- Privacy Policy
Buy Me a Coffee

Home » Research Methodology – Types, Examples and writing Guide
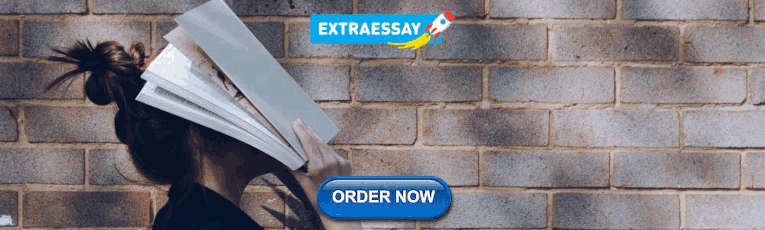
Research Methodology – Types, Examples and writing Guide
Table of Contents

Research Methodology
Definition:
Research Methodology refers to the systematic and scientific approach used to conduct research, investigate problems, and gather data and information for a specific purpose. It involves the techniques and procedures used to identify, collect , analyze , and interpret data to answer research questions or solve research problems . Moreover, They are philosophical and theoretical frameworks that guide the research process.
Structure of Research Methodology
Research methodology formats can vary depending on the specific requirements of the research project, but the following is a basic example of a structure for a research methodology section:
I. Introduction
- Provide an overview of the research problem and the need for a research methodology section
- Outline the main research questions and objectives
II. Research Design
- Explain the research design chosen and why it is appropriate for the research question(s) and objectives
- Discuss any alternative research designs considered and why they were not chosen
- Describe the research setting and participants (if applicable)
III. Data Collection Methods
- Describe the methods used to collect data (e.g., surveys, interviews, observations)
- Explain how the data collection methods were chosen and why they are appropriate for the research question(s) and objectives
- Detail any procedures or instruments used for data collection
IV. Data Analysis Methods
- Describe the methods used to analyze the data (e.g., statistical analysis, content analysis )
- Explain how the data analysis methods were chosen and why they are appropriate for the research question(s) and objectives
- Detail any procedures or software used for data analysis
V. Ethical Considerations
- Discuss any ethical issues that may arise from the research and how they were addressed
- Explain how informed consent was obtained (if applicable)
- Detail any measures taken to ensure confidentiality and anonymity
VI. Limitations
- Identify any potential limitations of the research methodology and how they may impact the results and conclusions
VII. Conclusion
- Summarize the key aspects of the research methodology section
- Explain how the research methodology addresses the research question(s) and objectives
Research Methodology Types
Types of Research Methodology are as follows:
Quantitative Research Methodology
This is a research methodology that involves the collection and analysis of numerical data using statistical methods. This type of research is often used to study cause-and-effect relationships and to make predictions.
Qualitative Research Methodology
This is a research methodology that involves the collection and analysis of non-numerical data such as words, images, and observations. This type of research is often used to explore complex phenomena, to gain an in-depth understanding of a particular topic, and to generate hypotheses.
Mixed-Methods Research Methodology
This is a research methodology that combines elements of both quantitative and qualitative research. This approach can be particularly useful for studies that aim to explore complex phenomena and to provide a more comprehensive understanding of a particular topic.
Case Study Research Methodology
This is a research methodology that involves in-depth examination of a single case or a small number of cases. Case studies are often used in psychology, sociology, and anthropology to gain a detailed understanding of a particular individual or group.
Action Research Methodology
This is a research methodology that involves a collaborative process between researchers and practitioners to identify and solve real-world problems. Action research is often used in education, healthcare, and social work.
Experimental Research Methodology
This is a research methodology that involves the manipulation of one or more independent variables to observe their effects on a dependent variable. Experimental research is often used to study cause-and-effect relationships and to make predictions.
Survey Research Methodology
This is a research methodology that involves the collection of data from a sample of individuals using questionnaires or interviews. Survey research is often used to study attitudes, opinions, and behaviors.
Grounded Theory Research Methodology
This is a research methodology that involves the development of theories based on the data collected during the research process. Grounded theory is often used in sociology and anthropology to generate theories about social phenomena.
Research Methodology Example
An Example of Research Methodology could be the following:
Research Methodology for Investigating the Effectiveness of Cognitive Behavioral Therapy in Reducing Symptoms of Depression in Adults
Introduction:
The aim of this research is to investigate the effectiveness of cognitive-behavioral therapy (CBT) in reducing symptoms of depression in adults. To achieve this objective, a randomized controlled trial (RCT) will be conducted using a mixed-methods approach.
Research Design:
The study will follow a pre-test and post-test design with two groups: an experimental group receiving CBT and a control group receiving no intervention. The study will also include a qualitative component, in which semi-structured interviews will be conducted with a subset of participants to explore their experiences of receiving CBT.
Participants:
Participants will be recruited from community mental health clinics in the local area. The sample will consist of 100 adults aged 18-65 years old who meet the diagnostic criteria for major depressive disorder. Participants will be randomly assigned to either the experimental group or the control group.
Intervention :
The experimental group will receive 12 weekly sessions of CBT, each lasting 60 minutes. The intervention will be delivered by licensed mental health professionals who have been trained in CBT. The control group will receive no intervention during the study period.
Data Collection:
Quantitative data will be collected through the use of standardized measures such as the Beck Depression Inventory-II (BDI-II) and the Generalized Anxiety Disorder-7 (GAD-7). Data will be collected at baseline, immediately after the intervention, and at a 3-month follow-up. Qualitative data will be collected through semi-structured interviews with a subset of participants from the experimental group. The interviews will be conducted at the end of the intervention period, and will explore participants’ experiences of receiving CBT.
Data Analysis:
Quantitative data will be analyzed using descriptive statistics, t-tests, and mixed-model analyses of variance (ANOVA) to assess the effectiveness of the intervention. Qualitative data will be analyzed using thematic analysis to identify common themes and patterns in participants’ experiences of receiving CBT.
Ethical Considerations:
This study will comply with ethical guidelines for research involving human subjects. Participants will provide informed consent before participating in the study, and their privacy and confidentiality will be protected throughout the study. Any adverse events or reactions will be reported and managed appropriately.
Data Management:
All data collected will be kept confidential and stored securely using password-protected databases. Identifying information will be removed from qualitative data transcripts to ensure participants’ anonymity.
Limitations:
One potential limitation of this study is that it only focuses on one type of psychotherapy, CBT, and may not generalize to other types of therapy or interventions. Another limitation is that the study will only include participants from community mental health clinics, which may not be representative of the general population.
Conclusion:
This research aims to investigate the effectiveness of CBT in reducing symptoms of depression in adults. By using a randomized controlled trial and a mixed-methods approach, the study will provide valuable insights into the mechanisms underlying the relationship between CBT and depression. The results of this study will have important implications for the development of effective treatments for depression in clinical settings.
How to Write Research Methodology
Writing a research methodology involves explaining the methods and techniques you used to conduct research, collect data, and analyze results. It’s an essential section of any research paper or thesis, as it helps readers understand the validity and reliability of your findings. Here are the steps to write a research methodology:
- Start by explaining your research question: Begin the methodology section by restating your research question and explaining why it’s important. This helps readers understand the purpose of your research and the rationale behind your methods.
- Describe your research design: Explain the overall approach you used to conduct research. This could be a qualitative or quantitative research design, experimental or non-experimental, case study or survey, etc. Discuss the advantages and limitations of the chosen design.
- Discuss your sample: Describe the participants or subjects you included in your study. Include details such as their demographics, sampling method, sample size, and any exclusion criteria used.
- Describe your data collection methods : Explain how you collected data from your participants. This could include surveys, interviews, observations, questionnaires, or experiments. Include details on how you obtained informed consent, how you administered the tools, and how you minimized the risk of bias.
- Explain your data analysis techniques: Describe the methods you used to analyze the data you collected. This could include statistical analysis, content analysis, thematic analysis, or discourse analysis. Explain how you dealt with missing data, outliers, and any other issues that arose during the analysis.
- Discuss the validity and reliability of your research : Explain how you ensured the validity and reliability of your study. This could include measures such as triangulation, member checking, peer review, or inter-coder reliability.
- Acknowledge any limitations of your research: Discuss any limitations of your study, including any potential threats to validity or generalizability. This helps readers understand the scope of your findings and how they might apply to other contexts.
- Provide a summary: End the methodology section by summarizing the methods and techniques you used to conduct your research. This provides a clear overview of your research methodology and helps readers understand the process you followed to arrive at your findings.
When to Write Research Methodology
Research methodology is typically written after the research proposal has been approved and before the actual research is conducted. It should be written prior to data collection and analysis, as it provides a clear roadmap for the research project.
The research methodology is an important section of any research paper or thesis, as it describes the methods and procedures that will be used to conduct the research. It should include details about the research design, data collection methods, data analysis techniques, and any ethical considerations.
The methodology should be written in a clear and concise manner, and it should be based on established research practices and standards. It is important to provide enough detail so that the reader can understand how the research was conducted and evaluate the validity of the results.
Applications of Research Methodology
Here are some of the applications of research methodology:
- To identify the research problem: Research methodology is used to identify the research problem, which is the first step in conducting any research.
- To design the research: Research methodology helps in designing the research by selecting the appropriate research method, research design, and sampling technique.
- To collect data: Research methodology provides a systematic approach to collect data from primary and secondary sources.
- To analyze data: Research methodology helps in analyzing the collected data using various statistical and non-statistical techniques.
- To test hypotheses: Research methodology provides a framework for testing hypotheses and drawing conclusions based on the analysis of data.
- To generalize findings: Research methodology helps in generalizing the findings of the research to the target population.
- To develop theories : Research methodology is used to develop new theories and modify existing theories based on the findings of the research.
- To evaluate programs and policies : Research methodology is used to evaluate the effectiveness of programs and policies by collecting data and analyzing it.
- To improve decision-making: Research methodology helps in making informed decisions by providing reliable and valid data.
Purpose of Research Methodology
Research methodology serves several important purposes, including:
- To guide the research process: Research methodology provides a systematic framework for conducting research. It helps researchers to plan their research, define their research questions, and select appropriate methods and techniques for collecting and analyzing data.
- To ensure research quality: Research methodology helps researchers to ensure that their research is rigorous, reliable, and valid. It provides guidelines for minimizing bias and error in data collection and analysis, and for ensuring that research findings are accurate and trustworthy.
- To replicate research: Research methodology provides a clear and detailed account of the research process, making it possible for other researchers to replicate the study and verify its findings.
- To advance knowledge: Research methodology enables researchers to generate new knowledge and to contribute to the body of knowledge in their field. It provides a means for testing hypotheses, exploring new ideas, and discovering new insights.
- To inform decision-making: Research methodology provides evidence-based information that can inform policy and decision-making in a variety of fields, including medicine, public health, education, and business.
Advantages of Research Methodology
Research methodology has several advantages that make it a valuable tool for conducting research in various fields. Here are some of the key advantages of research methodology:
- Systematic and structured approach : Research methodology provides a systematic and structured approach to conducting research, which ensures that the research is conducted in a rigorous and comprehensive manner.
- Objectivity : Research methodology aims to ensure objectivity in the research process, which means that the research findings are based on evidence and not influenced by personal bias or subjective opinions.
- Replicability : Research methodology ensures that research can be replicated by other researchers, which is essential for validating research findings and ensuring their accuracy.
- Reliability : Research methodology aims to ensure that the research findings are reliable, which means that they are consistent and can be depended upon.
- Validity : Research methodology ensures that the research findings are valid, which means that they accurately reflect the research question or hypothesis being tested.
- Efficiency : Research methodology provides a structured and efficient way of conducting research, which helps to save time and resources.
- Flexibility : Research methodology allows researchers to choose the most appropriate research methods and techniques based on the research question, data availability, and other relevant factors.
- Scope for innovation: Research methodology provides scope for innovation and creativity in designing research studies and developing new research techniques.
Research Methodology Vs Research Methods
About the author.
Muhammad Hassan
Researcher, Academic Writer, Web developer
You may also like

How to Cite Research Paper – All Formats and...

Data Collection – Methods Types and Examples

Delimitations in Research – Types, Examples and...

Research Paper Format – Types, Examples and...

Research Process – Steps, Examples and Tips

Research Design – Types, Methods and Examples
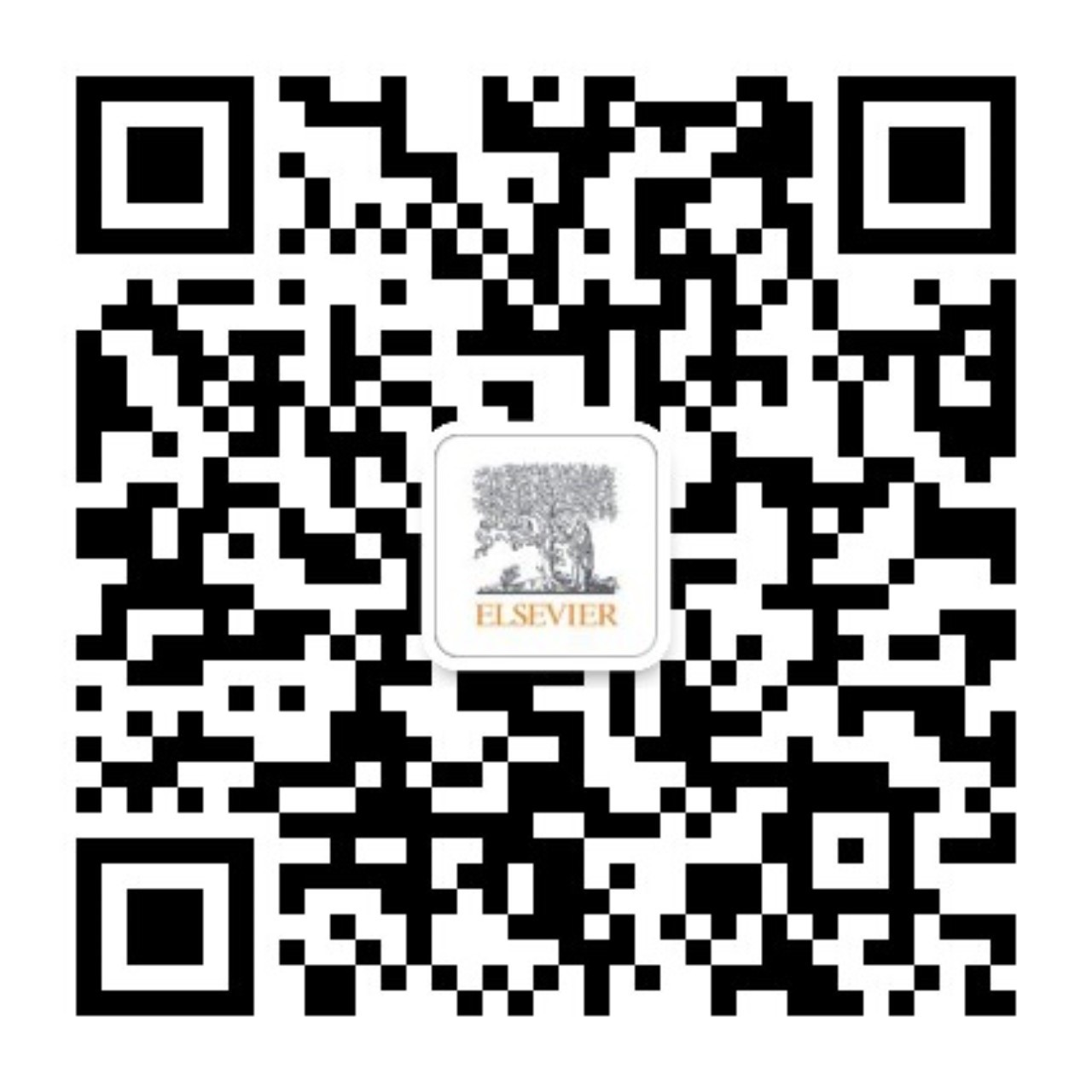
- Research Process
Choosing the Right Research Methodology: A Guide for Researchers
- 3 minute read
- 33.9K views
Table of Contents
Choosing an optimal research methodology is crucial for the success of any research project. The methodology you select will determine the type of data you collect, how you collect it, and how you analyse it. Understanding the different types of research methods available along with their strengths and weaknesses, is thus imperative to make an informed decision.
Understanding different research methods:
There are several research methods available depending on the type of study you are conducting, i.e., whether it is laboratory-based, clinical, epidemiological, or survey based . Some common methodologies include qualitative research, quantitative research, experimental research, survey-based research, and action research. Each method can be opted for and modified, depending on the type of research hypotheses and objectives.
Qualitative vs quantitative research:
When deciding on a research methodology, one of the key factors to consider is whether your research will be qualitative or quantitative. Qualitative research is used to understand people’s experiences, concepts, thoughts, or behaviours . Quantitative research, on the contrary, deals with numbers, graphs, and charts, and is used to test or confirm hypotheses, assumptions, and theories.
Qualitative research methodology:
Qualitative research is often used to examine issues that are not well understood, and to gather additional insights on these topics. Qualitative research methods include open-ended survey questions, observations of behaviours described through words, and reviews of literature that has explored similar theories and ideas. These methods are used to understand how language is used in real-world situations, identify common themes or overarching ideas, and describe and interpret various texts. Data analysis for qualitative research typically includes discourse analysis, thematic analysis, and textual analysis.
Quantitative research methodology:
The goal of quantitative research is to test hypotheses, confirm assumptions and theories, and determine cause-and-effect relationships. Quantitative research methods include experiments, close-ended survey questions, and countable and numbered observations. Data analysis for quantitative research relies heavily on statistical methods.
Analysing qualitative vs quantitative data:
The methods used for data analysis also differ for qualitative and quantitative research. As mentioned earlier, quantitative data is generally analysed using statistical methods and does not leave much room for speculation. It is more structured and follows a predetermined plan. In quantitative research, the researcher starts with a hypothesis and uses statistical methods to test it. Contrarily, methods used for qualitative data analysis can identify patterns and themes within the data, rather than provide statistical measures of the data. It is an iterative process, where the researcher goes back and forth trying to gauge the larger implications of the data through different perspectives and revising the analysis if required.
When to use qualitative vs quantitative research:
The choice between qualitative and quantitative research will depend on the gap that the research project aims to address, and specific objectives of the study. If the goal is to establish facts about a subject or topic, quantitative research is an appropriate choice. However, if the goal is to understand people’s experiences or perspectives, qualitative research may be more suitable.
Conclusion:
In conclusion, an understanding of the different research methods available, their applicability, advantages, and disadvantages is essential for making an informed decision on the best methodology for your project. If you need any additional guidance on which research methodology to opt for, you can head over to Elsevier Author Services (EAS). EAS experts will guide you throughout the process and help you choose the perfect methodology for your research goals.
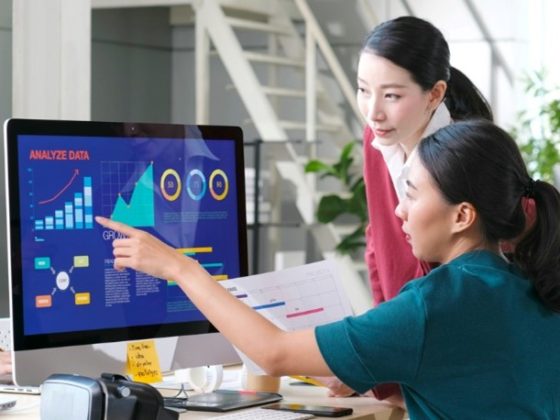
Why is data validation important in research?
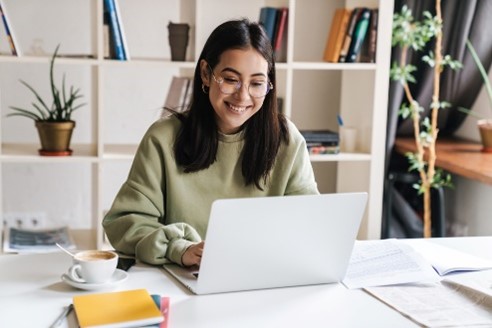
When Data Speak, Listen: Importance of Data Collection and Analysis Methods
You may also like.
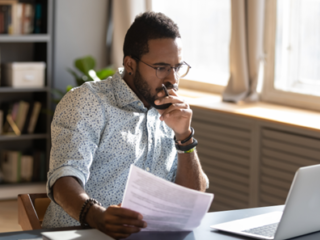
Descriptive Research Design and Its Myriad Uses
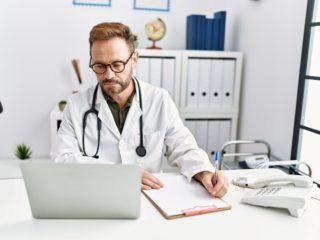
Five Common Mistakes to Avoid When Writing a Biomedical Research Paper
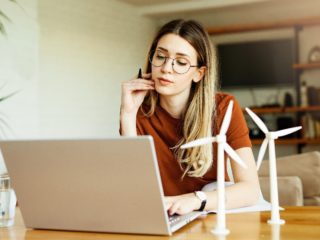
Making Technical Writing in Environmental Engineering Accessible
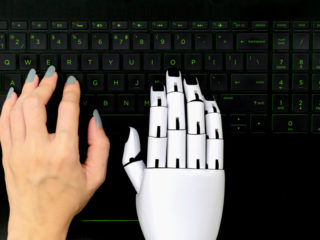
To Err is Not Human: The Dangers of AI-assisted Academic Writing
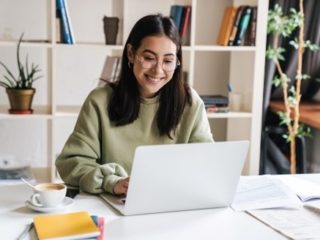
Writing a good review article
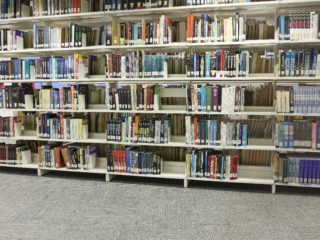
Scholarly Sources: What are They and Where can You Find Them?
Input your search keywords and press Enter.

What Is Research Methodology? A Plain-Language Explanation & Definition (With Examples)
By Derek Jansen (MBA) and Kerryn Warren (PhD) | June 2020 (Last updated April 2023)
If you’re new to formal academic research, it’s quite likely that you’re feeling a little overwhelmed by all the technical lingo that gets thrown around. And who could blame you – “research methodology”, “research methods”, “sampling strategies”… it all seems never-ending!
In this post, we’ll demystify the landscape with plain-language explanations and loads of examples (including easy-to-follow videos), so that you can approach your dissertation, thesis or research project with confidence. Let’s get started.
Research Methodology 101
- What exactly research methodology means
- What qualitative , quantitative and mixed methods are
- What sampling strategy is
- What data collection methods are
- What data analysis methods are
- How to choose your research methodology
- Example of a research methodology
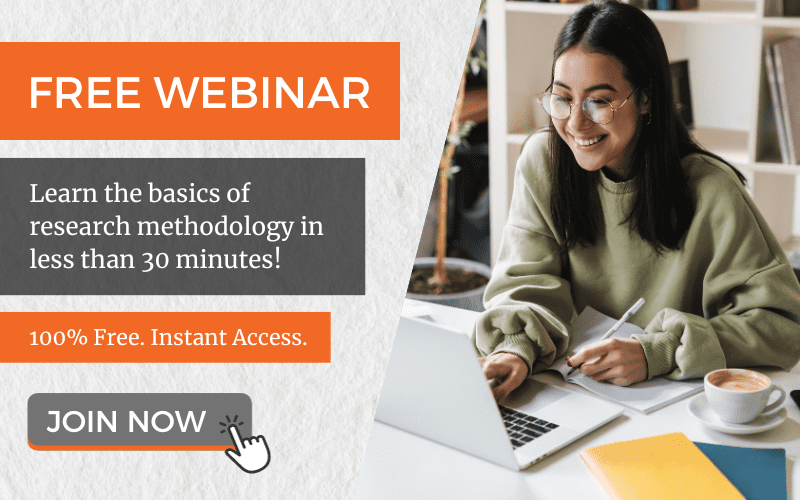
What is research methodology?
Research methodology simply refers to the practical “how” of a research study. More specifically, it’s about how a researcher systematically designs a study to ensure valid and reliable results that address the research aims, objectives and research questions . Specifically, how the researcher went about deciding:
- What type of data to collect (e.g., qualitative or quantitative data )
- Who to collect it from (i.e., the sampling strategy )
- How to collect it (i.e., the data collection method )
- How to analyse it (i.e., the data analysis methods )
Within any formal piece of academic research (be it a dissertation, thesis or journal article), you’ll find a research methodology chapter or section which covers the aspects mentioned above. Importantly, a good methodology chapter explains not just what methodological choices were made, but also explains why they were made. In other words, the methodology chapter should justify the design choices, by showing that the chosen methods and techniques are the best fit for the research aims, objectives and research questions.
So, it’s the same as research design?
Not quite. As we mentioned, research methodology refers to the collection of practical decisions regarding what data you’ll collect, from who, how you’ll collect it and how you’ll analyse it. Research design, on the other hand, is more about the overall strategy you’ll adopt in your study. For example, whether you’ll use an experimental design in which you manipulate one variable while controlling others. You can learn more about research design and the various design types here .
Need a helping hand?
What are qualitative, quantitative and mixed-methods?
Qualitative, quantitative and mixed-methods are different types of methodological approaches, distinguished by their focus on words , numbers or both . This is a bit of an oversimplification, but its a good starting point for understanding.
Let’s take a closer look.
Qualitative research refers to research which focuses on collecting and analysing words (written or spoken) and textual or visual data, whereas quantitative research focuses on measurement and testing using numerical data . Qualitative analysis can also focus on other “softer” data points, such as body language or visual elements.
It’s quite common for a qualitative methodology to be used when the research aims and research questions are exploratory in nature. For example, a qualitative methodology might be used to understand peoples’ perceptions about an event that took place, or a political candidate running for president.
Contrasted to this, a quantitative methodology is typically used when the research aims and research questions are confirmatory in nature. For example, a quantitative methodology might be used to measure the relationship between two variables (e.g. personality type and likelihood to commit a crime) or to test a set of hypotheses .
As you’ve probably guessed, the mixed-method methodology attempts to combine the best of both qualitative and quantitative methodologies to integrate perspectives and create a rich picture. If you’d like to learn more about these three methodological approaches, be sure to watch our explainer video below.
What is sampling strategy?
Simply put, sampling is about deciding who (or where) you’re going to collect your data from . Why does this matter? Well, generally it’s not possible to collect data from every single person in your group of interest (this is called the “population”), so you’ll need to engage a smaller portion of that group that’s accessible and manageable (this is called the “sample”).
How you go about selecting the sample (i.e., your sampling strategy) will have a major impact on your study. There are many different sampling methods you can choose from, but the two overarching categories are probability sampling and non-probability sampling .
Probability sampling involves using a completely random sample from the group of people you’re interested in. This is comparable to throwing the names all potential participants into a hat, shaking it up, and picking out the “winners”. By using a completely random sample, you’ll minimise the risk of selection bias and the results of your study will be more generalisable to the entire population.
Non-probability sampling , on the other hand, doesn’t use a random sample . For example, it might involve using a convenience sample, which means you’d only interview or survey people that you have access to (perhaps your friends, family or work colleagues), rather than a truly random sample. With non-probability sampling, the results are typically not generalisable .
To learn more about sampling methods, be sure to check out the video below.
What are data collection methods?
As the name suggests, data collection methods simply refers to the way in which you go about collecting the data for your study. Some of the most common data collection methods include:
- Interviews (which can be unstructured, semi-structured or structured)
- Focus groups and group interviews
- Surveys (online or physical surveys)
- Observations (watching and recording activities)
- Biophysical measurements (e.g., blood pressure, heart rate, etc.)
- Documents and records (e.g., financial reports, court records, etc.)
The choice of which data collection method to use depends on your overall research aims and research questions , as well as practicalities and resource constraints. For example, if your research is exploratory in nature, qualitative methods such as interviews and focus groups would likely be a good fit. Conversely, if your research aims to measure specific variables or test hypotheses, large-scale surveys that produce large volumes of numerical data would likely be a better fit.
What are data analysis methods?
Data analysis methods refer to the methods and techniques that you’ll use to make sense of your data. These can be grouped according to whether the research is qualitative (words-based) or quantitative (numbers-based).
Popular data analysis methods in qualitative research include:
- Qualitative content analysis
- Thematic analysis
- Discourse analysis
- Narrative analysis
- Interpretative phenomenological analysis (IPA)
- Visual analysis (of photographs, videos, art, etc.)
Qualitative data analysis all begins with data coding , after which an analysis method is applied. In some cases, more than one analysis method is used, depending on the research aims and research questions . In the video below, we explore some common qualitative analysis methods, along with practical examples.
Moving on to the quantitative side of things, popular data analysis methods in this type of research include:
- Descriptive statistics (e.g. means, medians, modes )
- Inferential statistics (e.g. correlation, regression, structural equation modelling)
Again, the choice of which data collection method to use depends on your overall research aims and objectives , as well as practicalities and resource constraints. In the video below, we explain some core concepts central to quantitative analysis.
How do I choose a research methodology?
As you’ve probably picked up by now, your research aims and objectives have a major influence on the research methodology . So, the starting point for developing your research methodology is to take a step back and look at the big picture of your research, before you make methodology decisions. The first question you need to ask yourself is whether your research is exploratory or confirmatory in nature.
If your research aims and objectives are primarily exploratory in nature, your research will likely be qualitative and therefore you might consider qualitative data collection methods (e.g. interviews) and analysis methods (e.g. qualitative content analysis).
Conversely, if your research aims and objective are looking to measure or test something (i.e. they’re confirmatory), then your research will quite likely be quantitative in nature, and you might consider quantitative data collection methods (e.g. surveys) and analyses (e.g. statistical analysis).
Designing your research and working out your methodology is a large topic, which we cover extensively on the blog . For now, however, the key takeaway is that you should always start with your research aims, objectives and research questions (the golden thread). Every methodological choice you make needs align with those three components.
Example of a research methodology chapter
In the video below, we provide a detailed walkthrough of a research methodology from an actual dissertation, as well as an overview of our free methodology template .
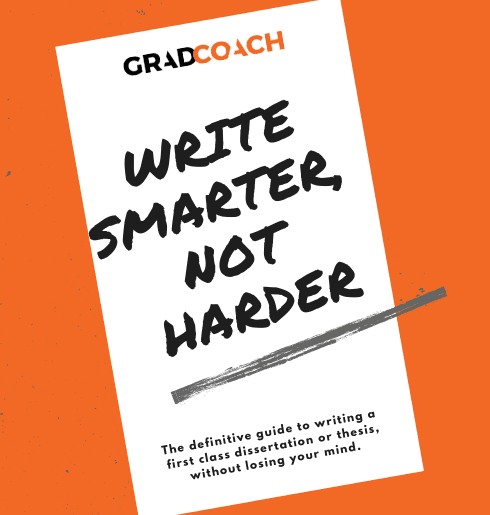
Psst… there’s more (for free)
This post is part of our dissertation mini-course, which covers everything you need to get started with your dissertation, thesis or research project.
You Might Also Like:
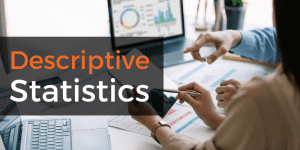
198 Comments
Thank you for this simple yet comprehensive and easy to digest presentation. God Bless!
You’re most welcome, Leo. Best of luck with your research!
I found it very useful. many thanks
This is really directional. A make-easy research knowledge.
Thank you for this, I think will help my research proposal
Thanks for good interpretation,well understood.
Good morning sorry I want to the search topic
Thank u more
Thank you, your explanation is simple and very helpful.
Very educative a.nd exciting platform. A bigger thank you and I’ll like to always be with you
That’s the best analysis
So simple yet so insightful. Thank you.
This really easy to read as it is self-explanatory. Very much appreciated…
Thanks for this. It’s so helpful and explicit. For those elements highlighted in orange, they were good sources of referrals for concepts I didn’t understand. A million thanks for this.
Good morning, I have been reading your research lessons through out a period of times. They are important, impressive and clear. Want to subscribe and be and be active with you.
Thankyou So much Sir Derek…
Good morning thanks so much for the on line lectures am a student of university of Makeni.select a research topic and deliberate on it so that we’ll continue to understand more.sorry that’s a suggestion.
Beautiful presentation. I love it.
please provide a research mehodology example for zoology
It’s very educative and well explained
Thanks for the concise and informative data.
This is really good for students to be safe and well understand that research is all about
Thank you so much Derek sir🖤🙏🤗
Very simple and reliable
This is really helpful. Thanks alot. God bless you.
very useful, Thank you very much..
thanks a lot its really useful
in a nutshell..thank you!
Thanks for updating my understanding on this aspect of my Thesis writing.
thank you so much my through this video am competently going to do a good job my thesis
Very simple but yet insightful Thank you
This has been an eye opening experience. Thank you grad coach team.
Very useful message for research scholars
Really very helpful thank you
yes you are right and i’m left
Research methodology with a simplest way i have never seen before this article.
wow thank u so much
Good morning thanks so much for the on line lectures am a student of university of Makeni.select a research topic and deliberate on is so that we will continue to understand more.sorry that’s a suggestion.
Very precise and informative.
Thanks for simplifying these terms for us, really appreciate it.
Thanks this has really helped me. It is very easy to understand.
I found the notes and the presentation assisting and opening my understanding on research methodology
Good presentation
Im so glad you clarified my misconceptions. Im now ready to fry my onions. Thank you so much. God bless
Thank you a lot.
thanks for the easy way of learning and desirable presentation.
Thanks a lot. I am inspired
Well written
I am writing a APA Format paper . I using questionnaire with 120 STDs teacher for my participant. Can you write me mthology for this research. Send it through email sent. Just need a sample as an example please. My topic is ” impacts of overcrowding on students learning
Thanks for your comment.
We can’t write your methodology for you. If you’re looking for samples, you should be able to find some sample methodologies on Google. Alternatively, you can download some previous dissertations from a dissertation directory and have a look at the methodology chapters therein.
All the best with your research.
Thank you so much for this!! God Bless
Thank you. Explicit explanation
Thank you, Derek and Kerryn, for making this simple to understand. I’m currently at the inception stage of my research.
Thnks a lot , this was very usefull on my assignment
excellent explanation
I’m currently working on my master’s thesis, thanks for this! I’m certain that I will use Qualitative methodology.
Thanks a lot for this concise piece, it was quite relieving and helpful. God bless you BIG…
I am currently doing my dissertation proposal and I am sure that I will do quantitative research. Thank you very much it was extremely helpful.
Very interesting and informative yet I would like to know about examples of Research Questions as well, if possible.
I’m about to submit a research presentation, I have come to understand from your simplification on understanding research methodology. My research will be mixed methodology, qualitative as well as quantitative. So aim and objective of mixed method would be both exploratory and confirmatory. Thanks you very much for your guidance.
OMG thanks for that, you’re a life saver. You covered all the points I needed. Thank you so much ❤️ ❤️ ❤️
Thank you immensely for this simple, easy to comprehend explanation of data collection methods. I have been stuck here for months 😩. Glad I found your piece. Super insightful.
I’m going to write synopsis which will be quantitative research method and I don’t know how to frame my topic, can I kindly get some ideas..
Thanks for this, I was really struggling.
This was really informative I was struggling but this helped me.
Thanks a lot for this information, simple and straightforward. I’m a last year student from the University of South Africa UNISA South Africa.
its very much informative and understandable. I have enlightened.
An interesting nice exploration of a topic.
Thank you. Accurate and simple🥰
This article was really helpful, it helped me understanding the basic concepts of the topic Research Methodology. The examples were very clear, and easy to understand. I would like to visit this website again. Thank you so much for such a great explanation of the subject.
Thanks dude
Thank you Doctor Derek for this wonderful piece, please help to provide your details for reference purpose. God bless.
Many compliments to you
Great work , thank you very much for the simple explanation
Thank you. I had to give a presentation on this topic. I have looked everywhere on the internet but this is the best and simple explanation.
thank you, its very informative.
Well explained. Now I know my research methodology will be qualitative and exploratory. Thank you so much, keep up the good work
Well explained, thank you very much.
This is good explanation, I have understood the different methods of research. Thanks a lot.
Great work…very well explanation
Thanks Derek. Kerryn was just fantastic!
Great to hear that, Hyacinth. Best of luck with your research!
Its a good templates very attractive and important to PhD students and lectuter
Thanks for the feedback, Matobela. Good luck with your research methodology.
Thank you. This is really helpful.
You’re very welcome, Elie. Good luck with your research methodology.
Well explained thanks
This is a very helpful site especially for young researchers at college. It provides sufficient information to guide students and equip them with the necessary foundation to ask any other questions aimed at deepening their understanding.
Thanks for the kind words, Edward. Good luck with your research!
Thank you. I have learned a lot.
Great to hear that, Ngwisa. Good luck with your research methodology!
Thank you for keeping your presentation simples and short and covering key information for research methodology. My key takeaway: Start with defining your research objective the other will depend on the aims of your research question.
My name is Zanele I would like to be assisted with my research , and the topic is shortage of nursing staff globally want are the causes , effects on health, patients and community and also globally
Thanks for making it simple and clear. It greatly helped in understanding research methodology. Regards.
This is well simplified and straight to the point
Thank you Dr
I was given an assignment to research 2 publications and describe their research methodology? I don’t know how to start this task can someone help me?
Sure. You’re welcome to book an initial consultation with one of our Research Coaches to discuss how we can assist – https://gradcoach.com/book/new/ .
Thanks a lot I am relieved of a heavy burden.keep up with the good work
I’m very much grateful Dr Derek. I’m planning to pursue one of the careers that really needs one to be very much eager to know. There’s a lot of research to do and everything, but since I’ve gotten this information I will use it to the best of my potential.
Thank you so much, words are not enough to explain how helpful this session has been for me!
Thanks this has thought me alot.
Very concise and helpful. Thanks a lot
Thank Derek. This is very helpful. Your step by step explanation has made it easier for me to understand different concepts. Now i can get on with my research.
I wish i had come across this sooner. So simple but yet insightful
really nice explanation thank you so much
I’m so grateful finding this site, it’s really helpful…….every term well explained and provide accurate understanding especially to student going into an in-depth research for the very first time, even though my lecturer already explained this topic to the class, I think I got the clear and efficient explanation here, much thanks to the author.
It is very helpful material
I would like to be assisted with my research topic : Literature Review and research methodologies. My topic is : what is the relationship between unemployment and economic growth?
Its really nice and good for us.
THANKS SO MUCH FOR EXPLANATION, ITS VERY CLEAR TO ME WHAT I WILL BE DOING FROM NOW .GREAT READS.
Short but sweet.Thank you
Informative article. Thanks for your detailed information.
I’m currently working on my Ph.D. thesis. Thanks a lot, Derek and Kerryn, Well-organized sequences, facilitate the readers’ following.
great article for someone who does not have any background can even understand
I am a bit confused about research design and methodology. Are they the same? If not, what are the differences and how are they related?
Thanks in advance.
concise and informative.
Thank you very much
How can we site this article is Harvard style?
Very well written piece that afforded better understanding of the concept. Thank you!
Am a new researcher trying to learn how best to write a research proposal. I find your article spot on and want to download the free template but finding difficulties. Can u kindly send it to my email, the free download entitled, “Free Download: Research Proposal Template (with Examples)”.
Thank too much
Thank you very much for your comprehensive explanation about research methodology so I like to thank you again for giving us such great things.
Good very well explained.Thanks for sharing it.
Thank u sir, it is really a good guideline.
so helpful thank you very much.
Thanks for the video it was very explanatory and detailed, easy to comprehend and follow up. please, keep it up the good work
It was very helpful, a well-written document with precise information.
how do i reference this?
MLA Jansen, Derek, and Kerryn Warren. “What (Exactly) Is Research Methodology?” Grad Coach, June 2021, gradcoach.com/what-is-research-methodology/.
APA Jansen, D., & Warren, K. (2021, June). What (Exactly) Is Research Methodology? Grad Coach. https://gradcoach.com/what-is-research-methodology/
Your explanation is easily understood. Thank you
Very help article. Now I can go my methodology chapter in my thesis with ease
I feel guided ,Thank you
This simplification is very helpful. It is simple but very educative, thanks ever so much
The write up is informative and educative. It is an academic intellectual representation that every good researcher can find useful. Thanks
Wow, this is wonderful long live.
Nice initiative
thank you the video was helpful to me.
Thank you very much for your simple and clear explanations I’m really satisfied by the way you did it By now, I think I can realize a very good article by following your fastidious indications May God bless you
Thanks very much, it was very concise and informational for a beginner like me to gain an insight into what i am about to undertake. I really appreciate.
very informative sir, it is amazing to understand the meaning of question hidden behind that, and simple language is used other than legislature to understand easily. stay happy.
This one is really amazing. All content in your youtube channel is a very helpful guide for doing research. Thanks, GradCoach.
research methodologies
Please send me more information concerning dissertation research.
Nice piece of knowledge shared….. #Thump_UP
This is amazing, it has said it all. Thanks to Gradcoach
This is wonderful,very elaborate and clear.I hope to reach out for your assistance in my research very soon.
This is the answer I am searching about…
realy thanks a lot
Thank you very much for this awesome, to the point and inclusive article.
Thank you very much I need validity and reliability explanation I have exams
Thank you for a well explained piece. This will help me going forward.
Very simple and well detailed Many thanks
This is so very simple yet so very effective and comprehensive. An Excellent piece of work.
I wish I saw this earlier on! Great insights for a beginner(researcher) like me. Thanks a mil!
Thank you very much, for such a simplified, clear and practical step by step both for academic students and general research work. Holistic, effective to use and easy to read step by step. One can easily apply the steps in practical terms and produce a quality document/up-to standard
Thanks for simplifying these terms for us, really appreciated.
Thanks for a great work. well understood .
This was very helpful. It was simple but profound and very easy to understand. Thank you so much!
Great and amazing research guidelines. Best site for learning research
hello sir/ma’am, i didn’t find yet that what type of research methodology i am using. because i am writing my report on CSR and collect all my data from websites and articles so which type of methodology i should write in dissertation report. please help me. i am from India.
how does this really work?
perfect content, thanks a lot
As a researcher, I commend you for the detailed and simplified information on the topic in question. I would like to remain in touch for the sharing of research ideas on other topics. Thank you
Impressive. Thank you, Grad Coach 😍
Thank you Grad Coach for this piece of information. I have at least learned about the different types of research methodologies.
Very useful content with easy way
Thank you very much for the presentation. I am an MPH student with the Adventist University of Africa. I have successfully completed my theory and starting on my research this July. My topic is “Factors associated with Dental Caries in (one District) in Botswana. I need help on how to go about this quantitative research
I am so grateful to run across something that was sooo helpful. I have been on my doctorate journey for quite some time. Your breakdown on methodology helped me to refresh my intent. Thank you.
thanks so much for this good lecture. student from university of science and technology, Wudil. Kano Nigeria.
It’s profound easy to understand I appreciate
Thanks a lot for sharing superb information in a detailed but concise manner. It was really helpful and helped a lot in getting into my own research methodology.
Comment * thanks very much
This was sooo helpful for me thank you so much i didn’t even know what i had to write thank you!
You’re most welcome 🙂
Simple and good. Very much helpful. Thank you so much.
This is very good work. I have benefited.
Thank you so much for sharing
This is powerful thank you so much guys
I am nkasa lizwi doing my research proposal on honors with the university of Walter Sisulu Komani I m on part 3 now can you assist me.my topic is: transitional challenges faced by educators in intermediate phase in the Alfred Nzo District.
Appreciate the presentation. Very useful step-by-step guidelines to follow.
I appreciate sir
wow! This is super insightful for me. Thank you!
Indeed this material is very helpful! Kudos writers/authors.
I want to say thank you very much, I got a lot of info and knowledge. Be blessed.
I want present a seminar paper on Optimisation of Deep learning-based models on vulnerability detection in digital transactions.
Need assistance
Dear Sir, I want to be assisted on my research on Sanitation and Water management in emergencies areas.
I am deeply grateful for the knowledge gained. I will be getting in touch shortly as I want to be assisted in my ongoing research.
The information shared is informative, crisp and clear. Kudos Team! And thanks a lot!
hello i want to study
Hello!! Grad coach teams. I am extremely happy in your tutorial or consultation. i am really benefited all material and briefing. Thank you very much for your generous helps. Please keep it up. If you add in your briefing, references for further reading, it will be very nice.
All I have to say is, thank u gyz.
Good, l thanks
thank you, it is very useful
Trackbacks/Pingbacks
- What Is A Literature Review (In A Dissertation Or Thesis) - Grad Coach - […] the literature review is to inform the choice of methodology for your own research. As we’ve discussed on the Grad Coach blog,…
- Free Download: Research Proposal Template (With Examples) - Grad Coach - […] Research design (methodology) […]
- Dissertation vs Thesis: What's the difference? - Grad Coach - […] and thesis writing on a daily basis – everything from how to find a good research topic to which…
Submit a Comment Cancel reply
Your email address will not be published. Required fields are marked *
Save my name, email, and website in this browser for the next time I comment.
- Print Friendly
News alert: UC Berkeley has announced its next university librarian
Secondary menu
- Log in to your Library account
- Hours and Maps
- Connect from Off Campus
- UC Berkeley Home
Search form
Research methods--quantitative, qualitative, and more: overview.
- Quantitative Research
- Qualitative Research
- Data Science Methods (Machine Learning, AI, Big Data)
- Text Mining and Computational Text Analysis
- Evidence Synthesis/Systematic Reviews
- Get Data, Get Help!
About Research Methods
This guide provides an overview of research methods, how to choose and use them, and supports and resources at UC Berkeley.
As Patten and Newhart note in the book Understanding Research Methods , "Research methods are the building blocks of the scientific enterprise. They are the "how" for building systematic knowledge. The accumulation of knowledge through research is by its nature a collective endeavor. Each well-designed study provides evidence that may support, amend, refute, or deepen the understanding of existing knowledge...Decisions are important throughout the practice of research and are designed to help researchers collect evidence that includes the full spectrum of the phenomenon under study, to maintain logical rules, and to mitigate or account for possible sources of bias. In many ways, learning research methods is learning how to see and make these decisions."
The choice of methods varies by discipline, by the kind of phenomenon being studied and the data being used to study it, by the technology available, and more. This guide is an introduction, but if you don't see what you need here, always contact your subject librarian, and/or take a look to see if there's a library research guide that will answer your question.
Suggestions for changes and additions to this guide are welcome!
START HERE: SAGE Research Methods
Without question, the most comprehensive resource available from the library is SAGE Research Methods. HERE IS THE ONLINE GUIDE to this one-stop shopping collection, and some helpful links are below:
- SAGE Research Methods
- Little Green Books (Quantitative Methods)
- Little Blue Books (Qualitative Methods)
- Dictionaries and Encyclopedias
- Case studies of real research projects
- Sample datasets for hands-on practice
- Streaming video--see methods come to life
- Methodspace- -a community for researchers
- SAGE Research Methods Course Mapping
Library Data Services at UC Berkeley
Library Data Services Program and Digital Scholarship Services
The LDSP offers a variety of services and tools ! From this link, check out pages for each of the following topics: discovering data, managing data, collecting data, GIS data, text data mining, publishing data, digital scholarship, open science, and the Research Data Management Program.
Be sure also to check out the visual guide to where to seek assistance on campus with any research question you may have!
Library GIS Services
Other Data Services at Berkeley
D-Lab Supports Berkeley faculty, staff, and graduate students with research in data intensive social science, including a wide range of training and workshop offerings Dryad Dryad is a simple self-service tool for researchers to use in publishing their datasets. It provides tools for the effective publication of and access to research data. Geospatial Innovation Facility (GIF) Provides leadership and training across a broad array of integrated mapping technologies on campu Research Data Management A UC Berkeley guide and consulting service for research data management issues
General Research Methods Resources
Here are some general resources for assistance:
- Assistance from ICPSR (must create an account to access): Getting Help with Data , and Resources for Students
- Wiley Stats Ref for background information on statistics topics
- Survey Documentation and Analysis (SDA) . Program for easy web-based analysis of survey data.
Consultants
- D-Lab/Data Science Discovery Consultants Request help with your research project from peer consultants.
- Research data (RDM) consulting Meet with RDM consultants before designing the data security, storage, and sharing aspects of your qualitative project.
- Statistics Department Consulting Services A service in which advanced graduate students, under faculty supervision, are available to consult during specified hours in the Fall and Spring semesters.
Related Resourcex
- IRB / CPHS Qualitative research projects with human subjects often require that you go through an ethics review.
- OURS (Office of Undergraduate Research and Scholarships) OURS supports undergraduates who want to embark on research projects and assistantships. In particular, check out their "Getting Started in Research" workshops
- Sponsored Projects Sponsored projects works with researchers applying for major external grants.
- Next: Quantitative Research >>
- Last Updated: Apr 3, 2023 3:14 PM
- URL: https://guides.lib.berkeley.edu/researchmethods
Have a language expert improve your writing
Run a free plagiarism check in 10 minutes, automatically generate references for free.
- Knowledge Base
- Methodology
Research Methods | Definition, Types, Examples
Research methods are specific procedures for collecting and analysing data. Developing your research methods is an integral part of your research design . When planning your methods, there are two key decisions you will make.
First, decide how you will collect data . Your methods depend on what type of data you need to answer your research question :
- Qualitative vs quantitative : Will your data take the form of words or numbers?
- Primary vs secondary : Will you collect original data yourself, or will you use data that have already been collected by someone else?
- Descriptive vs experimental : Will you take measurements of something as it is, or will you perform an experiment?
Second, decide how you will analyse the data .
- For quantitative data, you can use statistical analysis methods to test relationships between variables.
- For qualitative data, you can use methods such as thematic analysis to interpret patterns and meanings in the data.
Table of contents
Methods for collecting data, examples of data collection methods, methods for analysing data, examples of data analysis methods, frequently asked questions about methodology.
Data are the information that you collect for the purposes of answering your research question . The type of data you need depends on the aims of your research.
Qualitative vs quantitative data
Your choice of qualitative or quantitative data collection depends on the type of knowledge you want to develop.
For questions about ideas, experiences and meanings, or to study something that can’t be described numerically, collect qualitative data .
If you want to develop a more mechanistic understanding of a topic, or your research involves hypothesis testing , collect quantitative data .
You can also take a mixed methods approach, where you use both qualitative and quantitative research methods.
Primary vs secondary data
Primary data are any original information that you collect for the purposes of answering your research question (e.g. through surveys , observations and experiments ). Secondary data are information that has already been collected by other researchers (e.g. in a government census or previous scientific studies).
If you are exploring a novel research question, you’ll probably need to collect primary data. But if you want to synthesise existing knowledge, analyse historical trends, or identify patterns on a large scale, secondary data might be a better choice.
Descriptive vs experimental data
In descriptive research , you collect data about your study subject without intervening. The validity of your research will depend on your sampling method .
In experimental research , you systematically intervene in a process and measure the outcome. The validity of your research will depend on your experimental design .
To conduct an experiment, you need to be able to vary your independent variable , precisely measure your dependent variable, and control for confounding variables . If it’s practically and ethically possible, this method is the best choice for answering questions about cause and effect.
Prevent plagiarism, run a free check.
Your data analysis methods will depend on the type of data you collect and how you prepare them for analysis.
Data can often be analysed both quantitatively and qualitatively. For example, survey responses could be analysed qualitatively by studying the meanings of responses or quantitatively by studying the frequencies of responses.
Qualitative analysis methods
Qualitative analysis is used to understand words, ideas, and experiences. You can use it to interpret data that were collected:
- From open-ended survey and interview questions, literature reviews, case studies, and other sources that use text rather than numbers.
- Using non-probability sampling methods .
Qualitative analysis tends to be quite flexible and relies on the researcher’s judgement, so you have to reflect carefully on your choices and assumptions.
Quantitative analysis methods
Quantitative analysis uses numbers and statistics to understand frequencies, averages and correlations (in descriptive studies) or cause-and-effect relationships (in experiments).
You can use quantitative analysis to interpret data that were collected either:
- During an experiment.
- Using probability sampling methods .
Because the data are collected and analysed in a statistically valid way, the results of quantitative analysis can be easily standardised and shared among researchers.
Quantitative research deals with numbers and statistics, while qualitative research deals with words and meanings.
Quantitative methods allow you to test a hypothesis by systematically collecting and analysing data, while qualitative methods allow you to explore ideas and experiences in depth.
In mixed methods research , you use both qualitative and quantitative data collection and analysis methods to answer your research question .
A sample is a subset of individuals from a larger population. Sampling means selecting the group that you will actually collect data from in your research.
For example, if you are researching the opinions of students in your university, you could survey a sample of 100 students.
Statistical sampling allows you to test a hypothesis about the characteristics of a population. There are various sampling methods you can use to ensure that your sample is representative of the population as a whole.
The research methods you use depend on the type of data you need to answer your research question .
- If you want to measure something or test a hypothesis , use quantitative methods . If you want to explore ideas, thoughts, and meanings, use qualitative methods .
- If you want to analyse a large amount of readily available data, use secondary data. If you want data specific to your purposes with control over how they are generated, collect primary data.
- If you want to establish cause-and-effect relationships between variables , use experimental methods. If you want to understand the characteristics of a research subject, use descriptive methods.
Methodology refers to the overarching strategy and rationale of your research project . It involves studying the methods used in your field and the theories or principles behind them, in order to develop an approach that matches your objectives.
Methods are the specific tools and procedures you use to collect and analyse data (e.g. experiments, surveys , and statistical tests ).
In shorter scientific papers, where the aim is to report the findings of a specific study, you might simply describe what you did in a methods section .
In a longer or more complex research project, such as a thesis or dissertation , you will probably include a methodology section , where you explain your approach to answering the research questions and cite relevant sources to support your choice of methods.
Is this article helpful?
More interesting articles.
- A Quick Guide to Experimental Design | 5 Steps & Examples
- Between-Subjects Design | Examples, Pros & Cons
- Case Study | Definition, Examples & Methods
- Cluster Sampling | A Simple Step-by-Step Guide with Examples
- Confounding Variables | Definition, Examples & Controls
- Construct Validity | Definition, Types, & Examples
- Content Analysis | A Step-by-Step Guide with Examples
- Control Groups and Treatment Groups | Uses & Examples
- Controlled Experiments | Methods & Examples of Control
- Correlation vs Causation | Differences, Designs & Examples
- Correlational Research | Guide, Design & Examples
- Critical Discourse Analysis | Definition, Guide & Examples
- Cross-Sectional Study | Definitions, Uses & Examples
- Data Cleaning | A Guide with Examples & Steps
- Data Collection Methods | Step-by-Step Guide & Examples
- Descriptive Research Design | Definition, Methods & Examples
- Doing Survey Research | A Step-by-Step Guide & Examples
- Ethical Considerations in Research | Types & Examples
- Explanatory Research | Definition, Guide, & Examples
- Explanatory vs Response Variables | Definitions & Examples
- Exploratory Research | Definition, Guide, & Examples
- External Validity | Types, Threats & Examples
- Extraneous Variables | Examples, Types, Controls
- Face Validity | Guide with Definition & Examples
- How to Do Thematic Analysis | Guide & Examples
- How to Write a Strong Hypothesis | Guide & Examples
- Inclusion and Exclusion Criteria | Examples & Definition
- Independent vs Dependent Variables | Definition & Examples
- Inductive Reasoning | Types, Examples, Explanation
- Inductive vs Deductive Research Approach (with Examples)
- Internal Validity | Definition, Threats & Examples
- Internal vs External Validity | Understanding Differences & Examples
- Longitudinal Study | Definition, Approaches & Examples
- Mediator vs Moderator Variables | Differences & Examples
- Mixed Methods Research | Definition, Guide, & Examples
- Multistage Sampling | An Introductory Guide with Examples
- Naturalistic Observation | Definition, Guide & Examples
- Operationalisation | A Guide with Examples, Pros & Cons
- Population vs Sample | Definitions, Differences & Examples
- Primary Research | Definition, Types, & Examples
- Qualitative vs Quantitative Research | Examples & Methods
- Quasi-Experimental Design | Definition, Types & Examples
- Questionnaire Design | Methods, Question Types & Examples
- Random Assignment in Experiments | Introduction & Examples
- Reliability vs Validity in Research | Differences, Types & Examples
- Reproducibility vs Replicability | Difference & Examples
- Research Design | Step-by-Step Guide with Examples
- Sampling Methods | Types, Techniques, & Examples
- Semi-Structured Interview | Definition, Guide & Examples
- Simple Random Sampling | Definition, Steps & Examples
- Stratified Sampling | A Step-by-Step Guide with Examples
- Structured Interview | Definition, Guide & Examples
- Systematic Review | Definition, Examples & Guide
- Systematic Sampling | A Step-by-Step Guide with Examples
- Textual Analysis | Guide, 3 Approaches & Examples
- The 4 Types of Reliability in Research | Definitions & Examples
- The 4 Types of Validity | Types, Definitions & Examples
- Transcribing an Interview | 5 Steps & Transcription Software
- Triangulation in Research | Guide, Types, Examples
- Types of Interviews in Research | Guide & Examples
- Types of Research Designs Compared | Examples
- Types of Variables in Research | Definitions & Examples
- Unstructured Interview | Definition, Guide & Examples
- What Are Control Variables | Definition & Examples
- What Is a Case-Control Study? | Definition & Examples
- What Is a Cohort Study? | Definition & Examples
- What Is a Conceptual Framework? | Tips & Examples
- What Is a Double-Barrelled Question?
- What Is a Double-Blind Study? | Introduction & Examples
- What Is a Focus Group? | Step-by-Step Guide & Examples
- What Is a Likert Scale? | Guide & Examples
- What is a Literature Review? | Guide, Template, & Examples
- What Is a Prospective Cohort Study? | Definition & Examples
- What Is a Retrospective Cohort Study? | Definition & Examples
- What Is Action Research? | Definition & Examples
- What Is an Observational Study? | Guide & Examples
- What Is Concurrent Validity? | Definition & Examples
- What Is Content Validity? | Definition & Examples
- What Is Convenience Sampling? | Definition & Examples
- What Is Convergent Validity? | Definition & Examples
- What Is Criterion Validity? | Definition & Examples
- What Is Deductive Reasoning? | Explanation & Examples
- What Is Discriminant Validity? | Definition & Example
- What Is Ecological Validity? | Definition & Examples
- What Is Ethnography? | Meaning, Guide & Examples
- What Is Non-Probability Sampling? | Types & Examples
- What Is Participant Observation? | Definition & Examples
- What Is Peer Review? | Types & Examples
- What Is Predictive Validity? | Examples & Definition
- What Is Probability Sampling? | Types & Examples
- What Is Purposive Sampling? | Definition & Examples
- What Is Qualitative Observation? | Definition & Examples
- What Is Qualitative Research? | Methods & Examples
- What Is Quantitative Observation? | Definition & Examples
- What Is Quantitative Research? | Definition & Methods
- What Is Quota Sampling? | Definition & Examples
- What is Secondary Research? | Definition, Types, & Examples
- What Is Snowball Sampling? | Definition & Examples
- Within-Subjects Design | Explanation, Approaches, Examples
Reference management. Clean and simple.
What is research methodology?
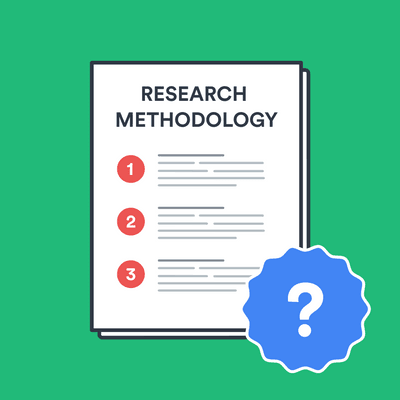
The basics of research methodology
Why do you need a research methodology, what needs to be included, why do you need to document your research method, what are the different types of research instruments, qualitative / quantitative / mixed research methodologies, how do you choose the best research methodology for you, frequently asked questions about research methodology, related articles.
When you’re working on your first piece of academic research, there are many different things to focus on, and it can be overwhelming to stay on top of everything. This is especially true of budding or inexperienced researchers.
If you’ve never put together a research proposal before or find yourself in a position where you need to explain your research methodology decisions, there are a few things you need to be aware of.
Once you understand the ins and outs, handling academic research in the future will be less intimidating. We break down the basics below:
A research methodology encompasses the way in which you intend to carry out your research. This includes how you plan to tackle things like collection methods, statistical analysis, participant observations, and more.
You can think of your research methodology as being a formula. One part will be how you plan on putting your research into practice, and another will be why you feel this is the best way to approach it. Your research methodology is ultimately a methodological and systematic plan to resolve your research problem.
In short, you are explaining how you will take your idea and turn it into a study, which in turn will produce valid and reliable results that are in accordance with the aims and objectives of your research. This is true whether your paper plans to make use of qualitative methods or quantitative methods.
The purpose of a research methodology is to explain the reasoning behind your approach to your research - you'll need to support your collection methods, methods of analysis, and other key points of your work.
Think of it like writing a plan or an outline for you what you intend to do.
When carrying out research, it can be easy to go off-track or depart from your standard methodology.
Tip: Having a methodology keeps you accountable and on track with your original aims and objectives, and gives you a suitable and sound plan to keep your project manageable, smooth, and effective.
With all that said, how do you write out your standard approach to a research methodology?
As a general plan, your methodology should include the following information:
- Your research method. You need to state whether you plan to use quantitative analysis, qualitative analysis, or mixed-method research methods. This will often be determined by what you hope to achieve with your research.
- Explain your reasoning. Why are you taking this methodological approach? Why is this particular methodology the best way to answer your research problem and achieve your objectives?
- Explain your instruments. This will mainly be about your collection methods. There are varying instruments to use such as interviews, physical surveys, questionnaires, for example. Your methodology will need to detail your reasoning in choosing a particular instrument for your research.
- What will you do with your results? How are you going to analyze the data once you have gathered it?
- Advise your reader. If there is anything in your research methodology that your reader might be unfamiliar with, you should explain it in more detail. For example, you should give any background information to your methods that might be relevant or provide your reasoning if you are conducting your research in a non-standard way.
- How will your sampling process go? What will your sampling procedure be and why? For example, if you will collect data by carrying out semi-structured or unstructured interviews, how will you choose your interviewees and how will you conduct the interviews themselves?
- Any practical limitations? You should discuss any limitations you foresee being an issue when you’re carrying out your research.
In any dissertation, thesis, or academic journal, you will always find a chapter dedicated to explaining the research methodology of the person who carried out the study, also referred to as the methodology section of the work.
A good research methodology will explain what you are going to do and why, while a poor methodology will lead to a messy or disorganized approach.
You should also be able to justify in this section your reasoning for why you intend to carry out your research in a particular way, especially if it might be a particularly unique method.
Having a sound methodology in place can also help you with the following:
- When another researcher at a later date wishes to try and replicate your research, they will need your explanations and guidelines.
- In the event that you receive any criticism or questioning on the research you carried out at a later point, you will be able to refer back to it and succinctly explain the how and why of your approach.
- It provides you with a plan to follow throughout your research. When you are drafting your methodology approach, you need to be sure that the method you are using is the right one for your goal. This will help you with both explaining and understanding your method.
- It affords you the opportunity to document from the outset what you intend to achieve with your research, from start to finish.
A research instrument is a tool you will use to help you collect, measure and analyze the data you use as part of your research.
The choice of research instrument will usually be yours to make as the researcher and will be whichever best suits your methodology.
There are many different research instruments you can use in collecting data for your research.
Generally, they can be grouped as follows:
- Interviews (either as a group or one-on-one). You can carry out interviews in many different ways. For example, your interview can be structured, semi-structured, or unstructured. The difference between them is how formal the set of questions is that is asked of the interviewee. In a group interview, you may choose to ask the interviewees to give you their opinions or perceptions on certain topics.
- Surveys (online or in-person). In survey research, you are posing questions in which you ask for a response from the person taking the survey. You may wish to have either free-answer questions such as essay-style questions, or you may wish to use closed questions such as multiple choice. You may even wish to make the survey a mixture of both.
- Focus Groups. Similar to the group interview above, you may wish to ask a focus group to discuss a particular topic or opinion while you make a note of the answers given.
- Observations. This is a good research instrument to use if you are looking into human behaviors. Different ways of researching this include studying the spontaneous behavior of participants in their everyday life, or something more structured. A structured observation is research conducted at a set time and place where researchers observe behavior as planned and agreed upon with participants.
These are the most common ways of carrying out research, but it is really dependent on your needs as a researcher and what approach you think is best to take.
It is also possible to combine a number of research instruments if this is necessary and appropriate in answering your research problem.
There are three different types of methodologies, and they are distinguished by whether they focus on words, numbers, or both.
➡️ Want to learn more about the differences between qualitative and quantitative research, and how to use both methods? Check out our guide for that!
If you've done your due diligence, you'll have an idea of which methodology approach is best suited to your research.
It’s likely that you will have carried out considerable reading and homework before you reach this point and you may have taken inspiration from other similar studies that have yielded good results.
Still, it is important to consider different options before setting your research in stone. Exploring different options available will help you to explain why the choice you ultimately make is preferable to other methods.
If proving your research problem requires you to gather large volumes of numerical data to test hypotheses, a quantitative research method is likely to provide you with the most usable results.
If instead you’re looking to try and learn more about people, and their perception of events, your methodology is more exploratory in nature and would therefore probably be better served using a qualitative research methodology.
It helps to always bring things back to the question: what do I want to achieve with my research?
Once you have conducted your research, you need to analyze it. Here are some helpful guides for qualitative data analysis:
➡️ How to do a content analysis
➡️ How to do a thematic analysis
➡️ How to do a rhetorical analysis
Research methodology refers to the techniques used to find and analyze information for a study, ensuring that the results are valid, reliable and that they address the research objective.
Data can typically be organized into four different categories or methods: observational, experimental, simulation, and derived.
Writing a methodology section is a process of introducing your methods and instruments, discussing your analysis, providing more background information, addressing your research limitations, and more.
Your research methodology section will need a clear research question and proposed research approach. You'll need to add a background, introduce your research question, write your methodology and add the works you cited during your data collecting phase.
The research methodology section of your study will indicate how valid your findings are and how well-informed your paper is. It also assists future researchers planning to use the same methodology, who want to cite your study or replicate it.
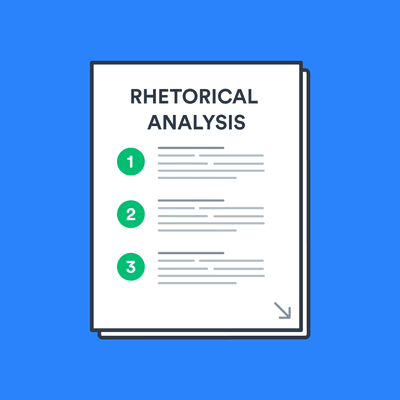
What is Research Methodology? Definition, Types, and Examples
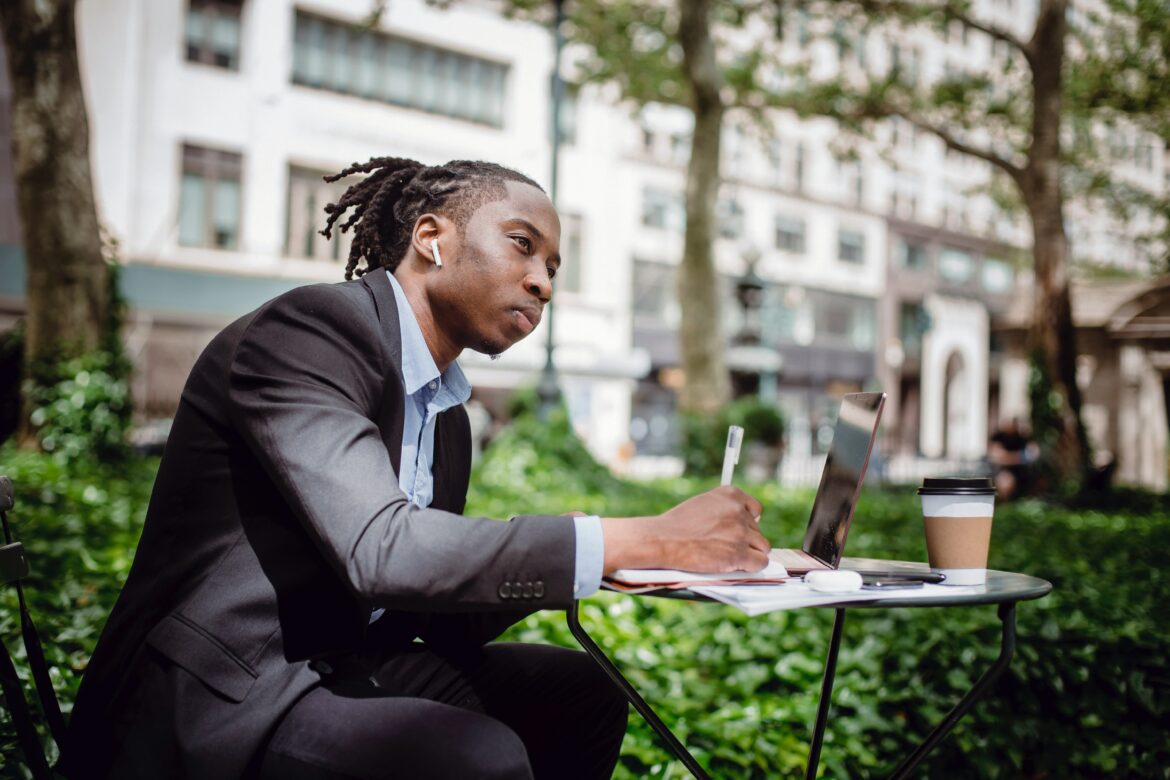
Research methodology 1,2 is a structured and scientific approach used to collect, analyze, and interpret quantitative or qualitative data to answer research questions or test hypotheses. A research methodology is like a plan for carrying out research and helps keep researchers on track by limiting the scope of the research. Several aspects must be considered before selecting an appropriate research methodology, such as research limitations and ethical concerns that may affect your research.
The research methodology section in a scientific paper describes the different methodological choices made, such as the data collection and analysis methods, and why these choices were selected. The reasons should explain why the methods chosen are the most appropriate to answer the research question. A good research methodology also helps ensure the reliability and validity of the research findings. There are three types of research methodology—quantitative, qualitative, and mixed-method, which can be chosen based on the research objectives.
What is research methodology ?
A research methodology describes the techniques and procedures used to identify and analyze information regarding a specific research topic. It is a process by which researchers design their study so that they can achieve their objectives using the selected research instruments. It includes all the important aspects of research, including research design, data collection methods, data analysis methods, and the overall framework within which the research is conducted. While these points can help you understand what is research methodology, you also need to know why it is important to pick the right methodology.
Why is research methodology important?
Having a good research methodology in place has the following advantages: 3
- Helps other researchers who may want to replicate your research; the explanations will be of benefit to them.
- You can easily answer any questions about your research if they arise at a later stage.
- A research methodology provides a framework and guidelines for researchers to clearly define research questions, hypotheses, and objectives.
- It helps researchers identify the most appropriate research design, sampling technique, and data collection and analysis methods.
- A sound research methodology helps researchers ensure that their findings are valid and reliable and free from biases and errors.
- It also helps ensure that ethical guidelines are followed while conducting research.
- A good research methodology helps researchers in planning their research efficiently, by ensuring optimum usage of their time and resources.
Writing the methods section of a research paper? Let Paperpal help you achieve perfection
Types of research methodology.
There are three types of research methodology based on the type of research and the data required. 1
- Quantitative research methodology focuses on measuring and testing numerical data. This approach is good for reaching a large number of people in a short amount of time. This type of research helps in testing the causal relationships between variables, making predictions, and generalizing results to wider populations.
- Qualitative research methodology examines the opinions, behaviors, and experiences of people. It collects and analyzes words and textual data. This research methodology requires fewer participants but is still more time consuming because the time spent per participant is quite large. This method is used in exploratory research where the research problem being investigated is not clearly defined.
- Mixed-method research methodology uses the characteristics of both quantitative and qualitative research methodologies in the same study. This method allows researchers to validate their findings, verify if the results observed using both methods are complementary, and explain any unexpected results obtained from one method by using the other method.
What are the types of sampling designs in research methodology?
Sampling 4 is an important part of a research methodology and involves selecting a representative sample of the population to conduct the study, making statistical inferences about them, and estimating the characteristics of the whole population based on these inferences. There are two types of sampling designs in research methodology—probability and nonprobability.
- Probability sampling
In this type of sampling design, a sample is chosen from a larger population using some form of random selection, that is, every member of the population has an equal chance of being selected. The different types of probability sampling are:
- Systematic —sample members are chosen at regular intervals. It requires selecting a starting point for the sample and sample size determination that can be repeated at regular intervals. This type of sampling method has a predefined range; hence, it is the least time consuming.
- Stratified —researchers divide the population into smaller groups that don’t overlap but represent the entire population. While sampling, these groups can be organized, and then a sample can be drawn from each group separately.
- Cluster —the population is divided into clusters based on demographic parameters like age, sex, location, etc.
- Convenience —selects participants who are most easily accessible to researchers due to geographical proximity, availability at a particular time, etc.
- Purposive —participants are selected at the researcher’s discretion. Researchers consider the purpose of the study and the understanding of the target audience.
- Snowball —already selected participants use their social networks to refer the researcher to other potential participants.
- Quota —while designing the study, the researchers decide how many people with which characteristics to include as participants. The characteristics help in choosing people most likely to provide insights into the subject.
What are data collection methods?
During research, data are collected using various methods depending on the research methodology being followed and the research methods being undertaken. Both qualitative and quantitative research have different data collection methods, as listed below.
Qualitative research 5
- One-on-one interviews: Helps the interviewers understand a respondent’s subjective opinion and experience pertaining to a specific topic or event
- Document study/literature review/record keeping: Researchers’ review of already existing written materials such as archives, annual reports, research articles, guidelines, policy documents, etc.
- Focus groups: Constructive discussions that usually include a small sample of about 6-10 people and a moderator, to understand the participants’ opinion on a given topic.
- Qualitative observation : Researchers collect data using their five senses (sight, smell, touch, taste, and hearing).
Quantitative research 6
- Sampling: The most common type is probability sampling.
- Interviews: Commonly telephonic or done in-person.
- Observations: Structured observations are most commonly used in quantitative research. In this method, researchers make observations about specific behaviors of individuals in a structured setting.
- Document review: Reviewing existing research or documents to collect evidence for supporting the research.
- Surveys and questionnaires. Surveys can be administered both online and offline depending on the requirement and sample size.
Let Paperpal help you write the perfect research methods section. Start now!
What are data analysis methods.
The data collected using the various methods for qualitative and quantitative research need to be analyzed to generate meaningful conclusions. These data analysis methods 7 also differ between quantitative and qualitative research.
Quantitative research involves a deductive method for data analysis where hypotheses are developed at the beginning of the research and precise measurement is required. The methods include statistical analysis applications to analyze numerical data and are grouped into two categories—descriptive and inferential.
Descriptive analysis is used to describe the basic features of different types of data to present it in a way that ensures the patterns become meaningful. The different types of descriptive analysis methods are:
- Measures of frequency (count, percent, frequency)
- Measures of central tendency (mean, median, mode)
- Measures of dispersion or variation (range, variance, standard deviation)
- Measure of position (percentile ranks, quartile ranks)
Inferential analysis is used to make predictions about a larger population based on the analysis of the data collected from a smaller population. This analysis is used to study the relationships between different variables. Some commonly used inferential data analysis methods are:
- Correlation: To understand the relationship between two or more variables.
- Cross-tabulation: Analyze the relationship between multiple variables.
- Regression analysis: Study the impact of independent variables on the dependent variable.
- Frequency tables: To understand the frequency of data.
- Analysis of variance: To test the degree to which two or more variables differ in an experiment.
Qualitative research involves an inductive method for data analysis where hypotheses are developed after data collection. The methods include:
- Content analysis: For analyzing documented information from text and images by determining the presence of certain words or concepts in texts.
- Narrative analysis: For analyzing content obtained from sources such as interviews, field observations, and surveys. The stories and opinions shared by people are used to answer research questions.
- Discourse analysis: For analyzing interactions with people considering the social context, that is, the lifestyle and environment, under which the interaction occurs.
- Grounded theory: Involves hypothesis creation by data collection and analysis to explain why a phenomenon occurred.
- Thematic analysis: To identify important themes or patterns in data and use these to address an issue.
How to choose a research methodology?
Here are some important factors to consider when choosing a research methodology: 8
- Research objectives, aims, and questions —these would help structure the research design.
- Review existing literature to identify any gaps in knowledge.
- Check the statistical requirements —if data-driven or statistical results are needed then quantitative research is the best. If the research questions can be answered based on people’s opinions and perceptions, then qualitative research is most suitable.
- Sample size —sample size can often determine the feasibility of a research methodology. For a large sample, less effort- and time-intensive methods are appropriate.
- Constraints —constraints of time, geography, and resources can help define the appropriate methodology.
Got writer’s block? Kickstart your research paper writing with Paperpal now!
How to write a research methodology .
A research methodology should include the following components: 3,9
- Research design —should be selected based on the research question and the data required. Common research designs include experimental, quasi-experimental, correlational, descriptive, and exploratory.
- Research method —this can be quantitative, qualitative, or mixed-method.
- Reason for selecting a specific methodology —explain why this methodology is the most suitable to answer your research problem.
- Research instruments —explain the research instruments you plan to use, mainly referring to the data collection methods such as interviews, surveys, etc. Here as well, a reason should be mentioned for selecting the particular instrument.
- Sampling —this involves selecting a representative subset of the population being studied.
- Data collection —involves gathering data using several data collection methods, such as surveys, interviews, etc.
- Data analysis —describe the data analysis methods you will use once you’ve collected the data.
- Research limitations —mention any limitations you foresee while conducting your research.
- Validity and reliability —validity helps identify the accuracy and truthfulness of the findings; reliability refers to the consistency and stability of the results over time and across different conditions.
- Ethical considerations —research should be conducted ethically. The considerations include obtaining consent from participants, maintaining confidentiality, and addressing conflicts of interest.
Streamline Your Research Paper Writing Process with Paperpal
The methods section is a critical part of the research papers, allowing researchers to use this to understand your findings and replicate your work when pursuing their own research. However, it is usually also the most difficult section to write. This is where Paperpal can help you overcome the writer’s block and create the first draft in minutes with Paperpal Copilot, its secure generative AI feature suite.
With Paperpal you can get research advice, write and refine your work, rephrase and verify the writing, and ensure submission readiness, all in one place. Here’s how you can use Paperpal to develop the first draft of your methods section.
- Generate an outline: Input some details about your research to instantly generate an outline for your methods section
- Develop the section: Use the outline and suggested sentence templates to expand your ideas and develop the first draft.
- P araph ras e and trim : Get clear, concise academic text with paraphrasing that conveys your work effectively and word reduction to fix redundancies.
- Choose the right words: Enhance text by choosing contextual synonyms based on how the words have been used in previously published work.
- Check and verify text : Make sure the generated text showcases your methods correctly, has all the right citations, and is original and authentic. .
You can repeat this process to develop each section of your research manuscript, including the title, abstract and keywords. Ready to write your research papers faster, better, and without the stress? Sign up for Paperpal and start writing today!
Frequently Asked Questions
Q1. What are the key components of research methodology?
A1. A good research methodology has the following key components:
- Research design
- Data collection procedures
- Data analysis methods
- Ethical considerations
Q2. Why is ethical consideration important in research methodology?
A2. Ethical consideration is important in research methodology to ensure the readers of the reliability and validity of the study. Researchers must clearly mention the ethical norms and standards followed during the conduct of the research and also mention if the research has been cleared by any institutional board. The following 10 points are the important principles related to ethical considerations: 10
- Participants should not be subjected to harm.
- Respect for the dignity of participants should be prioritized.
- Full consent should be obtained from participants before the study.
- Participants’ privacy should be ensured.
- Confidentiality of the research data should be ensured.
- Anonymity of individuals and organizations participating in the research should be maintained.
- The aims and objectives of the research should not be exaggerated.
- Affiliations, sources of funding, and any possible conflicts of interest should be declared.
- Communication in relation to the research should be honest and transparent.
- Misleading information and biased representation of primary data findings should be avoided.
Q3. What is the difference between methodology and method?
A3. Research methodology is different from a research method, although both terms are often confused. Research methods are the tools used to gather data, while the research methodology provides a framework for how research is planned, conducted, and analyzed. The latter guides researchers in making decisions about the most appropriate methods for their research. Research methods refer to the specific techniques, procedures, and tools used by researchers to collect, analyze, and interpret data, for instance surveys, questionnaires, interviews, etc.
Research methodology is, thus, an integral part of a research study. It helps ensure that you stay on track to meet your research objectives and answer your research questions using the most appropriate data collection and analysis tools based on your research design.
Accelerate your research paper writing with Paperpal. Try for free now!
- Research methodologies. Pfeiffer Library website. Accessed August 15, 2023. https://library.tiffin.edu/researchmethodologies/whatareresearchmethodologies
- Types of research methodology. Eduvoice website. Accessed August 16, 2023. https://eduvoice.in/types-research-methodology/
- The basics of research methodology: A key to quality research. Voxco. Accessed August 16, 2023. https://www.voxco.com/blog/what-is-research-methodology/
- Sampling methods: Types with examples. QuestionPro website. Accessed August 16, 2023. https://www.questionpro.com/blog/types-of-sampling-for-social-research/
- What is qualitative research? Methods, types, approaches, examples. Researcher.Life blog. Accessed August 15, 2023. https://researcher.life/blog/article/what-is-qualitative-research-methods-types-examples/
- What is quantitative research? Definition, methods, types, and examples. Researcher.Life blog. Accessed August 15, 2023. https://researcher.life/blog/article/what-is-quantitative-research-types-and-examples/
- Data analysis in research: Types & methods. QuestionPro website. Accessed August 16, 2023. https://www.questionpro.com/blog/data-analysis-in-research/#Data_analysis_in_qualitative_research
- Factors to consider while choosing the right research methodology. PhD Monster website. Accessed August 17, 2023. https://www.phdmonster.com/factors-to-consider-while-choosing-the-right-research-methodology/
- What is research methodology? Research and writing guides. Accessed August 14, 2023. https://paperpile.com/g/what-is-research-methodology/
- Ethical considerations. Business research methodology website. Accessed August 17, 2023. https://research-methodology.net/research-methodology/ethical-considerations/
Paperpal is a comprehensive AI writing toolkit that helps students and researchers achieve 2x the writing in half the time. It leverages 21+ years of STM experience and insights from millions of research articles to provide in-depth academic writing, language editing, and submission readiness support to help you write better, faster.
Get accurate academic translations, rewriting support, grammar checks, vocabulary suggestions, and generative AI assistance that delivers human precision at machine speed. Try for free or upgrade to Paperpal Prime starting at US$19 a month to access premium features, including consistency, plagiarism, and 30+ submission readiness checks to help you succeed.
Experience the future of academic writing – Sign up to Paperpal and start writing for free!
Related Reads:
- Dangling Modifiers and How to Avoid Them in Your Writing
- Webinar: How to Use Generative AI Tools Ethically in Your Academic Writing
- Research Outlines: How to Write An Introduction Section in Minutes with Paperpal Copilot
- How to Paraphrase Research Papers Effectively
Language and Grammar Rules for Academic Writing
Climatic vs. climactic: difference and examples, you may also like, word choice problems: how to use the right..., how to avoid plagiarism when using generative ai..., what are journal guidelines on using generative ai..., types of plagiarism and 6 tips to avoid..., how to write an essay introduction (with examples)..., similarity checks: the author’s guide to plagiarism and..., what is a master’s thesis: a guide for..., should you use ai tools like chatgpt for..., what are the benefits of generative ai for..., how to avoid plagiarism tips and advice for....
- Resources Home 🏠
- Try SciSpace Copilot
- Search research papers
- Add Copilot Extension
- Try AI Detector
- Try Paraphraser
- Try Citation Generator
- April Papers
- June Papers
- July Papers

Here's What You Need to Understand About Research Methodology

Table of Contents
Research methodology involves a systematic and well-structured approach to conducting scholarly or scientific inquiries. Knowing the significance of research methodology and its different components is crucial as it serves as the basis for any study.
Typically, your research topic will start as a broad idea you want to investigate more thoroughly. Once you’ve identified a research problem and created research questions , you must choose the appropriate methodology and frameworks to address those questions effectively.
What is the definition of a research methodology?
Research methodology is the process or the way you intend to execute your study. The methodology section of a research paper outlines how you plan to conduct your study. It covers various steps such as collecting data, statistical analysis, observing participants, and other procedures involved in the research process
The methods section should give a description of the process that will convert your idea into a study. Additionally, the outcomes of your process must provide valid and reliable results resonant with the aims and objectives of your research. This thumb rule holds complete validity, no matter whether your paper has inclinations for qualitative or quantitative usage.
Studying research methods used in related studies can provide helpful insights and direction for your own research. Now easily discover papers related to your topic on SciSpace and utilize our AI research assistant, Copilot , to quickly review the methodologies applied in different papers.

The need for a good research methodology
While deciding on your approach towards your research, the reason or factors you weighed in choosing a particular problem and formulating a research topic need to be validated and explained. A research methodology helps you do exactly that. Moreover, a good research methodology lets you build your argument to validate your research work performed through various data collection methods, analytical methods, and other essential points.
Just imagine it as a strategy documented to provide an overview of what you intend to do.
While undertaking any research writing or performing the research itself, you may get drifted in not something of much importance. In such a case, a research methodology helps you to get back to your outlined work methodology.
A research methodology helps in keeping you accountable for your work. Additionally, it can help you evaluate whether your work is in sync with your original aims and objectives or not. Besides, a good research methodology enables you to navigate your research process smoothly and swiftly while providing effective planning to achieve your desired results.
What is the basic structure of a research methodology?
Usually, you must ensure to include the following stated aspects while deciding over the basic structure of your research methodology:
1. Your research procedure
Explain what research methods you’re going to use. Whether you intend to proceed with quantitative or qualitative, or a composite of both approaches, you need to state that explicitly. The option among the three depends on your research’s aim, objectives, and scope.
2. Provide the rationality behind your chosen approach
Based on logic and reason, let your readers know why you have chosen said research methodologies. Additionally, you have to build strong arguments supporting why your chosen research method is the best way to achieve the desired outcome.
3. Explain your mechanism
The mechanism encompasses the research methods or instruments you will use to develop your research methodology. It usually refers to your data collection methods. You can use interviews, surveys, physical questionnaires, etc., of the many available mechanisms as research methodology instruments. The data collection method is determined by the type of research and whether the data is quantitative data(includes numerical data) or qualitative data (perception, morale, etc.) Moreover, you need to put logical reasoning behind choosing a particular instrument.
4. Significance of outcomes
The results will be available once you have finished experimenting. However, you should also explain how you plan to use the data to interpret the findings. This section also aids in understanding the problem from within, breaking it down into pieces, and viewing the research problem from various perspectives.
5. Reader’s advice
Anything that you feel must be explained to spread more awareness among readers and focus groups must be included and described in detail. You should not just specify your research methodology on the assumption that a reader is aware of the topic.
All the relevant information that explains and simplifies your research paper must be included in the methodology section. If you are conducting your research in a non-traditional manner, give a logical justification and list its benefits.
6. Explain your sample space
Include information about the sample and sample space in the methodology section. The term "sample" refers to a smaller set of data that a researcher selects or chooses from a larger group of people or focus groups using a predetermined selection method. Let your readers know how you are going to distinguish between relevant and non-relevant samples. How you figured out those exact numbers to back your research methodology, i.e. the sample spacing of instruments, must be discussed thoroughly.
For example, if you are going to conduct a survey or interview, then by what procedure will you select the interviewees (or sample size in case of surveys), and how exactly will the interview or survey be conducted.
7. Challenges and limitations
This part, which is frequently assumed to be unnecessary, is actually very important. The challenges and limitations that your chosen strategy inherently possesses must be specified while you are conducting different types of research.
The importance of a good research methodology
You must have observed that all research papers, dissertations, or theses carry a chapter entirely dedicated to research methodology. This section helps maintain your credibility as a better interpreter of results rather than a manipulator.
A good research methodology always explains the procedure, data collection methods and techniques, aim, and scope of the research. In a research study, it leads to a well-organized, rationality-based approach, while the paper lacking it is often observed as messy or disorganized.
You should pay special attention to validating your chosen way towards the research methodology. This becomes extremely important in case you select an unconventional or a distinct method of execution.
Curating and developing a strong, effective research methodology can assist you in addressing a variety of situations, such as:
- When someone tries to duplicate or expand upon your research after few years.
- If a contradiction or conflict of facts occurs at a later time. This gives you the security you need to deal with these contradictions while still being able to defend your approach.
- Gaining a tactical approach in getting your research completed in time. Just ensure you are using the right approach while drafting your research methodology, and it can help you achieve your desired outcomes. Additionally, it provides a better explanation and understanding of the research question itself.
- Documenting the results so that the final outcome of the research stays as you intended it to be while starting.
Instruments you could use while writing a good research methodology
As a researcher, you must choose which tools or data collection methods that fit best in terms of the relevance of your research. This decision has to be wise.
There exists many research equipments or tools that you can use to carry out your research process. These are classified as:
a. Interviews (One-on-One or a Group)
An interview aimed to get your desired research outcomes can be undertaken in many different ways. For example, you can design your interview as structured, semi-structured, or unstructured. What sets them apart is the degree of formality in the questions. On the other hand, in a group interview, your aim should be to collect more opinions and group perceptions from the focus groups on a certain topic rather than looking out for some formal answers.
In surveys, you are in better control if you specifically draft the questions you seek the response for. For example, you may choose to include free-style questions that can be answered descriptively, or you may provide a multiple-choice type response for questions. Besides, you can also opt to choose both ways, deciding what suits your research process and purpose better.
c. Sample Groups
Similar to the group interviews, here, you can select a group of individuals and assign them a topic to discuss or freely express their opinions over that. You can simultaneously note down the answers and later draft them appropriately, deciding on the relevance of every response.
d. Observations
If your research domain is humanities or sociology, observations are the best-proven method to draw your research methodology. Of course, you can always include studying the spontaneous response of the participants towards a situation or conducting the same but in a more structured manner. A structured observation means putting the participants in a situation at a previously decided time and then studying their responses.
Of all the tools described above, it is you who should wisely choose the instruments and decide what’s the best fit for your research. You must not restrict yourself from multiple methods or a combination of a few instruments if appropriate in drafting a good research methodology.
Types of research methodology
A research methodology exists in various forms. Depending upon their approach, whether centered around words, numbers, or both, methodologies are distinguished as qualitative, quantitative, or an amalgamation of both.
1. Qualitative research methodology
When a research methodology primarily focuses on words and textual data, then it is generally referred to as qualitative research methodology. This type is usually preferred among researchers when the aim and scope of the research are mainly theoretical and explanatory.
The instruments used are observations, interviews, and sample groups. You can use this methodology if you are trying to study human behavior or response in some situations. Generally, qualitative research methodology is widely used in sociology, psychology, and other related domains.
2. Quantitative research methodology
If your research is majorly centered on data, figures, and stats, then analyzing these numerical data is often referred to as quantitative research methodology. You can use quantitative research methodology if your research requires you to validate or justify the obtained results.
In quantitative methods, surveys, tests, experiments, and evaluations of current databases can be advantageously used as instruments If your research involves testing some hypothesis, then use this methodology.
3. Amalgam methodology
As the name suggests, the amalgam methodology uses both quantitative and qualitative approaches. This methodology is used when a part of the research requires you to verify the facts and figures, whereas the other part demands you to discover the theoretical and explanatory nature of the research question.
The instruments for the amalgam methodology require you to conduct interviews and surveys, including tests and experiments. The outcome of this methodology can be insightful and valuable as it provides precise test results in line with theoretical explanations and reasoning.
The amalgam method, makes your work both factual and rational at the same time.
Final words: How to decide which is the best research methodology?
If you have kept your sincerity and awareness intact with the aims and scope of research well enough, you must have got an idea of which research methodology suits your work best.
Before deciding which research methodology answers your research question, you must invest significant time in reading and doing your homework for that. Taking references that yield relevant results should be your first approach to establishing a research methodology.
Moreover, you should never refrain from exploring other options. Before setting your work in stone, you must try all the available options as it explains why the choice of research methodology that you finally make is more appropriate than the other available options.
You should always go for a quantitative research methodology if your research requires gathering large amounts of data, figures, and statistics. This research methodology will provide you with results if your research paper involves the validation of some hypothesis.
Whereas, if you are looking for more explanations, reasons, opinions, and public perceptions around a theory, you must use qualitative research methodology.The choice of an appropriate research methodology ultimately depends on what you want to achieve through your research.
Frequently Asked Questions (FAQs) about Research Methodology
1. how to write a research methodology.
You can always provide a separate section for research methodology where you should specify details about the methods and instruments used during the research, discussions on result analysis, including insights into the background information, and conveying the research limitations.
2. What are the types of research methodology?
There generally exists four types of research methodology i.e.
- Observation
- Experimental
- Derivational
3. What is the true meaning of research methodology?
The set of techniques or procedures followed to discover and analyze the information gathered to validate or justify a research outcome is generally called Research Methodology.
4. Where lies the importance of research methodology?
Your research methodology directly reflects the validity of your research outcomes and how well-informed your research work is. Moreover, it can help future researchers cite or refer to your research if they plan to use a similar research methodology.

You might also like

Consensus GPT vs. SciSpace GPT: Choose the Best GPT for Research
Literature Review and Theoretical Framework: Understanding the Differences

Using AI for research: A beginner’s guide
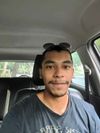

Research Methodology: Overview of Research Methodology
- Overview of Research Methodology
- General Encyclopedias on Research Methodology
- General Handbooks on Research Methodology
- Focus Groups
- Case Studies
- Cost Benefit Analysis
- Participatory Action Research
- Archival Research
- Data Analysis
Research Methods Overview
If you are planning to do research - whether you are doing a student research project, IQP, MQP, GPS project, thesis, or dissertation, you need to use valid approaches and tools to set up your study, gather your data, and make sense of your findings. This research methods guide will help you choose a methodology and launch into your research project.
Data collection and data analysis are research methods that can be applied to many disciplines. There is Qualitative research and Quantitative Research. The focus of this guide, includes most popular methods including:
focus groups
case studies
We are happy to answer questions about research methods and assist with choosing a method that is right for your research in person or online. below is a video on how to book a research consultation
"How-To": Booking a Research Consultation

" Research Data Management " by Peter Neish is marked with CC0 1.0 .
Research Design vs Research Method
What is the difference between Research Design and Research Method?
Research design is a plan to answer your research question. A research method is a strategy used to implement that plan. Research design and methods are different but closely related, because good research design ensures that the data you obtain will help you answer your research question more effectively.
Which research method should I choose ?
It depends on your research goal. It depends on what subjects (and who) you want to study. Let's say you are interested in studying what makes people happy, or why some students are more conscious about recycling on campus. To answer these questions, you need to make a decision about how to collect your data. Most frequently used methods include:
- Observation / Participant Observation
- Experiments
- Secondary Data Analysis / Archival Study
- Mixed Methods (combination of some of the above)
One particular method could be better suited to your research goal than others, because the data you collect from different methods will be different in quality and quantity. For instance, surveys are usually designed to produce relatively short answers, rather than the extensive responses expected in qualitative interviews.
What other factors should I consider when choosing one method over another?
Time for data collection and analysis is something you want to consider. An observation or interview method, so-called qualitative approach, helps you collect richer information, but it takes time. Using a survey helps you collect more data quickly, yet it may lack details. So, you will need to consider the time you have for research and the balance between strengths and weaknesses associated with each method (e.g., qualitative vs. quantitative).
Research Data Management
Research Data Management (RDM) refers to how you are going to keep and share your data over longer time frame - like after you graduate. It is defined as the organization, documentation, storage, and preservation of the data resulting from the research process, where data can be broadly defined as the outcome of experiments or observations that validate research findings, and can take a variety of forms including numerical output ( quantitative data ), qualitative data , documentation, images, audio, and video.
"Research Design" by George C Gordon Library is licensed under CC BY 4.0 / A derivative from the original work
- Next: General Encyclopedias on Research Methodology >>
- Last Updated: Jul 31, 2023 3:07 PM
- URL: https://libguides.wpi.edu/researchmethod
How can we help?
- [email protected]
- Consultation
- 508-831-5410

Top 100 Research Methodology Project Topics
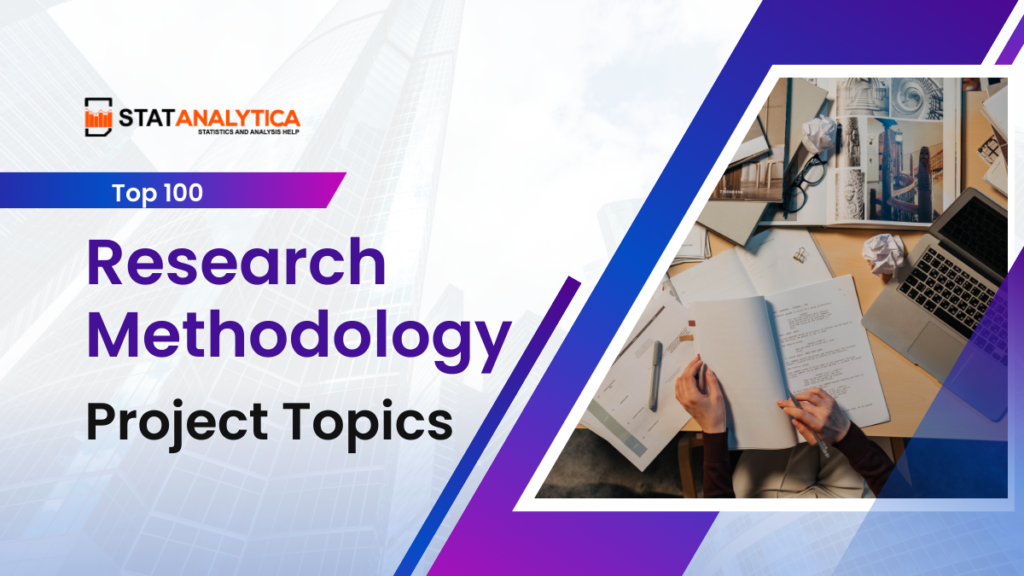
Research methodology might sound like a fancy term, but it’s simply the way researchers go about investigating a question or problem. Think of it as a roadmap for your project, guiding you through the steps to find answers. It’s crucial to pick the right methodology because it determines how you collect and analyze data, which affects the reliability of your findings. So, let’s check 100 research methodology project topics below.
Types of Research Methodologies
Table of Contents
There are mainly three types of research methodologies: quantitative, qualitative, and mixed-methods.
Quantitative Research Methodology
Quantitative research focuses on collecting numerical data and analyzing it statistically. It’s great for measuring things objectively.
For instance, if you’re studying how many people prefer coffee over tea, quantitative research can provide concrete numbers.
Qualitative Research Methodology
Qualitative research, on the other hand, dives deep into understanding people’s experiences, feelings, and behaviors. It’s like peeling an onion layer by layer to reveal the underlying emotions and motivations.
For example, if you want to explore why some students struggle with math, qualitative research can uncover personal stories and perspectives.
Mixed-Methods Research
Sometimes, researchers use a combination of quantitative and qualitative methods, known as mixed-methods research.
This approach offers a more comprehensive understanding of a topic by blending numerical data with rich narratives. It’s like having the best of both worlds.
Factors Influencing Choice of Research Methodology
Several factors influence the choice of research methodology:
- Nature of the research question: Is it about measuring something objectively or understanding complex human behaviors?
- Availability of resources: Do you have access to the tools and expertise needed for a particular methodology?
- Time constraints: How much time do you have to conduct the research?
- Ethical considerations: Are there any ethical concerns related to your research methods?
Steps Involved in Research Methodology for Project Topics
Regardless of the chosen methodology, research typically follows these steps:
- Problem Definition: Clearly define the research question or problem you want to address.
- Literature Review: Explore existing research and theories related to your topic to build a solid foundation.
- Selection of Research Design: Choose the appropriate methodology based on your research question and objectives.
- Data Collection: Gather relevant data using surveys, interviews, observations, or experiments.
- Data Analysis: Analyze the collected data using statistical tools (for quantitative research) or thematic analysis (for qualitative research).
- Interpretation of Results: Draw conclusions based on your analysis and discuss their implications.
Best Practices in Research Methodology for Project Topics
To ensure the quality and integrity of your research, follow these best practices:
- Ensuring validity and reliability of data: Use reliable measurement tools and sampling techniques to minimize errors.
- Ethical considerations in research: Obtain informed consent from participants, protect their privacy, and avoid any form of deception.
- Proper documentation and citation: Keep detailed records of your research process and cite all sources properly to avoid plagiarism.
- Peer review and feedback: Seek feedback from peers and experts in your field to improve the quality of your research.
- The impact of online surveys on response rates and data quality.
- Comparing the effectiveness of focus groups and individual interviews in marketing research.
- Analyzing the ethical considerations of using social media data for research.
- Exploring the potential of big data analytics in social science research.
- Evaluating the reliability and validity of mixed-methods research approaches.
- Examining the role of cultural sensitivity in international research projects.
- Investigating the challenges and opportunities of conducting research in conflict zones.
- Analyzing the effectiveness of different strategies for recruiting research participants.
- Exploring the use of action research methodologies in addressing real-world problems.
- Evaluating the impact of researcher bias on the research process and outcomes.
- Investigating the potential of citizen science for collecting and analyzing data.
- Exploring the use of virtual reality in conducting research studies.
- Analyzing the ethical considerations of conducting research with vulnerable populations.
- Evaluating the effectiveness of different strategies for disseminating research findings.
- Examining the role of storytelling in qualitative research.
- Investigating the use of visual methods in research, such as photography and video.
- Analyzing the challenges and opportunities of conducting longitudinal research studies.
- Exploring the use of case studies in research projects.
- Evaluating the effectiveness of different strategies for coding and analyzing qualitative data.
- Examining the role of theory in research design and analysis.
- Investigating the use of discourse analysis methodologies in research.
- Analyzing the strengths and limitations of quantitative research methods.
- Exploring the use of experimental research designs in social science research.
- Evaluating the effectiveness of different sampling techniques in research.
- Examining the role of research ethics committees in ensuring the ethical conduct of research.
- Investigating the challenges and opportunities of conducting research online.
- Analyzing the impact of social media on public perceptions of research.
- Exploring the use of gamification in research to increase participant engagement.
- Evaluating the effectiveness of different strategies for data visualization.
- Examining the role of open access in making research findings available to a wider audience.
- Investigating the challenges and opportunities of interdisciplinary research collaborations.
- Analyzing the impact of political and economic factors on research funding.
- Exploring the use of participatory action research methodologies to empower communities.
- Evaluating the effectiveness of different strategies for knowledge mobilization.
- Examining the role of research in informing policy and practice.
- Investigating the use of artificial intelligence in research methodologies.
- Analyzing the ethical considerations of using facial recognition technology in research.
- Exploring the potential of blockchain technology to improve data security and transparency in research.
- Evaluating the effectiveness of different strategies for engaging with stakeholders in research projects.
- Examining the role of reflexivity in qualitative research.
- Investigating the use of narrative inquiry methodologies in research.
- Analyzing the strengths and limitations of case studies as a research method.
- Exploring the use of secondary data analysis in research projects.
- Evaluating the effectiveness of different strategies for managing and storing research data.
- Examining the role of research assistants in the research process.
- Investigating the challenges and opportunities of conducting research in developing countries.
- Analyzing the impact of climate change on research methodologies.
- Exploring the use of citizen science for environmental monitoring.
- Evaluating the effectiveness of different strategies for conducting research with indigenous communities.
- Examining the role of research in promoting social justice.
- Investigating the historical development of research methodologies.
- Analyzing the impact of technological advancements on research practices.
- Exploring the use of mixed methods research approaches in different disciplines.
- Evaluating the effectiveness of different strategies for managing research projects.
- Examining the role of research funders in shaping research agendas.
- Investigating the challenges and opportunities of conducting research across different cultures.
- Analyzing the impact of language barriers on research communication.
- Exploring the use of collaborative online platforms for conducting research.
- Evaluating the effectiveness of different strategies for promoting research skills development.
- Examining the role of research misconduct in undermining public trust in research.
- Investigating the challenges and opportunities of conducting research with children.
- Analyzing the impact of research on mental health and well-being.
- Exploring the use of arts-based research methodologies.
- Evaluating the effectiveness of different strategies for recruiting and retaining research participants.
- Examining the role of research networks in supporting researchers.
- Investigating the challenges and opportunities of conducting research in the private sector.
- Exploring the use of open science practices to promote research transparency and reproducibility.
- Evaluating the effectiveness of different strategies for mentoring and supporting early-career researchers.
- Examining the role of research misconduct in retracting scientific articles.
- Investigating the challenges and opportunities of data sharing in research.
- Analyzing the impact of open data initiatives on scientific progress.
- Exploring the use of crowdsourcing in research to gather data and solve problems.
- Evaluating the effectiveness of different strategies for promoting research impact.
- Examining the role of alternative research metrics in evaluating the quality of research.
- Investigating the use of bibliometrics to analyze research trends and identify emerging areas.
- Analyzing the impact of research on public policy and decision-making.
- Exploring the use of participatory research methodologies to empower communities.
- Evaluating the effectiveness of different strategies for communicating research findings to the public.
- Examining the role of social media in disseminating research findings.
- Analyzing the impact of humanitarian aid on research practices in developing countries.
- Exploring the use of research methodologies to address global challenges, such as climate change and poverty.
- Evaluating the effectiveness of different strategies for building research capacity in developing countries.
- Examining the role of international research collaborations in promoting global research excellence.
- Investigating the challenges and opportunities of conducting research in the field of artificial intelligence.
- Analyzing the ethical considerations of using autonomous robots in research.
- Exploring the potential of artificial intelligence to automate research tasks.
- Evaluating the effectiveness of different strategies for mitigating the risks of bias in artificial intelligence-powered research.
- Examining the role of research in shaping the future of work.
- Investigating the impact of automation on research jobs.
- Exploring the use of new technologies to improve research efficiency and productivity.
- Evaluating the effectiveness of different strategies for developing transferable skills for researchers.
- Examining the role of lifelong learning in maintaining research expertise.
- Investigating the impact of research funding cuts on research quality and innovation.
- Exploring the use of alternative funding models, such as crowdfunding and philanthropy, to support research.
- Evaluating the effectiveness of different strategies for advocating for increased research funding.
- Examining the role of research universities in driving innovation and economic growth.
- Investigating the impact of research on social and cultural change.
- Exploring the future of research methodologies in an ever-changing world.
Examples of Research Methodology Project Topics
Here are some examples of project topics suited for different research methodologies:
Quantitative Research Topics
- The impact of social media usage on mental health among teenagers.
- Factors influencing customer satisfaction in the hospitality industry.
Qualitative Research Topics
- Exploring the experiences of first-generation college students.
- Understanding the challenges faced by small business owners during the COVID-19 pandemic.
Mixed-Methods Research Topics
- Assessing the effectiveness of a school bullying prevention program .
- Investigating the relationship between exercise habits and stress levels among working adults.
Research methodology is like a compass that guides you through the journey of inquiry. By understanding the different types of methodologies, factors influencing their choice, and best practices, you can embark on your research methodology project topics journey with confidence.
Remember, the key to successful research lies in asking the right questions and choosing the appropriate methodology to find the answers.
Related Posts
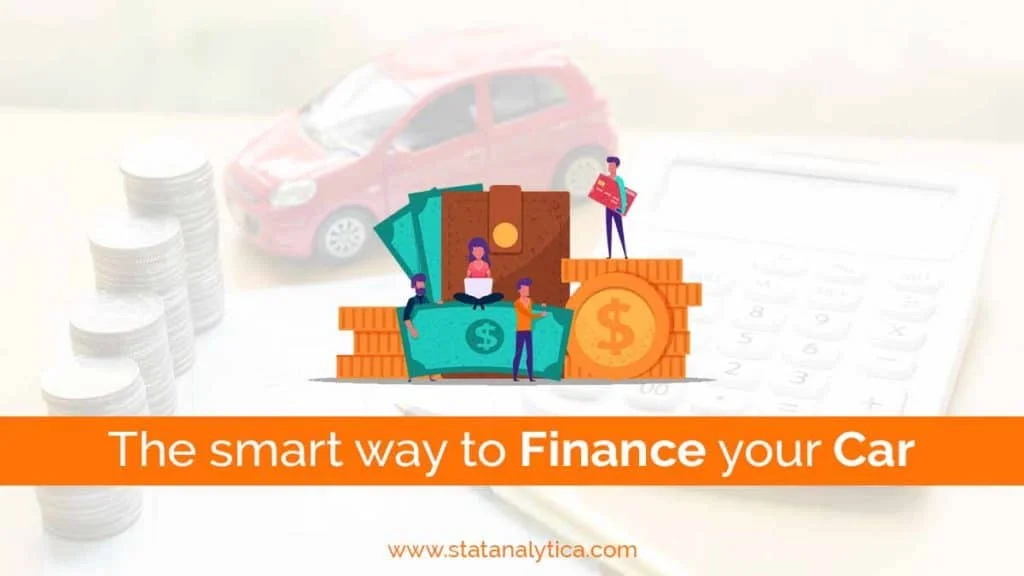
Step by Step Guide on The Best Way to Finance Car
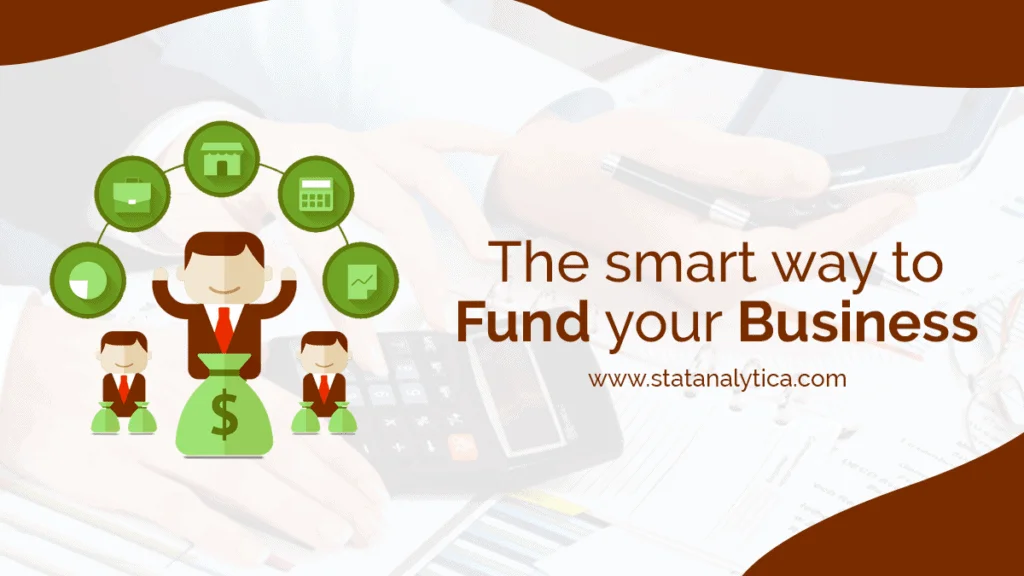
The Best Way on How to Get Fund For Business to Grow it Efficiently
Leave a comment cancel reply.
Your email address will not be published. Required fields are marked *
Have a language expert improve your writing
Run a free plagiarism check in 10 minutes, generate accurate citations for free.
- Knowledge Base
Methodology
- What Is a Research Design | Types, Guide & Examples
What Is a Research Design | Types, Guide & Examples
Published on June 7, 2021 by Shona McCombes . Revised on November 20, 2023 by Pritha Bhandari.
A research design is a strategy for answering your research question using empirical data. Creating a research design means making decisions about:
- Your overall research objectives and approach
- Whether you’ll rely on primary research or secondary research
- Your sampling methods or criteria for selecting subjects
- Your data collection methods
- The procedures you’ll follow to collect data
- Your data analysis methods
A well-planned research design helps ensure that your methods match your research objectives and that you use the right kind of analysis for your data.
Table of contents
Step 1: consider your aims and approach, step 2: choose a type of research design, step 3: identify your population and sampling method, step 4: choose your data collection methods, step 5: plan your data collection procedures, step 6: decide on your data analysis strategies, other interesting articles, frequently asked questions about research design.
- Introduction
Before you can start designing your research, you should already have a clear idea of the research question you want to investigate.
There are many different ways you could go about answering this question. Your research design choices should be driven by your aims and priorities—start by thinking carefully about what you want to achieve.
The first choice you need to make is whether you’ll take a qualitative or quantitative approach.
Qualitative research designs tend to be more flexible and inductive , allowing you to adjust your approach based on what you find throughout the research process.
Quantitative research designs tend to be more fixed and deductive , with variables and hypotheses clearly defined in advance of data collection.
It’s also possible to use a mixed-methods design that integrates aspects of both approaches. By combining qualitative and quantitative insights, you can gain a more complete picture of the problem you’re studying and strengthen the credibility of your conclusions.
Practical and ethical considerations when designing research
As well as scientific considerations, you need to think practically when designing your research. If your research involves people or animals, you also need to consider research ethics .
- How much time do you have to collect data and write up the research?
- Will you be able to gain access to the data you need (e.g., by travelling to a specific location or contacting specific people)?
- Do you have the necessary research skills (e.g., statistical analysis or interview techniques)?
- Will you need ethical approval ?
At each stage of the research design process, make sure that your choices are practically feasible.
Receive feedback on language, structure, and formatting
Professional editors proofread and edit your paper by focusing on:
- Academic style
- Vague sentences
- Style consistency
See an example
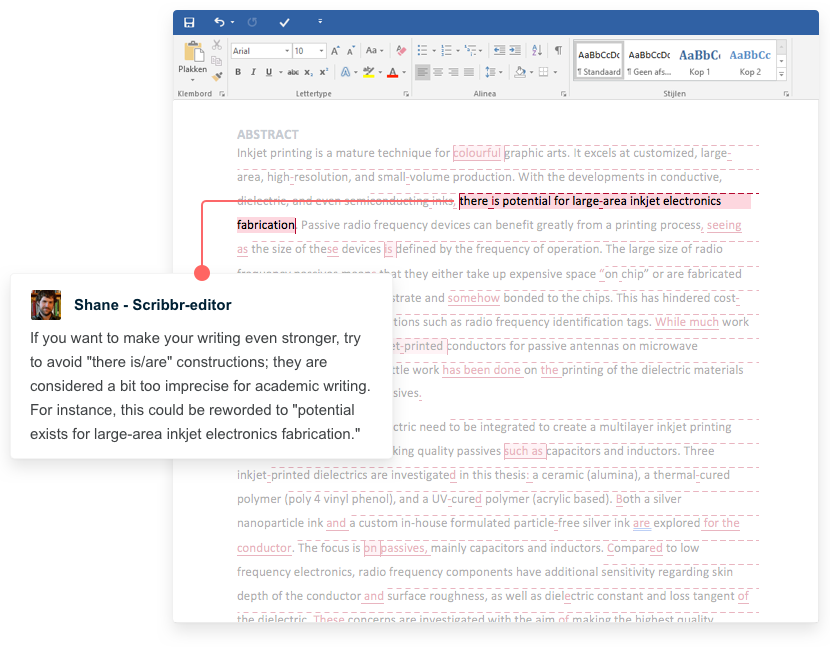
Within both qualitative and quantitative approaches, there are several types of research design to choose from. Each type provides a framework for the overall shape of your research.
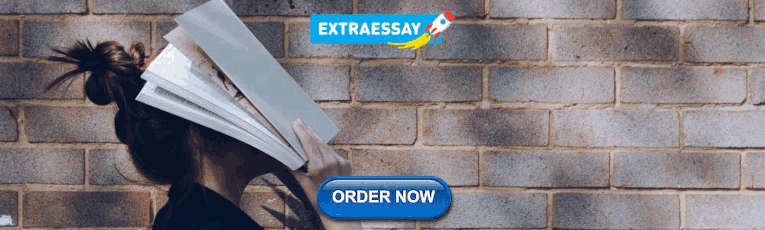
Types of quantitative research designs
Quantitative designs can be split into four main types.
- Experimental and quasi-experimental designs allow you to test cause-and-effect relationships
- Descriptive and correlational designs allow you to measure variables and describe relationships between them.
With descriptive and correlational designs, you can get a clear picture of characteristics, trends and relationships as they exist in the real world. However, you can’t draw conclusions about cause and effect (because correlation doesn’t imply causation ).
Experiments are the strongest way to test cause-and-effect relationships without the risk of other variables influencing the results. However, their controlled conditions may not always reflect how things work in the real world. They’re often also more difficult and expensive to implement.
Types of qualitative research designs
Qualitative designs are less strictly defined. This approach is about gaining a rich, detailed understanding of a specific context or phenomenon, and you can often be more creative and flexible in designing your research.
The table below shows some common types of qualitative design. They often have similar approaches in terms of data collection, but focus on different aspects when analyzing the data.
Your research design should clearly define who or what your research will focus on, and how you’ll go about choosing your participants or subjects.
In research, a population is the entire group that you want to draw conclusions about, while a sample is the smaller group of individuals you’ll actually collect data from.
Defining the population
A population can be made up of anything you want to study—plants, animals, organizations, texts, countries, etc. In the social sciences, it most often refers to a group of people.
For example, will you focus on people from a specific demographic, region or background? Are you interested in people with a certain job or medical condition, or users of a particular product?
The more precisely you define your population, the easier it will be to gather a representative sample.
- Sampling methods
Even with a narrowly defined population, it’s rarely possible to collect data from every individual. Instead, you’ll collect data from a sample.
To select a sample, there are two main approaches: probability sampling and non-probability sampling . The sampling method you use affects how confidently you can generalize your results to the population as a whole.
Probability sampling is the most statistically valid option, but it’s often difficult to achieve unless you’re dealing with a very small and accessible population.
For practical reasons, many studies use non-probability sampling, but it’s important to be aware of the limitations and carefully consider potential biases. You should always make an effort to gather a sample that’s as representative as possible of the population.
Case selection in qualitative research
In some types of qualitative designs, sampling may not be relevant.
For example, in an ethnography or a case study , your aim is to deeply understand a specific context, not to generalize to a population. Instead of sampling, you may simply aim to collect as much data as possible about the context you are studying.
In these types of design, you still have to carefully consider your choice of case or community. You should have a clear rationale for why this particular case is suitable for answering your research question .
For example, you might choose a case study that reveals an unusual or neglected aspect of your research problem, or you might choose several very similar or very different cases in order to compare them.
Data collection methods are ways of directly measuring variables and gathering information. They allow you to gain first-hand knowledge and original insights into your research problem.
You can choose just one data collection method, or use several methods in the same study.
Survey methods
Surveys allow you to collect data about opinions, behaviors, experiences, and characteristics by asking people directly. There are two main survey methods to choose from: questionnaires and interviews .
Observation methods
Observational studies allow you to collect data unobtrusively, observing characteristics, behaviors or social interactions without relying on self-reporting.
Observations may be conducted in real time, taking notes as you observe, or you might make audiovisual recordings for later analysis. They can be qualitative or quantitative.
Other methods of data collection
There are many other ways you might collect data depending on your field and topic.
If you’re not sure which methods will work best for your research design, try reading some papers in your field to see what kinds of data collection methods they used.
Secondary data
If you don’t have the time or resources to collect data from the population you’re interested in, you can also choose to use secondary data that other researchers already collected—for example, datasets from government surveys or previous studies on your topic.
With this raw data, you can do your own analysis to answer new research questions that weren’t addressed by the original study.
Using secondary data can expand the scope of your research, as you may be able to access much larger and more varied samples than you could collect yourself.
However, it also means you don’t have any control over which variables to measure or how to measure them, so the conclusions you can draw may be limited.
Here's why students love Scribbr's proofreading services
Discover proofreading & editing
As well as deciding on your methods, you need to plan exactly how you’ll use these methods to collect data that’s consistent, accurate, and unbiased.
Planning systematic procedures is especially important in quantitative research, where you need to precisely define your variables and ensure your measurements are high in reliability and validity.
Operationalization
Some variables, like height or age, are easily measured. But often you’ll be dealing with more abstract concepts, like satisfaction, anxiety, or competence. Operationalization means turning these fuzzy ideas into measurable indicators.
If you’re using observations , which events or actions will you count?
If you’re using surveys , which questions will you ask and what range of responses will be offered?
You may also choose to use or adapt existing materials designed to measure the concept you’re interested in—for example, questionnaires or inventories whose reliability and validity has already been established.
Reliability and validity
Reliability means your results can be consistently reproduced, while validity means that you’re actually measuring the concept you’re interested in.
For valid and reliable results, your measurement materials should be thoroughly researched and carefully designed. Plan your procedures to make sure you carry out the same steps in the same way for each participant.
If you’re developing a new questionnaire or other instrument to measure a specific concept, running a pilot study allows you to check its validity and reliability in advance.
Sampling procedures
As well as choosing an appropriate sampling method , you need a concrete plan for how you’ll actually contact and recruit your selected sample.
That means making decisions about things like:
- How many participants do you need for an adequate sample size?
- What inclusion and exclusion criteria will you use to identify eligible participants?
- How will you contact your sample—by mail, online, by phone, or in person?
If you’re using a probability sampling method , it’s important that everyone who is randomly selected actually participates in the study. How will you ensure a high response rate?
If you’re using a non-probability method , how will you avoid research bias and ensure a representative sample?
Data management
It’s also important to create a data management plan for organizing and storing your data.
Will you need to transcribe interviews or perform data entry for observations? You should anonymize and safeguard any sensitive data, and make sure it’s backed up regularly.
Keeping your data well-organized will save time when it comes to analyzing it. It can also help other researchers validate and add to your findings (high replicability ).
On its own, raw data can’t answer your research question. The last step of designing your research is planning how you’ll analyze the data.
Quantitative data analysis
In quantitative research, you’ll most likely use some form of statistical analysis . With statistics, you can summarize your sample data, make estimates, and test hypotheses.
Using descriptive statistics , you can summarize your sample data in terms of:
- The distribution of the data (e.g., the frequency of each score on a test)
- The central tendency of the data (e.g., the mean to describe the average score)
- The variability of the data (e.g., the standard deviation to describe how spread out the scores are)
The specific calculations you can do depend on the level of measurement of your variables.
Using inferential statistics , you can:
- Make estimates about the population based on your sample data.
- Test hypotheses about a relationship between variables.
Regression and correlation tests look for associations between two or more variables, while comparison tests (such as t tests and ANOVAs ) look for differences in the outcomes of different groups.
Your choice of statistical test depends on various aspects of your research design, including the types of variables you’re dealing with and the distribution of your data.
Qualitative data analysis
In qualitative research, your data will usually be very dense with information and ideas. Instead of summing it up in numbers, you’ll need to comb through the data in detail, interpret its meanings, identify patterns, and extract the parts that are most relevant to your research question.
Two of the most common approaches to doing this are thematic analysis and discourse analysis .
There are many other ways of analyzing qualitative data depending on the aims of your research. To get a sense of potential approaches, try reading some qualitative research papers in your field.
If you want to know more about the research process , methodology , research bias , or statistics , make sure to check out some of our other articles with explanations and examples.
- Simple random sampling
- Stratified sampling
- Cluster sampling
- Likert scales
- Reproducibility
Statistics
- Null hypothesis
- Statistical power
- Probability distribution
- Effect size
- Poisson distribution
Research bias
- Optimism bias
- Cognitive bias
- Implicit bias
- Hawthorne effect
- Anchoring bias
- Explicit bias
A research design is a strategy for answering your research question . It defines your overall approach and determines how you will collect and analyze data.
A well-planned research design helps ensure that your methods match your research aims, that you collect high-quality data, and that you use the right kind of analysis to answer your questions, utilizing credible sources . This allows you to draw valid , trustworthy conclusions.
Quantitative research designs can be divided into two main categories:
- Correlational and descriptive designs are used to investigate characteristics, averages, trends, and associations between variables.
- Experimental and quasi-experimental designs are used to test causal relationships .
Qualitative research designs tend to be more flexible. Common types of qualitative design include case study , ethnography , and grounded theory designs.
The priorities of a research design can vary depending on the field, but you usually have to specify:
- Your research questions and/or hypotheses
- Your overall approach (e.g., qualitative or quantitative )
- The type of design you’re using (e.g., a survey , experiment , or case study )
- Your data collection methods (e.g., questionnaires , observations)
- Your data collection procedures (e.g., operationalization , timing and data management)
- Your data analysis methods (e.g., statistical tests or thematic analysis )
A sample is a subset of individuals from a larger population . Sampling means selecting the group that you will actually collect data from in your research. For example, if you are researching the opinions of students in your university, you could survey a sample of 100 students.
In statistics, sampling allows you to test a hypothesis about the characteristics of a population.
Operationalization means turning abstract conceptual ideas into measurable observations.
For example, the concept of social anxiety isn’t directly observable, but it can be operationally defined in terms of self-rating scores, behavioral avoidance of crowded places, or physical anxiety symptoms in social situations.
Before collecting data , it’s important to consider how you will operationalize the variables that you want to measure.
A research project is an academic, scientific, or professional undertaking to answer a research question . Research projects can take many forms, such as qualitative or quantitative , descriptive , longitudinal , experimental , or correlational . What kind of research approach you choose will depend on your topic.
Cite this Scribbr article
If you want to cite this source, you can copy and paste the citation or click the “Cite this Scribbr article” button to automatically add the citation to our free Citation Generator.
McCombes, S. (2023, November 20). What Is a Research Design | Types, Guide & Examples. Scribbr. Retrieved April 1, 2024, from https://www.scribbr.com/methodology/research-design/
Is this article helpful?
Shona McCombes
Other students also liked, guide to experimental design | overview, steps, & examples, how to write a research proposal | examples & templates, ethical considerations in research | types & examples, "i thought ai proofreading was useless but..".
I've been using Scribbr for years now and I know it's a service that won't disappoint. It does a good job spotting mistakes”

- SUGGESTED TOPICS
- The Magazine
- Newsletters
- Managing Yourself
- Managing Teams
- Work-life Balance
- The Big Idea
- Data & Visuals
- Reading Lists
- Case Selections
- HBR Learning
- Topic Feeds
- Account Settings
- Email Preferences
Creating a Corporate Social Responsibility Program with Real Impact
- Emilio Marti,
- David Risi,
- Eva Schlindwein,
- Andromachi Athanasopoulou

Lessons from multinational companies that adapted their CSR practices based on local feedback and knowledge.
Exploring the critical role of experimentation in Corporate Social Responsibility (CSR), research on four multinational companies reveals a stark difference in CSR effectiveness. Successful companies integrate an experimental approach, constantly adapting their CSR practices based on local feedback and knowledge. This strategy fosters genuine community engagement and responsive initiatives, as seen in a mining company’s impactful HIV/AIDS program. Conversely, companies that rely on standardized, inflexible CSR methods often fail to achieve their goals, demonstrated by a failed partnership due to local corruption in another mining company. The study recommends encouraging broad employee participation in CSR and fostering a culture that values CSR’s long-term business benefits. It also suggests that sustainable investors and ESG rating agencies should focus on assessing companies’ experimental approaches to CSR, going beyond current practices to examine the involvement of diverse employees in both developing and adapting CSR initiatives. Overall, embracing a dynamic, data-driven approach to CSR is essential for meaningful social and environmental impact.
By now, almost all large companies are engaged in corporate social responsibility (CSR): they have CSR policies, employ CSR staff, engage in activities that aim to have a positive impact on the environment and society, and write CSR reports. However, the evolution of CSR has brought forth new challenges. A stark contrast to two decades ago, when the primary concern was the sheer neglect of CSR, the current issue lies in the ineffective execution of these practices. Why do some companies implement CSR in ways that create a positive impact on the environment and society, while others fail to do so? Our research reveals that experimentation is critical for impactful CSR, which has implications for both companies that implement CSR and companies that externally monitor these CSR activities, such as sustainable investors and ESG rating agencies.
- EM Emilio Marti is an assistant professor at the Rotterdam School of Management (RSM) at Erasmus University Rotterdam.
- DR David Risi is a professor at the Bern University of Applied Sciences and a habilitated lecturer at the University of St. Gallen. His research focuses on how companies organize CSR and sustainability.
- ES Eva Schlindwein is a professor at the Bern University of Applied Sciences and a postdoctoral fellow at the University of Oxford. Her research focuses on how organizations navigate tensions between business and society.
- AA Andromachi Athanasopoulou is an associate professor at Queen Mary University of London and an associate fellow at the University of Oxford. Her research focuses on how individuals manage their leadership careers and make ethically charged decisions.
Partner Center
- Course Description
- Course Info
- Calendar/Links
Theme by Anders Norén
Project: Final Report
Project report.
Due: Wednesday, 4/24
Example report in box folder, linked here
General Directions
The final report is intended to provide a comprehensive account of your collaborative course project in data science. The report should demonstrate your ability to apply the data science skills you have learned to a real-world project holistically, from posing research questions and gathering data to analysis, visualization, interpretation, and communication. The report should stand on its own so that it makes sense to someone who has not read your proposal or prototype.
The report should contain at least the parts defined below. In terms of length, it should be 5-7 pages using standard margins (1 in.), font (11-12 pt), and line spacing (1-1.5). A typical submission is around 3-4 pages of text and 5-7 pages overall with tables and figures. It is important to stay within the page limit, as practicing being succinct is an important skill. Your final report should also have a descriptive title, not “CS216 Project Report”. You should convert your written report to a pdf and upload it to Gradescope under the assignment “Project Final Report” by the due date, and assign the appropriate pages to questions in the grading rubric. Be sure to include your names and NetIDs in your final document and use the group submission feature on Gradescope. You do not need to upload your accompanying data, code, or other supplemental resources demonstrating your work to Gradescope; instead, your report should contain instructions on how to access these resources (see the Results and Methods section below for more details).
In general, your approach to this report should be to write as if you had “planned this as your project all along.” A report is not a chronological story of your project, it is a summary of what you did where the “story” serves the reader’s comprehension.
- E (Exemplary, 20pts) – Work that meets all requirements in terms of formatting and sections.
- S (Satisfactory, 19 pts) – Work that meets all requirements but is over 7 pages.
- N (Not yet, 12pts) – Does not meet all requirements.
- U (Unassessable, 4pts) – Missing at least one section.
Part 1: Introduction and Research Questions (15 points)
Your final report should begin by introducing your topic and restating your research question(s) as in your proposal. As before, your research question(s) should be (1) substantial, (2) feasible, and (3) relevant. In contrast to the prior reports, the final report does not need to explicitly justify that the research questions are substantial and feasible in the text ; your results should demonstrate both of these points. Therefore, you should remove that text to save space.
You should still explicitly justify how your research questions are relevant. In other words, be sure to explain the motivation of your research questions. Remember that relevant research questions address a subject of importance and interest within the scientific community or broader society. Additionally, we are looking for why your group believes this research project is worthwhile to your time in this course.
You can start with the text from your prototype, but you should update your introduction and research questions to reflect changes in or refinements of the project vision. You should not state specific updates, rather, write the report as the final product and the prior milestones do not exist. Pretend the readers are unaware of the prior milestones. If you feel like an explanation of changes since the prototype is warranted, place that in the appendix. Your introduction should be sufficient to provide context for the rest of your report.
- E (Exemplary, 15pts) – Comprehensive introduction with clearly labeled, up-to-date research questions and a justification for how the research questions are relevant. Report introduction can stand alone without references to prior versions of the project; no text for explicit justifications for “substantial” and “feasible” are made for the research question(s).
- S (Satisfactory, 14pts) – Comprehensive introduction with clearly labeled, updated research questions and a justification for how the research questions are relevant. The introduction and research questions may not have been refined from the prototype (they have still kept reasoning for why their research questions are substantial and feasible).
- N (Not yet, 9pts) – Incomplete introduction where the research questions or justification are missing pieces, but at least some of it is present. Or the justification is clearly not reasonable.
- U (Unassessable, 3pts) – Incomplete introduction where it is entirely missing the research questions or justification or does not demonstrate meaningful effort.
Part 2: Data Sources (15 points)
Discuss the data you have collected and are using to answer your research questions. Be specific: name the datasets you are using, the information they contain, and where they were collected from / how they were prepared. You can begin with the text from your prototype, but be sure to update it to fit the vision for your final project.
- E (Exemplary, 15pts) – Origins of data are properly specified, cited, and relevant to answering the research question(s). If any significant data wrangling, cleaning, or other data preparation was done, these processes are explained.
- S (Satisfactory, 14pts) – Origins of data are properly specified and cited. However, the justification is not clear why the data is relevant to the proposed research question(s). If any significant data wrangling, cleaning, or other data preparation was done, these processes are explained.
- N (Not yet, 9pts) – Poorly specified data sources or the justification for using that data set or the methods to acquire the data is lacking. No discussion of preparing the dataset.
- U (Unassessable, 3pts) – Data sources or methods to acquire data are missing or do not demonstrate meaningful effort.
Part 3: What Modules Are You Using? (15 points)
Your project should utilize concepts from modules we have covered in this course to answer your research question(s). We will assume you will use modules 1 (Python), 2 (Numpy/Pandas), and 5 (Probability). Your final report should state at least 3 more modules that you have utilized for your project. Each module should have a short description of how you used the knowledge in this module and a justification for that use. In addition, include what specific concepts from the module you used and at what stage of your project you mostly used this module. Potential stages include, but are not limited to: data gathering, data cleaning, data investigation, data analysis, and final report.
- Module 3: Visualization
- Module 4: Data Wrangling
- Module 6: Combining Data
- Module 7: Statistical Inference
- Module 8: Prediction & Supervised Machine Learning
- Module 9: Databases and SQL
- Module 10: Deep Learning
Your overall report should clearly show that you used the modules discussed in this section. You should add any additional modules used and update the existing modules to be more specific to the different tasks and stages of your projects that changed since your prototype.
- E (Exemplary, 15pts) – States at least 3 modules. For each module, they provide an updated (1) short description of how they used the module, (2) justification for using this module, (3) specific concepts they used, (4) what stage they used it, and (5) clearly implemented it in the final report.
- S (Satisfactory, 14pts) – States at least 3 modules. For each module, they provide an updated (1) short description of how they used the module, (2) justification for using this module, (3) what concepts they used and (4) what stage they used it. Less than 3 modules are clearly implemented in the final report.
- N (Not yet, 9pts) – States at least 3 modules. For each module, they provide an updated (1) short description of how they used the module, (2) justification for using this module, (3) what concepts they used and (4) what stage they used it. Only one module is clearly implemented in the final report.
- U (Unassessable, 3pts) – Does not meet the Not Yet criteria.
Part 4: Results and Methods (15 points)
This is likely to be the longest section of your paper at multiple pages. The results and methods section of your report should explain your detailed results and the methods used to obtain them. Where possible, results should be summarized using clearly labeled tables or figures and supplemented with written explanations of the significance of the results with respect to the research questions outlined previously. Please note that a screenshot of your dataset does not count as a table or figure and should not be included in your final report.
Your description of your methods should be specific. For example, if you scraped multiple web databases, merged them, and created a visualization, then you should explain how each step was conducted in enough detail that an informed reader could reasonably be expected to reproduce your results with time and effort. Just saying, “we cleaned the data and dealt with missing values” or “we built a predictive model” is insufficient detail.
Your report should also contain instructions on how to access your full implementation (that is, your code, data, and any other supplemental resources like additional charts or tables). The simplest way to do so is to include a link to the box folder, GitLab repo, or whatever other platforms your group is using to house your data and code.
- E (Exemplary, 15pts) – Results are thoroughly discussed using clearly labeled tables or figures followed by written descriptions. Specific explanation of how the results were generated and from what data. Link to code/data to create charts or visualizations is provided.
- S (Satisfactory, 14pts) – Results are thoroughly discussed using clearly labeled tables or figures followed by written descriptions. Explanation of how the results were generated may lack some specification or it is somewhat unclear as to what data the results are from. Link provided.
- N (Not yet, 9pts) – Results are discussed using tables with missing labels or lacking written descriptions. It is unclear how the results were generated and from what data.
- U (Unassessable, 3pts) – Results are missing or do not demonstrate meaningful effort.
Part 5: Limitations and Future Work (10 points)
In this part, you should discuss any important limitations or caveats to your results with respect to answering your research questions. For example, if you don’t have as much data as you would like or are unable to fairly evaluate the performance of a predictive model, explain and contextualize those limitations. You may want to consider any ethical implications or potential biases of your results as well.
Finally, provide a brief discussion of future work. This could explain how future research might address the limitations you outline, or it could pose additional follow-up research questions based on your results so far. In short, explain how an informed reader (such as a peer in the class) could improve on and extend your results.
- E (Exemplary, 10pts) – Comprehensive and explicit discussion of important limitations and caveats to results. Brief discussion of future work and how results could be extended and improved upon.
- S (Satisfactory, 9pts) – Discussion of important limitations and caveats to results could be improved or the discussion of future work and how results could be extended and improved upon lacks some specification.
- N (Not yet, 6pts) – Incomplete discussion of important limitations and caveats to results. Discussion of future work and how results could be extended and improved upon may lack some specification.
- U (Unassessable, 2pts) – Limitations and future work are missing or do not demonstrate meaningful effort.
Part 6: Conclusion (5 points)
Provide a brief (one or two paragraphs) summary of your results. This summary of results should address all of your research questions.
If one of your research questions was “Did COVID-19 result in bankruptcy in North Carolina during 2020?” then a possible (and purely hypothetical) summary of results might be:
We aggregate the public records disclosures of small businesses in North Carolina from January 2019 to December 2020 and find substantial evidence that COVID-19 did result in a moderate increase in bankruptcy during 2020. This increase is not geographically uniform and is concentrated during summer and fall 2020. We also examined the impact of federal stimulus but cannot provide an evaluation of its impact from the available data.
- E (Exemplary, 10pts) – Research questions are clearly and completely addressed through a summary of results.
- S (Satisfactory, 9pts) – Research questions are clearly addressed through a summary of results. The results may be lacking in completely answering the research questions.
- N (Not yet, 6pts) – Research questions are somewhat addressed through a summary of results. The results are lacking in completely answering the research questions. Or the results of one of the research questions is missing.
- U (Unassessable, 2pts) – Conclusion is missing or does not demonstrate meaningful effort.
(Optional) Part 7: Appendix of additional figures, tables, and updates summary.
If you are struggling to keep your report within the 5-7 page limit, you may move some (not all) of your figures and tables to an optional appendix that will not count against your page limit. However, your report should stand on its own without the appendix . The appendix is for adding more nuance to your results, not to give you more space to talk about your results. Succinctness is an important skill to practice when doing data science. Your grader is not expected to look at the appendix when grading.
If you strongly feel like a summary of project updates since the proposal is required, you may put them in this appendix as well and mention they are in the appendix in the introduction.
Checklist Before You Submit:
- 5-7 pages in length
- Standard margins (1 in.)
- Font size is 11-12 pt
- Line spacing is 1-1.5
- Final document is a pdf
- Descriptive project title
- Do you feel as if this part meets the requirements of E (Exemplary) or S (Satisfactory) ?
Author Joey Scarpa
Posted March 30, 2024 — 4:30 pm
Categories Project
Leave a Reply Cancel reply
Your email address will not be published. Required fields are marked *
Save my name, email, and website in this browser for the next time I comment.
This paper is in the following e-collection/theme issue:
Published on 1.4.2024 in Vol 10 (2024)
Using Project Extension for Community Healthcare Outcomes to Enhance Substance Use Disorder Care in Primary Care: Mixed Methods Study
Authors of this article:

Original Paper
- MacKenzie Koester, MPH ;
- Rosemary Motz, MPH, MA, RDN ;
- Ariel Porto, MPH ;
- Nikita Reyes Nieves, MPH ;
- Karen Ashley, EdD
Weitzman Institute, Moses Weitzman Health System, Washington, DC, United States
Corresponding Author:
MacKenzie Koester, MPH
Weitzman Institute
Moses Weitzman Health System
1575 I Street Northwest
Washington, DC, 20005
United States
Phone: 1 8603476971
Email: [email protected]
Background: Substance use and overdose deaths make up a substantial portion of injury-related deaths in the United States, with the state of Ohio leading the nation in rates of diagnosed substance use disorder (SUD). Ohio’s growing epidemic has indicated a need to improve SUD care in a primary care setting through the engagement of multidisciplinary providers and the use of a comprehensive approach to care.
Objective: The purpose of this study was to assess the ability of the Weitzman Extension for Community Healthcare Outcomes (ECHO): Comprehensive Substance Use Disorder Care program to both address and meet 7 series learning objectives and address substances by analyzing (1) the frequency of exposure to the learning objective topics and substance types during case discussions and (2) participants’ change in knowledge, self-efficacy, attitudes, and skills related to the treatment of SUDs pre- to postseries. The 7 series learning objective themes included harm reduction, team-based care, behavioral techniques, medication-assisted treatment, trauma-informed care, co-occurring conditions, and social determinants of health.
Methods: We used a mixed methods approach using a conceptual content analysis based on series learning objectives and substances and a 2-tailed paired-samples t test of participants’ self-reported learner outcomes. The content analysis gauged the frequency and dose of learning objective themes and illicit and nonillicit substances mentioned in participant case presentations and discussions, and the paired-samples t test compared participants’ knowledge, self-efficacy, attitudes, and skills associated with learning objectives and medication management of substances from pre- to postseries.
Results: The results of the content analysis indicated that 3 learning objective themes—team-based care, harm reduction, and social determinants of health—resulted in the highest frequencies and dose, appearing in 100% (n=22) of case presentations and discussions. Alcohol had the highest frequency and dose among the illicit and nonillicit substances, appearing in 81% (n=18) of case presentations and discussions. The results of the paired-samples t test indicated statistically significant increases in knowledge domain statements related to polysubstance use ( P =.02), understanding the approach other disciplines use in SUD care ( P =.02), and medication management strategies for nicotine ( P =.03) and opioid use disorder ( P =.003). Statistically significant increases were observed for 2 self-efficacy domain statements regarding medication management for nicotine ( P =.002) and alcohol use disorder ( P =.02). Further, 1 statistically significant increase in the skill domain was observed regarding using the stages of change theory in interventions ( P =.03).
Conclusions: These findings indicate that the ECHO program’s content aligned with its stated learning objectives; met its learning objectives for the 3 themes where significant improvements were measured; and met its intent to address multiple substances in case presentations and discussions. These results demonstrate that Project ECHO is a potential tool to educate multidisciplinary providers in a comprehensive approach to SUD care.
Introduction
In the United States, overdose deaths continue to be a major cause of injury-related deaths. Since the onset of the COVID-19 pandemic, numbers have only accelerated, and the state of Ohio has led the nation in high substance use disorder (SUD) rates, including drug use and prescription drug use. The Centers for Disease Control and Prevention ranks the state among the top 5 across the United States with the highest rates of opioid overdose deaths [ 1 ]. While research has shown an increase in the number of people enrolled in substance use treatment in Ohio between 2015 and 2019 there was still a notable high increase in the annual average prevalence of past-year illicit drug use disorder in Ohio (3.6%) compared to the regional average (3%) and the national average (2.9%) [ 2 ]. In addition, past-month alcohol use disorder (9.3%), cannabis use disorder (5.8%), and tobacco use disorder (35.2%) were higher than the national average among young adults aged 18-25 years [ 2 ]. Ohio’s growing epidemic has highlighted the need to improve SUD care in a primary care setting by training providers to better address differences in care and social determinants of health through the use of behavioral techniques, harm-reduction philosophy of care, medication management, and a team-based care approach.
Weitzman Extension for Community Healthcare Outcomes: Comprehensive Substance Use Disorder Care Program
Beginning in 2021, Buckeye Health Plan and Ohio University Heritage College of Osteopathic Medicine have partnered with the Weitzman Institute (WI), a national primary care research, policy, and education institute, to provide targeted support and education to Ohio primary care medical and behavioral health providers working with underserved patients, especially those in the rural, southeastern Appalachian region, using the evidence-based Project Extension for Community Healthcare Outcomes (ECHO) learning model. Project ECHO uses frequent videoconference sessions to connect a target audience of learners with subject matter experts for didactic and case-based instruction and engaged discussion [ 3 ]. Through regular attendance at these sessions, Project ECHO aims to equip learners with the knowledge, confidence, and skills to better manage complex cases.
WI has over 11 years of experience in developing and delivering Project ECHO programs to meet the needs of providers working in resource-limited settings. As an early adopter of the model in 2012, Weitzman ECHO programs have been offered in 22 topic areas to over 8000 health care professionals across all 50 states, Washington D.C., and Puerto Rico. Working in collaboration, Buckeye Health Plan and Ohio University aimed to leverage this expertise and offer multiple Project ECHO programs each year for providers in topics of the greatest need and interest.
As described, one of Ohio’s most dire population health needs is to improve outcomes for patients experiencing addiction. Thus, SUD was selected as the second ECHO program developed through this partnership. More specifically, opioids are a heightened concern throughout both Ohio and the United States, and the opioid epidemic has spurred significant funding allocations, such as the Biden Administration’s US $1.5 billion award to states and territories to end the epidemic [ 4 ]. However, there are many additional substances of concern, both illicit and nonillicit, such as alcohol, tobacco, cannabis, methamphetamine, and cocaine [ 5 ], which may receive less attention given the directed funding for opioids. For this reason, it was decided that the ECHO would address not only opioids, or any one substance, but rather be designed to provide techniques to help providers address SUD overall through a comprehensive, team-based lens and a harm reduction philosophy of care. Reflecting this broad topical approach, the program was titled the Weitzman ECHO: Comprehensive Substance Use Disorder Care (CSUDC ECHO) program.
CSUDC ECHO consisted of 24 twice-monthly sessions held between July 2021 and July 2022. Each 1-hour session included a 20- to 25-minute didactic presentation followed by 1 patient case submitted by a participant before the session and discussed live for the remaining 35-40 minutes. Textbox 1 outlines the didactic presentation topics for each session. A multidisciplinary core faculty facilitated each session and was comprised of 1 physician with dual board certification in family medicine and addiction medicine and experienced in treating SUDs at federally qualified health centers; 1 nurse practitioner who developed and leads a federally qualified health center medication-assisted treatment (MAT) program; 1 supervisory licensed counselor; and 1 population health expert. Together, the faculty built a 12-month curriculum covering diverse topics such as medication management, team-based care, trauma-informed care, stages of change and motivational interviewing, polysubstance use and co-occurring conditions, and coordinating levels of care.
Session and didactic topic
- Philosophy of care (no case presentation).
- Harm reduction strategies.
- Principals of medication management.
- Team-based care: care provision partners.
- Trauma-informed care: an overview.
- Motivational interviewing.
- Stages of change for addiction.
- Assessing stages of change and stage-based interventions.
- Medications for opioid use disorder basics.
- Behavioral health and primary care coordination.
- Transitions of care.
- Polysubstance use.
- Social determinants of health including barriers or challenges (no case presentation).
- Adverse childhood experiences.
- Legal factors and access.
- Mental health crisis and coordination of care.
- Medication-assisted treatment for alcohol and tobacco use disorders.
- Self-determination and strength-based approaches.
- Contingency management for substance use disorder.
- HIV and hepatitis C virus in patients with substance use disorder.
- Screening, brief intervention and referral to treatment into primary care.
- Stimulant use disorder treatment and medication management.
- Co-occurring mental health substance use disorder.
- Tobacco cessation for polysubstance patients.
Participants were recruited by email blasts targeted to each partner’s network of Ohio primary care providers and other members of the care team. A total of 109 participants attended at least one session, 16 participants attended between 7 and 11 sessions, and 23 participants attended over 12 (half) of the sessions. On average, there were 32 attendees at each session. Continuing education credits were offered to medical providers, behavioral health providers, and nurses.
Purpose of Study
The purpose of this study was to assess the ability of CSUDC ECHO to both address and meet 7 learning objectives ( Textbox 2 ) and address multiple substances by analyzing (1) the frequency of exposure to the learning objective topics and substance types during case discussions and (2) participants’ knowledge, self-efficacy, skills, and attitudes related to the treatment of SUDs pre- to postprogram.
- Project a harm reduction philosophy of care into your treatment of patients experiencing substance use disorders and explain this concept to peers.
- Use the care team more effectively to improve the management of patients experiencing substance use disorders.
- Use motivational interviewing and other behavioral techniques to improve patient outcomes related to substance use disorders.
- Better differentiate and implement medication management strategies for patients experiencing substance use disorders.
- Illustrate trauma-informed practices in the screening, assessment, and treatment of patients experiencing substance use disorders.
- Describe and manage common co-occurring conditions and polysubstance use more effectively in patients experiencing substance use disorders.
- Distinguish and address factors related to social determinants of health faced by specific populations experiencing substance use disorders.
Study Design and Data Collection
This study used a mixed methods design, using a conceptual content analysis [ 6 ] analyzing ECHO participant-led case presentations, as well as a 2-tailed paired-samples t test of participant self-reported learner outcomes. All ECHO attendees who registered and attended the Project ECHO CSUDC sessions are included in the deductive content analysis. All ECHO attendees who registered before and through the first session of the series were invited to complete a preseries survey (n=106) via Qualtrics survey software (Qualtrics). The preseries survey remained open for 3 weeks from June 25, 2021, to July 18, 2021. A total of 79 responses were received (n=79) for a response rate of 75%. Upon completion of the ECHO series, active attendees (ie, those that were still active at the conclusion of the series and did not officially drop from the series, as well as those who enrolled throughout the series) were invited to complete a postseries survey via Qualtrics Survey Software (n=90). The postseries survey remained open for 4 weeks from July 7, 2022, to August 2, 2022. A total of 25 responses were received (n=25) for a response rate of 28%. A total of 16 consented participants completed both the preseries and postseries surveys (n=16) and are included in the paired-samples t tests statistical analysis.
Ethical Considerations
This study was approved by the Community Health Center, Inc, Institutional Review Board (IRB; 1190) on January 6, 2022. Informed consent was accounted for by the authors through the administration of a consent form on the postseries survey gathering participant consent to use their deidentified survey data for the paired-samples t test analysis. The deductive content analysis was considered a secondary analysis and was given exempt status. All data used in this study were deidentified, accounting for privacy and confidentially. No compensation for participation in this study was deemed necessary by the IRB.
Survey Tools
The preseries and postseries surveys were internally created and based on the Consolidated Framework for Implementation Research (CFIR) [ 7 ] and Moore’s Model of Outcomes Assessment Framework [ 8 ]. The specific CFIR domains assessed for include intervention characteristics, outer setting, inner setting, characteristics of individuals, and process [ 7 ]. Additionally, the levels of Moore’s Model of Outcomes Assessment Framework assessed for include level 2 (satisfaction), level 3a (declarative knowledge), level 3b (procedural knowledge), level 4 (competence), level 5 (performance), and level 6 (patient health) [ 8 ]. The surveys assessed changes in participants’ self-reported knowledge, attitudes, self-efficacy, and skills through statements centered on the series’ learning objectives. The preseries survey also collected participant characteristics including provider type and years of experience working with patients diagnosed with SUDs, as well as team-based care practices. Additionally, the postseries survey collected information on engagement and practice changes. The preseries survey instrument is presented in Multimedia Appendix 1 and the postseries survey instrument is presented in Multimedia Appendix 2 .
While the preseries survey and postseries survey tools were based on CFIR [ 7 ] and Moore’s Model of Outcomes Assessment Framework [ 8 ], both surveys were internally designed. The internal research and evaluation and CSUDC ECHO programmatic teams created the survey tools through several iterations of the internal review, which also consisted of selecting the appropriate domain (ie, knowledge, attitudes, self-efficacy, and skills) to assess each series’ learning objective. Each domain used a 5-point Likert scale to assess responses. The surveys were then presented to the CSUDC ECHO series stakeholders and faculty for review and approval before administering the surveys to the ECHO attendees. See Multimedia Appendices 1 and 2 for the domain placement of learning objectives and the 5-point Likert scales.
Conceptual Content Analysis
To further evaluate Weitzman ECHO CSUDC aims, researchers conducted a conceptual content analysis [ 6 ] using a set of a priori themes extracted from the series’ learning objectives. Series’ learning objectives are detailed in Textbox 2 . To establish a priori themes, researchers met before the launch of the ECHO to examine the series’ 7 learning objectives and extracted 7 themes for the content analysis. The themes were: harm reduction, team-based care, behavioral techniques, MAT, trauma-informed care, co-occurring conditions, and social determinants of health. To assess the frequency to which multiple substances were discussed, the themes also included 5 illicit and nonillicit substances of concern: alcohol, stimulants, opioids, cannabis, tobacco, or nicotine, plus polysubstance use when any 2 or more of these substances were identified. A conceptual analysis approach was used to gauge the dose and frequency of all learning objective themes and selected illicit and nonillicit substances. The content analysis aimed to confirm the discussion of the series’ learning objectives during case presentations and to determine to what extent multiple substances were able to be addressed.
Researchers evaluated all 22 participant-led ECHO case presentations and discussions for the presence of the selected themes in the prepared participant cases, faculty recommendations, and participant recommendations. Case presentations and discussions consisted of participants independently preparing a patient case to present and receive participant and faculty guidance for a patient treatment plan. Case presentations were recorded and transcribed using Zoom videoconferencing software (Zoom Video Communications, Inc). The transcriptions were then used for the conceptual content analysis.
To ensure coding accuracy, 4 researchers independently coded 27% (n=6) of the case presentations and met to reconcile discrepancies and better establish coding parameters. After reconciling discrepancies, 1 researcher coded the remaining 16 case presentations and discussion transcripts. The content analysis themes and descriptions are presented in Table 1 .
Paired-Samples t Test
To determine if Project ECHO CSUDC affected participant learner outcomes, researchers calculated mean scores reported on a Likert scale of 1 to 5 and conducted a paired-samples t test to compare pre- and postseries scores at a .05 significance level. The surveys consisted of matching statements assessing knowledge, self-efficacy, attitudes, and skills associated with the series’ learning objectives. The data were assessed for normality and homogeneity of variance and the assumptions were met. The data analysis was conducted using SPSS Statistics for Windows (version 26.0; IBM Corp).
Participant Characteristics
CSUDC ECHO participants were asked to report their role type on the preseries survey. Of the participants that responded to the survey items (n=79), a majority were other care team members (n = 32; 41%) followed by behavioral health providers (n = 30; 38%) and medical providers (n = 16; 21%). Additionally, participants were asked to indicate their years of experience working with SUDs. Most participants had between 1 and 5 years of experience (n=23; 29%) followed by 6-10 years (n=15; 19%), 11-20 years (n=14; 18%), less than 1 year (n=13; 16%), 7 participants indicated they do not work directly with patients (n=7; 9%), 21-30 years (n=4; 5%), 31-40 years (n=2; 3%), and more than 40 years of experience (n=1; 1%). Full participant characteristics of the entire CSUDC ECHO attendees, excluding the paired-samples t test sample, the paired-samples t test sample only, and all combined CSUDC ECHO attendees are provided in Table 2 .
The attendance data of participants included in the paired-samples t test analysis were analyzed. Further, 6 (n=6; 38%) of the paired-samples t test participants attended 1% (n=1) to 25% (n=6) of the 24 CSUDC ECHO sessions, 3 (n = 3; 19%) attended 26% (n=7) to 49% (n=11) of the sessions, 4 (n = 4; 25%) attended 50% (n=12) to 75% (n=18) of the sessions, and 3 (n = 3; 19%) attended 76% (n=19) to 100% (n=24) of the sessions.
a ECHO: Extension for Community Healthcare Outcomes.
b CSUDC: Comprehensive Substance Use Disorder Care.
c SUD: substance use disorder.
The conceptual content analysis indicated that all of the a priori themes relating to the learning objectives resulted in high frequencies and doses, appearing in a majority of case presentations and discussions. Further, 3 themes appeared in 100% (n = 22) of case presentations and discussions, including team-based care at a frequency of 156, followed by harm reduction at a frequency of 152, and social determinants of health at a frequency of 135. In total, 4 themes appeared in less than 100% (n=22) of case presentations and discussions, but above 81% (n=18), including co-occurring conditions with a frequency of 118 and appearing in 95% (n = 21) of case presentations and discussions, followed by behavioral techniques at a frequency of 108 and appearing in 91% (n = 20) of case presentations and discussions, MAT at a frequency of 89 and appearing in 86% (n = 19) of case presentations and discussions, and trauma-informed care at a frequency of 79 and appearing in 82% (n=18) case presentations and discussions. Additionally, multiple substances were represented but at differing frequencies. The substance that resulted in the highest frequency and dose was alcohol at a frequency of 64 and appeared in 81% (n = 18) of case presentations and discussions, followed by stimulants at a frequency of 55 and 77% (n=17) of case presentations and discussions, opioids at a frequency of 49 and 59% (n = 13) of case presentations and discussions. Cannabis resulted with a frequency of 38 but appeared in 64% (n = 14) of case presentations and discussions. Finally, tobacco and nicotine resulted in the lowest frequency at 11 and dose appearing in 27% (n = 6) of case presentations and discussions. When evaluating polysubstance use, which was limited to the use of two or more of the listed substances, we found a dose of 95% (n = 21) of case presentations and discussions. The frequency of polysubstance use was not included in the conceptual content analysis since it was not a learning objective theme and the emphasis of the conceptual content analysis was focused on the specific illicit and nonillicit substance types. The results of the conceptual content analysis are presented in Table 3 .
a MAT: medication-assisted treatment.
b —: not available.
In total, 4 knowledge domain statements resulted in statistically significant increases: understanding polysubstance use in patients experiencing SUD ( P =.02), understanding the approach colleagues in other disciplines use to address SUD ( P =.02), knowledge of medication management strategies for nicotine use disorder ( P =.03), and knowledge of medication management strategies for opioid use disorder (OUD; P =.003). Additionally, all knowledge domain statements resulted in an increased change in mean score from preseries to postseries. The results of the knowledge domain preseries and postseries scores are presented in Table 4 .
No attitudes domain statements resulted as statistically significant. All attitudes domain statements resulted in an increased change in mean score from preseries to postseries except the statement about a treatment plan for a patient experiencing an illicit SUD only being successful if abstinence is maintained, which resulted in a negative change in mean score. The negative change in mean score from preseries to postseries was the appropriate direction of change for alignment with promoting a harm reduction philosophy. The results of the attitudes domain preseries and postseries scores are presented in Table 5 .
Self-Efficacy
In total, 2 self-efficacy statements resulted in statistically significant increases: choosing a medication management strategy for nicotine use disorder ( P =.002) and alcohol use disorder ( P =.02). Additionally, all self-efficacy domain statements resulted in an increased change in mean score from preseries to postseries. The results of the self-efficacy domain preseries and postseries scores are presented in Table 6 .
a SMART: specific, measurable, achievable, relevant, timely.
In total, 1 skill domain statement resulted in a statistically significant increase: using the stages of change theory to provide stage-based interventions to patients experiencing SUDs ( P =.03). Additionally, all skill domain statements resulted in an increased change in mean score from preseries to postseries. The results of the skill domain preseries and postseries scores are presented in Table 7 .
a IOP: intensive outpatient.
Principal Findings
Ohio’s annual average prevalence of tobacco use, heroin use, use of prescription pain relievers, OUDs, illicit drug use disorder, and SUD have been higher compared to both regional and national averages [ 2 ]. Considering the need to address this public health concern, CSUDC ECHO was implemented to train Ohio providers and care team members in substance use care. CSUDC ECHO enhanced the Project ECHO work in this field by focusing content and learning objectives on a comprehensive, team-based lens and a harm reduction philosophy of care to address multiple illicit and nonillicit substances including opioids, alcohol, nicotine, cannabis, and stimulants. To assess the ability of the CSUDC ECHO program to meet its 7 program learning objectives ( Textbox 2 ) and address multiple substances, this study analyzed (1) the frequency of exposure to learning objective themes and substance types during case presentations and discussions and (2) participating providers’ change in knowledge, attitudes, self-efficacy, and skills related to the treatment of SUDs.
Study results demonstrate that all 7 learning objectives were frequently addressed in the content of case presentations and discussions throughout the program, with team-based care being the most frequently mentioned, 3 objectives appearing in 100% (n=22) of case discussions (eg, team-based care, harm reduction, and co-occurring conditions), and all 7 objectives appearing in >81% (n=18) of all cases discussed. This may have resulted in the learner outcome improvement pre- to postprogram for multiple learner domains (eg, knowledge, self-efficacy, and skill) for the following themes: team-based care, MAT, polysubstance use, and behavioral techniques. No pattern emerged among the participants included in the paired-samples t test analysis exposure to didactic topics and changes in learner outcomes.
Alcohol, stimulants, opioids, cannabis, and nicotine were addressed in the content of case presentations and discussions throughout CSUDC ECHO with alcohol being the most frequently mentioned and most common substance appearing in cases, 4 substances appearing in >59% (n=13) of case discussions (eg, alcohol, stimulants, opioid, and cannabis), and all coded substances appearing in at least a quarter of cases. The dialogue about these substances during case discussions likely resulted in improvements to the following learner outcomes related to medication management: alcohol use disorder, OUD, and nicotine use disorder. Medication management of cannabis use disorder was not assessed in the pre- to postsurveys. Additionally, the didactic presentation topics that centered on alcohol, opioid, and nicotine use disorder resulted in a higher attendance rate with about 40% (n=6) to 50% (n=8) of the participants included in the paired-samples t test analysis attending the sessions, as compared to only 20% (n=3) of the aforementioned participant sample having attended the session centered on stimulant use disorder.
These findings indicate that the ECHO program’s content aligned with its stated learning objectives; met its learning objectives for the 3 themes where significant improvements were measured; and met its intent to address multiple substances in case presentations and discussions. While case presentations and discussions comprise from half to the majority of content in the sessions (30-35 minutes of a 60-minute session), content during sessions also includes faculty didactic presentations (20-25 minutes), which also addresses these 7 learning objectives and various substances but was not a part of the content analysis. Therefore, learner outcome improvements may also be a result of content addressed in didactic presentations.
While the Project ECHO model has been shown to be effective in training the primary care workforce [ 9 ], specifically on OUD [ 10 , 11 ] and addiction medicine [ 12 , 13 ], there has been no documentation, to our knowledge, of the ability of a team-based, comprehensive SUD and polysubstance-focused Project ECHO designed to improve learner outcomes (eg, knowledge, self-efficacy, and skills). Although Komaromy and colleagues [ 14 ] investigated the frequency of cases presented based on substance type in a comprehensive SUD-focused ECHO, a content analysis of the case presentation and discussion transcripts was not analyzed to either assess the frequency of substances or learning objectives. Furthermore, to our knowledge, this process has not been combined in a mixed method approach to compare learner outcomes with a content analysis to gauge the ability of an SUD-focused Project ECHO program to meet its stated learning objectives. Our results reported here align with this literature and expand to demonstrate that Project ECHO is a potential tool to effectively educate multidisciplinary providers in a comprehensive approach to SUD care.
This study has several strengths which promote the ability of the Project ECHO model in enhancing health care providers’ knowledge, self-efficacy, and skill associated with comprehensive SUD care. The focus of this study is unique as there is minimal research exploring the benefits and training ability of Project ECHO with a comprehensive SUD care focus. This study’s noteworthy strength is the use of a mixed methods design that presents a comprehensive evaluation correlating the content addressed in the case presentations and discussions to statistically significant learner outcomes to demonstrate how this telementoring continuing education series improved provider’s knowledge, skills, and self-efficacy to benefit participating providers and their practices.
Limitations
This study faced several limitations during data collection and analysis. The first limitation of this study was the limited sample size and low response rate. There was a decline between the number of participants who completed the preseries survey and postseries survey, resulting in a low comparative sample, which restricted the options for statistical analysis. Another limitation was generalizability; the results of this Project ECHO are limited to the target audience of medical providers, behavioral health providers, and care team members from the state of Ohio, which is not a representative sample of broader populations nationally. Additionally, participants self-selected to take part in the Project ECHO series, which presents the potential for self-selection bias. Another limitation this study faced was the lack of available or reliable data on Project ECHO and its ability to meet learning objectives and address multiple substances through providers’ knowledge, self-efficacy, skill, and attitudes. Furthermore, self-reported data to assess knowledge and skills, and self-reported data in general, could present participant biases and is difficult to corroborate with outcomes. The use of internally designed survey instruments instead of using validated instruments presents as a limitation. In light of these limitations, future studies in this subject matter should include a larger data set. Additionally, future studies using a nested analysis approach might provide more insight into how the learning objective themes coincide with the various illicit and nonillicit substance types and would be a useful analysis to contribute to the knowledge base. Another recommendation for future studies in this subject matter should include a deeper analysis of attendance dose and exposure to didactic topics to better understand the impact on changes in learner outcomes. Future research with greater validity will contribute to the significant gaps in literature regarding this subject.
Conclusions
The purpose of this research study was to assess the ability of CSUDC ECHO to both address and meet 7 learning objectives ( Textbox 2 ) and address multiple substances by analyzing (1) the frequency of exposure to the learning objective topics and substance types during case presentations and discussions and (2) participants’ knowledge, self-efficacy, skills, and attitudes related to the treatment of SUDs from pre- to postprogram. The results of this study indicate that CSUDC ECHO was able to both address and meet its learning objectives while addressing multiple substances, as demonstrated by improvements in learner knowledge, self-efficacy, and skills. All learning objective themes resulted in high frequencies and doses, appearing in a majority of case presentations throughout the series. These promising results suggest that Project ECHO is a potential tool to educate primary care providers, behavioral health providers, and care team members in a comprehensive approach to SUD assessment and treatment through complex case discussions combined with didactic learning for certain settings. As Project ECHO programs continue to be established globally and existing programs strengthen, further research examining the model’s ability to achieve positive learning outcomes and factors that may contribute to these outcomes (eg, frequency of topic dose) is needed to confirm the outcomes in larger population samples, additional topics of focus, and other geographical settings.
Acknowledgments
The authors would like to acknowledge our partners at Buckeye Health Plan and Ohio University Heritage College of Osteopathic Medicine. We would like to thank our funders, Centene Corporation through its subsidiary, Buckeye Health Plan; without their financial support, this work would not have been possible. We would like to thank the faculty that led the ECHO sessions, delivered didactic presentations, and provided high-quality case recommendations, including core faculty members Dana Vallangeon, doctor of medicine, Tracy Plouck, master of public administration, Amy Black, master of science in nursing, advanced practice registered nurse, nurse practitioner-certified, Ericka Ludwig, licensed professional clinical counselors applying for training supervision designation, licensed independent chemical dependency counselor, as well as guest faculty members. We would also like to thank our Weitzman Institute colleagues who helped with the content analysis: Zeba Kokan, Claire Newby, and Reilly Orner. To learn more about Weitzman Extension for Community Healthcare Outcomes programs, visit their website [ 15 ]. This project was supported by Buckeye Health Plan, a subsidiary of Centene Corporation. The views, opinions, and content expressed in this paper do not necessarily reflect the views, opinions, or policies of Buckeye Health Plan or Centene Corporation. The authors did not use generative artificial intelligence in any portion of this paper.
Data Availability
The data sets generated and analyzed during this study are not publicly available due to a portion of the data being deemed as exempt by the institutional review board and the institutional review board approving a waiver of informed consent for the exempt data, as well as the sensitive nature of the data, but are available from the corresponding author on reasonable request.
Authors' Contributions
MK wrote this paper, reviewed this paper, designed the evaluation plan, and performed the qualitative and statistical analyses. AP wrote this paper, reviewed this paper, and assisted with the evaluation design and approval. RM wrote this paper, reviewed this paper, performed the literature review, and assisted with the evaluation design and approval. NRN wrote this paper, reviewed this paper, and performed the literature review. KA critically reviewed this paper.
Conflicts of Interest
None declared.
Weitzman Extension for Community Healthcare Outcomes: Comprehensive Substance Use Disorder Care preseries survey instrument.
Weitzman Extension for Community Healthcare Outcomes: Comprehensive Substance Use Disorder Care postseries survey instrument.
- Drug overdose mortality by state. Centers for Disease Control and Prevention. 2023. URL: https://www.cdc.gov/nchs/pressroom/sosmap/drug_poisoning_mortality/drug_poisoning.htm [accessed 2023-01-30]
- Behavioral health barometer: Ohio, Volume 6: indicators as measured through the 2019 national survey on drug use and health and the national survey of substance abuse treatment services. Substance Abuse and Mental Health Services Administration. 2020. URL: https://www.samhsa.gov/data/sites/default/files/reports/rpt32852/Ohio-BH-Barometer_Volume6.pdf [accessed 2023-01-30]
- Arora S, Thornton K, Murata G, Deming P, Kalishman S, Dion D, et al. Outcomes of treatment for hepatitis C virus infection by primary care providers. N Engl J Med. 2011;364(23):2199-2207. [ FREE Full text ] [ CrossRef ] [ Medline ]
- Fact sheet: Biden-Harris administration announces new actions and funding to address the overdose epidemic and support recovery. The White House. 2022. URL: https://www.whitehouse.gov/briefing-room/statements-releases/2022/09/23/fact-sheet-biden-harris-administration-announces-new-actions-and-funding-to-address-the-overdose-epidemic-and-support-recovery/ [accessed 2023-01-27]
- Key substance use and mental health indicators in the United States: results from the 2019 national survey on drug use and health. Substance Abuse and Mental Health Services Administration. 2020. URL: https://www.samhsa.gov/data/sites/default/files/reports/rpt29393/2019NSDUHFFRPDFWHTML/2019NSDUHFFR1PDFW090120.pdf [accessed 2023-01-25]
- Abroms LC, Padmanabhan N, Thaweethai L, Phillips T. iPhone apps for smoking cessation: a content analysis. Am J Prev Med. 2011;40(3):279-285. [ FREE Full text ] [ CrossRef ] [ Medline ]
- Damschroder LJ, Aron DC, Keith RE, Kirsh SR, Alexander JA, Lowery JC. Fostering implementation of health services research findings into practice: a consolidated framework for advancing implementation science. Implement Sci. 2009;4:50. [ FREE Full text ] [ CrossRef ] [ Medline ]
- Moore DE, Green JS, Gallis HA. Achieving desired results and improved outcomes: integrating planning and assessment throughout learning activities. J Contin Educ Health Prof. 2009;29(1):1-15. [ CrossRef ] [ Medline ]
- Zhou C, Crawford A, Serhal E, Kurdyak P, Sockalingam S. The impact of project ECHO on participant and patient outcomes: a systematic review. Acad Med. 2016;91(10):1439-1461. [ FREE Full text ] [ CrossRef ] [ Medline ]
- Tofighi B, Isaacs N, Byrnes-Enoch H, Lakew R, Lee JD, Berry C, et al. Expanding treatment for opioid use disorder in publicly funded primary care clinics: exploratory evaluation of the NYC health + hospitals buprenorphine ECHO program. J Subst Abuse Treat. 2019;106:1-3. [ FREE Full text ] [ CrossRef ] [ Medline ]
- Alford DP, Zisblatt L, Ng P, Hayes SM, Peloquin S, Hardesty I, et al. SCOPE of pain: an evaluation of an opioid risk evaluation and mitigation strategy continuing education program. Pain Med. 2016;17(1):52-63. [ FREE Full text ] [ CrossRef ] [ Medline ]
- Sagi MR, Aurobind G, Chand P, Ashfak A, Karthick C, Kubenthiran N, et al. Innovative telementoring for addiction management for remote primary care physicians: a feasibility study. Indian J Psychiatry. 2018;60(4):461-466. [ FREE Full text ] [ CrossRef ] [ Medline ]
- Englander H, Patten A, Lockard R, Muller M, Gregg J. Spreading addictions care across Oregon's rural and community hospitals: mixed-methods evaluation of an interprofessional telementoring ECHO program. J Gen Intern Med. 2021;36(1):100-107. [ FREE Full text ] [ CrossRef ] [ Medline ]
- Komaromy M, Duhigg D, Metcalf A, Carlson C, Kalishman S, Hayes L, et al. Project ECHO (Extension for Community Healthcare Outcomes): A new model for educating primary care providers about treatment of substance use disorders. Subst Abus. 2016;37(1):20-24. [ FREE Full text ] [ CrossRef ] [ Medline ]
- Weitzman Institute. URL: https://www.weitzmaninstitute.org/education/weitzman-echo/ [accessed 2024-03-12]
Abbreviations
Edited by T de Azevedo Cardoso; submitted 12.04.23; peer-reviewed by A Arbabisarjou, J Ford Ii; comments to author 12.09.23; revised version received 06.11.23; accepted 29.02.24; published 01.04.24.
©MacKenzie Koester, Rosemary Motz, Ariel Porto, Nikita Reyes Nieves, Karen Ashley. Originally published in JMIR Medical Education (https://mededu.jmir.org), 01.04.2024.
This is an open-access article distributed under the terms of the Creative Commons Attribution License (https://creativecommons.org/licenses/by/4.0/), which permits unrestricted use, distribution, and reproduction in any medium, provided the original work, first published in JMIR Medical Education, is properly cited. The complete bibliographic information, a link to the original publication on https://mededu.jmir.org/, as well as this copyright and license information must be included.

News & Events
- Submit an Event
- Yale Engineering Magazine
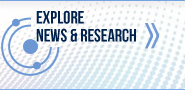
Roberts Innovation Fund to Support Inventions in AI, Quantum, Water
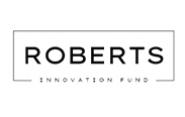
In line with Yale Engineering’s Strategic Vision, the Roberts Innovation Fund bolsters the School’s culture of innovation and entrepreneurship by advancing an ecosystem that brings to market discoveries that will benefit humanity.
Yale Engineering Dean Jeffrey Brock said the large number of excellent proposals this year demonstrates the continued need for the kind of opportunities that the Roberts Fund provides.
“Our faculty have long been producing groundbreaking research, and once again, the Roberts Innovation Fund is helping to get these inspired ideas out of the laboratories and to the market, and ultimately to the people and industries that will benefit the most from them,” Brock said. “The areas of research in this year’s selected projects emphasize Yale's priorities in quantum, artificial intelligence, and planetary solutions, demonstrating how Yale Engineering’s goals and our Strategic Vision are advancing the goals of the University.”
In its second year, the Roberts Innovation Fund has significantly expanded its reach and resources, setting the stage for a transformative impact on how Yale Engineering’s innovations make the leap from research to real-world applications.
"This year has been about expansion for the Roberts Innovation Fund,” said the Fund’s director, Claudia Reuter. “As we considered more ways to support the innovative work happening within Yale Engineering advance from ‘lab to market,’ we broadened the aperture of potential domains for consideration, added new members to our external advisory board with additional industry experience, and thanks to the leadership of Yale Engineering we were able to allocate more capital. We're now in the process of refining the types of support we provide to awardees and look forward to helping each project advance to their greatest potential impact."
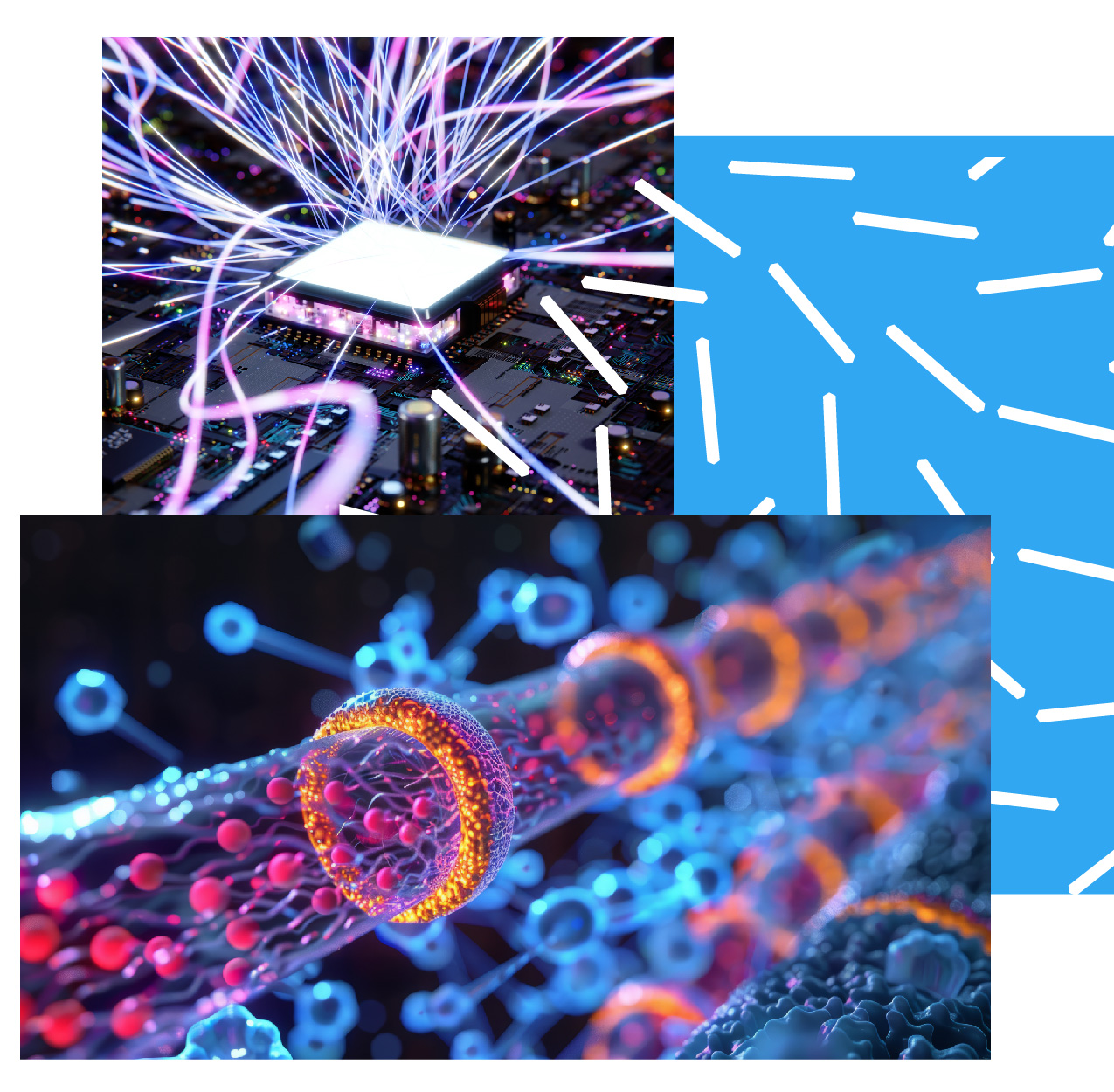
One of the awardees, Jaehong Kim, said he’s looking forward to working with the Roberts Fund on his project to develop a more efficient catalytic material and wastewater treatment method.
“Properly treating toxic wastewater and potentially recycling it are becoming more important for industries to remain cost-competitive and compliant to increasingly stringent environmental regulations,” said Kim, the Henry P. Becton Sr. Professor of Chemical & Environmental Engineering. “The Roberts Innovation Fund brings our research one step toward commercializing our technology and one step closer to making a major impact on this pivotal matter.”
In addition to providing $1 million in accelerator funding, the Roberts Innovation Fund also provided Phase II follow-on awards of $50,000 to two projects from last year. As the fund team works to support projects on their path from lab to market, they recognize that some projects may require additional runway to advance, and plan to provide a pathway for some Phase II follow on funding each year. This year's Phase II projects are "Foundational AI in Radiology: Transformative text-based tools for radiology,” led by Arman Cohan (Computer Science) and Sophie Chheang (Radiology and Biomedical Imaging) and “Combining DeFi and AI for intelligent credit scoring, led by Electrical Engineering faculty member Leandros Tassiulas, with Georgios Palaiokrassas, Jason Ofeidis, Aosong Feng, and Farooq Anjum.
This year’s awardees are:
Advancing Novel PFAS Treatment Technologies from Lab to Commercialization
Prof. John Fortner with Lydia Watt, Susanna Maisto, Seung Soo Lee, and Öze Durak Chemical & Environmental Engineering
PFAS pollution - the harmful substances also known as “forever chemicals” - is now a globally ubiquitous and a serious public health threat. Potentially, affecting millions of people through tap water alone, PFAS is one of the emerging water contaminants targeted by the U.S. government’s recent $10 billion management effort. The team led by John Fortner has developed technologies to separate and destroy PFAS at point-of-use water treatment technology companies, municipal water treatment facilities, and other locations. His technologies have significant potential to outperform conventional PFAS treatment approaches.
Scaling Catalytic Membranes for Oil & Gas Produced Water Treatment - Industrial wastewater treatment and reuse
Prof. Jaehong Kim with James Licato and Claire Chouinard Chemical & Environmental Engineering
As the cost of water rises due to global challenges, reusing and recycling water has become inevitable for industries in the U.S. and around the world for their sustainable growth. Technologies that can actually destroy contaminants are ideal, but they require major breakthroughs to become viable and cost-effective.
Commonly employed in many industries, advanced oxidation processes (AOPs) are designed to destroy water pollutants, but current AOPs can’t achieve complete destruction of contaminants. A team led by Jaehong Kim has developed a new catalytic material and treatment scheme that demonstrates near-complete destruction of organic pollutants with the highest-ever recorded efficiency at a low operating cost.
Data-Centric Quantum Computing
Prof. Yongshan Ding with Shifan Xu Computer Science
While quantum processing units (QPUs) have advanced significantly, the challenge of efficiently translating classical data into a format compatible with QPUs hinders the deployment of quantum applications in data-driven fields like machine learning, optimization, and scientific computing. Yongshan Ding has created a solution that provides user-friendly access to cutting-edge quantum random access memory (QRAM) - a technology that’s pivotal for converting classical data into quantum data, a crucial but challenging step for practical quantum computing. This unique platform serves as a valuable testing ground to explore the commercial potential of various quantum applications.
A manufacturable process for a material to combine logic and memory in semiconductor devices
Prof. Charles Ahn with Fred Walker, Kidae Shin Applied Physics and Mechanical Engineering & Materials Science
Materials technology for current microelectronics, based on conventional silicon and insulating oxides, is approaching its physical limits. At the same time, demands for electronic devices with superior processing capabilities and lower power consumption are ever increasing. Charles Ahn’s research group is developing materials technologies capable of being manufactured on a large-scale that would address current limitations of today's logic and memory devices. With such functionalities as non-volatility, extremely low energy consumption, and neuromorphic computing capabilities for AI applications, the approach has the potential to advance semiconductor technology, attracting the interest of semiconductor companies that are actively pursuing new materials systems.
AI in Your Pocket
Prof. Amin Karbasi with Amir Zandieh and Insu Ha Electrical Engineering and Computer Science
Despite significant advancements, democratizing large AI models remains a challenge. The development of such models demands thousands of GPU training hours, incurring costs in the hundreds of thousands of dollars, limiting powerful AI models to organizations with substantial resources. Led by Amin Karbasi, this project aims to make AI cost-effective, enabling individuals to run them on their edge devices offline, fostering broader accessibility and unlocking their full potential. The solution enables the development of larger AI models without increasing the computational budget. This expansion of model sizes, as demonstrated in generative models like ChatGPT, unlocks new emergent properties of AI, like human-like reasoning abilities, and more.
Krania: Decentralized Machine Learning as a Service
Prof. Ben Fisch with Josh Beal Computer Science
Due to the centralization risks of machine learning as a service (MLaaS) and the high cost of running large language models on consumer-grade hardware, there has been growing interest in decentralized inference networks, which crowdsource the computation over many participants. Two opposing challenges are incentivizing participation and trusting the results of computation. Participants may cut costs and claim the rewards by faking results rather than computing them accurately. Ben Fisch and his research team have created Krania – a system addressing these issues by employing verifiable distributed computation to validate results and streamline efficiency, demonstrating the potential for secure, decentralized MLaaS.
Towards manufacturable short-wave infrared (SWIR) vertical-cavity-surface-emitting lasers (VCSELs)
Prof. Jung Han with Jacob Tarn, Rebecca Levonian, Jin-ho Kang Electrical Engineering
Vertical Cavity Surface Emitting Lasers (VCSELs) are critical in 3D optical sensing and data communications but are currently limited to an 800-1000 nm near infrared (NIR) range due to material constraints. Extending VCSELs into the 1300-2300 nm Short-Wave Infrared (SWIR) range could revolutionize this technology, enhancing data transmission speeds, fiber optic reach, and improve eye safety in free-space applications. Jung Han aims to commercialize SWIR VCSELs by advancing photonic technologies, bringing significant improvements to fields like 3D sensing, facial recognition, augmented and virtual reality, autonomous vehicles, biophotonics, and high-speed optical links.
Prof. Ruzica Piskac with Prof. Scott Shapiro Computer Science and Yale Law School
As many have experienced, a paramount challenge with large language models is their propensity for "hallucinations," or generating plausible but false or misleading information. This is particularly problematic in the legal domain, where accuracy is critical. To address this, Piskac and collaborator Scott Shapiro from the Yale Law School are developing reliable and trustworthy legal chatbots (“lawbots”) to assist with legal inquiries by implementing formal verification methods to mathematically validate the responses. This verification aims to ensure that the lawbots are accurate and reliable, thereby establishing a new standard of dependability in AI assistance for legal experts and democratizing legal knowledge for ordinary citizens.
Geometric Deep Sensing Technology
Prof. Fengnian Xia with Ting Li Electrical Engineering
The rapid growth of autonomous vehicles, biometric systems, and robotics has created an urgent need for advanced optical sensors that are compact, capable of handling large amounts of data, and AI-compatible. Traditional sensors, however, fall short in meeting these modern requirements. Fengnian Xia’s innovative and proprietary technology – geometric deep sensing – looks to fill this void by bringing this versatile and intelligent sensing solution to market. The design and manufacturing of these cutting-edge semiconductor devices will meet the critical need for smaller, smarter, and highly adaptable optical sensors, unlocking new commercial opportunities in a rapidly evolving landscape.
Prof. Mark Saltzman with Fan Yang, Jennifer Ayres, Bruce Cao, and Gregory Kopf Biomedical Engineering and Chemical & Environmental Engineering
There is a pressing need for more convenient and efficient treatments for individuals who currently rely on frequent medications and injections. This is especially relevant for HIV-positive individuals, and those at risk of HIV exposure. Additionally, improved drug delivery systems could greatly benefit conditions like endometriosis and chronic diseases requiring sustained medication.
Saltzman’s Evershield technology offers a long-acting, biodegradable polymer implant that ensures consistent release of therapeutic agents over extended periods. This innovative approach utilizes a safe, degradable polymer compatible with multiple active pharmaceutical ingredients and has proven effective for delivering contraceptive agents and anti-retrovirals. While addressing reproductive health needs, this technology also holds promise for treatments for glaucoma, macular degeneration, osteoarthritis, chronic pain management, and other applications.
For further project details and more information about the Fund, visit https://ventures.yale.edu/2023-2024-roberts-awards
Pictured L-R, Front row: Amin Karbasi, Ruzica Piskac. Middle row: Kidae Shin, Fengnian Xia Back row: Fred Walker, John Fortner, Yongshan Ding, Dean Jeffrey Brock, Jaehong Kim, Mark Saltzman, Fund Director Claudia Reuter, Jung Han. Not pictured: Charles Ahn, Ben Fisch.
Help | Advanced Search
Computer Science > Machine Learning
Title: tfb: towards comprehensive and fair benchmarking of time series forecasting methods.
Abstract: Time series are generated in diverse domains such as economic, traffic, health, and energy, where forecasting of future values has numerous important applications. Not surprisingly, many forecasting methods are being proposed. To ensure progress, it is essential to be able to study and compare such methods empirically in a comprehensive and reliable manner. To achieve this, we propose TFB, an automated benchmark for Time Series Forecasting (TSF) methods. TFB advances the state-of-the-art by addressing shortcomings related to datasets, comparison methods, and evaluation pipelines: 1) insufficient coverage of data domains, 2) stereotype bias against traditional methods, and 3) inconsistent and inflexible pipelines. To achieve better domain coverage, we include datasets from 10 different domains: traffic, electricity, energy, the environment, nature, economic, stock markets, banking, health, and the web. We also provide a time series characterization to ensure that the selected datasets are comprehensive. To remove biases against some methods, we include a diverse range of methods, including statistical learning, machine learning, and deep learning methods, and we also support a variety of evaluation strategies and metrics to ensure a more comprehensive evaluations of different methods. To support the integration of different methods into the benchmark and enable fair comparisons, TFB features a flexible and scalable pipeline that eliminates biases. Next, we employ TFB to perform a thorough evaluation of 21 Univariate Time Series Forecasting (UTSF) methods on 8,068 univariate time series and 14 Multivariate Time Series Forecasting (MTSF) methods on 25 datasets. The benchmark code and data are available at this https URL .
Submission history
Access paper:.
- HTML (experimental)
- Other Formats

References & Citations
- Google Scholar
- Semantic Scholar
BibTeX formatted citation

Bibliographic and Citation Tools
Code, data and media associated with this article, recommenders and search tools.
- Institution
arXivLabs: experimental projects with community collaborators
arXivLabs is a framework that allows collaborators to develop and share new arXiv features directly on our website.
Both individuals and organizations that work with arXivLabs have embraced and accepted our values of openness, community, excellence, and user data privacy. arXiv is committed to these values and only works with partners that adhere to them.
Have an idea for a project that will add value for arXiv's community? Learn more about arXivLabs .
Thank you for visiting nature.com. You are using a browser version with limited support for CSS. To obtain the best experience, we recommend you use a more up to date browser (or turn off compatibility mode in Internet Explorer). In the meantime, to ensure continued support, we are displaying the site without styles and JavaScript.
- View all journals
- My Account Login
- Explore content
- About the journal
- Publish with us
- Sign up for alerts
- Open access
- Published: 26 March 2024
Predicting and improving complex beer flavor through machine learning
- Michiel Schreurs ORCID: orcid.org/0000-0002-9449-5619 1 , 2 , 3 na1 ,
- Supinya Piampongsant 1 , 2 , 3 na1 ,
- Miguel Roncoroni ORCID: orcid.org/0000-0001-7461-1427 1 , 2 , 3 na1 ,
- Lloyd Cool ORCID: orcid.org/0000-0001-9936-3124 1 , 2 , 3 , 4 ,
- Beatriz Herrera-Malaver ORCID: orcid.org/0000-0002-5096-9974 1 , 2 , 3 ,
- Christophe Vanderaa ORCID: orcid.org/0000-0001-7443-5427 4 ,
- Florian A. Theßeling 1 , 2 , 3 ,
- Łukasz Kreft ORCID: orcid.org/0000-0001-7620-4657 5 ,
- Alexander Botzki ORCID: orcid.org/0000-0001-6691-4233 5 ,
- Philippe Malcorps 6 ,
- Luk Daenen 6 ,
- Tom Wenseleers ORCID: orcid.org/0000-0002-1434-861X 4 &
- Kevin J. Verstrepen ORCID: orcid.org/0000-0002-3077-6219 1 , 2 , 3
Nature Communications volume 15 , Article number: 2368 ( 2024 ) Cite this article
41k Accesses
778 Altmetric
Metrics details
- Chemical engineering
- Gas chromatography
- Machine learning
- Metabolomics
- Taste receptors
The perception and appreciation of food flavor depends on many interacting chemical compounds and external factors, and therefore proves challenging to understand and predict. Here, we combine extensive chemical and sensory analyses of 250 different beers to train machine learning models that allow predicting flavor and consumer appreciation. For each beer, we measure over 200 chemical properties, perform quantitative descriptive sensory analysis with a trained tasting panel and map data from over 180,000 consumer reviews to train 10 different machine learning models. The best-performing algorithm, Gradient Boosting, yields models that significantly outperform predictions based on conventional statistics and accurately predict complex food features and consumer appreciation from chemical profiles. Model dissection allows identifying specific and unexpected compounds as drivers of beer flavor and appreciation. Adding these compounds results in variants of commercial alcoholic and non-alcoholic beers with improved consumer appreciation. Together, our study reveals how big data and machine learning uncover complex links between food chemistry, flavor and consumer perception, and lays the foundation to develop novel, tailored foods with superior flavors.
Similar content being viewed by others
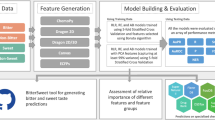
BitterSweet: Building machine learning models for predicting the bitter and sweet taste of small molecules
Rudraksh Tuwani, Somin Wadhwa & Ganesh Bagler
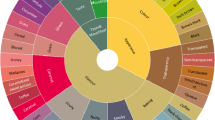
Sensory lexicon and aroma volatiles analysis of brewing malt
Xiaoxia Su, Miao Yu, … Tianyi Du
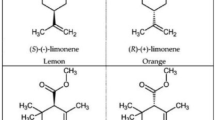
Predicting odor from molecular structure: a multi-label classification approach
Kushagra Saini & Venkatnarayan Ramanathan
Introduction
Predicting and understanding food perception and appreciation is one of the major challenges in food science. Accurate modeling of food flavor and appreciation could yield important opportunities for both producers and consumers, including quality control, product fingerprinting, counterfeit detection, spoilage detection, and the development of new products and product combinations (food pairing) 1 , 2 , 3 , 4 , 5 , 6 . Accurate models for flavor and consumer appreciation would contribute greatly to our scientific understanding of how humans perceive and appreciate flavor. Moreover, accurate predictive models would also facilitate and standardize existing food assessment methods and could supplement or replace assessments by trained and consumer tasting panels, which are variable, expensive and time-consuming 7 , 8 , 9 . Lastly, apart from providing objective, quantitative, accurate and contextual information that can help producers, models can also guide consumers in understanding their personal preferences 10 .
Despite the myriad of applications, predicting food flavor and appreciation from its chemical properties remains a largely elusive goal in sensory science, especially for complex food and beverages 11 , 12 . A key obstacle is the immense number of flavor-active chemicals underlying food flavor. Flavor compounds can vary widely in chemical structure and concentration, making them technically challenging and labor-intensive to quantify, even in the face of innovations in metabolomics, such as non-targeted metabolic fingerprinting 13 , 14 . Moreover, sensory analysis is perhaps even more complicated. Flavor perception is highly complex, resulting from hundreds of different molecules interacting at the physiochemical and sensorial level. Sensory perception is often non-linear, characterized by complex and concentration-dependent synergistic and antagonistic effects 15 , 16 , 17 , 18 , 19 , 20 , 21 that are further convoluted by the genetics, environment, culture and psychology of consumers 22 , 23 , 24 . Perceived flavor is therefore difficult to measure, with problems of sensitivity, accuracy, and reproducibility that can only be resolved by gathering sufficiently large datasets 25 . Trained tasting panels are considered the prime source of quality sensory data, but require meticulous training, are low throughput and high cost. Public databases containing consumer reviews of food products could provide a valuable alternative, especially for studying appreciation scores, which do not require formal training 25 . Public databases offer the advantage of amassing large amounts of data, increasing the statistical power to identify potential drivers of appreciation. However, public datasets suffer from biases, including a bias in the volunteers that contribute to the database, as well as confounding factors such as price, cult status and psychological conformity towards previous ratings of the product.
Classical multivariate statistics and machine learning methods have been used to predict flavor of specific compounds by, for example, linking structural properties of a compound to its potential biological activities or linking concentrations of specific compounds to sensory profiles 1 , 26 . Importantly, most previous studies focused on predicting organoleptic properties of single compounds (often based on their chemical structure) 27 , 28 , 29 , 30 , 31 , 32 , 33 , thus ignoring the fact that these compounds are present in a complex matrix in food or beverages and excluding complex interactions between compounds. Moreover, the classical statistics commonly used in sensory science 34 , 35 , 36 , 37 , 38 , 39 require a large sample size and sufficient variance amongst predictors to create accurate models. They are not fit for studying an extensive set of hundreds of interacting flavor compounds, since they are sensitive to outliers, have a high tendency to overfit and are less suited for non-linear and discontinuous relationships 40 .
In this study, we combine extensive chemical analyses and sensory data of a set of different commercial beers with machine learning approaches to develop models that predict taste, smell, mouthfeel and appreciation from compound concentrations. Beer is particularly suited to model the relationship between chemistry, flavor and appreciation. First, beer is a complex product, consisting of thousands of flavor compounds that partake in complex sensory interactions 41 , 42 , 43 . This chemical diversity arises from the raw materials (malt, yeast, hops, water and spices) and biochemical conversions during the brewing process (kilning, mashing, boiling, fermentation, maturation and aging) 44 , 45 . Second, the advent of the internet saw beer consumers embrace online review platforms, such as RateBeer (ZX Ventures, Anheuser-Busch InBev SA/NV) and BeerAdvocate (Next Glass, inc.). In this way, the beer community provides massive data sets of beer flavor and appreciation scores, creating extraordinarily large sensory databases to complement the analyses of our professional sensory panel. Specifically, we characterize over 200 chemical properties of 250 commercial beers, spread across 22 beer styles, and link these to the descriptive sensory profiling data of a 16-person in-house trained tasting panel and data acquired from over 180,000 public consumer reviews. These unique and extensive datasets enable us to train a suite of machine learning models to predict flavor and appreciation from a beer’s chemical profile. Dissection of the best-performing models allows us to pinpoint specific compounds as potential drivers of beer flavor and appreciation. Follow-up experiments confirm the importance of these compounds and ultimately allow us to significantly improve the flavor and appreciation of selected commercial beers. Together, our study represents a significant step towards understanding complex flavors and reinforces the value of machine learning to develop and refine complex foods. In this way, it represents a stepping stone for further computer-aided food engineering applications 46 .
To generate a comprehensive dataset on beer flavor, we selected 250 commercial Belgian beers across 22 different beer styles (Supplementary Fig. S1 ). Beers with ≤ 4.2% alcohol by volume (ABV) were classified as non-alcoholic and low-alcoholic. Blonds and Tripels constitute a significant portion of the dataset (12.4% and 11.2%, respectively) reflecting their presence on the Belgian beer market and the heterogeneity of beers within these styles. By contrast, lager beers are less diverse and dominated by a handful of brands. Rare styles such as Brut or Faro make up only a small fraction of the dataset (2% and 1%, respectively) because fewer of these beers are produced and because they are dominated by distinct characteristics in terms of flavor and chemical composition.
Extensive analysis identifies relationships between chemical compounds in beer
For each beer, we measured 226 different chemical properties, including common brewing parameters such as alcohol content, iso-alpha acids, pH, sugar concentration 47 , and over 200 flavor compounds (Methods, Supplementary Table S1 ). A large portion (37.2%) are terpenoids arising from hopping, responsible for herbal and fruity flavors 16 , 48 . A second major category are yeast metabolites, such as esters and alcohols, that result in fruity and solvent notes 48 , 49 , 50 . Other measured compounds are primarily derived from malt, or other microbes such as non- Saccharomyces yeasts and bacteria (‘wild flora’). Compounds that arise from spices or staling are labeled under ‘Others’. Five attributes (caloric value, total acids and total ester, hop aroma and sulfur compounds) are calculated from multiple individually measured compounds.
As a first step in identifying relationships between chemical properties, we determined correlations between the concentrations of the compounds (Fig. 1 , upper panel, Supplementary Data 1 and 2 , and Supplementary Fig. S2 . For the sake of clarity, only a subset of the measured compounds is shown in Fig. 1 ). Compounds of the same origin typically show a positive correlation, while absence of correlation hints at parameters varying independently. For example, the hop aroma compounds citronellol, and alpha-terpineol show moderate correlations with each other (Spearman’s rho=0.39 and 0.57), but not with the bittering hop component iso-alpha acids (Spearman’s rho=0.16 and −0.07). This illustrates how brewers can independently modify hop aroma and bitterness by selecting hop varieties and dosage time. If hops are added early in the boiling phase, chemical conversions increase bitterness while aromas evaporate, conversely, late addition of hops preserves aroma but limits bitterness 51 . Similarly, hop-derived iso-alpha acids show a strong anti-correlation with lactic acid and acetic acid, likely reflecting growth inhibition of lactic acid and acetic acid bacteria, or the consequent use of fewer hops in sour beer styles, such as West Flanders ales and Fruit beers, that rely on these bacteria for their distinct flavors 52 . Finally, yeast-derived esters (ethyl acetate, ethyl decanoate, ethyl hexanoate, ethyl octanoate) and alcohols (ethanol, isoamyl alcohol, isobutanol, and glycerol), correlate with Spearman coefficients above 0.5, suggesting that these secondary metabolites are correlated with the yeast genetic background and/or fermentation parameters and may be difficult to influence individually, although the choice of yeast strain may offer some control 53 .
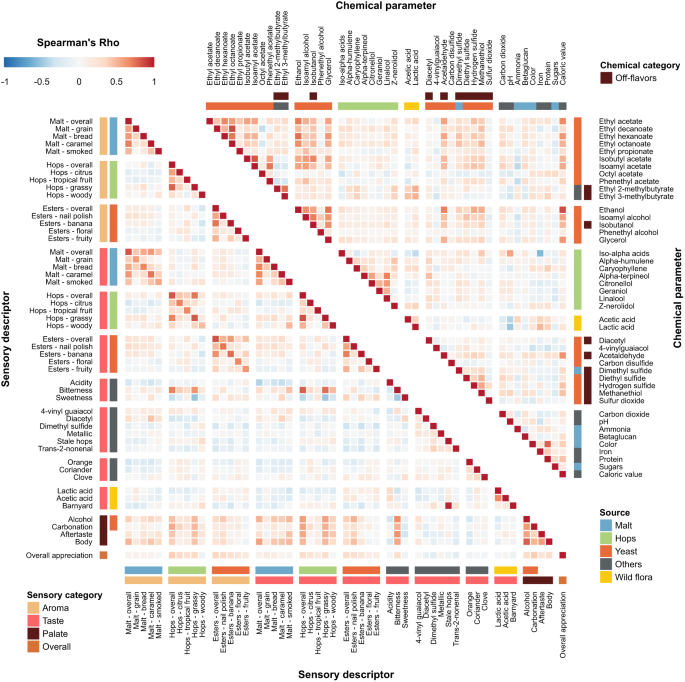
Spearman rank correlations are shown. Descriptors are grouped according to their origin (malt (blue), hops (green), yeast (red), wild flora (yellow), Others (black)), and sensory aspect (aroma, taste, palate, and overall appreciation). Please note that for the chemical compounds, for the sake of clarity, only a subset of the total number of measured compounds is shown, with an emphasis on the key compounds for each source. For more details, see the main text and Methods section. Chemical data can be found in Supplementary Data 1 , correlations between all chemical compounds are depicted in Supplementary Fig. S2 and correlation values can be found in Supplementary Data 2 . See Supplementary Data 4 for sensory panel assessments and Supplementary Data 5 for correlation values between all sensory descriptors.
Interestingly, different beer styles show distinct patterns for some flavor compounds (Supplementary Fig. S3 ). These observations agree with expectations for key beer styles, and serve as a control for our measurements. For instance, Stouts generally show high values for color (darker), while hoppy beers contain elevated levels of iso-alpha acids, compounds associated with bitter hop taste. Acetic and lactic acid are not prevalent in most beers, with notable exceptions such as Kriek, Lambic, Faro, West Flanders ales and Flanders Old Brown, which use acid-producing bacteria ( Lactobacillus and Pediococcus ) or unconventional yeast ( Brettanomyces ) 54 , 55 . Glycerol, ethanol and esters show similar distributions across all beer styles, reflecting their common origin as products of yeast metabolism during fermentation 45 , 53 . Finally, low/no-alcohol beers contain low concentrations of glycerol and esters. This is in line with the production process for most of the low/no-alcohol beers in our dataset, which are produced through limiting fermentation or by stripping away alcohol via evaporation or dialysis, with both methods having the unintended side-effect of reducing the amount of flavor compounds in the final beer 56 , 57 .
Besides expected associations, our data also reveals less trivial associations between beer styles and specific parameters. For example, geraniol and citronellol, two monoterpenoids responsible for citrus, floral and rose flavors and characteristic of Citra hops, are found in relatively high amounts in Christmas, Saison, and Brett/co-fermented beers, where they may originate from terpenoid-rich spices such as coriander seeds instead of hops 58 .
Tasting panel assessments reveal sensorial relationships in beer
To assess the sensory profile of each beer, a trained tasting panel evaluated each of the 250 beers for 50 sensory attributes, including different hop, malt and yeast flavors, off-flavors and spices. Panelists used a tasting sheet (Supplementary Data 3 ) to score the different attributes. Panel consistency was evaluated by repeating 12 samples across different sessions and performing ANOVA. In 95% of cases no significant difference was found across sessions ( p > 0.05), indicating good panel consistency (Supplementary Table S2 ).
Aroma and taste perception reported by the trained panel are often linked (Fig. 1 , bottom left panel and Supplementary Data 4 and 5 ), with high correlations between hops aroma and taste (Spearman’s rho=0.83). Bitter taste was found to correlate with hop aroma and taste in general (Spearman’s rho=0.80 and 0.69), and particularly with “grassy” noble hops (Spearman’s rho=0.75). Barnyard flavor, most often associated with sour beers, is identified together with stale hops (Spearman’s rho=0.97) that are used in these beers. Lactic and acetic acid, which often co-occur, are correlated (Spearman’s rho=0.66). Interestingly, sweetness and bitterness are anti-correlated (Spearman’s rho = −0.48), confirming the hypothesis that they mask each other 59 , 60 . Beer body is highly correlated with alcohol (Spearman’s rho = 0.79), and overall appreciation is found to correlate with multiple aspects that describe beer mouthfeel (alcohol, carbonation; Spearman’s rho= 0.32, 0.39), as well as with hop and ester aroma intensity (Spearman’s rho=0.39 and 0.35).
Similar to the chemical analyses, sensorial analyses confirmed typical features of specific beer styles (Supplementary Fig. S4 ). For example, sour beers (Faro, Flanders Old Brown, Fruit beer, Kriek, Lambic, West Flanders ale) were rated acidic, with flavors of both acetic and lactic acid. Hoppy beers were found to be bitter and showed hop-associated aromas like citrus and tropical fruit. Malt taste is most detected among scotch, stout/porters, and strong ales, while low/no-alcohol beers, which often have a reputation for being ‘worty’ (reminiscent of unfermented, sweet malt extract) appear in the middle. Unsurprisingly, hop aromas are most strongly detected among hoppy beers. Like its chemical counterpart (Supplementary Fig. S3 ), acidity shows a right-skewed distribution, with the most acidic beers being Krieks, Lambics, and West Flanders ales.
Tasting panel assessments of specific flavors correlate with chemical composition
We find that the concentrations of several chemical compounds strongly correlate with specific aroma or taste, as evaluated by the tasting panel (Fig. 2 , Supplementary Fig. S5 , Supplementary Data 6 ). In some cases, these correlations confirm expectations and serve as a useful control for data quality. For example, iso-alpha acids, the bittering compounds in hops, strongly correlate with bitterness (Spearman’s rho=0.68), while ethanol and glycerol correlate with tasters’ perceptions of alcohol and body, the mouthfeel sensation of fullness (Spearman’s rho=0.82/0.62 and 0.72/0.57 respectively) and darker color from roasted malts is a good indication of malt perception (Spearman’s rho=0.54).
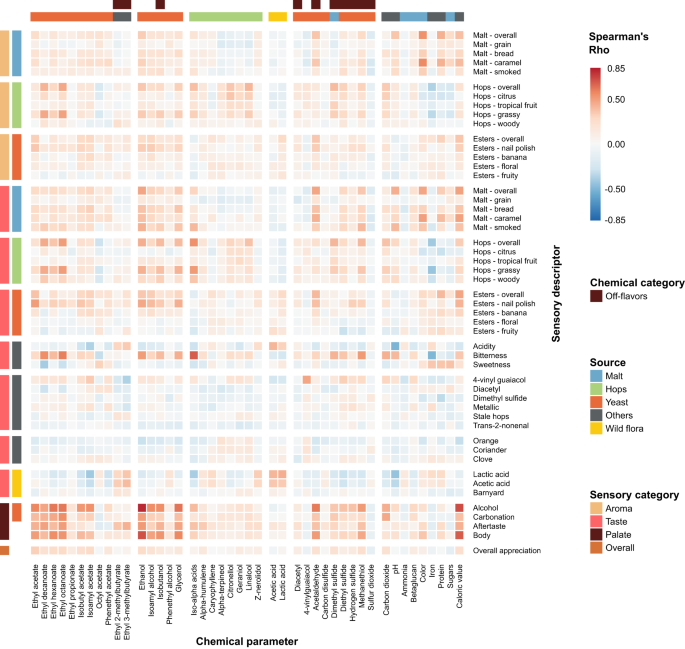
Heatmap colors indicate Spearman’s Rho. Axes are organized according to sensory categories (aroma, taste, mouthfeel, overall), chemical categories and chemical sources in beer (malt (blue), hops (green), yeast (red), wild flora (yellow), Others (black)). See Supplementary Data 6 for all correlation values.
Interestingly, for some relationships between chemical compounds and perceived flavor, correlations are weaker than expected. For example, the rose-smelling phenethyl acetate only weakly correlates with floral aroma. This hints at more complex relationships and interactions between compounds and suggests a need for a more complex model than simple correlations. Lastly, we uncovered unexpected correlations. For instance, the esters ethyl decanoate and ethyl octanoate appear to correlate slightly with hop perception and bitterness, possibly due to their fruity flavor. Iron is anti-correlated with hop aromas and bitterness, most likely because it is also anti-correlated with iso-alpha acids. This could be a sign of metal chelation of hop acids 61 , given that our analyses measure unbound hop acids and total iron content, or could result from the higher iron content in dark and Fruit beers, which typically have less hoppy and bitter flavors 62 .
Public consumer reviews complement expert panel data
To complement and expand the sensory data of our trained tasting panel, we collected 180,000 reviews of our 250 beers from the online consumer review platform RateBeer. This provided numerical scores for beer appearance, aroma, taste, palate, overall quality as well as the average overall score.
Public datasets are known to suffer from biases, such as price, cult status and psychological conformity towards previous ratings of a product. For example, prices correlate with appreciation scores for these online consumer reviews (rho=0.49, Supplementary Fig. S6 ), but not for our trained tasting panel (rho=0.19). This suggests that prices affect consumer appreciation, which has been reported in wine 63 , while blind tastings are unaffected. Moreover, we observe that some beer styles, like lagers and non-alcoholic beers, generally receive lower scores, reflecting that online reviewers are mostly beer aficionados with a preference for specialty beers over lager beers. In general, we find a modest correlation between our trained panel’s overall appreciation score and the online consumer appreciation scores (Fig. 3 , rho=0.29). Apart from the aforementioned biases in the online datasets, serving temperature, sample freshness and surroundings, which are all tightly controlled during the tasting panel sessions, can vary tremendously across online consumers and can further contribute to (among others, appreciation) differences between the two categories of tasters. Importantly, in contrast to the overall appreciation scores, for many sensory aspects the results from the professional panel correlated well with results obtained from RateBeer reviews. Correlations were highest for features that are relatively easy to recognize even for untrained tasters, like bitterness, sweetness, alcohol and malt aroma (Fig. 3 and below).
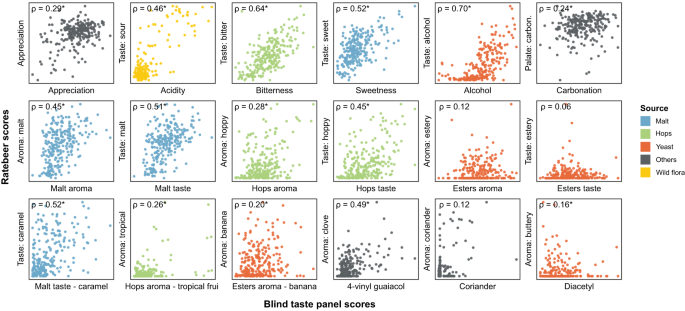
RateBeer text mining results can be found in Supplementary Data 7 . Rho values shown are Spearman correlation values, with asterisks indicating significant correlations ( p < 0.05, two-sided). All p values were smaller than 0.001, except for Esters aroma (0.0553), Esters taste (0.3275), Esters aroma—banana (0.0019), Coriander (0.0508) and Diacetyl (0.0134).
Besides collecting consumer appreciation from these online reviews, we developed automated text analysis tools to gather additional data from review texts (Supplementary Data 7 ). Processing review texts on the RateBeer database yielded comparable results to the scores given by the trained panel for many common sensory aspects, including acidity, bitterness, sweetness, alcohol, malt, and hop tastes (Fig. 3 ). This is in line with what would be expected, since these attributes require less training for accurate assessment and are less influenced by environmental factors such as temperature, serving glass and odors in the environment. Consumer reviews also correlate well with our trained panel for 4-vinyl guaiacol, a compound associated with a very characteristic aroma. By contrast, correlations for more specific aromas like ester, coriander or diacetyl are underrepresented in the online reviews, underscoring the importance of using a trained tasting panel and standardized tasting sheets with explicit factors to be scored for evaluating specific aspects of a beer. Taken together, our results suggest that public reviews are trustworthy for some, but not all, flavor features and can complement or substitute taste panel data for these sensory aspects.
Models can predict beer sensory profiles from chemical data
The rich datasets of chemical analyses, tasting panel assessments and public reviews gathered in the first part of this study provided us with a unique opportunity to develop predictive models that link chemical data to sensorial features. Given the complexity of beer flavor, basic statistical tools such as correlations or linear regression may not always be the most suitable for making accurate predictions. Instead, we applied different machine learning models that can model both simple linear and complex interactive relationships. Specifically, we constructed a set of regression models to predict (a) trained panel scores for beer flavor and quality and (b) public reviews’ appreciation scores from beer chemical profiles. We trained and tested 10 different models (Methods), 3 linear regression-based models (simple linear regression with first-order interactions (LR), lasso regression with first-order interactions (Lasso), partial least squares regressor (PLSR)), 5 decision tree models (AdaBoost regressor (ABR), extra trees (ET), gradient boosting regressor (GBR), random forest (RF) and XGBoost regressor (XGBR)), 1 support vector regression (SVR), and 1 artificial neural network (ANN) model.
To compare the performance of our machine learning models, the dataset was randomly split into a training and test set, stratified by beer style. After a model was trained on data in the training set, its performance was evaluated on its ability to predict the test dataset obtained from multi-output models (based on the coefficient of determination, see Methods). Additionally, individual-attribute models were ranked per descriptor and the average rank was calculated, as proposed by Korneva et al. 64 . Importantly, both ways of evaluating the models’ performance agreed in general. Performance of the different models varied (Table 1 ). It should be noted that all models perform better at predicting RateBeer results than results from our trained tasting panel. One reason could be that sensory data is inherently variable, and this variability is averaged out with the large number of public reviews from RateBeer. Additionally, all tree-based models perform better at predicting taste than aroma. Linear models (LR) performed particularly poorly, with negative R 2 values, due to severe overfitting (training set R 2 = 1). Overfitting is a common issue in linear models with many parameters and limited samples, especially with interaction terms further amplifying the number of parameters. L1 regularization (Lasso) successfully overcomes this overfitting, out-competing multiple tree-based models on the RateBeer dataset. Similarly, the dimensionality reduction of PLSR avoids overfitting and improves performance, to some extent. Still, tree-based models (ABR, ET, GBR, RF and XGBR) show the best performance, out-competing the linear models (LR, Lasso, PLSR) commonly used in sensory science 65 .
GBR models showed the best overall performance in predicting sensory responses from chemical information, with R 2 values up to 0.75 depending on the predicted sensory feature (Supplementary Table S4 ). The GBR models predict consumer appreciation (RateBeer) better than our trained panel’s appreciation (R 2 value of 0.67 compared to R 2 value of 0.09) (Supplementary Table S3 and Supplementary Table S4 ). ANN models showed intermediate performance, likely because neural networks typically perform best with larger datasets 66 . The SVR shows intermediate performance, mostly due to the weak predictions of specific attributes that lower the overall performance (Supplementary Table S4 ).
Model dissection identifies specific, unexpected compounds as drivers of consumer appreciation
Next, we leveraged our models to infer important contributors to sensory perception and consumer appreciation. Consumer preference is a crucial sensory aspects, because a product that shows low consumer appreciation scores often does not succeed commercially 25 . Additionally, the requirement for a large number of representative evaluators makes consumer trials one of the more costly and time-consuming aspects of product development. Hence, a model for predicting chemical drivers of overall appreciation would be a welcome addition to the available toolbox for food development and optimization.
Since GBR models on our RateBeer dataset showed the best overall performance, we focused on these models. Specifically, we used two approaches to identify important contributors. First, rankings of the most important predictors for each sensorial trait in the GBR models were obtained based on impurity-based feature importance (mean decrease in impurity). High-ranked parameters were hypothesized to be either the true causal chemical properties underlying the trait, to correlate with the actual causal properties, or to take part in sensory interactions affecting the trait 67 (Fig. 4A ). In a second approach, we used SHAP 68 to determine which parameters contributed most to the model for making predictions of consumer appreciation (Fig. 4B ). SHAP calculates parameter contributions to model predictions on a per-sample basis, which can be aggregated into an importance score.
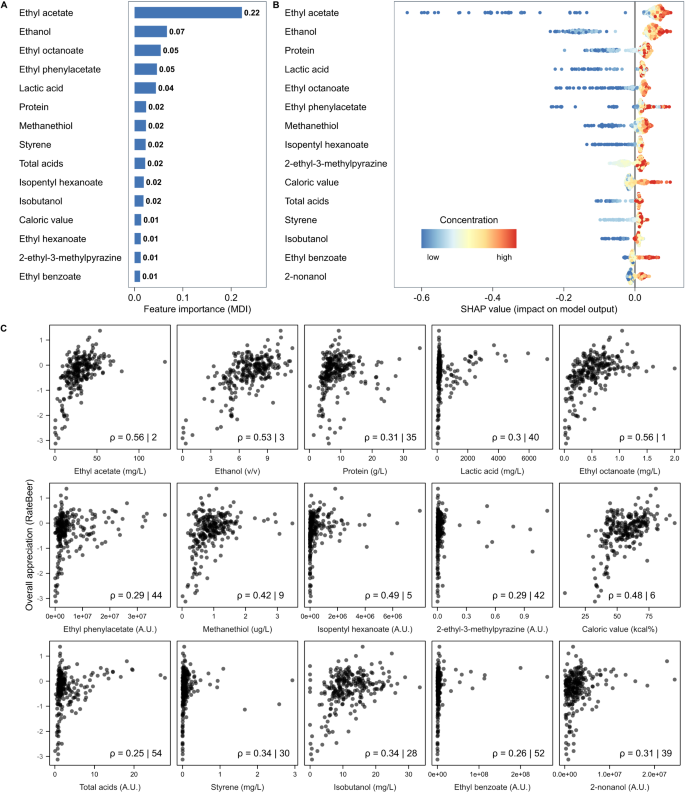
A The impurity-based feature importance (mean deviance in impurity, MDI) calculated from the Gradient Boosting Regression (GBR) model predicting RateBeer appreciation scores. The top 15 highest ranked chemical properties are shown. B SHAP summary plot for the top 15 parameters contributing to our GBR model. Each point on the graph represents a sample from our dataset. The color represents the concentration of that parameter, with bluer colors representing low values and redder colors representing higher values. Greater absolute values on the horizontal axis indicate a higher impact of the parameter on the prediction of the model. C Spearman correlations between the 15 most important chemical properties and consumer overall appreciation. Numbers indicate the Spearman Rho correlation coefficient, and the rank of this correlation compared to all other correlations. The top 15 important compounds were determined using SHAP (panel B).
Both approaches identified ethyl acetate as the most predictive parameter for beer appreciation (Fig. 4 ). Ethyl acetate is the most abundant ester in beer with a typical ‘fruity’, ‘solvent’ and ‘alcoholic’ flavor, but is often considered less important than other esters like isoamyl acetate. The second most important parameter identified by SHAP is ethanol, the most abundant beer compound after water. Apart from directly contributing to beer flavor and mouthfeel, ethanol drastically influences the physical properties of beer, dictating how easily volatile compounds escape the beer matrix to contribute to beer aroma 69 . Importantly, it should also be noted that the importance of ethanol for appreciation is likely inflated by the very low appreciation scores of non-alcoholic beers (Supplementary Fig. S4 ). Despite not often being considered a driver of beer appreciation, protein level also ranks highly in both approaches, possibly due to its effect on mouthfeel and body 70 . Lactic acid, which contributes to the tart taste of sour beers, is the fourth most important parameter identified by SHAP, possibly due to the generally high appreciation of sour beers in our dataset.
Interestingly, some of the most important predictive parameters for our model are not well-established as beer flavors or are even commonly regarded as being negative for beer quality. For example, our models identify methanethiol and ethyl phenyl acetate, an ester commonly linked to beer staling 71 , as a key factor contributing to beer appreciation. Although there is no doubt that high concentrations of these compounds are considered unpleasant, the positive effects of modest concentrations are not yet known 72 , 73 .
To compare our approach to conventional statistics, we evaluated how well the 15 most important SHAP-derived parameters correlate with consumer appreciation (Fig. 4C ). Interestingly, only 6 of the properties derived by SHAP rank amongst the top 15 most correlated parameters. For some chemical compounds, the correlations are so low that they would have likely been considered unimportant. For example, lactic acid, the fourth most important parameter, shows a bimodal distribution for appreciation, with sour beers forming a separate cluster, that is missed entirely by the Spearman correlation. Additionally, the correlation plots reveal outliers, emphasizing the need for robust analysis tools. Together, this highlights the need for alternative models, like the Gradient Boosting model, that better grasp the complexity of (beer) flavor.
Finally, to observe the relationships between these chemical properties and their predicted targets, partial dependence plots were constructed for the six most important predictors of consumer appreciation 74 , 75 , 76 (Supplementary Fig. S7 ). One-way partial dependence plots show how a change in concentration affects the predicted appreciation. These plots reveal an important limitation of our models: appreciation predictions remain constant at ever-increasing concentrations. This implies that once a threshold concentration is reached, further increasing the concentration does not affect appreciation. This is false, as it is well-documented that certain compounds become unpleasant at high concentrations, including ethyl acetate (‘nail polish’) 77 and methanethiol (‘sulfury’ and ‘rotten cabbage’) 78 . The inability of our models to grasp that flavor compounds have optimal levels, above which they become negative, is a consequence of working with commercial beer brands where (off-)flavors are rarely too high to negatively impact the product. The two-way partial dependence plots show how changing the concentration of two compounds influences predicted appreciation, visualizing their interactions (Supplementary Fig. S7 ). In our case, the top 5 parameters are dominated by additive or synergistic interactions, with high concentrations for both compounds resulting in the highest predicted appreciation.
To assess the robustness of our best-performing models and model predictions, we performed 100 iterations of the GBR, RF and ET models. In general, all iterations of the models yielded similar performance (Supplementary Fig. S8 ). Moreover, the main predictors (including the top predictors ethanol and ethyl acetate) remained virtually the same, especially for GBR and RF. For the iterations of the ET model, we did observe more variation in the top predictors, which is likely a consequence of the model’s inherent random architecture in combination with co-correlations between certain predictors. However, even in this case, several of the top predictors (ethanol and ethyl acetate) remain unchanged, although their rank in importance changes (Supplementary Fig. S8 ).
Next, we investigated if a combination of RateBeer and trained panel data into one consolidated dataset would lead to stronger models, under the hypothesis that such a model would suffer less from bias in the datasets. A GBR model was trained to predict appreciation on the combined dataset. This model underperformed compared to the RateBeer model, both in the native case and when including a dataset identifier (R 2 = 0.67, 0.26 and 0.42 respectively). For the latter, the dataset identifier is the most important feature (Supplementary Fig. S9 ), while most of the feature importance remains unchanged, with ethyl acetate and ethanol ranking highest, like in the original model trained only on RateBeer data. It seems that the large variation in the panel dataset introduces noise, weakening the models’ performances and reliability. In addition, it seems reasonable to assume that both datasets are fundamentally different, with the panel dataset obtained by blind tastings by a trained professional panel.
Lastly, we evaluated whether beer style identifiers would further enhance the model’s performance. A GBR model was trained with parameters that explicitly encoded the styles of the samples. This did not improve model performance (R2 = 0.66 with style information vs R2 = 0.67). The most important chemical features are consistent with the model trained without style information (eg. ethanol and ethyl acetate), and with the exception of the most preferred (strong ale) and least preferred (low/no-alcohol) styles, none of the styles were among the most important features (Supplementary Fig. S9 , Supplementary Table S5 and S6 ). This is likely due to a combination of style-specific chemical signatures, such as iso-alpha acids and lactic acid, that implicitly convey style information to the original models, as well as the low number of samples belonging to some styles, making it difficult for the model to learn style-specific patterns. Moreover, beer styles are not rigorously defined, with some styles overlapping in features and some beers being misattributed to a specific style, all of which leads to more noise in models that use style parameters.
Model validation
To test if our predictive models give insight into beer appreciation, we set up experiments aimed at improving existing commercial beers. We specifically selected overall appreciation as the trait to be examined because of its complexity and commercial relevance. Beer flavor comprises a complex bouquet rather than single aromas and tastes 53 . Hence, adding a single compound to the extent that a difference is noticeable may lead to an unbalanced, artificial flavor. Therefore, we evaluated the effect of combinations of compounds. Because Blond beers represent the most extensive style in our dataset, we selected a beer from this style as the starting material for these experiments (Beer 64 in Supplementary Data 1 ).
In the first set of experiments, we adjusted the concentrations of compounds that made up the most important predictors of overall appreciation (ethyl acetate, ethanol, lactic acid, ethyl phenyl acetate) together with correlated compounds (ethyl hexanoate, isoamyl acetate, glycerol), bringing them up to 95 th percentile ethanol-normalized concentrations (Methods) within the Blond group (‘Spiked’ concentration in Fig. 5A ). Compared to controls, the spiked beers were found to have significantly improved overall appreciation among trained panelists, with panelist noting increased intensity of ester flavors, sweetness, alcohol, and body fullness (Fig. 5B ). To disentangle the contribution of ethanol to these results, a second experiment was performed without the addition of ethanol. This resulted in a similar outcome, including increased perception of alcohol and overall appreciation.
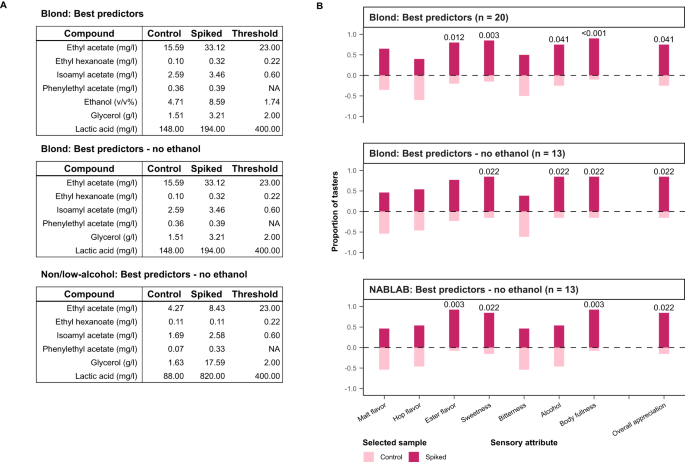
Adding the top chemical compounds, identified as best predictors of appreciation by our model, into poorly appreciated beers results in increased appreciation from our trained panel. Results of sensory tests between base beers and those spiked with compounds identified as the best predictors by the model. A Blond and Non/Low-alcohol (0.0% ABV) base beers were brought up to 95th-percentile ethanol-normalized concentrations within each style. B For each sensory attribute, tasters indicated the more intense sample and selected the sample they preferred. The numbers above the bars correspond to the p values that indicate significant changes in perceived flavor (two-sided binomial test: alpha 0.05, n = 20 or 13).
In a last experiment, we tested whether using the model’s predictions can boost the appreciation of a non-alcoholic beer (beer 223 in Supplementary Data 1 ). Again, the addition of a mixture of predicted compounds (omitting ethanol, in this case) resulted in a significant increase in appreciation, body, ester flavor and sweetness.
Predicting flavor and consumer appreciation from chemical composition is one of the ultimate goals of sensory science. A reliable, systematic and unbiased way to link chemical profiles to flavor and food appreciation would be a significant asset to the food and beverage industry. Such tools would substantially aid in quality control and recipe development, offer an efficient and cost-effective alternative to pilot studies and consumer trials and would ultimately allow food manufacturers to produce superior, tailor-made products that better meet the demands of specific consumer groups more efficiently.
A limited set of studies have previously tried, to varying degrees of success, to predict beer flavor and beer popularity based on (a limited set of) chemical compounds and flavors 79 , 80 . Current sensitive, high-throughput technologies allow measuring an unprecedented number of chemical compounds and properties in a large set of samples, yielding a dataset that can train models that help close the gaps between chemistry and flavor, even for a complex natural product like beer. To our knowledge, no previous research gathered data at this scale (250 samples, 226 chemical parameters, 50 sensory attributes and 5 consumer scores) to disentangle and validate the chemical aspects driving beer preference using various machine-learning techniques. We find that modern machine learning models outperform conventional statistical tools, such as correlations and linear models, and can successfully predict flavor appreciation from chemical composition. This could be attributed to the natural incorporation of interactions and non-linear or discontinuous effects in machine learning models, which are not easily grasped by the linear model architecture. While linear models and partial least squares regression represent the most widespread statistical approaches in sensory science, in part because they allow interpretation 65 , 81 , 82 , modern machine learning methods allow for building better predictive models while preserving the possibility to dissect and exploit the underlying patterns. Of the 10 different models we trained, tree-based models, such as our best performing GBR, showed the best overall performance in predicting sensory responses from chemical information, outcompeting artificial neural networks. This agrees with previous reports for models trained on tabular data 83 . Our results are in line with the findings of Colantonio et al. who also identified the gradient boosting architecture as performing best at predicting appreciation and flavor (of tomatoes and blueberries, in their specific study) 26 . Importantly, besides our larger experimental scale, we were able to directly confirm our models’ predictions in vivo.
Our study confirms that flavor compound concentration does not always correlate with perception, suggesting complex interactions that are often missed by more conventional statistics and simple models. Specifically, we find that tree-based algorithms may perform best in developing models that link complex food chemistry with aroma. Furthermore, we show that massive datasets of untrained consumer reviews provide a valuable source of data, that can complement or even replace trained tasting panels, especially for appreciation and basic flavors, such as sweetness and bitterness. This holds despite biases that are known to occur in such datasets, such as price or conformity bias. Moreover, GBR models predict taste better than aroma. This is likely because taste (e.g. bitterness) often directly relates to the corresponding chemical measurements (e.g., iso-alpha acids), whereas such a link is less clear for aromas, which often result from the interplay between multiple volatile compounds. We also find that our models are best at predicting acidity and alcohol, likely because there is a direct relation between the measured chemical compounds (acids and ethanol) and the corresponding perceived sensorial attribute (acidity and alcohol), and because even untrained consumers are generally able to recognize these flavors and aromas.
The predictions of our final models, trained on review data, hold even for blind tastings with small groups of trained tasters, as demonstrated by our ability to validate specific compounds as drivers of beer flavor and appreciation. Since adding a single compound to the extent of a noticeable difference may result in an unbalanced flavor profile, we specifically tested our identified key drivers as a combination of compounds. While this approach does not allow us to validate if a particular single compound would affect flavor and/or appreciation, our experiments do show that this combination of compounds increases consumer appreciation.
It is important to stress that, while it represents an important step forward, our approach still has several major limitations. A key weakness of the GBR model architecture is that amongst co-correlating variables, the largest main effect is consistently preferred for model building. As a result, co-correlating variables often have artificially low importance scores, both for impurity and SHAP-based methods, like we observed in the comparison to the more randomized Extra Trees models. This implies that chemicals identified as key drivers of a specific sensory feature by GBR might not be the true causative compounds, but rather co-correlate with the actual causative chemical. For example, the high importance of ethyl acetate could be (partially) attributed to the total ester content, ethanol or ethyl hexanoate (rho=0.77, rho=0.72 and rho=0.68), while ethyl phenylacetate could hide the importance of prenyl isobutyrate and ethyl benzoate (rho=0.77 and rho=0.76). Expanding our GBR model to include beer style as a parameter did not yield additional power or insight. This is likely due to style-specific chemical signatures, such as iso-alpha acids and lactic acid, that implicitly convey style information to the original model, as well as the smaller sample size per style, limiting the power to uncover style-specific patterns. This can be partly attributed to the curse of dimensionality, where the high number of parameters results in the models mainly incorporating single parameter effects, rather than complex interactions such as style-dependent effects 67 . A larger number of samples may overcome some of these limitations and offer more insight into style-specific effects. On the other hand, beer style is not a rigid scientific classification, and beers within one style often differ a lot, which further complicates the analysis of style as a model factor.
Our study is limited to beers from Belgian breweries. Although these beers cover a large portion of the beer styles available globally, some beer styles and consumer patterns may be missing, while other features might be overrepresented. For example, many Belgian ales exhibit yeast-driven flavor profiles, which is reflected in the chemical drivers of appreciation discovered by this study. In future work, expanding the scope to include diverse markets and beer styles could lead to the identification of even more drivers of appreciation and better models for special niche products that were not present in our beer set.
In addition to inherent limitations of GBR models, there are also some limitations associated with studying food aroma. Even if our chemical analyses measured most of the known aroma compounds, the total number of flavor compounds in complex foods like beer is still larger than the subset we were able to measure in this study. For example, hop-derived thiols, that influence flavor at very low concentrations, are notoriously difficult to measure in a high-throughput experiment. Moreover, consumer perception remains subjective and prone to biases that are difficult to avoid. It is also important to stress that the models are still immature and that more extensive datasets will be crucial for developing more complete models in the future. Besides more samples and parameters, our dataset does not include any demographic information about the tasters. Including such data could lead to better models that grasp external factors like age and culture. Another limitation is that our set of beers consists of high-quality end-products and lacks beers that are unfit for sale, which limits the current model in accurately predicting products that are appreciated very badly. Finally, while models could be readily applied in quality control, their use in sensory science and product development is restrained by their inability to discern causal relationships. Given that the models cannot distinguish compounds that genuinely drive consumer perception from those that merely correlate, validation experiments are essential to identify true causative compounds.
Despite the inherent limitations, dissection of our models enabled us to pinpoint specific molecules as potential drivers of beer aroma and consumer appreciation, including compounds that were unexpected and would not have been identified using standard approaches. Important drivers of beer appreciation uncovered by our models include protein levels, ethyl acetate, ethyl phenyl acetate and lactic acid. Currently, many brewers already use lactic acid to acidify their brewing water and ensure optimal pH for enzymatic activity during the mashing process. Our results suggest that adding lactic acid can also improve beer appreciation, although its individual effect remains to be tested. Interestingly, ethanol appears to be unnecessary to improve beer appreciation, both for blond beer and alcohol-free beer. Given the growing consumer interest in alcohol-free beer, with a predicted annual market growth of >7% 84 , it is relevant for brewers to know what compounds can further increase consumer appreciation of these beers. Hence, our model may readily provide avenues to further improve the flavor and consumer appreciation of both alcoholic and non-alcoholic beers, which is generally considered one of the key challenges for future beer production.
Whereas we see a direct implementation of our results for the development of superior alcohol-free beverages and other food products, our study can also serve as a stepping stone for the development of novel alcohol-containing beverages. We want to echo the growing body of scientific evidence for the negative effects of alcohol consumption, both on the individual level by the mutagenic, teratogenic and carcinogenic effects of ethanol 85 , 86 , as well as the burden on society caused by alcohol abuse and addiction. We encourage the use of our results for the production of healthier, tastier products, including novel and improved beverages with lower alcohol contents. Furthermore, we strongly discourage the use of these technologies to improve the appreciation or addictive properties of harmful substances.
The present work demonstrates that despite some important remaining hurdles, combining the latest developments in chemical analyses, sensory analysis and modern machine learning methods offers exciting avenues for food chemistry and engineering. Soon, these tools may provide solutions in quality control and recipe development, as well as new approaches to sensory science and flavor research.
Beer selection
250 commercial Belgian beers were selected to cover the broad diversity of beer styles and corresponding diversity in chemical composition and aroma. See Supplementary Fig. S1 .
Chemical dataset
Sample preparation.
Beers within their expiration date were purchased from commercial retailers. Samples were prepared in biological duplicates at room temperature, unless explicitly stated otherwise. Bottle pressure was measured with a manual pressure device (Steinfurth Mess-Systeme GmbH) and used to calculate CO 2 concentration. The beer was poured through two filter papers (Macherey-Nagel, 500713032 MN 713 ¼) to remove carbon dioxide and prevent spontaneous foaming. Samples were then prepared for measurements by targeted Headspace-Gas Chromatography-Flame Ionization Detector/Flame Photometric Detector (HS-GC-FID/FPD), Headspace-Solid Phase Microextraction-Gas Chromatography-Mass Spectrometry (HS-SPME-GC-MS), colorimetric analysis, enzymatic analysis, Near-Infrared (NIR) analysis, as described in the sections below. The mean values of biological duplicates are reported for each compound.
HS-GC-FID/FPD
HS-GC-FID/FPD (Shimadzu GC 2010 Plus) was used to measure higher alcohols, acetaldehyde, esters, 4-vinyl guaicol, and sulfur compounds. Each measurement comprised 5 ml of sample pipetted into a 20 ml glass vial containing 1.75 g NaCl (VWR, 27810.295). 100 µl of 2-heptanol (Sigma-Aldrich, H3003) (internal standard) solution in ethanol (Fisher Chemical, E/0650DF/C17) was added for a final concentration of 2.44 mg/L. Samples were flushed with nitrogen for 10 s, sealed with a silicone septum, stored at −80 °C and analyzed in batches of 20.
The GC was equipped with a DB-WAXetr column (length, 30 m; internal diameter, 0.32 mm; layer thickness, 0.50 µm; Agilent Technologies, Santa Clara, CA, USA) to the FID and an HP-5 column (length, 30 m; internal diameter, 0.25 mm; layer thickness, 0.25 µm; Agilent Technologies, Santa Clara, CA, USA) to the FPD. N 2 was used as the carrier gas. Samples were incubated for 20 min at 70 °C in the headspace autosampler (Flow rate, 35 cm/s; Injection volume, 1000 µL; Injection mode, split; Combi PAL autosampler, CTC analytics, Switzerland). The injector, FID and FPD temperatures were kept at 250 °C. The GC oven temperature was first held at 50 °C for 5 min and then allowed to rise to 80 °C at a rate of 5 °C/min, followed by a second ramp of 4 °C/min until 200 °C kept for 3 min and a final ramp of (4 °C/min) until 230 °C for 1 min. Results were analyzed with the GCSolution software version 2.4 (Shimadzu, Kyoto, Japan). The GC was calibrated with a 5% EtOH solution (VWR International) containing the volatiles under study (Supplementary Table S7 ).
HS-SPME-GC-MS
HS-SPME-GC-MS (Shimadzu GCMS-QP-2010 Ultra) was used to measure additional volatile compounds, mainly comprising terpenoids and esters. Samples were analyzed by HS-SPME using a triphase DVB/Carboxen/PDMS 50/30 μm SPME fiber (Supelco Co., Bellefonte, PA, USA) followed by gas chromatography (Thermo Fisher Scientific Trace 1300 series, USA) coupled to a mass spectrometer (Thermo Fisher Scientific ISQ series MS) equipped with a TriPlus RSH autosampler. 5 ml of degassed beer sample was placed in 20 ml vials containing 1.75 g NaCl (VWR, 27810.295). 5 µl internal standard mix was added, containing 2-heptanol (1 g/L) (Sigma-Aldrich, H3003), 4-fluorobenzaldehyde (1 g/L) (Sigma-Aldrich, 128376), 2,3-hexanedione (1 g/L) (Sigma-Aldrich, 144169) and guaiacol (1 g/L) (Sigma-Aldrich, W253200) in ethanol (Fisher Chemical, E/0650DF/C17). Each sample was incubated at 60 °C in the autosampler oven with constant agitation. After 5 min equilibration, the SPME fiber was exposed to the sample headspace for 30 min. The compounds trapped on the fiber were thermally desorbed in the injection port of the chromatograph by heating the fiber for 15 min at 270 °C.
The GC-MS was equipped with a low polarity RXi-5Sil MS column (length, 20 m; internal diameter, 0.18 mm; layer thickness, 0.18 µm; Restek, Bellefonte, PA, USA). Injection was performed in splitless mode at 320 °C, a split flow of 9 ml/min, a purge flow of 5 ml/min and an open valve time of 3 min. To obtain a pulsed injection, a programmed gas flow was used whereby the helium gas flow was set at 2.7 mL/min for 0.1 min, followed by a decrease in flow of 20 ml/min to the normal 0.9 mL/min. The temperature was first held at 30 °C for 3 min and then allowed to rise to 80 °C at a rate of 7 °C/min, followed by a second ramp of 2 °C/min till 125 °C and a final ramp of 8 °C/min with a final temperature of 270 °C.
Mass acquisition range was 33 to 550 amu at a scan rate of 5 scans/s. Electron impact ionization energy was 70 eV. The interface and ion source were kept at 275 °C and 250 °C, respectively. A mix of linear n-alkanes (from C7 to C40, Supelco Co.) was injected into the GC-MS under identical conditions to serve as external retention index markers. Identification and quantification of the compounds were performed using an in-house developed R script as described in Goelen et al. and Reher et al. 87 , 88 (for package information, see Supplementary Table S8 ). Briefly, chromatograms were analyzed using AMDIS (v2.71) 89 to separate overlapping peaks and obtain pure compound spectra. The NIST MS Search software (v2.0 g) in combination with the NIST2017, FFNSC3 and Adams4 libraries were used to manually identify the empirical spectra, taking into account the expected retention time. After background subtraction and correcting for retention time shifts between samples run on different days based on alkane ladders, compound elution profiles were extracted and integrated using a file with 284 target compounds of interest, which were either recovered in our identified AMDIS list of spectra or were known to occur in beer. Compound elution profiles were estimated for every peak in every chromatogram over a time-restricted window using weighted non-negative least square analysis after which peak areas were integrated 87 , 88 . Batch effect correction was performed by normalizing against the most stable internal standard compound, 4-fluorobenzaldehyde. Out of all 284 target compounds that were analyzed, 167 were visually judged to have reliable elution profiles and were used for final analysis.
Discrete photometric and enzymatic analysis
Discrete photometric and enzymatic analysis (Thermo Scientific TM Gallery TM Plus Beermaster Discrete Analyzer) was used to measure acetic acid, ammonia, beta-glucan, iso-alpha acids, color, sugars, glycerol, iron, pH, protein, and sulfite. 2 ml of sample volume was used for the analyses. Information regarding the reagents and standard solutions used for analyses and calibrations is included in Supplementary Table S7 and Supplementary Table S9 .
NIR analyses
NIR analysis (Anton Paar Alcolyzer Beer ME System) was used to measure ethanol. Measurements comprised 50 ml of sample, and a 10% EtOH solution was used for calibration.
Correlation calculations
Pairwise Spearman Rank correlations were calculated between all chemical properties.
Sensory dataset
Trained panel.
Our trained tasting panel consisted of volunteers who gave prior verbal informed consent. All compounds used for the validation experiment were of food-grade quality. The tasting sessions were approved by the Social and Societal Ethics Committee of the KU Leuven (G-2022-5677-R2(MAR)). All online reviewers agreed to the Terms and Conditions of the RateBeer website.
Sensory analysis was performed according to the American Society of Brewing Chemists (ASBC) Sensory Analysis Methods 90 . 30 volunteers were screened through a series of triangle tests. The sixteen most sensitive and consistent tasters were retained as taste panel members. The resulting panel was diverse in age [22–42, mean: 29], sex [56% male] and nationality [7 different countries]. The panel developed a consensus vocabulary to describe beer aroma, taste and mouthfeel. Panelists were trained to identify and score 50 different attributes, using a 7-point scale to rate attributes’ intensity. The scoring sheet is included as Supplementary Data 3 . Sensory assessments took place between 10–12 a.m. The beers were served in black-colored glasses. Per session, between 5 and 12 beers of the same style were tasted at 12 °C to 16 °C. Two reference beers were added to each set and indicated as ‘Reference 1 & 2’, allowing panel members to calibrate their ratings. Not all panelists were present at every tasting. Scores were scaled by standard deviation and mean-centered per taster. Values are represented as z-scores and clustered by Euclidean distance. Pairwise Spearman correlations were calculated between taste and aroma sensory attributes. Panel consistency was evaluated by repeating samples on different sessions and performing ANOVA to identify differences, using the ‘stats’ package (v4.2.2) in R (for package information, see Supplementary Table S8 ).
Online reviews from a public database
The ‘scrapy’ package in Python (v3.6) (for package information, see Supplementary Table S8 ). was used to collect 232,288 online reviews (mean=922, min=6, max=5343) from RateBeer, an online beer review database. Each review entry comprised 5 numerical scores (appearance, aroma, taste, palate and overall quality) and an optional review text. The total number of reviews per reviewer was collected separately. Numerical scores were scaled and centered per rater, and mean scores were calculated per beer.
For the review texts, the language was estimated using the packages ‘langdetect’ and ‘langid’ in Python. Reviews that were classified as English by both packages were kept. Reviewers with fewer than 100 entries overall were discarded. 181,025 reviews from >6000 reviewers from >40 countries remained. Text processing was done using the ‘nltk’ package in Python. Texts were corrected for slang and misspellings; proper nouns and rare words that are relevant to the beer context were specified and kept as-is (‘Chimay’,’Lambic’, etc.). A dictionary of semantically similar sensorial terms, for example ‘floral’ and ‘flower’, was created and collapsed together into one term. Words were stemmed and lemmatized to avoid identifying words such as ‘acid’ and ‘acidity’ as separate terms. Numbers and punctuation were removed.
Sentences from up to 50 randomly chosen reviews per beer were manually categorized according to the aspect of beer they describe (appearance, aroma, taste, palate, overall quality—not to be confused with the 5 numerical scores described above) or flagged as irrelevant if they contained no useful information. If a beer contained fewer than 50 reviews, all reviews were manually classified. This labeled data set was used to train a model that classified the rest of the sentences for all beers 91 . Sentences describing taste and aroma were extracted, and term frequency–inverse document frequency (TFIDF) was implemented to calculate enrichment scores for sensorial words per beer.
The sex of the tasting subject was not considered when building our sensory database. Instead, results from different panelists were averaged, both for our trained panel (56% male, 44% female) and the RateBeer reviews (70% male, 30% female for RateBeer as a whole).
Beer price collection and processing
Beer prices were collected from the following stores: Colruyt, Delhaize, Total Wine, BeerHawk, The Belgian Beer Shop, The Belgian Shop, and Beer of Belgium. Where applicable, prices were converted to Euros and normalized per liter. Spearman correlations were calculated between these prices and mean overall appreciation scores from RateBeer and the taste panel, respectively.
Pairwise Spearman Rank correlations were calculated between all sensory properties.
Machine learning models
Predictive modeling of sensory profiles from chemical data.
Regression models were constructed to predict (a) trained panel scores for beer flavors and quality from beer chemical profiles and (b) public reviews’ appreciation scores from beer chemical profiles. Z-scores were used to represent sensory attributes in both data sets. Chemical properties with log-normal distributions (Shapiro-Wilk test, p < 0.05 ) were log-transformed. Missing chemical measurements (0.1% of all data) were replaced with mean values per attribute. Observations from 250 beers were randomly separated into a training set (70%, 175 beers) and a test set (30%, 75 beers), stratified per beer style. Chemical measurements (p = 231) were normalized based on the training set average and standard deviation. In total, three linear regression-based models: linear regression with first-order interaction terms (LR), lasso regression with first-order interaction terms (Lasso) and partial least squares regression (PLSR); five decision tree models, Adaboost regressor (ABR), Extra Trees (ET), Gradient Boosting regressor (GBR), Random Forest (RF) and XGBoost regressor (XGBR); one support vector machine model (SVR) and one artificial neural network model (ANN) were trained. The models were implemented using the ‘scikit-learn’ package (v1.2.2) and ‘xgboost’ package (v1.7.3) in Python (v3.9.16). Models were trained, and hyperparameters optimized, using five-fold cross-validated grid search with the coefficient of determination (R 2 ) as the evaluation metric. The ANN (scikit-learn’s MLPRegressor) was optimized using Bayesian Tree-Structured Parzen Estimator optimization with the ‘Optuna’ Python package (v3.2.0). Individual models were trained per attribute, and a multi-output model was trained on all attributes simultaneously.
Model dissection
GBR was found to outperform other methods, resulting in models with the highest average R 2 values in both trained panel and public review data sets. Impurity-based rankings of the most important predictors for each predicted sensorial trait were obtained using the ‘scikit-learn’ package. To observe the relationships between these chemical properties and their predicted targets, partial dependence plots (PDP) were constructed for the six most important predictors of consumer appreciation 74 , 75 .
The ‘SHAP’ package in Python (v0.41.0) was implemented to provide an alternative ranking of predictor importance and to visualize the predictors’ effects as a function of their concentration 68 .
Validation of causal chemical properties
To validate the effects of the most important model features on predicted sensory attributes, beers were spiked with the chemical compounds identified by the models and descriptive sensory analyses were carried out according to the American Society of Brewing Chemists (ASBC) protocol 90 .
Compound spiking was done 30 min before tasting. Compounds were spiked into fresh beer bottles, that were immediately resealed and inverted three times. Fresh bottles of beer were opened for the same duration, resealed, and inverted thrice, to serve as controls. Pairs of spiked samples and controls were served simultaneously, chilled and in dark glasses as outlined in the Trained panel section above. Tasters were instructed to select the glass with the higher flavor intensity for each attribute (directional difference test 92 ) and to select the glass they prefer.
The final concentration after spiking was equal to the within-style average, after normalizing by ethanol concentration. This was done to ensure balanced flavor profiles in the final spiked beer. The same methods were applied to improve a non-alcoholic beer. Compounds were the following: ethyl acetate (Merck KGaA, W241415), ethyl hexanoate (Merck KGaA, W243906), isoamyl acetate (Merck KGaA, W205508), phenethyl acetate (Merck KGaA, W285706), ethanol (96%, Colruyt), glycerol (Merck KGaA, W252506), lactic acid (Merck KGaA, 261106).
Significant differences in preference or perceived intensity were determined by performing the two-sided binomial test on each attribute.
Reporting summary
Further information on research design is available in the Nature Portfolio Reporting Summary linked to this article.
Data availability
The data that support the findings of this work are available in the Supplementary Data files and have been deposited to Zenodo under accession code 10653704 93 . The RateBeer scores data are under restricted access, they are not publicly available as they are property of RateBeer (ZX Ventures, USA). Access can be obtained from the authors upon reasonable request and with permission of RateBeer (ZX Ventures, USA). Source data are provided with this paper.
Code availability
The code for training the machine learning models, analyzing the models, and generating the figures has been deposited to Zenodo under accession code 10653704 93 .
Tieman, D. et al. A chemical genetic roadmap to improved tomato flavor. Science 355 , 391–394 (2017).
Article ADS CAS PubMed Google Scholar
Plutowska, B. & Wardencki, W. Application of gas chromatography–olfactometry (GC–O) in analysis and quality assessment of alcoholic beverages – A review. Food Chem. 107 , 449–463 (2008).
Article CAS Google Scholar
Legin, A., Rudnitskaya, A., Seleznev, B. & Vlasov, Y. Electronic tongue for quality assessment of ethanol, vodka and eau-de-vie. Anal. Chim. Acta 534 , 129–135 (2005).
Loutfi, A., Coradeschi, S., Mani, G. K., Shankar, P. & Rayappan, J. B. B. Electronic noses for food quality: A review. J. Food Eng. 144 , 103–111 (2015).
Ahn, Y.-Y., Ahnert, S. E., Bagrow, J. P. & Barabási, A.-L. Flavor network and the principles of food pairing. Sci. Rep. 1 , 196 (2011).
Article CAS PubMed PubMed Central Google Scholar
Bartoshuk, L. M. & Klee, H. J. Better fruits and vegetables through sensory analysis. Curr. Biol. 23 , R374–R378 (2013).
Article CAS PubMed Google Scholar
Piggott, J. R. Design questions in sensory and consumer science. Food Qual. Prefer. 3293 , 217–220 (1995).
Article Google Scholar
Kermit, M. & Lengard, V. Assessing the performance of a sensory panel-panellist monitoring and tracking. J. Chemom. 19 , 154–161 (2005).
Cook, D. J., Hollowood, T. A., Linforth, R. S. T. & Taylor, A. J. Correlating instrumental measurements of texture and flavour release with human perception. Int. J. Food Sci. Technol. 40 , 631–641 (2005).
Chinchanachokchai, S., Thontirawong, P. & Chinchanachokchai, P. A tale of two recommender systems: The moderating role of consumer expertise on artificial intelligence based product recommendations. J. Retail. Consum. Serv. 61 , 1–12 (2021).
Ross, C. F. Sensory science at the human-machine interface. Trends Food Sci. Technol. 20 , 63–72 (2009).
Chambers, E. IV & Koppel, K. Associations of volatile compounds with sensory aroma and flavor: The complex nature of flavor. Molecules 18 , 4887–4905 (2013).
Pinu, F. R. Metabolomics—The new frontier in food safety and quality research. Food Res. Int. 72 , 80–81 (2015).
Danezis, G. P., Tsagkaris, A. S., Brusic, V. & Georgiou, C. A. Food authentication: state of the art and prospects. Curr. Opin. Food Sci. 10 , 22–31 (2016).
Shepherd, G. M. Smell images and the flavour system in the human brain. Nature 444 , 316–321 (2006).
Meilgaard, M. C. Prediction of flavor differences between beers from their chemical composition. J. Agric. Food Chem. 30 , 1009–1017 (1982).
Xu, L. et al. Widespread receptor-driven modulation in peripheral olfactory coding. Science 368 , eaaz5390 (2020).
Kupferschmidt, K. Following the flavor. Science 340 , 808–809 (2013).
Billesbølle, C. B. et al. Structural basis of odorant recognition by a human odorant receptor. Nature 615 , 742–749 (2023).
Article ADS PubMed PubMed Central Google Scholar
Smith, B. Perspective: Complexities of flavour. Nature 486 , S6–S6 (2012).
Pfister, P. et al. Odorant receptor inhibition is fundamental to odor encoding. Curr. Biol. 30 , 2574–2587 (2020).
Moskowitz, H. W., Kumaraiah, V., Sharma, K. N., Jacobs, H. L. & Sharma, S. D. Cross-cultural differences in simple taste preferences. Science 190 , 1217–1218 (1975).
Eriksson, N. et al. A genetic variant near olfactory receptor genes influences cilantro preference. Flavour 1 , 22 (2012).
Ferdenzi, C. et al. Variability of affective responses to odors: Culture, gender, and olfactory knowledge. Chem. Senses 38 , 175–186 (2013).
Article PubMed Google Scholar
Lawless, H. T. & Heymann, H. Sensory evaluation of food: Principles and practices. (Springer, New York, NY). https://doi.org/10.1007/978-1-4419-6488-5 (2010).
Colantonio, V. et al. Metabolomic selection for enhanced fruit flavor. Proc. Natl. Acad. Sci. 119 , e2115865119 (2022).
Fritz, F., Preissner, R. & Banerjee, P. VirtualTaste: a web server for the prediction of organoleptic properties of chemical compounds. Nucleic Acids Res 49 , W679–W684 (2021).
Tuwani, R., Wadhwa, S. & Bagler, G. BitterSweet: Building machine learning models for predicting the bitter and sweet taste of small molecules. Sci. Rep. 9 , 1–13 (2019).
Dagan-Wiener, A. et al. Bitter or not? BitterPredict, a tool for predicting taste from chemical structure. Sci. Rep. 7 , 1–13 (2017).
Pallante, L. et al. Toward a general and interpretable umami taste predictor using a multi-objective machine learning approach. Sci. Rep. 12 , 1–11 (2022).
Malavolta, M. et al. A survey on computational taste predictors. Eur. Food Res. Technol. 248 , 2215–2235 (2022).
Lee, B. K. et al. A principal odor map unifies diverse tasks in olfactory perception. Science 381 , 999–1006 (2023).
Mayhew, E. J. et al. Transport features predict if a molecule is odorous. Proc. Natl. Acad. Sci. 119 , e2116576119 (2022).
Niu, Y. et al. Sensory evaluation of the synergism among ester odorants in light aroma-type liquor by odor threshold, aroma intensity and flash GC electronic nose. Food Res. Int. 113 , 102–114 (2018).
Yu, P., Low, M. Y. & Zhou, W. Design of experiments and regression modelling in food flavour and sensory analysis: A review. Trends Food Sci. Technol. 71 , 202–215 (2018).
Oladokun, O. et al. The impact of hop bitter acid and polyphenol profiles on the perceived bitterness of beer. Food Chem. 205 , 212–220 (2016).
Linforth, R., Cabannes, M., Hewson, L., Yang, N. & Taylor, A. Effect of fat content on flavor delivery during consumption: An in vivo model. J. Agric. Food Chem. 58 , 6905–6911 (2010).
Guo, S., Na Jom, K. & Ge, Y. Influence of roasting condition on flavor profile of sunflower seeds: A flavoromics approach. Sci. Rep. 9 , 11295 (2019).
Ren, Q. et al. The changes of microbial community and flavor compound in the fermentation process of Chinese rice wine using Fagopyrum tataricum grain as feedstock. Sci. Rep. 9 , 3365 (2019).
Hastie, T., Friedman, J. & Tibshirani, R. The Elements of Statistical Learning. (Springer, New York, NY). https://doi.org/10.1007/978-0-387-21606-5 (2001).
Dietz, C., Cook, D., Huismann, M., Wilson, C. & Ford, R. The multisensory perception of hop essential oil: a review. J. Inst. Brew. 126 , 320–342 (2020).
CAS Google Scholar
Roncoroni, Miguel & Verstrepen, Kevin Joan. Belgian Beer: Tested and Tasted. (Lannoo, 2018).
Meilgaard, M. Flavor chemistry of beer: Part II: Flavor and threshold of 239 aroma volatiles. in (1975).
Bokulich, N. A. & Bamforth, C. W. The microbiology of malting and brewing. Microbiol. Mol. Biol. Rev. MMBR 77 , 157–172 (2013).
Dzialo, M. C., Park, R., Steensels, J., Lievens, B. & Verstrepen, K. J. Physiology, ecology and industrial applications of aroma formation in yeast. FEMS Microbiol. Rev. 41 , S95–S128 (2017).
Article PubMed PubMed Central Google Scholar
Datta, A. et al. Computer-aided food engineering. Nat. Food 3 , 894–904 (2022).
American Society of Brewing Chemists. Beer Methods. (American Society of Brewing Chemists, St. Paul, MN, U.S.A.).
Olaniran, A. O., Hiralal, L., Mokoena, M. P. & Pillay, B. Flavour-active volatile compounds in beer: production, regulation and control. J. Inst. Brew. 123 , 13–23 (2017).
Verstrepen, K. J. et al. Flavor-active esters: Adding fruitiness to beer. J. Biosci. Bioeng. 96 , 110–118 (2003).
Meilgaard, M. C. Flavour chemistry of beer. part I: flavour interaction between principal volatiles. Master Brew. Assoc. Am. Tech. Q 12 , 107–117 (1975).
Briggs, D. E., Boulton, C. A., Brookes, P. A. & Stevens, R. Brewing 227–254. (Woodhead Publishing). https://doi.org/10.1533/9781855739062.227 (2004).
Bossaert, S., Crauwels, S., De Rouck, G. & Lievens, B. The power of sour - A review: Old traditions, new opportunities. BrewingScience 72 , 78–88 (2019).
Google Scholar
Verstrepen, K. J. et al. Flavor active esters: Adding fruitiness to beer. J. Biosci. Bioeng. 96 , 110–118 (2003).
Snauwaert, I. et al. Microbial diversity and metabolite composition of Belgian red-brown acidic ales. Int. J. Food Microbiol. 221 , 1–11 (2016).
Spitaels, F. et al. The microbial diversity of traditional spontaneously fermented lambic beer. PLoS ONE 9 , e95384 (2014).
Blanco, C. A., Andrés-Iglesias, C. & Montero, O. Low-alcohol Beers: Flavor Compounds, Defects, and Improvement Strategies. Crit. Rev. Food Sci. Nutr. 56 , 1379–1388 (2016).
Jackowski, M. & Trusek, A. Non-Alcohol. beer Prod. – Overv. 20 , 32–38 (2018).
Takoi, K. et al. The contribution of geraniol metabolism to the citrus flavour of beer: Synergy of geraniol and β-citronellol under coexistence with excess linalool. J. Inst. Brew. 116 , 251–260 (2010).
Kroeze, J. H. & Bartoshuk, L. M. Bitterness suppression as revealed by split-tongue taste stimulation in humans. Physiol. Behav. 35 , 779–783 (1985).
Mennella, J. A. et al. A spoonful of sugar helps the medicine go down”: Bitter masking bysucrose among children and adults. Chem. Senses 40 , 17–25 (2015).
Wietstock, P., Kunz, T., Perreira, F. & Methner, F.-J. Metal chelation behavior of hop acids in buffered model systems. BrewingScience 69 , 56–63 (2016).
Sancho, D., Blanco, C. A., Caballero, I. & Pascual, A. Free iron in pale, dark and alcohol-free commercial lager beers. J. Sci. Food Agric. 91 , 1142–1147 (2011).
Rodrigues, H. & Parr, W. V. Contribution of cross-cultural studies to understanding wine appreciation: A review. Food Res. Int. 115 , 251–258 (2019).
Korneva, E. & Blockeel, H. Towards better evaluation of multi-target regression models. in ECML PKDD 2020 Workshops (eds. Koprinska, I. et al.) 353–362 (Springer International Publishing, Cham, 2020). https://doi.org/10.1007/978-3-030-65965-3_23 .
Gastón Ares. Mathematical and Statistical Methods in Food Science and Technology. (Wiley, 2013).
Grinsztajn, L., Oyallon, E. & Varoquaux, G. Why do tree-based models still outperform deep learning on tabular data? Preprint at http://arxiv.org/abs/2207.08815 (2022).
Gries, S. T. Statistics for Linguistics with R: A Practical Introduction. in Statistics for Linguistics with R (De Gruyter Mouton, 2021). https://doi.org/10.1515/9783110718256 .
Lundberg, S. M. et al. From local explanations to global understanding with explainable AI for trees. Nat. Mach. Intell. 2 , 56–67 (2020).
Ickes, C. M. & Cadwallader, K. R. Effects of ethanol on flavor perception in alcoholic beverages. Chemosens. Percept. 10 , 119–134 (2017).
Kato, M. et al. Influence of high molecular weight polypeptides on the mouthfeel of commercial beer. J. Inst. Brew. 127 , 27–40 (2021).
Wauters, R. et al. Novel Saccharomyces cerevisiae variants slow down the accumulation of staling aldehydes and improve beer shelf-life. Food Chem. 398 , 1–11 (2023).
Li, H., Jia, S. & Zhang, W. Rapid determination of low-level sulfur compounds in beer by headspace gas chromatography with a pulsed flame photometric detector. J. Am. Soc. Brew. Chem. 66 , 188–191 (2008).
Dercksen, A., Laurens, J., Torline, P., Axcell, B. C. & Rohwer, E. Quantitative analysis of volatile sulfur compounds in beer using a membrane extraction interface. J. Am. Soc. Brew. Chem. 54 , 228–233 (1996).
Molnar, C. Interpretable Machine Learning: A Guide for Making Black-Box Models Interpretable. (2020).
Zhao, Q. & Hastie, T. Causal interpretations of black-box models. J. Bus. Econ. Stat. Publ. Am. Stat. Assoc. 39 , 272–281 (2019).
Article MathSciNet Google Scholar
Hastie, T., Tibshirani, R. & Friedman, J. The Elements of Statistical Learning. (Springer, 2019).
Labrado, D. et al. Identification by NMR of key compounds present in beer distillates and residual phases after dealcoholization by vacuum distillation. J. Sci. Food Agric. 100 , 3971–3978 (2020).
Lusk, L. T., Kay, S. B., Porubcan, A. & Ryder, D. S. Key olfactory cues for beer oxidation. J. Am. Soc. Brew. Chem. 70 , 257–261 (2012).
Gonzalez Viejo, C., Torrico, D. D., Dunshea, F. R. & Fuentes, S. Development of artificial neural network models to assess beer acceptability based on sensory properties using a robotic pourer: A comparative model approach to achieve an artificial intelligence system. Beverages 5 , 33 (2019).
Gonzalez Viejo, C., Fuentes, S., Torrico, D. D., Godbole, A. & Dunshea, F. R. Chemical characterization of aromas in beer and their effect on consumers liking. Food Chem. 293 , 479–485 (2019).
Gilbert, J. L. et al. Identifying breeding priorities for blueberry flavor using biochemical, sensory, and genotype by environment analyses. PLOS ONE 10 , 1–21 (2015).
Goulet, C. et al. Role of an esterase in flavor volatile variation within the tomato clade. Proc. Natl. Acad. Sci. 109 , 19009–19014 (2012).
Article ADS CAS PubMed PubMed Central Google Scholar
Borisov, V. et al. Deep Neural Networks and Tabular Data: A Survey. IEEE Trans. Neural Netw. Learn. Syst. 1–21 https://doi.org/10.1109/TNNLS.2022.3229161 (2022).
Statista. Statista Consumer Market Outlook: Beer - Worldwide.
Seitz, H. K. & Stickel, F. Molecular mechanisms of alcoholmediated carcinogenesis. Nat. Rev. Cancer 7 , 599–612 (2007).
Voordeckers, K. et al. Ethanol exposure increases mutation rate through error-prone polymerases. Nat. Commun. 11 , 3664 (2020).
Goelen, T. et al. Bacterial phylogeny predicts volatile organic compound composition and olfactory response of an aphid parasitoid. Oikos 129 , 1415–1428 (2020).
Article ADS Google Scholar
Reher, T. et al. Evaluation of hop (Humulus lupulus) as a repellent for the management of Drosophila suzukii. Crop Prot. 124 , 104839 (2019).
Stein, S. E. An integrated method for spectrum extraction and compound identification from gas chromatography/mass spectrometry data. J. Am. Soc. Mass Spectrom. 10 , 770–781 (1999).
American Society of Brewing Chemists. Sensory Analysis Methods. (American Society of Brewing Chemists, St. Paul, MN, U.S.A., 1992).
McAuley, J., Leskovec, J. & Jurafsky, D. Learning Attitudes and Attributes from Multi-Aspect Reviews. Preprint at https://doi.org/10.48550/arXiv.1210.3926 (2012).
Meilgaard, M. C., Carr, B. T. & Carr, B. T. Sensory Evaluation Techniques. (CRC Press, Boca Raton). https://doi.org/10.1201/b16452 (2014).
Schreurs, M. et al. Data from: Predicting and improving complex beer flavor through machine learning. Zenodo https://doi.org/10.5281/zenodo.10653704 (2024).
Download references
Acknowledgements
We thank all lab members for their discussions and thank all tasting panel members for their contributions. Special thanks go out to Dr. Karin Voordeckers for her tremendous help in proofreading and improving the manuscript. M.S. was supported by a Baillet-Latour fellowship, L.C. acknowledges financial support from KU Leuven (C16/17/006), F.A.T. was supported by a PhD fellowship from FWO (1S08821N). Research in the lab of K.J.V. is supported by KU Leuven, FWO, VIB, VLAIO and the Brewing Science Serves Health Fund. Research in the lab of T.W. is supported by FWO (G.0A51.15) and KU Leuven (C16/17/006).
Author information
These authors contributed equally: Michiel Schreurs, Supinya Piampongsant, Miguel Roncoroni.
Authors and Affiliations
VIB—KU Leuven Center for Microbiology, Gaston Geenslaan 1, B-3001, Leuven, Belgium
Michiel Schreurs, Supinya Piampongsant, Miguel Roncoroni, Lloyd Cool, Beatriz Herrera-Malaver, Florian A. Theßeling & Kevin J. Verstrepen
CMPG Laboratory of Genetics and Genomics, KU Leuven, Gaston Geenslaan 1, B-3001, Leuven, Belgium
Leuven Institute for Beer Research (LIBR), Gaston Geenslaan 1, B-3001, Leuven, Belgium
Laboratory of Socioecology and Social Evolution, KU Leuven, Naamsestraat 59, B-3000, Leuven, Belgium
Lloyd Cool, Christophe Vanderaa & Tom Wenseleers
VIB Bioinformatics Core, VIB, Rijvisschestraat 120, B-9052, Ghent, Belgium
Łukasz Kreft & Alexander Botzki
AB InBev SA/NV, Brouwerijplein 1, B-3000, Leuven, Belgium
Philippe Malcorps & Luk Daenen
You can also search for this author in PubMed Google Scholar
Contributions
S.P., M.S. and K.J.V. conceived the experiments. S.P., M.S. and K.J.V. designed the experiments. S.P., M.S., M.R., B.H. and F.A.T. performed the experiments. S.P., M.S., L.C., C.V., L.K., A.B., P.M., L.D., T.W. and K.J.V. contributed analysis ideas. S.P., M.S., L.C., C.V., T.W. and K.J.V. analyzed the data. All authors contributed to writing the manuscript.
Corresponding author
Correspondence to Kevin J. Verstrepen .
Ethics declarations
Competing interests.
K.J.V. is affiliated with bar.on. The other authors declare no competing interests.
Peer review
Peer review information.
Nature Communications thanks Florian Bauer, Andrew John Macintosh and the other, anonymous, reviewer(s) for their contribution to the peer review of this work. A peer review file is available.
Additional information
Publisher’s note Springer Nature remains neutral with regard to jurisdictional claims in published maps and institutional affiliations.
Supplementary information
Supplementary information, peer review file, description of additional supplementary files, supplementary data 1, supplementary data 2, supplementary data 3, supplementary data 4, supplementary data 5, supplementary data 6, supplementary data 7, reporting summary, source data, source data, rights and permissions.
Open Access This article is licensed under a Creative Commons Attribution 4.0 International License, which permits use, sharing, adaptation, distribution and reproduction in any medium or format, as long as you give appropriate credit to the original author(s) and the source, provide a link to the Creative Commons licence, and indicate if changes were made. The images or other third party material in this article are included in the article’s Creative Commons licence, unless indicated otherwise in a credit line to the material. If material is not included in the article’s Creative Commons licence and your intended use is not permitted by statutory regulation or exceeds the permitted use, you will need to obtain permission directly from the copyright holder. To view a copy of this licence, visit http://creativecommons.org/licenses/by/4.0/ .
Reprints and permissions
About this article
Cite this article.
Schreurs, M., Piampongsant, S., Roncoroni, M. et al. Predicting and improving complex beer flavor through machine learning. Nat Commun 15 , 2368 (2024). https://doi.org/10.1038/s41467-024-46346-0
Download citation
Received : 30 October 2023
Accepted : 21 February 2024
Published : 26 March 2024
DOI : https://doi.org/10.1038/s41467-024-46346-0
Share this article
Anyone you share the following link with will be able to read this content:
Sorry, a shareable link is not currently available for this article.
Provided by the Springer Nature SharedIt content-sharing initiative
By submitting a comment you agree to abide by our Terms and Community Guidelines . If you find something abusive or that does not comply with our terms or guidelines please flag it as inappropriate.
Quick links
- Explore articles by subject
- Guide to authors
- Editorial policies
Sign up for the Nature Briefing: Translational Research newsletter — top stories in biotechnology, drug discovery and pharma.

Supervised machine learning algorithms for predicting student dropout and academic success: a comparative study
- Open access
- Published: 04 January 2024
- Volume 4 , article number 2 , ( 2024 )
Cite this article
You have full access to this open access article
- Alice Villar ORCID: orcid.org/0000-0001-8250-1340 1 &
- Carolina Robledo Velini de Andrade ORCID: orcid.org/0000-0002-5344-2991 2
1522 Accesses
Explore all metrics
Utilizing a dataset sourced from a higher education institution, this study aims to assess the efficacy of diverse machine learning algorithms in predicting student dropout and academic success. Our focus was on algorithms capable of effectively handling imbalanced data. To tackle class imbalance, we employed the SMOTE resampling technique. We applied a range of algorithms, including Decision Tree (DT), Support Vector Machine (SVM), Random Forest (RF), as well as boosting algorithms such as Gradient Boosting (GB), Extreme Gradient Boosting (XGBoost), CatBoost (CB), and Light Gradient Boosting Machine (LB). To enhance the models' performance, we conducted hyperparameter tuning using Optuna. Additionally, we employed the Isolation Forest (IF) method to identify outliers or anomalies within the dataset. Notably, our findings indicate that boosting algorithms, particularly LightGBM and CatBoost with Optuna, outperformed traditional classification methods. Our study's generalizability to other contexts is constrained due to its reliance on a single dataset, with inherent limitations. Nevertheless, this research provides valuable insights into the effectiveness of various machine learning algorithms for predicting student dropout and academic success. By benchmarking these algorithms, our project offers guidance to both researchers and practitioners in their choice of suitable approaches for similar predictive tasks.
Similar content being viewed by others
Early Prediction of student’s Performance in Higher Education: A Case Study
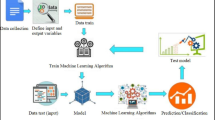
Predicting the percentage of student placement: A comparative study of machine learning algorithms
Erman Çakıt & Metin Dağdeviren
Predicting Student Retention Among a Homogeneous Population Using Data Mining
Avoid common mistakes on your manuscript.
1 Introduction
Predicting student behavior is a crucial task for educational institutions, as it can help to improve curriculum design and plan academic support interventions that are timely and personalized. As Chung and Lee [ 1 ] highlighted, “at-risk students” who drop out of school due to difficulties are more likely to adopt antisocial behaviors or face challenges in the labor market, making it harder for them to adapt to society.
Machine learning (ML) techniques, such as predictive modeling, have the potential to improve student retention by allowing educators to recognize students' weaknesses and provide learning metrics at any stage of educational progress [ 2 ]. The application of these techniques can be used to aid in the development of early warning systems, which can detect students who are at risk of dropping out in advance and offer the necessary support [ 3 ].
In this comparative study, we explore various methods for predicting student success and dropout in higher education institutions, building upon the work of Martins et al. [ 4 ], “Early Prediction of Student’s Performance in Higher Education: a Case Study.” Martins et al. used machine learning classification models to predict students who might be at risk of failing to complete their degrees on time at the Polytechnic Institute of Portalegre (IPP) in Portugal.
While their study focused solely on supervised classification algorithms, we expand upon their work by incorporating unsupervised classification algorithms into our analysis. We apply resampling methods such as SMOTE and ADASYN to enhance the representation of the minority class in the dataset. Additionally, we employ various machine learning algorithms, including cost-sensitive learning algorithms, ensemble algorithms, and unsupervised anomaly detection algorithms. Furthermore, we leverage SMOTE and ADASYN with the unsupervised classification algorithm Isolation Forest to identify patterns and clusters within the data.
In this paper, we present a comparative study that utilizes the dataset collected by Martins et al. [ 4 ].
Specifically, we aim to address the following research questions:
RQ1: how effective are different resampling techniques SMOTE and ADASYN in addressing class imbalance in the context of dropout prediction?
RQ2: which machine learning algorithms are most effective in predicting student dropout in our dataset?
RQ3: how do boosting algorithms (Gradient Boosting, Extreme Gradient Boosting, CatBoost, and LightGBM) compare to traditional machine learning algorithms in predicting student dropout?
RQ4: what are the key factors that contribute to student success or failure, as revealed by the application of SHAP (SHapley Additive exPlanations)?
The remainder of this paper is organized as follows. In Sect. 2 , we provide a condensed analysis of related work, focusing on the class imbalance problem in the context of dropout prediction. In this section, we also discuss the findings of Martins et al. [ 4 ], which serve as a foundation for our comparative study. In Sect. 2 , we offer a concise review of related research, with a specific focus on addressing the class imbalance problem within dropout prediction. Additionally, we discuss the key findings of Martins et al. [ 4 ], which serve as the basis for our comparative study. Later in Sect. 3 , we initiate our exploration with a bibliometric analysis, examining the knowledge structure surrounding the efficacy of boosting algorithms in forecasting student academic success. Subsequently, we transition into a systematic literature review, concentrating on the most highly cited papers in this domain. Section 4 describes the methodology used to build and evaluate the Machine Learning (ML) models, including the data description, methods used to handle the unbalanced dataset, and the procedures for training and evaluating the classification models. In Sect. 5 , we present the experimental results of Supervised and Unsupervised Machine Learning models. In this section, we compare F1-scores of Supervised ML Algorithms before and after Hyperparameter Optimization with Optina and apply the SHAP (SHapley Additive exPlanations) method to explain the output of the algorithms that performed best, specifically LightGBM and CatBoost with Optuna. Section 6 presents a comprehensive assessment of supervised machine learning algorithms' performance in predicting student outcomes. Section 7 concludes the paper and suggests future research directions.
2 Analyzing class imbalance in dropout prediction and building on prior research
Section 2 provides a condensed analysis of the related work. The first subsection discusses the class imbalance problem and the application of unsupervised machine learning techniques in classification, particularly in the context of dropout prediction. The second subsection provides a summary of Martins et al. ’s [ 4 ] research, which serves as a foundation for our comparative study.
2.1 Addressing class imbalance in machine learning for classification
In educational data mining (EDM), class imbalance is a common issue, particularly when dealing with student retention data. This occurs because the number of students who drop out is significantly smaller than the number of students who stay in school or have good academic performance.
Class imbalance negatively impacts the accuracy of predictive models. Due to the scarcity of data points on the minority class, they tend to be biased towards the majority class, resulting in poor predictions for the minority class. [ 2 , 5 ]. In such cases, standard classifier algorithms may not perform well, as they usually have a bias towards the majority class.
To address this issue, a number of techniques have been proposed in the literature. As noted by Islam et al. [ 6 ], in the past few decades, three popular approaches have been used to handle imbalanced data: data-driven, algorithm-based, and hybrid methods.
Data-driven methods focus on balancing the distribution of classes in the training set by either oversampling the minority class or undersampling the majority class (increasing the representation of the minority class or decreasing the representation of the majority class). Oversampling techniques include Random Over-Sampling Examples (ROSE), Synthetic Minority Over-Sampling Technique (SMOTE), and Adaptive Synthetic (ADASYN), among others. Undersampling techniques include Random Under-Sampling Examples (RUSE) and Tomek Links (TL).
Algorithm-based techniques, on the other hand, modify the learning algorithms to improve their performance on imbalanced data. Examples of such techniques include cost-sensitive learning, anomaly detection, and ensemble-based methods like bagging and boosting.
Hybrid methods combine data-driven and algorithm-based techniques to improve the performance of machine learning models on imbalanced data. For example, hybrid methods may use data-driven techniques to balance the distribution of classes in the training set and then apply algorithm-based techniques to further improve the performance of the model.
Class imbalance is a common problem in predicting student performance, and various techniques have been proposed in the literature to address it. Rastrollo-Guerrero et al. [ 7 ] conducted a review of nearly 70 papers to identify the modern techniques commonly used for predicting student performance. They found that supervised learning, particularly the Support Vector Machine algorithm, was the most widely used and provided accurate results. Decision Tree (DT), Naïve Bayes (NB), and Random Forest (RF) were also well-studied algorithms that produced good results.
According to Rastrollo-Guerrero et al. [ 7 ], unsupervised learning is often considered an unattractive technique for researchers due to its low accuracy in predicting students' behavior in certain cases. However, the authors suggest that this can serve as an incentive for further research to improve these techniques and obtain more reliable results.
Our study aims to contribute to this area by exploring the effectiveness of unsupervised machine learning using Isolation Forest (IF) for predicting student dropout, while also addressing class imbalance in the data. Despite our efforts, our study found that IF did not perform well in predicting student dropout. These findings highlight the need for continued research into alternative machine learning algorithms and techniques that can effectively handle class imbalance and improve dropout prediction.
Recent research has demonstrated the effectiveness of boosting algorithms in predicting student dropout [ 8 , 9 ]. These studies are in line with our findings, which demonstrate that boosting algorithms outperformed traditional classification methods.
2.2 Summary of Martins et al. [ 4 ] research
In this section, we briefly describe the purpose of their study, the methods they used, the datasets they worked with, and their findings. In addition, it shows the include limitations of their study and how our comparative study builds upon their work.
Martins et al. [ 4 ] aimed to develop a system that could identify students with potential difficulties in their academic path at an early stage, so that strategies to support the students could be put into place. Their research focused on building a system that generalizes to any course at IPP, rather than focusing on a specific field of study. The dataset included information from students enrolled in several courses from the four different schools belonging to IPP. Additionally, their paper only relied on information available at the time of enrollment and did not include any information on academic performance after enrollment. Another unique aspect of the paper was the use of a third intermediate class (relative success) in addition to the usual approach of restricting the model categories to failure/success. This allowed for different interventions for academic support and guidance for students who are at moderate risk versus those who are at high risk of being unsuccessful.
In their work, Martins et al. [ 4 ] highlighted the limitations of using accuracy as a performance metric for models trained on imbalanced datasets. This is because accuracy may give a false sense of good performance, as it tends to favor the majority class and can ignore the performance of the minority classes. To address this issue, Martins et al. used single-class metrics to evaluate the performance of the model on each class separately.
Specifically, they used the F1 measure, which takes into account the balance between precision and recall, as the performance metric for the three classes in the dataset. By computing F1 scores for each class, Martins et al. were able to gain insights into the performance of the model for both the majority and minority classes. They were then able to use the average F1 score for the three classes as the metric for hyperparameter tuning, ensuring that the model is optimized to perform well on all classes.
Additionally, Martins et al. computed the accuracy of the optimized model as an overall metric, which provides a useful summary of the model’s performance. By using both F1 scores and accuracy as performance metrics, Martins et al. were able to gain a more complete understanding of the performance of their model, especially for imbalanced datasets.
Martins et al. [ 4 ] aimed to improve classification performance using different algorithms. Initially, they applied four algorithms, namely DT, SVM, RL, and RF, to the dataset. Then, they applied the same four algorithms with hyperparameter tuning using grid search and compared the results. To deal with the class imbalance challenge, they applied data sampling techniques SMOTE (Synthetic Minority Over-sampling Technique) and ADASYN (Adaptive Synthetic Sampling) to the dataset prior to model training. They found that SMOTE outperformed ADASYN. Thus, they applied SMOTE and used the same four algorithms with hyperparameter tuning using grid search and compared the results. Furthermore, Martins et al. [ 4 ] applied four boosting algorithms, namely Gradient Boosting, Extreme Gradient Boosting, CatBoost, and LogitBoost, using SMOTE. They then applied the same four boosting algorithms with hyperparameter tuning using randomized grid search and compared the results, using SMOTE.
In the study conducted by Martins et al. [ 4 ], the findings suggest that these boosting algorithms outperform standard methods when dealing with particular classification tasks. Among the four boosting algorithms assessed, Extreme Gradient Boosting emerged as the top classifier, although very similar to Gradient Boosting. However, despite the use of boosting algorithms, Martins et al. found that these models still struggled to accurately classify the minority classes in the imbalanced dataset. This is a common issue with imbalanced datasets, where the majority class dominates the dataset and makes it difficult for the model to learn from the minority class samples.
3 Systematic review and bibliometric analysis: boosting algorithms in student success prediction
In this section, we conducted a comprehensive bibliometric analysis using the Bibliometrix R-package. Table 1 presents the assessment criteria and Table 2 outlines our search strategy methodology. Our search yielded a total of 203 relevant articles.
Expanding on the knowledge acquired from the Most Globally Cited Documents among the 203 articles, we conducted a Systematic Literature Review (SLR) detailed in Sect. 3.2 . Our focus was on the 15 most frequently cited papers in the field. These papers illuminate the present state of research regarding boosting algorithms, particularly Gradient Boosting (GB) and Extreme Gradient Boosting (XGBoost), in the context of student performance. These algorithms consistently exhibited superior performance, highlighting their potential as valuable tools for advancing educational research and enhancing student outcomes.
3.1 Overview of the literature landscape
This subsection presents two informative graphs: Annual Scientific Production (Fig. 1 ) and the Most Globally Cited Documents (Fig. 2 ), both based on our dataset of 203 documents. Additionally, Table 3 provides key statistics, revealing a notable annual growth rate of 78.18% and an average document age of just 1.42 years, emphasizing the timeliness of research in this domain. Notably, each document in our dataset received an average of 6.241 citations, underscoring the significance and influence of the literature.
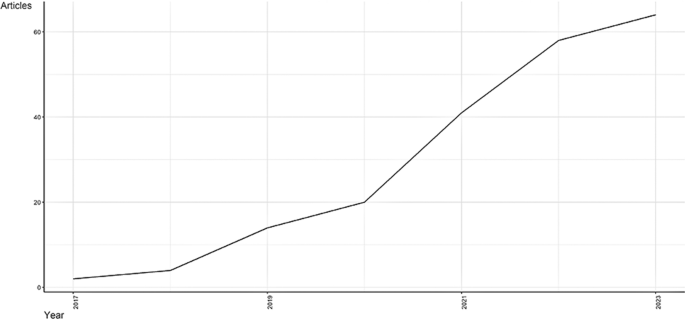
Annual scientific production
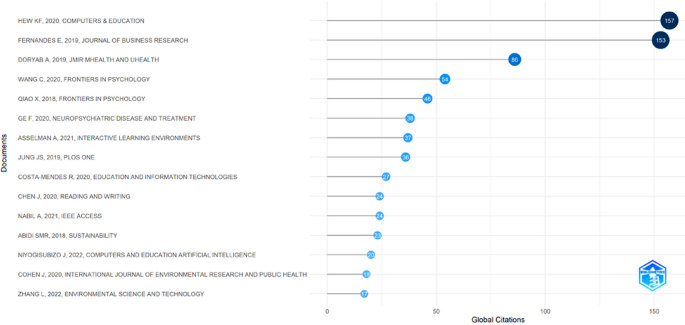
Most globally cited documents
3.2 Systematic literature review: leveraging boosting algorithms for precision in predicting student performance
This section presents our Systematic Literature Review (SLR) based on the 15 most frequently cited papers in the field (Table 4 ). This table provides additional details for each document in the ranked list of our bibliometric research (Fig. 2 ), including the full title, the total number of citations per year (TC per year), and the normalized citation impact scores (normalized TC).
In this section, we present the findings of our Systematic Literature Review (SLR) focused on the 15 most frequently cited papers in the field, which are presented in Table 4 . This table provides comprehensive details for each document in our bibliometric research (see Fig. 2 ). The information includes the full title, the total number of citations per year (TC per year), and the normalized citation impact scores (normalized TC).
In each of the following studies, boosting algorithms, particularly Gradient Boosting (GB) and Extreme Gradient Boosting (XGBoost), consistently showcased superior performance, underscoring their potential as valuable tools for enhancing educational research and improving student outcomes.
Predicting student satisfaction with MOOCs [ 10 ]
In their study, Hew et al. aimed to measure MOOC success based on student satisfaction, rather than conventional completion rates. Employing machine learning and sentiment analysis, the study examined 249 MOOCs and student perceptions. Notably, their research revealed that the gradient boosting trees model outperformed other candidate models, demonstrating acceptable values for accuracy, precision, recall, F1 score, and Cohen’s kappa, leading to its selection for the study.
Predicting academic performance in public schools [ 11 ]
Fernandes et al. developed classification models using the Gradient Boost Machine (GBM) algorithm to predict student performance and compared their predictive capabilities at different points in the academic cycle. They began with a descriptive statistical analysis of datasets from the State Department of Education in the Federal District of Brazil, creating two datasets—one with attributes collected before the school year commenced and another with additional variables like ‘absences,’ ‘grades,’ and ‘school subjects’ gathered two months into the school year. The choice of GBM classification models stemmed from its ability to combine multiple decision trees into a potent predictive model. Notably, the accuracy of these models was significantly enhanced through the boosting technique, which played a pivotal role in leveraging data and decision trees to offer valuable insights into student academic outcomes.
Identifying behavioral phenotypes of loneliness in college students [ 12 ]
Doryab et al. employed various analytic methods to understand loneliness in college students. They used machine learning classification to infer the level of loneliness and changes in loneliness levels, employing an ensemble of gradient boosting and logistic regression algorithms with feature selection. Notably, the boosting algorithm, gradient boosting, was chosen for its ability to perform well on noisy datasets and learn complex nonlinear decision boundaries. In their research, gradient boosting demonstrated superior performance in detecting outcomes related to loneliness, which highlights its effectiveness in analyzing and predicting behavioral phenotypes associated with loneliness and social isolation among college students.
Anxiety levels during COVID-19 in Chinese University students [ 13 ]
Wang et al. explored anxiety severity among Chinese non-graduating undergraduate students during the COVID-19 pandemic using machine learning techniques. The study applied machine learning techniques, specifically Extreme Gradient Boosting (XGBoost), to predict both the anxiety level and changes in anxiety levels four weeks later, using initial SAS (a sociodemographic questionnaire and the Self-Rating Anxiety Scale) scores from students’ first test. Remarkably, the XGBoost model demonstrated superior prediction accuracy when compared to the traditional multiple stepwise regression model in evaluating the anxiety status of university students.
Educational assessment process data analysis [ 14 ]
Qiao and Jiao addressed the application of data mining methods to analyze educational assessment process data. Among the supervised techniques used, the results indicated that gradient boosting outperformed the other methods, achieving the highest classification accuracy with a Kappa value of 0.94 and an overall accuracy of 0.96. Overall, all four methods demonstrated satisfactory classification accuracy, but the boosting algorithm, gradient boosting, exhibited superior performance in accurately analyzing the educational assessment process data, highlighting its efficacy in this context.
Predicting psychological state among Chinese undergraduate students [ 15 ]
Ge et al. aimed to develop predictive models for identifying adolescents at high risk of suicide during the COVID-19 using machine learning techniques. The gradient boosting algorithm demonstrated one of the highest accuracy rates among the models. Their study included 2009 students, with a response rate of 80.36%. The Extreme Gradient Boosting (XGBoost) algorithm demonstrated remarkable performance by accurately predicting 97.3% of students with probable anxiety and 96.2% of students with probable insomnia symptoms.
Enhancing student performance prediction with ensemble methods [ 16 ]
Asselman et al. aimed to improve the accuracy of predicting student performance by harnessing Ensemble Learning methods in the context of Knowledge Tracing (KT). They introduced a new Performance Factors Analysis (PFA) approach utilizing various models, including Random Forest, AdaBoost, and XGBoost. These models were evaluated across three distinct datasets, and the experimental results revealed that the scalable XGBoost algorithm outperformed the other models, leading to a substantial enhancement in performance prediction compared to the original PFA algorithm.
Predicting suicidal risk in Korean adolescents [ 17 ]
Jung et al. sought to develop predictive models for identifying adolescents at high risk of suicide using machine learning methods. They employed five different machine learning techniques, including logistic regression (LR), random forest (RF), support vector machine (SVM), artificial neural network (ANN), and extreme gradient boosting (XGB). Through their analysis, they found that factors such as sadness, violence, substance use, and stress were associated with an increased risk of suicidal ideation/attempt among adolescents. Using 26 predictor variables, the machine learning models demonstrated comparable accuracy to logistic regression, with XGB exhibiting the highest accuracy at 79.0%, followed by SVM at 78.7%, LR at 77.9%, RF at 77.8%, and ANN at 77.5%.
Predicting high school academic scores [ 18 ]
Costa-Mendes et al. aimed to predict high school academic scores using machine learning techniques. They employed several algorithms, including Random Forest (RF), support vector machine (SVM), artificial neural network (ANN), and extreme gradient boosting (XGB), stacking them as an ensemble. The results of their study demonstrated that all machine learning algorithms outperformed the classical multiple linear regression model in terms of predictive accuracy for high school academic scores. Specifically, XGB showed superior performance to RF, particularly when specific conditions related to the determinant of the relationship between matrices (Det [R]) were met.
Disparities in elementary students’ reading literacy [ 19 ]
Chen et al. examined disparities in reading literacy among elementary students and identify the key factors contributing to these disparities. They employed four machine learning algorithms: logistic regression, support vector machine (SVM), decision tree (DT), and extreme gradient boosting (XGBoost). These algorithms were utilized to classify and predict high- and low-proficiency readers while pinpointing the most influential factors in differentiating between high and low achievers. The study found that, compared to logistic regression and DT, both linear SVM and XGBoost exhibited outstanding and robust classification performances. Consequently, linear SVM and XGBoost were selected to identify the most important features or factors responsible for distinguishing high-achieving students from their low-achieving counterparts.
Early-stage prediction of student academic performance [ 20 ]
Nabil et al. addressed the challenge of early-stage prediction of student academic performance, with a focus on courses like “Programming” and “Data Structures” known for high failure and dropout rates. The study utilized various predictive models, including a Deep Neural Network (DNN), Decision Tree (DT), Random Forest (RF), Gradient Boosting (GB), Logistic Regression (LR), Support Vector Classifier, and K-Nearest Neighbor. They also tackled the issue of imbalanced datasets, employing resampling methods like SMOTE, ADASYN, ROS, and SMOTE-ENN to mitigate this challenge. Results from the experiments highlighted the effectiveness of the proposed DNN model in predicting students' performance in data structure courses and identifying students at risk of failure early in the semester, achieving an accuracy of 89%. This accuracy surpassed that of other models like decision tree, logistic regression, support vector classifier, and K-nearest neighbor. Notably, Gradient Boosting (GB) also exhibited strong performance, achieving one of the best accuracies at 91%, highlighting its effectiveness in addressing the imbalanced dataset issue and enhancing prediction results.
Identifying struggling students [ 21 ]
Abidi et al. used machine learning techniques to identify students who struggled with mastering algebra skills assigned as homework within an Intelligent Tutoring System (ITS). Gradient boosting, along with other models, exhibited high accuracy in classifying students attempting algebra homework. The study focused on ASSISTments, an ITS, and employed various machine learning techniques to analyze skill-builder data. Seven candidate models were utilized, including Naïve Bayes (NB), Generalized Linear Model (GLM), Logistic Regression (LR), Deep Learning (DL), Decision Tree (DT), Random Forest (RF), and Gradient Boosted Trees (XGBoost). The boosting algorithm, XGBoost, along with RF, GLM, and DL, exhibited high accuracy in classifying confused students attempting algebra homework within the ITS. The study emphasized the importance of leveraging machine learning methods to identify and support students who struggle with specific skills, ultimately contributing to their knowledge and promoting their role in sustainable development, with XGBoost showing one of the highest accuracies at 84.9%.
Predicting student dropout [ 22 ]
Niyogisubizo et al. proposed a novel stacked ensemble method for predicting student dropout, a pressing research topic. The authors proposed a novel stacked ensemble method, combining Random Forest (RF), Extreme Gradient Boosting (XGBoost), Gradient Boosting (GB), and Feed-forward Neural Networks (FNN) to achieve this prediction. Employing a dataset spanning from 2016 to 2020 from Constantine the Philosopher University in Nitra, their approach outperformed base models, as demonstrated by testing accuracy and the area under the curve (AUC) evaluation metrics. Particularly, XGBoost exhibited an AUC score of 0.978, emphasizing its strong performance in identifying students at risk of dropping out of university classes.
Predicting suicide risk based on language in therapy sessions [ 25 ]
Cohen et al. addressed the pressing issue of rising adolescent suicide rates by exploring the feasibility of using machine learning to identify suicide risk based on language samples from therapy sessions. This study involved the testing of natural language processing machine learning models within outpatient therapy sessions for adolescents. Data collection encompassed language samples, standardized depression and suicidality scale scores, and therapists' impressions of the client's mental state. Among the Machine Learning (ML) models tested, Extreme Gradient Boosting (XGBoost) demonstrated the best performance with an area under the curve (AUC) of 0.78 during external validation.
Monitoring health effects during haze pollution [ 23 ]
Zhang et al. investigated the potential of using breath-borne volatile organic compounds (VOCs) as biomarkers for monitoring health effects related to air pollution, specifically during haze pollution episodes. The study involved 47 healthy college students, and their exhaled breath samples were collected and analyzed for VOCs before, during, and after two separate haze pollution episodes using gas chromatography-ion mobility spectrometry (GC-IMS). Machine learning, specifically the Gradient Boosting Machine (GBM) model, was employed to differentiate between different exposure periods. The GBM model demonstrated excellent performance in distinguishing pre- and on-exposure to haze pollution, achieving precision rates of 90–100% for both haze episodes.
4 Methodology used to build and evaluate the machine learning models
This methodology section starts with an overview of the methodology used for building and evaluating unsupervised machine learning models, followed by detailed subsections that explain the data description and preprocessing, class imbalance treatment, model training and evaluation procedures, and experimental design. Finally, the section ends with a presentation and discussion of the experimental results.
4.1 Overview
The methodology employed in this study involved several sequential steps and algorithms to address the research objectives. An overview of our methodology is presented in Fig. 3 , outlining the key steps undertaken.
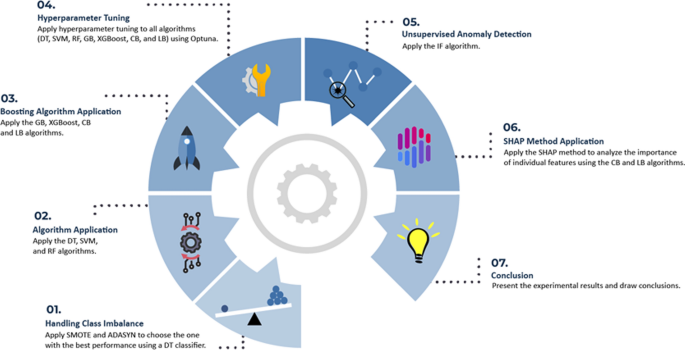
Methodology to build and evaluate the machine learning models
The first step involved applying the SMOTE (Synthetic Minority Over-sampling Technique) and ADASYN (Adaptive Synthetic Sampling) methods to resample the dataset. These techniques are commonly used for handling imbalanced datasets. To compare their performance, a decision tree classifier was employed, and the results were evaluated. Based on this comparison, the best-performing resampling technique was selected for further analysis.
Next, the preprocessed dataset was subjected to classification using three algorithms: Decision Tree (DT), Support Vector Machines (SVM), and Random Forest (RF). These algorithms were chosen for their effectiveness in classification tasks. The performance of each algorithm was evaluated to identify any significant differences.
To enhance the classification performance, boosting algorithms were employed, namely Gradient Boosting (GB), Extreme Gradient Boosting (XGBoost), CatBoost (CB), and LightGBM (LB). These algorithms are known for their ability to improve predictive power. By incorporating these boosting techniques, the study aimed to optimize the classification results obtained from the initial algorithms.
Furthermore, the study employed the Isolation Forests algorithm, an unsupervised anomaly detection technique, to identify outliers or anomalies within the dataset. This step focused on identifying instances that deviated significantly from the majority of the dataset.
To optimize the performance of all the algorithms used in this study, hyperparameter tuning was conducted using Optuna. Optuna is a hyperparameter optimization framework that assists in finding the best combination of hyperparameters for machine learning models. This step aimed to fine-tune the algorithms' performance by optimizing their respective hyperparameters. To ensure the reliability of our findings, we integrated Optuna with the cross-validation function, cross_val_score() . This approach not only enabled us to achieve hyperparameter optimization but also allowed for a rigorous assessment of the models' performance.
Additionally, the SHAP (SHapley Additive exPlanations) framework was applied to gain insights into the contribution of each feature in predicting the model's output. Specifically, SHAP was employed with the CATBoost (CB) and LightGBM (LB) algorithms to analyze the importance of individual features in the classification task. This analysis helped to enhance the understanding of how each feature influenced the model's predictions and identify key factors driving the classification results.
In the final phase of our study, we presented and thoroughly analyzed the experimental results obtained through various methods and algorithms. To evaluate the discriminative capabilities of our top-performing models, LightGBM (LB) and CatBoost (CB), we utilized ROC (Receiver Operating Characteristic) and AUC (Area Under the Curve) curves. Additionally, we conducted further assessments to explore potential significant differences in the results achieved by the LightGBM and CatBoost algorithms. To accomplish this, we employed the Statistical Tests for Algorithms Comparison (STAC) platform.
Table 5 compares the analysis methods we use in the present paper and the methodology used by Martins et al. [ 4 ], which was presented in Sect. 2.2 .
4.2 Data description
In this paper, we present a comparative study with Martins et al. [ 4 ], utilizing the same dataset that they collected. The dataset is in a CSV file format and contains information about 4424 students, with 35 columns. The dataset covers a range of factors that may influence a student’s academic success, including demographic, socio-economic, and academic factors. The data contains variables related to demographic factors (age at enrollment, gender, marital status, nationality, address code, special needs), socio-economic factors (whether the student works or not, the educational and employment background of their parents, whether they received a student grant or have student debt) and student’s academic path (admission grade, retention years at high school, their admission grade, how many years they spent in high school, the order of choice for their enrolled course, and the type, of course, they took in high school).
Martins et al. [ 4 ] classified each record into three categories based on how long it took the student to obtain their degree: Success, Relative Success, and Failure. Success means the student obtained their degree in due time, Relative Success means they took up to three extra years to obtain their degree, and Failure means they took more than three extra years to obtain their degree or did not obtain it at all. These categories correspond to different levels of risk, with low-risk students having a high probability of succeeding, medium-risk students who might benefit from institution measures to succeed, and high-risk students having a high probability of failing.
The imbalanced distribution of the records among the three categories presented challenges for developing accurate predictive models that could effectively identify high-risk students who might benefit from interventions. As a result, predictive models that are trained on such imbalanced datasets tend to be biased towards the majority class and may have lower accuracy in predicting the minority classes. As shown in Fig. 4 , in the distribution, “Failure” accounts for 32.15% of total records, and “Relative Success” accounts for 17.96% of total records, while the majority class, “Success”, accounts for 49.89% of the records.
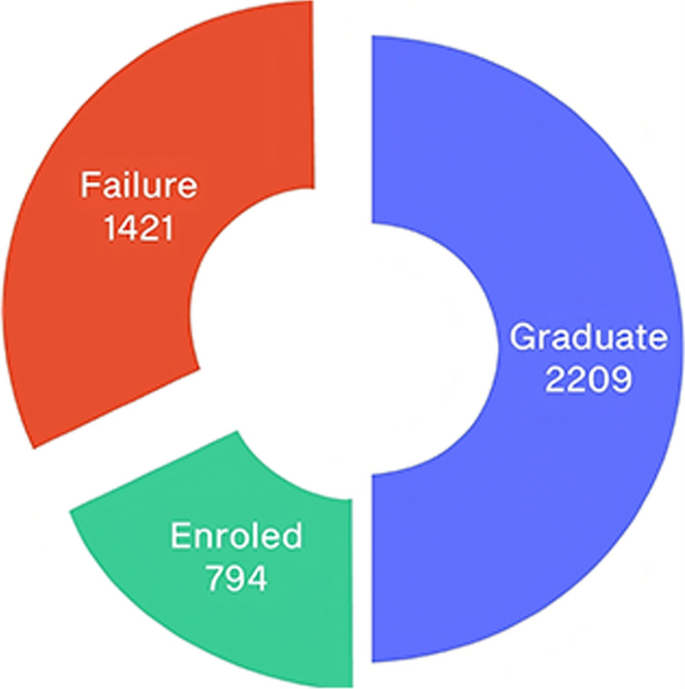
Data distribution
4.3 Resampling methods to address class imbalance
Class imbalance is a common challenge in classification tasks, such as predicting student dropout rates. To address this issue, we explore different resampling methods to improve the accuracy of our models in this study. Specifically, we compare the performance of two commonly used methods, SMOTE and ADASYN, to address the class imbalance in our dataset. We use decision trees to compare their performance, and it is worth noting that we do not use Logistic Regression due to the presence of multicollinearity in our data, as explained in Sect. 4.4 .
Our findings indicate that both SMOTE and Adasyn resampling methods effectively improve the accuracy of our models. However, SMOTE slightly outperforms Adasyn in addressing the class imbalance issue, as shown in Table 6 . It is important to note that the dataset we used in this study had no null and no missing data. This means that all the data points in the dataset were complete and did not require any imputation or handling of missing values.
4.4 Navigating challenges of data preprocessing
Martins et al. [ 4 ] employed a rigorous data preprocessing method to handle anomalies and unexplainable outliers, resulting in a final dataset consisting of 3623 records and 25 independent variables. In contrast, we decided not to remove anomalies in the preprocessing stage for several reasons, such as the potential impact on the representativeness of the dataset, the potential loss of valuable information, and the potential difficulty of distinguishing between true anomalies and rare but meaningful data points. As such, we have decided that the benefits of retaining anomalies outweighed the potential drawbacks.
Our preprocessing analysis revealed that there was significant Multicollinearity in our dataset. This means that predictor variables were highly correlated with each other, making it difficult to distinguish the individual effects of each variable on the outcome. Specifically, we employed the Variance Inflation Factor (VIF) method, which is a widely-used approach for detecting Multicollinearity in regression analysis. The VIF method calculates the degree of correlation between predictor variables, and if the correlation is high, it indicates that Multicollinearity is present in the data.
A VIF of 1 indicates that there is no multicollinearity, while a VIF of greater than 1 indicates that multicollinearity may be present. A VIF of 5 or greater is often considered high. Table 7 shows the variables with a VIF higher than 10. Table 8 shows the variables with a VIF between 5 and 10.
When Multicollinearity is present in a dataset, the regression coefficients can become unreliable or even meaningless, ultimately affecting the model's accuracy and validity. To mitigate this issue, we opted not to use Logistic Regression, which is not recommended in the presence of Multicollinearity and can lead to biased results. Instead, we used a range of machine learning algorithms that are better suited for handling Multicollinearity, such as decision trees, random forests, and gradient-boosting models.
5 Supervised ML algorithm comparison and Optuna hyperparameter optimization
This section presents the results obtained by applying three machine learning algorithms, namely Decision Trees, Support Vector Machines, and Random Forest, to predict student dropout and failure in our case study. Additionally, the section includes the results of Hyperparameter Optimization using Optuna.
5.1 Supervised algorithms
This section of the paper will present the F1-score results for seven different supervised machine learning algorithms: Decision Tree, Random Forest, Support Vector Machine, Gradient Boosting, Extreme Gradient Boosting, CatBoost, and LightGBM. The F1-score metric will be used to evaluate the performance of each algorithm. Additionally, the Optuna framework will be applied to optimize the hyperparameters of each model, and the F1-score after the application of Optuna will also be included in the table. The tables will provide a clear comparison of the performance of each algorithm, both before and after hyperparameter optimization.
The F1-score is a suitable evaluation metric for imbalanced datasets, as it balances precision and recall. In this study, the F1-score was chosen to assess the model’s accuracy, considering both false positives and false negatives. By combining precision and recall, the F1-score provides a comprehensive measure of the model's performance. Higher F1 scores indicate better classification performance for a specific class.
5.1.1 Traditional algorithms
This section provides an overview of the results obtained through the application of traditional algorithms, specifically Decision Tree (DT), Random Forest (RF), and Support Vector Machine (SVM), to predict student dropout and failure within our case study. Additionally, we present the outcomes of our Hyperparameter Optimization using Optuna. DT, RF, and SVM are machine learning algorithms used for various classification and regression tasks, but they differ in their underlying principles and how they make decisions.
Decision trees
Decision Trees are a supervised learning algorithm that partitions the data into subsets based on the values of different input features. It makes decisions by following a tree-like structure of binary decisions. Table 9 shows the F1 scores obtained from the Decision Tree (DT) model applied to three different classes: Graduate, Dropout, and Enrolled. The results reveal that the DT model achieved an F1 score of 0.75 for the Graduate class, 0.78 for the Dropout class, and 0.69 for the Enrolled class. These scores represent the RF model's ability to accurately classify instances from each respective class.
To further optimize the Decision Tree model's performance, the Optuna framework was applied. The “f1_weighted” metric was used to evaluate the hyperparameters, which takes into account the F1 scores for each class, considering the number of samples in each class and assigning higher weight to the classes with more samples. The hyperparameter optimization process was run for 20 trials. Comparing the F1 scores obtained from the DT model and the DT model with Optuna, it is observed that the F1 score for the three classes has increased slightly. This suggests that the hyperparameter optimization process was successful in improving the model's performance, especially for the Dropout class.
Random forest
Random Forest is an ensemble learning technique designed to enhance accuracy and mitigate overfitting by combining multiple decision trees. It achieves this by constructing numerous decision trees, each trained on a subset of the data, and then consolidating their predictions. This method, rooted in decision trees, finds utility in classification and regression tasks, distinguished for its robustness and adeptness in managing high-dimensional data.
Table 10 shows the F1 scores obtained from the Random Forest (RF) model applied to three different classes: Graduate, Dropout, and Enrolled. The results show that the Random Forest model achieved an F1 score of 0.84 for the Graduate class, 0.87 for the Dropout class, and 0.81 for the Enrolled class. These scores represent the RF model’s ability to accurately classify instances from each respective class. To further optimize the RF model’s performance, the Optuna framework was applied, using the “f1_weighted” metric to evaluate the hyperparameters. The hyperparameter optimization process was run for 20 trials. Comparing the F1 scores obtained from the RF model and the RF model with Optuna, it is observed that the F1 score remained practically the same. This suggests that the hyperparameter optimization process did not have a significant impact on the model's performance.
Support vector machines
Support Vector Machine (SVM) seeks the optimal hyperplane to separate data into distinct classes by maximizing the margin, which represents the distance between the hyperplane and the nearest data points of each class. This process entails mathematical optimization, where SVM strategically chooses support vectors, the data points closest to the hyperplane, to define the decision boundary. Table 11 presents the F1 scores obtained from the Support Vector Machines (SVM) model applied to three different classes: Graduate, Dropout, and Enrolled. Additionally, the table includes the results after applying the Optuna framework to optimize the SVM model's hyperparameters.
The initial SVM model achieved an F1 score of 0.79 for the Graduate class, 0.76 for the Dropout class, and 0.68 for the Enrolled class. These scores represent the SVM model's ability to accurately classify instances from each respective class. To further improve the SVM model's performance, the Optuna framework was employed to optimize its hyperparameters. Optuna conducts a search for the best combination of hyperparameters to enhance the model’s performance. The results reflect the F1 scores after applying Optuna to the SVM model. However, the F1 scores for the SVM model with Optuna remain the same as the initial SVM model. The F1 score for the Graduate class is 0.79, 0.76 for the Dropout class, and 0.68 for the Enrolled class. This indicates that the hyperparameter optimization process did not have a substantial impact on the model's performance in this case.
5.1.2 Boosting algorithms
This section presents the results obtained by applying four boosting algorithms, namely Gradient Boosting (GB), Extreme Gradient Boosting (XGBoost), CatBoost (CB), and LightGBM (LB) to predict student dropout and failure in our case study. Additionally, the section includes the results of Hyperparameter Optimization using Optuna. These four algorithms belong to the family of gradient boosting algorithms. They share the common purpose of improving predictive performance by combining weak learners through an ensemble approach, and they have each introduced unique optimizations to enhance their performance and usability. Additionally, each of these algorithms has introduced distinct optimizations to enhance their performance and usability.
Gradient boosting (GB)
Gradient Boosting (GB) serves as the foundational algorithm in this group of gradient boosting algorithms. It constructs an ensemble of decision trees sequentially, with each tree designed to rectify the errors of the preceding one. During training, it minimizes a loss function by adjusting the weights of data points.
Table 12 displays the F1 scores obtained from the Gradient Boosting (GB) model applied to three different classes: Graduate, Dropout, and Enrolled. Additionally, it includes the results after applying the Optuna framework to optimize the Gradient Boosting model’s hyperparameters. The initial Gradient Boosting model achieved an F1 score of 0.83 for the Graduate class, 0.85 for the Dropout class, and 0.76 for the Enrolled class. These scores represent the GB model's ability to accurately classify instances from each respective class.
To further improve the GB model’s performance, the Optuna framework was employed to optimize its hyperparameters. However, the F1 scores for the Gradient Boosting model with Optuna remain the same as the initial Gradient Boosting model. This suggests that the hyperparameter optimization process did not have a substantial impact on the model's performance in this case.
Extreme gradient boosting (XGBoost)
XGBoost represents an optimized and highly efficient implementation of gradient boosting, featuring several enhancements such as regularization, parallel processing, and a tree-pruning algorithm. These improvements contribute to its speed and often result in higher predictive accuracy compared to traditional gradient boosting.
Table 13 displays the F1 scores obtained from the Extreme Gradient Boosting (XGBoost) model applied to three different classes: Graduate, Dropout, and Enrolled. Additionally, the table includes the results after applying the Optuna framework to optimize the Gradient Boosting model's hyperparameters. The initial XGBoost model achieved an F1 score of 0.86 for the Graduate class, 0.88 for the Dropout class, and 0.83 for the Enrolled class. These scores represent the XGBoost model's ability to accurately classify instances from each respective class.
To further improve the XGBoost model's performance, the Optuna framework was utilized to optimize its hyperparameters. Notably, the F1 scores for the XGBoost model with Optuna differ from the initial XGBoost model. The F1 score for the Graduate class is 0.81, 0.82 for the Dropout class, and 0.75 for the Enrolled class. In this scenario, the hyperparameter optimization process led to a slight decrease in the F1 scores for the XGBoost model.
CatBoost (CB)
CatBoost (CB) is another gradient boosting algorithm tailored for categorical feature support. It can automatically handle categorical variables without one-hot encoding. CB also incorporates robust handling of missing data and employs ordered boosting to enhance its performance.
Table 14 presents the F1 scores obtained from the CB model applied to three different classes: Graduate, Dropout, and Enrolled. It also includes the results after applying the Optuna framework to optimize the CB model’s hyperparameters. The initial CB model achieved an F1 score of 0.86 for the Graduate class, 0.87 for the Dropout class, and 0.82 for the Enrolled class. These scores reflect the CatBoost model's ability to accurately classify instances from each respective class.
The results show that the F1 scores for the CB model with Optuna differ from the initial CatBoost model. The F1 score for the Graduate class is 0.86, 0.88 for the Dropout class, and 0.84 for the Enrolled class. This indicates that the hyperparameter optimization process had an impact on the model's performance, resulting in slightly higher F1 scores for the Dropout and Enrolled classes.
LightGBM (LB)
LightGBM (LB) is a machine learning algorithm prized for its remarkable speed and memory efficiency. This is achieved through its histogram-based tree-building approach and gradient-based one-sided sampling. LB proves invaluable for handling large datasets, where optimizing training times and resource utilization are critical factors.
Table 15 presents the F1 scores obtained from the LB model applied to three different classes: Graduate, Dropout, and Enrolled. The table also includes the results after applying the Optuna framework to optimize the LightGBM model’s hyperparameters. The initial LB model achieved an F1 score of 0.86 for the Graduate class, 0.87 for the Dropout class, and 0.83 for the Enrolled class. These scores reflect the LB model’s ability to accurately classify instances from each respective class.
The F1 scores for the LB model with Optuna remain the same as the initial LB model. The F1 score for the Graduate class is 0.86, 0.88 for the Dropout class, and 0.83 for the Enrolled class. This indicates that the hyperparameter optimization process did not have a substantial impact on the model’s performance in this case.
5.2 Unsupervised outlier detection with isolation forest
Isolation Forest (IF) is an anomaly detection algorithm frequently employed in machine learning and data mining to identify outliers or anomalies within a dataset. Its fundamental concept revolves around the isolation of anomalies by randomly selecting features and partitioning the data into subsets until the anomalies are singled out.
In our case study, we applied the Isolation Forest (IF) algorithm to assess its efficacy in outlier detection and classification within a dataset featuring three classes: failure, Enrolled, and Graduate. Our objective was to ascertain whether the minority classes, namely dropout and enrolled, would be identified as outliers. In order to evaluate the IF algorithm, we utilized the SMOTE test dataset, which consisted of 1325 instances. The application of the algorithm involved two stages. The first stage focuses on the overall results with the contamination as default. In the second stage, a contamination rate of 0.5 was applied to further evaluate the algorithm’s performance.
In the first stage, the following results were obtained:
Outlier detection:
Total number of outliers : The IF algorithm successfully identified 197 instances as outliers, representing data points that significantly deviated from the majority of the dataset. The outliers account for approximately 14.86% of the total dataset.
Minority classes:
Dropout Prediction and Enrolled Prediction : The IF algorithm predicted instances from the minority classes a total of 129 times. This highlights the algorithm's ability to identify and classify instances within these minority classes as outliers. These instances constitute approximately 9.73% of the total dataset.
Accurate Classification : The IF algorithm identified and classified instances as graduates 68 times. These instances account for approximately 5.13% of the total dataset.
In the second stage, the following results were obtained:
Anomalies Identified:
Dropout Prediction: The IF algorithm identified 357 instances of dropouts as anomalies, which represents 26.94%
Enrolled Prediction: the IF algorithm identified 155 instances of enrolled students as anomalies, which represents 11.69%.
Misclassifications:
The IF algorithm classified 181 instances as anomalies when they were actually graduates, which represents 13.66%. These indicate instances where the algorithm considered them to be anomalous, but in reality, they belong to the graduate class.
The performance of the IF algorithm in outlier detection and classification was promising. The algorithm showed effectiveness in identifying most of the minority classes as outliers. However, further analysis and refinement may be necessary to address the misclassification of graduates as anomalies while maintaining high accuracy in identifying other anomalies within the dataset.
5.3 SHAP method application
In order to gain a deeper understanding of the contribution of each feature in predicting the model’s output, we employed the SHAP (“SHapley Additive exPlanations”) method. This method provides insights into the importance and influence of different features on the model’s predictions.
We applied the SHAP method in conjunction with two algorithms: LightGBM (LB) and CatBoost. By leveraging SHAP with the LB algorithm (Fig. 5 ) and the CatBoost algorithm (Fig. 6 ), we obtained visual representations of the top ten variables and their respective importance scores. The outputs from both the LB and CatBoost algorithms demonstrated similar results, with the same variables consistently appearing at the top in terms of their contribution to the model’s predictions. This consistency indicates the robustness and reliability of these variables in influencing the model's output across different algorithms.
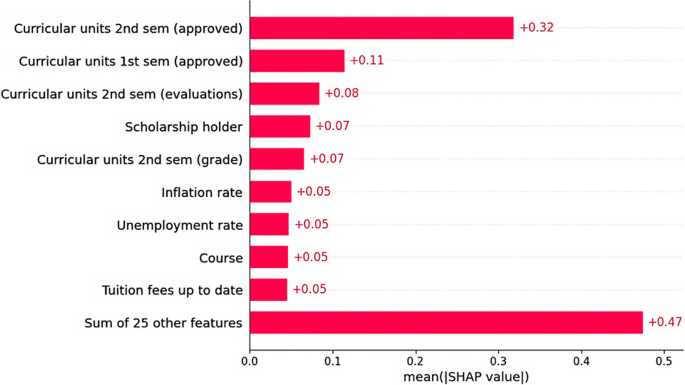
SHAP Method using LightGBM (LB)
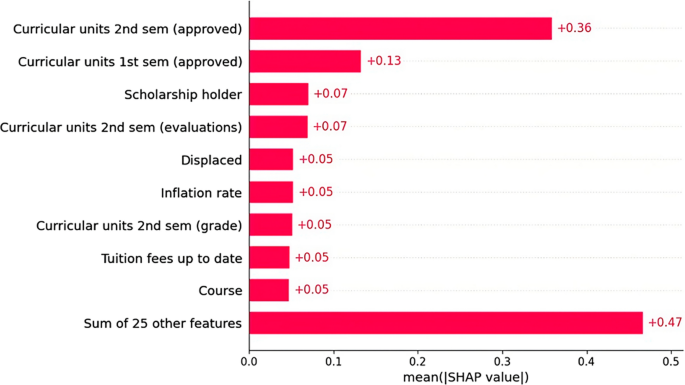
SHAP Method using CatBoost (CB)
By examining the top ten variables identified through SHAP analysis, we can gain valuable insights into the factors that have the most significant impact on the model’s predictions. These insights can guide us in understanding the underlying patterns and relationships within the dataset and help us make informed decisions related to feature selection, model interpretation, and potential areas for further investigation.
6 Discussion: performance evaluation and model comparison in predicting student outcomes
Table 16 summarizes the performance of the various supervised machine learning algorithms in the study. The models were evaluated based on their F1 scores for three target classes: Graduate, Dropout, and Enrolled. Boosting algorithms, specifically LightGBM (LB) and CatBoost (CB) with Optuna, consistently outperformed traditional classification algorithms in predicting student outcomes accurately, as evidenced by their higher F1 scores across the target classes. The success of boosting algorithms can be attributed to their ability to handle class imbalance effectively. Imbalanced class distributions are common in educational datasets, where minority classes, such as dropouts, require careful attention. Boosting algorithms, by nature, have mechanisms that address class imbalance issues and focus on learning from the minority classes, resulting in improved predictive performance.
To assess the discriminative capabilities of our top-performing models, LightGBM (LB) and CatBoost (CB), we employ ROC (Receiver Operating Characteristic) and AUC (Area Under the Curve) curves. The AUC value is a metric that ranges from 0 to 1, with higher values indicating superior model performance in classification tasks. In a broader context, an AUC value of 0.5 suggests random guessing (no discrimination), meaning the model struggles to distinguish between positive and negative cases. An AUC in the range of 0.7 to 0.8 is generally considered acceptable, while an AUC between 0.8 and 0.9 is regarded as excellent. Moreover, an AUC exceeding 0.9 is classified as outstanding, as indicated by Hosmer et al. [ 24 ].
Figure 7 (ROC Curve for Catboost—one-vs-one multiclass) and Fig. 8 (ROC Curve for LightGBM—one-vs-one multiclass) graphically represent the performance of CatBoost and LightGBM in a multi-class classification task. In such a scenario, ROC curves and AUC values are calculated for each class against every other class, providing insight into the model's ability to differentiate one class from the rest.
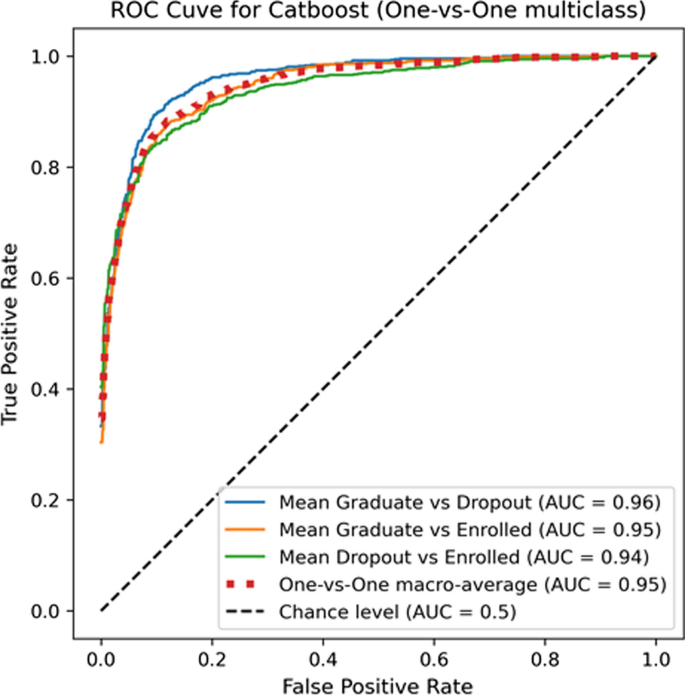
ROC Curve for Catboost (one-vs-one multiclass)
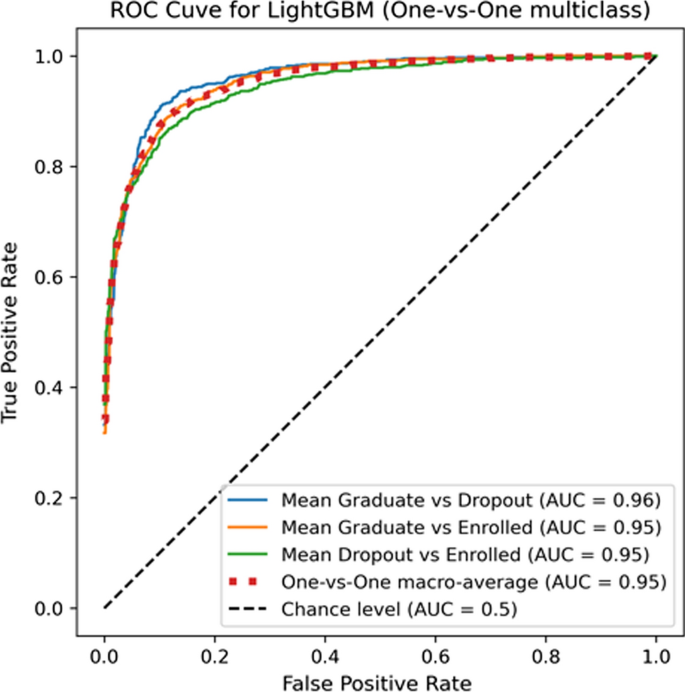
ROC Curve for LightGBM (one-vs-one multiclass)
Our findings, depicted in Figs. 7 and 8 , reveal an AUC exceeding 0.9. The position of the ROC curve on the graph reflects the model's performance. When the curve resides close to the top-left corner, it signifies that the model boasts a high true positive rate and a low false positive rate across various decision thresholds, a highly desirable trait. An AUC surpassing 0.9 is typically considered outstanding, indicating exceptional classification performance. This result underscores the exceptional discriminative prowess of these models, demonstrating their proficiency in distinguishing between different classes or groups within the dataset and affirming their reliability for the intended task.
To further evaluate potential significant differences between the results obtained using the LightGBM and Catboost algorithms, we employ the Statistical Tests for Algorithms Comparison (STAC) platform. This method serves to determine whether the disparity between the algorithm yielding the best results and other algorithms is statistically meaningful.
Based on the collected data, the STAC platform generates a decision tree to identify the most appropriate statistical test for the user-provided data. As presented in Fig. 9 , the STAC assistant advised us the use of a specific statistical test: the paired samples t-test.
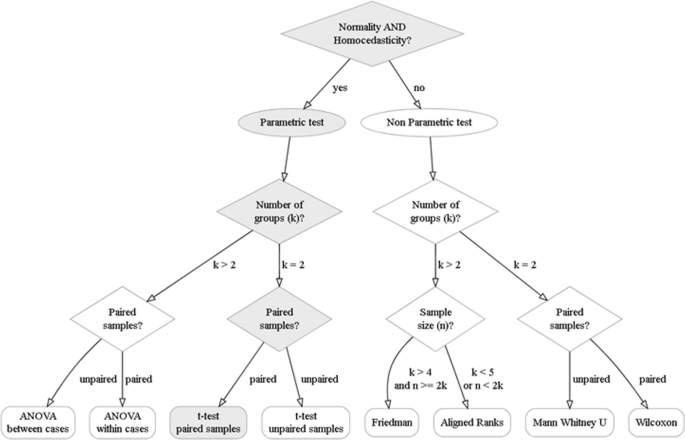
STAC assistant decision tree
The paired sample t-test is employed to assess whether the mean difference between two sets of observations is statistically different from zero. In this type of t-test, each subject or entity is measured twice, yielding pairs of observations. In our specific case, we utilize the paired sample t-test to compare the f1-scores of the LightGBM and Catboost algorithms, which are the top-performing algorithms in our models. Our objective is to determine whether there exists a statistically significant difference between their results or if one outperforms the other.
The outcome of this analysis yielded a p-value of 0.003, using a significance level of 0.05. This indicates that the null hypothesis is accepted, implying that the f1-scores of the LightGBM and Catboost algorithms have statistically identical average values. Consequently, we can confidently conclude that there is no substantial difference between the f1-scores of these two models.
7 Conclusion
This article presents a comprehensive study comparing supervised and unsupervised machine learning algorithms for predicting student dropout and academic success, with a primary focus on addressing class imbalance through SMOTE resampling. Notably, SMOTE significantly improved model accuracy. The study highlighted the exceptional performance of boosting algorithms, particularly LightGBM and CatBoost, surpassing traditional classification methods. However, these boosting algorithms often lack interpretability, which led us to employ the SHAP method for insightful feature analysis. We also explored the Isolation Forest algorithm for outlier detection, yielding promising results, although further refinement is needed to avoid misclassification.
These findings offer valuable guidance to researchers and practitioners in selecting suitable approaches for predicting student outcomes, optimizing model performance, and designing targeted interventions for at-risk students. Future research could explore alternative ensemble methods and advanced optimization techniques to enhance predictive model accuracy and interpretability in educational contexts.
As presented in our Systematic Literature Review (SLR) and corroborated in the present paper, Boosting algorithms consistently demonstrate their superiority in predicting various student performance aspects across diverse educational settings. Their consistent outperformance of traditional methods like logistic regression and decision trees underscores their potential as valuable tools for advancing educational research and enhancing student outcomes.
In the discussion section, we employ the ROC and AUC curves for the top-performing algorithms, LightGBM (LB) and CatBoost (CB). AUC values exceeded 0.9, which indicated outstanding classification performance, confirming these models' proficiency in distinguishing between different classes within the dataset. To assess differences between LightGBM and CatBoost, we employ the Statistical Tests for Algorithms Comparison (STAC), which shows that there is no substantial difference in their predictive performance.
It's important to acknowledge that our study has limitations, primarily its reliance on a single dataset, which may limit generalizability to other contexts. Future research avenues could explore hybrid ensemble methods, combining boosting algorithms with traditional classifiers, and investigate advanced optimization techniques beyond Optuna for hyperparameter fine-tuning to unlock further performance improvements.
Data availability
The authors utilized a dataset from a higher education institution, which is publicly available. They have also made the dataset and necessary code accessible through a GitHu, b repository, ensuring transparency and reproducibility of their research project.
Code availability
https://github.com/alicevillar/SML-Comparative-Study .
Chung JY, Lee S. Dropout early warning systems for high school students using machine learning. Child Youth Serv Rev. 2019;96:346–53.
Article Google Scholar
Gkontzis AF, Kotsiantis S, Panagiotakopoulos CT, Verykios VS. A predictive analytics framework as a countermeasure for attrition of students. Interact Learn Environ. 2022;30(6):1028–43.
Berens J, Schneider K, Görtz S, Oster S, Burghoff J. Early detection of students at risk–predicting student dropouts using administrative student data and machine learning methods. SSRN J. 2018. https://doi.org/10.2139/ssrn.3275433 .
Martins MV, Tolledo D, Machado J, Baptista LM, Realinho V. Early prediction of student’s performance in higher education: a case study. In: Rocha Á, Adeli H, Dzemyda G, Moreira F, Correia AMR, editors. Trends and applications in information systems and technologies, vol. 9. Berlin: Springer International Publishing; 2021. p. 166–75.
Chapter Google Scholar
Thammasiri D, Delen D, Meesad P, Kasap N. A critical assessment of imbalanced class distribution problem: the case of predicting freshmen student attrition. Expert Syst Appl. 2014;41(2):321–30.
Islam A, Belhaouari SB, Rehman AU, Bensmail H. KNNOR: An oversampling technique for imbalanced datasets. Appl Soft Comput. 2022;115:108288.
Rastrollo-Guerrero JL, Gómez-Pulido JA, Durán-Domínguez A. Analyzing and predicting students’ performance by means of machine learning: a review. Appl Sci. 2020;10(3):1042.
Hamim T, Benabbou F, Sael N. Student profile modeling using boosting algorithms. Int J Web-Based Learn Teach Technol. 2022;17(5):1–13.
Tenpipat W, Akkarajitsakul K. Student dropout prediction: a KMUTT case study. In: 2020 1st international conference on big data analytics and practices (IBDAP). IEEE. 2020. pp. 1–5.
Hew KF, Hu X, Qiao C, Tang Y. What predicts student satisfaction with MOOCs: a gradient boosting trees supervised machine learning and sentiment analysis approach. Comput Educ. 2020;145:103724.
Fernandes E, Holanda M, Victorino M, Borges V, Carvalho R, Van Erven G. Educational data mining: predictive analysis of academic performance of public school students in the capital of Brazil. J Bus Res. 2019;94:335–43.
Doryab A, Villalba DK, Chikersal P, Dutcher JM, Tumminia M, Liu X, Dey AK. Identifying behavioral phenotypes of loneliness and social isolation with passive sensing: statistical analysis, data mining and machine learning of smartphone and fitbit data. JMIR mHealth uHealth. 2019;7(7):e13209.
Wang C, Zhao H, Zhang H. Chinese college students have higher anxiety in new semester of online learning during COVID-19: a machine learning approach. Front Psychol. 2020;11:587413.
Qiao X, Jiao H. Data mining techniques in analyzing process data: a didactic. Front Psychol. 2018;9:2231.
Ge F, Zhang D, Wu L, Mu H. Predicting psychological state among Chinese undergraduate students in the COVID-19 epidemic: a longitudinal study using a machine learning. Neuropsychiatr Dis Treat. 2020;16:2111–8.
Asselman A, Khaldi M, Aammou S. Enhancing the prediction of student performance based on the machine learning XGBoost algorithm. Interact Learn Environ. 2021. https://doi.org/10.1080/10494820.2021.1928235 .
Jung JS, Park SJ, Kim EY, Na KS, Kim YJ, Kim KG. Prediction models for high risk of suicide in Korean adolescents using machine learning techniques. PLoS ONE. 2019;14(6):e0217639.
Costa-Mendes R, Oliveira T, Castelli M, Cruz-Jesus F. A machine learning approximation of the 2015 Portuguese high school student grades: a hybrid approach. Educ Inf Technol. 2021;26(2):1527–47.
Chen J, Zhang Y, Hu J. Synergistic effects of instruction and affect factors on high-and low-ability disparities in elementary students’ reading literacy. Read Writ. 2021;34:199–230.
Nabil A, Seyam M, Abou-Elfetouh A. Prediction of students’ academic performance based on courses’ grades using deep neural networks. IEEE Access. 2021;9:140731–46.
Abidi SMR, Hussain M, Xu Y, Zhang W. Prediction of confusion attempting algebra homework in an intelligent tutoring system through machine learning techniques for educational sustainable development. Sustainability. 2018;11(1):105.
Niyogisubizo J, Liao L, Nziyumva E, Murwanashyaka E, Nshimyumukiza PC. Predicting student’s dropout in university classes using two-layer ensemble machine learning approach: a novel stacked generalization. Comput Educ Artif Intell. 2022;3:100066.
Zhang L, Li X, Chen H, Wu Z, Hu M, Yao M. Haze air pollution health impacts of breath-borne VOCs. Environ Sci Technol. 2022;56(12):8541–51.
Hosmer DW Jr, Lemeshow S, Sturdivant RX. Applied logistic regression, vol. 398. Hoboken: John Wiley & Sons; 2013.
Book Google Scholar
Cohen J, Wright-Berryman J, Rohlfs L, Wright D, Campbell M, Gingrich D, Pestian J. A feasibility study using a machine learning suicide risk prediction model based on open-ended interview language in adolescent therapy sessions. Int J Environ Res Public Health. 2020;17(21):8187.
Download references
Acknowledgements
We would like to sincerely thank graphic designer Juan Leite Farias for his contributions to the artwork's graphic design for this paper.
The authors received no funding for this work.
Author information
Authors and affiliations.
Faculty of Computer Science, University of Essex, Colchester, Essex, UK
Alice Villar
Faculty of Applied Mathematics, University of Sao Paulo, Sao Paulo, Sao Paulo, Brazil
Carolina Robledo Velini de Andrade
You can also search for this author in PubMed Google Scholar
Contributions
Conception or design of the work: AV and CdA. Data collection: AV and CdA. Data analysis and interpretation: CdA and AV. Drafting the article: AV. Critical revision of the article: AV and CdA. Final approval of the version to be published: AV and CdA.
Corresponding authors
Correspondence to Alice Villar or Carolina Robledo Velini de Andrade .
Ethics declarations
Ethics approval and consent to participate.
Not applicable.
Consent for publication
Competing interests.
We declare that we have no significant competing financial, professional, or personal interests that might have influenced the performance or presentation of the work described in this manuscript.
Additional information
Publisher's note.
Springer Nature remains neutral with regard to jurisdictional claims in published maps and institutional affiliations.
Rights and permissions
Open Access This article is licensed under a Creative Commons Attribution 4.0 International License, which permits use, sharing, adaptation, distribution and reproduction in any medium or format, as long as you give appropriate credit to the original author(s) and the source, provide a link to the Creative Commons licence, and indicate if changes were made. The images or other third party material in this article are included in the article's Creative Commons licence, unless indicated otherwise in a credit line to the material. If material is not included in the article's Creative Commons licence and your intended use is not permitted by statutory regulation or exceeds the permitted use, you will need to obtain permission directly from the copyright holder. To view a copy of this licence, visit http://creativecommons.org/licenses/by/4.0/ .
Reprints and permissions
About this article
Villar, A., de Andrade, C.R.V. Supervised machine learning algorithms for predicting student dropout and academic success: a comparative study. Discov Artif Intell 4 , 2 (2024). https://doi.org/10.1007/s44163-023-00079-z
Download citation
Received : 27 June 2023
Accepted : 14 September 2023
Published : 04 January 2024
DOI : https://doi.org/10.1007/s44163-023-00079-z
Share this article
Anyone you share the following link with will be able to read this content:
Sorry, a shareable link is not currently available for this article.
Provided by the Springer Nature SharedIt content-sharing initiative
- Dropout prediction
- Supervised classification algorithms
- Unsupervised classification algorithms
- Class imbalance
- Boosting algorithms
- Hyperparameters
- Learning analytics
Advertisement
- Find a journal
- Publish with us
- Track your research
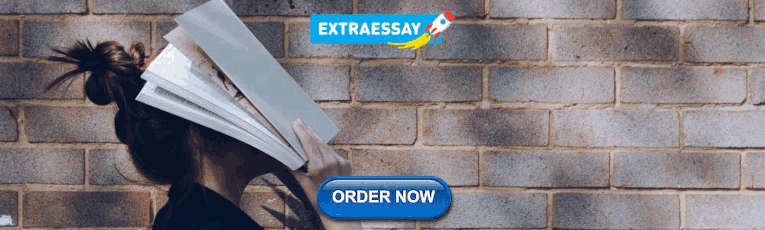
IMAGES
VIDEO
COMMENTS
Research methodology formats can vary depending on the specific requirements of the research project, but the following is a basic example of a structure for a research methodology section: ... Mixed-Methods Research Methodology. This is a research methodology that combines elements of both quantitative and qualitative research. This approach ...
Research methods are specific procedures for collecting and analyzing data. Developing your research methods is an integral part of your research design. When planning your methods, there are two key decisions you will make. First, decide how you will collect data. Your methods depend on what type of data you need to answer your research question:
To choose the right research methodology for your dissertation or thesis, you need to consider three important factors. Based on these three factors, you can decide on your overarching approach - qualitative, quantitative or mixed methods. Once you've made that decision, you can flesh out the finer details of your methodology, such as the ...
Step 1: Explain your methodological approach. Step 2: Describe your data collection methods. Step 3: Describe your analysis method. Step 4: Evaluate and justify the methodological choices you made. Tips for writing a strong methodology chapter. Other interesting articles.
Types of Research Designs Compared | Guide & Examples. Published on June 20, 2019 by Shona McCombes.Revised on June 22, 2023. When you start planning a research project, developing research questions and creating a research design, you will have to make various decisions about the type of research you want to do.. There are many ways to categorize different types of research.
Conclusion: Choosing an optimal research methodology is crucial for the success of any research project. The methodology you select will determine the type of data you collect, how you collect it, and how you analyse it. Understanding the different types of research methods available along with their strengths and weaknesses, is thus imperative ...
As we mentioned, research methodology refers to the collection of practical decisions regarding what data you'll collect, from who, how you'll collect it and how you'll analyse it. Research design, on the other hand, is more about the overall strategy you'll adopt in your study. For example, whether you'll use an experimental design ...
About Research Methods. This guide provides an overview of research methods, how to choose and use them, and supports and resources at UC Berkeley. As Patten and Newhart note in the book Understanding Research Methods, "Research methods are the building blocks of the scientific enterprise. They are the "how" for building systematic knowledge.
To analyse data collected in a statistically valid manner (e.g. from experiments, surveys, and observations). Meta-analysis. Quantitative. To statistically analyse the results of a large collection of studies. Can only be applied to studies that collected data in a statistically valid manner. Thematic analysis.
A research methodology encompasses the way in which you intend to carry out your research. This includes how you plan to tackle things like collection methods, statistical analysis, participant observations, and more. You can think of your research methodology as being a formula. One part will be how you plan on putting your research into ...
Definition, Types, and Examples. Research methodology 1,2 is a structured and scientific approach used to collect, analyze, and interpret quantitative or qualitative data to answer research questions or test hypotheses. A research methodology is like a plan for carrying out research and helps keep researchers on track by limiting the scope of ...
Provide the rationality behind your chosen approach. Based on logic and reason, let your readers know why you have chosen said research methodologies. Additionally, you have to build strong arguments supporting why your chosen research method is the best way to achieve the desired outcome. 3. Explain your mechanism.
This research methods guide will help you choose a methodology and launch into your research project. Data collection and data analysis are research methods that can be applied to many disciplines. There is Qualitative research and Quantitative Research. The focus of this guide, includes most popular methods including: surveys. interviews ...
Research methodology is classified based on different categories. They include a general category, nature of the study, purpose, research design, and data type. There are also interviews and case studies based on research methodology. In some research, the researcher combines more than two and very few methods.
A research methodology is a series of steps you take when creating a research project. These are effectively a framework that defines how you conduct your research going forwards, acting as a procedure that you follow when selecting, processing and analysing information about a topic. A research methodologist designs methodologies to increase ...
By understanding the different types of methodologies, factors influencing their choice, and best practices, you can embark on your research methodology project topics journey with confidence. Remember, the key to successful research lies in asking the right questions and choosing the appropriate methodology to find the answers.
Categories of Research Methods and Types of Research Results Illustrated with a Continuous Project. In Proceedings of the 21st International Conference on Enterprise Information Systems (ICEIS ...
A research design is a strategy for answering your research question using empirical data. Creating a research design means making decisions about: Your overall research objectives and approach. Whether you'll rely on primary research or secondary research. Your sampling methods or criteria for selecting subjects. Your data collection methods.
Exploring the critical role of experimentation in Corporate Social Responsibility (CSR), research on four multinational companies reveals a stark difference in CSR effectiveness. Successful ...
Additionally, we are looking for why your group believes this research project is worthwhile to your time in this course. You can start with the text from your prototype, but you should update your introduction and research questions to reflect changes in or refinements of the project vision. ... Part 4: Results and Methods (15 points) This is ...
Background: Substance use and overdose deaths make up a substantial portion of injury-related deaths in the United States, with the state of Ohio leading the nation in rates of diagnosed substance use disorder (SUD). Ohio's growing epidemic has indicated a need to improve SUD care in a primary care setting through the engagement of multidisciplinary providers and the use of a comprehensive ...
In an increasingly volatile environment, organizational learning plays a crucial role in helping organizations turn crises into opportunities and enhance organizational resilience. However, the existing research remains unclear on how organizational learning affects the formation of organizational resilience. The aim of this study is to explore the pathways by which organizational learning ...
In this work, we discuss building performant Multimodal Large Language Models (MLLMs). In particular, we study the importance of various architecture components and data choices. Through careful and comprehensive ablations of the image encoder, the vision language connector, and various pre-training data choices, we identified several crucial design lessons. For example, we demonstrate that ...
New methods to purify our water, advance quantum computing, democratize artificial intelligence, and deliver life-saving drugs are just some of the projects funded by the 2024 Roberts Innovation Fund Awards, providing $1 million in accelerator funding to support 10 new inventions led by faculty from Yale's School of Engineering & Applied Science.
Time series are generated in diverse domains such as economic, traffic, health, and energy, where forecasting of future values has numerous important applications. Not surprisingly, many forecasting methods are being proposed. To ensure progress, it is essential to be able to study and compare such methods empirically in a comprehensive and reliable manner. To achieve this, we propose TFB, an ...
Interestingly, different beer styles show distinct patterns for some flavor compounds (Supplementary Fig. S3).These observations agree with expectations for key beer styles, and serve as a control ...
Nevertheless, this research provides valuable insights into the effectiveness of various machine learning algorithms for predicting student dropout and academic success. By benchmarking these algorithms, our project offers guidance to both researchers and practitioners in their choice of suitable approaches for similar predictive tasks.