- - Google Chrome
Intended for healthcare professionals
- Access provided by Google Indexer
- My email alerts
- BMA member login
- Username * Password * Forgot your log in details? Need to activate BMA Member Log In Log in via OpenAthens Log in via your institution

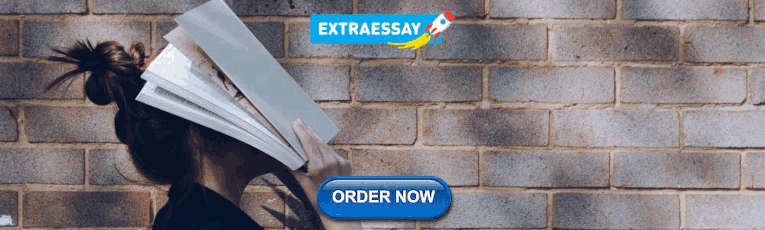
Search form
- Advanced search
- Search responses
- Search blogs
- Beauty sleep:...
Beauty sleep: experimental study on the perceived health and attractiveness of sleep deprived people
- Related content
- Peer review
- John Axelsson , researcher 1 2 ,
- Tina Sundelin , research assistant and MSc student 2 ,
- Michael Ingre , statistician and PhD student 3 ,
- Eus J W Van Someren , researcher 4 ,
- Andreas Olsson , researcher 2 ,
- Mats Lekander , researcher 1 3
- 1 Osher Center for Integrative Medicine, Department of Clinical Neuroscience, Karolinska Institutet, 17177 Stockholm, Sweden
- 2 Division for Psychology, Department of Clinical Neuroscience, Karolinska Institutet
- 3 Stress Research Institute, Stockholm University, Stockholm
- 4 Netherlands Institute for Neuroscience, an Institute of the Royal Netherlands Academy of Arts and Sciences, and VU Medical Center, Amsterdam, Netherlands
- Correspondence to: J Axelsson john.axelsson{at}ki.se
- Accepted 22 October 2010
Objective To investigate whether sleep deprived people are perceived as less healthy, less attractive, and more tired than after a normal night’s sleep.
Design Experimental study.
Setting Sleep laboratory in Stockholm, Sweden.
Participants 23 healthy, sleep deprived adults (age 18-31) who were photographed and 65 untrained observers (age 18-61) who rated the photographs.
Intervention Participants were photographed after a normal night’s sleep (eight hours) and after sleep deprivation (31 hours of wakefulness after a night of reduced sleep). The photographs were presented in a randomised order and rated by untrained observers.
Main outcome measure Difference in observer ratings of perceived health, attractiveness, and tiredness between sleep deprived and well rested participants using a visual analogue scale (100 mm).
Results Sleep deprived people were rated as less healthy (visual analogue scale scores, mean 63 (SE 2) v 68 (SE 2), P<0.001), more tired (53 (SE 3) v 44 (SE 3), P<0.001), and less attractive (38 (SE 2) v 40 (SE 2), P<0.001) than after a normal night’s sleep. The decrease in rated health was associated with ratings of increased tiredness and decreased attractiveness.
Conclusion Our findings show that sleep deprived people appear less healthy, less attractive, and more tired compared with when they are well rested. This suggests that humans are sensitive to sleep related facial cues, with potential implications for social and clinical judgments and behaviour. Studies are warranted for understanding how these effects may affect clinical decision making and can add knowledge with direct implications in a medical context.
Introduction
The recognition [of the case] depends in great measure on the accurate and rapid appreciation of small points in which the diseased differs from the healthy state Joseph Bell (1837-1911)
Good clinical judgment is an important skill in medical practice. This is well illustrated in the quote by Joseph Bell, 1 who demonstrated impressive observational and deductive skills. Bell was one of Sir Arthur Conan Doyle’s teachers and served as a model for the fictitious detective Sherlock Holmes. 2 Generally, human judgment involves complex processes, whereby ingrained, often less consciously deliberated responses from perceptual cues are mixed with semantic calculations to affect decision making. 3 Thus all social interactions, including diagnosis in clinical practice, are influenced by reflexive as well as reflective processes in human cognition and communication.
Sleep is an essential homeostatic process with well established effects on an individual’s physiological, cognitive, and behavioural functionality 4 5 6 7 and long term health, 8 but with only anecdotal support of a role in social perception, such as that underlying judgments of attractiveness and health. As illustrated by the common expression “beauty sleep,” an individual’s sleep history may play an integral part in the perception and judgments of his or her attractiveness and health. To date, the concept of beauty sleep has lacked scientific support, but the biological importance of sleep may have favoured a sensitivity to perceive sleep related cues in others. It seems warranted to explore such sensitivity, as sleep disorders and disturbed sleep are increasingly common in today’s 24 hour society and often coexist with some of the most common health problems, such as hypertension 9 10 and inflammatory conditions. 11
To describe the relation between sleep deprivation and perceived health and attractiveness we asked untrained observers to rate the faces of people who had been photographed after a normal night’s sleep and after a night of sleep deprivation. We chose facial photographs as the human face is the primary source of information in social communication. 12 A perceiver’s response to facial cues, signalling the bearer’s emotional state, intentions, and potential mate value, serves to guide actions in social contexts and may ultimately promote survival. 13 14 15 We hypothesised that untrained observers would perceive sleep deprived people as more tired, less healthy, and less attractive compared with after a normal night’s sleep.
Using an experimental design we photographed the faces of 23 adults (mean age 23, range 18-31 years, 11 women) between 14.00 and 15.00 under two conditions in a balanced design: after a normal night’s sleep (at least eight hours of sleep between 23.00-07.00 and seven hours of wakefulness) and after sleep deprivation (sleep 02.00-07.00 and 31 hours of wakefulness). We advertised for participants at four universities in the Stockholm area. Twenty of 44 potentially eligible people were excluded. Reasons for exclusion were reported sleep disturbances, abnormal sleep requirements (for example, sleep need out of the 7-9 hour range), health problems, or availability on study days (the main reason). We also excluded smokers and those who had consumed alcohol within two days of the protocol. One woman failed to participate in both conditions. Overall, we enrolled 12 women and 12 men.
The participants slept in their own homes. Sleep times were confirmed with sleep diaries and text messages. The sleep diaries (Karolinska sleep diary) included information on sleep latency, quality, duration, and sleepiness. Participants sent a text message to the research assistant by mobile phone (SMS) at bedtime and when they got up on the night before sleep deprivation. They had been instructed not to nap. During the normal sleep condition the participants’ mean duration of sleep, estimated from sleep diaries, was 8.45 (SE 0.20) hours. The sleep deprivation condition started with a restriction of sleep to five hours in bed; the participants sent text messages (SMS) when they went to sleep and when they woke up. The mean duration of sleep during this night, estimated from sleep diaries and text messages, was 5.06 (SE 0.04) hours. For the following night of total sleep deprivation, the participants were monitored in the sleep laboratory at all times. Thus, for the sleep deprivation condition, participants came to the laboratory at 22.00 (after 15 hours of wakefulness) to be monitored, and stayed awake for a further 16 hours. We therefore did not observe the participants during the first 15 hours of wakefulness, when they had had a slightly restricted sleep, but had good control over the last 16 hours of wakefulness when sleepiness increased in magnitude. For the sleep condition, participants came to the laboratory at 12.00 (after five hours of wakefulness). They were kept indoors two hours before being photographed to avoid the effects of exposure to sunlight and the weather. We had a series of five or six photographs (resolution 3872×2592 pixels) taken in a well lit room, with a constant white balance (×900l; colour temperature 4200 K, Nikon D80; Nikon, Tokyo). The white balance was differently set during the two days of the study and affected seven photographs (four taken during sleep deprivation and three during a normal night’s sleep). Removing these participants from the analyses did not affect the results. The distance from camera to head was fixed, as was the focal length, within 14 mm (between 44 and 58 mm). To ensure a fixed surface area of each face on the photograph, the focal length was adapted to the head size of each participant.
For the photo shoot, participants wore no makeup, had their hair loose (combed backwards if long), underwent similar cleaning or shaving procedures for both conditions, and were instructed to “sit with a straight back and look straight into the camera with a neutral, relaxed facial expression.” Although the photographer was not blinded to the sleep conditions, she followed a highly standardised procedure during each photo shoot, including minimal interaction with the participants. A blinded rater chose the most typical photograph from each series of photographs. This process resulted in 46 photographs; two (one from each sleep condition) of each of the 23 participants. This part of the study took place between June and September 2007.
In October 2007 the photographs were presented at a fixed interval of six seconds in a randomised order to 65 observers (mainly students at the Karolinska Institute, mean age 30 (range 18-61) years, 40 women), who were unaware of the conditions of the study. They rated the faces for attractiveness (very unattractive to very attractive), health (very sick to very healthy), and tiredness (not at all tired to very tired) on a 100 mm visual analogue scale. After every 23 photographs a brief intermission was allowed, including a working memory task lasting 23 seconds to prevent the faces being memorised. To ensure that the observers were not primed to tiredness when rating health and attractiveness they rated the photographs for attractiveness and health in the first two sessions and tiredness in the last. To avoid the influence of possible order effects we presented the photographs in a balanced order between conditions for each session.
Statistical analyses
Data were analysed using multilevel mixed effects linear regression, with two crossed independent random effects accounting for random variation between observers and participants using the xtmixed procedure in Stata 9.2. We present the effect of condition as a percentage of change from the baseline condition as the reference using the absolute value in millimetres (rated on the visual analogue scale). No data were missing in the analyses.
Sixty five observers rated each of the 46 photographs for attractiveness, health, and tiredness: 138 ratings by each observer and 2990 ratings for each of the three factors rated. When sleep deprived, people were rated as less healthy (visual analogue scale scores, mean 63 (SE 2) v 68 (SE 2)), more tired (53 (SE 3) v 44 (SE 3)), and less attractive (38 (SE 2) v 40 (SE 2); P<0.001 for all) than after a normal night’s sleep (table 1 ⇓ ). Compared with the normal sleep condition, perceptions of health and attractiveness in the sleep deprived condition decreased on average by 6% and 4% and tiredness increased by 19%.
Multilevel mixed effects regression on effect of how sleep deprived people are perceived with respect to attractiveness, health, and tiredness
- View inline
A 10 mm increase in tiredness was associated with a −3.0 mm change in health, a 10 mm increase in health increased attractiveness by 2.4 mm, and a 10 mm increase in tiredness reduced attractiveness by 1.2 mm (table 2 ⇓ ). These findings were also presented as correlation, suggesting that faces with perceived attractiveness are positively associated with perceived health (r=0.42, fig 1 ⇓ ) and negatively with perceived tiredness (r=−0.28, fig 1). In addition, the average decrease (for each face) in attractiveness as a result of deprived sleep was associated with changes in tiredness (−0.53, n=23, P=0.03) and in health (0.50, n=23, P=0.01). Moreover, a strong negative association was found between the respective perceptions of tiredness and health (r=−0.54, fig 1). Figure 2 ⇓ shows an example of observer rated faces.
Associations between health, tiredness, and attractiveness
Fig 1 Relations between health, tiredness, and attractiveness of 46 photographs (two each of 23 participants) rated by 65 observers on 100 mm visual analogue scales, with variation between observers removed using empirical Bayes’ estimates
- Download figure
- Open in new tab
- Download powerpoint
Fig 2 Participant after a normal night’s sleep (left) and after sleep deprivation (right). Faces were presented in a counterbalanced order
To evaluate the mediation effects of sleep loss on attractiveness and health, tiredness was added to the models presented in table 1 following recommendations. 16 The effect of sleep loss was significantly mediated by tiredness on both health (P<0.001) and attractiveness (P<0.001). When tiredness was added to the model (table 1) with an estimated coefficient of −2.9 (SE 0.1; P<0.001) the independent effect of sleep loss on health decreased from −4.2 to −1.8 (SE 0.5; P<0.001). The effect of sleep loss on attractiveness decreased from −1.6 (table 1) to −0.62 (SE 0.4; P=0.133), with tiredness estimated at −1.1 (SE 0.1; P<0.001). The same approach applied to the model of attractiveness and health (table 2), with a decrease in the association from 2.4 to 2.1 (SE 0.1; P<0.001) with tiredness estimated at −0.56 (SE 0.1; P<0.001).
Sleep deprived people are perceived as less attractive, less healthy, and more tired compared with when they are well rested. Apparent tiredness was strongly related to looking less healthy and less attractive, which was also supported by the mediating analyses, indicating that a large part of the found effects and relations on appearing healthy and attractive were mediated by looking tired. The fact that untrained observers detected the effects of sleep loss in others not only provides evidence for a perceptual ability not previously subjected to experimental control, but also supports the notion that sleep history gives rise to socially relevant signals that provide information about the bearer. The adaptiveness of an ability to detect sleep related facial cues resonates well with other research, showing that small deviations from the average sleep duration in the long term are associated with an increased risk of health problems and with a decreased longevity. 8 17 Indeed, even a few hours of sleep deprivation inflict an array of physiological changes, including neural, endocrinological, immunological, and cellular functioning, that if sustained are relevant for long term health. 7 18 19 20 Here, we show that such physiological changes are paralleled by detectable facial changes.
These results are related to photographs taken in an artificial setting and presented to the observers for only six seconds. It is likely that the effects reported here would be larger in real life person to person situations, when overt behaviour and interactions add further information. Blink interval and blink duration are known to be indicators of sleepiness, 21 and trained observers are able to evaluate reliably the drowsiness of drivers by watching their videotaped faces. 22 In addition, a few of the people were perceived as healthier, less tired, and more attractive during the sleep deprived condition. It remains to be evaluated in follow-up research whether this is due to random error noise in judgments, or associated with specific characteristics of observers or the sleep deprived people they judge. Nevertheless, we believe that the present findings can be generalised to a wide variety of settings, but further studies will have to investigate the impact on clinical studies and other social situations.
Importantly, our findings suggest a prominent role of sleep history in several domains of interpersonal perception and judgment, in which sleep history has previously not been considered of importance, such as in clinical judgment. In addition, because attractiveness motivates sexual behaviour, collaboration, and superior treatment, 13 sleep loss may have consequences in other social contexts. For example, it has been proposed that facial cues perceived as attractive are signals of good health and that this recognition has been selected evolutionarily to guide choice of mate and successful transmission of genes. 13 The fact that good sleep supports a healthy look and poor sleep the reverse may be of particular relevance in the medical setting, where health estimates are an essential part. It is possible that people with sleep disturbances, clinical or otherwise, would be judged as more unhealthy, whereas those who have had an unusually good night’s sleep may be perceived as rather healthy. Compared with the sleep deprivation used in the present investigation, further studies are needed to investigate the effects of less drastic acute reductions of sleep as well as long term clinical effects.
Conclusions
People are capable of detecting sleep loss related facial cues, and these cues modify judgments of another’s health and attractiveness. These conclusions agree well with existing models describing a link between sleep and good health, 18 23 as well as a link between attractiveness and health. 13 Future studies should focus on the relevance of these facial cues in clinical settings. These could investigate whether clinicians are better than the average population at detecting sleep or health related facial cues, and whether patients with a clinical diagnosis exhibit more tiredness and are less healthy looking than healthy people. Perhaps the more successful doctors are those who pick up on these details and act accordingly.
Taken together, our results provide important insights into judgments about health and attractiveness that are reminiscent of the anecdotal wisdom harboured in Bell’s words, and in the colloquial notion of “beauty sleep.”
What is already known on this topic
Short or disturbed sleep and fatigue constitute major risk factors for health and safety
Complaints of short or disturbed sleep are common among patients seeking healthcare
The human face is the main source of information for social signalling
What this study adds
The facial cues of sleep deprived people are sufficient for others to judge them as more tired, less healthy, and less attractive, lending the first scientific support to the concept of “beauty sleep”
By affecting doctors’ general perception of health, the sleep history of a patient may affect clinical decisions and diagnostic precision
Cite this as: BMJ 2010;341:c6614
We thank B Karshikoff for support with data acquisition and M Ingvar for comments on an earlier draft of the manuscript, both without compensation and working at the Department for Clinical Neuroscience, Karolinska Institutet, Sweden.
Contributors: JA designed the data collection, supervised and monitored data collection, wrote the statistical analysis plan, carried out the statistical analyses, obtained funding, drafted and revised the manuscript, and is guarantor. TS designed and carried out the data collection, cleaned the data, drafted, revised the manuscript, and had final approval of the manuscript. JA and TS contributed equally to the work. MI wrote the statistical analysis plan, carried out the statistical analyses, drafted the manuscript, and critically revised the manuscript. EJWVS provided statistical advice, advised on data handling, and critically revised the manuscript. AO provided advice on the methods and critically revised the manuscript. ML provided administrative support, drafted the manuscript, and critically revised the manuscript. All authors approved the final version of the manuscript.
Funding: This study was funded by the Swedish Society for Medical Research, Rut and Arvid Wolff’s Memory Fund, and the Osher Center for Integrative Medicine.
Competing interests: All authors have completed the Unified Competing Interest form at www.icmje.org/coi_disclosure.pdf (available on request from the corresponding author) and declare: no support from any company for the submitted work; no financial relationships with any companies that might have an interest in the submitted work in the previous 3 years; no other relationships or activities that could appear to have influenced the submitted work.
Ethical approval: This study was approved by the Karolinska Institutet’s ethical committee. Participants were compensated for their participation.
Participant consent: Participant’s consent obtained.
Data sharing: Statistical code and dataset of ratings are available from the corresponding author at john.axelsson{at}ki.se .
This is an open-access article distributed under the terms of the Creative Commons Attribution Non-commercial License, which permits use, distribution, and reproduction in any medium, provided the original work is properly cited, the use is non commercial and is otherwise in compliance with the license. See: http://creativecommons.org/licenses/by-nc/2.0/ and http://creativecommons.org/licenses/by-nc/2.0/legalcode .
- ↵ Deten A, Volz HC, Clamors S, Leiblein S, Briest W, Marx G, et al. Hematopoietic stem cells do not repair the infarcted mouse heart. Cardiovasc Res 2005 ; 65 : 52 -63. OpenUrl Abstract / FREE Full Text
- ↵ Doyle AC. The case-book of Sherlock Holmes: selected stories. Wordsworth, 1993.
- ↵ Lieberman MD, Gaunt R, Gilbert DT, Trope Y. Reflection and reflexion: a social cognitive neuroscience approach to attributional inference. Adv Exp Soc Psychol 2002 ; 34 : 199 -249. OpenUrl CrossRef
- ↵ Drummond SPA, Brown GG, Gillin JC, Stricker JL, Wong EC, Buxton RB. Altered brain response to verbal learning following sleep deprivation. Nature 2000 ; 403 : 655 -7. OpenUrl CrossRef PubMed
- ↵ Harrison Y, Horne JA. The impact of sleep deprivation on decision making: a review. J Exp Psychol Appl 2000 ; 6 : 236 -49. OpenUrl CrossRef PubMed Web of Science
- ↵ Huber R, Ghilardi MF, Massimini M, Tononi G. Local sleep and learning. Nature 2004 ; 430 : 78 -81. OpenUrl CrossRef PubMed Web of Science
- ↵ Spiegel K, Leproult R, Van Cauter E. Impact of sleep debt on metabolic and endocrine function. Lancet 1999 ; 354 : 1435 -9. OpenUrl CrossRef PubMed Web of Science
- ↵ Kripke DF, Garfinkel L, Wingard DL, Klauber MR, Marler MR. Mortality associated with sleep duration and insomnia. Arch Gen Psychiatry 2002 ; 59 : 131 -6. OpenUrl CrossRef PubMed Web of Science
- ↵ Olson LG, Ambrogetti A. Waking up to sleep disorders. Br J Hosp Med (Lond) 2006 ; 67 : 118 , 20. OpenUrl PubMed
- ↵ Rajaratnam SM, Arendt J. Health in a 24-h society. Lancet 2001 ; 358 : 999 -1005. OpenUrl CrossRef PubMed Web of Science
- ↵ Ranjbaran Z, Keefer L, Stepanski E, Farhadi A, Keshavarzian A. The relevance of sleep abnormalities to chronic inflammatory conditions. Inflamm Res 2007 ; 56 : 51 -7. OpenUrl CrossRef PubMed Web of Science
- ↵ Haxby JV, Hoffman EA, Gobbini MI. The distributed human neural system for face perception. Trends Cogn Sci 2000 ; 4 : 223 -33. OpenUrl CrossRef PubMed Web of Science
- ↵ Rhodes G. The evolutionary psychology of facial beauty. Annu Rev Psychol 2006 ; 57 : 199 -226. OpenUrl CrossRef PubMed Web of Science
- ↵ Todorov A, Mandisodza AN, Goren A, Hall CC. Inferences of competence from faces predict election outcomes. Science 2005 ; 308 : 1623 -6. OpenUrl Abstract / FREE Full Text
- ↵ Willis J, Todorov A. First impressions: making up your mind after a 100-ms exposure to a face. Psychol Sci 2006 ; 17 : 592 -8. OpenUrl Abstract / FREE Full Text
- ↵ Krull JL, MacKinnon DP. Multilevel modeling of individual and group level mediated effects. Multivariate Behav Res 2001 ; 36 : 249 -77. OpenUrl CrossRef Web of Science
- ↵ Ayas NT, White DP, Manson JE, Stampfer MJ, Speizer FE, Malhotra A, et al. A prospective study of sleep duration and coronary heart disease in women. Arch Intern Med 2003 ; 163 : 205 -9. OpenUrl CrossRef PubMed Web of Science
- ↵ Bryant PA, Trinder J, Curtis N. Sick and tired: does sleep have a vital role in the immune system. Nat Rev Immunol 2004 ; 4 : 457 -67. OpenUrl CrossRef PubMed Web of Science
- ↵ Cirelli C. Cellular consequences of sleep deprivation in the brain. Sleep Med Rev 2006 ; 10 : 307 -21. OpenUrl CrossRef PubMed Web of Science
- ↵ Irwin MR, Wang M, Campomayor CO, Collado-Hidalgo A, Cole S. Sleep deprivation and activation of morning levels of cellular and genomic markers of inflammation. Arch Intern Med 2006 ; 166 : 1756 -62. OpenUrl CrossRef PubMed Web of Science
- ↵ Schleicher R, Galley N, Briest S, Galley L. Blinks and saccades as indicators of fatigue in sleepiness warnings: looking tired? Ergonomics 2008 ; 51 : 982 -1010. OpenUrl CrossRef PubMed Web of Science
- ↵ Wierwille WW, Ellsworth LA. Evaluation of driver drowsiness by trained raters. Accid Anal Prev 1994 ; 26 : 571 -81. OpenUrl CrossRef PubMed Web of Science
- ↵ Horne J. Why we sleep—the functions of sleep in humans and other mammals. Oxford University Press, 1988.
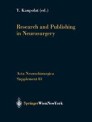
Research and Publishing in Neurosurgery pp 109–113 Cite as
How to Write an Experimental Research Paper
- M. N. Pamir M.D. 2 , 3
- Conference paper
436 Accesses
1 Citations
Part of the book series: Acta Neurochirurgica Supplements ((NEUROCHIRURGICA,volume 83))
The art and practice of academic neurosurgery are mastered by defining and learning the pertinent basic principles and skills. This article aims to present general guidelines to one of the many roles of a neurosurgeon: Writing an experimental research paper.
Every research report must use the “IMRAD formula: introduction, methods, results and discussion”. After the IMRAD is finished, abstract should be written and the title should be “created”. Your abstract should answer these questions: “Why did you start?, what did you do?, what answer did you get?, and what does it mean?”. Title of the research paper should be short enough to catch glance and memory of the reader and be long enough to give the essential information of what the paper is about.
Writing about the results of the experiment is no easier than the research itself. As surgery, writing a scientific paper is also an improvisation, but general principles should be learned and used in practice. The most effective style of learning basic skills to construct a research paper is the “trial and error” type.
- Neurosurgical education
This is a preview of subscription content, log in via an institution .
Buying options
- Available as PDF
- Read on any device
- Instant download
- Own it forever
- Available as EPUB and PDF
- Compact, lightweight edition
- Dispatched in 3 to 5 business days
- Free shipping worldwide - see info
- Durable hardcover edition
Tax calculation will be finalised at checkout
Purchases are for personal use only
Unable to display preview. Download preview PDF.
Ad Hoc Working Group for Clinical Appraisal of the Medical Literature (1987) A proposal for more informative abstracts of clinical articles. Ann Intern Med 106: 598–604.
Google Scholar
Balch C, McKneally M, McPeek B, Mulder D, Spitzer W, Troidl H, Wechsler A (1997) Pentathlete: the many roles of academic surgeon. In: Troidl H, McKneally M, Mulder D, Wechsler A, McPeek B, Spitzer W (eds) Surgical research. New York: Springer, Berlin Heidelberg New York Tokyo.
Condon R (1986) Type III Error. Arch Surg 121: 877–878.
PubMed CAS Google Scholar
Fleming A (1929) On the antimicrobial action of cultures of a penicillium, with special reference to their use in the isolation of B. influenzae. Br J Exper Pathol 10: 226–236.
CAS Google Scholar
Hill A (1965) The reasons for writing. Br Med J 2: 626–627.
Article Google Scholar
Hugh E (1987) Structured abstracts for papers reporting clinical articles. Ann Intern Med 106: 626–627.
International Committee of Medical Journal Editors (1997) Uniform requirements for manuscripts submitted to biomedical journals. New Engl J Med 336: 309–315.
Pollock A, Evans M (1997) Writing a scientific paper. In: Troidl H, McKneally M, Mulder D, Wechsler A, McPeek B, Spitzer W (eds) Surgical research. Springer, Berlin Heidelberg New York Tokyo.
Tufte E (1983) The visual display of quantitative information. CT: Graphics, Cheshire.
Watson J, Crick F (1953) Molecular structure of nucleic acids. A structure for deoxyribose nucleic acid. Nature 4356: 737–738.
Download references
Author information
Authors and affiliations.
Institute of Neurological Sciences, Marmara University, Istanbul, Turkey
M. N. Pamir M.D.
Norolojik Bilimler Enstitusu, Marmara University, PK 53 Maltepe, Istanbul, Turkey
You can also search for this author in PubMed Google Scholar
Editor information
Editors and affiliations.
Ankara, Turkey
Y. Kanpolat
Rights and permissions
Reprints and permissions
Copyright information
© 2002 Springer-Verlag/Wien
About this paper
Cite this paper.
Pamir, M.N. (2002). How to Write an Experimental Research Paper. In: Kanpolat, Y. (eds) Research and Publishing in Neurosurgery. Acta Neurochirurgica Supplements, vol 83. Springer, Vienna. https://doi.org/10.1007/978-3-7091-6743-4_18
Download citation
DOI : https://doi.org/10.1007/978-3-7091-6743-4_18
Publisher Name : Springer, Vienna
Print ISBN : 978-3-7091-7399-2
Online ISBN : 978-3-7091-6743-4
eBook Packages : Springer Book Archive
Share this paper
Anyone you share the following link with will be able to read this content:
Sorry, a shareable link is not currently available for this article.
Provided by the Springer Nature SharedIt content-sharing initiative
- Publish with us
Policies and ethics
- Find a journal
- Track your research
- How it works
A Complete Guide to Experimental Research
Published by Carmen Troy at August 14th, 2021 , Revised On August 25, 2023
A Quick Guide to Experimental Research
Experimental research refers to the experiments conducted in the laboratory or observation under controlled conditions. Researchers try to find out the cause-and-effect relationship between two or more variables.
The subjects/participants in the experiment are selected and observed. They receive treatments such as changes in room temperature, diet, atmosphere, or given a new drug to observe the changes. Experiments can vary from personal and informal natural comparisons. It includes three types of variables ;
- Independent variable
- Dependent variable
- Controlled variable
Before conducting experimental research, you need to have a clear understanding of the experimental design. A true experimental design includes identifying a problem , formulating a hypothesis , determining the number of variables, selecting and assigning the participants, types of research designs , meeting ethical values, etc.
There are many types of research methods that can be classified based on:
- The nature of the problem to be studied
- Number of participants (individual or groups)
- Number of groups involved (Single group or multiple groups)
- Types of data collection methods (Qualitative/Quantitative/Mixed methods)
- Number of variables (single independent variable/ factorial two independent variables)
- The experimental design
Types of Experimental Research
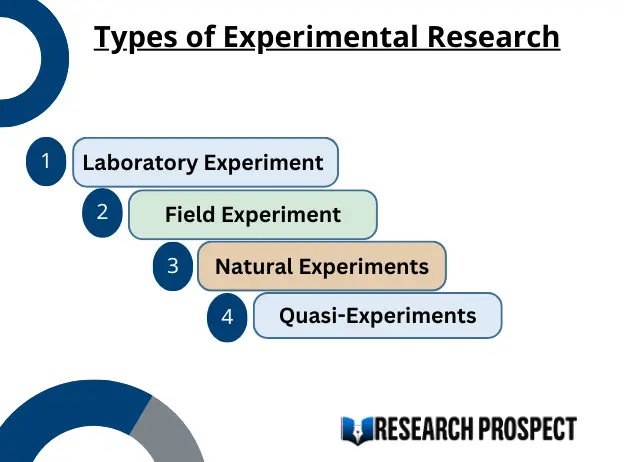
Laboratory Experiment
It is also called experimental research. This type of research is conducted in the laboratory. A researcher can manipulate and control the variables of the experiment.
Example: Milgram’s experiment on obedience.
Field Experiment
Field experiments are conducted in the participants’ open field and the environment by incorporating a few artificial changes. Researchers do not have control over variables under measurement. Participants know that they are taking part in the experiment.
Natural Experiments
The experiment is conducted in the natural environment of the participants. The participants are generally not informed about the experiment being conducted on them.
Examples: Estimating the health condition of the population. Did the increase in tobacco prices decrease the sale of tobacco? Did the usage of helmets decrease the number of head injuries of the bikers?
Quasi-Experiments
A quasi-experiment is an experiment that takes advantage of natural occurrences. Researchers cannot assign random participants to groups.
Example: Comparing the academic performance of the two schools.
Does your Research Methodology Have the Following?
- Great Research/Sources
- Perfect Language
- Accurate Sources
If not, we can help. Our panel of experts makes sure to keep the 3 pillars of Research Methodology strong.
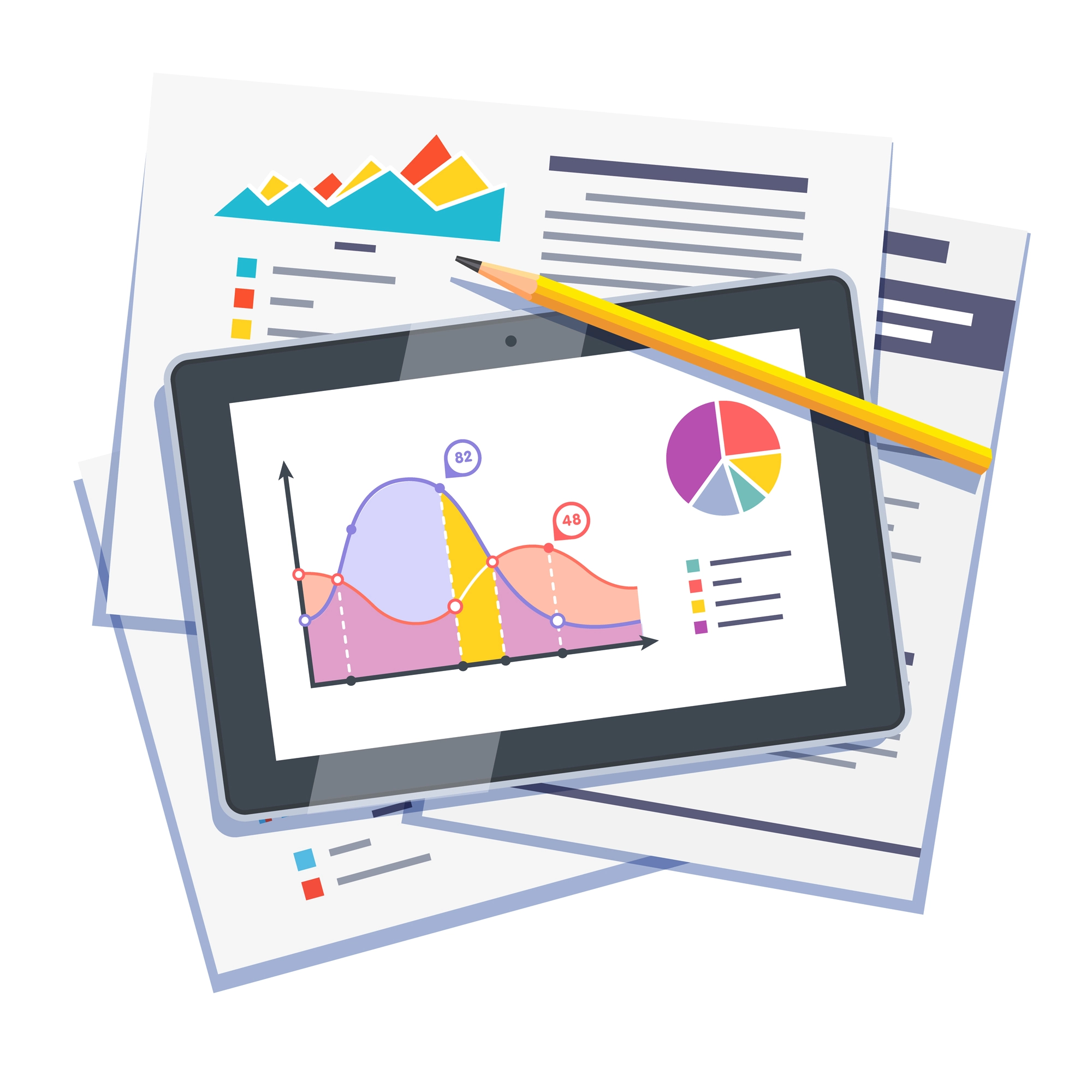
How to Conduct Experimental Research?
Step 1. identify and define the problem.
You need to identify a problem as per your field of study and describe your research question .
Example: You want to know about the effects of social media on the behavior of youngsters. It would help if you found out how much time students spend on the internet daily.
Example: You want to find out the adverse effects of junk food on human health. It would help if you found out how junk food frequent consumption can affect an individual’s health.
Step 2. Determine the Number of Levels of Variables
You need to determine the number of variables . The independent variable is the predictor and manipulated by the researcher. At the same time, the dependent variable is the result of the independent variable.
In the first example, we predicted that increased social media usage negatively correlates with youngsters’ negative behaviour.
In the second example, we predicted the positive correlation between a balanced diet and a good healthy and negative relationship between junk food consumption and multiple health issues.
Step 3. Formulate the Hypothesis
One of the essential aspects of experimental research is formulating a hypothesis . A researcher studies the cause and effect between the independent and dependent variables and eliminates the confounding variables. A null hypothesis is when there is no significant relationship between the dependent variable and the participants’ independent variables. A researcher aims to disprove the theory. H0 denotes it. The Alternative hypothesis is the theory that a researcher seeks to prove. H1or HA denotes it.
Why should you use a Plagiarism Detector for your Paper?
It ensures:
- Original work
- Structure and Clarity
- Zero Spelling Errors
- No Punctuation Faults
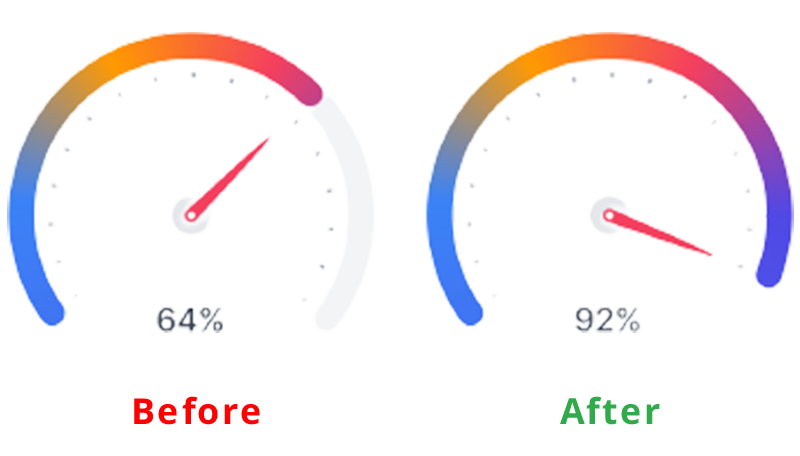
Step 4. Selection and Assignment of the Subjects
It’s an essential feature that differentiates the experimental design from other research designs . You need to select the number of participants based on the requirements of your experiment. Then the participants are assigned to the treatment group. There should be a control group without any treatment to study the outcomes without applying any changes compared to the experimental group.
Randomisation: The participants are selected randomly and assigned to the experimental group. It is known as probability sampling. If the selection is not random, it’s considered non-probability sampling.
Stratified sampling : It’s a type of random selection of the participants by dividing them into strata and randomly selecting them from each level.
Matching: Even though participants are selected randomly, they can be assigned to the various comparison groups. Another procedure for selecting the participants is ‘matching.’ The participants are selected from the controlled group to match the experimental groups’ participants in all aspects based on the dependent variables.
What is Replicability?
When a researcher uses the same methodology and subject groups to carry out the experiments, it’s called ‘replicability.’ The results will be similar each time. Researchers usually replicate their own work to strengthen external validity.
Step 5. Select a Research Design
You need to select a research design according to the requirements of your experiment. There are many types of experimental designs as follows.
Step 6. Meet Ethical and Legal Requirements
- Participants of the research should not be harmed.
- The dignity and confidentiality of the research should be maintained.
- The consent of the participants should be taken before experimenting.
- The privacy of the participants should be ensured.
- Research data should remain confidential.
- The anonymity of the participants should be ensured.
- The rules and objectives of the experiments should be followed strictly.
- Any wrong information or data should be avoided.
Tips for Meeting the Ethical Considerations
To meet the ethical considerations, you need to ensure that.
- Participants have the right to withdraw from the experiment.
- They should be aware of the required information about the experiment.
- It would help if you avoided offensive or unacceptable language while framing the questions of interviews, questionnaires, or Focus groups.
- You should ensure the privacy and anonymity of the participants.
- You should acknowledge the sources and authors in your dissertation using any referencing styles such as APA/MLA/Harvard referencing style.
Step 7. Collect and Analyse Data.
Collect the data by using suitable data collection according to your experiment’s requirement, such as observations, case studies , surveys , interviews , questionnaires, etc. Analyse the obtained information.
Step 8. Present and Conclude the Findings of the Study.
Write the report of your research. Present, conclude, and explain the outcomes of your study .
Frequently Asked Questions
What is the first step in conducting an experimental research.
The first step in conducting experimental research is to define your research question or hypothesis. Clearly outline the purpose and expectations of your experiment to guide the entire research process.
You May Also Like
A meta-analysis is a formal, epidemiological, quantitative study design that uses statistical methods to generalise the findings of the selected independent studies.
A survey includes questions relevant to the research topic. The participants are selected, and the questionnaire is distributed to collect the data.
A confounding variable can potentially affect both the suspected cause and the suspected effect. Here is all you need to know about accounting for confounding variables in research.
USEFUL LINKS
LEARNING RESOURCES
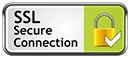
COMPANY DETAILS
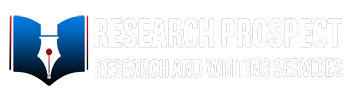
- How It Works

An official website of the United States government
The .gov means it’s official. Federal government websites often end in .gov or .mil. Before sharing sensitive information, make sure you’re on a federal government site.
The site is secure. The https:// ensures that you are connecting to the official website and that any information you provide is encrypted and transmitted securely.
- Publications
- Account settings
Preview improvements coming to the PMC website in October 2024. Learn More or Try it out now .
- Advanced Search
- Journal List
- Front Psychol
Experimental Research on Dreaming: State of the Art and Neuropsychoanalytic Perspectives
Perrine m. ruby.
1 INSERM U1028, Lyon Neuroscience Research Center, Brain Dynamics and Cognition Team, Lyon, France
2 CNRS UMR5292, Lyon Neuroscience Research Center, Brain Dynamics and Cognition Team, Lyon, France
3 University Lyon 1, Lyon, France
Dreaming is still a mystery of human cognition, although it has been studied experimentally for more than a century. Experimental psychology first investigated dream content and frequency. The neuroscientific approach to dreaming arose at the end of the 1950s and soon proposed a physiological substrate of dreaming: rapid eye movement sleep. Fifty years later, this hypothesis was challenged because it could not explain all of the characteristics of dream reports. Therefore, the neurophysiological correlates of dreaming are still unclear, and many questions remain unresolved. Do the representations that constitute the dream emerge randomly from the brain, or do they surface according to certain parameters? Is the organization of the dream’s representations chaotic or is it determined by rules? Does dreaming have a meaning? What is/are the function(s) of dreaming? Psychoanalysis provides hypotheses to address these questions. Until now, these hypotheses have received minimal attention in cognitive neuroscience, but the recent development of neuropsychoanalysis brings new hopes of interaction between the two fields. Considering the psychoanalytical perspective in cognitive neuroscience would provide new directions and leads for dream research and would help to achieve a comprehensive understanding of dreaming. Notably, several subjective issues at the core of the psychoanalytic approach, such as the concept of personal meaning, the concept of unconscious episodic memory and the subject’s history, are not addressed or considered in cognitive neuroscience. This paper argues that the focus on singularity and personal meaning in psychoanalysis is needed to successfully address these issues in cognitive neuroscience and to progress in the understanding of dreaming and the psyche.
The word “dream” is commonly used to express an unattainable ideal or a very deep and strong desire:
I have a dream that my four little children will one day live in a nation where they will not be judged by the color of their skin, but by the content of their character. Martin Luther King
In dream reports, however, one often notices banal situations, strange scenes, or even frightening events. Why is there such a contrast between the popular meaning of the word “dream” and the content of dream reports? Why are some dream scenes so bizarre? Are dreams built from images that arise randomly from the sleeping brain? Or is the emergence and organization of dream images controlled by currently unknown parameters? Does dreaming have a function?
Answering these questions is not easy because dreaming is elusive. We still do not know when it happens during the night, how long it lasts, whether we can recall its entire content, or how to control it. For more than a century, such limited understanding of dreaming has seriously hampered experimental investigations. Nonetheless, scientific research has managed to produce considerable information about the phenomenology and physiology of dreaming and has improved our understanding of this fascinating phenomenon.
Experimental Research on Dreaming
Dreaming and experimental psychology, dream content.
Dreaming was first investigated on an experimental level in the nineteenth century. Calkins ( 1893 ) published the first statistical results about dreaming and argued that some aspects of dream content could be quantified. Later, questionnaires and automatic analysis of the lexical content of dream reports allowed psychologists to show that dream content has some precise phenomenological characteristics. According to psychological studies (Hall and Van de Castle, 1966 ; Schwartz, 1999 ), visual imagery occurs more frequently in dreams than imagery of other senses (audition, olfaction, touch, and taste); the dream drama is mostly lived by the dreamer from a first-person perspective; some elements of real-life events previously experienced by the dreamer often contribute to the scene of the dream; most often, the dream sequence is not within the dreamer’s voluntary control (i.e., the dreamer may be convinced during the dream that the dream’s story is really happening); temporal and spatial incoherencies can occur in the dream story; the dream report is often full of people interacting with each other (e.g., discussions, fights, pursuit, sexuality); and finally, the dream report often contains strong emotions.
Substantial variability of content exists, however, among the same individual’s dreams and among the dreams of different individuals. Further, psychological studies have shown that many internal and external parameters can influence dream content. For example, males report more aggression and violence in their dreams than do females (Nielsen et al., 2003 ; Schredl et al., 2004 ). External stimulation perceived by the dreamer can be incorporated into dreams (Koulack, 1969 ; Saint-Denys, 1867; Hoelscher et al., 1981 ), as illustrated by the famous Dali painting Dream Caused by the Flight of a Bee around a Pomegranate a Second before Awakening . The current concerns of the subject may also be found in the content of his/her dreams (Schwartz, 1999 ; Domhoff and Schneider, 2008 ), and many aspects of the subject’s daily life were found to influence dream content, including news events (Bulkeley and Kahan, 2008 ), musical practice (Uga et al., 2006 ), religious beliefs (Domhoff and Schneider, 2008 ), chronic pain (Raymond et al., 2002 ), mood (Cartwright et al., 1998a ), or a violent living environment (Valli et al., 2005 ). By contrast, congenital or acquired malformations do not seem to significantly influence dream content (Voss et al., 2010 ; Saurat et al., 2011 ).
Based on these results, two opposing hypotheses were formulated: the continuity hypothesis (Schredl and Hofmann, 2003 ) and the discontinuity hypothesis (Rechtschaffen, 1978 ; Kahn et al., 1997 ; Stickgold et al., 2001 ). The former relies on results showing that the themes of an individual’s thoughts during waking life and dreaming are similar; the latter focuses on the fundamentally different structures of thoughts during waking life and dreaming. Voss et al. ( 2010 ) stressed in their recent paper that these hypotheses represent oversimplified approaches to dream analysis and argued that waking and dreaming thoughts were related but structurally independent; in other words, she argued in favor of merging the continuity and discontinuity hypotheses.
Dream report frequency
Dream report frequency (DRF) can vary within subjects and varies substantially among subjects. In a study of 900 German subjects with a large age range from various socioprofessional categories, the mean DRF was approximately 1 dream report per week (Schredl, 2008 ). This result shows that the dream experience is common and familiar to everyone. Psychological studies have demonstrated that many parameters covary with DRF and may thus influence it.
Sleep parameters
First, DRF varies according to the sleep stage preceding awakening (e.g., Dement and Kleitman, 1957b ; Nielsen, 2000 , for a review). More dream reports are obtained after an awakening during rapid eye movement (REM) sleep than after an awakening during non-REM (NREM) sleep. These results inspired the REM sleep hypothesis of dreaming (see the section Dreaming and Neuroscience). Second, DRF increases with the number of awakenings during sleep, according to retrospective self-evaluations of awakenings (Cory and Ormiston, 1975 ; Schredl et al., 2003 ). Such studies showed that the more the subjects tended to awaken during sleep, the higher their DRF. These results support the hypothesis of Koulack and Goodenough ( 1976 ), which proposes that nocturnal awakenings facilitate the encoding of the dream in memory and thus facilitate dream recall upon awakening. However, this hypothesis has not been tested by measuring awakenings with polysomnographic recordings in healthy subjects with various DRFs. Finally, DRF varies according to the method of awakening. Abrupt awakenings lead to more dream reports than gradual awakenings (Shapiro et al., 1963 , 1965 ; Goodenough et al., 1965 ).
Physiological and environmental parameters
Dream report frequency deceases with age (e.g., Schredl, 2008 ) and tends to be slightly higher among females than males (e.g., Schredl, 2008 ; Schredl and Reinhard, 2008 ). Remarkably, Schredl’s ( 2008 ) results revealed that DRF also varied according to the size of the subject’s place of residence.
Psychological parameters
First, increased professional stress or interpersonal stress resulted in an increase in DRF (for a review, see Schredl, 1999 ). Second, an interest in dreams or a positive attitude toward dreams clearly covaries with DRF (Hill et al., 1997 ; Schredl, 1999 ; Schredl et al., 2003 ). The greater an individual’s interest in dreams, the higher his/her DRF. Third, several cognitive abilities have been found to covary with DRF. Contradictory results have been reported for the correlation between DRF and memory abilities (short-term, long-term, visual, verbal, implicit, and explicit; significant positive correlation: Cory and Ormiston, 1975 ; Belicki et al., 1978 ; Butler and Watson, 1985 ; Schredl et al., 1995 ; Solms, 1997 ; no significant correlation: Cohen, 1971 ; Belicki et al., 1978 ; Schredl et al., 1995 , 1997 , 2003 ; Solms, 1997 ) and the correlation between DRF and visual imagery ( significant positive correlation : Hiscock and Cohen, 1973 ; Richardson, 1979 ; Okada et al., 2000 ; no significant correlation : Hill et al., 1997 ; Okada et al., 2000 ). However, several studies have consistently shown that DRF is positively correlated with creativity (Fitch and Armitage, 1989 ; Schredl, 1999 ; Schredl et al., 2003 ) and intelligence scales (multiple-choice vocabulary test, Schonbar, 1959 ; Shipley Intelligence Scale, Connor and Boblitt, 1970 ). Finally, many authors have reported a correlation between DRF and personality traits. Subjects with a high DRF are more likely to have a personality with thinner boundaries (Hartmann described people with thin boundaries as being open, trustworthy, vulnerable, and sensitive; Hartmann, 1989 ; Hartmann et al., 1991 ; Schredl et al., 2003 ), to be more anxious (Schonbar, 1959 ; Tart, 1962 ), to have a higher level of absorption (the absorption scale measures the capacity to become absorptively involved in imaginative and esthetic experiences; Hill et al., 1997 ; Schredl, 1999 ; Schredl et al., 2003 ), to be more open to experience (Hill et al., 1997 ; Schredl et al., 2003 ), and to be less alexithymic (alexithymia is a personality variable that incorporates difficulty identifying and describing feelings, difficulty distinguishing between feelings and the physical sensation of emotional arousal, limited imaginative processes, and an externally oriented cognitive style; De Gennaro et al., 2003 ; Nielsen et al., 2011 ) compared to subjects with a low dream recall frequency. However, those results have not always been reproducible (e.g., Schredl, 2002 for openness to experience; Cory and Ormiston, 1975 ; Hill et al., 1997 for anxiety; Nielsen et al., 1997 for alexithymia) and, according to the recent review by Blagrove and Pace-Schott ( 2010 ), it is difficult to draw conclusions about a possible link between personality traits and DRF.
In conclusion, numerous parameters have been identified that covary with DRF. Schredl stressed in many of his papers that the studied parameters usually explain only a small percentage of the total variance (e.g., Schredl, 2008 ). Thus, the DRF variation profile suggests that the production, encoding and recall of dreams are influenced by numerous parameters that probably interact with each other.
Dreaming and neuroscience
The neuroscientific approach to dreaming arose at the end of the 1950s with the discovery of REM during human sleep by the American physiologist Nathaniel Kleitman and his team (Aserinsky and Kleitman, 1953 ; Dement and Kleitman, 1957a ). During these sleep episodes with saccades, the researchers noticed a decrease in voltage and an increase in frequency in the EEG, accompanied by an increase in cardiac frequency variability and a decrease in body movements. They concluded that these physiological modifications indicate a particular sleep stage, which they called REM sleep. A few years later, the French team led by neurobiologist Michel Jouvet discovered that the lack of movement during REM sleep in cats was due to a general muscular atonia, controlled notably by the locus coeruleus α in the brainstem (Jouvet and Michel, 1959 ; Berger, 1961 later showed that muscular atonia during REM sleep also occurs in humans). Interestingly, the inability to move during REM sleep indicates deep sleep and paradoxically, the fast EEG activity of REM sleep resembles EEG activity in wakefulness. Jouvet concluded that this particular physiological state is associated with a “third state” of the brain (in addition to the brain states associated with wakefulness and NREM sleep) which he called “paradoxical sleep” instead of “REM sleep” (Jouvet et al., 1959 ; Jouvet, 1992 ). Several years later, Fisher et al. ( 1965 ) discovered another physiological characteristic of REM sleep: the penile erection.
During the same period, the American team noticed that a subject awakened during REM sleep very often reported a dream (80% of awakenings in REM sleep vs. 6% of awakenings in NREM sleep are followed by a dream report, according to Dement and Kleitman, 1957b ). Researchers concluded that dreaming occurs during REM sleep. The eye movements of REM sleep would allow the dreamer to scan the imaginary scene of the dream (the scanning hypothesis); the cerebral cortex activation revealed by the rapid EEG would allow intense cognitive activity, creating the complex stories of a dream; and the lack of muscle tone would prevent the dreamer from acting out his dreams. From that time on, researchers investigated REM sleep to obtain answers about dreaming.
In the 1990s, researchers used functional neuroimaging techniques such as positron emission tomography (PET) to investigate brain activity during REM sleep in humans. This new approach enabled researchers to demonstrate that the functional organization of the brain during REM sleep is different from the functional organization of the brain during wakefulness (Maquet et al., 1996 ; Braun et al., 1998 ). In comparison to wakefulness, brain activity during REM sleep is decreased in some brain regions (e.g., in the dorsolateral prefrontal cortex; Braun et al., 1998 ) and increased in other regions (e.g., in the occipital and temporal cortex, the hippocampus and parahippocampus, the anterior cingulate, the precentral and postcentral gyri, the superior parietal cortex, and the pons; Braun et al., 1998 ; Maquet et al., 2000 ). Looking more generally for brain activity correlating with REM sleep (the vigilance states considered included wakefulness, slow-wave sleep, and REM sleep), Maquet et al. ( 1996 ) found negative correlations in the precuneus, posterior cingulate cortex, temporoparietal junction, and dorsolateral prefrontal cortex and positive correlations in the amygdala, anterior cingulate, postcentral gyrus, thalamus, and pons (see Schwartz and Maquet, 2002 ; Maquet et al., 2005 ; Nir and Tononi, 2010 for reviews). Based on these results, researchers argued that the particular functional organization of the brain during REM sleep could explain the phenomenological characteristics of dream reports (Hobson and Pace-Schott, 2002 ; Schwartz and Maquet, 2002 ; Maquet et al., 2005 ; Nir and Tononi, 2010 ). They considered that brain activity increases and decreases during REM sleep could be interpreted on the basis of what we know about brain activity during wakefulness. In this context, the increased occipital cortex activity during REM sleep could explain the visual component of dream reports because neuroimaging results during wakefulness showed that visual imagery with the eyes closed activates the occipital cortex (Kosslyn and Thompson, 2003 ). The decreased activity in the temporoparietal junction during REM sleep may explain why dreams are mainly experienced in the egocentric coordinates of the first-person; indeed, during wakefulness, activity in the temporoparietal junction was reported to be greater for allocentric vs. egocentric representation (e.g., Ruby and Decety, 2001 ; Zacks et al., 2003 ) and for third- vs. first-person perspective (e.g., Ruby and Decety, 2003 , 2004 ). The increased activity in the hippocampus during REM sleep could explain why dreams are often composed of known images or characters, as the hippocampus is known to be associated with the encoding and retrieval of lived events during wakefulness (e.g., Piolino et al., 2009 ). The decreased activity in the lateral prefrontal cortex during REM sleep could explain why dream stories lack consistency, why the dreamer’s perception of time is altered, why the dream story is beyond the control of the dreamer and why the dreamer is convinced that the dream story is really happening. Indeed, during wakefulness, the lateral prefrontal cortex is involved in executive function, cognitive control, and working memory (Petrides, 2005 ; Koechlin and Hyafil, 2007 ). The increased activity in the medial prefrontal cortex during REM sleep could explain the attribution of thoughts, beliefs, and emotions to the characters in the dream because, during wakefulness, the medial prefrontal cortex is known to participate in mind reading (Ruby et al., 2007 , 2009 ; Legrand and Ruby, 2009 ). The increased activity in the motor cortex (precentral gyrus) during REM sleep could explain the movements of the characters’ bodies in the dream because, during wakefulness, motor imagery, and the imagination of someone’s action from the third-person perspective involve the precentral gyrus (Decety et al., 1994 ; Ruby and Decety, 2001 ). Finally, the amygdala’s activity during REM sleep could explain why emotions, especially fear, are often mentioned in dream reports; indeed, the amygdala is involved in the processing of emotional stimuli during wakefulness (Adolphs, 2008 ).
In conclusion, results from experimental psychology and neuroscience allow us to better understand the phenomenology of dreaming and the cerebral correlates of some characteristics of dream reports. Still, what do they tell us about the role of dreaming? What are the current hypotheses about dream function(s)?
Hypotheses about dream function(s)
No function.
At the end of the twentieth century, the neurologist Alan Hobson, who was profoundly anti-psychoanalysis, proposed a theory that deprived dreaming of any function. Hobson argued that dreaming is an epiphenomenon of REM sleep: “Because dreams are so difficult to remember, it seems unlikely that attention to their content could afford much in the way of high-priority survival value. Indeed, it might instead be assumed that dreaming is an epiphenomenon of REM sleep whose cognitive content is so ambiguous as to invite misleading or even erroneous interpretation” (Hobson et al., 1998 ).
Psychological individualism
In contrast, other teams, like Michel Jouvet’s, believed that dreaming serves a vital function. In 1979, Jouvet’s team blocked muscular atonia during REM sleep in a cat by damaging the locus coeruleus α in its brainstem. This lesion resulted in the appearance of movements during REM sleep. Movies from the Jouvet lab show sleeping cats performing complex motor actions (with altered control and coordination) resembling those of wakefulness, such as fur licking, growling, chasing prey, mastication, and fighting. From these videos, the authors concluded that the cat was acting out its dream, and they called this non-physiological state “oneiric behavior” (Sastre and Jouvet, 1979 ). These results led Jouvet to propose that dreaming plays a role in reinforcing a species’ typical behavior. Later in his career, Jouvet moved toward a hypothesis focusing on the role of dreaming in the individual dimension. He speculated that dreams (note that, for Jouvet, dreams and paradoxical sleep were equivalent) could be involved in psychological individualism and in the stability of the dreamer’s personality (Jouvet, 1991 , 1992 , 1998 ). According to Jouvet, “the brain is the sole organ of homeotherms that do not undergo cell division. We thus have to explain how certain aspects of psychological heredity (found in homozygote twins raised in different surroundings) may persist for a whole life (psychological individuation). A definitive genetic programming during development (by neurogenesis) is unlikely due to the plasticity of the nervous system. That is why we have to consider the possibility of an iterative genetic programming. The internal mechanisms (synchronous) of paradoxical sleep (SP) are particularly adapted to such programming. This would activate an endogenous system of stimulation that would stimulate and stabilize receptors genetically programmed by DNA in some neuronal circuits. The excitation of these neurons during SP leads to oniric behaviors that could be experimentally revealed – the lists of these behaviors are specific to each individual and indirect data suggest a genetic component of this programming. Amongst the mechanisms allowing the iterative programming of SP, sleep is particularly important. Security – and hence the inhibition of the arousal system – is a sine qua non-condition for genetic programming to take place. In that sense, sleep could very well be the guardian of dreaming” (Jouvet, 1991 ). In other words, Jouvet’s hypothesis is that paradoxical sleep restores neuronal circuitry that was modified during the day to preserve the expression of the genetic program that codes for psychological characteristics. This process would ensure the stability of personality across time.
The threat simulation theory
The Finnish psychologist Antti Revonsuo recently proposed a hypothesis called threat simulation theory, which explains the fearful characteristics of dream content (Revonsuo, 2000 ; Valli and Revonsuo, 2009 ). According to this theory, dreams serve as virtual training places to improve threat avoidance or threat fighting ability. The theory postulates that such nocturnal training makes the dreamer more efficient at resolving threatening situations during wakefulness.
Emotional regulation
Cartwright et al. ( 1998a , b ) defended the idea that dreaming is involved in emotional regulation. Her team showed that, in healthy subjects, the depression level before sleep was significantly correlated with affect in the first REM report. Her team also observed that low scorers on the depression scale displayed a flat distribution of positive and negative affect in dreams, whereas those with a depressed mood before sleep showed a pattern of decreasing negative and increasing positive affect in dreams reported from successive REM periods (Cartwright et al., 1998a ). These results led Cartwright’s team to suggest that dreaming may actively moderate mood overnight in normal subjects. The team strengthened this hypothesis by showing that among subjects who were depressed because of a divorce, those who reported more negative dreams at the beginning of sleep and fewer at the night’s end were more likely to be in remission 1 year later than subjects who had fewer negative dreams at the beginning of sleep and more at the end of the night (Cartwright et al., 1998b ). The researchers concluded that negative dreams early in the night may reflect a within-sleep mood regulation process, whereas those that occur later may indicate a failure in the completion of this process.
Memory consolidation
Finally, a current mainstream hypothesis in cognitive neuroscience credits sleep and dreaming with a role in memory consolidation (for a recent review, see Diekelmann and Born, 2010 ). Numerous studies have shown that brain activity during training is replayed during post-training sleep (e.g., using a serial reaction time task Maquet et al., 2000 , demonstrated replay during REM sleep; using a maze exploration task Peigneux et al., 2004 , demonstrated replay during slow-wave sleep). Decreased performance during the post-training day in sleep-deprived subjects further suggested that the replay of brain activity at night contributes to memory consolidation (e.g., Maquet et al., 2003 ). Only recently, however, have experimental results in humans argued in favor of a role of dreaming per se in memory consolidation. In one study, subjects were trained on a virtual navigation task before taking a nap. Post-nap tests showed that subjects who dreamed about the task performed better than subjects who did not dream (note that only 4 out of 50 subjects dreamed about the task in this study; Wamsley et al., 2010 ). Using a different approach, Nielsen and colleagues provided additional arguments supporting a link between dreams and memory (Nielsen et al., 2004 ; Nielsen and Stenstrom, 2005 ). This team demonstrated that dreams preferably incorporate events that the dreamer lived the day before and events that the dreamer lived 7 days before the dream (U shaped curve). Animal studies have shown that after associative learning, the excitability of hippocampal cells increases (which leads to an increase in neuronal plasticity) and then returns to baseline 7 days after training (Thompson et al., 1996 ). The similarity between the delay of episodic event incorporation into dreams and the delay of post-training cellular plasticity in the hippocampus led the Canadian team to suggest a link between dreaming and episodic memory consolidation.
In summary, the preceding section describes the current state of the art on dreaming, its phenomenology and cerebral correlates and hypotheses about its functions. Some substantial advances have been made, but much remains to be understood.
Unresolved Issues
The link between oneiric behaviors and dream reports.
A piece of evidence in favor of a strong link between REM sleep and dreaming is the oneiric behavior (the appearance of complex motor behaviors when motor inhibition is suppressed during REM sleep) discovered by Sastre and Jouvet ( 1979 ) in cats and reproduced by Sanford et al. ( 2001 ) in rats. Researchers interpreted these results as the animal acting out its dream. However, as animals do not talk, the link between oneiric behavior and dream recall cannot be tested experimentally. This limitation seriously hampers our understanding of dreaming. In humans, complex motor behaviors (e.g., talking, grabbing, and manipulating imaginary objects, walking, and running) can also occur during REM sleep in a pathological context. This syndrome is called REM sleep behavior disorder (RBD). It can be caused by substance withdrawal (e.g., alcohol, Nitrazepam) or intoxication (e.g., caffeine, tricyclic antidepressants) or by various diseases (e.g., Parkinson’s and Alzheimer’s diseases, pontine neoplasms). According to physicians experts on this syndrome, some patients report dreams that are consistent with their behaviors in REM sleep (Mahowald and Schenck, 2000 ). According to the literature, however, such matches seem to be loose and not systematic. Only one study has tested whether observers can link dream content to sleep behaviors in RBD (Valli et al., 2011 ). In this study, each video recording of motor manifestations was combined with four dream reports, and seven judges had to match the video clip with the correctly reported dream content. The authors found that reported dream content can be linked to motor behaviors at a level better than chance. However, only 39.5% of video-dream pairs were correctly identified. Note, however, that because the authors obtained only movements and not behavioral episodes for many RBD patients, the link between videos and dream reports was unfairly difficult to make.
It is important to note that motor behavior during sleep can happen outside of REM sleep. Sleepwalking and sleep terrors, which occur during NREM sleep, are usually not considered dream enactments. However, we know that dreams can happen during NREM sleep, and many patients report dreamlike mentation after awakening from sleepwalking or sleep terrors (71%, according to Oudiette et al., 2009 ). In addition, Oudiette et al. ( 2009 ) reported that the dreamlike mentation can correspond with the sleep behavior in NREM sleep. Consequently, the authors concluded that sleepwalking may represent an acting out of corresponding dreamlike mentation.
Recent research suggests that any kind of motor behavior during sleep can be considered an oneiric behavior. One of the challenges for future research is to test the strength of the link between these oneiric behaviors and dream reports in a controlled and systematic way.
Neurophysiological correlates of dreaming
Despite the numerous neuroimaging studies of sleep in humans, the neurophysiological correlates of dreaming remain unclear.
Indeed, dreaming can happen during NREM sleep, and although NREM brain activity differs substantially from REM sleep brain activity (Maquet et al., 2000 ; Buchsbaum et al., 2001 ), some NREM dreams are phenomenologically indistinguishable from REM dreams (Hobson, 1988 ; Cavallero et al., 1992 ; Cicogna et al., 1998 ; Wittmann et al., 2004 ). This phenomenon is difficult to understand given what we currently know about the sleeping brain and about dreaming. One explanation may rely on the possibility that brain activity during sleep is not as stable as we think.
Brain activity during REM sleep in humans is considered to be well understood (Hobson and Pace-Schott, 2002 ; Schwartz and Maquet, 2002 ; Nir and Tononi, 2010 ), but several results question this notion. First, contrary to the common belief that dorsolateral prefrontal cortex activity decreases during REM sleep, several studies have reported increased activity in the dorsolateral prefrontal cortex during REM sleep (Hong et al., 1995 , 2009 ; Nofzinger et al., 1997 ; Kubota et al., 2011 ). Second, brain activity during REM sleep is heterogeneous. The mean regional cerebral blood flow during 1 min of REM sleep (e.g., as reported in Maquet et al., 1996 ) and the regional cerebral blood flow associated with the rapid eye movements of REM sleep (Hong et al., 2009 ; Miyauchi et al., 2009 ) highlight different brain regions. Finally, few congruencies have been noted in the results of studies investigating brain activity during REM sleep (Hong et al., 1995 , 2009 ; Maquet et al., 1996 , 2000 ; Braun et al., 1997 , 1998 ; Nofzinger et al., 1997 ; Peigneux et al., 2001 ; Wehrle et al., 2005 ; Miyauchi et al., 2009 ; Kubota et al., 2011 ), even between studies using the same technique and the same contrasts (e.g., Braun et al., 1998 ; Maquet et al., 2000 ), or between studies investigating the same REM event (e.g., brain activity associated with rapid eyes movements, as in Peigneux et al., 2001 ; Wehrle et al., 2005 ; Hong et al., 2009 ; Miyauchi et al., 2009 ). Furthermore, few brain regions are consistently reported across the majority of the studies. This inconsistency suggests great intra- and intersubject variability in brain activity during REM sleep in humans. A challenge for future research will be to find out whether the variability in brain activity during REM sleep can be explained by the variability in dream content.
Because dream reports can be collected after awakenings from any sleep stage, one may hypothesize that the brain activity that subserves dreaming (if such brain activity is reproducible across dreams) is quite constant throughout the night and can be observed during all sleep stages. Some results have supported this hypothesis and encouraged further attention in this direction. Buchsbaum et al. ( 2001 ), for example, reported that metabolism in the primary visual areas and certain parts of the lateral temporal cortex does not fluctuate much across REM and slow-wave sleep. Similarly, Nielsen’s team found that dream recall (vs. no dream recall) was associated with decreased alpha (8–12 Hz) power in the EEG preceding awakening, regardless of the sleep stage (Stage 2 or REM sleep; Esposito et al., 2004 ). Interestingly, some authors have suggested that decreased power in the alpha band during wakefulness reflects search and retrieval processes in long-term memory (for a review, see Klimesch, 1999 ).
Processes of selection and organization of dream representations
Nielsen’s team found that episodic events from the 1, 7, and 8 days before a dream were more often incorporated into the dream than were events from 2 or 6 days before the dream (Nielsen et al., 2004 ; results reproduced by Blagrove et al., 2011 ). This result tells us that internal processes control and shape dream content and thus help us to constrain and shape hypotheses about the function and biological basis of dreaming.
At the end of the nineteenth century, Saint-Denys (1867) showed that a sensory stimulus (e.g., the scent of lavender) presented to a sleeping subject without his or her knowledge could induce the incorporation of an event associated with the stimulus (e.g., holidays spent near a lavender field) into the dream, regardless of the delay between the dream and the association stimulus/events (lavender scent/holidays). The author demonstrated that the external world can influence dream content in a direct or indirect way.
Finally, it appears that both external and internal parameters can shape or govern dream content. Nonetheless, few of these parameters are known, and some regularities in the phenomenology of dreams suggest that more influencing parameters remain to be discovered. For example, some individuals experience recurring themes, characters, or places in their dreams. In line with this observation, Michael Schredl’s team showed that the content and style of a person’s life strongly influence dream content (Schredl and Hofmann, 2003 ). However, the rule(s) governing which lived events are incorporated into dreams remain unknown. Do the representations constituting the dream emerge randomly from the brain, or do they surface according to certain parameters? Similarly, is the organization of the dream’s representations chaotic, or is it determined by rules? Does dreaming have a meaning? What is/are the function(s) of dreaming?
Dreaming, Psychoanalysis, and Neuropsychoanalysis
Psychoanalysis, which was developed by the neurologist Sigmund Freud in the beginning of the twentieth century, proposes answers to the questions raised above. Indeed, his theory of the human mind comprises hypotheses about the rules of selection and organization of the representations that constitute dreams.
At the beginning of the twentieth century, Freud presented the concept of the unconscious. He proposed that a part of our mind is made up of thoughts, desires, emotions, and knowledge that we are not aware of, but that nevertheless profoundly influence and guide our behaviors. In his books (e.g., Freud, 1900, 1920 ), Freud proposes that the unconscious mind comes out in slips and dreams. Its expression, however, is coded within dreams (the work of dream), and unconscious thoughts are distorted before they emerge in the conscious mind of the sleeping subject (manifest content of the dream). As a consequence, the dreamer is not disturbed by repressed and unacceptable thoughts (latent content of the dream) and can continue sleeping (this is the reason why Freud considered dreams the guardians of sleep). Hence, according to Freud, decoding dreams’ latent content provides an access to the unconscious mind.
In Freud’s theory of the mind, unconscious thoughts and feelings may cause the patient to experience life difficulties and/or maladjustment, and free unconscious thoughts can help the patient gain insight into his/her situation. As a consequence, Freud developed techniques to decode dreams and provide a way for an analyst to look inside the words and unconscious images of the patient, and to free them through patient insight. One of these techniques is called free association, and is regarded as an essential part of the psychoanalytic therapy process. In order for an analyst to get to the latent content of a dream, he requires the patient to discuss the dream’s manifest content and encourage free association about the dream. Free association is the principle that the patient is to say anything and everything that comes to mind. This includes decensoring his/her own speech so that he/she truly expresses everything. Over time, the therapist or analyst will draw associations between the many trains of uncensored speech the patient shares during each session. This can lead to patient insight into their unconscious thoughts or repressed memories, and the accomplishment of their ultimate goal of “freedom from the oppression of the unconscious” (Trull, 2005 ).
Hence, Freud considered that dreams, as well as slips, have a meaning and can be interpreted, so that one is justified in inferring from them the presence of restrained or repressed intentions (Freud, 1900, 1920 ). Note that, in Freud’s theory of the mind, the words “meaning” and “intention” are closely linked: “Let us agree once more on what we understand by the ‘meaning’ of a psychic process. A psychic process is nothing more than the purpose which it serves and the position which it holds in a psychic sequence. We can also substitute the word ‘purpose’ or ‘intention’ for ‘meaning’ in most of our investigations” (Freud, 1920 ).
In other words, according to Freud, decoding dreams with the free association method provides an access to what makes each of us so special, uncorvering the forces that guide one’s behavior. It gives access to an unknown dimension of ourselves that is fundamental in understanding who we are. It provides access to personal meaning.
This hypothesis, attributing significant importance and meaning to dreams, has rarely been considered by neuroscientists who often consider Freud’s work and theory unscientific.
However, this situation may change as the relationship between psychoanalysis and neuroscience evolves. The starting point was the creation of the International Society for Neuropsychoanalysis in 2000. It was founded by neuropsychologist and psychoanalyst Mark Solms with the intention to promote interactions and collaborations between psychoanalysis and neuroscience. The challenge was serious, as illustrated by neuroscientist Alan Hobson’s aggressiveness in the famous dream debate (Alan Hobson vs. Mark Solms) entitled “Should Freud’s dream theory be abandoned?” held in Tucson, Arizona, in 2006 during the Towards a Science of Consciousness meeting (scientific arguments can be found in Solms, 2000 and Hobson et al., 2000 ). Alan Hobson tried to convince the assembly that Freud was 100% wrong and that Freud’s dream theory was misguided and misleading and should be abandoned. He aimed to demonstrate that Freud’s dream theory is incompatible with what we know about how the brain works. He added that Freud’s dream theory was not scientific because it was not testable or falsifiable. Finally, he presented his model of dreaming, the activation-synthesis hypothesis (Hobson and McCarley, 1977 ; Hobson et al., 2000 ): “The Activation-Synthesis model of dream construction proposed that the phasic signals arising in the pontine brainstem during REM sleep and impinging upon the cortex and limbic forebrain led directly to the visual and motor hallucinations, emotion, and distinctively bizarre cognition that characterize dream mentation. In doing so, these chaotically generated signals arising from the brain stem acted as a physiological Rorschach test, initiating a process of image and narrative synthesis involving associative and language regions of the brain and resulting in the construction of the dream scenarios.” In contrast, Mark Solms demonstrated that what is currently known about the dreaming brain is at least broadly consistent with Freud’s dream theory. He argued that it is generally accepted that brain stem activation is necessary, but not sufficient, to explain the particular characteristics of dream consciousness. What does explain the particular characteristics of dream consciousness, according to Solms, are the following features of brain activity during REM sleep (Braun et al., 1997 ): the activation of core forebrain emotion and instinctual drive mechanisms, i.e., the limbic and paralimbic brain areas (the anterior cingulate, insula, hippocampus, parahippocampal gyrus, and temporal pole), and of the posterior perceptual system (the fusiform gyrus, superior, inferior and middle temporal gyrus, and angular gyrus) and the deactivation of executive dorsolateral frontal control mechanisms (the dorsolateral prefrontal cortex). He further argued that his lesion studies (Solms, 1997 ) are congruent with neuroimaging results because they showed that a total cessation of dreaming results from lesions in the medial part of the frontal lobe and in the temporoparietal junction (whereas no cessation of dreaming was observed for core brainstem lesions or for dorsolateral prefrontal lesions). Finally he emphasized that the activation of motivational mechanisms (such as drives and basic emotions) and of posterior perceptual system associated with deactivation of the executive control (i.e., reality oriented regulatory mechanism) during REM sleep, is broadly consistent with Freud’s dream theory which claims that our instinctual drive states (notably appetitive and libidinal drive system) are relatively disinhibited during sleep. Note that experimental results demonstrating the existence of unconscious representations that guide behavior (e.g., Shevrin and Fritzler, 1968 ; Bunce et al., 1999 ; Arminjon, 2011 , for a review) could also have been cited in support of Freud’s dream theory. This debate was a success for Mark Solms and neuropsychoanalysis. Indeed, at the end of the debate, approximately 100 people voted “no” (i.e., “Freud’s dream theory should not be abandoned”), approximately 50 people voted “yes” and 50 voted “I don’t know”.
Solms’ ( 1997 , 2000 ) approach to dreaming and his experimental results fundamentally challenged our current understanding of dreaming. He proposes that dreaming and REM sleep are controlled by different brain mechanisms. According to Solms, REM sleep is controlled by cholinergic brain stem mechanisms, whereas dreaming is mediated by forebrain mechanisms that are probably dopaminergic. This implies that dreaming can be activated by a variety of NREM triggers. Several experimental results support this hypothesis.
First, behavioral studies have demonstrated that the link between REM sleep and dream reports is lax. Subjects awakened during NREM sleep can recall dreams at a high rate (Foulkes, 1962 : 74% of awakenings in NREM sleep were followed by dream reports; Cavallero et al., 1992 : 64%; Wittmann et al., 2004 : 60%); dreams can be recalled after a nap consisting only of NREM sleep (Salzarulo, 1971 ; Palagini et al., 2004 ); and some individuals never recall dreams, even when awakened from REM sleep (Pagel, 2003 ). In addition, in healthy subjects with a normal dream recall frequency (around 1 dream recall per week, Schredl, 2008 ), dream recall after an awakening during REM sleep is not systematic: 5–30% of awakenings in REM sleep are not followed by a dream recall, according to the literature (e.g., Dement and Kleitman, 1957a , b ; Foulkes, 1962 ; Hobson, 1988 ). Finally, 5–10% of NREM dreams cannot be distinguished from REM dreams based on their content (Hobson, 1988 ; Cavallero et al., 1992 ; Cicogna et al., 1998 ; Wittmann et al., 2004 ).
Second, as Solms ( 2000 ) argued, the amount of dream recall can be modulated by dopamine agonists (Scharf et al., 1978 ; Nausieda et al., 1982 ) without concomitant modification of the duration and frequency of REM sleep (Hartmann et al., 1980 ). Dream recall can be suppressed by focal brain lesions (at the temporo-parieto-occipital junction and ventromedial prefrontal cortex; Solms, 1997 , 2000 ). These lesions do not have any appreciable effects on REM frequency, duration, or density (Kerr et al., 1978 ; Michel and Sieroff, 1981 ). Finally, some clinical studies suggest that a dream can be triggered by nocturnal seizures in NREM sleep, i.e., by focal brain stimulation. Some cases of recurring nightmares caused by epileptiform activity in the temporal lobe have indeed been reported (Solms, 2000 ).
Conclusion: Collaboration between Neuroscience and Psychoanalysis Would Benefit Dream Research
Considering the issues that remain unresolved (e.g., neurophysiologic variability, parameter(s) influencing the emergence of representations in dreams, the meaning of dreams), a psychoanalytic perspective would certainly benefit dream research by providing new directions/leads and helping to reach a comprehensive understanding of dreaming.
On the one hand, psychological research has demonstrated that dream content is influenced by one’s personal life, especially personal concerns (Schwartz, 1999 ; Schwartz and Maquet, 2002 ; Schredl and Hofmann, 2003 ), and some neuroscientists have hypothesized that dreaming is involved in psychological individualism. Thus, both psychology and neuroscience have provided results and hypotheses that validate the possibility that dreaming has something to do with personal and meaningful issues. On the other hand, Freud argued that the unconscious, which guides behaviors and desires, express itself during dreams. The two disciplines’ (cognitive neuroscience and psychoanalysis) convergence on dreaming thus seems obvious; however, very little collaboration has occurred to date.
Note that some experimental studies in psychology have considered the psychoanalytic perspective. For example, Greenberg et al. ( 1992 ) attempted “a research-based reconsideration of the psychoanalytical theory of dreaming.” They evaluated the presence of problems (defined as an expression of negative feeling or any situation evoking such feeling or requiring some change or adaptation) during dreaming and pre- and post-sleep wakefulness in two subjects. They showed that problems occurred very frequently in the manifest dream content and that these problems were nearly systematically related to the problems noted during pre-sleep wakefulness. In addition, they observed that effective dreams (i.e., dreams that presented some solution to the individuals’ problems) were followed by a waking state in which the impact of the problems was diminished, whereas ineffective dreams were followed by the persistence of the problems. This study thus confirmed that personal concerns influence dream content. In addition it provided new results suggesting that dreaming may have some psychological problem-solving function (this result recalls the neuroscientific findings that sleep has a cognitive problem-solving function associated with brain reorganization; e.g., Wagner et al., 2004 ; Darsaud et al., 2011 ). Greenberg et al.’s ( 1992 ) study managed to quantify personal issues and clearly broadened the cognitive neuroscience perspective on dreaming. To proceed further, approaches integrating psychoanalysis and neuroscience must now be developed. Several subjective issues at the core of the psychoanalytic approach, such as the concept of personal meaning, the concept of unconscious episodic memory and the subject’s history, are not addressed or considered in cognitive neuroscience. This limitation hampers the understanding of psychological and neurophysiological functioning in humans. These issues must be addressed, and the expertise of psychoanalysts in singularity and personal meaning is needed to do so in neuroscience and to further the understanding of dreaming and of the psyche.
Conflict of Interest Statement
The author declares that the research was conducted in the absence of any commercial or financial relationships that could be construed as a potential conflict of interest.
- Adolphs R. (2008). Fear, faces, and the human amygdala . Curr. Opin. Neurobiol. 18 , 166–172 10.1016/j.conb.2008.06.006 [ PMC free article ] [ PubMed ] [ CrossRef ] [ Google Scholar ]
- Arminjon M. (2011). The four postulates of Freudian unconscious neurocognitive convergences . Front. Psychol. 2 :125. 10.3389/fpsyg.2011.00125 [ PMC free article ] [ PubMed ] [ CrossRef ] [ Google Scholar ]
- Aserinsky E., Kleitman N. (1953). Regularly occurring periods of eye motility, and concomitant phenomena, during sleep . Science 118 , 273–274 10.1126/science.118.3062.273 [ PubMed ] [ CrossRef ] [ Google Scholar ]
- Belicki K., Hunt H., Kelly P. (1978). The function of dream and dreamer variables in the question of dream recall . Sleep Res. 7 , 167 [ Google Scholar ]
- Berger R. J. (1961). Tonus of extrinsic laryngeal muscles during sleep and dreaming . Science 134 , 840. 10.1126/science.134.3482.840 [ PubMed ] [ CrossRef ] [ Google Scholar ]
- Blagrove M., Henley-Einion J., Barnett A., Edwards D., Heidi Seage C. (2011). A replication of the 5-7 day dream-lag effect with comparison of dreams to future events as control for baseline matching . Conscious. Cogn. 20 , 384–391 10.1016/j.concog.2010.07.006 [ PubMed ] [ CrossRef ] [ Google Scholar ]
- Blagrove M., Pace-Schott E. F. (2010). Trait and neurobiological correlates of individual differences in dream recall and dream content . Int. Rev. Neurobiol. 92 , 155–180 10.1016/S0074-7742(10)92008-4 [ PubMed ] [ CrossRef ] [ Google Scholar ]
- Braun A. R., Balkin T. J., Wesensten N. J., Gwadry F., Carson R. E., Varga M., Baldwin P., Belenky G., Herscovitch P. (1998). Dissociated pattern of activity in visual cortices and their projections during human rapid eye movement sleep . Science 279 , 91–95 10.1126/science.279.5347.91 [ PubMed ] [ CrossRef ] [ Google Scholar ]
- Braun A. R., Balkin T. J., Wesenten N. J., Carson R. E., Varga M., Baldwin P., Selbie S., Belenky G., Herscovitch P. (1997). Regional cerebral blood flow throughout the sleep-wake cycle. An H215O PET study . Brain 120 ( Pt 7 ), 1173–1197 10.1093/brain/120.5.761 [ PubMed ] [ CrossRef ] [ Google Scholar ]
- Buchsbaum M. S., Hazlett E. A., Wu J., Bunney W. E., Jr. (2001). Positron emission tomography with deoxyglucose-F18 imaging of sleep . Neuropsychopharmacology 25 , S50–S56 10.1016/S0893-133X(01)00339-6 [ PubMed ] [ CrossRef ] [ Google Scholar ]
- Bulkeley K., Kahan T. L. (2008). The impact of September 11 on dreaming . Conscious. Cogn. 17 , 1248–1256 10.1016/j.concog.2008.07.001 [ PubMed ] [ CrossRef ] [ Google Scholar ]
- Bunce S. C., Bernat E., Wong P. S., Shevrin H. (1999). Further evidence for unconscious learning: preliminary support for the conditioning of facial EMG to subliminal stimuli . J. Psychiatr. Res. 33 , 341–347 10.1016/S0022-3956(99)00003-5 [ PubMed ] [ CrossRef ] [ Google Scholar ]
- Butler S. F., Watson R. (1985). Individual differences in memory for dreams: the role of cognitive skills . Percept. Mot. Skills 61 , 823–828 10.2466/pms.1985.61.3.823 [ CrossRef ] [ Google Scholar ]
- Calkins M. W. (1893). Statistics of dreams . Am. J. Psychol. 5 , 311–343 10.2307/1411924 [ CrossRef ] [ Google Scholar ]
- Cartwright R., Luten A., Young M., Mercer P., Bears M. (1998a). Role of REM sleep and dream affect in overnight mood regulation: a study of normal volunteers . Psychiatry Res. 81 , 1–8 10.1016/S0165-1781(98)00089-4 [ PubMed ] [ CrossRef ] [ Google Scholar ]
- Cartwright R., Young M. A., Mercer P., Bears M. (1998b). Role of REM sleep and dream variables in the prediction of remission from depression . Psychiatry Res. 80 , 249–255 10.1016/S0165-1781(98)00071-7 [ PubMed ] [ CrossRef ] [ Google Scholar ]
- Cavallero C., Cicogna P., Natale V., Occhionero M., Zito A. (1992). Slow wave sleep dreaming . Sleep 15 , 562–566 [ PubMed ] [ Google Scholar ]
- Cicogna P. C., Natale V., Occhionero M., Bosinelli M. (1998). A comparison of mental activity during sleep onset and morning awakening . Sleep 21 , 462–470 [ PubMed ] [ Google Scholar ]
- Cohen D. B. (1971). Dream recall and short-term memory . Percept. Mot. Skills 33 , 867–871 10.2466/pms.1971.33.1.101 [ PubMed ] [ CrossRef ] [ Google Scholar ]
- Connor G. N., Boblitt W. M. E. (1970). Reported frequency of dream recall as a function of intelligence and various personality test factors . J. Clin. Psychol. 26 , 438–439 10.1002/1097-4679(197010)26:4<438::AID-JCLP2270260410>3.0.CO;2-X [ PubMed ] [ CrossRef ] [ Google Scholar ]
- Cory T. L., Ormiston D. W. (1975). Predicting the frequency of dream recall . J. Abnorm. Psychol. 84 , 261–266 10.1037/h0076653 [ PubMed ] [ CrossRef ] [ Google Scholar ]
- Darsaud A., Wagner U., Balteau E., Desseilles M., Sterpenich V., Vandewalle G., Albouy G., Dang-Vu T., Collette F., Boly M., Schabus M., Degueldre C., Luxen A., Maquet P. (2011). Neural precursors of delayed insight . J. Cogn. Neurosci. 23 , 1900–1910 10.1162/jocn.2010.21448 [ PubMed ] [ CrossRef ] [ Google Scholar ]
- De Gennaro L., Ferrara M., Cristiani R., Curcio G., Martiradonna V., Bertini M. (2003). Alexithymia and dream recall upon spontaneous morning awakening . Psychosom. Med. 65 , 301–306 10.1097/01.PSY.0000058373.50240.71 [ PubMed ] [ CrossRef ] [ Google Scholar ]
- Decety J., Perani D., Jeannerod M., Bettinardi V., Tadary B., Woods R., Mazziotta J. C., Fazio F. (1994). Mapping motor representations with positron emission tomography . Nature 371 , 600–602 10.1038/371600a0 [ PubMed ] [ CrossRef ] [ Google Scholar ]
- Dement W., Kleitman N. (1957a). Cyclic variations in EEG during sleep and their relation to eye movements, body motility, and dreaming . Electroencephalogr. Clin. Neurophysiol. 9 , 673–690 10.1016/0013-4694(57)90088-3 [ PubMed ] [ CrossRef ] [ Google Scholar ]
- Dement W., Kleitman N. (1957b). The relation of eye movements during sleep to dream activity: an objective method for the study of dreaming . J. Exp. Psychol. 53 , 339–346 10.1037/h0048189 [ PubMed ] [ CrossRef ] [ Google Scholar ]
- Diekelmann S., Born J. (2010). The memory function of sleep . Nat. Rev. Neurosci. 11 , 114–126 10.1038/nrn2762-c2 [ PubMed ] [ CrossRef ] [ Google Scholar ]
- Domhoff G. W., Schneider A. (2008). Studying dream content using the archive and search engine on DreamBank.net . Conscious. Cogn. 17 , 1238–1247 10.1016/j.concog.2008.06.010 [ PubMed ] [ CrossRef ] [ Google Scholar ]
- Esposito M. J., Nielsen T. A., Paquette T. (2004). Reduced alpha power associated with the recall of mentation from Stage 2 and Stage REM sleep . Psychophysiology 41 , 288–297 10.1111/j.1469-8986.00143.x [ PubMed ] [ CrossRef ] [ Google Scholar ]
- Fisher C., Gorss J., Zuch J. (1965). Cycle of penile erection synchronous with dreaming (Rem) sleep. Preliminary report . Arch. Gen. Psychiatry 12 , 29–45 [ PubMed ] [ Google Scholar ]
- Fitch T., Armitage R. (1989). Variations in cognitive style among high and low frequency dream recallers . Pers. Individ. dif. 10 , 869–875 10.1016/0191-8869(89)90022-6 [ CrossRef ] [ Google Scholar ]
- Foulkes W. D. (1962). Dream reports from different stages of sleep . J. Abnorm. Soc. Psychol. 65 , 14–25 10.1037/h0040431 [ PubMed ] [ CrossRef ] [ Google Scholar ]
- Freud S. (1967). L’interprétation des rẽves (I. Meyerson, Trans.) . Paris: PUF. (Original work published 1900) [ Google Scholar ]
- Freud S. (1920). A General Introduction to Psychoanalysis . New York: Boni and Liveright publishers [ Google Scholar ]
- Goodenough D. R., Lewis H. B., Shapiro A., Jaret L., Sleser I. (1965). Dream reporting following abrupt and gradual awakenings from different types of sleep . J. Pers. Soc. Psychol. 56 , 170–179 [ PubMed ] [ Google Scholar ]
- Greenberg R., Katz H., Schwartz W., Pearlman C. (1992). A research-based reconsideration of the psychoanalytic theory of dreaming . J. Am. Psychoanal. Assoc. 40 , 531–550 [ PubMed ] [ Google Scholar ]
- Hall C. S., Van de Castle R. L. (1966). The Content Analysis of Dreams . New York: Appleton-Century-Crofts [ Google Scholar ]
- Hartmann E. (1989). Boundaries of dreams, boundaries of dreamers: thin and thick boundaries as a new personality measure . Psychiatr. J. Univ. Ott. 14 , 557–560 [ PubMed ] [ Google Scholar ]
- Hartmann E., Elkin R., Garg M. (1991). Personality and dreaming: the dreams of people with very thick or very thin boundaries . Dreaming 1 , 311–324 [ Google Scholar ]
- Hartmann E., Russ D., Oldfield M., Falke R., Skoff B. (1980). Dream content: effects of l-DOPA . Sleep Res. 9 , 153 [ Google Scholar ]
- Hill C. E., Diemer R. A., Heaton K. J. (1997). Dream interpretation sessions: who volunteers, who benefits, and what volunteer clients view as most and least helpful . J. Couns. Psychol. 44 , 53–62 10.1037/0022-0167.44.1.53 [ CrossRef ] [ Google Scholar ]
- Hiscock M., Cohen D. (1973). Visual imagery and dream recall . J. Res. Pers. 7 , 179–188 10.1016/0092-6566(73)90051-2 [ CrossRef ] [ Google Scholar ]
- Hobson J. A. (1988). The Dreaming Brain . New York: Basic Books [ Google Scholar ]
- Hobson J. A., McCarley R. W. (1977). The brain as a dream-state generator: an activation-synthesis hypothesis of the dream process . Am. J. Psychiatry 134 , 1335–1348 [ PubMed ] [ Google Scholar ]
- Hobson J. A., Pace-Schott E. F. (2002). The cognitive neuroscience of sleep: neuronal systems, consciousness and learning . Nat. Rev. Neurosci. 3 , 679–693 10.1038/nrn915 [ PubMed ] [ CrossRef ] [ Google Scholar ]
- Hobson J. A., Pace-Schott E. F., Stickgold R. (2000). Dreaming and the brain: toward a cognitive neuroscience of conscious states . Behav. Brain Sci. 23 , 793–842 10.1017/S0140525X00003976 [ PubMed ] [ CrossRef ] [ Google Scholar ]
- Hobson J. A., Stickgold R., Pace-Schott E. F. (1998). The neuropsychology of REM sleep dreaming . Neuroreport 9 , R1–R14 10.1097/00001756-199807130-00051 [ PubMed ] [ CrossRef ] [ Google Scholar ]
- Hoelscher T. J., Klinger E., Barta S. G. (1981). Incorporation of concern- and nonconcern-related verbal stimuli into dream content . J. Abnorm. Psychol. 90 , 88–91 10.1037/0021-843X.90.1.88 [ CrossRef ] [ Google Scholar ]
- Hong C. C., Gillin J. C., Dow B. M., Wu J., Buchsbaum M. S. (1995). Localized and lateralized cerebral glucose metabolism associated with eye movements during REM sleep and wakefulness: a positron emission tomography (PET) study . Sleep 18 , 570–580 [ PubMed ] [ Google Scholar ]
- Hong C. C., Harris J. C., Pearlson G. D., Kim J. S., Calhoun V. D., Fallon J. H., Golay X., Gillen J. S., Simmonds D. J., van Zijl P. C., Zee D. S., Pekar J. J. (2009). fMRI evidence for multisensory recruitment associated with rapid eye movements during sleep . Hum. Brain Mapp. 30 , 1705–1722 10.1002/hbm.20635 [ PMC free article ] [ PubMed ] [ CrossRef ] [ Google Scholar ]
- Jouvet M. (1991). Le sommeil paradoxal: est-il le gardien de l’individuation psychologique? Can. J. Psychol. 45 , 148–168 10.1037/h0084291 [ PubMed ] [ CrossRef ] [ Google Scholar ]
- Jouvet M. (1992). Le sommeil et le rẽve . Paris: Odile Jacob [ Google Scholar ]
- Jouvet M. (1998). Paradoxical sleep as a programming system . J. Sleep Res. 7 ( Suppl. 1 ), 1–5 10.1046/j.1365-2869.7.s1.1.x [ PubMed ] [ CrossRef ] [ Google Scholar ]
- Jouvet M., Michel F. (1959). Corrélations électromyographique du sommeil chez le Chat décortiqué et mésencéphalique chronique . C. R. Seances Soc. Biol. Fil. 153 , 422–425 [ PubMed ] [ Google Scholar ]
- Jouvet M., Michel F., Courjon J. (1959). Sur un stade d’activité électrique cérébrale rapide au cours du sommeil physiologique . C. R. Seances Soc. Biol. Fil. 153 , 1024–1028 [ PubMed ] [ Google Scholar ]
- Kahn D., Pace-Schott E. F., Hobson J. A. (1997). Consciousness in waking and dreaming: the roles of neuronal oscillation and neuromodulation in determining similarities and differences . Neuroscience 78 , 13–38 10.1016/S0306-4522(96)00550-7 [ PubMed ] [ CrossRef ] [ Google Scholar ]
- Kerr N., Foulkes D., Jurkovic G. (1978). Reported absence of visual dream imagery in a normally sighted subject with Turner’s syndrome . J. Ment. Imagery 2 , 247–264 [ Google Scholar ]
- Klimesch W. (1999). EEG alpha and theta oscillations reflect cognitive and memory performance: a review and analysis . Brain Res. Brain Res. Rev. 29 , 169–195 10.1016/S0165-0173(98)00056-3 [ PubMed ] [ CrossRef ] [ Google Scholar ]
- Koechlin E., Hyafil A. (2007). Anterior prefrontal function and the limits of human decision-making . Science 318 , 594–598 10.1126/science.1142995 [ PubMed ] [ CrossRef ] [ Google Scholar ]
- Kosslyn S. M., Thompson W. L. (2003). When is early visual cortex activated during visual mental imagery? Psychol. Bull. 129 , 723–746 10.1037/0033-2909.129.5.723 [ PubMed ] [ CrossRef ] [ Google Scholar ]
- Koulack D. (1969). Effects of somatosensory stimulation on dream content . Arch. Gen. Psychiatry 20 , 718–725 [ PubMed ] [ Google Scholar ]
- Koulack D., Goodenough D. R. (1976). Dream recall and dream recall failure: an arousal-retrieval model . Psychol. Bull. 83 , 975–984 10.1037/0033-2909.83.5.975 [ CrossRef ] [ Google Scholar ]
- Kubota Y., Takasu N. N., Horita S., Kondo M., Shimizu M., Okada T., Wakamura T., Toichi M. (2011). Dorsolateral prefrontal cortical oxygenation during REM sleep in humans . Brain Res. 1389 , 83–92 10.1016/j.brainres.2011.02.061 [ PubMed ] [ CrossRef ] [ Google Scholar ]
- Legrand D., Ruby P. (2009). What is self-specific? Theoretical investigation, and critical review of neuroimaging results . Psychol. Rev. 116 , 252–282 10.1037/a0014172 [ PubMed ] [ CrossRef ] [ Google Scholar ]
- Mahowald M. W., Schenck C. H. (2000). “REM sleep parasomnias,” in Principles and Practice of Sleep Medicine , eds Kryger M. H., Roth T., Dement W. C. (Philadelphia: W.B. Saunders; ), 724–741 [ Google Scholar ]
- Maquet P., Laureys S., Peigneux P., Fuchs S., Petiau C., Phillips C., Aerts J., Del Fiore G., Degueldre C., Meulemans T., Luxen A., Franck G., Van Der Linden M., Smith C., Cleeremans A. (2000). Experience-dependent changes in cerebral activation during human REM sleep . Nat. Neurosci. 3 , 831–836 10.1038/77744 [ PubMed ] [ CrossRef ] [ Google Scholar ]
- Maquet P., Peters J., Aerts J., Delfiore G., Degueldre C., Luxen A., Franck G. (1996). Functional neuroanatomy of human rapid-eye-movement sleep and dreaming . Nature 383 , 163–166 10.1038/383163a0 [ PubMed ] [ CrossRef ] [ Google Scholar ]
- Maquet P., Ruby P., Maudoux A., Albouy G., Sterpenich V., Dang-Vu T., Desseilles M., Boly M., Perrin F., Peigneux P., Laureys S. (2005). Human cognition during REM sleep and the activity profile within frontal and parietal cortices: a reappraisal of functional neuroimaging data . Prog. Brain Res. 150 , 219–227 10.1016/S0079-6123(05)50016-5 [ PubMed ] [ CrossRef ] [ Google Scholar ]
- Maquet P., Schwartz S., Passingham R., Frith C. (2003). Sleep-related consolidation of a visuomotor skill: brain mechanisms as assessed by functional magnetic resonance imaging . J. Neurosci. 23 , 1432–1440 [ PMC free article ] [ PubMed ] [ Google Scholar ]
- Michel F., Sieroff E. (1981). Une approche anatomo-clinique des déficits de l’imagerie onirique, est-elle possible? Sleep: Proceedings of an International Colloquium . Milan: Carlo Erba Formitala [ Google Scholar ]
- Miyauchi S., Misaki M., Kan S., Fukunaga T., Koike T. (2009). Human brain activity time-locked to rapid eye movements during REM sleep . Exp. Brain Res. 192 , 657–667 10.1007/s00221-008-1579-2 [ PubMed ] [ CrossRef ] [ Google Scholar ]
- Nausieda P., Weiner W., Kaplan L., Weber S., Klawans H. (1982). Sleep disruption in the course of chronic levodopa therapy: an early feature of the levodopa psychosis . Clin. Neuropharmacol. 5 , 183–194 10.1097/00002826-198205020-00003 [ PubMed ] [ CrossRef ] [ Google Scholar ]
- Nielsen T. A. (2000). A review of mentation in REM and NREM sleep: “covert” REM sleep as a possible reconciliation of two opposing models . Behav. Brain Sci. 23 , 851–866 10.1017/S0140525X00974028 [ PubMed ] [ CrossRef ] [ Google Scholar ]
- Nielsen T. A., Kuiken D., Alain G., Stenstrom P., Powell R. A. (2004). Immediate and delayed incorporations of events into dreams: further replication and implications for dream function . J. Sleep Res. 13 , 327–336 10.1111/j.1365-2869.2004.00421.x [ PubMed ] [ CrossRef ] [ Google Scholar ]
- Nielsen T. A., Levrier K., Montplaisir J. (2011). Dreaming correlates of alexithymia among sleep-disordered patients . Dreaming 21 , 16–31 10.1037/a0022861 [ CrossRef ] [ Google Scholar ]
- Nielsen T. A., Ouellet L., Warnes H., Cartier A., Malo J. L., Montplaisir J. (1997). Alexithymia and impoverished dream recall in asthmatic patients: evidence from self-report measures . J. Psychosom. Res. 42 , 53–59 10.1016/S0022-3999(96)00230-9 [ PubMed ] [ CrossRef ] [ Google Scholar ]
- Nielsen T. A., Stenstrom P. (2005). What are the memory sources of dreaming? Nature 437 , 1286–1289 10.1038/nature04288 [ PubMed ] [ CrossRef ] [ Google Scholar ]
- Nielsen T. A., Zadra A. L., Simard V., Saucier S., Stenstrom P., Smith C., Kuiken D. (2003). The typical dreams of Canadian university students . Dreaming 13 , 211–235 10.1023/B:DREM.0000003144.40929.0b [ CrossRef ] [ Google Scholar ]
- Nir Y., Tononi G. (2010). Dreaming and the brain: from phenomenology to neurophysiology . Trends Cogn. Sci. (Regul. Ed.) 14 , 88–100 10.1016/j.tics.2009.12.001 [ PMC free article ] [ PubMed ] [ CrossRef ] [ Google Scholar ]
- Nofzinger E. A., Mintun M. A., Wiseman M., Kupfer D. J., Moore R. Y. (1997). Forebrain activation in REM sleep: an FDG PET study . Brain Res. 770 , 192–201 10.1016/S0006-8993(97)00807-X [ PubMed ] [ CrossRef ] [ Google Scholar ]
- Okada H., Matsuoka K., Hatakeyama T. (2000). Dream-recall frequency and waking imagery . Percept. Mot. Skills 91 ( 3 Pt 1 ), 759–766 10.2466/pms.2000.91.3.759 [ PubMed ] [ CrossRef ] [ Google Scholar ]
- Oudiette D., Leu S., Pottier M., Buzare M. A., Brion A., Arnulf I. (2009). Dreamlike mentations during sleepwalking and sleep terrors in adults . Sleep 32 , 1621–1627 [ PMC free article ] [ PubMed ] [ Google Scholar ]
- Pagel J. F. (2003). Non-dreamers . Sleep Med. 4 , 235–241 10.1016/S1389-9457(02)00255-1 [ PubMed ] [ CrossRef ] [ Google Scholar ]
- Palagini L., Gemignani A., Feinberg I., Guazzelli M., Campbell I. G. (2004). Mental activity after early afternoon nap awakenings in healthy subjects . Brain Res. Bull. 63 , 361–368 10.1016/j.brainresbull.2003.12.008 [ PubMed ] [ CrossRef ] [ Google Scholar ]
- Peigneux P., Laureys S., Fuchs S., Collette F., Perrin F., Reggers J., Phillips C., Degueldre C., Del Fiore G., Aerts J., Luxen A., Maquet P. (2004). Are spatial memories strengthened in the human hippocampus during slow wave sleep? Neuron 44 , 535–545 10.1016/j.neuron.2004.10.007 [ PubMed ] [ CrossRef ] [ Google Scholar ]
- Peigneux P., Laureys S., Fuchs S., Delbeuck X., Degueldre C., Aerts J., Delfiore G., Luxen A., Maquet P. (2001). Generation of rapid eye movements during paradoxical sleep in humans . Neuroimage 14 , 701–708 10.1006/nimg.2001.0874 [ PubMed ] [ CrossRef ] [ Google Scholar ]
- Petrides M. (2005). Lateral prefrontal cortex: architectonic and functional organization . Philos. Trans. R. Soc. Lond. B Biol. Sci. 360 , 781–795 10.1098/rstb.2005.1631 [ PMC free article ] [ PubMed ] [ CrossRef ] [ Google Scholar ]
- Piolino P., Desgranges B., Eustache F. (2009). Episodic autobiographical memories over the course of time: cognitive, neuropsychological and neuroimaging findings . Neuropsychologia 47 , 2314–2329 10.1016/j.neuropsychologia.2009.01.020 [ PubMed ] [ CrossRef ] [ Google Scholar ]
- Raymond I., Nielsen T. A., Lavigne G., Choinière M. (2002). Incorporation of pain in dreams of hospitalized burn victims . Sleep 25 , 765–770 [ PubMed ] [ Google Scholar ]
- Rechtschaffen A. (1978). The single-mindedness and isolation of dreams . Sleep 1 , 97–109 [ PubMed ] [ Google Scholar ]
- Revonsuo A. (2000). The reinterpretation of dreams: an evolutionary hypothesis of the function of dreaming . Behav. Brain Sci. 23 , 877–901; discussion 904–1121. 10.1017/S0140525X00814028 [ PubMed ] [ CrossRef ] [ Google Scholar ]
- Richardson A. (1979). Dream recall frequency and vividness of visual imagery . J. Ment. Imagery 3 , 65–72 [ Google Scholar ]
- Ruby P., Collette F., D’Argembeau A., Peters F., Degueldre C., Balteau E., Luxen A., Maquet P., Salmon E. (2009). Perspective taking to assess self personality: what is modified in Alzheimer’s disease? Neurobiol. Aging 30 , 1637–1651 10.1016/j.neurobiolaging.2007.12.014 [ PubMed ] [ CrossRef ] [ Google Scholar ]
- Ruby P., Decety J. (2001). Effect of subjective perspective taking during simulation of action: a PET investigation of agency . Nat. Neurosci. 4 , 546–550 [ PubMed ] [ Google Scholar ]
- Ruby P., Decety J. (2003). What you believe versus what you think they believe: a neuroimaging study of conceptual perspective-taking . Eur. J. Neurosci. 17 , 2475–2480 10.1046/j.1460-9568.2003.02673.x [ PubMed ] [ CrossRef ] [ Google Scholar ]
- Ruby P., Decety J. (2004). How would you feel versus how do you think she would feel? A neuroimaging study of perspective-taking with social emotions . J. Cogn. Neurosci. 16 , 988–999 10.1162/0898929041502661 [ PubMed ] [ CrossRef ] [ Google Scholar ]
- Ruby P., Schmidt C., Hogge M., D’Argembeau A., Collette F., Salmon E. (2007). Social mind representation: where does it fail in frontotemporal dementia? J. Cogn. Neurosci. 19 , 1–13 10.1162/jocn.2007.19.1.1 [ PubMed ] [ CrossRef ] [ Google Scholar ]
- Saint-Denys H. (1977). Les Rẽves et les moyens de les diriger . Paris: D’Aujourd’hui; (Originally published in 1867). [ Google Scholar ]
- Salzarulo P. (1971). Electroencephalographic and polygraphic study of afternoon sleep in normal subjects . Electroencephalogr. Clin. Neurophysiol. 30 , 399–407 10.1016/0013-4694(71)90254-9 [ PubMed ] [ CrossRef ] [ Google Scholar ]
- Sanford L. D., Cheng C. S., Silvestri A. J., Mann G. L., Morrison A. R. (2001). Sleep and behavior in rats with pontine lesions producing REM without atonia . Sleep Res. Online 4 , 1–5 [ Google Scholar ]
- Sastre J. P., Jouvet M. (1979). Le comportement onirique du chat . Physiol. Behav. 22 , 979–989 10.1016/0031-9384(79)90344-5 [ PubMed ] [ CrossRef ] [ Google Scholar ]
- Saurat M. T., Agbakou M., Attigui P., Golmard J. L., Arnulf I. (2011). Walking dreams in congenital and acquired paraplegia . Conscious. Cogn. 20 , 1425–1432 10.1016/j.concog.2011.05.015 [ PubMed ] [ CrossRef ] [ Google Scholar ]
- Scharf B., Moskowitz C., Lupton M., Klawans H. (1978). Dream phenomena induced by chronic Levodopa therapy . J. Neural. Transm. 43 , 143–151 10.1007/BF01579073 [ PubMed ] [ CrossRef ] [ Google Scholar ]
- Schonbar R. A. (1959). Some manifest characteristics of recallers and nonrecallers of dreams . J. Consult. Psychol. 23 , 414–418 10.1037/h0047400 [ CrossRef ] [ Google Scholar ]
- Schredl M. (1999). Dream recall: research, clinical implications and future directions . Sleep Hypn. 1 , 72–81 [ Google Scholar ]
- Schredl M. (2002). Dream recall frequency and openness to experience: a negative finding . Pers. Individ. Dif. 33 , 1285–1289 10.1016/S0191-8869(02)00013-2 [ CrossRef ] [ Google Scholar ]
- Schredl M. (2008). Dream recall frequency in a representative German sample . Percept. Mot. Skills 106 , 699–702 10.2466/pms.106.3.690-692 [ PubMed ] [ CrossRef ] [ Google Scholar ]
- Schredl M., Ciric P., Gotz S., Wittmann L. (2004). Typical dreams: stability and gender differences . J. Psychol. 138 , 485–494 10.3200/JRLP.138.6.485-494 [ PubMed ] [ CrossRef ] [ Google Scholar ]
- Schredl M., Frauscher S., Shendi A. (1995). Dream recall and visual memory . Percept. Mot. Skills 81 , 256–258 10.2466/pms.1995.81.1.256 [ PubMed ] [ CrossRef ] [ Google Scholar ]
- Schredl M., Hofmann F. (2003). Continuity between waking activities and dream activities . Conscious. Cogn. 12 , 298–308 10.1016/S1053-8100(02)00072-7 [ PubMed ] [ CrossRef ] [ Google Scholar ]
- Schredl M., Reinhard I. (2008). Gender differences in dream recall: a meta-analysis . J. Sleep Res. 17 , 125–131 10.1111/j.1365-2869.2008.00626.x [ PubMed ] [ CrossRef ] [ Google Scholar ]
- Schredl M., Jochum S., Souguenet S. (1997). Dream recall, visual memory, and absorption in imaginings . Pers. Individ. Dif. 2 , 291–292 10.1016/S0191-8869(96)00192-4 [ CrossRef ] [ Google Scholar ]
- Schredl M., Wittmann L., Ciric P., Gotz S. (2003). Factors of home dream recall: a structural equation model . J. Sleep Res. 12 , 133–141 10.1046/j.1365-2869.2003.00344.x [ PubMed ] [ CrossRef ] [ Google Scholar ]
- Schwartz S. (1999). Exploration statistique et neuropsychologique des phénomènes oniriques au travers des textes et des images de rẽves . Ph.D. thesis, University of Lausanne, Lausanne, 375 [ Google Scholar ]
- Schwartz S., Maquet P. (2002). Sleep imaging and the neuro-psychological assessment of dreams . Trends Cogn. Sci. (Regul. Ed.) 6 , 23–30 10.1016/S1364-6613(00)01818-0 [ PubMed ] [ CrossRef ] [ Google Scholar ]
- Shapiro A., Goodenough D. R., Gryler R. B. (1963). Dream recall as a function of method of awakening . Psychosom. Med. 25 , 174–180 [ PubMed ] [ Google Scholar ]
- Shapiro A., Goodenough D. R., Lewis H. B., Sleser I. (1965). Gradual arousal from sleep: a determinant of thinking reports . Psychosom. Med. 27 , 342–349 [ PubMed ] [ Google Scholar ]
- Shevrin H., Fritzler D. E. (1968). Visual evoked response correlates of unconscious mental processes . Science 161 , 295–298 10.1126/science.161.3838.295 [ PubMed ] [ CrossRef ] [ Google Scholar ]
- Solms M. (1997). The Neuropsychology of Dreaming: A Clinico-Anatomical Study . Mahwah, NJ: Lawrence Erlbaum Associates [ Google Scholar ]
- Solms M. (2000). Dreaming and REM sleep are controlled by different brain mechanisms . Behav. Brain Sci. 23 , 843–850 10.1017/S0140525X00964021 [ PubMed ] [ CrossRef ] [ Google Scholar ]
- Stickgold R., Hobson J. A., Fosse R., Fosse M. (2001). Sleep, learning, and dreams: off-line memory reprocessing . Science 294 , 1052–1057 10.1126/science.1063530 [ PubMed ] [ CrossRef ] [ Google Scholar ]
- Tart C. T. (1962). Frequency of dream recall and some personality measures . J. Consult. Psychol. 26 , 467–470 10.1037/h0046056 [ PubMed ] [ CrossRef ] [ Google Scholar ]
- Thompson L. T., Moyer J. R., Jr., Disterhoft J. F. (1996). Transient changes in excitability of rabbit CA3 neurons with a time course appropriate to support memory consolidation . J. Neurophysiol. 76 , 1836–1849 [ PubMed ] [ Google Scholar ]
- Trull T. (2005). Clinical Psychology , 7th Edn Belmont, CA: Thomson Wadsworth [ Google Scholar ]
- Uga V., Lemut M. C., Zampi C., Zilli I., Salzarulo P. (2006). Music in dreams . Conscious. Cogn. 15 , 351–357 10.1016/j.concog.2005.09.003 [ PubMed ] [ CrossRef ] [ Google Scholar ]
- Valli K., Frauscher B., Gschliesser V., Wolf E., Falkenstetter T., Schönwald S. V., Ehrmann L., Zangerl A., Marti I., Boesch S. M., Revonsuo A., Poewe W., Högl B. (2011). Can observers link dream content to behaviours in rapid eye movement sleep behaviour disorder? A cross-sectional experimental pilot study . J. Sleep Res. [Epub ahead of print]. 10.1111/j.1365-2869.2011.00938.x [ PubMed ] [ CrossRef ] [ Google Scholar ]
- Valli K., Revonsuo A. (2009). The threat simulation theory in light of recent empirical evidence: a review . Am. J. Psychol. 122 , 17–38 [ PubMed ] [ Google Scholar ]
- Valli K., Revonsuo A., Pälkäs O., Ismail K. H., Ali K. J., Punamäki R. L. (2005). The threat simulation theory of the evolutionary function of dreaming: eidence from dreams of traumatized children . Conscious. Cogn. 14 , 188–218 10.1016/S1053-8100(03)00019-9 [ PubMed ] [ CrossRef ] [ Google Scholar ]
- Voss U., Tuin I., Schermelleh-Engel K., Hobson A. (2010). Waking and dreaming: related but structurally independent. Dream reports of congenitally paraplegic and deaf-mute persons . Conscious. Cogn. 20 , 673–687 10.1016/j.concog.2010.10.020 [ PubMed ] [ CrossRef ] [ Google Scholar ]
- Wagner U., Gais S., Haider H., Verleger R., Born J. (2004). Sleep inspires insight . Nature 427 , 352–355 10.1038/nature02223 [ PubMed ] [ CrossRef ] [ Google Scholar ]
- Wamsley E. J., Tucker M., Payne J. D., Benavides J. A., Stickgold R. (2010). Dreaming of a learning task is associated with enhanced sleep-dependant memory consolidation . Curr. Biol. 20 , 850–855 10.1016/j.cub.2010.08.012 [ PMC free article ] [ PubMed ] [ CrossRef ] [ Google Scholar ]
- Wehrle R., Czisch M., Kaufmann C., Wetter T. C., Holsboer F., Auer D. P., Pollmächer T. (2005). Rapid eye movement-related brain activation in human sleep: a functional magnetic resonance imaging study . Neuroreport 16 , 853–857 10.1097/00001756-200505310-00015 [ PubMed ] [ CrossRef ] [ Google Scholar ]
- Wittmann L., Palmy C., Schredl M. (2004). NREM sleep dream recall, dream report length and cortical activation . Sleep Hypn. 6 , 53–57 [ Google Scholar ]
- Zacks J. M., Vettel J. M., Michelon P. (2003). Imagined viewer and object rotations dissociated with event-related FMRI . J. Cogn. Neurosci. 15 , 1002–1018 10.1162/089892903770007399 [ PubMed ] [ CrossRef ] [ Google Scholar ]

Writing Center: Experimental Research Papers
- How to Set Up an Appointment Online
- Documentation Styles
- Parts of Speech
- Types of Clauses
- Punctuation
- Spelling & Mechanics
- Usage & Styles
- Resources for ESL Students
- How to Set up an APA Paper
- How to Set up an MLA Paper
- Adapt to Academic Learning
- Audience Awareness
- Learn Touch Typing
- Getting Started
- Thesis Statement
- The First Draft
- Proofreading
- Writing Introductions
- Writing Conclusions
- Chicago / Turabian Style
- CSE / CBE Style
- Avoiding Plagiarism
- Cross-Cultural Understanding
- Writing Resources
- Research Paper - General Guidelines
- Annotated Bibliographies
- History Papers
- Science Papers
- Experimental Research Papers
- Exegetical Papers
- FAQs About Creative Writing
- Tips For Creative Writing
- Exercises To Develop Creative Writing Skills
- Checklist For Creative Writing
- Additional Resources For Creative Writing
- FAQs About Creating PowerPoints
- Tips For Creating PowerPoints
- Exercises to Improve PowerPoint Skills
- Checklist For PowerPoints
- Structure For GRE Essay
- Additional Resources For PowerPoints
- Additional Resources For GRE Essay Writing
- FAQs About Multimodal Assignments
- Tips For Creating Multimodal Assignments
- Checklist For Multimodal Assignments
- Additional Resources For Multimodal Assignments
- GRE Essay Writing FAQ
- Tips for GRE Essay Writing
- Sample GRE Essay Prompts
- Checklist For GRE Essays
- Cover Letter
- Personal Statements
- Resources for Tutors
- Chapter 2: Theoretical Perspectives on Learning a Second Language
- Chapter 4: Reading an ESL Writer's Text
- Chapter 5: Avoiding Appropriation
- Chapter 6: 'Earth Aches by Midnight': Helping ESL Writers Clarify Their Intended Meaning
- Chapter 7: Looking at the Whole Text
- Chapter 8: Meeting in the Middle: Bridging the Construction of Meaning with Generation 1.5 Learners
- Chapter 9: A(n)/The/Ø Article About Articles
- Chapter 10: Editing Line by Line
- Chapter 14: Writing Activities for ESL Writers
- Resources for Faculty
- Writing Center Newsletter
- Writing Center Survey
FAQs About Experimental Research Papers (APA)
What is a research paper?
A researcher uses a research paper to explain how they conducted a research study to answer a question or test a hypothesis. They explain why they conducted the study, the research question or hypothesis they tested, how they conducted the study, the results of their study, and the implications of these results.
What is the purpose of an experimental research paper?
A research paper is intended to inform others about advancement in a particular field of study. The researcher who wrote the paper identified a gap in the research in a field of study and used their research to help fill this gap. The researcher uses their paper to inform others about the knowledge that the results of their study contribute.
What sections are included in an experimental research paper?
A typical research paper contains a Title Page, Abstract, Introduction, Methods, Results, Discussion, and References section. Some also contain a Table and Figures section and Appendix section.
What citation style is used for experimental research papers?
APA (American Psychological Association) style is most commonly used for research papers.
Structure Of Experimental Research Papers (APA)
- Answers the question of “What is this paper about and who wrote it?”
- Located on the first page of the paper
- The author’s note acknowledges any support that the authors received from others
- A student paper also includes the course number and name, instructor’s name, and assignment due date
- Contains a title that summarizes the purpose and content of the research study and engages the audience
- No longer than 250 words
- Summarizes important background information, the research questions and/or hypothesis, methods, key findings, and implications of the findings
- Explains what the topic of the research is and why the topic is worth studying
- Summarizes and discusses prior research conducted on the topic
- Identifies unresolved issues and gaps in past research that the current research will address
- Ends with an overview of the current research study, including how the independent and dependent variables, the research questions or hypotheses, and the objective of the research
- Explains how the research study was conducted
- Typically includes 3 sections: Participants, Materials, and Procedure
- Includes characteristics of the subjects, how the subjects were selected and recruited, how their anonymity was protected, and what feedback was provided to the participants
- Describes any equipment, surveys, tests, questionnaires, informed consent forms, and observational techniques
- Describes the independent and dependent variables, the type of research design, and how the data was collected
- Explains what results were found in the research study
- Describes the data that was collected and the results of statistical tests
- Explains the significance of the results
- Accepts or denies the hypotheses
- Details the implications of these findings
- Addresses the limitations of the study and areas for future research
- Includes all sources that were mentioned in the research study
- Adheres to APA citation styles
- Includes all tables and/or figures that were used in the research study
- Each table and figure is placed on a separate page
- Tables are included before figures
- Begins with a bolded, centered header such as “ Table 1 ”
- Appends all forms, surveys, tests, etc. that were used in the study
- Only includes documents that were referenced in the Methods section
- Each entry is placed on a separate page
- Begins with a bolded, centered header such as “ Appendix A ”
Tips For Experimental Research Papers (APA)
- Initial interest will motivate you to complete your study
- Your entire study will be centered around this question or statement
- Use only verifiable sources that provide accurate information about your topic
- You need to thoroughly understand the field of study your topic is on to help you recognize the gap your research will fill and the significance of your results
- This will help you identify what you should study and what the significance of your study will be
- Create an outline before you begin writing to help organize your thoughts and direct you in your writing
- This will prevent you from losing the source or forgetting to cite the source
- Work on one section at a time, rather than trying to complete multiple sections at once
- This information can be easily referred to as your write your various sections
- When conducting your research, working general to specific will help you narrow your topic and fully understand the field your topic is in
- When writing your literature review, writing from general to specific will help the audience understand your overall topic and the narrow focus of your research
- This will prevent you from losing sources you may need later
- Incorporate correct APA formatting as you write, rather than changing the formatting at the end of the writing process
Checklist For Experimental Research Papers (APA)
- If the paper is a student paper, it contains the title of the project, the author’s name(s), the instructor's name, course number and name, and assignment due date
- If the paper is a professional paper, it includes the title of the paper, the author’s name(s), the institutional affiliation, and the author note
- Begins on the first page of the paper
- The title is typed in upper and lowercase letters, four spaces below the top of the paper, and written in boldface
- Other information is separated by a space from the title
Title (found on title page)
- Informs the audience about the purpose of the paper
- Captures the attention of the audience
- Accurately reflects the purpose and content of the research paper
Abstract
- Labeled as “ Abstract ”
- Begins on the second page
- Provides a short, concise summary of the content of the research paper
- Includes background information necessary to understand the topic
- Background information demonstrates the purpose of the paper
- Contains the hypothesis and/or research questions addressed in the paper
- Has a brief description of the methods used
- Details the key findings and significance of the results
- Illustrates the implications of the research study
- Contains less than 250 words
Introduction
- Starts on the third page
- Includes the title of the paper in bold at the top of the page
- Contains a clear statement of the problem that the paper sets out to address
- Places the research paper within the context of previous research on the topic
- Explains the purpose of the research study and what you hope to find
- Describes the significance of the study
- Details what new insights the research will contribute
- Concludes with a brief description of what information will be mentioned in the literature review
Literature Review
- Labeled as “ Literature Review”
- Presents a general description of the problem area
- Defines any necessary terms
- Discusses and summarizes prior research on the selected topic
- Identifies any unresolved issues or gaps in research that the current research plans to address
- Concludes with a summary of the current research study, including the independent and dependent variables, the research questions or hypotheses, and the objective of the research
- Labeled as “ Methods ”
- Efficiently explains how the research study was conducted
- Appropriately divided into sections
- Describes the characteristics of the participants
- Explains how the participants were selected
- Details how the anonymity of the participants was protected
- Notes what feedback the participants will be provided
- Describes all materials and instruments that were used
- Mentions how the procedure was conducted and data collected
- Notes the independent and dependent variables
- Includes enough information that another researcher could duplicate the research
Results
- Labeled as “ Results ”
- Describes the data was collected
- Explains the results of statistical tests that were performed
- Omits any analysis or discussion of the implications of the study
Discussion
- Labeled as “ Discussion ”
- Describes the significance of the results
- Relates the results to the research questions and/or hypotheses
- States whether the hypotheses should be rejected or accepted
- Addresses limitations of the study, including potential bias, confounds, imprecision of measures, and limits to generalizability
- Explains how the study adds to the knowledge base and expands upon past research
- Labeled as “ References ”
- Correctly cites sources according to APA formatting
- Orders sources alphabetically
- All sources included in the study are cited in the reference section
Table and Figures (optional)
- Each table and each figure is placed on a separate page
- Tables and figures are included after the reference page
- Tables and figures are correctly labeled
- Each table and figure begins with a bolded, centered header such as “ Table 1 ,” “ Table 2 ,”
Appendix (optional)
- Any forms, surveys, tests, etc. are placed in the Appendix
- All appendix entries are mentioned in the Methods section
- Each appendix begins on a new page
- Each appendix begins with a bolded, centered header such as “ Appendix A, ” “ Appendix B ”
Additional Resources For Experimental Research Papers (APA)
- https://www.mcwritingcenterblog.org/single-post/how-to-conduct-research-using-the-library-s-resources
- https://www.mcwritingcenterblog.org/single-post/how-to-read-academic-articles
- https://researchguides.ben.edu/source-evaluation
- https://researchguides.library.brocku.ca/external-analysis/evaluating-sources
- https://writing.wisc.edu/handbook/assignments/planresearchpaper/
- https://nmu.edu/writingcenter/tips-writing-research-paper
- https://writingcenter.gmu.edu/guides/how-to-write-a-research-question
- https://www.unr.edu/writing-speaking-center/student-resources/writing-speaking-resources/guide-to-writing-research-papers
- https://drive.google.com/drive/folders/1F4DFWf85zEH4aZvm10i8Ahm_3xnAekal?usp=sharing
- https://owl.purdue.edu/owl/research_and_citation/apa_style/apa_formatting_and_style_guide/general_format.html
- https://libguides.elmira.edu/research
- https://www.nhcc.edu/academics/library/doing-library-research/basic-steps-research-process
- https://libguides.wustl.edu/research
- << Previous: Science Papers
- Next: Exegetical Papers >>
- Last Updated: Sep 14, 2023 10:30 AM
- URL: https://mc.libguides.com/writingcenter
Purdue Online Writing Lab Purdue OWL® College of Liberal Arts
APA Sample Paper: Experimental Psychology
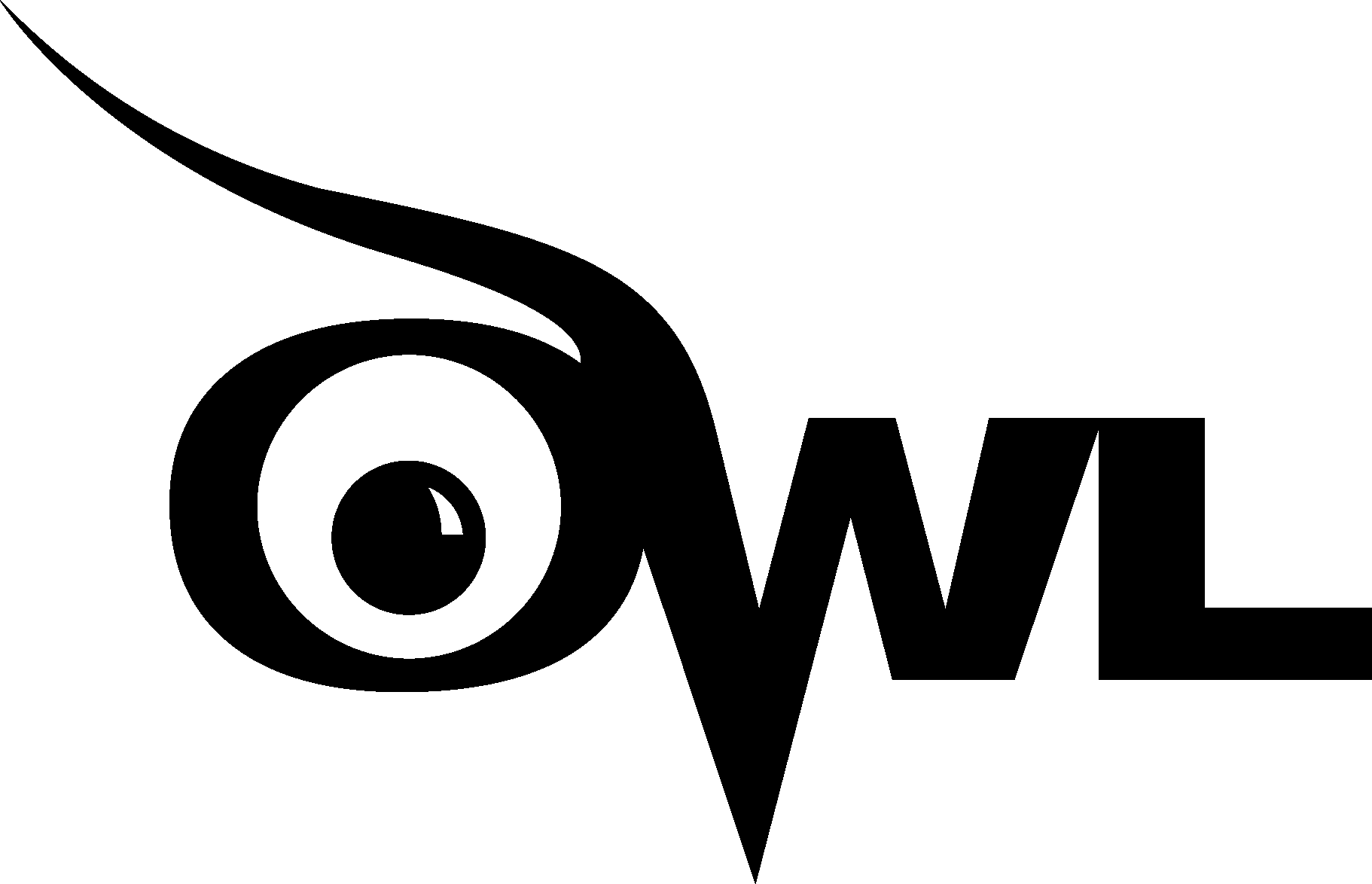
Welcome to the Purdue OWL
This page is brought to you by the OWL at Purdue University. When printing this page, you must include the entire legal notice.
Copyright ©1995-2018 by The Writing Lab & The OWL at Purdue and Purdue University. All rights reserved. This material may not be published, reproduced, broadcast, rewritten, or redistributed without permission. Use of this site constitutes acceptance of our terms and conditions of fair use.
Media File: APA Sample Paper: Experimental Psychology
This resource is enhanced by an Acrobat PDF file. Download the free Acrobat Reader

Experimental Research Design — 6 mistakes you should never make!
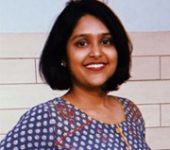
Since school days’ students perform scientific experiments that provide results that define and prove the laws and theorems in science. These experiments are laid on a strong foundation of experimental research designs.
An experimental research design helps researchers execute their research objectives with more clarity and transparency.
In this article, we will not only discuss the key aspects of experimental research designs but also the issues to avoid and problems to resolve while designing your research study.
Table of Contents
What Is Experimental Research Design?
Experimental research design is a framework of protocols and procedures created to conduct experimental research with a scientific approach using two sets of variables. Herein, the first set of variables acts as a constant, used to measure the differences of the second set. The best example of experimental research methods is quantitative research .
Experimental research helps a researcher gather the necessary data for making better research decisions and determining the facts of a research study.
When Can a Researcher Conduct Experimental Research?
A researcher can conduct experimental research in the following situations —
- When time is an important factor in establishing a relationship between the cause and effect.
- When there is an invariable or never-changing behavior between the cause and effect.
- Finally, when the researcher wishes to understand the importance of the cause and effect.
Importance of Experimental Research Design
To publish significant results, choosing a quality research design forms the foundation to build the research study. Moreover, effective research design helps establish quality decision-making procedures, structures the research to lead to easier data analysis, and addresses the main research question. Therefore, it is essential to cater undivided attention and time to create an experimental research design before beginning the practical experiment.
By creating a research design, a researcher is also giving oneself time to organize the research, set up relevant boundaries for the study, and increase the reliability of the results. Through all these efforts, one could also avoid inconclusive results. If any part of the research design is flawed, it will reflect on the quality of the results derived.
Types of Experimental Research Designs
Based on the methods used to collect data in experimental studies, the experimental research designs are of three primary types:
1. Pre-experimental Research Design
A research study could conduct pre-experimental research design when a group or many groups are under observation after implementing factors of cause and effect of the research. The pre-experimental design will help researchers understand whether further investigation is necessary for the groups under observation.
Pre-experimental research is of three types —
- One-shot Case Study Research Design
- One-group Pretest-posttest Research Design
- Static-group Comparison
2. True Experimental Research Design
A true experimental research design relies on statistical analysis to prove or disprove a researcher’s hypothesis. It is one of the most accurate forms of research because it provides specific scientific evidence. Furthermore, out of all the types of experimental designs, only a true experimental design can establish a cause-effect relationship within a group. However, in a true experiment, a researcher must satisfy these three factors —
- There is a control group that is not subjected to changes and an experimental group that will experience the changed variables
- A variable that can be manipulated by the researcher
- Random distribution of the variables
This type of experimental research is commonly observed in the physical sciences.
3. Quasi-experimental Research Design
The word “Quasi” means similarity. A quasi-experimental design is similar to a true experimental design. However, the difference between the two is the assignment of the control group. In this research design, an independent variable is manipulated, but the participants of a group are not randomly assigned. This type of research design is used in field settings where random assignment is either irrelevant or not required.
The classification of the research subjects, conditions, or groups determines the type of research design to be used.
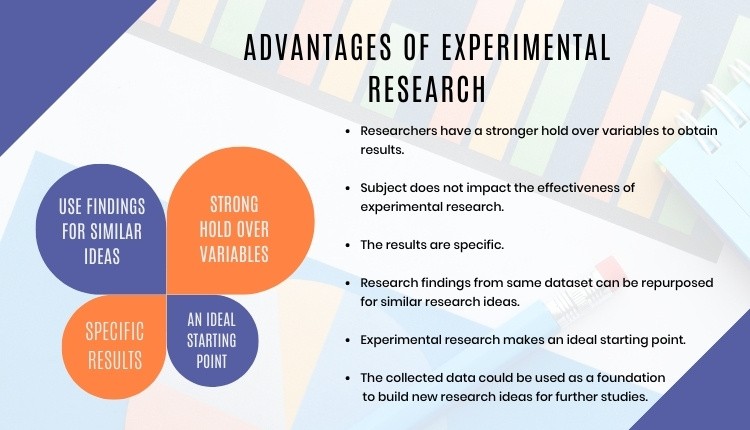
Advantages of Experimental Research
Experimental research allows you to test your idea in a controlled environment before taking the research to clinical trials. Moreover, it provides the best method to test your theory because of the following advantages:
- Researchers have firm control over variables to obtain results.
- The subject does not impact the effectiveness of experimental research. Anyone can implement it for research purposes.
- The results are specific.
- Post results analysis, research findings from the same dataset can be repurposed for similar research ideas.
- Researchers can identify the cause and effect of the hypothesis and further analyze this relationship to determine in-depth ideas.
- Experimental research makes an ideal starting point. The collected data could be used as a foundation to build new research ideas for further studies.
6 Mistakes to Avoid While Designing Your Research
There is no order to this list, and any one of these issues can seriously compromise the quality of your research. You could refer to the list as a checklist of what to avoid while designing your research.
1. Invalid Theoretical Framework
Usually, researchers miss out on checking if their hypothesis is logical to be tested. If your research design does not have basic assumptions or postulates, then it is fundamentally flawed and you need to rework on your research framework.
2. Inadequate Literature Study
Without a comprehensive research literature review , it is difficult to identify and fill the knowledge and information gaps. Furthermore, you need to clearly state how your research will contribute to the research field, either by adding value to the pertinent literature or challenging previous findings and assumptions.
3. Insufficient or Incorrect Statistical Analysis
Statistical results are one of the most trusted scientific evidence. The ultimate goal of a research experiment is to gain valid and sustainable evidence. Therefore, incorrect statistical analysis could affect the quality of any quantitative research.
4. Undefined Research Problem
This is one of the most basic aspects of research design. The research problem statement must be clear and to do that, you must set the framework for the development of research questions that address the core problems.
5. Research Limitations
Every study has some type of limitations . You should anticipate and incorporate those limitations into your conclusion, as well as the basic research design. Include a statement in your manuscript about any perceived limitations, and how you considered them while designing your experiment and drawing the conclusion.
6. Ethical Implications
The most important yet less talked about topic is the ethical issue. Your research design must include ways to minimize any risk for your participants and also address the research problem or question at hand. If you cannot manage the ethical norms along with your research study, your research objectives and validity could be questioned.
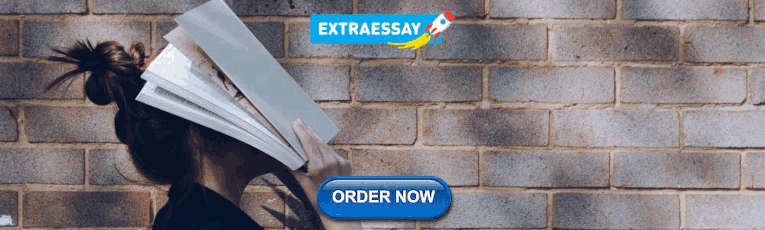
Experimental Research Design Example
In an experimental design, a researcher gathers plant samples and then randomly assigns half the samples to photosynthesize in sunlight and the other half to be kept in a dark box without sunlight, while controlling all the other variables (nutrients, water, soil, etc.)
By comparing their outcomes in biochemical tests, the researcher can confirm that the changes in the plants were due to the sunlight and not the other variables.
Experimental research is often the final form of a study conducted in the research process which is considered to provide conclusive and specific results. But it is not meant for every research. It involves a lot of resources, time, and money and is not easy to conduct, unless a foundation of research is built. Yet it is widely used in research institutes and commercial industries, for its most conclusive results in the scientific approach.
Have you worked on research designs? How was your experience creating an experimental design? What difficulties did you face? Do write to us or comment below and share your insights on experimental research designs!
Frequently Asked Questions
Randomization is important in an experimental research because it ensures unbiased results of the experiment. It also measures the cause-effect relationship on a particular group of interest.
Experimental research design lay the foundation of a research and structures the research to establish quality decision making process.
There are 3 types of experimental research designs. These are pre-experimental research design, true experimental research design, and quasi experimental research design.
The difference between an experimental and a quasi-experimental design are: 1. The assignment of the control group in quasi experimental research is non-random, unlike true experimental design, which is randomly assigned. 2. Experimental research group always has a control group; on the other hand, it may not be always present in quasi experimental research.
Experimental research establishes a cause-effect relationship by testing a theory or hypothesis using experimental groups or control variables. In contrast, descriptive research describes a study or a topic by defining the variables under it and answering the questions related to the same.

good and valuable
Very very good
Good presentation.
Rate this article Cancel Reply
Your email address will not be published.
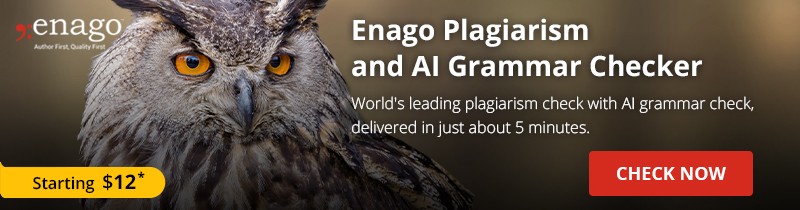
Enago Academy's Most Popular Articles
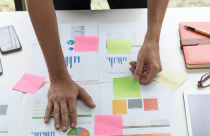
- Publishing Research
- Reporting Research
How to Optimize Your Research Process: A step-by-step guide
For researchers across disciplines, the path to uncovering novel findings and insights is often filled…
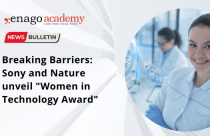
- Industry News
- Trending Now
Breaking Barriers: Sony and Nature unveil “Women in Technology Award”
Sony Group Corporation and the prestigious scientific journal Nature have collaborated to launch the inaugural…
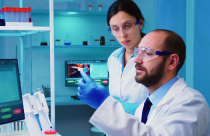
Achieving Research Excellence: Checklist for good research practices
Academia is built on the foundation of trustworthy and high-quality research, supported by the pillars…
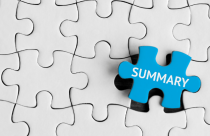
- Promoting Research
Plain Language Summary — Communicating your research to bridge the academic-lay gap
Science can be complex, but does that mean it should not be accessible to the…
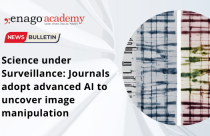
Science under Surveillance: Journals adopt advanced AI to uncover image manipulation
Journals are increasingly turning to cutting-edge AI tools to uncover deceitful images published in manuscripts.…
Choosing the Right Analytical Approach: Thematic analysis vs. content analysis for…
Comparing Cross Sectional and Longitudinal Studies: 5 steps for choosing the right…
Research Recommendations – Guiding policy-makers for evidence-based decision making

Sign-up to read more
Subscribe for free to get unrestricted access to all our resources on research writing and academic publishing including:
- 2000+ blog articles
- 50+ Webinars
- 10+ Expert podcasts
- 50+ Infographics
- 10+ Checklists
- Research Guides
We hate spam too. We promise to protect your privacy and never spam you.
I am looking for Editing/ Proofreading services for my manuscript Tentative date of next journal submission:
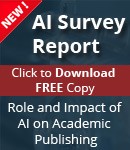
What should universities' stance be on AI tools in research and academic writing?
Have a language expert improve your writing
Run a free plagiarism check in 10 minutes, generate accurate citations for free.
- Knowledge Base
Methodology
- What Is a Research Design | Types, Guide & Examples
What Is a Research Design | Types, Guide & Examples
Published on June 7, 2021 by Shona McCombes . Revised on November 20, 2023 by Pritha Bhandari.
A research design is a strategy for answering your research question using empirical data. Creating a research design means making decisions about:
- Your overall research objectives and approach
- Whether you’ll rely on primary research or secondary research
- Your sampling methods or criteria for selecting subjects
- Your data collection methods
- The procedures you’ll follow to collect data
- Your data analysis methods
A well-planned research design helps ensure that your methods match your research objectives and that you use the right kind of analysis for your data.
Table of contents
Step 1: consider your aims and approach, step 2: choose a type of research design, step 3: identify your population and sampling method, step 4: choose your data collection methods, step 5: plan your data collection procedures, step 6: decide on your data analysis strategies, other interesting articles, frequently asked questions about research design.
- Introduction
Before you can start designing your research, you should already have a clear idea of the research question you want to investigate.
There are many different ways you could go about answering this question. Your research design choices should be driven by your aims and priorities—start by thinking carefully about what you want to achieve.
The first choice you need to make is whether you’ll take a qualitative or quantitative approach.
Qualitative research designs tend to be more flexible and inductive , allowing you to adjust your approach based on what you find throughout the research process.
Quantitative research designs tend to be more fixed and deductive , with variables and hypotheses clearly defined in advance of data collection.
It’s also possible to use a mixed-methods design that integrates aspects of both approaches. By combining qualitative and quantitative insights, you can gain a more complete picture of the problem you’re studying and strengthen the credibility of your conclusions.
Practical and ethical considerations when designing research
As well as scientific considerations, you need to think practically when designing your research. If your research involves people or animals, you also need to consider research ethics .
- How much time do you have to collect data and write up the research?
- Will you be able to gain access to the data you need (e.g., by travelling to a specific location or contacting specific people)?
- Do you have the necessary research skills (e.g., statistical analysis or interview techniques)?
- Will you need ethical approval ?
At each stage of the research design process, make sure that your choices are practically feasible.
Receive feedback on language, structure, and formatting
Professional editors proofread and edit your paper by focusing on:
- Academic style
- Vague sentences
- Style consistency
See an example
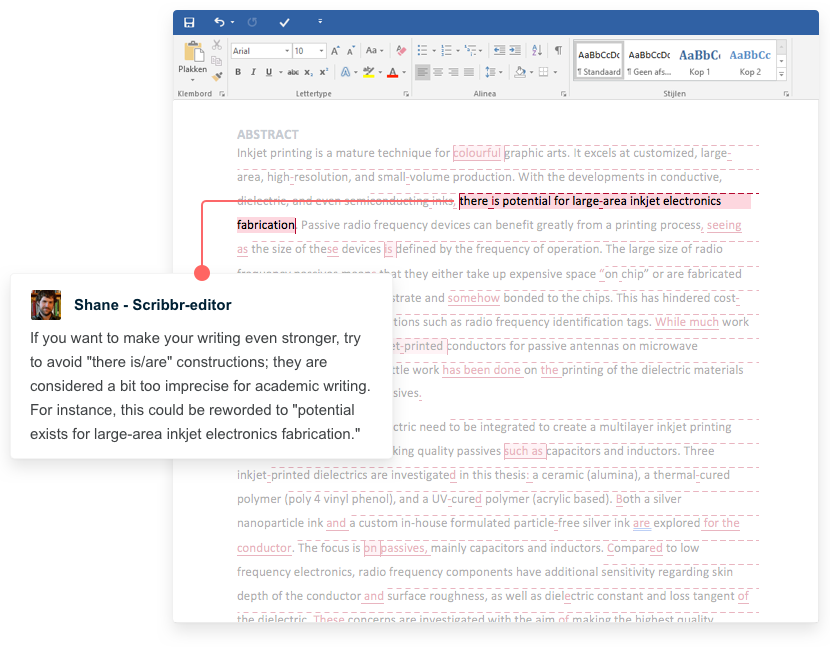
Within both qualitative and quantitative approaches, there are several types of research design to choose from. Each type provides a framework for the overall shape of your research.
Types of quantitative research designs
Quantitative designs can be split into four main types.
- Experimental and quasi-experimental designs allow you to test cause-and-effect relationships
- Descriptive and correlational designs allow you to measure variables and describe relationships between them.
With descriptive and correlational designs, you can get a clear picture of characteristics, trends and relationships as they exist in the real world. However, you can’t draw conclusions about cause and effect (because correlation doesn’t imply causation ).
Experiments are the strongest way to test cause-and-effect relationships without the risk of other variables influencing the results. However, their controlled conditions may not always reflect how things work in the real world. They’re often also more difficult and expensive to implement.
Types of qualitative research designs
Qualitative designs are less strictly defined. This approach is about gaining a rich, detailed understanding of a specific context or phenomenon, and you can often be more creative and flexible in designing your research.
The table below shows some common types of qualitative design. They often have similar approaches in terms of data collection, but focus on different aspects when analyzing the data.
Your research design should clearly define who or what your research will focus on, and how you’ll go about choosing your participants or subjects.
In research, a population is the entire group that you want to draw conclusions about, while a sample is the smaller group of individuals you’ll actually collect data from.
Defining the population
A population can be made up of anything you want to study—plants, animals, organizations, texts, countries, etc. In the social sciences, it most often refers to a group of people.
For example, will you focus on people from a specific demographic, region or background? Are you interested in people with a certain job or medical condition, or users of a particular product?
The more precisely you define your population, the easier it will be to gather a representative sample.
- Sampling methods
Even with a narrowly defined population, it’s rarely possible to collect data from every individual. Instead, you’ll collect data from a sample.
To select a sample, there are two main approaches: probability sampling and non-probability sampling . The sampling method you use affects how confidently you can generalize your results to the population as a whole.
Probability sampling is the most statistically valid option, but it’s often difficult to achieve unless you’re dealing with a very small and accessible population.
For practical reasons, many studies use non-probability sampling, but it’s important to be aware of the limitations and carefully consider potential biases. You should always make an effort to gather a sample that’s as representative as possible of the population.
Case selection in qualitative research
In some types of qualitative designs, sampling may not be relevant.
For example, in an ethnography or a case study , your aim is to deeply understand a specific context, not to generalize to a population. Instead of sampling, you may simply aim to collect as much data as possible about the context you are studying.
In these types of design, you still have to carefully consider your choice of case or community. You should have a clear rationale for why this particular case is suitable for answering your research question .
For example, you might choose a case study that reveals an unusual or neglected aspect of your research problem, or you might choose several very similar or very different cases in order to compare them.
Data collection methods are ways of directly measuring variables and gathering information. They allow you to gain first-hand knowledge and original insights into your research problem.
You can choose just one data collection method, or use several methods in the same study.
Survey methods
Surveys allow you to collect data about opinions, behaviors, experiences, and characteristics by asking people directly. There are two main survey methods to choose from: questionnaires and interviews .
Observation methods
Observational studies allow you to collect data unobtrusively, observing characteristics, behaviors or social interactions without relying on self-reporting.
Observations may be conducted in real time, taking notes as you observe, or you might make audiovisual recordings for later analysis. They can be qualitative or quantitative.
Other methods of data collection
There are many other ways you might collect data depending on your field and topic.
If you’re not sure which methods will work best for your research design, try reading some papers in your field to see what kinds of data collection methods they used.
Secondary data
If you don’t have the time or resources to collect data from the population you’re interested in, you can also choose to use secondary data that other researchers already collected—for example, datasets from government surveys or previous studies on your topic.
With this raw data, you can do your own analysis to answer new research questions that weren’t addressed by the original study.
Using secondary data can expand the scope of your research, as you may be able to access much larger and more varied samples than you could collect yourself.
However, it also means you don’t have any control over which variables to measure or how to measure them, so the conclusions you can draw may be limited.
Here's why students love Scribbr's proofreading services
Discover proofreading & editing
As well as deciding on your methods, you need to plan exactly how you’ll use these methods to collect data that’s consistent, accurate, and unbiased.
Planning systematic procedures is especially important in quantitative research, where you need to precisely define your variables and ensure your measurements are high in reliability and validity.
Operationalization
Some variables, like height or age, are easily measured. But often you’ll be dealing with more abstract concepts, like satisfaction, anxiety, or competence. Operationalization means turning these fuzzy ideas into measurable indicators.
If you’re using observations , which events or actions will you count?
If you’re using surveys , which questions will you ask and what range of responses will be offered?
You may also choose to use or adapt existing materials designed to measure the concept you’re interested in—for example, questionnaires or inventories whose reliability and validity has already been established.
Reliability and validity
Reliability means your results can be consistently reproduced, while validity means that you’re actually measuring the concept you’re interested in.
For valid and reliable results, your measurement materials should be thoroughly researched and carefully designed. Plan your procedures to make sure you carry out the same steps in the same way for each participant.
If you’re developing a new questionnaire or other instrument to measure a specific concept, running a pilot study allows you to check its validity and reliability in advance.
Sampling procedures
As well as choosing an appropriate sampling method , you need a concrete plan for how you’ll actually contact and recruit your selected sample.
That means making decisions about things like:
- How many participants do you need for an adequate sample size?
- What inclusion and exclusion criteria will you use to identify eligible participants?
- How will you contact your sample—by mail, online, by phone, or in person?
If you’re using a probability sampling method , it’s important that everyone who is randomly selected actually participates in the study. How will you ensure a high response rate?
If you’re using a non-probability method , how will you avoid research bias and ensure a representative sample?
Data management
It’s also important to create a data management plan for organizing and storing your data.
Will you need to transcribe interviews or perform data entry for observations? You should anonymize and safeguard any sensitive data, and make sure it’s backed up regularly.
Keeping your data well-organized will save time when it comes to analyzing it. It can also help other researchers validate and add to your findings (high replicability ).
On its own, raw data can’t answer your research question. The last step of designing your research is planning how you’ll analyze the data.
Quantitative data analysis
In quantitative research, you’ll most likely use some form of statistical analysis . With statistics, you can summarize your sample data, make estimates, and test hypotheses.
Using descriptive statistics , you can summarize your sample data in terms of:
- The distribution of the data (e.g., the frequency of each score on a test)
- The central tendency of the data (e.g., the mean to describe the average score)
- The variability of the data (e.g., the standard deviation to describe how spread out the scores are)
The specific calculations you can do depend on the level of measurement of your variables.
Using inferential statistics , you can:
- Make estimates about the population based on your sample data.
- Test hypotheses about a relationship between variables.
Regression and correlation tests look for associations between two or more variables, while comparison tests (such as t tests and ANOVAs ) look for differences in the outcomes of different groups.
Your choice of statistical test depends on various aspects of your research design, including the types of variables you’re dealing with and the distribution of your data.
Qualitative data analysis
In qualitative research, your data will usually be very dense with information and ideas. Instead of summing it up in numbers, you’ll need to comb through the data in detail, interpret its meanings, identify patterns, and extract the parts that are most relevant to your research question.
Two of the most common approaches to doing this are thematic analysis and discourse analysis .
There are many other ways of analyzing qualitative data depending on the aims of your research. To get a sense of potential approaches, try reading some qualitative research papers in your field.
If you want to know more about the research process , methodology , research bias , or statistics , make sure to check out some of our other articles with explanations and examples.
- Simple random sampling
- Stratified sampling
- Cluster sampling
- Likert scales
- Reproducibility
Statistics
- Null hypothesis
- Statistical power
- Probability distribution
- Effect size
- Poisson distribution
Research bias
- Optimism bias
- Cognitive bias
- Implicit bias
- Hawthorne effect
- Anchoring bias
- Explicit bias
A research design is a strategy for answering your research question . It defines your overall approach and determines how you will collect and analyze data.
A well-planned research design helps ensure that your methods match your research aims, that you collect high-quality data, and that you use the right kind of analysis to answer your questions, utilizing credible sources . This allows you to draw valid , trustworthy conclusions.
Quantitative research designs can be divided into two main categories:
- Correlational and descriptive designs are used to investigate characteristics, averages, trends, and associations between variables.
- Experimental and quasi-experimental designs are used to test causal relationships .
Qualitative research designs tend to be more flexible. Common types of qualitative design include case study , ethnography , and grounded theory designs.
The priorities of a research design can vary depending on the field, but you usually have to specify:
- Your research questions and/or hypotheses
- Your overall approach (e.g., qualitative or quantitative )
- The type of design you’re using (e.g., a survey , experiment , or case study )
- Your data collection methods (e.g., questionnaires , observations)
- Your data collection procedures (e.g., operationalization , timing and data management)
- Your data analysis methods (e.g., statistical tests or thematic analysis )
A sample is a subset of individuals from a larger population . Sampling means selecting the group that you will actually collect data from in your research. For example, if you are researching the opinions of students in your university, you could survey a sample of 100 students.
In statistics, sampling allows you to test a hypothesis about the characteristics of a population.
Operationalization means turning abstract conceptual ideas into measurable observations.
For example, the concept of social anxiety isn’t directly observable, but it can be operationally defined in terms of self-rating scores, behavioral avoidance of crowded places, or physical anxiety symptoms in social situations.
Before collecting data , it’s important to consider how you will operationalize the variables that you want to measure.
A research project is an academic, scientific, or professional undertaking to answer a research question . Research projects can take many forms, such as qualitative or quantitative , descriptive , longitudinal , experimental , or correlational . What kind of research approach you choose will depend on your topic.
Cite this Scribbr article
If you want to cite this source, you can copy and paste the citation or click the “Cite this Scribbr article” button to automatically add the citation to our free Citation Generator.
McCombes, S. (2023, November 20). What Is a Research Design | Types, Guide & Examples. Scribbr. Retrieved April 5, 2024, from https://www.scribbr.com/methodology/research-design/
Is this article helpful?
Shona McCombes
Other students also liked, guide to experimental design | overview, steps, & examples, how to write a research proposal | examples & templates, ethical considerations in research | types & examples, "i thought ai proofreading was useless but..".
I've been using Scribbr for years now and I know it's a service that won't disappoint. It does a good job spotting mistakes”
Help | Advanced Search
Computer Science > Computation and Language
Title: realm: reference resolution as language modeling.
Abstract: Reference resolution is an important problem, one that is essential to understand and successfully handle context of different kinds. This context includes both previous turns and context that pertains to non-conversational entities, such as entities on the user's screen or those running in the background. While LLMs have been shown to be extremely powerful for a variety of tasks, their use in reference resolution, particularly for non-conversational entities, remains underutilized. This paper demonstrates how LLMs can be used to create an extremely effective system to resolve references of various types, by showing how reference resolution can be converted into a language modeling problem, despite involving forms of entities like those on screen that are not traditionally conducive to being reduced to a text-only modality. We demonstrate large improvements over an existing system with similar functionality across different types of references, with our smallest model obtaining absolute gains of over 5% for on-screen references. We also benchmark against GPT-3.5 and GPT-4, with our smallest model achieving performance comparable to that of GPT-4, and our larger models substantially outperforming it.
Submission history
Access paper:.
- HTML (experimental)
- Other Formats
References & Citations
- Google Scholar
- Semantic Scholar
BibTeX formatted citation

Bibliographic and Citation Tools
Code, data and media associated with this article, recommenders and search tools.
- Institution
arXivLabs: experimental projects with community collaborators
arXivLabs is a framework that allows collaborators to develop and share new arXiv features directly on our website.
Both individuals and organizations that work with arXivLabs have embraced and accepted our values of openness, community, excellence, and user data privacy. arXiv is committed to these values and only works with partners that adhere to them.
Have an idea for a project that will add value for arXiv's community? Learn more about arXivLabs .
Thank you for visiting nature.com. You are using a browser version with limited support for CSS. To obtain the best experience, we recommend you use a more up to date browser (or turn off compatibility mode in Internet Explorer). In the meantime, to ensure continued support, we are displaying the site without styles and JavaScript.
- View all journals
- My Account Login
- Explore content
- About the journal
- Publish with us
- Sign up for alerts
- Open access
- Published: 26 March 2024
Predicting and improving complex beer flavor through machine learning
- Michiel Schreurs ORCID: orcid.org/0000-0002-9449-5619 1 , 2 , 3 na1 ,
- Supinya Piampongsant 1 , 2 , 3 na1 ,
- Miguel Roncoroni ORCID: orcid.org/0000-0001-7461-1427 1 , 2 , 3 na1 ,
- Lloyd Cool ORCID: orcid.org/0000-0001-9936-3124 1 , 2 , 3 , 4 ,
- Beatriz Herrera-Malaver ORCID: orcid.org/0000-0002-5096-9974 1 , 2 , 3 ,
- Christophe Vanderaa ORCID: orcid.org/0000-0001-7443-5427 4 ,
- Florian A. Theßeling 1 , 2 , 3 ,
- Łukasz Kreft ORCID: orcid.org/0000-0001-7620-4657 5 ,
- Alexander Botzki ORCID: orcid.org/0000-0001-6691-4233 5 ,
- Philippe Malcorps 6 ,
- Luk Daenen 6 ,
- Tom Wenseleers ORCID: orcid.org/0000-0002-1434-861X 4 &
- Kevin J. Verstrepen ORCID: orcid.org/0000-0002-3077-6219 1 , 2 , 3
Nature Communications volume 15 , Article number: 2368 ( 2024 ) Cite this article
49k Accesses
847 Altmetric
Metrics details
- Chemical engineering
- Gas chromatography
- Machine learning
- Metabolomics
- Taste receptors
The perception and appreciation of food flavor depends on many interacting chemical compounds and external factors, and therefore proves challenging to understand and predict. Here, we combine extensive chemical and sensory analyses of 250 different beers to train machine learning models that allow predicting flavor and consumer appreciation. For each beer, we measure over 200 chemical properties, perform quantitative descriptive sensory analysis with a trained tasting panel and map data from over 180,000 consumer reviews to train 10 different machine learning models. The best-performing algorithm, Gradient Boosting, yields models that significantly outperform predictions based on conventional statistics and accurately predict complex food features and consumer appreciation from chemical profiles. Model dissection allows identifying specific and unexpected compounds as drivers of beer flavor and appreciation. Adding these compounds results in variants of commercial alcoholic and non-alcoholic beers with improved consumer appreciation. Together, our study reveals how big data and machine learning uncover complex links between food chemistry, flavor and consumer perception, and lays the foundation to develop novel, tailored foods with superior flavors.
Similar content being viewed by others
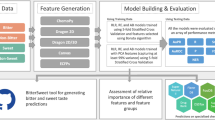
BitterSweet: Building machine learning models for predicting the bitter and sweet taste of small molecules
Rudraksh Tuwani, Somin Wadhwa & Ganesh Bagler
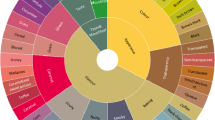
Sensory lexicon and aroma volatiles analysis of brewing malt
Xiaoxia Su, Miao Yu, … Tianyi Du
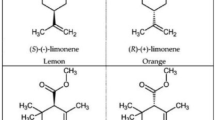
Predicting odor from molecular structure: a multi-label classification approach
Kushagra Saini & Venkatnarayan Ramanathan
Introduction
Predicting and understanding food perception and appreciation is one of the major challenges in food science. Accurate modeling of food flavor and appreciation could yield important opportunities for both producers and consumers, including quality control, product fingerprinting, counterfeit detection, spoilage detection, and the development of new products and product combinations (food pairing) 1 , 2 , 3 , 4 , 5 , 6 . Accurate models for flavor and consumer appreciation would contribute greatly to our scientific understanding of how humans perceive and appreciate flavor. Moreover, accurate predictive models would also facilitate and standardize existing food assessment methods and could supplement or replace assessments by trained and consumer tasting panels, which are variable, expensive and time-consuming 7 , 8 , 9 . Lastly, apart from providing objective, quantitative, accurate and contextual information that can help producers, models can also guide consumers in understanding their personal preferences 10 .
Despite the myriad of applications, predicting food flavor and appreciation from its chemical properties remains a largely elusive goal in sensory science, especially for complex food and beverages 11 , 12 . A key obstacle is the immense number of flavor-active chemicals underlying food flavor. Flavor compounds can vary widely in chemical structure and concentration, making them technically challenging and labor-intensive to quantify, even in the face of innovations in metabolomics, such as non-targeted metabolic fingerprinting 13 , 14 . Moreover, sensory analysis is perhaps even more complicated. Flavor perception is highly complex, resulting from hundreds of different molecules interacting at the physiochemical and sensorial level. Sensory perception is often non-linear, characterized by complex and concentration-dependent synergistic and antagonistic effects 15 , 16 , 17 , 18 , 19 , 20 , 21 that are further convoluted by the genetics, environment, culture and psychology of consumers 22 , 23 , 24 . Perceived flavor is therefore difficult to measure, with problems of sensitivity, accuracy, and reproducibility that can only be resolved by gathering sufficiently large datasets 25 . Trained tasting panels are considered the prime source of quality sensory data, but require meticulous training, are low throughput and high cost. Public databases containing consumer reviews of food products could provide a valuable alternative, especially for studying appreciation scores, which do not require formal training 25 . Public databases offer the advantage of amassing large amounts of data, increasing the statistical power to identify potential drivers of appreciation. However, public datasets suffer from biases, including a bias in the volunteers that contribute to the database, as well as confounding factors such as price, cult status and psychological conformity towards previous ratings of the product.
Classical multivariate statistics and machine learning methods have been used to predict flavor of specific compounds by, for example, linking structural properties of a compound to its potential biological activities or linking concentrations of specific compounds to sensory profiles 1 , 26 . Importantly, most previous studies focused on predicting organoleptic properties of single compounds (often based on their chemical structure) 27 , 28 , 29 , 30 , 31 , 32 , 33 , thus ignoring the fact that these compounds are present in a complex matrix in food or beverages and excluding complex interactions between compounds. Moreover, the classical statistics commonly used in sensory science 34 , 35 , 36 , 37 , 38 , 39 require a large sample size and sufficient variance amongst predictors to create accurate models. They are not fit for studying an extensive set of hundreds of interacting flavor compounds, since they are sensitive to outliers, have a high tendency to overfit and are less suited for non-linear and discontinuous relationships 40 .
In this study, we combine extensive chemical analyses and sensory data of a set of different commercial beers with machine learning approaches to develop models that predict taste, smell, mouthfeel and appreciation from compound concentrations. Beer is particularly suited to model the relationship between chemistry, flavor and appreciation. First, beer is a complex product, consisting of thousands of flavor compounds that partake in complex sensory interactions 41 , 42 , 43 . This chemical diversity arises from the raw materials (malt, yeast, hops, water and spices) and biochemical conversions during the brewing process (kilning, mashing, boiling, fermentation, maturation and aging) 44 , 45 . Second, the advent of the internet saw beer consumers embrace online review platforms, such as RateBeer (ZX Ventures, Anheuser-Busch InBev SA/NV) and BeerAdvocate (Next Glass, inc.). In this way, the beer community provides massive data sets of beer flavor and appreciation scores, creating extraordinarily large sensory databases to complement the analyses of our professional sensory panel. Specifically, we characterize over 200 chemical properties of 250 commercial beers, spread across 22 beer styles, and link these to the descriptive sensory profiling data of a 16-person in-house trained tasting panel and data acquired from over 180,000 public consumer reviews. These unique and extensive datasets enable us to train a suite of machine learning models to predict flavor and appreciation from a beer’s chemical profile. Dissection of the best-performing models allows us to pinpoint specific compounds as potential drivers of beer flavor and appreciation. Follow-up experiments confirm the importance of these compounds and ultimately allow us to significantly improve the flavor and appreciation of selected commercial beers. Together, our study represents a significant step towards understanding complex flavors and reinforces the value of machine learning to develop and refine complex foods. In this way, it represents a stepping stone for further computer-aided food engineering applications 46 .
To generate a comprehensive dataset on beer flavor, we selected 250 commercial Belgian beers across 22 different beer styles (Supplementary Fig. S1 ). Beers with ≤ 4.2% alcohol by volume (ABV) were classified as non-alcoholic and low-alcoholic. Blonds and Tripels constitute a significant portion of the dataset (12.4% and 11.2%, respectively) reflecting their presence on the Belgian beer market and the heterogeneity of beers within these styles. By contrast, lager beers are less diverse and dominated by a handful of brands. Rare styles such as Brut or Faro make up only a small fraction of the dataset (2% and 1%, respectively) because fewer of these beers are produced and because they are dominated by distinct characteristics in terms of flavor and chemical composition.
Extensive analysis identifies relationships between chemical compounds in beer
For each beer, we measured 226 different chemical properties, including common brewing parameters such as alcohol content, iso-alpha acids, pH, sugar concentration 47 , and over 200 flavor compounds (Methods, Supplementary Table S1 ). A large portion (37.2%) are terpenoids arising from hopping, responsible for herbal and fruity flavors 16 , 48 . A second major category are yeast metabolites, such as esters and alcohols, that result in fruity and solvent notes 48 , 49 , 50 . Other measured compounds are primarily derived from malt, or other microbes such as non- Saccharomyces yeasts and bacteria (‘wild flora’). Compounds that arise from spices or staling are labeled under ‘Others’. Five attributes (caloric value, total acids and total ester, hop aroma and sulfur compounds) are calculated from multiple individually measured compounds.
As a first step in identifying relationships between chemical properties, we determined correlations between the concentrations of the compounds (Fig. 1 , upper panel, Supplementary Data 1 and 2 , and Supplementary Fig. S2 . For the sake of clarity, only a subset of the measured compounds is shown in Fig. 1 ). Compounds of the same origin typically show a positive correlation, while absence of correlation hints at parameters varying independently. For example, the hop aroma compounds citronellol, and alpha-terpineol show moderate correlations with each other (Spearman’s rho=0.39 and 0.57), but not with the bittering hop component iso-alpha acids (Spearman’s rho=0.16 and −0.07). This illustrates how brewers can independently modify hop aroma and bitterness by selecting hop varieties and dosage time. If hops are added early in the boiling phase, chemical conversions increase bitterness while aromas evaporate, conversely, late addition of hops preserves aroma but limits bitterness 51 . Similarly, hop-derived iso-alpha acids show a strong anti-correlation with lactic acid and acetic acid, likely reflecting growth inhibition of lactic acid and acetic acid bacteria, or the consequent use of fewer hops in sour beer styles, such as West Flanders ales and Fruit beers, that rely on these bacteria for their distinct flavors 52 . Finally, yeast-derived esters (ethyl acetate, ethyl decanoate, ethyl hexanoate, ethyl octanoate) and alcohols (ethanol, isoamyl alcohol, isobutanol, and glycerol), correlate with Spearman coefficients above 0.5, suggesting that these secondary metabolites are correlated with the yeast genetic background and/or fermentation parameters and may be difficult to influence individually, although the choice of yeast strain may offer some control 53 .
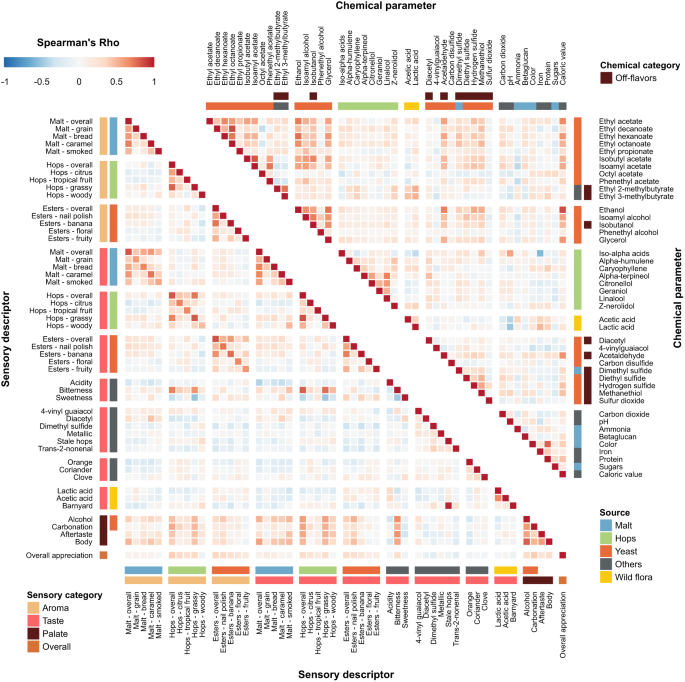
Spearman rank correlations are shown. Descriptors are grouped according to their origin (malt (blue), hops (green), yeast (red), wild flora (yellow), Others (black)), and sensory aspect (aroma, taste, palate, and overall appreciation). Please note that for the chemical compounds, for the sake of clarity, only a subset of the total number of measured compounds is shown, with an emphasis on the key compounds for each source. For more details, see the main text and Methods section. Chemical data can be found in Supplementary Data 1 , correlations between all chemical compounds are depicted in Supplementary Fig. S2 and correlation values can be found in Supplementary Data 2 . See Supplementary Data 4 for sensory panel assessments and Supplementary Data 5 for correlation values between all sensory descriptors.
Interestingly, different beer styles show distinct patterns for some flavor compounds (Supplementary Fig. S3 ). These observations agree with expectations for key beer styles, and serve as a control for our measurements. For instance, Stouts generally show high values for color (darker), while hoppy beers contain elevated levels of iso-alpha acids, compounds associated with bitter hop taste. Acetic and lactic acid are not prevalent in most beers, with notable exceptions such as Kriek, Lambic, Faro, West Flanders ales and Flanders Old Brown, which use acid-producing bacteria ( Lactobacillus and Pediococcus ) or unconventional yeast ( Brettanomyces ) 54 , 55 . Glycerol, ethanol and esters show similar distributions across all beer styles, reflecting their common origin as products of yeast metabolism during fermentation 45 , 53 . Finally, low/no-alcohol beers contain low concentrations of glycerol and esters. This is in line with the production process for most of the low/no-alcohol beers in our dataset, which are produced through limiting fermentation or by stripping away alcohol via evaporation or dialysis, with both methods having the unintended side-effect of reducing the amount of flavor compounds in the final beer 56 , 57 .
Besides expected associations, our data also reveals less trivial associations between beer styles and specific parameters. For example, geraniol and citronellol, two monoterpenoids responsible for citrus, floral and rose flavors and characteristic of Citra hops, are found in relatively high amounts in Christmas, Saison, and Brett/co-fermented beers, where they may originate from terpenoid-rich spices such as coriander seeds instead of hops 58 .
Tasting panel assessments reveal sensorial relationships in beer
To assess the sensory profile of each beer, a trained tasting panel evaluated each of the 250 beers for 50 sensory attributes, including different hop, malt and yeast flavors, off-flavors and spices. Panelists used a tasting sheet (Supplementary Data 3 ) to score the different attributes. Panel consistency was evaluated by repeating 12 samples across different sessions and performing ANOVA. In 95% of cases no significant difference was found across sessions ( p > 0.05), indicating good panel consistency (Supplementary Table S2 ).
Aroma and taste perception reported by the trained panel are often linked (Fig. 1 , bottom left panel and Supplementary Data 4 and 5 ), with high correlations between hops aroma and taste (Spearman’s rho=0.83). Bitter taste was found to correlate with hop aroma and taste in general (Spearman’s rho=0.80 and 0.69), and particularly with “grassy” noble hops (Spearman’s rho=0.75). Barnyard flavor, most often associated with sour beers, is identified together with stale hops (Spearman’s rho=0.97) that are used in these beers. Lactic and acetic acid, which often co-occur, are correlated (Spearman’s rho=0.66). Interestingly, sweetness and bitterness are anti-correlated (Spearman’s rho = −0.48), confirming the hypothesis that they mask each other 59 , 60 . Beer body is highly correlated with alcohol (Spearman’s rho = 0.79), and overall appreciation is found to correlate with multiple aspects that describe beer mouthfeel (alcohol, carbonation; Spearman’s rho= 0.32, 0.39), as well as with hop and ester aroma intensity (Spearman’s rho=0.39 and 0.35).
Similar to the chemical analyses, sensorial analyses confirmed typical features of specific beer styles (Supplementary Fig. S4 ). For example, sour beers (Faro, Flanders Old Brown, Fruit beer, Kriek, Lambic, West Flanders ale) were rated acidic, with flavors of both acetic and lactic acid. Hoppy beers were found to be bitter and showed hop-associated aromas like citrus and tropical fruit. Malt taste is most detected among scotch, stout/porters, and strong ales, while low/no-alcohol beers, which often have a reputation for being ‘worty’ (reminiscent of unfermented, sweet malt extract) appear in the middle. Unsurprisingly, hop aromas are most strongly detected among hoppy beers. Like its chemical counterpart (Supplementary Fig. S3 ), acidity shows a right-skewed distribution, with the most acidic beers being Krieks, Lambics, and West Flanders ales.
Tasting panel assessments of specific flavors correlate with chemical composition
We find that the concentrations of several chemical compounds strongly correlate with specific aroma or taste, as evaluated by the tasting panel (Fig. 2 , Supplementary Fig. S5 , Supplementary Data 6 ). In some cases, these correlations confirm expectations and serve as a useful control for data quality. For example, iso-alpha acids, the bittering compounds in hops, strongly correlate with bitterness (Spearman’s rho=0.68), while ethanol and glycerol correlate with tasters’ perceptions of alcohol and body, the mouthfeel sensation of fullness (Spearman’s rho=0.82/0.62 and 0.72/0.57 respectively) and darker color from roasted malts is a good indication of malt perception (Spearman’s rho=0.54).
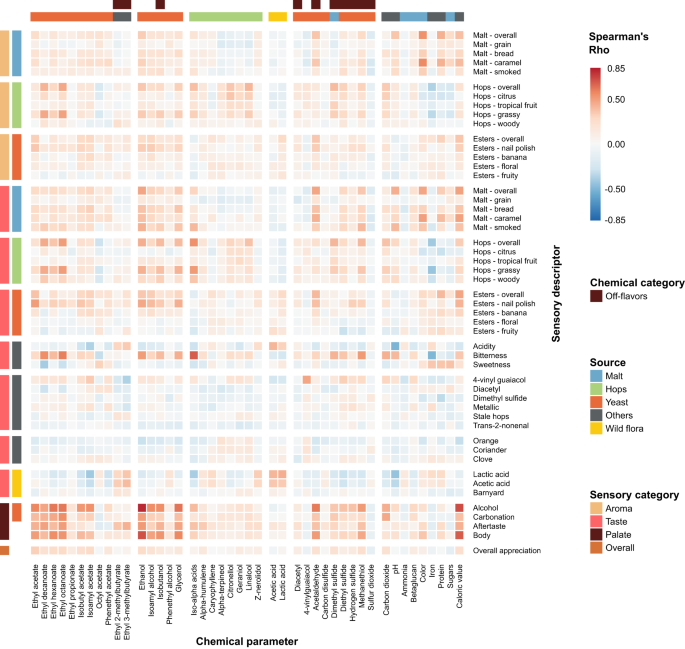
Heatmap colors indicate Spearman’s Rho. Axes are organized according to sensory categories (aroma, taste, mouthfeel, overall), chemical categories and chemical sources in beer (malt (blue), hops (green), yeast (red), wild flora (yellow), Others (black)). See Supplementary Data 6 for all correlation values.
Interestingly, for some relationships between chemical compounds and perceived flavor, correlations are weaker than expected. For example, the rose-smelling phenethyl acetate only weakly correlates with floral aroma. This hints at more complex relationships and interactions between compounds and suggests a need for a more complex model than simple correlations. Lastly, we uncovered unexpected correlations. For instance, the esters ethyl decanoate and ethyl octanoate appear to correlate slightly with hop perception and bitterness, possibly due to their fruity flavor. Iron is anti-correlated with hop aromas and bitterness, most likely because it is also anti-correlated with iso-alpha acids. This could be a sign of metal chelation of hop acids 61 , given that our analyses measure unbound hop acids and total iron content, or could result from the higher iron content in dark and Fruit beers, which typically have less hoppy and bitter flavors 62 .
Public consumer reviews complement expert panel data
To complement and expand the sensory data of our trained tasting panel, we collected 180,000 reviews of our 250 beers from the online consumer review platform RateBeer. This provided numerical scores for beer appearance, aroma, taste, palate, overall quality as well as the average overall score.
Public datasets are known to suffer from biases, such as price, cult status and psychological conformity towards previous ratings of a product. For example, prices correlate with appreciation scores for these online consumer reviews (rho=0.49, Supplementary Fig. S6 ), but not for our trained tasting panel (rho=0.19). This suggests that prices affect consumer appreciation, which has been reported in wine 63 , while blind tastings are unaffected. Moreover, we observe that some beer styles, like lagers and non-alcoholic beers, generally receive lower scores, reflecting that online reviewers are mostly beer aficionados with a preference for specialty beers over lager beers. In general, we find a modest correlation between our trained panel’s overall appreciation score and the online consumer appreciation scores (Fig. 3 , rho=0.29). Apart from the aforementioned biases in the online datasets, serving temperature, sample freshness and surroundings, which are all tightly controlled during the tasting panel sessions, can vary tremendously across online consumers and can further contribute to (among others, appreciation) differences between the two categories of tasters. Importantly, in contrast to the overall appreciation scores, for many sensory aspects the results from the professional panel correlated well with results obtained from RateBeer reviews. Correlations were highest for features that are relatively easy to recognize even for untrained tasters, like bitterness, sweetness, alcohol and malt aroma (Fig. 3 and below).
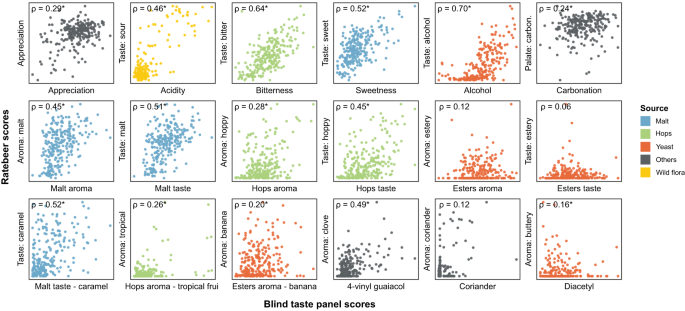
RateBeer text mining results can be found in Supplementary Data 7 . Rho values shown are Spearman correlation values, with asterisks indicating significant correlations ( p < 0.05, two-sided). All p values were smaller than 0.001, except for Esters aroma (0.0553), Esters taste (0.3275), Esters aroma—banana (0.0019), Coriander (0.0508) and Diacetyl (0.0134).
Besides collecting consumer appreciation from these online reviews, we developed automated text analysis tools to gather additional data from review texts (Supplementary Data 7 ). Processing review texts on the RateBeer database yielded comparable results to the scores given by the trained panel for many common sensory aspects, including acidity, bitterness, sweetness, alcohol, malt, and hop tastes (Fig. 3 ). This is in line with what would be expected, since these attributes require less training for accurate assessment and are less influenced by environmental factors such as temperature, serving glass and odors in the environment. Consumer reviews also correlate well with our trained panel for 4-vinyl guaiacol, a compound associated with a very characteristic aroma. By contrast, correlations for more specific aromas like ester, coriander or diacetyl are underrepresented in the online reviews, underscoring the importance of using a trained tasting panel and standardized tasting sheets with explicit factors to be scored for evaluating specific aspects of a beer. Taken together, our results suggest that public reviews are trustworthy for some, but not all, flavor features and can complement or substitute taste panel data for these sensory aspects.
Models can predict beer sensory profiles from chemical data
The rich datasets of chemical analyses, tasting panel assessments and public reviews gathered in the first part of this study provided us with a unique opportunity to develop predictive models that link chemical data to sensorial features. Given the complexity of beer flavor, basic statistical tools such as correlations or linear regression may not always be the most suitable for making accurate predictions. Instead, we applied different machine learning models that can model both simple linear and complex interactive relationships. Specifically, we constructed a set of regression models to predict (a) trained panel scores for beer flavor and quality and (b) public reviews’ appreciation scores from beer chemical profiles. We trained and tested 10 different models (Methods), 3 linear regression-based models (simple linear regression with first-order interactions (LR), lasso regression with first-order interactions (Lasso), partial least squares regressor (PLSR)), 5 decision tree models (AdaBoost regressor (ABR), extra trees (ET), gradient boosting regressor (GBR), random forest (RF) and XGBoost regressor (XGBR)), 1 support vector regression (SVR), and 1 artificial neural network (ANN) model.
To compare the performance of our machine learning models, the dataset was randomly split into a training and test set, stratified by beer style. After a model was trained on data in the training set, its performance was evaluated on its ability to predict the test dataset obtained from multi-output models (based on the coefficient of determination, see Methods). Additionally, individual-attribute models were ranked per descriptor and the average rank was calculated, as proposed by Korneva et al. 64 . Importantly, both ways of evaluating the models’ performance agreed in general. Performance of the different models varied (Table 1 ). It should be noted that all models perform better at predicting RateBeer results than results from our trained tasting panel. One reason could be that sensory data is inherently variable, and this variability is averaged out with the large number of public reviews from RateBeer. Additionally, all tree-based models perform better at predicting taste than aroma. Linear models (LR) performed particularly poorly, with negative R 2 values, due to severe overfitting (training set R 2 = 1). Overfitting is a common issue in linear models with many parameters and limited samples, especially with interaction terms further amplifying the number of parameters. L1 regularization (Lasso) successfully overcomes this overfitting, out-competing multiple tree-based models on the RateBeer dataset. Similarly, the dimensionality reduction of PLSR avoids overfitting and improves performance, to some extent. Still, tree-based models (ABR, ET, GBR, RF and XGBR) show the best performance, out-competing the linear models (LR, Lasso, PLSR) commonly used in sensory science 65 .
GBR models showed the best overall performance in predicting sensory responses from chemical information, with R 2 values up to 0.75 depending on the predicted sensory feature (Supplementary Table S4 ). The GBR models predict consumer appreciation (RateBeer) better than our trained panel’s appreciation (R 2 value of 0.67 compared to R 2 value of 0.09) (Supplementary Table S3 and Supplementary Table S4 ). ANN models showed intermediate performance, likely because neural networks typically perform best with larger datasets 66 . The SVR shows intermediate performance, mostly due to the weak predictions of specific attributes that lower the overall performance (Supplementary Table S4 ).
Model dissection identifies specific, unexpected compounds as drivers of consumer appreciation
Next, we leveraged our models to infer important contributors to sensory perception and consumer appreciation. Consumer preference is a crucial sensory aspects, because a product that shows low consumer appreciation scores often does not succeed commercially 25 . Additionally, the requirement for a large number of representative evaluators makes consumer trials one of the more costly and time-consuming aspects of product development. Hence, a model for predicting chemical drivers of overall appreciation would be a welcome addition to the available toolbox for food development and optimization.
Since GBR models on our RateBeer dataset showed the best overall performance, we focused on these models. Specifically, we used two approaches to identify important contributors. First, rankings of the most important predictors for each sensorial trait in the GBR models were obtained based on impurity-based feature importance (mean decrease in impurity). High-ranked parameters were hypothesized to be either the true causal chemical properties underlying the trait, to correlate with the actual causal properties, or to take part in sensory interactions affecting the trait 67 (Fig. 4A ). In a second approach, we used SHAP 68 to determine which parameters contributed most to the model for making predictions of consumer appreciation (Fig. 4B ). SHAP calculates parameter contributions to model predictions on a per-sample basis, which can be aggregated into an importance score.
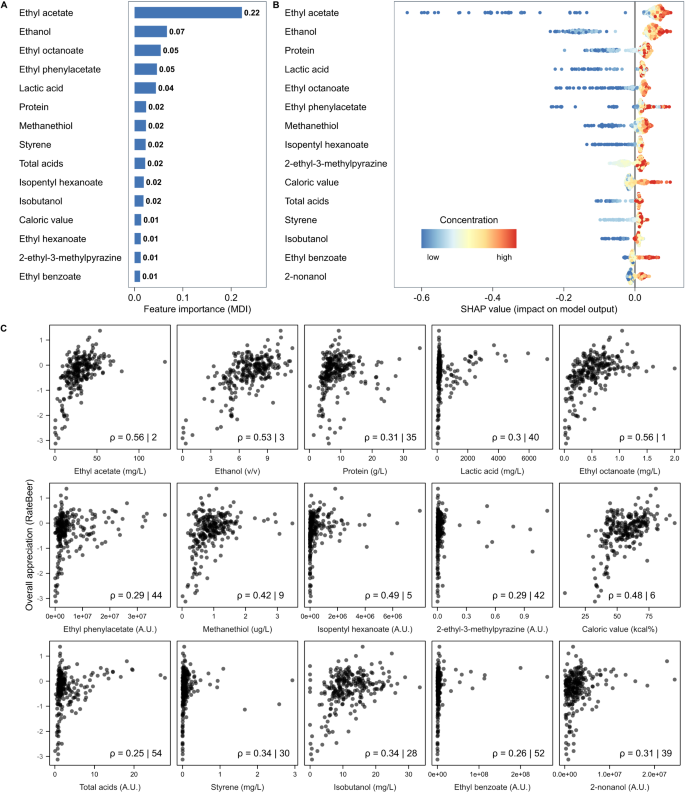
A The impurity-based feature importance (mean deviance in impurity, MDI) calculated from the Gradient Boosting Regression (GBR) model predicting RateBeer appreciation scores. The top 15 highest ranked chemical properties are shown. B SHAP summary plot for the top 15 parameters contributing to our GBR model. Each point on the graph represents a sample from our dataset. The color represents the concentration of that parameter, with bluer colors representing low values and redder colors representing higher values. Greater absolute values on the horizontal axis indicate a higher impact of the parameter on the prediction of the model. C Spearman correlations between the 15 most important chemical properties and consumer overall appreciation. Numbers indicate the Spearman Rho correlation coefficient, and the rank of this correlation compared to all other correlations. The top 15 important compounds were determined using SHAP (panel B).
Both approaches identified ethyl acetate as the most predictive parameter for beer appreciation (Fig. 4 ). Ethyl acetate is the most abundant ester in beer with a typical ‘fruity’, ‘solvent’ and ‘alcoholic’ flavor, but is often considered less important than other esters like isoamyl acetate. The second most important parameter identified by SHAP is ethanol, the most abundant beer compound after water. Apart from directly contributing to beer flavor and mouthfeel, ethanol drastically influences the physical properties of beer, dictating how easily volatile compounds escape the beer matrix to contribute to beer aroma 69 . Importantly, it should also be noted that the importance of ethanol for appreciation is likely inflated by the very low appreciation scores of non-alcoholic beers (Supplementary Fig. S4 ). Despite not often being considered a driver of beer appreciation, protein level also ranks highly in both approaches, possibly due to its effect on mouthfeel and body 70 . Lactic acid, which contributes to the tart taste of sour beers, is the fourth most important parameter identified by SHAP, possibly due to the generally high appreciation of sour beers in our dataset.
Interestingly, some of the most important predictive parameters for our model are not well-established as beer flavors or are even commonly regarded as being negative for beer quality. For example, our models identify methanethiol and ethyl phenyl acetate, an ester commonly linked to beer staling 71 , as a key factor contributing to beer appreciation. Although there is no doubt that high concentrations of these compounds are considered unpleasant, the positive effects of modest concentrations are not yet known 72 , 73 .
To compare our approach to conventional statistics, we evaluated how well the 15 most important SHAP-derived parameters correlate with consumer appreciation (Fig. 4C ). Interestingly, only 6 of the properties derived by SHAP rank amongst the top 15 most correlated parameters. For some chemical compounds, the correlations are so low that they would have likely been considered unimportant. For example, lactic acid, the fourth most important parameter, shows a bimodal distribution for appreciation, with sour beers forming a separate cluster, that is missed entirely by the Spearman correlation. Additionally, the correlation plots reveal outliers, emphasizing the need for robust analysis tools. Together, this highlights the need for alternative models, like the Gradient Boosting model, that better grasp the complexity of (beer) flavor.
Finally, to observe the relationships between these chemical properties and their predicted targets, partial dependence plots were constructed for the six most important predictors of consumer appreciation 74 , 75 , 76 (Supplementary Fig. S7 ). One-way partial dependence plots show how a change in concentration affects the predicted appreciation. These plots reveal an important limitation of our models: appreciation predictions remain constant at ever-increasing concentrations. This implies that once a threshold concentration is reached, further increasing the concentration does not affect appreciation. This is false, as it is well-documented that certain compounds become unpleasant at high concentrations, including ethyl acetate (‘nail polish’) 77 and methanethiol (‘sulfury’ and ‘rotten cabbage’) 78 . The inability of our models to grasp that flavor compounds have optimal levels, above which they become negative, is a consequence of working with commercial beer brands where (off-)flavors are rarely too high to negatively impact the product. The two-way partial dependence plots show how changing the concentration of two compounds influences predicted appreciation, visualizing their interactions (Supplementary Fig. S7 ). In our case, the top 5 parameters are dominated by additive or synergistic interactions, with high concentrations for both compounds resulting in the highest predicted appreciation.
To assess the robustness of our best-performing models and model predictions, we performed 100 iterations of the GBR, RF and ET models. In general, all iterations of the models yielded similar performance (Supplementary Fig. S8 ). Moreover, the main predictors (including the top predictors ethanol and ethyl acetate) remained virtually the same, especially for GBR and RF. For the iterations of the ET model, we did observe more variation in the top predictors, which is likely a consequence of the model’s inherent random architecture in combination with co-correlations between certain predictors. However, even in this case, several of the top predictors (ethanol and ethyl acetate) remain unchanged, although their rank in importance changes (Supplementary Fig. S8 ).
Next, we investigated if a combination of RateBeer and trained panel data into one consolidated dataset would lead to stronger models, under the hypothesis that such a model would suffer less from bias in the datasets. A GBR model was trained to predict appreciation on the combined dataset. This model underperformed compared to the RateBeer model, both in the native case and when including a dataset identifier (R 2 = 0.67, 0.26 and 0.42 respectively). For the latter, the dataset identifier is the most important feature (Supplementary Fig. S9 ), while most of the feature importance remains unchanged, with ethyl acetate and ethanol ranking highest, like in the original model trained only on RateBeer data. It seems that the large variation in the panel dataset introduces noise, weakening the models’ performances and reliability. In addition, it seems reasonable to assume that both datasets are fundamentally different, with the panel dataset obtained by blind tastings by a trained professional panel.
Lastly, we evaluated whether beer style identifiers would further enhance the model’s performance. A GBR model was trained with parameters that explicitly encoded the styles of the samples. This did not improve model performance (R2 = 0.66 with style information vs R2 = 0.67). The most important chemical features are consistent with the model trained without style information (eg. ethanol and ethyl acetate), and with the exception of the most preferred (strong ale) and least preferred (low/no-alcohol) styles, none of the styles were among the most important features (Supplementary Fig. S9 , Supplementary Table S5 and S6 ). This is likely due to a combination of style-specific chemical signatures, such as iso-alpha acids and lactic acid, that implicitly convey style information to the original models, as well as the low number of samples belonging to some styles, making it difficult for the model to learn style-specific patterns. Moreover, beer styles are not rigorously defined, with some styles overlapping in features and some beers being misattributed to a specific style, all of which leads to more noise in models that use style parameters.
Model validation
To test if our predictive models give insight into beer appreciation, we set up experiments aimed at improving existing commercial beers. We specifically selected overall appreciation as the trait to be examined because of its complexity and commercial relevance. Beer flavor comprises a complex bouquet rather than single aromas and tastes 53 . Hence, adding a single compound to the extent that a difference is noticeable may lead to an unbalanced, artificial flavor. Therefore, we evaluated the effect of combinations of compounds. Because Blond beers represent the most extensive style in our dataset, we selected a beer from this style as the starting material for these experiments (Beer 64 in Supplementary Data 1 ).
In the first set of experiments, we adjusted the concentrations of compounds that made up the most important predictors of overall appreciation (ethyl acetate, ethanol, lactic acid, ethyl phenyl acetate) together with correlated compounds (ethyl hexanoate, isoamyl acetate, glycerol), bringing them up to 95 th percentile ethanol-normalized concentrations (Methods) within the Blond group (‘Spiked’ concentration in Fig. 5A ). Compared to controls, the spiked beers were found to have significantly improved overall appreciation among trained panelists, with panelist noting increased intensity of ester flavors, sweetness, alcohol, and body fullness (Fig. 5B ). To disentangle the contribution of ethanol to these results, a second experiment was performed without the addition of ethanol. This resulted in a similar outcome, including increased perception of alcohol and overall appreciation.
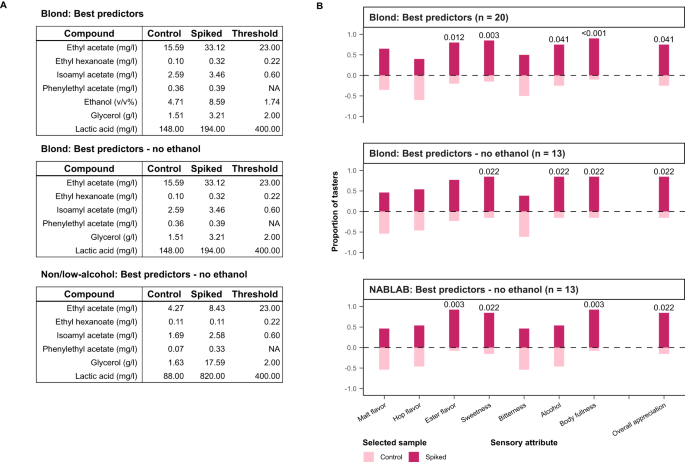
Adding the top chemical compounds, identified as best predictors of appreciation by our model, into poorly appreciated beers results in increased appreciation from our trained panel. Results of sensory tests between base beers and those spiked with compounds identified as the best predictors by the model. A Blond and Non/Low-alcohol (0.0% ABV) base beers were brought up to 95th-percentile ethanol-normalized concentrations within each style. B For each sensory attribute, tasters indicated the more intense sample and selected the sample they preferred. The numbers above the bars correspond to the p values that indicate significant changes in perceived flavor (two-sided binomial test: alpha 0.05, n = 20 or 13).
In a last experiment, we tested whether using the model’s predictions can boost the appreciation of a non-alcoholic beer (beer 223 in Supplementary Data 1 ). Again, the addition of a mixture of predicted compounds (omitting ethanol, in this case) resulted in a significant increase in appreciation, body, ester flavor and sweetness.
Predicting flavor and consumer appreciation from chemical composition is one of the ultimate goals of sensory science. A reliable, systematic and unbiased way to link chemical profiles to flavor and food appreciation would be a significant asset to the food and beverage industry. Such tools would substantially aid in quality control and recipe development, offer an efficient and cost-effective alternative to pilot studies and consumer trials and would ultimately allow food manufacturers to produce superior, tailor-made products that better meet the demands of specific consumer groups more efficiently.
A limited set of studies have previously tried, to varying degrees of success, to predict beer flavor and beer popularity based on (a limited set of) chemical compounds and flavors 79 , 80 . Current sensitive, high-throughput technologies allow measuring an unprecedented number of chemical compounds and properties in a large set of samples, yielding a dataset that can train models that help close the gaps between chemistry and flavor, even for a complex natural product like beer. To our knowledge, no previous research gathered data at this scale (250 samples, 226 chemical parameters, 50 sensory attributes and 5 consumer scores) to disentangle and validate the chemical aspects driving beer preference using various machine-learning techniques. We find that modern machine learning models outperform conventional statistical tools, such as correlations and linear models, and can successfully predict flavor appreciation from chemical composition. This could be attributed to the natural incorporation of interactions and non-linear or discontinuous effects in machine learning models, which are not easily grasped by the linear model architecture. While linear models and partial least squares regression represent the most widespread statistical approaches in sensory science, in part because they allow interpretation 65 , 81 , 82 , modern machine learning methods allow for building better predictive models while preserving the possibility to dissect and exploit the underlying patterns. Of the 10 different models we trained, tree-based models, such as our best performing GBR, showed the best overall performance in predicting sensory responses from chemical information, outcompeting artificial neural networks. This agrees with previous reports for models trained on tabular data 83 . Our results are in line with the findings of Colantonio et al. who also identified the gradient boosting architecture as performing best at predicting appreciation and flavor (of tomatoes and blueberries, in their specific study) 26 . Importantly, besides our larger experimental scale, we were able to directly confirm our models’ predictions in vivo.
Our study confirms that flavor compound concentration does not always correlate with perception, suggesting complex interactions that are often missed by more conventional statistics and simple models. Specifically, we find that tree-based algorithms may perform best in developing models that link complex food chemistry with aroma. Furthermore, we show that massive datasets of untrained consumer reviews provide a valuable source of data, that can complement or even replace trained tasting panels, especially for appreciation and basic flavors, such as sweetness and bitterness. This holds despite biases that are known to occur in such datasets, such as price or conformity bias. Moreover, GBR models predict taste better than aroma. This is likely because taste (e.g. bitterness) often directly relates to the corresponding chemical measurements (e.g., iso-alpha acids), whereas such a link is less clear for aromas, which often result from the interplay between multiple volatile compounds. We also find that our models are best at predicting acidity and alcohol, likely because there is a direct relation between the measured chemical compounds (acids and ethanol) and the corresponding perceived sensorial attribute (acidity and alcohol), and because even untrained consumers are generally able to recognize these flavors and aromas.
The predictions of our final models, trained on review data, hold even for blind tastings with small groups of trained tasters, as demonstrated by our ability to validate specific compounds as drivers of beer flavor and appreciation. Since adding a single compound to the extent of a noticeable difference may result in an unbalanced flavor profile, we specifically tested our identified key drivers as a combination of compounds. While this approach does not allow us to validate if a particular single compound would affect flavor and/or appreciation, our experiments do show that this combination of compounds increases consumer appreciation.
It is important to stress that, while it represents an important step forward, our approach still has several major limitations. A key weakness of the GBR model architecture is that amongst co-correlating variables, the largest main effect is consistently preferred for model building. As a result, co-correlating variables often have artificially low importance scores, both for impurity and SHAP-based methods, like we observed in the comparison to the more randomized Extra Trees models. This implies that chemicals identified as key drivers of a specific sensory feature by GBR might not be the true causative compounds, but rather co-correlate with the actual causative chemical. For example, the high importance of ethyl acetate could be (partially) attributed to the total ester content, ethanol or ethyl hexanoate (rho=0.77, rho=0.72 and rho=0.68), while ethyl phenylacetate could hide the importance of prenyl isobutyrate and ethyl benzoate (rho=0.77 and rho=0.76). Expanding our GBR model to include beer style as a parameter did not yield additional power or insight. This is likely due to style-specific chemical signatures, such as iso-alpha acids and lactic acid, that implicitly convey style information to the original model, as well as the smaller sample size per style, limiting the power to uncover style-specific patterns. This can be partly attributed to the curse of dimensionality, where the high number of parameters results in the models mainly incorporating single parameter effects, rather than complex interactions such as style-dependent effects 67 . A larger number of samples may overcome some of these limitations and offer more insight into style-specific effects. On the other hand, beer style is not a rigid scientific classification, and beers within one style often differ a lot, which further complicates the analysis of style as a model factor.
Our study is limited to beers from Belgian breweries. Although these beers cover a large portion of the beer styles available globally, some beer styles and consumer patterns may be missing, while other features might be overrepresented. For example, many Belgian ales exhibit yeast-driven flavor profiles, which is reflected in the chemical drivers of appreciation discovered by this study. In future work, expanding the scope to include diverse markets and beer styles could lead to the identification of even more drivers of appreciation and better models for special niche products that were not present in our beer set.
In addition to inherent limitations of GBR models, there are also some limitations associated with studying food aroma. Even if our chemical analyses measured most of the known aroma compounds, the total number of flavor compounds in complex foods like beer is still larger than the subset we were able to measure in this study. For example, hop-derived thiols, that influence flavor at very low concentrations, are notoriously difficult to measure in a high-throughput experiment. Moreover, consumer perception remains subjective and prone to biases that are difficult to avoid. It is also important to stress that the models are still immature and that more extensive datasets will be crucial for developing more complete models in the future. Besides more samples and parameters, our dataset does not include any demographic information about the tasters. Including such data could lead to better models that grasp external factors like age and culture. Another limitation is that our set of beers consists of high-quality end-products and lacks beers that are unfit for sale, which limits the current model in accurately predicting products that are appreciated very badly. Finally, while models could be readily applied in quality control, their use in sensory science and product development is restrained by their inability to discern causal relationships. Given that the models cannot distinguish compounds that genuinely drive consumer perception from those that merely correlate, validation experiments are essential to identify true causative compounds.
Despite the inherent limitations, dissection of our models enabled us to pinpoint specific molecules as potential drivers of beer aroma and consumer appreciation, including compounds that were unexpected and would not have been identified using standard approaches. Important drivers of beer appreciation uncovered by our models include protein levels, ethyl acetate, ethyl phenyl acetate and lactic acid. Currently, many brewers already use lactic acid to acidify their brewing water and ensure optimal pH for enzymatic activity during the mashing process. Our results suggest that adding lactic acid can also improve beer appreciation, although its individual effect remains to be tested. Interestingly, ethanol appears to be unnecessary to improve beer appreciation, both for blond beer and alcohol-free beer. Given the growing consumer interest in alcohol-free beer, with a predicted annual market growth of >7% 84 , it is relevant for brewers to know what compounds can further increase consumer appreciation of these beers. Hence, our model may readily provide avenues to further improve the flavor and consumer appreciation of both alcoholic and non-alcoholic beers, which is generally considered one of the key challenges for future beer production.
Whereas we see a direct implementation of our results for the development of superior alcohol-free beverages and other food products, our study can also serve as a stepping stone for the development of novel alcohol-containing beverages. We want to echo the growing body of scientific evidence for the negative effects of alcohol consumption, both on the individual level by the mutagenic, teratogenic and carcinogenic effects of ethanol 85 , 86 , as well as the burden on society caused by alcohol abuse and addiction. We encourage the use of our results for the production of healthier, tastier products, including novel and improved beverages with lower alcohol contents. Furthermore, we strongly discourage the use of these technologies to improve the appreciation or addictive properties of harmful substances.
The present work demonstrates that despite some important remaining hurdles, combining the latest developments in chemical analyses, sensory analysis and modern machine learning methods offers exciting avenues for food chemistry and engineering. Soon, these tools may provide solutions in quality control and recipe development, as well as new approaches to sensory science and flavor research.
Beer selection
250 commercial Belgian beers were selected to cover the broad diversity of beer styles and corresponding diversity in chemical composition and aroma. See Supplementary Fig. S1 .
Chemical dataset
Sample preparation.
Beers within their expiration date were purchased from commercial retailers. Samples were prepared in biological duplicates at room temperature, unless explicitly stated otherwise. Bottle pressure was measured with a manual pressure device (Steinfurth Mess-Systeme GmbH) and used to calculate CO 2 concentration. The beer was poured through two filter papers (Macherey-Nagel, 500713032 MN 713 ¼) to remove carbon dioxide and prevent spontaneous foaming. Samples were then prepared for measurements by targeted Headspace-Gas Chromatography-Flame Ionization Detector/Flame Photometric Detector (HS-GC-FID/FPD), Headspace-Solid Phase Microextraction-Gas Chromatography-Mass Spectrometry (HS-SPME-GC-MS), colorimetric analysis, enzymatic analysis, Near-Infrared (NIR) analysis, as described in the sections below. The mean values of biological duplicates are reported for each compound.
HS-GC-FID/FPD
HS-GC-FID/FPD (Shimadzu GC 2010 Plus) was used to measure higher alcohols, acetaldehyde, esters, 4-vinyl guaicol, and sulfur compounds. Each measurement comprised 5 ml of sample pipetted into a 20 ml glass vial containing 1.75 g NaCl (VWR, 27810.295). 100 µl of 2-heptanol (Sigma-Aldrich, H3003) (internal standard) solution in ethanol (Fisher Chemical, E/0650DF/C17) was added for a final concentration of 2.44 mg/L. Samples were flushed with nitrogen for 10 s, sealed with a silicone septum, stored at −80 °C and analyzed in batches of 20.
The GC was equipped with a DB-WAXetr column (length, 30 m; internal diameter, 0.32 mm; layer thickness, 0.50 µm; Agilent Technologies, Santa Clara, CA, USA) to the FID and an HP-5 column (length, 30 m; internal diameter, 0.25 mm; layer thickness, 0.25 µm; Agilent Technologies, Santa Clara, CA, USA) to the FPD. N 2 was used as the carrier gas. Samples were incubated for 20 min at 70 °C in the headspace autosampler (Flow rate, 35 cm/s; Injection volume, 1000 µL; Injection mode, split; Combi PAL autosampler, CTC analytics, Switzerland). The injector, FID and FPD temperatures were kept at 250 °C. The GC oven temperature was first held at 50 °C for 5 min and then allowed to rise to 80 °C at a rate of 5 °C/min, followed by a second ramp of 4 °C/min until 200 °C kept for 3 min and a final ramp of (4 °C/min) until 230 °C for 1 min. Results were analyzed with the GCSolution software version 2.4 (Shimadzu, Kyoto, Japan). The GC was calibrated with a 5% EtOH solution (VWR International) containing the volatiles under study (Supplementary Table S7 ).
HS-SPME-GC-MS
HS-SPME-GC-MS (Shimadzu GCMS-QP-2010 Ultra) was used to measure additional volatile compounds, mainly comprising terpenoids and esters. Samples were analyzed by HS-SPME using a triphase DVB/Carboxen/PDMS 50/30 μm SPME fiber (Supelco Co., Bellefonte, PA, USA) followed by gas chromatography (Thermo Fisher Scientific Trace 1300 series, USA) coupled to a mass spectrometer (Thermo Fisher Scientific ISQ series MS) equipped with a TriPlus RSH autosampler. 5 ml of degassed beer sample was placed in 20 ml vials containing 1.75 g NaCl (VWR, 27810.295). 5 µl internal standard mix was added, containing 2-heptanol (1 g/L) (Sigma-Aldrich, H3003), 4-fluorobenzaldehyde (1 g/L) (Sigma-Aldrich, 128376), 2,3-hexanedione (1 g/L) (Sigma-Aldrich, 144169) and guaiacol (1 g/L) (Sigma-Aldrich, W253200) in ethanol (Fisher Chemical, E/0650DF/C17). Each sample was incubated at 60 °C in the autosampler oven with constant agitation. After 5 min equilibration, the SPME fiber was exposed to the sample headspace for 30 min. The compounds trapped on the fiber were thermally desorbed in the injection port of the chromatograph by heating the fiber for 15 min at 270 °C.
The GC-MS was equipped with a low polarity RXi-5Sil MS column (length, 20 m; internal diameter, 0.18 mm; layer thickness, 0.18 µm; Restek, Bellefonte, PA, USA). Injection was performed in splitless mode at 320 °C, a split flow of 9 ml/min, a purge flow of 5 ml/min and an open valve time of 3 min. To obtain a pulsed injection, a programmed gas flow was used whereby the helium gas flow was set at 2.7 mL/min for 0.1 min, followed by a decrease in flow of 20 ml/min to the normal 0.9 mL/min. The temperature was first held at 30 °C for 3 min and then allowed to rise to 80 °C at a rate of 7 °C/min, followed by a second ramp of 2 °C/min till 125 °C and a final ramp of 8 °C/min with a final temperature of 270 °C.
Mass acquisition range was 33 to 550 amu at a scan rate of 5 scans/s. Electron impact ionization energy was 70 eV. The interface and ion source were kept at 275 °C and 250 °C, respectively. A mix of linear n-alkanes (from C7 to C40, Supelco Co.) was injected into the GC-MS under identical conditions to serve as external retention index markers. Identification and quantification of the compounds were performed using an in-house developed R script as described in Goelen et al. and Reher et al. 87 , 88 (for package information, see Supplementary Table S8 ). Briefly, chromatograms were analyzed using AMDIS (v2.71) 89 to separate overlapping peaks and obtain pure compound spectra. The NIST MS Search software (v2.0 g) in combination with the NIST2017, FFNSC3 and Adams4 libraries were used to manually identify the empirical spectra, taking into account the expected retention time. After background subtraction and correcting for retention time shifts between samples run on different days based on alkane ladders, compound elution profiles were extracted and integrated using a file with 284 target compounds of interest, which were either recovered in our identified AMDIS list of spectra or were known to occur in beer. Compound elution profiles were estimated for every peak in every chromatogram over a time-restricted window using weighted non-negative least square analysis after which peak areas were integrated 87 , 88 . Batch effect correction was performed by normalizing against the most stable internal standard compound, 4-fluorobenzaldehyde. Out of all 284 target compounds that were analyzed, 167 were visually judged to have reliable elution profiles and were used for final analysis.
Discrete photometric and enzymatic analysis
Discrete photometric and enzymatic analysis (Thermo Scientific TM Gallery TM Plus Beermaster Discrete Analyzer) was used to measure acetic acid, ammonia, beta-glucan, iso-alpha acids, color, sugars, glycerol, iron, pH, protein, and sulfite. 2 ml of sample volume was used for the analyses. Information regarding the reagents and standard solutions used for analyses and calibrations is included in Supplementary Table S7 and Supplementary Table S9 .
NIR analyses
NIR analysis (Anton Paar Alcolyzer Beer ME System) was used to measure ethanol. Measurements comprised 50 ml of sample, and a 10% EtOH solution was used for calibration.
Correlation calculations
Pairwise Spearman Rank correlations were calculated between all chemical properties.
Sensory dataset
Trained panel.
Our trained tasting panel consisted of volunteers who gave prior verbal informed consent. All compounds used for the validation experiment were of food-grade quality. The tasting sessions were approved by the Social and Societal Ethics Committee of the KU Leuven (G-2022-5677-R2(MAR)). All online reviewers agreed to the Terms and Conditions of the RateBeer website.
Sensory analysis was performed according to the American Society of Brewing Chemists (ASBC) Sensory Analysis Methods 90 . 30 volunteers were screened through a series of triangle tests. The sixteen most sensitive and consistent tasters were retained as taste panel members. The resulting panel was diverse in age [22–42, mean: 29], sex [56% male] and nationality [7 different countries]. The panel developed a consensus vocabulary to describe beer aroma, taste and mouthfeel. Panelists were trained to identify and score 50 different attributes, using a 7-point scale to rate attributes’ intensity. The scoring sheet is included as Supplementary Data 3 . Sensory assessments took place between 10–12 a.m. The beers were served in black-colored glasses. Per session, between 5 and 12 beers of the same style were tasted at 12 °C to 16 °C. Two reference beers were added to each set and indicated as ‘Reference 1 & 2’, allowing panel members to calibrate their ratings. Not all panelists were present at every tasting. Scores were scaled by standard deviation and mean-centered per taster. Values are represented as z-scores and clustered by Euclidean distance. Pairwise Spearman correlations were calculated between taste and aroma sensory attributes. Panel consistency was evaluated by repeating samples on different sessions and performing ANOVA to identify differences, using the ‘stats’ package (v4.2.2) in R (for package information, see Supplementary Table S8 ).
Online reviews from a public database
The ‘scrapy’ package in Python (v3.6) (for package information, see Supplementary Table S8 ). was used to collect 232,288 online reviews (mean=922, min=6, max=5343) from RateBeer, an online beer review database. Each review entry comprised 5 numerical scores (appearance, aroma, taste, palate and overall quality) and an optional review text. The total number of reviews per reviewer was collected separately. Numerical scores were scaled and centered per rater, and mean scores were calculated per beer.
For the review texts, the language was estimated using the packages ‘langdetect’ and ‘langid’ in Python. Reviews that were classified as English by both packages were kept. Reviewers with fewer than 100 entries overall were discarded. 181,025 reviews from >6000 reviewers from >40 countries remained. Text processing was done using the ‘nltk’ package in Python. Texts were corrected for slang and misspellings; proper nouns and rare words that are relevant to the beer context were specified and kept as-is (‘Chimay’,’Lambic’, etc.). A dictionary of semantically similar sensorial terms, for example ‘floral’ and ‘flower’, was created and collapsed together into one term. Words were stemmed and lemmatized to avoid identifying words such as ‘acid’ and ‘acidity’ as separate terms. Numbers and punctuation were removed.
Sentences from up to 50 randomly chosen reviews per beer were manually categorized according to the aspect of beer they describe (appearance, aroma, taste, palate, overall quality—not to be confused with the 5 numerical scores described above) or flagged as irrelevant if they contained no useful information. If a beer contained fewer than 50 reviews, all reviews were manually classified. This labeled data set was used to train a model that classified the rest of the sentences for all beers 91 . Sentences describing taste and aroma were extracted, and term frequency–inverse document frequency (TFIDF) was implemented to calculate enrichment scores for sensorial words per beer.
The sex of the tasting subject was not considered when building our sensory database. Instead, results from different panelists were averaged, both for our trained panel (56% male, 44% female) and the RateBeer reviews (70% male, 30% female for RateBeer as a whole).
Beer price collection and processing
Beer prices were collected from the following stores: Colruyt, Delhaize, Total Wine, BeerHawk, The Belgian Beer Shop, The Belgian Shop, and Beer of Belgium. Where applicable, prices were converted to Euros and normalized per liter. Spearman correlations were calculated between these prices and mean overall appreciation scores from RateBeer and the taste panel, respectively.
Pairwise Spearman Rank correlations were calculated between all sensory properties.
Machine learning models
Predictive modeling of sensory profiles from chemical data.
Regression models were constructed to predict (a) trained panel scores for beer flavors and quality from beer chemical profiles and (b) public reviews’ appreciation scores from beer chemical profiles. Z-scores were used to represent sensory attributes in both data sets. Chemical properties with log-normal distributions (Shapiro-Wilk test, p < 0.05 ) were log-transformed. Missing chemical measurements (0.1% of all data) were replaced with mean values per attribute. Observations from 250 beers were randomly separated into a training set (70%, 175 beers) and a test set (30%, 75 beers), stratified per beer style. Chemical measurements (p = 231) were normalized based on the training set average and standard deviation. In total, three linear regression-based models: linear regression with first-order interaction terms (LR), lasso regression with first-order interaction terms (Lasso) and partial least squares regression (PLSR); five decision tree models, Adaboost regressor (ABR), Extra Trees (ET), Gradient Boosting regressor (GBR), Random Forest (RF) and XGBoost regressor (XGBR); one support vector machine model (SVR) and one artificial neural network model (ANN) were trained. The models were implemented using the ‘scikit-learn’ package (v1.2.2) and ‘xgboost’ package (v1.7.3) in Python (v3.9.16). Models were trained, and hyperparameters optimized, using five-fold cross-validated grid search with the coefficient of determination (R 2 ) as the evaluation metric. The ANN (scikit-learn’s MLPRegressor) was optimized using Bayesian Tree-Structured Parzen Estimator optimization with the ‘Optuna’ Python package (v3.2.0). Individual models were trained per attribute, and a multi-output model was trained on all attributes simultaneously.
Model dissection
GBR was found to outperform other methods, resulting in models with the highest average R 2 values in both trained panel and public review data sets. Impurity-based rankings of the most important predictors for each predicted sensorial trait were obtained using the ‘scikit-learn’ package. To observe the relationships between these chemical properties and their predicted targets, partial dependence plots (PDP) were constructed for the six most important predictors of consumer appreciation 74 , 75 .
The ‘SHAP’ package in Python (v0.41.0) was implemented to provide an alternative ranking of predictor importance and to visualize the predictors’ effects as a function of their concentration 68 .
Validation of causal chemical properties
To validate the effects of the most important model features on predicted sensory attributes, beers were spiked with the chemical compounds identified by the models and descriptive sensory analyses were carried out according to the American Society of Brewing Chemists (ASBC) protocol 90 .
Compound spiking was done 30 min before tasting. Compounds were spiked into fresh beer bottles, that were immediately resealed and inverted three times. Fresh bottles of beer were opened for the same duration, resealed, and inverted thrice, to serve as controls. Pairs of spiked samples and controls were served simultaneously, chilled and in dark glasses as outlined in the Trained panel section above. Tasters were instructed to select the glass with the higher flavor intensity for each attribute (directional difference test 92 ) and to select the glass they prefer.
The final concentration after spiking was equal to the within-style average, after normalizing by ethanol concentration. This was done to ensure balanced flavor profiles in the final spiked beer. The same methods were applied to improve a non-alcoholic beer. Compounds were the following: ethyl acetate (Merck KGaA, W241415), ethyl hexanoate (Merck KGaA, W243906), isoamyl acetate (Merck KGaA, W205508), phenethyl acetate (Merck KGaA, W285706), ethanol (96%, Colruyt), glycerol (Merck KGaA, W252506), lactic acid (Merck KGaA, 261106).
Significant differences in preference or perceived intensity were determined by performing the two-sided binomial test on each attribute.
Reporting summary
Further information on research design is available in the Nature Portfolio Reporting Summary linked to this article.
Data availability
The data that support the findings of this work are available in the Supplementary Data files and have been deposited to Zenodo under accession code 10653704 93 . The RateBeer scores data are under restricted access, they are not publicly available as they are property of RateBeer (ZX Ventures, USA). Access can be obtained from the authors upon reasonable request and with permission of RateBeer (ZX Ventures, USA). Source data are provided with this paper.
Code availability
The code for training the machine learning models, analyzing the models, and generating the figures has been deposited to Zenodo under accession code 10653704 93 .
Tieman, D. et al. A chemical genetic roadmap to improved tomato flavor. Science 355 , 391–394 (2017).
Article ADS CAS PubMed Google Scholar
Plutowska, B. & Wardencki, W. Application of gas chromatography–olfactometry (GC–O) in analysis and quality assessment of alcoholic beverages – A review. Food Chem. 107 , 449–463 (2008).
Article CAS Google Scholar
Legin, A., Rudnitskaya, A., Seleznev, B. & Vlasov, Y. Electronic tongue for quality assessment of ethanol, vodka and eau-de-vie. Anal. Chim. Acta 534 , 129–135 (2005).
Loutfi, A., Coradeschi, S., Mani, G. K., Shankar, P. & Rayappan, J. B. B. Electronic noses for food quality: A review. J. Food Eng. 144 , 103–111 (2015).
Ahn, Y.-Y., Ahnert, S. E., Bagrow, J. P. & Barabási, A.-L. Flavor network and the principles of food pairing. Sci. Rep. 1 , 196 (2011).
Article CAS PubMed PubMed Central Google Scholar
Bartoshuk, L. M. & Klee, H. J. Better fruits and vegetables through sensory analysis. Curr. Biol. 23 , R374–R378 (2013).
Article CAS PubMed Google Scholar
Piggott, J. R. Design questions in sensory and consumer science. Food Qual. Prefer. 3293 , 217–220 (1995).
Article Google Scholar
Kermit, M. & Lengard, V. Assessing the performance of a sensory panel-panellist monitoring and tracking. J. Chemom. 19 , 154–161 (2005).
Cook, D. J., Hollowood, T. A., Linforth, R. S. T. & Taylor, A. J. Correlating instrumental measurements of texture and flavour release with human perception. Int. J. Food Sci. Technol. 40 , 631–641 (2005).
Chinchanachokchai, S., Thontirawong, P. & Chinchanachokchai, P. A tale of two recommender systems: The moderating role of consumer expertise on artificial intelligence based product recommendations. J. Retail. Consum. Serv. 61 , 1–12 (2021).
Ross, C. F. Sensory science at the human-machine interface. Trends Food Sci. Technol. 20 , 63–72 (2009).
Chambers, E. IV & Koppel, K. Associations of volatile compounds with sensory aroma and flavor: The complex nature of flavor. Molecules 18 , 4887–4905 (2013).
Pinu, F. R. Metabolomics—The new frontier in food safety and quality research. Food Res. Int. 72 , 80–81 (2015).
Danezis, G. P., Tsagkaris, A. S., Brusic, V. & Georgiou, C. A. Food authentication: state of the art and prospects. Curr. Opin. Food Sci. 10 , 22–31 (2016).
Shepherd, G. M. Smell images and the flavour system in the human brain. Nature 444 , 316–321 (2006).
Meilgaard, M. C. Prediction of flavor differences between beers from their chemical composition. J. Agric. Food Chem. 30 , 1009–1017 (1982).
Xu, L. et al. Widespread receptor-driven modulation in peripheral olfactory coding. Science 368 , eaaz5390 (2020).
Kupferschmidt, K. Following the flavor. Science 340 , 808–809 (2013).
Billesbølle, C. B. et al. Structural basis of odorant recognition by a human odorant receptor. Nature 615 , 742–749 (2023).
Article ADS PubMed PubMed Central Google Scholar
Smith, B. Perspective: Complexities of flavour. Nature 486 , S6–S6 (2012).
Pfister, P. et al. Odorant receptor inhibition is fundamental to odor encoding. Curr. Biol. 30 , 2574–2587 (2020).
Moskowitz, H. W., Kumaraiah, V., Sharma, K. N., Jacobs, H. L. & Sharma, S. D. Cross-cultural differences in simple taste preferences. Science 190 , 1217–1218 (1975).
Eriksson, N. et al. A genetic variant near olfactory receptor genes influences cilantro preference. Flavour 1 , 22 (2012).
Ferdenzi, C. et al. Variability of affective responses to odors: Culture, gender, and olfactory knowledge. Chem. Senses 38 , 175–186 (2013).
Article PubMed Google Scholar
Lawless, H. T. & Heymann, H. Sensory evaluation of food: Principles and practices. (Springer, New York, NY). https://doi.org/10.1007/978-1-4419-6488-5 (2010).
Colantonio, V. et al. Metabolomic selection for enhanced fruit flavor. Proc. Natl. Acad. Sci. 119 , e2115865119 (2022).
Fritz, F., Preissner, R. & Banerjee, P. VirtualTaste: a web server for the prediction of organoleptic properties of chemical compounds. Nucleic Acids Res 49 , W679–W684 (2021).
Tuwani, R., Wadhwa, S. & Bagler, G. BitterSweet: Building machine learning models for predicting the bitter and sweet taste of small molecules. Sci. Rep. 9 , 1–13 (2019).
Dagan-Wiener, A. et al. Bitter or not? BitterPredict, a tool for predicting taste from chemical structure. Sci. Rep. 7 , 1–13 (2017).
Pallante, L. et al. Toward a general and interpretable umami taste predictor using a multi-objective machine learning approach. Sci. Rep. 12 , 1–11 (2022).
Malavolta, M. et al. A survey on computational taste predictors. Eur. Food Res. Technol. 248 , 2215–2235 (2022).
Lee, B. K. et al. A principal odor map unifies diverse tasks in olfactory perception. Science 381 , 999–1006 (2023).
Mayhew, E. J. et al. Transport features predict if a molecule is odorous. Proc. Natl. Acad. Sci. 119 , e2116576119 (2022).
Niu, Y. et al. Sensory evaluation of the synergism among ester odorants in light aroma-type liquor by odor threshold, aroma intensity and flash GC electronic nose. Food Res. Int. 113 , 102–114 (2018).
Yu, P., Low, M. Y. & Zhou, W. Design of experiments and regression modelling in food flavour and sensory analysis: A review. Trends Food Sci. Technol. 71 , 202–215 (2018).
Oladokun, O. et al. The impact of hop bitter acid and polyphenol profiles on the perceived bitterness of beer. Food Chem. 205 , 212–220 (2016).
Linforth, R., Cabannes, M., Hewson, L., Yang, N. & Taylor, A. Effect of fat content on flavor delivery during consumption: An in vivo model. J. Agric. Food Chem. 58 , 6905–6911 (2010).
Guo, S., Na Jom, K. & Ge, Y. Influence of roasting condition on flavor profile of sunflower seeds: A flavoromics approach. Sci. Rep. 9 , 11295 (2019).
Ren, Q. et al. The changes of microbial community and flavor compound in the fermentation process of Chinese rice wine using Fagopyrum tataricum grain as feedstock. Sci. Rep. 9 , 3365 (2019).
Hastie, T., Friedman, J. & Tibshirani, R. The Elements of Statistical Learning. (Springer, New York, NY). https://doi.org/10.1007/978-0-387-21606-5 (2001).
Dietz, C., Cook, D., Huismann, M., Wilson, C. & Ford, R. The multisensory perception of hop essential oil: a review. J. Inst. Brew. 126 , 320–342 (2020).
CAS Google Scholar
Roncoroni, Miguel & Verstrepen, Kevin Joan. Belgian Beer: Tested and Tasted. (Lannoo, 2018).
Meilgaard, M. Flavor chemistry of beer: Part II: Flavor and threshold of 239 aroma volatiles. in (1975).
Bokulich, N. A. & Bamforth, C. W. The microbiology of malting and brewing. Microbiol. Mol. Biol. Rev. MMBR 77 , 157–172 (2013).
Dzialo, M. C., Park, R., Steensels, J., Lievens, B. & Verstrepen, K. J. Physiology, ecology and industrial applications of aroma formation in yeast. FEMS Microbiol. Rev. 41 , S95–S128 (2017).
Article PubMed PubMed Central Google Scholar
Datta, A. et al. Computer-aided food engineering. Nat. Food 3 , 894–904 (2022).
American Society of Brewing Chemists. Beer Methods. (American Society of Brewing Chemists, St. Paul, MN, U.S.A.).
Olaniran, A. O., Hiralal, L., Mokoena, M. P. & Pillay, B. Flavour-active volatile compounds in beer: production, regulation and control. J. Inst. Brew. 123 , 13–23 (2017).
Verstrepen, K. J. et al. Flavor-active esters: Adding fruitiness to beer. J. Biosci. Bioeng. 96 , 110–118 (2003).
Meilgaard, M. C. Flavour chemistry of beer. part I: flavour interaction between principal volatiles. Master Brew. Assoc. Am. Tech. Q 12 , 107–117 (1975).
Briggs, D. E., Boulton, C. A., Brookes, P. A. & Stevens, R. Brewing 227–254. (Woodhead Publishing). https://doi.org/10.1533/9781855739062.227 (2004).
Bossaert, S., Crauwels, S., De Rouck, G. & Lievens, B. The power of sour - A review: Old traditions, new opportunities. BrewingScience 72 , 78–88 (2019).
Google Scholar
Verstrepen, K. J. et al. Flavor active esters: Adding fruitiness to beer. J. Biosci. Bioeng. 96 , 110–118 (2003).
Snauwaert, I. et al. Microbial diversity and metabolite composition of Belgian red-brown acidic ales. Int. J. Food Microbiol. 221 , 1–11 (2016).
Spitaels, F. et al. The microbial diversity of traditional spontaneously fermented lambic beer. PLoS ONE 9 , e95384 (2014).
Blanco, C. A., Andrés-Iglesias, C. & Montero, O. Low-alcohol Beers: Flavor Compounds, Defects, and Improvement Strategies. Crit. Rev. Food Sci. Nutr. 56 , 1379–1388 (2016).
Jackowski, M. & Trusek, A. Non-Alcohol. beer Prod. – Overv. 20 , 32–38 (2018).
Takoi, K. et al. The contribution of geraniol metabolism to the citrus flavour of beer: Synergy of geraniol and β-citronellol under coexistence with excess linalool. J. Inst. Brew. 116 , 251–260 (2010).
Kroeze, J. H. & Bartoshuk, L. M. Bitterness suppression as revealed by split-tongue taste stimulation in humans. Physiol. Behav. 35 , 779–783 (1985).
Mennella, J. A. et al. A spoonful of sugar helps the medicine go down”: Bitter masking bysucrose among children and adults. Chem. Senses 40 , 17–25 (2015).
Wietstock, P., Kunz, T., Perreira, F. & Methner, F.-J. Metal chelation behavior of hop acids in buffered model systems. BrewingScience 69 , 56–63 (2016).
Sancho, D., Blanco, C. A., Caballero, I. & Pascual, A. Free iron in pale, dark and alcohol-free commercial lager beers. J. Sci. Food Agric. 91 , 1142–1147 (2011).
Rodrigues, H. & Parr, W. V. Contribution of cross-cultural studies to understanding wine appreciation: A review. Food Res. Int. 115 , 251–258 (2019).
Korneva, E. & Blockeel, H. Towards better evaluation of multi-target regression models. in ECML PKDD 2020 Workshops (eds. Koprinska, I. et al.) 353–362 (Springer International Publishing, Cham, 2020). https://doi.org/10.1007/978-3-030-65965-3_23 .
Gastón Ares. Mathematical and Statistical Methods in Food Science and Technology. (Wiley, 2013).
Grinsztajn, L., Oyallon, E. & Varoquaux, G. Why do tree-based models still outperform deep learning on tabular data? Preprint at http://arxiv.org/abs/2207.08815 (2022).
Gries, S. T. Statistics for Linguistics with R: A Practical Introduction. in Statistics for Linguistics with R (De Gruyter Mouton, 2021). https://doi.org/10.1515/9783110718256 .
Lundberg, S. M. et al. From local explanations to global understanding with explainable AI for trees. Nat. Mach. Intell. 2 , 56–67 (2020).
Ickes, C. M. & Cadwallader, K. R. Effects of ethanol on flavor perception in alcoholic beverages. Chemosens. Percept. 10 , 119–134 (2017).
Kato, M. et al. Influence of high molecular weight polypeptides on the mouthfeel of commercial beer. J. Inst. Brew. 127 , 27–40 (2021).
Wauters, R. et al. Novel Saccharomyces cerevisiae variants slow down the accumulation of staling aldehydes and improve beer shelf-life. Food Chem. 398 , 1–11 (2023).
Li, H., Jia, S. & Zhang, W. Rapid determination of low-level sulfur compounds in beer by headspace gas chromatography with a pulsed flame photometric detector. J. Am. Soc. Brew. Chem. 66 , 188–191 (2008).
Dercksen, A., Laurens, J., Torline, P., Axcell, B. C. & Rohwer, E. Quantitative analysis of volatile sulfur compounds in beer using a membrane extraction interface. J. Am. Soc. Brew. Chem. 54 , 228–233 (1996).
Molnar, C. Interpretable Machine Learning: A Guide for Making Black-Box Models Interpretable. (2020).
Zhao, Q. & Hastie, T. Causal interpretations of black-box models. J. Bus. Econ. Stat. Publ. Am. Stat. Assoc. 39 , 272–281 (2019).
Article MathSciNet Google Scholar
Hastie, T., Tibshirani, R. & Friedman, J. The Elements of Statistical Learning. (Springer, 2019).
Labrado, D. et al. Identification by NMR of key compounds present in beer distillates and residual phases after dealcoholization by vacuum distillation. J. Sci. Food Agric. 100 , 3971–3978 (2020).
Lusk, L. T., Kay, S. B., Porubcan, A. & Ryder, D. S. Key olfactory cues for beer oxidation. J. Am. Soc. Brew. Chem. 70 , 257–261 (2012).
Gonzalez Viejo, C., Torrico, D. D., Dunshea, F. R. & Fuentes, S. Development of artificial neural network models to assess beer acceptability based on sensory properties using a robotic pourer: A comparative model approach to achieve an artificial intelligence system. Beverages 5 , 33 (2019).
Gonzalez Viejo, C., Fuentes, S., Torrico, D. D., Godbole, A. & Dunshea, F. R. Chemical characterization of aromas in beer and their effect on consumers liking. Food Chem. 293 , 479–485 (2019).
Gilbert, J. L. et al. Identifying breeding priorities for blueberry flavor using biochemical, sensory, and genotype by environment analyses. PLOS ONE 10 , 1–21 (2015).
Goulet, C. et al. Role of an esterase in flavor volatile variation within the tomato clade. Proc. Natl. Acad. Sci. 109 , 19009–19014 (2012).
Article ADS CAS PubMed PubMed Central Google Scholar
Borisov, V. et al. Deep Neural Networks and Tabular Data: A Survey. IEEE Trans. Neural Netw. Learn. Syst. 1–21 https://doi.org/10.1109/TNNLS.2022.3229161 (2022).
Statista. Statista Consumer Market Outlook: Beer - Worldwide.
Seitz, H. K. & Stickel, F. Molecular mechanisms of alcoholmediated carcinogenesis. Nat. Rev. Cancer 7 , 599–612 (2007).
Voordeckers, K. et al. Ethanol exposure increases mutation rate through error-prone polymerases. Nat. Commun. 11 , 3664 (2020).
Goelen, T. et al. Bacterial phylogeny predicts volatile organic compound composition and olfactory response of an aphid parasitoid. Oikos 129 , 1415–1428 (2020).
Article ADS Google Scholar
Reher, T. et al. Evaluation of hop (Humulus lupulus) as a repellent for the management of Drosophila suzukii. Crop Prot. 124 , 104839 (2019).
Stein, S. E. An integrated method for spectrum extraction and compound identification from gas chromatography/mass spectrometry data. J. Am. Soc. Mass Spectrom. 10 , 770–781 (1999).
American Society of Brewing Chemists. Sensory Analysis Methods. (American Society of Brewing Chemists, St. Paul, MN, U.S.A., 1992).
McAuley, J., Leskovec, J. & Jurafsky, D. Learning Attitudes and Attributes from Multi-Aspect Reviews. Preprint at https://doi.org/10.48550/arXiv.1210.3926 (2012).
Meilgaard, M. C., Carr, B. T. & Carr, B. T. Sensory Evaluation Techniques. (CRC Press, Boca Raton). https://doi.org/10.1201/b16452 (2014).
Schreurs, M. et al. Data from: Predicting and improving complex beer flavor through machine learning. Zenodo https://doi.org/10.5281/zenodo.10653704 (2024).
Download references
Acknowledgements
We thank all lab members for their discussions and thank all tasting panel members for their contributions. Special thanks go out to Dr. Karin Voordeckers for her tremendous help in proofreading and improving the manuscript. M.S. was supported by a Baillet-Latour fellowship, L.C. acknowledges financial support from KU Leuven (C16/17/006), F.A.T. was supported by a PhD fellowship from FWO (1S08821N). Research in the lab of K.J.V. is supported by KU Leuven, FWO, VIB, VLAIO and the Brewing Science Serves Health Fund. Research in the lab of T.W. is supported by FWO (G.0A51.15) and KU Leuven (C16/17/006).
Author information
These authors contributed equally: Michiel Schreurs, Supinya Piampongsant, Miguel Roncoroni.
Authors and Affiliations
VIB—KU Leuven Center for Microbiology, Gaston Geenslaan 1, B-3001, Leuven, Belgium
Michiel Schreurs, Supinya Piampongsant, Miguel Roncoroni, Lloyd Cool, Beatriz Herrera-Malaver, Florian A. Theßeling & Kevin J. Verstrepen
CMPG Laboratory of Genetics and Genomics, KU Leuven, Gaston Geenslaan 1, B-3001, Leuven, Belgium
Leuven Institute for Beer Research (LIBR), Gaston Geenslaan 1, B-3001, Leuven, Belgium
Laboratory of Socioecology and Social Evolution, KU Leuven, Naamsestraat 59, B-3000, Leuven, Belgium
Lloyd Cool, Christophe Vanderaa & Tom Wenseleers
VIB Bioinformatics Core, VIB, Rijvisschestraat 120, B-9052, Ghent, Belgium
Łukasz Kreft & Alexander Botzki
AB InBev SA/NV, Brouwerijplein 1, B-3000, Leuven, Belgium
Philippe Malcorps & Luk Daenen
You can also search for this author in PubMed Google Scholar
Contributions
S.P., M.S. and K.J.V. conceived the experiments. S.P., M.S. and K.J.V. designed the experiments. S.P., M.S., M.R., B.H. and F.A.T. performed the experiments. S.P., M.S., L.C., C.V., L.K., A.B., P.M., L.D., T.W. and K.J.V. contributed analysis ideas. S.P., M.S., L.C., C.V., T.W. and K.J.V. analyzed the data. All authors contributed to writing the manuscript.
Corresponding author
Correspondence to Kevin J. Verstrepen .
Ethics declarations
Competing interests.
K.J.V. is affiliated with bar.on. The other authors declare no competing interests.
Peer review
Peer review information.
Nature Communications thanks Florian Bauer, Andrew John Macintosh and the other, anonymous, reviewer(s) for their contribution to the peer review of this work. A peer review file is available.
Additional information
Publisher’s note Springer Nature remains neutral with regard to jurisdictional claims in published maps and institutional affiliations.
Supplementary information
Supplementary information, peer review file, description of additional supplementary files, supplementary data 1, supplementary data 2, supplementary data 3, supplementary data 4, supplementary data 5, supplementary data 6, supplementary data 7, reporting summary, source data, source data, rights and permissions.
Open Access This article is licensed under a Creative Commons Attribution 4.0 International License, which permits use, sharing, adaptation, distribution and reproduction in any medium or format, as long as you give appropriate credit to the original author(s) and the source, provide a link to the Creative Commons licence, and indicate if changes were made. The images or other third party material in this article are included in the article’s Creative Commons licence, unless indicated otherwise in a credit line to the material. If material is not included in the article’s Creative Commons licence and your intended use is not permitted by statutory regulation or exceeds the permitted use, you will need to obtain permission directly from the copyright holder. To view a copy of this licence, visit http://creativecommons.org/licenses/by/4.0/ .
Reprints and permissions
About this article
Cite this article.
Schreurs, M., Piampongsant, S., Roncoroni, M. et al. Predicting and improving complex beer flavor through machine learning. Nat Commun 15 , 2368 (2024). https://doi.org/10.1038/s41467-024-46346-0
Download citation
Received : 30 October 2023
Accepted : 21 February 2024
Published : 26 March 2024
DOI : https://doi.org/10.1038/s41467-024-46346-0
Share this article
Anyone you share the following link with will be able to read this content:
Sorry, a shareable link is not currently available for this article.
Provided by the Springer Nature SharedIt content-sharing initiative
By submitting a comment you agree to abide by our Terms and Community Guidelines . If you find something abusive or that does not comply with our terms or guidelines please flag it as inappropriate.
Quick links
- Explore articles by subject
- Guide to authors
- Editorial policies
Sign up for the Nature Briefing: Translational Research newsletter — top stories in biotechnology, drug discovery and pharma.

“Something Works” in U.S. Jails: Misconduct and Recidivism Effects of the IGNITE Program
A longstanding and influential view in U.S. correctional policy is that “nothing works” when it comes to rehabilitating incarcerated individuals. We revisit this hypothesis by studying an innovative law-enforcement-led program launched in the county jail of Flint, Michigan: Inmate Growth Naturally and Intentionally Through Education (IGNITE). We develop an instrumental variable approach to estimate the effects of IGNITE exposure, which leverages quasi-random court delays that cause individuals to spend more time in jail both before and after the program’s launch. Holding time in jail fixed, we find that one additional month of IGNITE exposure reduces within-jail misconduct by 49% and reduces three-month recidivism by 18%, with the recidivism effects growing over time. Surveys of staff and community members, along with administrative test score records and within-jail text messages, suggest that cultural change and improved literacy and numeracy scores are key contributing mechanisms.
We thank Mauricio Cáceres Bravo, Jennifer Doleac, Sara Heller, Adriana Lleras-Muney, Matthew Mizel, Michael Mueller-Smith, Matthew Pecenco, Evan Rose, Nico Rotundo, Yotam Shem-Tov, and seminar participants at Harvard University, Opportunity Insights, the University of Connecticut, the University of Michigan, the NBER Economics of Crime Working Group, and the University of California Los Angeles for helpful comments. We are grateful to Meghan Beal at the National Sheriffs’ Association, Superintendent Mickie Kujat and Principal Brad Basista of Mt. Morris Consolidated Schools, and to the administration and incarcerated individuals of Genesee County and Saginaw County Jails—especially Deputy Annie Bueche, Undersheriff Mike Gomez, Major Jason Gould, Lieutenant Ebony Rasco, and Sheriff Christopher Swanson. We gratefully acknowledge funding from Arnold Ventures. Xingyou Ye, Miguel Purroy, Meera Mody, Anne Fogarty, Jeongmook Lim, and Michelle Wu provided expert research assistance. The views expressed herein are those of the authors and do not necessarily reflect the views of the National Bureau of Economic Research.
MARC RIS BibTeΧ
Download Citation Data
Working Groups
Conferences, more from nber.
In addition to working papers , the NBER disseminates affiliates’ latest findings through a range of free periodicals — the NBER Reporter , the NBER Digest , the Bulletin on Retirement and Disability , the Bulletin on Health , and the Bulletin on Entrepreneurship — as well as online conference reports , video lectures , and interviews .
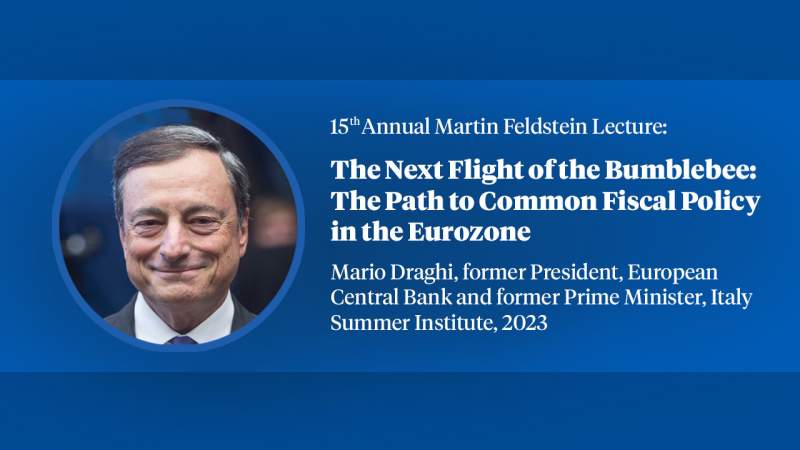
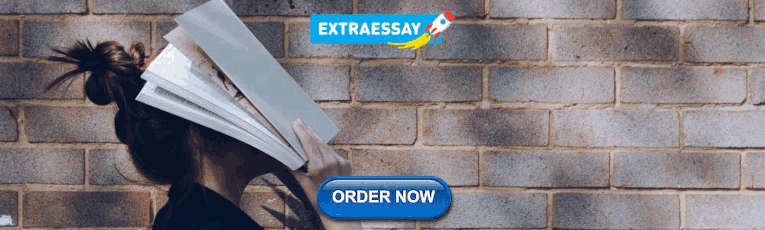
COMMENTS
Experimental research serves as a fundamental scientific method aimed at unraveling. cause-and-effect relationships between variables across various disciplines. This. paper delineates the key ...
Study, experimental, or research design is the backbone of good research. It directs the experiment by orchestrating data collection, defines the statistical analysis of the resultant data, and guides the interpretation of the results. When properly described in the written report of the experiment, it serves as a road map to readers, 1 helping ...
Abstract. Experimental research is a study that strictly adheres to a scientific research design. It includes a hypothesis, a variable that can be manipulated by the researcher, and variables that can be measured, calculated and compared. Most importantly, experimental research is completed in a controlled environment.
Step 1: Define your variables. You should begin with a specific research question. We will work with two research question examples, one from health sciences and one from ecology: Example question 1: Phone use and sleep. You want to know how phone use before bedtime affects sleep patterns.
Experimental science is the queen of sciences and the goal of all speculation. Roger Bacon (1214-1294) Download chapter PDF. Experiments are part of the scientific method that helps to decide the fate of two or more competing hypotheses or explanations on a phenomenon. The term 'experiment' arises from Latin, Experiri, which means, 'to ...
Writing an experimental research paper follows the principles and structure detailed in Chap. 4. However, there are some aspects especially important for reports about experimental research projects or (partly) different from reports about other research projects. We address these idiosyncrasies following the standard structure of a scientific ...
Methods. Using an experimental design we photographed the faces of 23 adults (mean age 23, range 18-31 years, 11 women) between 14.00 and 15.00 under two conditions in a balanced design: after a normal night's sleep (at least eight hours of sleep between 23.00-07.00 and seven hours of wakefulness) and after sleep deprivation (sleep 02.00-07.00 and 31 hours of wakefulness).
Experimental research design is centrally concerned with constructing research that is high in causal (internal) validity. Randomized experimental designs provide the highest levels of causal validity. Quasi-experimental designs have a number of potential threats to their causal validity. Yet, new quasi-experimental designs adopted from fields ...
present paper is to explicate the logic of experimental design and why it is so vital to questions that demand causal conclusions. In addition, types of internal ... relationships among variables as experimental research" (Gall et al., 2005, p. 249). There are three designs that meet the characteristics of true experimental
Abstract. Experimental protocols are key when planning, performing and publishing research in many disciplines, especially in relation to the reporting of materials and methods. However, they vary in their content, structure and associated data elements. This article presents a guideline for describing key content for reporting experimental ...
This article aims to present general guidelines to one of the many roles of a neurosurgeon: Writing an experimental research paper. Every research report must use the "IMRAD formula: introduction, methods, results and discussion". After the IMRAD is finished, abstract should be written and the title should be "created".
Collect the data by using suitable data collection according to your experiment's requirement, such as observations, case studies , surveys , interviews, questionnaires, etc. Analyse the obtained information. Step 8. Present and Conclude the Findings of the Study. Write the report of your research.
Dreaming is still a mystery of human cognition, although it has been studied experimentally for more than a century. Experimental psychology first investigated dream content and frequency. The neuroscientific approach to dreaming arose at the end of the 1950s and soon proposed a physiological substrate of dreaming: rapid eye movement sleep.
What is the purpose of an experimental research paper? A research paper is intended to inform others about advancement in a particular field of study. The researcher who wrote the paper identified a gap in the research in a field of study and used their research to help fill this gap. The researcher uses their paper to inform others about the ...
Writing the Experimental Report: Overview, Introductions, and Literature Reviews; Writing the Experimental Report: Methods, Results, and Discussion; Tables, Appendices, Footnotes and Endnotes; References and Sources for More Information; APA Sample Paper: Experimental Psychology; Suggested Resources
Summary. When research psychologists talk about writing a paper, they are talking about a lengthy and complicated chain of events that includes a great deal more than just reporting research results. In this chapter, we outline these events from start to finish: PLANNING EXPERIMENTAL RESEARCH. Getting an Idea.
There are 3 types of experimental research designs. These are pre-experimental research design, true experimental research design, and quasi experimental research design. 1. The assignment of the control group in quasi experimental research is non-random, unlike true experimental design, which is randomly assigned. 2.
A research design is a strategy for answering your research question using empirical data. Creating a research design means making decisions about: Your overall research objectives and approach. Whether you'll rely on primary research or secondary research. Your sampling methods or criteria for selecting subjects. Your data collection methods.
View a PDF of the paper titled ReALM: Reference Resolution As Language Modeling, by Joel Ruben Antony Moniz and 7 other authors. View PDF HTML (experimental) Abstract: Reference resolution is an important problem, one that is essential to understand and successfully handle context of different kinds. This context includes both previous turns ...
To our knowledge, no previous research gathered data at this scale (250 samples, 226 chemical parameters, 50 sensory attributes and 5 consumer scores) to disentangle and validate the chemical ...
We know from more recent ecological research that the relationships between black and brown rats are complex and influenced by a wide range of biotic and abiotic factors (13-15).For instance, in some areas of the globe, the larger and more aggressive brown rat is thought to outcompete its smaller cousin [leading to geographical species distribution patterns driven by competitive exclusion of ...
The huge transverse Lorentz force experienced by CORC cable during the operation of fusion projects may cause irreversible degradation of its current-carrying capacity. Therefore, it is particularly important to study the influence of cable parameters on their transverse compression performance, and use these influence laws to adjust cable parameters to improve the critical value of transverse ...
We analyze how expropriation risk reduces incentives for innovation and reallocates resources from the innovative sector, building on Romer's(1990) model. Our framework predicts the R&D expenditure, the share of human capital in R&D, the number of patents, technical progress, and economic growth ...
Founded in 1920, the NBER is a private, non-profit, non-partisan organization dedicated to conducting economic research and to disseminating research findings among academics, public policy makers, and business professionals.
Seattle's AI2 Incubator launched a new online forum called Harmonious for discussing research papers and advances related to artificial intelligence, saying the aim was to cut through the glut ...
Our experimental results demonstrate that our strategy significantly surpasses existing methods in enhancing the reconstruction of sparse marine scenes. ... Feature papers represent the most advanced research with significant potential for high impact in the field. A Feature Paper should be a substantial original Article that involves several ...