Advertisement
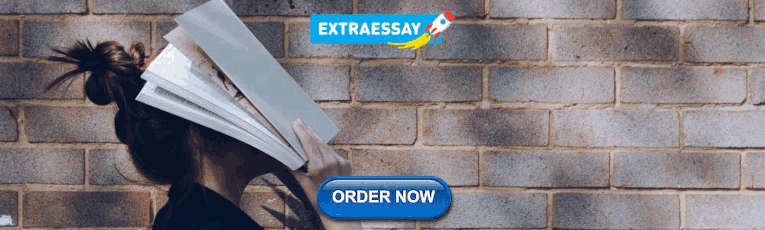
The effects of online education on academic success: A meta-analysis study
- Published: 06 September 2021
- Volume 27 , pages 429–450, ( 2022 )
Cite this article
- Hakan Ulum ORCID: orcid.org/0000-0002-1398-6935 1
77k Accesses
26 Citations
11 Altmetric
Explore all metrics
The purpose of this study is to analyze the effect of online education, which has been extensively used on student achievement since the beginning of the pandemic. In line with this purpose, a meta-analysis of the related studies focusing on the effect of online education on students’ academic achievement in several countries between the years 2010 and 2021 was carried out. Furthermore, this study will provide a source to assist future studies with comparing the effect of online education on academic achievement before and after the pandemic. This meta-analysis study consists of 27 studies in total. The meta-analysis involves the studies conducted in the USA, Taiwan, Turkey, China, Philippines, Ireland, and Georgia. The studies included in the meta-analysis are experimental studies, and the total sample size is 1772. In the study, the funnel plot, Duval and Tweedie’s Trip and Fill Analysis, Orwin’s Safe N Analysis, and Egger’s Regression Test were utilized to determine the publication bias, which has been found to be quite low. Besides, Hedge’s g statistic was employed to measure the effect size for the difference between the means performed in accordance with the random effects model. The results of the study show that the effect size of online education on academic achievement is on a medium level. The heterogeneity test results of the meta-analysis study display that the effect size does not differ in terms of class level, country, online education approaches, and lecture moderators.
Avoid common mistakes on your manuscript.
1 Introduction
Information and communication technologies have become a powerful force in transforming the educational settings around the world. The pandemic has been an important factor in transferring traditional physical classrooms settings through adopting information and communication technologies and has also accelerated the transformation. The literature supports that learning environments connected to information and communication technologies highly satisfy students. Therefore, we need to keep interest in technology-based learning environments. Clearly, technology has had a huge impact on young people's online lives. This digital revolution can synergize the educational ambitions and interests of digitally addicted students. In essence, COVID-19 has provided us with an opportunity to embrace online learning as education systems have to keep up with the rapid emergence of new technologies.
Information and communication technologies that have an effect on all spheres of life are also actively included in the education field. With the recent developments, using technology in education has become inevitable due to personal and social reasons (Usta, 2011a ). Online education may be given as an example of using information and communication technologies as a consequence of the technological developments. Also, it is crystal clear that online learning is a popular way of obtaining instruction (Demiralay et al., 2016 ; Pillay et al., 2007 ), which is defined by Horton ( 2000 ) as a way of education that is performed through a web browser or an online application without requiring an extra software or a learning source. Furthermore, online learning is described as a way of utilizing the internet to obtain the related learning sources during the learning process, to interact with the content, the teacher, and other learners, as well as to get support throughout the learning process (Ally, 2004 ). Online learning has such benefits as learning independently at any time and place (Vrasidas & MsIsaac, 2000 ), granting facility (Poole, 2000 ), flexibility (Chizmar & Walbert, 1999 ), self-regulation skills (Usta, 2011b ), learning with collaboration, and opportunity to plan self-learning process.
Even though online education practices have not been comprehensive as it is now, internet and computers have been used in education as alternative learning tools in correlation with the advances in technology. The first distance education attempt in the world was initiated by the ‘Steno Courses’ announcement published in Boston newspaper in 1728. Furthermore, in the nineteenth century, Sweden University started the “Correspondence Composition Courses” for women, and University Correspondence College was afterwards founded for the correspondence courses in 1843 (Arat & Bakan, 2011 ). Recently, distance education has been performed through computers, assisted by the facilities of the internet technologies, and soon, it has evolved into a mobile education practice that is emanating from progress in the speed of internet connection, and the development of mobile devices.
With the emergence of pandemic (Covid-19), face to face education has almost been put to a halt, and online education has gained significant importance. The Microsoft management team declared to have 750 users involved in the online education activities on the 10 th March, just before the pandemic; however, on March 24, they informed that the number of users increased significantly, reaching the number of 138,698 users (OECD, 2020 ). This event supports the view that it is better to commonly use online education rather than using it as a traditional alternative educational tool when students do not have the opportunity to have a face to face education (Geostat, 2019 ). The period of Covid-19 pandemic has emerged as a sudden state of having limited opportunities. Face to face education has stopped in this period for a long time. The global spread of Covid-19 affected more than 850 million students all around the world, and it caused the suspension of face to face education. Different countries have proposed several solutions in order to maintain the education process during the pandemic. Schools have had to change their curriculum, and many countries supported the online education practices soon after the pandemic. In other words, traditional education gave its way to online education practices. At least 96 countries have been motivated to access online libraries, TV broadcasts, instructions, sources, video lectures, and online channels (UNESCO, 2020 ). In such a painful period, educational institutions went through online education practices by the help of huge companies such as Microsoft, Google, Zoom, Skype, FaceTime, and Slack. Thus, online education has been discussed in the education agenda more intensively than ever before.
Although online education approaches were not used as comprehensively as it has been used recently, it was utilized as an alternative learning approach in education for a long time in parallel with the development of technology, internet and computers. The academic achievement of the students is often aimed to be promoted by employing online education approaches. In this regard, academicians in various countries have conducted many studies on the evaluation of online education approaches and published the related results. However, the accumulation of scientific data on online education approaches creates difficulties in keeping, organizing and synthesizing the findings. In this research area, studies are being conducted at an increasing rate making it difficult for scientists to be aware of all the research outside of their expertise. Another problem encountered in the related study area is that online education studies are repetitive. Studies often utilize slightly different methods, measures, and/or examples to avoid duplication. This erroneous approach makes it difficult to distinguish between significant differences in the related results. In other words, if there are significant differences in the results of the studies, it may be difficult to express what variety explains the differences in these results. One obvious solution to these problems is to systematically review the results of various studies and uncover the sources. One method of performing such systematic syntheses is the application of meta-analysis which is a methodological and statistical approach to draw conclusions from the literature. At this point, how effective online education applications are in increasing the academic success is an important detail. Has online education, which is likely to be encountered frequently in the continuing pandemic period, been successful in the last ten years? If successful, how much was the impact? Did different variables have an impact on this effect? Academics across the globe have carried out studies on the evaluation of online education platforms and publishing the related results (Chiao et al., 2018 ). It is quite important to evaluate the results of the studies that have been published up until now, and that will be published in the future. Has the online education been successful? If it has been, how big is the impact? Do the different variables affect this impact? What should we consider in the next coming online education practices? These questions have all motivated us to carry out this study. We have conducted a comprehensive meta-analysis study that tries to provide a discussion platform on how to develop efficient online programs for educators and policy makers by reviewing the related studies on online education, presenting the effect size, and revealing the effect of diverse variables on the general impact.
There have been many critical discussions and comprehensive studies on the differences between online and face to face learning; however, the focus of this paper is different in the sense that it clarifies the magnitude of the effect of online education and teaching process, and it represents what factors should be controlled to help increase the effect size. Indeed, the purpose here is to provide conscious decisions in the implementation of the online education process.
The general impact of online education on the academic achievement will be discovered in the study. Therefore, this will provide an opportunity to get a general overview of the online education which has been practiced and discussed intensively in the pandemic period. Moreover, the general impact of online education on academic achievement will be analyzed, considering different variables. In other words, the current study will allow to totally evaluate the study results from the related literature, and to analyze the results considering several cultures, lectures, and class levels. Considering all the related points, this study seeks to answer the following research questions:
What is the effect size of online education on academic achievement?
How do the effect sizes of online education on academic achievement change according to the moderator variable of the country?
How do the effect sizes of online education on academic achievement change according to the moderator variable of the class level?
How do the effect sizes of online education on academic achievement change according to the moderator variable of the lecture?
How do the effect sizes of online education on academic achievement change according to the moderator variable of the online education approaches?
This study aims at determining the effect size of online education, which has been highly used since the beginning of the pandemic, on students’ academic achievement in different courses by using a meta-analysis method. Meta-analysis is a synthesis method that enables gathering of several study results accurately and efficiently, and getting the total results in the end (Tsagris & Fragkos, 2018 ).
2.1 Selecting and coding the data (studies)
The required literature for the meta-analysis study was reviewed in July, 2020, and the follow-up review was conducted in September, 2020. The purpose of the follow-up review was to include the studies which were published in the conduction period of this study, and which met the related inclusion criteria. However, no study was encountered to be included in the follow-up review.
In order to access the studies in the meta-analysis, the databases of Web of Science, ERIC, and SCOPUS were reviewed by utilizing the keywords ‘online learning and online education’. Not every database has a search engine that grants access to the studies by writing the keywords, and this obstacle was considered to be an important problem to be overcome. Therefore, a platform that has a special design was utilized by the researcher. With this purpose, through the open access system of Cukurova University Library, detailed reviews were practiced using EBSCO Information Services (EBSCO) that allow reviewing the whole collection of research through a sole searching box. Since the fundamental variables of this study are online education and online learning, the literature was systematically reviewed in the related databases (Web of Science, ERIC, and SCOPUS) by referring to the keywords. Within this scope, 225 articles were accessed, and the studies were included in the coding key list formed by the researcher. The name of the researchers, the year, the database (Web of Science, ERIC, and SCOPUS), the sample group and size, the lectures that the academic achievement was tested in, the country that the study was conducted in, and the class levels were all included in this coding key.
The following criteria were identified to include 225 research studies which were coded based on the theoretical basis of the meta-analysis study: (1) The studies should be published in the refereed journals between the years 2020 and 2021, (2) The studies should be experimental studies that try to determine the effect of online education and online learning on academic achievement, (3) The values of the stated variables or the required statistics to calculate these values should be stated in the results of the studies, and (4) The sample group of the study should be at a primary education level. These criteria were also used as the exclusion criteria in the sense that the studies that do not meet the required criteria were not included in the present study.
After the inclusion criteria were determined, a systematic review process was conducted, following the year criterion of the study by means of EBSCO. Within this scope, 290,365 studies that analyze the effect of online education and online learning on academic achievement were accordingly accessed. The database (Web of Science, ERIC, and SCOPUS) was also used as a filter by analyzing the inclusion criteria. Hence, the number of the studies that were analyzed was 58,616. Afterwards, the keyword ‘primary education’ was used as the filter and the number of studies included in the study decreased to 3152. Lastly, the literature was reviewed by using the keyword ‘academic achievement’ and 225 studies were accessed. All the information of 225 articles was included in the coding key.
It is necessary for the coders to review the related studies accurately and control the validity, safety, and accuracy of the studies (Stewart & Kamins, 2001 ). Within this scope, the studies that were determined based on the variables used in this study were first reviewed by three researchers from primary education field, then the accessed studies were combined and processed in the coding key by the researcher. All these studies that were processed in the coding key were analyzed in accordance with the inclusion criteria by all the researchers in the meetings, and it was decided that 27 studies met the inclusion criteria (Atici & Polat, 2010 ; Carreon, 2018 ; Ceylan & Elitok Kesici, 2017 ; Chae & Shin, 2016 ; Chiang et al. 2014 ; Ercan, 2014 ; Ercan et al., 2016 ; Gwo-Jen et al., 2018 ; Hayes & Stewart, 2016 ; Hwang et al., 2012 ; Kert et al., 2017 ; Lai & Chen, 2010 ; Lai et al., 2015 ; Meyers et al., 2015 ; Ravenel et al., 2014 ; Sung et al., 2016 ; Wang & Chen, 2013 ; Yu, 2019 ; Yu & Chen, 2014 ; Yu & Pan, 2014 ; Yu et al., 2010 ; Zhong et al., 2017 ). The data from the studies meeting the inclusion criteria were independently processed in the second coding key by three researchers, and consensus meetings were arranged for further discussion. After the meetings, researchers came to an agreement that the data were coded accurately and precisely. Having identified the effect sizes and heterogeneity of the study, moderator variables that will show the differences between the effect sizes were determined. The data related to the determined moderator variables were added to the coding key by three researchers, and a new consensus meeting was arranged. After the meeting, researchers came to an agreement that moderator variables were coded accurately and precisely.
2.2 Study group
27 studies are included in the meta-analysis. The total sample size of the studies that are included in the analysis is 1772. The characteristics of the studies included are given in Table 1 .
2.3 Publication bias
Publication bias is the low capability of published studies on a research subject to represent all completed studies on the same subject (Card, 2011 ; Littell et al., 2008 ). Similarly, publication bias is the state of having a relationship between the probability of the publication of a study on a subject, and the effect size and significance that it produces. Within this scope, publication bias may occur when the researchers do not want to publish the study as a result of failing to obtain the expected results, or not being approved by the scientific journals, and consequently not being included in the study synthesis (Makowski et al., 2019 ). The high possibility of publication bias in a meta-analysis study negatively affects (Pecoraro, 2018 ) the accuracy of the combined effect size, causing the average effect size to be reported differently than it should be (Borenstein et al., 2009 ). For this reason, the possibility of publication bias in the included studies was tested before determining the effect sizes of the relationships between the stated variables. The possibility of publication bias of this meta-analysis study was analyzed by using the funnel plot, Orwin’s Safe N Analysis, Duval and Tweedie’s Trip and Fill Analysis, and Egger’s Regression Test.
2.4 Selecting the model
After determining the probability of publication bias of this meta-analysis study, the statistical model used to calculate the effect sizes was selected. The main approaches used in the effect size calculations according to the differentiation level of inter-study variance are fixed and random effects models (Pigott, 2012 ). Fixed effects model refers to the homogeneity of the characteristics of combined studies apart from the sample sizes, while random effects model refers to the parameter diversity between the studies (Cumming, 2012 ). While calculating the average effect size in the random effects model (Deeks et al., 2008 ) that is based on the assumption that effect predictions of different studies are only the result of a similar distribution, it is necessary to consider several situations such as the effect size apart from the sample error of combined studies, characteristics of the participants, duration, scope, and pattern of the study (Littell et al., 2008 ). While deciding the model in the meta-analysis study, the assumptions on the sample characteristics of the studies included in the analysis and the inferences that the researcher aims to make should be taken into consideration. The fact that the sample characteristics of the studies conducted in the field of social sciences are affected by various parameters shows that using random effects model is more appropriate in this sense. Besides, it is stated that the inferences made with the random effects model are beyond the studies included in the meta-analysis (Field, 2003 ; Field & Gillett, 2010 ). Therefore, using random effects model also contributes to the generalization of research data. The specified criteria for the statistical model selection show that according to the nature of the meta-analysis study, the model should be selected just before the analysis (Borenstein et al., 2007 ; Littell et al., 2008 ). Within this framework, it was decided to make use of the random effects model, considering that the students who are the samples of the studies included in the meta-analysis are from different countries and cultures, the sample characteristics of the studies differ, and the patterns and scopes of the studies vary as well.
2.5 Heterogeneity
Meta-analysis facilitates analyzing the research subject with different parameters by showing the level of diversity between the included studies. Within this frame, whether there is a heterogeneous distribution between the studies included in the study or not has been evaluated in the present study. The heterogeneity of the studies combined in this meta-analysis study has been determined through Q and I 2 tests. Q test evaluates the random distribution probability of the differences between the observed results (Deeks et al., 2008 ). Q value exceeding 2 value calculated according to the degree of freedom and significance, indicates the heterogeneity of the combined effect sizes (Card, 2011 ). I 2 test, which is the complementary of the Q test, shows the heterogeneity amount of the effect sizes (Cleophas & Zwinderman, 2017 ). I 2 value being higher than 75% is explained as high level of heterogeneity.
In case of encountering heterogeneity in the studies included in the meta-analysis, the reasons of heterogeneity can be analyzed by referring to the study characteristics. The study characteristics which may be related to the heterogeneity between the included studies can be interpreted through subgroup analysis or meta-regression analysis (Deeks et al., 2008 ). While determining the moderator variables, the sufficiency of the number of variables, the relationship between the moderators, and the condition to explain the differences between the results of the studies have all been considered in the present study. Within this scope, it was predicted in this meta-analysis study that the heterogeneity can be explained with the country, class level, and lecture moderator variables of the study in terms of the effect of online education, which has been highly used since the beginning of the pandemic, and it has an impact on the students’ academic achievement in different lectures. Some subgroups were evaluated and categorized together, considering that the number of effect sizes of the sub-dimensions of the specified variables is not sufficient to perform moderator analysis (e.g. the countries where the studies were conducted).
2.6 Interpreting the effect sizes
Effect size is a factor that shows how much the independent variable affects the dependent variable positively or negatively in each included study in the meta-analysis (Dinçer, 2014 ). While interpreting the effect sizes obtained from the meta-analysis, the classifications of Cohen et al. ( 2007 ) have been utilized. The case of differentiating the specified relationships of the situation of the country, class level, and school subject variables of the study has been identified through the Q test, degree of freedom, and p significance value Fig. 1 and 2 .
3 Findings and results
The purpose of this study is to determine the effect size of online education on academic achievement. Before determining the effect sizes in the study, the probability of publication bias of this meta-analysis study was analyzed by using the funnel plot, Orwin’s Safe N Analysis, Duval and Tweedie’s Trip and Fill Analysis, and Egger’s Regression Test.
When the funnel plots are examined, it is seen that the studies included in the analysis are distributed symmetrically on both sides of the combined effect size axis, and they are generally collected in the middle and lower sections. The probability of publication bias is low according to the plots. However, since the results of the funnel scatter plots may cause subjective interpretations, they have been supported by additional analyses (Littell et al., 2008 ). Therefore, in order to provide an extra proof for the probability of publication bias, it has been analyzed through Orwin’s Safe N Analysis, Duval and Tweedie’s Trip and Fill Analysis, and Egger’s Regression Test (Table 2 ).
Table 2 consists of the results of the rates of publication bias probability before counting the effect size of online education on academic achievement. According to the table, Orwin Safe N analysis results show that it is not necessary to add new studies to the meta-analysis in order for Hedges g to reach a value outside the range of ± 0.01. The Duval and Tweedie test shows that excluding the studies that negatively affect the symmetry of the funnel scatter plots for each meta-analysis or adding their exact symmetrical equivalents does not significantly differentiate the calculated effect size. The insignificance of the Egger tests results reveals that there is no publication bias in the meta-analysis study. The results of the analysis indicate the high internal validity of the effect sizes and the adequacy of representing the studies conducted on the relevant subject.
In this study, it was aimed to determine the effect size of online education on academic achievement after testing the publication bias. In line with the first purpose of the study, the forest graph regarding the effect size of online education on academic achievement is shown in Fig. 3 , and the statistics regarding the effect size are given in Table 3 .
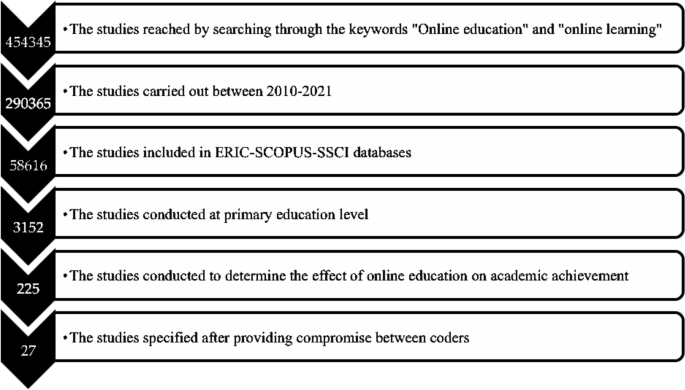
The flow chart of the scanning and selection process of the studies
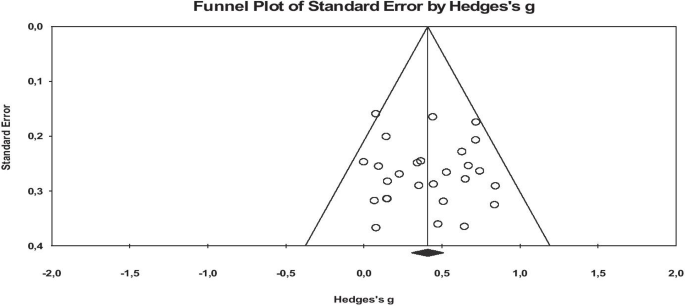
Funnel plot graphics representing the effect size of the effects of online education on academic success
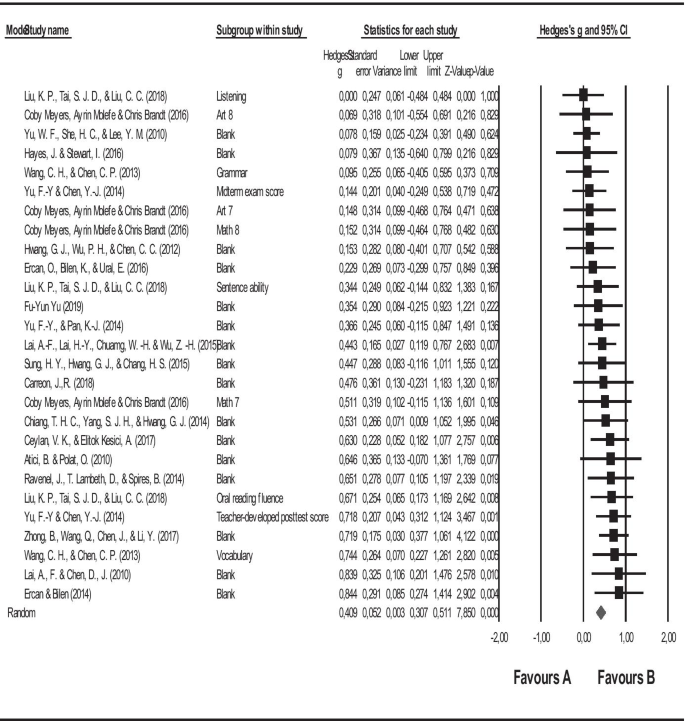
Forest graph related to the effect size of online education on academic success
The square symbols in the forest graph in Fig. 3 represent the effect sizes, while the horizontal lines show the intervals in 95% confidence of the effect sizes, and the diamond symbol shows the overall effect size. When the forest graph is analyzed, it is seen that the lower and upper limits of the combined effect sizes are generally close to each other, and the study loads are similar. This similarity in terms of study loads indicates the similarity of the contribution of the combined studies to the overall effect size.
Figure 3 clearly represents that the study of Liu and others (Liu et al., 2018 ) has the lowest, and the study of Ercan and Bilen ( 2014 ) has the highest effect sizes. The forest graph shows that all the combined studies and the overall effect are positive. Furthermore, it is simply understood from the forest graph in Fig. 3 and the effect size statistics in Table 3 that the results of the meta-analysis study conducted with 27 studies and analyzing the effect of online education on academic achievement illustrate that this relationship is on average level (= 0.409).
After the analysis of the effect size in the study, whether the studies included in the analysis are distributed heterogeneously or not has also been analyzed. The heterogeneity of the combined studies was determined through the Q and I 2 tests. As a result of the heterogeneity test, Q statistical value was calculated as 29.576. With 26 degrees of freedom at 95% significance level in the chi-square table, the critical value is accepted as 38.885. The Q statistical value (29.576) counted in this study is lower than the critical value of 38.885. The I 2 value, which is the complementary of the Q statistics, is 12.100%. This value indicates that the accurate heterogeneity or the total variability that can be attributed to variability between the studies is 12%. Besides, p value is higher than (0.285) p = 0.05. All these values [Q (26) = 29.579, p = 0.285; I2 = 12.100] indicate that there is a homogeneous distribution between the effect sizes, and fixed effects model should be used to interpret these effect sizes. However, some researchers argue that even if the heterogeneity is low, it should be evaluated based on the random effects model (Borenstein et al., 2007 ). Therefore, this study gives information about both models. The heterogeneity of the combined studies has been attempted to be explained with the characteristics of the studies included in the analysis. In this context, the final purpose of the study is to determine the effect of the country, academic level, and year variables on the findings. Accordingly, the statistics regarding the comparison of the stated relations according to the countries where the studies were conducted are given in Table 4 .
As seen in Table 4 , the effect of online education on academic achievement does not differ significantly according to the countries where the studies were conducted in. Q test results indicate the heterogeneity of the relationships between the variables in terms of countries where the studies were conducted in. According to the table, the effect of online education on academic achievement was reported as the highest in other countries, and the lowest in the US. The statistics regarding the comparison of the stated relations according to the class levels are given in Table 5 .
As seen in Table 5 , the effect of online education on academic achievement does not differ according to the class level. However, the effect of online education on academic achievement is the highest in the 4 th class. The statistics regarding the comparison of the stated relations according to the class levels are given in Table 6 .
As seen in Table 6 , the effect of online education on academic achievement does not differ according to the school subjects included in the studies. However, the effect of online education on academic achievement is the highest in ICT subject.
The obtained effect size in the study was formed as a result of the findings attained from primary studies conducted in 7 different countries. In addition, these studies are the ones on different approaches to online education (online learning environments, social networks, blended learning, etc.). In this respect, the results may raise some questions about the validity and generalizability of the results of the study. However, the moderator analyzes, whether for the country variable or for the approaches covered by online education, did not create significant differences in terms of the effect sizes. If significant differences were to occur in terms of effect sizes, we could say that the comparisons we will make by comparing countries under the umbrella of online education would raise doubts in terms of generalizability. Moreover, no study has been found in the literature that is not based on a special approach or does not contain a specific technique conducted under the name of online education alone. For instance, one of the commonly used definitions is blended education which is defined as an educational model in which online education is combined with traditional education method (Colis & Moonen, 2001 ). Similarly, Rasmussen ( 2003 ) defines blended learning as “a distance education method that combines technology (high technology such as television, internet, or low technology such as voice e-mail, conferences) with traditional education and training.” Further, Kerres and Witt (2003) define blended learning as “combining face-to-face learning with technology-assisted learning.” As it is clearly observed, online education, which has a wider scope, includes many approaches.
As seen in Table 7 , the effect of online education on academic achievement does not differ according to online education approaches included in the studies. However, the effect of online education on academic achievement is the highest in Web Based Problem Solving Approach.
4 Conclusions and discussion
Considering the developments during the pandemics, it is thought that the diversity in online education applications as an interdisciplinary pragmatist field will increase, and the learning content and processes will be enriched with the integration of new technologies into online education processes. Another prediction is that more flexible and accessible learning opportunities will be created in online education processes, and in this way, lifelong learning processes will be strengthened. As a result, it is predicted that in the near future, online education and even digital learning with a newer name will turn into the main ground of education instead of being an alternative or having a support function in face-to-face learning. The lessons learned from the early period online learning experience, which was passed with rapid adaptation due to the Covid19 epidemic, will serve to develop this method all over the world, and in the near future, online learning will become the main learning structure through increasing its functionality with the contribution of new technologies and systems. If we look at it from this point of view, there is a necessity to strengthen online education.
In this study, the effect of online learning on academic achievement is at a moderate level. To increase this effect, the implementation of online learning requires support from teachers to prepare learning materials, to design learning appropriately, and to utilize various digital-based media such as websites, software technology and various other tools to support the effectiveness of online learning (Rolisca & Achadiyah, 2014 ). According to research conducted by Rahayu et al. ( 2017 ), it has been proven that the use of various types of software increases the effectiveness and quality of online learning. Implementation of online learning can affect students' ability to adapt to technological developments in that it makes students use various learning resources on the internet to access various types of information, and enables them to get used to performing inquiry learning and active learning (Hart et al., 2019 ; Prestiadi et al., 2019 ). In addition, there may be many reasons for the low level of effect in this study. The moderator variables examined in this study could be a guide in increasing the level of practical effect. However, the effect size did not differ significantly for all moderator variables. Different moderator analyzes can be evaluated in order to increase the level of impact of online education on academic success. If confounding variables that significantly change the effect level are detected, it can be spoken more precisely in order to increase this level. In addition to the technical and financial problems, the level of impact will increase if a few other difficulties are eliminated such as students, lack of interaction with the instructor, response time, and lack of traditional classroom socialization.
In addition, COVID-19 pandemic related social distancing has posed extreme difficulties for all stakeholders to get online as they have to work in time constraints and resource constraints. Adopting the online learning environment is not just a technical issue, it is a pedagogical and instructive challenge as well. Therefore, extensive preparation of teaching materials, curriculum, and assessment is vital in online education. Technology is the delivery tool and requires close cross-collaboration between teaching, content and technology teams (CoSN, 2020 ).
Online education applications have been used for many years. However, it has come to the fore more during the pandemic process. This result of necessity has brought with it the discussion of using online education instead of traditional education methods in the future. However, with this research, it has been revealed that online education applications are moderately effective. The use of online education instead of face-to-face education applications can only be possible with an increase in the level of success. This may have been possible with the experience and knowledge gained during the pandemic process. Therefore, the meta-analysis of experimental studies conducted in the coming years will guide us. In this context, experimental studies using online education applications should be analyzed well. It would be useful to identify variables that can change the level of impacts with different moderators. Moderator analyzes are valuable in meta-analysis studies (for example, the role of moderators in Karl Pearson's typhoid vaccine studies). In this context, each analysis study sheds light on future studies. In meta-analyses to be made about online education, it would be beneficial to go beyond the moderators determined in this study. Thus, the contribution of similar studies to the field will increase more.
The purpose of this study is to determine the effect of online education on academic achievement. In line with this purpose, the studies that analyze the effect of online education approaches on academic achievement have been included in the meta-analysis. The total sample size of the studies included in the meta-analysis is 1772. While the studies included in the meta-analysis were conducted in the US, Taiwan, Turkey, China, Philippines, Ireland, and Georgia, the studies carried out in Europe could not be reached. The reason may be attributed to that there may be more use of quantitative research methods from a positivist perspective in the countries with an American academic tradition. As a result of the study, it was found out that the effect size of online education on academic achievement (g = 0.409) was moderate. In the studies included in the present research, we found that online education approaches were more effective than traditional ones. However, contrary to the present study, the analysis of comparisons between online and traditional education in some studies shows that face-to-face traditional learning is still considered effective compared to online learning (Ahmad et al., 2016 ; Hamdani & Priatna, 2020 ; Wei & Chou, 2020 ). Online education has advantages and disadvantages. The advantages of online learning compared to face-to-face learning in the classroom is the flexibility of learning time in online learning, the learning time does not include a single program, and it can be shaped according to circumstances (Lai et al., 2019 ). The next advantage is the ease of collecting assignments for students, as these can be done without having to talk to the teacher. Despite this, online education has several weaknesses, such as students having difficulty in understanding the material, teachers' inability to control students, and students’ still having difficulty interacting with teachers in case of internet network cuts (Swan, 2007 ). According to Astuti et al ( 2019 ), face-to-face education method is still considered better by students than e-learning because it is easier to understand the material and easier to interact with teachers. The results of the study illustrated that the effect size (g = 0.409) of online education on academic achievement is of medium level. Therefore, the results of the moderator analysis showed that the effect of online education on academic achievement does not differ in terms of country, lecture, class level, and online education approaches variables. After analyzing the literature, several meta-analyses on online education were published (Bernard et al., 2004 ; Machtmes & Asher, 2000 ; Zhao et al., 2005 ). Typically, these meta-analyzes also include the studies of older generation technologies such as audio, video, or satellite transmission. One of the most comprehensive studies on online education was conducted by Bernard et al. ( 2004 ). In this study, 699 independent effect sizes of 232 studies published from 1985 to 2001 were analyzed, and face-to-face education was compared to online education, with respect to success criteria and attitudes of various learners from young children to adults. In this meta-analysis, an overall effect size close to zero was found for the students' achievement (g + = 0.01).
In another meta-analysis study carried out by Zhao et al. ( 2005 ), 98 effect sizes were examined, including 51 studies on online education conducted between 1996 and 2002. According to the study of Bernard et al. ( 2004 ), this meta-analysis focuses on the activities done in online education lectures. As a result of the research, an overall effect size close to zero was found for online education utilizing more than one generation technology for students at different levels. However, the salient point of the meta-analysis study of Zhao et al. is that it takes the average of different types of results used in a study to calculate an overall effect size. This practice is problematic because the factors that develop one type of learner outcome (e.g. learner rehabilitation), particularly course characteristics and practices, may be quite different from those that develop another type of outcome (e.g. learner's achievement), and it may even cause damage to the latter outcome. While mixing the studies with different types of results, this implementation may obscure the relationship between practices and learning.
Some meta-analytical studies have focused on the effectiveness of the new generation distance learning courses accessed through the internet for specific student populations. For instance, Sitzmann and others (Sitzmann et al., 2006 ) reviewed 96 studies published from 1996 to 2005, comparing web-based education of job-related knowledge or skills with face-to-face one. The researchers found that web-based education in general was slightly more effective than face-to-face education, but it is insufficient in terms of applicability ("knowing how to apply"). In addition, Sitzmann et al. ( 2006 ) revealed that Internet-based education has a positive effect on theoretical knowledge in quasi-experimental studies; however, it positively affects face-to-face education in experimental studies performed by random assignment. This moderator analysis emphasizes the need to pay attention to the factors of designs of the studies included in the meta-analysis. The designs of the studies included in this meta-analysis study were ignored. This can be presented as a suggestion to the new studies that will be conducted.
Another meta-analysis study was conducted by Cavanaugh et al. ( 2004 ), in which they focused on online education. In this study on internet-based distance education programs for students under 12 years of age, the researchers combined 116 results from 14 studies published between 1999 and 2004 to calculate an overall effect that was not statistically different from zero. The moderator analysis carried out in this study showed that there was no significant factor affecting the students' success. This meta-analysis used multiple results of the same study, ignoring the fact that different results of the same student would not be independent from each other.
In conclusion, some meta-analytical studies analyzed the consequences of online education for a wide range of students (Bernard et al., 2004 ; Zhao et al., 2005 ), and the effect sizes were generally low in these studies. Furthermore, none of the large-scale meta-analyzes considered the moderators, database quality standards or class levels in the selection of the studies, while some of them just referred to the country and lecture moderators. Advances in internet-based learning tools, the pandemic process, and increasing popularity in different learning contexts have required a precise meta-analysis of students' learning outcomes through online learning. Previous meta-analysis studies were typically based on the studies, involving narrow range of confounding variables. In the present study, common but significant moderators such as class level and lectures during the pandemic process were discussed. For instance, the problems have been experienced especially in terms of eligibility of class levels in online education platforms during the pandemic process. It was found that there is a need to study and make suggestions on whether online education can meet the needs of teachers and students.
Besides, the main forms of online education in the past were to watch the open lectures of famous universities and educational videos of institutions. In addition, online education is mainly a classroom-based teaching implemented by teachers in their own schools during the pandemic period, which is an extension of the original school education. This meta-analysis study will stand as a source to compare the effect size of the online education forms of the past decade with what is done today, and what will be done in the future.
Lastly, the heterogeneity test results of the meta-analysis study display that the effect size does not differ in terms of class level, country, online education approaches, and lecture moderators.
*Studies included in meta-analysis
Ahmad, S., Sumardi, K., & Purnawan, P. (2016). Komparasi Peningkatan Hasil Belajar Antara Pembelajaran Menggunakan Sistem Pembelajaran Online Terpadu Dengan Pembelajaran Klasikal Pada Mata Kuliah Pneumatik Dan Hidrolik. Journal of Mechanical Engineering Education, 2 (2), 286–292.
Article Google Scholar
Ally, M. (2004). Foundations of educational theory for online learning. Theory and Practice of Online Learning, 2 , 15–44. Retrieved on the 11th of September, 2020 from https://eddl.tru.ca/wp-content/uploads/2018/12/01_Anderson_2008-Theory_and_Practice_of_Online_Learning.pdf
Arat, T., & Bakan, Ö. (2011). Uzaktan eğitim ve uygulamaları. Selçuk Üniversitesi Sosyal Bilimler Meslek Yüksek Okulu Dergisi , 14 (1–2), 363–374. https://doi.org/10.29249/selcuksbmyd.540741
Astuti, C. C., Sari, H. M. K., & Azizah, N. L. (2019). Perbandingan Efektifitas Proses Pembelajaran Menggunakan Metode E-Learning dan Konvensional. Proceedings of the ICECRS, 2 (1), 35–40.
*Atici, B., & Polat, O. C. (2010). Influence of the online learning environments and tools on the student achievement and opinions. Educational Research and Reviews, 5 (8), 455–464. Retrieved on the 11th of October, 2020 from https://academicjournals.org/journal/ERR/article-full-text-pdf/4C8DD044180.pdf
Bernard, R. M., Abrami, P. C., Lou, Y., Borokhovski, E., Wade, A., Wozney, L., et al. (2004). How does distance education compare with classroom instruction? A meta- analysis of the empirical literature. Review of Educational Research, 3 (74), 379–439. https://doi.org/10.3102/00346543074003379
Borenstein, M., Hedges, L. V., Higgins, J. P. T., & Rothstein, H. R. (2009). Introduction to meta-analysis . Wiley.
Book Google Scholar
Borenstein, M., Hedges, L., & Rothstein, H. (2007). Meta-analysis: Fixed effect vs. random effects . UK: Wiley.
Card, N. A. (2011). Applied meta-analysis for social science research: Methodology in the social sciences . Guilford.
Google Scholar
*Carreon, J. R. (2018 ). Facebook as integrated blended learning tool in technology and livelihood education exploratory. Retrieved on the 1st of October, 2020 from https://files.eric.ed.gov/fulltext/EJ1197714.pdf
Cavanaugh, C., Gillan, K. J., Kromrey, J., Hess, M., & Blomeyer, R. (2004). The effects of distance education on K-12 student outcomes: A meta-analysis. Learning Point Associates/North Central Regional Educational Laboratory (NCREL) . Retrieved on the 11th of September, 2020 from https://files.eric.ed.gov/fulltext/ED489533.pdf
*Ceylan, V. K., & Elitok Kesici, A. (2017). Effect of blended learning to academic achievement. Journal of Human Sciences, 14 (1), 308. https://doi.org/10.14687/jhs.v14i1.4141
*Chae, S. E., & Shin, J. H. (2016). Tutoring styles that encourage learner satisfaction, academic engagement, and achievement in an online environment. Interactive Learning Environments, 24(6), 1371–1385. https://doi.org/10.1080/10494820.2015.1009472
*Chiang, T. H. C., Yang, S. J. H., & Hwang, G. J. (2014). An augmented reality-based mobile learning system to improve students’ learning achievements and motivations in natural science inquiry activities. Educational Technology and Society, 17 (4), 352–365. Retrieved on the 11th of September, 2020 from https://www.researchgate.net/profile/Gwo_Jen_Hwang/publication/287529242_An_Augmented_Reality-based_Mobile_Learning_System_to_Improve_Students'_Learning_Achievements_and_Motivations_in_Natural_Science_Inquiry_Activities/links/57198c4808ae30c3f9f2c4ac.pdf
Chiao, H. M., Chen, Y. L., & Huang, W. H. (2018). Examining the usability of an online virtual tour-guiding platform for cultural tourism education. Journal of Hospitality, Leisure, Sport & Tourism Education, 23 (29–38), 1. https://doi.org/10.1016/j.jhlste.2018.05.002
Chizmar, J. F., & Walbert, M. S. (1999). Web-based learning environments guided by principles of good teaching practice. Journal of Economic Education, 30 (3), 248–264. https://doi.org/10.2307/1183061
Cleophas, T. J., & Zwinderman, A. H. (2017). Modern meta-analysis: Review and update of methodologies . Switzerland: Springer. https://doi.org/10.1007/978-3-319-55895-0
Cohen, L., Manion, L., & Morrison, K. (2007). Observation. Research Methods in Education, 6 , 396–412. Retrieved on the 11th of September, 2020 from https://www.researchgate.net/profile/Nabil_Ashraf2/post/How_to_get_surface_potential_Vs_Voltage_curve_from_CV_and_GV_measurements_of_MOS_capacitor/attachment/5ac6033cb53d2f63c3c405b4/AS%3A612011817844736%401522926396219/download/Very+important_C-V+characterization+Lehigh+University+thesis.pdf
Colis, B., & Moonen, J. (2001). Flexible Learning in a Digital World: Experiences and Expectations. Open & Distance Learning Series . Stylus Publishing.
CoSN. (2020). COVID-19 Response: Preparing to Take School Online. CoSN. (2020). COVID-19 Response: Preparing to Take School Online. Retrieved on the 3rd of September, 2021 from https://www.cosn.org/sites/default/files/COVID-19%20Member%20Exclusive_0.pdf
Cumming, G. (2012). Understanding new statistics: Effect sizes, confidence intervals, and meta-analysis. New York, USA: Routledge. https://doi.org/10.4324/9780203807002
Deeks, J. J., Higgins, J. P. T., & Altman, D. G. (2008). Analysing data and undertaking meta-analyses . In J. P. T. Higgins & S. Green (Eds.), Cochrane handbook for systematic reviews of interventions (pp. 243–296). Sussex: John Wiley & Sons. https://doi.org/10.1002/9780470712184.ch9
Demiralay, R., Bayır, E. A., & Gelibolu, M. F. (2016). Öğrencilerin bireysel yenilikçilik özellikleri ile çevrimiçi öğrenmeye hazır bulunuşlukları ilişkisinin incelenmesi. Eğitim ve Öğretim Araştırmaları Dergisi, 5 (1), 161–168. https://doi.org/10.23891/efdyyu.2017.10
Dinçer, S. (2014). Eğitim bilimlerinde uygulamalı meta-analiz. Pegem Atıf İndeksi, 2014(1), 1–133. https://doi.org/10.14527/pegem.001
*Durak, G., Cankaya, S., Yunkul, E., & Ozturk, G. (2017). The effects of a social learning network on students’ performances and attitudes. European Journal of Education Studies, 3 (3), 312–333. 10.5281/zenodo.292951
*Ercan, O. (2014). Effect of web assisted education supported by six thinking hats on students’ academic achievement in science and technology classes . European Journal of Educational Research, 3 (1), 9–23. https://doi.org/10.12973/eu-jer.3.1.9
Ercan, O., & Bilen, K. (2014). Effect of web assisted education supported by six thinking hats on students’ academic achievement in science and technology classes. European Journal of Educational Research, 3 (1), 9–23.
*Ercan, O., Bilen, K., & Ural, E. (2016). “Earth, sun and moon”: Computer assisted instruction in secondary school science - Achievement and attitudes. Issues in Educational Research, 26 (2), 206–224. https://doi.org/10.12973/eu-jer.3.1.9
Field, A. P. (2003). The problems in using fixed-effects models of meta-analysis on real-world data. Understanding Statistics, 2 (2), 105–124. https://doi.org/10.1207/s15328031us0202_02
Field, A. P., & Gillett, R. (2010). How to do a meta-analysis. British Journal of Mathematical and Statistical Psychology, 63 (3), 665–694. https://doi.org/10.1348/00071010x502733
Geostat. (2019). ‘Share of households with internet access’, National statistics office of Georgia . Retrieved on the 2nd September 2020 from https://www.geostat.ge/en/modules/categories/106/information-and-communication-technologies-usage-in-households
*Gwo-Jen, H., Nien-Ting, T., & Xiao-Ming, W. (2018). Creating interactive e-books through learning by design: The impacts of guided peer-feedback on students’ learning achievements and project outcomes in science courses. Journal of Educational Technology & Society., 21 (1), 25–36. Retrieved on the 2nd of October, 2020 https://ae-uploads.uoregon.edu/ISTE/ISTE2019/PROGRAM_SESSION_MODEL/HANDOUTS/112172923/CreatingInteractiveeBooksthroughLearningbyDesignArticle2018.pdf
Hamdani, A. R., & Priatna, A. (2020). Efektifitas implementasi pembelajaran daring (full online) dimasa pandemi Covid-19 pada jenjang Sekolah Dasar di Kabupaten Subang. Didaktik: Jurnal Ilmiah PGSD STKIP Subang, 6 (1), 1–9.
Hart, C. M., Berger, D., Jacob, B., Loeb, S., & Hill, M. (2019). Online learning, offline outcomes: Online course taking and high school student performance. Aera Open, 5(1).
*Hayes, J., & Stewart, I. (2016). Comparing the effects of derived relational training and computer coding on intellectual potential in school-age children. The British Journal of Educational Psychology, 86 (3), 397–411. https://doi.org/10.1111/bjep.12114
Horton, W. K. (2000). Designing web-based training: How to teach anyone anything anywhere anytime (Vol. 1). Wiley Publishing.
*Hwang, G. J., Wu, P. H., & Chen, C. C. (2012). An online game approach for improving students’ learning performance in web-based problem-solving activities. Computers and Education, 59 (4), 1246–1256. https://doi.org/10.1016/j.compedu.2012.05.009
*Kert, S. B., Köşkeroğlu Büyükimdat, M., Uzun, A., & Çayiroğlu, B. (2017). Comparing active game-playing scores and academic performances of elementary school students. Education 3–13, 45 (5), 532–542. https://doi.org/10.1080/03004279.2016.1140800
*Lai, A. F., & Chen, D. J. (2010). Web-based two-tier diagnostic test and remedial learning experiment. International Journal of Distance Education Technologies, 8 (1), 31–53. https://doi.org/10.4018/jdet.2010010103
*Lai, A. F., Lai, H. Y., Chuang W. H., & Wu, Z.H. (2015). Developing a mobile learning management system for outdoors nature science activities based on 5e learning cycle. Proceedings of the International Conference on e-Learning, ICEL. Proceedings of the International Association for Development of the Information Society (IADIS) International Conference on e-Learning (Las Palmas de Gran Canaria, Spain, July 21–24, 2015). Retrieved on the 14th November 2020 from https://files.eric.ed.gov/fulltext/ED562095.pdf
Lai, C. H., Lin, H. W., Lin, R. M., & Tho, P. D. (2019). Effect of peer interaction among online learning community on learning engagement and achievement. International Journal of Distance Education Technologies (IJDET), 17 (1), 66–77.
Littell, J. H., Corcoran, J., & Pillai, V. (2008). Systematic reviews and meta-analysis . Oxford University.
*Liu, K. P., Tai, S. J. D., & Liu, C. C. (2018). Enhancing language learning through creation: the effect of digital storytelling on student learning motivation and performance in a school English course. Educational Technology Research and Development, 66 (4), 913–935. https://doi.org/10.1007/s11423-018-9592-z
Machtmes, K., & Asher, J. W. (2000). A meta-analysis of the effectiveness of telecourses in distance education. American Journal of Distance Education, 14 (1), 27–46. https://doi.org/10.1080/08923640009527043
Makowski, D., Piraux, F., & Brun, F. (2019). From experimental network to meta-analysis: Methods and applications with R for agronomic and environmental sciences. Dordrecht: Springer. https://doi.org/10.1007/978-94-024_1696-1
* Meyers, C., Molefe, A., & Brandt, C. (2015). The Impact of the" Enhancing Missouri's Instructional Networked Teaching Strategies"(eMINTS) Program on Student Achievement, 21st-Century Skills, and Academic Engagement--Second-Year Results . Society for Research on Educational Effectiveness. Retrieved on the 14 th November, 2020 from https://files.eric.ed.gov/fulltext/ED562508.pdf
OECD. (2020). ‘A framework to guide an education response to the COVID-19 Pandemic of 2020 ’. https://doi.org/10.26524/royal.37.6
Pecoraro, V. (2018). Appraising evidence . In G. Biondi-Zoccai (Ed.), Diagnostic meta-analysis: A useful tool for clinical decision-making (pp. 99–114). Cham, Switzerland: Springer. https://doi.org/10.1007/978-3-319-78966-8_9
Pigott, T. (2012). Advances in meta-analysis . Springer.
Pillay, H. , Irving, K., & Tones, M. (2007). Validation of the diagnostic tool for assessing Tertiary students’ readiness for online learning. Higher Education Research & Development, 26 (2), 217–234. https://doi.org/10.1080/07294360701310821
Prestiadi, D., Zulkarnain, W., & Sumarsono, R. B. (2019). Visionary leadership in total quality management: efforts to improve the quality of education in the industrial revolution 4.0. In the 4th International Conference on Education and Management (COEMA 2019). Atlantis Press
Poole, D. M. (2000). Student participation in a discussion-oriented online course: a case study. Journal of Research on Computing in Education, 33 (2), 162–177. https://doi.org/10.1080/08886504.2000.10782307
Rahayu, F. S., Budiyanto, D., & Palyama, D. (2017). Analisis penerimaan e-learning menggunakan technology acceptance model (Tam)(Studi Kasus: Universitas Atma Jaya Yogyakarta). Jurnal Terapan Teknologi Informasi, 1 (2), 87–98.
Rasmussen, R. C. (2003). The quantity and quality of human interaction in a synchronous blended learning environment . Brigham Young University Press.
*Ravenel, J., T. Lambeth, D., & Spires, B. (2014). Effects of computer-based programs on mathematical achievement scores for fourth-grade students. i-manager’s Journal on School Educational Technology, 10 (1), 8–21. https://doi.org/10.26634/jsch.10.1.2830
Rolisca, R. U. C., & Achadiyah, B. N. (2014). Pengembangan media evaluasi pembelajaran dalam bentuk online berbasis e-learning menggunakan software wondershare quiz creator dalam mata pelajaran akuntansi SMA Brawijaya Smart School (BSS). Jurnal Pendidikan Akuntansi Indonesia, 12(2).
Sitzmann, T., Kraiger, K., Stewart, D., & Wisher, R. (2006). The comparative effective- ness of Web-based and classroom instruction: A meta-analysis . Personnel Psychology, 59 (3), 623–664. https://doi.org/10.1111/j.1744-6570.2006.00049.x
Stewart, D. W., & Kamins, M. A. (2001). Developing a coding scheme and coding study reports. In M. W. Lipsey & D. B. Wilson (Eds.), Practical metaanalysis: Applied social research methods series (Vol. 49, pp. 73–90). Sage.
Swan, K. (2007). Research on online learning. Journal of Asynchronous Learning Networks, 11 (1), 55–59.
*Sung, H. Y., Hwang, G. J., & Chang, Y. C. (2016). Development of a mobile learning system based on a collaborative problem-posing strategy. Interactive Learning Environments, 24 (3), 456–471. https://doi.org/10.1080/10494820.2013.867889
Tsagris, M., & Fragkos, K. C. (2018). Meta-analyses of clinical trials versus diagnostic test accuracy studies. In G. Biondi-Zoccai (Ed.), Diagnostic meta-analysis: A useful tool for clinical decision-making (pp. 31–42). Cham, Switzerland: Springer. https://doi.org/10.1007/978-3-319-78966-8_4
UNESCO. (2020, Match 13). COVID-19 educational disruption and response. Retrieved on the 14 th November 2020 from https://en.unesco.org/themes/education-emergencies/ coronavirus-school-closures
Usta, E. (2011a). The effect of web-based learning environments on attitudes of students regarding computer and internet. Procedia-Social and Behavioral Sciences, 28 (262–269), 1. https://doi.org/10.1016/j.sbspro.2011.11.051
Usta, E. (2011b). The examination of online self-regulated learning skills in web-based learning environments in terms of different variables. Turkish Online Journal of Educational Technology-TOJET, 10 (3), 278–286. Retrieved on the 14th November 2020 from https://files.eric.ed.gov/fulltext/EJ944994.pdf
Vrasidas, C. & MsIsaac, M. S. (2000). Principles of pedagogy and evaluation for web-based learning. Educational Media International, 37 (2), 105–111. https://doi.org/10.1080/095239800410405
*Wang, C. H., & Chen, C. P. (2013). Effects of facebook tutoring on learning english as a second language. Proceedings of the International Conference e-Learning 2013, (2009), 135–142. Retrieved on the 15th November 2020 from https://files.eric.ed.gov/fulltext/ED562299.pdf
Wei, H. C., & Chou, C. (2020). Online learning performance and satisfaction: Do perceptions and readiness matter? Distance Education, 41 (1), 48–69.
*Yu, F. Y. (2019). The learning potential of online student-constructed tests with citing peer-generated questions. Interactive Learning Environments, 27 (2), 226–241. https://doi.org/10.1080/10494820.2018.1458040
*Yu, F. Y., & Chen, Y. J. (2014). Effects of student-generated questions as the source of online drill-and-practice activities on learning . British Journal of Educational Technology, 45 (2), 316–329. https://doi.org/10.1111/bjet.12036
*Yu, F. Y., & Pan, K. J. (2014). The effects of student question-generation with online prompts on learning. Educational Technology and Society, 17 (3), 267–279. Retrieved on the 15th November 2020 from http://citeseerx.ist.psu.edu/viewdoc/download?doi=10.1.1.565.643&rep=rep1&type=pdf
*Yu, W. F., She, H. C., & Lee, Y. M. (2010). The effects of web-based/non-web-based problem-solving instruction and high/low achievement on students’ problem-solving ability and biology achievement. Innovations in Education and Teaching International, 47 (2), 187–199. https://doi.org/10.1080/14703291003718927
Zhao, Y., Lei, J., Yan, B, Lai, C., & Tan, S. (2005). A practical analysis of research on the effectiveness of distance education. Teachers College Record, 107 (8). https://doi.org/10.1111/j.1467-9620.2005.00544.x
*Zhong, B., Wang, Q., Chen, J., & Li, Y. (2017). Investigating the period of switching roles in pair programming in a primary school. Educational Technology and Society, 20 (3), 220–233. Retrieved on the 15th November 2020 from https://repository.nie.edu.sg/bitstream/10497/18946/1/ETS-20-3-220.pdf
Download references
Author information
Authors and affiliations.
Primary Education, Ministry of Turkish National Education, Mersin, Turkey
You can also search for this author in PubMed Google Scholar
Corresponding author
Correspondence to Hakan Ulum .
Additional information
Publisher's note.
Springer Nature remains neutral with regard to jurisdictional claims in published maps and institutional affiliations.
Rights and permissions
Reprints and permissions
About this article
Ulum, H. The effects of online education on academic success: A meta-analysis study. Educ Inf Technol 27 , 429–450 (2022). https://doi.org/10.1007/s10639-021-10740-8
Download citation
Received : 06 December 2020
Accepted : 30 August 2021
Published : 06 September 2021
Issue Date : January 2022
DOI : https://doi.org/10.1007/s10639-021-10740-8
Share this article
Anyone you share the following link with will be able to read this content:
Sorry, a shareable link is not currently available for this article.
Provided by the Springer Nature SharedIt content-sharing initiative
- Online education
- Student achievement
- Academic success
- Meta-analysis
- Find a journal
- Publish with us
- Track your research
Thank you for visiting nature.com. You are using a browser version with limited support for CSS. To obtain the best experience, we recommend you use a more up to date browser (or turn off compatibility mode in Internet Explorer). In the meantime, to ensure continued support, we are displaying the site without styles and JavaScript.
- View all journals
- My Account Login
- Explore content
- About the journal
- Publish with us
- Sign up for alerts
- Open access
- Published: 04 September 2018
The stability of educational achievement across school years is largely explained by genetic factors
- Kaili Rimfeld ORCID: orcid.org/0000-0001-5139-065X 1 na1 ,
- Margherita Malanchini 1 , 2 na1 ,
- Eva Krapohl 1 ,
- Laurie J. Hannigan ORCID: orcid.org/0000-0003-3123-5411 1 ,
- Philip S. Dale ORCID: orcid.org/0000-0002-7697-8510 3 &
- Robert Plomin 1
npj Science of Learning volume 3 , Article number: 16 ( 2018 ) Cite this article
25k Accesses
48 Citations
269 Altmetric
Metrics details
- Human behaviour
Little is known about the etiology of developmental change and continuity in educational achievement. Here, we study achievement from primary school to the end of compulsory education for 6000 twin pairs in the UK-representative Twins Early Development Study sample. Results showed that educational achievement is highly heritable across school years and across subjects studied at school (twin heritability ~60%; SNP heritability ~30%); achievement is highly stable (phenotypic correlations ~0.70 from ages 7 to 16). Twin analyses, applying simplex and common pathway models, showed that genetic factors accounted for most of this stability (70%), even after controlling for intelligence (60%). Shared environmental factors also contributed to the stability, while change was mostly accounted for by individual-specific environmental factors. Polygenic scores, derived from a genome-wide association analysis of adult years of education, also showed stable effects on school achievement. We conclude that the remarkable stability of achievement is largely driven genetically even after accounting for intelligence.
Similar content being viewed by others
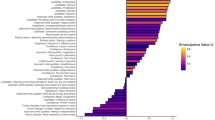
Worldwide divergence of values
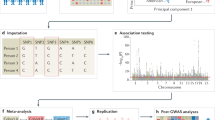
Genome-wide association studies
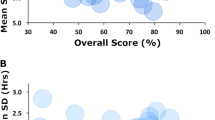
Sleep quality, duration, and consistency are associated with better academic performance in college students
Introduction.
Educational achievement is important to society and to children as individuals. In fact, educational achievement has been shown to be a good predictor of many life outcomes, such as occupational status, happiness, health, and even life expectancy. 1 , 2 , 3 , 4 , 5 Influences on educational achievement, including genetic and environmental etiologies, can best be studied during the period of compulsory education when the full range of family characteristics is represented. Compulsory education in the UK culminates with standardized nation-wide exams, the General Certificate of Secondary Education (GCSE). GCSE grades are a gateway to further education, university acceptance, and even later employment, shaping individuals’ life-long educational and professional trajectories. Previous twin research has shown that GCSE performance is highly heritable, and to a lesser extent explained by environmental factors. 6 However, little is known about whether the same or different genetic and environmental effects contribute to individual differences in achievement over the course of compulsory education. In the present paper, quantitative (twin) and molecular genetic (DNA) methods are used to examine the etiology and developmental course of educational achievement during the primary and secondary education period, culminating in GCSE grades.
There is now converging evidence for the heritability of educational achievement across school years using family designs, such as twin and adoption studies, and DNA-based methods. Twin studies have shown that around 60% of individual differences in school achievement are explained by inherited differences in children’s DNA sequence. 6 , 7 , 8 , 9 , 10 , 11 , 12 , 13 , 14 , 15 , 16 This holds when considering overall achievement scores as well as separate school subjects, from Sciences to Humanities. 11 , 12 It is also possible to estimate heritability using DNA of unrelated individuals, where small DNA differences between individuals (single-nucleotide polymorphisms (SNPs)) are associated with the individuals’ scores in a trait of interest. Rather than estimating the association between each SNP and the trait, this method estimates the association between the trait and all the SNPs combined. 17 , 18 This so-called SNP heritability for educational achievement has been shown to be around 20–30%. 12 , 19 , 20 , 21 The SNP heritability is less than that estimated by twin studies partly because SNP heritability is limited to additive effects of common SNPs that are included in current arrays used to genotype SNPs. Because genome-wide association (GWA) studies have the same limitations as SNP heritability, SNP heritability is the current ceiling for the phenotypic variance that GWA studies can explain.
These univariate genetic analyses have shown that the heritability of educational achievement is substantial and consistent across school years, from primary to secondary education and culminating in the GCSEs. 6 , 9 However, that conclusion is agnostic about the extent to which the same or different genetic factors contribute to individual differences in educational achievement longitudinally from age to age, that is, to stability and change. Understanding the developmental etiology of educational achievement in this way has considerable potential for illuminating the mechanisms that trigger differences in GCSE performance and, consequently, in educational and professional outcomes.
Multivariate genetic methods can be used to address this question of the etiology of age-to-age stability and change. Using a multivariate twin design we have previously demonstrated that, during the primary school years, genetic and shared environmental factors show substantial stability in English, Mathematics, and Science, while non-shared environmental factors contribute to change. 9 However, the genetic and environmental etiology of stability and change of educational achievement across the longer span of school years, from primary school to secondary education and beyond, remains unexplored. Only a few longitudinal studies of reading ability have been reported. In one study, the stability of reading, measured as word recognition, was explained largely by genetic factors (around 70%) from primary through secondary school. 22 Another study found that the etiology of reading fluency across the first five years of schooling, an important developmental time when students transition from ‘learning to read’ to ‘reading to learn’, was characterized by stable genetic and shared environmental influences. 23 Two additional longitudinal analyses of reading comprehension in two different samples from the UK 24 and US 25 also showed substantial genetic stability. However, school achievement involves much more than reading.
To our knowledge, no longitudinal analysis has been conducted to assess the genetic and environmental etiology of continuity and change of educational achievement throughout compulsory education, for specific subjects as well as for general educational achievement. This is the purpose of the current study, which uses longitudinal data from age 7 to 16 on educational achievement from a UK-representative sample of 6000 twin pairs participating in the Twins Early Development Study (TEDS). 26
We also addressed the issue of stability and change in school achievement, for the first time using DNA-based analyses. In addition to SNP heritability, which was described earlier, another recently developed method predicts academic achievement directly from DNA using specific SNPs that have been shown to be associated with the trait in GWA analyses. This method aggregates thousands of SNP associations, which individually have very small effects, into a genome-wide polygenic score (GPS) 27 with effects weighted by results from the GWA discovery sample. A GPS can be used to predict variance in a trait for unrelated individuals in a sample independent of the GWA discovery sample. We will refer to this estimate as GPS heritability. It explains less variance than SNP heritability or twin study heritability because GPS heritability predicts educational achievement from specific SNPs.
Our EduYears GPS was derived from a GWA study of years of education for 300,000 individuals. 28 We used the GWA summary data to create an EduYears GPS for each of 6000 unrelated individuals (one member of a twin pair) in our TEDS sample 26 in the UK. We correlated EduYears GPS with achievement measures at ages 7, 9, 12, and 16. We have previously shown that EduYears GPS predicts up to 9% of the variance in GCSE scores; 29 here we extend this analysis and investigate results for specific subjects in addition to general achievement. The focus of our present analyses is the extent to which the EduYears GPS contributes to stability of educational achievement.
Genetic stability of school achievement might be explained fully or in part by general cognitive ability ( g ), which has also been shown to be substantially heritable 10 , 30 , 31 and developmentally stable, 32 and is one of the strongest predictors of school achievement. 33 , 34 , 35 , 36 Moreover, the links between achievement and g have shown to be explained by genetic factors. 7 , 33 , 37 Because g is a likely candidate to explain stability of school achievement across compulsory education, we also investigate the role of g in the stability of educational achievement, using both the twin design and DNA-based methods.
In summary, in this study we use twin analyses and GPS analyses of longitudinal data from TEDS from age 7 to age 16, including GCSE scores, to investigate three issues—the stability of general educational achievement, the stability of achievement in specific subjects, and the contribution of g to the stability of educational achievement.
Phenotypic analyses
Means and standard deviations were calculated for school achievement across compulsory education for the whole sample, males and females separately, and for all five sex and zygosity groups: monozygotic (MZ) males, dizygotic (DZ) males, MZ females, DZ females, and DZ opposite-sex twin pairs. One twin per pair was randomly selected for phenotypic analyses to maintain independence of data. Analyses of variance (ANOVA) were used to test the significance of these group differences. ANOVA results showed some significant sex differences, however, sex and zygosity together explain only 1% of variance in achievement on average (Supplementary Table 1 ). For subsequent analyses, the data were corrected for mean sex differences, as described in the Methods section.
Genetic analyses
Univariate genetic analyses.
Figure 1a presents the twin ACE estimates for achievement across development. All achievement measures show substantial heritability (A ~60%). Shared (C) and non-shared (E) environmental factors both explained about 20% of the variance. Estimates did not vary systematically across subjects or school years. Twin intra-class correlations and parameter estimates with confidence intervals are presented in Supplementary Table 2 , which shows that parameter estimates were also similar for teacher ratings, exam performance, and achievement scores that combined teacher ratings and exam performance.
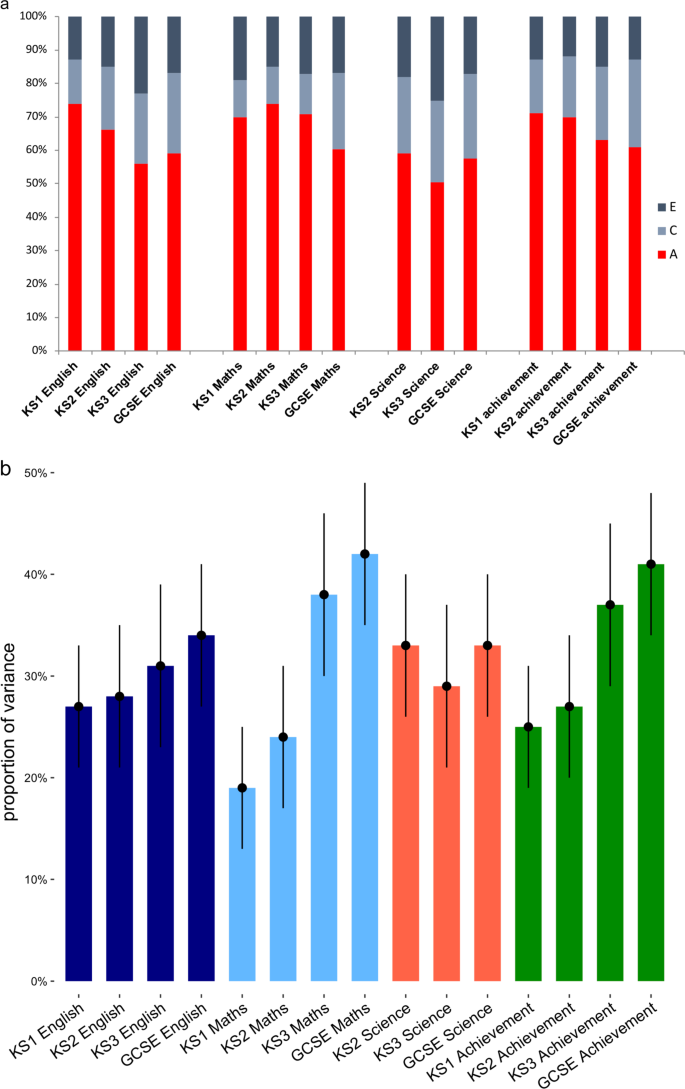
a Twin model-fitting results for univariate analyses of educational achievement. A = additive genetic, C = shared environmental, E = non-shared environmental proportions of the variance. b SNP heritability estimates of the proportion of variance explained by the additive effects of common SNPs (standard errors as error bars) for the same measures of educational achievement. SNP heritabilities were calculated following adjustment for sex and population stratification. Note: KS1 age 7; KS2 age 11; KS3 age 14; GCSE age 16; Note: Achievement is a composite score of English and Mathematics
SNP heritabilities were calculated for the same achievement measures using the GCTA package (see Methods). Figure 1b shows that SNP heritabilities were substantial (~30%) but, as expected, only about half as large as the twin estimates, although there was a trend towards increasing SNP heritability with age. For example, the SNP heritability of Mathematics achievement (composite of test scores and teacher ratings) was 19% (SE = 0.06) in KS1 and 38% (SE = 0.08) in KS3 and 42% (SE = 0.07) for GCSE. Twin heritabilities and SNP heritabilities did not differ much across age after the variance accounted for by general congitive ability ( g ) was controlled for by means of linear regression (Supplementary Figure 1 ). The trend towards increasing SNP heritability with age seen in Fig. 1 disappeared when controlling for g (Supplementary Figure 1(b) ), which shows increasing heritability with age. 38
Multivariate genetic analyses of age-to-age stability
Academic achievement (measured as the mean of English and Mathematics) was highly stable, with age-to-age correlations ranging from 0.66 to 0.85 (Fig. 2a ). In bivariate twin analyses comparing each pair of ages, genetic factors accounted for a substantial proportion of the covariance between ages, explaining from 63 to 79% of the phenotypic correlations (Fig. 2a ). Controlling for g only slightly reduced the phenotypic stability (range = 0.50–0.78) and genetic stability (range = 0.53–0.82) of the correlations (Fig. 2b ). The phenotypic stability from age to age was still mostly accounted for by genetic factors, even after controlling for g (52–72%; Fig. 2b ). Supplementary Table 3 presents the phenotypic and genetic correlations with 95% confidence intervals for the overall achievement and for separate subjects.
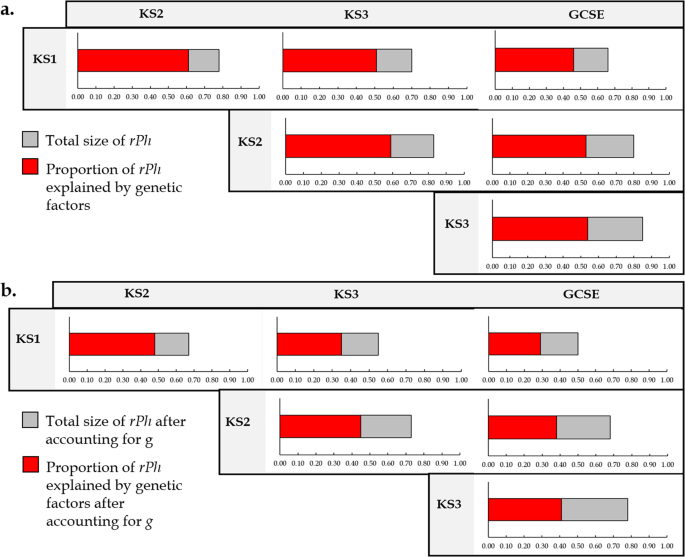
a Proportion of the phenotypic correlation ( r Ph) across ages accounted for by genetic factors . b Proportion of the phenotypic correlation across ages accounted for by genetic factors after controlling for g . Note: KS1 age 7; KS2 age 11; KS3 age 14; GCSE age 16; Note: Achievement is a composite score of English and Mathematics
Etiological contributions to stability and change were assessed using multivariate models encompassing all ages of assessment. The first was a simplex longitudinal model 39 (see Methods and Supplementary Figure 2 for details). The results, presented in Fig. 3 , indicate that the stability of core academic achievement was largely explained by additive genetic (A) factors—the genetic paths from age to age are 0.86, 0.84, and 0.86. C was also stable from age to age, accounting for a smaller proportion of variance in academic achievement, amounting to around one-third of the proportion of variance explained by A. E contributed variance that was unique to the measurement occasion, and did not influence subsequent academic achievement across school years, as indicated by the residuals (age-specific effects; E s2 , E S3 , and E i4 ). (See Supplementary Figure 3 for the results of simplex model for English, Mathematics and Science separately.)
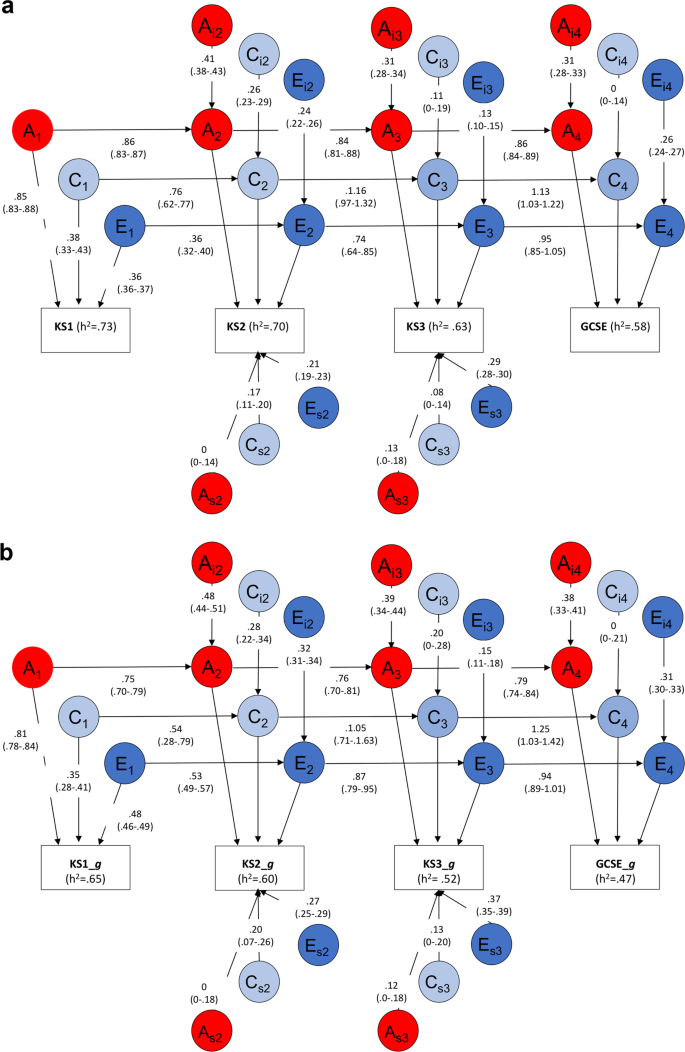
a Simplex model-fitting results for stability and change of overall achievement across compulsory education. b Simplex model-fitting results for stability and change of overall achievement across compulsory education after controlling for g . Note: KS1 age 7; KS2 age 11; KS3 age 14; GCSE age 16; Note: Achievement is a composite score of English and Mathematics, Note: The path estimates are reported rather than standardized variance components
The proportion of heritability at each age that is accounted for by genetic effects different from those operating at the previous age can be calculated by dividing the sum of the innovation path squared (A i ) and the age-specific genetic path squared (A s ) by the overall heritability. For example, for GCSE in Fig. 3a , 17% (i.e., 0.31 2 /0.58) of the heritability of core GCSE performance is innovation (there is no age-specific genetic path); the rest of the heritability (83%) is transmitted from previous achievement ages. For KS3 core achievement, 78% (i.e., 0.70 (heritability of KS2) ×0.84 2 (genetic transmission)/0.63 (heritability of KS3)) of the genetic variance was transmitted from KS2, and for KS2 77% (0.73 × 0.86 2 /0.70) of the genetic variance was transmitted from KS1. There was substantial innovative genetic influence at each age (A i )—24%, 15%, and 17% at ages 12, 14, and 16, respectively. To investigate whether the new genetic influence was due to increasing use of test assessments and decreasing use of teacher assessments across the four ages, we repeated the analyses using only standardized test scores across the school years (Supplementary Figure S4 ), but the results were highly similar. The remaining genetic variance (0% at age 12 and 3% at age 14) was age specific (path A s ), in other words, not operating at the previous age and not transmitted to the next age. These paths were not significant as indicated by their 95% confidence intervals.
We also repeated the simplex models statistically controlling for g (Fig. 3b ). The heritability of core school achievement was somewhat lower after controlling for g , comparable to the bivariate genetic results shown in Fig. 2 . Nonetheless, educational achievement continued to be highly stable and its stability was still largely accounted for by genetic factors; genetic paths from age to age are 0.75, 0.76, and 0.79.
In order to assess how much variance in the stability of core educational achievement is explained by a single genetic factor, a genetic common pathway model was used (See Methods and Supplementary Figure 5 ). The results of the common pathway model are presented in Fig. 4 . Seventy percent of the overall stability of core educational achievement across compulsory education (heritability of the latent factor) was explained by genetic factors; 24% of the stability of educational achievement was explained by shared environmental factors (Fig. 4a ). The results were similar when we controlled for g— genetic factors explained 59% of the stability in core educational achievement after controlling for intelligence, 21% of the stability was explained by shared environmental factors (Fig. 4b ).
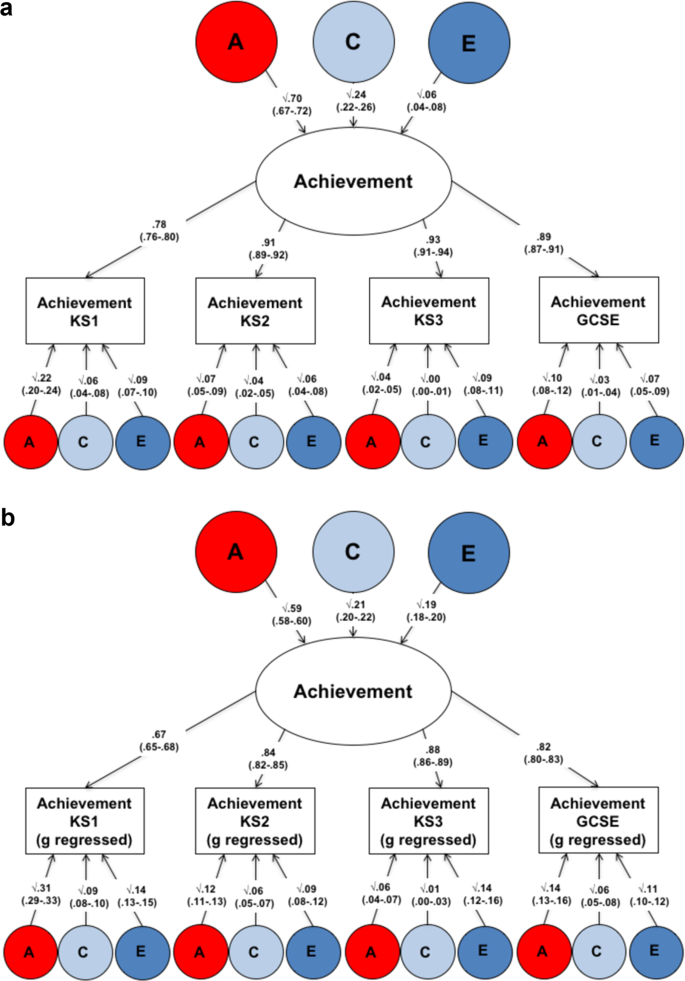
a Common pathway model presenting the standardized squared path estimates for overall achievement. b and for overall achievement when controlling for g . A = additive genetic, C = shared environmental, and E = non-shared environmental components of variance. Note: KS1 age 7; KS2 age 11; KS3 age 14; and GCSE age 16; Note: Achievement is a composite score of English and Mathematics
GPS analyses
As a complement to our twin results, we investigated genetic stability for core educational achievement using a different methodological approach: EduYears GPS. EduYears GPS increasingly predicted core educational achievement—about 4% for KS1, 6% for KS2, 8% for KS3, and 10% for GCSE. In order to address the question of genetic stability and innovation, we explored the age-specificity of the EduYears GPS prediction, after accounting for the variance explained at all preceding ages. In line with the multivariate twin analyses, EduYears GPS’ prediction of educational achievement was largely stable from age to age (Fig. 5 ). That is, our regression analyses indicated little (<1%) age-specific genetic prediction once the stable prediction of EduYears GPS from all previous ages was taken into account. Details of these analyses for core achievement, for subjects separately, and controlling for g and previous achievement are presented in Supplementary Table 4 . In summary, results were similar for separate subjects and after controlling for g and previous achievement. However, EduYears GPS still predicts educational achievement when only controlling for g , explaining around 4% in GCSE performance, as illustrated in Supplementary Figure 6 .
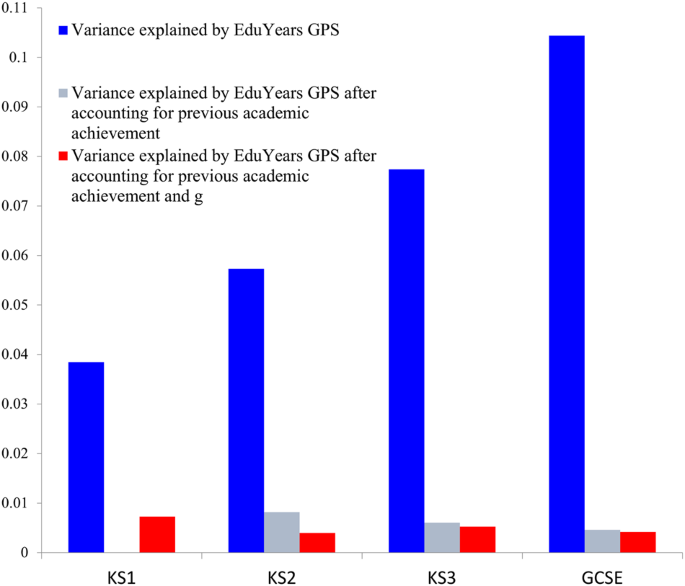
Variance explained by GPS ( EduYears ) using Gaussian mixture weights of 1.0 for overall educational achievement across compulsory education. Note: KS1 age 7; KS2 age 11; KS3 age 14; GCSE age 16; Note: Achievement is a composite score of English and Mathematics
The present study shows that individual differences in educational achievement are highly stable across the years of compulsory schooling from primary through secondary school. Children who do well at the beginning of primary school also tend to do well at the end of compulsory education for much the same reasons. The very high stability of academic achievement across compulsory school years is an interesting finding, particularly when considering that children go through major cognitive and emotional changes from childhood to adolescence, as well as experiencing changes in teachers, friends, and schools.
In addition, the nature of educational achievement also changes during the school years as children are exposed to more subjects and more complex subjects. For reading, children move from learning to read to using reading to learn. The present twin analyses address, for the first time, the etiology of the stability of academic outcomes over compulsory education, showing that genetic factors are largely responsible for this stability. In other words, the same genetic factors largely shape individual differences in achievement from primary through secondary school. Shared environmental factors were also largely stable, although they explained a smaller proportion of overall variance in achievement. However, it has been suggested that shared environmental effects might actually be driven genetically. 40 , 41 We show that age-to-age change in achievement scores was to a large extent explained by non-shared environmental factors. This is another example of the general rubric of behavioral genetics, “genetic stability, environmental change”. 22 , 30 , 42 , 43 , 44 We also noted some genetic innovation (change) at each stage of assessment, but, consistent with an overall pattern of stability, all of these new genetic influences were transmitted to the next achievement stage.
A reasonable assumption is that the substantial genetic stability observed here is explained by general cognitive ability ( g , intelligence). Importantly, however, we showed that the heritability of educational achievement over school years and its stability is not explained by g alone. The results of our twin analyses showed that when g was controlled for, educational achievement remained highly heritable and stable and the stability of educational achievement independent of g was still explained by genetic factors. Although there was evidence for some specific (new) genetic influence at each age, again these new genetic influences were not age specific but were transmitted to the next assessment stage. This is in line with our earlier reports in which we showed that educational achievement at age 16 is not explained by intelligence alone. 10 , 12 The EduYears GPS regression analysis yielded similar results showing genetic stability, even after controlling for g . This GPS result is not exactly analogous to the twin study results, as we tested the effect of the same genetic variants over time. Nevertheless, our multi-method approach yielded similar results indicating that the substantial stability of educational achievement is to a large extent explained by genetic factors.
As new, more powerful GWA studies are conducted, the predictive power of the EduYears GPS prediction is likely to increase. The GPS calculated using the 2013 EduYears GWA summary statistics with a sample size of 126,000 45 predicted around 3% of variance in educational achievement in TEDS, 20 compared to 10% of variance explained in the current study based on the 2016 EduYears GWAS with a sample size of 330,000. Another more powerful GWA of educational attainment was recently published, involving over one million participants, this is likely to be a game changer in terms of predictive power. 46
It should be noted that EduYears GPS predicts only about 4% of the variance in adult years of education (educational attainment) 28 in independent samples, but it predicts more than twice as much variance in GCSE scores at age 16. We are not aware of any other example in which a GPS predicts less variance in the GWA target trait (educational attainment) than in another trait (GCSE scores). We suggest that the reason for this unusual finding is that educational attainment is a much coarser measure than GCSE scores, which are the result of hours of standardized assessment. In support of this hypothesis, we find that EduYears GPS also predicts 4% of the variance when we analyzed a similarly coarse dichotomous item about whether or not TEDS participants planned to go to university. Furthermore, EduYears GPS also predicts 4% of the variance in a cruder measure of GCSE achievement—5 passes at grades A* to C, which is often used in government statistics, and used for selection purposes by many employers and educational institutions (Supplementary Table 4 ).
The limitations of this study include the usual assumptions of the twin design, which are described in detail elsewhere. 43 , 47 One of these limitations involves assortative mating, in which mate selection is not at random but is instead based on trait similarity. Assortative mating on cognitive abilities and educational achievement has been shown to be substantial (~0.40). 36 , 43 , 48 In the twin design, assortative mating increases DZ correlations relative to MZ correlations and could therefore lead to underestimating heritability and overestimating shared environmental influence; in effect this makes the present findings concerning heritability conservative. GCTA and GPS methods also have their limitations. Notably, both of these DNA-based methods rely on the additive effects of common SNPs genotyped on SNP arrays, and do not capture gene-gene or gene-environment interplay or the effects of less common SNPs. 49 However, since the main limitations are different for each method used in the current study, the fact that our multi-method approach yielded similar results is a strength.
Our multi-method analyses corroborated previous findings showing that individual differences in educational achievement are largely explained by inherited differences in DNA sequence. The novel contribution of our study is to show that the substantial stability of educational achievement across compulsory education is to a large extent explained by genetic factors, even after controlling for g .
Our finding of genetically driven stability of educational achievement should provide additional motivation to identify children in need of interventions as early as possible, as the problems are likely to remain throughout the school years. GPS prediction, specifically, might in the future provide a tool to identify children with educational problems very early in life and aid in providing both individualized prevention and individualized learning programs. We hope that with GPS, we can move towards precision education, just as medicine is moving towards precision medicine. 50 , 51 For example, GPS could be used to identify children at birth at genetic risk for developing reading problems, thus enabling early intervention. As preventive interventions have greater chances of succeeding early in life, a great strength of GPS is that they can predict at birth just as well as later in life, which enables early intervention, particularly for those children who are likely to struggle the most.
Participants
The present study used the TEDS sample. TEDS is a large twin study that recruited over 16,000 twin pairs born between 1994 and 1996 in England and Wales. More than 10,000 twin pairs are still actively involved in the study. Rich cognitive and behavioral data, including educational achievement, have been collected from the twins, their parents and teachers, over compulsory education and beyond. Importantly, TEDS was a representative sample of the UK population at first contact, and remains representative in terms of family socioeconomic status and ethinicity. 26 , 52 Ethical approval for this study was received from King’s College London Ethics Committee.
The sample for the present study included all twins with available academic achievement measures over the school years. Participants who had major medical or psychiatric conditions, or those with severe perinatal complications, were removed from the analyses. Zygosity was assessed by the parent-reported questionnaire of physical similarity. This measure has been shown to be highly reliable. 53 Nevertheless, DNA testing was conducted when zygosity was unclear from the questionnaire. The sample size per academic achievement measure is shown in Supplementary Table S1 .
DNA has been genotyped for a subsample of unrelated individuals from TEDS (one twin per pair). We processed genotypes for 6710 individuals using the standard quality control procedure followed by imputation of genetic variants to the Haplotype Reference Consortium 54 (see Supplementary Methods). We then matched the individuals with genotyped data to those participants with available academic achievement data.
Measures of educational achievement obtained by TEDS
TEDS has obtained assessments of academic achievement directly from the twins’ teachers who reported grades following the UK National Curriculum guidelines, a standardized core academic curriculum formulated by the National Foundation for Educational Research (NFER) and the Qualifications and Curriculum Authority (QCA) (NFER: http://www.nfer.ac.uk/index.cfm ; QCA: http://www.qca.org.uk ). Data were obtained directly from teachers. At age 7 data are available for English and Mathematics; at ages 12 and 14 data are available for English, Mathematics, and Science. The teacher rating of English used a combined rating of students’ reading, writing, and speaking and listening; Mathematics used a combined score of knowledge in numbers, shapes, space, using and applying mathematics, and measures; and Science used a score combining life process, scientific enquiry, and physical process. These teacher ratings were found to be highly reliable when compared to the achievement measures collected by the UK National Pupil Database (NPD), as described later.
GCSE exam results were obtained from twins themselves or from their parents via questionnaires sent over mail or via telephone. GCSEs are UK-wide standardized examinations taken at age of 16 at the end of compulsory education. Children choose from a variety of different subjects, while English, Mathematics, and Science are compulsory. We used exam grades from English, Mathematics, and Science for the current analyses. Composite measures were created for English (mean of English language and English literature grades), Science (mean of single or double-weighted Science or, when taken separately Chemistry, Physics, and Biology grade), and Mathematics.
Measures of educational achievement obtained from the NPD
The TEDS dataset was linked to the NPD for every participant for whom we received written informed consent from either the twin or the parent. NPD is a rich UK database collecting data about students’ academic achievement across the school years ( https://www.gov.uk/government/collections/national-pupil-database ). Data are available for each Key Stage (KS) completed in the UK following the National Curriculum (NC). Teachers provide NC ratings for every student at the end of each KS (similarly to data collected at TEDS for the NC ratings in English, Mathematics, and Science). Exam scores as well as teacher ratings are available from KS1–KS3; and exam scores only are available for KS4 and KS5. Children’s ages for KS1, KS2, and KS3 are about 7, 11, and 14 years. KS4 marks the end of compulsory education with GCSE testing at about age 16. Sample size and descriptive characteristics for each measure are provided in Supplementary Table S1 .
Composite scores of educational achievement
Composite scores were calculated at each KS combining the teacher ratings (both TEDS and NPD) with the exam scores for English, Mathematics, and Science separately by taking a mean of the three scores. The average correlation between NPD and TEDS teacher ratings was 0.70 (see Supplementary Table S5 ), and the average correlation between teacher ratings and exam scores was 0.80 (see Supplementary Table S6 ). For GCSE performance at the end of compulsory education, GCSE grades collected by TEDS and by NPD correlated 0.98 for English, 0.99 for Mathematics, and >0.95 for all Sciences. A mean score for NPD and TEDS was created to increase the sample size; when fewer measures were available we used any available data to calculate the composite score of educational achievement.
The overall achievement measure (core achievement) was calculated at each KS by taking a mean of English NC teacher ratings, Mathematics NC teacher rating (for both NPD and TEDS), English exam score, and Mathematics exam score. We did not include Science grades in overall achievement scores to make a more direct comparison across age because Science is not part of the National Curriculum at KS1.
Measures of general cognitive ability ( g )
General cognitive ability ( g ; intelligence) was assessed in TEDS at ages 7, 9, 10, 12, 14, and 16. For the present analyses we created a longitudinal composite measure of g as a mean of these six assessments. See Supplementary Methods for a more detailed description of g measures.
The measures were described in terms of means and variance, comparing males and females and identical and non-identical twins; mean differences for age and sex and their interaction were tested using univariate ANOVA. Phenotypic correlations were calculated between academic achievement measures across development. The academic achievement measures were corrected for the small mean effects of age and sex (Supplementary Table S1 ) by rescoring the variable as a standardized residual correcting for age and sex, because in the analysis of twin data members of a twin pair are identical in age and MZ twins are identical for sex, and this would otherwise inflate twin estimates of shared environment. 55 Full sex limitation genetic modeling has previously been reported for academic achievement and found only very minor sex differences in genetic and environmental estimates. 6 , 9 , 12 For these reasons, and to increase power in the present analyses, the full sample was used, combining males and females and including opposite-sex pairs.
Finally, before conducting twin analyses, the achievement measures were corrected for skew because they were slightly negatively skewed. The achievement measures were corrected for skew by mapping it on to a standard normal distribution using the rank-based van der Waerden’s transformation. 56
Twin design
The twin design was used for univariate and multivariate genetic analyses. The twin method offers a natural experiment capitalizing on the known genetic relatedness of MZ and DZ twin pairs. MZ twins are genetically identical and share 100% of their genes, while DZ twins share on average 50% of their segregating genes. Both MZ and DZ twins are assumed to share 100% of their shared environmental influences growing up in the same family. Non-shared environmental influences are unique to individuals, not contributing to similarity between twins. Using these known family relatedness coefficients, it is possible to estimate the relative contribution of additive genetic (A), shared environmental (C), and non-shared environmental (E) effects on the variance and covariance of the phenotypes, by comparing MZ correlations to DZ correlations. Heritability can be roughly calculated by doubling the difference between MZ and DZ correlations, C can be calculated by deducting heritability from MZ correlation and E can be estimated by deducting MZ correlation from unity (following Falconer’s formula). 47 These parameters can be estimated more accurately using structural equation modeling, which also provides 95% confidence intervals and estimates of model fit. The structural equation modeling program OpenMx was used for all model-fitting analyses. 57
These univariate analyses can be extended to multivariate analyses to study the etiology of covariance between multiple traits. Multivariate genetic method decomposes the covariance between traits into additive genetic (A), shared environmental (C), and non-shared environmental (E) components by comparing the cross-trait cross-twin correlations between MZ and DZ twin pairs. This method also enables estimation of the genetic correlation ( r G), which is an index of pleiotropy, indicating the extent to which the same genetic variants influence two traits or measures of the same trait at two times. The shared environmental correlation ( r C) and non-shared environmental correlation ( r E) are estimated in a similar manner. 43 , 47
We used two longitudinal models to study the issue of age-to-age stability of educational achievement.
The simplex model is a multivariate genetic model that estimates the extent to which the genetic and environmental influences on a trait are transmitted from age to age, and the extent to which innovative and age-specific influences emerge. 58 The covariance or correlation matrix for such data is called simplex because the strength of the associations tends to correspond to differences between ages, that is, they are often highest along the diagonal and fall systematically as the difference between ages increases. 58 The simplex model is illustrated in Supplementary Figure S2 .
The common pathway model is a multivariate genetic model in which the variance common to all measures included in the analysis can be reduced to a common latent factor, for which the A, C, and E components are estimated. As well as estimating the etiology of the common latent factor, the model allows for the estimation of the A, C, and E components of the residual variance in each measure that is not captured by the latent construct. 59 The common pathway model estimates the extent to which the stable variance in educational achievement across compulsory education (the latent factor of achievement) is explained by A, C, and E. The common pathway model is illustrated in Supplementary Figure S6 .
SNP heritability
The genome-wide complex trait analysis (GCTA) software package enables estimates of the proportion of phenotypic variance or covariance that is explained by all SNPs that are available on genotype arrays, without testing the association of any single SNP individually. 17 , 49 , 60 This estimate is often called SNP heritability. This method does not use known genetic relatedness coefficients but estimates heritability from DNA using only unrelated individuals. SNP heritability is calculated using restricted maximum likelihood and the variance and covariance is decomposed using mixed linear models.
First, the genetic relatedness matrix is calculated by weighting genetic similarities between all possible pairs of individuals with the allele frequencies across all SNPs on the DNA array. Individuals who are found to be even remotely related (greater than fifth cousins) are removed from the analyses as they would otherwise bias the results, which rely on chance genetic similarity between pairs of individuals. 17 , 18 , 61 The matrix of pair-by-pair genetic similarity is compared to the matrix of pair-by-pair phenotypic similarity using the residual maximum likelihood estimation. SNP heritabilities were calculated for overall achievement across compulsory education, as well as for specific subjects.
Genome-wide polygenic scores
GPSs aggregate the effects of individual SNPs shown to be associated with the trait in a GWA study. 62 GPSs were calculated for 6710 participants using summary statistics from Okbay et al. 28 GWA analysis of years of education ( EduYears ). 28 Of the 293,723 participants in the EduYears GWA discovery sample, the summary statistics excluded 23andMe participants, for legal reasons. Polygenic scores were constructed as the weighted sums of each individual’s genotype across all SNPs using the LDpred method 63 (see Supplementary Methods for details). Delta R 2 is reported as the estimate of variance explained by the GPS. These delta R 2 estimates were obtained by comparing the incremental increase in the model R 2 after adding the GPS to the regression model, and comparing this to the model that included 10 principal components in order to control for population stratification. See Supplementary Methods for genetic quality control and further information about GPS calculation.
We correlated EduYears with general educational achievement composites, as well as with performance in specific subjects at each age to estimate EduYears GPS heritability. Delta R 2 are reported as the estimates of variance explained by adding the GPS to the regression model that included the academic achievement from all earlier ages to assess the extent to which EduYears contributes to age-to-age stability.
Data availability
For information on data availability, please see the TEDS data access policy. This can be found at: http://www.teds.ac.uk/research/collaborators-and-data/teds-data-access-policy . All relevant data are available from the authors according to the TEDS data access policy.
Arendt, J. N. Does education cause better health? A panel data analysis using school reforms for identification. Econ. Educ. Rev. 24 , 149–160 (2005).
Article Google Scholar
Cutler, D. M. & Lleras-Muney, A. Education and health: insights from international comparison s. NBER working paper no. 17738. (The National Bureau of Economic Research, Cambridge, MA, 2012).
Gottfredson, L. S. & Deary, I. J. Intelligence predicts health and longevity, but why? Curr. Dir. Psychol. Sci. 13 , 1–4 (2004).
Oreopoulos, P. & Salvanes, K. G. Priceless: the nonpecuniary benefits of schooling. J. Econ. Perspect. 25 , 159–184 (2011).
Furnham, A. & Cheng, H. Childhood cognitive ability predicts adult financial well-being. J. Intell. 5 , 3 (2016).
Shakeshaft, N. G. et al. Strong genetic influence on a UK nationwide test of educational achievement at the end of compulsory education at age 16. PLoS ONE 8 , e80341 (2013).
Article PubMed PubMed Central CAS Google Scholar
Bartels, M., Rietveld, M. J. H., Van Baal, G. C. M. & Boomsma, D. I. Heritability of educational achievement in 12-year-olds and the overlap with cognitive ability. Twin Res. 5 , 544–553 (2002).
Article PubMed Google Scholar
Coventry, W. et al. The etiology of individual differences in second language acquisition in Australian school students: a behavior-genetic study. Lang. Learn. 62 , 880–901 (2012).
Kovas, Y., Haworth, C. M. A., Dale, P. S. & Plomin, R. The genetic and environmental origins of learning abilities and disabilities in the early school years. Monogr. Soc. Res. Child Dev. 72 , 1–144 (2007).
Google Scholar
Krapohl, E. et al. The high heritability of educational achievement reflects many genetically influenced traits, not just intelligence. Proc. Natl Acad. Sci. USA 111 , 15273–15278 (2014).
Article PubMed CAS Google Scholar
Rimfeld, K., Ayorech, Z., Dale, P. S., Kovas, Y. & Plomin, R. Genetics affects choice of academic subjects as well as achievement. Sci. Rep. 6 , 26373 (2016).
Rimfeld, K., Kovas, Y., Dale, P. S. & Plomin, R. Pleiotropy across academic subjects at the end of compulsory education. Sci. Rep. 5 , 11713 (2015).
Article PubMed PubMed Central Google Scholar
Petrill, S. A. et al. Genetic and environmental influences on the growth of early reading skills. J. Child Psychol. Psychiatry Allied Discip. 51 , 660–667 (2010).
Wadsworth, S. J., DeFries, J. C., Fulker, D. W. & Plomin, R. Cognitive ability and academic achievement in the Colorado adoption project: A multivariate genetic analysis of parent-offspring and sibling data. Behav. Genet. 25 , 1–15 (1995).
Wainwright, M. A., Wright, M. J., Geffen, G. M., Luciano, M. & Martin, N. G. The genetic basis of academic achievement on the Queensland Core Skills Test and its shared genetic variance with IQ. Behav. Genet. 35 , 133–145 (2005).
Martin, N. G. & Martin, P. G. The inheritance of scholastric abilities in a sample of twins. I. Ascertainments of the sample and diagnosis of zygosity. Ann. Hum. Genet. 39 , 213–218 (1975).
Yang, J., Lee, S. H., Goddard, M. E. & Visscher, P. M. GCTA: a tool for genome-wide complex trait analysis. Am. J. Hum. Genet. 88 , 76–82 (2011).
Yang, J., Lee, S. H., Goddard, M. E. & Visscher, P. M. Genome-wide complex trait analysis (GCTA): Methods, data analyses, and interpretations. Methods Mol. Biol. 1019 , 215–236 (2013).
Trzaskowski, M. et al. DNA evidence for strong genome-wide pleiotropy of cognitive and learning abilities. Behav. Genet. 43 , 267–273 (2013).
Krapohl, E. & Plomin, R. Genetic link between family socioeconomic status and children’s educational achievement estimated from genome-wide SNPs. Mol. Psychiatry 21 , 437–443 (2016).
Davis, O. S. P. et al. The correlation between reading and mathematics ability at age twelve has a substantial genetic component. Nat. Commun. 5 , 4204 (2014).
Wadsworth, S. J., Corley, R. P., Hewitt, J. K. & DeFries, J. C. Stability of genetic and environmental influences on reading performance at 7, 12, and 16 years of age in the Colorado Adoption Project. Behav. Genet. 31 , 353–359 (2001).
Hart, S. A. et al. Exploring how nature and nurture affect the development of reading: an analysis of the Florida twin project on reading. Dev. Psychol. 49 , 1971–1981 (2013).
Malanchini, M. et al. Reading self-perceived ability, enjoyment and achievement: a genetically informative study of their reciprocal links over time. Dev. Psychol. 53 , 698–712 (2017).
Soden, B. et al. Longitudinal stability in reading comprehension is largely heritable from grades 1 to 6. PLoS ONE 10 , e0113807 (2015).
Haworth, C. M. A., Davis, O. S. P. & Plomin, R. Twins Early Development Study (TEDS): a genetically sensitive investigation of cognitive and behavioral development from childhood to young adulthood. Twin Res. Hum. Genet. 16 , 117–125 (2013).
Palla, L. & Dudbridge, F. A fast method that uses polygenic scores to estimate the variance explained by genome-wide marker panels and the proportion of variants affecting a trait. Am. J. Hum. Genet. 97 , 250–259 (2015).
Okbay, A. et al. Genome-wide association study identifies 74 loci associated with educational attainment. Nature 533 , 539–542 (2016).
Selzam, S. et al. Predicting educational achievement from DNA. Mol. Psychiatry 22 , 267–272 (2017).
Deary, I. J. et al. Genetic contributions to stability and change in intelligence from childhood to old age. Nature 482 , 212 (2012).
Deary, I. J., Spinath, F. M. & Bates, T. C. Genetics of intelligence. Eur. J. Hum. Genet. 14 , 690–700 (2006).
Gow, A. J. et al. Stability and change in intelligence from age 11 to ages 70, 79, and 87: the Lothian Birth Cohorts of 1921 and 1936. Psychol. Aging 26 , 232–240 (2011).
Calvin, C. M. et al. Multivariate genetic analyses of cognition and academic achievement from two population samples of 174,000 and 166,000 school children. Behav. Genet. 42 , 699–710 (2012).
Deary, I. J., Strand, S., Smith, P. & Fernandes, C. Intelligence and educational achievement. Intelligence 35 , 13–21 (2007).
Deary, I. J. Intelligence. Annu. Rev. Psychol. 63 , 453–482 (2012).
Plomin, R. & Deary, I. J. Genetics and intelligence differences: five special findings. Mol. Psychiatry 20 , 98–108 (2015).
Petrill, S. A. & Wilkerson, B. Intelligence and achievement: a behavioral genetic perspective. Educ. Psychol. Rev. 12 , 185–199 (2000).
Haworth, C. M. A. et al. The heritability of general cognitive ability increases linearly from childhood to young adulthood. Mol. Psychiatry 15 , 1112–1120 (2010).
Eaves, L. J., Long, J. & Heath, A. C. A theory of developmental change in quantitative phenotypes applied to cognitive development. Behav. Genet. 16 , 143–162 (1986).
Kong, A. et al. The nature of nurture: effects of parental genotypes. Science 359 , 424–428 (2018).
Bates, T. C. et al. The nature of nurture: using a virtual-parent design to test parenting effects on children’s educational attainment in genotyped families. Twin Res. Hum. Genet. 21 , 73–83 (2018).
DeFries, J. C., Plomin, R. & LaBuda, M. C. Genetic stability of cognitive development from childhood to adulthood. Dev. Psychol. 23 , 4–12 (1987).
Knopik, V. S., Neiderhiser, J. M., DeFries, J. C. & Plomin, R. Behavioral Genetics. (Worth Publishers, New York, 2017).
Plomin, R., Pedersen, N. L., Lichtenstein, P. & McClearn, G. E. Variability and stability in cognitive abilities are largely genetic later in life. Behav. Genet. 24 , 207–215 (1994).
Rietveld, C. A. et al. GWAS of 126,559 individuals identifies genetic variants associated with educational attainment. Sci. (80-.). 340 , 1467–1471 (2013).
Article CAS Google Scholar
Lee, J. J. et al. Gene discovery and polygenic prediction from a genome-wide association study ofeducational attainment in 1.1 million individuals. Nat. Genet 50 , 1112–1121 (2018).
Rijsdijk, F. V. & Sham, P. C. Analytic approaches to twin data using structural equation models. Brief. Bioinform. 3 , 119–133 (2002).
Hugh-Jones, D., Verweij, K. J. H. St, Pourcain, B. & Abdellaoui, A. Assortative mating on educational attainment leads to genetic spousal resemblance for polygenic scores. Intelligence 59 , 103–108 (2016).
Yang, J. et al. Common SNPs explain a large proportion of the heritability for human height. Nat. Genet. 42 , 565–569 (2010).
Plomin, R. & Von Stumm, S. The new genetics of intelligence. Nat. Rev. Genet. 19 , 148–159 (2018).
Article PubMed CAS PubMed Central Google Scholar
Aronson, S. J. & Rehm, H. L. Building the foundation for genomics in precision medicine. Nature 526 , 336–342 (2015).
Oliver, B. R. & Plomin, R. Twins’ Early Development Study (TEDS): a multivariate, longitudinal genetic investigation of language, cognition and behavior problems from childhood through adolescence. Twin Res. Hum. Genet. 10 , 96–105 (2007).
Price, T. S. et al. Infant zygosity can be assigned by parental report questionnaire data. Twin Res. 3 , 129–133 (2000).
McCarthy, S. et al. A reference panel of 64,976 haplotypes for genotype imputation. Nat Genet 48 , 1279–83 (2016).
McGue, M. & Bouchard, T. J. Adjustment of twin data for the effects of age and sex. Behav. Genet. 14 , 325–343 (1984).
Van Der Waerden, B. L. On the sources of my book Moderne Algebra. Hist. Math. 2, (31–40 (1975).
Boker, S. et al. OpenMx: an open source extended structural equation modeling framework. Psychometrika 76 , 306–317 (2011).
Boomsma, D. I. & Molenaar, P. C. M. The genetic analysis of repeated measures. I. Simplex models. Behav. Genet. 17 , 111–123 (1987).
Rijsdijk, F. V. Encyclopedia of Statistics in Behavioral Science (eds Everitt, B. S. & Howell, D. C.) 1, 330–331 (John Wiley & Sons Ltd., New Jersey, 2005).
Lee, S. H., Yang, J., Goddard, M. E., Visscher, P. M. & Wray, N. R. Estimation of pleiotropy between complex diseases using single-nucleotide polymorphism-derived genomic relationships and restricted maximum likelihood. Bioinformatics 28 , 2540–2542 (2012).
Trzaskowski, M. et al. Genetic influence on family socioeconomic status and children’s intelligence. Intelligence 42 , 83–88 (2014).
Dudbridge, F. Power and predictive accuracy of polygenic risk scores. PLoS Genet. 9 , e1003348 (2013).
Vilhjálmsson, B. J. et al. Modeling linkage disequilibrium increases accuracy of polygenic risk scores. Am. J. Hum. Genet. 97 , 576–592 (2015).
Download references
Acknowledgements
We gratefully acknowledge the ongoing contribution of the participants in the Twins Early Development Study (TEDS) and their families. TEDS is supported by a program grant to R.P. from the UK Medical Research Council (MR/M021475/1 and previously G0901245), with additional support from the US National Institutes of Health (AG046938). The research leading to these results has also received funding from the European Research Council under the European Union’s Seventh Framework Programme (FP7/2007-2013)/ grant agreement n° 602768 and ERC grant agreement n° 295366. R.P. is supported by a Medical Research Council Professorship award (G19/2). L.J.H. is supported by an ESRC multidisciplinary studentship. M.M. is partly supported by the David Wechsler early career grant for innovative work in cognition.
Author information
These authors jointly supervised this work: Kaili Rimfeld, Margherita Malanchini
Authors and Affiliations
Social, Genetic and Developmental Psychiatry Centre, Institute of Psychiatry, Psychology and Neuroscience, King’s College London, London, UK
Kaili Rimfeld, Margherita Malanchini, Eva Krapohl, Laurie J. Hannigan & Robert Plomin
Department of Psychology, University of Texas at Austin, Austin, USA
Margherita Malanchini
Department of Speech and Hearing Sciences, University of New Mexico, Albuquerque, USA
Philip S. Dale
You can also search for this author in PubMed Google Scholar
Contributions
Conceived and designed the experiments: K.R., M.M., R.P. Analyzed the data: K.R., M.M., L.J.H., E.K. Wrote the paper: K.R., M.M., P.S.D., R.P. All authors approved the final draft of the paper.
Corresponding author
Correspondence to Kaili Rimfeld .
Ethics declarations
Competing interests.
The authors declare no competing interests.
Additional information
Publisher's note: Springer Nature remains neutral with regard to jurisdictional claims in published maps and institutional affiliations.
Electronic supplementary material
Supplemental material, supplementary methods, rights and permissions.
Open Access This article is licensed under a Creative Commons Attribution 4.0 International License, which permits use, sharing, adaptation, distribution and reproduction in any medium or format, as long as you give appropriate credit to the original author(s) and the source, provide a link to the Creative Commons license, and indicate if changes were made. The images or other third party material in this article are included in the article’s Creative Commons license, unless indicated otherwise in a credit line to the material. If material is not included in the article’s Creative Commons license and your intended use is not permitted by statutory regulation or exceeds the permitted use, you will need to obtain permission directly from the copyright holder. To view a copy of this license, visit http://creativecommons.org/licenses/by/4.0/ .
Reprints and permissions
About this article
Cite this article.
Rimfeld, K., Malanchini, M., Krapohl, E. et al. The stability of educational achievement across school years is largely explained by genetic factors. npj Science Learn 3 , 16 (2018). https://doi.org/10.1038/s41539-018-0030-0
Download citation
Received : 05 December 2017
Revised : 10 July 2018
Accepted : 18 July 2018
Published : 04 September 2018
DOI : https://doi.org/10.1038/s41539-018-0030-0
Share this article
Anyone you share the following link with will be able to read this content:
Sorry, a shareable link is not currently available for this article.
Provided by the Springer Nature SharedIt content-sharing initiative
This article is cited by
Infrastructuring educational genomics: associations, architectures, and apparatuses.
- Ben Williamson
- Dimitra Kotouza
- Jessica Pykett
Postdigital Science and Education (2024)
Conventional twin studies overestimate the environmental differences between families relevant to educational attainment
- Tobias Wolfram
- Damien Morris
npj Science of Learning (2023)
Delayed tracking and inequality of opportunity: Gene-environment interactions in educational attainment
- Antonie Knigge
- Dorret I. Boomsma
npj Science of Learning (2022)
Investigating how the accuracy of teacher expectations of pupil performance relate to socioeconomic and genetic factors
- Ciarrah-Jane Shannon Barry
- Neil M. Davies
- Tim T. Morris
Scientific Reports (2022)
The practical utility of genetic screening in school settings
- W. van Dijk
npj Science of Learning (2021)
Quick links
- Explore articles by subject
- Guide to authors
- Editorial policies
Sign up for the Nature Briefing newsletter — what matters in science, free to your inbox daily.

- Review article
- Open access
- Published: 10 February 2020
Predicting academic success in higher education: literature review and best practices
- Eyman Alyahyan 1 &
- Dilek Düştegör ORCID: orcid.org/0000-0003-2980-1314 2
International Journal of Educational Technology in Higher Education volume 17 , Article number: 3 ( 2020 ) Cite this article
107k Accesses
193 Citations
10 Altmetric
Metrics details
Student success plays a vital role in educational institutions, as it is often used as a metric for the institution’s performance. Early detection of students at risk, along with preventive measures, can drastically improve their success. Lately, machine learning techniques have been extensively used for prediction purpose. While there is a plethora of success stories in the literature, these techniques are mainly accessible to “computer science”, or more precisely, “artificial intelligence” literate educators. Indeed, the effective and efficient application of data mining methods entail many decisions, ranging from how to define student’s success , through which student attributes to focus on , up to which machine learning method is more appropriate to the given problem . This study aims to provide a step-by-step set of guidelines for educators willing to apply data mining techniques to predict student success. For this, the literature has been reviewed, and the state-of-the-art has been compiled into a systematic process, where possible decisions and parameters are comprehensively covered and explained along with arguments. This study will provide to educators an easier access to data mining techniques, enabling all the potential of their application to the field of education.
Introduction
Computers have become ubiquitous, especially in the last three decades, and are significantly widespread. This has led to the collection of vast volumes of heterogeneous data, which can be utilized for discovering unknown patterns and trends (Han et al., 2011 ), as well as hidden relationships (Sumathi & Sivanandam, 2006 ), using data mining techniques and tools (Fayyad & Stolorz, 1997 ). The analysis methods of data mining can be roughly categorized as: 1) classical statistics methods (e.g. regression analysis, discriminant analysis, and cluster analysis) (Hand, 1998 ), 2) artificial intelligence (Zawacki-Richter, Marín, Bond, & Gouverneur, 2019 ) (e.g. genetic algorithms, neural computing, and fuzzy logic), and 3) machine learning (e.g. neural networks, symbolic learning, and swarm optimization) (Kononenko & Kukar, 2007 ). The latter consists of a combination of advanced statistical methods and AI heuristics. These techniques can benefit various fields through different objectives, such as extracting patterns, predicting behavior, or describing trends. A standard data mining process starts by integrating raw data – from different data sources – which is cleaned to remove noise, duplicated or inconsistent data. After that, the cleaned data is transformed into a concise format that can be understood by data mining tools, through filtering and aggregation techniques. Then, the analysis step identifies the existing interesting patterns, which can be displayed for a better visualization (Han et al., 2011 ) (Fig. 1 ).
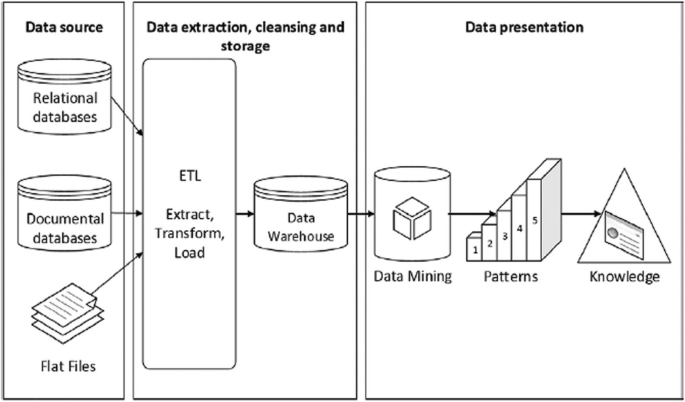
standard data mining process (Han et al. 2011 )
Recently data mining has been applied to various fields like healthcare (Kavakiotis et al., 2017 ), business (Massaro, Maritati, & Galiano, 2018 ), and also education (Adekitan, 2018 ). Indeed, the development of educational database management systems created a large number of educational databases, which enabled the application of data mining to extract useful information from this data. This led to the emergence of Education Data Mining (EDM) (Calvet Liñán & Juan Pérez, 2015 ; Dutt, Ismail, & Herawan, 2017 ) as an independent research field. Nowadays, EDM plays a significant role in discovering patterns of knowledge about educational phenomena and the learning process (Anoopkumar & Rahman, 2016 ), including understanding performance (Baker, 2009 ). Especially, data mining has been used for predicting a variety of crucial educational outcomes, like performance (Xing, 2019 ), retention (Parker, Hogan, Eastabrook, Oke, & Wood, 2006 ), success (Martins, Miguéis, Fonseca, & Alves, 2019 ; Richard-Eaglin, 2017 ), satisfaction (Alqurashi, 2019 ), achievement (Willems, Coertjens, Tambuyzer, & Donche, 2018 ), and dropout rate (Pérez, Castellanos, & Correal, 2018 ).
The process of EDM (see Fig. 2 ) is an iterative knowledge discovery process that consists of hypothesis formulation, testing, and refinement (Moscoso-Zea et al., 2016 ; Sarala & Krishnaiah, 2015 ). Despite many publications, including case studies, on educational data mining, it is still difficult for educators – especially if they are a novice to the field of data mining – to effectively apply these techniques to their specific academic problems. Every step described in Fig. 2 necessitates several decisions and set-up of parameters, which directly affect the quality of the obtained result.
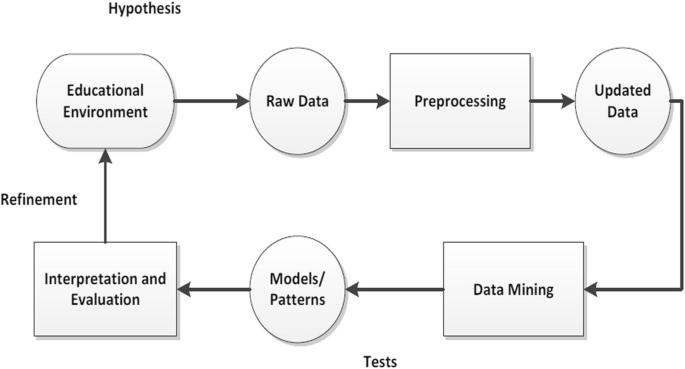
Knowledge discovery process in educational institutions (Moscoso-Zea, Andres-Sampedro, & Lujan-Mora, 2016 )
This study aims to fill the described gap, by providing a complete guideline, providing easier access to data mining techniques and enabling all the potential of their application to the field of education. In this study, we specifically focus on the problem of predicting the academic success of students in higher education. For this, the state-of-the-art has been compiled into a systematic process, where all related decisions and parameters are comprehensively covered and explained along with arguments.
In the following, first, section 2 clarifies what is academic success and how it has been defined and measured in various studies with a focus on the factors that can be used for predicting academic success. Then, section 3 presents the methodology adopted for the literature review. Section 4 reviews data mining techniques used in predicting students’ academic success, and compares their predictive accuracy based on various case studies. Section 5 concludes the review, with a recapitulation of the whole process. Finally, section 6 concludes this paper and outlines the future work.
Academic success definition
Student success is a crucial component of higher education institutions because it is considered as an essential criterion for assessing the quality of educational institutions (National Commission for Academic Accreditation &, 2015 ). There are several definitions of student success in the literature. In (Kuh, Kinzie, Buckley, Bridges, & Hayek, 2006 ), a definition of student success is synthesized from the literature as “Student success is defined as academic achievement, engagement in educationally purposeful activities, satisfaction, acquisition of desired knowledge, skills and competencies, persistence, attainment of educational outcomes, and post-college performance”. While this is a multi-dimensional definition, authors in (York, Gibson, & Rankin, 2015 ) gave an amended definition concentrating on the most important six components, that is to say “Academic achievement, satisfaction, acquisition of skills and competencies, persistence, attainment of learning objectives, and career success” (Fig. 3 ).
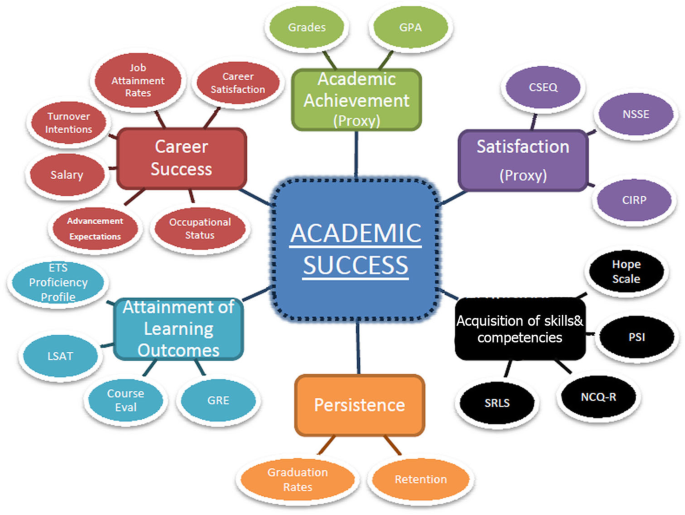
Defining academic success and its measurements (York et al., 2015 )
Despite reports calling for more detailed views of the term, the bulk of published researchers measure academic success narrowly as academic achievement. Academic achievement itself is mainly based on Grade Point Average (GPA), or Cumulative Grade Point Average (CGPA) (Parker, Summerfeldt, Hogan, & Majeski, 2004 ), which are grade systems used in universities to assign an assessment scale for students’ academic performance (Choi, 2005 ), or grades (Bunce & Hutchinson, 2009 ). The academic success has also been defined related to students’ persistence, also called academic resilience (Finn & Rock, 1997 ), which in turn is also mainly measured through the grades and GPA, measures of evaluations by far the most widely available in institutions.
Review methodology
Early prediction of students’ performance can help decision makers to provide the needed actions at the right moment, and to plan the appropriate training in order to improve the student’s success rate. Several studies have been published in using data mining methods to predict students’ academic success. One can observe several levels targeted:
Degree level: predicting students’ success at the time of obtention of the degree.
Year level: predicting students’ success by the end of the year.
Course level: predicting students’ success in a specific course.
Exam level: predicting students’ success in an exam for a specific course.
In this study, the literature related to the exam level is excluded as the outcome of a single exam does not necessarily imply a negative outcome.
In terms of coverage, section 4 and 5 only covers articles published within the last 5 years. This restriction was necessary to scale down the search space, due to the popularity of EDM. The literature was searched from Science Direct, ProQuest, IEEE Xplore, Springer Link, EBSCO, JSTOR, and Google Scholar databases, using academic success , academic achievement , student success , educational data mining , data mining techniques , data mining process and predicting students’ academic performance as keywords. While we acknowledge that there may be articles not included in this review, seventeen key articles about data mining techniques that were reviewed in sections 4 and 5 .
Influential factors in predicting academic success
One important decision related to the prediction of students’ academic success in higher education is to clearly define what is academic success. After that, one can think about the potential influential factors, which are dictating the data that needs to be collected and mined.
While a broad variety of factors have been investigated in the literature with respect to their impact on the prediction of students’ academic success (Fig. 4 ), we focus here on prior-academic achievement , student demographics , e-learning activity , psychological attributes , and environments , as our investigation revealed that they are the most commonly reported factors (summarized in Table 1 ). As a matter of fact, the top 2 factors, namely, prior-academic achievement , and student demographics , were presented in 69% of the research papers. This observation is aligned with the results of The previous literature review which emphasized that the grades of internal assessment and CGPA are the most common factors used to predict student performance in EDM (Shahiri, Husain, & Rashid, 2015 ). With more than 40%, prior academic achievement is the most important factor. This is basically the historical baggage of students. It is commonly identified as grades (or any other academic performance indicators) that students obtained in the past (pre-university data, and university-data). The pre-university data includes high school results that help understand the consistency in students’ performance (Anuradha & Velmurugan, 2015 ; Asif et al., 2015 ; Asif et al., 2017 ; Garg, 2018 ; Mesarić & Šebalj, 2016 ; Mohamed & Waguih, 2017 ; Singh & Kaur, 2016 ). They also provide insight into their interest in different topics (i.e., courses grade (Asif et al., 2015 ; Asif et al., 2017 ; Oshodi et al., 2018 ; Singh & Kaur, 2016 )). Additionally, this can also include pre-admission data which is the university entrance test results (Ahmad et al., 2015 ; Mesarić & Šebalj, 2016 ; Oshodi et al., 2018 ). The university-data consists of grades already obtained by the students since entering the university, including semesters GPA or CGPA (Ahmad et al., 2015 ; Almarabeh, 2017 ; Hamoud et al., 2018 ; Mueen et al., 2016 ; Singh & Kaur, 2016 ), courses marks (Al-barrak & Al-razgan, 2016 ; Almarabeh, 2017 ; Anuradha & Velmurugan, 2015 ; Asif et al., 2015 ; Asif et al., 2017 ; Hamoud et al., 2018 ; Mohamed & Waguih, 2017 ; Mueen et al., 2016 ; Singh & Kaur, 2016 ; Sivasakthi, 2017 ) and course assessment grades (e.g. assignment (Almarabeh, 2017 ; Anuradha & Velmurugan, 2015 ; Mueen et al., 2016 ; Yassein et al., 2017 ); quizzes (Almarabeh, 2017 ; Anuradha & Velmurugan, 2015 ; Mohamed & Waguih, 2017 ; Yassein et al., 2017 ); lab-work (Almarabeh, 2017 ; Mueen et al., 2016 ; Yassein et al., 2017 ); and attendance (Almarabeh, 2017 ; Anuradha & Velmurugan, 2015 ; Garg, 2018 ; Mueen et al., 2016 ; Putpuek et al., 2018 ; Yassein et al., 2017 )).
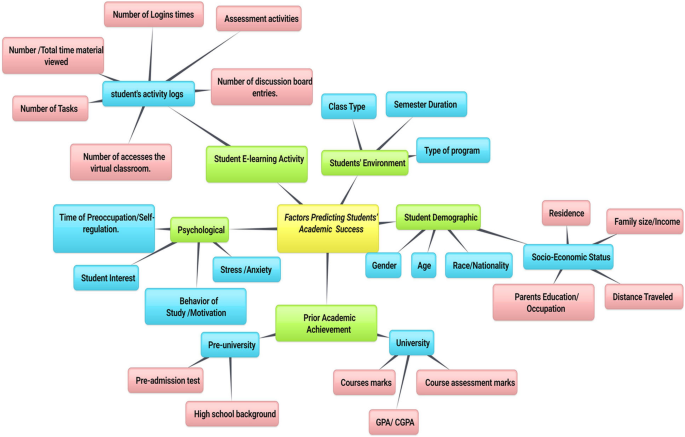
a broad variety of factors potentially impacting the prediction of students’ academic success
Students’ demographic is a topic of divergence in the literature. Several studies indicated its impact on students’ success, for example, gender (Ahmad et al., 2015 ; Almarabeh, 2017 ; Anuradha & Velmurugan, 2015 ; Garg, 2018 ; Hamoud et al., 2018 ; Mohamed & Waguih, 2017 ; Putpuek et al., 2018 ; Sivasakthi, 2017 ), age (Ahmad et al., 2015 ; Hamoud et al., 2018 ; Mueen et al., 2016 ), race/ethnicity (Ahmad et al., 2015 ), socioeconomic status (Ahmad et al., 2015 ; Anuradha & Velmurugan, 2015 ; Garg, 2018 ; Hamoud et al., 2018 ; Mohamed & Waguih, 2017 ; Mueen et al., 2016 ; Putpuek et al., 2018 ), and father’s and mother’s background (Hamoud et al., 2018 ; Mohamed & Waguih, 2017 ; Singh & Kaur, 2016 ) have been shown to be important. Yet, few studies also reported just the opposite, for gender in particular (Almarabeh, 2017 ; Garg, 2018 ).
Some attributes related to the student’s environment were found to be impactful information such as program type (Hamoud et al., 2018 ; Mohamed & Waguih, 2017 ), class type (Mueen et al., 2016 ; Sivasakthi, 2017 ) and semester period (Mesarić & Šebalj, 2016 ).
Among the reviewed papers, also many researchers used Student E-learning Activity information, such as a number of login times, number of discussion board entries, number / total time material viewed (Hamoud et al., 2018 ), as influential attributes and their impact, though minor, were reported.
The psychological attributes are determined as the interests and personal behavior of the student; several studies have shown them to be impactful on students’ academic success. To be more precise, student interest (Hamoud et al., 2018 ), the behavior towards study (Hamoud et al., 2018 ; Mueen et al., 2016 ), stress and anxiety (Hamoud et al., 2018 ; Putpuek et al., 2018 ), self-regulation and time of preoccupation (Garg, 2018 ; Hamoud et al., 2018 ), and motivation (Mueen et al., 2016 ), were found to influence success.
Data mining techniques for prediction of students’ academic success
The design of a prediction model using data mining techniques requires the instantiation of many characteristics, like the type of the model to build, or methods and techniques to apply (Witten, Frank, Hall, & Pal, 2016 ). This section defines these attributes, provide some of their instances, and reveal the statistics of their occurrence among the reviewed papers grouped by the target variable in the student success prediction, that is to say, degree level, year level, and course level.
Degree level
Several case studies have been published, seeking prediction of academic success at the degree level. One can observe two main approaches in term of the model to build: classification where CGPA that is targeted is a category as multi class problem such as (a letter grade (Adekitan & Salau, 2019 ; Asif et al., 2015 ; Asif et al., 2017 ) or overall rating (Al-barrak & Al-razgan, 2016 ; Putpuek et al., 2018 )) or binary class problem such as (pass/fail (Hamoud et al., 2018 ; Oshodi et al., 2018 )). As for the other approach, it is the regression where the numerical value of CGPA is predicted (Asif et al., 2017 ). We can also observe a broad variety in terms of the department students belongs to, from architecture (Oshodi et al., 2018 ), to education (Putpuek et al., 2018 ), with a majority in technical fields (Adekitan & Salau, 2019 ; Al-barrak & Al-razgan, 2016 ; Asif et al., 2015 ; Hamoud et al., 2018 ). An interesting finding is related to predictors: studies that included university-data, especially grades from first 2 years of the program, yielded better performance than studies that included only demographics (Putpuek et al., 2018 ), or only pre-university data (Oshodi et al., 2018 ). Details regarding the algorithm used, the sample size, the best accuracy and corresponding method, as well as the software environment that was used are all in Table 2 .
Less case studies have been reported, seeking prediction of academic success at the year level. Yet, the observations regarding these studies are very similar to the one related to degree level (reported in previous section). Similar to previous sub-section, studies that included only social conditions and pre-university data gave the worse accuracy (Singh & Kaur, 2016 ), while including university-data improved results (Anuradha & Velmurugan, 2015 ). Nevertheless, it is interesting to note that even the best accuracy in (Anuradha & Velmurugan, 2015 ) is inferior to the accuracy in (Adekitan & Salau, 2019 ; Asif et al., 2015 ; Asif et al., 2017 ) reported in previous section. This can be explained by the fact that in (Anuradha & Velmurugan, 2015 ), only 1 year of past university-data is included while in (Asif et al., 2015 ; Asif et al., 2017 ), 2 years of past university-data and in (Adekitan & Salau, 2019 ) 3 years of past university-data is covered. Other details for these methods are in Table 3 .
Course level
Finally, some studies can be reported, seeking the prediction of academic success at the course level. As already mentioned in degree level and year level sections, the comparative work gives accuracies of 62% to 89% while predicting success at a course level can give accuracies more than 89%, which can be seen as a more straightforward task than predicting success at degree level or year level. The best accuracy is obtained in course level with 93%. In (Garg, 2018 ), the target course was an advanced programming course while the influential factor was a previous programming course, also a prerequisite course. This demonstrates how important it is to have a field knowledge and use this knowledge to guide the decisions in the process and target important features. All other details for these methods are in Table 4 .
Data mining process model for student success prediction
This section compiles as a set of guidelines the various steps to take while using educational data mining techniques for student success prediction; all decisions needed to be taken at various stages of the process are explained, along with a shortlist of best practices collected from the literature. The proposed framework (Fig. 5 ) has been derived from well-known processes (Ahmad et al., 2015 ; Huang, 2011 ; Pittman, 2008 ). It consists of six main stages: 1) data collection, 2) data initial preparation, 3) statistical analysis, 4) data preprocessing, 5) data mining implementation, and 6) result evaluation. These stages are detailed in the next subsections.

Stages of the EDM framework
Data collection
In educational data mining, the needed information can be extracted from multiple sources. As indicated in Table 1 , the most influential factor observed in the literature is Prior Academic Achievement. Related data, that is to say, pre-university or university-data, can easily be retrieved from the university Student Information System (SIS) that are so widely used nowadays. SIS can also provide some student demographics (e.g. age, gender, ethnicity), but socio-economic status might not be available explicitly. In that case, this could either be deduced from existing data, or it might be directly acquired from students through surveys. Similarly, students’ environment related information also can be extracted from the SIS, while psychological data would probably need the student to fill a survey. Finally, students’ e-learning activities can be obtained from e-learning system logs (Table 5 ).
Initial preparation of data
In its original form, the data (also called raw data) is usually not ready for analysis and modeling. Data sets that are mostly obtained from merging tables in the various systems cited in Table 5 might contain missing data, inconsistent data, incorrect data, miscoded data, and duplicate data. This is why the raw data needs to go through an initial preparation (Fig. 6 ), consisting of 1) selection, 2) cleaning, and 3) derivation of new variables. This is a vital step, and usually the most time consuming (CrowdFlower, 2016 ).
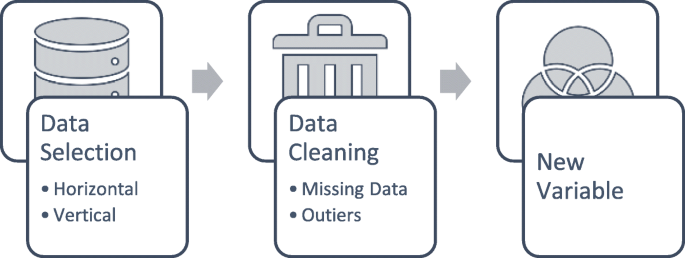
Initial Preparation of Data
Data selection
The dimension of the data gathered can be significant, especially while using prior academic achievements (e.g. if all past courses are included both from high-school and completed undergraduate years). This can negatively impact the computational complexity. Furthermore, including all the gathered data in the analysis can yield below optimal prediction results, especially in case of data redundancy, or data dependency. Thus, it is crucial to determine which attributes are important, or needs to be included in the analysis. This requires a good understanding of the data mining goals as well as the data itself (Pyle, Editor, & Cerra, 1999 ). Data selection, also called “Dimensionality Reduction” (Liu & Motoda, 1998 ), consists in vertical (attributes/variables) selection and horizontal (instance/records) selection (García, Luengo, & Herrera, 2015 ; Nisbet, Elder, & Miner, 2009 ; Pérez et al., 2015 ) (Table 6 ). Also, it is worth noticing that models obtained from a reduced number of features will be easier to understand (Pyle et al., 1999 ).
Data cleaning
Data sources tend to be inconsistent, contain noises, and usually suffer from missing values (Linoff & Berry, 2011 ). When a value is not stored for a variable, it is considered as missing data. When a value is in an abnormal distance from the other values in the dataset, it is called an outlier. Literature reveals that missing values and outliers are very common in the field of EDM. Thus, it is important to know how to handle them without compromising the quality of the prediction. All things considered, dealing with missing values or outliers cannot be done by a general procedure, and several methods need to be considered within the context of the problem. Nevertheless, we try to here to summarize the main approaches observed in the literature and Table 7 provides a succinct summary of them.
If not treated, missing value becomes a problem for some classifiers. For example, Support Vector Machines (SVMs), Neural Networks (NN), Naive Bayes, and Logistic Regression require full observation (Pelckmans, De Brabanter, Suykens, & De Moor, 2005 ; Salman & Vomlel, 2017 ; Schumacker, 2012 ), however, decision trees and random forests can handle missing data (Aleryani, Wang, De, & Iglesia, 2018 ). There are two strategies to deal with missing values. The first one is a listwise deletion, and it consists in deleting either the record (row deletion, when missing values are few) or the attribute/variable (column deletion, when missing values are too many). The second strategy, imputation, that derives the missing value from the remainder of the data (e.g. median, mean, a constant value for numerical value, or randomly selected value from missing values distribution (McCarthy, McCarthy, Ceccucci, & Halawi, 2019 ; Nisbet et al., 2009 )).
Outliers data are also known as anomalies, can easily be identified by visual means, creating a histogram, stem and leaf plots or box plots and looking for very high or very low values. Once identified, outliers can be removed from the modeling data. Another possibility is to converts the numeric variable to a categorical variable (i.e. bin the data) or leaves the outliers in the data (McCarthy et al., 2019 ).
Derivation of new variables
New variables can be derived from existing variables by combining them (Nisbet et al., 2009 ). When done based on domain knowledge, this can improve the data mining system (Feelders, Daniels, & Holsheimer, 2000 ). For example, GPA is a common variable that can be obtained from SIS system. If taken as it is, a student’s GPA reflects his/her average in a given semester. However, this does not explicitly say anything about this student’s trend over several semesters. For the same GPA, one student could be in a steady state, going through an increasing trend, or experiencing a drastic performance drop. Thus, calculating the difference in GPA between consecutive semesters will add an extra information. While there is no systematic method for deriving new variables, Table 8 recapitulates the instances that we observed in the EDM literature dedicated to success prediction.
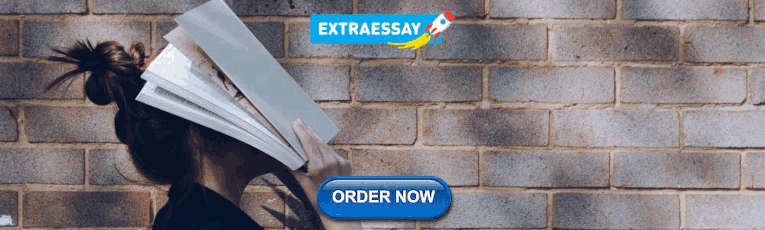
Statistical analysis
Preliminary statistical analysis, especially through visualization, allows to better understand the data before moving to more sophisticated data mining tasks and algorithms (McCarthy et al., 2019 ). Table 9 summarizes the statistics commonly derived depending on the data type. Data mining tools contain descriptive statistical capabilities. Dedicated tools like STATISTICA (Jascaniene, Nowak, Kostrzewa-Nowak, & Kolbowicz, 2013 ) and SPSS (L. A. D. of S. University of California and F. Foundation for Open Access Statistics, 2004 ) can also provide tremendous insight.
It is important to note that this step can especially help planning further steps in DM process, including data pre-processing to identify the outliers, determining the patterns of missing data, study the distribution of each variable and identify the relationship between independent variables and the target variable (see Table 10 ). Furthermore, statistical analysis is used in the interpreting stage to explain the results of the DM model (Pyle et al., 1999 ).
Data preprocessing
The last step before the analysis of the data and modeling is preprocessing, which consists of 1) data transformation, 2) how to handle imbalanced data sets, and 3) feature selection (Fig. 7 ).
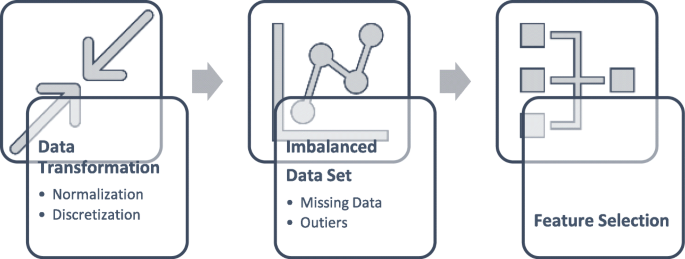
Data Preprocessing
Data transformation
Data transformation is a necessary process to eliminate dissimilarities in the dataset, thus it becomes more appropriate for data mining (Osborne, 2002 ). In EDM for success prediction, we can observe the following operations:
Normalization of numeric attributes: this is a scaling technique used when the data includes varying scales, and the used data mining algorithm cannot provide a clear assumptions of the data distribution (Patro & Sahu, 2015 ). We can cite K-nearest neighbors and artificial neural networks (How to Normalize and Standardize Your Machine Learning Data in Weka, n.d. ) as examples of such algorithms. Normalizing the data may improve the accuracy and the efficiency of the mining algorithms, and provide better results (Shalabi & Al-Kasasbeh, 2006 ). The common normalization techniques are min-max (MM), decimal scaling, Z-score (ZS), median and MAD, double sigmoid (DS), tanh, and bi-weight normalizations (Kabir, Ahmad, & Swamy, 2015 ).
Discretization: The simplest method of discretization binning (García et al., 2015 ), converts a continuous numeric variable into a series of categories by creating a finite number of bins and assigning a specific number of values to each attribute in each bin. Discretization is a necessary step when using DM techniques that allow only for categorical variables (Liu, Hussain, Tan, & Dash, 2002 ; Maimon & Rokach, 2005 ) such as C4.5 (Quinlan, 2014 ), Apriori (Agrawal, 2005 ) and Naïve Bayes (Flores, Gámez, Martínez, & Puerta, 2011 ). Discretization also increases the accuracy of the models by overcoming noisy data, and by identifying outliers’ values. Finally, discrete features are easier to understand, handle, and explain.
Convert to numeric variables: Most DM algorithms offer better results using a numeric variable. Therefore, data needs to be converted into numerical variables, using any of these methods:
Encode labels using a value between [0 and N (class-1)34 ] where N is the number of labels (Why One-Hot Encode Data in Machine Learning, n.d. ).
A dummy variable is a binary variable denoted as (0 or 1) to represent one level of a categorical variable, where (1) reflects the presence of level and (0) reflects the absence of level. One dummy variable will be created for each present level (Mayhew & Simonoff, 2015 ).
Combining levels: this allows reducing the number of levels in categorical variables and improving model performance. This is done by simply combining similar levels into alike groups through domain (Simple Methods to deal with Categorical Variables in Predictive Modeling, n.d. ).
However, note that all these methods do not necessarily lead to improved results. Therefore, it is important to repeat the modeling process by trying different preprocessing scenarios, evaluate the performance of the model, and identify the best results. Table 11 . recapitulates the various EDM application of preprocessing methods.
Imbalanced datasets
It is common in EDM applications that the dataset is imbalanced, meaning that the number of samples from one class is significantly less than the samples from other classes (e.g. number of failing students vs passing students) (El-Sayed, Mahmood, Meguid, & Hefny, 2015 ; Qazi & Raza, 2012 ). This lack of balance may negatively impact the performance of data mining algorithms (Chotmongkol & Jitpimolmard, 1993 ; Khoshgoftaar, Golawala, & Van Hulse, 2007 ; Maheshwari, Jain, & Jadon, 2017 ; Qazi & Raza, 2012 ). Re-sampling (under or over-sampling) is the solution of choice (Chotmongkol & Jitpimolmard, 1993 ; Kaur & Gosain, 2018 ; Maheshwari et al., 2017 ). Under-sampling consists in removing instances from the major class, either randomly or by some techniques to balance the classes. Oversampling consists of increasing the number of instances in the minor class, either by randomly duplicating some samples, or by synthetically generating samples (Chawla, Bowyer, Hall, & Kegelmeyer, 2002 ) (see Table 12 ).
Feature selection
When the data set is prepared and ready for modeling, then the important variables can be chosen and submitted to the modeling algorithm. This step, called feature selection, is an important strategy to be followed to mining the data (Liu & Motoda, 1998 ). Feature selection aims to choose a subset of attributes from the input data with the capability of giving an efficient description for the input data while reducing effects from unrelated variables while preserving sufficient prediction results (Guyon & Elisseeff, 2003 ). Feature selection enables reduced computation time, improved prediction performance while allowing a better understanding of the data (Chandrashekar & Sahin, 2014 ). Feature selection methods are classified into filter and wrapper methods (Kohavi & John, 1997 ). Filter methods work as preprocessing to rank the features, so high-ranking features are identified and applied to the predictor. In wrapper methods, the criterion for selecting the feature is the performance of the forecasting device, meaning that the predictor is wrapped on a search algorithm which will find a subset that gives the highest predictor performance. Moreover, there are embedded methods (Blum & Langley, 1997 ; Guyon & Elisseeff, 2003 ; P. (Institute for the S. of L. and E. Langley, 1994 ) which include variable selection as part of the training process without the need for splitting the data into training and testing sets. However, most data mining tools contains embedded feature selection methods making it easy to try them and chose the best one.
Data mining implementation
Data mining models.
Two types of data mining models are commonly used in EDM applications for success prediction: predictive and descriptive (Kantardzic, 2003 ). Predictive models apply supervised learning functions to provide estimation for expected values of dependent variables according to the features of relevant independent variables (Bramer, 2016 ). Descriptive models are used to produce patterns that describe the fundamental structure, relations, and interconnectedness of the mined data by applying unsupervised learning functions on it (Peng, Kou, Shi, & Chen, 2008 ). Typical examples of predictive models are classification (Umadevi & Marseline, 2017 ) and regression (Bragança, Portela, & Santos, 2018 ), while clustering (Dutt et al., 2017 ) and association (Zhang, Niu, Li, & Zhang, 2018 ), produce descriptive models. As stated in section 4 , classification is the most used method, followed by regression and clustering. The most commonly used classification techniques are Bayesian networks, neural networks, decision trees (Romero & Ventura, 2010 ). Common regression techniques are linear regression and logistic regression analysis (Siguenza-Guzman, Saquicela, Avila-Ordóñez, Vandewalle, & Cattrysse, 2015 ). Clustering uses techniques like neural networks, K-means algorithms, fuzzy clustering and discrimination analysis (Dutt et al., 2017 ). Table 13 shows the recurrence of specific algorithms based on the literature review that we performed.
In the process, first one needs to choose a model, namely predictive or descriptive. Then, the algorithms to build the models are chosen from the 10 techniques considered as the top 10 in DM in terms of performance, always prefer models that are interpretable and understandable such as DT and linear models (Wu et al., 2008 ). Once the algorithms have been chosen, they require to be configured before they are applied. The user must provide suitable values for the parameters in advance in order to obtain good results for the models. There are various strategies to tune parameters for EDM algorithms, used to find the most useful performing parameters. The trial and error approach is one of the simplest and easiest methods for non-expert users (Ruano, Ribes, Sin, Seco, & Ferrer, 2010 ). It consists of performing numerous experiments by modifying the parameters’ values until finding the most beneficial performing parameters.
Data mining tools
Data mining has a stack of open source tools such as machine learning tools which supports the researcher in analyzing the dataset using several algorithms. Such tools are vastly used for predictive analysis, visualization, and statistical modeling. WEKA is the most used tool for predictive modeling (Jayaprakash, 2018 ). This can be explained by its many pre-built tools for data pre-processing, classification, association rules, regression, and visualization, as well as its user-friendliness, and accessibility even to a novice in programming or data mining. But we can also cite RapidMiner and Clementine as stated in Table 4 .
Results evaluation
As several models are usually built, it is important to evaluate them and select the most appropriate. While evaluating the performance of classification algorithms, normally the confusion matrix as shown in Table 14 is used. This table gathers four important metrics related to a given success prediction model:
True Positive (TP): number of successful students classified correctly as “successful”.
False Positive (FP): number of successful students incorrectly classified as “non-successful”.
True Negative (TN): number of did not successful students classified correctly as “non-successful”.
False Negative (FN): number of did not successful students classified incorrectly as “successful”.
Different performance measures are included to evaluate the model of each classifier, almost all measures of performance are based on the confusion matrix and the numbers in it. To produce more accurate results, these measures are evaluated together. In this research, we’ll focus on the measures used in the classification problems. The measures commonly used in the literature are provided in Table 15 .
Early student performance prediction can help universities to provide timely actions, like planning for appropriate training to improve students’ success rate. Exploring educational data can certainly help in achieving the desired educational goals. By applying EDM techniques, it is possible to develop prediction models to improve student success. However, using data mining techniques can be daunting and challenging for non-technical persons. Despite the many dedicated software’s, this is still not a straightforward process, involving many decisions. This study presents a clear set of guidelines to follow for using EDM for success prediction. The study was limited to undergraduate level, however the same principles can be easily adapted to graduate level. It has been prepared for those people who are novice in data mining, machine learning or artificial intelligence.
A variety of factors have been investigated in the literature related to its impact on predicting students ‘academic success which was measured as academic achievement, as our investigation showed that prior-academic achievement, student demographics, e-learning activity, psychological attributes, are the most common factors reported. In terms of prediction techniques, many algorithms have been applied to predict student success under the classification technique.
Moreover, a six stages framework is proposed, and each stage is presented in detail. While technical background is kept to a minimum, as this not the scope of this study, all possible design and implementation decisions are covered, along with best practices compiled from the relevant literature.
It is an important implication of this review that educators and non-proficient users are encouraged to applied EDM techniques for undergraduate students from any discipline (e.g. social sciences). While reported findings are based on the literature (e.g. potential definition of academic success, features to measure it, important factors), any available additional data can easily be included in the analysis, including faculty data (e.g. competence, criteria of recruitment, academic qualifications) may be to discover new determinants.
Availability of data and materials
Not applicable.
Abbreviations
(Probabilistic) neural network
Classification
- Data mining
Decision tree
Educational data mining
K-nearest neighbors
Logistic regression
Naive Bayes
Neural network
Random forest
Rule induction
Random tree
Tree ensemble
Adekitan, A. I. (2018). “Data mining approach to predicting the performance of first year student in a university using the admission requirements,” no. Aina 2002 .
Google Scholar
Adekitan, A. I., & Salau, O. (2019). The impact of engineering students’ performance in the first three years on their graduation result using educational data mining. Heliyon , 5 (2), e01250.
Article Google Scholar
Agrawal, S. (2005). Database Management Systems Fast Algorithms for Mining Association Rules. In In Proc. 20th int. conf. very large data bases, VLDB , (pp. 487–499).
Ahmad, F., Ismail, N. H., & Aziz, A. A. (2015). The Prediction of Students ’ Academic Performance Using Classification Data Mining Techniques, 9 (129), 6415–6426.
Al-barrak, M. A., & Al-razgan, M. (2016). Predicting Students Final GPA Using Decision Trees : A Case Study. International Journal of Information and Education Technology, 6 (7), 528–533.
Aleryani, A., Wang, W., De, B., & Iglesia, L. (2018). Dealing with missing data and uncertainty in the context of data mining. In International Conference on Hybrid Artificial Intelligence Systems .
Almarabeh, H. (2017). Analysis of students’ performance by using different data mining classifiers. International Journal of Modern Education and Computer Science , 9 (8), 9–15.
Alqurashi, E. (2019). Predicting student satisfaction and perceived learning within online learning environments. Distance Education , 40 (1), 133–148.
Anoopkumar, M., & Rahman, A. M. J. M. Z. (2016). A Review on Data Mining techniques and factors used in Educational Data Mining to predict student amelioration. In 2016 International Conference on Data Mining and Advanced Computing (SAPIENCE) , (pp. 122–133).
Chapter Google Scholar
Anuradha, C., & Velmurugan, T. (2015). A Comparative Analysis on the Evaluation of Classification Algorithms in the Prediction of Students Performance. Indian Journal of Science and Technology , 8 (July), 1–12.
Asif, R., Merceron, A., Abbas, S., & Ghani, N. (2017). Analyzing undergraduate students ’ performance using educational data mining. Computers in Education , 113 , 177–194.
Asif, R., Merceron, A., & Pathan, M. K. (2015). Predicting student academic performance at degree level: A case study. International Journal of Intelligent Systems and Applications , 7 (1), 49–61.
Baker, R. Y. A. N. S. J. D. (2009). The State of Educational Data Mining in 2009: A Review and Future Visions. Journal of Educational Data Mining , 5(8), 3–16.
Blum, A. L., & Langley, P. (1997). Selection of relevant features and examples in machine learning. Artificial Intelligence , 97 (1–2), 245–271.
Article MathSciNet MATH Google Scholar
Bragança, R., Portela, F., & Santos, M. (2019). A regression data mining approach in Lean Production. Concurrency and Computation: Practice and Experience, 31(22), e4449.
Bramer, M. (2016). Principles of data mining . London: Springer London.
Book MATH Google Scholar
Bunce, D. M., & Hutchinson, K. D. (2009). The use of the GALT (Group Assessment of Logical Thinking) as a predictor of academic success in college chemistry. Journal of Chemical Education , 70 (3), 183.
Calvet Liñán, L., & Juan Pérez, Á. A. (2015). Educational Data Mining and Learning Analytics: differences, similarities, and time evolution. International Journal of Educational Technology in Higher Education , 12 (3), 98.
Chandrashekar, G., & Sahin, F. (2014). A survey on feature selection methods. Computers & Electrical Engineering , 40 (1), 16–28.
Chawla, N. V., Bowyer, K. W., Hall, L. O., & Kegelmeyer, W. P. (2002). SMOTE: Synthetic minority over-sampling technique. Journal of Artificial Intelligence Research , 16 , 321–357.
Article MATH Google Scholar
Choi, N. (2005). Self-efficacy and self-concept as predictors of college students’ academic performance. Psychology in the Schools , 42 (2), 197–205.
Chotmongkol, V., & Jitpimolmard, S. (1993). Cryptococcal intracerebral mass lesions associated with cryptococcal meningitis. The Southeast Asian Journal of Tropical Medicine and Public Health , 24 (1), 94–98.
CrowdFlower (2016). Data Science Report , (pp. 8–9).
Dutt, A., Ismail, M. A., & Herawan, T. (2017). A systematic review on educational data mining. IEEE Access , 5 , 15991–16005.
El-Sayed, A. A., Mahmood, M. A. M., Meguid, N. A., & Hefny, H. A. (2015). Handling autism imbalanced data using synthetic minority over-sampling technique (SMOTE). In 2015 Third World Conference on Complex Systems (WCCS) , (pp. 1–5).
Fayyad, U., & Stolorz, P. (1997). Data mining and KDD: Promise and challenges. Future Generation Computer Systems , 13 (2–3), 99–115.
Feelders, A., Daniels, H., & Holsheimer, M. (2000). Methodological and practical aspects of data mining. Information Management , 37 (5), 271–281.
Finn, J. D., & Rock, D. A. (1997). Academic success among students at risk for school failure. The Journal of Applied Psychology , 82 (2), 221–234.
Flores, M. J., Gámez, J. A., Martínez, A. M., & Puerta, J. M. (2011). Handling numeric attributes when comparing Bayesian network classifiers: Does the discretization method matter? Applied Intelligence , 34 (3), 372–385.
García, S., Luengo, J., & Herrera, F. (2015). Data preprocessing in data mining , (vol. 72). Cham: Springer International Publishing.
Garg, R. (2018). Predict Sudent performance in different regions of Punjab. International Journal of Advanced Research in Computer Science , 9 (1), 236–241.
Guyon, I., & Elisseeff, A. (2003). An Introduction to Variable and Feature Selection. Journal of Machine Learning Research , 3 (Mar), 1157–1182.
MATH Google Scholar
Hamoud, A. K., Hashim, A. S., & Awadh, W. A. (2018). Predicting Student Performance in Higher Education Institutions Using Decision Tree Analysis. International Journal of Interactive Multimedia and Artificial Intelligence , inPress, 1.
Han, J., Kamber, M., & Pei, J. (2011). Data mining : concepts and techniques. Elsevier Science. Retrieved from https://www.elsevier.com/books/data-mining-concepts-and-techniques/han/978-0-12-381479-1 .
Hand, D. J. (1998). Data mining: Statistics and more? The American Statistician , 52 (2), 112–118.
“How to Normalize and Standardize Your Machine Learning Data in Weka.”n.d. [Online]. Available: https://machinelearningmastery.com/normalize-standardize-machine-learning-data-weka/ . [Accessed: 11 Jun 2019].
S. Huang, “Predictive modeling and analysis of student academic performance in an engineering dynamics course,” All Grad. Theses Diss., 2011.
Jascaniene, N., Nowak, R., Kostrzewa-Nowak, D., & Kolbowicz, M. (2013). Selected aspects of statistical analyses in sport with the use of STATISTICA software. Central European Journal of Sport Sciences and Medicine, 3(3), 3–11.
Jayaprakash, S. (2018). A Survey on Academic Progression of Students in Tertiary Education using Classification Algorithms. International Journal of Engineering Technology Science and Research IJETSR, 5(2), 136–142.
Kabir, W., Ahmad, M. O., & Swamy, M. N. S. (2015). A novel normalization technique for multimodal biometric systems. In 2015 IEEE 58th International Midwest Symposium on Circuits and Systems (MWSCAS) , (pp. 1–4).
Kantardzic, M. (2003). Data mining : concepts, models, methods, and algorithms. Wiley-Interscience. Retrieved from https://ieeexplore-ieee-org.library.iau.edu.sa/book/5265979 .
Kaur, P., & Gosain, A. (2018). Comparing the behavior of oversampling and Undersampling approach of class imbalance learning by combining class imbalance problem with noise , (pp. 23–30). Singapore: Springer.
Kavakiotis, I., Tsave, O., Salifoglou, A., Maglaveras, N., Vlahavas, I., & Chouvarda, I. (2017). Machine learning and data mining methods in diabetes research. Computational and Structural Biotechnology Journal , 15 , 104–116.
Khoshgoftaar, T. M., Golawala, M., & Van Hulse, J. (2007). An Empirical Study of Learning from Imbalanced Data Using Random Forest. In 19th IEEE International Conference on Tools with Artificial Intelligence (ICTAI 2007) , (pp. 310–317).
Kohavi, R., & John, G. H. (1997). Wrappers for feature subset selection. Artificial Intelligence , 97 (1–2), 273–324.
Kononenko, I., & Kukar, M. (2007b). Machine learning and data mining. Machine Learning and Data Mining. Woodhead Publishing Limited. https://doi.org/10.1533/9780857099440 .
Kuh, G. D., Kinzie, J., Buckley, J. A., Bridges, B. K., & Hayek, J. C. (2006). What matters to student success: A review of the literature commissioned report for the National Symposium on postsecondary student success: Spearheading a dialog on student success .
L. A. D. of S. University of California and F. Foundation for Open Access Statistics., F. (2004). A Handbook of Statistical Analyses using SPSS. Journal of Statistical Software (Vol. 11). Foundation for Open Access Statistics. Retrieved from https://doaj.org/article/d7d17defdbea412f9b8c6a74789d735e .
Linoff, G., & Berry, M. J. A. (2011). Data mining techniques : for marketing, sales, and customer relationship management. Wiley. Retrieved from https://www.wiley.com/en-us/Data+Mining+Techniques%3A+For+Marketing%2C+Sales%2C+and+Customer+Relationship+Management%2C+3rd+Edition-p-9781118087459 .
Liu, H., Hussain, F., Tan, C. L., & Dash, M. (2002). Discretization: An enabling technique. Data Mining and Knowledge Discovery , 6 (4), 393–423.
Article MathSciNet Google Scholar
Liu, H., & Motoda, H. (1998). Feature selection for knowledge discovery and data mining . US: Springer.
Maheshwari, S., Jain, R. C., & Jadon, R. S. (2017). A Review on Class Imbalance Problem: Analysis and Potential Solutions. International Journal of Computer Science Issues (IJCSI), 14(6), 43-51.
Maimon, Oded and Rokach, L. (2005). Data mining and knowledge discovery handbook. Journal of Experimental Psychology: General (Vol. 136). Springer. Retrieved from https://www.springer.com/gp/book/9780387254654 .
Martins, M. P. G., Miguéis, V. L., Fonseca, D. S. B., & Alves, A. (2019). A data mining approach for predicting academic success – A case study , (pp. 45–56). Cham: Springer.
Massaro, A., Maritati, V., & Galiano, A. (2018). Data mining model performance of sales predictive algorithms based on Rapidminer workflows. International Journal of Computer Science & Information Technology , 10 (3), 39–56.
Mayhew, M. J., & Simonoff, J. S. (2015). Non-white, no more: Effect coding as an alternative to dummy coding with implications for higher education researchers. Journal of College Student Development , 56 (2), 170–175.
McCarthy, R. V., McCarthy, M. M., Ceccucci, W., & Halawi, L. (2019). Introduction to Predictive Analytics. In Applying Predictive Analytics. Springer International Publishing. https://doi.org/10.1007/978-3-030-14038-0 .
Book Google Scholar
Mesarić, J., & Šebalj, D. (2016). Decision trees for predicting the academic success of students. Croatian Operational Research Review , 7 (2), 367–388.
Mohamed, M. H., & Waguih, H. M. (2017). Early prediction of student success using a data mining classification technique. International Journal of Science and Research , 6 (10), 126–131.
Moscoso-Zea, O., Andres-Sampedro, & Lujan-Mora, S. (2016). Datawarehouse design for educational data mining. In 2016 15th International Conference on Information Technology Based Higher Education and Training (ITHET) , (pp. 1–6).
Mueen, A., Zafar, B., & Manzoor, U. (2016). Modeling and predicting students’ academic performance using data mining techniques. International Journal of Modern Education and Computer Science , 8 (11), 36–42.
“National Commission for Academic Accreditation & Assessment Standards for Quality Assurance and Accreditation of Higher Education Institutions,” 2015.
Nisbet, R., Elder, J. F. (John F., & Miner, G. (2009). Handbook of statistical analysis and data mining applications. Academic Press/Elsevier. Retrieved from https://www.elsevier.com/books/handbook-of-statistical-analysis-and-data-mining-applications/nisbet/978-0-12-416632-5 .
Osborne, J. (2002). Notes on the Use of Data Transformation. Practical Assessment, Research, and Evaluation, 8(6), 1–7.
Oshodi, O. S., Aluko, R. O., Daniel, E. I., Aigbavboa, C. O., & Abisuga, A. O. (2018). Towards reliable prediction of academic performance of architecture students using data mining techniques. Journal of Engineering, Design and Technology , 16(3), 385–397.
P. Institute for the S. of L. and E. Langley (1994). Selection of Relevant Features in Machine Learning. In Proceedings of the AAAI Fall symposium on relevance , (pp. 140–144).
Parker, J. D., Hogan, M. J., Eastabrook, J. M., Oke, A., & Wood, L. M. (2006). Emotional intelligence and student retention: Predicting the successful transition from high school to university. Personality and Individual differences , 41 (7), 1329–1336.
Parker, J. D. A., Summerfeldt, L. J., Hogan, M. J., & Majeski, S. A. (2004). Emotional intelligence and academic success: Examining the transition from high school to university. Personality and individual differences , 36 (1), 163–172.
Patro, S. G. K., & Sahu, K. K. (2015). Normalization: A preprocessing stage. International Advanced Research Journal in Science, Engineering and Technology, 2(3), 20–22.
Pelckmans, K., De Brabanter, J., Suykens, J. A. K., & De Moor, B. (2005). Handling missing values in support vector machine classifiers. Neural Networks , 18 (5–6), 684–692.
Peng, Y., Kou, G., Shi, Y., & Chen, Z. (2008). A descriptive framework for the field of data mining and knowledge discovery. International Journal of Information Technology and Decision Making , 7 (4), 639–682.
Pérez, B., Castellanos, C., & Correal, D. (2018). Predicting student drop-out rates using data mining techniques: A case study , (pp. 111–125). Cham: Springer.
Pérez, J., Iturbide, E., Olivares, V., Hidalgo, M., Almanza, N., & Martínez, A. (2015). A data preparation methodology in data mining applied to mortality population databases. Advances in Intelligent Systems and Computing , 353 , 1173–1182.
Pittman, K. (2008). Comparison of Data Mining Techniques used to Predict Student Retention ,” ProQuest Diss. Publ, (vol. 3297573).
Putpuek, N., Rojanaprasert, N., Atchariyachanvanich, K., & Thamrongthanyawong, T. (2018). Comparative Study of Prediction Models for Final GPA Score : A Case Study of Rajabhat Rajanagarindra University. In 2018 IEEE/ACIS 17th International Conference on Computer and Information Science , (pp. 92–97).
Pyle, D., Editor, S., & Cerra, D. D. (1999). Data preparation for data mining. Applied Artificial Intelligence , 17 (5), 375–381.
Qazi, N., & Raza, K. (2012). Effect of Feature Selection, SMOTE and under Sampling on Class Imbalance Classification. In 2012 UKSim 14th International Conference on Computer Modelling and Simulation , (pp. 145–150).
Quinlan, J. R. (2014). C4. 5: programs for machine learning. Elsevier. Retrieved from https://www.elsevier.com/books/c45/quinlan/978-0-08-050058-4 .
Richard-Eaglin, A. (2017). Predicting student success in nurse practitioner programs. Journal of the American Association of Nurse Practitioners , 29 (10), 600–605.
Romero, C., & Ventura, S. (2010). Educational data mining: A review of the state of the art. IEEE Transactions on Systems, Man, and Cybernetics, Part C (Applications and Reviews) , 40 (6), 601–618.
Ruano, M. V., Ribes, J., Sin, G., Seco, A., & Ferrer, J. (2010). A systematic approach for fine-tuning of fuzzy controllers applied to WWTPs. Environmental Modelling & Software , 25 (5), 670–676.
Salman, I., & Vomlel, J. (2017). A machine learning method for incomplete and imbalanced medical data .
Sarala, V., & Krishnaiah, J. (2015). Empirical study of data mining techniques in education system. International Journal of Advances in Computer Science and Technology (IJACST) , 4(1), 15–21.
Schumacker, R. (2012). Predicting Student Graduation in Higher Education Using Data Mining Models: a Comparison. University of Alabama Libraries. Retrieved from https://ir.ua.edu/bitstream/handle/123456789/1395/file_1.pdf?sequence=1&isAllowed=y .
Shahiri, A. M., Husain, W., & Rashid, N. A. (2015). A review on predicting Student’s performance using data mining techniques. Procedia Computer Science , 72 , 414–422.
Shalabi, L., Shaaban, Z., & Kasasbeh, B. (2006). Data mining: A preprocessing engine. Journal of Computer Science , 2(9), 735–739.
Siguenza-Guzman, L., Saquicela, V., Avila-Ordóñez, E., Vandewalle, J., & Cattrysse, D. (2015). Literature review of data mining applications in academic libraries. Journal of Academic of Librarianship , 41 (4), 499–510.
“Simple Methods to deal with Categorical Variables in Predictive Modeling.” n.d. [Online]. Available: https://www.analyticsvidhya.com/blog/2015/11/easy-methods-deal-categorical-variables-predictive-modeling/ . Accessed 4 July 2019.
Singh, W., & Kaur, P. (2016). Comparative Analysis of Classification Techniques for Predicting Computer Engineering Students’ Academic Performance. International Journal of Advanced Research in Computer Science , 7(6), 31–36.
M. Sivasakthi, “Classification and Prediction based Data Mining Algorithms to Predict Students ’ Introductory programming Performance,” Icici, 0–4, 2017.
Sumathi, S., & Sivanandam, S. N. (2006). Introduction to data mining and its applications. Springer. Retrieved from https://www.springer.com/gp/book/9783540343509 .
Umadevi, S., & Marseline, K. S. J. (2017). A survey on data mining classification algorithms. In 2017 International Conference on Signal Processing and Communication (ICSPC) , (pp. 264–268).
“Why One-Hot Encode Data in Machine Learning?” n.d. [Online]. Available: https://machinelearningmastery.com/why-one-hot-encode-data-in-machine-learning/ . Accessed 4 July 2019.
Willems, J., Coertjens, L., Tambuyzer, B., & Donche, V. (2019). Identifying science students at risk in the first year of higher education: the incremental value of non-cognitive variables in predicting early academic achievement. European Journal of Psychology of Education , 34(4), 847–872.
Witten, I. H., Frank, E., Hall, M. A., & Pal, C. J. (2016). Data Mining: Practical Machine Learning Tools and Techniques. Data Mining: Practical Machine Learning Tools and Techniques (3rd ed.). Elsevier Inc. https://doi.org/10.1016/c2009-0-19715-5 .
Wu, X., et al. (2008). Top 10 algorithms in data mining. Knowledge and Information Systems , 14 (1), 1–37.
Xing, W. (2019). Exploring the influences of MOOC design features on student performance and persistence. Distance Education , 40 (1), 98–113.
Yassein, N. A., Helali, R. G. M., & Mohomad, S. B. (2017). Information Technology & Software Engineering Predicting Student Academic Performance in KSA using Data Mining Techniques. Journal of Information Technology and Software Engineering , 7(5), 1–5.
York, T. T., Gibson, C., & Rankin, S. (2015). Defining and Measuring Academic Success. Practical Assessment, Research & Evaluation , 20 , 5.
Zawacki-Richter, O., Marín, V. I., Bond, M., & Gouverneur, F. (2019). Systematic review of research on artificial intelligence applications in higher education – where are the educators? International Journal of Educational Technology in Higher Education , 16(1), 16–39 Springer Netherlands.
Zhang, L., Niu, D., Li, Y., & Zhang, Z. (2018). A Survey on Privacy Preserving Association Rule Mining. In 2018 5th International Conference on Information Science and Control Engineering (ICISCE) , (pp. 93–97).
Download references
Acknowledgments
Not applicable .
Author information
Authors and affiliations.
Department of Computer Science, College of Sciences and Humanities, Imam Abdulrahman Bin Faisal University, 12020, Jubail, 31961, Saudi Arabia
Eyman Alyahyan
Department of Computer Science, College of Computer Science and Information Technology, Imam Abdulrahman Bin Faisal University, 2435, Dammam, 31441, Saudi Arabia
Dilek Düştegör
You can also search for this author in PubMed Google Scholar
Contributions
This study is part of EA’s MS studies requirements under the supervision of DD. EA carried out the literature review, while DD is responsible of the conceptualization of the paper. EA prepared an initial draft of the manuscript, that DD thoroughly re-organized and corrected. Both authors read and approved the final manuscript.
Corresponding author
Correspondence to Dilek Düştegör .
Ethics declarations
Competing interests.
The authors declare that they have no competing interests.
Additional information
Publisher’s note.
Springer Nature remains neutral with regard to jurisdictional claims in published maps and institutional affiliations.
Rights and permissions
Open Access This article is distributed under the terms of the Creative Commons Attribution 4.0 International License ( http://creativecommons.org/licenses/by/4.0/ ), which permits unrestricted use, distribution, and reproduction in any medium, provided you give appropriate credit to the original author(s) and the source, provide a link to the Creative Commons license, and indicate if changes were made.
Reprints and permissions
About this article
Cite this article.
Alyahyan, E., Düştegör, D. Predicting academic success in higher education: literature review and best practices. Int J Educ Technol High Educ 17 , 3 (2020). https://doi.org/10.1186/s41239-020-0177-7
Download citation
Received : 09 October 2019
Accepted : 21 January 2020
Published : 10 February 2020
DOI : https://doi.org/10.1186/s41239-020-0177-7
Share this article
Anyone you share the following link with will be able to read this content:
Sorry, a shareable link is not currently available for this article.
Provided by the Springer Nature SharedIt content-sharing initiative
- Higher education
- Student success

An official website of the United States government
The .gov means it’s official. Federal government websites often end in .gov or .mil. Before sharing sensitive information, make sure you’re on a federal government site.
The site is secure. The https:// ensures that you are connecting to the official website and that any information you provide is encrypted and transmitted securely.
- Publications
- Account settings
Preview improvements coming to the PMC website in October 2024. Learn More or Try it out now .
- Advanced Search
- Journal List
- CBE Life Sci Educ
- v.21(2); Summer 2022
Reframing Educational Outcomes: Moving beyond Achievement Gaps
Sarita y. shukla.
† School of Educational Studies, University of Washington, Bothell, Bothell, WA 98011-8246
Elli J. Theobald
‡ Department of Biology, University of Washington, Seattle, Seattle, WA 98195
Joel K. Abraham
§ Department of Biological Science, California State University–Fullerton, Fullerton, CA 92831
Rebecca M. Price
ǁ School of Interdisciplinary Arts & Sciences, University of Washington, Bothell, Bothell, WA 98011-8246
The term “achievement gap” has a negative and racialized history, and using the term reinforces a deficit mindset that is ingrained in U.S. educational systems. In this essay, we review the literature that demonstrates why “achievement gap” reflects deficit thinking. We explain why biology education researchers should avoid using the phrase and also caution that changing vocabulary alone will not suffice. Instead, we suggest that researchers explicitly apply frameworks that are supportive, name racially systemic inequities and embrace student identity. We review four such frameworks—opportunity gaps, educational debt, community cultural wealth, and ethics of care—and reinterpret salient examples from biology education research as an example of each framework. Although not exhaustive, these descriptions form a starting place for biology education researchers to explicitly name systems-level and asset-based frameworks as they work to end educational inequities.
INTRODUCTION
Inequities plague educational systems in the United States, from pre-K through graduate school. Many of these inequities exist along racial, gender, and socioeconomic lines ( Kozol, 2005 ; Sadker et al. , 2009 ), and they impact the educational outcomes of students. For decades, education research has focused on comparisons of these educational outcomes, particularly with respect to test scores of students across racial and ethnic identities. The persistent differences in these test scores or other outcomes are often referred to as “achievement gaps,” which in turn serve as the basis for numerous educational policy and structural changes ( Carey, 2014 ).
A recent essay in CBE—Life Sciences Education ( LSE ) questioned narrowly defining “success” in educational settings ( Weatherton and Schussler, 2021 ). The authors posit that success must be defined and contextualized, and they asked the community to recognize the racial undercurrents associated with defining success as limited to high test scores and grade point averages (GPAs; Weatherton and Schussler, 2021 ). In this essay, we make a complementary point. We contend that the term “achievement gap” is misaligned with the intent and focus of recent biology education research. We base this realization on the fact that the term “achievement gap” can have a deeper meaning than documenting a difference among otherwise equal groups ( Kendi, 2019 ; Gouvea, 2021 ). It triggers deficit thinking ( Quinn, 2020 ); unnecessarily centers middle and upper class, White, male students as the norm ( Milner, 2012 ); and downplays the impact of structural inequities ( Ladson-Billings, 2006 ; Carter and Welner, 2013 ).
This essay unpacks the negative consequences of using the term “achievement gap” when comparing student learning across different racial groups. We advocate for abandoning the term. Similarly, we suggest that, in addition to changing our terminology, biology education researchers can explicitly apply theoretical frameworks that are more appropriate for interrogating inequities among educational outcomes across students from different demographics. We emphasize that the idea that a simple “find and replace,” swapping out the term “achievement gap” for other phrases, is not sufficient.
In the heart of this essay, we review some of these systems-level and asset-based frameworks for research that explores differences in academic performance ( Figure 1 ): opportunity gaps ( Carter and Welner, 2013 ), educational debt ( Ladson-Billings, 2006 ), community cultural wealth ( Yosso, 2005 ), and ethics of care ( Noddings, 1988 ). Within each of these frameworks, we review examples of biology education literature that we believe rely on them, explicitly or implicitly. We conclude by reiterating the need for education researchers to name explicitly the systems-level and asset-based frameworks used in future research.

Research frameworks highlighted in the essay. The column in gray summarizes deficit-based frameworks that focus on achievement gaps. The middle column (in gold) includes examples of systems-based frameworks that acknowledge that student learning is associated with society-wide habits. The rightmost columns (in peach) include examples of asset-based models that associate student learning with students’ strengths. The columns are not mutually exclusive, in that studies can draw from multiple frameworks simultaneously or sequentially.
We will use the phrase “students from historically or currently marginalized groups” to describe the students who have been and still are furthest from the center of educational justice. However, when discussing work of other researchers, we will use the terminology they use in their papers. Our conceptualization of this phrase matches, as near as we can tell, Asai’s phrase “PEERs—persons excluded for their ethnicity or race” ( Asai, 2020 , p. 754). We also choose to capitalize “White” to acknowledge that people in this category have a visible racial identity ( Painter, 2020 ).
Positionality
Our positionalities—our unique life experiences and identities—mediate our understanding of the world ( Takacs, 2003 ). What we see as salient in our research situation arises from our own life experiences. Choices in our research, including the types of data we collect and how we clean the data and prepare it for analysis, adopt analytical tools, and make sense of these analyses are important decision points that affect study results and our findings ( Huntington-Klein et al. , 2021 ). We recognize that it is impossible to be free of bias ( Noble, 2018 ; Obermeyer et al. , 2019 ). Therefore, we put forth our positionality to acknowledge the lenses through which we make decisions as researchers and to forefront the impact of our identities on our research. Still, the breadth of our experiences cannot be described fully in a few sentences.
The four authors of this essay have unique and complementary life experiences that contribute to the sense-making presented in this essay. S.Y.S. has been teaching since 2003 and teaching in higher education since 2012. She is a South Asian immigrant to the United States, and a cisgender woman. E.J.T. has taught middle school, high school, and college science since 2006. She is a cisgender White woman. J.K.A. is a cisgender Black mixed-race man who comes from a family of relatively recent immigrants with different educational paths. He has worked in formal and informal education since 2000. R.M.P. is a cisgender Jewish, White woman, and she has been teaching college since 2006. We represent a team of people who explicitly acknowledge that our experiences influence the lenses through which we work. Our guiding principles are 1) progress over perfection, 2) continual reflection and self-improvement, and 3) deep care for students. These principles guide our research and teaching, impacting our interactions with colleagues (faculty and staff) as well as students. Ultimately, these principles motivate us to make ourselves aware of, reflect on, and learn from our mistakes.
Simply Changing Vocabulary Does Not Suffice
The term “achievement gap” is used in research that examines differences in achievement—commonly defined as differences in test scores—across students from different demographic groups ( Coleman et al. , 1966 ). Some studies replace “achievement gap” with “score gap” (e.g., Jencks and Phillips, 2006 ), because it defines the type of achievement under consideration; others use “opportunity gap,” because it emphasizes differences in opportunities students have had throughout their educational history (e.g., Carter and Welner, 2013 ; more on opportunity gaps later). The shift for which we advocate, however, does not reside only with terminology. Instead, we call for a deeper shift of using research frameworks that acknowledge and respect students’ histories and empower them now.
The underlying framework in research that uses “achievement gap” or even “score gap” may not be immediately apparent. Take for example two studies that both use the seemingly benign term, “score gap.” A close read indicates that one study attributed the difference in test scores between Black and White students to deficient “culture and child-rearing practices” ( Farkas, 2004 , p. 18). Thus, even though the researcher uses what can be considered to be more neutral terminology, the phrase in this context represents deficit thinking and blame. On the other hand, another study uses the term “score gap” to explore differences that have been historically studied through cultures of poverty, genetic, and familial backgrounds ( Jencks and Phillips, 2006 ). While these researchers discuss the Black–White score gap, they present evidence that examines this phenomenon with nuanced constructs, such as stereotype threat ( Steele, 2011 ) and resources available. These authors also mention ways to reduce score gaps, such as smaller class sizes and high teacher expectations ( Jencks and Phillips, 2006 ).
Some researchers who use the phrase “achievement gap” explicitly avoid deficit thinking and instead embrace an asset-based framework. Jordt et al. (2017) address systemic racism, just as Jencks and Phillips (2006) do. Specifically, Jordt et al. (2017) identified an intervention that affirmed student values that might also be a potential tool for increasing underrepresented minority (URM) student exam scores in college-level introductory science courses. The researchers found that this intervention produced a 4.2% increase in exam performance for male URM students and a 2.2% increase for female URM students. Thus, while they use “achievement gap” throughout the paper to refer to racial and gender differences in exam scores, the study focused on ways to support URM student success.
In pursuit of improved language and clarity of intent, the term “achievement gap” should be replaced to reflect the research framework used to interrogate educational outcomes within and across demographic groups.
DEFICIT THINKING
Deficit thinking describes a mindset, or research framework, in which differences in outcomes between members of different groups, generally a politically and economically dominant group and an oppressed group, are attributed to a quality that is lacking in the biology, culture, or mindset of the oppressed group ( Valencia, 1997 ). Deficit thinking has pervaded public and academic discourse about the education of students from different races and ethnicities in the United States for centuries ( Menchaca, 1997 ).
Tenacious deficit-based explanations blame students from historically or currently marginalized groups for lower educational attainment. These falsities include biological inferiority due to brain size or structure ( Menchaca, 1997 ), negative cultural attributes such as inferior language acquisition ( Dudley-Marling, 2007 ), and accumulated deficits due to a “culture of poverty” ( Pearl, 1997 ; Gorski, 2016 ). More recently, lower achievement has been attributed to a lack of “grit” ( Ris, 2015 ) or the propensity for a “fixed” mindset ( Gorski, 2016 ; Tewell, 2020 ). While ideas around grit and mindset have demonstrable value in certain circumstances (e.g., Hacisalihoglu et al. , 2020 ), they fall short as primary explanations for differences in educational outcomes, because they focus attention on perceived deficits of students while providing little information about structural influences on failure and success, including how we define those constructs ( Harper, 2010 ; Gorski, 2016 ). In other words, deficit models often posit students as the people responsible for improving their own educational outcomes ( Figure 1 ).
Deficit thinking, regardless of intent, blames individuals, their families, their schools, or their greater communities for the consequences of societal inequities ( Yosso, 2006 ; Figure 1 ). This blame ignores the historic and structural drivers of inequity in our society, placing demands on members of underserved groups to adapt to unfair systems ( Valencia, 1997 ). A well-documented example of structural inequity is the consistent underresourcing of public schools that serve primarily students of color and children from lower socioeconomic backgrounds ( Darling-Hammond, 2013 ; Rothstein, 2013 ). Because learning is heavily influenced by factors outside the school environment, such as food security, trauma, and health ( Rothstein, 2013 ), schools themselves reflect gross disparities in resourcing based on historic discrimination ( Darling-Hammond, 2013 ). Deficit thinking focuses on student or cultural characteristics to explain performance differences and tends to overlook or minimize the impacts of systemic disparities. Deficit thinking also strengthens the narrative around student groups in terms of shortcomings, reinforces negative stereotypes, and ignores successes or drivers of success in those same groups ( Harper, 2015 ).
Achievement Gaps
The term “achievement gap” has historically described the difference in scores attained by students from racial and ethnic minority groups compared with White students on standardized tests or course exams ( Coleman et al. , 1966 ). As students from other historically or currently marginalized groups, such as female or first-generation students, are increasingly centered in research, the term is now used more broadly to compare any student population to White, middle and upper class, men ( Harper, 2010 ; Milner, 2012 ). Using White men as the basis for comparison comes at the expense of students from other groups ( Harper, 2010 ; Milner, 2012 ). Basing comparisons on the cultural perspectives of a single dominant group leads to “differences” being interpreted as “deficits,” which risks dehumanizing people in the marginalized groups ( Dinishak, 2016 ). Furthermore, centering White, wealthy, male performance means that even students from groups that tend to have higher test scores, like Asian-American students, risk dehumanization as “model minorities” or “just good at math” ( Shah, 2019 ).
Many researchers have highlighted the fact that the term “achievement gap” is a part of broader deficit-thinking models and rooted in racial hierarchy ( Ladson-Billings, 2006 ; Gutiérrez, 2008 ; Martin, 2009 ; Milner, 2012 ; Kendi, 2019 ). Focusing on achievement gaps emphasizes between-group differences over within-group differences ( Young et al. , 2017 ), reifies sociopolitical and historical groupings of people ( Martin, 2009 ), and minimizes attention to structural inequalities in education ( Ladson-Billings, 2006 ; Alliance to Reclaim Our Schools, 2018 ). Gutiérrez (2008) names this obsession with achievement gaps as a “gap-gazing fetish” that draws attention away from finding solutions that promote equitable learning ( Gutiérrez, 2008 ). Under a deficit-thinking model, achievement gaps are viewed as the primary problem, rather than a symptom of the problem ( Gutiérrez, 2008 ), and for decades they have been attributed to different characteristics of the demographics being compared ( Valencia, 1997 ). As such, proposed solutions tend to be couched in terms of remediation for students ( Figure 1 ).
Ignoring the social context of students’ education necessarily limits inferences that can be drawn about their success. Limiting measures of educational success, also conceptualized as achievement, to performance on exams or overall college GPA, often leaves out consideration of other potential data sources ( Weatherton and Schussler, 2021 ; Figure 2 ). This narrow perspective tends to perpetuate the systems of power and privilege that are already in place ( Gutiérrez, 2008 ). The biology education research community can instead broaden its sense of success to recognize the underlying historical and current contexts and the intersections of identities (e.g., racial, gender, socioeconomic) that contribute to those differences ( Weatherton and Schussler, 2021 ).

A selection of potential data sources that could inform researchers about within- and between-group differences in educational outcomes. This list does not encompass the full range of possible data sources, nor does it imply a hierarchy to the data. Instead, it reflects some of the diversity of quantitative and qualitative data that are directly linked to student outcomes and that are used under multiple research frameworks.
In biology education research, many papers still use the language of “achievement gap,” even in instances when researchers explicitly or implicitly use other nondeficit frameworks. While some may argue that this language merely describes a pattern, its origin and history is explicitly and inextricably linked to deficit-thinking models ( Gutiérrez, 2008 ; Milner, 2012 ). Thus, we join others in the choice to abandon the term “achievement gap” in favor of language—and frameworks—that align better to the goals of our research and to avoid the limitations and harm that can arise through its use.
Example: Focusing on Achievement Gaps Can Reinforce Racial Stereotypes
Messages of perpetual underachievement can inadvertently reinforce negative stereotypes. For example, Quinn (2020) demonstrated that, when participants watched a 2-minute video of a newscast using the term “achievement gap,” they disproportionately underpredicted the graduation rate of Black students relative to White students, even more so than participants in a control group who watched a counter-stereotypical video. They also scored significantly higher on an instrument measuring bias. Because bias is dynamic and affected by the environment, Quinn concludes that the video discussing the achievement gap likely heightened the bias of the participants ( Quinn, 2020 ).
Education researchers, just like the participants in Quinn’s (2020) study, inadvertently carry implicit bias against students from the different groups they study, and those biases can shift depending on context. Quinn (2020) demonstrates that just using the term “achievement gap” can reinforce the pervasive racial hierarchy that places Black students at the bottom. Researchers, without intending to, can be complicit in a system of White privilege and power if the language and frameworks underlying their study design, data collection, and/or data interpretation are aligned with bias and stereotype. If the goal is to dismantle inequities in our educational systems and research on those systems, the biology education research community must consider the historical and social weight of its literature to address racism head on, as progressive articles have been doing (e.g., Eddy and Hogan, 2014 ; Canning et al. , 2019 ; Theobald et al. , 2020 ).
SYSTEMS-LEVEL FRAMEWORKS
To move away from the achievement gap discourse—because of the history of the term, the perceived blame toward individual students, as well as the deficit thinking the term may imbue and provoke—we highlight some of the other frameworks for understanding student outcomes. We conclude discussion of each framework with an example from education research that can be reinterpreted within it, keeping in mind that multiple frameworks can be applied to different studies. We acknowledge two caveats about these reinterpretations: first, we are adding another layer of interpretation to the original studies, and we cannot claim that the original authors agree with these interpretations; second, each example could be interpreted through multiple frameworks, especially because these frameworks overlap ( Figure 1 ).
In this section, we begin at the systems level by examining opportunity gaps and educational debt. Rather than blaming students or their cultures for deficits in performance, these systems-level perspectives name white supremacy and the concomitant policies that maintain power imbalances as the cause of disparate student experiences.
Opportunity Gaps
The framework of opportunity gaps shifts the onus of differential student performance away from individual deficiencies and assigns solutions to actions that address systemic racism ( Milner, 2012 ; Figure 1 ). Specifically, opportunity gaps embody the difference in performance between students from historically and currently marginalized groups and middle and upper class, White, male students, with primary emphasis on opportunities that students have or have not had, rather than on their current performance (i.e., achievement) in a class ( Milner, 2012 ). Compared with deficit models, the focus shifts from assigning responsibility for the gap from the individual to society ( Figure 1 ).
Some researchers explore opportunity gaps by discussing the structural challenges that students from historically and currently marginalized groups have been facing (e.g., Rothstein, 2013 ). For example, poor funding in K–12 schools leads to inconsistent, poorly qualified, and poorly compensated teachers; few and outdated textbooks ( Darling-Hammond, 2013 ); limited field trips; a lack of extracurricular resources ( Rothstein, 2013 ); and inadequately supplied and cleaned bathrooms ( Darling-Hammond, 2013 ). Additional structural challenges that occur outside school buildings, but impact learning, include poor health and lack of medical care, food and housing insecurity, lead poisoning and iron deficiency, asthma, and depression ( Rothstein, 2013 ).
While the literature about opportunity gaps focuses more on K–12 than higher education ( Carter and Welner, 2013 ), college instructors can exacerbate opportunity gaps by biasing who has privilege (i.e., opportunities) in their classrooms. For example, some biology education literature focuses on how instructors’ implicit biases impact our students, such as by unconsciously elevating the status of males in the classroom ( Eddy et al. , 2014 ; Grunspan et al. , 2016 ).
Example: CUREs Can Prevent Opportunity Gaps.
Course-based undergraduate research experiences (CUREs) are one way to prevent opportunity gaps (e.g., Bangera and Brownell, 2014 ; CUREnet, n.d. ). Specifically, we interpret the suggestions that Bangera and Brownell (2014) make about building CUREs as a way to recognize that some students have the opportunity to participate in undergraduate research experiences while others do not. For example, students who access extracurricular research opportunities are likely relatively comfortable talking to faculty and, in many cases, have the financial resources to pursue unpaid laboratory positions ( Bangera and Brownell, 2014 ). More broadly, when research experiences occur outside the curriculum, they privilege students who know how to pursue and gain access to them. However, CUREs institutionalize the opportunity to conduct research, so that every student benefits from conducting research while pursuing an undergraduate degree.
Educational Debt
Ladson-Billings (2006) submits that American society has an educational debt, rather than an educational deficit. This framework shifts the work of finding solutions to educational inequities away from individuals and onto systems ( Figure 1 ). The metaphor is economic: A deficit refers to current mismanagement of funds, but a debt is the systematic accumulation of mismanagement over time. Therefore, differences in student performances are framed by a history that reflects amoral, systemic, sociopolitical, and economic inequities. Ladson-Billing ( 2006 ) suggests that focusing on debts highlights injustices that Black, Latina/o, and recent immigrant students have incurred: Focusing on student achievement in the absence of a discussion of past injustices does not redress the ways in which students and their parents have been denied access to educational opportunities, nor does it redress the ways in which structural and institutional racism dictate differences in performance. This approach begins by acknowledging the structural and institutional barriers to achievement in order to dismantle existing inequities. This reframing helps set the scope of the problem and identify a more accurate and just lens through which we make sense of the problem ( Cho et al. , 2013 ).
Example: NSF Supports Historically Black Colleges and Universities.
One program that aims to repay educational debt is the NSF’s Historically Black Colleges and Universities Undergraduate Program ( National Science Foundation, 2020 ). This grant program supports HBCUs in ways intended to have far-reaching consequences; among the multiple strands are opportunities to begin research projects and to fund specific, short-term goals to improve science, technology, engineering, and mathematics (STEM) education. Another strand establishes broadening participation research centers. Financial resources aimed specifically at historically Black colleges and universities, and other minority-serving institutions acknowledge and address the stresses that marginalized students experience at primarily White campuses. Supporting HBCUs in turn supports students. As former NSF program officer Claudia Rankins reports:
From my own (yet to be published) research, a participant described the HBCU where he studied physics as providing a “dome of security and safety.” In contrast, he recounted that when he attended a predominantly White institution, he constantly needed to be guarded and employ “his body sense,” an act that made him tense, defensive, and unable to listen. ( Rankins, 2019 , p. 50)
Example: Institutions Can Repay Educational Debt.
Institutions can repay educational debt by ensuring that their students have the resources and support structures necessary to succeed. The Biology Scholars Program at the University of California, Berkeley, is a prime example ( Matsui et al. , 2003 ; Estrada et al. , 2019 ). This program, begun in 1992 ( Matsui et al. , 2003 ) and still going strong ( Berkeley Biology Scholars Program, n.d. ), creates physical and psychological spaces that support learning: a study space and study groups, paid research experiences, and thoughtful mentoring. The students recruited to the program are from first-generation, low-socioeconomic status backgrounds and from groups that are historically underrepresented. When the students enter college, they have lower GPAs and Scholastic Aptitude Test scores than their counterparts with the same demographic profile who are not in the program. And yet, when they graduate, students in the Biology Scholars Program have higher GPAs and higher retention in biology majors than their counterparts ( Matsui et al. , 2003 ), perhaps because of the extended social support they receive from peers ( Estrada et al. , 2021 ). Moreover, students in this program report lower levels of stress and a greater sense of well-being ( Estrada et al. , 2019 ).
ASSET-BASED FRAMEWORKS
In this section, we continue to explore frameworks that move away from the achievement gap discourse, now focusing on models that build from students’ strengths. We have chosen two frameworks whose implications seem particularly relevant to and coincident with anti-racist research in biology education: community cultural wealth ( Yosso, 2005 ) and ethics of care ( Noddings, 1988 ). As before, we reinterpret articles from the education literature to illustrate these frameworks, and we once again include the caveats that we extend beyond the authors’ original interpretations and that other frameworks could also be used to reinterpret the examples.
Community Cultural Wealth
One asset-based way to frame student outcomes is to begin with the strengths that people from different demographic groups hold ( Yosso, 2005 ). Rather than focusing on racism, this approach focuses on community cultural wealth. The premise is that everyone can contribute a wealth of knowledge and approaches from their own cultures ( Yosso, 2005 ).
Community cultural wealth begins with critical race theory (CRT; Yosso, 2005 ). CRT illuminates the impact of race and racism embedded in all aspects of life within U.S. society ( Omi and Winant, 2014 ). CRT acknowledges that racism is interconnected with the founding of the United States. Race is viewed in tandem with intersecting identities that oppose dominant ones, and the constructs of CRT emerge by attending to the experiences of people from communities of color ( Yosso, 2005 ). Therefore, the experiences of students of color are central to transformative education that addresses the overrepresentation of White philosophies. CRT calls on research to validate and center these perspectives to develop a critical understanding about racism.
Community cultural wealth builds on these ideas by viewing communities of color as a source of students’ strength ( Yosso, 2005 ). The purpose of schooling is to build on the strengths that students have when they arrive, rather than to treat students as voids that need to be filled: students’ cultural wealth must be acknowledged, affirmed, and amplified through their education. This approach is consistent with those working to decolonize scientific knowledge (e.g., Howard and Kern, 2019 ).
Example: Community Cultural Wealth Can Improve Mentoring.
Thompson and Jensen-Ryan (2018) offer advice to mentors about how to use cultural wealth to mentor undergraduate students in research. They identify the forms of scientific cultural capital that research mentors typically value, finding that these aspects of a scientific identity are closely associated with majority culture. They challenge mentors to broaden the forms of recognizable capital. For example, members of the faculty can actively recruit students into their labs from programs aimed to promote the diversity of scientists, rather than insisting that students approach them with their interest to work in the lab ( Thompson and Jensen-Ryan, 2018 ). They can recognize that undergraduate students may not express an interest in a research career–especially initially—but that research experience is still formative. They can recognize that students who are strong mentors to their peers are valuable members of a research team and that this skill is a form of scientific capital. They can value the diverse backgrounds of students in their labs, rather than insisting that they come from families that have prioritized scientific thinking and research. In sum, the gaps that Thompson and Jensen-Ryan (2018) identify are in research mentors’ attitudes, rather than in student performance.
Assets can also be developed in the classroom. We interpret Parnes et al. ’s (2020) analysis of the Connected Scholars program as stemming from community cultural wealth. The Connected Scholars program normalized help-seeking and increased the help network available to first-generation college students, 90% of whom were racial or ethnic minorities, in a 6-week summer program that bridged students from high school to college. First-generation college students were provided explicit instruction on how to sustain these two types of support. The Connected Scholars intervention promoted help-seeking behaviors and seemed to mediate higher GPAs. Additionally, students in the intervention reported through a survey that they had better relationships with their instructors than students in the control group ( Parnes et al. , 2020 ). In other words, cultural wealth can be amplified in college for first-generation students (see also the Biology Scholars Program, discussed in the Opportunity Gaps section; Matsui et al. , 2003 ; Estrada et al. , 2019 ).
Ethics of Care
As a framework, ethics of care complements community cultural wealth, in that both are asset-based. A key difference is that community cultural wealth focuses on the assets that students bring, and ethics of care focuses on the assets that an instructor brings to create a classroom of respect and confidence in students.
A foundation of biology education research is that instructors want their students to learn, and it is buttressed by literature concerning students’ emotional well-being. For example, the field considers how students with disabilities experience active learning ( Gin et al. , 2020 ) and how group work promotes collaboration and learning ( Wilson et al. , 2018 ). Studies like these echo the philosophy of ethics of care developed by Noddings (1988) .
The premises of teaching through the ethics of care are that everyone—including students and instructors—has both an innate desire to learn and the capacity to nurture ( Pang et al. , 2000 ). In teaching, these premises form the basis for student–instructor relationships. Nieto and Bode (2012) caution against the oversimplification that caring means being nice: the ethics of care encompasses niceness, in addition to articulating high standards of performance. Instructors must also support and respect students as they meet those standards, especially when students did not recognize that they could meet those goals at the outset. This framework is about nurturing students to accomplish more than they thought possible.
Combining an inclusive culture, for example, through positive instructor talk ( Seidel et al. , 2015 ; Harrison et al. , 2019 ; Seah et al. , 2021 ), growth mindset ( Canning et al. , 2019 ), or increased course structure ( Eddy and Hogan, 2014 ), with evidence-based practices for teaching content ( Freeman et al. , 2014 ; Theobald et al. , 2020 ) has garnered recent attention as a way to create a powerful ethic of care in classrooms. For example, instructor talk, that is, what instructors say in class other than the content they are teaching, addresses student affect. Seidel et al. (2015) and Harrison et al. (2019) analyzed classroom transcripts to identify different categories of instructor talk. While further research can probe the impacts of instructor talk on student outcomes, the idea is consistent with the principles of ethics of care: for example, one category of talk describes the instructor–student relationship as one of respect, fostered through statements such as “People are bringing different pieces of experience and knowledge into this question and I want to kind of value the different kinds of experience and knowledge that you bring in” ( Seidel et al. , 2015 , p. 6). Instructor talk also generates a classroom culture of support and validation for marginalized students and overall builds classroom community ( Ladson-Billings, 2013 ).
Example: Departments Can Implement Care.
Gutiérrez (2000) presents an example of an entire department applying ethics of care to support how African-American students learn math. This study is an ethnography of a particularly successful STEM magnet program in a public high school with a population that is majority African American. In her analysis of the math department, Gutiérrez avoids the phrase “achievement gap,” while also recognizing that people outside the school assume a deficit model when considering the students . Instead, she illustrates how researchers can use an asset-based lens to build from knowledge about differences in performance ( Gutiérrez, 2000 ).
Gutierrez ( 2000 ) examines pedagogy that supports African-American students. She documents how a culture of excellence is developed within a school setting that promotes student achievement. This culture is complex, in that there are multiple layers of support that provide students with repertoires for advancement ( Gutiérrez, 2000 )—the emphasis is on how teachers create an environment where students are both challenged through the curriculum and supported along the way. The teachers in this study have a dynamic conception of their students, and they demonstrate a unified commitment to support the broadest array of students at their school. The institution itself, represented in part through the departmental chair, has values that empower teachers to support students, proactive commitment from teachers to find innovative practices to serve students, and a supportive chairperson.
The math department exhibited a student-centered approach that epitomizes ethics of care. The teachers in the math department rotated through all of the courses and were therefore familiar with the entire curriculum. This knowledge helped them support one another, sharing successful strategies and working to improve the courses. It set up an environment in which they prioritized making decisions collectively. This collaboration led to a sense of togetherness among teachers and a sense of investment in individual students’ successes. As a result, the teachers decided to remove less-challenging courses from the curriculum and replaced them with more advanced courses—against the recommendations of the school district. The chair of the department worked with the faculty to support student learning, consider course assignments, and choose topics for and frequency of faculty meetings. The chair also attended to teachers’ emotional needs, for example, by talking to teachers every day, working with teachers to determine the best strategies for evaluating teaching practices, and enacting a teaching philosophy that valued problem solving over achieving correct answers.
The support that the teachers provided each other coincided with strong support for students. For example, students attended the magnet program because they were interested in science; they notably did not have to take entrance exams or maintain a certain GPA. If students struggled with a subject, they received tutoring. The teachers also invited graduates of the program to come back and visit, keeping the students motivated by showing them success.
Example: Biology Instructors Can Adopt an Ethics of Care.
In much of the research on differential performance in our field, researchers focus on identifying strategies that help students, regardless of their histories, in their learning success. This asset-based approach acknowledges that students start at different places, but also that instructors can implement strategies that support all students in a trajectory toward common learning goals. This argument is often posited in terms of inclusive teaching (e.g., Dewsbury and Brame, 2019 ).
Some papers that measure the effect of inclusive teaching practices may use “gap” language, perhaps as a historical artifact of our discipline. These papers emphasize the just mission to “close the gap”—or, in anti-deficit language, for all students to learn the material and perform well on assessments. For example, Theobald et al. (2020) conducted a meta-analysis of undergraduate STEM classes, drawing on 26 studies of courses reporting failure rates (44,606 students) and 15 studies (9238 students) that reported exam scores. Within these samples, they compared instruction in lecture format with instruction using active-learning strategies. The analysis compared the success of students from minoritized groups using these two teaching strategies and found conclusive evidence of the efficacy of active teaching for underrepresented student success in STEM courses. The powerful implication of this study is that college STEM instructors can mitigate some of the effects of oppression that students have experienced in their lifetime.
In another study demonstrating the philosophy of ethics of care, Canning et al. (2019) found narrower racial disparities in performance in courses taught by instructors who had a growth mindset about their students’ ability to learn, compared with instructors who viewed level of achievement as fixed. In fact, they found that the instructor mindset had a bigger impact on student performance than other faculty characteristics ( Canning et al. , 2019 ). While they focused on the negative consequences of instructors’ fixed mindset, the corollary is that a growth mindset can reflect an ethics of care that both motivates students and generates a positive classroom environment.
The successful instructors will also work to recognize their implicit biases and to ensure that they support a growth mindset for all students, regardless of demographic. This is particularly relevant, because implicit biases have “more to do with associations we’ve absorbed through history and culture than with explicit racial animus” ( Eberhardt, 2019 , p. 160). Realizing how our own socialization may have conditioned us to automatically produce harmful but hidden narratives warrants our attention ( Eberhardt, 2019 ).
MOVING FORWARD
Ladson-Billings (2006) reframed the performance of students from historically and currently marginalized groups from achievement gap to educational debt; this reframing has contributed to a movement to critically examine the term. At the same time, however, the term “achievement gap” has become a catchall used by researchers untethered from its deeper historical context.
Researchers choose words to describe their research that reflect their personal worldviews and research frameworks; in turn, these worldviews and frameworks influence future researchers. Every discipline grapples with terminology, and phrases that were common historically may fall out of use. In some instances, the terms themselves no longer suffice, so a simple “search and replace” may be all that is required to address the issue. The term “achievement gap,” however, is tied to specific frameworks that need to be acknowledged and redressed; it affects how research is designed, how results are interpreted, and what conclusions are drawn. Simply replacing “achievement gap” would not address the undermining nature of deficit-based research frameworks.
Researchers who used the term “achievement gap” may not have intended to use a deficit-thinking framework in their study. In fact, as we have demonstrated with our examples, some powerful articles exist in biology education research that used the term and also implicitly used one of the systems-level or asset-based frameworks we identified.
In these examples, we have reinterpreted the results of primary research with the frameworks we identified. This leads to two points of caution. The first is that we are adding another layer of interpretation, one that the original authors may not have intended. The second is that each example could be interpreted through multiple frameworks, especially because these frameworks overlap ( Figure 1 ). For example, Bangera and Brownell (2014) identify barriers to participating in independent undergraduate research experiences. Course-based undergraduate research experiences (CUREs) offer research opportunities to students who previously could not access them. As discussed earlier, we posited CUREs as an example of a way to reduce opportunity gaps. However, we could also have interpreted the act of implementing a CURE as repaying an educational debt by repairing a form of bias typical within the academy ( Figure 1 ).
Addressing educational inequities requires that biology education researchers quantify differences in performance across demographic groups ( Figure 2 ) and must be done with the utmost care. Disaggregating data is necessary, as is analyzing those data with a just framework that dismantles racial hierarchies and carefully considers the sources of data used to understand those inequities. The frameworks we choose affect our analysis; we must avoid the common trap of assuming that quantitative data and data analysis are free from bias. To illustrate the degree of subjectivity that enters data analysis, Huntington-Klein et al. (2021) found that when seven different researchers received copies of the same data set, each reported different levels of statistical significance, including one researcher who found an effect that was opposite to what the others found. Moving away from analyses based on the phrase “achievement gap” will avoid unintentionally reinforcing the racial bias and better reflect the intention of disaggregating data to quantify differences in performance across demographic groups to actively dismantle persistent educational inequities.
In addition to disaggregating and diversifying data on outcomes ( Figure 2 ), the biology education research community must consider how definitions of success may center White, middle-class ways of knowing and performing ( Weatherton and Schussler, 2021 ). In their recent essay, Weatherton and Schussler (2021) reported that, in articles published in LSE between the years 2015 and 2020, the word “success,” when defined, largely meant high GPAs and exam scores. This narrow definition of success prioritizes scientific content, whereas there are additional admirable goals by which success could be measured ( Figure 2 ; see also Weatherton and Schussler, 2021 and references therein). Moreover, the scientific skills that are valued are Eurocentric, rather embodying a diversity of scientific approaches ( Howard and Kern, 2019 ). In addition to the limitations of narrowly defining success as exam performance, it should be noted that tests themselves are not always fair or equitable across all student populations ( Martinková et al. , 2017 ); success measured in this way should be interpreted with caution, particularly when comparing students across different courses, institutions, or identities.
As we discussed earlier, instructors’ and researchers’ deep beliefs about educational success and achievement necessarily impact their actions. For this reason, we propose that interrogating the frameworks we use is necessary and that such interrogation should acknowledge harm that may have been inflicted. While writing this essay, for example, our understandings of the frameworks underlying our own research, teaching, and other engagements have grown. Much like the research studies we discuss, our intentions, actions, and frameworks can be and have been out of alignment. For example, our own actions with respect to departmental policies, course designs, and program structures have not always reflected the principles to which we subscribe. Although this essay focuses on frameworks in research, we provide a list of some questions that we have asked of ourselves and that could catalyze reflection in all areas of our professional work ( Table 1 ).
A list of questions that individuals or groups could use to adopt frameworks that achieve a more equitable and just educational system
In conclusion, we have presented four ways to frame differences in academic performance across students from different demographic groups that firmly reject deficit-based thinking ( Figure 1 ). The notions of opportunity gaps and educational debt demonstrate how systems thinking can recognize socio-environmental barriers to student learning. Asset-based frameworks that include community cultural wealth and ethics of care can help identify actions that institutions, instructors, and students can take to meet learning goals. We hope that researchers in the field move forward by 1) avoiding, or at least minimizing, deficit thinking; 2) explicitly stating asset-based and systems-level frameworks that celebrate students’ accomplishments and move toward justice; and 3) using language consistent with their frameworks.
Acknowledgments
We thank Starlette Sharp and our external reviewers for helpful feedback on this article. We live and work on the lands of the Kizh/Tongva/Gabrieleño, Duwamish, and Willow (Sammamish) People past, present, and future. We also acknowledge the people whose uncompensated labor built this country, including many of its academic institutions.
- Alliance to Reclaim Our Schools. (2018). Confronting the education debt: We owe billions to Black, Brown and low-income students and their schools (p. 25). Retrieved February 23, 2022, from http://educationdebt.reclaimourschools.org/wp-content/uploads/2018/08/Confronting-the-Education-Debt_FullReport.pdf [ Google Scholar ]
- Asai, D. J. (2020). Race matters . Cell , 181 ( 4 ), 754–757. 10.1016/j.cell.2020.03.044 [ PubMed ] [ CrossRef ] [ Google Scholar ]
- Bangera, G., Brownell, S. E. (2014). Course-based undergraduate research experiences can make scientific research more inclusive . CBE—Life Sciences Education , 13 , 602–606. 10.1187/cbe.14-06-0099 [ PMC free article ] [ PubMed ] [ CrossRef ] [ Google Scholar ]
- Berkeley Biology Scholars Program. (n.d.). Home . Retrieved March 25, 2021, from https://bsp.berkeley.edu/home
- Canning, E. A., Muenks, K., Green, D. J., Murphy, M. C. (2019). STEM faculty who believe ability is fixed have larger racial achievement gaps and inspire less student motivation in their classes . Science Advances , 5 ( 2 ), eaau4734. 10.1126/sciadv.aau4734 [ PMC free article ] [ PubMed ] [ CrossRef ] [ Google Scholar ]
- Carey, R. L. (2014). A cultural analysis of the achievement gap discourse: Challenging the language and labels used in the work of school reform . Urban Education , 49 ( 4 ), 440–468. 10.1177/0042085913507459 [ CrossRef ] [ Google Scholar ]
- Carter, P. L., Welner, K. G. (2013). Closing the opportunity gap: What America must do to give every child an even chance . New York, NY: Oxford University Press. [ Google Scholar ]
- Cho, S., Crenshaw, K. W., McCall, L. (2013). Toward a field of intersectionality studies: Theory, applications, and praxis . Signs: Journal of Women in Culture and Society , 38 , 785–810. [ Google Scholar ]
- Coleman, J. S., Campbell, E. Q., Hobson, C. J., McPartland, J., Weinfeld, F. D., York, R. L. (1966). Equality of educational opportunity . Washington, DC: U.S. Department of Health, Education, and Welfare. [ Google Scholar ]
- CUREnet. (n.d.). Home page . Retrieved May 28, 2021, from https://serc.carleton.edu/curenet/index.html
- Darling-Hammond, L. (2013). Inequality and school resources: What it will take to close the opportunity gap? In Carter, P. L., Welner, K. G. (Eds.), Closing the opportunity gap: What America must do to give every child an even chance (pp. 77–97). : Oxford University Press. [ Google Scholar ]
- Dewsbury, B. M., Brame, C. J. (2019). Inclusive Teaching . CBE—Life Sciences Education , 18 ( 2 ), fe2. 10.1187/cbe.19-01-0021 [ PMC free article ] [ PubMed ] [ CrossRef ] [ Google Scholar ]
- Dinishak, J. (2016). The deficit view and its critics . Disability Studies Quarterly , 36 ( 4 ). 10.1080/1361332052000341006 [ CrossRef ] [ Google Scholar ]
- Dudley-Marling, C. (2007). Return of the deficit . Journal of Educational Controversy , 2 ( 1 ), 14. [ Google Scholar ]
- Eberhardt, J. (2019). Biased: Uncovering the hidden prejudice that shapes what we see, think, and do . New York, NY: Viking. [ Google Scholar ]
- Eddy, S. L., Brownell, S. E., Wenderoth, M. P. (2014). Gender gaps in achievement and participation in multiple introductory biology classrooms . CBE—Life Sciences Education , 13 ( 3 ), 478–492. 10.1187/cbe.13-10-0204 [ PMC free article ] [ PubMed ] [ CrossRef ] [ Google Scholar ]
- Eddy, S. L., Hogan, K. A. (2014). Getting under the hood: How and for whom does increasing course structure work? CBE—Life Sciences Education , 13 ( 3 ), 453–468. 10.1187/cbe.14-03-0050 [ PMC free article ] [ PubMed ] [ CrossRef ] [ Google Scholar ]
- Estrada, M., Eppig, A., Flores, L., Matsui, J. T. (2019). A longitudinal study of the Biology Scholars Program: Maintaining student integration and intention to persist in science career pathways . Understanding Interventions , 10 , 26. [ Google Scholar ]
- Estrada, M., Young, G. R., Flores, L., Yu, B., Matsui, J. (2021). Content and quality of science training programs matter: Longitudinal study of the Biology Scholars Program . CBE—Life Sciences Education , 20 ( 3 ), ar44. 10.1187/cbe.21-01-0011 [ PMC free article ] [ PubMed ] [ CrossRef ] [ Google Scholar ]
- Farkas, G. (2004). The Black-White test score gap . Context , 3 , 12–19. 10.1525/ctx.2004.3.2.12 [ CrossRef ] [ Google Scholar ]
- Freeman, S., Eddy, S. L., McDonough, M., Smith, M. K., Okoroafor, N., Jordt, H., Wenderoth, M. P. (2014). Active learning increases student performance in science, engineering, and mathematics . Proceedings of the National Academy of Sciences USA , 111 ( 23 ), 8410–8415. 10.1073/pnas.1319030111 [ PMC free article ] [ PubMed ] [ CrossRef ] [ Google Scholar ]
- Gin, L. E., Guerrero, F. A., Cooper, K. M., Brownell, S. E. (2020). Is active learning accessible? Exploring the process of providing accommodations to students with disabilities . CBE—Life Sciences Education , 19 ( 4 ), es12. 10.1187/cbe.20-03-0049 [ PMC free article ] [ PubMed ] [ CrossRef ] [ Google Scholar ]
- Gorski, P. C. (2016). Poverty and the ideological imperative: A call to unhook from deficit and grit ideology and to strive for structural ideology in teacher education ,. Journal of Education for Teaching , 42 ( 4 ), 378–386. 10.1080/02607476.2016.1215546 [ CrossRef ] [ Google Scholar ]
- Gouvea, J. S. (2021). Antiracism and the problems with “achievement gaps” in STEM education . CBE—Life Sciences Education , 20 ( 1 ), fe2. 10.1187/cbe.20-12-0291 [ PMC free article ] [ PubMed ] [ CrossRef ] [ Google Scholar ]
- Grunspan, D. Z., Eddy, S. L., Brownell, S. E., Wiggins, B. L., Crowe, A. J., Goodreau, S. M. (2016). Males under-estimate academic performance of their female peers in undergraduate biology classrooms . PLoS ONE , 16. [ PMC free article ] [ PubMed ] [ Google Scholar ]
- Gutiérrez, R. (2000). Advancing African-American, urban youth in mathematics: Unpacking the success of one math department . American Journal of Education , 109 ( 1 ), 63–111. 10.1086/444259 [ CrossRef ] [ Google Scholar ]
- Gutiérrez, R. (2008). A “gap-gazing” fetish in mathematics education? Problematizing research on the achievement gap . Journal for Research in Mathematics Education , 39 ( 4 ), 357–364. [ Google Scholar ]
- Hacisalihoglu, G., Stephens, D., Stephens, S., Johnson, L., Edington, M. (2020). Enhancing undergraduate student success in stem fields through growth-mindset and grit . Education Sciences , 10 ( 10 ), 279. 10.3390/educsci10100279 [ CrossRef ] [ Google Scholar ]
- Harper, S. R. (2010). An anti-deficit achievement framework for research on students of color in STEM . New Directions for Institutional Research 148 , 63–74. [ Google Scholar ]
- Harper, S. R. (2015). Success in these schools? Visual counternarratives of young men of color and urban high schools they attend . Urban Education , 50 , 139–169. 10.1177/0042085915569738 [ CrossRef ] [ Google Scholar ]
- Harrison, C. D., Nguyen, T. A., Seidel, S. B., Escobedo, A. M., Hartman, C., Lam, K., ... & Tanner, K. D. (2019). Investigating instructor talk in novel contexts: Widespread use, unexpected categories, and an emergent sampling strategy . CBE—Life Sciences Education , 18 ( 3 ), ar47. 10.1187/cbe.18-10-0215 [ PMC free article ] [ PubMed ] [ CrossRef ] [ Google Scholar ]
- Howard, M. A., Kern, A. L. (2019). Conceptions of wayfinding: Decolonizing science education in pursuit of Native American success . Cultural Studies of Science Education , 14 , 1135–1148. 10.1007/s11422-018-9889-6 [ CrossRef ] [ Google Scholar ]
- Huntington-Klein, N., Arenas, A., Beam, E., Bertoni, M., Bloem, J. R., Burli, P., ... & Stopnitzky, Y. (2021). The influence of hidden researcher decisions in applied microeconomics . Economic Inquiry , 59 ( 3 ), 944–960. 10.1111/ecin.12992 [ CrossRef ] [ Google Scholar ]
- Jencks, C., Phillips, M. (2006). The Black-White test score gap: An introduction . In The Black-White test score gap (pp. 1–51). Washington, DC: Brookings Institution Press. [ Google Scholar ]
- Jordt, H., Eddy, S. L., Brazil, R., Lau, I., Mann, C., Brownell, S. E., ... & Freeman, S. (2017). Values affirmation intervention reduces achievement gap between underrepresented minority and white students in introductory biology classes . CBE—Life Sciences Education , 16 ( 3 ), ar41. 10.1187/cbe.16-12-0351 [ PMC free article ] [ PubMed ] [ CrossRef ] [ Google Scholar ]
- Kendi, I. X. (2019). How to be an antiracist . New York, NY: One World. [ Google Scholar ]
- Kozol, J. (2005). The shame of the nation . New York, NY: Crown Publishing. [ Google Scholar ]
- Ladson-Billings, G. (2006). From the achievement gap to the education debt: Understanding achievement in U.S. schools . Educational Researcher , 35 ( 7 ), 3–12. 10.3102/0013189X035007003 [ CrossRef ] [ Google Scholar ]
- Ladson-Billings, G. (2013). The dreamkeepers (2nd ed.). San Francisco, CA: Jossey-Bass. [ Google Scholar ]
- Martin, D. B. (2009). Researching race in mathematics education . Teachers College Record , 111 , 295–338. [ Google Scholar ]
- Martinková, P., Drabinová, A., Liaw, Y.-L., Sanders, E. A., McFarland, J. L., Price, R. M. (2017). Checking equity: Why differential item functioning analysis should be a routine part of developing conceptual assessments . CBE—Life Sciences Education , 16 ( 2 ), rm2. 10.1187/cbe.16-10-0307 [ PMC free article ] [ PubMed ] [ CrossRef ] [ Google Scholar ]
- Matsui, J. T., Liu, R., Kane, C. M. (2003). Evaluating a science diversity program at UC Berkeley: More questions than answers . Cell Biology Education , 2 ( 2 ), 117–121. 10.1187/cbe.02-10-0050 [ PMC free article ] [ PubMed ] [ CrossRef ] [ Google Scholar ]
- Menchaca, M. (1997). Early racist discourses: The roots of deficit thinking . In Valencia, R. R. (Ed.), The evolution of deficit thinking: Educational thought and practice ( The Stanford series on education and public policy) (pp. 13–40). Washington, DC: Falmer Press/Taylor & Francis. [ Google Scholar ]
- Milner, H. R. (2012). Beyond a test score: Explaining opportunity gaps in educational practice . Journal of Black Studies , 43 ( 6 ), 693–718. 10.1177/0021934712442539 [ CrossRef ] [ Google Scholar ]
- National science Foundation. (2020. ). Historically Black Colleges and Universities - Undergraduate Program (HBCU-UP) . Retrieved February 23, 2022, from https://beta.nsf.gov/funding/opportunities/historically-black-colleges-and-universities-undergraduate-program-hbcu
- Nieto, S., Bode, P. (2012). Affirming diversity: The sociopolitical context of multicultural education (6th ed.). Boston, MA: Pearson Education. [ Google Scholar ]
- Noble, S. (2018). Algorithms of oppression (Illustrated ed.). New York: NYU Press. [ Google Scholar ]
- Noddings, N. (1988). An ethic of caring and its implications for instructional arrangements . American Journal of Education , 96 ( 2 ), 215–230. 10.1086/443894 [ CrossRef ] [ Google Scholar ]
- Obermeyer, Z., Powers, B., Vogeli, C., Mullainathan, S. (2019). Dissecting racial bias in an algorithm used to manage the health of populations . Science , 366 , 447–453. 10.1126/science.aax2342 [ PubMed ] [ CrossRef ] [ Google Scholar ]
- Omi, M., Winant, H. (2014). Racial formation in the United States (3rd ed.). New York, NY: Routledge. [ Google Scholar ]
- Painter, N. I. (2020, July 22). Why “White” should be capitalized, too . Washington Post . Retrieved February 23, 2022, from www.washingtonpost.com/opinions/2020/07/22/why-white-should-be-capitalized [ Google Scholar ]
- Pang, V. O., Rivera, J., Mora, J. K. (2000). The ethic of caring: Clarifying the foundation of multicultural education . Educational Forum , 64 , 25–32. [ Google Scholar ]
- Parnes, M. F., Kanchewa, S. S., Marks, A. K., Schwartz, S. E. O. (2020). Closing the college achievement gap: Impacts and processes of a help-seeking intervention . Journal of Applied Developmental Psychology , 67 , 101121. 10.1016/j.appdev.2020.101121 [ CrossRef ] [ Google Scholar ]
- Pearl, A. (1997). Cultural and accumulated environmental models . In Valencia, R. R. (Ed.), The evolution of deficit thinking: Educational thought and practice ( The Stanford series on education and public policy) (pp. 132–159). Washington, DC: Falmer Press/Taylor & Francis. [ Google Scholar ]
- Quinn, D. M. (2020). Experimental effects of “achievement gap” news reporting on viewers’ racial stereotypes, inequality explanations, and inequality prioritization . Educational Researcher , 49 ( 7 ), 482–492. 10.3102/0013189X20932469 [ CrossRef ] [ Google Scholar ]
- Rankins, C. (2019). HBCUs and Black STEM student success . Peer Review , 21 , 50–51. [ Google Scholar ]
- Ris, E. W. (2015). Grit: A short history of a useful concept . Journal of Educational Controversy , 10 ( 1 ). Retrieved February 23, 2022, from https://cedar.wwu.edu/jec/vol10/iss1/3 [ Google Scholar ]
- Rothstein, R. (2013). Why children from lower socioeconomic classes, on average, have lower academic achievement than middle-class children . In Carter, P. L., Welner, K. G. (Eds.), Closing the opportunity gap: What America must do to give every child an even chance (pp. 61–74). New York, NY: Oxford University Press. [ Google Scholar ]
- Sadker, D., Sadker, M. P., Zittleman, K. R. (2009). Still failing at fairness: How gender bias cheats girls and boys in school and what we can do about it . New York, NY: Scribner. [ Google Scholar ]
- Seah, Y. M., Chang, A. M., Dabee, S., Davidge, B., Erickson, J. R., Olanrewaju, A. O., Price, R. M. (2021). Pandemic-related instructor talk: How new instructors supported students at the onset of the COVID-19 pandemic . Journal of Microbiology & Biology Education , 22 . 10.1128/jmbe.v22i1.2401 [ PMC free article ] [ PubMed ] [ CrossRef ] [ Google Scholar ]
- Seidel, S. B., Reggi, A. L., Schinske, J. N., Burrus, L. W., Tanner, K. D. (2015). Beyond the biology: A systematic investigation of noncontent instructor talk in an introductory biology course . CBE—Life Sciences Education , 14 ( 4 ), ar43. 10.1187/cbe.15-03-0049 [ PMC free article ] [ PubMed ] [ CrossRef ] [ Google Scholar ]
- Shah, N. (2019). “Asians are good at math” is not a compliment: STEM success as a threat to personhood . Harvard Educational Review , 89 , 661–686. 10.17763/1943-5045-89.4.661 [ CrossRef ] [ Google Scholar ]
- Steele, C. M. (2011). Whistling Vivaldi: How stereotypes affect us and what we can do (Reprint ed.). New York: Norton. [ Google Scholar ]
- Takacs, D. (2003). How does your positionality bias your epistemology? Thought & Action , 19 , 27–38. [ Google Scholar ]
- Tewell, E. (2020). The problem with grit: Dismantling deficit thinking in library instruction . Portal: Libraries and the Academy , 20 , 137–159. [ Google Scholar ]
- Theobald, E. J., Hill, M. J., Tran, E., Agrawal, S., Arroyo, E. N., Behling, S., ... & Freeman, S. (2020). Active learning narrows achievement gaps for underrepresented students in undergraduate science, technology, engineering, and math . Proceedings of the National Academy of Sciences USA , 117 ( 12 ), 6476–6483. 10.1073/pnas.1916903117 [ PMC free article ] [ PubMed ] [ CrossRef ] [ Google Scholar ]
- Thompson, J. J., Jensen-Ryan, D. (2018). Becoming a “science person”: Faculty recognition and the development of cultural capital in the context of undergraduate biology research . CBE—Life Sciences Education , 17 ( 4 ), ar62. 10.1187/cbe.17-11-0229 [ PMC free article ] [ PubMed ] [ CrossRef ] [ Google Scholar ]
- Valencia, R. R. (1997). The evolution of deficit thinking: Educational thought and practice ( The Stanford series on education and public policy). Washington, DC: Falmer Press/Taylor & Francis. [ Google Scholar ]
- Weatherton, M., Schussler, E. E. (2021). Success for all? A call to re-examine how student success is defined in higher education . CBE—Life Sciences Education , 20 ( 1 ), es3. 10.1187/cbe.20-09-0223 [ PMC free article ] [ PubMed ] [ CrossRef ] [ Google Scholar ]
- Wilson, K. J., Brickman, P., Brame, C. J. (2018). Group Work . CBE—Life Sciences Education , 17 ( 1 ), fe1. 10.1187/cbe.17-12-0258 [ PMC free article ] [ PubMed ] [ CrossRef ] [ Google Scholar ]
- Yosso, T. J. (2005). Whose culture has capital? A critical race theory discussion of community cultural wealth . Race Ethnicity and Education , 8 ( 1 ), 69–91. 10.1080/1361332052000341006 [ CrossRef ] [ Google Scholar ]
- Yosso, T. J. (2006). Critical race counterstories along the Chicana/Chicano educational pipeline . New York, NY: Routledge. [ Google Scholar ]
- Young, J. L., Young, J. R., Ford, D. Y. (2017). Standing in the gaps: Examining the effects of early gifted education on black girl achievement in STEM . Journal of Advanced Academics , 28 ( 4 ), 290–312. 10.1177/1932202X17730549 [ CrossRef ] [ Google Scholar ]
Change Password
Your password must have 8 characters or more and contain 3 of the following:.
- a lower case character,
- an upper case character,
- a special character
Password Changed Successfully
Your password has been changed
- Sign in / Register
Request Username
Can't sign in? Forgot your username?
Enter your email address below and we will send you your username
If the address matches an existing account you will receive an email with instructions to retrieve your username
Reframing Educational Outcomes: Moving beyond Achievement Gaps
- Sarita Y. Shukla
- Elli J. Theobald
- Joel K. Abraham
- Rebecca M. Price
School of Educational Studies, University of Washington, Bothell, Bothell, WA 98011-8246
Search for more papers by this author
Department of Biology, University of Washington, Seattle, Seattle, WA 98195
Department of Biological Science, California State University–Fullerton, Fullerton, CA 92831
*Address correspondence to: Rebecca M. Price ( E-mail Address: [email protected] )
School of Interdisciplinary Arts & Sciences, University of Washington, Bothell, Bothell, WA 98011-8246
The term “achievement gap” has a negative and racialized history, and using the term reinforces a deficit mindset that is ingrained in U.S. educational systems. In this essay, we review the literature that demonstrates why “achievement gap” reflects deficit thinking. We explain why biology education researchers should avoid using the phrase and also caution that changing vocabulary alone will not suffice. Instead, we suggest that researchers explicitly apply frameworks that are supportive, name racially systemic inequities and embrace student identity. We review four such frameworks—opportunity gaps, educational debt, community cultural wealth, and ethics of care—and reinterpret salient examples from biology education research as an example of each framework. Although not exhaustive, these descriptions form a starting place for biology education researchers to explicitly name systems-level and asset-based frameworks as they work to end educational inequities.
INTRODUCTION
Inequities plague educational systems in the United States, from pre-K through graduate school. Many of these inequities exist along racial, gender, and socioeconomic lines ( Kozol, 2005 ; Sadker et al. , 2009 ), and they impact the educational outcomes of students. For decades, education research has focused on comparisons of these educational outcomes, particularly with respect to test scores of students across racial and ethnic identities. The persistent differences in these test scores or other outcomes are often referred to as “achievement gaps,” which in turn serve as the basis for numerous educational policy and structural changes ( Carey, 2014 ).
A recent essay in CBE—Life Sciences Education ( LSE ) questioned narrowly defining “success” in educational settings ( Weatherton and Schussler, 2021 ). The authors posit that success must be defined and contextualized, and they asked the community to recognize the racial undercurrents associated with defining success as limited to high test scores and grade point averages (GPAs; Weatherton and Schussler, 2021 ). In this essay, we make a complementary point. We contend that the term “achievement gap” is misaligned with the intent and focus of recent biology education research. We base this realization on the fact that the term “achievement gap” can have a deeper meaning than documenting a difference among otherwise equal groups ( Kendi, 2019 ; Gouvea, 2021 ). It triggers deficit thinking ( Quinn, 2020 ); unnecessarily centers middle and upper class, White, male students as the norm ( Milner, 2012 ); and downplays the impact of structural inequities ( Ladson-Billings, 2006 ; Carter and Welner, 2013 ).
This essay unpacks the negative consequences of using the term “achievement gap” when comparing student learning across different racial groups. We advocate for abandoning the term. Similarly, we suggest that, in addition to changing our terminology, biology education researchers can explicitly apply theoretical frameworks that are more appropriate for interrogating inequities among educational outcomes across students from different demographics. We emphasize that the idea that a simple “find and replace,” swapping out the term “achievement gap” for other phrases, is not sufficient.
In the heart of this essay, we review some of these systems-level and asset-based frameworks for research that explores differences in academic performance ( Figure 1 ): opportunity gaps ( Carter and Welner, 2013 ), educational debt ( Ladson-Billings, 2006 ), community cultural wealth ( Yosso, 2005 ), and ethics of care ( Noddings, 1988 ). Within each of these frameworks, we review examples of biology education literature that we believe rely on them, explicitly or implicitly. We conclude by reiterating the need for education researchers to name explicitly the systems-level and asset-based frameworks used in future research.
FIGURE 1. Research frameworks highlighted in the essay. The column in gray summarizes deficit-based frameworks that focus on achievement gaps. The middle column (in gold) includes examples of systems-based frameworks that acknowledge that student learning is associated with society-wide habits. The rightmost columns (in peach) include examples of asset-based models that associate student learning with students’ strengths. The columns are not mutually exclusive, in that studies can draw from multiple frameworks simultaneously or sequentially.
We will use the phrase “students from historically or currently marginalized groups” to describe the students who have been and still are furthest from the center of educational justice. However, when discussing work of other researchers, we will use the terminology they use in their papers. Our conceptualization of this phrase matches, as near as we can tell, Asai’s phrase “PEERs—persons excluded for their ethnicity or race” ( Asai, 2020 , p. 754). We also choose to capitalize “White” to acknowledge that people in this category have a visible racial identity ( Painter, 2020 ).
Positionality
Our positionalities—our unique life experiences and identities—mediate our understanding of the world ( Takacs, 2003 ). What we see as salient in our research situation arises from our own life experiences. Choices in our research, including the types of data we collect and how we clean the data and prepare it for analysis, adopt analytical tools, and make sense of these analyses are important decision points that affect study results and our findings ( Huntington-Klein et al. , 2021 ). We recognize that it is impossible to be free of bias ( Noble, 2018 ; Obermeyer et al. , 2019 ). Therefore, we put forth our positionality to acknowledge the lenses through which we make decisions as researchers and to forefront the impact of our identities on our research. Still, the breadth of our experiences cannot be described fully in a few sentences.
The four authors of this essay have unique and complementary life experiences that contribute to the sense-making presented in this essay. S.Y.S. has been teaching since 2003 and teaching in higher education since 2012. She is a South Asian immigrant to the United States, and a cisgender woman. E.J.T. has taught middle school, high school, and college science since 2006. She is a cisgender White woman. J.K.A. is a cisgender Black mixed-race man who comes from a family of relatively recent immigrants with different educational paths. He has worked in formal and informal education since 2000. R.M.P. is a cisgender Jewish, White woman, and she has been teaching college since 2006. We represent a team of people who explicitly acknowledge that our experiences influence the lenses through which we work. Our guiding principles are 1) progress over perfection, 2) continual reflection and self-improvement, and 3) deep care for students. These principles guide our research and teaching, impacting our interactions with colleagues (faculty and staff) as well as students. Ultimately, these principles motivate us to make ourselves aware of, reflect on, and learn from our mistakes.
Simply Changing Vocabulary Does Not Suffice
The term “achievement gap” is used in research that examines differences in achievement—commonly defined as differences in test scores—across students from different demographic groups ( Coleman et al. , 1966 ). Some studies replace “achievement gap” with “score gap” (e.g., Jencks and Phillips, 2006 ), because it defines the type of achievement under consideration; others use “opportunity gap,” because it emphasizes differences in opportunities students have had throughout their educational history (e.g., Carter and Welner, 2013 ; more on opportunity gaps later). The shift for which we advocate, however, does not reside only with terminology. Instead, we call for a deeper shift of using research frameworks that acknowledge and respect students’ histories and empower them now.
The underlying framework in research that uses “achievement gap” or even “score gap” may not be immediately apparent. Take for example two studies that both use the seemingly benign term, “score gap.” A close read indicates that one study attributed the difference in test scores between Black and White students to deficient “culture and child-rearing practices” ( Farkas, 2004 , p. 18). Thus, even though the researcher uses what can be considered to be more neutral terminology, the phrase in this context represents deficit thinking and blame. On the other hand, another study uses the term “score gap” to explore differences that have been historically studied through cultures of poverty, genetic, and familial backgrounds ( Jencks and Phillips, 2006 ). While these researchers discuss the Black–White score gap, they present evidence that examines this phenomenon with nuanced constructs, such as stereotype threat ( Steele, 2011 ) and resources available. These authors also mention ways to reduce score gaps, such as smaller class sizes and high teacher expectations ( Jencks and Phillips, 2006 ).
Some researchers who use the phrase “achievement gap” explicitly avoid deficit thinking and instead embrace an asset-based framework. Jordt et al. (2017) address systemic racism, just as Jencks and Phillips (2006) do. Specifically, Jordt et al. (2017) identified an intervention that affirmed student values that might also be a potential tool for increasing underrepresented minority (URM) student exam scores in college-level introductory science courses. The researchers found that this intervention produced a 4.2% increase in exam performance for male URM students and a 2.2% increase for female URM students. Thus, while they use “achievement gap” throughout the paper to refer to racial and gender differences in exam scores, the study focused on ways to support URM student success.
In pursuit of improved language and clarity of intent, the term “achievement gap” should be replaced to reflect the research framework used to interrogate educational outcomes within and across demographic groups.
DEFICIT THINKING
Deficit thinking describes a mindset, or research framework, in which differences in outcomes between members of different groups, generally a politically and economically dominant group and an oppressed group, are attributed to a quality that is lacking in the biology, culture, or mindset of the oppressed group ( Valencia, 1997 ). Deficit thinking has pervaded public and academic discourse about the education of students from different races and ethnicities in the United States for centuries ( Menchaca, 1997 ).
Tenacious deficit-based explanations blame students from historically or currently marginalized groups for lower educational attainment. These falsities include biological inferiority due to brain size or structure ( Menchaca, 1997 ), negative cultural attributes such as inferior language acquisition ( Dudley-Marling, 2007 ), and accumulated deficits due to a “culture of poverty” ( Pearl, 1997 ; Gorski, 2016 ). More recently, lower achievement has been attributed to a lack of “grit” ( Ris, 2015 ) or the propensity for a “fixed” mindset ( Gorski, 2016 ; Tewell, 2020 ). While ideas around grit and mindset have demonstrable value in certain circumstances (e.g., Hacisalihoglu et al. , 2020 ), they fall short as primary explanations for differences in educational outcomes, because they focus attention on perceived deficits of students while providing little information about structural influences on failure and success, including how we define those constructs ( Harper, 2010 ; Gorski, 2016 ). In other words, deficit models often posit students as the people responsible for improving their own educational outcomes ( Figure 1 ).
Deficit thinking, regardless of intent, blames individuals, their families, their schools, or their greater communities for the consequences of societal inequities ( Yosso, 2006 ; Figure 1 ). This blame ignores the historic and structural drivers of inequity in our society, placing demands on members of underserved groups to adapt to unfair systems ( Valencia, 1997 ). A well-documented example of structural inequity is the consistent underresourcing of public schools that serve primarily students of color and children from lower socioeconomic backgrounds ( Darling-Hammond, 2013 ; Rothstein, 2013 ). Because learning is heavily influenced by factors outside the school environment, such as food security, trauma, and health ( Rothstein, 2013 ), schools themselves reflect gross disparities in resourcing based on historic discrimination ( Darling-Hammond, 2013 ). Deficit thinking focuses on student or cultural characteristics to explain performance differences and tends to overlook or minimize the impacts of systemic disparities. Deficit thinking also strengthens the narrative around student groups in terms of shortcomings, reinforces negative stereotypes, and ignores successes or drivers of success in those same groups ( Harper, 2015 ).
Achievement Gaps
The term “achievement gap” has historically described the difference in scores attained by students from racial and ethnic minority groups compared with White students on standardized tests or course exams ( Coleman et al. , 1966 ). As students from other historically or currently marginalized groups, such as female or first-generation students, are increasingly centered in research, the term is now used more broadly to compare any student population to White, middle and upper class, men ( Harper, 2010 ; Milner, 2012 ). Using White men as the basis for comparison comes at the expense of students from other groups ( Harper, 2010 ; Milner, 2012 ). Basing comparisons on the cultural perspectives of a single dominant group leads to “differences” being interpreted as “deficits,” which risks dehumanizing people in the marginalized groups ( Dinishak, 2016 ). Furthermore, centering White, wealthy, male performance means that even students from groups that tend to have higher test scores, like Asian-American students, risk dehumanization as “model minorities” or “just good at math” ( Shah, 2019 ).
Many researchers have highlighted the fact that the term “achievement gap” is a part of broader deficit-thinking models and rooted in racial hierarchy ( Ladson-Billings, 2006 ; Gutiérrez, 2008 ; Martin, 2009 ; Milner, 2012 ; Kendi, 2019 ). Focusing on achievement gaps emphasizes between-group differences over within-group differences ( Young et al. , 2017 ), reifies sociopolitical and historical groupings of people ( Martin, 2009 ), and minimizes attention to structural inequalities in education ( Ladson-Billings, 2006 ; Alliance to Reclaim Our Schools, 2018 ). Gutiérrez (2008) names this obsession with achievement gaps as a “gap-gazing fetish” that draws attention away from finding solutions that promote equitable learning ( Gutiérrez, 2008 ). Under a deficit-thinking model, achievement gaps are viewed as the primary problem, rather than a symptom of the problem ( Gutiérrez, 2008 ), and for decades they have been attributed to different characteristics of the demographics being compared ( Valencia, 1997 ). As such, proposed solutions tend to be couched in terms of remediation for students ( Figure 1 ).
Ignoring the social context of students’ education necessarily limits inferences that can be drawn about their success. Limiting measures of educational success, also conceptualized as achievement, to performance on exams or overall college GPA, often leaves out consideration of other potential data sources ( Weatherton and Schussler, 2021 ; Figure 2 ). This narrow perspective tends to perpetuate the systems of power and privilege that are already in place ( Gutiérrez, 2008 ). The biology education research community can instead broaden its sense of success to recognize the underlying historical and current contexts and the intersections of identities (e.g., racial, gender, socioeconomic) that contribute to those differences ( Weatherton and Schussler, 2021 ).
FIGURE 2. A selection of potential data sources that could inform researchers about within- and between-group differences in educational outcomes. This list does not encompass the full range of possible data sources, nor does it imply a hierarchy to the data. Instead, it reflects some of the diversity of quantitative and qualitative data that are directly linked to student outcomes and that are used under multiple research frameworks.
In biology education research, many papers still use the language of “achievement gap,” even in instances when researchers explicitly or implicitly use other nondeficit frameworks. While some may argue that this language merely describes a pattern, its origin and history is explicitly and inextricably linked to deficit-thinking models ( Gutiérrez, 2008 ; Milner, 2012 ). Thus, we join others in the choice to abandon the term “achievement gap” in favor of language—and frameworks—that align better to the goals of our research and to avoid the limitations and harm that can arise through its use.
Example: Focusing on Achievement Gaps Can Reinforce Racial Stereotypes
Messages of perpetual underachievement can inadvertently reinforce negative stereotypes. For example, Quinn (2020) demonstrated that, when participants watched a 2-minute video of a newscast using the term “achievement gap,” they disproportionately underpredicted the graduation rate of Black students relative to White students, even more so than participants in a control group who watched a counter-stereotypical video. They also scored significantly higher on an instrument measuring bias. Because bias is dynamic and affected by the environment, Quinn concludes that the video discussing the achievement gap likely heightened the bias of the participants ( Quinn, 2020 ).
Education researchers, just like the participants in Quinn’s (2020) study, inadvertently carry implicit bias against students from the different groups they study, and those biases can shift depending on context. Quinn (2020) demonstrates that just using the term “achievement gap” can reinforce the pervasive racial hierarchy that places Black students at the bottom. Researchers, without intending to, can be complicit in a system of White privilege and power if the language and frameworks underlying their study design, data collection, and/or data interpretation are aligned with bias and stereotype. If the goal is to dismantle inequities in our educational systems and research on those systems, the biology education research community must consider the historical and social weight of its literature to address racism head on, as progressive articles have been doing (e.g., Eddy and Hogan, 2014 ; Canning et al. , 2019 ; Theobald et al. , 2020 ).
SYSTEMS-LEVEL FRAMEWORKS
To move away from the achievement gap discourse—because of the history of the term, the perceived blame toward individual students, as well as the deficit thinking the term may imbue and provoke—we highlight some of the other frameworks for understanding student outcomes. We conclude discussion of each framework with an example from education research that can be reinterpreted within it, keeping in mind that multiple frameworks can be applied to different studies. We acknowledge two caveats about these reinterpretations: first, we are adding another layer of interpretation to the original studies, and we cannot claim that the original authors agree with these interpretations; second, each example could be interpreted through multiple frameworks, especially because these frameworks overlap ( Figure 1 ).
In this section, we begin at the systems level by examining opportunity gaps and educational debt. Rather than blaming students or their cultures for deficits in performance, these systems-level perspectives name white supremacy and the concomitant policies that maintain power imbalances as the cause of disparate student experiences.
Opportunity Gaps
The framework of opportunity gaps shifts the onus of differential student performance away from individual deficiencies and assigns solutions to actions that address systemic racism ( Milner, 2012 ; Figure 1 ). Specifically, opportunity gaps embody the difference in performance between students from historically and currently marginalized groups and middle and upper class, White, male students, with primary emphasis on opportunities that students have or have not had, rather than on their current performance (i.e., achievement) in a class ( Milner, 2012 ). Compared with deficit models, the focus shifts from assigning responsibility for the gap from the individual to society ( Figure 1 ).
Some researchers explore opportunity gaps by discussing the structural challenges that students from historically and currently marginalized groups have been facing (e.g., Rothstein, 2013 ). For example, poor funding in K–12 schools leads to inconsistent, poorly qualified, and poorly compensated teachers; few and outdated textbooks ( Darling-Hammond, 2013 ); limited field trips; a lack of extracurricular resources ( Rothstein, 2013 ); and inadequately supplied and cleaned bathrooms ( Darling-Hammond, 2013 ). Additional structural challenges that occur outside school buildings, but impact learning, include poor health and lack of medical care, food and housing insecurity, lead poisoning and iron deficiency, asthma, and depression ( Rothstein, 2013 ).
While the literature about opportunity gaps focuses more on K–12 than higher education ( Carter and Welner, 2013 ), college instructors can exacerbate opportunity gaps by biasing who has privilege (i.e., opportunities) in their classrooms. For example, some biology education literature focuses on how instructors’ implicit biases impact our students, such as by unconsciously elevating the status of males in the classroom ( Eddy et al. , 2014 ; Grunspan et al. , 2016 ).
Example: CUREs Can Prevent Opportunity Gaps.
Course-based undergraduate research experiences (CUREs) are one way to prevent opportunity gaps (e.g., Bangera and Brownell, 2014 ; CUREnet, n.d. ). Specifically, we interpret the suggestions that Bangera and Brownell (2014) make about building CUREs as a way to recognize that some students have the opportunity to participate in undergraduate research experiences while others do not. For example, students who access extracurricular research opportunities are likely relatively comfortable talking to faculty and, in many cases, have the financial resources to pursue unpaid laboratory positions ( Bangera and Brownell, 2014 ). More broadly, when research experiences occur outside the curriculum, they privilege students who know how to pursue and gain access to them. However, CUREs institutionalize the opportunity to conduct research, so that every student benefits from conducting research while pursuing an undergraduate degree.
Educational Debt
Ladson-Billings (2006) submits that American society has an educational debt, rather than an educational deficit. This framework shifts the work of finding solutions to educational inequities away from individuals and onto systems ( Figure 1 ). The metaphor is economic: A deficit refers to current mismanagement of funds, but a debt is the systematic accumulation of mismanagement over time. Therefore, differences in student performances are framed by a history that reflects amoral, systemic, sociopolitical, and economic inequities. Ladson-Billing ( 2006 ) suggests that focusing on debts highlights injustices that Black, Latina/o, and recent immigrant students have incurred: Focusing on student achievement in the absence of a discussion of past injustices does not redress the ways in which students and their parents have been denied access to educational opportunities, nor does it redress the ways in which structural and institutional racism dictate differences in performance. This approach begins by acknowledging the structural and institutional barriers to achievement in order to dismantle existing inequities. This reframing helps set the scope of the problem and identify a more accurate and just lens through which we make sense of the problem ( Cho et al. , 2013 ).
Example: NSF Supports Historically Black Colleges and Universities.
From my own (yet to be published) research, a participant described the HBCU where he studied physics as providing a “dome of security and safety.” In contrast, he recounted that when he attended a predominantly White institution, he constantly needed to be guarded and employ “his body sense,” an act that made him tense, defensive, and unable to listen. ( Rankins, 2019 , p. 50)
Example: Institutions Can Repay Educational Debt.
Institutions can repay educational debt by ensuring that their students have the resources and support structures necessary to succeed. The Biology Scholars Program at the University of California, Berkeley, is a prime example ( Matsui et al. , 2003 ; Estrada et al. , 2019 ). This program, begun in 1992 ( Matsui et al. , 2003 ) and still going strong ( Berkeley Biology Scholars Program, n.d. ), creates physical and psychological spaces that support learning: a study space and study groups, paid research experiences, and thoughtful mentoring. The students recruited to the program are from first-generation, low-socioeconomic status backgrounds and from groups that are historically underrepresented. When the students enter college, they have lower GPAs and Scholastic Aptitude Test scores than their counterparts with the same demographic profile who are not in the program. And yet, when they graduate, students in the Biology Scholars Program have higher GPAs and higher retention in biology majors than their counterparts ( Matsui et al. , 2003 ), perhaps because of the extended social support they receive from peers ( Estrada et al. , 2021 ). Moreover, students in this program report lower levels of stress and a greater sense of well-being ( Estrada et al. , 2019 ).
ASSET-BASED FRAMEWORKS
In this section, we continue to explore frameworks that move away from the achievement gap discourse, now focusing on models that build from students’ strengths. We have chosen two frameworks whose implications seem particularly relevant to and coincident with anti-racist research in biology education: community cultural wealth ( Yosso, 2005 ) and ethics of care ( Noddings, 1988 ). As before, we reinterpret articles from the education literature to illustrate these frameworks, and we once again include the caveats that we extend beyond the authors’ original interpretations and that other frameworks could also be used to reinterpret the examples.
Community Cultural Wealth
One asset-based way to frame student outcomes is to begin with the strengths that people from different demographic groups hold ( Yosso, 2005 ). Rather than focusing on racism, this approach focuses on community cultural wealth. The premise is that everyone can contribute a wealth of knowledge and approaches from their own cultures ( Yosso, 2005 ).
Community cultural wealth begins with critical race theory (CRT; Yosso, 2005 ). CRT illuminates the impact of race and racism embedded in all aspects of life within U.S. society ( Omi and Winant, 2014 ). CRT acknowledges that racism is interconnected with the founding of the United States. Race is viewed in tandem with intersecting identities that oppose dominant ones, and the constructs of CRT emerge by attending to the experiences of people from communities of color ( Yosso, 2005 ). Therefore, the experiences of students of color are central to transformative education that addresses the overrepresentation of White philosophies. CRT calls on research to validate and center these perspectives to develop a critical understanding about racism.
Community cultural wealth builds on these ideas by viewing communities of color as a source of students’ strength ( Yosso, 2005 ). The purpose of schooling is to build on the strengths that students have when they arrive, rather than to treat students as voids that need to be filled: students’ cultural wealth must be acknowledged, affirmed, and amplified through their education. This approach is consistent with those working to decolonize scientific knowledge (e.g., Howard and Kern, 2019 ).
Example: Community Cultural Wealth Can Improve Mentoring.
Thompson and Jensen-Ryan (2018) offer advice to mentors about how to use cultural wealth to mentor undergraduate students in research. They identify the forms of scientific cultural capital that research mentors typically value, finding that these aspects of a scientific identity are closely associated with majority culture. They challenge mentors to broaden the forms of recognizable capital. For example, members of the faculty can actively recruit students into their labs from programs aimed to promote the diversity of scientists, rather than insisting that students approach them with their interest to work in the lab ( Thompson and Jensen-Ryan, 2018 ). They can recognize that undergraduate students may not express an interest in a research career–especially initially—but that research experience is still formative. They can recognize that students who are strong mentors to their peers are valuable members of a research team and that this skill is a form of scientific capital. They can value the diverse backgrounds of students in their labs, rather than insisting that they come from families that have prioritized scientific thinking and research. In sum, the gaps that Thompson and Jensen-Ryan (2018) identify are in research mentors’ attitudes, rather than in student performance.
Assets can also be developed in the classroom. We interpret Parnes et al. ’s (2020) analysis of the Connected Scholars program as stemming from community cultural wealth. The Connected Scholars program normalized help-seeking and increased the help network available to first-generation college students, 90% of whom were racial or ethnic minorities, in a 6-week summer program that bridged students from high school to college. First-generation college students were provided explicit instruction on how to sustain these two types of support. The Connected Scholars intervention promoted help-seeking behaviors and seemed to mediate higher GPAs. Additionally, students in the intervention reported through a survey that they had better relationships with their instructors than students in the control group ( Parnes et al. , 2020 ). In other words, cultural wealth can be amplified in college for first-generation students (see also the Biology Scholars Program, discussed in the Opportunity Gaps section; Matsui et al. , 2003 ; Estrada et al. , 2019 ).
Ethics of Care
As a framework, ethics of care complements community cultural wealth, in that both are asset-based. A key difference is that community cultural wealth focuses on the assets that students bring, and ethics of care focuses on the assets that an instructor brings to create a classroom of respect and confidence in students.
A foundation of biology education research is that instructors want their students to learn, and it is buttressed by literature concerning students’ emotional well-being. For example, the field considers how students with disabilities experience active learning ( Gin et al. , 2020 ) and how group work promotes collaboration and learning ( Wilson et al. , 2018 ). Studies like these echo the philosophy of ethics of care developed by Noddings (1988) .
The premises of teaching through the ethics of care are that everyone—including students and instructors—has both an innate desire to learn and the capacity to nurture ( Pang et al. , 2000 ). In teaching, these premises form the basis for student–instructor relationships. Nieto and Bode (2012) caution against the oversimplification that caring means being nice: the ethics of care encompasses niceness, in addition to articulating high standards of performance. Instructors must also support and respect students as they meet those standards, especially when students did not recognize that they could meet those goals at the outset. This framework is about nurturing students to accomplish more than they thought possible.
Combining an inclusive culture, for example, through positive instructor talk ( Seidel et al. , 2015 ; Harrison et al. , 2019 ; Seah et al. , 2021 ), growth mindset ( Canning et al. , 2019 ), or increased course structure ( Eddy and Hogan, 2014 ), with evidence-based practices for teaching content ( Freeman et al. , 2014 ; Theobald et al. , 2020 ) has garnered recent attention as a way to create a powerful ethic of care in classrooms. For example, instructor talk, that is, what instructors say in class other than the content they are teaching, addresses student affect. Seidel et al. (2015) and Harrison et al. (2019) analyzed classroom transcripts to identify different categories of instructor talk. While further research can probe the impacts of instructor talk on student outcomes, the idea is consistent with the principles of ethics of care: for example, one category of talk describes the instructor–student relationship as one of respect, fostered through statements such as “People are bringing different pieces of experience and knowledge into this question and I want to kind of value the different kinds of experience and knowledge that you bring in” ( Seidel et al. , 2015 , p. 6). Instructor talk also generates a classroom culture of support and validation for marginalized students and overall builds classroom community ( Ladson-Billings, 2013 ).
Example: Departments Can Implement Care.
Gutiérrez (2000) presents an example of an entire department applying ethics of care to support how African-American students learn math. This study is an ethnography of a particularly successful STEM magnet program in a public high school with a population that is majority African American. In her analysis of the math department, Gutiérrez avoids the phrase “achievement gap,” while also recognizing that people outside the school assume a deficit model when considering the students . Instead, she illustrates how researchers can use an asset-based lens to build from knowledge about differences in performance ( Gutiérrez, 2000 ).
Gutierrez ( 2000 ) examines pedagogy that supports African-American students. She documents how a culture of excellence is developed within a school setting that promotes student achievement. This culture is complex, in that there are multiple layers of support that provide students with repertoires for advancement ( Gutiérrez, 2000 )—the emphasis is on how teachers create an environment where students are both challenged through the curriculum and supported along the way. The teachers in this study have a dynamic conception of their students, and they demonstrate a unified commitment to support the broadest array of students at their school. The institution itself, represented in part through the departmental chair, has values that empower teachers to support students, proactive commitment from teachers to find innovative practices to serve students, and a supportive chairperson.
The math department exhibited a student-centered approach that epitomizes ethics of care. The teachers in the math department rotated through all of the courses and were therefore familiar with the entire curriculum. This knowledge helped them support one another, sharing successful strategies and working to improve the courses. It set up an environment in which they prioritized making decisions collectively. This collaboration led to a sense of togetherness among teachers and a sense of investment in individual students’ successes. As a result, the teachers decided to remove less-challenging courses from the curriculum and replaced them with more advanced courses—against the recommendations of the school district. The chair of the department worked with the faculty to support student learning, consider course assignments, and choose topics for and frequency of faculty meetings. The chair also attended to teachers’ emotional needs, for example, by talking to teachers every day, working with teachers to determine the best strategies for evaluating teaching practices, and enacting a teaching philosophy that valued problem solving over achieving correct answers.
The support that the teachers provided each other coincided with strong support for students. For example, students attended the magnet program because they were interested in science; they notably did not have to take entrance exams or maintain a certain GPA. If students struggled with a subject, they received tutoring. The teachers also invited graduates of the program to come back and visit, keeping the students motivated by showing them success.
Example: Biology Instructors Can Adopt an Ethics of Care.
In much of the research on differential performance in our field, researchers focus on identifying strategies that help students, regardless of their histories, in their learning success. This asset-based approach acknowledges that students start at different places, but also that instructors can implement strategies that support all students in a trajectory toward common learning goals. This argument is often posited in terms of inclusive teaching (e.g., Dewsbury and Brame, 2019 ).
Some papers that measure the effect of inclusive teaching practices may use “gap” language, perhaps as a historical artifact of our discipline. These papers emphasize the just mission to “close the gap”—or, in anti-deficit language, for all students to learn the material and perform well on assessments. For example, Theobald et al. (2020) conducted a meta-analysis of undergraduate STEM classes, drawing on 26 studies of courses reporting failure rates (44,606 students) and 15 studies (9238 students) that reported exam scores. Within these samples, they compared instruction in lecture format with instruction using active-learning strategies. The analysis compared the success of students from minoritized groups using these two teaching strategies and found conclusive evidence of the efficacy of active teaching for underrepresented student success in STEM courses. The powerful implication of this study is that college STEM instructors can mitigate some of the effects of oppression that students have experienced in their lifetime.
In another study demonstrating the philosophy of ethics of care, Canning et al. (2019) found narrower racial disparities in performance in courses taught by instructors who had a growth mindset about their students’ ability to learn, compared with instructors who viewed level of achievement as fixed. In fact, they found that the instructor mindset had a bigger impact on student performance than other faculty characteristics ( Canning et al. , 2019 ). While they focused on the negative consequences of instructors’ fixed mindset, the corollary is that a growth mindset can reflect an ethics of care that both motivates students and generates a positive classroom environment.
The successful instructors will also work to recognize their implicit biases and to ensure that they support a growth mindset for all students, regardless of demographic. This is particularly relevant, because implicit biases have “more to do with associations we’ve absorbed through history and culture than with explicit racial animus” ( Eberhardt, 2019 , p. 160). Realizing how our own socialization may have conditioned us to automatically produce harmful but hidden narratives warrants our attention ( Eberhardt, 2019 ).
MOVING FORWARD
Ladson-Billings (2006) reframed the performance of students from historically and currently marginalized groups from achievement gap to educational debt; this reframing has contributed to a movement to critically examine the term. At the same time, however, the term “achievement gap” has become a catchall used by researchers untethered from its deeper historical context.
Researchers choose words to describe their research that reflect their personal worldviews and research frameworks; in turn, these worldviews and frameworks influence future researchers. Every discipline grapples with terminology, and phrases that were common historically may fall out of use. In some instances, the terms themselves no longer suffice, so a simple “search and replace” may be all that is required to address the issue. The term “achievement gap,” however, is tied to specific frameworks that need to be acknowledged and redressed; it affects how research is designed, how results are interpreted, and what conclusions are drawn. Simply replacing “achievement gap” would not address the undermining nature of deficit-based research frameworks.
Researchers who used the term “achievement gap” may not have intended to use a deficit-thinking framework in their study. In fact, as we have demonstrated with our examples, some powerful articles exist in biology education research that used the term and also implicitly used one of the systems-level or asset-based frameworks we identified.
In these examples, we have reinterpreted the results of primary research with the frameworks we identified. This leads to two points of caution. The first is that we are adding another layer of interpretation, one that the original authors may not have intended. The second is that each example could be interpreted through multiple frameworks, especially because these frameworks overlap ( Figure 1 ). For example, Bangera and Brownell (2014) identify barriers to participating in independent undergraduate research experiences. Course-based undergraduate research experiences (CUREs) offer research opportunities to students who previously could not access them. As discussed earlier, we posited CUREs as an example of a way to reduce opportunity gaps. However, we could also have interpreted the act of implementing a CURE as repaying an educational debt by repairing a form of bias typical within the academy ( Figure 1 ).
Addressing educational inequities requires that biology education researchers quantify differences in performance across demographic groups ( Figure 2 ) and must be done with the utmost care. Disaggregating data is necessary, as is analyzing those data with a just framework that dismantles racial hierarchies and carefully considers the sources of data used to understand those inequities. The frameworks we choose affect our analysis; we must avoid the common trap of assuming that quantitative data and data analysis are free from bias. To illustrate the degree of subjectivity that enters data analysis, Huntington-Klein et al. (2021) found that when seven different researchers received copies of the same data set, each reported different levels of statistical significance, including one researcher who found an effect that was opposite to what the others found. Moving away from analyses based on the phrase “achievement gap” will avoid unintentionally reinforcing the racial bias and better reflect the intention of disaggregating data to quantify differences in performance across demographic groups to actively dismantle persistent educational inequities.
In addition to disaggregating and diversifying data on outcomes ( Figure 2 ), the biology education research community must consider how definitions of success may center White, middle-class ways of knowing and performing ( Weatherton and Schussler, 2021 ). In their recent essay, Weatherton and Schussler (2021) reported that, in articles published in LSE between the years 2015 and 2020, the word “success,” when defined, largely meant high GPAs and exam scores. This narrow definition of success prioritizes scientific content, whereas there are additional admirable goals by which success could be measured ( Figure 2 ; see also Weatherton and Schussler, 2021 and references therein). Moreover, the scientific skills that are valued are Eurocentric, rather embodying a diversity of scientific approaches ( Howard and Kern, 2019 ). In addition to the limitations of narrowly defining success as exam performance, it should be noted that tests themselves are not always fair or equitable across all student populations ( Martinková et al. , 2017 ); success measured in this way should be interpreted with caution, particularly when comparing students across different courses, institutions, or identities.
As we discussed earlier, instructors’ and researchers’ deep beliefs about educational success and achievement necessarily impact their actions. For this reason, we propose that interrogating the frameworks we use is necessary and that such interrogation should acknowledge harm that may have been inflicted. While writing this essay, for example, our understandings of the frameworks underlying our own research, teaching, and other engagements have grown. Much like the research studies we discuss, our intentions, actions, and frameworks can be and have been out of alignment. For example, our own actions with respect to departmental policies, course designs, and program structures have not always reflected the principles to which we subscribe. Although this essay focuses on frameworks in research, we provide a list of some questions that we have asked of ourselves and that could catalyze reflection in all areas of our professional work ( Table 1 ).
In conclusion, we have presented four ways to frame differences in academic performance across students from different demographic groups that firmly reject deficit-based thinking ( Figure 1 ). The notions of opportunity gaps and educational debt demonstrate how systems thinking can recognize socio-environmental barriers to student learning. Asset-based frameworks that include community cultural wealth and ethics of care can help identify actions that institutions, instructors, and students can take to meet learning goals. We hope that researchers in the field move forward by 1) avoiding, or at least minimizing, deficit thinking; 2) explicitly stating asset-based and systems-level frameworks that celebrate students’ accomplishments and move toward justice; and 3) using language consistent with their frameworks.
ACKNOWLEDGMENTS
We thank Starlette Sharp and our external reviewers for helpful feedback on this article. We live and work on the lands of the Kizh/Tongva/Gabrieleño, Duwamish, and Willow (Sammamish) People past, present, and future. We also acknowledge the people whose uncompensated labor built this country, including many of its academic institutions.
- Alliance to Reclaim Our Schools . ( 2018 ). Confronting the education debt: We owe billions to Black, Brown and low-income students and their schools (p. 25). Retrieved February 23, 2022, from http://educationdebt.reclaimourschools.org/wp-content/uploads/2018/08/Confronting-the-Education-Debt_FullReport.pdf Google Scholar
- Asai, D. J. ( 2020 ). Race matters . Cell , 181 (4), 754–757. https://doi.org/10.1016/j.cell.2020.03.044 Medline , Google Scholar
- Bangera, G., & Brownell, S. E. ( 2014 ). Course-based undergraduate research experiences can make scientific research more inclusive . CBE—Life Sciences Education , 13 , 602–606. https://doi.org/10.1187/cbe.14-06-0099 Link , Google Scholar
- Berkeley Biology Scholars Program . ( n.d. ). Home . Retrieved March 25, 2021, from https://bsp.berkeley.edu/home Google Scholar
- Canning, E. A., Muenks, K., Green, D. J., & Murphy, M. C. ( 2019 ). STEM faculty who believe ability is fixed have larger racial achievement gaps and inspire less student motivation in their classes . Science Advances , 5 (2), eaau4734. https://doi.org/10.1126/sciadv.aau4734 Medline , Google Scholar
- Carey, R. L. ( 2014 ). A cultural analysis of the achievement gap discourse: Challenging the language and labels used in the work of school reform . Urban Education , 49 (4), 440–468. https://doi.org/10.1177/0042085913507459 Google Scholar
- Carter, P. L., & Welner, K. G. ( 2013 ). Closing the opportunity gap: What America must do to give every child an even chance . New York, NY: Oxford University Press. Google Scholar
- Cho, S., Crenshaw, K. W., & McCall, L. ( 2013 ). Toward a field of intersectionality studies: Theory, applications, and praxis . Signs: Journal of Women in Culture and Society , 38 , 785–810. Google Scholar
- Coleman, J. S., Campbell, E. Q., Hobson, C. J., McPartland, J., Weinfeld, F. D., & York, R. L. ( 1966 ). Equality of educational opportunity . Washington, DC: U.S. Department of Health, Education, and Welfare. Google Scholar
- CUREnet . ( n.d. ). Home page . Retrieved May 28, 2021, from https://serc.carleton.edu/curenet/index.html Google Scholar
- Darling-Hammond, L. ( 2013 ). Inequality and school resources: What it will take to close the opportunity gap? In Carter, P. L.Welner, K. G. (Eds.), Closing the opportunity gap: What America must do to give every child an even chance (pp. 77–97). : Oxford University Press. Google Scholar
- Dewsbury, B. M., & Brame, C. J. ( 2019 ). Inclusive Teaching . CBE—Life Sciences Education , 18 (2), fe2. https://doi.org/10.1187/cbe.19-01-0021 Link , Google Scholar
- Dinishak, J. ( 2016 ). The deficit view and its critics . Disability Studies Quarterly , 36 (4). http://dsq-sds.org/article/view/5236/4475 Google Scholar
- Dudley-Marling, C. ( 2007 ). Return of the deficit . Journal of Educational Controversy , 2 (1), 14. Google Scholar
- Eberhardt, J. ( 2019 ). Biased: Uncovering the hidden prejudice that shapes what we see, think, and do . New York, NY: Viking. Google Scholar
- Eddy, S. L., Brownell, S. E., & Wenderoth, M. P. ( 2014 ). Gender gaps in achievement and participation in multiple introductory biology classrooms . CBE—Life Sciences Education , 13 (3), 478–492. https://doi.org/10.1187/cbe.13-10-0204 Link , Google Scholar
- Eddy, S. L., & Hogan, K. A. ( 2014 ). Getting under the hood: How and for whom does increasing course structure work? CBE—Life Sciences Education , 13 (3), 453–468. https://doi.org/10.1187/cbe.14-03-0050 Link , Google Scholar
- Estrada, M., Eppig, A., Flores, L., & Matsui, J. T. ( 2019 ). A longitudinal study of the Biology Scholars Program: Maintaining student integration and intention to persist in science career pathways . Understanding Interventions , 10 , 26. Google Scholar
- Estrada, M., Young, G. R., Flores, L., Yu, B., & Matsui, J. ( 2021 ). Content and quality of science training programs matter: Longitudinal study of the Biology Scholars Program . CBE—Life Sciences Education , 20 (3), ar44. https://doi.org/10.1187/cbe.21-01-0011 Link , Google Scholar
- Farkas, G. ( 2004 ). The Black-White test score gap . Context , 3 , 12–19. https://doi.org/10.1525/ctx.2004.3.2.12 Google Scholar
- Freeman, S., Eddy, S. L., McDonough, M., Smith, M. K., Okoroafor, N., Jordt, H., & Wenderoth, M. P. ( 2014 ). Active learning increases student performance in science, engineering, and mathematics . Proceedings of the National Academy of Sciences USA , 111 (23), 8410–8415. https://doi.org/10.1073/pnas.1319030111 Medline , Google Scholar
- Gin, L. E., Guerrero, F. A., Cooper, K. M., & Brownell, S. E. ( 2020 ). Is active learning accessible? Exploring the process of providing accommodations to students with disabilities . CBE—Life Sciences Education , 19 (4), es12. https://doi.org/10.1187/cbe.20-03-0049 Link , Google Scholar
- Gorski, P. C. ( 2016 ). Poverty and the ideological imperative: A call to unhook from deficit and grit ideology and to strive for structural ideology in teacher education ,. Journal of Education for Teaching , 42 (4), 378–386. https://doi.org/10.1080/02607476.2016.1215546 Google Scholar
- Gouvea, J. S. ( 2021 ). Antiracism and the problems with “achievement gaps” in STEM education . CBE—Life Sciences Education , 20 (1), fe2. https://doi.org/10.1187/cbe.20-12-0291 Link , Google Scholar
- Grunspan, D. Z., Eddy, S. L., Brownell, S. E., Wiggins, B. L., Crowe, A. J., & Goodreau, S. M. ( 2016 ). Males under-estimate academic performance of their female peers in undergraduate biology classrooms . PLoS ONE , 16. Google Scholar
- Gutiérrez, R. ( 2000 ). Advancing African-American, urban youth in mathematics: Unpacking the success of one math department . American Journal of Education , 109 (1), 63–111. https://doi.org/10.1086/444259 Google Scholar
- Gutiérrez, R. ( 2008 ). A “gap-gazing” fetish in mathematics education? Problematizing research on the achievement gap . Journal for Research in Mathematics Education , 39 (4), 357–364. Google Scholar
- Hacisalihoglu, G., Stephens, D., Stephens, S., Johnson, L., & Edington, M. ( 2020 ). Enhancing undergraduate student success in stem fields through growth-mindset and grit . Education Sciences , 10 (10), 279. https://doi.org/10.3390/educsci10100279 Google Scholar
- Harper, S. R. ( 2010 ). An anti-deficit achievement framework for research on students of color in STEM . New Directions for Institutional Research 148 , 63–74. Google Scholar
- Harper, S. R. ( 2015 ). Success in these schools? Visual counternarratives of young men of color and urban high schools they attend . Urban Education , 50 , 139–169. https://doi.org/10.1177/0042085915569738 Google Scholar
- Harrison, C. D., Nguyen, T. A., Seidel, S. B., Escobedo, A. M., Hartman, C., Lam, K. , ... & Tanner, K. D. ( 2019 ). Investigating instructor talk in novel contexts: Widespread use, unexpected categories, and an emergent sampling strategy . CBE—Life Sciences Education , 18 (3), ar47. https://doi.org/10.1187/cbe.18-10-0215 Link , Google Scholar
- Howard, M. A., & Kern, A. L. ( 2019 ). Conceptions of wayfinding: Decolonizing science education in pursuit of Native American success . Cultural Studies of Science Education , 14 , 1135–1148. https://doi.org/10.1007/s11422-018-9889-6 Google Scholar
- Huntington-Klein, N., Arenas, A., Beam, E., Bertoni, M., Bloem, J. R., Burli, P. , ... & Stopnitzky, Y. ( 2021 ). The influence of hidden researcher decisions in applied microeconomics . Economic Inquiry , 59 (3), 944–960. https://doi.org/10.1111/ecin.12992 Google Scholar
- Jencks, C., & Phillips, M. ( 2006 ). The Black-White test score gap: An introduction . In The Black-White test score gap (pp. 1–51). Washington, DC: Brookings Institution Press. Google Scholar
- Jordt, H., Eddy, S. L., Brazil, R., Lau, I., Mann, C., Brownell, S. E. , ... & Freeman, S. ( 2017 ). Values affirmation intervention reduces achievement gap between underrepresented minority and white students in introductory biology classes . CBE—Life Sciences Education , 16 (3), ar41. https://doi.org/10.1187/cbe.16-12-0351 Link , Google Scholar
- Kendi, I. X. ( 2019 ). How to be an antiracist . New York, NY: One World. Google Scholar
- Kozol, J. ( 2005 ). The shame of the nation . New York, NY: Crown Publishing. Google Scholar
- Ladson-Billings, G. ( 2006 ). From the achievement gap to the education debt: Understanding achievement in U.S. schools . Educational Researcher , 35 (7), 3–12. https://doi.org/10.3102/0013189X035007003 Google Scholar
- Ladson-Billings, G. ( 2013 ). The dreamkeepers (2nd ed.). San Francisco, CA: Jossey-Bass. Google Scholar
- Martin, D. B. ( 2009 ). Researching race in mathematics education . Teachers College Record , 111 , 295–338. Google Scholar
- Martinková, P., Drabinová, A., Liaw, Y.-L., Sanders, E. A., McFarland, J. L., & Price, R. M. ( 2017 ). Checking equity: Why differential item functioning analysis should be a routine part of developing conceptual assessments . CBE—Life Sciences Education , 16 (2), rm2. https://doi.org/10.1187/cbe.16-10-0307 Link , Google Scholar
- Matsui, J. T., Liu, R., & Kane, C. M. ( 2003 ). Evaluating a science diversity program at UC Berkeley: More questions than answers . Cell Biology Education , 2 (2), 117–121. https://doi.org/10.1187/cbe.02-10-0050 Link , Google Scholar
- Menchaca, M. ( 1997 ). Early racist discourses: The roots of deficit thinking . In Valencia, R. R. (Ed.), The evolution of deficit thinking: Educational thought and practice ( The Stanford series on education and public policy ) (pp. 13–40). Washington, DC: Falmer Press/Taylor & Francis. Google Scholar
- Milner, H. R. ( 2012 ). Beyond a test score: Explaining opportunity gaps in educational practice . Journal of Black Studies , 43 (6), 693–718. https://doi.org/10.1177/0021934712442539 Google Scholar
- National science Foundation . ( 2020 ). Historically Black Colleges and Universities - Undergraduate Program (HBCU-UP) . Retrieved February 23, 2022, from https://beta.nsf.gov/funding/opportunities/historically-black-colleges-and-universities-undergraduate-program-hbcu Google Scholar
- Nieto, S., & Bode, P. ( 2012 ). Affirming diversity: The sociopolitical context of multicultural education (6th ed.). Boston, MA: Pearson Education. Google Scholar
- Noble, S. ( 2018 ). Algorithms of oppression (Illustrated ed.). New York: NYU Press. Google Scholar
- Noddings, N. ( 1988 ). An ethic of caring and its implications for instructional arrangements . American Journal of Education , 96 (2), 215–230. https://doi.org/10.1086/443894 Google Scholar
- Obermeyer, Z., Powers, B., Vogeli, C., & Mullainathan, S. ( 2019 ). Dissecting racial bias in an algorithm used to manage the health of populations . Science , 366 , 447–453. https://doi.org/10.1126/science.aax2342 Medline , Google Scholar
- Omi, M., & Winant, H. ( 2014 ). Racial formation in the United States (3rd ed.). New York, NY: Routledge. Google Scholar
- Painter, N. I. ( 2020 , July 22). Why “White” should be capitalized, too . Washington Post . Retrieved February 23, 2022, from www.washingtonpost.com/opinions/2020/07/22/why-white-should-be-capitalized Google Scholar
- Pang, V. O., Rivera, J., & Mora, J. K. ( 2000 ). The ethic of caring: Clarifying the foundation of multicultural education . Educational Forum , 64 , 25–32. Google Scholar
- Parnes, M. F., Kanchewa, S. S., Marks, A. K., & Schwartz, S. E. O. ( 2020 ). Closing the college achievement gap: Impacts and processes of a help-seeking intervention . Journal of Applied Developmental Psychology , 67 , 101121. https://doi.org/10.1016/j.appdev.2020.101121 Google Scholar
- Pearl, A. ( 1997 ). Cultural and accumulated environmental models . In Valencia, R. R. (Ed.), The evolution of deficit thinking: Educational thought and practice ( The Stanford series on education and public policy ) (pp. 132–159). Washington, DC: Falmer Press/Taylor & Francis. Google Scholar
- Quinn, D. M. ( 2020 ). Experimental effects of “achievement gap” news reporting on viewers’ racial stereotypes, inequality explanations, and inequality prioritization . Educational Researcher , 49 (7), 482–492. https://doi.org/10.3102/0013189X20932469 Google Scholar
- Rankins, C. ( 2019 ). HBCUs and Black STEM student success . Peer Review , 21 , 50–51. Google Scholar
- Ris, E. W. ( 2015 ). Grit: A short history of a useful concept . Journal of Educational Controversy , 10 (1). Retrieved February 23, 2022, from https://cedar.wwu.edu/jec/vol10/iss1/3 Google Scholar
- Rothstein, R. ( 2013 ). Why children from lower socioeconomic classes, on average, have lower academic achievement than middle-class children . In Carter, P. L.Welner, K. G. (Eds.), Closing the opportunity gap: What America must do to give every child an even chance (pp. 61–74). New York, NY: Oxford University Press. Google Scholar
- Sadker, D., Sadker, M. P., & Zittleman, K. R. ( 2009 ). Still failing at fairness: How gender bias cheats girls and boys in school and what we can do about it . New York, NY: Scribner. Google Scholar
- Seah, Y. M., Chang, A. M., Dabee, S., Davidge, B., Erickson, J. R., Olanrewaju, A. O., & Price, R. M. ( 2021 ). Pandemic-related instructor talk: How new instructors supported students at the onset of the COVID-19 pandemic . Journal of Microbiology & Biology Education , 22 . https://doi.org/10.1128/jmbe.v22i1.2401 Medline , Google Scholar
- Seidel, S. B., Reggi, A. L., Schinske, J. N., Burrus, L. W., & Tanner, K. D. ( 2015 ). Beyond the biology: A systematic investigation of noncontent instructor talk in an introductory biology course . CBE—Life Sciences Education , 14 (4), ar43. https://doi.org/10.1187/cbe.15-03-0049 Link , Google Scholar
- Shah, N. ( 2019 ). “Asians are good at math” is not a compliment: STEM success as a threat to personhood . Harvard Educational Review , 89 , 661–686. https://doi.org/10.17763/1943-5045-89.4.661 Google Scholar
- Steele, C. M. ( 2011 ). Whistling Vivaldi: How stereotypes affect us and what we can do (Reprint ed.). New York: Norton. Google Scholar
- Takacs, D. ( 2003 ). How does your positionality bias your epistemology? Thought & Action , 19 , 27–38. Google Scholar
- Tewell, E. ( 2020 ). The problem with grit: Dismantling deficit thinking in library instruction . Portal: Libraries and the Academy , 20 , 137–159. Google Scholar
- Theobald, E. J., Hill, M. J., Tran, E., Agrawal, S., Arroyo, E. N., Behling, S. , ... & Freeman, S. ( 2020 ). Active learning narrows achievement gaps for underrepresented students in undergraduate science, technology, engineering, and math . Proceedings of the National Academy of Sciences USA , 117 (12), 6476–6483. https://doi.org/10.1073/pnas.1916903117 Medline , Google Scholar
- Thompson, J. J., & Jensen-Ryan, D. ( 2018 ). Becoming a “science person”: Faculty recognition and the development of cultural capital in the context of undergraduate biology research . CBE—Life Sciences Education , 17 (4), ar62. https://doi.org/10.1187/cbe.17-11-0229 Link , Google Scholar
- Valencia, R. R. ( 1997 ). The evolution of deficit thinking: Educational thought and practice ( The Stanford series on education and public policy ). Washington, DC: Falmer Press/Taylor & Francis. Google Scholar
- Weatherton, M., & Schussler, E. E. ( 2021 ). Success for all? A call to re-examine how student success is defined in higher education . CBE—Life Sciences Education , 20 (1), es3. https://doi.org/10.1187/cbe.20-09-0223 Link , Google Scholar
- Wilson, K. J., Brickman, P., & Brame, C. J. ( 2018 ). Group Work . CBE—Life Sciences Education , 17 (1), fe1. https://doi.org/10.1187/cbe.17-12-0258 Link , Google Scholar
- Yosso, T. J. ( 2005 ). Whose culture has capital? A critical race theory discussion of community cultural wealth . Race Ethnicity and Education , 8 (1), 69–91. https://doi.org/10.1080/1361332052000341006 Google Scholar
- Yosso, T. J. ( 2006 ). Critical race counterstories along the Chicana/Chicano educational pipeline . New York, NY: Routledge. Google Scholar
- Young, J. L., Young, J. R., & Ford, D. Y. ( 2017 ). Standing in the gaps: Examining the effects of early gifted education on black girl achievement in STEM . Journal of Advanced Academics , 28 (4), 290–312. https://doi.org/10.1177/1932202X17730549 Google Scholar
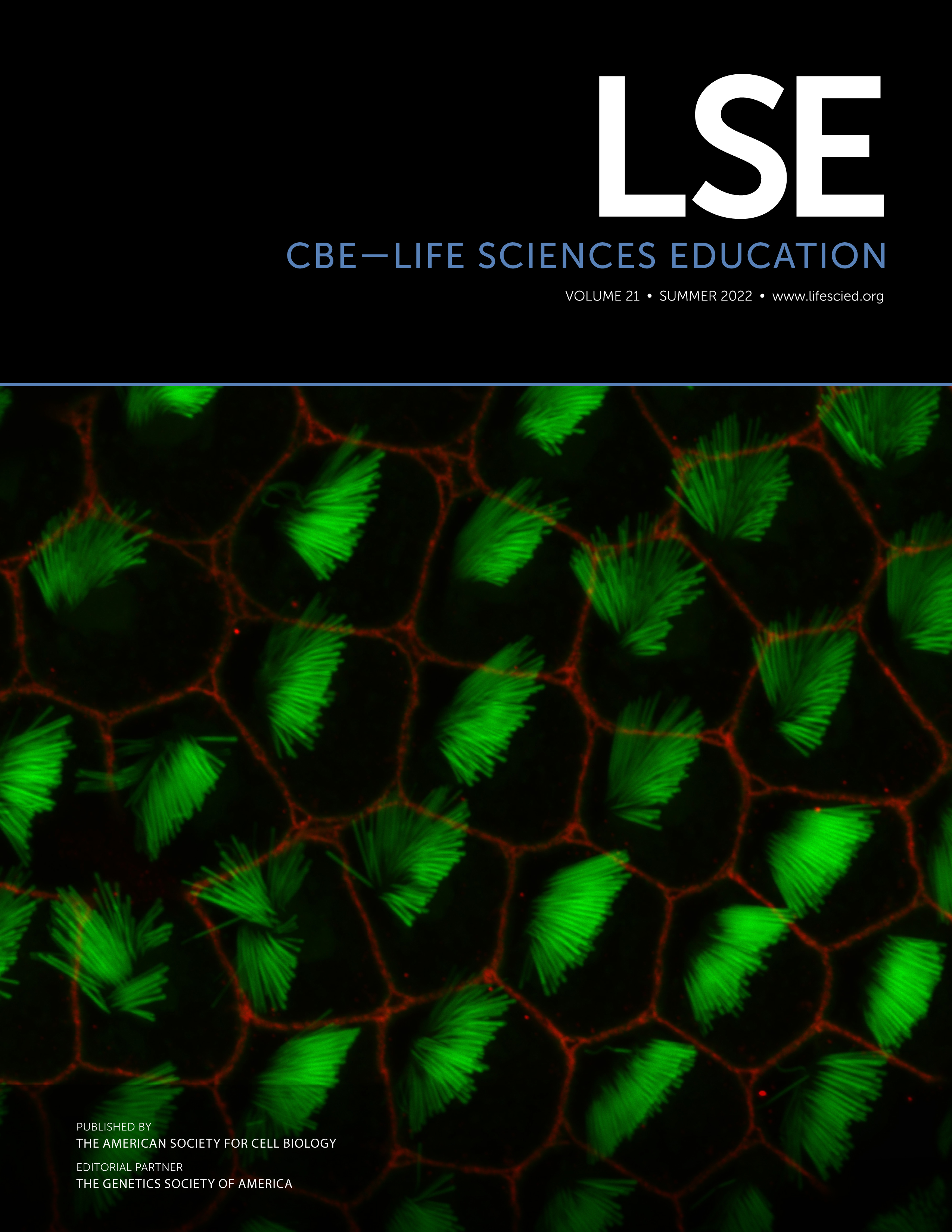
Submitted: 3 June 2021 Revised: 20 December 2021 Accepted: 2 February 2022
© 2022 S. Y. Shukla et al. CBE—Life Sciences Education © 2022 The American Society for Cell Biology. This article is distributed by The American Society for Cell Biology under license from the author(s). It is available to the public under an Attribution–Noncommercial–Share Alike 3.0 Unported Creative Commons License (http://creativecommons.org/licenses/by-nc-sa/3.0).
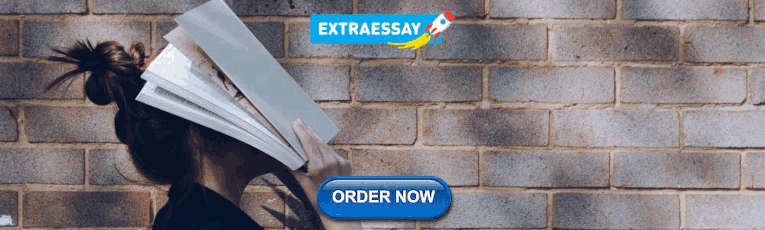
IMAGES
VIDEO
COMMENTS
Educational achievement, and its relationship with socioeconomic background, is one of the enduring issues in educational research. The influential Coleman Report 1 concluded that schools ...
Phillip J. Moore. Academic achievement was once thought to be the most important outcome of formal educational experiences and while there is little doubt as to the vital role such achievements play in student life and later (Kell, Lubinski, & Benbow, 2013 ), researchers and policy makers are ever increasingly turning to social and emotional ...
Academic achievement plays an important role in child development because academic skills, especially in reading and mathematics, affect many outcomes, including educational attainment, performance and income at work, physical and mental health, and longevity (Calvin et al., 2017; Kuncel & Hezlett, 2010; Wrulich et al., 2014).Not surprisingly, much research in the past several decades has ...
student, education and teaching are discussed (Voltmer & von Salisch, 2017). Candidates are firstly compared in terms of academic achievement in transition into an upper-level education or in employment (von Stumm, Hell, & Chamorro-Premuzic, 2011). Thus, academic achievement is important and determining the future education of individuals and job
Introduction. The family has been recognised as one of the primary contributors to children's and adolescents' success in school. In one of the earliest and best-known studies about the influence of families and schools on student achievement and educational opportunities, Coleman et al. (Citation 1966) concluded that family background matters most, whereas there are few differences ...
Abstract. The foundations of socioeconomic inequities and the educational outcomes of efforts to reduce gaps in socioeconomic status are of great interest to researchers around the world, and narrowing the achievement gap is a common goal for most education systems. This review of the literature focuses on socioeconomic status (SES) and its ...
The purpose of this study is to analyze the effect of online education, which has been extensively used on student achievement since the beginning of the pandemic. In line with this purpose, a meta-analysis of the related studies focusing on the effect of online education on students' academic achievement in several countries between the years 2010 and 2021 was carried out. Furthermore, this ...
Educational achievement is important to society and to children as individuals. In fact, educational achievement has been shown to be a good predictor of many life outcomes, such as occupational ...
Ample evidence indicates that school absences are detrimental to pupils' academic achievement (Aucejo and Romano 2016; Gershenson, Jacknowitz, and Brannegan 2017; Gottfried 2010, 2011; Gottfried and Kirksey 2017; Klein, Sosu, and Dare 2022; Morrissey, Hutchison, and Winsler 2014; Smerillo et al. 2018).For instance, children who were frequently absent during early kindergarten had lower ...
income and minority students who struggle with low achievement and high dropout rates. There has been an explosion of academic interest in incentive based education pro-grams in recent years.3 Our program is closest in design to a series of randomized ex-1Related research finds evidence that high discount rates among adolescents can partially ...
researchers around the world, and narrowing the achievement gap is a common goal. for most education systems. This review of the literature focuses on socioeconomic. status (SES) and its related ...
Background. Historical research shows that influencing student motivation is a factor in achieving academic success. The use of a competency-based educational (CBE) model provides a challenge in recognition of students who exceed the competency requirements of a given task or written paper.
Second, research shows that children in single-parent households score below children in two-parent households, on average, on measures of educational achievement (Amato, 2005; Brown, 2010; McLanahan and Sandefur, 1994). The combination of these two observations suggests that the rise in single parenthood has lowered (or slowed improvements in ...
Abstract. Academic achievement represents performance outcomes that indicate the extent to which a person has accomplished specific goals that were the focus of activities in instructional ...
1. Introduction. Over the past years, the student population applying to and entering university has become more diverse in terms of social, cultural and economic capital, age, nationality (Morlaix & Suchaut, 2014), prior education, and academic achievement (Anderton, Evans, & Chivers, 2016).Moreover, in many countries, social changes have also contributed to changes in higher education ...
Abstract. This paper is a quantitative synthesis of research into parental involvement and academic achievement through a meta-analysis of 37 studies in kindergarten, primary and secondary schools carried out between 2000 and 2013. Effect size estimations were obtained by transforming Fisher's correlation coefficient.
As a matter of fact, the top 2 factors, namely, prior-academic achievement, and student demographics, were presented in 69% of the research papers. This observation is aligned with the results of The previous literature review which emphasized that the grades of internal assessment and CGPA are the most common factors used to predict student ...
Introduction. Achievement motivation energizes and directs behavior toward achievement and therefore is known to be an important determinant of academic success (e.g., Robbins et al., 2004; Hattie, 2009; Plante et al., 2013; Wigfield et al., 2016).Achievement motivation is not a single construct but rather subsumes a variety of different constructs like motivational beliefs, task values, goals ...
In biology education research, many papers still use the language of "achievement gap," even in instances when researchers explicitly or implicitly use other nondeficit frameworks. ... From the achievement gap to the education debt: Understanding achievement in U.S. schools. Educational Researcher, 35 (7), 3-12. 10.3102/0013189X035007003 ...
Review of Educational Research. The Review of Educational Research (RER) publishes critical, integrative reviews of research literature bearing on education, including conceptualizations, interpretations, and syntheses of literature and scholarly work in a field broadly relevant to … | View full journal description.
This study suggests focused treatments for low-income students. Policymakers and educators may reduce the achievement gap and promote fair education by understanding how Socioeconomic status ...
The research results indicated that school bullying exists in all schools regardless if they are governmental or private ones. The study also concluded that school bullying affect student's academic achievement either victims or the bullies. Keywords: school bullying, academic achievement, teachers 1. Introduction
In biology education research, many papers still use the language of "achievement gap," even in instances when researchers explicitly or implicitly use other nondeficit frameworks. While some may argue that this language merely describes a pattern, its origin and history is explicitly and inextricably linked to deficit-thinking models ...