- Open access
- Published: 21 June 2022
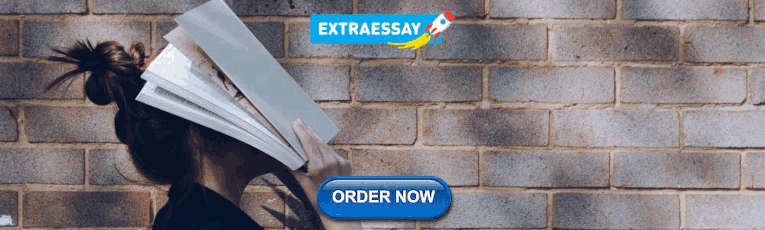
The impact of macroeconomic factors on food price inflation: an evidence from India
- Asharani Samal ORCID: orcid.org/0000-0001-8275-0267 1 ,
- Mallesh Ummalla 2 &
- Phanindra Goyari 1
Future Business Journal volume 8 , Article number: 15 ( 2022 ) Cite this article
15k Accesses
4 Citations
Metrics details
The present study investigates the impact of macroeconomic factors on food price inflation in India utilizing the monthly time series during January 2006–March 2019. The long-run relationship is confirmed among the variables using the ARDL bounds testing approach to cointegration. The coefficients of long-run estimates show that per capita income, money supply, global food prices, and agricultural wages are positively and significantly impacted food price inflation in both the short and long-run. While food grain availability has a negative and significant impact on food price inflation in both the short-run and long run. Further, the short-run estimates revealed that real exchange rate positively impacts food price inflation. However, the coefficient is insignificant in the short-run. The Granger causality estimates show that a short-run bidirectional causality is confirmed among per capita income, the exchange rate, per capita net availability of food grain and food price inflation. Further, there is evidence of unidirectional causality running from global food prices to food price inflation. However, there is no causal relationship running from money supply and agricultural wages to food price inflation in the short-run.
Introduction
The main objective of monetary policy in any economy is to maintain price stability. However, high food price inflation affects not only macroeconomic stability but also small farmers and poor consumers of the developing country where poor people spend their massive portion of income on food consumption. Agricultural commodity price volatility negatively impacts all societies by causing macroeconomic instability; specifically, it affects the impoverished that spend a large portion of their resources on food and fuel [ 47 ]. Therefore, high food price inflation has become a significant concern among the researchers and policymakers in determining responsible factors to surge in food price inflation. The high food price inflation has been experienced in the recent period due to increasing demand for biofuels in many developed countries, increasing demand for various diets among newly prosperous populations as compared to the production of such foodstuff, rise in minimum support prices, rapid regional economic growth, increasing the cost of fertilizers and other inputs, rising oil prices, etc.
Agriculture is very competitive in producing homogenous goods, given its vulnerability and high dependence on monsoon. It also contributes 17% of gross domestic product and employs more than 50% of the population. However, the contribution of the agricultural sector to GDP has been declining substantially since 2014, and the growth of agriculture is likely to increase by 2.1% in 2018–19 [ 23 ]. Further, price of agricultural products is more volatile than the non-agricultural sector due to high dependence on climate change. Therefore, attention should be given to the agricultural sector and the behavior of agriculture prices, especially for developing countries like India, where the majority of the population depends on agriculture. The persistent and high food price inflation over the period has gained more extensive attention in India by the researchers and policymakers as food price inflation has been the major contributor behind the increase in overall Wholesale Price Index (WPI) inflation in India [ 2 ]. Further, agricultural price is susceptible to relative changes in input prices, supply factors, etc.
Theoretically, rising food prices are basically due to two factors in the literature, i.e., real and monetary shocks. These are explained by structuralist and monetarist approaches, respectively. According to structuralists, the money supply is passive, and the real shocks in a particular sector tend to upsurge in food price inflation. Hence, inflation occurs in the prices of other goods. However, monetarists argued that inflation could arise through an autonomous increase in money supply via generating aggregate demand, which increases the relative price of commodities. Therefore, an increase in money supply is a cause for inflation, not necessarily by real shocks.
However, the developing countries like India are not exceptional from higher food prices and macro-economic instability. Since the 1991 economic reforms, the Indian economy has maintained a single-digit economic growth rate and moderate inflation. However, in recent years, one of the major problems that the Indian economy is facing is higher food price inflation. The WPI food price inflation was documented 10.20% during January 2008–July 2010 [ 33 ]. Further, CPI-IW for food was experienced at 8.05% during 2006–2019 while it was recorded at 13%, especially in 2013. However, the growth rate of gross food grain production was 2.66% during this period. The demand for food commodities increases at a higher rate due to the high economic growth rate (7–9%) per annum. In contrast, the annual growth of agriculture is relatively low (1.5%) compared to the service sector and GDP growth [ 40 ]. The total investment in agriculture has been reduced from 2.43 to 1.28% during 1979–80 to 2007–08 period [ 28 ]. The expenditure on subsidies, maintenance of existing projects, the relatively lower allocation for irrigation, rural infrastructure and research, lack of adequate credit support, and credit infrastructure in rural areas are the drivers of slow growth in public investment in agriculture [ 43 ]. Given this high food price inflation, researchers and policymakers have raised severe concern about reducing the food price inflation because most of the population spend half of the income on food expenditure, and food containing a larger share in the CPI basket. Therefore, it is necessary to find the causes and suitable majors to reduce food price inflation.
The present study contributes to food price inflation literature in several ways. First, a wide range of studies has investigated the drivers of food price inflation in India. The various demand and supply-side factors, namely, per capita income, growth of money supply, changing patterns of the consumer's dietary habits, high agricultural wages, speculations, and low growth of agricultural productions, are accountable for high food inflation. However, the results are ambiguous and vary considerably across countries due to different data periods and econometric methodologies applied in their studies. Second, the change in macroeconomic factors may have a substantial effect on food price inflation. For instance, if the food prices positively impact money supply, the consumer suffers from welfare loss. If it negatively effects on food prices, the producer suffers from welfare loss. However, this relationship of macroeconomic factors has not empirically analyzed significantly with respect to food price inflation in India. Third, various studies have explored the impact of macroeconomic factors on food price inflation across the world. For example, Kargbo [ 24 ] for Eastern and Southern Africa, Kargbo [ 25 ] for West Africa, Reziti [ 38 ] for Greek, Kargbo [ 26 ] for South Africa, Yu [ 46 ] for China and Sasmal [ 40 ]. Nevertheless, few studies have empirically examined the impact of selected macroeconomic factors on food price inflation by incorporating a control variable like per capita net availability of food grain into account. To the best of my knowledge, there is no study existing in the context of India. Fourth, most of the studies have taken WPI food indices, food items from only primary food articles or some of the index of selected food items, such as cereals and pulses, fruits and vegetables, milk and milk products, egg, meat, and fish as a measure of food price inflation. However, the present study has used the combined price index for industrial worker food (CPI-IW-F). Fifth, numerous studies have concluded that food price inflation is triggered by supply-side factors (see, [ 11 , 17 , 33 ]). However, to examine the rise in food price inflation, we have included both demand and supply-side factors in our study. Six, the present study also considered that food price inflation is not only influenced by domestic factors but also by global factors. More specifically, changes in global food prices and the exchange rate might positively and significantly impact food price inflation. However, the effect of these external factors on food price inflation does not explain the extent of food price inflation driven by domestic supply-side factors. For this purpose, we have included the per capita net availability of food grain as a control variable in the model. Suppose the demand for agricultural food items rises remarkably owing to a surge in macroeconomic factors. However, the supply of food items failed to meet the demand proportionately, then food items prices will go up. Therefore, the goal of the present study is to analyze the long-run and short-run impact of macroeconomic factors on food price inflation and verify the causal relationship aspect of these variables in the case of India over the period January 2006–March 2019.
The remaining of the paper is structured as follows. “ Literature review ” section follows the review of the literature on the relationship between macroeconomic factors and food price inflation. “ Methods ” section represents data and methodology. “ Results and discussion ” section discusses the results of the study. “ Conclusions ” section provides conclusive remarks and policy implications.
Literature review
This paper aims to examine the impact of macroeconomic factors on food price inflation. This section provides a review of the literature to establish the empirical basis of the link between macroeconomic factors and food price inflation.
Per capita income and food price inflation
Per capita income has a positive impact on food price inflation via increasing purchasing power of the money in the hands of the people, which leads to a surge in demand for food items resulting in a rise in food prices. Carrasco and Mukhopadhyay [ 10 ] argued that per capita income is positively affected food prices in three South Asian economies. However, the decline in agricultural production increases food prices up, and magnitudes are varying across countries. Agrawal and Kumarasamy [ 1 ] documented that food price inflation rose with the response to increases in India’s per capita income. They also suggested a 1% surge in per capita income upsurges the demand for fruits, vegetables, milk, and edible oil by 0.55–0.65%, and animal products by 0.38%. However, it reduces the demand for cereals and pulses by 0.05% and 0.20%, respectively. Joiya and Shahzad [ 22 ] and Sasmal [ 40 ] reported the same findings in Pakistan and India, respectively. In contrast, the study also found a negative association between food price inflation and per capita income. Kargbo [ 24 ] in Ethiopia and Malawi among Eastern and Southern African countries, Kargbo [ 25 ] for Cote d'Ivoire of West African countries.
Money supply and food price inflation
An increase in the money supply positively affects food price inflation through market credit facility by generating aggregate demand. However, it reduces food prices by creating investment via availing credit to the producer. Numerous studies have investigated the impact of money supply on food price inflation across the world. For example, Mellor and Dar [ 29 ] found that the expansion of money supply largely determines upward pressure on food grains prices. Barnett et al. [ 5 ] found that money supply positively affects food inflation and agricultural commodity prices in the U.S. Similar results were found by Kargbo [ 26 ] and Asfaha and Jooste [ 3 ] for South Africa. Further, Bhattacharya and Jain [ 8 ] concluded that an unexpected monetary tightening induces food price inflation in emerging and developed countries. However, a negative relationship is established between money supply and food prices for Kargbo [ 24 ], Kargbo [ 25 ], and Yu [ 46 ] for Eastern and Southern African countries and West African countries and China.
Exchange rate and food price inflation
The depreciation of the real exchange rate increases food price inflation by expanding the cost of importing petroleum products, fertilizer, and other finished products relating to agricultural commodities, leading to rising domestic market prices. In other words, depreciation of the exchange rate directly affects the agricultural sectors vi changing the prices of tradable and non-tradable goods resulting in a change in the prices of agricultural products in favor of the farmer. Taylor and Spriggs [ 45 ] showed that the exchange rate has a greater influence on volatility of agricultural prices in Canada. Similarly, Mitchell [ 30 ] and Mushtaq et al. [ 32 ] and Iddrisua and Alagidede [ 19 ] also concluded that the depreciation of the exchange rate is positively affected food prices in the U.S., Pakistan and South Africa, respectively. In contrast to this, Cho et al. [ 12 ] confirmed that change in the exchange rate has a negative impact on relative agricultural prices. However, Sasmal [ 40 ] found no significant relationship between the exchange rate and food price inflation in India during 1971–2012.
Global food price and food price inflation
The increase in the global food price of the commodity can influence the domestic price via international trade mechanism. The export increases as the global food price increases resulting in a decrease in domestic market supply followed by a hike in prices. On the contrary, the rise in import raises the domestic substitute food item’s price followed by a surge in domestic market price. Robles [ 39 ] indicated that the international prices transmission has a positive impact on the domestic agricultural market in Asian and Latin American countries. Gulati and Saini [ 16 ] revealed that the global food price index is positively impacted food price inflation in India. Similarly, Baltzer [ 4 ] states that an increase in international prices promotes domestic prices in the case of Brazil and South Africa. However, the price.
transmission is very limited in China and India. Lee and Park [ 27 ] confirmed that the lagged values of global food price inflation are positively impacted food price inflation in 72 countries. Selliah et al. [ 41 ] revealed that an increase in global food price increases domestic food prices in both the short and long run in Sri Lanka. Similarly, Holtemöller and Mallick [ 17 ], Bhattacharya and Sen Gupta [ 6 ], and Huria and Pathania [ 18 ] also documented that global food price shocks have a significant and positive inflationary trend on food inflation in India. However, Rajmal and Mishra [ 37 ] pointed out a limited transmission of prices from international food prices to domestic prices in India.
Agricultural wage and food price inflation
One of the significant public work programs is the National Rural Employment Guarantee (NREG), which promotes the real daily agricultural wage rates. On the one hand, an increase in rural wages can induce food prices by increasing production costs. On the other hand, it raises food prices via higher purchasing power, resulting in higher wages, which boosts the demand for food items. Gulati and Saini [ 16 ] revealed that domestic farm wages are positively associated with food price inflation in India. Goyal and Baikar [ 15 ] showed that the rapid increase in MGNREGA wages when it merged with inflation boosts agricultural wages rather than the implementation of MGNREGA across India. Bhattacharya and Sen Gupta [ 6 , 7 ] examined the drivers of food price inflation in India over the period 2006–2013. The structural vector error correction model (SVECM) showed that agricultural wage inflation promotes food price inflation after the implementation of MNGREGS.
The above literature review shows that both demand and supply-side factors have contributed to food price inflation. Many studies have investigated the impact of macroeconomic factors on food price inflation across the globe. However, only a few studies have been directed, which empirically examined the effect of macroeconomic factors on food price inflation by incorporating per capita net availability of food grain and agricultural wages in a multivariate framework. So far as we know, there is no study available in the case of India in this regard employing monthly data over the period January 2006–March 2019. Hence, our study attempts to fill this gap.
The present study makes use of monthly time series data on per capita GDP (Y), real exchange rate (EX), money supply (M3), global food price index (GF), per capita net availability of food grain (NFG), agricultural wages (AW) and combined price index-industrial workers for food (CPI-IW-F) indices as a proxy for food price index (FP) during January 2006–March 2019. The data on per capita GDP, real exchange rate, money supply is collected from the Reserve Bank of India (RBI), whereas combined price index-industrial workers for food and agricultural wages are retrieved from the Ministry of Labor Bureau, Government of India. The data on per capita net availability of food grain and real global food price index are obtained from the Directorate of Economics and Statistics, Department of Agriculture & Farmers Welfare, Government of India, and the Food and Agriculture Organization of the United Nations, respectively. Monthly data on per capita net availability of food grain and per capita GDP is not available in the case of India. Therefore, we have used the linear interpolation method to get the monthly data for this variable. Footnote 1 The selection of the data period has been considered based on the availability of uniform and consistent monthly data over a period of time. We use high-frequency data while working on macroeconomic variables to capture the true impact of it [ 31 ]. Further, data on food price inflation is volatile, measuring the impact of macroeconomic factors on food inflation using high-frequency data, namely, weekly and monthly, provides accurate estimates rather than annual series. Since, data on a targeted variable i.e., food inflation is not available on a weekly basis for a longer period in the case of India. Therefore, we have used monthly data for this purpose.
The real exchange rate (EX) is measured as the real effective exchange rate which is trade based weighted average value of Indian currency against 36-currency bilateral weights, per capita income (Y) is measured as the percentage change in per capita gross domestic product, money supply (MS) is measured as broad money (MS), global food prices (GF) are measured as a real global food price index, and agricultural wages (AW) is measured as average daily wage rates from agricultural occupations; per capita net availability of food grain (NFG) is measured as gross production plus net imports plus stocks. Finally, food price inflation (FP) is measured as combined price index-industrial workers for food index (CPI-IW-F). The food inflation was experienced in India from 2006 onwards. However, the CPI-combined series is used and available from 2014 onwards to measure the official inflation rate. To get a more extended frequency of data on food inflation series, we have used consumer price index-industrial workers for food (CPI-IW-F) as a proxy for food inflation measures. We select to use CPI-IW-F because Bicchal and Durai [ 9 ] and Goyal [ 14 ] established that CPI-IW and CPI-combined have similar properties with CPI-IW is available for a more extended period. All the variables are seasonally adjusted using CENSUS X13 and converted into the natural logarithm form except per capita GDP.
Unit root tests
One should necessarily check the properties of all the variables before commencing any econometric techniques as it gives spurious and invalid results. The ARDL technique requires to check the integration properties of the selected variables to confirm that none of the variables should follow I (2) process, which seems to be invalid and unsuitable for applying the ARDL approach. Therefore, we use the ADF and PP tests to check the order of integration of the variables.
ARDL bounds testing approach to cointegration
We employ the ARDL bounds testing approach to cointegration propounded by Pesaran and Shin [ 35 ] and Pesaran et al. [ 36 ] in order to examine the long-run and short-run association between macroeconomic factors and food price inflation in India. This method is superior over other traditional approaches of Johansen and Juselius [ 21 ] and Johansen [ 20 ] cointegration on the following grounds. First, it is one of the most popular and flexible methods. It does not impose any restriction on any nature of data and can be applied irrespective of all the order of integration I (1) or I (0) or both mix. Second, as noted by Pesaran and Shin [ 35 ] that ARDL estimators give the true parameters, and coefficients are super consistent as compared to other long-run estimates, especially when the sample size is small. Third, it also helps to eradicate the problem of the endogeneity that appears in the model. Fourth, it is even able to capture both short-run and long-run estimates simultaneously. The unrestricted error correction models (UECM) of the ARDL model can be represented as follows:
where ∆ denotes first difference operator; \({\varepsilon}_{t}\) is the error term; \({\alpha }_{1}\) , \({\alpha }_{2}\) , \({\alpha }_{3}\) , \({\alpha }_{4}\) , \({\alpha }_{5}\) , \({\alpha }_{6}\) , and \({\alpha }_{7}\) are the constant; \({\alpha }_{F}\) , \({\alpha }_{Y}\) , \({\alpha }_{M}\) , \({\alpha }_{E}\) , \({\alpha }_{G}\) , \({\alpha }_{NF}\) , and \({\alpha }_{A}\) are the long-run coefficients; \({\beta }_{h}\) , \({\beta }_{i}\) , \({\beta }_{j}\) , \({\beta }_{k},{\beta }_{l}, {\beta }_{m}\) and \({\beta }_{n}\) are the short-run coefficients.
The optimal lag selection has been made based on the Akaike Information Criteria (AIC). The primary step in the ARDL model is to estimate the Eqs. ( 1 – 7 ) by ordinary least squares (OLS). The long-run relationship is determined based on the F test or Wald test for the coefficient of the lagged levels of the variables. The null hypothesis of no long-run relationship, \({H}_{0}:{\alpha }_{F}={\alpha }_{Y}={\alpha }_{M}={\alpha }_{E}={\alpha }_{G}={\alpha }_{NF}={\alpha }_{A}=0\) against the alternative hypothesis of the long-run, \({H}_{1}:{\alpha }_{F}\ne {\alpha }_{Y}\ne {\alpha }_{M}\ne {\alpha }_{E}\ne {\alpha }_{G}\ne {\alpha }_{NF}\ne {\alpha }_{A}=0\) referred to the equation follows as (FP/Y, MS, EX, GF, NFG, AW). According to Pesaran et al. [ 37 ], the null hypothesis of no long-run association can be rejected if F -statistics is greater than the upper critical bound (UCB). It suggests that there is a long-run association among the variables. While, test statistics falls below the lower critical bound (LCB), null hypothesis cannot be rejected. It suggests that there is no long-run association among the variables. If the calculated value falls between the lower and upper critical points, the result is inconclusive. Because the two asymptotic critical values bound lower value (assuming the regressors are I (0)) and upper (assuming purely I (1) regressors) provide a test for cointegration.
After identifying the long-run relationship among the variables, our next step is to apply the vector error correction model to examine the directions of causality among the variables in both the short-run and long-run. The model of VECM can be written as follows.
where \(\Delta\) is the difference operator; \({ECM}_{t-1}\) is the lagged error correction term, which is derived from the long-run cointegration relationship; \({\varepsilon}_{1t}\) , \({\varepsilon}_{2t}\) , \({\varepsilon}_{3t}, {{\varepsilon}_{4t}, \varepsilon}_{5t}, {\varepsilon}_{6t}\) and \({\varepsilon}_{7t}\) are the random errors; \({\gamma }_{1}\) , \({\gamma }_{2}\) , \({\gamma }_{3, }{\gamma }_{4, }{\gamma }_{5, }{ \gamma }_{6}\) and \({\gamma }_{7}\) are the speed of adjustments. The long-run relationship among the variables indicates that there is a presence of Granger-causality at least one direction, which is determined by F -statistics and lagged error correction term. The short-run causal relationship is represented by F- statistics on the explanatory variables while long-run causal relationship is represented by t-statistics on the coefficient of the lagged error correction term. The error correction term ( \({ECT}_{t-1}\) ) is negative and statistically significant (t-statistic) at the 1% significance level.
Results and discussion
Preliminary analysis.
A preliminary analysis is conducted using commonly used descriptive statistics. We also reported the summary of descriptive statistics of all the considered variables during the study period in Table 1 . The results revealed that the average food price index and the real exchange rate is 5.375% and 4.687% during the study period. However, the average money supply and real global food price index is 11.185% and 4.619%. The per capita net availability of food grain and agricultural wages is 5.130% and 6.931% whereas, per capita income is 0.445%, which is lower than other variables across the sample period. The results of the correlation matrix are represented in Table 2 . The correlation analysis results revealed that per capita income, money supply, real exchange rate, real global food price index, per capita net availability of food grain and agricultural wages are positively associated with food price inflation. For instance, food price inflation is highly correlated with per capita income, money supply, real exchange rate, per capita net availability of food grain, and agricultural wages. It suggests that macroeconomic factors might be promoting food price inflation in India. Similarly, per capita income is positively correlated with money supply, exchange rate, and per capita net availability of food grain, and agricultural wages. Further, there is a high positive correlation between agricultural wages and per capita net availability of food grain.
Results of unit root tests
To avoid spurious and invalid results of all the non-stationary data, we have checked the integration properties of all the variables and confirm that none of the variables follows the I (2) process. Therefore, the ADF and PP unit root tests are used to check the order of integrations of the variables. The results of unit root tests are reported in Table 3 . It indicates that food price inflation (FP), per capita income (Y), money supply (MS), real exchange rate (EX), real global food price index (GF), per capita net availability of food grain (FG) and agricultural wages (AW) are integrated of order I (1).
Results of ARDL cointegration tests
The above unit root test results show that all variables follow a same order of integration, i.e., I (1). Therefore, we apply the ARDL technique to check the long-run relationship among the variables using Eqs. ( 1 )-( 7 ) during January 2006–March 2019. Here, the optimal lag length is 2, according to VAR lag order selection criteria. The results of the ARDL model are presented in Table 4 . The result shows that calculated F -statistics (4.155) is larger than UCB at the 5% level of significance when food price inflation is considered a dependent variable (FP/Y, MS, EX, GF, NFG, WA). It indicates that there is a long-run relationship among food price inflation (FP) and per capita income (Y), money supply (MS), real exchange rate (EX)), global food prices (GF), per capita net availability of food grain (NFG), and agricultural wages (WA). Likewise, calculated F- statistics (11.043) is also larger than UCB at the 5% level of significance when per capita income is considered a dependent variable and integrated order (1). Therefore, UCB is applied to establish a long-run relationship among the variables. Likewise, calculated F- statistics (10.239) is also larger than UCB at the 5% level of significance when money supply (MS) is considered a dependent variable. Similarly, calculated F- statistics (3.335) is also larger than UCB at the 10% level of significance when global food price (GF) is considered a dependent variable. However, calculated F -statistics is lower than UCB when the exchange rate (EX), per capita net availability of food grain (NFG), and agricultural wages (AW) serve as dependent variables. It suggests a there is no long-run relationship among the variables when the exchange rate, per capita net availability of food grain and agricultural wages are the dependent variables.
Results of long-run and short-run estimates
The cointegration test results based on the ARDL model revealed the long-run equilibrium relationship among the variables. However, these results do not explain the cause-and-effect association among the food price inflation and macroeconomic factors, namely, per capita income, money supply, exchange rate, global food prices, per capita net availability of food grains, and agricultural wages. Hence, we have investigated the impact of macroeconomic factors on food price inflation in this part. It is better to check the long-run effect of macroeconomic factors on food price inflation after confirming the cointegration relationship among the variables when food price inflation is considered the dependent variable. The results of the long-run analysis are reported in Table 5 in panel-I. The long-run results illustrate that per capita income is positively and significantly impacted food price inflation. It implies that a one unit increase in per capita income induces food price inflation by 0.14 unit. The rise in per capita income raises the purchasing power of the money, which leads to a surge in demand for food items resulting in a hike in food prices. The results of our study similar to Carrasco and Mukhopadhyay [ 10 ] in three South Asian economies, Agrawal and Kumarasamy [ 1 ] in India, Joiya and Shahzad [ 22 ] in Pakistan and Sasmal [ 40 ] in India. However, our result is inconsistent with Kargbo [ 24 ] and Kargbo [ 25 ], who revealed a negative relation between the variables in Ethiopia and Malawi, and in Cote d'Ivoire, respectively.
Similarly, a 1% increase in money supply increases food price inflation by 0.36%. It implies that the rise in money supply puts upward pressure on food price inflation and is significant at the 1% level of significance. Money supply is positively affecting food price inflation by generating aggregate demand in the market, which pushes the food prices up. This finding is consistent with Kargbo [ 24 ] for Kenya, Sudan, and Tanzania among the Eastern and Southern African countries and contradictory with Sasmal [ 40 ] who did not find any long-run relationship between money supply and food price inflation in India and Yu [ 46 ] for China who confirmed that monetary policy expansion has a negative impact on prices of seven major food products in the long-run. Similarly, a rise in the real exchange rate has a downward pressure on food price inflation. It indicates that a 1% increase in the real exchange rate will have a negative impact on food price inflation by 0.30%. The increase in the real exchange rate reduces food prices by lowering the import of petroleum products, fertilizer, and other products relating to agricultural commodities in the long run. Hence, organic fertilizers can be used to produce commercial food products to reduce the dependency on fertilizers, which may maintain price stability and reduce the negative welfare impact on food prices. This outcome is consistent with Cho et al. [ 12 ] and is inconsistent with Iddrisua and Alagidede [ 19 ] in South Africa, Durevall et al. [ 13 ] in Ethiopia. Further, per capita net availability of food grain has a negative impact on food price inflation. In other words, there is an inverse relationship between per capita net availability of food grains and food price inflation in India. It suggests that a 1% increase in per capita net availability of food grains reduces food price inflation by 0.69%. The increase in the supply of net food availability in the domestic market by increasing food production can reduce food price inflation. On the other hand, if the supply of food grain availability declines due to crop failure, it increases food price inflation. Therefore, the government should increase domestic food production and reduce the exports of commodities at the time of food inflation to maintain stability in prices. Further, agricultural production is seasonal, and it is highly correlated to the month of food harvest. The stock of food grain during harvest season can avoid the off seasonal food price inflation. Increasing the stock of food items by establishing a larger cold storage system and strengthen and widening the existing warehouses can also help to control food inflation in India. This result is similar to Kargbo [ 25 ] in Cote d’Ivoire and Nigeria and Carrasco and Mukhopadhyay [ 10 ] in three South Asian economies and inconsistent with Sasmal [ 40 ], who failed to establish a significant relationship between agricultural food production and food price inflation in India in the long-run. Furthermore, our results revealed that food price inflation rose with the response to increases in global food prices. It suggests that a 1% surge in global food price upsurges food price inflation by 0.13%. This result is consistent with Selliah et al. [ 41 ] for Sri Lanka, Holtemöller and Mallick [ 17 ] for India, and Huria and Pathania [ 18 ] for India. However, Rajmal and Mishra [ 37 ] and Baltzer [ 4 ] pointed out a limited transmission of prices from international food prices to domestic prices in India. The extent of transmission of global food prices on price hike in the domestic market depends on at which magnitudes commodity’s international trade takes place. Finally, our study results also found that agricultural wages have a positive and significant impact on food price inflation at the 1% level of significance. It implies that a 1% surge in agricultural wages increases the food price inflation by 0.31% in the long-run. The rise in wage rate via welfare-oriented-schemes like MNGREGS increases the bargaining and purchasing power of money, increasing in demand for food items followed by increased food inflation. The increase in the agricultural wage rate should be substituted with food price inflation by increasing productivity. Hence, the increase in demand for food originated by a hike in the agricultural wage rate can be substituted by raising the productivity of each worker. A similar result is found by Bhattacharya and Sen Gupta [ 7 ] for India.
After having discussed long-run results, we shall move in to discuss with reference to the short-run. The results of the short-run analysis are reported in Table 5 in panel-II. The short-run analysis indicates an increase in per capita income and money supply is positively related to food price inflation in the short-run as the coefficients of these variables are statistically significant. Similarly, food price inflation rises with the increase in global food prices. Further, the real exchange rate has a positive impact on food price inflation in the short-run. However, the result is not significant. Moreover, agricultural wages have a positive impact on food price inflation. It implies that an increase in agricultural wages raises food price inflation in the short run. The outcome is consistent with Huria and Pathania [ 18 ] for India. However, per capita net availability of food grain is negatively and significantly impacted food price inflation. It suggests that a 1% increase in food grain availability decreases food price inflation by 0.11% in the short-run. In contrast, a decrease in the growth rate of food grain availability increases food price inflation. This finding is similar to Kargbo [ 25 ] in Cote d’Ivoire and Senegal among West African countries. Finally, the results also documented lagged food price inflation positively impacts present food price inflation. It suggests that a 1% increase in lagged food price upsurges food price inflation by 0.36% in the short run.
The sign of lagged ECT is negative and significant at the 1% level, which implies that short-run deviation from food prices can be restored toward the long-run equilibrium with a 16.8% speed. The model has satisfied all the diagnostic tests. This model is free from autoregressive conditional heteroscedasticity; the functional form of the model is well specified, which is represented by the Ramsey RESET coefficient.
Results of VECM Granger causality test
After identifying the long-run association between macroeconomic factors and food price inflation, we have employed the VECM Granger causality test to examine the directions of causality among the variables in both the short-run and long-run. The Granger causality results are represented in Table 6 . The outcomes of the short-run causality tests are obtained from the F -statistics of lagged independent variables, while the results of long-run causality are obtained from the negative and significant coefficients of t-statistics of lagged error correction term. The results are reported in Table 6 and show that a short-run bidirectional causality is confirmed between per capita income, exchange rate, and food price inflation at a 1% level. This finding is opposite of Sasmal [ 40 ], who reported a unidirectional causality running from per capita income to food price inflation in India. Similarly, a bidirectional causality is existed between percapita net availability of food grain and food price inflation in the short run. It implies that the increase in food grain availability reduces food price inflation by increasing domestic food grain production on the one hand. Whereas on the other hand, an increase in food price inflation also leads to a rise in food grain availability by rising demand for food products. Further, there is a unidirectional causality running from global food prices to food price inflation. It suggests that an increase in global price attracts exporters to increase their supply of food items to the global market to get high profit, which eventually decreases the domestic market supply, resulting an in a price increase. However, there is no causal relationship running from money supply and agricultural wages to food price inflation in the short run.
There is an existence of a bidirectional causal relationship between global food prices and per capita income. However, no causality runs from money supply, exchange rate, per capita net availability of food grain, and agricultural wages to per capita income. A short run unidirectional causality is established from food price inflation, exchange rate, global food prices, and per capita net availability of food grain to money supply. A bidirectional causality has existed between agricultural wages and money supply in the short run. Further, unidirectional causality is running from per capita income, the exchange rate to global food prices. A unidirectional causal relationship is found from per capita income, money supply, the exchange rate, and global food prices to per capita net availability of food grain in the short run. Moreover, short-run unidirectional causality is confirmed from food price inflation, per capita income, the exchange rate and global food prices to agricultural wages.
Moving to the long-run causality, the coefficients of \({ECM}_{t-1}\) are negative and statistically significant in Eq. ( 8 ), where money supply, global food prices, and per capita net availability of food grain are the dependent variables. Therefore, results revealed a bidirectional causality among the money supply, global food prices, and per capita net availability of food grain production in the long-run.
Conclusions
This study aimed to examine the impact of macroeconomic factors on food price inflation in India during January 2006–March 2019. To consider the short-run dynamics and the long-run analysis and directions of causality among the variables, we have applied the ARDL model and Granger causality test in our study. The ARDL results have shown evidence of the long-run association among the macroeconomic factors and food price inflation. The long-run result show that percapita income, money supply, global food price, and agricultural wages have a positive and significant impact on food price inflation of India in both the long-run and short run. However, the per capita net availability of food grain negatively impacts food price inflation. It implies that an increase in food grain availability reduces food price inflation in both the short and long run. Further, the real exchange rate is positively affecting food price inflation. However, it is insignificant in the short-run. The Granger causality estimates show that a short-run bidirectional causality is confirmed among per capita income, the exchange rate, per capita net availability of food grain, and food price inflation. Further, there is evidence of unidirectional causality running from global food prices to food price inflation. However, there is no causal relationship running from money supply and agricultural wages to food price inflation in the short run. The long-run results revealed a bidirectional causality among the money supply, global food prices and per capita net availability of food grain.
Given these results, the paper makes important contribution to the macroeconomic factors and food price inflation in India. The significant policy suggestions are that the growth in money supply promotes food price inflation in the long-run, which affects the welfare of the poor consumer as the majority of the people depend on agriculture. It also positively affects market credit facility by generating aggregate demand followed by changes in relative prices across commodities, which push the food prices up. Therefore, the government should adopt effective policy measures to protect consumers from higher food prices. These are the effectively implementation of public distribution systems, policies for food security, and reducing the money supply via adopting a contractionary monetary policy, which eventually reduces food price inflation by reducing demand for food items. Further, the increase in global food inflation triggers food price inflation by international trade channels. However, the influence of global food inflation on food price inflation can be moderated by introducing a flexible tariff structure. Hence, the government should introduce stable and liberal trade policies that reduce food price inflation without compromising farmers remuneration values.
Moreover, our result also revealed that an increase in the net availability of food grain reduces food price inflation in both the short and long run. Therefore, the government should take necessary steps in favor of an increase in domestic food production. The high yielding variety (HYV) seeds, easily accessible credit facilities should be available to the farmer, increasing the domestic agricultural food production, thereby reducing the import of agricultural goods through the exchange rate and their adverse impact on food inflation. The stock of food grain during harvest season can avoid off seasonal food inflation. The increasing the stock of food items by establishing an extensive cold storage system and strengthening large warehouses can control food inflation in India. Furthermore, the rise in agricultural wages boosts food price inflation. The increase in the agricultural wage rate should be substituted with food price inflation by increasing labor productivity. Hence, the increase in demand originated by a hike in the agricultural wage rate can be substituted by raising each workers productivity.
Our results also found that per capita income Granger causes food price inflation both in the short-run. In this respect, we can say that there is a huge sectoral imbalance among the sectors. The government should be more focused on the agricultural sector and its growth and productivity by allocating massive funds in the irrigation, agricultural research, and innovation of modern technology and its adaptation in agriculture instead of spending on the name of social security and welfare of the poor. Therefore, balanced and sustainable growth and stability can be achieved for a developing country like India. The real exchange rate and food price inflation Granger causes to each other. The depreciation of the real exchange rate increases the food price inflation via expanding the import of petroleum products, fertilizer, and other finished products relating to agricultural commodities, which are very expensive. The increasing import of these products promotes food price inflation by raising domestic prices. Hence, to reduce the food price inflation, the government should increase the domestic agricultural production to meet our demand for food items rather than importing from other countries.
Availability of data and materials
Data used in the study are available in the Reserve Bank of India, Ministry of Labor Bureau, Directorate of Economics and Statistics, Department of Agriculture & Farmers Welfare, Government of India, and the Food and Agriculture Organization of the United Nations.
By using the linear interpolation method, we have converted the annual data into the monthly time series data. Because the high-frequency data increases the power of a statistical test and provide robust results [ 48 ]. The interpolation method has been widely used in the empirical analysis [ 34 , 42 , 44 ].
Abbreviations
Autoregressive distributed lag
Combined price index
Wholesale price index
Reserve Bank of India
Agrawal P, Kumarasamy D (2014) Food price inflation in India: causes and cures. Indian Econ Rev 49(1):57–84
Google Scholar
Anand R, Ding D, Tulin V (2014) Food inflation in India: the role of monetary policy. IMF working paper-14/178
Asfaha TA, Jooste A (2007) The effect of monetary changes on relative agricultural prices. Agrekon 46(4):460–474
Article Google Scholar
Baltzer K (2013) International to domestic price transmission in fourteen developing countries during the 2007–08. [In] Food crisis food price policy in an era of market instability. Oxford University Press, Oxford
Barnett RC, Bessler DA, Thompson RL (1983) The money supply and nominal agricultural prices. Am J Agric Econ 65(2):303–307
Bhattacharya R, Sen Gupta A (2017) What role did rising demand play in driving food prices up? South Asian J Macroecon Public Finance 6(1):59–81
Bhattacharya R, Sen Gupta A (2018) Drivers and impact of food inflation in India. Macroecon Finance Emerg Mark Econ 11(2):146–168
Bhattacharya R, Jain R (2020) Can monetary policy stabilise food inflation? Evidence from advanced and emerging economies. Econ Model 89:122–141
Bicchal M, Durai SRS (2019) Rationality of inflation expectations: an interpretation of Google Trends data. Macroecon Finance Emerg Mark Econ 12(3):229–239
Carrasco B, Mukhopadhyay H (2012) Food price escalation in South Asia. Econ Polit Wkly 47(46):59–70
Chand R (2010) Understanding the nature and causes of food inflation. Econ Polit Wkly 9XLV(9):10–13
Cho G, Kim M, Koo WW (2005) Macro effects on agricultural prices in different time horizons. Meeting of the American Agricultural Economics Association, Providence, Rhode Island
Durevall D, Loening J, Birru Y (2013) Inflation dynamics and food prices in Ethiopia. J Dev Econ 104:89–106
Goyal A (2015) Understanding high inflation trend in India. South Asian J Macroecon Public Finance 4(1):1–42
Goyal A, Baikar AK (2015) Psychology, cyclicality or social programmes: Rural wage and inflation dynamics in India. Econ Pol Wkly 50:116–125
Gulati A, Saini S (2013) Taming food inflation in India. Commission for Agricultural Cost and Prices, Discussion paper 4. Ministry of Agriculture, Government of India
Holtemöller O, Mallick S (2016) Global food prices and monetary policy in an emerging market economy: the case of India. J Asian Econ 46:56–70
Huria S, Pathania K (2018) Dynamics of food inflation: assessing the role of intermediaries. Glob Bus Rev 19(5):1363–1378
Iddrisu AA, Alagidede IA (2020) Monetary policy and food inflation in South Africa: A quantile regression analysis. Food Policy 91: 101816.
Johansen S (1991) Estimation and hypothesis testing of cointegration vectors in Gaussian vector autoregressive models. Econometrica 59(6):1551–1580
Johansen S, Juselius K (1990) Maximum likelihood estimation and inference on cointegration with applications to the demand for money. Oxf Bull Econ Stat 52(2):169–210
Joiya S, Shahzad A (2013) Determinants of high food prices: the case of Pakistan. Pak Econ Soc Rev 51(1):93–107
Kapoor A (2018) Competitiveness in agriculture will boost other sectors, Business standard, June 05, retrieved from https://www.business-standard.com/article/news-ians/competitiveness-in-agriculture-will-boost-other-sectors-column-active-voice-118060500301_1.html
Kargbo JM (2000) Impacts of monetary and macroeconomic factors on food prices in eastern and Southern Africa. Appl Econ 32(11):1373–1389
Kargbo JM (2005) Impacts of monetary and macroeconomic factors on food prices in West Africa. Agrekon 44(2):205–224
Kargbo JM (2007) The effects of macroeconomic factors on South African agriculture. Appl Econ 39(17):2211–2230
Lee HH, Park C Y (2013) International transmission of food prices and volatilities: a panel analysis. Asian Development Bank economics working paper series (373). https://doi.org/10.2139/ssrn.2323056
Mani H, Bhalachandran G, Pandit VN (2011) Public investment in agriculture and GDP growth: Another look at the intersectoral linkages and policy implications. Working paper no. 201, Centre for Development Economics, Delhi School of Economics
Mellor JW, Dar AK (1968) Determinants and development implications of food grains prices in India, 1949–1964. Am J Agric Econ 50(4):962–974
Mitchell D (2008) A note on rising food prices. World Bank policy research working paper series no. 4682
Modugno M (2013) Now-casting inflation using high frequency data. Int J Forecast 29(4):664–675
Mushtaq K, Awan DA, Abedullah A, Ahmad F (2011) Impact of monetary and macroeconomic factors on wheat prices in Pakistan: implications for food security. Lahore J Econ 16(1):95–110
Nair S, Eapen L (2012) Food price inflation in India (2008 to 2010). Econ Polit Wkly 47(20):46–54
Paramati SR, Bhattacharya M, Ozturk I, Zakari A (2018) Determinants of energy demand in African frontier market economies: an empirical investigation. Energy 148:123–133
Pesaran MH, Shin Y (1999) An autoregressive distributed lag modelling approach to cointegration analysis. In: Strom S (ed) Econometrics and economic theory in 20th century: the Ragnar Frisch centennial symposium, Chapter 11. Cambridge University Press, Cambridge
Pesaran MH, Shin Y, Smith RJ (2001) Bounds testing approaches to the analysis of level relationships. J Appl Economet 16(3):289–326
Rajmal, Mishra S (2009) Transmission of international food prices to domestic food prices The Indian evidence. RBI staff studies, Department of Economic Analysis and Policy, S S (DEAP):6/2009
Reziti I (2005) The relationship between macroeconomic variables and relative price variability in Greek Agriculture. Int Adv Econ Res 11(1):111–119
Robles M (2011) Price transmission from international agricultural commodity markets to domestic food prices: case studies in Asia and Latin America. IFPRI, Washington, DC
Sasmal J (2015) Food price inflation in India: the growing economy with sluggish agriculture. J Econ Finance Admin Sci 20(38):30–40
Selliah S, Applanaidu SD, Hassan S (2015) Transmission of global food prices to domestic prices: evidence from Sri Lanka. Asian Soc Sci 11(12)
Shahbaz M, Arouri M, Teulon F (2014) Short- and long-run relationships between natural gas consumption and economic growth: evidence from Pakistan. Econ Model 41:219–226
Sivagnanam KJ, Murugan K (2016) Impact of public investment on agriculture sector in India. J Econ Soc Dev 12(2):45–51
Tang CF, Chua SY (2012) The savings-growth nexus for the Malaysian economy: a view through rolling sub-samples. Appl Econ 44(32):4173–4185
Taylor J, Spriggs J (1989) Effects of the monetary macro-economy on Canadian agricultural prices. Can J Econ 22(2):278–289
Yu X (2014) Monetary easing policy and long-run food prices: evidence from China. Econ Model 40:175–183
Zhang Z, Lohr L, Escalante C, Wetzstein M (2010) Food versus fuel: What do prices tell us? Energy Policy 38(1):445–451
Zhou S (2001) The power of cointegration tests versus data frequency and time spans. South Econ J 67(4):906–921
Download references
Acknowledgements
Not applicable.
The authors declare that no funding from any agencies was received for this study.
Author information
Authors and affiliations.
School of Economics, University of Hyderabad, Hyderabad, 500046, India
Asharani Samal & Phanindra Goyari
School of Business, Woxsen University, Hyderabad, 502345, India
Mallesh Ummalla
You can also search for this author in PubMed Google Scholar
Contributions
AS: Conceptualization, methodology, software, formal analysis, data curation, writing—original draft, reviewing and editing. MU: Resources, data curation, writing—reviewing and editing. PG: Resources, supervision and editing. All authors have read and approved the final manuscript.
Corresponding author
Correspondence to Asharani Samal .
Ethics declarations
Ethics approval and consent to participate, consent for publication, competing interests.
The authors declare that they have no competing interests.
Additional information
Publisher's note.
Springer Nature remains neutral with regard to jurisdictional claims in published maps and institutional affiliations.
Rights and permissions
Open Access This article is licensed under a Creative Commons Attribution 4.0 International License, which permits use, sharing, adaptation, distribution and reproduction in any medium or format, as long as you give appropriate credit to the original author(s) and the source, provide a link to the Creative Commons licence, and indicate if changes were made. The images or other third party material in this article are included in the article's Creative Commons licence, unless indicated otherwise in a credit line to the material. If material is not included in the article's Creative Commons licence and your intended use is not permitted by statutory regulation or exceeds the permitted use, you will need to obtain permission directly from the copyright holder. To view a copy of this licence, visit http://creativecommons.org/licenses/by/4.0/ .
Reprints and permissions
About this article
Cite this article.
Samal, A., Ummalla, M. & Goyari, P. The impact of macroeconomic factors on food price inflation: an evidence from India. Futur Bus J 8 , 15 (2022). https://doi.org/10.1186/s43093-022-00127-7
Download citation
Received : 02 July 2021
Accepted : 15 May 2022
Published : 21 June 2022
DOI : https://doi.org/10.1186/s43093-022-00127-7
Share this article
Anyone you share the following link with will be able to read this content:
Sorry, a shareable link is not currently available for this article.
Provided by the Springer Nature SharedIt content-sharing initiative
- Food price inflation
- Macroeconomic factors
- ARDL bounds testing approach
- Causality test
JEL Classification
- Business News
- India Business News
- Explained in charts: India's inflation situation and its impact on households
Explained in charts: India's inflation situation and its impact on households
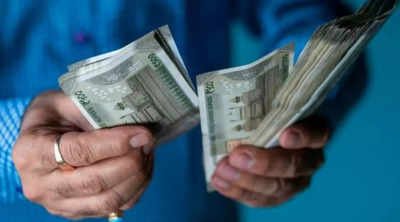
Visual Stories
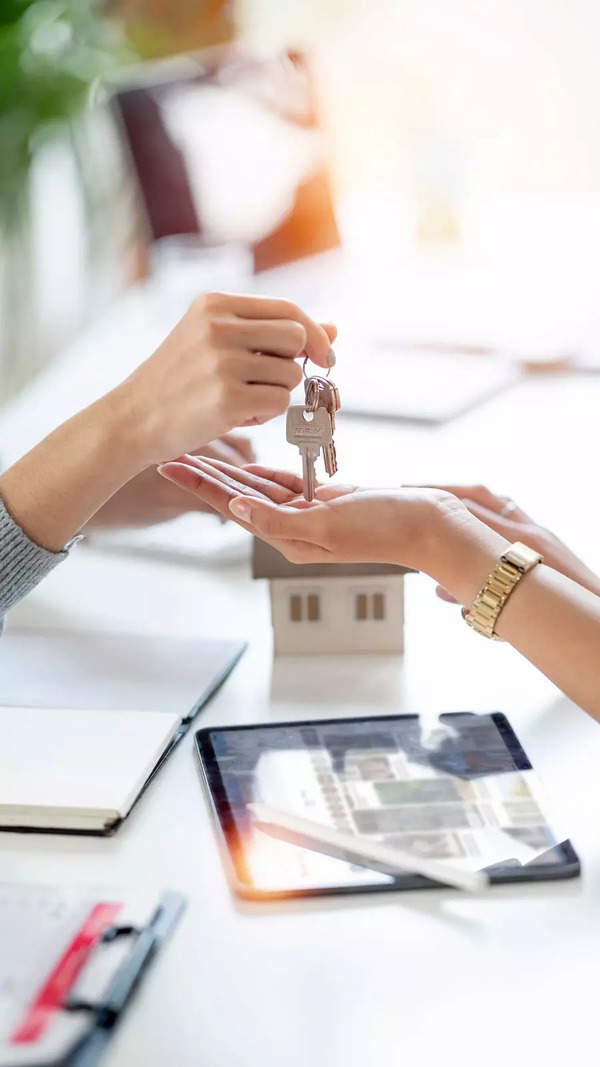
PPF Calculator
This financial tool allows one to resolve their queries related to Public Provident Fund account.
FD Calculator
When investing in a fixed deposit, the amount you deposit earns interest as per the prevailing...
NPS Calculator
The National Pension System or NPS is a measure to introduce a degree of financial stability...
Mutual Fund Calculator
Mutual Funds are one of the most incredible investment strategies that offer better returns...
Other Times Group News Sites
Popular categories, hot on the web, trending topics, living and entertainment, latest news.

- Occasional Papers
- RBI-Occasional Papers-Vol. 42, No.2, 2021
Market arrivals of the TOP crops in the regional wholesale markets or “mandis” are therefore tracked to form a view about expected price movements in the near-term, such that lower/higher arrivals indicate higher/ lower prices ( Chart 4 and Annex Table A8). Such information is available through various government sources like the Agmarknet portal, National Horticulture Board (NHB), National Horticultural Research and Development Foundation (NHRDF) and a few private agencies which track these items. Yet, with fruits and vegetables being delisted from the Agriculture Produce Market Committee (APMC) Act in many states, arrivals data from APMC mandis,may not indicate the true picture with respect to prices 6 .
At the same time, another important source of price information for these items is the Department of Consumer Affairs (DCA) under the Ministry of Consumer Affairs, which collects daily data on prices of essential fooditems from 167 centres across the country 7 . In case of tomato, onion and potato, these prices have a high correlation of around 99 per cent with corresponding CPI indices (Annex Table A7). Thus, DCA data provide a good indication of price movement of these items for the current month as the data is released on a daily basis and can be used for nowcasting food inflation.
But this data cannot be used for understanding the nature of price pressures, for which researchers often rely on news articles, announcements by ministry officials, information from traders and retailers and private agencies that track ground-level information for these commodities. This additional information on factors behind sudden price changes can be utilised to estimate the possible direction and duration of price shock and the magnitude of expected price changes.
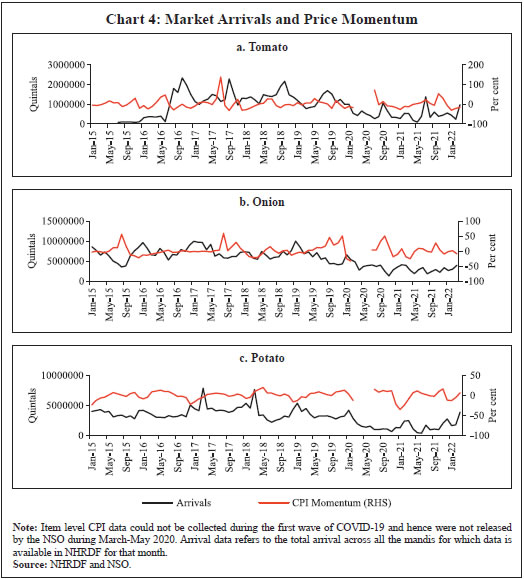
Since TOP items have a high contribution to food inflation volatility and their prices are subject to supply shocks often induced by localised extreme weather events, farmers’ protests, transporters’ strikes, storage losses and sometimes speculative stocking by traders – all of which are covered by local newspapers – news articles related to TOP can provide additional information at an early stage of accumulating price pressures. Such information, which is usually in the form of unstructured data, can be used to create sentiment scores. If these commodity sentiments have lead information about future prices, they can be helpful in nowcasting and forecasting food inflation.
Section III Data and Methodology
For the construction of a news-based sentiment indicator, we develop a novel dataset of daily news items related to TOP commodities from nine leading English news dailies published during January 2011 - August 2021. The newspapers are selected based on their national coverage and reporting on events and issues related to agri-commodities. In the first step, we use generic search terms, such as the name of a given commodity, to extract newsarticles related to a given commodity at scale 8 . Such text-mining applications are prone to ‘noise’ wherein articles unrelated to the topic of interest might also creep in, thereby lowering the signal-to-noise ratio of the data. Therefore, to avoid noise in the data, we create a set of keywords – ‘supply’, ‘demand’ and ‘prices’ – that capture the market dynamics of TOP commodities. We then filter and retain only those news items which contain at least one keyword each from the set of commodity, supply-demand and price-related keywords. 9
This filtering step ensures that the dataset contains only the most contextual and meaningful information for our analysis, to the best extent possible ( Chart 5 ). Finally, the text data are subjected to routine data cleaning procedure, such as removal of stopwords, numbers, white spaces and word stemming, etc., to organise the final dataset containing day-wise news items.
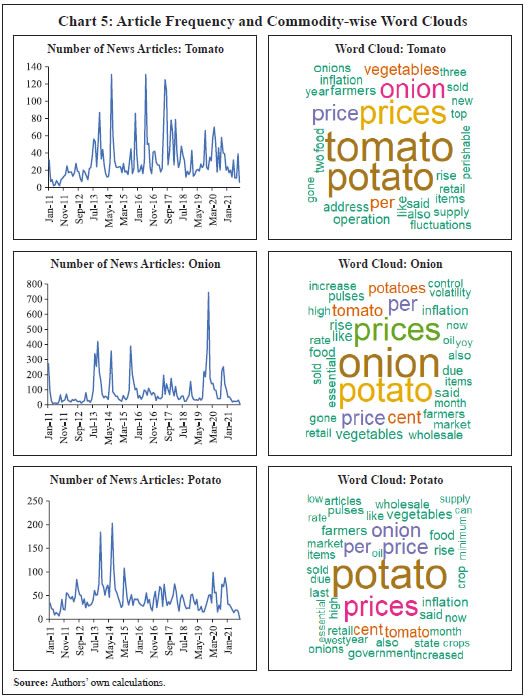
Next, we use the framework laid down by Ardia et al., (2021) for thecomputation of sentiment indices using newspaper text data 10 . While there are several approaches for sentiment computation, we adopt a lexicon-based approach, in particular a valence-shifting bigrams approach for the computation of commodity-wise sentiment indices. The lexicon-based approach is generally considered flexible, transparent, and computationally convenient as compared to other alternatives (Algaba et al., 2020). Briefly put, this approach matches words (or group of words) occurring in a document with a pre-defined wordlist of polarized (positive and negative) words and assigns quantitative scores to each matching word depending on whether its tonality is ‘positive’ or ‘negative’. For our purpose, the Loughran-McDonald lexicon – designed specifically for analysing economic and financial texts –was used (Loughran and McDonald, 2011) 11 . More specifically:
1. For each commodity-specific news item, the Loughran-McDonaldlexicon was used to assign a sentiment score {v i S i,n,t } to each polarizedword occurring in a news article d n published at time t. The term v i captures the impact of valence shifters or keywords that may negate, amplify or de-amplify polarized words in the given document.
2. Thus, ‘positive’ and ‘negative’ words were assigned a sentiment score of (+1) and (-1), respectively. The scores were then adjusted for valence shifting words depending on whether such words appear before polarized words in the document. It may be noted that this computation occurs at the sentence-level to better account for such valence-shiftingkeywords 12 .

We assume that a positive (negative) sentiment score is indicative of an expected fall (increase) in the prices of the given commodity. This interpretation is corroborated by the analysis presented in the next section that sheds light on the historical relationship between our constructed sentiments and actual price movements of TOP commodities.
Section IV TOP Sentiment Indices - Preliminary Data Analysis
TOP prices exhibit a seasonal pattern which is based on crop sowing and market arrivals across the country. However, they occasionally undergo major spikes due to localised factors, inducing high volatility to overall food inflation. The three vegetables were thus made part of essential commodities and were covered under the Essential Commodities Act, 1955 and hence their prices are monitored regularly by the government. Onion and potato, however, were removed from the list of essential commodities in September 2020 through an amendment to the Act with a rider that these items can be included in the list again only under extraordinary circumstances like wars, famines, or other natural calamities.
As expected, the derived monthly net sentiment score of TOP and changes in their prices as reflected in CPI showcase an inverse relationshipbetween them 13 ( Chart 6 ). Large increases in TOP prices seen after major supply shocks coincide with large fall in sentiment related to each of the three commodities. Major price spikes observed in TOP in the recent past were often associated with supply shocks. During the period of our study i.e., from January 2011 to August 2021 (more than 10 years), tomato prices have undergone four major price spikes while both onion and potato have undergone five major price spikes.
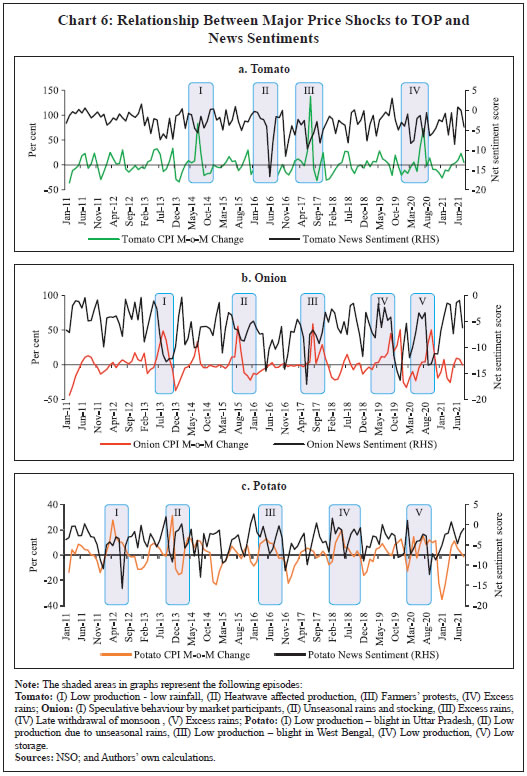
The major causes of these sharp spikes include farmers’ protests, blight disease in potato, speculative stock holding by traders, heatwave, excess rains, etc., but the major and recurring source is related to weather related shocks. Unseasonal, excess or deficit rains affect the production of these perishable vegetables. The extent of price spikes, as measured by the month-on-month (m-o-m) change in CPI indices of these vegetables, i.e., momentum, indicate that the maximum spike was observed in tomato prices in July 2017 (138 per cent), which was the result of farmers’ protests in response to low prices of tomatoes being received by them. The extent of spikes in potato prices, however, is much lower compared to tomatoes and onions (maximum momentum of 31.4 per cent in November 2013). It can also be observed that the sharp spikes in prices usually last for one to two months in case of tomatoes and around three months in case of onions and potatoes. Given the importance of TOP, the government often resorts to supply management measures – like restricting exports, imposing condition of minimum export price (MEP), increasing imports, placing stockholding limits on traders, wholesalers and retailers – to ensure availability in the domestic market and stabilise prices.
The inverse relationship between TOP prices as well as their sentiments is also corroborated by the pair-wise statistical correlations for the most recent five-year daily data sample from 2016-2021 ( Table 2 ). For instance, while onion and potato prices have a correlation of 0.45, the correlation between their sentiments is 0.67. Similarly, correlation between prices of tomato with prices of potato and onion is 0.40 and 0.28, respectively, whereas related sentiments exhibit a much stronger correlation of 0.56 and 0.39, respectively. Correlation between the price-sentiment pair of tomato, onion and potato is (-)0.20, (-)0.42 and (-)0.33, respectively. Overall, the correlation between TOP sentiments and prices is negative signifying that a decrease in TOP sentiments is associated with an increase in related prices.
As a next step, we subject the daily TOP prices and sentiment indices to a Granger Causality test to ascertain whether news-based sentiments are helpful in capturing the change in prices. Our results show that sentiment Granger causes prices for all the three commodities supporting the argument that the constructed sentiment indices are indeed helpful in capturing the future change in prices ( Table 3 ).
Section V Empirical Analysis
The preliminary statistical analysis in the previous sections is indicative of useful forward-looking information contained in the commodity sentiment indices. To formally analyse this, we undertake a time-series forecasting analysis. This section lays down the details of our forecasting analysis conducted using monthly and daily high-frequency data. In the first part of our forecasting analysis, we augment various univariate and multivariate time-series models with our sentiment indices and test their forecasting performance against a benchmark model. Like the news-based sentiment data, DCA provides an alternative set of high-frequency information on prices of food commodities across different centres in India. This data can be regarded as ‘hard’ information which can also be used in inflation projections. Therefore, the second part of our analysis focuses on comparing the forecasting performance of models augmented with sentiment data vis-à-vis DCA price data for TOP commodities. In the final part of our analysis, we showcase how daily high-frequency sentiment indices can be used to forecast CPI-Food inflation in a mixed-frequency sample framework.
V.1. Forecasting using Monthly Data
For the formal forecasting analysis, we consider monthly changes in CPI-Vegetables and CPI-Food & beverages, both in month-on-month (m-o-m) and year-on-year (y-o-y) per cent change terms, as our target variables. Thus, we have four different target variables. In line with standard practice, we divide the full sample of data into a training and a testing sample. The train sample, from January 2011 to August 2019, was used for estimation of the models. The test sample, from September 2019 to August 2021, was used for comparing model forecast performance in terms of the root mean-squared error (RMSE) of forecasts generated by different models.
We consider different specifications of autoregressive integrated movingaverage (ARIMA) models 14 . Taking the approach of parsimony, we combine all TOP-related news articles and use the sentiment scoring method described in section III to construct a composite TOP sentiment index. We introduce this index into our suite of ARIMA models to assess whether inclusion of such news-based information leads to gains in forecasting accuracy. Following Jose et al., (2021), other drivers of domestic food prices, such as global food prices, rainfall and minimum support prices (MSP), were also included as control variables in the forecasting model for robustness (additional results provided in the Appendix ).
The out-of-sample forecast performance of various models is provided in Tables 4-7 . To capture the performance of models augmented with sentiment data over time, we combine the standard out-of-sample forecasting approach with a rolling window to ensure a dynamic and robust evaluation of model forecast performance across horizons. For ease of comparison, we present the results in terms of relative performance vis-à-vis a benchmark AR(1) model, where relative performance is defined as RMSE of model m at horizon h scaled by the RMSE of the benchmark model for the same horizon. Any value less than one suggests gains in forecasting accuracy as it indicates that the actual RMSE value for a given model is lower than that of the benchmark model.
Beginning with Table 4 , which presents results for the m-o-m changes in CPI-Vegetables series, almost all models from M2-M6, are able to outperform the benchmark AR model (M1) across different horizons, particularly less than 6-months. However, addition of sentiment data is seen to improve forecasting performance across all horizons. For instance, augmenting a simple AR(1) model with NSS (M2) results in forecasting gains of about 2-6 per cent across horizons. Similarly, adding NSS to seasonal ARIMA model (M6 vs. M5) leads to better forecast performance, such that M6 is able to generate accuracy gains to the tune of 7-10 per cent over the benchmark across horizons.
Forecasting performance in case of y-o-y measure of CPI-Vegetables showcases the benefits of incorporating news-based information even further, especially at the near-term horizon ( Table 5 ). While not much improvement is seen in case of ARIMA models (M2-M4), SARIMA models deliver better forecast performance.
The forecasting performance of models for the m-o-m changes in CPI-Food and beverages, however, are mixed ( Table 6 ). The AR(1) model augmented with NSS delivers better forecast performance compared to the benchmark model, although these gains are comparatively modest (2-5 per cent) relative to CPI-Vegetables. Rest of the models fails to outperform the benchmark.
Lastly, in case of CPI-Food and beverages y-o-y series, SARIMA model (M5) and SARIMA model with sentiment information (M6) provides the best forecasts ( Table 7 ). As seen in the earlier cases, adding sentiment information to forecasting models leads to a general improvement in forecasting accuracy.
V.2. Forecast Comparison between Sentiment data and DCA Data
To compare the extent of forward-looking information embedded in news-based ‘soft’ data and ‘hard’ DCA data, we estimate separate bivariate vector autoregression (VAR) models containing each set of indicators and compare their out-of-sample forecasting performance. A bivariate VAR model can be generally expressed as follows:
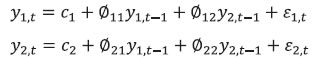
where y i,t represents a set of endogenous variables whereas Ɛ 1,t and Ɛ 2,t are white noise processes that may be contemporaneously correlated. Our basic framework, including the target indicators, forecast horizon and train-test sample, remains the same as in the last subsection 15 . We estimate the VAR model using ordinary least squares (OLS) method while choosing optimal lag structure via the AIC criterion. The forecasting performance based on rolling out-of-sample forecasts, in the form of relative RMSE with respect to a benchmark AR(1) model, is provided in Table 8 .
In case of CPI-Vegetables inflation, both set of indicators showcase a similar forecasting ability across time horizons. In some cases, such as m-o-m CPI-Vegetables model augmented with sentiment or DCA data fail to outperform the benchmark. However, in case of CPI-Food & beverages inflation, bivariate VAR model with sentiment data outperforms both the benchmark and DCA data-based model across horizons. In case of m-o-m measure, the gains in forecasting accuracy range from 13 to 32 per cent, whereas even higher gains ranging from 56 to 75 per cent accrue when sentiment data is used to forecast the CPI-Food & beverages y-o-y measure of inflation. This highlights the efficacy of sentiment data for forecasting inflation over and above the only other existing high-frequency prices data provided by DCA.
V.3. Forecasting using Mixed-frequency Data: A MIDAS Approach
Having access to high-frequency news data, we exploit the information embedded in daily news sentiments to forecast monthly inflation series. A mixed data sampling (MIDAS) regression approach comes in handy by allowing the use of data sampled at different frequencies in the same regression. In particular, the MIDAS methodology proposed by Ghysels et al. (2002; 2006; 2007) and Andreou et al. (2010) allows the estimation of regression models where the dependent variable is sampled at a lower frequency compared to one or more of the independent variables. Thus, MIDAS helps in incorporating the information in higher-frequency data into the lower frequency regression model in a flexible and parsimonious way. A MIDAS regression model can be generally specified as follows:
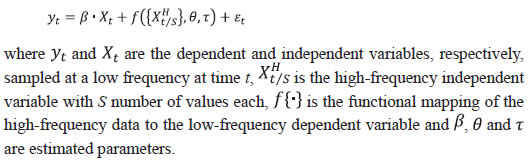
Traditional approaches to mixed-frequency regression either introduce a sum/average of the high-frequency data with a single coefficient (implicitly equal weights) or include individual components of the high-frequency variable in the model allowing for separate coefficients. On the other hand, by allowing for several different weighting functions to decide optimal weights and reducing the number of estimated parameters by placing adequate constraints, the MIDAS approach offers a flexible framework to incorporate high-frequency information into a regression model.
Various weighting schemes available under this approach are (a) step-weighting; (b) polynomial distributed lag (PDL) weighting; (c) exponential PDL weighting; (d) normalised beta function weighting; and (e) individual coefficients weighting technique (U-MIDAS). Adopting a similar train-test approach as in the previous sub-section, we use a simple AR(1) model estimated using the MIDAS framework with PDL and U-MIDAS weighting techniques for predicting our target variable. We incorporate the high-frequency information by introducing up to 30 lags of combined TOP net sentiment score computed at a daily frequency. The out-of-sample forecasts on test data are generated using the dynamic rolling window approach from one-to 12-months ahead horizon. The forecast performance, in the form of relative RMSE of forecasts generated by best-performing MIDAS model compared to the AR(1) benchmark, is presented in Table 9 .
As is evident from Table 9 , leveraging daily news-based sentiment data has clear benefits in terms of gains in forecasting accuracy in m-o-m space. In case of m-o-m changes in CPI-Vegetables and CPI-Food and beverages, the MIDAS model with daily data outperforms the benchmark model at the one-month ahead horizon. In addition to these one-month ahead predictions or nowcasts, the MIDAS model outperforms the benchmark for two- to six-months ahead horizon, where the gains range from 2-5 per cent for m-o-m changes in CPI-Vegetables and CPI-Food and beverages series. On the other hand, sentiment augmented model fails to outperform the benchmark model in case of inflation measures taken in y-o-y terms across different horizons. Thus, overall, it can be said that daily news-based sentiment indicators for TOP commodities can help near-term month-on-month projections of price index for CPI Vegetables and CPI Food and beverages over different forecast horizons.
Section VI Conclusion and the Way Forward
Recurrent supply disruptions driven by unseasonal rainfall, floods, droughts, pest attacks, protests by farmers/transport operators, etc., makes the task of inflation forecasting an arduous challenge in India. In this study, therefore, we develop a novel dataset consisting of news articles related to three main agricultural commodities viz., tomato, onion and potato or TOP to forecast CPI-based inflation in vegetables and food & beverages. We quantify the information content of news articles using natural language processing (NLP) techniques to assess whether news-based alternate data can help in achieving better forecasts. Through various forecasting methods premised on monthly and daily data, we provide empirical evidence to conclude that news-based data in the form of sentiment indices provides gains in forecasting accuracy. The forward-looking information content embedded in news data, therefore, suggests the use of news-based sentiment indicators as an additional source of information for inflation forecasting. This is crucial from a policy perspective in an environment of highly uncertain food price dynamics that are increasingly becoming climate dependent.
While this study is a first step in the direction of using news-based big data for food inflation forecasting, the analysis can be extended in several ways. From the perspective of forecasting headline inflation and its components, a larger number of commodities and other items corresponding with the official CPI basket can be included in the forecasting framework. More nuanced NLP techniques, such as those based on supervised machine-learning methods for sentiment quantification, or unsupervised topic modelling approach can be used to derive more granular information on topics such as supply and demand for various items. The suite of time-series methods can also be expanded to experiment with other advanced techniques, such as dynamic factor models, penalized regressions and deep learning models which might help achieve better forecasting accuracy. Moreover, given the forward-looking content of news-based data, it can also be used for turning point analysis of inflationary shocks to the economy. Finally, while we have focused on point forecasts in this paper, it remains to be seen whether news-based information can also help in reducing the uncertainty around forecasts. We leave these issues for future research.
Aprigliano, V., Emiliozzi, S., Guaitoli, G., Luciani, A., Marcucci, J., & Monteforte, L. (2022). The power of text-based indicators in forecasting Italian economic activity. International Journal of Forecasting.
Algaba, A., Ardia, D., Bluteau, K., Borms, S., & Boudt, K. (2020). Econometrics meets sentiment: An overview of methodology and applications. Journal of Economic Surveys, 34(3), 512-547.
Andreou, E., Ghysels, E., & Kourtellos, A. (2013). Should macroeconomic forecasters use daily financial data and how? Journal of Business & Economic Statistics, 31(2), 240-251.
Ardia, D., Bluteau, K., Borms, S., & Boudt, K. (2021). The R package sentometrics to compute, aggregate and predict with textual sentiment. Journal of Statistical Software, 99(2), 1-40.
Banerjee, A., Kanodia, A., & Ray, P. (2021). Deciphering Indian inflationary expectations through text mining: an exploratory approach. Indian Economic Review, 1-18.
Barbaglia, L., Consoli, S., & Manzan, S. (2022). Forecasting with economic news. Journal of Business & Economic Statistics, 1-12.
Bholat, D., Hansen, S., Santos, P., & Schonhardt-Bailey, C. (2015). Text mining for central banks. Available at SSRN 2624811.
Ellingsen, J., Larsen, V. H., & Thorsrud, L. A. (2022). News media versus FRED-MD for macroeconomic forecasting. Journal of Applied Econometrics, 37(1), 63-81.
Gandomi, A., & Haider, M. (2015). Beyond the hype: Big data concepts, methods, and analytics. International Journal of Information Management, 35(2), 137-144.
Gentzkow, M., Kelly, B., & Taddy, M. (2019). Text as data. Journal of Economic Literature, 57(3), 535-74.
Ghosh, S. , Kundu, S., & Dilip, A. (2021). Green Swans and their Economic Impact on Indian Coastal States. Reserve Bank of India Occasional Papers, 42(1).
Ghysels, E., Santa-Clara, P., & Valkanov, R. (2002). The MIDAS touch: Mixed data sampling regression models. Working Paper, UNC and UCLA.
Ghysels, E., Santa-Clara, P., & Valkanov, R. (2006). Predicting volatility: getting the most out of return data sampled at different frequencies. Journal of Econometrics, 131(1-2), 59-95.
Ghysels, E., Sinko, A., & Valkanov, R. (2007). MIDAS regressions: Further results and new directions. Econometric Reviews, 26(1), 53-90.
Goshima, K., Ishijima, H., Shintani, M., & Yamamoto, H. (2021). Forecasting Japanese inflation with a news-based leading indicator of economic activities. Studies in Nonlinear Dynamics & Econometrics, 25(4), 111-133.
John, J., Singh, S., & Kapur, M. (2020). Inflation forecast combinations – The Indian experience. RBI Working Paper Series, No. 11/2020, Reserve Bank of India, Mumbai, India.
Jose, J., Shekhar, H., Kundu, S., Kishore, V., & Bhoi, B. B. (2021). Alternative Inflation Forecasting Models for India–What Performs Better in Practice?. Reserve Bank of India Occasional Papers, 42(1).
Kalamara, E., Turrell, A., Redl, C., Kapetanios, G., & Kapadia, S. (2020). Making Text Count: Economic Forecasting Using Newspaper Text. Bank of England Working Paper No. 865, Available at SSRN: https://ssrn.com/abstract=3610770 or http://dx.doi.org/10.2139/ssrn.3610770
Kapur, M. (2013). Revisiting the Phillips curve for India and inflation forecasting. Journal of Asian Economics. 25(1), 17-27
Kishore, V. and Shekhar, H. (2022), Extreme weather events and vegetables inflation in India. Economic and Political Weekly. Forthcoming.
Kumari, S., & Giddi, G. (2020). Inflation decoded through the power of words. RBI Monthly Bulletin, May, Reserve Bank of India, Mumbai, India.
Larsen, V. H., & Thorsrud, L. A. (2019). The value of news for economic developments. Journal of Econometrics, 210(1), 203-218.
Lei, C., Lu, Z., & Zhang, C. (2015). News on inflation and the epidemiology of inflation expectations in China. Economic Systems, 39(4), 644-653.
Liu, B. (2020). Sentiment analysis: Mining opinions, sentiments, and emotions. Cambridge University Press.
Loughran, T., & McDonald, B. (2011). When is a liability not a liability? Textual analysis, dictionaries, and 10-Ks. The Journal of Finance, 66(1), 35-65.
Maji, B., & Das, A. (2016). Forecasting inflation with mixed frequency data in India. Calcutta Statistical Association Bulletin, 68(1-2), 92-110.
Munezero, M., Montero, C. S., Sutinen, E., & Pajunen, J. (2014). Are they different? Affect, feeling, emotion, sentiment, and opinion detection in text. IEEE Transactions on Affective Computing, 5(2), 101-111.
Priyaranjan, N., & Pratap, B. (2020). Macroeconomic Effects of Uncertainty: A Big Data Analysis for India. RBI Working Paper Series (No. 04/2020), Reserve Bank of India, Mumbai, India.
Pratap, B., & Sengupta, S. (2019). Macroeconomic forecasting in India: Does machine learning hold the key to better forecasts?. RBI Working Paper Series, No. 04/2019, Reserve Bank of India, Mumbai, India.
Rambaccussing, D., & Kwiatkowski, A. (2020). Forecasting with news sentiment: Evidence with UK newspapers. International Journal of Forecasting, 36(4), 1501-1516.
Ravi, K., & Ravi, V. (2015). A survey on opinion mining and sentiment analysis: tasks, approaches and applications. Knowledge-based Systems, 89, 14-46.
Reserve Bank of India. (2021). Monetary Policy Report, October.
Sahoo, S., Kumar S. and Gupta B. (2020). Pass-through of international food prices to emerging market economies: A revisit. Reserve Bank of India Occasional Papers, Vol. 41, No. 1: 2020
Sahu, S., & Chattopadhyay, S. (2020). Epidemiology of inflation expectations and internet search: an analysis for India. Journal of Economic Interaction and Coordination, 15(3), 649-671.
Shapiro, A. H., Sudhof, M., & Wilson, D. J. (2020). Measuring news sentiment. Journal of Econometrics, 228 (2), 221-243.
Taboada, M. (2016). Sentiment analysis: An overview from linguistics. Annual Review of Linguistics, 2, 325-347.
Thakur, G. S. M., Bhattacharyya, R., & Mondal, S. S. (2016). Artificial neural network-based model for forecasting of inflation in India. Fuzzy Information and Engineering, 8(1), 87-100.
Tilly, S., Ebner, M., & Livan, G. (2021). Macroeconomic forecasting through news, emotions and narrative. Expert Systems with Applications, 175, 114760.
* Bhanu Pratap ( [email protected] ), Abhishek Ranjan ( [email protected] ), Vimal Kishore ( [email protected] ) and Binod B. Bhoi ( [email protected] ) are from the Department of Economic and Policy Research (DEPR), Reserve Bank of India, Mumbai. The authors are thankful to Harendra Kumar Behera, Shweta Kumari and an anonymous referee for their helpful comments and suggestions on the paper. The views expressed in the paper are those of the authors and do not represent the views of the Reserve Bank of India.
1 Headline inflation is measured by year-on-year changes in the all-India CPI-Combined (Rural + Urban) with base year: 2012=100 released by the National Statistical Office (NSO), Ministry of Statistics and Programme Implementation, Government of India.
2 PIB link - https://static.pib.gov.in/WriteReadData/specificdocs/documents/2021/oct/doc2021102961.pdf
3 According to Munezero et al. (2014), sentiment is one of the so-called human subjectivity terms that may reflect a person’s desires, beliefs, and feelings that are features of a person’s private state of mind which can only be observed through textual, audio, or visual communication. Algaba et al. (2020) define sentiment as “the disposition of an entity toward an entity, expressed via a certain medium”.
4 The share of food and beverages in overall consumer price index - combined basket is 45.86 per cent (Base: 2012=100).
5 Contribution of subgroup (say, A) to variance in total (A+B) is calculated using the following formula: Contribution (A) = W(A)W(A) Cov(A, A) + W(A)W(B) Cov(A, B), where W is the weight of the sub-group and Cov is covariance.
6 For instance, Assam and Meghalaya delisted fruits and vegetables in January 2014, Delhi in September 2014, Odisha in February 2015, Gujarat in April 2015, Maharashtra in July 2016, etc.
7 Coverage of centres keep changing, earlier it was around 120.
8 The news dataset has been constructed using application developed by Meltwater Inc., https://www.meltwater.com/en .
9 The keyword sets are as follows: commodity = (onion/tomato/potato, onions/tomatoes/ potatoes); prices = (price, prices, inflation); supply-demand keywords = (increase, increases, increased, hike, hikes, rise, rose, rising, surge, surges, surged, soar, soaring, climbed, climbing, skyrocketed, skyrocketing, shoot, push, peak, peaked, fall, fell, decrease, decreases, decreased, crash, crashed, plunge, plunged, drop, dropped, decline, declined, down, cool, cooling, tumbled, slipped, harvest, rain, rainfall, flood, floods, flooding, rain damage, destroy, destroyed, stock, stocking, market arrival, market arrivals, arrivals, arrival, production, supply, bottleneck, supply chain, shortage, shortages, export, sowing, sowing delay, spoiled crop, rotten, hoarding, cold storage, transport strike, truckers strike, pest attack, drought, demand, increased demand, high demand, festival demand, bumper crop, supply, supply glut, higher production, supply boost, import, export ban, fresh crop arrivals, increased market arrivals, stock limit, stock limits, hoarding, buffer stock, low demand, sluggish demand, weak demand, lack of demand, hailstorm, unseasonal, protest, farmer protest, protests).
10 We use the R sentometrics package for end-to-end computational purpose.
11 The dictionary can be accessed here - https://sraf.nd.edu/loughranmcdonald-master-dictionary/ .
12 Valence-shifting keywords tend to negate, amplify or de-amplify the meaning of other words thereby changing the tone of the sentence. For instance, “this is not good” would be assigned a score of (+1) under the normal sentiment scoring approach. However, it would be assigned a score of (-1) due to the presence of a negating word ‘not’ under the approach adopted by in our case.
13 As mentioned earlier, CPI item level data were not released by NSO during March-May 2020. To create a continuous series of CPI indices for tomato, onion and potato – for comparison with sentiment indices and for charts – momentums of DCA for tomato, onion and potato were used to impute corresponding CPI indices for the missing months, given the high correlation between the two.
14 ARIMA and SARIMA are popular time-series models due to faster computation, interpretation and better predictive ability for short-term forecasting. ARIMA and seasonal ARIMA methods are frequently used for inflation forecasting in the Indian context (Jose et al., 2021). Model selection in case ARIMA(p,d,q) and SARIMA(p,d,q)(P,D,Q) was done using the Akaike Information Criterion (AIC). Model estimation was done using maximum likelihood estimation (MLE) technique.
15 To ensure the stationarity condition, CPI-Vegetables, CPI-Food & beverages and DCA price data are taken in y-o-y (per cent) or m-o-m (per cent), as applicable for a given model and target indicator. The composite TOP sentiment index is taken in level form since it was found to follow an I(0) process.
The dynamics of survey-based household inflation expectations in India
IIM Ranchi Journal of Management Studies
ISSN : 2754-0138
Article publication date: 13 December 2021
Issue publication date: 1 March 2022
The author analyzes households' inflation expectations data for India, collected quarterly by the RBI for more than a decade. The contribution of this paper lies in two folds. First, this study examines the relationship between relatively recent inflation expectations survey of households (IESH) and the actual inflation for India. Secondly, the author employs a structural VAR with the time period 2006 Q2 to 2020 Q2 on inflation expectation survey data of India. A short-term non-recursive restriction is imposed in the model in order to capture the simultaneous co-dependence causal effect of inflation expectation and realized inflation.
Design/methodology/approach
This paper studies the dynamic behavior of inflation expectations survey data in two folds. First, the author analyzes the time series property of the survey data. The author begins with testing the stationarity property of the series, followed by the casual relationship between the expected and actual inflation. The author further examines the short-run and long-run behavior of the IESH with actual inflation. Employing autoregressive distributed lag and Johansen co-integration, the author tested if a long-run relationship exists between the variables. In the second approach, the author investigates the determinants of inflation expectations by employing a non-recursive SVAR model.
The preliminary explanatory test reveals that inflation expectation is a policy variable and should be used in monetary policy as an instrument variable. The model identifies the price puzzle for India. The author finds that the response of inflation to a monetary policy shock is neutral. The results also indicate that the expectations of the general public are self-fulfilling.
Originality/value
IESH has only commenced from September 2005, hence is relatively new as compared to other survey in developed countries. Being a new data set so far, the author could not locate any study devoted in analyzing the behavior of the data with other macroeconomic variables.
- Inflation expectations
- Monetary policy
Impulse response function
Jha, S. (2022), "The dynamics of survey-based household inflation expectations in India", IIM Ranchi Journal of Management Studies , Vol. 1 No. 1, pp. 38-54. https://doi.org/10.1108/IRJMS-08-2021-0109
Emerald Publishing Limited
Copyright © 2021, Saakshi Jha
Published in IIM Ranchi Journal of Management Studies . Published by Emerald Publishing Limited. This article is published under the Creative Commons Attribution (CC BY 4.0) licence. Anyone may reproduce, distribute, translate and create derivative works of this article (for both commercial and non-commercial purposes), subject to full attribution to the original publication and authors. The full terms of this licence may be seen at http://creativecommons.org/licences/by/4.0/legalcode
1. Introduction
Every central bank faces the challenge of keeping the inflation rate within reasonable limits. One of the main factors that determine the rate of inflation is the inflation expectations of various macroeconomic agents in an economy. Thus, central banks try to keep the inflation expectations “well-anchored” primarily by making their policy for targeting inflation public and by sharing the data on the inflation expectations of professional forecasters and the general public. While these policies have been an inherent part of the central banks in developed countries, it is only recently that India has begun following a similar path.
Inflation expectations can influence the behavior of economic agents. The inter-temporal decisions like savings, investments, wage negotiations, etc., made by economic agents are highly dependent on their expectations about future inflation. These inter-temporal decisions, in turn, affect economic activity, which further influences actual inflation. If the current inflation rate creates expectations for future inflation, which itself is induced by the expectations of the economic agents, it leads to the creation of an “inflation expectations spiral” in the economy. The effect of the “inflation expectations spiral” can cause high and persistent inflation, thereby reducing the effectiveness of monetary policy for controlling inflation.
In order to control inflation and to avoid the trap of “inflation expectations spiral,” the monetary policymakers must know the pattern and behavior of expected inflation. Berk (2002) indicates that any effect of monetary policy on inflation expectations depends upon the direction and intensity of the causality between the inflation expectations and actual inflation. Ball et al. (2005) point out that the dynamic correlation between inflation expectations and realized inflation will help anchor inflation expectations and strengthen the credibility of central banks. In other words, a strong co-relationship between the realized and expected inflation will allow monetary policy to achieve price stability for the economy.
Several studies have presented different conclusions on the relationship between inflation expectations and actual inflation. Among those finding inflation expectations to be self-fulfilling, Leduc et al. (2007) prove inflation expectations to be the cause of the high and persistent US inflation rate of the 1970s. Mavroeidis et al. (2014) study shows that inflation expectations could cause inflation in a self-fulfilling spiral. Other studies, on the other hand, conclude that actual inflation has significant effects on inflation expectations. The expectations-augmented Phillips Curve predicts that actual and expected inflation would move in a coordinated and balanced relationship ( Phelps, 1967 ). Furthermore, studies like Chen (2008) demonstrate a significant positive correlation between the expectations of future inflation and current actual inflation. Feng and Zhu (2012) study document a causal relationship from expected inflation to actual inflation.
In addition to the unidirectional nexus indicated by the above literature, other studies support a bi-directional relationship between inflation expectations and actual inflation. Patra and Ray (2010) certify bidirectional causality between the inflation expectations and the actual inflation. They state that the increase in inflation may cause an increase in inflation expectations, which will further drive up inflation. Reid (2015) finds a causal relationship between realized inflation and inflation expectations in South Africa. The change in inflation occurs first, followed by a similarly adjusted change in expectations. Using impulse responses, Kim and Lee (2013) illustrate the dynamic effects on actual inflation due to the shocks of the inflation expectation for Asian countries.
Previous research provides evidence of opposing and supporting the lead-lag relationship between expected and actual inflation and even supporting the bi-directional linkages. The existence of such vivid literature indicates the importance of the studies. Our study, highly intertwined with the existing literature, attempts to identify the relationship between actual and expected inflation for India.
The contribution of this paper to the existing literature is as follows. First, this study examines the relationship between relatively recent inflation expectations survey of households (IESH) and the actual inflation for India. The survey has only commenced from September 2005; hence it is relatively new than other surveys in developed countries. Being a new data set so far, we could not locate any study devoted to analyzing the behavior of the data with other macroeconomic variables. Second, like other developed countries, India recently (June 2016) has formally adopted inflation targeting as its framework. With inflation targeting as its framework, analyzing the dynamic behavior of inflation expectations becomes of paramount importance.
This paper studies the dynamic behavior of inflation expectations survey data in two folds. First, we analyze the time series property of the survey data. We begin with testing the stationarity property of the series, followed by the casual relationship between the expected and actual inflation. Our study reveals inflation expectations survey data to be stationary at the first difference and indicates a causal relationship between actual consumer price index (CPI) and wholesale price index (WPI) inflation and the expected inflation. Second, we proceed further by examining the short-run and long-run behavior of the IESH with actual inflation. Employing autoregressive distributed lag (ARDL) and Johansen co-integration, we test if a long-run relationship exists between actual CPI and WPI inflation and households' inflation expectations survey data.
Further, use structural vector auto regressive (SVAR) model to study the various determinants of inflation expectation and their effects on economic variables and inflation. We construct a four-variable VAR with the output gap, nominal interest rate, inflation and inflation expectation as our endogenous variable. We impose a non-recursive restriction in our VAR, as inflation expectation attributes simultaneous co-dependence causal effect with realized inflation.
Inflation in India is measured by the WPI and CPI. WPI measures the inflation from the producer side as its basket constitutes wholesale goods. The CPI is mainly considered as consumer side inflation as the basket consists of consumer goods. In our analysis for both the dynamic nature of inflation expectations and the SVAR, we use CPI as our realized inflation. Since inflation expectations collected by RBI are household survey data, we believe that a lot of general public expectations must be based on the price of their day-to-day consumption. Hence CPI comes into play. Secondly, we find a long-run correlation of survey data with CPI and not WPI inflation. Moreover, the lagged CPI inflation shows a better correlation with survey data than the lagged WPI.
This paper is organized in the following manner: Section 2 explains the data set used for the analyses. Next, Section 3 reports on the unit root test results for analyzing the stationarity of the data and the Granger causality results. Further, Section 4 explains the long-run relationship between realized inflation and the IESH. Section 5 provides the genesis of structural vector autoregressive (SVAR), Section 6 presents the results, and Section 7 concludes.
2.1 Inflation expectations survey of households [1]
Reserve Bank of India (RBI) initiated a survey of the inflation expectations of the general public (IESH) since September 2005. The survey has both qualitative and quantitative responses regarding the changes in price levels and the rate of inflation for three months and one year ahead.
The survey data has been published in the public domain since September 2006. Recently, the 63rd round of the survey concluded, collecting responses from around 6,000 households. A visual representation of survey-based inflation expectations variable and actual inflation gives us a quick idea about how these series move over time.
Figure 1 reveals the relationship between household inflation expectations and actual inflation: CPI and WPI. In the initial period, Figure 1 depicts a lag relationship of inflation expectations with the CPI inflation. It could be said; initially, the inflation CPI is leading the public perception in a very early stage of the survey. This pattern of households' inflation expectations and actual CPI inflation can only be witnessed till the 2008 financial crisis. During the global recession of 2007–08, the public expectations about inflation were higher than the actual CPI inflation.
Similarly, the highest peak in actual inflation was witnessed in the second quarter of 2009 (15.2%), whereas the expectations were at their highest four-quarters earlier, i.e. in the second quarter of 2008 (13.5%). Since that time, the expectations have always responded to the CPI with lags. The trend that is clear from the figure is that the expectations, no matter the situation in the economy, have always remained higher than the actual CPI inflation.
Unlike the CPI inflation, the earlier observations show that expectations are in line with the WPI inflation. Until the second quarter of 2008, the general public's expectations followed the WPI inflation, i.e. during the same period the highest peak was obtained for all of the variables. The divergence in the data is seen after this, i.e. when the WPI inflation drops, the expectations fall in response, but then they rise again. One reason for this rise in inflation expectations may be CPI inflation. The CPI inflation increased to 15.3% in quarter two of 2009. Hence, this may have pulled the inflation expectations up. Looking at the data, we believe that since WPI has been the policy variable in India for a long time, people's expectations in the starting quarters were based on it. Gradually, as the survey proceeded, the public began relating their expectations to the prices of the basket of goods consumed by them rather than WPI. Hence, we find that expectations started to connect more to CPI rather than WPI, despite the latter being the policy variable until 2016.
Table 1 contains the statistical summaries of the variables of our interest. Both CPI and WPI are positively and significantly correlated with the three-month-ahead inflation expectations. A quick observation of the results shows inflation CPI (mean – 7.49 and std. dev. – 2.89) to be closely associated with the three-months ahead mean households' inflation expectations (mean – 9.60 and std. dev. – 2.31) than the WPI inflation (mean – 4.58 and std. dev. – 3.71).
2.2 Output gap
To generate the output gap, we collect the Gross Domestic Product (GDP) at factor cost from the RBI database on the Indian economy. The output gap is the difference between the seasonally adjusted real GDP series using the X-11 ARIMA method and the trend computed through the HP filter.
All the other data like CPI inflation, WPI inflation and repo rate is obtained from the database of the Indian economy (DBIE), RBI. The analysis runs from the second quarter of 2006 until the second quarter of 2020. The survey data on inflation expectations is available in the public domain from the second quarter of 2006 onward; hence, we commence our analysis from this period.
3. Empirical analysis
This part of our study is an attempt to better understand the survey-based inflation expectations of the general public. We begin by exploring the economic relation of inflation expectations with actual or realized inflation by investigating the time-series properties of the former. We start by testing the stationarity property for both CPI and WPI of inflation, with a particular focus on the tests meant for small samples; then we analyze the causality relation between the two series on realized and survey-based inflation expectations, and, finally, examine the existence of a long-run relationship between the series.
3.1 Stationarity property
As a first step in determining the time-series properties of survey-based inflation expectations, we investigate its stationarity property. A stationary time series has a constant mean and variance over time. We check the hypothesis that IESH has no systematic trend, no systematic change in the variance and no periodicity or seasonality to the series.
We investigate the presence of a unit root with the following four tests – augmented Dickey–Fuller (ADF), Phillips Perron (PP), Dickey–Fuller generalized least square (DF-GLS), and Ng-Perron. The reason for using all four tests is as follows. The ADF and PP tests are widely used in the unit root literature, though they suffer from drawbacks. The ADF test is known to have low power, and the PP test does not perform well with small samples ( Davidson and Mackinnon, 2004 ).
Perron and Ng (1996) , Elliot et al. (1996) and Ng and Perron (2001) have modified the traditional ADF and PP tests to mitigate the size distortion and to increase the power of each test for every persistent alternative. We incorporate these tests for our time series data since the sample size is small and provides the robustness check.
Elliot et al. (1996) proposed an efficient test modifying the Dickey–Fuller test statistic using a generalized least square (GLS) method. They demonstrate that the modified test has the best overall performance in small sample size and power tests, conclusively dominating the standard Dickey–Fuller test. In particular, Elliott et al. (1996) find that their “DF-GLS” test “has substantially improved power when an unknown mean or trend is present.”( 1996 , p. 813).
Therefore, the DF-GLS and NG-Perron [2] unit root tests are also conducted, as they perform better with small samples. All these tests deliver robust results, suggesting that the series is stationary.
Table 2 indicates that all the variables are stationary at first difference.
3.2 Pairwise Granger causality
As outlined in the Introduction section, inflation expectations are an essential variable for policymakers since this variable might both affect and get affected by actual or realized inflation. Therefore, a two-way causal relationship is suspected between these two variables. We investigate whether such a relationship prevails between survey-based inflation expectations and actual CPI and WPI inflations in India. The results of the Granger causality tests are presented in Table 3 .
Results from Table 3 support the hypothesis that a causal relationship exists between the CPI inflation and inflation expectations. CPI inflation comprises the prices of consumer goods and services; a two-way relationship between CPI inflation and inflation expectations of the general public is justified. For WPI inflation, we find a mixed result. Table 3 first row indicates a weak acceptance of the null hypothesis of inflation expectations with WPI inflation. At the same time, the vice-versa does hold. One of the reasons for such a discrepancy could be that the general public relates more to the prices of the goods they regularly consume rather than the wholesale prices relevant to the producers. This was perhaps the primary reason why the Urjit Patel Committee Report in 2014 recommended that CPI inflation be the official measure of inflation instead of the WPI inflation. It was formally accepted as an official measure of inflation in 2016 by RBI.
On identifying the causal relationship between the actual and expected inflation, we try to identify the short- and long-run relationship between them. The two econometric approaches widely used to determine the short and long-run relationship are the ARDL – ECM and vector error correcting method (VECM) approach.
Both approaches mentioned above have their specifications like ARDL – ECM can only be used when anyone series is stationary at I(0). In contrast, VECM is used when series are integrated at I(1) and are co-integrated in the long run. In our case, all our series are stationary at I(1) (as depicted in Table 2 ). However, in the literature, there is debate regarding the stationarity of the inflation series. Juselius (2006) mentions that inflation series can be stationary at a level if the series is considered for more extended periods; otherwise, the series remains non-stationary. Since the time period of survey-based inflation data is limited in the Indian context, it is not surprising that the data series is stationary at the first difference and not at levels. However, Reid (2015) estimates the ARDL –ECM (error correction model) and VECM model for measuring the stickiness of survey-based inflation expectations for South Africa for 40 observations. The study considers inflation to be stationary at both levels and the first difference. Following the same line, we also analyze the long-run relationship of households' survey-based inflation expectations with actual inflation using the ARDL and VECM approach.
3.3 ARDL bound testing approach
Considering that the actual inflation can also be stationary at the level, we examine the long-run relationship between inflation expectations and realized inflation following the ARDL bound test approach to co-integration developed by Pesaran et al. (2001) . ARDL is considered a better way of testing a long-run relationship. Firstly, it can be used for series that are not stationary at the same level, i.e. it can be applied to the series irrespective of I(0) or I(1). Secondly, it can simultaneously estimate short-run and long-run parameters. Thirdly, it has better small sample properties ( Smyth and Narayan, 2006 ). The following unrestricted error correction model is estimated for ARDL: (1) Δ IESH t = α 01 + α 12 IESH t − 1 + α 21 InfCPI t − 1 + ∑ i = 0 p α 1 i Δ IESH t − i + ∑ j = 0 q α 1 j Δ InfCPI t − j + ε t (2) Δ IESH t = α 02 + α 12 IESH t − 1 + α 22 InfWPI t − 1 + ∑ i = 0 p α 1 i Δ IESH t − i + ∑ j = 0 q α 1 j Δ InfWPI t − j + μ t Where, Δ is the first difference operator, α 01 is the constant, α 12 and α 21 are the long-run coefficients and α i and α j represents the short-run coefficients. The ε t is the white noise error term. The optimal lag structure for the ARDL approach is determined by Schwarz Bayesian information criterion. We estimate equation 2 to determine the long-run relation of IESH with inflation WPI.
To determine the long-run relationship between the variables, two separate bound tests are performed: a Wald or F -test for the joint null hypothesis H 0 : α 12 = α 21 = 0 and a t -test on lagged dependent variable. The asymptotic distribution of the F -test is non-standard; one can use the value of the critical bound provided by Pesaran et al. (2001) . There are two asymptotic critical values computed by Pesaran et al. (2001) ; one at I(0), that variables are considered not to be co-integrated, and second at I(1) when variables are co-integrated. The I(0) is regarded as lower critical bound (LCB) and I(1) as upper critical bound (UCB). If the test statistic exceeds the UCB, then the variables are co-integrated in the long run. Additionally, if the test statistic is below the LCB, the null hypothesis of no co-integration is not rejected. On the other hand, if test statistics lie between LCB and UCB, the results are inconclusive. equation (1) and equation (2) in the ARDL version of the error correction model can be expressed as equation (3) and (4) , respectively below. (3) Δ IESH t = α 01 + ∑ i = 0 p α 1 i Δ IESH t − i + ∑ j = 0 q α 1 j Δ InfCPI t − j + λ ECM t − 1 + ϵ t (4) Δ IESH t = α 02 + ∑ i = 0 p α 1 i Δ IESH t − i + ∑ j = 0 q α 1 j Δ InfWPI t − j + λ ECM t − 1 + ε t Where, λ is the speed of adjustment parameter, and ECM is the residuals obtained from the estimated co-integration model of equations (1) and (2) . The α 1 i and α 1 j if significant, provides evidence on the direction of the short-run causation while a significant t -statistic for the ECM depicts the presence of significant long-run causation.
Table 4 presents the bound test result. The calculated F -statistics (5.53) is greater than the UCB at a 10% significance level for CPI inflation. Whereas for WPI inflation, the F -statistics is lower than UCB for all the significance levels. Hence we conclude based on the results that there exists no co-integration relation between IESH and WPI inflation. This inspection of IESH with CPI and WPI inflation separately provides us with some insight, but because the results obtained are not significant at a higher degree as well as the unit root result proves the actual inflation series to be stationary at I(1), we avoid the ARDL regression. In order to establish the long-run relationship, we run a VECM. The result of which is present in the next section.
4. Long-run co-integration between inflation expectations and realized inflation
The causality test above indicates the actual CPI and WPI inflation cause households’ inflation expectation. The linear relationship between the two variables can be expressed as equation 5 . (5) π t e = α + β π t + e t Where, π t e is the inflation expectations and π t is the actual inflation. The econometric analysis of equation 5 is possible only when the relationship as established is stable. For a stable relationship, the variables should be stationary. Our unit root test suggests that the households' inflation expectations and actual inflation are non-stationary at levels, thereby stationary at I(1). Being non-stationary, there is a high tendency that these variables may not converge to equilibrium in the long run.
Moreover, the deviations from the equilibrium will not be eliminated in the long run. However, if the series are co-integrated, linked up in the long run, then the linear combination of the two series shall be stationary. Solving for the error term, we can rewrite equation 5 as equation 6 below. (6) e t = π t e − β π t
Since the error term is stationary, the linear term of the right-hand side variable of equation 6 must also be stationary. Thus the time path of two non-stationary variables must be linked, that is, they must be co-integrated.
A characteristic of co-integrated variables is that their time path depends upon the extent of deviations from equilibrium, for if such deviations are temporary, at least one of the variables has to move to restore the equilibrium. From the following system of equations, equilibrium can restore at period t either by a decline in expected inflation, an increase in actual inflation, or a combination of both. Δ π t e = δ π e ( π t − 1 e − β π t − 1 ) + ε t π e , δ π e > 0 (7) Δ π t = δ π ( π t − 1 e − β π t − 1 ) + ε t π , δ π < 0
Equation 7 above represents the ECM. In an ECM, the deviations from the equilibrium influence the short-term dynamics of the variables. The inflation expectations and actual inflation change in response to the stochastic shocks ( ε t π e and ε t π ) and to pervious' period deviations from the long-run equilibrium. If the long-run deviation ( π t − 1 e − β π t − 1 > 0 ) is positive, then the inflation expectations would rise, and actual inflation would fall to equilibrium. We further incorporate the lagged term of each variable in both the above equations. Δ π t e = α 10 + α π e ( π t − 1 e − β π t − 1 ) + ∑ α 11 ( i ) Δ π t − i e + ∑ α 12 ( i ) Δ π t − i + ε t π e Δ π t = α 20 − α π ( π t − 1 e − β π t − 1 ) + ∑ α 21 ( i ) Δ π t − i e + ∑ α 22 ( i ) Δ π t − i + ϵ t π
The two variable error correction equations above is a bivariate VAR in first difference augmented by error correction terms α π e ( π t − 1 e − β π t − 1 ) and α π ( π t − 1 e − β π t − 1 ) coined as a vector error correction model (VECM). The parameters α π e and α π is termed as the speed of adjustment parameters. The larger the α π e , the greater the response of inflation expectations to the previous period deviations from long-run equilibrium. At the opposite extreme, a very small value of α π e i ndicate that the inflation expectations are unresponsive to long-run deviations.
We test the co-integration between the series using Johansen co-integration. The lag length for each series is based on Schwartz–Bayes lag selection criterion. The chosen lag structure for CPI and WPI inflation is 1 lag each. The results of Johansen co-integration identify both the actual inflation to be co-integrated with households’ inflation expectations in the long run. In order to understand the short-run and long-run dynamics of the variables, we estimate a VECM model. Table 5 presents the result of the model.
The first and second column of Table 5 presents the VECM results of households' inflation expectations with CPI inflation and WPI inflation. The first panel of the table indicates the long-run dynamics. When interpreted in terms of long-run elasticity, the results indicate a 0.40% change in CPI inflation and 0.51% changes in WPI inflation in response to a 1% change in inflation expectations.
The second panel of Table 5 reports the short-run dynamics of the model. The lagged coefficient of the inflation expectations and actual inflation is not significant but are of correct signs. The “speed of adjustment” coefficient, which depicts the speed at which the deviation is adjusted in the long run, is statistically significant at the 1% significance level. The sign of the speed of adjustment is in accord with the convergence to the long-run equilibrium. The previous period deviation from the long-run equilibrium is corrected in the current period at the speed of 28 and 14% for CPI and WPI inflation, respectively.
The last panel of Table 5 describes the diagnostic test of the model. The null hypothesis of no serial correlation fails to reject as the p -value of chi-square is higher than the 5% level. Hence there is a presence of no serial correlation in residuals of the model. The residuals are all free from heteroskedasticity as the null hypothesis of the presence is rejected with a p -value of 0.16% for CPI inflation, whereas for WPI inflation, the null is not rejected (0.05).
The model diagnostic test in the last row of the table notes the p -value of the test. The LM test is done for the presence of serial correlation in the residual of the model. The null of the test states absence of serial correlation. p -value is greater than 5% indicates acceptance of the null hypothesis. Hence our model is free from serial correlation. Further, the p -value of the chi-square for the absence of heteroskedasticity is done in the last row. The p -value greater than and equal to 5% indicates that the residuals are all homoscedasticity.
5. SVAR methodology
We use a SVAR methodology to examine the determinants of inflation expectations of households in India. We write the SVAR model in the following way for p order: (8) A 0 X t = A 1 X t − 1 + A 2 X t − 1 + ⋯ + A p X t − p + e t Where, X t is a vector of n endogenous variables at time period t . The structured shock in the model is given by e t , is assumed to follow white noise innovation, i.e. uncorrelated, and the variance and covariance matrix are of the n * n identity matrix. The A 0 is defined by A 0 = [ 1 − a 12 0 … − a n 1 0 ⋮ ⋮ ⋮ ⋮ … … ⋮ ⋮ − a n 1 0 − a n 2 0 … 1 ] Where, A 0 is n × n matrix whose row i and column j element is a i j s for s = 1,2,3 …. p .
Now, if each side of the equation is pre-multiplied by A 0 − 1 , the result will be: (9) x t = γ 1 x t − 1 + γ 2 x t − 1 + … … … … + γ p x t − p + v t Where, γ s = A 0 − 1 A s , for s = 1,2,3, …. p v t = A 0 − 1 e t .
Thus equation 9 above is the reduced form of dynamic SVAR of equation 8 . The structural form error e t term and the reduced form residuals v t are related as follows: e t = A 0 v t
To estimate the parameters from the structural form of the model, the models must be exactly or over-identified. A restriction is now imposed to identify the mutually independent structural shocks that will cause the independent variable to fluctuate. The number of restrictions that are imposed for any VAR model is n ( n −1)/2.
The literature widely supports the imposition of recursive restriction on the VAR model, especially in monetary policy [3] . Leduc et al. (2007) use the recursive model restriction to identify the structural shocks. Mishra and Mishra (2010) use the recursive VAR model to identify and measure the monetary policy shock on the real side of the economy with respect to India.
A recursive model has all casual effects in a unidirectional framework; hence there lacks the bidirectional impact of the variable. In a non-recursive model, the causal effect can be represented as both unidirectional and reciprocal. Sims (1986) employs the VAR model for policy analysis. He argues that the policy-making decision consists of some identifying assumptions, and these assumptions in the econometric policy-making model may not be certain. Hence, a VAR model can be used as it can incorporate the uncertainty in the identification issue. Other works that include non-recursive restrictions in SVAR are Gordon and Leeper (1994) , Sims and Zha (1998) and Leeper et al. (1996) . Ueda (2010) employs a non-recursive restriction on the reduced form of the SVAR model for understanding the determinants of inflation expectation. He emphasizes using non-recursive restrictions as inflation expectation has a dual casual effect, i.e. it is affected by and affects inflation. Hence we too employ a non-recursive limitation in our model.
We estimate a VAR model with four endogenous variables. The output gap ( y t ), the short-term nominal interest rate ( i t ), the past inflation rate ( π t ) and the inflation expectation rate at time period t (π t e ). We consider the repo rate to be our short-term nominal interest rate. The inflation rate is the CPI, which constitutes the consumer basket. Also, CPI does show a long-run correlation with household expectations. The real GDP at factor cost is taken for computing the output gap. The output gap is the difference between the actual and potential GDP. So for our analysis, we compute the output gap by differencing the real GDP (seasonally adjusted) by its trend obtained by the HP filter. For de-seasoning, we use the X -11 algorithm from the US Commerce Department. The inflation expectations are three months mean household expectation survey data that RBI quarterly collects for 18 cities presently. The sample period for our analysis is 2006Q2 to 2020Q2.
The zero restriction imposed on our model is described below. The four variables that are represented by X t = { y t , π t − 1 , i t , π t e } , the A 0 the coefficient matrix in the equation is: A 0 = [ X 0 0 0 0 X 0 X X X 0 X X X X X ]
The number of restrictions that we impose is equal to n ( n − 1)/2. So as we have four variables, the restrictions imposed in the model are 4(4 − 1)/2 = 6 zero restrictions. By imposing these restrictions, the equation yields: y t = A 1 ( L ) X t − 1 + e t y i t = β 1 π t e + A 2 ( L ) X t − 1 + e t i π t − 1 = β 2 y t + β 3 π t e + A 3 ( L ) X t − 1 + e t p π t e = β 4 y t − β 5 i t + β 6 π t − 1 + A 4 ( L ) X t − 1 + e t p e
These restrictions imply the following rationale:
The output gap response only to the lagged variable and does not affect any of the contemporaneous variables. The shock associated with the equation implies the demand shock. The second equation represents the interest rate equation. The central bank sets the interest rate. Since the other economic variables like output gap and inflation rate affect the economy with a lag, only inflation expectations are a proxy for their own expectation ( Ueda, 2010 ). The inclusion of the expectations variable in the equation determines the forward-looking behavior of the policymakers. Kim (1999) and Sims and Zha (2006) assume this to be an essential non-recursive restriction. The corresponding shock is the interest rate or monetary policy shock by the central bank. The coefficient of β 1 is expected to be positive. The third equation, past inflation CPI, is not contemporaneously related to interest rate because of lagged effect of monetary policy. This equation is comparable to new Keynesian Phillips Curve. The coefficient of β 2 and β 3 are expected to be positive, and in the case of purely forward-looking NKPC the β 3 is expected to be less than or close to unity. The corresponding shock is interpreted as an unexpected shock in the Phillips Curve. There is no restriction imposed in the fourth equation. Inflation expectation is an unobserved component; hence what effect more is indecisive. Moreover, the model's objective is to understand the determinants of inflation expectation; hence there was no restriction imposed. Also, while making expectation general public do consider all information past or in contemporaneous form. The corresponding shock is interpreted as inflation expectation shock.
Figure 2 represents the non-recursive restriction that is imposed in the model.
6. Estimated results
6.1 variance decomposition.
Table 6 presents the variance decomposition results for all the four endogenous variables at the horizon of 1, 2, 3, 4, 8 and 12 quarters.
The contribution of the monetary policy shock to the expected inflation is about 30% in the short run. Its contribution to realized inflation is relatively negligible, primarily due to price stickiness. Secondly, the contribution of the demand shock to the realized inflation is less than 6% up to four quarters but close to 10% up to the 12 quarters. The immediate impact of the demand shock on inflation expectations is large, which slowly mitigates in the long run. Thirdly, the contribution of inflation shocks to the inflation expectations is huge and is close to 30% in the long run. These findings indicate that realized and expected inflation changes are mainly caused by the monetary policy and the output in the long run.
6.2 Robust analysis
6.2.1 impulse response function.
Figure 3 illustrates the impulse responses of four endogenous variables to four structural shocks. Each column represents a structural shock of one-standard-error magnitude, and each row represents the responses of the endogenous variable.
The first column shows the positive demand shock (e_y) lowers the output gap, does not affect the interest rate and increases the inflation and inflation expectation. The demand shock is expected to increase the output gap when hitting the economy, which is not proven in this Figure. However, the demand shock does increase inflation and, therefore, inflation expectations on the shock, which reduces down the horizon. These features also indicate that the effect of a demand shock on inflation is not persistent in the long run.
The second column represents the monetary policy (interest rate) shock. The positive monetary policy shock does not impact the output gap, whereas it decreases the interest rate. A tightened monetary policy shock does not have an immediate impact on actual inflation. However, an increase in inflation is witnessed from the 4th period onward. With inflation expectations, the shock creates an outward hump shape impact. The “price puzzle” theory, which indicates an increase in interest rate, is witnessed in the analysis here. Thereby, it could be stated here that the price puzzle for the Indian economy even holds in the presence of inflation expectations.
The third column represents that the positive inflation shock lowers the inflation and output gap; however, the shock to output dies quickly. The inflation shock increases the interest rate. For inflation expectations, the shocks firstly increase the inflation expectations, which lowers down and converges to equilibrium in the long run.
The fourth column represents positive inflation expectation shock to the four variables. The shock lowers the interest rate, inflation and inflation expectation immediately, increasing the output gap.
6.2.2 Model diagnostic test
As the last exercise, we run the diagnostic test to prove the stability of the VAR model. We run our SVAR model on lag 1 based on the Akaike information criterion (AIC). To check the stability of the model, we check the stability condition by checking the inverse root. Table 7 provides the result of the inverse root.
The results of the inverse root have the module less than and lie inside the unit circle; hence the VAR model is stable. We then check for the presence of autocorrelation in the residuals. We perform the LM test up to lag 4. Table 8 presents the result of the LM test.
The null hypothesis of the LM test indicates the absence of serial correlation among the residuals. The probability of the test value should lie above 5% to accept the null hypothesis. As shown in Table 8 , our result proves the absence of serial correlation up to lag 6, indicating the estimated VAR model free from autocorrelation.
At last, we check if the residuals are normally distributed. We run the Cholskey orthogonalization, normality test. Table 9 presents the results of the Jarque–Bera test. The null hypothesis of the test considers the residuals to be multivariate normal. We reject the null hypothesis if the p -value lies below 5% and do not reject it if it lies above 5%.
7. Conclusion
We analyze households' inflation expectation data for India, collected quarterly by the RBI for more than a decade. In this work, we explore the time-series properties of the survey data and investigate further the determinants of inflation expectation. The preliminary explanatory test reveals that inflation expectation is a policy variable and should be used in monetary policy as an instrument variable. The realized CPI inflation exhibits both short- and long-run relationships with the inflation expectations, indicating a strong co-relationship between the realized and expected inflation. This established relationship will further help policymakers in anchoring inflation expectations, which will enhance the central bank's credibility. To investigate the determinants of inflation expectations, we employ the SVAR model. We impose non-recursive restrictions on the model, considering the reciprocal relation between inflation and inflation expectations. Inflation expectation adjusts to the change in response to interest rate, inflation and the output gap. Hence while framing the monetary policy, inflation expectations do become an important variable to consider.
Households inflation expectations and actual inflation
Non recursive restriction for SVAR model
Statistical summary of realized inflation and survey-based inflation expectations in India
Autocorrelation test
Residual normality test
A detail discussion of this could be traced from Saakshi (2019) .
The results of the Ng–Perron test are available upon request.
For example: Sims (1980 , 1992) , Bernanke and Mihov (1998) , Christiano et al . (1999) , and Leduc et al . (2007) .
Ball , L. , Mankiw , G. and Reis , R. ( 2005 ), “ Monetary policy for inattentive economies ”, Journal of Monetary Economics , Vol. 52 , pp. 703 - 725 .
Berk , J.M. ( 2002 ), “ Consumers' inflation expectations and monetary policy in Europe ”, DNB Staff Reports 55/2000 , De Nederlandsche Bank , Amsterdam .
Bernanke , B.S. and Mihov , I. ( 1998 ), “ Measuring monetary policy ”, Quarterly Journal of Economics , Vol. 113 , pp. 869 - 902 .
Chen , Y. ( 2008 ), “ Research on new Keynesian Philips Curve in China ”, Economic Research Journal , Vol. 12 , pp. 50 - 64 .
Christiano , L.J. , Eichenbaum , M. and Evans , C.L. ( 1999 ), “ Monetary policy shocks: what have we learned and to what end? ”, in Taylor , J.B. and Woodford , M. (Eds), Handbook of Macroeconomics , Elsevier Science , Amsterdam , Vol. 1A , pp. 65 - 178 .
Davidson , R. and Mackinnon , J.G. ( 2004 ), Econometric Theory and Methods , Oxford University Press , Oxford .
Elliott , G. , Rothenberg , T. and Stock , J. ( 1996 ), “ Efficient tests for an autoregressive unit root ”, Econometrica , Vol. 64 , pp. 813 - 836 .
Feng , S. and Zhu , H. ( 2012 ), “ An analysis of interaction mechanism between inflation expectations and actual inflation in China ”, Journal of Nanjing Normal University , Vol. 6 , pp. 35 - 41 .
Gordon , D.B. and Leeper , E.M. ( 1994 ), “ The dynamic impacts of monetary policy: an exercise in tentative identification ”, Journal of Political Economy , Vol. 102 No. 6 , pp. 1228 - 1247 .
Juselius , K. ( 2006 ), The Cointegrated VAR Model: Methodology and Applications , Oxford University Press , Oxford .
Kim , S. ( 1999 ), “ Do monetary policy shocks matter in the G-7 countries? Using common identifying assumptions about monetary policy across countries ”, Journal of International Economics , Vol. 48 , pp. 387 - 412 .
Kim , J.I. and Lee , J. ( 2013 ), “ How important are inflation expectations in driving Asian inflation? ”, Globalisation and Inflation Dynamics in Asia and the Pacific, Bank for International Settlements , Vol. 70 , pp. 41 - 63 .
Leduc , S. , Sill , K. and Stark , T. ( 2007 ), “ Self-fulfilling expectations and the inflation of the 1970s: evidence from the Livingston survey ”, Journal of Monetary Economics , Vol. 54 , pp. 433 - 459 .
Leeper , E.M. , Sims , C.A. and Zha , T. ( 1996 ), “ What does monetary policy do? ”, Brookings Papers on Economic Activity , Vol. 2 , pp. 1 - 78 .
Mavroeidis , S. , Plagborg-Moller , M. and Stock , J.H. ( 2014 ), “ Empirical evidence on inflation expectations in the new Keynesian Phillips Curve ”, Journal of Economic Literature , Vol. 52 , pp. 124 - 188 .
Mishra , A. and Mishra , V. ( 2010 ), “ A VAR model of monetary policy and hypothetical case of inflation targeting in India ”, Discussion Paper Series 15/10 , Monash University .
Ng , S. and Perron , P. ( 2001 ), “ Lag length selection and the construction of unit root tests with good size and power ”, Econometrica , Vol. 69 , pp. 1519 - 1554 .
Patra , M. and Ray , P. ( 2010 ). Inflation expectations and monetary policy in India: an empirical exploration . IMF Working Paper No. 2010/084 .
Perron , P. and Ng , S. ( 1996 ), “ Useful modifications to some unit root tests with dependent errors and their local asymptotic properties ”, Review of Economic Studies , Vol. 63 , pp. 435 - 463 .
Pesaran , M.H. , Shin , Y. and Smith , R.J. ( 2001 ), “ Bounds testing approaches to the analysis of level relationships ”, Journal of Applied Econometrics , Vol. 16 , pp. 289 - 326 .
Phelps , E. ( 1967 ), “ Phillips curves, expectations of inflation and optimal unemployment over Time ”, Economica , Vol. 34 No. 135 , pp. 254 - 281 , doi: 10.2307/2552025 .
Reid , M. ( 2015 ), “ Inflation expectations of the inattentive general public ”, Economic Modelling , Vol. 46 , pp. 157 - 166 .
Saakshi ( 2019 ), “ A macroeconomic analysis of survey based inflation expectations in India ”, Dissertation , Indian Institute of Technology Kanpur .
Sims , C.A. ( 1980 ), “ Macroeconomics and reality ”, Econometrica , Vol. 48 , pp. 1 - 48 .
Sims , C.A. ( 1986 ), “ Are forecasting models useable for policy analysis? ”, Quarterly Review , Vol. 1011 , pp. 2 - 16 , Federal Reserve Bank of Minneapolis .
Sims , C.A. ( 1992 ), “ Interpreting the macroeconomic time series facts: the effects of monetary policy ”, European Economic Review , Vol. 36 , pp. 975 - 1000 .
Sims , C.A. and Zha , T. ( 1998 ), “ Bayesian methods for dynamic multivariate models ”, International Economic Review , Vol. 39 , pp. 949 - 968 .
Sims , C. and Zha , T. ( 2006 ), “ Does monetary policy generate recessions? ”, Macroeconomic Dynamics , Vol. 10 No. 2 , pp. 231 - 272 , doi: 10.1017/S136510050605019X .
Smyth , R. and Narayan , P. ( 2006 ), “ Dead man walking: an empirical reassessment of the deterrent effect of capital punishment using the bounds testing approach to cointegration ”, Applied Economics , Vol. 38 No. 17 , pp. 1975 - 1989 .
Ueda , K. ( 2010 ), “ Determinants of households' inflation expectations in Japan and the United States ”, Journal of the Japanese and International Economies , Vol. 24 , pp. 503 - 518 .
Further reading
Reserve Bank of India ( 2009 ), Report of the Technical Advisory Committee on Surveys .
Reserve Bank of India ( 2010 ), RBI Inflation Expectations Survey of Households: September 2010 , Round 21 .
Reserve Bank of India ( 2014 ), Report of the Expert Committee to Revise and Strengthen the Monetary Policy Framework .
Acknowledgements
The author expresses gratitude to the Editor-in-charge, the Associate Editor and the anonymous referees for their helpful comments. The author is also thankful to Sohini Sahu and Sunny Bhushan for their valuable comments and suggestions.
Corresponding author
About the author.
Saakshi Jha is an Assistant Professor in Economics at the Indian Institute of Management Ranchi (IIM Ranchi), India. She received her Doctor of Philosophy degree in Economics from the Indian Institute of Technology Kanpur (IITK), India, and was awarded the Outstanding Ph.D. Thesis Award. She earned her Master of Arts degree in Economics from Banaras Hindu University (BHU), India and obtained her Bachelor of Arts degree from St. Aloysius College (Jabalpur), India. She works in macroeconomics, and her current research focuses primarily on monetary economics and economic growth.
Related articles
We’re listening — tell us what you think, something didn’t work….
Report bugs here
All feedback is valuable
Please share your general feedback
Join us on our journey
Platform update page.
Visit emeraldpublishing.com/platformupdate to discover the latest news and updates
Questions & More Information
Answers to the most commonly asked questions here
- Search Menu
- Browse content in Arts and Humanities
- Browse content in Archaeology
- Anglo-Saxon and Medieval Archaeology
- Archaeological Methodology and Techniques
- Archaeology by Region
- Archaeology of Religion
- Archaeology of Trade and Exchange
- Biblical Archaeology
- Contemporary and Public Archaeology
- Environmental Archaeology
- Historical Archaeology
- History and Theory of Archaeology
- Industrial Archaeology
- Landscape Archaeology
- Mortuary Archaeology
- Prehistoric Archaeology
- Underwater Archaeology
- Urban Archaeology
- Zooarchaeology
- Browse content in Architecture
- Architectural Structure and Design
- History of Architecture
- Residential and Domestic Buildings
- Theory of Architecture
- Browse content in Art
- Art Subjects and Themes
- History of Art
- Industrial and Commercial Art
- Theory of Art
- Biographical Studies
- Byzantine Studies
- Browse content in Classical Studies
- Classical History
- Classical Philosophy
- Classical Mythology
- Classical Literature
- Classical Reception
- Classical Art and Architecture
- Classical Oratory and Rhetoric
- Greek and Roman Papyrology
- Greek and Roman Epigraphy
- Greek and Roman Law
- Greek and Roman Archaeology
- Late Antiquity
- Religion in the Ancient World
- Digital Humanities
- Browse content in History
- Colonialism and Imperialism
- Diplomatic History
- Environmental History
- Genealogy, Heraldry, Names, and Honours
- Genocide and Ethnic Cleansing
- Historical Geography
- History by Period
- History of Emotions
- History of Agriculture
- History of Education
- History of Gender and Sexuality
- Industrial History
- Intellectual History
- International History
- Labour History
- Legal and Constitutional History
- Local and Family History
- Maritime History
- Military History
- National Liberation and Post-Colonialism
- Oral History
- Political History
- Public History
- Regional and National History
- Revolutions and Rebellions
- Slavery and Abolition of Slavery
- Social and Cultural History
- Theory, Methods, and Historiography
- Urban History
- World History
- Browse content in Language Teaching and Learning
- Language Learning (Specific Skills)
- Language Teaching Theory and Methods
- Browse content in Linguistics
- Applied Linguistics
- Cognitive Linguistics
- Computational Linguistics
- Forensic Linguistics
- Grammar, Syntax and Morphology
- Historical and Diachronic Linguistics
- History of English
- Language Evolution
- Language Reference
- Language Acquisition
- Language Variation
- Language Families
- Lexicography
- Linguistic Anthropology
- Linguistic Theories
- Linguistic Typology
- Phonetics and Phonology
- Psycholinguistics
- Sociolinguistics
- Translation and Interpretation
- Writing Systems
- Browse content in Literature
- Bibliography
- Children's Literature Studies
- Literary Studies (Romanticism)
- Literary Studies (American)
- Literary Studies (Asian)
- Literary Studies (European)
- Literary Studies (Eco-criticism)
- Literary Studies (Modernism)
- Literary Studies - World
- Literary Studies (1500 to 1800)
- Literary Studies (19th Century)
- Literary Studies (20th Century onwards)
- Literary Studies (African American Literature)
- Literary Studies (British and Irish)
- Literary Studies (Early and Medieval)
- Literary Studies (Fiction, Novelists, and Prose Writers)
- Literary Studies (Gender Studies)
- Literary Studies (Graphic Novels)
- Literary Studies (History of the Book)
- Literary Studies (Plays and Playwrights)
- Literary Studies (Poetry and Poets)
- Literary Studies (Postcolonial Literature)
- Literary Studies (Queer Studies)
- Literary Studies (Science Fiction)
- Literary Studies (Travel Literature)
- Literary Studies (War Literature)
- Literary Studies (Women's Writing)
- Literary Theory and Cultural Studies
- Mythology and Folklore
- Shakespeare Studies and Criticism
- Browse content in Media Studies
- Browse content in Music
- Applied Music
- Dance and Music
- Ethics in Music
- Ethnomusicology
- Gender and Sexuality in Music
- Medicine and Music
- Music Cultures
- Music and Media
- Music and Religion
- Music and Culture
- Music Education and Pedagogy
- Music Theory and Analysis
- Musical Scores, Lyrics, and Libretti
- Musical Structures, Styles, and Techniques
- Musicology and Music History
- Performance Practice and Studies
- Race and Ethnicity in Music
- Sound Studies
- Browse content in Performing Arts
- Browse content in Philosophy
- Aesthetics and Philosophy of Art
- Epistemology
- Feminist Philosophy
- History of Western Philosophy
- Metaphysics
- Moral Philosophy
- Non-Western Philosophy
- Philosophy of Language
- Philosophy of Mind
- Philosophy of Perception
- Philosophy of Science
- Philosophy of Action
- Philosophy of Law
- Philosophy of Religion
- Philosophy of Mathematics and Logic
- Practical Ethics
- Social and Political Philosophy
- Browse content in Religion
- Biblical Studies
- Christianity
- East Asian Religions
- History of Religion
- Judaism and Jewish Studies
- Qumran Studies
- Religion and Education
- Religion and Health
- Religion and Politics
- Religion and Science
- Religion and Law
- Religion and Art, Literature, and Music
- Religious Studies
- Browse content in Society and Culture
- Cookery, Food, and Drink
- Cultural Studies
- Customs and Traditions
- Ethical Issues and Debates
- Hobbies, Games, Arts and Crafts
- Lifestyle, Home, and Garden
- Natural world, Country Life, and Pets
- Popular Beliefs and Controversial Knowledge
- Sports and Outdoor Recreation
- Technology and Society
- Travel and Holiday
- Visual Culture
- Browse content in Law
- Arbitration
- Browse content in Company and Commercial Law
- Commercial Law
- Company Law
- Browse content in Comparative Law
- Systems of Law
- Competition Law
- Browse content in Constitutional and Administrative Law
- Government Powers
- Judicial Review
- Local Government Law
- Military and Defence Law
- Parliamentary and Legislative Practice
- Construction Law
- Contract Law
- Browse content in Criminal Law
- Criminal Procedure
- Criminal Evidence Law
- Sentencing and Punishment
- Employment and Labour Law
- Environment and Energy Law
- Browse content in Financial Law
- Banking Law
- Insolvency Law
- History of Law
- Human Rights and Immigration
- Intellectual Property Law
- Browse content in International Law
- Private International Law and Conflict of Laws
- Public International Law
- IT and Communications Law
- Jurisprudence and Philosophy of Law
- Law and Politics
- Law and Society
- Browse content in Legal System and Practice
- Courts and Procedure
- Legal Skills and Practice
- Primary Sources of Law
- Regulation of Legal Profession
- Medical and Healthcare Law
- Browse content in Policing
- Criminal Investigation and Detection
- Police and Security Services
- Police Procedure and Law
- Police Regional Planning
- Browse content in Property Law
- Personal Property Law
- Study and Revision
- Terrorism and National Security Law
- Browse content in Trusts Law
- Wills and Probate or Succession
- Browse content in Medicine and Health
- Browse content in Allied Health Professions
- Arts Therapies
- Clinical Science
- Dietetics and Nutrition
- Occupational Therapy
- Operating Department Practice
- Physiotherapy
- Radiography
- Speech and Language Therapy
- Browse content in Anaesthetics
- General Anaesthesia
- Neuroanaesthesia
- Clinical Neuroscience
- Browse content in Clinical Medicine
- Acute Medicine
- Cardiovascular Medicine
- Clinical Genetics
- Clinical Pharmacology and Therapeutics
- Dermatology
- Endocrinology and Diabetes
- Gastroenterology
- Genito-urinary Medicine
- Geriatric Medicine
- Infectious Diseases
- Medical Toxicology
- Medical Oncology
- Pain Medicine
- Palliative Medicine
- Rehabilitation Medicine
- Respiratory Medicine and Pulmonology
- Rheumatology
- Sleep Medicine
- Sports and Exercise Medicine
- Community Medical Services
- Critical Care
- Emergency Medicine
- Forensic Medicine
- Haematology
- History of Medicine
- Browse content in Medical Skills
- Clinical Skills
- Communication Skills
- Nursing Skills
- Surgical Skills
- Browse content in Medical Dentistry
- Oral and Maxillofacial Surgery
- Paediatric Dentistry
- Restorative Dentistry and Orthodontics
- Surgical Dentistry
- Medical Ethics
- Medical Statistics and Methodology
- Browse content in Neurology
- Clinical Neurophysiology
- Neuropathology
- Nursing Studies
- Browse content in Obstetrics and Gynaecology
- Gynaecology
- Occupational Medicine
- Ophthalmology
- Otolaryngology (ENT)
- Browse content in Paediatrics
- Neonatology
- Browse content in Pathology
- Chemical Pathology
- Clinical Cytogenetics and Molecular Genetics
- Histopathology
- Medical Microbiology and Virology
- Patient Education and Information
- Browse content in Pharmacology
- Psychopharmacology
- Browse content in Popular Health
- Caring for Others
- Complementary and Alternative Medicine
- Self-help and Personal Development
- Browse content in Preclinical Medicine
- Cell Biology
- Molecular Biology and Genetics
- Reproduction, Growth and Development
- Primary Care
- Professional Development in Medicine
- Browse content in Psychiatry
- Addiction Medicine
- Child and Adolescent Psychiatry
- Forensic Psychiatry
- Learning Disabilities
- Old Age Psychiatry
- Psychotherapy
- Browse content in Public Health and Epidemiology
- Epidemiology
- Public Health
- Browse content in Radiology
- Clinical Radiology
- Interventional Radiology
- Nuclear Medicine
- Radiation Oncology
- Reproductive Medicine
- Browse content in Surgery
- Cardiothoracic Surgery
- Gastro-intestinal and Colorectal Surgery
- General Surgery
- Neurosurgery
- Paediatric Surgery
- Peri-operative Care
- Plastic and Reconstructive Surgery
- Surgical Oncology
- Transplant Surgery
- Trauma and Orthopaedic Surgery
- Vascular Surgery
- Browse content in Science and Mathematics
- Browse content in Biological Sciences
- Aquatic Biology
- Biochemistry
- Bioinformatics and Computational Biology
- Developmental Biology
- Ecology and Conservation
- Evolutionary Biology
- Genetics and Genomics
- Microbiology
- Molecular and Cell Biology
- Natural History
- Plant Sciences and Forestry
- Research Methods in Life Sciences
- Structural Biology
- Systems Biology
- Zoology and Animal Sciences
- Browse content in Chemistry
- Analytical Chemistry
- Computational Chemistry
- Crystallography
- Environmental Chemistry
- Industrial Chemistry
- Inorganic Chemistry
- Materials Chemistry
- Medicinal Chemistry
- Mineralogy and Gems
- Organic Chemistry
- Physical Chemistry
- Polymer Chemistry
- Study and Communication Skills in Chemistry
- Theoretical Chemistry
- Browse content in Computer Science
- Artificial Intelligence
- Computer Architecture and Logic Design
- Game Studies
- Human-Computer Interaction
- Mathematical Theory of Computation
- Programming Languages
- Software Engineering
- Systems Analysis and Design
- Virtual Reality
- Browse content in Computing
- Business Applications
- Computer Security
- Computer Games
- Computer Networking and Communications
- Digital Lifestyle
- Graphical and Digital Media Applications
- Operating Systems
- Browse content in Earth Sciences and Geography
- Atmospheric Sciences
- Environmental Geography
- Geology and the Lithosphere
- Maps and Map-making
- Meteorology and Climatology
- Oceanography and Hydrology
- Palaeontology
- Physical Geography and Topography
- Regional Geography
- Soil Science
- Urban Geography
- Browse content in Engineering and Technology
- Agriculture and Farming
- Biological Engineering
- Civil Engineering, Surveying, and Building
- Electronics and Communications Engineering
- Energy Technology
- Engineering (General)
- Environmental Science, Engineering, and Technology
- History of Engineering and Technology
- Mechanical Engineering and Materials
- Technology of Industrial Chemistry
- Transport Technology and Trades
- Browse content in Environmental Science
- Applied Ecology (Environmental Science)
- Conservation of the Environment (Environmental Science)
- Environmental Sustainability
- Environmentalist Thought and Ideology (Environmental Science)
- Management of Land and Natural Resources (Environmental Science)
- Natural Disasters (Environmental Science)
- Nuclear Issues (Environmental Science)
- Pollution and Threats to the Environment (Environmental Science)
- Social Impact of Environmental Issues (Environmental Science)
- History of Science and Technology
- Browse content in Materials Science
- Ceramics and Glasses
- Composite Materials
- Metals, Alloying, and Corrosion
- Nanotechnology
- Browse content in Mathematics
- Applied Mathematics
- Biomathematics and Statistics
- History of Mathematics
- Mathematical Education
- Mathematical Finance
- Mathematical Analysis
- Numerical and Computational Mathematics
- Probability and Statistics
- Pure Mathematics
- Browse content in Neuroscience
- Cognition and Behavioural Neuroscience
- Development of the Nervous System
- Disorders of the Nervous System
- History of Neuroscience
- Invertebrate Neurobiology
- Molecular and Cellular Systems
- Neuroendocrinology and Autonomic Nervous System
- Neuroscientific Techniques
- Sensory and Motor Systems
- Browse content in Physics
- Astronomy and Astrophysics
- Atomic, Molecular, and Optical Physics
- Biological and Medical Physics
- Classical Mechanics
- Computational Physics
- Condensed Matter Physics
- Electromagnetism, Optics, and Acoustics
- History of Physics
- Mathematical and Statistical Physics
- Measurement Science
- Nuclear Physics
- Particles and Fields
- Plasma Physics
- Quantum Physics
- Relativity and Gravitation
- Semiconductor and Mesoscopic Physics
- Browse content in Psychology
- Affective Sciences
- Clinical Psychology
- Cognitive Psychology
- Cognitive Neuroscience
- Criminal and Forensic Psychology
- Developmental Psychology
- Educational Psychology
- Evolutionary Psychology
- Health Psychology
- History and Systems in Psychology
- Music Psychology
- Neuropsychology
- Organizational Psychology
- Psychological Assessment and Testing
- Psychology of Human-Technology Interaction
- Psychology Professional Development and Training
- Research Methods in Psychology
- Social Psychology
- Browse content in Social Sciences
- Browse content in Anthropology
- Anthropology of Religion
- Human Evolution
- Medical Anthropology
- Physical Anthropology
- Regional Anthropology
- Social and Cultural Anthropology
- Theory and Practice of Anthropology
- Browse content in Business and Management
- Business Ethics
- Business Strategy
- Business History
- Business and Technology
- Business and Government
- Business and the Environment
- Comparative Management
- Corporate Governance
- Corporate Social Responsibility
- Entrepreneurship
- Health Management
- Human Resource Management
- Industrial and Employment Relations
- Industry Studies
- Information and Communication Technologies
- International Business
- Knowledge Management
- Management and Management Techniques
- Operations Management
- Organizational Theory and Behaviour
- Pensions and Pension Management
- Public and Nonprofit Management
- Strategic Management
- Supply Chain Management
- Browse content in Criminology and Criminal Justice
- Criminal Justice
- Criminology
- Forms of Crime
- International and Comparative Criminology
- Youth Violence and Juvenile Justice
- Development Studies
- Browse content in Economics
- Agricultural, Environmental, and Natural Resource Economics
- Asian Economics
- Behavioural Finance
- Behavioural Economics and Neuroeconomics
- Econometrics and Mathematical Economics
- Economic History
- Economic Systems
- Economic Methodology
- Economic Development and Growth
- Financial Markets
- Financial Institutions and Services
- General Economics and Teaching
- Health, Education, and Welfare
- History of Economic Thought
- International Economics
- Labour and Demographic Economics
- Law and Economics
- Macroeconomics and Monetary Economics
- Microeconomics
- Public Economics
- Urban, Rural, and Regional Economics
- Welfare Economics
- Browse content in Education
- Adult Education and Continuous Learning
- Care and Counselling of Students
- Early Childhood and Elementary Education
- Educational Equipment and Technology
- Educational Strategies and Policy
- Higher and Further Education
- Organization and Management of Education
- Philosophy and Theory of Education
- Schools Studies
- Secondary Education
- Teaching of a Specific Subject
- Teaching of Specific Groups and Special Educational Needs
- Teaching Skills and Techniques
- Browse content in Environment
- Applied Ecology (Social Science)
- Climate Change
- Conservation of the Environment (Social Science)
- Environmentalist Thought and Ideology (Social Science)
- Natural Disasters (Environment)
- Social Impact of Environmental Issues (Social Science)
- Browse content in Human Geography
- Cultural Geography
- Economic Geography
- Political Geography
- Browse content in Interdisciplinary Studies
- Communication Studies
- Museums, Libraries, and Information Sciences
- Browse content in Politics
- African Politics
- Asian Politics
- Chinese Politics
- Comparative Politics
- Conflict Politics
- Elections and Electoral Studies
- Environmental Politics
- European Union
- Foreign Policy
- Gender and Politics
- Human Rights and Politics
- Indian Politics
- International Relations
- International Organization (Politics)
- International Political Economy
- Irish Politics
- Latin American Politics
- Middle Eastern Politics
- Political Behaviour
- Political Economy
- Political Institutions
- Political Methodology
- Political Communication
- Political Philosophy
- Political Sociology
- Political Theory
- Politics and Law
- Public Policy
- Public Administration
- Quantitative Political Methodology
- Regional Political Studies
- Russian Politics
- Security Studies
- State and Local Government
- UK Politics
- US Politics
- Browse content in Regional and Area Studies
- African Studies
- Asian Studies
- East Asian Studies
- Japanese Studies
- Latin American Studies
- Middle Eastern Studies
- Native American Studies
- Scottish Studies
- Browse content in Research and Information
- Research Methods
- Browse content in Social Work
- Addictions and Substance Misuse
- Adoption and Fostering
- Care of the Elderly
- Child and Adolescent Social Work
- Couple and Family Social Work
- Developmental and Physical Disabilities Social Work
- Direct Practice and Clinical Social Work
- Emergency Services
- Human Behaviour and the Social Environment
- International and Global Issues in Social Work
- Mental and Behavioural Health
- Social Justice and Human Rights
- Social Policy and Advocacy
- Social Work and Crime and Justice
- Social Work Macro Practice
- Social Work Practice Settings
- Social Work Research and Evidence-based Practice
- Welfare and Benefit Systems
- Browse content in Sociology
- Childhood Studies
- Community Development
- Comparative and Historical Sociology
- Economic Sociology
- Gender and Sexuality
- Gerontology and Ageing
- Health, Illness, and Medicine
- Marriage and the Family
- Migration Studies
- Occupations, Professions, and Work
- Organizations
- Population and Demography
- Race and Ethnicity
- Social Theory
- Social Movements and Social Change
- Social Research and Statistics
- Social Stratification, Inequality, and Mobility
- Sociology of Religion
- Sociology of Education
- Sport and Leisure
- Urban and Rural Studies
- Browse content in Warfare and Defence
- Defence Strategy, Planning, and Research
- Land Forces and Warfare
- Military Administration
- Military Life and Institutions
- Naval Forces and Warfare
- Other Warfare and Defence Issues
- Peace Studies and Conflict Resolution
- Weapons and Equipment

- < Previous chapter

4 Inflation In India: What do the Data Reveal about Regional Dimensions?
- Published: June 2012
- Cite Icon Cite
- Permissions Icon Permissions
In this study, the regional variation in inflation is examined using national income deflator, wholesale price index, and consumer price indices of agricultural labour, industrial workers, and urban non-manual workers. All partial cost of living indices show a decline in inflation after liberalization. The same trend is shown by the national income deflators. Among the three broad sectors of the economy, the decline is highest for the secondary sector but remains more or less the same for the primary and tertiary sectors. The σ-convergence of inflation among states for the entire period shows that there is convergence in all indicators except in the cost of living index of urban non-manual workers. Further statistical analysis using the dummy variable method does not support the convergence after liberalization. Finally, the aggregate index of all the partial indices using Borda Rank do not show any stable pattern for the states between the two periods, that is, post- and pre-liberalization period. Two notable extremes are West Bengal and Karnataka.
Signed in as
Institutional accounts.
- GoogleCrawler [DO NOT DELETE]
- Google Scholar Indexing
Personal account
- Sign in with email/username & password
- Get email alerts
- Save searches
- Purchase content
- Activate your purchase/trial code
Institutional access
- Sign in with a library card Sign in with username/password Recommend to your librarian
- Institutional account management
- Get help with access
Access to content on Oxford Academic is often provided through institutional subscriptions and purchases. If you are a member of an institution with an active account, you may be able to access content in one of the following ways:
IP based access
Typically, access is provided across an institutional network to a range of IP addresses. This authentication occurs automatically, and it is not possible to sign out of an IP authenticated account.
Sign in through your institution
Choose this option to get remote access when outside your institution. Shibboleth/Open Athens technology is used to provide single sign-on between your institution’s website and Oxford Academic.
- Click Sign in through your institution.
- Select your institution from the list provided, which will take you to your institution's website to sign in.
- When on the institution site, please use the credentials provided by your institution. Do not use an Oxford Academic personal account.
- Following successful sign in, you will be returned to Oxford Academic.
If your institution is not listed or you cannot sign in to your institution’s website, please contact your librarian or administrator.
Sign in with a library card
Enter your library card number to sign in. If you cannot sign in, please contact your librarian.
Society Members
Society member access to a journal is achieved in one of the following ways:
Sign in through society site
Many societies offer single sign-on between the society website and Oxford Academic. If you see ‘Sign in through society site’ in the sign in pane within a journal:
- Click Sign in through society site.
- When on the society site, please use the credentials provided by that society. Do not use an Oxford Academic personal account.
If you do not have a society account or have forgotten your username or password, please contact your society.
Sign in using a personal account
Some societies use Oxford Academic personal accounts to provide access to their members. See below.
A personal account can be used to get email alerts, save searches, purchase content, and activate subscriptions.
Some societies use Oxford Academic personal accounts to provide access to their members.
Viewing your signed in accounts
Click the account icon in the top right to:
- View your signed in personal account and access account management features.
- View the institutional accounts that are providing access.
Signed in but can't access content
Oxford Academic is home to a wide variety of products. The institutional subscription may not cover the content that you are trying to access. If you believe you should have access to that content, please contact your librarian.
For librarians and administrators, your personal account also provides access to institutional account management. Here you will find options to view and activate subscriptions, manage institutional settings and access options, access usage statistics, and more.
Our books are available by subscription or purchase to libraries and institutions.
- About Oxford Academic
- Publish journals with us
- University press partners
- What we publish
- New features
- Open access
- Rights and permissions
- Accessibility
- Advertising
- Media enquiries
- Oxford University Press
- Oxford Languages
- University of Oxford
Oxford University Press is a department of the University of Oxford. It furthers the University's objective of excellence in research, scholarship, and education by publishing worldwide
- Copyright © 2024 Oxford University Press
- Cookie settings
- Cookie policy
- Privacy policy
- Legal notice
This Feature Is Available To Subscribers Only
Sign In or Create an Account
This PDF is available to Subscribers Only
For full access to this pdf, sign in to an existing account, or purchase an annual subscription.
MBA Knowledge Base
Business • Management • Technology
Home » Managerial Economics » Case Study: Inflation in India
Case Study: Inflation in India
Knowing Inflation
By inflation one generally means rise in prices. To be more correct inflation is persistent rise in the general price level rather than a once-for-all rise in it, while deflation is persistent falling price. A situation is described as inflationary when either the prices or the supply of money are rising, but in practice both will rise together. These days economies of all countries whether underdeveloped, developing as well developed suffers from inflation. Inflation or persistent rising prices are major problem today in world. Because of many reasons, first, the rate of inflation these years are much high than experienced earlier periods. Second, Inflation in these years coexists with high rate of unemployment, which is a new phenomenon and made it difficult to control inflation.
An inflationary situation is where there is ‘too much money chasing too few goods’. As products/services are scarce in relation to the money available in the hands of buyers, prices of the products/services rise to adjust for the larger quantum of money chasing them.
Read More: Definition of inflation and it’s types
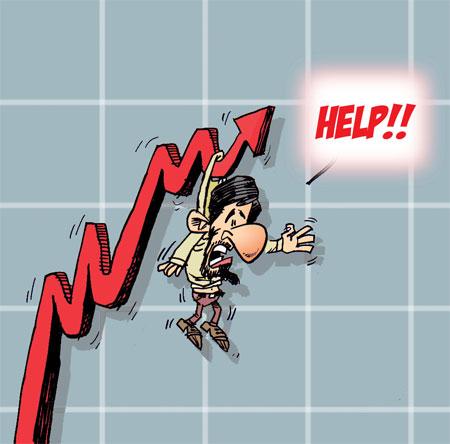
Inflation is no stranger to the Indian economy. The Indian economy has been registering stupendous growth after the liberalization of Indian economy. In fact, till the early nineties Indians were used to ignore inflation. But, since the mid-nineties controlling inflation has become a priority. The natural fallout of this has been that we, as a nation, have become virtually intolerant to inflation. The opening up of the Indian economy in the early 1990s had increased India’s industrial output and consequently has raised the India Inflation Rate. While inflation was primarily caused by domestic factors (supply usually was unable to meet demand, resulting in the classical definition of inflation of too much money chasing too few goods), today the situation has changed significantly.
Inflation today is caused more by global rather than by domestic factors. Naturally, as the Indian economy undergoes structural changes, the causes of domestic inflation too have undergone tectonic changes. The main cause of rise in the rate of inflation rate in India is the pricing disparity of agricultural products between the producer and consumers in the Indian market. Moreover, the sky-rocketing of prices of food products, manufacturing products, and essential commodities have also catapulted the inflation rate in India. Furthermore, the unstable international crude oil prices have worsened the situation.
Defining causes of Inflation
What exactly is the nature of this inflation which has the nation in its grip? The different causes of inflation which are experienced in Indian economy in a large proportion would be:-
- Demand-pull inflation: This is basically when the aggregate demand in an economy exceeds the aggregate supply. It is also defined as `too much money chasing too few goods’. Bare-boned, it means that a country is capable of producing only 100 items but the demand is for 105 items. It’s a very simple demand-supply issue. The more demand there is, the costlier it becomes. Much the same as the way real estate in the country is rising.
- Cost-push inflation: This is caused when there is a supply shock. This represents the condition where, even though there is no increase in Aggregate Demand, prices may still rise. I.e. non availability of a commodity would lead to increase in prices. This may happen if the costs of especially wage cost rise.
- Imported Inflation: This is inflation due to increases in the prices of imports. Increases in the prices of imported final products directly affect any expenditure-based measure of inflation. They play an important role in driving the rise in domestic prices. The rise in the global prices of crude oil and agricultural commodities, including food grains, and industrial products, and setbacks to global economy resulting from sub-prime mortgage disaster and US recession have contributed to India’s inflation.
Other Causes:
- When the government of a country print money in excess, prices increase to keep up with the increase in currency, leading to inflation.
- Increase in production and labor costs, have a direct impact on the price of the final product, resulting in inflation.
- When countries borrow money, they have to cope with the interest burden. This interest burden results in inflation.
- High taxes on consumer products, can also lead to inflation. An increase in indirect taxes can also lead to increased production costs.
- Inflation can artificially be created through a circular increase in wage earners demands and then the subsequent increase in producer costs which will drive up the prices of their goods and services. This will then translate back into higher prices for the wage earners or consumers. As demands go higher from each side, inflation will continue to rise.
- Debt, war and other issues that cause a drastic financial blunder can also cause the inflation.
Measuring Inflation
Inflation in India is mainly estimated on the basis of fluctuations in the wholesale price index (WPI). The wholesale price index comprises of the following indices:
- Domestic Wholesale Price Index (DWPI)
- Export Price Index (EPI)
- Import Price Index (IPI)
- Overall Wholesale Price Index (OWPI)
The WPI consists of about 435 items and has three broad categories. They are:-
- Primary Articles (weight of 22.0253) — 22% Index
- Fuel, Power, Light, and Lubricants (weight of 14.2262) – 14% Index
- Manufactured Products (weight of 63.7485) — 64% Index
The base year of the WPI is 1993-94. The base year usually chosen is one where there has been fairly less volatility. The Indian WPI figure is released weekly on every Thursday. But recently the government has approved the proposal to release a wholesale price based inflation data on a monthly basis, instead of every week. The new series of WPI based inflation with 2004-05 as the base year would be launched soon. The move is aimed at improving the accuracy of the inflation data.
The monthly release of WPI is a widely-followed international practice. And, it is expected to improve the quality of data. Collection of price data of manufactured products will, accordingly, have a monthly frequency consistent with the practice of release of WPI. The new series of WPI based inflation with 2004-05 as the base year would be launched soon. However, the government will continue to release a weekly index for primary articles, and commodities in the fuel, power, light and lubricants groups. The weekly index will facilitate monitoring of prices of agricultural commodities and petroleum products, which are sensitive in nature.
Problems of Inflation
It has been reported that the manufacturing capacity in India is running around 95 per cent, which usually means it is running at full capacity. Therefore, when the price of manufactured products is increasing, it means that demand is usually higher than supply and that is a clear case of demand-pull inflation.
On the primary goods front, which consists of fruits, vegetables, food-grains etc, it is not that straight-forward. It has certainly been all over the news that the prices of fruits and vegetables are increasing and a trip to the supermarket or local grocery shop will testify to that. Although it is a clear case of demand-pull inflation, on the other, it is also a bit of a supply shock when one considers the fact that there is an abnormally high percentage of fruits and vegetables that goes to waste because of the lack of cold-storage facilities. Some estimates say 50 per cent of produce goes to waste and that is a conservative number.
The fuel price hike is a straight example of cost push inflation. When OPEC (The Organization of the Petroleum Exporting Countries) was formed, it squeezed the supply of oil and this caused oil prices to rise, contributing to higher inflation. Since oil is used in every industry, a sharp rise in the price of oil leads to an increase in the prices of all commodities.
The in depth problems due to inflation would be:
- When the balance between supply and demand goes out of control, consumers could change their buying habits, forcing manufacturers to cut down production.
- Inflation can create major problems in the economy. Price increase can worsen the poverty affecting low income household.
- Inflation creates economic uncertainty and is a dampener to the investment climate slowing growth and finally it reduce savings and thereby consumption.
- The producers would not be able to control the cost of raw material and labor and hence the price of the final product. This could result in less profit or in some extreme case no profit, forcing them out of business.
- Manufacturers would not have an incentive to invest in new equipment and new technology.
- Uncertainty would force people to withdraw money from the bank and convert it into product with long lasting value like gold, artifacts.
The imbalances inflation has created in the Indian economy:-
- It has created a new rich class in social and political lives who are corrupt themselves and also corrupt the overall society.
- The increased prices reduced the capacity to save and people preferred present consumption to future consumption.
- It has provided protection and subsides to industries which bred inefficiency.
- It has lead to misallocation of resources due to distortion of relative prices and finally a redistribution of wealth from the poor to the rich.
- It disturbs balance of payments.
Curbing Inflation
There are several reasons why we should worry about the spike in the inflation rate. Inflation is a tax on the poor and long-term lenders. Inflation is already too high, though it is definitely not at economy-wrecking levels. But it’s best to be serious about the threat it poses. Inflation has emerged as the biggest risk to the global outlook, having risen to very high levels across the world, levels that have not been generally seen for a couple of decades.
Currently, in India, we go through boom-and-bust cycles; sometimes GDP growth rates are very high and sometimes GDP growth rates drop sharply. This boom-and-bust cycle is unpleasant for every household. There is a powerful international consensus that stabilizing inflation reduces this boom-and-bust cycle of GDP growth.
India is facing the problem of inflationary pressure because of the increase in Aggregate Demand while Aggregate Supply is respectively constant. The inflationary pressure faced by Indian Economy is due to Demand-Pull inflation i.e. Aggregate Demand > Aggregate Supply. Thus to curb inflation need to fill the gap between Aggregate Demand and Aggregate Supply. For this either we need to increase Aggregate Supply or decrease Aggregate Demand that can hamper economic development. To increase Aggregate Supply either there is a need to increase production capacity of all current production units or to build new production plants.
But as quoted in a survey done by RBI that all the production plants are running at their full production capacity thus all resources are full employed. The other way is to build new plant but to do this will take at least 18months to 2years. Thus meanwhile we need to decrease Money Supply, which is opted by RBI. Increasing production of useful goods and services is what India should focus on.
As in the short run it is not possible to meet the gap between Aggregate Demand and Aggregate Supply thus RBI is planning to decrease liquidity by reducing Money Supply from the market. RBI planned that Liquidity from the market can be drained by decreasing money supply and to do so it is increasing CRR, repo rate, reverse repo rate and taking other measure like that.
CRR i.e. Cash Reserve Ratio (Liquidity Ratio) is the percentage of deposit that a commercial bank needs to keep with RBI by which RBI control liquidity in the market and create Money Supply. Repo Rate is the rate at which RBI lends money to other commercial Banks.
The Reserve Bank said that such decisions had been taken to curb inflation in India. RBI is taking positive steps to reduce the inflation since inflations rates are going up week by week. By raising the reserve rate, a deflationary pressure can be put on the economy, since the money multiplier has been reduced. People will therefore save more. But in this hike, there is negative impact in terms of higher interest rates and personal loans, vehicle loans and other loans become costly. RBI may hike the rate to reduce the money circulation in the country but it also decreased the sales of all loan items and further it reduces the manufacturing activity of many industries. Now the public and private sector banks may raise the interest rate at which they lend money to borrowers.
Produce more exports than imports than another country, then your money deflates with respect to that currency. Exporting becomes a problem cause buyers from outside feel that the goods are expensive so they prefer buying some other country’s goods with cheaper rate. Thus money does not come in. in the same way, when public has more money they buy foreign goods, thus money goes out which is bad. There is a need to encourage people to purchase goods produced within the country.
It is important for policymakers to make credible announcements and degrade interest rates. Private agents must believe that these announcements will reflect actual future policy. If an announcement about low-level inflation targets is made but not believed by private agents, wage-setting will anticipate high-level inflation and so wages will be higher and inflation will rise. A high wage will increase a consumer’s demand (demand pull inflation) and a firm’s costs (cost push inflation), so inflation rises. Hence, if a policymaker’s announcements regarding monetary policy are not credible, policy will not have the desired effect.
Keynesians emphasize reducing demand in general, often through fiscal policy, using increased taxation or reduced government spending to reduce demand as well as by using monetary policy. Supply-side economists advocate fighting inflation by fixing the exchange rate between the currency and some reference currency such as gold. This would be a return to the gold standard. All of these policies are achieved in practice through a process of open market operations.
As individuals what can we do to stop Inflation?
Firstly save!!! As much of your money as possible should be saved. This will reduce the demand on the economy and hopefully reduce inflation. Do not overuse daily essentials like cooking gas, electricity etc. Cut down on inessentials when buying groceries. Look for cheaper alternatives to products that you normally buy.
Keep roads, highways, sidewalks, etc., beautified to help attract tourism and bring additional monetary into a growing economy. Stop illegal immigration. Illegal activities reap the benefits of the country but don’t pay taxes. Government-backed investment schemes such as Post Office Savings Schemes, Public Provident Funds (PPF) and National Savings Certificates (NSC) are best to invest in when inflation is slowly inching up and you are only looking at safety, not returns. Invest in short term deposits and funds, commodities and property. This will help you to slowly reach your financial goals while safeguarding your hard-earned money.
Read More: Treasury bills and inflation control
Related Posts:
- Deflation - Meaning, Effects and Modes of Control
- Correction of Balance of Payments (BoP) Deficit
- Effects of Inflation on Different Groups of Society
- Factors determining spot exchange rates in Forex Markets
- Effects of Black Money on Economy
- Role of Fiscal Policy in Economic Development
- Keynesian and Classical Economists Views about Disequilibrium
- National Income Statistics: Meaning and Uses
- The Stages of Inflation
- Causes and Effects of Inflation
Leave a Reply Cancel reply
Your email address will not be published. Required fields are marked *
Browse Econ Literature
- Working papers
- Software components
- Book chapters
- JEL classification
More features
- Subscribe to new research
RePEc Biblio
Author registration.
- Economics Virtual Seminar Calendar NEW!
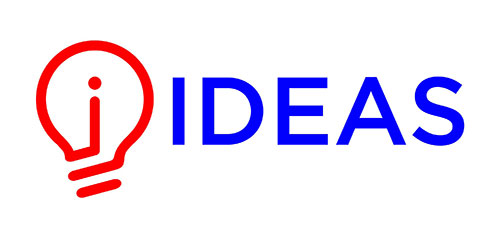
Inflation in India: causes and anti-inflationary policy perception
- Author & abstract
- 36 References
- Most related
- Related works & more
Corrections
(University of Gour Banga)
- Biswajit Maitra
Suggested Citation
Download full text from publisher, references listed on ideas.
Follow serials, authors, keywords & more
Public profiles for Economics researchers
Various research rankings in Economics
RePEc Genealogy
Who was a student of whom, using RePEc
Curated articles & papers on economics topics
Upload your paper to be listed on RePEc and IDEAS
New papers by email
Subscribe to new additions to RePEc
EconAcademics
Blog aggregator for economics research
Cases of plagiarism in Economics
About RePEc
Initiative for open bibliographies in Economics
News about RePEc
Questions about IDEAS and RePEc
RePEc volunteers
Participating archives
Publishers indexing in RePEc
Privacy statement
Found an error or omission?
Opportunities to help RePEc
Get papers listed
Have your research listed on RePEc
Open a RePEc archive
Have your institution's/publisher's output listed on RePEc
Get RePEc data
Use data assembled by RePEc
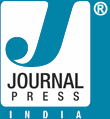
Journal Press India ®
- Our Journals
- PRAGATI: Journal of Indian Economy
- Vol 8 , Issue 2 , July - December 2021
- 10.17492/jpi.pragati.v8i2.822104
A Graphical Case Study of Inflation Beliefs in India: Key Socio-Demographics
Vol 8 , Issue 2 , July - December 2021 | Pages: 62-98 | Research Paper

Published Online: December 15, 2021
- Author Affiliations
- Article Metrics
Author Details
This paper studies micro-level data from the Reserve Bank of India’s ‘Inflation Expectations Survey of Households’ with a nuanced focus on socio-demographics of gender, age, occupation, and city of residence. We document inflation expectations and perceptions relating to this representative sample from four distinct Indian cities, with an intercity comparison shaping the heart of our research. Our study reveals that women, younger respondents, financial sector employees, other category employees as well as cities from higher GSDP states have a more insightful understanding of inflation trends and are more accurate in their inflation rate deductions. Periods of economic un-certainty and volatility are marked with a conspicuous upward bias, polarisation, and inaccuracy in household inflation beliefs. Important policy recommendations are highlighted in our paper as well. This has strong implications with regards to helping central banks and policymakers in their decision-making processes.
IESH, Inflation expectations; Economic uncertainty; Demographics.
- Arioli, R., Bates, C., Dieden, H., Duca, L., Friz, R., Gayer, C., Kenny, G., Meyler, A., & Pavlova, I. (2017). EU consumers' quantitative inflation perceptions and expectations: An evaluation. European Central Bank Occasional, 186 . 44-64. DOI: http://dx.doi.org/10.2866/953408
- Armantier, O., Koşar, G., Pomerantz, R., Skandalis, D., Smith, K., Topa, G., & Klaauw, V. D. W. (2020). How economic crises affect inflation beliefs: Evidence from the COVID-19 pandemic. Journal of Economic Behavior & Organization, 189 . 443-469. DOI: http://dx.doi.org/10.2139/ssrn.3742949
- Ashton, M. J. (2012). “ Real-Feel” inflation: Quantitative estimation of inflation perceptions. Business Economics , 47 (1), 14–26. Retrieved from http://www.jstor.org/stable/23491574
- Campelo, A., Malgarini, M., Bittencourt, V., & Velho, V. (2019) Inflation expectations of Brazilian consumers: an analysis based on the FGV survey . International Review of Applied Economics , 33:4, 505-522, DOI: 10.1080/02692171.2018.1515897
- Das, A., Lahiri, K., Zhao, Y. (2017). Inflation expectations in India: Learning from household tendency surveys. International Journal of Forecasting . 35 (3). 980-993. DOI: https://doi.org/10.1016/j.ijforecast.2019.03.007
- Ehrmann, M., Pfajfar, D., Santoro, E. (2015). Consumers' Attitudes and Their Inflation Expectations. FED Working Paper No. 2015 015. Retrieved from http://dx.doi.org/ 10.17016/FEDS.2015.015
- Evans, M., & Wachtel, P. (1993). Inflation Regimes and the Sources of Inflation Uncertainty. Journal of Money, Credit and Banking , 25(3), 475–511. https://doi.org/10.2307/2077719
- Gali, J. (2008). Monetary Policy, Inflation, and the Business Cycle: An Introduction to the New Keynesian Framework . Princeton University Press: New Jersey.
- Inflation Expectation Survey of Households. (2010, April 19). Retrieved from https://rbi.org.in/Scripts/PublicationsView.aspLx?id=14036
- Kamdar, R. (2019). The Inattentive Consumer: Sentiment and Expectations, No 647, 2019. Meeting Papers, Society for Economic Dynamics.
- Katona, G., (1975). Psychological Economics . New York: Elsevier
- Kershoff, George & Smit, B. (2002). Conducting Inflation Expectation Surveys in South Africa. South African Journal of Economics, 70 (3), 205-212. DOI: 10.1111/j.1813-6982.2002.tb01299.x
- Manski, C. F. (2004). Statistical treatment rules for heterogeneous populations, Econometrica , 72(4), 1221-1246.
- Pfajfar, D., & Santoro, E. (2008). Asymmetries in Inflation Expectation Formation Across Demographic Groups. Cambridge Working Papers in Economics. Retrieved from https://doi.org/10.17863/CAM.5612
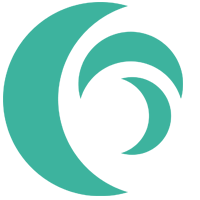
News/Events
Indira Institute of ...
Indira Institute of Management, Pune Organizing International Confe...
D. Y. Patil Internat...
D. Y. Patil International University, Akurdi-Pune Organizing Nation...
ISBM College of Engi...
ISBM College of Engineering, Pune Organizing International Conferen...
Periyar Maniammai In...
Department of Commerce Periyar Maniammai Institute of Science &...
Institute of Managem...
Vivekanand Education Society's Institute of Management Studies ...
Deccan Education Society Institute of Management Development and Re...
S.B. Patil Institute...
Pimpri Chinchwad Education Trust's S.B. Patil Institute of Mana...
D. Y. Patil IMCAM, A...
D. Y. Patil Institute of Master of Computer Applications & Managem...
Vignana Jyothi Insti...
Vignana Jyothi Institute of Management International Conference on ...
Department of Commer...
Department of Commerce, Faculty of Commerce & Business, University...
Suggested Citation
By continuing to use this website, you consent to the use of cookies in accordance with our Cookie Policy.
India inflation likely cooled in March, albeit slightly- Reuters poll
- Medium Text
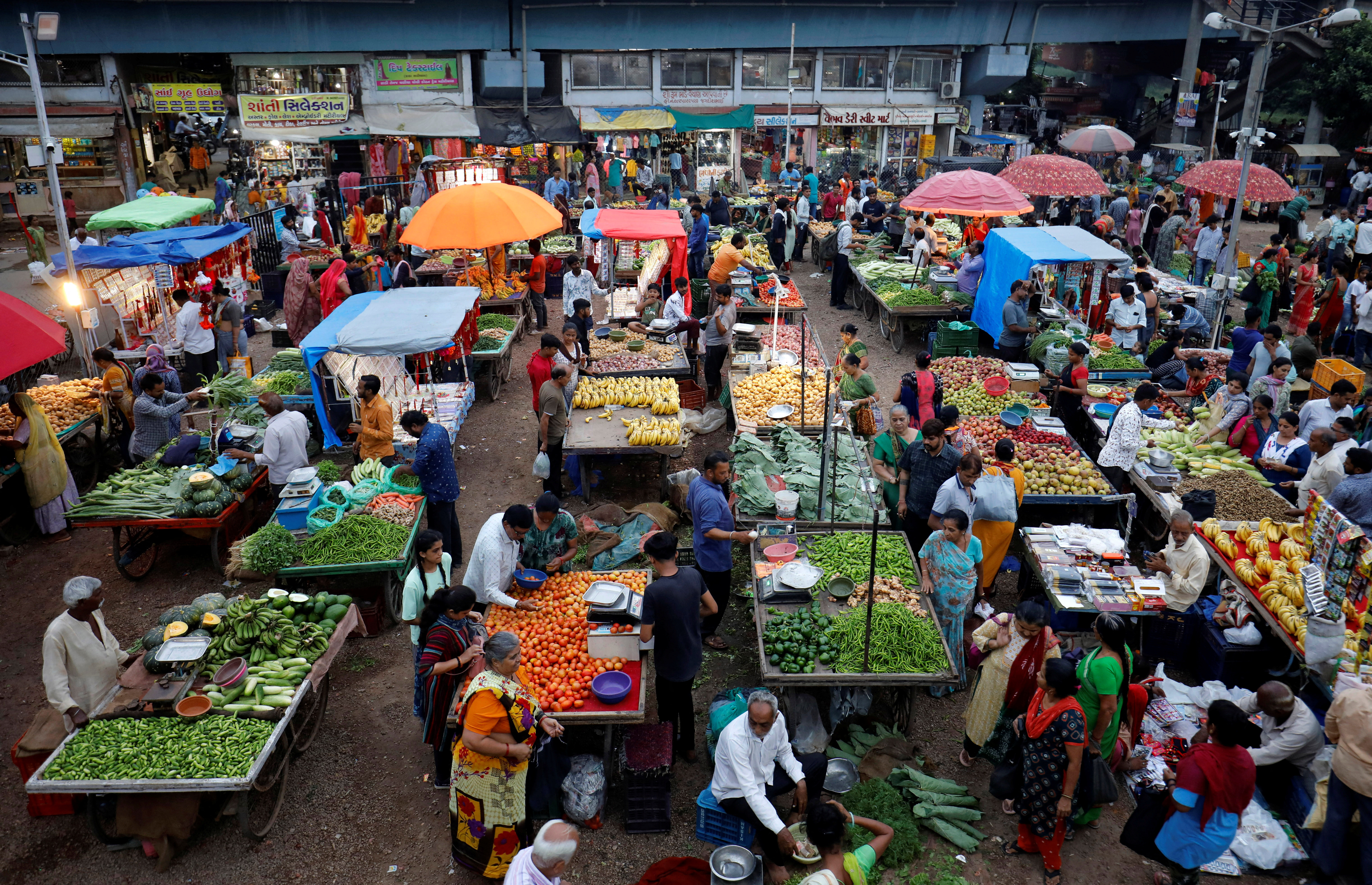
- Reuters Poll graphic on India inflation
- Data due at 1200 GMT, April 12
The Reuters Daily Briefing newsletter provides all the news you need to start your day. Sign up here.
Reporting by Milounee Purohit; Polling by Anant Chandak and Susobhan Sarkar; Editing by Ross Finley and Jonathan Cable, Kirsten Donovan
Our Standards: The Thomson Reuters Trust Principles. New Tab , opens new tab
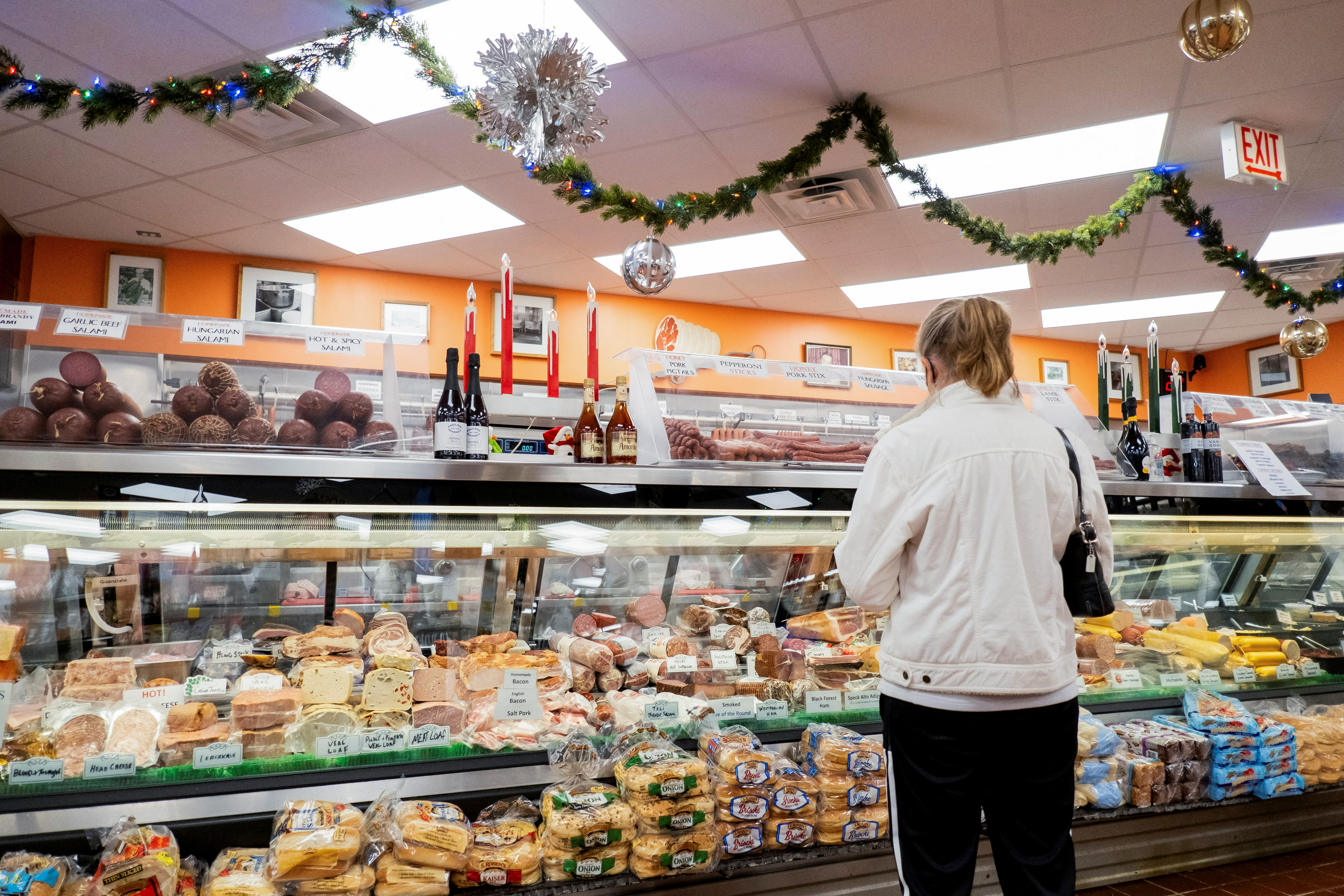
World Chevron
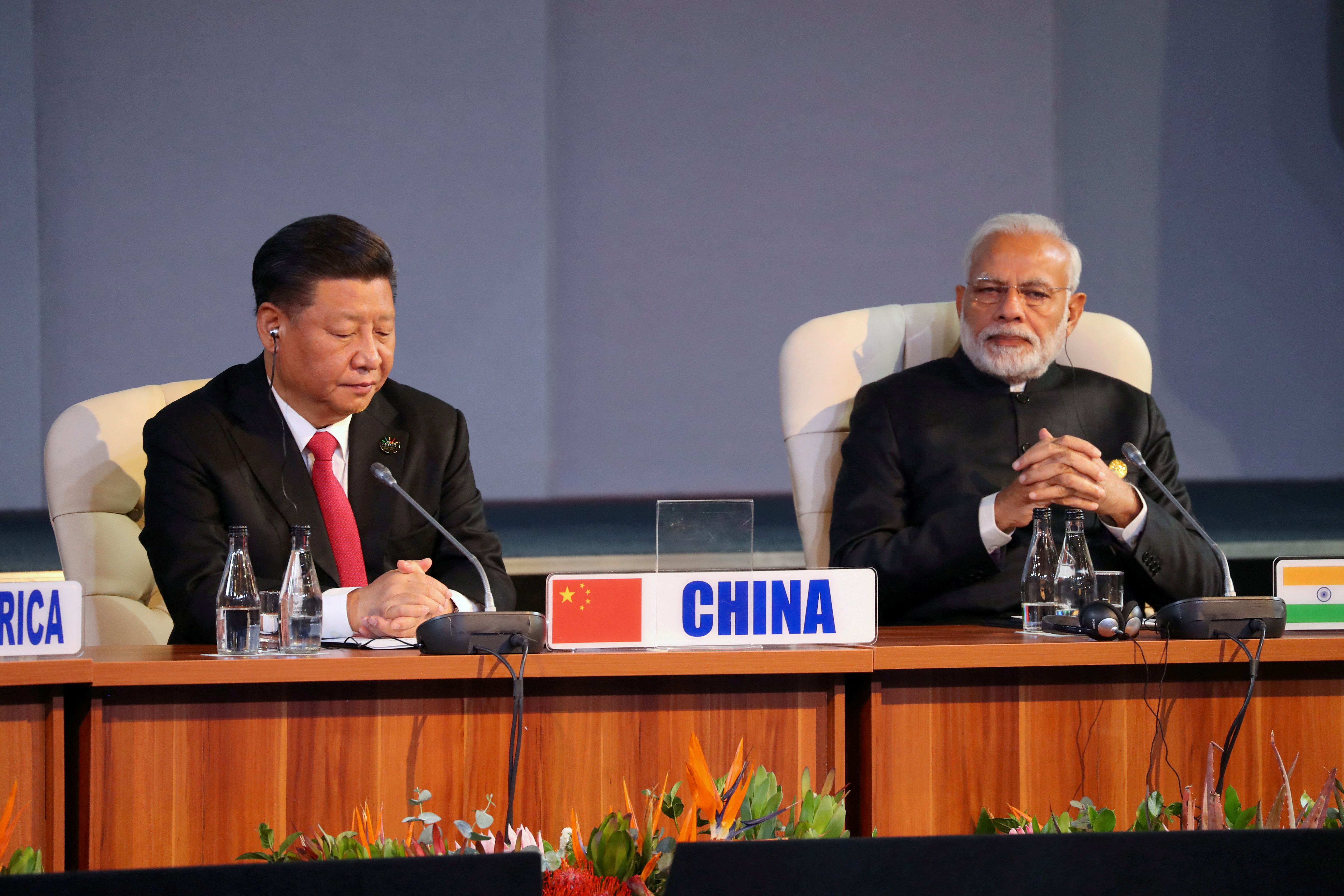
India, China should urgently address 'prolonged situation' on borders, Modi says
India and China should urgently address the "prolonged situation" on their border, Indian Prime Minister Narendra Modi said in remarks published on Wednesday, in an apparent softening of tone on bilateral issues between the two nuclear-armed neighbours.
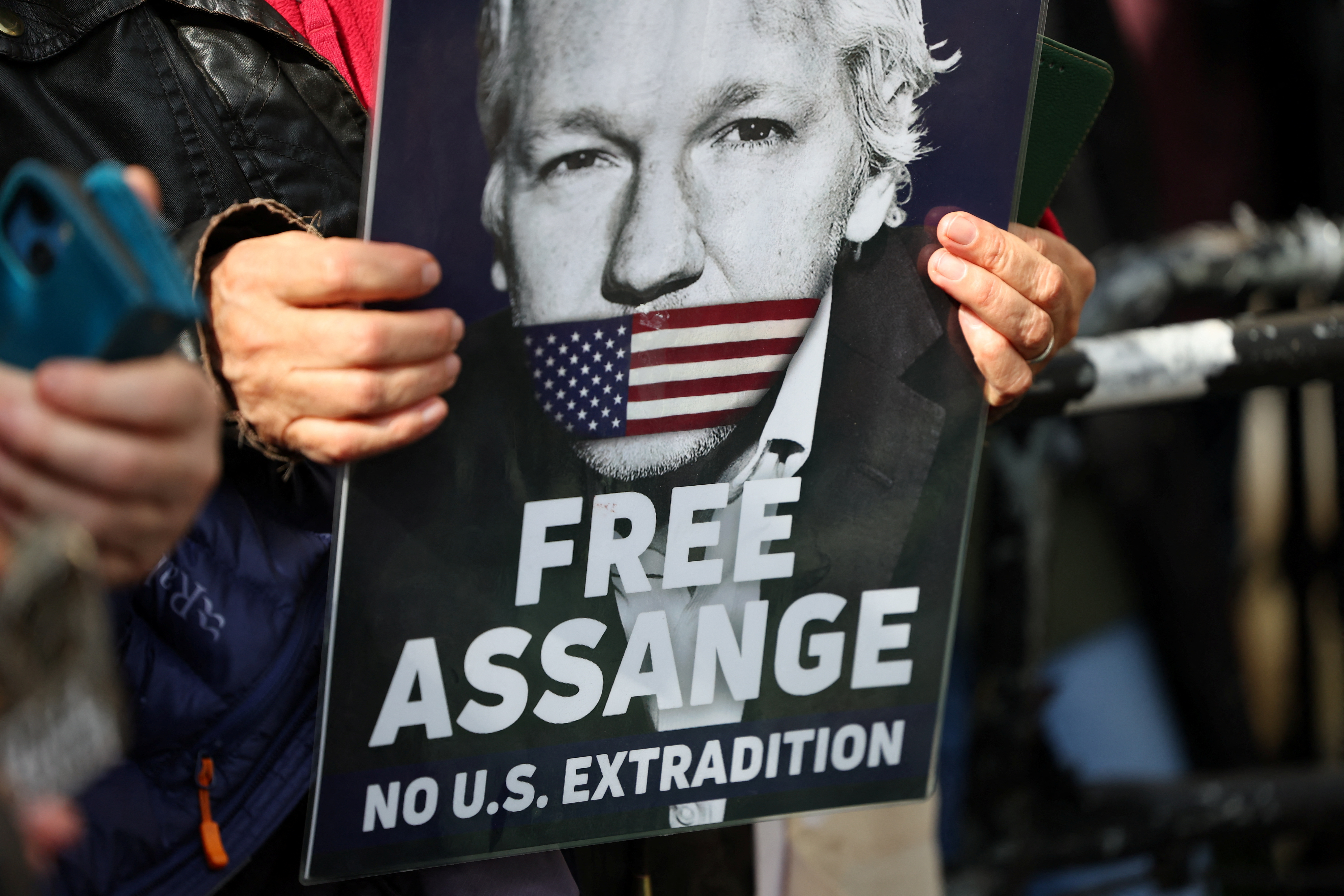

Inflation Rate in India 2024: A closer look at economic trends
Stay informed about the latest trends and developments regarding inflation rates in india in 2024.
- " class="general-icons icon-sq-whatsapp">
- " class="general-icons icon-sq-googleplus popup">
India's current inflation rate
Related stories.

Top 10 largest economies in the world in 2024

Why India will replace China as the world's growth engine this decade

Poverty rate in India: Trend over the years and causes
India inflation rate (cpi) - historical data, what is cpi, what is wpi, how is inflation calculated, types of inflation.
- Demand-Pull Inflation: This occurs when aggregate demand in an economy outpaces the supply of goods and services. It is typically fueled by factors such as increased consumer spending, expansionary fiscal policies, or excessive money supply. Demand-pull inflation leads to rising prices as businesses struggle to meet the high demand.
- Cost-Push Inflation: Cost-push inflation arises from increases in production costs, such as wages, raw materials, or taxes. When businesses face higher input costs, they pass on the additional expenses to consumers through higher prices. Cost-push inflation can be triggered by factors like rising energy prices, changes in government regulations, or supply chain disruptions.
- Built-In Inflation: Built-in inflation is a self-perpetuating cycle of price increases driven by inflation expectations. It occurs when workers and businesses anticipate future inflation and negotiate higher wages and prices to protect their purchasing power. This leads to an upward spiral of wages, production costs, and prices.
- Hyperinflation: Hyperinflation is an extreme and rapid form of inflation where prices skyrocket uncontrollably. It typically occurs due to a severe collapse in confidence in the currency, often caused by excessive money printing, political instability, or economic crises. Hyperinflation erodes the value of money and can have devastating effects on an economy.
- Stagflation: Stagflation refers to a combination of stagnant economic growth, high unemployment , and inflationary pressures. It presents a challenging situation for policymakers because traditional measures to stimulate economic growth, such as lowering interest rates or increasing government spending, can exacerbate inflation.
- Disinflation: It refers to a decrease in the rate of inflation. It means that while prices are still rising, they are doing so at a slower rate compared to the past. Disinflation is often seen as a positive development as it brings relief from rapid price increases, but it is different from deflation, where overall prices decline.
Frequently Asked Questions (FAQs)
- " class="general-icons icon-sq-youtube">
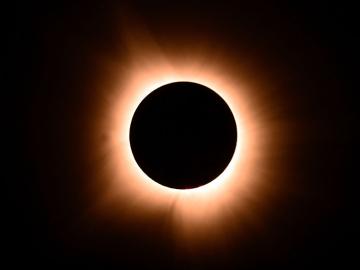
Encircling the dark: Eyecatching photos this week

How Mokobara shed its baggage
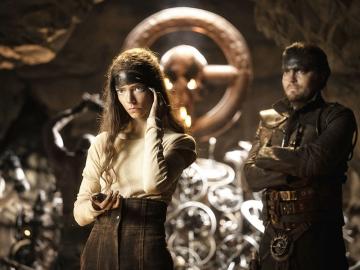
George Miller readies for 'Furiosa: A Mad Max Saga' in 'addictive' series
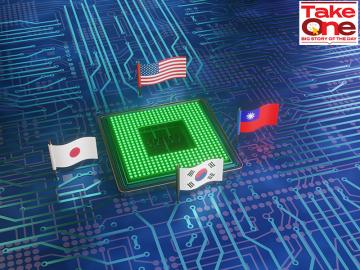
The global race for AI chips intensifies. Where does India stand?
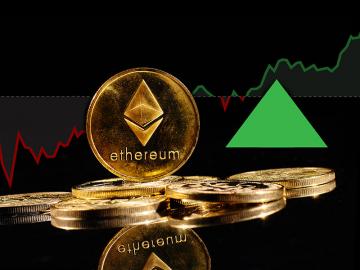
Ethereum picks up pace in the second week of April
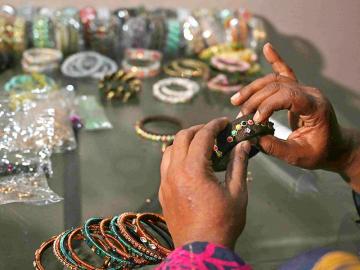
From furnace to forearms: A story of Pakistan's delicate Eid bangles
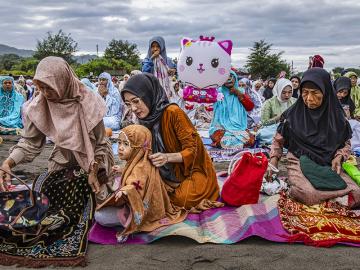
Photo of the day: Eid Al-Fitr on the 'sea of sands'

Is study abroad turning burden from boon?
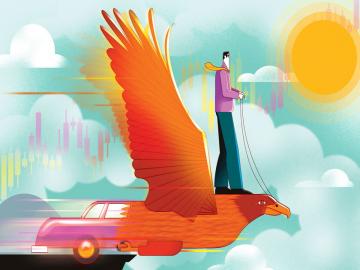
$1 trillion market cap not just a possibility but a vision for future of startups: Redseer Strategy
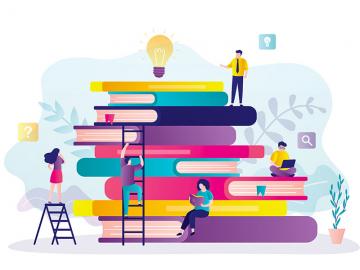
Publish or perish: What the research says about productivity in academia

A team's internal focus is only half the story: Henrik Bresman

Bitcoin clears $70,000 resistance: how sustainable is it?
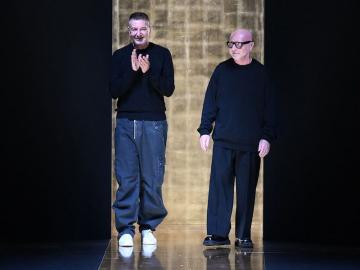
Dolce & Gabbana and the artisans who inspired them on show in Milan

Born to help: Syria bikers deliver Ramadan meals
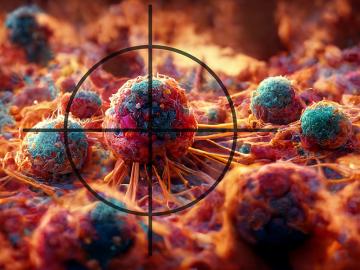
India can become the new cancer capital of the world: Apollo report

Inflation in India: Causes and Consequences
According to data released by the National Statistical Office (NSO), retail inflation increased to 6.5% in January 2023.
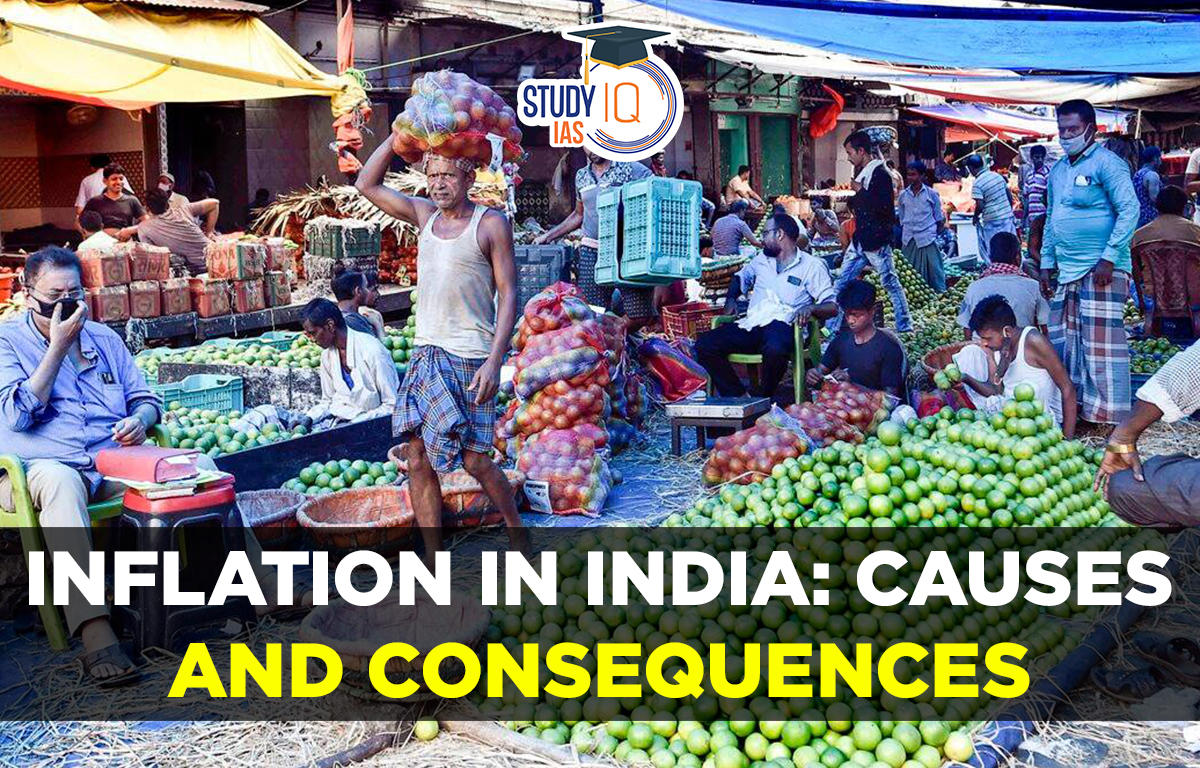
Table of Contents
Context: According to data released by the National Statistical Office (NSO) , retail inflation increased to 6.5% in January 2023.
About Retail Inflation
It is measured by the consumer price index (CPI). The change in the price index over a period of time is referred to as CPI-based inflation, or retail inflation.
- CPI measures retail inflation by collecting data on the prices of goods and services that are consumed by the retail population of the country.
- The CPI monitors retail prices at a certain level for a particular commodity; price movement of goods and services at rural, urban and all-India levels .
- Among the major States , Telangana recorded the highest inflation in January at 8.6%, followed by Andhra Pradesh (8.25%), Madhya Pradesh (8.13%), Uttar Pradesh (7.45%) and Haryana (7.05%).
Causes for Spike in Inflation
- Cereals inflation surged from 13.8% in December to 16.1%;
- Milk products accelerated from 8.5% in the previous month to 8.8%;
- Spices jumped from 20.3% inflation to 21.1% in January.
- Inflation in eggs sped from 6.9% in December to 8.8% in January, while the pace of price rise in meat and fish rose from 5.1% to 6.04%.
- It is a measure of inflation arrived at by removing the prices of food and fuel.
CPI and Repo Rate
- CPI is an essential tool for RBI as it looks after the monetary policies of the country.
- The framework by RBI requires it to keep the inflation rate around 4% however it can fluctuate anywhere between 2% and 6%.
- The primary motive of RBI is inflation control, while it may change from time to time depending on the decision of the monetary policy committee.
Impact of Increase in Inflation
- RBI raises the repo rate when it believes that inflation is not in control.
- Higher interest rates drag down overall demand for goods and services by making loans costlier. Lower demand is expected to cool down inflation.
- If inflation stays persistently high (‘sticky’), it would necessitate the RBI to keep raising interest rates
Sticky Inflation
- Inflation being sticky essentially means that inflation is taking longer than expected to fall.
- Higher food and fuel prices have spiralling impact on the broader economy and made other things costlier.
- However, India is not the only country facing sticky inflation.
- Many other countries such as the US and countries in the euro zone — are also struggling to come out from sticky inflation.
CPI vs. WPI

Sharing is caring!
How Retail Inflation measured?
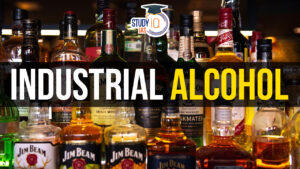
Leave a comment
Your email address will not be published. Required fields are marked *
Save my name, email, and website in this browser for the next time I comment.

- UPSC Online Coaching
- UPSC Exam 2024
- UPSC Syllabus 2024
- UPSC Prelims Syllabus 2024
- UPSC Mains Syllabus 2024
- UPSC Exam Pattern 2024
- UPSC Age Limit 2024
- UPSC Calendar 2024
- UPSC Syllabus in Hindi
- UPSC Full Form

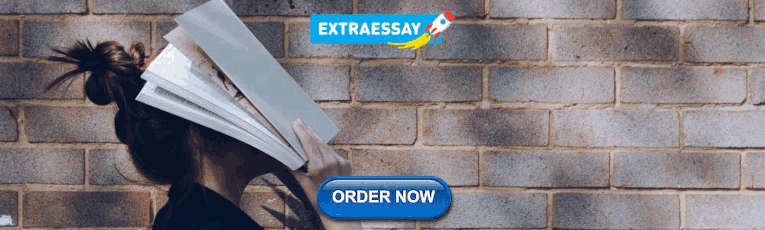
Recent Posts
- UPPSC Exam 2024
- UPPSC Calendar
- UPPSC Syllabus 2024
- UPPSC Exam Pattern 2024
- UPPSC Application Form 2024
- UPPSC Eligibility Criteria 2024
- UPPSC Admit card 2024
- UPPSC Salary And Posts
- UPPSC Cut Off
- UPPSC Previous Year Paper
BPSC Exam 2024
- BPSC 70th Notification
- BPSC 69th Exam Analysis
- BPSC Admit Card
- BPSC Syllabus
- BPSC Exam Pattern
- BPSC Cut Off
- BPSC Question Papers
IB ACIO Exam
- IB ACIO Salary
- IB ACIO Syllabus
CSIR SO ASO Exam
- CSIR SO ASO Exam 2024
- CSIR SO ASO Result 2024
- CSIR SO ASO Exam Date
- CSIR SO ASO Question Paper
- CSIR SO ASO Answer key 2024
- CSIR SO ASO Exam Date 2024
- CSIR SO ASO Syllabus 2024
Study Material Categories
- Daily The Hindu Analysis
- Daily Practice Quiz for Prelims
- Daily Answer Writing
- Daily Current Affairs
- Indian Polity
- Environment and Ecology
- Art and Culture
- General Knowledge
- Biographies

IMPORTANT EXAMS

- Terms & Conditions
- Return & Refund Policy
- Privacy Policy
We've detected unusual activity from your computer network
To continue, please click the box below to let us know you're not a robot.
Why did this happen?
Please make sure your browser supports JavaScript and cookies and that you are not blocking them from loading. For more information you can review our Terms of Service and Cookie Policy .
For inquiries related to this message please contact our support team and provide the reference ID below.
Inflation in India 2023 – Causes, Impact & What to Expect for Indian Stock Market & Investors

Visiting a subway outlet revealed that sandwiches no longer come with a free slice of cheese. McDonald’s and Burger King had also dropped tomatoes from some of their burgers. Not just international fast-food chains, local restaurants had also increased the prices of tomato-based dishes.
India’s Retail Inflation Falls
In July, vegetable prices increased by a staggering 37% year on year, with tomato prices at some wholesale markets increasing 1,400% over the 3 months . Annual food-inflation rate hit 11.5% in July, highest in over 3 years, pushing the overall inflation rate to a 15-month high of 7.4%.
India’s CPI (Consumer Price Index) or retail inflation, fell to around 6.83% from its 15-month high of 7.44% in July. Ministry of Statistics and Programme Implementation (MoSPI)’s data on food inflation has slowed from 11.51% in July to 9.94% in August.
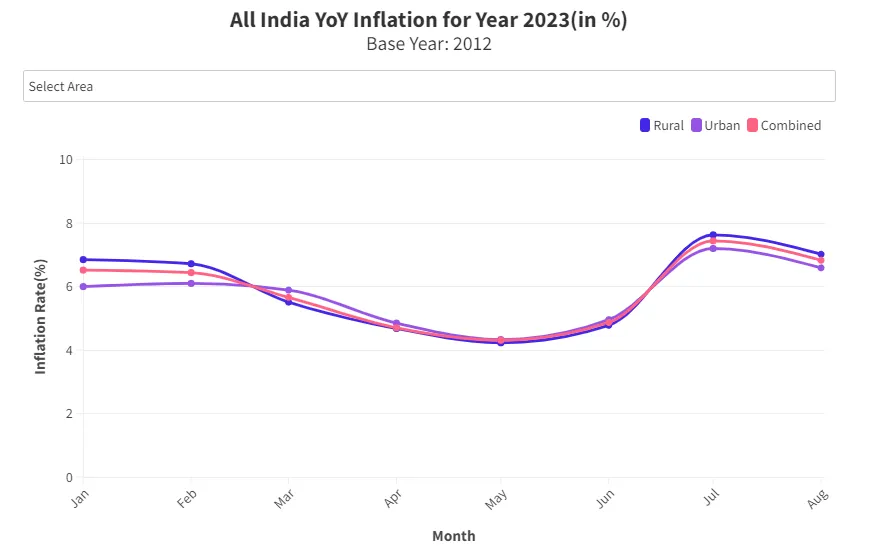
This moderation was primarily due to falling prices of vegetables along with cost reduction in housing, clothing, non-essential commodities etc. In terms of food inflation, some items like potatoes showed a year-on-year decline of 14.3%, and refined oil prices are down by 24.5% year-on-year.
However, tomatoes saw prices rise by a staggering 180% from a year ago. This was around 201% in July on a year-on-year basis – so on a month-on-month basis tomato prices have fallen around 21.7% which is a positive sign. Potato, Onion, Rice, Atta and Dals – basically staple items, all saw sequential increase between July and August.
Food prices between June and July increased by 8% which should’ve been around 2% looking at long term averages. In August, food prices have fallen by around 0.5% month on month and expectation is for September to show continued decrease of upto 2.5% month-on-month for food prices.
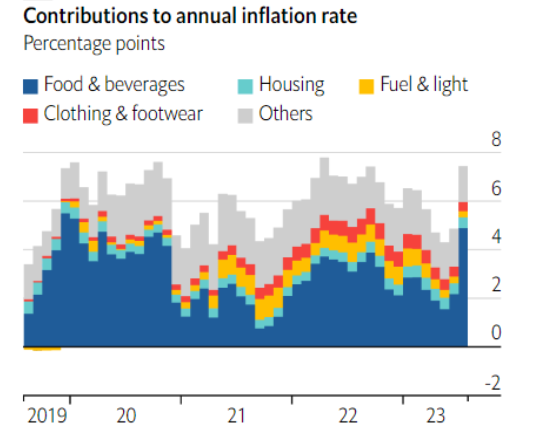
Going forward, a good monsoon for September would help Kharif production yields which could help reduce CPI further. There is no significant month on month change in inflation for utilities – with minor decreases being seen for airfares in the month of August.
At the same time turbulence in the crude oil markets and late monsoon hurting pulse crop production could hurt any improvements in the inflation figures . Analysts are carefully monitoring other commodities like oil, pulse, and wheat.
Notably, the CPI is still above RBI’s target of 2-6% for India. But for now a reduction in inflation and slowing down of food inflation appears to be a positive development. Last month RBI maintained its repo rate at 6.5% and didn’t pay too much heed to the 15-month high inflation number of July – accounting it to a mismatch of demand and supply which spurred the vegetable prices and hence the food inflation figures.
Read this article on RBI’s Rate Pause, Inflation and Why Tomatoes are making Farmers Crorepatis .
India’s Wholesale Price Index Deflation Eases
India’s Wholesale Price Index (WPI) continued in deflationary mode for the fifth consecutive month in August. However, the rate of deflation has eased to -0.52% from -1.36% in July. Moderation in deflation rate was driven by reduction in fuel and power prices.
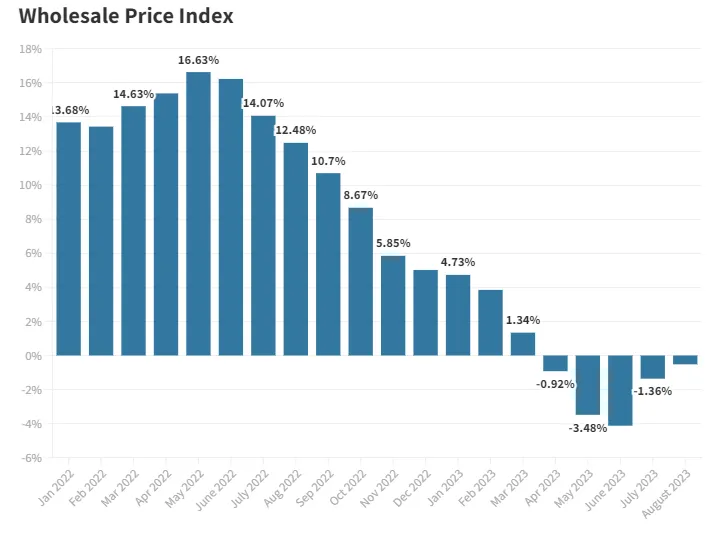
- Year-on-year decline in fuel and power costs decreased from -12.8% in July to -6% in August. On a month-to-month basis, these costs rose 3%, marking a reversal from several months of decline.
- Deflation in manufactured products remained almost stable, dropping slightly from -2.5% in July to -2.4% in August. For the first time in four months, prices of manufactured products increased marginally by 0.14%.
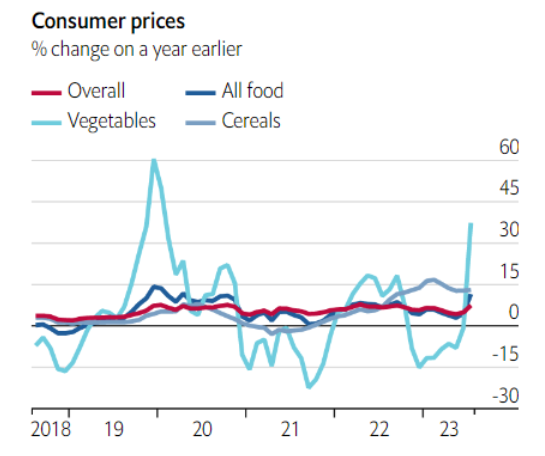
Deflationary trend in wholesale prices will likely reverse in the coming months. Factors like rising crude oil prices, cereals, and pulses could maintain Consumer Price Index (CPI) inflation within the range of 5.5%-6.5% over the next two quarters.
Why is Food Inflation so High?
Unpredictable weather conditions have wreaked havoc on Indian agriculture. Heavy rains in several regions have led to the submerging of farmlands and disrupting supply chains. Conversely, excessive heat has damaged crops. By the end of July, Indian farmers had sown 40% less than the typical cultivation for that period. August recorded a 36% deficit in rainfall, making it the driest August in over 120 years.
- Monsoon Rainfall: Monsoon in 2023 is expected to be below normal, with officials recording 90 to 95% of Long Period Average (LPA). IMD had earlier forecast a normal monsoon at 96% LPA with an error margin of +/- 4%. IMD predicts a rainfall of 91-109% of long period average (LPA) for September, which may not be sufficient to cover the existing deficit. Rainfall tracking for 2 weeks of September is 5.8% below long term averages, but Skymet expects it to improve for the remainder which could help reduce the deficit.
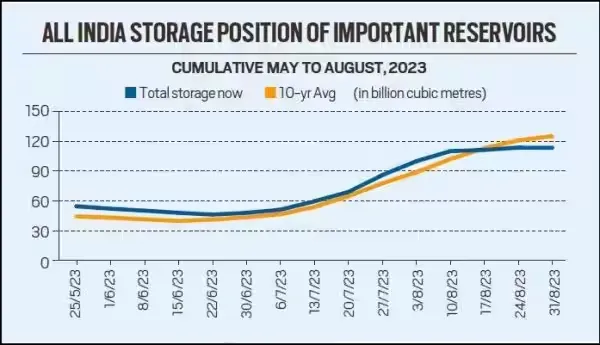
- Geographical Disparity: Central India had a rainfall deficit of 47%, and South India had a 60% shortfall in August.
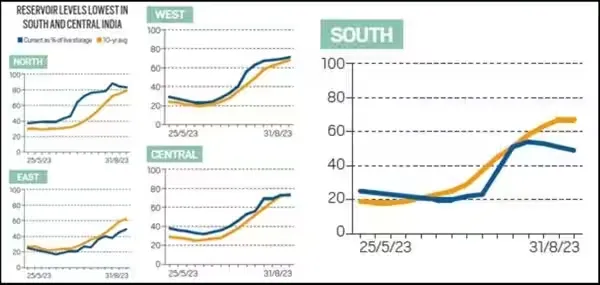
- Agriculture: Uneven and erratic monsoons affected the Kharif sowing. Lower rainfall levels in August have the potential to affect flowering of crops, leading to concerns over food inflation and production levels. Area under Kharif crop sowing is 3.6% higher year-on-year but significantly lags in pulses, cotton, and oilseeds.
- Temperature: Both the average maximum and mean temperatures in August were the highest ever recorded since 1901. Above-normal maximum temperatures are expected over most parts of India.
Poor monsoon also meant that power demand increased unexpectedly, mostly for irrigation. A record high power generation was recorded in August, with coal contributing to 66.7% of the total power generated—the highest for the month in 6 years. Monsoon rains contribute to nearly 75% of India’s annual rainfall – imperative for our agricultural economy since more than 50% of India’s arable land relies on these monsoon rains – all of this impacts not just food production, but also employment opportunities for migrant workers, farmers and countless people involved in the food supply chain.
India’s Strategy to Control Inflation: Food Export Bans
Indian government & RBI have been proactive in their response to curb retail inflation & food inflation. RBI has maintained interest rates for 3 consecutive months since April 2023 – attributing high inflation to temporary mismatch of supply demand constraints and expects prices to stabilize from September onwards. The Indian Government has imposed a 40% tax on onion exports, imposed export restrictions on rice and removed import restrictions on tomatoes from Nepal – all to ensure sufficient domestic supply and push down prices. It is also releasing grain from its godams to restrain prices from spiralling out of control and to offer subsidized food items to impoverished households – expecting to spend a $12 billion to contain food prices. With election year approaching, inflation is a stickler in the government’s hopes for a continued term.
What does a Food Export Ban do? The logic is straightforward: by restricting exports, the aim is to increase domestic supply, which should theoretically lead to lower prices. Food security is not just a political issue it can become a volatile societal issue rapidly when the majority of the country is engaged in agriculture. There are consequences to such actions:
- Food Hoarding: Food export bans may exacerbate the very problem they aim to solve. Farmers may choose to hoard their produce until the ban is lifted, which can lead to even more volatile domestic prices.
- Impact on Agricultural Livelihoods: Even if domestic prices do fall due to an export ban, the repercussions can be harmful. Lower domestic prices can mean reduced income for the large majority of families engaged in agriculture, especially when you consider the high margin taken by several stakeholders in the food supply chain, which can worsen poverty.
- Political Biases: Benefits of lower food prices are disproportionately skewed toward urban cities who usually wield more political influence than their rural counterparts.
Global Implications of India’s Food Export Bans
India’s aggressive domestic policies have already triggered a hike in global food prices. India is the world’s largest exporter of rice with a 40% market share – the government has halted export of non-basmati white rice. It has increased prices for export of onions and a ban on sugar exports being more likely as production numbers appear to be lower. China, another major exporter of staples, especially rice, has also experienced erratic weather with torrential rains in July and floods damaging rice production. All of this has put pressure on the price of staples (rice, onion, sugar etc.) globally. Since then, prices of rice in Thailand have increased 20%. The UN’s Rice Price Index has increased by 2.8% in July, its highest level since 2011.
And it’s not just India, according to the International Food Policy Research Institute, at least 20 countries have imposed export restrictions since the Ukraine-Russia war. Another contributor to rising prices is the erratic climate change situation that has hampered yields. This could have a domino effect as around 80% of the world’s population lives in countries that are net importers of food. Political exigencies, especially in a politically sensitive period in India, might make food self-sufficiency and inflation control more immediate concerns than the global repercussions.
Impact of Crude Oil Prices on Inflation & Indian Economy
Saudi Arabia has reduced its oil supply by 1 million barrels per day, Russia has restricted output by 300,000 barrels per day. Soon after the announcement, Brent crude futures rose 1.2% closing above the $90 mark for the first time since November 16, 2022. US WTI futures had gained 1.3% settling above a 10-month high.
1. High Import Bill
India’s heavy dependence on crude oil imports to meet its energy needs means that any international price fluctuation will substantially affect its import bill. According to the Petroleum Planning & Analysis Cell, the net import bill for oil and gas was $9.8 billion in July 2023, compared to $15.8 billion in the same month last year. High international crude oil prices are expected to significantly inflate this figure, impacting the country’s current account.
2. Soaring Fuel Prices
Domestic oil marketing companies, including Indian Oil, BPCL, and HPCL, may find it increasingly untenable to maintain current petrol and diesel prices. Last year, despite international crude oil prices peaking at $140 per barrel, OMCs refrained from significantly increasing retail fuel prices. This inaction led to substantial losses for these refiners, which now will likely pass on to consumers.
3. Depreciation of Indian Currency
Rising crude prices often strengthen the U.S. dollar against other currencies, including the Indian rupee. This depreciation makes oil imports even more expensive, creating a vicious circle. Recently, the Indian rupee touched a 10-month low, declining to 83.18 to the US dollar, largely due to a rally in crude oil prices and a strong dollar index.
4. Widening Fiscal Deficit
A surge in crude oil prices will significantly increase the subsidy burden for the Indian government, which covers the difference between the market and controlled prices of essential fuel like kerosene, diesel, and LPG. This additional burden could potentially widen India’s fiscal deficit beyond the estimated ₹17.9 trillion for FY24.
5. Unfavourable Trade Balance
Increased dependence on imported oil could affect India’s trade balance and expose the economy to currency fluctuations and external shocks. Higher crude prices also pose a threat to trade agreements, impacting the overall economic stability.
6. Inflationary Pressures
Rising fuel prices directly contribute to inflation by increasing the cost of goods and services. According to the Reserve Bank of India (RBI), such a surge could decrease aggregate demand due to lower disposable incomes, affecting the broader economic activity.
7. Lower Economic Growth
Elevated crude oil prices lead to higher production and transportation costs across sectors , negatively affecting profitability and competitiveness. This could result in a decline in discretionary spending, thereby affecting consumer behaviour and, by extension, the overall economy.
Impact of Inflation on Investors & Markets
High inflation affects all of us – investors and markets. RBI expects inflation to remain above % until Q1 2024-25 on account of persistent food price pressures & rising crude oil prices. What does high inflation outlook mean for interest rates? Well it looks unlikely that RBI will significantly reverse its 250 bps increase in interest rates over the last year. For 3 consecutive months, it has maintained the repo rate at 6.50%. Expectations are that nay rate cut is unlikely in the short term, until inflation is under RBI’s control. RBI has revised its inflation expectation upwards for the year from 5.1% to 5.4%.
Impact of Inflation on Indian Markets
- Equities: High inflation and interest rates raise the cost of capital, thereby affecting company valuations. High interest rates mean cost of borrowing goes up which impacts companies future cashflow projections. Stock prices are usually discounted by future cash flows; higher discount rates mean lower valuations.
- Gold: Traditionally gains ground during high inflation, serving as a hedge against devalued currency.
- Bonds: Rising inflation pushes bond yields higher, making them less attractive. India’s benchmark 10-year bond yields increased by 10 basis points to 7.20% in the last month.
Impact of Inflation on Investor Strategies
- Stocks: Long-term investors should focus on companies with strong earnings and growth potential. Given the inflation and interest rate scenario, equities that are fundamentally strong and well-priced could be good additions.
- IPOs: Exercising caution is crucial. With inflation and interest rates high, there could be a rush to launch IPOs at inflated valuations. Only invest if the valuations are justifiable.
- Mutual Funds: In a high-inflation environment, long-term investing in equity mutual funds is advisable. The focus should be on the right fund selection and disciplined asset allocation – using SIPs to average out your entry and exit points.
New India’s Growth Opportunity
Fitch has maintained India’s growth forecast at 6.3% for the current fiscal year, citing resilience in the economy despite a tighter monetary policy and weakened exports – with growth forecast for next fiscal year at 6.5%. While the Indian economy grew by 7.8% in the Q1, there could be moderation in growth for Q2 since food export bans have weakened exports, credit growth has stagnated, and diminished consumer confidence. With Diwali & other festivals around the corner, there could be an uptick in discretionary spending on consumption which helps boost the growth of the economy however this counterbalanced with inflation playing spoilsport and dampening any household spending.
Explore Wright Research smallcases here
Disclaimer : Investment in securities market are subject to market risks. Read all the related documents carefully before investing. Registration granted by SEBI, membership of BASL (in case of IAs) and certification from NISM in no way guarantee performance of the intermediary or provide any assurance of returns to investors. Visit bit.ly/sc-wc for more disclosures.

You may want to read
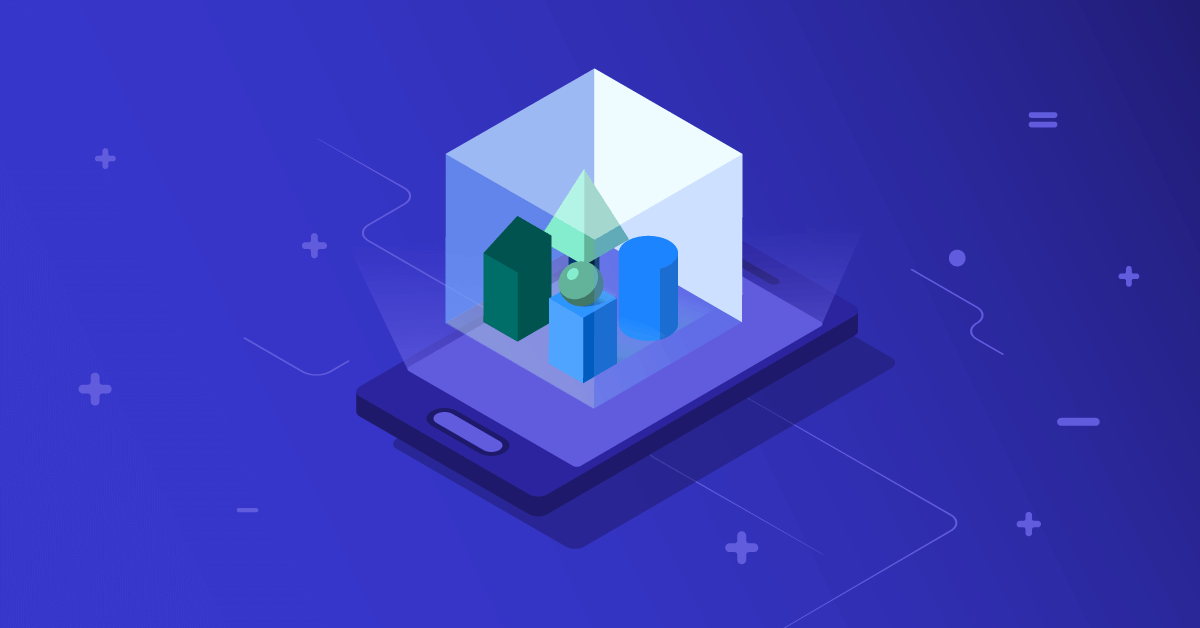
Cancel reply
Your email address will not be published. Required fields are marked *
Save my name, email, and website in this browser for the next time I comment.
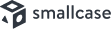
smallcase Technologies Private Limited #51, 3rd Floor, Le Parc Richmonde, Richmond Road, Shanthala Nagar, Richmond Town, Bangalore - 560025
Download App
Popular smallcases
- All Weather Investing
- Top 100 Stocks
- Equity & Gold
- Green Energy Portfolio
smallcase Guides
- What is smallcase?
- smallcase fees & charges
- smallcase subscription
smallcase Calculators
- SIP Calculator
- Lumpsum Calculator
- Compound Interest Calculator
- Post Office RD Calculator
- NPS Calculator
- RD Calculator
- SSY Calculator
Stock Portfolio Collections
- Green Energy Stocks
- Chemical Stocks
- Electric Vehicle (EV) Stocks
- Dividend Stocks
- REIT Stocks
- Fertilizer Stocks
- Semi Conductor Stocks
- Search Stocks
Company: About | Disclosures | Terms & Conditions | Privacy Policy | User Created smallcases | Investment Tools | Press | For Businesses
Investment 101: Portfolio Investing | Dow Theory | Coffee Can Investing | Stocks SIP | NIFTY Index
Star Investors: Rakesh Jhunjhunwala Portfolio | Rekha Jhunjhunwala Portfolio | Azim Premji Portfolio | Radhakishan Damani Portfolio | Vijay Kedia Portfolio | Dolly Khanna Portfolio | Sunil Singhania Portfolio | Mukul Agrawal Portfolio | Ashish Dhawan Portfolio | Shankar Sharma Portfolio | Ashish Kacholia Portfolio
Stocks on smallcase: EKI Energy (EKI) | Bhansali Engineering (BHAN) | Max Financial Services LTD (MAXI) | AU Small Finance Bank (AUFI) | Reliance Industries (RELI) | Infosys (INFY) | Ambica Agarbathies (AAAI) | Mos Utility (MSS) | Gautam Gems | Infollion Research Services Ltd (INFOL) | Tata Motors (TAMO) | Sg Finserve Ltd (SGFIN) | See All Stocks
Dislaimer: This platform is intended for informational purposes only and is not intended to provide investment advice. The stock prices displayed are delayed and may not reflect the most current market conditions. Investment in securities market are subject to market risks. Read all the related documents carefully before investing. You can also consider consulting a financial advisor before making any investment decisions. Smallcase Technologies Private Limited shall not be responsible for any losses that may occur as a result of using this platform.
The views expressed in this article are those of the author and do not necessarily reflect the views of Smallcase Technologies Private Limited (STPL) or any of its associates. The information provided in this article is for educational and informational purposes only. Investors are responsible for their investment decisions and are responsible to validate all the information used to make the investment decision. Investors should understand that his/her investment decision is based on personal investment needs and risk tolerance, and performance information available on here is one amongst many other things that should be considered while making an investment decision. Past performance does not guarantee future returns.
Investments in securities market are subject to market risks. Read all the related documents carefully before investing. Registration granted by SEBI, membership of BASL (in case of IAs) and certification from NISM in no way guarantee performance of the intermediary or provide any assurance of returns to investors. The examples and/or scurities quoted (if any) are for illustration only and are not recommendatory.
The content and data available on the website, including but not limited to index value, return numbers and rationale are for information and illustration purposes only. Charts and performance numbers do not include the impact of transaction fee and other related costs. Past performance does not guarantee future returns and performances of the portfolios are subject to market risk. Data used for calculation of live returns and other information is provided by exchange approved third party vendors and has neither been audited nor validated by the Company. Detailed return calculation methodology is available here . Detailed volatility calculation methodology is available here .
The user agrees to assume complete and full responsibility for the outcomes of all of his/her investment decisions that he/she makes, including any direct, indirect, incidental, consequential, special, punitive or any other losses/damages if any that may be incurred by him/her. Smallcase Technologies shall not be responsible or liable for any direct, indirect, incidental, consequential, special, punitive or any other losses/damages arising out of the recipient's investments. For disclosures related to Smallcase Technologies Pvt Ltd, please visit this page .
All smallcases present in the articles are created by SEBI licensed entities. The disclosure of these entities can be found below:
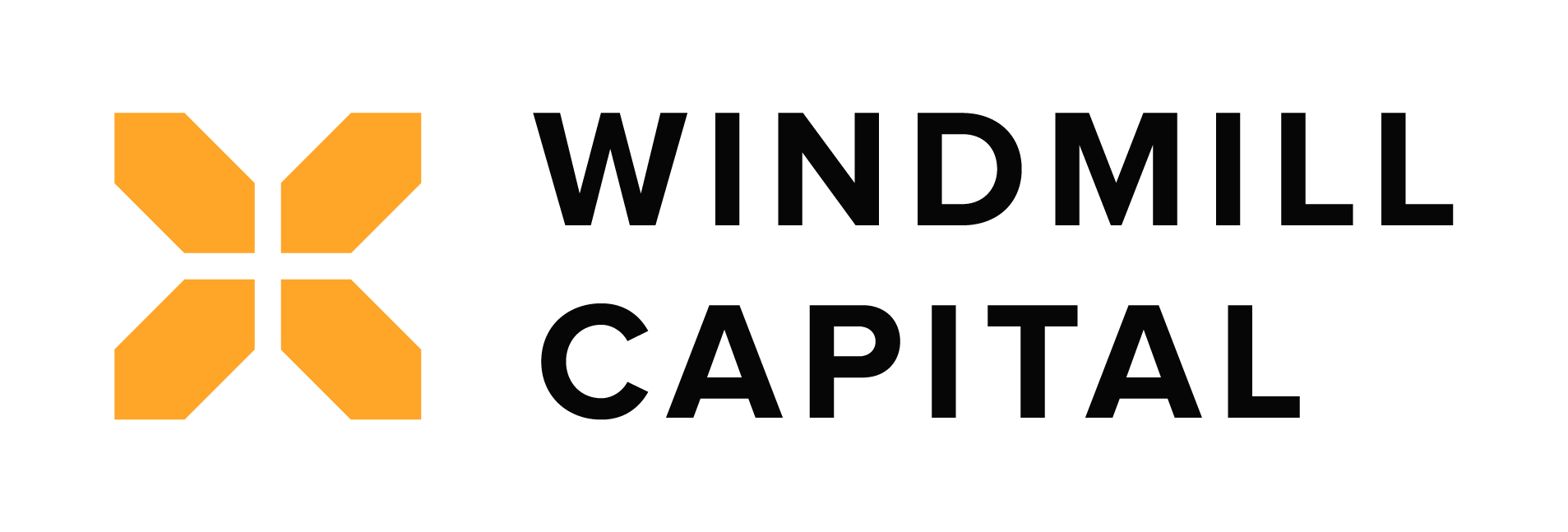
INH200007645
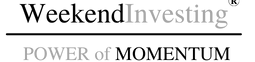
INH100008717
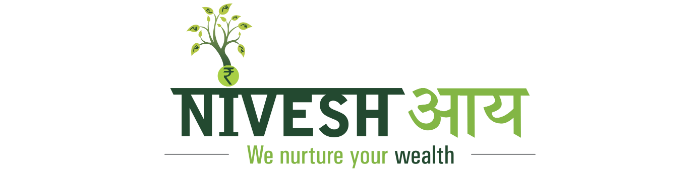
INA000017541
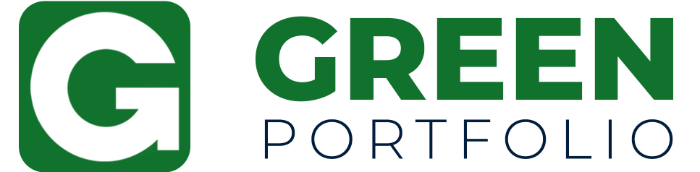
INH100008513
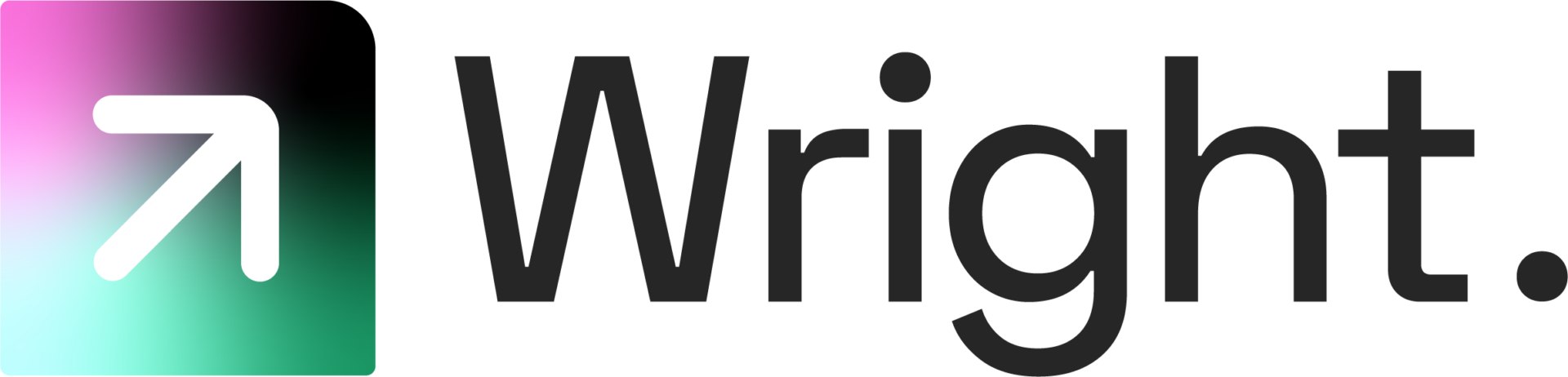
INA100015717
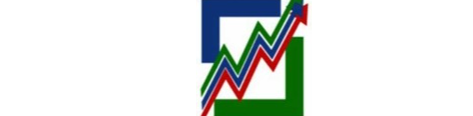
INP000004946
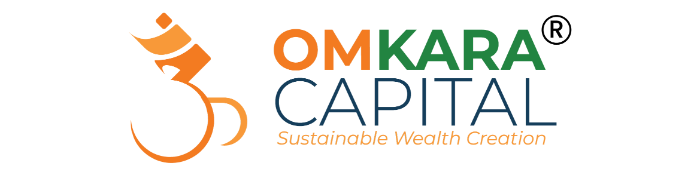
INA000016825
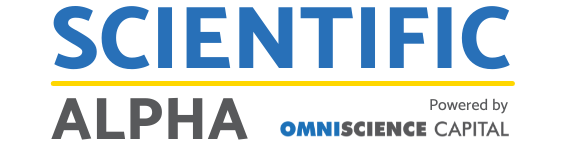
INA000007623
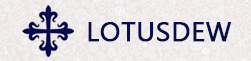
INA200013770
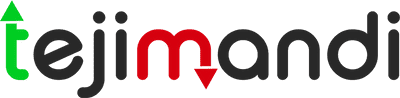
INH000009445
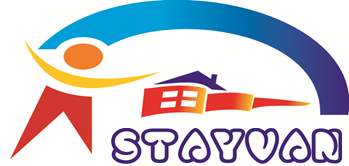
INH000006077
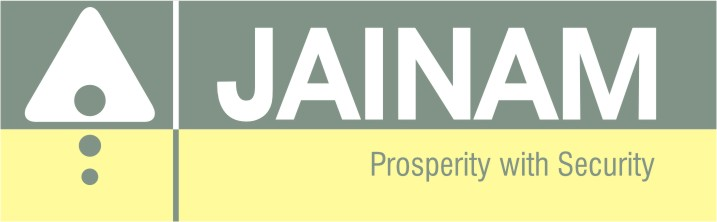
INH000006448
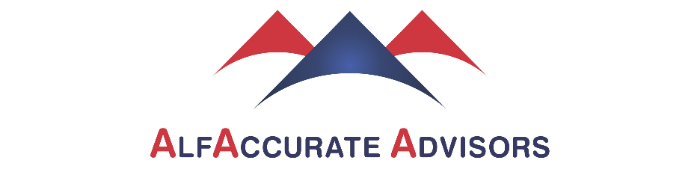
INA000015701
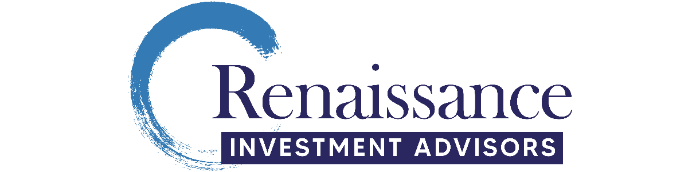
INA000016436
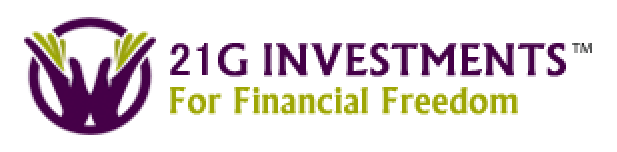
INA000017231
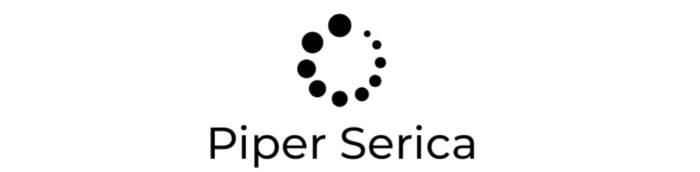
INP000006749
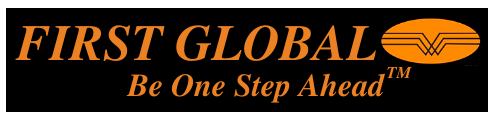
INH000004635
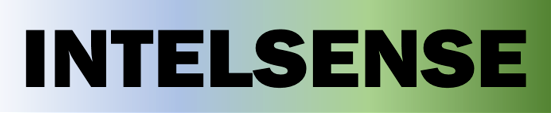
INH300006607
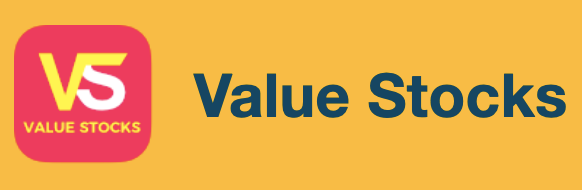
INA300002022
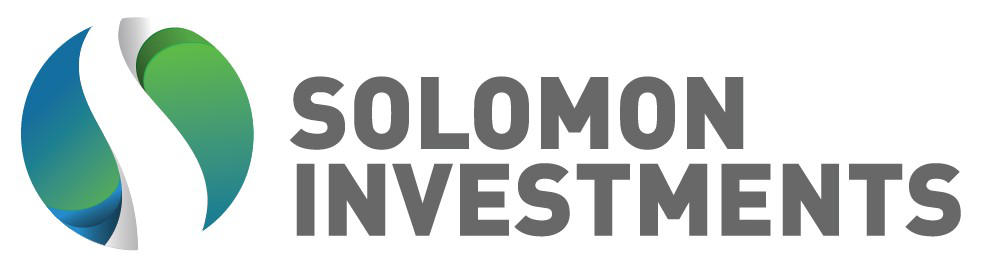
INH000009630
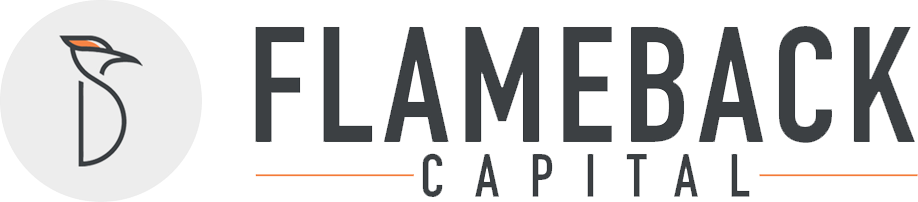
INA200013798
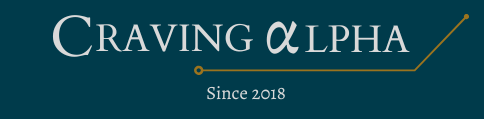
INA300017038
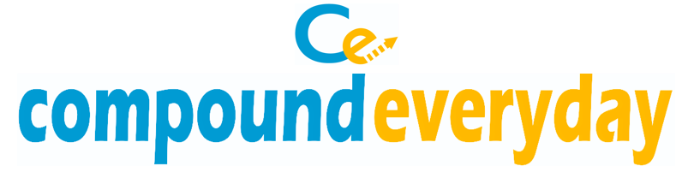
INA000017198
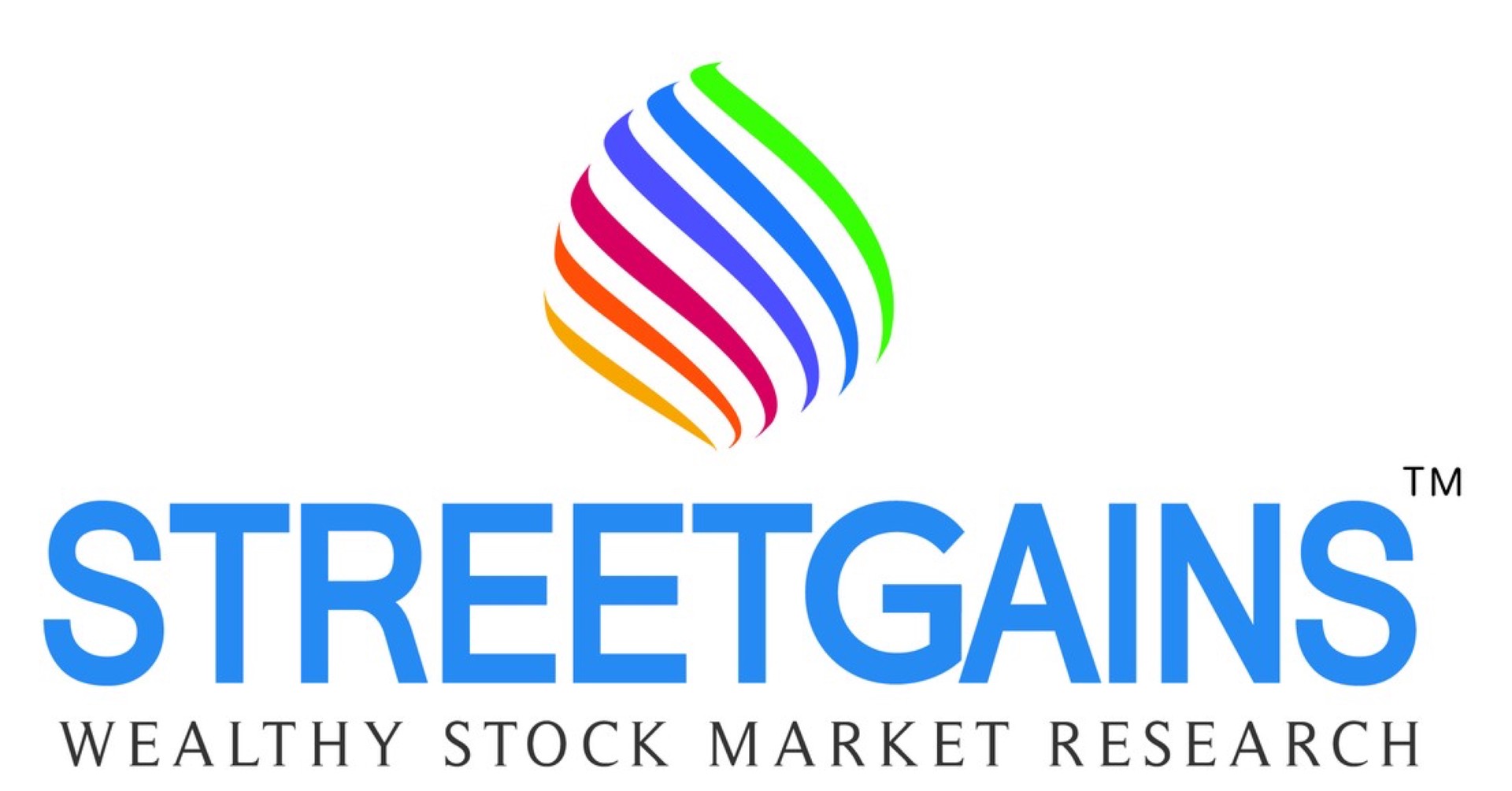
INH200003208
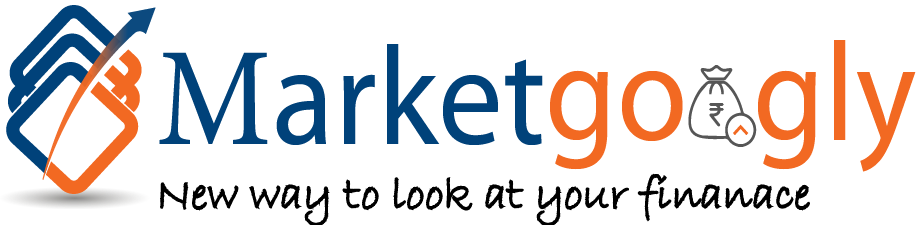
INA300012547
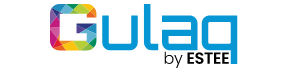
INA000016463
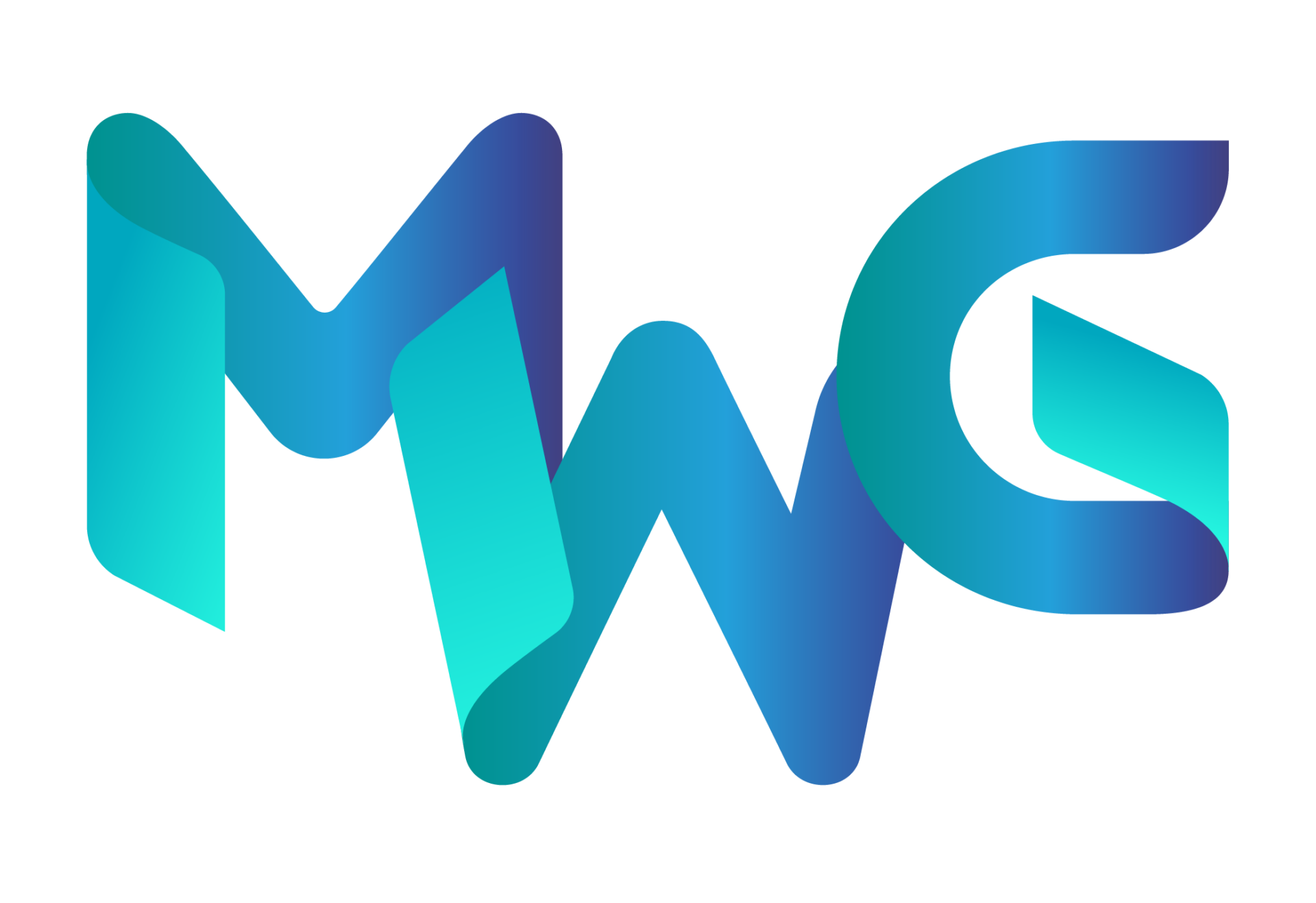
INH000008677
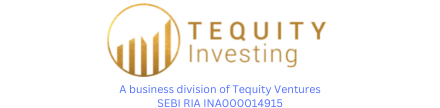
INA000014915
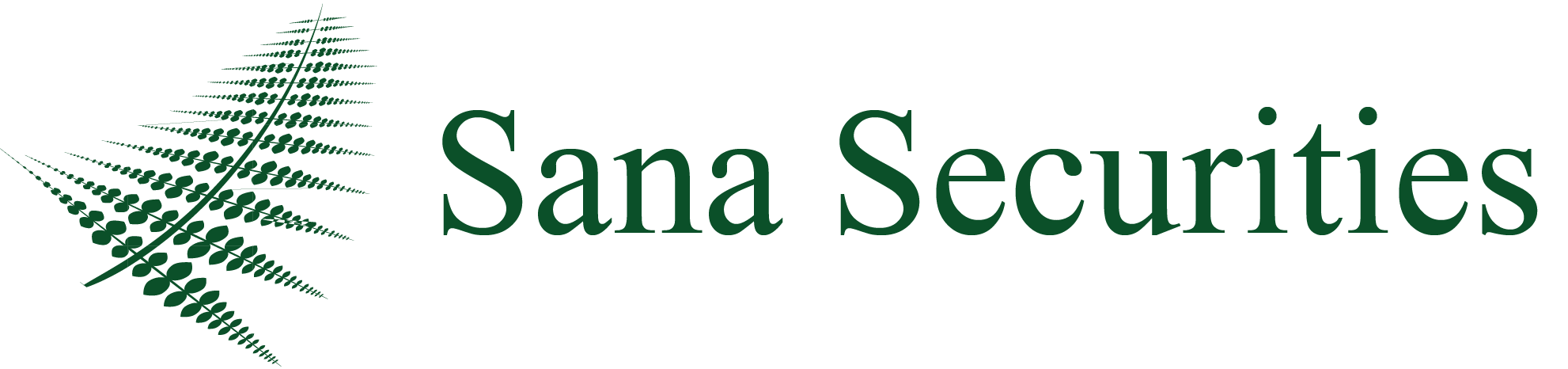
INA100004608
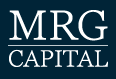
INP000006253
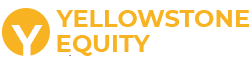
INH000007216
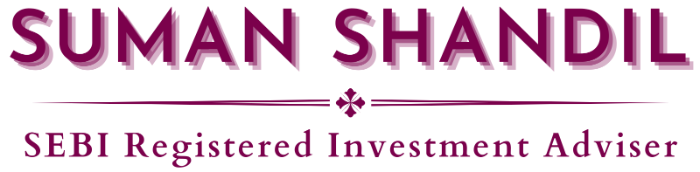
INA100013205
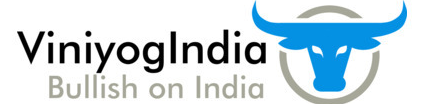
INA300008614
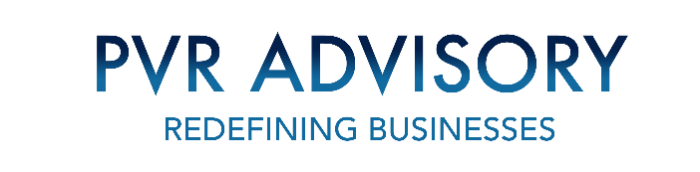
INA200010904
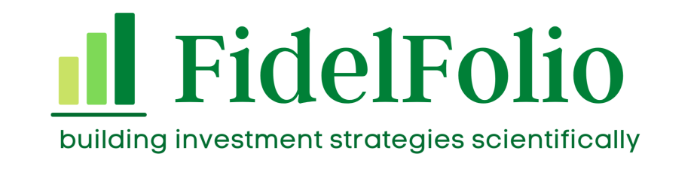
INA000016056
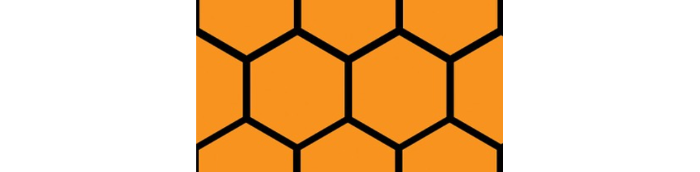
INH000001139
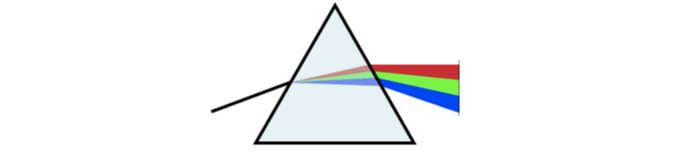
INA000010584
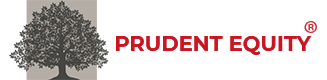
INH100009488
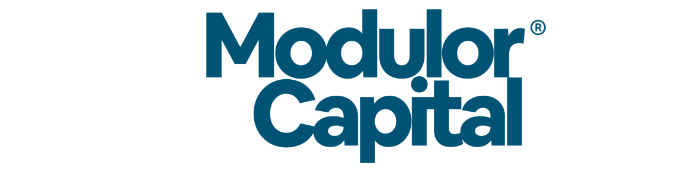
INA100015115
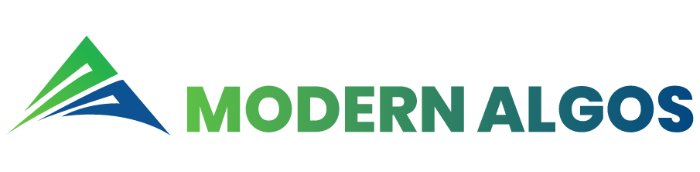
INH200009935
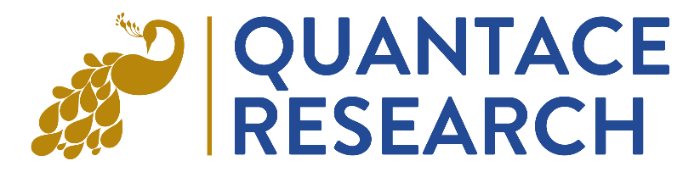
INH000008312
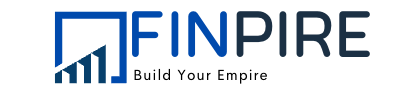
INH100008638
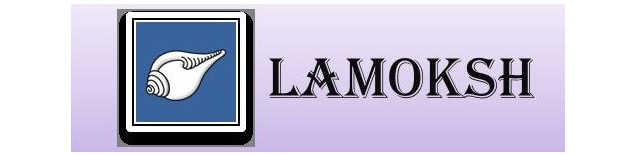
INA100014426
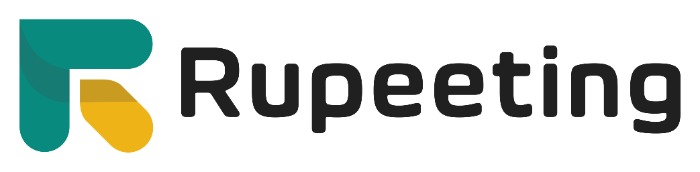
INA000015747
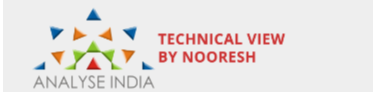
INH000008075
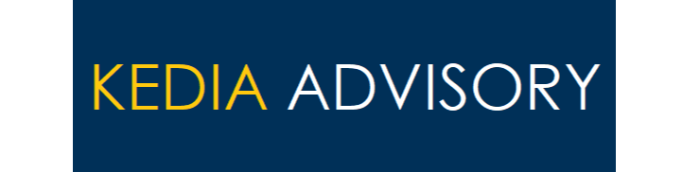
INH000006156
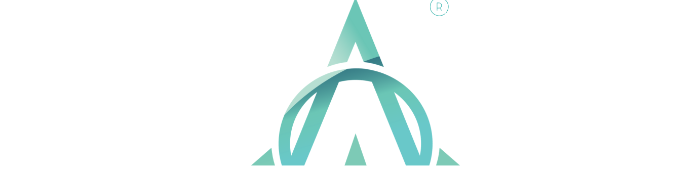
INA300003616
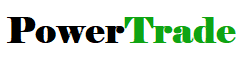
INH000008552
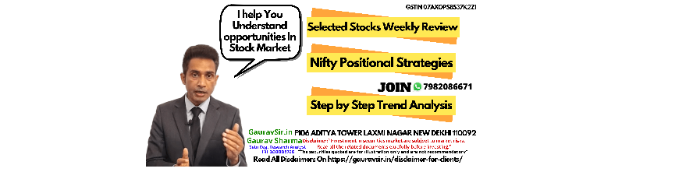
INH100008726
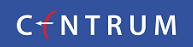
INH000001469
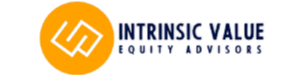
INH000009047
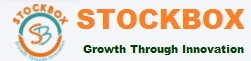
INH100008799
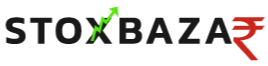
INH100008939
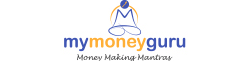
INA100010402
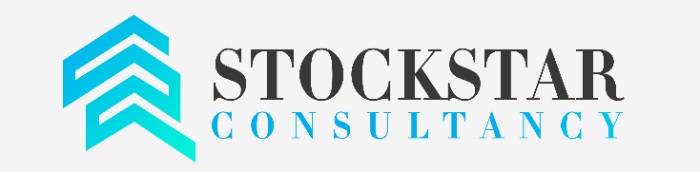
INH200009032
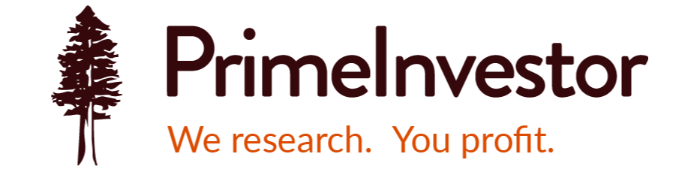
INH200008653
New study uncovers lesser-discussed yet major driver of inflation: 'The numbers are rather striking'
R ising temperatures and extreme weather events will drive food prices and inflation higher within the next decade, according to a new study.
A joint product of climate scientist Max Kotz and the European Central Bank, the paper was published in Communications Earth & Environment on March 21. Kotz works at Germany's Potsdam Institute for Climate Impact Research.
"The physical impacts of climate change are going to have a persistent effect on inflation," he told Phys.org . "This is really from my perspective another example of one of the ways in which climate change can undermine human welfare, economic welfare."
Which of these factors would most effectively motivate you to buy an induction stove?
Healthier indoor air, superior cooking results, helping the planet, i wouldn't buy an induction stove.
One reason for the projected increases is that hot weather and natural disasters, including floods and droughts, can crush food crops. These events are getting more intense and happening more frequently because of rising global temperatures, which are "basically steroids for weather," as one climate expert says .
"There are these productivity shocks that we know about from climate change, from the weather phenomena caused by climate change, from heat waves and so forth to reduce agricultural productivity," Kotz said. "Those also then have a knock-on effect on food inflation, on headline inflation."
In the study, researchers calculated that "weather and climate shocks" will increase food costs by 1.5 to 1.8 percentage points annually by 2035 and inflation by 0.8 to 0.9 percentage points, Phys.org reported, noting the figures may seem small but are "significant."
Watch now: The most sustainable thing about the new Rivian? Its price tag
The study used "national exposure to different weather conditions" and monthly prices of goods and services from 121 countries from 1996 to 2021.
"Pressures are largest at low latitudes and show strong seasonality at high latitudes, peaking in summer," the authors wrote , meaning the problem will be worse for countries close to the equator. Those nearer the poles will have issues when the heat is hottest.
"Climateflation" is "all too real and the numbers are rather striking," Columbia University climate economist Gernot Wagner told Phys.org .
"Beyond 2035 the magnitude of estimated pressures on inflation diverges strongly across emission scenarios, suggesting that decisive mitigation of greenhouse gases could substantially reduce them," according to the study. "By 2060, there is a strong and robust difference in the average global pressures on food inflation between the highest and lowest emission scenarios."
The scientists estimated that by 2060, food inflation will rise by 2.1 percentage points per year. But if countries cut their pollution production to a best-case scenario, climate-driven inflation will be "only marginally larger in 2060 than in 2035," they said . A worst-case scenario, however, would lead to food inflation of more than 4 percentage points per year "across large parts of the world."
To help decrease the amount of pollution we release into the atmosphere — where it surrounds the planet like a blanket, trapping heat — you can take steps such as switching to solar or wind energy to power your home , changing your buying habits to support companies that don't use plastic , and reducing your red meat intake . The Commons app will even help you do it, and you can earn money in the process.
Join our free newsletter for cool news and actionable info that makes it easy to help yourself while helping the planet.
New study uncovers lesser-discussed yet major driver of inflation: 'The numbers are rather striking' first appeared on The Cool Down .
Modelling Inflation in India
- Original Article
- Published: 30 June 2021
- Volume 19 , pages 555–581, ( 2021 )
Cite this article
- Pulapre Balakrishnan 1 &
- M. Parameswaran ORCID: orcid.org/0000-0001-8338-0376 2
337 Accesses
3 Citations
1 Altmetric
Explore all metrics
The Reserve Bank of India pursues inflation targeting, which is based on the premise that inflation is related to the ‘output gap’, defined as the deviation of output from its natural level. We present an alternative model of inflation derived from structuralist macroeconomics, the highlight of which is an agricultural sector prone to fluctuating output. A distinctive feature of the model is that inflation is related to the relative price of agricultural goods, in addition to other determining variables. We then subject both the models to econometric testing that involved alternative measures of inflation, sample periods and data frequencies. The output-gap model is rejected while the structuralist model is statistically validated in the tests. This evidence points strongly to the need to take agricultural prices into account when designing anti-inflationary policy in India.
This is a preview of subscription content, log in via an institution to check access.
Access this article
Price includes VAT (Russian Federation)
Instant access to the full article PDF.
Rent this article via DeepDyve
Institutional subscriptions
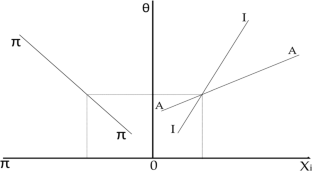
Similar content being viewed by others
Dynamics and Determinants of Inflation in Ethiopia
Inflation dynamics in india: relative role of structural and monetary factors.
Biswajit Maitra
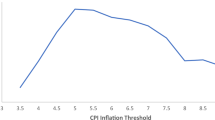
Inflation-Growth Relationship: New Evidence for India
Barendra Kumar Bhoi & Gulapsha Tabasum
See, for instance, Romer ( 2018 ).
See RBI ( 2014 ).
See Taylor ( 1984 ).
See Palma ( 1987 ).
Kaldor ( 1971 , 1976 ) describes this process. The condition for non-inflationary growth in a developing economy had already been identified by Kalecki ( 1955 , 1971 ).
See Basu ( 2003 ).
It may be noted that once θ and P i are known P a follows.
RBI ( 2014 ).
This is not surprising. In one of the earliest tests of the output gap model of inflation for India Nachane and Lakshmi ( 2002 ) had found that the output gap is mostly of “perverse sign” and statistically not significant.
In the light of our preceding comment on the method adopted by Srinivasan et al. it may be said that Ball et al. adopt the standard procedure, i.e., they use the current dated output and not lags of the same in a regression of the Phillips Curve.
See Srinivasan et al. ( 2006 ).
See Eq. 10.
See Patra and Kapur ( 2010 ) and Guimaraes and Papi ( 2016 ).
See Paul ( 2009 ).
See Canavese ( 1982 ).
For instance, in the case of output gap in quarterly series, the instruments chosen were the 2nd, 4th, 6th and 8th lags, the respective correlation coefficients being − 0.84, − 0.94, 0.86, and 0.93 (see Roodman, 2009 ; Murray, 2006 and Reed, 2015 ).
Schreiber ( 2008 ) shows that non-PSD of the estimated variance differences in a Hausman test can be systematic and is not only a finite sample issue.
Hatekar, et al. ( 2011 ) is an exception but their study is confined to manufacturing price inflation.
See Fuhrer ( 1997 ) and Rudd and Whelan ( 2007 ).
Ball, L., A. Chari, and P. Mishra. 2016. Understanding Inflation in India, NBER Working Paper No. 22948.
Basu, K. 2003. Analytical development economics—the less developed economy . Cambridge: MIT Press.
Google Scholar
Canavese, A.J. 1982. The structuralist explanation in the theory of inflation. World Development 10: 523–529.
Article Google Scholar
Canova, F., and B.E. Hansen. 1995. Are seasonal patterns constant over time? A Test for Seasonal Stability. Journal of Business & Economic Statistics 13: 237–252.
Fuhrer, J. 1997. The (Un)importance of forward-looking behaviour in price specifications. Journal of Money, Credit and Banking 29: 338–350.
Fuhrer, J., Y. Kodrzycki, J.S. Little, and G.P. Olivei. 2009. The Philips curve in historical context. In Understanding inflation and the implications for monetary policy: a Philips curve retrospective , ed. J. Fuhrer, Y. Kodrzycki, J.S. Little, and G.P. Olivei. Cambridge: MIT Press.
Chapter Google Scholar
Gali, J., and M. Gertler. 1999. Inflation dynamics: a structural econometric analysis. Journal of Monetary Economics. 44: 195.
Guimarães, R.F., and L. Papi. 2016. Inflation dynamics in india: what can we learn from Phillips curves? In Taming indian inflation , ed. R. Anand and P. Cashin. Washington, D.C: International Monetary Fund.
Hamilton, J.D. 2018. Why you should never use the Hodrick-Prescott filter. The Review of Economics and Statistics. 100: 831–843.
Hatekar, N., A. Sharma, and S. Kulkarni. 2011. What drives inflation in India: overheating or input costs? Economic and Political Weekly. 46: 46–51.
Hylleberg, S., R.F. Engle, C.W.J. Granger, and B.S. Yoo. 1990. Seasonal integration and cointegration. Journal of Econometrics. 44: 215–238.
Kaldor, N., 1971. The role of industrialisation in Latin American inflations, reprinted . In: Further Essays in Applied Economics, Duckworth, London.
Kaldor, N. 1976. Inflation and recession in the world economy. The Economic Journal. 86: 703–714.
Kalecki, M. 1955. The problem of financing economic development. Indian Economic Review. 3: 1–22.
Kwiatkowski, D., P.C.B. Phillips, P. Schmidt, and Y. Shin. 1992. Testing the null hypothesis of stationarity against the alternative of a unit root: how sure are we that economic time series have a unit root? Journal of Econometrics. 54: 159–178.
Murray, M.P. 2006. Avoiding invalid instruments and coping with weak instruments. Journal of Economic Perspectives. 20: 111–132.
Nachane, D.M., and R. Lakshmi. 2002. Dynamics of inflation in India: a P-Star approach. Applied Economics. 34: 101–110.
Palma, J.G. 1987. Structuralism. The New Palgrave: A Dictionary of Economics 4: 527–531.
Patra, M. D, and M. Kapur. 2010. A monetary policy model without money for India, Working Paper 10/183. International Monetary Fund, Washington, D.C.
Paul, B.P. 2009. In search of the Phillips curve for India. Journal of Asian Economics. 20: 479–488.
Reed, W.R. 2015. On the practice of lagging variables to avoid simultaneity. Oxford Bulletin of Economics and Statistics. 77: 897–905.
Reserve Bank of India. 2014. Report of the expert committee to revise and strengthen the monetary policy framework . Mumbai: RBI.
Roberts, J.M. 1995. New Keynesian economics and the Phillips curve. Journal of Money, Credit and Banking 27: 975–984.
Romer, P. 2018. Advanced macroeconomics . McGraw-Hill Economics.
Roodman, D. 2009. A note on the theme of too many instruments. Oxford Bulletin of Economics and Statistics. 71: 135–158.
Rudd, J., and K. Whelan. 2007. Modeling inflation dynamics: a critical review of recent research. Journal of Money, Credit and Banking. 39: 155–170.
Schreiber, Sven. 2008. The Hausman test statistic can be negative even asymptotically. Journal of Economics and Statistics 228 (4): 394–405.
Srinivasan, N., V. Mahambare, and M. Ramachandran. 2006. Modelling inflation in India: critique of the structuralist approach. Journal of Quantitative Economics, New Series. 4: 45–58.
Taylor, L. 1984. Structuralist macroeconomics . New York: Basic Books.
Download references
Acknowledgements
We thank Abhijit Manik Sen, Dipankar Dasgupta, Susanto Basu, Mausumi Das, Ajit Kumar Ghose, Dilip Mookherjee, Bharat Ramaswami, Partha Ray, Bhaskar Dutta, A. Amanullah, Anjan Mukherji, Regupathy Venkatachalam and Rathin Roy for discussions and seminar audiences at the National Institute of Public Finance and Policy, Indian Institute of Management Calcutta, South Asian University, Azim Premji University, Ashoka University, the Centre for Development Studies and the Delhi School of Economics for comments. Gurdeep Lamba assisted with the initial estimation and Dennis Rajakumar helped us track down some crucial data. We record financial assistance from Ashoka University and the Centre for Development Studies. The comments of four anonymous referees of this journal and of the Editor are gratefully acknowledged. Errors that may yet remain would be ours.
Author information
Authors and affiliations.
Ashoka University, Sonipat, Haryana, 131029, India
Pulapre Balakrishnan
Centre for Development Studies, Thiruvananthapuram, Kerala, 695011, India
M. Parameswaran
You can also search for this author in PubMed Google Scholar
Corresponding author
Correspondence to M. Parameswaran .
Additional information
Publisher's note.
Springer Nature remains neutral with regard to jurisdictional claims in published maps and institutional affiliations.
A1. Testing for a Unit Root and Seasonal Stability
Unit root properties of the time series were tested using the Augmented Dickey–Fuller (ADF) test, the Kwiatkowski, Phillips, Schmidt, and Shin (KPSS) test, and the Zivot and Andrews test. The Zivot–Andrews test is used because a trend stationary series with a break in the trend can be wrongly diagnosed as an I(1) process by both the ADF and KPSS tests. This test allows for an unknown break in trend and intercept when testing for a unit root. The lag length for the ADF test was selected on the basis of the Bayesian Information Criteria (BIC) and lag length in KPSS test was fixed at 1, as the simulation results reported in Kwiatkowski et al. ( 1992 ), showed that for a sample size similar to ours, a lag length of one provides correct size of the test.
In the case of quarterly data, we also test for a seasonal unit root or seasonal stability using the tests developed in Canova and Hansen ( 1995 ) and Hylleberg et al. ( 1990 ), respectively known in the literature as the Canova–Hansen test and HEGY test.
The test results are presented in Tables 16 , 17 , 18 , 19 , 20 , 21 and summary measures of variables are presented in Tables 22 and 23 .
Rights and permissions
Reprints and permissions
About this article
Balakrishnan, P., Parameswaran, M. Modelling Inflation in India. J. Quant. Econ. 19 , 555–581 (2021). https://doi.org/10.1007/s40953-021-00238-y
Download citation
Accepted : 15 June 2021
Published : 30 June 2021
Issue Date : September 2021
DOI : https://doi.org/10.1007/s40953-021-00238-y
Share this article
Anyone you share the following link with will be able to read this content:
Sorry, a shareable link is not currently available for this article.
Provided by the Springer Nature SharedIt content-sharing initiative
- Inflation in India
- New Keynesian Phillips Curve
- Structuralist macroeconomics
JEL Classification
- Find a journal
- Publish with us
- Track your research
10 popular McDonald's menu items that cost up to 168% more now than a decade ago
- McDonald's has increased prices the quickest of 12 fast-food chains since 2014, a new report found.
- Collectively, the cost of a group of the most popular McDonald's items has doubled in that time.
- Price increases at Starbucks and Subway were much closer to inflation.

Your favorite McDonald's meal might cost twice as much as it did 10 years ago.
Collectively, a selection of McDonald's most popular items, from the Big Mac to a medium order of fries, doubled in price between 2014 and 2024, a report by FinanceBuzz found. That increase was the most of several major fast-food chains that FinanceBuzz, a finance-news and -research website, examined.
Over the same period, inflation rose 31%, per the report.
Among the biggest McDonald's price hikes was for the McDouble sandwich, which went from an average price of $1.19 in 2014 to $3.19 in 2024 — a 168% increase. The cost of a Quarter Pounder with cheese meal also more than doubled, from an average of $5.39 in 2014 to $11.99 this year, according to the report.
A McDonald's spokesperson said the numbers were "not an accurate representation of pricing at McDonald's restaurants" but did not provide alternative estimates of how much the chain had raised prices.
"As the article itself notes, pricing is set by individual franchisees and varies by restaurant," the spokesperson said, referencing the FinanceBuzz report.
Burger King told Business Insider that it had "always offered quality food at affordable prices, and we will continue to be very thoughtful about making sure our guests receive great value for the money they spend with us."
Related stories
A Starbucks spokesperson said that the chain was "evaluating and adjusting prices on an ongoing basis."
Spokespeople for the other nine restaurants mentioned in the study did not immediately respond to requests for comment from BI.
FinanceBuzz looked at the prices of 10 menu items from each restaurant examined in the study. It collected historical pricing data and, in the case of McDonald's, adjusted for the variety of pricing strategies used by franchisees, it said.
McDonald's has increased prices multiple times over the past decade. One of the most visible examples is the demise of its dollar menu , which now includes items that cost up to $3 — and few, if any, that actually sell for $1.
Executives acknowledged in February that McDonald's price increases had put off customers who made $45,000 a year or below. Many are ordering less at the chain's restaurants and eating at home more often, they said.
But other restaurants raised prices nearly as much during the decade. The fried-chicken chain Popeyes increased prices by 86%, while Taco Bell hiked them by 81%, the study found.
Not all chains were as aggressive with their increases. Both Subway and Starbucks increased prices by 39%. At Starbucks, the menu price of some products rose slower than broader inflation: The average cost of a caramel macchiato went up just 17% over the decade, for example.
Inflation has slowed in recent months, and some restaurant chains have indicated they plan to slow price increases in 2024.
But other costs, such as employee pay , remain high.
Inflation is still increasing, which means higher prices at many fast-food restaurants are here to stay, Daniel Roccato, a clinical professor of finance at the University of San Diego's Knauss School of Business, said.
"We won't see prices drop, but we can expect a pause," he said in FinanceBuzz's report.
Do you work at McDonald's or another restaurant chain and have a story idea? Reach out to this reporter at [email protected].
Watch: US vs India McDonald's | Food Wars
- Main content
- Share full article
For more audio journalism and storytelling, download New York Times Audio , a new iOS app available for news subscribers.

- April 10, 2024 • 22:49 Trump’s Abortion Dilemma
- April 9, 2024 • 30:48 How Tesla Planted the Seeds for Its Own Potential Downfall
- April 8, 2024 • 30:28 The Eclipse Chaser
- April 7, 2024 The Sunday Read: ‘What Deathbed Visions Teach Us About Living’
- April 5, 2024 • 29:11 An Engineering Experiment to Cool the Earth
- April 4, 2024 • 32:37 Israel’s Deadly Airstrike on the World Central Kitchen
- April 3, 2024 • 27:42 The Accidental Tax Cutter in Chief
- April 2, 2024 • 29:32 Kids Are Missing School at an Alarming Rate
- April 1, 2024 • 36:14 Ronna McDaniel, TV News and the Trump Problem
- March 29, 2024 • 48:42 Hamas Took Her, and Still Has Her Husband
- March 28, 2024 • 33:40 The Newest Tech Start-Up Billionaire? Donald Trump.
- March 27, 2024 • 28:06 Democrats’ Plan to Save the Republican House Speaker
How Tesla Planted the Seeds for Its Own Potential Downfall
Elon musk’s factory in china saved his company and made him ultrarich. now, it may backfire..
Hosted by Katrin Bennhold
Featuring Mara Hvistendahl
Produced by Rikki Novetsky and Mooj Zadie
With Rachelle Bonja
Edited by Lisa Chow and Alexandra Leigh Young
Original music by Marion Lozano , Diane Wong , Elisheba Ittoop and Sophia Lanman
Engineered by Chris Wood
Listen and follow The Daily Apple Podcasts | Spotify | Amazon Music
When Elon Musk set up Tesla’s factory in China, he made a bet that brought him cheap parts and capable workers — a bet that made him ultrarich and saved his company.
Mara Hvistendahl, an investigative reporter for The Times, explains why, now, that lifeline may have given China the tools to beat Tesla at its own game.
On today’s episode

Mara Hvistendahl , an investigative reporter for The New York Times.

Background reading
A pivot to China saved Elon Musk. It also bound him to Beijing .
Mr. Musk helped create the Chinese electric vehicle industry. But he is now facing challenges there as well as scrutiny in the West over his reliance on China.
There are a lot of ways to listen to The Daily. Here’s how.
We aim to make transcripts available the next workday after an episode’s publication. You can find them at the top of the page.
Fact-checking by Susan Lee .
The Daily is made by Rachel Quester, Lynsea Garrison, Clare Toeniskoetter, Paige Cowett, Michael Simon Johnson, Brad Fisher, Chris Wood, Jessica Cheung, Stella Tan, Alexandra Leigh Young, Lisa Chow, Eric Krupke, Marc Georges, Luke Vander Ploeg, M.J. Davis Lin, Dan Powell, Sydney Harper, Mike Benoist, Liz O. Baylen, Asthaa Chaturvedi, Rachelle Bonja, Diana Nguyen, Marion Lozano, Corey Schreppel, Rob Szypko, Elisheba Ittoop, Mooj Zadie, Patricia Willens, Rowan Niemisto, Jody Becker, Rikki Novetsky, John Ketchum, Nina Feldman, Will Reid, Carlos Prieto, Ben Calhoun, Susan Lee, Lexie Diao, Mary Wilson, Alex Stern, Dan Farrell, Sophia Lanman, Shannon Lin, Diane Wong, Devon Taylor, Alyssa Moxley, Summer Thomad, Olivia Natt, Daniel Ramirez and Brendan Klinkenberg.
Our theme music is by Jim Brunberg and Ben Landsverk of Wonderly. Special thanks to Sam Dolnick, Paula Szuchman, Lisa Tobin, Larissa Anderson, Julia Simon, Sofia Milan, Mahima Chablani, Elizabeth Davis-Moorer, Jeffrey Miranda, Renan Borelli, Maddy Masiello, Isabella Anderson and Nina Lassam.
Katrin Bennhold is the Berlin bureau chief. A former Nieman fellow at Harvard University, she previously reported from London and Paris, covering a range of topics from the rise of populism to gender. More about Katrin Bennhold
Mara Hvistendahl is an investigative reporter for The Times focused on Asia. More about Mara Hvistendahl
Advertisement
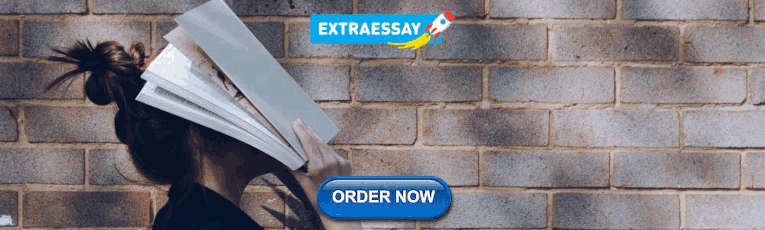
IMAGES
VIDEO
COMMENTS
Worries grew as the inflation rate (measured as the twelve-month change in the consumer price index) rose from 3.7% to 12.1% over 2001-2010. The inflation rate has since fallen to 5.2% in early 2015, leading to a debate about whether this moderation is likely to endure or inflation will rise again.
Our study complements the existing literature by providing a more recent assessment of the inflation process in India and highlights the role of domestic factors in shifting this process. There is also a large strand of literature that examines the role played by inflation targeting in driving inflation outcomes.
This paper revisits the threshold level of inflation for India. The empirical analysis follows spline regression for the period 1996-97Q1 to 2019-20Q4. The results suggest the existence of a statistically significant threshold level of inflation at 5 to 5.5% in terms of both CPI and WPI. Below this level, the impact of inflation on growth is generally positive whereas it is negative above ...
It has subsequently been adopted in India by former RBI Governor Raghuram Rajan in 2016 as a means to keep price levels in check while boosting growth. Thus, currently India has targeted inflation at 4 per cent (with an upper and lower limit of 6% and 2%, respectively).
The present study investigates the impact of macroeconomic factors on food price inflation in India utilizing the monthly time series during January 2006-March 2019. The long-run relationship is confirmed among the variables using the ARDL bounds testing approach to cointegration. The coefficients of long-run estimates show that per capita income, money supply, global food prices, and ...
Studies enquiring role of the fiscal factors on inflation in India is limited. However, Ramu and Gayithri have identified some channel through which fiscal deficit passes on and impacts inflation in India. Besides, the results of Romer , Lane study have justified the view that more open economies have lower inflation. According to these authors ...
The present study aims to empirically understand the behaviour of core inflation in India. A measure of core inflation is developed, and it is empirically estimated for the time period of January 2012-December 2019 based on consumer price index data. Then, we study the response of core inflation with respect to certain macroeconomic variables.
India's retail inflation spikes to 6.07% in February, government data revealed. Today, most economists are in favour of low and stable rate of inflation. So, it is important to control inflation in order to minimise its impact on the economy. Key words:Inflation, Purchasing power, Consumer Price Index, Economy.
India's retail inflation for the month of March jumped to a 17-month high of 6.95 per cent. ... In case of a rise of 100 bps to 8 per cent, it amounts to Rs 41,822.
Indeed, if we look at the peaks of monthly year-over-year inflation reached during a given year, the three peaks were higher—20.8 percent in 1991, 18.1 percent in 1998, and 20.2 percent in 2009-10 ( Figure 3.5, panel 1). Panel 2 presents the number of months for which food inflation was higher than nonfood inflation.
While there are several studies on inflation forecasting in India, such as Thakur et al., (2016), Maji and Das (2017), Pratap and Sengupta (2019), John et al., (2020) and Jose et al., (2021), none of the studies have explored the predictive ability of news data for forecasting inflation in India. Our study aims to add to this literature through ...
The contribution of this paper lies in two folds. First, this study examines the relationship between relatively recent inflation expectations survey of households (IESH) and the actual inflation for India. Secondly, the author employs a structural VAR with the time period 2006 Q2 to 2020 Q2 on inflation expectation survey data of India.
Abstract. Using the quarterly data on WPI inflation and its components, the study examines the trends and patterns of inflation in India from 1991. After disaggregation of WPI index into its ...
This is true in the case of India also. This may be because data on prices are not comparable at the state level 1 and because they are assumed to be determined by national-level demand and supply factors; national level inflation is taken as a proxy for state level price behaviour. Price formation at the national level has a lot to do with ...
Case Study: Inflation in India. Knowing Inflation. By inflation one generally means rise in prices. To be more correct inflation is persistent rise in the general price level rather than a once-for-all rise in it, while deflation is persistent falling price. A situation is described as inflationary when either the prices or the supply of money ...
We study the individual price behaviour in India using monthly item-wise CPI prices during 2011-2019 and explore the impact of implementation of inflation targeting on price behaviour. We find that inflation targeting has significantly reduced the frequency of price increases and increased the frequency of price decreases in India; however, it has not been successful in reducing the ...
Downloadable (with restrictions)! A comprehensive strategy for price stability, particularly for the long-run, requires coordination between monetary and fiscal policy. To our knowledge, the fiscal initiative to control inflation in India is abstracted. The targets and executions of the fiscal policy of different state governments are independent, lop-sided, and also distinct from the fiscal ...
This paper studies micro-level data from the Reserve Bank of India's 'Inflation Expectations Survey of Households' with a nuanced focus on socio-demographics of gender, age, occupation, and city of residence.
India's consumer price inflation likely eased to a five-month low of 4.91% in March but was still above the Reserve Bank of India's 4% medium-term target as food price rises persist, according to ...
The retail inflation rate registered a slight drop of 0.01 percent in one month, as it stood at 5.10 percent in January 2024. The current inflation remains in the Reserve Bank of India (RBI ...
Causes for Spike in Inflation. Higher Food Inflation: Consumer food prices increased to 5.94% from 4.2% in December 2022 . Spices jumped from 20.3% inflation to 21.1% in January. Inflation in eggs sped from 6.9% in December to 8.8% in January, while the pace of price rise in meat and fish rose from 5.1% to 6.04%.
The RBI's hawkish line may be a further drag on growth, they say. "With food inflation being the prime driver of headline inflation while core inflation is at its pandemic low and well below ...
To identify the role played by inflation targeting in lowering inflation in India, we start by first studying its recent history. Figure 1 may be seen the trajectory of annual inflation on a quarterly basis from the year 2000 onwards, including the period from the first quarter of the financial year 2016. Details of the sources of data used in this study are given in the Appendix A1 and ...
India's Retail Inflation Falls. In July, vegetable prices increased by a staggering 37% year on year, with tomato prices at some wholesale markets increasing 1,400% over the 3 months . Annual food-inflation rate hit 11.5% in July, highest in over 3 years, pushing the overall inflation rate to a 15-month high of 7.4%.
The Indian central bank's key interest rate was kept unchanged for a seventh straight policy meeting on Friday as growth in the economy is expected to remain robust while inflation stays above ...
A worst-case scenario would lead to food inflation of more than 4 percentage points per year "across large parts of the world." New study uncovers lesser-discussed yet major driver of inflation ...
Recent Studies of Inflation in India we undertake a brief survey of the econometric studies on inflation in India. ... From the comparative statics of Figs. 2 and 3, it can be seen that in the structuralist model, inflation can arise in the case of both an expansion or contraction of the economy, depending upon the origin of the shock. It ...
FinanceBuzz. Among the biggest McDonald's price hikes was for the McDouble sandwich, which went from an average price of $1.19 in 2014 to $3.19 in 2024 — a 168% increase. The cost of a Quarter ...
29. Hosted by Katrin Bennhold. Featuring Mara Hvistendahl. Produced by Rikki Novetsky and Mooj Zadie. With Rachelle Bonja. Edited by Lisa Chow and Alexandra Leigh Young. Original music by Marion ...