 30–50 percent of the overall course grade.
Once cases are graded, debrief with the class on what they did right and areas needing improvement (30- to 40-minute in-person or Zoom session).
Encourage faculty teams that teach common courses to build appropriate instructional materials, grading rubrics, videos, sample cases, and teaching notes.
When selecting case studies, we have found that the best ones for PACADI analyses are about 15 pages long and revolve around a focal management decision. This length provides adequate depth yet is not protracted. Some of our tested and favorite marketing cases include Brand W , Hubspot , Kraft Foods Canada , TRSB(A) , and Whiskey & Cheddar .
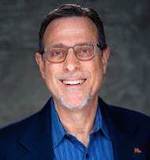
Art Weinstein , Ph.D., is a professor of marketing at Nova Southeastern University, Fort Lauderdale, Florida. He has published more than 80 scholarly articles and papers and eight books on customer-focused marketing strategy. His latest book is Superior Customer Value—Finding and Keeping Customers in the Now Economy . Dr. Weinstein has consulted for many leading technology and service companies.
Herbert V. Brotspies , D.B.A., is an adjunct professor of marketing at Nova Southeastern University. He has over 30 years’ experience as a vice president in marketing, strategic planning, and acquisitions for Fortune 50 consumer products companies working in the United States and internationally. His research interests include return on marketing investment, consumer behavior, business-to-business strategy, and strategic planning.
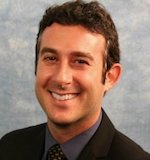
John T. Gironda , Ph.D., is an assistant professor of marketing at the University of North Carolina Wilmington. His research has been published in Industrial Marketing Management, Psychology & Marketing , and Journal of Marketing Management . He has also presented at major marketing conferences including the American Marketing Association, Academy of Marketing Science, and Society for Marketing Advances.
Related Articles
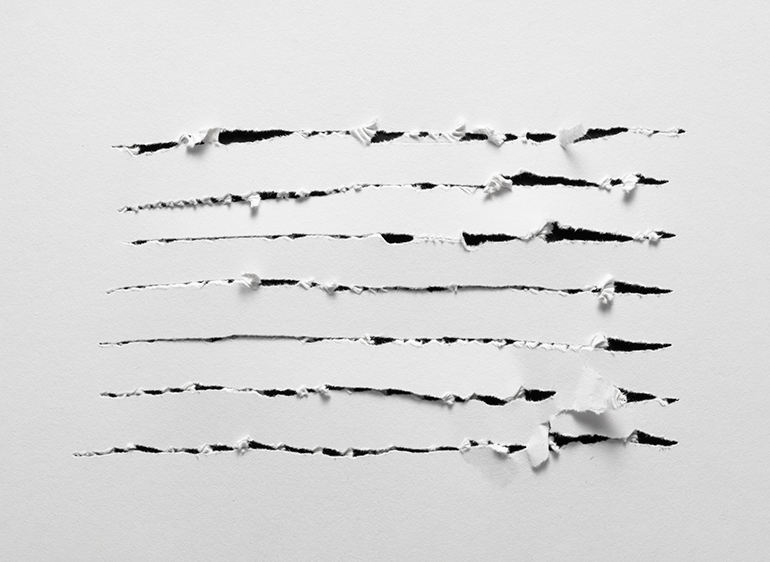
We use cookies to understand how you use our site and to improve your experience, including personalizing content. Learn More . By continuing to use our site, you accept our use of cookies and revised Privacy Policy .
Sales CRM Terms
What are Decision Criteria? (Explained With Examples)
Oct 11, 2023

Decision criteria are essential factors that individuals or organizations consider when making a decision. They serve as the basis for evaluating different options and ultimately choosing the best course of action. In this article, we will delve into the definition of decision criteria, explore their advantages and disadvantages, and provide practical examples in various contexts, such as startups, consulting, and digital marketing agencies. We will also utilize analogies to help illustrate the concept further.
1. What are Decision Criteria?
Decision criteria encompass the specific factors that individuals or organizations prioritize when making a decision. These factors can include financial considerations, strategic objectives, customer preferences, technological feasibility, and many other relevant aspects. By establishing decision criteria, individuals or organizations can systematically evaluate different options and determine the best course of action.
When it comes to decision-making, having clear and well-defined criteria is crucial. Decision criteria serve as a set of guidelines or standards that help individuals or organizations assess and compare different alternatives. These criteria can be quantitative or qualitative, depending on the nature of the decision and the available information.
1.1 Definition of Decision Criteria
Decision criteria refer to the specific standards or benchmarks used to assess different alternatives during a decision-making process. These criteria can be quantitative or qualitative and are often tailored to the specific context or goals of the decision-making process.
Quantitative decision criteria involve measurable factors that can be assigned numerical values. For example, in a financial decision, criteria such as return on investment (ROI), net present value (NPV), or payback period may be used. These criteria provide a clear and objective basis for evaluating options.
On the other hand, qualitative decision criteria involve subjective factors that are difficult to quantify but still play a significant role in the decision-making process. These criteria can include factors such as brand reputation, customer satisfaction, or ethical considerations. While they may not have precise numerical values, they are essential for capturing the intangible aspects that influence decision outcomes.
1.2 Advantages of Decision Criteria
Using decision criteria provides several benefits. Firstly, decision criteria help ensure that all relevant aspects are taken into account, leading to more comprehensive and informed decisions. By considering a range of factors, decision-makers can avoid overlooking critical aspects that could impact the success of the chosen option.
Secondly, decision criteria enable individuals or organizations to compare and prioritize different options based on their importance. By assigning weights or rankings to each criterion, decision-makers can objectively assess the pros and cons of each alternative. This allows for a more structured decision-making process and reduces the likelihood of relying on subjective judgments solely.
Lastly, decision criteria facilitate stakeholder collaboration and transparency as they provide a clear framework for evaluating options and aligning diverse perspectives. When decision criteria are well-defined and communicated, stakeholders can understand the rationale behind the chosen option and feel more engaged in the decision-making process.
1.3 Disadvantages of Decision Criteria
While decision criteria offer significant advantages, they are not without their limitations. One potential disadvantage is that decision criteria can oversimplify complex problems or situations. By focusing on specific factors, decision criteria may overlook critical nuances or underlying complexities that could impact the final decision. It is important for decision-makers to be aware of this limitation and consider additional information or expert opinions to supplement the criteria.
Additionally, decision criteria can be influenced by biases or limited perspectives, potentially leading to suboptimal outcomes. For example, if decision criteria heavily prioritize short-term financial gains, long-term sustainability or ethical considerations may be overlooked. To mitigate this risk, decision-makers should strive for a diverse and inclusive decision-making process that incorporates a wide range of perspectives and expertise.
In conclusion, decision criteria play a vital role in the decision-making process. They provide a structured framework for evaluating alternatives and help ensure that all relevant aspects are considered. However, decision criteria should be used judiciously, taking into account the limitations and potential biases they may introduce. By combining decision criteria with broader considerations and expert insights, individuals and organizations can make more informed and effective decisions.
2. Examples of Decision Criteria
Examining practical examples will further illuminate the concept of decision criteria. Let's explore how decision criteria can be applied in different contexts:
2.1 Example in a Startup Context
In a startup context, decision criteria could include factors such as market potential, cost-effectiveness, scalability, and alignment with the company's vision and mission. By prioritizing these criteria, startup founders can evaluate potential business opportunities and make data-driven decisions that maximize chances of success.
For example, let's consider a tech startup that is developing a new mobile app. The decision criteria for this startup could involve analyzing the size of the target market, assessing the potential demand for the app, and evaluating the competition in the app market. Additionally, the startup may consider the cost-effectiveness of developing and maintaining the app, as well as its scalability potential in terms of user growth and revenue generation.
By carefully considering these decision criteria, the startup can make informed choices about whether to proceed with the app development, how to allocate resources, and how to position the app in the market.
2.2 Example in a Consulting Context
When working on a consulting project, decision criteria might involve client requirements, industry best practices, profitability, and long-term sustainability. By utilizing these criteria, consultants can assess alternative solutions and recommend the most suitable strategy to address the client's challenges and achieve their goals.
For instance, let's imagine a consulting firm that is tasked with helping a manufacturing company optimize its production processes. The decision criteria in this context could include analyzing the client's specific requirements, benchmarking against industry best practices, evaluating the potential profitability of proposed solutions, and considering the long-term sustainability of the implemented changes.
By carefully evaluating these decision criteria, the consulting firm can provide the client with a well-informed recommendation on how to streamline their production processes, improve efficiency, and ultimately achieve their desired business outcomes.
2.3 Example in a Digital Marketing Agency Context
In the context of a digital marketing agency, decision criteria could include factors such as target audience reach, cost per acquisition, return on investment, and campaign performance metrics. By incorporating these criteria, digital marketers can make data-informed decisions on the optimal marketing channels, strategies, and campaigns to maximize client's online presence and achieve desired business outcomes.
For example, let's consider a digital marketing agency that is working with an e-commerce client to increase their online sales. The decision criteria for this agency could involve analyzing the potential reach of different marketing channels, calculating the cost per acquisition for each channel, evaluating the expected return on investment for various marketing strategies, and monitoring campaign performance metrics such as click-through rates and conversion rates.
By carefully considering these decision criteria, the digital marketing agency can develop a tailored marketing plan that focuses on the most effective channels, strategies, and campaigns to drive targeted traffic to the client's website, increase conversions, and ultimately boost online sales.
2.4 Example with Analogies
To grasp the concept of decision criteria further, let's consider an analogy. Imagine you are choosing a holiday destination. The decision criteria might encompass factors such as travel costs, weather preferences, available activities, and cultural experiences. By evaluating these criteria, you can prioritize destinations that align with your preferences and make an informed decision on the best holiday spot.
For instance, let's say you are planning a vacation and have several potential destinations in mind. The decision criteria you might consider could include analyzing the cost of travel and accommodation, assessing the weather conditions and climate preferences, evaluating the availability of activities and attractions, and considering the cultural experiences each destination offers.
By carefully evaluating these decision criteria, you can narrow down your options and choose a holiday destination that suits your budget, weather preferences, desired activities, and cultural interests.
In conclusion, decision criteria play a crucial role in the decision-making process. They allow individuals or organizations to assess alternatives systematically, considering crucial factors and aligning decisions with their goals. While decision criteria have advantages such as comprehensiveness and clarity, it is important to be aware of their limitations and use them judiciously alongside broader considerations. By examining practical examples and analogies, we can better understand how decision criteria apply in various contexts and enhance our decision-making capabilities.
About the author

Arnaud Belinga

Close deals x2 faster with
Breakcold sales crm.
SEE PRICING
*No credit card required
Related Articles
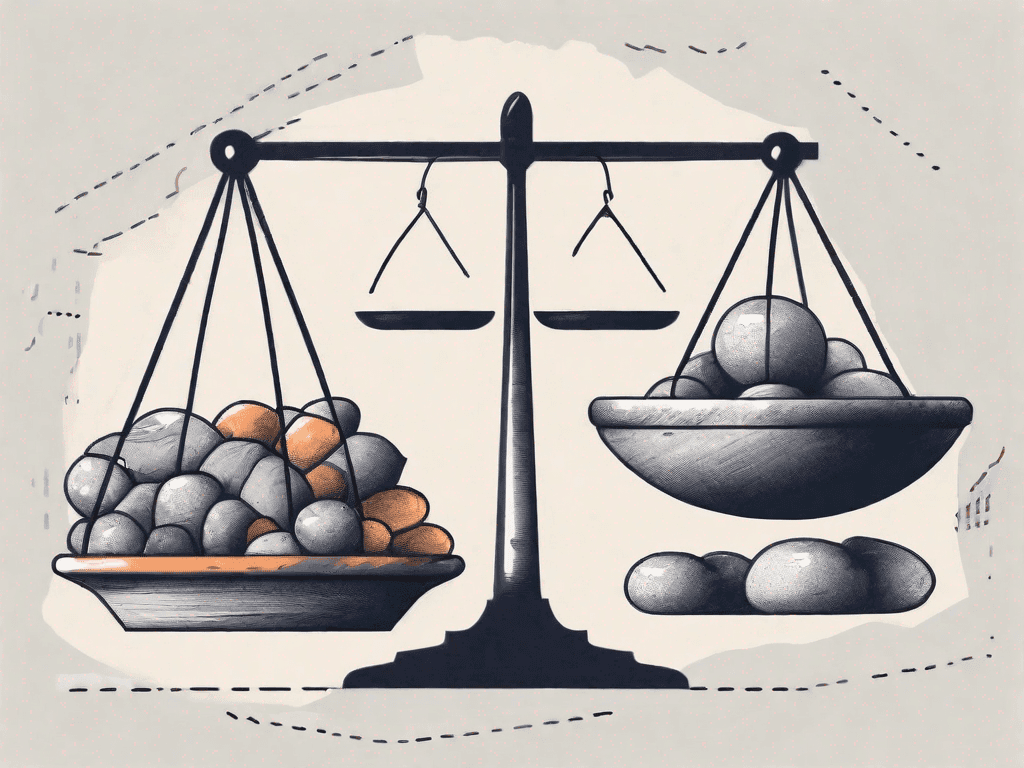
What is the 80-20 rule? (Explained With Examples)

What is the ABCD Sales Method? (Explained With Examples)
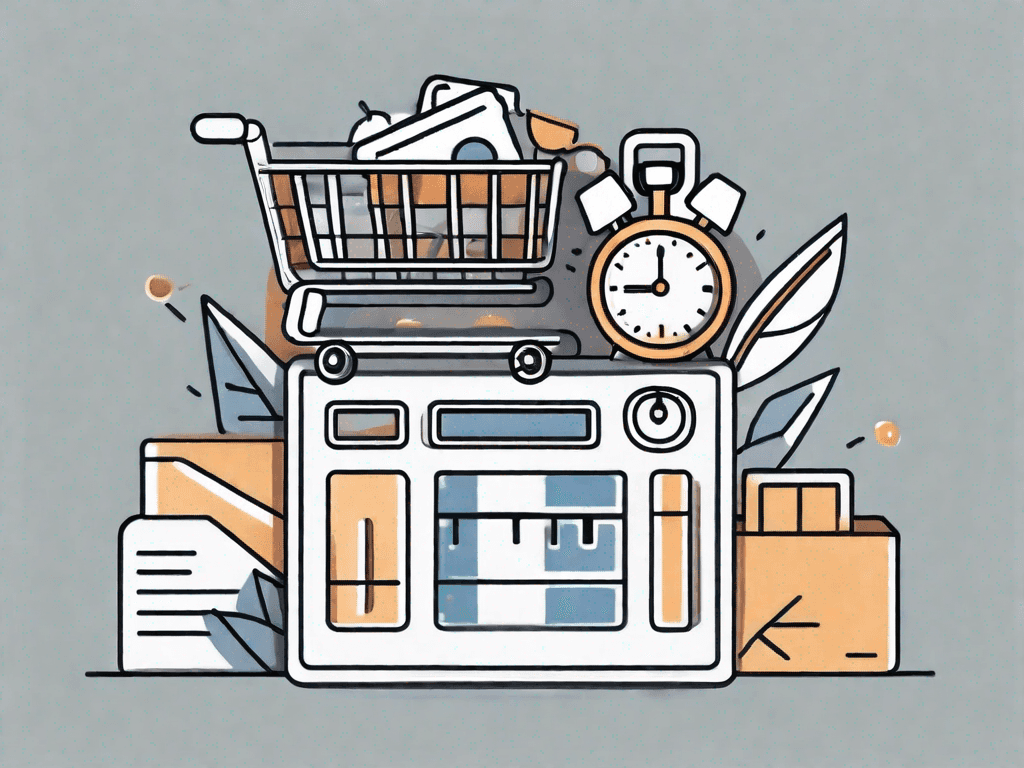
What is an Accelerated Sales Cycle? (Explained With Examples)

What is Account-Based Marketing (ABM)? (Explained With Examples)

What is an Account Manager? (Explained With Examples)
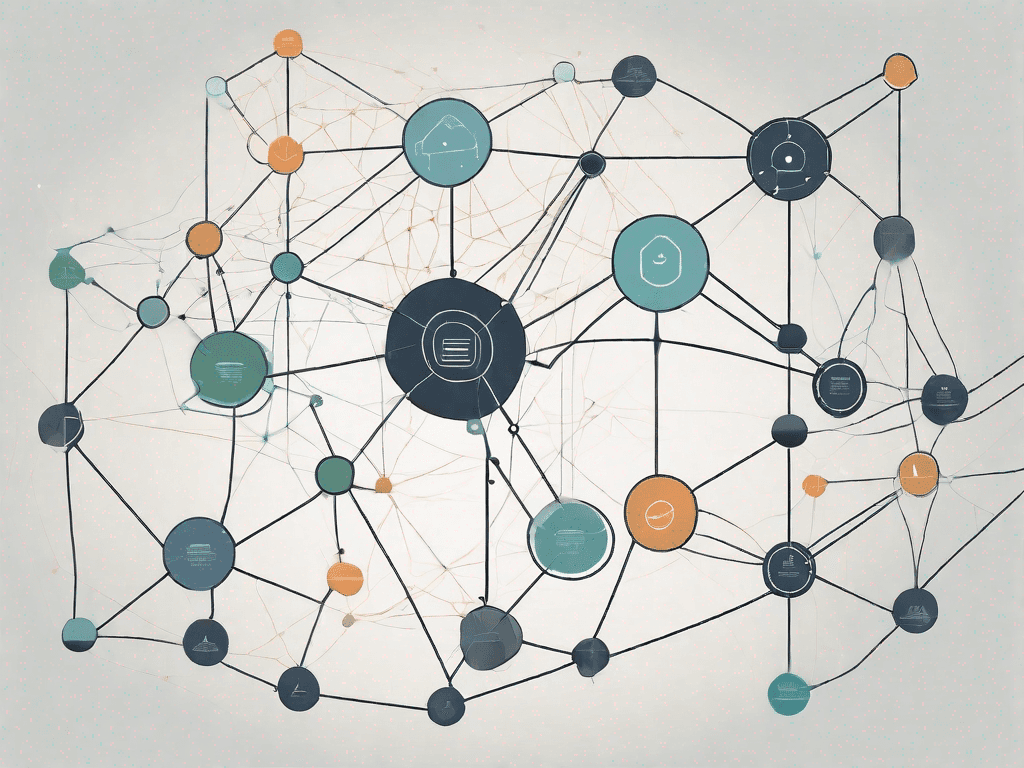
What is Account Mapping? (Explained With Examples)

What is Account-Based Selling? (Explained With Examples)
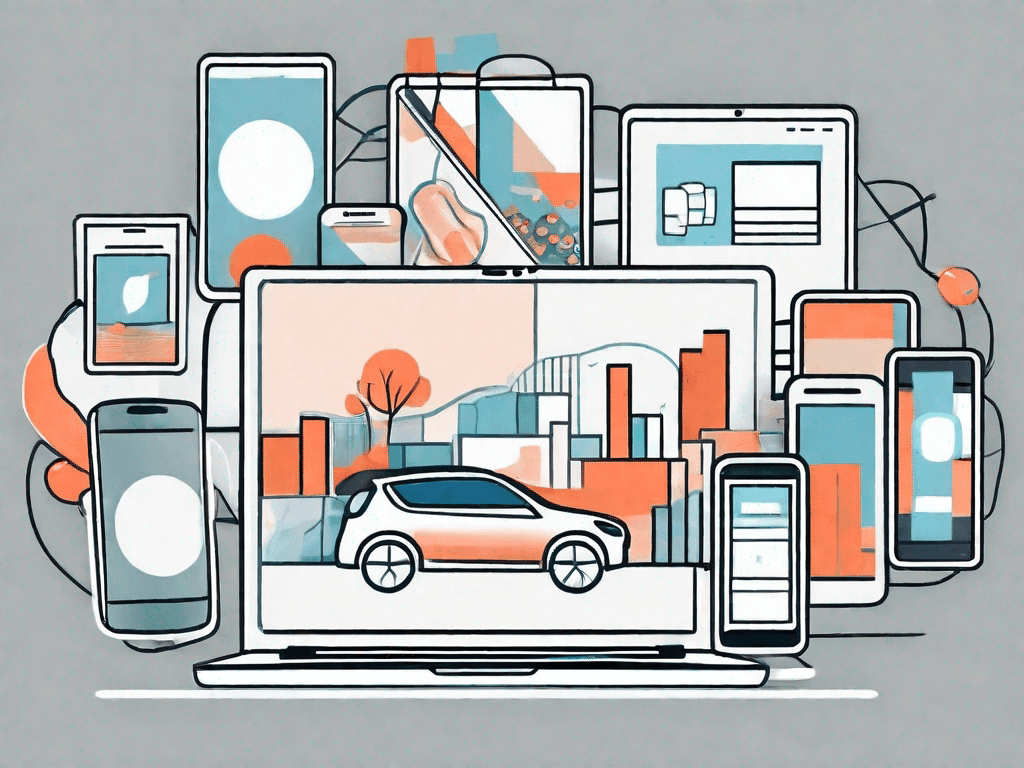
What is Ad Targeting? (Explained With Examples)

What is the Addressable Market? (Explained With Examples)

What is the Adoption Curve? (Explained With Examples)
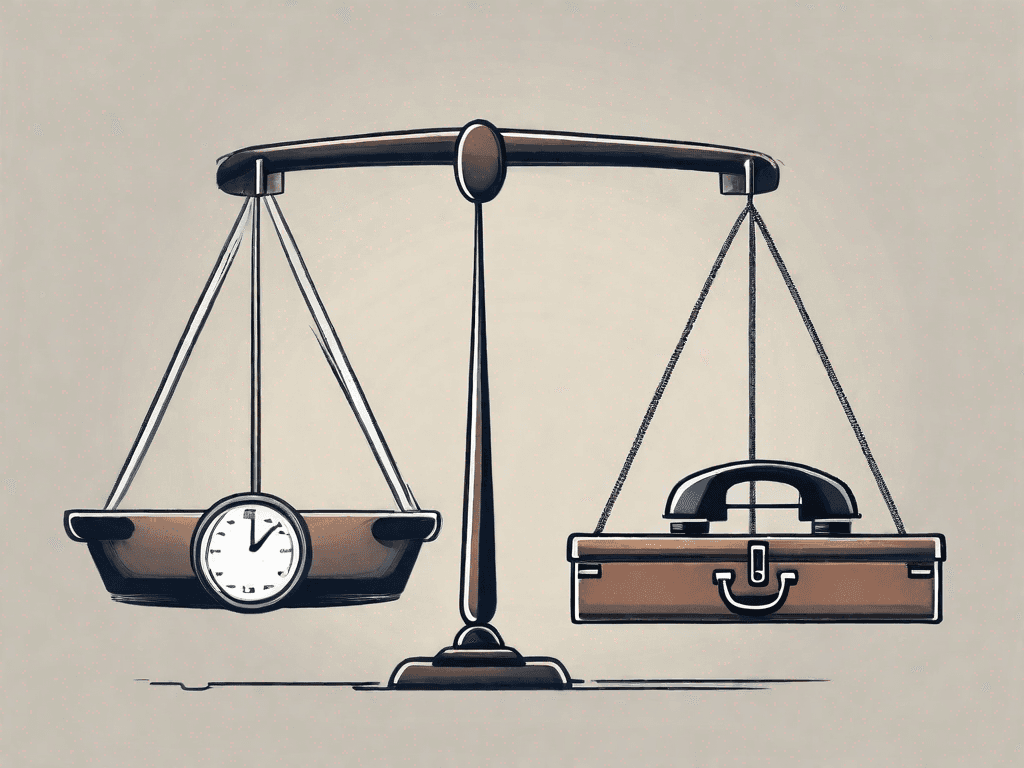
What is an AE (Account Executive)? (Explained With Examples)

What is Affiliate Marketing? (Explained With Examples)

What is AI in Sales? (Explained With Examples)
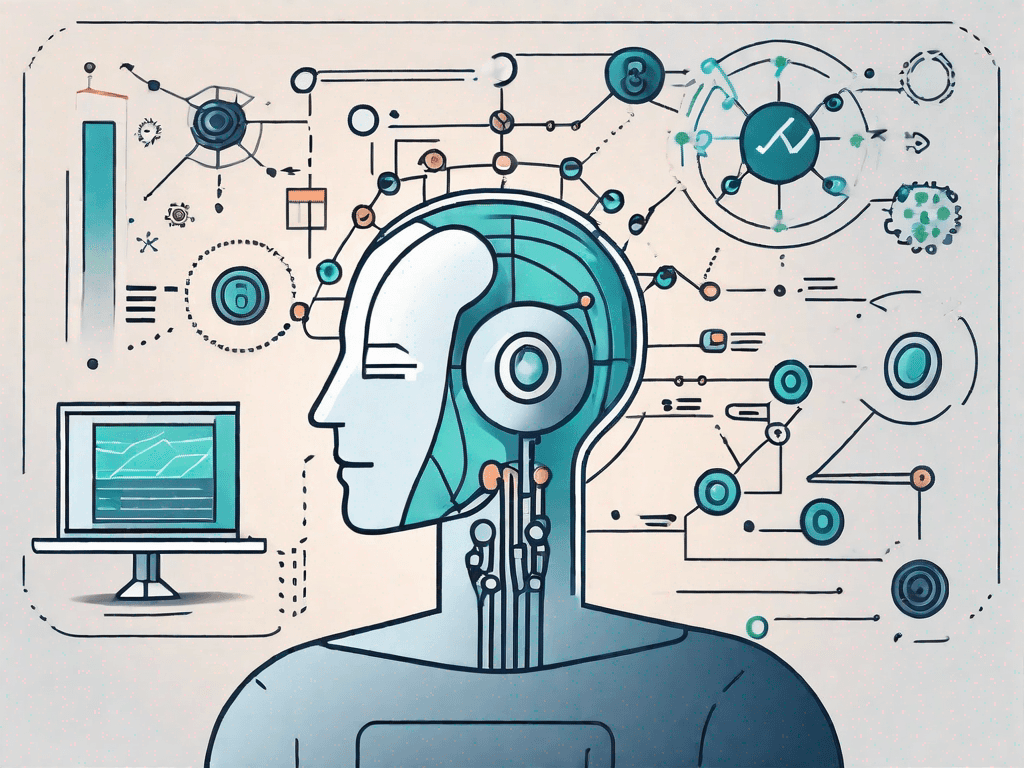
What is an AI-Powered CRM? (Explained With Examples)
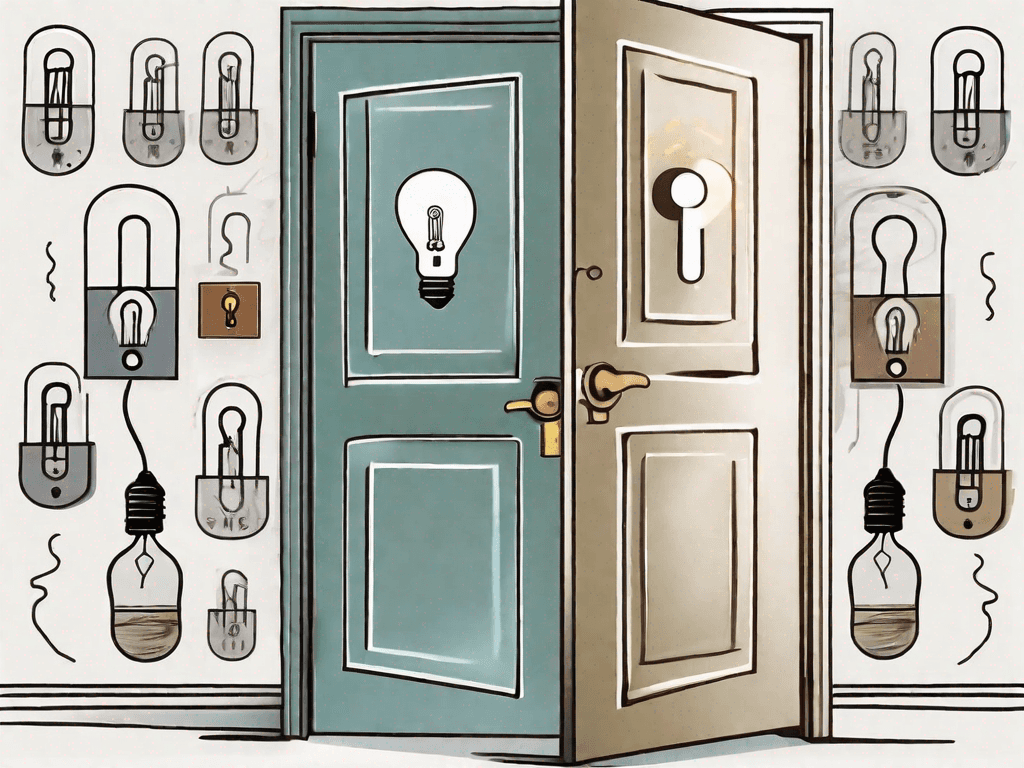
What is an Alternative Close? (Explained With Examples)

What is the Annual Contract Value? (ACV - Explained With Examples)

What are Appointments Set? (Explained With Examples)

What is an Assumptive Close? (Explained With Examples)

What is Automated Outreach? (Explained With Examples)

What is Average Revenue Per Account (ARPA)? (Explained With Examples)

What is B2B (Business-to-Business)? (Explained With Examples)

What is B2G (Business-to-Government)? (Explained With Examples)

What is B2P (Business-to-Partner)? (Explained With Examples)

What is BANT (Budget, Authority, Need, Timing)? (Explained With Examples)

What is Behavioral Economics in Sales? (Explained With Examples)

What is Benchmark Data? (Explained With Examples)

What is Benefit Selling? (Explained With Examples)
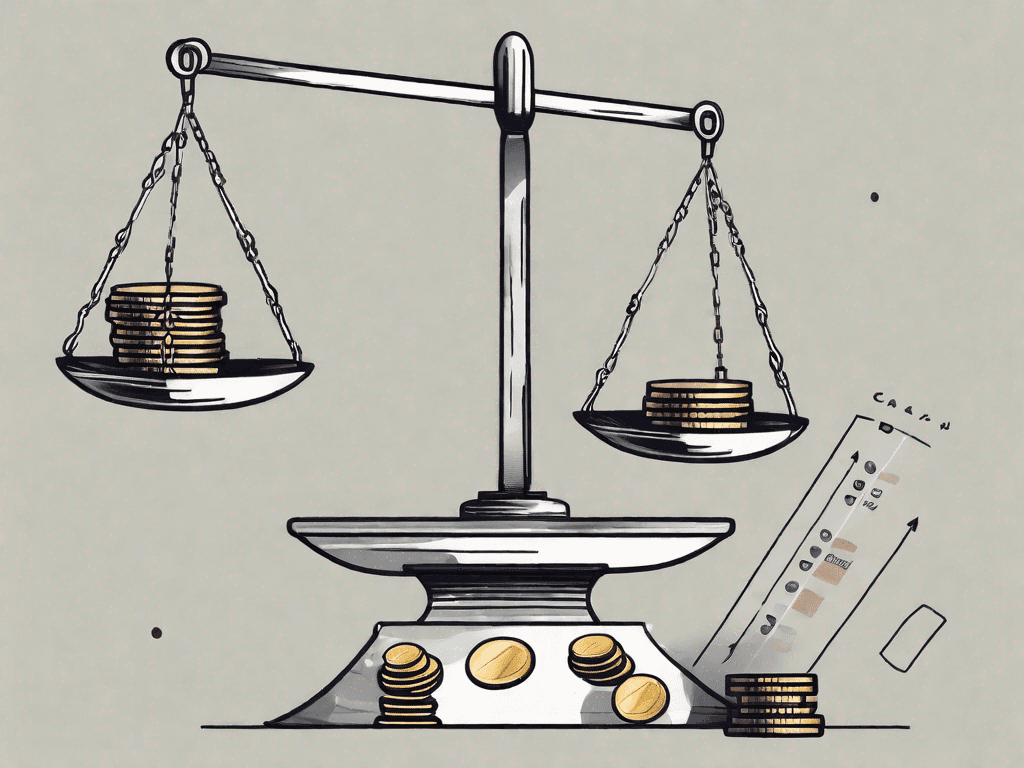
What are Benefit Statements? (Explained With Examples)

What is Beyond the Obvious? (Explained With Examples)

What is a Bootstrapped Startup? (Explained With Examples)

What is the Bottom of the Funnel (BOFU)? (Explained With Examples)

What is Bounce Rate? (Explained With Examples)

What is Brand Awareness? (Explained With Examples)

What is the Break-Even Point? (Explained With Examples)

What is a Breakup Email? (Explained With Examples)

What is Business Development? (Explained With Examples)

What are Business Insights? (Explained With Examples)

What is Business Process Automation? (Explained With Examples)

What is a Buyer Persona? (Explained With Examples)

What is the Buyer's Journey? (Explained With Examples)

What is the Buying Cycle? (Explained With Examples)

What is a Buying Signal? (Explained With Examples)
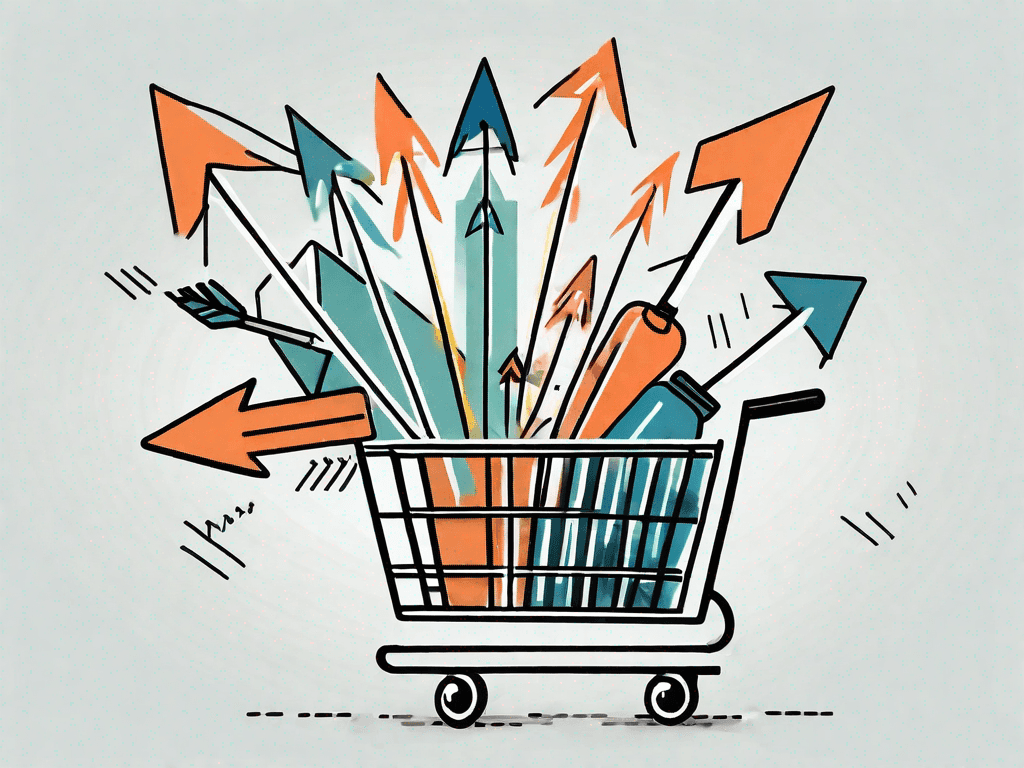
What is a Buying Team? (Explained With Examples)

What is a C-Level Executive? (Explained With Examples)
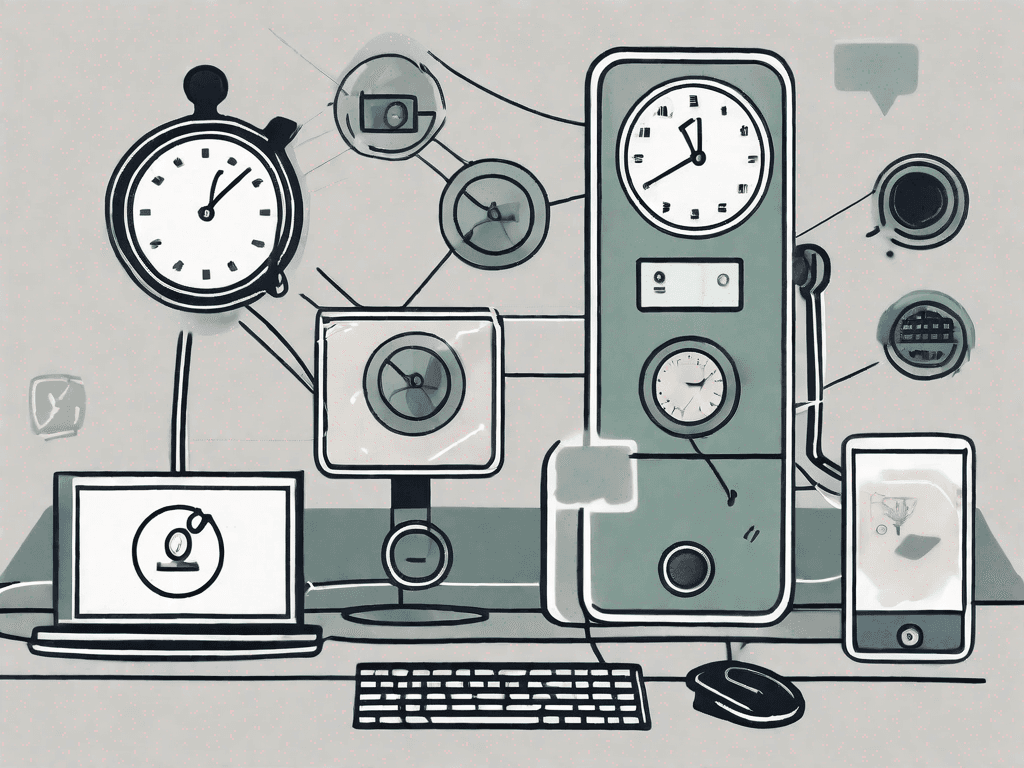
What is Call Logging? (Explained With Examples)

What is Call Recording? (Explained With Examples)
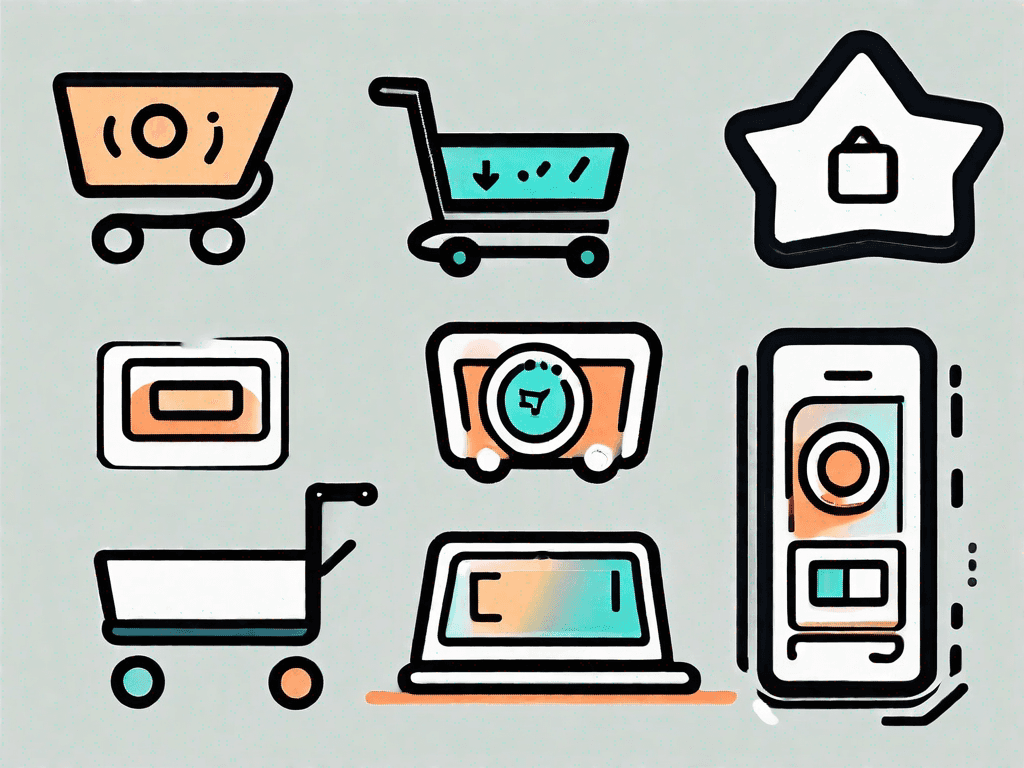
What is a Call-to-Action (CTA)? (Explained With Examples)
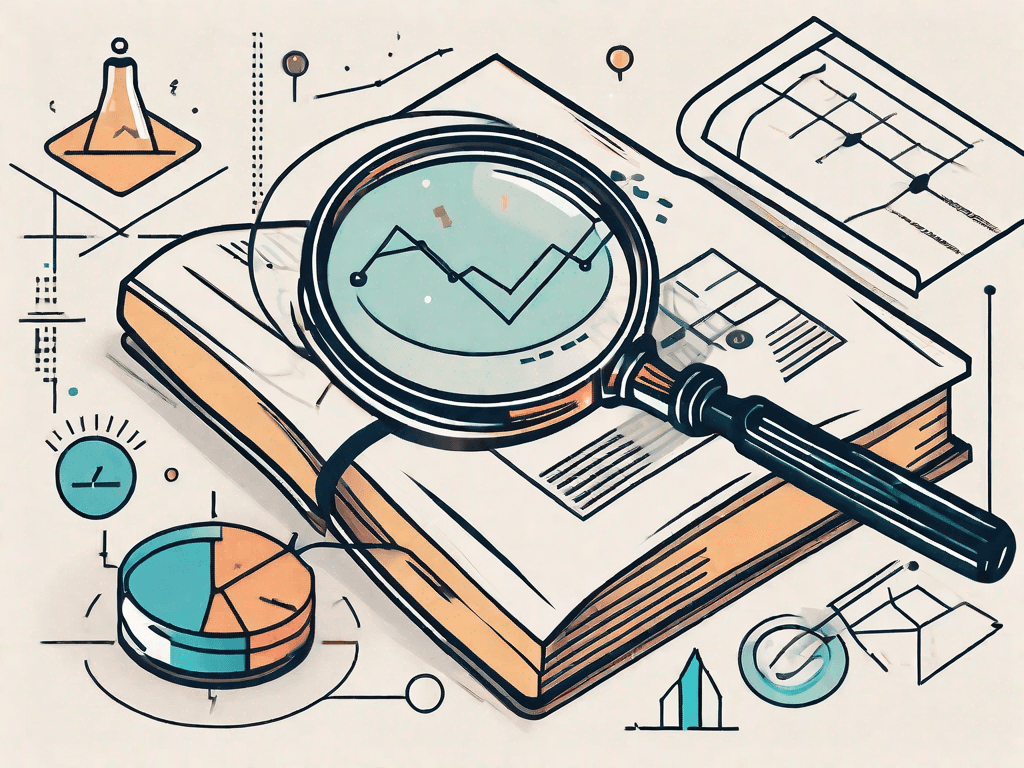
What is Case Study Analysis? (Explained With Examples)

What is Challenger Sales? (Explained With Examples)
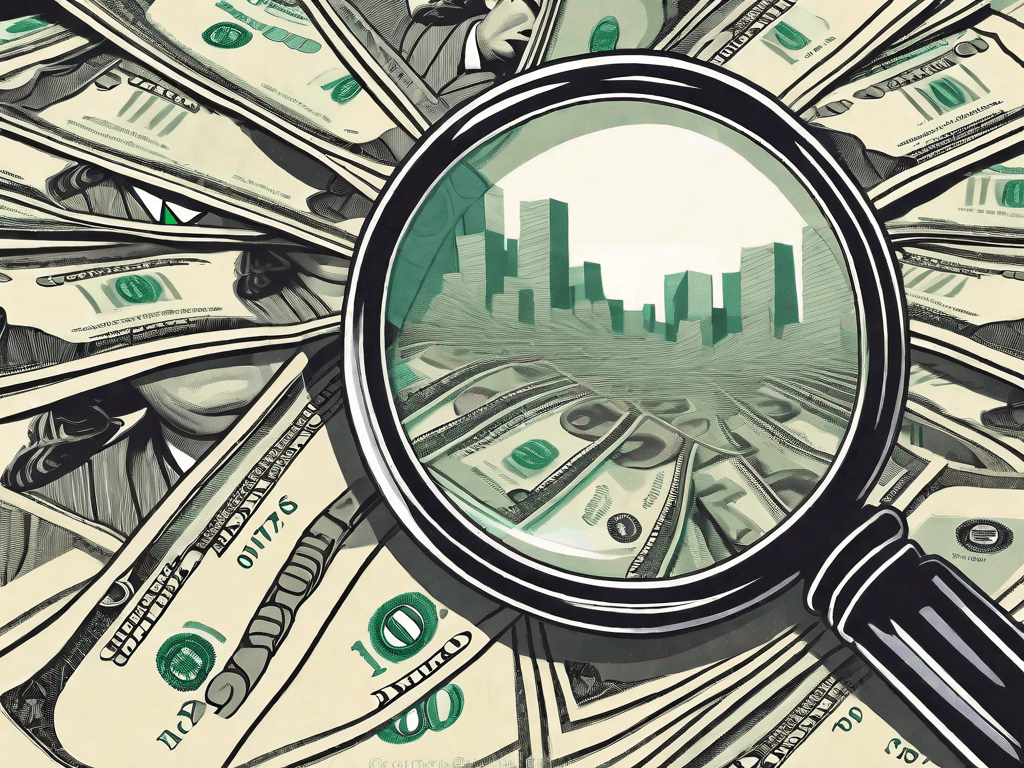
What is Chasing Lost Deals? (Explained With Examples)
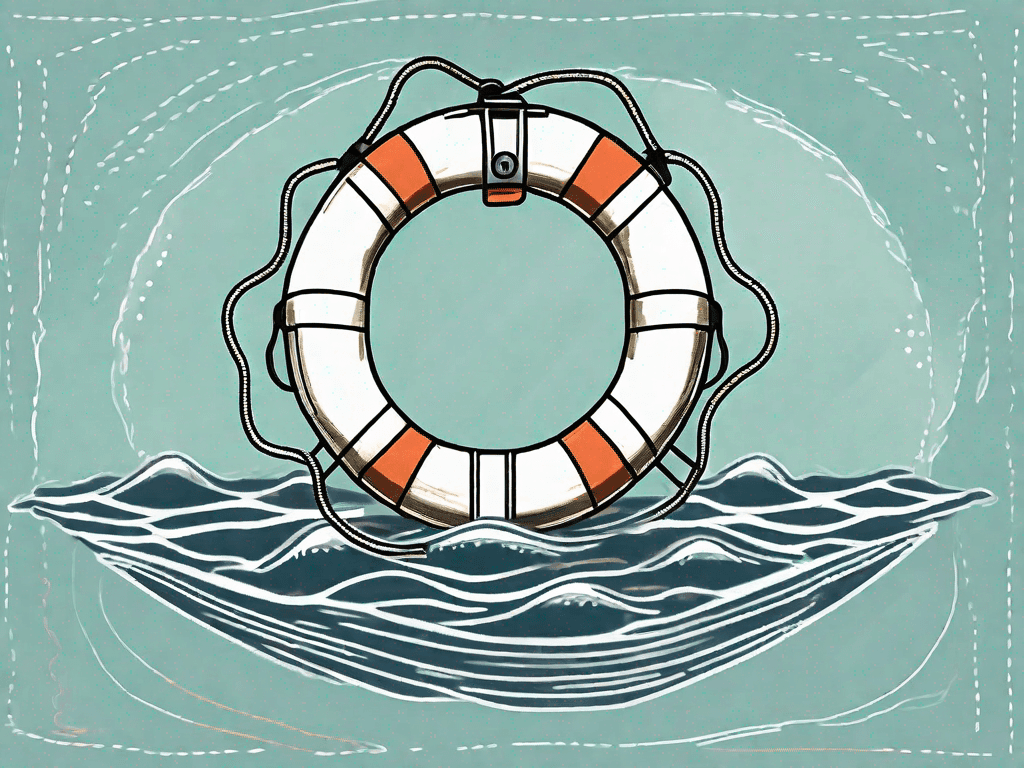
What is Churn Prevention? (Explained With Examples)

What is Churn Rate? (Explained With Examples)
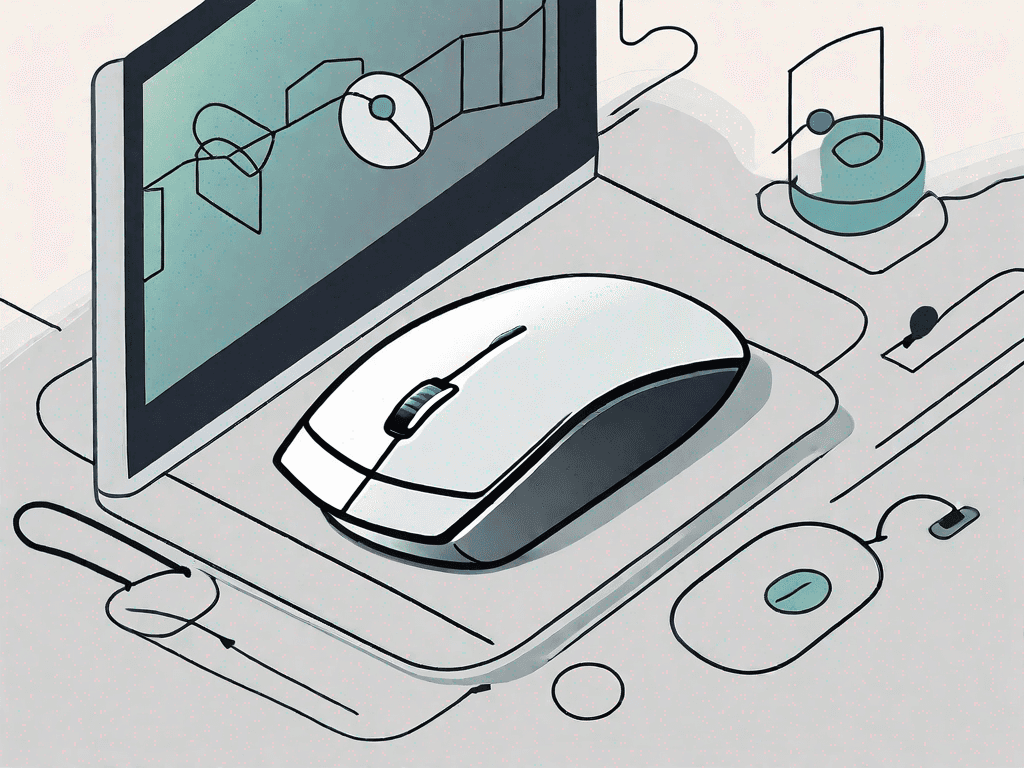
What is Click-Through Rate (CTR)? (Explained With Examples)

What is Client Acquisition? (Explained With Examples)
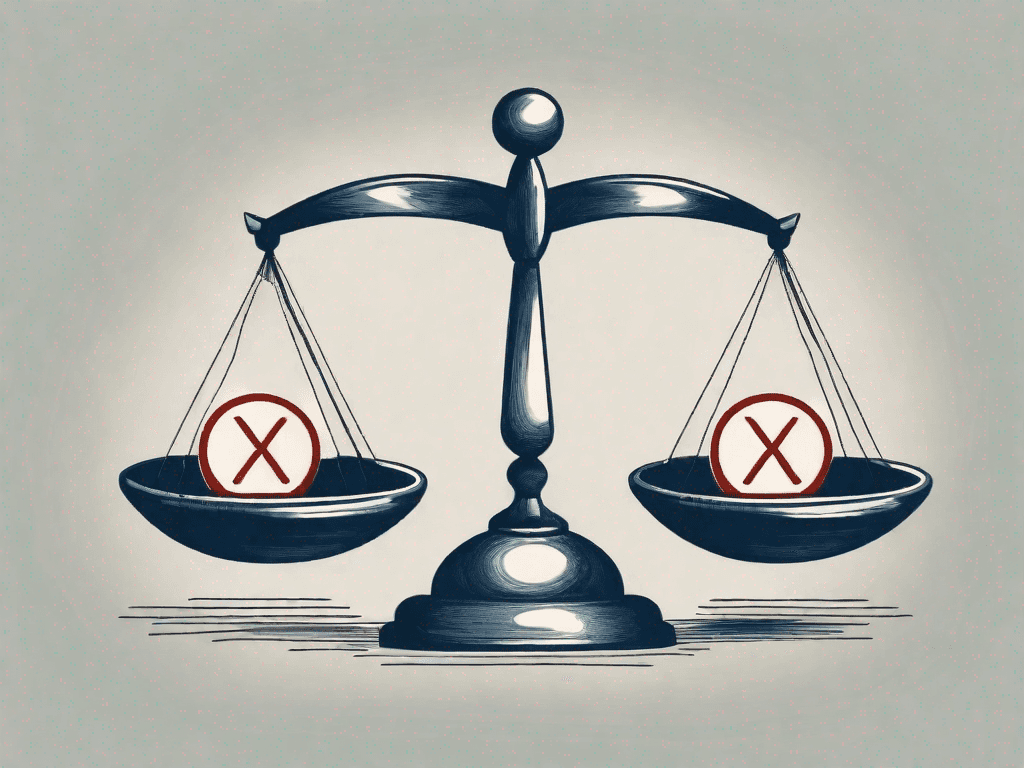
What is the Closing Ratio? (Explained With Examples)
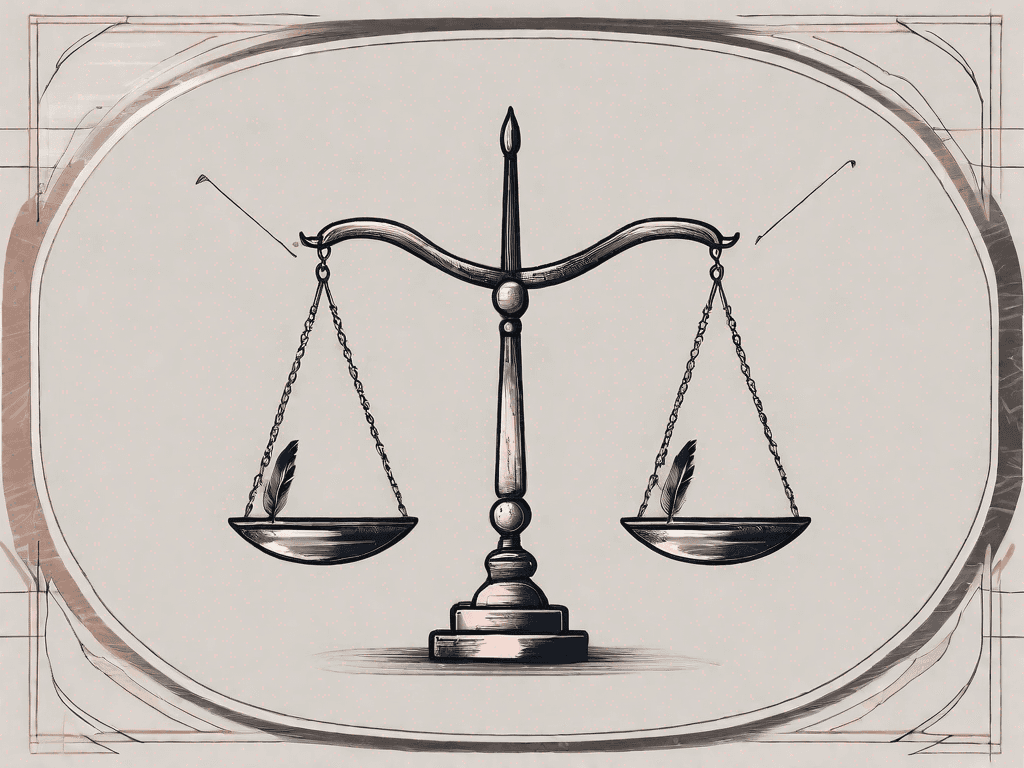
What is the Ben Franklin Close? (Explained With Examples)
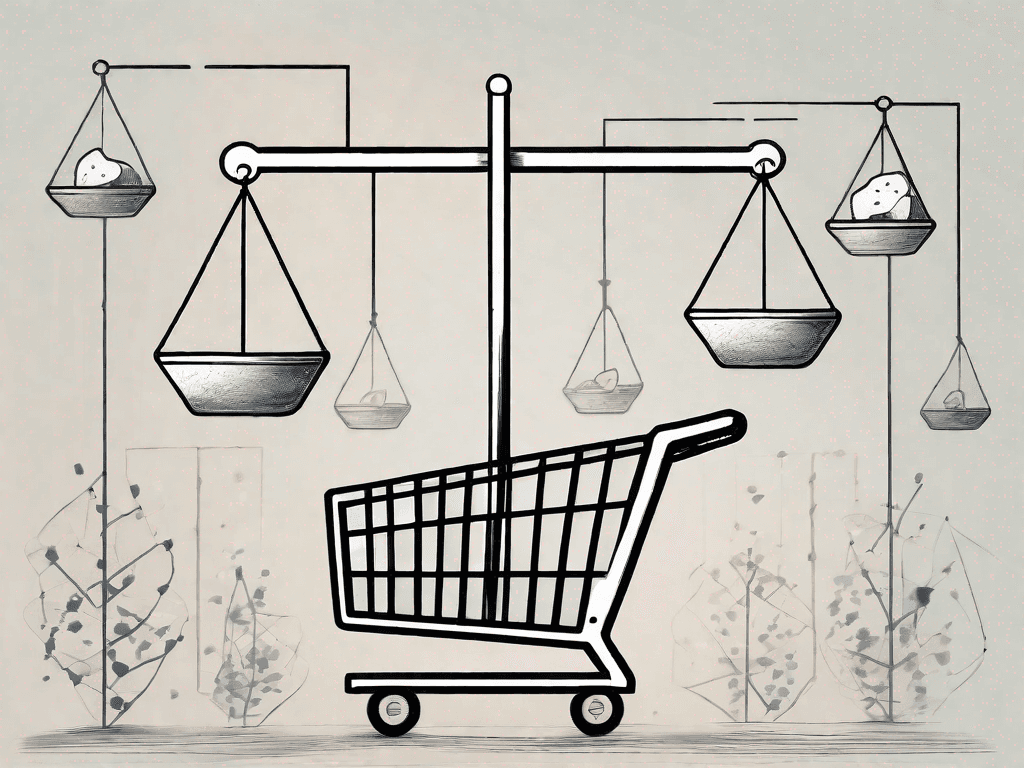
What is Cognitive Bias in Sales? (Explained With Examples)

What is Cognitive Dissonance in Sales? (Explained With Examples)

What is Cold Calling? (Explained With Examples)

What is Cold Outreach? (Explained With Examples)

What is a Competitive Advantage? (Explained With Examples)

What is a Competitive Analysis? (Explained With Examples)

What is Competitive Positioning? (Explained With Examples)

What is Conceptual Selling? (Explained With Examples)

What is Consultative Closing? (Explained With Examples)

What is Consultative Negotiation? (Explained With Examples)

What is Consultative Prospecting? (Explained With Examples)
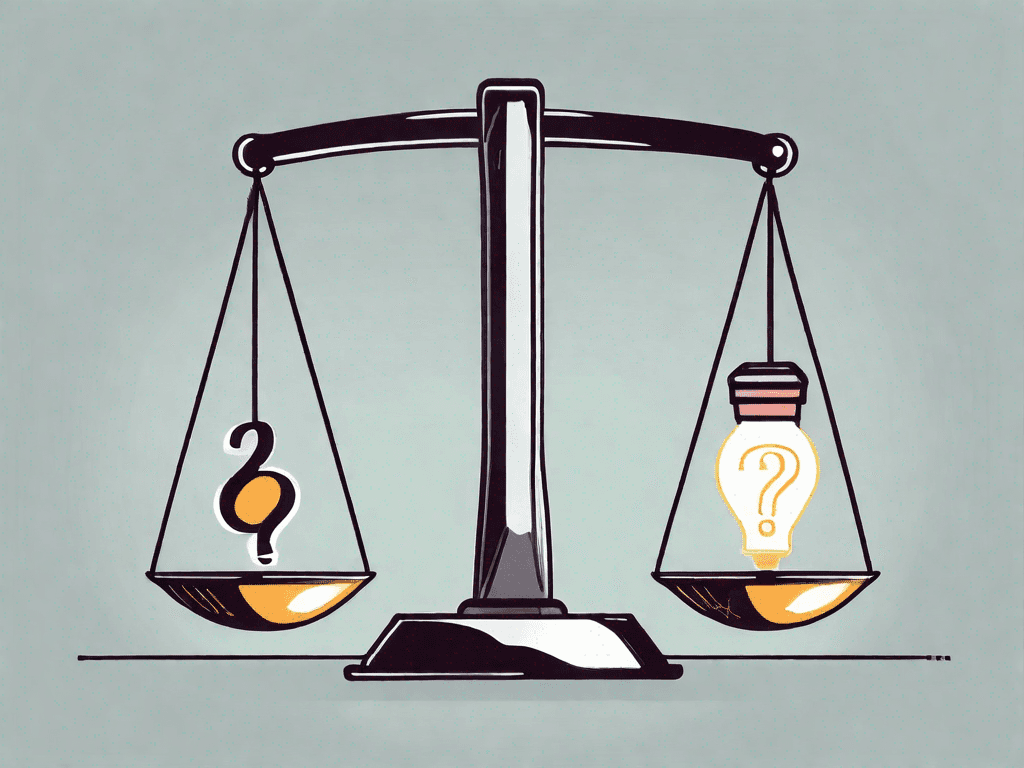
What is Consultative Selling? (Explained With Examples)

What is Content Marketing? (Explained With Examples)

What is Content Syndication? (Explained With Examples)

What is a Conversion Funnel? (Explained With Examples)

What is Conversion Optimization? (Explained With Examples)

What is a Conversion Path? (Explained With Examples)

What is Conversion Rate? (Explained With Examples)

What is Cost-Per-Click (CPC)? (Explained With Examples)

What is a CRM (Customer Relationship Management)? (Explained With Examples)

What is Cross-Cultural Selling? (Explained With Examples)
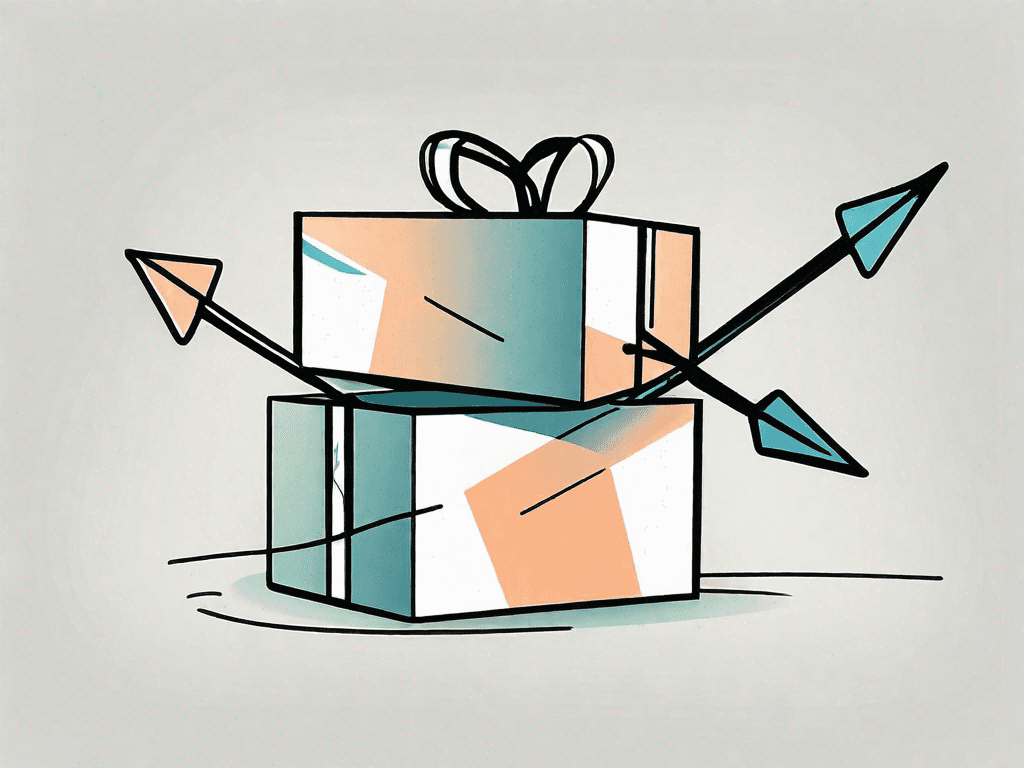
What is a Cross-Sell Ratio? (Explained With Examples)

What is Cross-Selling? (Explained With Examples)

What is Customer Acquisition Cost (CAC)? (Explained With Examples)

What is Customer-Centric Marketing? (Explained With Examples)

What is Customer-Centric Selling? (Explained With Examples)

What is Customer Journey Mapping? (Explained With Examples)

What is the Customer Journey? (Explained With Examples)
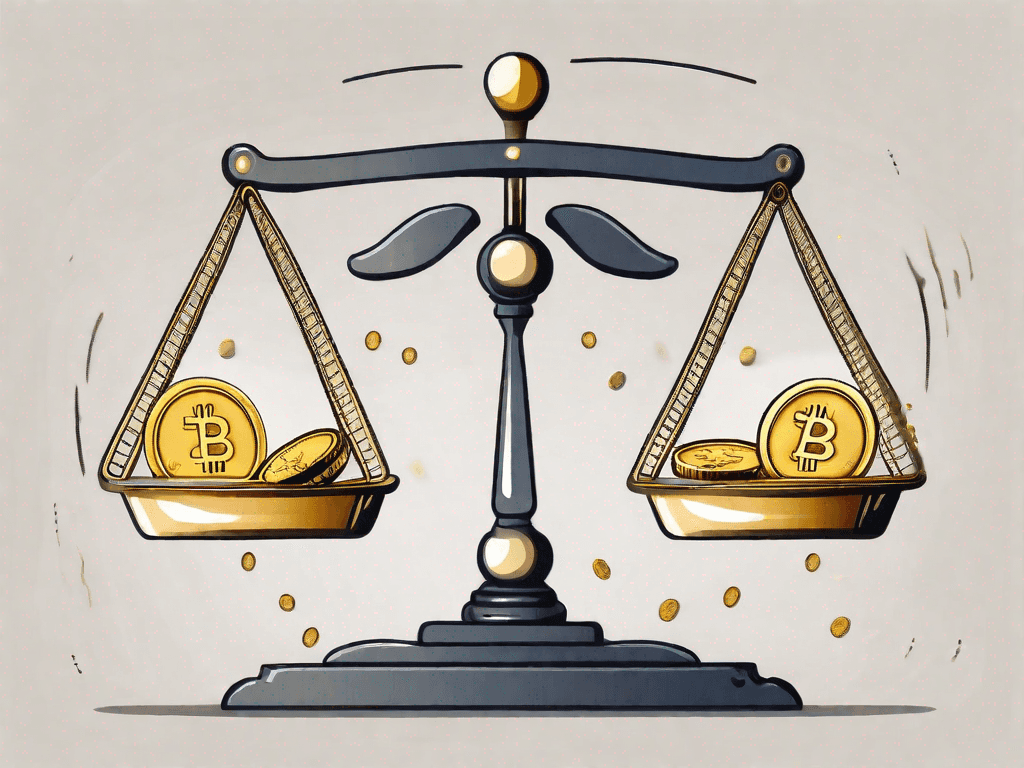
What is the Customer Lifetime Value (CLV)? (Explained With Examples)
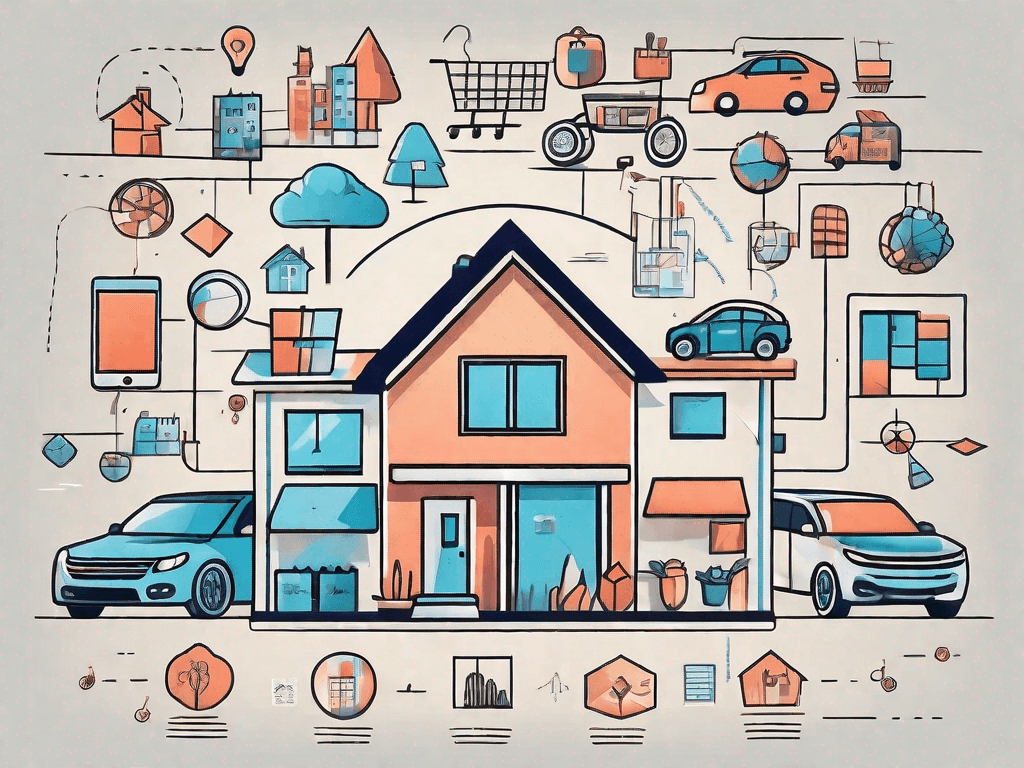
What is Customer Profiling? (Explained With Examples)

What is Customer Retention? (Explained With Examples)

What is Dark Social? (Explained With Examples)
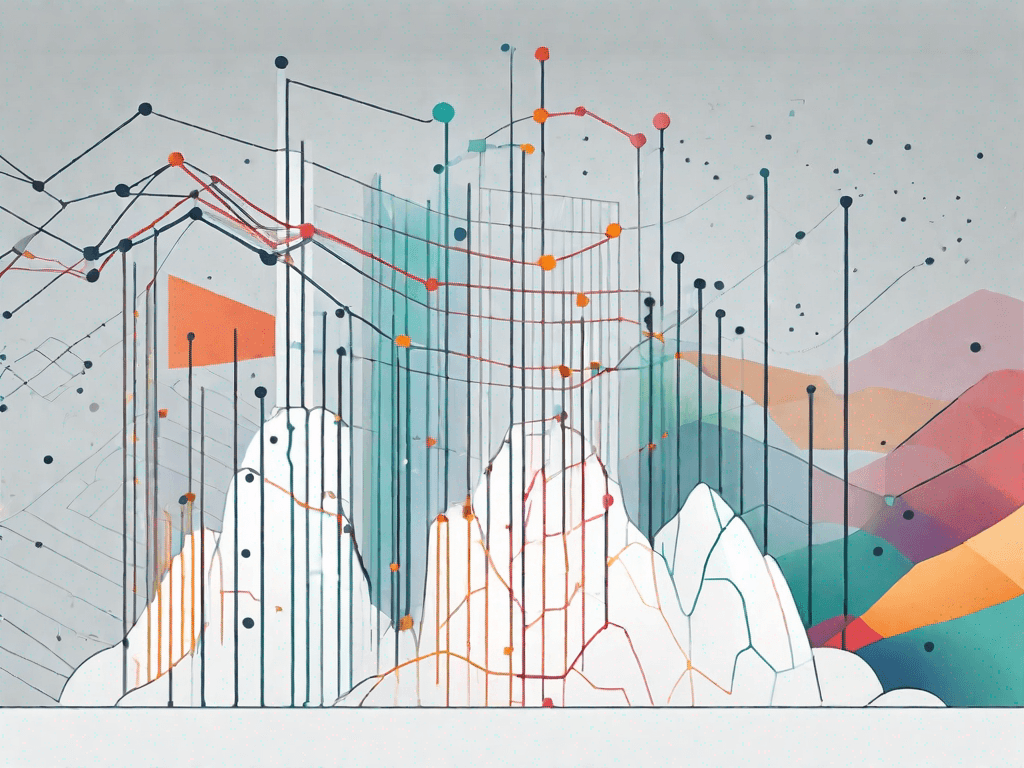
What is Data Enrichment? (Explained With Examples)

What is Data Segmentation? (Explained With Examples)
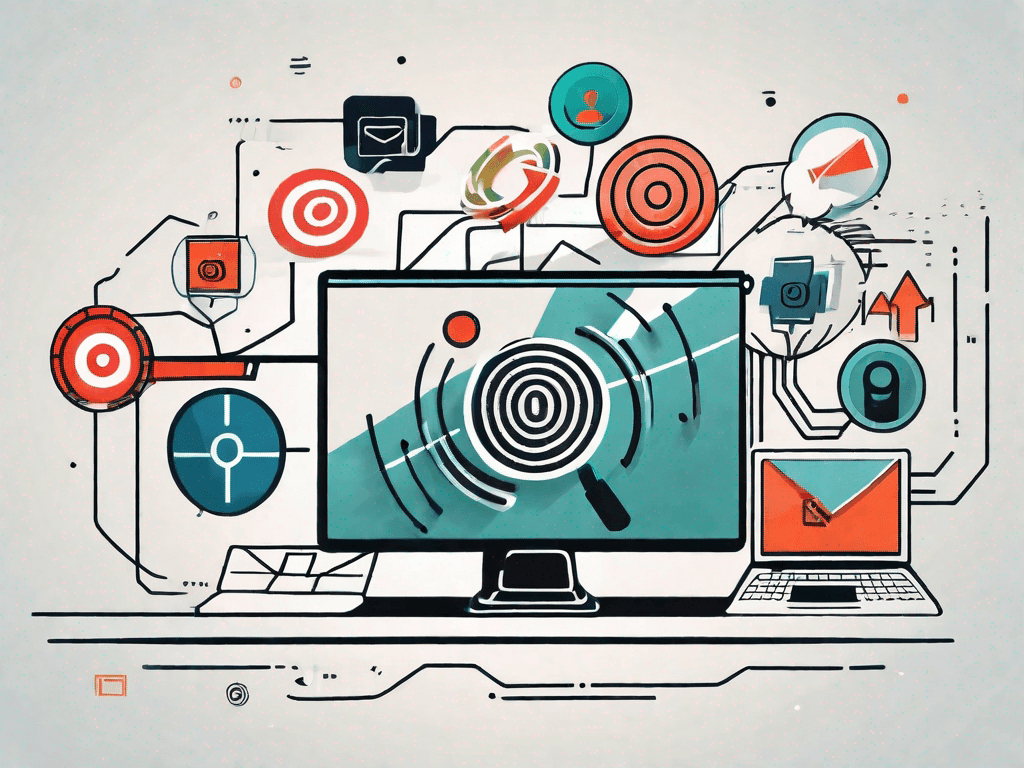
What is Database Marketing? (Explained With Examples)
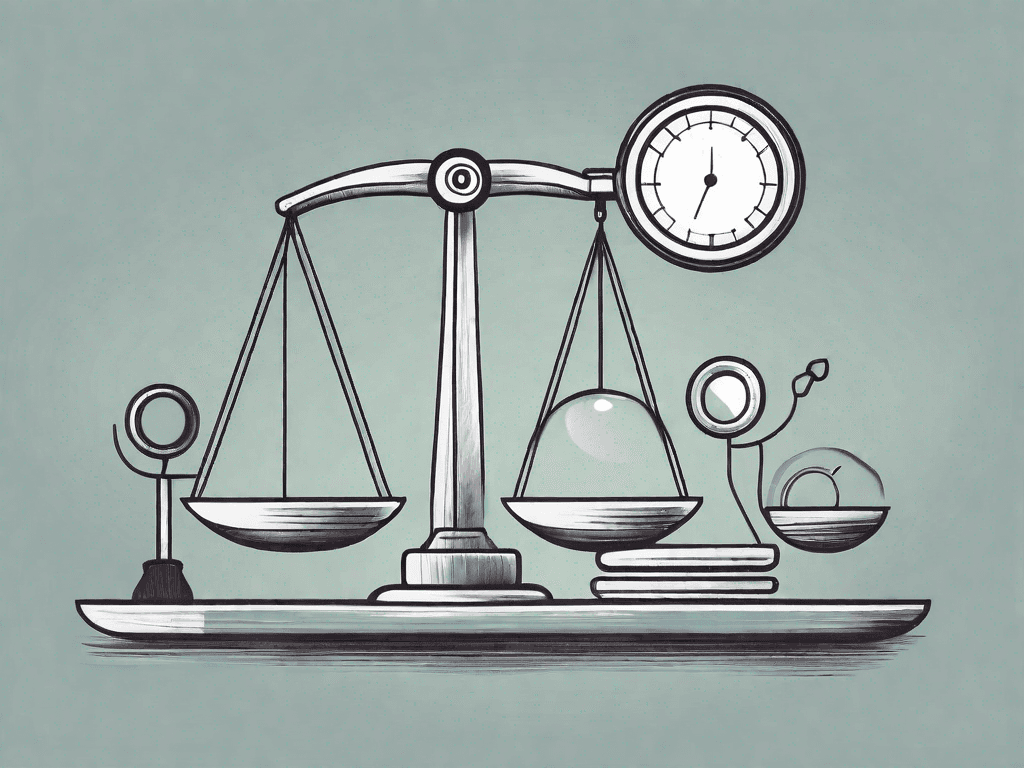
What is a Decision Maker? (Explained With Examples)

What is a Decision-Making Unit (DMU)? (Explained With Examples)

What is Demand Generation? (Explained With Examples)
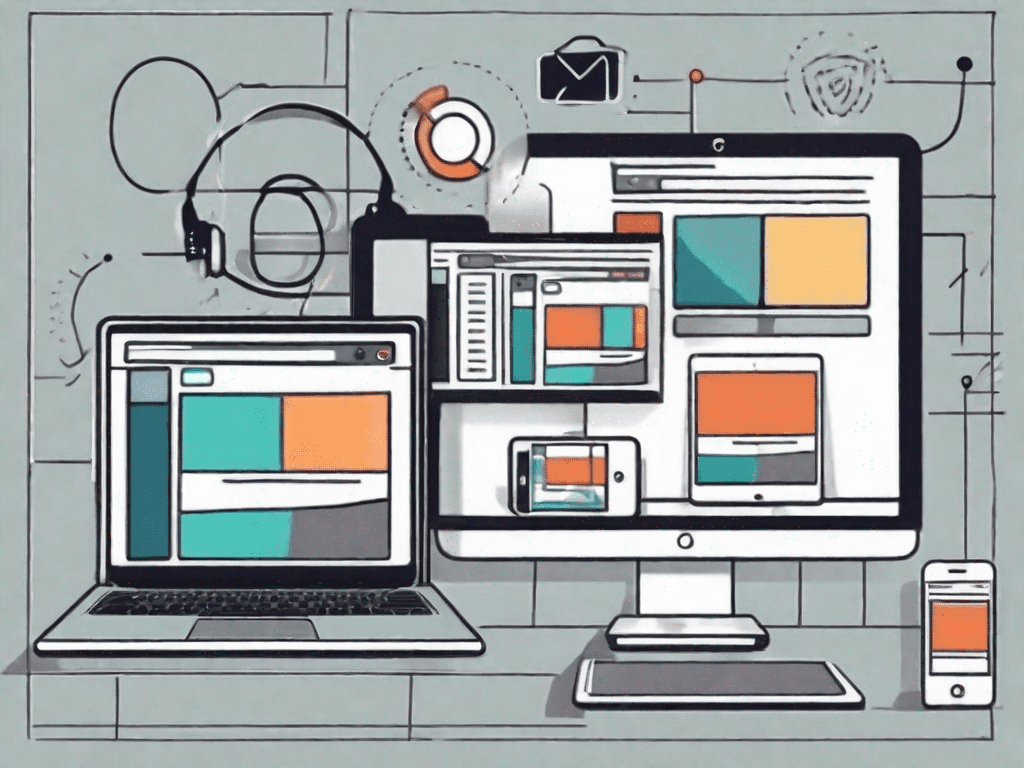
What is Digital Marketing? (Explained With Examples)

What is Direct Marketing? (Explained With Examples)
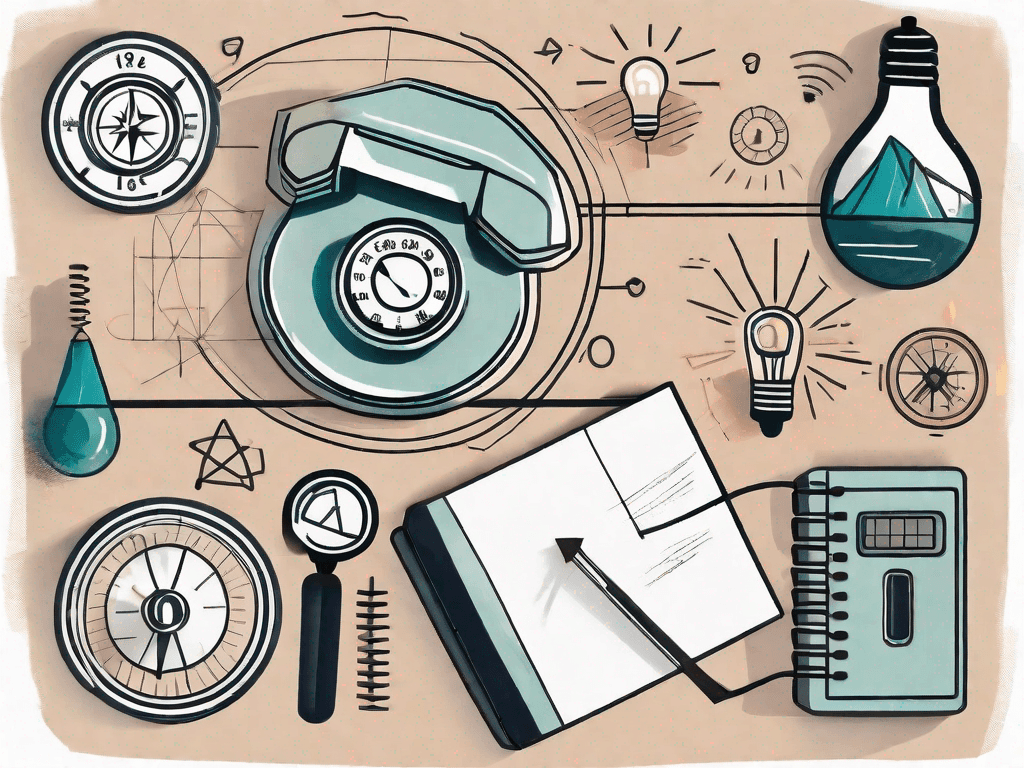
What is a Discovery Call? (Explained With Examples)
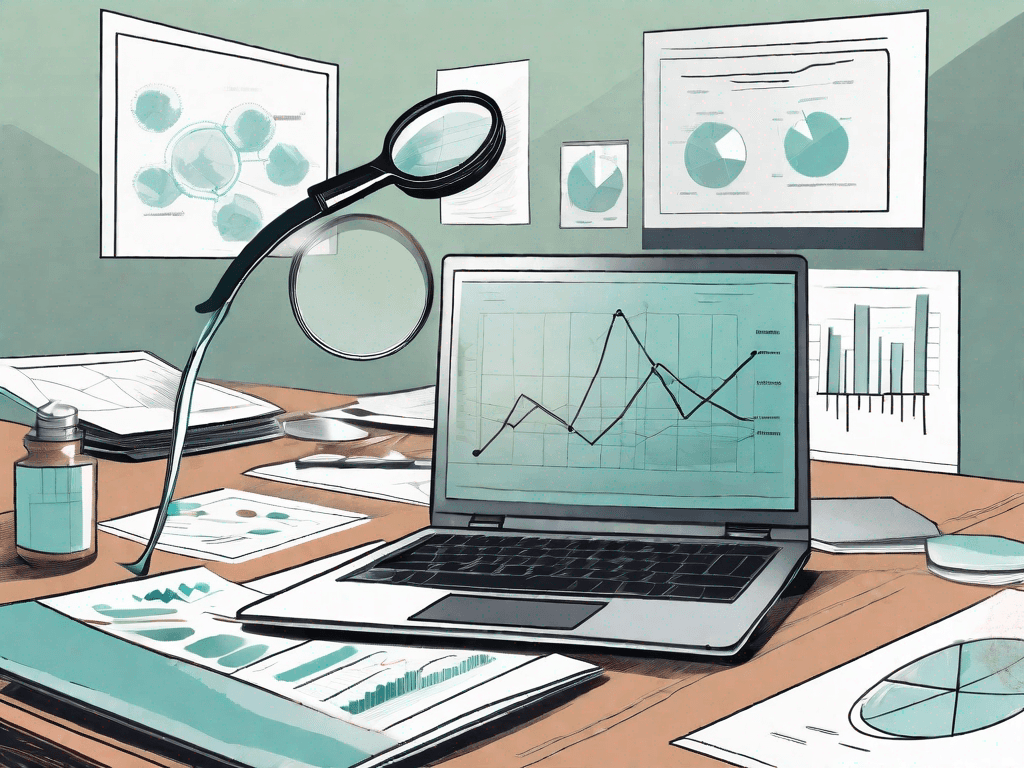
What is a Discovery Meeting? (Explained With Examples)
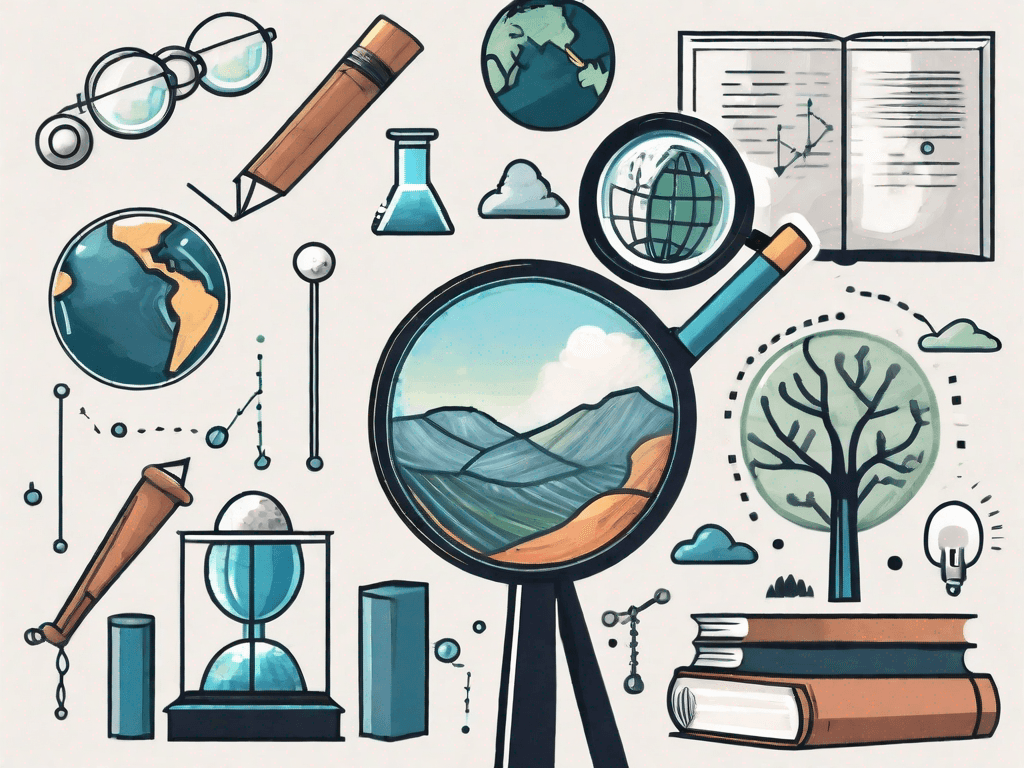
What are Discovery Questions? (Explained With Examples)

What is Door-to-Door Sales? (Explained With Examples)

What is a Drip Campaign? (Explained With Examples)

What is Dunning? (Explained With Examples)

What is an Early Adopter? (Explained With Examples)

What is Elevator Pitch? (Explained With Examples)

What is Email Hygiene? (Explained With Examples)
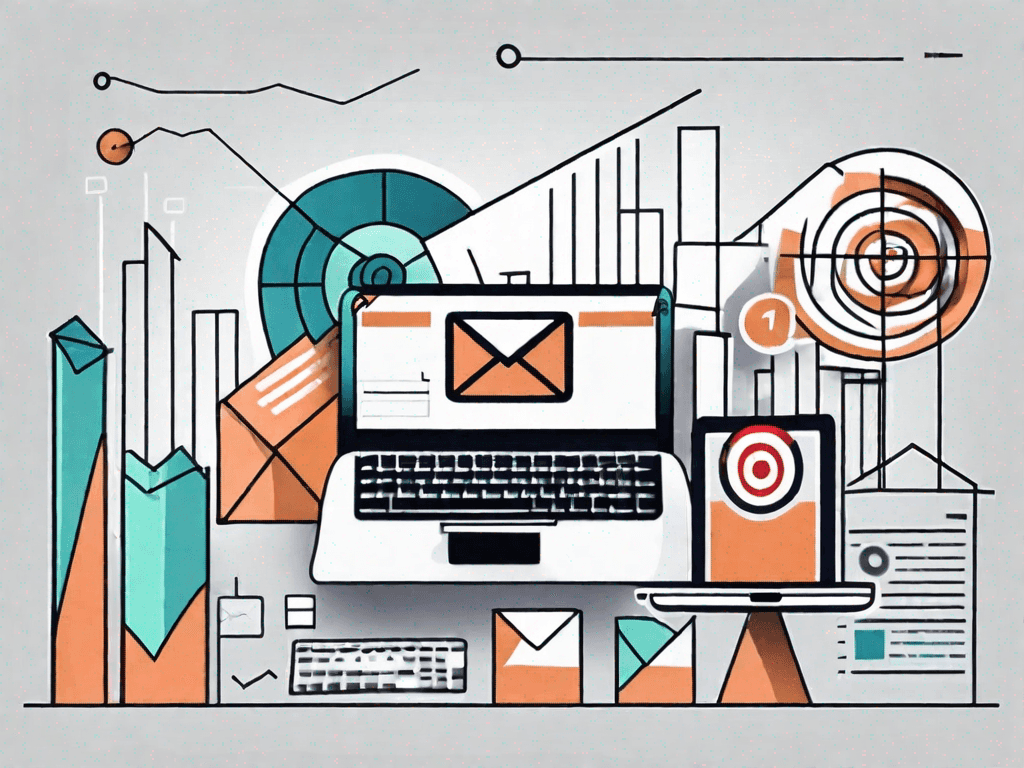
What is Email Marketing? (Explained With Examples)

What is Emotional Intelligence Selling? (Explained With Examples)
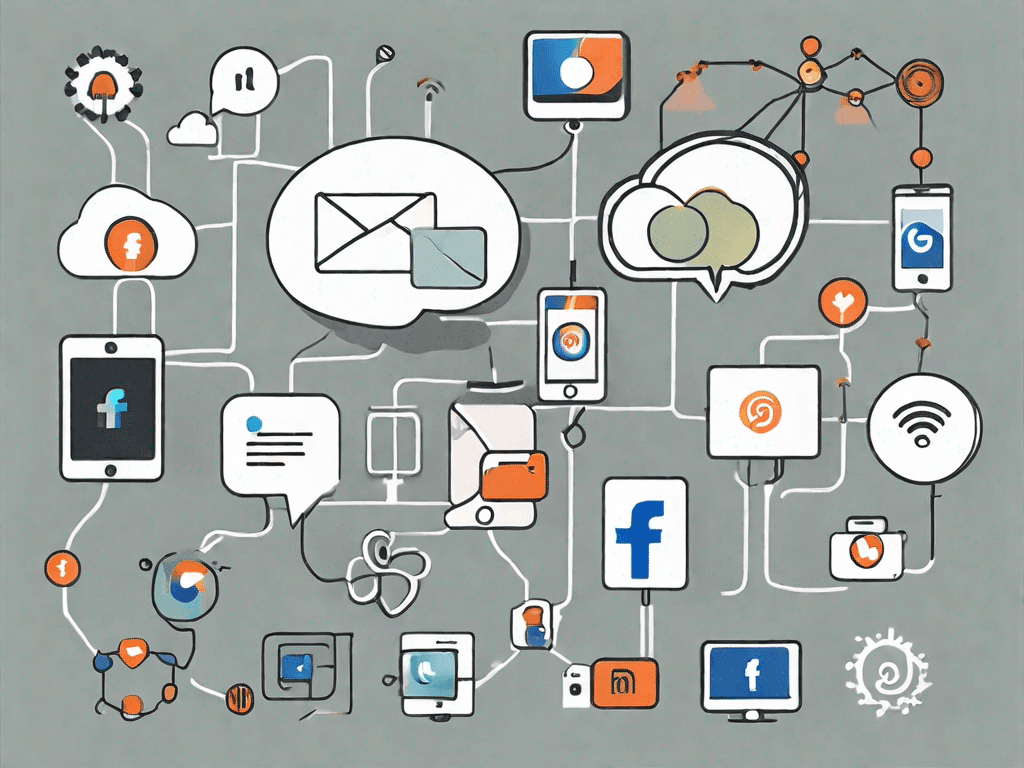
What is Engagement Marketing? (Explained With Examples)

What is Engagement Rate? (Explained With Examples)

What is Engagement Strategy? (Explained With Examples)

What is Feature-Benefit Selling? (Explained With Examples)

What is Field Sales? (Explained With Examples)

What is a Follow-Up? (Explained With Examples)

What is Forecast Accuracy? (Explained With Examples)

What is a Funnel? (Explained With Examples)
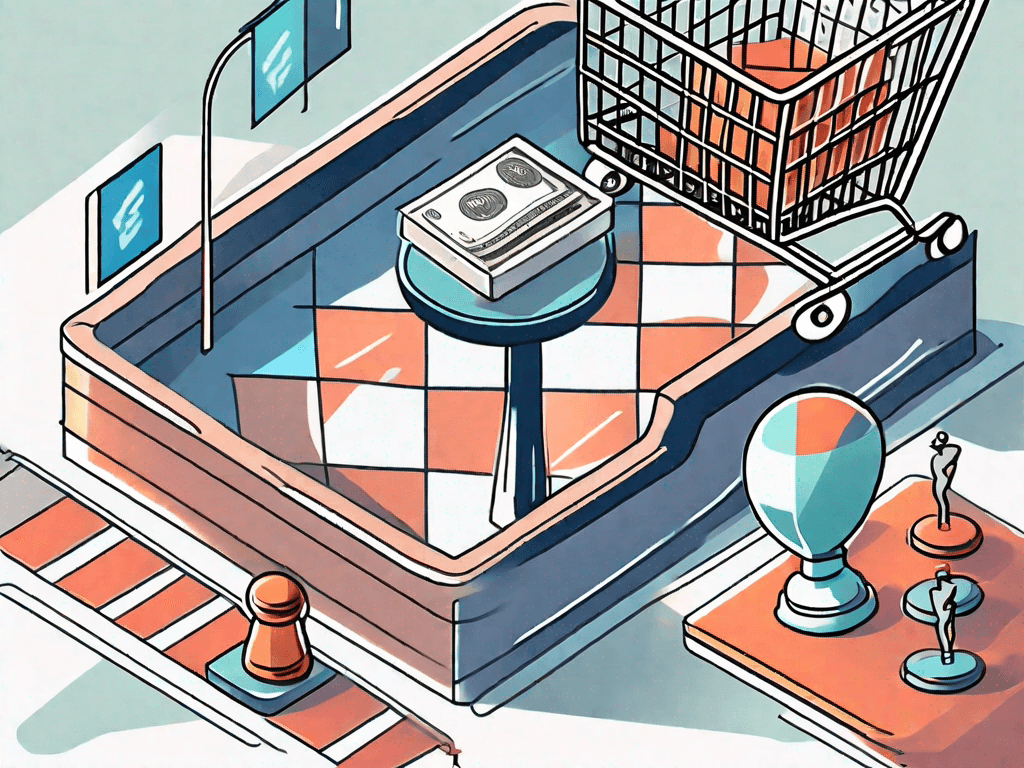
What is Gamification in Sales? (Explained With Examples)

What is Gatekeeper Strategy? (Explained With Examples)

What is Gatekeeper? (Explained With Examples)

What is a Go-to Market Strategy? (Explained With Examples)

What is Growth Hacking? (Explained With Examples)
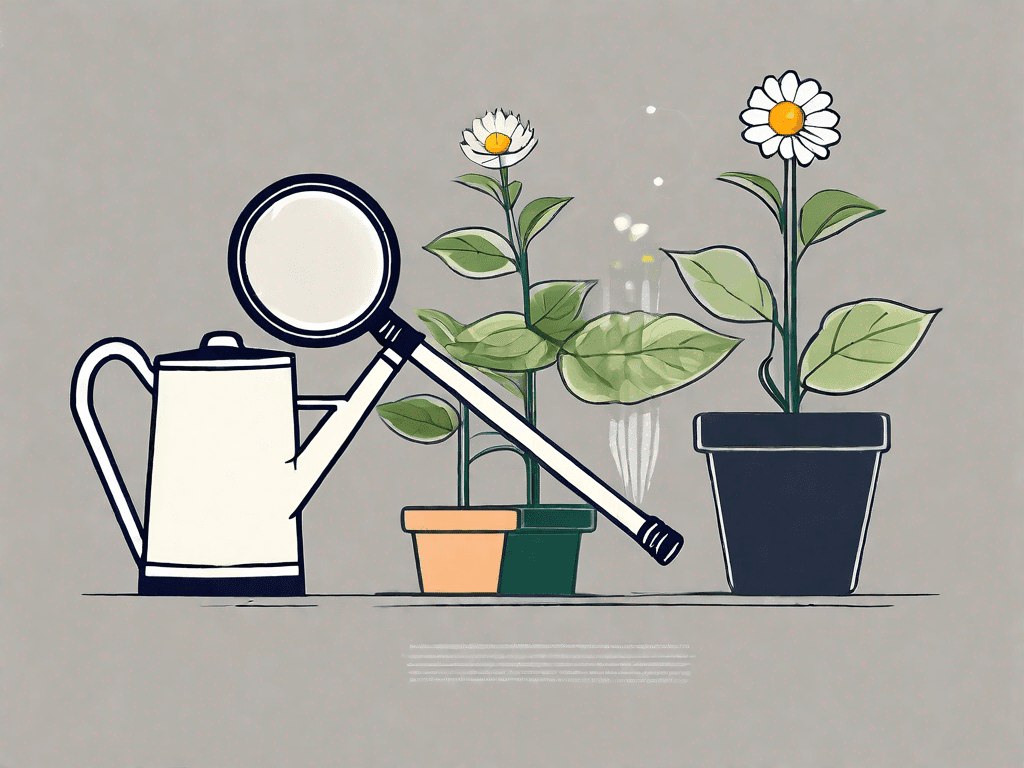
What is Growth Marketing? (Explained With Examples)

What is Guerrilla Marketing? (Explained With Examples)

What is High-Ticket Sales? (Explained With Examples)

What is Holistic Selling? (Explained With Examples)

What is Ideal Customer Profile (ICP)? (Explained With Examples)

What is Inbound Lead Generation? (Explained With Examples)

What is an Inbound Lead? (Explained With Examples)
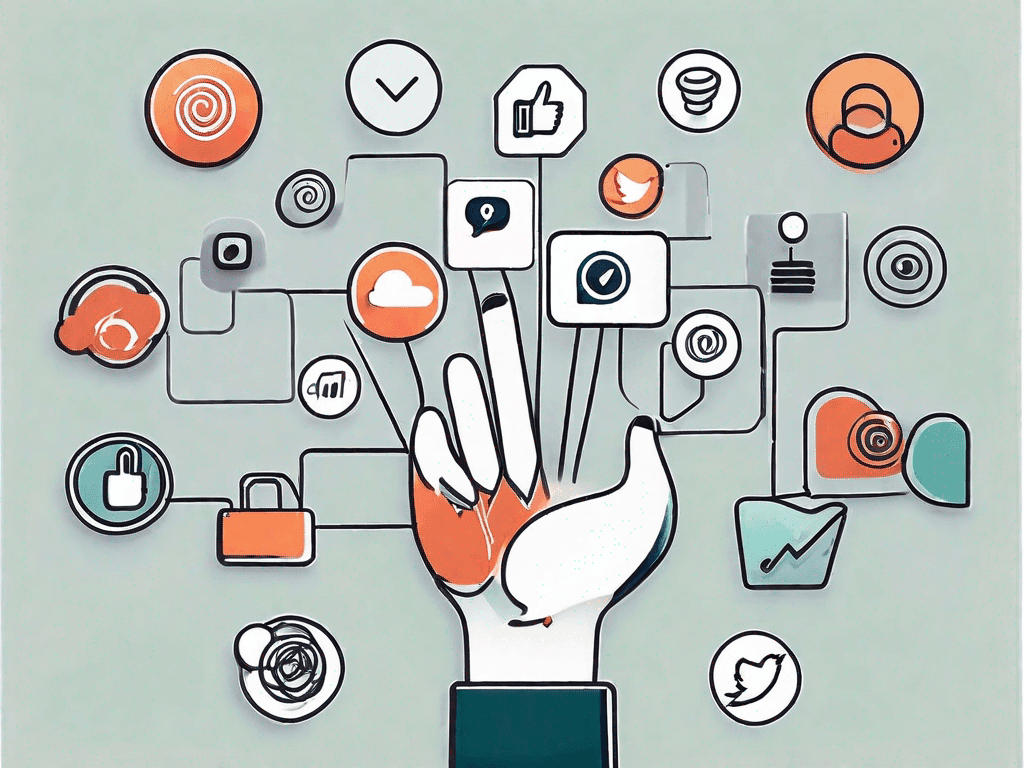
What is Inbound Marketing? (Explained With Examples)
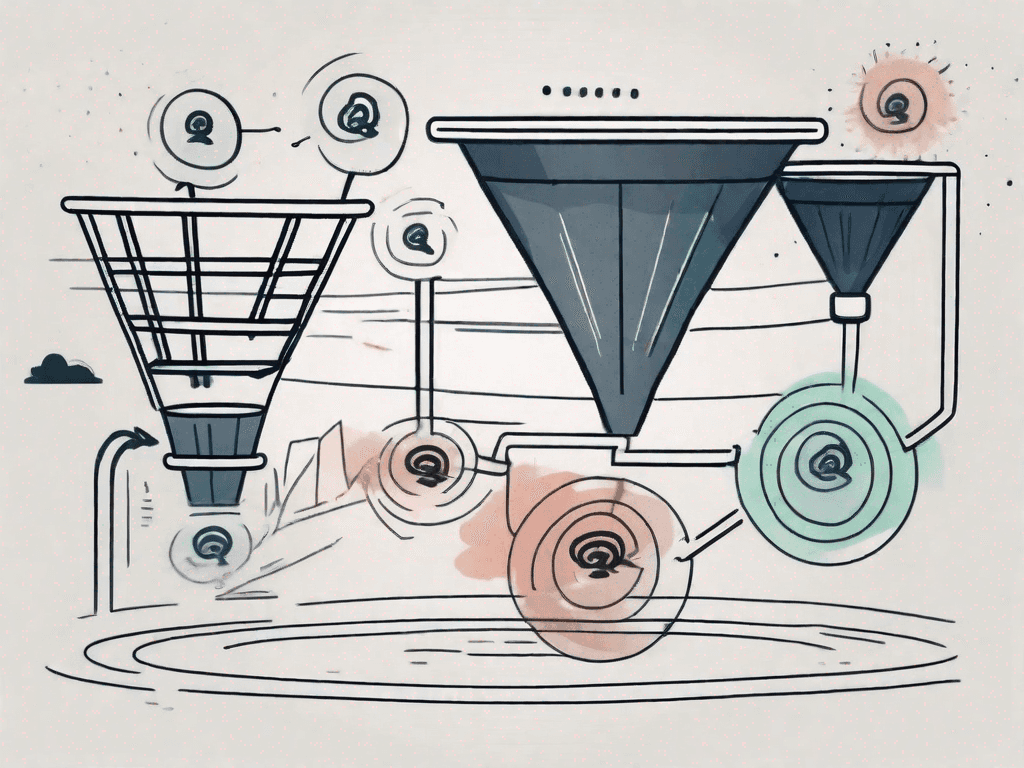
What is Inbound Sales? (Explained With Examples)

What is Influencer Marketing? (Explained With Examples)

What is Inside Sales Representative? (Explained With Examples)

What is Inside Sales? (Explained With Examples)

What is Insight Selling? (Explained With Examples)

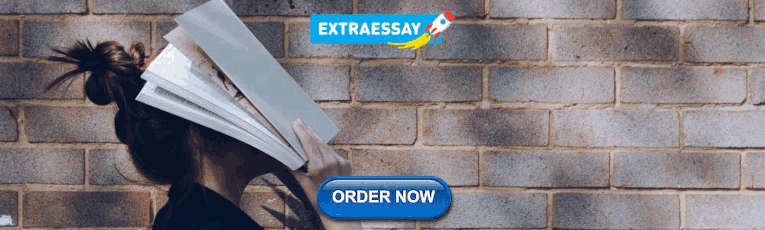
What is a Key Account? (Explained With Examples)

What is a Key Performance Indicator (KPI)? (Explained With Examples)

What is a Landing Page? (Explained With Examples)
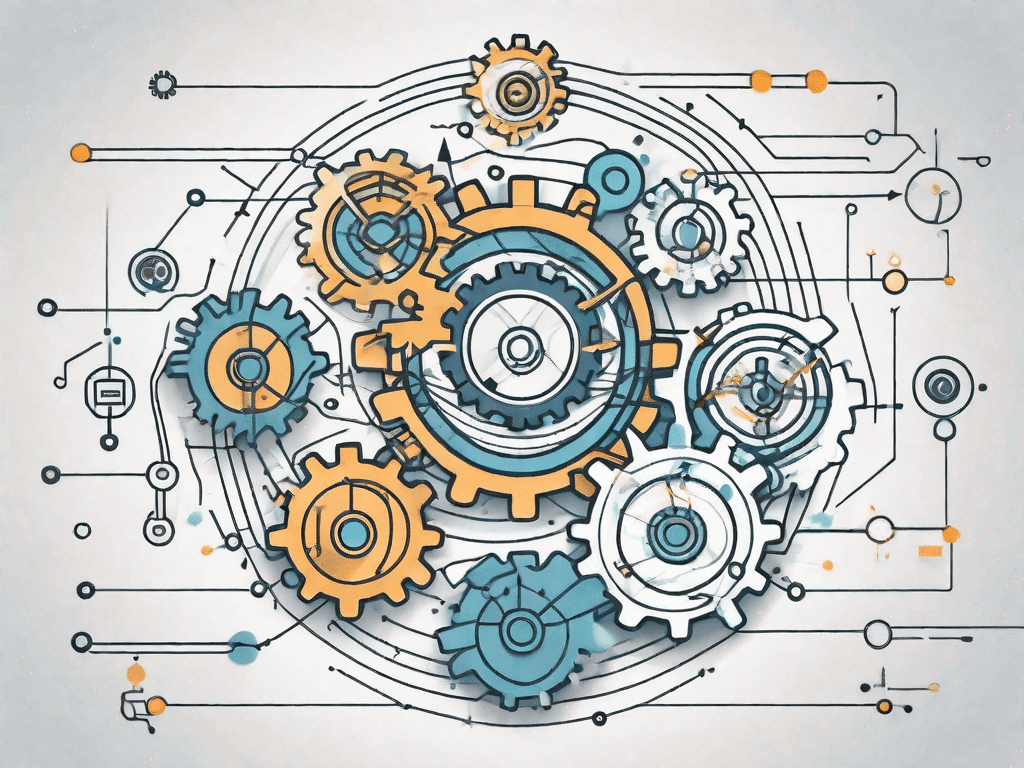
What is Lead Database? (Explained With Examples)

What is a Lead Enrichment? (Explained With Examples)

What is Lead Generation? (Explained With Examples)

What is Lead Nurturing? (Explained With Examples)

What is Lead Qualification? (Explained With Examples)
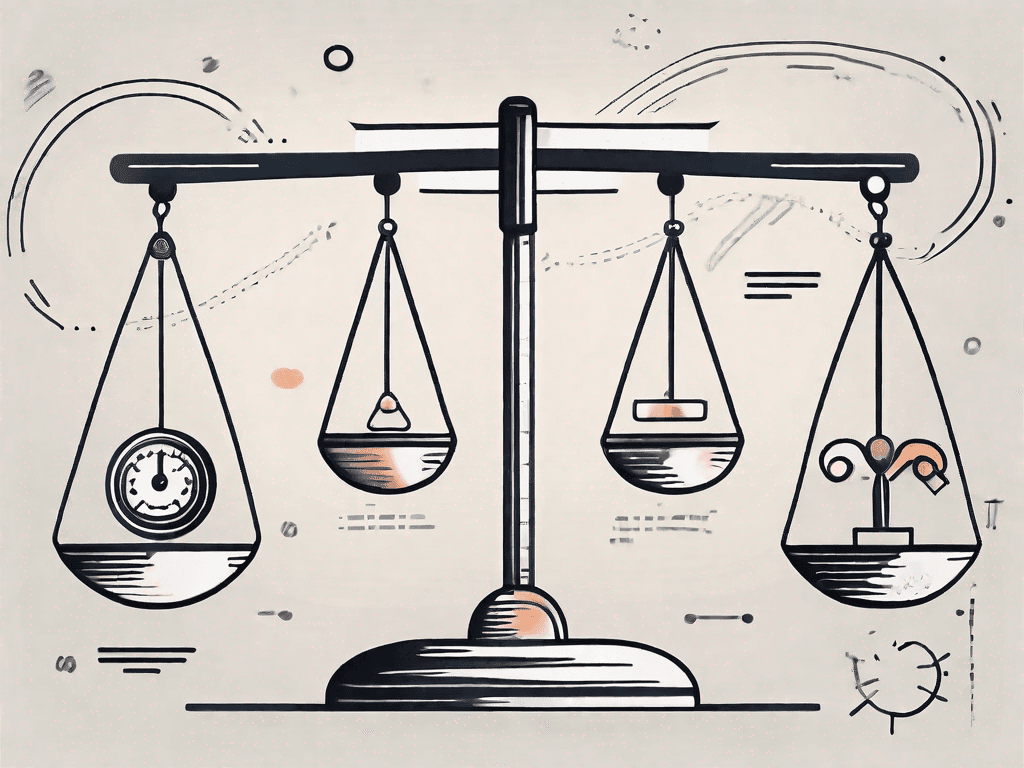
What is Lead Scoring? (Explained With Examples)
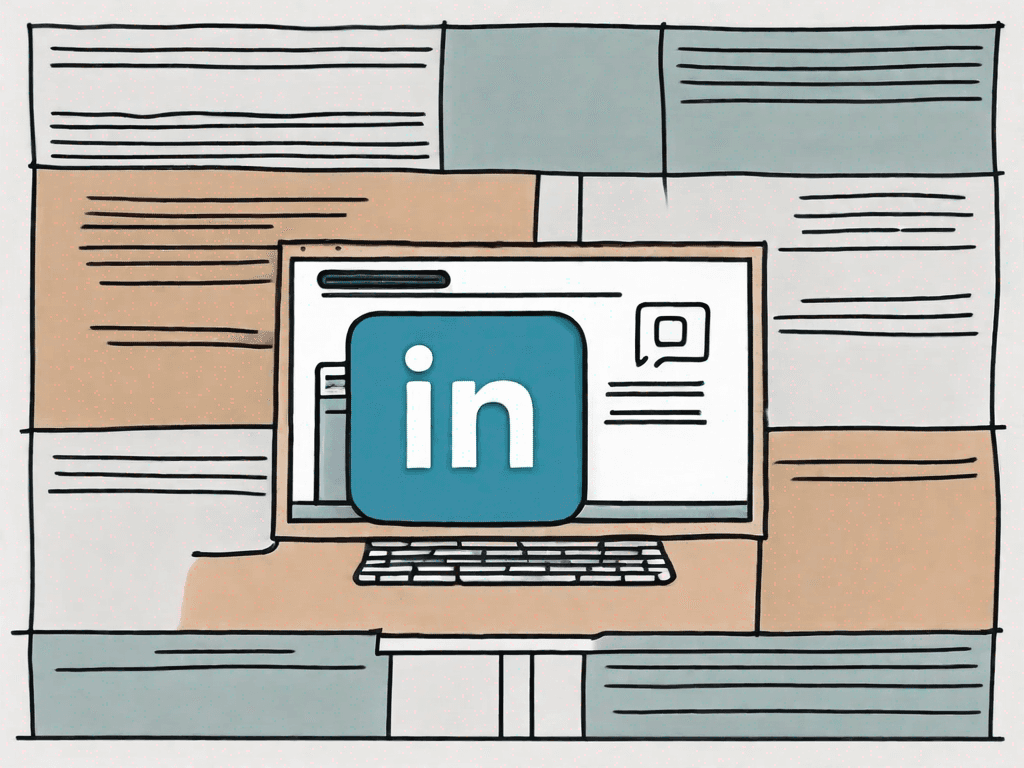
What are LinkedIn InMails? (Explained With Examples)

What is LinkedIn Sales Navigator? (Explained With Examples)

What is Lost Opportunity? (Explained With Examples)

What is Market Positioning? (Explained With Examples)

What is Market Research? (Explained With Examples)
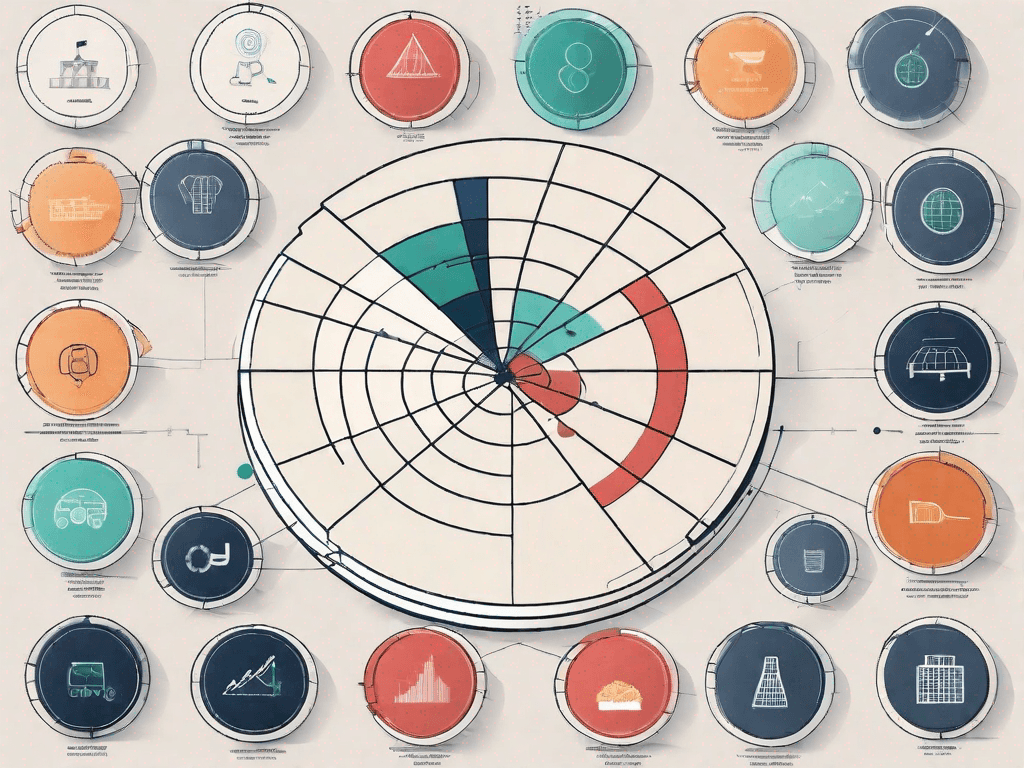
What is Market Segmentation? (Explained With Examples)

What is MEDDIC? (Explained With Examples)

What is Middle Of The Funnel (MOFU)? (Explained With Examples)

What is Motivational Selling? (Explained With Examples)

What is a MQL (Marketing Qualified Lead)? (Explained With Examples)

What is MRR Growth? (Explained With Examples)
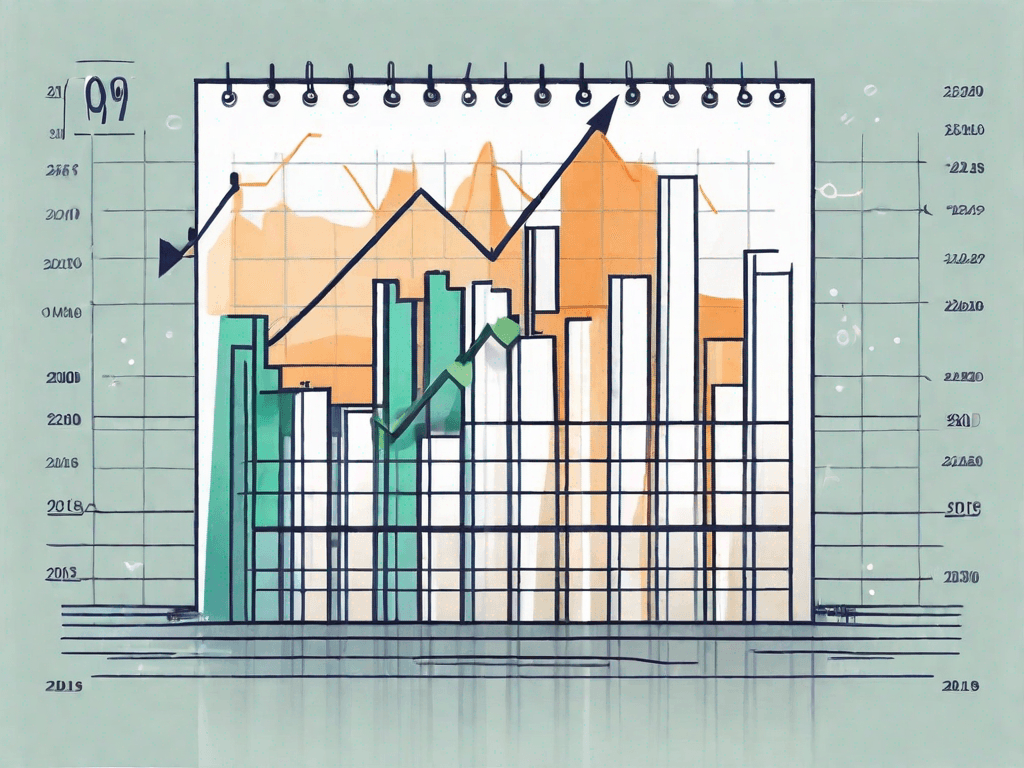
What is MRR (Monthly Recurring Revenue)? (Explained With Examples)
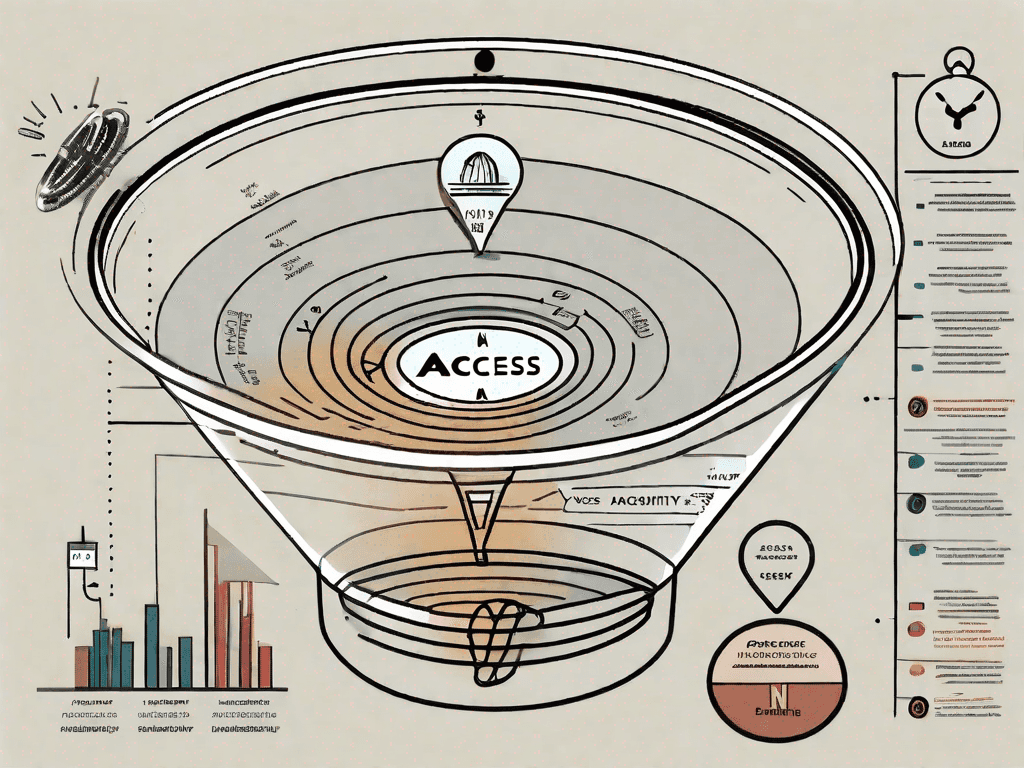
What is N.E.A.T. Selling? (Explained With Examples)

What is Neil Rackham's Sales Tactics? (Explained With Examples)
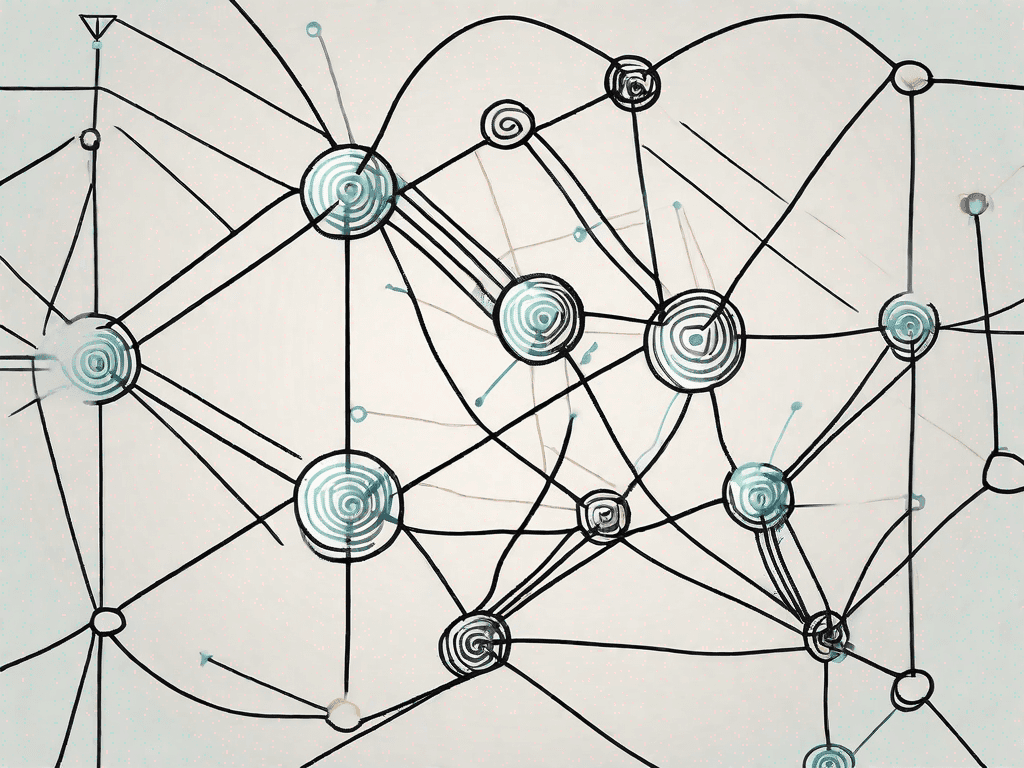
What is Networking? (Explained With Examples)

What is NLP Sales Techniques? (Explained With Examples)

What is the Net Promotion Score? (NPS - Explained With Examples)
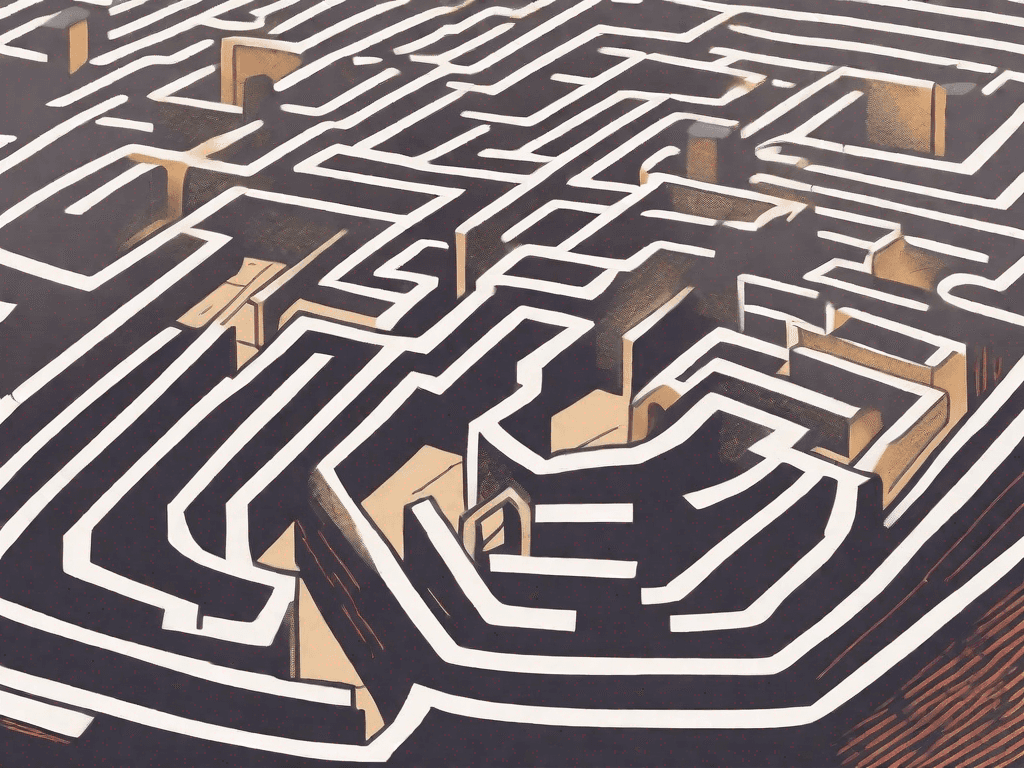
What is Objection Handling Framework? (Explained With Examples)

What is On-Hold Messaging? (Explained With Examples)

What is Onboarding in Sales? (Explained With Examples)

What is Online Advertising? (Explained With Examples)

What is Outbound Sales? (Explained With Examples)

What is Pain Points Analysis? (Explained With Examples)

What is Permission Marketing? (Explained With Examples)

What is Personality-Based Selling? (Explained With Examples)
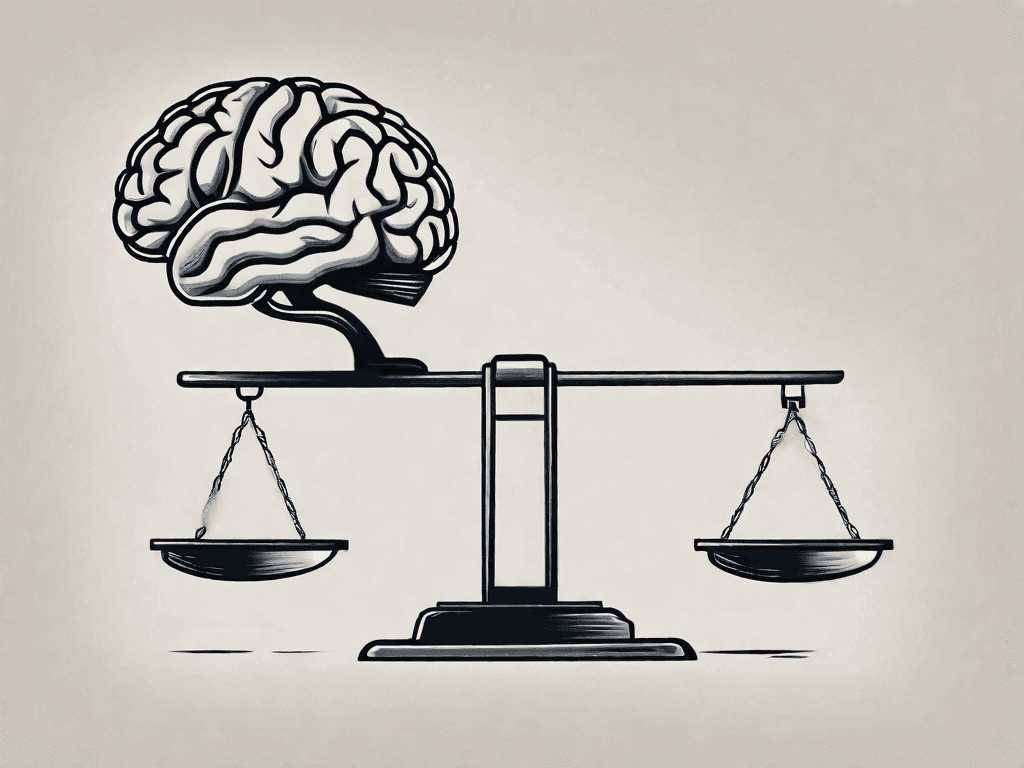
What is Persuasion Selling? (Explained With Examples)
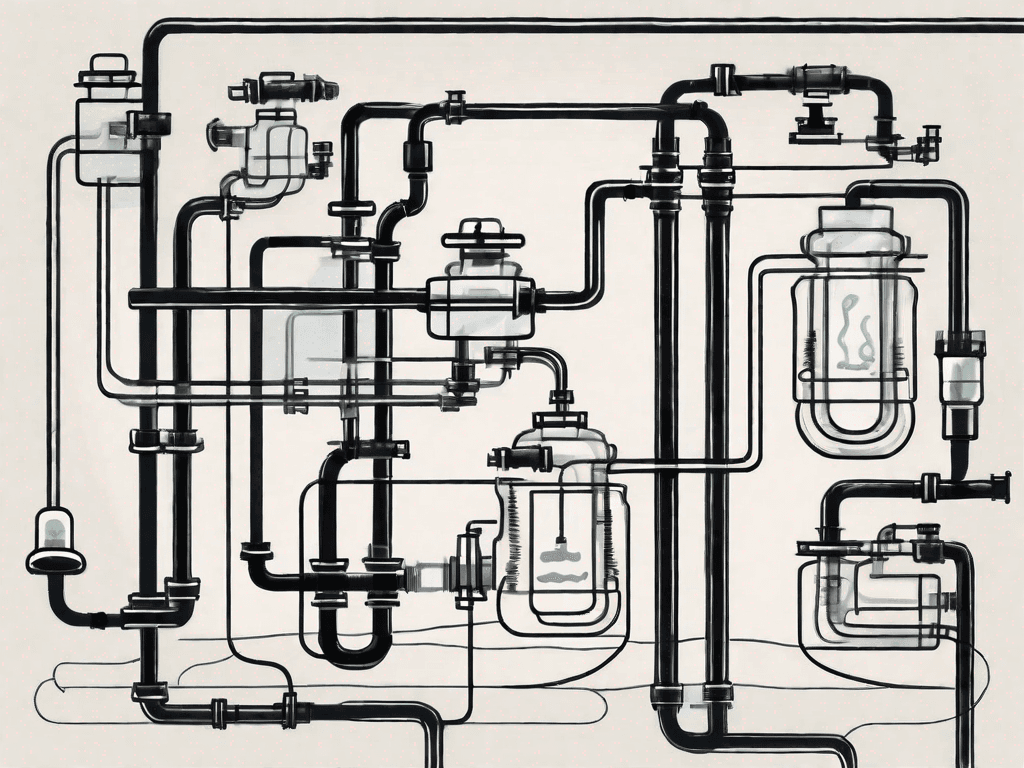
What is Pipeline Management? (Explained With Examples)

What is Pipeline Velocity? (Explained With Examples)

What is Predictive Lead Scoring? (Explained With Examples)

What is Price Negotiation? (Explained With Examples)
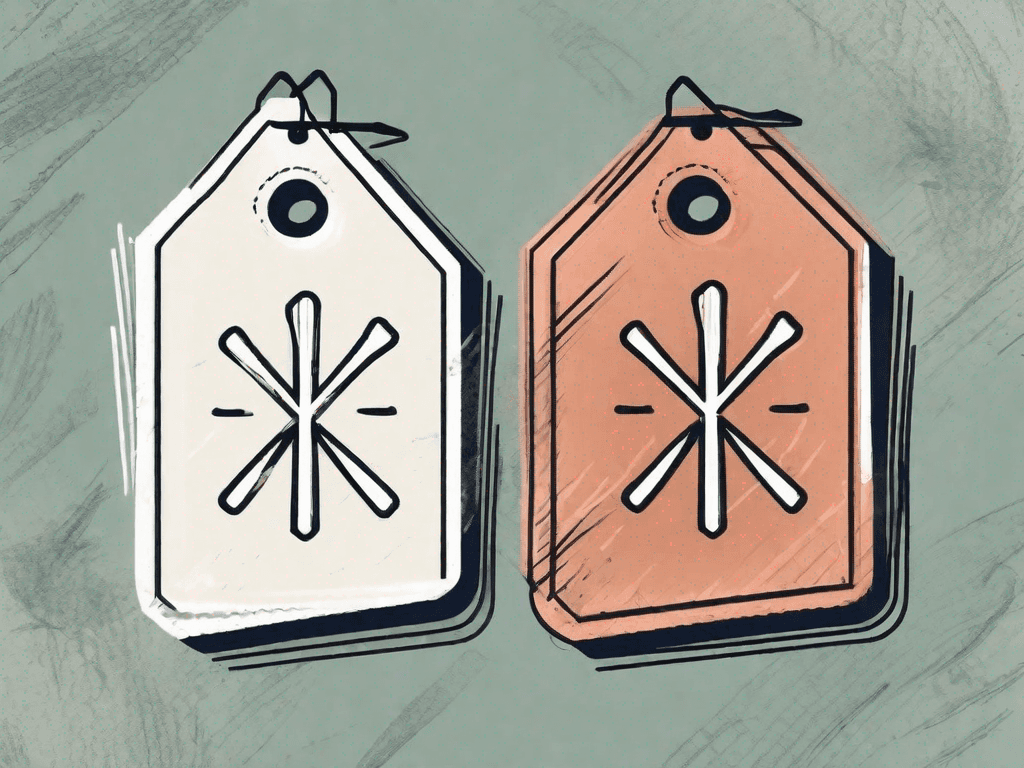
What is Price Objection? (Explained With Examples)

What is Price Sensitivity? (Explained With Examples)

What is Problem-Solution Selling? (Explained With Examples)
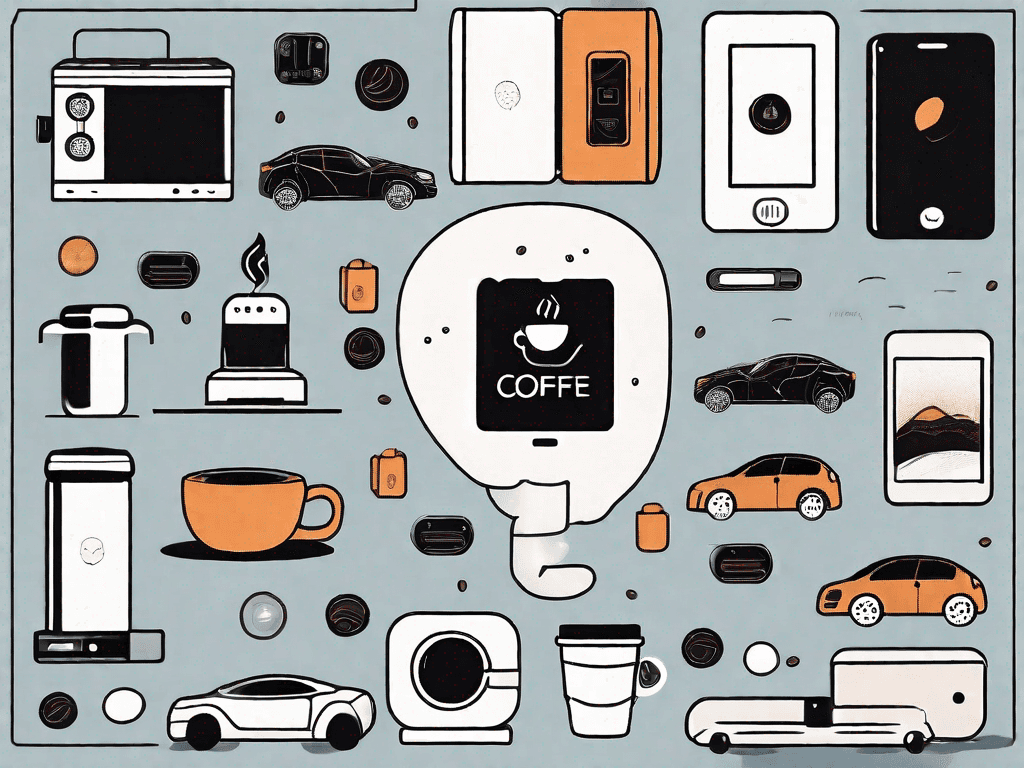
What is Product Knowledge? (Explained With Examples)

What is Product-Led-Growth? (Explained With Examples)
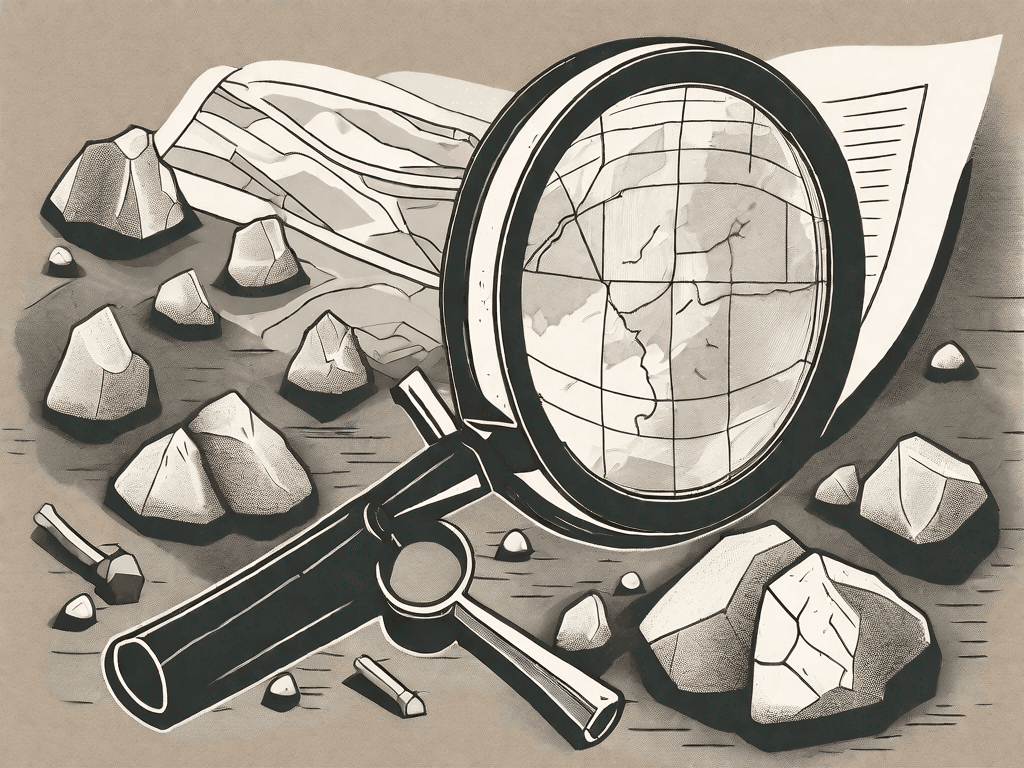
What is Prospecting? (Explained With Examples)

What is a Qualified Lead? (Explained With Examples)

What is Question-Based Selling? (Explained With Examples)
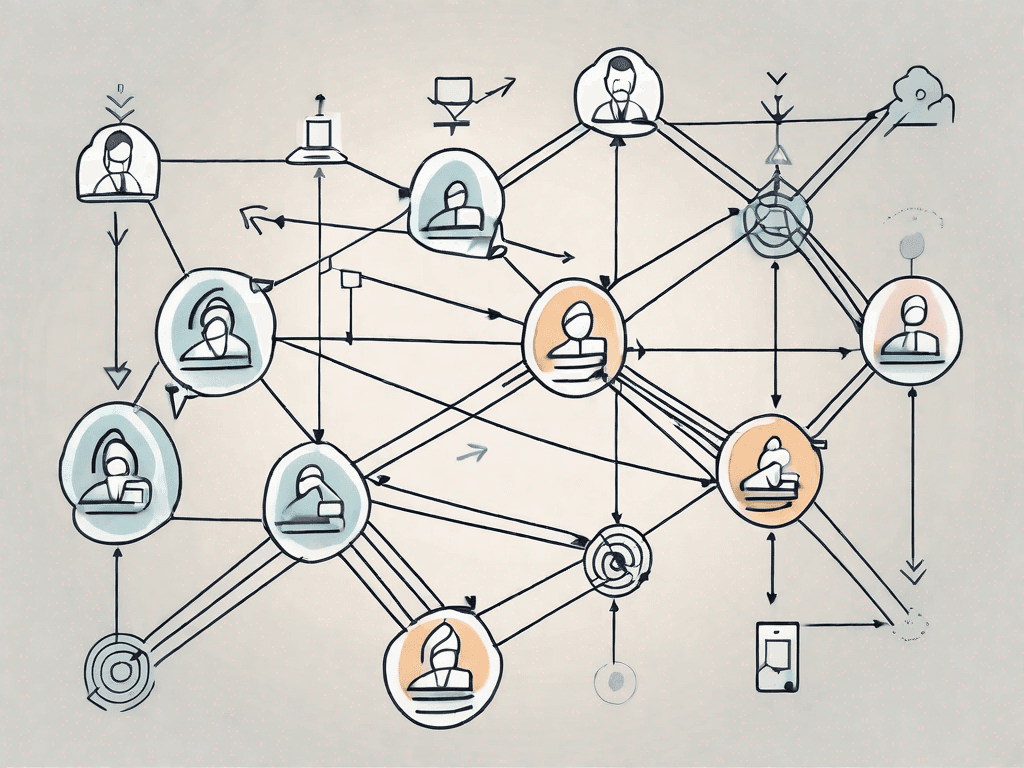
What is Referral Marketing? (Explained With Examples)

What is Relationship Building? (Explained With Examples)
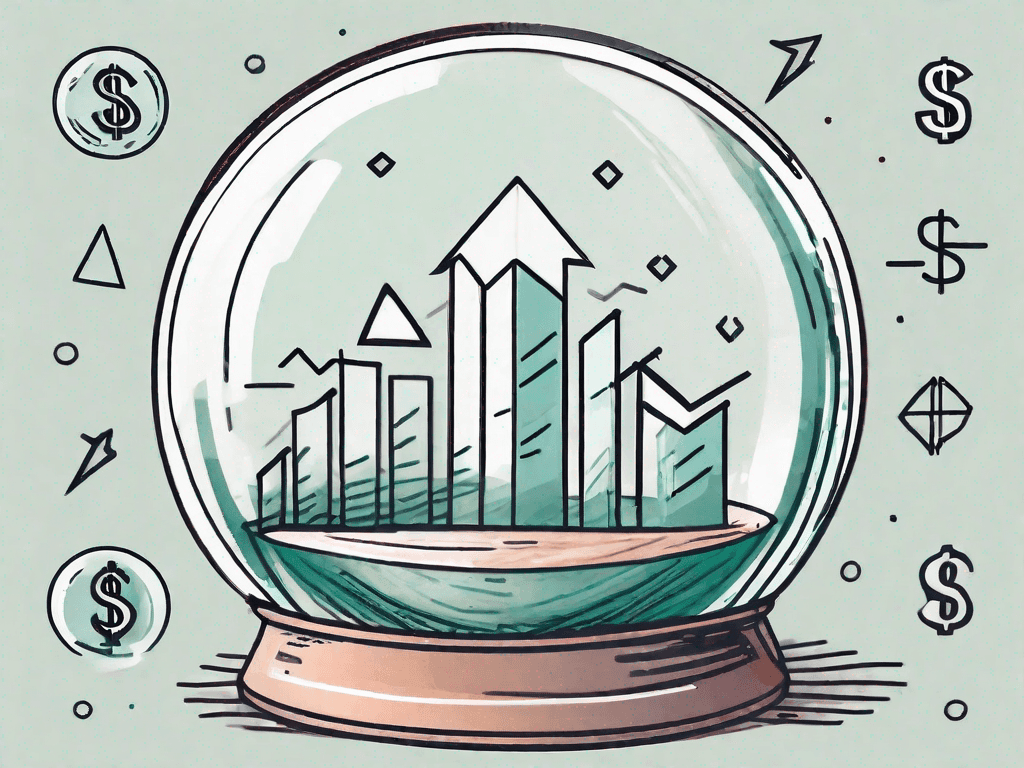
What is Revenue Forecast? (Explained With Examples)

What is a ROI? (Explained With Examples)

What is Sales Automation? (Explained With Examples)

What is a Sales Bonus Plan? (Explained With Examples)

What is a Sales Champion? (Explained With Examples)

What is a Sales Collateral? (Explained With Examples)

What is a Sales Commission Structure Plan? (Explained With Examples)

What is a Sales CRM? (Explained With Examples)

What is a Sales Cycle? (Explained With Examples)
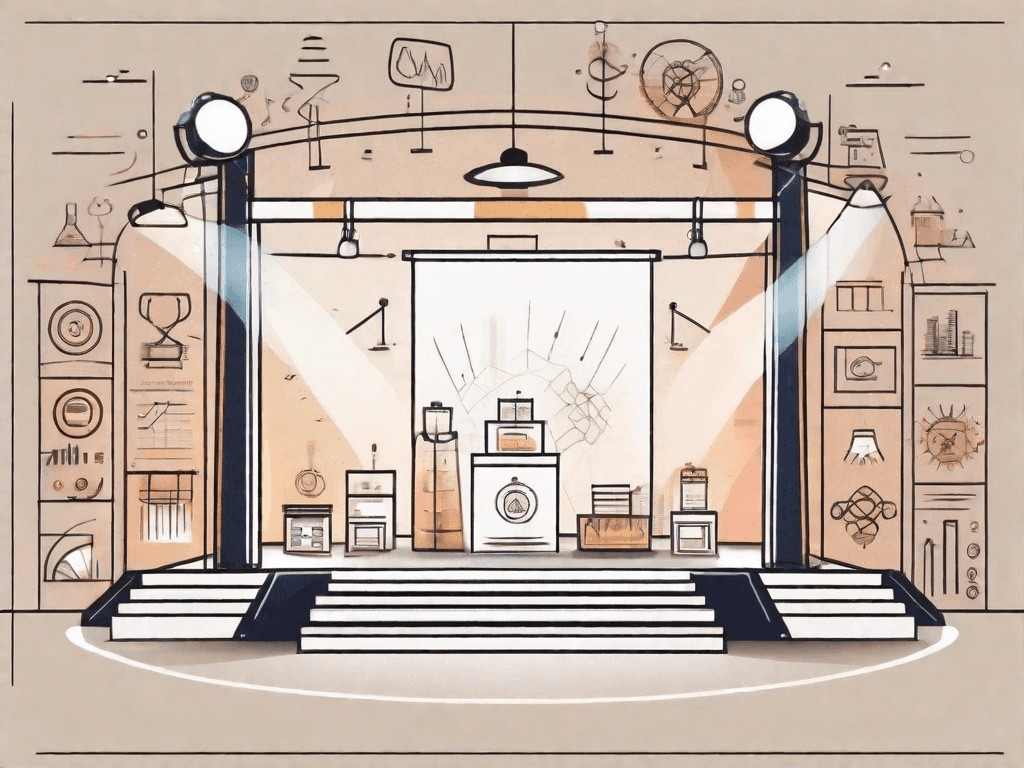
What is a Sales Demo? (Explained With Examples)
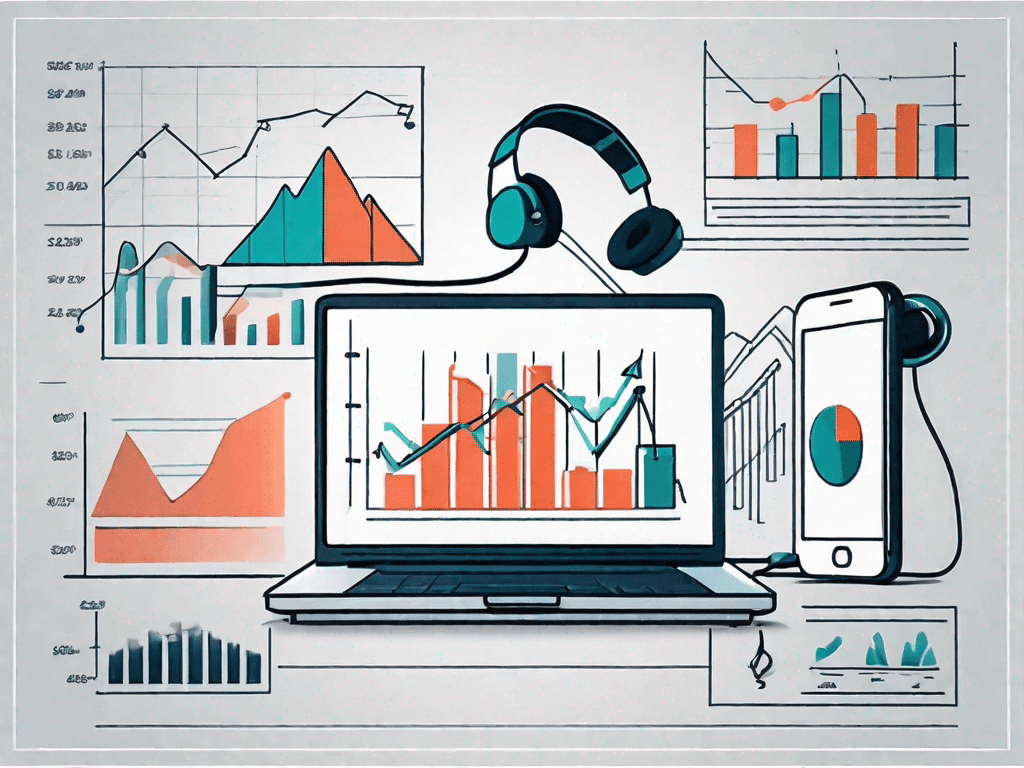
What is Sales Enablement? (Explained With Examples)

What is a Sales Flywheel? (Explained With Examples)

What is a Sales Funnel? (Explained With Examples)
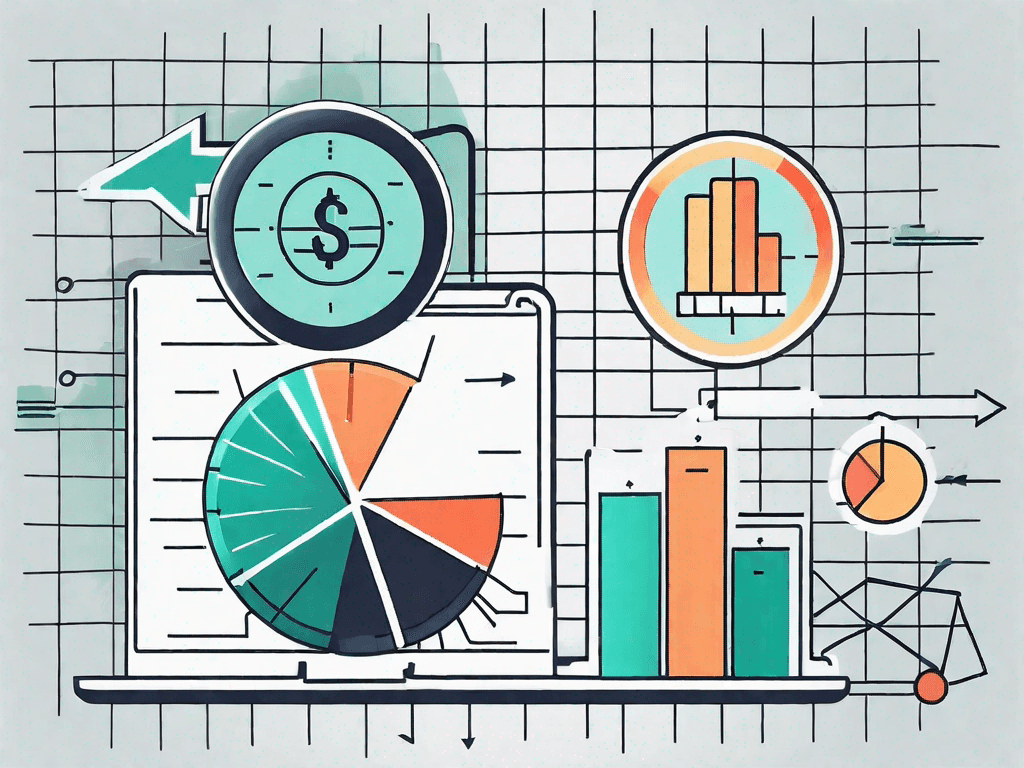
What are Sales KPIs? (Explained With Examples)

What is a Sales Meetup? (Explained With Examples)

What is a Sales Pipeline? (Explained With Examples)

What is a Sales Pitch? (Explained With Examples)

What is a Sales Playbook? (Explained With Examples)
Try breakcold now, are you ready to accelerate your sales pipeline.
Join over +1000 agencies, startups & consultants closing deals with Breakcold Sales CRM
Get Started for free
Sales CRM Features
Sales CRM Software
Sales Pipeline
Sales Lead Tracking
CRM with social media integrations
Social Selling Software
Contact Management
CRM Unified Email LinkedIn Inbox
Breakcold works for many industries
CRM for Agencies
CRM for Startups
CRM for Consultants
CRM for Small Business
CRM for LinkedIn
CRM for Coaches
Sales CRM & Sales Pipeline Tutorials
The 8 Sales Pipeline Stages
The Best CRMs for Agencies
The Best CRMs for Consultants
The Best LinkedIn CRMs
How to close deals in 2024, not in 2010
CRM automation: from 0 to PRO in 5 minutes
LinkedIn Inbox Management
LinkedIn Account-Based Marketing (2024 Tutorial with video)
Tools & more
Sales Pipeline Templates
Alternatives
Integrations
CRM integration with LinkedIn
© 2024 Breakcold
Privacy Policy
Terms of Service
- Search Menu
- Browse content in Arts and Humanities
- Browse content in Archaeology
- Anglo-Saxon and Medieval Archaeology
- Archaeological Methodology and Techniques
- Archaeology by Region
- Archaeology of Religion
- Archaeology of Trade and Exchange
- Biblical Archaeology
- Contemporary and Public Archaeology
- Environmental Archaeology
- Historical Archaeology
- History and Theory of Archaeology
- Industrial Archaeology
- Landscape Archaeology
- Mortuary Archaeology
- Prehistoric Archaeology
- Underwater Archaeology
- Urban Archaeology
- Zooarchaeology
- Browse content in Architecture
- Architectural Structure and Design
- History of Architecture
- Residential and Domestic Buildings
- Theory of Architecture
- Browse content in Art
- Art Subjects and Themes
- History of Art
- Industrial and Commercial Art
- Theory of Art
- Biographical Studies
- Byzantine Studies
- Browse content in Classical Studies
- Classical Literature
- Classical Reception
- Classical History
- Classical Philosophy
- Classical Mythology
- Classical Art and Architecture
- Classical Oratory and Rhetoric
- Greek and Roman Papyrology
- Greek and Roman Archaeology
- Greek and Roman Epigraphy
- Greek and Roman Law
- Late Antiquity
- Religion in the Ancient World
- Digital Humanities
- Browse content in History
- Colonialism and Imperialism
- Diplomatic History
- Environmental History
- Genealogy, Heraldry, Names, and Honours
- Genocide and Ethnic Cleansing
- Historical Geography
- History by Period
- History of Emotions
- History of Agriculture
- History of Education
- History of Gender and Sexuality
- Industrial History
- Intellectual History
- International History
- Labour History
- Legal and Constitutional History
- Local and Family History
- Maritime History
- Military History
- National Liberation and Post-Colonialism
- Oral History
- Political History
- Public History
- Regional and National History
- Revolutions and Rebellions
- Slavery and Abolition of Slavery
- Social and Cultural History
- Theory, Methods, and Historiography
- Urban History
- World History
- Browse content in Language Teaching and Learning
- Language Learning (Specific Skills)
- Language Teaching Theory and Methods
- Browse content in Linguistics
- Applied Linguistics
- Cognitive Linguistics
- Computational Linguistics
- Forensic Linguistics
- Grammar, Syntax and Morphology
- Historical and Diachronic Linguistics
- History of English
- Language Evolution
- Language Reference
- Language Variation
- Language Families
- Language Acquisition
- Lexicography
- Linguistic Anthropology
- Linguistic Theories
- Linguistic Typology
- Phonetics and Phonology
- Psycholinguistics
- Sociolinguistics
- Translation and Interpretation
- Writing Systems
- Browse content in Literature
- Bibliography
- Children's Literature Studies
- Literary Studies (Romanticism)
- Literary Studies (American)
- Literary Studies (Modernism)
- Literary Studies (Asian)
- Literary Studies (European)
- Literary Studies (Eco-criticism)
- Literary Studies - World
- Literary Studies (1500 to 1800)
- Literary Studies (19th Century)
- Literary Studies (20th Century onwards)
- Literary Studies (African American Literature)
- Literary Studies (British and Irish)
- Literary Studies (Early and Medieval)
- Literary Studies (Fiction, Novelists, and Prose Writers)
- Literary Studies (Gender Studies)
- Literary Studies (Graphic Novels)
- Literary Studies (History of the Book)
- Literary Studies (Plays and Playwrights)
- Literary Studies (Poetry and Poets)
- Literary Studies (Postcolonial Literature)
- Literary Studies (Queer Studies)
- Literary Studies (Science Fiction)
- Literary Studies (Travel Literature)
- Literary Studies (War Literature)
- Literary Studies (Women's Writing)
- Literary Theory and Cultural Studies
- Mythology and Folklore
- Shakespeare Studies and Criticism
- Browse content in Media Studies
- Browse content in Music
- Applied Music
- Dance and Music
- Ethics in Music
- Ethnomusicology
- Gender and Sexuality in Music
- Medicine and Music
- Music Cultures
- Music and Media
- Music and Culture
- Music and Religion
- Music Education and Pedagogy
- Music Theory and Analysis
- Musical Scores, Lyrics, and Libretti
- Musical Structures, Styles, and Techniques
- Musicology and Music History
- Performance Practice and Studies
- Race and Ethnicity in Music
- Sound Studies
- Browse content in Performing Arts
- Browse content in Philosophy
- Aesthetics and Philosophy of Art
- Epistemology
- Feminist Philosophy
- History of Western Philosophy
- Metaphysics
- Moral Philosophy
- Non-Western Philosophy
- Philosophy of Language
- Philosophy of Mind
- Philosophy of Perception
- Philosophy of Action
- Philosophy of Law
- Philosophy of Religion
- Philosophy of Science
- Philosophy of Mathematics and Logic
- Practical Ethics
- Social and Political Philosophy
- Browse content in Religion
- Biblical Studies
- Christianity
- East Asian Religions
- History of Religion
- Judaism and Jewish Studies
- Qumran Studies
- Religion and Education
- Religion and Health
- Religion and Politics
- Religion and Science
- Religion and Law
- Religion and Art, Literature, and Music
- Religious Studies
- Browse content in Society and Culture
- Cookery, Food, and Drink
- Cultural Studies
- Customs and Traditions
- Ethical Issues and Debates
- Hobbies, Games, Arts and Crafts
- Lifestyle, Home, and Garden
- Natural world, Country Life, and Pets
- Popular Beliefs and Controversial Knowledge
- Sports and Outdoor Recreation
- Technology and Society
- Travel and Holiday
- Visual Culture
- Browse content in Law
- Arbitration
- Browse content in Company and Commercial Law
- Commercial Law
- Company Law
- Browse content in Comparative Law
- Systems of Law
- Competition Law
- Browse content in Constitutional and Administrative Law
- Government Powers
- Judicial Review
- Local Government Law
- Military and Defence Law
- Parliamentary and Legislative Practice
- Construction Law
- Contract Law
- Browse content in Criminal Law
- Criminal Procedure
- Criminal Evidence Law
- Sentencing and Punishment
- Employment and Labour Law
- Environment and Energy Law
- Browse content in Financial Law
- Banking Law
- Insolvency Law
- History of Law
- Human Rights and Immigration
- Intellectual Property Law
- Browse content in International Law
- Private International Law and Conflict of Laws
- Public International Law
- IT and Communications Law
- Jurisprudence and Philosophy of Law
- Law and Society
- Law and Politics
- Browse content in Legal System and Practice
- Courts and Procedure
- Legal Skills and Practice
- Primary Sources of Law
- Regulation of Legal Profession
- Medical and Healthcare Law
- Browse content in Policing
- Criminal Investigation and Detection
- Police and Security Services
- Police Procedure and Law
- Police Regional Planning
- Browse content in Property Law
- Personal Property Law
- Study and Revision
- Terrorism and National Security Law
- Browse content in Trusts Law
- Wills and Probate or Succession
- Browse content in Medicine and Health
- Browse content in Allied Health Professions
- Arts Therapies
- Clinical Science
- Dietetics and Nutrition
- Occupational Therapy
- Operating Department Practice
- Physiotherapy
- Radiography
- Speech and Language Therapy
- Browse content in Anaesthetics
- General Anaesthesia
- Neuroanaesthesia
- Clinical Neuroscience
- Browse content in Clinical Medicine
- Acute Medicine
- Cardiovascular Medicine
- Clinical Genetics
- Clinical Pharmacology and Therapeutics
- Dermatology
- Endocrinology and Diabetes
- Gastroenterology
- Genito-urinary Medicine
- Geriatric Medicine
- Infectious Diseases
- Medical Toxicology
- Medical Oncology
- Pain Medicine
- Palliative Medicine
- Rehabilitation Medicine
- Respiratory Medicine and Pulmonology
- Rheumatology
- Sleep Medicine
- Sports and Exercise Medicine
- Community Medical Services
- Critical Care
- Emergency Medicine
- Forensic Medicine
- Haematology
- History of Medicine
- Browse content in Medical Skills
- Clinical Skills
- Communication Skills
- Nursing Skills
- Surgical Skills
- Medical Ethics
- Browse content in Medical Dentistry
- Oral and Maxillofacial Surgery
- Paediatric Dentistry
- Restorative Dentistry and Orthodontics
- Surgical Dentistry
- Medical Statistics and Methodology
- Browse content in Neurology
- Clinical Neurophysiology
- Neuropathology
- Nursing Studies
- Browse content in Obstetrics and Gynaecology
- Gynaecology
- Occupational Medicine
- Ophthalmology
- Otolaryngology (ENT)
- Browse content in Paediatrics
- Neonatology
- Browse content in Pathology
- Chemical Pathology
- Clinical Cytogenetics and Molecular Genetics
- Histopathology
- Medical Microbiology and Virology
- Patient Education and Information
- Browse content in Pharmacology
- Psychopharmacology
- Browse content in Popular Health
- Caring for Others
- Complementary and Alternative Medicine
- Self-help and Personal Development
- Browse content in Preclinical Medicine
- Cell Biology
- Molecular Biology and Genetics
- Reproduction, Growth and Development
- Primary Care
- Professional Development in Medicine
- Browse content in Psychiatry
- Addiction Medicine
- Child and Adolescent Psychiatry
- Forensic Psychiatry
- Learning Disabilities
- Old Age Psychiatry
- Psychotherapy
- Browse content in Public Health and Epidemiology
- Epidemiology
- Public Health
- Browse content in Radiology
- Clinical Radiology
- Interventional Radiology
- Nuclear Medicine
- Radiation Oncology
- Reproductive Medicine
- Browse content in Surgery
- Cardiothoracic Surgery
- Gastro-intestinal and Colorectal Surgery
- General Surgery
- Neurosurgery
- Paediatric Surgery
- Peri-operative Care
- Plastic and Reconstructive Surgery
- Surgical Oncology
- Transplant Surgery
- Trauma and Orthopaedic Surgery
- Vascular Surgery
- Browse content in Science and Mathematics
- Browse content in Biological Sciences
- Aquatic Biology
- Biochemistry
- Bioinformatics and Computational Biology
- Developmental Biology
- Ecology and Conservation
- Evolutionary Biology
- Genetics and Genomics
- Microbiology
- Molecular and Cell Biology
- Natural History
- Plant Sciences and Forestry
- Research Methods in Life Sciences
- Structural Biology
- Systems Biology
- Zoology and Animal Sciences
- Browse content in Chemistry
- Analytical Chemistry
- Computational Chemistry
- Crystallography
- Environmental Chemistry
- Industrial Chemistry
- Inorganic Chemistry
- Materials Chemistry
- Medicinal Chemistry
- Mineralogy and Gems
- Organic Chemistry
- Physical Chemistry
- Polymer Chemistry
- Study and Communication Skills in Chemistry
- Theoretical Chemistry
- Browse content in Computer Science
- Artificial Intelligence
- Computer Architecture and Logic Design
- Game Studies
- Human-Computer Interaction
- Mathematical Theory of Computation
- Programming Languages
- Software Engineering
- Systems Analysis and Design
- Virtual Reality
- Browse content in Computing
- Business Applications
- Computer Games
- Computer Security
- Computer Networking and Communications
- Digital Lifestyle
- Graphical and Digital Media Applications
- Operating Systems
- Browse content in Earth Sciences and Geography
- Atmospheric Sciences
- Environmental Geography
- Geology and the Lithosphere
- Maps and Map-making
- Meteorology and Climatology
- Oceanography and Hydrology
- Palaeontology
- Physical Geography and Topography
- Regional Geography
- Soil Science
- Urban Geography
- Browse content in Engineering and Technology
- Agriculture and Farming
- Biological Engineering
- Civil Engineering, Surveying, and Building
- Electronics and Communications Engineering
- Energy Technology
- Engineering (General)
- Environmental Science, Engineering, and Technology
- History of Engineering and Technology
- Mechanical Engineering and Materials
- Technology of Industrial Chemistry
- Transport Technology and Trades
- Browse content in Environmental Science
- Applied Ecology (Environmental Science)
- Conservation of the Environment (Environmental Science)
- Environmental Sustainability
- Environmentalist Thought and Ideology (Environmental Science)
- Management of Land and Natural Resources (Environmental Science)
- Natural Disasters (Environmental Science)
- Nuclear Issues (Environmental Science)
- Pollution and Threats to the Environment (Environmental Science)
- Social Impact of Environmental Issues (Environmental Science)
- History of Science and Technology
- Browse content in Materials Science
- Ceramics and Glasses
- Composite Materials
- Metals, Alloying, and Corrosion
- Nanotechnology
- Browse content in Mathematics
- Applied Mathematics
- Biomathematics and Statistics
- History of Mathematics
- Mathematical Education
- Mathematical Finance
- Mathematical Analysis
- Numerical and Computational Mathematics
- Probability and Statistics
- Pure Mathematics
- Browse content in Neuroscience
- Cognition and Behavioural Neuroscience
- Development of the Nervous System
- Disorders of the Nervous System
- History of Neuroscience
- Invertebrate Neurobiology
- Molecular and Cellular Systems
- Neuroendocrinology and Autonomic Nervous System
- Neuroscientific Techniques
- Sensory and Motor Systems
- Browse content in Physics
- Astronomy and Astrophysics
- Atomic, Molecular, and Optical Physics
- Biological and Medical Physics
- Classical Mechanics
- Computational Physics
- Condensed Matter Physics
- Electromagnetism, Optics, and Acoustics
- History of Physics
- Mathematical and Statistical Physics
- Measurement Science
- Nuclear Physics
- Particles and Fields
- Plasma Physics
- Quantum Physics
- Relativity and Gravitation
- Semiconductor and Mesoscopic Physics
- Browse content in Psychology
- Affective Sciences
- Clinical Psychology
- Cognitive Psychology
- Cognitive Neuroscience
- Criminal and Forensic Psychology
- Developmental Psychology
- Educational Psychology
- Evolutionary Psychology
- Health Psychology
- History and Systems in Psychology
- Music Psychology
- Neuropsychology
- Organizational Psychology
- Psychological Assessment and Testing
- Psychology of Human-Technology Interaction
- Psychology Professional Development and Training
- Research Methods in Psychology
- Social Psychology
- Browse content in Social Sciences
- Browse content in Anthropology
- Anthropology of Religion
- Human Evolution
- Medical Anthropology
- Physical Anthropology
- Regional Anthropology
- Social and Cultural Anthropology
- Theory and Practice of Anthropology
- Browse content in Business and Management
- Business Ethics
- Business History
- Business Strategy
- Business and Technology
- Business and Government
- Business and the Environment
- Comparative Management
- Corporate Governance
- Corporate Social Responsibility
- Entrepreneurship
- Health Management
- Human Resource Management
- Industrial and Employment Relations
- Industry Studies
- Information and Communication Technologies
- International Business
- Knowledge Management
- Management and Management Techniques
- Operations Management
- Organizational Theory and Behaviour
- Pensions and Pension Management
- Public and Nonprofit Management
- Strategic Management
- Supply Chain Management
- Browse content in Criminology and Criminal Justice
- Criminal Justice
- Criminology
- Forms of Crime
- International and Comparative Criminology
- Youth Violence and Juvenile Justice
- Development Studies
- Browse content in Economics
- Agricultural, Environmental, and Natural Resource Economics
- Asian Economics
- Behavioural Finance
- Behavioural Economics and Neuroeconomics
- Econometrics and Mathematical Economics
- Economic History
- Economic Methodology
- Economic Systems
- Economic Development and Growth
- Financial Markets
- Financial Institutions and Services
- General Economics and Teaching
- Health, Education, and Welfare
- History of Economic Thought
- International Economics
- Labour and Demographic Economics
- Law and Economics
- Macroeconomics and Monetary Economics
- Microeconomics
- Public Economics
- Urban, Rural, and Regional Economics
- Welfare Economics
- Browse content in Education
- Adult Education and Continuous Learning
- Care and Counselling of Students
- Early Childhood and Elementary Education
- Educational Equipment and Technology
- Educational Strategies and Policy
- Higher and Further Education
- Organization and Management of Education
- Philosophy and Theory of Education
- Schools Studies
- Secondary Education
- Teaching of a Specific Subject
- Teaching of Specific Groups and Special Educational Needs
- Teaching Skills and Techniques
- Browse content in Environment
- Applied Ecology (Social Science)
- Climate Change
- Conservation of the Environment (Social Science)
- Environmentalist Thought and Ideology (Social Science)
- Natural Disasters (Environment)
- Social Impact of Environmental Issues (Social Science)
- Browse content in Human Geography
- Cultural Geography
- Economic Geography
- Political Geography
- Browse content in Interdisciplinary Studies
- Communication Studies
- Museums, Libraries, and Information Sciences
- Browse content in Politics
- African Politics
- Asian Politics
- Chinese Politics
- Comparative Politics
- Conflict Politics
- Elections and Electoral Studies
- Environmental Politics
- European Union
- Foreign Policy
- Gender and Politics
- Human Rights and Politics
- Indian Politics
- International Relations
- International Organization (Politics)
- International Political Economy
- Irish Politics
- Latin American Politics
- Middle Eastern Politics
- Political Behaviour
- Political Economy
- Political Institutions
- Political Theory
- Political Methodology
- Political Communication
- Political Philosophy
- Political Sociology
- Politics and Law
- Public Policy
- Public Administration
- Quantitative Political Methodology
- Regional Political Studies
- Russian Politics
- Security Studies
- State and Local Government
- UK Politics
- US Politics
- Browse content in Regional and Area Studies
- African Studies
- Asian Studies
- East Asian Studies
- Japanese Studies
- Latin American Studies
- Middle Eastern Studies
- Native American Studies
- Scottish Studies
- Browse content in Research and Information
- Research Methods
- Browse content in Social Work
- Addictions and Substance Misuse
- Adoption and Fostering
- Care of the Elderly
- Child and Adolescent Social Work
- Couple and Family Social Work
- Developmental and Physical Disabilities Social Work
- Direct Practice and Clinical Social Work
- Emergency Services
- Human Behaviour and the Social Environment
- International and Global Issues in Social Work
- Mental and Behavioural Health
- Social Justice and Human Rights
- Social Policy and Advocacy
- Social Work and Crime and Justice
- Social Work Macro Practice
- Social Work Practice Settings
- Social Work Research and Evidence-based Practice
- Welfare and Benefit Systems
- Browse content in Sociology
- Childhood Studies
- Community Development
- Comparative and Historical Sociology
- Economic Sociology
- Gender and Sexuality
- Gerontology and Ageing
- Health, Illness, and Medicine
- Marriage and the Family
- Migration Studies
- Occupations, Professions, and Work
- Organizations
- Population and Demography
- Race and Ethnicity
- Social Theory
- Social Movements and Social Change
- Social Research and Statistics
- Social Stratification, Inequality, and Mobility
- Sociology of Religion
- Sociology of Education
- Sport and Leisure
- Urban and Rural Studies
- Browse content in Warfare and Defence
- Defence Strategy, Planning, and Research
- Land Forces and Warfare
- Military Administration
- Military Life and Institutions
- Naval Forces and Warfare
- Other Warfare and Defence Issues
- Peace Studies and Conflict Resolution
- Weapons and Equipment
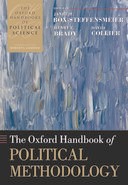
- < Previous chapter
- Next chapter >
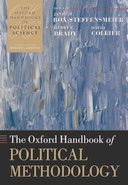
28 Case Selection for Case‐Study Analysis: Qualitative and Quantitative Techniques
John Gerring is Professor of Political Science, Boston University.
- Published: 02 September 2009
- Cite Icon Cite
- Permissions Icon Permissions
This article presents some guidance by cataloging nine different techniques for case selection: typical, diverse, extreme, deviant, influential, crucial, pathway, most similar, and most different. It also indicates that if the researcher is starting from a quantitative database, then methods for finding influential outliers can be used. In particular, the article clarifies the general principles that might guide the process of case selection in case-study research. Cases are more or less representative of some broader phenomenon and, on that score, may be considered better or worse subjects for intensive analysis. The article then draws attention to two ambiguities in case-selection strategies in case-study research. The first concerns the admixture of several case-selection strategies. The second concerns the changing status of a case as a study proceeds. Some case studies follow only one strategy of case selection.
Case ‐study analysis focuses on one or several cases that are expected to provide insight into a larger population. This presents the researcher with a formidable problem of case selection: Which cases should she or he choose?
In large‐sample research, the task of case selection is usually handled by some version of randomization. However, in case‐study research the sample is small (by definition) and this makes random sampling problematic, for any given sample may be wildly unrepresentative. Moreover, there is no guarantee that a few cases, chosen randomly, will provide leverage into the research question of interest.
In order to isolate a sample of cases that both reproduces the relevant causal features of a larger universe (representativeness) and provides variation along the dimensions of theoretical interest (causal leverage), case selection for very small samples must employ purposive (nonrandom) selection procedures. Nine such methods are discussed in this chapter, each of which may be identified with a distinct case‐study “type:” typical, diverse, extreme, deviant, influential, crucial, pathway, most‐similar , and most‐different . Table 28.1 summarizes each type, including its general definition, a technique for locating it within a population of potential cases, its uses, and its probable representativeness.
While each of these techniques is normally practiced on one or several cases (the diverse, most‐similar, and most‐different methods require at least two), all may employ additional cases—with the proviso that, at some point, they will no longer offer an opportunity for in‐depth analysis and will thus no longer be “case studies” in the usual sense ( Gerring 2007 , ch. 2 ). It will also be seen that small‐ N case‐selection procedures rest, at least implicitly, upon an analysis of a larger population of potential cases (as does randomization). The case(s) identified for intensive study is chosen from a population and the reasons for this choice hinge upon the way in which it is situated within that population. This is the origin of the terminology—typical, diverse, extreme, et al. It follows that case‐selection procedures in case‐study research may build upon prior cross‐case analysis and that they depend, at the very least, upon certain assumptions about the broader population.
In certain circumstances, the case‐selection procedure may be structured by a quantitative analysis of the larger population. Here, several caveats must be satisfied. First, the inference must pertain to more than a few dozen cases; otherwise, statistical analysis is problematic. Second, relevant data must be available for that population, or a significant sample of that population, on key variables, and the researcher must feel reasonably confident in the accuracy and conceptual validity of these variables. Third, all the standard assumptions of statistical research (e.g. identification, specification, robustness) must be carefully considered, and wherever possible, tested. I shall not dilate further on these familiar issues except to warn the researcher against the unreflective use of statistical techniques. 1 When these requirements are not met, the researcher must employ a qualitative approach to case selection.
The point of this chapter is to elucidate general principles that might guide the process of case selection in case‐study research, building upon earlier work by Harry Eckstein, Arend Lijphart, and others. Sometimes, these principles can be applied in a quantitative framework and sometimes they are limited to a qualitative framework. In either case, the logic of case selection remains quite similar, whether practiced in small‐ N or large‐ N contexts.
Before we begin, a bit of notation is necessary. In this chapter “ N ” refers to cases, not observations. Here, I am concerned primarily with causal inference, rather than inferences that are descriptive or predictive in nature. Thus, all hypotheses involve at least one independent variable ( X ) and one dependent variable ( Y ). For convenience, I shall label the causal factor of special theoretical interest X 1 , and the control variable, or vector of controls (if there are any), X 2 . If the writer is concerned to explain a puzzling outcome, but has no preconceptions about its causes, then the research will be described as Y‐centered . If a researcher is concerned to investigate the effects of a particular cause, with no preconceptions about what these effects might be, the research will be described as X‐centered . If a researcher is concerned to investigate a particular causal relationship, the research will be described as X 1 / Y‐centered , for it connects a particular cause with a particular outcome. 2 X ‐ or Y ‐centered research is exploratory; its purpose is to generate new hypotheses. X 1 / Y‐centered research, by contrast, is confirmatory/disconfirmatory; its purpose is to test an existing hypothesis.
1 Typical Case
In order for a focused case study to provide insight into a broader phenomenon it must be representative of a broader set of cases. It is in this context that one may speak of a typical‐case approach to case selection. The typical case exemplifies what is considered to be a typical set of values, given some general understanding of a phenomenon. By construction, the typical case is also a representative case.
Some typical cases serve an exploratory role. Here, the author chooses a case based upon a set of descriptive characteristics and then probes for causal relationships. Robert and Helen Lynd (1929/1956) selected a single city “to be as representative as possible of contemporary American life.” Specifically, they were looking for a city with
1) a temperate climate; 2) a sufficiently rapid rate of growth to ensure the presence of a plentiful assortment of the growing pains accompanying contemporary social change; 3) an industrial culture with modern, high‐speed machine production; 4) the absence of dominance of the city's industry by a single plant (i.e., not a one‐industry town); 5) a substantial local artistic life to balance its industrial activity …; and 6) the absence of any outstanding peculiarities or acute local problems which would mark the city off from the midchannel sort of American community. ( Lynd and Lynd 1929/1956 , quoted in Yin 2004 , 29–30)
After examining a number of options the Lynds decided that Muncie, Indiana, was more representative than, or at least as representative as, other midsized cities in America, thus qualifying as a typical case.
This is an inductive approach to case selection. Note that typicality may be understood according to the mean, median, or mode on a particular dimension; there may be multiple dimensions (as in the foregoing example); and each may be differently weighted (some dimensions may be more important than others). Where the selection criteria are multidimensional and a large sample of potential cases is in play, some form of factor analysis may be useful in identifying the most‐typical case(s).
However, the more common employment of the typical‐case method involves a causal model of some phenomenon of theoretical interest. Here, the researcher has identified a particular outcome ( Y ), and perhaps a specific X 1 / Y hypothesis, which she wishes to investigate. In order to do so, she looks for a typical example of that causal relationship. Intuitively, one imagines that a case selected according to the mean values of all parameters must be a typical case relative to some causal relationship. However, this is by no means assured.
Suppose that the Lynds were primarily interested in explaining feelings of trust/distrust among members of different social classes (one of the implicit research goals of the Middletown study). This outcome is likely to be affected by many factors, only some of which are included in their six selection criteria. So choosing cases with respect to a causal hypothesis involves, first of all, identifying the relevant parameters. It involves, secondly, the selection of a case that has a “typical” value relative to the overall causal model; it is well explained. Cases with untypical scores on a particular dimension (e.g. very high or very low) may still be typical examples of a causal relationship. Indeed, they may be more typical than cases whose values lie close to the mean. Thus, a descriptive understanding of typicality is quite different from a causal understanding of typicality. Since it is the latter version that is more common, I shall adopt this understanding of typicality in the remainder of the discussion.
From a qualitative perspective, causal typicality involves the selection of a case that conforms to expectations about some general causal relationship. It performs as expected. In a quantitative setting, this notion is measured by the size of a case's residual in a large‐ N cross‐case model. Typical cases lie on or near the regression line; their residuals are small. Insofar as the model is correctly specified, the size of a case's residual (i.e. the number of standard deviations that separate the actual value from the fitted value) provides a helpful clue to how representative that case is likely to be. “Outliers” are unlikely to be representative of the target population.
Of course, just because a case has a low residual does not necessarily mean that it is a representative case (with respect to the causal relationship of interest). Indeed, the issue of case representativeness is an issue that can never be definitively settled. When one refers to a “typical case” one is saying, in effect, that the probability of a case's representativeness is high, relative to other cases. This test of typicality is misleading if the statistical model is mis‐specified. And it provides little insurance against errors that are purely stochastic. A case may lie directly on the regression line but still be, in some important respect, atypical. For example, it might have an odd combination of values; the interaction of variables might be different from other cases; or additional causal mechanisms might be at work. For this reason, it is important to supplement a statistical analysis of cases with evidence drawn from the case in question (the case study itself) and with our deductive knowledge of the world. One should never judge a case solely by its residual. Yet, all other things being equal, a case with a low residual is less likely to be unusual than a case with a high residual, and to this extent the method of case selection outlined here may be a helpful guide to case‐study researchers faced with a large number of potential cases.
By way of conclusion, it should be noted that because the typical case embodies a typical value on some set of causally relevant dimensions, the variance of interest to the researcher must lie within that case. Specifically, the typical case of some phenomenon may be helpful in exploring causal mechanisms and in solving identification problems (e.g. endogeneity between X 1 and Y , an omitted variable that may account for X 1 and Y , or some other spurious causal association). Depending upon the results of the case study, the author may confirm an existing hypothesis, disconfirm that hypothesis, or reframe it in a way that is consistent with the findings of the case study. These are the uses of the typical‐case study.
2 Diverse Cases
A second case‐selection strategy has as its primary objective the achievement of maximum variance along relevant dimensions. I refer to this as a diverse‐case method. For obvious reasons, this method requires the selection of a set of cases—at minimum, two—which are intended to represent the full range of values characterizing X 1 , Y , or some particular X 1 / Y relationship. 3
Where the individual variable of interest is categorical (on/off, red/black/blue, Jewish/Protestant/Catholic), the identification of diversity is readily apparent. The investigator simply chooses one case from each category. For a continuous variable, the choices are not so obvious. However, the researcher usually chooses both extreme values (high and low), and perhaps the mean or median as well. The researcher may also look for break‐points in the distribution that seem to correspond to categorical differences among cases. Or she may follow a theoretical hunch about which threshold values count, i.e. which are likely to produce different values on Y .
Another sort of diverse case takes account of the values of multiple variables (i.e. a vector), rather than a single variable. If these variables are categorical, the identification of causal types rests upon the intersection of each category. Two dichotomous variables produce a matrix with four cells. Three trichotomous variables produce a matrix of eight cells. And so forth. If all variables are deemed relevant to the analysis, the selection of diverse cases mandates the selection of one case drawn from within each cell. Let us say that an outcome is thought to be affected by sex, race (black/white), and marital status. Here, a diverse‐case strategy of case selection would identify one case within each of these intersecting cells—a total of eight cases. Things become slightly more complicated when one or more of the factors is continuous, rather than categorical. Here, the diversity of case values do not fall neatly into cells. Rather, these cells must be created by fiat—e.g. high, medium, low.
It will be seen that where multiple variables are under consideration, the logic of diverse‐case analysis rests upon the logic of typological theorizing—where different combinations of variables are assumed to have effects on an outcome that vary across types ( Elman 2005 ; George and Bennett 2005 , 235; Lazarsfeld and Barton 1951 ). George and Smoke, for example, wish to explore different types of deterrence failure—by “fait accompli,” by “limited probe,” and by “controlled pressure.” Consequently, they wish to find cases that exemplify each type of causal mechanism. 4
Diversity may thus refer to a range of variation on X or Y , or to a particular combination of causal factors (with or without a consideration of the outcome). In each instance, the goal of case selection is to capture the full range of variation along the dimension(s) of interest.
Since diversity can mean many things, its employment in a large‐ N setting is necessarily dependent upon how this key term is defined. If it is understood to pertain only to a single variable ( X 1 or Y ), then the task is fairly simple. A categorical variable mandates the choice of at least one case from each category—two if dichotomous, three if trichotomous, and so forth. A continuous variable suggests the choice of at least one “high” and “low” value, and perhaps one drawn from the mean or median. But other choices might also be justified, according to one's hunch about the underlying causal relationship or according to natural thresholds found in the data, which may be grouped into discrete categories. Single‐variable traits are usually easy to discover in a large‐ N setting through descriptive statistics or through visual inspection of the data.
Where diversity refers to particular combinations of variables, the relevant cross‐ case technique is some version of stratified random sampling (in a probabilistic setting) or Qualitative Comparative Analysis (in a deterministic setting) ( Ragin 2000 ). If the researcher suspects that a causal relationship is affected not only by combinations of factors but also by their sequencing , then the technique of analysis must incorporate temporal elements ( Abbott 2001 ; Abbott and Forrest 1986 ; Abbott and Tsay 2000 ). Thus, the method of identifying causal types rests upon whatever method of identifying causal relationships is employed in the large‐ N sample.
Note that the identification of distinct case types is intended to identify groups of cases that are internally homogeneous (in all respects that might affect the causal relationship of interest). Thus, the choice of cases within each group should not be problematic, and may be accomplished through random sampling or purposive case selection. However, if there is suspected diversity within each category, then measures should be taken to assure that the chosen cases are typical of each category. A case study should not focus on an atypical member of a subgroup.
Indeed, considerations of diversity and typicality often go together. Thus, in a study of globalization and social welfare systems, Duane Swank (2002) first identifies three distinctive groups of welfare states: “universalistic” (social democratic), “corporatist conservative,” and “liberal.” Next, he looks within each group to find the most‐typical cases. He decides that the Nordic countries are more typical of the universalistic model than the Netherlands since the latter has “some characteristics of the occupationally based program structure and a political context of Christian Democratic‐led governments typical of the corporatist conservative nations” ( Swank 2002 , 11; see also Esping‐Andersen 1990 ). Thus, the Nordic countries are chosen as representative cases within the universalistic case type, and are accompanied in the case‐study portion of his analysis by other cases chosen to represent the other welfare state types (corporatist conservative and liberal).
Evidently, when a sample encompasses a full range of variation on relevant parameters one is likely to enhance the representativeness of that sample (relative to some population). This is a distinct advantage. Of course, the inclusion of a full range of variation may distort the actual distribution of cases across this spectrum. If there are more “high” cases than “low” cases in a population and the researcher chooses only one high case and one low case, the resulting sample of two is not perfectly representative. Even so, the diverse‐case method probably has stronger claims to representativeness than any other small‐ N sample (including the standalone typical case). The selection of diverse cases has the additional advantage of introducing variation on the key variables of interest. A set of diverse cases is, by definition, a set of cases that encompasses a range of high and low values on relevant dimensions. There is, therefore, much to recommend this method of case selection. I suspect that these advantages are commonly understood and are applied on an intuitive level by case‐study researchers. However, the lack of a recognizable name—and an explicit methodological defense—has made it difficult for case‐study researchers to utilize this method of case selection, and to do so in an explicit and self‐conscious fashion. Neologism has its uses.
3 Extreme Case
The extreme‐case method selects a case because of its extreme value on an independent ( X 1 ) or dependent ( Y ) variable of interest. Thus, studies of domestic violence may choose to focus on extreme instances of abuse ( Browne 1987 ). Studies of altruism may focus on those rare individuals who risked their lives to help others (e.g. Holocaust resisters) ( Monroe 1996 ). Studies of ethnic politics may focus on the most heterogeneous societies (e.g. Papua New Guinea) in order to better understand the role of ethnicity in a democratic setting ( Reilly 2000–1 ). Studies of industrial policy often focus on the most successful countries (i.e. the NICS) ( Deyo 1987 ). And so forth. 5
Often an extreme case corresponds to a case that is considered to be prototypical or paradigmatic of some phenomena of interest. This is because concepts are often defined by their extremes, i.e. their ideal types. Italian Fascism defines the concept of Fascism, in part, because it offered the most extreme example of that phenomenon. However, the methodological value of this case, and others like it, derives from its extremity (along some dimension of interest), not its theoretical status or its status in the literature on a subject.
The notion of “extreme” may now be defined more precisely. An extreme value is an observation that lies far away from the mean of a given distribution. This may be measured (if there are sufficient observations) by a case's “Z score”—the number of standard deviations between a case and the mean value for that sample. Extreme cases have high Z scores, and for this reason may serve as useful subjects for intensive analysis.
For a continuous variable, the distance from the mean may be in either direction (positive or negative). For a dichotomous variable (present/absent), extremeness may be interpreted as unusual . If most cases are positive along a given dimension, then a negative case constitutes an extreme case. If most cases are negative, then a positive case constitutes an extreme case. It should be clear that researchers are not simply concerned with cases where something “happened,” but also with cases where something did not. It is the rareness of the value that makes a case valuable, in this context, not its positive or negative value. 6 Thus, if one is studying state capacity, a case of state failure is probably more informative than a case of state endurance simply because the former is more unusual. Similarly, if one is interested in incest taboos a culture where the incest taboo is absent or weak is probably more useful than a culture where it is present or strong. Fascism is more important than nonfascism. And so forth. There is a good reason, therefore, why case studies of revolution tend to focus on “revolutionary” cases. Theda Skocpol (1979) had much more to learn from France than from Austro‐Hungary since France was more unusual than Austro‐Hungary within the population of nation states that Skocpol was concerned to explain. The reason is quite simple: There are fewer revolutionary cases than nonrevolutionary cases; thus, the variation that we explore as a clue to causal relationships is encapsulated in these cases, against a background of nonrevolutionary cases.
Note that the extreme‐case method of case selection appears to violate the social science folk wisdom warning us not to “select on the dependent variable.” 7 Selecting cases on the dependent variable is indeed problematic if a number of cases are chosen, all of which lie on one end of a variable's spectrum (they are all positive or negative), and if the researcher then subjects this sample to cross‐case analysis as if it were representative of a population. 8 Results for this sort of analysis would almost assuredly be biased. Moreover, there will be little variation to explain since the values of each case are explicitly constrained.
However, this is not the proper employment of the extreme‐case method. (It is more appropriately labeled an extreme‐ sample method.) The extreme‐case method actually refers back to a larger sample of cases that lie in the background of the analysis and provide a full range of variation as well as a more representative picture of the population. It is a self‐conscious attempt to maximize variance on the dimension of interest, not to minimize it. If this population of cases is well understood— either through the author's own cross‐case analysis, through the work of others, or through common sense—then a researcher may justify the selection of a single case exemplifying an extreme value for within‐case analysis. If not, the researcher may be well advised to follow a diverse‐case method, as discussed above.
By way of conclusion, let us return to the problem of representativeness. It will be seen that an extreme case may be typical or deviant. There is simply no way to tell because the researcher has not yet specified an X 1 / Y causal proposition. Once such a causal proposition has been specified one may then ask whether the case in question is similar to some population of cases in all respects that might affect the X 1 / Y relationship of interest (i.e. unit homogeneous). It is at this point that it becomes possible to say, within the context of a cross‐case statistical model, whether a case lies near to, or far from, the regression line. However, this sort of analysis means that the researcher is no longer pursuing an extreme‐case method. The extreme‐case method is purely exploratory—a way of probing possible causes of Y , or possible effects of X , in an open‐ended fashion. If the researcher has some notion of what additional factors might affect the outcome of interest, or of what relationship the causal factor of interest might have with Y , then she ought to pursue one of the other methods explored in this chapter. This also implies that an extreme‐case method may transform into a different kind of approach as a study evolves; that is, as a more specific hypothesis comes to light. Useful extreme cases at the outset of a study may prove less useful at a later stage of analysis.
4 Deviant Case
The deviant‐case method selects that case(s) which, by reference to some general understanding of a topic (either a specific theory or common sense), demonstrates a surprising value. It is thus the contrary of the typical case. Barbara Geddes (2003) notes the importance of deviant cases in medical science, where researchers are habitually focused on that which is “pathological” (according to standard theory and practice). The New England Journal of Medicine , one of the premier journals of the field, carries a regular feature entitled Case Records of the Massachusetts General Hospital. These articles bear titles like the following: “An 80‐Year‐Old Woman with Sudden Unilateral Blindness” or “A 76‐Year‐Old Man with Fever, Dyspnea, Pulmonary Infiltrates, Pleural Effusions, and Confusion.” 9 Another interesting example drawn from the field of medicine concerns the extensive study now devoted to a small number of persons who seem resistant to the AIDS virus ( Buchbinder and Vittinghoff 1999 ; Haynes, Pantaleo, and Fauci 1996 ). Why are they resistant? What is different about these people? What can we learn about AIDS in other patients by observing people who have built‐in resistance to this disease?
Likewise, in psychology and sociology case studies may be comprised of deviant (in the social sense) persons or groups. In economics, case studies may consist of countries or businesses that overperform (e.g. Botswana; Microsoft) or underperform (e.g. Britain through most of the twentieth century; Sears in recent decades) relative to some set of expectations. In political science, case studies may focus on countries where the welfare state is more developed (e.g. Sweden) or less developed (e.g. the United States) than one would expect, given a set of general expectations about welfare state development. The deviant case is closely linked to the investigation of theoretical anomalies. Indeed, to say deviant is to imply “anomalous.” 10
Note that while extreme cases are judged relative to the mean of a single distribution (the distribution of values along a single variable), deviant cases are judged relative to some general model of causal relations. The deviant‐case method selects cases which, by reference to some (presumably) general relationship, demonstrate a surprising value. They are “deviant” in that they are poorly explained by the multivariate model. The important point is that deviant‐ness can only be assessed relative to the general (quantitative or qualitative) model. This means that the relative deviant‐ness of a case is likely to change whenever the general model is altered. For example, the United States is a deviant welfare state when this outcome is gauged relative to societal wealth. But it is less deviant—and perhaps not deviant at all—when certain additional (political and societal) factors are included in the model, as discussed in the epilogue. Deviance is model dependent. Thus, when discussing the concept of the deviant case it is helpful to ask the following question: Relative to what general model (or set of background factors) is Case A deviant?
Conceptually, we have said that the deviant case is the logical contrary of the typical case. This translates into a directly contrasting statistical measurement. While the typical case is one with a low residual (in some general model of causal relations), a deviant case is one with a high residual. This means, following our previous discussion, that the deviant case is likely to be an un representative case, and in this respect appears to violate the supposition that case‐study samples should seek to reproduce features of a larger population.
However, it must be borne in mind that the primary purpose of a deviant‐case analysis is to probe for new—but as yet unspecified—explanations. (If the purpose is to disprove an extant theory I shall refer to the study as crucial‐case, as discussed below.) The researcher hopes that causal processes identified within the deviant case will illustrate some causal factor that is applicable to other (more or less deviant) cases. This means that a deviant‐case study usually culminates in a general proposition, one that may be applied to other cases in the population. Once this general proposition has been introduced into the overall model, the expectation is that the chosen case will no longer be an outlier. Indeed, the hope is that it will now be typical , as judged by its small residual in the adjusted model. (The exception would be a circumstance in which a case's outcome is deemed to be “accidental,” and therefore inexplicable by any general model.)
This feature of the deviant‐case study should help to resolve questions about its representativeness. Even if it is not possible to measure the new causal factor (and thus to introduce it into a large‐ N cross‐case model), it may still be plausible to assert (based on general knowledge of the phenomenon) that the chosen case is representative of a broader population.
5 Influential Case
Sometimes, the choice of a case is motivated solely by the need to verify the assumptions behind a general model of causal relations. Here, the analyst attempts to provide a rationale for disregarding a problematic case or a set of problematic cases. That is to say, she attempts to show why apparent deviations from the norm are not really deviant, or do not challenge the core of the theory, once the circumstances of the special case or cases are fully understood. A cross‐case analysis may, after all, be marred by several classes of problems including measurement error, specification error, errors in establishing proper boundaries for the inference (the scope of the argument), and stochastic error (fluctuations in the phenomenon under study that are treated as random, given available theoretical resources). If poorly fitting cases can be explained away by reference to these kinds of problems, then the theory of interest is that much stronger. This sort of deviant‐case analysis answers the question, “What about Case A (or cases of type A)? How does that, seemingly disconfirming, case fit the model?”
Because its underlying purpose is different from the usual deviant‐case study, I offer a new term for this method. The influential case is a case that casts doubt upon a theory, and for that reason warrants close inspection. This investigation may reveal, after all, that the theory is validated—perhaps in some slightly altered form. In this guise, the influential case is the “case that proves the rule.” In other instances, the influential‐case analysis may contribute to disconfirming, or reconceptualizing, a theory. The key point is that the value of the case is judged relative to some extant cross‐case model.
A simple version of influential‐case analysis involves the confirmation of a key case's score on some critical dimension. This is essentially a question of measurement. Sometimes cases are poorly explained simply because they are poorly understood. A close examination of a particular context may reveal that an apparently falsifying case has been miscoded. If so, the initial challenge presented by that case to some general theory has been obviated.
However, the more usual employment of the influential‐case method culminates in a substantive reinterpretation of the case—perhaps even of the general model. It is not just a question of measurement. Consider Thomas Ertman's (1997) study of state building in Western Europe, as summarized by Gerardo Munck. This study argues
that the interaction of a) the type of local government during the first period of statebuilding, with b) the timing of increases in geopolitical competition, strongly influences the kind of regime and state that emerge. [Ertman] tests this hypothesis against the historical experience of Europe and finds that most countries fit his predictions. Denmark, however, is a major exception. In Denmark, sustained geopolitical competition began relatively late and local government at the beginning of the statebuilding period was generally participatory, which should have led the country to develop “patrimonial constitutionalism.” But in fact, it developed “bureaucratic absolutism.” Ertman carefully explores the process through which Denmark came to have a bureaucratic absolutist state and finds that Denmark had the early marks of a patrimonial constitutionalist state. However, the country was pushed off this developmental path by the influence of German knights, who entered Denmark and brought with them German institutions of local government. Ertman then traces the causal process through which these imported institutions pushed Denmark to develop bureaucratic absolutism, concluding that this development was caused by a factor well outside his explanatory framework. ( Munck 2004 , 118)
Ertman's overall framework is confirmed insofar as he has been able to show, by an in‐depth discussion of Denmark, that the causal processes stipulated by the general theory hold even in this apparently disconfirming case. Denmark is still deviant, but it is so because of “contingent historical circumstances” that are exogenous to the theory ( Ertman 1997 , 316).
Evidently, the influential‐case analysis is similar to the deviant‐case analysis. Both focus on outliers. However, as we shall see, they focus on different kinds of outliers. Moreover, the animating goals of these two research designs are quite different. The influential‐case study begins with the aim of confirming a general model, while the deviant‐case study has the aim of generating a new hypothesis that modifies an existing general model. The confusion stems from the fact that the same case study may fulfill both objectives—qualifying a general model and, at the same time, confirming its core hypothesis.
Thus, in their study of Roberto Michels's “iron law of oligarchy,” Lipset, Trow, and Coleman (1956) choose to focus on an organization—the International Typographical Union—that appears to violate the central presupposition. The ITU, as noted by one of the authors, has “a long‐term two‐party system with free elections and frequent turnover in office” and is thus anything but oligarchic ( Lipset 1959 , 70). As such, it calls into question Michels's grand generalization about organizational behavior. The authors explain this curious result by the extraordinarily high level of education among the members of this union. Michels's law is shown to be true for most organizations, but not all. It is true, with qualifications. Note that the respecification of the original model (in effect, Lipset, Trow, and Coleman introduce a new control variable or boundary condition) involves the exploration of a new hypothesis. In this instance, therefore, the use of an influential case to confirm an existing theory is quite similar to the use of a deviant case to explore a new theory.
In a quantitative idiom, influential cases are those that, if counterfactually assigned a different value on the dependent variable, would most substantially change the resulting estimates. They may or may not be outliers (high‐residual cases). Two quantitative measures of influence are commonly applied in regression diagnostics ( Belsey, Kuh, and Welsch 2004 ). The first, often referred to as the leverage of a case, derives from what is called the hat matrix . Based solely on each case's scores on the independent variables, the hat matrix tells us how much a change in (or a measurement error on) the dependent variable for that case would affect the overall regression line. The second is Cook's distance , a measure of the extent to which the estimates of all the parameters would change if a given case were omitted from the analysis. Cases with a large leverage or Cook's distance contribute quite a lot to the inferences drawn from a cross‐case analysis. In this sense, such cases are vital for maintaining analytic conclusions. Discovering a significant measurement error on the dependent variable or an important omitted variable for such a case may dramatically revise estimates of the overall relationships. Hence, it may be quite sensible to select influential cases for in‐depth study.
Note that the use of an influential‐case strategy of case selection is limited to instances in which a researcher has reason to be concerned that her results are being driven by one or a few cases. This is most likely to be true in small to moderate‐sized samples. Where N is very large—greater than 1,000, let us say—it is extremely unlikely that a small set of cases (much less an individual case) will play an “influential” role. Of course, there may be influential sets of cases, e.g. countries within a particular continent or cultural region, or persons of Irish extraction. Sets of influential observations are often problematic in a time‐series cross‐section data‐set where each unit (e.g. country) contains multiple observations (through time), and hence may have a strong influence on aggregate results. Still, the general rule is: the larger the sample, the less important individual cases are likely to be and, hence, the less likely a researcher is to use an influential‐case approach to case selection.
6 Crucial Case
Of all the extant methods of case selection perhaps the most storied—and certainly the most controversial—is the crucial‐case method, introduced to the social science world several decades ago by Harry Eckstein. In his seminal essay, Eckstein (1975 , 118) describes the crucial case as one “that must closely fit a theory if one is to have confidence in the theory's validity, or, conversely, must not fit equally well any rule contrary to that proposed.” A case is crucial in a somewhat weaker—but much more common—sense when it is most, or least, likely to fulfill a theoretical prediction. A “most‐likely” case is one that, on all dimensions except the dimension of theoretical interest, is predicted to achieve a certain outcome, and yet does not. It is therefore used to disconfirm a theory. A “least‐likely” case is one that, on all dimensions except the dimension of theoretical interest, is predicted not to achieve a certain outcome, and yet does so. It is therefore used to confirm a theory. In all formulations, the crucial‐case offers a most‐difficult test for an argument, and hence provides what is perhaps the strongest sort of evidence possible in a nonexperimental, single‐case setting.
Since the publication of Eckstein's influential essay, the crucial‐case approach has been claimed in a multitude of studies across several social science disciplines and has come to be recognized as a staple of the case‐study method. 11 Yet the idea of any single case playing a crucial (or “critical”) role is not widely accepted among most methodologists (e.g. Sekhon 2004 ). (Even its progenitor seems to have had doubts.)
Let us begin with the confirmatory (a.k.a. least‐likely) crucial case. The implicit logic of this research design may be summarized as follows. Given a set of facts, we are asked to contemplate the probability that a given theory is true. While the facts matter, to be sure, the effectiveness of this sort of research also rests upon the formal properties of the theory in question. Specifically, the degree to which a theory is amenable to confirmation is contingent upon how many predictions can be derived from the theory and on how “risky” each individual prediction is. In Popper's (1963 , 36) words, “Confirmations should count only if they are the result of risky predictions ; that is to say, if, unenlightened by the theory in question, we should have expected an event which was incompatible with the theory—and event which would have refuted the theory. Every ‘good’ scientific theory is a prohibition; it forbids certain things to happen. The more a theory forbids, the better it is” (see also Popper 1934/1968 ). A risky prediction is therefore one that is highly precise and determinate, and therefore unlikely to be achieved by the product of other causal factors (external to the theory of interest) or through stochastic processes. A theory produces many such predictions if it is fully elaborated, issuing predictions not only on the central outcome of interest but also on specific causal mechanisms, and if it is broad in purview. (The notion of riskiness may also be conceptualized within the Popperian lexicon as degrees of falsifiability .)
These points can also be articulated in Bayesian terms. Colin Howson and Peter Urbach explain: “The degree to which h [a hypothesis] is confirmed by e [a set of evidence] depends … on the extent to which P(eČh) exceeds P (e) , that is, on how much more probable e is relative to the hypothesis and background assumptions than it is relative just to background assumptions.” Again, “confirmation is correlated with how much more probable the evidence is if the hypothesis is true than if it is false” ( Howson and Urlbach 1989 , 86). Thus, the stranger the prediction offered by a theory—relative to what we would normally expect—the greater the degree of confirmation that will be afforded by the evidence. As an intuitive example, Howson and Urbach (1989 , 86) offer the following:
If a soothsayer predicts that you will meet a dark stranger sometime and you do in fact, your faith in his powers of precognition would not be much enhanced: you would probably continue to think his predictions were just the result of guesswork. However, if the prediction also gave the correct number of hairs on the head of that stranger, your previous scepticism would no doubt be severely shaken.
While these Popperian/Bayesian notions 12 are relevant to all empirical research designs, they are especially relevant to case‐study research designs, for in these settings a single case (or, at most, a small number of cases) is required to bear a heavy burden of proof. It should be no surprise, therefore, that Popper's idea of “riskiness” was to be appropriated by case‐study researchers like Harry Eckstein to validate the enterprise of single‐case analysis. (Although Eckstein does not cite Popper the intellectual lineage is clear.) Riskiness, here, is analogous to what is usually referred to as a “most‐ difficult” research design, which in a case‐study research design would be understood as a “least‐likely” case. Note also that the distinction between a “must‐fit” case and a least‐likely case—that, in the event, actually does fit the terms of a theory—is a matter of degree. Cases are more or less crucial for confirming theories. The point is that, in some circumstances, a paucity of empirical evidence may be compensated by the riskiness of the theory.
The crucial‐case research design is, perforce, a highly deductive enterprise; much depends on the quality of the theory under investigation. It follows that the theories most amenable to crucial‐case analysis are those which are lawlike in their precision, degree of elaboration, consistency, and scope. The more a theory attains the status of a causal law, the easier it will be to confirm, or to disconfirm, with a single case. Indeed, risky predictions are common in natural science fields such as physics, which in turn served as the template for the deductive‐nomological (“covering‐law”) model of science that influenced Eckstein and others in the postwar decades (e.g. Hempel 1942 ).
A frequently cited example is the first important empirical demonstration of the theory of relativity, which took the form of a single‐event prediction on the occasion of the May 29, 1919, solar eclipse ( Eckstein 1975 ; Popper 1963 ). Stephen Van Evera (1997 , 66–7) describes the impact of this prediction on the validation of Einstein's theory.
Einstein's theory predicted that gravity would bend the path of light toward a gravity source by a specific amount. Hence it predicted that during a solar eclipse stars near the sun would appear displaced—stars actually behind the sun would appear next to it, and stars lying next to the sun would appear farther from it—and it predicted the amount of apparent displacement. No other theory made these predictions. The passage of this one single‐case‐study test brought the theory wide acceptance because the tested predictions were unique—there was no plausible competing explanation for the predicted result—hence the passed test was very strong.
The strength of this test is the extraordinary fit between the theory and a set of facts found in a single case, and the corresponding lack of fit between all other theories and this set of facts. Einstein offered an explanation of a particular set of anomalous findings that no other existing theory could make sense of. Of course, one must assume that there was no—or limited—measurement error. And one must assume that the phenomenon of interest is largely invariant; light does not bend differently at different times and places (except in ways that can be understood through the theory of relativity). And one must assume, finally, that the theory itself makes sense on other grounds (other than the case of special interest); it is a plausible general theory. If one is willing to accept these a priori assumptions, then the 1919 “case study” provides a very strong confirmation of the theory. It is difficult to imagine a stronger proof of the theory from within an observational (nonexperimental) setting.
In social science settings, by contrast, one does not commonly find single‐case studies offering knockout evidence for a theory. This is, in my view, largely a product of the looseness (the underspecification) of most social science theories. George and Bennett point out that while the thesis of the democratic peace is as close to a “law” as social science has yet seen, it cannot be confirmed (or refuted) by looking at specific causal mechanisms because the causal pathways mandated by the theory are multiple and diverse. Under the circumstances, no single‐case test can offer strong confirmation of the theory ( George and Bennett 2005 , 209).
However, if one adopts a softer version of the crucial‐case method—the least‐likely (most difficult) case—then possibilities abound. Indeed, I suspect that, implicitly , most case‐study work that makes a positive argument focusing on a single case (without a corresponding cross‐case analysis) relies largely on the logic of the least‐ likely case. Rarely is this logic made explicit, except perhaps in a passing phrase or two. Yet the deductive logic of the “risky” prediction is central to the case‐study enterprise. Whether a case study is convincing or not often rests on the reader's evaluation of how strong the evidence for an argument might be, and this in turn—wherever cross‐ case evidence is limited and no manipulated treatment can be devised—rests upon an estimation of the degree of “fit” between a theory and the evidence at hand, as discussed.
Lily Tsai's (2007) investigation of governance at the village level in China employs several in‐depth case studies of villages which are chosen (in part) because of their least‐likely status relative to the theory of interest. Tsai's hypothesis is that villages with greater social solidarity (based on preexisting religious or familial networks) will develop a higher level of social trust and mutual obligation and, as a result, will experience better governance. Crucial cases, therefore, are villages that evidence a high level of social solidarity but which, along other dimensions, would be judged least likely to develop good governance, e.g. they are poor, isolated, and lack democratic institutions or accountability mechanisms from above. “Li Settlement,” in Fujian province, is such a case. The fact that this impoverished village nonetheless boasts an impressive set of infrastructural accomplishments such as paved roads with drainage ditches (a rarity in rural China) suggests that something rather unusual is going on here. Because her case is carefully chosen to eliminate rival explanations, Tsai's conclusions about the special role of social solidarity are difficult to gainsay. How else is one to explain this otherwise anomalous result? This is the strength of the least‐likely case, where all other plausible causal factors for an outcome have been minimized. 13
Jack Levy (2002 , 144) refers to this, evocatively, as a “Sinatra inference:” if it can make it here, it can make it anywhere (see also Khong 1992 , 49; Sagan 1995 , 49; Shafer 1988 , 14–6). Thus, if social solidarity has the hypothesized effect in Li Settlement it should have the same effect in more propitious settings (e.g. where there is greater economic surplus). The same implicit logic informs many case‐study analyses where the intent of the study is to confirm a hypothesis on the basis of a single case.
Another sort of crucial case is employed for the purpose of dis confirming a causal hypothesis. A central Popperian insight is that it is easier to disconfirm an inference than to confirm that same inference. (Indeed, Popper doubted that any inference could be fully confirmed, and for this reason preferred the term “corroborate.”) This is particularly true of case‐study research designs, where evidence is limited to one or several cases. The key proviso is that the theory under investigation must take a consistent (a.k.a. invariant, deterministic) form, even if its predictions are not terrifically precise, well elaborated, or broad.
As it happens, there are a fair number of invariant propositions floating around the social science disciplines (Goertz and Levy forthcoming; Goertz and Starr 2003 ). It used to be argued, for example, that political stability would occur only in countries that are relatively homogeneous, or where existing heterogeneities are mitigated by cross‐cutting cleavages ( Almond 1956 ; Bentley 1908/1967 ; Lipset 1960/1963 ; Truman 1951 ). Arend Lijphart's (1968) study of the Netherlands, a peaceful country with reinforcing social cleavages, is commonly viewed as refuting this theory on the basis of a single in‐depth case analysis. 14
Granted, it may be questioned whether presumed invariant theories are really invariant; perhaps they are better understood as probabilistic. Perhaps, that is, the theory of cross‐cutting cleavages is still true, probabilistically, despite the apparent Dutch exception. Or perhaps the theory is still true, deterministically, within a subset of cases that does not include the Netherlands. (This sort of claim seems unlikely in this particular instance, but it is quite plausible in many others.) Or perhaps the theory is in need of reframing; it is true, deterministically, but applies only to cross‐ cutting ethnic/racial cleavages, not to cleavages that are primarily religious. One can quibble over what it means to “disconfirm” a theory. The point is that the crucial case has, in all these circumstances, provided important updating of a theoretical prior.
Heretofore, I have treated causal factors as dichotomous. Countries have either reinforcing or cross‐cutting cleavages and they have regimes that are either peaceful or conflictual. Evidently, these sorts of parameters are often matters of degree. In this reading of the theory, cases are more or less crucial. Accordingly, the most useful—i.e. most crucial—case for Lijphart's purpose is one that has the most segregated social groups and the most peaceful and democratic track record. In these respects, the Netherlands was a very good choice. Indeed, the degree of disconfirmation offered by this case study is probably greater than the degree of disconfirmation that might have been provided by other cases such as India or Papua New Guinea—countries where social peace has not always been secure. The point is that where variables are continuous rather than dichotomous it is possible to evaluate potential cases in terms of their degree of crucialness .
Note that the crucial‐case method of case‐selection, whether employed in a confirmatory or disconfirmatory mode, cannot be employed in a large‐ N context. This is because an explicit cross‐case model would render the crucial‐case study redundant. Once one identifies the relevant parameters and the scores of all cases on those parameters, one has in effect constructed a cross‐case model that confirms or disconfirms the theory in question. The case study is thenceforth irrelevant, at least as a means of decisive confirmation or disconfirmation. 15 It remains highly relevant as a means of exploring causal mechanisms, of course. Yet, because this objective is quite different from that which is usually associated with the term, I enlist a new term for this technique.
7 Pathway Case
One of the most important functions of case‐study research is the elucidation of causal mechanisms. But which sort of case is most useful for this purpose? Although all case studies presumably shed light on causal mechanisms, not all cases are equally transparent. In situations where a causal hypothesis is clear and has already been confirmed by cross‐case analysis, researchers are well advised to focus on a case where the causal effect of X 1 on Y can be isolated from other potentially confounding factors ( X 2 ). I shall call this a pathway case to indicate its uniquely penetrating insight into causal mechanisms. In contrast to the crucial case, this sort of method is practicable only in circumstances where cross‐case covariational patterns are well studied and where the mechanism linking X 1 and Y remains dim. Because the pathway case builds on prior cross‐case analysis, the problem of case selection must be situated within that sample. There is no standalone pathway case.
The logic of the pathway case is clearest in situations of causal sufficiency—where a causal factor of interest, X 1 , is sufficient by itself (though perhaps not necessary) to account for Y 's value (0 or 1). The other causes of Y , about which we need make no assumptions, are designated as a vector, X 2 .
Note that wherever various causal factors are substitutable for one another, each factor is conceptualized (individually) as sufficient ( Braumoeller 2003 ). Thus, situations of causal equifinality presume causal sufficiency on the part of each factor or set of conjoint factors. An example is provided by the literature on democratization, which stipulates three main avenues of regime change: leadership‐initiated reform, a controlled opening to opposition, or the collapse of an authoritarian regime ( Colomer 1991 ). The case‐study format constrains us to analyze one at a time, so let us limit our scope to the first one—leadership‐initiated reform. So considered, a causal‐pathway case would be one with the following features: (a) democratization, (b) leadership‐initiated reform, (c) no controlled opening to the opposition, (d) no collapse of the previous authoritarian regime, and (e) no other extraneous factors that might affect the process of democratization. In a case of this type, the causal mechanisms by which leadership‐initiated reform may lead to democratization will be easiest to study. Note that it is not necessary to assume that leadership‐initiated reform always leads to democratization; it may or may not be a deterministic cause. But it is necessary to assume that leadership‐initiated reform can sometimes lead to democratization on its own (given certain background features).
Now let us move from these examples to a general‐purpose model. For heuristic purposes, let us presume that all variables in that model are dichotomous (coded as 0 or 1) and that the model is complete (all causes of Y are included). All causal relationships will be coded so as to be positive: X 1 and Y covary as do X 2 and Y . This allows us to visualize a range of possible combinations at a glance.
Recall that the pathway case is always focused, by definition, on a single causal factor, denoted X 1 . (The researcher's focus may shift to other causal factors, but may only focus on one causal factor at a time.) In this scenario, and regardless of how many additional causes of Y there might be (denoted X 2 , a vector of controls), there are only eight relevant case types, as illustrated in Table 28.2 . Identifying these case types is a relatively simple matter, and can be accomplished in a small‐ N sample by the construction of a truth‐table (modeled after Table 28.2 ) or in a large‐ N sample by the use of cross‐tabs.
Notes : X 1 = the variable of theoretical interest. X 2 = a vector of controls (a score of 0 indicates that all control variables have a score of 0, while a score of 1 indicates that all control variables have a score of 1). Y = the outcome of interest. A–H = case types (the N for each case type is indeterminate). G, H = possible pathway cases. Sample size = indeterminate.
Assumptions : (a) all variables can be coded dichotomously (a binary coding of the concept is valid); (b) all independent variables are positively correlated with Y in the general case; ( c ) X 1 is (at least sometimes) a sufficient cause of Y .
Note that the total number of combinations of values depends on the number of control variables, which we have represented with a single vector, X 2 . If this vector consists of a single variable then there are only eight case types. If this vector consists of two variables ( X 2a , X 2b ) then the total number of possible combinations increases from eight (2 3 ) to sixteen (2 4 ). And so forth. However, none of these combinations is relevant for present purposes except those where X 2a and X 2b have the same value (0 or 1). “Mixed” cases are not causal pathway cases, for reasons that should become clear.
The pathway case, following the logic of the crucial case, is one where the causal factor of interest, X 1 , correctly predicts Y while all other possible causes of Y (represented by the vector, X 2 ) make “wrong” predictions. If X 1 is—at least in some circumstances—a sufficient cause of Y , then it is these sorts of cases that should be most useful for tracing causal mechanisms. There are only two such cases in Ta b l e 28.2—G and H. In all other cases, the mechanism running from X 1 to Y would be difficult to discern either because X 1 and Y are not correlated in the usual way (constituting an unusual case, in the terms of our hypothesis) or because other confounding factors ( X 2 ) intrude. In case A, for example, the positive value on Y could be a product of X 1 or X 2 . An in‐depth examination of this case is not likely to be very revealing.
Keep in mind that because the researcher already knows from her cross‐case examination what the general causal relationships are, she knows (prior to the case‐ study investigation) what constitutes a correct or incorrect prediction. In the crucial‐ case method, by contrast, these expectations are deductive rather than empirical. This is what differentiates the two methods. And this is why the causal pathway case is useful principally for elucidating causal mechanisms rather than verifying or falsifying general propositions (which are already more or less apparent from the cross‐case evidence). Of course, we must leave open the possibility that the investigation of causal mechanisms would invalidate a general claim, if that claim is utterly contingent upon a specific set of causal mechanisms and the case study shows that no such mechanisms are present. However, this is rather unlikely in most social science settings. Usually, the result of such a finding will be a reformulation of the causal processes by which X 1 causes Y —or, alternatively, a realization that the case under investigation is aberrant (atypical of the general population of cases).
Sometimes, the research question is framed as a unidirectional cause: one is interested in why 0 becomes 1 (or vice versa) but not in why 1 becomes 0. In our previous example, we asked why democracies fail, not why countries become democratic or authoritarian. So framed, there can be only one type of causal‐pathway case. (Whether regime failure is coded as 0 or 1 is a matter of taste.) Where researchers are interested in bidirectional causality—a movement from 0 to 1 as well as from 1 to 0—there are two possible causal‐pathway cases, G and H. In practice, however, one of these case types is almost always more useful than the other. Thus, it seems reasonable to employ the term “pathway case” in the singular. In order to determine which of these two case types will be more useful for intensive analysis the researcher should look to see whether each case type exhibits desirable features such as: (a) a rare (unusual) value on X 1 or Y (designated “extreme” in our previous discussion), (b) observable temporal variation in X 1 , ( c ) an X 1 / Y relationship that is easier to study (it has more visible features; it is more transparent), or (d) a lower residual (thus indicating a more typical case, within the terms of the general model). Usually, the choice between G and H is intuitively obvious.
Now, let us consider a scenario in which all (or most) variables of concern to the model are continuous, rather than dichotomous. Here, the job of case selection is considerably more complex, for causal “sufficiency” (in the usual sense) cannot be invoked. It is no longer plausible to assume that a given cause can be entirely partitioned, i.e. rival factors eliminated. However, the search for a pathway case may still be viable. What we are looking for in this scenario is a case that satisfies two criteria: (1) it is not an outlier (or at least not an extreme outlier) in the general model and (2) its score on the outcome ( Y ) is strongly influenced by the theoretical variable of interest ( X 1 ), taking all other factors into account ( X 2 ). In this sort of case it should be easiest to “see” the causal mechanisms that lie between X 1 and Y .
Achieving the second desiderata requires a bit of manipulation. In order to determine which (nonoutlier) cases are most strongly affected by X 1 , given all the other parameters in the model, one must compare the size of the residuals for each case in a reduced form model, Y = Constant + X 2 + Res reduced , with the size of the residuals for each case in a full model, Y = Constant + X 2 + X 1 + Res full . The pathway case is that case, or set of cases, which shows the greatest difference between the residual for the reduced‐form model and the full model (ΔResidual). Thus,
Note that the residual for a case must be smaller in the full model than in the reduced‐ form model; otherwise, the addition of the variable of interest ( X 1 ) pulls the case away from the regression line. We want to find a case where the addition of X 1 pushes the case towards the regression line, i.e. it helps to “explain” that case.
As an example, let us suppose that we are interested in exploring the effect of mineral wealth on the prospects for democracy in a society. According to a good deal of work on this subject, countries with a bounty of natural resources—particularly oil—are less likely to democratize (or once having undergone a democratic transition, are more likely to revert to authoritarian rule) ( Barro 1999 ; Humphreys 2005 ; Ross 2001 ). The cross‐country evidence is robust. Yet as is often the case, the causal mechanisms remain rather obscure. In order to better understand this phenomenon it may be worthwhile to exploit the findings of cross‐country regression models in order to identify a country whose regime type (i.e. its democracy “score” on some general index) is strongly affected by its natural‐research wealth, all other things held constant. An analysis of this sort identifies two countries— the United Arab Emirates and Kuwait—with high Δ Residual values and modest residuals in the full model (signifying that these cases are not outliers). Researchers seeking to explore the effect of oil wealth on regime type might do well to focus on these two cases since their patterns of democracy cannot be well explained by other factors—e.g. economic development, religion, European influence, or ethnic fractionalization. The presence of oil wealth in these countries would appear to have a strong independent effect on the prospects for democratization in these cases, an effect that is well modeled by general theory and by the available cross‐case evidence.
To reiterate, the logic of causal “elimination” is much more compelling where variables are dichotomous and where causal sufficiency can be assumed ( X 1 is sufficient by itself, at least in some circumstances, to cause Y ). Where variables are continuous, the strategy of the pathway case is more dubious, for potentially confounding causal factors ( X 2 ) cannot be neatly partitioned. Even so, we have indicated why the selection of a pathway case may be a logical approach to case‐study analysis in many circumstances.
The exceptions may be briefly noted. Sometimes, where all variables in a model are dichotomous, there are no pathway cases, i.e. no cases of type G or H (in Table 28.2 ). This is known as the “empty cell” problem, or a problem of severe causal multicollinearity. The universe of observational data does not always oblige us with cases that allow us to independently test a given hypothesis. Where variables are continuous, the analogous problem is that of a causal variable of interest ( X 1 ) that has only minimal effects on the outcome of interest. That is, its role in the general model is quite minor. In these situations, the only cases that are strongly affected by X 1 —if there are any at all—may be extreme outliers, and these sorts of cases are not properly regarded as providing confirmatory evidence for a proposition, for reasons that are abundantly clear by now.
Finally, it should be clarified that the identification of a causal pathway case does not obviate the utility of exploring other cases. One might, for example, want to compare both sorts of potential pathway cases—G and H—with each other. Many other combinations suggest themselves. However, this sort of multi‐case investigation moves beyond the logic of the causal‐pathway case.
8 Most‐similar Cases
The most‐similar method employs a minimum of two cases. 16 In its purest form, the chosen pair of cases is similar in all respects except the variable(s) of interest. If the study is exploratory (i.e. hypothesis generating), the researcher looks for cases that differ on the outcome of theoretical interest but are similar on various factors that might have contributed to that outcome, as illustrated in Table 28.3 (A) . This is a common form of case selection at the initial stage of research. Often, fruitful analysis begins with an apparent anomaly: two cases are apparently quite similar, and yet demonstrate surprisingly different outcomes. The hope is that intensive study of these cases will reveal one—or at most several—factors that differ across these cases. These differing factors ( X 1 ) are looked upon as putative causes. At this stage, the research may be described by the second diagram in Table 28.3 (B) . Sometimes, a researcher begins with a strong hypothesis, in which case her research design is confirmatory (hypothesis testing) from the get‐go. That is, she strives to identify cases that exhibit different outcomes, different scores on the factor of interest, and similar scores on all other possible causal factors, as illustrated in the second (hypothesis‐testing) diagram in Table 28.3 (B) .
The point is that the purpose of a most‐similar research design, and hence its basic setup, often changes as a researcher moves from an exploratory to a confirmatory mode of analysis. However, regardless of where one begins, the results, when published, look like a hypothesis‐testing research design. Question marks have been removed: (A) becomes (B) in Table 28.3 .
As an example, let us consider Leon Epstein's classic study of party cohesion, which focuses on two “most‐similar” countries, the United States and Canada. Canada has highly disciplined parties whose members vote together on the floor of the House of Commons while the United States has weak, undisciplined parties, whose members often defect on floor votes in Congress. In explaining these divergent outcomes, persistent over many years, Epstein first discusses possible causal factors that are held more or less constant across the two cases. Both the United States and Canada inherited English political cultures, both have large territories and heterogeneous populations, both are federal, and both have fairly loose party structures with strong regional bases and a weak center. These are the “control” variables. Where they differ is in one constitutional feature: Canada is parliamentary while the United States is presidential. And it is this institutional difference that Epstein identifies as the crucial (differentiating) cause. (For further examples of the most‐similar method see Brenner 1976 ; Hamilton 1977 ; Lipset 1968 ; Miguel 2004 ; Moulder 1977 ; Posner 2004 .)
X 1 = the variable of theoretical interest. X 2 = a vector of controls. Y = the outcome of interest.
Several caveats apply to any most‐similar analysis (in addition to the usual set of assumptions applying to all case‐study analysis). First, each causal factor is understood as having an independent and additive effect on the outcome; there are no “interaction” effects. Second, one must code cases dichotomously (high/low, present/absent). This is straightforward if the underlying variables are also dichotomous (e.g. federal/unitary). However, it is often the case that variables of concern in the model are continuous (e.g. party cohesion). In this setting, the researcher must “dichotomize” the scoring of cases so as to simplify the two‐case analysis. (Some flexibility is admissible on the vector of controls ( X 2 ) that are “held constant” across the cases. Nonidentity is tolerable if the deviation runs counter to the predicted hypothesis. For example, Epstein describes both the United States and Canada as having strong regional bases of power, a factor that is probably more significant in recent Canadian history than in recent American history. However, because regional bases of power should lead to weaker parties, rather than stronger parties, this element of nonidentity does not challenge Epstein's conclusions. Indeed, it sets up a most‐difficult research scenario, as discussed above.)
In one respect the requirements for case control are not so stringent. Specifically, it is not usually necessary to measure control variables (at least not with a high degree of precision) in order to control for them. If two countries can be assumed to have similar cultural heritages one needn't worry about constructing variables to measure that heritage. One can simply assert that, whatever they are, they are more or less constant across the two cases. This is similar to the technique employed in a randomized experiment, where the researcher typically does not attempt to measure all the factors that might affect the causal relationship of interest. She assumes, rather, that these unknown factors have been neutralized across the treatment and control groups by randomization or by the choice of a sample that is internally homogeneous.
The most useful statistical tool for identifying cases for in‐depth analysis in a most‐ similar setting is probably some variety of matching strategy—e.g. exact matching, approximate matching, or propensity‐score matching. 17 The product of this procedure is a set of matched cases that can be compared in whatever way the researcher deems appropriate. These are the “most‐similar” cases. Rosenbaum and Silber (2001 , 223) summarize:
Unlike model‐based adjustments, where [individuals] vanish and are replaced by the coefficients of a model, in matching, ostensibly comparable patterns are compared directly, one by one. Modern matching methods involve statistical modeling and combinatorial algorithms, but the end result is a collection of pairs or sets of people who look comparable, at least on average. In matching, people retain their integrity as people, so they can be examined and their stories can be told individually.
Matching, conclude the authors, “facilitates, rather than inhibits, thick description” ( Rosenbaum and Silber 2001 , 223).
In principle, the same matching techniques that have been used successfully in observational studies of medical treatments might also be adapted to the study of nation states, political parties, cities, or indeed any traditional paired cases in the social sciences. Indeed, the current popularity of matching among statisticians—relative, that is, to garden‐variety regression models—rests upon what qualitative researchers would recognize as a “case‐based” approach to causal analysis. If Rosenbaum and Silber are correct, it may be perfectly reasonable to appropriate this large‐ N method of analysis for case‐study purposes.
As with other methods of case selection, the most‐similar method is prone to problems of nonrepresentativeness. If employed in a qualitative fashion (without a systematic cross‐case selection strategy), potential biases in the chosen case must be addressed in a speculative way. If the researcher employs a matching technique of case selection within a large‐ N sample, the problem of potential bias can be addressed by assuring the choice of cases that are not extreme outliers, as judged by their residuals in the full model. Most‐similar cases should also be “typical” cases, though some scope for deviance around the regression line may be acceptable for purposes of finding a good fit among cases.
X 1 = the variable of theoretical interest. X 2a–d = a vector of controls. Y = the outcome of interest.
9 Most‐different Cases
A final case‐selection method is the reverse image of the previous method. Here, variation on independent variables is prized, while variation on the outcome is eschewed. Rather than looking for cases that are most‐similar, one looks for cases that are most‐ different . Specifically, the researcher tries to identify cases where just one independent variable ( X 1 ), as well as the dependent variable ( Y ), covary, while all other plausible factors ( X 2a–d ) show different values. 18
The simplest form of this two‐case comparison is illustrated in Table 28.4 . Cases A and B are deemed “most different,” though they are similar in two essential respects— the causal variable of interest and the outcome.
As an example, I follow Marc Howard's (2003) recent work, which explores the enduring impact of Communism on civil society. 19 Cross‐national surveys show a strong correlation between former Communist regimes and low social capital, controlling for a variety of possible confounders. It is a strong result. Howard wonders why this relationship is so strong and why it persists, and perhaps even strengthens, in countries that are no longer socialist or authoritarian. In order to answer this question, he focuses on two most‐different cases, Russia and East Germany. These two countries were quite different—in all ways other than their Communist experience— prior to the Soviet era, during the Soviet era (since East Germany received substantial subsidies from West Germany), and in the post‐Soviet era, as East Germany was absorbed into West Germany. Yet, they both score near the bottom of various cross‐ national indices intended to measure the prevalence of civic engagement in the current era. Thus, Howard's (2003 , 6–9) case selection procedure meets the requirements of the most‐different research design: Variance is found on all (or most) dimensions aside from the key factor of interest (Communism) and the outcome (civic engagement).
What leverage is brought to the analysis from this approach? Howard's case studies combine evidence drawn from mass surveys and from in‐depth interviews of small, stratified samples of Russians and East Germans. (This is a good illustration, incidentally, of how quantitative and qualitative evidence can be fruitfully combined in the intensive study of several cases.) The product of this analysis is the identification of three causal pathways that, Howard (2003 , 122) claims, help to explain the laggard status of civil society in post‐Communist polities: “the mistrust of communist organizations, the persistence of friendship networks, and the disappointment with post‐communism.” Simply put, Howard (2003 , 145) concludes, “a great number of citizens in Russia and Eastern Germany feel a strong and lingering sense of distrust of any kind of public organization, a general satisfaction with their own personal networks (accompanied by a sense of deteriorating relations within society overall), and disappointment in the developments of post‐communism.”
The strength of this most‐different case analysis is that the results obtained in East Germany and Russia should also apply in other post‐Communist polities (e.g. Lithuania, Poland, Bulgaria, Albania). By choosing a heterogeneous sample, Howard solves the problem of representativeness in his restricted sample. However, this sample is demonstrably not representative across the population of the inference, which is intended to cover all countries of the world.
More problematic is the lack of variation on key causal factors of interest— Communism and its putative causal pathways. For this reason, it is difficult to reach conclusions about the causal status of these factors on the basis of the most‐different analysis alone. It is possible, that is, that the three causal pathways identified by Howard also operate within polities that never experienced Communist rule.
Nor does it seem possible to conclusively eliminate rival hypotheses on the basis of this most‐different analysis. Indeed, this is not Howard's intention. He wishes merely to show that whatever influence on civil society might be attributed to economic, cultural, and other factors does not exhaust this subject.
My considered judgment is that the most‐different research design provides minimal leverage into the problem of why Communist systems appear to suppress civic engagement, years after their disappearance. Fortunately, this is not the only research design employed by Howard in his admirable study. Indeed, the author employs two other small‐ N cross‐case methods, as well as a large‐ N cross‐country statistical analysis. These methods do most of the analytic work. East Germany may be regarded as a causal pathway case (see above). It has all the attributes normally assumed to foster civic engagement (e.g. a growing economy, multiparty competition, civil liberties, a free press, close association with Western European culture and politics), but nonetheless shows little or no improvement on this dimension during the post‐ transition era ( Howard 2003 , 8). It is plausible to attribute this lack of change to its Communist past, as Howard does, in which case East Germany should be a fruitful case for the investigation of causal mechanisms. The contrast between East and West Germany provides a most‐similar analysis since the two polities share virtually everything except a Communist past. This variation is also deftly exploited by Howard.
I do not wish to dismiss the most‐different research method entirely. Surely, Howard's findings are stronger with the intensive analysis of Russia than they would be without. Yet his book would not stand securely on the empirical foundation provided by most‐different analysis alone. If one strips away the pathway‐case (East Germany) and the most‐similar analysis (East/West Germany) there is little left upon which to base an analysis of causal relations (aside from the large‐ N cross‐national analysis). Indeed, most scholars who employ the most‐different method do so in conjunction with other methods. 20 It is rarely, if ever, a standalone method. 21
Generalizing from this discussion of Marc Howard's work, I offer the following summary remarks on the most‐different method of case analysis. (I leave aside issues faced by all case‐study analyses, issues that are explored in Gerring 2007 .)
Let us begin with a methodological obstacle that is faced by both Millean styles of analysis—the necessity of dichotomizing every variable in the analysis. Recall that, as with most‐similar analysis, differences across cases must generally be sizeable enough to be interpretable in an essentially dichotomous fashion (e.g. high/low, present/absent) and similarities must be close enough to be understood as essentially identical (e.g. high/high, present/present). Otherwise the results of a Millean style analysis are not interpretable. The problem of “degrees” is deadly if the variables under consideration are, by nature, continuous (e.g. GDP). This is a particular concern in Howard's analysis, where East Germany scores somewhat higher than Russia in civic engagement; they are both low, but Russia is quite a bit lower. Howard assumes that this divergence is minimal enough to be understood as a difference of degrees rather than of kinds, a judgment that might be questioned. In these respects, most‐different analysis is no more secure—but also no less—than most‐similar analysis.
In one respect, most‐different analysis is superior to most‐similar analysis. If the coding assumptions are sound, the most‐different research design may be quite useful for eliminating necessary causes . Causal factors that do not appear across the chosen cases—e.g. X 2a–d in Table 28.4 —are evidently unnecessary for the production of Y . However, it does not follow that the most‐different method is the best method for eliminating necessary causes. Note that the defining feature of this method is the shared element across cases— X 1 in Table 28.4 . This feature does not help one to eliminate necessary causes. Indeed, if one were focused solely on eliminating necessary causes one would presumably seek out cases that register the same outcomes and have maximum diversity on other attributes. In Table 28.4 , this would be a set of cases that satisfy conditions X 2a–d , but not X 1 . Thus, even the presumed strength of the most‐different analysis is not so strong.
Usually, case‐study analysis is focused on the identification (or clarification) of causal relations, not the elimination of possible causes. In this setting, the most‐ different technique is useful, but only if assumptions of causal uniqueness hold. By “causal uniqueness,” I mean a situation in which a given outcome is the product of only one cause: Y cannot occur except in the presence of X . X is necessary, and in some situations (given certain background conditions) sufficient, to cause Y . 22
Consider the following hypothetical example. Suppose that a new disease, about which little is known, has appeared in Country A. There are hundreds of infected persons across dozens of affected communities in that country. In Country B, located at the other end of the world, several new cases of the disease surface in a single community. In this setting, we can imagine two sorts of Millean analyses. The first examines two similar communities within Country A, one of which has developed the disease and the other of which has not. This is the most‐similar style of case comparison, and focuses accordingly on the identification of a difference between the two cases that might account for variation across the sample. A second approach focuses on communities where the disease has appeared across the two countries and searches for any similarities that might account for these similar outcomes. This is the most‐different research design.
Both are plausible approaches to this particular problem, and we can imagine epidemiologists employing them simultaneously. However, the most‐different design demands stronger assumptions about the underlying factors at work. It supposes that the disease arises from the same cause in any setting. This is often a reasonable operating assumption when one is dealing with natural phenomena, though there are certainly many exceptions. Death, for example, has many causes. For this reason, it would not occur to us to look for most‐different cases of high mortality around the world. In order for the most‐different research design to effectively identify a causal factor at work in a given outcome, the researcher must assume that X 1 —the factor held constant across the diverse cases—is the only possible cause of Y (see Table 28.4 ). This assumption rarely holds in social‐scientific settings. Most outcomes of interest to anthropologists, economists, political scientists, and sociologists have multiple causes. There are many ways to win an election, to build a welfare state, to get into a war, to overthrow a government, or—returning to Marc Howard's work—to build a strong civil society. And it is for this reason that most‐different analysis is rarely applied in social science work and, where applied, is rarely convincing.
If this seems a tad severe, there is a more charitable way of approaching the most‐different method. Arguably, this is not a pure “method” at all but merely a supplement, a way of incorporating diversity in the sub‐sample of cases that provide the unusual outcome of interest. If the unusual outcome is revolutions, one might wish to encompass a wide variety of revolutions in one's analysis. If the unusual outcome is post‐Communist civil society, it seems appropriate to include a diverse set of post‐Communist polities in one's sample of case studies, as Marc Howard does. From this perspective, the most‐different method (so‐called) might be better labeled a diverse‐case method, as explored above.
10 Conclusions
In order to be a case of something broader than itself, the chosen case must be representative (in some respects) of a larger population. Otherwise—if it is purely idiosyncratic (“unique”)—it is uninformative about anything lying outside the borders of the case itself. A study based on a nonrepresentative sample has no (or very little) external validity. To be sure, no phenomenon is purely idiosyncratic; the notion of a unique case is a matter that would be difficult to define. One is concerned, as always, with matters of degree. Cases are more or less representative of some broader phenomenon and, on that score, may be considered better or worse subjects for intensive analysis. (The one exception, as noted, is the influential case.)
Of all the problems besetting case‐study analysis, perhaps the most persistent— and the most persistently bemoaned—is the problem of sample bias ( Achen and Snidal 1989 ; Collier and Mahoney 1996 ; Geddes 1990 ; King, Keohane, and Verba 1994 ; Rohlfing 2004 ; Sekhon 2004 ). Lisa Martin (1992 , 5) finds that the overemphasis of international relations scholars on a few well‐known cases of economic sanctions— most of which failed to elicit any change in the sanctioned country—“has distorted analysts view of the dynamics and characteristics of economic sanctions.” Barbara Geddes (1990) charges that many analyses of industrial policy have focused exclusively on the most successful cases—primarily the East Asian NICs—leading to biased inferences. Anna Breman and Carolyn Shelton (2001) show that case‐study work on the question of structural adjustment is systematically biased insofar as researchers tend to focus on disaster cases—those where structural adjustment is associated with very poor health and human development outcomes. These cases, often located in sub‐Saharan Africa, are by no means representative of the entire population. Consequently, scholarship on the question of structural adjustment is highly skewed in a particular ideological direction (against neoliberalism) (see also Gerring, Thacker, and Moreno 2005) .
These examples might be multiplied many times. Indeed, for many topics the most‐studied cases are acknowledged to be less than representative. It is worth reflecting upon the fact that our knowledge of the world is heavily colored by a few “big” (populous, rich, powerful) countries, and that a good portion of the disciplines of economics, political science, and sociology are built upon scholars' familiarity with the economics, political science, and sociology of one country, the United States. 23 Case‐study work is particularly prone to problems of investigator bias since so much rides on the researcher's selection of one (or a few) cases. Even if the investigator is unbiased, her sample may still be biased simply by virtue of “random” error (which may be understood as measurement error, error in the data‐generation process, or as an underlying causal feature of the universe).
There are only two situations in which a case‐study researcher need not be concerned with the representativeness of her chosen case. The first is the influential case research design, where a case is chosen because of its possible influence on a cross‐case model, and hence is not expected to be representative of a larger sample. The second is the deviant‐case method, where the chosen case is employed to confirm a broader cross‐case argument to which the case stands as an apparent exception. Yet even here the chosen case is expected to be representative of a broader set of cases—those, in particular, that are poorly explained by the extant model.
In all other circumstances, cases must be representative of the population of interest in whatever ways might be relevant to the proposition in question. Note that where a researcher is attempting to disconfirm a deterministic proposition the question of representativeness is perhaps more appropriately understood as a question of classification: Is the chosen case appropriately classified as a member of the designated population? If so, then it is fodder for a disconfirming case study.
If the researcher is attempting to confirm a deterministic proposition, or to make probabilistic arguments about a causal relationship, then the problem of representativeness is of the more usual sort: Is case A unit‐homogeneous relative to other cases in the population? This is not an easy matter to test. However, in a large‐ N context the residual for that case (in whatever model the researcher has greatest confidence in) is a reasonable place to start. Of course, this test is only as good as the model at hand. Any incorrect specifications or incorrect modeling procedures will likely bias the results and give an incorrect assessment of each case's “typicality.” In addition, there is the possibility of stochastic error, errors that cannot be modeled in a general framework. Given the explanatory weight that individual cases are asked to bear in a case‐study analysis, it is wise to consider more than just the residual test of representativeness. Deductive logic and an in‐depth knowledge of the case in question are often more reliable tools than the results of a cross‐case model.
In any case, there is no dispensing with the question. Case studies (with the two exceptions already noted) rest upon an assumed synecdoche: The case should stand for a population. If this is not true, or if there is reason to doubt this assumption, then the utility of the case study is brought severely into question.
Fortunately, there is some safety in numbers. Insofar as case‐study evidence is combined with cross‐case evidence the issue of sample bias is mitigated. Indeed, the suspicion of case‐study work that one finds in the social sciences today is, in my view, a product of a too‐literal interpretation of the case‐study method. A case study tout court is thought to mean a case study tout seul . Insofar as case studies and cross‐case studies can be enlisted within the same investigation (either in the same study or by reference to other studies in the same subfield), problems of representativeness are less worrisome. This is the virtue of cross‐level work, a.k.a. “triangulation.”
11 Ambiguities
Before concluding, I wish to draw attention to two ambiguities in case‐selection strategies in case‐study research. The first concerns the admixture of several case‐ selection strategies. The second concerns the changing status of a case as a study proceeds.
Some case studies follow only one strategy of case selection. They are typical , diverse , extreme , deviant , influential , crucial , pathway , most‐similar , or most‐different research designs, as discussed. However, many case studies mix and match among these case‐selection strategies. Indeed, insofar as all case studies seek representative samples, they are always in search of “typical” cases. Thus, it is common for writers to declare that their case is, for example, both extreme and typical; it has an extreme value on X 1 or Y but is not, in other respects, idiosyncratic. There is not much that one can say about these combinations of strategies except that, where the cases allow for a variety of empirical strategies, there is no reason not to pursue them. And where the same cases can serve several functions at once (without further effort on the researcher's part), there is little cost to a multi‐pronged approach to case analysis.
The second issue that deserves emphasis is the changing status of a case during the course of a researcher's investigation—which may last for years, if not decades. The problem is acute wherever a researcher begins in an exploratory mode and proceeds to hypothesis‐testing (that is, she develops a specific X 1 / Y proposition) or where the operative hypothesis or key control variable changes (a new causal factor is discovered or another outcome becomes the focus of analysis). Things change. And it is the mark of a good researcher to keep her mind open to new evidence and new insights. Too often, methodological discussions give the misleading impression that hypotheses are clear and remain fixed over the course of a study's development. Nothing could be further from the truth. The unofficial transcripts of academia— accessible in informal settings, where researchers let their guards down (particularly if inebriated)—are filled with stories about dead‐ends, unexpected findings, and drastically revised theory chapters. It would be interesting, in this vein, to compare published work with dissertation prospectuses and fellowship applications. I doubt if the correlation between these two stages of research is particularly strong.
Research, after all, is about discovery, not simply the verification or falsification of static hypotheses. That said, it is also true that research on a particular topic should move from hypothesis generating to hypothesis‐testing. This marks the progress of a field, and of a scholar's own work. As a rule, research that begins with an open‐ended ( X ‐ or Y ‐centered) analysis should conclude with a determinate X 1 / Y hypothesis.
The problem is that research strategies that are ideal for exploration are not always ideal for confirmation. The extreme‐case method is inherently exploratory since there is no clear causal hypothesis; the researcher is concerned merely to explore variation on a single dimension ( X or Y ). Other methods can be employed in either an open‐ ended (exploratory) or a hypothesis‐testing (confirmatory/disconfirmatory) mode. The difficulty is that once the researcher has arrived at a determinate hypothesis the originally chosen research design may no longer appear to be so well designed.
This is unfortunate, but inevitable. One cannot construct the perfect research design until (a) one has a specific hypothesis and (b) one is reasonably certain about what one is going to find “out there” in the empirical world. This is particularly true of observational research designs, but it also applies to many experimental research designs: Usually, there is a “good” (informative) finding, and a finding that is less insightful. In short, the perfect case‐study research design is usually apparent only ex post facto .
There are three ways to handle this. One can explain, straightforwardly, that the initial research was undertaken in an exploratory fashion, and therefore not constructed to test the specific hypothesis that is—now—the primary argument. Alternatively, one can try to redesign the study after the new (or revised) hypothesis has been formulated. This may require additional field research or perhaps the integration of additional cases or variables that can be obtained through secondary sources or through consultation of experts. A final approach is to simply jettison, or de‐emphasize, the portion of research that no longer addresses the (revised) key hypothesis. A three‐case study may become a two‐case study, and so forth. Lost time and effort are the costs of this downsizing.
In the event, practical considerations will probably determine which of these three strategies, or combinations of strategies, is to be followed. (They are not mutually exclusive.) The point to remember is that revision of one's cross‐case research design is normal and perhaps to be expected. Not all twists and turns on the meandering trail of truth can be anticipated.
12 Are There Other Methods of Case Selection?
At the outset of this chapter I summarized the task of case selection as a matter of achieving two objectives: representativeness (typicality) and variation (causal leverage). Evidently, there are other objectives as well. For example, one wishes to identify cases that are independent of each other. If chosen cases are affected by each other (sometimes known as Galton's problem or a problem of diffusion), this problem must be corrected before analysis can take place. I have neglected this issue because it is usually apparent to the researcher and, in any case, there are no simple techniques that might be utilized to correct for such biases. (For further discussion of this and other factors impinging upon case selection see Gerring 2001 , 178–81.)
I have also disregarded pragmatic/logistical issues that might affect case selection. Evidently, case selection is often influenced by a researcher's familiarity with the language of a country, a personal entrée into that locale, special access to important data, or funding that covers one archive rather than another. Pragmatic considerations are often—and quite rightly—decisive in the case‐selection process.
A final consideration concerns the theoretical prominence of a particular case within the literature on a subject. Researchers are sometimes obliged to study cases that have received extensive attention in previous studies. These are sometimes referred to as “paradigmatic” cases or “exemplars” ( Flyvbjerg 2004 , 427).
However, neither pragmatic/logistical utility nor theoretical prominence qualifies as a methodological factor in case selection. That is, these features of a case have no bearing on the validity of the findings stemming from a study. As such, it is appropriate to grant these issues a peripheral status in this chapter.
One final caveat must be issued. While it is traditional to distinguish among the tasks of case selection and case analysis, a close look at these processes shows them to be indistinct and overlapping. One cannot choose a case without considering the sort of analysis that it might be subjected to, and vice versa. Thus, the reader should consider choosing cases by employing the nine techniques laid out in this chapter along with any considerations that might be introduced by virtue of a case's quasi‐experimental qualities, a topic taken up elsewhere ( Gerring 2007 , ch. 6 ).
Abadie, A. , Drukker, D. , Herr, J. L. , and Imbens, G. W. 2001 . Implementing matching estimators for average treatment effects in Stata. Stata Journal , 1: 1–18.
Google Scholar
Abbott, A. 2001 . Time Matters: On Theory and Method . Chicago: University of Chicago Press.
Google Preview
—— and Tsay, A. 2000 . Sequence analysis and optimal matching methods in sociology. Sociological Methods and Research , 29: 3–33. 10.1177/0049124100029001001
—— and Forrest, J. 1986 . Optimal matching methods for historical sequences. Journal of Interdisciplinary History , 16: 471–94. 10.2307/204500
Achen, C. H. , and Snidal, D. 1989 . Rational deterrence theory and comparative case studies. World Politics , 41: 143–69. 10.2307/2010405
Allen, W. S. 1965 . The Nazi Seizure of Power: The Experience of a Single German Town, 1930–1935 . New York: Watts.
Almond, G. A. 1956 . Comparative political systems. Journal of Politics , 18: 391–409.
Amenta, E. 1991 . Making the most of a case study: theories of the welfare state and the American experience. Pp. 172–94 in Issues and Alternatives in Comparative Social Research ed. C. C. Ragin . Leiden: E. J. Brill.
Barro, R. J. 1999 . Determinants of democracy. Journal of Political Economy , 107: 158–83. 10.1086/250107
Belsey, D. A. , Kuh, E. , and Welsch, R. E. 2004 . Regression Diagnostics: Identifying Influential Data and Sources of Collinearity . New York: Wiley.
Bennett, A. , Lepgold, J. , and Unger, D. 1994 . Burden‐sharing in the Persian Gulf War. International Organization , 48: 39–75. 10.1017/S0020818300000813
Bentley, A. 1908/ 1967 . The Process of Government . Cambridge, Mass.: Harvard University Press.
Brady, H. E. , and Collier, D. (eds.) 2004 . Rethinking Social Inquiry: Diverse Tools, Shared Standards . Lanham, Md.: Rowman and Littlefield.
Braumoeller, B. F. 2003 . Causal complexity and the study of politics. Political Analysis , 11: 209–33. 10.1093/pan/mpg012
Breman, A. , and Shelton, C. 2001. Structural adjustment and health: a literature review of the debate, its role‐players and presented empirical evidence. CMH Working Paper Series, Paper No. WG6: 6. WHO, Commission on Macroeconomics and Health.
Brenner, R. 1976 . Agrarian class structure and economic development in pre‐industrial Europe. Past and Present , 70: 30–75. 10.1093/past/70.1.30
Browne, A. 1987 . When Battered Women Kill . New York: Free Press.
Buchbinder, S. , and Vittinghoff, E. 1999 . HIV‐infected long‐term nonprogressors: epidemiology, mechanisms of delayed progression, and clinical and research implications. Microbes Infect , 1: 1113–20. 10.1016/S1286-4579(99)00204-X
Cohen, M. R. , and Nagel, E. 1934 . An Introduction to Logic and Scientific Method . New York: Harcourt, Brace and Company.
Collier, D. , and Mahoney, J. 1996 . Insights and pitfalls: selection bias in qualitative research. World Politics , 49: 56–91. 10.1353/wp.1996.0023
Collier, R. B. , and Collier, D. 1991/ 2002 . Shaping the Political Arena: Critical Junctures, the Labor Movement, and Regime Dynamics in Latin America . Notre Dame, Ind.: University of Notre Dame Press.
Colomer, J. M. 1991 . Transitions by agreement: modeling the Spanish way. American Political Science Review , 85: 1283–302. 10.2307/1963946
Converse, P. E. , and Dupeux, G. 1962 . Politicization of the electorate in France and the United States. Public Opinion Quarterly , 16: 1–23. 10.1086/267067
Coppedge, M. J. 2004. The conditional impact of the economy on democracy in Latin America. Presented at the conference “Democratic Advancements and Setbacks: What Have We Learnt?”, Uppsala University, June 11–13.
De Felice, E. G. 1986 . Causal inference and comparative methods. Comparative Political Studies , 19: 415–37. 10.1177/0010414086019003005
Desch, M. C. 2002 . Democracy and victory: why regime type hardly matters. International Security , 27: 5–47. 10.1162/016228802760987815
Deyo, F. (ed.) 1987 . The Political Economy of the New Asian Industrialism . Ithaca, NY: Cornell University Press.
Dion, D. 1998 . Evidence and inference in the comparative case study. Comparative Politics , 30: 127–45. 10.2307/422284
Eckstein, H. 1975 . Case studies and theory in political science. In Handbook of Political Science , vii: Political Science: Scope and Theory , ed. F. I. Greenstein and N. W. Polsby . Reading, Mass.: Addison‐Wesley.
Eggan, F. 1954 . Social anthropology and the method of controlled comparison. American Anthropologist , 56: 743–63. 10.1525/aa.1954.56.5.02a00020
Elman, C. 2003 . Lessons from Lakatos. In Progress in International Relations Theory: Appraising the Field , ed. C. Elman and M. F. Elman . Cambridge, Mass.: MIT Press.
—— 2005 . Explanatory typologies in qualitative studies of international politics. International Organization , 59: 293–326.
Emigh, R. 1997 . The power of negative thinking: the use of negative case methodology in the development of sociological theory. Theory and Society , 26: 649–84. 10.1023/A:1006896217647
Epstein, L. D. 1964 . A comparative study of Canadian parties. American Political Science Review , 58: 46–59. 10.2307/1952754
Ertman, T. 1997 . Birth of the Leviathan: Building States and Regimes in Medieval and Early Modern Europe . Cambridge: Cambridge University Press.
Esping‐Andersen, G. 1990 . The Three Worlds of Welfare Capitalism . Princeton, NJ: Princeton University Press.
Flyvbjerg, B. 2004 . Five misunderstandings about case‐study research. Pp. 420–34 in Qualitative Research Practice , ed. C. Seale , G. Gobo , J. F. Gubrium , and D. Silverman . London: Sage.
Geddes, B. 1990 . How the cases you choose affect the answers you get: selection bias in comparative politics. In Political Analysis , vol. ii, ed. J. A. Stimson . Ann Arbor: University of Michigan Press.
—— 2003 . Paradigms and Sand Castles: Theory Building and Research Design in Comparative Politics . Ann Arbor: University of Michigan Press.
George, A. L. , and Bennett, A. 2005 . Case Studies and Theory Development . Cambridge, Mass.: MIT Press.
—— and Smoke, R. 1974 . Deterrence in American Foreign Policy: Theory and Practice . New York: Columbia University Press.
Gerring, J. 2001 . Social Science Methodology: A Criterial Framework . Cambridge: Cambridge University Press.
—— 2007 . Case Study Research: Principles and Practices . Cambridge: Cambridge University Press.
—— Thacker, S. and Moreno, C. 2005. Do neoliberal policies save lives? Unpublished manuscript.
Goertz, G. and Starr, H. (eds.) 2003 . Necessary Conditions: Theory, Methodology and Applications . New York: Rowman and Littlefield.
—— and Levy, J. (eds.) forthcoming. Causal explanations, necessary conditions, and case studies: World War I and the end of the Cold War. Manuscript.
Goodin, R. E. and Smitsman, A. 2000 . Placing welfare states: the Netherlands as a crucial test case. Journal of Comparative Policy Analysis , 2: 39–64. 10.1080/13876980008412635
Gujarati, D. N. 2003 . Basic Econometrics , 4th edn. New York: McGraw‐Hill.
Hamilton, G. G. 1977 . Chinese consumption of foreign commodities: a comparative perspective. American Sociological Review , 42: 877–91. 10.2307/2094574
Haynes, B. F. Pantaleo, G. and Fauci, A. S. 1996 . Toward an understanding of the correlates of protective immunity to HIV infection. Science , 271: 324–8. 10.1126/science.271.5247.324
Hempel, C. G. 1942 . The function of general laws in history. Journal of Philosophy , 39: 35–48. 10.2307/2017635
Ho, D. E. Imai, K. King, G. and Stuart, E. A. 2004. Matching as nonparametric preprocessing for reducing model dependence in parametric causal inference. Manuscript.
Howard, M. M. 2003 . The Weakness of Civil Society in Post‐Communist Europe . Cambridge: Cambridge University Press.
Howson, C. and Urbach, P. 1989 . Scientific Reasoning: The Bayesian Approach . La Salle, Ill.: Open Court.
Humphreys, M. 2005 . Natural resources, conflict, and conflict resolution: uncovering the mechanisms. Journal of Conflict Resolution , 49: 508–37. 10.1177/0022002705277545
Jenicek, M. 2001 . Clinical Case Reporting in Evidence‐Based Medicine , 2nd edn. Oxford: Oxford University Press.
Karl, T. L. 1997 . The Paradox of Plenty: Oil Booms and Petro‐states . Berkeley: University of California Press.
Kazancigil, A. 1994 . The deviant case in comparative analysis: high stateness in comparative analysis. Pp. 213–38 in Comparing Nations: Concepts, Strategies, Substance , ed. M. Dogan and A. Kazancigil . Cambridge: Blackwell.
Kemp, K. A. 1986 . Race, ethnicity, class and urban spatial conflict: Chicago as a crucial case Urban Studies , 23: 197–208. 10.1080/00420988620080231
Kendall, P. L. and Wolf, K. M. 1949/ 1955 . The analysis of deviant cases in communications research. In Communications Research, 1948–1949 , ed. P. F. Lazarsfeld and F. N. Stanton. New York: Harper and Brothers. Reprinted as pp. 167–70 in The Language of Social Research , ed. P. F. Lazarsfeld and M. Rosenberg . New York: Free Press.
Kennedy, C. H. 2005 . Single‐case Designs for Educational Research . Boston: Allyn and Bacon.
Kennedy, P. 2003 . A Guide to Econometrics , 5th edn. Cambridge, Mass.: MIT Press.
Khong, Y. F. 1992 . Analogies at War: Korea, Munich, Dien Bien Phu, and the Vietnam Decisions of 1965 . Princeton, NJ: Princeton University Press.
King, G. Keohane, R. O. and Verba, S. 1994 . Designing Social Inquiry: Scientific Inference in Qualitative Research . Princeton, NJ: Princeton University Press.
Lakatos, I. 1978 . The Methodology of Scientific Research Programmes . Cambridge: Cambridge University Press.
Lazarsfeld, P. F. and Barton, A. H. 1951 . Qualitative measurement in the social sciences: classification, typologies, and indices. In The Policy Sciences , ed. D. Lerner and H. D. Lass‐ well. Stanford, Calif.: Stanford University Press.
Levy, J. S. 2002 . Qualitative methods in international relations. In Evaluating Methodology in International Studies , ed. F. P. Harvey and M. Brecher. Ann Arbor: University of Michigan Press.
Lijphart, A. 1968 . The Politics of Accommodation: Pluralism and Democracy in the Netherlands . Berkeley: University of California Press.
—— 1969 . Consociational democracy. World Politics , 21: 207–25. 10.2307/2009820
—— 1971 . Comparative politics and the comparative method. American Political Science Review , 65: 682–93.
—— 1975 . The comparable cases strategy in comparative research. Comparative Political Studies , 8: 158–77.
Lipset, S. M. 1959 . Some social requisites of democracy: economic development and political development. American Political Science Review , 53: 69–105. 10.2307/1951731
—— 1960/ 1963 . Political Man: The Social Bases of Politics . Garden City, NY: Anchor.
—— 1968 . Agrarian Socialism: The Cooperative Commonwealth Federation in Saskatchewan. A Study in Political Sociology . Garden City, NY: Doubleday.
—— Trow, M. A. and Coleman, J. S. 1956 . Union Democracy: The Internal Politics of the International Typographical Union . New York: Free Press.
Lynd, R. S. and Lynd, H. M. 1929/ 1956 . Middletown: A Study in American Culture . New York: Harcourt, Brace.
Mahoney, J. and Goertz, G. 2004 . The possibility principle: choosing negative cases in comparative research. American Political Science Review , 98: 653–69.
Martin, L. L. 1992 . Coercive Cooperation: Explaining Multilateral Economic Sanctions .Princeton, NJ: Princeton University Press.
Mayo, D. G. 1996 . Error and the Growth of Experimental Knowledge . Chicago: University of Chicago Press.
Meckstroth, T. 1975 . “Most different systems” and “most similar systems:” a study in the logic of comparative inquiry. Comparative Political Studies , 8: 133–77.
Miguel, E. 2004 . Tribe or nation: nation‐building and public goods in Kenya versus Tanzania. World Politics , 56: 327–62. 10.1353/wp.2004.0018
Mill, J. S. 1843/ 1872 . The System of Logic , 8th edn. London: Longmans, Green.
Monroe, K. R. 1996 . The Heart of Altruism: Perceptions of a Common Humanity . Princeton, NJ: Princeton University Press.
Moore, B., Jr. 1966 . Social Origins of Dictatorship and Democracy: Lord and Peasant in the Making of the Modern World . Boston: Beacon Press.
Morgan, S. L. and Harding, D. J. 2005. Matching estimators of causal effects: from stratification and weighting to practical data analysis routines. Manuscript.
Moulder, F. V. 1977 . Japan, China and the Modern World Economy: Toward a Reinterpretation of East Asian Development ca. 1600 to ca. 1918 . Cambridge: Cambridge University Press.
Munck, G. L. 2004 . Tools for qualitative research. Pp. 105–21 in Rethinking Social Inquiry: Diverse Tools, Shared Standards , ed. H. E. Brady and D. Collier . Lanham, Md. : Rowman and Littlefield.
Njolstad, O. 1990 . Learning from history? Case studies and the limits to theory‐building. Pp. 220–46 in Arms Races: Technological and Political Dynamics , ed. O. Njolstad . Thousand Oaks, Calif.: Sage.
Patton, M. Q. 2002 . Qualitative Evaluation and Research Methods . Newbury Park, Calif.: Sage.
Popper, K. 1934/ 1968 . The Logic of Scientific Discovery . New York: Harper and Row.
—— 1963 . Conjectures and Refutations . London: Routledge and Kegan Paul.
Posner, D. 2004 . The political salience of cultural difference: why Chewas and Tumbukas are allies in Zambia and adversaries in Malawi. American Political Science Review , 98: 529–46.
Przeworski, A. and Teune, H. 1970 . The Logic of Comparative Social Inquiry . New York: John Wiley.
Queen, S. 1928 . Round table on the case study in sociological research. Publications of the American Sociological Society, Papers and Proceedings , 22: 225–7.
Ragin, C. C. 2000 . Fuzzy‐set Social Science . Chicago: University of Chicago Press.
—— 2004 . Turning the tables. Pp. 123–38 in Rethinking Social Inquiry: Diverse Tools, Shared Standards , ed. H. E. Brady and D. Collier. Lanham, Md. : Rowman and Littlefield.
Reilly, B. 2000 –1. Democracy, ethnic fragmentation, and internal conflict: confused theories, faulty data, and the “crucial case” of Papua New Guinea. International Security , 25: 162–85. 10.1162/016228800560552
—— and Phillpot, R. 2003 . “Making democracy work” in Papua New Guinea: social capital and provincial development in an ethnically fragmented society. Asian Survey , 42: 906–27. 10.1525/as.2002.42.6.906
Rogowski, R. 1995 . The role of theory and anomaly in social‐scientific inference. American Political Science Review , 89: 467–70. 10.2307/2082443
Rohlfing, I. 2004. Have you chosen the right case? Uncertainty in case selection for single case studies. Working Paper, International University, Bremen.
Rosenbaum, P. R. 2004 . Matching in observational studies. In Applied Bayesian Modeling and Causal Inference from an Incomplete‐data Perspective , ed. A. Gelman and X.‐L. Meng . New York: John Wiley.
—— and Silber, J. H. 2001 . Matching and thick description in an observational study of mortality after surgery. Biostatistics , 2: 217–32. 10.1093/biostatistics/2.2.217
Ross, M. 2001 . Does oil hinder democracy? World Politics , 53: 325–61. 10.1353/wp.2001.0011
Sagan, S. D. 1995 . Limits of Safety: Organizations, Accidents, and Nuclear Weapons . Princeton, NJ: Princeton University Press.
Sekhon, J. S. 2004 . Quality meets quantity: case studies, conditional probability and counter‐ factuals. Perspectives in Politics , 2: 281–93.
Shafer, M. D. 1988 . Deadly Paradigms: The Failure of U.S. Counterinsurgency Policy . Princeton, NJ: Princeton University Press.
Skocpol, T. 1979 . States and Social Revolutions: A Comparative Analysis of France, Russia, and China . Cambridge: Cambridge University Press.
—— and Somers, M. 1980 . The uses of comparative history in macrosocial inquiry. Comparative Studies in Society and History , 22: 147–97.
Stinchcombe, A. L. 1968 . Constructing Social Theories . New York: Harcourt, Brace.
Swank, D. H. 2002 . Global Capital, Political Institutions, and Policy Change in Developed Welfare States . Cambridge: Cambridge University Press.
Tendler, J. 1997 . Good Government in the Tropics . Baltimore: Johns Hopkins University Press.
Truman, D. B. 1951 . The Governmental Process . New York: Alfred A. Knopf.
Tsai, L. 2007 . Accountability without Democracy: How Solidary Groups Provide Public Goods in Rural China . Cambridge: Cambridge University Press.
Van Evera, S. 1997 . Guide to Methods for Students of Political Science . Ithaca, NY: Cornell University Press.
Wahlke, J. C. 1979 . Pre‐behavioralism in political science. American Political Science Review , 73: 9–31. 10.2307/1954728
Yashar, D. J. 2005 . Contesting Citizenship in Latin America: The Rise of Indigenous Movements and the Postliberal Challenge . Cambridge: Cambridge University Press.
Yin, R. K. 2004 . Case Study Anthology . Thousand Oaks, Calif.: Sage.
Gujarati (2003) ; Kennedy (2003) . Interestingly, the potential of cross‐case statistics in helping to choose cases for in‐depth analysis is recognized in some of the earliest discussions of the case‐study method (e.g. Queen 1928 , 226).
This expands on Mill (1843/1872 , 253), who wrote of scientific enquiry as twofold: “either inquiries into the cause of a given effect or into the effects or properties of a given cause.”
This method has not received much attention on the part of qualitative methodologists; hence, the absence of a generally recognized name. It bears some resemblance to J. S. Mill's Joint Method of Agreement and Difference ( Mill 1843/1872 ), which is to say a mixture of most‐similar and most‐different analysis, as discussed below. Patton (2002 , 234) employs the concept of “maximum variation (heterogeneity) sampling.”
More precisely, George and Smoke (1974 , 534, 522–36, ch. 18 ; see also discussion in Collier and Mahoney 1996 , 78) set out to investigate causal pathways and discovered, through the course of their investigation of many cases, these three causal types. Yet, for our purposes what is important is that the final sample includes at least one representative of each “type.”
For further examples see Collier and Mahoney (1996) ; Geddes (1990) ; Tendler (1997) .
Traditionally, methodologists have conceptualized cases as having “positive” or “negative” values (e.g. Emigh 1997 ; Mahoney and Goertz 2004 ; Ragin 2000 , 60; 2004 , 126).
Geddes (1990) ; King, Keohane, and Verba (1994) . See also discussion in Brady and Collier (2004) ; Collier and Mahoney (1996) ; Rogowski (1995) .
The exception would be a circumstance in which the researcher intends to disprove a deterministic argument ( Dion 1998 ).
Geddes (2003 , 131). For other examples of casework from the annals of medicine see “Clinical reports” in the Lancet , “Case studies” in Canadian Medical Association Journal , and various issues of the Journal of Obstetrics and Gynecology , often devoted to clinical cases (discussed in Jenicek 2001 , 7). For examples from the subfield of comparative politics see Kazancigil (1994) .
For a discussion of the important role of anomalies in the development of scientific theorizing see Elman (2003) ; Lakatos (1978) . For examples of deviant‐case research designs in the social sciences see Amenta (1991) ; Coppedge (2004) ; Eckstein (1975) ; Emigh (1997) ; Kendall and Wolf (1949/1955) .
For examples of the crucial‐case method see Bennett, Lepgold, and Unger (1994) ; Desch (2002) ; Goodin and Smitsman (2000) ; Kemp (1986) ; Reilly and Phillpot (2003) . For general discussion see George and Bennett (2005) ; Levy (2002) ; Stinchcombe (1968 , 24–8).
A third position, which purports to be neither Popperian or Bayesian, has been articulated by Mayo (1996 , ch. 6 ). From this perspective, the same idea is articulated as a matter of “severe tests.”
It should be noted that Tsai's conclusions do not rest solely on this crucial case. Indeed, she employs a broad range of methodological tools, encompassing case‐study and cross‐case methods.
See also the discussion in Eckstein (1975) and Lijphart (1969) . For additional examples of case studies disconfirming general propositions of a deterministic nature see Allen (1965); Lipset, Trow, and Coleman (1956) ; Njolstad (1990) ; Reilly (2000–1) ; and discussion in Dion (1998) ; Rogowski (1995) .
Granted, insofar as case‐study analysis provides a window into causal mechanisms, and causal mechanisms are integral to a given theory, a single case may be enlisted to confirm or disconfirm a proposition. However, if the case study upholds a posited pattern of X/Y covariation, and finds fault only with the stipulated causal mechanism, it would be more accurate to say that the study forces the reformulation of a given theory, rather than its confirmation or disconfirmation. See further discussion in the following section.
Sometimes, the most‐similar method is known as the “method of difference,” after its inventor ( Mill 1843/1872 ). For later treatments see Cohen and Nagel (1934) ; Eggan (1954) ; Gerring (2001 , ch. 9 ); Lijphart (1971 ; 1975) ; Meckstroth (1975) ; Przeworski and Teune (1970) ; Skocpol and Somers (1980) .
For good introductions see Ho et al. (2004) ; Morgan and Harding (2005) ; Rosenbaum (2004) ; Rosenbaum and Silber (2001) . For a discussion of matching procedures in Stata see Abadie et al. (2001) .
The most‐different method is also sometimes referred to as the “method of agreement,” following its inventor, J. S. Mill (1843/1872) . See also De Felice (1986) ; Gerring (2001 , 212–14); Lijphart (1971 ; 1975) ; Meckstroth (1975) ; Przeworski and Teune (1970) ; Skocpol and Somers (1980) . For examples of this method see Collier and Collier (1991/2002) ; Converse and Dupeux (1962) ; Karl (1997) ; Moore (1966) ; Skocpol (1979) ; Yashar (2005 , 23). However, most of these studies are described as combining most‐similar and most‐different methods.
In the following discussion I treat the terms social capital, civil society, and civic engagement interchangeably.
E.g. Collier and Collier (1991/2002) ; Karl (1997) ; Moore (1966) ; Skocpol (1979) ; Yashar (2005 , 23). Karl (1997) , which affects to be a most‐different system analysis (20), is a particularly clear example of this. Her study, focused ostensibly on petro‐states (states with large oil reserves), makes two sorts of inferences. The first concerns the (usually) obstructive role of oil in political and economic development. The second sort of inference concerns variation within the population of petro‐states, showing that some countries (e.g. Norway, Indonesia) manage to avoid the pathologies brought on elsewhere by oil resources. When attempting to explain the constraining role of oil on petro‐states, Karl usually relies on contrasts between petro‐states and nonpetro‐states (e.g. ch. 10 ). Only when attempting to explain differences among petro‐states does she restrict her sample to petro‐states. In my opinion, very little use is made of the most‐different research design.
This was recognized, at least implicitly, by Mill (1843/1872 , 258–9). Skepticism has been echoed by methodologists in the intervening years (e.g. Cohen and Nagel 1934 , 251–6; Gerring 2001 ; Skocpol and Somers 1980 ). Indeed, explicit defenses of the most‐different method are rare (but see De Felice 1986 ).
Another way of stating this is to say that X is a “nontrivial necessary condition” of Y .
Wahlke (1979 , 13) writes of the failings of the “behavioralist” mode of political science analysis: “It rarely aims at generalization; research efforts have been confined essentially to case studies of single political systems, most of them dealing …with the American system.”
- About Oxford Academic
- Publish journals with us
- University press partners
- What we publish
- New features
- Open access
- Institutional account management
- Rights and permissions
- Get help with access
- Accessibility
- Advertising
- Media enquiries
- Oxford University Press
- Oxford Languages
- University of Oxford
Oxford University Press is a department of the University of Oxford. It furthers the University's objective of excellence in research, scholarship, and education by publishing worldwide
- Copyright © 2024 Oxford University Press
- Cookie settings
- Cookie policy
- Privacy policy
- Legal notice
This Feature Is Available To Subscribers Only
Sign In or Create an Account
This PDF is available to Subscribers Only
For full access to this pdf, sign in to an existing account, or purchase an annual subscription.
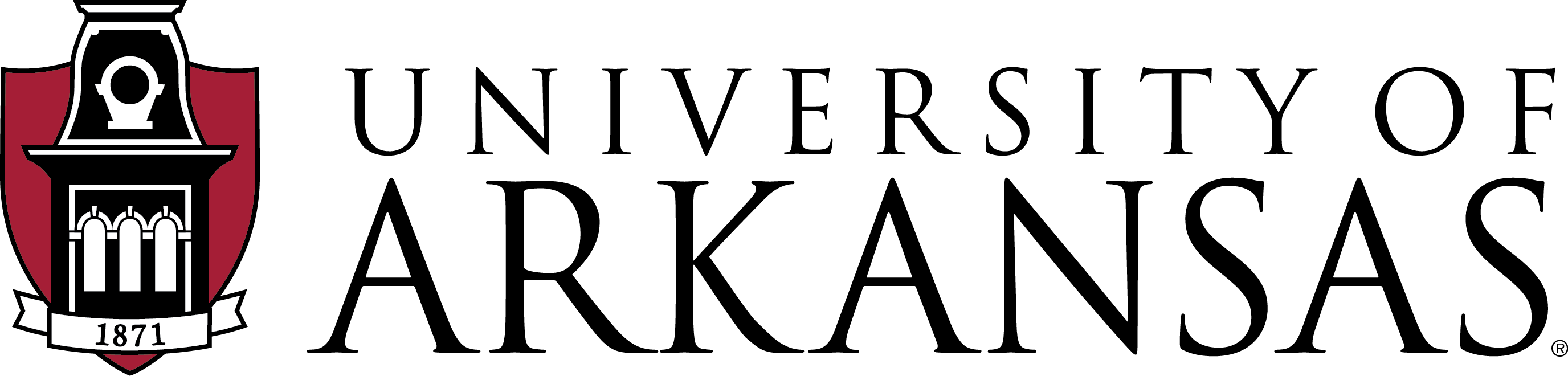
Want to create or adapt books like this? Learn more about how Pressbooks supports open publishing practices.
Criteria for Case Scenario Analysis
Define the problem : Students should focus on defining the problem by determining the root cause, not the underlying symptom(s).
Develop reasonable alternatives : Students should develop three to four reasonable alternatives to deal with the problem. Most laws are written around the concept of what a reasonable person would do.
Evaluate each alternative: Generally, any alternative has both advantages and disadvantages. Students should provide at least two advantages and two disadvantages for each alternative.
Select the preferred alternative: Students should select one alternative or a combination of alternatives to resolve the underlying problem. Additionally, students should provide a reasonable and logical explanation as to why one alternative or combination of alternatives is better than another alternative.
Support the decision with empirical evidence : Students should support their decisions with empirical evidence as applicable. Not all empirical evidence is generalizable to every problem.
Critical Employment, Ethical, and Legal Scenarios in Human Resource Development Copyright © 2020 by Claretha Hughes is licensed under a Creative Commons Attribution-NonCommercial-ShareAlike 4.0 International License , except where otherwise noted.
Share This Book

- Introduction
- About Case Study Reports
- Section A: Overview
- Section B: Planning and Researching
- Section C: Parts of a Case Study
- Section D: Reviewing and Presenting
- Section E: Revising Your Work
- Section F: Resources
- Your Workspace
- Guided Writing Tools

- About Lab Reports
- Section C: Critical Features
- Section D: Parts of a Lab Report

- About Literature Review
- Section C: Parts of a Literature Review
- Section D: Critical Writing Skills

- About Reflective Writing
- Section B: How Can I Reflect?
- Section C: How Do I Get Started?
- Section D: Writing a Reflection

Case Study Report Annotated Example: Alternatives and Decision Criteria
Use the arrows that appear in this window to navigate through the annotations. Read the corresponding highlighted text in the content box below. Click Begin Activity to start.
Present all viable, mutually exclusive alternatives.
Outline the criteria that will be systematically applied in order to determine the best solution to the problem being faced.
Be sure to include ranking and weighting information associated with the decision criteria.
There are two separate organizational structures that are consistent with the recommended strategy and that fit Company XYZ’s needs. Each structure takes a different approach to balancing innovation with a focus on profitability and efficiency.
Alternative 1
The first alternative organizational structure, referred to as a matrix structure, is a unique structure associated with an equal balance of profitability and innovation. This includes having two separate groups of managers; one group will be responsible for the functional aspects of the organization, including marketing, accounting, and any other activities necessary for Company XYZ’s operations to continue. The second group of managers will be responsible for specific product lines including innovation and development. This structure will likely see one employee with two different managers; one for their product line and one for their associated functional department.
Decision Criteria
Each of the aforementioned alternatives can be evaluated using the following decision criteria (listed in order of importance and including the weighting of each criterion):
- The recommendation must increase both sales and employee morale ( 60% );
- The recommendation must align with a strategy that promotes innovation and product development (25%); and
- The recommendation must be able to absorb Company XYZ’s growth in the future, including the ability to add or remove product lines as needed (15%).
- Executive Summary
- Alternatives
- Recommendations
- Privacy Policy
Case Study Format
Following are the main Components/Parts of a Case Study;
Executive Summary
Introduction, alternatives and decision criteria, recommendations and implementation plan, conclusion and references, citing sources.
Writing a case study is not a simple process as it can take several months to write it successfully. There are many stages you need to complete first and after that, you finally come at the stage of writing your case study like selecting the topic, a lot of research ( qualitative research, quantitative research or interview with subjects etc), etc. While writing a case study, bear in mind that all the case studies aren’t the same for sure and vary largely in size, type, and design. It is necessary for a writer to follow a proper case study format while writing it, no matter what.
If you are going to write your very first case study, you need to know that every case study has a specific format, as mentioned above. Students or businesses all over the globe must follow that format in order to make their case study successful. However, one may choose to be a little bit different but the basics remain the same for everyone. Here we are going to discuss different case study sections. The purpose of every section in case study format is different from other and comprise of several unique key elements.
You may also study: How To Write a Case Study
It will definitely assist you to write your own case study with the utmost ease. The sections that are included in the case study are executive summary, introduction, analysis, alternatives and decision criteria, recommendations and implementation plan, conclusions and references, citing sources.
Before moving towards a detailed view of the case study format , let us have a look at the case study benefits.
Value of Case Study
Have you ever think why case studies are developed? This is the question you must ask yourself before writing your very first case study. Knowing the answers and keeping all that stuff in mind will let you write a successful and worth-reading case study.
It is the best way to put the students or learners in an active learning mode. Instead of just testing their memory, case study challenges the students to test their learning via practice, which is usually the easiest approach. In short, case studies proffer students a great opportunity to analyze and resolve the real-world problem with a practical approach. It makes the interpretation as well as problem-solving tasks easy for the learners.
A detailed look at the Case Study Format
Executive summary for a case study is usually similar to the general summary. It is basically a short snapshot that shed the image of your entire case precisely, which consists of a page, most of the time. It doesn’t include too much detail about your case but focuses on key elements or main highlights of your case study. Reading the executive summary of your case study must give the reader an idea about the entire case study and its key elements. There are two approaches adopted to write case studies.
- The first approach is to write the case study’s executive summary in short paragraphs.
- On the other hand, the second approach is to write it in form of points.
Key Points to Cover in an Executive Summary
There are a few things which are necessary to include in your case study’s executive summary i.e. problem statement, recommendation, evidence and supporting arguments, and last but not the least conclusion.
All these things comprise to form a perfect executive summary, which let the reader walk through the entire case study, just by reading it.
- The first thing to include in a case study format is an executive summary, as mentioned above. The very first thing to add in executive summary is problem statement. It let the reader know about the key issue discussed in the entire case in just a few lines. Problem statement usually comprises of one or two statement but may vary according to the case.
- The second thing to add is recommendation after stating the problem statement. What is this recommendation about? It presents one or a few ideas to resolve the problem stated in problem statement.
- The next thing to add in the executive summary is supporting arguments and evidence. It is all about highlighting key areas of your entire case and the arguments of the case. Moreover, it also states one or a few pieces of evidence that support your recommendation section.
- This is the last thing to add in the executive summary is conclusion that definitely concludes everything stated here in this portion. You must let the reader know the key message you want to deliver. Also, state why it is essential to resolve this problem and what are the expected outcomes if the reader follows your recommendations you stated in your case study.
The introduction section of the case study is somehow different from the introduction section of research paper . What is this section intended to have? It is usually here to formulate the stage for your entire case study. It must not only introduce the report of your case but also should state the key problem being faced and discussed thoroughly in a clear and accurate tone.
One thing which is worth-mentioning here is that case study is not like a scientific research report, which is only read by the experts or scientists. It must be written in such a way that a layperson could read and understand it well. Reading the introduction section of case study must let the user know about full case study i.e. what it is about, what are the key areas discussed in this and how the reader will get benefit from it etc. It must not be short enough to miss the necessary details. On the other hand, it must not be long enough that it becomes boring.
Don’t include irrelevant or unnecessary details in it. Just be precise and accurate, and try to include the following:
- A perfect and well-written introductory sentence.
- A short but precise problem statement.
- All necessary problem details.
- The best recommendations for the stated problem.
- And last but not the least: roadmap of the entire case study.
The next section of the case study, when it comes to case study format is analysis. It is usually a detailed section of your case study and it is supposed to examine the problem (which is identified in the previous section) in detail.
When it comes to the right way to structure the analysis section, make sure to ask from your instructor about this, whether there is any format to follow specifically when writing it i.e. SWOT or PEST etc? If your instructor tells you to write it generally, here are a few important things you need to know.
- Start with examining the problem and try to focus on its most crucial or sensitive parts. Here, you are not meant to include any irrelevant or unnecessary details. Your main focus should be the main problem and its critical areas.
- Make sure to mention the causes as well as effects, or any other detail you think is necessary to include. Also, make use of headings to highlight every single portion.
- Here, you are also meant to provide a meaningful conclusion to your analysis. It must conclude all the points, ideas and thoughts you discussed previously into some meaningful ending.
This section of the case study format addresses two key areas. The first one is alternatives and the second one is the decision criteria.
As the name suggests, alternatives must mention all the potential ways the identified problem can be addressed. It let the reader think about the different directions (which are successful as well) to solve the problem. Knowing all the alternatives or the available options to solve the problem, the reader can definitely identify the best possible solution to the problem, as per knowledge and thinking criteria.
One thing which is worth mentioning here is that all the presented solutions to the problems in the alternatives portion must be mutually exclusive. Why is it important to present the mutually exclusive alternatives? What are basically mutually exclusive alternatives?
Mutually exclusive alternatives refer to the situation in which selecting one alternative eliminate all others. There is a specific and a single solution to the identified problem. Mutually exclusive alternatives prevent a scenario in which it becomes essential to implement several available alternatives. When the alternatives will be mutually exclusive, it means that choosing one will eliminate the chances of selection of all others and thus, one alternative will be implemented.
The second thing which needs to be stated here in this portion is decision criteria. It means that you must state precisely your decisive factor i.e. key requirements one need to meet successfully for solving the problem. It is the most important thing here in this portion and you must state it in easy to read and simple words so that the reader could understand it well.
In this section of the case study format , the reader is well aware of all the recommendations for sure. So, there is no need to introduce the reader to the basics of the recommendations again. Rather, you are supposed to let the reader know the specifics of recommendation for solving the identified problem. In this regard, the reader will automatically get all the aspects of the recommended solution to the problem and will see how it will take you to the path of success i.e. towards the path of resolving the problem. For executing the recommendation in a successful manner, here you need to proffer the reader a well thought-out and a comprehensive implementation plan so that the reader could execute the recommended solution, making sure the success.
The recommendations and implementation plan is supposed to include a few things must, which are the following:
A detailed overview of what your recommendation entails, which are necessary steps to follow to implement this successfully and also, the required expertise or a list of equipment needed.
When it comes to the implementation plan, here are a few things which are essential to state here:
- The most important parts of the entire plan of implementation, and who will be accountable for those parts separately.
- Whether it is short term, long term or medium term implementation plan.
- The overall cost required to implement the recommendation.
- The effects of the implementation of recommendation on the entire organization.
- The last but not the least thing to mention here is the potential things which could fail while implementation and plan to recover that failure, if any.
This is the portion of your case study where you are going to make a final ending note for your reader in a few easy to understand yet powerful statements. These statements must emphasize the proposed recommendations. As per common observation, a few instructors don’t suggest you to include this portion of conclusion in your case study but it is helpful in providing a strong endnote to your case study.
There are a few things which are essential to add in this conclusion section, which are the following:
- If the purpose of your case is complex, make sure to summarize it here, in point form, so that the reader could have a review at the entire case again, before approaching the conclusion.
- If you haven’t yet stated the importance of your findings, make sure to do it here in this conclusion section.
- A few concluding sentences that shed the case’s summary and let the reader know what he has learned from this. Moreover, choose to finalize with a few memorable and impactful sentences.
However, the conclusion is the most important section of your case study as you are going to give your entire case an end note, so here are a few things you must keep in your mind while writing this portion.
- A few people give an abrupt ending to the case study, which is one of the biggest mistakes ever. The reason is that the ending must be impactful and must not leave the reader disappointed. So, formulate a few sentences to create a path towards a natural close.
- While stating your recommendation, try to summarize the ways problem will be resolved at hand.
- Make sure everything you write in conclusion portion is convincing enough to persuade the reader to believe that the recommended solution will work the best for solving the particular problem.
As per universal rule, you must cite any idea, though, or expression that is not yours and is presented by someone else. These citations are must to include at the end of your case study. The plagiarism policies or academic misconduct policies vary from one institute to another so you must familiarize yourself with the ones of your institute. Other than this, try your level best to make your case study written in a perfect manner and make sure to cite all of the following:
- Ideas presented by others, which are originally not by you.
- Use of quotations is not recommended while writing the case study. But, if you do, make sure to cite it properly.
- Any summarized work by any other writer.
- Definitions, models or theories etc presented by others must also be properly cited.
- Any information from company websites, annual reports, or press releases must also be cited in a proper manner.
What is the Proper Way to Write Citations?
If you are going to write the citation for the very first time, you need to know that these aren’t written generally. Rather, there is a specific format to follow while writing them. There are a few citations styles which are used by the students universally but before finalizing, you must ask your instructor to suggest you the best one.
A few most commonly used citation styles are the following:
An Ideal Case Study should be LOGICAL, ALL INCLUSIVE & THOROUGH
There are a few characteristics your case study must possess, which are the following:
The first and foremost thing is that each and every section or part of your case study must be logical. Any guesses or estimations must not be included in your case study as everything is supposed to be logical and authentic. However, you can choose to write your observation generally in your case study but it must not state any sort of assumptions made from that.
All INCLUSIVE
The next thing is that your case study must not miss any data or findings. It should be all inclusive i.e. you aren’t given the authority to choose data or findings to include or skip. You are liable to put everything in it. Otherwise, it could fail to be a successful case study.
The last thing to mention here is that your case study must be thorough. You aren’t just meant to write down all the observations during your research but rather, you must proffer the in-depth detail to every observation as well.
So, this is all about writing your case study as well as the right case study format to follow while writing it. Keep all the above-listed things in mind and start your writing process now.
Case Study Format Example 01:
Case study format example 02:, case study format example 03:.
Lots of Luck!
Related Posts
Research paper example, how to write a motivational statement, how to write a good psychology research proposal, how to write a mechanical engineering research paper, apa research paper parts and sections, how to research a topic, how to write a personal essay, research project outline example, research paper format, what is a dissertation paper, leave a comment cancel reply.
Please enter an answer in digits:
Alternative Courses of Action in Case Study: Examples and How To Write
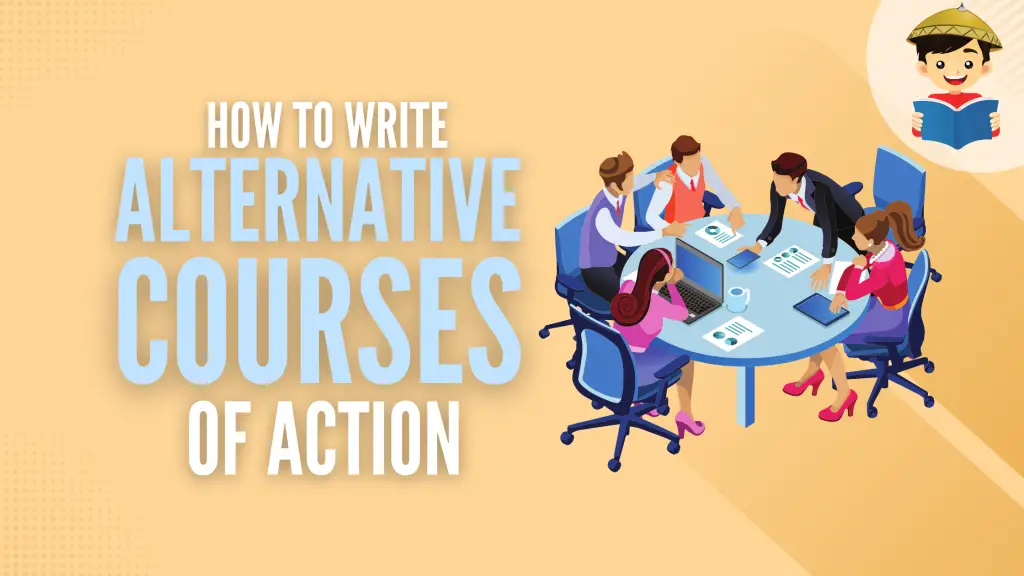
The ultimate goal of creating a case study is to develop a feasible action that can solve the problem it raised.
One way to achieve this is by enumerating all the possible solutions for your case study’s subject. The portion of the case study where you perform this is called ACA or Alternative Courses of Action.
Are you struggling with writing your case study’s ACA? Do not worry; we have provided you with the most detailed guide on writing the Alternative Courses of Action (ACA) of a case study.
Table of Contents
What are alternative courses of action (aca) in a case study.
Alternative Courses of Action (ACA) are the possible actions a firm or organization can implement to address the problem indicated in the case study. These are suggested actions that a firm can consider to arrive at the most feasible and effective solution to the problem.
This portion doesn’t provide the actual and optimal solution yet. Instead, it contains proposed alternatives that will still undergo an evaluation of their respective advantages and disadvantages to help you come up with the best solution.
The ACA you will offer and indicate will be based on your case study’s SWOT analysis in the “ Areas of Consideration ” portion. Thus, a SWOT analysis is performed first before writing the ACA.
What Is the Importance of Alternative Courses of Action (ACA) in a Case Study?
Given the financial, logistical, and operational limitations, developing solutions that the firm can perform can be challenging. By enumerating and evaluating the ACA of your case study, you can filter out the alternatives that can be a potential solution to the problem, given the business’s constraints 1 . This makes your proposed solutions feasible and more meaningful.
How To Write Alternative Courses of Action in Case Study
Here are the steps on how to write the Alternative Courses of Action for your case study:
1. Analyze the Results of Your SWOT Analysis
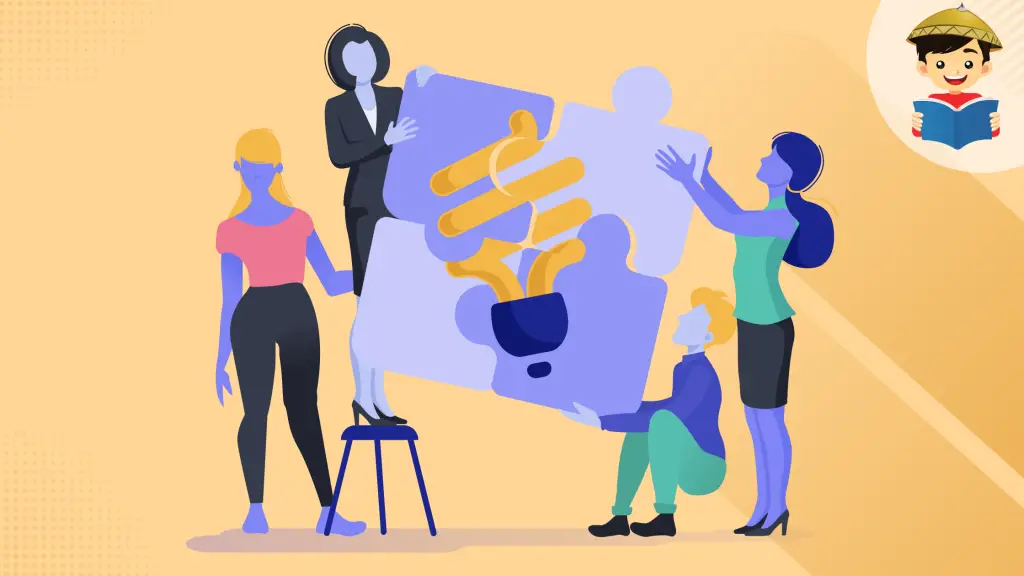
Using the SWOT analysis, consider how the firm can use its strengths and opportunities to address its weaknesses, mitigate threats, and eventually solve the case study’s problem.
Suppose that the case study’s problem is declining monthly sales, and the SWOT analysis showed the following:
- Strength : Creative marketing team
- Opportunity : Increasing trend of using social media to promote products
Then, you may include an ACA about developing the digital marketing arm of the firm to attract more customers and boost monthly sales. This can also address one of the possible threats the firm faces, which is increasing direct marketing costs.
2. Write Your Proposed Solutions/Alternative Courses of Action (ACA) for Your Case Study’s Problem
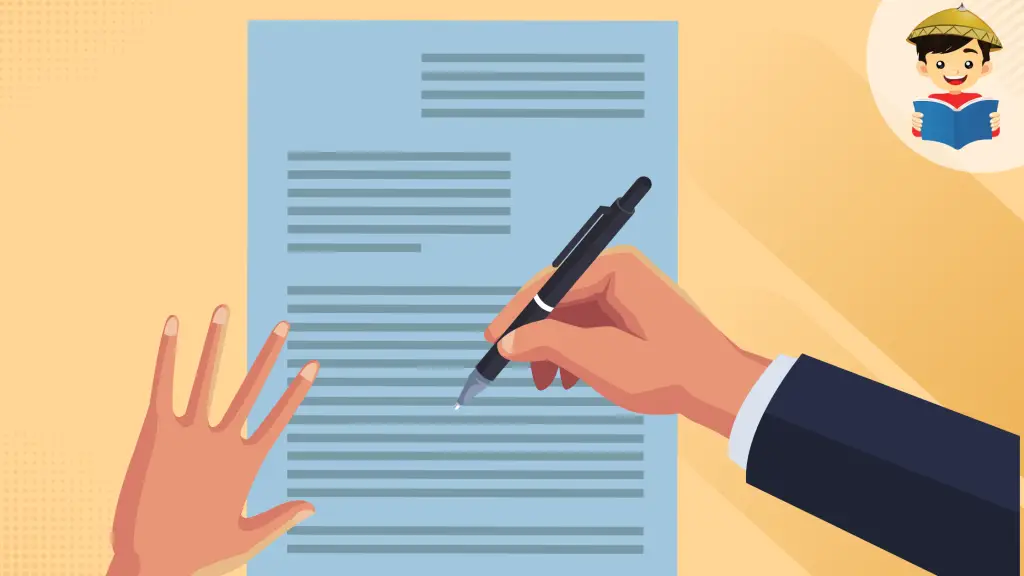
Once you have reviewed your SWOT analysis and come up with possible solutions, it’s time to write them formally in your manuscript. Each solution does not have to be too detailed and wordy. State the specific action that the firm must perform concisely.
Going back to our previous example in Step 1, here is one of the possible ACA that can be included:
ACA #1: Utilize digital platforms such as web pages and social media sites as an alternative marketing platform to reach a wider potential customer base. Digital marketing, together with the traditional direct marketing strategy currently employed, maximizes the business’ market presence, attracting more customers, and potentially driving revenues upward.
In our example above, there is a clear statement of the firm’s action: to use web pages and social media sites to reach more potential customers and increase market presence. Notice how the ACA above provides only an overview of “what to do” and not a complete elaboration on “how to do it.”
3. Identify the Advantages and Disadvantages of Each ACA You Have Proposed
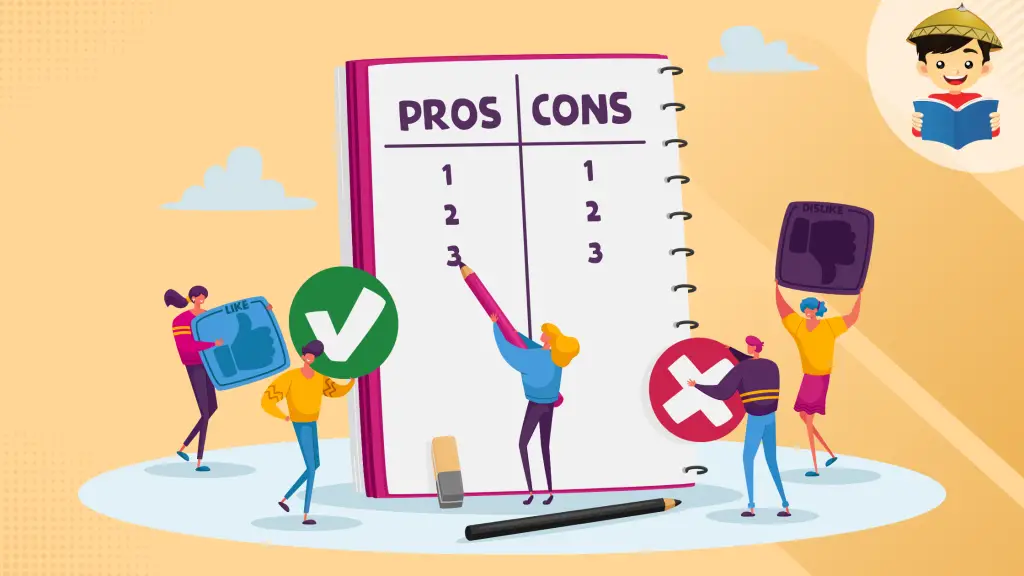
After specifying the ACA, you must evaluate them by stating their respective advantages (pros) and disadvantages (cons). In other words, you must state how your ACA favors the firm (advantages) and its downsides and limitations (disadvantages).
Again, your evaluation does not have to be too detailed but make sure that it is relevant to the ACA that it pertains to.
Let’s return to the ACA we developed from step 2, utilizing digital platforms (e.g., social media sites) to reach more potential customers. What do you think will be the pros and cons of this ACA?
Let’s start with its potential benefits (advantages). Using digital platforms is cheaper than using print ads or direct marketing. So, this will save some funds for the firm. In short, it is cost-effective.
Second, digital platforms offer analytical tools to measure your ads’ reach, making it easier to evaluate people’s perceptions of your offering.
Third, using social media sites makes communicating with any potential customer easier. You can quickly respond to their queries, especially if they are interested in your product.
Lastly, you can reach as many types of people as possible by taking advantage of the internet algorithm.
Now, let us consider its disadvantages 2 . First, using digital marketing takes time and effort to learn, and you must be able to adapt quickly to the changes in trends and new strategies to keep up with the competition.
Second, you must deal with the increasing market competition, as many businesses already use digital platforms.
Third, you have to deal with negative feedback from your customers that are visible to the public and may affect their perception of your brand.
After pondering over the pros and cons of your ACA, it’s time to write them concisely in your manuscript. You can present it in two ways: by tabulating it or by simply listing them.
Example in Table Form:
Examples of Alternative Courses of Action (ACA) in a Case Study
Case Study Problem: Xenon Pastries faces a problem handling larger orders as Christmas Day approaches. With an estimated 15% increase in customer demand, this is the most significant increase in their daily orders since 2012. The management aims to maximize profit opportunities given the rise in customer demand.
ACA #1: Hire part-time workers to increase staff numbers and meet the overwhelming seasonal increase in customer orders. Currently, Xenon Pastries has a total of 9 workers who are responsible for the accommodation of orders, preparation, and delivery of products, and addressing customers’ inquiries and complaints. Hiring 2 – 3 part-time workers can increase productivity and meet the daily order volume.
- Do not require too much effort to implement since hiring announcements only require signages or social media postings
- High certainty of finding potential workers due to the high unemployment rate
- Improve overall productivity of the business and the well-being of other workers since their workload will be lessened
Disadvantages
- Increase in operating expense in the form of wages to the new workers
- Managing more employees and monitoring their performance can be challenging
- New workers might find it challenging to adapt essential skills required in the operation of the business
ACA #2: Increase the prices of Xenon pastries’ products to increase revenues . This option can maximize Xenon Pastries’ profit even if not all customers’ orders are accommodated.
- Cost-effective
- Easy to implement since it only requires changing the price tags of the products
- If customers’ desire to buy the products does not change, the price increase will certainly increase the business’ revenue
- Some customers might be discouraged from buying because of an increase in prices
- There’s a possibility that the increase in the price of the products will make it more expensive relative to competitors’ products
Case Study Problem: Delta Motors has been manufacturing motorcycles for ten years. Recently, the business suffered a gradual shrink in its quarterly revenues due to the increasing popularity of traditional and newly-developed electric bikes. Delta Motors seeks a long-term strategy to attract potential customers to bounce back sales.
ACA #1: Develop a “regular installment payment” scheme to attract customers who wish to purchase motorcycles but have insufficient lump-sum money to acquire one. This payment scheme allows customers to pay an initial deposit and the remaining amount through smaller monthly payments.
- Enticing for middle to low-income individuals who comprise a large chunk of the population
- Requires low initial capital to implement
- Provides a new source of monthly income streams that can benefit the financial standing of the company
- Risk of default or delays in installment payments
- Requires additional human resources to manage and collect installment payments
- The payment scheme requires time to gain returns due to the periodic flow of funds
- Requires a careful creation of guidelines and terms and conditions to ensure smooth facilitation of the installment payment scheme
ACA #2: Introduce new motorcycle models that can entice different types of customers. These models will feature popular designs and more efficient engines.
- This may capture the public’s interest in Delta Motors, which can lead to an increase in the number of potential customers and earning opportunities
- Enables the business to keep up with the intense market competition by providing something “fresh” to the public
- Provides more alternatives for those who already support Delta Motors, strengthening their loyalty to the brand
- Conceptualization of a new model takes a lot of brainstorming to test its feasibility and effectiveness
- Requires sufficient funds to sustain the investment for the development of a new model
- It requires effective marketing strategies to promote the new model to the public
Tips and Warnings
- Do not include in this portion your case study’s conclusion . Think of ACA as a list of possible ways to address the problem. In other words, you suggest the possible alternatives to be selected here. The “ Recommendation ” portion of your case study is where you pick the most appropriate way to solve the problem.
- Use statistical data to support the advantages and disadvantages of each ACA. Although this is optional, presenting numerical data makes your analysis more concrete and factual than just stating them descriptively.
- Do not fall into the “meat sandwich” trap. This happens when you intently make some of the alternatives less desirable so that your preferred choice stands out. This can be done by refusing to elaborate on their benefits or excessively concentrating on their disadvantages. Make sure that each ACA has potential and can be implemented realistically.
Frequently Asked Questions
1. how many alternative courses of action (aca) can a case study have.
Sometimes your instructor or teacher will tell you the required number of ACA that must be included in your case study . However, there’s no “standard” limit to how many ACA you can indicate.
2. What is the difference between Alternative Courses of Action (ACA) and Recommendations?
As mentioned earlier, the case study’s ACA aims to enumerate all possible solutions to the problem. It is not the stage where you state the “final” action you deem most appropriate to address the issue. The case study portion where you explicitly mention your “best” alternative is called the “Recommendation.”
To help you understand the point above, let’s return to our Delta Motors example. In our previous section, we have provided two ACA that can solve the problem, namely (1) developing a regular installment payment plan and (2) introducing a new motorcycle model.
Suppose that upon careful analysis and evaluation of these ACA, you came up with ACA #2 as the more fitting solution to the problem. When you write your case study’s recommendation, you must indicate the ACA you chose and your reasons for selecting it.
Here’s an example of the Recommendation of the case study:
Recommendation
Introducing new motorcycle models that feature popular designs and more efficient engines to entice different types of customers is the most promising alternative course of action that Delta Motors can implement to bounce back its quarterly revenues and keep up with the competitive market. This creates a strong impression on the public of the company’s dedication to promoting high-quality motorcycles that can withstand changes in consumer preferences and market trends. Furthermore, this action proves that the company is continuously evolving to offer a variety of alternative models to suit everyone’s tastes. With proper promotion, these models can rekindle the company’s popularity in the automotive and motorcycle industry.
- How to Analyze a Case Study. Retrieved 23 May 2022, from https://wps.prenhall.com/bp_laudon_essbus_7/48/12303/3149605.cw/content/index.html
- Develop a Digital Marketing Plan. Retrieved 23 May 2022, from https://www.nibusinessinfo.co.uk/content/advantages-and-disadvantages-digital-marketing
Written by Jewel Kyle Fabula
in Career and Education , Juander How
Last Updated July 8, 2023 08:29 PM
Jewel Kyle Fabula
Jewel Kyle Fabula is a Bachelor of Science in Economics student at the University of the Philippines Diliman. His passion for learning mathematics developed as he competed in some mathematics competitions during his Junior High School years. He loves cats, playing video games, and listening to music.
Browse all articles written by Jewel Kyle Fabula
Copyright Notice
All materials contained on this site are protected by the Republic of the Philippines copyright law and may not be reproduced, distributed, transmitted, displayed, published, or broadcast without the prior written permission of filipiknow.net or in the case of third party materials, the owner of that content. You may not alter or remove any trademark, copyright, or other notice from copies of the content. Be warned that we have already reported and helped terminate several websites and YouTube channels for blatantly stealing our content. If you wish to use filipiknow.net content for commercial purposes, such as for content syndication, etc., please contact us at legal(at)filipiknow(dot)net
Best–Worst Method and Simple Additive Weighting for Selection Problems in Process Systems Engineering
- Short communication
- Published: 22 April 2024
Cite this article
- Maria Victoria Migo-Sumagang 1 ,
- Kathleen B. Aviso ORCID: orcid.org/0000-0002-9994-5172 2 &
- Raymond R. Tan 2
The best–worst method (BWM) is a relatively new multi-criterion decision-making (MCDM) technique that was developed to overcome some of the drawbacks of the analytic hierarchy process (AHP). Like its predecessor, BWM also relies on the strategy of decomposing complex decision problems into tractable sub-problems, while also providing a quantitative framework for synthesizing their results into a coherent global solution. However, BWM does not require the tedious pairwise comparison of all problem elements (i.e., criteria and alternatives). Instead, a small subset of all possible pairwise comparisons is used to formulate a linear programming (LP) model whose solution gives weights that are comparable to those generated by AHP. This feature allows more rapid and less error-prone elicitation of expert opinion to calibrate the decision model. However, there are few published works to date on the use of BWM in process systems engineering (PSE). We address this research gap by demonstrating the applicability of BWM coupled with simple additive weighting (SAW) using case studies on the selection of chemical reactors, plastic waste management processes, and 3D printers.
This is a preview of subscription content, log in via an institution to check access.
Access this article
Price includes VAT (Russian Federation)
Instant access to the full article PDF.
Rent this article via DeepDyve
Institutional subscriptions
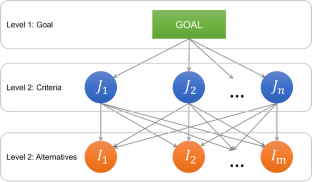
Data and Code Availability
All data used in this work are given in the main text. The optimization models are available upon request.
Abbreviations
Analytic hierarchy process
Build time estimation
Best-worst method
Cost of material consumed
Construction problems
Dimensional accuracy
Economic aspects
Environmental
Energy usage
Functionality
Geometric accuracy
Isothermality
Linear programming
Material consumption estimation
Multi-criterion decision-making
Non-linear programming
Processing cost
Process systems engineering
Residence-contact time
Sampling and analysis
Simple additive weighting
Software capabilities
Selectivity disguise-decay
Surface roughness
Sustainability
Technical performance
Set of alternatives
Set of criteria
Index for the best alternative (or most important criterion)
Index for alternative option
Index for criteria
Index for the worst alternative (or least important criterion)
Vector which contains the relative preference of the “best” criterion against all other criteria
Relative preference of the “best” criterion against criterion j
Relative preference of the “worst” criterion against criterion j
Vector which contains the relative preference of the “worst” criterion against all other criteria
Score of alternative \(i\) in criterion \(j\)
Measure of consistency between the pairwise comparisons
Overall score or value of alternative \(i\)
Weight of the “best” criterion
Optimal weight of criterion \(j\)
Weight of the “worst” criterion
Bandyopadhyay S (2020) Interval pinch analysis for resource conservation networks with epistemic uncertainties. Ind Eng Chem Res 59:13669–13681
Article Google Scholar
Best-Worst Method https://bestworstmethod.com . Accessed 27 Dec 2023
Couper JR, Penney WR, Fair JR (2012) Chemical process equipment, 3rd edn. Elsevier, Amsterdam, Netherlands
Google Scholar
Gibson I, Rosen D, Stucker B, Khorasani M (2020) Additive manufacturing technologies. Springer, Cham, Switzerland
Hanratty PJ, Joseph B (1992) Decision-making in chemical engineering and expert systems: application of the analytic hierarchy process to reactor selection. Comput Chem Eng 16:849–860
Jahan A, Ismail MY, Mustapha F, Sapuan SM (2010) Material selection based on ordinal data. Mater Des 31:3180–3187
Jia X, Zhou T, Li Z, Aviso KB, Tan RR, Jia X, Klemeš JJ, Wang F (2021) Integrated approach for sectoral carbon drawdown solutions. Chem Eng Trans 88:1117–1122
Justino Netto JM, Ragoni IG, Frezzatto Santos LE, Silveira ZC (2019) Selecting low-cost 3D printers using the AHP method: a case study. SN Appl Sci 1:335
Li Z, Jia X, Jin H, ...Xu C, Wei H (2021) Determining optimal municipal solid waste management scenario based on best-worst method. J Environ Eng Landsc Manag 29:150–161
MacCrimmon KR (1968) Decision making among multiple attribute alternatives: a survey and consolidated approach, RAND Memorandum, RM-4823-ARPA
Mohammadi M, Rezaei J (2020) Bayesian best-worst method: a probabilistic group decision making model. Omega 96:102075
Promentilla MAB, Aviso KB, Lucas RIG, Razon LF, Tan RR (2018) Teaching analytic hierarchy process (AHP) in undergraduate chemical engineering courses. Educ Chem Eng 23:34–41
Rezaei J (2015) Best-worst multi-criteria decision-making method. Omega 53:49–57
Rezaei J (2016) Best-worst multi-criteria decision-making method: some properties and a linear model. Omega 64:126–130
Saaty TL (1990) How to make a decision: the analytic hierarchy process. Eur J Oper Res 48:9–26
Serna J, Díaz EN, Narváez PC, Camargo M, Gálvez D, Orjuela Á (2016) Multi-criteria decision analysis for the selection of sustainable chemical process routes during early design stages. Chem Eng Res Des 113:28–49
Sikdar SK (2009) On aggregating multiple indicators into a single metric for sustainability. Clean Technol Environ Policy 11:157–161
Sindhwani K, Gupta P, Kumar A, Srivastava R (2022) Evaluation of plastic waste management methods using multi criteria decision making tool †AHP. Adv Transdiscip Eng 27:453–461
Tan RR, Promentilla MAB (2013) A methodology for augmenting sparse pairwise comparison matrices in AHP: applications to energy systems. Clean Technol Environ Policy 15:713–719
Tan RR, Almario EMR, Aviso KB, Cruz JB, Promentilla MAB (2019) A methodology for tracing the rank invariance region in multi-criterion selection problems: application to negative emission technologies. Process Integr Optim Sustain 3:533–536
Wang F, Wang J, Ren J, Li Z, Nie X, Tan RR, Jia X (2020) Continuous improvement strategies for environmental risk mitigation in chemical plants, Resources. Conserv Recycl 160:104885
Wang Z, Rangaiah GP, Wang X (2021) Preference ranking on the basis of ideal-average distance method for multi-criteria decision-making. Ind Eng Chem Res 60:11216–11230
Weekman VW (1974) Laboratory reactors and their limitations. AIChE J 20:833–840
Wu Q, Liu X, Zhou L, Qin J, Rezaei J (2024) An analytical framework for the best–worst method. Omega 123:102974
Download references
Author information
Authors and affiliations.
Department of Chemical Engineering, University of the Philippines, Los Baños College, Los Baños, Laguna, 4031, Philippines
Maria Victoria Migo-Sumagang
Department of Chemical Engineering, De La Salle University, 2401 Taft Avenue, 0922, Manila, Philippines
Kathleen B. Aviso & Raymond R. Tan
You can also search for this author in PubMed Google Scholar
Contributions
MVMS—writing, editing, and computations; KBA—writing, editing, and computations; RRT—writing, editing, and computations.
Corresponding author
Correspondence to Kathleen B. Aviso .
Ethics declarations
Conflict of interest.
The authors declare no competing interests.
Additional information
Publisher's note.
Springer Nature remains neutral with regard to jurisdictional claims in published maps and institutional affiliations.
Rights and permissions
Reprints and permissions
About this article
Migo-Sumagang, M.V., Aviso, K.B. & Tan, R.R. Best–Worst Method and Simple Additive Weighting for Selection Problems in Process Systems Engineering. Process Integr Optim Sustain (2024). https://doi.org/10.1007/s41660-024-00414-6
Download citation
Received : 04 January 2024
Revised : 23 March 2024
Accepted : 12 April 2024
Published : 22 April 2024
DOI : https://doi.org/10.1007/s41660-024-00414-6
Share this article
Anyone you share the following link with will be able to read this content:
Sorry, a shareable link is not currently available for this article.
Provided by the Springer Nature SharedIt content-sharing initiative
- Selection problems
- Decision analysis
- Mathematical programming
- Laboratory equipment
- Plastic pollution
- Additive manufacturing
- Find a journal
- Publish with us
- Track your research
- Architecture and Design
- Asian and Pacific Studies
- Business and Economics
- Classical and Ancient Near Eastern Studies
- Computer Sciences
- Cultural Studies
- Engineering
- General Interest
- Geosciences
- Industrial Chemistry
- Islamic and Middle Eastern Studies
- Jewish Studies
- Library and Information Science, Book Studies
- Life Sciences
- Linguistics and Semiotics
- Literary Studies
- Materials Sciences
- Mathematics
- Social Sciences
- Sports and Recreation
- Theology and Religion
- Publish your article
- The role of authors
- Promoting your article
- Abstracting & indexing
- Publishing Ethics
- Why publish with De Gruyter
- How to publish with De Gruyter
- Our book series
- Our subject areas
- Your digital product at De Gruyter
- Contribute to our reference works
- Product information
- Tools & resources
- Product Information
- Promotional Materials
- Orders and Inquiries
- FAQ for Library Suppliers and Book Sellers
- Repository Policy
- Free access policy
- Open Access agreements
- Database portals
- For Authors
- Customer service
- People + Culture
- Journal Management
- How to join us
- Working at De Gruyter
- Mission & Vision
- De Gruyter Foundation
- De Gruyter Ebound
- Our Responsibility
- Partner publishers

Your purchase has been completed. Your documents are now available to view.
Interval-valued T-spherical fuzzy extended power aggregation operators and their application in multi-criteria decision-making
As an effective tool to show the fuzziness of qualitative information, the interval-valued T-spherical fuzzy set can utilize three kinds of information, namely, membership, abstinence, and non-membership, to show the opinions of decision-maker. Given this advantage, many interval-valued T-spherical fuzzy multi-criteria decision-making (IVTSF-MCDM) methods have been designed. However, most of the existing IVTSF-MCDM methods have a common limitation that the inability to effectively show the impacts of extreme data. To address this limitation, this study develops a novel MCDM method based on interval-valued T-spherical fuzzy extended power aggregation operator. First, interval-valued T-spherical fuzzy cross-entropy (CE) and interval-valued T-spherical fuzzy symmetrical CE are defined to measure the difference between two interval-valued T-spherical fuzzy numbers, which are used to determine criteria weights in MCDM. Second, interval-valued T-spherical fuzzy extended power average operator and interval-valued T-spherical fuzzy extended power geometric operator are proposed, and their properties are investigated. Moreover, in view of that criteria may be assigned to different weights, this study defines interval-valued T-spherical fuzzy extended power weighted average operator and interval-valued T-spherical fuzzy extended power weighted geometric operator to derive the order of alternatives. Finally, the applicability of the proposed method is validated by the case about investment country selection, while the sensitivity and comparison analyses are also conducted to further prove its advantages and effectiveness.
1 Introduction
Multi-criteria decision-making (MCDM) is an important research field in decision science. Since the evaluation information provided by decision-makers (DMs) is usually ambiguous and uncertain in MCDM, some fuzzy sets have been adopted to express uncertain evaluation information, including fuzzy set intuitionistic fuzzy set, Pythagorean fuzzy set, and q-rung orthopair fuzzy set [ 1 , 2 , 3 , 4 ]. In actual situations, DMs’ opinions involve more answers of types: yes, no, abstain, and refusal. Voting is a suitable example of such situation as human voters may be classified into four types: vote for, abstain, vote against, and refusal of the voting. To deal with this situation, Cuong presented the picture fuzzy set [ 5 ]. The picture fuzzy set includes three kinds of degrees, namely, the membership degree μ ( 0 ≤ μ ≤ 1 ) , abstinence degree η ( 0 ≤ η ≤ 1 ) , and non-membership degree v ( 0 ≤ v ≤ 1 ) , and the restriction is that the sum of the three degrees must not exceed one, i.e., μ + η + v ≤ 1 . Subsequently, inspired by ideas of q-rung orthopair fuzzy set, Mahmood et al. [ 6 ] extended picture fuzzy set to define T-spherical fuzzy set (TSFS), which satisfies the condition μ q + η q + v q ≤ 1 ( q ≥ 1 ) . However, DMs may choose interval numbers to express their opinions since their cognitions of the evaluation object are uncertain. Thus, Ullah et al. [ 7 ] proposed interval-valued TSFS (IVTSFS) on the basis of TSFS, in which the membership degree, abstinence degree, and non-membership degree are expressed in form of interval numbers. Obviously, IVTSFS can more intuitively show the perceptions of DMs by comparing with the aforementioned fuzzy sets.
Taking the advantages of IVTSFS, some interval-valued T-spherical fuzzy MCDM (IVTSF-MCDM) methods have been proposed [ 6 , 7 , 8 , 9 , 10 , 11 , 12 , 13 ]. For example, Ullah et al. proposed interval-valued T-spherical fuzzy weighted average (IVTSFWA) operator and interval-valued T-spherical fuzzy weighted geometric (IVTSFWG) operator, and used these aggregation operators to handle MCDM problems [ 7 ]. Subsequently, Jin et al. [ 8 ] developed some Hamacher aggregation operators in the environment of IVTSFSs, including interval-valued T-spherical fuzzy Hamacher weighted average (IVTSFHWA) and interval-valued T-spherical fuzzy Hamacher weighted geometric (IVTSFHWG) operators, and utilized these operators to design MCDM method. Considering that Dombi t-norm and Dombi t-conorm have more flexibility, Ullah et al. [ 9 ] presented the concepts of interval-valued T-spherical fuzzy Dombi weighted average (IVTSFDWA) and interval-valued T-spherical fuzzy Dombi weighted geometric (IVTSFDWG) operators, and applied these operators into MCDM. Inspired by Frank t-norm and Frank t-conorm, Hussain et al. [ 10 ] defined interval-valued T-spherical fuzzy Frank aggregation operators, which are used to assess the business proposals. In view of the aforementioned operators that cannot reflect the interrelationships between two criteria in MCDM, Akram et al. [ 11 ] extended Bonferroni mean operators to interval-valued T-spherical fuzzy context. In fact, there are some relationships among more than two criteria. Thus, Yang, et al. [ 12 ] proposed interval-valued T-spherical fuzzy weighted Muirhead mean (IVTSFWMM) operator and established the decision-making method of digitalization solutions of medical system based on this operator. Although these interval-valued T-spherical fuzzy aggregation operators are from different mathematical perspective, they ignore the impacts of extreme data, such as too high and too low evaluation results, on the final result in MCDM. To address this issue, Xu and Yager [ 13 ] proposed the power average (PA) operator and power geometric (PG) operator, which regard extreme data as erroneous or biased information. Thus, PA and PG operators assign smaller weights to extreme data. Some scholars [ 14 , 15 , 16 , 17 , 18 ] have applied PA and PG operators to solve MCDM problems with different fuzzy sets. However, extreme data may be important in some cases. For example, five professors’ assessments about the innovativeness of an article are expressed in form of interval value T-spherical fuzzy numbers (IVTSFNs), where the full professor gives a IVTSFN ([0.1, 0.2], [0.6, 0.6], [0.3, 0.4]), and four assistant professors’ evaluations are ([0.3, 0.5], [0.4, 0.6], [0.2, 0.6]). Although the full professor’ opinion differs from that of assistant professors, his opinion is more important than that of others. It is worth mentioning that full professor’ opinions are regarded as biased information and assigned to a smaller weight when using PA or PG operator. Obviously, PA or PG operator may lead to unreasonable results in some cases. To overcome this shortcoming, Xiong et al. [ 19 ] and Xiong et al. [ 20 ] introduced the extended power average (EPA) operator and extended power geometric (EPG) operator, respectively, which set an adjustable parameter to reflect the preferences of DMs on extreme data on the basic of PA and PG operators. Obviously, extended power aggregation operators can describe more important information than PA and PG. However, there are few studies on extended power aggregation operators in IVTSF-MCDM problems. Hence, it is valuable to employ EPA and EPG operators to solve IVTSF-MCDM problems.
In IVTSF-MCDM, different criteria usually have different impacts on the decision results. In other words, different criteria should be assigned with different weights. The maximizing deviation approach is very effective in determining criteria weights, which relies on the differences between DMs’ opinions under each criterion [ 21 ]. Therefore, how to choose a suitable tool to measure the differences between data is material for the maximizing deviation approach. As an important concept of information theory, cross-entropy (CE) can measure the difference between two probability distributions. In other words, CE can reflect the gap of the data to some extent. Therefore, it is very effective to combine CE with the maximizing deviation approach to determine criteria weights. The CE has been extended to the different fuzzy environment, such as intuitionistic fuzzy CE [ 22 ], Pythagorean fuzzy CE [ 23 ], q-rung orthopair fuzzy CE [ 24 ], picture fuzzy CE [ 25 ], and T-spherical fuzzy CE [ 26 ]. These existing CEs have been successfully applied into MCDM problems. However, few scholars explore CE under interval-valued T-spherical fuzzy context. Therefore, this article designs interval-valued T-spherical fuzzy CE (IVTSFCE) and interval-valued T-spherical fuzzy symmetric CE (IVTSFSCE) to calculate the differences between two IVTSFNs. Given this characteristic of IVTSFSCE, it can be combined with the maximizing deviation approach to derive criteria weights in IVTSF-MCDM problems, and it provides the necessary support for the proposed MCDM method.
The previous researches of CE [ 23 , 24 , 25 , 26 ] cannot deal with interval-valued T-spherical fuzzy information.
Some existing IVTSF-MCDM methods [ 7 , 8 , 9 , 10 , 11 ] cannot consider the influence of extreme data on the order of alternatives, which leads to an unreasonable result.
The IVTSFCE and IVTSFSCE are developed to measure the differences between two IVTSFNs.
Some interval-valued T-spherical fuzzy extended power aggregation operators, such as IVTSFEPA, IVTSFEPG, IVTSFEPWA and IVTSFEPWG operators, are presented to show the effect of extreme data.
A novel MCDM method is designed using IVTSFCE and interval-valued T-spherical fuzzy extended power aggregation operators.
The framework of this article includes: in Section 2 , some basic knowledge about IVTSFS, PA, and PG operators is recalled, while IVTSFCE and IVTSFSCE are defined. In Section 3 , some interval-valued T-spherical fuzzy extended power aggregation operators are presented and their properties are investigated. In Section 4 , a novel MCDM method is proposed on the basis of IVTSFCE and interval-valued T-spherical fuzzy extended power aggregation operators. Section 5 presents an application of the proposed method into an illustrative case. Additionally, the sensitivity and comparison analyses prove the merit of the proposed method. In Section 6 , some conclusions are given.
2 Preliminaries
In this section, the IVTSFS, PA, and PG operators are recalled, and the concepts of IVTSFCE and IVTSFSCE are defined.
Definition 1
(Ullah et al. [ 7 ]) Let X be a finite set, and the IVTSFS on X is expressed as:
where [ μ x L , μ x U ] , [ η x L , η x U ] , and [ v x L , v x U ] denote the elements x ∈ X satisfying the degree of membership, abstinence, and non-membership of the set T, respectively, and meet 0 ≤ μ x L ≤ μ x U ≤ 1 , 0 ≤ η x L ≤ η x U ≤ 1 , 0 ≤ v x L ≤ v x U ≤ 1 and 0 ≤ ( μ x U ) q + ( η x U ) q + v x U ) q ≤ 1 ( q ≥ 1 ) .
For the convenience of expression, we denote ( [ μ x L , μ x U ] , [ η x L , η x U ] , [ v x L , v x U ] ) as α = ( [ μ L , μ U ] , [ η L , η U ] , [ v L , v U ] ) and call α the interval T - spherical fuzzy number (IVTSFN).
Definition 2
α 1 ⊕ α 2 = ( [ ( ( μ 1 L ) q + ( μ 2 L ) q − ( μ 1 L μ 2 L ) q ) 1 / q , ( ( μ 1 U ) q + ( μ 2 U ) q − ( μ 1 U μ 2 U ) q ) 1 / q ] , [ η 1 L η 2 L , η 1 U η 2 U ] , [ v 1 L v 2 L , v 1 U v 2 U ] )
α 1 ⊗ α 2 = ( [ μ 1 L μ 2 L , μ 1 U μ 2 U ] , [ ( ( η 1 L ) q + ( η 2 L ) q − ( η 1 L η 2 L ) q ) 1 / q , ( ( η 1 U ) q + ( η 2 U ) q − ( η 1 U η 2 U ) q ) 1 / q ] , [ ( ( v 1 L ) q + ( v 2 L ) q − ( v 1 L v 2 L ) q ) 1 / q , ( ( v 1 U ) q + ( v 2 U ) q − ( v 1 U v 2 U ) q ) 1 / q ] )
λ α 1 = ( [ ( 1 − ( 1 − ( μ 1 L ) q ) λ ) 1 / q , ( 1 − ( 1 − ( μ 1 U ) q ) λ ) 1 / q ] , [ ( η 1 L ) λ , ( η 1 U ) λ ] , [ ( v 1 L ) λ , ( v 1 U ) λ ] )
( α 1 ) λ = ( [ ( μ 1 L ) λ , ( μ 1 U ) λ ] , [ ( 1 − ( 1 − ( η 1 L ) q ) λ ) 1 / q , ( 1 − ( 1 − ( η 1 U ) q ) λ ) 1 / q ] , [ ( 1 − ( 1 − ( v 1 L ) q ) λ ) 1 / q , ( 1 − ( 1 − ( v 1 U ) q ) λ ) 1 / q ] )
Definition 3
(Ullah et al. [ 7 ]) Let an IVTSFN be α = ( [ μ L , μ U ] , [ η L , η U ] , [ v L , v U ] ) , then its score function sc ( α ) is as follows:
According to the score function of IVTSFN, Ullah et al. [ 7 ] presented the comparison laws for two IVTSFNs, which is as follows: (1) if sc ( α 1 ) > sc ( α 2 ) , then α 1 > α 2 ; (2) if sc ( α 1 ) = sc ( α 2 ) , then α 1 ≈ α 2 .
2.2 Extended power aggregation operators
To depict the effect of extreme data, Xiong et al. [ 19 ] and Xiong et al. [ 20 ] proposed EPA operator and EPG operator, respectively.
Definition 4
The EPA operator is defined as:
The EPG operator is defined as:
where the parameter ξ ∈ ( − ∞ , 1 − n ] ∪ [ 0 , + ∞ ) , t ( a k ) = ∑ l = 1 , l ≠ k n Sup ( a k , a l ) , Sup ( a k , a l ) denotes the support of a l for a k and satisfies three conditions: (1) Sup ( a k , a l ) ∈ [ 0 , 1 ] ; (2) Sup ( a k , a l ) = Sup ( a l , a k ) ; (3) if | a k − a l | ≤ | a p − a q | , then Sup ( a k , a l ) ≥ Sup ( a p , a q ) .
It is worth noting that if DMs consider the extreme values to be error or bias information, the parameter takes ξ ∈ [ 0 , + ∞ ) . If DMs regard the extreme values as important information, the parameter takes ξ ∈ ( − ∞ , 1 − n ] .
2.3 IVTSFCE
To measure the difference between two IVISFNs, this subsection proposes the concept of IVTSFCE.
Definition 5
Let two IVISFNs be α 1 = ( [ μ 1 L , μ 1 U ] , [ η 1 L , η 1 U ] , [ v 1 L , v 1 U ] ) and α 2 = ( [ μ 2 L , μ 2 U ] , [ η 2 L , η 2 U ] , [ v 2 L , v 2 U ] ) , then IVTSFCE is calculated as:
It is worth noting that CE ( α 1 , α 2 ) does not satisfy symmetry. Thus, this study proposes IVTSFSCE.
Definition 6
Let two IVISFNs be α 1 = ( [ μ 1 L , μ 1 U ] , [ η 1 L , η 1 U ] , [ v 1 L , v 1 U ] ) and α 2 = ( [ μ 2 L , μ 2 U ] , [ η 2 L , η 2 U ] , [ v 2 L , v 2 U ] ) , then IVTSFSCE can be defined as:
Let DE ( α 1 , α 2 ) be the symmetric CE between α 1 and α 2 , then it meets the following properties:
(1) DE ( α 1 , α 2 ) = DE ( α 2 , α 1 ) ;
(2) 0 ≤ DE ( α 1 , α 2 ) ≤ 1 ;
(3) DE ( α 1 , α 2 ) = 0 if and only if α 1 = α 2 .
Property (1) is obvious; thus, this article proves properties (2) and (3).
Property (2): It is easy to have
Thus, we can derive
Furthermore, we can have 0 ≤ DE ( α 1 , α 2 ) ≤ 1 .
Property (3): Let the function be f ( t ) = − ln t . Then, its first-order and second-order derivatives are f ′ ( t ) = − 1 / t < 0 and f ″ ( t ) = 1 / t 2 > 0 , respectively, which implies that f ( x ) is a concave function. According to the properties of concave functions, there must exist − ln ( λ 1 t 1 + λ 2 t 2 ) ≤ − λ 1 ln t 1 − λ 2 ln t 2 , with λ 1 + λ 2 = 1 and λ 1 , λ 2 ∈ ( 0 , 1 ) . Moreover, − ln ( λ 1 t 1 + λ 2 t 2 ) = − λ 1 ln t 1 − λ 2 ln t 2 if and only if t 1 = t 2 .
Assume t 1 = ( μ 1 L + μ 2 L ) / 2 μ 1 L , t 2 = ( 2 − μ 1 L − μ 2 L ) / ( 2 − 2 μ 1 L ) , λ 1 = μ 1 L and λ 2 = 1 − μ 1 L . Then, we can deduce that μ 1 L ln ( 2 μ 1 L / ( μ 1 L + μ 2 L ) ) + ( 1 − μ 1 L ) ln ( 2 ( 1 − μ 1 L ) / ( 2 − μ 1 L − μ 2 L ) ) = 0 if and only if ( μ 1 L + μ 2 L ) / μ 1 L = ( 2 − μ 1 L − μ 2 L ) / ( 2 − 2 μ 1 L ) ⇒ μ 1 L = μ 2 L . Furthermore, we can have DE ( α 1 , α 2 ) = 0 if and only if α 1 = α 2 .□
3 Interval-valued T-spherical fuzzy extended power aggregation operators
Inspired by EPA and EPG operators, this section proposes four interval-valued T-spherical fuzzy extended power aggregation operators, such as IVTSFEPA, IVTSFEPG, IVTSFEPWA, and IVTSFEPWG operators.
3.1 IVTSFEPA and IVTSFEPG operators
Definition 7.
The IVTSFEPA operator is expressed as:
The IVTSFEPG operator is defined as:
where the parameter ξ ∈ ( − ∞ , 1 − n ] ∪ [ 0 , + ∞ ) , , Sup ( α k , α l ) denotes the support of α l for α k and satisfies three conditions: (1) Sup ( α k , α l ) ∈ [ 0 , 1 ] ; (2) Sup ( α k , α l ) = Sup ( α l , α k ) ; (3) if DE ( α k , α l ) ≤ DE ( α p , α q ) , then Sup ( α k , α l ) ≥ Sup ( α p , α q ) .
According to Definition 7, the support Sup ( α k , α l ) is similar to the similarity between two IVTSFNs. If two IVTSFNs are more similar, their support degree is larger. Thus, this study assumes Sup ( α k , α l ) = 1 − DE ( α k , α l ) .
Let a set of IVTSFNs be α k = ( [ μ k L , μ k U ] , [ η k L , η k U ] , [ v k L , v k U ] ) ( k = 1 , 2 , … , n ) , then the results obtained by IVTSFEPA and IVTSFEPG operators are still an IVTSFN. The corresponding calculation results are
According to the operator law of IVTSFE in Definition 2, we have
Based on the first mathematical induction, we obtain
Then, we can obtain
Furthermore, we assume equation ( 9 ) holds when n = r , and obtain
Final, when n = r + 1 , we have
Therefore, equation ( 11 ) holds. Furthermore, we can obtain
In short, equation ( 10 ) holds. Since μ k L ≤ μ k U , η k L ≤ η k U , and v k L ≤ v k U , there must be
In addition, we obtain
Hence, the calculation result of IVTSFEPA operator is still an IVTSFN. The proof for IVTSFEPA operator meeting Theorem 1 is similar to the proof for IVTSFEPG operator meeting Theorem 1, which is omitted in this study.□
(Idempotence) Let α 1 , α 2 , … , α n be a set of IVTSFNs with α 1 = α 2 = … = α n = α , then
(Boundedness) Let α 1 , α 2 , … , α n be a set of IVTSFNs. Then, we have α − = ( [ min k μ k L , min k μ k U ] , [ max k η k L , max k η k U ] , [ max k v k L , max k v k U ] ) and α + = ( [ max k μ k L , max k μ k U ] , [ min k η k L , min k η k U ] , [ min k v k L , min k v k U ] ) . There exists
Let α 1 , α 2 , … , α n be a set of IVTSFNs, and α ′ 1 , α ′ 2 , … , α ′ n be any replacement of α 1 , α 2 , … , α n . Then, we have
(Monotonicity) Let the two sets of IVTSFNs be α 1 , α 2 , … , α n and β 1 , β 2 , … , β n , respectively, with α k ≤ β k ( k = 1 , 2 , … , n ) . Then, we have
3.2 IVTSFEPWA and IVTSFEPWG operators
In practice, different IVTSFNs may be assigned to different weights, and the IVTSFEPA and IVTSFEPG operators do not take into account the weights of IVTSFNs. Therefore, this sub-section proposes IVTSFEPWA and IVTSFEPWG operators.
Definition 8
The IVTSFEPWA operator is expressed as:
The IVTSFEPWG operator is expressed as:
Clearly, IVTSFEPWA and IVTSFEPWG operators still satisfy Theorem 1, as well as properties 2–5. Moreover, if w 1 = w 2 = … = w n = 1 / n , then IVTSFEPWA and IVTSFEPWG operators reduce to IVTSFEPA and IVTSFEPG operators, respectively.
4 MCDM model based on interval-valued T-spherical fuzzy extended power aggregation operators and IVTSFCE
This section proposes a novel MCDM model using interval-valued T-spherical fuzzy extended power aggregation operators and IVTSFCE. First, with the aid of maximizing deviation approach and IVTSFCE, the criteria weights are determined. Moreover, IVTSFEPWA and IVTSFEPWG operators are used to obtain comprehensive evaluation results of alternatives. In the end, the ranking of alternative is determined according to their comprehensive evaluation results.
4.1 Problem description
Suppose that an IVTSF-MCDM problem involves m alternatives X = { x i | i = 1 , 2 , … , m } and n criteria C = { c j | j = 1 , 2 , … , n } . Let M = ( α i j ) m × n be a decision matrix given by DMs, where the IVTSFN α i j = ( [ μ i j L , μ i j U ] , [ η i j L , η i j U ] , [ v i j L , v i j U ] ) represents the evaluation of alternative x i under criterion c j . The decision matrix M can be shown as:
4.2 Determination of criteria weights by maximizing deviation approach and IVTSFCE
In this sub-section, motivated by the maximizing deviation approach (Farrokhizadeh et al. [ 21 ]), we develop a maximizing CE to obtain the criteria weights under interval-valued T-spherical fuzzy environments.
First, the CE between the alternative x i and other alternatives x k ( k = 1 , 2 , … , m , k ≠ i ) with respect to the criteria c j is calculated as follows:
Next, the overall CE of all alternatives concerning the criteria c j is calculated as follows:
The weighted CE function is then constructed as follows:
Then, the following optimization model is constructed to compute the optimal weight vector of criteria, as follows:
The Lagrange function is constructed to obtain the solution of the aforementioned model as follows:
The criteria weight vector after solving the Lagrange function is obtained as follows:
4.3 Aggregation of the evaluation results of alternatives by using interval-valued T-spherical fuzzy extended power aggregation operators
Calculate the support degrees of criteria values by the following equation:
Calculate the total support degrees of criteria values by the following equation:
Calculate the weight information of criteria values by the following equation:
Utilize IVTSFPWA and IVTSFPWG operators to aggregate the evaluation results of alternatives, as follows:
Calculate the score values of α i ∈ { α i A , α i G } ( i = 1 , 2 , … , m ) . In general, a higher score value of an alternative indicates that its performance is better.
4.4 Process of the proposed IVTSF-MCDM model
According to the former analysis, the procedure of the proposed IVTSF-MCDM model is as follows:
Stage 1. Collect the evaluation results given by DMs.
Step 1.1: Determine m alternatives { x i | i = 1 , 2 , … , m } and n criteria { c j ∣ j = 1 , 2 , … , n } .
Step 1.2: Ask DMs to provide their assessments of alternatives under criteria, and adopt IVTSFS to express these assessments; thus, obtain the interval-valued T-spherical fuzzy decision matrix M = ( α i j ) m × n .
Stage 2 . Determine criteria weights by maximizing deviation approach and IVTSFCE.
Step 2.1: Compute the overall CE of all alternatives under each criterion through equations ( 22 )–( 24 ).
Step 2.2: Construct optimization model with aid of equations ( 25 ) and ( 26 ).
Step 2.3: Calculate the weights of criteria according to equations ( 27 ) and ( 28 ).
Stage 3. Calculate the comprehensive evaluation results of alternatives using interval-valued T-spherical fuzzy extended power aggregation operators.
Step 3.1: Calculate the total support degrees of criteria values based on equations ( 29 ) and ( 30 ).
Step 3.2: Calculate the weight information of criteria values, see equation ( 31 ).
Step 3.3: Aggregate the criteria values of an alternative to obtain the comprehensive evaluation result of this alternative by utilizing IVTSFEPWA or IVTSFEPWG operator, see equations ( 32 ) and ( 33 ).
Step 3.4: Rank the comprehensive evaluation results of alternatives to obtain the order of them by using the ranking method of IVTSFN.
5 Case analysis
5.1 case description.
Comfort zone ( c 1 ), which indicates the economic condition and the degree of social stability of the investee country.
Government regulations ( c 2 ), which represents the stability and rationality of relevant policies and regulations of the investee country.
People’s interest ( c 3 ), which is the degree of fit between the interests of the people of the invested country and the investment project.
Market competition ( c 4 ), which refers to the market competition in the invested country.
5.2 Solving the aforementioned case by the proposed method
In this subsection, the proposed method is employed to select the optimal invest country.
Step 1.1–1.2 : The board members evaluate four countries according to the aforementioned four evaluation criteria, and the relevant evaluation results are expressed in IVTSFNs. Then, Table 1 shows the assessment results of board members.
Decision matrix
Step 2.1: According to equations ( 22 )–( 24 ), the overall CE of all alternatives under each criterion are computed as:
DE 1 = 0.2524 , DE 2 = 0.1726 , DE 3 = 0.1550 , DE 4 = 0.1504
Step 2.2–2.3: With the aid of equations ( 25 )–( 28 ), the weights of criteria are determined, which are as follows:
w 1 = 0.3456 , w 2 = 0.2363 , w 3 = 0.2122 , w 4 = 0.2059
Stage 3. Calculate the comprehensive evaluation results of alternatives by using interval-valued T-spherical fuzzy extended power aggregation operators.
Step 3.1: The total support degrees of criteria values are calculated based on equations ( 29 ) and ( 30 ), which are shown in Table 2 .
Total support degree
Step 3.2: Without losing generality, let ξ = 1 . Then, the weight information of criteria values is derived by using equation ( 31 ). The results are shown in Table 3 .
Weight information of criteria values
Step 3.3: Let q = 5 . Then, we utilize IVTSFEPWA or IVTSFEPWG operator to aggregate the evaluation results of alternatives.
If adopting the IVTSFEPWA operator, then we have
If adopting the IVTSFEPWG operator, then we have
Step 3.4: The score values of α 1 A , α 2 A , α 3 A , α 4 A , α 1 G , α 2 G , α 3 G , α 4 G are calculated by equation ( 2 ), respectively, such as sc ( α 1 A ) = 0.0649 , sc ( α 2 A ) = 0.0613 , sc ( α 3 A ) = 0.1471 , sc ( α 4 A ) = 0.0867 , sc ( α 1 G ) = 0.0235 , sc ( α 2 G ) = 0.0332 , sc ( α 3 G ) = 0.0454 , and sc ( α 4 G ) = 0.0089 .
Thus, according to the aforementioned score values, the order of alternatives is x 3 ≻ x 4 ≻ x 1 ≻ x 2 when adopting the IVTSFEPWA operator, whereas the order of alternatives is x 3 ≻ x 2 ≻ x 1 ≻ x 4 when adopting the IVTSFEPWG operator.
Obviously, The United Arab Emirates ( x 3 ) is the best country to invest in when adopting two aggregation operators. However, the order of other countries derived by the IVTSFEPWA operator is inconsistent with that derived by the IVTSFEPWG operator. The reason for this gap is their focus is different. The IVTSFEPWA operator emphasizes the overall level of alternatives, whereas the IVTSFEPWG operator pays attention to the strengths of alternatives in a single criterion.
5.3 Sensitivity analysis
According to the proposed method, different values of parameters ξ and q may cause different score values of alternatives, thus affecting the ranking result of alternatives. Therefore, this sub-section calculates the score values of countries with different values of ξ and q to validate their influence on the sorting results based on the aforementioned case.
The parameter ξ represents DMs’ preferences on extreme data. If the value of ξ is larger than zero, it means extreme data belonging to error or bias information. On the contrary, it implies that extreme data are very important when the value of ξ is smaller than zero. In case of q = 5 , the values of ξ are set from interval [ − 13 , − 4 ] ∪ [ 0 , 9 ] in increments of 1 for sensitivity analysis. Figure 1 shows the effect of different ξ values on the score values of countries.

Score values of countries with the different values of ξ : (a) score values of countries derived by the IVTSEFPWA operator under different ξ and (b) score values of countries derived by the IVTSFEPWG operator under different ξ .
As can be seen from Figure 1 , when using the IVTSFEPWA operator with different values of ξ , the sorting result of countries is still x 3 ≻ x 4 ≻ x 1 ≻ x 2 . Meanwhile, if employing the IVTSFEPWG operator with different values of ξ , the order of countries is x 3 ≻ x 2 ≻ x 1 ≻ x 4 . Therefore, we can conclude that parameter ξ does not affect the final decision result.
The parameter q reflects the space range of DMs’ beliefs about membership degree, abstinence degree, and non-membership degree. Generally, a larger value of q indicates the larger range of the space of DMs’ beliefs. In case of ξ = 1 , the values of parameter q are set from interval [ 1 , 20 ] in increments of 1 for calculating the score values of countries, which are shown in Figure 2 .

Score values of countries with the different values of q : (a) score values of countries derived by the IVTSFEPWA operator under different q and (b) score values of countries derived by the IVTSFEPWG operator under different q .
when q ∈ [ 1 , 3 ] and using the IVTSFEPWA operator, the sorting result is x 3 ≻ x 4 ≻ x 2 ≻ x 1 ;
when q ∈ [ 4 , 20 ] and using the IVTSFEPWA operator, the sorting result is x 3 ≻ x 4 ≻ x 1 ≻ x 2 ;
when q = 1 and using the IVTSFEPWG operator, the sorting result is x 3 ≻ x 4 ≻ x 1 ≻ x 2 ;
when q ∈ [ 2 , 20 ] and using the IVTSFEPWG operator, the sorting result is x 3 ≻ x 2 ≻ x 1 ≻ x 4 .
Thus, the change of the value of q will have an impact on the final sorting result, but it will not result in the change of the optimal solution.
5.4 Comparative analysis
The MCDM method-based IVTSFWA or IVTSFWG operator (namely the integrated operation-based method) [ 7 ]. This method adopts IVTSFWA and IVTSFWG operator to obtain the total evaluation result of each alternative. Then, the score values of total evaluation results are calculated to rank alternatives.
The IVTSF-MCDM method based on technique for order performance by similarity to ideal solution (TOPSIS) (namely, IVTSF-TOPSIS) [ 27 ]. This method depends on the relative closeness of alternatives to sort alternatives.
The IVTSF-MCDM method based on TODIM (an acronym in Portuguese of interactive and MCDM) (namely, IVTSF-TODIM) (Ju et al. [ 28 ]). This method relies on the dominance of an alternative over other alternatives to compute the overall prospect value of this alternative that is used to rank alternatives.
For ensuring the rationality of the comparison, all methods use the evaluation information and criteria weight in the aforementioned case. The comparison details and the ranking of countries obtained by the aforementioned three methods are shown in Table 4 and Figure 3 .
Results obtained by different methods

Sorting results of different methods.
Both IVTSFWA and IVTSFWG operators simply aggregate the evaluations of alternatives, which ignores the effects of extreme values on the final result. The IVTSFEPWA and IVTSFEPWG operator utilize the parameter ξ to effectively show the impact of extreme values on the ranking of alternatives. Therefore, the calculation results derived by the proposed method are more reasonable than that derived by IVTSFWA and IVTSFWG operators.
The ranking index of IVTSF-TOPSIS and IVTSF-TODIM differs from that of the proposed method. The IVTSF-TOPSIS adopts the relative closeness to rank alternatives, which focus on the utility values of alternatives through reference points. The IVTSF-TODIM employs the overall prospect value to sort alternatives, which depends on the preference, indifference, and incomparability relations among alternatives to determine the priorities of alternatives. However, both IVTSF-TOPSIS and IVTSF-TODIM do not consider too high or too low evaluation results may affect the performances of alternatives. Conversely, the proposed method uses the IVTSFEPWA and IVTSFEPWG operator to overcome this shortcoming of IVTSF-TOPSIS and IVTSF-TODIM. Thus, the proposed method can provide more realistic results by comparing with IVTSF-TOPSIS and IVTSF-TODIM.
According to the aforementioned comparative analysis, we can conclude that the proposed method has a main advantage that the impact of extreme data is effectively considered under interval-valued T-spherical fuzzy environment.
6 Conclusion
In view of most of the existing IVTSF-MCDM methods that neglect the impacts of extreme data, this study presents a novel MCDM method based on interval-valued T-spherical fuzzy extended power aggregation operator. With the aid of EPA and EPG operators, IVTSFEPA, IVTSFEPG, IVTSFEPWA, and IVTSFEPWG operators are defined to rank alternatives for MCDM problems. Moreover, IVTSFCE and IVTSFSCE are proposed to measure the difference between two IVTSFNs and are used to derive the weights of criteria in MCDM. Final, we prove that the proposed method can reflect the effect of too high or too low evaluation results on the ranking of alternatives by comparing with other IVTSF-MCDM methods.
There are several research directions for future studies. The maximizing deviation approach is used to calculate the objective weights of criteria, and it can be combined with subjective weighting methods to derive the importance of criteria in the future. In addition, the DMs’ irrational behaviors also can affect the order of alternatives. Therefore, the IVTSF-MCDM method considering DMs’ irrational behaviors is our next work.
Acknowledgments
The author thank the anonymous reviewers for their advice, which has led to an improvement of this article.
Funding information: The author states no funding invloved.
Author contributions: Lu Chen has performed the data analysis and the validation, and wrote the manuscript.
Conflict of interest: The author has no relevant financial or non-financial interests to disclose.
Data availability statement: No data associated in the manuscript.
[1] Zadeh LA. Fuzzy sets. Inf Control. 1965;8(3):338–53. 10.1016/S0019-9958(65)90241-X Search in Google Scholar
[2] Atanassov K. Intuitionistic fuzzy sets. Fuzzy Sets Syst. 1986;20(1):87–96. 10.1016/S0165-0114(86)80034-3 Search in Google Scholar
[3] Yager RR. Pythagorean membership grades, complex numbers and decision making. Int J Intell Syst. 2013;28(5):436–52. 10.1002/int.21584 Search in Google Scholar
[4] Yager RR, Alajlan N. Approximate reasoning with generalized orthopair fuzzy sets. Inf Fusion. 2017;3(8):65–73. 10.1016/j.inffus.2017.02.005 Search in Google Scholar
[5] Cuong BC. Picture fuzzy sets. J Comput Sci Cybern. 2013;30:409–20. Search in Google Scholar
[6] Mahmood T, Ullah K, Khan Q, Jan N. An approach toward decision-making and medical diagnosis problems using the concept of spherical fuzzy sets. Neural Comput Appl. 2019;31(11):7041–53. 10.1007/s00521-018-3521-2 Search in Google Scholar
[7] Ullah K, Hassan N, Mahmood T, Jan N, Hassan M. Evaluation of investment policy based on multi-attribute decision-making using interval valued T-spherical fuzzy aggregation operators. Symmetry. 2019;11(3):357. 10.3390/sym11030357 Search in Google Scholar
[8] Jin Y, Kousar Z, Ullah K, Mahmood T, Pehlivan NY, Ali Z. Approach to multi-attribute decision-making methods for performance evaluation process using interval-valued T-spherical fuzzy Hamacher aggregation information. Axioms. 2021;10(3):145. 10.3390/axioms10030145 Search in Google Scholar
[9] Ullah K, Garg H, Gul Z, Mahmood T, Khan Q, Ali Z. Interval valued T-spherical fuzzy information aggregation based on Dombi t-norm and Dombi t-conorm for multi-attribute decision making problems. Symmetry. 2021;13(6):1053. 10.3390/sym13061053 Search in Google Scholar
[10] Hussain A, Ullah K, Wang H, Bari M. Assessment of the business proposals using Frank aggregation operators based on interval-valued T-spherical fuzzy information. J Funct Spaces. 2022;28880340:1–24. 10.1155/2022/2880340 Search in Google Scholar
[11] Akram M, Ullah K, Pamucar D. Performance evaluation of solar energy cells using the interval-valued T-spherical fuzzy Bonferroni mean operators. Energies. 2022;15(1):292. 10.3390/en15010292 Search in Google Scholar
[12] Yang Z, Zhang T, Garg H, et al. A multi-criteria framework for addressing digitalization solutions of medical system under interval-valued T-spherical fuzzy information. Appl Soft Comput. 2022;130:109635. 10.1016/j.asoc.2022.109635 Search in Google Scholar
[13] Xu ZS, Yager RR. Power-geometric operators and their use in group decision making. IEEE Trans Fuzzy Syst. 2010;18(1):94–105. 10.1109/TFUZZ.2009.2036907 Search in Google Scholar
[14] Xu ZS. Approaches to multiple attribute group decision making based on intuitionistic fuzzy power aggregation operators. Knowl Syst. 2011;24(6):749–60. 10.1016/j.knosys.2011.01.011 Search in Google Scholar
[15] Wei GW, Lu M. Pythagorean fuzzy power aggregation operators in multiple attribute decision making. Int J Intell Syst. 2018;33(1):169–86. 10.1002/int.21946 Search in Google Scholar
[16] Ju YB, Luo C, Ma J, Wang AH. A novel multiple-attribute group decision-making method based on q-rung orthopair fuzzy generalized power weighted aggregation operators. Int J Intell Syst. 2019;34(9):2077–103. 10.1002/int.22132 Search in Google Scholar
[17] Liu PD, Khan Q, Mahmood T, Hassan N. T-spherical fuzzy power Muirhead mean operator based on novel operational laws and their application in multi-attribute group decision making. IEEE Access. 2019;7:22613–32. 10.1109/ACCESS.2019.2896107 Search in Google Scholar
[18] Zhang HY, Wei GW, Chen XD. Spherical fuzzy Dombi power Heronian mean aggregation operators for multiple attribute group decision-making. Comput Appl Math. 2022;41(3):98. 10.1007/s40314-022-01785-7 Search in Google Scholar
[19] Xiong SH, Chen ZS, Chang JP, Chin KS. On extended power average operators for decision-making: A case study in emergency response plan selection of civil aviation. Computers Ind Eng. 2019;130:258–71. 10.1016/j.cie.2019.02.027 Search in Google Scholar
[20] Xiong SH, Zhu CY, Chen ZS, Deveci M, Chiclana F, Skibniewski MJ. On extended power geometric operator for proportional hesitant fuzzy linguistic large-scale group decision-making. Inf Sci. 2023;632:637–63. 10.1016/j.ins.2023.03.001 Search in Google Scholar
[21] Farrokhizadeh E, Seyfi-Shishavan SA, Gündoğdu FK, Donyatalab Y, Kahraman C, Seifi SH. A spherical fuzzy methodology integrating maximizing deviation and TOPSIS methods. Eng Appl Artif Intell. 2021;101:104212. 10.1016/j.engappai.2021.104212 Search in Google Scholar
[22] Verma R. On intuitionistic fuzzy order-α divergence and entropy measures with MABAC method for multiple attribute group decision-making. J Intell Fuzzy Syst. 2021;40(1):1191–217. 10.3233/JIFS-201540 Search in Google Scholar
[23] Li LM, Zheng TT, Wu QY. Novel Pythagorean fuzzy entropy and Pythagorean fuzzy cross-entropy measures and their applications. J Intell Fuzzy Syst. 2021;41(6):6527–46. 10.3233/JIFS-210365 Search in Google Scholar
[24] Verma R. Multiple attribute group decision‐making based on order‐α divergence and entropy measures under q‐rung orthopair fuzzy environment. Int J Intell Syst. 2020;35(4):718–50. 10.1002/int.22223 Search in Google Scholar
[25] Wei GW. Picture fuzzy cross-entropy for multiple attribute decision making problems. J Bus Econ Manag. 2016;17(4):491–502. 10.3846/16111699.2016.1197147 Search in Google Scholar
[26] Yang W, Pang YF. T-Spherical fuzzy ORESTE method based on cross-entropy measures and its application in multiple attribute decision-making. Soft Comput. 2022;26(1):10371–87. 10.1007/s00500-022-07287-2 Search in Google Scholar
[27] Aydoğdu E, Güner E, Aldemir B, Aygün H. Complex spherical fuzzy TOPSIS based on entropy. Expert Syst Appl. 2023;215(1):119331. 10.1016/j.eswa.2022.119331 Search in Google Scholar
[28] Ju YB, Liang YY, Luo C, Dong PW, Santibanez EDR, Wang AH. T-spherical fuzzy TODIM method for multi-criteria group decision-making problem with incomplete weight information. Soft Comput. 2021;25(4):2981–3001. 10.1007/s00500-020-05357-x Search in Google Scholar
© 2024 the author(s), published by De Gruyter
This work is licensed under the Creative Commons Attribution 4.0 International License.
- X / Twitter
Supplementary Materials
Please login or register with De Gruyter to order this product.
Journal and Issue
Articles in the same issue.
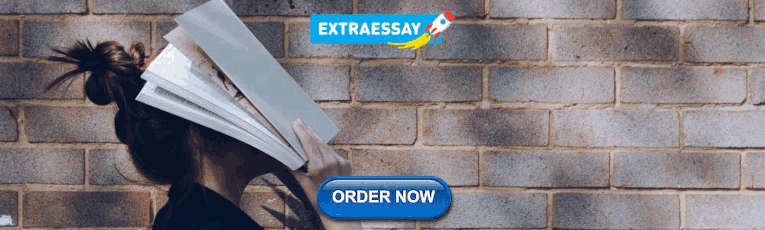
IMAGES
VIDEO
COMMENTS
Provide an in-depth analysis of each alternative based on the criteria chosen in step three. Decision tables using criteria as columns and alternatives as rows can be helpful. The pros and cons of the various choices as well as the short- and long-term implications of each may be evaluated. Best, worst, and most likely scenarios can also be ...
Organization of decision essays: 1. recommended decision (position statement) 2. decision options (alternatives to your decision) 3. decision criteria (explicit and relevant) 4. proof of recommended options (apply criteria to each option to show how good the fit is between your option and the information in the case) 5.
1.1 Definition of Decision Criteria. Decision criteria refer to the specific standards or benchmarks used to assess different alternatives during a decision-making process. These criteria can be quantitative or qualitative and are often tailored to the specific context or goals of the decision-making process.
Identify, prioritize, and deal with issues and problems; 2. Judge the quality and relevance of information — fact, opinion, hearsay, lies, and so on; 3. Make and assess necessary assumptions; 4. Relate the information to the issues, problems, and decisions in the case; 5. Resolve conflicting information;
The authors' decision to conduct case study research with qualitative methods was based on various reasons. ... Reliability and generalizability are the two main criteria for assessing a research study. ... Richardson J., Manroop L. (2011). Alternative paradigms and the study and practice of performance management and evaluation. Human ...
The following is a list of tips and strategies for writing the alternatives and decision section of a case study report: Your instructor may make the alternatives section of a case study report optional; however, if you can think of at least one reasonable and viable alternative in addition to your recommendation, then this section should be ...
Alternatives, Criteria, Analysis, Decision, Implementation); it can improve learning outcomes by helping students better solve and analyze business problems, make decisions, ... Customer Value—A Case Study," Back to the Future: Revisiting the Foundations of Marketing from Society for Marketing Advances, West Palm Beach, FL (November 2, 2018).
º Representativeness: After the case study is conducted it may be corroborated by a cross‐case test, which includes a general hypothesis (a new variable) based on the case‐study research. If the case is now an on‐lier, it may be considered representative of the new relationship. 5. Influential. º Definition: Cases (1 or more) with ...
Criteria for Case Scenario Analysis Define the problem: Students should focus on defining the problem by determining the root cause, not the underlying symptom(s). Develop reasonable alternatives: Students should develop three to four reasonable alternatives to deal with the problem. Most laws are written around the concept of what a reasonable ...
How to approach a case study 3.10. E. Evaluation of alternatives and selecting the preferred one ... Often you can set up a simple table with key decision criteria as columns and alternatives as rows, and write this section based on the table. Each alternative must be compared to each criteria and its suitability ranked in some way, such as met ...
Abstract. Establishing alternatives and criteria is essential to multi-criteria evaluation. In this, the first question is: what knowledge can be derived from theory and research about an optimum number of alternatives and criteria? Does the quality of decision-making improve in relation to the amount of information used to solve a policy problem?
Case Study Report Annotated Example: Alternatives and Decision Criteria. Use the arrows that appear in this window to navigate through the annotations. Read the corresponding highlighted text in the content box below. Click Begin Activity to start. There are two separate organizational structures that are consistent with the recommended ...
Alternatives and Decision Criteria. This section of the case study format addresses two key areas. The first one is alternatives and the second one is the decision criteria. As the name suggests, alternatives must mention all the potential ways the identified problem can be addressed.
We compare how several forms of multi-criteria decision analysis (MCDA) can enhance the practice of alternatives assessment (AA). We report on a workshop in which 12 practitioners from US corporations, government agencies, NGOs, and consulting organizations applied different MCDA techniques to three AA case studies to understand
Alternatives analysis is a method used in regulation and product design to identify, compare, and evaluate the safety and viability of potential substitutes for hazardous chemicals. Objectives: We assessed whether decision science may assist the alternatives analysis decision maker in comparing alternatives across a range of metrics. Methods: A ...
Step 2: Identify Decision-Making Criteria. The next step is to identify factors that must . be considered when generating alternatives. Decision-making criteria should include the needs and interests of relevant stakeholders as well as external environmental factors that may influence the feasibility of alternative solutions. Examples might
Here are the steps on how to write the Alternative Courses of Action for your case study: 1. Analyze the Results of Your SWOT Analysis. Using the SWOT analysis, consider how the firm can use its strengths and opportunities to address its weaknesses, mitigate threats, and eventually solve the case study's problem.
The CR is used to measure the quality of the judgments made by the members of the decision group, and it is considered that a CR of less than 0.10 is acceptable. If it is higher, the decision-maker should redo his estimates and make a new judgment. The CI and CR are estimated according to Eqs. 10.3 and 10.4.
This finding is consistent with previous studies (Rivett, 1977, Stewart, 1981), and it fulfilled expectations that insights about the structure of the multidimensional decision could be discussed without directly assigning preferences to the alternatives and criteria. As mentioned earlier, this is an often-overlooked area of the MCDA process.
IV. ALTERNATIVES AND DECISION CRITERIA. Alternatives. Carbon Capture and Storage Technology (CCS) is one of the innovative machines that can capture and store present Carbon Dioxide (CO2) in the atmosphere. However, a large amount of money is needed to have this CCS because this technology is expensive.
To conduct such a study, actual decisions were needed to get the researcher close to the phenomena of interest: the evaluation practices of strategic decision makers, the complexity of the task, and decision outcomes. A case study approach was applied to uncover what decision makers do to evaluate altematives, determine the diffi-
What are decision criteria? Decision criteria are the principles, values, rules, variables, and conditions that an organization or team uses to select an option or make a decision. These criteria guide teams in selecting a course of action among several alternatives. They improve the quality, rationality, and fairness of the team's decisions.
Business. Decision criteria that are used by businesses to make decisions such as which projects to invest in at a point in time. Brand. Budget. Business strategy. Capital. Company culture. Competitive advantage. Competitive landscape.
The best-worst method (BWM) is a relatively new multi-criterion decision-making (MCDM) technique that was developed to overcome some of the drawbacks of the analytic hierarchy process (AHP). Like its predecessor, BWM also relies on the strategy of decomposing complex decision problems into tractable sub-problems, while also providing a quantitative framework for synthesizing their results ...
The TOPSIS multi-criteria decision-making method was used to identify the most desirable food basket. The results of this study show that the VFB could be the most desirable alternative to the CIFB. Replacing the CIFB with the VFB causes a 10% and 21% lower water and carbon footprint, respectively.
As an effective tool to show the fuzziness of qualitative information, the interval-valued T-spherical fuzzy set can utilize three kinds of information, namely, membership, abstinence, and non-membership, to show the opinions of decision-maker. Given this advantage, many interval-valued T-spherical fuzzy multi-criteria decision-making (IVTSF-MCDM) methods have been designed. However, most of ...