- Open access
- Published: 21 June 2022
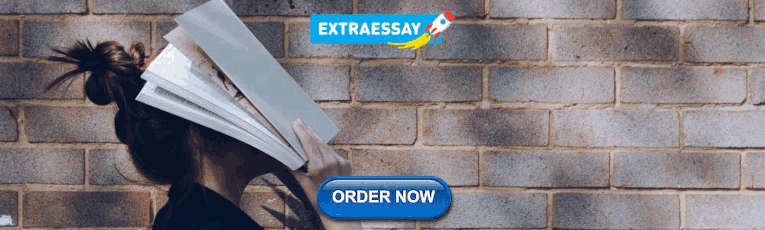
The impact of macroeconomic factors on food price inflation: an evidence from India
- Asharani Samal ORCID: orcid.org/0000-0001-8275-0267 1 ,
- Mallesh Ummalla 2 &
- Phanindra Goyari 1
Future Business Journal volume 8 , Article number: 15 ( 2022 ) Cite this article
15k Accesses
4 Citations
Metrics details
The present study investigates the impact of macroeconomic factors on food price inflation in India utilizing the monthly time series during January 2006–March 2019. The long-run relationship is confirmed among the variables using the ARDL bounds testing approach to cointegration. The coefficients of long-run estimates show that per capita income, money supply, global food prices, and agricultural wages are positively and significantly impacted food price inflation in both the short and long-run. While food grain availability has a negative and significant impact on food price inflation in both the short-run and long run. Further, the short-run estimates revealed that real exchange rate positively impacts food price inflation. However, the coefficient is insignificant in the short-run. The Granger causality estimates show that a short-run bidirectional causality is confirmed among per capita income, the exchange rate, per capita net availability of food grain and food price inflation. Further, there is evidence of unidirectional causality running from global food prices to food price inflation. However, there is no causal relationship running from money supply and agricultural wages to food price inflation in the short-run.
Introduction
The main objective of monetary policy in any economy is to maintain price stability. However, high food price inflation affects not only macroeconomic stability but also small farmers and poor consumers of the developing country where poor people spend their massive portion of income on food consumption. Agricultural commodity price volatility negatively impacts all societies by causing macroeconomic instability; specifically, it affects the impoverished that spend a large portion of their resources on food and fuel [ 47 ]. Therefore, high food price inflation has become a significant concern among the researchers and policymakers in determining responsible factors to surge in food price inflation. The high food price inflation has been experienced in the recent period due to increasing demand for biofuels in many developed countries, increasing demand for various diets among newly prosperous populations as compared to the production of such foodstuff, rise in minimum support prices, rapid regional economic growth, increasing the cost of fertilizers and other inputs, rising oil prices, etc.
Agriculture is very competitive in producing homogenous goods, given its vulnerability and high dependence on monsoon. It also contributes 17% of gross domestic product and employs more than 50% of the population. However, the contribution of the agricultural sector to GDP has been declining substantially since 2014, and the growth of agriculture is likely to increase by 2.1% in 2018–19 [ 23 ]. Further, price of agricultural products is more volatile than the non-agricultural sector due to high dependence on climate change. Therefore, attention should be given to the agricultural sector and the behavior of agriculture prices, especially for developing countries like India, where the majority of the population depends on agriculture. The persistent and high food price inflation over the period has gained more extensive attention in India by the researchers and policymakers as food price inflation has been the major contributor behind the increase in overall Wholesale Price Index (WPI) inflation in India [ 2 ]. Further, agricultural price is susceptible to relative changes in input prices, supply factors, etc.
Theoretically, rising food prices are basically due to two factors in the literature, i.e., real and monetary shocks. These are explained by structuralist and monetarist approaches, respectively. According to structuralists, the money supply is passive, and the real shocks in a particular sector tend to upsurge in food price inflation. Hence, inflation occurs in the prices of other goods. However, monetarists argued that inflation could arise through an autonomous increase in money supply via generating aggregate demand, which increases the relative price of commodities. Therefore, an increase in money supply is a cause for inflation, not necessarily by real shocks.
However, the developing countries like India are not exceptional from higher food prices and macro-economic instability. Since the 1991 economic reforms, the Indian economy has maintained a single-digit economic growth rate and moderate inflation. However, in recent years, one of the major problems that the Indian economy is facing is higher food price inflation. The WPI food price inflation was documented 10.20% during January 2008–July 2010 [ 33 ]. Further, CPI-IW for food was experienced at 8.05% during 2006–2019 while it was recorded at 13%, especially in 2013. However, the growth rate of gross food grain production was 2.66% during this period. The demand for food commodities increases at a higher rate due to the high economic growth rate (7–9%) per annum. In contrast, the annual growth of agriculture is relatively low (1.5%) compared to the service sector and GDP growth [ 40 ]. The total investment in agriculture has been reduced from 2.43 to 1.28% during 1979–80 to 2007–08 period [ 28 ]. The expenditure on subsidies, maintenance of existing projects, the relatively lower allocation for irrigation, rural infrastructure and research, lack of adequate credit support, and credit infrastructure in rural areas are the drivers of slow growth in public investment in agriculture [ 43 ]. Given this high food price inflation, researchers and policymakers have raised severe concern about reducing the food price inflation because most of the population spend half of the income on food expenditure, and food containing a larger share in the CPI basket. Therefore, it is necessary to find the causes and suitable majors to reduce food price inflation.
The present study contributes to food price inflation literature in several ways. First, a wide range of studies has investigated the drivers of food price inflation in India. The various demand and supply-side factors, namely, per capita income, growth of money supply, changing patterns of the consumer's dietary habits, high agricultural wages, speculations, and low growth of agricultural productions, are accountable for high food inflation. However, the results are ambiguous and vary considerably across countries due to different data periods and econometric methodologies applied in their studies. Second, the change in macroeconomic factors may have a substantial effect on food price inflation. For instance, if the food prices positively impact money supply, the consumer suffers from welfare loss. If it negatively effects on food prices, the producer suffers from welfare loss. However, this relationship of macroeconomic factors has not empirically analyzed significantly with respect to food price inflation in India. Third, various studies have explored the impact of macroeconomic factors on food price inflation across the world. For example, Kargbo [ 24 ] for Eastern and Southern Africa, Kargbo [ 25 ] for West Africa, Reziti [ 38 ] for Greek, Kargbo [ 26 ] for South Africa, Yu [ 46 ] for China and Sasmal [ 40 ]. Nevertheless, few studies have empirically examined the impact of selected macroeconomic factors on food price inflation by incorporating a control variable like per capita net availability of food grain into account. To the best of my knowledge, there is no study existing in the context of India. Fourth, most of the studies have taken WPI food indices, food items from only primary food articles or some of the index of selected food items, such as cereals and pulses, fruits and vegetables, milk and milk products, egg, meat, and fish as a measure of food price inflation. However, the present study has used the combined price index for industrial worker food (CPI-IW-F). Fifth, numerous studies have concluded that food price inflation is triggered by supply-side factors (see, [ 11 , 17 , 33 ]). However, to examine the rise in food price inflation, we have included both demand and supply-side factors in our study. Six, the present study also considered that food price inflation is not only influenced by domestic factors but also by global factors. More specifically, changes in global food prices and the exchange rate might positively and significantly impact food price inflation. However, the effect of these external factors on food price inflation does not explain the extent of food price inflation driven by domestic supply-side factors. For this purpose, we have included the per capita net availability of food grain as a control variable in the model. Suppose the demand for agricultural food items rises remarkably owing to a surge in macroeconomic factors. However, the supply of food items failed to meet the demand proportionately, then food items prices will go up. Therefore, the goal of the present study is to analyze the long-run and short-run impact of macroeconomic factors on food price inflation and verify the causal relationship aspect of these variables in the case of India over the period January 2006–March 2019.
The remaining of the paper is structured as follows. “ Literature review ” section follows the review of the literature on the relationship between macroeconomic factors and food price inflation. “ Methods ” section represents data and methodology. “ Results and discussion ” section discusses the results of the study. “ Conclusions ” section provides conclusive remarks and policy implications.
Literature review
This paper aims to examine the impact of macroeconomic factors on food price inflation. This section provides a review of the literature to establish the empirical basis of the link between macroeconomic factors and food price inflation.
Per capita income and food price inflation
Per capita income has a positive impact on food price inflation via increasing purchasing power of the money in the hands of the people, which leads to a surge in demand for food items resulting in a rise in food prices. Carrasco and Mukhopadhyay [ 10 ] argued that per capita income is positively affected food prices in three South Asian economies. However, the decline in agricultural production increases food prices up, and magnitudes are varying across countries. Agrawal and Kumarasamy [ 1 ] documented that food price inflation rose with the response to increases in India’s per capita income. They also suggested a 1% surge in per capita income upsurges the demand for fruits, vegetables, milk, and edible oil by 0.55–0.65%, and animal products by 0.38%. However, it reduces the demand for cereals and pulses by 0.05% and 0.20%, respectively. Joiya and Shahzad [ 22 ] and Sasmal [ 40 ] reported the same findings in Pakistan and India, respectively. In contrast, the study also found a negative association between food price inflation and per capita income. Kargbo [ 24 ] in Ethiopia and Malawi among Eastern and Southern African countries, Kargbo [ 25 ] for Cote d'Ivoire of West African countries.
Money supply and food price inflation
An increase in the money supply positively affects food price inflation through market credit facility by generating aggregate demand. However, it reduces food prices by creating investment via availing credit to the producer. Numerous studies have investigated the impact of money supply on food price inflation across the world. For example, Mellor and Dar [ 29 ] found that the expansion of money supply largely determines upward pressure on food grains prices. Barnett et al. [ 5 ] found that money supply positively affects food inflation and agricultural commodity prices in the U.S. Similar results were found by Kargbo [ 26 ] and Asfaha and Jooste [ 3 ] for South Africa. Further, Bhattacharya and Jain [ 8 ] concluded that an unexpected monetary tightening induces food price inflation in emerging and developed countries. However, a negative relationship is established between money supply and food prices for Kargbo [ 24 ], Kargbo [ 25 ], and Yu [ 46 ] for Eastern and Southern African countries and West African countries and China.
Exchange rate and food price inflation
The depreciation of the real exchange rate increases food price inflation by expanding the cost of importing petroleum products, fertilizer, and other finished products relating to agricultural commodities, leading to rising domestic market prices. In other words, depreciation of the exchange rate directly affects the agricultural sectors vi changing the prices of tradable and non-tradable goods resulting in a change in the prices of agricultural products in favor of the farmer. Taylor and Spriggs [ 45 ] showed that the exchange rate has a greater influence on volatility of agricultural prices in Canada. Similarly, Mitchell [ 30 ] and Mushtaq et al. [ 32 ] and Iddrisua and Alagidede [ 19 ] also concluded that the depreciation of the exchange rate is positively affected food prices in the U.S., Pakistan and South Africa, respectively. In contrast to this, Cho et al. [ 12 ] confirmed that change in the exchange rate has a negative impact on relative agricultural prices. However, Sasmal [ 40 ] found no significant relationship between the exchange rate and food price inflation in India during 1971–2012.
Global food price and food price inflation
The increase in the global food price of the commodity can influence the domestic price via international trade mechanism. The export increases as the global food price increases resulting in a decrease in domestic market supply followed by a hike in prices. On the contrary, the rise in import raises the domestic substitute food item’s price followed by a surge in domestic market price. Robles [ 39 ] indicated that the international prices transmission has a positive impact on the domestic agricultural market in Asian and Latin American countries. Gulati and Saini [ 16 ] revealed that the global food price index is positively impacted food price inflation in India. Similarly, Baltzer [ 4 ] states that an increase in international prices promotes domestic prices in the case of Brazil and South Africa. However, the price.
transmission is very limited in China and India. Lee and Park [ 27 ] confirmed that the lagged values of global food price inflation are positively impacted food price inflation in 72 countries. Selliah et al. [ 41 ] revealed that an increase in global food price increases domestic food prices in both the short and long run in Sri Lanka. Similarly, Holtemöller and Mallick [ 17 ], Bhattacharya and Sen Gupta [ 6 ], and Huria and Pathania [ 18 ] also documented that global food price shocks have a significant and positive inflationary trend on food inflation in India. However, Rajmal and Mishra [ 37 ] pointed out a limited transmission of prices from international food prices to domestic prices in India.
Agricultural wage and food price inflation
One of the significant public work programs is the National Rural Employment Guarantee (NREG), which promotes the real daily agricultural wage rates. On the one hand, an increase in rural wages can induce food prices by increasing production costs. On the other hand, it raises food prices via higher purchasing power, resulting in higher wages, which boosts the demand for food items. Gulati and Saini [ 16 ] revealed that domestic farm wages are positively associated with food price inflation in India. Goyal and Baikar [ 15 ] showed that the rapid increase in MGNREGA wages when it merged with inflation boosts agricultural wages rather than the implementation of MGNREGA across India. Bhattacharya and Sen Gupta [ 6 , 7 ] examined the drivers of food price inflation in India over the period 2006–2013. The structural vector error correction model (SVECM) showed that agricultural wage inflation promotes food price inflation after the implementation of MNGREGS.
The above literature review shows that both demand and supply-side factors have contributed to food price inflation. Many studies have investigated the impact of macroeconomic factors on food price inflation across the globe. However, only a few studies have been directed, which empirically examined the effect of macroeconomic factors on food price inflation by incorporating per capita net availability of food grain and agricultural wages in a multivariate framework. So far as we know, there is no study available in the case of India in this regard employing monthly data over the period January 2006–March 2019. Hence, our study attempts to fill this gap.
The present study makes use of monthly time series data on per capita GDP (Y), real exchange rate (EX), money supply (M3), global food price index (GF), per capita net availability of food grain (NFG), agricultural wages (AW) and combined price index-industrial workers for food (CPI-IW-F) indices as a proxy for food price index (FP) during January 2006–March 2019. The data on per capita GDP, real exchange rate, money supply is collected from the Reserve Bank of India (RBI), whereas combined price index-industrial workers for food and agricultural wages are retrieved from the Ministry of Labor Bureau, Government of India. The data on per capita net availability of food grain and real global food price index are obtained from the Directorate of Economics and Statistics, Department of Agriculture & Farmers Welfare, Government of India, and the Food and Agriculture Organization of the United Nations, respectively. Monthly data on per capita net availability of food grain and per capita GDP is not available in the case of India. Therefore, we have used the linear interpolation method to get the monthly data for this variable. Footnote 1 The selection of the data period has been considered based on the availability of uniform and consistent monthly data over a period of time. We use high-frequency data while working on macroeconomic variables to capture the true impact of it [ 31 ]. Further, data on food price inflation is volatile, measuring the impact of macroeconomic factors on food inflation using high-frequency data, namely, weekly and monthly, provides accurate estimates rather than annual series. Since, data on a targeted variable i.e., food inflation is not available on a weekly basis for a longer period in the case of India. Therefore, we have used monthly data for this purpose.
The real exchange rate (EX) is measured as the real effective exchange rate which is trade based weighted average value of Indian currency against 36-currency bilateral weights, per capita income (Y) is measured as the percentage change in per capita gross domestic product, money supply (MS) is measured as broad money (MS), global food prices (GF) are measured as a real global food price index, and agricultural wages (AW) is measured as average daily wage rates from agricultural occupations; per capita net availability of food grain (NFG) is measured as gross production plus net imports plus stocks. Finally, food price inflation (FP) is measured as combined price index-industrial workers for food index (CPI-IW-F). The food inflation was experienced in India from 2006 onwards. However, the CPI-combined series is used and available from 2014 onwards to measure the official inflation rate. To get a more extended frequency of data on food inflation series, we have used consumer price index-industrial workers for food (CPI-IW-F) as a proxy for food inflation measures. We select to use CPI-IW-F because Bicchal and Durai [ 9 ] and Goyal [ 14 ] established that CPI-IW and CPI-combined have similar properties with CPI-IW is available for a more extended period. All the variables are seasonally adjusted using CENSUS X13 and converted into the natural logarithm form except per capita GDP.
Unit root tests
One should necessarily check the properties of all the variables before commencing any econometric techniques as it gives spurious and invalid results. The ARDL technique requires to check the integration properties of the selected variables to confirm that none of the variables should follow I (2) process, which seems to be invalid and unsuitable for applying the ARDL approach. Therefore, we use the ADF and PP tests to check the order of integration of the variables.
ARDL bounds testing approach to cointegration
We employ the ARDL bounds testing approach to cointegration propounded by Pesaran and Shin [ 35 ] and Pesaran et al. [ 36 ] in order to examine the long-run and short-run association between macroeconomic factors and food price inflation in India. This method is superior over other traditional approaches of Johansen and Juselius [ 21 ] and Johansen [ 20 ] cointegration on the following grounds. First, it is one of the most popular and flexible methods. It does not impose any restriction on any nature of data and can be applied irrespective of all the order of integration I (1) or I (0) or both mix. Second, as noted by Pesaran and Shin [ 35 ] that ARDL estimators give the true parameters, and coefficients are super consistent as compared to other long-run estimates, especially when the sample size is small. Third, it also helps to eradicate the problem of the endogeneity that appears in the model. Fourth, it is even able to capture both short-run and long-run estimates simultaneously. The unrestricted error correction models (UECM) of the ARDL model can be represented as follows:
where ∆ denotes first difference operator; \({\varepsilon}_{t}\) is the error term; \({\alpha }_{1}\) , \({\alpha }_{2}\) , \({\alpha }_{3}\) , \({\alpha }_{4}\) , \({\alpha }_{5}\) , \({\alpha }_{6}\) , and \({\alpha }_{7}\) are the constant; \({\alpha }_{F}\) , \({\alpha }_{Y}\) , \({\alpha }_{M}\) , \({\alpha }_{E}\) , \({\alpha }_{G}\) , \({\alpha }_{NF}\) , and \({\alpha }_{A}\) are the long-run coefficients; \({\beta }_{h}\) , \({\beta }_{i}\) , \({\beta }_{j}\) , \({\beta }_{k},{\beta }_{l}, {\beta }_{m}\) and \({\beta }_{n}\) are the short-run coefficients.
The optimal lag selection has been made based on the Akaike Information Criteria (AIC). The primary step in the ARDL model is to estimate the Eqs. ( 1 – 7 ) by ordinary least squares (OLS). The long-run relationship is determined based on the F test or Wald test for the coefficient of the lagged levels of the variables. The null hypothesis of no long-run relationship, \({H}_{0}:{\alpha }_{F}={\alpha }_{Y}={\alpha }_{M}={\alpha }_{E}={\alpha }_{G}={\alpha }_{NF}={\alpha }_{A}=0\) against the alternative hypothesis of the long-run, \({H}_{1}:{\alpha }_{F}\ne {\alpha }_{Y}\ne {\alpha }_{M}\ne {\alpha }_{E}\ne {\alpha }_{G}\ne {\alpha }_{NF}\ne {\alpha }_{A}=0\) referred to the equation follows as (FP/Y, MS, EX, GF, NFG, AW). According to Pesaran et al. [ 37 ], the null hypothesis of no long-run association can be rejected if F -statistics is greater than the upper critical bound (UCB). It suggests that there is a long-run association among the variables. While, test statistics falls below the lower critical bound (LCB), null hypothesis cannot be rejected. It suggests that there is no long-run association among the variables. If the calculated value falls between the lower and upper critical points, the result is inconclusive. Because the two asymptotic critical values bound lower value (assuming the regressors are I (0)) and upper (assuming purely I (1) regressors) provide a test for cointegration.
After identifying the long-run relationship among the variables, our next step is to apply the vector error correction model to examine the directions of causality among the variables in both the short-run and long-run. The model of VECM can be written as follows.
where \(\Delta\) is the difference operator; \({ECM}_{t-1}\) is the lagged error correction term, which is derived from the long-run cointegration relationship; \({\varepsilon}_{1t}\) , \({\varepsilon}_{2t}\) , \({\varepsilon}_{3t}, {{\varepsilon}_{4t}, \varepsilon}_{5t}, {\varepsilon}_{6t}\) and \({\varepsilon}_{7t}\) are the random errors; \({\gamma }_{1}\) , \({\gamma }_{2}\) , \({\gamma }_{3, }{\gamma }_{4, }{\gamma }_{5, }{ \gamma }_{6}\) and \({\gamma }_{7}\) are the speed of adjustments. The long-run relationship among the variables indicates that there is a presence of Granger-causality at least one direction, which is determined by F -statistics and lagged error correction term. The short-run causal relationship is represented by F- statistics on the explanatory variables while long-run causal relationship is represented by t-statistics on the coefficient of the lagged error correction term. The error correction term ( \({ECT}_{t-1}\) ) is negative and statistically significant (t-statistic) at the 1% significance level.
Results and discussion
Preliminary analysis.
A preliminary analysis is conducted using commonly used descriptive statistics. We also reported the summary of descriptive statistics of all the considered variables during the study period in Table 1 . The results revealed that the average food price index and the real exchange rate is 5.375% and 4.687% during the study period. However, the average money supply and real global food price index is 11.185% and 4.619%. The per capita net availability of food grain and agricultural wages is 5.130% and 6.931% whereas, per capita income is 0.445%, which is lower than other variables across the sample period. The results of the correlation matrix are represented in Table 2 . The correlation analysis results revealed that per capita income, money supply, real exchange rate, real global food price index, per capita net availability of food grain and agricultural wages are positively associated with food price inflation. For instance, food price inflation is highly correlated with per capita income, money supply, real exchange rate, per capita net availability of food grain, and agricultural wages. It suggests that macroeconomic factors might be promoting food price inflation in India. Similarly, per capita income is positively correlated with money supply, exchange rate, and per capita net availability of food grain, and agricultural wages. Further, there is a high positive correlation between agricultural wages and per capita net availability of food grain.
Results of unit root tests
To avoid spurious and invalid results of all the non-stationary data, we have checked the integration properties of all the variables and confirm that none of the variables follows the I (2) process. Therefore, the ADF and PP unit root tests are used to check the order of integrations of the variables. The results of unit root tests are reported in Table 3 . It indicates that food price inflation (FP), per capita income (Y), money supply (MS), real exchange rate (EX), real global food price index (GF), per capita net availability of food grain (FG) and agricultural wages (AW) are integrated of order I (1).
Results of ARDL cointegration tests
The above unit root test results show that all variables follow a same order of integration, i.e., I (1). Therefore, we apply the ARDL technique to check the long-run relationship among the variables using Eqs. ( 1 )-( 7 ) during January 2006–March 2019. Here, the optimal lag length is 2, according to VAR lag order selection criteria. The results of the ARDL model are presented in Table 4 . The result shows that calculated F -statistics (4.155) is larger than UCB at the 5% level of significance when food price inflation is considered a dependent variable (FP/Y, MS, EX, GF, NFG, WA). It indicates that there is a long-run relationship among food price inflation (FP) and per capita income (Y), money supply (MS), real exchange rate (EX)), global food prices (GF), per capita net availability of food grain (NFG), and agricultural wages (WA). Likewise, calculated F- statistics (11.043) is also larger than UCB at the 5% level of significance when per capita income is considered a dependent variable and integrated order (1). Therefore, UCB is applied to establish a long-run relationship among the variables. Likewise, calculated F- statistics (10.239) is also larger than UCB at the 5% level of significance when money supply (MS) is considered a dependent variable. Similarly, calculated F- statistics (3.335) is also larger than UCB at the 10% level of significance when global food price (GF) is considered a dependent variable. However, calculated F -statistics is lower than UCB when the exchange rate (EX), per capita net availability of food grain (NFG), and agricultural wages (AW) serve as dependent variables. It suggests a there is no long-run relationship among the variables when the exchange rate, per capita net availability of food grain and agricultural wages are the dependent variables.
Results of long-run and short-run estimates
The cointegration test results based on the ARDL model revealed the long-run equilibrium relationship among the variables. However, these results do not explain the cause-and-effect association among the food price inflation and macroeconomic factors, namely, per capita income, money supply, exchange rate, global food prices, per capita net availability of food grains, and agricultural wages. Hence, we have investigated the impact of macroeconomic factors on food price inflation in this part. It is better to check the long-run effect of macroeconomic factors on food price inflation after confirming the cointegration relationship among the variables when food price inflation is considered the dependent variable. The results of the long-run analysis are reported in Table 5 in panel-I. The long-run results illustrate that per capita income is positively and significantly impacted food price inflation. It implies that a one unit increase in per capita income induces food price inflation by 0.14 unit. The rise in per capita income raises the purchasing power of the money, which leads to a surge in demand for food items resulting in a hike in food prices. The results of our study similar to Carrasco and Mukhopadhyay [ 10 ] in three South Asian economies, Agrawal and Kumarasamy [ 1 ] in India, Joiya and Shahzad [ 22 ] in Pakistan and Sasmal [ 40 ] in India. However, our result is inconsistent with Kargbo [ 24 ] and Kargbo [ 25 ], who revealed a negative relation between the variables in Ethiopia and Malawi, and in Cote d'Ivoire, respectively.
Similarly, a 1% increase in money supply increases food price inflation by 0.36%. It implies that the rise in money supply puts upward pressure on food price inflation and is significant at the 1% level of significance. Money supply is positively affecting food price inflation by generating aggregate demand in the market, which pushes the food prices up. This finding is consistent with Kargbo [ 24 ] for Kenya, Sudan, and Tanzania among the Eastern and Southern African countries and contradictory with Sasmal [ 40 ] who did not find any long-run relationship between money supply and food price inflation in India and Yu [ 46 ] for China who confirmed that monetary policy expansion has a negative impact on prices of seven major food products in the long-run. Similarly, a rise in the real exchange rate has a downward pressure on food price inflation. It indicates that a 1% increase in the real exchange rate will have a negative impact on food price inflation by 0.30%. The increase in the real exchange rate reduces food prices by lowering the import of petroleum products, fertilizer, and other products relating to agricultural commodities in the long run. Hence, organic fertilizers can be used to produce commercial food products to reduce the dependency on fertilizers, which may maintain price stability and reduce the negative welfare impact on food prices. This outcome is consistent with Cho et al. [ 12 ] and is inconsistent with Iddrisua and Alagidede [ 19 ] in South Africa, Durevall et al. [ 13 ] in Ethiopia. Further, per capita net availability of food grain has a negative impact on food price inflation. In other words, there is an inverse relationship between per capita net availability of food grains and food price inflation in India. It suggests that a 1% increase in per capita net availability of food grains reduces food price inflation by 0.69%. The increase in the supply of net food availability in the domestic market by increasing food production can reduce food price inflation. On the other hand, if the supply of food grain availability declines due to crop failure, it increases food price inflation. Therefore, the government should increase domestic food production and reduce the exports of commodities at the time of food inflation to maintain stability in prices. Further, agricultural production is seasonal, and it is highly correlated to the month of food harvest. The stock of food grain during harvest season can avoid the off seasonal food price inflation. Increasing the stock of food items by establishing a larger cold storage system and strengthen and widening the existing warehouses can also help to control food inflation in India. This result is similar to Kargbo [ 25 ] in Cote d’Ivoire and Nigeria and Carrasco and Mukhopadhyay [ 10 ] in three South Asian economies and inconsistent with Sasmal [ 40 ], who failed to establish a significant relationship between agricultural food production and food price inflation in India in the long-run. Furthermore, our results revealed that food price inflation rose with the response to increases in global food prices. It suggests that a 1% surge in global food price upsurges food price inflation by 0.13%. This result is consistent with Selliah et al. [ 41 ] for Sri Lanka, Holtemöller and Mallick [ 17 ] for India, and Huria and Pathania [ 18 ] for India. However, Rajmal and Mishra [ 37 ] and Baltzer [ 4 ] pointed out a limited transmission of prices from international food prices to domestic prices in India. The extent of transmission of global food prices on price hike in the domestic market depends on at which magnitudes commodity’s international trade takes place. Finally, our study results also found that agricultural wages have a positive and significant impact on food price inflation at the 1% level of significance. It implies that a 1% surge in agricultural wages increases the food price inflation by 0.31% in the long-run. The rise in wage rate via welfare-oriented-schemes like MNGREGS increases the bargaining and purchasing power of money, increasing in demand for food items followed by increased food inflation. The increase in the agricultural wage rate should be substituted with food price inflation by increasing productivity. Hence, the increase in demand for food originated by a hike in the agricultural wage rate can be substituted by raising the productivity of each worker. A similar result is found by Bhattacharya and Sen Gupta [ 7 ] for India.
After having discussed long-run results, we shall move in to discuss with reference to the short-run. The results of the short-run analysis are reported in Table 5 in panel-II. The short-run analysis indicates an increase in per capita income and money supply is positively related to food price inflation in the short-run as the coefficients of these variables are statistically significant. Similarly, food price inflation rises with the increase in global food prices. Further, the real exchange rate has a positive impact on food price inflation in the short-run. However, the result is not significant. Moreover, agricultural wages have a positive impact on food price inflation. It implies that an increase in agricultural wages raises food price inflation in the short run. The outcome is consistent with Huria and Pathania [ 18 ] for India. However, per capita net availability of food grain is negatively and significantly impacted food price inflation. It suggests that a 1% increase in food grain availability decreases food price inflation by 0.11% in the short-run. In contrast, a decrease in the growth rate of food grain availability increases food price inflation. This finding is similar to Kargbo [ 25 ] in Cote d’Ivoire and Senegal among West African countries. Finally, the results also documented lagged food price inflation positively impacts present food price inflation. It suggests that a 1% increase in lagged food price upsurges food price inflation by 0.36% in the short run.
The sign of lagged ECT is negative and significant at the 1% level, which implies that short-run deviation from food prices can be restored toward the long-run equilibrium with a 16.8% speed. The model has satisfied all the diagnostic tests. This model is free from autoregressive conditional heteroscedasticity; the functional form of the model is well specified, which is represented by the Ramsey RESET coefficient.
Results of VECM Granger causality test
After identifying the long-run association between macroeconomic factors and food price inflation, we have employed the VECM Granger causality test to examine the directions of causality among the variables in both the short-run and long-run. The Granger causality results are represented in Table 6 . The outcomes of the short-run causality tests are obtained from the F -statistics of lagged independent variables, while the results of long-run causality are obtained from the negative and significant coefficients of t-statistics of lagged error correction term. The results are reported in Table 6 and show that a short-run bidirectional causality is confirmed between per capita income, exchange rate, and food price inflation at a 1% level. This finding is opposite of Sasmal [ 40 ], who reported a unidirectional causality running from per capita income to food price inflation in India. Similarly, a bidirectional causality is existed between percapita net availability of food grain and food price inflation in the short run. It implies that the increase in food grain availability reduces food price inflation by increasing domestic food grain production on the one hand. Whereas on the other hand, an increase in food price inflation also leads to a rise in food grain availability by rising demand for food products. Further, there is a unidirectional causality running from global food prices to food price inflation. It suggests that an increase in global price attracts exporters to increase their supply of food items to the global market to get high profit, which eventually decreases the domestic market supply, resulting an in a price increase. However, there is no causal relationship running from money supply and agricultural wages to food price inflation in the short run.
There is an existence of a bidirectional causal relationship between global food prices and per capita income. However, no causality runs from money supply, exchange rate, per capita net availability of food grain, and agricultural wages to per capita income. A short run unidirectional causality is established from food price inflation, exchange rate, global food prices, and per capita net availability of food grain to money supply. A bidirectional causality has existed between agricultural wages and money supply in the short run. Further, unidirectional causality is running from per capita income, the exchange rate to global food prices. A unidirectional causal relationship is found from per capita income, money supply, the exchange rate, and global food prices to per capita net availability of food grain in the short run. Moreover, short-run unidirectional causality is confirmed from food price inflation, per capita income, the exchange rate and global food prices to agricultural wages.
Moving to the long-run causality, the coefficients of \({ECM}_{t-1}\) are negative and statistically significant in Eq. ( 8 ), where money supply, global food prices, and per capita net availability of food grain are the dependent variables. Therefore, results revealed a bidirectional causality among the money supply, global food prices, and per capita net availability of food grain production in the long-run.
Conclusions
This study aimed to examine the impact of macroeconomic factors on food price inflation in India during January 2006–March 2019. To consider the short-run dynamics and the long-run analysis and directions of causality among the variables, we have applied the ARDL model and Granger causality test in our study. The ARDL results have shown evidence of the long-run association among the macroeconomic factors and food price inflation. The long-run result show that percapita income, money supply, global food price, and agricultural wages have a positive and significant impact on food price inflation of India in both the long-run and short run. However, the per capita net availability of food grain negatively impacts food price inflation. It implies that an increase in food grain availability reduces food price inflation in both the short and long run. Further, the real exchange rate is positively affecting food price inflation. However, it is insignificant in the short-run. The Granger causality estimates show that a short-run bidirectional causality is confirmed among per capita income, the exchange rate, per capita net availability of food grain, and food price inflation. Further, there is evidence of unidirectional causality running from global food prices to food price inflation. However, there is no causal relationship running from money supply and agricultural wages to food price inflation in the short run. The long-run results revealed a bidirectional causality among the money supply, global food prices and per capita net availability of food grain.
Given these results, the paper makes important contribution to the macroeconomic factors and food price inflation in India. The significant policy suggestions are that the growth in money supply promotes food price inflation in the long-run, which affects the welfare of the poor consumer as the majority of the people depend on agriculture. It also positively affects market credit facility by generating aggregate demand followed by changes in relative prices across commodities, which push the food prices up. Therefore, the government should adopt effective policy measures to protect consumers from higher food prices. These are the effectively implementation of public distribution systems, policies for food security, and reducing the money supply via adopting a contractionary monetary policy, which eventually reduces food price inflation by reducing demand for food items. Further, the increase in global food inflation triggers food price inflation by international trade channels. However, the influence of global food inflation on food price inflation can be moderated by introducing a flexible tariff structure. Hence, the government should introduce stable and liberal trade policies that reduce food price inflation without compromising farmers remuneration values.
Moreover, our result also revealed that an increase in the net availability of food grain reduces food price inflation in both the short and long run. Therefore, the government should take necessary steps in favor of an increase in domestic food production. The high yielding variety (HYV) seeds, easily accessible credit facilities should be available to the farmer, increasing the domestic agricultural food production, thereby reducing the import of agricultural goods through the exchange rate and their adverse impact on food inflation. The stock of food grain during harvest season can avoid off seasonal food inflation. The increasing the stock of food items by establishing an extensive cold storage system and strengthening large warehouses can control food inflation in India. Furthermore, the rise in agricultural wages boosts food price inflation. The increase in the agricultural wage rate should be substituted with food price inflation by increasing labor productivity. Hence, the increase in demand originated by a hike in the agricultural wage rate can be substituted by raising each workers productivity.
Our results also found that per capita income Granger causes food price inflation both in the short-run. In this respect, we can say that there is a huge sectoral imbalance among the sectors. The government should be more focused on the agricultural sector and its growth and productivity by allocating massive funds in the irrigation, agricultural research, and innovation of modern technology and its adaptation in agriculture instead of spending on the name of social security and welfare of the poor. Therefore, balanced and sustainable growth and stability can be achieved for a developing country like India. The real exchange rate and food price inflation Granger causes to each other. The depreciation of the real exchange rate increases the food price inflation via expanding the import of petroleum products, fertilizer, and other finished products relating to agricultural commodities, which are very expensive. The increasing import of these products promotes food price inflation by raising domestic prices. Hence, to reduce the food price inflation, the government should increase the domestic agricultural production to meet our demand for food items rather than importing from other countries.
Availability of data and materials
Data used in the study are available in the Reserve Bank of India, Ministry of Labor Bureau, Directorate of Economics and Statistics, Department of Agriculture & Farmers Welfare, Government of India, and the Food and Agriculture Organization of the United Nations.
By using the linear interpolation method, we have converted the annual data into the monthly time series data. Because the high-frequency data increases the power of a statistical test and provide robust results [ 48 ]. The interpolation method has been widely used in the empirical analysis [ 34 , 42 , 44 ].
Abbreviations
Autoregressive distributed lag
Combined price index
Wholesale price index
Reserve Bank of India
Agrawal P, Kumarasamy D (2014) Food price inflation in India: causes and cures. Indian Econ Rev 49(1):57–84
Google Scholar
Anand R, Ding D, Tulin V (2014) Food inflation in India: the role of monetary policy. IMF working paper-14/178
Asfaha TA, Jooste A (2007) The effect of monetary changes on relative agricultural prices. Agrekon 46(4):460–474
Article Google Scholar
Baltzer K (2013) International to domestic price transmission in fourteen developing countries during the 2007–08. [In] Food crisis food price policy in an era of market instability. Oxford University Press, Oxford
Barnett RC, Bessler DA, Thompson RL (1983) The money supply and nominal agricultural prices. Am J Agric Econ 65(2):303–307
Bhattacharya R, Sen Gupta A (2017) What role did rising demand play in driving food prices up? South Asian J Macroecon Public Finance 6(1):59–81
Bhattacharya R, Sen Gupta A (2018) Drivers and impact of food inflation in India. Macroecon Finance Emerg Mark Econ 11(2):146–168
Bhattacharya R, Jain R (2020) Can monetary policy stabilise food inflation? Evidence from advanced and emerging economies. Econ Model 89:122–141
Bicchal M, Durai SRS (2019) Rationality of inflation expectations: an interpretation of Google Trends data. Macroecon Finance Emerg Mark Econ 12(3):229–239
Carrasco B, Mukhopadhyay H (2012) Food price escalation in South Asia. Econ Polit Wkly 47(46):59–70
Chand R (2010) Understanding the nature and causes of food inflation. Econ Polit Wkly 9XLV(9):10–13
Cho G, Kim M, Koo WW (2005) Macro effects on agricultural prices in different time horizons. Meeting of the American Agricultural Economics Association, Providence, Rhode Island
Durevall D, Loening J, Birru Y (2013) Inflation dynamics and food prices in Ethiopia. J Dev Econ 104:89–106
Goyal A (2015) Understanding high inflation trend in India. South Asian J Macroecon Public Finance 4(1):1–42
Goyal A, Baikar AK (2015) Psychology, cyclicality or social programmes: Rural wage and inflation dynamics in India. Econ Pol Wkly 50:116–125
Gulati A, Saini S (2013) Taming food inflation in India. Commission for Agricultural Cost and Prices, Discussion paper 4. Ministry of Agriculture, Government of India
Holtemöller O, Mallick S (2016) Global food prices and monetary policy in an emerging market economy: the case of India. J Asian Econ 46:56–70
Huria S, Pathania K (2018) Dynamics of food inflation: assessing the role of intermediaries. Glob Bus Rev 19(5):1363–1378
Iddrisu AA, Alagidede IA (2020) Monetary policy and food inflation in South Africa: A quantile regression analysis. Food Policy 91: 101816.
Johansen S (1991) Estimation and hypothesis testing of cointegration vectors in Gaussian vector autoregressive models. Econometrica 59(6):1551–1580
Johansen S, Juselius K (1990) Maximum likelihood estimation and inference on cointegration with applications to the demand for money. Oxf Bull Econ Stat 52(2):169–210
Joiya S, Shahzad A (2013) Determinants of high food prices: the case of Pakistan. Pak Econ Soc Rev 51(1):93–107
Kapoor A (2018) Competitiveness in agriculture will boost other sectors, Business standard, June 05, retrieved from https://www.business-standard.com/article/news-ians/competitiveness-in-agriculture-will-boost-other-sectors-column-active-voice-118060500301_1.html
Kargbo JM (2000) Impacts of monetary and macroeconomic factors on food prices in eastern and Southern Africa. Appl Econ 32(11):1373–1389
Kargbo JM (2005) Impacts of monetary and macroeconomic factors on food prices in West Africa. Agrekon 44(2):205–224
Kargbo JM (2007) The effects of macroeconomic factors on South African agriculture. Appl Econ 39(17):2211–2230
Lee HH, Park C Y (2013) International transmission of food prices and volatilities: a panel analysis. Asian Development Bank economics working paper series (373). https://doi.org/10.2139/ssrn.2323056
Mani H, Bhalachandran G, Pandit VN (2011) Public investment in agriculture and GDP growth: Another look at the intersectoral linkages and policy implications. Working paper no. 201, Centre for Development Economics, Delhi School of Economics
Mellor JW, Dar AK (1968) Determinants and development implications of food grains prices in India, 1949–1964. Am J Agric Econ 50(4):962–974
Mitchell D (2008) A note on rising food prices. World Bank policy research working paper series no. 4682
Modugno M (2013) Now-casting inflation using high frequency data. Int J Forecast 29(4):664–675
Mushtaq K, Awan DA, Abedullah A, Ahmad F (2011) Impact of monetary and macroeconomic factors on wheat prices in Pakistan: implications for food security. Lahore J Econ 16(1):95–110
Nair S, Eapen L (2012) Food price inflation in India (2008 to 2010). Econ Polit Wkly 47(20):46–54
Paramati SR, Bhattacharya M, Ozturk I, Zakari A (2018) Determinants of energy demand in African frontier market economies: an empirical investigation. Energy 148:123–133
Pesaran MH, Shin Y (1999) An autoregressive distributed lag modelling approach to cointegration analysis. In: Strom S (ed) Econometrics and economic theory in 20th century: the Ragnar Frisch centennial symposium, Chapter 11. Cambridge University Press, Cambridge
Pesaran MH, Shin Y, Smith RJ (2001) Bounds testing approaches to the analysis of level relationships. J Appl Economet 16(3):289–326
Rajmal, Mishra S (2009) Transmission of international food prices to domestic food prices The Indian evidence. RBI staff studies, Department of Economic Analysis and Policy, S S (DEAP):6/2009
Reziti I (2005) The relationship between macroeconomic variables and relative price variability in Greek Agriculture. Int Adv Econ Res 11(1):111–119
Robles M (2011) Price transmission from international agricultural commodity markets to domestic food prices: case studies in Asia and Latin America. IFPRI, Washington, DC
Sasmal J (2015) Food price inflation in India: the growing economy with sluggish agriculture. J Econ Finance Admin Sci 20(38):30–40
Selliah S, Applanaidu SD, Hassan S (2015) Transmission of global food prices to domestic prices: evidence from Sri Lanka. Asian Soc Sci 11(12)
Shahbaz M, Arouri M, Teulon F (2014) Short- and long-run relationships between natural gas consumption and economic growth: evidence from Pakistan. Econ Model 41:219–226
Sivagnanam KJ, Murugan K (2016) Impact of public investment on agriculture sector in India. J Econ Soc Dev 12(2):45–51
Tang CF, Chua SY (2012) The savings-growth nexus for the Malaysian economy: a view through rolling sub-samples. Appl Econ 44(32):4173–4185
Taylor J, Spriggs J (1989) Effects of the monetary macro-economy on Canadian agricultural prices. Can J Econ 22(2):278–289
Yu X (2014) Monetary easing policy and long-run food prices: evidence from China. Econ Model 40:175–183
Zhang Z, Lohr L, Escalante C, Wetzstein M (2010) Food versus fuel: What do prices tell us? Energy Policy 38(1):445–451
Zhou S (2001) The power of cointegration tests versus data frequency and time spans. South Econ J 67(4):906–921
Download references
Acknowledgements
Not applicable.
The authors declare that no funding from any agencies was received for this study.
Author information
Authors and affiliations.
School of Economics, University of Hyderabad, Hyderabad, 500046, India
Asharani Samal & Phanindra Goyari
School of Business, Woxsen University, Hyderabad, 502345, India
Mallesh Ummalla
You can also search for this author in PubMed Google Scholar
Contributions
AS: Conceptualization, methodology, software, formal analysis, data curation, writing—original draft, reviewing and editing. MU: Resources, data curation, writing—reviewing and editing. PG: Resources, supervision and editing. All authors have read and approved the final manuscript.
Corresponding author
Correspondence to Asharani Samal .
Ethics declarations
Ethics approval and consent to participate, consent for publication, competing interests.
The authors declare that they have no competing interests.
Additional information
Publisher's note.
Springer Nature remains neutral with regard to jurisdictional claims in published maps and institutional affiliations.
Rights and permissions
Open Access This article is licensed under a Creative Commons Attribution 4.0 International License, which permits use, sharing, adaptation, distribution and reproduction in any medium or format, as long as you give appropriate credit to the original author(s) and the source, provide a link to the Creative Commons licence, and indicate if changes were made. The images or other third party material in this article are included in the article's Creative Commons licence, unless indicated otherwise in a credit line to the material. If material is not included in the article's Creative Commons licence and your intended use is not permitted by statutory regulation or exceeds the permitted use, you will need to obtain permission directly from the copyright holder. To view a copy of this licence, visit http://creativecommons.org/licenses/by/4.0/ .
Reprints and permissions
About this article
Cite this article.
Samal, A., Ummalla, M. & Goyari, P. The impact of macroeconomic factors on food price inflation: an evidence from India. Futur Bus J 8 , 15 (2022). https://doi.org/10.1186/s43093-022-00127-7
Download citation
Received : 02 July 2021
Accepted : 15 May 2022
Published : 21 June 2022
DOI : https://doi.org/10.1186/s43093-022-00127-7
Share this article
Anyone you share the following link with will be able to read this content:
Sorry, a shareable link is not currently available for this article.
Provided by the Springer Nature SharedIt content-sharing initiative
- Food price inflation
- Macroeconomic factors
- ARDL bounds testing approach
- Causality test
JEL Classification
Swami P Saxena India
Archana Singh India
MIAR ICDS Rank: 5.7 (2019)
(Calculated & maintained by School of Library and Information Science, University of Barcelona, Spain. )
Click HERE to know more!
Journal's copyright & self-archiving policies are available in SHERPA/RoMEO , click the logo below to know more
DETERMINANTS OF INFLATION IN INDIA: AN ECONOMETRIC ANALYSIS
Dr. SWAMI P SAXENA 1
Ms. ARCHANA SINGH 2
1 Professor, Department of Applied Business Economics, Dayalbagh Educational Institute (Deemed University) Agra, India
2 Research Scholar, Department of Applied Business Economics, Dayalbagh Educational Institute (Deemed University) Agra, India
Inflation is a continual increase in general price level of goods and services in an economy over a period of time. It is caused by many factors, important among them are excess of demand of goods and services over supply, macroeconomic performance, money supply, economic policies implications, environmental factors etc. A number of researchers in the past made attempts to identify determinants of inflation and to investigate the impact of identified variables on inflation in European and also in some Asian economies. But, in context of India, not many studies can be traced in the literature. The purpose of this paper is to shed some light on the impact of selected variables on inflation in India. The paper considers CPI (Consumer Price Index) inflation as dependent variable and a set of independent macroeconomic variables, which includes Gross Domestic Product, Money Supply, Deposit Rate, Prime Lending Rate, Exchange Rate, Trade Volume (Value of Imports and Exports) and Crude Oil Prices. The empirical analysis covers the quarterly data series for ten financial years from 2002Q1 to 2012Q1. The collected data is analyzed using ADF Unit root test, Granger Causality test, and the Ordinary Least Square (OLS) technique.
KEYWORDS: Inflation, Economic Growth, Trade Volume, Investment, OLS
JEL Classification Codes: E31, E32, E51
INTRODUCTION
Inflation is one is of the most dreaded and misunderstood economic phenomena. It is a persistent increase in general price level of goods and services in an economy over a period of time, thus reflects a decrease in the purchasing power or a loss in real value per unit of money within an economy. The most well known measures of inflation are the CPI, which measures consumer prices; and the GDP deflator, which measures inflation in the whole domestic economy. Macroeconomists believes that high rates of inflation are caused by an excessive growth of money supply and the price rise. But, in this era of globalization, effects of economic inflation cross borders and percolate both developed and developing countries. Whether it is due to increased money supply, or increasing fuel prices, or increase in demand, it is needless to emphasize, that the causes of today's inflation are complicated.
The level of inflation is an aspect of major concerns to government, businesses, and especially to individual consumers. Inflation management is one of the most difficult jobs an economic policymaker has to carry out. The goal of each and every Government is to maintain relatively stable and low levels of inflation. In India, the average inflation rate from 1969 to 2013 is measured at 7.73 percent with historical high of 34.68 Percent (September 1974) and a record low of -11.31 Percent (May 1976). The inflation rate in India measured by the Ministry of Commerce and Industry in August 2013 was 6.10 percent.
REVIEW OF LITERATURE
Liu and Adedeji (2000) studied the determinants of inflation in the Islamic Republic of Iran for data covering the period from 1989 to 1999. By applying Johansen co-integration test and vector error correction model, they concluded that lag value of money supply, monetary growth, four years previous expected rate of inflation are positively contributed towards inflation while two years previous value of exchange premium is negatively correlated with inflation. Mallik and Chowdhury (2001) examined the short-run and long-run dynamics of the relationship between inflation and economic growth for four South Asian economies: Bangladesh, India, Pakistan, and Sri Lanka. By applying co-integration and error correction models to the annual data retrieved from IMF, they found two motivating results, viz., the relationship between inflation and economic growth is positive and statistically significant for all four countries, and the sensitivity of growth to changes in inflation rates is smaller than that of inflation to changes in growth rates.
Faria and Carneiro (2001) examined the relationship between inflation and economic growth in Brazil. Using bivariate time series model on annual data for the period 1980 – 1995, they observed a short-run negative association between inflation and economic growth, but no association in long run. Nachane and Lakshmi (2002) in their study employed P-Star model of dynamics of inflation in India. The authors found that velocity in India is trend stationary. Using cointegration techniques, the paper explored possibilities to develop a model to gauge inflationary pressures in the economy. The model developed by authors’ significantly outperformed seasonal ARMA benchmark model. John (2003) used post liberalisation data to study the causality between monetary aggregates and exchange rates. The paper employed VAR framework to find out as to which monetary aggregate explains the inflation in a better way. The authors observed that the explanatory power of selected variables in explaining inflation is not significantly high.
Srinivasan, Mahambare and Ramachandran (2006), estimated an augmented Phillips curve to examine the effect of supply shocks on inflation in India. In an OLS framework the authors found that supply shocks have only a transitory effect on both headline inflation and core inflation. Jan, Kalonji, and Miyajima (2008) used annual data to examine the determinants of inflation in Sierra Leone. They used a structural VAR approach to help forecast inflation for operational purposes. Andersson et al. (2009) analyzed the determinants of inflation differentials and price levels in the euro countries. Using dynamical panel analysis the researchers concluded that inflation differentials are primarily determined by cyclical positions and the inflation persistence. Kandil and Morsy (2009) also studied determinants of inflation with special reference to Gulf Cooperation Council (GCC) since 2003. Using an empirical model that included domestic and external factors, the authors found that inflation in major trading partners of GCC appears the most relevant to domestic inflation in GCC.
Kishor (2009) studied the role of real money gap and the deviation of real money balance from its long-run equilibrium level for predicting inflation in India. He found real money gap a significant predictor of inflation in India. Greenidge and DaCosta (2009) used unrestricted error-correction model and bounds test for co integrating analysis to capture new developments in the inflationary process in selected Caribbean economies (Jamaica, Guyana, Barbados and Trinidad and Tobago). The findings indicate that the determinants for inflation in the Caribbean are both cost-push and demand-pull. Dua and Gaur (2009) investigated determination of inflation in the framework of an open economy forward-looking as well as conventional backward-looking Phillips curve for eight Asian countries. Using quarterly data from 1990 to 2005 and applying the instrumental variables estimation technique, they found that the output gap, and at least one measure of international competitiveness to be significant in explaining the inflation rate in almost all the countries.
Xufang (2010) examined the association between China stock market and macroeconomic indicators like interest rate, GDP and inflation. They used (EGARCH) model for each variable, to estimate volatility, and then take second step to examine the causal relationship between the volatility of stock market returns and macroeconomic variables using LA-VAR model. Dlamini and Nxumalo (2011) used annual data from 1974 to 2000 and analyzed the determinants of inflation in Swaziland by employing the econometric technique of cointegration and error correction model (ECM). More recently, Francis and Godfried (2013) used annual data covering period from 1990 to 2009 to analyse determinants of inflation in Ghana by employing various diagnostic, evaluation tests. The findings show that real output and money supply were the strongest forces exerting pressure on the price level.
RESEARCH OBJECTIVES AND METHODOLOGY
This paper intends to develop an econometric model of the determinants of inflation in India. Accordingly, it focuses on (i) understanding of the dynamics of inflation, (ii) identification of major macroeconomic determinants of inflation, and (iii) econometric modelling of inflation in India. The paper is based on statistical database of selected variables for the period of ten years from 2002-Q1 to 2012-Q1.
The study considers Consumer Price Index as dependent variable, and the set of independent macroeconomic variables viz., Gross Domestic Product, Deposit Rate, Exchange Rate, Money Supply, Crude Oil Prices, Prime Lending Rate, and Trading Volume (Value of Imports and Exports). The brief description of selected variables with their source is given in table 1.
The description of econometrics tools used for analysis is as follows.
The Stationarity Test (Unit Root Test): Before using the time series data for further investigation (testing Cointegration and implementing the Granger Causality Test) it must be tested for unit root and stationarity. If we do not test and regress a time series variable on another time series variables using OLS, estimation can obtain a very high r 2 (though meaningful relationship between the variables may not exist). This situation reflects the problem of spurious regression between totally unrelated variables generated by a non-stationary process. A variable is said to be integrated of order one, or I(1), if it is stationary after differencing once, or of order two, I(2) if differenced twice. If the variable is stationary without differencing, then it is integrated of order zero, I(0). Thus, a series is said to be stationary if the mean and variance are time invariant.
Several tests of non-stationarity called unit root tests have been developed in the time series econometrics literature, like Dickey-Fuller (DF), Augmented Dickey-Fuller (ADF) and Phillips and Perron (PP) tests, where in most of these tests the null hypothesis is that there is a unit root, and it is rejected only when there is strong evidence against it. So for the purpose of modelling we need to testify the time series non stationarity. Accordingly, the researchers established stationarity of data using the Augmented Dickey-Fuller (ADF) Unit Root Test.
Granger Causality Test: Causality is a kind of statistical feedback concept which is widely used in the building of forecasting models. Historically, Granger (1969) and Sim (1972) were the ones who formalized the application of causality in economics. Granger causality test is a technique for determining whether one time series is significant in forecasting another (Granger, 1969). The standard Granger causality test (Granger, 1988) seeks to determine whether past values of a variable helps to predict changes in another variable. The definition states that in the conditional distribution, lagged values of Y t add no information to explanation of movements of X t beyond that provided by lagged values of X t itself (Green, 2003). We should take note of the fact that the Granger causality technique measures the information given by one variable in explaining the latest value of another variable. In addition, it also says that variable Y is Granger caused by variable X if variable X assists in predicting the value of variable Y. If this is the case, it means that the lagged values of variable X are statistically significant in explaining variable Y. The null hypothesis (H0) that we test in this case is that the X variable does not Granger cause variable Y, and variable Y does not Granger cause variable X. In nutshell, one variable (X t ) is said to granger cause another variable (Y t ) if the lagged values of X t can predict Y t and vice-versa. The spirit of Engle and Granger (1987) lies in the idea that if the two variables are integrated as order one, I(1), and both residuals are I(0), this indicates that the two variables are co integrated. The following model has been estimated in order to determine the direction of causality.
Let y and x be stationary time series. To test the null hypothesis that x does not Granger cause y, one first finds the proper lagged values of y to include in a univariate auto regression of y:
y_t=a_o+a_1 y_(t-1)+a_2 y_(t-2)+ ⋯ +a_m y_(t-m)+ residual_t
Next, the auto regression is augmented by including lagged values of x:
y_t=a_o+a_1 y_(t-1)+a_2 y_(t-2)+ ⋯ +a_m y_(t-m)+b_1 x_(t-1)+ ⋯ +b_q x_(t-q)+residual_t
One retains in this regression all lagged values of x that are individually significant according to their t-statistics, provided that collectively they add explanatory power to the regression according to an F-test (whose null hypothesis is no explanatory power jointly added by the x's). In the notation of the above augmented regression, p is the shortest, and q is the longest lag length for which the lagged value of x is significant.
The null hypothesis that x does not Granger-cause y is not rejected if and only if no lagged values of x are retained in the regression. Granger causality is not necessarily true causality. If both X and Y is driven by a common third process with different lags, one might still accept the alternative hypothesis of Granger causality. Yet, manipulation of one of the variables would not change the other. Indeed, the Granger test is designed to handle pairs of variables, and may produce misleading results when the true relationship involves three or more variables. A similar test involving more variables can be applied with vector auto regression .
ANALYSIS AND EMPIRICAL RESULTS
TRENDS OF INFLATION: Following figure reflects the changes in inflation in India from the year 2002 Q1 to 2012 Q1.
Figure 1: Inflation in India: (Consumer Prices Index % Change)

The above graph indicates that the inflation rate in the first quarter of 2001 was 5.10% which decreased up to quarter two of 2004 (except in 2003 quarter two) and after that severe fluctuations have been seen up to quarter four of 2009. In 2010 quarter one, inflation rate was at its highest peak of 15.32% and then decreased continuously up to quarter one of 2012.
BASIC DESCRIPTIVES : Basic descriptives of selected dependent and independent variables presented in (table-2) indicates that out of all variables only PLR has negative growth rate during the period of study. The value of standard deviation is very high in case of M2 which indicates very high degree dispersion in data. When we see the values of skewness, the variables that have positively skewed distribution are DR, EXR and M2, while negatively skewed distribution is observed in case of remaining variables.
A peaked curve is called leptokurtic, if kurtosis value is greater than 3, Mesokurtic, if kurtosis value is equals to 3, and Platykurtic, if the value of kurtosis is lesser than 3. The values of kurtosis indicates that EXPORT is the only variable which is platykurtic, while the distribution of variables CPI, DR, EXR, GDP, IMPORT, M2, PLR and OIL is leptokurtic.
Jarque Bera (JB) test for normality states that the distribution is normal if JB probability is more than 0.05, otherwise the distribution is considered non-normal. Among the variables under consideration DR, IMPORT, M2, PLR, and OIL have the non-normal distribution, while CPI, EXR, GDP and EXPORT are normally distributed.
CORRELATIONS : The correlation matrix of selected variables in (table-3) indicates that CPI has negative low degree correlation with M2, OIL and DR while with other variables has low degree positive correlation and only PLR is the variable with which it has moderate degree positive correlation.
RESULTS OF UNIT ROOT TEST : Before applying causality analysis on the selected variables, it is must to apply a formal test to confirm whether time series is stationary or not. For this purpose researchers applied Augmented Dickey Fuller (ADF) test of unit root. The lag length based on the Akaike Information Criterion (AIC) selected is four. In ADF test, the null hypothesis is that a variable contains a unit root/ are generated by a non-stationary process, and alternative hypothesis is that the variables are generated by a stationary process/ does not contains unit root. The results of ADF test contained in table 4 show that ‘t’ value of all the variables is less than critical value. It rejects the null hypotheses at 1 percent level of significance. Hence, it can be said that all the variables are stationary at level except, CPI which has been made stationary after differencing once. Thus, researchers made all variables stationary after taking first differences with lag order four (selected on basis of Akaike Information Criteria).
RESULTS OF GRANGER CAUSALITY TEST: The results of causality analysis reported in table 5 (see appendix) indicate that there exists bidirectional causality between EXPORT and EXR, unidirectional causality between CPI and IMPORT, CPI and PLR, M2 and DR, IMPORT and EXR, OIL and EXR, M2 and IMPORT, OIL and IMPORT, EXPORT and IMPORT, OIL and M2, and EXPORT and OIL at 5 percent level of significance. There exists no causality among remaining variables.
RESULTS OF OLS (ORDINARY LEAST SQUARE): The results of OLS applied on selected variables (table 6) show Durbin Watson statistics (DW) between 1.5 and 2.5, which indicates that there is no autocorrelation in the series. The DW statistics close to 2 indicates that the model is better fit. The lesser AIC value (3.22) also indicates that the lag length selected for analysis purpose is also correct. There exist opposite relationship between Durbin Watson (DW) statistic and Akaike Information Criteria (AIC). High difference between r 2 and adjusted r 2 indicates that there are some other significant variables which may influence the variations in dependent variable. The P value of F statistics is more than 0.05, which proves that independent variables have significant impact on the identified dependent variable.
The fundamental multiple OLS equation for selected variables can be written as:
CPI t = β o +β 1 DR t +β 2 EXR t +β 3 GDP t +β 4 IMPORT t +β 5 M2 t + β 6 OIL t +β 7 PLR t +β 8 EXPORT t + ε t
Where: β o, β 1, β 2, β 3, β 4, β 5, β 6, β 7, and β 8 ≠ 0
Based on results of OLS analysis (table 6), the equation for examining combined effect of selected variables on CPI is as follows.
CPI t = -1.106 - 0.013 DR t + 0.026 EXR t + 0.155 GDP t - 0.003 IMPORT t - 0.010 M2 t - 0.014 OIL t + 0.054 PLR t + 0.022 EXPORT t + ε t
The above equation of selected independent variables (DR, EXR, GDP, IMPORT, M2, OIL, PLR, and EXPORT) on dependent variable (CPI) indicates that the DR, IMPORT, M2 and OIL have the negative impact on CPI while other variables have the positive impact on CPI.
This paper is a modest effort for identifying major macroeconomic determinants of inflation and examining the trend and pattern of inflation in India. The results of analysis show F-value more than 0.05 indicating that selected independent variables have significant impact on CPI. However, the high difference between r 2 and adjusted r 2 indicates that there are some other significant variables which may influence the variations in dependent variables. To conclude, we can say that though identified independent variables have significant impact on the inflation, there are some other variables which may have impact on inflation.
- Abidemi, OI and Malik, SAA. 2010. “Analysis of Inflation and its Determinant in Nigeria”, Pakistan Journal of Social Sciences, 7(2).
- Acharya Shankar. 2012. “India: After the Global Crisis”, Orient BlackSwan Private Limited, Hyderabad.
- Andersson M, Masuch K and Schiffbauer M. 2009. “Determinants of Inflation and Price Level Differentials across the Euro Area Countries”, European Central Bank, Working Paper No. 1129.
- Batura, N. 2008. “Understanding Recent Trends in Inflation”, Economic & Political Weekly, Vol. XLIII(24), June 14-20.
- Bishnoi, TR and TP Koirala. 2006. “Stability and Robustness of Inflation Model”, Journal of Quantitative Economics, Volume 4(2), June.
- Cheng Hoon Lim and Laura Papi. 1997. “An Econometric Analysis of the Determinants of Inflation in Turkey”, IMF Working Paper No. 97/170.
- Dua Pami and Upasna Gaur. 2009. “Determination of Inflation in an Open Economy Phillips Curve Framework: The Case of Developed and Developing Asian Countries”, Working Paper No. 178, Centre for Development Economics, Delhi School of Economics, Delhi, April.
- Experts and Scholars from BRICS Countries. 2012. “The BRICS Report”, First Edition, Oxford University Press, New Delhi.
- Gary G Moser. 1995. “The Main Determinants of Inflation in Nigeria”, IMF Staff Papers, Vol. 42(2).
- Government of India. 2012. Economic Survey, Ministry of Finance, New Delhi.
- Greenidge Kevin and Dianna DaCosta. 2009. “Determinants of Inflation in Selected Caribbean Countries”, Business, Finance & Economics in Emerging Economies, Vol. 4(2).
- Ilker Domaç. 1998. “The Main Determinants of inflation in Albania”, World Bank Policy Research Working Paper No. 1930.
- Jan Gottschalk, Kadima Kalonji, and Ken Miyajima. 2008. “Analyzing Determinants of Inflation When There Are Data Limitations: The Case of Sierra Leone”, IMF Working Paper.
- John, RM. 2003. “Inflation in India: An Analysis Using Post Liberalized Data”, IGIDR Working Paper.
- Kandil M and Morsy H. 2009. “Determinants of Inflation in GCC”, IMF Working Paper No. 09/82.
- Kishor N Kundan. 2009. “Modeling Inflation in India: The Role of Money”, MPRA Paper No. 16098.
- Kuijs, L. 1998. “Determinants of Inflation, Exchange Rate and Output in Nigeria”. IMF working Paper No. 160.
- Lakshmi R. 2002. “Dynamics of Inflation in India: A P-Star Approach”, Applied Economics, 34(1).
- Lim, CH, and Papi, L. 1997. “An Econometric Analysis of the Determinants of Inflation in Turkey”, IMF Working Paper No. 170.
- Liu, O, and Adedeji, OS. 2000. “Determinants of Inflation in the Islamic Republic of Iran: A Macroeconomic Analysis”, IMF Working Paper No. 127.
- Maddala GS. 2001. “Introduction to Econometrics”, Third Edition, John Wiley and Sons, Singapore.
- Mallik G and Chowdhury A. 2001. “Inflation and Economic Growth: Evidence from South Asian Countries”, Asian Pacific Development Journal, Vol. 8(1).
- Srinivasan, NV, Mahambare, and M Ramachandran. 2006. “Modelling Inflation in India: A Critique of the Structural Approach”, Journal of Quantitative Economics, Vol. 4(2), June.
- Wang X. 2010. “The Relationship between Stock Market Volatility and Macroeconomic Volatility: Evidence from China”, International Research Journal of Finance, 7396 African Journal of Business Management Economics, 49.
WorldWide Indexing, Abstracting and Readership. Peer Reviewed- Refereed International Publication available at http://thescholedge.org ©Scholedge R&D Center

ISSN: 2394-3378
Reviewed & Published by SCHOLEDGE Publishing .
Listed & indexed in : BASE- Bielefeild Academic Search Engine | PKP Index | EBSCO Academic Complete Upgraded | EBSCO Business Source Corporate Plus | WorldCat Discovery Service | CrossRef Metadata Search | CiteseerX | JournalTOC UK | Airiti | CNK Scholar | CNKI | ECONBIZ | J Gate | OCLC | Scilit | EZB | ZDB | ZBW and many other academic source databases.

- Login To RMS System
- About JETIR URP
- About All Approval and Licence
- Conference/Special Issue Proposal
- Book and Dissertation/Thesis Publication
- How start New Journal & Software
- Best Papers Award
- Mission and Vision
- Reviewer Board
- Join JETIR URP
- Call For Paper
- Research Areas
- Publication Guidelines
- Sample Paper Format
- Submit Paper Online
- Processing Charges
- Hard Copy and DOI Charges
- Check Your Paper Status
- Current Issue
- Past Issues
- Special Issues
- Conference Proposal
- Recent Conference
- Published Thesis
Contact Us Click Here
Whatsapp contact click here, published in:.
Volume 10 Issue 1 January-2023 eISSN: 2349-5162
UGC and ISSN approved 7.95 impact factor UGC Approved Journal no 63975
Unique identifier.
Published Paper ID: JETIR2301285
Registration ID: 504941
Page Number
Post-publication.
- Downlaod eCertificate, Confirmation Letter
- editor board member
- JETIR front page
- Journal Back Page
- UGC Approval 14 June W.e.f of CARE List UGC Approved Journal no 63975
Share This Article
Important links:.
- Call for Paper
- Submit Manuscript online
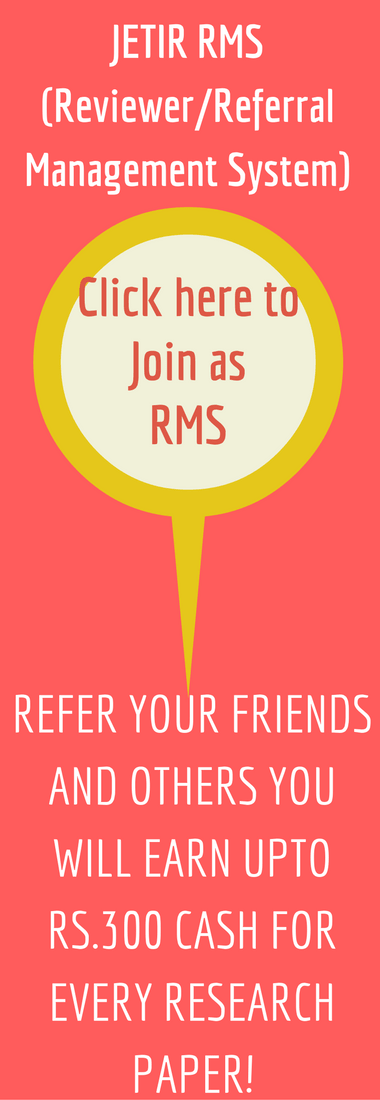
- Karan Deshwal
Cite This Article
2349-5162 | Impact Factor 7.95 Calculate by Google Scholar An International Scholarly Open Access Journal, Peer-Reviewed, Refereed Journal Impact Factor 7.95 Calculate by Google Scholar and Semantic Scholar | AI-Powered Research Tool, Multidisciplinary, Monthly, Multilanguage Journal Indexing in All Major Database & Metadata, Citation Generator
Publication Details
Download paper / preview article.

Download Paper
Preview this article, download pdf, print this page.

Impact Factor:
Impact factor calculation click here current call for paper, call for paper cilck here for more info important links:.
- Follow Us on

- Developed by JETIR
India's March retail inflation eases to five-month low
- Medium Text
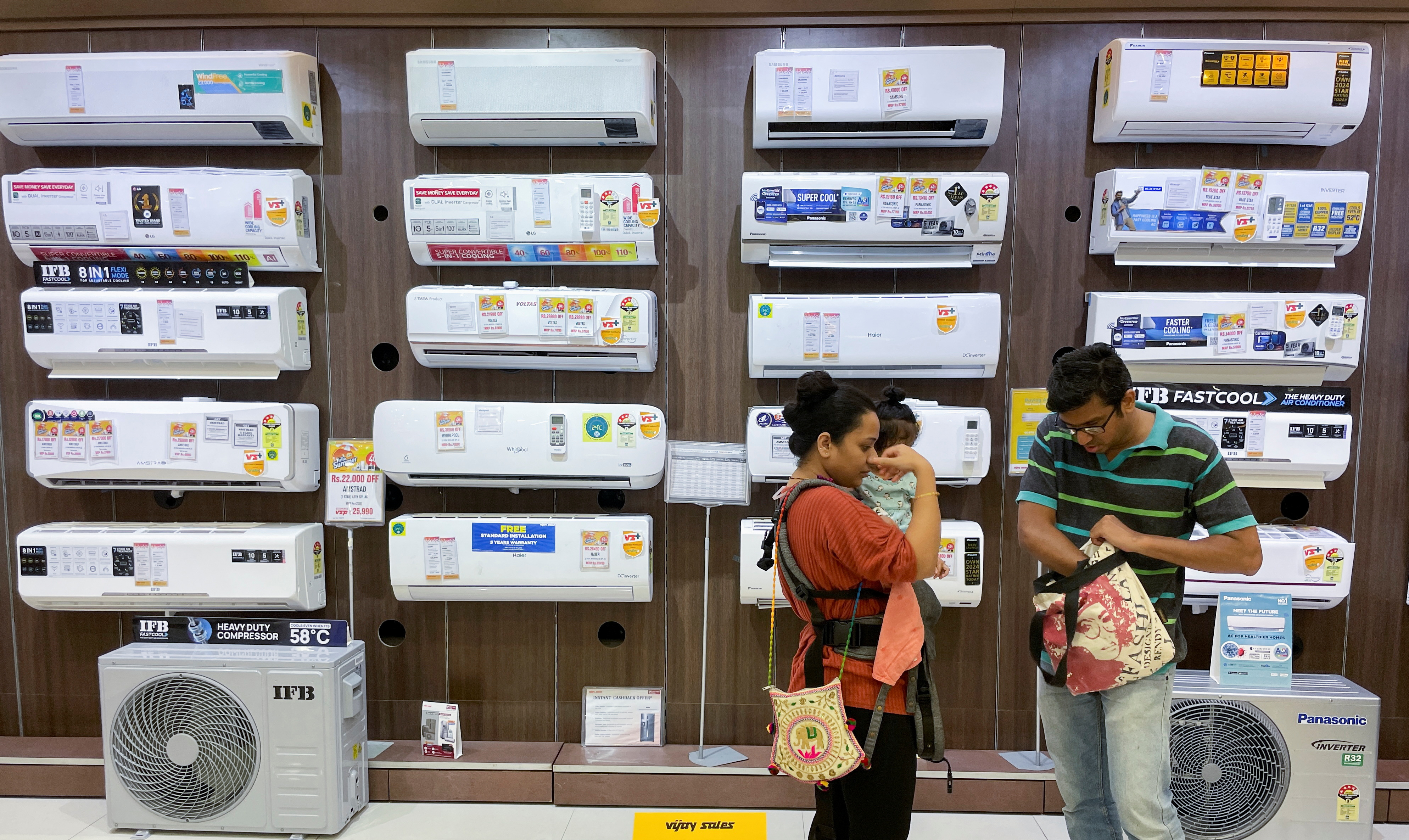
The Reuters Daily Briefing newsletter provides all the news you need to start your day. Sign up here.
Reporting by Sarita Chaganti Singh; Editing by Sharon Singleton and Susan Fenton
Our Standards: The Thomson Reuters Trust Principles. New Tab , opens new tab
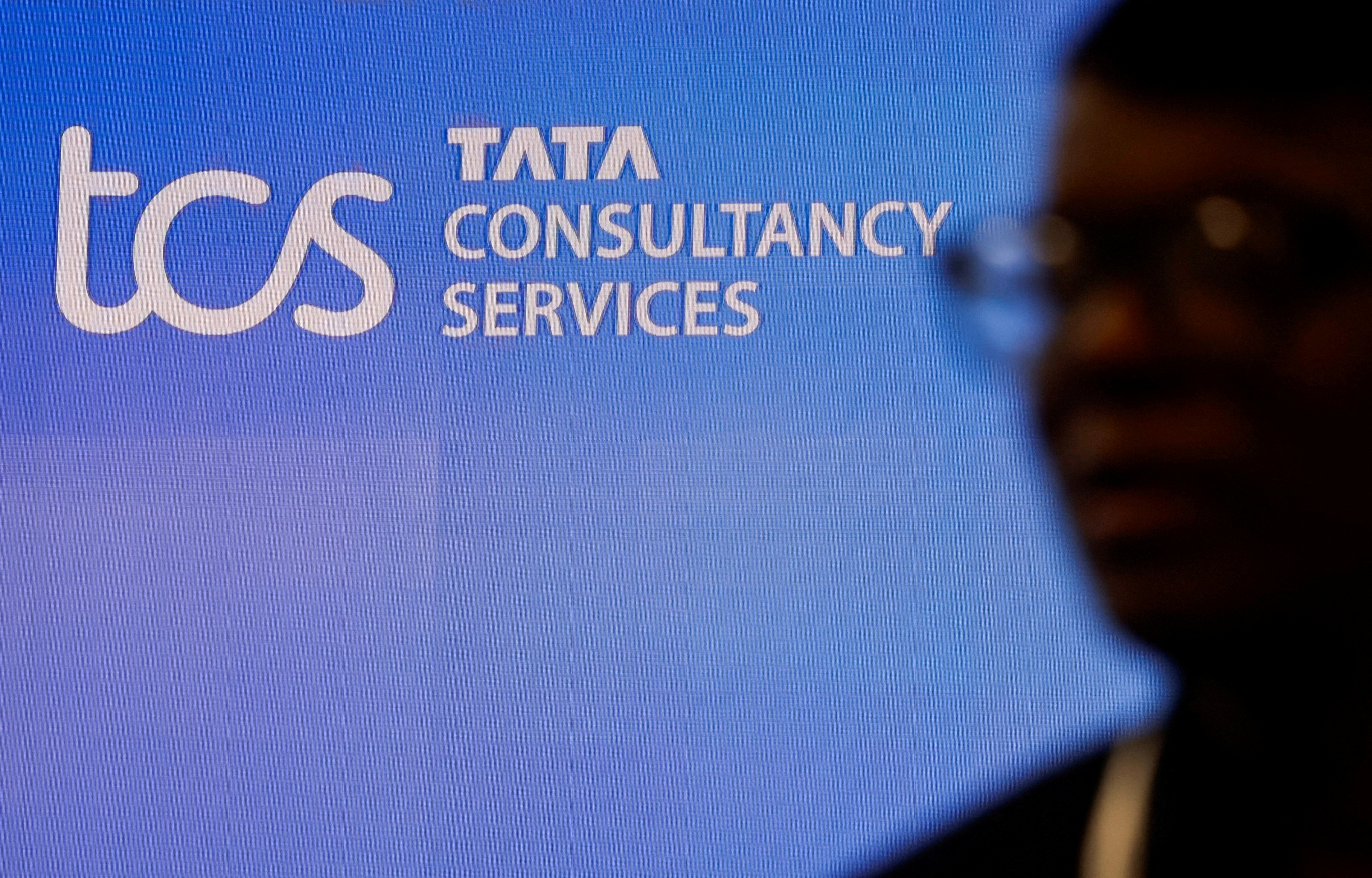
World Chevron
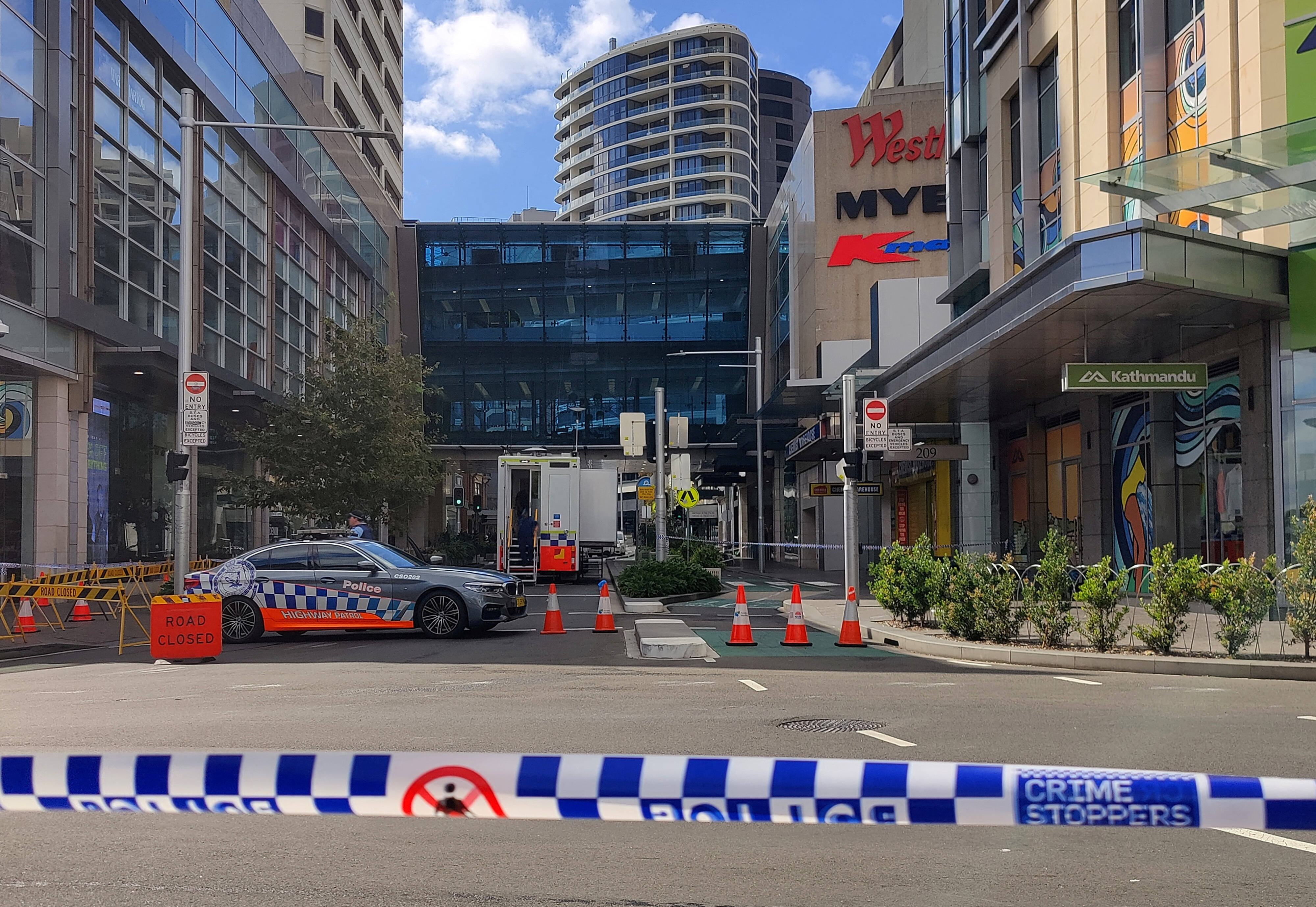
Australian police say Sydney knife attacker may have targeted women
Australian police on Monday said the attacker who fatally stabbed six people at a busy shopping centre in Sydney's beach suburb of Bondi may have targeted women, as the country mourned the victims and hundreds of people laid flowers near the scene.
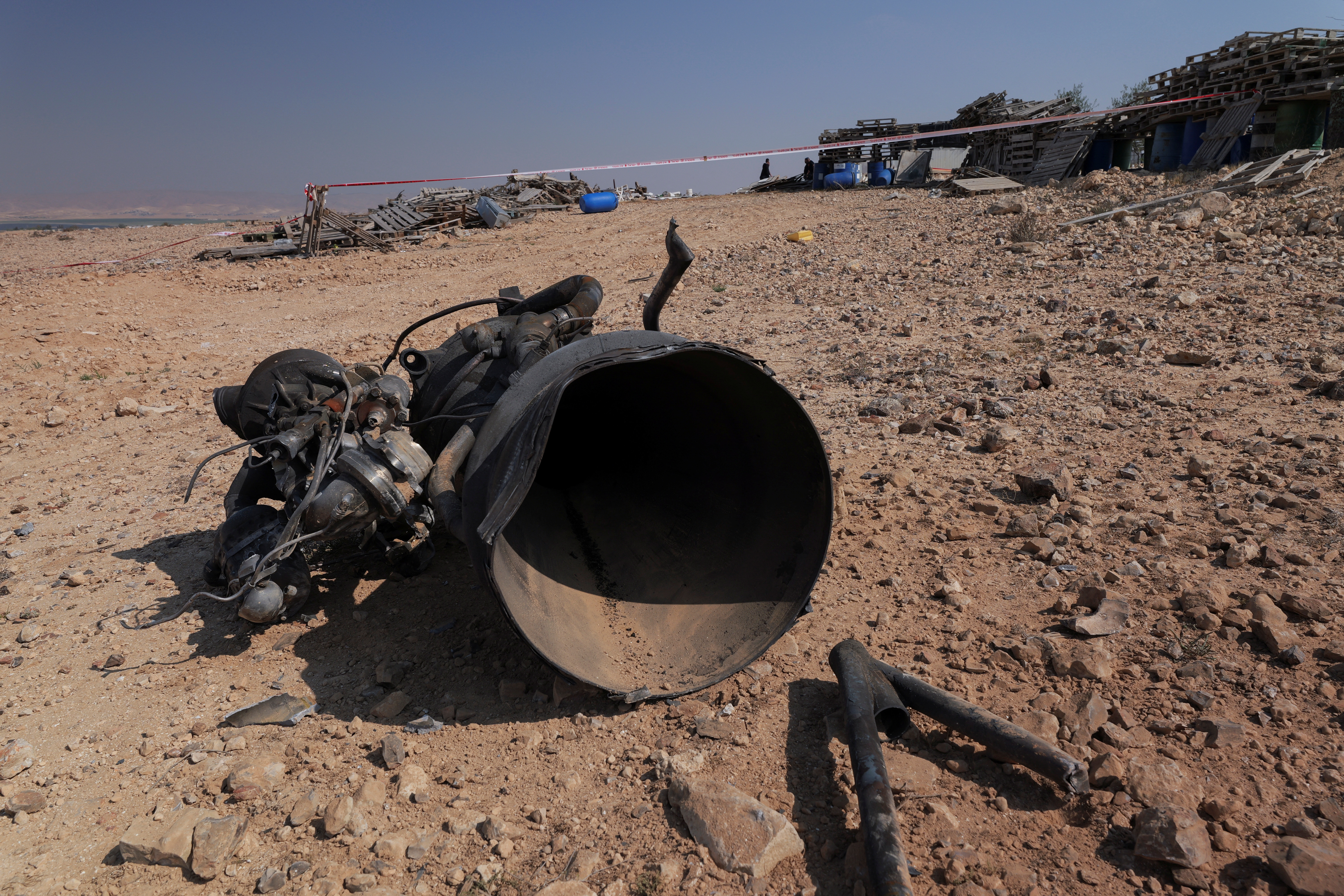
- International
- Today’s Paper
- Expresso Live
- Premium Stories
- Express Shorts
- Health & Wellness
- Board Exam Results
GDP growth may slow to 6.1% in 2024: Moody’s
Moody’s analytics said the inflation outlooks for china and india, asia’s two largest economies, are more uncertain.

India’s economy will likely grow 6.1% in 2024, lower than 7.7% in 2023, Moody’s Analytics on Friday projected, adding that inflation outlook remained more uncertain.
Looking at GDP relative to its trajectory prior to the Covid-19 pandemic showed that India and Southeast Asia have seen some of the largest output losses worldwide and are only beginning to recover, Moody’s Analytics said in its report titled ‘APAC Outlook: Listening Through the Noise’.

“Output in India remains 4%lower than it would have been without the pandemic and its various aftershocks—from supply snags to military conflicts abroad. Things are worse in ASEAN , where GDP is 6% below pre-pandemic projections. This compares with an output loss of about 2% for the world economy as a whole,” it said. “We expect India’s GDP to grow 6.1% in 2024 after 7.7% last year.”
Moody’s Analytics said the inflation outlooks for China and India, Asia’s two largest economies, are more uncertain. “Inflation in India is at the opposite extreme, with recent consumer price inflation rates hovering around 5%, close to the upper end of the Reserve Bank of India’s target range of 2% to 6% and without clear evidence of a trend towards slowing price pressures,” it said.
India’s retail inflation declined to a five-month low of 4.85% in March mainly due to cooling food prices, according to official data released on Friday.

In its report, Moody’s Analytics said the region overall is doing better than other parts of the world. The APAC (Asia Pacific) economy will grow 3.8% this year, which compares with a growth of 2.5% for the world economy, it said.
The Reserve Bank recently said food price uncertainties continue to weigh on the inflation trajectory going forward, and retained 4.5% retail inflation projection for 2024-25.
Continuing geopolitical tensions also pose upside risk to commodity prices and supply chains, RBI said.
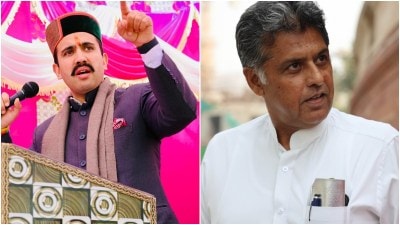
The Congress on Saturday released its list of 16 candidates for the upcoming Lok Sabha elections, fielding Vikramaditya Singh, son of former Chief Minister Virbhadra Singh, from Mandi. Congress MP Manish Tewari has been fielded from Chandigarh.
- Israel Iran News Live Updates: Iran mounts aerial attack on Israel with drones, missiles; ‘minor damage’ to army base reported 20 mins ago
- Candidates Chess 2024 Live Updates: Vidit resigns against Gukesh; Nakamura defeats Caruana 3 mins ago
- Lok Sabha Elections 2024 Live Updates: Andhra CM Jagan Reddy injured in stone-pelting incident during Vijayawada roadshow 10 hours ago
- Delhi News Live Updates: Light rains lash parts of Capital; 15 flights diverted due to bad weather 13 hours ago
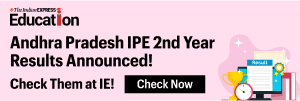
Best of Express
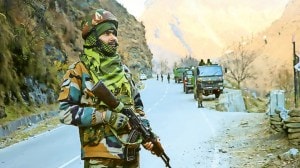
Buzzing Now
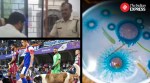
Apr 14: Latest News
- 01 Kejriwal doesn’t want to meet Sanjay Singh: BJP slams AAP Rajya Sabha MP over ‘CM not allowed to meet family in Tihar’ remark
- 02 Rains continue in Marathwada, Vidarbha; heaviest in Yavatmal at 63 mm
- 03 River barges break loose in Pittsburgh, causing damage and closing bridge
- 04 IPL 2024 Purple Cap: Yuzvendra Chahal back on top, Kagiso Rabada makes his way into the race
- 05 Israel says monitoring ‘planned attack’ against it by Iran, proxies
- Elections 2024
- Political Pulse
- Entertainment
- Movie Review
- Newsletters
- Gold Rate Today
- Silver Rate Today
- Petrol Rate Today
- Diesel Rate Today
- Web Stories
How Quickly Do Prices Respond to Monetary Policy?
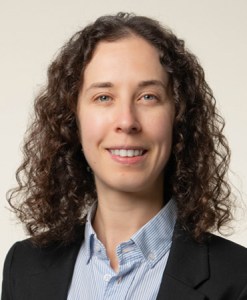
Download PDF (238 KB)
FRBSF Economic Letter 2024-10 | April 8, 2024
With inflation still above the Federal Reserve’s 2% objective, there is renewed interest in understanding how quickly federal funds rate hikes typically affect inflation. Beyond monetary policy’s well-known lagged effect on the economy overall, new analysis highlights that not all prices respond with the same strength or speed. Results suggest that inflation for the most responsive categories of goods and services has come down substantially from recent highs, likely due in part to more restrictive monetary policy. As a result, the contributions of these categories to overall inflation have fallen.
Monetary policy affects inflation with a lag. This means that, although interest rates react quickly when the Federal Reserve raises the federal funds rate, the effects on inflation are slower and indirect. Higher interest rates increase borrowing costs, slowing investment and overall demand, which ultimately eases the pressure on prices. Understanding the timing and strength of this mechanism is key for policymakers.
Many researchers have estimated the speed and strength of the economy’s response to monetary policy, notably Romer and Romer (2004). The focus is typically a broader measure of inflation, such as headline or core, which reflects an average across many goods and services. However, not all prices of the component goods and services react to monetary policy in the same way. For example, food and energy prices, which are excluded from core but included in headline inflation, often move more in response to global market fluctuations, such as changes in international oil prices, rather than to changes in domestic monetary policy.
In this Economic Letter , we estimate how prices of different goods and services respond to changes in the federal funds rate and use those estimates to build a monetary policy-responsive inflation index. We find substantial variation in how prices react to monetary policy, which suggests that understanding the makeup of overall inflation can provide insights into the transmission of monetary policy to inflation. The extent to which categories that are more responsive to the federal funds rate contribute to inflation affects how much slowing in economic activity is needed to reduce overall inflation. Our analysis indicates that recent ups and downs of inflation have been focused in categories that are most sensitive to monetary policy. Inflation rates for the most sensitive categories—and their contributions to headline inflation—rose from the first half of 2020 through mid-2022, reaching a higher peak than headline inflation, and then began to decline. The inflation rate for this most responsive group of goods and services categories is now close to its pre-2020 rate. Our findings suggest that the Fed’s rate hikes that began in March 2022 are exerting downward pressure on prices and will continue to do so in the near term. Our estimated lags are consistent with the view that the full effects of past policy tightening are still working their way through the economy.
Measuring how prices react to monetary policy
To understand which goods and services are most responsive to monetary policy, we need to determine how their prices react to changes in the federal funds rate, the Federal Reserve’s main policy rate. Because the Federal Reserve adjusts the federal funds rate target in response to macroeconomic developments, including inflation, we use a transformation of the federal funds rate in our estimation. This transformed series, developed by Romer and Romer (2004) and updated by Wieland and Yang (2020), captures the differences between Federal Reserve staff forecasts and the chosen target rate, leaving only policy shocks, or movements in the federal funds rate that are not driven by actual or anticipated changes in economic conditions. We use this series as a so-called instrument for the federal funds rate, such that our results can account for how the federal funds rate itself, rather than its transformation, affects inflation.
We use an approach developed by Jordà (2005) that compares two forecasts—with and without rate shocks—to estimate how the federal funds rate affects price movements over time. Specifically, we estimate the relationship between the federal funds rate and the cumulative percent change in prices, controlling for recent trends in the federal funds rate, inflation, and economic activity. Repeating this estimation over multiple horizons produces a forecast comparison, or impulse response function, that gives us an estimate of the expected percent change in prices following a rate increase. For example, applying this method to the headline personal consumption expenditures (PCE) price index indicates that four years after a 1 percentage point increase in the federal funds rate, overall prices are typically about 2.5% below what they would have been without the rate increase.
Creating a policy-responsive inflation index
We estimate impulse response functions separately for the 136 goods and services categories that collectively make up headline PCE inflation. Figure 1 shows examples of the largest cumulative percent price declines over a four-year period in response to a 1 percentage point increase in the federal funds rate. The goods and services categories selected as examples account for large shares of total expenditures in headline PCE inflation. We also include one example of the few categories where prices do not decline, higher education, shown as a small positive value.
Figure 1 Reaction to a policy rate increase: Selected PCE categories
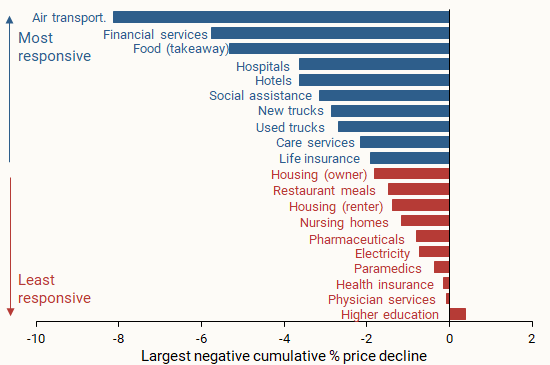
The takeaway from Figure 1 is that headline PCE inflation is made up of categories that differ in their responsiveness to increases in the federal funds rate. Some respond more strongly, such as those with larger typical cumulative price declines, while others respond less strongly, such as those with smaller typical price declines. Focusing on the most responsive categories can shed light on how monetary policy has influenced the path of inflation over the post-pandemic period. We use our results to divide the categories into two groups of goods and services. The most responsive group (blue bars) contains goods and services whose largest cumulative percent price decline over a four-year window is in the top 50% of all such declines. The least responsive group (red bars) contains goods and services in the bottom 50%.
Following the methods in Shapiro (2022), we use these two groups, along with the share of total expenditures for each good or service, to create two new aggregate PCE inflation measures. Figure 2 shows their 12-month percent changes over time. The blue shading marks the period from mid-2019 until early 2020 when the Federal Reserve lowered the federal funds rate. The vertical yellow line marks the start of the most recent tightening cycle in March 2022. Inflation in the most responsive categories (blue line) is more volatile than overall headline PCE inflation (green line) from the Bureau of Economic Analysis (BEA), and inflation in the least responsive categories is less volatile (red line).
Figure 2 Most and least responsive inflation rates
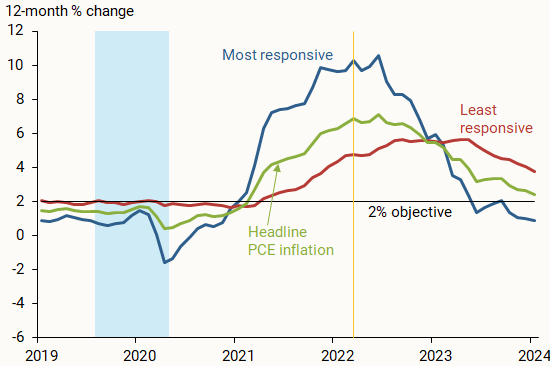
After the start of the 2020 recession, inflation rates for both categories rose but have since come down from their recent peaks. This pattern is particularly pronounced for the most responsive inflation group, for which inflation peaked at 10.5% in mid-2022 and has fallen to 0.9% as of January 2024; this is just under its average of 1% from 2012, when the Federal Reserve officially adopted a numerical inflation objective, to 2019. Inflation in the least responsive group peaked later, in early 2023, and has fallen only slightly to 3.8% as of January 2024; it remains well above its 2012–2019 average of 1.8%.
How does policy-responsive inflation react to rate increases?
The inflation rates of categories in the most and least responsive groups can move for reasons beyond changes in the federal funds rate, such as global or national macroeconomic developments. To assess the specific role of policy rate increases, we use the methodology described earlier to estimate how the most and least responsive inflation groups tend to react to rate hikes.
The results in Figure 3 suggest that an increase in the federal funds rate typically starts exerting downward pressure on the most responsive prices after about 18 months, when the line showing the impulse response function falls below zero. Month-to-month price changes start falling after a little over a year, depicted when the slope drops below zero and stays negative. This is quicker than the response of overall headline prices from the BEA (not shown), which becomes negative after a little over 24 months and shows month-to-month declines after about 18 months.
Figure 3 Reaction of most and least responsive prices to rate hikes
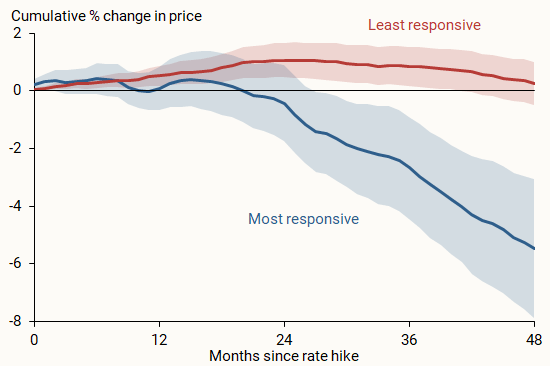
Because we grouped inflation categories based on the size of their response, there is not necessarily a tie-in to the speed of each categories’ change. However, our results suggest that looking at the most responsive goods and services may also be a useful way of assessing how quickly monetary policy affects inflation.
Applying the typical impact timing of the most responsive group of goods and services to the most recent tightening cycle, shown by the federal funds rate line in Figure 4, leads to several conclusions. First, rate cuts from 2019 to early 2020 could have contributed upward price pressures starting in mid- to late 2020 and thus could explain some of the rise in inflation over this period. Second, the tightening cycle that began in March 2022 likely started putting downward pressure on prices in mid-2023 and will continue to do so in the near term. This is consistent with the view that the full effects of monetary policy tightening have yet to be felt. Finally, though inflation for the most responsive categories has been falling since mid-2022, the early part of this decline was likely to have been driven more by changes in prevailing economic conditions than by policy tightening, given estimated policy lags. Some research has considered whether policy lags have shortened (see, for example, Doh and Foerster 2021); however, because inflation began falling mere months after the first rate hike, the drop in inflation may have been too soon to be caused by policy action.
Figure 4 Headline inflation contributions and the federal funds rate
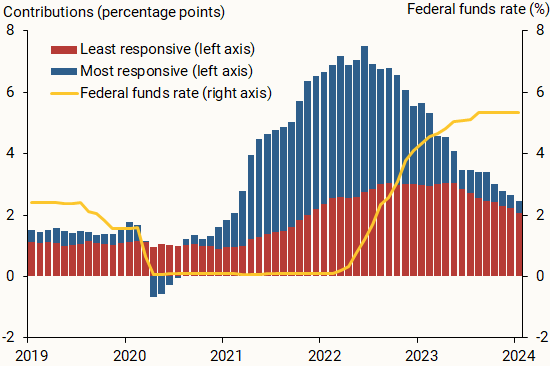
Our findings in this Letter are useful for broadening our understanding of how monetary policy affects inflation. For example, if inflation and the contributions to overall headline inflation are high in a set of categories that are more responsive to monetary policy, as was the case in early 2022, then rate hikes during the most recent tightening cycle are likely to continue to reduce inflation due to policy lags. On the other hand, though inflation in the least responsive categories may come down because of other economic forces, less inflation is currently coming from categories that are most responsive to monetary policy, perhaps limiting policy impacts going forward.
Doh, Taeyoung, and Andrew T. Foerster. 2022. “ Have Lags in Monetary Policy Transmission Shortened? ” FRB Kansas City Economic Bulletin (December 21).
Jordà, Òscar. 2005. “Estimation and Inference of Impulse Responses by Local Projections.” American Economic Review 95(1), pp. 161–182.
Romer, Christina, and David Romer. 2004. “A New Measure of Monetary Shocks: Derivation and Implications.” American Economic Review 94(4), pp. 1,055–1,084.
Shapiro, Adam. 2022. “ A Simple Framework to Monitor Inflation .” FRB San Francisco Working Paper 2020-29.
Wieland, Johannes, and Mu‐Jeung Yang. 2020. “Financial Dampening.” Journal of Money, Credit and Banking 52(1), pp. 79–113.
Opinions expressed in FRBSF Economic Letter do not necessarily reflect the views of the management of the Federal Reserve Bank of San Francisco or of the Board of Governors of the Federal Reserve System. This publication is edited by Anita Todd and Karen Barnes. Permission to reprint portions of articles or whole articles must be obtained in writing. Please send editorial comments and requests for reprint permission to [email protected]
Why Do We Dislike Inflation?
This paper provides new evidence on a long-standing question asked by Shiller (1997): Why do we dislike inflation? I conducted two surveys on representative samples of the US population to elicit people’s perceptions about the impacts of inflation and their reactions to it. The predominant reason for people’s aversion to inflation is the widespread belief that it diminishes their buying power, as neither personal nor general wage increases seem to match the pace of rising prices. As a result, respondents report having to make costly adjustments in their budgets and behaviors, especially among lower-income groups. Inflation also provokes stress, emotional responses, and a sense of inequity, as the wages of high-income individuals are perceived to grow more rapidly amidst inflation. Many respondents believe that firms have considerable discretion in setting wages, opting not to raise them in order to boost profits, rather than being compelled by market dynamics. The potential positive associations of inflation, such as with reduced unemployment or enhanced economic activity, are typically not recognized by respondents. Inflation ranks high in priority among various economic and social issues, with respondents blaming the government and businesses for it. I also highlight a substantial polarization in attitudes towards inflation along partisan lines, as well as across income groups.
This is an earlier version of the paper prepared for the Spring 2024 Brookings Papers on Economic Activity (BPEA) conference and the final version of this paper will be published in the Spring 2024 BPEA issue. I thank Carola Binder, Janice Eberly, Yuriy Gorodnichenko, Francesco Nuzzi, and Jon Steinsson for helpful comments and feedback. I am deeply grateful to Alberto Binetti, Filippo Giorgis, and Alfonso Merendino for excellent research assistance. The views expressed herein are those of the author and do not necessarily reflect the views of the National Bureau of Economic Research.
MARC RIS BibTeΧ
Download Citation Data
Working Groups
More from nber.
In addition to working papers , the NBER disseminates affiliates’ latest findings through a range of free periodicals — the NBER Reporter , the NBER Digest , the Bulletin on Retirement and Disability , the Bulletin on Health , and the Bulletin on Entrepreneurship — as well as online conference reports , video lectures , and interviews .
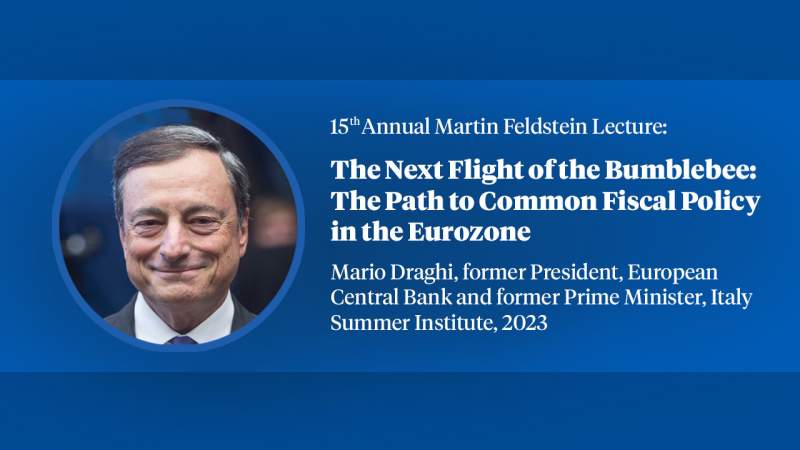

An official website of the United States government
The .gov means it’s official. Federal government websites often end in .gov or .mil. Before sharing sensitive information, make sure you’re on a federal government site.
The site is secure. The https:// ensures that you are connecting to the official website and that any information you provide is encrypted and transmitted securely.
- Publications
- Account settings
Preview improvements coming to the PMC website in October 2024. Learn More or Try it out now .
- Advanced Search
- Journal List
- Springer Nature - PMC COVID-19 Collection

What lowered inflation in India: monetary policy or commodity prices?
Pulapre balakrishnan.
1 Ashoka University, Sonipat, Haryana 131029 India
2 IIM Kozhikode, Kozhikode, India
M. Parameswaran
3 Centre for Development Studies, Thiruvananthapuram, Kerala India
India has seen lower inflation by historical standards for the past 6 years. This has been attributed to the adoption of inflation targeting by the central bank, the Reserve Bank of India in 2016. In particular, it has been asserted that the lower inflation reflects the anchoring of expectations. We evaluate these claims. An econometric investigation indicates that there is no basis to the claim that inflation has been lowered due to the anchoring of expectations. On the other hand, we are able to account for the trajectory of inflation in India after 2016 in terms of an alternative explanation of inflation, namely the structuralist.
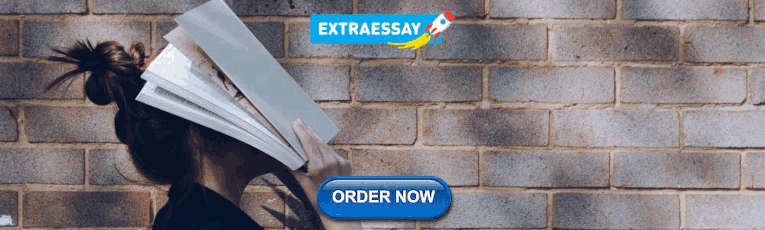
Introduction
In 2015, India’s parliament amended the Reserve Bank of India (RBI) Act of 1933 to make inflation control the sole objective of monetary policy. The amendment mandated RBI to pursue an inflation target of 4%, measured in terms of the Consumer Price Index (CPI), with a tolerance band of ± 2 percentage point. This arrangement came into effect from August 5, 2016 and was to last till March 31, 2021. With it, the RBI made the transition to an inflation targeting (IT) central bank. In the 5 years, inflation has mostly remained within the target range. As per the original terms of agreement between the India’s government and its central bank, the policy of inflation targeting was reviewed after 5 years and the original mandate was renewed. Prior to the official verdict, academic evaluations had appeared. These had concluded that inflation targeting in India had succeeded and that this had been achieved by the anchoring of inflation expectations by the RBI. 1 We investigate this claim. Our view is that for the claim that inflation targeting has succeeded, it is necessary to first establish that the central bank has actually controlled inflation. This would require demonstrating that the inflation model on which the policy of inflation targeting is based, namely the New Keynesian Phillips Curve (NKPC), with its emphasis on forward-looking expectations is empirically valid in the Indian case. Evaluations claiming the success of inflation targeting in India have not been accompanied by such an exercise. In its absence, there would be no basis for the claim that it is inflation targeting that is responsible for the observed path of inflation in India since 2016. In this paper, we first investigate the claimed effectiveness of inflation targeting in having lowered the inflation rate in India. Next, we provide an explanation of the observed trajectory of inflation after the adoption of inflation targeting.
Background: anti-inflation policy before inflation targeting
Inflation targeting is only one of a set of possible inflation control policies. Thus, skepticism about the efficacy of inflation targeting, which is a particular strategy, does not imply rejection of inflation control as a legitimate objective of economic policy. This is worth emphasizing as it is not well understood. Even before inflation targeting was advanced, Friedman had brought inflation control to the center of macroeconomics through repeated references to the dangers of inflation. As a monetarist, Friedman had prescribed money supply-targeting as the means to control inflation. On the other hand, under inflation targeting, it is the use of the interest rate to target inflation that is prescribed. Therefore, what is new about inflation targeting is only the instrument chosen, not the goal itself. 2 There is, however, the implicit suggestion that inflation targeting is more effective than the erstwhile monetarist approach, as the instrument—the policy interest rate—is directly under the control of the central bank, in a way that the money supply is not. However, what has mostly remained hidden in the public discourse is the empirical validity of the economic model that underlies inflation targeting. Given our objective in this paper, it is worth repeating that it cannot simply be assumed that this model is a valid representation of the inflationary process. This must be demonstrated.
Recent inflation in India
To identify the role played by inflation targeting in lowering inflation in India, we start by first studying its recent history. Figure 1 may be seen the trajectory of annual inflation on a quarterly basis from the year 2000 onwards, including the period from the first quarter of the financial year 2016. Details of the sources of data used in this study are given in the Appendix A1 and Appendix A2 presents the results on the unit root properties of the variables used in this study.

The trajectory of inflation in India
When interpreting the data, it would be useful to bear in mind that inflation targeting was adopted in 2016. Five observations may be made on the basis of this history. First, it can be asserted that inflation has been lowered very significantly in India over the past decade. From a high of 15% in 2009–10 (Q4), inflation hit a low of 4% in 2014–15 (Q3). Second, since 2016, the inflation rate has mostly remained within the prescribed band. Third, it begins to rise about 3 years into the experiment, in September 2019. As this is before the lockdown in response to the COVID-19 pandemic, the increase cannot be put down to an extraordinary event. Fourthly, even if it is said that inflation has remained within the band for much of the time since inflation targeting was adopted, it had entered the prescribed band some 7 quarters before 2016, having been on a downward trend for at least a year before entering the band. This should alert us to the possibility that factors other than inflation targeting may have been responsible for lowering inflation in India. At least, it would be premature to claim a role for inflation targeting without investigating the matter. Finally, inflation has remained within the band of 4 ± 2%, and for a longer period, even in the distant past, in the early 2000s, once again suggesting a role for other factors in its determination.
In a preliminary attempt to understand the determinants of inflation, we study movements in the principal components of the consumer price index. From the data in Table Table1, 1 , we can see a steady decline in food-price inflation since 2012–13. In fact, this decline is greater than the decline in the inflation rate, implying a decline in the relative price of food. It gives reason to believe that declining food-price inflation has had a role in the decline in inflation. The behavior of ‘core’ inflation, or inflation excluding food and fuel price movements, also declining in this period, though not as sharply, suggests that it may not be autonomous of the behavior of food prices. In fact, it may have been driven by it. If that is so, the case for targeting core instead of headline inflation, a proposition always on the agenda of international policy entrepreneurs and repeated by India’s government economists, weakens. The data in Table Table1 1 establish that inflation in India was trending downward well before 2016 and had settled into the band, or stabilized, a full 2 years before IT was adopted.
Inflation by components of the consumer price index
Source: Reserve Bank of India; https://www.rbi.org
Can anchored expectations describe recent inflation in India?
It is asserted that inflation targeting works via the anchoring of inflation expectations by the central bank. Accordingly, it has been claimed that inflation in India has been contained within the target range since 2016 because the RBI successfully anchored the expectation of inflation. 3 We now investigate the claim. Figure 2 presents 3-month ahead and 1-year ahead inflation expectations of households and actual inflation. The inflation expectation data are from the households’ inflation expectations surveys conducted periodically by the RBI. The expected inflation rates are averages across households. Visual inspection of the graphs suggests the following. First, for the period from 2016—when inflation targeting was adopted—while there is slight upward movement in the 3-month ahead inflation expectations, and none for 1-year ahead inflation expectations, actual inflation has a distinctly cyclical trajectory. Second, while expectations have been relatively steady since 2016, suggesting that they have been anchored, they had been revised downwards dramatically in the 2 years prior to that, when inflation targeting had not yet been adopted. Third, even if it were to be asserted that expectations have been anchored after 2016 they have remained higher than the upper end of the target range and at times far higher than the target itself. 4 Finally, actual inflation has fluctuated considerably more than expectations, implying that the latter are unlikely to have been a major factor in driving inflation. These facts make it difficult to sustain the argument that inflation in India has been tamed by anchoring expectations.

Households’ inflation expectations and actual inflation
We now investigate econometrically the role of inflation expectations in the dynamics of inflation in India using the “canonical form” 5 of the NKPC,
where ∆p t stands for the inflation rate, E t ∆p t + 1 is the expectation at time t of inflation in the next period, y t is the output gap, and β and ϒ are positive constants.
Note that in this specification, expectations are forward-looking. Thus, there is no ‘intrinsic’ inertia to inflation. However, motivated by the discovery of inertia in practice, Gali & Gertler, ( 1999 ) recommend augmentation of the NKPC by including the one period lagged inflation rate. Inclusion of lagged inflation along with forward inflation rate implies that sub-set of firms is using “a backward-looking rule of thumb to set prices”.
The resulting model, specified as follows:
is termed by them “the hybrid New Keynesian Phillips Curve”. 6 We find this classification of firms into two categories according to their expectation formation mechanism to be ad hoc. However, as it is often found in the literature, we use this very specification so that our estimates are comparable.
Two considerations arise when estimating Eq. (1). First, we need a measure of the output gap. We follow the standard practice of using the Hodrick–Prescott (H–P) filter to estimate the trend and treat the deviation of log of actual output from its trend value for each year as the output gap. However, as the H–P filter has been subjected to criticism, we also use a second measure of the output gap, identified as D–T, which is the deviation of output from a polynomial trend with quarterly dummies. 7 Here, the underlying assumption is that the natural level of output evolves along a trend, and deviations from it are considered as the cycle. 8 Second, we would need data on expectations, which are unobservable. For this variable, we use survey data on expected inflation of households released by the RBI. The model was estimated by OLS and GMM-IV alternately. In the GMM-IV estimation, the output gap and expected inflation were treated as endogenous, and, therefore, instrumented. Instruments are the lagged values of the output gap selected on the basis of its correlation with the current value of the output gap. The results are presented in Table Table2. In 2 . In the Table, columns numbered (1) and (2) report the OLS estimates and columns (3) and (4) report GMM-IV estimates. Note that the estimated coefficients of expectations are not statistically significant. 9 The measures of output gap are significant in two cases, though with a negative sign, thus contradicting the theory underlying the NKPC.
Estimates of the NKPC with survey-based expectations
(1) t statistics in parentheses, (2) ** and * indicate significance at the 1 and 5 percent level, respectively, (3) q1 … q3 are quarterly dummies
These estimates of the NKPC for India point conclusively to it being a poor representation of inflation in the country. 10 Our finding of the lack of validity of the output gap model for Indian data is in line with the findings of other researchers, see Paul ( 2009 ) and Hatekar, Sharma and Kulkarni ( 2011 ). Before it may be assumed that this finding reflects some developing-economy pathology, it may be noted that the output gap model is not always validated for the United States economy either. 11 Our finding of the insignificance of inflation expectations when the NKPC is estimated for India is consistent with the argument of Rudd, ( 2021 ) that the theoretical basis for the role of expectations in inflation is weak. That is, it has simply been assumed.
We have used headline inflation as our measure. The output gap model has been validated for India using core inflation as the measure (see: Ball and Mishra, 2016 ). Two comments would be in order here. First, India’s central bank targets headline inflation, so it is headline inflation that needs to be addressed when evaluating the record of IT in the country. Second, in a separate investigation, we have found that core inflation is related to agricultural-price inflation which is part of headline inflation. This association has two implications. First, core inflation has no autonomous status. Second, the RBI cannot control core inflation without first stabilizing agricultural-price inflation.
Does the finding that the NKPC is without statistical validity when confronted with Indian data leave us without an explanation of inflation in the country? We now turn to this issue.
Commodity price movements and recent inflation in India
An explanation of inflation other than that implied by the New Keynesian Phillips Curve has actually existed for far longer. Though developed several decades prior to the emergence of inflation targeting, it did not receive attention in the mainstream, because the framework within which it was embedded was developed for the analysis of the developing economies. 12 Specifically, this explanation of inflation was originally meant for Latin America’s economies, but it has applicability for much of the developing world, especially India. This structuralist model of inflation is a sub-set of a larger model of the economy that can explain both output and inflation. Crucially, it generates outcomes that are observed in India which cannot be explained by the Phillips Curve. These are disinflationary expansions and inflationary recessions. They stem from the presence of an agricultural sector, with characteristics different from that of industry, as demonstrated by Balakrishnan and Parameswaran ( 2021 ).
In structuralist macroeconomics, the economy is modeled as consisting of two sectors, agriculture and industry, with price and output determination mechanisms varying between the two. The agricultural price clears the market in each period, i.e., it is determined by supply and demand, while the industrial price is cost-determined, with a fixed mark-up. The output of agriculture is considered exogenous, as it is driven by weather, while industrial output is demand-determined. Industrial costs are made up of labor and material costs, notably the price of imported oil. The price of oil is determined in the global market while the wage is related to the general price level, though with a lag. In this model, it is seen 13 that inflation (π) is positively related to the relative price of the agricultural good (θ), industrial costs ( γ W t + τ e P m , t ) and lagged inflation as follows 14 :
Comparative statics applied to this model show that a rise in the inflation rate can occur from either an expansion of industry or a decline in agricultural output. This feature has the implication that we can make no definite judgment about the level of activity, in particular, whether actual output exceeds the natural level, by observing the change in the inflation rate. Surely, inflation due to a negative agricultural shock cannot reasonably be interpreted as a case of the economy ‘over-heating’ due to output expansion.
Estimates of the structuralist model of inflation for India
We estimated the structuralist inflation model for India using quarterly data and over the same sample period as we had earlier done with the NKPC. The choice of variables in the econometric specification of the structuralist model is based on Eq. ( 3 ). The relative price of the agricultural good is the ratio of implicit GDP deflator of Agriculture, Forestry and Fishing to that of non-agriculture sector, which includes Construction, Manufacturing and Services. As the relative price was found to be non-stationary, its log difference (growth rate) was used. 15 Allowing for the possibility that relative price is endogenous in the econometric model, the model has been estimated using both OLS and GMM-IV. The instruments used are the lagged values of the growth rate of relative price and growth rate of agricultural GDP. All lags up to ten with absolute value of correlation coefficient of 0.30 or above with the current value of growth rate of relative price are taken as instruments. The results are presented in Table Table3. 3 . As the OLS and GMM-IV estimates are, mostly, very close to one another we make no distinction between them when discussing the results. First, the coefficient on the relative price of agricultural goods is statistically significant and quite high. As in the theoretical model, the price of oil matters for inflation, though the coefficient is far lower than that for the relative price. Lagged inflation matters for current inflation, implying inertia. Inflation inertia has the implication that inflation cannot be ended merely through central bank announcements termed “communication”. Finally, the explanatory power of the model—seen in the adjusted R 2 —is quite high for a model in changes. These results justify the conclusion that structuralist inflation model is a valid description of the inflationary process in India, in particular that the relative price of agricultural goods is the principal driver of inflation.
Estimates of the structuralist model of inflation
Dependent variable is CPI inflation; period is 1996–97(Q1) to 2020–21(Q1)
(1) t statistics in parentheses
(2) ** and * indicate significance at the 1 and 5% level, respectively
(3) q1 … q3 are quarterly dummies
Interpreting lower inflation in India since 2016
On the basis of the econometric evidence for the two models of inflation in the Indian context, it may be surmised that the stable inflation since the adoption of inflation targeting in 2016 owes to the behavior of the relative price of agricultural goods and the price of imported oil. This is confirmed by the following exercise. We use the OLS estimate of the structuralist inflation model estimated using data upto 2016–17(Q2), to predict inflation after inflation targeting was adopted up to 2020–21 (Q1), being the last year for which data were available when the exercise was conducted. The time series of the actual and predicted inflation rates are graphed in Fig. 3 . A close fit may be seen. 16 In particular, that the predictions from a structuralist inflation model show a downward trend after 2016, the year in which inflation targeting was adopted. This implies that the observed trajectory of inflation can be fully understood within the framework of structuralist macroeconomics. This combined with our finding that expectations do not drive inflation, and that the output gap in the NKPC is not statistically significant, implies that it would be wrong to attribute this trajectory to the monetary policy of the RBI, in particular inflation targeting.

Predictions based on the structuralist model
As the main determining variable of inflation in the structuralist model is the relative price of agricultural goods, we investigated the change in its growth rate for the period under consideration. Rates of growth for sub-periods established by the Bai-Perron ( 1998 ) method are presented in Table Table4. 4 . A statistically significant reduction in the rate of growth of the relative price is evident from 2011 to 12. The reduction is very strong from 2017 to 18 onwards. The results of our econometric investigation, taken together, lend high credence to the conclusion that the lowering of inflation in India since 2011, and particularly since 2016, owes overwhelmingly to the slower growth of commodity prices.
Growth rates of the relative price of agricultural goods
The exponential growth rate is reported. The breakdates have been estimated
Finally, in Fig. 4 , is presented a plot of inflation and the growth of the relative price of agricultural goods. A co-movement is evident, with the relative price change leading inflation. The correlation coefficient is 0.59.

Inflation and the relative price of agricultural goods
In 2021, India completed 5 years of inflation targeting. Reviews have asserted that the fact of inflation remaining within the mandated band is evidence of the success of inflation targeting. We have argued here that such an approach cannot exclude observational equivalence, that observed inflation may have been generated by a process other than the one assumed under inflation targeting, the New Keynesian Phillips Curve. Accordingly, we investigated which of two alternative models of inflation account for the Indian experience. It was found the New Keynesian Phillips curve, on which central banks across the world base inflation targeting, is not validated for Indian data but the structuralist model of inflation, based on the relative price of agricultural goods, is. Further, the latter model was found to predict, upto a considerable degree of accuracy, the trajectory of inflation after inflation targeting was adopted. This implies that subdued inflation in India, observed for almost a decade by now, can be put down to the behavior of commodity prices. Now, control of inflation would require a different set of instruments than what is available to central banks.
Appendix: A1. Data sources
The definition and construction of variables have been explained as and when they appear in the text. Here we state the sources of the data used.
Output: The study used quarterly GDP data for the period 1996–97 Q1 to 2020–21 Q1. The quarterly GDP data in current and constant prices were collected from the website of the Central Statistical Office (CSO).
Prices: Following the RBI, the inflation rate is measured by the rate of change in the consumer price index (CPI). For the years for which a single consumer price index is unavailable a combined index has been formed by combining the CPI for Industrial Workers and the CPI for Agricultural Workers. Oil price has been measured by the wholesale price index for mineral oils. All price data are from the EPW Research Foundation’s ‘India Time Series’ database. The GDP deflators for the agricultural and non-agricultural sectors, used in the construction of the relative price, are computed from data on sectoral GDP at current and constant prices taken from the national accounts statistics.
Inflation Expectations : Expectations data are from the Household’s Inflation Expectations Survey of RBI, available for downloading from the website www.rbi.org.in .
Averages of the expected inflation across households are used in the econometric estimation.
A2. Testing for a unit root and seasonal stability
Unit root properties of the time series were tested using the Augmented Dickey–Fuller (ADF) test, the Kwiatkowski, Phillips, Schmidt, and Shin (KPSS) test, and the Zivot and Andrews test. The Zivot-Andrews test is used because a trend stationary series with a break in the trend can be wrongly diagnosed as an I(1) process by both the ADF and KPSS tests. This test allows for an unknown break in trend and intercept when testing for a unit root. The lag length for the ADF test was selected on the basis of the Bayesian Information Criteria (BIC) and lag length in KPSS test was fixed at 1, as the simulation results reported in Kwiatkowski, Phillips, Schmidt, and Shin (1992), 17 showed that for a sample size similar to ours, a lag length of one provides correct size of the test. As the data is quarterly, we also test for a seasonal unit root or seasonal stability using the tests developed in Canova and Hansen (1995) 18 and Hylleberg, Engle, Granger, and Yoo (HEGY, 1990), 19 respectively known in the literature as the Canova–Hansen test and HEGY test. The test results are reported in Tables Tables5, 5 , ,6, 6 , ,7, 7 , ,8 8 .
Unit Root test: quarterly data, level
Note: Critical values at 5 percent level are given in parentheses. The null hypothesis in the ADF and Zivot and Andrews tests is that the series is I(1) and alternative is that it is I(0). In the KPSS test the null hypothesis I(0) and alternative I(1). In all the cases, the alternative hypothesis is trend stationarity, except in the case inflation, where the plot against time showed no trend and hence the alternative of stationarity around the mean was chosen
Unit Root test: quarterly data, first -difference
Note: Critical values at the 5 percent level are given in parentheses. The null hypothesis in the ADF test is that the series is I(1) and the alternative is that it is I(0). In the KPSS test the null hypothesis is that the series is I(0) and the alternative is that it is I(1). In all the cases, the alternative hypothesis is stationarity around mean, as the plots revealed no trend
Testing for a seasonal unit root: quarterly data, level
Note: The null hypothesis in the Canova–Hansen test is stationarity of the series and the null hypothesis of the HEGY test is that the series has a unit root. In both cases, the joint-F statistics is reported. P values are given in parentheses. For the HEGY test, the p values are bootstrapped
Testing for a seasonal unit root: quarterly data, first-difference
Note: The null hypothesis in the Canova–Hansen test is stationarity of the series and the null hypothesis in the HEGY test is that it contains a unit root. In both cases the joint-F statistic is reported. P values are given in parentheses and in HEGY test the p values are bootstrapped
Acknowledgements
Support from Ashoka University, the Centre for Development Studies and the Indian Institute of Management Kozhikode is gratefully acknowledged. We thank Bharat Ramaswami, Dipankar Dasgupta, Mausumi Das, Ajit Kumar Ghose, Partha Ray, Alex Thomas, Arjun Jaidev, Binoy Goswami and Ajit Mishra, and seminar audiences at Ashoka University, National Institute of Public Finance and Policy, Indian Institute of Management Calcutta, South Asian University, Azim Premji University, Delhi School of Economics, Centre for Development Studies, EGROW Foundation and the Institute of Economic Growth for discussions. Errors that may remain would be ours.
The authors declare that no funds, grants, or other support were received during the preparation of this manuscript.
Declarations
On behalf of all the authors, the corresponding author states that there is no conflict of interest.
1 See Eichengreen, Gupta and Choudhary ( 2020 ).
2 For an account of the evolution of monetary policy in India and the practices of inflation targeting, see Dua ( 2020 ).
3 See, the statement “The inflation process in India has become increasingly sensitive to forward-looking expectations.” in RBI ( 2021 ).
4 The current Governor of the Reserve Bank of India has stated "… we (also) want to anchor inflation expectations within the tolerance band and closer to the inflation target in the medium term." ‘Economic Times’, ( 2021 ).
5 See Fuhrer et al., ( 2009 ). This is also the form that is found in RBI, ( 2014 ), a report that had recommended inflation targeting for India.
6 Gali & Gertler, ( 1999 ).
7 The results were similar when deviations from a linear trend with quarterly dummies are used.
8 See Okun, ( 1980 ).
9 The results were similar when 1-year ahead inflation expectations were used.
10 Results of extensive testing of the NKPC using different measures of inflation and the output gap, and data at alternative frequencies and of varying sample periods are reported in Balakrishnan and Parameswaran ( 2021 ).
11 See Rudd and Whelan ( 2007 ).
12 See Taylor ( 1984 ) for an exposition of structuralist macroeconomics, Cardoso ( 1981 ) for a model of inflation within the framework and Basu ( 1997 ) for a discussion of the structuralist explanation of inflation and its origins.
13 See Balakrishnan and Parameswaran ( 2021 ).
14 In (3) W a n d P m are the wage and the price of imported material, respectively, γ and τ the respective input coefficients, and eis the exchange rate.
15 It may be noted that Canavese ( 1984 ) presents a structuralist model of inflation in which the rate of inflation is a function of the growth rate of the relative price of the agricultural good.
16 A similar out-of-sample prediction using information from the GMM-IV estimates reported above gave the same prediction. Specifically, under OLS, the correlation coefficient between actual inflation and predicted inflation is 0.80 for the entire period and 0.78 for the period of inflation targeting. The corresponding estimates for GMM-IV estimates are 0.79 and 0.77, respectively.
17 Kwiatkowski, D.; Phillips, P. C. B.; Schmidt, P. & Shin, Y. (1992), 'Testing the null hypothesis of stationarity against the alternative of a unit root: How sure are we that economic time series have a unit root?', Journal of Econometrics 54 , 159–178.
18 Canova, F. & Hansen, B. E. (1995), 'Are Seasonal Patterns Constant Over Time? A Test for Seasonal Stability', Journal of Business & Economic Statistics 13 (3), 237-252.
19 Hylleberg, S.; Engle, R. F.; Granger, C. W. J. & Yoo, B. S. (1990) 'Seasonal integration and cointegration', Journal of Econometrics, 44(1), 215—238.
Publisher's Note
Springer Nature remains neutral with regard to jurisdictional claims in published maps and institutional affiliations.
Contributor Information
Pulapre Balakrishnan, Email: [email protected] .
M. Parameswaran, Email: ude.sdc@narawsemarap .
- Bai J, Perron P. Estimating and testing linear models with multiple structural changes. ‘Econometrica’ 1998; 66 (1):47–78. doi: 10.2307/2998540. [ CrossRef ] [ Google Scholar ]
- Balakrishnan P, Parameswaran M. Modelling inflation in India. Journal of Quantitative Economics. 2021; 19 :555–581. doi: 10.1007/s40953-021-00238-y. [ CrossRef ] [ Google Scholar ]
- Ball, L., A. Chari & P. Mishra (2016) Understanding Inflation in India . NBER Working Paper No. 22948.
- Basu K. Analytical development economics. MIT Press; 1997. [ Google Scholar ]
- Canavese A. The structuralist explanation in the theory of inflation. World Development. 1984; 10 :523–529. doi: 10.1016/0305-750X(82)90053-5. [ CrossRef ] [ Google Scholar ]
- Cardoso EA. Food supply and inflation. Journal of Development Economics. 1981; 8 (3):269–284. doi: 10.1016/0304-3878(81)90016-X. [ CrossRef ] [ Google Scholar ]
- Dua P. Monetary policy framework in India. Indian Economic Review. 2020; 55 :117–154. doi: 10.1007/s41775-020-00085-3. [ PMC free article ] [ PubMed ] [ CrossRef ] [ Google Scholar ]
- Economic Times. (2021). RBI’s Shaktikanta Das wants to support growth, keep inflation expectations anchored , July 8.
- Eichengreen B, Gupta P, Choudhary R. Inflation targeting in India: An interim assessment, policy research working paper no. 9422. Washington, DC: The World Bank; 2020. [ Google Scholar ]
- Fuhrer, J., Kodrzycki, Y., Little, J. S., & Olivei, G. P. (Eds.). (2009). Understanding inflation and the implications for monetary policy: A Phillips curve retrospective . Cambridge: MIT Press.
- Gali J, Gertler M. Inflation dynamics: A structural econometric analysis. Journal of Monetary Economics. 1999; 44 :195–222. doi: 10.1016/S0304-3932(99)00023-9. [ CrossRef ] [ Google Scholar ]
- Hatekar N, Sharma A, Kulkarni S. What drives inflation in India: Overheating or input costs? Economic & Political Weekly. 2011; 34 :46–51. [ Google Scholar ]
- Okun AM. Rational expectations-with-misperceptions as a theory of the business cycle. Journal of Money, Credit and Banking. 1980; 12 (4):817–825. doi: 10.2307/1992036. [ CrossRef ] [ Google Scholar ]
- Paul BP. In search of the Phillips curve for India. Journal of Asian Economics. 2009; 20 :479–488. doi: 10.1016/j.asieco.2009.04.007. [ CrossRef ] [ Google Scholar ]
- RBI. (2014). Report of the expert committee to revise and strengthen the monetary policy framework . Reserve Bank of India, Mumbai. https://rbi.org.in/Scripts/PublicationReportDetails.aspx?ID=743 .
- RBI. (2021). Is the Phillips curve in India dead, inert and stirring to life or alive and well ?. RBI Bulletin
- Rudd, J.B. (2021). Why do we think that inflation expectations matter for inflation? (And should we?), Finance and economics discussion series 2021–062 . Washington: Board of Governors of the Federal Reserve System. 10.17016/FEDS.2021.062.
- Rudd JB, Whelan K. Modeling inflation dynamics: A critical review of recent research. Journal of Money Credit and Banking. 2007; 39 :155–170. doi: 10.1111/j.1538-4616.2007.00019.x. [ CrossRef ] [ Google Scholar ]
- Taylor L. Structuralist macroeconomics. Basic Books; 1984. [ Google Scholar ]
You think you earned your raise and you think inflation is unfair: New economic paper sums up Biden’s dilemma

“ It’s the economy, stupid ,” has been the unofficial mantra of all U.S. presidents seeking reelection since the 1990s and that immortal phrase from the ragin’ cajun himself, James Carville . But Joe Biden may be the first incumbent to test that theory, as poll after poll finds that, despite the economy firing on all cylinders , Americans are steadfastly pessimistic about it.
In other words, it’s shaping up to be not the economy, stupid, in 2024. So what is it? Maybe it’s inflation after all.
Price hikes are still top of mind for voters despite peaking 18 months ago. In a March YouGov poll, Americans named it as the top issue facing the country, while inflation worries just sent business owners’ optimism to its lowest point in a decade . And while it has long been known that consumers hate rising prices, but the depths of that hate—and just how differently regular voters think when compared to economists—becomes clear in a new Brookings Institution paper that aims to answer the question posed in its title: “ Why Do We Dislike Inflation ?”
Its findings reveal that normal people are not like economists when it comes to this issue.
The real reaction to inflation
Ask economists about the post-pandemic recovery, and they will cite some combination of COVID-induced shortages and supply-chain snarls , mass business closures and layoffs , hefty government spending to encourage a fast economic recovery and get more people back to work, and several years of ultralow interest rates, although left- and right-leaning economists disagree on whether the government made the right choices.
Normal people, though, see “the economy” as a collection of different factors, only the worst of which are the government’s fault. Specifically, when it comes to inflation, people blame the government; when it comes to their wage increases, people believe they’ve been earned.
“[I]ndividuals rarely ascribe the raises they receive during inflationary periods to adjustments for inflation. Rather, they attribute these increases to job performance or career progression,” writes study author Stefanie Stantcheva, a professor of political economy at Harvard University.
Nearly half of the respondents to the survey reported getting a pay increase. But when they were asked about the reasons for the increase, people were more than twice as likely to credit their own on-the-job performance than inflation. (Among the highest-paid respondents, those making $125,000 or more, the difference was nearly three times.)
“When workers get pay increases, they think it’s their own work; when they see inflation, they think that’s bad policy,” Dean Baker, cofounder and senior economist at the Center for Economic and Policy Research, told Fortune . “As an economist, it’s annoying.”
Baker noted that this latest paper confirms and updates research dating back to the 1990s, when Robert Shiller found people angry about inflation, pessimistic about their ability to make up inflation gains with pay raises, and mistrustful of concepts that economists take for granted.
Both Baker and Shiller are worth listening to, for similar but different reasons. Baker has been blogging about economics, and media coverage of it, for decades now at Beat the Press , advocating a generally progressive viewpoint but also regularly questioning political economy, especially the employment of copyright. He was one of the few economists to spot the housing bubble before it burst in late 2007. Shiller, a Nobel laureate, also spotted the 2000s housing bubble, and his name graces one of the housing market’s key indexes, the Case-Shiller .
What is inflation, anyway?
What’s more troubling is that only half of the respondents to Stantcheva’s study could correctly define inflation as a general increase in the price of goods. (A sample incorrect definition: “The hiking of prices of consumer goods to offset the country’s debt due to elites overspending and throwing money away.”)
Respondents were also hazy on other economic concepts, such as the relationship between inflation and unemployment. Balancing the two is the job of the Federal Reserve, whose mandate requires it to balance “maximum employment” and “stable prices.” The Fed painstakingly tries to reconcile these two opposite forces, knowing that a tight job market can lead to slightly higher inflation (since workers have more bargaining power to demand higher pay, which can get passed on to consumers), while focusing on ultralow inflation can keep the economy underperforming.
While most respondents in Stantcheva’s survey said there was a relationship between unemployment and inflation, only one in four correctly identified the tradeoff between high inflation and low unemployment.
When respondents were asked an open-ended question about the cause of inflation, the top answer was “Biden and the administration,” followed by “greed,” “monetary policy,” and “fiscal policy.” There is a partisan divide in these answers, with Democrats much more likely to answer “greed” while Republicans were prone to point to “Biden and the administration.” Respondents making under $40,000 a year were twice as likely to blame Biden as those making $125,000 and over.
Nearly no one discussed COVID-19 as a reason for inflation, although some people said “supply and demand” was a cause.
In general, inflation, or rising prices, happens when there is too much demand in relation to supply. And when that happens in several industries , like oil and gas, transportation, food, and manufacturing, higher prices can be reflected nearly across the board, according to a Harvard Business Review report . A drop in supply can also be caused by significant disruptions to resources, like labor and energy, used to fuel the economy—such as the drop in oil production and disruption in global food supplies after Russia’s invasion of Ukraine in 2022, or a year before that, the shortfall in manufacturing of semiconductor chips and the hiccups in shipping that characterized global reopening. Many economists have cited these global “ supply shocks ” as a key reason inflation soared to 40-year highs.
Another school of thought, famously given definition by a quote from another Nobel laureate, Milton Friedman : “Inflation is always and everywhere a monetary phenomenon.” In May 2023, Fortune ’s Shawn Tully reported on a relation of this theory from the generally Friedmanite “money doctor” Steve Hanke, who said the “ monetary bathtub ” had simply grown too full during the pandemic. Inflation can also occur when worker wages are rising fast and companies pass on those wages in the form of higher prices.
When it comes to the job market, though, there are clear links to policy decisions made by Congress and the states during and after the pandemic, said Elise Gould, senior economist at the Economics Policy Institute, a left-leaning think tank. After employers laid off tens of millions of workers in 2020, Congress and many states “provided more adequate unemployment benefits, and disproportionately supported those low-wage workers who lost their jobs,” she told Fortune . “They could stay afloat and were less desperate, and businesses had to work harder to attract them,” leading to today’s tight labor market. Stimulus checks to people and businesses and funding for local government also contributed, as did minimum-wage hikes in 13 states that index wages to inflation.
But among the general public, Gould said, “there's a disconnect. I don't think people always see how things happen in the same way [as economists].”
That’s bad news for the Biden camp, since—despite the rate of inflation falling to just one-third of its peak two years ago—Americans seem more worried about it than ever. One in four respondents to an Economist/YouGov poll in March cited “inflation/prices” as the top issue facing the nation, a higher portion than the one in five who gave the same answer 18 months ago . Perhaps reelection depends on the 10% of respondents to Stantcheva’s survey who blamed “the system.”
“No one, just the prices,” said one survey taker when asked who they were angry at. “Can’t tell if it’s the stores or the government.”
Latest in Finance
- 0 minutes ago

Investors don’t seem that worried, for now, about a wider conflict in the Middle East after Iran’s unprecedented attack on Israel

Israel-Iran conflict will add to Fed’s caution on rate cuts as oil prices may disrupt inflation fight—but China and OPEC+ could ease pressure, Capital Economics says

Bitcoin ‘halving’ will cost crypto miners $10 billion a year in lost revenue and ‘could well determine who comes out ahead and who gets left behind’

Traveling between Europe and Asia will take longer as airlines reroute flights after Iran’s attack on Israel

Here’s what Wall Street expects from financial markets after Iran’s attack on Israel. ‘The risk is if this situation escalates and there is contagion in the region’

Markets flash early signs of risk from wider Mideast war as crypto prices sink after Iran launches wave of drones at Israel
Most popular.

Founder of Toms shoes went on a men’s retreat with other entrepreneurs to combat his loneliness and depression: ‘I lost a lot of my clear meaning and purpose’

Workers at Elon Musk’s Boring Co. accidentally dug too close to a supporting column of the Las Vegas monorail last year, forcing officials to briefly halt service

The ‘Oracle of Wall Street’ expands on why the ‘crisis of the American male’ will send home prices crashing 30%: Gaming, rampant loneliness, and not enough single women homebuyers

Boeing’s CEO search has a new frontrunner—and insiders say it could mean a radical change for the $104 billion ailing planemaker

Vietnam’s ‘blazing furnace’ sees real estate mogul sentenced to death for $12 billion fraud. It’s the biggest eruption yet in Asia’s volcanic housing bust

Car dealership owner turned politician goes back to college to study AI, machine learning: ‘There won’t be robots with red eyes coming after us any time soon’
Inflation targeting and price behaviour: evidence from India
- Published: 23 December 2022
- Volume 57 , pages 265–284, ( 2022 )
Cite this article
- Amlendu Dubey ORCID: orcid.org/0000-0003-3423-7607 1 &
- Juhi Lohani 1
1162 Accesses
1 Altmetric
Explore all metrics
We study the individual price behaviour in India using monthly item-wise CPI prices during 2011–2019 and explore the impact of implementation of inflation targeting on price behaviour. We find that inflation targeting has significantly reduced the frequency of price increases and increased the frequency of price decreases in India; however, it has not been successful in reducing the dispersions in price increases or price decreases, a measure of welfare cost of inflation. We find that another monetary policy event during our period of analysis, demonetization, led to fall in the spread of price increases and rise in the spread of price decreases. Further, we find that increase in monthly inflation increases the frequency of price increases and decreases the frequency of price decreases which is as per the expectations. Overall, our results have important implications for the effectiveness of monetary policy in India in achieving price stability.
Similar content being viewed by others
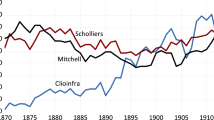
European consumer price indices since 1870
Jonas Ljungberg
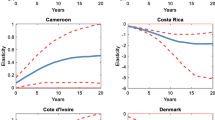
Inflation and growth: the role of institutions
Hakan Yilmazkuday
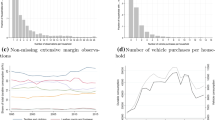
Inflation expectations and consumer spending: the role of household balance sheets
Lenard Lieb & Johannes Schuffels
Avoid common mistakes on your manuscript.
1 Introduction
Consumer Price Index (CPI) is instrumental in measuring inflation and monitoring price stability by central banks worldwide. In May 2016, India officially adopted inflation targeting (IT) as its monetary policy framework. The inflation target in India is set at 4% CPI inflation with the upper tolerance limit of 6% and lower limit of 2%. The important features of IT policy include preannouncement of inflation targets in public, transparency and accountability of central banks to achieve the objective of low and stable inflation (Roger, 2010 ).
As far as relationship between inflation targeting and price stability is concerned, a section of literature shows that inflation targeting reduces inflation (Creel & Hubert, 2015 ; Mishkin, 2000 ; Mishkin & Schmidt-Hebbel, 2007 ; Neumann & von Hagen, 2002 ; Vega & Winkelried, 2005 ; Wu, 2004 ) while others argue that there is no significant impact of IT on inflation (Angeriz & Arestis, 2007 ; Ball & Sheridan, 2005 ; Johnson, 2002 ; Lin & Ye, 2007 ). Svensson, ( 1997 ) shows that inflation targeting helps in reducing inflation variability while Bernanke et al., ( 1999 ) and Civcir & Akcaglayan, ( 2010 ) explain the role of IT regime in anchoring inflationary expectations. Similarly, King, ( 2002 ) shows that the inflation rates in the IT countries are less persistent and less volatile while Fititi & Hichri, ( 2014 ) show that IT is a relevant policy in achieving price stability.
However, we could not find any study which specifically explores the implications of inflation targeting (IT) on frequency of price increases, decreases or overall price changes. The issue is important because high inflation increases price dispersion and distorts the allocative role of the price system (Nakamura et al., 2018 ). In economies with consistently high inflation, relative prices fluctuate, as prices move away frequently from their optimal value during the price adjustment process. Consequently, relative prices no longer give correct signals regarding relative cost of production leading to production inefficiency and significant welfare cost of inflation (Nakamura et al., 2018 ). Also, at low inflation rates, a significant portion of the price changes should consist of price decreases. As inflation increases, the frequency of price decreases should fall, and frequency of price increases should increase (Gagnon, 2009 ; Nakamura et al., 2018 ). In this paper, taking the case of CPI prices in India, we study the implications of inflation targeting on this aspect of price behaviour.
We find that the implementation of inflation targeting has led to significant reduction in the frequency of price increases and increased the frequency of price decreases in India; however, it does not have any impact on the dispersion of price increases or price decreases. This result suggests that although inflation targeting has been successful in stabilizing the inflation rate, it has not been successful in reducing the price dispersion emanating from high inflation. This may be because of targeting a relatively wider inflation range of 2% to 6% in India as compared to other inflation targeting economies.
During our period of analysis, another important policy of Demonetization was announced in 2016. Demonetization affected various sectors of the economy with varying degrees. Chodorow-Reich et al., ( 2020 ) show that the decline in cash led to the fall in the growth rate of economic activity by at least 2 percentage points during the quarter demonetization was implemented. Other studies have used descriptive statistics (Krishnan & Siegel, 2017 ; RBI, 2017 ) or time series methods (Aggarwal & Narayanan, 2021 ; Banerjee & Kala, 2017 ) to understand the impact of demonetization on the various sectors of Indian economy. Aggarwal and Narayanan ( 2021 ) studied the impact of demonetization on domestic agricultural trade and found the trade got displaced considerably following demonetization because of the collapse in prices. Currency squeeze due to demonetization along with seasonal factors pushed food inflation significantly down but did not show much impact on inflation excluding food and fuel (RBI, 2017 ). Our results show that demonetization seems to have significantly reduced the dispersion of price increases and increased the dispersion of price decreases. It also seems to have reduced the kurtosis in price decreases.
Further, we find that increase in monthly inflation increased the frequency of price increases and decreased the frequency of price decreases which is as per the literature. Overall, we find that CPI Prices in India are highly flexible which is similar to the price behaviour found in other emerging market economies (Barros et al., 2009 ; Kovanen, 2006 ; Medina et al., 2007 ).
Rest of the paper is organized as follows. In Sect. 2 , we discuss the individual CPI price behaviour in India. In Sect. 3 , we discuss the methodology adopted and the results. Section 4 concludes the paper.
2 Individual CPI prices in India
Behaviour of individual prices has important implications for monetary policy. There has been several studies analysing micro-level CPI and PPI data, such as Bils & Klenow, ( 2004 ) and Nakamura & Steinsson, ( 2008 ) for the USA, the Inflation Persistence Network (IPN) country-level studies for the Euro area (Dhyne et al., 2006 and Vermeulen et al., 2007 ), and Bunn & Ellis, ( 2009 , 2012a , 2012b ) for the UK. Other empirical studies such as Alvarez & Burriel, ( 2010 ), Alvarez et al., ( 2014 ), Alvarez & Lippi ( 2014 ), Costain & Nakov ( 2011 ), Vavra, ( 2014 ), Kara, ( 2015 ), and Dixon & Tian, ( 2017 ) have added more variations in the study of price behaviour by estimating price models and comparing them with the standard pricing theories. Dhyne et al., ( 2009 ) show that the degree of price stickiness, regarded as price-setting behaviour, varies across countries and sectors. For instance, the frequency of price changes is lower in the Euro region than in the USA. Moreover, in developing countries like Brazil, Mexico, Slovakia, and Chile, the frequency of price changes are higher. Morande & Tejada, ( 2008 ) and Kovanon, ( 2006 ) have further found evidence of sectoral heterogeneity in price stickiness in the developing countries of Latin America and Sierra Leone, respectively.
In order to understand the nature of price changes in India, we collect item-wise monthly CPI price data from Ministry of Statistics and Programme Implementation (MOSPI), Govt. of India, for the years 2011–2019. Data of other variables used in the study such as Money Supply and Index of Industrial Production have been collected from the Reserve Bank of India’s Database on Indian Economy.
The data collected for the CPI are classified as item-wise price quotes concerning their product type and location. These price quotes were utilized to estimate any change in price for each product in a particular month. We found that over a period of 8 years during 2011 – 2019, on an average, percentage of CPI goods changing prices each month is very high, i.e., 94.1%. Moreover, the increases in CPI prices are more than the price decreases and there is a significant difference between the increases (71.6%) and decreases (22.5%). The data suggest that Indian CPI prices change much more often than advanced countries like the USA where 26% of prices are estimated to change each month (Bils & Klenow, 2004 ) and other emerging market economies like Chile where 46.1% of prices change each month (Medina et al., 2007 ).
The distribution of the size of CPI price changes is summarized in Table 5 in Appendix. The magnitude of the price changes range from -9% to 13%. In Fig. 4 in Appendix, it can be seen that there are a significant number of price changes that are relatively in the range of -1% to 1%. The width of the distribution depicts that approximately 95% of all the price changes are found to be less than 8.5%. 25% of all the price cuts are less than 1% and go up to 9%. 25% of all price increases are above 6% and range up to 13%. There are spikes in the distribution of price changes at percentage figures like 4% or 7%. Nearly 95% of the distribution is covered by the interval between -10% and 10%. The median price change is 0.32% rise. A higher proportion of price changes, as can be seen in the distribution are small price changes and lie between -2% to 0% and 0% to 2%.
Figure 5 in Appendix depicts the distribution of the number of months between price changes per item in CPI. It can be observed that on an average, 22% of the products change prices in less than a month. Almost 95% of products have an average duration between price changes of less than six months. Hence, it can be observed that a lesser percentage of products take a long time to change prices in India.
When we analyse the distribution of the magnitude of price changes in Table 6 , it is observed that price changes of vegetables have the maximum interquartile range (IQR). In the case of services, only price changes for transport and communication have a wide distribution, all other services’ price changes show low interquartile range.
Zhou and Dixon ( 2019 ) for the UK and Gaarder ( 2009 ) for Norway highlight that prices of goods such as alcoholic beverages, narcotics, and tobacco remain stable for a significant period. They tend to fluctuate less, and thereby, the price stickiness is higher for these categories. In our results, however, the price changes in the tobacco is considerably higher when we compare it to the results found in the UK and Norway. Further, the prices of beverages also show significant fluctuation.
In Fig. 6 in Appendix we plot the duration of a price since its previous change against the size of that price change to study the link between the magnitude and frequency of price changes. It can be observed that the prices of most products are found to have changed within a period of one month since the last change. However, both the decreases and increases in median percentage price changes in a period of one month are almost uniform. Moreover, the majority of the prices are found to be either stagnant or increasing in the second month. Overall, we find that the increases in prices are more than the decreases in prices.
Overall, we find that prices are very flexible in India, except the food prices as the frequency of price changes for food is much lesser than the frequency of other goods.
Now we analyse the monthly frequency of price changes. Figure 1 shows the monthly frequency of all price changes along with the monthly frequencies of price increases and price decreases. We find that after the implementation of inflation targeting in India in May 2016, percentage of increases have fallen, and the percentage of price decreases have risen significantly. In our econometric estimation as discussed in the next section we empirically test whether inflation targeting has any statistically significant impact on the frequency of price increases and decreases.
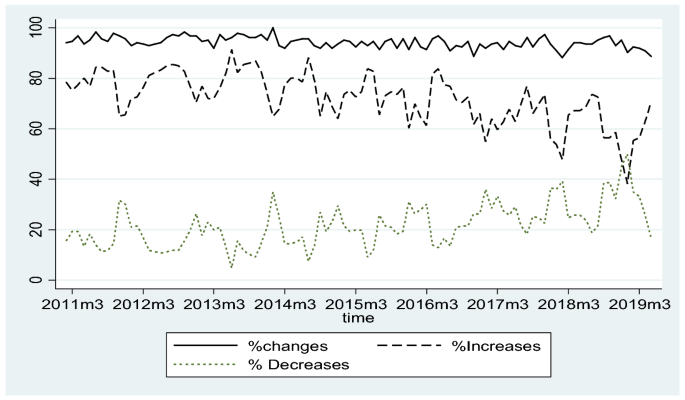
Time series of frequency of price changes
Further, in Fig. 2 , we use interquartile range and standard deviation to measure dispersion of price changes. Interquartile range (75th Percentile-25th Percentile) considers only the middle 50% of the distribution whereas standard deviation takes into account all the values including the extreme values. Both the distributions are very noisy and volatile.
Midrigan, ( 2011 ), Alvarez and Lippi ( 2014 ) and Alvarez et al. ( 2016 ) stress the importance of kurtosis of the price growth distribution in understanding the price behaviour. Empirically, it has been seen that the distribution of size of price changes exhibits a large number of big as well as small price changes. A kurtosis that is large depicts this varying price changes feature of the distribution. High kurtosis emphasizes the presence of flexible prices in the economy as flexible prices only will react even to the small shocks. In Fig. 3 , we plot time series of monthly kurtosis of all price changes in a month.
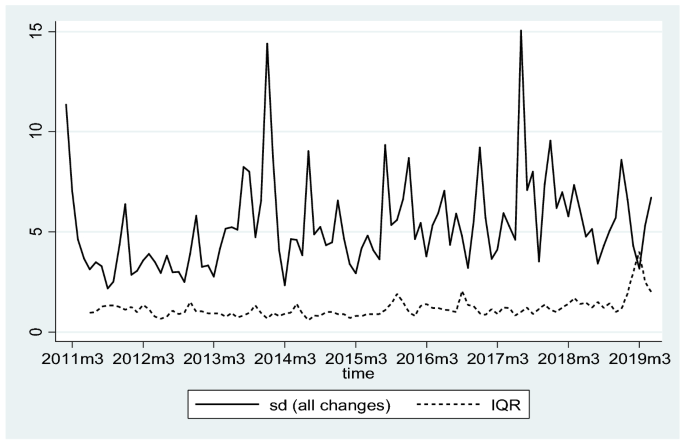
Times series of price-level dispersion
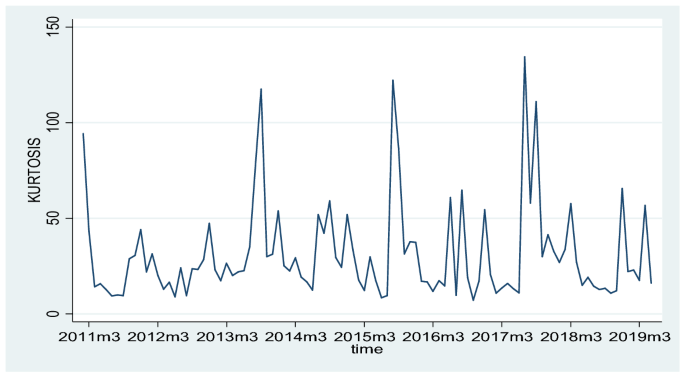
Time series of Kurtosis
In the next section, we empirically test whether the implementation of inflation targeting has affected the consumer price behaviour in India as measured by frequency, dispersion, and kurtosis of the price changes apart from other macroeconomic variables such as inflation, money supply, and output growth.
3 Econometric estimation
We study the relationship between the frequency of price changes and other macroeconomic variables of inflation, output growth, and M3 growth in India. We have considered monthly inflation that is based on month on month increase in the CPI price level following Dixon et al., ( 2020 ). We include inflation targeting and demonetization dummies in our analysis to analyse implications of these policies. Inflation targeting dummy takes value 1 post-inflation targeting implementation in May 2016 and 0 prior to it. We consider the period from November 2016 to February 2017 as demonetization period as the money supply which fell considerably in this period returned to pre-November 2016 levels by February 2017. Demonetization variable takes 1 in this period and 0 otherwise. To measure the output growth, we use Index of Industrial Production (IIP) where we take monthly output growth variable.
We adopt ordinary least squares (OLS) and instrument variable (IV) estimation techniques for our work following Dixon et al., ( 2020 ). Endogeneity bias and measurement errors can arise in OLS estimation because of which an IV estimator is employed to address these concerns. Taking into account residual autocorrelation and heteroscedasticity, we compute Newey–West standard errors. Our dependent variables are overall frequency of price changes, frequency of price increases, and frequency of price decreases. The list of our independent variables has monthly output growth, monthly inflation, money supply (M3) growth, and dummies for inflation targeting and demonetization. All the variables used in the analysis are stationary. In the case of IV estimation, we use monthly inflation and output growth as endogenous covariates. The instruments used in the case of IV regression are the first and second-order lags of these four regressors.
Here we present the results of IV regression in Table 1 . OLS results are reported in appendix in Table 7 . The results show that an increase in monthly inflation leads to an increase in the frequency of price increases and decrease in the frequency of price decreases both in OLS and IV estimation which is as per expectation. The negative sign of the IT coefficient for the frequency of price changes and price increases shows that the inflation targeting policy has helped reduce the frequency of price changes and price increases. Similarly, the positive sign of IT coefficient in case of frequency of price decreases shows that it has increased the frequency of price decreases. Demonetization seems to have no statistically significant impact on frequency of price changes, increases or decreases.
3.1 Dispersion and kurtosis of price changes
In this section, to understand the implications of inflation targeting and other macroeconomic variables on dispersion of price changes, price increases and price decreases, we regress the monthly standard deviation, interquartile range, and kurtosis of theses variables on macroeconomic variables and dummies for policies. In Table 2 , we report the results of IV estimation when the dependent variables are standard deviation, interquartile range and kurtosis of overall price changes. OLS results are reported in appendix in Table 8 . The IT variable is positive and significant both in the case of standard deviation and interquartile range of prices changes which shows that implementation of inflation targeting has increased dispersion in price changes. The demonetization variable is negative and statistically significant for the interquartile range showing a fall in the variability of price changes as an effect of demonetization. Money growth (M3) is negative and significant for the interquartile range of price changes showing that an increase in M3 growth led to a fall in the spread of price changes.
3.2 Dispersion and kurtosis of price increases and decreases
We further segregate the price changes into price increases and price decreases and run similar regressions for increases and decreases separately. Table 3 shows the results of IV regressions for price increases. Table 9 shows the results of OLS regressions. Money growth is found to be negative and statistically significant for all the measures of price dispersion in IV regressions. Negative coefficients suggest that a rise in money growth leads to a fall in the dispersion of price increases. IT variable is positive and significant in the case of interquartile range of price increases suggesting that implementation of inflation targeting has increased the spread of price increases. Demonetization variable is negative and significant for standard deviation and interquartile range suggesting that demonetization reduced the spread of price increases. Monthly inflation is positive and significant in case of standard deviation and interquartile range of price increases in OLS regression.
Table 4 shows the IV regression results for the price decreases. OLS results are reported in Table 10 . We find that monthly inflation reduces the spread of price decreases. Inflation targeting seems to have reduced the interquartile range of prices decreases and increased the kurtosis of price decreases. Demonetization clearly increased the spread of price decreases as measured by interquartile range and reduced the possibility of outliers as measured by kurtosis.
4 Conclusion
In this paper, we study the nature of CPI prices in India and the implications of adoption of inflation targeting on price behaviour. Our results show that prices in India are highly flexible as compared to advanced economies. We find almost 94% of CPI goods in India change their prices every month. Our results suggest that inflation targeting has led to significant reduction in the frequency of price increases and increased the frequency of price decreases stabilizing inflation; however, it has not been successful in reducing the price dispersion arising out of high inflation. This may be because of relatively wider band of inflation target (2% to 6%) adopted in India as compared to other inflation targeting economies.
Demonetization seems to have significantly reduced the dispersion of price increases and increased the dispersion of price decreases. Further we find that increase in monthly inflation increases the frequency of price increases and decreases the frequency of price decreases which is as per expectations. We also find some evidence that monthly inflation increases the dispersion in price increases and reduces the dispersion in price decreases.
The relationship between inflation targeting and the frequency of price changes lets us comment on the policy's ability to stabilize prices in the economy. With inflation targeting, our results show that monetary policy has become effective in stabilizing inflation; however, it has not been successful in reducing the welfare cost of high inflation as shown by inefficient price dispersions.
Data availability
The data that support the findings of this study are openly available in Ministry of Statistics and Programme Implementation webpage at https://training.niip.gov.in/web/niip/themes-categories/-/report/view/1101 .
Aggarwal, N., & Narayanan, S. (2021). Impact of India's demonetization on domestic agricultural markets. Available at SSRN 3066042.
Alvarez, L. J., & Burriel, P. (2010). Is a Calvo price setting model consistent with individual price data? The BE journal of Macroeconomics . https://doi.org/10.2202/1935-1690.1748
Article Google Scholar
Alvarez, F., & Lippi, F. (2014). Price setting with menu cost for multiproduct firms. Econometrica, 82 (1), 89–135.
Alvarez, F., Le Bihan, H., & Lippi, F. (2014). Small and large price changes and the propagation of monetary shocks (No. w20155). National Bureau of Economic Research.
Alvarez, F., Le Bihan, H., & Lippi, F. (2016). The real effects of monetary shocks in sticky price models: A sufficient statistic approach. American Economic Review, 106 (10), 2817–2851.
Angeriz, A., & Arestis, P. (2007). Assessing the performance of ‘inflation targeting lite’countries. World Economy, 30 (11), 1621–1645.
Ball, L., & Sheridan, N. (2005). Does inflation targeting matter? The inflation targeting debate (pp. 249–276). University of Chicago Press.
Google Scholar
Banerjee, A., & Kala, N. (2017). The economic and political consequences of India’s demonetisation. VoxDev, https://voxdev.org/topic/institutions-political-economy/economic-and-political-consequences-india-s-demonetisation , 6 .
Barros, R., Bonomo, M., Carvalho, C., & Matos, S. (2009). Price setting in a variable macroeconomic environment: Evidence from Brazilian CPI. Unpublished paper, Getulio Vargas Foundation and Federal Reserve Bank of New York.
Bernanke, B. S., Laubach, T., Mishkin, F. S., & Posen, A. S. (1999). Missing the mark-the truth about inflation targeting. Foreign Affairs, 78 , 158.
Bils, M., & Klenow, P. J. (2004). Some evidence on the importance of sticky prices. Journal of Political Economy, 112 (5), 947–985.
Bunn, P., & Ellis, C. (2009). Price-setting behaviour in the United Kingdom: a microdata approach. Bank of England Quarterly Bulletin, 49 (1), 28–36.
Bunn, P., & Ellis, C. (2012a). Examining the behaviour of individual UK consumer prices. The Economic Journal, 122 (558), F35–F55.
Bunn, P., & Ellis, C. (2012b). How do individual UK producer prices behave? The Economic Journal, 122 (558), F16–F34.
Chodorow-Reich, G., Gopinath, G., Mishra, P., & Narayanan, A. (2020). Cash and the economy: Evidence from India’s demonetization. The Quarterly Journal of Economics, 135 (1), 57–103.
Civcir, I., & Akçağlayan, A. (2010). Inflation targeting and the exchange rate: Does it matter in Turkey? Journal of Policy Modeling, 32 (3), 339–354.
Costain, J., & Nakov, A. (2011). Distributional dynamics under smoothly state-dependent pricing. Journal of Monetary Economics, 58 (6–8), 646–665.
Creel, J., & Hubert, P. (2015). Has inflation targeting changed the conduct of monetary policy? Macroeconomic Dynamics, 19 (1), 1–21.
Dhyne, E., Alvarez, L. J., Le Bihan, H., Veronese, G., Dias, D., Hoffmann, J., & Vilmunen, J. (2006). Price changes in the euro area and the United States: Some facts from individual consumer price data. Journal of Economic Perspectives, 20 (2), 171–192.
Dhyne, E., Konieczny, J., Rumler, F., & Sevestre, P. (2009). Price rigidity in the euro area-An assessment (No. 380). Directorate General Economic and Financial Affairs (DG ECFIN), European Commission.
Dixon, H. D., & Tian, K. (2017). What we can learn about the behaviour of firms from the average monthly frequency of price-changes: an application to the UK CPI data. Oxford Bulletin of Economics and Statistics, 79 (6), 907–932.
Dixon, H., Luintel, K., & Tian, K. (2020). The impact of the 2008 crisis on UK prices: What we can learn from the CPI microdata 1. Oxford Bulletin of Economics and Statistics, 82 (6), 1322–1341.
Ftiti, Z., & Hichri, W. (2014). The price stability under inflation targeting regime: An analysis with a new intermediate approach. Economic Modelling, 38 , 23–32.
Gaarder, I. B. (2009). A Survival Analysis of Consumer Prices in Norway 1975–2004 (Master's thesis).
Gagnon, E. (2009). Price setting during low and high inflation: Evidence from Mexico. The Quarterly Journal of Economics, 124 (3), 1221–1263.
Johnson, D. R. (2002). The effect of inflation targeting on the behavior of expected inflation: Evidence from an 11 country panel. Journal of monetary economics, 49 (8), 1521–1538.
Kara, E. (2015). The reset inflation puzzle and the heterogeneity in price stickiness. Journal of Monetary Economics, 76 , 29–37.
King, M. (2002). The inflation target ten years on. Available at SSRN 709004.
Kovanen, A. (2006). Why do prices in Sierra Leone change so often? A case study using micro-level price data. SSRN Electronic Journal . https://doi.org/10.2139/ssrn.892944
Krishnan, D., & Siegel, S. (2017). Effects of demonetization: Evidence from 28 slum neighborhoods in mumbai. Available at SSRN 2896026.
Lin, S., & Ye, H. (2007). Does inflation targeting really make a difference? Evaluating the treatment effect of inflation targeting in seven industrial countries. Journal of Monetary Economics, 54 (8), 2521–2533.
Medina, J. P., Rappoport, D., & Soto, C. (2007). Dynamics of price adjustments: Evidence from micro level data for Chile. Central Bank of Chile Working Paper, 432.
Midrigan, V. (2011). Menu costs, multiproduct firms, and aggregate fluctuations. Econometrica, 79 (4), 1139–1180.
Mishkin, F. S. (2000). Inflation targeting in emerging-market countries. American Economic Review, 90 (2), 105–109.
Mishkin, F. S., & Schmidt-Hebbel, K. (2007). Does inflation targeting make a difference? . NBER working paper series no. 12876.
Morandé, F., & Tejada, M. (2008). Price stickiness in emerging economies: Empirical evidence for four Latin-American countries . University of Chile.
Nakamura, E., & Steinsson, J. (2008). Five facts about prices: A reevaluation of menu cost models. The Quarterly Journal of Economics, 123 (4), 1415–1464.
Nakamura, E., Steinsson, J., Sun, P., & Villar, D. (2018). The elusive costs of inflation: Price dispersion during the US great inflation. The Quarterly Journal of Economics, 133 (4), 1933–1980.
Neumann, M. J., & Von Hagen, J. (2002). Does inflation targeting matter? (No. B 01–2002). ZEI working paper.
RBI (2017). Macroeconomic impact of demonetisation - a preliminary assessment. https://rbidocs.rbi.org.in/rdocs/Publications/PDFs/MID10031760E85BDAFEFD497193995BB1B6DBE602.PDF .
Roger, S. (2010). Inflation targeting turns 20: A growing number of countries are making a specific inflation rate the primary goal of monetary policy, with success. Finance & Development, 47 (001), 59.
Svensson, L. E. (1997). Inflation forecast targeting: Implementing and monitoring inflation targets. European Economic Review, 41 (6), 1111–1146.
Vavra, J. (2014). Inflation dynamics and time-varying volatility: New evidence and an Ss interpretation. Quarterly Journal of Economics, 129 , 215–258.
Vega, M., & Winkelried, D. (2005). Inflation targeting and inflation behavior: A successful story? International Journal of Central Banking, 1 (3), 153–175.
Vermeulen, P. (2007). Macro Price setting in the euro area: Some stylised facts from Individual Producer Price. SSRN Electronic Journal . https://doi.org/10.2139/ssrn.1694544
Wu, T. Y. (2004). Does inflation targeting reduce inflation? An analysis for the OECD industrial countries. Working papers series 83, Central Bank of Brazil, Research Department.
Zhou, P., & Dixon, H. (2019). The determinants of price rigidity in the UK: Analysis of the CPI and PPI microdata and application to macrodata modelling. The Manchester School, 87 (5), 640–677.
Download references
No funding was received for conducting this study.
Author information
Authors and affiliations.
Indian Institute of Technology Delhi, New Delhi, 110016, India
Amlendu Dubey & Juhi Lohani
You can also search for this author in PubMed Google Scholar
Corresponding author
Correspondence to Amlendu Dubey .
Ethics declarations
Conflict of interest.
The authors report there are no competing interests to declare.
Additional information
Publisher's note.
Springer Nature remains neutral with regard to jurisdictional claims in published maps and institutional affiliations.
Tables 5 , 6 , 7 , 8 , 9 and 10
See Figs. 4 , 5 and 6
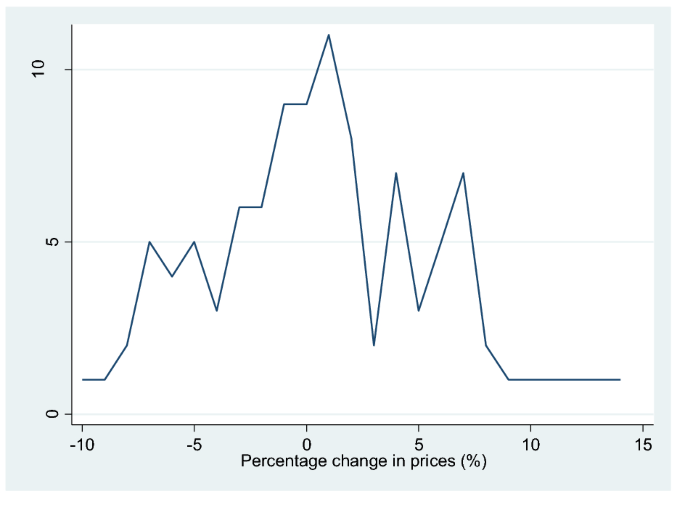
Distribution of the magnitude of price changes
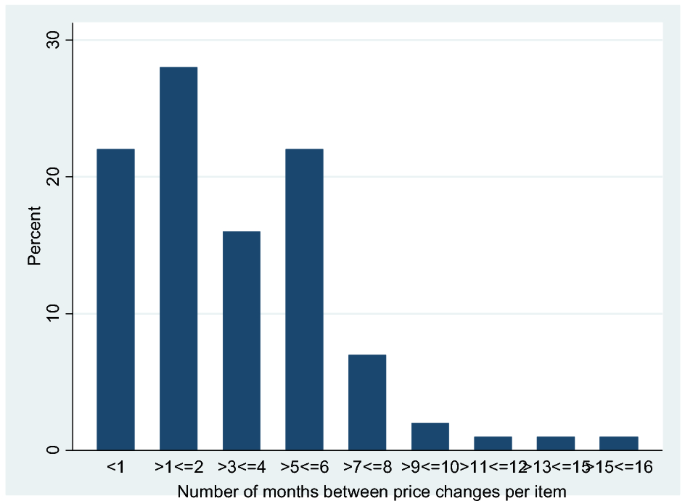
Distribution of number of months between price changes per item
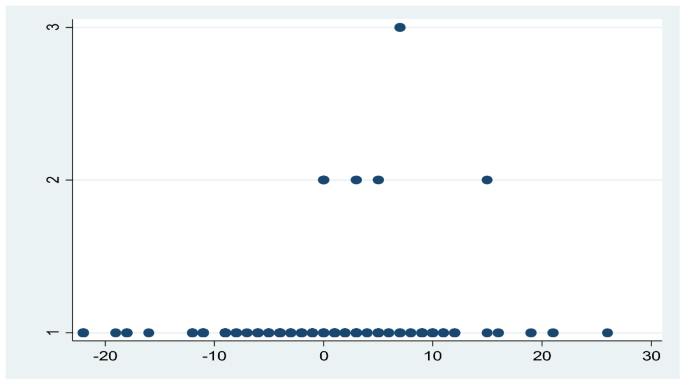
Distribution of size of consumer price changes and duration since previous price
Rights and permissions
Springer Nature or its licensor (e.g. a society or other partner) holds exclusive rights to this article under a publishing agreement with the author(s) or other rightsholder(s); author self-archiving of the accepted manuscript version of this article is solely governed by the terms of such publishing agreement and applicable law.
Reprints and permissions
About this article
Dubey, A., Lohani, J. Inflation targeting and price behaviour: evidence from India. Ind. Econ. Rev. 57 , 265–284 (2022). https://doi.org/10.1007/s41775-022-00148-7
Download citation
Accepted : 04 December 2022
Published : 23 December 2022
Issue Date : December 2022
DOI : https://doi.org/10.1007/s41775-022-00148-7
Share this article
Anyone you share the following link with will be able to read this content:
Sorry, a shareable link is not currently available for this article.
Provided by the Springer Nature SharedIt content-sharing initiative
- Inflation targeting
- Demonetization
- Frequency of price changes
JEL Classification
- Find a journal
- Publish with us
- Track your research
Why the price of chocolate exploded: How climate change drives inflation
Chocolate prices are soaring — and believe it or not, climate change is the big reason why, by matthew rozsa.
When you blend raw cocoa with sugar, it yields one of the most universally desired products on the planet, whether as part of a candy bar at the corner store or a high-end artisanal chocolate at a price point many times higher. But there's a problem: The cocoa beans indispensable to making real chocolate have become increasingly expensive, with the price rising by 136% between July 2022 and February 2024.
This is primarily about the large plantations of cocoa trees in West Africa that provide most of the global supply. As climate change causes increasingly erratic and extreme weather — especially heat waves, shifting rainfall patterns and other climate-related risks — becomes more common, the trees suffer and the harvest becomes unpredictable. The 2023-2024 season is expected to yield 374,000 tons less cocoa than usual, a big drop from the previous season, which was 74,000 tons below normal levels.
While chocolate is a luxury good not essential to survival, it's nonetheless a major global commodity, and the ripple effects of a poor cocoa harvest will be significant. And that's just one example: Climate change is causing prices to rise rapidly for many of the things we buy. According to a recent study in the journal Communications Earth & Environment, the heat extremes caused by global warming will "enhance inflationary pressures" on a wide range of products.
Researchers studied more than 27,000 observations of monthly consumer price indices around the world, and then ran those figures through a fixed-effects regressions model to ascertain how climate change impacts inflation. They found that as temperatures increased, prices for food consistently rose as well with them, and determined that this inflation was persistent for over 12 months in both affluent and low-income countries.
"Our study looks at how the price of food responds to climate anomalies in historical data for over 121 countries between 1996 and 2020," Max Kotz , the corresponding author of the study and a post-doctoral research scientist at the Potsdam Institute for Climate Impact Research, told Salon. "Our main finding is that food prices increase in response to higher temperatures in places and seasons that are already warm. We find very similar responses across wealthy and less-developed countries."
Previous studies have identified links between rising temperatures and inflation using historical data, this study is unique in extrapolating about future inflation by analyzing historical data and applying that analysis to projections of future climate conditions.
"We find very robust evidence that future climate change will amplify inflation across all countries of the world," Kotz said. "Our central estimate is for impacts on food inflation of approximately 1.5 percentage points per year by 2035, on average, across the world." Although nations in the Global South will be disproportionately impacted, residents of higher-latitude countries in North America and Europe will still feel the hurt, especially during the increasingly warm summer months.
Want more health and science stories in your inbox? Subscribe to Salon's weekly newsletter Lab Notes .
"We look at how exceptionally hot summers such as that in Europe in 2022 impacted inflation, finding that food inflation was 0.67 percentage points higher and that this could be amplified by 30-50% due to future climate change by 2035," Kotz said.
This is not the only recent study to link climate change and inflation. The Reserve Bank of India cautioned Monday that ongoing climate change could cause frequent inflationary shocks of inflation that might lead the Indian government to tighten monetary policy. Both rising average temperatures and more frequent extreme weather, the bank reported, have ripple effects on the economy throughout the country.
"Frequent and overlapping adverse climate shocks pose key upside risks to the outlook on international and domestic food prices," RBI governor Shaktikanta Das said in a policy statement accompanying the report.
"We see clear evidence that unmitigated climate change would cause even larger inflation impacts later in the century, and that reducing greenhouse gas emissions to keep within the Paris Climate Agreement would substantially reduce these impacts."
University of North Texas economist Alla Semenova published a paper in the journal Globalizations which found similar evidence that inflation will worsen as climate change-caused events — particularly heat waves, wildfires and severe storms — become more common. Semenova also argued that Green New Deal-type policies that attempt to mitigate the effects of climate change will not reduce this inflation, and that only "degrowth" (a term for sustainable economic policies not focused on increasing GDP) can prevent this from happening.
"Growth-based Green New Deal and related fiscal policies will be ineffective at mitigating climate change and reducing its inflationary impacts," Semenova writes. "Unless degrowth solutions are sought, climate change and its price effects will only intensify over time."
Kotz echoed Semenova's observations about the role of climate change in causing future inflation.
"We see clear evidence that unmitigated climate change would cause even larger inflation impacts later in the century, and by contrast that reducing greenhouse gas emissions to keep within the Paris Climate Agreement would substantially reduce these impacts," Kotz said. "We also show that adaptation could theoretically reduce impacts, but we do not see any evidence that this has occurred historically. "
One way to interpret these reports is that until governments and bankers can craft monetary policies that address or correct the inflationary impact of climate change, prices will continue to rise on numerous categories of goods, including chocolate. This disruption in the supply chain of the world's favorite sweet demonstrates “the importance for all consumers around the world that climate change is tackled, and that climate targets and climate change mitigation measures like nationally determined contributions are met,” said Rodrigo Carcamo , senior economist at the U.N. Conference on Trade and Development, in a press statement.
about the climate struggle:
- Sunrise Movement's Varshini Prakash on not losing hope on climate change: "I'm still here fighting"
- If Congress can pass it, Build Back Better could be a second "Green New Deal"
- Grade inflation: Does Biden deserve an "A" from progressives? Let's get real
Matthew Rozsa is a staff writer at Salon. He received a Master's Degree in History from Rutgers-Newark in 2012 and was awarded a science journalism fellowship from the Metcalf Institute in 2022.
Related Topics ------------------------------------------
Related articles.
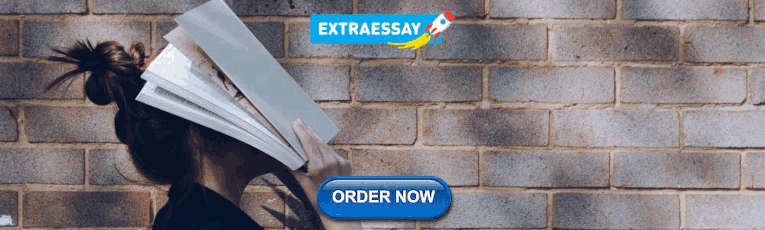
IMAGES
VIDEO
COMMENTS
Worries grew as the inflation rate (measured as the twelve-month change in the consumer price index) rose from 3.7% to 12.1% over 2001-2010. The inflation rate has since fallen to 5.2% in early 2015, leading to a debate about whether this moderation is likely to endure or inflation will rise again.
inflation in India also botto med to (-)11% in two years . after 1974 in ye ar 1976. ... This research paper discovers the impact of INR and USD variations of currency on Indian economy. The ...
This paper revisits the threshold level of inflation for India. The empirical analysis follows spline regression for the period 1996-97Q1 to 2019-20Q4. The results suggest the existence of a statistically significant threshold level of inflation at 5 to 5.5% in terms of both CPI and WPI. Below this level, the impact of inflation on growth is generally positive whereas it is negative above ...
The present study investigates the impact of macroeconomic factors on food price inflation in India utilizing the monthly time series during January 2006-March 2019. The long-run relationship is confirmed among the variables using the ARDL bounds testing approach to cointegration. The coefficients of long-run estimates show that per capita income, money supply, global food prices, and ...
The paper explores the causes of inflation in India over the period 1981-82 to 2016-17. The causal impact of some potential variables like monetary, fiscal, structural, and trade openness on the CPI and WPI inflation, are assessed involving the vector autoregressive model.
The primary objective of this paper is to measure persistence in overall inflation measured by WPI and CPI-IW and their components for the Indian economy over the period 1996:4 to 2017:02. The study first tests for persistence and then quantifies it using various univariate and multivariate approach. The univariate approach estimates persistence using sum of autoregressive coefficient, largest ...
India's Inflation Process Before and After Flexible Inflation Targeting. by Patrick Blagrave and Weicheng Lian. IMF Working Papers describe research in progress by the author(s) and are published to elicit comments and to encourage debate. The views expressed in IMF Working Papers are those of the author(s) and do not necessarily represent ...
Abstract. Purpose - Inflation and its related uncertainty can impose costs on real economic output in any economy. This paper aims to analyze the relationship between inflation and inflation ...
Accordingly, it focuses on (i) understanding of the dynamics of inflation, (ii) identification of major macroeconomic determinants of inflation, and (iii) econometric modelling of inflation in India. The paper is based on statistical database of selected variables for the period of ten years from 2002-Q1 to 2012-Q1.
But, there is no research paper, which has analyzed the content of the RBI policy statements. ... Inflation data used for the concerned month is the CPI from the Ministry of Statistics and Programme Implementation of India. The CPI inflation data for the related months are shown in Table 3. The calibrating scale is shown in Table 2. Table 3 ...
and average r ate of infla tion was 6.05 and during 1971-1980, average rate of inflation was. 8.68 from 1980-1990, inflation ranges from 3.3 to 13.1 and in 1991 India experienced highest ...
Abstract. This paper explores the effects of India's adoption of inflation targeting (IT) as a monetary policy framework in 2016 on long-term inflation expectations in the private sector.
This paper provides an assessment of India's inflation-targeting regime. It shows that the Reserve Bank of India is best characterized as a flexible inflation targeter: contrary to criticism, it does not neglect changes in the output gap when setting policy rates. The paper does not find that the Reserve Bank of India became more hawkish ...
The present study intends to find out the causal relationship between inflation measured on Wholesale Price Index (WPI) and a host of its determinants like Gross Domestic Product (GDP), money supply, interest rate and velocity of money in Indian context using time series data set of 46 years starting from 1970-71 to 2015-16.
Inflation in India has declined steadily from an average of 10.3 % between 1990-1994, to 8.9 % during 1995-1999 and to. 4.3 % in this decade. Similarly in South Africa, inflation has declined from an average of 12.5 %, to. 7.3 % and to 5.1 % over the same time periods.
that inflation is something that needs to be addressed with great urgency. [Dr. Manmohan Singh, Prime Minister of India, 4 February 2011, New Delhi]. Inflation is the rate of price growth over a given period of time. Inflation is usually a broad measure, such as an overall increase in prices or an increase in the cost of living in a country.
The inflation has turned out to be more volatile during the subsequent period (2001-2022) than the previous sub-periods. The instability and uncertainty in the economy are dangerous for the growth of the economy. Monetary and fiscal measures should be adopted to stabilized the irregular behavior of inflation in India. The study has established that the growth rate in CPI was higher than the ...
Research on inflation in India has also come from the Reserve Bank of India, naturally, and the IMF. ... Patra, M. D, and M. Kapur. 2010. A monetary policy model without money for India, Working Paper 10/183. International Monetary Fund, Washington, D.C. Paul, B.P. 2009. In search of the Phillips curve for India. Journal of Asian Economics. 20: ...
Changing Central Bank Pressures and Inflation. Hassan Afrouzi, Marina Halac, Kenneth S. Rogoff & Pierre Yared. Working Paper 32308. DOI 10.3386/w32308. Issue Date April 2024. We present a simple long-run aggregate demand and supply framework for evaluating long-run inflation. The framework illustrates how exogenous economic and political ...
NEW DELHI, April 12 (Reuters) - India's retail inflation (INCPIY=ECI) , opens new tab in March eased to 4.85% from 5.09% in February, government data showed on Friday. A Reuters poll of 50 ...
Moody's Analytics said the inflation outlooks for China and India, Asia's two largest economies, are more uncertain. "Inflation in India is at the opposite extreme, with recent consumer price inflation rates hovering around 5%, close to the upper end of the Reserve Bank of India's target range of 2% to 6% and without clear evidence of a trend towards slowing price pressures," it said.
ADB projects India's economy to remain robust with growth expected to slow slightly before improving in 2025-26. ... Retail inflation to ease to 4.6% this year, food inflation to cool to 5.7% if ...
This paper surveys research on the welfare cost of inflation. New estimates are provided, based on U.S. time series for 1900-94, interpreted in a variety of ways.
With inflation still above the Federal Reserve's 2% objective, there is renewed interest in understanding how quickly federal funds rate hikes typically affect inflation. Beyond monetary policy's well-known lagged effect on the economy overall, new analysis highlights that not all prices respond with the same strength or speed. Results suggest that inflation for the most responsive ...
The predominant reason for people's aversion to inflation is the widespread belief that it diminishes their buying power, as neither personal nor general wage increases seem to match the pace of rising prices. As a result, respondents report having to make costly adjustments in their budgets and behaviors, especially among lower-income groups.
Abstract. India has seen lower inflation by historical standards for the past 6 years. This has been attributed to the adoption of inflation targeting by the central bank, the Reserve Bank of India in 2016. In particular, it has been asserted that the lower inflation reflects the anchoring of expectations. We evaluate these claims.
The Indian central bank's key interest rate was kept unchanged for a seventh straight policy meeting on Friday as growth in the economy is expected to remain robust while inflation stays above ...
Baker noted that this latest paper confirms and updates research dating back to the 1990s, when Robert Shiller found people angry about inflation, pessimistic about their ability to make up ...
We study the individual price behaviour in India using monthly item-wise CPI prices during 2011-2019 and explore the impact of implementation of inflation targeting on price behaviour. We find that inflation targeting has significantly reduced the frequency of price increases and increased the frequency of price decreases in India; however, it has not been successful in reducing the ...
The Reserve Bank of India cautioned Monday that ongoing climate change could cause frequent inflationary shocks of inflation that might lead the Indian government to tighten monetary policy. Both ...