- Living reference work entry
- First Online: 02 October 2020
- Cite this living reference work entry
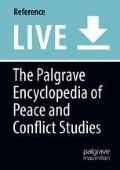
- Sara Hellmüller 3
415 Accesses
1 Citations
12 Altmetric
In 2017, United Nations (UN) Secretary-General António Guterres recognized mediation as “essential, flexible and effective tool which is utilized for conflict prevention, management and resolution by the United Nations and a wide range of other organizations and actors” (UN Secretary-General 2017, p 6). Mediation is defined as “a process of conflict management, related to but distinct from the parties’ own negotiations, where those in conflict seek the assistance of, or accept an offer of help from, an outsider […] without resorting to physical force or invoking the authority of law” (Bercovitch 2009, p. 343). Thus, mediation, at a minimum, denotes the assistance by a third party to a negotiation between two or more conflict parties to find a peaceful agreement (see also “ Negotiation ,” “ Peace Agreements ”). It has become a standard international response to armed conflicts since the end of the Cold War (Gowan and Stedman 2018; Greig and Diehl 2012). This is reflected in research, which has produced relevant knowledge on how to render mediation more effective. It has thereby strongly relied on positivist approaches with rationalist conceptual frameworks and quantitative methodologies. While producing important findings, it has left three main gaps, calling for more disaggregated findings, a stronger focus on ideational aspects, and awareness of changing macro-level factors in the broader world political context of mediation.
- Peacemaking
- Conflict resolution
This is a preview of subscription content, log in via an institution to check access.
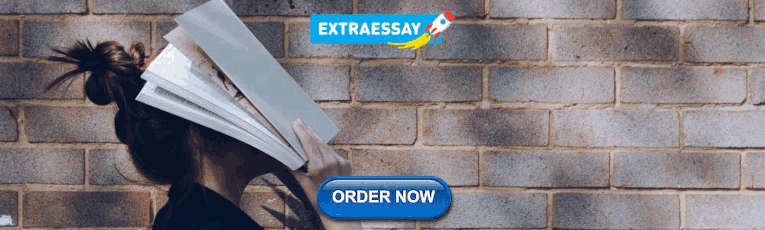
Access this chapter
Institutional subscriptions
Examples include the United States, in the Middle East and Southern Africa, the Soviet Union in Tashkent and between India and Pakistan over Kashmir, and the UN between Iran and Iraq and in Cyprus (Crocker et al. 1999 , p. 6).
Lundgren and Svensson ( 2020 ) note a reverse trend with a declining number of armed conflicts receiving mediation in the last 15 years.
For instance, the UN Mediation Support Unit, the European Union (EU) Mediation Support Team, the Organization for Security and Co-operation in Europe (OSCE) Mediation Support Team, the Inter-Governmental Authority on Development (IGAD) Mediation Support Team, and specialized mediation teams in Switzerland, Germany, Sweden, and other countries.
I use the terms effectiveness and success of mediation interchangeably. Some authors distinguish success and effectiveness and take the latter to refer to instances in which the outcomes of a mediation process correspond to the objectives set by the mediator (Kleiboer 1996 ).
He later added a third condition, which is that the conflict parties must have legitimate spokespersons to represent them in the mediation process, but he classifies it as being of secondary importance (Zartman 2001 , pp. 10–11).
The absence of a ripe moment for mediation does not mean that mediators cannot do anything. As Crocker ( 1992 , p. 471) states, “the absence of ‘ripeness’ does not tell us to walk away and do nothing. Rather, it helps us to identify obstacles and suggests ways of handling them and managing the problem until resolution becomes possible.” Mediators can use different strategies to “ripen” a conflict by helping conflict parties realize that a ripe moment is at hand or contribute to ripening it (Pruitt 2005 , p. 7).
Touval and Zartman ( 1985 ) distinguish between communication, formulation, and manipulation; (Bercovitch 2009 ) between communication, procedural, and directive styles; Kressel and Pruitt ( 1985 ) between reflexive, substantive, and contextual mediation styles; and Curran et al. ( 2004 ) distinguish between forcing and fostering.
For a critical view on this materialistic focus, see Duursma ( 2020 ) and Hellmüller et al. ( 2020 ).
Kraus et al. ( 2019 ) provide a noteworthy exception.
Crocker et al. ( 2015a ) and Zartman ( 2020 )) are exceptions.
African Union. (2014). African Union mediation support handbook . Umhlanga Rocks: African Centre for the Constructive Resolution of Disputes (ACCORD).
Google Scholar
Allansson, M., Melander, E., & Themnér, L. (2017). Organized violence, 1989–2016. Journal of Peace Research, 54 (4), 574–587.
Article Google Scholar
Assefa, H. (1987). Mediation of civil wars: Approaches and strategies: The Sudan Conflict . London: Westview.
Avruch, K. (2004). Culture and conflict resolution . Washington, DC: United States Institute of Peace Press.
Beardsley, K. (2008). Agreement without Peace? International mediation and time inconsistency problems. American Journal of Political Science, 52 (4), 723–740.
Beardsley, K. (2011). The mediation dilemma . New York: Cornell University Press.
Book Google Scholar
Beardsley, K. (2013). Using the right tool for the job: Mediator leverage and conflict resolution. Penn State Journal of Law & International Affairs, 2 (1), 57–65.
Beardsley, K., & Danneman, N. (2015). Mediation in international conflicts. In R. A. S. Scott & M. C. Buchmann (Eds.), Emerging trends in the social and behavioral sciences: An interdisciplinary, searchable, and linkable resource (pp. 1–13). New York: Wiley.
Beardsley, K., Quinn, D. M., Biswas, B., & Wilkenfeld, J. (2006). Mediation style and crisis outcomes. The Journal of Conflict Resolution, 50 (1), 58–86.
Bercovitch, J. (2002). Introduction: Putting mediation into context. In J. Bercovitch (Ed.), Studies in international mediation (pp. 15–34). New York: Palgrave.
Bercovitch, J. (2009). Mediation and conflict resolution. In J. Bercovitch, V. Kremenyuk, & I. W. Zartman (Eds.), The SAGE handbook of conflict resolution (pp. 340–354). London: SAGE.
Chapter Google Scholar
Bercovitch, J., & Gartner, S. S. (2006). Is there method in the madness of mediation? Some lessons for mediators from quantitative studies of mediation. International Interactions, 32 (4), 329–354.
Bercovitch, J., Anagnoson, J. T., & Wille, D. (1991). Some conceptual issues and empirical trends in the study of successful mediation in international relations. Journal of Peace Research, 28 (1), 7–17.
Black, P. W., & Avruch, K. (1998). Culture, power and international negotiations: Understanding Palau-US status negotiations. In D. Jacquin-Berdal, A. Oros, & M. Verweij (Eds.), Culture in world politics (pp. 34–60). Houndmills: Macmillan.
Burton, J. W. (1990). Conflict: Resolution and prevention . New York: St. Martin’s Press.
Convergne, E. (2016a). Learning to mediate? The mediation support unit and the production of expertise by the UN. Journal of Intervention and Statebuilding, 10 (2), 181–199.
Convergne, E. (2016b). UN mediators’ collaboration with scholars and expert NGOs: Explaining the need for knowledge-based communities in today’s conflicts. International Negotiation, 21 (1), 135–164.
Council of the European Union. (2009). Concept on strengthening EU mediation and dialogue capacities (Vol. 15779/09). Brussels.
Crocker, C. A. (1992). High Noon in Southern Africa: making peace in a rough neighbourhood . New York/London: W. W. Norton and Company.
Crocker, C. A., Hampson, F. O., & Aall, P. (1999). Introduction. In C. A. Crocker, F. O. Hampson, & P. Aal (Eds.), Herding cats: Multiparty mediation in a complex world (pp. 3–18). Washington, DC: United States Institute of Peace Press.
Crocker, C., Osler Hampson, F., & Aall, P. (2004). Taming intractable conflicts: Mediation in the hardest cases . Washington, DC: U.S. Institute of Peace Press.
Crocker, C. A., Hampson, F. O., & Aall, P. (2015a). The shifting sands of peacemaking: Challenges of multiparty mediation. International Negotiation, 20 (3), 363–388.
Crocker, C. A., Hampson, F. O., Aall, P., & Palamar, S. (2015b). Why is mediation so hard? The case of Syria. In M. Galluccio (Ed.), Handbook of international negotiation: Interpersonal, intercultural, and diplomatic perspectives (pp. 139–156). Cham: Springer.
Curran, D., Sebenius, J. K., & Watkins, M. (2004). Two paths to peace: Contrasting George Mitchell in Northern Ireland with Richard Holbrooke in Bosnia–Herzegovina. Negotiation Journal, 20 (4), 513–537.
DeRouen, K., Jr., Bercovitch, J., & Pospieszna, P. (2011). Introducing the Civil Wars Mediation (CWM) dataset. Journal of Peace Research, 48 (5), 663–672.
Duursma, A. (2014). A current literature review of international mediation. International Journal of Conflict Management, 25 (1), 81–98.
Duursma, A. (2020). African solutions to African challenges: The role of legitimacy in mediating Civil Wars in Africa. International Organization, 74 (2), 295–330.
Favretto, K. (2009). Should peacemakers take Sides? major power mediation, coercion, and bias. American Political Science Review, 103 (2), 248–263.
Fearon, J. D. (1995). Rationalist explanations for War. International Organization, 49 (3), 379–414.
Frei, D. (1976). Conditions affecting the effectiveness of international mediation. Peace Science Society (International), 26 (1), 67–84.
Gartner, S. S. (2011). Signs of trouble: Regional organization mediation and Civil War agreement durability. The Journal of Politics, 73 (2), 380–390.
Goodby, J. (1996). When war won out: Bosnian peace plans before Dayton. International Negotiation, 1 (3), 501–523.
Greig, M. (2001). Moments of opportunity: Recognizing conditions of ripeness for international mediation between enduring rivals. Journal of Conflict Resolution, 45 (6), 691–718.
Greig, M. J., & Diehl, P. F. (2012). International mediation . Cambridge: Polity.
Greig, J. M., & Regan, P. M. (2008). When do they say yes? An analysis of the willingness to offer and accept mediation in Civil Wars. International Studies Quarterly, 52 (4), 759–781.
Haass, R. N. (1990). Conflicts unending: The United States and regional disputes . New Haven: Yale University Press.
Harbom, L., Högbladh, S., & Wallensteen, P. (2006). Armed conflict and peace agreements. Journal of Peace Research, 43 (5), 617–631.
Hellmüller, S. (2019). Beyond buzzwords: Civil Society inclusion in mediation. In H.-W. Jeong (Ed.), Conflict intervention and transformation: Theory and practice (pp. 47–63). Lanham: Rowman & Littlefield International.
Hellmüller, S., Palmiano Federer, J., & Pring, J. (2017). Are mediators norm entrepreneurs? Bern: Swisspeace.
Hellmüller, S., Pring, J., & Richmond, O. (2020). Norms in International Peace Mediation. Swiss Political Science Review, (forthcoming) .
Hirblinger, A. T., & Landau, D. M. (2020). Daring to differ: Strategies of inclusion in peacemaking. Security Dialogue, 51 (4), 305–322.
Iji, T. (2005). Cooperation, coordination and complementarity in international peacemaking: The Tajikistan experience. International Peacekeeping, 12 (2), 189–204.
Inman, M., Kishi, R., Wilkenfeld, J., Gelfand, M., & Salmon, E. (2013). Cultural Influences on Mediation in International Crises. Journal of Conflict Resolution, 58 (4), 685–712.
Kastner, P. (2015). Legal normativity in the resolution of internal armed conflict . New York: Cambridge University Press.
Kaufman, S. J. (2006). Escaping the symbolic politics trap: Reconciliation initiatives and conflict resolution in ethnic wars. Journal of Peace Research, 43 (2), 201–218.
Kelman, H. C. (2005). Building trust among enemies: The central challenge for international conflict resolution. International Journal of Intercultural Relations, 29 (6), 639–650.
Kleiboer, M. (1996). Understanding success and failure of international mediation. The Journal of Conflict Resolution, 40 (2), 360–389.
Kraus, A. I., Frazer, O., Kirchhoff, L., Kyselova, T., Mason, S. J. A., & Palmiano Federer, J. (2019). Dilemmas and trade-offs in peacemaking: A framework for navigating difficult decisions. Politics and Governance, 7 (4), 331–342.
Kressel, K., & Pruitt, D. G. (1985). Themes in the mediation of social conflict. Journal of Social Issues, 41 (2), 179–198.
Kriesberg, L., & Thorson, S. (Eds.). (1991). Timing the de-escalation of international conflicts . Syracuse: Syracuse University Press.
Kydd, A. H. (2003). Which side are you on? Bias, credibility and mediation. American Journal of Political Science, 47 (4), 597–611.
Kydd, A. H. (2006). When can mediators build trust? American Political Science Review, 100 (3), 449–462.
Lanz, D., & Gasser, R. (2013). A crowded field: Competition and coordination in international peace mediation . Pretoria: Centre for Mediation in Africa / University of Pretoria.
Lundgren, M., & Svensson, I. (2020). The surprising decline of international mediation in armed conflicts. Research and Politics, 7 (2), 1–7.
Mandell, B. S., & Tomlin, B. W. (1991). Mediation in the development of norms to manage conflict: Kissinger in the Middle East. Journal of Peace Research, 28 (1), 43–55.
Maundi, M., Khadiagala, G., Nuemah, K., Touval, S., & Zartman, I. W. (2000). Entry and access in mediation . Washington, DC: United States Institute of Peace.
Maundi, M. O., Zartman, I. W., Khadiagala, G. M., & Nuamah, K. (2006). Getting in: Mediators’ entry into the settlement of African conflicts . Washington, DC: United States Institute of Peace Press.
Mediation Support Network. (2012). Regional Intergovernmental Organizations in mediation efforts: Lessons from West Africa. Discussion Points of the MSN, 3 .
Melander, E., Möller, F., & Öberg, M. (2009). Managing intrastate low-intensity armed conflict 1993–2004: A new dataset. International Interactions, 35 (1), 58–85.
Melander, E., Pettersson, T., & Themnér, L. (2016). Organized violence, 1989–2015. Journal of Peace Research, 53 (5), 727–742.
Moore, C. (1986). The mediation process: Practical strategies for resolving conflict . San Francisco: Jossey-Bass Publishers.
Nathan, L. (1999). When push comes to shove: The failure of international mediation in African civil wars. Track Two, 8 (2), 1–23.
OSCE. (2014). Mediation and dialogue facilitation in the OSCE: Reference guide . Vienna: Organization for Security and Co-operation in Europe.
Paffenholz, T. (2014). Civil society and peace negotiations: Beyond the inclusion–exclusion dichotomy. Negotiation Journal, 30 (1), 69–91.
Pettersson, T., & Eck, K. (2018). Organized violence, 1989–2017. Journal of Peace Research, 55 (4), 535–547.
Pettersson, T., Högbladh, S., & Öberg, M. (2019). Organized violence, 1989–2018 and peace agreements. Journal of Peace Research, 56 (4), 589–603.
Pruitt, D. G. (2005). Whither ripeness theory? Washington, DC: George Mason University.
Rauchhaus, R. W. (2006). Asymmetric information, mediation and conflict management. World Politics, 58 (2), 207–241.
Regan, P. M. (1996). Conditions of successful third-party intervention in intrastate conflicts. The Journal of Conflict Resolution, 40 (2), 336–359.
Regan, P., & Aydin, A. (2006). Diplomacy and other forms of intervention in Civil War. Journal of Conflict Resolution, 50 (5), 736–756.
Regan, P. M., & Stam, A. C. (2000). In the nick of time: Conflict management, mediation timing, and the duration of interstate disputes. International Studies Quarterly, 44 (2), 239–260.
Regan, P. M., Frank, R. W., & Aydi, A. (2009). Diplomatic interventions and Civil War: A new dataset. Journal of Peace Research, 46 (1), 135–146.
Richmond, O. (1998). Devious objectives and the disputants’ view of international mediation: A theoretical framework. Journal of Peace Research, 35 (6), 707–722.
Savun, B. (2008). Information, bias, and mediation success. International Studies Quarterly, 52 (1), 25–47.
Savun, B. (2009). Mediator types and the effectiveness of information provision strategies in the resolution of international conflict. In J. Bercovitch & S. Gartner (Eds.), New approaches to mediation (pp. 96–114). London: Routledge.
Silbey, S. S., & Merry, S. E. (1986). Mediator settlement strategies. Law & Policy, 8 (1), 7–32.
Sisk, T. (2009). International mediation in Civil Wars: Bargaining with bullets . New York: Routledge.
Smith, W. P. (1985). Effectiveness of the biased mediator. Negotiation Journal, 1 (4), 363–372.
Spencer, D. E., & Yang, H. (1993). Lessons from the field of intra-national conflict resolution. Notre Dame Law Review, 67 (5), 1495–1512.
Stedman, S. J. (1991). Peacemaking in Civil War: International Mediation in Zimbabwe, 1974–1980 . Boulder: Lynne Rienner Publishers.
Svensson, I. (2007). Bargaining, bias and peace brokers: How rebels commit to peace. Journal of Peace Research, 44 (2), 177–194.
Svensson, I. (2009). Who brings which peace? Biased versus neutral mediation and institutional peace arrangements in civil wars. Journal of Conflict Resolution, 53 (3), 446–469.
Touval, S. (1975). Biased intermediaries: Theoretical and historical considerations. Jerusalem Journal of International Relations, 1 , 51–69.
Touval, S. (1992). The Superpowers as Mediators. In J. Bercovitch & J. Z. Rubin (Eds.), Mediation in international relations (Vol. 41, pp. 232–248). London: Palgrave Macmillan UK.
Touval, S., & Zartman, I. W. (1985). International mediation in theory and practice . Boulder: Westview Press.
United Nations. (2012). UN guidance for effective mediation . New York: United Nations.
Vuković, S. (2014). Three degrees of success in international mediation. Millennium: Journal of International Studies, 42 (3), 966–976.
Vuković, S. (2015a). International multiparty mediation and conflict management – Challenges of cooperation and coordination . London: Routledge.
Vuković, S. (2015b). Soft power, bias and manipulation of international organizations in international mediation. International Negotiation, 20 (3), 414–443.
Wagner, R. H. (2000). Bargaining and war. American Journal of Political Science, 44 (3), 469–484.
Wall, J. A., & Rude, D. E. (1985). Judicial mediation: Techniques, strategies, and situational effects. Journal of Social Issues, 41 (4), 47–64.
Wallensteen, P., & Pettersson, T. (2015). Armed conflicts, 1946-2014. Journal of Peace Research, 52 (4), 536–550.
Wallensteen, P., & Svensson, I. (2014). Talking peace: International mediation in armed conflicts. Journal of Peace Research, 51 (2), 315–327.
Wehr, P. (1979). Conflict regulation . Boulder: Westview.
Werner, S., & Yuen, A. (2005). Making and keeping peace. International Organization, 59 (2), 261–292.
Zartman, I. W. (1985). Ripe for resolution: Conflict and intervention in Africa . New York: Oxford University Press.
Zartman, I. W. (2001). The timing of peace initiatives: Hurting stalemates and ripe moments. The Global Review of Ethnopolitics, 1 (1), 8–18.
Zartman, I. W. (2008). Negotiation and conflict management . New York: Routledge.
Zartman, I. W. (2020). Structuring in a vacuum: Negotiating in the current world disorder. International Negotiation, 25 , 1–13.
Download references
Author information
Authors and affiliations.
Centre on Conflict, Development and Peacebuilding, Graduate Institute of International and Development Studies, Geneva, Switzerland
Sara Hellmüller
You can also search for this author in PubMed Google Scholar
Corresponding author
Correspondence to Sara Hellmüller .
Section Editor information
No affiliation provided
Sandra Pogodda
Rights and permissions
Reprints and permissions
Copyright information
© 2020 The Author(s), under exclusive licence to Springer Nature Switzerland AG
About this entry
Cite this entry.
Hellmüller, S. (2020). Mediation. In: The Palgrave Encyclopedia of Peace and Conflict Studies. Palgrave Macmillan, Cham. https://doi.org/10.1007/978-3-030-11795-5_135-1
Download citation
DOI : https://doi.org/10.1007/978-3-030-11795-5_135-1
Received : 06 August 2020
Accepted : 20 August 2020
Published : 02 October 2020
Publisher Name : Palgrave Macmillan, Cham
Print ISBN : 978-3-030-11795-5
Online ISBN : 978-3-030-11795-5
eBook Packages : Springer Reference Political Science and International Studies Reference Module Humanities and Social Sciences Reference Module Business, Economics and Social Sciences
- Publish with us
Policies and ethics
- Find a journal
- Track your research
To read this content please select one of the options below:
Please note you do not have access to teaching notes, a current literature review of international mediation.
International Journal of Conflict Management
ISSN : 1044-4068
Article publication date: 4 February 2014
A rapidly expanding body of literature on international mediation, as well as the central role international mediation plays in modern-day conflict resolution, make it necessary to review and analyze this vastly evolving field of study. This study seeks to review the most significant trends and debates in the literature on international mediation, with an emphasis on the literature of the past six years.
Design/methodology/approach
Reflecting Wall et al. 's staged conceptualization of the mediation process; this review essay is divided in three sections that cover the antecedents of mediation, possible mediation approaches, and the outcomes these approaches yield – making it possible to review and analyze the diverse sets of theories within the field of mediation, as well the various methodological approaches employed to test these theories.
Much research to date has focused on how international mediation in armed conflicts affects the likelihood of reaching a negotiated agreement, while other possible outcomes of mediation have been understudied. Accordingly, research needs to be done on the effects of mediation attempts that did not lead to a peace agreement, as well as the accumulative effect of peace agreements. Furthermore, the relation between negative peace and mediation has been studied extensively, but how mediation affects the degree of positive peace has received scant scholarly attention. Finally, the interlinkages between the different phases of the mediation process need to be examined more extensively.
Originality/value
This review identifies the state of the art knowledge concerning the international mediation process, which allows peacemakers to make informed decisions in order to prevent and resolve armed conflict in the twenty-first century.
- International mediation
- Mediation occurrence
- Mediation outcomes
- Mediation strategy
- Mediator type
Duursma, A. (2014), "A current literature review of international mediation", International Journal of Conflict Management , Vol. 25 No. 1, pp. 81-98. https://doi.org/10.1108/IJCMA-02-2012-0020
Emerald Group Publishing Limited
Copyright © 2014, Emerald Group Publishing Limited
Related articles
We’re listening — tell us what you think, something didn’t work….
Report bugs here
All feedback is valuable
Please share your general feedback
Join us on our journey
Platform update page.
Visit emeraldpublishing.com/platformupdate to discover the latest news and updates
Questions & More Information
Answers to the most commonly asked questions here
A current literature review of international mediation
Purpose – A rapidly expanding body of literature on international mediation, as well as the central role international mediation plays in modern-day conflict resolution, make it necessary to review and analyze this vastly evolving field of study. This study seeks to review the most significant trends and debates in the literature on international mediation, with an emphasis on the literature of the past six years.
Mediators’ strategies for a successful mediation : a literature review of conflict mediation
Access full-text files, journal title, journal issn, volume title.
In a conflict mediation, a mediator works as a third party to help disputants negotiate their problems and facilitate them to reach a consensus. The goal of a conflict mediations is more than solving problems. From a mediator’s point of view, the key issue in a conflict mediation is to produce results that can benefit each party in the conflict. Previous research examined mediators’ practice in conflict mediations theoretically and empirically. However, there is a lack of specific exploration on mediation strategies that mediators can apply in practice for a successful mediation. The aim of this report is to find feasible strategies that can be used by mediators through reviewing past literature on conflict mediation and rethinking a three-month mediation training the author experienced. Findings indicate that strategies such as creating a comfortable environment, active listening, being aware of disputants’ nonverbal behaviors, and showing empathy are useful and can lead to successful mediation. In addition, in conflict mediations, mediators should be selective to these strategies depending on context. The mediators’ strategies that are summarized in this report are general and possible for a successful mediation; in other words, if mediator use these strategies it will lead to more satisfied parties, so that they can ensure a smoother mediation rather than guarantee an outcome
Description
Lcsh subject headings, collections.

An official website of the United States government
The .gov means it’s official. Federal government websites often end in .gov or .mil. Before sharing sensitive information, make sure you’re on a federal government site.
The site is secure. The https:// ensures that you are connecting to the official website and that any information you provide is encrypted and transmitted securely.
- Publications
- Account settings
Preview improvements coming to the PMC website in October 2024. Learn More or Try it out now .
- Advanced Search
- Journal List
- HHS Author Manuscripts

Evaluating mediation and moderation effects in school psychology: A presentation of methods and review of current practice
Third variable effects elucidate the relation between two other variables, and can describe why they are related or under what conditions they are related. This article demonstrates methods to analyze two third-variable effects: moderation and mediation. The utility of examining moderation and mediation effects in school psychology is described and current use of the analyses in applied school psychology research is reviewed and evaluated. Proper statistical methods to test the effects are presented, and different effect size measures for the models are provided. Extensions of the basic moderator and mediator models are also described.
Across disciplines, researchers are recognizing the advantage of integrating more sophisticated methodology into their statistical toolkits. Advanced statistical models allow the investigation of complex research hypotheses that may better approximate the multifaceted reality from which data are drawn, permitting for example, the investigation of differential growth trajectories of behavioral change, nested data structures such as individuals within schools, or multivariate processes that underlie or modify other bivariate relations. This latter class of models, sometimes referred to as third variable effect models, has received particular emphasis recently because these models allow for an understanding of the factors that may modify or inform the relation of two other variables. However, the utility of these methods is limited if their implementation does not reflect best practices currently advocated in the methodological literature.
The purpose of this paper is to describe two third-variable effect models, specifically mediation analysis and moderation analysis, for the school psychology audience and to present current methodological recommendations for their implementation in applied research. We first describe the utility of implementing the models in school psychology research and provide an example application of the models. We then conduct a literature review of how mediation analysis and moderation analysis have been applied in three top school psychology journals over the past 23 years to gauge current practice. We follow with a presentation of the basic mediation and moderation models, illustrating the most recent recommendations for their statistical estimation, and briefly discuss advanced models that involve the effects.
Why study mediation and moderation?
Although evaluating bivariate relations between variables can lend insight into whether a hypothesized relation holds or whether a program appears to work, it cannot address questions of why, how, and for whom the relation holds, or the program works. Investigating third variables such as moderators and mediators permits the investigation of such questions, informing both theory and evidence-based practice in school psychology. A moderator variable is a third variable ( Z ) that changes the relation between a predictor ( X) and an outcome ( Y ), thereby affecting the strength and/or direction of the relation between the two variables. Moderators demonstrate the generalizability of the relation between X and Y , illustrating the context(s) under which the relation holds. Moderation effects are often referred to as statistical “interactions” in the social science literature; the terms are interchangeable and refer to the same phenomenon. A mediator variable ( M ) is a third variable that explains how or why two other variables (i.e., X and Y ) are related. In a mediation model, the independent variable ( X ) predicts the mediator variable ( M ) which in turn predicts the outcome ( Y ). Thus, a mediator is intermediate in the relation between X and Y . By modeling an intermediate variable in the X – Y relation, the overall effect between X and Y can be decomposed into component parts called the direct effect of X on Y and the indirect effect of X on Y through M (i.e., the mediated effect). Investigating both direct and indirect effects often provides more insight than simply evaluating the bivariate X – Y relation alone, and researchers have proposed several different ways to statistically test mediation using the component parts.
Studying mechanisms of change by investigating mediator variables has the potential to direct and refine the development of evidence-based interventions because it can shed light on how an intervention achieves its effects (or alternatively why it fails to achieve effects). Additionally, studying contextual effects by investigating moderator variables has the potential to extend the generalizability and external validity of evidence-based treatment programs to different cultural groups or in different settings. By analyzing mediation and moderation effects in this way, researchers can promote theory refinement and positively affect practice.
Investigating mediation and moderation effects also works toward fulfilling several recent federal mandates and legislation in education. Legislative movements such as the No Child Left Behind Act ( U.S. Department of Education, 2002 ) and the reauthorization of the Individuals With Disabilities Education Improvement Act (IDEA, 2004) dictate the use of evidence-based practice in the assessment and intervention of students in both general and special education. Investigating moderators in the development of evidence-based programs can preemptively direct practice by targeting individuals that are likely to respond to treatment or individuals that may need additional support ( Kazdin & Nock, 2003 ). This is particularly important given the recent movements towards the Response-to-Intervention (RTI) model of identification and intervention, where education and placement decisions are based on the degree to which specific students respond to evidence-based treatment. Additionally, analyzing mediation in the development and evaluation of evidence-based programs can identify the effectiveness of individual components of treatment packages. Thus, the investigation of mediation and moderation effects can refine treatment or intervention programs by removing components that do not work, and/or promoting components that do work.
Example application of mediation and moderation analysis in school psychology
Most school-based treatment or intervention programs, regardless of the target outcome, consist of a molar treatment package that consists of various treatment components which have some hypothesized connection to the primary outcome of interest ( Shadish, Cook, & Campbell, 2001 ). Programs aim to manipulate these various components in an effort to change the outcome variable. Statistical mediation analysis can be implemented to evaluate hypothesized mechanisms of change in such intervention processes. Consider a reading program that is aimed at increasing overall reading competence in elementary school students with learning disabilities. Program researchers may hypothesize that oral reading fluency underlies the acquisition of reading competence, such that the program aims to improve fluency which, in turn, influences tests scores. One can formally test this hypothesis with a mediation analysis where oral reading fluency is modeled as a mediator of the relation between the reading program and reading competency. The bottom panel of Fig. 1 shows a conceptual diagram of the mediation analysis, where squares in the figure correspond to observed variables in the model and single-headed directional arrows indicate regression coefficients. By investigating statistical mediation, a researcher can examine underlying hypotheses for why or how the reading intervention works. Note that mediation hypotheses cannot be tested or falsified through a simple examination of bivariate correlations or research that only investigates reading fluency as a primary outcome.

Conceptual path diagram of the mediation reading program example.
If the reading treatment program from the previous example was specifically aimed at improving reading skills in students with learning disabilities, researchers may be interested in determining whether the program generalizes to students in the broader school population. A researcher could formally test this hypothesis by examining differential treatment effects of the program by learning disability status in a statistical moderation analysis. Fig. 2 shows a conceptual diagram of a moderation analysis. The arrow that connects the Reading Program box to the Reading Competence box represents the effect of the reading program on reading competence (e.g., a regression coefficient), and the arrow from the LD Status box to the arrow denotes the moderating effect that learning disability status has on this association. Non-significant results from such a moderation analysis would indicate that the program generalized to the larger school population; whereas significant results would indicate that the program affected students with and without learning disabilities differently.

Conceptual path diagram of the moderation reading program example.
Literature review
To guide our presentation of methods for this paper, we conducted a literature review to examine how school psychologists have used and applied mediation and moderation models in their research over the past 23 years. We examined articles in three predominant journals: (a) Journal of School Psychology , (b) School Psychology Review , and (c) School Psychology Quarterly . The full text of these journals was searched between 1986 and 2008 across four sources: Science Direct , Psych Info , and EBSCO Psychology and the Behavioral Sciences , respectively. Search terms for each journal were mediation , mediator , indirect effect , moderation , moderator , interaction term , and statistical interaction . The term “interaction” was not explicitly searched for by itself to avoid a deluge of articles involving personal or social interactions. Thus the results from this search are not exhaustive and should only be used to obtain a general point of reference to the current state of practice of statistical mediation and moderation analysis in school psychology.
The literature survey identified 48 studies that examined mediation and 50 studies that examined moderation resulting in a pool of 98 articles. Seven of these studies investigated both mediation and moderation and were counted twice due to interest in separate methodological aspects of each technique. Thus, 91 separate articles were identified within the literature review. Fig. 3 displays a frequency histogram of publication date for the articles examined. The noticeable negative skew in the graph illustrates increased use of both mediation and moderation over time, likely fostered by seminal methodological publications on the methods in later years (e.g., Aiken & West, 1991 ; MacKinnon, Lockwood, Hoffman, West, & Sheets, 2002 ).

Frequency histogram of moderation and mediation publications in selected school psychology journals over time.
Articles were coded based on the method used to analyze data to understand how the each of the models was used and evaluated. Articles that tested mediation were broken down into those that used (a) a causal step approach ( Baron & Kenny, 1986 ), (b) normal theory point estimation ( Sobel, 1982 ), and (c) structural equation modeling (SEM). Articles that used SEM were further coded into those that used a test of overall model fit to test mediation versus those that directly estimated a mediated effect point estimate. Articles that tested moderation were broken down into those that used (a) moderated multiple regression (i.e. Aiken & West, 1991 ), (b) ANOVA, and (c) moderated multiple regression in hierarchal linear models (i.e. models controlling for multilevel data). Table 1 illustrates descriptive statistics from the review as well as the median sample size employed in each method.
Literature survey results: methods used to test mediation and moderation effects.
Note: descriptive statistics are reported separately for mediation and moderation analyses.
Eight of the studies (8%) in the review were manipulated program evaluations with three examining mediation and five examining moderation. Programs that were evaluated included various academic and behavior prevention and intervention programs, parenting programs, and a subjective well-being intervention program. Of the studies in the review, 52 (53%) were cross-sectional, with approximately half of those investigating mediation. Thirty two of the studies (32%) used two waves of data in their analysis, and 15 of the studies (15%) used three or more waves of data. Although substantive areas of the studies varied widely, patterns emerged that were consistent with general school psychology research. Roughly half of the review articles investigated ( n =45) academic achievement as a key outcome variable. Other studies examined predictors of negative interpersonal interactions and classroom behavior, social relations among adolescents and children, school climate, subjective well-being and school engagement, cognitive processes, and Attention Deficit Hyperactivity Disorder. Given the purpose of this paper, no formal method of categorization was developed to evaluate substantive area of interest. Thus the preceding must be considered in light of the fact that these are rather arbitrary and nonexclusive categories.
Evaluating results from the literature review reveals key points. First, the use and application of moderation analysis appears generally based on best practices, namely moderated multiple regression. This is not unexpected given that key methodological resources on the method were published many years ago (e.g., Aiken & West, 1991 ; Cohen & Cohen, 1983 ). Conversely, it appears that the use and application of statistical mediation analysis consists primarily of outdated methods, namely using underpowered methods (e.g., causal steps approach and normal theory point estimation) to examine mediation. Given this discrepancy and the larger need to address appropriate analyses for investigating mediation, we first review the proper methods to test moderation effects and focus the remainder of the article on describing contemporary statistical methods to test mediation.
Moderation analysis
What is a moderator.
As stated earlier, a moderator is a third variable ( Z ) that changes the relation between a predictor ( X ) and an outcome ( Y ), thereby affecting the strength and/or direction of the relation between the two variables. The moderator (typically a covariate or secondary predictor of interest) interacts with the primary predictor variable to influence the outcome, such that any effects of the primary predictor on the dependent variable are conditional on, or dependent on, values of the moderator. In a regression context, this dependency yields different bivariate regression lines predicting Y from X for different values of the moderator variable, Z . In an ANOVA context (or alternatively in a regression with categorical predictor variables), this dependency can be illustrated by non-parallel lines for moderator-based subgroups. Moderators may enhance, reduce, or directionally change the effect of the predictor on an outcome, such that the effects of one variable depend on levels of the other variable in analysis. In the presence of moderation, the main effects are no longer additive and need to be interpreted with reference to the moderator variable, because the outcome variable is explained by the simultaneous effect of the variables.
In general, moderator effects are one of two types: ordinal or disordinal interactions ( Cohen et al., 2003 ). In plots of the data, ordinal interactions are illustrated by lines that do not cross one another in the plot, whereas disordinal interactions are illustrated by lines that do cross in the plot (see Fig. 4 ). Ordinal interactions may be of two subtypes. A synergistic interaction effect occurs when a change in the level of the moderator variable enhances the bivariate relation between X and Y ( Cohen et al., 2003 ). A buffering interaction effect occurs when a change in the level of the moderator variable reduces the magnitude of the bivariate relation between X and Y . Fig. 4 illustrates two such examples, where Panel 2 corresponds to a synergistic interaction such that the effect of treatment is greater for students with learning disabilities versus those with none, and Panel 3 corresponds to a buffering interaction such that the effect of treatment is less for students with learning disabilities versus those with none. Detailed descriptions of different moderator effects and a framework for their estimation and interpretation were originally presented in Aiken and West (1991) . More recently, Aguinis (2004) has also written a book on moderator effects.

Differentiating interaction effects. The solid line in each graph across the panels pertains to the X – Y relation at one level of the moderator variable (i.e., learning disabled). The dashed line in each graph across the panels pertains to the X – Y relation at the other level of the moderator variable (i.e., not learning disabled).
Estimating the basic moderation model
To demonstrate estimation of the basic moderation model, we provide a numerical example for the aforementioned hypothetical school psychology study where the efficacy of a reading program is potentially influenced by a student’s learning disability status. The data for the numerical example are presented in Table 2 . Throughout this section the reading program acts as the primary predictor variable (i.e., X ), learning disability status acts as the moderator variable (i.e., Z ), and reading performance serves as the outcome variable (i.e., Y ).
Dataset for numerical examples ( N =20).
Note. For the reading program variable a value of −.5 corresponds to the control group and a value of .5 corresponds to the treatment group. For the learning disability status variable a value of −.5 corresponds to students with no learning disabilities and a value of .5 corresponds to students with a learning disability.
The basic moderation model is estimated with the following multiple regression equation
where Ŷ is the predicted value of a student’s reading performance, X represents group assignment to the reading program (i.e., treatment vs. control), Z corresponds to a student’s learning disability status (i.e., learning disabled vs. not learning disabled), and XZ is the interaction term formed by multiplying the reading program and learning disability codes. Recall that coding schemes such as dummy coding or effects coding are a way of representing nominal or ordinal variables in a regression model ( Cohen et al., 2003 ). For details on implementing different coding schemes to accommodate categorical variables in a multiple regression model, see Cohen et al. (2003) or Aiken and West (1991) .
Eq. (1) is the preferred statistical method for testing moderation effects with observed (i.e., not latent) variables because it allows for categorical or continuous predictors and it provides tests of the main effects and the interaction effect ( Aguinis, 2004 ; Aiken & West, 1991 ). Note that inclusion of the main effects (i.e., the lower order predictors X and Z ) is critical because the failure to include non-significant lower order terms in analysis confounds effects in the model. Effects are confounded because the variance associated with the lower order terms is not independent of the variance associated with the interaction term. If the lower order main effects are not included in the regression model, they are not statistically partialled out of the interaction, and the significance of the interaction term may be artificially inflated ( Aiken & West, 1991 ).
The multiple regression equation corresponding to the reading program example is as follows
The regression coefficients in the equation quantify the effect of the variable on reading performance while controlling for the effect of other variables in the model. Sample estimates of the population regression parameters (i.e., the β s) in the moderation model are denoted by β ^ in the following paragraphs. For example, β ^ 1 = 1.098 ( t = 2.410 , p = .028 ) is the regression coefficient relating the reading program to reading performance controlling for both a student’s learning disability status and the interaction of the reading program and learning disability status; β ^ = − .142 , ( t =−.311, p =.760) is the regression coefficient relating learning disability status to reading performance controlling for the effects of the reading program and the interaction of the reading program and learning disability status in the model; and β ^ 3 = 1.995 ( t = 2.188 , p = .044 ) is the regression coefficient relating the interactive effective of the reading program and students’ learning disability status on reading performance controlling for each effect independently. Finally, β ^ 0 = 0.45 ( t = .199 , p = .845 ) represents the intercept in the equation, or the predicted reading score value when all predictors in the model equal zero. The β ^ 3 coefficient corresponding to the interactive effect of the reading program and learning disability status is considered a higher-order term in the model as it is created by multiplying other variables in the equation.
Fig. 5 depicts the moderated multiple regression equation for the numerical example as a path diagram, where double-headed curved lines denote correlations among the predictor variables. Sample estimates of the regression coefficients and their corresponding significance levels appear above the paths to which they correspond. Note that the model in Fig. 5 is somewhat different from the depiction of an interaction effect in Fig. 2 . Fig. 2 is conceptual in nature, whereas Fig. 5 corresponds to the moderated multiple regression in Eq. (2) .

The basic moderation model. X =the independent variable, Y =the dependent variable, Z =the moderator variable, XZ =the interaction of the independent and moderator variables, β ^ 1 = the effect of the independent variable on the outcome controlling for Z and XZ , β ^ 2 = the effect of the moderator on the outcome controlling for X and XZ , and β ^ 3 = the effect of the XZ interaction on the outcome controlling for the lower order effects. *Corresponds to p <.05 and ns corresponds to not significant. Correlations among the predictor variables are approximately zero because of the balanced design in the example.
Testing for moderation
A t -test of the regression coefficient associated with the XZ interaction term (i.e., β ^ 3 ) is one way to determine if there is statistical moderation. If the β ^ 3 coefficient is significant, then there is a significant moderation effect. The parameter estimate, its standard error, and the significance level of the coefficient associated with the interaction are provided in any standard output of a regression analysis in conventional statistical software packages such as SAS or SPSS. As shown in the numerical example, the regression coefficient associated with the interactive effect of the reading program and students’ learning disability status on reading performance was significant at an alpha level of .05 ( β ^ 3 = 1.995 , t = 2.188 , p = .044 ) . The significant finding suggests that the efficacy of the reading program on students’ reading performance was affected by, or moderated by , their learning disability status (i.e., there was significant moderation).
In lieu of the t -test, one can also use an R 2 change test (denoted R 2 Δ) to evaluate moderation ( Aguinis, 2004 ; Aiken & West, 1991 ). The R 2 Δtest, which is distributed as an F -statistic, quantifies variance accounted for by the XZ interaction, above and beyond the variance accounted for in the model without the interaction term. The F -statistic for the R 2 Δ change is computed with the following equation
where R 2 2 is the R 2 associated with the model that includes the main effects and the interaction term (i.e., Eq. (1) ), R 1 2 is the R 2 associated with a regression model that includes only the main effects for X and Z , k 2 is the number of predictors in the equation that includes the interaction term, k 1 is the number of predictors in the equation that includes only the main effects, and N is the sample size. The R 2 Δ is easily requested in conventional statistical software packages, so there is no need to compute the statistic by hand. Results from the R 2 Δ test of moderation will be identical to results from a t -test of the β ^ 3 interaction term in Eq. (1) , such that the square root of the R 2 Δ F -statistic will equal the value of the t -statistic, and the p -values of the two tests will be identical. Returning to the numerical example, the R 2 Δ statistic comparing a model with only the reading program and learning disability status predicting reading performance ( R 2 =.221) to a model with both predictors and their interaction ( R 2 =.400) is R 2 Δ=.179( F =3.980, p =.044). Note that the square root of the F -statistic equals the value of the t -statistic for the interaction term in Eq. (2) (i.e., 3.980 = 1.995 ), and the p -values from the two tests are identical (i.e., p =0.44).
Multicollinearity and centering predictor variables in moderation analysis
Entering the product of X and Z into the moderated multiple regression equation to model the effect of their interaction on the outcome introduces multicollinearity, such that X and Z and highly correlated with the XZ product term. The implication of this multicollinearity is that standard errors of the regression coefficients become inflated, resulting in unstable estimates. Much of this excessive correlation is due to scaling of the variables (i.e., differences in the means). Multicollinearity due to such scaling is termed non-essential multicollinearity and can be reduced by centering the predictor variables (i.e., subtracting the mean from each score; Aiken & West, 1991 ). Centering the predictors about their mean also improves interpretation of lower order terms in the equation (Aiken & West).
In a balanced design where there are equal sample sizes across groups, a researcher can mean center dummy coded dichotomous variables by subtracting the mean of the codes (i.e., the mean of 0 and 1=.5) from each observation to yield effect codes with the values of −.5 and .5. Note that one can also center in unbalanced designs where the sample size across groups is unequal, but the resulting codes will no longer be .5 and −.5. The researcher can mean center continuous predictor variables by computing the mean of the predictor (e.g., X or Z ) and subtracting this value from each observed score in the data for that variable ( Aiken & West, 1991 )
Mean centering the interaction term is achieved by forming the product of the two centered variables in Eqs. (4) and (5)
Note that this approach to centering of the interaction term is not equivalent to first forming the product of the observed X and Z variables, computing the mean of the XZ term and then subtracting this from the observed values. The proper way to mean center the interaction is as described in Eq. (6) .
Mean centering predictor variables aids interpretation of lower order coefficients (i.e., X and Z ) in a moderation analysis because the transformation moves the zero point of the predictors to a meaningful location (the mean of each variable). By moving the zero point of the predictors to their means, the intercept in the moderation model becomes the expected value of the outcome variable at the average of all the predictor variables. The interpretation of the β 1 parameter becomes the regression of Y on X at the mean of Z , or alternatively the average regression of Y on X across all values of Z . Returning to the numerical example, the β ^ 1 coefficient ( β ^ 1 = 1.098 ) represents the average effect of the reading program on student reading performance across students with and without learning disabilities. Similarly, the interpretation of the β 2 parameter becomes the regression of Y on Z at the mean of X , or alternatively the average regression of Y on Z across all values of X . In the numerical example, the β ^ 2 coefficient ( β ^ 2 = − .142 ) represents the average effect of learning disability status on student reading performance across the treatment and the control groups. The interpretation of the higher-order interaction term parameter, β 3 , remains the same across centered and uncentered solutions: the interactive effect of X and Z on Y .
Interpreting moderation effects
The main effect of the primary predictor does not fully characterize the relation between X and Y in the presence of significant moderation. Instead, the relation between X and Y must be analyzed at different values of the moderator variable, Z , to fully understand the association. Plotting the interaction aids interpretation of the interaction effect and provides a way to investigate how the relation between Y and X changes across levels of the moderator variable. Fig. 6 demonstrates a plot of the interaction for the numerical reading program example and illustrates that students with a learning disability have greater positive reading gains from the program than do students without a learning disability. Specifically, students with a learning disability have lower reading performance at baseline relative to non-learning disabled controls and show better reading performance after participating in the treatment.

Plot of the interaction for the numerical reading program example.
When plotting interactions, specific values of the moderator variable, Z , must be chosen at which to graph the regression of Y on X . For categorical moderator variables, values of Z should correspond to the codes for the groups that you wish to plot (as shown in the plot of the numerical example). For continuous moderator variables, when theory or other important criteria provide meaningful values of Z , those values can be chosen. In cases where specific values of Z are less meaningful, Aiken and West (1991) recommend plotting simple slopes for scores at ±1 standard deviation from the mean of Z and at the mean of Z itself. These values represent a range in which most observed scores should fall in a normal distribution. However, it is important to make sure the data actually contain score values at ±1 standard deviation from the mean, otherwise the graph represents an extrapolation beyond the data.
Rearranging the terms in Eq. (1) facilitates plotting of the interaction ( Aiken & West, 1991 ) and illustrates how the slope of Y on X is dependent on the value of the moderator variable, Z
If there is no interaction effect then the β 3 coefficient equals zero and drops out of the equation. If there is no β 3 coefficient in Eq. (7) , then the regression of Y on X depends wholly on β 1 . Contrast this to a case where there is a non-zero interaction effect (i.e., β 3 is less than or greater than zero). If β 3 is non-zero then the term remains in the equation and the X – Y relation depends on both β 1 and β 3 . The larger the absolute value of β 3 , the more the relation between X and Y is affected by the moderator variable.
Regression slopes that correspond to the prediction of Y from X at a single value of Z are termed simple slopes. The estimation and interpretation of simple slopes is described in detail in Aiken and West (1991) . Tests of statistical significance for these simple slopes, or simple effects, are available and indicate whether the regression of Y on X at a given value of Z significantly differs from zero (see pp. 14–18 in Aiken & West). Aiken and West also demonstrate how comparisons may be made among the simple effects to examine which pairs of slopes significantly differ from one another. Note that for continuous moderators, the presence of a significant XZ interaction implies that simple slopes vary across values of Z ( Aiken & West, 1991 ). The simple slope equation for students with learning disabilities (i.e., X =.5) in the numerical example is
and the simple slope equation for students without learning disabilities (i.e., X =−.5) in the numerical example is
These simple regression equations are consistent with the graph of the interaction in Fig. 6 that showed students with learning disabilities obtain better benefits from the reading program than do students without learning disabilities. That is, the regression coefficient associated with students who have learning disabilities is larger than that of the regression coefficient associated with students who do not have a learning disability.
It is important to note that the moderation example demonstrated in this article makes use of unstandardized coefficients from the regression model. Although standardized coefficients are sometimes advocated as a means to compare the magnitude of effects across samples, there are several statistical and interpretational issues that preclude presentation of standardized coefficients in a moderation analysis. First, the utility of comparing standardized coefficients across studies (in the presence or absence of an interaction effect) is itself questionable as the coefficients depend on the variance of the sample from which they are derived. Problems with these comparisons escalate with an increase in multicollinearity among the predictor variables. Second, standardization introduces additional error variance into the regression coefficient due to the inclusion of the variances of X and Y as a stochastic scaling adjustment in computation. Moreover, unlike unstandardized regression coefficients, the numerical value of the interaction term changes across centered and uncentered solutions in a moderation analysis. Values of any simple slopes and their associated level of significance also change. Finally, SAS and SPSS do not properly standardize the interaction term in analysis, treating it as an individual variable rather than the product of two random coefficients. For all of these reasons, it is inappropriate to report and interpret standardized coefficients when an interaction is present in a multiple regression model.
Effect sizes in moderation models
Estimates of effect size describe the practical significance of an effect, independent of sample size. Effect size measures are important when interpreting analysis results because they can inform non-significant findings and can enhance understanding of the practical utility of statistically significant effects. It is increasingly common that journal outlets require the reporting of effect size in addition to the results from null hypothesis significance tests. Squared partial correlation coefficients, or partial r 2 measures, are an example of effect size measures for moderation analysis (and more generally multiple regression analysis) and are equivalent to the R 2 Δ described earlier.
R 2 Δ illustrates the unique portion of variance explained by a predictor in the criterion variable that is not explained by other predictors in the model ( Cohen et al., 2003 ). Although originally only shown for the interaction effect in the Testing for Moderation section of the paper, R 2 Δ can be computed for any effect in the moderation model such as the effect of X on Y , the effect of Z on Y , or the effect of the interaction of X and Z on Y . When assessing Partial r 2 values for the numerical example were .266, .006, and .230 for the effect of X , Z , and the XZ interaction, respectively. Cohen (1988) defined small, moderate, and large effect sizes of squared partial correlation coefficients (such as the R 2 Δ) as .02, .13, and .26, respectively. Partial r 2 effect size measures can be requested as output options from a regression analysis in standard statistical software packages and may be useful to report in analysis.
Power in moderation models
Power is often low in moderation analyses because of the small effect sizes that are typically observed in the social sciences ( Aiken & West, 1991 ; Stone-Romero & Liakhovitski, 2002 ). Reviews of substantive literature in the social sciences demonstrate that interaction effects in real data typically explain between 1% and 3% of the variance in the dependent variable ( Champoux & Peters, 1987 ). Thus, interactions explaining even 1% of the variance may be meaningful ( Abelson, 1985 ; Evans, 1985 ; McClelland & Judd, 1993 ). To maximize the power of detecting moderator effects, researchers may use the largest sample available, consider the use of extreme groups to increase variance in the design (e.g., oversample participants that are either very high-scoring or very low-scoring on a non-manipulated independent variable), and choose measures that have high reliability (Aiken & West; Stone-Romero & Liakhovitski).
Assumptions of the moderation model
Several statistical assumptions underlie the moderation model. Most of these assumptions correspond to those associated with ordinary least squares regression, such as correct model specification, error-free measurement, and normally distributed residuals ( Cohen et al., 2003 ). One of the major assumptions of the moderated multiple regression model is homogeneity of error variance, also called homoscedascity. Homoscedascity requires that the residual variance that remains after predicting Y from X is constant across values of the moderator variable, Z , such that the predicted scores, Ŷ , are distributed similarly along the simple slopes. Bartlett’s M statistic provides a formal statistical test of whether homogeneity of error variance has been violated (non-significant results indicate that no violation has occurred). The statistical test is available in both SAS and SPSS. In the presence of heteroskedastic error variance, there are nonparametric tests available to test for moderation in both ANOVA (e.g., the Kruskal–Wallis test; Kruskal & Wallis, 1952 ) and regression frameworks (e.g, nonparametric regression; e.g., Takezawa, 2005 ).
Advanced moderation models
The interaction effects described here represent the basic moderation model. Models that incorporate more complex, higher-order moderator effects are also possible. For example, it may be of interest to model three-way interaction effects where the effect of a two-way interaction on an outcome can be explored across levels of a fourth variable in the model. As well, curvilinear by linear interactions may also be investigated where quadratic terms are entered into the equation. Although the methods described here for the basic moderation model extend to these more complex scenarios, interpretation of effects becomes increasingly challenging with the introduction of more than one moderator variable or the presence of curvilinear relations ( Aiken & West, 1991 ).
Mediation analysis
What is a mediator.
As previously described, a mediator is a third variable that explains how or why two other variables are related. The mediation model is a three variable model where an independent variable ( X ) predicts a mediator variable ( M ), which in turn predicts an outcome ( Y ). The mediator intervenes in the relation between X and Y illustrating the mechanism through which the two variables are related. By modeling an intermediary variable in the X – Y relation, the researcher is able to decompose the bivariate relation between the two variables into direct and indirect (i.e., mediated) effects. If the mediator accounts for the total effect of X on Y , such that there is no relation between the variables once the mediator is partialled out of their relation and the direct effect is equal to zero, then the mediating process is termed complete mediation . If instead a direct effect of X on Y remains once the mediator is partialled out of the X – Y relation, then the mediating process is termed partial mediation (e.g., Shrout & Bolger, 2002 ).
Differentiating mediators from other third variable effects
There are several other third variables that also elucidate the relation between two other variables, but these variables are not mediators ( MacKinnon, Krull, & Lockwood, 2000 ). A confounder is a variable that is related to other variables, such that it artificially enhances their relation. The difference between a confounder and a mediator variable is that a confounder is not intermediate in a causal sequence, as is hypothesized in the mediation model. An example of a confounder variable is prior subject knowledge in a study that evaluates the effectiveness of different learning strategies. If prior subject knowledge is not accounted for, it may artificially enhance or detract from the effects of a given learning approach making the bivariate relation noticeably change from what it would be otherwise.
A covariate is a variable that is related to a predictor and outcome variable but does not appreciably change the bivariate relation between the two when included in a model. Covariates are often used in analysis to reduce unexplained variability in an outcome variable. Like a confounder, a covariate is not hypothesized to occur in a causal sequence between X and Y . Examples of covariates might be demographic variables such as gender or socioeconomic status.
Estimating the basic mediation model
To demonstrate estimation of the basic mediation model, data from a numerical example are evaluated (see Table 2 ), where the aforementioned reading program was hypothesized to achieve its effects by increasing the oral reading fluency of subjects. Throughout the following sections the reading program is the primary predictor variable (i.e., X ), oral reading fluency is the mediator variable (i.e., M ), and reading performance is the outcome variable (i.e., Y ). Fig. 1 shows a path diagram of this mediation model.
The basic mediation model is defined by three equations. First, the overall effect of X (the primary predictor variable) on Y is
where Ŷ is the predicted value of a student’s reading performance, X represents group assignment to the reading program (i.e., treatment vs. control), β 0 Y is the regression intercept, and β 1 Y is the slope coefficient representing the relation between the program and reading performance. The overall effect represents the relationship between X and Y without consideration of the mediator. The top panel of Fig. 1 shows this overall effect (specifically the β 1 Y regression coefficient) as a solid line. The dashed lines in Panel 1 of the Figure indicate that although there is an implicit underlying process by which X and Y are related in the mediation model, this process is not directly estimated in the overall effect regression equation. Instead, the overall effect regression equation in the top panel of the Figure combines the direct and indirect effect into a single regression coefficient. This overall effect is important because it plays a role in defining some of the test statistics and effect sizes in the mediation model.
The mediation model uses two regression equations to decompose the overall effect into its direct and indirect components as follows
where M ̂ is the predicted value of a student’s oral reading fluency, M corresponds to a student’s observed oral reading fluency, β 0 Y * and β 0 M are regression intercepts, and the β 1 Y * , β 2 Y , and β 1 M terms are regression slopes. The asterisks in Eq. (11) denote the fact that the coefficients are different from those in Eq. (10) . Specifically in Eq. (11) , β 1 Y * represents the influence of X on Y partialling out the mediator, whereas β 1 Y in Eq. (10) is not a partial regression coefficient; it is simply the bivariate relationship between X and Y not controlling for other variables.
Returning to the small data set in Table 2 , the estimates of the previous equations are
The regression coefficients in the equations quantify various effects of predictors on the mediator and outcome variables. Specifically, in Eq. (13) where reading performance is only predicted by the reading program, β ^ 1 Y = 1.098 ( t = 2.237 , p = .038 ) is the regression coefficient corresponding to the overall effect (i.e., the total effect) of the reading program on reading performance. In Eq. (14) where reading performance is predicted by both the reading program and oral reading fluency, β ^ 1 Y ∗ = 1.686 ( t = 1.702 , p = .107 ) is the regression coefficient relating the direct effect of the reading program ( X ) to reading performance ( Y ), controlling for oral reading fluency ( M ), and β ^ 2 Y = .232 ( t = − .686 , p = .502 ) is the regression coefficient relating oral reading fluency to reading performance, controlling for the effect of the reading program. Turning to the equation where the mediator is the outcome variable, β ^ 1 M = 2.527 ( t = 7.284 , p < .001 ) is the regression coefficient relating the reading program to oral reading fluency. Finally, β ^ 0 Y , β ^ 0 Y ∗ and β ^ 0 M represent the intercepts in each equation; these terms do not play a role in a mediation analysis. Various coefficients from the three mediation regression equations are used to define different statistical tests of mediation, which are outlined in subsequent sections. Fig. 7 illustrates the mediation regression equations and the estimates from the numerical example in a path diagram. Recall that in path diagrams squares correspond to observed variables in the model and single-headed directional arrows indicate regression coefficients. In line with the numerical example for the moderation model, sample estimates of the regression coefficients and their corresponding significance levels appear above the paths to which they correspond in the Figure.

The basic mediation model. X =the independent variable, Y =the dependent variable, M =the mediator variable, β ^ 1 M =the effect of the independent variable on the mediator, β ^ 2 Y =the effect of the mediator on the outcome controlling for X , and β ^ 1 Y =the direct effect of the X on Y controlling for M . *Corresponds to p <.05, **corresponds to p <.001 and ns corresponds to not significant.
Causal steps
Several different methods have been proposed to test mediation. Early articles on mediation analysis illustrated causal step methods to test for mediation, where the mediation regression equations were evaluated sequentially (e.g., Baron & Kenny, 1986 ; Judd & Kenny, 1981 ). First, a significant overall effect of the independent variable ( X ) on the dependent variable ( Y ) is required. In other words, the β ^ 1 Y regression coefficient in Eq. (10) must be significant. Second, the mediator ( M ) must affect Y , and X must affect M . Thus the β 2 Y and β 1 M slope coefficients from Eqs. (11) and (12) must also both be significant for mediation to hold. Finally, the magnitude of the overall effect of X on Y must be greater than the direct effect of X on Y that remains after partialling out the mediator. If all of these criteria are satisfied, then there is evidence for mediation. Recall that this was the most common approach to testing mediation hypotheses found in our previous literature review.
Although researchers frequently use the causal steps approach, it has several critical limitations. First, the method is underpowered ( MacKinnon et al., 2002 ). The low power is primarily due to the requirement that X and Y are significantly related. Fritz and MacKinnon (2007) showed that with small effect sizes of mediation regression parameters (i.e., β ^ 1 M and β ^ 2 Y ), 20,886 subjects are needed to detect effects with .8 power in complete mediation models. Results from the literature review presented at the beginning of this article indicate that the median sample size of mediation studies conducted with causal steps methodology in the school psychology domain is 173, substantially below the optimal sample size. Second, the causal steps method does not provide a point estimate of the mediated effect, and thus precludes formation of confidence intervals or evaluation of standard errors. Finally, the requirement that there be a significant overall effect of X on Y in order for there to be significant mediation is debatable ( MacKinnon & Fairchild, 2009 ). Although the overall effect of X on Y is unquestionably of interest in most studies, it is not a test of mediation. There are several scenarios in which significant mediation can occur without a significant overall effect of X on Y (e.g., mediation effects that differ in sign or in magnitude across different subgroups).
Difference in coefficients
The difference in coefficients estimator quantifies the reduction in the overall effect of the independent variable on the dependent variable after accounting for the mediator. A researcher forms the estimate by subtracting the direct effect of X on Y when M is partialled out of the relation (i.e., β ^ 1 Y ∗ ), from the overall effect of X on Y when M is not included in the model (i.e., β ^ 1 Y ). Consulting the path diagram in Fig. 7 , the estimator takes the path connecting X and Y in Panel 1 of the Figure, or the coefficient that results from simply regressing Y on X , and subtracts the path connecting X and Y in Panel 2 of the Figure, or the coefficient that results from regressing Y on both X and M . The logic behind the estimator is that, if the mediator accounts for all or part of the relation between X and Y , then when it is accounted for in their relation the magnitude of their association should reduce. Significance testing of the mediated effect point estimate is conducted by dividing the difference in coefficients by an estimate of its normal theory standard error (e.g., McGuigan & Langholtz, 1988 ). Although the difference in coefficients may be an intuitive framework to understand the logic of mediation, the estimator does not easily generalize to more complicated models such as those that involve estimating effects on categorical variables or multiple mediators. There are preferable estimators of the mediated effect.
Product of coefficients
The product of coefficients estimator is derived from the estimation of indirect effects in path analysis, where the effects of one variable on another are transmitted through an intermediary predictor variable. The product of coefficients estimator of mediation is more flexible than the difference in coefficients, extending to more complex mediation models such as those with multiple outcomes. Alwin and Hauser (1975) describe how indirect effects “tell us how much of a given effect occurs because the manipulation of the antecedent variable of interest leads to changes in other variables which in turn change the consequent variable” (p.39). Applied to mediation, this means that the mediated effect depends on the extent to which the independent variable changes the mediator and on the extent to which the mediator subsequently affects the outcome variable. With this logic, the product of coefficients estimator multiplies the β ^ 1 M coefficient connecting the X and M variables in Fig. 7 by the β ^ 2 Y coefficient connecting the M and Y variables in the Figure. Thus, the product of coefficients estimator defines the mediated effect as β ^ 1 M β ^ 2 Y . As with the difference in coefficients estimator, significance testing of the mediated effect point estimate is conducted by dividing the product of coefficients by an estimate of its normal theory standard error ( Sobel, 1982 ). This standard error is
where s β ^ 1 M 2 is the sampling variance (i.e., squared standard error) of the β ^ 1 M coefficient and s β ^ 2 Y 2 is the sampling variance of the β ^ 2 Y coefficient. The product of coefficients estimate of the mediated effect for the reading program example is (2.527)(−.232)=−.586. The standard errors of the two coefficients are .347 and .339, respectively. Substituting the regression coefficients and their standard errors into Eq. (16) yields s β ^ 1 M β ^ 2 Y = .853 . Dividing the point estimate by its standard error gives a z statistic of z =−.687, which is not significant ( p =.493).
Asymmetric confidence limits and resampling
Although both the difference in coefficients and the product of coefficients estimators allow for null hypothesis significance testing and confidence interval estimation, both have conservative Type 1 error rates and limited power to detect mediation in small sample sizes ( MacKinnon et al., 2002 ). Conventional standard error estimators based on normality assumptions (e.g., Eq. (16) ) do not accurately capture the sampling distribution of the mediated effect and thus are limited in making accurate statistical inferences. Normal theory standard errors are imperfect for mediation because the sampling distribution of the product of two normally distributed variables (e.g., the regression coefficients used to compute the mediated effect β ^ 1 M β ^ 2 Y ) is not normally distributed. Rather, the sampling distributions of the mediated effect are often asymmetric and kurtotic. The implication of this asymmetry is that critical values associated with a given level of confidence from the standard normal distribution, or the z -score value at which an estimate will be considered significant, are no longer associated with the correct area under the curve. For example, 1.96 is no longer the z -value that is associated with p =.05 in a two-tailed test. Methodologists have derived the sampling distribution of the product of two normally distributed variables ( Meeker, Cornwell, & Aroian, 1981 ), so it is possible to obtain adjusted critical values that yield correct p -values. Using statistical tests for mediation based on adjusted critical values that account for asymmetry in the distribution of the mediated effect will have greater power because they more accurately capture the shape of the sampling distribution.
An automated computer program ( PRODCLIN; MacKinnon, Fritz, Williams, & Lockwood, 2007 ) has been developed to estimate asymmetric confidence limits for the mediated effect, β ^ 1 M β ^ 2 Y , based on Meeker et al.’s (1981) derivations and is freely accessible at http://www.public.asu.edu/~davidpm/ripl/Prodclin/ . The program computes asymmetric confidence limits for any mediated effect with a single mediator and a single outcome variable. The user need only provide sample estimates of the β ^ 1 M coefficient, the β ^ 2 Y coefficient, and their respective standard errors. All of these estimates are provided in the output of any standard statistical software package such as SAS or SPSS. Inputting sample estimates of the β ^ 1 M and β ^ 2 Y regression coefficients from the previous numerical example into the PRODCLIN program yields asymmetric confidence limits of [−2.316, 1.083] for the mediated effect. Because zero falls within the interval, we know that the estimate is not significant.
The PRODCLIN program does not extend beyond the single mediator model in which a single mediated effect is estimated for a continuous outcome variable. Thus asymmetric confidence limits based on the distribution of the product are the preferred method to estimate mediation in the basic single mediator model only, or to describe individual mediated effects in a multiple mediator model with continuous outcomes. When more complex mediation models are estimated, such as those that consider more than one dependent variable or more than one mediator variable, asymmetric confidence intervals for the mediated effect can be formed with resampling methods such as the bootstrap (see Efron & Tibshirani, 1993 ). Bootstrapping procedures form empirical confidence limits for the mediated effect using the sampling distribution of the data itself. The conventional method of bootstrapping is called the “percentile bootstrap”. In short, bootstrapping utilizes the sample data as a population from which random samples of size N are drawn with replacement a large number of times. Estimates of mediation are then computed in each of the bootstrapped samples and an empirical sampling distribution of the mediated effect estimates is formed. The collection of mediation effects from the bootstrap samples forms a frequency distribution, and the 5th and 95th percentiles from this frequency distribution serve as the confidence interval limits for the mediated effect. The percentile bootstrap (as well as more advanced resampling methods such as the bias-corrected bootstrap) is available in several different SEM software packages, including AMOS, EQS, LISREL, and MPLUS. In packages like SAS or SPSS, automated macros to obtain bootstrap confidence limits for the mediated effect can be created. Several such macros for a variety of different mediation models are available for use at Dr. Kris Preacher’s website: http://quantpsy.org .
Mediation analysis in program evaluation
Mediation analysis is especially useful in program evaluation where it is used to understand the underlying mechanisms of a program. Whether a manipulation was effective or unsuccessful, mediation analysis is able to identify components of the program that contributed to its success or failure. By evaluating specific components of a program that produce intended or unintended change, mediation analysis can identify: (a) supportive elements, or those components that encouraged intended behavior, (b) ineffective elements, or those components that did not contribute to changing the behavioral outcome, and/or (c) iatrogenic elements, or those components that promoted unintended effects of the program. To that end, it is important to differentiate overall program effect hypotheses from mediation hypotheses. Although testing the overall relation between X and Y is vital in its own right, a non-significant overall program effect does not preclude a statistically significant mediation effect ( MacKinnon & Fairchild, 2009 ). This emphasizes why the causal steps approach to investigating mediation, which requires a significant overall effect of X on Y , is limiting. By identifying the successful and unsuccessful components of a program, interventions can be iteratively refined to be more efficient and cost effective.
When considered in the context of program evaluation, component paths in the mediation model have distinct interpretations. The path relating the program to the mediator variable (i.e., β ^ 1 M ) corresponds to the action theory of the model, defining what components of the program are designed to manipulate mechanisms of change ( Chen, 1990 ; MacKinnon, 2008 ). This part of the model outlines pieces of the program curriculum related to hypothesized mediator(s) and illustrates how the intervention intends to change the mediating variable(s). The path relating the mediator variable to the outcome (i.e., β ^ 2 Y ) corresponds to the conceptual theory of the model, describing how hypothesized mediators are theoretically linked to outcomes of interest ( Chen, 1990 ; MacKinnon, 2008 ). Relationships examined in this part of the model are driven by previous research or theory presented in the literature that explain motivations for, or antecedents of, behavioral outcomes.
Power in mediation models
The power to detect mediation effects is conventionally lower than the power to detect main effects because the magnitude of the mediated effect is bounded by the individual coefficients from which it is formed. Recent research has shown that causal steps tests for mediation and normal theory point estimators of the mediated effect are underpowered ( Fritz & MacKinnon, 2007 ; MacKinnon et al., 2002 ). As presented earlier, with small effect sizes for the mediation regression parameters (i.e., β ^ 1 M and β ^ 2 Y ), the causal steps method requires 20,886 subjects to achieve .80 power (Fritz & MacKinnon). Although the sample size requirement for normal theory standard error estimators is smaller, the methods are still underpowered relative to newer methods that account for asymmetry in the sampling distribution of the mediated effect. Methodological work has shown that asymmetric confidence limits based on the distribution of the product and resampling methods such as the percentile bootstrap and the bias-corrected bootstrap give the best combination of low Type 1 error rates and power to detect effects in mediation models (Fritz & MacKinnon; MacKinnon et al.). Consequently, these methods should be implemented to test mediation hypotheses in substantive research.
Effect size in mediation models
Currently, effect size measures for mediation have only been outlined for the single mediator model. Effect sizes of two types have been described: (a) measures for individual paths of the mediation model and (b) measures for the mediated effect itself. Effect size measures for individual paths in the model are useful for understanding what parts of the model work and what parts need to be improved. One such measure, squared correlations (or squared partial correlations), quantify the amount of variance explained in the mediation model that is uniquely attributable to a single piece of the design (as described in the Effect Sizes in Moderation Models section). By identifying the relative contribution of individual paths in the mediation model, programs can be iteratively refined to promote stronger components and to remove or reduce weaker ones. Squared correlations and squared partial correlations from the numerical example are: r M X 2 = .746 , r M Y . X 2 = .027 , and r X Y . M 2 = .146 . More specifically, r M X 2 represents the squared correlation between the M and X variables in the model, r M Y . X 2 corresponds to the squared partial correlation between the M and Y variables with the influence of X removed, and r X Y . M 2 denotes the squared partial correlation between X and Y variables in the model with the influence of M removed. The measures illustrate that the weakest part of the mediation model is the b 2 Y coefficient, demonstrating that oral reading fluency (i.e., the mediator) is only weakly related to reading performance (i.e., the outcome variable). However, recall that there was significant moderation in the reading example dataset, such that learning disability status impacted the efficacy of the reading program. Rather than oral reading fluency truly being unrelated to reading performance, it may be possible that learning disability status also moderates the impact of oral reading fluency on reading performance. Ignoring this moderation effect can attenuate the overall relation between the variables. Squared partial correlation effect size measures for the mediation model are further discussed and statistically evaluated in Fairchild, MacKinnon, Taborga, and Taylor (2009) .
Two effect size measures for the overall mediated effect have been developed and evaluated: an R 2 mediated measure and the proportion mediated. The R 2 mediated measure quantifies the proportion of variance in the outcome that is common to both X and M but can be attributable to neither predictor alone ( Fairchild et al., 2009 ). In this way, the measure is able to illustrate the practical significance of a mediated effect. The R 2 mediated effect size measure is computed by using squared bivariate correlations and the overall model R 2 from a model where Y is predicted from both X and M , as follows
where r M Y 2 is the squared correlation between the outcome and the mediator, R Y , M X 2 is the overall model R 2 from the regression equation where Y is predicted from X and M (i.e., Eq. (11) ), and r X Y 2 is the squared correlation between the outcome and the independent variable (i.e., X ). The R 2 mediated effect size measure for the numerical example is .109−(.239−.217)=.087. Applying Cohen’s (1988) benchmark values for R 2 Δ (i.e., .02, .13, and 26), the effect size of the non-significant mediated effect in the example is in the small range. Although the R 2 mediated measure can be used with smaller sample sizes, it is possible that estimates from the measure can be negative in some circumstances (a mathematical artifact of the equation).
The proportion mediated effect size is an intuitive measure that quantifies the proportion of a total effect that is mediated (e.g., Ditlevsen, Christensen, Lynch, Damsgaard, & Keiding, 2005 ). The proportion mediated has also been used as a test of mediation itself in some research studies (e.g., Foshee et al., 1998 ). The measure is computed by taking the ratio of the mediated effect to the total effect, as follows
The estimate of the proportion mediated effect for the numerical example is: (2.527*−.189)/[(2.527*−.189)+1.477]=−.244. The estimate is negative for the example simply because the direct and indirect effects in the model are of opposite sign. Such mediation models are sometimes called inconsistent mediation models . Discussion of inconsistent mediation models is beyond the scope of this paper, but MacKinnon (2008) describes the phenomenon in detail for readers who are interested. To improve interpretability of the proportion mediated estimate in such circumstances, Alwin and Hauser (1975) recommend taking the absolute value of the regression coefficients before computing the effect size measure. Doing so for the numerical example yields a proportion mediated effect of .244, or approximately 24%. Because absolute values were used in computation, interpretation of the measure changes slightly and becomes the proportion of the absolute overall effect that is mediated. So for the numerical example, 24% of the absolute overall effect is mediated. Although the proportion mediated was computed here, there is evidence that the effect size measure should only be used with sample sizes that are greater than or equal to 500 due to instability in the estimate ( MacKinnon, Fairchild, Yoon, & Ryu, 2007 ). Thus, presentation of the effect size estimate with the numerical example data where N =20 is for illustrative purposes only. At present, there are no suggested benchmarks to formally gauge the magnitude of the proportion mediation. Because there are various advantages and disadvantages to each mediation effect size measure, it may be useful to implement more than one to gain the clearest picture of effects in a mediation model.
Assumptions
A number of assumptions underlie estimation of the mediation model. The majority of these assumptions attend to those associated with ordinary least squares regression (OLS), namely: (a) correct specification of the model’s functional form, (b) no omitted variables from the model, (c) no measurement error, (d) homoscedascity of residuals, (e) uncorrelated error terms across equations, and (f) normally distributed residuals ( Cohen et al., 2003 ). Beyond these OLS estimation assumptions, mediation analyses also assume correct causal ordering of the variables, no reverse causality effects, and no XM interaction.
Causal inference in mediation analysis
Issues of causality and limitations of causal inference in the statistical mediation model have been widely discussed in the literature. Model-implied causal relations are plausible when both the independent variable and the mediator are experimentally manipulated, but this scenario is unusual. Non-randomized studies make interpretation of causal relations in the mediation model tentative, and statistical frameworks such as structural equation modeling do not guard against this limitation. Random assignment of the independent variable allows for the causal effect estimation of the β 1 M path in the mediation model, but unless subjects are also randomly assigned to levels of the mediator, the β 2 Y and β 1 Y paths in the model are not causal parameters. Because measurement of the mediating variable occurs subsequent to the assignment of treatment conditions, values of the mediating variable may be confounded with the unknown assignment mechanism. Consequently, in non-experimental contexts, it is critical to have a strong theoretical rationale for relations among variables in the mediation model.
Advanced mediation models
Although many interesting research questions can be evaluated with the basic single mediator model, research studies often introduce design elements or variable attributes that are not accommodated by the conventional single mediator model. In the following sections, we briefly describe several advanced mediation models that extend to some of these situations. All of these models require additional considerations in their statistical estimation that go beyond those described for the single mediator model. Citations for methodological articles on each model are provided to direct further reading.
Multilevel mediation models
In many prevention or intervention studies, particularly in school-based settings, individual participants are clustered within larger groups such as a classroom, school, or a school district. In these situations, the assumption of independent observations underlying conventional statistical models such as ANOVA and regression is violated, and Type 1 error rates of the models are often increased (see the Peugh article in this issue). Methods to estimate multilevel mediation models that accurately model dependency among observations in the data are available (e.g., Kenny, Korchmaros, & Bolger, 2003 ; Krull & MacKinnon, 2001 ) and should be implemented in these cases. These methods are often appropriate when a program is randomized at the group level (such as a school) and the mediator and outcome variables are measured at the individual level (such as students).
Mediation in SEM
When modeling more than one dependent variable, a detailed modeling approach is required due to the possibility of correlated dependent variables and/or correlated error terms. Path analysis, a variation of structural equation modeling (SEM), is an extension of multiple regression that simultaneously estimates the equations in a mediation model. In SEM, the fit of an observed covariance matrix of the variables is compared to a model-implied covariance matrix, and goodness-of-fit statistics are calculated to analyze discrepancies between the two. Though more than one mediated effect may be estimated in these models, methods described for the single mediator model can be implemented to test individual mediated effects. Tests of overall fit of the model can also provide evidence for a hypothesized mediation relation, or relations ( James, Mulaik, & Brett, 2006 ; MacKinnon, 2008 ).
Categorical mediation models
It may also be of interest to explore mediation effects on a categorical outcome variable, such as whether students pass a grade level, or whether they drop out of school. In both circumstances, a researcher uses information from available predictor variables to predict whether a student passes or does not pass, or alternatively whether a student remains in or drops out of school. Because in these cases the dependent variable takes on only two possible values, one models the probability that the given event will occur. When modeling the probability of an event occurrence, the use of standard OLS regression procedures can lead to incorrect conclusions because assumptions underlying the model are violated. Instead, logistic or probit regression frameworks should be utilized. MacKinnon, Lockwood, Brown, Wang, & Hoffman (2007) describe and compare methods to test mediation in these types of models.
Simultaneously analyzing mediation and moderation effects
Combining the mediation and moderation models into a single framework allows the investigation of complex research hypotheses. There are many different types of relations that can be explored in such models. For example, is the mechanism by which a program or manipulation achieves its effects the same across different groups? This question asks if a mediated effect differs across levels of a moderator variable, and the analysis that addresses this question has been often referred to as moderated mediation in the literature. It may also be of interest to ask if the reason an overall effect is moderated can be explained by a mediation process. This question considers whether the presence of an interaction effect can be explained by a mediating mechanism, a phenomenon that has been called mediated moderation in the literature. Finally, it may be informative to examine moderation in individual paths of the mediation model to investigate contextual effects in different pieces of the model. Plotting simple mediated effects in these models to understand the mediation relation at different levels of the moderator variable has been proposed in the literature ( Tein et al., 2004 ). Several other sources explain these modeling extensions and present methods for testing all such hypotheses ( Edwards & Lambert, 2007 ; Fairchild & MacKinnon, 2009 ; MacKinnon, 2008 ; Preacher, Rucker, & Hayes, 2007 ).
Longitudinal mediation models
Cross-sectional data only provide a snapshot of concurrent relations, limiting the ability to compute unbiased estimates of the mediated effect ( Cole & Maxwell, 2003 ; Gollob & Reichardt, 1991 ; Maxwell & Cole, 2007 ). Since mediation seeks to explain the mechanisms by which a causal effect occurs, and causes must precede effects in time, it makes sense that longitudinal data are well suited to test mediation. There are several benefits of utilizing longitudinal data to examine mediation, including an increased power relative to cross-sectional designs (due to the ability to reduce within-subject variability) and the ability to investigate the assumption of correct causal ordering of the X to M to Y relation.
Two-wave longitudinal mediation models that examine pre–post data can quantify the overall amount of change, but these models do not accommodate questions about intra-individual variability of change or potential curvilinear change because doing so requires at least three waves of data. Three models are generally used to assess change in 2-wave longitudinal models: (a) difference score models, (b) residualized change scores, and (c) ANCOVA (analysis of covariance). Models with two waves of data are often considered half-longitudinal mediation models because some relations in the data are cross-sectional (e.g., both M and Y are measured at post-test). MacKinnon (2008) describes two-wave longitudinal mediation models in detail.
Longitudinal data with three or more time points allow for the investigation of change over time in mediating processes as well as true longitudinal mediation relations where the independent variable, the mediator, and the outcome occur in sequential time points. Most of these models require estimation in a structural equation modeling software program due to the complexity of model parameters. Latent growth curve models are an example of such models and provide the flexibility to investigate heterogeneity in mediational growth processes across participants. Cheong, MacKinnon, and Khoo (2003) , Maxwell and Cole (2007) , and Cole and Maxwell (2003) all describe methods to test longitudinal mediation models with three or more waves of data. MacKinnon (2008) also illustrates these models.
Multiple mediator models
Most behaviors are affected by multiple variables, so it is sensible that there are multiple mediator models of behavioral outcomes. Models that consider the effect of more than one mediator in a relationship are called multiple mediator models. Multiple mediator models are a straightforward extension of the single mediator case, but their interpretation can be more difficult when considering the large number of possible relations among variables in the model. Such models introduce the idea of a total mediated effect , or the sum of all individual mediated effects together. There are multiple mediator models where the effects of the mediator variables occur in sequence, such that one mediator temporally precedes another. In addition, it is possible to estimate models where the effects of several mediators are “stacked”, such that the mediators are hypothesized to affect an outcome contemporaneously. In these models, statistical contrasts among different mediated effects in the model can be used to determine whether two mediation effects significantly differ from one another. MacKinnon (2008) provides detailed guidance on how to estimate these models, and Taylor, MacKinnon, and Tein (2008) describe three-path mediation analysis (appropriate for serial mediation) in detail.
Concluding remarks
The investigation of third variables such as moderators and mediators contributes to understanding why or under what conditions two variables are related. When conducted correctly, these analyses have the potential to inform the evaluation of prevention and intervention programs, as well as to refine program curricula. Given recent federal mandates for evidence-based practice in education and beyond, these models will likely continue to be valuable to the school psychologist and other substantive researchers in the future. Investigators can conduct both the basic moderation and mediation models in a multiple regression analysis framework using conventional statistical software packages such as SAS or SPSS. Varied methodological resources that extend these basic models are also available and enable the exploration of interesting and advanced research hypotheses that involve moderator and mediator effects. Though such extensions often require estimation in structural equation modeling software to accommodate complex data structures, core assumptions of the models and their underlying basic frameworks remain as described here.
This paper sought to describe contemporary methods for testing moderation and mediation effects in school psychology. We described the basic moderation and mediation models for the substantive researcher, and demonstrated methods to estimate and explore effects in the models. The literature review conducted at the beginning of this paper illustrated that, although school psychology researchers generally implemented proper methods to conduct moderation analyses, investigators have been using imperfect methods to test mediation effects. Thus in an effort to facilitate the examination of valuable mediation research hypotheses in school psychology, we offer specific recommendations for conducting statistical mediation analyses to conclude this article.
The product of coefficients estimator of mediation is the most generalizable estimator of the mediated effect and should be implemented in substantive research. Because the sampling distribution of the mediated effect is asymmetric, the utility of normal theory variance estimators of mediation which do not accurately capture the asymmetry in the mediated effect sampling distribution is limited. Asymmetric confidence limits based on resampling methods, as well as those based on the distribution of the product of two random variables, have greater power and more accurate Type 1 error rates relative to normal theory methods and should be utilized. In addition to significance testing of the mediated effect, there are several effect size measures available to describe effects in a single mediator model. Such measures provide useful information on the magnitude of mediation effects that are independent of sample size or statistical significance and should be reported when possible. When available, longitudinal data should be used to examine mediation hypotheses as assumptions of the mediation model are more feasible (and testable) in this context. The product of coefficients estimator and asymmetric confidence limits for the mediated effect as described in the paper can be equivalently applied to longitudinal data where X , M , and Y come from sequential time points. Finally, although the original causal steps approach to testing mediation (i.e., Baron & Kenny, 1986 ; Judd & Kenny, 1981 ) required an overall significant effect of X and Y to provide evidence for mediation, the requirement is restrictive and is not itself a test of mediation. Non-significant overall effects do not preclude the investigation of mediation. This is especially critical to recognize in program evaluation where investigating mediation in spite of a non-significant overall program effect will likely promote understanding of what parts of the program contributed to its failure or detracted from its success.
SPSS code to compute the basic mediation and moderation models
- * Comments in the code are denoted by lines that start with an asterisk (∗). These comments are not commands, but provide guidance or additional notes for the reader. We do not recommend cutting and pasting the comments into SPSS command lines as they contain special characters in some circumstances.
- * Throughout the Appendix, X references the independent variable, Z references the moderator variable, XZ references the product of the independent variable and the moderator, M references the mediator variable, and Y references the dependent variable (i.e., the outcome).
- * The XZ interaction variable was computed prior to running analysis by creating a new variable that multiplied values of X and Z for each subject in the data.
- * The researcher can substitute out all or any variable names to correspond with variable names that appear in his or her own data, as well as alter the code to request additional statistics if desired. This demonstration only serves to illustrate the basic structure of the single mediator model and the moderation model.
- * The following code illustrates how to estimate a basic single mediator model. Recall that three regression equations defined the single mediator model.
- /STATISTICS COEFF CI
- /DEPENDENT Y
- /METHOD=ENTER X.
- /METHOD=ENTER X M.
- /DEPENDENT M
*** THIS COMPLETES ESTIMATION OF THE SINGLE MEDIATOR MODEL.***
- * The following code illustrates how to estimate the basic moderation model. Recall that one regression equation defined the moderation model.
- /METHOD=ENTER X Z XZ.
***** THIS COMPLETES ESTIMATION OF THE MODERATION MODEL. *****
- Abelson RP. A variance explanation paradox: When a little is a lot. Psychological Bulletin. 1985; 97 :129–133. [ Google Scholar ]
- Aguinis H. Regression analysis for categorical moderators. New York, NY: The Guilford Press; 2004. [ Google Scholar ]
- Aiken LS, West SG. Multiple regression: Testing and interpreting interactions. Thousand Oaks, CA: Sage Publications; 1991. [ Google Scholar ]
- Alwin DF, Hauser RM. The decomposition of effects in path analysis. American Sociological Review. 1975; 40 :37–47. [ Google Scholar ]
- Baron RM, Kenny DA. The moderator–mediator variable distinction in social psychological research: Conceptual, strategic, and statistical considerations. Journal of Personality and Social Psychology. 1986; 51 :1173–1182. [ PubMed ] [ Google Scholar ]
- Champoux JE, Peters WS. Form, effect size, and power in moderated regression analysis. Journal of Occupational Psychology. 1987; 60 :243–255. [ Google Scholar ]
- Chen HT. Theory driven evaluations. Newbury Park, CA: Sage; 1990. [ Google Scholar ]
- Cheong J, MacKinnon DP, Khoo S-T. Investigation of mediational processes using parallel process latent growth curve modeling. Structural Equation Modeling. 2003; 10 :238–262. [ PMC free article ] [ PubMed ] [ Google Scholar ]
- Cohen J. Statistical power analysis for the behavioral sciences. New York, NY: Lawrence Erlbaum Associates; 1988. [ Google Scholar ]
- Cohen J, Cohen P. Applied multiple regression/correlation analysis for the behavioral sciences. 2nd. Hillsdale, NJ: Erlbaum; 1983. [ Google Scholar ]
- Cohen J, Cohen P, West SG, Aiken LS. Applied multiple regression/correlation analysis for the behavioural sciences. 3rd. Mahwah, NJ: Lawrence Earlbaum Associates; 2003. [ Google Scholar ]
- Cole DA, Maxwell SE. Testing mediational models with longitudinal data: Questions and tips in the use of structural equation modeling. Journal of Abnormal Psychology. 2003; 112 :558–577. [ PubMed ] [ Google Scholar ]
- Ditlevsen S, Christensen U, Lynch J, Damsgaard MT, Keiding N. The mediation proportion: A structural equation approach for estimating the proportion of exposure effect on outcome explained by an intermediate variable. Epidemiology. 2005; 16 :114–120. [ PubMed ] [ Google Scholar ]
- Edwards JR, Lambert LS. Methods for integrating moderation and mediation: A general analytical framework using moderated path analysis. Psychological Methods. 2007; 12 :1–22. [ PubMed ] [ Google Scholar ]
- Efron B, Tibshirani RJ. An introduction to the bootstrap. London: Chapman & Hall; 1993. [ Google Scholar ]
- Evans MG. A Monte Carlo study of the effects of correlated method variance in moderated multiple regression analysis. Organizational Behavior and Human Decisions Processes. 1985; 36 :305–323. [ Google Scholar ]
- Fairchild AJ, MacKinnon DP. A general model for testing mediation and moderation effects. Prevention Science. 2009; 10 :87–99. [ PMC free article ] [ PubMed ] [ Google Scholar ]
- Fairchild AJ, MacKinnon DP, Taborga MP, Taylor AB. R 2 effect size measures for mediation analysis. Behavioral Research Methods. 2009; 41 :486–498. [ PMC free article ] [ PubMed ] [ Google Scholar ]
- Foshee VA, Bauman KE, Arriaga XB, Helms RW, Koch GG, Linder GF. An evaluation of safe dates, an adolescent dating violence prevention program. American Journal of Public Health. 1998; 88 (1):45–50. [ PMC free article ] [ PubMed ] [ Google Scholar ]
- Fritz MS, MacKinnon DP. Required sample size to detect the mediated effect. Psychological Science. 2007; 18 :233–239. [ PMC free article ] [ PubMed ] [ Google Scholar ]
- Gollob HF, Reichardt CS. Interpreting and estimating indirect effects assuming time lags really matter. In: Collins LM, Horn JL, editors. Best methods for the analysis of change: Recent advances, unanswered questions, future directions. Washington, D. C: American Psychological Association; 1991. pp. 243–259. [ Google Scholar ]
- Individuals with Disabilities Education Act (IDEA), 20 U.S.C. § 1414 et seq.
- James LR, Mulaik SA, Brett JM. A tale of two methods. Organizational Research Methods. 2006; 9 :233–244. [ Google Scholar ]
- Judd CM, Kenny DA. Process analysis: Estimating mediation in treatment evaluations. Evaluation Review. 1981; 5 :602–619. [ Google Scholar ]
- Kazdin A, Nock M. Delineating mechanisms of change in child and adolescent therapy: Methodological issues and research recommendations. Journal of Child Psychology and Psychiatry. 2003; 44 :1116–1129. [ PubMed ] [ Google Scholar ]
- Kenny DA, Korchmaros JD, Bolger N. Lower level mediation in multilevel models. Psychological Methods. 2003; 8 :115–128. [ PubMed ] [ Google Scholar ]
- Krull JL, MacKinnon DP. Multilevel modeling of individual and group level mediated effects. Multivariate Behavioral Research. 2001; 36 :249–277. [ PubMed ] [ Google Scholar ]
- Kruskal WH, Wallis WA. Use of ranks in one-criterion variance analysis. Journal of the American Statistical Association. 1952; 47 :583–621. [ Google Scholar ]
- MacKinnon DP. Introduction to statistical mediation analysis. Mahwah, NJ: Earlbaum; 2008. [ Google Scholar ]
- MacKinnon DP, Fairchild AJ. Current directions in mediation analysis. Current Directions in Psychology. 2009; 18 :16–20. [ PMC free article ] [ PubMed ] [ Google Scholar ]
- MacKinnon DP, Fritz MS, Williams J, Lockwood CM. Distribution of the product confidence intervals for the indirect effect: Program PRODCLIN. Behavior Research Methods. 2007; 39 :384–389. [ PMC free article ] [ PubMed ] [ Google Scholar ]
- MacKinnon DP, Lockwood CM, Brown CH, Wang W, Hoffman JM. The intermediate endpoint effect in logistic and probit regression. Clinical Trials. 2007; 4 :499–513. [ PMC free article ] [ PubMed ] [ Google Scholar ]
- MacKinnon DP, Krull JL, Lockwood CM. Equivalence of the mediation, confounding, and suppression effect. Prevention Science. 2000; 1 (4):173–181. [ PMC free article ] [ PubMed ] [ Google Scholar ]
- MacKinnon DP, Lockwood CM, Hoffman JM, West SG, Sheets V. A comparison of methods to test the significance of mediation and other intervening variable effects. Psychological Methods. 2002; 7 :83–104. [ PMC free article ] [ PubMed ] [ Google Scholar ]
- MacKinnon DP, Fairchild AJ, Yoon M, Ryu E. Evaluation of the proportion mediated effect size measure of mediation Unpublished manuscript 2007 [ Google Scholar ]
- Maxwell SE, Cole DA. A bias in cross-sectional analyses of longitudinal mediation. Psychological Methods. 2007; 12 :23–44. [ PubMed ] [ Google Scholar ]
- McClelland GH, Judd CM. Statistical difficulties of detecting interactions and moderator effects. Psychological Bulletin. 1993; 114 :376–390. [ PubMed ] [ Google Scholar ]
- McGuigan K, Langholtz B. A note on testing mediation paths using ordinary least-squares regression. 1988 Unpublished note. [ Google Scholar ]
- Meeker WQ, Jr, Cornwell LW, Aroian LA. In: The product of two normally distributed random variablesSelected tables in mathematical statistics. Kennedy WJ, Odeh RE, editors. VII. Providence, RI: American Mathematical Society; 1981. [ Google Scholar ]
- Preacher KJ, Rucker DD, Hayes RF. Addressing moderated mediation hypotheses: Theory, methods, and prescriptions. Multivariate Behavioral Research. 2007; 42 :185–227. [ PubMed ] [ Google Scholar ]
- Shadish W, Cook T, Campbell D. Experimental and quasi-experimental designs for generalized causal inference. Boston: Houghton-Mifflin; 2001. [ Google Scholar ]
- Shrout PE, Bolger N. Mediation in experimental and nonexperimental studies: New procedures and recommendations. Psychological Methods. 2002; 7 (4):422–445. [ PubMed ] [ Google Scholar ]
- Sobel ME. Asymptotic confidence intervals for indirect effects in structural equation models. Sociological Methodology. 1982; 13 :290–312. [ Google Scholar ]
- Stone-Romero EF, Liakhovitski D. Strategies for detecting moderator variables: A review of conceptual and empirical issues. Research in Personnel and Human Resources Management. 2002; 21 :333–372. [ Google Scholar ]
- Takezawa K. Introduction to nonparametric regression. Hoboken, NJ: Wiley; 2005. [ Google Scholar ]
- Taylor AB, MacKinnon DP, Tein J-Y. Tests of the three-path mediated effect. Organizational Research Methods. 2008; 11 :241–269. [ Google Scholar ]
- Tein J, Sandler IN, MacKinnon DP, Wolchik SA. How did it work? Who did it work for? Mediation in the context of a moderated prevention effect for children of divorce. Journal of Consulting and Clinical Psychology. 2004; 72 :617–624. [ PMC free article ] [ PubMed ] [ Google Scholar ]
- U.S. Department of Education. No child left behind: A desktop reference. Washington DC: Education Publications; 2002. PDF available at www.ed.gov/admins/lead/account/cvlbreference/index.html . [ Google Scholar ]
Click through the PLOS taxonomy to find articles in your field.
For more information about PLOS Subject Areas, click here .
Loading metrics
Open Access
Peer-reviewed
Research Article
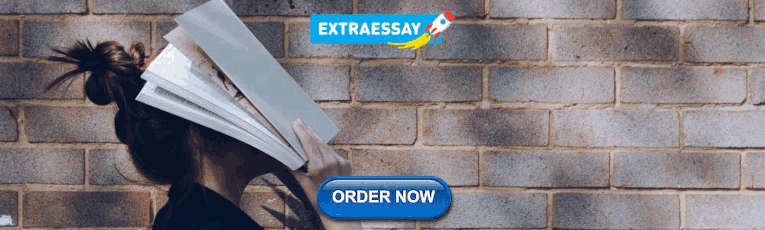
Anxiety, Affect, Self-Esteem, and Stress: Mediation and Moderation Effects on Depression
Affiliations Department of Psychology, University of Gothenburg, Gothenburg, Sweden, Network for Empowerment and Well-Being, University of Gothenburg, Gothenburg, Sweden
Affiliation Network for Empowerment and Well-Being, University of Gothenburg, Gothenburg, Sweden
Affiliations Department of Psychology, University of Gothenburg, Gothenburg, Sweden, Network for Empowerment and Well-Being, University of Gothenburg, Gothenburg, Sweden, Department of Psychology, Education and Sport Science, Linneaus University, Kalmar, Sweden
* E-mail: [email protected]
Affiliations Network for Empowerment and Well-Being, University of Gothenburg, Gothenburg, Sweden, Center for Ethics, Law, and Mental Health (CELAM), University of Gothenburg, Gothenburg, Sweden, Institute of Neuroscience and Physiology, The Sahlgrenska Academy, University of Gothenburg, Gothenburg, Sweden
- Ali Al Nima,
- Patricia Rosenberg,
- Trevor Archer,
- Danilo Garcia
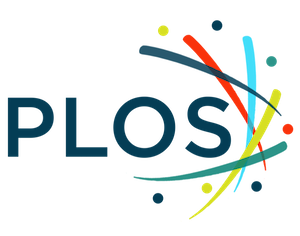
- Published: September 9, 2013
- https://doi.org/10.1371/journal.pone.0073265
- Reader Comments
23 Sep 2013: Nima AA, Rosenberg P, Archer T, Garcia D (2013) Correction: Anxiety, Affect, Self-Esteem, and Stress: Mediation and Moderation Effects on Depression. PLOS ONE 8(9): 10.1371/annotation/49e2c5c8-e8a8-4011-80fc-02c6724b2acc. https://doi.org/10.1371/annotation/49e2c5c8-e8a8-4011-80fc-02c6724b2acc View correction
Mediation analysis investigates whether a variable (i.e., mediator) changes in regard to an independent variable, in turn, affecting a dependent variable. Moderation analysis, on the other hand, investigates whether the statistical interaction between independent variables predict a dependent variable. Although this difference between these two types of analysis is explicit in current literature, there is still confusion with regard to the mediating and moderating effects of different variables on depression. The purpose of this study was to assess the mediating and moderating effects of anxiety, stress, positive affect, and negative affect on depression.
Two hundred and two university students (males = 93, females = 113) completed questionnaires assessing anxiety, stress, self-esteem, positive and negative affect, and depression. Mediation and moderation analyses were conducted using techniques based on standard multiple regression and hierarchical regression analyses.
Main Findings
The results indicated that (i) anxiety partially mediated the effects of both stress and self-esteem upon depression, (ii) that stress partially mediated the effects of anxiety and positive affect upon depression, (iii) that stress completely mediated the effects of self-esteem on depression, and (iv) that there was a significant interaction between stress and negative affect, and between positive affect and negative affect upon depression.
The study highlights different research questions that can be investigated depending on whether researchers decide to use the same variables as mediators and/or moderators.
Citation: Nima AA, Rosenberg P, Archer T, Garcia D (2013) Anxiety, Affect, Self-Esteem, and Stress: Mediation and Moderation Effects on Depression. PLoS ONE 8(9): e73265. https://doi.org/10.1371/journal.pone.0073265
Editor: Ben J. Harrison, The University of Melbourne, Australia
Received: February 21, 2013; Accepted: July 22, 2013; Published: September 9, 2013
Copyright: © 2013 Nima et al. This is an open-access article distributed under the terms of the Creative Commons Attribution License, which permits unrestricted use, distribution, and reproduction in any medium, provided the original author and source are credited.
Funding: The authors have no support or funding to report.
Competing interests: The authors have declared that no competing interests exist.
Introduction
Mediation refers to the covariance relationships among three variables: an independent variable (1), an assumed mediating variable (2), and a dependent variable (3). Mediation analysis investigates whether the mediating variable accounts for a significant amount of the shared variance between the independent and the dependent variables–the mediator changes in regard to the independent variable, in turn, affecting the dependent one [1] , [2] . On the other hand, moderation refers to the examination of the statistical interaction between independent variables in predicting a dependent variable [1] , [3] . In contrast to the mediator, the moderator is not expected to be correlated with both the independent and the dependent variable–Baron and Kenny [1] actually recommend that it is best if the moderator is not correlated with the independent variable and if the moderator is relatively stable, like a demographic variable (e.g., gender, socio-economic status) or a personality trait (e.g., affectivity).
Although both types of analysis lead to different conclusions [3] and the distinction between statistical procedures is part of the current literature [2] , there is still confusion about the use of moderation and mediation analyses using data pertaining to the prediction of depression. There are, for example, contradictions among studies that investigate mediating and moderating effects of anxiety, stress, self-esteem, and affect on depression. Depression, anxiety and stress are suggested to influence individuals' social relations and activities, work, and studies, as well as compromising decision-making and coping strategies [4] , [5] , [6] . Successfully coping with anxiety, depressiveness, and stressful situations may contribute to high levels of self-esteem and self-confidence, in addition increasing well-being, and psychological and physical health [6] . Thus, it is important to disentangle how these variables are related to each other. However, while some researchers perform mediation analysis with some of the variables mentioned here, other researchers conduct moderation analysis with the same variables. Seldom are both moderation and mediation performed on the same dataset. Before disentangling mediation and moderation effects on depression in the current literature, we briefly present the methodology behind the analysis performed in this study.
Mediation and moderation
Baron and Kenny [1] postulated several criteria for the analysis of a mediating effect: a significant correlation between the independent and the dependent variable, the independent variable must be significantly associated with the mediator, the mediator predicts the dependent variable even when the independent variable is controlled for, and the correlation between the independent and the dependent variable must be eliminated or reduced when the mediator is controlled for. All the criteria is then tested using the Sobel test which shows whether indirect effects are significant or not [1] , [7] . A complete mediating effect occurs when the correlation between the independent and the dependent variable are eliminated when the mediator is controlled for [8] . Analyses of mediation can, for example, help researchers to move beyond answering if high levels of stress lead to high levels of depression. With mediation analysis researchers might instead answer how stress is related to depression.
In contrast to mediation, moderation investigates the unique conditions under which two variables are related [3] . The third variable here, the moderator, is not an intermediate variable in the causal sequence from the independent to the dependent variable. For the analysis of moderation effects, the relation between the independent and dependent variable must be different at different levels of the moderator [3] . Moderators are included in the statistical analysis as an interaction term [1] . When analyzing moderating effects the variables should first be centered (i.e., calculating the mean to become 0 and the standard deviation to become 1) in order to avoid problems with multi-colinearity [8] . Moderating effects can be calculated using multiple hierarchical linear regressions whereby main effects are presented in the first step and interactions in the second step [1] . Analysis of moderation, for example, helps researchers to answer when or under which conditions stress is related to depression.
Mediation and moderation effects on depression
Cognitive vulnerability models suggest that maladaptive self-schema mirroring helplessness and low self-esteem explain the development and maintenance of depression (for a review see [9] ). These cognitive vulnerability factors become activated by negative life events or negative moods [10] and are suggested to interact with environmental stressors to increase risk for depression and other emotional disorders [11] , [10] . In this line of thinking, the experience of stress, low self-esteem, and negative emotions can cause depression, but also be used to explain how (i.e., mediation) and under which conditions (i.e., moderation) specific variables influence depression.
Using mediational analyses to investigate how cognitive therapy intervations reduced depression, researchers have showed that the intervention reduced anxiety, which in turn was responsible for 91% of the reduction in depression [12] . In the same study, reductions in depression, by the intervention, accounted only for 6% of the reduction in anxiety. Thus, anxiety seems to affect depression more than depression affects anxiety and, together with stress, is both a cause of and a powerful mediator influencing depression (See also [13] ). Indeed, there are positive relationships between depression, anxiety and stress in different cultures [14] . Moreover, while some studies show that stress (independent variable) increases anxiety (mediator), which in turn increased depression (dependent variable) [14] , other studies show that stress (moderator) interacts with maladaptive self-schemata (dependent variable) to increase depression (independent variable) [15] , [16] .
The present study
In order to illustrate how mediation and moderation can be used to address different research questions we first focus our attention to anxiety and stress as mediators of different variables that earlier have been shown to be related to depression. Secondly, we use all variables to find which of these variables moderate the effects on depression.
The specific aims of the present study were:
- To investigate if anxiety mediated the effect of stress, self-esteem, and affect on depression.
- To investigate if stress mediated the effects of anxiety, self-esteem, and affect on depression.
- To examine moderation effects between anxiety, stress, self-esteem, and affect on depression.
Ethics statement
This research protocol was approved by the Ethics Committee of the University of Gothenburg and written informed consent was obtained from all the study participants.
Participants
The present study was based upon a sample of 206 participants (males = 93, females = 113). All the participants were first year students in different disciplines at two universities in South Sweden. The mean age for the male students was 25.93 years ( SD = 6.66), and 25.30 years ( SD = 5.83) for the female students.
In total, 206 questionnaires were distributed to the students. Together 202 questionnaires were responded to leaving a total dropout of 1.94%. This dropout concerned three sections that the participants chose not to respond to at all, and one section that was completed incorrectly. None of these four questionnaires was included in the analyses.
Instruments
Hospital anxiety and depression scale [17] ..
The Swedish translation of this instrument [18] was used to measure anxiety and depression. The instrument consists of 14 statements (7 of which measure depression and 7 measure anxiety) to which participants are asked to respond grade of agreement on a Likert scale (0 to 3). The utility, reliability and validity of the instrument has been shown in multiple studies (e.g., [19] ).
Perceived Stress Scale [20] .
The Swedish version [21] of this instrument was used to measures individuals' experience of stress. The instrument consist of 14 statements to which participants rate on a Likert scale (0 = never , 4 = very often ). High values indicate that the individual expresses a high degree of stress.
Rosenberg's Self-Esteem Scale [22] .
The Rosenberg's Self-Esteem Scale (Swedish version by Lindwall [23] ) consists of 10 statements focusing on general feelings toward the self. Participants are asked to report grade of agreement in a four-point Likert scale (1 = agree not at all, 4 = agree completely ). This is the most widely used instrument for estimation of self-esteem with high levels of reliability and validity (e.g., [24] , [25] ).
Positive Affect and Negative Affect Schedule [26] .
This is a widely applied instrument for measuring individuals' self-reported mood and feelings. The Swedish version has been used among participants of different ages and occupations (e.g., [27] , [28] , [29] ). The instrument consists of 20 adjectives, 10 positive affect (e.g., proud, strong) and 10 negative affect (e.g., afraid, irritable). The adjectives are rated on a five-point Likert scale (1 = not at all , 5 = very much ). The instrument is a reliable, valid, and effective self-report instrument for estimating these two important and independent aspects of mood [26] .
Questionnaires were distributed to the participants on several different locations within the university, including the library and lecture halls. Participants were asked to complete the questionnaire after being informed about the purpose and duration (10–15 minutes) of the study. Participants were also ensured complete anonymity and informed that they could end their participation whenever they liked.
Correlational analysis
Depression showed positive, significant relationships with anxiety, stress and negative affect. Table 1 presents the correlation coefficients, mean values and standard deviations ( sd ), as well as Cronbach ' s α for all the variables in the study.
- PPT PowerPoint slide
- PNG larger image
- TIFF original image
https://doi.org/10.1371/journal.pone.0073265.t001
Mediation analysis
Regression analyses were performed in order to investigate if anxiety mediated the effect of stress, self-esteem, and affect on depression (aim 1). The first regression showed that stress ( B = .03, 95% CI [.02,.05], β = .36, t = 4.32, p <.001), self-esteem ( B = −.03, 95% CI [−.05, −.01], β = −.24, t = −3.20, p <.001), and positive affect ( B = −.02, 95% CI [−.05, −.01], β = −.19, t = −2.93, p = .004) had each an unique effect on depression. Surprisingly, negative affect did not predict depression ( p = 0.77) and was therefore removed from the mediation model, thus not included in further analysis.
The second regression tested whether stress, self-esteem and positive affect uniquely predicted the mediator (i.e., anxiety). Stress was found to be positively associated ( B = .21, 95% CI [.15,.27], β = .47, t = 7.35, p <.001), whereas self-esteem was negatively associated ( B = −.29, 95% CI [−.38, −.21], β = −.42, t = −6.48, p <.001) to anxiety. Positive affect, however, was not associated to anxiety ( p = .50) and was therefore removed from further analysis.
A hierarchical regression analysis using depression as the outcome variable was performed using stress and self-esteem as predictors in the first step, and anxiety as predictor in the second step. This analysis allows the examination of whether stress and self-esteem predict depression and if this relation is weaken in the presence of anxiety as the mediator. The result indicated that, in the first step, both stress ( B = .04, 95% CI [.03,.05], β = .45, t = 6.43, p <.001) and self-esteem ( B = .04, 95% CI [.03,.05], β = .45, t = 6.43, p <.001) predicted depression. When anxiety (i.e., the mediator) was controlled for predictability was reduced somewhat but was still significant for stress ( B = .03, 95% CI [.02,.04], β = .33, t = 4.29, p <.001) and for self-esteem ( B = −.03, 95% CI [−.05, −.01], β = −.20, t = −2.62, p = .009). Anxiety, as a mediator, predicted depression even when both stress and self-esteem were controlled for ( B = .05, 95% CI [.02,.08], β = .26, t = 3.17, p = .002). Anxiety improved the prediction of depression over-and-above the independent variables (i.e., stress and self-esteem) (Δ R 2 = .03, F (1, 198) = 10.06, p = .002). See Table 2 for the details.
https://doi.org/10.1371/journal.pone.0073265.t002
A Sobel test was conducted to test the mediating criteria and to assess whether indirect effects were significant or not. The result showed that the complete pathway from stress (independent variable) to anxiety (mediator) to depression (dependent variable) was significant ( z = 2.89, p = .003). The complete pathway from self-esteem (independent variable) to anxiety (mediator) to depression (dependent variable) was also significant ( z = 2.82, p = .004). Thus, indicating that anxiety partially mediates the effects of both stress and self-esteem on depression. This result may indicate also that both stress and self-esteem contribute directly to explain the variation in depression and indirectly via experienced level of anxiety (see Figure 1 ).
Changes in Beta weights when the mediator is present are highlighted in red.
https://doi.org/10.1371/journal.pone.0073265.g001
For the second aim, regression analyses were performed in order to test if stress mediated the effect of anxiety, self-esteem, and affect on depression. The first regression showed that anxiety ( B = .07, 95% CI [.04,.10], β = .37, t = 4.57, p <.001), self-esteem ( B = −.02, 95% CI [−.05, −.01], β = −.18, t = −2.23, p = .03), and positive affect ( B = −.03, 95% CI [−.04, −.02], β = −.27, t = −4.35, p <.001) predicted depression independently of each other. Negative affect did not predict depression ( p = 0.74) and was therefore removed from further analysis.
The second regression investigated if anxiety, self-esteem and positive affect uniquely predicted the mediator (i.e., stress). Stress was positively associated to anxiety ( B = 1.01, 95% CI [.75, 1.30], β = .46, t = 7.35, p <.001), negatively associated to self-esteem ( B = −.30, 95% CI [−.50, −.01], β = −.19, t = −2.90, p = .004), and a negatively associated to positive affect ( B = −.33, 95% CI [−.46, −.20], β = −.27, t = −5.02, p <.001).
A hierarchical regression analysis using depression as the outcome and anxiety, self-esteem, and positive affect as the predictors in the first step, and stress as the predictor in the second step, allowed the examination of whether anxiety, self-esteem and positive affect predicted depression and if this association would weaken when stress (i.e., the mediator) was present. In the first step of the regression anxiety ( B = .07, 95% CI [.05,.10], β = .38, t = 5.31, p = .02), self-esteem ( B = −.03, 95% CI [−.05, −.01], β = −.18, t = −2.41, p = .02), and positive affect ( B = −.03, 95% CI [−.04, −.02], β = −.27, t = −4.36, p <.001) significantly explained depression. When stress (i.e., the mediator) was controlled for, predictability was reduced somewhat but was still significant for anxiety ( B = .05, 95% CI [.02,.08], β = .05, t = 4.29, p <.001) and for positive affect ( B = −.02, 95% CI [−.04, −.01], β = −.20, t = −3.16, p = .002), whereas self-esteem did not reach significance ( p < = .08). In the second step, the mediator (i.e., stress) predicted depression even when anxiety, self-esteem, and positive affect were controlled for ( B = .02, 95% CI [.08,.04], β = .25, t = 3.07, p = .002). Stress improved the prediction of depression over-and-above the independent variables (i.e., anxiety, self-esteem and positive affect) (Δ R 2 = .02, F (1, 197) = 9.40, p = .002). See Table 3 for the details.
https://doi.org/10.1371/journal.pone.0073265.t003
Furthermore, the Sobel test indicated that the complete pathways from the independent variables (anxiety: z = 2.81, p = .004; self-esteem: z = 2.05, p = .04; positive affect: z = 2.58, p <.01) to the mediator (i.e., stress), to the outcome (i.e., depression) were significant. These specific results might be explained on the basis that stress partially mediated the effects of both anxiety and positive affect on depression while stress completely mediated the effects of self-esteem on depression. In other words, anxiety and positive affect contributed directly to explain the variation in depression and indirectly via the experienced level of stress. Self-esteem contributed only indirectly via the experienced level of stress to explain the variation in depression. In other words, stress effects on depression originate from “its own power” and explained more of the variation in depression than self-esteem (see Figure 2 ).
https://doi.org/10.1371/journal.pone.0073265.g002
Moderation analysis
Multiple linear regression analyses were used in order to examine moderation effects between anxiety, stress, self-esteem and affect on depression. The analysis indicated that about 52% of the variation in the dependent variable (i.e., depression) could be explained by the main effects and the interaction effects ( R 2 = .55, adjusted R 2 = .51, F (55, 186) = 14.87, p <.001). When the variables (dependent and independent) were standardized, both the standardized regression coefficients beta (β) and the unstandardized regression coefficients beta (B) became the same value with regard to the main effects. Three of the main effects were significant and contributed uniquely to high levels of depression: anxiety ( B = .26, t = 3.12, p = .002), stress ( B = .25, t = 2.86, p = .005), and self-esteem ( B = −.17, t = −2.17, p = .03). The main effect of positive affect was also significant and contributed to low levels of depression ( B = −.16, t = −2.027, p = .02) (see Figure 3 ). Furthermore, the results indicated that two moderator effects were significant. These were the interaction between stress and negative affect ( B = −.28, β = −.39, t = −2.36, p = .02) (see Figure 4 ) and the interaction between positive affect and negative affect ( B = −.21, β = −.29, t = −2.30, p = .02) ( Figure 5 ).
https://doi.org/10.1371/journal.pone.0073265.g003
Low stress and low negative affect leads to lower levels of depression compared to high stress and high negative affect.
https://doi.org/10.1371/journal.pone.0073265.g004
High positive affect and low negative affect lead to lower levels of depression compared to low positive affect and high negative affect.
https://doi.org/10.1371/journal.pone.0073265.g005
The results in the present study show that (i) anxiety partially mediated the effects of both stress and self-esteem on depression, (ii) that stress partially mediated the effects of anxiety and positive affect on depression, (iii) that stress completely mediated the effects of self-esteem on depression, and (iv) that there was a significant interaction between stress and negative affect, and positive affect and negative affect on depression.
Mediating effects
The study suggests that anxiety contributes directly to explaining the variance in depression while stress and self-esteem might contribute directly to explaining the variance in depression and indirectly by increasing feelings of anxiety. Indeed, individuals who experience stress over a long period of time are susceptible to increased anxiety and depression [30] , [31] and previous research shows that high self-esteem seems to buffer against anxiety and depression [32] , [33] . The study also showed that stress partially mediated the effects of both anxiety and positive affect on depression and that stress completely mediated the effects of self-esteem on depression. Anxiety and positive affect contributed directly to explain the variation in depression and indirectly to the experienced level of stress. Self-esteem contributed only indirectly via the experienced level of stress to explain the variation in depression, i.e. stress affects depression on the basis of ‘its own power’ and explains much more of the variation in depressive experiences than self-esteem. In general, individuals who experience low anxiety and frequently experience positive affect seem to experience low stress, which might reduce their levels of depression. Academic stress, for instance, may increase the risk for experiencing depression among students [34] . Although self-esteem did not emerged as an important variable here, under circumstances in which difficulties in life become chronic, some researchers suggest that low self-esteem facilitates the experience of stress [35] .
Moderator effects/interaction effects
The present study showed that the interaction between stress and negative affect and between positive and negative affect influenced self-reported depression symptoms. Moderation effects between stress and negative affect imply that the students experiencing low levels of stress and low negative affect reported lower levels of depression than those who experience high levels of stress and high negative affect. This result confirms earlier findings that underline the strong positive association between negative affect and both stress and depression [36] , [37] . Nevertheless, negative affect by itself did not predicted depression. In this regard, it is important to point out that the absence of positive emotions is a better predictor of morbidity than the presence of negative emotions [38] , [39] . A modification to this statement, as illustrated by the results discussed next, could be that the presence of negative emotions in conjunction with the absence of positive emotions increases morbidity.
The moderating effects between positive and negative affect on the experience of depression imply that the students experiencing high levels of positive affect and low levels of negative affect reported lower levels of depression than those who experience low levels of positive affect and high levels of negative affect. This result fits previous observations indicating that different combinations of these affect dimensions are related to different measures of physical and mental health and well-being, such as, blood pressure, depression, quality of sleep, anxiety, life satisfaction, psychological well-being, and self-regulation [40] – [51] .
Limitations
The result indicated a relatively low mean value for depression ( M = 3.69), perhaps because the studied population was university students. These might limit the generalization power of the results and might also explain why negative affect, commonly associated to depression, was not related to depression in the present study. Moreover, there is a potential influence of single source/single method variance on the findings, especially given the high correlation between all the variables under examination.
Conclusions
The present study highlights different results that could be arrived depending on whether researchers decide to use variables as mediators or moderators. For example, when using meditational analyses, anxiety and stress seem to be important factors that explain how the different variables used here influence depression–increases in anxiety and stress by any other factor seem to lead to increases in depression. In contrast, when moderation analyses were used, the interaction of stress and affect predicted depression and the interaction of both affectivity dimensions (i.e., positive and negative affect) also predicted depression–stress might increase depression under the condition that the individual is high in negative affectivity, in turn, negative affectivity might increase depression under the condition that the individual experiences low positive affectivity.
Acknowledgments
The authors would like to thank the reviewers for their openness and suggestions, which significantly improved the article.
Author Contributions
Conceived and designed the experiments: AAN TA. Performed the experiments: AAN. Analyzed the data: AAN DG. Contributed reagents/materials/analysis tools: AAN TA DG. Wrote the paper: AAN PR TA DG.
- View Article
- Google Scholar
- 3. MacKinnon DP, Luecken LJ (2008) How and for Whom? Mediation and Moderation in Health Psychology. Health Psychol 27 (2 Suppl.): s99–s102.
- 4. Aaroe R (2006) Vinn över din depression [Defeat depression]. Stockholm: Liber.
- 5. Agerberg M (1998) Ut ur mörkret [Out from the Darkness]. Stockholm: Nordstedt.
- 6. Gilbert P (2005) Hantera din depression [Cope with your Depression]. Stockholm: Bokförlaget Prisma.
- 8. Tabachnick BG, Fidell LS (2007) Using Multivariate Statistics, Fifth Edition. Boston: Pearson Education, Inc.
- 10. Beck AT (1967) Depression: Causes and treatment. Philadelphia: University of Pennsylvania Press.
- 21. Eskin M, Parr D (1996) Introducing a Swedish version of an instrument measuring mental stress. Stockholm: Psykologiska institutionen Stockholms Universitet.
- 22. Rosenberg M (1965) Society and the Adolescent Self-Image. Princeton, NJ: Princeton University Press.
- 23. Lindwall M (2011) Självkänsla – Bortom populärpsykologi & enkla sanningar [Self-Esteem – Beyond Popular Psychology and Simple Truths]. Lund:Studentlitteratur.
- 25. Blascovich J, Tomaka J (1991) Measures of self-esteem. In: Robinson JP, Shaver PR, Wrightsman LS (Red.) Measures of personality and social psychological attitudes San Diego: Academic Press. 161–194.
- 30. Eysenck M (Ed.) (2000) Psychology: an integrated approach. New York: Oxford University Press.
- 31. Lazarus RS, Folkman S (1984) Stress, Appraisal, and Coping. New York: Springer.
- 32. Johnson M (2003) Självkänsla och anpassning [Self-esteem and Adaptation]. Lund: Studentlitteratur.
- 33. Cullberg Weston M (2005) Ditt inre centrum – Om självkänsla, självbild och konturen av ditt själv [Your Inner Centre – About Self-esteem, Self-image and the Contours of Yourself]. Stockholm: Natur och Kultur.
- 34. Lindén M (1997) Studentens livssituation. Frihet, sårbarhet, kris och utveckling [Students' Life Situation. Freedom, Vulnerability, Crisis and Development]. Uppsala: Studenthälsan.
- 35. Williams S (1995) Press utan stress ger maximal prestation [Pressure without Stress gives Maximal Performance]. Malmö: Richters förlag.
- 37. Garcia D, Kerekes N, Andersson-Arntén A–C, Archer T (2012) Temperament, Character, and Adolescents' Depressive Symptoms: Focusing on Affect. Depress Res Treat. DOI:10.1155/2012/925372.
- 40. Garcia D, Ghiabi B, Moradi S, Siddiqui A, Archer T (2013) The Happy Personality: A Tale of Two Philosophies. In Morris EF, Jackson M-A editors. Psychology of Personality. New York: Nova Science Publishers. 41–59.
- 41. Schütz E, Nima AA, Sailer U, Andersson-Arntén A–C, Archer T, Garcia D (2013) The affective profiles in the USA: Happiness, depression, life satisfaction, and happiness-increasing strategies. In press.
- 43. Garcia D, Nima AA, Archer T (2013) Temperament and Character's Relationship to Subjective Well- Being in Salvadorian Adolescents and Young Adults. In press.
- 44. Garcia D (2013) La vie en Rose: High Levels of Well-Being and Events Inside and Outside Autobiographical Memory. J Happiness Stud. DOI: 10.1007/s10902-013-9443-x.
- 48. Adrianson L, Djumaludin A, Neila R, Archer T (2013) Cultural influences upon health, affect, self-esteem and impulsiveness: An Indonesian-Swedish comparison. Int J Res Stud Psychol. DOI: 10.5861/ijrsp.2013.228.
- Open access
- Published: 29 March 2024
Insomnia and creativity in Chinese adolescents: mediation through need for cognition
- Xiaoyang Ren 1 ,
- Min Shi 1 &
BMC Psychology volume 12 , Article number: 180 ( 2024 ) Cite this article
69 Accesses
Metrics details
Creativity is an essential cognitive ability that plays a crucial role in advanced thinking. While previous research has demonstrated the impact of insomnia on cognitive function, its effects on creativity in Chinese adolescents remain unclear. This study explored the relationship between insomnia (specifically, daytime and nighttime disturbances) and creativity in adolescents. Additionally, it examined the potential mediating effect of the need for cognition on this relationship.
Questionnaires were administered to 302 adolescents to measure their creativity, need for cognition, and insomnia levels using the Williams Creative Tendencies Scale, Need for Cognition Scale, and Bergen Insomnia Scale, respectively. Regression analysis was conducted to examine the direct impact of insomnia on creativity. Furthermore, a mediation model was constructed to investigate the role of the need for cognition in mediating the relationship between insomnia and creativity.
The findings of the present study indicated that insomnia had a direct impact on the creativity of adolescents, demonstrating a time-of-day effect. Daytime disturbances were found to have a positive correlation with overall creativity and imagination, whereas no significant direct effect was found between nighttime disturbances and creativity. Further analysis revealed that insomnia, specifically daytime disturbances, might influence creativity by affecting the individual’s need for cognition. However, no similar indirect effects were observed for the relationship between nighttime disturbances and creativity.
Conclusions
Our findings indicate that adolescents might experience improved creativity as a result of daytime disruptions, and the level of need for cognition could play a crucial role in understanding the link between insomnia and creativity in adolescents.
Peer Review reports
Introduction
Insomnia is a condition characterized by an individual’s self-reported difficulties in sleeping [ 1 , 2 ]. It is characterized by symptoms such as taking a long time to fall asleep, waking up frequently during the night, experiencing prolonged periods of wakefulness during sleep, and frequent brief awakenings [ 3 ]. In recent years, issues like staying up late, not getting enough sleep, and struggling to fall asleep have become increasingly prevalent among adolescents [ 4 ]. The White Paper 2023 China Youth and Children’s Sleep Index, released by the China Sleep Research Association, reveals concerning statistics about the sleep patterns of junior high school students in China. According to the report, only 18.9% of these students manage to sleep for more than 8 h, while a staggering 59.4% sleep for less than 7 h. On average, these students only get 6.82 h of sleep, indicating that the majority of them do not meet the recommended amount of sleep. A study conducted in the Shandong Province of China explored the prevalence of sleep problems among adolescents. The findings revealed that 37.44% of adolescents suffered from insufficient sleep, while 26.89% reported experiencing poor sleep quality [ 5 ]. Another meta-analysis, which included 63 studies and a total of 430,422 Chinese adolescents, discovered that 104,802 adolescents experienced sleep disturbances. The overall prevalence of sleep problems was found to be 26%, with junior high school students having a detection rate of 20% [ 6 ]. As widely known, adolescents go through a crucial stage of psychological transformation. Issues such as sleep deprivation and sleep disorders appear to have a significant influence on their mental well-being, especially in terms of cognition and personality development [ 7 , 8 ].
Can tired minds generate creative ideas? Some researchers have found that the cognitive processes utilized before sleep by individuals with insomnia, such as rehearsing, planning, and problem-solving, are similar to the stages involved in creative thinking, such as preparation and incubation [ 9 , 10 ]. As a result, a hypothesis has emerged suggesting that individuals with disrupted sleep might exhibit greater creativity. In addition, it should be noted that disrupted sleep and the widely recognized consequences of sleep deprivation are symptoms of depression and anxiety [ 11 , 12 ], while depression and anxiety have also been associated with creativity [ 13 ]. This suggests that sleep issues could have been prevalent among individuals who are highly creative. However, it is important to consider that sleep problems have been shown to negatively affect cognitive function as well. For example, a study using fMRI have demonstrated that lack of sleep reduced the communication between various brain regions such as the amygdala, dorsolateral prefrontal cortex, dorsal anterior cingulate gyrus, and right inferior frontal gyrus. This weakened functional connectivity could result in a negative bias when it comes to encoding memories [ 14 ]. Additionally, research has found that sleep deprivation could also impact the activity of brain regions involved in fearful learning, namely the prefrontal cortex, hippocampus, and amygdala [ 15 ]. Since the activity of the aforementioned brain regions is crucial for individual creativity, some researchers have also suggested that problems such as sleep deprivation and sleep disorders may produce impairments in cognition, memory, etc., which in turn interfere with creativity [ 16 ].
It is noteworthy that only two studies have delved into the connection between insomnia and creativity until now. Firstly, researchers discovered a positive correlation between insomnia and creativity by comparing the prevalence of sleep disturbances in 30 creative children versus 30 control children. Notably, the highly creative children exhibited a higher incidence of sleep disturbances than the control group [ 17 ]. Subsequently, a recent study indicated a minor direct impact of a global insomnia factor on divergent thinking, implying time-of-day effects where nighttime sleep disturbances positively predicted divergent thinking more strongly than daytime disturbances [ 18 ]. These findings suggest that sleep disturbances may possess some beneficial predictive effects on creativity among children and adolescents. However, there could be disparities in the impact of sleep disturbances during the day and night. Despite this, the majority of existing studies have focused on the influence of insomnia on creative thinking, leaving a gap in research evidence regarding its effects on creative personality. It is established that insomnia is linked to personality traits [ 19 ]. Therefore, the primary objective of this study is to investigate the relationship between adolescents’ creativity (specifically creative personality) and insomnia. Building on the outcomes of previous studies, we hypothesized that insomnia would significantly and positively predict adolescents’ creativity (creative personality).
Although a tenuous link has been established between insomnia and creativity, it is postulated that additional variables might influence this relationship. Taking these observations into account, a crucial question arises: How does insomnia impact creativity? Since coming up with original and useful ideas requires several cognitively demanding processes [ 20 , 21 ], the need for cognition may also play an important role in creativity. The need for cognition refers to an individual’s tendency to engage in and derive pleasure from tasks that require cognitive effort [ 22 ]. Individuals with a strong need for cognition are more prone to innovate and have a deeper interest in addressing challenging problems. For instance, research suggested that those with a high cognitive need were more likely to generate ideas for ambiguous scenarios [ 23 ]. Furthermore, individuals with a strong need for cognition exhibited heightened creativity in problem-solving and possessed more pronounced creative personalities [ 24 , 25 ]. Therefore, the need for cognition might serve as a significant and positive predictor of creative personality [ 26 ]. In considering the role of insomnia in creativity, it is plausible that the need for cognition could act as a mediator, influencing the association between the two variables.
However, there was evidence that insomnia could impact individuals’ willingness to invest more time and effort when faced with complex tasks. The microanalytic model of insomnia highlighted hyperarousal as a key regulatory feature, which could distort perceptions of time and exacerbate the challenges associated with falling asleep and experiencing distress. As a result, the consequences of insomnia on the following day could include fatigue, mood disturbances such as irritability, cognitive impairments, and a reduced ability to engage in or enjoy mentally demanding tasks [ 27 ]. Furthermore, the maintained cognitive model of insomnia suggested that insomniacs tend to worry excessively about sleep and its consequences. This negative cognition leaded to emotional distress, and the resulting anxiety prompted individuals to hyperfocus on internal and external cues related to sleep-related threats. Consequently, this state of anxiety could lead to a lack of interest and motivation in solving complex problems, as well as crowding out the time needed for engaging in mentally challenging tasks [ 28 ]. Supported by neuroimaging and neurobiochemistry evidence, researchers have found that individuals with insomnia often exhibit impairments in various cognitive functions, including episodic memory, working memory, and certain aspects of executive functioning [ 7 ]. Given these findings, it is likely that insomnia can reduce an individual’s cognitive engagement and motivation to seek new knowledge, thereby suppressing the anticipated effect of insomnia on creativity. Therefore, the second objective of our study was to further investigate the psychological mechanisms that underlie the impact of insomnia on creativity. Drawing from the aforementioned theoretical and empirical evidence, we hypothesized that the need for cognition played a mediating role in the relationship between insomnia and creativity.
Taking into account that previous research primarily focused on young adults or children, who exhibited distinct sleep patterns compared to adolescents, the relationship between insomnia and the creativity of adolescents, particularly their creative personality, remained enigmatic. The objective of this study was to explore the impact of insomnia on adolescents’ creativity, specifically their creative personality, and to unravel the underlying mechanisms. Drawing from existing theoretical and empirical research, we postulated that: (1) insomnia, encompassing both daytime and nighttime disturbances, was associated with creativity in adolescents, and there might exist time-of-day effects (H1); and (2) the need for cognition might serve as a mediator between insomnia and creativity (H2).
Materials and methods
After a thorough literature review and consideration of previous research, the research questions and hypotheses were formulated in January 2023. Utilizing a cross-sectional research design, questionnaires were administered to a cohort of middle school students in Jinan, Shandong Province, in April of the same year. These questionnaires aimed to capture data on all the relevant research variables, including creativity, insomnia, and the need for cognition at the same time. Subsequently, the collected data was entered into a database and subjected to rigorous checking and analysis.
Participants and procedure
To ensure the validity and relevance of our study, we collaborated closely with a local school in the recruitment process. Initially, we liaised with the school’s head to disseminate recruitment details. Leveraging the assistance of class teachers, we carefully selected participants based on the following criteria: all participants were required to be native Chinese speakers with normal or corrected vision, exhibit no signs of mental or physical health issues, possess normal intellectual development, not encounter any reading difficulties, and not consume psychotropic drugs. Only students who expressed a willingness to participate and fulfilled the study’s criteria were ultimately chosen to participate in the testing process. This meticulous approach ensured that our sample population was representative and well-suited for the objectives of our research.
In this study, 318 junior high school students participated, of whom 302 were included in the primary analysis due to having complete datasets, yielding an effective participation rate of 94.97%. Participants’ ages ranged from 12 to 14, with an average of 12.97 years ( SD = 0.49). Specifically, 41 were 12 years old, 229 were 13, and 32 were 14. 147 were females (48.7%) and 155 were males (51.3%). Regarding the parents’ educational backgrounds, the survey revealed that 31 fathers (10.3%) and 34 mothers (11.3%) held university degrees or higher qualifications. Notably, most parents had completed their education at the middle or high school level (70.2%). When it came to parental occupations, the survey found that the fathers’ top three professions were doctors (25.5%), self-employed individuals (11.6%), and drivers (8.3%). Meanwhile, for mothers, the most common occupations were self-employed (19.2%), salespeople (11.6%), and laborers (8.9%).
The Institutional Review Board of Shandong Normal University has granted approval for this study, ensuring that all measurements adhere strictly to the pertinent guidelines and regulations for psychological research. The group tests were conducted within the classroom setting, led by a psychology-major researcher as the primary tester. Initially, we secured the authorization and support of the school’s teaching department. Subsequently, we utilized the students’ self-study period to clarify the purpose of the research and underscore the principles of voluntariness, anonymity, and honesty. Ultimately, the participants were required to complete a psychological test within approximately 30 min, assessing various aspects such as creativity, need for cognition, insomnia, along with personal family information.
An adapted version of the Williams Creative Tendencies Scale (WCTS) was utilized to assess the creativity of the participants [ 29 ]. This scale was widely employed in numerous prior creativity studies and exhibited strong reliability [ 30 , 31 ]. The adapted version included 11 items to measure adventurousness, 14 items to measure curiosity, 13 items to measure imagination, and 12 items to measure challenge. Each item was rated on a Likert scale ranging from 1 (strongly disagree) to 3 (strongly agree). By compiling the total scores, we can effectively evaluate the creativity of the students. Notably, all the items demonstrated good reliability, with a Cronbach’s α value of 0.86.
- Need for cognition
The Need for Cognition Scale (NCS) [ 22 ] was employed in its shortened version to assess participants’ need for cognition. The 18-item Chinese version of the NCS was initially introduced [ 32 ] and subsequently validated as suitable for both adolescents and young adults in subsequent studies [ 33 ]. Participants were instructed to answer the questions based on their actual circumstances. Each item was rated using a Likert-type scale ranging from 1 (strongly opposed) to 5 (strongly agreed). The total score was calculated by summing up the responses to all 18 items, with higher scores indicating a stronger need for cognition. This measurement demonstrated good reliability in the current study, with a Cronbach’s α value of 0.76.
We utilized the Bergen Insomnia Scale (BIS) to assess insomnia among the participants [ 34 ]. The scale comprises six items, all aligned with the Diagnostic and Statistical Manual of Mental Disorders (DSM-IV-TR) criteria for clinical insomnia. The validity of these items has been confirmed through subjective reports and polysomnographic data, encompassing sleep-stage progression, limb movement, and physiological measurements of respiration during controlled laboratory sleep. Three items focus on nighttime disturbances, such as “How many days a week did it take you over 30 minutes to fall asleep after switching off the lights in the past month?”; the other three items target daytime disturbances, like “How many days a week did you feel rested upon waking up in the past month?”. Participants were asked to rate their symptoms on a weekly basis using an eight-point scale ranging from 0 to 7. The total score for the first three items represents nighttime disturbances, while the last three items reflect daytime disturbances. This measurement demonstrated strong reliability for daytime disturbances (Cronbach’s α = 0.86), nighttime disturbances (Cronbach’s α = 0.62), and the overall insomnia score (Cronbach’s α = 0.80) in the Chinese population. According to previous literature, this scale demonstrated good reliability among the Chinese population [ 35 ].
Socioeconomic status (SES)
Recognizing the challenges in precisely measuring income, domestic researchers often turn to an alternative method: assessing a family’s socioeconomic status (SES) through a detailed analysis of their parents’ occupation and education level [ 36 ]. In the present study, we utilized the SES questionnaire to gather participants’ reports on their parents’ occupational and educational backgrounds [ 37 ]. These reports were then coded and graded, following established occupational classification standards, to ensure consistency and comparability across respondents. The occupational classification system employed in this study encompassed five distinct levels: (1) those engaged in temporary, unskilled, agricultural, or non-technical work; (2) self-employed individuals, manual laborers, and technicians; (3) general management and professional technical personnel, including clerks and employees in the commercial service industry; (4) middle-level professionals, managers, and technical personnel, as well as auxiliary professionals specializing in various fields of science, technology, and enterprise work; and (5) senior professional technicians, executives, and leading cadres exercising administrative functions in government, institutions, and social organizations, as well as high- and middle-level managers in large and medium-sized enterprises and private business owners. By utilizing this graded classification system, we aimed to capture a comprehensive representation of participants’ SES backgrounds, ensuring the validity and reliability of our findings.
Furthermore, the educational attainment of parents was categorized into distinct levels:“no schooling or primary education”, “junior middle school”, “high school or technical secondary school”, “junior college”, “university (undergraduate)”, and “graduate”. Participants were required to select the most appropriate category based on their parents’ educational qualifications, and each choice was assigned a numerical score ranging from 1 to 6 during the coding process.
Ultimately, the cumulative score served as an indicator of the family’s socioeconomic status, with a potential range spanning from 4 to 22. Notably, in this research endeavor, the SES scores for both mothers and fathers were computed separately, allowing for a nuanced understanding of each parent’s contribution to the overall socioeconomic profile of the family.
Data analysis
First, we employed the Pearson correlation to assess the relationships between the research variables in the present study. To explore the direct impact of insomnia (independent variables) on creativity (dependent variables), we resorted to multiple linear regression analysis. Specifically, gender, age, socioeconomic status of both parents, and insomnia total score (or daytime and nighttime disturbances) were simultaneously entered into the regression equation. Additionally, we utilized the mediation model to delve into the intricate relationships between insomnia, need for cognition, and creativity. To validate the mediation effects, we relied on the bootstrapping method. From the data, 5000 bootstrap samples were drawn, and 95% bootstrap confidence intervals (CI) were computed. For these statistical analyses, we employed SPSS 17.0 process SPSS macro PROCESS (model 4) ( http://www.afhayes.com ) [ 38 ]. This macro has been extensively used and developed for testing complex models incorporating mediating variables [ 39 ].
Common method deviation test
While the self-report method is a popular choice for data collection, it can potentially lead to common method variance (CMV) issues. To mitigate these concerns, we implemented various control measures to safeguard participants’ anonymity. Among these measures, we ensured that the collected data was strictly limited to scientific research purposes and employed reverse expressions for certain items [ 40 ]. Additionally, to enhance the study’s precision, we utilized the Harman single factor test to process the data. Specifically, we conducted a non-rotating principal component factor analysis on the aforementioned items. The results indicated that the first factor explained only 13.66% of the variation (falling below the 40% threshold). Consequently, this study did not exhibit significant common method variance issues in the collected data.
Descriptive statistics of study variables
Table 1 presents the means, standard deviations, bivariate correlations and gender differences among study variables. The independent samples t-test results revealed that females significantly scored higher than males on measures of insomnia, daytime disturbances, and imagination. Our findings further indicated a positive correlation between insomnia and daytime disturbances with imagination, whereas a negative correlation was observed with the need for cognition. Moreover, the need for cognition demonstrated positive associations with the creativity total score, adventure, curiosity, imagination, and challenge. Mother’s socioeconomic status (SES) exhibited a positive association with imagination. The data for all variables had no outliers and were within three standard deviations. The distributions of all variables approached normality, with skewness and kurtosis ranging from − 1 to 1.
Direct effect tests
The collinearity diagnosis revealed that the tolerance values for the variables of insomnia, daytime and nighttime disturbances, and need for cognition were greater than 0.2, ranging from 0.78 to 0.98, indicating the absence of significant collinearity issues.
The regression analysis results demonstrated that insomnia ( β = 0.19, p < 0.01) and daytime disturbances ( β = 0.24, p < 0.01) positively predicted imagination when controlling for gender, age, father’s SES, and mother’s SES. However, no significant direct effect of nighttime disturbances was observed on the creativity total score, adversity, curiosity, imagination, and challenge (Tables 2 and 3 ). Therefore, H1 was supported. Based on previous research, effect sizes of 0.10, 0.30, and 0.50 are considered small, medium, and large, respectively [ 18 , 41 ]. Consequently, insomnia ( β = 0.19) and daytime disturbances ( β = 0.24) exhibited small-to-medium positive effects on creativity, particularly in terms of imagination.
Indirect effect tests
The indirect effect of need for cognition between insomnia and creativity.
Firstly, the total effect of insomnia on creativity was tested, and it was demonstrated that the path coefficient was not significant. Subsequently, the mediating variable of cognition was added to the model to obtain the path type shown in Fig. 1 . The results showed that insomnia had a direct effect on creativity, and the need for cognition played an indirect role between insomnia and creativity (Table 4 ). The bootstrap test was utilized, and 5000 repeated samples were taken to test the mediating effect and estimate the confidence interval. The absence of 0 in the 95% confidence interval suggested that the indirect effect was significant (see Table 5 ). Therefore, H2 was supported. Similar results were found for imagination, while only indirect effects were found for adventure, curiosity, and challenge. According to the recently proposed mediation effect test method [ 42 ], the indirect effect of need for cognition on the relationship between insomnia and creativity was established, which manifested suppression effects. In other words, the inclusion of the need for cognition enhanced the relationship between insomnia and creativity.
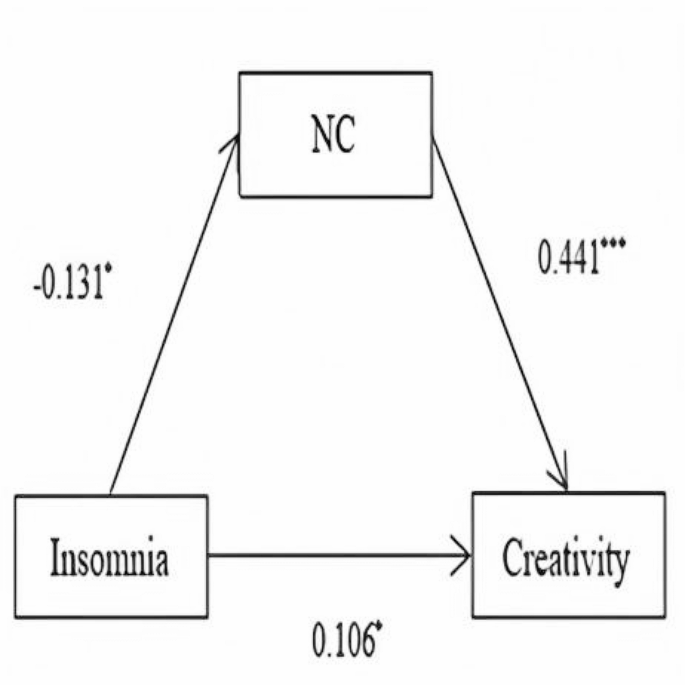
Mediation analysis model testing relationships among insomnia, need for cognition (NC) and creativity
The indirect effect of need for cognition between daytime disturbances and creativity
Similar analysis processes were also conducted to investigate the relationship between daytime disturbances and creativity. Testing the total effect of daytime disturbances on creativity revealed that the path coefficient was not significant. The mediating variable, need for cognition, was then added to the model to obtain the path type shown in Fig. 2 . The results showed that daytime disturbances had a direct effect on creativity, and the need for cognition played an indirect role in the relationship between daytime disturbances and creativity (Table 6 ). Finally, the bootstrap test was employed, and 5000 replicated samples were taken to test the mediating effect and establish the confidence interval. The exclusion of 0 from the 95% confidence interval indicated a statistically significant indirect effect (see Table 7 ). Similar results were found for imagination, while for adventure, curiosity, and challenge, only indirect effects were found. Also, the inclusion of the need for cognition enhanced the relationship between daytime disturbances and creativity.
The indirect effect of need for cognition between nighttime disturbances and creativity
Although s imilar analysis processes were also conducted to examine the relationship between nighttime disturbances and creativity, no significant direct of nighttime disturbances or indirect effects of the need for cognition were found (see Supplementary Table S1 , Table S2 ).
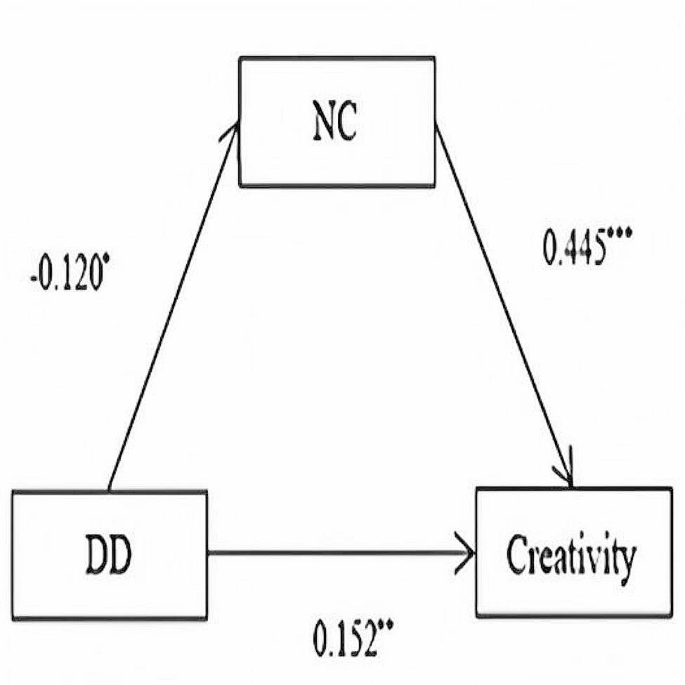
Mediation analysis model testing relationships among daytime disturbances (DD), need for cognition (NC) and creativity
Insomnia and creativity in Chinese adolescents
The current study firstly examined the direct effect of insomnia (daytime disturbances, nighttime disturbances) on adolescents’ creativity. Based on our preliminary findings, insomnia was found to have a beneficial impact on the overall creativity score and imagination, aligning with prior research (H1 was supported). Further analysis showed that there indeed existed time-of-day effects: disturbances during the day had a significant effect on imagination, whereas the effect of disturbances during the night was not significant.
It’s worth nothing that the direct impact of insomnia on creativity was limited to imagination. Imagination is the ability to imagine things that have not yet happened and speculate intuitively, transcending the boundaries of the senses and reality [ 43 ]. It is the basis of all creative activities and a crucial part of culture life [ 44 ]. In a state of insomnia, individuals’ minds may be active, which may enhance individuals’ ability to visualize and increase their openness to new ideas and perspectives.
Moreover, our findings revealed a significant direct impact of daytime disturbances solely on imagination, with no comparable effect observed for nighttime disturbances. This seemed to contrast previous research conducted on young adults, indicating that ‘evening types’ - individuals who typically prefer staying up late and waking up late - tend to perform slightly better on certain measures of creativity [ 45 ]. However, it’s crucial to note that the sleep patterns of adolescents differ from those of young adults. Even if they stay up late, adolescents have less chance of waking up late. Consequently, nighttime disturbances may not be advantageous for them. Conversely, daytime disturbances resulting in fatigue and mood swings might lead to less stringent cognitive control, fostering opportunities for unconventional thinking. Hence, it becomes evident that the investigation of insomnia’s influence on creativity should take into account the time-of-day effects. Daytime disturbances appeared to positively predict creativity more strongly than nighttime disturbances in adolescents.
Mediation of need for cognition
Although the direct impact of insomnia on creativity was notable, the majority of the observed effects were of small-to-medium magnitude. Researchers postulated the existence of a third variable that could potentially mediate the relationship between sleep and creativity [ 46 ]. To delve deeper into the influence of insomnia on creativity, we investigated the intermediary role of the need for cognition. Our findings generally indicated that insomnia might exert its influence on creativity by modulating the need for cognition (H2 was supported). The introduction of need for cognition as a variable strengthened the predictive power of insomnia-related factors (such as daytime disturbances) on creative outcomes (like imagination). These observations suggests the emergence of a suppression effect, which refers to a scenario where a third variable attenuates the relationship between an independent variable (X) and a dependent variable (Y), even when the null hypothesis is true. In psychological research, the absence of a direct relationship between X and Y often poses a challenge. The suppression effect offered a valuable framework for addressing such scenarios and elucidating why significant relationships might not be immediately apparent [ 47 ]. Similarly, our results revealed that need for cognition acted as a suppressor, mitigating the effects of insomnia on creativity.
Despite the absence of a significant direct effect of insomnia, the need for cognition was supported as an indirect influence on adventure, curiosity, and challenge. Adventurousness, curiosity, and challenge-seeking all involve cognitive endeavors such as facing failure or criticism, inquiring into the root cause of problems, engaging in confusing situations, and making order out of chaos [ 43 ]. These creative personalities are strongly influenced by their need for cognition, and insomnia may influence them indirectly by altering their need for cognition.
Limitations
Although these findings offer valuable insights, it’s important to acknowledge several limitations. Firstly, the study employed a cross-sectional design, which assessed variables simultaneously, thus lacking evidence of a temporal link between insomnia and creativity. Longitudinal studies are needed to establish a definitive cause-and-effect relationship between these variables. Secondly, the current study primarily focused on creative personality, overlooking the impact of creative cognition. Given that insomnia is a small-to-medium predictor of divergent thinking [ 18 ], it’s crucial to investigate whether the need for cognition mediates this relationship, enhancing our understanding of the factors that truly influence insomnia’s predictive power over creativity. Finally, the findings of this study have not been replicated in other samples, limiting their generalizability. Future research should aim to replicate these results in diverse enrollment groups, particularly those experiencing severe insomnia, to gain a more comprehensive understanding of the phenomenon.
Despite the limitations described, the present study has two strengths. The primary strength is to first reveal the time-of-day effect associated with insomnia and adolescents’ creativity. These preliminary findings offer profound insights into the impact of sleep disturbances on adolescents’ creativity, thereby aiding in the development of accurate sleep concepts and promoting mental well-being. Secondly, insomnia was found to be more likely to influence creativity through affecting need for cognition. These revelations contribute to establishing scientific frameworks for understanding adolescents’ sleep patterns and suggest that the need for cognition is a crucial aspect in examining the link between insomnia and creativity. Notably, the suppression effect of the need for cognition offers an explanation for the tenuous association between insomnia and creativity, providing a theoretical foundation for fostering the emergence and development of creativity among adolescents with insomnia.
Data availability
Data is provided within the manuscript.
Abbreviations
Williams Creative Tendencies Scale
Need for Cognition Scale
Bergen Insomnia Scale
Socioeconomic Status
Common Method Variance
Father Socioeconomic Status
Mother Socioeconomic Status
Daytime Disturbances
Nighttime Disturbances
Need for Cognition
Standard Error
Savard MH, Savard J, Simard S, Ivers H. Empirical validation of the insomnia severity index in cancer patients. Psychol Oncol. 2005;14(6):429–41.
Article Google Scholar
Liu S, Zhang B. Interpretation of Chinese guidelines for the diagnosis and treatment of insomnia. Chin J Mod Neurol. 2017;17(9):633–8.
Google Scholar
Sateia MJ, Doghramji K, Hauri PJ, Morin CM. Evaluation of chronic insomnia. An American Academy of Sleep Medicine review. Sleep. 2000;23:243–308.
Article PubMed Google Scholar
Li L, Sun H. Review of research on bedtime procrastination. China J Health Psychol. 2020;28(2):316–20.
Pei Y, Wang X, Kong L, Ran X, Wei M, Gao J. The correlation between sleep and mental health of primary and secondary school students in Shandong Province. Chin School Health. 2023;44(11):1674–8.
Meng J, Ling G, Jing H, Gou l. Prevalence of sleep disturbances in Chinese adolescents: a systematic review and meta-analysis. PLoS ONE. 2021; 6(3), e0247333.
Fortier-Brochu É, Beaulieu-Bonneau S, Ivers H, Morin CM. Insomnia and daytime cognitive performance: a meta-analysis. Sleep Med Rev. 2012;16(1):83–94.
Kronholm E, Puusniekka R, Jokela J, et al. Trends in self-reported sleep problems, tiredness and related school performance among Finnish adolescents from 1984 to 2011. J Sleep Res. 2015;24:3–10.
Runco MA. Problem finding, problem solving, and creativity. Norwood, NJ: Ablex; 1994.
Wallas G. The art of thought. London: J. Cape; 1926.
Johnson EO, Roth T, Breslau N. The association of insomnia with anxiety disorders and depression: exploration of the direction of risk. J Psychiatr Res. 2006;40(8):700–8.
Neckelmann D, Mykletun A, Dahl AA. Chronic insomnia as a risk factor for developing anxiety and depression. Sleep. 2007;30(7):873–80.
Article PubMed PubMed Central Google Scholar
Carson SH. Creativity and psychopathology: a shared vulnerability model. Can J Psychiatry. 2011;56(3):144–53.
Shao Y, Lei Y, Wang L et al. Altered resting-state amygdala functional connectivity after 36 hours of total sleep deprivation. PLoS ONE 2014; 9(11), e112222.
Zhang J, Zhang H, Li H, Lei Y. The effect of sleep on fear learning and its cognitive neural mechanism. Adv Psychol Sci. 2023;31(4):631–40.
Dirk DC, Renato P. Sleepy but creative? How affective commitment, knowledge sharing and organizational forgiveness mitigate the dysfunctional effect of insomnia on creative behaviors. Personnel Rev. 2020;50(1):108–28.
Healey D, Runco MA. Could creativity be associated with insomnia? Creativity Res J. 2006;18(1):39–43.
Beaty RE, Silvia PJ, Nusbaum EC, Vartanian O. Tired minds, tired ideas? Exploring insomnia and creativity. Think Skills Creativity. 2013;9:69–75.
Ma G, Cui F. Research progress on the relationship between personality traits and insomnia. Chin J Health Med. 2017;19(1):87–9.
Amabile TM. A model of creativity and innovation in organizations. In Staw BM, Cummings LL, editors. Research in organizational behavior. Greenwich. 1988, 123–167.
Mumford MD, Mobley MI, Reiter-Palmon R, et al. Process analytic models of creative capacities. Creativity Res J. 1991;4:91–122.
Cacioppo JT, Petty RE. The need for cognition. J Personal Soc Psychol. 1982;42:116–31.
Dollinger SJ. Need for uniqueness, need for cognition, and creativity. J Creative Behav. 2003;37:99–116.
Du Z, Zhang L, Wang Y. Relationship between college students cognitive need, specialty commitment and innovative personality. Chin J School Health. 2011;32(4):454–6.
Hahn MH, Lee KC. Exploring the role of self-confidence, need-for-cognition, and the degree of IT support on individual creativity: multilevel analysis approach. Curr Psychol. 2017;36:565–76.
Du Z, Zhang L, Wang Y. The relationship between college students’ cognitive needs, professional commitment and innovative personality. Chin School Health. 2011;32(4):454–6.
Morin CM. Insomnia: psychological assessment and management. Guilford Press. 1993; 56–60.
Harvey AG. A cognitive model of insomnia. Behav Res Ther. 2002;40(8):869–93.
Lin X, Wang M. Williams creative thinking activity manual. Taiwan: Psychology publishing house; 1997.
Li WF, Li XT, Huang LJ, et al. Brain structure links trait creativity to openness to experience. Soc Cognit Affect Neurosci. 2015;10(2):191–8.
Tang G, Zou H, Hou K, et al. The characteristics of family innovation environment and their effects on everyday creative behavior: the mediating role of personality. J Psychol Sci. 2014;37(5):1125–31.
Gao Q. Conceptualization and measurement of need for cognition. Chin J Psychol. 1994;36(1):1–20.
Yang L, Zhang Q. An experimental study on Relationship between intrinsic-extrinsic motivation and Creativity of 1-Grade Junior High School Students. J Southwest China Normal Univ (Natural Science). 2004;29(1):123–6.
Pallesen S, Bjorvatn B, Nordhus IH, et al. A new scale for measuring insomnia: the Bergen Insomnia Scale. Percept Mot Skills. 2008;107(3):691–706.
Wei S, Chen B, Zhao Q, Bai Y. Reliability and validity of the Chinese version of the Bergen Shift Sleep Questionnaire. Chin J Mod Nurs. 2018;24(29):3508–12.
Qiao N, Zhang J, Liu G, Lin C. Effects of family socioeconomic status and parental involvement on junior students’ academic achievements: the moderating role of students’ perception of teachers’ support. Psychol Dev Educ. 2013;29:507–14.
Shi B, Shen J. The relationship between family socioeconomic status, intelligence, internal motivation and creativity. Psychol Dev Educ. 2007;23:30–4.
Hayes AF. Introduction to mediation, moderation, and conditional process analysis. New York: Guilford Press; 2013.
Carciofo R. Morningness–eveningness and affect: the mediating roles of sleep quality and metacognitive beliefs. Sleep Biol Rhythms. 2020;18(1):17–26.
Zhou H, Long L. Statistical remedies for common method biases. Adv Psychol Sci. 2004;12:942–50.
Cohen J. Statistical power for the behavioral sciences. Hillsdale, NJ: Erlbaum; 1988.
Wen Z, Ye B. Mediation effects analysis: methodology and model development. Adv Psychol Sci. 2014;22(5):731–45.
Liu C, Zhang H, Zhang L, et al. The influence of parental rearing style on students’ creativity tendency and self-efficacy. China J Health Psychol. 2013;21(4):589–91.
Vygotsky LS. Imagination and creativity in childhood. J Russian East Eur Psychol. 2004;42(1):7–97.
Giampietro M, Cavallera GM. Morning and evening types and creative thinking. Pers Indiv Differ. 2006;42:453–63.
Liu N, Yuan Y. Relationship between creativity and insomnia in children and adolescents. China J Health Psychol. 2022;30(5):790–4.
Liu Z, Liu T, Mu S. Statistical analysis framework of suppression effect and its application. Psychology: Techniques Appl. 2021;9(10):610–8.
Download references
Acknowledgements
Not applicable.
This research did not receive any specifc grant from funding agencies in the public, commercial, or not-for-proft sectors.
Author information
Authors and affiliations.
Department of Psychology, Shandong Normal University, No.88 East Wenhua Road, 250014, Jinan, China
Xiaoyang Ren, Min Shi & Si Si
You can also search for this author in PubMed Google Scholar
Contributions
Xiaoyang Ren and Si Si wrote the main manuscript text and Min Shi prepared figures and tables. All authors reviewed the manuscript.Author Contributions Statement.
Corresponding author
Correspondence to Si Si .
Ethics declarations
Ethical approval and consent.
Ethical approval for the study was provided by the Institutional Review Board of Shandong Normal University, Jinan, China. Informed consent from their legal guardian for study participation was obtained. All measurements were performed in accordance with relevant guidelines and regulations of psychological study.
Competing interests
The authors declare no competing interests.
Conflict of interest
The author declared that the research was conducted in the absence of any commercial or financial relationships that could be construed as a potential conflict of interest.
Consent for publication
Additional information, publisher’s note.
Springer Nature remains neutral with regard to jurisdictional claims in published maps and institutional affiliations.
Electronic supplementary material
Below is the link to the electronic supplementary material.
Supplementary Material 1
Rights and permissions.
Open Access This article is licensed under a Creative Commons Attribution 4.0 International License, which permits use, sharing, adaptation, distribution and reproduction in any medium or format, as long as you give appropriate credit to the original author(s) and the source, provide a link to the Creative Commons licence, and indicate if changes were made. The images or other third party material in this article are included in the article’s Creative Commons licence, unless indicated otherwise in a credit line to the material. If material is not included in the article’s Creative Commons licence and your intended use is not permitted by statutory regulation or exceeds the permitted use, you will need to obtain permission directly from the copyright holder. To view a copy of this licence, visit http://creativecommons.org/licenses/by/4.0/ . The Creative Commons Public Domain Dedication waiver ( http://creativecommons.org/publicdomain/zero/1.0/ ) applies to the data made available in this article, unless otherwise stated in a credit line to the data.
Reprints and permissions
About this article
Cite this article.
Ren, X., Shi, M. & Si, S. Insomnia and creativity in Chinese adolescents: mediation through need for cognition. BMC Psychol 12 , 180 (2024). https://doi.org/10.1186/s40359-024-01663-3
Download citation
Received : 03 January 2024
Accepted : 15 March 2024
Published : 29 March 2024
DOI : https://doi.org/10.1186/s40359-024-01663-3
Share this article
Anyone you share the following link with will be able to read this content:
Sorry, a shareable link is not currently available for this article.
Provided by the Springer Nature SharedIt content-sharing initiative
- Time-of-day effect
- Indirect effect
BMC Psychology
ISSN: 2050-7283
- General enquiries: [email protected]
- Election 2024
- Entertainment
- Newsletters
- Photography
- Personal Finance
- AP Buyline Personal Finance
- Press Releases
- Israel-Hamas War
- Russia-Ukraine War
- Global elections
- Asia Pacific
- Latin America
- Middle East
- Election Results
- Delegate Tracker
- AP & Elections
- March Madness
- AP Top 25 Poll
- Movie reviews
- Book reviews
- Personal finance
- Financial Markets
- Business Highlights
- Financial wellness
- Artificial Intelligence
- Social Media
Book Review: Short story anthology ‘The Black Girl Survives in This One’ challenges the horror canon
This cover image released by Flatiron shows “The Black Girl Survives in This One” horror stories edited by Desiree S. Evans and Saraciea J. Fennell. (Flatiron via AP)
- Copy Link copied

Ahh, the Final Girl — a point of pride, a point of contention. Too often, the white, virginal, Western ideal. But not this time.
“The Black Girl Survives in This One,” a short story anthology edited by Saraciea J. Fennell and Desiree S. Evans, is changing the literary horror canon. As self-proclaimed fans of “Scary Stories to Tell in the Dark” and “Goosebumps,” the editors have upped the ante with a new collection spotlighting Black women and girls, defying the old tropes that would box Black people in as support characters or victims.
The 15 stories are introduced with an excellent forward by Tananarive Due laying out the groundwork with a brief history of Black women in horror films and literature, and of her own experiences. She argues with an infallible persuasiveness that survival is the thread that connects Black women and the genre that has largely shunned them for so long.
These are the kind of stories that stick with you long after you’ve read them.
“Queeniums for Greenium!” by Brittney Morris features a cult-ish smoothie MLM with a deadly level of blind faith that had my heart pounding and my eyes watering with laughter at intervals. And “The Skittering Thing” by Monica Brashears captures the sheer panic of being hunted in the dark, with some quirky twists.
Many of the stories are set in the most terrifying real-life place there is: high school. As such, there are teen crushes and romance aplenty, as well as timely slang that’s probably already outdated.
Honestly, this was one of the best parts: seeing 15 different authors’ takes on a late-teens Black girl. How does she wear her hair, who are her friends, is she religious, where does she live, does she like boys or girls or no one at all? Is she a bratty teen or a goody-two-shoes or a bookworm or just doing her best to get through it? Each protagonist is totally unique and the overall cast of both characters and writers diverse.
And even though we know the Black girl survives, the end is still a shock, because the real question is how.
The anthology has something for everyone, from a classic zombie horror in “Cemetery Dance Party” by Saraciea J. Fennell to a spooky twist on Afrofuturism in “Welcome Back to The Cosmos” by Kortney Nash. Two of the stories have major “Get Out” vibes that fans of Jordan Peele will appreciate (“Black Girl Nature Group” by Maika Moulite and Maritza Moulite and “Foxhunt” by Charlotte Nicole Davies). If your flavor is throwbacks and cryptids, Justina Ireland’s “Black Pride” has you covered. Or if you like slow-burn psychological thrillers and smart protagonists, “TMI” by Zakiya Delila Harris.
Overall, it’s a bit long and the anthology could stand to drop a couple of the weaker stories. But it’s well worth adding to any scary book collection, and horror fans are sure to find some new favorites.
AP book reviews: https://apnews.com/hub/book-reviews
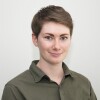
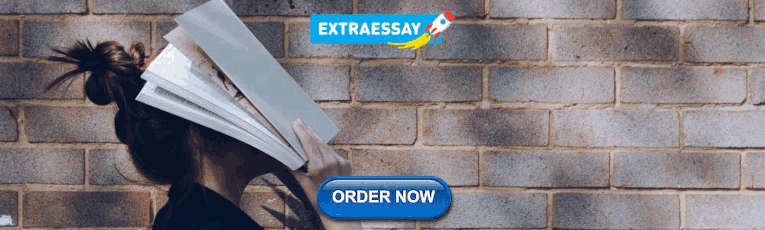
IMAGES
VIDEO
COMMENTS
Abstract. Purpose - A rapidly expanding body of literature on international mediation, as well as the central role international mediation plays in modern-day conflict resolution, make it ...
Literature review: Mediation or peacekeeping. As the main forms of non-coercive international intervention, mediation and peacekeeping have received extensive scholarly attention. Notably, a large number of quantitative studies find that both policy instruments reduce the severity and duration of conflict, as well as the likelihood of conflict ...
The literature that describes mediation per se, mediation approaches, and outcomes is very descriptive rather than theoretical. The literature that deals with the determinants of the mediation, approaches, and outcomes is quite descriptive but also provides an ample base for theory development.
The mediation literature of the past decade is organized into six topical areas: the determinants of media-tion, mediation per se, approaches employed by mediators, determinants of the mediation approaches, out- ... to review and analyze the mediation literature during this period. In the following pages, we proffer such a review, with an ...
The mediation literature does not provide an unanimous definition of success (Kleiboer 1996, p. 361; ... Duursma, A. (2014). A current literature review of international mediation. International Journal of Conflict Management, 25(1), 81-98. Article Google Scholar
In this article, we review the mediation literature from the past decade, utilizing a cybernetic mediation paradigm to organize the material. In this paradigm, we note that the type of conflict, country, culture, and mediation institutions affect the mediation process. Within this process, the mediator and disputants interact with each other ...
A rapidly expanding body of literature on international mediation, as well as the central role international mediation plays in modern-day conflict resolution, make it necessary to review and analyze this vastly evolving field of study. This study seeks to review the most significant trends and debates in the literature on international ...
ANN LYNN. University of Missouri. This article reviews the mediation literature over the past decade. Initially the literature is organized and integrated in a framework that focuses on the mediator's decision to mediate, choice of mediation techniques, the outcomes of mediation, and the determinants of these factors.
This article reviews the mediation literature over the past decade. Initially the literature is organized and integrated in a framework that focuses on the mediator's decision to mediate, the choice of mediation techniques, the outcomes of mediation, and the determinants of these factors. Subsequently, the authors comment on the reviewed literature and offer suggestions for future research.
Mediation Research: A Current Review. In this article, we review the mediation literature from the past decade, utilizing a cybernetic mediation paradigm to organize the material. In this paradigm, we note that the type of conflict, country, culture, and mediation institutions affect the mediation process. Within this process, the mediator and ...
This article reviews the mediation literature over the past decade. Initially the literature is organized and integrated in a framework that focuses on the mediator's decision to mediate, the choice of mediation techniques, the outcomes of mediation, and the determinants of these factors. Subsequently, the authors comment on the reviewed ...
Purpose - A rapidly expanding body of literature on international mediation, as well as the central role international mediation plays in modern-day conflict resolution, make it necessary to review and analyze this vastly evolving field of study. This study seeks to review the most significant trends and debates in the literature on international mediation, with an emphasis on the literature ...
A vectorial perspective on the work of conflict mediation. Relational ontologies have been receiving increased attention in fields such as organization studies (Orlikowski, 2007; Vosselman, 2014) and communication studies (Cooren, 2015b; Cooren and Sandler, 2014; Ganesh and Wang, 2015; Jahn, 2018), because they "can facilitate novel ways of attending to social problems" (Kuhn et al., 2017: 4).
This study seeks to review the most significant trends and debates in the literature on international mediation, with an emphasis on the literature of the past six years. Purpose - A rapidly expanding body of literature on international mediation, as well as the central role international mediation plays in modern-day conflict resolution ...
reviewed literature using such terms as mediation, workplace mediation, workplace con ict and alternative dispute resolution. In addition to a general search of peer revie w literature, search was ...
However, there is a lack of specific exploration on mediation strategies that mediators can apply in practice for a successful mediation. The aim of this report is to find feasible strategies that can be used by mediators through reviewing past literature on conflict mediation and rethinking a three-month mediation training the author experienced.
The review focuses on mediation analysis in quantitative empirical studies. Therefore, we excluded qualitative, mixed-method, and case studies, literature reviews, meta-analyses, methodological papers (e.g., the development of an instrument), and theoretical papers. 2.2. Literature search. We searched the literature in November and December 2019.
Fritz and MacKinnon (2007) showed that with small effect sizes of mediation regression parameters (i.e., β ^ 1 M and β ^ 2 Y), 20,886 subjects are needed to detect effects with .8 power in complete mediation models. Results from the literature review presented at the beginning of this article indicate that the median sample size of mediation ...
151 THE NEWCASTLE LAW REVIEW [VOL12 of the broader mediation literature suggests that the focus on simple effectiveness may have influenced the design of many mediation programs and services.3 So widespread is its application in research and practice, simple effectiveness could be said to be an "industry
Background Mediation analysis investigates whether a variable (i.e., mediator) changes in regard to an independent variable, in turn, affecting a dependent variable. Moderation analysis, on the other hand, investigates whether the statistical interaction between independent variables predict a dependent variable. Although this difference between these two types of analysis is explicit in ...
This was also surprising considering the mediation literature which points towards facilitative mediation being the norm rather than a more evaluative approach. It appeared that the mediator in this case study drew on his own legal experience to even provide an opinion at one stage. ... Moreton K. Literature Review: Disagreements in the Care of ...
Furthermore, a mediation model was constructed to investigate the role of the need for cognition in mediating the relationship between insomnia and creativity. The findings of the present study indicated that insomnia had a direct impact on the creativity of adolescents, demonstrating a time-of-day effect. ... After a thorough literature review ...
An organizational ethical climate enhances the degree of collaboration and cohesion among employees and facilitates the development and interests of organizations. Such roles lead to organizational sustainable development and survival. Therefore, the importance of ethical climate in organizations is becoming increasingly apparent. In this background, this study aims to explore whether an ...
In a conflict mediation, a mediator works as a third party to help disputants negotiate their problems and facilitate them to reach a consensus. The goal of a conflict mediations is more than solving problems. From a mediator's point of view, the key issue in a conflict mediation is to produce results that can benefit each party in the conflict. Previous research examined mediators ...
We conducted an extensive systematic literature review to understand the trends and efficacy of AI-based services in preventing and tracing child sexual abuse. The selection of research studies was performed in accordance with the PRISMA standards. Relevant studies were extracted from databases such as ScienceDirect, Springer, IEEE, and MDPI. ...
Objective: An exploratory review of the literature was carried out regarding the benefits of palliative care in patients with advanced heart failure, in order to synthesize the available and updated evidence. Methodology: Searched for articles published from 2017 to 2022 related to palliative care in patients with heart failure and using the ...
The trilogy opened with "City on Fire" (2021) as Ryan and a handful of allies fled Providence, Rhode Island, after losing a gang war to the Italian Mafia. It continued with "City of Dreams" (2023) as Ryan tried, and failed, to build a new life in Los Angeles. As "City in Ruins" opens, we find an older Ryan operating as a silent ...
The present PRISMA-guided article systematically reviews the current state of research on the autonomous sensory meridian response (ASMR). A systematic literature search was conducted in Pubmed, SCOPUS, and Web of Science (last search: March 2022) selecting all studies that conducted quantitative scientific research on the ASMR phenomenon. Fifty-four studies focusing on ASMR were retrieved ...
This article presents and assesses the state of the art in research on the course and outcomes of international mediation. The review consists of three parts. First, the elusive notion of mediation success is addressed. Second, the key contextual and process factors thought to explain international mediation outcomes are discussed.
Ahh, the Final Girl — a point of pride, a point of contention. Too often, the white, virginal, Western ideal. But not this time. "The Black Girl Survives in This One," a short story anthology edited by Saraciea J. Fennell and Desiree S. Evans, is changing the literary horror canon.