Click through the PLOS taxonomy to find articles in your field.
For more information about PLOS Subject Areas, click here .
Loading metrics
Open Access
Peer-reviewed
Research Article
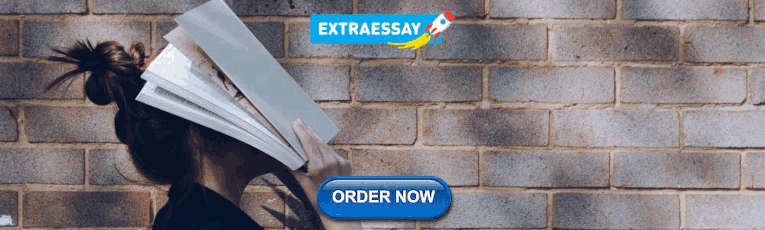
The contrasting effects of body image and self-esteem in the makeup usage
Roles Data curation, Formal analysis, Funding acquisition, Investigation, Project administration, Visualization, Writing – original draft, Writing – review & editing
* E-mail: [email protected]
Affiliation Department of Experimental Psychology, University of São Paulo, São Paulo, Brazil

Roles Data curation, Funding acquisition, Writing – review & editing
Roles Conceptualization, Funding acquisition, Methodology, Writing – review & editing
Roles Conceptualization, Funding acquisition, Methodology, Project administration, Resources, Supervision, Writing – review & editing
- Anthonieta Looman Mafra,
- Caio S. A. Silva,
- Marco A. C. Varella,
- Jaroslava V. Valentova
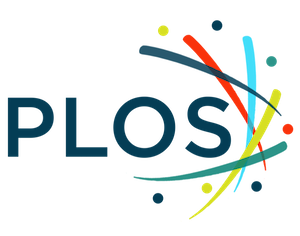
- Published: March 25, 2022
- https://doi.org/10.1371/journal.pone.0265197
- Peer Review
- Reader Comments
Women wearing makeup are perceived by others as more attractive, competent, dominant, and more socially prestigious. Individuals differ in how much and how frequently they use makeup. Some studies show that women with lower self-esteem use more makeup, probably to hide imperfections. However, women with higher self-esteem can also use makeup to attract attention. This study verified whether social and general self-esteem and body image are associated with makeup usage in Brazilian women. We collected data from 1,483 women ( M age = 31.08; SD = 11.15) about body image (appearance orientation and appearance evaluation), social self-esteem, general self-esteem, and makeup usage (frequency of makeup usage, time spent applying makeup per day, and money spent on makeup per month). Appearance orientation positively predicted frequency of makeup usage, time spent applying makeup, and money spent on makeup, whereas appearance evaluation inversely predicted money spent on makeup per month. Social self-esteem and general self-esteem also positively predicted money spent on makeup, but in different directions. The results suggest that the significance given to appearance and social interactions are importantly associated with makeup usage in women.
Citation: Mafra AL, Silva CSA, Varella MAC, Valentova JV (2022) The contrasting effects of body image and self-esteem in the makeup usage. PLoS ONE 17(3): e0265197. https://doi.org/10.1371/journal.pone.0265197
Editor: Piotr Sorokowski, University of Wroclaw, POLAND
Received: July 22, 2021; Accepted: February 26, 2022; Published: March 25, 2022
Copyright: © 2022 Mafra et al. This is an open access article distributed under the terms of the Creative Commons Attribution License , which permits unrestricted use, distribution, and reproduction in any medium, provided the original author and source are credited.
Data Availability: Data used to this paper can be found by accessing the following link: https://osf.io/d2z7e/ (DOI: 10.17605/OSF.IO/D2Z7E ).
Funding: AM was supported by the FAPESP (Fundação de amparo à pesquisa do estado de são paulo, Grant number: 2018/16370-5), CS was supported by CNPq (Conselho nacional de desenvolvimento científico e tecnológico, Grant number 143811/2019-3), MV was supported by the CAPES (Coordenação de Aperfeiçoamento de Pessoal de Nível Superior, Grant number 33002010037P0—MEC/CAPES).
Competing interests: The authors have declared that no competing interests exist.
Introduction
Surveys with American women showed that 78% spent one hour per day on their appearance (e.g., hair treatments, dressing up, and makeup). Taking on average 55 minutes of women’s day, hair and makeup seemed to need more time invested than other appearance related behaviors. [ 1 ] American women did not only spend time on active appearance enhancing behaviors, but between the most watched categories by women on YouTube, the top two are appearance related [ 1 ]. Another study showed that American women spent, on average, 10 minutes on makeup in the morning and 85% tended to apply at least 16 products on their faces before leaving home. The results also concluded that New York women spent around 300,000 US dollars during their lifetime on facial cosmetics [ 2 ]. These examples illustrate the importance American women attribute to physical appearance and self-care [e.g., 3 ].
Women’s attractiveness is an important factor in their lives, affecting how they feel about themselves. Several studies found a positive relationship between attractiveness and self-esteem in women [e.g., 4 , 5 ]. Cash et al. [ 6 ] found that physical appearance is positively correlated with appearance satisfaction, and Grilo et al. [ 7 ] found a positive relation between appearance evaluation and general self-esteem. However, these studies have focused on general self-esteem.
Whereas general self-esteem reflects how a person feels about themselves and their value in comparison to others [ 8 ], social self-esteem is how individuals feel about themselves during social interactions with others, and how those interactions affect their social value [ 9 ]. In this way, social self-esteem is more affected by social interactions than general self-esteem. Social self-esteem is positively linked to use of social network sites [ 10 , 11 ] whereas general self-esteem appears to be inversely proportional to social network sites [ 12 , 13 ], especially in women [ 14 ]. Vogel and colleagues [ 15 ] found that social comparison negatively affected general self-esteem negatively. Thus, the increase in digital media usage increased the pressure to look as good as possible because people were increasingly exposed to images and videos of very good looking individuals [ 16 ]. On the other hand, Steinsbekk and colleagues [ 14 ] found that self-oriented social network sites use was not related to general self-esteem. Therefore, social network sites might increase social self-esteem by promoting more social interactions.
Although appearance can be manipulated for better or for worse [ 17 ], the most common direction of appearance manipulation is appearance enhancement rather than worsening. Appearance enhancement is considered to be a self-promotion strategy in which one may capture more attention from others [ 18 ]. To enhance their attractiveness and to look better than same sex peers, women in Western societies use various tactics, such as high heels [e.g., 19 ], cosmetic surgeries [e.g., 20 ], and/or makeup [e.g., 21 ]. Interestingly, Kelley [ 16 ] interviewed 132 American college women and found that 37% of them reported they started using makeup because they were unsatisfied with their appearance. In sixth grade girls with negative self-esteem, using makeup increases pleasure pursuit [ 22 ]. Gentina et al. [ 23 ] found that makeup can also serve as a ritual of transition to adulthood among adolescents.
A recent experimental study compared women’s self-perception without makeup and with professionally applied makeup and showed that made up women considered themselves more feminine, attractive, more satisfied with appearance, and as having higher self-esteem [ 24 ]. Similarly, women wearing their usual facial cosmetics rated themselves as more attractive than when the cosmetics were removed [ 6 ]. A cross-sectional study further reported that women who rated themselves lower on physical attractiveness used more makeup [ 25 ].
Although individual differences in physical facial attractiveness are larger than intra-individual differences caused by facial cosmetics [ 26 ], makeup is used to improve evaluation by others [ 6 , 27 ] and may enhance prosocial feelings [ 25 ]. However, contrasting results were found regarding self-esteem and their relationship with makeup usage. Robertson and colleagues [ 25 ] found that self-esteem is negatively related to cosmetic usage whereas Al-Samydai et al. [ 28 ] findings pointed to a positive association. Nevertheless, the contrasting results may be due to the characteristics of the samples: the first study was conducted on 30 British undergraduate women and the latter on 606 Jordanian women. Studies investigating the association between self-esteem and makeup usage in different sociocultural settings are needed.
There are several ways to measure appearance, including perception of physical attractiveness (e.g., facial or body attractiveness rated by others), morphological measures (e.g., muscularity, body shape), and body image (e.g., individuals’ attitude toward appearance). Body image is broadly used to measure appearance because it is the reflection of individual satisfaction with their physical appearance and the importance placed on always looking good [ 28 ]. Whereas appearance orientation measures the importance attributed to their own appearance, such as how important they think it is to always look good, appearance evaluation measures how attractive the individual considers themselves, how satisfied they are with their own body [ 28 ]. Thus, body image measures self-perception of their attractiveness and also the pressure they put on themselves to always look good. Researchers relating makeup and body image tend to approach only the appearance evaluation factor, leaving out appearance orientation [e.g. 6 , 29 ], despite several studies showing the impact social pressure exerts on individuals’ self-perception [ 7 ], self-esteem [ 10 ], and even well-being [ 11 ].
Thus, our goal was to test if makeup usage in women (frequency of makeup usage, money spent on makeup, and time spent applying makeup per day) is predicted by general and social self-esteems, and body image (self-perceived attractiveness and importance one gives to tidiness). Despite some contrasting findings, Al-Samydai et al. [ 28 ] pointed out that makeup enhances women’s social interactions and performance and Robertson et al. [ 25 ] found a positive relationship between makeup usage and self-presentation and self-consciousness. Therefore, we expected that makeup usage would be predicted by social-related aspects (appearance orientation and social self-esteem) rather than by appearance evaluation and general self-esteem.
Materials and methods
Participants.
A total of 1,651 Brazilian women took part in the research. For the present study, 1,483 women between 18 and 75 years old ( M age = 31.08; SD = 11.15) entered in the final analyses. All the 168 participants younger than 18 years had their data excluded. This sample was comprised 32.2% of women with graduate degrees, 26.1% undergraduate students, 20.6% women with completed undergraduate degrees, 10.0% women with secondary education, 9.3% graduate students, and 1.7% with no education or unfinished secondary education. Most of the women considered themselves White (73.0%), 17.8% indicated mixed ethnicity ( pardo ), 4.8% identified as Black, 2.8% as Asian, and 1.6% indicated “Other” ethnicity.
About family income, most of the participants (27.4%) declared from approximately US$ 499 to 998 (exchange rate of the day December 28 2021), 19.4% declared from US$ 998 to 1,496, 15.4% declared from US$ 166 to 498, 15% declared more than US$ 2,494, 11.8% declared from US$ 1,497 to 1,995, 8% from US$ 1,996 to 2,493, and 3.1% up to US$ 165. The average Brazilian income per capita was US$ 313 in 2018 (when most of the data was collected) [ 30 ]. Most participants were from Southeast Brazil.
Instruments
Participants completed a Qualtrics online questionnaire (Qualtrics, Provo, UT), containing sociodemographic questions, Cosmetics Use Inventory and additional questions, Social self-esteem questionnaire, General self-esteem questionnaire, and Body image scale.
Sociodemographic questions.
This section included questions such as age, sex, gender, sexual orientation, ethnicity, relationship status, current pregnancy, and current socioeconomic status (educational level and family income).
Cosmetics use inventory [ 31 ].
We used a part of an adapted version of the inventory [ 32 ] in which participants rate on a 7-point-scale the frequency they use from “never” to “always”: 1. base, concealer, and/or powder; 2. mascara; 3. eyeliner or eye pencil; 4. shade; and 5. lipstick and/or gloss. Higher averaged scores correspond to higher levels of facial cosmetic use. We used the version translated (and back translated) into Brazilian Portuguese. All the variables were positively associated (i.e., women who use more one type of makeup tend to use the other types of makeup more frequently, too) (See S1 Table in S1 File ).
Further, participants responded about their monthly expenses with makeup using the following options (in our survey in Brazilian Reals): USD 0, up to USD 2.50, USD 2.50–6, USD 6–10, USD 10–15, USD 15–20, USD 20–25, USD 25–50, more than USD 50. Time spent applying makeup per day was responded using the following options: less than 5 minutes, 5–10 minutes, 10–20 minutes, 20–30 minutes, more than 30 minutes.
Social self-esteem questionnaire [ 9 ].
This is a 30-item instrument composed of phrases describing one’s ability to deal with different social situations. The participant answers how accurately each sentence describes what her behavior or feelings would be in each situation on a six-point Likert scale. The higher the score, the greater the participant’s ease in dealing with social situations (e.g., “I make friends easily”). We used the version translated (and back translated) into Brazilian Portuguese (Cronbach α = 0.95).
General self-esteem questionnaire [ 8 ].
This is a 10-item instrument with a four-point Likert response scale. It contains affirmations about individuals’ feelings and beliefs about themselves (e.g., “On the whole, I am satisfied with myself”). We used the version translated and adapted into Portuguese and validated for the Brazilian population (Cronbach α = 0.91) [ 33 ].
Body image scale [ 28 ].
This is an attitudinal body image instrument composed of two subscales measuring appearance evaluation and appearance orientation with a five-point Likert response scale. The subscales are composed of 17 statements, 11 of them related to appearance orientation (e.g. “It is important that I always look good”) and six related to appearance evaluation (e.g. “I like my looks just the way they are”). We used the version translated (and back translated) into Brazilian Portuguese (Cronbach α = 0.82).
After written ethical approval by the local Institutional Review Board of Anhembi Morumbi University (nr. 2.960.684), participants were recruited through social media and institutional e-mails. Thus, it was a sample based on convenience, and does not represent the Brazilian population. Participants completed informed consent and then responded to anonymous online questionnaires. Inclusion criteria were to have access to the Internet and to be a Brazilian woman 18 years old or older. Participants took 30 minutes on average to complete the survey.
Data analyses
First, using IBM SPSS Statistics for Windows, version 21 (IBM Corp., Armonk, N.Y., USA), we checked data normality (See S2 Table in S1 File ). Most data were not normally distributed, and we thus conducted exploratory non-parametric correlations among makeup usage, social and general self-esteems, and body image in order to verify correlations among the independent variables and test for multicollinearity. The independent variables were weakly and moderately associated, with low risk of multicollinearity (VIF ranged from 1.002 to 2.002).
Second, to test for a possible effect on makeup usage, social and general self-esteems, and body image entered as independent variables into categorical regressions (CATREG). We chose to use this analysis because it uses an optimal scaling feature that solves the problem of lack of linearity of the scales and it calculates an optimal regression equation and the effect of each independent variable (appearance orientation, appearance evaluation, general self-esteem, and social self-esteem) on the dependent variables (frequency of makeup usage, money spent on makeup, and time spent doing makeup per day). All statistical tests were performed with the significance level indicated at .05.
Makeup usage descriptives
Most participants use makeup half of the time (26.2%) or sometimes (24.9%) and 44.9% spend less than five minutes applying makeup per day. Also, 19.6% spent nothing and 19.6% spent up to USD 2.50 on makeup per month. See S3 to S5 Tables in S1 File for detailed data.
Correlations between makeup usage and social and general self-esteem, and body image attitudes
Kendall correlation indicated that money spent on makeup per month, time spent applying makeup per day, and frequency of makeup usage are moderately and positively correlated (See Table 1 ). Further, these three measures of makeup usage are moderately and positively correlated to appearance orientation. Money spent on makeup per month and frequency of makeup usage are weakly and positively related to social self-esteem. Frequency of makeup usage also presented a positive and weak correlation with general self-esteem. Social self-esteem was moderately and positively correlated with general self-esteem, weakly and positively associated with appearance evaluation and appearance orientation. General self-esteem was moderately and positively associated with appearance evaluation.
- PPT PowerPoint slide
- PNG larger image
- TIFF original image
https://doi.org/10.1371/journal.pone.0265197.t001
The sociodemographic variables (age, family income, and educational level) were weakly and positively associated with money spent on makeup, frequency of makeup usage, general self-esteem, social self-esteem, and appearance evaluation. There were no associations among the sociodemographic variables and time spent on makeup and appearance orientation.
The effect of general and social self-esteems and body image on makeup usage
To test for a possible effect of social and personal self-esteems, and body image on makeup usage, we conducted three categorical regression models, with money spent on makeup per month, time spent applying makeup per day, and frequency of makeup usage as dependent variables. We also included the sociodemographic variables age, family income, and educational level in the analyses in order to control the variability of our sample ( Table 2 ).
https://doi.org/10.1371/journal.pone.0265197.t002
Only appearance orientation and age predicted time spent applying makeup per day and frequency of makeup usage, whereas all variables except educational level predicted money spent on makeup.
The aim of the study was to verify if general and social self-esteems and body image (i.e., appearance orientation and appearance evaluation) were associated with makeup usage among Brazilian women. Altogether, our findings suggested that women who feel comfortable with their appearance and have higher general self-esteem spent less money on makeup whereas women with higher social self-esteem spent more money on makeup; and women who allocated more importance to the way they looked not only spent more money on makeup but spent more time applying makeup and using makeup more frequently.
Appearance orientation was a significant predictor of makeup usage. Thus, women who give more importance to their appearance and are always neat, use makeup more often, spend more time applying makeup, and spend more money on makeup. Similarly, Robertson et al. [ 25 ] found a positive relationship between cosmetic usage and self-presentation. In women, appearance orientation is also linked to neuroticism and narcissism [ 34 ], eating disorders [ 35 ], and drive for muscularity in men [ 36 ]. Women frequently have their bodies objectified, i.e. treated like an object that exists to please others. Through self-objectification, they disconnect their bodies from their persons, and sometimes internalize this perspective and start evaluating and treating themselves as mere bodies [ 37 ], highlighting the visual assessment. Women with higher levels of self-objectification would place more attention to their appearance and grooming instead of other aspects, such as identity development. Therefore, appearance orientation may be used as a measure of self-objectification [ 34 ]. A deeper investigation about appearance orientation and self-objectification should be conducted in order to clarify if they are in fact measuring similar traits.
Further, when adolescents with positive body image were interviewed, they claimed their family and friends used to talk about their appearance, but not about their bodies (i.e., they comment about their clothing, hair style, makeup, etc., but not about their physical traits, such as how fat they are [ 38 ]). Thus, someone’s appearance orientation is more liable in commentaries than someone’s body. It suggests that appearance orientation would be more susceptible to social influences than appearance evaluation, and consequently, would be positively related to appearance modifications, including makeup usage. This would also explain why accepting oneself is negatively related to purchasing makeup.
Corroborating Frisén and Holmqvist’s [ 38 ] results, we found that women with higher appearance evaluation, i.e. women who were more satisfied with their appearance, tended to spend less money on makeup. Our study supported findings of Robertson and colleagues [ 25 ] who reported an inverse association between cosmetic usage and self-rated physical appearance. For these individuals, makeup usage may not be related to satisfaction with their body, so it does not make them feel physically more attractive. Indeed, cosmetics have a smaller impact on individuals higher in attractiveness than on less attractive individuals [ 26 ].
Frederick and Reynolds [ 39 ] presented the cognitive behavioral model in which makeup would be an appearance fixing strategy, being a response to emotions and thoughts related to body image. That is, factors experienced throughout an individual’s life influenced one’s body image by associating their appearance schema. Future experiences can activate this model, influencing how this new information is processed. The thoughts and emotions related to one’s schema will respond by adjusting self-regulatory processes. Makeup usage, thus, would be a way to improve body image through fixing imperfections in people who are not satisfied with their appearance (appearance schema) and are concerned about it.
Additionally, Mafra et al. [ 40 ] conducted a study on Brazilian men and women with low socioeconomic status and found that spending more money on cosmetics did not make women feel better about themselves (e.g., more attractive). According to a review by Tylka and Wood-Barcalow [ 41 ], positive body image is the acceptance of one’s own body, feeling happy and complete even knowing its imperfections and that it is not consistent with idealized images. Nevertheless, others’ perceptions also may influence individuals’ positive body image [ 39 ] as well as the type of watched advertisement may influence women’s self-esteem, body image, and mood [ 42 ].
Social self-esteem is a positive predictor of money spent on makeup per month. Although Robertson et al. [ 25 ] found a negative association between cosmetic usage and social confidence, adolescent girls reported to use makeup because they wanted to feel admired by the public [ 23 ], suggesting that makeup functions improve social impressions. In a recent review, Davis and Arnocky [ 18 ] argued that makeup may be used as a strategy to enhance social status. In fact, besides bringing advantages in attracting mates and competing with rivals [ 21 ], makeup usage was positively associated with social interaction and performance [ 43 ], with women who use makeup being perceived as more competent [ 44 ], more dominant, and higher in social prestige [ 45 ]. As social interactions are important for people with high social self-esteem levels, makeup may be a tool to increase confidence in interpersonal relationships. On the other hand, women who feel good about themselves (i.e., with high general self-esteem) tended to spend less money on makeup usage. This result also corroborated Robertson et al. [ 25 ], in which a negative correlation between makeup usage and general self-esteem was found.
According to a recent study, makeup use also affects other women. After being exposed to pictures of same-sex peers wearing makeup, Australian undergraduate women reported willingness to change their appearance, for example, hair, skin [ 29 ]. Possibly, women who give more importance to physical appearance invest more money also on other beauty products, not just makeup. Future research investigating how other products that can enhance women’s appearance relate to appearance orientation and ideal stereotypes of beauty could make a great contribution to the field.
Overall, our study suggested that women with greater self-esteem associated with social interactions would attribute higher importance to their appearance, resulting in more makeup usage. Makeup usage may enhance women’s confidence to deal with social situations. However, our study was cross-sectional, thus an experimental study could complement our approach by testing if social-related aspects influence the consumption of makeup more than intrinsic-related aspects. Another important point to highlight is that the social influence on makeup usage is rather speculative since we have not directly asked the participants about the possible impact of the media and social networks on their body image. Finally, our sample was composed by a majority of highly educated Caucasian women of medium to high family incomes, which does not fully represent the Brazilian population (mostly composed by Black and mixed ethnicities in low educational levels and low family income earnings). A study trying to reach more women outside the university may better represent the Brazilian population.
Supporting information
S1 file. additional analyses..
https://doi.org/10.1371/journal.pone.0265197.s001
Acknowledgments
We are grateful for English proofreading and helpful suggestions made by Prof. Daniel J. Kruger. We are also grateful to the participants and researchers who donated their time for this to become a reality.
- 1. Wonder. How much time or money do women spend researching or test driving skincare and/or beauty products in a month or a year? Wonder. 2017 April 27 [Cited 2021 March 5]. https://askwonder.com/research/time-money-women-spend-researching-test-driving-skincare-and-beauty-products-9tso6hkti
- 2. Barettom. How Much Is Your Face Worth? Our Survey Results Revealed! Skinstore. 2017 [Cited 2021 July 20]. https://www.skinstore.com/blog/skincare/womens-face-worth-survey-2017/
- 3. Varella MAC, Valentova JV, Fernández AM. Evolution of artistic and aesthetic propensities through female competitive ornamentation. In: Fisher ML, editor. The Oxford Handbook of Female Competition. New York: Oxford University Press; 2017. pp. 757–783.
- View Article
- Google Scholar
- PubMed/NCBI
- 8. Rosenberg M. Society and the adolescent self-image. Revised edition. Middletown, CT: Wesleyan University Press; 1989.
- 16. Kelley KE. Societal Pressure and Makeup Usage. M.Sc. Thesis, University of Houston-Clear Lake. 2019. https://uhcl-ir.tdl.org/handle/10657.1/1465
- 20. Ponchio MC, Martins CG, Vieira CBM, Menezes D. Fatores determinantes da propensão ao consumo de cirurgias plásticas estéticas [Determinants of the propensity to consume aesthetic plastic surgery]. Revista Brasileira de Marketing [Journal of Brazilian Marketing]. 2013;
- 26. Agência IBGE. POF 2017–2018: cerca de ¼ da renda disponível das famílias brasileiras é não monetária. Agência IBGE Notícias. 2020 November 26 [Cited 2022 February 1]. https://agenciadenoticias.ibge.gov.br/agencia-sala-de-imprensa/2013-agencia-de-noticias/releases/29490-pof-2017-2018-cerca-de-da-renda-disponivel-das-familias-brasileiras-e-nao-monetaria
- 32. Worsley RE. The effect of goal orientation, model idealization, and message framing on the effectiveness of cosmetics advertising. Doctoral Dissertation, University of Canterbury. 2015. https://ir.canterbury.ac.nz/handle/10092/10574
- Share full article
Advertisement
Supported by
Up the Career Ladder, Lipstick In Hand

By Catherine Saint Louis
- Oct. 12, 2011
WANT more respect, trust and affection from your co-workers?
Wearing makeup — but not gobs of Gaga-conspicuous makeup — apparently can help. It increases people’s perceptions of a woman’s likability, her competence and (provided she does not overdo it) her trustworthiness, according to a new study, which also confirmed what is obvious: that cosmetics boost a woman’s attractiveness.
It has long been known that symmetrical faces are considered more comely, and that people assume that handsome folks are intelligent and good. There is also some evidence that women feel more confident when wearing makeup, a kind of placebo effect, said Nancy Etcoff, the study’s lead author and an assistant clinical professor of psychology at Harvard University (yes, scholars there study eyeshadow as well as stem cells). But no research, till now, has given makeup credit for people inferring that a woman was capable, reliable and amiable.
The study was paid for by Procter & Gamble, which sells CoverGirl and Dolce & Gabbana makeup, but researchers like Professor Etcoff and others from Boston University and the Dana-Farber Cancer Institute were responsible for its design and execution.
The study’s 25 female subjects, aged 20 to 50 and white, African-American and Hispanic, were photographed barefaced and in three looks that researchers called natural, professional and glamorous. They were not allowed to look in a mirror, lest their feelings about the way they looked affect observers’ impressions.
One hundred forty-nine adults (including 61 men) judged the pictures for 250 milliseconds each, enough time to make a snap judgment. Then 119 different adults (including 30 men) were given unlimited time to look at the same faces.
The participants judged women made up in varying intensities of luminance contrast (fancy words for how much eyes and lips stand out compared with skin) as more competent than barefaced women, whether they had a quick glance or a longer inspection.
“I’m a little surprised that the relationship held for even the glamour look,” said Richard Russell, an assistant professor of psychology at Gettysburg College in Gettysburg, Pa. “If I call to mind a heavily competent woman like, say, Hillary Clinton, I don’t think of a lot of makeup. Then again, she’s often onstage so for all I know she is wearing a lot.”
However, the glamour look wasn’t all roses.
“If you wear a glam look, you should know you look very attractive” at quick glance, said Professor Etcoff, the author of “Survival of the Prettiest” (Doubleday, 1999), which argued that the pursuit of beauty is a biological as well as a cultural imperative. But over time, “there may be a lowering of trust, so if you are in a situation where you need to be a trusted source, perhaps you should choose a different look.”
Just as boardroom attire differs from what you would wear to a nightclub, so can makeup be chosen strategically depending on the agenda.
“There are times when you want to give a powerful ‘I’m in charge here’ kind of impression, and women shouldn’t be afraid to do that,” by, say, using a deeper lip color that could look shiny, increasing luminosity, said Sarah Vickery, another author of the study and a Procter & Gamble scientist. “Other times you want to give off a more balanced, more collaborative appeal.”
In that case, she suggested, opt for lip tones that are light to moderate in color saturation, providing contrast to facial skin, but not being too glossy.
But some women did not view the study’s findings as progress.
“I don’t wear makeup, nor do I wish to spend 20 minutes applying it,” said Deborah Rhode, a law professor at Stanford University who wrote “The Beauty Bias” (Oxford University Press, 2010), which details how appearance unjustly affects some workers. “The quality of my teaching shouldn’t depend on the color of my lipstick or whether I’ve got mascara on.”
She is no “beauty basher,” she said. “I’m against our preoccupation, and how judgments about attractiveness spill over into judgments about competence and job performance. We like individuals in the job market to be judged on the basis of competence, not cosmetics.”
But Professor Etcoff argued that there has been a cultural shift in ideas about self adornment, including makeup. “Twenty or 30 years ago, if you got dressed up, it was simply to please men, or it was something you were doing because society demands it,” she said. “Women and feminists today see this is their own choice, and it may be an effective tool.”
Dr. Vickery, whose Ph.D. is in chemistry, added that cosmetics “can significantly change how people see you, how smart people think you are on first impression, or how warm and approachable, and that look is completely within a woman’s control, when there are so many things you cannot control.”
Bobbi Brown, the founder of her namesake cosmetics line, suggested that focusing on others’ perceptions misses the point of what makes makeup powerful.
“We are able to transform ourselves, not only how we are perceived, but how we feel,” she said.
Ms. Brown also said that the wrong color on a subject may have caused some testers to conclude that women with high-contrasting makeup were more “untrustworthy.” “People will have a bad reaction if it’s not the right color, not the right texture, or if the makeup is not enhancing your natural beauty,” she said.
Daniel Hamermesh, an economics professor at the University of Texas at Austin, said the conclusion that makeup makes women look more likable — or more socially cooperative — made sense to him because “we conflate looks and a willingness to take care of yourself with a willingness to take care of people.”
Professor Hamermesh, the author of “Beauty Pays” (Princeton University Press, 2011), which lays out the leg-up the beautiful get, said he wished that good-looking people were not treated differently, but said he was a realist.
“Like any other thing that society rewards, people will take advantage of it,” he said of makeup’s benefits. “I’m an economist, so I say, why not? But I wish society didn’t reward this. I think we’d be a fairer world if beauty were not rewarded, but it is.”
Explore Our Style Coverage
The latest in fashion, trends, love and more..
An Unusual Path to Hollywood: Sobhita Dhulipala has taken on risky roles in her acting career, outside of India’s blockbuster hits . Now, she’s starring in Dev Patel’s “Monkey Man.”
These Scientists Rock, Literally: The Pasteur Institute in Paris, known for its world-altering scientific research , has been making advancements in another field: the musical arts.
JoJo Siwa Grows Up: Siwa, the child star turned children’s entertainer, who at first modeled her career on Hannah Montana, is now after her own Miley (Cyrus) moment .
Jill Biden Makes an Entrance: The first lady was glittering in crystals — days after Melania Trump stepped out in pink at a Palm Beach fund-raiser. Together, the pictures offer a harbinger of what is to come .
Creating Works of Ephemeral Beauty: A YouTube rabbit hole led Blanka Amezkua to a small Mexican town and the centuries-old craft of papel picado — chiseling intricate patterns into colorful paper flags.
New York Bridal Fashion Week: Reimagined classic silhouettes, a play on textures and interactive presentations brought fresh takes to the spring and summer 2025 bridal collections.
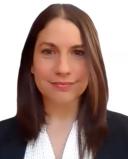
How Makeup Changes the Way We're Perceived
Our new research demonstrates the visual effects of cosmetics..
Posted November 18, 2022 | Reviewed by Tyler Woods
- The persistence and ubiquity of makeup is due, at least in part, to its perceptual potency.
- Faces wearing makeup are perceived as more attractive and they activate reward centers in the brain.
- Makeup makes facial skin look more even in part by increasing contrast between the skin and adjacent facial features.
Modern forms of makeup have been used at least since the ancient Egyptians, and pigments believed to be used on the body are among the earliest known human artifacts. The persistence and ubiquity of makeup is due, at least in part, to its perceptual potency. For instance, faces wearing makeup are perceived as more attractive and they activate reward centers in the brain .
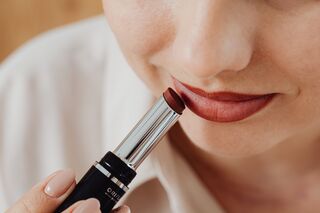
A common purpose of makeup is to make the skin appear more even. This is the purpose of complexion-oriented makeup products, such as foundation and concealer. Using two physical measurements of skin evenness, researchers have found that the skin does, indeed, have greater physical homogeneity with makeup than without makeup. The same study also reported evidence that cosmetics influence perceived skin evenness, with the same faces rated by participants as having more even skin when presented wearing makeup compared to not.
Interestingly, the effect size of the perceptual judgment was larger than the effect sizes of the physical measurements, suggesting that there are factors affecting the perception of skin evenness that are not captured by the physical measurements of isolated skin patches.
Therefore, a new scientific article published today (on which I am a co-author) aimed to explore the possibility that makeup makes facial skin look more even in part by increasing contrast between the skin and adjacent facial features.
To test this hypothesis, we ran two studies in which participants rated how even skin appeared in faces with and without makeup. The first study used digitally-applied makeup and the second study used actual cosmetic products applied by a professional makeup artist.
Critically, the faces in the makeup condition had cosmetics applied to the lips, eyes, and eyebrows, but no products applied to the skin. Thus, the skin was physically identical in both conditions; only the facial features differed.
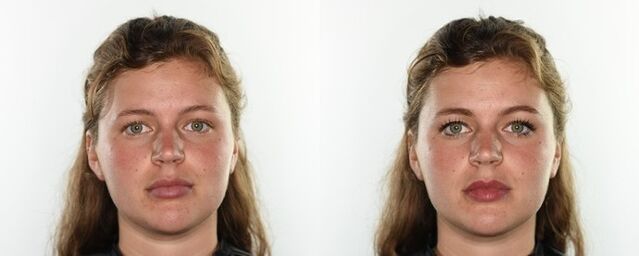
Whether makeup was applied digitally (Study 1) or by a professional makeup artist (Study 2), participants rated the skin as appearing more even in the condition with makeup applied to the eyebrows, eyes, and lips. These results demonstrate that cosmetics make skin appear more even not only through products like foundation and concealer that are applied directly to the skin, but also through products like lipstick and mascara that are applied to the features.
So, why does the skin appear more even when makeup is applied only to the features? We believe the appearance of spots and wrinkles may be suppressed by the increase in facial contrast from cosmetics. This study demonstrates that makeup affects skin appearance not only through the application of products to the skin, but also by modifying the visual context of the skin.
The study, “ Makeup applied to facial features increases perceived skin evenness ” was published today in the scientific journal Vision Research .
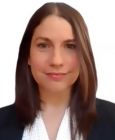
Carlota Batres, Ph.D. , is a psychology professor at Franklin and Marshall College and the director of the Preferences Lab. Her research focuses on understanding the information that faces convey and how it affects social outcomes.
- Find a Therapist
- Find a Treatment Centre
- Find Online Therapy
- Calgary, AB
- Edmonton, AB
- Hamilton, ON
- Montréal, QC
- Toronto, ON
- Vancouver, BC
- Winnipeg, MB
- Mississauga, ON
- Oakville, ON
- Asperger's
- Bipolar Disorder
- Chronic Pain
- Eating Disorders
- Passive Aggression
- Personality
- Goal Setting
- Positive Psychology
- Stopping Smoking
- Low Sexual Desire
- Relationships
- Child Development
- Therapy Center NEW
- Diagnosis Dictionary
- Types of Therapy
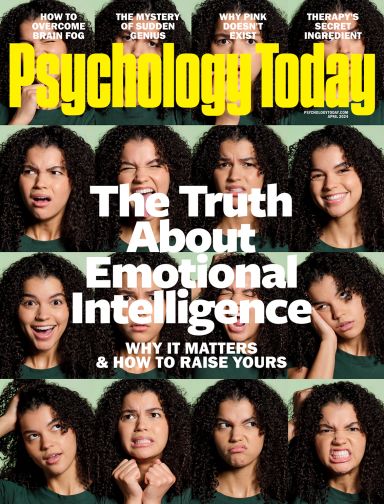
Understanding what emotional intelligence looks like and the steps needed to improve it could light a path to a more emotionally adept world.
- Coronavirus Disease 2019
- Affective Forecasting
- Neuroscience

An official website of the United States government
The .gov means it’s official. Federal government websites often end in .gov or .mil. Before sharing sensitive information, make sure you’re on a federal government site.
The site is secure. The https:// ensures that you are connecting to the official website and that any information you provide is encrypted and transmitted securely.
- Publications
- Account settings
Preview improvements coming to the PMC website in October 2024. Learn More or Try it out now .
- Advanced Search
- Journal List
- Springer Nature - PMC COVID-19 Collection

Changes in consumers’ awareness and interest in cosmetic products during the pandemic
Yeong-hyeon choi.
1 Dept. of Clothing and Textiles, Hanyang University, 222 Wangsimni-ro, Seongdong-gu, Seoul, Korea
Seong Eun Kim
2 Department of Textile and Apparel Management, University of Missouri, Columbia, Missouri USA
Kyu-Hye Lee
Associated data.
Please contact author for data requests.
This research investigates the impact of the COVID-19 pandemic on consumers’ perspectives of beauty and individual cosmetic products. Since the first confirmed case of COVID-19 was announced on December 31st, 2019, the search volumes of Google News have been updated and information on confirmed cases of the disease has been collected. This study used Python 3.7, NodeXL 1.0.1, and Smart PLS 3.0 to analyze consumer awareness of cosmetic products during the pandemic. The results reveal that consumers’ perspectives of beauty are impacted by a pandemic. Global consumers perceive skincare as an important aspect during the pandemic, while the importance of makeup fell after the outbreak. The awareness of skincare and makeup products has changed. The spread of the pandemic (SOP) has a positive impact on skincare products, but a negative impact on makeup products, except for eye makeup products, which was positive. Finally, the SOP was not significant in terms of consumers’ interest in masks. Fifth, interest in masks showed a positive relationship with interest in skincare products, such as cleansing products, while a negative relationship was observed with interest in makeup products. Overall, this study concludes that pandemics certainly have an impact on global consumers’ perspectives. As a pandemic spread, interest in skincare products increases, while interest in makeup products decreases. This study has academic significance in that it investigates the effects of consumption of cosmetic products during the stay-at-home rules. It can be used as standard information for setting marketing strategies in pandemic-like situations in the future.
Introduction
The coronavirus disease‑19 (COVID‑19) is an infectious disease caused by the severe acute respiratory syndrome coronavirus‑2 (SARS-CoV‑2) (Babu, 2020 ). As the number of confirmed cases of COVID-19 increased, the World Health Organization (WHO) announced a pandemic on March 11th, 2020. In an attempt to slow the pandemic, the WHO announced personal hygiene guidelines. Governments all over the world have induced people to follow the guidelines, which include regularly washing hands, social distancing, and wearing a medical mask (Cartaud et al., 2020 ). As the period of social distancing has been prolonged and people are wearing personal protective equipment (PPE) to prevent themselves from being infected, the overall demand for cosmetics has declined (Wischhover, 2020 ).
Since consumers now have to stay in their homes longer, they are stressed over difficulties in terms of their appearance (Pikoos et al., 2020 ). This situation led to people feeling less need or opportunity to wear makeup; hence, the overall demand for makeup products has dropped (Biskanaki et al., 2020 ). However, not all signals for the cosmetic industry are negative. Due to the current situation being conducive to wearing masks for prolonged periods, consumers are suffering from acne, and they are focusing more on skincare products (Rubin, 2020 ; Schiffer, 2020 ). Although the interest in makeup products has decreased, that for skincare products has shown a different direction in terms of sales. Interestingly, this behavior is a global phenomenon. Given that the preference for makeup products, such as eyeshadows or lipstick, is different from beauty awareness in each country, the present skincare-focused phenomena follow similar reactions and patterns. This indicates that a pandemic-like situation can make consumer’s attitude toward cosmetic products somewhat different to what it was before such a situation.
This behavior raises another intriguing point. The motivation of applying makeup is to express one’s identity, gain confidence, or be seen as a well-mannered person (Karabulut et al., 2020 ). No matter how little makeup consumers wear due to the PPE and stay-home policy, they still engage in social interactions. If consumers perceive wearing PPE as a tool for hiding themselves, they would not have an intention to use certain products. On the other hand, if consumers are only focused on survival during the pandemic, the results of cosmetic behavior would be more complicated.
Our research goal is to determine consumers’ cosmetic behavior in the COVID-19 pandemic situation. To do so, we (1) compare the perceptions of cosmetic products before and after the outbreak of COVID-19 using social big data analysis. (2) We establish the causal relationship between COVID-19 variances and purchase behavior of cosmetic products using partial least squares structural equation modeling (PLS-SEM). From a macro perspective, this study reveals the impact of COVID-19 on consumers’ attitude toward cosmetic and skincare products. The multi-dimensional approaches used herein contribute to an in-depth understanding of consumer behavior in the COVID-19 situation.
Literature review
The social self is “a tug-of-war” between societal similarity and individual uniqueness (Brewer, 1991 ). It is a human need to conform with others while simultaneously maintaining their individual presence. There are various ways to reveal one’s social self to society. Of these, makeup is a method to reveal and express one’s social self to society (Lee & Oh, 2018 ). In addition, according to which shades and the degree of makeup (from bare face to full makeup) a person wears, their social appearance could be easily and instantly changed. Due to this salience of makeup, the degree of makeup status is not only someone’s style but a metaphor for their feelings or intentions. However, COVID-19 has forced everyone to wear masks, which is a critical obstacle in constructing a social self through makeup. Thus, the decision of whether to apply makeup is an important indicator of cosmetic behavior, perception of a pandemic situation, and maintenance of societal appearance.
Social self and maskne
In the past, makeup was considered the preserve of women (Cash & Cash, 1982 ; Miller & Cox, 1982 ). However, male consumers have recently begun to show increased interest in caring for their appearances, which has led to a growth in the male cosmetic products market (Iida, 2006 ). Generation Y men tend to express their self-esteem through fashion products and are concerned about their bodies and weight (Sung & Yan, 2020 ). This phenomenon could provide the reason why male consumers have an increased interest in makeup, which is commonly viewed as an aesthetic concept. In fact, men want to be seen as more charming and masculine, not feminine (Souiden & Diagne, 2009 ). Thus, the context of makeup has shifted from being seen as more feminine to being able to express oneself to society (Kim & Choi, 2020 ).
There is now a dilemma regarding using makeup to reveal one’s social self. Due to COVID-19, wearing a mask is a normal state or mandatory regulation in some countries when people go out, meet someone, or are in a public space. This regulation obliges people to cover half of their facial area, which has induced consumers to practice a passive attitude toward cosmetics (Han et al., 2020 ). The fact that masks can cause flare-ups of acne is considered one of the main reasons for this attitude. As the inner temperature of the mask causes flare-up in the facial area, many consumers have suffered from acne due to prolonged periods of wearing masks (Park et al., 2020 ). Given the significantly positive correlation between cosmetics and acne (Perera et al., 2018 ), the sealing effect of the mask makes the wearer more susceptible to acne flare-ups. It is popularly known as “maskne or mask acne” (Kosasih, 2020 ).
The dilemma of makeup starts here. To some, applying makeup could be a really important ritual that helps them prepare to present their social self. However, due to the COVID-19 pandemic, there is an adverse synergy between makeup and wearing a mask for an extended period due to the aforementioned side effects, like acne or flushing (Yu, 2020 ). It presents an awkward situation in which wearing makeup causes bad skin conditions and going bare faced is somewhat embarrassing. If someone decides to wear makeup for social activity, they make it their priority to maintain their social self. On the other hand, if someone does not apply makeup when they are going out, they put more weight on caring for their skin conditions. The side effects of wearing a mask for long periods could be considered a minor problem when the benefit is maintaining our health. Given that makeup is one of the ways to present a good social self, a no-makeup situation may induce feelings of shame. In particular, consumers who are very aware of their surroundings and others’ evaluation may regard going bare faced as a shameful situation. It leads to degradation of their social self-esteem and also increases stress (Gruenewald et al., 2004 ).
In a pandemic-like situation, people experience death, anxiety, and depression (Lee et al., 2020 ). Anxiety regarding appearance management occurs. Pikoos et al. ( 2020 ) revealed that during COVID-19 restrictions, societal appearance pressure persisted in individuals with high dysmorphic concerns. Pikoos et al. ( 2020 ) found that a highly appearance-concerned group displayed feelings of pain and suffering due to the shutdown of beauty services, and the demand for cosmetics once restrictions are lifted has increased. This is the result of the anxiety that they cannot maintain their appearance and worry about it when they go out. This study regards pandemic anxiety variance as the number of confirmed cases. It means that as more confirmed cases occur and COVID-19 spreads, this leads to higher levels of anxiety (Elbay et al., 2020 ). Therefore, it is expected that if the spread of the pandemic (SOP) becomes more severe, anxiety will increase. As a result, the interest in all cosmetic product categories (skincare and makeup products) would show an incremental relationship.
- Research question 1 (R1): Pandemics will change consumers’ awareness of cosmetic products (skincare and makeup).
- Hypothesis 1 (H1): Spread of the pandemic (SOP) will positively influence interest in skincare products.
- Hypothesis 2 (H2): Spread of the pandemic (SOP) will positively influence interest in makeup products.
As one of the preventive measures, wearing a mask has become a default option during outdoor activities. In the early phase of the pandemic, there was significant interest in wearing a mask, as it was not a default option. However, before the outbreak of COVID-19, it was considered unusual behavior. More than a year since the outbreak began, mask wearing is no longer considered abnormal or special behavior. In a pandemic situation, the period during which a disease remains contagious is long time, and the interest in preventive measures shows a declining tendency (Bults et al., 2011 ). Consumers concentrate on their discomfort. In particular, wearing a mask for prolonged durations causes maskne, which is one of the direct and immediate side effects of the activity. This study expects that the interest in masks leads to incremental interest in skincare products but not in makeup products.
- Hypothesis 3 (H3): Spread of the pandemic (SOP) will not influence interest in face masks.
- Hypothesis 4 (H4): Interest in face masks will positively influence interest in skincare products.
- Hypothesis 5 (H5): Interest in face masks will negatively influence interest in makeup products.
The main variables and hypotheses of this study are illustrated in Fig. 1 .

Conceptual research framework
Social network analysis
One of the characteristics of social network and big data analysis is being able to investigate the potentially key elements of a study. This is done by extracting essential data and analyzing it through the bottom-up approach. It is cost effective and consumes less time as it does not require surveys or interviews to be conducted and this method is now being used in various fields (Niyirora & Argones, 2019 ; Wu et al., 2017 ). Social network analysis, the most commonly used method of analyzing big data, deduces the characteristics of the network by determining occurrence and regularity through appearance frequency and relationships between words (Park & Leydesdorff, 2004 ). Centrality, a measure of the central location of a node within a network, is used in the interpretation of data (Hanneman & Riddle, 2005 ).
Ahmed et al. ( 2020 ) investigated the COVID-19 situation using social network analysis. The authors examined Twitter posts and investigated the motive of the 5G COVID-19 conspiracy theory. They confirmed that the most popular web sources shared by users are fake news websites. Based on Twitter posts, Yum ( 2020 ) analyzed the role of public and political administrators in COVID-19 pandemic situation using social network analysis. Choi and Lee ( 2020 ) used text mining and social network analysis to examine the changes in consumers’ perspectives of fashion products during the pandemic. Korean fashion consumers were mainly concerned with precautions, home life, digital and cosmetic products, online channels, and consumption. This indicates a stronger stance on precaution than fashion during COVID-19 than in previous pandemics (SARS, MERS).
Measurement: Tf-idf and centralities
Term frequency-inverse document frequency ( Tf-idf ) and central value were measured and then analyzed. In PLS-SEM, development of worldwide confirmed cases was used as an exogenous variable, and search volumes of individual cosmetic products was used as an endogenous variable. Python 3.7 was used to measure Tf-idf value, and NodeXL 1.0.1 was used in the network analysis stage to measure centrality. Tf-idf , a measuring standard that supplements the frequency, is normally used to judge the importance of a certain word in a corpus. Tf-idf weighting is term frequency (tf) × inverse document frequency (idf) . Given a collection of terms t ∈ T that appear in a set of N documents d ∈ D , each of length n d , Tf-idf, weighting is computed as shown in Eq. 1 (Choi & Lee, 2021 ; Yahav et al., 2019 ).
Centrality analysis defines the meanings of each keyword in the context of a document (Choi et al., 2021 ), which are indicated in terms of degree, betweenness, closeness, and eigenvector centrality (Kwahk, 2014 ), as shown in Eqs. 2 – 5 . The centrality measure can be summarized by standardizing the equation as follows: (1) C x ( N i ) is the centrality of actor i in each calculation. (2) Every g is the number of actor i in networks (Kwahk, 2014 ; Wasserman & Faust, 1994 ). (3) The standardized actor betweenness centrality is then divided by the maximum value (( g − 1)( g − 2)/2) of the theoretical betweenness centrality (Freeman, 1979; Wasserman & Faust, 1994 ). (4) The value of closeness centrality for the number of actors g is C c ( N i ) =1/( g − 1), and the standardized actor closeness centrality is multiplied by g − 1 to consider the size of the entire network (Beauchamp, 1965 ; Freeman, 1978 ). (5) In eigenvector centrality, actor i is the i th element of unit eigenvector e , and e represents the largest eigenvalue of the adjacency matrix, with x as an element. X is an adjacency matrix with X ij as an element, and λ is an array of eigen values (Bonacich, 2007 ; Kwahk, 2014 ). To obtain eigenvector centrality, multiple steps of computation are performed. In the first step, the centrality value is calculated as the sum of the degree for g . The centrality value of each actor is then calculated as the sum of the first-stage centrality values of each actor. The final centrality values are calculated as the sum of the results of the second stage. This step-by-step computation process is repeated until the centrality value no longer changes.
Equation 1 Calculation of Tf-idf
Equation 2 Degree centrality of actor i
Equation 3 Betweenness centrality of actor i
Equation 4 Closeness centrality of actor i
Equation 5 Eigenvector centrality of actor i.
Preliminary investigation
The occurrence of the first recorded case of COVID-19 on December 31st, 2019 until September 30th 2020 was set as the standard period for collecting data. To compare consumers’ awareness, data between December 31st, 2018 and September 30th, 2019 were also collected. Preliminary investigation was conducted before selecting keywords to be investigated. We analyzed Google News, Twitter posts, and YouTube comments containing the words “corona” and “beauty” for nine months following the outbreak of COVID-19 (total: 8192).
As a result of preliminary investigation, according to appearance frequency, “mask” (687), “skincare” (490), “makeup” (459), and “haircare” (330) were identified as the beauty keywords related to COVID-19. In the network analysis, “skincare,” “makeup,” and “beauty” were set as the keywords. “Mask” showed a relationship with “corona,” but it also showed a strong relationship with “face” and “facial.” This suggests it may relate to medical masks and skin management masks, so it was excluded from the analysis. Google News was selected as the collecting channel as the data contained relatively less noise (Table (Table1 1 ).
Volume of data for each keyword
All data were collected from Google News
Data collection and analysis for PLS-SEM
PLS-SEM analysis was used to identify the impact of the spread of infectious disease on consumers’ interest in cosmetic products. To determine the development of the spread, worldwide confirmed cases were collected from the conoraboard.kr website, which provides real-time information about COVID-19. The data collection period was from January 21st, 2020 to September 30th, 2020, the range provided by the website. Consumers’ interest in cosmetic products is based on data on search volumes for keywords in Google Trends. The data were collected globally and limited to the category of “beauty & fitness.” In contrast, previous studies use “mask” as the main beauty keyword (e.g. Choi & Lee, 2020 ). Therefore, we identified the search volume data of the keywords “mask” and “acne.” Sub products of the specific cosmetic products are set according to social big data analysis in the Tf-idf standard.
To verify the model, we used development of coronavirus confirmed cases and search volume of keywords in Google Trends. Spread of disease is defined as the development of coronavirus confirmed cases. The measurement tool is the number of daily confirmed cases from January 21st to September 30th, 2020 obtained from conoraboard.kr. Mask interest is defined as consumers’ interest in masks, measured by the search volume of “mask” in Google. Interest in skincare and makeup are defined as consumers’ interest in individual products, measured by the search volume of all relative keywords in Google. As per the results of social network analysis, keywords with high Tf-idf are selected as representative items. Data on representative keywords are also collected within the same amount of time, using the same methods. A total of 253 records were collected and 13 items were measured. Smart PLS 3.0 was used to determine the influencing relationship. In PLS-SEM, the sampling was conducted 5000 times via bootstrapping. After verifying path coefficients and significance, it was evaluated by identifying the R 2 , f 2 , and Q 2 values.
Results and discussion
Comparison of consumers’ beauty awareness in the pandemic, based on tf-idf value.
Since the announcement of the first confirmed case of coronavirus on December 31st, 2019, social data including the keyword “beauty” have been collected. Keywords were distilled and centrality score and Tf-idf , which can complement simple frequency, were measured. The top 50 keywords were used in the analysis and the top 30 keywords according to the Tf-idf are reported in Table Table2 2 .
Consumers’ cosmetic awareness before and after COVID-19 outbreak
a , b , c , d Degree, betweenness, closeness, and eigenvector centrality, respectively
Before the COVID-19 pandemic, keywords such as “makeup” ( Tf-idf = 369.68), “product” (315.68), “brand” (276.77), “busy” (215.22), “routine” (200.66), “industry” (142.08), “natural” (140.15), “fashion” (138.77), “trick” (133.73), and “skincare” (132.91) appeared in the upper ranks of consumers’ awareness. Although keywords such as “product” (410.57), “makeup” (389.54), “brand” (328.95), “routine” (221.11), and “skincare” (191.71) appeared in the upper ranks, keywords such as “coronavirus” (191.71) and “pandemic” (85.51) appeared in the infectious disease keywords. These results indicate that a pandemic impacts general consumers’ awareness of cosmetics. Makeup remains the most significant product that composes consumers’ cosmetic awareness, irrespective of the pandemic situation. In the case of skincare, there has been an increase in rank since the pandemic.
Analysis of network structure and centrality
We extracted the top 50 keywords based on the co-occurrence frequency and then clustered them using the Wakita-Tsurumi algorithm. Consumer awareness of cosmetic behavior before COVID-19 outbreak is illustrated in Fig. 2 . Figure 3 represents the beauty behavior after the COVID-19 outbreak. In Fig. 3 , new keywords in relation to COVID-19 have emerged after the outbreak. The comparison of the network size is as follows. The maximum geodesic distance (diameter) of the networks for 2019 and 2020 is 3, and the overall networks were similar in size because both were equally limited to the top 50 keywords. In 2019, the total number of edges of the network was 590, the average geodesic distance was 1.76, and the graph density was 0.24. The total number of edges for 2020 was 706, the average geodesic distance was 1.72, and the graph density was 0.28. Compared to 2019, the significant increase in the number of edges in 2020 means that the correlation between keywords has increased within the context. As a result, we can see that the average geodesic distance of the graph has decreased, and the graph density has increased.

Consumers’ cosmetic awareness before COVID-19 (Wakita-Tsurumi algorithm)

Consumers’ cosmetic awareness after COVID-19 (Wakita-Tsurumi algorithm)
For centrality measurement, in the case of makeup, both degree and betweenness centrality declined in consumers’ cosmetic awareness after, rather than before, the pandemic. On the other hand, skincare showed an increase in all centrality items (C d , C b , and C e ) except closeness centrality after the outbreak. On this basis, while the influence of makeup products has decreased, that of skincare products has increased. Moreover, in the results of Tf-idf and centrality measurement, keywords of color cosmetics, such as “lipstick,” “foundation,” and “eyeshadow,” which appeared as representative keywords, did not appear in the upper ranks after the outbreak.
Before COVID-19, skincare was classified in the compact groups such as “salon,” “versatile,” and “home,” but after the outbreak, skincare joined larger groups comprising “global,” “digital,” “clean,” and “technology,” which account for 28% of the whole group. Furthermore, before the pandemic, makeup was classified into groups containing the words “celebrity,” “fashion,” “Fenty,” and “tutorial.” After the pandemic, it was reclassified into groups containing “pandemic,” “change,” “face,” “treatment,” “skin,” and so on. During the pandemic, skincare was considered as cleansing to prevent coronavirus infection, and makeup was considered in terms of skin problems due to the pandemic situation.
According to the network analysis, the appearance of keywords related to coronavirus determined that pandemics affect consumers’ cosmetic awareness. After the outbreak, global consumers recognized skincare as extremely important. Makeup was also regarded as being important; however, its importance importance showed a declining trend.
Consumers’ awareness of skincare and makeup
The results of preliminary investigation revealed that skincare and makeup appear as the main cosmetic behaviors and products. We therefore conducted a comparison of consumers’ awareness based on the keywords before and after pandemic (Table (Table3). 3 ). After the outbreak, “coronavirus” appeared as an upper ranked Tf-idf keyword in every cosmetic product. This highlights the fact that the COVID-19 pandemic impacts consumers’ cosmetic awareness.
Consumers’ cosmetic awareness before and after COVID-19
Words in bold = major cosmetic products. Italics = COVID-19 related words
The results of comparing representative keywords of individual cosmetic products before and after the pandemic are shown below. First, in the case of skincare, “self” ( Tf-idf = 55.17), “mask” (47.81), “prevention” (47.50), “maskne” (46.92), “acne” (38.39), and “lockdown” (36.75) appeared as the representative keywords. In terms of consumer’s cosmetic awareness, this indicates the appearance of skin problems (or acne) caused due to wearing masks to prevent infection and the phenomena of self-care at home due to lockdown. The main products are serum (56.61/31.43), sunscreen (41.37/45.55), cream (37.61/28.58), and cleansers (24.29/45.30). Additionally, keywords such as “mask” (47.81) and “Maskne” (46.92) appear as representative keywords in cosmetic awareness, include skincare products for “acne” (38.39).
Second, in terms of consumers’ awareness on makeup, keywords such as “mask” (43.98), “lockdown” (32.80), “home” (26.39), “COVID-19” (26.39), “distance” (26.39), “maskne” (25.05), and “washing” (25.05) appeared after the outbreak. As with skincare, skin problems caused by wearing masks and cleansing the face appeared as the main interest after the spread of the disease. In addition, words related to social distancing and lockdown phenomena related to makeup started to appear. The representative products are “lipstick” (48.85/60.95), “eyeliner” (38.86/ 53.80), and “foundation” (35.07/45.26).
The analysis of consumers’ cosmetic awareness of skincare and makeup products before and after the outbreak of the COVID-19 pandemic revealed keywords related to infectious disease (coronavirus, mask, lockdown). Therefore, it can be argued that consumers’ awareness regarding individual cosmetic products has been affected by the pandemic.
Spread of the pandemic and consumers’ interest in cosmetic products
Model evaluation.
Since the confirmed cases of COVID-19 and search volume of individual cosmetic products’ keywords are measured with a single measurement object, significance and suitability, such as convergent validity, multi-collinearity, outer weights, and outer loading do not need to be judged. The PLS-SEM model is assessed by R 2 , f 2 , and Q 2 values (Shin, 2018 ). In investigation of consumer behaviors, if the coefficient of determination for the endogenous variable is larger than R 2 = 0.20, it is judged to have a very high estimated suitability (Hair et al., 2017 ). The values of R 2 in the endogenous variable are skincare products ( R 2 serum = 0.31, R 2 sunscreen = 0.21, R 2 acne = 0.32, R 2 cleanser = 0.15, and R 2 cream = 0.25), makeup products ( R 2 eyeliner = 0.21, R 2 foundation = 0.36, and R 2 lipstick = 0.27), and mask ( R 2 = 0.20). The values of R 2 for acne, serum, and foundation are larger than R 2 = 0.30. The majority of the other endogenous variables were larger than R 2 = 0.20, indicating a high level of suitability.
If the effect size ( f 2 ) in PLS-SEM is greater than f 2 = 0.02, it indicates a small effect size for latent exogenous variables on latent endogenous variables. If it is greater than f 2 = 0.15, it indicates a medium effect size. If it is greater than f 2 = 0.35, it indicates a large effect size. The interest in cosmetic products due to the spread of disease showed foundation as ( f 2 = 0.53), which has an effect size larger than f 2 = 0.35 and it was identified as having the largest contribution. Serum ( f 2 = 0.17), acne ( f 2 = 0.25), lipstick ( f 2 = 0.28), and mask ( f 2 = 0.25) have medium effect sizes. Sunscreen ( f 2 = 0.09), cleanser ( f 2 = 0.07), and eyeliner ( f 2 = 0.04) have small effect sizes. Masks, cream ( f 2 = 0.21), foundation ( f 2 = 0.24), and lipstick ( f 2 = 0.26) showed medium effect sizes, and the others showed small effect sizes ( f 2 serum = 0.08, f 2 sunscreen = 0.05, f 2 acne = 0.03, f 2 cleansing = 0.03, and f 2 eyeliner = 0.11). Next, by identifying the estimated suitability ( Q 2 ) through the blindfolding process, all Q 2 values of the latent endogenous variables were larger than 0, indicating the suitability of the entire constitutive model.
Hypothesis testing
Sop and interest in cosmetic products.
The impact of the spread of the COVID-19 pandemic on global consumers’ awareness regarding individual cosmetic products is as follows (Table (Table4; 4 ; Fig. 4 ). The SOP had a positively influencing relationship with all skincare products: serum ( β = 0.50, p < 0.001), sunscreen ( β = 0.40, p < 0.001), cream ( β = 0.33, p < 0.001), cleanser ( β = 0.34, p < 0.001), and acne ( β = 0.52, p < 0.001). Therefore, it was clear that as the number of confirmed cases increased, global consumers’ interest in skincare products also increased. This result statistically proves news reports and interviews about changes in cosmetic behaviors due to the pandemic (Cerullo, 2020 ; Wallace & CNN Business, 2020 ). Therefore, H1 (H1a, H1b, H1c, H1d, H1e) was supported.
Direct path coefficients of SOP and interest in mask and individual cosmetic products
SOP spread of pandemic
* p < 0.05, ** p < 0.01, *** p < 0.001, respectively

Results of partial least squares structural equation modeling (PLS-SEM)
The SOP had a negatively influencing relationship with lipstick ( β = − 0.23, p < 0.001) and foundation ( β = − 0.41, p < 0.001). This is statistical proof that the sales of makeup products have decreased (Cerullo, 2020 ; Halliday, 2020 ). This decrease is due to wearing masks or staying at home to prevent infection and spreading of the virus. Since people decide not to apply makeup to the area of the face covered by a mask does not need to have makeup applied, interest in makeup has naturally fallen. Meanwhile, eyeliner ( β = 0.38, p < 0.001) showed a positively influencing relationship with regards to interest in the product. Opposite to the case of lipsticks and foundations, the eyes are still visible when wearing masks, so consumers tend to pay more attention to eye makeup, leading to the increased interest in eyeliners (see Table Table4 4 ).
“Maskne” behavior manifests in that consumers tend to focus their efforts on the exposed area of their face. This is also similar to Muslim females’ cosmetic behavior, in which they pay more attention to eye makeup as they cover the rest of their face due to their religious ideology. In each case, the social setting forces people to hide their facial area, which leads to the limited application of makeup, that is, eye makeup. Hill et al. ( 2012 ) argued cosmetics such as lipsticks are a decorative product. In a pandemic situation, since a mask covers the nose, lips, and chin, eye makeup products are considered a decorative product. Therefore, H2c was accepted, and H2a and H2b were rejected.
Interest in face mask and cosmetic products
The SOP and consumers’ interest in masks were β = 0.11, p > 0.05, indicating that it does not have a significantly influencing relationship; H3 is therefore rejected (Table (Table5). 5 ). However, the result of dividing the confirmed cases of COVID-19 by the timing of the spread was interesting. In the early phase from January to April 2020, it presented a high coefficient ( β = 0.80, p < 0.001). In the next phase from May to July 2020, SOP also revealed a positive impact on masks ( β = 0.57, p < 0.001), but it was lower than the previous phase. However, since the pandemic has been prolonged, the interest in masks has fallen and it seems to have levelled off. Consequently, the increase of confirmed cases may have influenced the relationship with the interest in masks. However, as the pandemic continues, it does not have a significantly influencing relationship; H3 was therefore accepted.
Direct path coefficients of SOP and interest in mask according to three phases of COVID-19 pandemic
Classification: based on the overall data and the spread of COVID-19
Although the interest in face masks did not form significantly influencing relationships with serum, sunscreen, and cream, it showed a positively influencing relationship with acne ( β = 0.15, p < 0.001) and cleansing ( β = 0.11, p < 0.05). This is similar to the background of the appearance of the word “maskne,” which proves the interest in masks has a relationship with skin problems, such as acne, caused by wearing masks. On the other hand, cream ( β = − 0.12, p < 0.05) had a significantly negative influencing relationship. Since “cream” can include moisturizing cream, BB cream, sun cream, and so on, it can be assumed that this affected the result.
Therefore, H4a, H4b, and H4c were rejected, and H4d and H4e were accepted. Meanwhile, interest in masks showed a negatively influencing relationship with lipstick ( β = − 0.46, p < 0.001), foundation ( β = − 0.36, p < 0.001), and eyeliner ( β = − 0.25, p < 0.001). As consumers’ interest in masks increases, interest in makeup products for the entire face decreases. Therefore, H5 (H5a, H5b, H5c) was accepted.
Conclusions
This research explored the changes in consumers’ awareness of cosmetic products during the pandemic. The results suggest that epidemiological situations, such as COVID-19, affect global consumers’ awareness of cosmetics and each cosmetic category (skincare and makeup). The SOP has increased consumers’ interest in skincare products, while interest in makeup products (lipstick and foundation) has decreased except for eyeliner products. This suggests that anxiety regarding of severity of COVID-19 not only affects interest in skin conditions but also that consumers seek to maintain their social self-esteem by wearing makeup on the exposed areas of their face (Gruenewald et al., 2004 ). However, interest in makeup products was shown to have a negative influencing relationship with masks. Obligation to wear a mask for an extended period may cause discomfort experiences (Park et al., 2020 ). In terms of masks and makeup products, consumers have expressed complaints and discussed the side effects of makeup and wearing PPE in the online community.
The SOP did not have a significantly influencing relationship with consumers’ interest in face masks. In the early stages of increased confirmed cases, a positively influencing relationship with the interest in face masks was observed, but it did not form a significantly influencing relationship as the pandemic progressed. It is obvious that the prolonged pandemic situation makes the preventive measure ineffective compared to the early phase (Bults et al., 2011 ). The outbreak has lasted more than a year and mask wearing has become the norm. Therefore, regardless of whether the number of confirmed cases is on the rise, the interest in mask wearing would not follow a similar trend. A summary of the results of this study is shown in (Table (Table6 6 ).
Summary of changes in consumers’ cosmetic awareness and interest after COVID-19 outbreak
In terms of practical contribution, this study analyzed global consumers’ macro awareness, allowing cosmetic companies to examine comprehensive opinions about consumers’ beauty habits during a pandemic. Therefore, if future pandemics have similar characteristics to the current COVID-19 outbreak, beauty companies have to pay more attention to the production of sanitary, cleanliness, and so-called trouble-care products. As consumers also consider their social self and social self-esteem as important as skincare despite the spread of pandemic, it is necessary to establish a niche makeup strategy to enable consumers to represent their own identity.
This study has the following limitations. We collected data in English, which is the global official language; therefore, it is difficult to apply this study to non-English speaking countries. Newspaper articles and industrial reports were used as references to best measure the social phenomena occurring in real time, and macro data were used to encompass all consumers. Future research should be conducted with much more elaborate theories and accurate measurements. From another viewpoint, demand for cosmetic products decreased in the early stage of the outbreak, and people created new forms of beauty behaviors such as “stay-home makeup” and “makeup for Zoom meetings.” Moreover, consumers’ preferences or behaviors regarding cosmetic products are subject to change, depending on the characteristics of infectious diseases. For example, if a new pandemic is not caused by a respiratory infection, the results derived from this study cannot be applied. Subsequent studies need to analyze new beauty behaviors after COVID-19.
Despite the aforementioned limitations, this study is considered important as it has statistically demonstrated that in a pandemic situation, interest in cosmetics may vary depending on their function and category. In addition, the motivations for wearing makeup could be construed as behavior of maintaining the social self. This provides the cosmetic industry some insights into the fact that consumers’ concerns about appearance in pandemic situations are diverse. Consumers are diverse and every case has customized needs for managing their societal appearance. Thus, the results have some managerial implications for the cosmetic industry in terms of which products they should focus on for production and establishing marketing strategies during pandemic situations. Moreover, as a new approach, this study expanded the generalizable scope by using macroscopic data such as social media text and volume of Google keyword searches.
Authors’ contributions
YHC and SEK designed the study and developed the theoretical framework and conducted analysis. SEK contributed data collection and analysis. KHL guided the development of the theoretical framework, results, and conclusion and reviewed the manuscript. All authors read and approved the final manuscript.
Authors’ information
Yeong-Hyeon Choi, Ph.D. Candidate, Department of Clothing and Textiles, Hanyang University, Seoul, Korea. Seong Eun Kim, Doctoral Student, Department of Textiles and Apparel Management, University of Missouri, Columbia, Missouri, USA. Kyu-Hye Lee, Professor, Human-Tech Convergence Major, Dept of Clothing and Textiles, Hanyang University, Korea.
No funding information
Availability of data and materials
Declarations.
The authors declare that they have no competing interests.
Publisher's Note
Springer Nature remains neutral with regard to jurisdictional claims in published maps and institutional affiliations.
Contributor Information
Yeong-Hyeon Choi, Email: rk.ca.gnaynah@hyiohc .
Seong Eun Kim, Email: ude.iruossim@xggks .
Kyu-Hye Lee, Email: rk.ca.gnaynah@eelhk .
- Ahmed W, Vidal-Alaball J, Downing J, Seguí FL. COVID-19 and the 5G conspiracy theory: Social network analysis of Twitter data. Journal of Medical Internet Research. 2020; 22 (5):e19458. doi: 10.2196/19458. [ PMC free article ] [ PubMed ] [ CrossRef ] [ Google Scholar ]
- Babu M. Face masks in coronavirus disease-19 infection. International Journal of Health & Allied Sciences. 2020; 9 (4):311–315. doi: 10.4103/ijhas.IJHAS_76_20. [ CrossRef ] [ Google Scholar ]
- Beauchamp MA. An improved index of centrality. Behavioral Science. 1965; 10 (2):161–163. doi: 10.1002/bs.3830100205. [ PubMed ] [ CrossRef ] [ Google Scholar ]
- Biskanaki F, Rallis Ε, Andreou Ε, Sfyri Ε, Tertipi Ν, Kefala V. Social-economic impact of COVID-19 pandemic on aesthetic centers in Greece. Journal of Cosmetic Dermatology. 2020; 19 (9):2165–2168. doi: 10.1111/jocd.13517. [ PMC free article ] [ PubMed ] [ CrossRef ] [ Google Scholar ]
- Bonacich P. Some unique properties of eigenvector centrality. Social Networks. 2007; 29 (4):555–564. doi: 10.1016/j.socnet.2007.04.002. [ CrossRef ] [ Google Scholar ]
- Brewer MB. The social self: On being the same and different at the same time. Personality and Social Psychology Bulletin. 1991; 17 (5):475–482. doi: 10.1177/0146167291175001. [ CrossRef ] [ Google Scholar ]
- Bults M, Beaujean DJ, de Zwart O, Kok G, van Empelen P, van Steenbergen JE, Voeten HA. Perceived risk, anxiety, and behavioural responses of the general public during the early phase of the Influenza A (H1N1) pandemic in the Netherlands: Results of three consecutive online surveys. BMC Public Health. 2011; 11 :2. doi: 10.1186/1471-2458-11-2. [ PMC free article ] [ PubMed ] [ CrossRef ] [ Google Scholar ]
- Cartaud A, Quesque F, Coello Y. Wearing a face mask against Covid-19 results in a reduction of social distancing. PLoS ONE. 2020; 15 (12):e0243023. doi: 10.1371/journal.pone.0243023. [ PMC free article ] [ PubMed ] [ CrossRef ] [ Google Scholar ]
- Cash TF, Cash DW. Women’s use of cosmetics: Psychosocial correlates and consequences. International Journal of Cosmetic Science. 1982; 4 (1):1–14. doi: 10.1111/j.1467-2494.1982.tb00295.x. [ PubMed ] [ CrossRef ] [ Google Scholar ]
- Cerullo, M. (2020, Mar 27). Sales of hair-dye kits jump as stuck-at-home workers cut off from salons. CBS News . https://www.cbsnews.com/news/women-hair-color-dye-coronavirus-quarantine-zoom
- Choi YH, Lee KH. Changes in consumer perception of fashion products in a pandemic: Effects of COVID-19 spread. The Research Journal of the Costume Culture. 2020; 28 (3):285–298. doi: 10.29049/rjcc.20.28.3.285. [ CrossRef ] [ Google Scholar ]
- Choi YH, Lee KH. Ethical consumers’ awareness of vegan materials: Focused on fake fur and fake leather. Sustainability. 2021; 13 (1):436. doi: 10.3390/su13010436. [ CrossRef ] [ Google Scholar ]
- Choi YH, Kim SE, Lee KH. Faux fur trade networks using macroscopic data: A social network approach. Sustainability. 2021; 13 (3):1427. doi: 10.3390/su13031427. [ CrossRef ] [ Google Scholar ]
- Elbay RY, Kurtulmuş A, Arpacıoğlu S, Karadere E. Depression, anxiety, stress levels of physicians and associated factors in Covid-19 pandemics. Psychiatry Research. 2020; 290 :113130. doi: 10.1016/j.psychres.2020.113130. [ PMC free article ] [ PubMed ] [ CrossRef ] [ Google Scholar ]
- Freeman LC. Centrality in social networks conceptual clarification. Social Networks. 1978; 1 (3):215–239. doi: 10.1016/0378-8733(78)90021-7. [ CrossRef ] [ Google Scholar ]
- Gruenewald TL, Kemeny ME, Aziz N, Fahey JL. Acute threat to the social self: Shame, social self-esteem, and cortisol activity. Psychosomatic Medicine. 2004; 66 (6):915–924. doi: 10.1097/01.psy.0000143639.61693.ef. [ PubMed ] [ CrossRef ] [ Google Scholar ]
- Hair JF, Jr, Hult GT, Ringle C, Sarstedt M. A primer on partial least squares structural equations modeling (PLS-SEM) 2. Sage Publications; 2017. [ Google Scholar ]
- Halliday, S. (2020, Apr 4). Nail care is the UK’s “lipstick effect” in the Covid-19 crisis, French brands benefit. Fashion Network . https://ww.fashionnetwork.com/news/Nail-care-is-the-uk-s-lipstick-effect-in-covid-19-crisis-french-brands-benefit,1205575.html
- Han C, Shi J, Chen Y, Zhang Z. Increased flare of acne caused by long-time mask wearing during COVID-19 pandemic among general population. Dermatologic Therapy. 2020; 33 (4):e13704. doi: 10.1111/dth.13704. [ PMC free article ] [ PubMed ] [ CrossRef ] [ Google Scholar ]
- Hanneman RA, Riddle M. Introduction to social network methods. University of California; 2005. [ Google Scholar ]
- Hill SE, Rodeheffer CD, Griskevicius V, Durante K, White AE. Boosting beauty in an economic decline: Mating, spending, and the lipstick effect. Journal of Personality and Social Psychology. 2012; 103 (2):275–291. doi: 10.1037/a0028657. [ PubMed ] [ CrossRef ] [ Google Scholar ]
- Iida Y. Beyond the ‘feminization of masculinity’: Transforming patriarchy with the ‘feminine’ in contemporary Japanese youth culture. Inter Asia Cultural Studies. 2006; 6 (1):56–74. doi: 10.1080/1462394042000326905. [ CrossRef ] [ Google Scholar ]
- Karabulut F, Aytac MB, Akin E. Makeup consumption and Islamic religiosity. Human Arenas. 2020; 3 :534–551. doi: 10.1007/s42087-020-00106-w. [ CrossRef ] [ Google Scholar ]
- Kim, J. H., & Choi, S. H. (2020). A study on the men's make-up motivation and their make-up attitudes. Korean Journal of Consumer and Advertising Psychology, 21(3), 453-470. 10.21074/kjlcap.2020.21.3.453
- Kosasih LP. MASKNE: Mask-induced acne flare during Coronavirus disease-19. What is it and how to manage it? Open Access Macedonian Journal of Medical Sciences. 2020; 8 (T1):411–415. doi: 10.3889/oamjms.2020.5388. [ CrossRef ] [ Google Scholar ]
- Kwahk KY. Social network analysis. CR Books; 2014. [ Google Scholar ]
- Lee H, Oh H. The effects of self-esteem on makeup involvement and makeup satisfaction among elementary students. Archives of Design Research. 2018; 31 (2):87–94. doi: 10.15187/adr.2018.05.31.2.87. [ CrossRef ] [ Google Scholar ]
- Lee SA, Jobe MC, Mathis AA, Gibbons JA. Incremental validity of coronaphobia: Coronavirus anxiety explains depression, generalized anxiety, and death anxiety. Journal of Anxiety Disorders. 2020; 74 :102268. doi: 10.1016/j.janxdis.2020.102268. [ PMC free article ] [ PubMed ] [ CrossRef ] [ Google Scholar ]
- Miller LC, Cox CL. For appearances’ sake: Public self-consciousness and makeup use. Personality and Social Psychology Bulletin. 1982; 8 (4):748–751. doi: 10.1177/0146167282084023. [ CrossRef ] [ Google Scholar ]
- Niyirora J, Argones O. Network analysis of medical care services. Health Informatics Journal. 2019; 26 (3):1631–1658. doi: 10.1177/1460458219887047. [ PubMed ] [ CrossRef ] [ Google Scholar ]
- Park HW, Leydesdorff L. Understanding the KrKwic: A computer program for the analysis of Korean text. Journal of the Korean Data Analysis Society. 2004; 6 (5):1377–1387. [ Google Scholar ]
- Park SR, Han J, Yeong YM, Kang NY, Kim EJ. Effect of face mask on skin characteristics changes during the COVID-19 pandemic. Skin Research and Technology. 2020 doi: 10.1111/srt.12983. [ PMC free article ] [ PubMed ] [ CrossRef ] [ Google Scholar ]
- Perera MPN, Peiris WMDM, Pathmanathan D, Mallawaarachchi S, Karunathilake IM. Relationship between acne vulgaris and cosmetic usage in Sri Lankan urban adolescent females. Journal of Cosmetic Dermatology. 2018; 17 (3):431–436. doi: 10.1111/jocd.12431. [ PubMed ] [ CrossRef ] [ Google Scholar ]
- Pikoos TD, Buzwell S, Sharp G, Rossell SL. The COVID-19 pandemic: Psychological and behavioral responses to the shutdown of the beauty industry. International Journal of Eating Disorders. 2020; 53 (12):1993–2002. doi: 10.1002/eat.23385. [ PubMed ] [ CrossRef ] [ Google Scholar ]
- Rubin, C. (2020, June 17). Maskne is the new acne, and here’s what is causing it. New York Times . https://www.nytimes.com/article/maskne-acne.html
- Schiffer, J. (2020, Aug 12). The beauty trends customers are buying during Covid-19. Vogue Business . https://www.voguebusiness.com/beauty/the-beauty-trends-customers-are-buying-during-covid-19
- Shin GK. Partial least squares structural equation modeling with SmartPLS 3.0, SPSS, G*Power. CR Books; 2018. [ Google Scholar ]
- Souiden N, Diagne M. Canadian and French men’s consumption of cosmetics: A comparison of their attitudes and motivations. Journal of Consumer Marketing. 2009; 26 (2):97–109. doi: 10.1108/07363760910940465. [ CrossRef ] [ Google Scholar ]
- Sung J, Yan RN. Predicting clothing behaviors of Generation Y men through self-esteem and body dissatisfaction. Fashion and Textiles. 2020; 7 :10. doi: 10.1186/s40691-019-0200-6. [ CrossRef ] [ Google Scholar ]
- Wallace, A., & CNN Business. (2020, Apr 11). Walmart CEO says we’re in the “hair color” phase of panic buying. CNN Business . https://edition.cnn.com/2020/04/11/business/panic-buying-walmart-hair-color-coronavirus/index.html
- Wasserman S, Faust K. Social network analysis: Methods and applications. Cambridge University Press; 1994. [ Google Scholar ]
- Wischhover, C. (2020, Aug 11). How the beauty industry is surviving the pandemic. Vox . https://www.vox.com/the-goods/21352703/beauty-industry-pandemic-cosmetics-makeup-skincare-lipstick-nuface
- Wu SH, Huang SCT, Tsai CYD, Lin PY. Customer citizenship behavior on social networking sites. Internet Research. 2017; 27 (2):428–448. doi: 10.1108/IntR-12-2015-0331. [ CrossRef ] [ Google Scholar ]
- Yahav I, Shehory O, Schwartz D. Comments mining with TF-IDF: The inherent bias and its removal. IEEE Transactions on Knowledge and Data Engineering. 2019; 31 (3):437–450. doi: 10.1109/TKDE.2018.2840127. [ CrossRef ] [ Google Scholar ]
- Yu, J. (2020, Mar 5)). How COVID-19 is impacting personal care and beauty in China. KANTAR . https://www.kantar.com/inspiration/coronavirus/how-covid-19-is-impacting-personal-care-and-beauty-in-china
- Yum S. Social network analysis for coronavirus (COVID-19) in the United States. Social Science Quarterly. 2020; 101 (4):1642–1647. doi: 10.1111/ssqu.12808. [ PMC free article ] [ PubMed ] [ CrossRef ] [ Google Scholar ]
Thank you for visiting nature.com. You are using a browser version with limited support for CSS. To obtain the best experience, we recommend you use a more up to date browser (or turn off compatibility mode in Internet Explorer). In the meantime, to ensure continued support, we are displaying the site without styles and JavaScript.
- View all journals
- My Account Login
- Explore content
- About the journal
- Publish with us
- Sign up for alerts
- Open access
- Published: 10 December 2021
Light makeup decreases receivers’ negative emotional experience
- Ling Zhang 1 na1 ,
- Wenfeng Chen 1 na1 ,
- Menghan Liu 1 ,
- Yuxiao Ou 1 ,
- Erjia Xu 1 &
- Ping Hu 1
Scientific Reports volume 11 , Article number: 23802 ( 2021 ) Cite this article
3089 Accesses
5 Citations
Metrics details
- Human behaviour
Makeup is widely used in modern society and has a positive effect on perceived attractiveness. However, little is known about the other possible outcomes of makeup use. In this study, we investigated whether makeup enhances a receiver’s emotional experience. Dynamic faces with or without makeup are presented in Experiments 1 and 2. Participants were asked to imagine themselves video chatting with a target person (expresser) with different expressions: neutral, angry, sad, or happy, and then to appraise their own subjective emotional experience. Emotional valence, arousal, and willingness to communicate were also assessed in Experiment 2. The results showed that makeup improved perceived facial attractiveness and increased the willingness to communicate. More importantly, it revealed that wearing makeup could weaken receivers’ negative experiences arising from the angry and sad conditions, which is not the case for the non-makeup condition, but could not affect the happy contagion. Furthermore, incremental changes in the amount of makeup were not accompanied by incremental changes in emotional appraisal (valence and arousal). Overall, we found that makeup may affect emotional contagion and interpersonal communication. Whether the alleviated negative experience due to makeup is adaptive may need further discussion.
Similar content being viewed by others
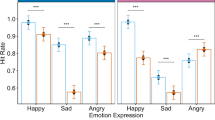
Influence of child and adult faces with face masks on emotion perception and facial mimicry
Till Kastendieck, Nele Dippel, … Ursula Hess
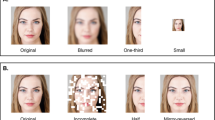
Humans judge faces in incomplete photographs as physically more attractive
Diana Orghian & César A. Hidalgo
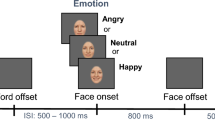
Deepfake smiles matter less—the psychological and neural impact of presumed AI-generated faces
Anna Eiserbeck, Martin Maier, … Rasha Abdel Rahman
Introduction
Video chat is a widely used communication medium due to its efficiency and convenience. In China, there is a phenomenon wherein individuals attach importance to their own facial attractiveness and attempt to improve their appearance through methods such as the use of the makeup mode during video calls. This led us to the question of whether there is a different emotional experience when a person with or without makeup expresses emotions such as happiness or anger.
Makeup is prevalent in daily life regardless of a person’s appearance and age; its use is encouraged in many situations, such as at work or for appointments. Its most common purpose is to improve facial attractiveness 1 , 2 , 3 , which refers to the positive and joyful emotional experience induced by attractive faces that motivates others to approach the person 4 . Wearing makeup is an intentionally guided strategy of self-presentation 5 , and it is used to cover up facial imperfections and make one appear more charming 6 . People who wear makeup are considered healthier and more confident than those without makeup 7 . Moreover, many benefits associated with natural facial attractiveness can also be experienced with the use of makeup 8 , 9 . This means that although makeup is artificial, it can achieve psychological consequences similar to those brought on by natural high attractiveness, such as a more positive evaluation of personality traits (e.g., self-confidence, sociability) and the perception of having higher economic or educational status 7 , 10 , 11 , 12 , 13 .
However, it is unknown if this effect also applies to emotional contagion, which refers to the process of transferring an emotion from one individual (expresser) to another (receiver) 14 . The receiver is influenced by the emotion of the expresser, which ultimately results in the receiver’s emotions becoming consistent with those of the expresser 15 , 16 . Despite the lack of direct evidence, previous research has indicated that individuals with higher attractiveness are more popular in social interactions 17 , 18 . For example, participants were more willing to participate in games with more attractive partners 19 . By contrast, individuals with lower attractiveness may experience negative treatment and evaluations in their social interactions. They may be subjected to dishonesty 20 , or considered less social or altruistic, or as having lower intelligence 21 . Facial attractiveness not only affects social interactions but also modulates emotional perception and may directly impact emotional experience 22 , 23 . Nevertheless, there is insufficient evidence to expound on the relationship between makeup and emotional contagion.
Based on the perspective that improved facial attractiveness is associated with certain benefits, it is reasonable to infer that makeup may facilitate emotional contagion via enhanced willingness for interaction and approachability. Facial attractiveness may have a reward value and lead to individuals experiencing positive feelings 24 , 25 . For example, highly attractive faces are considered to exhibit more positive expressivity than less attractive faces, such that even attractive faces with neutral expressions are usually rated as having positive expressions 26 , 27 , 28 . However, unattractive faces induce negative emotional responses 24 . When participants were required to observe highly attractive and less attractive faces while facial electromyography was simultaneously recorded, researchers found that less attractive faces triggered greater responses in the levator labialis muscle (associated with the disgust emotion) regardless of age (children or adults), implying that faces with low attractiveness may result in receivers’ negative emotions 24 . However, these studies indicate that natural facial attractiveness has more positively correlated social consequences, while beauty achieved artificially through makeup may not always have the same effect 1 , 2 . Excessive makeup may signify low morality 29 and trustworthiness 2 as well as less restricted sociosexuality 30 , but this is a false signal. It remains unknown whether makeup can modulate emotional experiences.
Furthermore, it remains unclear whether the role of makeup varies with the type of emotion. Evidence has supported that interpersonal interactions are affected by facial attractiveness, emotional expression, and the interaction between these two 22 , 27 . Highly attractive faces enhance the processing of positive emotions and exhibit more advantages associated with happy faces than angry faces 22 , while less attractive individuals are perceived to have more negative expressivity, although their expressions are actually neutral 27 .
Based on the above, four predictions for the role of makeup in emotional contagion may be made. First, emotional contagion will occur; expressers’ positive (or negative) emotions will evoke participants’ positive (or negative) experiences with or without makeup. Second, makeup will affect the degree of emotional contagion by enhancing the perceived valence of emotional expressions, thereby enhancing positive emotional contagion and weakening negative emotional contagion. Third, we hypothesize the effect of makeup on emotions may be unbalanced. Evidence from related studies has indicated an asymmetrical mutual influence of different emotional expressions and facial attractiveness 22 , 31 , 32 , which suggests that wearing makeup may only affect positive or negative emotional contagion. Moreover, for neutral expressions, we propose an open hypothesis. On the one hand, neutral expressions with higher attractiveness are usually considered to have positive valence 27 , 28 . On the other hand, maybe different from emotional perception—emotional contagion is an interactive process, and if there was no obvious intention to transfer positive or negative emotions to the participants, the emotional experience of the receiver may remain unchanged. Fourth, makeup, which increases facial attractiveness and the positive expressivity of faces, may lead to enhanced willingness for interpersonal approachability.
To examine these predictions, we measured the emotional experiences of participants (receivers), who were shown pictures of emotional expressers with or without makeup. Our main purpose was (1) to examine whether makeup can evoke the receivers’ positive appraisal of facial attractiveness, (2) whether makeup affected emotional contagion, and (3) whether this effect was modulated by emotional categories. In light of findings that facial attractiveness and emotional expressions (the magnitude of facial muscle activities evoked by emotions) may affect perceived emotional valence and arousal 27 , 31 , 32 , we maintained the same facial expression before and after makeup to ensure that only the makeup was being manipulated. Meanwhile, given that heavy and excessive makeup may have negative effects 1 , 2 and that light makeup is often considered more attractive and suitable 33 , 34 , we ensured the use of light makeup in the current study over heavy makeup.
In addition, dynamic facial expressions (and not static expressions) were employed for the following reasons. First, dynamic facial expressions are more appropriate and effective than static facial expressions for simulating emotional contagion in real life 35 , 36 . Second, using dynamic video can make receivers more sensitive towards facial attractiveness, thus more easily invoking emotional responses 37 , even though there is no significant difference in the evaluation of attractiveness between static and dynamic pictures 38 . In particular, the materials included both female and male faces displaying various emotions, both of which were treated according the same make-up standard. However, there may be a difference in the perception of men wearing makeup, as this does not conform to gender stereotypes. Although not mainstream, an emerging phenomenon has been occurring wherein young males in China use light makeup to modify their appearance, especially among those seen on TV. Therefore, male faces were also taken into consideration.
Experiment 1
Participants.
Previous studies have observed reliable effects of facial attractiveness on emotion perception or empathy across tasks with approximately 30 participants 23 , 39 . Considering the uncertainty of whether a potential makeup effect might be weaker and sample sizes recommended by Simmons et al. 40 and Brysbaert et al. 41 , we adopted a conservative approach and recruited at least 40 participants per condition. Therefore, 48 university students (16 males, age range 18–30 years, M = 21.83 ± 2.70 years, sample C, see Table 1 ) participated in this experiment and were paid ¥50 for their participation.
The emotional expression video clips were selected from the Dynamic FACES database 42 and comprised clips of 38 young actors (23 males) who each performed four two-second facial expression videos, including neutral, happy, sad, and angry expressions, gradually progressing from a neutral face to the maximum emotion. Reactions to the video, such as emotional valence and arousal, were pre-tested on another group of participants (Sample A, 27 students, 10 males, M = 21.37 ± 2.44 years old) to ensure effective selection of emotional materials. The participants were required to rate the videos according to the maximum intensity of emotions, as the emotions expressed in the videos gradually increased in intensity. Descriptive statistics (Sample A) are presented in Table 2 . Meanwhile, a repeated-measures ANOVA was conducted on valence and arousal; the specific analysis is presented in Supplementary Analysis 1.1 . For valence, happy videos were more positive than neutral videos; sad and angry videos were more negative than neutral videos. For arousal, neutral videos resulted in significantly lower arousal than the other videos; happy and angry videos were similar, but sad videos were relatively less arousing than happy and angry videos. In summary, the results showed the manipulation of the emotional materials to be valid.
Subsequently, the faces in these videos were processed with makeup. For doing this, each video clip was broken down into 50 pictures at 25 frames per second. All 50 facial pictures from the same clip were lightly made up following a uniform beauty standard using Photoshop, which included slight whitening and smoothing of the skin and application of pale pink lipstick (see Fig. 1 ). These treatments were based on the outcomes of makeup applications in real life, such as skin whitening 43 and smoothing 44 . The edited pictures were then recomposed into a two-second video clip.
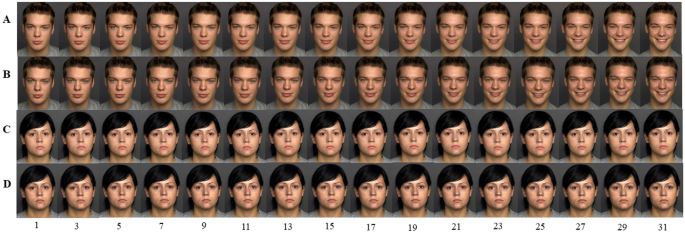
The make-up treatment of emotional videos in Experiment 1. The makeup treatment is shown in Figure. ( A,B ) (happy video) or ( C,D ) (neutral video) were the same emotional videos, ( A,C ) were lightly made up, but ( B,D ) not. The number below indicates the location of this picture in the video (25 frames per second). The original emotional expression video clips were selected from the Dynamic FACES database (No. 066 and No. 140) 42 , and have been permitted to use by its authors.
The original videos were composed via morphing according to the descriptions in the database. Therefore, both the original and makeup versions may look unnatural. To confirm that both versions matched in terms of how natural they looked and the extent to which makeup enhanced facial attractiveness, another 23 participants (Sample B, nine males, M = 19.78 ± 1.88 years old) were asked to rate how natural all the video clips were and how attractive the models were in all neutral video clips—this was done using a nine-point Likert scale, where 1 = extremely unnatural/unattractive, 9 = extremely natural/attractive . The pre-rating scores are presented in Table 2 (Sample B). Moreover, a repeated-measures ANOVA was conducted on naturalness (see Supplementary Analysis 1.2 ), which indicated that neutral videos were considered more natural than other videos. However, happy videos were the most unnatural clips, although they did not reach a significant level when compared with sad videos. As previously mentioned, the original videos were edited; therefore, the variations in the teeth may have led to the happy videos being considered the most unnatural. However, applying makeup did not alter the naturalness of videos, which could be demonstrated through a non-significant main effect of treatment and its interaction with emotion. Accordingly, although the manipulation of naturalness was not balanced among emotions, it did not interfere with the effect of makeup and its interaction with emotions on emotional contagion.
Five minutes before the main task, the participants filled in the Positive and Negative Affect Schedule (PANAS) 45 to assess their current emotional state.
The participants sat in a quiet room directly facing the center of a screen at 140 cd/m 2 brightness, where an emotional video of 330 × 430 pixels was presented for 2000 ms. The participants were asked to pretend that they were in a video chat with the person in the video and were informed that “The person in the video may express an emotion to you, and after the video ends, you will be asked to evaluate your own immediate and real emotional experience”, The evaluation was to be made on a nine-point Likert scale, ranging from negative to positive, where 1 = Extremely negative, 5 = Neutral, 9 = Extremely positive. The scenario aimed to simulate the emotional contagion process in a video chat as accurately as possible. The experiment included four blocks with 76 trials each, comprising 19 trials for each of the four emotions. The introduction was reiterated before each block began. The original version of each video did not appear in the same block. The order among blocks and trials was randomized, with a 2-min break between two consecutive blocks (see Fig. 2 ).
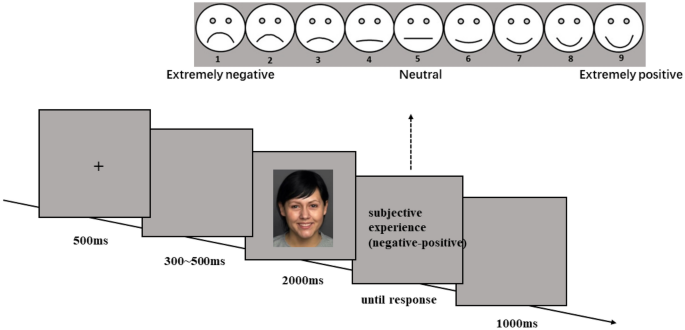
Experimental procedure of Experiment 1. The emotional expression video clips (No. 140) was selected from the dynamic FACES database 42 , and have been permitted to use by its authors.
After the experiment, the participants were asked about its purpose. None of them realized that there were makeup and no makeup conditions, and they were unable to determine the experimental goal.
Results and discussion
Manipulation check: the effect of makeup on facial attractiveness (only under neutral conditions).
A paired-sample t -test (two-tailed, Sample B) was used to test whether makeup had an effect on attractiveness. As opposed to the non-makeup condition ( M = 4.32, SD = 0.73), the makeup condition ( M = 4.63, SD = 0.75) significantly improved facial attractiveness, t 22 = 5.391, p < 0.001, Cohen’s d = 1.108.
Participants’ emotional state before experiment
The PANAS was measured five minutes before the emotional contagion tasks to confirm the participants’ emotional state before the experiment. The average PANAS scores were as follows: 30.06 ( SD = 5.95) for positive affect and 17.85 ( SD = 5.65) for negative affect. A paired-sample t test (Sample C) showed that participants were in a moderately positive emotional state, and the positive state scores were higher than those of the negative state before the emotional contagion experiment, t 47 = 10.285, p < 0.001, Cohen’s d = 2.198.
The influence of makeup on emotional contagion
To determine whether makeup affects emotional contagion, we performed a 4 × 2 repeated-measures ANOVA on participants’ self-reported emotional experience (Sample C), with emotion (neutral, angry, happy, sad) and treatment (makeup, non-makeup) as within-participant independent variables. Mauchly’s test of sphericity was adopted to test homogeneity of variance. If Mauchly’s test of sphericity was significant, the Greenhouse–Geisser was employed to correct the results (the same correction method was used for subsequent analysis).
Compared with non-makeup, the makeup condition gave rise to more positive experiences regardless of emotions, with a significant main effect of treatment ( F 1, 47 = 13.17, p = 0.001, η p 2 = 0.219). Meanwhile, a significant main effect of emotion was uncovered ( F 3,141 = 368.198, p < 0.001, η p 2 = 0.887). Post-hoc tests indicated that regardless of makeup, the emotional experiences of the angry ( MD angry-neutral = − 1.776, SE = 0.117, p < 0.001) and sad expressions ( MD sad-neutral = − 1.392, SE = 0.097, p < 0.001) were more negative than the neutral condition, while happy ( MD happy-neutral = 2.388, SE = 0.116, p < 0.001) was more positive than the neutral condition. Consistent with the pre-test of emotional valence, angry expressions evoked more negative experiences than sad expressions ( MD angry-sad = − 0.384, SE = 0.045, p < 0.001). The results proved that the corresponding emotional experiences of participants were evoked by different emotional expressions.
Moreover, the interaction between treatment and emotion was significant ( F 3,141 = 4.803, p = 0.003, η p 2 = 0.093). A simple effect test revealed that the emotional experiences evoked by faces with makeup were less negative than those evoked by non-makeup faces (angry: MD = 0.093, SE = 0.041, p = 0.027; sad: MD = 0.175, SE = 0.032, p < 0.001). However, this was not the case for the happy and neutral conditions (happy: MD = 0.025, SE = 0.044, p = 0.569; neutral: MD = 0.055, SE = 0.037, p = 0.141; see Fig. 3 a; the difference value of makeup minus non-makeup conditions among different emotions was calculated and compared in Fig. 3 b, see Supplementary Analysis 6 for detailed analysis).
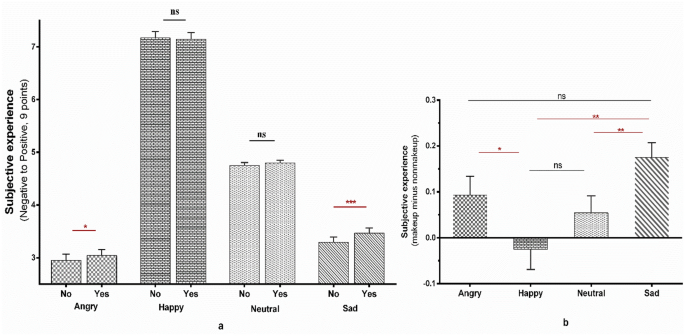
The effect of makeup on emotional contagion in Experiment 1. ( a ) The effect of makeup on different emotional contagion. “Yes” refers to makeup conditions, and “No” indicated non-makeup conditions. ( b ) The differences in increments induced by makeup among emotions. The error bar represents standard error. “*” p < 0.05, “**” p < 0.01, “***” p < 0.001.
Overall, Experiment 1 found that makeup weakened the negative emotional contagion under the angry and sad conditions, while the emotional contagion under the neutral and happy conditions remained unchanged. However, it is noteworthy that the video clips in Experiment 1 were morphed, and the happy videos were considered relatively more unnatural than other emotions, which may have affected the impact of makeup on the emotional contagion of happy expressions.
Experiment 2
Given the exploratory nature of this study and little direct evidence about the interaction of makeup and emotions in the past, Experiment 2 was conducted to verify the results repeatedly with changed materials and participants. As previously mentioned, the stimuli in Experiment 1 were made using morphing technology, which may cause some expressions to look unnatural and could interfere with the experimental results. Therefore, natural emotional expression videos were presented in Experiment 2. In Experiment 1 (Sample A), only the emotional valence and arousal of non-makeup conditions (including happy, neutral, sad, and angry) were rated before to check whether the manipulation of emotions was workable. However, the effect of makeup on emotional evaluation (valence and arousal) and whether it affected the relationship between makeup and emotional contagion is unknown, although previous studies have pointed out that facial attractiveness may modulate emotional perceptions 23 . Therefore, the participants (Sample D) were also asked to complete the emotional evaluation task, for both the makeup and non-makeup conditions, after the emotional contagion task in Experiment 2. In addition to the emotional evaluation and emotional contagion tasks, an extra choice task about further exchanges was also presented to explore the preference for makeup in interpersonal communication.
Considering that Experiment 2 also explored the correlation between emotional contagion and emotional evaluation, we adopted G*power 3.1.9.2 to estimate the required sample of participants using one-tails, with moderate correlation ρ H1 = 0.4, α = 0.05, power (1 − β err prob) = 0.8; accordingly, at least 37 participants were required. Moreover, as mentioned in Experiment 1, a sample size reaching at least 40 participants per condition would be a more conservative consideration; therefore, another 40 university students (Sample D, 10 males, M = 20.98 ± 2.84 years old) were recruited and paid ¥50 for participating. All participants had normal or corrected vision and had experienced no mental or mood disorders in recent days.
Video clips with the highest emotional intensity were selected from the Amsterdam Dynamic Facial Expression Set–Bath Intensity Variations (ADFES-BIV) 46 . There were a total of 48 video clips made up of 13 actors (six males) performing four two-second facial expressions with different emotions (neutral, happy, sad, angry). The progression of emotional intensity in these videos was recorded naturally, rather than being morphed.
The makeup treatments were the same as in Experiment 1, and the same group of subjects (Sample B) were recruited to rate the naturalness of all the video clips and the attractiveness of all the neutral video clips, to maintain consistency between Experiment 1 and Experiment 2 in the evaluation of naturalness and attractiveness.
The pre-rating scores are shown in Table 3 (Sample B), and a repeated-measures ANOVA on naturalness (Supplementary Analysis 4 ) showed that the naturalness of the neutral expressions was highest, while the other emotional expressions were similar in scores. Moreover, there was no significant interaction between emotion and treatment, nor a main effect of treatment, which implied that makeup did not affect naturalness.
The procedure was identical to that of Experiment 1, except for the following. A total of 96 trials were split into four blocks, with 24 trials in each block. Five minutes after the emotional contagion task, the participants were asked to perform an emotional evaluation task that required them to rate the valence and arousal they experienced after watching all the videos. After the emotional evaluation, the participants were asked about the purpose of the experiment, and no one guessed it. Then, the participants completed a choice task. In the choice task, two images of the same person with a neutral expression were presented simultaneously on the screen: one was the made-up version and the other was the original version. The positions (left or right) of the photos were counterbalanced. The participants were told that they were seeing the same person in two different states, and they were asked to choose which image they would be more willing to communicate with.
Manipulation check: the effect of makeup on facial attractiveness (only under the neutral condition)
A paired-sample t -test (two-tailed, Sample B) was conducted to test the effect of makeup on attractiveness. As opposed to the non-makeup condition ( M = 4.46, SD = 0.759), the makeup condition increased facial attractiveness ( M = 4.86, SD = 0.926), t 22 = 3.712, p < 0.001, Cohen’s d = 0.774.
Consistent with Experiment 1, the participants’ positive state (Sample D, M = 30.43, SD = 6.07) was higher than the negative state ( M = 15.35, SD = 3.76), t 39 = 14.66, p < 0.001, Cohen’s d = 2.305.
As in Experiment 1 , a 4 × 2 repeated-measures ANOVA was conducted (Sample D) to explore the effect of makeup on emotional contagion. The main effects of emotion ( F 3,117 = 341.767, p < 0.001, η p 2 = 0.898) were significant, with angry (MD angry-neutral = − 1.664, SE = 0.135) and sad expressions ( MD sad-neutral = − 1.124, SE = 0.115) arousing more negative experiences and happy expressions ( MD happy-neutral = 2.481, SE = 0.094) evoking more positive experiences when compared with neutral expressions (all p s < 0.001) regardless of makeup. Meanwhile, a significant main effect of treatment was also found ( F 1,39 = 14.125, p = 0.001, η p 2 = 0.266). The faces wearing makeup significantly induced more positive experiences than non-makeup faces regardless of emotions ( MD makeup-nonmakeup = 0.134, SE = 0.036, p = 0.001).
Furthermore, the interaction effect between treatment and emotion was significant ( F 3,117 = 3.062, p = 0.031, η p 2 = 0.073). A simple effect analysis showed that the emotional experience in the non-makeup condition was significantly negative compared to the makeup condition (angry: MD = − 0.237, SE = 0.06, p < 0.001; sad: MD = − 0.169, SE = 0.053, p = 0.003). In contrast, there were no significant differences between the makeup and non-makeup conditions for either the happy or neutral conditions (happy: F 1,39 = 1.703, p = 0.2, η p 2 = 0.042; neutral: F 1,39 = 1.293, p = 0.262, η p 2 = 0.032) (Fig. 4 a; the difference value of makeup minus non-makeup conditions among different emotions was calculated and compared in Fig. 4 b, see Supplementary Analysis 7 for detailed analysis).
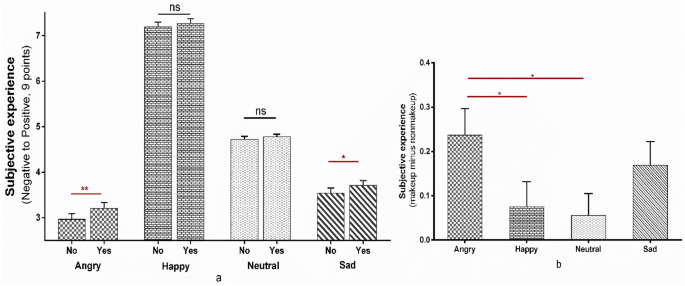
The effect of makeup on emotional contagion in Experiment 2. ( a ) The effect of makeup on different emotional contagion. “Yes” refers to makeup conditions, and “No” refers to non-makeup conditions. ( b ) The differences of increments induced by makeup among emotions. The error bar represents standard error. “*” p < 0.05, “**” p < 0.01, “***” p < 0.001.
The influence of makeup on emotional evaluation
Considering that makeup may change participants’ perceptions and evaluation to match the emotional attributes (valence and arousal) of the expressers, resulting in differences in emotional experiences between the makeup and non-makeup conditions, a repeated-measures ANOVA was conducted with emotion and treatment as within-subject independent variables and valence and arousal as dependent variables. The data of one participant that had been incompletely recorded were excluded (sample D).
For valence, emotion ( F 3,114 = 439.12, p < 0.001, η p 2 = 0.92) and treatment ( F 1,38 = 11.46, p = 0.002, η p 2 = 0.231) had significant main effects, and their significant interaction ( F 3,114 = 5.22, p = 0.002, η p 2 = 0.121) showed that for the angry and sad conditions, the emotional valence under the non-makeup condition was significantly lower than that of the makeup condition (angry: MD = − 0.169, SE = 0.032, p < 0.001; sad: MD = − 0.093, SE = 0.045, p = 0.046). This suggested that there was a more negative emotional valence for anger and sadness for non-makeup expressers. By contrast, no significant differences were found between the makeup and non-makeup conditions under the happy ( MD = − 0.004, SE = 0.043, p = 0.928) and neutral conditions ( MD = − 0.006, SE = 0.024, p = 0.809).
With respect to arousal, emotion ( F 3,114 = 72.24, p < 0.001, η p 2 = 0.655) and treatment ( F 1,38 = 5.384, p = 0.026, η p 2 = 0.124) had significant main effects, and the interaction effect ( F 3,114 = 4.858, p < 0.001, η p 2 = 0.113) was also significant. A simple effect analysis showed that for the angry and sad expressions, emotional arousal under the non-makeup condition was higher than that under the makeup condition (angry: MD = 0.218, SE = 0.075, p = 0.006; sad: MD = 0.181, SE = 0.062, p = 0.006). However, no significant differences were observed between the makeupand non-makeup conditions under the happy ( MD = − 0.037, SE = 0.072, p = 0.605) and neutral conditions ( MD = 0.01, SE = 0.039, p = 0.801).
In summary, we found that wearing makeup increased emotional valence and decreased emotional arousal, which supported our prior hypothesis that applying makeup can change the perception of emotional attributes. However, Song et al. 47 found that facial attractiveness enhanced the perception of emotional intensity for both neutral and positive emotions rather than anger when using artificial expressions, which is inconsistent with the current study; this may be related to the materials used. The current study used dynamic videos instead of photos and controlled the amplitudes of facial muscle activities under conditions with or without makeup.
The relationship between emotional contagion and emotion evaluation
As previously mentioned, we assumed that the different effect of makeup on emotional evaluation may ultimately result in different emotional contagion. Based on the above analysis, we also found a similar result pattern in the simple effect test (after a significant interaction of treatment and emotion) between emotional contagion and evaluation. Therefore, to explore whether the effect of makeup on emotional contagion was related to the change in participants’ perceptions and evaluation of expressers’ emotional expressions caused by makeup, we calculated respectively the differences between the scores of emotional contagion, valence, and arousal under the makeup and non-makeup conditions. We then standardized the increments and used Z-scores to calculate the Pearson product-moment correlation between emotional experience and valence and emotional experience and arousal, respectively, and found that the increments of emotional experience were irrelevant to the increments of emotional valence or arousal regardless of the emotional conditions of angry ( r valence = 0.087, r arousal = 0.117), neutral ( r valence = − 0.289, r arousal = 0.196), happy ( r valence = 0.292, r arousal = − 0.065), and sad ( r valence = 0.017, r arousal = − 0.05) (all ps > 0.05).
We previously presumed that emotional contagion may be affected by emotional evaluation and that the increments of makeup on emotional contagion may be due to the influence of facial attractiveness on emotional appraisal. If the increments of emotional contagion caused by makeup are induced by the increments of emotional evaluation, then the increments brought by makeup should be positively correlated. However, the results did not support the original hypothesis. Perhaps the increments of emotional contagion and of valence or arousal are asynchronous or nonlinear. Another explanation may be that participants’ emotional experiences were affected not only by the emotions expressed by others, but also by factors such as motivation and relationship affinity 48 , 49 , 50 , all of which may result in asynchronous variations.
Makeup and further communication choice
In Experiment 2, the participants were tasked with choosing which face they would communicate with more, and it was found that an average of 80.19% of make-up objects were preferred by participants for further communication. The average proportion of participants choosing makeup in the total trials ranged from 38.46% to 100.00%, SD = 19.15%. We calculated the differences in the makeup and non-makeup conditions in terms of facial attractiveness and employed an item analysis to calculate the Pearson product-moment correlation between the differences and the proportion of participants who selected faces with high attractiveness ( r = 0.668, p = 0.023). This finding confirmed the common phenomenon that attractive individuals have more opportunity in job applications and interview processes 51 , 52 .
General discussion
In brief, Experiments 1 and 2 consistently demonstrated that wearing light makeup can significantly improve perceived facial attractiveness and attenuate negative emotional contagion, as in the angry and sad conditions. The effect of makeup on emotional contagion may be partially mediated by facial attractiveness. We confirmed that perceived facial attractiveness increased when viewing a face wearing makeup through a manipulation test of attractiveness, which was consistent with previous research 8 . The effect of makeup vanished when facial attractiveness was included as a covariate in the analysis of covariance (see Supplementary Analysis 2.5 ).
Consistent with previous findings, this study indicates that emotions are contagious in social communication. By facial mimicry or social appraisal 53 , the expresser’s emotions can be effectively transmitted to the receiver and evoke the receiver’s similar emotional experience. Emotional contagion is regarded as an adhesive of social relations 54 and forms one of the bases of empathy 55 , which is an emotional process shared by animals and human beings and has evolutionary significance 16 , 56 , 57 , 58 .
Furthermore, this study is the first to prove that makeup can affect emotional contagion, similar to its impact on other interpersonal processes 22 , 27 . In particular, makeup primarily and significantly affected receivers’ negative emotions by reducing the negative emotions felt by them. There are several possible explanations for these results. First, attractive faces brought about by makeup can evoke pleasurable feelings 24 and may weaken the intensity of negative emotions, thereby resulting in fewer negative experiences. If this is the case, the participants’ emotional experiences would vary according to changes in emotional valence or arousal. However, the analysis of the emotional evaluation in Experiment 2 demonstrated that the increments of emotional experience were irrelevant to both valence and arousal. Therefore, this explanation was inconsistent with our results. Second, the expressers’ facial expressions dynamically and gradually changed from neutral to maximum emotion; therefore, the participants may have appraised facial attractiveness before they detected the negative emotion 9 , 19 , 28 , 59 , 60 . Consequently, attractive faces may divert more attention from emotional processes, resulting in inadequate processing of negative emotions and subsequently reducing participants’ negative experiences 61 . Third, the preference for attractiveness may have potentially promoted the prosocial motivation of receivers 19 , 62 . Previous research has pointed out that when more attractive expressers express anger, receivers tend to automatically regulate their negative emotions and impulsive responses to relieve the tense atmosphere, which is regarded as a vital factor in further exchange and cooperation 63 . Our data also indicated that receivers displayed more intent to communicate with individuals wearing makeup.
However, makeup did not affect contagious experiences under the neutral and happy conditions. Evidence on emotional imitation 64 , 65 has suggested that positive emotional expressions usually shape a relatively friendly atmosphere; receivers rarely evaluate extra information and automatically imitate it, then rapidly respond with a positive response such as smiling 66 . The effect of makeup on positive emotional contagion may thereby be ignored compared with affinity intention 67 . By contrast, when experiencing negative emotions in which adverse signals are often conveyed, the receivers may appraise the expressers’ personality, intentions, status, and relationships to make appropriate decisions and responses 68 , 69 . Therefore, negative emotional contagion may be more affected by external information 70 , such as facial attractiveness. Nevertheless, this issue remains controversial 70 , 71 , and more research is still required to explore and explain why positive contagion is not affected by makeup. Furthermore, for the neutral expressions, because emotional expressers do not obviously transmit positive or negative emotions to receivers in interpersonal contact, no clear affinity or non-affinity motivation was expressed to participants 72 . However, contexts where neutral expressions are expressed can be more formal and serious; therefore, a similar neutral expression may be a more appropriate emotional response in this situation regardless of makeup. However, an analysis based on item (see Supplementary Analysis 2 ) indicated that made-up faces may cause some positive feelings in neutral conditions and should therefore be further explored.
This study provides evidence for the relationship between makeup and emotional contagion and an available reference for the application scenarios of makeup in social communication, such as election, jury decisions, and service sales. For emotional expressers, individuals can choose to wear cosmetics to adjust facial attractiveness according to their intentions or situation, thereby partially influencing others’ emotional experiences. For example, makeup may be a good choice for concealing emotions when individuals are reluctant to let others feel sadness or pity, such as body makeup at a funeral parlor. On the other hand, if individuals are seeking others’ sympathy or help, wearing beautiful makeup may be futile. Because higher attractiveness is often associated with better survival conditions 9 , it may lead others to misjudge real distress and weaken sympathy and emotional contagion from sad experiences 73 , 74 . Furthermore, emotional receivers (judges) can realize the attractiveness preference brought about by makeup and then modulate their responses to decrease prejudice or discrimination 75 .
Nevertheless, this study had some limitations that should be considered in future research. First, gender differences were not explored in detail, even though a plethora of research on facial attractiveness has emphasized the importance of gender 8 , 76 , 77 . In fact, participants may pay more attention to the opposite gender due to the biological purpose of reproduction 78 , 79 . However, the evolutionary byproduct explanation supports the idea that attractiveness preference evolved into a cross-gender feature as socialization became more complex and then turned into an indicator of overall quality 80 , 81 . Although it is not the main concern, our study suggested that the gender of participants and expressers does not obviously change the role of makeup on emotional contagion in Experiment 1 (see Supplementary Analysis 3 ). Future studies should explore this phenomenon further. Second, the ethnic differences in this study did not account for the participants being Mongolian, as Caucasian faces were used in the videos. The group and cultural differences between the receivers and the expressers in the current study may limit the generalizability of the findings. We are currently collecting and attempting to create a Chinese Facial Expression Video Database and hope that this limitation can be addressed in follow-up research. Third, we performed digital makeup according to the presentation of real makeup, yet there could be some differences between the two. For example, digital makeup may not look as natural as real makeup. Finally, these results are mainly applicable to those wearing light makeup; therefore, whether the findings apply to those wearing heavier makeup requires further discussion.
Ethics approval and consent to participate
This research was approved by the Renmin University of China research ethics committee. All participants had signed informed consent after being given a complete description of the study and agreed to publish their data publicly. The ethics committee approved this consent procedure and all methods were performed in accordance with the relevant guidelines and regulations.
Data availability
Raw data associated with this article can be found in the online version at https://pan.baidu.com/s/10TnMdsoIUAVvzkKhmNh5fQ , extraction code “AB01”. As long as you mentioned our works, you can free access to the data, materials for academic purposes, but not for commercial purposes or for profit. Using the third party material in this article need to contact the appropriate copyright owner for permission.
Etcoff, N. L., Stock, S., Haley, L. E., Vickery, S. A. & House, D. M. Cosmetics as a feature of the extended human phenotype: Modulation of the perception of biologically important facial signals. PLoS ONE . https://doi.org/10.1371/journal.pone.0025656 (2011).
Article PubMed PubMed Central Google Scholar
Cox, C. L. & Glick, W. H. Resume evaluations and cosmetics use: When more is not better. Sex Roles 14 , 51–58. https://doi.org/10.1007/BF00287847 (1986).
Article Google Scholar
Graham, J. A. & Jouhar, A. The effects of cosmetics on person perception. Int. J. Cosmetic Sci. 3 , 199–210. https://doi.org/10.1111/j.1467-2494.1981.tb00283.x (1981).
Article CAS Google Scholar
Hatfield, E. & Sprecher, S. Mirror, Mirror: The Importance of Looks in Everyday Life (Suny Press, 1986).
Google Scholar
Osborn, D. R. Beauty is as beauty does?: Makeup and posture effects on physical attractiveness judgments. J. Appl. Soc. Psychol. 26 , 31–51. https://doi.org/10.1111/j.1559-1816.1996.tb01837.x (2010).
Korichi, R., Pelle-De-Queral, D., Gazano, G. & Aubert, A. Why women use makeup: Implication of psychological traits in makeup functions. J. Cosmet. Sci. 59 , 127–137. https://doi.org/10.1111/j.1468-2494.2008.00452_3.x (2008).
Article PubMed Google Scholar
Nash, R., Fieldman, G., Hussey, T., Lévêque, J. L. & Pineau, P. Cosmetics: They influence more than Caucasian female facial attractiveness. J. Appl. Soc. Psychol. 36 , 493–504. https://doi.org/10.1111/j.0021-9029.2006.00016.x (2006).
Póvoa, A. C. S., Pech, W., Viacava, J. & Schwartz, M. T. Is the beauty premium accessible to all? An experimental analysis. J. Econ. Psychol. https://doi.org/10.1016/j.joep.2020.102252 (2020).
Wong, J. S. & Penner, A. M. Gender and the returns to attractiveness. Res. Soc. Stratif. Mobil. 44 , 113–123. https://doi.org/10.1016/j.rssm.2016.04.002 (2016).
Cross, N. et al. Facial attractiveness, weight status, and personality trait attribution: The role of attractiveness in weight stigma. Obes. Res. Clin. Pract. https://doi.org/10.1016/j.orcp.2016.03.007 (2016).
Foo, Y. Z., Simmons, L. W. & Rhodes, G. Predictors of facial attractiveness and health in humans. Sci. Rep. 7 , 1–12. https://doi.org/10.1038/srep39731 (2017).
Lee, H. & Oh, H. The effects of self-esteem on makeup involvement and makeup satisfaction among elementary students. Arch. Des Res. 31 , 87–94. https://doi.org/10.15187/adr.2018.05.31.2.87 (2018).
Scholz, J. K. & Sicinski, K. Facial attractiveness and lifetime earnings: Evidence from a cohort study. Rev. Econ. Stat. 97 , 14–28. https://doi.org/10.1162/REST_a_00435 (2015).
Belkin, L. Y. Emotional contagion in the electronic communication context: Conceptualizing the dynamics and implications of electronic emotional encounters in organizations. J. Organ. Cult. Commun. Conf. 13 , 105–122 (2009).
Bruder, M., Dosmukhambetova, D., Nerb, J. & Manstead, A. S. Emotional signals in nonverbal interaction: Dyadic facilitation and convergence in expressions, appraisals, and feelings. Cogn. Emot. 26 , 480–502. https://doi.org/10.1080/02699931.2011.645280 (2012).
Peters, K. & Kashima, Y. A multimodal theory of affect diffusion. Psychol. Bull. 141 , 966–992. https://doi.org/10.1037/bul0000020 (2015).
Damon, F., Mottier, H., Méary, D. & Pascalis, O. A review of attractiveness preferences in infancy: From faces to objects. Adapt. Hum. Behav. Physiol. 3 , 321–336. https://doi.org/10.1007/s40750-017-0071-2 (2017).
Langlois, J. H. et al. Maxims or myths of beauty? A meta-analytic and theoretical review. Psychol. Bull. 126 , 390–423. https://doi.org/10.1037/0033-2909.126.3.390 (2000).
Article CAS PubMed Google Scholar
Chen, J., Zhong, J., Zhang, Y., Peng, L. & Hong, L. Electrophysiological correlates of processing facial attractiveness and its influence on cooperative behavior. Neurosci. Lett. 517 , 65–70. https://doi.org/10.1016/j.neulet.2012.02.082 (2012).
Jing, W., Xia, T., Xu, L., Ru, T. & Lei, M. What is beautiful brings out what is good in you: The effect of facial attractiveness on individuals’ honesty. Int. J. Psychol. 52 , 197–204. https://doi.org/10.1002/ijop.12218 (2017).
Griffin, A. M. & Langlois, J. H. Stereotype directionality and attractiveness stereotyping: Is beauty good or is ugly bad? Soc. Cogn. 24 , 187–206. https://doi.org/10.1521/soco.2006.24.2.187 (2006).
Lindeberg, S., Craig, B. M. & Lipp, O. V. You look pretty happy: Attractiveness moderates emotion perception. Emotion 19 , 1070–1080. https://doi.org/10.1037/emo0000513 (2019).
Mertens, A., Hepp, J., Voss, A. & Hische, A. Pretty crowds are happy crowds: The influence of attractiveness on mood perception. Psychol. Res. https://doi.org/10.1007/s00426-020-01360-x (2020).
Principe, C. P. & Langlois, J. H. Faces differing in attractiveness elicit corresponding affective responses. Cogn. Emot. 25 , 140–148. https://doi.org/10.1080/02699931003612098 (2011).
Winston, J. S., O’Doherty, J., Kilner, J. M., Perrett, D. I. & Dolan, R. J. Brain systems for assessing facial attractiveness. Neuropsychologia 45 , 195–206. https://doi.org/10.1016/j.neuropsychologia.2006.05.009 (2007).
Mueser, K. T., Grau, B. W., Sussman, S. & Rosen, A. J. You’re only as pretty as you feel: Facial expression as a determinant of physical attractiveness. J. Abnorm. Soc. Psychol. 46 , 469–478. https://doi.org/10.1037/0022-3514.46.2.469 (1984).
Rennels, J. L. & Kayl, A. J. Differences in expressivity based on attractiveness: Target or perceiver effects? J. Exp. Soc. Psychol. 60 , 163–172. https://doi.org/10.1016/j.jesp.2015.05.012 (2015).
Werheid, K., Schacht, A. & Sommer, W. Facial attractiveness modulates early and late event-related brain potentials. Biol. Psychol. 76 , 100–108. https://doi.org/10.1016/j.biopsycho.2007.06.008 (2007).
Workman, J. E. & Johnson, K. The role of cosmetics in impression formation. Clothing. Text. Res. J. 10 , 63–67. https://doi.org/10.1177/0887302X9101000109 (1991).
Batres, C. et al. Evidence that makeup is a false signal of sociosexuality. Pers. Individ. Differ. 122 , 148–154. https://doi.org/10.1016/j.paid.2017.10.023 (2018).
Tomas, J. & Španić, A. M. Angry and beautiful: The interactive effect of facial expression and attractiveness on time perception. Psihologijske teme 25 , 299–315 (2016).
Ueda, R., Kuraguchi, K. & Ashida, H. Asymmetric effect of expression intensity on evaluations of facial attractiveness. SAGE Open 6 , 215824401667756. https://doi.org/10.1177/2158244016677569 (2016).
Tagai, K., Shimakura, H., Isobe, H. & Nittono, H. The light-makeup advantage in facial processing: Evidence from event-related potentials. PLoS ONE 12 , e0172489. https://doi.org/10.1371/journal.pone.0172489 (2017).
Article CAS PubMed PubMed Central Google Scholar
Tagai, K., Ohtaka, H. & Nittono, H. Faces with light makeup are better recognized than faces with heavy makeup. Front. Psychol. 7 , 226. https://doi.org/10.3389/fpsyg.2016.00226 (2016).
Rymarczyk, K., Żurawski, L., Jankowiak-Siuda, K. & Szatkowska, I. Do dynamic compared to static facial expressions of happiness and anger reveal enhanced facial mimicry? PLoS ONE 11 , e0158534. https://doi.org/10.1371/journal.pone.0158534 (2016).
Rymarczyk, K., Żurawski, L., Jankowiak-Siuda, K. & Szatkowska, I. Emotional empathy and facial mimicry for static and dynamic facial expressions of fear and disgust. Front. Psychol. 7 , 1853. https://doi.org/10.3389/fpsyg.2016.01853 (2016).
Rubenstein, A. J. Variation in perceived attractiveness: Differences between dynamic and static faces. Psychol. Sci. 16 , 759–762. https://doi.org/10.1111/j.1467-9280.2005.01610.x (2005).
Rhodes, G., Lie, H. C., Thevaraja, N., Taylor, L. & Simmons, L. W. Facial attractiveness ratings from video-clips and static images tell the same story. PLoS ONE 6 , e26653. https://doi.org/10.1371/journal.pone.0026653 (2011).
Article ADS CAS PubMed PubMed Central Google Scholar
Meng, J., Li, X., Peng, W., Li, Z. & Shen, L. The interaction between pain and attractiveness perception in others. Sci. Rep. 10 , 1–11. https://doi.org/10.1038/s41598-020-62478-x (2020).
Article ADS CAS Google Scholar
Simmons, J. P., Nelson, L. D. & Simonsohn, U. False-positive psychology: Undisclosed flexibility in data collection and analysis allows presenting anything as significant. Psychol. Sci. 22 , 1359–1366. https://doi.org/10.1177/0956797611417632 (2011).
Brysbaert, M. & Stevens, M. Power analysis and effect size in mixed effects models: A tutorial. J. Cogn. https://doi.org/10.5334/joc.10 (2018).
Holland, C. A., Ebner, N. C., Lin, T. & Samanez-Larkin, G. R. Emotion identification across adulthood using the dynamic FACES database of emotional expressions in younger, middle aged, and older adults. Cogn. Emot. 33 , 245–257. https://doi.org/10.1080/02699931.2018.1445981 (2019).
Pauline, B., Anne, L., Stéphane, A., Thomas, M. & Xavier, F. Skin whitening cosmetics: Feedback and challenges in the development of natural skin lighteners. Cosmetics 3 , 36. https://doi.org/10.3390/cosmetics3040036 (2016).
Batres, C., Porcheron, A., Latreille, J., Roche, M. & Russell, R. Cosmetics increase skin evenness: Evidence from perceptual and physical measures. Skin Res. Technol. 25 , 672–676. https://doi.org/10.1111/srt.12700 (2019).
Watson, D., Clark, L. A. & Tellegen, A. Development and validation of brief measures of positive and negative affect: The PANAS scales. J. Pers. Soc. Psychol. 54 , 1063–1070. https://doi.org/10.1037/0022-3514.54.6.1063 (1988).
Wingenbach, T., Ashwin, C. & Brosnan, M. Validation of the amsterdam dynamic facial expression set–bath intensity variations (ADFES-BIV): A set of videos expressing low, intermediate, and high intensity emotions. PLoS ONE . https://doi.org/10.1371/journal.pone.0147112 (2016).
Song, M., Shinomori, K. & Qian, Q. Perception and expressing habits of smiling and angry expressions modulated by facial physical attractiveness in Asian female persons. Jpn. Psychol. Res. 61 , 12–24. https://doi.org/10.1111/jpr.12198 (2019).
Berscheid, E. & Ammazzalorso, H. Emotional experience in close relationships. In Blackwell Handbook of Social Psychology: Interpersonal Processes: Fletcher/Blackwell (eds Fletcher, G. J. O. & Clark, M. S.) 308–330 (Blackwell Publishers Ltd, 2001).
Kaczmarek, L. D. et al. Splitting the affective atom: Divergence of valence and approach-avoidance motivation during a dynamic emotional experience. Curr. Psychol. https://doi.org/10.1007/s12144-019-00264-3 (2019).
Venaglia, R. B. & Lemay, E. P. Jr. Accurate and biased perceptions of partner’s conflict behaviors shape emotional experience. J. Soc. Pers. Relatsh. 36 , 3293–3312. https://doi.org/10.1177/0265407518818771 (2019).
Bóo, F. L., Rossi, M. A. & Urzúa, S. S. The labor market return to an attractive face: Evidence from a field experiment. Econ. Lett. 118 , 170–172. https://doi.org/10.1016/j.econlet.2012.10.016 (2013).
Ruffle, B. J. & Shtudiner, Z. Are good-looking people more employable? Manage. Sci. 61 , 1760–1776. https://doi.org/10.1287/mnsc.2014.1927 (2015).
Palagi, E., Celeghin, A., Tamietto, M., Winkielman, P. & Norscia, I. The neuroethology of spontaneous mimicry and emotional contagion in human and non-human animals. Neurosci. Biobehav. Rev. 111 , 149. https://doi.org/10.1016/j.neubiorev.2020.01.020 (2020).
Barsade, S. G. The ripple effect: Emotional contagion and its influence on group behavior. Adm. Sci. Q 47 (4), 644–675. https://doi.org/10.2307/3094912 (2002).
De Waal, F. B. The antiquity of empathy. Science 336 , 874–876. https://doi.org/10.1126/science.1220999 (2012).
Article ADS CAS PubMed Google Scholar
Briefer, E. F. Vocal contagion of emotions in non-human animals. Proc. R. Soc. B Biol. Sci. 285 , 20172783. https://doi.org/10.1098/rspb.2017.2783 (2018).
Park, S., Choi, S. J., Mun, S. & Whang, M. Measurement of emotional contagion using synchronization of heart rhythm pattern between two persons: Application to sales managers and sales force synchronization. Physiol. Behav. 200 , 148–158. https://doi.org/10.1016/j.physbeh.2018.04.022 (2019).
Nakahashi, W. & Ohtsuki, H. Evolution of emotional contagion in group-living animals. J. Theor. Biol. 440 , 12–20. https://doi.org/10.1016/j.jtbi.2017.12.015 (2018).
Article ADS MathSciNet PubMed MATH Google Scholar
Claus-Christian, C., Faerber, S. J., Dorothee, A. M., Bernhard, M. & Florian, H. First gender, then attractiveness: Indications of gender-specific attractiveness processing via ERP onsets. Neurosci. Lett. 686 , 186–192. https://doi.org/10.1016/j.neulet.2018.09.009 (2018).
Hung, S. M., Nieh, C. H. & Hsieh, P. J. Unconscious processing of facial attractiveness: Invisible attractive faces orient visual attention. Sci. Rep. 6 , 37117. https://doi.org/10.1038/srep37117 (2016).
Sun, D., Chan, C., Fan, J., Yi, W. & Lee, T. Are happy faces attractive? The roles of early vs. late processing. Front. Psychol. 6 , 1812. https://doi.org/10.3389/fpsyg.2015.01812 (2015).
Hurlemann, R., Scheele, D., Maier, W. & Schultz, J. Oxytocin drives prosocial biases in favor of attractive people. Behav. Brain Sci. 40 , e30. https://doi.org/10.1017/S0140525X16000510 (2017).
Watkins, L. E., Dilillo, D., Hoffman, L. & Templin, J. Do self-control depletion and negative emotion contribute to intimate partner aggression? A lab-based study. Psychol. Violence 5 , 35–45. https://doi.org/10.1037/a0033955 (2013).
Deng, H. & Ping, H. Matching your face or appraising the situation: Two paths to emotional contagion. Front. Psychol. 8 , 2278. https://doi.org/10.3389/fpsyg.2017.02278 (2017).
Prochazkova, E. & Kret, M. E. Connecting minds and sharing emotions through mimicry: A neurocognitive model of emotional contagion. Neurosci. Biobehav. Rev. 80 , 99–114. https://doi.org/10.1016/j.neubiorev.2017.05.013 (2017).
Bourgeois, P. & Hess, U. The impact of social context on mimicry. Biol. Psychol. 77 , 343–352. https://doi.org/10.1016/j.biopsycho.2007.11.008 (2008).
Hess, U. & Fischer, A. Emotional mimicry as social regulation. Pers. Soc. Psychol. Rev. 17 , 142–157. https://doi.org/10.1177/1088868312472607 (2013).
Sachisthal, M. S., Sauter, D. A. & Fischer, A. H. Mimicry of ingroup and outgroup emotional expressions. Compr. Results Soc. Psychol. 1 , 86–105. https://doi.org/10.1080/23743603.2017.1298355 (2016).
Wróbel, M. & Imbir, K. K. Broadening the perspective on emotional contagion and emotional mimicry: The correction hypothesis. Perspect. Psychol. Sci. 14 , 437–451. https://doi.org/10.1177/1745691618808523 (2019).
Rauchbauer, B., Majdandžić, J., Hummer, A., Windischberger, C. & Lamm, C. Distinct neural processes are engaged in the modulation of mimicry by social group-membership and emotional expressions. Cortex 70 , 49–67. https://doi.org/10.1016/j.cortex.2015.03.007 (2015).
Kelly, J. R., Iannone, N. E. & McCarty, M. K. Emotional contagion of anger is automatic: An evolutionary explanation. Br. J. Soc. Psychol. 55 , 182–191. https://doi.org/10.1111/bjso.12134 (2016).
Hess, U. & Fischer, A. Emotional mimicry: Why and when we mimic emotions. Soc. Pers. Psychol. Compass 8 , 45–57. https://doi.org/10.1111/spc3.12083 (2014).
Fisher, R. J. & Ma, Y. The price of being beautiful: Negative effects of attractiveness on empathy for children in need. J. Consum. Res. 41 (2), 436–450. https://doi.org/10.1086/676967 (2014).
Jankowiak-Siuda, K. et al. Empathic responses for pain in facial muscles are modulated by actor’s attractiveness and gender, and perspective taken by observer. Front. Psychol. 10 , 642. https://doi.org/10.3389/fpsyg.2019.00624 (2019).
Hamermesh, D. S. & Abrevaya, J. Beauty is the promise of happiness? Eur. Econ. Rev. 64 , 351–368. https://doi.org/10.1016/j.euroecorev.2013.09.005 (2013).
Hester, N. Perceived negative emotion in neutral faces: Gender-dependent effects on attractiveness and threat. Emotion 19 , 1490–1494. https://doi.org/10.1037/emo0000525 (2019).
Klümper, L., Wühr, P., Hassebrauck, M. & Schwarz, S. Automaticity of facial attractiveness perception and sex-specific mating strategies. Cognition 204 , 104379. https://doi.org/10.1016/j.cognition.2020.104379 (2020).
Halberstadt, J. & Rhodes, G. The attractiveness of nonface averages: Implications for an evolutionary explanation of the attractiveness of average faces. Psychol. Sci. 11 , 285–289. https://doi.org/10.1111/1467-9280.00257 (2000).
Van Vugt, M. & Iredale, W. Men behaving nicely: Public goods as peacock tails. Br. J. Psychol. 104 , 3–13. https://doi.org/10.1111/j.2044-8295.2011.02093.x (2013).
Chen, B.-B. Explanations for attractiveness-related positive biases in an evolutionary perspective of life history theory. Behav. Brain Sci. https://doi.org/10.1017/S0140525X16000455 (2017).
Maestripieri, D., Henry, A. & Nickels, N. Explaining financial and prosocial biases in favor of attractive people: Interdisciplinary perspectives from economics, social psychology, and evolutionary psychology. Behav. Brain Sci. https://doi.org/10.1017/S0140525X16000340 (2017).
Download references
Acknowledgements
This work was supported by supported by National Social Science Foundation of China (Major Program) [Grant Numbers: 19ZDA021], and fund for building world-class universities (disciplines) of Renmin University of China.
Author information
These authors contributed equally: Ling Zhang and Wenfeng Chen.
Authors and Affiliations
Department of Psychology, Renmin University of China, Beijing, 100872, China
Ling Zhang, Wenfeng Chen, Menghan Liu, Yuxiao Ou, Erjia Xu & Ping Hu
You can also search for this author in PubMed Google Scholar
Contributions
L.Z. designed and conducted the experiment, completed data analysis and wrote the paper. W.C. and P.H. supervised the whole research and revised the paper. M.L., Y.O. and E.X. assisted in the treatment of experimental materials. All authors involved in revising the manuscript critically for important intellectual content and approved the final version of the manuscript.
Corresponding author
Correspondence to Ping Hu .
Ethics declarations
Competing interests.
The authors declare no competing interests.
Additional information
Publisher's note.
Springer Nature remains neutral with regard to jurisdictional claims in published maps and institutional affiliations.
Supplementary Information
Supplementary information., rights and permissions.
Open Access This article is licensed under a Creative Commons Attribution 4.0 International License, which permits use, sharing, adaptation, distribution and reproduction in any medium or format, as long as you give appropriate credit to the original author(s) and the source, provide a link to the Creative Commons licence, and indicate if changes were made. The images or other third party material in this article are included in the article's Creative Commons licence, unless indicated otherwise in a credit line to the material. If material is not included in the article's Creative Commons licence and your intended use is not permitted by statutory regulation or exceeds the permitted use, you will need to obtain permission directly from the copyright holder. To view a copy of this licence, visit http://creativecommons.org/licenses/by/4.0/ .
Reprints and permissions
About this article
Cite this article.
Zhang, L., Chen, W., Liu, M. et al. Light makeup decreases receivers’ negative emotional experience. Sci Rep 11 , 23802 (2021). https://doi.org/10.1038/s41598-021-03129-7
Download citation
Received : 29 May 2021
Accepted : 25 November 2021
Published : 10 December 2021
DOI : https://doi.org/10.1038/s41598-021-03129-7
Share this article
Anyone you share the following link with will be able to read this content:
Sorry, a shareable link is not currently available for this article.
Provided by the Springer Nature SharedIt content-sharing initiative
This article is cited by
Effect of makeup use on depressive symptoms: an open, randomized and controlled trial.
- Marcos C. Veçoso
- Souvenir Zalla
- Vânia R. Leite-Silva
Dermatology and Therapy (2024)
Facial attractiveness facilitates other-race faces recognizing: the role of facial attractiveness in other-race effect
- Hongyun Guo
Current Psychology (2023)
By submitting a comment you agree to abide by our Terms and Community Guidelines . If you find something abusive or that does not comply with our terms or guidelines please flag it as inappropriate.
Quick links
- Explore articles by subject
- Guide to authors
- Editorial policies
Sign up for the Nature Briefing newsletter — what matters in science, free to your inbox daily.

share this!
November 18, 2022
New research on how makeup applied only to facial features increases perceived skin evenness
by Franklin & Marshall College
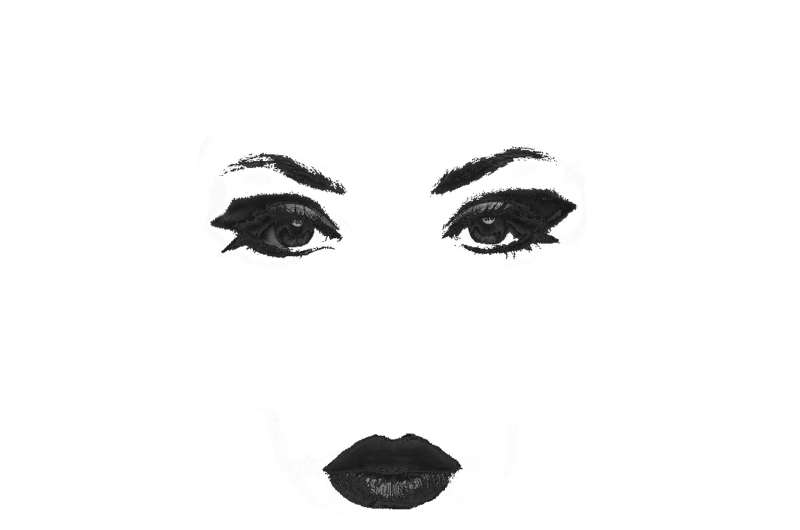
Provided by Franklin & Marshall College
Explore further
Feedback to editors

Liquid droplets shape how cells respond to change, shows study
8 hours ago

Rice bran nanoparticles show promise as affordable and targeted anticancer agent
9 hours ago

Advance in forensic fingerprint research provides new hope for cold cases

How spicy does mustard get depending on the soil?

Electron videography captures moving dance between proteins and lipids

New findings shed light on how bella moths use poison to attract mates

AI tool creates 'synthetic' images of cells for enhanced microscopy analysis

Announcing the birth of QUIONE, a unique analog quantum processor
10 hours ago

World's oases threatened by desertification, even as humans expand them
11 hours ago

NASA's Voyager 1 resumes sending engineering updates to Earth
Relevant physicsforums posts, cover songs versus the original track, which ones are better, biographies, history, personal accounts.
Apr 21, 2024
Interesting anecdotes in the history of physics?
History of railroad safety - spotlight on current derailments, for ww2 buffs.
Apr 20, 2024
Who is your favorite Jazz musician and what is your favorite song?
Apr 19, 2024
More from Art, Music, History, and Linguistics
Related Stories

Macho makeup: New research on how cosmetics increase attractiveness in men
Nov 3, 2022

Lead-based cosmetics poisoned 18th-century European socialites in search of whiter skin
Feb 28, 2022

Twelve-week treatment regimen reduces facial redness
Jul 1, 2022

FDA warns of dangers from skin lightening creams
Sep 1, 2022

Expert tips for taming oily skin
Apr 10, 2018

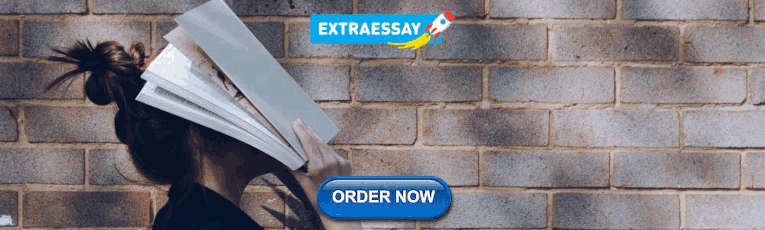
Study shows smiling makes you look older, unless you're old already
Nov 29, 2021
Recommended for you

Saturday Citations: Irrationality modeled; genetic basis for PTSD; Tasmanian devils still endangered

Many prisoners go years without touching a smartphone—it means they struggle to navigate life on the outside

Data-driven music: Converting climate measurements into music
Apr 18, 2024

Researchers find lower grades given to students with surnames that come later in alphabetical order
Apr 17, 2024

Study reveals how humanity could unite to address global challenges
Apr 16, 2024

Saturday Citations: Listening to bird dreams, securing qubits, imagining impossible billiards
Apr 13, 2024
Let us know if there is a problem with our content
Use this form if you have come across a typo, inaccuracy or would like to send an edit request for the content on this page. For general inquiries, please use our contact form . For general feedback, use the public comments section below (please adhere to guidelines ).
Please select the most appropriate category to facilitate processing of your request
Thank you for taking time to provide your feedback to the editors.
Your feedback is important to us. However, we do not guarantee individual replies due to the high volume of messages.
E-mail the story
Your email address is used only to let the recipient know who sent the email. Neither your address nor the recipient's address will be used for any other purpose. The information you enter will appear in your e-mail message and is not retained by Phys.org in any form.
Newsletter sign up
Get weekly and/or daily updates delivered to your inbox. You can unsubscribe at any time and we'll never share your details to third parties.
More information Privacy policy
Donate and enjoy an ad-free experience
We keep our content available to everyone. Consider supporting Science X's mission by getting a premium account.
E-mail newsletter
Differential effects of makeup on perceived age
Affiliations.
- 1 Department of Psychology, Gettysburg College, Gettysburg, Pennsylvania, USA.
- 2 CHANEL Fragrance & Beauty Research & Innovation, Pantin, France.
- 3 Département Psychologie Cognitive, Ergonomie, Université de Toulouse, France.
- 4 Institut Universitaire de France, Paris, France.
- 5 Société Lincoln, Boulogne-Billancourt, France.
- 6 Laboratoire de Psychologie et NeuroCognition, Université Grenoble Alpes, France.
- PMID: 30073658
- DOI: 10.1111/bjop.12337
Makeup accentuates three youth-related visual features - skin homogeneity, facial contrast, and facial feature size. By manipulating these visual features, makeup should make faces appear younger. We tested this hypothesis in an experiment in which participants estimated the age of carefully controlled photographs of faces with and without makeup. We found that 40- and especially 50-year-old women did appear significantly younger when wearing makeup. Contrary to our hypothesis, 30-year-old women looked no different in age with or without makeup, while 20-year-old women looked older with makeup. Two further studies replicated these results, finding that makeup made middle-aged women look younger, but made young women look older. Seeking to better understand why makeup makes young women look older, we ran a final study and found evidence that people associate makeup use with adulthood. By activating associations with adulthood, makeup may provide an upward bias on age estimations of women who are not clearly adult. We propose that makeup affects social perceptions through bottom-up routes, by modifying visual cues such as facial contrast, facial feature size, and skin homogeneity, and also through top-down routes, by activating social representations and norms associated with makeup use.
Keywords: age; cosmetics; face perception; makeup; person perception.
© 2018 The British Psychological Society.
- Age Factors*
- Face* / anatomy & histology
- Middle Aged
- Perception*
- Photic Stimulation
- Skin / anatomy & histology
- Social Norms*
- Young Adult
- Australia (AUD $)
- Austria (EUR €)
- Belgium (EUR €)
- Canada (CAD $)
- Czechia (CZK Kč)
- Denmark (DKK kr.)
- Finland (EUR €)
- France (EUR €)
- Germany (EUR €)
- Hong Kong SAR (HKD $)
- Ireland (EUR €)
- Israel (ILS ₪)
- Italy (EUR €)
- Japan (JPY ¥)
- Malaysia (MYR RM)
- Netherlands (EUR €)
- New Zealand (NZD $)
- Norway (USD $)
- Poland (PLN zł)
- Portugal (EUR €)
- Singapore (SGD $)
- South Korea (KRW ₩)
- Spain (EUR €)
- Sweden (SEK kr)
- Switzerland (CHF CHF)
- United Arab Emirates (AED د.إ)
- United Kingdom (GBP £)
- United States (USD $)
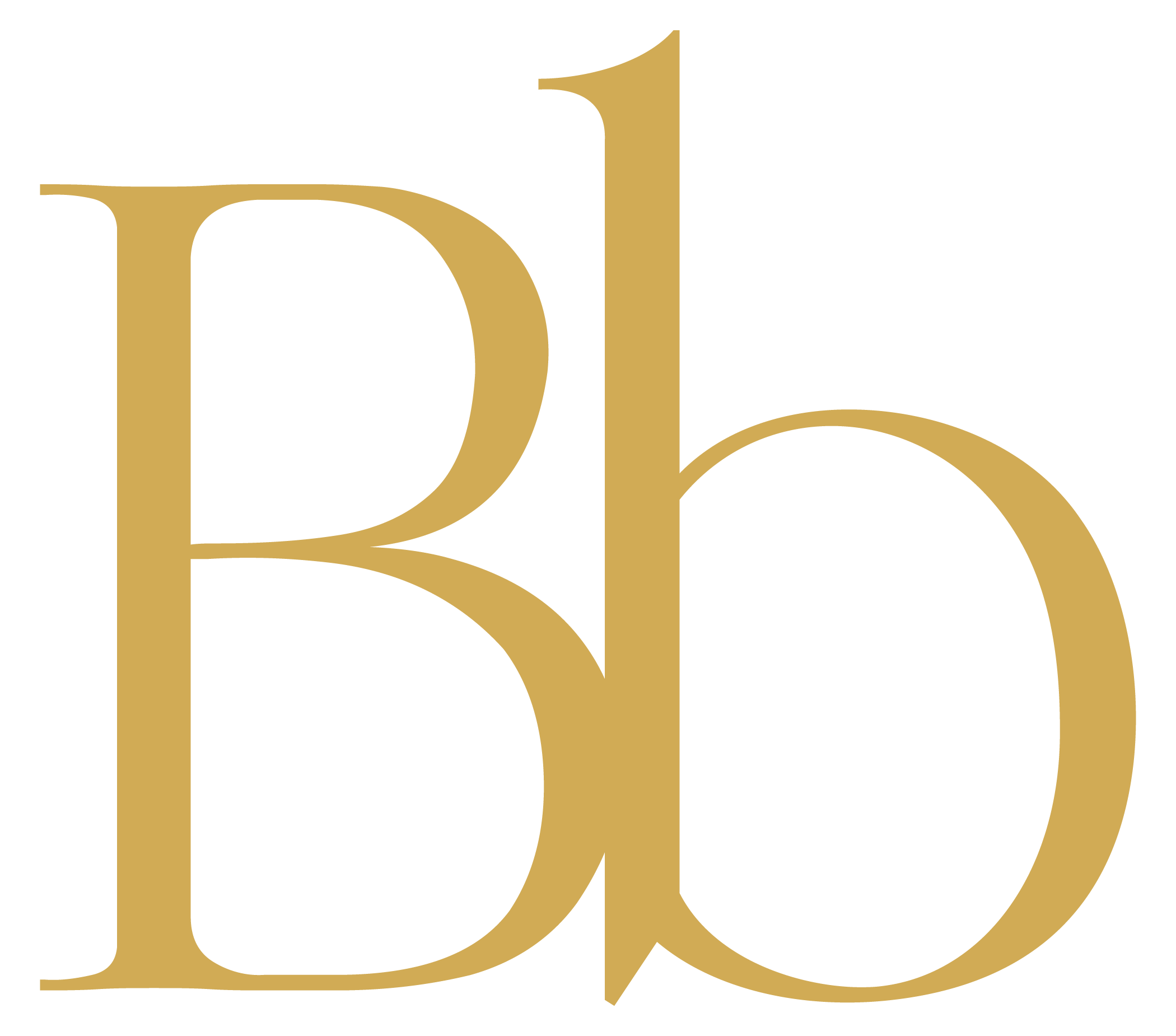
Sign up and save
Sign up for our mailing list to receive discounts and exclusive offers.
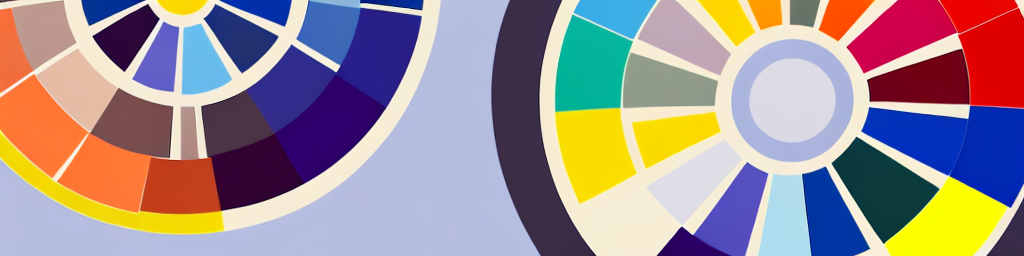
Understanding the Basics of Color Theory in Makeup
Color theory may seem like a daunting concept to grasp, but it is an essential element of any makeup artist's skill set. In this article, we will cover the basics of color theory, what it is, and why it matters in makeup artistry. We will delve into the science behind color theory and makeup and how to choose the perfect colors for your skin tone. Additionally, we will explore primary colors as opposed to secondary colors, warm vs. cool colors, and how to make complementing colors work in your favor. We will also go into detail about triadic color schemes and the significance of neutrals in makeup and color theory.
Why Color Theory Matters in Makeup Artistry
Color theory plays an essential role in makeup artistry, and it can dictate whether a makeup look succeeds or fails. If the colors are not combined correctly, the makeup may end up looking unflattering and stark.
One of the most important aspects of color theory in makeup artistry is understanding the color wheel. The color wheel is a tool that helps artists determine which colors complement each other and which ones clash. By using the color wheel, makeup artists can create harmonious and balanced looks that enhance their clients' natural beauty.
Another reason why color theory is crucial in makeup artistry is that it can help artists create specific moods and emotions with their makeup looks. For example, warm colors like red and orange can create a sense of energy and excitement, while cool colors like blue and green can evoke a feeling of calmness and serenity. By understanding the psychology of color, makeup artists can use their skills to create looks that not only look beautiful but also convey a specific message or feeling.
The Science Behind Color Theory and Makeup
The science behind color theory lies in the color wheel, which is a visual representation of how different colors work together. Makeup artists need to familiarize themselves with the color wheel to create makeup looks that complement the model's skin tone while achieving the desired effect.
Understanding the color wheel is essential for makeup artists to create a harmonious and balanced look. Complementary colors, which are opposite each other on the color wheel, can be used to create a bold and striking effect. Analogous colors, which are next to each other on the color wheel, can be used to create a more subtle and natural look. By using the principles of color theory, makeup artists can enhance the natural beauty of their clients and create stunning makeup looks.
How to Choose the Right Colors for Your Skin Tone
Choosing the right colors for a makeup look depends on the skin tone of the model. Skin tone can be classified into warm undertones or cool undertones. If the skin has yellow or olive undertones, it is considered warm, whereas if it has pink or blue undertones, it is cool.
It is important to note that certain colors can complement or clash with different skin tones. For warm undertones, earthy tones such as browns, oranges, and yellows can enhance the natural warmth of the skin. Cool undertones, on the other hand, can benefit from cooler colors such as blues, purples, and greens. However, it is also important to consider personal preferences and the occasion when choosing makeup colors.
Primary Colors vs. Secondary Colors: What's the Difference?
Primary colors are red, blue, and yellow, which are the building blocks of all the other colors on the color wheel. On the other hand, secondary colors are made by combining two primary colors, and they include purple, green, and orange.
It's important to note that primary and secondary colors are not the only types of colors. There are also tertiary colors, which are made by combining a primary color with a secondary color. Examples of tertiary colors include yellow-green, blue-green, red-orange, and yellow-orange.
Understanding the differences between primary, secondary, and tertiary colors is essential for artists, designers, and anyone working with color. By knowing how colors are created and how they interact with each other, you can create visually appealing and harmonious designs that effectively communicate your message.
Warm vs. Cool Colors: Which Ones Work Best for You?
Warm colors, including reds, oranges, and yellows, generally complement warm skin tones, while cool colors, such as blues, purples, and greens, highlight cool skin tones. However, makeup artists usually mix warm and cool tones to achieve a balance in a makeup look.
It's important to note that warm and cool colors can also have different psychological effects on individuals. Warm colors are often associated with energy, passion, and excitement, while cool colors are associated with calmness, relaxation, and serenity. This is why warm colors are often used in marketing to create a sense of urgency or excitement, while cool colors are used in healthcare settings to promote a sense of calmness and relaxation.
Complementary Colors: How to Make Them Work in Your Favor
Complementary colors are opposite colors on the color wheel, such as purple and yellow. Combining complementary colors creates a bold, eye-catching makeup look that stands out.
However, it's important to use complementary colors in moderation. Too much of a bold color combination can be overwhelming and distracting. Instead, try using complementary colors as accents, such as a pop of color on the lips or a bold eyeliner.
Another way to make complementary colors work in your favor is to consider your skin tone. For example, if you have warm undertones, you may want to opt for complementary colors with warm undertones as well, such as orange and blue. If you have cool undertones, complementary colors with cool undertones, such as green and pink, may be more flattering.
Triadic Color Schemes: A Guide to Creating Balanced Looks
A triadic color scheme is created by selecting three colors equally spaced on the color wheel. Makeup artists can use this scheme to create a balanced makeup look that doesn't overwhelm the model's skin tone.
The Role of Neutrals in Makeup and Color Theory
Neutrals, such as browns, grays, and black, can balance out a colorful makeup look. They can also be used to create a subtle look, either alone or as a base shadow.
Another important role of neutrals in makeup is their ability to contour and define facial features. By using a matte brown shade, for example, you can create the illusion of a more defined cheekbone or a slimmer nose. Neutrals can also be used to soften harsh lines and edges, creating a more natural and blended look.
When it comes to color theory, neutrals are considered to be universal shades that can complement any skin tone or eye color. They can be used to tone down bright or bold colors, making them more wearable and suitable for everyday looks. Additionally, neutrals can be mixed and matched with each other to create a variety of looks, from smoky eyes to natural, no-makeup makeup.
Tips for Experimenting with Bold and Bright Colors
Playing around with bold and bright colors can be fun, but it also requires skill and knowledge of color theory. Makeup artists can experiment with colors by taking baby steps and building up their confidence gradually.
One way to start experimenting with bold and bright colors is to choose a single color and use it as a statement piece. For example, a bright red lipstick can be paired with neutral eye makeup and a simple outfit to create a bold and confident look. Another tip is to use complementary colors, such as blue and orange or purple and yellow, to create a striking contrast. Remember to also consider the skin tone and undertones of the client when selecting colors. With practice and experimentation, makeup artists can master the art of using bold and bright colors to create stunning looks.
Common Mistakes to Avoid When Applying Colorful Makeup
Some common mistakes to avoid when applying colorful makeup include wearing the wrong colors for your skin tone and over-application. Over-application can make the makeup overpowering and unflattering.
Another mistake to avoid is not blending the colors properly. When using multiple colors, it is important to blend them seamlessly to create a cohesive look. Harsh lines and uneven blending can make the makeup look messy and unprofessional.
Additionally, not considering the occasion or setting can also be a mistake. While bold and colorful makeup can be fun for a night out, it may not be appropriate for a professional setting or a more formal event. It is important to consider the context and dress accordingly.
How to Use Color Theory to Enhance Your Natural Beauty
Using color theory to enhance natural beauty involves using colors that complement the model's skin tone and creating a natural makeup look that accentuates the features.
One way to determine which colors complement a person's skin tone is to identify their undertones. Undertones can be warm, cool, or neutral. Warm undertones have a yellow or golden hue, while cool undertones have a pink or blue hue. Neutral undertones have a mix of both warm and cool tones. Once you have identified the undertones, you can choose colors that complement them. For example, if someone has warm undertones, they may look best in earthy tones like browns, oranges, and yellows. If someone has cool undertones, they may look best in jewel tones like blues, greens, and purples.
Applying Color Theory Principles to Different Makeup Products (e.g., lipsticks, eyeshadows, blushes)
Applying color theory principles to different makeup products involves understanding which colors work for which products. For example, red lipstick might work well with coral blush, while blue eyeshadow can complement peach blush.
It is also important to consider the undertones of the products being used. For instance, warm-toned eyeshadows like gold and copper pair well with warm-toned lipsticks like orange and red, while cool-toned eyeshadows like silver and blue work better with cool-toned lipsticks like pink and purple.
Another factor to consider is the occasion and the desired look. For a natural, everyday look, it is best to stick to neutral colors that complement the skin tone. However, for a bold and dramatic look, contrasting colors can be used to create a striking effect. It is important to experiment with different color combinations to find what works best for each individual's unique features and preferences.
Advanced Techniques for Mixing and Matching Colors in Makeup Artistry
Advanced techniques for mixing and matching colors involve playing with pigments, textures, and brush techniques. For instance, a makeup artist can play with the color pay-off of two products to create a unique, one-of-a-kind color.
Another technique is to use complementary colors to create a bold and striking look. Complementary colors are opposite each other on the color wheel, such as red and green or blue and orange. When used together, they create a high contrast and can make the eyes or lips pop. It's important to blend the colors well to avoid a harsh or jarring effect.
Mastering Color Theory: Practical Exercises and Challenges for Makeup Artists
One way to master color theory is to practice. Makeup artists can take up challenges that stretch their skills in color theory. They can also create mood boards and color swatches to hone their knowledge of color theory.
Another effective way for makeup artists to master color theory is to study the color wheel and understand the relationships between colors. Complementary colors, for example, are opposite each other on the color wheel and can create a striking contrast when used together. Analogous colors, on the other hand, are next to each other on the color wheel and can create a harmonious and cohesive look. By understanding these relationships, makeup artists can create stunning and well-balanced looks for their clients.
Next Article >
< previous article.
© Brave in Bloom, 2023
Other Journal Entries
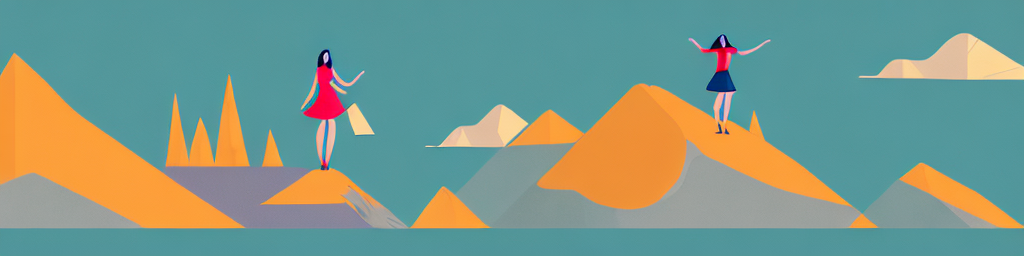
Our Best Skincare Products
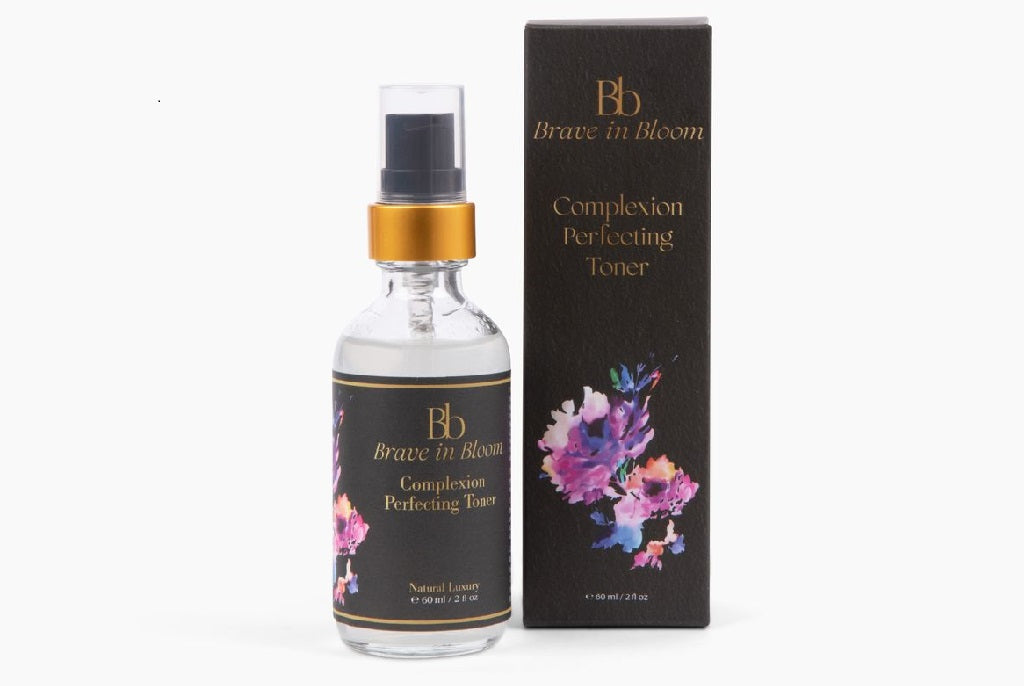
Our Best Aging Prevention Products
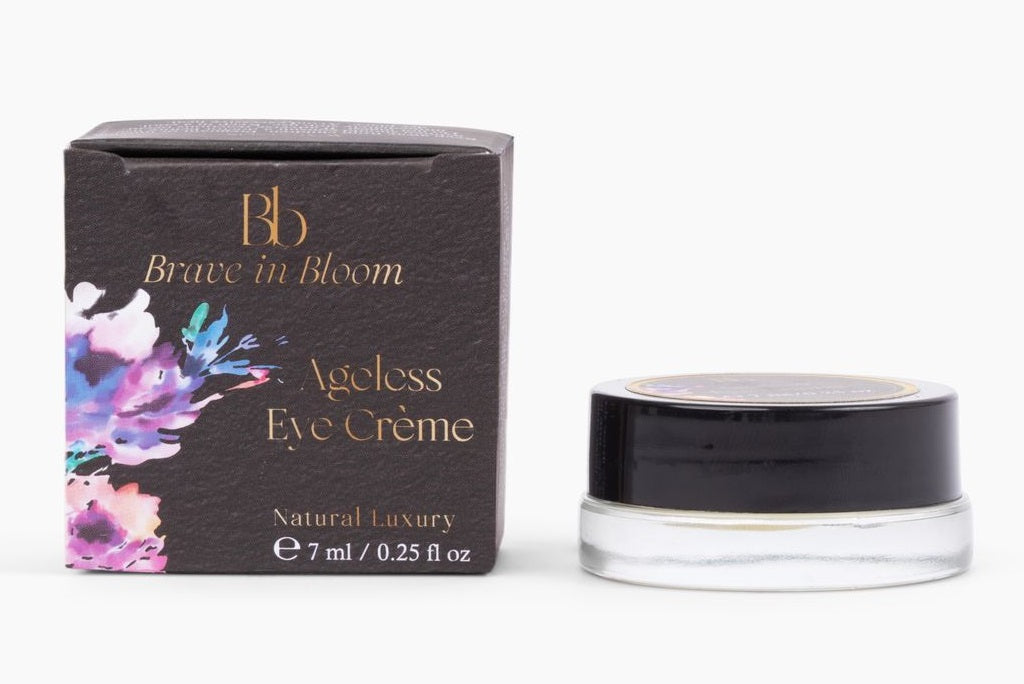
- Research article
- Open access
- Published: 12 June 2019
The impact of skin care products on skin chemistry and microbiome dynamics
- Amina Bouslimani 1 na1 ,
- Ricardo da Silva 1 na1 ,
- Tomasz Kosciolek 2 ,
- Stefan Janssen 2 , 3 ,
- Chris Callewaert 2 , 4 ,
- Amnon Amir 2 ,
- Kathleen Dorrestein 1 ,
- Alexey V. Melnik 1 ,
- Livia S. Zaramela 2 ,
- Ji-Nu Kim 2 ,
- Gregory Humphrey 2 ,
- Tara Schwartz 2 ,
- Karenina Sanders 2 ,
- Caitriona Brennan 2 ,
- Tal Luzzatto-Knaan 1 ,
- Gail Ackermann 2 ,
- Daniel McDonald 2 ,
- Karsten Zengler 2 , 5 , 6 ,
- Rob Knight 2 , 5 , 6 , 7 &
- Pieter C. Dorrestein 1 , 2 , 5 , 8
BMC Biology volume 17 , Article number: 47 ( 2019 ) Cite this article
96k Accesses
90 Citations
122 Altmetric
Metrics details
Use of skin personal care products on a regular basis is nearly ubiquitous, but their effects on molecular and microbial diversity of the skin are unknown. We evaluated the impact of four beauty products (a facial lotion, a moisturizer, a foot powder, and a deodorant) on 11 volunteers over 9 weeks.
Mass spectrometry and 16S rRNA inventories of the skin revealed decreases in chemical as well as in bacterial and archaeal diversity on halting deodorant use. Specific compounds from beauty products used before the study remain detectable with half-lives of 0.5–1.9 weeks. The deodorant and foot powder increased molecular, bacterial, and archaeal diversity, while arm and face lotions had little effect on bacterial and archaeal but increased chemical diversity. Personal care product effects last for weeks and produce highly individualized responses, including alterations in steroid and pheromone levels and in bacterial and archaeal ecosystem structure and dynamics.
Conclusions
These findings may lead to next-generation precision beauty products and therapies for skin disorders.
The human skin is the most exposed organ to the external environment and represents the first line of defense against external chemical and microbial threats. It harbors a microbial habitat that is person-specific and varies considerably across the body surface [ 1 , 2 , 3 , 4 ]. Recent findings suggested an association between the use of antiperspirants or make-up and skin microbiota composition [ 5 , 6 , 7 ]. However, these studies were performed for a short period (7–10 days) and/or without washing out the volunteers original personal care products, leading to incomplete evaluation of microbial alterations because the process of skin turnover takes 21–28 days [ 5 , 6 , 7 , 8 , 9 ]. It is well-established that without intervention, most adult human microbiomes, skin or other microbiomes, remain stable compared to the differences between individuals [ 3 , 10 , 11 , 12 , 13 , 14 , 15 , 16 ].
Although the skin microbiome is stable for years [ 10 ], little is known about the molecules that reside on the skin surface or how skin care products influence this chemistry [ 17 , 18 ]. Mass spectrometry can be used to detect host molecules, personalized lifestyles including diet, medications, and personal care products [ 18 , 19 ]. However, although the impact of short-term dietary interventions on the gut microbiome has been assessed [ 20 , 21 ], no study has yet tested how susceptible the skin chemistry and Microbiome are to alterations in the subjects’ personal care product routine.
In our recent metabolomic/microbiome 3D cartography study [ 18 ], we observed altered microbial communities where specific skin care products were present. Therefore, we hypothesized that these products might shape specific skin microbial communities by changing their chemical environment. Some beauty product ingredients likely promote or inhibit the growth of specific bacteria: for example, lipid components of moisturizers could provide nutrients and promote the growth of lipophilic bacteria such as Staphylococcus and Propionibacterium [ 18 , 22 , 23 ]. Understanding both temporal variations of the skin microbiome and chemistry is crucial for testing whether alterations in personal habits can influence the human skin ecosystem and, perhaps, host health. To evaluate these variations, we used a multi-omics approach integrating metabolomics and microbiome data from skin samples of 11 healthy human individuals. Here, we show that many compounds from beauty products persist on the skin for weeks following their use, suggesting a long-term contribution to the chemical environment where skin microbes live. Metabolomics analysis reveals temporal trends correlated to discontinuing and resuming the use of beauty products and characteristic of variations in molecular composition of the skin. Although highly personalized, as seen with the microbiome, the chemistry, including hormones and pheromones such as androstenone and androsterone, were dramatically altered. Similarly, by experimentally manipulating the personal care regime of participants, bacterial and molecular diversity and structure are altered, particularly for the armpits and feet. Interestingly, a high person-to-person molecular and bacterial variability is maintained over time even though personal care regimes were modified in exactly the same way for all participants.
Skin care and hygiene products persist on the skin
Systematic strategies to influence both the skin chemistry and microbiome have not yet been investigated. The outermost layer of the skin turns over every 3 to 4 weeks [ 8 , 9 ]. How the microbiome and chemistry are influenced by altering personal care and how long the chemicals of personal care products persist on the skin are essentially uncharacterized. In this study, we collected samples from skin of 12 healthy individuals—six males and six females—over 9 weeks. One female volunteer had withdrawn due to skin irritations that developed, and therefore, we describe the remaining 11 volunteers. Samples were collected from each arm, armpit, foot, and face, including both the right and left sides of the body (Fig. 1 a). All participants were asked to adhere to the same daily personal care routine during the first 6 weeks of this study (Fig. 1 b). The volunteers were asked to refrain from using any personal care product for weeks 1–3 except a mild body wash (Fig. 1 b). During weeks 4–6, in addition to the body wash, participants were asked to apply selected commercial skin care products at specific body parts: a moisturizer on the arm, a sunscreen on the face, an antiperspirant on the armpits, and a soothing powder on the foot (Fig. 1 b). To monitor adherence of participants to the study protocol, molecular features found in the antiperspirant, facial lotion, moisturizer, and foot powder were directly tracked with mass spectrometry from the skin samples. For all participants, the mass spectrometry data revealed the accumulation of specific beauty product ingredients during weeks 4–6 (Additional file 1 : Figure S1A-I, Fig. 2 a orange arrows). Examples of compounds that were highly abundant during T4–T6 in skin samples are avobenzone (Additional file 1 : Figure S1A), dexpanthenol (Additional file 1 : Figure S1B), and benzalkonium chloride (Additional file 1 : Figure S1C) from the facial sunscreen; trehalose 6-phosphate (Additional file 1 : Figure S1D) and glycerol stearate (Additional file 1 : Figure S1E) from the moisturizer applied on arms; indolin (Additional file 1 : Figure S1F) and an unannotated compound ( m/z 233.9, rt 183.29 s) (Additional file 1 : Figure S1G) from the foot powder; and decapropylene glycol (Additional file 1 : Figure S1H) and nonapropylene glycol (Additional file 1 : Figure S1I) from the antiperspirant. These results suggest that there is likely a compliance of all individuals to study requirements and even if all participants confirmed using each product every day, the amount of product applied by each individual may vary. Finally, for weeks 7–9, the participants were asked to return to their normal routine by using the same personal care products they used prior to the study. In total, excluding all blanks and personal care products themselves, we analyzed 2192 skin samples for both metabolomics and microbiome analyses.
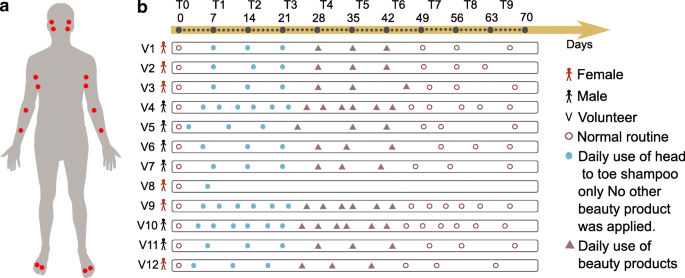
Study design and representation of changes in personal care regime over the course of 9 weeks. a Six males and six females were recruited and sampled using swabs on two locations from each body part (face, armpits, front forearms, and between toes) on the right and left side. The locations sampled were the face—upper cheek bone and lower jaw, armpit—upper and lower area, arm—front of elbow (antecubitis) and forearm (antebrachium), and feet—in between the first and second toe and third and fourth toe. Volunteers were asked to follow specific instructions for the use of skin care products. b Following the use of their personal skin care products (brown circles), all volunteers used only the same head to toe shampoo during the first 3 weeks (week 1–week 3) and no other beauty product was applied (solid blue circle). The following 3 weeks (week 4–week 6), four selected commercial beauty products were applied daily by all volunteers on the specific body part (deodorant antiperspirant for the armpits, soothing foot powder for the feet between toes, sunscreen for the face, and moisturizer for the front forearm) (triangles) and continued to use the same shampoo. During the last 3 weeks (week 7–week 9), all volunteers went back to their normal routine and used their personal beauty products (circles). Samples were collected once a week (from day 0 to day 68—10 timepoints from T0 to T9) for volunteers 1, 2, 3, 4, 5, 6, 7, 9, 10, 11, and 12, and on day 0 and day 6 for volunteer 8, who withdraw from the study after day 6. For 3 individuals (volunteers 4, 9, 10), samples were collected twice a week (19 timepoints total). Samples collected for 11 volunteers during 10 timepoints: 11 volunteers × 10 timepoints × 4 samples × 4 body sites = 1760. Samples collected from 3 selected volunteers during 9 additional timepoints: 3 volunteers × 9 timepoints × 4 samples × 4 body sites = 432. See also the “ Subject recruitment and sample collection ” section in the “ Methods ” section
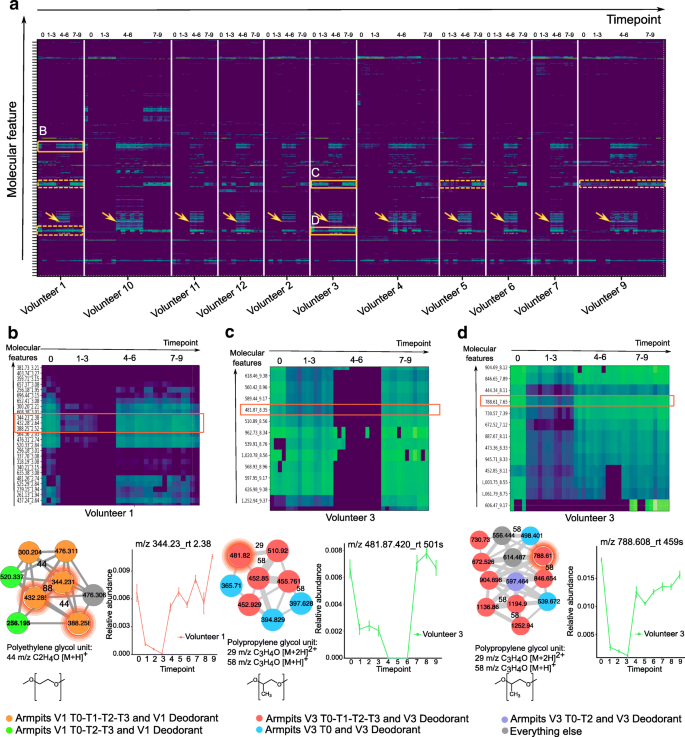
Monitoring the persistence of personal care product ingredients in the armpits over a 9-week period. a Heatmap representation of the most abundant molecular features detected in the armpits of all individuals during the four phases (0: initial, 1–3: no beauty products, 4–6: common products, and 7–9: personal products). Green color in the heatmap represents the highest molecular abundance and blue color the lowest one. Orange boxes with plain lines represent enlargement of cluster of molecules that persist on the armpits of volunteer 1 ( b ) and volunteer 3 ( c , d ). Orange clusters with dotted lines represent same clusters of molecules found on the armpits of other volunteers. Orange arrows represent the cluster of compounds characteristic of the antiperspirant used during T4–T6. b Polyethylene glycol (PEG) molecular clusters that persist on the armpits of individual 1. The molecular subnetwork, representing molecular families [ 24 ], is part of a molecular network ( http://gnps.ucsd.edu/ProteoSAFe/status.jsp?task=f5325c3b278a46b29e8860ec5791d5ad ) generated from MS/MS data collected from the armpits of volunteer 1 (T0–T3) MSV000081582 and MS/MS data collected from the deodorant used by volunteer 1 before the study started (T0) MSV000081580. c , d Polypropylene glycol (PPG) molecular families that persist on the armpits of individual 3, along with the corresponding molecular subnetwork that is part of the molecular network accessible here http://gnps.ucsd.edu/ProteoSAFe/status.jsp?task=aaa1af68099d4c1a87e9a09f398fe253 . Subnetworks were generated from MS/MS data collected from the armpits of volunteer 3 (T0–T3) MSV000081582 and MS/MS data collected from the deodorant used by volunteer 3 at T0 MSV000081580. The network nodes were annotated with colors. Nodes represent MS/MS spectra found in armpit samples of individual 1 collected during T0, T1, T2, and T3 and in personal deodorant used by individual 1 (orange nodes); armpit samples of individual 1 collected during T0, T2, and T3 and personal deodorant used by individual 1 (green nodes); armpit samples of individual 3 collected during T0, T1, T2, and T3 and in personal deodorant used by individual 3 (red nodes); armpit samples of individual 3 collected during T0 and in personal deodorant used by individual 3 (blue nodes); and armpit samples of individual 3 collected during T0 and T2 and in personal deodorant used by individual 3 (purple nodes). Gray nodes represent everything else. Error bars represent standard error of the mean calculated at each timepoint from four armpit samples collected from the right and left side of each individual separately. See also Additional file 1 : Figure S1
To understand how long beauty products persist on the skin, we monitored compounds found in deodorants used by two volunteers—female 1 and female 3—before the study (T0), over the first 3 weeks (T1–T3) (Fig. 1 b). During this phase, all participants used exclusively the same body wash during showering, making it easier to track ingredients of their personal care products. The data in the first 3 weeks (T1–T3) revealed that many ingredients of deodorants used on armpits (Fig. 2 a) persist on the skin during this time and were still detected during the first 3 weeks or at least during the first week following the last day of use. Each of the compounds detected in the armpits of individuals exhibited its own unique half-life. For example, the polyethylene glycol (PEG)-derived compounds m/z 344.227, rt 143 s (Fig. 2 b, S1J); m/z 432.279, rt 158 s (Fig. 2 b, S1K); and m/z 388.253, rt 151 s (Fig. 2 b, S1L) detected on armpits of volunteer 1 have a calculated half-life of 0.5 weeks (Additional file 1 : Figure S1J-L, all p values < 1.81e−07), while polypropylene glycol (PPG)-derived molecules m/z 481.87, rt 501 s (Fig. 2 c, S1M); m/z 560.420, rt 538 s (Fig. 2 c, S1N); m/z 788.608, rt 459 s (Fig. 2 d, S1O); m/z 846.650, rt 473 s (Fig. 2 d, S1P); and m/z 444.338, rt 486 s (Fig. 2 d, S1Q) found on armpits of volunteers 3 and 1 (Fig. 2 a) have a calculated half-life ranging from 0.7 to 1.9 weeks (Additional file 1 : Figure S1M-Q, all p values < 0.02), even though they originate from the same deodorant used by each individual. For some ingredients of deodorant used by volunteer 3 on time 0 (Additional file 1 : Figure S1M, N), a decline was observed during the first week, then little to no traces of these ingredients were detected during weeks 4–6 (T4–T6), then finally these ingredients reappear again during the last 3 weeks of personal product use (T7–T9). This suggests that these ingredients are present exclusively in the personal deodorant used by volunteer 3 before the study. Because a similar deodorant (Additional file 1 : Figure S1O-Q) and a face lotion (Additional file 1 : Figure S1R) was used by volunteer 3 and volunteer 2, respectively, prior to the study, there was no decline or absence of their ingredients during weeks 4–6 (T4–T6).
Polyethylene glycol compounds (Additional file 1 : Figure S1J-L) wash out faster from the skin than polypropylene glycol (Additional file 1 : Figure S1M-Q)(HL ~ 0.5 weeks vs ~ 1.9 weeks) and faster than fatty acids used in lotions (HL ~ 1.2 weeks) (Additional file 1 : Figure S1R), consistent with their hydrophilic (PEG) and hydrophobic properties (PPG and fatty acids) [ 25 , 26 ]. This difference in hydrophobicity is also reflected in the retention time as detected by mass spectrometry. Following the linear decrease of two PPG compounds from T0 to T1, they accumulated noticeably during weeks 2 and 3 (Additional file 1 : Figure S1M, N). This accumulation might be due to other sources of PPG such as the body wash used during this period or the clothes worn by person 3. Although PPG compounds were not listed in the ingredient list of the shampoo, we manually inspected the LC-MS data collected from this product and confirmed the absence of PPG compounds in the shampoo. The data suggest that this trend is characteristic of accumulation of PPG from additional sources. These could be clothes, beds, or sheets, in agreement with the observation of these molecules found in human habitats [ 27 ] but also in the public GNPS mass spectrometry dataset MSV000079274 that investigated the chemicals from dust collected from 1053 mattresses of children.
Temporal molecular and bacterial diversity in response to personal care use
To assess the effect of discontinuing and resuming the use of skin care products on molecular and microbiota dynamics, we first evaluated their temporal diversity. Skin sites varied markedly in their initial level (T0) of molecular and bacterial diversity, with higher molecular diversity at all sites for female participants compared to males (Fig. 3 a, b, Wilcoxon rank-sum-WR test, p values ranging from 0.01 to 0.0001, from foot to arm) and higher bacterial diversity in face (WR test, p = 0.0009) and armpits (WR test, p = 0.002) for females (Fig. 3 c, d). Temporal diversity was similar across the right and left sides of each body site of all individuals (WR test, molecular diversity: all p values > 0.05; bacterial diversity: all p values > 0.20). The data show that refraining from using beauty products (T1–T3) leads to a significant decrease in molecular diversity at all sites (Fig. 3 a, b, WR test, face: p = 8.29e−07, arm: p = 7.08e−09, armpit: p = 1.13e−05, foot: p = 0.002) and bacterial diversity mainly in armpits (WR test, p = 0.03) and feet (WR test, p = 0.04) (Fig. 3 c, d). While molecular diversity declined (Fig. 3 a, b) for arms and face, bacterial diversity (Fig. 3 c, d) was less affected in the face and arms when participants did not use skin care products (T1–T3). The molecular diversity remained stable in the arms and face of female participants during common beauty products use (T4–T6) to immediately increase as soon as the volunteers went back to their normal routines (T7–T9) (WR test, p = 0.006 for the arms and face)(Fig. 3 a, b). A higher molecular (Additional file 1 : Figure S2A) and community (Additional file 1 : Figure S2B) diversity was observed for armpits and feet of all individuals during the use of antiperspirant and foot powder (T4–T6) (WR test, molecular diversity: armpit p = 8.9e−33, foot p = 1.03e−11; bacterial diversity: armpit p = 2.14e−28, foot p = 1.26e−11), followed by a molecular and bacterial diversity decrease in the armpits when their regular personal beauty product use was resumed (T7–T9) (bacterial diversity: WR test, p = 4.780e−21, molecular diversity: WR test, p = 2.159e−21). Overall, our data show that refraining from using beauty products leads to lower molecular and bacterial diversity, while resuming the use increases their diversity. Distinct variations between male and female molecular and community richness were perceived at distinct body parts (Fig. 3 a–d). Although the chemical diversity of personal beauty products does not explain these variations (Additional file 1 : Figure S2C), differences observed between males and females may be attributed to many environmental and lifestyle factors including different original skin care and different frequency of use of beauty products (Additional file 2 : Table S1), washing routines, and diet.

Molecular and bacterial diversity over a 9-week period, comparing samples based on their molecular (UPLC-Q-TOF-MS) or bacterial (16S rRNA amplicon) profiles. Molecular and bacterial diversity using the Shannon index was calculated from samples collected from each body part at each timepoint, separately for female ( n = 5) and male ( n = 6) individuals. Error bars represent standard error of the mean calculated at each timepoint, from up to four samples collected from the right and left side of each body part, of females ( n = 5) and males ( n = 6) separately. a , b Molecular alpha diversity measured using the Shannon index from five females (left panel) and six males (right panel), over 9 weeks, from four distinct body parts (armpits, face, arms, feet). c , d Bacterial alpha diversity measured using the Shannon index, from skin samples collected from five female (left panel) and six male individuals (right panel), over 9 weeks, from four distinct body parts (armpits, face, arms, feet). See also Additional file 1 : Figure S2
Longitudinal variation of skin metabolomics signatures
To gain insights into temporal metabolomics variation associated with beauty product use, chemical inventories collected over 9 weeks were subjected to multivariate analysis using the widely used Bray–Curtis dissimilarity metric (Fig. 4 a–c, S3A). Throughout the 9-week period, distinct molecular signatures were associated to each specific body site: arm, armpit, face, and foot (Additional file 1 : Figure S3A, Adonis test, p < 0.001, R 2 0.12391). Mass spectrometric signatures displayed distinct individual trends at each specific body site (arm, armpit, face, and foot) over time, supported by their distinct locations in PCoA (principal coordinate analysis) space (Fig. 4 a, b) and based on the Bray–Curtis distances between molecular profiles (Additional file 1 : Figure S3B, WR test, all p values < 0.0001 from T0 through T9). This suggests a high molecular inter-individual variability over time despite similar changes in personal care routines. Significant differences in molecular patterns associated to ceasing (T1–T3) (Fig. 4 b, Additional file 1 : Figure S3C, WR test, T0 vs T1–T3 p < 0.001) and resuming the use of common beauty products (T4–T6) (Additional file 1 : Figure S3C) were observed in the arm, face, and foot (Fig. 4 b), although the armpit exhibited the most pronounced changes (Fig. 4 b, Additional file 1 : Figure S3D, E, random forest highlighting that 100% of samples from each phase were correctly predicted). Therefore, we focused our analysis on this region. Molecular changes were noticeable starting the first week (T1) of discontinuing beauty product use. As shown for armpits in Fig. 4 c, these changes at the chemical level are specific to each individual, possibly due to the extremely personalized lifestyles before the study and match their original use of deodorant. Based on the initial use of underarm products (T0) (Additional file 2 : Table S1), two groups of participants can be distinguished: a group of five volunteers who used stick deodorant as evidenced by the mass spectrometry data and another group of volunteers where we found few or no traces suggesting they never or infrequently used stick deodorants (Additional file 2 : Table S1). Based on this criterion, the chemical trends shown in Fig. 4 c highlight that individuals who used stick deodorant before the beginning of the study (volunteers 1, 2, 3, 9, and 12) displayed a more pronounced shift in their armpits’ chemistries as soon as they stopped using deodorant (T1–T3), compared to individuals who had low detectable levels of stick deodorant use (volunteers 4, 6, 7, and 10), or “rarely-to-never” (volunteers 5 and 11) use stick deodorants as confirmed by the volunteers (Additional file 1 : Figure S3F, WR test, T0 vs T1–T3 all p values < 0.0001, with greater distance for the group of volunteers 1, 2, 3, 9, and 12, compared to volunteers 4, 5, 6, 7, 10, and 11). The most drastic shift in chemical profiles was observed during the transition period, when all participants applied the common antiperspirant on a daily basis (T4–T6) (Additional file 1 : Figure S3D, E). Finally, the molecular profiles became gradually more similar to those collected before the experiment (T0) as soon as the participants resumed using their personal beauty products (T7–T9) (Additional file 1 : Figure S3C), although traces of skin care products did last through the entire T7–T9 period in people who do not routinely apply these products (Fig. 4 c).
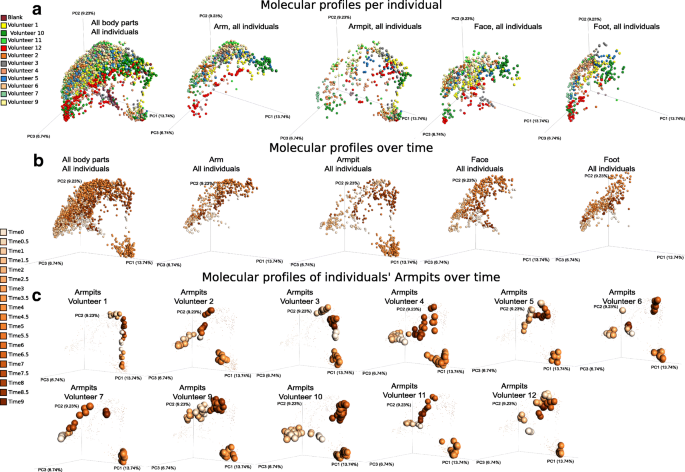
Individualized influence of beauty product application on skin metabolomics profiles over time. a Multivariate statistical analysis (principal coordinate analysis (PCoA)) comparing mass spectrometry data collected over 9 weeks from the skin of 11 individuals, all body parts, combined (first plot from the left) and then displayed separately (arm, armpits, face, feet). Color scale represents volunteer ID. The PCoA was calculated on all samples together, and subsets of the data are shown in this shared space and the other panels. b The molecular profiles collected over 9 weeks from all body parts, combined then separately (arm, armpits, face, feet). c Representative molecular profiles collected over 9 weeks from armpits of 11 individuals (volunteers 1, 2, 3, 4, 5, 6, 7, 9, 10, 11, 12). Color gradient in b and c represents timepoints (time 0 to time 9), ranging from the lightest orange color to the darkest one that represent the earliest (time 0) to the latest (time 9) timepoint, respectively. 0.5 timepoints represent additional timepoints where three selected volunteers were samples (volunteers 4, 9, and 10). PCoA plots were generated using the Bray–Curtis dissimilarity matrix and visualized in Emperor [ 28 ]. See also Additional file 1 : Figure S3
Comparing chemistries detected in armpits at the end timepoints—when no products were used (T3) and during product use (T6)—revealed distinct molecular signatures characteristic of each phase (random forest highlighting that 100% of samples from each group were correctly predicted, see Additional file 1 : Figure S3D, E). Because volunteers used the same antiperspirant during T4–T6, molecular profiles converged during that time despite individual patterns at T3 (Fig. 4 b, c, Additional file 1 : Figure S3D). These distinct chemical patterns reflect the significant impact of beauty products on skin molecular composition. Although these differences may in part be driven by beauty product ingredients detected on the skin (Additional file 1 : Figure S1), we anticipated that additional host- and microbe-derived molecules may also be involved in these molecular changes.
To characterize the chemistries that vary over time, we used molecular networking, a MS visualization approach that evaluates the relationship between MS/MS spectra and compares them to reference MS/MS spectral libraries of known compounds [ 29 , 30 ]. We recently showed that molecular networking can successfully organize large-scale mass spectrometry data collected from the human skin surface [ 18 , 19 ]. Briefly, molecular networking uses the MScluster algorithm [ 31 ] to merge all identical spectra and then compares and aligns all unique pairs of MS/MS spectra based on their similarities where 1.0 indicates a perfect match. Similarities between MS/MS spectra are calculated using a similarity score, and are interpreted as molecular families [ 19 , 24 , 32 , 33 , 34 ]. Here, we used this method to compare and characterize chemistries found in armpits, arms, face, and foot of 11 participants. Based on MS/MS spectral similarities, chemistries highlighted through molecular networking (Additional file 1 : Figure S4A) were associated with each body region with 8% of spectra found exclusively in the arms, 12% in the face, 14% in the armpits, and 2% in the foot, while 18% of the nodes were shared between all four body parts and the rest of spectra were shared between two body sites or more (Additional file 1 : Figure S4B). Greater spectral similarities were highlighted between armpits, face, and arm (12%) followed by the arm and face (9%) (Additional file 1 : Figure S4B).
Molecules were annotated with Global Natural Products Social Molecular Networking (GNPS) libraries [ 29 ], using accurate parent mass and MS/MS fragmentation patterns, according to level 2 or 3 of annotation defined by the 2007 metabolomics standards initiative [ 35 ]. Through annotations, molecular networking revealed that many compounds derived from steroids (Fig. 5 a–d), bile acids (Additional file 1 : Figure S5A-D), and acylcarnitines (Additional file 1 : Figure S5E-F) were exclusively detected in the armpits. Using authentic standards, the identity of some pheromones and bile acids were validated to a level 1 identification with matched retention times (Additional file 1 : Figure S6B, S7A, C, D). Other steroids and bile acids were either annotated using standards with identical MS/MS spectra but slightly different retention times (Additional file 1 : Figure S6A) or annotated with MS/MS spectra match with reference MS/MS library spectra (Additional file 1 : Figure S6C, D, S7B, S6E-G). These compounds were therefore classified as level 3 [ 35 ]. Acylcarnitines were annotated to a family of possible acylcarnitines (we therefore classify as level 3), as the positions of double bonds or cis vs trans configurations are unknown (Additional file 1 : Figure S8A, B).
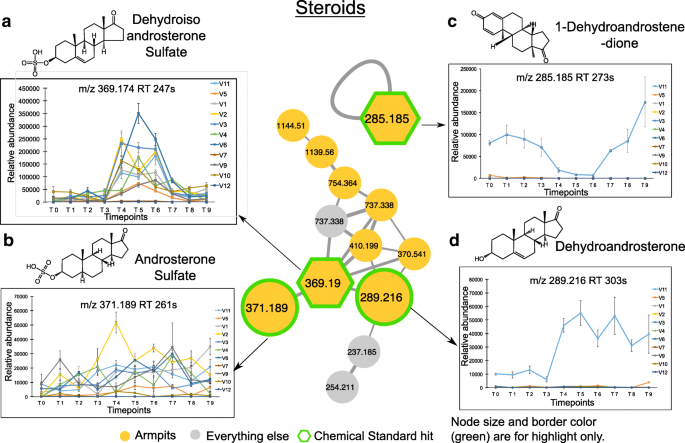
Underarm steroids and their longitudinal abundance. a – d Steroid molecular families in the armpits and their relative abundance over a 9-week period. Molecular networking was applied to characterize chemistries from the skin of 11 healthy individuals. The full network is shown in Additional file 1 : Figure S4A, and networking parameters can be found here http://gnps.ucsd.edu/ProteoSAFe/status.jsp?task=284fc383e4c44c4db48912f01905f9c5 for MS/MS datasets MSV000081582. Each node represents a consensus of a minimum of 3 identical MS/MS spectra. Yellow nodes represent MS/MS spectra detected in armpits samples. Hexagonal shape represents MS/MS spectra match between skin samples and chemical standards. Plots are representative of the relative abundance of each compound over time, calculated separately from LC-MS1 data collected from the armpits of each individual. Steroids detected in armpits are a , dehydroisoandrosterone sulfate ( m/z 369.190, rt 247 s), b androsterone sulfate ( m/z 371.189, rt 261 s), c 1-dehydroandrostenedione ( m/z 285.185, rt 273 s), and d dehydroandrosterone ( m/z 289.216, rt 303 s). Relative abundance over time of each steroid compound is represented. Error bars represent the standard error of the mean calculated at each timepoint from four armpit samples from the right and left side of each individual separately. See also Additional file 1 : Figures S4-S8
Among the steroid compounds, several molecular families were characterized: androsterone (Fig. 5 a, b, d), androstadienedione (Fig. 5 c), androstanedione (Additional file 1 : Figure S6E), androstanolone (Additional file 1 : Figure S6F), and androstenedione (Additional file 1 : Figure S6G). While some steroids were detected in the armpits of several individuals, such as dehydroisoandrosterone sulfate ( m/z 369.19, rt 247 s) (9 individuals) (Fig. 5 a, Additional file 1 : Figure S6A), androsterone sulfate ( m/z 371.189, rt 261 s) (9 individuals) (Fig. 5 b, Additional file 1 : Figure S6C), and 5-alpha-androstane-3,17-dione ( m/z 271.205, rt 249 s) (9 individuals) (Additional file 1 : Figure S6E), other steroids including 1-dehydroandrostenedione ( m/z 285.185, rt 273 s) (Fig. 5 c, Additional file 1 : Figure S6B), dehydroandrosterone ( m/z 289.216, rt 303 s) (Fig. 5 d, Additional file 1 : Figure S6D), and 5-alpha-androstan-17.beta-ol-3-one ( m/z 291.231, rt 318 s) (Additional file 1 : Figure S6F) were only found in the armpits of volunteer 11 and 4-androstene-3,17-dione ( m/z 287.200, rt 293 s) in the armpits of volunteer 11 and volunteer 5, both are male that never applied stick deodorants (Additional file 1 : Figure S6G). Each molecular species exhibited a unique pattern over the 9-week period. The abundance of dehydroisoandrosterone sulfate (Fig. 5 a, WR test, p < 0.01 for 7 individuals) and dehydroandrosterone (Fig. 5 a, WR test, p = 0.00025) significantly increased during the use of antiperspirant (T4–T6), while androsterone sulfate (Fig. 5 b) and 5-alpha-androstane-3,17-dione (Additional file 1 : Figure S6E) display little variation over time. Unlike dehydroisoandrosterone sulfate (Fig. 5 a) and dehydroandrosterone (Fig. 5 d), steroids including 1-dehydroandrostenedione (Fig. 5 c, WR test, p = 0.00024) and 4-androstene-3,17-dione (Additional file 1 : Figure S6G, WR test, p = 0.00012) decreased in abundance during the 3 weeks of antiperspirant application (T4–T6) in armpits of male 11, and their abundance increased again when resuming the use of his normal skin care routines (T7–T9). Interestingly, even within the same individual 11, steroids were differently impacted by antiperspirant use as seen for 1-dehydroandrostenedione that decreased in abundance during T4–T6 (Fig. 5 c, WR test, p = 0.00024), while dehydroandrosterone increased in abundance (Fig. 5 d, WR test, p = 0.00025), and this increase was maintained during the last 3 weeks of the study (T7–T9).
In addition to steroids, many bile acids (Additional file 1 : Figure S5A-D) and acylcarnitines (Additional file 1 : Figure S5E-F) were detected on the skin of several individuals through the 9-week period. Unlike taurocholic acid found only on the face (Additional file 1 : Figures S5A, S7A) and tauroursodeoxycholic acid detected in both armpits and arm samples (Additional file 1 : Figures S5B, S7B), other primary bile acids such as glycocholic (Additional file 1 : Figures S5C, S7C) and chenodeoxyglycocholic acid (Additional file 1 : Figures S5D, S7D) were exclusively detected in the armpits. Similarly, acylcarnitines were also found either exclusively in the armpits (hexadecanoyl carnitines) (Additional file 1 : Figures S5E, S8A) or in the armpits and face (tetradecenoyl carnitine) (Additional file 1 : Figures S5F, S8B) and, just like the bile acids, they were also stably detected during the whole 9-week period.
Bacterial communities and their variation over time
Having demonstrated the impact of beauty products on the chemical makeup of the skin, we next tested the extent to which skin microbes are affected by personal care products. We assessed temporal variation of bacterial communities detected on the skin of healthy individuals by evaluating dissimilarities of bacterial collections over time using unweighted UniFrac distance [ 36 ] and community variation at each body site in association to beauty product use [ 3 , 15 , 37 ]. Unweighted metrics are used for beta diversity calculations because we are primarily concerned with changes in community membership rather than relative abundance. The reason for this is that skin microbiomes can fluctuate dramatically in relative abundance on shorter timescales than that assessed here. Longitudinal variations were revealed for the armpits (Fig. 6 a) and feet microbiome by their overall trend in the PCoA plots (Fig. 6 b), while the arm (Fig. 6 c) and face (Fig. 6 d) displayed relatively stable bacterial profiles over time. As shown in Fig. 6 a–d, although the microbiome was site-specific, it varied more between individuals and this inter-individual variability was maintained over time despite same changes in personal care routine (WR test, all p values at all timepoints < 0.05, T5 p = 0.07), in agreement with previous findings that individual differences in the microbiome are large and stable over time [ 3 , 4 , 10 , 37 ]. However, we show that shifts in the microbiome can be induced by changing hygiene routine and therefore skin chemistry. Changes associated with using beauty products (T4–T6) were more pronounced for the armpits (Fig. 6 a, WR test, p = 1.61e−52) and feet (Fig. 6 b, WR test, p = 6.15e−09), while little variations were observed for the face (Fig. 6 d, WR test, p = 1.402.e−83) and none for the arms (Fig. 6 c, WR test, p = 0.296).
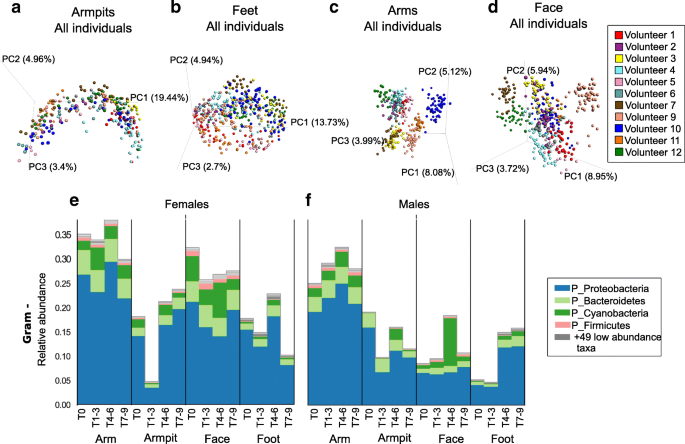
Longitudinal variation of skin bacterial communities in association with beauty product use. a - d Bacterial profiles collected from skin samples of 11 individuals, over 9 weeks, from four distinct body parts a) armpits, b) feet, c) arms and d) face, using multivariate statistical analysis (Principal Coordinates Analysis PCoA) and unweighted Unifrac metric. Each color represents bacterial samples collected from an individual. PCoA were calculated separately for each body part. e , f Representative Gram-negative (Gram -) bacteria collected from arms, armpits, face and feet of e) female and f) male participants. See also Additional file 1 : Figure S9A, B showing Gram-negative bacterial communities represented at the genus level
A significant increase in abundance of Gram-negative bacteria including the phyla Proteobacteria and Bacteroidetes was noticeable for the armpits and feet of both females (Fig. 6 e; Mann–Whitney U , p = 8.458e−07) and males (Fig. 6 f; Mann–Whitney U , p = 0.0004) during the use of antiperspirant (T4–T6), while their abundance remained stable for the arms and face during that time (Fig. 6 e, f; female arm p = 0.231; female face p value = 0.475; male arm p = 0.523;male face p = 6.848751e−07). These Gram-negative bacteria include Acinetobacter and Paracoccus genera that increased in abundance in both armpits and feet of females (Additional file 1 : Figure S9A), while a decrease in abundance of Enhydrobacter was observed in the armpits of males (Additional file 1 : Figure S9B). Cyanobacteria, potentially originating from plant material (Additional file 1 : Figure S9C) also increased during beauty product use (T4–T6) especially in males, in the armpits and face of females (Fig. 6 e) and males (Fig. 6 f). Interestingly, although chloroplast sequences (which group phylogenetically within the cyanobacteria [ 38 ]) were only found in the facial cream (Additional file 1 : Figure S9D), they were detected in other locations as well (Fig. 6 e, f. S9E, F), highlighting that the application of a product in one region will likely affect other regions of the body. For example, when showering, a face lotion will drip down along the body and may be detected on the feet. Indeed, not only did the plant material from the cream reveal this but also the shampoo used for the study for which molecular signatures were readily detected on the feet as well (Additional file 1 : Figure S10A). Minimal average changes were observed for Gram-positive organisms (Additional file 1 : Figure S10B, C), although in some individuals the variation was greater than others (Additional file 1 : Figure S10D, E) as discussed for specific Gram-positive taxa below.
At T0, the armpit’s microflora was dominated by Staphylococcus (26.24%, 25.11% of sequencing reads for females and 27.36% for males) and Corynebacterium genera (26.06%, 17.89% for females and 34.22% for males) (Fig. 7 a—first plot from left and Additional file 1 : Figure S10D, E). They are generally known as the dominant armpit microbiota and make up to 80% of the armpit microbiome [ 39 , 40 ]. When no deodorants were used (T1–T3), an overall increase in relative abundance of Staphylococcus (37.71%, 46.78% for females and 30.47% for males) and Corynebacterium (31.88%, 16.50% for females and 44.15% for males) genera was noticeable (WR test, p < 3.071e−05) (Fig. 7 a—first plot from left), while the genera Anaerococcus and Peptoniphilus decreased in relative abundance (WR test, p < 0.03644) (Fig. 7 a—first plot from left and Additional file 1 : Figure S10D, E). When volunteers started using antiperspirants (T4–T6), the relative abundance of Staphylococcus (37.71%, 46.78% females and 30.47% males, to 21.71%, 25.02% females and 19.25% males) and Corynebacterium (31.88%, 16.50% females and 44.15% males, to 15.83%, 10.76% females and 19.60% males) decreased (WR test, p < 3.071e−05) (Fig. 7 a, Additional file 1 : Figure S10D, E) and at the same time, the overall alpha diversity increased significantly (WR test, p = 3.47e−11) (Fig. 3 c, d). The microbiota Anaerococcus (WR test, p = 0.0006018) , Peptoniphilus (WR test, p = 0.008639), and Micrococcus (WR test, p = 0.0377) increased significantly in relative abundance, together with a lot of additional low-abundant species that lead to an increase in Shannon alpha diversity (Fig. 3 c, d). When participants went back to normal personal care products (T7–T9), the underarm microbiome resembled the original underarm community of T0 (WR test, p = 0.7274) (Fig. 7 a). Because armpit bacterial communities are person-specific (inter-individual variability: WR test, all p values at all timepoints < 0.05, besides T5 p n.s), variation in bacterial abundance upon antiperspirant use (T4–T6) differ between individuals and during the whole 9-week period (Fig. 7a —taxonomic plots per individual). For example, the underarm microbiome of male 5 exhibited a unique pattern, where Corynebacterium abundance decreased drastically during the use of antiperspirant (82.74 to 11.71%, WR test, p = 3.518e−05) while in the armpits of female 9 a huge decrease in Staphylococcus abundance was observed (Fig. 7 a) (65.19 to 14.85%, WR test, p = 0.000113). Unlike other participants, during T0–T3, the armpits of individual 11 were uniquely characterized by the dominance of a sequence that matched most closely to the Enhydrobacter genera . The transition to antiperspirant use (T4–T6) induces the absence of Enhydrobacter (30.77 to 0.48%, WR test, p = 0.01528) along with an increase of Corynebacterium abundance (26.87 to 49.74%, WR test, p = 0.1123) (Fig. 7 a—male 11).
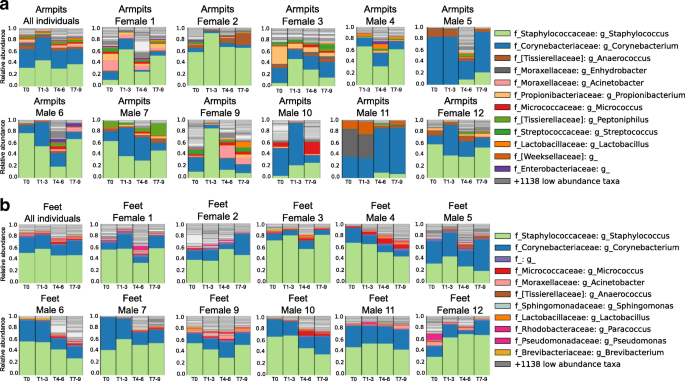
Person-to-person bacterial variabilities over time in the armpits and feet. a Armpit microbiome changes when stopping personal care product use, then resuming. Armpit bacterial composition of the 11 volunteers combined, then separately, (female 1, female 2, female 3, male 4, male 5, male 6, male 7, female 9, male 10, male 11, female 12) according to the four periods within the experiment. b Feet bacterial variation over time of the 12 volunteers combined, then separately (female 1, female 2, female 3, male 4, male 5, male 6, male 7, female 9, male 10, male 11, female 12) according to the four periods within the experiment. See also Additional file 1 : Figure S9-S13
In addition to the armpits, a decline in abundance of Staphylococcus and Corynebacterium was perceived during the use of the foot powder (46.93% and 17.36%, respectively) compared to when no beauty product was used (58.35% and 22.99%, respectively) (WR test, p = 9.653e−06 and p = 0.02032, respectively), while the abundance of low-abundant foot bacteria significantly increased such as Micrococcus (WR test, p = 1.552e−08), Anaerococcus (WR test, p = 3.522e−13), Streptococcus (WR test, p = 1.463e−06), Brevibacterium (WR test, p = 6.561e−05), Moraxellaceae (WR test, p = 0.0006719), and Acinetobacter (WR test, p = 0.001487), leading to a greater bacterial diversity compared to other phases of the study (Fig. 7 b first plot from left, Additional file 1 : Figure S10D, E, Fig. 3 c, d).
We further evaluated the relationship between the two omics datasets by superimposing the principal coordinates calculated from metabolome and microbiome data (Procrustes analysis) (Additional file 1 : Figure S11) [ 34 , 41 , 42 ]. Metabolomics data were more correlated with patterns observed in microbiome data in individual 3 (Additional file 1 : Figure S11C, Mantel test, r = 0.23, p < 0.001), individual 5 (Additional file 1 : Figure S11E, r = 0.42, p < 0.001), individual 9 (Additional file 1 : Figure S11H, r = 0.24, p < 0.001), individual 10 (Additional file 1 : Figure S11I, r = 0.38, p < 0.001), and individual 11 (Additional file 1 : Figure S11J, r = 0.35, p < 0.001) when compared to other individuals 1, 2, 4, 6, 7, and 12 (Additional file 1 : Figure S11A, B, D, F, G, K, respectively) (Mantel test, all r < 0.2, all p values < 0.002, for volunteer 2 p n.s). Furthermore, these correlations were individually affected by ceasing (T1–T3) or resuming the use of beauty products (T4–T6 and T7–T9) (Additional file 1 : Figure S11A-K).
Overall, metabolomics–microbiome correlations were consistent over time for the arms, face, and feet although alterations were observed in the arms of volunteers 7 (Additional file 1 : Figure S11G) and 10 (Additional file 1 : Figure S11I) and the face of volunteer 7 (Additional file 1 : Figure S11G) during product use (T4–T6). Molecular–bacterial correlations were mostly affected in the armpits during antiperspirant use (T4–T6), as seen for volunteers male 7 (Additional file 1 : Figure S11G) and 11 (Additional file 1 : Figure S11J) and females 2 (Additional file 1 : Figure S11B), 9 (Additional file 1 : Figure S11H), and 12 (Additional file 1 : Figure S11K). This perturbation either persisted during the last 3 weeks (Additional file 1 : Figure S11D, E, H, I, K) when individuals went back to their normal routine (T7–T9) or resembled the initial molecular–microbial correlation observed in T0 (Additional file 1 : Figure S11C, G, J). These alterations in molecular–bacterial correlation are driven by metabolomics changes during antiperspirant use as revealed by metabolomics shifts on the PCoA space (Additional file 1 : Figure S11), partially due to the deodorant’s chemicals (Additional file 1 : Figure S1J, K) but also to changes observed in steroid levels in the armpits (Fig. 5A, C, D , Additional file 1 : Figure S6G), suggesting metabolome-dependant changes of the skin microbiome. In agreement with previous findings that showed efficient biotransformation of steroids by Corynebacterium [ 43 , 44 ], our correlation analysis associates specific steroids that were affected by antiperspirant use in the armpits of volunteer 11 (Fig. 5 c, d, Additional file 1 : Figure S6G) with microbes that may produce or process them: 1-dehydroandrostenedione, androstenedione, and dehydrosterone with Corynebacterium ( r = − 0.674, p = 6e−05; r = 0.671, p = 7e−05; r = 0.834, p < 1e−05, respectively) (Additional file 1 : Figure S12A, B, C, respectively) and Enhydrobacter ( r = 0.683, p = 4e−05; r = 0.581, p = 0.00095; r = 0.755, p < 1e−05 respectively) (Additional file 1 : Figure S12D, E, F, respectively).
Despite the widespread use of skin care and hygiene products, their impact on the molecular and microbial composition of the skin is poorly studied. We established a workflow that examines individuals to systematically study the impact of such lifestyle characteristics on the skin by taking a broad look at temporal molecular and bacterial inventories and linking them to personal skin care product use. Our study reveals that when the hygiene routine is modified, the skin metabolome and microbiome can be altered, but that this alteration depends on product use and location on the body. We also show that like gut microbiome responses to dietary changes [ 20 , 21 ], the responses are individual-specific.
We recently reported that traces of our lifestyle molecules can be detected on the skin days and months after the original application [ 18 , 19 ]. Here, we show that many of the molecules associated with our personal skin and hygiene products had a half-life of 0.5 to 1.9 weeks even though the volunteers regularly showered, swam, or spent time in the ocean. Thus, a single application of some of these products has the potential to alter the microbiome and skin chemistry for extensive periods of time. Our data suggests that although host genetics and diet may play a role, a significant part of the resilience of the microbiome that has been reported [ 10 , 45 ] is due to the resilience of the skin chemistry associated with personal skin and hygiene routines, or perhaps even continuous re-exposure to chemicals from our personal care routines that are found on mattresses, furniture, and other personal objects [ 19 , 27 , 46 ] that are in constant contact. Consistent with this observation is that individuals in tribal regions and remote villages that are infrequently exposed to the types of products used in this study have very different skin microbial communities [ 47 , 48 ] and that the individuals in this study who rarely apply personal care products had a different starting metabolome. We observed that both the microbiome and skin chemistry of these individuals were most significantly affected by these products. This effect by the use of products at T4–T6 on the volunteers that infrequently used them lasted to the end phase of the study even though they went back to infrequent use of personal care products. What was notable and opposite to what the authors originally hypothesized is that the use of the foot powder and antiperspirant increased the diversity of microbes and that some of this diversity continued in the T7–T9 phase when people went back to their normal skin and hygiene routines. It is likely that this is due to the alteration in the nutrient availability such as fatty acids and moisture requirements, or alteration of microbes that control the colonization via secreted small molecules, including antibiotics made by microbes commonly found on the skin [ 49 , 50 ].
We detected specific molecules on the skin that originated from personal care products or from the host. One ingredient that lasts on the skin is propylene glycol, which is commonly used in deodorants and antiperspirants and added in relatively large amounts as a humectant to create a soft and sleek consistency [ 51 ]. As shown, daily use of personal care products is leading to high levels of exposure to these polymers. Such polymers cause contact dermatitis in a subset of the population [ 51 , 52 ]. Our data reveal a lasting accumulation of these compounds on the skin, suggesting that it may be possible to reduce their dose in deodorants or frequency of application and consequently decrease the degree of exposure to such compounds. Formulation design of personal care products may be influenced by performing detailed outcome studies. In addition, longer term impact studies are needed, perhaps in multiple year follow-up studies, to assess if the changes we observed are permanent or if they will recover to the original state.
Some of the host- and microbiome-modified molecules were also detected consistently, such as acylcarnitines, bile acids, and certain steroids. This means that a portion of the molecular composition of a person’s skin is not influenced by the beauty products applied to the skin, perhaps reflecting the level of exercise for acylcarnitines [ 53 , 54 ] or the liver (dominant location where they are made) or gallbladder (where they are stored) function for bile acids. The bile acid levels are not related to sex and do not change in amount during the course of this study. While bile acids are typically associated with the human gut microbiome [ 34 , 55 , 56 , 57 , 58 ], it is unclear what their role is on the skin and how they get there. One hypothesis is that they are present in the sweat that is excreted through the skin, as this is the case for several food-derived molecules such as caffeine or drugs and medications that have been previously reported on the human skin [ 19 ] or that microbes synthesize them de novo [ 55 ]. The only reports we could find on bile acids being associated with the skin describe cholestasis and pruritus diseases. Cholestasis and pruritus in hepatobiliary disease have symptoms of skin bile acid accumulation that are thought to be responsible for severe skin itching [ 59 , 60 ]. However, since bile acids were found in over 50% of the healthy volunteers, their detection on the skin is likely a common phenotype among the general population and not only reflective of disease, consistent with recent reports challenging these molecules as biomarkers of disease [ 59 ]. Other molecules that were detected consistently came from personal care products.
Aside from molecules that are person-specific and those that do not vary, there are others that can be modified via personal care routines. Most striking is how the personal care routines influenced changes in hormones and pheromones in a personalized manner. This suggests that there may be personalized recipes that make it possible to make someone more or less attractive to others via adjustments of hormonal and pheromonal levels through alterations in skin care.
Here, we describe the utilization of an approach that combines metabolomics and microbiome analysis to assess the effect of modifying personal care regime on skin chemistry and microbes. The key findings are as follows: (1) Compounds from beauty products last on the skin for weeks after their first use despite daily showering. (2) Beauty products alter molecular and bacterial diversity as well as the dynamic and structure of molecules and bacteria on the skin. (3) Molecular and bacterial temporal variability is product-, site-, and person-specific, and changes are observed starting the first week of beauty product use. This study provides a framework for future investigations to understand how lifestyle characteristics such as diet, outdoor activities, exercise, and medications shape the molecular and microbial composition of the skin. These factors have been studied far more in their impact on the gut microbiome and chemistry than in the skin. Revealing how such factors can affect skin microbes and their associated metabolites may be essential to define long-term skin health by restoring the appropriate microbes particularly in the context of skin aging [ 61 ] and skin diseases [ 49 ] as has shown to be necessary for amphibian health [ 62 , 63 ], or perhaps even create a precision skin care approach that utilizes the proper care ingredients based on the microbial and chemical signatures that could act as key players in host defense [ 49 , 64 , 65 ].
Subject recruitment and sample collection
Twelve individuals between 25 and 40 years old were recruited to participate in this study, six females and six males. Female volunteer 8 dropped out of the study as she developed a skin irritation during the T1–T3 phase. All volunteers signed a written informed consent in accordance with the sampling procedure approved by the UCSD Institutional Review Board (Approval Number 161730). Volunteers were required to follow specific instructions during 9 weeks. They were asked to bring in samples of their personal care products they used prior to T0 so they could be sampled as well. Following the initial timepoint time 0 and during the first 3 weeks (week 1–week 3), volunteers were asked not to use any beauty products (Fig. 1 b). During the next 3 weeks (week 4–week 6), four selected commercial beauty products provided to all volunteers were applied once a day at specific body part (deodorant for the armpits, soothing foot powder between the toes, sunscreen for the face, and moisturizer for front forearms) (Fig. 1 b, Additional file 3 : Table S2 Ingredient list of beauty products). During the first 6 weeks, volunteers were asked to shower with a head to toe shampoo. During the last 3 weeks (week 7–week 9), all volunteers went back to their normal routine and used the personal care products used before the beginning of the study (Fig. 1 b). Volunteers were asked not to shower the day before sampling. Samples were collected by the same three researchers to ensure consistency in sampling and the area sampled. Researchers examined every subject together and collected metabolomics and microbiome samples from each location together. Samples were collected once a week (from day 0 to day 68—10 timepoints total) for volunteers 1, 2, 3, 4, 5, 6, 7, 9, 10, 11, and 12, and on day 0 and day 6 for volunteer 8. For individuals 4, 9, and 10, samples were collected twice a week. Samples collected for 11 volunteers during 10 timepoints: 11 volunteers × 10 timepoints × 4 samples × 4 body sites = 1760. Samples collected from 3 selected volunteers during 9 additional timepoints: 3 volunteers × 9 timepoints × 4 samples × 4 body sites = 432. All samples were collected following the same protocol described in [ 18 ]. Briefly, samples were collected over an area of 2 × 2 cm, using pre-moistened swabs in 50:50 ethanol/water solution for metabolomics analysis or in Tris-EDTA buffer for 16S rRNA sequencing. Four samples were collected from each body part right and left side. The locations sampled were the face—upper cheek bone and lower jaw, armpit—upper and lower area, arm—front of the elbow (antecubitis) and forearm (antebrachium), and feet—in between the first and second toe and third and fourth toe. Including personal care product references, a total of 2275 samples were collected over 9 weeks and were submitted to both metabolomics and microbial inventories.
Metabolite extraction and UPLC-Q-TOF mass spectrometry analysis
Skin swabs were extracted and analyzed using a previously validated workflow described in [ 18 , 19 ]. All samples were extracted in 200 μl of 50:50 ethanol/water solution for 2 h on ice then overnight at − 20 °C. Swab sample extractions were dried down in a centrifugal evaporator then resuspended by vortexing and sonication in a 100 μl 50:50 ethanol/water solution containing two internal standards (fluconazole 1 μM and amitriptyline 1 μM). The ethanol/water extracts were then analyzed using a previously validated UPLC-MS/MS method [ 18 , 19 ]. We used a ThermoScientific UltiMate 3000 UPLC system for liquid chromatography and a Maxis Q-TOF (Quadrupole-Time-of-Flight) mass spectrometer (Bruker Daltonics), controlled by the Otof Control and Hystar software packages (Bruker Daltonics) and equipped with ESI source. UPLC conditions of analysis are 1.7 μm C18 (50 × 2.1 mm) UHPLC Column (Phenomenex), column temperature 40 °C, flow rate 0.5 ml/min, mobile phase A 98% water/2% acetonitrile/0.1% formic acid ( v / v ), mobile phase B 98% acetonitrile/2% water/0.1% formic acid ( v / v ). A linear gradient was used for the chromatographic separation: 0–2 min 0–20% B, 2–8 min 20–99% B, 8–9 min 99–99% B, 9–10 min 0% B. Full-scan MS spectra ( m/z 80–2000) were acquired in a data-dependant positive ion mode. Instrument parameters were set as follows: nebulizer gas (nitrogen) pressure 2 Bar, capillary voltage 4500 V, ion source temperature 180 °C, dry gas flow 9 l/min, and spectra rate acquisition 10 spectra/s. MS/MS fragmentation of 10 most intense selected ions per spectrum was performed using ramped collision induced dissociation energy, ranged from 10 to 50 eV to get diverse fragmentation patterns. MS/MS active exclusion was set after 4 spectra and released after 30 s.
Mass spectrometry data collected from the skin of 12 individuals can be found here MSV000081582.
LC-MS data processing
LC-MS raw data files were converted to mzXML format using Compass Data analysis software (Bruker Daltonics). MS1 features were selected for all LC-MS datasets collected from the skin of 12 individuals and blank samples (total 2275) using the open-source software MZmine [ 66 ]—see Additional file 4 : Table S3 for parameters. Subsequent blank filtering, total ion current, and internal standard normalization were performed (Additional file 5 : Table S4) for representation of relative abundance of molecular features (Fig. 2 , Additional file 1 : Figure S1), principal coordinate analysis (PCoA) (Fig. 4 ). For steroid compounds in Fig. 5 a–d, bile acids (Additional file 1 : Figure S5A-D), and acylcarnitines (Additional file 1 : Figure S5E, F) compounds, crop filtering feature available in MZmine [ 66 ] was used to identify each feature separately in all LC-MS data collected from the skin of 12 individuals (see Additional file 4 : Table S3 for crop filtering parameters and feature finding in Additional file 6 : Table S5).
Heatmap in Fig. 2 was constructed from the bucket table generated from LC-MS1 features (Additional file 7 : Table S6) and associated metadata (Additional file 8 : Table S7) using the Calour command line available here: https://github.com/biocore/calour . Calour parameters were as follows: normalized read per sample 5000 and cluster feature minimum reads 50. Procrustes and Pearson correlation analyses in Additional file 1 : Figures S10 and S11 were performed using the feature table in Additional file 9 : Table S8, normalized using the probabilistic quotient normalization method [ 67 ].
16S rRNA amplicon sequencing
16S rRNA sequencing was performed following the Earth Microbiome Project protocols [ 68 , 69 ], as described before [ 18 ]. Briefly, DNA was extracted using MoBio PowerMag Soil DNA Isolation Kit and the V4 region of the 16S rRNA gene was amplified using barcoded primers [ 70 ]. PCR was performed in triplicate for each sample, and V4 paired-end sequencing [ 70 ] was performed using Illumina HiSeq (La Jolla, CA). Raw sequence reads were demultiplexed and quality controlled using the defaults, as provided by QIIME 1.9.1 [ 71 ]. The primary OTU table was generated using Qiita ( https://qiita.ucsd.edu/ ), using UCLUST ( https://academic.oup.com/bioinformatics/article/26/19/2460/230188 ) closed-reference OTU picking method against GreenGenes 13.5 database [ 72 ]. Sequences can be found in EBI under accession number EBI: ERP104625 or in Qiita ( qiita.ucsd.edu ) under Study ID 10370. Resulting OTU tables were then rarefied to 10,000 sequences/sample for downstream analyses (Additional file 10 Table S9). See Additional file 11 : Table S10 for read count per sample and Additional file 1 : Figure S13 representing the samples that fall out with rarefaction at 10,000 threshold. The dataset includes 35 blank swab controls and 699 empty controls. The blank samples can be accessed through Qiita ( qiita.ucsd.edu ) as study ID 10370 and in EBI with accession number EBI: ERP104625. Blank samples can be found under the metadata category “sample_type” with the name “empty control” and “Swabblank.” These samples fell below the rarefaction threshold at 10,000 (Additional file 11 : Table S10).
To rule out the possibility that personal care products themselves contained the microbes that induced the changes in the armpit and foot microbiomes that were observed in this study (Fig. 7 ), we subjected the common personal care products that were used in this study during T4–T6 also to 16S rRNA sequencing. The data revealed that within the limit of detectability of the current experiment, few 16S signatures were detected. One notable exception was the most dominant plant-originated bacteria chloroplast detected in the sunscreen lotion applied on the face (Additional file 1 : Figure S9D), that was also detected on the face of individuals and at a lower level on their arms, sites where stable microbial communities were observed over time (Additional file 1 : Figure S9E, F). This finding is in agreement with our previous data from the 3D cartographical skin maps that revealed the presence of co-localized chloroplast and lotion molecules [ 18 ]. Other low-abundant microbial signatures found in the sunscreen lotion include additional plant-associated bacteria: mitochondria [ 73 ], Bacillaceae [ 74 , 75 ], Planococcaceae [ 76 ], and Ruminococcaceae family [ 77 ], but all these bacteria are not responsible for microbial changes associated to beauty product use, as they were poorly detected in the armpits and feet (Fig. 7 ).
To assess the origin of Cyanobacteria detected in skin samples, each Greengenes [ 72 ] 13_8 97% OTU table (per lane; obtained from Qiita [ 78 ] study 10,370) was filtered to only features with a p__Cyanobacteria phylum. The OTU maps for these tables—which relate each raw sequence to an OTU ID—were then filtered to only those observed p__Cyanobacteria OTU IDs. The filtered OTU map was used to extract the raw sequences into a single file. Separately, the unaligned Greengenes 13_8 99% representative sequences were filtered into two sets, first the set of representatives associated with c__Chloroplast (our interest database), and second the set of sequences associated with p__Cyanobacteria without the c__Chloroplast sequences (our background database). Platypus Conquistador [ 79 ] was then used to determine what reads were observed exclusively in the interest database and not in the background database. Of the 4,926,465 raw sequences associated with a p__Cyanobacteria classification (out of 318,686,615 total sequences), at the 95% sequence identity level with 100% alignment, 4,860,258 sequences exclusively recruit to full-length chloroplast 16S by BLAST [ 80 ] with the bulk recruiting to streptophytes (with Chlorophyta and Stramenopiles to a lesser extent). These sequences do not recruit non-chloroplast Cyanobacteria full length 16S.
Half-life calculation for metabolomics data
In order to estimate the biological half-life of molecules detected in the skin, the first four timepoints of the study (T0, T1, T2, T3) were considered for the calculation to allow the monitoring of personal beauty products used at T0. The IUPAC’s definition of biological half-life as the time required to a substance in a biological system to be reduced to half of its value, assuming an approximately exponential removal [ 81 ] was used. The exponential removal can be described as C ( t ) = C 0 e − tλ where t represents the time in weeks, C 0 represents the initial concentration of the molecule, C ( t ) represents the concentration of the molecule at time t , and λ is the rate of removal [ http://onlinelibrary.wiley.com/doi/10.1002/9780470140451.ch2/summary ]. The parameter λ was estimated by a mixed linear effects model in order to account for the paired sample structure. The regression model tests the null hypothesis that λ is equal to zero and only the significant ( p value < 0.05) parameters were considered.
Principal coordinate analysis
We performed principal coordinate analysis (PCoA) on both metabolomics and microbiome data. For metabolomics, we used MS1 features (Additional file 5 : Table S4) and calculated Bray–Curtis dissimilarity metric using ClusterApp ( https://github.com/mwang87/q2_metabolomics ).
For microbiome data, we used rarefied OTU table (Additional file 10 : Table S9) and used unweighted UniFrac metric [ 36 ] to calculate beta diversity distance matrix using QIIME2 (https://qiime2.org). Results from both data sources were visualized using Emperor ( https://biocore.github.io/emperor/ ) [ 28 ].
Molecular networking
Molecular networking was generated from LC-MS/MS data collected from skin samples of 11 individuals MSV000081582, using the Global Natural Products Social Molecular Networking platform (GNPS) [ 29 ]. Molecular network parameters for MS/MS data collected from all body parts of 11 individuals during T0–T9 MSV000081582 are accessible here http://gnps.ucsd.edu/ProteoSAFe/status.jsp?task=284fc383e4c44c4db48912f01905f9c5 . Molecular network parameters for MS/MS data collected from armpits T0–T3 MSV000081582 and deodorant used by individual 1 and 3 MSV000081580 can be found here http://gnps.ucsd.edu/ProteoSAFe/status.jsp?task=f5325c3b278a46b29e8860ec57915ad and here http://gnps.ucsd.edu/ProteoSAFe/status.jsp?task=aaa1af68099d4c1a87e9a09f398fe253 , respectively. Molecular networks were exported and visualized in Cytoscape 3.4.0. [ 82 ]. Molecular networking parameters were set as follows: parent mass tolerance 1 Da, MS/MS fragment ion tolerance 0.5 Da, and cosine threshold 0.65 or greater, and only MS/MS spectral pairs with at least 4 matched fragment ions were included. Each MS/MS spectrum was only allowed to connect to its top 10 scoring matches, resulting in a maximum of 10 connections per node. The maximum size of connected components allowed in the network was 600, and the minimum number of spectra required in a cluster was 3. Venn diagrams were generated from Cytoscape data http://gnps.ucsd.edu/ProteoSAFe/status.jsp?task=284fc383e4c44c4db48912f01905f9c5 using Cytoscape [ 82 ] Venn diagram app available here http://apps.cytoscape.org/apps/all .
Shannon molecular and bacterial diversity
The diversity analysis was performed separately for 16S rRNA data and LC-MS data. For each sample in each feature table (LC-MS data and microbiome data), we calculated the value of the Shannon diversity index. For LC-MS data, we used the full MZmine feature table (Additional file 5 : Table S4). For microbiome data, we used the closed-reference BIOM table rarefied to 10,000 sequences/sample. For diversity changes between timepoints, we aggregated Shannon diversity values across groups of individuals (all, females, males) and calculated mean values and standard errors. All successfully processed samples (detected features in LC-MS or successful sequencing with 10,000 or more sequences/sample) were considered.
Beauty products and chemical standards
Samples (10 mg) from personal care products used during T0 and T7–T9 MSV000081580 (Additional file 2 : Table S1) and common beauty products used during T4–T6 MSV000081581 (Additional file 3 : Table S2) were extracted in 1 ml 50:50 ethanol/water. Sample extractions were subjected to the same UPLC-Q-TOF MS method used to analyze skin samples and described above in the section “ Metabolite extraction and UPLC-Q-TOF mass spectrometry analysis .” Authentic chemical standards MSV000081583 including 1-dehydroandrostenedion (5 μM), chenodeoxyglycocholic acid (5 μM), dehydroisoandrosterone sulfate (100 μM), glycocholic acid (5 μM), and taurocholic acid (5 μM) were analyzed using the same mass spectrometry workflow used to run skin and beauty product samples.
Monitoring beauty product ingredients in skin samples
In order to monitor beauty product ingredients used during T4–T6, we selected only molecular features present in each beauty product sample (antiperspirant, facial lotion, body moisturizer, soothing powder) and then filtered the aligned MZmine feature table (Additional file 5 : Table S4) for the specific feature in specific body part samples. After feature filtering, we selected all features that had a higher average intensity on beauty product phase (T4–T6) compared to non-beauty product phase (T1–T3). The selected features were annotated using GNPS dereplication output http://gnps.ucsd.edu/ProteoSAFe/status.jsp?task=69319caf219642a5a6748a3aba8914df , plotted using R package ggplot2 ( https://cran.r-project.org/web/packages/ggplot2/index.html ) and visually inspected for meaningful patterns.
Random forest analysis
Random forest analysis was performed in MetaboAnalyst 3.0 online platform http://www.metaboanalyst.ca/faces/home.xhtml . Using LC-MS1 features found in armpit samples collected on T3 and T6. Random forest parameters were set as follows: top 1000 most abundant features, number of predictors to try for each node 7, estimate of error rate (0.0%).
BugBase analysis
To determine the functional potential of microbial communities within our samples, we used BugBase [ 83 ]. Because we do not have direct access to all of the gene information due to the use of 16S rRNA marker gene sequencing, we can only rely on phylogenetic information inferred from OTUs. BugBase takes advantage of this information to predict microbial phenotypes by associating OTUs with gene content using PICRUSt [ 84 ]. Thus, using BugBase, we can predict such phenotypes as Gram staining, or oxidative stress tolerance at each timepoint or each phase. All statistical analyses in BugBase are performed using non-parametric differentiation tests (Mann–Whitney U ).
Taxonomic plots
Rarefied OTU counts were collapsed according to the OTU’s assigned family and genus name per sample, with a single exception for the class of chloroplasts. Relative abundances of each family-genus group are obtained by dividing by overall reads per sample, i.e., 10,000. Samples are grouped by volunteer, body site, and time/phase. Abundances are aggregated by taking the mean overall samples, and resulting abundances are again normalized to add up to 1. Low-abundant taxa are not listed in the legend and plotted in grayscale. Open-source code is available at https://github.com/sjanssen2/ggmap/blob/master/ggmap/snippets.py
Dissimilarity-based analysis
Pairwise dissimilarity matrices were generated for metabolomics and 16S metagenomics quantification tables, described above, using Bray–Curtis dissimilarity through QIIME 1.9.1 [ 71 ]. Those distance matrices were used to perform Procrustes analysis (QIIME 1.9.1), and Mantel test (scikit-bio version 0.5.1) to measure the correlation between the metabolome and microbiome over time. The metabolomics dissimilarities were used to perform the PERMANOVA test to assess the significance of body part grouping. The PCoA and Procrustes plots were visualized in EMPeror. The dissimilarity matrices were also used to perform distance tests, comparing the distances within and between individuals and distances from time 0 to times 1, 2, and 3 using Wilcoxon rank-sum tests (SciPy version 0.19.1) [ 19 ].
Statistical analysis for molecular and microbial data
Statistical analyses were performed in R and Python (R Core Team 2018). Monotonic relationships between two variables were tested using non-parametric Spearman correlation tests. The p values for correlation significance were subsequently corrected using Benjamini and Hochberg false discovery rate control method. The relationship between two groups was tested using non-parametric Wilcoxon rank-sum tests. The relationship between multiple groups was tested using non-parametric Kruskal–Wallis test. The significance level was set to 5%, unless otherwise mentioned, and all tests were performed as two-sided tests.
Oh J, Byrd AL, Deming C, Conlan S, Kong HH, Segre JA. Biogeography and individuality shape function in the human skin metagenome. Nature. 2014;514(7520):59–64.
Article CAS PubMed PubMed Central Google Scholar
Grice EA, Segre JA. The skin microbiome. Nat Rev Microbiol. 2011;9(4):244–53.
Costello EK, Lauber CL, Hamady M, Fierer N, Gordon JI, Knight R. Bacterial community variation in human body habitats across space and time. Science. 2009;326(5960):1694–7.
Grice EA, Kong HH, Conlan S, Deming CB, Davis J, Young AC, et al. Topographical and temporal diversity of the human skin microbiome. Science. 2009;324(5931):1190–2.
Urban J, Fergus DJ, Savage AM, Ehlers M, Menninger HL, Dunn RR, et al. The effect of habitual and experimental antiperspirant and deodorant product use on the armpit microbiome. PeerJ. 2016;4:e1605.
Article PubMed PubMed Central Google Scholar
Callewaert C, Hutapea P, Van de Wiele T, Boon N. Deodorants and antiperspirants affect the axillary bacterial community. Arch Dermatol Res. 2014;306(8):701–10.
Article CAS PubMed Google Scholar
Staudinger T, Pipal A, Redl B. Molecular analysis of the prevalent microbiota of human male and female forehead skin compared to forearm skin and the influence of make-up. J Appl Microbiol. 2011;110(6):1381–9.
Houben E, De Paepe K, Rogiers V. A keratinocyte’s course of life. Skin Pharmacol Physiol. 2007;20(3):122–32.
Hoath SB, Leahy DG. The organization of human epidermis: functional epidermal units and phi proportionality. J Invest Dermatol. 2003;121(6):1440–6.
Oh J, Byrd AL, Park M, Kong HH, Segre JA. Temporal stability of the human skin microbiome. Cell. 2016;165(4):854–66.
Schloissnig S, Arumugam M, Sunagawa S, Mitreva M, Tap J, Zhu A, et al. Genomic variation landscape of the human gut microbiome. Nature. 2013;493(7430):45–50.
Article PubMed Google Scholar
Faith JJ, Guruge JL, Charbonneau M, Subramanian S, Seedorf H, Goodman AL, et al. The long-term stability of the human gut microbiota. Science. 2013;341(6141):1237439.
Hall MW, Singh N, Ng KF, Lam DK, Goldberg MB, Tenenbaum HC, et al. Inter-personal diversity and temporal dynamics of dental, tongue, and salivary microbiota in the healthy oral cavity. NPJ Biofilms Microbiomes. 2017;3:2.
Utter DR, Mark Welch JL, Borisy GG. Individuality, stability, and variability of the plaque microbiome. Front Microbiol. 2016;7:564.
Flores GE, Caporaso JG, Henley JB, Rideout JR, Domogala D, Chase J, et al. Temporal variability is a personalized feature of the human microbiome. Genome Biol. 2014;15(12):531.
The Human Microbiome Project C. Structure, function and diversity of the healthy human microbiome. Nature [Article]. 2012;486:207.
Article Google Scholar
Dorrestein PC, Gallo RL, Knight R. Microbial skin inhabitants: friends forever. Cell. 2016;165(4):771–2.
Bouslimani A, Porto C, Rath CM, Wang M, Guo Y, Gonzalez A, et al. Molecular cartography of the human skin surface in 3D. Proc Natl Acad Sci U S A. 2015;112(17):E2120–9.
Bouslimani A, Melnik AV, Xu Z, Amir A, da Silva RR, Wang M, et al. Lifestyle chemistries from phones for individual profiling. Proc Natl Acad Sci U S A. 2016;113(48):E7645–E54.
David LA, Maurice CF, Carmody RN, Gootenberg DB, Button JE, Wolfe BE, et al. Diet rapidly and reproducibly alters the human gut microbiome. Nature. 2014;505(7484):559–63.
Wu GD, Chen J, Hoffmann C, Bittinger K, Chen YY, Keilbaugh SA, et al. Linking long-term dietary patterns with gut microbial enterotypes. Science. 2011;334(6052):105–8.
Unno M, Cho O, Sugita T. Inhibition of Propionibacterium acnes lipase activity by the antifungal agent ketoconazole. Microbiol Immunol. 2017;61(1):42–4.
Holland C, Mak TN, Zimny-Arndt U, Schmid M, Meyer TF, Jungblut PR, et al. Proteomic identification of secreted proteins of Propionibacterium acnes. BMC Microbiol. 2010;10:230.
Nguyen DD, Wu CH, Moree WJ, Lamsa A, Medema MH, Zhao X, et al. MS/MS networking guided analysis of molecule and gene cluster families. Proc Natl Acad Sci U S A. 2013;110(28):E2611–20.
Soltanpour S, Jouyban A. Solubility of acetaminophen and ibuprofen in polyethylene glycol 600, propylene glycol and water mixtures at 25°C. J Mol Liq. 2010;155(2):80–4.
Article CAS Google Scholar
Haglund BO. Solubility studies of polyethylene glycols in ethanol and water. Thermochimica Acta. 1987;114(1):97–102.
Petras D, Nothias LF, Quinn RA, Alexandrov T, Bandeira N, Bouslimani A, et al. Mass spectrometry-based visualization of molecules associated with human habitats. Anal Chem. 2016;88(22):10775–84.
Vazquez-Baeza Y, Pirrung M, Gonzalez A, Knight R. EMPeror: a tool for visualizing high-throughput microbial community data. Gigascience. 2013;2(1):16.
Wang M, Carver JJ, Phelan VV, Sanchez LM, Garg N, Peng Y, et al. Sharing and community curation of mass spectrometry data with Global Natural Products Social Molecular Networking. Nat Biotechnol. 2016;34(8):828–37.
Watrous J, Roach P, Alexandrov T, Heath BS, Yang JY, Kersten RD, et al. Mass spectral molecular networking of living microbial colonies. Proc Natl Acad Sci U S A. 2012;109(26):E1743–52.
Frank AM, Monroe ME, Shah AR, Carver JJ, Bandeira N, Moore RJ, et al. Spectral archives: extending spectral libraries to analyze both identified and unidentified spectra. Nat Methods. 2011;8(7):587–91.
Quinn RA, Nothias LF, Vining O, Meehan M, Esquenazi E, Dorrestein PC. Molecular networking as a drug discovery, drug metabolism, and precision medicine strategy. Trends Pharmacol Sci. 2017;38(2):143–54.
Luzzatto-Knaan T, Garg N, Wang M, Glukhov E, Peng Y, Ackermann G, et al. Digitizing mass spectrometry data to explore the chemical diversity and distribution of marine cyanobacteria and algae. Elife. 2017;6:e24214.
Melnik AV, da Silva RR, Hyde ER, Aksenov AA, Vargas F, Bouslimani A, et al. Coupling targeted and untargeted mass spectrometry for metabolome-microbiome-wide association studies of human fecal samples. Anal Chem. 2017;89(14):7549–59.
Sumner LW, Amberg A, Barrett D, Beale MH, Beger R, Daykin CA, et al. Proposed minimum reporting standards for chemical analysis Chemical Analysis Working Group (CAWG) Metabolomics Standards Initiative (MSI). Metabolomics. 2007;3(3):211–21.
Lozupone C, Knight R. UniFrac: a new phylogenetic method for comparing microbial communities. Appl Environ Microbiol. 2005;71(12):8228–35.
Caporaso JG, Lauber CL, Costello EK, Berg-Lyons D, Gonzalez A, Stombaugh J, et al. Moving pictures of the human microbiome. Genome Biol. 2011;12(5):R50.
Green BR. Chloroplast genomes of photosynthetic eukaryotes. Plant J. 2011;66(1):34–44.
Callewaert C, Kerckhof FM, Granitsiotis MS, Van Gele M, Van de Wiele T, Boon N. Characterization of Staphylococcus and Corynebacterium clusters in the human axillary region. PLoS One. 2013;8(8):e70538.
Callewaert C, Lambert J, Van de Wiele T. Towards a bacterial treatment for armpit malodour. Exp Dermatol. 2017;26(5):388–91.
Tripathi A, Melnik AV, Xue J, Poulsen O, Meehan MJ, Humphrey G, et al. Intermittent hypoxia and hypercapnia, a hallmark of obstructive sleep apnea, alters the gut microbiome and metabolome. mSystems. 2018;3(3):e00020-18.
Gower JC. Generalized procrustes analysis. Psychometrika [journal article]. 1975;40(1):33–51.
Decreau RA, Marson CM, Smith KE, Behan JM. Production of malodorous steroids from androsta-5,16-dienes and androsta-4,16-dienes by Corynebacteria and other human axillary bacteria. J Steroid Biochem Mol Biol. 2003;87(4–5):327–36.
Austin C, Ellis J. Microbial pathways leading to steroidal malodour in the axilla. J Steroid Biochem Mol Biol. 2003;87(1):105–10.
Lloyd-Price J, Mahurkar A, Rahnavard G, Crabtree J, Orvis J, Hall AB, et al. Strains, functions and dynamics in the expanded Human Microbiome Project. Nature. 2017;550(7674):61-6.
Kapono CA, Morton JT, Bouslimani A, Melnik AV, Orlinsky K, Knaan TL, et al. Creating a 3D microbial and chemical snapshot of a human habitat. Sci Rep. 2018;8(1):3669.
Clemente JC, Pehrsson EC, Blaser MJ, Sandhu K, Gao Z, Wang B, et al. The microbiome of uncontacted Amerindians. Sci Adv. 2015;1(3):e1500183.
Blaser MJ, Dominguez-Bello MG, Contreras M, Magris M, Hidalgo G, Estrada I, et al. Distinct cutaneous bacterial assemblages in a sampling of South American Amerindians and US residents. ISME J. 2013;7(1):85–95.
Nakatsuji T, Chen TH, Narala S, Chun KA, Two AM, Yun T, et al. Antimicrobials from human skin commensal bacteria protect against Staphylococcus aureus and are deficient in atopic dermatitis. Sci Transl Med 2017;9(378)eaah4680.
Hollands A, Gonzalez D, Leire E, Donald C, Gallo RL, Sanderson-Smith M, et al. A bacterial pathogen co-opts host plasmin to resist killing by cathelicidin antimicrobial peptides. J Biol Chem. 2012;287(49):40891–7.
Zirwas MJ, Moennich J. Antiperspirant and deodorant allergy: diagnosis and management. J Clin Aesthet Dermatol. 2008;1(3):38–43.
PubMed PubMed Central Google Scholar
Funk JO, Maibach HI. Propylene glycol dermatitis: re-evaluation of an old problem. Contact Dermatitis. 1994;31(4):236–41.
Lehmann R, Zhao X, Weigert C, Simon P, Fehrenbach E, Fritsche J, et al. Medium chain acylcarnitines dominate the metabolite pattern in humans under moderate intensity exercise and support lipid oxidation. PLoS One. 2010;5(7):e11519.
Hiatt WR, Regensteiner JG, Wolfel EE, Ruff L, Brass EP. Carnitine and acylcarnitine metabolism during exercise in humans. Dependence on skeletal muscle metabolic state. J Clin Invest. 1989;84(4):1167–73.
Fischbach MA, Segre JA. Signaling in host-associated microbial communities. Cell. 2016;164(6):1288–300.
Devlin AS, Fischbach MA. A biosynthetic pathway for a prominent class of microbiota-derived bile acids. Nat Chem Biol [Article]. 2015;11(9):685–90.
Ridlon JM, Kang DJ, Hylemon PB, Bajaj JS. Bile acids and the gut microbiome. Curr Opin Gastroenterol. 2014;30(3):332–8.
Humbert L, Maubert MA, Wolf C, Duboc H, Mahe M, Farabos D, et al. Bile acid profiling in human biological samples: comparison of extraction procedures and application to normal and cholestatic patients. J Chromatogr B Analyt Technol Biomed Life Sci. 2012;899:135–45.
Ghent CN, Bloomer JR. Itch in liver disease: facts and speculations. Yale J Biol Med. 1979;52(1):77–82.
CAS PubMed PubMed Central Google Scholar
Herndon JH Jr. Pathophysiology of pruritus associated with elevated bile acid levels in serum. Arch Intern Med. 1972;130(4):632–7.
Zapata HJ, Quagliarello VJ. The microbiota and microbiome in aging: potential implications in health and age-related diseases. J Am Geriatr Soc. 2015;63(4):776–81.
Kueneman JG, Woodhams DC, Harris R, Archer HM, Knight R, McKenzie VJ. Probiotic treatment restores protection against lethal fungal infection lost during amphibian captivity. Proc Biol Sci. 2016;283(1839):e20161553.
Woodhams DC, Brandt H, Baumgartner S, Kielgast J, Kupfer E, Tobler U, et al. Interacting symbionts and immunity in the amphibian skin mucosome predict disease risk and probiotic effectiveness. PLoS One. 2014;9(4):e96375.
Belkaid Y, Tamoutounour S. The influence of skin microorganisms on cutaneous immunity. Nat Rev Immunol. 2016;16(6):353–66.
Belkaid Y, Segre JA. Dialogue between skin microbiota and immunity. Science. 2014;346(6212):954–9.
Pluskal T, Castillo S, Villar-Briones A, Oresic M. MZmine 2: modular framework for processing, visualizing, and analyzing mass spectrometry-based molecular profile data. BMC Bioinformatics. 2010;11:395.
Dieterle F, Ross A, Schlotterbeck G, Senn H. Probabilistic quotient normalization as robust method to account for dilution of complex biological mixtures. Application in 1H NMR metabonomics. Anal Chem. 2006;78(13):4281–90.
Gilbert JA, Jansson JK, Knight R. The Earth Microbiome project: successes and aspirations. BMC Biol. 2014;12:69.
Caporaso JG, Lauber CL, Walters WA, Berg-Lyons D, Huntley J, Fierer N, et al. Ultra-high-throughput microbial community analysis on the Illumina HiSeq and MiSeq platforms. ISME J. 2012;6(8):1621–4.
Walters W, Hyde ER, Berg-Lyons D, Ackermann G, Humphrey G, Parada A, et al. Improved bacterial 16S rRNA gene (V4 and V4-5) and fungal internal transcribed spacer marker gene primers for microbial community surveys. mSystems. 2016;1(1):e00009-15.
Caporaso JG, Kuczynski J, Stombaugh J, Bittinger K, Bushman FD, Costello EK, et al. QIIME allows analysis of high-throughput community sequencing data. Nat Methods. 2010;7(5):335–6.
McDonald D, Price MN, Goodrich J, Nawrocki EP, DeSantis TZ, Probst A, et al. An improved Greengenes taxonomy with explicit ranks for ecological and evolutionary analyses of bacteria and archaea. ISME J. 2012;6(3):610–8.
Haferkamp I. The diverse members of the mitochondrial carrier family in plants. FEBS Lett. 2007;581(12):2375–9.
Burgess SA, Flint SH, Lindsay D, Cox MP, Biggs PJ. Insights into the Geobacillus stearothermophilus species based on phylogenomic principles. BMC Microbiol. 2017;17(1):140.
Goh KM, Gan HM, Chan KG, Chan GF, Shahar S, Chong CS, et al. Analysis of Anoxybacillus genomes from the aspects of lifestyle adaptations, prophage diversity, and carbohydrate metabolism. PLoS One. 2014;9(6):e90549.
Carvalhais LC, Dennis PG, Badri DV, Tyson GW, Vivanco JM, Schenk PM. Activation of the jasmonic acid plant defence pathway alters the composition of rhizosphere bacterial communities. PLoS One. 2013;8(2):e56457.
Barelli C, Albanese D, Donati C, Pindo M, Dallago C, Rovero F, et al. Habitat fragmentation is associated to gut microbiota diversity of an endangered primate: implications for conservation. Sci Rep. 2015;5:14862.
Gonzalez A, Navas-Molina JA, Kosciolek T, McDonald D, Vazquez-Baeza Y, Ackermann G, et al. Qiita: rapid, web-enabled microbiome meta-analysis. Nat Methods. 2018;15(10):796–8.
Gonzalez A, Vazquez-Baeza Y, Pettengill JB, Ottesen A, McDonald D, Knight R. Avoiding pandemic fears in the subway and conquering the platypus. mSystems. 2016;1(3):e00050-16.
Altschul SF, Gish W, Miller W, Myers EW, Lipman DJ. Basic local alignment search tool. J Mol Biol. 1990;215(3):403–10.
Wilkinson ADMaA. IUPAC. Compendium of chemical terminology, 2nd ed. (the "Gold Book": Blackwell Scientific Publications, Oxford 1997.
Smoot ME, Ono K, Ruscheinski J, Wang PL, Ideker T. Cytoscape 2.8: new features for data integration and network visualization. Bioinformatics. 2011;27(3):431–2.
Ward T, Larson J, Meulemans J, Hillmann B, Lynch J, Sidiropoulos D, et al. BugBase Predicts Organism Level Microbiome Phenotypes. bioRxiv. 2017;133462. https://doi.org/10.1101/133462 .
Langille MGI, Zaneveld J, Caporaso JG, McDonald D, Knights D, Reyes JA, et al. Predictive functional profiling of microbial communities using 16S rRNA marker gene sequences. Nat Biotech [Computational Biology]. 2013;31(9):814–21.
Download references
Acknowledgements
We thank all volunteers who were recruited in this study for their participation and Carla Porto for discussions regarding beauty products selected in this study. We further acknowledge Bruker for the support of the shared instrumentation infrastructure that enabled this work.
This work was partially supported by US National Institutes of Health (NIH) Grant. P.C.D. acknowledges funding from the European Union’s Horizon 2020 Programme (Grant 634402). A.B was supported by the National Institute of Justice Award 2015-DN-BX-K047. C.C. was supported by a fellowship of the Belgian American Educational Foundation and the Research Foundation Flanders. L.Z., J.K, and K.Z. acknowledge funding from the US National Institutes of Health under Grant No. AR071731. TLK was supported by Vaadia-BARD Postdoctoral Fellowship Award No. FI-494-13.
Availability of data and materials
The mass spectrometry data have been deposited in the MassIVE database (MSV000081582, MSV000081580 and MSV000081581). Molecular network parameters for MS/MS data collected from all body parts of 11 individuals during T0-T9 MSV000081582 are accessible here http://gnps.ucsd.edu/ProteoSAFe/status.jsp?task=284fc383e4c44c4db48912f01905f9c5 . Molecular network parameters for MS/MS data collected from armpits T0–T3 MSV000081582 and deodorant used by individual 1 and 3 MSV000081580 can be found here http://gnps.ucsd.edu/ProteoSAFe/status.jsp?task=f5325c3b278a46b29e8860ec5791d5ad and here http://gnps.ucsd.edu/ProteoSAFe/status.jsp?task=aaa1af68099d4c1a87e9a09f398fe253 , respectively. OTU tables can be found in Qiita ( qiita.ucsd.edu ) as study ID 10370, and sequences can be found in EBI under accession number EBI: ERP104625.
Author information
Amina Bouslimani and Ricardo da Silva contributed equally to this work.
Authors and Affiliations
Collaborative Mass Spectrometry Innovation Center, Skaggs School of Pharmacy and Pharmaceutical Sciences, San Diego, USA
Amina Bouslimani, Ricardo da Silva, Kathleen Dorrestein, Alexey V. Melnik, Tal Luzzatto-Knaan & Pieter C. Dorrestein
Department of Pediatrics, University of California, San Diego, La Jolla, CA, 92037, USA
Tomasz Kosciolek, Stefan Janssen, Chris Callewaert, Amnon Amir, Livia S. Zaramela, Ji-Nu Kim, Gregory Humphrey, Tara Schwartz, Karenina Sanders, Caitriona Brennan, Gail Ackermann, Daniel McDonald, Karsten Zengler, Rob Knight & Pieter C. Dorrestein
Department for Pediatric Oncology, Hematology and Clinical Immunology, University Children’s Hospital, Medical Faculty, Heinrich-Heine-University Düsseldorf, Düsseldorf, Germany
Stefan Janssen
Center for Microbial Ecology and Technology, Ghent University, 9000, Ghent, Belgium
Chris Callewaert
Center for Microbiome Innovation, University of California, San Diego, La Jolla, CA, 92307, USA
Karsten Zengler, Rob Knight & Pieter C. Dorrestein
Department of Bioengineering, University of California, San Diego, La Jolla, CA, 92093, USA
Karsten Zengler & Rob Knight
Department of Computer Science and Engineering, University of California, San Diego, La Jolla, CA, 92093, USA
Department of Pharmacology, University of California, San Diego, La Jolla, CA, 92037, USA
Pieter C. Dorrestein
You can also search for this author in PubMed Google Scholar
Contributions
AB and PCD contributed to the study and experimental design. AB, KD, and TLK contributed to the metabolite and microbial sample collection. AB contributed to the mass spectrometry data collection. AB, RS, and AVM contributed to the mass spectrometry data analysis. RS contributed to the metabolomics statistical analysis and microbial–molecular correlations. GH, TS, KS, and CB contributed to the 16S rRNA sequencing. AB and GA contributed to the metadata organization. TK, SJ, CC, AA, and DMD contributed to the microbial data analysis and statistics. LZ, JK, and KZ contributed to the additional data analysis. AB, PCD, and RK wrote the manuscript. All authors read and approved the final manuscript.
Corresponding authors
Correspondence to Rob Knight or Pieter C. Dorrestein .
Ethics declarations
Ethics approval and consent to participate.
All participants signed a written informed consent in accordance with the sampling procedure approved by the UCSD Institutional Review Board (Approval Number 161730).
Competing interests
Dorrestein is on the advisory board for SIRENAS, a company that aims to find therapeutics from ocean environments. There is no overlap between this research and the company. The other authors declare that they have no competing interests.
Publisher’s Note
Springer Nature remains neutral with regard to jurisdictional claims in published maps and institutional affiliations.
Additional files
Additional file 1:.
Figure S1. Beauty products ingredients persist on skin of participants. Figure S2. Beauty product application impacts the molecular and bacterial diversity on skin of 11 individuals while the chemical diversity from personal beauty products used by males and females on T0 is similar. Figure S3. Longitudinal impact of ceasing and resuming the use of beauty products on the molecular composition of the skin over time. Figure S4. Molecular networking to highlight MS/MS spectra found in each body part. Figure S5. Longitudinal abundance of bile acids and acylcarnitines in skin samples. Figure S6. Characterization of steroids in armpits samples. Figure S7. Characterization of bile acids in armpit samples. Figure S8. Characterization of Acylcarnitine family members in skin samples. Figure S9. Beauty products applied at one body part might affect other areas of the body, while specific products determine stability versus variability of microflora at each body site. Figure S10. Representation of Gram-positive bacteria over time and the molecular features from the shampoo detected on feet. Figure S11. Procrustes analysis to correlate the skin microbiome and metabolome over time. Figure S12. Correlation between specific molecules and bacteria that change over time in armpits of individual 11. Figure S13. Representation of the number of samples that were removed (gray) and those retained (blue) after rarefaction at 10,000 threshold. (DOCX 1140 kb)
Additional file 2:
Table S1. List of personal (T0 and T7–9) beauty products and their frequency of use. (XLSX 30 kb)
Additional file 3:
Table S2. List of ingredients of common beauty products used during T4–T6. (PDF 207 kb)
Additional file 4:
Table S3. Mzmine feature finding and crop filtering parameters. (XLSX 4 kb)
Additional file 5:
Table S4. Feature table for statistical analysis with blank filtering and total ion current normalization. (CSV 150242 kb)
Additional file 6:
Table S5. Feature table for individual feature abundance in armpits. (XLSX 379 kb)
Additional file 7:
Table S6. Feature table for Calour analysis. (CSV 91651 kb)
Additional file 8:
Table S7. Metadata for Calour analysis. (TXT 129 kb)
Additional file 9:
Table S8. feature table with Probabilistic quotient normalization for molecular–microbial analysis. (ZIP 29557 kb)
Additional file 10:
Table S9. OTU table rarefied to 10,000 sequences per sample. (BIOM 9493 kb)
Additional file 11:
Table S10. 16S rRNA sequencing read counts per sample. (TSV 2949 kb)
Rights and permissions
Open Access This article is distributed under the terms of the Creative Commons Attribution 4.0 International License ( http://creativecommons.org/licenses/by/4.0/ ), which permits unrestricted use, distribution, and reproduction in any medium, provided you give appropriate credit to the original author(s) and the source, provide a link to the Creative Commons license, and indicate if changes were made. The Creative Commons Public Domain Dedication waiver ( http://creativecommons.org/publicdomain/zero/1.0/ ) applies to the data made available in this article, unless otherwise stated.
Reprints and permissions
About this article
Cite this article.
Bouslimani, A., da Silva, R., Kosciolek, T. et al. The impact of skin care products on skin chemistry and microbiome dynamics. BMC Biol 17 , 47 (2019). https://doi.org/10.1186/s12915-019-0660-6
Download citation
Received : 20 February 2019
Accepted : 30 April 2019
Published : 12 June 2019
DOI : https://doi.org/10.1186/s12915-019-0660-6
Share this article
Anyone you share the following link with will be able to read this content:
Sorry, a shareable link is not currently available for this article.
Provided by the Springer Nature SharedIt content-sharing initiative
- Skin care products
- Mass spectrometry
- Metabolomics
- 16S rRNA sequencing
BMC Biology
ISSN: 1741-7007
- Submission enquiries: [email protected]
- General enquiries: [email protected]
Sciencing_Icons_Science SCIENCE
Sciencing_icons_biology biology, sciencing_icons_cells cells, sciencing_icons_molecular molecular, sciencing_icons_microorganisms microorganisms, sciencing_icons_genetics genetics, sciencing_icons_human body human body, sciencing_icons_ecology ecology, sciencing_icons_chemistry chemistry, sciencing_icons_atomic & molecular structure atomic & molecular structure, sciencing_icons_bonds bonds, sciencing_icons_reactions reactions, sciencing_icons_stoichiometry stoichiometry, sciencing_icons_solutions solutions, sciencing_icons_acids & bases acids & bases, sciencing_icons_thermodynamics thermodynamics, sciencing_icons_organic chemistry organic chemistry, sciencing_icons_physics physics, sciencing_icons_fundamentals-physics fundamentals, sciencing_icons_electronics electronics, sciencing_icons_waves waves, sciencing_icons_energy energy, sciencing_icons_fluid fluid, sciencing_icons_astronomy astronomy, sciencing_icons_geology geology, sciencing_icons_fundamentals-geology fundamentals, sciencing_icons_minerals & rocks minerals & rocks, sciencing_icons_earth scructure earth structure, sciencing_icons_fossils fossils, sciencing_icons_natural disasters natural disasters, sciencing_icons_nature nature, sciencing_icons_ecosystems ecosystems, sciencing_icons_environment environment, sciencing_icons_insects insects, sciencing_icons_plants & mushrooms plants & mushrooms, sciencing_icons_animals animals, sciencing_icons_math math, sciencing_icons_arithmetic arithmetic, sciencing_icons_addition & subtraction addition & subtraction, sciencing_icons_multiplication & division multiplication & division, sciencing_icons_decimals decimals, sciencing_icons_fractions fractions, sciencing_icons_conversions conversions, sciencing_icons_algebra algebra, sciencing_icons_working with units working with units, sciencing_icons_equations & expressions equations & expressions, sciencing_icons_ratios & proportions ratios & proportions, sciencing_icons_inequalities inequalities, sciencing_icons_exponents & logarithms exponents & logarithms, sciencing_icons_factorization factorization, sciencing_icons_functions functions, sciencing_icons_linear equations linear equations, sciencing_icons_graphs graphs, sciencing_icons_quadratics quadratics, sciencing_icons_polynomials polynomials, sciencing_icons_geometry geometry, sciencing_icons_fundamentals-geometry fundamentals, sciencing_icons_cartesian cartesian, sciencing_icons_circles circles, sciencing_icons_solids solids, sciencing_icons_trigonometry trigonometry, sciencing_icons_probability-statistics probability & statistics, sciencing_icons_mean-median-mode mean/median/mode, sciencing_icons_independent-dependent variables independent/dependent variables, sciencing_icons_deviation deviation, sciencing_icons_correlation correlation, sciencing_icons_sampling sampling, sciencing_icons_distributions distributions, sciencing_icons_probability probability, sciencing_icons_calculus calculus, sciencing_icons_differentiation-integration differentiation/integration, sciencing_icons_application application, sciencing_icons_projects projects, sciencing_icons_news news.
- Share Tweet Email Print
- Home ⋅
- Science Fair Project Ideas for Kids, Middle & High School Students ⋅
Eighth Grade Science Fair Projects Involving Makeup
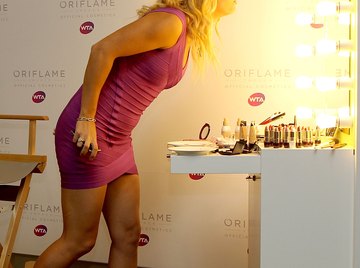
Makeup & Science Fair Ideas
According to American Demographics, nearly 90 percent of U.S. women wear makeup at least some of the time. However, many know little about the dizzying array of products available, the history of makeup, how it's made, its physiological effects and its societal significance. The products are such an intrinsic part of our society that they lend themselves to a wide range of science fair projects and many of those are achievable for 8th graders.
Biology/Medical Projects
Like many household items, makeup attracts and grows bacteria. An interesting project would be to test brand-new makeup for bacteria content, and then test makeup of the same brand used by you and your friends and track the differences. Another idea would be to introduce bacteria to samples of five different brands of the same type of makeup and measure growth over time to discover which are the most likely to support bacteria growth. Finally, you could test makeup past its use-by date to find out if it promotes bacteria growth more than fresh makeup of the same brand.
Chemistry Projects
Become a cosmetic chemist by developing your own cosmetics for a project. Lip balm, lipstick and mascara were all made at home at one point. You can make your own makeup items and compare them to commercial products by determining if they last as long, adhere correctly and do their jobs as well. Then you could have your friends wear your product and report on the results, which you can compile into informative charts and graphs. Alternatively, you can test several versions of the same product using variables like different dyes, flavors and ratios to see if you are able to improve on commercial products. You can report on your successes and failures and attempt to explain the factors that led up to the outcome.
Psychology Projects
Some studies say that about a third of British women won't even leave the house without wearing makeup, leading to speculation that cosmetics are often linked to self-esteem. You can explore whether makeup improves the self-esteem of women and girls by asking your friends who use makeup — and their moms — to go without it for a week. They can keep a daily diary about anything that happens related to the absence of makeup. Similarly, you could have friends who wear little or no makeup use it regularly for a week and keep a diary.
Sociology Projects
The use of makeup ranges back to at least 10000 B.C. For an informative science project, you can research the history of makeup and its significance in the societies of the past. You can prepare short reports about what societies used makeup to indicate status, beauty, wealth and healthiness. Another approach would be to report on ritual makeup in ceremonies and the everyday life of women (and sometimes men) in societies throughout history, and how these roles apply today in various societies, from advanced industrial nations to relatively primitive tribes in remote areas.
Related Articles
Makeup science projects, food technology project topics, chemistry research topic ideas, what is the difference between reverse engineering..., teenage girl science fair ideas, citric acid powder uses, how to calculate a test average, girly science fair project ideas, experiments on which mouthwash kills bacteria, dielectric breakdown vs. insulation resistance test, science fair projects on chewing gum, forensic science projects for high school students, forgotten women in science: esther lederberg, girly science fair projects for kids, why should you only test for one variable at a time..., how does marijuana help people's health, cosmetic science projects, a science project on which nail polish lasts longer, cosmetic science fair project ideas.
- Science Buddies: Potions and Lotions — Lessons in Cosmetic Chemistry
- Julian Trubin: Cosmetics and Cosmetology
- American Demographics: Women at the Cosmetics Counter — Demographic Trends in the Cosmetics Industry
- Daily Mail: One in Three Women Will Never Leave Home Without Make-up
- Cosmetics Info: A History of Cosmetics From Ancient Times
About the Author
Pete Hisey has been a writer and editor for over 25 years, primarily in the business-to-business field. He has expertise in many areas, including retail, consumer electronics, banking, dining, agriculture and entertainment media.
Photo Credits
Matthew Stockman/Getty Images Sport/Getty Images
Find Your Next Great Science Fair Project! GO
We can help you reset your password using the email address linked to your BioOne Complete account.

- BioOne Complete Titles
- BioOne eBook Titles
- About BioOne Digital Library
- How to Subscribe & Access
- Library Resources
- Publisher Resources
- Instructor Resources
- FIGURES & TABLES
- DOWNLOAD PAPER SAVE TO MY LIBRARY
A consistent challenge for undergraduate instructors is how to properly and objectively assess students who cannot attend regularly scheduled exams. Though many alternatives exist, perhaps the most common strategy is to allow students to take a makeup exam at a different time. Many instructors avoid this option for fear of the students gaining an unfair advantage in their exam preparations. I assessed student performance on makeup exams in relation to their typical performance on other exams throughout the course, to determine if there was any signal of score improvement or decline on makeup exams. I analyzed the data in regard to when students took the makeup exam, what their excuse for missing the regularly scheduled exam was, and the type of course in which they took the makeup. Students' makeup-exam scores were not significantly different from their regular-exam scores, though students who took a makeup due to a school-sponsored activity scored worse than students taking a makeup due to family emergencies or illness. While this research cannot definitively state that makeup exams do not provide some sort of advantage to student scores, it suggests that if students are trying to “game the system,” at the very least, they aren't winning.

KEYWORDS/PHRASES
Publication title:, collection title:, publication years.
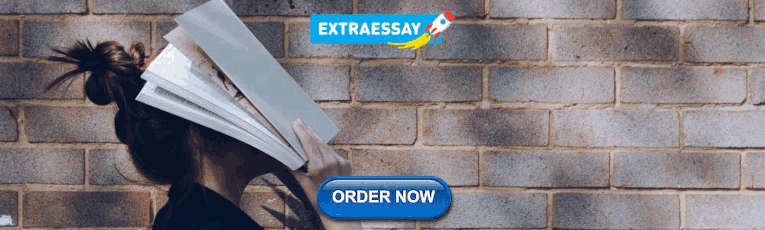
IMAGES
VIDEO
COMMENTS
Research has demonstrated a positive effect of makeup on facial attractiveness (Cash et al., 1989; Russell, 2003; Etcoff et al., 2011).Makeup has also been found to influence social perceptions (Etcoff et al., 2011; Klatt et al., 2016).While researchers have typically compared faces with makeup to faces without makeup, we propose that perceived effects will differ based on the amount of makeup ...
To test the hypothesis that professional makeup is heavier than self-applied makeup, we investigated perceptions of the amount of makeup worn, as impression formation has been found to be affected by heavy versus moderate application of makeup (Aguinaldo & Peissig, 2021; Etcoff et al., 2011; Workman & Johnson, 1991).We also investigated perceptions of makeup naturality (naturalness) because ...
To test this hypothesis, we ran two studies in which participants rated how even skin appeared in faces with and without makeup. The first study used digitally-applied makeup and the second study ...
Women wearing makeup are perceived by others as more attractive, competent, dominant, and more socially prestigious. Individuals differ in how much and how frequently they use makeup. Some studies show that women with lower self-esteem use more makeup, probably to hide imperfections. However, women with higher self-esteem can also use makeup to attract attention. This study verified whether ...
In a study, women were photographed wearing varying amounts of makeup, from left: barefaced, natural, professional and glamorous. Viewers considered the women wearing more makeup to be more competent.
For Jafari and Maclaran (2014) makeup is recognized as a tool for enriching lives thorough creativity, and for Tran et al, (2020) whilst recognizing "the duplexity of make up use… on self esteem" (p.8), there is explicit recognition of the impact of cosmetics on self-esteem if internally motivated and used as a tool for creativity ...
To test this hypothesis, we ran two studies in which participants rated how even skin appeared in faces with and without makeup. The first study used digitally-applied makeup and the second study ...
Social self and maskne. In the past, makeup was considered the preserve of women (Cash & Cash, 1982; Miller & Cox, 1982).However, male consumers have recently begun to show increased interest in caring for their appearances, which has led to a growth in the male cosmetic products market (Iida, 2006).Generation Y men tend to express their self-esteem through fashion products and are concerned ...
The make-up treatment of emotional videos in Experiment 1. The makeup treatment is shown in Figure. ... which supported our prior hypothesis that applying makeup can change the perception of ...
Makeup is a form of body art that has been used for millennia to change facial appearance. Though styles of makeup have varied through time and between cultures, the actual modification of the ...
2019) a study showed that make-up has a. positive impact on the self-esteem of 56.1% of. women sample, and a positive impact on the self-. confidence of 58.4% of them (Kosmala et al., 2019) More ...
By manipulating these visual features, makeup should make faces appear younger. We tested this hypothesis in an experiment in which participants estimated the age of carefully controlled photographs of faces with and without makeup. We found that 40- and especially 50-year-old women did appear significantly younger when wearing makeup.
Despite making only superficial changes to the face, makeup had large effects on apparent age. Makeup made the 50-year-old women appear around 1.5 years younger and made the 20-year-old women look ...
A secondary purpose of Experiment 4 was to examine whether eye makeup and lipstick increase the focus on face parts (Hypothesis 4), with a heightened focus on the eyes in the eye makeup condition (Hypothesis 4a) and with a heightened focus on the mouth in the lipstick condition (Hypothesis 4b) as compared to the no makeup condition. Method
An estimated 44 percent of American women do not like to leave their homes without makeup on. Research shows there are two primary reasons why women wear makeup: Camouflage - Women who are anxious and insecure tend to use makeup to appear less noticeable. Seduction - Women who want to be noticeably more attractive tend to use makeup to be ...
ness to women wearing heavy makeup (vs. no makeup) because heavy makeup causes perceivers to focus more on their sexiness and sexual appeal rather than their inter-nal characteristics. Consistent with this possibility, re-search has found that women'sfaceswithheavymakeup prompt perceptions of promiscuity and sexual availability
These findings, therefore, do not support the hypothesis that makeup use predicts actual sociosexuality. The results of Studies 1 and 2 suggest that even though makeup is perceived as a valid signal of sociosexuality, it is not. Further research needs to determine why makeup use is perceived to signal sociosexuality when, in fact, it does not ...
One of the most important aspects of color theory in makeup artistry is understanding the color wheel. The color wheel is a tool that helps artists determine which colors complement each other and which ones clash. By using the color wheel, makeup artists can create harmonious and balanced looks that enhance their clients' natural beauty.
Background Use of skin personal care products on a regular basis is nearly ubiquitous, but their effects on molecular and microbial diversity of the skin are unknown. We evaluated the impact of four beauty products (a facial lotion, a moisturizer, a foot powder, and a deodorant) on 11 volunteers over 9 weeks. Results Mass spectrometry and 16S rRNA inventories of the skin revealed decreases in ...
The use of makeup ranges back to at least 10000 B.C. For an informative science project, you can research the history of makeup and its significance in the societies of the past. You can prepare short reports about what societies used makeup to indicate status, beauty, wealth and healthiness. Another approach would be to report on ritual makeup ...
We have thus tested our hypothesis that makeup influences attribution of human-like traits with well-controlled images of faces and with extreme variations of makeup (no makeup versus heavy makeup)," Bernard explained. "Future researchers might want to replicate our findings in a more nuanced way, e.g., by gradually manipulating the makeup ...
Objectification theory suggests that sexualization has significant dehumanizing consequences for how perceivers see women. To date, research has mostly documented how sexualized bodies in the mass media are objectified and dehumanized. The purpose of the present work was to test the novel cosmetics dehumanization hypothesis (CDH), that is, that subtler manifestations of sexualization, such as ...
Students taking the makeup exam early had a significantly higher regular-exam z -score (0.136) than students taking the makeup exam late (-0.428), represented by a P -value <0.05. There was no significant difference in makeup-exam scores ( P = 0.14) or in the change in z -score for these two groups ( P = 0.61).