Incorporate STEM journalism in your classroom
- Exercise type: Activity
- Topic: Science & Society
- Category: Research & Design
- Category: Diversity in STEM
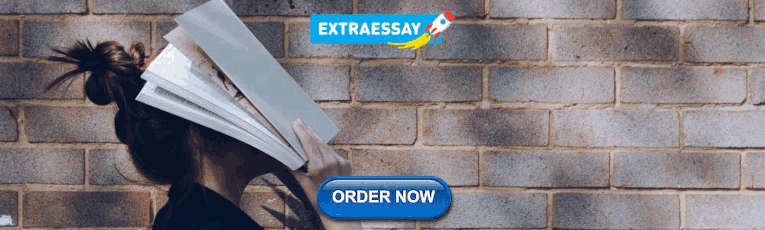
How bias affects scientific research
- Download Student Worksheet
Purpose: Students will work in groups to evaluate bias in scientific research and engineering projects and to develop guidelines for minimizing potential biases.
Procedural overview: After reading the Science News for Students article “ Think you’re not biased? Think again ,” students will discuss types of bias in scientific research and how to identify it. Students will then search the Science News archive for examples of different types of bias in scientific and medical research. Students will read the National Institute of Health’s Policy on Sex as a Biological Variable and analyze how this policy works to reduce bias in scientific research on the basis of sex and gender. Based on their exploration of bias, students will discuss the benefits and limitations of research guidelines for minimizing particular types of bias and develop guidelines of their own.
Approximate class time: 2 class periods
How Bias Affects Scientific Research student guide
Computer with access to the Science News archive
Interactive meeting and screen-sharing application for virtual learning (optional)
Directions for teachers:
One of the guiding principles of scientific inquiry is objectivity. Objectivity is the idea that scientific questions, methods and results should not be affected by the personal values, interests or perspectives of researchers. However, science is a human endeavor, and experimental design and analysis of information are products of human thought processes. As a result, biases may be inadvertently introduced into scientific processes or conclusions.
In scientific circles, bias is described as any systematic deviation between the results of a study and the “truth.” Bias is sometimes described as a tendency to prefer one thing over another, or to favor one person, thing or explanation in a way that prevents objectivity or that influences the outcome of a study or the understanding of a phenomenon. Bias can be introduced in multiple points during scientific research — in the framing of the scientific question, in the experimental design, in the development or implementation of processes used to conduct the research, during collection or analysis of data, or during the reporting of conclusions.
Researchers generally recognize several different sources of bias, each of which can strongly affect the results of STEM research. Three types of bias that often occur in scientific and medical studies are researcher bias, selection bias and information bias.
Researcher bias occurs when the researcher conducting the study is in favor of a certain result. Researchers can influence outcomes through their study design choices, including who they choose to include in a study and how data are interpreted. Selection bias can be described as an experimental error that occurs when the subjects of the study do not accurately reflect the population to whom the results of the study will be applied. This commonly happens as unequal inclusion of subjects of different races, sexes or genders, ages or abilities. Information bias occurs as a result of systematic errors during the collection, recording or analysis of data.
When bias occurs, a study’s results may not accurately represent phenomena in the real world, or the results may not apply in all situations or equally for all populations. For example, if a research study does not address the full diversity of people to whom the solution will be applied, then the researchers may have missed vital information about whether and how that solution will work for a large percentage of a target population.
Bias can also affect the development of engineering solutions. For example, a new technology product tested only with teenagers or young adults who are comfortable using new technologies may have user experience issues when placed in the hands of older adults or young children.
Want to make it a virtual lesson? Post the links to the Science News for Students article “ Think you’re not biased? Think again ,” and the National Institutes of Health information on sickle-cell disease . A link to additional resources can be provided for the students who want to know more. After students have reviewed the information at home, discuss the four questions in the setup and the sickle-cell research scenario as a class. When the students have a general understanding of bias in research, assign students to breakout rooms to look for examples of different types of bias in scientific and medical research, to discuss the Science News article “ Biomedical studies are including more female subjects (finally) ” and the National Institute of Health’s Policy on Sex as a Biological Variable and to develop bias guidelines of their own. Make sure the students have links to all articles they will need to complete their work. Bring the groups back together for an all-class discussion of the bias guidelines they write.
Assign the Science News for Students article “ Think you’re not biased? Think again ” as homework reading to introduce students to the core concepts of scientific objectivity and bias. Request that they answer the first two questions on their guide before the first class discussion on this topic. In this discussion, you will cover the idea of objective truth and introduce students to the terminology used to describe bias. Use the background information to decide what level of detail you want to give to your students.
As students discuss bias, help them understand objective and subjective data and discuss the importance of gathering both kinds of data. Explain to them how these data differ. Some phenomena — for example, body temperature, blood type and heart rate — can be objectively measured. These data tend to be quantitative. Other phenomena cannot be measured objectively and must be considered subjectively. Subjective data are based on perceptions, feelings or observations and tend to be qualitative rather than quantitative. Subjective measurements are common and essential in biomedical research, as they can help researchers understand whether a therapy changes a patient’s experience. For instance, subjective data about the amount of pain a patient feels before and after taking a medication can help scientists understand whether and how the drug works to alleviate pain. Subjective data can still be collected and analyzed in ways that attempt to minimize bias.
Try to guide student discussion to include a larger context for bias by discussing the effects of bias on understanding of an “objective truth.” How can someone’s personal views and values affect how they analyze information or interpret a situation?
To help students understand potential effects of biases, present them with the following scenario based on information from the National Institutes of Health :
Sickle-cell disease is a group of inherited disorders that cause abnormalities in red blood cells. Most of the people who have sickle-cell disease are of African descent; it also appears in populations from the Mediterranean, India and parts of Latin America. Males and females are equally likely to inherit the condition. Imagine that a therapy was developed to treat the condition, and clinical trials enlisted only male subjects of African descent. How accurately would the results of that study reflect the therapy’s effectiveness for all people who suffer from sickle-cell disease?
In the sickle-cell scenario described above, scientists will have a good idea of how the therapy works for males of African descent. But they may not be able to accurately predict how the therapy will affect female patients or patients of different races or ethnicities. Ask the students to consider how they would devise a study that addressed all the populations affected by this disease.
Before students move on, have them answer the following questions. The first two should be answered for homework and discussed in class along with the remaining questions.
1.What is bias?
In common terms, bias is a preference for or against one idea, thing or person. In scientific research, bias is a systematic deviation between observations or interpretations of data and an accurate description of a phenomenon.
2. How can biases affect the accuracy of scientific understanding of a phenomenon? How can biases affect how those results are applied?
Bias can cause the results of a scientific study to be disproportionately weighted in favor of one result or group of subjects. This can cause misunderstandings of natural processes that may make conclusions drawn from the data unreliable. Biased procedures, data collection or data interpretation can affect the conclusions scientists draw from a study and the application of those results. For example, if the subjects that participate in a study testing an engineering design do not reflect the diversity of a population, the end product may not work as well as desired for all users.
3. Describe two potential sources of bias in a scientific, medical or engineering research project. Try to give specific examples.
Researchers can intentionally or unintentionally introduce biases as a result of their attitudes toward the study or its purpose or toward the subjects or a group of subjects. Bias can also be introduced by methods of measuring, collecting or reporting data. Examples of potential sources of bias include testing a small sample of subjects, testing a group of subjects that is not diverse and looking for patterns in data to confirm ideas or opinions already held.
4. How can potential biases be identified and eliminated before, during or after a scientific study?
Students should brainstorm ways to identify sources of bias in the design of research studies. They may suggest conducting implicit bias testing or interviews before a study can be started, developing guidelines for research projects, peer review of procedures and samples/subjects before beginning a study, and peer review of data and conclusions after the study is completed and before it is published. Students may focus on the ideals of transparency and replicability of results to help reduce biases in scientific research.
Obtain and evaluate information about bias
Students will now work in small groups to select and analyze articles for different types of bias in scientific and medical research. Students will start by searching the Science News or Science News for Students archives and selecting articles that describe scientific studies or engineering design projects. If the Science News or Science News for Students articles chosen by students do not specifically cite and describe a study, students should consult the Citations at the end of the article for links to related primary research papers. Students may need to read the methods section and the conclusions of the primary research paper to better understand the project’s design and to identify potential biases. Do not assume that every scientific paper features biased research.
Student groups should evaluate the study or engineering design project outlined in the article to identify any biases in the experimental design, data collection, analysis or results. Students may need additional guidance for identifying biases. Remind them of the prior discussion about sources of bias and task them to review information about indicators of bias. Possible indicators include extreme language such as all , none or nothing ; emotional appeals rather than logical arguments; proportions of study subjects with specific characteristics such as gender, race or age; arguments that support or refute one position over another and oversimplifications or overgeneralizations. Students may also want to look for clues related to the researchers’ personal identity such as race, religion or gender. Information on political or religious points of view, sources of funding or professional affiliations may also suggest biases.
Students should also identify any deliberate attempts to reduce or eliminate bias in the project or its results. Then groups should come back together and share the results of their analysis with the class.
If students need support in searching the archives for appropriate articles, encourage groups to brainstorm search terms that may turn up related articles. Some potential search terms include bias , study , studies , experiment , engineer , new device , design , gender , sex , race , age , aging , young , old , weight , patients , survival or medical .
If you are short on time or students do not have access to the Science News or Science News for Students archive, you may want to provide articles for students to review. Some suggested articles are listed in the additional resources below.
Once groups have selected their articles, students should answer the following questions in their groups.
1. Record the title and URL of the article and write a brief summary of the study or project.
Answers will vary, but students should accurately cite the article evaluated and summarize the study or project described in the article. Sample answer: We reviewed the Science News article “Even brain images can be biased,” which can be found at www.sciencenews.org/blog/scicurious/even-brain-images-can-be-biased. This article describes how scientific studies of human brains that involve electronic images of brains tend to include study subjects from wealthier and more highly educated households and how researchers set out to collect new data to make the database of brain images more diverse.
2. What sources of potential bias (if any) did you identify in the study or project? Describe any procedures or policies deliberately included in the study or project to eliminate biases.
The article “Even brain images can be biased” describes how scientists identified a sampling bias in studies of brain images that resulted from the way subjects were recruited. Most of these studies were conducted at universities, so many college students volunteer to participate, which resulted in the samples being skewed toward wealthier, educated, white subjects. Scientists identified a database of pediatric brain images and evaluated the diversity of the subjects in that database. They found that although the subjects in that database were more ethnically diverse than the U.S. population, the subjects were generally from wealthier households and the parents of the subjects tended to be more highly educated than average. Scientists applied statistical methods to weight the data so that study samples from the database would more accurately reflect American demographics.
3. How could any potential biases in the study or design project have affected the results or application of the results to the target population?
Scientists studying the rate of brain development in children were able to recognize the sampling bias in the brain image database. When scientists were able to apply statistical methods to ensure a better representation of socioeconomically diverse samples, they saw a different pattern in the rate of brain development in children. Scientists learned that, in general, children’s brains matured more quickly than they had previously thought. They were able to draw new conclusions about how certain factors, such as family wealth and education, affected the rate at which children’s brains developed. But the scientsits also suggested that they needed to perform additional studies with a deliberately selected group of children to ensure true diversity in the samples.
In this phase, students will review the Science News article “ Biomedical studies are including more female subjects (finally) ” and the NIH Policy on Sex as a Biological Variable , including the “ guidance document .” Students will identify how sex and gender biases may have affected the results of biomedical research before NIH instituted its policy. The students will then work with their group to recommend other policies to minimize biases in biomedical research.
To guide their development of proposed guidelines, students should answer the following questions in their groups.
1. How have sex and gender biases affected the value and application of biomedical research?
Gender and sex biases in biomedical research have diminished the accuracy and quality of research studies and reduced the applicability of results to the entire population. When girls and women are not included in research studies, the responses and therapeutic outcomes of approximately half of the target population for potential therapies remain unknown.
2. Why do you think the NIH created its policy to reduce sex and gender biases?
In the guidance document, the NIH states that “There is a growing recognition that the quality and generalizability of biomedical research depends on the consideration of key biological variables, such as sex.” The document goes on to state that many diseases and conditions affect people of both sexes, and restricting diversity of biological variables, notably sex and gender, undermines the “rigor, transparency, and generalizability of research findings.”
3. What impact has the NIH Policy on Sex as a Biological Variable had on biomedical research?
The NIH’s policy that sex is factored into research designs, analyses and reporting tries to ensure that when developing and funding biomedical research studies, researchers and institutes address potential biases in the planning stages, which helps to reduce or eliminate those biases in the final study. Including females in biomedical research studies helps to ensure that the results of biomedical research are applicable to a larger proportion of the population, expands the therapies available to girls and women and improves their health care outcomes.
4. What other policies do you think the NIH could institute to reduce biases in biomedical research? If you were to recommend one set of additional guidelines for reducing bias in biomedical research, what guidelines would you propose? Why?
Students could suggest that the NIH should have similar policies related to race, gender identity, wealth/economic status and age. Students should identify a category of bias or an underserved segment of the population that they think needs to be addressed in order to improve biomedical research and health outcomes for all people and should recommend guidelines to reduce bias related to that group. Students recommending guidelines related to race might suggest that some populations, such as African Americans, are historically underserved in terms of access to medical services and health care, and they might suggest guidelines to help reduce the disparity. Students might recommend that a certain percentage of each biomedical research project’s sample include patients of diverse racial and ethnic backgrounds.
5. What biases would your suggested policy help eliminate? How would it accomplish that goal?
Students should describe how their proposed policy would address a discrepancy in the application of biomedical research to the entire human population. Race can be considered a biological variable, like sex, and race has been connected to higher or lower incidence of certain characteristics or medical conditions, such as blood types or diabetes, which sometimes affect how the body reponds to infectious agents, drugs, procedures or other therapies. By ensuring that people from diverse racial and ethnic groups are included in biomedical research studies, scientists and medical professionals can provide better medical care to members of those populations.
Class discussion about bias guidelines
Allow each group time to present its proposed bias-reducing guideline to another group and to receive feedback. Then provide groups with time to revise their guidelines, if necessary. Act as a facilitator while students conduct the class discussion. Use this time to assess individual and group progress. Students should demonstrate an understanding of different biases that may affect patient outcomes in biomedical research studies and in practical medical settings. As part of the group discussion, have students answer the following questions.
1. Why is it important to identify and eliminate biases in research and engineering design?
The goal of most scientific research and engineering projects is to improve the quality of life and the depth of understanding of the world we live in. By eliminating biases, we can better serve the entirety of the human population and the planet .
2. Were there any guidelines that were suggested by multiple groups? How do those actions or policies help reduce bias?
Answers will depend on the guidelines developed and recommended by other groups. Groups could suggest policies related to race, gender identity, wealth/economic status and age. Each group should clearly identify how its guidelines are designed to reduce bias and improve the quality of human life.
3. Which guidelines developed by your classmates do you think would most reduce the effects of bias on research results or engineering designs? Support your selection with evidence and scientific reasoning.
Answers will depend on the guidelines developed and recommended by other groups. Students should agree that guidelines that minimize inequities and improve health care outcomes for a larger group are preferred. Guidelines addressing inequities of race and wealth/economic status are likely to expand access to improved medical care for the largest percentage of the population. People who grow up in less economically advantaged settings have specific health issues related to nutrition and their access to clean water, for instance. Ensuring that people from the lowest economic brackets are represented in biomedical research improves their access to medical care and can dramatically change the length and quality of their lives.
Possible extension
Challenge students to honestly evaluate any biases they may have. Encourage them to take an Implicit Association Test (IAT) to identify any implicit biases they may not recognize. Harvard University has an online IAT platform where students can participate in different assessments to identify preferences and biases related to sex and gender, race, religion, age, weight and other factors. You may want to challenge students to take a test before they begin the activity, and then assign students to take a test after completing the activity to see if their preferences have changed. Students can report their results to the class if they want to discuss how awareness affects the expression of bias.
Additional resources
If you want additional resources for the discussion or to provide resources for student groups, check out the links below.
Additional Science News articles:
Even brain images can be biased
Data-driven crime prediction fails to erase human bias
What we can learn from how a doctor’s race can affect Black newborns’ survival
Bias in a common health care algorithm disproportionately hurts black patients
Female rats face sex bias too
There’s no evidence that a single ‘gay gene’ exists
Positive attitudes about aging may pay off in better health
What male bias in the mammoth fossil record says about the animal’s social groups
The man flu struggle might be real, says one researcher
Scientists may work to prevent bias, but they don’t always say so
The Bias Finders
Showdown at Sex Gap
University resources:
Project Implicit (Take an Implicit Association Tests)
Catalogue of Bias
Understanding Health Research
Log in using your username and password
- Search More Search for this keyword Advanced search
- Latest content
- Current issue
- Write for Us
- BMJ Journals More You are viewing from: Google Indexer
You are here
- Volume 17, Issue 4
- Bias in research
- Article Text
- Article info
- Citation Tools
- Rapid Responses
- Article metrics

- Joanna Smith 1 ,
- Helen Noble 2
- 1 School of Human and Health Sciences, University of Huddersfield , Huddersfield , UK
- 2 School of Nursing and Midwifery, Queens's University Belfast , Belfast , UK
- Correspondence to : Dr Joanna Smith , School of Human and Health Sciences, University of Huddersfield, Huddersfield HD1 3DH, UK; j.e.smith{at}hud.ac.uk
https://doi.org/10.1136/eb-2014-101946
Statistics from Altmetric.com
Request permissions.
If you wish to reuse any or all of this article please use the link below which will take you to the Copyright Clearance Center’s RightsLink service. You will be able to get a quick price and instant permission to reuse the content in many different ways.
The aim of this article is to outline types of ‘bias’ across research designs, and consider strategies to minimise bias. Evidence-based nursing, defined as the “process by which evidence, nursing theory, and clinical expertise are critically evaluated and considered, in conjunction with patient involvement, to provide the delivery of optimum nursing care,” 1 is central to the continued development of the nursing professional. Implementing evidence into practice requires nurses to critically evaluate research, in particular assessing the rigour in which methods were undertaken and factors that may have biased findings.
What is bias in relation to research and why is understanding bias important?
Although different study designs have specific methodological challenges and constraints, bias can occur at each stage of the research process ( table 1 ). In quantitative research, the validity and reliability are assessed using statistical tests that estimate the size of error in samples and calculating the significance of findings (typically p values or CIs). The tests and measures used to establish the validity and reliability of quantitative research cannot be applied to qualitative research. However, in the broadest context, these terms are applicable, with validity referring to the integrity and application of the methods and the precision in which the findings accurately reflect the data, and reliability referring to the consistency within the analytical processes. 4
- View inline
Types of research bias
How is bias minimised when undertaken research?
Bias exists in all study designs, and although researchers should attempt to minimise bias, outlining potential sources of bias enables greater critical evaluation of the research findings and conclusions. Researchers bring to each study their experiences, ideas, prejudices and personal philosophies, which if accounted for in advance of the study, enhance the transparency of possible research bias. Clearly articulating the rationale for and choosing an appropriate research design to meet the study aims can reduce common pitfalls in relation to bias. Ethics committees have an important role in considering whether the research design and methodological approaches are biased, and suitable to address the problem being explored. Feedback from peers, funding bodies and ethics committees is an essential part of designing research studies, and often provides valuable practical guidance in developing robust research.
In quantitative studies, selection bias is often reduced by the random selection of participants, and in the case of clinical trials randomisation of participants into comparison groups. However, not accounting for participants who withdraw from the study or are lost to follow-up can result in sample bias or change the characteristics of participants in comparison groups. 7 In qualitative research, purposeful sampling has advantages when compared with convenience sampling in that bias is reduced because the sample is constantly refined to meet the study aims. Premature closure of the selection of participants before analysis is complete can threaten the validity of a qualitative study. This can be overcome by continuing to recruit new participants into the study during data analysis until no new information emerges, known as data saturation. 8
In quantitative studies having a well-designed research protocol explicitly outlining data collection and analysis can assist in reducing bias. Feasibility studies are often undertaken to refine protocols and procedures. Bias can be reduced by maximising follow-up and where appropriate in randomised control trials analysis should be based on the intention-to-treat principle, a strategy that assesses clinical effectiveness because not everyone complies with treatment and the treatment people receive may be changed according to how they respond. Qualitative research has been criticised for lacking transparency in relation to the analytical processes employed. 4 Qualitative researchers must demonstrate rigour, associated with openness, relevance to practice and congruence of the methodological approach. Although other researchers may interpret the data differently, appreciating and understanding how the themes were developed is an essential part of demonstrating the robustness of the findings. Reducing bias can include respondent validation, constant comparisons across participant accounts, representing deviant cases and outliers, prolonged involvement or persistent observation of participants, independent analysis of the data by other researchers and triangulation. 4
In summary, minimising bias is a key consideration when designing and undertaking research. Researchers have an ethical duty to outline the limitations of studies and account for potential sources of bias. This will enable health professionals and policymakers to evaluate and scrutinise study findings, and consider these when applying findings to practice or policy.
- Wakefield AJ ,
- Anthony A ,
- ↵ The Lancet . Retraction—ileal-lymphoid-nodular hyperplasia, non-specific colitis, and pervasive developmental disorder in children . Lancet 2010 ; 375 : 445 . OpenUrl CrossRef PubMed Web of Science
- Easterbrook PJ ,
- Berlin JA ,
- Gopalan R ,
- Petticrew M ,
- Thomson H ,
- Francis J ,
- Johnston M ,
- Robertson C ,
Competing interests None.
Read the full text or download the PDF:
How to present limitations in research
Last updated
30 January 2024
Reviewed by
Limitations don’t invalidate or diminish your results, but it’s best to acknowledge them. This will enable you to address any questions your study failed to answer because of them.
In this guide, learn how to recognize, present, and overcome limitations in research.
- What is a research limitation?
Research limitations are weaknesses in your research design or execution that may have impacted outcomes and conclusions. Uncovering limitations doesn’t necessarily indicate poor research design—it just means you encountered challenges you couldn’t have anticipated that limited your research efforts.
Does basic research have limitations?
Basic research aims to provide more information about your research topic. It requires the same standard research methodology and data collection efforts as any other research type, and it can also have limitations.
- Common research limitations
Researchers encounter common limitations when embarking on a study. Limitations can occur in relation to the methods you apply or the research process you design. They could also be connected to you as the researcher.
Methodology limitations
Not having access to data or reliable information can impact the methods used to facilitate your research. A lack of data or reliability may limit the parameters of your study area and the extent of your exploration.
Your sample size may also be affected because you won’t have any direction on how big or small it should be and who or what you should include. Having too few participants won’t adequately represent the population or groups of people needed to draw meaningful conclusions.
Research process limitations
The study’s design can impose constraints on the process. For example, as you’re conducting the research, issues may arise that don’t conform to the data collection methodology you developed. You may not realize until well into the process that you should have incorporated more specific questions or comprehensive experiments to generate the data you need to have confidence in your results.
Constraints on resources can also have an impact. Being limited on participants or participation incentives may limit your sample sizes. Insufficient tools, equipment, and materials to conduct a thorough study may also be a factor.
Common researcher limitations
Here are some of the common researcher limitations you may encounter:
Time: some research areas require multi-year longitudinal approaches, but you might not be able to dedicate that much time. Imagine you want to measure how much memory a person loses as they age. This may involve conducting multiple tests on a sample of participants over 20–30 years, which may be impossible.
Bias: researchers can consciously or unconsciously apply bias to their research. Biases can contribute to relying on research sources and methodologies that will only support your beliefs about the research you’re embarking on. You might also omit relevant issues or participants from the scope of your study because of your biases.
Limited access to data : you may need to pay to access specific databases or journals that would be helpful to your research process. You might also need to gain information from certain people or organizations but have limited access to them. These cases require readjusting your process and explaining why your findings are still reliable.
- Why is it important to identify limitations?
Identifying limitations adds credibility to research and provides a deeper understanding of how you arrived at your conclusions.
Constraints may have prevented you from collecting specific data or information you hoped would prove or disprove your hypothesis or provide a more comprehensive understanding of your research topic.
However, identifying the limitations contributing to your conclusions can inspire further research efforts that help gather more substantial information and data.
- Where to put limitations in a research paper
A research paper is broken up into different sections that appear in the following order:
Introduction
Methodology
The discussion portion of your paper explores your findings and puts them in the context of the overall research. Either place research limitations at the beginning of the discussion section before the analysis of your findings or at the end of the section to indicate that further research needs to be pursued.
What not to include in the limitations section
Evidence that doesn’t support your hypothesis is not a limitation, so you shouldn’t include it in the limitation section. Don’t just list limitations and their degree of severity without further explanation.
- How to present limitations
You’ll want to present the limitations of your study in a way that doesn’t diminish the validity of your research and leave the reader wondering if your results and conclusions have been compromised.
Include only the limitations that directly relate to and impact how you addressed your research questions. Following a specific format enables the reader to develop an understanding of the weaknesses within the context of your findings without doubting the quality and integrity of your research.
Identify the limitations specific to your study
You don’t have to identify every possible limitation that might have occurred during your research process. Only identify those that may have influenced the quality of your findings and your ability to answer your research question.
Explain study limitations in detail
This explanation should be the most significant portion of your limitation section.
Link each limitation with an interpretation and appraisal of their impact on the study. You’ll have to evaluate and explain whether the error, method, or validity issues influenced the study’s outcome and how.
Propose a direction for future studies and present alternatives
In this section, suggest how researchers can avoid the pitfalls you experienced during your research process.
If an issue with methodology was a limitation, propose alternate methods that may help with a smoother and more conclusive research project. Discuss the pros and cons of your alternate recommendation.
Describe steps taken to minimize each limitation
You probably took steps to try to address or mitigate limitations when you noticed them throughout the course of your research project. Describe these steps in the limitation section.
- Limitation example
“Approaches like stem cell transplantation and vaccination in AD [Alzheimer’s disease] work on a cellular or molecular level in the laboratory. However, translation into clinical settings will remain a challenge for the next decade.”
The authors are saying that even though these methods showed promise in helping people with memory loss when conducted in the lab (in other words, using animal studies), more studies are needed. These may be controlled clinical trials, for example.
However, the short life span of stem cells outside the lab and the vaccination’s severe inflammatory side effects are limitations. Researchers won’t be able to conduct clinical trials until these issues are overcome.
- How to overcome limitations in research
You’ve already started on the road to overcoming limitations in research by acknowledging that they exist. However, you need to ensure readers don’t mistake weaknesses for errors within your research design.
To do this, you’ll need to justify and explain your rationale for the methods, research design, and analysis tools you chose and how you noticed they may have presented limitations.
Your readers need to know that even when limitations presented themselves, you followed best practices and the ethical standards of your field. You didn’t violate any rules and regulations during your research process.
You’ll also want to reinforce the validity of your conclusions and results with multiple sources, methods, and perspectives. This prevents readers from assuming your findings were derived from a single or biased source.
- Learning and improving starts with limitations in research
Dealing with limitations with transparency and integrity helps identify areas for future improvements and developments. It’s a learning process, providing valuable insights into how you can improve methodologies, expand sample sizes, or explore alternate approaches to further support the validity of your findings.
Get started today
Go from raw data to valuable insights with a flexible research platform
Editor’s picks
Last updated: 21 December 2023
Last updated: 16 December 2023
Last updated: 6 October 2023
Last updated: 25 November 2023
Last updated: 12 May 2023
Last updated: 15 February 2024
Last updated: 11 March 2024
Last updated: 12 December 2023
Last updated: 18 May 2023
Last updated: 6 March 2024
Last updated: 10 April 2023
Last updated: 20 December 2023
Latest articles
Related topics, log in or sign up.
Get started for free
Thank you for visiting nature.com. You are using a browser version with limited support for CSS. To obtain the best experience, we recommend you use a more up to date browser (or turn off compatibility mode in Internet Explorer). In the meantime, to ensure continued support, we are displaying the site without styles and JavaScript.
- View all journals
- My Account Login
- Explore content
- About the journal
- Publish with us
- Sign up for alerts
- Open access
- Published: 10 November 2022
Large studies reveal how reference bias limits policy applications of self-report measures
- Benjamin Lira 1 na1 ,
- Joseph M. O’Brien 2 na1 ,
- Pablo A. Peña 3 ,
- Brian M. Galla 4 ,
- Sidney D’Mello 5 ,
- David S. Yeager 2 ,
- Amy Defnet 6 ,
- Tim Kautz 6 ,
- Kate Munkacsy 6 &
- Angela L. Duckworth 1
Scientific Reports volume 12 , Article number: 19189 ( 2022 ) Cite this article
6400 Accesses
7 Citations
26 Altmetric
Metrics details
- Human behaviour
There is growing policy interest in identifying contexts that cultivate self-regulation. Doing so often entails comparing groups of individuals (e.g., from different schools). We show that self-report questionnaires—the most prevalent modality for assessing self-regulation—are prone to reference bias , defined as systematic error arising from differences in the implicit standards by which individuals evaluate behavior. In three studies, adolescents ( N = 229,685) whose peers performed better academically rated themselves lower in self-regulation and held higher standards for self-regulation. This effect was not observed for task measures of self-regulation and led to paradoxical predictions of college persistence 6 years later. These findings suggest that standards for self-regulation vary by social group, limiting the policy applications of self-report questionnaires.
Similar content being viewed by others
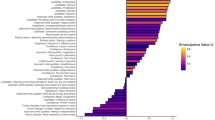
Worldwide divergence of values
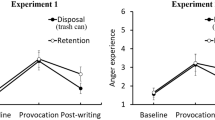
Anger is eliminated with the disposal of a paper written because of provocation
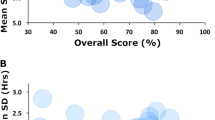
Sleep quality, duration, and consistency are associated with better academic performance in college students
Introduction.
Self-regulation refers to a diverse set of personal qualities, distinct from cognitive ability, that enable individuals to set and pursue goals. The terminology favored for self-regulation and its facets varies across the literatures of child development (e.g., effortful control, ego strength) 1 , 2 , 3 , adult personality (e.g., Big Five conscientiousness) 4 , psychopathology (e.g., impulse control) 5 , and economics (e.g., temporal discounting) 6 , 7 . Such diverse traditions in behavioral science have directed this attention because individual differences in self-regulation predict later life outcomes, including academic performance 8 , 9 , 10 ; physical and mental health 11 , 12 , 13 ; well-being and life satisfaction 14 ; civic and social behavior 12 , 15 ; job performance 16 ; earnings 12 , 17 , 18 , 19 ; and wealth 12 , 17 . Moreover, the effects of self-regulation are independent of, and comparable in magnitude to, cognitive ability and family socioeconomic status (SES) 8 , 12 .
A half-century of basic research suggests that self-regulation develops optimally in caring environments that encourage adaptive goal-relevant knowledge (e.g., strategies for managing attention), beliefs (e.g., that emotion and motivation can be regulated), and values (e.g., that self-regulation is important) 20 . This development extends far beyond early childhood, when children are mostly in the company and care of parents. Indeed, adolescence may be particularly important for supporting self-regulation because of the rapid growth, learning, adaptation, and neurobiological development that mark this period of life 21 , 22 , 23 . Further, impulsive choices in adolescence (e.g., to start smoking, to drop out of school) can alter life trajectories in ways that are difficult to reverse 12 .
Schools are a natural target for policy because of their potential to provide equal access to environments that support the development of self-regulation 24 , 25 . Not only is school where young people spend most of their waking hours outside the home, it is also where they experience a multitude of factors that have been shown to either scaffold or stymie the development of self-regulation, including adult role models 26 , 27 and peers 28 , 29 . Recently, a growing chorus of policymakers has urged schools to extend their purview beyond traditional academic coursework and into the domain of social-emotional skills such as self-regulation—a trend that is reflected in the expanded scope of federal and state standards and accountability systems 30 , 31 , 32 .
In this investigation, we identify a pervasive measurement bias that, if not remedied, may thwart policymakers’ efforts to evaluate, measure, and improve the effectiveness of schools that foster adolescent self-regulation. The possibility of this measurement bias has led to serious questions from policymakers about “whether we can make [self-regulation skills] visible, comparable, and therefore amenable to deliberate policy action in a similar way that traditional tests do with academic knowledge and skills” 33 . As a result, education systems have been left with great interest in self-regulation and related constructs—but insufficient scientific guidance.
The empirical starting point for our research is the mixed and often counterintuitive evidence regarding school effects on self-regulation. On one hand, Jackson et al. 34 show encouraging evidence that schools can differ in how much they improve students’ scores on a self-report measure of hard work, and these school differences predicted students’ later college enrollment and persistence. On the other hand, evaluations of charter schools show that they fail to raise self-reports of self-regulation, despite raising report card grades, standardized test scores, attendance rates, and college enrollment levels while reducing incarceration and unplanned pregnancies 35 , 36 , 37 , 38 . Are high-performing schools whose cultures explicitly emphasize hard work and high expectations 39 , 40 in fact having no impact on students’ self-regulation—or is there a problem in how self-regulation is measured?
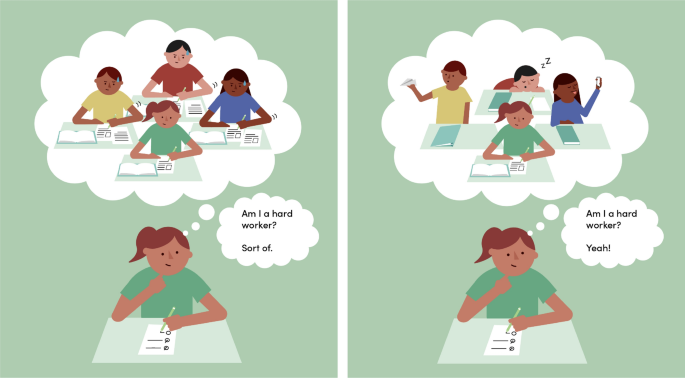
Peers influence the standards by which an individual judges their own behavior, resulting in a “reference bias” effect that distorts cross-context comparisons of self-reported self-regulation. Illustration by Tom McQuaid.
We suggest that reference bias, the systematic error that arises when respondents refer to different implicit standards when answering the same questions 41 , is a legitimate threat to between-school comparisons and can help explain the conflicting evidence of school effects on self-regulation. Moreover, we contend that even within a school, comparisons of students are biased when different subgroups of students rely on different standards when answering the same questions. In the present policy context, reference bias is especially pernicious because it is difficult to detect and diagnose. Unlike social desirability bias, modesty bias 42 , faking, and response style biases 43 , reference bias can emerge even when respondents answer truthfully, and it can coexist with otherwise strong validity associations at the individual level. This is because reference bias can distort inferences any time there are comparisons of self-regulation across groups who differ in their frames of references—for example, schools with very different peer cultures with respect to effort, or even subcultures within a school.
Why might self-report questionnaires be subject to reference bias? Dominant models in survey methodology identify a multi-stage cognitive response process: students first read and interpret the question; then they identify relevant information in memory, form a summary judgment, and translate this judgment into one of the response options; finally, they edit their response if motivated to do so 44 , 45 , 46 . As illustrated in Fig. 1 , a student may interpret a questionnaire item and its response options differently depending on their peers’ typical behaviors 47 . If they have high-achieving classmates who, for example, study for hours each evening and consistently arrive prepared for class, they might judge themselves against higher standards and rate themselves lower in self-regulation than an equally industrious student whose lower-achieving peers study and prepare less. While schools might be effective in increasing self-regulated behavior, they might at the same time increase the standards, leading to lower self-reported self-regulation.
A well-established research literature has demonstrated that the subjective view students hold of themselves, both in general terms (i.e., self-esteem) and in the realm of academic performance (i.e., academic self-concept) depends upon peer comparisons 42 , 48 , 49 . In particular, the Big Fish Little Pond Effect (BFLPE) refers to the lower academic self-concept of students in higher-achieving schools 50 . A related and older literature on social comparison has demonstrated that in general, people spontaneously compare themselves to other people, especially to people who are superior to them in some way, which can lower their subjective appraisal of their own ability 51 . Finally, there is evidence that academic self-concept and standardized test scores are positively correlated within countries but inversely correlated between countries—a phenomenon dubbed the attitude-achievement paradox 42 , 52 . In sum, there is ample evidence for the influence of peers on inherently subjective constructs.
In contrast, evidence that reference bias distorts comparisons of self-regulation across social groups has been indirect. A handful of cross-cultural studies have yielded paradoxical findings (e.g., Asian countries such as Japan and South Korea ranking lower in self-reported conscientiousness than other countries that are typically thought to be less conscientious 53 ), but none of these studies directly measured standards for behavior, relying instead on experts’ ratings of cultural stereotypes or indirect proxies for self-regulation (e.g., the average walking speed in downtown locations of a convenience sample of a country’s residents, as a proxy for the nation’s conscientiousness).
In the educational literature, studies that compare the test scores and average self-regulation scores for different schools have not ruled out unobserved confounds, such as the possibility that school factors (e.g., average family income) that increase test scores (e.g., due to investment in educational opportunities) also decrease self-regulation (e.g., by shielding children from responsibilities that could cultivate self-regulation). Therefore, the research literature to date has not been able to distinguish biases in self-reports from potentially true group differences in self-regulation.
In this investigation, we overcome these limitations by using three complementary methods to examine reference bias more directly than has been possible previously. Our approach is motivated by the basic finding that people judge themselves compared to salient and similar others 47 . Therefore we exploit (Studies 1 and 2) or work around (Study 3) variation in people’s reference groups.
In Study 1 (total N = 206,589 students in k = 562 Mexican high schools), we show that the reference bias effect appears even within the same school in a year-over-year comparison. When students are surrounded by higher-achieving peers relative to other students at the same school in a different year, they rate themselves lower in self-regulation. Study 2 addresses an additional confound that could remain in Study 1’s analysis, which is the possibility that year-over-year fluctuations in test scores are not random but are due to choices made by families about the academic trajectory of the school. In Study 2 ( N = 21,818 students in k = 62 U.S. secondary schools), we rule this out with an analysis rooted in the purported psychological explanation for reference bias, which is that people’s self-judgments should be more influenced by the peers whose behaviors they observe rather than peers whose behaviors they do not observe. We show that reference bias is evident in data from a single school year only when administrative data showed that the peers shared classes and therefore had an opportunity to observe each other’s self-regulated behavior. Furthermore, Study 2 examined the theorized, but typically unmeasured, explanation for reference bias: differences in students’ implicit standards for self-regulation (i.e., how many hours of homework constitute “a lot of homework” and how often it means to “sometimes” forget what they need for class).
Studies 1 and 2 argue against school-level alternative explanations for reference bias but nevertheless allowed for the possibility that high-achieving peers reduce a student’s real capacity for self-regulation. Study 3 ( N = 1278 seniors in k = 15 U.S. high schools) addressed this possibility with a workaround: an objective behavioral task that involves no self-reports and therefore is not subject to biases due to differences in frames of reference. By matching self-regulation data collected in high school to records of college graduation, we show that there is no evidence of reference bias when a behavioral task is used. This evidence is bolstered by Study 3’s use of a measure of school achievement that is independent of the high school peer group: graduation from college within 6 years after high school completion.
Study 1: Evidence for reference bias in a country-wide natural experiment
In 2012 and 2013, the Secretariat of Public Education administered questionnaires measuring grit (the passion and perseverance for long term-term goals 54 ) and collected data on academic performance from high school seniors in a nationally representative sample of 10% of high schools in Mexico. We analyzed data from the 1% of all schools that, by chance, were selected in both years. This enabled us to exploit exogenous variation in the academic performance of the 2013 high school cohort when compared to the performance of the 2012 cohort. Reference bias was quantified as the effect on self-reported grit uniquely attributable to peer academic performance (i.e., the cohort-wide averages of GPA, standardized math test scores, and standardized reading test scores, respectively, excluding said student from the average), after controlling for differences between schools, cohort year, and each student’s own academic performance.
Sample and procedure
High school seniors in two representative random samples, each comprising 10% of schools in Mexico, completed standardized achievement tests of math and reading and, separately, self-report questionnaires late in the spring term of the 2011–2012 and 2012–2013 academic years, respectively. By chance, about 1% ( k = 562) of high schools were included in both years. Our final sample includes 97.8% of the students in these high schools ( N = 206,589) who completed a questionnaire measure of grit. There were slightly more girls than boys in our sample (53.49% female). On average, students in our sample were 17.61 years old (SD = 0.79).
Self-reported grit
The Technical Committee for Background Questionnaires at the National Center of Evaluation for Higher Education in Mexico (Centro Nacional de Evaluación para la Educación Superior) translated all 8 items of the Short Grit Scale 55 as well as its 5-point Likert-type response scale (1 = Not at all like me to 5 = Very much like me ) into Spanish. The observed reliability was \(\alpha = 0.62\) . All reported reliabilities are Cronbach’s alphas.
Grade point average (GPA)
Students reported their overall, verbal, and math GPAs using a categorical scale which ranged from less than 5.9 to 10 in half-point increments (i.e., < 5.9, 6.0–6.4, 6.5–6.9, etc.). We used the midpoint of the range in our analyses (i.e., 5.7, 6.2, 6.7, etc.). Although official GPAs were not available, meta-analytic estimates of the correlation between self-reported and objectively recorded GPA is \(r =0.82\) 56 . To avoid any issues with multicollinearity, we ran separate models for each GPA measure.
Standardized test scores
The Mexican Secretariat of Public Education provided standardized math and reading scores.
Analytic strategy
We used ordinary least squares (OLS) regression with clustered standard errors to predict self-reported grit from student’s own and peer’s academic performance:
where \(G_{ist}\) is the self-reported grit for student i who was in 12th grade in school s at time t (2012 or 2013). Term \(a_{ist}\) is that student’s own academic performance, operationalized as self-reported GPA, standardized math scores, or standardized verbal scores, respectively. Term \(b_{-ist}\) represents the average academic performance of students sharing a school with each student i , excluding student i . Term \(\theta _s\) represents fixed effects for each student’s school and captures ways in which schools might differ from each other—including such differences as teachers, curricula, school policies, and regional populations from which schools draw their members. Term \(\eta _{t}\) (fixed effect for year), captures how cohorts for each school systematically differ from each other. \(\epsilon _i\) represents error.
Students surrounded by higher-performing classmates rate themselves lower in grit
Consistent with prior research, among students in the same school, self-reported grit correlated positively with GPA ( \(\beta\) = 0.43, p < 0.001), standardized math test scores ( \(\beta\) = 0.16, p < 0.001), and standardized reading test scores ( \(\beta\) = 0.16, p < 0.001). However, consistent with reference bias, self-reported grit correlated inversely with schoolmates’ GPA ( \(\beta = -0.25\) , p < 0.001), peer standardized math test scores ( \(\beta = -0.09\) , p \(< 0.001\) ), and peer standardized reading test scores ( \(\beta = -0.07\) , \(p = 0.004\) ). See Fig. 2 and Supporting Information for details.
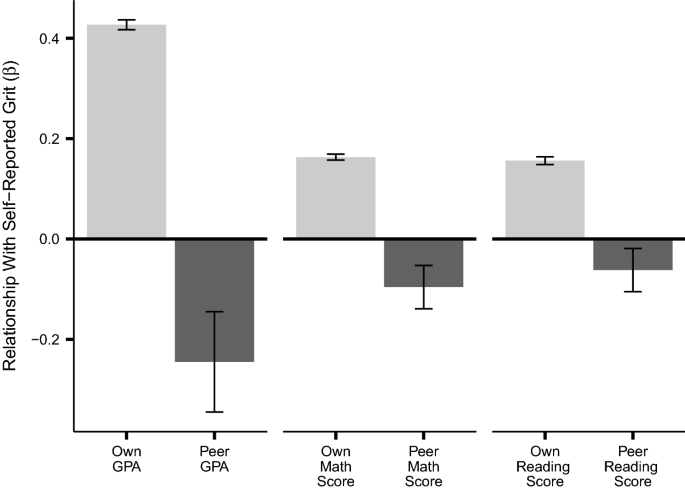
In Study 1, self-reported grit correlated positively with a student’s own academic performance but inversely with the performance of their schoolmates. OLS models included demographic controls and school fixed effects. Error bars represent 95% confidence intervals. Model \(R^2\) s for GPA, math score, and language score were 0.124, 0.071, and 0.071, respectively.
Evidence for reference bias was consistent across demographic subgroups
Capitalizing on the size and representativeness of our sample, we explored moderators of reference bias. Regression coefficients for peer academic performance were not significantly different across subgroups defined by gender, mother’s educational level, school type (public or private), or school size. See Tables S6 and S7 in Supporting Information for details.
Study 2: Replication and extension in a single large school district
In Study 2, we partnered with the nonprofit organization Character Lab to replicate and extend Study 1 with a sample of students in grades 8 through 12 in a large, diverse school district in the United States. This partnership enabled us to obtain official class schedules for each student, which we used to distinguish near- versus far-peers as students who did or didn’t share daily academic classes, respectively. Whereas GPA was self-reported in Study 1, in Study 2 we obtained GPA from official school records. As part of a larger survey administered by Character Lab, students completed a self-report questionnaire of conscientiousness (the tendency to be organized, responsible, and hardworking 57 ) as well as two questions we developed to directly assess self-regulation standards.
This study included data from N = 21,818 (50% female, \(M_{age}\) = 15.60, \(SD_{age}\) = 1.54) students attending k = 62 middle and high schools in a large public school district in the United States who completed surveys in either October 2019 or February 2020. This district was part of Character Lab Research Network (CLRN), a consortium of school partners committed to advancing scientific insights that help children thrive. According to school records, the race/ethnicity of our sample was: Hispanic/Latinx (41%), White (28%), Black (23%), and other (8%). About half (49%) of students were eligible for free and reduced-price meals.
Self-reported conscientiousness
Students completed 12 items from the Big Five Inventory-2 58 assessing conscientiousness (e.g., “I am someone who is persistent, works until the task is finished”) using a 5-point Likert-type scale ranging from 1 = Not like me at all to 5 = Totally like me . The observed reliability was \(\alpha = 0.83\) .
Standards for hard work and preparedness
We included two questions to measure implicit standards for self-regulation. One question assessed norms for hard work: “If a student in your grade says they did ‘a lot of homework’ on a weeknight, how long would you guess they mean?” Eight response options ranged from 15 min (coded as 0.25 hours) to 3 or more hours (coded as 3 hours). The second question assessed norms for preparedness: “If a student in your grade says they ‘sometimes’ forget something they need for class, how often would you guess they mean?” Seven response options ranged from once a month to three times or more per day (coded as 66 times per month). We reverse-coded these values such that higher numbers indicated stricter standards for preparedness. These items were created for this study and used here for the first time.
From school administrative records, we calculated GPAs on a 100-point scale by averaging final grades in students’ academic courses (English language arts, math, science, social studies) for the quarter in which students took the survey during the 2019–2020 school year.
Near-peer and far-peer GPAs
For each student, we designated near-peers as those students who took at least one academic course with the target student during the quarter in which they took the survey. We designated far-peers as students in the same school who did not share any academic courses. For the average student in our sample, 38% of schoolmates were near-peers and 62% were far-peers.
To examine whether self-regulation standards and conscientiousness related to students’ own and peers’ performance, we fit OLS regression models with standard errors clustered by school to estimate the following equation:
where \(S_{is}\) is a survey measure of conscientiousness or self-regulation standards for student i in school s , \(a_{is}\) is a student’s own GPA, \(b_{-is}\) is the average GPA of students in the same school sharing at least one academic course with student i , \(c_{-is}\) is the average GPA of students in the same school but not sharing any academic courses with student i , \(x_{is}\) is a vector of student characteristics (age, gender, race/ethnicity, grade level, free or reduced-price meal status, English language learner status, special-education status, home language, and timing of the survey), \(\theta _{s}\) represents school fixed effects, and \(\epsilon _i\) is is a random error term.
Reference bias replicates: students whose classmates perform better academically rate themselves as lower in conscientiousness. As expected, this effect is driven by near-peers rather than far-peers
If implicit standards for self-regulation are determined by social comparison, reference bias should be driven by the individuals with whom individuals are in direct contact. As shown in Fig. 3 , consistent with Study 1, self-reported conscientiousness was correlated positively with a student’s own GPA ( \(\beta = 0.29\) , \(p< 0.001\) ), negatively with the GPA of near-peers ( \(\beta = - 0.06\) , \(p < 0.001\) ), and not at all with the GPA of far-peers ( \(\beta = 0.01\) , \(p = 0.395\) ). See Table S10 in Supporting Information for details.
Students whose near-peers perform better academically hold higher self-regulation standards
As expected, standards for hard work were predicted by a student’s own GPA ( \(\beta = 0.07\) , \(p < 0.001\) ) and the GPA of their near-peers ( \(\beta = 0.23\) , \(p < 0.001\) ), but not the GPA of their far-peers ( \(\beta = -0.03\) , \(p = 0.198\) ). The same pattern emerged for preparedness norms, which were predicted by students own GPA ( \(\beta = 0.05\) , \(p < 0.001\) ) and the GPA of their near-peers ( \(\beta = 0.14\) , \(p < 0.001\) ), but not far-peers ( \(\beta = -0.02\) , \(p = 0.080\) ). As in Study 1, the patterns of findings were generally similar across subgroups. See Tables S11 – S16 in Supporting Information for details.
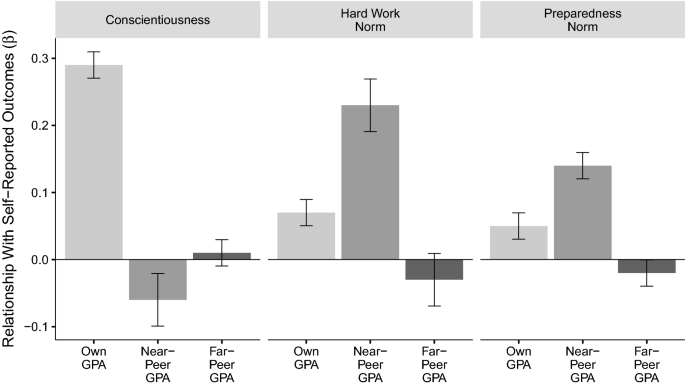
In Study 2, self-reported conscientiousness correlated positively with a student’s own GPA and negatively with the GPA of near-peers. In contrast, standards for what constitutes hard work and preparedness correlated positively with both own and near-peer GPA. As expected, there was no effect of far-peer GPA. OLS models included demographic controls and school fixed effects. Error bars represent 95% confidence intervals. Model \(R^2\) s for conscientiousness, hard work norms, and preparedness norms were 0.095, 0.159, and 0.059, respectively.
Study 3: In a longitudinal study of college graduation, evidence of reference bias in questionnaire but not in task measures of self-regulation
In Study 3, we sought evidence of discriminant validity. Unlike questionnaires, which require participants to make subjective judgments of their behavior, task measures assay behavior directly. In a prospective, longitudinal study of N = 1278 students attending k = 15 different college-preparatory charter schools in the United States, we tested the prediction that reference bias should be evident in questionnaire but not behavioral task measures of self-regulation. In their senior year of high school, students self-reported their grit and self-control (the ability to be in command of one’s behavior and to inhibit one’s impulses 57 ). In addition, they completed the Academic Diligence Task, a behavioral task in which students voluntarily allocate attention to either good-for-me-later math problems or fun-for-me-now games and videos. The Academic Diligence Task has previously been validated as indexing self-control and grit 59 , 60 . Six years later, we used the National Student Clearinghouse database to identify students who successfully obtained their college diploma.
A few weeks before graduation, N = 1278 (55% female, \(M_{age}\) = 18.01, \(SD_{age}\) = 1.01) high school seniors responded to self-report questionnaires and task measures in school computer labs. Students attended k = 15 charter schools located in various urban centers in the United States. Between 76 and 98% of the students at each school participated in the study. Most students were socioeconomically disadvantaged (84% of students’ mothers had less than a 4-year degree, 68% qualified for free or reduced-priced meals), and were mostly Latinx (46%) and African American (40%).
Students completed a 4-item version of the Grit Scale developed specifically for adolescents 61 . Students responded on a 5-point Likert-type scale ranging from 1 = Not at all true to 5 = Completely true . The observed reliability was \(\alpha = 0.78\) .
Self-control
Students completed four items from the Domain-Specific Impulsivity Scale 59 , 62 assessing academic self-control (e.g., “I forgot something needed for school”). Students responded on a 5-point Likert-type scale ranging from Not at all true to Completely true. The observed reliability was \(\alpha = 0.72\) .
Academic Diligence Task (ADT)
A subset ( n = 802) of students in our sample completed the Academic Diligence Task, a behavioral assessment of self-regulation that has been validated in separate research 59 . This computer-based task begins with screens explaining that practicing simple mathematical skills like subtraction can aid in further enhancing overall math abilities. Then, they completed three 3-min timed task blocks. In each, they chose between “Do math” and “Play game or watch movie.” Clicking “Do math” displayed a math task involving single-digit subtraction with multiple-choice responses. On the other hand, clicking “Play game or watch movie” allowed students to play Tetris or watch entertaining videos. Students could freely switch between them during each block. See Supporting Information for details. The key metric from the ADT was the mean number of problems correctly answered over the three blocks. Basic subtraction is very easy for most 12th grade students, so attentive engagement with the task resulted almost exclusively in correct answers: The median rate of correct responses was 98.3%. Due to positive skew and some clustering of data at 0 (i.e., students who did no math problems), we applied a square-root transformation to minimize bias from extremely high scores; this created an approximately normal distribution, which we used in subsequent calculations. Models using raw (i.e., non-transformed) ADT scores are shown in Table S19 . Across the three blocks, the observed reliability was \(\alpha = .78\) .
General cognitive ability
During the online survey, students completed a brief (12-item) version of Raven’s Progressive Matrices as an assessment of general cognitive ability 63 . The ability variable was calculated as the sum of correctly answered questions out of 12, with any missing questions marked as incorrect. The observed reliability was \(\alpha = 0.73\) .
College graduation
We matched our data to the National Student Clearinghouse, a public database that includes enrollment and graduation data for over 97% of students in 2022 64 , 65 . We coded six-year college graduation as 1 = obtained degree within 6 years of enrollment and 0 = did not obtain degree within 6 years of enrollment.
Because we were interested in both individual-level and school-level differences in self-regulation, we used multilevel modeling to analyze how the Academic Diligence Task and self-reported grit and self-control, predict college graduation. Specifically, we expected the ADT to positively predict college graduation at both the within- and between-school levels. We expected the relationship to be positive because prior research shows that students who obtain higher ADT scores tend to perform better academically 59 . Moreover, we expected the relationship to be positive at both levels because, as a task measure, it does not involve comparative judgment and thus cannot be influenced by reference bias. In contrast, we expected self-reported grit and self-control to positively predict college graduation within a school but negatively between schools. We used a missing dummy variable coding approach to deal with missing data and included controls for general cognitive ability in our models.
Evidence of reference bias in longitudinal predictions of college graduation from self-reported, but not objectively measured, self-regulation
As shown in Fig. 4 , among seniors in the same high school, higher scores on self-report questionnaires of self-control ( b = 0.16, OR = 1.17, p = 0.022) and grit ( b = 0.16, OR = 1.18, p = 0.020) each predicted greater odds of earning a college diploma 6 years later. However, college graduation rates were actually lower for schools with higher self-reported self-control and grit scores ( \(b = -0.44\) , OR = 0.64, p = 0.001; \(b = -0.39\) , OR = 0.68, p = 0.005, for self-control and grit, respectively).
This paradoxical pattern was not evident when self-regulation was assessed objectively using the Academic Diligence Task 59 . Among seniors in the same school, college graduation was predicted by higher scores on the Academic Diligence Task ( b = 0.15, OR = 1.17, p = 0.031). Likewise, when comparing across schools, college graduation rates were higher for schools whose students performed better on the Academic Diligence Task ( b = 0.46, OR = 1.58, p < 0.001).
Taken as a whole these findings suggest that reference bias reversed the relationship between self-regulation and graduation across schools. See Supporting Information for summaries of multilevel logistic regression models, robustness checks, and a replication of the own versus peer performance models in Studies 1 and 2.
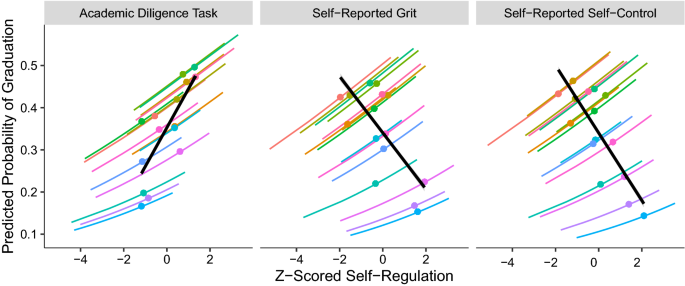
In Study 3, comparing students within schools (colored lines), higher self-regulation predicted higher odds of college graduation, whether measured by self-report questionnaires for grit and self-control or by a behavioral task called the Academic Diligence Task. When comparing schools to each other, however, higher self-reported grit and self-control scores predicted lower graduation rates, whereas the behavioral task positively predicted college graduation, as shown in the solid black lines. Plots show predicted probabilities of graduation from multilevel logistic regression models. AUC s for models predicting the academic diligence task, self-reported grit, and self-reported self-control were 0.694, 0.693, and, 0.676, respectively.
The three studies in this investigation provide direct evidence for reference bias in self-reported self-regulation. In Study 1, high school seniors rated themselves lower in grit when their schoolmates earned higher GPAs and standardized achievement test scores. In Study 2, we replicated this effect using self-report questionnaires of conscientiousness and showed that it was driven by near-peers rather than by far-peers. Further, we showed that the GPA of near-peers (but not far-peers) correlates positively with self-regulation standards. Finally, in Study 3, we found that using self-report questionnaires of grit and self-control to predict college graduation 6 years later produced paradoxical results: Within a high school, students with higher self-reported self-regulation were more likely to graduate from college 6 years later, but across schools, average levels of self-regulation negatively predicted graduation. In contrast, an objective task measure of self-regulation—which indexed performance directly and did not ask students to judge themselves—positively predicted college graduation both within and across schools.
How big are reference bias effects? Studies 1 and 2 provide estimates in the range of r = 0.06 to 0.25. All else being equal, a student in our samples whose peers’ academic achievement is one standard deviation above the mean is predicted to rate their own self-regulation as 10–20% of a standard deviation lower. Assuming that higher standards for self-regulation depress self-report ratings while at the same time, via social norms and modeling, encourage more self-regulated behavior, these are actually lower-bound estimates. Consistent with this possibility, when we use a behavioral task to assess self-regulation, we observe results consistent with positive peer effects (Study 3), which have also been previously reported in the literature 66 , 67 , 68 . Taken together, our findings suggest that reference bias effects, even across social groups in the same country, can be at least small-to-medium in size by contemporary benchmarks 69 and comparable to the effect sizes for faking on self-regulation questionnaires in workplace settings 70 .
Several limitations of the current investigation suggest promising directions for future research.
First, we must be cautious about drawing strong causal inferences from the non-experimental data in our three field studies. In Study 1, variation in peer quality could have influenced self-reported self-regulation for reasons other than reference bias. Against this, we found direct evidence for near-peer influence on self-regulation standards provided in Study 2. However, in Study 2, there is the possibility of reverse-causality. For example, rather than near-peers determining self-regulation standards, it is possible that self-regulation standards determined patterns of enrollment (e.g., students with higher standards self-selecting into the same difficult classes). In Study 3, we cannot rule out the possibility that some unmeasured confound gave rise to contradictory within-school versus between-school results on self-report (but not objective task) measures of self-regulation. In sum, it is important to confirm our observational findings by experimentally manipulating peer groups and/or standards of self-regulation.
Second, there are limits to the external validity of our conclusions. In particular, we examined reference bias in adolescence, a developmental period in which sensitivity to peers is at its apogee 71 . The adolescents in our investigation lived in Mexico (Study 1) and the United States. (Studies 2 and 3). Further research on children and adults, in a wider sample of countries, and in contexts outside formal schooling, is needed to establish boundary conditions and moderators of reference bias. In general, effect sizes for reference bias are expected to be smaller when comparing social groups with more similar standards.
Third, we did not collect nuanced data on social networks (e.g., friendships, acquaintances). Indeed, our operationalization of peer groups was quite crude—students in the same grade and attending the same school in Study 1 and 3, and students in the same grade and school who share at least one academic class (i.e., near-peers) in Study 2. Given the increasing prevalence of social-network studies and the continued popularity of self-report questionnaires in behavioral science, it should be possible to identify the influence of prominent social referents and close friends on reference bias.
Finally, while we collected information about student’s standards for self-regulation (in Study 2) and an objective measure of self-regulation (in Study 3), we have yet to collect both types of measures in the same sample. Doing so in a future study would enable us to test a mediation model in which peers influence standards for self-regulation which, in turn, diminish self-reported self-regulation relative to performance on a behavioral task of self-regulation. More generally, additional research is needed to establish the mediators, moderators, and boundary conditions of reference bias in the measurement of self-regulation.
Unfortunately, the problem of reference bias is not easily corrected. The most commonly suggested solution is anchoring vignettes 72 . This technique entails asking participants to rate detailed descriptions of hypothetical characters. These ratings are then used to adjust self-report questionnaire scores upward or downward depending on the stringency or leniency with which participants evaluated the hypothetical characters. Anchoring vignettes can increase the reliability and validity of self-reports 73 but do not always work as intended 74 . They also increase the time, effort, and literacy required from survey respondents, which may limit their utility at scale 73 , 75 .
A related possibility is to use behaviorally anchored 76 or act-frequency rating scales 77 , which ask respondents to rate themselves on more specific, contextualized behaviors than is typical in traditional questionnaires. For example, while students at over-subscribed charter schools do not rate themselves as more self-regulated, they and their parents do report more “minutes of homework completed” in an open-ended question in the same questionnaire 38 . In our view, such questions might mitigate response bias but probably do not eliminate it altogether. Why not? Because all subjective judgments rely, at least to some degree, on implicit standards that can differ (e.g., What level of effort is sufficient to consider yourself to be “doing homework”?).
As shown in Study 3, self-regulation can be assessed with behavioral tasks, which appear immune to reference bias. However, task measures have their own limitations, including a dramatically lower signal-to-noise ratio when compared to questionnaires and, relatedly, surprisingly modest associations with other measures of self-regulation 46 , 78 , 79 , 80 , 81 .
Perhaps the best means of obviating reference bias is to take a multi-method, multi-informant approach to assessment, including trained observers who can rate behavior across multiple occasions 12 . Observers who have seen hundreds, if not thousands, of cases typically have a wider reference frame than the individuals they are evaluating, which might explain why teacher ratings of behavior are more reliable and predictive of future outcomes than either parental reports or student self-reports 82 . The rarity of multi-method and multi-informant approaches suggests that, unfortunately, few researchers have the necessary resources or expertise to implement it, particularly at scale.
What are the implications of reference bias for researchers and policymakers?
Reference bias could suppress, or even reverse, the measured effects of interventions if the standards by which people judge their own behavior on pre- and post-questionnaires shift as a function of the intervention 83 . In one study, participants were asked to rate their interviewing skills before training ( pre ). Afterward, participants rated themselves again ( post ) and, in addition, retrospectively estimated what their skills had been at baseline ( then ). Even though questionnaire items were identical for all assessments, then ratings were lower than pre ratings—suggesting that participants adopted higher standards as a result of the intervention. Moreover, third-party judges’ ratings of performance matched then - post change better than pre - post differences 84 .
The implications of reference bias extend beyond intervention research. Consider, for example, mean-level increases in conscientiousness from adolescence through midlife 85 , 86 , 87 . If adults in their 50s hold higher standards for what it means to be courteous, rule-abiding, and self-controlled than teenagers, then age differences in conscientiousness may be even larger than we now think. In fact, to the extent that implicit standards and actual behavior are inversely correlated, reference bias should be expected to attenuate associations of self-regulation with groups of any kind.
While the importance of personal qualities like self-regulation is incontrovertible, the specter of reference bias argues against relying on self-report questionnaires when comparing students attending different schools, citizens who live in different countries, or indeed any of the members of any social group whose standards could differ from one another. Are you a hard worker? Responding to such a question requires looking within to identify the patterns of our behavior. In addition, the evidence for reference bias presented here suggests that knowingly or not, we also look around when we decide how to respond.
Ethics statement
All methods were carried out in accordance with relevant guidelines and regulations. Participants in Studies 2 and 3 completed written informed consent prior to participation in this study. Participants in Study 1 were completing country-mandated educational assessments, and thus did not complete written informed consent. We accessed this secondary dataset with authorization from the Mexican Secretariat of Education. Study 1 was approved by the Mexican Secretariat of Education. Study 2 was approved by Advarra IRB. Study 3 was approved by Stanford University IRB.
Data availability
The data that support the findings of Study 1 are available from the Mexican Ministry of Education but restrictions apply to the availability of these data, which were used under license for the current study, and so are not publicly available. Data are however available from the authors upon reasonable request and with permission of the Mexican Ministry of Education. Data for Study 2 and Study 3 are included in this published article’s Supporting Information .
Rothbart, M. K. Temperament, development, and personality. Curr. Dir. Psychol. Sci. 16 , 207–212. https://doi.org/10.1111/j.1467-8721.2007.00505.x (2007).
Article Google Scholar
Mischel, W., Shoda, Y. & Rodriguez, M. L. Delay of gratification in children. Science 244 , 933–938. https://doi.org/10.1126/science.2658056 (1989).
Article ADS CAS PubMed Google Scholar
Freud, S. Beyond the Pleasure Principle 90 (The International Psycho-Analytical Press, 1922).
Book Google Scholar
Roberts, B. W. & Yoon, H. J. Personality psychology. Annu. Rev. Psychol. 73 , 489–516 (2022).
Article PubMed Google Scholar
Nigg, J. T. Annual research review: On the relations among self-regulation, self-control, executive functioning, effortful control, cognitive control, impulsivity, risk-taking, and inhibition for developmental psychopathology. J. Child Psychol. Psychiatry 58 , 361–383. https://doi.org/10.1111/jcpp.12675 (2017).
Berns, G. S., Laibson, D. & Loewenstein, G. Intertemporal choice—toward an integrative framework. Trends Cogn. Sci. 11 , 482–488. https://doi.org/10.1016/j.tics.2007.08.011 (2007).
Heckman, J. J. & Kautz, T. Hard evidence on soft skills. Labour Econ. 19 , 451–464. https://doi.org/10.1016/j.labeco.2012.05.014 (2012).
Article PubMed PubMed Central Google Scholar
Duckworth, A. L., Taxer, J. L., Eskreis-Winkler, L., Galla, B. M. & Gross, J. J. Self-control and academic achievement. Annu. Rev. Psychol. 70 , 373–399. https://doi.org/10.1146/annurev-psych-010418-103230 (2019).
Bierman, K. L., Nix, R. L., Greenberg, M. T., Blair, C. & Domitrovich, C. E. Executive functions and school readiness intervention: Impact, moderation, and mediation in the Head Start REDI program. Dev. Psychopathol. 20 , 821–843. https://doi.org/10.1017/S0954579408000394 (2008).
Vedel, A. The Big Five and tertiary academic performance: A systematic review and meta-analysis. Personal. Individ. Differ. 71 , 66–76. https://doi.org/10.1016/j.paid.2014.07.011 (2014).
Daly, M., Egan, M., Quigley, J., Delaney, L. & Baumeister, R. F. Childhood self-control predicts smoking throughout life: Evidence from 21,000 cohort study participants. Health Psychol. 35 , 1254–1263. https://doi.org/10.1037/hea0000393 (2016).
Moffitt, T. E. et al. A gradient of childhood self-control predicts health, wealth, and public safety. Proc. Natl. Acad. Sci. 108 , 2693–2698. https://doi.org/10.1073/pnas.1010076108 (2011).
Article ADS PubMed PubMed Central Google Scholar
Bogg, T. & Roberts, B. W. Conscientiousness and health-related behaviors: A meta-analysis of the leading behavioral contributors to mortality. Psychol. Bull. 130 , 887–919. https://doi.org/10.1037/0033-2909.130.6.887 (2004).
Hofmann, W., Luhmann, M., Fisher, R. R., Vohs, K. D. & Baumeister, R. F. Yes, but are they happy? Effects of trait self-control on affective well-being and life satisfaction: Trait self-control and well-being. J. Pers. 82 , 265–277. https://doi.org/10.1111/jopy.12050 (2014).
Hirschi, T. Self-control and crime. In Handbook of Self-Regulation , 537–552.
Barrick, M. R. & Mount, M. K. The big five personality dimensions and job performance: A meta-analysis. Pers. Psychol. 44 , 1–26. https://doi.org/10.1111/j.1744-6570.1991.tb00688.x (1991).
Duckworth, A. L., Weir, D., Tsukayama, E. & Kwok, D. Who does well in life? Conscientious adults excel in both objective and subjective success. Front. Psychol. https://doi.org/10.3389/fpsyg.2012.00356 (2012).
Wiersma, U. J. & Kappe, R. Selecting for extroversion but rewarding for conscientiousness. Eur. J. Work Organ. Psychol. 26 , 314–323. https://doi.org/10.1080/1359432X.2016.1266340 (2017).
Denissen, J. J. A. et al. Uncovering the power of personality to shape income. Psychol. Sci. 29 , 3–13 (2018).
Doebel, S. Rethinking executive function and its development. Perspect. Psychol. Sci. 15 , 942–956. https://doi.org/10.1177/1745691620904771 (2020).
Casey, B. J. Beyond simple models of self-control to circuit-based accounts of adolescent behavior. Annu. Rev. Psychol. 66 , 295–319. https://doi.org/10.1146/annurev-psych-010814-015156 (2015).
Article CAS PubMed Google Scholar
Dahl, R. E., Allen, N. B., Wilbrecht, L. & Suleiman, A. B. Importance of investing in adolescence from a developmental science perspective. Nature 554 , 441–450. https://doi.org/10.1038/nature25770 (2018).
Steinberg, L. Cognitive and affective development in adolescence. Trends Cogn. Sci. 9 , 69–74. https://doi.org/10.1016/j.tics.2004.12.005 (2005).
Bailey, R., Meland, E. A., Brion-Meisels, G. & Jones, S. M. Getting developmental science back into schools: Can what we know about self-regulation help change how we think about “No Excuses’’?. Front. Psychol. 10 , 1885. https://doi.org/10.3389/fpsyg.2019.01885 (2019).
Hamilton, S. F. Chapter 6: The secondary school in the ecology of adolescent development. Rev. Res. Educ. 11 , 227–258. https://doi.org/10.3102/0091732X011001227 (1984).
Leonard, J. A., Lee, Y. & Schulz, L. E. Infants make more attempts to achieve a goal when they see adults persist. Science 357 , 1290–1294. https://doi.org/10.1126/science.aan2317 (2017).
Bandura, A. & Mischel, W. Modification of Self-Imposed delay of reward through exposure to live and symbolic models. J. Pers. Soc. Psychol. 2 , 698–705 (1965).
King, K. M., McLaughlin, K. A., Silk, J. & Monahan, K. C. Peer effects on self-regulation in adolescence depend on the nature and quality of the peer interaction. Dev. Psychopathol. 30 , 1389–1401. https://doi.org/10.1017/S0954579417001560 (2018).
Doebel, S. & Munakata, Y. Group influences on engaging self-control: Children delay gratification and value it more when their in-group delays and their out-group doesn’t. Psychol. Sci. 29 , 738–748. https://doi.org/10.1177/0956797617747367 (2018).
Bertling, J. P., Marksteiner, T. & Kyllonen, P. C. General noncognitive outcomes. In Assessing Contexts of Learning (eds Kuger, S. et al. ) 255–281 (Springer, 2016). https://doi.org/10.1007/978-3-319-45357-6_10 .
Chapter Google Scholar
U.S. Department of Education. Every Student Succeeds Act (ESSA) (2015).
Center for Disease Control and Prevention. Whole School, Whole Community, Whole Child (WSCC) (2021).
OECD. Beyond Academic Learning: First Results from the Survey of Social and Emotional Skills (OECD, 2021).
Jackson, C. K., Porter, S. C., Easton, J. Q., Blanchard, A. & Kiguel, S. School effects on socioemotional development, school-based arrests, and educational attainment. Am. Econ. Rev. Insights 2 , 491–508 (2020).
West, M. R. et al. Promise and paradox: Measuring students’ non-cognitive skills and the impact of schooling. Educ. Eval. Policy Anal. 38 , 148–170. https://doi.org/10.3102/0162373715597298 (2016).
Dobbie, W. & Fryer, R. G. The medium-term impacts of high-achieving charter schools. J. Polit. Econ. 123 , 985–1037. https://doi.org/10.1086/682718 (2015).
Tuttle, C. C. et al. Understanding the Effect of KIPP as it Scales, Volume I, Impacts on Achievement and Other Outcomes. Tech. Rep, Mathematica Policy Research (2015).
Tuttle, C. C. et al. KIPP Middle Schools: Impacts on Achievement and Other Outcomes. Tech. Rep, Mathematica Policy Research (2013).
Angrist, J. D., Pathak, P. A. & Walters, C. R. Explaining charter school effectiveness. Am. Econ. J. Appl. Econ. 5 , 1–27. https://doi.org/10.1257/app.5.4.1 (2013).
Dobbie, W. & Fryer, R. G. Getting beneath the veil of effective schools: Evidence from New York City. Am. Econ. J. Appl. Econ. 5 , 28–60. https://doi.org/10.1257/app.5.4.28 (2013).
Heine, S. J., Lehman, D. R., Peng, K. & Greenholtz, J. What’s wrong with cross-cultural comparisons of subjective Likert scales?: The reference-group effect. J. Pers. Soc. Psychol. 82 , 903–918. https://doi.org/10.1037/0022-3514.82.6.903 (2002).
Van de Gaer, E., Grisay, A., Schulz, W. & Gebhardt, E. The reference group effect. Cult. Psychol. 43 , 24 (2012).
Google Scholar
Van Vaerenbergh, Y. & Thomas, T. D. Response styles in survey research: A literature review of antecedents, consequences, and remedies. Int. J. Public Opin. Res. 25 , 195–217. https://doi.org/10.1093/ijpor/eds021 (2013).
Schwarz, N. & Oyserman, D. Asking questions about behavior: Cognition, communication, and questionnaire construction. Am. J. Eval. 22 , 127–160 (2001).
Tourangeau, R., Rips, L. J. & Rasinski, K. The Psychology of Survey Response (Cambridge University Press, 2000).
Duckworth, A. L. & Yeager, D. S. Measurement matters: Assessing personal qualities other than cognitive ability for educational purposes. Educ. Res. 44 , 237–251. https://doi.org/10.3102/0013189X15584327 (2015).
Morina, N. Comparisons Inform Me Who I Am: A general comparative-processing model of self-perception. Perspect. Psychol. Sci. 16 , 1281–1299 (2021).
Marsh, H. W. & Craven, R. G. Reciprocal effects of self-concept and performance from a multidimensional perspective: Beyond seductive pleasure and unidimensional perspectives. Perspect. Psychol. Sci. 1 , 133–163. https://doi.org/10.1111/j.1745-6916.2006.00010.x (2006).
Marsh, H. W. et al. The Big-fish-little-pond-effect stands up to critical scrutiny: Implications for theory, methodology, and future research. Educ. Psychol. Rev. 20 , 319–350. https://doi.org/10.1007/s10648-008-9075-6 (2008).
Marsh, H. W. The Big-Fish-Little-Pond effect on academic self-concept. J. Educ. Psychol. 79 , 280–295 (1987).
Gerber, J. P., Wheeler, L. & Suls, J. A social comparison theory meta-analysis 60+ years on. Psychol. Bull. 144 , 177–197. https://doi.org/10.1037/bul0000127 (2018).
Bybee, R. & McCrae, B. Scientific literacy and student attitudes: Perspectives from PISA 2006 science. Int. J. Sci. Educ. 33 , 7–26. https://doi.org/10.1080/09500693.2010.518644 (2011).
Schmitt, D. P., Allik, J., McCrae, R. R. & Benet-Martínez, V. The geographic distribution of Big Five personality traits: Patterns and profiles of human self-description across 56 nations. J. Cross-Cultural Psychol. 38 , 173–212. https://doi.org/10.1177/0022022106297299 (2007).
Duckworth, A. L., Peterson, C., Matthews, M. D. & Kelly, D. R. Grit: Perseverance and passion for long-term goals. J. Pers. Soc. Psychol. 92 , 1087–1101. https://doi.org/10.1037/0022-3514.92.6.1087 (2007).
Duckworth, A. L. & Quinn, P. D. Development and validation of the Short Grit scale (Grit-S). J. Pers. Assess. 91 , 166–174. https://doi.org/10.1080/00223890802634290 (2009).
Kuncel, N. R., Credé, M. & Thomas, L. L. The validity of self-reported grade point averages, class ranks, and test scores: A meta-analysis and review of the literature. Rev. Educ. Res. 75 , 63–82. https://doi.org/10.3102/00346543075001063 (2005).
American Psychological Association. APA Dictionary of Psychology 1st edn. (American Psychological Association, 2007).
Soto, C. J. & John, O. P. Short and extra-short forms of the Big Five Inventory-2: The BFI-2-S and BFI-2-XS. J. Res. Pers. 68 , 69–81. https://doi.org/10.1016/j.jrp.2017.02.004 (2017).
Galla, B. M. et al. The Academic Diligence Task (ADT): Assessing individual differences in effort on tedious but important schoolwork. Contemp. Educ. Psychol. 39 , 314–325. https://doi.org/10.1016/j.cedpsych.2014.08.001 (2014).
Zamarro, G., Nichols, M., Duckworth, A. & D’Mello, S. Further Validation of Survey Effort Measures of Relevant Character Skills: Results from a Sample of High School Students. EDRE Working Paper2018-07. https://doi.org/10.2139/ssrn.3265332 (2018).
Galla, B. M. et al. Why high school grades are better predictors of on-time college graduation than are admissions test scores: The roles of self-regulation and cognitive ability. Am. Educ. Res. J. 56 , 2077–2115. https://doi.org/10.3102/0002831219843292 (2019).
Tsukayama, E., Duckworth, A. L. & Kim, B. Domain-specific impulsivity in school-age children. Dev. Sci. 16 , 879–893. https://doi.org/10.1111/desc.12067 (2013).
Raven, J. & Raven, J. Raven progressive matrices. In Handbook of Nonverbal Assessment (ed. McCallum, R. S.) 223–237 (Kluwer Academic, 2003).
Dynarski, S. M., Hemelt, S. W. & Hyman, J. M. The missing manual: Using National Student Clearinghouse data to track postsecondary outcomes. Educ. Eval. Policy Anal. 37 , 53S-79S (2015).
Schoenecker, C. & Reeves, R. The National Student Clearinghouse: The largest current student tracking database. New Directions Community Coll. 143 , 47–57. https://doi.org/10.1002/cc.335 (2008).
Cialdini, R. B. Descriptive social norms as underappreciated sources of social control. Psychometrika 72 , 263–268. https://doi.org/10.1007/s11336-006-1560-6 (2007).
Article MathSciNet MATH Google Scholar
Bandura, A. Social Learning Theory (General Learning Press, 1971).
Sacerdote, B. Peer effects in education: How might they work, how big are they and how much do we know thus far?. Handb. Econ. Educ. 3 , 249–277. https://doi.org/10.1016/B978-0-444-53429-3.00004-1 (2011).
Funder, D. C. & Ozer, D. J. Evaluating effect size in psychological research: Sense and nonsense. Adv. Methods Pract. Psychol. Sci. 2 , 156–168. https://doi.org/10.1177/2515245919847202 (2019).
Martínez, A. & Salgado, J. F. A meta-analysis of the faking resistance of forced-choice personality inventories. Front. Psychol. 12 , 732241. https://doi.org/10.3389/fpsyg.2021.732241 (2021).
Steinberg, L. & Amanda, S. M. Adolescent development. Annu. Rev. Psychol. 52 , 83–110 (2000).
King, G., Murray, C. J. L., Salomon, J. A. & Tandon, A. Enhancing the validity and cross-cultural comparability of measurement in survey research. Am. Polit. Sci. Rev. 98 , 191–207. https://doi.org/10.1017/S000305540400108X (2004).
Primi, R., Zanon, C., Santos, D., De Fruyt, F. & John, O. P. Anchoring vignettes: Can they make adolescent self-reports of social-emotional skills more reliable, discriminant, and criterion-valid?. Eur. J. Psychol. Assess. 32 , 39–51. https://doi.org/10.1027/1015-5759/a000336 (2016).
Grol-Prokopczyk, H., Verdes-Tennant, E., McEniry, M. & Ispány, M. Promises and pitfalls of anchoring vignettes in health survey research. Demography 52 , 1703–1728. https://doi.org/10.1007/s13524-015-0422-1 (2015).
Bertling, J. P., Borgonovi, F. & Almonte, D. E. Psychosocial skills in large-scale assessments: Trends, challenges, and policy implications. In Psychosocial Skills and School Systems in the 21st Century: Theory, Research, and Practice. The Springer Series on Human Exceptionality (eds Lipnevich, A. A. et al. ) (Springer, 2016). https://doi.org/10.1007/978-3-319-28606-8 .
Schwab, D. P., Heneman, H. G. & DeCotiis, T. A. Behaviorally anchored rating scales: A review of the literature. Pers. Psychol. 28 , 549–562. https://doi.org/10.1111/j.1744-6570.1975.tb01392.x (1975).
Buss, D. M. & Craik, K. H. The act frequency approach to personality. Psychol. Rev. 90 , 105–126 (1983).
Enkavi, A. Z. et al. Large-scale analysis of test-retest reliabilities of self-regulation measures. Proc. Natl. Acad. Sci. 116 , 5472–5477. https://doi.org/10.1073/pnas.1818430116 (2019).
Article ADS CAS PubMed PubMed Central Google Scholar
Duckworth, A. L. & Kern, M. L. A meta-analysis of the convergent validity of self-control measures. J. Res. Pers. 45 , 259–268. https://doi.org/10.1016/j.jrp.2011.02.004 (2011).
Sharma, L., Kohl, K., Morgan, T. A. & Clark, L. A. “Impulsivity’’: Relations between self-report and behavior. J. Pers. Soc. Psychol. 104 , 559–575. https://doi.org/10.1037/a0031181 (2013).
Friedman, N. P. & Gustavson, D. E. Do rating and task measures of control abilities assess the same thing?. Curr. Dir. Psychol. Sci. 31 , 262–271. https://doi.org/10.1177/09637214221091824 (2022).
Feng, S., Han, Y., Heckman, J. J. & Kautz, T. Comparing the reliability and predictive power of child, teacher, and guardian reports of noncognitive skills. Proc. Natl. Acad. Sci. 119 , e2113992119. https://doi.org/10.1073/pnas.2113992119 (2022).
Article CAS PubMed PubMed Central Google Scholar
Howard, G. S. Response-shift bias: A problem in evaluating interventions with pre/post self-reports. Eval. Rev. 4 , 93–106 (1980).
Howard, G. S. & Dailey, P. R. Response-Shift Bias: A source of contamination of self-report measures. J. Appl. Psychol. 64 , 144–150 (1979).
Roberts, B. W., Walton, K. E. & Viechtbauer, W. Patterns of mean-level change in personality traits across the life course: A meta-analysis of longitudinal studies. Psychol. Bull. 132 , 1–25. https://doi.org/10.1037/0033-2909.132.1.1 (2006).
Damian, R. I. & Spengler, M. Sixteen going on sixty-six: A longitudinal study of personality stability and change across 50 years. J. Pers. Soc. Psychol. 117 , 674–695 (2018).
Roberts, B. W. & DelVecchio, W. F. The rank-order consistency of personality traits from childhood to old age: A quantitative review of longitudinal studies. Psychol. Bull. 126 , 3–25. https://doi.org/10.1037/0033-2909.126.1.3 (2000).
Download references
Acknowledgements
This research received support from the Bill & Melinda Gates Foundation, the Raikes Foundation, the William T. Grant Foundation, and a fellowship from the Center for Advanced Study in the Behavioral Sciences (CASBS) to the sixth author and grants from the John Templeton Foundation, the Walton Family Foundation, and National Science Foundation to the last author. This research was supported by the National Institute of Child Health and Human Development (Grant No. 10.13039/100000071 R01HD084772-01). The authors wish to thank Donald Kamentz, Laura Keane, and the schools and students who participated in the research.
Author information
These authors contributed equally: Benjamin Lira and Joseph M. O’Brien.
Authors and Affiliations
University of Pennsylvania, Philadelphia, USA
Benjamin Lira & Angela L. Duckworth
University of Texas at Austin, Austin, USA
Joseph M. O’Brien & David S. Yeager
University of Chicago, Chicago, USA
Pablo A. Peña
University of Pittsburgh, Pittsburgh, USA
Brian M. Galla
University of Colorado-Boulder, Boulder, USA
Sidney D’Mello
Mathematica, Inc., Princeton, USA
Amy Defnet, Tim Kautz & Kate Munkacsy
You can also search for this author in PubMed Google Scholar
Contributions
A.L.D., D.S.Y., J.M.O., and P.A.P. conceptualized the study and developed the methodology; B.M.G. developed methodology; A.L.D., D.S.Y., and J.M.O. collected data; B.L., J.M.O., P.A.P., A.D., T.K., K.M., A.L.D., and D.S.Y. analyzed and interpreted data; B.L., A.L.D., D.S.Y., J.M.O., P.A.P., A.D., T.K., and K.M. wrote the paper; all authors revised and approved the final draft .
Corresponding author
Correspondence to Benjamin Lira .
Ethics declarations
Competing interests.
The authors declare no competing interests.
Additional information
Publisher's note.
Springer Nature remains neutral with regard to jurisdictional claims in published maps and institutional affiliations.
Supplementary Information
Supplementary information., rights and permissions.
Open Access This article is licensed under a Creative Commons Attribution 4.0 International License, which permits use, sharing, adaptation, distribution and reproduction in any medium or format, as long as you give appropriate credit to the original author(s) and the source, provide a link to the Creative Commons licence, and indicate if changes were made. The images or other third party material in this article are included in the article's Creative Commons licence, unless indicated otherwise in a credit line to the material. If material is not included in the article's Creative Commons licence and your intended use is not permitted by statutory regulation or exceeds the permitted use, you will need to obtain permission directly from the copyright holder. To view a copy of this licence, visit http://creativecommons.org/licenses/by/4.0/ .
Reprints and permissions
About this article
Cite this article.
Lira, B., O’Brien, J.M., Peña, P.A. et al. Large studies reveal how reference bias limits policy applications of self-report measures. Sci Rep 12 , 19189 (2022). https://doi.org/10.1038/s41598-022-23373-9
Download citation
Received : 17 May 2022
Accepted : 31 October 2022
Published : 10 November 2022
DOI : https://doi.org/10.1038/s41598-022-23373-9
Share this article
Anyone you share the following link with will be able to read this content:
Sorry, a shareable link is not currently available for this article.
Provided by the Springer Nature SharedIt content-sharing initiative
This article is cited by
Is irritability multidimensional: psychometrics of the irritability and dysregulation of emotion scale (tides-13).
- Andrew S. Dissanayake
- Annie Dupuis
European Child & Adolescent Psychiatry (2024)
By submitting a comment you agree to abide by our Terms and Community Guidelines . If you find something abusive or that does not comply with our terms or guidelines please flag it as inappropriate.
Quick links
- Explore articles by subject
- Guide to authors
- Editorial policies
Sign up for the Nature Briefing newsletter — what matters in science, free to your inbox daily.


Research Bias 101: What You Need To Know
By: Derek Jansen (MBA) | Expert Reviewed By: Dr Eunice Rautenbach | September 2022
If you’re new to academic research, research bias (also sometimes called researcher bias) is one of the many things you need to understand to avoid compromising your study. If you’re not careful, research bias can ruin the credibility of your study.
In this post, we’ll unpack the thorny topic of research bias. We’ll explain what it is , look at some common types of research bias and share some tips to help you minimise the potential sources of bias in your research.
Overview: Research Bias 101
- What is research bias (or researcher bias)?
- Bias #1 – Selection bias
- Bias #2 – Analysis bias
- Bias #3 – Procedural (admin) bias
So, what is research bias?
Well, simply put, research bias is when the researcher – that’s you – intentionally or unintentionally skews the process of a systematic inquiry , which then of course skews the outcomes of the study . In other words, research bias is what happens when you affect the results of your research by influencing how you arrive at them.
For example, if you planned to research the effects of remote working arrangements across all levels of an organisation, but your sample consisted mostly of management-level respondents , you’d run into a form of research bias. In this case, excluding input from lower-level staff (in other words, not getting input from all levels of staff) means that the results of the study would be ‘biased’ in favour of a certain perspective – that of management.
Of course, if your research aims and research questions were only interested in the perspectives of managers, this sampling approach wouldn’t be a problem – but that’s not the case here, as there’s a misalignment between the research aims and the sample .
Now, it’s important to remember that research bias isn’t always deliberate or intended. Quite often, it’s just the result of a poorly designed study, or practical challenges in terms of getting a well-rounded, suitable sample. While perfect objectivity is the ideal, some level of bias is generally unavoidable when you’re undertaking a study. That said, as a savvy researcher, it’s your job to reduce potential sources of research bias as much as possible.
To minimize potential bias, you first need to know what to look for . So, next up, we’ll unpack three common types of research bias we see at Grad Coach when reviewing students’ projects . These include selection bias , analysis bias , and procedural bias . Keep in mind that there are many different forms of bias that can creep into your research, so don’t take this as a comprehensive list – it’s just a useful starting point.
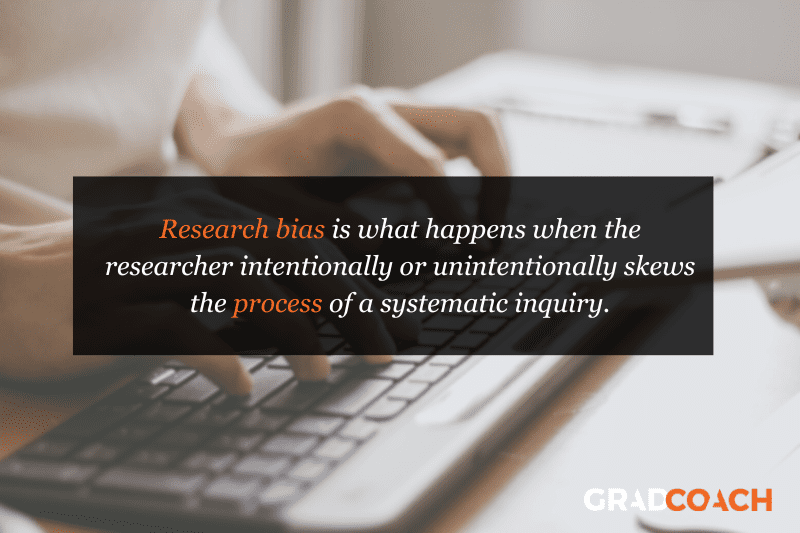
Bias #1 – Selection Bias
First up, we have selection bias . The example we looked at earlier (about only surveying management as opposed to all levels of employees) is a prime example of this type of research bias. In other words, selection bias occurs when your study’s design automatically excludes a relevant group from the research process and, therefore, negatively impacts the quality of the results.
With selection bias, the results of your study will be biased towards the group that it includes or favours, meaning that you’re likely to arrive at prejudiced results . For example, research into government policies that only includes participants who voted for a specific party is going to produce skewed results, as the views of those who voted for other parties will be excluded.
Selection bias commonly occurs in quantitative research , as the sampling strategy adopted can have a major impact on the statistical results . That said, selection bias does of course also come up in qualitative research as there’s still plenty room for skewed samples. So, it’s important to pay close attention to the makeup of your sample and make sure that you adopt a sampling strategy that aligns with your research aims. Of course, you’ll seldom achieve a perfect sample, and that okay. But, you need to be aware of how your sample may be skewed and factor this into your thinking when you analyse the resultant data.
Need a helping hand?
Bias #2 – Analysis Bias
Next up, we have analysis bias . Analysis bias occurs when the analysis itself emphasises or discounts certain data points , so as to favour a particular result (often the researcher’s own expected result or hypothesis). In other words, analysis bias happens when you prioritise the presentation of data that supports a certain idea or hypothesis , rather than presenting all the data indiscriminately .
For example, if your study was looking into consumer perceptions of a specific product, you might present more analysis of data that reflects positive sentiment toward the product, and give less real estate to the analysis that reflects negative sentiment. In other words, you’d cherry-pick the data that suits your desired outcomes and as a result, you’d create a bias in terms of the information conveyed by the study.
Although this kind of bias is common in quantitative research, it can just as easily occur in qualitative studies, given the amount of interpretive power the researcher has. This may not be intentional or even noticed by the researcher, given the inherent subjectivity in qualitative research. As humans, we naturally search for and interpret information in a way that confirms or supports our prior beliefs or values (in psychology, this is called “confirmation bias”). So, don’t make the mistake of thinking that analysis bias is always intentional and you don’t need to worry about it because you’re an honest researcher – it can creep up on anyone .
To reduce the risk of analysis bias, a good starting point is to determine your data analysis strategy in as much detail as possible, before you collect your data . In other words, decide, in advance, how you’ll prepare the data, which analysis method you’ll use, and be aware of how different analysis methods can favour different types of data. Also, take the time to reflect on your own pre-conceived notions and expectations regarding the analysis outcomes (in other words, what do you expect to find in the data), so that you’re fully aware of the potential influence you may have on the analysis – and therefore, hopefully, can minimize it.
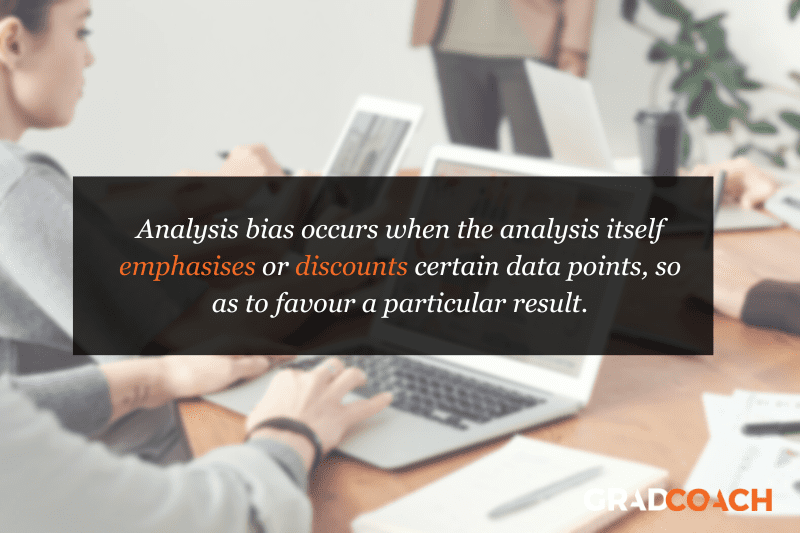
Bias #3 – Procedural Bias
Last but definitely not least, we have procedural bias , which is also sometimes referred to as administration bias . Procedural bias is easy to overlook, so it’s important to understand what it is and how to avoid it. This type of bias occurs when the administration of the study, especially the data collection aspect, has an impact on either who responds or how they respond.
A practical example of procedural bias would be when participants in a study are required to provide information under some form of constraint. For example, participants might be given insufficient time to complete a survey, resulting in incomplete or hastily-filled out forms that don’t necessarily reflect how they really feel. This can happen really easily, if, for example, you innocently ask your participants to fill out a survey during their lunch break.
Another form of procedural bias can happen when you improperly incentivise participation in a study. For example, offering a reward for completing a survey or interview might incline participants to provide false or inaccurate information just to get through the process as fast as possible and collect their reward. It could also potentially attract a particular type of respondent (a freebie seeker), resulting in a skewed sample that doesn’t really reflect your demographic of interest.
The format of your data collection method can also potentially contribute to procedural bias. If, for example, you decide to host your survey or interviews online, this could unintentionally exclude people who are not particularly tech-savvy, don’t have a suitable device or just don’t have a reliable internet connection. On the flip side, some people might find in-person interviews a bit intimidating (compared to online ones, at least), or they might find the physical environment in which they’re interviewed to be uncomfortable or awkward (maybe the boss is peering into the meeting room, for example). Either way, these factors all result in less useful data.
Although procedural bias is more common in qualitative research, it can come up in any form of fieldwork where you’re actively collecting data from study participants. So, it’s important to consider how your data is being collected and how this might impact respondents. Simply put, you need to take the respondent’s viewpoint and think about the challenges they might face, no matter how small or trivial these might seem. So, it’s always a good idea to have an informal discussion with a handful of potential respondents before you start collecting data and ask for their input regarding your proposed plan upfront.
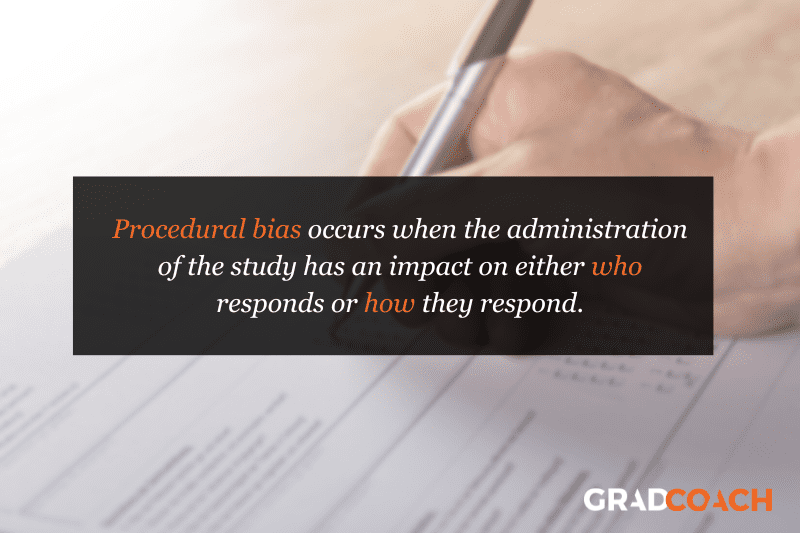
Let’s Recap
Ok, so let’s do a quick recap. Research bias refers to any instance where the researcher, or the research design , negatively influences the quality of a study’s results, whether intentionally or not.
The three common types of research bias we looked at are:
- Selection bias – where a skewed sample leads to skewed results
- Analysis bias – where the analysis method and/or approach leads to biased results – and,
- Procedural bias – where the administration of the study, especially the data collection aspect, has an impact on who responds and how they respond.
As I mentioned, there are many other forms of research bias, but we can only cover a handful here. So, be sure to familiarise yourself with as many potential sources of bias as possible to minimise the risk of research bias in your study.
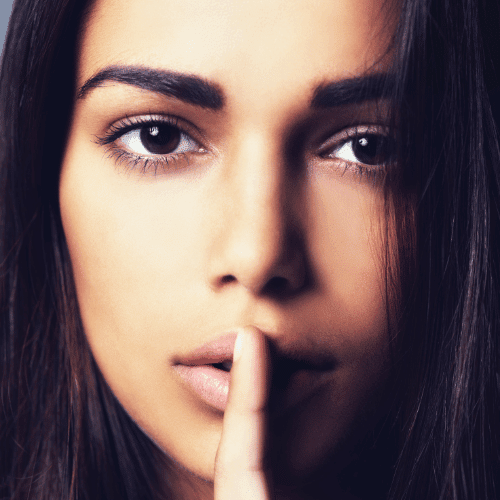
Psst... there’s more!
This post was based on one of our popular Research Bootcamps . If you're working on a research project, you'll definitely want to check this out ...
You Might Also Like:
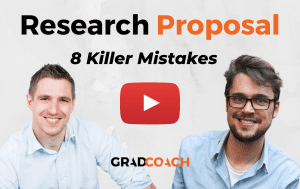
This is really educational and I really like the simplicity of the language in here, but i would like to know if there is also some guidance in regard to the problem statement and what it constitutes.
Do you have a blog or video that differentiates research assumptions, research propositions and research hypothesis?
Submit a Comment Cancel reply
Your email address will not be published. Required fields are marked *
Save my name, email, and website in this browser for the next time I comment.
- Print Friendly
How to Write Limitations of the Study (with examples)
This blog emphasizes the importance of recognizing and effectively writing about limitations in research. It discusses the types of limitations, their significance, and provides guidelines for writing about them, highlighting their role in advancing scholarly research.
Updated on August 24, 2023

No matter how well thought out, every research endeavor encounters challenges. There is simply no way to predict all possible variances throughout the process.
These uncharted boundaries and abrupt constraints are known as limitations in research . Identifying and acknowledging limitations is crucial for conducting rigorous studies. Limitations provide context and shed light on gaps in the prevailing inquiry and literature.
This article explores the importance of recognizing limitations and discusses how to write them effectively. By interpreting limitations in research and considering prevalent examples, we aim to reframe the perception from shameful mistakes to respectable revelations.
What are limitations in research?
In the clearest terms, research limitations are the practical or theoretical shortcomings of a study that are often outside of the researcher’s control . While these weaknesses limit the generalizability of a study’s conclusions, they also present a foundation for future research.
Sometimes limitations arise from tangible circumstances like time and funding constraints, or equipment and participant availability. Other times the rationale is more obscure and buried within the research design. Common types of limitations and their ramifications include:
- Theoretical: limits the scope, depth, or applicability of a study.
- Methodological: limits the quality, quantity, or diversity of the data.
- Empirical: limits the representativeness, validity, or reliability of the data.
- Analytical: limits the accuracy, completeness, or significance of the findings.
- Ethical: limits the access, consent, or confidentiality of the data.
Regardless of how, when, or why they arise, limitations are a natural part of the research process and should never be ignored . Like all other aspects, they are vital in their own purpose.
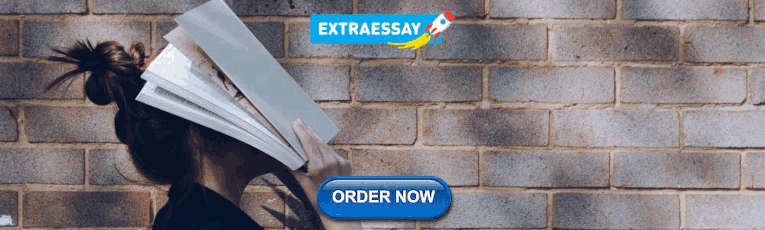
Why is identifying limitations important?
Whether to seek acceptance or avoid struggle, humans often instinctively hide flaws and mistakes. Merging this thought process into research by attempting to hide limitations, however, is a bad idea. It has the potential to negate the validity of outcomes and damage the reputation of scholars.
By identifying and addressing limitations throughout a project, researchers strengthen their arguments and curtail the chance of peer censure based on overlooked mistakes. Pointing out these flaws shows an understanding of variable limits and a scrupulous research process.
Showing awareness of and taking responsibility for a project’s boundaries and challenges validates the integrity and transparency of a researcher. It further demonstrates the researchers understand the applicable literature and have thoroughly evaluated their chosen research methods.
Presenting limitations also benefits the readers by providing context for research findings. It guides them to interpret the project’s conclusions only within the scope of very specific conditions. By allowing for an appropriate generalization of the findings that is accurately confined by research boundaries and is not too broad, limitations boost a study’s credibility .
Limitations are true assets to the research process. They highlight opportunities for future research. When researchers identify the limitations of their particular approach to a study question, they enable precise transferability and improve chances for reproducibility.
Simply stating a project’s limitations is not adequate for spurring further research, though. To spark the interest of other researchers, these acknowledgements must come with thorough explanations regarding how the limitations affected the current study and how they can potentially be overcome with amended methods.
How to write limitations
Typically, the information about a study’s limitations is situated either at the beginning of the discussion section to provide context for readers or at the conclusion of the discussion section to acknowledge the need for further research. However, it varies depending upon the target journal or publication guidelines.
Don’t hide your limitations
It is also important to not bury a limitation in the body of the paper unless it has a unique connection to a topic in that section. If so, it needs to be reiterated with the other limitations or at the conclusion of the discussion section. Wherever it is included in the manuscript, ensure that the limitations section is prominently positioned and clearly introduced.
While maintaining transparency by disclosing limitations means taking a comprehensive approach, it is not necessary to discuss everything that could have potentially gone wrong during the research study. If there is no commitment to investigation in the introduction, it is unnecessary to consider the issue a limitation to the research. Wholly consider the term ‘limitations’ and ask, “Did it significantly change or limit the possible outcomes?” Then, qualify the occurrence as either a limitation to include in the current manuscript or as an idea to note for other projects.
Writing limitations
Once the limitations are concretely identified and it is decided where they will be included in the paper, researchers are ready for the writing task. Including only what is pertinent, keeping explanations detailed but concise, and employing the following guidelines is key for crafting valuable limitations:
1) Identify and describe the limitations : Clearly introduce the limitation by classifying its form and specifying its origin. For example:
- An unintentional bias encountered during data collection
- An intentional use of unplanned post-hoc data analysis
2) Explain the implications : Describe how the limitation potentially influences the study’s findings and how the validity and generalizability are subsequently impacted. Provide examples and evidence to support claims of the limitations’ effects without making excuses or exaggerating their impact. Overall, be transparent and objective in presenting the limitations, without undermining the significance of the research.
3) Provide alternative approaches for future studies : Offer specific suggestions for potential improvements or avenues for further investigation. Demonstrate a proactive approach by encouraging future research that addresses the identified gaps and, therefore, expands the knowledge base.
Whether presenting limitations as an individual section within the manuscript or as a subtopic in the discussion area, authors should use clear headings and straightforward language to facilitate readability. There is no need to complicate limitations with jargon, computations, or complex datasets.
Examples of common limitations
Limitations are generally grouped into two categories , methodology and research process .
Methodology limitations
Methodology may include limitations due to:
- Sample size
- Lack of available or reliable data
- Lack of prior research studies on the topic
- Measure used to collect the data
- Self-reported data

The researcher is addressing how the large sample size requires a reassessment of the measures used to collect and analyze the data.
Research process limitations
Limitations during the research process may arise from:
- Access to information
- Longitudinal effects
- Cultural and other biases
- Language fluency
- Time constraints

The author is pointing out that the model’s estimates are based on potentially biased observational studies.
Final thoughts
Successfully proving theories and touting great achievements are only two very narrow goals of scholarly research. The true passion and greatest efforts of researchers comes more in the form of confronting assumptions and exploring the obscure.
In many ways, recognizing and sharing the limitations of a research study both allows for and encourages this type of discovery that continuously pushes research forward. By using limitations to provide a transparent account of the project's boundaries and to contextualize the findings, researchers pave the way for even more robust and impactful research in the future.
Charla Viera, MS
See our "Privacy Policy"
Ensure your structure and ideas are consistent and clearly communicated
Pair your Premium Editing with our add-on service Presubmission Review for an overall assessment of your manuscript.
Open Access is an initiative that aims to make scientific research freely available to all. To date our community has made over 100 million downloads. It’s based on principles of collaboration, unobstructed discovery, and, most importantly, scientific progression. As PhD students, we found it difficult to access the research we needed, so we decided to create a new Open Access publisher that levels the playing field for scientists across the world. How? By making research easy to access, and puts the academic needs of the researchers before the business interests of publishers.
We are a community of more than 103,000 authors and editors from 3,291 institutions spanning 160 countries, including Nobel Prize winners and some of the world’s most-cited researchers. Publishing on IntechOpen allows authors to earn citations and find new collaborators, meaning more people see your work not only from your own field of study, but from other related fields too.
Brief introduction to this section that descibes Open Access especially from an IntechOpen perspective
Want to get in touch? Contact our London head office or media team here
Our team is growing all the time, so we’re always on the lookout for smart people who want to help us reshape the world of scientific publishing.
Home > Books > Cohort Studies in Health Sciences
Limitations and Biases in Cohort Studies
Submitted: 26 October 2017 Reviewed: 23 January 2018 Published: 28 February 2018
DOI: 10.5772/intechopen.74324
Cite this chapter
There are two ways to cite this chapter:
From the Edited Volume
Cohort Studies in Health Sciences
Edited by R. Mauricio Barría
To purchase hard copies of this book, please contact the representative in India: CBS Publishers & Distributors Pvt. Ltd. www.cbspd.com | [email protected]
Chapter metrics overview
5,883 Chapter Downloads
Impact of this chapter
Total Chapter Downloads on intechopen.com

Total Chapter Views on intechopen.com
Overall attention for this chapters
Good practice in research involves considering diverse sources of biases when designing a study for later validation of results. If they are recognized beforehand, it is possible to minimize or avoid them. Selection biases may originate at the time of enrolling the subjects of study, making it necessary to clearly state the selection criteria of the exposed and nonexposed individuals. If people get lost from the original sample, bias may be introduced by the consequences of reducing the sample. Biases of information could originate in loss of evidence at the moment of recording the data. The definition of follow-up protocols may also help to keep registers of all variables, so information will not be missed from the individuals under study or from the observers who conduct the follow-up. It is necessary to apply the same protocols and instruments for measuring and evaluating the health outcomes in exposed and nonexposed individuals in order to avoid biases of miss-classification. Confusion biases can be avoided at the time of designing the study, with the inclusion of confounding variables from the onset. Matching by age and gender is strongly recommended, and finally, adjustment techniques are used at the time of the data analysis.
- systematic error
- selection bias
- information bias
- interaction
- cohort studies
Author Information
Muriel ramirez-santana *.
- Public Health Department, Faculty of Medicine, Universidad Catolica del Norte, Coquimbo, Chile
*Address all correspondence to: [email protected]
1. Introduction
The external validity of the results of an analytical study (including cohort studies) is determined by the possibility that the results can be extrapolated to larger populations, making the representativeness and randomness of the sample(s) important. However, there is controversy about the real need of representativeness when other situations are more relevant in the study, for example, some practical reasons, restrictions in the selection criteria or focus in certain population groups [ 1 ].
Internal validity, however, is determined by a series of factors that can lead to systematic errors or biases [ 2 ]. Bias can originate both in the design stage of the study, such as sample selection, data collection or analysis, but can be minimized with good planning of the study protocol or using statistical analysis techniques in this phase of the study [ 3 ]. The sample size will determine the validity in terms of the statistical power necessary to reject or approve the working hypothesis. An adequate sample size will make it easier to avoid random errors in the results of the study.
Although cohort studies have a lower risk of presenting biases than other types of epidemiological studies (ecological, cross-sectional or prevalence studies, cases and controls), they are not free of them. This chapter highlights the types of biases, their origin, their effects on the validity of the study and ways to avoid or minimize them. The chapter also gives examples that allow better understanding of the concepts as well as practical advice when carrying out a cohort study.
1.1. Feasibility considerations
Study protocols should always adhere to the evaluation of duly accredited Scientific Ethics Committees. Ethical principles indicate that all participants must adhere to informed consent before beginning to participate in the study, being able to understand all the implications of participating and to decline his/her participation at any moment. Authorizations of the managers in charge of the administration of any institution (healthcare centers, schools, municipalities, hospitals or others) are usually required to access the registered data or to collect the health information of the users. In studies of occupational health, authorization of the workplaces is required to perform the evaluations of jobs and workers exposed to occupational hazards. In studies about infants or children, framed in the educational sector, the assent of the minors is required, in addition to informed consent of the parents/guardians/proxies, and authorization of the executives of participating educational facilities. Collaboration agreements, purchase of laboratory services, transport, locations, surveyors, data analysis, computer support and other technical and logistical requirements that involve carrying out a follow-up study of people, usually for several years, must also be managed. When a large research team is involved, protocols must be in place for recruitment, evaluations, transporting and storage of samples and materials, laboratory procedures, recording data, backing up information and so on.
2. Bias in cohort studies
Certainly, among analytical epidemiological research, cohort studies are less prone to have bias than the case-control ones, specifically regarding memory bias. But as any other epidemiological study, several biases could be present in cohort studies. In this sense, researchers must be aware of those biases in advance and take them into account at the moment of selecting participants, designing the study (collection tools/instruments), when registering the data during field work (data base design) and, later on, at the moment of analyzing and interpreting the data (statistical analysis).
We understand bias as systematic errors that can lead to mistaken results or interpretation regarding the association under study, when the purpose of a study is assessing the association of certain factors toward supporting the causality of a health event or outcome [ 4 ].
There are several ways of classification of biases. For academic proposes, we will use the following classification [ 2 , 4 ]:
Selection bias: originated from the way the participants of the study are selected or followed and can affect the apparent association between the exposure and outcome.
Information biases: could originate in the observed individuals, in the observers or in the instruments used to assess the outcomes.
Confusion bias: their origin is in the relationship that other variables that are not the exposition are related to the outcome, and can modulate the effect(s) of the exposition, contributing to a spurious association.
We will now review each kind of bias in detail and with some examples.
2.1. Selection bias
In cohort studies, the researcher must select exposed and nonexposed individuals. In the first place, it should be understood that both groups are representative of the general population from where they are taken, in order to facilitate the external validity of the study (basic condition to generalize the results in order to support causality). This condition, however, would not necessarily affect the internal validity. In other words, the internal validity is due to systematic errors sourced in stubborn participation of individuals.
The appropriated assessment of the exposure is the first crucial step. Auto-selection is one of the circumstances that could lead to inaccurate selection. As an example, a study conducted among pregnant women in Norway intended to evaluate auto-selection bias by comparing two cohorts; one group was taken from the Medical Birth Registry (2000–2006) as a population-based cohort, and the second group was from women who agreed to participate in the Norwegian Mother and Child Cohort Study. The results suggested that the prevalence estimates of exposures and the outcomes were biased due to self-selection in the Norwegian Mother and Child Cohort Study. Nevertheless, the estimates of exposure-outcome associations were not biased [ 5 ]. But in other cases, the associations could also be flawed.
Another example for selection bias might occur when the compared cohorts are part of a population who receive public health interventions, so the exposure can be misled by this influence. That is the example given by researchers who studied the association of bad water quality (measured by E. coli burden) and development of diarrhea in Bangladesh. Interventions to purify water (use of chlorine) may interfere by reducing the pathogens and misclassify the exposure [ 6 ].
Selection criteria must be clearly defined from the beginning of the study in a way that ensures that biases are avoided. For example, a research was conducted with the objective of assessing the association between exposure to pesticides and neurocognitive impairment, including fine motor coordination [ 7 ]. The researchers used Purdue Pegboard and MOART reaction time tests to measure the outcome. If any right- or left-handed people were selected, bias may be introduced when evaluating the outcomes due to the way that the tests are performed. Both tests have separated evaluation of left and right hands, giving certain scoring to the performance. Then, one important inclusion criterion to consider was right-handed people only; so the responses were standardized under the same criteria, and bias was eluded.
Another example of selection bias can happen in large multicenter cohort studies evaluating the association between diet and cancer. In this case, systematic errors may originate in the measurement of the exposure by dietary questionnaires that are not easy to standardize for all locations. Researchers suggest to use the calibration approach for such cases [ 8 ].
Another type of selection bias is known as the nonresponders or no-participation bias, which are less frequent in prospective cohort studies due to the need for strict following-up of the participants, strengthening the evaluations during the follow-up visits, encouraging participants and evaluators (observers) to always respond and/or register the records properly. Nevertheless, missing data could be present in retrospective cohort studies, where previously registered data are used. This will be explained in detail later, related to the information biases (Section 2.2).
In prospective cohort studies, loss of follow-up may occur, giving rise to selection bias. Loss of follow-up bias is caused by the loss of individuals from one or more exposure groups. Because cohort studies take normally several months or years of following the participants, it is expected that life situations will vary from time to time, causing some of the participants to get lost during the development of the study. Individuals can be lost homogeneously in the groups to be compared, causing bias of poor global miss-classification, which generally leads the estimate toward the null value [ 9 ]. Or individuals from a single group can be lost, causing bias of poor differential miss-classification. In the first case, the estimated risk would not be severely affected, because the incidence rates would keep similar in both groups, but the power of the results may be lost. In the latter case, the results to be obtained may be underestimating or overestimating the association. For example, if the people who are exposed and develop the outcome (disease) are lost, the incidence rate may be lower among the exposed individuals and the relative risk (RR) would be underestimated. On the other hand, if people who are not exposed and do not get the disease after time of follow-up get lost, then the incidence rate among the nonexposed will be higher and the RR would be overestimated.
Here is a hypothetic example showing the four possibilities of losing individuals:
Original data :
Size of the exposed cohort = 1000.
Size of the nonexposed cohort = 1000.
Number of individuals with the outcome among the exposed = 100.
Number of individuals with the outcome among the nonexposed = 10.
Correct results:
Incidence rate in exposed = 100/1000 = 0.1.
Incidence rate in nonexposed = 10/1000 = 0.01.
Relative risk = 10.
Loss of 50 individuals during follow-up with the disease among the exposed:
Incidence rate in exposed = 50/1000 = 0.05.
Relative risk = 5.
L oss of five individuals during follow-up with the disease among the nonexposed:
Incidence rate in nonexposed = 5/1000 = 0.005.
Relative risk = 20.
Loss of 100 individuals during follow-up without the disease among the exposed:
Incidence rate in exposed = 100/800 = 0.125.
Relative risk = 12.5.
Loss of 200 individuals during follow-up without the disease among the nonexposed:
Incidence rate in nonexposed = 10/800 = 0.0125.
Relative risk = 8.
As you can see, the estimated association variation is given by the number of people who completed the follow-up schedule. The general recommendation is that 60–80% of the individuals complete the timeframe defined originally, but a study that simulated a cohort of 500 observations with 1000 replications in computer found utterly biased estimates of the risks with low ranks of loss to follow-up [ 10 ]. On the other hand, as was already said, the results of the diminution in the number of subjects can also affect the statistical power of the results. Then, in the design of the study, at least 10% sample loss must be considered, so this proportion must be added to the minimum calculated sample size for the study. During the field work phase, measures need to be taken in advance in order to avoid losing individuals. To ensure the permanence of the individuals during the follow-up time, it is suggested to include incentives for the participants. These incentives do not necessarily have to be monetary, and a food and transportation voucher can be offered for those who attend scheduled evaluations.
In addition, it may happen that nonexposed individuals enter into the exposed group or vice versa. An example of this could occur when studying the association of tobacco consumption and a certain outcome. Then, during the study, people who smoke can leave the consumption and/or people who do not smoke can start smoking. In those cases, it is suggested to use the incidence density indicator instead of the cumulative incidence. The incidence density is interpreted as exposure measured in units of person-time , for example, person-weeks or person-days. Person-time is the sum of the time periods of observation of each person who has been observed for all or part of the entire time period [ 4 ].
The incidence density is calculated as follows:
It is important to mention that the person-time unit is not in all occasions equivalent to the person-time of all individuals. For example, one person-year could represent one person being followed for 1 year or two people being followed for 6 months. But in any case, this is a way of measuring incidence that is very useful in cohort studies because it avoids the issue of subjects shifting form one exposure group to the other.
Finally, we have the selective survival bias. This bias is known in occupational health as the healthy worker effect and occurs when workers who have the health effect (disease or outcome) abandon the work, so a greater proportion of healthy exposed workers finally lead to underestimation of the health effect or outcome. This situation may happen when the exposed individuals have the condition already for certain time (prevalent cohort), so the probability to express the outcome is greater than individuals who were recently exposed (incident cohort). That effect is known as left truncation bias or time related (immortal time bias, time lag bias) [ 11 , 12 ]. This influence has been described in several studies: occupational settings, development of AIDS among HIV patients, cancer survival, obstetric research, use of acetylsalicylic acid and myocardial infarction [ 13 , 14 , 15 ]. The last is a good example, showing how the use of a cohort recently diagnosed with myocardial infarction has differences in baseline characteristics and prognosis compared to the group that has had the disease for some time (prevalent cohort), even though they were taken from the same population. Then, the researcher suggested studying incident cohorts when estimating survival of a defined outcome [ 14 ]. Another example of occupational health has been published utilizing simulation with the Monte Carlo technique. Results showed that prevalent jobs contribute to descendant bias in an occupational cohort. This arises because individuals who are less susceptible to the exposure’s effect continue to be exposed, thus undervaluing the association [ 13 ].
2.2. Information biases
Loss during follow-up may cause information bias that was already explained in detail in Section 2.1 [ 10 ].
Usually in prospective cohorts, information bias is easy to elude, because measures may be taken during the design by including all variables in the registration forms (instruments), in order to not miss variables of interest. On the other hand, in retrospective cohorts, already existing records may be used. In that case, there could be missing data due to poor registration quality or due to variables that were not considered to be registered in advance. In both cases, the origin of missing information can lead to information bias. To minimize this effect on large population-based cohorts, it is possible to exclude individuals who have missing data from the analyses. But, this is a decision that researchers can take when the size of the remaining cohort still allows for sufficient statistical power to validate the results. That was the case presented in a large study conducted among the Danish population assessing the association between lifestyle and colorectal cancer [ 16 ]. From a total of 160,725 potential participants, several hundreds were not included due to nonresponse, cancer diagnosis and missing data (N = 997). Finally, a cohort of over 55,000 people was included in the investigation.
One important source of bias in cohort studies can occur when diagnosing the health event or outcome. It is necessary to apply the same protocol for measuring or evaluating the health outcomes in exposed and nonexposed individuals in order to avoid the biases of misclassification [ 9 ]. Similarly to what happen in the previously explained bias caused by loss of follow-up, the final effect of misclassification will depend on whether the inaccuracy in the evaluated outcome influences both exposure groups (global misclassification bias) or only affects one of them (differential misclassification bias).
Let us have a look at a hypothetical example in a study that evaluates the risk of having myocardial infarction due to exposure to a high-fat diet. Table 1 shows the correct classification.
High-fat diet and acute myocardial infarction, correct classification .
RR = (250/700)/(100/1000) = 0.357/0.1 = 3.57.
If the evaluation of the exposure is misled in both groups due to the mistakes in the daily food register, this results in a non-differential misclassification. Imagining that 20% of the exposed people go to the nonexposed group and 20% of nonexposed goes to the exposed group, we could have the following situation ( Table 2 ).
High-fat diet and acute myocardial infarction, non-differential misclassification.
RR = (290/700)/(260/1000) = 0.414/0.26 = 1.59.
In that case, the relative risk is diminished due to a higher incidence among the nonexposed group.
Now, suppose that the evaluators applied two diagnostic tests to the exposed that resulted in an increased diagnosis of myocardial infarction among the exposed group. This will result in a differential misclassification due to the mistaken diagnosis in the outcomes ( Table 3 ).
High-fat diet and acute myocardial infarction, differential misclassification.
RR = (295/700)/(100/1000) = 0.421/0.1 = 4.21.
In this last case, when 10% of the exposed people without myocardial infarction moved to the disease group, the result is a higher relative risk due to a higher incidence among the exposed group.
A good example of this kind of misclassification bias could be given regarding the use of mortality records, which are frequently used in epidemiological studies. The registered codes of the diagnoses may be mistaken and lead to misclassification of the outcomes. That was studied recently by Deckert, who reported the results of a simulation study based on real data of cardiovascular disease mortality [ 17 ]. He reported that non-differential bias can to lead to a null hypothesis, whereas differential misclassification leads the observed Standardized Mortality Ratios to be incorrect, in either direction or magnitude. Differences were from 10 to 30%, depending on the sensitivity and specificity characteristics of the diagnosis of cardiovascular disease [ 17 ]. Statistic techniques like quantitative bias analysis (QBA) or bootstrapping disease status imputation could be used to correct misclassification bias due to correct diagnostic codes [ 18 ]. Although statistical adjustments are possible to do in cases where standard information is available, these techniques are not always enough to overcome the bias. An example is reported by Candice Johnson et al., related to the misclassification of self-reported obesity and diabetes, adjusted by the National Health and Nutrition Examination Survey [ 19 ].
Regarding the accuracy in gathering information during the follow-up visits, one could have the temptation to assess more strictly the exposed individuals than the nonexposed or to evaluate the exposed persons more frequently than the nonexposed. The advice is to apply the same protocol and instruments to both groups of people, and in that way, bias introduced by the observer or the instruments is avoided. We understand as instruments the questionnaires, weighting scale, sphygmomanometer, altimeter, laboratory tests/techniques and others. Additionally, if the person(s) who observe and diagnose the outcome are aware of the exposure status, a preconception may lead to overdiagnose the exposed people and/or underdiagnose the nonexposed people. Alike in randomized trials, the best way to avoid this bias is blinding the observers.
There is also a possibility that bias may originate from the observed individuals. It can happen that people, who know they are under observation, change their behavior. This has been called the Hawthorne effect and is due to the effects that the research can produce in the participants (observers and/or studied individuals). This was first described in a factory near Chicago between 1924 and 1936, in which a group of workers who knew they were under strict supervision significantly improved their productivity, compared to workers who were not aware of being observed [ 20 ]. There is still some controversy about the real predisposition effect of the participant’s observation and the amount of bias that could cause. Some studies have found such an effect, but others have not [ 20 , 21 ]. For example, a study conducted in Tanzania regarding malaria treatment did find a modest suggestion that the health professionals maintained better practice during the study [ 22 ].
Finally, all types of epidemiological studies may be affected by partiality in the phase of the analysis. The way to avoid this analytical bias is by masking or blinding the statistician. That means the statistician, or the person performing the analysis, does not know the exposure condition at the time of the analysis.
2.3. Confusion bias and interaction
We understand a confounder as a variable that is associated with the exposure as well as to the health event or outcome, but not being necessarily a cause of the event. For example, an inaccurate causal inference can be made between drinking coffee and pancreatic cancer, when drinking coffee has been associated with a smoking habit [ 4 ]. This is known as spurious association .
The most common confusion variables to be considered during the design of any epidemiological study are gender and age. As cohort studies are observational, people are not randomly assigned to the exposure and nonexposure group; it is not always possible to match both groups by certain variables such as sex, age, or other confounders. Depending on the exposure or events being studied, other variables could work as confounders; therefore, before designing any study, it is important for researchers to read previous studies and develop the design with all evidence that highlight confounders.
Several examples can be given in this matter: (1) In the aforementioned study about acetylsalicylic acid exposure and major bleeding, confounders considered were age, sex, previous hospitalization for alcoholism, non-bleeding ulcer disease, other non-bleeding conditions, and comorbidities [ 15 ]; then the researchers could adjust the risk ratios according to those variables. (2) In the study relating endometriosis and infertility, the considered confounders were menstrual cycle pattern, hirsutism, participant’s birthweight, race, household income, husband’s education, BMI at age 18 years, alcohol consumption, oral contraception use, any analgesic use, health screening behavior, personal history of cardiovascular disease, and personal history of diabetes [ 23 ]. In that case, researchers could evaluate if any of those variables truly acted as confounders or not. (3) In a study relating air pollution and mortality risks, past exposure to pollution and habit of tobacco consumption were not considered; because they may act as confounders for the results of the association and causal inference could be misled [ 24 ]. For a better understanding, please refer to Figure 1 .

Scheme of confounding (smoking) in relation to the exposure (air pollution) and the outcome (acute myocardial infarction).
There are some cases in which the dose of exposure may introduce confusion. Such is the case of age, smoking, or drinking alcohol. Age involves the quantity of years (or months) itself, and for the analysis, it will be possible to use as a continuous variable or create ranges of age for stratification analysis. On the other hand, for consumption, it is recommended to consider registering the quantities being consumed by the individuals, so strata can be made during the analysis phase. Tobacco may be registered by number of cigarettes per day. The case of alcohol is rather difficult. The suggestion is to ask for quantity (number of glasses) and types of drinks consumed and then transform it into grams of pure alcohol consumed daily or weekly.
Confounding variables can be controlled in several ways: restriction, matching, stratification and more sophisticated multivariate techniques [ 2 ].
Restriction is a simple way of avoiding the introduction of already known confounders, by excluding people who present that factor from the beginning. The problem is that this could limit recruitment and the representativeness of certain population groups. So, while increasing the internal validity, this may reduce external validity [ 2 ].
As it was said before, matching is rather difficult to do in prospective studies because the first enrolment criterion is the exposure. Matching normally is used in case control studies, but researchers could emphasize that the proportion of women and men would be 50% each or that a ratio of young/old people was similar in the exposure groups.
Stratification is a simple statistic technique that could be used during analysis, but that requires forethought concerning the possible confusion variables and registering them.
The technique consists of separating the analysis of association, according to strata of the confusing factor, for example, perform separate analyses of men and women when it is suspected that gender may be a confounder. Then, when the difference between the calculated raw risk and the risk calculated by strata is over 15%, we could say that confusion is present.
Let’s see an example. In a rural area, a study proposed to evaluate the relationship between indoor exposure to smoke—from the combustion of wood stoves—and the occurrence of tuberculosis (TB). The results obtained are presented in Table 4 .
Tuberculosis and indoor exposure to smoke from wood burning.
The RR calculation is presented as: RR = (50/71)/(238/762) = 0.704/0.312 = 2.25.
Therefore, the factor indoor exposure to wood smoke for food cooking turned out to be positively associated with the disease. In other words, the incidence of the disease among the exposed group was significantly more than two times greater than that among the nonexposure group.
Given that there is a suspicion that cigarette smoking could modify the effect of indoor contamination on the risk of acquiring tuberculosis, smoking habits were considered. Then, it was possible to assess if this condition acted as a confounder in the association between tuberculosis and indoor smoke exposure using stratification.
The stratification is shown below, where the smoking habit was coded as “never” or “past or present” ( Tables 5 and 6 ).
TB and indoor exposure to smoke from wood burning of never smokers .
Risk ratio calculation among never smokers : RR = (33/50)/(186/597) = 0.66/0.311 = 2.12.
TB and indoor exposure to smoke from wood burning of past or present smokers .
Risk ratio calculation among smokers past or present : RR = (17/21)/(52/165) = 0.81/0.315 = 2.57.
As a conclusion of the stratification results, the factor indoor exposure to smoke was found to be positively and significantly associated with the disease, both in nonsmokers and in past or present smokers. However, in past or present smokers, the risk of suffering from tuberculosis is 44% higher than in nonsmokers (from 2.57 to 2.12), when the indoor pollution was present, confirming that smoking habit acts like a confounder in the association between indoor smoke and tuberculosis incidence.
In addition to confusion, we have the concept of interaction that refers to the effect that two of more factors have by increasing or reducing the incidence of a disease when they are together. Then, the incidence resulting when the factors are together differs from the incidence when the factors are isolated.
Let us try to find interaction in the same example.
In order to assess interaction, it will be necessary to calculate the association between smoking and tuberculosis alone (without the indoor exposure to wood burning smoke) Table 7 .
TB and smoking habits (without indoor exposure to smoke from wood burning).
Risk calculation of smoking among nonexposed to smoke from wood burning: RR = (52/165)/(186/597) = 0.315/0.311 = 1.01.
The result shows that the relative risk of developing TB due exclusively to the habit of smoking is almost nil. But, to know if there is interaction, we should estimate if the presence of both exposures together differs or not from the expected effects if the two exposures were simply the sum of both.
Incidence rate of TB without any smoke exposure = 31.1%
Incidence rate of TB with smoking only = 31.5%
Incidence rate of TB with indoor pollution only = 66%
Incidence rate of TB with smoking and indoor pollution = 81%
AR to smoking = (TB incidence due to smoking−baseline TB incidence) = 31.5–31.1 = 0.4.
AR to indoor pollution = (TB incidence if indoor pollution−baseline TB incidence) = 66–31.1 = 34.9.
The expected attributable risk to both factors would be the addition of the TB incidence of (smoking + indoor contamination) = 34.9 + 0.4 = 35.3%. Then, the expected incidence will be (31.1 + 35.3) = 66.4%.
But the real TB incidence with both exposure factors was 81%. The difference between 81 and 66.4 would be attributable to the interaction, which is 14.6%.
In other way, the incidence when both factors are together is higher than the addition of incidences when the factors are alone, taking into consideration that we have to clear the underground risk (incidence of TB in population free of exposures).
Effectively, we have shown that interaction is present, because the incidences of both exposures together differ from the expected effects if the two exposures were simply the sum of both. As a conclusion, the indoor pollution is a risk factor to develop TB in that setting, but this risk increases substantially more if people smoke indoors. For a better understanding, please refer to Figure 2 .

Incidence rates and attributable risk to factors related to TB incidence and their interaction.
Coming back to the control of confusion bias, adjustment techniques using statistical models require computer training and have the advantage of working with two or more possible confounding variables; opposite to stratification that permits working on one factor only. When using modeling multivariate techniques, logistic regression or proportional hazard regression might be used, but researchers must be aware of how to interpret the results properly [ 2 ].
An important comment about the confusion is that finding a confounder is not always an issue to be worried about. It could also be useful. For example, in the mentioned study about pesticide exposure in agricultural workers and cognitive impairment, gender turned out to be a confounder [ 7 ]. This resulted from the type of work performed differing between men and women. Men used to perform tasks like mixing, blending and applying pesticides; while women proned to collecting fruits, so men were directly exposed to the toxins. Then, knowing that men were more exposed and, consequently, more susceptible to the health damage, the preventive measures may be oriented by strengthening them toward men, but still keeping care on women.
Finally, confounders are not a mistake in the research, but a phenomenon that is present must be understood by the investigators in order to finally consider them when interpreting the results of the study [ 4 ].
3. Conclusions
As shown, biases can be present in any study, originating from multiple steps of the investigation. Their presence should not be grounds for rejection of the results due to the poor quality of the study, but careful attention is required when interpreting the results. To the extent that the researcher is able to recognize the biases, he/she can be proactive in mitigating them, either by way of improving the design or applying statistical techniques (stratification or multivariate adjustment) when analyzing the results. Therefore, when clinicians or researchers look for good quality of articles to read and use as references, they must recognize them when interpreting the results and acknowledge the limitations that the studies may have.
It should be noted that biases are more frequent among retrospective cohort, given by missing information when using existing records (information bias) or by selection bias, because individuals are selected after the outcome has occurred, so both conditions (exposure and outcome) are present at the moment of enrollment. In that case, it is easier that exposed or unexposed subjects would be related to the result of interest, causing selection bias. On the other hand, a prospective cohort design could be affected by the loss of follow-up. Both types of cohort studies may be influenced by information bias, confusion or interaction.
Interesting tools for weighting quality and predisposition to unfairness in observational studies have been gathered and reported by Sanderson et al. [ 25 ]. Those included items for selection methods, measurement of study variables, design-specific sources of bias, control of confounding variables and use of statistics.
Finally, it is considered that cohort studies are used normally as a source of information of systematic reviews and meta-analysis. In those cases, publication bias and outcome reporting bias must be taken into consideration. This is because the journals are prone to publish positive results rather than negative ones, a situation that has been shown [ 26 ].
Conflict of interest
The author of this chapter declares no conflict of interest.
- 1. Richiardi L, Pizzi C, Pearce N. Commentary: Representativeness is usually not necessary and often should be avoided. International Journal of Epidemiology. 2017; 42 :1018-1022
- 2. Grimes DA, Schulz KF. Bias and causal associations in observational research. Lancet. 2002; 359 :248-252
- 3. Mantel N. Avoidance of bias in cohort studies. National Cancer Institute Monograph. May 1985; 67 :169-172
- 4. Gordis L. Epidemiology. 4th ed. Philadelphia: Elsevier; 2009
- 5. Roy MN, Vollset SE, Gjessing HK, Skjærven R, Melve KK, Schreuder P, Alsaker ER, Haug K, Daltveit AK, Per Magnus. Self selection bias in a large prospective pregnancy cohort in Norway. Paediatric and Perinatal Epidemiology 2009; 23 :507‐608
- 6. Ercumen A, Arnold BF, Naser AM, Unicomb L, Colford JM, Luby SP. Potential sources of bias in the use of Escherichia coli to measure waterborne diarrhoea risk in low-income settings. Tropical Medicine & International Health. 2017; 22 :2-11
- 7. Ramírez-Santana M, Zúñiga L, Corral S, Sandoval R, Scheepers PT, Van Der Velden K, Roeleveld N, Pancetti F. Assessing biomarkers and neuropsychological outcomes in rural populations exposed to organophosphate pesticides in Chile—Study design and protocol Environmental and occupational health. BMC Public Health. 2015; 15 :116. DOI: 10.1186/s12889-015-1463-5
- 8. Kaaks R, Plummer M, Riboli E, Estève J, Van Staveren W. Adjustment for bias due to errors in exposure assessments in multicenter cohort studies on diet and cancer: A calibration approach. The American Journal of Clinical Nutrition. 1994; 59 :2455-2505
- 9. Copeland KT, Checkoway H, McMichael AJ, Holbrook RH. Bias due to misclassification in the estimation of relative risk. American Journal of Epidemiology. 1997; 105 (5):188-495. DOI: https://doi.org/10.1093/oxfordjournals.aje.a112408
- 10. Kristman V, Manno M, Côte P. Loss to follow-up in cohort studies: how much is too much? European Journal of Epidemiology. 2004; 19 :751-760
- 11. Lévesque Linda E, Hanley James A, Kezouh Abbas SS. Problem of immortal time bias in cohort studies: Example using statins for preventing progression of diabetes. British Medical Journal. 2010; 340 :b5087. DOI: https://doi.org/10.1136/bmj.b5087
- 12. Suissa S. Lower risk of death with SGLT2 inhibitors in observational studies: Real or bias? Diabetes Care. Jan. 2018; 41 :6-10
- 13. Applebaum KM, Malloy EJ, Eisen EA. Left truncation, susceptibility, and bias in occupational cohort studies. NIH Public Access. 2014; 22 :599-606
- 14. Buckley BS, Simpson CR, McLernon DJ, Hannaford PC, Murphy AW. Considerable differences exist between prevalent and incident myocardial infarction cohorts derived from the same population. Journal of Clinical Epidemiology. 2010; 63 :1351-1357
- 15. Pedersen L, Stürmer T. Conditioning on future exposure to define study cohorts can induce bias: The case of low-dose acetylsalicylic acid and risk of major bleeding. Clinical Epidemiology. 2017; 9 :611-626
- 16. Sedgwick P. Cohort studies: Souce of bias. British Medical Journal. 2011; 343 :d7839. DOI: https://doi.org/10.1136/bmj.d7839
- 17. Deckert A. The existence of standard-biased mortality ratios due to death certificate misclassification - A simulation study based on a true story. BMC Medical Research Methodology. 2016; 16 :1-9
- 18. Walraven C. A comparison of methods to correct for misclassification bias from administrative database diagnostic codes. International Journal of Epidemiology. 2017; 0 :1-12
- 19. Johnson CY, Flanders WD, Strickland MJ, Honein MA, Howards PP. Potential sensitivity of bias analysis results to incorrect assumptions of nondifferential or differential binary exposures misclassification. Epidemiology. 2014; 15 :902-909
- 20. McCambridge J, Witton J, Elbourne DR. Systematic review of the Hawthorne effect: New concepts are needed to study research participation effects. Journal of Clinical Epidemiology. 2014; 67 :267-277
- 21. Henry SG, Jerant A, Iosif A-M, Feldman MD, Cipri C, Kravitz RL. Analysis of threats to research validity introduced by audio recording clinic visits: Selection bias, Hawthorne effect, both, or neither? Diagnostic Microbiology and Infectious Disease. 2016; 28 :1304-1314
- 22. Leurent B, Reyburn H, Muro F, Mbakilwa H, Schellenberg D. Monitoring patient care through health facility exit interviews: An assessment of the Hawthorne effect in a trial of adherence to malaria treatment guidelines in Tanzania. BMC Infectious Diseases. 2016; 16 (59):1-9
- 23. Prescott J, Farland LV, Tobias DK, Gaskins AJ, Spiegelman D, Chavarro JE, Rich-Edwards JW, Barbieri RL, Missmer SA. A prospective cohort study of endometriosis and subsequent risk of infertility. Human Reproduction. 2016; 31 :1475-1482
- 24. Hansell A, Ghosh RE, Blangiardo M, Perkins C, Vienneau D, Goffe K, Briggs D, Gulliver J. Historic air pollution exposure and Long-term mortality risks in England and Wales: Prospective longitudinal cohort study. Thorax. 2016; 71 :330-338
- 25. Sanderson S, Tatt ID, Higgins JPT. Tools for assessing quality and susceptibility to bias in observational studies in epidemiology : A systematic review and annotated bibliography. International Journal of Epidemiology. 2007; 36 :666-676
- 26. Dwan K, Altman DG, Arnaiz JA, et al. Systematic Review of the empirical evidence of study publication bias and outcome reporting bias. PLoS One. 2008; 3 :1-30
© 2018 The Author(s). Licensee IntechOpen. This chapter is distributed under the terms of the Creative Commons Attribution 3.0 License , which permits unrestricted use, distribution, and reproduction in any medium, provided the original work is properly cited.
Continue reading from the same book
Edited by René Mauricio Barría
Published: 19 September 2018
By Cristhian Saavedra Santiesteban
1104 downloads
By René Mauricio Barría
1832 downloads
By Samer Hammoudeh, Wessam Gadelhaq and Ibrahim Janah...
3670 downloads
- USC Libraries
- Research Guides
Organizing Your Social Sciences Research Paper
- Limitations of the Study
- Purpose of Guide
- Design Flaws to Avoid
- Independent and Dependent Variables
- Glossary of Research Terms
- Reading Research Effectively
- Narrowing a Topic Idea
- Broadening a Topic Idea
- Extending the Timeliness of a Topic Idea
- Academic Writing Style
- Applying Critical Thinking
- Choosing a Title
- Making an Outline
- Paragraph Development
- Research Process Video Series
- Executive Summary
- The C.A.R.S. Model
- Background Information
- The Research Problem/Question
- Theoretical Framework
- Citation Tracking
- Content Alert Services
- Evaluating Sources
- Primary Sources
- Secondary Sources
- Tiertiary Sources
- Scholarly vs. Popular Publications
- Qualitative Methods
- Quantitative Methods
- Insiderness
- Using Non-Textual Elements
- Common Grammar Mistakes
- Writing Concisely
- Avoiding Plagiarism
- Footnotes or Endnotes?
- Further Readings
- Generative AI and Writing
- USC Libraries Tutorials and Other Guides
- Bibliography
The limitations of the study are those characteristics of design or methodology that impacted or influenced the interpretation of the findings from your research. Study limitations are the constraints placed on the ability to generalize from the results, to further describe applications to practice, and/or related to the utility of findings that are the result of the ways in which you initially chose to design the study or the method used to establish internal and external validity or the result of unanticipated challenges that emerged during the study.
Price, James H. and Judy Murnan. “Research Limitations and the Necessity of Reporting Them.” American Journal of Health Education 35 (2004): 66-67; Theofanidis, Dimitrios and Antigoni Fountouki. "Limitations and Delimitations in the Research Process." Perioperative Nursing 7 (September-December 2018): 155-163. .
Importance of...
Always acknowledge a study's limitations. It is far better that you identify and acknowledge your study’s limitations than to have them pointed out by your professor and have your grade lowered because you appeared to have ignored them or didn't realize they existed.
Keep in mind that acknowledgment of a study's limitations is an opportunity to make suggestions for further research. If you do connect your study's limitations to suggestions for further research, be sure to explain the ways in which these unanswered questions may become more focused because of your study.
Acknowledgment of a study's limitations also provides you with opportunities to demonstrate that you have thought critically about the research problem, understood the relevant literature published about it, and correctly assessed the methods chosen for studying the problem. A key objective of the research process is not only discovering new knowledge but also to confront assumptions and explore what we don't know.
Claiming limitations is a subjective process because you must evaluate the impact of those limitations . Don't just list key weaknesses and the magnitude of a study's limitations. To do so diminishes the validity of your research because it leaves the reader wondering whether, or in what ways, limitation(s) in your study may have impacted the results and conclusions. Limitations require a critical, overall appraisal and interpretation of their impact. You should answer the question: do these problems with errors, methods, validity, etc. eventually matter and, if so, to what extent?
Price, James H. and Judy Murnan. “Research Limitations and the Necessity of Reporting Them.” American Journal of Health Education 35 (2004): 66-67; Structure: How to Structure the Research Limitations Section of Your Dissertation. Dissertations and Theses: An Online Textbook. Laerd.com.
Descriptions of Possible Limitations
All studies have limitations . However, it is important that you restrict your discussion to limitations related to the research problem under investigation. For example, if a meta-analysis of existing literature is not a stated purpose of your research, it should not be discussed as a limitation. Do not apologize for not addressing issues that you did not promise to investigate in the introduction of your paper.
Here are examples of limitations related to methodology and the research process you may need to describe and discuss how they possibly impacted your results. Note that descriptions of limitations should be stated in the past tense because they were discovered after you completed your research.
Possible Methodological Limitations
- Sample size -- the number of the units of analysis you use in your study is dictated by the type of research problem you are investigating. Note that, if your sample size is too small, it will be difficult to find significant relationships from the data, as statistical tests normally require a larger sample size to ensure a representative distribution of the population and to be considered representative of groups of people to whom results will be generalized or transferred. Note that sample size is generally less relevant in qualitative research if explained in the context of the research problem.
- Lack of available and/or reliable data -- a lack of data or of reliable data will likely require you to limit the scope of your analysis, the size of your sample, or it can be a significant obstacle in finding a trend and a meaningful relationship. You need to not only describe these limitations but provide cogent reasons why you believe data is missing or is unreliable. However, don’t just throw up your hands in frustration; use this as an opportunity to describe a need for future research based on designing a different method for gathering data.
- Lack of prior research studies on the topic -- citing prior research studies forms the basis of your literature review and helps lay a foundation for understanding the research problem you are investigating. Depending on the currency or scope of your research topic, there may be little, if any, prior research on your topic. Before assuming this to be true, though, consult with a librarian! In cases when a librarian has confirmed that there is little or no prior research, you may be required to develop an entirely new research typology [for example, using an exploratory rather than an explanatory research design ]. Note again that discovering a limitation can serve as an important opportunity to identify new gaps in the literature and to describe the need for further research.
- Measure used to collect the data -- sometimes it is the case that, after completing your interpretation of the findings, you discover that the way in which you gathered data inhibited your ability to conduct a thorough analysis of the results. For example, you regret not including a specific question in a survey that, in retrospect, could have helped address a particular issue that emerged later in the study. Acknowledge the deficiency by stating a need for future researchers to revise the specific method for gathering data.
- Self-reported data -- whether you are relying on pre-existing data or you are conducting a qualitative research study and gathering the data yourself, self-reported data is limited by the fact that it rarely can be independently verified. In other words, you have to the accuracy of what people say, whether in interviews, focus groups, or on questionnaires, at face value. However, self-reported data can contain several potential sources of bias that you should be alert to and note as limitations. These biases become apparent if they are incongruent with data from other sources. These are: (1) selective memory [remembering or not remembering experiences or events that occurred at some point in the past]; (2) telescoping [recalling events that occurred at one time as if they occurred at another time]; (3) attribution [the act of attributing positive events and outcomes to one's own agency, but attributing negative events and outcomes to external forces]; and, (4) exaggeration [the act of representing outcomes or embellishing events as more significant than is actually suggested from other data].
Possible Limitations of the Researcher
- Access -- if your study depends on having access to people, organizations, data, or documents and, for whatever reason, access is denied or limited in some way, the reasons for this needs to be described. Also, include an explanation why being denied or limited access did not prevent you from following through on your study.
- Longitudinal effects -- unlike your professor, who can literally devote years [even a lifetime] to studying a single topic, the time available to investigate a research problem and to measure change or stability over time is constrained by the due date of your assignment. Be sure to choose a research problem that does not require an excessive amount of time to complete the literature review, apply the methodology, and gather and interpret the results. If you're unsure whether you can complete your research within the confines of the assignment's due date, talk to your professor.
- Cultural and other type of bias -- we all have biases, whether we are conscience of them or not. Bias is when a person, place, event, or thing is viewed or shown in a consistently inaccurate way. Bias is usually negative, though one can have a positive bias as well, especially if that bias reflects your reliance on research that only support your hypothesis. When proof-reading your paper, be especially critical in reviewing how you have stated a problem, selected the data to be studied, what may have been omitted, the manner in which you have ordered events, people, or places, how you have chosen to represent a person, place, or thing, to name a phenomenon, or to use possible words with a positive or negative connotation. NOTE : If you detect bias in prior research, it must be acknowledged and you should explain what measures were taken to avoid perpetuating that bias. For example, if a previous study only used boys to examine how music education supports effective math skills, describe how your research expands the study to include girls.
- Fluency in a language -- if your research focuses , for example, on measuring the perceived value of after-school tutoring among Mexican-American ESL [English as a Second Language] students and you are not fluent in Spanish, you are limited in being able to read and interpret Spanish language research studies on the topic or to speak with these students in their primary language. This deficiency should be acknowledged.
Aguinis, Hermam and Jeffrey R. Edwards. “Methodological Wishes for the Next Decade and How to Make Wishes Come True.” Journal of Management Studies 51 (January 2014): 143-174; Brutus, Stéphane et al. "Self-Reported Limitations and Future Directions in Scholarly Reports: Analysis and Recommendations." Journal of Management 39 (January 2013): 48-75; Senunyeme, Emmanuel K. Business Research Methods. Powerpoint Presentation. Regent University of Science and Technology; ter Riet, Gerben et al. “All That Glitters Isn't Gold: A Survey on Acknowledgment of Limitations in Biomedical Studies.” PLOS One 8 (November 2013): 1-6.
Structure and Writing Style
Information about the limitations of your study are generally placed either at the beginning of the discussion section of your paper so the reader knows and understands the limitations before reading the rest of your analysis of the findings, or, the limitations are outlined at the conclusion of the discussion section as an acknowledgement of the need for further study. Statements about a study's limitations should not be buried in the body [middle] of the discussion section unless a limitation is specific to something covered in that part of the paper. If this is the case, though, the limitation should be reiterated at the conclusion of the section.
If you determine that your study is seriously flawed due to important limitations , such as, an inability to acquire critical data, consider reframing it as an exploratory study intended to lay the groundwork for a more complete research study in the future. Be sure, though, to specifically explain the ways that these flaws can be successfully overcome in a new study.
But, do not use this as an excuse for not developing a thorough research paper! Review the tab in this guide for developing a research topic . If serious limitations exist, it generally indicates a likelihood that your research problem is too narrowly defined or that the issue or event under study is too recent and, thus, very little research has been written about it. If serious limitations do emerge, consult with your professor about possible ways to overcome them or how to revise your study.
When discussing the limitations of your research, be sure to:
- Describe each limitation in detailed but concise terms;
- Explain why each limitation exists;
- Provide the reasons why each limitation could not be overcome using the method(s) chosen to acquire or gather the data [cite to other studies that had similar problems when possible];
- Assess the impact of each limitation in relation to the overall findings and conclusions of your study; and,
- If appropriate, describe how these limitations could point to the need for further research.
Remember that the method you chose may be the source of a significant limitation that has emerged during your interpretation of the results [for example, you didn't interview a group of people that you later wish you had]. If this is the case, don't panic. Acknowledge it, and explain how applying a different or more robust methodology might address the research problem more effectively in a future study. A underlying goal of scholarly research is not only to show what works, but to demonstrate what doesn't work or what needs further clarification.
Aguinis, Hermam and Jeffrey R. Edwards. “Methodological Wishes for the Next Decade and How to Make Wishes Come True.” Journal of Management Studies 51 (January 2014): 143-174; Brutus, Stéphane et al. "Self-Reported Limitations and Future Directions in Scholarly Reports: Analysis and Recommendations." Journal of Management 39 (January 2013): 48-75; Ioannidis, John P.A. "Limitations are not Properly Acknowledged in the Scientific Literature." Journal of Clinical Epidemiology 60 (2007): 324-329; Pasek, Josh. Writing the Empirical Social Science Research Paper: A Guide for the Perplexed. January 24, 2012. Academia.edu; Structure: How to Structure the Research Limitations Section of Your Dissertation. Dissertations and Theses: An Online Textbook. Laerd.com; What Is an Academic Paper? Institute for Writing Rhetoric. Dartmouth College; Writing the Experimental Report: Methods, Results, and Discussion. The Writing Lab and The OWL. Purdue University.
Writing Tip
Don't Inflate the Importance of Your Findings!
After all the hard work and long hours devoted to writing your research paper, it is easy to get carried away with attributing unwarranted importance to what you’ve done. We all want our academic work to be viewed as excellent and worthy of a good grade, but it is important that you understand and openly acknowledge the limitations of your study. Inflating the importance of your study's findings could be perceived by your readers as an attempt hide its flaws or encourage a biased interpretation of the results. A small measure of humility goes a long way!
Another Writing Tip
Negative Results are Not a Limitation!
Negative evidence refers to findings that unexpectedly challenge rather than support your hypothesis. If you didn't get the results you anticipated, it may mean your hypothesis was incorrect and needs to be reformulated. Or, perhaps you have stumbled onto something unexpected that warrants further study. Moreover, the absence of an effect may be very telling in many situations, particularly in experimental research designs. In any case, your results may very well be of importance to others even though they did not support your hypothesis. Do not fall into the trap of thinking that results contrary to what you expected is a limitation to your study. If you carried out the research well, they are simply your results and only require additional interpretation.
Lewis, George H. and Jonathan F. Lewis. “The Dog in the Night-Time: Negative Evidence in Social Research.” The British Journal of Sociology 31 (December 1980): 544-558.
Yet Another Writing Tip
Sample Size Limitations in Qualitative Research
Sample sizes are typically smaller in qualitative research because, as the study goes on, acquiring more data does not necessarily lead to more information. This is because one occurrence of a piece of data, or a code, is all that is necessary to ensure that it becomes part of the analysis framework. However, it remains true that sample sizes that are too small cannot adequately support claims of having achieved valid conclusions and sample sizes that are too large do not permit the deep, naturalistic, and inductive analysis that defines qualitative inquiry. Determining adequate sample size in qualitative research is ultimately a matter of judgment and experience in evaluating the quality of the information collected against the uses to which it will be applied and the particular research method and purposeful sampling strategy employed. If the sample size is found to be a limitation, it may reflect your judgment about the methodological technique chosen [e.g., single life history study versus focus group interviews] rather than the number of respondents used.
Boddy, Clive Roland. "Sample Size for Qualitative Research." Qualitative Market Research: An International Journal 19 (2016): 426-432; Huberman, A. Michael and Matthew B. Miles. "Data Management and Analysis Methods." In Handbook of Qualitative Research . Norman K. Denzin and Yvonna S. Lincoln, eds. (Thousand Oaks, CA: Sage, 1994), pp. 428-444; Blaikie, Norman. "Confounding Issues Related to Determining Sample Size in Qualitative Research." International Journal of Social Research Methodology 21 (2018): 635-641; Oppong, Steward Harrison. "The Problem of Sampling in qualitative Research." Asian Journal of Management Sciences and Education 2 (2013): 202-210.
- << Previous: 8. The Discussion
- Next: 9. The Conclusion >>
- Last Updated: Apr 22, 2024 9:12 AM
- URL: https://libguides.usc.edu/writingguide
- Privacy Policy
Buy Me a Coffee

Home » Limitations in Research – Types, Examples and Writing Guide
Limitations in Research – Types, Examples and Writing Guide
Table of Contents

Limitations in Research
Limitations in research refer to the factors that may affect the results, conclusions , and generalizability of a study. These limitations can arise from various sources, such as the design of the study, the sampling methods used, the measurement tools employed, and the limitations of the data analysis techniques.
Types of Limitations in Research
Types of Limitations in Research are as follows:
Sample Size Limitations
This refers to the size of the group of people or subjects that are being studied. If the sample size is too small, then the results may not be representative of the population being studied. This can lead to a lack of generalizability of the results.
Time Limitations
Time limitations can be a constraint on the research process . This could mean that the study is unable to be conducted for a long enough period of time to observe the long-term effects of an intervention, or to collect enough data to draw accurate conclusions.
Selection Bias
This refers to a type of bias that can occur when the selection of participants in a study is not random. This can lead to a biased sample that is not representative of the population being studied.
Confounding Variables
Confounding variables are factors that can influence the outcome of a study, but are not being measured or controlled for. These can lead to inaccurate conclusions or a lack of clarity in the results.
Measurement Error
This refers to inaccuracies in the measurement of variables, such as using a faulty instrument or scale. This can lead to inaccurate results or a lack of validity in the study.
Ethical Limitations
Ethical limitations refer to the ethical constraints placed on research studies. For example, certain studies may not be allowed to be conducted due to ethical concerns, such as studies that involve harm to participants.
Examples of Limitations in Research
Some Examples of Limitations in Research are as follows:
Research Title: “The Effectiveness of Machine Learning Algorithms in Predicting Customer Behavior”
Limitations:
- The study only considered a limited number of machine learning algorithms and did not explore the effectiveness of other algorithms.
- The study used a specific dataset, which may not be representative of all customer behaviors or demographics.
- The study did not consider the potential ethical implications of using machine learning algorithms in predicting customer behavior.
Research Title: “The Impact of Online Learning on Student Performance in Computer Science Courses”
- The study was conducted during the COVID-19 pandemic, which may have affected the results due to the unique circumstances of remote learning.
- The study only included students from a single university, which may limit the generalizability of the findings to other institutions.
- The study did not consider the impact of individual differences, such as prior knowledge or motivation, on student performance in online learning environments.
Research Title: “The Effect of Gamification on User Engagement in Mobile Health Applications”
- The study only tested a specific gamification strategy and did not explore the effectiveness of other gamification techniques.
- The study relied on self-reported measures of user engagement, which may be subject to social desirability bias or measurement errors.
- The study only included a specific demographic group (e.g., young adults) and may not be generalizable to other populations with different preferences or needs.
How to Write Limitations in Research
When writing about the limitations of a research study, it is important to be honest and clear about the potential weaknesses of your work. Here are some tips for writing about limitations in research:
- Identify the limitations: Start by identifying the potential limitations of your research. These may include sample size, selection bias, measurement error, or other issues that could affect the validity and reliability of your findings.
- Be honest and objective: When describing the limitations of your research, be honest and objective. Do not try to minimize or downplay the limitations, but also do not exaggerate them. Be clear and concise in your description of the limitations.
- Provide context: It is important to provide context for the limitations of your research. For example, if your sample size was small, explain why this was the case and how it may have affected your results. Providing context can help readers understand the limitations in a broader context.
- Discuss implications : Discuss the implications of the limitations for your research findings. For example, if there was a selection bias in your sample, explain how this may have affected the generalizability of your findings. This can help readers understand the limitations in terms of their impact on the overall validity of your research.
- Provide suggestions for future research : Finally, provide suggestions for future research that can address the limitations of your study. This can help readers understand how your research fits into the broader field and can provide a roadmap for future studies.
Purpose of Limitations in Research
There are several purposes of limitations in research. Here are some of the most important ones:
- To acknowledge the boundaries of the study : Limitations help to define the scope of the research project and set realistic expectations for the findings. They can help to clarify what the study is not intended to address.
- To identify potential sources of bias: Limitations can help researchers identify potential sources of bias in their research design, data collection, or analysis. This can help to improve the validity and reliability of the findings.
- To provide opportunities for future research: Limitations can highlight areas for future research and suggest avenues for further exploration. This can help to advance knowledge in a particular field.
- To demonstrate transparency and accountability: By acknowledging the limitations of their research, researchers can demonstrate transparency and accountability to their readers, peers, and funders. This can help to build trust and credibility in the research community.
- To encourage critical thinking: Limitations can encourage readers to critically evaluate the study’s findings and consider alternative explanations or interpretations. This can help to promote a more nuanced and sophisticated understanding of the topic under investigation.
When to Write Limitations in Research
Limitations should be included in research when they help to provide a more complete understanding of the study’s results and implications. A limitation is any factor that could potentially impact the accuracy, reliability, or generalizability of the study’s findings.
It is important to identify and discuss limitations in research because doing so helps to ensure that the results are interpreted appropriately and that any conclusions drawn are supported by the available evidence. Limitations can also suggest areas for future research, highlight potential biases or confounding factors that may have affected the results, and provide context for the study’s findings.
Generally, limitations should be discussed in the conclusion section of a research paper or thesis, although they may also be mentioned in other sections, such as the introduction or methods. The specific limitations that are discussed will depend on the nature of the study, the research question being investigated, and the data that was collected.
Examples of limitations that might be discussed in research include sample size limitations, data collection methods, the validity and reliability of measures used, and potential biases or confounding factors that could have affected the results. It is important to note that limitations should not be used as a justification for poor research design or methodology, but rather as a way to enhance the understanding and interpretation of the study’s findings.
Importance of Limitations in Research
Here are some reasons why limitations are important in research:
- Enhances the credibility of research: Limitations highlight the potential weaknesses and threats to validity, which helps readers to understand the scope and boundaries of the study. This improves the credibility of research by acknowledging its limitations and providing a clear picture of what can and cannot be concluded from the study.
- Facilitates replication: By highlighting the limitations, researchers can provide detailed information about the study’s methodology, data collection, and analysis. This information helps other researchers to replicate the study and test the validity of the findings, which enhances the reliability of research.
- Guides future research : Limitations provide insights into areas for future research by identifying gaps or areas that require further investigation. This can help researchers to design more comprehensive and effective studies that build on existing knowledge.
- Provides a balanced view: Limitations help to provide a balanced view of the research by highlighting both strengths and weaknesses. This ensures that readers have a clear understanding of the study’s limitations and can make informed decisions about the generalizability and applicability of the findings.
Advantages of Limitations in Research
Here are some potential advantages of limitations in research:
- Focus : Limitations can help researchers focus their study on a specific area or population, which can make the research more relevant and useful.
- Realism : Limitations can make a study more realistic by reflecting the practical constraints and challenges of conducting research in the real world.
- Innovation : Limitations can spur researchers to be more innovative and creative in their research design and methodology, as they search for ways to work around the limitations.
- Rigor : Limitations can actually increase the rigor and credibility of a study, as researchers are forced to carefully consider the potential sources of bias and error, and address them to the best of their abilities.
- Generalizability : Limitations can actually improve the generalizability of a study by ensuring that it is not overly focused on a specific sample or situation, and that the results can be applied more broadly.
About the author
Muhammad Hassan
Researcher, Academic Writer, Web developer
You may also like

How to Cite Research Paper – All Formats and...

Data Collection – Methods Types and Examples

Delimitations in Research – Types, Examples and...

Research Paper Format – Types, Examples and...

Research Process – Steps, Examples and Tips

Research Design – Types, Methods and Examples

Maintenance work is planned for Wednesday 1st May 2024 from 9:00am to 11:00am (BST).
During this time, the performance of our website may be affected - searches may run slowly and some pages may be temporarily unavailable. If this happens, please try refreshing your web browser or try waiting two to three minutes before trying again.
We apologise for any inconvenience this might cause and thank you for your patience.
Chemical Society Reviews
Bridging the gap between academic research and industrial development in advanced all-solid-state lithium–sulfur batteries.

* Corresponding authors
a Chemical Sciences and Engineering Division, Argonne National Laboratory, 9700 S Cass Ave, Lemont, IL 60439, USA E-mail: [email protected] , [email protected]
b Eastern Institute for Advanced Study, Eastern Institute of Technology, Ningbo, Zhejiang, P. R. China
c Laurel Heights Secondary School, 650 Laurelwood Dr, Waterloo, ON, Canada
The energy storage and vehicle industries are heavily investing in advancing all-solid-state batteries to overcome critical limitations in existing liquid electrolyte-based lithium-ion batteries, specifically focusing on mitigating fire hazards and improving energy density. All-solid-state lithium–sulfur batteries (ASSLSBs), featuring earth-abundant sulfur cathodes, high-capacity metallic lithium anodes, and non-flammable solid electrolytes, hold significant promise. Despite these appealing advantages, persistent challenges like sluggish sulfur redox kinetics, lithium metal failure, solid electrolyte degradation, and manufacturing complexities hinder their practical use. To facilitate the transition of these technologies to an industrial scale, bridging the gap between fundamental scientific research and applied R&D activities is crucial. Our review will address the inherent challenges in cell chemistries within ASSLSBs, explore advanced characterization techniques, and delve into innovative cell structure designs. Furthermore, we will provide an overview of the recent trends in R&D and investment activities from both academia and industry. Building on the fundamental understandings and significant progress that has been made thus far, our objective is to motivate the battery community to advance ASSLSBs in a practical direction and propel the industrialized process.
Article information
Download citation, permissions.

J. Lee, C. Zhao, C. Wang, A. Chen, X. Sun, K. Amine and G. Xu, Chem. Soc. Rev. , 2024, Advance Article , DOI: 10.1039/D3CS00439B
To request permission to reproduce material from this article, please go to the Copyright Clearance Center request page .
If you are an author contributing to an RSC publication, you do not need to request permission provided correct acknowledgement is given.
If you are the author of this article, you do not need to request permission to reproduce figures and diagrams provided correct acknowledgement is given. If you want to reproduce the whole article in a third-party publication (excluding your thesis/dissertation for which permission is not required) please go to the Copyright Clearance Center request page .
Read more about how to correctly acknowledge RSC content .
Social activity
Search articles by author.
This article has not yet been cited.
Advertisements
Numbers, Facts and Trends Shaping Your World
Read our research on:
Full Topic List
Regions & Countries
- Publications
- Our Methods
- Short Reads
- Tools & Resources
Read Our Research On:
Discrimination Experiences Shape Most Asian Americans’ Lives
4. asian americans and discrimination during the covid-19 pandemic, table of contents.
- Key findings from the survey
- Most Asian Americans have been treated as foreigners in some way, no matter where they were born
- Most Asian Americans have been subjected to ‘model minority’ stereotypes, but many haven’t heard of the term
- Experiences with other daily and race-based discrimination incidents
- In their own words: Key findings from qualitative research on Asian Americans and discrimination experiences
- Discrimination in interpersonal encounters with strangers
- Racial discrimination at security checkpoints
- Encounters with police because of race or ethnicity
- Racial discrimination in the workplace
- Quality of service in restaurants and stores
- Discrimination in neighborhoods
- Experiences with name mispronunciation
- Discrimination experiences of being treated as foreigners
- In their own words: How Asian Americans would react if their friend was told to ‘go back to their home country’
- Awareness of the term ‘model minority’
- Views of the term ‘model minority’
- How knowledge of Asian American history impacts awareness and views of the ‘model minority’ label
- Most Asian Americans have experienced ‘model minority’ stereotypes
- In their own words: Asian Americans’ experiences with the ‘model minority’ stereotype
- Asian adults who personally know an Asian person who has been threatened or attacked since COVID-19
- In their own words: Asian Americans’ experiences with discrimination during the COVID-19 pandemic
- Experiences with talking about racial discrimination while growing up
- Is enough attention being paid to anti-Asian racism in the U.S.?
- Acknowledgments
- Sample design
- Data collection
- Weighting and variance estimation
- Methodology: 2021 focus groups of Asian Americans
- Appendix: Supplemental tables
Following the coronavirus outbreak, reports of discrimination and violence toward Asian Americans increased. A previous Pew Research Center survey of English-speaking Asian adults showed that as of 2021, one-third said they feared someone might threaten or physically attack them. English-speaking Asian adults in 2022 were also more likely than other racial or ethnic groups to say they had changed their daily routines due to concerns they might be threatened or attacked. 19
In this new 2022-23 survey, Asian adults were asked if they personally know another Asian person in the U.S. who had been attacked since the pandemic began.
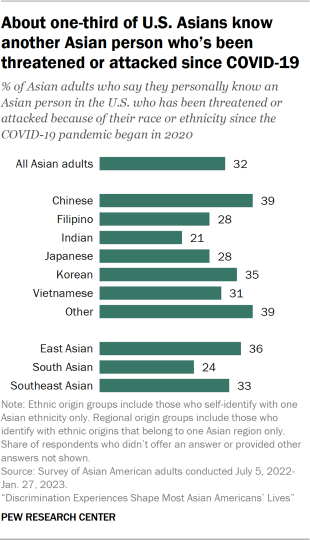
About one-third of Asian adults (32%) say they personally know an Asian person in the U.S. who has been threatened or attacked because of their race or ethnicity since the COVID-19 pandemic began in 2020.
Whether Asian adults know someone with this experience varies across Asian ethnic origin groups:
- About four-in-ten Chinese adults (39%) say they personally know another Asian person who has been threatened or attacked since the coronavirus outbreak. Similar shares of Korean adults (35%) and those who belong to less populous Asian origin groups (39%) – those categorized as “other” in this report – say the same.
- About three-in-ten Vietnamese (31%), Japanese (28%) and Filipino (28%) Americans and about two-in-ten Indian adults (21%) say they know another Asian person in the U.S. who has been the victim of a racially motivated threat or attack.
Additionally, there are some differences by regional origin groups:
- Overall, similar shares of East and Southeast Asian adults say they know another Asian person who’s been threatened or attacked because of their race or ethnicity (36% and 33%, respectively).
- A somewhat smaller share of South Asian adults say the same (24%).
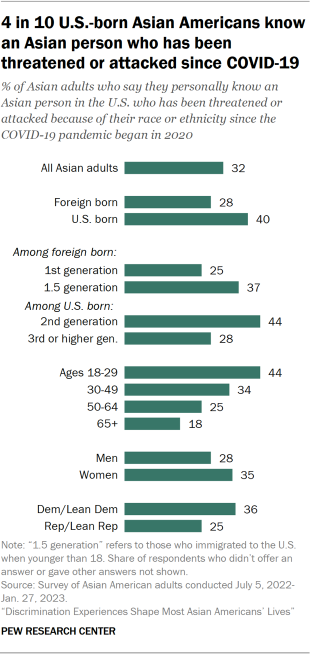
There are also differences across nativity and immigrant generations:
- U.S.-born Asian adults are more likely than Asian immigrants to say they know another Asian person who has been threatened or attacked during the COVID-19 pandemic (40% vs. 28%, respectively).
- Among immigrants, those who are 1.5 generation – those who came to the U.S. as children – are more likely than the first generation – those who immigrated as adults – to say they know someone with this experience (37% vs. 25%).
- And among U.S.-born Asian Americans, 44% of second-generation adults say this, compared with 28% of third- or higher-generation Asian adults.
Whether Asian Americans personally know another Asian person who was threatened or attacked because of their race or ethnicity since the beginning of the pandemic also varies across other demographic groups:
- Age: 44% of Asian adults under 30 years old say they know someone who has been threatened or attacked during the pandemic, compared with 18% of those 65 and older.
- Gender: Asian women are somewhat more likely than men to say they know an Asian person in the U.S. who has been threatened or attacked during the COVID-19 pandemic (35% vs. 28%, respectively).
- Party: 36% of Asian Democrats and Democratic leaners say they know another Asian person who has been threatened or attacked because of their race or ethnicity, higher than the share among Republicans and Republican leaners (25%).
Heightened anti-Asian discrimination during the COVID-19 pandemic
These survey findings follow a spike in reports of discrimination against Asian Americans during the COVID-19 pandemic. The number of federally recognized hate crime incidents of anti-Asian bias increased from 158 in 2019 to 279 in 2020 and 746 in 2021, according to hate crime statistics published by the FBI . In 2022, the number of anti-Asian hate crimes decreased for the first time since the coronavirus outbreak, to 499 incidents. Between March 2020 and May 2023, the organization Stop AAPI Hate received more than 11,000 self-reported incidents of anti-Asian bias, the vast majority of which involved harassment, bullying, shunning and other discrimination incidents.
Additionally, previous research found that calling COVID-19 the “Chinese Virus,” “Asian Virus” or other names that attach location or ethnicity to the disease was associated with anti-Asian sentiment in online discourse. Use of these phrases by politicians or other prominent public officials, such as by former President Donald Trump , coincided with greater use among the general public and more frequent instances of bias against Asian Americans.
In the 2021 Pew Research Center focus groups of Asian Americans, participants discussed their experiences of being discriminated against because of their race or ethnicity during the COVID-19 pandemic.
Participants talked about being shamed in both public and private spaces. Some Asian immigrant participants talked about being afraid to speak out because of how it might impact their immigration status:
“I was walking in [the city where I live], and a White old woman was poking me in the face saying, ‘You are disgusting,’ and she was trying to hit me. I ran away crying. … At the time, I was with my boyfriend, but he also just came to the U.S., so we ran away together thinking that if we cause trouble, we could be deported.”
–Immigrant woman of Korean origin in late 20s (translated from Korean)
“[A very close friend of mine] lived at [a] school dormitory, and when the pandemic just happened … his room was directly pasted with the adhesive tape saying things like ‘Chinese virus quarantine.’”
–Immigrant man of Chinese origin in early 30s (translated from Mandarin)
Many participants talked about being targeted because others perceive them as Chinese , regardless of their ethnicity:
“I think the crimes [that happened] against other Asian people can happen to me while going through COVID-19. When I see a White person, I don’t know if their ancestors are Scottish or German, so they will look at me and think the same. It seems that they can’t distinguish between Korean and Chinese and think that we are from Asia and the onset of COVID-19 is our fault. This is something that can happen to all of us. So I think Asian Americans should come together and let people know that we are also human and we have rights. I came to think about Asian Americans that they shouldn’t stay still even if they’re trampled on.”
–Immigrant woman of Korean origin in early 50s (translated from Korean)
“Even when I was just getting on the bus, [people acted] as if I was carrying the virus. People would not sit with me, they would sit a bit far. It was because I look Chinese.”
–Immigrant woman of Bhutanese origin in early 30s (translated from Dzongkha)
Amid these incidents, some participants talked about feeling in community and kinship with other Asian people:
“[When I hear stories about Asian people in the news,] I feel like automatically you just have a sense of connection to someone that’s Asian. … [I]t makes me and my family and everyone else that I know that is Asian super mad and upset that this is happening. [For example,] the subway attacks where there was a mother who got dragged down the stairs for absolutely no reason. It just kind of makes you scared because you are Asian, and I would tell my mom, ‘You’re not going anywhere without me.’ We got pepper spray and all of that. But there is definitely a difference because you just feel a connection with them no matter if you don’t know them.”
–U.S.-born woman of Taiwanese origin in early 20s
“[A]s a result of the pandemic, I think we saw an increase in Asian hate in the media. I think that was one time where I realized as an Asian person, I felt a lot of pain. I felt a lot of fear, I felt a lot of anger and frustration for my community. … I think it was just at that specific moment when I saw the Asian hate, Asian hate crimes, and I realized, ‘Oh, they’re targeting my people.’ I don’t know how to explain it exactly. I never really referred to myself just plainly as an Asian American, but when I saw it in that media and I saw people who looked like me or people who I related with getting hurt and mistreated, I felt anger for that community, for my community.””
–U.S.-born woman of Korean origin in late teens
Some connected discrimination during the pandemic to other times of heightened anti-Asian discrimination . For example, one woman connected anti-Asian discrimination during COVID-19 to the period after Sept. 11:
“[T]he hate crimes I’m reading about now are towards Chinese [people] because of COVID, but I remember after 9/11, that was – I remember the looks that people would give me on the subway but also reading the violent acts committed towards Indians of all types, just the confusion – I mean, I say confusion but I mean really they wanted to attack Muslims, but they didn’t care, they were just looking for a brown person to attack. So there’s always something that happens that then suddenly falls on one community or another.”
–U.S.-born man of Indian origin in late 40s
- Pew Research Center’s American Trends Panel surveys of Asian adults were conducted only in English and are representative of the English-speaking Asian adult population. In 2021, 70% of Asian adults spoke only English or said they speak English “very well,” according to a Pew Research Center analysis of the 2021 American Community Survey. By contrast, the Center’s 2022-23 survey of Asian Americans was conducted in six languages, including Chinese (Simplified and Traditional), English, Hindi, Korean, Tagalog and Vietnamese. ↩
Sign up for our weekly newsletter
Fresh data delivery Saturday mornings
Sign up for The Briefing
Weekly updates on the world of news & information
- Asian Americans
- Discrimination & Prejudice
- Immigration Issues
- Race Relations
- Racial Bias & Discrimination
Key facts about Asian Americans living in poverty
Methodology: 2023 focus groups of asian americans, 1 in 10: redefining the asian american dream (short film), the hardships and dreams of asian americans living in poverty, key facts about asian american eligible voters in 2024, most popular, report materials.
1615 L St. NW, Suite 800 Washington, DC 20036 USA (+1) 202-419-4300 | Main (+1) 202-857-8562 | Fax (+1) 202-419-4372 | Media Inquiries
Research Topics
- Age & Generations
- Coronavirus (COVID-19)
- Economy & Work
- Family & Relationships
- Gender & LGBTQ
- Immigration & Migration
- International Affairs
- Internet & Technology
- Methodological Research
- News Habits & Media
- Non-U.S. Governments
- Other Topics
- Politics & Policy
- Race & Ethnicity
- Email Newsletters
ABOUT PEW RESEARCH CENTER Pew Research Center is a nonpartisan fact tank that informs the public about the issues, attitudes and trends shaping the world. It conducts public opinion polling, demographic research, media content analysis and other empirical social science research. Pew Research Center does not take policy positions. It is a subsidiary of The Pew Charitable Trusts .
Copyright 2024 Pew Research Center
Terms & Conditions
Privacy Policy
Cookie Settings
Reprints, Permissions & Use Policy

Superradiant atoms could push the boundaries of how precisely time can be measured
Superradiant atoms can help us measure time more precisely than ever. In a new study, researchers from the University of Copenhagen present a new method for measuring the time interval, the second, mitigating some of the limitations that today's most advanced atomic clocks encounter. The result could have broad implications in areas such as space travel, volcanic eruptions and GPS systems.
The second is the most precisely-defined unit of measurement, compared to other base units such as the kilogram, meter, and degree Kelvin. Time is currently measured by atomic clocks in different places around the world, which together, tell us what time it is. Using radio waves, atomic clocks continuously send signals that synchronize our computers, phones and wristwatches.
Oscillations are the key to keeping time. In a grandfather clock, these oscillations are from a pendulum's swinging from side to side every second, while in an atomic clock, it is a laser beam which corresponds to an energy transition in strontium and oscillates about a million billion times per second.
But according to PhD fellow Eliot Bohr from the Niels Bohr Institute -- great-grandson of Niels Bohr -- even atomic clocks could become more precise. This is because the detection laser, used by most modern atomic clocks to read the oscillation of atoms, heats up the atoms so much that they escape -- which degrades precision.
"Because the atoms constantly need to be replaced with fresh new atoms, while new atoms are being prepared, the clock loses time ever so slightly.Therefore, we are attempting to overcome some of the current challenges and limitations of the world's best atomic clocks by, among other things, reusing the atoms so that they don't need to be replaced as often," explains Eliot Bohr who was employed at the Niels Bohr Institute when he did the research, but who is now PhD fellow at the University of Colorado.
He is the lead author of a new study published in the scientific journal Nature Communications , which uses an innovative and perhaps more efficient way of measuring time.
Superradiance and cooling to absolute zero
The current methodology consists of a hot oven that spits roughly 300 million strontium atoms into an extraordinarily chilly ball of cold atoms known as a magneto-optical trap, or MOT. The temperature of these atoms is approximately -273 °C -- very near absolute zero -- and there are two mirrors with a light field in between them to enhance the atomic interactions. Together with his research colleagues, Bohr has developed a new method to read out the atoms.
"When the atoms land in the vacuum chamber, they lie completely still because it is so cold, which makes it possible to register their oscillations with the two mirrors at opposing ends of the chamber," explains Eliot Bohr.
The reason why the researchers don't need to heat the atoms with a laser and destroy them is thanks to a quantum physical phenomenon known as 'superradiance'. The phenomenon occurs when the group of strontium atoms is entangled and at the same time emits light in the field between the two mirrors.
"Themirrors cause the atoms to behave as a single unit. Collectively, they emit a powerful light signal that we can use to read out the atomic state, a crucial step for measuring time. This method heats up the atoms minimally, so It all happens without replacing the atoms, and this has the potential to make it a more precise measurement method," explains Bohr.
GPS, space missions and volcanic eruptions
According to Eliot Bohr, the new research result may be beneficial for developing a more accurate GPS system. Indeed, the roughly 30 satellites that constantly circle Earth and tell us where we are need atomic clocks to measure time.
"Whenever satellites determine the position of your phone or GPS, you are using an atomic clock in a satellite. The precision of the atomic clocks is so important that If that atomic clock is off by a microsecond, it means an inaccuracy of about 100 meters on the Earth's surface," explains Eliot Bohr.
Future space missions are another area where the researcher foresees more precise atomic clocks making a significant impact.
"When people and crafts are sent out into space, they venture even further away from our satellites. Consequently, the requirements for precise time measurements to navigate in space are much greater," he says.
The result could also be helpful in the development of a new generation of smaller, portable atomic clocks that could be used for more than "just" measuring time.
"Atomic clocks are sensitive to gravitational changes and can therefore be used to detect changes in Earth's mass and gravity, and this could help us predict when volcanic eruptions and earthquakes will occur," says Bohr.
Bohr emphasizes that while the new method using superradiant atoms is very promising, it is still a "proof of concept" which needs further refinement. .
The research was conducted by the team of Jörg Helge Müller and Jan Thomsen at the Niels Bohr Institute, in collaboration with PhD students Sofus Laguna Kristensen and Julian Robinson-Tait, and postdoc Stefan Alaric Schäffer. The project also included contributions from theorists Helmut Ritsch and Christoph Hotter from the University of Innsbruck, as well as Tanya Zelevinsky from Columbia University.
- Engineering
- Weapons Technology
- Nanotechnology
- Quantum Physics
- Global Positioning System
- Electron configuration
- Time in physics
- Oscillation
- Special relativity
- Constructal theory
Story Source:
Materials provided by University of Copenhagen - Faculty of Science . Note: Content may be edited for style and length.
Journal Reference :
- Eliot A. Bohr, Sofus L. Kristensen, Christoph Hotter, Stefan A. Schäffer, Julian Robinson-Tait, Jan W. Thomsen, Tanya Zelevinsky, Helmut Ritsch, Jörg H. Müller. Collectively enhanced Ramsey readout by cavity sub- to superradiant transition . Nature Communications , 2024; 15 (1) DOI: 10.1038/s41467-024-45420-x
Cite This Page :
Explore More
- Making Diamonds at Ambient Pressure
- Eruption of Mega-Magnetic Star
- Clean Fuel Generation With Simple Twist
- Bioluminescence in Animals 540 Million Years Ago
- Fossil Frogs Share Their Skincare Secrets
- Fussy Eater? Most Parents Play Short Order Cook
- Precise Time Measurement: Superradiant Atoms
- Artificial Cells That Act Like Living Cells
- Affordable and Targeted Anticancer Agent
- This Alloy Is Kinky
Trending Topics
Strange & offbeat.

An official website of the United States government
The .gov means it’s official. Federal government websites often end in .gov or .mil. Before sharing sensitive information, make sure you’re on a federal government site.
The site is secure. The https:// ensures that you are connecting to the official website and that any information you provide is encrypted and transmitted securely.
- Publications
- Account settings
Preview improvements coming to the PMC website in October 2024. Learn More or Try it out now .
- Advanced Search
- Journal List
- Biochem Med (Zagreb)
- v.23(1); 2013 Feb
Bias in research
By writing scientific articles we communicate science among colleagues and peers. By doing this, it is our responsibility to adhere to some basic principles like transparency and accuracy. Authors, journal editors and reviewers need to be concerned about the quality of the work submitted for publication and ensure that only studies which have been designed, conducted and reported in a transparent way, honestly and without any deviation from the truth get to be published. Any such trend or deviation from the truth in data collection, analysis, interpretation and publication is called bias. Bias in research can occur either intentionally or unintentionally. Bias causes false conclusions and is potentially misleading. Therefore, it is immoral and unethical to conduct biased research. Every scientist should thus be aware of all potential sources of bias and undertake all possible actions to reduce or minimize the deviation from the truth. This article describes some basic issues related to bias in research.
Introduction
Scientific papers are tools for communicating science between colleagues and peers. Every research needs to be designed, conducted and reported in a transparent way, honestly and without any deviation from the truth. Research which is not compliant with those basic principles is misleading. Such studies create distorted impressions and false conclusions and thus can cause wrong medical decisions, harm to the patient as well as substantial financial losses. This article provides the insight into the ways of recognizing sources of bias and avoiding bias in research.
Definition of bias
Bias is any trend or deviation from the truth in data collection, data analysis, interpretation and publication which can cause false conclusions. Bias can occur either intentionally or unintentionally ( 1 ). Intention to introduce bias into someone’s research is immoral. Nevertheless, considering the possible consequences of a biased research, it is almost equally irresponsible to conduct and publish a biased research unintentionally.
It is worth pointing out that every study has its confounding variables and limitations. Confounding effect cannot be completely avoided. Every scientist should therefore be aware of all potential sources of bias and undertake all possible actions to reduce and minimize the deviation from the truth. If deviation is still present, authors should confess it in their articles by declaring the known limitations of their work.
It is also the responsibility of editors and reviewers to detect any potential bias. If such bias exists, it is up to the editor to decide whether the bias has an important effect on the study conclusions. If that is the case, such articles need to be rejected for publication, because its conclusions are not valid.
Bias in data collection
Population consists of all individuals with a characteristic of interest. Since, studying a population is quite often impossible due to the limited time and money; we usually study a phenomenon of interest in a representative sample. By doing this, we hope that what we have learned from a sample can be generalized to the entire population ( 2 ). To be able to do so, a sample needs to be representative of the population. If this is not the case, conclusions will not be generalizable, i.e. the study will not have the external validity.
So, sampling is a crucial step for every research. While collecting data for research, there are numerous ways by which researchers can introduce bias in the study. If, for example, during patient recruitment, some patients are less or more likely to enter the study than others, such sample would not be representative of the population in which this research is done. In that case, these subjects who are less likely to enter the study will be under-represented and those who are more likely to enter the study will be over-represented relative to others in the general population, to which conclusions of the study are to be applied to. This is what we call a selection bias . To ensure that a sample is representative of a population, sampling should be random, i.e. every subject needs to have equal probability to be included in the study. It should be noted that sampling bias can also occur if sample is too small to represent the target population ( 3 ).
For example, if the aim of the study is to assess the average hsCRP (high sensitive C-reactive protein) concentration in healthy population in Croatia, the way to go would be to recruit healthy individuals from a general population during their regular annual health check up. On the other hand, a biased study would be one which recruits only volunteer blood donors because healthy blood donors are usually individuals who feel themselves healthy and who are not suffering from any condition or illness which might cause changes in hsCRP concentration. By recruiting only healthy blood donors we might conclude that hsCRP is much lower that it really is. This is a kind of sampling bias, which we call a volunteer bias .
Another example for volunteer bias occurs by inviting colleagues from a laboratory or clinical department to participate in the study on some new marker for anemia. It is very likely that such study would preferentially include those participants who might suspect to be anemic and are curious to learn it from this new test. This way, anemic individuals might be over-represented. A research would then be biased and it would not allow generalization of conclusions to the rest of the population.
Generally speaking, whenever cross-sectional or case control studies are done exclusively in hospital settings, there is a good chance that such study will be biased. This is called admission bias . Bias exists because the population studied does not reflect the general population.
Another example of sampling bias is the so called survivor bias which usually occurs in cross-sectional studies. If a study is aimed to assess the association of altered KLK6 (human Kallikrein-6) expression with a 10 year incidence of Alzheimer’s disease, subjects who died before the study end point might be missed from the study.
Misclassification bias is a kind of sampling bias which occurs when a disease of interest is poorly defined, when there is no gold standard for diagnosis of the disease or when a disease might not be easy detectable. This way some subjects are falsely classified as cases or controls whereas they should have been in another group. Let us say that a researcher wants to study the accuracy of a new test for an early detection of the prostate cancer in asymptomatic men. Due to absence of a reliable test for the early prostate cancer detection, there is a chance that some early prostate cancer cases would go misclassified as disease-free causing the under- or over-estimation of the accuracy of this new marker.
As a general rule, a research question needs to be considered with much attention and all efforts should be made to ensure that a sample is as closely matched to the population, as possible.
Bias in data analysis
A researcher can introduce bias in data analysis by analyzing data in a way which gives preference to the conclusions in favor of research hypothesis. There are various opportunities by which bias can be introduced during data analysis, such as by fabricating, abusing or manipulating the data. Some examples are:
- reporting non-existing data from experiments which were never done (data fabrication);
- eliminating data which do not support your hypothesis (outliers, or even whole subgroups);
- using inappropriate statistical tests to test your data;
- performing multiple testing (“fishing for P”) by pair-wise comparisons ( 4 ), testing multiple endpoints and performing secondary or subgroup analyses, which were not part of the original plan in order “to find” statistically significant difference regardless to hypothesis.
For example, if the study aim is to show that one biomarker is associated with another in a group of patients, and this association does not prove significant in a total cohort, researchers may start “torturing the data” by trying to divide their data into various subgroups until this association becomes statistically significant. If this sub-classification of a study population was not part of the original research hypothesis, such behavior is considered data manipulation and is neither acceptable nor ethical. Such studies quite often provide meaningless conclusions such as:
- CRP was statistically significant in a subgroup of women under 37 years with cholesterol concentration > 6.2 mmol/L;
- lactate concentration was negatively associated with albumin concentration in a subgroup of male patients with a body mass index in the lowest quartile and total leukocyte count below 4.00 × 10 9 /L.
Besides being biased, invalid and illogical, those conclusions are also useless, since they cannot be generalized to the entire population.
There is a very often quoted saying (attributed to Ronald Coase, but unpublished to the best of my knowledge), which says: “If you torture the data long enough, it will confess to anything”. This actually means that there is a good chance that statistical significance will be reached only by increasing the number of hypotheses tested in the work. The question is then: is this significant difference real or did it occur by pure chance?
Actually, it is well known that if 20 tests are performed on the same data set, at least one Type 1 error (α) is to be expected. Therefore, the number of hypotheses to be tested in a certain study needs to determined in advance. If multiple hypotheses are tested, correction for multiple testing should be applied or study should be declared as exploratory.
Bias in data interpretation
By interpreting the results, one needs to make sure that proper statistical tests were used, that results were presented correctly and that data are interpreted only if there was a statistical significance of the observed relationship ( 5 ). Otherwise, there may be some bias in a research.
However, wishful thinking is not rare in scientific research. Some researchers tend to believe so much in their original hypotheses that they tend to neglect the original findings and interpret them in favor of their beliefs. Examples are:
- discussing observed differences and associations even if they are not statistically significant (the often used expression is “borderline significance”);
- discussing differences which are statistically significant but are not clinically meaningful;
- drawing conclusions about the causality, even if the study was not designed as an experiment;
- drawing conclusions about the values outside the range of observed data (extrapolation);
- overgeneralization of the study conclusions to the entire general population, even if a study was confined to the population subset;
- Type I (the expected effect is found significant, when actually there is none) and type II (the expected effect is not found significant, when it is actually present) errors ( 6 ).
Even if this is done as an honest error or due to the negligence, it is still considered a serious misconduct.
Publication bias
Unfortunately, scientific journals are much more likely to accept for publication a study which reports some positive than a study with negative findings. Such behavior creates false impression in the literature and may cause long-term consequences to the entire scientific community. Also, if negative results would not have so many difficulties to get published, other scientists would not unnecessarily waste their time and financial resources by re-running the same experiments.
Journal editors are the most responsible for this phenomenon. Ideally, a study should have equal opportunity to be published regardless of the nature of its findings, if designed in a proper way, with valid scientific assumptions, well conducted experiments and adequate data analysis, presentation and conclusions. However, in reality, this is not the case. To enable publication of studies reporting negative findings, several journals have already been launched, such as Journal of Pharmaceutical Negative Results, Journal of Negative Results in Biomedicine, Journal of Interesting Negative Results and some other. The aim of such journals is to counterbalance the ever-increasing pressure in the scientific literature to publish only positive results.
It is our policy at Biochemia Medica to give equal consideration to submitted articles, regardless to the nature of its findings.
One sort of publication bias is the so called funding bias which occurs due to the prevailing number of studies funded by the same company, related to the same scientific question and supporting the interests of the sponsoring company. It is absolutely acceptable to receive funding from a company to perform a research, as long as the study is run independently and not being influenced in any way by the sponsoring company and as long as the funding source is declared as a potential conflict of interest to the journal editors, reviewers and readers.
It is the policy of our Journal to demand such declaration from the authors during submission and to publish this declaration in the published article ( 7 ). By this we believe that scientific community is given an opportunity to judge on the presence of any potential bias in the published work.
There are many potential sources of bias in research. Bias in research can cause distorted results and wrong conclusions. Such studies can lead to unnecessary costs, wrong clinical practice and they can eventually cause some kind of harm to the patient. It is therefore the responsibility of all involved stakeholders in the scientific publishing to ensure that only valid and unbiased research conducted in a highly professional and competent manner is published ( 8 ).
Potential conflict of interest
None declared.
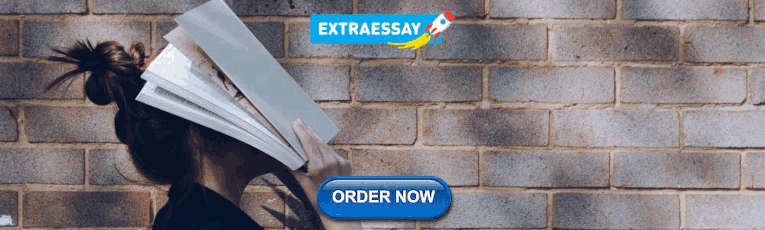
IMAGES
VIDEO
COMMENTS
Rather, it must be understood that bias is always present to some degree due to inherent limitations in research, its design, implementation, and ethical considerations. Therefore, it is instead crucial to evaluate how much bias is present in a study and how the researchers attempted to minimize any sources of bias. [5]
Information bias occurs during the data collection step and is common in research studies that involve self-reporting and retrospective data collection. It can also result from poor interviewing techniques or differing levels of recall from participants. The main types of information bias are: Recall bias. Observer bias.
Bias—commonly understood to be any influence that provides a distortion in the results of a study (Polit & Beck, 2014)—is a term drawn from the quantitative research paradigm.Most (though perhaps not all) of us would recognize the concept as being incompatible with the philosophical underpinnings of qualitative inquiry (Thorne, Stephens, & Truant, 2016).
Abstract. This narrative review provides an overview on the topic of bias as part of Plastic and Reconstructive Surgery 's series of articles on evidence-based medicine. Bias can occur in the planning, data collection, analysis, and publication phases of research. Understanding research bias allows readers to critically and independently review ...
Introduction. Bias, perhaps best described as 'any process at any stage of inference which tends to produce results or conclusions that differ systematically from the truth,' can pollute the entire spectrum of research, including its design, analysis, interpretation and reporting. 1 It can taint entire bodies of research as much as it can individual studies. 2 3 Given this extensive ...
In terms of research, "bias is any trend or deviation from the truth in data collection, data analysis, interpretation and publication which can cause false conclusions" (Simundic, 2013, p. 12). From this definition it can be determined that bias may occur in any part of the research process. Furthermore, it must be acknowledged that bias ...
Future research is needed to refine our methodology, but our empirically grounded form of bias-adjusted meta-analysis could be implemented as follows: 1.) collate studies for the same true effect ...
Reporting bias may occur when there are concerns with regard to the outcomes reported within the results of a study [ 1 ]. Selective outcome reporting is the primary concern in this form of bias ...
Based on their exploration of bias, students will discuss the benefits and limitations of research guidelines for minimizing particular types of bias and develop guidelines of their own ...
The aim of this article is to outline types of 'bias' across research designs, and consider strategies to minimise bias. Evidence-based nursing, defined as the "process by which evidence, nursing theory, and clinical expertise are critically evaluated and considered, in conjunction with patient involvement, to provide the delivery of optimum nursing care,"1 is central to the continued ...
Here's an example of a limitation explained in a research paper about the different options and emerging solutions for delaying memory decline. These statements appeared in the first two sentences of the discussion section: "Approaches like stem cell transplantation and vaccination in AD [Alzheimer's disease] work on a cellular or molecular level in the laboratory.
The implications of reference bias extend beyond intervention research. Consider, for example, mean-level increases in conscientiousness from adolescence through midlife 85,86,87. If adults in ...
Research bias refers to any instance where the researcher, or the research design, negatively influences the quality of a study's results, whether intentionally or not. The three common types of research bias we looked at are: Selection bias - where a skewed sample leads to skewed results. Analysis bias - where the analysis method and/or ...
Common types of limitations and their ramifications include: Theoretical: limits the scope, depth, or applicability of a study. Methodological: limits the quality, quantity, or diversity of the data. Empirical: limits the representativeness, validity, or reliability of the data. Analytical: limits the accuracy, completeness, or significance of ...
Good practice in research involves considering diverse sources of biases when designing a study for later validation of results. If they are recognized beforehand, it is possible to minimize or avoid them. Selection biases may originate at the time of enrolling the subjects of study, making it necessary to clearly state the selection criteria of the exposed and nonexposed individuals. If ...
Limitations generally fall into some common categories, and in a sense we can make a checklist for authors here. Price and Murnan ( 2004) gave an excellent and detailed summary of possible research limitations in their editorial for the American Journal of Health Education. They discussed limitations affecting internal and external validity ...
The limitations of any research study will be rooted in the validity of its results—specifically threats to internal or external validity . ... Pannucci CJ, Wilkins EG. Identifying and avoiding bias in research. Plast Reconstr Surg. 2010; 126:619-625. doi: 10.1097/PRS.0b013e3181de24bc. [PMC free article] [Google Scholar] 27. Bagg A ...
Such bias potentially undermines the validity of many research findings. However, more recent studies have found little evidence in support of the phenomenon. To investigate the robustness of the initial elevation phenomenon, we conducted the largest experiments to date in diverse online samples ( N = 5,285 across three studies, from Prolific.co).
In other words, you have to the accuracy of what people say, whether in interviews, focus groups, or on questionnaires, at face value. However, self-reported data can contain several potential sources of bias that you should be alert to and note as limitations. These biases become apparent if they are incongruent with data from other sources.
Identify the limitations: Start by identifying the potential limitations of your research. These may include sample size, selection bias, measurement error, or other issues that could affect the validity and reliability of your findings. Be honest and objective: When describing the limitations of your research, be honest and objective.
In this report, the authors discuss methodological and data challenges for studying racial bias in police shootings and implications for assessing available evidence (as of early 2024) on this topic. They explore the importance of thinking of racial bias as a process with a series of stages, the data that are available to study bias in ...
A new analysis from Pew Research Center examines the accuracy of polling on more than 20 topics, ranging from Americans' employment and vaccination status to whether they've served in the military or experienced financial hardship. The analysis shows that, despite low response rates, national polls like the Center's come within a few ...
The energy storage and vehicle industries are heavily investing in advancing all-solid-state batteries to overcome critical limitations in existing liquid electrolyte-based lithium-ion batteries, specifically focusing on mitigating fire hazards and improving energy density. All-solid-state lithium-sulfur batteries
The share who say inaccuracy is the aspect they dislike most has increased from 31% to 40% in the past five years. These findings come from a broader Center survey of U.S. adults' news habits. The survey asked Americans who get news on social media to describe - in their own words - the things they like and dislike most about getting news ...
Overcoming social desirability bias . The main strategy to prevent social desirability bias is to validate the self-reporting instrument before implementing it for data collection. 6 - 11 Such validation can be either internal or external. In internal validation, the responses collected from the self-reporting instrument are compared with other data collection methods, such as laboratory ...
These survey findings follow a spike in reports of discrimination against Asian Americans during the COVID-19 pandemic. The number of federally recognized hate crime incidents of anti-Asian bias increased from 158 in 2019 to 279 in 2020 and 746 in 2021, according to hate crime statistics published by the FBI.
Sean McDowell (Ph.D.) is an associate professor in the Christian Apologetics program at Talbot School of Theology, Biola University. He is a gifted communicator with a passion for equipping the Church, and in particular young people, to make the case for the Christian faith. Sean travels throughout the United States and abroad, speaking at ...
Researchers interested in intergroup biases could benefit from understanding the promises and limitations of applying findings in the debiasing literature to the issue of discrimination. ... Imhoff R. (2023). Toward parsimony in bias research: A proposed common framework of belief-consistent information processing for a set of biases ...
Superradiant atoms can help us measure time more precisely than ever. In a new study, researchers present a new method for measuring the time interval, the second, mitigating some of the ...
Definition of bias. Bias is any trend or deviation from the truth in data collection, data analysis, interpretation and publication which can cause false conclusions. Bias can occur either intentionally or unintentionally ( 1 ). Intention to introduce bias into someone's research is immoral.