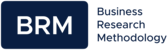
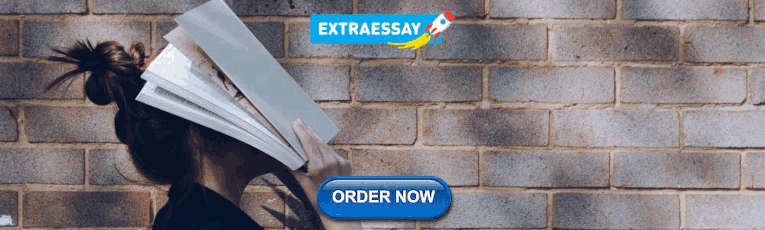
Questionnaires
Questionnaires can be classified as both, quantitative and qualitative method depending on the nature of questions. Specifically, answers obtained through closed-ended questions (also called restricted questions) with multiple choice answer options are analyzed using quantitative methods. Research findings in this case can be illustrated using tabulations, pie-charts, bar-charts and percentages.
Answers obtained to open-ended questionnaire questions (also known as unrestricted questions), on the other hand, are analyzed using qualitative methods. Primary data collected using open-ended questionnaires involve discussions and critical analyses without use of numbers and calculations.
There are following types of questionnaires:
Computer questionnaire . Respondents are asked to answer the questionnaire which is sent by mail. The advantages of the computer questionnaires include their inexpensive price, time-efficiency, and respondents do not feel pressured, therefore can answer when they have time, giving more accurate answers. However, the main shortcoming of the mail questionnaires is that sometimes respondents do not bother answering them and they can just ignore the questionnaire.
Telephone questionnaire . Researcher may choose to call potential respondents with the aim of getting them to answer the questionnaire. The advantage of the telephone questionnaire is that, it can be completed during the short amount of time. The main disadvantage of the phone questionnaire is that it is expensive most of the time. Moreover, most people do not feel comfortable to answer many questions asked through the phone and it is difficult to get sample group to answer questionnaire over the phone.
In-house survey . This type of questionnaire involves the researcher visiting respondents in their houses or workplaces. The advantage of in-house survey is that more focus towards the questions can be gained from respondents. However, in-house surveys also have a range of disadvantages which include being time consuming, more expensive and respondents may not wish to have the researcher in their houses or workplaces for various reasons.
Mail Questionnaire . This sort of questionnaires involve the researcher to send the questionnaire list to respondents through post, often attaching pre-paid envelope. Mail questionnaires have an advantage of providing more accurate answer, because respondents can answer the questionnaire in their spare time. The disadvantages associated with mail questionnaires include them being expensive, time consuming and sometimes they end up in the bin put by respondents.
Questionnaires can include the following types of questions:
Open question questionnaires . Open questions differ from other types of questions used in questionnaires in a way that open questions may produce unexpected results, which can make the research more original and valuable. However, it is difficult to analyze the results of the findings when the data is obtained through the questionnaire with open questions.
Multiple choice question s. Respondents are offered a set of answers they have to choose from. The downsize of questionnaire with multiple choice questions is that, if there are too many answers to choose from, it makes the questionnaire, confusing and boring, and discourages the respondent to answer the questionnaire.
Dichotomous Questions . Thes type of questions gives two options to respondents – yes or no, to choose from. It is the easiest form of questionnaire for the respondent in terms of responding it.
Scaling Questions . Also referred to as ranking questions, they present an option for respondents to rank the available answers to questions on the scale of given range of values (for example from 1 to 10).
For a standard 15,000-20,000 word business dissertation including 25-40 questions in questionnaires will usually suffice. Questions need be formulated in an unambiguous and straightforward manner and they should be presented in a logical order.
Questionnaires as primary data collection method offer the following advantages:
- Uniformity: all respondents are asked exactly the same questions
- Cost-effectiveness
- Possibility to collect the primary data in shorter period of time
- Minimum or no bias from the researcher during the data collection process
- Usually enough time for respondents to think before answering questions, as opposed to interviews
- Possibility to reach respondents in distant areas through online questionnaire
At the same time, the use of questionnaires as primary data collection method is associated with the following shortcomings:
- Random answer choices by respondents without properly reading the question.
- In closed-ended questionnaires no possibility for respondents to express their additional thoughts about the matter due to the absence of a relevant question.
- Collecting incomplete or inaccurate information because respondents may not be able to understand questions correctly.
- High rate of non-response
Survey Monkey represents one of the most popular online platforms for facilitating data collection through questionnaires. Substantial benefits offered by Survey Monkey include its ease to use, presentation of questions in many different formats and advanced data analysis capabilities.

Survey Monkey as a popular platform for primary data collection
There are other alternatives to Survey Monkey you might want to consider to use as a platform for your survey. These include but not limited to Jotform, Google Forms, Lime Survey, Crowd Signal, Survey Gizmo, Zoho Survey and many others.
My e-book, The Ultimate Guide to Writing a Dissertation in Business Studies: a step by step approach contains a detailed, yet simple explanation of quantitative methods. The e-book explains all stages of the research process starting from the selection of the research area to writing personal reflection. Important elements of dissertations such as research philosophy, research approach, research design, methods of data collection and data analysis are explained in simple words.
John Dudovskiy
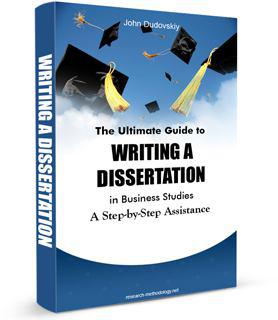
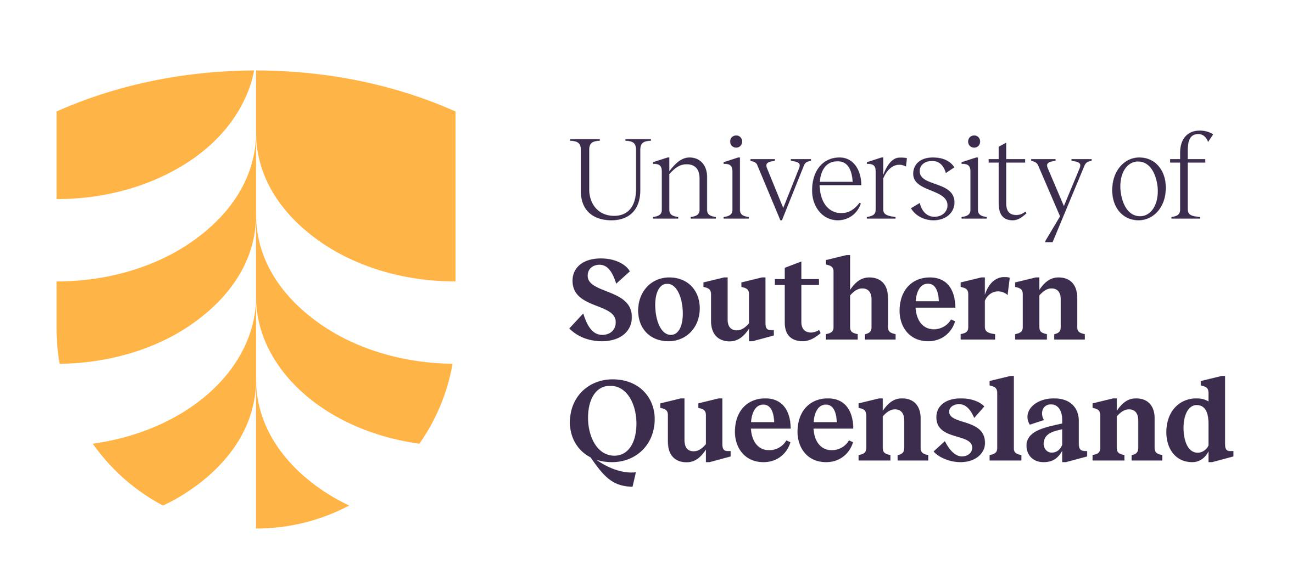
Want to create or adapt books like this? Learn more about how Pressbooks supports open publishing practices.
9 Survey research
Survey research is a research method involving the use of standardised questionnaires or interviews to collect data about people and their preferences, thoughts, and behaviours in a systematic manner. Although census surveys were conducted as early as Ancient Egypt, survey as a formal research method was pioneered in the 1930–40s by sociologist Paul Lazarsfeld to examine the effects of the radio on political opinion formation of the United States. This method has since become a very popular method for quantitative research in the social sciences.
The survey method can be used for descriptive, exploratory, or explanatory research. This method is best suited for studies that have individual people as the unit of analysis. Although other units of analysis, such as groups, organisations or dyads—pairs of organisations, such as buyers and sellers—are also studied using surveys, such studies often use a specific person from each unit as a ‘key informant’ or a ‘proxy’ for that unit. Consequently, such surveys may be subject to respondent bias if the chosen informant does not have adequate knowledge or has a biased opinion about the phenomenon of interest. For instance, Chief Executive Officers may not adequately know employees’ perceptions or teamwork in their own companies, and may therefore be the wrong informant for studies of team dynamics or employee self-esteem.
Survey research has several inherent strengths compared to other research methods. First, surveys are an excellent vehicle for measuring a wide variety of unobservable data, such as people’s preferences (e.g., political orientation), traits (e.g., self-esteem), attitudes (e.g., toward immigrants), beliefs (e.g., about a new law), behaviours (e.g., smoking or drinking habits), or factual information (e.g., income). Second, survey research is also ideally suited for remotely collecting data about a population that is too large to observe directly. A large area—such as an entire country—can be covered by postal, email, or telephone surveys using meticulous sampling to ensure that the population is adequately represented in a small sample. Third, due to their unobtrusive nature and the ability to respond at one’s convenience, questionnaire surveys are preferred by some respondents. Fourth, interviews may be the only way of reaching certain population groups such as the homeless or illegal immigrants for which there is no sampling frame available. Fifth, large sample surveys may allow detection of small effects even while analysing multiple variables, and depending on the survey design, may also allow comparative analysis of population subgroups (i.e., within-group and between-group analysis). Sixth, survey research is more economical in terms of researcher time, effort and cost than other methods such as experimental research and case research. At the same time, survey research also has some unique disadvantages. It is subject to a large number of biases such as non-response bias, sampling bias, social desirability bias, and recall bias, as discussed at the end of this chapter.
Depending on how the data is collected, survey research can be divided into two broad categories: questionnaire surveys (which may be postal, group-administered, or online surveys), and interview surveys (which may be personal, telephone, or focus group interviews). Questionnaires are instruments that are completed in writing by respondents, while interviews are completed by the interviewer based on verbal responses provided by respondents. As discussed below, each type has its own strengths and weaknesses in terms of their costs, coverage of the target population, and researcher’s flexibility in asking questions.
Questionnaire surveys
Invented by Sir Francis Galton, a questionnaire is a research instrument consisting of a set of questions (items) intended to capture responses from respondents in a standardised manner. Questions may be unstructured or structured. Unstructured questions ask respondents to provide a response in their own words, while structured questions ask respondents to select an answer from a given set of choices. Subjects’ responses to individual questions (items) on a structured questionnaire may be aggregated into a composite scale or index for statistical analysis. Questions should be designed in such a way that respondents are able to read, understand, and respond to them in a meaningful way, and hence the survey method may not be appropriate or practical for certain demographic groups such as children or the illiterate.
Most questionnaire surveys tend to be self-administered postal surveys , where the same questionnaire is posted to a large number of people, and willing respondents can complete the survey at their convenience and return it in prepaid envelopes. Postal surveys are advantageous in that they are unobtrusive and inexpensive to administer, since bulk postage is cheap in most countries. However, response rates from postal surveys tend to be quite low since most people ignore survey requests. There may also be long delays (several months) in respondents’ completing and returning the survey, or they may even simply lose it. Hence, the researcher must continuously monitor responses as they are being returned, track and send non-respondents repeated reminders (two or three reminders at intervals of one to one and a half months is ideal). Questionnaire surveys are also not well-suited for issues that require clarification on the part of the respondent or those that require detailed written responses. Longitudinal designs can be used to survey the same set of respondents at different times, but response rates tend to fall precipitously from one survey to the next.
A second type of survey is a group-administered questionnaire . A sample of respondents is brought together at a common place and time, and each respondent is asked to complete the survey questionnaire while in that room. Respondents enter their responses independently without interacting with one another. This format is convenient for the researcher, and a high response rate is assured. If respondents do not understand any specific question, they can ask for clarification. In many organisations, it is relatively easy to assemble a group of employees in a conference room or lunch room, especially if the survey is approved by corporate executives.
A more recent type of questionnaire survey is an online or web survey. These surveys are administered over the Internet using interactive forms. Respondents may receive an email request for participation in the survey with a link to a website where the survey may be completed. Alternatively, the survey may be embedded into an email, and can be completed and returned via email. These surveys are very inexpensive to administer, results are instantly recorded in an online database, and the survey can be easily modified if needed. However, if the survey website is not password-protected or designed to prevent multiple submissions, the responses can be easily compromised. Furthermore, sampling bias may be a significant issue since the survey cannot reach people who do not have computer or Internet access, such as many of the poor, senior, and minority groups, and the respondent sample is skewed toward a younger demographic who are online much of the time and have the time and ability to complete such surveys. Computing the response rate may be problematic if the survey link is posted on LISTSERVs or bulletin boards instead of being emailed directly to targeted respondents. For these reasons, many researchers prefer dual-media surveys (e.g., postal survey and online survey), allowing respondents to select their preferred method of response.
Constructing a survey questionnaire is an art. Numerous decisions must be made about the content of questions, their wording, format, and sequencing, all of which can have important consequences for the survey responses.
Response formats. Survey questions may be structured or unstructured. Responses to structured questions are captured using one of the following response formats:
Dichotomous response , where respondents are asked to select one of two possible choices, such as true/false, yes/no, or agree/disagree. An example of such a question is: Do you think that the death penalty is justified under some circumstances? (circle one): yes / no.
Nominal response , where respondents are presented with more than two unordered options, such as: What is your industry of employment?: manufacturing / consumer services / retail / education / healthcare / tourism and hospitality / other.
Ordinal response , where respondents have more than two ordered options, such as: What is your highest level of education?: high school / bachelor’s degree / postgraduate degree.
Interval-level response , where respondents are presented with a 5-point or 7-point Likert scale, semantic differential scale, or Guttman scale. Each of these scale types were discussed in a previous chapter.
Continuous response , where respondents enter a continuous (ratio-scaled) value with a meaningful zero point, such as their age or tenure in a firm. These responses generally tend to be of the fill-in-the blanks type.
Question content and wording. Responses obtained in survey research are very sensitive to the types of questions asked. Poorly framed or ambiguous questions will likely result in meaningless responses with very little value. Dillman (1978) [1] recommends several rules for creating good survey questions. Every single question in a survey should be carefully scrutinised for the following issues:
Is the question clear and understandable ?: Survey questions should be stated in very simple language, preferably in active voice, and without complicated words or jargon that may not be understood by a typical respondent. All questions in the questionnaire should be worded in a similar manner to make it easy for respondents to read and understand them. The only exception is if your survey is targeted at a specialised group of respondents, such as doctors, lawyers and researchers, who use such jargon in their everyday environment. Is the question worded in a negative manner ?: Negatively worded questions such as ‘Should your local government not raise taxes?’ tend to confuse many respondents and lead to inaccurate responses. Double-negatives should be avoided when designing survey questions.
Is the question ambiguous ?: Survey questions should not use words or expressions that may be interpreted differently by different respondents (e.g., words like ‘any’ or ‘just’). For instance, if you ask a respondent, ‘What is your annual income?’, it is unclear whether you are referring to salary/wages, or also dividend, rental, and other income, whether you are referring to personal income, family income (including spouse’s wages), or personal and business income. Different interpretation by different respondents will lead to incomparable responses that cannot be interpreted correctly.
Does the question have biased or value-laden words ?: Bias refers to any property of a question that encourages subjects to answer in a certain way. Kenneth Rasinky (1989) [2] examined several studies on people’s attitude toward government spending, and observed that respondents tend to indicate stronger support for ‘assistance to the poor’ and less for ‘welfare’, even though both terms had the same meaning. In this study, more support was also observed for ‘halting rising crime rate’ and less for ‘law enforcement’, more for ‘solving problems of big cities’ and less for ‘assistance to big cities’, and more for ‘dealing with drug addiction’ and less for ‘drug rehabilitation’. A biased language or tone tends to skew observed responses. It is often difficult to anticipate in advance the biasing wording, but to the greatest extent possible, survey questions should be carefully scrutinised to avoid biased language.
Is the question double-barrelled ?: Double-barrelled questions are those that can have multiple answers. For example, ‘Are you satisfied with the hardware and software provided for your work?’. In this example, how should a respondent answer if they are satisfied with the hardware, but not with the software, or vice versa? It is always advisable to separate double-barrelled questions into separate questions: ‘Are you satisfied with the hardware provided for your work?’, and ’Are you satisfied with the software provided for your work?’. Another example: ‘Does your family favour public television?’. Some people may favour public TV for themselves, but favour certain cable TV programs such as Sesame Street for their children.
Is the question too general ?: Sometimes, questions that are too general may not accurately convey respondents’ perceptions. If you asked someone how they liked a certain book and provided a response scale ranging from ‘not at all’ to ‘extremely well’, if that person selected ‘extremely well’, what do they mean? Instead, ask more specific behavioural questions, such as, ‘Will you recommend this book to others, or do you plan to read other books by the same author?’. Likewise, instead of asking, ‘How big is your firm?’ (which may be interpreted differently by respondents), ask, ‘How many people work for your firm?’, and/or ‘What is the annual revenue of your firm?’, which are both measures of firm size.
Is the question too detailed ?: Avoid unnecessarily detailed questions that serve no specific research purpose. For instance, do you need the age of each child in a household, or is just the number of children in the household acceptable? However, if unsure, it is better to err on the side of details than generality.
Is the question presumptuous ?: If you ask, ‘What do you see as the benefits of a tax cut?’, you are presuming that the respondent sees the tax cut as beneficial. Many people may not view tax cuts as being beneficial, because tax cuts generally lead to lesser funding for public schools, larger class sizes, and fewer public services such as police, ambulance, and fire services. Avoid questions with built-in presumptions.
Is the question imaginary ?: A popular question in many television game shows is, ‘If you win a million dollars on this show, how will you spend it?’. Most respondents have never been faced with such an amount of money before and have never thought about it—they may not even know that after taxes, they will get only about $640,000 or so in the United States, and in many cases, that amount is spread over a 20-year period—and so their answers tend to be quite random, such as take a tour around the world, buy a restaurant or bar, spend on education, save for retirement, help parents or children, or have a lavish wedding. Imaginary questions have imaginary answers, which cannot be used for making scientific inferences.
Do respondents have the information needed to correctly answer the question ?: Oftentimes, we assume that subjects have the necessary information to answer a question, when in reality, they do not. Even if a response is obtained, these responses tend to be inaccurate given the subjects’ lack of knowledge about the question being asked. For instance, we should not ask the CEO of a company about day-to-day operational details that they may not be aware of, or ask teachers about how much their students are learning, or ask high-schoolers, ‘Do you think the US Government acted appropriately in the Bay of Pigs crisis?’.
Question sequencing. In general, questions should flow logically from one to the next. To achieve the best response rates, questions should flow from the least sensitive to the most sensitive, from the factual and behavioural to the attitudinal, and from the more general to the more specific. Some general rules for question sequencing:
Start with easy non-threatening questions that can be easily recalled. Good options are demographics (age, gender, education level) for individual-level surveys and firmographics (employee count, annual revenues, industry) for firm-level surveys.
Never start with an open ended question.
If following a historical sequence of events, follow a chronological order from earliest to latest.
Ask about one topic at a time. When switching topics, use a transition, such as, ‘The next section examines your opinions about…’
Use filter or contingency questions as needed, such as, ‘If you answered “yes” to question 5, please proceed to Section 2. If you answered “no” go to Section 3′.
Other golden rules . Do unto your respondents what you would have them do unto you. Be attentive and appreciative of respondents’ time, attention, trust, and confidentiality of personal information. Always practice the following strategies for all survey research:
People’s time is valuable. Be respectful of their time. Keep your survey as short as possible and limit it to what is absolutely necessary. Respondents do not like spending more than 10-15 minutes on any survey, no matter how important it is. Longer surveys tend to dramatically lower response rates.
Always assure respondents about the confidentiality of their responses, and how you will use their data (e.g., for academic research) and how the results will be reported (usually, in the aggregate).
For organisational surveys, assure respondents that you will send them a copy of the final results, and make sure that you follow up with your promise.
Thank your respondents for their participation in your study.
Finally, always pretest your questionnaire, at least using a convenience sample, before administering it to respondents in a field setting. Such pretesting may uncover ambiguity, lack of clarity, or biases in question wording, which should be eliminated before administering to the intended sample.
Interview survey
Interviews are a more personalised data collection method than questionnaires, and are conducted by trained interviewers using the same research protocol as questionnaire surveys (i.e., a standardised set of questions). However, unlike a questionnaire, the interview script may contain special instructions for the interviewer that are not seen by respondents, and may include space for the interviewer to record personal observations and comments. In addition, unlike postal surveys, the interviewer has the opportunity to clarify any issues raised by the respondent or ask probing or follow-up questions. However, interviews are time-consuming and resource-intensive. Interviewers need special interviewing skills as they are considered to be part of the measurement instrument, and must proactively strive not to artificially bias the observed responses.
The most typical form of interview is a personal or face-to-face interview , where the interviewer works directly with the respondent to ask questions and record their responses. Personal interviews may be conducted at the respondent’s home or office location. This approach may even be favoured by some respondents, while others may feel uncomfortable allowing a stranger into their homes. However, skilled interviewers can persuade respondents to co-operate, dramatically improving response rates.
A variation of the personal interview is a group interview, also called a focus group . In this technique, a small group of respondents (usually 6–10 respondents) are interviewed together in a common location. The interviewer is essentially a facilitator whose job is to lead the discussion, and ensure that every person has an opportunity to respond. Focus groups allow deeper examination of complex issues than other forms of survey research, because when people hear others talk, it often triggers responses or ideas that they did not think about before. However, focus group discussion may be dominated by a dominant personality, and some individuals may be reluctant to voice their opinions in front of their peers or superiors, especially while dealing with a sensitive issue such as employee underperformance or office politics. Because of their small sample size, focus groups are usually used for exploratory research rather than descriptive or explanatory research.
A third type of interview survey is a telephone interview . In this technique, interviewers contact potential respondents over the phone, typically based on a random selection of people from a telephone directory, to ask a standard set of survey questions. A more recent and technologically advanced approach is computer-assisted telephone interviewing (CATI). This is increasing being used by academic, government, and commercial survey researchers. Here the interviewer is a telephone operator who is guided through the interview process by a computer program displaying instructions and questions to be asked. The system also selects respondents randomly using a random digit dialling technique, and records responses using voice capture technology. Once respondents are on the phone, higher response rates can be obtained. This technique is not ideal for rural areas where telephone density is low, and also cannot be used for communicating non-audio information such as graphics or product demonstrations.
Role of interviewer. The interviewer has a complex and multi-faceted role in the interview process, which includes the following tasks:
Prepare for the interview: Since the interviewer is in the forefront of the data collection effort, the quality of data collected depends heavily on how well the interviewer is trained to do the job. The interviewer must be trained in the interview process and the survey method, and also be familiar with the purpose of the study, how responses will be stored and used, and sources of interviewer bias. They should also rehearse and time the interview prior to the formal study.
Locate and enlist the co-operation of respondents: Particularly in personal, in-home surveys, the interviewer must locate specific addresses, and work around respondents’ schedules at sometimes undesirable times such as during weekends. They should also be like a salesperson, selling the idea of participating in the study.
Motivate respondents: Respondents often feed off the motivation of the interviewer. If the interviewer is disinterested or inattentive, respondents will not be motivated to provide useful or informative responses either. The interviewer must demonstrate enthusiasm about the study, communicate the importance of the research to respondents, and be attentive to respondents’ needs throughout the interview.
Clarify any confusion or concerns: Interviewers must be able to think on their feet and address unanticipated concerns or objections raised by respondents to the respondents’ satisfaction. Additionally, they should ask probing questions as necessary even if such questions are not in the script.
Observe quality of response: The interviewer is in the best position to judge the quality of information collected, and may supplement responses obtained using personal observations of gestures or body language as appropriate.
Conducting the interview. Before the interview, the interviewer should prepare a kit to carry to the interview session, consisting of a cover letter from the principal investigator or sponsor, adequate copies of the survey instrument, photo identification, and a telephone number for respondents to call to verify the interviewer’s authenticity. The interviewer should also try to call respondents ahead of time to set up an appointment if possible. To start the interview, they should speak in an imperative and confident tone, such as, ‘I’d like to take a few minutes of your time to interview you for a very important study’, instead of, ‘May I come in to do an interview?’. They should introduce themself, present personal credentials, explain the purpose of the study in one to two sentences, and assure respondents that their participation is voluntary, and their comments are confidential, all in less than a minute. No big words or jargon should be used, and no details should be provided unless specifically requested. If the interviewer wishes to record the interview, they should ask for respondents’ explicit permission before doing so. Even if the interview is recorded, the interviewer must take notes on key issues, probes, or verbatim phrases
During the interview, the interviewer should follow the questionnaire script and ask questions exactly as written, and not change the words to make the question sound friendlier. They should also not change the order of questions or skip any question that may have been answered earlier. Any issues with the questions should be discussed during rehearsal prior to the actual interview sessions. The interviewer should not finish the respondent’s sentences. If the respondent gives a brief cursory answer, the interviewer should probe the respondent to elicit a more thoughtful, thorough response. Some useful probing techniques are:
The silent probe: Just pausing and waiting without going into the next question may suggest to respondents that the interviewer is waiting for more detailed response.
Overt encouragement: An occasional ‘uh-huh’ or ‘okay’ may encourage the respondent to go into greater details. However, the interviewer must not express approval or disapproval of what the respondent says.
Ask for elaboration: Such as, ‘Can you elaborate on that?’ or ‘A minute ago, you were talking about an experience you had in high school. Can you tell me more about that?’.
Reflection: The interviewer can try the psychotherapist’s trick of repeating what the respondent said. For instance, ‘What I’m hearing is that you found that experience very traumatic’ and then pause and wait for the respondent to elaborate.
After the interview is completed, the interviewer should thank respondents for their time, tell them when to expect the results, and not leave hastily. Immediately after leaving, they should write down any notes or key observations that may help interpret the respondent’s comments better.
Biases in survey research
Despite all of its strengths and advantages, survey research is often tainted with systematic biases that may invalidate some of the inferences derived from such surveys. Five such biases are the non-response bias, sampling bias, social desirability bias, recall bias, and common method bias.
Non-response bias. Survey research is generally notorious for its low response rates. A response rate of 15-20 per cent is typical in a postal survey, even after two or three reminders. If the majority of the targeted respondents fail to respond to a survey, this may indicate a systematic reason for the low response rate, which may in turn raise questions about the validity of the study’s results. For instance, dissatisfied customers tend to be more vocal about their experience than satisfied customers, and are therefore more likely to respond to questionnaire surveys or interview requests than satisfied customers. Hence, any respondent sample is likely to have a higher proportion of dissatisfied customers than the underlying population from which it is drawn. In this instance, not only will the results lack generalisability, but the observed outcomes may also be an artefact of the biased sample. Several strategies may be employed to improve response rates:
Advance notification: Sending a short letter to the targeted respondents soliciting their participation in an upcoming survey can prepare them in advance and improve their propensity to respond. The letter should state the purpose and importance of the study, mode of data collection (e.g., via a phone call, a survey form in the mail, etc.), and appreciation for their co-operation. A variation of this technique may be to ask the respondent to return a prepaid postcard indicating whether or not they are willing to participate in the study.
Relevance of content: People are more likely to respond to surveys examining issues of relevance or importance to them.
Respondent-friendly questionnaire: Shorter survey questionnaires tend to elicit higher response rates than longer questionnaires. Furthermore, questions that are clear, non-offensive, and easy to respond tend to attract higher response rates.
Endorsement: For organisational surveys, it helps to gain endorsement from a senior executive attesting to the importance of the study to the organisation. Such endorsement can be in the form of a cover letter or a letter of introduction, which can improve the researcher’s credibility in the eyes of the respondents.
Follow-up requests: Multiple follow-up requests may coax some non-respondents to respond, even if their responses are late.
Interviewer training: Response rates for interviews can be improved with skilled interviewers trained in how to request interviews, use computerised dialling techniques to identify potential respondents, and schedule call-backs for respondents who could not be reached.
Incentives : Incentives in the form of cash or gift cards, giveaways such as pens or stress balls, entry into a lottery, draw or contest, discount coupons, promise of contribution to charity, and so forth may increase response rates.
Non-monetary incentives: Businesses, in particular, are more prone to respond to non-monetary incentives than financial incentives. An example of such a non-monetary incentive is a benchmarking report comparing the business’s individual response against the aggregate of all responses to a survey.
Confidentiality and privacy: Finally, assurances that respondents’ private data or responses will not fall into the hands of any third party may help improve response rates
Sampling bias. Telephone surveys conducted by calling a random sample of publicly available telephone numbers will systematically exclude people with unlisted telephone numbers, mobile phone numbers, and people who are unable to answer the phone when the survey is being conducted—for instance, if they are at work—and will include a disproportionate number of respondents who have landline telephone services with listed phone numbers and people who are home during the day, such as the unemployed, the disabled, and the elderly. Likewise, online surveys tend to include a disproportionate number of students and younger people who are constantly on the Internet, and systematically exclude people with limited or no access to computers or the Internet, such as the poor and the elderly. Similarly, questionnaire surveys tend to exclude children and the illiterate, who are unable to read, understand, or meaningfully respond to the questionnaire. A different kind of sampling bias relates to sampling the wrong population, such as asking teachers (or parents) about their students’ (or children’s) academic learning, or asking CEOs about operational details in their company. Such biases make the respondent sample unrepresentative of the intended population and hurt generalisability claims about inferences drawn from the biased sample.
Social desirability bias . Many respondents tend to avoid negative opinions or embarrassing comments about themselves, their employers, family, or friends. With negative questions such as, ‘Do you think that your project team is dysfunctional?’, ‘Is there a lot of office politics in your workplace?’, ‘Or have you ever illegally downloaded music files from the Internet?’, the researcher may not get truthful responses. This tendency among respondents to ‘spin the truth’ in order to portray themselves in a socially desirable manner is called the ‘social desirability bias’, which hurts the validity of responses obtained from survey research. There is practically no way of overcoming the social desirability bias in a questionnaire survey, but in an interview setting, an astute interviewer may be able to spot inconsistent answers and ask probing questions or use personal observations to supplement respondents’ comments.
Recall bias. Responses to survey questions often depend on subjects’ motivation, memory, and ability to respond. Particularly when dealing with events that happened in the distant past, respondents may not adequately remember their own motivations or behaviours, or perhaps their memory of such events may have evolved with time and no longer be retrievable. For instance, if a respondent is asked to describe his/her utilisation of computer technology one year ago, or even memorable childhood events like birthdays, their response may not be accurate due to difficulties with recall. One possible way of overcoming the recall bias is by anchoring the respondent’s memory in specific events as they happened, rather than asking them to recall their perceptions and motivations from memory.
Common method bias. Common method bias refers to the amount of spurious covariance shared between independent and dependent variables that are measured at the same point in time, such as in a cross-sectional survey, using the same instrument, such as a questionnaire. In such cases, the phenomenon under investigation may not be adequately separated from measurement artefacts. Standard statistical tests are available to test for common method bias, such as Harmon’s single-factor test (Podsakoff, MacKenzie, Lee & Podsakoff, 2003), [3] Lindell and Whitney’s (2001) [4] market variable technique, and so forth. This bias can potentially be avoided if the independent and dependent variables are measured at different points in time using a longitudinal survey design, or if these variables are measured using different methods, such as computerised recording of dependent variable versus questionnaire-based self-rating of independent variables.
- Dillman, D. (1978). Mail and telephone surveys: The total design method . New York: Wiley. ↵
- Rasikski, K. (1989). The effect of question wording on public support for government spending. Public Opinion Quarterly , 53(3), 388–394. ↵
- Podsakoff, P. M., MacKenzie, S. B., Lee, J.-Y., & Podsakoff, N. P. (2003). Common method biases in behavioral research: A critical review of the literature and recommended remedies. Journal of Applied Psychology , 88(5), 879–903. http://dx.doi.org/10.1037/0021-9010.88.5.879. ↵
- Lindell, M. K., & Whitney, D. J. (2001). Accounting for common method variance in cross-sectional research designs. Journal of Applied Psychology , 86(1), 114–121. ↵
Social Science Research: Principles, Methods and Practices (Revised edition) Copyright © 2019 by Anol Bhattacherjee is licensed under a Creative Commons Attribution-NonCommercial-ShareAlike 4.0 International License , except where otherwise noted.
Share This Book
Imperial College London Imperial College London
Latest news.

Imperial celebrates its international scholars and continues to grow programmes

Enterprise Lab and The Greenhouse highly ranked in FT list of top startup hubs

Nigeria expands school food programme following work with Imperial
- Centre for Higher Education Research and Scholarship
- Research and Innovation
- Educational research methods
- Methods in educational research
Questionnaires
Questionnaires can be used qualitatively or quantitatively. As with all other methods, the value of the questionnaire depends on its ability to provide data which can answer the research question, and the way that a questionnaire is designed and worded can be significant in this. A questionnaire designed to capture levels of student satisfaction may well provide information to this end, but for researchers interested in more than this, such measures could amount to little more than superficial data. Careful consideration needs to be given to what the questionnaire is intended to elicit, and so – depending on their study – some researchers might find it more useful to use pre-existing standardised questionnaires based on validated scales such as those used to measure self-efficacy (Bandura, 2006) or agency (Tapal et al., 2017).
Guidance for developing questionnaires using self-efficacy scales [pdf]
"The questionnaire is a widely used and useful instrument for collecting survey information, providing structured – often numerical – data, able to be administrated without the presence of the researcher and often comparatively straightforward to analyse. These attractions have to be counterbalanced by the time taken to develop, pilot and refine the questionnaire, by the possible unsophistication and limited and superficial scope of the data that are collected […]. The researcher will have to judge the appropriateness of using a questionnaire for data collection, and, if so, what kind of questionnaire it should be." Cohen, Manion and Morrison, 2018, p.471
Cohen, Manion and Morrison (2018) provide a comprehensive overview of the different issues and stages involved in questionnaire design and it is important that each of these is given full consideration from the outset. These issues include:
- Intended population/sample – as this can influence the form, wording and means of administrating the questionnaire
- Intended method of data analysis – to ensure that questions are framed appropriately
- Type of questionnaire: structured/closed, semi-structured or “unstructured”
- Question/response types – e.g. dichotomous questions, multiple choice, Likert/rating scales, constant sum, rank ordering, open ended
- Wording of questions – e.g. need for clarity, risk of leading responses
- Opportunity to pilot and revise questionnaire
As with all educational research, attention must be given to the particular ethical and practical considerations involved in this particular type of research. For many researchers, online survey tools such as Qualtrics provide a convenient means of administering questionnaires but these require attention to particular considerations – which Cohen, Manion and Morrison (2018) provide further detailed guidance on in Chapter 18.
Quantitative questionnaire design
A key priority with quantitative questionnaire design is to be clear from the outset exactly what it is you want to measure, why you want to do this and whether your proposed design is actually going to generate the sort of data you need. Do you want, for instance, to generate inferential or just descriptive statistics? Different question types lend themselves to different scales of data (rating scales to ordinal data, for instance) so thinking ahead to the analysis is an essential part of the design phase. Equally, if a pre- and post- study design is deemed appropriate, then the essential principles of experimental design need to be factored into the design and administration of the questionnaires.
Qualitative questionnaire design
If your area of research renders it necessary to obtain qualitative data, it might be worth considering in the first instance if interviews or focus groups might provide a more appropriate means of eliciting this. Self-completion questionnaires do not provide scope for probing further if questions are left unanswered or incomplete, and participants can vary enormously in terms of the time they are prepared to devote and the amount they are prepared to write in completing open text questionnaires. If questionnaires are most appropriate, however, then the general principles of good questionnaire design (layout, wording, ordering and so on) need to be considered alongside the practicality and feasibility of completing the questionnaire from the participants’ point of view.
Chapter 9 Survey Research
Survey research a research method involving the use of standardized questionnaires or interviews to collect data about people and their preferences, thoughts, and behaviors in a systematic manner. Although census surveys were conducted as early as Ancient Egypt, survey as a formal research method was pioneered in the 1930-40s by sociologist Paul Lazarsfeld to examine the effects of the radio on political opinion formation of the United States. This method has since become a very popular method for quantitative research in the social sciences.
The survey method can be used for descriptive, exploratory, or explanatory research. This method is best suited for studies that have individual people as the unit of analysis. Although other units of analysis, such as groups, organizations or dyads (pairs of organizations, such as buyers and sellers), are also studied using surveys, such studies often use a specific person from each unit as a “key informant” or a “proxy” for that unit, and such surveys may be subject to respondent bias if the informant chosen does not have adequate knowledge or has a biased opinion about the phenomenon of interest. For instance, Chief Executive Officers may not adequately know employee’s perceptions or teamwork in their own companies, and may therefore be the wrong informant for studies of team dynamics or employee self-esteem.
Survey research has several inherent strengths compared to other research methods. First, surveys are an excellent vehicle for measuring a wide variety of unobservable data, such as people’s preferences (e.g., political orientation), traits (e.g., self-esteem), attitudes (e.g., toward immigrants), beliefs (e.g., about a new law), behaviors (e.g., smoking or drinking behavior), or factual information (e.g., income). Second, survey research is also ideally suited for remotely collecting data about a population that is too large to observe directly. A large area, such as an entire country, can be covered using mail-in, electronic mail, or telephone surveys using meticulous sampling to ensure that the population is adequately represented in a small sample. Third, due to their unobtrusive nature and the ability to respond at one’s convenience, questionnaire surveys are preferred by some respondents. Fourth, interviews may be the only way of reaching certain population groups such as the homeless or illegal immigrants for which there is no sampling frame available. Fifth, large sample surveys may allow detection of small effects even while analyzing multiple variables, and depending on the survey design, may also allow comparative analysis of population subgroups (i.e., within-group and between-group analysis). Sixth, survey research is economical in terms of researcher time, effort and cost than most other methods such as experimental research and case research. At the same time, survey research also has some unique disadvantages. It is subject to a large number of biases such as non-response bias, sampling bias, social desirability bias, and recall bias, as discussed in the last section of this chapter.
Depending on how the data is collected, survey research can be divided into two broad categories: questionnaire surveys (which may be mail-in, group-administered, or online surveys), and interview surveys (which may be personal, telephone, or focus group interviews). Questionnaires are instruments that are completed in writing by respondents, while interviews are completed by the interviewer based on verbal responses provided by respondents. As discussed below, each type has its own strengths and weaknesses, in terms of their costs, coverage of the target population, and researcher’s flexibility in asking questions.
Questionnaire Surveys
Invented by Sir Francis Galton, a questionnaire is a research instrument consisting of a set of questions (items) intended to capture responses from respondents in a standardized manner. Questions may be unstructured or structured. Unstructured questions ask respondents to provide a response in their own words, while structured questions ask respondents to select an answer from a given set of choices. Subjects’ responses to individual questions (items) on a structured questionnaire may be aggregated into a composite scale or index for statistical analysis. Questions should be designed such that respondents are able to read, understand, and respond to them in a meaningful way, and hence the survey method may not be appropriate or practical for certain demographic groups such as children or the illiterate.
Most questionnaire surveys tend to be self-administered mail surveys , where the same questionnaire is mailed to a large number of people, and willing respondents can complete the survey at their convenience and return it in postage-prepaid envelopes. Mail surveys are advantageous in that they are unobtrusive, and they are inexpensive to administer, since bulk postage is cheap in most countries. However, response rates from mail surveys tend to be quite low since most people tend to ignore survey requests. There may also be long delays (several months) in respondents’ completing and returning the survey (or they may simply lose it). Hence, the researcher must continuously monitor responses as they are being returned, track and send reminders to non-respondents repeated reminders (two or three reminders at intervals of one to 1.5 months is ideal). Questionnaire surveys are also not well-suited for issues that require clarification on the part of the respondent or those that require detailed written responses. Longitudinal designs can be used to survey the same set of respondents at different times, but response rates tend to fall precipitously from one survey to the next.
A second type of survey is group-administered questionnaire . A sample of respondents is brought together at a common place and time, and each respondent is asked to complete the survey questionnaire while in that room. Respondents enter their responses independently without interacting with each other. This format is convenient for the researcher, and high response rate is assured. If respondents do not understand any specific question, they can ask for clarification. In many organizations, it is relatively easy to assemble a group of employees in a conference room or lunch room, especially if the survey is approved by corporate executives.
A more recent type of questionnaire survey is an online or web survey. These surveys are administered over the Internet using interactive forms. Respondents may receive an electronic mail request for participation in the survey with a link to an online website where the survey may be completed. Alternatively, the survey may be embedded into an e-mail, and can be completed and returned via e-mail. These surveys are very inexpensive to administer, results are instantly recorded in an online database, and the survey can be easily modified if needed. However, if the survey website is not password-protected or designed to prevent multiple submissions, the responses can be easily compromised. Furthermore, sampling bias may be a significant issue since the survey cannot reach people that do not have computer or Internet access, such as many of the poor, senior, and minority groups, and the respondent sample is skewed toward an younger demographic who are online much of the time and have the time and ability to complete such surveys. Computing the response rate may be problematic, if the survey link is posted on listservs or bulletin boards instead of being e-mailed directly to targeted respondents. For these reasons, many researchers prefer dual-media surveys (e.g., mail survey and online survey), allowing respondents to select their preferred method of response.
Constructing a survey questionnaire is an art. Numerous decisions must be made about the content of questions, their wording, format, and sequencing, all of which can have important consequences for the survey responses.
Response formats. Survey questions may be structured or unstructured. Responses to structured questions are captured using one of the following response formats:
- Dichotomous response , where respondents are asked to select one of two possible choices, such as true/false, yes/no, or agree/disagree. An example of such a question is: Do you think that the death penalty is justified under some circumstances (circle one): yes / no.
- Nominal response , where respondents are presented with more than two unordered options, such as: What is your industry of employment: manufacturing / consumer services / retail / education / healthcare / tourism & hospitality / other.
- Ordinal response , where respondents have more than two ordered options, such as: what is your highest level of education: high school / college degree / graduate studies.
- Interval-level response , where respondents are presented with a 5-point or 7-point Likert scale, semantic differential scale, or Guttman scale. Each of these scale types were discussed in a previous chapter.
- Continuous response , where respondents enter a continuous (ratio-scaled) value with a meaningful zero point, such as their age or tenure in a firm. These responses generally tend to be of the fill-in-the blanks type.
Question content and wording. Responses obtained in survey research are very sensitive to the types of questions asked. Poorly framed or ambiguous questions will likely result in meaningless responses with very little value. Dillman (1978) recommends several rules for creating good survey questions. Every single question in a survey should be carefully scrutinized for the following issues:
- Is the question clear and understandable: Survey questions should be stated in a very simple language, preferably in active voice, and without complicated words or jargon that may not be understood by a typical respondent. All questions in the questionnaire should be worded in a similar manner to make it easy for respondents to read and understand them. The only exception is if your survey is targeted at a specialized group of respondents, such as doctors, lawyers and researchers, who use such jargon in their everyday environment.
- Is the question worded in a negative manner: Negatively worded questions, such as should your local government not raise taxes, tend to confuse many responses and lead to inaccurate responses. Such questions should be avoided, and in all cases, avoid double-negatives.
- Is the question ambiguous: Survey questions should not words or expressions that may be interpreted differently by different respondents (e.g., words like “any” or “just”). For instance, if you ask a respondent, what is your annual income, it is unclear whether you referring to salary/wages, or also dividend, rental, and other income, whether you referring to personal income, family income (including spouse’s wages), or personal and business income? Different interpretation by different respondents will lead to incomparable responses that cannot be interpreted correctly.
- Does the question have biased or value-laden words: Bias refers to any property of a question that encourages subjects to answer in a certain way. Kenneth Rasinky (1989) examined several studies on people’s attitude toward government spending, and observed that respondents tend to indicate stronger support for “assistance to the poor” and less for “welfare”, even though both terms had the same meaning. In this study, more support was also observed for “halting rising crime rate” (and less for “law enforcement”), “solving problems of big cities” (and less for “assistance to big cities”), and “dealing with drug addiction” (and less for “drug rehabilitation”). A biased language or tone tends to skew observed responses. It is often difficult to anticipate in advance the biasing wording, but to the greatest extent possible, survey questions should be carefully scrutinized to avoid biased language.
- Is the question double-barreled: Double-barreled questions are those that can have multiple answers. For example, are you satisfied with the hardware and software provided for your work? In this example, how should a respondent answer if he/she is satisfied with the hardware but not with the software or vice versa? It is always advisable to separate double-barreled questions into separate questions: (1) are you satisfied with the hardware provided for your work, and (2) are you satisfied with the software provided for your work. Another example: does your family favor public television? Some people may favor public TV for themselves, but favor certain cable TV programs such as Sesame Street for their children.
- Is the question too general: Sometimes, questions that are too general may not accurately convey respondents’ perceptions. If you asked someone how they liked a certain book and provide a response scale ranging from “not at all” to “extremely well”, if that person selected “extremely well”, what does he/she mean? Instead, ask more specific behavioral questions, such as will you recommend this book to others, or do you plan to read other books by the same author? Likewise, instead of asking how big is your firm (which may be interpreted differently by respondents), ask how many people work for your firm, and/or what is the annual revenues of your firm, which are both measures of firm size.
- Is the question too detailed: Avoid unnecessarily detailed questions that serve no specific research purpose. For instance, do you need the age of each child in a household or is just the number of children in the household acceptable? However, if unsure, it is better to err on the side of details than generality.
- Is the question presumptuous: If you ask, what do you see are the benefits of a tax cut, you are presuming that the respondent sees the tax cut as beneficial. But many people may not view tax cuts as being beneficial, because tax cuts generally lead to lesser funding for public schools, larger class sizes, and fewer public services such as police, ambulance, and fire service. Avoid questions with built-in presumptions.
- Is the question imaginary: A popular question in many television game shows is “if you won a million dollars on this show, how will you plan to spend it?” Most respondents have never been faced with such an amount of money and have never thought about it (most don’t even know that after taxes, they will get only about $640,000 or so in the United States, and in many cases, that amount is spread over a 20-year period, so that their net present value is even less), and so their answers tend to be quite random, such as take a tour around the world, buy a restaurant or bar, spend on education, save for retirement, help parents or children, or have a lavish wedding. Imaginary questions have imaginary answers, which cannot be used for making scientific inferences.
- Do respondents have the information needed to correctly answer the question: Often times, we assume that subjects have the necessary information to answer a question, when in reality, they do not. Even if a response is obtained, in such case, the responses tend to be inaccurate, given their lack of knowledge about the question being asked. For instance, we should not ask the CEO of a company about day-to-day operational details that they may not be aware of, or asking teachers about how much their students are learning, or asking high-schoolers “Do you think the US Government acted appropriately in the Bay of Pigs crisis?”
Question sequencing. In general, questions should flow logically from one to the next. To achieve the best response rates, questions should flow from the least sensitive to the most sensitive, from the factual and behavioral to the attitudinal, and from the more general to the more specific. Some general rules for question sequencing:
- Start with easy non-threatening questions that can be easily recalled. Good options are demographics (age, gender, education level) for individual-level surveys and firmographics (employee count, annual revenues, industry) for firm-level surveys.
- Never start with an open ended question.
- If following an historical sequence of events, follow a chronological order from earliest to latest.
- Ask about one topic at a time. When switching topics, use a transition, such as “The next section examines your opinions about …”
- Use filter or contingency questions as needed, such as: “If you answered “yes” to question 5, please proceed to Section 2. If you answered “no” go to Section 3.”
Other golden rules . Do unto your respondents what you would have them do unto you. Be attentive and appreciative of respondents’ time, attention, trust, and confidentiality of personal information. Always practice the following strategies for all survey research:
- People’s time is valuable. Be respectful of their time. Keep your survey as short as possible and limit it to what is absolutely necessary. Respondents do not like spending more than 10-15 minutes on any survey, no matter how important it is. Longer surveys tend to dramatically lower response rates.
- Always assure respondents about the confidentiality of their responses, and how you will use their data (e.g., for academic research) and how the results will be reported (usually, in the aggregate).
- For organizational surveys, assure respondents that you will send them a copy of the final results, and make sure that you follow up with your promise.
- Thank your respondents for their participation in your study.
- Finally, always pretest your questionnaire, at least using a convenience sample, before administering it to respondents in a field setting. Such pretesting may uncover ambiguity, lack of clarity, or biases in question wording, which should be eliminated before administering to the intended sample.
Interview Survey
Interviews are a more personalized form of data collection method than questionnaires, and are conducted by trained interviewers using the same research protocol as questionnaire surveys (i.e., a standardized set of questions). However, unlike a questionnaire, the interview script may contain special instructions for the interviewer that is not seen by respondents, and may include space for the interviewer to record personal observations and comments. In addition, unlike mail surveys, the interviewer has the opportunity to clarify any issues raised by the respondent or ask probing or follow-up questions. However, interviews are time-consuming and resource-intensive. Special interviewing skills are needed on part of the interviewer. The interviewer is also considered to be part of the measurement instrument, and must proactively strive not to artificially bias the observed responses.
The most typical form of interview is personal or face-to-face interview , where the interviewer works directly with the respondent to ask questions and record their responses.
Personal interviews may be conducted at the respondent’s home or office location. This approach may even be favored by some respondents, while others may feel uncomfortable in allowing a stranger in their homes. However, skilled interviewers can persuade respondents to cooperate, dramatically improving response rates.
A variation of the personal interview is a group interview, also called focus group . In this technique, a small group of respondents (usually 6-10 respondents) are interviewed together in a common location. The interviewer is essentially a facilitator whose job is to lead the discussion, and ensure that every person has an opportunity to respond. Focus groups allow deeper examination of complex issues than other forms of survey research, because when people hear others talk, it often triggers responses or ideas that they did not think about before. However, focus group discussion may be dominated by a dominant personality, and some individuals may be reluctant to voice their opinions in front of their peers or superiors, especially while dealing with a sensitive issue such as employee underperformance or office politics. Because of their small sample size, focus groups are usually used for exploratory research rather than descriptive or explanatory research.
A third type of interview survey is telephone interviews . In this technique, interviewers contact potential respondents over the phone, typically based on a random selection of people from a telephone directory, to ask a standard set of survey questions. A more recent and technologically advanced approach is computer-assisted telephone interviewing (CATI), increasing being used by academic, government, and commercial survey researchers, where the interviewer is a telephone operator, who is guided through the interview process by a computer program displaying instructions and questions to be asked on a computer screen. The system also selects respondents randomly using a random digit dialing technique, and records responses using voice capture technology. Once respondents are on the phone, higher response rates can be obtained. This technique is not ideal for rural areas where telephone density is low, and also cannot be used for communicating non-audio information such as graphics or product demonstrations.
Role of interviewer. The interviewer has a complex and multi-faceted role in the interview process, which includes the following tasks:
- Prepare for the interview: Since the interviewer is in the forefront of the data collection effort, the quality of data collected depends heavily on how well the interviewer is trained to do the job. The interviewer must be trained in the interview process and the survey method, and also be familiar with the purpose of the study, how responses will be stored and used, and sources of interviewer bias. He/she should also rehearse and time the interview prior to the formal study.
- Locate and enlist the cooperation of respondents: Particularly in personal, in-home surveys, the interviewer must locate specific addresses, and work around respondents’ schedule sometimes at undesirable times such as during weekends. They should also be like a salesperson, selling the idea of participating in the study.
- Motivate respondents: Respondents often feed off the motivation of the interviewer. If the interviewer is disinterested or inattentive, respondents won’t be motivated to provide useful or informative responses either. The interviewer must demonstrate enthusiasm about the study, communicate the importance of the research to respondents, and be attentive to respondents’ needs throughout the interview.
- Clarify any confusion or concerns: Interviewers must be able to think on their feet and address unanticipated concerns or objections raised by respondents to the respondents’ satisfaction. Additionally, they should ask probing questions as necessary even if such questions are not in the script.
- Observe quality of response: The interviewer is in the best position to judge the quality of information collected, and may supplement responses obtained using personal observations of gestures or body language as appropriate.
Conducting the interview. Before the interview, the interviewer should prepare a kit to carry to the interview session, consisting of a cover letter from the principal investigator or sponsor, adequate copies of the survey instrument, photo identification, and a telephone number for respondents to call to verify the interviewer’s authenticity. The interviewer should also try to call respondents ahead of time to set up an appointment if possible. To start the interview, he/she should speak in an imperative and confident tone, such as “I’d like to take a few minutes of your time to interview you for a very important study,” instead of “May I come in to do an interview?” He/she should introduce himself/herself, present personal credentials, explain the purpose of the study in 1-2 sentences, and assure confidentiality of respondents’ comments and voluntariness of their participation, all in less than a minute. No big words or jargon should be used, and no details should be provided unless specifically requested. If the interviewer wishes to tape-record the interview, he/she should ask for respondent’s explicit permission before doing so. Even if the interview is recorded, the interview must take notes on key issues, probes, or verbatim phrases.
During the interview, the interviewer should follow the questionnaire script and ask questions exactly as written, and not change the words to make the question sound friendlier. They should also not change the order of questions or skip any question that may have been answered earlier. Any issues with the questions should be discussed during rehearsal prior to the actual interview sessions. The interviewer should not finish the respondent’s sentences. If the respondent gives a brief cursory answer, the interviewer should probe the respondent to elicit a more thoughtful, thorough response. Some useful probing techniques are:
- The silent probe: Just pausing and waiting (without going into the next question) may suggest to respondents that the interviewer is waiting for more detailed response.
- Overt encouragement: Occasional “uh-huh” or “okay” may encourage the respondent to go into greater details. However, the interviewer must not express approval or disapproval of what was said by the respondent.
- Ask for elaboration: Such as “can you elaborate on that?” or “A minute ago, you were talking about an experience you had in high school. Can you tell me more about that?”
- Reflection: The interviewer can try the psychotherapist’s trick of repeating what the respondent said. For instance, “What I’m hearing is that you found that experience very traumatic” and then pause and wait for the respondent to elaborate.
After the interview in completed, the interviewer should thank respondents for their time, tell them when to expect the results, and not leave hastily. Immediately after leaving, they should write down any notes or key observations that may help interpret the respondent’s comments better.
Biases in Survey Research
Despite all of its strengths and advantages, survey research is often tainted with systematic biases that may invalidate some of the inferences derived from such surveys. Five such biases are the non-response bias, sampling bias, social desirability bias, recall bias, and common method bias.
Non-response bias. Survey research is generally notorious for its low response rates. A response rate of 15-20% is typical in a mail survey, even after two or three reminders. If the majority of the targeted respondents fail to respond to a survey, then a legitimate concern is whether non-respondents are not responding due to a systematic reason, which may raise questions about the validity of the study’s results. For instance, dissatisfied customers tend to be more vocal about their experience than satisfied customers, and are therefore more likely to respond to questionnaire surveys or interview requests than satisfied customers. Hence, any respondent sample is likely to have a higher proportion of dissatisfied customers than the underlying population from which it is drawn. In this instance, not only will the results lack generalizability, but the observed outcomes may also be an artifact of the biased sample. Several strategies may be employed to improve response rates:
- Advance notification: A short letter sent in advance to the targeted respondents soliciting their participation in an upcoming survey can prepare them in advance and improve their propensity to respond. The letter should state the purpose and importance of the study, mode of data collection (e.g., via a phone call, a survey form in the mail, etc.), and appreciation for their cooperation. A variation of this technique may request the respondent to return a postage-paid postcard indicating whether or not they are willing to participate in the study.
- Relevance of content: If a survey examines issues of relevance or importance to respondents, then they are more likely to respond than to surveys that don’t matter to them.
- Respondent-friendly questionnaire: Shorter survey questionnaires tend to elicit higher response rates than longer questionnaires. Furthermore, questions that are clear, non-offensive, and easy to respond tend to attract higher response rates.
- Endorsement: For organizational surveys, it helps to gain endorsement from a senior executive attesting to the importance of the study to the organization. Such endorsement can be in the form of a cover letter or a letter of introduction, which can improve the researcher’s credibility in the eyes of the respondents.
- Follow-up requests: Multiple follow-up requests may coax some non-respondents to respond, even if their responses are late.
- Interviewer training: Response rates for interviews can be improved with skilled interviewers trained on how to request interviews, use computerized dialing techniques to identify potential respondents, and schedule callbacks for respondents who could not be reached.
- Incentives : Response rates, at least with certain populations, may increase with the use of incentives in the form of cash or gift cards, giveaways such as pens or stress balls, entry into a lottery, draw or contest, discount coupons, promise of contribution to charity, and so forth.
- Non-monetary incentives: Businesses, in particular, are more prone to respond to non-monetary incentives than financial incentives. An example of such a non-monetary incentive is a benchmarking report comparing the business’s individual response against the aggregate of all responses to a survey.
- Confidentiality and privacy: Finally, assurances that respondents’ private data or responses will not fall into the hands of any third party, may help improve response rates.
Sampling bias. Telephone surveys conducted by calling a random sample of publicly available telephone numbers will systematically exclude people with unlisted telephone numbers, mobile phone numbers, and people who are unable to answer the phone (for instance, they are at work) when the survey is being conducted, and will include a disproportionate number of respondents who have land-line telephone service with listed phone numbers and people who stay home during much of the day, such as the unemployed, the disabled, and the elderly. Likewise, online surveys tend to include a disproportionate number of students and younger people who are constantly on the Internet, and systematically exclude people with limited or no access to computers or the Internet, such as the poor and the elderly. Similarly, questionnaire surveys tend to exclude children and the illiterate, who are unable to read, understand, or meaningfully respond to the questionnaire. A different kind of sampling bias relate to sampling the wrong population, such as asking teachers (or parents) about academic learning of their students (or children), or asking CEOs about operational details in their company. Such biases make the respondent sample unrepresentative of the intended population and hurt generalizability claims about inferences drawn from the biased sample.
Social desirability bias . Many respondents tend to avoid negative opinions or embarrassing comments about themselves, their employers, family, or friends. With negative questions such as do you think that your project team is dysfunctional, is there a lot of office politics in your workplace, or have you ever illegally downloaded music files from the Internet, the researcher may not get truthful responses. This tendency among respondents to “spin the truth” in order to portray themselves in a socially desirable manner is called the “social desirability bias”, which hurts the validity of response obtained from survey research. There is practically no way of overcoming the social desirability bias in a questionnaire survey, but in an interview setting, an astute interviewer may be able to spot inconsistent answers and ask probing questions or use personal observations to supplement respondents’ comments.
Recall bias. Responses to survey questions often depend on subjects’ motivation, memory, and ability to respond. Particularly when dealing with events that happened in the distant past, respondents may not adequately remember their own motivations or behaviors or perhaps their memory of such events may have evolved with time and no longer retrievable. For instance, if a respondent to asked to describe his/her utilization of computer technology one year ago or even memorable childhood events like birthdays, their response may not be accurate due to difficulties with recall. One possible way of overcoming the recall bias is by anchoring respondent’s memory in specific events as they happened, rather than asking them to recall their perceptions and motivations from memory.
Common method bias. Common method bias refers to the amount of spurious covariance shared between independent and dependent variables that are measured at the same point in time, such as in a cross-sectional survey, using the same instrument, such as a questionnaire. In such cases, the phenomenon under investigation may not be adequately separated from measurement artifacts. Standard statistical tests are available to test for common method bias, such as Harmon’s single-factor test (Podsakoff et al. 2003), Lindell and Whitney’s (2001) market variable technique, and so forth. This bias can be potentially avoided if the independent and dependent variables are measured at different points in time, using a longitudinal survey design, of if these variables are measured using different methods, such as computerized recording of dependent variable versus questionnaire-based self-rating of independent variables.
- Social Science Research: Principles, Methods, and Practices. Authored by : Anol Bhattacherjee. Provided by : University of South Florida. Located at : http://scholarcommons.usf.edu/oa_textbooks/3/ . License : CC BY-NC-SA: Attribution-NonCommercial-ShareAlike
- Skip to main content
- Skip to primary sidebar
- Skip to footer
- QuestionPro

- Solutions Industries Gaming Automotive Sports and events Education Government Travel & Hospitality Financial Services Healthcare Cannabis Technology Use Case NPS+ Communities Audience Contactless surveys Mobile LivePolls Member Experience GDPR Positive People Science 360 Feedback Surveys
- Resources Blog eBooks Survey Templates Case Studies Training Help center

Home Market Research
Questionnaire: The ultimate guide, advantages & examples
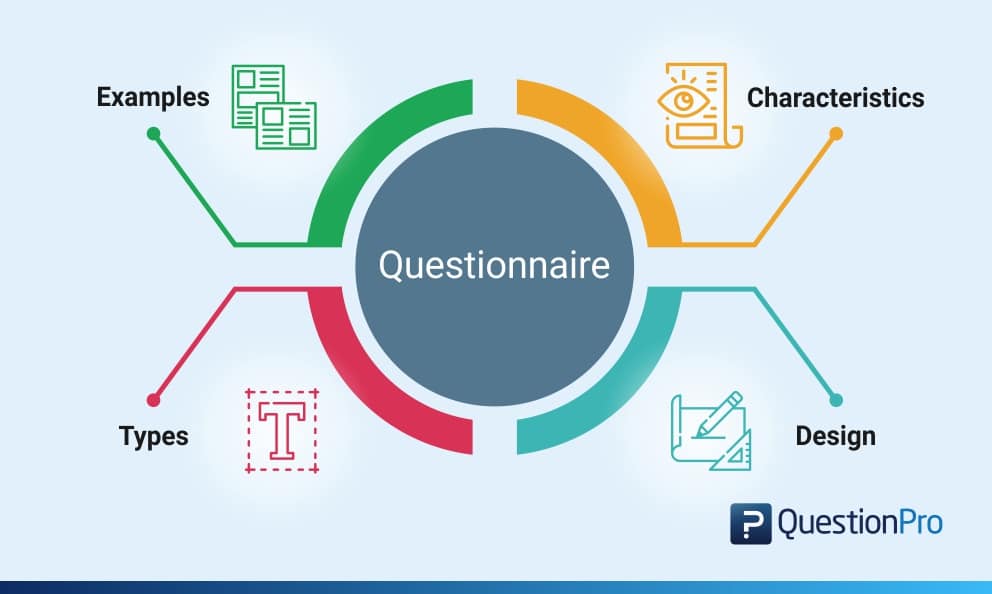
What is a Questionnaire?
A questionnaire is a research instrument that consists of a set of questions or other types of prompts that aims to collect information from a respondent. A research questionnaire is typically a mix of close-ended questions and open-ended questions .
Open-ended, long-form questions offer the respondent the ability to elaborate on their thoughts. Research questionnaires were developed in 1838 by the Statistical Society of London.
LEARN ABOUT: Candidate Experience Survey
The data collected from a data collection questionnaire can be both qualitative as well as quantitative in nature. A questionnaire may or may not be delivered in the form of a survey , but a survey always consists of a questionnaire.
LEARN ABOUT: Testimonial Questions
Advantages of a good questionnaire design
- With a survey questionnaire, you can gather a lot of data in less time.
- There is less chance of any bias(like selection bias ) creeping if you have a standard set of questions to be used for your target audience. You can apply logic to questions based on the respondents’ answers, but the questionnaire will remain standard for a group of respondents that fall in the same segment.
- Surveying online survey software is quick and cost-effective. It offers you a rich set of features to design, distribute, and analyze the response data.
- It can be customized to reflect your brand voice. Thus, it can be used to reinforce your brand image.
- The responses can be compared with the historical data and understand the shift in respondents’ choices and experiences.
- Respondents can answer the questionnaire without revealing their identity. Also, many survey software complies with significant data security and privacy regulations.
LEARN ABOUT: Structured Questionnaire
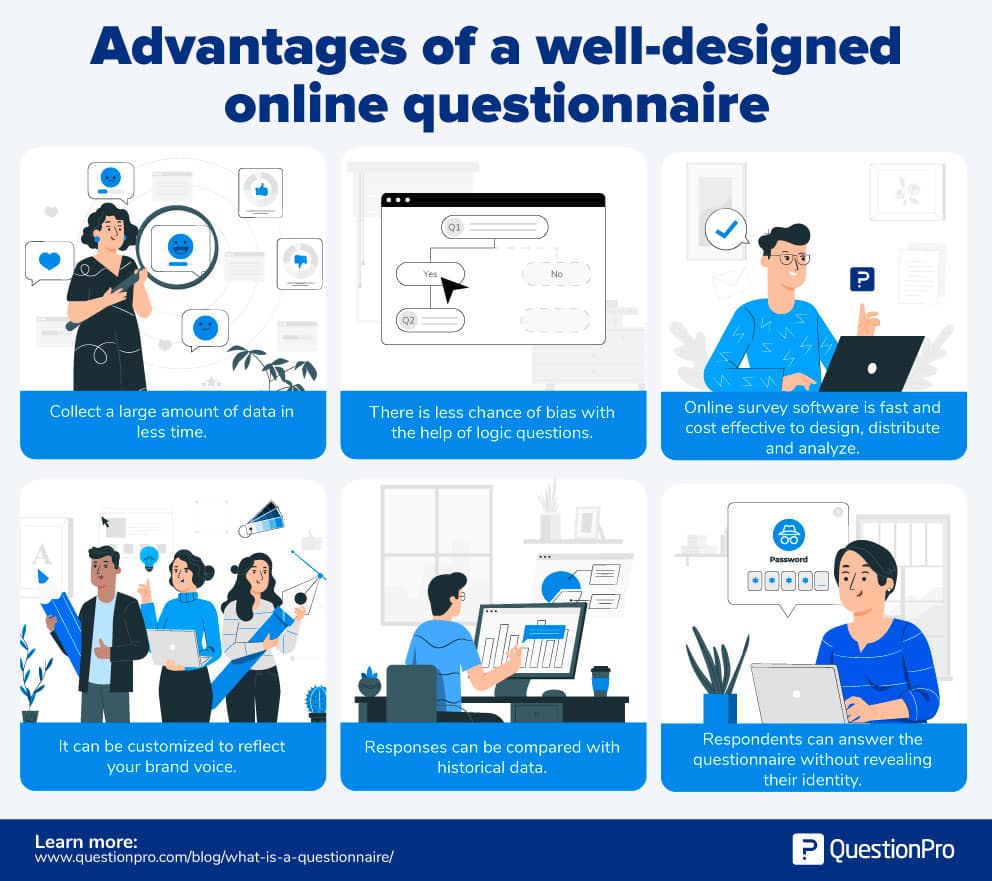
Characteristics of a good questionnaire
Your survey design depends on the type of information you need to collect from respondents. Qualitative questionnaires are used when there is a need to collect exploratory information to help prove or disprove a hypothesis. Quantitative questionnaires are used to validate or test a previously generated hypothesis. However, most questionnaires follow some essential characteristics:
- Uniformity: Questionnaires are very useful to collect demographic information, personal opinions, facts, or attitudes from respondents. One of the most significant attributes of a research form is uniform design and standardization. Every respondent sees the same questions. This helps in data collection and statistical analysis of this data. For example, the retail store evaluation questionnaire template contains questions for evaluating retail store experiences. Questions relate to purchase value, range of options for product selections, and quality of merchandise. These questions are uniform for all customers.
LEARN ABOUT: Research Process Steps
- Exploratory: It should be exploratory to collect qualitative data. There is no restriction on questions that can be in your questionnaire. For example, you use a data collection questionnaire and send it to the female of the household to understand her spending and saving habits relative to the household income. Open-ended questions give you more insight and allow the respondents to explain their practices. A very structured question list could limit the data collection.
LEARN ABOUT: Best Data Collection Tools
- Question Sequence: It typically follows a structured flow of questions to increase the number of responses. This sequence of questions is screening questions , warm-up questions, transition questions, skip questions, challenging questions, and classification questions. For example, our motivation and buying experience questionnaire template covers initial demographic questions and then asks for time spent in sections of the store and the rationale behind purchases.
Types & Definitions
As we explored before, questionnaires can be either structured or free-flowing. Let’s take a closer look at what that entails for your surveys.
- Structured Questionnaires: Structured questionnaires collect quantitative data . The questionnaire is planned and designed to gather precise information. It also initiates a formal inquiry, supplements data, checks previously accumulated data, and helps validate any prior hypothesis.
- Unstructured Questionnaires: Unstructured questionnaires collect qualitative data . They use a basic structure and some branching questions but nothing that limits the responses of a respondent. The questions are more open-ended to collect specific data from participants.
Types of questions in a questionnaire
You can use multiple question types in a questionnaire. Using various question types can help increase responses to your research questionnaire as they tend to keep participants more engaged. The best customer satisfaction survey templates are the most commonly used for better insights and decision-making.
Some of the widely used types of questions are:
- Open-Ended Questions: Open-ended questions help collect qualitative data in a questionnaire where the respondent can answer in a free form with little to no restrictions.
- Dichotomous Questions: The dichotomous question is generally a “yes/no” close-ended question . This question is usually used in case of the need for necessary validation. It is the most natural form of a questionnaire.
- Multiple-Choice Questions: Multiple-choice questions are a close-ended question type in which a respondent has to select one (single-select multiple-choice question) or many (multi-select multiple choice question) responses from a given list of options. The multiple-choice question consists of an incomplete stem (question), right answer or answers, incorrect answers, close alternatives, and distractors. Of course, not all multiple-choice questions have all of the answer types. For example, you probably won’t have the wrong or right answers if you’re looking for customer opinion.
- Scaling Questions: These questions are based on the principles of the four measurement scales – nominal, ordinal, interval, and ratio . A few of the question types that utilize these scales’ fundamental properties are rank order questions , Likert scale questions , semantic differential scale questions , and Stapel scale questions .
LEARN ABOUT: System Usability Scale
- Pictorial Questions: This question type is easy to use and encourages respondents to answer. It works similarly to a multiple-choice question. Respondents are asked a question, and the answer choices are images. This helps respondents choose an answer quickly without over-thinking their answers, giving you more accurate data.
Types of Questionnaires
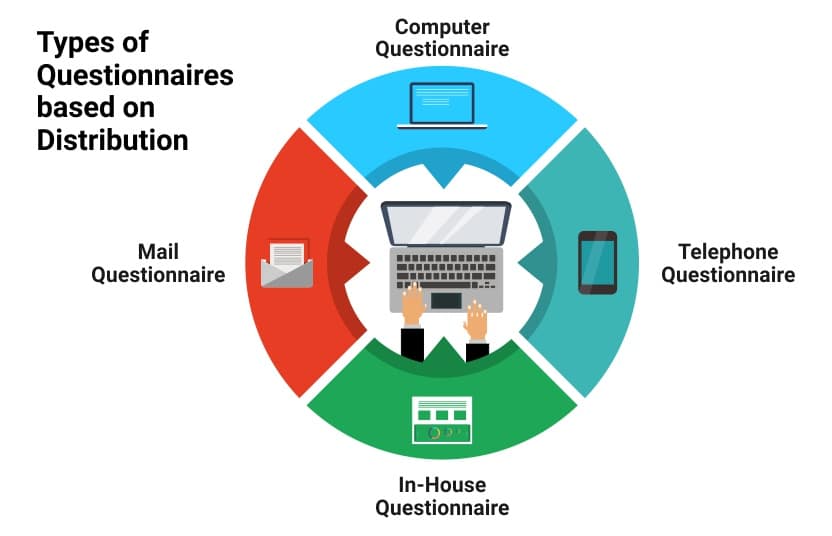
Questionnaires can be administered or distributed in the following forms:
- Online Questionnaire : In this type, respondents are sent the questionnaire via email or other online mediums. This method is generally cost-effective and time-efficient. Respondents can also answer at leisure. Without the pressure to respond immediately, responses may be more accurate. The disadvantage, however, is that respondents can easily ignore these questionnaires. Read more about online surveys .
- Telephone Questionnaire: A researcher makes a phone call to a respondent to collect responses directly. Responses are quick once you have a respondent on the phone. However, a lot of times, the respondents hesitate to give out much information over the phone. It is also an expensive way of conducting research. You’re usually not able to collect as many responses as other types of questionnaires, so your sample may not represent the broader population.
- In-House Questionnaire: This type is used by a researcher who visits the respondent’s home or workplace. The advantage of this method is that the respondent is in a comfortable and natural environment, and in-depth data can be collected. The disadvantage, though, is that it is expensive and slow to conduct.
LEARN ABOUT: Survey Sample Sizes
- Mail Questionnaire: These are starting to be obsolete but are still being used in some market research studies. This method involves a researcher sending a physical data collection questionnaire request to a respondent that can be filled in and sent back. The advantage of this method is that respondents can complete this on their own time to answer truthfully and entirely. The disadvantage is that this method is expensive and time-consuming. There is also a high risk of not collecting enough responses to make actionable insights from the data.
How to design a Questionnaire
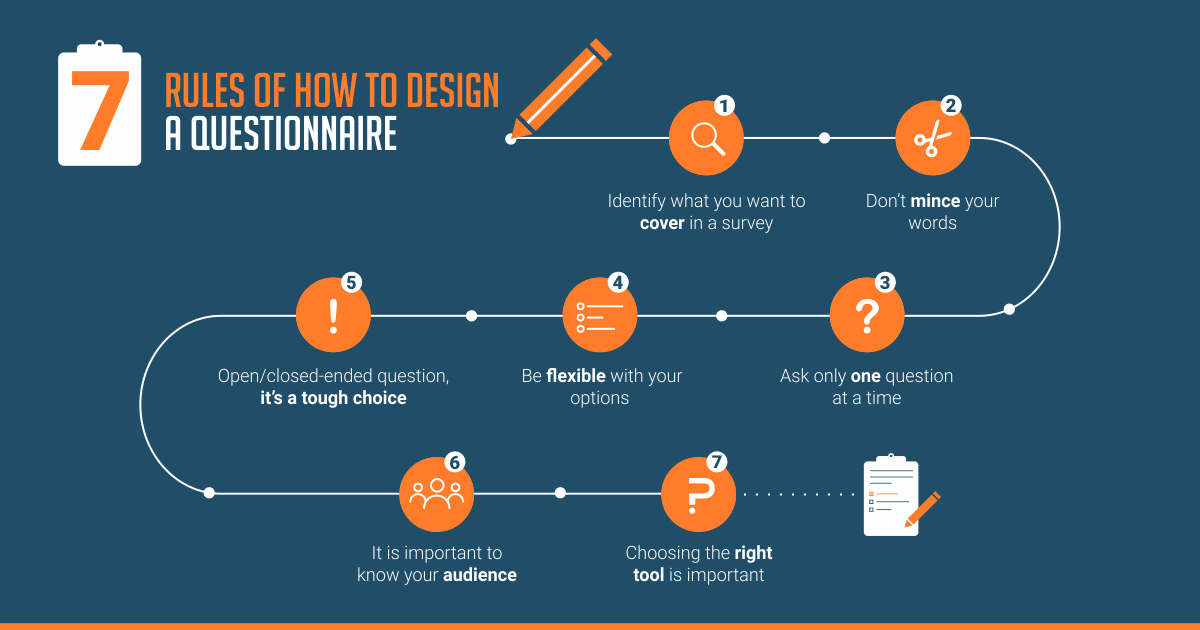
Questionnaire design is a multistep process that requires attention to detail at every step.
Researchers are always hoping that the responses received for a survey questionnaire yield useable data. If the questionnaire is too complicated, there is a fair chance that the respondent might get confused and will drop out or answer inaccurately.
LEARN ABOUT: Easy Test Maker
As a survey creator , you may want to pre-test the survey by administering it to a focus group during development. You can try out a few different questionnaire designs to determine which resonates best with your target audience. Pre-testing is a good practice as the survey creator can comprehend the initial stages if there are any changes required in the survey .
Steps Involved in Questionnaire Design
1. identify the scope of your research:.
Think about what your questionnaire is going to include before you start designing the look of it. The clarity of the topic is of utmost importance as this is the primary step in creating the questionnaire. Once you are clear on the purpose of the questionnaire, you can begin the design process.
LEARN ABOUT: Social Communication Questionnaire
2. Keep it simple:
The words or phrases you use while writing the questionnaire must be easy to understand. If the questions are unclear, the respondents may simply choose any answer and skew the data you collect.
3. Ask only one question at a time:
At times, a researcher may be tempted to add two similar questions. This might seem like an excellent way to consolidate answers to related issues, but it can confuse your respondents or lead to inaccurate data. If any of your questions contain the word “and,” take another look. This question likely has two parts, which can affect the quality of your data.
4. Be flexible with your options:
While designing, the survey creator needs to be flexible in terms of “option choice” for the respondents. Sometimes the respondents may not necessarily want to choose from the answer options provided by the survey creator. An “other” option often helps keep respondents engaged in the survey.
5. The open-ended or closed-ended question is a tough choice:
The survey creator might end up in a situation where they need to make distinct choices between open or close-ended questions. The question type should be carefully chosen as it defines the tone and importance of asking the question in the first place.
If the questionnaire requires the respondents to elaborate on their thoughts, an open-ended q u estion is the best choice. If the surveyor wants a specific response, then close-ended questions should be their primary choice. The key to asking closed-ended questions is to generate data that is easy to analyze and spot trends.
6. It is essential to know your audience:
A researcher should know their target audience. For example, if the target audience speaks mostly Spanish, sending the questionnaire in any other language would lower the response rate and accuracy of data. Something that may seem clear to you may be confusing to your respondents. Use simple language and terminology that your respondents will understand, and avoid technical jargon and industry-specific language that might confuse your respondents.
For efficient market research, researchers need a representative sample collected using one of the many sampling techniques , such as a sample questionnaire. It is imperative to plan and define these target respondents based on the demographics required.
7. Choosing the right tool is essential:
QuestionPro is a simple yet advanced survey software platform that the surveyors can use to create a questionnaire or choose from the already existing 300+ questionnaire templates.
Always save personal questions for last. Sensitive questions may cause respondents to drop off before completing. If these questions are at the end, the respondent has had time to become more comfortable with the interview and are more likely to answer personal or demographic questions.
Differences between a Questionnaire and a Survey
Read more: Difference between a survey and a questionnaire
Questionnaire Examples
The best way to understand how questionnaires work is to see the types of questionnaires available. Some examples of a questionnaire are:
USE THIS FREE TEMPLATE
The above survey questions are typically easy to use, understand, and execute. Additionally, the standardized answers of a survey questionnaire instead of a person-to-person conversation make it easier to compile useable data.
The most significant limitation of a data collection questionnaire is that respondents need to read all of the questions and respond to them. For example, you send an invitation through email asking respondents to complete the questions on social media. If a target respondent doesn’t have the right social media profiles, they can’t answer your questions.
Learn More: 350+ Free Survey Examples and Templates
MORE LIKE THIS
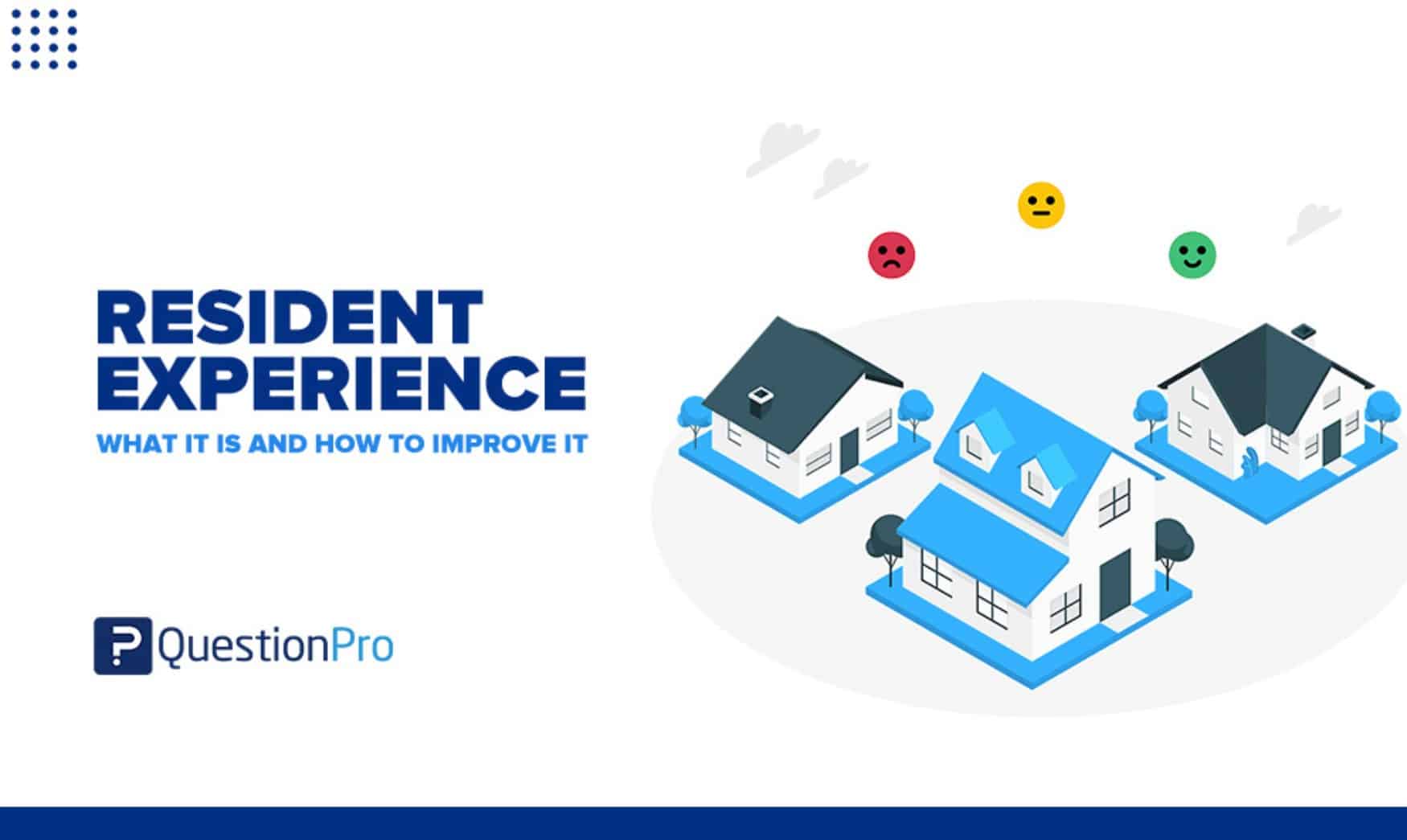
Resident Experience: What It Is and How to Improve It
Mar 27, 2024
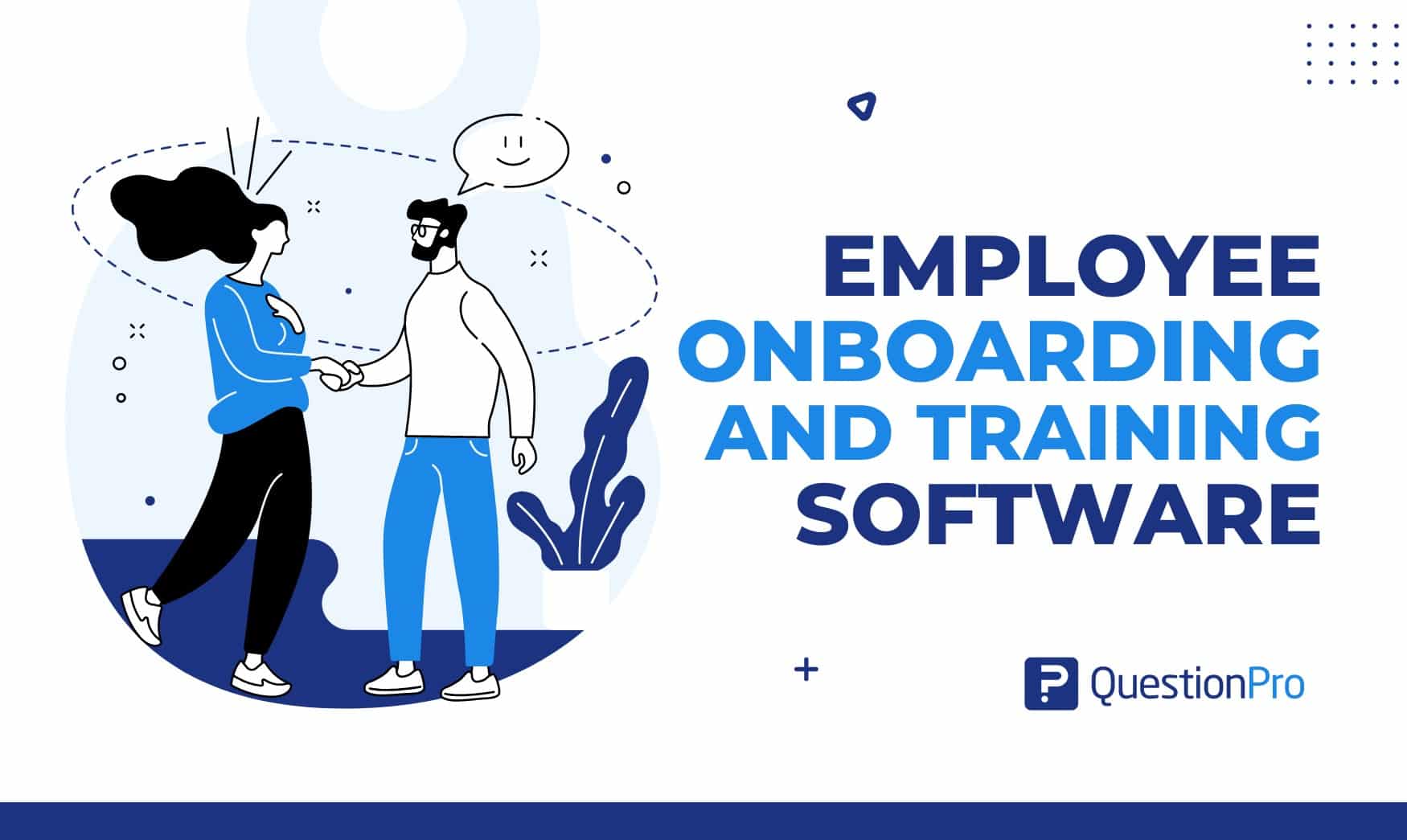
11 Best Employee Onboarding and Training Software in 2024
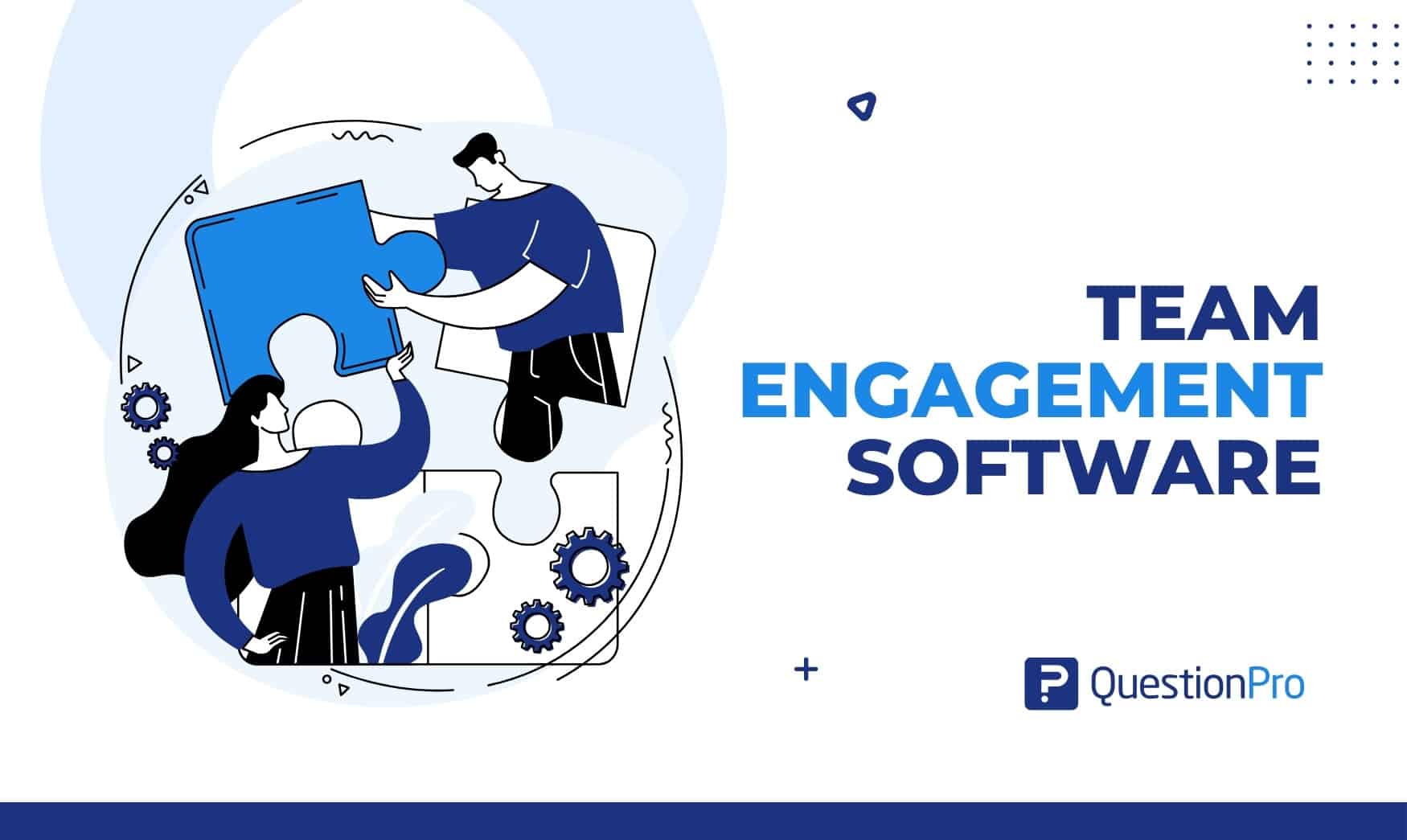
Top 11 Team Engagement Software in 2024
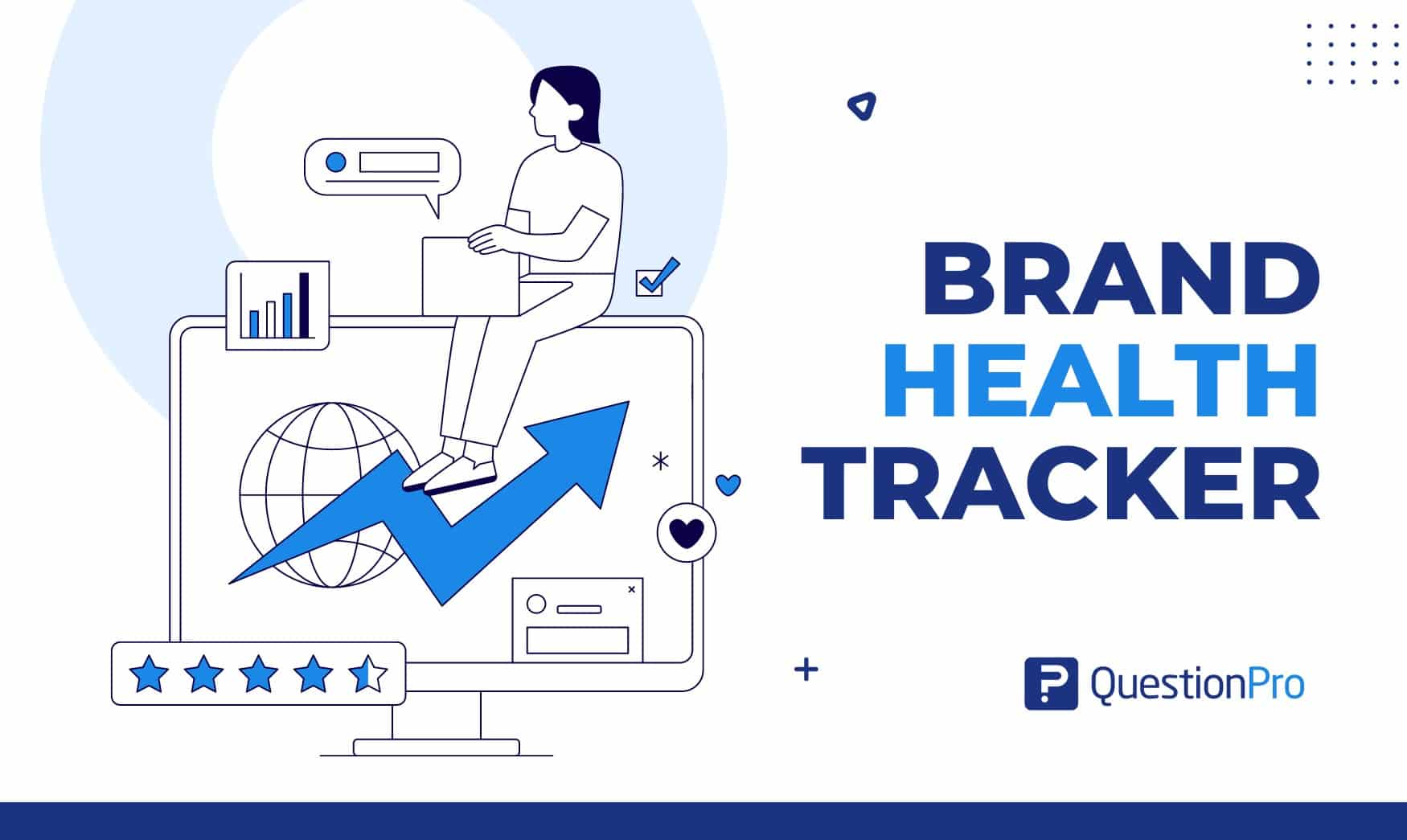
8 Leading Brand Health Tracker to Track Your Brand Reputation
Mar 26, 2024
Other categories
- Academic Research
- Artificial Intelligence
- Assessments
- Brand Awareness
- Case Studies
- Communities
- Consumer Insights
- Customer effort score
- Customer Engagement
- Customer Experience
- Customer Loyalty
- Customer Research
- Customer Satisfaction
- Employee Benefits
- Employee Engagement
- Employee Retention
- Friday Five
- General Data Protection Regulation
- Insights Hub
- Life@QuestionPro
- Market Research
- Mobile diaries
- Mobile Surveys
- New Features
- Online Communities
- Question Types
- Questionnaire
- QuestionPro Products
- Release Notes
- Research Tools and Apps
- Revenue at Risk
- Survey Templates
- Training Tips
- Uncategorized
- Video Learning Series
- What’s Coming Up
- Workforce Intelligence

An official website of the United States government
The .gov means it’s official. Federal government websites often end in .gov or .mil. Before sharing sensitive information, make sure you’re on a federal government site.
The site is secure. The https:// ensures that you are connecting to the official website and that any information you provide is encrypted and transmitted securely.
- Publications
- Account settings
Preview improvements coming to the PMC website in October 2024. Learn More or Try it out now .
- Advanced Search
- Journal List
- J Adv Pract Oncol
- v.6(2); Mar-Apr 2015
Understanding and Evaluating Survey Research
A variety of methodologic approaches exist for individuals interested in conducting research. Selection of a research approach depends on a number of factors, including the purpose of the research, the type of research questions to be answered, and the availability of resources. The purpose of this article is to describe survey research as one approach to the conduct of research so that the reader can critically evaluate the appropriateness of the conclusions from studies employing survey research.
SURVEY RESEARCH
Survey research is defined as "the collection of information from a sample of individuals through their responses to questions" ( Check & Schutt, 2012, p. 160 ). This type of research allows for a variety of methods to recruit participants, collect data, and utilize various methods of instrumentation. Survey research can use quantitative research strategies (e.g., using questionnaires with numerically rated items), qualitative research strategies (e.g., using open-ended questions), or both strategies (i.e., mixed methods). As it is often used to describe and explore human behavior, surveys are therefore frequently used in social and psychological research ( Singleton & Straits, 2009 ).
Information has been obtained from individuals and groups through the use of survey research for decades. It can range from asking a few targeted questions of individuals on a street corner to obtain information related to behaviors and preferences, to a more rigorous study using multiple valid and reliable instruments. Common examples of less rigorous surveys include marketing or political surveys of consumer patterns and public opinion polls.
Survey research has historically included large population-based data collection. The primary purpose of this type of survey research was to obtain information describing characteristics of a large sample of individuals of interest relatively quickly. Large census surveys obtaining information reflecting demographic and personal characteristics and consumer feedback surveys are prime examples. These surveys were often provided through the mail and were intended to describe demographic characteristics of individuals or obtain opinions on which to base programs or products for a population or group.
More recently, survey research has developed into a rigorous approach to research, with scientifically tested strategies detailing who to include (representative sample), what and how to distribute (survey method), and when to initiate the survey and follow up with nonresponders (reducing nonresponse error), in order to ensure a high-quality research process and outcome. Currently, the term "survey" can reflect a range of research aims, sampling and recruitment strategies, data collection instruments, and methods of survey administration.
Given this range of options in the conduct of survey research, it is imperative for the consumer/reader of survey research to understand the potential for bias in survey research as well as the tested techniques for reducing bias, in order to draw appropriate conclusions about the information reported in this manner. Common types of error in research, along with the sources of error and strategies for reducing error as described throughout this article, are summarized in the Table .

Sources of Error in Survey Research and Strategies to Reduce Error
The goal of sampling strategies in survey research is to obtain a sufficient sample that is representative of the population of interest. It is often not feasible to collect data from an entire population of interest (e.g., all individuals with lung cancer); therefore, a subset of the population or sample is used to estimate the population responses (e.g., individuals with lung cancer currently receiving treatment). A large random sample increases the likelihood that the responses from the sample will accurately reflect the entire population. In order to accurately draw conclusions about the population, the sample must include individuals with characteristics similar to the population.
It is therefore necessary to correctly identify the population of interest (e.g., individuals with lung cancer currently receiving treatment vs. all individuals with lung cancer). The sample will ideally include individuals who reflect the intended population in terms of all characteristics of the population (e.g., sex, socioeconomic characteristics, symptom experience) and contain a similar distribution of individuals with those characteristics. As discussed by Mady Stovall beginning on page 162, Fujimori et al. ( 2014 ), for example, were interested in the population of oncologists. The authors obtained a sample of oncologists from two hospitals in Japan. These participants may or may not have similar characteristics to all oncologists in Japan.
Participant recruitment strategies can affect the adequacy and representativeness of the sample obtained. Using diverse recruitment strategies can help improve the size of the sample and help ensure adequate coverage of the intended population. For example, if a survey researcher intends to obtain a sample of individuals with breast cancer representative of all individuals with breast cancer in the United States, the researcher would want to use recruitment strategies that would recruit both women and men, individuals from rural and urban settings, individuals receiving and not receiving active treatment, and so on. Because of the difficulty in obtaining samples representative of a large population, researchers may focus the population of interest to a subset of individuals (e.g., women with stage III or IV breast cancer). Large census surveys require extremely large samples to adequately represent the characteristics of the population because they are intended to represent the entire population.
DATA COLLECTION METHODS
Survey research may use a variety of data collection methods with the most common being questionnaires and interviews. Questionnaires may be self-administered or administered by a professional, may be administered individually or in a group, and typically include a series of items reflecting the research aims. Questionnaires may include demographic questions in addition to valid and reliable research instruments ( Costanzo, Stawski, Ryff, Coe, & Almeida, 2012 ; DuBenske et al., 2014 ; Ponto, Ellington, Mellon, & Beck, 2010 ). It is helpful to the reader when authors describe the contents of the survey questionnaire so that the reader can interpret and evaluate the potential for errors of validity (e.g., items or instruments that do not measure what they are intended to measure) and reliability (e.g., items or instruments that do not measure a construct consistently). Helpful examples of articles that describe the survey instruments exist in the literature ( Buerhaus et al., 2012 ).
Questionnaires may be in paper form and mailed to participants, delivered in an electronic format via email or an Internet-based program such as SurveyMonkey, or a combination of both, giving the participant the option to choose which method is preferred ( Ponto et al., 2010 ). Using a combination of methods of survey administration can help to ensure better sample coverage (i.e., all individuals in the population having a chance of inclusion in the sample) therefore reducing coverage error ( Dillman, Smyth, & Christian, 2014 ; Singleton & Straits, 2009 ). For example, if a researcher were to only use an Internet-delivered questionnaire, individuals without access to a computer would be excluded from participation. Self-administered mailed, group, or Internet-based questionnaires are relatively low cost and practical for a large sample ( Check & Schutt, 2012 ).
Dillman et al. ( 2014 ) have described and tested a tailored design method for survey research. Improving the visual appeal and graphics of surveys by using a font size appropriate for the respondents, ordering items logically without creating unintended response bias, and arranging items clearly on each page can increase the response rate to electronic questionnaires. Attending to these and other issues in electronic questionnaires can help reduce measurement error (i.e., lack of validity or reliability) and help ensure a better response rate.
Conducting interviews is another approach to data collection used in survey research. Interviews may be conducted by phone, computer, or in person and have the benefit of visually identifying the nonverbal response(s) of the interviewee and subsequently being able to clarify the intended question. An interviewer can use probing comments to obtain more information about a question or topic and can request clarification of an unclear response ( Singleton & Straits, 2009 ). Interviews can be costly and time intensive, and therefore are relatively impractical for large samples.
Some authors advocate for using mixed methods for survey research when no one method is adequate to address the planned research aims, to reduce the potential for measurement and non-response error, and to better tailor the study methods to the intended sample ( Dillman et al., 2014 ; Singleton & Straits, 2009 ). For example, a mixed methods survey research approach may begin with distributing a questionnaire and following up with telephone interviews to clarify unclear survey responses ( Singleton & Straits, 2009 ). Mixed methods might also be used when visual or auditory deficits preclude an individual from completing a questionnaire or participating in an interview.
FUJIMORI ET AL.: SURVEY RESEARCH
Fujimori et al. ( 2014 ) described the use of survey research in a study of the effect of communication skills training for oncologists on oncologist and patient outcomes (e.g., oncologist’s performance and confidence and patient’s distress, satisfaction, and trust). A sample of 30 oncologists from two hospitals was obtained and though the authors provided a power analysis concluding an adequate number of oncologist participants to detect differences between baseline and follow-up scores, the conclusions of the study may not be generalizable to a broader population of oncologists. Oncologists were randomized to either an intervention group (i.e., communication skills training) or a control group (i.e., no training).
Fujimori et al. ( 2014 ) chose a quantitative approach to collect data from oncologist and patient participants regarding the study outcome variables. Self-report numeric ratings were used to measure oncologist confidence and patient distress, satisfaction, and trust. Oncologist confidence was measured using two instruments each using 10-point Likert rating scales. The Hospital Anxiety and Depression Scale (HADS) was used to measure patient distress and has demonstrated validity and reliability in a number of populations including individuals with cancer ( Bjelland, Dahl, Haug, & Neckelmann, 2002 ). Patient satisfaction and trust were measured using 0 to 10 numeric rating scales. Numeric observer ratings were used to measure oncologist performance of communication skills based on a videotaped interaction with a standardized patient. Participants completed the same questionnaires at baseline and follow-up.
The authors clearly describe what data were collected from all participants. Providing additional information about the manner in which questionnaires were distributed (i.e., electronic, mail), the setting in which data were collected (e.g., home, clinic), and the design of the survey instruments (e.g., visual appeal, format, content, arrangement of items) would assist the reader in drawing conclusions about the potential for measurement and nonresponse error. The authors describe conducting a follow-up phone call or mail inquiry for nonresponders, using the Dillman et al. ( 2014 ) tailored design for survey research follow-up may have reduced nonresponse error.
CONCLUSIONS
Survey research is a useful and legitimate approach to research that has clear benefits in helping to describe and explore variables and constructs of interest. Survey research, like all research, has the potential for a variety of sources of error, but several strategies exist to reduce the potential for error. Advanced practitioners aware of the potential sources of error and strategies to improve survey research can better determine how and whether the conclusions from a survey research study apply to practice.
The author has no potential conflicts of interest to disclose.
Final dates! Join the tutor2u subject teams in London for a day of exam technique and revision at the cinema. Learn more →
Reference Library
Collections
- See what's new
- All Resources
- Student Resources
- Assessment Resources
- Teaching Resources
- CPD Courses
- Livestreams
Study notes, videos, interactive activities and more!
Sociology news, insights and enrichment
Currated collections of free resources
Browse resources by topic
- All Sociology Resources
Resource Selections
Currated lists of resources
Study Notes
Research Methods: Questionnaires
Last updated 15 Jun 2020
- Share on Facebook
- Share on Twitter
- Share by Email
A questionnaire, or social survey, is a popular research method that consists of a list of questions.
If administered directly by the researcher to the subject in person then this is the same as a structured interview ; however, questionnaires can also be completed independently (self-completion questionnaires) and therefore administered in bulk, through the post or electronically for example. The method can use closed or open questions or indeed a mixture of the two, depending on what sort of data is desired and how the researcher intends to analyse it.
Reliability and Validity of Questionnaires
In the context of research, the reliability of a method refers to the extent to which, were the same study to be repeated, it would produce the same results. For this to be the case, samples need to be representative, questions or processes need to be uniform and data would generally need to be quantitative. Researchers need to be confident that if they repeat the same research and the result is different that what they are studying has genuinely changed and not just that their original method was not sufficiently reliable. If you take the example of opinion polls on people's voting preferences: if the support for parties changes by several points, the researchers (and their "customers") need to be confident that this is because people are really changing their minds about how they intend to vote; that it is not simply that the research method is unreliable and therefore changes between polls are likely and unpredictable. If that were the case it would render their data useless.
Questionnaires are generally considered to be high in reliability . This is because it is possible to ask a uniform set of questions. Any problems in the design of the survey can be ironed out after a pilot study . The more closed questions used, the more reliable the research.
Valid research reveals a true picture. Data that is high in validity tends to be qualitative and is often described as "rich". It seeks to provide the researcher with verstehen - a deep, true understanding of their research object. The validity of data produced by questionnaires can be undermined by the use of closed questions which limit respondents' answers.
In a questionnaire (or structured interview ) it is possible to ask open questions or closed questions. Closed questions are those with a limited number of possible responses, often "yes" or "no". Closed questions help to make data easier to analyse and more reliable. This is because closed questions produce quantitative data. However, restricting responses can impact validity. To try to overcome this, sociologists often broaden possible responses to closed questions, by, for example, ranking possible responses or indicating the degree of agreement with a statement. The latter is known as the Likert Scale, and is a way of quantifying qualitative data for ease of analysis. It is also possible to mix closed questions with an open "other (please specify)" option.
Open questions do not limit the possible answers that the responder can give, producing qualitative data which is generally considered to be higher in validity. This is because it can be detailed and the respondent can give their own views, rather than be limited by the assumptions of the researcher. However, such data can be very difficult to analyse. There is also the danger that options are simply limited during analysis rather than design (ie. the researcher puts the wide range of responses into a smaller number of categories in order to analyse them). This depends on the researcher's interpretation of the respondent's response which could be affected by subjectivity or the researcher's values.
Because questionnaires are usually used to produce quantitative data, they are generally thought to be more reliable than valid. However, they do have the advantage of being able to produce a mixture of reliable and valid data, known as triangulation .
- Questionnaire
- Closed Questions
- Open Question
- Primary Data
- Response Rate
You might also like
Research methods - "card drop" activity.
Quizzes & Activities
Research Design: Choice of Research Method
The british census.
18th February 2020
Money really can buy happiness and recessions can take it away
22nd July 2020
Tikly et al, ‘Evaluation of Aiming High: African Caribbean Achievement Project’, (2006)
Quantitative research methods (online lesson).
Online Lessons
Methods in Context: Researching Cultural Factors (Online Lesson)
A new model of social class findings from the bbc’s great british class survey experiment, our subjects.
- › Criminology
- › Economics
- › Geography
- › Health & Social Care
- › Psychology
- › Sociology
- › Teaching & learning resources
- › Student revision workshops
- › Online student courses
- › CPD for teachers
- › Livestreams
- › Teaching jobs
Boston House, 214 High Street, Boston Spa, West Yorkshire, LS23 6AD Tel: 01937 848885
- › Contact us
- › Terms of use
- › Privacy & cookies
© 2002-2024 Tutor2u Limited. Company Reg no: 04489574. VAT reg no 816865400.

How to Design Effective Research Questionnaires for Robust Findings
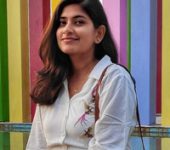
As a staple in data collection, questionnaires help uncover robust and reliable findings that can transform industries, shape policies, and revolutionize understanding. Whether you are exploring societal trends or delving into scientific phenomena, the effectiveness of your research questionnaire can make or break your findings.
In this article, we aim to understand the core purpose of questionnaires, exploring how they serve as essential tools for gathering systematic data, both qualitative and quantitative, from diverse respondents. Read on as we explore the key elements that make up a winning questionnaire, the art of framing questions which are both compelling and rigorous, and the careful balance between simplicity and depth.
Table of Contents
The Role of Questionnaires in Research
So, what is a questionnaire? A questionnaire is a structured set of questions designed to collect information, opinions, attitudes, or behaviors from respondents. It is one of the most commonly used data collection methods in research. Moreover, questionnaires can be used in various research fields, including social sciences, market research, healthcare, education, and psychology. Their adaptability makes them suitable for investigating diverse research questions.
Questionnaire and survey are two terms often used interchangeably, but they have distinct meanings in the context of research. A survey refers to the broader process of data collection that may involve various methods. A survey can encompass different data collection techniques, such as interviews , focus groups, observations, and yes, questionnaires.
Pros and Cons of Using Questionnaires in Research:
While questionnaires offer numerous advantages in research, they also come with some disadvantages that researchers must be aware of and address appropriately. Careful questionnaire design, validation, and consideration of potential biases can help mitigate these disadvantages and enhance the effectiveness of using questionnaires as a data collection method.
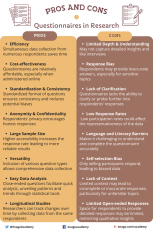
Structured vs Unstructured Questionnaires
Structured questionnaire:.
A structured questionnaire consists of questions with predefined response options. Respondents are presented with a fixed set of choices and are required to select from those options. The questions in a structured questionnaire are designed to elicit specific and quantifiable responses. Structured questionnaires are particularly useful for collecting quantitative data and are often employed in surveys and studies where standardized and comparable data are necessary.
Advantages of Structured Questionnaires:
- Easy to analyze and interpret: The fixed response options facilitate straightforward data analysis and comparison across respondents.
- Efficient for large-scale data collection: Structured questionnaires are time-efficient, allowing researchers to collect data from a large number of respondents.
- Reduces response bias: The predefined response options minimize potential response bias and maintain consistency in data collection.
Limitations of Structured Questionnaires:
- Lack of depth: Structured questionnaires may not capture in-depth insights or nuances as respondents are limited to pre-defined response choices. Hence, they may not reveal the reasons behind respondents’ choices, limiting the understanding of their perspectives.
- Limited flexibility: The fixed response options may not cover all potential responses, therefore, potentially restricting respondents’ answers.
Unstructured Questionnaire:
An unstructured questionnaire consists of questions that allow respondents to provide detailed and unrestricted responses. Unlike structured questionnaires, there are no predefined response options, giving respondents the freedom to express their thoughts in their own words. Furthermore, unstructured questionnaires are valuable for collecting qualitative data and obtaining in-depth insights into respondents’ experiences, opinions, or feelings.
Advantages of Unstructured Questionnaires:
- Rich qualitative data: Unstructured questionnaires yield detailed and comprehensive qualitative data, providing valuable and novel insights into respondents’ perspectives.
- Flexibility in responses: Respondents have the freedom to express themselves in their own words. Hence, allowing for a wide range of responses.
Limitations of Unstructured Questionnaires:
- Time-consuming analysis: Analyzing open-ended responses can be time-consuming, since, each response requires careful reading and interpretation.
- Subjectivity in interpretation: The analysis of open-ended responses may be subjective, as researchers interpret and categorize responses based on their judgment.
- May require smaller sample size: Due to the depth of responses, researchers may need a smaller sample size for comprehensive analysis, making generalizations more challenging.
Types of Questions in a Questionnaire
In a questionnaire, researchers typically use the following most common types of questions to gather a variety of information from respondents:
1. Open-Ended Questions:
These questions allow respondents to provide detailed and unrestricted responses in their own words. Open-ended questions are valuable for gathering qualitative data and in-depth insights.
Example: What suggestions do you have for improving our product?
2. Multiple-Choice Questions
Respondents choose one answer from a list of provided options. This type of question is suitable for gathering categorical data or preferences.
Example: Which of the following social media/academic networking platforms do you use to promote your research?
- ResearchGate
- Academia.edu
3. Dichotomous Questions
Respondents choose between two options, typically “yes” or “no”, “true” or “false”, or “agree” or “disagree”.
Example: Have you ever published in open access journals before?
4. Scaling Questions
These questions, also known as rating scale questions, use a predefined scale that allows respondents to rate or rank their level of agreement, satisfaction, importance, or other subjective assessments. These scales help researchers quantify subjective data and make comparisons across respondents.
There are several types of scaling techniques used in scaling questions:
i. Likert Scale:
The Likert scale is one of the most common scaling techniques. It presents respondents with a series of statements and asks them to rate their level of agreement or disagreement using a range of options, typically from “strongly agree” to “strongly disagree”.For example: Please indicate your level of agreement with the statement: “The content presented in the webinar was relevant and aligned with the advertised topic.”
- Strongly Agree
- Strongly Disagree
ii. Semantic Differential Scale:
The semantic differential scale measures respondents’ perceptions or attitudes towards an item using opposite adjectives or bipolar words. Respondents rate the item on a scale between the two opposites. For example:
- Easy —— Difficult
- Satisfied —— Unsatisfied
- Very likely —— Very unlikely
iii. Numerical Rating Scale:
This scale requires respondents to provide a numerical rating on a predefined scale. It can be a simple 1 to 5 or 1 to 10 scale, where higher numbers indicate higher agreement, satisfaction, or importance.
iv. Ranking Questions:
Respondents rank items in order of preference or importance. Ranking questions help identify preferences or priorities.
Example: Please rank the following features of our app in order of importance (1 = Most Important, 5 = Least Important):
- User Interface
- Functionality
- Customer Support
By using a mix of question types, researchers can gather both quantitative and qualitative data, providing a comprehensive understanding of the research topic and enabling meaningful analysis and interpretation of the results. The choice of question types depends on the research objectives , the desired depth of information, and the data analysis requirements.
Methods of Administering Questionnaires
There are several methods for administering questionnaires, and the choice of method depends on factors such as the target population, research objectives , convenience, and resources available. Here are some common methods of administering questionnaires:
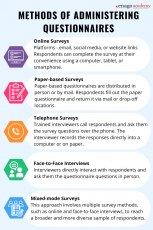
Each method has its advantages and limitations. Online surveys offer convenience and a large reach, but they may be limited to individuals with internet access. Face-to-face interviews allow for in-depth responses but can be time-consuming and costly. Telephone surveys have broad reach but may be limited by declining response rates. Researchers should choose the method that best suits their research objectives, target population, and available resources to ensure successful data collection.
How to Design a Questionnaire
Designing a good questionnaire is crucial for gathering accurate and meaningful data that aligns with your research objectives. Here are essential steps and tips to create a well-designed questionnaire:
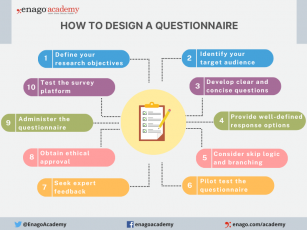
1. Define Your Research Objectives : Clearly outline the purpose and specific information you aim to gather through the questionnaire.
2. Identify Your Target Audience : Understand respondents’ characteristics and tailor the questionnaire accordingly.
3. Develop the Questions :
- Write Clear and Concise Questions
- Avoid Leading or Biasing Questions
- Sequence Questions Logically
- Group Related Questions
- Include Demographic Questions
4. Provide Well-defined Response Options : Offer exhaustive response choices for closed-ended questions.
5. Consider Skip Logic and Branching : Customize the questionnaire based on previous answers.
6. Pilot Test the Questionnaire : Identify and address issues through a pilot study .
7. Seek Expert Feedback : Validate the questionnaire with subject matter experts.
8. Obtain Ethical Approval : Comply with ethical guidelines , obtain consent, and ensure confidentiality before administering the questionnaire.
9. Administer the Questionnaire : Choose the right mode and provide clear instructions.
10. Test the Survey Platform : Ensure compatibility and usability for online surveys.
By following these steps and paying attention to questionnaire design principles, you can create a well-structured and effective questionnaire that gathers reliable data and helps you achieve your research objectives.
Characteristics of a Good Questionnaire
A good questionnaire possesses several essential elements that contribute to its effectiveness. Furthermore, these characteristics ensure that the questionnaire is well-designed, easy to understand, and capable of providing valuable insights. Here are some key characteristics of a good questionnaire:
1. Clarity and Simplicity : Questions should be clear, concise, and unambiguous. Avoid using complex language or technical terms that may confuse respondents. Simple and straightforward questions ensure that respondents interpret them consistently.
2. Relevance and Focus : Each question should directly relate to the research objectives and contribute to answering the research questions. Consequently, avoid including extraneous or irrelevant questions that could lead to data clutter.
3. Mix of Question Types : Utilize a mix of question types, including open-ended, Likert scale, and multiple-choice questions. This variety allows for both qualitative and quantitative data collections .
4. Validity and Reliability : Ensure the questionnaire measures what it intends to measure (validity) and produces consistent results upon repeated administration (reliability). Validation should be conducted through expert review and previous research.
5. Appropriate Length : Keep the questionnaire’s length appropriate and manageable to avoid respondent fatigue or dropouts. Long questionnaires may result in incomplete or rushed responses.
6. Clear Instructions : Include clear instructions at the beginning of the questionnaire to guide respondents on how to complete it. Explain any technical terms, formats, or concepts if necessary.
7. User-Friendly Format : Design the questionnaire to be visually appealing and user-friendly. Use consistent formatting, adequate spacing, and a logical page layout.
8. Data Validation and Cleaning : Incorporate validation checks to ensure data accuracy and reliability. Consider mechanisms to detect and correct inconsistent or missing responses during data cleaning.
By incorporating these characteristics, researchers can create a questionnaire that maximizes data quality, minimizes response bias, and provides valuable insights for their research.
In the pursuit of advancing research and gaining meaningful insights, investing time and effort into designing effective questionnaires is a crucial step. A well-designed questionnaire is more than a mere set of questions; it is a masterpiece of precision and ingenuity. Each question plays a vital role in shaping the narrative of our research, guiding us through the labyrinth of data to meaningful conclusions. Indeed, a well-designed questionnaire serves as a powerful tool for unlocking valuable insights and generating robust findings that impact society positively.
Have you ever designed a research questionnaire? Reflect on your experience and share your insights with researchers globally through Enago Academy’s Open Blogging Platform . Join our diverse community of 1000K+ researchers and authors to exchange ideas, strategies, and best practices, and together, let’s shape the future of data collection and maximize the impact of questionnaires in the ever-evolving landscape of research.
Frequently Asked Questions
A research questionnaire is a structured tool used to gather data from participants in a systematic manner. It consists of a series of carefully crafted questions designed to collect specific information related to a research study.
Questionnaires play a pivotal role in both quantitative and qualitative research, enabling researchers to collect insights, opinions, attitudes, or behaviors from respondents. This aids in hypothesis testing, understanding, and informed decision-making, ensuring consistency, efficiency, and facilitating comparisons.
Questionnaires are a versatile tool employed in various research designs to gather data efficiently and comprehensively. They find extensive use in both quantitative and qualitative research methodologies, making them a fundamental component of research across disciplines. Some research designs that commonly utilize questionnaires include: a) Cross-Sectional Studies b) Longitudinal Studies c) Descriptive Research d) Correlational Studies e) Causal-Comparative Studies f) Experimental Research g) Survey Research h) Case Studies i) Exploratory Research
A survey is a comprehensive data collection method that can include various techniques like interviews and observations. A questionnaire is a specific set of structured questions within a survey designed to gather standardized responses. While a survey is a broader approach, a questionnaire is a focused tool for collecting specific data.
The choice of questionnaire type depends on the research objectives, the type of data required, and the preferences of respondents. Some common types include: • Structured Questionnaires: These questionnaires consist of predefined, closed-ended questions with fixed response options. They are easy to analyze and suitable for quantitative research. • Semi-Structured Questionnaires: These questionnaires combine closed-ended questions with open-ended ones. They offer more flexibility for respondents to provide detailed explanations. • Unstructured Questionnaires: These questionnaires contain open-ended questions only, allowing respondents to express their thoughts and opinions freely. They are commonly used in qualitative research.
Following these steps ensures effective questionnaire administration for reliable data collection: • Choose a Method: Decide on online, face-to-face, mail, or phone administration. • Online Surveys: Use platforms like SurveyMonkey • Pilot Test: Test on a small group before full deployment • Clear Instructions: Provide concise guidelines • Follow-Up: Send reminders if needed

Thank you, Riya. This is quite helpful. As discussed, response bias is one of the disadvantages in the use of questionnaires. One way to help limit this can be to use scenario based questions. These type of questions may help the respondents to be more reflective and active in the process.
Thank you, Dear Riya. This is quite helpful.
Rate this article Cancel Reply
Your email address will not be published.
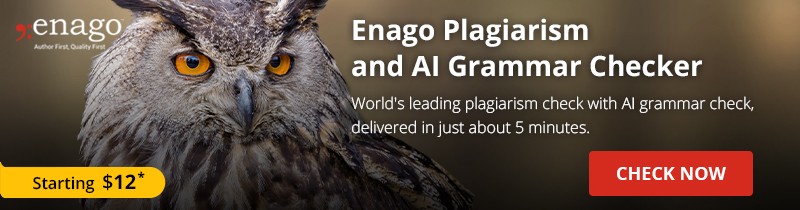
Enago Academy's Most Popular Articles
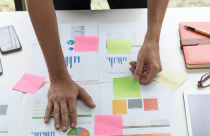
- Publishing Research
- Reporting Research
How to Optimize Your Research Process: A step-by-step guide
For researchers across disciplines, the path to uncovering novel findings and insights is often filled…
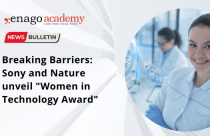
- Industry News
- Trending Now
Breaking Barriers: Sony and Nature unveil “Women in Technology Award”
Sony Group Corporation and the prestigious scientific journal Nature have collaborated to launch the inaugural…
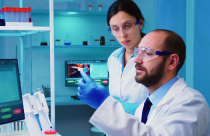
Achieving Research Excellence: Checklist for good research practices
Academia is built on the foundation of trustworthy and high-quality research, supported by the pillars…
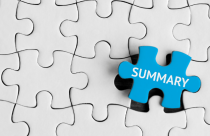
- Promoting Research
Plain Language Summary — Communicating your research to bridge the academic-lay gap
Science can be complex, but does that mean it should not be accessible to the…
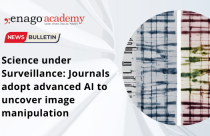
Science under Surveillance: Journals adopt advanced AI to uncover image manipulation
Journals are increasingly turning to cutting-edge AI tools to uncover deceitful images published in manuscripts.…
Choosing the Right Analytical Approach: Thematic analysis vs. content analysis for…
Comparing Cross Sectional and Longitudinal Studies: 5 steps for choosing the right…
Research Recommendations – Guiding policy-makers for evidence-based decision making

Sign-up to read more
Subscribe for free to get unrestricted access to all our resources on research writing and academic publishing including:
- 2000+ blog articles
- 50+ Webinars
- 10+ Expert podcasts
- 50+ Infographics
- 10+ Checklists
- Research Guides
We hate spam too. We promise to protect your privacy and never spam you.
I am looking for Editing/ Proofreading services for my manuscript Tentative date of next journal submission:
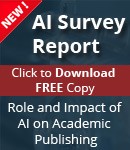
What should universities' stance be on AI tools in research and academic writing?
- Privacy Policy
Buy Me a Coffee

Home » Survey Instruments – List and Their Uses
Survey Instruments – List and Their Uses
Table of Contents

Survey Instruments
Definition:
Survey instruments are tools used to collect data from a sample of individuals or a population. They typically consist of a series of questions designed to gather information on a particular topic or issue.
List of Survey Instruments
Types of Survey Instruments are as follows:
- Questionnaire : A questionnaire is a survey instrument consisting of a series of questions designed to gather information from a large number of respondents.
- Interview Schedule : An interview schedule is a survey instrument that is used to collect data from a small number of individuals through a face-to-face conversation or online communication.
- Focus Group Discussion Guide: A focus group discussion guide is a survey instrument used to facilitate a group discussion on a particular topic to collect opinions, attitudes, and perceptions of participants.
- Observation Checklist : An observation checklist is a survey instrument that is used to observe and record behaviors, events, or processes in a systematic and organized manner.
- Rating Scale: A rating scale is a survey instrument that is used to measure the extent to which an individual agrees or disagrees with a particular statement, or rates the quality of a product, service, or experience.
- Likert Scale: A Likert scale is a survey instrument that is used to measure attitudes, opinions, or perceptions of individuals towards a particular topic or statement.
- Semantic Differential Scale : A semantic differential scale is a survey instrument that is used to measure the connotative meaning of a particular concept, product, or service.
- Checklist: A checklist is a survey instrument that is used to systematically gather information on a specific topic or subject.
- Diaries and Logs: Diaries and logs are survey instruments that are used to record behaviors, activities, and experiences of participants over a period of time.
- Case Study: A case study is a survey instrument that is used to investigate a particular phenomenon, process, or event in-depth by analyzing the data from multiple sources.
- Ethnographic Field Notes : Ethnographic field notes are survey instruments used by ethnographers to record their observations and reflections during fieldwork, often in the form of detailed descriptions of people, places, and events.
- Psychometric Tests : Psychometric tests are survey instruments used to measure cognitive abilities, aptitudes, and personality traits.
- Exit Interviews : Exit interviews are survey instruments used to gather feedback from departing employees about their experiences working for a company, organization, or institution.
- Needs Assessment Surveys: Needs assessment surveys are survey instruments used to identify the needs, priorities, and preferences of a target population to inform program development and resource allocation.
- Community Needs Assessments : Community needs assessments are survey instruments used to gather information about the needs and priorities of a particular community, including its demographics, resources, and challenges.
- Performance Appraisal Forms: Performance appraisal forms are survey instruments used to evaluate the performance of employees against specific job-related criteria.
- Customer Needs Assessment Surveys: Customer needs assessment surveys are survey instruments used to identify the needs and preferences of customers to inform product development and marketing strategies.
- Learning Style Inventories : Learning style inventories are survey instruments used to identify an individual’s preferred learning style, such as visual, auditory, or kinesthetic.
- Team Performance Assessments: Team performance assessments are survey instruments used to evaluate the effectiveness of teams in achieving their goals and objectives.
- Organizational Climate Surveys: Organizational climate surveys are survey instruments used to gather information about the perceptions, attitudes, and values of employees towards their workplace.
- Employee Engagement Surveys: Employee engagement surveys are survey instruments used to measure the level of engagement, satisfaction, and commitment of employees towards their job and the organization.
- Self-Report Measures: Self-report measures are survey instruments used to gather information directly from participants about their own thoughts, feelings, and behaviors.
- Personality Inventories: Personality inventories are survey instruments used to measure individual differences in personality traits such as extroversion, conscientiousness, and openness to experience.
- Achievement Tests : Achievement tests are survey instruments used to measure the knowledge or skills acquired by individuals in a specific subject area or academic discipline.
- Attitude Scales: Attitude scales are survey instruments used to measure the degree to which an individual holds a particular attitude or belief towards a specific object, person, or idea.
- Customer Satisfaction Surveys: Customer satisfaction surveys are survey instruments used to gather feedback from customers about their experience with a product or service.
- Market Research Surveys: Market research surveys are survey instruments used to collect data on consumer behavior, market trends, and preferences to inform business decisions.
- Health Assessments: Health assessments are survey instruments used to gather information about an individual’s physical and mental health status, including medical history, symptoms, and lifestyle factors.
- Environmental Surveys: Environmental surveys are survey instruments used to gather information about environmental conditions and the impact of human activities on the natural world.
- Program Evaluation Surveys : Program evaluation surveys are survey instruments used to assess the effectiveness of programs and interventions in achieving their intended outcomes.
- Culture Assessments: Culture assessments are survey instruments used to gather information about the culture of an organization, including its values, beliefs, and practices.
- Customer Feedback Forms: Customer feedback forms are survey instruments used to gather feedback from customers about their experience with a product, service, or company.
- User Acceptance Testing (UAT) Forms: User acceptance testing (UAT) forms are survey instruments used to gather feedback from users about the functionality and usability of a software application or system.
- Stakeholder Surveys: Stakeholder surveys are survey instruments used to gather feedback from stakeholders, such as customers, employees, investors, and partners, about their perceptions and expectations of an organization or project.
- Social Network Analysis (SNA) Surveys: Social network analysis (SNA) surveys are survey instruments used to map and analyze social networks and relationships within a group or community.
- Leadership Assessments: Leadership assessments are survey instruments used to evaluate the leadership skills, styles, and behaviors of individuals in a leadership role.
- Exit Polls : Exit polls are survey instruments used to gather data on voting patterns and preferences in an election or referendum.
- Customer Loyalty Surveys : Customer loyalty surveys are survey instruments used to measure the level of loyalty and advocacy of customers towards a brand or company.
- Online Feedback Forms : Online feedback forms are survey instruments used to gather feedback from website visitors, customers, or users about their experience with a website, application, or digital product.
- Needs Analysis Surveys: Needs analysis surveys are survey instruments used to identify the training and development needs of employees or students to inform curriculum design and professional development programs.
- Career Assessments: Career assessments are survey instruments used to evaluate an individual’s interests, values, and skills to inform career decision-making and planning.
- Customer Perception Surveys: Customer perception surveys are survey instruments used to gather information about how customers perceive a product, service, or brand.
- Employee Satisfaction Surveys: Employee satisfaction surveys are survey instruments used to measure the level of job satisfaction, engagement, and motivation of employees.
- Conflict Resolution Assessments: Conflict resolution assessments are survey instruments used to identify the causes and sources of conflict in a group or organization and to inform conflict resolution strategies.
- Cultural Competence Assessments: Cultural competence assessments are survey instruments used to evaluate an individual’s ability to work effectively with people from diverse cultural backgrounds.
- Job Analysis Surveys: Job analysis surveys are survey instruments used to gather information about the tasks, responsibilities, and requirements of a particular job or position.
- Employee Turnover Surveys : Employee turnover surveys are survey instruments used to gather information about the reasons why employees leave a company or organization.
- Quality of Life Assessments: Quality of life assessments are survey instruments used to gather information about an individual’s physical, emotional, and social well-being.
- User Satisfaction Surveys: User satisfaction surveys are survey instruments used to gather feedback from users about their satisfaction with a product, service, or application.
- Data Collection Forms: Data collection forms are survey instruments used to gather information about a specific research question or topic, often used in quantitative research.
- Program Evaluation Forms: Program evaluation forms are survey instruments used to assess the effectiveness, efficiency, and impact of a program or intervention.
- Cultural Awareness Surveys: Cultural awareness surveys are survey instruments used to assess an individual’s knowledge and understanding of different cultures and customs.
- Employee Perception Surveys: Employee perception surveys are survey instruments used to gather information about how employees perceive their work environment, management, and colleagues.
- Leadership 360 Assessments: Leadership 360 assessments are survey instruments used to evaluate the leadership skills, styles, and behaviors of individuals from multiple perspectives, including self-assessment, peer feedback, and supervisor evaluation.
- Health Needs Assessments: Health needs assessments are survey instruments used to gather information about the health needs and priorities of a population to inform public health policies and programs.
- Social Capital Surveys: Social capital surveys are survey instruments used to measure the social networks and relationships within a community and their impact on social and economic outcomes.
- Psychosocial Assessments: Psychosocial assessments are survey instruments used to evaluate an individual’s psychological, social, and emotional well-being.
- Training Evaluation Forms: Training evaluation forms are survey instruments used to assess the effectiveness and impact of a training program on knowledge, skills, and behavior.
- Patient Satisfaction Surveys: Patient satisfaction surveys are survey instruments used to gather feedback from patients about their experience with healthcare services and providers.
- Program Needs Assessments : Program needs assessments are survey instruments used to identify the needs, goals, and expectations of stakeholders for a program or intervention.
- Community Needs Assessments: Community needs assessments are survey instruments used to gather information about the needs, challenges, and assets of a community to inform community development programs and policies.
- Environmental Assessments : Environmental assessments are survey instruments used to evaluate the environmental impact of a project, program, or policy.
- Stakeholder Analysis Surveys: Stakeholder analysis surveys are survey instruments used to identify and prioritize the needs, interests, and influence of stakeholders in a project or initiative.
- Performance Appraisal Forms: Performance appraisal forms are survey instruments used to evaluate the performance and contribution of employees to inform promotions, rewards, and career development plans.
- Consumer Behavior Surveys : Consumer behavior surveys are survey instruments used to gather information about the attitudes, beliefs, and behaviors of consumers towards products, brands, and services.
- Audience Feedback Forms : Audience feedback forms are survey instruments used to gather feedback from audience members about their experience with a performance, event, or media content.
- Market Research Surveys: Market research surveys are survey instruments used to gather information about market trends, customer preferences, and competition to inform business strategy and decision-making.
- Health Risk Assessments: Health risk assessments are survey instruments used to identify an individual’s health risks and to provide personalized recommendations for preventive care and lifestyle changes.
- Employee Engagement Surveys : Employee engagement surveys are survey instruments used to measure the level of employee engagement, commitment, and motivation in a company or organization.
- Social Impact Assessments: Social impact assessments are survey instruments used to evaluate the social, economic, and environmental impact of a project or policy on stakeholders and the community.
- Needs Assessment Forms : Needs assessment forms are survey instruments used to identify the needs, expectations, and priorities of stakeholders for a particular program, service, or project.
- Organizational Climate Surveys: Organizational climate surveys are survey instruments used to measure the overall culture, values, and climate of an organization, including the level of trust, communication, and support.
- Risk Assessment Forms: Risk assessment forms are survey instruments used to identify and evaluate potential risks associated with a project, program, or activity.
- Customer Service Surveys: Customer service surveys are survey instruments used to gather feedback from customers about the quality of customer service provided by a company or organization.
- Performance Evaluation Forms : Performance evaluation forms are survey instruments used to evaluate the performance and contribution of employees to inform promotions, rewards, and career development plans.
- Community Impact Assessments : Community impact assessments are survey instruments used to evaluate the social, economic, and environmental impact of a project or policy on the community.
- Health Status Surveys : Health status surveys are survey instruments used to gather information about an individual’s health status, including physical, mental, and emotional well-being.
- Organizational Effectiveness Surveys: Organizational effectiveness surveys are survey instruments used to measure the overall effectiveness and performance of an organization, including the alignment of goals, strategies, and outcomes.
- Program Implementation Surveys: Program implementation surveys are survey instruments used to evaluate the implementation process of a program or intervention, including the quality, fidelity, and sustainability.
- Social Support Surveys : Social support surveys are survey instruments used to measure the level of social support and connectedness within a community or group and their impact on health and well-being.
Survey Instruments in Research Methods
The following are some commonly used survey instruments in research methods:
- Questionnaires : A questionnaire is a set of standardized questions designed to collect information about a specific topic. Questionnaires can be administered in different ways, including in person, over the phone, or online.
- Interviews : Interviews involve asking participants a series of questions in a face-to-face or phone conversation. Interviews can be structured, semi-structured, or unstructured depending on the research question and the researcher’s goals.
- Surveys : Surveys are used to collect data from a large number of participants through self-report. Surveys can be administered through various mediums, including paper-based, phone-based, and online surveys.
- Focus Groups : A focus group is a qualitative research method where a group of individuals is brought together to discuss a particular topic. The goal is to gather in-depth information about participants’ perceptions, attitudes, and beliefs.
- Case Studies: A case study is an in-depth analysis of an individual, group, or organization. The researcher collects data through various methods, including interviews, observation, and document analysis.
- Observations : Observations involve watching participants in their natural setting and recording their behavior. Observations can be structured or unstructured, and the data collected can be qualitative or quantitative.
Survey Instruments in Qualitative Research
In qualitative research , survey instruments are used to gather data from participants through structured or semi-structured questionnaires. These instruments are used to gather information on a wide range of topics, including attitudes, beliefs, perceptions, experiences, and behaviors.
Here are some commonly used survey instruments in qualitative research:
- Focus groups
- Questionnaires
- Observation
- Document analysis
Survey Instruments in Quantitative Research
Survey instruments are commonly used in quantitative research to collect data from a large number of respondents. The following are some commonly used survey instruments:
- Self-Administered Surveys:
- Telephone Surveys
- Online Surveys
- Focus Groups
- Observations
Importance of Survey Instruments
Here are some reasons why survey instruments are important:
- Provide valuable insights : Survey instruments help researchers gather accurate data and provide valuable insights into various phenomena. Researchers can use the data collected through surveys to analyze trends, patterns, and relationships between variables, leading to a better understanding of the topic at hand.
- Measure changes over time: By using survey instruments, researchers can measure changes in attitudes, beliefs, or behaviors over time. This allows them to identify trends and patterns, which can inform policy decisions and interventions.
- Inform decision-making: Survey instruments can provide decision-makers with information on the opinions, preferences, and needs of a particular group. This information can be used to make informed decisions and to tailor programs and policies to meet the specific needs of a population.
- Cost-effective: Compared to other research methods, such as focus groups or in-depth interviews, survey instruments are relatively cost-effective. They can be administered to a large number of participants at once, and data can be collected and analyzed quickly and efficiently.
- Standardization : Survey instruments can be standardized to ensure that all participants are asked the same questions in the same way. This helps to ensure that the data collected is consistent and reliable.
Applications of Survey Instruments
The data collected through surveys can be used for various purposes, including:
- Market research : Surveys can be used to collect data on consumer preferences, habits, and opinions, which can help businesses make informed decisions about their products or services.
- Social research: Surveys can be used to collect data on social issues such as public opinion, political preferences, and attitudes towards social policies.
- Health research: Surveys can be used to collect data on health-related issues such as disease prevalence, risk factors, and health behaviors.
- Education research : Surveys can be used to collect data on education-related issues such as student satisfaction, teacher performance, and educational outcomes.
- Customer satisfaction: Surveys can be used to collect data on customer satisfaction, which can help businesses improve their products and services.
- Employee satisfaction : Surveys can be used to collect data on employee satisfaction, which can help employers improve their workplace policies and practices.
- Program evaluation : Surveys can be used to collect data on program outcomes and effectiveness, which can help organizations improve their programs.
About the author
Muhammad Hassan
Researcher, Academic Writer, Web developer
You may also like

Data Collection – Methods Types and Examples

Delimitations in Research – Types, Examples and...

Research Process – Steps, Examples and Tips

Research Design – Types, Methods and Examples

Institutional Review Board – Application Sample...

Evaluating Research – Process, Examples and...
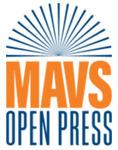
Want to create or adapt books like this? Learn more about how Pressbooks supports open publishing practices.
12.1 What is survey research, and when should you use it?
Learning objectives.
Learners will be able to…
- Distinguish between survey as a research design and questionnaires used to measure concepts
- Identify the strengths and weaknesses of surveys
- Evaluate whether survey design fits with their research question
Pre-awareness check (Knowledge)
Have you ever been selected as a participant to complete a survey? How were you contacted? Would you incorporate the researchers’ methods into your research design?
Researchers quickly learn that there is more to constructing a good survey than meets the eye. Survey design takes a great deal of thoughtful planning and often many rounds of revision, but it is worth the effort. As we’ll learn in this section, there are many benefits to choosing survey research as your data collection method. We’ll discuss what a survey is, its potential benefits and drawbacks, and what research projects are the best fit for survey design.
Is survey research right for your project?
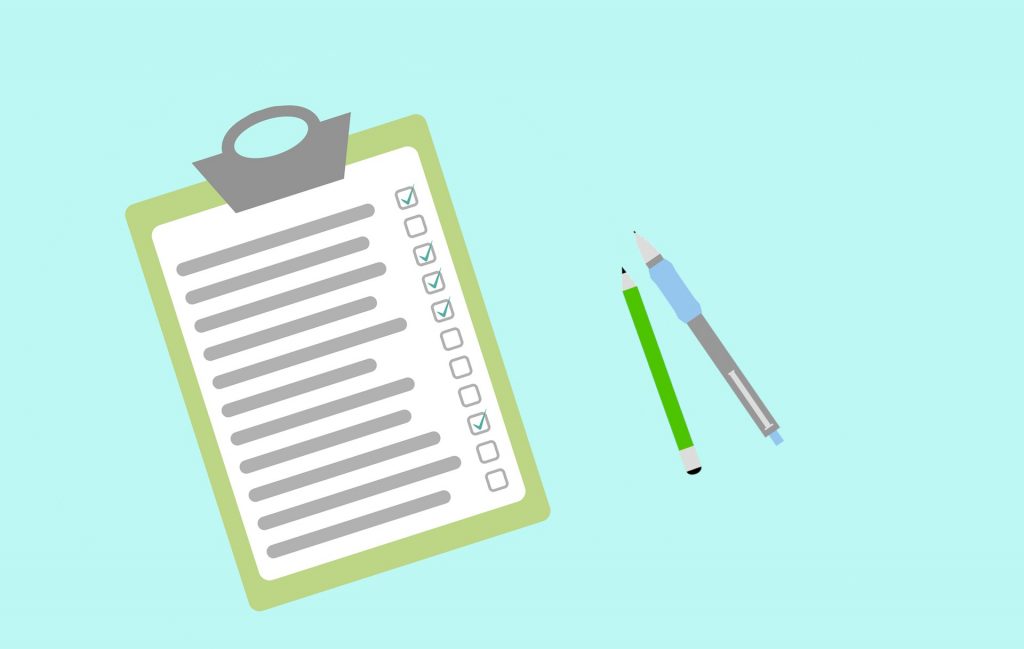
Questionnaires are completed by individual people, so the unit of observation is almost always individuals, rather than groups or organizations. Generally speaking, individuals provide the most informed data about their own lives and experiences, so surveys often also use individuals as the unit of analysis . Surveys are also helpful in analyzing dyads, families, groups, organizations, and communities, but regardless of the unit of analysis, the unit of observation for surveys is usually individuals.
In some cases, getting the most-informed person to complete the questionnaire may not be feasible . As we discussed in Chapter 2 and Chapter 6 , ethical duties to protect clients and vulnerable community members is important. The ethical supervision needed via the IRB to complete projects that pose significant risks to participants takes time and effort. Sometimes researchers rely on key informants and gatekeepers like clinicians, teachers, and administrators who are less likely to be harmed by the survey. Key informants are people who are especially knowledgeable about the topic. If your study is about nursing, you would probably consider nurses as your key informants. These considerations are more thoroughly addressed in Chapter 10 . Sometimes, participants complete surveys on behalf of people in your target population who are infeasible to survey for some reason. Some examples of key informants include a head of household completing a survey about family finances or an administrator completing a survey about staff morale on behalf of their employees. In this case, the survey respondent is a proxy , providing their best informed guess about the responses other people might have chosen if they were able to complete the survey independently. You are relying on an individual unit of observation (one person filling out a self-report questionnaire) and group or organization unit of analysis (the family or organization the researcher wants to make conclusions about). Proxies are commonly used when the target population is not capable of providing consent or appropriate answers, as in young children.
Proxies are relying on their best judgment of another person’s experiences, and while that is valuable information, it may introduce bias and error into the research process. For instance, If you are planning to conduct a survey of people with second-hand knowledge of your topic, consider reworking your research question to be about something they have more direct knowledge about and can answer easily.
Remember, every project has limitations. Social work researchers look for the most favorable choices in design and methodology, as there are no perfect projects. A missed opportunity is when researchers who want to understand client outcomes (unit of analysis) by surveying practitioners (unit of observation). If a practitioner has a caseload of 30 clients, it’s not really possible to answer a question like “how much progress have your clients made?” on a survey. Would they just average all 30 clients together? Instead, design a survey that asks them about their education, professional experience, and other things they know about first-hand. By making your unit of analysis and unit of observation the same, you can ensure the people completing your survey are able to provide informed answers.
Researchers may introduce measurement error if the person completing the questionnaire does not have adequate knowledge or has a biased opinion about the phenomenon of interest. [ INSERT SOME DISCUSSION HERE, FOR EXAMPLE GALLUP OPINION POLLS, ELECTION POLLING ]
In summary, survey design tends to be used in quantitative research and best fits with research projects that have the following attributes:
- Researchers plan to collect their own raw data, rather than secondary analysis of existing data.
- Researchers have access to the most knowledgeable people (that you can feasibly and ethically sample) to complete the questionnaire.
- Individuals are the unit of observation, and in many cases, the unit of analysis.
- Researchers will try to observe things objectively and try not to influence participants to respond differently.
- Research questions asks about indirect observables—things participants can self-report on a questionnaire.
- There are valid, reliable, and commonly used scales (or other self-report measures) for the variables in the research question.
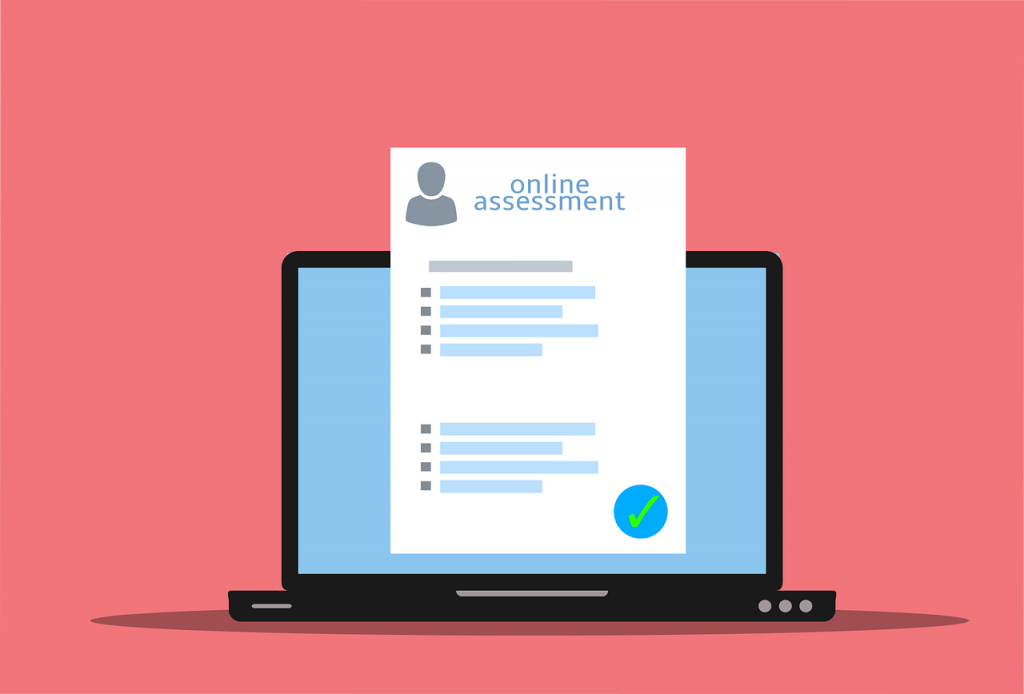
Strengths of survey methods
Researchers employing survey research as a research design enjoy a number of benefits. First, surveys are an excellent way to gather lots of information from many people and is cost-effective due to its potential for generalizability. Related to the benefit of cost-effectiveness is a survey’s potential for generalizability. Because surveys allow researchers to collect data from very large samples for a relatively low cost, survey methods lend themselves to probability sampling techniques, which we discussed in Chapter 10 . When used with probability sampling approaches, survey research is the best method to use when one hopes to gain a representative picture of the attitudes and characteristics of a large group.
Survey research is particularly adept at investigating indirect observables or constructs . Indirect observables (e.g., income, place of birth, or smoking behavior) are things we have to ask someone to self-report because we cannot observe them directly. Constructs such as people’s preferences (e.g., political orientation), traits (e.g., self-esteem), attitudes (e.g., toward immigrants), or beliefs (e.g., about a new law) are also often best collected through multi-item instruments such as scales. Unlike qualitative studies in which these beliefs and attitudes would be detailed in unstructured conversations, survey design seeks to systematize answers so researchers can make apples-to-apples comparisons across participants. Questionnaires used in survey design are flexible because you can ask about anything, and the variety of questions allows you to expand social science knowledge beyond what is naturally observable.
Survey research also tends to use reliable instruments within their method of inquiry, many scales in survey questionnaires are standardized instruments. Other methods, such as qualitative interviewing, which we’ll learn about in Chapter 18 , do not offer the same consistency that a quantitative survey offers. This is not to say that all surveys are always reliable. A poorly phrased question can cause respondents to interpret its meaning differently, which can reduce that question’s reliability. Assuming well-constructed questions and survey design, one strength of this methodology is its potential to produce reliable results.
The versatility of survey research is also an asset. Surveys are used by all kinds of people in all kinds of professions. They can measure anything that people can self-report. Surveys are also appropriate for exploratory, descriptive, and explanatory research questions (though exploratory projects may benefit more from qualitative methods). Moreover, they can be delivered in a number of flexible ways, including via email, mail, text, and phone. We will describe the many ways to implement a survey later on in this chapter.
In sum, the following are benefits of survey research:
- Cost-effectiveness
- Generalizability
- Reliability
- Versatility
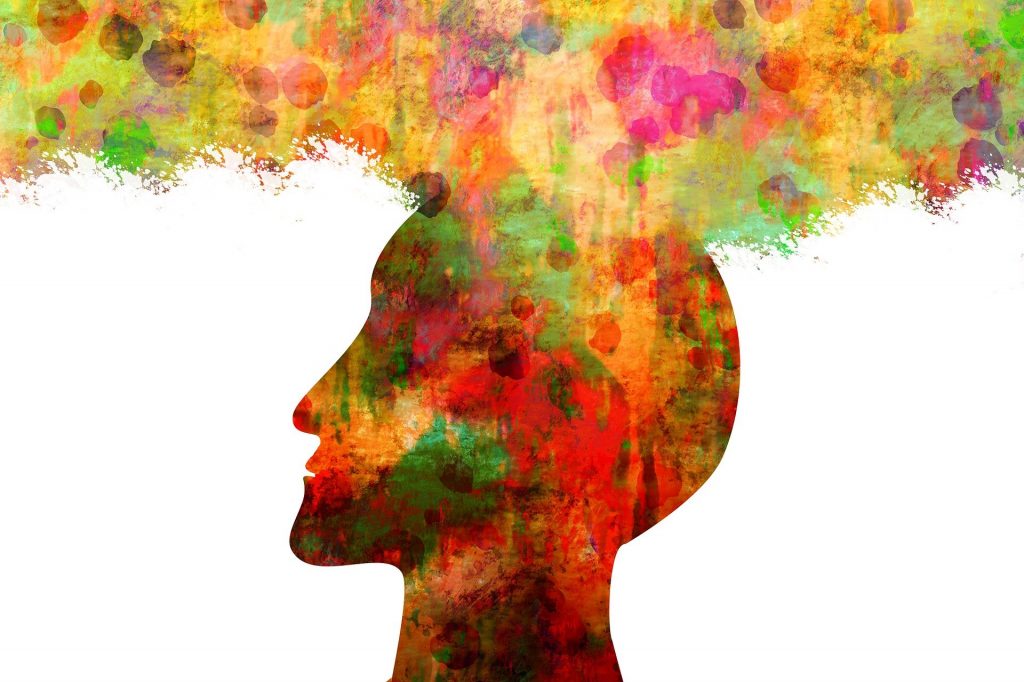
Weaknesses of survey methods
As with all methods of data collection, survey research also comes with a few drawbacks. First, while one might argue that surveys are flexible in the sense that we can ask any kind of question about any topic we want, once the survey is given to the first participant, there is nothing you can do to change the survey without biasing your results. Because surveys want to minimize the amount of influence that a researcher has on the participants, everyone gets the same questionnaire. Let’s say you mail a questionnaire out to 1,000 people and then discover, as responses start coming in, that your phrasing on a particular question seems to be confusing a number of respondents. At this stage, it’s too late for a do-over or to change the question for the respondents who haven’t yet returned their questionnaires. When conducting qualitative interviews or focus groups, on the other hand, a researcher can provide respondents further explanation if they’re confused by a question and can tweak their questions as they learn more about how respondents seem to understand them. Survey researchers often ask colleagues, students, and others to pilot test their questionnaire and catch any errors prior to sending it to participants; however, once researchers distribute the survey to participants, there is little they can do to change anything.
Depth can also be a problem with surveys. Survey questions are standardized; thus, it can be difficult to ask anything other than very general questions that a broad range of people will understand. Because of this, survey results may not provide as detailed of an understanding as results obtained using methods of data collection that allow a researcher to more comprehensively examine whatever topic is being studied. Let’s say, for example, that you want to learn something about voters’ willingness to elect an African American president. General Social Survey respondents were asked, “If your party nominated an African American for president, would you vote for him if he were qualified for the job?” (Smith, 2009). [2] Respondents were then asked to respond either yes or no to the question. But what if someone’s opinion was more complex than could be answered with a simple yes or no? What if, for example, a person was willing to vote for an African American man, but only if that person was a conservative, moderate, anti-abortion, antiwar, etc. Then we would miss out on that additional detail when the participant responded “yes,” to our question. Of course, you could add a question to your survey about moderate vs. radical candidates, but could you do that for all of the relevant attributes of candidates for all people? Moreover, how do you know that moderate or antiwar means the same thing to everyone who participates in your survey? Without having a conversation with someone and asking them follow up questions, survey research can lack enough detail to understand how people truly think.
In sum, potential drawbacks to survey research include the following:
- Inflexibility
- Lack of depth
- Problems specific to cross-sectional surveys, which we will address in the next section.
Secondary analysis of survey data
This chapter is designed to help you conduct your own survey, but that is not the only option for social work researchers. Look back to Chapter 2 and recall our discussion of secondary data analysis . As we talked about previously, using data collected by another researcher can have a number of benefits. Well-funded researchers have the resources to recruit a large representative sample and ensure their measures are valid and reliable prior to sending them to participants. Before you get too far into designing your own data collection, make sure there are no existing data sets out there that you can use to answer your question. We refer you to Chapter 2 for all full discussion of the strengths and challenges of using secondary analysis of survey data.
Key Takeaways
- Strengths of survey research include its cost effectiveness, generalizability, variety, reliability, and versatility.
- Weaknesses of survey research include inflexibility and lack of potential depth. There are also weaknesses specific to cross-sectional surveys, the most common type of survey.
TRACK 1 (IF YOU ARE CREATING A RESEARCH PROPOSAL FOR THIS CLASS):
If you are using quantitative methods in a student project, it is very likely that you are going to use survey design to collect your data.
- Check to make sure that your research question and study fit best with survey design using the criteria in this section
- Remind yourself of any limitations to generalizability based on your sampling frame.
- Refresh your memory on the operational definitions you will use for your dependent and independent variables.
TRACK 2 (IF YOU AREN’T CREATING A RESEARCH PROPOSAL FOR THIS CLASS):
You are interested in understanding more about the needs of unhoused individuals in rural communities, including how these needs vary based on demographic characteristics and personal identities.
- Develop a working research question for this topic.
- Using the criteria for survey design described in this section, do you think a survey would be appropriate to answer your research question? Why or why not?
- What are the potential limitations to generalizability if you select survey design to answer this research question?
- Unless researchers change the order of questions as part of their methodology and ensuring accurate responses to questions ↵
- Smith, T. W. (2009). Trends in willingness to vote for a Black and woman for president, 1972–2008. GSS Social Change Report No. 55 . Chicago, IL: National Opinion Research Center ↵
The use of questionnaires to gather data from multiple participants.
the group of people you successfully recruit from your sampling frame to participate in your study
A research instrument consisting of a set of questions (items) intended to capture responses from participants in a standardized manner
a participant answers questions about themselves
the entities that a researcher actually observes, measures, or collects in the course of trying to learn something about her unit of analysis (individuals, groups, or organizations)
entity that a researcher wants to say something about at the end of her study (individual, group, or organization)
whether you can practically and ethically complete the research project you propose
Someone who is especially knowledgeable about a topic being studied.
a person who completes a survey on behalf of another person
things that require subtle and complex observations to measure, perhaps we must use existing knowledge and intuition to define.
Conditions that are not directly observable and represent states of being, experiences, and ideas.
The degree to which an instrument reflects the true score rather than error. In statistical terms, reliability is the portion of observed variability in the sample that is accounted for by the true variability, not by error. Note : Reliability is necessary, but not sufficient, for measurement validity.
analyzing data that has been collected by another person or research group
Doctoral Research Methods in Social Work Copyright © by Mavs Open Press. All Rights Reserved.
Share This Book

Research Methodologies
- What are research designs?
- What are research methodologies?
What are research methods?
Quantitative research methods, qualitative research methods, mixed method approach, selecting the best research method.
- Additional Sources
Research methods are different from research methodologies because they are the ways in which you will collect the data for your research project. The best method for your project largely depends on your topic, the type of data you will need, and the people or items from which you will be collecting data. The following boxes below contain a list of quantitative, qualitative, and mixed research methods.
- Closed-ended questionnaires/survey: These types of questionnaires or surveys are like "multiple choice" tests, where participants must select from a list of premade answers. According to the content of the question, they must select the one that they agree with the most. This approach is the simplest form of quantitative research because the data is easy to combine and quantify.
- Structured interviews: These are a common research method in market research because the data can be quantified. They are strictly designed for little "wiggle room" in the interview process so that the data will not be skewed. You can conduct structured interviews in-person, online, or over the phone (Dawson, 2019).
Constructing Questionnaires
When constructing your questions for a survey or questionnaire, there are things you can do to ensure that your questions are accurate and easy to understand (Dawson, 2019):
- Keep the questions brief and simple.
- Eliminate any potential bias from your questions. Make sure that they do not word things in a way that favor one perspective over another.
- If your topic is very sensitive, you may want to ask indirect questions rather than direct ones. This prevents participants from being intimidated and becoming unwilling to share their true responses.
- If you are using a closed-ended question, try to offer every possible answer that a participant could give to that question.
- Do not ask questions that assume something of the participant. The question "How often do you exercise?" assumes that the participant exercises (when they may not), so you would want to include a question that asks if they exercise at all before asking them how often.
- Try and keep the questionnaire as short as possible. The longer a questionnaire takes, the more likely the participant will not complete it or get too tired to put truthful answers.
- Promise confidentiality to your participants at the beginning of the questionnaire.
Quantitative Research Measures
When you are considering a quantitative approach to your research, you need to identify why types of measures you will use in your study. This will determine what type of numbers you will be using to collect your data. There are four levels of measurement:
- Nominal: These are numbers where the order of the numbers do not matter. They aim to identify separate information. One example is collecting zip codes from research participants. The order of the numbers does not matter, but the series of numbers in each zip code indicate different information (Adamson and Prion, 2013).
- Ordinal: Also known as rankings because the order of these numbers matter. This is when items are given a specific rank according to specific criteria. A common example of ordinal measurements include ranking-based questionnaires, where participants are asked to rank items from least favorite to most favorite. Another common example is a pain scale, where a patient is asked to rank their pain on a scale from 1 to 10 (Adamson and Prion, 2013).
- Interval: This is when the data are ordered and the distance between the numbers matters to the researcher (Adamson and Prion, 2013). The distance between each number is the same. An example of interval data is test grades.
- Ratio: This is when the data are ordered and have a consistent distance between numbers, but has a "zero point." This means that there could be a measurement of zero of whatever you are measuring in your study (Adamson and Prion, 2013). An example of ratio data is measuring the height of something because the "zero point" remains constant in all measurements. The height of something could also be zero.
Focus Groups
This is when a select group of people gather to talk about a particular topic. They can also be called discussion groups or group interviews (Dawson, 2019). They are usually lead by a moderator to help guide the discussion and ask certain questions. It is critical that a moderator allows everyone in the group to get a chance to speak so that no one dominates the discussion. The data that are gathered from focus groups tend to be thoughts, opinions, and perspectives about an issue.
Advantages of Focus Groups
- Only requires one meeting to get different types of responses.
- Less researcher bias due to participants being able to speak openly.
- Helps participants overcome insecurities or fears about a topic.
- The researcher can also consider the impact of participant interaction.
Disadvantages of Focus Groups
- Participants may feel uncomfortable to speak in front of an audience, especially if the topic is sensitive or controversial.
- Since participation is voluntary, not every participant may contribute equally to the discussion.
- Participants may impact what others say or think.
- A researcher may feel intimidated by running a focus group on their own.
- A researcher may need extra funds/resources to provide a safe space to host the focus group.
- Because the data is collective, it may be difficult to determine a participant's individual thoughts about the research topic.
Observation
There are two ways to conduct research observations:
- Direct Observation: The researcher observes a participant in an environment. The researcher often takes notes or uses technology to gather data, such as a voice recorder or video camera. The researcher does not interact or interfere with the participants. This approach is often used in psychology and health studies (Dawson, 2019).
- Participant Observation: The researcher interacts directly with the participants to get a better understanding of the research topic. This is a common research method when trying to understand another culture or community. It is important to decide if you will conduct a covert (participants do not know they are part of the research) or overt (participants know the researcher is observing them) observation because it can be unethical in some situations (Dawson, 2019).
Open-Ended Questionnaires
These types of questionnaires are the opposite of "multiple choice" questionnaires because the answer boxes are left open for the participant to complete. This means that participants can write short or extended answers to the questions. Upon gathering the responses, researchers will often "quantify" the data by organizing the responses into different categories. This can be time consuming because the researcher needs to read all responses carefully.
Semi-structured Interviews
This is the most common type of interview where researchers aim to get specific information so they can compare it to other interview data. This requires asking the same questions for each interview, but keeping their responses flexible. This means including follow-up questions if a subject answers a certain way. Interview schedules are commonly used to aid the interviewers, which list topics or questions that will be discussed at each interview (Dawson, 2019).
Theoretical Analysis
Often used for nonhuman research, theoretical analysis is a qualitative approach where the researcher applies a theoretical framework to analyze something about their topic. A theoretical framework gives the researcher a specific "lens" to view the topic and think about it critically. it also serves as context to guide the entire study. This is a popular research method for analyzing works of literature, films, and other forms of media. You can implement more than one theoretical framework with this method, as many theories complement one another.
Common theoretical frameworks for qualitative research are (Grant and Osanloo, 2014):
- Behavioral theory
- Change theory
- Cognitive theory
- Content analysis
- Cross-sectional analysis
- Developmental theory
- Feminist theory
- Gender theory
- Marxist theory
- Queer theory
- Systems theory
- Transformational theory
Unstructured Interviews
These are in-depth interviews where the researcher tries to understand an interviewee's perspective on a situation or issue. They are sometimes called life history interviews. It is important not to bombard the interviewee with too many questions so they can freely disclose their thoughts (Dawson, 2019).
- Open-ended and closed-ended questionnaires: This approach means implementing elements of both questionnaire types into your data collection. Participants may answer some questions with premade answers and write their own answers to other questions. The advantage to this method is that you benefit from both types of data collection to get a broader understanding of you participants. However, you must think carefully about how you will analyze this data to arrive at a conclusion.
Other mixed method approaches that incorporate quantitative and qualitative research methods depend heavily on the research topic. It is strongly recommended that you collaborate with your academic advisor before finalizing a mixed method approach.
How do you determine which research method would be best for your proposal? This heavily depends on your research objective. According to Dawson (2019), there are several questions to ask yourself when determining the best research method for your project:
- Are you good with numbers and mathematics?
- Would you be interested in conducting interviews with human subjects?
- Would you enjoy creating a questionnaire for participants to complete?
- Do you prefer written communication or face-to-face interaction?
- What skills or experiences do you have that might help you with your research? Do you have any experiences from past research projects that can help with this one?
- How much time do you have to complete the research? Some methods take longer to collect data than others.
- What is your budget? Do you have adequate funding to conduct the research in the method you want?
- How much data do you need? Some research topics need only a small amount of data while others may need significantly larger amounts.
- What is the purpose of your research? This can provide a good indicator as to what research method will be most appropriate.
- << Previous: What are research methodologies?
- Next: Additional Sources >>
- Last Updated: Aug 2, 2022 2:36 PM
- URL: https://library.tiffin.edu/researchmethodologies
Research Methods In Psychology
Saul Mcleod, PhD
Editor-in-Chief for Simply Psychology
BSc (Hons) Psychology, MRes, PhD, University of Manchester
Saul Mcleod, PhD., is a qualified psychology teacher with over 18 years of experience in further and higher education. He has been published in peer-reviewed journals, including the Journal of Clinical Psychology.
Learn about our Editorial Process
Olivia Guy-Evans, MSc
Associate Editor for Simply Psychology
BSc (Hons) Psychology, MSc Psychology of Education
Olivia Guy-Evans is a writer and associate editor for Simply Psychology. She has previously worked in healthcare and educational sectors.
Research methods in psychology are systematic procedures used to observe, describe, predict, and explain behavior and mental processes. They include experiments, surveys, case studies, and naturalistic observations, ensuring data collection is objective and reliable to understand and explain psychological phenomena.
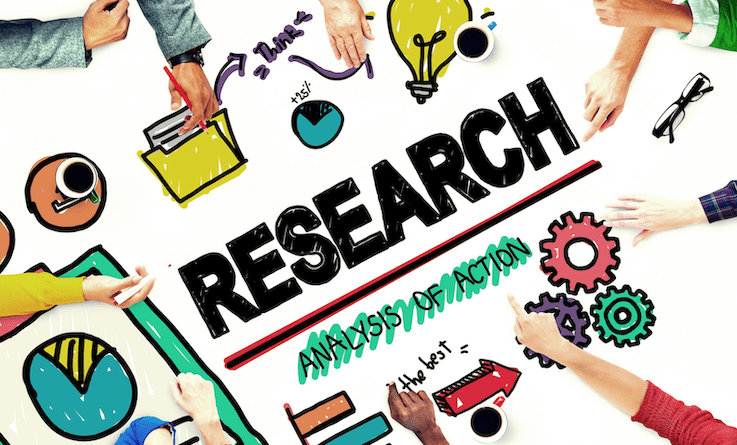
Hypotheses are statements about the prediction of the results, that can be verified or disproved by some investigation.
There are four types of hypotheses :
- Null Hypotheses (H0 ) – these predict that no difference will be found in the results between the conditions. Typically these are written ‘There will be no difference…’
- Alternative Hypotheses (Ha or H1) – these predict that there will be a significant difference in the results between the two conditions. This is also known as the experimental hypothesis.
- One-tailed (directional) hypotheses – these state the specific direction the researcher expects the results to move in, e.g. higher, lower, more, less. In a correlation study, the predicted direction of the correlation can be either positive or negative.
- Two-tailed (non-directional) hypotheses – these state that a difference will be found between the conditions of the independent variable but does not state the direction of a difference or relationship. Typically these are always written ‘There will be a difference ….’
All research has an alternative hypothesis (either a one-tailed or two-tailed) and a corresponding null hypothesis.
Once the research is conducted and results are found, psychologists must accept one hypothesis and reject the other.
So, if a difference is found, the Psychologist would accept the alternative hypothesis and reject the null. The opposite applies if no difference is found.
Sampling techniques
Sampling is the process of selecting a representative group from the population under study.
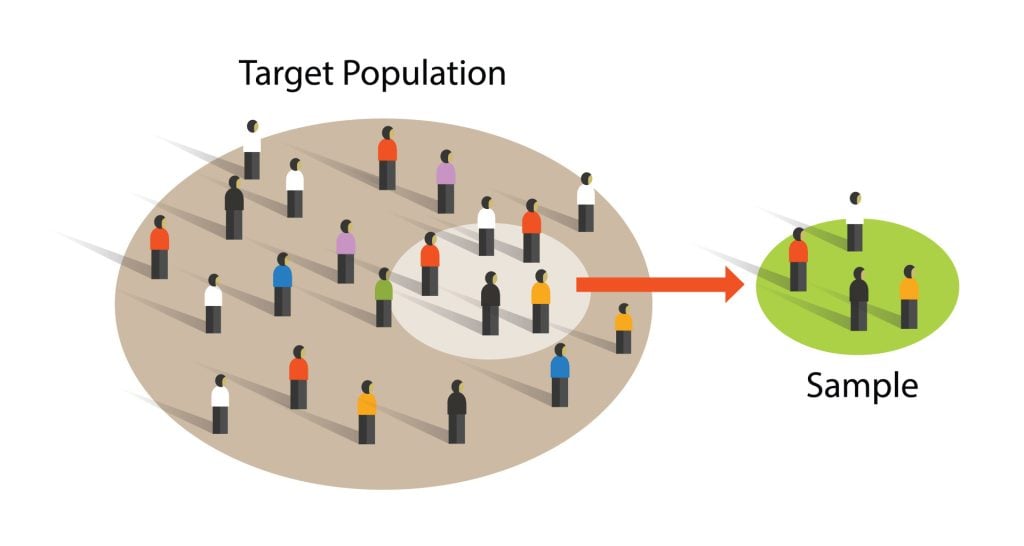
A sample is the participants you select from a target population (the group you are interested in) to make generalizations about.
Representative means the extent to which a sample mirrors a researcher’s target population and reflects its characteristics.
Generalisability means the extent to which their findings can be applied to the larger population of which their sample was a part.
- Volunteer sample : where participants pick themselves through newspaper adverts, noticeboards or online.
- Opportunity sampling : also known as convenience sampling , uses people who are available at the time the study is carried out and willing to take part. It is based on convenience.
- Random sampling : when every person in the target population has an equal chance of being selected. An example of random sampling would be picking names out of a hat.
- Systematic sampling : when a system is used to select participants. Picking every Nth person from all possible participants. N = the number of people in the research population / the number of people needed for the sample.
- Stratified sampling : when you identify the subgroups and select participants in proportion to their occurrences.
- Snowball sampling : when researchers find a few participants, and then ask them to find participants themselves and so on.
- Quota sampling : when researchers will be told to ensure the sample fits certain quotas, for example they might be told to find 90 participants, with 30 of them being unemployed.
Experiments always have an independent and dependent variable .
- The independent variable is the one the experimenter manipulates (the thing that changes between the conditions the participants are placed into). It is assumed to have a direct effect on the dependent variable.
- The dependent variable is the thing being measured, or the results of the experiment.
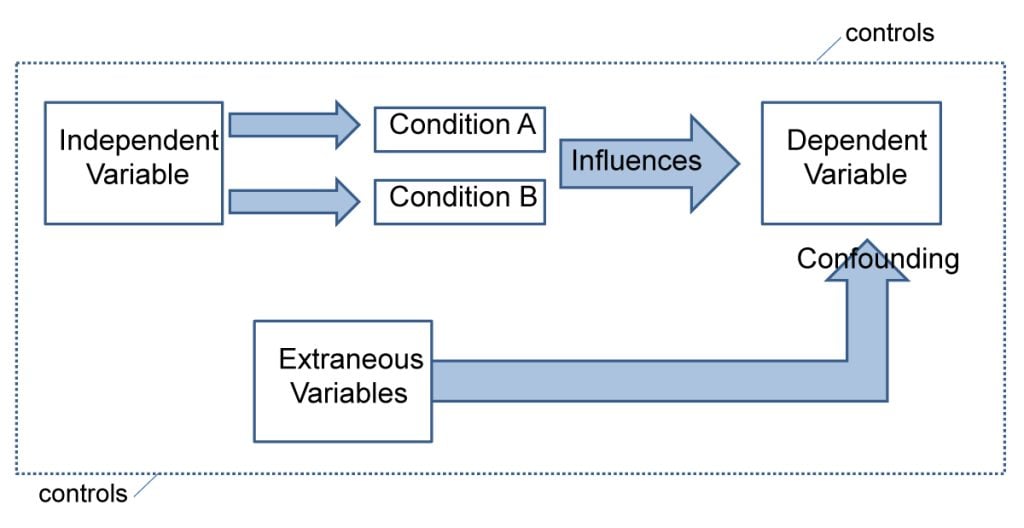
Operationalization of variables means making them measurable/quantifiable. We must use operationalization to ensure that variables are in a form that can be easily tested.
For instance, we can’t really measure ‘happiness’, but we can measure how many times a person smiles within a two-hour period.
By operationalizing variables, we make it easy for someone else to replicate our research. Remember, this is important because we can check if our findings are reliable.
Extraneous variables are all variables which are not independent variable but could affect the results of the experiment.
It can be a natural characteristic of the participant, such as intelligence levels, gender, or age for example, or it could be a situational feature of the environment such as lighting or noise.
Demand characteristics are a type of extraneous variable that occurs if the participants work out the aims of the research study, they may begin to behave in a certain way.
For example, in Milgram’s research , critics argued that participants worked out that the shocks were not real and they administered them as they thought this was what was required of them.
Extraneous variables must be controlled so that they do not affect (confound) the results.
Randomly allocating participants to their conditions or using a matched pairs experimental design can help to reduce participant variables.
Situational variables are controlled by using standardized procedures, ensuring every participant in a given condition is treated in the same way
Experimental Design
Experimental design refers to how participants are allocated to each condition of the independent variable, such as a control or experimental group.
- Independent design ( between-groups design ): each participant is selected for only one group. With the independent design, the most common way of deciding which participants go into which group is by means of randomization.
- Matched participants design : each participant is selected for only one group, but the participants in the two groups are matched for some relevant factor or factors (e.g. ability; sex; age).
- Repeated measures design ( within groups) : each participant appears in both groups, so that there are exactly the same participants in each group.
- The main problem with the repeated measures design is that there may well be order effects. Their experiences during the experiment may change the participants in various ways.
- They may perform better when they appear in the second group because they have gained useful information about the experiment or about the task. On the other hand, they may perform less well on the second occasion because of tiredness or boredom.
- Counterbalancing is the best way of preventing order effects from disrupting the findings of an experiment, and involves ensuring that each condition is equally likely to be used first and second by the participants.
If we wish to compare two groups with respect to a given independent variable, it is essential to make sure that the two groups do not differ in any other important way.
Experimental Methods
All experimental methods involve an iv (independent variable) and dv (dependent variable)..
- Field experiments are conducted in the everyday (natural) environment of the participants. The experimenter still manipulates the IV, but in a real-life setting. It may be possible to control extraneous variables, though such control is more difficult than in a lab experiment.
- Natural experiments are when a naturally occurring IV is investigated that isn’t deliberately manipulated, it exists anyway. Participants are not randomly allocated, and the natural event may only occur rarely.
Case studies are in-depth investigations of a person, group, event, or community. It uses information from a range of sources, such as from the person concerned and also from their family and friends.
Many techniques may be used such as interviews, psychological tests, observations and experiments. Case studies are generally longitudinal: in other words, they follow the individual or group over an extended period of time.
Case studies are widely used in psychology and among the best-known ones carried out were by Sigmund Freud . He conducted very detailed investigations into the private lives of his patients in an attempt to both understand and help them overcome their illnesses.
Case studies provide rich qualitative data and have high levels of ecological validity. However, it is difficult to generalize from individual cases as each one has unique characteristics.
Correlational Studies
Correlation means association; it is a measure of the extent to which two variables are related. One of the variables can be regarded as the predictor variable with the other one as the outcome variable.
Correlational studies typically involve obtaining two different measures from a group of participants, and then assessing the degree of association between the measures.
The predictor variable can be seen as occurring before the outcome variable in some sense. It is called the predictor variable, because it forms the basis for predicting the value of the outcome variable.
Relationships between variables can be displayed on a graph or as a numerical score called a correlation coefficient.
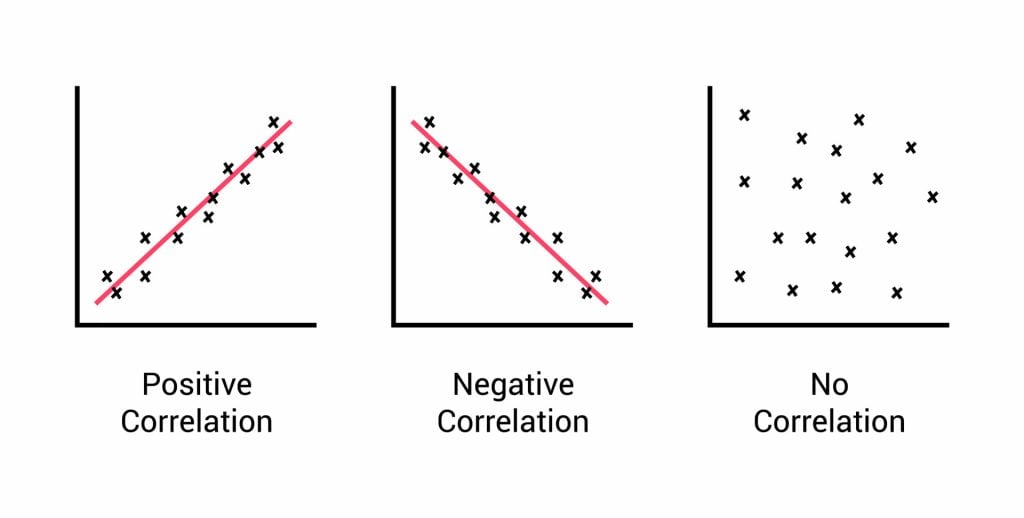
- If an increase in one variable tends to be associated with an increase in the other, then this is known as a positive correlation .
- If an increase in one variable tends to be associated with a decrease in the other, then this is known as a negative correlation .
- A zero correlation occurs when there is no relationship between variables.
After looking at the scattergraph, if we want to be sure that a significant relationship does exist between the two variables, a statistical test of correlation can be conducted, such as Spearman’s rho.
The test will give us a score, called a correlation coefficient . This is a value between 0 and 1, and the closer to 1 the score is, the stronger the relationship between the variables. This value can be both positive e.g. 0.63, or negative -0.63.
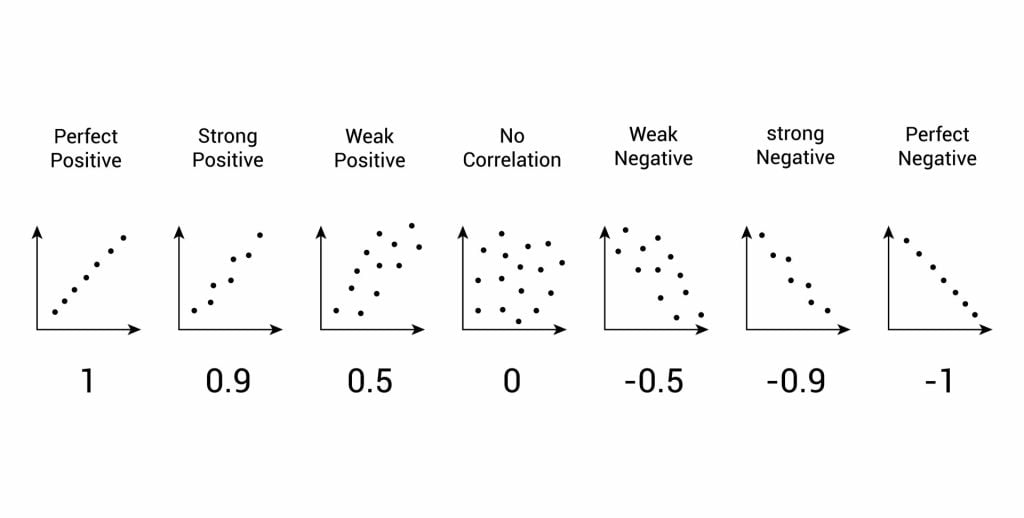
A correlation between variables, however, does not automatically mean that the change in one variable is the cause of the change in the values of the other variable. A correlation only shows if there is a relationship between variables.
Correlation does not always prove causation, as a third variable may be involved.
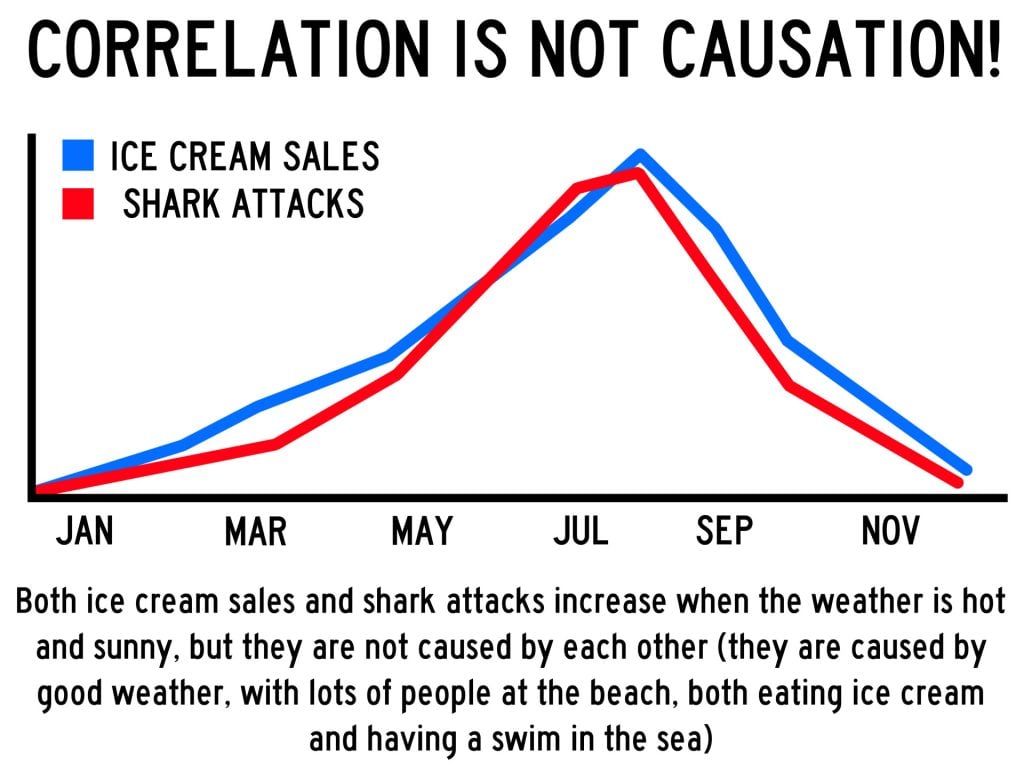
Interview Methods
Interviews are commonly divided into two types: structured and unstructured.
A fixed, predetermined set of questions is put to every participant in the same order and in the same way.
Responses are recorded on a questionnaire, and the researcher presets the order and wording of questions, and sometimes the range of alternative answers.
The interviewer stays within their role and maintains social distance from the interviewee.
There are no set questions, and the participant can raise whatever topics he/she feels are relevant and ask them in their own way. Questions are posed about participants’ answers to the subject
Unstructured interviews are most useful in qualitative research to analyze attitudes and values.
Though they rarely provide a valid basis for generalization, their main advantage is that they enable the researcher to probe social actors’ subjective point of view.
Questionnaire Method
Questionnaires can be thought of as a kind of written interview. They can be carried out face to face, by telephone, or post.
The choice of questions is important because of the need to avoid bias or ambiguity in the questions, ‘leading’ the respondent or causing offense.
- Open questions are designed to encourage a full, meaningful answer using the subject’s own knowledge and feelings. They provide insights into feelings, opinions, and understanding. Example: “How do you feel about that situation?”
- Closed questions can be answered with a simple “yes” or “no” or specific information, limiting the depth of response. They are useful for gathering specific facts or confirming details. Example: “Do you feel anxious in crowds?”
Its other practical advantages are that it is cheaper than face-to-face interviews and can be used to contact many respondents scattered over a wide area relatively quickly.
Observations
There are different types of observation methods :
- Covert observation is where the researcher doesn’t tell the participants they are being observed until after the study is complete. There could be ethical problems or deception and consent with this particular observation method.
- Overt observation is where a researcher tells the participants they are being observed and what they are being observed for.
- Controlled : behavior is observed under controlled laboratory conditions (e.g., Bandura’s Bobo doll study).
- Natural : Here, spontaneous behavior is recorded in a natural setting.
- Participant : Here, the observer has direct contact with the group of people they are observing. The researcher becomes a member of the group they are researching.
- Non-participant (aka “fly on the wall): The researcher does not have direct contact with the people being observed. The observation of participants’ behavior is from a distance
Pilot Study
A pilot study is a small scale preliminary study conducted in order to evaluate the feasibility of the key s teps in a future, full-scale project.
A pilot study is an initial run-through of the procedures to be used in an investigation; it involves selecting a few people and trying out the study on them. It is possible to save time, and in some cases, money, by identifying any flaws in the procedures designed by the researcher.
A pilot study can help the researcher spot any ambiguities (i.e. unusual things) or confusion in the information given to participants or problems with the task devised.
Sometimes the task is too hard, and the researcher may get a floor effect, because none of the participants can score at all or can complete the task – all performances are low.
The opposite effect is a ceiling effect, when the task is so easy that all achieve virtually full marks or top performances and are “hitting the ceiling”.
Research Design
In cross-sectional research , a researcher compares multiple segments of the population at the same time
Sometimes, we want to see how people change over time, as in studies of human development and lifespan. Longitudinal research is a research design in which data-gathering is administered repeatedly over an extended period of time.
In cohort studies , the participants must share a common factor or characteristic such as age, demographic, or occupation. A cohort study is a type of longitudinal study in which researchers monitor and observe a chosen population over an extended period.
Triangulation means using more than one research method to improve the study’s validity.
Reliability
Reliability is a measure of consistency, if a particular measurement is repeated and the same result is obtained then it is described as being reliable.
- Test-retest reliability : assessing the same person on two different occasions which shows the extent to which the test produces the same answers.
- Inter-observer reliability : the extent to which there is an agreement between two or more observers.
Meta-Analysis
A meta-analysis is a systematic review that involves identifying an aim and then searching for research studies that have addressed similar aims/hypotheses.
This is done by looking through various databases, and then decisions are made about what studies are to be included/excluded.
Strengths: Increases the conclusions’ validity as they’re based on a wider range.
Weaknesses: Research designs in studies can vary, so they are not truly comparable.
Peer Review
A researcher submits an article to a journal. The choice of the journal may be determined by the journal’s audience or prestige.
The journal selects two or more appropriate experts (psychologists working in a similar field) to peer review the article without payment. The peer reviewers assess: the methods and designs used, originality of the findings, the validity of the original research findings and its content, structure and language.
Feedback from the reviewer determines whether the article is accepted. The article may be: Accepted as it is, accepted with revisions, sent back to the author to revise and re-submit or rejected without the possibility of submission.
The editor makes the final decision whether to accept or reject the research report based on the reviewers comments/ recommendations.
Peer review is important because it prevent faulty data from entering the public domain, it provides a way of checking the validity of findings and the quality of the methodology and is used to assess the research rating of university departments.
Peer reviews may be an ideal, whereas in practice there are lots of problems. For example, it slows publication down and may prevent unusual, new work being published. Some reviewers might use it as an opportunity to prevent competing researchers from publishing work.
Some people doubt whether peer review can really prevent the publication of fraudulent research.
The advent of the internet means that a lot of research and academic comment is being published without official peer reviews than before, though systems are evolving on the internet where everyone really has a chance to offer their opinions and police the quality of research.
Types of Data
- Quantitative data is numerical data e.g. reaction time or number of mistakes. It represents how much or how long, how many there are of something. A tally of behavioral categories and closed questions in a questionnaire collect quantitative data.
- Qualitative data is virtually any type of information that can be observed and recorded that is not numerical in nature and can be in the form of written or verbal communication. Open questions in questionnaires and accounts from observational studies collect qualitative data.
- Primary data is first-hand data collected for the purpose of the investigation.
- Secondary data is information that has been collected by someone other than the person who is conducting the research e.g. taken from journals, books or articles.
Validity means how well a piece of research actually measures what it sets out to, or how well it reflects the reality it claims to represent.
Validity is whether the observed effect is genuine and represents what is actually out there in the world.
- Concurrent validity is the extent to which a psychological measure relates to an existing similar measure and obtains close results. For example, a new intelligence test compared to an established test.
- Face validity : does the test measure what it’s supposed to measure ‘on the face of it’. This is done by ‘eyeballing’ the measuring or by passing it to an expert to check.
- Ecological validit y is the extent to which findings from a research study can be generalized to other settings / real life.
- Temporal validity is the extent to which findings from a research study can be generalized to other historical times.
Features of Science
- Paradigm – A set of shared assumptions and agreed methods within a scientific discipline.
- Paradigm shift – The result of the scientific revolution: a significant change in the dominant unifying theory within a scientific discipline.
- Objectivity – When all sources of personal bias are minimised so not to distort or influence the research process.
- Empirical method – Scientific approaches that are based on the gathering of evidence through direct observation and experience.
- Replicability – The extent to which scientific procedures and findings can be repeated by other researchers.
- Falsifiability – The principle that a theory cannot be considered scientific unless it admits the possibility of being proved untrue.
Statistical Testing
A significant result is one where there is a low probability that chance factors were responsible for any observed difference, correlation, or association in the variables tested.
If our test is significant, we can reject our null hypothesis and accept our alternative hypothesis.
If our test is not significant, we can accept our null hypothesis and reject our alternative hypothesis. A null hypothesis is a statement of no effect.
In Psychology, we use p < 0.05 (as it strikes a balance between making a type I and II error) but p < 0.01 is used in tests that could cause harm like introducing a new drug.
A type I error is when the null hypothesis is rejected when it should have been accepted (happens when a lenient significance level is used, an error of optimism).
A type II error is when the null hypothesis is accepted when it should have been rejected (happens when a stringent significance level is used, an error of pessimism).
Ethical Issues
- Informed consent is when participants are able to make an informed judgment about whether to take part. It causes them to guess the aims of the study and change their behavior.
- To deal with it, we can gain presumptive consent or ask them to formally indicate their agreement to participate but it may invalidate the purpose of the study and it is not guaranteed that the participants would understand.
- Deception should only be used when it is approved by an ethics committee, as it involves deliberately misleading or withholding information. Participants should be fully debriefed after the study but debriefing can’t turn the clock back.
- All participants should be informed at the beginning that they have the right to withdraw if they ever feel distressed or uncomfortable.
- It causes bias as the ones that stayed are obedient and some may not withdraw as they may have been given incentives or feel like they’re spoiling the study. Researchers can offer the right to withdraw data after participation.
- Participants should all have protection from harm . The researcher should avoid risks greater than those experienced in everyday life and they should stop the study if any harm is suspected. However, the harm may not be apparent at the time of the study.
- Confidentiality concerns the communication of personal information. The researchers should not record any names but use numbers or false names though it may not be possible as it is sometimes possible to work out who the researchers were.

Can you tell people’s cognitive ability level from their response patterns in questionnaires?
- Original Manuscript
- Open access
- Published: 25 March 2024
Cite this article
You have full access to this open access article
- Stefan Schneider 1 , 2 , 3 ,
- Raymond Hernandez 1 ,
- Doerte U. Junghaenel 1 , 2 , 3 ,
- Haomiao Jin 4 ,
- Pey-Jiuan Lee 1 ,
- Hongxin Gao 4 ,
- Danny Maupin 4 ,
- Bart Orriens 5 ,
- Erik Meijer 5 &
- Arthur A. Stone 1 , 2
96 Accesses
3 Altmetric
Explore all metrics
Questionnaires are ever present in survey research. In this study, we examined whether an indirect indicator of general cognitive ability could be developed based on response patterns in questionnaires. We drew on two established phenomena characterizing connections between cognitive ability and people’s performance on basic cognitive tasks, and examined whether they apply to questionnaires responses. (1) The worst performance rule (WPR) states that people’s worst performance on multiple sequential tasks is more indicative of their cognitive ability than their average or best performance. (2) The task complexity hypothesis (TCH) suggests that relationships between cognitive ability and performance increase with task complexity. We conceptualized items of a questionnaire as a series of cognitively demanding tasks. A graded response model was used to estimate respondents’ performance for each item based on the difference between the observed and model-predicted response (“response error” scores). Analyzing data from 102 items (21 questionnaires) collected from a large-scale nationally representative sample of people aged 50+ years, we found robust associations of cognitive ability with a person’s largest but not with their smallest response error scores (supporting the WPR), and stronger associations of cognitive ability with response errors for more complex than for less complex questions (supporting the TCH). Results replicated across two independent samples and six assessment waves. A latent variable of response errors estimated for the most complex items correlated .50 with a latent cognitive ability factor, suggesting that response patterns can be utilized to extract a rough indicator of general cognitive ability in survey research.
Similar content being viewed by others
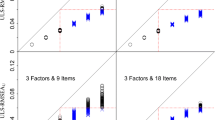
RMSEA, CFI, and TLI in structural equation modeling with ordered categorical data: The story they tell depends on the estimation methods
Yan Xia & Yanyun Yang
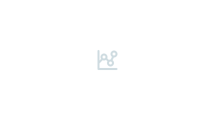
Statistical tests, P values, confidence intervals, and power: a guide to misinterpretations
Sander Greenland, Stephen J. Senn, … Douglas G. Altman
A Critical Review of the Job Demands-Resources Model: Implications for Improving Work and Health
Avoid common mistakes on your manuscript.
Introduction
Standardized self-report questionnaires are an ever-present research method in the social and medical sciences. Questionnaires are administered in many cross-sectional and longitudinal studies to collect information on a broad range of topics, including people’s behaviors and feelings, attitudes and opinions, health status, personality, environmental conditions, life events, and well-being (Groth-Marnat, 2003 ). Compared to other forms of data collection, such as behavioral observations or laboratory-based experiments, an advantage of self-report questionnaires is that they allow researchers to gather information from large numbers of respondents via paper and pencil or over the internet quickly, easily, and inexpensively.
Cognitive capacities are relevant to a wide range of behaviors, experiences, and everyday functions assessed in survey research (Jokela, 2022 ; Llewellyn et al., 2008 ). However, standardized cognitive assessments are relatively expensive, burdensome to respondents, and sometimes difficult to implement in large survey research. For this reason, many survey studies do not administer formal tests of cognitive abilities alongside questionnaires. In this study, we ask whether there are alternatives to formal cognitive tests that could be used to infer people’s cognitive ability levels from their behaviors in self-report surveys.
It is widely acknowledged that completing self-report questionnaires is a cognitively demanding task that involves various mental processes, including perception, attention, decision-making, and executive control. For each question in a survey, respondents need to read and understand the question, search the relevant information from memory, integrate the information into a summary judgment, and select a response that best reflects their judgment based on the retrieved information (Tourangeau, 1984 , 2018 ), paralleling steps that are assumed to govern many cognitive problem-solving tasks (e.g., encoding, inference, mapping, application, justification, response; Sternberg, 1979 ). If objective indicators could be developed based on people’s response patterns that reflect how well they perform at the task of completing questionnaires, then an indirect measurement of cognitive ability would become available as a by-product of a survey. This could provide an opportunity to measure cognitive abilities from questionnaires that are already administered as part of a survey study without any additional costs or respondent burden.
Prior research relating questionnaire response quality to cognitive ability
Several previous studies have provided evidence for empirical relationships between people’s cognitive abilities and the quality of their questionnaire responses. For example, respondents with lower cognitive abilities have been found to show more acquiescent responses (i.e., “yeah-saying” or agreeing with statements regardless of item content) in personality assessments (Lechner & Rammstedt, 2015 ; Schneider et al., 2021 ), to display more extreme response tendencies in emotional well-being assessments (Schneider, 2018 ), and to provide more indifferent (“don’t know”) responses in population-based surveys (Colsher & Wallace, 1989 ; Knäuper et al., 1997 ). Moreover, lower cognitive abilities have been associated with a greater tendency to provide conflicting answers to similar questions (Colsher & Wallace, 1989 ), with greater response outliers (Schneider et al., 2022 , 2021 ), and with more random (e.g., internally inconsistent) response patterns (Conijn et al., 2020 ; Schneider et al., 2022 ).
Challenges with using questionnaire response patterns as cognitive ability indicators
Even though these studies support the idea that the quality of people’s responses in questionnaires may at least partially reflect their cognitive capacity, to our knowledge, there have not been any formal attempts to use response patterns in questionnaires as an indirect measure of cognitive ability. Indeed, there are several significant challenges that make it difficult to unambiguously link questionnaire response patterns to cognitive ability levels. First, whereas standardized cognitive tests of intelligence or ability level are often constructed to assess the number of test items a person solves correctly (Kyllonen & Zu, 2016 ), many self-report questionnaires are intended to measure subjective attitudes and experiences, and there generally are no objectively correct and incorrect answers to these questions. In the absence of a “ground truth” for response accuracy, attempts to measure cognitive abilities from the quality of responses in questionnaires, therefore, must typically rely on psychometric indicators that quantify presumable “abnormalities” in response patterns, such as the extent to which a response is improbable given other information, inconsistent with other responses, or deviating from statistically expected responses (Schneider et al., 2022 ).
Second, many factors can influence observed response patterns, and it is usually impossible to attribute a pattern uniquely to a particular causal factor. For example, a low-quality answer pattern might reflect low cognitive ability or it might reflect carelessness, such that a respondent is not motivated enough to read the questions or does not attempt to find answers that reflect his or her true attitudes or opinions (Ward & Meade, 2023 ). Responses of lower quality could also result when a participant is distracted for a moment, gets fatigued when answering long questionnaire batteries, or is unfamiliar with the topic asked in the questions (Krosnick, 1991 ). These ambiguities are not unique to the measurement of cognitive abilities from questionnaire responses, and they similarly apply to other indirect indicators of cognitive ability such as response times on reaction time tasks (Kyllonen & Zu, 2016 ).
Third, even though prior research has documented consistent relationships between questionnaire response patterns and respondents’ cognitive ability, the strength of these relationships has generally been modest. The magnitude of the observed associations resembles the reliable, but not impressively high, correlations that have been observed between reaction times and measures of general intelligence (Schmiedek et al., 2007 ). To date, there have been no concentrated efforts to examine whether the signal that links questionnaire response patterns with cognitive ability can be meaningfully enhanced.
The present study
The goal of this study is to more firmly establish evidence supporting the idea that response patterns in questionnaires can be potentially useful as indirect indicators of cognitive ability, and to investigate which information from questionnaire response patterns is most closely associated with people’s cognitive ability levels. To do this, we draw on two key findings in intelligence research that have been reliably documented to characterize connections between general cognitive ability and people’s performance on elementary cognitive tasks (e.g., simple choice tasks): the worst performance rule (WPR) and the task complexity hypothesis (TCH).
The WPR states that people’s worst performance on multiple sequential tasks is more indicative of their cognitive ability than their average or best performance (Larson & Alderton, 1990 ). The most prominent theoretical explanation for this phenomenon is based on the idea that temporary lapses of attention or working memory lead to momentarily poor performance and that individuals of higher general cognitive ability have a better capacity for attentional control, thus preventing such lapses (Jensen, 1992 ; Larson & Alderton, 1990 ).
The TCH suggests that people’s performance on more complex versions of an elementary cognitive task correlates more highly with general cognitive abilities than less complex versions of the same task, presumably due to greater “g saturation” of more complex tasks (Kranzler et al., 1994 ; Larson et al., 1988 ; Stankov, 2000 ). The importance of the TCH for information processing is emphasized by Jensen ( 2006 ), who considered the observation that “individual differences in learning and performance increase monotonically as a function of increasing task complexity” (p. 205) as so central to label it the first law of individual differences.
Even though the predictions by the WPR and TCH have been most prominently studied in the measurement of cognitive processing speed by means of reaction times (i.e., “mental chronometry”), they have been viewed as universal and key phenomena that any process theory of cognitive ability has to account for (Jensen, 2006 ; Schubert, 2019 ). Thus, we argue that if response patterns on questionnaires reveal information about individual differences in general cognitive ability, they should also follow the WPR and TCH.
To test the predictions of the WPR and TCH, we conceptualize each item of a self-report questionnaire as a separate task and utilize a psychometric procedure based on item response theory (IRT) to capture the quality of responses on an item-by-item basis for each person. Obtaining an indicator of response quality separately for each questionnaire item is necessary in order to distinguish for which items an individual performed better versus worse (to test the WPR) and to evaluate people’s performance on less versus more complex items (to test the TCH). Specifically, we propose to measure item response quality as the extent to which the selected response differs from the statistically expected response given an IRT model, that is, the estimated response “ error ” score for each item. Following the prediction of the WPR, we hypothesize that the largest response error scores for each person reflect their worst performance in a self-report survey and are, therefore, most highly correlated with their general cognitive ability. Following the prediction of the TCH, we hypothesize that response error scores for more complex questionnaire items (i.e., items with a greater information load; Jensen, 2006 ) are more strongly associated with cognitive ability than response error scores for less complex questions. Finally, we investigate to what extent an indirect measurement of cognitive ability derived from response patterns in questionnaires can be meaningfully enhanced by taking the complexity of survey items into account. To evaluate the robustness of the results, we examine their replicability across two independent samples and across multiple measurement waves using survey data from a large US population-based panel study.
Study sample
The sample for the present analyses was drawn from the Health and Retirement Study (HRS), a longitudinal study that has been collecting data on the health, social, and economic well-being of older Americans since 1992 (Juster & Suzman, 1995 ). HRS participants are interviewed in two-year intervals. Initial HRS respondents were individuals between 51 and 61 years of age and their spouses. New cohorts were added over time to render the participant sample representative of the US population 50 years and older (Sonnega et al., 2014 ).
Since 2006, the HRS has administered the Participant Lifestyle Questionnaire (PLQ), also referred to as “leave-behind survey” (Smith et al., 2013 ). This self-administered questionnaire package was handed to respondents following the core interview, to be returned by mail. The PLQ has been administered every two years to a rotating 50% randomly selected subsample of participants who completed the core interviews. Half of the participants were assigned to complete the PLQ in Waves 8, 10, and 12, and the remaining (nonoverlapping) 50% were assigned to complete it in Waves 9, 11, 13, and so on. For the present study, we included the Wave 8 subsample (PLQ administered in 2006) as the primary analysis sample. We repeated the analyses in the Wave 9 subsample (PLQ administered in 2008) to examine the replicability of findings in an independent sample. In secondary analyses shown in the online appendix, we also repeated the analyses for subsequent Waves 10 to 13 to examine the robustness and replicability of results over time and with repeated assessments. We included only respondents who completed the PLQ by themselves; individuals whose questionnaires were completed by proxy respondents (between 1% and 2% per wave) were not included. All participants provided informed consent as part of the HRS, and the research was approved by the relevant institutional review boards.
Demographic characteristics of the analyzed Wave 8 and Wave 9 HRS samples are shown in Table 1 . A total of n = 7296 (Wave 8) and n = 6646 (Wave 9) participants were analyzed. Participants’ mean ages were 68.7 years ( SD = 10.0, Wave 8) and 69.9 years ( SD = 9.8, Wave 9). About three fifths of participants in each sample were female, four fifths were White, and about three fifths were married. Participants had on average completed 12.6 years of education, and the median income was about $37,000.
The analyses excluded respondents from the larger HRS cohorts who had participated in the core interview but did not return the paper-and-pencil PLQ by mail or did not complete the PLQ by themselves. This excluded n = 1129 (13.40%, Wave 8) and n = 1487 (18.28%, Wave 9) HRS respondents. Compared with the analysis samples, excluded HRS respondents were on average (across both waves) 1.92 years older, were 5.65% less likely women, 12.03% less likely White, and 8.07% less likely married, had 1.12 fewer years of education, and had $10,000 lower median income.
Cognitive ability measurement
Participants’ cognitive ability was measured with a composite of five standardized cognitive tests administered in the HRS to each participant at each wave. The test battery is based on the widely used Telephone Interview for Cognitive Status (Ofstedal et al., 2005 ) and it includes immediate free recall (0–10 points) and delayed free recall (0–10 points) to measure memory, a serial sevens subtraction test to measure attention and working memory (0–5 points), and backward counting from 20 to measure general mental processing (0–2 points). We followed previous studies (Crimmins et al., 2016 ) for calculating an overall cognitive ability score by summing the scores for all five subtests (possible score range: 0–27). A small percentage of participants (0.8–3.1%) did not provide scores for immediate and delayed free recall and serial sevens subtraction tests. HRS has developed an imputation algorithm for cognitive variables for all waves (McCammon et al., 2019 ), and we used the imputed data to accommodate missing scores on subtests. The overall cognitive ability score had mean = 15.26, SD = 4.35, composite reliability omega = .73 at Wave 8, and mean = 15.13, SD = 4.33, omega = .74 at Wave 9.
Self-report questionnaires
We analyzed responses for 102 self-report questions included in 21 multi-item psychometric rating scales assessed in the HRS PLQ. We only analyzed responses to questionnaires that were applicable to all respondents (i.e., questionnaires on participants’ experiences with their spouse, children, or work environment that were relevant only to respondent subgroups were excluded). Specifically, the constructs addressed by the questionnaires were life satisfaction (5 items), cynical hostility (5 items), optimism (6 items), hopelessness (4 items), loneliness (3 items), neighborhood physical disorder (4 items), neighborhood social cohesion (4 items), constraints on personal control (5 items), perceived mastery (5 items), religiosity/spirituality (4 items), everyday discrimination (5 items), social effort/reward balance (3 items), neuroticism (4 items), extraversion (5 items), conscientiousness (5 items), agreeableness (5 items), openness to experience (7 items), purpose in life (7 items), anxiety (5 items), anger-in (4 items), and anger-out (7 items). All items were rated on ordinal rating scales (e.g., strongly agree – strongly disagree) with between three and seven response options. For details on the questionnaires and their psychometric properties, see Smith et al. ( 2013 ).
Indicators of question complexity
Following Jensen ( 2006 ), we refer to the term complexity as the information load involved in answering a self-report question. Information load cannot be assessed with a single attribute, and we coded 10 different characteristics of each question that are likely related to information load based on prior literature (Bais et al., 2019 ; Knäuper et al., 1997 ; Schneider, Jin et al., 2023a ; Yan & Tourangeau, 2008 ) using four approaches, as described below.
Indicator 1
We counted the number of words in each item as a simple indicator of the number of task elements a respondent needed to attend to when answering the question. A binary word count (WC) variable was then created based on a median split of the observed item word counts: Items that consisted of 10 or more words were categorized as “longer” items, and items with fewer than 10 words were categorized as “shorter” items.
Indicator 2
Questions requiring greater verbal ability were coded using the Dale–Chall (DC) word list. The list contains approximately 3000 words that fourth grade students can generally reliably understand (Chall & Dale, 1995 ). Based on a median split across all items, we coded items containing two or more words that were not on the Dale–Chall word list as requiring greater verbal ability, and items with all words on the list or only one word not on the list as requiring less verbal ability.
Indicators 3 to 5
The Question Understanding Aid (QUAID; http://quaid.cohmetrix.com/ ), an online software tool for survey developers, was used to identify item wordings that may reduce the clarity of survey questions and increase the degree of uncertainty about the required response. The validity and utility of the QUAID has been previously established (Graesser et al., 2006 ; Graesser et al., 2000 ). Each item was categorized based on three types of potentially problematic wordings: presence or absence of (3) unfamiliar technical terms (UTT), (4) vague or imprecise relative terms (VRT), and (5) vague or ambiguous noun phrases (VNP).
Indicators 6 to 10
The Linguistic Inquiry and Word Count program (LIWC; Pennebaker et al., 2015 ) was used to identify item wordings that increase the degree of stimulus discrimination involved in reading and understanding a survey question. For each item, we coded whether or not it contained any (6) conjunctions (CON; e.g., if, whereas, because), (7) negations (NEG; e.g., no, not, never), (8) words indicating discrepancies (DIS; e.g., should, would, could), (9) words indicating tentative statements (TEN; e.g., maybe, perhaps), and (10) words indicating differentiation or exclusion (EXC; e.g., has not, but, else).
A composite measure of item complexity was computed by taking the sum of all 10 individual complexity indicators (possible range of the composite measure = 0 to 10).
Data analysis
We first describe the statistical methods to derive the proposed indicator of response quality (i.e., estimated response “error” scores) for the questionnaire item responses. Subsequently, we describe the analysis strategy to test the predictions of the WPR and TCH.
Response error scores derived from item response theory model
Whereas multi-item rating scales are typically used in research to estimate people’s actual scores on the underlying construct targeted by each scale (e.g., optimism, personality traits), our goal was to develop indicators that captured how closely people’s responses on each item reflected their presumable true scores on the underlying constructs. We used an IRT framework to estimate these true scores, which then served as a reference point for estimating response error scores for each item.
In a first modeling step, we fitted a unidimensional graded response model (GRM; Samejima, 1969 ) to each of the 21 PLQ scales at each assessment wave to estimate people’s presumable true scores for each of the scales. The GRM is a popular and flexible IRT model for ordered categorical responses. Let θ be the latent construct underlying the responses to the items in a scale, and suppose the items have m ordered response options. Let P* ijk ( θ ) be the probability that the i th respondent with a latent score θ i on the construct chooses response category k or higher on the j th item of the scale. The GRM then specifies P* ijk ( θ ) as a monotonically increasing function of the latent score θ :
where Y ij is the item response of person i to item j (responses can take values of 1, 2, . . . , m j ), a j is the item discrimination parameter, and b jk ( k = 1, 2, . . . , m j - 1) are threshold parameters for item j that separate two adjacent response categories k and k + 1. The probability of choosing a particular response category k at a given level of θ is given by the item category response function:
The GRM allows for item discrimination and threshold parameters to differ across items. The discrimination parameter indicates the strength of the relationship between an item and the measured construct. The threshold parameters provide information on the extent to which an item targets higher or lower levels of the construct (e.g., the “severity” level of a problem addressed by the question). For each of the 21 PLQ scales, we evaluated the fit of a unidimensional GRM by means of the comparative fit index (CFI >.95 for good model fit), Tucker–Lewis index (TLI >.95 for good fit), root mean square error of approximation (RMSEA < .06 for good fit), and standardized root mean square residual (SRMR < .08 for good fit). Across scales, the average model fit values were CFI = .970 (range = .868–1.00), TLI = .942 (range = .790–1.00), RMSEA = .104 (range = .000–.237), and SRMR = .038 (range = .000–.152) in the Wave 8 sample, and CFI = .969 (range = .879–1.00), TLI = .941 (range = .799–1.00), RMSEA = .116 (range = .000–.226), and SRMR = .041 (range = .000–.139) in the Wave 9 sample. For details, see Table S1 in the online Supplemental Appendix.
In a second step, we obtained latent variable estimates ( \(\widehat{\theta }\) ) of each person’s “true” scores on the underlying constructs using expected a posteriori parameter estimation, and calculated the response error scores by comparing the observed response for each item with the statistically expected response. The expected response was calculated as the weighted sum of the probabilities of all response categories given the person’s level of \(\widehat{\theta }\) , where the weights represent the response category value (e.g., values 1 to 5 on a five-point rating scale):
we then calculated response error scores in the following form:
The numerator is the absolute value of the usual residual term, that is, the absolute difference between observed score y and the expected score. The absolute value of the difference was used because we were interested only in the magnitude of the residual, and not its direction, as an indicator of response quality. For an individual giving optimal responses, the observed score should be close to the expected score predicted by the model. Larger absolute deviations from the expected score are indicative of low response quality. The denominator in Eq. 4 rescales the values of the absolute residuals by the number of threshold parameters (i.e., number of response categories m minus 1 ) for a given item. For each response, the resulting values of the response error scores can range from 0 (i.e., the response perfectly matches the statistically expected response) to 1 (the observed response is maximally different from the expected response). Response error scores were computed from all scales with no missing responses per person; the overall rate of missing item responses was 2.98% in the Wave 8 sample and 2.80% in Wave 9 sample.
Analysis of worst performance rule
To test the prediction of the WPR, we first divided each participant’s response error scores for all questionnaire items into deciles such that the first category comprised the 10% smallest error scores and the 10th category contained the 10% largest error scores for each person. We then averaged the error scores in each decile so that each participant had 10 mean error scores (one per decile) and estimated the correlation between people’s cognitive test scores and their mean error scores in each decile. According to the WPR, the correlations should differ in magnitude from the lowest to the highest decile. To test this, we needed to consider that the correlations were nonindependent (i.e., correlated). Accordingly, we estimated a correlation matrix consisting of the correlations among the mean error scores for each decile and their corresponding correlations with the cognitive test scores. We then Fisher z -transformed the correlations and conducted an omnibus Wald test examining whether the (dependent) correlations between the mean error scores and cognitive ability scores differed across the 10 deciles. Significant omnibus tests were followed by post hoc comparisons between correlation pairs conducted using the delta method.
Analysis of task complexity hypothesis
Multilevel regression models were estimated to test the TCH. The respondents’ response error scores for all 102 items (nested in individuals) served as outcome variable in the multilevel models. The error scores were regressed on item complexity scores at Level 1, allowing for random intercepts and random regression slopes across individuals, and the intercepts and slopes were regressed on cognitive test scores at Level 2. For respondent i and item j , the model equation was as follows:
where \({r}_{ij}\sim N\left(0, {\sigma }^{2}\right)\) and \(\left(\begin{array}{c}{u}_{0i} \\ {u}_{1i} \end{array}\right)\sim MVN\left(\left(\begin{array}{c}0\\ 0\end{array}\right),\left(\begin{array}{cc}{\tau }_{00}& \\ {\tau }_{10}& {\tau }_{11}\end{array}\right)\right)\)
The reduced-form equation of the same model is as follows:
The item complexity by cognitive ability cross-level interaction term tests the prediction by the THC. A significant interaction indicates that the relationship between cognitive ability and the response error scores depends on item complexity. The primary multilevel models were tested using the composite measure of item complexity (i.e., the sum of all complexity indicators). Secondary analyses used each of the individual indicators of item complexity in separate models to examine the robustness of the results across different complexity indicators.
A final set of analyses addressed the question to what extent the correlation between cognitive ability and people’s response error scores would be meaningfully different for surveys with less versus more complex items. To examine this, we reparameterized the model in Eq. 5 such that it used neither a traditional intercept nor regression slope, but rather parameters representing individual differences in response errors at lower versus higher item complexity levels (see Singer & Willett, 2003 , p. 187):
At Level 2, the parameter β 0ij represents latent individual differences in response errors for items with low complexity (complexity = 0) and β 1ij represents latent individual differences in response errors for items with higher complexity (complexity = 9; the highest observed score, see Results). The two parameters were allowed to correlate with each other at Level 2 and were simultaneously correlated with people’s cognitive ability scores in the multilevel model:
where \({r}_{ij}\sim N\left(0, {\sigma }^{2}\right)\) and \(\left(\begin{array}{c}{u}_{0i}\\ {u}_{1i}\\ {u}_{2i}\end{array}\right)\sim MVN\left(\left(\begin{array}{c}0\\ 0\\ 0\end{array}\right),\left(\begin{array}{ccc}{\tau }_{00}& & \\ {\tau }_{10}& {\tau }_{11}& \\ {\tau }_{20}& {\tau }_{21}& {\tau }_{22}\end{array}\right)\right)\)
We also tested the same model but instead of using a cognitive ability sum score, we fitted a latent cognitive ability factor underlying the four cognitive subtests (immediate recall, delayed recall, serial 7s, backward counting). The correlation between this latent cognitive ability factor and people’s latent response errors for more complex items represents our best estimate of the maximal correspondence between people’s response errors in questionnaires and participants’ true cognitive ability level. The model fit of the cognitive ability factor was first evaluated in a separate model; because scores on immediate- and delayed-recall tests are highly correlated, we allowed for a residual correlation between these subtests in the factor model. The cognitive ability factor was then incorporated at Level 2 of a multilevel structural equation model where it was correlated with the random effects of response errors for less complex (complexity = 0) and more complex (complexity = 9) items, respectively.
We used the R package mirt (Chalmers, 2012 ) for the IRT models used to derive the estimated response error scores. Analyses testing the WPR and THC were conducted in M plus version 8.10 (Muthén & Muthén, 2017 ) via the R package MplusAutomation (Hallquist & Wiley, 2018 ) and using maximum likelihood parameter estimation with standard errors robust to non-normality.
Distribution of estimated response error scores
The top panel in Fig. 1 shows histograms of the (absolute) response error scores estimated from the GRMs in each sample. Even though the distributions of the estimated response errors covered almost the full possible range, with observed values ranging from <.001 (observed responses nearly exactly matching the expected response) to >.990 (observed responses nearly maximally deviating from the expected response), the distributions were notably positively skewed. The median estimated response errors were .082 (interquartile range [IQR] = .030 to .175; Wave 8 sample) and .081 (IQR = .029 to .175; Wave 9 sample), indicating that the large majority of responses closely matched the statistically expected responses. Because skewed performance distributions have been suggested to distort results from analyses involving the WPR (see Coyle, 2003 ), we applied a cube root transformation to the response error scores, after which the scores approximated a normal distribution (see Fig. 1 , lower panel).
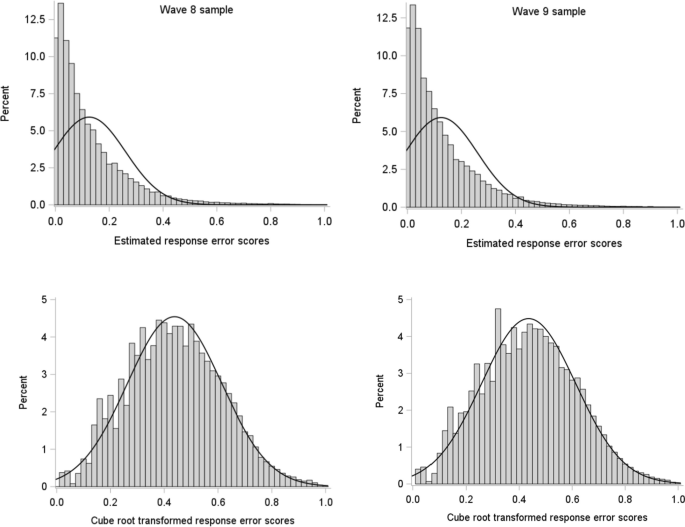
Histograms and normal density curves of estimated response error scores (top) and cube-root-transformed response error scores (bottom) in Wave 8 (left) and Wave 9 (right) samples
- Worst performance rule
To test the WPR, the transformed response error scores were used to compute mean error scores per respondent decile. As shown in Table 2 , the (grand) mean response error scores increased from the lowest (i.e., first) decile (means ranging from .15 to .16 across samples) to the highest (i.e., 10th) decile (mean = .74 in each sample) as expected (i.e., as a logical consequence of the grouping of scores into deciles). The standard deviations of response error scores were comparable across deciles ( SD s ranging from .04 to .06). As shown in the upper part of Table 2 , the correlations between response error scores in different deciles showed a systematic pattern whereby error scores in adjacent deciles were substantially correlated with each other ( r s ranging between .84 and .97). Error scores at opposite ends of the distribution were only modestly associated with each other (correlations between error scores in first and 10th decile of r = .14 in Wave 8 and r = .16 in Wave 9 samples, respectively), suggesting that these error scores had the potential to differ in their relationships with cognitive ability.
The correlations between people’s cognitive ability scores and their mean response error scores in each decile are shown in Table 3 . Omnibus Wald tests indicated that correlations with cognitive ability differed significantly across the 10 deciles ( p < .001 in each sample). Response error scores in the first decile (i.e., the smallest response errors) were not significantly correlated with cognitive ability scores ( r = −.01, p = .50 for Wave 8 sample; r = −.02, p = .16 for Wave 9 sample). Response errors for all other deciles were significantly associated with cognitive ability ( p s < .001), but the magnitude of the correlations increased monotonically with increasing deciles. Supporting the prediction by the WPR, response error scores in the 10th decile showed the highest correlations with people’s cognitive ability scores ( r = −.33, p < .001 for Wave 8 sample; r = −.35, p < .001 for Wave 9 sample), significantly exceeding the correlations for all other deciles ( p s < .01).
Task complexity hypothesis
Descriptive statistics for the various indicators of item complexity are shown in Table 4 . The occurrence of different aspects of item complexity across the 102 analyzed PLQ questions ranged from 15.7% for items containing negations to 50.0% for items with a word count of 10 or more words. With the exception of the indicator of unfamiliar technical terms, which showed small negative correlations with most other indicators, all item complexity indicators were positively intercorrelated ( r s ranging from .02 to .66; median r = .28), which means that different aspects of complexity tended to co-occur for a given item. A one-factor model for binary indicators showed the following fit: χ 2 [ df = 35] = 53.36, p = .02; CFI = .971, TLI = .963, RMSEA = .072, SRMR = .123. A composite measure of item complexity created as the sum of all binary indicators had a reliability of categorical omega = .82 (Green & Yang, 2009 ). The mean score of the composite complexity measure was 3.25 (SD = 2.65; median = 4.00), with a range of 0 to 9 (no item received the maximum possible complexity score of 10).
Results for the moderated multilevel regression models predicting people’s (cube-root-transformed) response error scores from the interaction between their cognitive test scores and item complexity are shown in Table 5 (Wave 8 sample) and Table 6 (Wave 9 sample). The first column in each table presents results for the composite measure of item complexity. Higher scores on the composite measure were significantly ( p < .001) associated with larger response errors for any cognitive ability level (Tables 5 and 6 show “simple slopes” of item complexity for a cognitive test score of 0), and higher cognitive scores were associated with smaller response errors at any item complexity level (Tables 5 and 6 show simple slopes of cognitive ability for an item complexity score of 0). The interaction between item complexity and cognitive ability was significant ( p < .001) in both samples. As shown in Fig. 2 , the association between cognitive ability and response error scores became more pronounced as item complexity increased, supporting the TCH. Specifically, for each point increase in composite item complexity above 0 on the 0–9 scale, the relationship (regression slope) between cognitive ability and response error scores increased by 27% in the Wave 8 sample and by 16% in the Wave 9 sample. Footnote 1
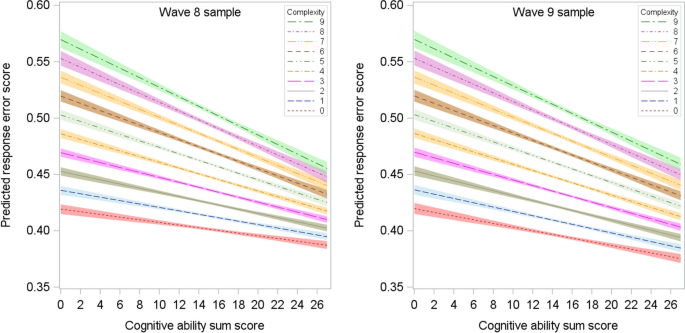
Relationship between cognitive ability sum scores and predicted response error scores by item complexity composite scores in the Wave 8 (left) and Wave 9 (right) samples. Colored bands represent 95% confidence intervals
Sensitivity analyses were conducted to explore potential nonlinearities in the extent to which the continuous composite item complexity measure moderated the relationship between cognitive ability and response error scores. To this end, we entered the composite complexity measure as a categorical (rather than continuous) predictor in the moderated multilevel regression models. To facilitate model convergence (with multiple correlated random regression slopes for the effects of the complexity categories on response error scores), the categories of the composite complexity measure were entered as five bins, where bin 1 consisted of complexity scores 0–1, bin 2 of scores 2–3, bin 3 of scores 4–5, bin 4 of scores 6–7, and bin 5 of scores 8–9. Compared to the relationship (regression slope) between cognitive ability and response error scores for the bin with the lowest item complexity (i.e., bin 1), the relationships increased by 64% (for bin 2), 207% (bin 3), 232% (bin 4), and 323% (bin 5) in the Wave 8 sample, and by 65% (bin 2), 96% (bin 3), 122% (bin 4), and 198% (bin 5) in the Wave 9 sample, suggesting approximately linear increases across item complexity bins.
Results for the secondary moderated multilevel regression analyses conducted for each of the individual indicators of item complexity are also shown in Tables 5 and 6 . In both samples, the cognitive ability by item complexity interaction term was significant ( p < .001) for 9 of 10 individual indicators in the expected direction. The numerically strongest moderation effects were evident for items containing 10 or more words, items containing negations, and items containing discrepancies, the presence of which increased the association (regression slope) between cognitive ability and response error scores by 118%, 103%, and 94% in the Wave 8 sample and by 77%, 85%, and 61% in the Wave 9 sample. The exception was the index of unfamiliar technical terms, which did not significantly moderate the relationship between cognitive ability and response errors ( p = .75 for Wave 8 and p = .87 for Wave 9 sample, respectively).
Given that the 102 PLQ items examined were included in 21 scales that may differ in overall item complexity, we also explored the correlations between cognitive ability scores and people’s average response errors on a scale-by-scale basis. The mean item composite complexities ranged from 0.00 to 6.33 across the 21 scales. The correlations between the cognitive ability scores and people’s average response errors ranged from r = −.228 to r = .115 across scales in Wave 8, and from r = −.257 to r = .137 in Wave 9. Differences in the mean item complexity between scales were significantly negatively associated with the magnitude of correlations between cognitive ability and response errors for corresponding scales, r = −.500 (95% CI = −.759 to −.070) for Wave 8 and r = −.478 (95% CI = −.748 to −.046) for Wave 9, respectively, indicating that response errors in scales comprising more complex items showed a stronger negative relationship with cognitive ability than response errors in scales with less complex items (see Figure S1 in the online appendix).
The final analyses examined the correlations between people’s cognitive ability scores and latent variables representing individual differences in the magnitude of response errors for less versus more complex questions. As shown in Fig. 3 (left panel), the correlations between the cognitive ability sum score and latent response errors at low composite item complexity of 0 were r = −.115 (95% CI = −.142 to −.087; Wave 8 sample) and r = −.145 (95% CI = −.173 to −.118; Wave 9 sample). By contrast, the correlations between the cognitive ability sum score and latent response errors at high item complexity of 9 (middle panel in Fig. 3 ) were r = −.391 (95% CI = −.419 to −.367; Wave 8 sample) and r = −.374 (95% CI = −.403 to −.345; Wave 9 sample). Footnote 2
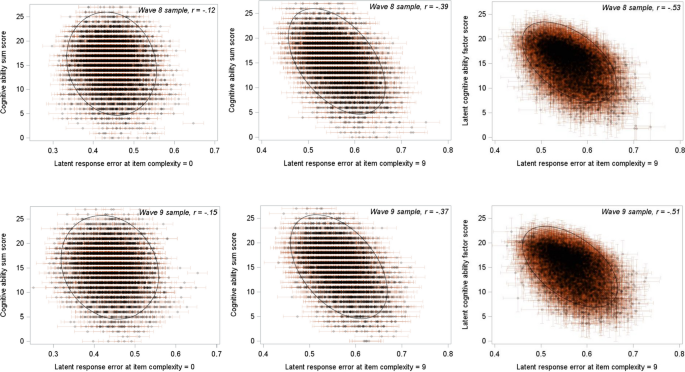
Scatter plots of the relationships between latent response errors at varying item complexity levels and cognitive ability scores in the Wave 8 (upper panel) and Wave 9 (lower panel) samples. Latent response errors are shown for item complexity levels of 0 (left panel) and 9 (middle and right panel). Cognitive ability scores are manifest sum scores (left and middle panel) and latent factor scores (right panel). Error bars represent standard errors for the factor scores of latent variables
The correlations between a latent cognitive ability factor and latent response errors were also estimated. A cognitive ability factor comprising the four cognitive subtests demonstrated adequate model fit in the Wave 8 sample (goodness of fit χ 2 [ df = 1] = 1.14, p = .28; CFI = 1.0, TLI = 1.0, RMSEA = .004, SRMR = .002) and in the Wave 9 sample (χ 2 [ df = 1] = 0.01, p = .91; CFI = 1.0, TLI = 1.0, RMSEA = .001, SRMR = .001). The correlations between the latent cognitive factor and latent response errors at low item complexity of 0 were r = −.154 (95% CI = −.192 to −.116; Wave 8 sample) and r = −.199 (95% CI = −.236 to −.163; Wave 9 sample). By contrast, the correlations between the latent cognitive factor and latent response errors at high item complexity of 9 were r = −.532 (95% CI = −.571 to −.493; Wave 8 sample) and r = −.513 (95% CI = −.554 to −.473; Wave 9 sample); see Fig. 3 (right panel). Footnote 3
Replication in subsequent HRS waves
The replication analyses involved repeated assessments of the same questionnaires in subsequent HRS waves. This allowed us to examine the long-term (4- and 8-year) retest correlations of the response error scores derived from the same samples. The mean retest correlation of respondents’ average response error scores across items was r = .625 (range = .607 to .646) for 4-year and r = .546 (range = .525 to .567) for 8-year intervals between assessment waves, respectively. Table S2 in the online appendix shows retest correlations involving the mean response error scores in each of the decile bins used for the analyses of the WPR as well as the response error scores estimated for less versus more complex questions; response errors in lower deciles showed somewhat lower retest correlations compared to those in the highest deciles (decile 1: mean r = .480 for 4-year and r = .417 for 8-year intervals; decile 10: mean r = .580 for 4-year and r = .505 for 8-year intervals).
Analyses of estimated response error scores and their relationships with cognitive ability scores in subsequent waves of HRS data yielded results that were almost identical to those in the Wave 8 and 9 samples and closely replicated the expected patterns based on the WPR and TCH. Details are shown in the online appendix (Tables S3 – S7 ).
The idea that people’s patterns of performance across a series of trials of cognitively demanding tasks can reveal important aspects of their cognitive abilities has a long history and has been extensively pursued in research on reaction time tasks (Kyllonen & Zu, 2016 ; Schmiedek et al., 2007 ). The WPR and TCH are widely replicated in reaction time research and have been regarded as pointing to universal basic mental processes underlying individual differences in cognitive ability (Jensen, 2006 ; Schubert, 2019 ). The present study results provide robust support for the hypothesis that predictions by the WPR and TCH translate to patterns of response errors derived from questionnaires, highlighting that low-quality survey response patterns should not routinely be viewed as a lack of respondent effort for the task, but instead suggesting the possibility that people’s questionnaire response patterns may serve as an indirect indicator of cognitive ability in survey research.
As predicted by the WPR, when response error scores estimated from the 102 PLQ item responses were divided into decile bins based on each person’s distribution of error scores, we found the strongest associations of cognitive ability with a person’s largest response errors, whereas a person’s smallest response errors were virtually uncorrelated with cognitive ability. This is in line with the idea that the largest response error scores estimated from a GRM represent an individual’s worst performance on a questionnaire and that these error scores reveal more about cognitive ability than do other portions of the response error distribution. The largest response errors (worst performance) showed correlations with cognitive ability ranging from r = −.33 to r = −.35; an intriguing observation is that these correlations are nearly identical in magnitude to those obtained in a recent meta-analysis of the WPR in reaction time studies (Schubert, 2019 ), where general cognitive ability showed an overall correlation of r = −.33 with people’s slowest responses, suggesting close convergence across diverse task domains (response errors in questionnaires versus reaction times). Additionally, response error scores in the higher decile bins showed somewhat greater long-term retest reliability than response error scores in lower decile bins, suggesting that people’s worst performance on questionnaires is temporally more stable than their average or best performance.
To date, the exact psychological, cognitive, or biological processes underlying the WPR are still speculative. Conceptual frameworks that have been proposed in the reaction time literature include the attentional control account and the drift–diffusion model account of the WPR (see Coyle, 2003 ; Schmiedek et al., 2007 ; Schubert, 2019 ). According to the attentional control account, the WPR can be attributed to attentional variability and occasional lapses in sustained attention. Attentional lapses disrupt the representation and maintenance of task-relevant information in working memory, and they are thought to occur more frequently and to be more pronounced in people with lower cognitive abilities (Jensen, 1992 ; Larson & Alderton, 1990 ; Welhaf et al., 2020 ). The drift–diffusion model is a mathematical model for two-choice decision-making processes (Ratcliff & Rouder, 1998 ). One essential parameter in the diffusion model is the “drift rate” parameter, which captures the rate at which individuals accumulate information necessary for decision-making, and which has been assumed to reflect individual differences in general information-processing efficiency (Schmiedek et al., 2007 ). Consistent with the WPR, studies have shown that the drift rate strongly affects the shape of the distribution of an individual’s response times, in that it impacts the worst performance (longest reaction times) more than average or best performance (Ratcliff et al., 2008 ).
To what extent the same mechanisms underlying the WPR for reaction times on elementary cognitive tasks translate to response errors in questionnaires is currently unknown. However, Coyle ( 2001 ) found that the WPR applies to measures of performance accuracy in a strategic memory task, extending beyond reaction times in elementary cognitive tasks. Coyle ( 2001 ) suggested that neural transmission errors may result in general cognitive slowing and occasional cognitive disruptions, resulting in more pronounced dips in task performance. In the context of a person completing a questionnaire, deficits in attentional control and/or information-processing efficiency may similarly lead to more pronounced fluctuations in response quality and a higher rate of responses that deviate substantially from the statistically expected response.
Our findings also showed robust support for the predictions of the TCH, with high consistency across the analyzed samples and measurement waves. Response error scores for questions that were coded as overall more complex were much more strongly associated with cognitive ability than those for less complex questionnaire items. This finding corresponds with the idea that more complex items or tasks with a greater information load require more cognitive effort and are more likely to strain a person’s working memory, such that they have greater potential to clearly differentiate between individuals with higher and lower cognitive ability (Jensen, 2006 ). In secondary analyses that examined each aspect of item complexity individually, nearly all individual item complexity indicators showed the same pattern of results (with variation in the magnitude of effects) while being moderately correlated with each other, suggesting that the individual indicators tapped partially overlapping and partially complementary aspects of complexity that may contribute to the overall information load associated with each item.
There are limits to the expectation that the association between people’s cognitive ability and their performance steadily increases for progressively more complex tasks. That is, at some level of complexity, there necessarily is a turning point after which further complexity increases yield lower associations with cognitive ability as the cognitive load of the task approaches or exceeds the capacities of many individuals to perform well (see Lindley et al., 1995 ). Our sensitivity analyses did not yield evidence of such a “turning point” but rather suggested approximately linear increases in the association between cognitive ability and response error scores for increasing item complexity levels. To what extent this finding generalizes to other surveys beyond those in the present study is an open question. It is possible that the rating scale items examined in this study fell within a relatively narrow complexity range, or that the information load in most self-report questions is generally at a level low enough that this turning point is rarely reached or exceeded.
The present evidence has direct implications for researchers interested in obtaining an indirect indicator of participants’ cognitive abilities from self-report surveys. To extract information that is indicative of people’s cognitive ability level, one strategy is to select each person’s largest response error scores (i.e., their worst performance), or to extract response error scores specifically from selected items or scales with a higher average item complexity level. Another strategy is to estimate individual differences in response errors that are predicted for a relatively high item complexity level using the multilevel modeling procedures outlined above (see Eq. 7a and 7b ) and based on all items administered. We think that the latter strategy may be preferrable because it does not discard items and because it statistically controls for the heterogeneity in complexity levels across items. A working example with step-by-step instructions and annotated software code is available at https://osf.io/vja3t/ for readers who wish to apply the required procedures to their own data. Our results showed that a latent variable of response errors estimated for the most complex PLQ items correlated at about .50 with a latent cognitive ability factor, suggesting that this strategy yields an individual differences measure that shares about 25% of the variance with people’s true cognitive ability.
Limitations and directions for future research
This study has several limitations that should be noted. First, even though we found robust effects supporting the WPR and TCH that were replicated in two independent samples and across multiple waves of a large longitudinal aging study, the two samples were drawn from the same population of older adults in the United States who participated in the HRS, and the same questionnaire items were administered to all respondents. Additional research is required to examine whether the current findings generalize to younger respondent samples and to surveys with different self-report questions.
Second, although the use of IRT provided a theoretically sound basis for assessing the quality of individual item responses, the method rests on the assumption that the model fits the data well and that the IRT model parameters are themselves not excessively biased by overall low response quality. We applied a simple unidimensional GRM to all 21 PLQ scales, which yielded a well-fitting model for many scales but also showed expected variation in model fit statistics as the PLQ scales were not originally developed using IRT principles. To improve the estimation of response error scores from IRT models, future research could consider the utility of multidimensional IRT models fitted to the items of multiple scales simultaneously, as well as iterative scale purification procedures that have been shown to reduce potential bias in the estimation of GRM item parameters when some respondents have overall low response quality (Hong & Cheng, 2019 ; Qiu et al., 2024 ). Moreover, our IRT modeling strategy implicitly assumed that the same measurement model used to assess the constructs underlying the PLQ scales holds equally well for all people. In fact, individuals or participant subgroups may use different implicit theories about the constructs assessed or may interpret specific items on a scale differently. This may have confounded the measurement of response error scores with actual differences in the way people’s true scores on a construct related to the probability of their responses. Tests for measurement invariance and adjustments for differential item functioning (Zumbo, 2007 ) applied to each scale before estimating response error scores could be used to reduce this potential confound.
Third, the cognitive measures in the HRS were designed with a focus on the detection of cognitive deficits at older ages (Crimmins et al., 2016 ). Even though it has been acknowledged that there is no universally optimal way to define the constituents of general cognitive ability or intelligence (van der Maas et al., 2014 ), the specific composition of cognitive subtests in the HRS may have impacted the results. In future research, it will be important to replicate the present findings with a different composition of cognitive tests to evaluate the generalizability of the present results. Moreover, it will be beneficial to examine data from studies that assess a broader range of cognitive domains than that available in the HRS data analyzed here to better understand whether specific cognitive functions are more closely related to response errors in questionnaires than other cognitive functions.
Fourth, even though we included multiple indicators of item complexity in our analysis, they were not without limitations. We considered only aspects of the questions themselves and did not code the complexity of the response scales, because the items in the PLQ consistently used ordinal response scales with little variation in response format and number of response options. We also relied exclusively on complexity indicators that can be automatically derived from text analysis software (QUAID, Graesser et al., 2000 ; LIWC, Pennebaker et al., 2015 ). Potentially important aspects of information load that require judgment by human coders (e.g., to what extent answering a question involves retrieval of information from memory) were not included, as these have been shown to suffer from low inter-coder reliability (Bais et al., 2019 ). More work is required to develop an optimal (composite) measure of question complexity.
Fifth, the present study was limited to questionnaires administered in paper-and-pencil format. Self-report surveys are increasingly administered electronically over the internet, and the results should be replicated with responses from web-administered surveys. Web-based data collection also provides access to additional sources of survey response behaviors that were not considered here, including item response latencies recorded passively as paradata alongside the actual item responses, which have previously proven useful as indicators of people’s cognitive ability (Junghaenel et al., 2023 ; Schneider, Junghaenel et al., 2023b ).
Finally, our analyses did not consider changes in response errors over the course of the questions within a survey and across multiple repeated assessment waves. Within a given survey, respondents with lower cognitive ability may be especially prone to lapses in concentration (with a possible increase in the likelihood of larger response errors) toward the end of the survey and after having expended potentially significant amounts of cognitive effort on previous survey questions (see Bowling et al., 2021 ). Across multiple assessment waves, participants are repeatedly exposed to the same questions, which may increase response quality (a possible decrease in response errors) due to practice effects and increasing familiarity with the questions (Kartsounidou et al., 2023 ), and the extent to which people benefit from practice may itself be a marker of individual differences in cognitive ability (Jensen, 2006 ; Jutten et al., 2020 ; Minear et al., 2018 ). Examining these dynamics is an interesting avenue for future research in that this could facilitate the development of strategies to further augment the usefulness of questionnaire response patterns as indirect cognitive ability indicators.
Conclusions
The present study results support the idea that response patterns in questionnaires reveal meaningful information about individual differences in general cognitive ability and provide new strategies for developing indirect indicators of questionnaire response quality that are most closely associated with people’s cognitive ability levels. Even though indirect performance indicators derived from survey response patterns are not a surrogate for formal cognitive tests, and should not be viewed as such, our results suggest that they might supplement cognitive test scores or serve as a rough indicator to examine group differences in cognitive ability in survey studies that have no cognitive test data available.
The percentage differences in the relationship (regression slope) between cognitive ability and response error scores per point increase in item complexity were computed as 100 * (interaction term / regression slope of cognitive ability for an item complexity score of 0).
We also estimated the correlations between the cognitive ability sum score and the latent response errors for other item complexity levels by centering the continuous item complexity predictor variable at intermediate levels in the multilevel model. The resulting correlations for integer item complexity scores of 1 to 8 were −.155, −.197, −.240, −.281, −.317, −.346, −.368, −.382 (Wave 8 sample) and −.182, −.220, −.259, −.296, −.327, −.350, −.365, −.371 (Wave 9 sample), respectively.
Correlations for integer item complexity scores of 1 to 8 were −.209, −.267, −.326, −.381, −.430, −.470, −.500, −.520 (Wave 8 sample) and −.249, −.302, −.356, −.406, −.448, −.480, −.501, −.511 (Wave 9 sample), respectively.
Bais, F., Schouten, B., Lugtig, P., Toepoel, V., Arends-Tòth, J., Douhou, S., ..., Vis, C. (2019). Can survey item characteristics relevant to measurement error be coded reliably? a case study on 11 dutch general population surveys. Sociological Methods & Research, 48 , 263–295.
Bowling, N. A., Gibson, A. M., Houpt, J. W., & Brower, C. K. (2021). Will the questions ever end? Person-level increases in careless responding during questionnaire completion. Organizational Research Methods, 24 , 718–738.
Article Google Scholar
Chall, J. S., & Dale, E. (1995). Readability revisited: The new dale-chall readability formula . Brookline Books.
Google Scholar
Chalmers, R. P. (2012). mirt: A multidimensional item response theory package for the R environment. Journal of statistical Software, 48 , 1–29.
Colsher, P. L., & Wallace, R. B. (1989). Data quality and age: Health and psychobehavioral correlates of item nonresponse and inconsistent responses. Journal of Gerontology, 44 , P45–P52.
Article PubMed Google Scholar
Conijn, J. M., van der Ark, L. A., & Spinhoven, P. (2020). Satisficing in mental health care patients: The effect of cognitive symptoms on self-report data quality. Assessment, 27 , 178–193.
Coyle, T. R. (2001). IQ is related to the worst performance rule in a memory task involving children. Intelligence, 29 , 117–129.
Coyle, T. R. (2003). A review of the worst performance rule: Evidence, theory, and alternative hypotheses. Intelligence, 31 , 567–587.
Crimmins, E. M., Saito, Y., & Kim, J. K. (2016). Change in cognitively healthy and cognitively impaired life expectancy in the United States 2000–2010. SSM - Population Health, 2 , 793–797.
Article PubMed PubMed Central Google Scholar
Graesser, A. C., Wiemer-Hastings, K., Kreuz, R., Wiemer-Hastings, P., & Marquis, K. (2000). QUAID: A questionnaire evaluation aid for survey methodologists. Behavior Research Methods, Instruments, & Computers, 32 , 254–262.
Graesser, A. C., Cai, Z., Louwerse, M. M., & Daniel, F. (2006). Question Understanding Aid (QUAID) a web facility that tests question comprehensibility. Public Opinion Quarterly, 70 , 3–22.
Green, S. B., & Yang, Y. (2009). Reliability of summed item scores using structural equation modeling: An alternative to coefficient alpha. Psychometrika, 74 , 155–167.
Groth-Marnat, G. (2003). Handbook of psychological assessment . John Wiley & Sons.
Hallquist, M. N., & Wiley, J. F. (2018). MplusAutomation: An R package for facilitating large-scale latent variable analyses in M plus. Structural Equation Modeling: A Multidisciplinary Journal, 25 , 621–638.
Hong, M. R., & Cheng, Y. (2019). Robust maximum marginal likelihood (RMML) estimation for item response theory models. Behavior Research Methods, 51 , 573–588.
Jensen, A. R. (1992). The importance of intraindividual variation in reaction time. Personality and Individual Differences, 13 , 869–881.
Jensen, A. R. (2006). Clocking the mind: Mental chronometry and individual differences . Elsevier.
Jokela, M. (2022). Why is cognitive ability associated with psychological distress and wellbeing? Exploring psychological, biological, and social mechanisms. Personality and Individual Differences, 192 , 111592.
Junghaenel, D. U., Schneider, S., Orriens, B., Jin, H., Lee, P.-J., Kapteyn, A., ..., Stone, A. A. (2023). Inferring Cognitive Abilities from Response Times to Web-Administered Survey Items in a Population-Representative Sample. Journal of Intelligence, 11 , 3.
Juster, F. T., & Suzman, R. (1995). An overview of the Health and Retirement Study. Journal of Human Resources, 30 , S7–S56.
Jutten, R. J., Grandoit, E., Foldi, N. S., Sikkes, S. A., Jones, R. N., Choi, S. E., ..., Tommet, D. (2020). Lower practice effects as a marker of cognitive performance and dementia risk: a literature review. Alzheimer's & Dementia: Diagnosis, Assessment & Disease Monitoring, 12 , e12055.
Kartsounidou, E., Kluge, R., Silber, H., & Gummer, T. (2023). Survey experience and its positive impact on response behavior in longitudinal surveys: Evidence from the probability-based GESIS Panel. International Journal of Social Research Methodology . https://doi.org/10.1080/13645579.2022.2163104
Knäuper, B., Belli, R. F., Hill, D. H., & Herzog, A. R. (1997). Question difficulty and respondents’ cognitive ability: The effect on data quality. Journal of Official Statistics, 13 , 181–199.
Kranzler, J. H., Whang, P. A., & Jensen, A. R. (1994). Task complexity and the speed and efficiency of elemental information processing: Another look at the nature of intellectual giftedness. Contemporary Educational Psychology, 19 , 447–459.
Krosnick, J. A. (1991). Response strategies for coping with the cognitive demands of attitude measures in surveys. Applied Cognitive Psychology, 5 , 213–236.
Kyllonen, P. C., & Zu, J. (2016). Use of response time for measuring cognitive ability. Journal of Intelligence, 4 , 14.
Larson, G. E., & Alderton, D. L. (1990). Reaction time variability and intelligence: A “worst performance” analysis of individual differences. Intelligence, 14 , 309–325.
Larson, G. E., Merritt, C. R., & Williams, S. E. (1988). Information processing and intelligence: Some implications of task complexity. Intelligence, 12 , 131–147.
Lechner, C. M., & Rammstedt, B. (2015). Cognitive ability, acquiescence, and the structure of personality in a sample of older adults. Psychological Assessment, 27 , 1301–1311.
Lindley, R. H., Wilson, S. M., Smith, W. R., & Bathurst, K. (1995). Reaction time (RT) and IQ: Shape of the task complexity function. Personality and individual Differences, 18 , 339–345.
Llewellyn, D. J., Lang, I. A., Langa, K. M., & Huppert, F. A. (2008). Cognitive function and psychological well-being: Findings from a population-based cohort. Age and ageing, 37 , 685–689.
McCammon, R. J., Fisher, G. G., Hassan, H., Faul, J. D., Rogers, W., & Weir, D. R. (2019). Health and retirement study imputation of cognitive functioning measures: 1992–2016 . University of Michigan.
Minear, M., Coane, J. H., Boland, S. C., Cooney, L. H., & Albat, M. (2018). The benefits of retrieval practice depend on item difficulty and intelligence. Journal of Experimental Psychology: Learning, Memory, and Cognition, 44 , 1474.
PubMed Google Scholar
Muthén, L. K., & Muthén, B. O. (2017). Mplus: Statistical Analysis with Latent Variables: User’s Guide (Version 8) . Muthén & Muthén.
Ofstedal, M. B., Fisher, G. G., & Herzog, A. R. (2005). Documentation of cognitive function measures in the health and retirement study . University of Michigan.
Book Google Scholar
Pennebaker, J. W., Boyd, R. L., Jordan, K., & Blackburn, K. (2015). The development and psychometric properties of LIWC2015 . University of Texas.
Qiu, X., Huang, S.-Y., Wang, W.-C., & Wang, Y.-G. (2024). An iterative scale purification procedure on lz for the detection of aberrant responses. Multivariate Behavioral Research, 59 , 62–77.
Ratcliff, R., & Rouder, J. N. (1998). Modeling response times for two-choice decisions. Psychological Science, 9 , 347–356.
Ratcliff, R., Schmiedek, F., & McKoon, G. (2008). A diffusion model explanation of the worst performance rule for reaction time and IQ. Intelligence, 36 , 10–17.
Samejima, F. (1969). Estimation of latent ability using a response pattern of graded scores. Psychometrika, 34 , 100–114.
Schmiedek, F., Oberauer, K., Wilhelm, O., Süß, H.-M., & Wittmann, W. W. (2007). Individual differences in components of reaction time distributions and their relations to working memory and intelligence. Journal of Experimental Psychology: General, 136 , 414–429.
Schneider, S. (2018). Extracting response style bias from measures of positive and negative affect in aging research. The Journals of Gerontology: Series B, 73 , 64–74.
Schneider, S., Junghaenel, D. U., Zelinski, E. M., Meijer, E., Stone, A. A., Langa, K. M., & Kapteyn, A. (2021). Subtle mistakes in self-report surveys predict future transition to dementia. Alzheimer’s and Dementia: Diagnosis, Assessment and Disease Monitoring, 13 , e12252.
PubMed PubMed Central Google Scholar
Schneider, S., Junghaenel, D. U., Meijer, E., Zelinski, E. M., Jin, H., Lee, P.-J., & Stone, A. A. (2022). Quality of survey responses at older ages predicts cognitive decline and mortality risk. Innovation in Aging, 6 , igac27.
Schneider, S., Jin, H., Orriens, B., Junghaenel, D. U., Kapteyn, A., Meijer, E., & Stone, A. A. (2023a). Using attributes of survey items to predict response times may benefit survey research. Field Methods, 35 , 87–99.
Schneider, S., Junghaenel, D. U., Meijer, E., Stone, A. A., Orriens, B., Jin, H., ..., Kapteyn, A. (2023b). Using item response times in online questionnaires to detect mild cognitive impairment. The Journals of Gerontology: Series B, 78 , 1278–1283.
Schubert, A.-L. (2019). A meta-analysis of the worst performance rule. Intelligence, 73 , 88–100.
Singer, J., & Willett, J. B. (2003). Applied longitudinal data analysis: Modeling change and event occurence . Oxford University Press.
Smith, J., Fisher, G. G., Ryan, L., Clarke, P., House, J., & Weir, D. (2013). Health and retirement study psychosocial and lifestyle questionnaire 2006–2010: Documentation report . University of Michigan.
Sonnega, A., Faul, J. D., Ofstedal, M. B., Langa, K. M., Phillips, J. W., & Weir, D. R. (2014). Cohort profile: The health and retirement study (HRS). International Journal of Epidemiology, 43 , 576–585.
Stankov, L. (2000). Complexity, metacognition, and fluid intelligence. Intelligence, 28 , 121–143.
Sternberg, R. J. (1979). The nature of mental abilities. American Psychologist, 34 , 214–230.
Tourangeau, R. (1984). Cognitive science and survey methods: a cognitive perspective. In T. Jabine, M. Straf, J. Tanur, & R. Tourangeau (Eds.), Cognitive aspects of survey design: Building a bridge between disciplines (pp. 73–100). National Academy Press.
Tourangeau, R. (2018). The survey response process from a cognitive viewpoint. Quality Assurance in Education, 26 , 169–181.
Van der Maas, H. L., Kan, K.-J., & Borsboom, D. (2014). Intelligence is what the intelligence test measures. Seriously. Journal of Intelligence, 2 , 12–15.
Ward, M., & Meade, A. W. (2023). Dealing with careless responding in survey data: Prevention, identification, and recommended best practices. Annual review of psychology, 74 , 577–596.
Welhaf, M. S., Smeekens, B. A., Meier, M. E., Silvia, P. J., Kwapil, T. R., & Kane, M. J. (2020). The worst performance rule, or the not-best performance rule? Latent-variable analyses of working memory capacity, mind-wandering propensity, and reaction time. Journal of Intelligence, 8 , 25.
Yan, T., & Tourangeau, R. (2008). Fast times and easy questions: The effects of age, experience and question complexity on web survey response times. Applied Cognitive Psychology: The Official Journal of the Society for Applied Research in Memory and Cognition, 22 , 51–68.
Zumbo, B. D. (2007). Three generations of DIF analyses: Considering where it has been, where it is now, and where it is going. Language assessment quarterly, 4 , 223–233.
Download references
Open access funding provided by SCELC, Statewide California Electronic Library Consortium This work was supported by a grant from the National Institute on Aging (R01AG068190). The Health and Retirement Study is funded by the National Institute on Aging (U01 AG009740) and the Social Security Administration, and performed at the Institute for Social Research, University of Michigan.
Author information
Authors and affiliations.
Dornsife Center for Self-Report Science, and Center for Economic & Social Research, University of Southern California, 635 Downey Way, Los Angeles, CA, 90089-3332, USA
Stefan Schneider, Raymond Hernandez, Doerte U. Junghaenel, Pey-Jiuan Lee & Arthur A. Stone
Department of Psychology, University of Southern California, Los Angeles, CA, USA
Stefan Schneider, Doerte U. Junghaenel & Arthur A. Stone
Leonard Davis School of Gerontology, University of Southern California, Los Angeles, CA, USA
Stefan Schneider & Doerte U. Junghaenel
School of Health Sciences, Faculty of Health and Medical Sciences, University of Surrey, Guildford, UK
Haomiao Jin, Hongxin Gao & Danny Maupin
Center for Economic and Social Research, University of Southern California, Los Angeles, CA, USA
Bart Orriens & Erik Meijer
You can also search for this author in PubMed Google Scholar
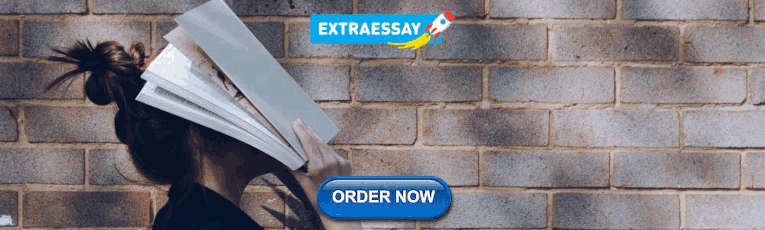
Corresponding author
Correspondence to Stefan Schneider .
Ethics declarations
Financial interests.
A.A. Stone is a Senior Scientist with the Gallup Organization and a consultant with HRA Pharma, IQVIA, and Adelphi Values, Inc. The remaining authors declared that there were no conflicts of interest with respect to the authorship or the publication of this article.
Ethics approval
Approval was obtained from the Institutional Review Board of the University of Southern California. The procedures used in this study adhere to the tenets of the Declaration of Helsinki.
Consent to participate
Informed consent was obtained from all individual participants included in the study.
Additional information
Publisher's note.
Springer Nature remains neutral with regard to jurisdictional claims in published maps and institutional affiliations.
Open Practices Statement
The data used in this study are freely available from the Health and Retirement Study website https://hrs.isr.umich.edu for registered users. For readers who wish to apply the proposed analyses to their own data, a full working example including step-by-step instructions and annotated software code is available at https://osf.io/vja3t/ . The study was not preregistered.
Supplementary Information
Below is the link to the electronic supplementary material.
Supplementary file1 (DOCX 56 KB)
Rights and permissions.
Open Access This article is licensed under a Creative Commons Attribution 4.0 International License, which permits use, sharing, adaptation, distribution and reproduction in any medium or format, as long as you give appropriate credit to the original author(s) and the source, provide a link to the Creative Commons licence, and indicate if changes were made. The images or other third party material in this article are included in the article's Creative Commons licence, unless indicated otherwise in a credit line to the material. If material is not included in the article's Creative Commons licence and your intended use is not permitted by statutory regulation or exceeds the permitted use, you will need to obtain permission directly from the copyright holder. To view a copy of this licence, visit http://creativecommons.org/licenses/by/4.0/ .
Reprints and permissions
About this article
Schneider, S., Hernandez, R., Junghaenel, D.U. et al. Can you tell people’s cognitive ability level from their response patterns in questionnaires?. Behav Res (2024). https://doi.org/10.3758/s13428-024-02388-2
Download citation
Accepted : 02 March 2024
Published : 25 March 2024
DOI : https://doi.org/10.3758/s13428-024-02388-2
Share this article
Anyone you share the following link with will be able to read this content:
Sorry, a shareable link is not currently available for this article.
Provided by the Springer Nature SharedIt content-sharing initiative
- Cognitive ability
- Questionnaire responding
- Item response theory
- Task complexity
- Find a journal
- Publish with us
- Track your research
- Open access
- Published: 22 March 2024
Translation and measurement properties of pregnancy and childbirth questionnaire in Iranian postpartum women
- Somayeh Abdolalipour 1 ,
- Shamsi Abbasalizadeh 2 ,
- Sakineh Mohammad-Alizadeh-Charandabi 1 ,
- Fatemeh Abbasalizadeh 2 ,
- Shayesteh Jahanfar 3 ,
- Mohammad Asghari Jafarabadi 4 , 5 , 6 ,
- Kosar Abdollahi 7 &
- Mojgan Mirghafourvadsnd 8
BMC Health Services Research volume 24 , Article number: 365 ( 2024 ) Cite this article
149 Accesses
Metrics details
Perceived care quality and patient satisfaction have been important care quality indicators in recent decades, and healthcare professionals have been influential on women’s childbirth experience. This study investigated the measurement properties of the Persian version of the Pregnancy and Childbirth Questionnaire (PCQ), designed to measure mothers’ satisfaction with the quality of healthcare services provided during pregnancy and childbirth.
This is a cross-sectional methodological study. Instrument translation, face validity, content validity, structural validity, and reliability evaluation were performed to determine the measurement properties of the PCQ’s Persian version. A backward-forward approach was employed for the translation process. Impact scores were selected based on the items’ importance to measure face validity. Content validity index (CVI) and content validity ratio (CVR) were calculated to measure content validity, and exploratory and confirmatory factor analyses were used to measure structural validity. The cluster random sampling method was used, resulting in a sample of 250 eligible women referred to the health centers of Tabriz, Iran, who were 4 to 6 weeks after giving birth. Cronbach’s alpha coefficient and Intraclass Correlation Coefficient (ICC) using a test-retest approach were used to determine the questionnaire’s reliability.
The impact scores of all items were above 1.5, which indicates a suitable face validity. The content validity was also favorable (CVR = 0.95, CVI = 0.90). Exploratory factor analysis on 25 items led to the removal of item 2 due to a factor loading of less than 0.3 and the extraction of three factors explaining 65.07% of the variances. The results of the sample adequacy size were significant (< 0.001, and Kaiser-Meyer-Olkin = 0.886). The model’s validity was confirmed based on the confirmatory factor analysis fit indicators (i.e., RMSEA = 0.08, SRMR = 0.09, TLI = 0.91, CFI = 0.93, x 2 /df = 4.65). The tool’s reliability was also confirmed (Cronbach’s alpha = 0.88, and ICC (95% CI) = 0.93 (0.88 to 0.95)).
The validity and reliability of the PCQ’s Persian version were suitable to measure the extent to which Iranian women are satisfied with the quality of prenatal and intrapartum care.
Peer Review reports
Healthcare systems are primarily concerned with delivering effective evidence-based services to meet clients’ clinical/medical needs and their expectations of good quality care [ 1 ]. Therefore, in recent decades, perceived care quality and patient satisfaction have become important indicators of care quality [ 2 ]. Pregnant women often refer to different care providers, which may interfere with personal treatment and continuity of care and negatively affect women’s satisfaction with the care they receive [ 3 ]. Birth attendants’ continuous support during childbirth improves the childbirth experience [ 4 ].
Mother’s satisfaction and childbirth experience are essential factors with significant short-term and long-term consequences on mother and child, such as postpartum depression, post-traumatic stress disorder, breastfeeding ability, and child abuse. Healthcare professionals influence women’s childbirth experience [ 5 ].
Millions of women around the world still fail to access prenatal, intrapartum, and postpartum health services [ 6 ]. Many healthcare problems are due to poor quality of care [ 7 ]. The percentage of perinatal care quality in a study on pregnant women in Iran was as follows: 50.8% inadequate, 16.1% average, 27.7% adequate, and 5.4% excellent [ 8 ]. Therefore, it is necessary to analyze and monitor the quality of the care provided during pregnancy and childbirth.
Assessing the quality of care should be primarily based on the experience of the target group. Many tools are designed to examine women’s satisfaction with the care provided by health systems. For example, the questionnaire Measuring Satisfaction with Maternal and Newborn Health Care Following Childbirth, published in 2011 in the United States, was proposed to measure the mothers’ satisfaction with the postpartum health care provided to mothers and their babies until two months after childbirth [ 9 ].
The Quality of Prenatal Care Questionnaire (QPCQ) was designed in Canada in 2014 to measure the quality of prenatal care 4 to 6 weeks after childbirth [ 10 ]. The pregnancy- and maternity-care patients’ experiences questionnaire (PreMaPEQ) with 16 items and 145 items was designed in 2015 to measure the pregnancy, childbirth, and postpartum care, as well as public health clinics’ care provided to pregnant women in Norway. The questionnaire should be completed about 4 to 12 months after delivery. This relatively long period may affect the results’ accuracy during pregnancy and childbirth due to the memory limitations of the respondents [ 11 ]. In addition, the Measurement of Midwifery Quality Postpartum (MMAY postpartum) questionnaire with 16 items was designed in Germany in 2021 to measure the quality of midwifery care after childbirth from the mothers’ perspective. It measures only the quality of care provided at home until about four months after delivery [ 12 ].
Truijens et al. (2014) designed the Pregnancy and Childbirth Questionnaire (PCQ), which is a valid (good face validity and structural validity) and reliable (high internal consistency) instrument. This questionnaire has 25 items and measures Dutch mothers’ satisfaction with the quality of care provided during pregnancy in health centers and childbirth in hospitals [ 3 ]. The questionnaire should be filled out about 4–6 weeks after childbirth. Eighteen items examine the experiences and perceptions of pregnant women regarding the quality of prenatal care, divided into personal treatment (11 items) and educational information (7 items). The rest (7 items) reflect mothers’ satisfaction with intrapartum care.
The PCQ was prepared for countries where childbirth happens at home and in hospitals. Since the perceived quality of care is a general concept and is not limited to a specific care system, this questionnaire applies to any system. Measuring the quality of healthcare provided to pregnant women during pregnancy and childbirth and responding to their needs and expectations is increasingly essential. Therefore, this study was conducted to investigate the measurement properties of the PCQ as a valid and reliable tool to measure the quality of care provided to pregnant women in Iran during pregnancy and childbirth.
Study design
This cross-sectional methodological study follows five translation stages: content validity, face validity, structural validity, and reliability evaluation to determine the measurement characteristics of the PCQ’s Persian version. The target population includes pregnant women referring to health centers in Tabriz, Iran.
Sample size
The structural validity in factor analysis requires at least 5 to 10 participants per the questionnaire’s items [ 13 ]. This study selects participants per each of the questionnaire’s 25 items, leading to a sample of 125 participants. Considering the design effect of 2 due to cluster sampling, the sample was calculated as 250.
Eligibility criteria
Inclusion criteria include women who had vaginal childbirth in the last 4–6 weeks. The exclusion criteria include underlying diseases such as cardiovascular disease, diabetes, mental disabilities, or other mental disorders, the death of a loved one in the past three months, and the unwillingness to participate in the study.
Sampling and data collection
We used the www.random.org website and randomly selected a quarter of the public health centers of Tabriz City from their list in the SIB system ( https://sib.iums.ac.ir ). The SIB system is an integrated health system and was designed to register, maintain, and update the electronic health record information of Iranians. Also, the type of health care services needed in community health centers are entered and recorded in this system. Then, we identified and called women who had given birth in the last 4 to 6 weeks. The research objectives were explained to them, and they consented to participate in face-to-face meetings in the health center at a given time. In the meetings, participants were informed comprehensively about the research, their written consent was obtained, and the researcher filled out the sociodemographic and obstetric characteristics questionnaire and the PCQ. Because some participants were illiterate or had low educational levels, to ensure the uniformity of the data collection method, the interview method was conducted to obtain the data. All interviews were conducted by a researcher (K.A.). Because only women with vaginal childbirth in the last 4 to 6 weeks were selected from the list in the SIB system (which was the main inclusion criterion for this study), the number of people who were excluded was small. Three people due to gestational hypertension, two people due to s diabetes mellitus, and six people due to unwillingness to participate in the study were not invited for the interview.
The research tools
Sociodemographic and obstetric characteristics questionnaire.
This questionnaire is a researcher-made tool that includes some questions used to describe participants’ characteristics such as age, education, occupation, income, number of pregnancies and deliveries, and participation in childbirth preparation classes. The validity of this questionnaire was measured through qualitative content and face validity.
The PCQ questionnaire
includes 25 items, of which 18 items measure the quality of perinatal care and seven measure the quality of childbirth care. The questionnaire uses a five-point Likert scale from completely agree (1) to completely disagree (5). The PCQ scores can vary from 25 to 125, with higher scores correlated with higher satisfaction levels [ 3 ]. The original version is available as a supplementary file 1 .
The translation processes
After obtaining permission from the initial designers of PCQ, Truijens et al. [ 3 ], the translation process was carried out using a five-step forward and backward translation approach [ 14 ]. First, the questionnaire items were translated separately by at least two translators fluent in Persian and English using semantic translation and a forward-translation approach. Contrary to literal translation, semantic translation transfers the essential meanings to the destination language. In other words, the translated questionnaire’s questions and words should be the same as those of the original. Second, the forward versions were compared by another supervisor translator, the existing contradictions were corrected, and a consolidated version was created from the forward versions. Third, the consolidated version was translated using a backward-translation approach into English by two translators fluent in Persian and English, who were blind to the questionnaires. Fourth, the expert committee, including three to four translators fluent in both languages, revised the forward, consolidated, and backward versions. The committee included one expert in language, one expert in questionnaire translation, one expert familiar with the concepts, and one coordinator. The experts investigated semantic, terminology, experimental, and perceptual equalities. The fifth stage includes the pre-test, where the pre-final version is provided to the target group.
Face validity
Once the questionnaire’s final version was prepared, the face validity determination form was given to 10 women who had a delivery in the last 4–6 weeks. The items were evaluated in terms of difficulty, relevance, and ambiguity by ten eligible women to confirm the questionnaire’s qualitative face validity. In addition, the item impact method using a 5-point Likert scale from unimportant (1) to very important (5) was used to calculate the impact scores and confirm the questionnaire’s quantitative face validity, retaining items with an impact score of greater than 1.5 [ 15 ].
Content validity
The content validity form was given to 10 midwifery and reproductive health specialists to check its content validity. The content validity of the tool was evaluated from a qualitative perspective based on the experts’ opinions on the questionnaire’s overall structure, the items’ contents, Persian grammar, and accurate scoring were received, and the necessary corrections were made. Moreover, the content validity index (CVI) and content validity ratio (CVR) were calculated in the quantitative part. The experts were asked to determine the items’ relevance, clarity, and simplicity using a 4-point Likert scale to calculate the CVI. The CVI varies between 0 and 1 [ 16 ]; items with CVI > 0.79 were kept, items with 0.79 > CVI > 0.70 were revised, and items with CVI < 0.70 were removed. The CVR was performed based on the experts’ opinions about each of the tool’s items using a 3-point Likert scale in terms of the necessity (i.e., necessary, useful but unnecessary, and unnecessary). According to the experts’ opinions and using the Lawshe table, items with CVR > 0.62 were kept, and the rest were removed [ 17 ].
Structural validity
Evaluation of structural validity was done by exploratory factor analysis (EFA) and confirmatory factor analysis (CFA) [ 18 ]. Employing both exploratory and confirmatory factor analyses was rooted in the nature of our study’s theoretical framework. While our initial approach was to test a pre-specified model based on existing theories, we also recognized that the solidity of the underlying theoretical structure could benefit from a more exploratory examination. The exploratory factor analysis allowed us to explore potential underlying structures in an unbiased manner, given the evolving nature of the research domain and the potential for undiscovered dimensions. Subsequently, the confirmatory factor analysis aimed to validate and confirm the proposed model, aligning with established theories as much as possible [ 19 ]. The EFA with Kaiser-Meyer Olkin criteria and Bartlett’s Sphericity test using the principal component analysis method with Varimax rotation (direct noblemen) were used to examine structural validity and extract factors with a factor loading of more than 0.3 [ 20 ]. Moreover, CFA uses the fit indices to check the model fit. The desired fit indices and their acceptable values to confirm the model are as follows: root mean score error of approximation (RMSEA) < 0.08, standardized root mean square residual (SRMR) < 0.10, Baseline vs. Saturated (Chi 2 /df) < 5, comparative fit index (CFI) > 0.90 and Tucker-Lewis Index (TLI) > 0.90 [ 21 ].
Reliability
Test-retest reliability and internal consistency were used to determine the questionnaire’s reliability [ 22 ]. The questionnaire completion process involved two stages separated by a two-week interval, during which 30 eligible participants took part to assess retesting stability. Participants were conveniently sampled, and data for this stage were gathered through in-person interviews. Internal consistency was determined based on Cronbach’s alpha coefficient. An intra-class correlation coefficient (ICC) greater than 0.7 was considered favorable [ 23 ].
Statistical analyses
SPSS Statistics 25 (IBM Corp, Armonk, NY, USA) and STATA 15 (Statcorp, College Station, Texas, USA) were used to analyze data. Descriptive statistics such as frequency (percentage) and mean (standard deviation) were used to describe the sociodemographic and obstetric characteristics data, which were normally distributed. Content validity using CVR and CVI, face validity using the impact score, structural validity using EFA and CFA, and the reliability of the research tool using Cronbach’s alpha coefficient and ICC were examined.
Ethical considerations
The required permissions were first obtained from the Ethics Committee of Tabriz University of Medical Sciences (IR.TBZMED.REC.1401.093). All ethical principles, including obtaining the necessary permission from the initial designers of the tool, obtaining written informed consent from all the participants, ensuring the confidentiality of their information, and the freedom to exit the study, were observed throughout the research.
The cluster sampling method was used, and 250 postpartum women were studied from August to December 2022. The PCQ descriptive characteristics are given in Table 1 .
In investigating the qualitative face validity, all the questionnaire items were described appropriately and without ambiguity or difficulty. All of them scored at least 1.5 when investigating the quantitative face validity. The CVI and CVR were equal to 0.9 and 0.95, respectively, which indicates the acceptability of the content validity. Table 2 presents the results of face and content validities.
Three factors were extracted using the exploratory factor analysis on 25 items of the questionnaire. The first factor, i.e., prenatal care-personal treatment, included 11 items and explained 49.49% of the total variance. The second factor, i.e., prenatal care-educational information, included seven items and explained 10.98% of the total variance, and the third factor, i.e., intrapartum care, included eight items and accounted for 4.59% of the total variance (Table 3 ). Moreover, the second item during the exploratory factor analysis was removed due to its factor loading of less than 0.3, and the number of items was reduced from 25 to 24 (Fig. 1 ).
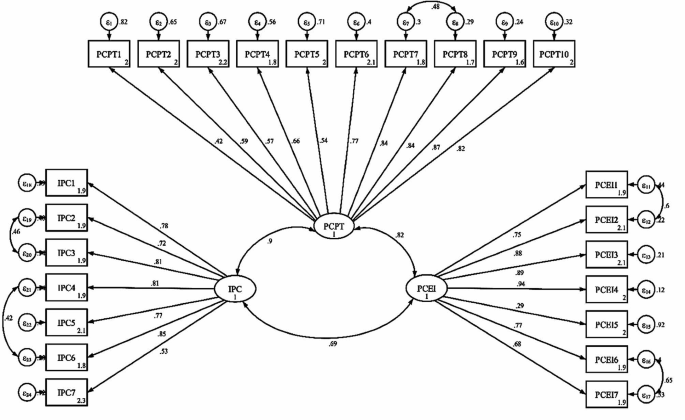
Factor structure model of the PCQ based on CFA. (All factor loadings are significant at P < 0.001). PCPT: Prenatal Care-Personal Treatment; PCEI: Prenatal Care Educational Information; IPC: Intrapartum Care
The KMO (0.886) was appropriate, indicating the adequacy of the sample size. The result of Bartlett’s Test of Sphericity (P˂ 0.001) was significant, indicating acceptable implementation of factor analysis concerning the correlation matrix on the study sample.
The results of CFA confirm a good fit of the model, and the model’s factor structure is confirmed (RMSEA (95% CI) = 0.081 (0.074 to 0.180), SRMR = 0.09, TLI = 0.91, CFI = 0.93, x 2 /df = 4.65, x2_ms = 1137.25, see Table 4 ).
Cronbach’s alpha was 0.88 for the whole tool, 0.78, 0.83, and 0.84 for the subdomains PCPT, PCEI, and IPC, in order. In addition, ICC (95% CI) for the whole tool was 0.93 (0.88 to 0.95) and 0.94 (0.90 to 0.96), 0.89 (0.83 to 0.93), and 0.86 (0.78 to 0.91) for above subdomains (Table 5 ). The final Persian version is available as a supplementary file 2 .
Women’s satisfaction with maternity services, especially care during childbirth and delivery, has become increasingly important to healthcare providers, managers, and policymakers, so increasing satisfaction is suggested to improve healthcare [ 24 ]. Measuring the quality of prenatal and intrapartum care is an essential step to evaluating its effectiveness more completely [ 10 ]. Therefore, appropriate measurement tools are needed to properly assess satisfaction with care during pregnancy and childbirth. This study investigates the measurement characteristics of the PCQ in Iranian women. The validity of this questionnaire was confirmed by face, content, and structural validity, and the reliability was also confirmed by test-retest and internal consistency in Iranian women.
Women who had received prenatal and intrapartum care completed the questionnaire, which is consistent with the growing consumer’s perspective in assessing healthcare quality. There are various maternity satisfaction measures, including single-item measures to extensive surveys of all aspects of maternity care [ 24 – 25 ].
The questionnaire’s items examine aspects such as communication, independence, participation, professionalism, educational information, teamwork, and spouse participation. Women, especially during childbirth, believe that the provided care should not be limited to providing information, but professionals with empathy and personal commitment should also understand their feelings and values [ 3 , 26 ].
The questionnaire’s CVI and CVR were appropriate, and no item was removed. The model adequacy was confirmed using the value obtained for KMO and the significance of Bartlett’s test. Three factors similar to the original version were extracted for this tool, but the second item was removed from the subscale prenatal care-personal treatment. The removed item relates to the wife’s participation in prenatal care, which was removed due to its factor loading of less than 0.3. Moreover, the exploratory factor analysis extracted three factors, explaining 65.07% of the variance, which is higher compared to that of the original tool (56.2%) [ 3 ].
Cronbach’s alpha coefficient for the whole tool and subscales PCPT, PCEI, and IPC was equal to 0.88, 0.78, 0.83, and 0.84, respectively, which were acceptable, but compared to the original tool, with corresponding values of 0.92, 0.89, 0.83 and 0.86, were lower [ 3 ].
Many studies have examined satisfaction with maternal care, but there are a few valid tools with a particular focus on satisfaction during pregnancy and childbirth. The Labor and Delivery Satisfaction Index (LADSI) questionnaire with 38 items is frequently used to measure women’s satisfaction with prenatal and intrapartum care. However, the reliability of the whole tool (α = 0.34) and its subscales (i.e., caring component α = 0.11, and technical component α = 0.78) is low [ 27 ]. The Maternal Satisfaction for Caesarean Section (MSCS) questionnaire with 22 items has good validity and reliability but is limited to women giving birth by cesarean delivery [ 28 ]. Moreover, Intrapartal care concerning WHO recommendations (IC-WHO) questionnaire with 63 items was proposed to measure the quality of care during childbirth based on WHO recommendations [ 29 ]. Compared to other questionnaires, this tool focuses on women’s understanding of the care’s safety factor, and measurement is based on this. Intrapartal-Specific QPP-questionnaire (QPP-I) with 32 items asks women to evaluate the provided care during childbirth in terms of the perceived reality and the subjective importance of care [ 30 ]. This tool has good content and structure validity, but its reliability is reported to be low in some subscales (i.e., perceived reality subscales α range = 0.50 to 0.92; and subjective importance subscales α range = 0.49 to 0.93).
The pregnancy and maternity care patients’ experiences questionnaire (PreMaPEQ) is very comprehensive and measures women’s experiences during pregnancy, childbirth, and postpartum, as well as the care provided in public health clinics. Its content and structure validity are suitable, but its reliability in three scales was less than 0.7 [ 11 ]. This questionnaire has 145 items, which answers to them may be difficult for mothers. Moreover, the questionnaire is provided to women for completion about 4 to 12 months after childbirth, which limits its results’ accuracy due to possible memory limitations of mothers about their pregnancy and childbirth experiences. Some indications suggest that surveying time to measure satisfaction affects satisfaction ratings. For example, satisfaction with care can change even over a short period [ 24 ]. Moreover, women’s satisfaction at hospitals can significantly vary from those after discharge. Assessing satisfaction with childbirth at a certain time after childbirth seems to be more appropriate because mothers have enough time to review their experience and determine their satisfaction. However, the long periods may result in some biases in answering the questions. The PCQ is given to women about 4 to 6 weeks after childbirth, which seems a suitable time to measure mothers’ satisfaction with the quality of care during pregnancy and delivery.
The research strengths and limitations
This study has some strengths including using the same data collection method (interview), a random selection of women from the health centers of Tabriz city with different socio-economic characteristics, and conducting interviews in the same time range (4 to 6 weeks) after childbirth. The studied women had only vaginal delivery and other childbirth types such as cesarean delivery were not included. Future research is suggested to apply this tool to women with cesarean delivery. Moreover, another research weakness was using the same set of data for exploratory and confirmatory factor analyses.
The results confirmed the validity and reliability of the PCQ’s Persian version to measure the satisfaction level with the quality of prenatal and intrapartum care among Iranian women. Face, content, and structural validity and calculation of internal consistency and intra-class correlation coefficient were done for the assessment of measurement properties. This tool helps specialists and medical staff to evaluate the quality of care provided during pregnancy and childbirth from the women’s perspective and, if necessary, carry out the necessary interventions to improve the quality of the care.
Data availability
The datasets generated and/or analyzed during the current study are not publicly available due to the limitations of ethical approval involving the patient data and anonymity but are available from the corresponding author upon reasonable requests.
Abbreviations
Pregnancy and childbirth questionnaire
Prenatal care-personal treatment
Prenatal care educational information
- Intrapartum care
Exploratory factor analysis
Confirmatory factor analysis
Intra-class Correlation Coefficient
Standard deviation
Content validity index
Content validity ratio
Degree of freedom
Kaiser-Meyer-Olkin
Root mean squared error of approximation
Comparative fit index
Tucker–Lewis index
Kyei-Nimakoh M, Carolan-Olah M, McCann TV. Access barriers to obstetric care at health facilities in sub-saharan Africa—a systematic review. Syst Reviews. 2017;6(1):1–6. https://doi.org/10.1186/s13643-017-0503-x .
Article Google Scholar
Truijens SE, Banga FR, Fransen AF, Pop VJ, van Runnard Heimel PJ, Oei SG. The effect of multi-professional simulation-based obstetric team training on patient-reported quality of care: a pilot study. Simul Healthc. 2015;10(4):210–6. https://doi.org/10.1097/SIH.0000000000000099 .
Article PubMed Google Scholar
Truijens SE, Pommer AM, van Runnard Heimel PJ, Verhoeven CJ, Oei SG, Pop VJ. Development of the pregnancy and Childbirth Questionnaire (PCQ): evaluating quality of care as perceived by women who recently gave birth. Eur J Obstet Gynecol Reprod Biol. 2014;174:35–40. https://doi.org/10.1016/j.ejogrb.2013.11.019 .
Perdok H, Verhoeven CJ, Van Dillen J, Schuitmaker TJ, Hoogendoorn K, Colli J, Schellevis FG, De Jonge A. Continuity of care is an important and distinct aspect of childbirth experience: findings of a survey evaluating experienced continuity of care, experienced quality of care and women’s perception of labor. BMC Pregnancy Childbirth. 2018;18(1):1–9. https://doi.org/10.1186/s12884-017-1615-y .
Lemmens SM, van Montfort P, Meertens LJ, Spaanderman ME, Smits LJ, de Vries RG, Scheepers HC. Perinatal factors related to pregnancy and childbirth satisfaction: a prospective cohort study. J Psychosom Obstet Gynecol. 2021;42(3):181–9. https://doi.org/10.1080/0167482X.2019.1708894 .
Khayat S, Dolatian M, Navidian A, Mahmoodi Z. Factors Affecting Adequacy of Prenatal Care in Suburban women of Southeast Iran: A Cross -sectional study. J Clin Diagn Res. 2018;12(4):QC01–5.
Google Scholar
Bahmani S, Shahoie R, Rahmani K. The quality of prenatal care from the perspective of the service recipients using the Servqual pattern during the COVID-19 pandemic in Sanandaj Comprehensive Health Centers. Nurs Midwife J. 2022;20(4):324–33. (Persian).
Simbar M, Nahidi F, Akbarzadeh A. Assessment of quality of prenatal care in Shahid Beheshti University of Medical Sciences health centers. Payesh J. 2012;11(4):529–44. (Persian).
Camacho FT, Weisman CS, Anderson RT, Hillemeier MM, Schaefer EW, Paul IM. Development and validation of a scale measuring satisfaction with maternal and newborn health care following childbirth. Matern Child Health J. 2012;16(5):997–1007. https://doi.org/10.1007/s10995-011-0823-8 .
Heaman MI, Sword WA, Akhtar-Danesh N, Bradford A, Tough S, Janssen PA, Young DC, Kingston DA, Hutton EK, Helewa ME. Quality of prenatal care questionnaire: instrument development and testing. BMC Pregnancy Childbirth. 2014;14(1):1–6.
Sjetne IS, Iversen HH, Kjøllesdal JG. A questionnaire to measure women’s experiences with pregnancy, birth, and postnatal care: instrument development and assessment following a national survey in Norway. BMC Pregnancy Childbirth. 2015;15(1):1–1. https://doi.org/10.1186/s12884-015-0611-3 .
Peters M, Kolip P, Schäfers R. A questionnaire to measure the quality of midwifery care in the postpartum period from women’s point of view: development and psychometric testing of MMAY postpartum. BMC Pregnancy Childbirth. 2021;21(1):1–0. https://doi.org/10.1186/s12884-021-03857-8 .
Comrey A, Lee H. A first course in factor analysis: psychology press. New York: Taylor and Francis Group; 2013.
Book Google Scholar
Lee WL, Chinna K. The forward-backward and dual-panel translation methods are comparable in producing semantic equivalent versions of a heart quality of life questionnaire. Int J Nurs Pract. 2019;25(1):e12715. https://doi.org/10.1111/ijn.12715 .
Setia MS. Methodology Series Module 9: Designing questionnaires and Clinical Record forms - Part II. Indian J Dermatol. 2017;62(3):258–61. https://doi.org/10.4103/ijd.IJD_200_17 .
Article PubMed PubMed Central Google Scholar
Polit DF, Beck CT, Owen SV. Is the CVI an acceptable indicator of content validity? Appraisal and recommendations. Res Nurs Health. 2007;30(4):459–67. https://doi.org/10.1002/nur.20199 .
Lawshe CH. A quantitative approach to content validity. Pers Psychol. 1975;28(4):563–75.
Mokkink LB, Terwee CB, Knol DL, Stratford PW, Alonso J, Patrick DL, Bouter LM, de Vet HC. The COSMIN checklist for evaluating the methodological quality of studies on measurement properties: a clarification of its content. BMC Med Res Methodol. 2010;10:22. https://doi.org/10.1186/1471-2288-10-22 .
Manapat PD, Anderson SF, Edwards MC. Evaluating avoidable heterogeneity in exploratory factor analysis results. Psychol Methods. 2023 May;11. https://doi.org/10.1037/met0000589 .
Harerimana A, Mtshali NG. Using exploratory and Confirmatory Factor Analysis to understand the role of technology in nursing education. Nurse Educ Today. 2020;92:104490. https://doi.org/10.1016/j.nedt.2020.104490 .
Schreiber J, Nora A, Stage F, Barlow L, King J. Confirmatory factor analyses and structural equations modeling: an introduction and review. J Educ Res. 2006;99(6).
Rousson V, Gasser T, Seifert B. Assessing interarater, interrater and test–retest reliability of continuous measurements. Stat Med. 2002;21(22):3431–46.
Cicchetti DV. Guidelines, criteria, and rules of thumb for evaluating normed and standardized assessment instruments in psychology. Psychol Assess. 1994;6(4):284. https://doi.org/10.1037/1040-3590.6.4.284 .
Sawyer A, Ayers S, Abbott J, Gyte G, Rabe H, Duley L. Measures of satisfaction with care during labor and birth: a comparative review. BMC Pregnancy Childbirth. 2013;13(1):1–0.
Britton J. The assessment of satisfaction with care in the perinatal period. J Psychosom Obstet Gynecol. 2012;33:37–44. https://doi.org/10.3109/0167482X.2012.658464 .
Goberna-Tricas J, Banu´ s-Gime´nez MR, Palacio-Tauste A. Satisfaction with pregnancy and birth services: the quality of maternity care services as experienced by women. Midwifery. 2011;27:231–7. https://doi.org/10.1016/j.midw.2010.10.004 .
Lomas J, Dore S, Enkin M, Mitchell A. The labor and delivery satisfaction index– the development and evaluation of a soft outcome measure. Birth. 1987;14:125–9. https://doi.org/10.1111/j.1523-536X.1987.tb01472.x .
Article CAS PubMed Google Scholar
Morgan PJ, Halpern S, Lo J. The development of a maternal satisfaction scale for cesarean section. Int J Obstet Anesth. 1999;8:165–70. https://doi.org/10.1016/S0959-289X(99)80132-0 .
Sandin-Bojo AK, Larsson BW, Hall-Lord ML. Women’s perception of intrapartal care in relation to WHO recommendations. J Clin Nurs. 2008;17:2993–3003. https://doi.org/10.1111/j.1365-2702.2007.02123.x .
Wilde Larsson B, Larsson G, Kvist LJ, Sandin-Bojo AK. Women’s opinions on intrapartal care: development of a theory-based questionnaire. J Clin Nurs. 2010;19:1748–60. https://doi.org/10.1111/j.1365-2702.2009.03055.x .
Download references
Acknowledgments
We should thank the Vice-Chancellor for Research of Tabriz University of Medical Sciences for their financial support and the invaluable participation of women would be appreciated.
This study was funded by Tabriz University of Medical Sciences (grant number: 69307). The funding source had no role in the design and conduct of the study, and decision to this manuscript writing and submission.
Author information
Authors and affiliations.
Department of Midwifery, Faculty of Nursing and Midwifery, Tabriz University of Medical Sciences, Tabriz, IR, Iran
Somayeh Abdolalipour & Sakineh Mohammad-Alizadeh-Charandabi
Women’s Reproductive Health Research Center, Tabriz University of Medical Sciences, Tabriz, Iran
Shamsi Abbasalizadeh & Fatemeh Abbasalizadeh
Department of Public Health and Community Medicine, Tufts School of Medicine, Boston, USA
Shayesteh Jahanfar
Cabrini Research, Cabrini Health, 3144, Melbourne, VIC, Australia
Mohammad Asghari Jafarabadi
School of Public Health and Preventative Medicine, Faculty of Medicine, Nursing and Health Sciences, Monash University, 3800, Melbourne, VIC, Australia
Road Traffic Injury Research Center, Tabriz University of Medical Sciences, Tabriz, Iran
Students Research Committee, Midwifery Department, Faculty of Nursing and Midwifery, Tabriz University of Medical Sciences, Tabriz, Iran
Kosar Abdollahi
Social determinants of Health Research Center, Tabriz University of Medical Sciences, Tabriz, IR, Iran
Mojgan Mirghafourvadsnd
You can also search for this author in PubMed Google Scholar
Contributions
SA, MM, ShA, SMA, FA, SJ, MAJ and KA contributed to the design of the study. SA and MM has written the first draft of this article and MAJ Analyzed and data. All authors have critically read the text and contributed with inputs and revisions and all authors gave their final approval of this version to be published.
Corresponding author
Correspondence to Mojgan Mirghafourvadsnd .
Ethics declarations
Competing interests.
The authors declare no competing interests.
Ethics approval and consent to participate
The current study was approved by the Ethics Committee of Tabriz University of Medical Sciences [ref: IR.TBZMED.REC.1400.093]. Written Informed consent to participate in the study was obtained from all the participants before enrolment. All methods were carried out following relevant guidelines and regulations.
Consent for publication
Not applicable.
Additional information
Publisher’s note.
Springer Nature remains neutral with regard to jurisdictional claims in published maps and institutional affiliations.
Electronic supplementary material
Below is the link to the electronic supplementary material.
Supplementary Material 1
Rights and permissions.
Open Access This article is licensed under a Creative Commons Attribution 4.0 International License, which permits use, sharing, adaptation, distribution and reproduction in any medium or format, as long as you give appropriate credit to the original author(s) and the source, provide a link to the Creative Commons licence, and indicate if changes were made. The images or other third party material in this article are included in the article’s Creative Commons licence, unless indicated otherwise in a credit line to the material. If material is not included in the article’s Creative Commons licence and your intended use is not permitted by statutory regulation or exceeds the permitted use, you will need to obtain permission directly from the copyright holder. To view a copy of this licence, visit http://creativecommons.org/licenses/by/4.0/ . The Creative Commons Public Domain Dedication waiver ( http://creativecommons.org/publicdomain/zero/1.0/ ) applies to the data made available in this article, unless otherwise stated in a credit line to the data.
Reprints and permissions
About this article
Cite this article.
Abdolalipour, S., Abbasalizadeh, S., Mohammad-Alizadeh-Charandabi, S. et al. Translation and measurement properties of pregnancy and childbirth questionnaire in Iranian postpartum women. BMC Health Serv Res 24 , 365 (2024). https://doi.org/10.1186/s12913-024-10689-7
Download citation
Received : 05 August 2023
Accepted : 06 February 2024
Published : 22 March 2024
DOI : https://doi.org/10.1186/s12913-024-10689-7
Share this article
Anyone you share the following link with will be able to read this content:
Sorry, a shareable link is not currently available for this article.
Provided by the Springer Nature SharedIt content-sharing initiative
- Quality of care
- Prenatal care
- Satisfaction
BMC Health Services Research
ISSN: 1472-6963
- General enquiries: [email protected]
Have a language expert improve your writing
Run a free plagiarism check in 10 minutes, automatically generate references for free.
- Knowledge Base
- Methodology
- Questionnaire Design | Methods, Question Types & Examples
Questionnaire Design | Methods, Question Types & Examples
Published on 6 May 2022 by Pritha Bhandari . Revised on 10 October 2022.
A questionnaire is a list of questions or items used to gather data from respondents about their attitudes, experiences, or opinions. Questionnaires can be used to collect quantitative and/or qualitative information.
Questionnaires are commonly used in market research as well as in the social and health sciences. For example, a company may ask for feedback about a recent customer service experience, or psychology researchers may investigate health risk perceptions using questionnaires.
Table of contents
Questionnaires vs surveys, questionnaire methods, open-ended vs closed-ended questions, question wording, question order, step-by-step guide to design, frequently asked questions about questionnaire design.
A survey is a research method where you collect and analyse data from a group of people. A questionnaire is a specific tool or instrument for collecting the data.
Designing a questionnaire means creating valid and reliable questions that address your research objectives, placing them in a useful order, and selecting an appropriate method for administration.
But designing a questionnaire is only one component of survey research. Survey research also involves defining the population you’re interested in, choosing an appropriate sampling method , administering questionnaires, data cleaning and analysis, and interpretation.
Sampling is important in survey research because you’ll often aim to generalise your results to the population. Gather data from a sample that represents the range of views in the population for externally valid results. There will always be some differences between the population and the sample, but minimising these will help you avoid sampling bias .
Prevent plagiarism, run a free check.
Questionnaires can be self-administered or researcher-administered . Self-administered questionnaires are more common because they are easy to implement and inexpensive, but researcher-administered questionnaires allow deeper insights.
Self-administered questionnaires
Self-administered questionnaires can be delivered online or in paper-and-pen formats, in person or by post. All questions are standardised so that all respondents receive the same questions with identical wording.
Self-administered questionnaires can be:
- Cost-effective
- Easy to administer for small and large groups
- Anonymous and suitable for sensitive topics
But they may also be:
- Unsuitable for people with limited literacy or verbal skills
- Susceptible to a nonreponse bias (most people invited may not complete the questionnaire)
- Biased towards people who volunteer because impersonal survey requests often go ignored
Researcher-administered questionnaires
Researcher-administered questionnaires are interviews that take place by phone, in person, or online between researchers and respondents.
Researcher-administered questionnaires can:
- Help you ensure the respondents are representative of your target audience
- Allow clarifications of ambiguous or unclear questions and answers
- Have high response rates because it’s harder to refuse an interview when personal attention is given to respondents
But researcher-administered questionnaires can be limiting in terms of resources. They are:
- Costly and time-consuming to perform
- More difficult to analyse if you have qualitative responses
- Likely to contain experimenter bias or demand characteristics
- Likely to encourage social desirability bias in responses because of a lack of anonymity
Your questionnaire can include open-ended or closed-ended questions, or a combination of both.
Using closed-ended questions limits your responses, while open-ended questions enable a broad range of answers. You’ll need to balance these considerations with your available time and resources.
Closed-ended questions
Closed-ended, or restricted-choice, questions offer respondents a fixed set of choices to select from. Closed-ended questions are best for collecting data on categorical or quantitative variables.
Categorical variables can be nominal or ordinal. Quantitative variables can be interval or ratio. Understanding the type of variable and level of measurement means you can perform appropriate statistical analyses for generalisable results.
Examples of closed-ended questions for different variables
Nominal variables include categories that can’t be ranked, such as race or ethnicity. This includes binary or dichotomous categories.
It’s best to include categories that cover all possible answers and are mutually exclusive. There should be no overlap between response items.
In binary or dichotomous questions, you’ll give respondents only two options to choose from.
White Black or African American American Indian or Alaska Native Asian Native Hawaiian or Other Pacific Islander
Ordinal variables include categories that can be ranked. Consider how wide or narrow a range you’ll include in your response items, and their relevance to your respondents.
Likert-type questions collect ordinal data using rating scales with five or seven points.
When you have four or more Likert-type questions, you can treat the composite data as quantitative data on an interval scale . Intelligence tests, psychological scales, and personality inventories use multiple Likert-type questions to collect interval data.
With interval or ratio data, you can apply strong statistical hypothesis tests to address your research aims.
Pros and cons of closed-ended questions
Well-designed closed-ended questions are easy to understand and can be answered quickly. However, you might still miss important answers that are relevant to respondents. An incomplete set of response items may force some respondents to pick the closest alternative to their true answer. These types of questions may also miss out on valuable detail.
To solve these problems, you can make questions partially closed-ended, and include an open-ended option where respondents can fill in their own answer.
Open-ended questions
Open-ended, or long-form, questions allow respondents to give answers in their own words. Because there are no restrictions on their choices, respondents can answer in ways that researchers may not have otherwise considered. For example, respondents may want to answer ‘multiracial’ for the question on race rather than selecting from a restricted list.
- How do you feel about open science?
- How would you describe your personality?
- In your opinion, what is the biggest obstacle to productivity in remote work?
Open-ended questions have a few downsides.
They require more time and effort from respondents, which may deter them from completing the questionnaire.
For researchers, understanding and summarising responses to these questions can take a lot of time and resources. You’ll need to develop a systematic coding scheme to categorise answers, and you may also need to involve other researchers in data analysis for high reliability .
Question wording can influence your respondents’ answers, especially if the language is unclear, ambiguous, or biased. Good questions need to be understood by all respondents in the same way ( reliable ) and measure exactly what you’re interested in ( valid ).
Use clear language
You should design questions with your target audience in mind. Consider their familiarity with your questionnaire topics and language and tailor your questions to them.
For readability and clarity, avoid jargon or overly complex language. Don’t use double negatives because they can be harder to understand.
Use balanced framing
Respondents often answer in different ways depending on the question framing. Positive frames are interpreted as more neutral than negative frames and may encourage more socially desirable answers.
Use a mix of both positive and negative frames to avoid bias , and ensure that your question wording is balanced wherever possible.
Unbalanced questions focus on only one side of an argument. Respondents may be less likely to oppose the question if it is framed in a particular direction. It’s best practice to provide a counterargument within the question as well.
Avoid leading questions
Leading questions guide respondents towards answering in specific ways, even if that’s not how they truly feel, by explicitly or implicitly providing them with extra information.
It’s best to keep your questions short and specific to your topic of interest.
- The average daily work commute in the US takes 54.2 minutes and costs $29 per day. Since 2020, working from home has saved many employees time and money. Do you favour flexible work-from-home policies even after it’s safe to return to offices?
- Experts agree that a well-balanced diet provides sufficient vitamins and minerals, and multivitamins and supplements are not necessary or effective. Do you agree or disagree that multivitamins are helpful for balanced nutrition?
Keep your questions focused
Ask about only one idea at a time and avoid double-barrelled questions. Double-barrelled questions ask about more than one item at a time, which can confuse respondents.
This question could be difficult to answer for respondents who feel strongly about the right to clean drinking water but not high-speed internet. They might only answer about the topic they feel passionate about or provide a neutral answer instead – but neither of these options capture their true answers.
Instead, you should ask two separate questions to gauge respondents’ opinions.
Strongly Agree Agree Undecided Disagree Strongly Disagree
Do you agree or disagree that the government should be responsible for providing high-speed internet to everyone?
You can organise the questions logically, with a clear progression from simple to complex. Alternatively, you can randomise the question order between respondents.
Logical flow
Using a logical flow to your question order means starting with simple questions, such as behavioural or opinion questions, and ending with more complex, sensitive, or controversial questions.
The question order that you use can significantly affect the responses by priming them in specific directions. Question order effects, or context effects, occur when earlier questions influence the responses to later questions, reducing the validity of your questionnaire.
While demographic questions are usually unaffected by order effects, questions about opinions and attitudes are more susceptible to them.
- How knowledgeable are you about Joe Biden’s executive orders in his first 100 days?
- Are you satisfied or dissatisfied with the way Joe Biden is managing the economy?
- Do you approve or disapprove of the way Joe Biden is handling his job as president?
It’s important to minimise order effects because they can be a source of systematic error or bias in your study.
Randomisation
Randomisation involves presenting individual respondents with the same questionnaire but with different question orders.
When you use randomisation, order effects will be minimised in your dataset. But a randomised order may also make it harder for respondents to process your questionnaire. Some questions may need more cognitive effort, while others are easier to answer, so a random order could require more time or mental capacity for respondents to switch between questions.
Follow this step-by-step guide to design your questionnaire.
Step 1: Define your goals and objectives
The first step of designing a questionnaire is determining your aims.
- What topics or experiences are you studying?
- What specifically do you want to find out?
- Is a self-report questionnaire an appropriate tool for investigating this topic?
Once you’ve specified your research aims, you can operationalise your variables of interest into questionnaire items. Operationalising concepts means turning them from abstract ideas into concrete measurements. Every question needs to address a defined need and have a clear purpose.
Step 2: Use questions that are suitable for your sample
Create appropriate questions by taking the perspective of your respondents. Consider their language proficiency and available time and energy when designing your questionnaire.
- Are the respondents familiar with the language and terms used in your questions?
- Would any of the questions insult, confuse, or embarrass them?
- Do the response items for any closed-ended questions capture all possible answers?
- Are the response items mutually exclusive?
- Do the respondents have time to respond to open-ended questions?
Consider all possible options for responses to closed-ended questions. From a respondent’s perspective, a lack of response options reflecting their point of view or true answer may make them feel alienated or excluded. In turn, they’ll become disengaged or inattentive to the rest of the questionnaire.
Step 3: Decide on your questionnaire length and question order
Once you have your questions, make sure that the length and order of your questions are appropriate for your sample.
If respondents are not being incentivised or compensated, keep your questionnaire short and easy to answer. Otherwise, your sample may be biased with only highly motivated respondents completing the questionnaire.
Decide on your question order based on your aims and resources. Use a logical flow if your respondents have limited time or if you cannot randomise questions. Randomising questions helps you avoid bias, but it can take more complex statistical analysis to interpret your data.
Step 4: Pretest your questionnaire
When you have a complete list of questions, you’ll need to pretest it to make sure what you’re asking is always clear and unambiguous. Pretesting helps you catch any errors or points of confusion before performing your study.
Ask friends, classmates, or members of your target audience to complete your questionnaire using the same method you’ll use for your research. Find out if any questions were particularly difficult to answer or if the directions were unclear or inconsistent, and make changes as necessary.
If you have the resources, running a pilot study will help you test the validity and reliability of your questionnaire. A pilot study is a practice run of the full study, and it includes sampling, data collection , and analysis.
You can find out whether your procedures are unfeasible or susceptible to bias and make changes in time, but you can’t test a hypothesis with this type of study because it’s usually statistically underpowered .
A questionnaire is a data collection tool or instrument, while a survey is an overarching research method that involves collecting and analysing data from people using questionnaires.
Closed-ended, or restricted-choice, questions offer respondents a fixed set of choices to select from. These questions are easier to answer quickly.
Open-ended or long-form questions allow respondents to answer in their own words. Because there are no restrictions on their choices, respondents can answer in ways that researchers may not have otherwise considered.
A Likert scale is a rating scale that quantitatively assesses opinions, attitudes, or behaviours. It is made up of four or more questions that measure a single attitude or trait when response scores are combined.
To use a Likert scale in a survey , you present participants with Likert-type questions or statements, and a continuum of items, usually with five or seven possible responses, to capture their degree of agreement.
You can organise the questions logically, with a clear progression from simple to complex, or randomly between respondents. A logical flow helps respondents process the questionnaire easier and quicker, but it may lead to bias. Randomisation can minimise the bias from order effects.
Questionnaires can be self-administered or researcher-administered.
Researcher-administered questionnaires are interviews that take place by phone, in person, or online between researchers and respondents. You can gain deeper insights by clarifying questions for respondents or asking follow-up questions.
Cite this Scribbr article
If you want to cite this source, you can copy and paste the citation or click the ‘Cite this Scribbr article’ button to automatically add the citation to our free Reference Generator.
Bhandari, P. (2022, October 10). Questionnaire Design | Methods, Question Types & Examples. Scribbr. Retrieved 25 March 2024, from https://www.scribbr.co.uk/research-methods/questionnaire-design/
Is this article helpful?
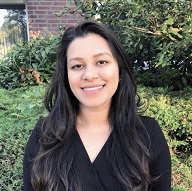
Pritha Bhandari
Other students also liked, doing survey research | a step-by-step guide & examples, what is a likert scale | guide & examples, reliability vs validity in research | differences, types & examples.
This paper is in the following e-collection/theme issue:
Published on 27.3.2024 in Vol 26 (2024)
Effectiveness of the Minder Mobile Mental Health and Substance Use Intervention for University Students: Randomized Controlled Trial
Authors of this article:

Original Paper
- Melissa Vereschagin 1 , BSc ;
- Angel Y Wang 1 , BA, MPhil ;
- Chris G Richardson 2 , PhD ;
- Hui Xie 3 , PhD ;
- Richard J Munthali 1 , PhD ;
- Kristen L Hudec 1 , PhD ;
- Calista Leung 1 , BA ;
- Katharine D Wojcik 4 , PhD ;
- Lonna Munro 1 , BSc ;
- Priyanka Halli 1 , MPH, MD ;
- Ronald C Kessler 5 , PhD ;
- Daniel V Vigo 1, 2 , LicPs, MD, DrPH
1 Department of Psychiatry, Faculty of Medicine, University of British Columbia, Vancouver, BC, Canada
2 School of Population and Public Health, Faculty of Medicine, University of British Columbia, Vancouver, BC, Canada
3 Faculty of Health Sciences, Simon Fraser University, Burnaby, BC, Canada
4 Menninger Department of Psychiatry & Behavioural Sciences, Baylor College of Medicine, Houston, TX, United States
5 Department of Health Care Policy, Harvard Medical School, Boston, MA, United States
Corresponding Author:
Daniel V Vigo, LicPs, MD, DrPH
Department of Psychiatry
Faculty of Medicine
University of British Columbia
2255 Wesbrook Mall
Vancouver, BC, V6T2A1
Phone: 1 6048228048
Email: [email protected]
Background: University attendance represents a transition period for students that often coincides with the emergence of mental health and substance use challenges. Digital interventions have been identified as a promising means of supporting students due to their scalability, adaptability, and acceptability. Minder is a mental health and substance use mobile app that was codeveloped with university students.
Objective: This study aims to examine the effectiveness of the Minder mobile app in improving mental health and substance use outcomes in a general population of university students.
Methods: A 2-arm, parallel-assignment, single-blinded, 30-day randomized controlled trial was used to evaluate Minder using intention-to-treat analysis. In total, 1489 participants were recruited and randomly assigned to the intervention (n=743, 49.9%) or waitlist control (n=746, 50.1%) condition. The Minder app delivers evidence-based content through an automated chatbot and connects participants with services and university social groups. Participants are also assigned a trained peer coach to support them. The primary outcomes were measured through in-app self-assessments and included changes in general anxiety symptomology, depressive symptomology, and alcohol consumption risk measured using the 7-item General Anxiety Disorder scale, 9-item Patient Health Questionnaire, and US Alcohol Use Disorders Identification Test–Consumption Scale, respectively, from baseline to 30-day follow-up. Secondary outcomes included measures related to changes in the frequency of substance use (cannabis, alcohol, opioids, and nonmedical stimulants) and mental well-being. Generalized linear mixed-effects models were used to examine each outcome.
Results: In total, 79.3% (589/743) of participants in the intervention group and 83% (619/746) of participants in the control group completed the follow-up survey. The intervention group had significantly greater average reductions in anxiety symptoms measured using the 7-item General Anxiety Disorder scale (adjusted group mean difference=−0.85, 95% CI −1.27 to −0.42; P <.001; Cohen d =−0.17) and depressive symptoms measured using the 9-item Patient Health Questionnaire (adjusted group mean difference=−0.63, 95% CI −1.08 to −0.17; P =.007; Cohen d =−0.11). A reduction in the US Alcohol Use Disorders Identification Test–Consumption Scale score among intervention participants was also observed, but it was not significant ( P =.23). Statistically significant differences in favor of the intervention group were found for mental well-being and reductions in the frequency of cannabis use and typical number of drinks consumed. A total of 77.1% (573/743) of participants in the intervention group accessed at least 1 app component during the study period.
Conclusions: In a general population sample of university students, the Minder app was effective in reducing symptoms of anxiety and depression, with provisional support for increasing mental well-being and reducing the frequency of cannabis and alcohol use. These findings highlight the potential ability of e-tools focused on prevention and early intervention to be integrated into existing university systems to support students’ needs.
Trial Registration: ClinicalTrials.gov NCT05606601; https://clinicaltrials.gov/ct2/show/NCT05606601
International Registered Report Identifier (IRRID): RR2-10.2196/49364
Introduction
University attendance is a transitional period in which many students experience novel stressors related to moving away from home, navigating new social environments, and managing increased educational and financial demands in the absence of their traditional support systems [ 1 , 2 ]. The transition to attending university also coincides with the peak period of onset of many mental disorders, including mood, anxiety, and substance use disorders [ 3 , 4 ]. Studies have documented the high rates of mental health and substance use problems experienced by university students [ 5 ], with research also indicating that students with preexisting mental health problems can experience a worsening of their conditions following the transition to attending university [ 6 ].
Despite this high need, most students experiencing mental health problems do not receive treatment [ 7 ]. Research investigating help seeking among university students indicates that, compared to structural barriers, attitudinal barriers are the most important reasons for not seeking help [ 8 ]. The most commonly cited reason students give for not seeking help is a preference for handling things on their own [ 7 , 8 ]. One way of adapting interventions to align with this preference is to provide students with tools that are self-guided and allow them autonomy over how and when to use the tools provided. e-Interventions can be accessed by users at any time and have been demonstrated to be effective in improving various mental health [ 9 ] and substance use outcomes among university students [ 10 ]. These interventions have also been identified as key components in proposed models of care for universities [ 11 ]. While much of the literature on e-interventions has been focused on web-based tools, mobile apps have been identified as a promising means of delivering mental health interventions due to not only the increase in smartphone use but also the wide range of interventions that can be delivered through mobile platforms [ 12 , 13 ].
Given the range of challenges faced by university students, including the high rates of disorder-level and subclinical mental health and substance use problems, transdiagnostic approaches to early intervention and prevention may be beneficial for this population [ 14 ]. Developing this type of intervention requires the use of a holistic, student-centered design approach to identify evidence-based condition-specific and cross-cutting opportunities for intervention. Furthermore, the intervention needs to be aligned with the perceived needs and preferences of students to ensure meaningful engagement [ 15 ]. On the basis of these requirements, we codeveloped a mental health and substance use mobile app called Minder for Canadian university students. This participatory codevelopment process involved significant input from students through the creation of a Student Advisory Committee, usability testing via a virtual boot camp (ie, individual user-testing combined with a web-based survey), focus groups, and a pilot feasibility study [ 16 ].
The objective of this study was to test the effectiveness of the Minder mobile app in improving mental health and substance use outcomes in a general population of university students.
Trial Design
This study was based on a 2-arm, parallel-assignment, single-blinded (the statistician was blinded), 30-day randomized controlled trial with 1 intervention group and 1 waitlist control group. The study was registered at ClinicalTrials.gov (NCT05606601), and a full study protocol has been published [ 17 ]. No significant changes to the trial protocol or intervention content were made during the trial period; however, several minor adjustments, along with a description of minor technical issues, can be found in Multimedia Appendix 1 .
Ethical Considerations
Ethics approval was obtained from the University of British Columbia (UBC) Behavioural Research Ethics Board on January 6, 2022 (ethics ID: H21-03248). Informed consent was obtained through a web-based self-assessment questionnaire at the beginning of the study. Participants were informed of their ability to opt out at any point within the study by emailing the research team. Identifiable data were stored in data files within the app backend, which were separate from all deidentified app use and survey data. This information can only be linked using a unique study ID number. Participants received a CAD $10 (US $7.4) gift card for completion of the baseline survey and an additional CAD $10 (US $7.4) gift card for completion of the 30-day follow-up survey.
Participants
The study was conducted at the UBC Point Grey (Vancouver, British Columbia, Canada) campus. Participants needed to confirm their eligibility using a web-based self-assessment questionnaire before registering and consenting to the study. The inclusion criteria were as follows: students currently enrolled at the UBC Vancouver campus, aged ≥17 years, having access to and being able to use a smartphone with Wi-Fi or cellular data, and speaking English. The only exclusion criterion was based on a single screening question assessing suicidality risk (“We want to make sure that this app is appropriate for you at this time. Do you have a current suicidal plan [i.e., a plan to end your life]?”). Anyone endorsing a current suicidal plan (ie, answering “yes”) was prevented from registering and was instead provided with a list of local crisis resources. The eligibility criterion of being a current UBC student was confirmed using a unique student log-in checkpoint as part of the registration process. This process also ensured that each student could only enroll once.
Recruitment and Consent
Given the large sample size needed for this study, many different recruitment methods were used. Online recruitment occurred through various social media platforms and a linked ongoing Student E-Mental Health trend study [ 18 ]. Recruitment also occurred through in-person and on-campus engagements, such as setting up informational booths at the university, displaying posters about the study, visiting in-person and online classes, having professors share study information with their classes, and contacting student groups to share information with their members. Paid bus and bus stop advertisements at the university were also used. A more detailed description of the recruitment methods can be found in the study protocol for this trial [ 17 ].
Participants’ consent was obtained using Qualtrics (Qualtrics International Inc), a web-based form, after completing the eligibility screening. The consent form indicated that participants would either gain access to the full app immediately or in 30 days following completion of the final survey. Individual accounts were created for each participant and sent to them with a link to download the app. Upon downloading the app and completing the baseline survey, participants were randomly assigned through the app to the intervention group, which received full access to the Minder app, or to the control group, which only had access to a restricted version of the app that included a short introduction video and the baseline and follow-up surveys. Participants received a CAD $10 (US $7.40) gift card for completion of the baseline survey and an additional CAD $10 (US $7.40) gift card for completion of the 30-day follow-up survey; however, the use of the app itself was not remunerated.
Randomization and Intervention
Participants were randomized using a custom-developed automated process incorporated directly into the mobile app following completion of the baseline survey. The system assigned participants to either the intervention or control group using a predetermined block randomization list (1:1 randomization in blocks of 10) stratified for past drug use (any lifetime use of opioids or nonmedical stimulants). Stratification by past drug use was used to account for the low number of students using these substances and the need to ensure that they were evenly distributed across the study groups. The randomization lists (1 for each stratification group) were generated using the web-based stratified block randomization list creator in the clinical trial software Sealed Envelope (Sealed Envelope Ltd) [ 19 ]. The intervention and control groups completed the main assessments of the primary and secondary outcomes at baseline and the 30-day follow-up. The intervention group was also prompted to complete a short survey at 2 weeks that consisted of a limited set of questions on anxiety and depression symptoms.
The Minder mobile app was codeveloped with university students and professionals with the goal of creating an engaging self-directed tool for students to improve their mental health and manage substance use. The intervention is designed for a general population of students and, thus, addresses a wide range of challenges related to postsecondary student life, including managing emotions, relationships, well-being, and university life. The self-directed nature of the app also allows students to access features when needed. The codevelopment process consisted of ongoing student input through student staff members and volunteers along with several phases of purposeful student engagement and feedback. Further details on the codevelopment process can be found in the study by Vereschagin et al [ 16 ].
Participants who were randomized to the intervention group were given full access to the Minder app and instructed to use it as they wanted. They were also presented with a tutorial video outlining the different features of the app. The Minder intervention consists of 4 main components: Chatbot Activities, Services, Community, and Peer Coaching. The chatbot activities consist of an automated preprogrammed chatbot that delivers evidence-based messages and videos. The content is based primarily on cognitive behavioral therapy and psychoeducation; however, there is also content adapted from dialectical behavioral therapy, mindfulness, metacognitive training, and motivational interviewing. The content sections are presented on a home page map with several islands: University Life , Wellbeing , Relationships , Sadness , Stress & Anxiety , and Substance Use ( Figure 1 ). There is also an Explore Chat located on the home page map that guides participants to select an activity that may be relevant to their current needs. A full list of the content included can be found in Multimedia Appendix 2 [ 16 ]. Most of the chat activities also contain a summary page that is unlocked upon completion of the chat activity and allows participants to review content they learned at a later time. In addition, several chat activities contain specific practice components that also become unlocked after the main activity is complete.
The Peer Coaching component consists of trained volunteers assigned to each participant. These peer coaches reach out to participants at the beginning of the trial and midway through. They can provide support in navigating the app or nonclinical peer support based on active listening and problem-solving. Peer coaches can communicate with participants through an in-app chat asynchronously or synchronously through scheduled appointments delivered over in-app chat message or audio or video call. Before engaging with peer coaches, participants must provide a phone number that can be used to contact them in a crisis situation and affirm that they are not currently at risk of self-harm or having suicidal thoughts, are not under the influence of substances, and understand the circumstances in which confidentiality would need to be broken (ie, crisis situations or abuse of a minor; Figure 2 A).

The Services component consists of a 10-question survey tool that provides participants with recommendations for resources based on their current needs and preferences ( Figure 2 B). The survey tool and recommendations were adapted from a previously developed tool for university students [ 20 ] and can be completed multiple times to receive new recommendations. Recommendations are provided for 6 areas related to student well-being: mental health and relationships, substance use, abuse, sexual wellness, housing, and education and activities. An additional safety component was added so that participants who based on the services survey were considered to be at high risk of suicidality (ie, plan for suicide or recent attempt and thoughts of hurting others) were asked to consent to provide their contact information and receive an expedited appointment with the university counseling services.
The Community component consists of a searchable directory of student groups or clubs at the university that are sorted by interest (eg, volunteering, arts, and advocacy; Figure 2 C).
The Minder app also contains several other general features. An SOS button appears in the top corner of the home page and provides a list of crisis resources if needed ( Figure 1 ). The settings page allows participants to update their username and password as well as change their avatar. Several types of push notifications were delivered through the app. General notifications were sent on days 4, 18, and 24. Reminders to complete the 2-week and 30-day follow-up surveys were sent as push notifications and via automated email reminders on those dates. Additional email reminders were sent on days 35 and 41 to remind participants to complete the follow-up survey if they had not done so already.
Participants who were randomized to the control group had access to a locked version of the app that only allowed them to complete the baseline survey and view a short introduction video that appears before the log-in screen. Following completion of the baseline survey, they received a pop-up message telling them that they would be notified when it was time to complete the next survey. The app was then locked so that control participants were not able to access any other areas of the app. At 30 days, participants were notified that it was time to complete the 30-day follow-up survey within the app, and this survey became unlocked. The app provided push notifications and automated email reminders to complete the follow-up survey on day 30 as well as additional email reminders on days 35 and 41 if the survey had not yet been completed.
Individuals who consented to participate in the study and received an invitation email to download the app but did not complete the baseline survey received additional email reminders at 7 days, at approximately 20 days, and several months later following the creation of their account.
The participants’ use of the app components was recorded through the app back-end system. This included starting each of the chatbot activities, completing the services survey and receiving recommendations, viewing community groups, and communicating with a peer coach.
The main assessments of the primary and secondary outcomes were collected through self-assessment within the Minder app at baseline and at the 30-day follow-up. The 30-day follow-up survey had to be completed within 44 days of the beginning of the baseline survey (30 days plus 2 weeks to accommodate the use of reminders) for the participants to be included in the analysis.
Primary Outcomes
The primary outcomes assessed in this study were changes in general anxiety symptomology, depressive symptomology, and alcohol consumption risk from baseline to follow-up at 30 days. All outcomes were assessed using self-report questionnaires completed directly in the mobile app.
Anxiety symptoms were assessed using the 7-item General Anxiety Disorder scale (GAD-7) assessment—a commonly used self-report scale that assesses symptoms of generalized anxiety [ 21 ]. Each GAD-7 question is scored from 0 ( not at all ) to 3 ( nearly every day ), with total scores ranging from 0 to 21 and higher scores indicating a worse outcome (ie, greater frequency of anxiety symptoms).
Depressive symptoms were assessed using the 9-item Patient Health Questionnaire (PHQ-9) self-report scale [ 22 ]. Each of the 9 questions is scored from 0 ( not at all ) to 3 ( nearly every day ). The total scores range from 0 to 29, with higher scores indicating a worse outcome (ie, a greater frequency of depressive symptoms).
Alcohol consumption risk was assessed using the US Alcohol Use Disorders Identification Test–Consumption Scale (USAUDIT-C) [ 23 ]. The USAUDIT-C is a 3-item self-report scale adapted from the consumption questions in the Alcohol Use Disorders Identification Test (AUDIT) [ 24 ]. Compared to the AUDIT, the USAUDIT-C includes expanded response options for the first 3 AUDIT questions—from 5 to 7 categories—to allow for more precise measurements when accounting for differences in standard drink sizes and cutoff limits. The higher the total score on the USAUDIT-C, the greater the respondent’s alcohol consumption and related risk [ 23 ].
Secondary Outcomes
A range of secondary outcomes, including reduced use of other substances and additional mental health constructs that the Minder app was theorized to affect, were also assessed. For the purposes of this study, we focused on examining changes from baseline to follow-up at 30 days in frequency of substance use (cannabis, alcohol, opioids, and nonmedical stimulants) and mental well-being.
Frequency of cannabis use was assessed using a single self-report question on frequency of cannabis consumption in the previous 30 days. The 3 questions on the USAUDIT-C assessed unique dimensions of alcohol consumption. Frequency of alcohol use was assessed using responses to the first question on the USAUDIT-C, which asks how often participants have a drink containing alcohol. The number of drinks consumed in a typical drinking session was assessed using the second question on the USAUDIT-C, which asks participants how many drinks containing alcohol they have on a typical day when drinking. Frequency of binge drinking was assessed using the third question in the USAUDIT-C, which asks participants how often they have ≥5 (if sex at birth was male) or ≥4 (if sex at birth was female) drinks on 1 occasion.
Frequency of any opioid use in the previous 30 days was assessed using self-reported questions that asked about any pharmaceutical opioid (eg, oxycodone; morphine; hydromorphone; meperidine; fentanyl patches; and codeine or codeine-containing products such as Tylenol 1, 2, or 3) with a physician’s prescription and taken as prescribed; any pharmaceutical opioid (eg, oxycodone; morphine; hydromorphone; meperidine; fentanyl patches; and codeine or codeine-containing products such as Tylenol 1, 2, or 3) either without a physician’s prescription or in larger doses than prescribed to get high, buzzed, or numbed out; and any street opioid (eg, heroin and fentanyl) or any other opioid obtained “on the street.” The final opioid use outcome was defined as the most frequent number among any prescribed, nonprescribed, and street opioids.
Frequency of nonmedical stimulant use in the previous 30 days was assessed using self-report questions that asked about the frequency of using any street stimulant (eg, cocaine, crack, methamphetamines, and crystal meth) or prescription stimulant (eg, amphetamine, methylphenidate, and modafinil) either without a physician’s prescription or in larger doses than prescribed to get high, buzzed, numbed out, or help them study or for any other reason. The final nonmedical stimulant use outcome was defined as the most frequent number among any prescription stimulant without a prescription or not as prescribed and any street stimulant. The exact wording and response options for the substance use secondary outcome assessments can be found in Multimedia Appendix 3 .
Mental well-being was assessed using the Short Warwick-Edinburgh Mental Wellbeing Scale. The Short Warwick-Edinburgh Mental Wellbeing Scale is a 7-item scale that has been widely validated [ 25 ], with total scores ranging from 7 to 35 and higher scores indicating a better outcome (ie, higher positive mental well-being).
Sample Size Estimation
The a priori sample size calculation for a small effect assessed using the PHQ-9, GAD-7, and USAUDIT-C was performed using an effect size defined by Cohen d =Δ/σ, where Δ is the group mean difference at the completion of the study and σ is the (pooled) within-group SD [ 17 ]. For a small effect size (Cohen d =0.2), the sample size required to have 80% power at a P =.02 level of significance (ie, 0.05/3 primary outcomes) was 524 in each group. After incorporating a 30% attrition rate, we anticipated requiring 748 participants in each group for a total of 1496 participants for the trial to be adequately powered.
Statistical Analysis
The study used a single-blinded approach in which only the statistician, who was external to the team, was blinded to the treatment group assignment when examining the primary hypotheses. The primary analysis was intention-to-treat (ITT) including all participants who completed the baseline assessment and were randomized to either the control or intervention group following the analysis plan prespecified in our protocol paper [ 17 ]. The analysis considered the following 2 features of the trial design: 3 primary end points and the use of block randomization.
For the 3 primary end points (GAD-7, PHQ-9, and USAUDIT-C scores), a global test for the null hypothesis of no treatment difference in all primary end points between the control and treatment groups was conducted first using a multivariate analysis of covariance for correlated data on GAD-7, PHQ-9, and USAUDIT-C scores at 30 days after the baseline, adjusting for their values at baseline and randomization blocks. Compared with testing each outcome separately, the advantages of the global test from joint modeling included more parsimonious hypothesis tests and mitigated concerns related to multiple testing [ 26 - 28 ] as well as pooling of the information over the correlated outcomes to increase study power, especially with missing outcomes [ 29 ]. If the global test rejected the null hypothesis and we concluded that there was an intervention effect on at least 1 of the 3 end points, we would then analyze each end point separately to identify which of the 3 study end points were affected by the intervention. We then used the sequential Hochberg correction method [ 30 ] to control the overall familywise error rate at α=.05 when testing the hypothesis for each individual primary end point. For baseline characteristics, 2-sample t tests (2-tailed) were used to compare means, chi-square tests were used to compare proportions, and the Kruskal-Wallis test was used to test for differences in medians.
The general approach for analyzing all the types of individual study outcomes separately (including the 3 primary end points and the secondary end points) was generalized linear mixed-effects models (GLMMs) for clustered measures with the randomization block as the clustering variable. These models can handle a wide range of outcome types, including continuous, binary, ordinal, and count, and can account for the correlations among observations within the same cluster. GLMMs have been widely used for conducting ITT analysis in randomized controlled trials with missing outcome data and can account for data missing at random (MAR) without the need to model why data are missing or to perform explicit imputations of the missing values [ 31 ]. Specifically, we analyzed each primary end point using a linear mixed-effects model, a special case of the GLMM.
For secondary outcomes, we used linear mixed-effects models to analyze mental well-being measures and a mixed-effects quasi–Poisson regression model with a log link (a special case of GLMM) for substance use frequency measures, and the zero-inflated Poisson was used for the number of drinks to deal with the excess zero counts. The treatment effect on an outcome at 30 days was assessed in these models with the treatment allocation as the main explanatory variable and with adjustment for baseline outcome assessment value as a fixed effect and the randomization block as a random effect. Robust empirical sandwich SE estimates that are robust to model misspecifications (eg, nonnormal error terms) were used for statistical inference.
The results from these GLMMs for the analysis are reported in the Results section as the model-adjusted differences in the group mean values of the continuous end points between the intervention and control groups. These intervention effect estimates, 95% CIs, and P values were obtained from the aforementioned GLMMs, clustering on randomization block effects and adjusting for the baseline outcome values. Standardized effect sizes were calculated for continuous scores by dividing the adjusted mean differences by the SDs across all participants at baseline. The incidence rate ratios (IRRs) from Poisson regression through GLMM and zero inflation for the number of drinks are also reported.
To evaluate the robustness of the results to alternative assumptions regarding missing data, sensitivity analyses were conducted on the primary outcomes via (1) using selection models to measure the potential impacts of data missing not at random [ 32 , 33 ] and (2) adjusting analysis for additional baseline covariates potentially predictive of missing data. All the primary analyses were conducted in SAS (version 9.4; SAS Institute), except for the sensitivity analysis of the selection models, which was conducted in the isni package in R (version 3.4; R Foundation for Statistical Computing) [ 34 ], whereas secondary analyses were conducted in Stata (version 15.1; StataCorp) [ 35 ].
Data and Privacy
Many steps were taken to ensure the privacy of participants. Each participant received an individual account that had a unique username and password. They were then able to change this password upon logging in. Only participant emails and phone numbers (if provided through access to peer coaching) were stored within the app; names were used only for consent and were never entered into the app. Instead, participants could create a unique username within the app that they were informed should not include their full name. Identifiable data (email and phone number) were stored in data files within the app back end separate from all the app use and survey data, which were recorded with only a study ID number.
Recruitment
Recruitment initially began on September 4, 2022, during an on-campus student orientation event where interested students were asked to provide contact information to receive a follow-up email; however, participants were not provided with app download information and user accounts to begin the study until September 28, 2022, due to technical delays. Recruitment concluded on June 2, 2023, with the last participant beginning the trial on June 11, 2023.
Participant Flow
In total, 2293 individuals were invited to participate in the trial following eligibility screening and provision of informed consent. Of those 2293 individuals, 1489 (64.9%) participants completed the baseline survey and were randomized into the trial, with 743 (49.9%) of the 1489 participants in the intervention group and 746 (50.1%) in the control group. A total of 79.3% (589/743) of participants in the intervention group and 83% (619/746) of participants in the control group completed the 30-day follow-up survey within the specified 44-day period to be included in the analysis (ie, 279/1489, 18.7% of participants who completed the baseline survey did not complete the 30-day follow-up survey within 44 days and were therefore considered lost to follow-up). Additional information on the participant flow is shown in Figure 3 .

Participant Characteristics
The baseline characteristics of the participants in the intervention and control groups are presented in Table 1 . The median age of the participants was 20 years, and 70.3% (1045/1487) self-identified as women. In terms of mental health, 33.8% (455/1347) reported a history of anxiety, and 38.9% (576/1481) reported moderate or greater levels of recent anxiety (ie, total score of ≥10) based on the GAD-7. A history of depression was reported by 28.4% (382/1347) of participants, with 43.8% (645/1474) reporting moderate or greater levels of recent depressive symptomology (ie, total score of ≥10) on the PHQ-9. The intervention group had higher baseline scores on both the GAD-7 ( P =.02) and PHQ-9 ( P =.02) . No other statistically significant differences in baseline characteristics were found between the intervention and control groups ( Table 1 ).
a Variations in the total n values for each characteristic were due to missing responses. We used 2-sample t tests (2-tailed) to compare means, chi-square tests to compare proportions, and the Kruskal-Wallis test to test for differences in medians.
b GAD-7: 7-item General Anxiety Disorder scale.
c PHQ-9: 9-item Patient Health Questionnaire.
d USAUDIT-C: US Alcohol Use Disorders Identification Test–Consumption Scale.
e SWEMWS: Short Warwick-Edinburgh Mental Wellbeing Scale.
Among those in the intervention group (743/1489, 49.9%), 77.1% (573/743) accessed at least 1 app component during the 30-day study period. More specifically, 73.8% (548/743) engaged in 1 or more chatbot activities, 21.8% (162/743) accessed the community component, 27.9% (207/743) completed the services survey, and 17.2% (128/743) accessed a peer coach.
For the 3 primary end points (GAD-7, PHQ-9, and USAUDIT-C scores), a global Wald test with the null hypothesis of no treatment difference in all primary end points between the control and treatment groups was conducted using a marginal multivariate analysis of covariance model for correlated data. This multivariate test of overall group differences was statistically significant (Hotelling test=0.02; P =.001; Table 2 ), indicating that there were group differences in at least 1 of the primary outcomes. We then tested each of the primary outcomes using a GLMM for clustered measures, adjusting for baseline values with the randomization block as the clustering variable, and applied a sequential Hochberg correction method [ 30 ] to control the overall familywise error rate at .05 when testing the hypothesis for each individual primary end point. The results of these GLMMs indicated that participants in the intervention group had significantly greater reductions in anxiety (adjusted group mean difference=−0.85, 95% CI −1.27 to −0.42; P <.001; Cohen d =−0.17, 95% CI −0.26 to −0.09) and depressive (adjusted group mean difference=−0.63, 95% CI −1.08 to −0.17; P =.007; Cohen d =−0.11, 95% CI −0.19 to −0.03) symptoms than those in the control group ( Table 2 ). Although participants in the intervention group also demonstrated a greater reduction in alcohol risk scores on the USAUDIT-C, this difference was not statistically significant (adjusted group mean difference=−0.13, 95% CI −0.34 to 0.08; P =.23).
We conducted the following analysis to quantify the robustness of primary findings to the assumption of data MAR. First, baseline covariates potentially predictive of missing data were added to the GLMM outcome models, and the intervention effect estimates remained similar and yielded qualitatively similar P values ( Table 2 , last 2 columns). Second, selection models were used that permit the missingness probability to depend on the unobserved outcome values after conditioning on the observed data, after which we computed the index of local sensitivity to nonignorability [ 34 ]. The index of local sensitivity to nonignorability analysis results are reported in Table 3 . The ISNI/SD (ISNI divided by SD) column in Table 3 estimates the change in intervention effect estimates for a moderate size of nonrandom missingness, where a 1-SD (SD of the outcome) increase in the outcome is associated with an e 1 =2.7–fold increase in the odds of being observed conditioned on the same values of the observed predictors for missingness. For such moderately sized nonrandom missingness, the changes in the intervention effect estimates were small for both GAD-7 and PHQ-9 scores ( Table 3 ). The MinNI column in Table 3 computes the minimum magnitude of nonignorable missingness needed for substantial sensitivity such that the selection bias due to data missing not at random is of the same size as the SE. The smaller the value of the minimum nonignorable missingness, the greater the sensitivity. A minimum nonignorable missingness of 1 is suggested as the cutoff value for important sensitivity [ 32 ]. The minimum nonignorable missingness values for both the GAD-7 and PHQ-9 far exceeded 1, indicating that no sensitivity to potential missingness not at random was present for the primary findings. Table 3 shows that both the control and intervention groups had moderate and comparable missing data percentages for both the GAD-7 and PHQ-9, which can explain why our analysis results were insensitive to the MAR assumption.
a Adjusting for block numbers and baseline outcome values.
b Global test: P =.001.
c Adjusting for age, gender, student status, race, substance use at baseline, block numbers, and baseline outcome values.
d Global test: P =.003.
e GAD-7: 7-item General Anxiety Disorder scale.
h N/A: not applicable.
k PHQ-9: 9-item Patient Health Questionnaire.
p USAUDIT-C: US Alcohol Use Disorders Identification Test–Consumption Scale.
a In the missing data percentage (n m /n), n m is the number of participants not completing the outcome assessment at 30 days, and n is the number of participants in the intention-to-treat sample.
b ISNI: index of sensitivity to nonignorability; SD refers to the SD of the outcomes.
c MinNI: minimum magnitude of nonignorable missingness. A MinNI of <1 indicates sensitivity to data missing not at random, and a MinNI of >1 indicates no sensitivity to data missing not at random.
d GAD-7: 7-item General Anxiety Disorder scale.
e PHQ-9: 9-item Patient Health Questionnaire.
f USAUDIT-C: US Alcohol Use Disorders Identification Test–Consumption Scale.
The GLMM for clustered measures with the randomization block as the clustering variable was also used to test for differences between the intervention and control groups on secondary outcomes without any adjustment for multiple testing. The results of these GLMMs indicated that ( Table 4 ), compared to those in the control group, participants in the intervention group had significantly greater improvements in mental well-being (adjusted mean difference=0.73, 95% CI 0.35-1.11; P <.001; Cohen d =0.17, 95% CI 0.08-0.26) and were significantly associated with a 20% reduction in their frequency of cannabis use (IRR=0.80, 95% CI 0.66-0.96; P =.02) and a 13% reduction in the typical number of drinks consumed when drinking (IRR=0.87, 95% CI 0.77-0.98; P =.03). No significant differences were found in frequency of binge drinking (IRR=0.98, 95% CI 0.86-1.13; P =.83), frequency of drinking (IRR=0.97, 95% CI 0.88-1.06; P =.48), or frequency of any opioid use (IRR=0.62, 95% CI 0.16-2.31; P =.48). The impact of the intervention on nonmedical stimulant use could not be assessed due to the small number of nonmedical stimulant users at baseline and follow-up.
a IRR: incidence rate ratio.
b Adjusted difference.
d N/A: not applicable.
g Refer to Multimedia Appendix 3 for the category definitions for each secondary measure.
Principal Findings
Minder was codeveloped with students to provide them with a set of self-guided tools to manage their mental health and substance use. In this study, we found that participants in the intervention group who had access to the Minder app reported significantly greater average reductions in symptoms of anxiety (GAD-7) and depression (PHQ-9) than those in the control group. This finding aligns with the presentation of “stress and anxiety” and “sadness” as key topic areas within the Minder app and each topic having its own dedicated content island. Although these findings showed small effects on average in our sample, this may be related to the fact that, at baseline, only 38.9% (576/1481) of the participants had moderate or greater levels of anxiety and 43.8% (645/1474) had moderate or greater levels of depressive symptoms. Many participants in our sample reported only mild or no symptoms of anxiety or depression, which may have contributed to the finding of a small effect on average. Recent reviews on the effects of smartphone apps on anxiety and depression have found larger effects than those reported in this study; however, most studies recruit participants with clinical-level problems [ 12 , 13 ]. A meta-analysis examining internet interventions for university students found small effect sizes for anxiety and depression [ 36 ]. The Minder intervention group also demonstrated significant improvements in mental well-being in our analysis of secondary outcomes, which reflects a more general positive domain of mental health that may be more relevant to students without existing mental health concerns.
One of the key decisions in this trial was to include students with few or no symptoms of anxiety or depression. Providing interventions to nonclinical populations makes them more accessible to the large proportion of the population who may not meet clinical diagnostic criteria but may still experience occasional mental health challenges that can be addressed using existing tools (eg, via app-based cognitive behavioral therapy) [ 37 ]. In addition, this study used an ITT analysis that included all participants who were randomized regardless of whether they used the intervention. There was also no minimum amount of required content for participants to complete, nor were they remunerated for their use of the app. This pragmatic approach provided an approximation of the average effect of Minder on mental health and substance use outcomes in a university population if it were to be made available to everyone. As outlined in our study protocol, we plan to complement these findings with additional secondary analyses to examine the impact of Minder in subgroups of participants defined by the extent of their app use and baseline mental health and sociodemographic characteristics.
Although we did not find evidence of an effect on overall alcohol consumption risk in our primary outcome analyses of the USAUDIT-C, we did find significant reductions in cannabis use frequency and the typical number of alcoholic drinks consumed in a drinking session in our analysis of secondary outcomes. Reduction in number of drinks consumed when drinking and reducing alcohol-related harms were a main focus of the alcohol intervention content in the Minder app. For example, there was an activity in the app that encouraged users to set a goal for how many drinks they would consume in a drinking session and then track the number consumed in real time using the app. In addition, psychoeducational and motivational interviewing content focused on reducing harms associated with drinking, including following lower-risk drinking guidelines or cutting back on alcohol use. Similarly, cannabis use content focused on following lower-risk cannabis use guidelines, such as reducing the frequency of use to once a week or weekends [ 38 ]. We did not find significant reductions in measures of opioid use or nonmedical stimulant use frequency. However, there were low numbers of participants who used these substances in the study, particularly nonmedical stimulants. This finding may be related to the way in which the current Minder app allows users to select whatever content they think is most relevant to them regardless of their current mental health or substance use status. Substance use is often perceived by students to be higher than it actually is among their peers, thus normalizing its use on university campuses [ 39 , 40 ]. As a result, students may not be as motivated to address substance use compared to other aspects of their lives in which they may be experiencing distress. Previous studies have found that attitudes and norms surrounding drinking predict alcohol use behaviors among college students [ 41 , 42 ]. Addressing existing positive attitudes regarding commonly used substances (eg, alcohol and cannabis), as well as variations in norms such as for different genders [ 43 ], may be important in improving engagement with this content and tailoring the app to the needs of users. Future versions of the Minder app could include more nuanced approaches to address potentially harmful norms by providing tailored recommendations for substance use content within the app.
These findings are promising when considering the potential benefits of the Minder app as a tool in more comprehensive approaches to campus mental health that include early intervention and prevention. Given the extensive mental health needs of university students, stepped-care approaches have been identified as an efficient strategy to organize the delivery of campus mental health services in Canada [ 44 ]. The Minder intervention, which requires few resources (support is limited to online formats and provided by volunteer student coaches), could be readily integrated into such systems to support self-screening (via the existing services component and completion of the PHQ-9 and GAD-7) and the provision of immediate support and resources for students without higher levels of clinical concerns. It is also important to note that the Minder app was co-designed with students to connect users with the broader campus mental health systems through the Services component as well as with the greater student body using the Community component. In this way, it can be used to strengthen the connections between these existing systems and fill gaps in services, particularly in the area of prevention and treatment for mild to moderate symptomology.
A major strength of the Minder app is that it has been meaningfully codeveloped with university students and campus health care providers. Many mobile apps are not able to retain users after initial download and have low engagement rates overall [ 45 ]. Co-design processes have been identified as an effective means of ensuring that e-tools meet the needs of the end users they are trying to help [ 15 ]. The Minder app used an extensive codevelopment process that allowed for many improvements to be made with direct input from students and the clinicians currently supporting them [ 16 ]. The positive impact and low rates of loss to follow-up in this trial provide some support for the general acceptability of Minder . Previous studies on internet-based interventions for university students have also demonstrated their effectiveness in reducing mental health outcomes and that these interventions are generally acceptable to students; however, acceptability is often not reported [ 9 ].
Another strength of this study is the pragmatic trial design, which included a large nonclinical sample. Remuneration was only provided to participants for completion of the baseline and follow-up surveys, not for use of the intervention itself. Participants were also not told to use the app in any specific way or for any given amount of time when starting the study. Although this approach may have contributed to the finding of small average effects, it does increase the generalizability of our findings to other populations and provides an estimate of the impact of the app if it were made available to all students.
Limitations
Several limitations should be considered when interpreting the results of our study. As with many trials for online interventions, the participants were not blinded to what condition they were in, leading to a potential for placebo effects. However, control group participants did download the app and completed the surveys within it, which may have helped mitigate these effects on the trial. There were also several minor technical issues throughout the trial that may have impacted the participants’ experience with the app; however, these were resolved quickly by the research team. An explanation of these issues can be found in Multimedia Appendix 1 . There were some participants in the intervention group who did not use the app at all apart from completing the surveys. Given the ITT trial design, all randomized participants were included in the analysis; however, future analyses are planned to examine the effects of the intervention for those who actually engaged with the app content, along with the identification of subgroups that may benefit the most from this type of intervention.
Implications for Future Research
Further research will be conducted to better understand how to optimize the Minder intervention for different needs and additional populations. By better understanding who benefitted from the intervention and what content they used, we plan to make the app more personalized (including recommendations and features). In addition, future codevelopment processes will be needed to further improve app use and incorporation into existing systems of care. There were some participants who did not use the app outside the surveys, so trying to make the intervention more appealing for these users will be important. This may involve gamification strategies, refinement of content and enhancement of the chatbot using artificial intelligence tools, and the development of new features.
Conclusions
The Minder app was effective in reducing symptoms of anxiety and depression, with provisional support for increasing mental well-being and reducing the frequency of cannabis and alcohol use in a general population of university students. These findings support our use of a codevelopment approach and provide evidence of the potential of digital intervention tools such as Minder to support prevention and early intervention efforts for university students.
Acknowledgments
This work was supported by the Health Canada Substance Use and Addictions Program (arrangement: 1920-HQ-000069; University of British Columbia ID: F19-02914).
Conflicts of Interest
For the past 3 years, RCK has been a consultant for the Cambridge Health Alliance; Canandaigua Veterans Affairs Medical Center; Holmusk; Partners Healthcare, Inc; RallyPoint Networks, Inc; and Sage Therapeutics. He has stock options in Cerebral Inc, Mirah, PYM, Roga Sciences, and Verisense Health.
Technical issues and protocol changes.
Chatbot activity content overview.
Identified secondary outcomes with scoring.
CONSORT-eHEALTH checklist (V 1.6.1).
- Cunningham S, Duffy A. Investing in our future: importance of postsecondary student mental health research. Can J Psychiatry. Feb 2019;64(2):79-81. [ FREE Full text ] [ CrossRef ] [ Medline ]
- Chan V, Moore J, Derenne J, Fuchs DC. Transitional age youth and college mental health. Child Adolesc Psychiatr Clin N Am. Jul 2019;28(3):363-375. [ FREE Full text ] [ CrossRef ] [ Medline ]
- de Girolamo G, Dagani J, Purcell R, Cocchi A, McGorry PD. Age of onset of mental disorders and use of mental health services: needs, opportunities and obstacles. Epidemiol Psychiatr Sci. Mar 2012;21(1):47-57. [ CrossRef ] [ Medline ]
- Kessler RC, Amminger GP, Aguilar-Gaxiola S, Alonso J, Lee S, Ustün TB. Age of onset of mental disorders: a review of recent literature. Curr Opin Psychiatry. Jul 2007;20(4):359-364. [ FREE Full text ] [ CrossRef ] [ Medline ]
- Auerbach RP, Mortier P, Bruffaerts R, Alonso J, Benjet C, Cuijpers P, et al. WHO World Mental Health Surveys International College Student Project: prevalence and distribution of mental disorders. J Abnorm Psychol. Oct 2018;127(7):623-638. [ FREE Full text ] [ CrossRef ] [ Medline ]
- Duffy A, Keown-Stoneman C, Goodday S, Horrocks J, Lowe M, King N, et al. Predictors of mental health and academic outcomes in first-year university students: identifying prevention and early-intervention targets. BJPsych Open. May 08, 2020;6(3):e46. [ FREE Full text ] [ CrossRef ] [ Medline ]
- Eisenberg D, Hunt J, Speer N, Zivin K. Mental health service utilization among college students in the United States. J Nerv Ment Dis. May 2011;199(5):301-308. [ CrossRef ] [ Medline ]
- Ebert DD, Mortier P, Kaehlke F, Bruffaerts R, Baumeister H, Auerbach RP, et al. Barriers of mental health treatment utilization among first-year college students: first cross-national results from the WHO World Mental Health International College Student Initiative. Int J Methods Psychiatr Res. Jun 2019;28(2):e1782. [ FREE Full text ] [ CrossRef ] [ Medline ]
- Lattie EG, Adkins EC, Winquist N, Stiles-Shields C, Wafford QE, Graham AK. Digital mental health interventions for depression, anxiety, and enhancement of psychological well-being among college students: systematic review. J Med Internet Res. Jul 22, 2019;21(7):e12869. [ FREE Full text ] [ CrossRef ] [ Medline ]
- Carey KB, Scott-Sheldon LA, Elliott JC, Bolles JR, Carey MP. Computer-delivered interventions to reduce college student drinking: a meta-analysis. Addiction. Nov 2009;104(11):1807-1819. [ FREE Full text ] [ CrossRef ] [ Medline ]
- Newfoundland and Labrador stepped care 2.0© e-mental health demonstration project final report. Mental Health Commission of Canada. URL: https://www.mentalhealthcommission.ca/wp-content/uploads/drupal/2019-09/emental_health_report_eng_0.pdf [accessed 2023-09-28]
- Firth J, Torous J, Nicholas J, Carney R, Pratap A, Rosenbaum S, et al. The efficacy of smartphone-based mental health interventions for depressive symptoms: a meta-analysis of randomized controlled trials. World Psychiatry. Oct 2017;16(3):287-298. [ FREE Full text ] [ CrossRef ] [ Medline ]
- Firth J, Torous J, Nicholas J, Carney R, Rosenbaum S, Sarris J. Can smartphone mental health interventions reduce symptoms of anxiety? a meta-analysis of randomized controlled trials. J Affect Disord. Aug 15, 2017;218:15-22. [ FREE Full text ] [ CrossRef ] [ Medline ]
- Mei C, Nelson B, Hartmann J, Spooner R, McGorry PD. Transdiagnostic early intervention, prevention, and prediction in psychiatry. In: Personalized Psychiatry. London, UK. Academic Press; 2020;27-37.
- Torous J, Nicholas J, Larsen ME, Firth J, Christensen H. Clinical review of user engagement with mental health smartphone apps: evidence, theory and improvements. Evid Based Ment Health. Aug 2018;21(3):116-119. [ FREE Full text ] [ CrossRef ] [ Medline ]
- Vereschagin M, Wang AY, Leung C, Richardson CG, Hudec KL, Doan Q, et al. Co-developing tools to support student mental health and substance use: minder app development from conceptualization to realization. J Behav Cogn Ther. Mar 2023;33(1):35-49. [ CrossRef ]
- Wang AY, Vereschagin M, Richardson CG, Xie H, Hudec KL, Munthali RJ, et al. Evaluating the effectiveness of a codeveloped e-mental health intervention for university students: protocol for a randomized controlled trial. JMIR Res Protoc. Aug 30, 2023;12:e49364. [ FREE Full text ] [ CrossRef ] [ Medline ]
- Jones LB, Judkowicz C, Hudec KL, Munthali RJ, Prescivalli AP, Wang AY, et al. The World Mental Health International College Student Survey in Canada: protocol for a mental health and substance use trend study. JMIR Res Protoc. Jul 29, 2022;11(7):e35168. [ FREE Full text ] [ CrossRef ] [ Medline ]
- Randomisation and online databases for clinical trials. Sealed Envelope. URL: https://www.sealedenvelope.com/ [accessed 2023-09-28]
- Virk P, Arora R, Burt H, Gadermann A, Barbic S, Nelson M, et al. HEARTSMAP-U: adapting a psychosocial self-screening and resource navigation support tool for use by post-secondary students. Front Psychiatry. Feb 22, 2022;13:812965. [ FREE Full text ] [ CrossRef ] [ Medline ]
- Spitzer RL, Kroenke K, Williams JB, Löwe B. A brief measure for assessing generalized anxiety disorder: the GAD-7. Arch Intern Med. May 22, 2006;166(10):1092-1097. [ CrossRef ] [ Medline ]
- Kroenke K, Spitzer RL, Williams JB. The PHQ-9: validity of a brief depression severity measure. J Gen Intern Med. Sep 2001;16(9):606-613. [ FREE Full text ] [ CrossRef ] [ Medline ]
- Higgins-Biddle JC, Babor TF. A review of the Alcohol Use Disorders Identification Test (AUDIT), AUDIT-C, and USAUDIT for screening in the United States: past issues and future directions. Am J Drug Alcohol Abuse. 2018;44(6):578-586. [ FREE Full text ] [ CrossRef ] [ Medline ]
- Saunders JB, Aasland OG, Babor TF, de la Fuente JR, Grant M. Development of the alcohol use disorders identification test (AUDIT): WHO collaborative project on early detection of persons with harmful alcohol consumption--II. Addiction. Jun 1993;88(6):791-804. [ CrossRef ] [ Medline ]
- Stewart-Brown S, Tennant A, Tennant R, Platt S, Parkinson J, Weich S. Internal construct validity of the Warwick-Edinburgh Mental Well-being Scale (WEMWBS): a Rasch analysis using data from the Scottish Health education population survey. Health Qual Life Outcomes. Feb 19, 2009;7:15. [ FREE Full text ] [ CrossRef ] [ Medline ]
- Guo L, Qian Y, Xie H. Assessing complier average causal effects from longitudinal trials with multiple endpoints and treatment noncompliance: an application to a study of Arthritis Health Journal. Stat Med. Jun 15, 2022;41(13):2448-2465. [ FREE Full text ] [ CrossRef ] [ Medline ]
- O'Brien PC. Procedures for comparing samples with multiple endpoints. Biometrics. Dec 1984;40(4):1079-1087. [ CrossRef ]
- Pocock SJ, Geller NL, Tsiatis AA. The analysis of multiple endpoints in clinical trials. Biometrics. Sep 1987;43(3):487-498. [ CrossRef ]
- Teixeira-Pinto A, Siddique J, Gibbons R, Normand SL. Statistical approaches to modeling multiple outcomes in psychiatric studies. Psychiatr Ann. Jul 01, 2009;39(7):729-735. [ FREE Full text ] [ CrossRef ] [ Medline ]
- Hochberg Y. A sharper Bonferroni procedure for multiple tests of significance. Biometrika. Dec 1988;75(4):800-802. [ CrossRef ]
- Diggle P, Heagerty P, Liang KY, Zeger S. Analysis of Longitudinal Data. Oxford, UK. Oxford University Press; 2013.
- Jing B, Qian Y, Heitjan DF, Xie H. Tutorial: assessing the impact of nonignorable missingness on regression analysis using index of local sensitivity to nonignorability. Psychol Methods. Nov 16, 2023. (forthcoming). [ CrossRef ] [ Medline ]
- Xie H, Qian Y. Measuring the impact of nonignorability in panel data with non-monotone nonresponse. J Appl Econom. Jan 18, 2012;27(1):129-159. [ CrossRef ]
- Xie H, Gao W, Xing B, Heitjan DF, Hedeker D, Yuan C. Measuring the impact of nonignorable missingness using the R package isni. Comput Methods Programs Biomed. Oct 2018;164:207-220. [ FREE Full text ] [ CrossRef ] [ Medline ]
- Stata Corp. Stata statistical software: release 15.1. StataCorp LLC. 2017. URL: https://www.stata.com/stata15/ [accessed 2024-03-11]
- Harrer M, Adam SH, Baumeister H, Cuijpers P, Karyotaki E, Auerbach RP, et al. Internet interventions for mental health in university students: a systematic review and meta-analysis. Int J Methods Psychiatr Res. Jun 2019;28(2):e1759. [ FREE Full text ] [ CrossRef ] [ Medline ]
- Bakker D, Kazantzis N, Rickwood D, Rickard N. Mental health smartphone apps: review and evidence-based recommendations for future developments. JMIR Ment Health. Mar 01, 2016;3(1):e7. [ FREE Full text ] [ CrossRef ] [ Medline ]
- Canada's lower-risk cannabis use guidelines. Government of Canada. URL: https://www.canada.ca/en/health-canada/services/drugs-medication/cannabis/resources/lower-risk-cannabis-use-guidelines.html [accessed 2023-09-28]
- Baer JS, Stacy A, Larimer M. Biases in the perception of drinking norms among college students. J Stud Alcohol. Nov 1991;52(6):580-586. [ CrossRef ] [ Medline ]
- Lewis MA, Neighbors C. Gender-specific misperceptions of college student drinking norms. Psychol Addict Behav. Dec 2004;18(4):334-339. [ CrossRef ] [ Medline ]
- DiBello AM, Miller MB, Neighbors C, Reid A, Carey KB. The relative strength of attitudes versus perceived drinking norms as predictors of alcohol use. Addict Behav. May 2018;80:39-46. [ FREE Full text ] [ CrossRef ] [ Medline ]
- Neighbors C, Lee CM, Lewis MA, Fossos N, Larimer ME. Are social norms the best predictor of outcomes among heavy-drinking college students? J Stud Alcohol Drugs. Jul 2007;68(4):556-565. [ FREE Full text ] [ CrossRef ] [ Medline ]
- Hathaway A, Mostaghim A, Kolar K, Erickson PG, Osborne G. A nuanced view of normalisation: attitudes of cannabis non-users in a study of undergraduate students at three Canadian universities. Drugs Educ Prev Policy. 2016;23(3):238-246. [ CrossRef ]
- Duffy A, Saunders KE, Malhi GS, Patten S, Cipriani A, McNevin SH, et al. Mental health care for university students: a way forward? Lancet Psychiatry. Nov 2019;6(11):885-887. [ CrossRef ] [ Medline ]
- Baumel A, Muench F, Edan S, Kane JM. Objective user engagement with mental health apps: systematic search and panel-based usage analysis. J Med Internet Res. Sep 25, 2019;21(9):e14567. [ FREE Full text ] [ CrossRef ] [ Medline ]
Abbreviations
Edited by G Eysenbach; submitted 05.11.23; peer-reviewed by S Gordon; comments to author 30.11.23; revised version received 05.12.23; accepted 17.02.24; published 27.03.24.
©Melissa Vereschagin, Angel Y Wang, Chris G Richardson, Hui Xie, Richard J Munthali, Kristen L Hudec, Calista Leung, Katharine D Wojcik, Lonna Munro, Priyanka Halli, Ronald C Kessler, Daniel V Vigo. Originally published in the Journal of Medical Internet Research (https://www.jmir.org), 27.03.2024.
This is an open-access article distributed under the terms of the Creative Commons Attribution License (https://creativecommons.org/licenses/by/4.0/), which permits unrestricted use, distribution, and reproduction in any medium, provided the original work, first published in the Journal of Medical Internet Research, is properly cited. The complete bibliographic information, a link to the original publication on https://www.jmir.org/, as well as this copyright and license information must be included.
Help | Advanced Search
Computer Science > Computer Vision and Pattern Recognition
Title: mm1: methods, analysis & insights from multimodal llm pre-training.
Abstract: In this work, we discuss building performant Multimodal Large Language Models (MLLMs). In particular, we study the importance of various architecture components and data choices. Through careful and comprehensive ablations of the image encoder, the vision language connector, and various pre-training data choices, we identified several crucial design lessons. For example, we demonstrate that for large-scale multimodal pre-training using a careful mix of image-caption, interleaved image-text, and text-only data is crucial for achieving state-of-the-art (SOTA) few-shot results across multiple benchmarks, compared to other published pre-training results. Further, we show that the image encoder together with image resolution and the image token count has substantial impact, while the vision-language connector design is of comparatively negligible importance. By scaling up the presented recipe, we build MM1, a family of multimodal models up to 30B parameters, including both dense models and mixture-of-experts (MoE) variants, that are SOTA in pre-training metrics and achieve competitive performance after supervised fine-tuning on a range of established multimodal benchmarks. Thanks to large-scale pre-training, MM1 enjoys appealing properties such as enhanced in-context learning, and multi-image reasoning, enabling few-shot chain-of-thought prompting.
Submission history
Access paper:.
- Download PDF
- Other Formats
References & Citations
- Google Scholar
- Semantic Scholar
BibTeX formatted citation

Bibliographic and Citation Tools
Code, data and media associated with this article, recommenders and search tools.
- Institution
arXivLabs: experimental projects with community collaborators
arXivLabs is a framework that allows collaborators to develop and share new arXiv features directly on our website.
Both individuals and organizations that work with arXivLabs have embraced and accepted our values of openness, community, excellence, and user data privacy. arXiv is committed to these values and only works with partners that adhere to them.
Have an idea for a project that will add value for arXiv's community? Learn more about arXivLabs .
Have a language expert improve your writing
Run a free plagiarism check in 10 minutes, generate accurate citations for free.
- Knowledge Base
Methodology
- What Is Qualitative Research? | Methods & Examples
What Is Qualitative Research? | Methods & Examples
Published on June 19, 2020 by Pritha Bhandari . Revised on June 22, 2023.
Qualitative research involves collecting and analyzing non-numerical data (e.g., text, video, or audio) to understand concepts, opinions, or experiences. It can be used to gather in-depth insights into a problem or generate new ideas for research.
Qualitative research is the opposite of quantitative research , which involves collecting and analyzing numerical data for statistical analysis.
Qualitative research is commonly used in the humanities and social sciences, in subjects such as anthropology, sociology, education, health sciences, history, etc.
- How does social media shape body image in teenagers?
- How do children and adults interpret healthy eating in the UK?
- What factors influence employee retention in a large organization?
- How is anxiety experienced around the world?
- How can teachers integrate social issues into science curriculums?
Table of contents
Approaches to qualitative research, qualitative research methods, qualitative data analysis, advantages of qualitative research, disadvantages of qualitative research, other interesting articles, frequently asked questions about qualitative research.
Qualitative research is used to understand how people experience the world. While there are many approaches to qualitative research, they tend to be flexible and focus on retaining rich meaning when interpreting data.
Common approaches include grounded theory, ethnography , action research , phenomenological research, and narrative research. They share some similarities, but emphasize different aims and perspectives.
Note that qualitative research is at risk for certain research biases including the Hawthorne effect , observer bias , recall bias , and social desirability bias . While not always totally avoidable, awareness of potential biases as you collect and analyze your data can prevent them from impacting your work too much.
Prevent plagiarism. Run a free check.
Each of the research approaches involve using one or more data collection methods . These are some of the most common qualitative methods:
- Observations: recording what you have seen, heard, or encountered in detailed field notes.
- Interviews: personally asking people questions in one-on-one conversations.
- Focus groups: asking questions and generating discussion among a group of people.
- Surveys : distributing questionnaires with open-ended questions.
- Secondary research: collecting existing data in the form of texts, images, audio or video recordings, etc.
- You take field notes with observations and reflect on your own experiences of the company culture.
- You distribute open-ended surveys to employees across all the company’s offices by email to find out if the culture varies across locations.
- You conduct in-depth interviews with employees in your office to learn about their experiences and perspectives in greater detail.
Qualitative researchers often consider themselves “instruments” in research because all observations, interpretations and analyses are filtered through their own personal lens.
For this reason, when writing up your methodology for qualitative research, it’s important to reflect on your approach and to thoroughly explain the choices you made in collecting and analyzing the data.
Qualitative data can take the form of texts, photos, videos and audio. For example, you might be working with interview transcripts, survey responses, fieldnotes, or recordings from natural settings.
Most types of qualitative data analysis share the same five steps:
- Prepare and organize your data. This may mean transcribing interviews or typing up fieldnotes.
- Review and explore your data. Examine the data for patterns or repeated ideas that emerge.
- Develop a data coding system. Based on your initial ideas, establish a set of codes that you can apply to categorize your data.
- Assign codes to the data. For example, in qualitative survey analysis, this may mean going through each participant’s responses and tagging them with codes in a spreadsheet. As you go through your data, you can create new codes to add to your system if necessary.
- Identify recurring themes. Link codes together into cohesive, overarching themes.
There are several specific approaches to analyzing qualitative data. Although these methods share similar processes, they emphasize different concepts.
Qualitative research often tries to preserve the voice and perspective of participants and can be adjusted as new research questions arise. Qualitative research is good for:
- Flexibility
The data collection and analysis process can be adapted as new ideas or patterns emerge. They are not rigidly decided beforehand.
- Natural settings
Data collection occurs in real-world contexts or in naturalistic ways.
- Meaningful insights
Detailed descriptions of people’s experiences, feelings and perceptions can be used in designing, testing or improving systems or products.
- Generation of new ideas
Open-ended responses mean that researchers can uncover novel problems or opportunities that they wouldn’t have thought of otherwise.
Here's why students love Scribbr's proofreading services
Discover proofreading & editing
Researchers must consider practical and theoretical limitations in analyzing and interpreting their data. Qualitative research suffers from:
- Unreliability
The real-world setting often makes qualitative research unreliable because of uncontrolled factors that affect the data.
- Subjectivity
Due to the researcher’s primary role in analyzing and interpreting data, qualitative research cannot be replicated . The researcher decides what is important and what is irrelevant in data analysis, so interpretations of the same data can vary greatly.
- Limited generalizability
Small samples are often used to gather detailed data about specific contexts. Despite rigorous analysis procedures, it is difficult to draw generalizable conclusions because the data may be biased and unrepresentative of the wider population .
- Labor-intensive
Although software can be used to manage and record large amounts of text, data analysis often has to be checked or performed manually.
If you want to know more about statistics , methodology , or research bias , make sure to check out some of our other articles with explanations and examples.
- Chi square goodness of fit test
- Degrees of freedom
- Null hypothesis
- Discourse analysis
- Control groups
- Mixed methods research
- Non-probability sampling
- Quantitative research
- Inclusion and exclusion criteria
Research bias
- Rosenthal effect
- Implicit bias
- Cognitive bias
- Selection bias
- Negativity bias
- Status quo bias
Quantitative research deals with numbers and statistics, while qualitative research deals with words and meanings.
Quantitative methods allow you to systematically measure variables and test hypotheses . Qualitative methods allow you to explore concepts and experiences in more detail.
There are five common approaches to qualitative research :
- Grounded theory involves collecting data in order to develop new theories.
- Ethnography involves immersing yourself in a group or organization to understand its culture.
- Narrative research involves interpreting stories to understand how people make sense of their experiences and perceptions.
- Phenomenological research involves investigating phenomena through people’s lived experiences.
- Action research links theory and practice in several cycles to drive innovative changes.
Data collection is the systematic process by which observations or measurements are gathered in research. It is used in many different contexts by academics, governments, businesses, and other organizations.
There are various approaches to qualitative data analysis , but they all share five steps in common:
- Prepare and organize your data.
- Review and explore your data.
- Develop a data coding system.
- Assign codes to the data.
- Identify recurring themes.
The specifics of each step depend on the focus of the analysis. Some common approaches include textual analysis , thematic analysis , and discourse analysis .
Cite this Scribbr article
If you want to cite this source, you can copy and paste the citation or click the “Cite this Scribbr article” button to automatically add the citation to our free Citation Generator.
Bhandari, P. (2023, June 22). What Is Qualitative Research? | Methods & Examples. Scribbr. Retrieved March 28, 2024, from https://www.scribbr.com/methodology/qualitative-research/
Is this article helpful?
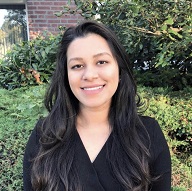
Pritha Bhandari
Other students also liked, qualitative vs. quantitative research | differences, examples & methods, how to do thematic analysis | step-by-step guide & examples, unlimited academic ai-proofreading.
✔ Document error-free in 5minutes ✔ Unlimited document corrections ✔ Specialized in correcting academic texts
Thank you for visiting nature.com. You are using a browser version with limited support for CSS. To obtain the best experience, we recommend you use a more up to date browser (or turn off compatibility mode in Internet Explorer). In the meantime, to ensure continued support, we are displaying the site without styles and JavaScript.
- View all journals
- My Account Login
- Explore content
- About the journal
- Publish with us
- Sign up for alerts
- Open access
- Published: 26 March 2024
Predicting and improving complex beer flavor through machine learning
- Michiel Schreurs ORCID: orcid.org/0000-0002-9449-5619 1 , 2 , 3 na1 ,
- Supinya Piampongsant 1 , 2 , 3 na1 ,
- Miguel Roncoroni ORCID: orcid.org/0000-0001-7461-1427 1 , 2 , 3 na1 ,
- Lloyd Cool ORCID: orcid.org/0000-0001-9936-3124 1 , 2 , 3 , 4 ,
- Beatriz Herrera-Malaver ORCID: orcid.org/0000-0002-5096-9974 1 , 2 , 3 ,
- Christophe Vanderaa ORCID: orcid.org/0000-0001-7443-5427 4 ,
- Florian A. Theßeling 1 , 2 , 3 ,
- Łukasz Kreft ORCID: orcid.org/0000-0001-7620-4657 5 ,
- Alexander Botzki ORCID: orcid.org/0000-0001-6691-4233 5 ,
- Philippe Malcorps 6 ,
- Luk Daenen 6 ,
- Tom Wenseleers ORCID: orcid.org/0000-0002-1434-861X 4 &
- Kevin J. Verstrepen ORCID: orcid.org/0000-0002-3077-6219 1 , 2 , 3
Nature Communications volume 15 , Article number: 2368 ( 2024 ) Cite this article
7171 Accesses
681 Altmetric
Metrics details
- Chemical engineering
- Gas chromatography
- Machine learning
- Metabolomics
- Taste receptors
The perception and appreciation of food flavor depends on many interacting chemical compounds and external factors, and therefore proves challenging to understand and predict. Here, we combine extensive chemical and sensory analyses of 250 different beers to train machine learning models that allow predicting flavor and consumer appreciation. For each beer, we measure over 200 chemical properties, perform quantitative descriptive sensory analysis with a trained tasting panel and map data from over 180,000 consumer reviews to train 10 different machine learning models. The best-performing algorithm, Gradient Boosting, yields models that significantly outperform predictions based on conventional statistics and accurately predict complex food features and consumer appreciation from chemical profiles. Model dissection allows identifying specific and unexpected compounds as drivers of beer flavor and appreciation. Adding these compounds results in variants of commercial alcoholic and non-alcoholic beers with improved consumer appreciation. Together, our study reveals how big data and machine learning uncover complex links between food chemistry, flavor and consumer perception, and lays the foundation to develop novel, tailored foods with superior flavors.
Similar content being viewed by others
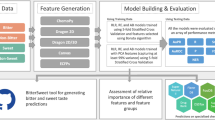
BitterSweet: Building machine learning models for predicting the bitter and sweet taste of small molecules
Rudraksh Tuwani, Somin Wadhwa & Ganesh Bagler
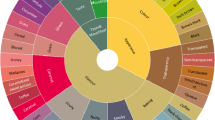
Sensory lexicon and aroma volatiles analysis of brewing malt
Xiaoxia Su, Miao Yu, … Tianyi Du
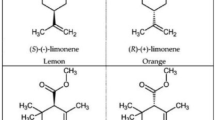
Predicting odor from molecular structure: a multi-label classification approach
Kushagra Saini & Venkatnarayan Ramanathan
Introduction
Predicting and understanding food perception and appreciation is one of the major challenges in food science. Accurate modeling of food flavor and appreciation could yield important opportunities for both producers and consumers, including quality control, product fingerprinting, counterfeit detection, spoilage detection, and the development of new products and product combinations (food pairing) 1 , 2 , 3 , 4 , 5 , 6 . Accurate models for flavor and consumer appreciation would contribute greatly to our scientific understanding of how humans perceive and appreciate flavor. Moreover, accurate predictive models would also facilitate and standardize existing food assessment methods and could supplement or replace assessments by trained and consumer tasting panels, which are variable, expensive and time-consuming 7 , 8 , 9 . Lastly, apart from providing objective, quantitative, accurate and contextual information that can help producers, models can also guide consumers in understanding their personal preferences 10 .
Despite the myriad of applications, predicting food flavor and appreciation from its chemical properties remains a largely elusive goal in sensory science, especially for complex food and beverages 11 , 12 . A key obstacle is the immense number of flavor-active chemicals underlying food flavor. Flavor compounds can vary widely in chemical structure and concentration, making them technically challenging and labor-intensive to quantify, even in the face of innovations in metabolomics, such as non-targeted metabolic fingerprinting 13 , 14 . Moreover, sensory analysis is perhaps even more complicated. Flavor perception is highly complex, resulting from hundreds of different molecules interacting at the physiochemical and sensorial level. Sensory perception is often non-linear, characterized by complex and concentration-dependent synergistic and antagonistic effects 15 , 16 , 17 , 18 , 19 , 20 , 21 that are further convoluted by the genetics, environment, culture and psychology of consumers 22 , 23 , 24 . Perceived flavor is therefore difficult to measure, with problems of sensitivity, accuracy, and reproducibility that can only be resolved by gathering sufficiently large datasets 25 . Trained tasting panels are considered the prime source of quality sensory data, but require meticulous training, are low throughput and high cost. Public databases containing consumer reviews of food products could provide a valuable alternative, especially for studying appreciation scores, which do not require formal training 25 . Public databases offer the advantage of amassing large amounts of data, increasing the statistical power to identify potential drivers of appreciation. However, public datasets suffer from biases, including a bias in the volunteers that contribute to the database, as well as confounding factors such as price, cult status and psychological conformity towards previous ratings of the product.
Classical multivariate statistics and machine learning methods have been used to predict flavor of specific compounds by, for example, linking structural properties of a compound to its potential biological activities or linking concentrations of specific compounds to sensory profiles 1 , 26 . Importantly, most previous studies focused on predicting organoleptic properties of single compounds (often based on their chemical structure) 27 , 28 , 29 , 30 , 31 , 32 , 33 , thus ignoring the fact that these compounds are present in a complex matrix in food or beverages and excluding complex interactions between compounds. Moreover, the classical statistics commonly used in sensory science 34 , 35 , 36 , 37 , 38 , 39 require a large sample size and sufficient variance amongst predictors to create accurate models. They are not fit for studying an extensive set of hundreds of interacting flavor compounds, since they are sensitive to outliers, have a high tendency to overfit and are less suited for non-linear and discontinuous relationships 40 .
In this study, we combine extensive chemical analyses and sensory data of a set of different commercial beers with machine learning approaches to develop models that predict taste, smell, mouthfeel and appreciation from compound concentrations. Beer is particularly suited to model the relationship between chemistry, flavor and appreciation. First, beer is a complex product, consisting of thousands of flavor compounds that partake in complex sensory interactions 41 , 42 , 43 . This chemical diversity arises from the raw materials (malt, yeast, hops, water and spices) and biochemical conversions during the brewing process (kilning, mashing, boiling, fermentation, maturation and aging) 44 , 45 . Second, the advent of the internet saw beer consumers embrace online review platforms, such as RateBeer (ZX Ventures, Anheuser-Busch InBev SA/NV) and BeerAdvocate (Next Glass, inc.). In this way, the beer community provides massive data sets of beer flavor and appreciation scores, creating extraordinarily large sensory databases to complement the analyses of our professional sensory panel. Specifically, we characterize over 200 chemical properties of 250 commercial beers, spread across 22 beer styles, and link these to the descriptive sensory profiling data of a 16-person in-house trained tasting panel and data acquired from over 180,000 public consumer reviews. These unique and extensive datasets enable us to train a suite of machine learning models to predict flavor and appreciation from a beer’s chemical profile. Dissection of the best-performing models allows us to pinpoint specific compounds as potential drivers of beer flavor and appreciation. Follow-up experiments confirm the importance of these compounds and ultimately allow us to significantly improve the flavor and appreciation of selected commercial beers. Together, our study represents a significant step towards understanding complex flavors and reinforces the value of machine learning to develop and refine complex foods. In this way, it represents a stepping stone for further computer-aided food engineering applications 46 .
To generate a comprehensive dataset on beer flavor, we selected 250 commercial Belgian beers across 22 different beer styles (Supplementary Fig. S1 ). Beers with ≤ 4.2% alcohol by volume (ABV) were classified as non-alcoholic and low-alcoholic. Blonds and Tripels constitute a significant portion of the dataset (12.4% and 11.2%, respectively) reflecting their presence on the Belgian beer market and the heterogeneity of beers within these styles. By contrast, lager beers are less diverse and dominated by a handful of brands. Rare styles such as Brut or Faro make up only a small fraction of the dataset (2% and 1%, respectively) because fewer of these beers are produced and because they are dominated by distinct characteristics in terms of flavor and chemical composition.
Extensive analysis identifies relationships between chemical compounds in beer
For each beer, we measured 226 different chemical properties, including common brewing parameters such as alcohol content, iso-alpha acids, pH, sugar concentration 47 , and over 200 flavor compounds (Methods, Supplementary Table S1 ). A large portion (37.2%) are terpenoids arising from hopping, responsible for herbal and fruity flavors 16 , 48 . A second major category are yeast metabolites, such as esters and alcohols, that result in fruity and solvent notes 48 , 49 , 50 . Other measured compounds are primarily derived from malt, or other microbes such as non- Saccharomyces yeasts and bacteria (‘wild flora’). Compounds that arise from spices or staling are labeled under ‘Others’. Five attributes (caloric value, total acids and total ester, hop aroma and sulfur compounds) are calculated from multiple individually measured compounds.
As a first step in identifying relationships between chemical properties, we determined correlations between the concentrations of the compounds (Fig. 1 , upper panel, Supplementary Data 1 and 2 , and Supplementary Fig. S2 . For the sake of clarity, only a subset of the measured compounds is shown in Fig. 1 ). Compounds of the same origin typically show a positive correlation, while absence of correlation hints at parameters varying independently. For example, the hop aroma compounds citronellol, and alpha-terpineol show moderate correlations with each other (Spearman’s rho=0.39 and 0.57), but not with the bittering hop component iso-alpha acids (Spearman’s rho=0.16 and −0.07). This illustrates how brewers can independently modify hop aroma and bitterness by selecting hop varieties and dosage time. If hops are added early in the boiling phase, chemical conversions increase bitterness while aromas evaporate, conversely, late addition of hops preserves aroma but limits bitterness 51 . Similarly, hop-derived iso-alpha acids show a strong anti-correlation with lactic acid and acetic acid, likely reflecting growth inhibition of lactic acid and acetic acid bacteria, or the consequent use of fewer hops in sour beer styles, such as West Flanders ales and Fruit beers, that rely on these bacteria for their distinct flavors 52 . Finally, yeast-derived esters (ethyl acetate, ethyl decanoate, ethyl hexanoate, ethyl octanoate) and alcohols (ethanol, isoamyl alcohol, isobutanol, and glycerol), correlate with Spearman coefficients above 0.5, suggesting that these secondary metabolites are correlated with the yeast genetic background and/or fermentation parameters and may be difficult to influence individually, although the choice of yeast strain may offer some control 53 .
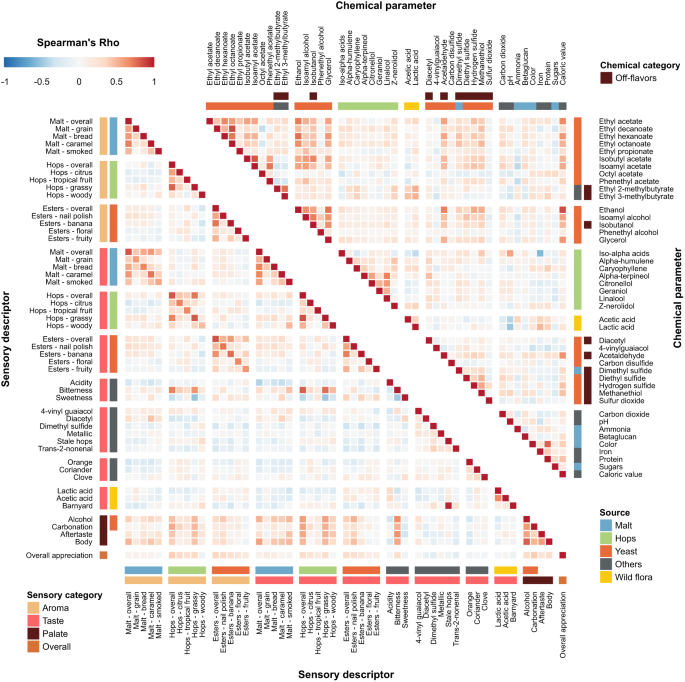
Spearman rank correlations are shown. Descriptors are grouped according to their origin (malt (blue), hops (green), yeast (red), wild flora (yellow), Others (black)), and sensory aspect (aroma, taste, palate, and overall appreciation). Please note that for the chemical compounds, for the sake of clarity, only a subset of the total number of measured compounds is shown, with an emphasis on the key compounds for each source. For more details, see the main text and Methods section. Chemical data can be found in Supplementary Data 1 , correlations between all chemical compounds are depicted in Supplementary Fig. S2 and correlation values can be found in Supplementary Data 2 . See Supplementary Data 4 for sensory panel assessments and Supplementary Data 5 for correlation values between all sensory descriptors.
Interestingly, different beer styles show distinct patterns for some flavor compounds (Supplementary Fig. S3 ). These observations agree with expectations for key beer styles, and serve as a control for our measurements. For instance, Stouts generally show high values for color (darker), while hoppy beers contain elevated levels of iso-alpha acids, compounds associated with bitter hop taste. Acetic and lactic acid are not prevalent in most beers, with notable exceptions such as Kriek, Lambic, Faro, West Flanders ales and Flanders Old Brown, which use acid-producing bacteria ( Lactobacillus and Pediococcus ) or unconventional yeast ( Brettanomyces ) 54 , 55 . Glycerol, ethanol and esters show similar distributions across all beer styles, reflecting their common origin as products of yeast metabolism during fermentation 45 , 53 . Finally, low/no-alcohol beers contain low concentrations of glycerol and esters. This is in line with the production process for most of the low/no-alcohol beers in our dataset, which are produced through limiting fermentation or by stripping away alcohol via evaporation or dialysis, with both methods having the unintended side-effect of reducing the amount of flavor compounds in the final beer 56 , 57 .
Besides expected associations, our data also reveals less trivial associations between beer styles and specific parameters. For example, geraniol and citronellol, two monoterpenoids responsible for citrus, floral and rose flavors and characteristic of Citra hops, are found in relatively high amounts in Christmas, Saison, and Brett/co-fermented beers, where they may originate from terpenoid-rich spices such as coriander seeds instead of hops 58 .
Tasting panel assessments reveal sensorial relationships in beer
To assess the sensory profile of each beer, a trained tasting panel evaluated each of the 250 beers for 50 sensory attributes, including different hop, malt and yeast flavors, off-flavors and spices. Panelists used a tasting sheet (Supplementary Data 3 ) to score the different attributes. Panel consistency was evaluated by repeating 12 samples across different sessions and performing ANOVA. In 95% of cases no significant difference was found across sessions ( p > 0.05), indicating good panel consistency (Supplementary Table S2 ).
Aroma and taste perception reported by the trained panel are often linked (Fig. 1 , bottom left panel and Supplementary Data 4 and 5 ), with high correlations between hops aroma and taste (Spearman’s rho=0.83). Bitter taste was found to correlate with hop aroma and taste in general (Spearman’s rho=0.80 and 0.69), and particularly with “grassy” noble hops (Spearman’s rho=0.75). Barnyard flavor, most often associated with sour beers, is identified together with stale hops (Spearman’s rho=0.97) that are used in these beers. Lactic and acetic acid, which often co-occur, are correlated (Spearman’s rho=0.66). Interestingly, sweetness and bitterness are anti-correlated (Spearman’s rho = −0.48), confirming the hypothesis that they mask each other 59 , 60 . Beer body is highly correlated with alcohol (Spearman’s rho = 0.79), and overall appreciation is found to correlate with multiple aspects that describe beer mouthfeel (alcohol, carbonation; Spearman’s rho= 0.32, 0.39), as well as with hop and ester aroma intensity (Spearman’s rho=0.39 and 0.35).
Similar to the chemical analyses, sensorial analyses confirmed typical features of specific beer styles (Supplementary Fig. S4 ). For example, sour beers (Faro, Flanders Old Brown, Fruit beer, Kriek, Lambic, West Flanders ale) were rated acidic, with flavors of both acetic and lactic acid. Hoppy beers were found to be bitter and showed hop-associated aromas like citrus and tropical fruit. Malt taste is most detected among scotch, stout/porters, and strong ales, while low/no-alcohol beers, which often have a reputation for being ‘worty’ (reminiscent of unfermented, sweet malt extract) appear in the middle. Unsurprisingly, hop aromas are most strongly detected among hoppy beers. Like its chemical counterpart (Supplementary Fig. S3 ), acidity shows a right-skewed distribution, with the most acidic beers being Krieks, Lambics, and West Flanders ales.
Tasting panel assessments of specific flavors correlate with chemical composition
We find that the concentrations of several chemical compounds strongly correlate with specific aroma or taste, as evaluated by the tasting panel (Fig. 2 , Supplementary Fig. S5 , Supplementary Data 6 ). In some cases, these correlations confirm expectations and serve as a useful control for data quality. For example, iso-alpha acids, the bittering compounds in hops, strongly correlate with bitterness (Spearman’s rho=0.68), while ethanol and glycerol correlate with tasters’ perceptions of alcohol and body, the mouthfeel sensation of fullness (Spearman’s rho=0.82/0.62 and 0.72/0.57 respectively) and darker color from roasted malts is a good indication of malt perception (Spearman’s rho=0.54).
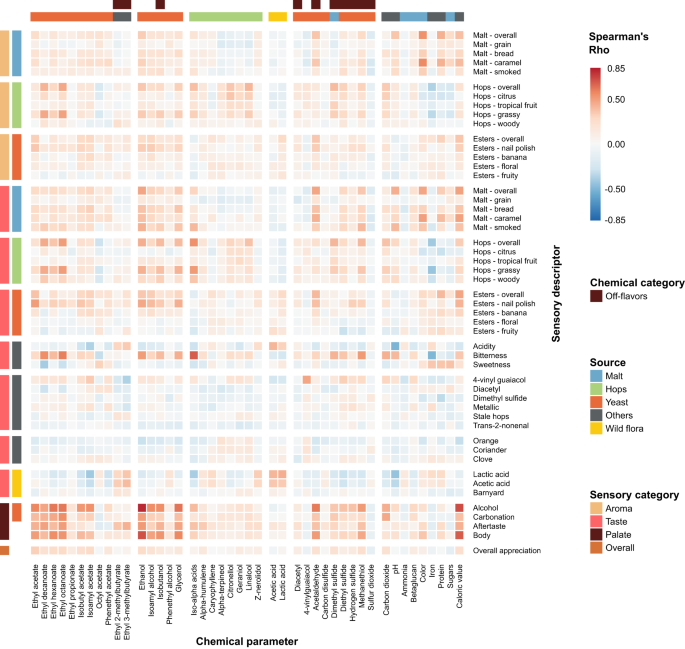
Heatmap colors indicate Spearman’s Rho. Axes are organized according to sensory categories (aroma, taste, mouthfeel, overall), chemical categories and chemical sources in beer (malt (blue), hops (green), yeast (red), wild flora (yellow), Others (black)). See Supplementary Data 6 for all correlation values.
Interestingly, for some relationships between chemical compounds and perceived flavor, correlations are weaker than expected. For example, the rose-smelling phenethyl acetate only weakly correlates with floral aroma. This hints at more complex relationships and interactions between compounds and suggests a need for a more complex model than simple correlations. Lastly, we uncovered unexpected correlations. For instance, the esters ethyl decanoate and ethyl octanoate appear to correlate slightly with hop perception and bitterness, possibly due to their fruity flavor. Iron is anti-correlated with hop aromas and bitterness, most likely because it is also anti-correlated with iso-alpha acids. This could be a sign of metal chelation of hop acids 61 , given that our analyses measure unbound hop acids and total iron content, or could result from the higher iron content in dark and Fruit beers, which typically have less hoppy and bitter flavors 62 .
Public consumer reviews complement expert panel data
To complement and expand the sensory data of our trained tasting panel, we collected 180,000 reviews of our 250 beers from the online consumer review platform RateBeer. This provided numerical scores for beer appearance, aroma, taste, palate, overall quality as well as the average overall score.
Public datasets are known to suffer from biases, such as price, cult status and psychological conformity towards previous ratings of a product. For example, prices correlate with appreciation scores for these online consumer reviews (rho=0.49, Supplementary Fig. S6 ), but not for our trained tasting panel (rho=0.19). This suggests that prices affect consumer appreciation, which has been reported in wine 63 , while blind tastings are unaffected. Moreover, we observe that some beer styles, like lagers and non-alcoholic beers, generally receive lower scores, reflecting that online reviewers are mostly beer aficionados with a preference for specialty beers over lager beers. In general, we find a modest correlation between our trained panel’s overall appreciation score and the online consumer appreciation scores (Fig. 3 , rho=0.29). Apart from the aforementioned biases in the online datasets, serving temperature, sample freshness and surroundings, which are all tightly controlled during the tasting panel sessions, can vary tremendously across online consumers and can further contribute to (among others, appreciation) differences between the two categories of tasters. Importantly, in contrast to the overall appreciation scores, for many sensory aspects the results from the professional panel correlated well with results obtained from RateBeer reviews. Correlations were highest for features that are relatively easy to recognize even for untrained tasters, like bitterness, sweetness, alcohol and malt aroma (Fig. 3 and below).
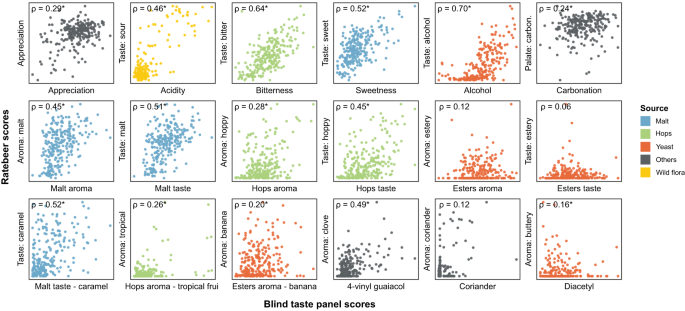
RateBeer text mining results can be found in Supplementary Data 7 . Rho values shown are Spearman correlation values, with asterisks indicating significant correlations ( p < 0.05, two-sided). All p values were smaller than 0.001, except for Esters aroma (0.0553), Esters taste (0.3275), Esters aroma—banana (0.0019), Coriander (0.0508) and Diacetyl (0.0134).
Besides collecting consumer appreciation from these online reviews, we developed automated text analysis tools to gather additional data from review texts (Supplementary Data 7 ). Processing review texts on the RateBeer database yielded comparable results to the scores given by the trained panel for many common sensory aspects, including acidity, bitterness, sweetness, alcohol, malt, and hop tastes (Fig. 3 ). This is in line with what would be expected, since these attributes require less training for accurate assessment and are less influenced by environmental factors such as temperature, serving glass and odors in the environment. Consumer reviews also correlate well with our trained panel for 4-vinyl guaiacol, a compound associated with a very characteristic aroma. By contrast, correlations for more specific aromas like ester, coriander or diacetyl are underrepresented in the online reviews, underscoring the importance of using a trained tasting panel and standardized tasting sheets with explicit factors to be scored for evaluating specific aspects of a beer. Taken together, our results suggest that public reviews are trustworthy for some, but not all, flavor features and can complement or substitute taste panel data for these sensory aspects.
Models can predict beer sensory profiles from chemical data
The rich datasets of chemical analyses, tasting panel assessments and public reviews gathered in the first part of this study provided us with a unique opportunity to develop predictive models that link chemical data to sensorial features. Given the complexity of beer flavor, basic statistical tools such as correlations or linear regression may not always be the most suitable for making accurate predictions. Instead, we applied different machine learning models that can model both simple linear and complex interactive relationships. Specifically, we constructed a set of regression models to predict (a) trained panel scores for beer flavor and quality and (b) public reviews’ appreciation scores from beer chemical profiles. We trained and tested 10 different models (Methods), 3 linear regression-based models (simple linear regression with first-order interactions (LR), lasso regression with first-order interactions (Lasso), partial least squares regressor (PLSR)), 5 decision tree models (AdaBoost regressor (ABR), extra trees (ET), gradient boosting regressor (GBR), random forest (RF) and XGBoost regressor (XGBR)), 1 support vector regression (SVR), and 1 artificial neural network (ANN) model.
To compare the performance of our machine learning models, the dataset was randomly split into a training and test set, stratified by beer style. After a model was trained on data in the training set, its performance was evaluated on its ability to predict the test dataset obtained from multi-output models (based on the coefficient of determination, see Methods). Additionally, individual-attribute models were ranked per descriptor and the average rank was calculated, as proposed by Korneva et al. 64 . Importantly, both ways of evaluating the models’ performance agreed in general. Performance of the different models varied (Table 1 ). It should be noted that all models perform better at predicting RateBeer results than results from our trained tasting panel. One reason could be that sensory data is inherently variable, and this variability is averaged out with the large number of public reviews from RateBeer. Additionally, all tree-based models perform better at predicting taste than aroma. Linear models (LR) performed particularly poorly, with negative R 2 values, due to severe overfitting (training set R 2 = 1). Overfitting is a common issue in linear models with many parameters and limited samples, especially with interaction terms further amplifying the number of parameters. L1 regularization (Lasso) successfully overcomes this overfitting, out-competing multiple tree-based models on the RateBeer dataset. Similarly, the dimensionality reduction of PLSR avoids overfitting and improves performance, to some extent. Still, tree-based models (ABR, ET, GBR, RF and XGBR) show the best performance, out-competing the linear models (LR, Lasso, PLSR) commonly used in sensory science 65 .
GBR models showed the best overall performance in predicting sensory responses from chemical information, with R 2 values up to 0.75 depending on the predicted sensory feature (Supplementary Table S4 ). The GBR models predict consumer appreciation (RateBeer) better than our trained panel’s appreciation (R 2 value of 0.67 compared to R 2 value of 0.09) (Supplementary Table S3 and Supplementary Table S4 ). ANN models showed intermediate performance, likely because neural networks typically perform best with larger datasets 66 . The SVR shows intermediate performance, mostly due to the weak predictions of specific attributes that lower the overall performance (Supplementary Table S4 ).
Model dissection identifies specific, unexpected compounds as drivers of consumer appreciation
Next, we leveraged our models to infer important contributors to sensory perception and consumer appreciation. Consumer preference is a crucial sensory aspects, because a product that shows low consumer appreciation scores often does not succeed commercially 25 . Additionally, the requirement for a large number of representative evaluators makes consumer trials one of the more costly and time-consuming aspects of product development. Hence, a model for predicting chemical drivers of overall appreciation would be a welcome addition to the available toolbox for food development and optimization.
Since GBR models on our RateBeer dataset showed the best overall performance, we focused on these models. Specifically, we used two approaches to identify important contributors. First, rankings of the most important predictors for each sensorial trait in the GBR models were obtained based on impurity-based feature importance (mean decrease in impurity). High-ranked parameters were hypothesized to be either the true causal chemical properties underlying the trait, to correlate with the actual causal properties, or to take part in sensory interactions affecting the trait 67 (Fig. 4A ). In a second approach, we used SHAP 68 to determine which parameters contributed most to the model for making predictions of consumer appreciation (Fig. 4B ). SHAP calculates parameter contributions to model predictions on a per-sample basis, which can be aggregated into an importance score.
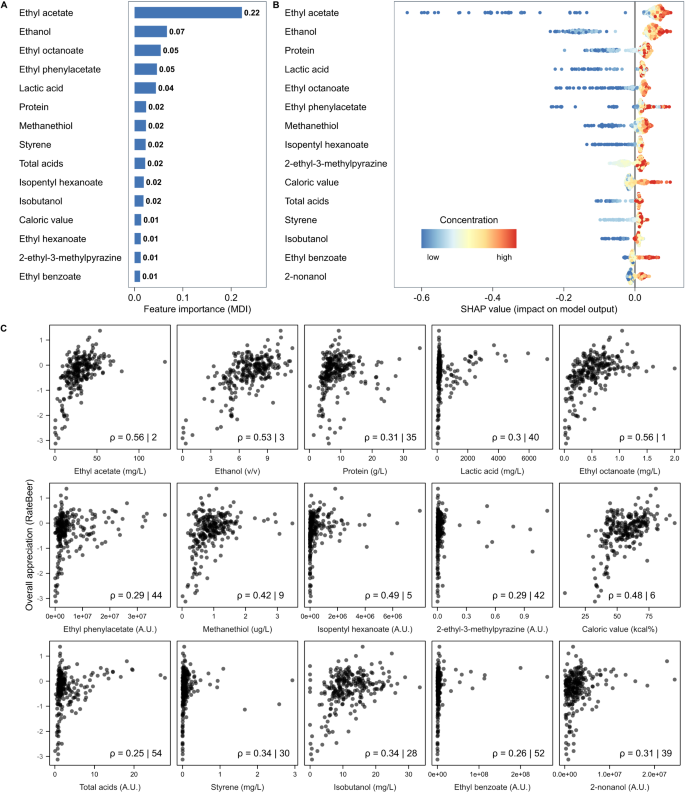
A The impurity-based feature importance (mean deviance in impurity, MDI) calculated from the Gradient Boosting Regression (GBR) model predicting RateBeer appreciation scores. The top 15 highest ranked chemical properties are shown. B SHAP summary plot for the top 15 parameters contributing to our GBR model. Each point on the graph represents a sample from our dataset. The color represents the concentration of that parameter, with bluer colors representing low values and redder colors representing higher values. Greater absolute values on the horizontal axis indicate a higher impact of the parameter on the prediction of the model. C Spearman correlations between the 15 most important chemical properties and consumer overall appreciation. Numbers indicate the Spearman Rho correlation coefficient, and the rank of this correlation compared to all other correlations. The top 15 important compounds were determined using SHAP (panel B).
Both approaches identified ethyl acetate as the most predictive parameter for beer appreciation (Fig. 4 ). Ethyl acetate is the most abundant ester in beer with a typical ‘fruity’, ‘solvent’ and ‘alcoholic’ flavor, but is often considered less important than other esters like isoamyl acetate. The second most important parameter identified by SHAP is ethanol, the most abundant beer compound after water. Apart from directly contributing to beer flavor and mouthfeel, ethanol drastically influences the physical properties of beer, dictating how easily volatile compounds escape the beer matrix to contribute to beer aroma 69 . Importantly, it should also be noted that the importance of ethanol for appreciation is likely inflated by the very low appreciation scores of non-alcoholic beers (Supplementary Fig. S4 ). Despite not often being considered a driver of beer appreciation, protein level also ranks highly in both approaches, possibly due to its effect on mouthfeel and body 70 . Lactic acid, which contributes to the tart taste of sour beers, is the fourth most important parameter identified by SHAP, possibly due to the generally high appreciation of sour beers in our dataset.
Interestingly, some of the most important predictive parameters for our model are not well-established as beer flavors or are even commonly regarded as being negative for beer quality. For example, our models identify methanethiol and ethyl phenyl acetate, an ester commonly linked to beer staling 71 , as a key factor contributing to beer appreciation. Although there is no doubt that high concentrations of these compounds are considered unpleasant, the positive effects of modest concentrations are not yet known 72 , 73 .
To compare our approach to conventional statistics, we evaluated how well the 15 most important SHAP-derived parameters correlate with consumer appreciation (Fig. 4C ). Interestingly, only 6 of the properties derived by SHAP rank amongst the top 15 most correlated parameters. For some chemical compounds, the correlations are so low that they would have likely been considered unimportant. For example, lactic acid, the fourth most important parameter, shows a bimodal distribution for appreciation, with sour beers forming a separate cluster, that is missed entirely by the Spearman correlation. Additionally, the correlation plots reveal outliers, emphasizing the need for robust analysis tools. Together, this highlights the need for alternative models, like the Gradient Boosting model, that better grasp the complexity of (beer) flavor.
Finally, to observe the relationships between these chemical properties and their predicted targets, partial dependence plots were constructed for the six most important predictors of consumer appreciation 74 , 75 , 76 (Supplementary Fig. S7 ). One-way partial dependence plots show how a change in concentration affects the predicted appreciation. These plots reveal an important limitation of our models: appreciation predictions remain constant at ever-increasing concentrations. This implies that once a threshold concentration is reached, further increasing the concentration does not affect appreciation. This is false, as it is well-documented that certain compounds become unpleasant at high concentrations, including ethyl acetate (‘nail polish’) 77 and methanethiol (‘sulfury’ and ‘rotten cabbage’) 78 . The inability of our models to grasp that flavor compounds have optimal levels, above which they become negative, is a consequence of working with commercial beer brands where (off-)flavors are rarely too high to negatively impact the product. The two-way partial dependence plots show how changing the concentration of two compounds influences predicted appreciation, visualizing their interactions (Supplementary Fig. S7 ). In our case, the top 5 parameters are dominated by additive or synergistic interactions, with high concentrations for both compounds resulting in the highest predicted appreciation.
To assess the robustness of our best-performing models and model predictions, we performed 100 iterations of the GBR, RF and ET models. In general, all iterations of the models yielded similar performance (Supplementary Fig. S8 ). Moreover, the main predictors (including the top predictors ethanol and ethyl acetate) remained virtually the same, especially for GBR and RF. For the iterations of the ET model, we did observe more variation in the top predictors, which is likely a consequence of the model’s inherent random architecture in combination with co-correlations between certain predictors. However, even in this case, several of the top predictors (ethanol and ethyl acetate) remain unchanged, although their rank in importance changes (Supplementary Fig. S8 ).
Next, we investigated if a combination of RateBeer and trained panel data into one consolidated dataset would lead to stronger models, under the hypothesis that such a model would suffer less from bias in the datasets. A GBR model was trained to predict appreciation on the combined dataset. This model underperformed compared to the RateBeer model, both in the native case and when including a dataset identifier (R 2 = 0.67, 0.26 and 0.42 respectively). For the latter, the dataset identifier is the most important feature (Supplementary Fig. S9 ), while most of the feature importance remains unchanged, with ethyl acetate and ethanol ranking highest, like in the original model trained only on RateBeer data. It seems that the large variation in the panel dataset introduces noise, weakening the models’ performances and reliability. In addition, it seems reasonable to assume that both datasets are fundamentally different, with the panel dataset obtained by blind tastings by a trained professional panel.
Lastly, we evaluated whether beer style identifiers would further enhance the model’s performance. A GBR model was trained with parameters that explicitly encoded the styles of the samples. This did not improve model performance (R2 = 0.66 with style information vs R2 = 0.67). The most important chemical features are consistent with the model trained without style information (eg. ethanol and ethyl acetate), and with the exception of the most preferred (strong ale) and least preferred (low/no-alcohol) styles, none of the styles were among the most important features (Supplementary Fig. S9 , Supplementary Table S5 and S6 ). This is likely due to a combination of style-specific chemical signatures, such as iso-alpha acids and lactic acid, that implicitly convey style information to the original models, as well as the low number of samples belonging to some styles, making it difficult for the model to learn style-specific patterns. Moreover, beer styles are not rigorously defined, with some styles overlapping in features and some beers being misattributed to a specific style, all of which leads to more noise in models that use style parameters.
Model validation
To test if our predictive models give insight into beer appreciation, we set up experiments aimed at improving existing commercial beers. We specifically selected overall appreciation as the trait to be examined because of its complexity and commercial relevance. Beer flavor comprises a complex bouquet rather than single aromas and tastes 53 . Hence, adding a single compound to the extent that a difference is noticeable may lead to an unbalanced, artificial flavor. Therefore, we evaluated the effect of combinations of compounds. Because Blond beers represent the most extensive style in our dataset, we selected a beer from this style as the starting material for these experiments (Beer 64 in Supplementary Data 1 ).
In the first set of experiments, we adjusted the concentrations of compounds that made up the most important predictors of overall appreciation (ethyl acetate, ethanol, lactic acid, ethyl phenyl acetate) together with correlated compounds (ethyl hexanoate, isoamyl acetate, glycerol), bringing them up to 95 th percentile ethanol-normalized concentrations (Methods) within the Blond group (‘Spiked’ concentration in Fig. 5A ). Compared to controls, the spiked beers were found to have significantly improved overall appreciation among trained panelists, with panelist noting increased intensity of ester flavors, sweetness, alcohol, and body fullness (Fig. 5B ). To disentangle the contribution of ethanol to these results, a second experiment was performed without the addition of ethanol. This resulted in a similar outcome, including increased perception of alcohol and overall appreciation.
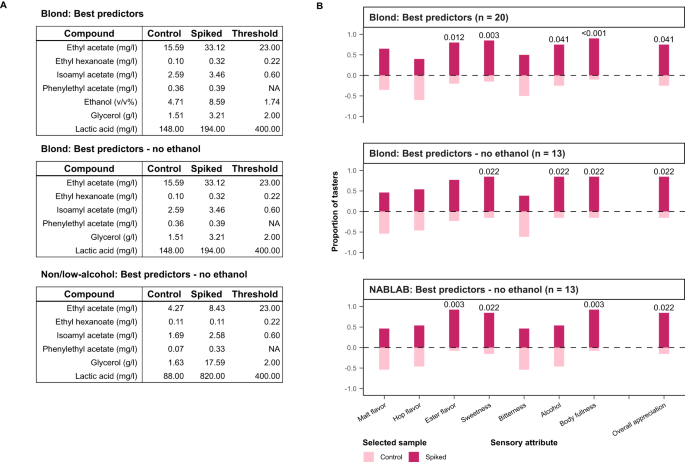
Adding the top chemical compounds, identified as best predictors of appreciation by our model, into poorly appreciated beers results in increased appreciation from our trained panel. Results of sensory tests between base beers and those spiked with compounds identified as the best predictors by the model. A Blond and Non/Low-alcohol (0.0% ABV) base beers were brought up to 95th-percentile ethanol-normalized concentrations within each style. B For each sensory attribute, tasters indicated the more intense sample and selected the sample they preferred. The numbers above the bars correspond to the p values that indicate significant changes in perceived flavor (two-sided binomial test: alpha 0.05, n = 20 or 13).
In a last experiment, we tested whether using the model’s predictions can boost the appreciation of a non-alcoholic beer (beer 223 in Supplementary Data 1 ). Again, the addition of a mixture of predicted compounds (omitting ethanol, in this case) resulted in a significant increase in appreciation, body, ester flavor and sweetness.
Predicting flavor and consumer appreciation from chemical composition is one of the ultimate goals of sensory science. A reliable, systematic and unbiased way to link chemical profiles to flavor and food appreciation would be a significant asset to the food and beverage industry. Such tools would substantially aid in quality control and recipe development, offer an efficient and cost-effective alternative to pilot studies and consumer trials and would ultimately allow food manufacturers to produce superior, tailor-made products that better meet the demands of specific consumer groups more efficiently.
A limited set of studies have previously tried, to varying degrees of success, to predict beer flavor and beer popularity based on (a limited set of) chemical compounds and flavors 79 , 80 . Current sensitive, high-throughput technologies allow measuring an unprecedented number of chemical compounds and properties in a large set of samples, yielding a dataset that can train models that help close the gaps between chemistry and flavor, even for a complex natural product like beer. To our knowledge, no previous research gathered data at this scale (250 samples, 226 chemical parameters, 50 sensory attributes and 5 consumer scores) to disentangle and validate the chemical aspects driving beer preference using various machine-learning techniques. We find that modern machine learning models outperform conventional statistical tools, such as correlations and linear models, and can successfully predict flavor appreciation from chemical composition. This could be attributed to the natural incorporation of interactions and non-linear or discontinuous effects in machine learning models, which are not easily grasped by the linear model architecture. While linear models and partial least squares regression represent the most widespread statistical approaches in sensory science, in part because they allow interpretation 65 , 81 , 82 , modern machine learning methods allow for building better predictive models while preserving the possibility to dissect and exploit the underlying patterns. Of the 10 different models we trained, tree-based models, such as our best performing GBR, showed the best overall performance in predicting sensory responses from chemical information, outcompeting artificial neural networks. This agrees with previous reports for models trained on tabular data 83 . Our results are in line with the findings of Colantonio et al. who also identified the gradient boosting architecture as performing best at predicting appreciation and flavor (of tomatoes and blueberries, in their specific study) 26 . Importantly, besides our larger experimental scale, we were able to directly confirm our models’ predictions in vivo.
Our study confirms that flavor compound concentration does not always correlate with perception, suggesting complex interactions that are often missed by more conventional statistics and simple models. Specifically, we find that tree-based algorithms may perform best in developing models that link complex food chemistry with aroma. Furthermore, we show that massive datasets of untrained consumer reviews provide a valuable source of data, that can complement or even replace trained tasting panels, especially for appreciation and basic flavors, such as sweetness and bitterness. This holds despite biases that are known to occur in such datasets, such as price or conformity bias. Moreover, GBR models predict taste better than aroma. This is likely because taste (e.g. bitterness) often directly relates to the corresponding chemical measurements (e.g., iso-alpha acids), whereas such a link is less clear for aromas, which often result from the interplay between multiple volatile compounds. We also find that our models are best at predicting acidity and alcohol, likely because there is a direct relation between the measured chemical compounds (acids and ethanol) and the corresponding perceived sensorial attribute (acidity and alcohol), and because even untrained consumers are generally able to recognize these flavors and aromas.
The predictions of our final models, trained on review data, hold even for blind tastings with small groups of trained tasters, as demonstrated by our ability to validate specific compounds as drivers of beer flavor and appreciation. Since adding a single compound to the extent of a noticeable difference may result in an unbalanced flavor profile, we specifically tested our identified key drivers as a combination of compounds. While this approach does not allow us to validate if a particular single compound would affect flavor and/or appreciation, our experiments do show that this combination of compounds increases consumer appreciation.
It is important to stress that, while it represents an important step forward, our approach still has several major limitations. A key weakness of the GBR model architecture is that amongst co-correlating variables, the largest main effect is consistently preferred for model building. As a result, co-correlating variables often have artificially low importance scores, both for impurity and SHAP-based methods, like we observed in the comparison to the more randomized Extra Trees models. This implies that chemicals identified as key drivers of a specific sensory feature by GBR might not be the true causative compounds, but rather co-correlate with the actual causative chemical. For example, the high importance of ethyl acetate could be (partially) attributed to the total ester content, ethanol or ethyl hexanoate (rho=0.77, rho=0.72 and rho=0.68), while ethyl phenylacetate could hide the importance of prenyl isobutyrate and ethyl benzoate (rho=0.77 and rho=0.76). Expanding our GBR model to include beer style as a parameter did not yield additional power or insight. This is likely due to style-specific chemical signatures, such as iso-alpha acids and lactic acid, that implicitly convey style information to the original model, as well as the smaller sample size per style, limiting the power to uncover style-specific patterns. This can be partly attributed to the curse of dimensionality, where the high number of parameters results in the models mainly incorporating single parameter effects, rather than complex interactions such as style-dependent effects 67 . A larger number of samples may overcome some of these limitations and offer more insight into style-specific effects. On the other hand, beer style is not a rigid scientific classification, and beers within one style often differ a lot, which further complicates the analysis of style as a model factor.
Our study is limited to beers from Belgian breweries. Although these beers cover a large portion of the beer styles available globally, some beer styles and consumer patterns may be missing, while other features might be overrepresented. For example, many Belgian ales exhibit yeast-driven flavor profiles, which is reflected in the chemical drivers of appreciation discovered by this study. In future work, expanding the scope to include diverse markets and beer styles could lead to the identification of even more drivers of appreciation and better models for special niche products that were not present in our beer set.
In addition to inherent limitations of GBR models, there are also some limitations associated with studying food aroma. Even if our chemical analyses measured most of the known aroma compounds, the total number of flavor compounds in complex foods like beer is still larger than the subset we were able to measure in this study. For example, hop-derived thiols, that influence flavor at very low concentrations, are notoriously difficult to measure in a high-throughput experiment. Moreover, consumer perception remains subjective and prone to biases that are difficult to avoid. It is also important to stress that the models are still immature and that more extensive datasets will be crucial for developing more complete models in the future. Besides more samples and parameters, our dataset does not include any demographic information about the tasters. Including such data could lead to better models that grasp external factors like age and culture. Another limitation is that our set of beers consists of high-quality end-products and lacks beers that are unfit for sale, which limits the current model in accurately predicting products that are appreciated very badly. Finally, while models could be readily applied in quality control, their use in sensory science and product development is restrained by their inability to discern causal relationships. Given that the models cannot distinguish compounds that genuinely drive consumer perception from those that merely correlate, validation experiments are essential to identify true causative compounds.
Despite the inherent limitations, dissection of our models enabled us to pinpoint specific molecules as potential drivers of beer aroma and consumer appreciation, including compounds that were unexpected and would not have been identified using standard approaches. Important drivers of beer appreciation uncovered by our models include protein levels, ethyl acetate, ethyl phenyl acetate and lactic acid. Currently, many brewers already use lactic acid to acidify their brewing water and ensure optimal pH for enzymatic activity during the mashing process. Our results suggest that adding lactic acid can also improve beer appreciation, although its individual effect remains to be tested. Interestingly, ethanol appears to be unnecessary to improve beer appreciation, both for blond beer and alcohol-free beer. Given the growing consumer interest in alcohol-free beer, with a predicted annual market growth of >7% 84 , it is relevant for brewers to know what compounds can further increase consumer appreciation of these beers. Hence, our model may readily provide avenues to further improve the flavor and consumer appreciation of both alcoholic and non-alcoholic beers, which is generally considered one of the key challenges for future beer production.
Whereas we see a direct implementation of our results for the development of superior alcohol-free beverages and other food products, our study can also serve as a stepping stone for the development of novel alcohol-containing beverages. We want to echo the growing body of scientific evidence for the negative effects of alcohol consumption, both on the individual level by the mutagenic, teratogenic and carcinogenic effects of ethanol 85 , 86 , as well as the burden on society caused by alcohol abuse and addiction. We encourage the use of our results for the production of healthier, tastier products, including novel and improved beverages with lower alcohol contents. Furthermore, we strongly discourage the use of these technologies to improve the appreciation or addictive properties of harmful substances.
The present work demonstrates that despite some important remaining hurdles, combining the latest developments in chemical analyses, sensory analysis and modern machine learning methods offers exciting avenues for food chemistry and engineering. Soon, these tools may provide solutions in quality control and recipe development, as well as new approaches to sensory science and flavor research.
Beer selection
250 commercial Belgian beers were selected to cover the broad diversity of beer styles and corresponding diversity in chemical composition and aroma. See Supplementary Fig. S1 .
Chemical dataset
Sample preparation.
Beers within their expiration date were purchased from commercial retailers. Samples were prepared in biological duplicates at room temperature, unless explicitly stated otherwise. Bottle pressure was measured with a manual pressure device (Steinfurth Mess-Systeme GmbH) and used to calculate CO 2 concentration. The beer was poured through two filter papers (Macherey-Nagel, 500713032 MN 713 ¼) to remove carbon dioxide and prevent spontaneous foaming. Samples were then prepared for measurements by targeted Headspace-Gas Chromatography-Flame Ionization Detector/Flame Photometric Detector (HS-GC-FID/FPD), Headspace-Solid Phase Microextraction-Gas Chromatography-Mass Spectrometry (HS-SPME-GC-MS), colorimetric analysis, enzymatic analysis, Near-Infrared (NIR) analysis, as described in the sections below. The mean values of biological duplicates are reported for each compound.
HS-GC-FID/FPD
HS-GC-FID/FPD (Shimadzu GC 2010 Plus) was used to measure higher alcohols, acetaldehyde, esters, 4-vinyl guaicol, and sulfur compounds. Each measurement comprised 5 ml of sample pipetted into a 20 ml glass vial containing 1.75 g NaCl (VWR, 27810.295). 100 µl of 2-heptanol (Sigma-Aldrich, H3003) (internal standard) solution in ethanol (Fisher Chemical, E/0650DF/C17) was added for a final concentration of 2.44 mg/L. Samples were flushed with nitrogen for 10 s, sealed with a silicone septum, stored at −80 °C and analyzed in batches of 20.
The GC was equipped with a DB-WAXetr column (length, 30 m; internal diameter, 0.32 mm; layer thickness, 0.50 µm; Agilent Technologies, Santa Clara, CA, USA) to the FID and an HP-5 column (length, 30 m; internal diameter, 0.25 mm; layer thickness, 0.25 µm; Agilent Technologies, Santa Clara, CA, USA) to the FPD. N 2 was used as the carrier gas. Samples were incubated for 20 min at 70 °C in the headspace autosampler (Flow rate, 35 cm/s; Injection volume, 1000 µL; Injection mode, split; Combi PAL autosampler, CTC analytics, Switzerland). The injector, FID and FPD temperatures were kept at 250 °C. The GC oven temperature was first held at 50 °C for 5 min and then allowed to rise to 80 °C at a rate of 5 °C/min, followed by a second ramp of 4 °C/min until 200 °C kept for 3 min and a final ramp of (4 °C/min) until 230 °C for 1 min. Results were analyzed with the GCSolution software version 2.4 (Shimadzu, Kyoto, Japan). The GC was calibrated with a 5% EtOH solution (VWR International) containing the volatiles under study (Supplementary Table S7 ).
HS-SPME-GC-MS
HS-SPME-GC-MS (Shimadzu GCMS-QP-2010 Ultra) was used to measure additional volatile compounds, mainly comprising terpenoids and esters. Samples were analyzed by HS-SPME using a triphase DVB/Carboxen/PDMS 50/30 μm SPME fiber (Supelco Co., Bellefonte, PA, USA) followed by gas chromatography (Thermo Fisher Scientific Trace 1300 series, USA) coupled to a mass spectrometer (Thermo Fisher Scientific ISQ series MS) equipped with a TriPlus RSH autosampler. 5 ml of degassed beer sample was placed in 20 ml vials containing 1.75 g NaCl (VWR, 27810.295). 5 µl internal standard mix was added, containing 2-heptanol (1 g/L) (Sigma-Aldrich, H3003), 4-fluorobenzaldehyde (1 g/L) (Sigma-Aldrich, 128376), 2,3-hexanedione (1 g/L) (Sigma-Aldrich, 144169) and guaiacol (1 g/L) (Sigma-Aldrich, W253200) in ethanol (Fisher Chemical, E/0650DF/C17). Each sample was incubated at 60 °C in the autosampler oven with constant agitation. After 5 min equilibration, the SPME fiber was exposed to the sample headspace for 30 min. The compounds trapped on the fiber were thermally desorbed in the injection port of the chromatograph by heating the fiber for 15 min at 270 °C.
The GC-MS was equipped with a low polarity RXi-5Sil MS column (length, 20 m; internal diameter, 0.18 mm; layer thickness, 0.18 µm; Restek, Bellefonte, PA, USA). Injection was performed in splitless mode at 320 °C, a split flow of 9 ml/min, a purge flow of 5 ml/min and an open valve time of 3 min. To obtain a pulsed injection, a programmed gas flow was used whereby the helium gas flow was set at 2.7 mL/min for 0.1 min, followed by a decrease in flow of 20 ml/min to the normal 0.9 mL/min. The temperature was first held at 30 °C for 3 min and then allowed to rise to 80 °C at a rate of 7 °C/min, followed by a second ramp of 2 °C/min till 125 °C and a final ramp of 8 °C/min with a final temperature of 270 °C.
Mass acquisition range was 33 to 550 amu at a scan rate of 5 scans/s. Electron impact ionization energy was 70 eV. The interface and ion source were kept at 275 °C and 250 °C, respectively. A mix of linear n-alkanes (from C7 to C40, Supelco Co.) was injected into the GC-MS under identical conditions to serve as external retention index markers. Identification and quantification of the compounds were performed using an in-house developed R script as described in Goelen et al. and Reher et al. 87 , 88 (for package information, see Supplementary Table S8 ). Briefly, chromatograms were analyzed using AMDIS (v2.71) 89 to separate overlapping peaks and obtain pure compound spectra. The NIST MS Search software (v2.0 g) in combination with the NIST2017, FFNSC3 and Adams4 libraries were used to manually identify the empirical spectra, taking into account the expected retention time. After background subtraction and correcting for retention time shifts between samples run on different days based on alkane ladders, compound elution profiles were extracted and integrated using a file with 284 target compounds of interest, which were either recovered in our identified AMDIS list of spectra or were known to occur in beer. Compound elution profiles were estimated for every peak in every chromatogram over a time-restricted window using weighted non-negative least square analysis after which peak areas were integrated 87 , 88 . Batch effect correction was performed by normalizing against the most stable internal standard compound, 4-fluorobenzaldehyde. Out of all 284 target compounds that were analyzed, 167 were visually judged to have reliable elution profiles and were used for final analysis.
Discrete photometric and enzymatic analysis
Discrete photometric and enzymatic analysis (Thermo Scientific TM Gallery TM Plus Beermaster Discrete Analyzer) was used to measure acetic acid, ammonia, beta-glucan, iso-alpha acids, color, sugars, glycerol, iron, pH, protein, and sulfite. 2 ml of sample volume was used for the analyses. Information regarding the reagents and standard solutions used for analyses and calibrations is included in Supplementary Table S7 and Supplementary Table S9 .
NIR analyses
NIR analysis (Anton Paar Alcolyzer Beer ME System) was used to measure ethanol. Measurements comprised 50 ml of sample, and a 10% EtOH solution was used for calibration.
Correlation calculations
Pairwise Spearman Rank correlations were calculated between all chemical properties.
Sensory dataset
Trained panel.
Our trained tasting panel consisted of volunteers who gave prior verbal informed consent. All compounds used for the validation experiment were of food-grade quality. The tasting sessions were approved by the Social and Societal Ethics Committee of the KU Leuven (G-2022-5677-R2(MAR)). All online reviewers agreed to the Terms and Conditions of the RateBeer website.
Sensory analysis was performed according to the American Society of Brewing Chemists (ASBC) Sensory Analysis Methods 90 . 30 volunteers were screened through a series of triangle tests. The sixteen most sensitive and consistent tasters were retained as taste panel members. The resulting panel was diverse in age [22–42, mean: 29], sex [56% male] and nationality [7 different countries]. The panel developed a consensus vocabulary to describe beer aroma, taste and mouthfeel. Panelists were trained to identify and score 50 different attributes, using a 7-point scale to rate attributes’ intensity. The scoring sheet is included as Supplementary Data 3 . Sensory assessments took place between 10–12 a.m. The beers were served in black-colored glasses. Per session, between 5 and 12 beers of the same style were tasted at 12 °C to 16 °C. Two reference beers were added to each set and indicated as ‘Reference 1 & 2’, allowing panel members to calibrate their ratings. Not all panelists were present at every tasting. Scores were scaled by standard deviation and mean-centered per taster. Values are represented as z-scores and clustered by Euclidean distance. Pairwise Spearman correlations were calculated between taste and aroma sensory attributes. Panel consistency was evaluated by repeating samples on different sessions and performing ANOVA to identify differences, using the ‘stats’ package (v4.2.2) in R (for package information, see Supplementary Table S8 ).
Online reviews from a public database
The ‘scrapy’ package in Python (v3.6) (for package information, see Supplementary Table S8 ). was used to collect 232,288 online reviews (mean=922, min=6, max=5343) from RateBeer, an online beer review database. Each review entry comprised 5 numerical scores (appearance, aroma, taste, palate and overall quality) and an optional review text. The total number of reviews per reviewer was collected separately. Numerical scores were scaled and centered per rater, and mean scores were calculated per beer.
For the review texts, the language was estimated using the packages ‘langdetect’ and ‘langid’ in Python. Reviews that were classified as English by both packages were kept. Reviewers with fewer than 100 entries overall were discarded. 181,025 reviews from >6000 reviewers from >40 countries remained. Text processing was done using the ‘nltk’ package in Python. Texts were corrected for slang and misspellings; proper nouns and rare words that are relevant to the beer context were specified and kept as-is (‘Chimay’,’Lambic’, etc.). A dictionary of semantically similar sensorial terms, for example ‘floral’ and ‘flower’, was created and collapsed together into one term. Words were stemmed and lemmatized to avoid identifying words such as ‘acid’ and ‘acidity’ as separate terms. Numbers and punctuation were removed.
Sentences from up to 50 randomly chosen reviews per beer were manually categorized according to the aspect of beer they describe (appearance, aroma, taste, palate, overall quality—not to be confused with the 5 numerical scores described above) or flagged as irrelevant if they contained no useful information. If a beer contained fewer than 50 reviews, all reviews were manually classified. This labeled data set was used to train a model that classified the rest of the sentences for all beers 91 . Sentences describing taste and aroma were extracted, and term frequency–inverse document frequency (TFIDF) was implemented to calculate enrichment scores for sensorial words per beer.
The sex of the tasting subject was not considered when building our sensory database. Instead, results from different panelists were averaged, both for our trained panel (56% male, 44% female) and the RateBeer reviews (70% male, 30% female for RateBeer as a whole).
Beer price collection and processing
Beer prices were collected from the following stores: Colruyt, Delhaize, Total Wine, BeerHawk, The Belgian Beer Shop, The Belgian Shop, and Beer of Belgium. Where applicable, prices were converted to Euros and normalized per liter. Spearman correlations were calculated between these prices and mean overall appreciation scores from RateBeer and the taste panel, respectively.
Pairwise Spearman Rank correlations were calculated between all sensory properties.
Machine learning models
Predictive modeling of sensory profiles from chemical data.
Regression models were constructed to predict (a) trained panel scores for beer flavors and quality from beer chemical profiles and (b) public reviews’ appreciation scores from beer chemical profiles. Z-scores were used to represent sensory attributes in both data sets. Chemical properties with log-normal distributions (Shapiro-Wilk test, p < 0.05 ) were log-transformed. Missing chemical measurements (0.1% of all data) were replaced with mean values per attribute. Observations from 250 beers were randomly separated into a training set (70%, 175 beers) and a test set (30%, 75 beers), stratified per beer style. Chemical measurements (p = 231) were normalized based on the training set average and standard deviation. In total, three linear regression-based models: linear regression with first-order interaction terms (LR), lasso regression with first-order interaction terms (Lasso) and partial least squares regression (PLSR); five decision tree models, Adaboost regressor (ABR), Extra Trees (ET), Gradient Boosting regressor (GBR), Random Forest (RF) and XGBoost regressor (XGBR); one support vector machine model (SVR) and one artificial neural network model (ANN) were trained. The models were implemented using the ‘scikit-learn’ package (v1.2.2) and ‘xgboost’ package (v1.7.3) in Python (v3.9.16). Models were trained, and hyperparameters optimized, using five-fold cross-validated grid search with the coefficient of determination (R 2 ) as the evaluation metric. The ANN (scikit-learn’s MLPRegressor) was optimized using Bayesian Tree-Structured Parzen Estimator optimization with the ‘Optuna’ Python package (v3.2.0). Individual models were trained per attribute, and a multi-output model was trained on all attributes simultaneously.
Model dissection
GBR was found to outperform other methods, resulting in models with the highest average R 2 values in both trained panel and public review data sets. Impurity-based rankings of the most important predictors for each predicted sensorial trait were obtained using the ‘scikit-learn’ package. To observe the relationships between these chemical properties and their predicted targets, partial dependence plots (PDP) were constructed for the six most important predictors of consumer appreciation 74 , 75 .
The ‘SHAP’ package in Python (v0.41.0) was implemented to provide an alternative ranking of predictor importance and to visualize the predictors’ effects as a function of their concentration 68 .
Validation of causal chemical properties
To validate the effects of the most important model features on predicted sensory attributes, beers were spiked with the chemical compounds identified by the models and descriptive sensory analyses were carried out according to the American Society of Brewing Chemists (ASBC) protocol 90 .
Compound spiking was done 30 min before tasting. Compounds were spiked into fresh beer bottles, that were immediately resealed and inverted three times. Fresh bottles of beer were opened for the same duration, resealed, and inverted thrice, to serve as controls. Pairs of spiked samples and controls were served simultaneously, chilled and in dark glasses as outlined in the Trained panel section above. Tasters were instructed to select the glass with the higher flavor intensity for each attribute (directional difference test 92 ) and to select the glass they prefer.
The final concentration after spiking was equal to the within-style average, after normalizing by ethanol concentration. This was done to ensure balanced flavor profiles in the final spiked beer. The same methods were applied to improve a non-alcoholic beer. Compounds were the following: ethyl acetate (Merck KGaA, W241415), ethyl hexanoate (Merck KGaA, W243906), isoamyl acetate (Merck KGaA, W205508), phenethyl acetate (Merck KGaA, W285706), ethanol (96%, Colruyt), glycerol (Merck KGaA, W252506), lactic acid (Merck KGaA, 261106).
Significant differences in preference or perceived intensity were determined by performing the two-sided binomial test on each attribute.
Reporting summary
Further information on research design is available in the Nature Portfolio Reporting Summary linked to this article.
Data availability
The data that support the findings of this work are available in the Supplementary Data files and have been deposited to Zenodo under accession code 10653704 93 . The RateBeer scores data are under restricted access, they are not publicly available as they are property of RateBeer (ZX Ventures, USA). Access can be obtained from the authors upon reasonable request and with permission of RateBeer (ZX Ventures, USA). Source data are provided with this paper.
Code availability
The code for training the machine learning models, analyzing the models, and generating the figures has been deposited to Zenodo under accession code 10653704 93 .
Tieman, D. et al. A chemical genetic roadmap to improved tomato flavor. Science 355 , 391–394 (2017).
Article ADS CAS PubMed Google Scholar
Plutowska, B. & Wardencki, W. Application of gas chromatography–olfactometry (GC–O) in analysis and quality assessment of alcoholic beverages – A review. Food Chem. 107 , 449–463 (2008).
Article CAS Google Scholar
Legin, A., Rudnitskaya, A., Seleznev, B. & Vlasov, Y. Electronic tongue for quality assessment of ethanol, vodka and eau-de-vie. Anal. Chim. Acta 534 , 129–135 (2005).
Loutfi, A., Coradeschi, S., Mani, G. K., Shankar, P. & Rayappan, J. B. B. Electronic noses for food quality: A review. J. Food Eng. 144 , 103–111 (2015).
Ahn, Y.-Y., Ahnert, S. E., Bagrow, J. P. & Barabási, A.-L. Flavor network and the principles of food pairing. Sci. Rep. 1 , 196 (2011).
Article CAS PubMed PubMed Central Google Scholar
Bartoshuk, L. M. & Klee, H. J. Better fruits and vegetables through sensory analysis. Curr. Biol. 23 , R374–R378 (2013).
Article CAS PubMed Google Scholar
Piggott, J. R. Design questions in sensory and consumer science. Food Qual. Prefer. 3293 , 217–220 (1995).
Article Google Scholar
Kermit, M. & Lengard, V. Assessing the performance of a sensory panel-panellist monitoring and tracking. J. Chemom. 19 , 154–161 (2005).
Cook, D. J., Hollowood, T. A., Linforth, R. S. T. & Taylor, A. J. Correlating instrumental measurements of texture and flavour release with human perception. Int. J. Food Sci. Technol. 40 , 631–641 (2005).
Chinchanachokchai, S., Thontirawong, P. & Chinchanachokchai, P. A tale of two recommender systems: The moderating role of consumer expertise on artificial intelligence based product recommendations. J. Retail. Consum. Serv. 61 , 1–12 (2021).
Ross, C. F. Sensory science at the human-machine interface. Trends Food Sci. Technol. 20 , 63–72 (2009).
Chambers, E. IV & Koppel, K. Associations of volatile compounds with sensory aroma and flavor: The complex nature of flavor. Molecules 18 , 4887–4905 (2013).
Pinu, F. R. Metabolomics—The new frontier in food safety and quality research. Food Res. Int. 72 , 80–81 (2015).
Danezis, G. P., Tsagkaris, A. S., Brusic, V. & Georgiou, C. A. Food authentication: state of the art and prospects. Curr. Opin. Food Sci. 10 , 22–31 (2016).
Shepherd, G. M. Smell images and the flavour system in the human brain. Nature 444 , 316–321 (2006).
Meilgaard, M. C. Prediction of flavor differences between beers from their chemical composition. J. Agric. Food Chem. 30 , 1009–1017 (1982).
Xu, L. et al. Widespread receptor-driven modulation in peripheral olfactory coding. Science 368 , eaaz5390 (2020).
Kupferschmidt, K. Following the flavor. Science 340 , 808–809 (2013).
Billesbølle, C. B. et al. Structural basis of odorant recognition by a human odorant receptor. Nature 615 , 742–749 (2023).
Article ADS PubMed PubMed Central Google Scholar
Smith, B. Perspective: Complexities of flavour. Nature 486 , S6–S6 (2012).
Pfister, P. et al. Odorant receptor inhibition is fundamental to odor encoding. Curr. Biol. 30 , 2574–2587 (2020).
Moskowitz, H. W., Kumaraiah, V., Sharma, K. N., Jacobs, H. L. & Sharma, S. D. Cross-cultural differences in simple taste preferences. Science 190 , 1217–1218 (1975).
Eriksson, N. et al. A genetic variant near olfactory receptor genes influences cilantro preference. Flavour 1 , 22 (2012).
Ferdenzi, C. et al. Variability of affective responses to odors: Culture, gender, and olfactory knowledge. Chem. Senses 38 , 175–186 (2013).
Article PubMed Google Scholar
Lawless, H. T. & Heymann, H. Sensory evaluation of food: Principles and practices. (Springer, New York, NY). https://doi.org/10.1007/978-1-4419-6488-5 (2010).
Colantonio, V. et al. Metabolomic selection for enhanced fruit flavor. Proc. Natl. Acad. Sci. 119 , e2115865119 (2022).
Fritz, F., Preissner, R. & Banerjee, P. VirtualTaste: a web server for the prediction of organoleptic properties of chemical compounds. Nucleic Acids Res 49 , W679–W684 (2021).
Tuwani, R., Wadhwa, S. & Bagler, G. BitterSweet: Building machine learning models for predicting the bitter and sweet taste of small molecules. Sci. Rep. 9 , 1–13 (2019).
Dagan-Wiener, A. et al. Bitter or not? BitterPredict, a tool for predicting taste from chemical structure. Sci. Rep. 7 , 1–13 (2017).
Pallante, L. et al. Toward a general and interpretable umami taste predictor using a multi-objective machine learning approach. Sci. Rep. 12 , 1–11 (2022).
Malavolta, M. et al. A survey on computational taste predictors. Eur. Food Res. Technol. 248 , 2215–2235 (2022).
Lee, B. K. et al. A principal odor map unifies diverse tasks in olfactory perception. Science 381 , 999–1006 (2023).
Mayhew, E. J. et al. Transport features predict if a molecule is odorous. Proc. Natl. Acad. Sci. 119 , e2116576119 (2022).
Niu, Y. et al. Sensory evaluation of the synergism among ester odorants in light aroma-type liquor by odor threshold, aroma intensity and flash GC electronic nose. Food Res. Int. 113 , 102–114 (2018).
Yu, P., Low, M. Y. & Zhou, W. Design of experiments and regression modelling in food flavour and sensory analysis: A review. Trends Food Sci. Technol. 71 , 202–215 (2018).
Oladokun, O. et al. The impact of hop bitter acid and polyphenol profiles on the perceived bitterness of beer. Food Chem. 205 , 212–220 (2016).
Linforth, R., Cabannes, M., Hewson, L., Yang, N. & Taylor, A. Effect of fat content on flavor delivery during consumption: An in vivo model. J. Agric. Food Chem. 58 , 6905–6911 (2010).
Guo, S., Na Jom, K. & Ge, Y. Influence of roasting condition on flavor profile of sunflower seeds: A flavoromics approach. Sci. Rep. 9 , 11295 (2019).
Ren, Q. et al. The changes of microbial community and flavor compound in the fermentation process of Chinese rice wine using Fagopyrum tataricum grain as feedstock. Sci. Rep. 9 , 3365 (2019).
Hastie, T., Friedman, J. & Tibshirani, R. The Elements of Statistical Learning. (Springer, New York, NY). https://doi.org/10.1007/978-0-387-21606-5 (2001).
Dietz, C., Cook, D., Huismann, M., Wilson, C. & Ford, R. The multisensory perception of hop essential oil: a review. J. Inst. Brew. 126 , 320–342 (2020).
CAS Google Scholar
Roncoroni, Miguel & Verstrepen, Kevin Joan. Belgian Beer: Tested and Tasted. (Lannoo, 2018).
Meilgaard, M. Flavor chemistry of beer: Part II: Flavor and threshold of 239 aroma volatiles. in (1975).
Bokulich, N. A. & Bamforth, C. W. The microbiology of malting and brewing. Microbiol. Mol. Biol. Rev. MMBR 77 , 157–172 (2013).
Dzialo, M. C., Park, R., Steensels, J., Lievens, B. & Verstrepen, K. J. Physiology, ecology and industrial applications of aroma formation in yeast. FEMS Microbiol. Rev. 41 , S95–S128 (2017).
Article PubMed PubMed Central Google Scholar
Datta, A. et al. Computer-aided food engineering. Nat. Food 3 , 894–904 (2022).
American Society of Brewing Chemists. Beer Methods. (American Society of Brewing Chemists, St. Paul, MN, U.S.A.).
Olaniran, A. O., Hiralal, L., Mokoena, M. P. & Pillay, B. Flavour-active volatile compounds in beer: production, regulation and control. J. Inst. Brew. 123 , 13–23 (2017).
Verstrepen, K. J. et al. Flavor-active esters: Adding fruitiness to beer. J. Biosci. Bioeng. 96 , 110–118 (2003).
Meilgaard, M. C. Flavour chemistry of beer. part I: flavour interaction between principal volatiles. Master Brew. Assoc. Am. Tech. Q 12 , 107–117 (1975).
Briggs, D. E., Boulton, C. A., Brookes, P. A. & Stevens, R. Brewing 227–254. (Woodhead Publishing). https://doi.org/10.1533/9781855739062.227 (2004).
Bossaert, S., Crauwels, S., De Rouck, G. & Lievens, B. The power of sour - A review: Old traditions, new opportunities. BrewingScience 72 , 78–88 (2019).
Google Scholar
Verstrepen, K. J. et al. Flavor active esters: Adding fruitiness to beer. J. Biosci. Bioeng. 96 , 110–118 (2003).
Snauwaert, I. et al. Microbial diversity and metabolite composition of Belgian red-brown acidic ales. Int. J. Food Microbiol. 221 , 1–11 (2016).
Spitaels, F. et al. The microbial diversity of traditional spontaneously fermented lambic beer. PLoS ONE 9 , e95384 (2014).
Blanco, C. A., Andrés-Iglesias, C. & Montero, O. Low-alcohol Beers: Flavor Compounds, Defects, and Improvement Strategies. Crit. Rev. Food Sci. Nutr. 56 , 1379–1388 (2016).
Jackowski, M. & Trusek, A. Non-Alcohol. beer Prod. – Overv. 20 , 32–38 (2018).
Takoi, K. et al. The contribution of geraniol metabolism to the citrus flavour of beer: Synergy of geraniol and β-citronellol under coexistence with excess linalool. J. Inst. Brew. 116 , 251–260 (2010).
Kroeze, J. H. & Bartoshuk, L. M. Bitterness suppression as revealed by split-tongue taste stimulation in humans. Physiol. Behav. 35 , 779–783 (1985).
Mennella, J. A. et al. A spoonful of sugar helps the medicine go down”: Bitter masking bysucrose among children and adults. Chem. Senses 40 , 17–25 (2015).
Wietstock, P., Kunz, T., Perreira, F. & Methner, F.-J. Metal chelation behavior of hop acids in buffered model systems. BrewingScience 69 , 56–63 (2016).
Sancho, D., Blanco, C. A., Caballero, I. & Pascual, A. Free iron in pale, dark and alcohol-free commercial lager beers. J. Sci. Food Agric. 91 , 1142–1147 (2011).
Rodrigues, H. & Parr, W. V. Contribution of cross-cultural studies to understanding wine appreciation: A review. Food Res. Int. 115 , 251–258 (2019).
Korneva, E. & Blockeel, H. Towards better evaluation of multi-target regression models. in ECML PKDD 2020 Workshops (eds. Koprinska, I. et al.) 353–362 (Springer International Publishing, Cham, 2020). https://doi.org/10.1007/978-3-030-65965-3_23 .
Gastón Ares. Mathematical and Statistical Methods in Food Science and Technology. (Wiley, 2013).
Grinsztajn, L., Oyallon, E. & Varoquaux, G. Why do tree-based models still outperform deep learning on tabular data? Preprint at http://arxiv.org/abs/2207.08815 (2022).
Gries, S. T. Statistics for Linguistics with R: A Practical Introduction. in Statistics for Linguistics with R (De Gruyter Mouton, 2021). https://doi.org/10.1515/9783110718256 .
Lundberg, S. M. et al. From local explanations to global understanding with explainable AI for trees. Nat. Mach. Intell. 2 , 56–67 (2020).
Ickes, C. M. & Cadwallader, K. R. Effects of ethanol on flavor perception in alcoholic beverages. Chemosens. Percept. 10 , 119–134 (2017).
Kato, M. et al. Influence of high molecular weight polypeptides on the mouthfeel of commercial beer. J. Inst. Brew. 127 , 27–40 (2021).
Wauters, R. et al. Novel Saccharomyces cerevisiae variants slow down the accumulation of staling aldehydes and improve beer shelf-life. Food Chem. 398 , 1–11 (2023).
Li, H., Jia, S. & Zhang, W. Rapid determination of low-level sulfur compounds in beer by headspace gas chromatography with a pulsed flame photometric detector. J. Am. Soc. Brew. Chem. 66 , 188–191 (2008).
Dercksen, A., Laurens, J., Torline, P., Axcell, B. C. & Rohwer, E. Quantitative analysis of volatile sulfur compounds in beer using a membrane extraction interface. J. Am. Soc. Brew. Chem. 54 , 228–233 (1996).
Molnar, C. Interpretable Machine Learning: A Guide for Making Black-Box Models Interpretable. (2020).
Zhao, Q. & Hastie, T. Causal interpretations of black-box models. J. Bus. Econ. Stat. Publ. Am. Stat. Assoc. 39 , 272–281 (2019).
Article MathSciNet Google Scholar
Hastie, T., Tibshirani, R. & Friedman, J. The Elements of Statistical Learning. (Springer, 2019).
Labrado, D. et al. Identification by NMR of key compounds present in beer distillates and residual phases after dealcoholization by vacuum distillation. J. Sci. Food Agric. 100 , 3971–3978 (2020).
Lusk, L. T., Kay, S. B., Porubcan, A. & Ryder, D. S. Key olfactory cues for beer oxidation. J. Am. Soc. Brew. Chem. 70 , 257–261 (2012).
Gonzalez Viejo, C., Torrico, D. D., Dunshea, F. R. & Fuentes, S. Development of artificial neural network models to assess beer acceptability based on sensory properties using a robotic pourer: A comparative model approach to achieve an artificial intelligence system. Beverages 5 , 33 (2019).
Gonzalez Viejo, C., Fuentes, S., Torrico, D. D., Godbole, A. & Dunshea, F. R. Chemical characterization of aromas in beer and their effect on consumers liking. Food Chem. 293 , 479–485 (2019).
Gilbert, J. L. et al. Identifying breeding priorities for blueberry flavor using biochemical, sensory, and genotype by environment analyses. PLOS ONE 10 , 1–21 (2015).
Goulet, C. et al. Role of an esterase in flavor volatile variation within the tomato clade. Proc. Natl. Acad. Sci. 109 , 19009–19014 (2012).
Article ADS CAS PubMed PubMed Central Google Scholar
Borisov, V. et al. Deep Neural Networks and Tabular Data: A Survey. IEEE Trans. Neural Netw. Learn. Syst. 1–21 https://doi.org/10.1109/TNNLS.2022.3229161 (2022).
Statista. Statista Consumer Market Outlook: Beer - Worldwide.
Seitz, H. K. & Stickel, F. Molecular mechanisms of alcoholmediated carcinogenesis. Nat. Rev. Cancer 7 , 599–612 (2007).
Voordeckers, K. et al. Ethanol exposure increases mutation rate through error-prone polymerases. Nat. Commun. 11 , 3664 (2020).
Goelen, T. et al. Bacterial phylogeny predicts volatile organic compound composition and olfactory response of an aphid parasitoid. Oikos 129 , 1415–1428 (2020).
Article ADS Google Scholar
Reher, T. et al. Evaluation of hop (Humulus lupulus) as a repellent for the management of Drosophila suzukii. Crop Prot. 124 , 104839 (2019).
Stein, S. E. An integrated method for spectrum extraction and compound identification from gas chromatography/mass spectrometry data. J. Am. Soc. Mass Spectrom. 10 , 770–781 (1999).
American Society of Brewing Chemists. Sensory Analysis Methods. (American Society of Brewing Chemists, St. Paul, MN, U.S.A., 1992).
McAuley, J., Leskovec, J. & Jurafsky, D. Learning Attitudes and Attributes from Multi-Aspect Reviews. Preprint at https://doi.org/10.48550/arXiv.1210.3926 (2012).
Meilgaard, M. C., Carr, B. T. & Carr, B. T. Sensory Evaluation Techniques. (CRC Press, Boca Raton). https://doi.org/10.1201/b16452 (2014).
Schreurs, M. et al. Data from: Predicting and improving complex beer flavor through machine learning. Zenodo https://doi.org/10.5281/zenodo.10653704 (2024).
Download references
Acknowledgements
We thank all lab members for their discussions and thank all tasting panel members for their contributions. Special thanks go out to Dr. Karin Voordeckers for her tremendous help in proofreading and improving the manuscript. M.S. was supported by a Baillet-Latour fellowship, L.C. acknowledges financial support from KU Leuven (C16/17/006), F.A.T. was supported by a PhD fellowship from FWO (1S08821N). Research in the lab of K.J.V. is supported by KU Leuven, FWO, VIB, VLAIO and the Brewing Science Serves Health Fund. Research in the lab of T.W. is supported by FWO (G.0A51.15) and KU Leuven (C16/17/006).
Author information
These authors contributed equally: Michiel Schreurs, Supinya Piampongsant, Miguel Roncoroni.
Authors and Affiliations
VIB—KU Leuven Center for Microbiology, Gaston Geenslaan 1, B-3001, Leuven, Belgium
Michiel Schreurs, Supinya Piampongsant, Miguel Roncoroni, Lloyd Cool, Beatriz Herrera-Malaver, Florian A. Theßeling & Kevin J. Verstrepen
CMPG Laboratory of Genetics and Genomics, KU Leuven, Gaston Geenslaan 1, B-3001, Leuven, Belgium
Leuven Institute for Beer Research (LIBR), Gaston Geenslaan 1, B-3001, Leuven, Belgium
Laboratory of Socioecology and Social Evolution, KU Leuven, Naamsestraat 59, B-3000, Leuven, Belgium
Lloyd Cool, Christophe Vanderaa & Tom Wenseleers
VIB Bioinformatics Core, VIB, Rijvisschestraat 120, B-9052, Ghent, Belgium
Łukasz Kreft & Alexander Botzki
AB InBev SA/NV, Brouwerijplein 1, B-3000, Leuven, Belgium
Philippe Malcorps & Luk Daenen
You can also search for this author in PubMed Google Scholar
Contributions
S.P., M.S. and K.J.V. conceived the experiments. S.P., M.S. and K.J.V. designed the experiments. S.P., M.S., M.R., B.H. and F.A.T. performed the experiments. S.P., M.S., L.C., C.V., L.K., A.B., P.M., L.D., T.W. and K.J.V. contributed analysis ideas. S.P., M.S., L.C., C.V., T.W. and K.J.V. analyzed the data. All authors contributed to writing the manuscript.
Corresponding author
Correspondence to Kevin J. Verstrepen .
Ethics declarations
Competing interests.
K.J.V. is affiliated with bar.on. The other authors declare no competing interests.
Peer review
Peer review information.
Nature Communications thanks Florian Bauer, Andrew John Macintosh and the other, anonymous, reviewer(s) for their contribution to the peer review of this work. A peer review file is available.
Additional information
Publisher’s note Springer Nature remains neutral with regard to jurisdictional claims in published maps and institutional affiliations.
Supplementary information
Supplementary information, peer review file, description of additional supplementary files, supplementary data 1, supplementary data 2, supplementary data 3, supplementary data 4, supplementary data 5, supplementary data 6, supplementary data 7, reporting summary, source data, source data, rights and permissions.
Open Access This article is licensed under a Creative Commons Attribution 4.0 International License, which permits use, sharing, adaptation, distribution and reproduction in any medium or format, as long as you give appropriate credit to the original author(s) and the source, provide a link to the Creative Commons licence, and indicate if changes were made. The images or other third party material in this article are included in the article’s Creative Commons licence, unless indicated otherwise in a credit line to the material. If material is not included in the article’s Creative Commons licence and your intended use is not permitted by statutory regulation or exceeds the permitted use, you will need to obtain permission directly from the copyright holder. To view a copy of this licence, visit http://creativecommons.org/licenses/by/4.0/ .
Reprints and permissions
About this article
Cite this article.
Schreurs, M., Piampongsant, S., Roncoroni, M. et al. Predicting and improving complex beer flavor through machine learning. Nat Commun 15 , 2368 (2024). https://doi.org/10.1038/s41467-024-46346-0
Download citation
Received : 30 October 2023
Accepted : 21 February 2024
Published : 26 March 2024
DOI : https://doi.org/10.1038/s41467-024-46346-0
Share this article
Anyone you share the following link with will be able to read this content:
Sorry, a shareable link is not currently available for this article.
Provided by the Springer Nature SharedIt content-sharing initiative
By submitting a comment you agree to abide by our Terms and Community Guidelines . If you find something abusive or that does not comply with our terms or guidelines please flag it as inappropriate.
Quick links
- Explore articles by subject
- Guide to authors
- Editorial policies
Sign up for the Nature Briefing: Translational Research newsletter — top stories in biotechnology, drug discovery and pharma.


- SUGGESTED TOPICS
- The Magazine
- Newsletters
- Managing Yourself
- Managing Teams
- Work-life Balance
- The Big Idea
- Data & Visuals
- Reading Lists
- Case Selections
- HBR Learning
- Topic Feeds
- Account Settings
- Email Preferences
How People Are Really Using GenAI
- Marc Zao-Sanders

The top 100 use cases as reported by users on Reddit, Quora, and other forums.
There are many use cases for generative AI, spanning a vast number of areas of domestic and work life. Looking through thousands of comments on sites such as Reddit and Quora, the author’s team found that the use of this technology is as wide-ranging as the problems we encounter in our lives. The 100 categories they identified can be divided into six top-level themes, which give an immediate sense of what generative AI is being used for: Technical Assistance & Troubleshooting (23%), Content Creation & Editing (22%), Personal & Professional Support (17%), Learning & Education (15%), Creativity & Recreation (13%), Research, Analysis & Decision Making (10%).
It’s been a little over a year since ChatGPT brought generative AI into the mainstream. In that time, we’ve ridden a wave of excitement about the current utility and future impact of large language models (LLMs). These tools already have hundreds of millions of weekly users, analysts are projecting a multi-trillion dollar contribution to the economy, and there’s now a growing array of credible competitors to OpenAI.

- Marc Zao-Sanders is CEO and co-founder of filtered.com , which develops algorithmic technology to make sense of corporate skills and learning content. He’s the author of Timeboxing – The Power of Doing One Thing at a Time . Find Marc on LinkedIn or at www.marczaosanders.com .
Partner Center
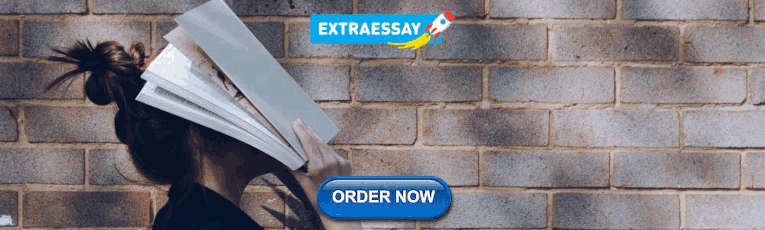
COMMENTS
Questionnaires vs. surveys. A survey is a research method where you collect and analyze data from a group of people. A questionnaire is a specific tool or instrument for collecting the data.. Designing a questionnaire means creating valid and reliable questions that address your research objectives, placing them in a useful order, and selecting an appropriate method for administration.
A questionnaire is a research instrument consisting of a series of questions for the purpose of gathering information from respondents. Questionnaires can be thought of as a kind of written interview. They can be carried out face to face, by telephone, computer, or post. Questionnaires provide a relatively cheap, quick, and efficient way of ...
Questionnaires can be classified as both, quantitative and qualitative method depending on the nature of questions. Specifically, answers obtained through closed-ended questions (also called restricted questions) with multiple choice answer options are analyzed using quantitative methods. Research findings in this case can be illustrated using ...
A Questionnaire is a research tool or survey instrument that consists of a set of questions or prompts designed to gather information from individuals or groups of people. It is a standardized way of collecting data from a large number of people by asking them a series of questions related to a specific topic or research objective.
Survey research means collecting information about a group of people by asking them questions and analyzing the results. To conduct an effective survey, follow these six steps: Determine who will participate in the survey. Decide the type of survey (mail, online, or in-person) Design the survey questions and layout.
Survey research is a research method involving the use of standardised questionnaires or interviews to collect data about people and their preferences, thoughts, and behaviours in a systematic manner. Although census surveys were conducted as early as Ancient Egypt, survey as a formal research method was pioneered in the 1930-40s by sociologist Paul Lazarsfeld to examine the effects of the ...
Questionnaires. Questionnaires can be used qualitatively or quantitatively. As with all other methods, the value of the questionnaire depends on its ability to provide data which can answer the research question, and the way that a questionnaire is designed and worded can be significant in this. A questionnaire designed to capture levels of ...
Chapter 9 Survey Research. Survey research a research method involving the use of standardized questionnaires or interviews to collect data about people and their preferences, thoughts, and behaviors in a systematic manner. Although census surveys were conducted as early as Ancient Egypt, survey as a formal research method was pioneered in the ...
People often decide to use a questionnaire for research questions that need a different method. Sometimes, a questionnaire will be appropriate only if used within a mixed methodology study—for example, to extend and quantify the findings of an initial exploratory phase. Table A on bmj.com gives some real examples where questionnaires were ...
In research studies, questionnaires are commonly used as data collection tools, either as the only source of information or in combination with other techniques in mixed-method studies. However, the quality and accuracy of data collected using a questionnaire depend on how it is designed, used, and validated.
A questionnaire is a research instrument that consists of a set of questions or other types of prompts that aims to collect information from a respondent. A research questionnaire is typically a mix of close-ended questions and open-ended questions. Open-ended, long-form questions offer the respondent the ability to elaborate on their thoughts.
Survey research is defined as "the collection of information from a sample of individuals through their responses to questions" ( Check & Schutt, 2012, p. 160 ). This type of research allows for a variety of methods to recruit participants, collect data, and utilize various methods of instrumentation. Survey research can use quantitative ...
The online questionnaire was an efficient and fast way to reach a large number of academics. I used the online survey platform, which does not require entering data or coding as data is input by the participants and answers are saved automatically (Sills & Song, 2002). Using questionnaires as a data collection tool has some drawbacks.
A questionnaire, or social survey, is a popular research method that consists of a list of questions. If administered directly by the researcher to the subject in person then this is the same as a structured interview; however, questionnaires can also be completed independently (self-completion questionnaires) and therefore administered in bulk ...
10. Test the Survey Platform: Ensure compatibility and usability for online surveys. By following these steps and paying attention to questionnaire design principles, you can create a well-structured and effective questionnaire that gathers reliable data and helps you achieve your research objectives.
The research methods you use depend on the type of data you need to answer your research question. If you want to measure something or test a hypothesis, use quantitative methods. If you want to explore ideas, thoughts and meanings, use qualitative methods. If you want to analyze a large amount of readily-available data, use secondary data.
Survey Instruments in Research Methods. The following are some commonly used survey instruments in research methods: Questionnaires: A questionnaire is a set of standardized questions designed to collect information about a specific topic. Questionnaires can be administered in different ways, including in person, over the phone, or online.
The most common method used in social and management sciences is the survey questionnaire because it is a cost-effective means to gather the data. It is inexpensive and allows quick responses and ...
Survey research also tends to use reliable instruments within their method of inquiry, many scales in survey questionnaires are standardized instruments. Other methods, such as qualitative interviewing, which we'll learn about in Chapter 18, do not offer the same consistency that a quantitative survey offers. This is not to say that all ...
Closed-ended questionnaires/survey: These types of questionnaires or surveys are like "multiple choice" tests, where participants must select from a list of premade answers.According to the content of the question, they must select the one that they agree with the most. This approach is the simplest form of quantitative research because the data is easy to combine and quantify.
Olivia Guy-Evans, MSc. Research methods in psychology are systematic procedures used to observe, describe, predict, and explain behavior and mental processes. They include experiments, surveys, case studies, and naturalistic observations, ensuring data collection is objective and reliable to understand and explain psychological phenomena.
Standardized self-report questionnaires are an ever-present research method in the social and medical sciences. Questionnaires are administered in many cross-sectional and longitudinal studies to collect information on a broad range of topics, including people's behaviors and feelings, attitudes and opinions, health status, personality, environmental conditions, life events, and well-being ...
The Best-Worst Method (BWM) is an MCDA tool that has proven useful due to its simplicity and computational capability. This research aims to test the applicability of this method to a population with low levels of education and determine whether a questionnaire with a continuous visual aid, a slider, is more suitable than the standard ...
The cluster random sampling method was used, resulting in a sample of 250 eligible women referred to the health centers of Tabriz, Iran, who were 4 to 6 weeks after giving birth. Cronbach's alpha coefficient and Intraclass Correlation Coefficient (ICC) using a test-retest approach were used to determine the questionnaire's reliability. Results
Questionnaires vs surveys. A survey is a research method where you collect and analyse data from a group of people. A questionnaire is a specific tool or instrument for collecting the data.. Designing a questionnaire means creating valid and reliable questions that address your research objectives, placing them in a useful order, and selecting an appropriate method for administration.
Objective: This study aims to examine the effectiveness of the Minder mobile app in improving mental health and substance use outcomes in a general population of university students. Methods: A 2-arm, parallel-assignment, single-blinded, 30-day randomized controlled trial was used to evaluate Minder using intention-to-treat analysis.
In this work, we discuss building performant Multimodal Large Language Models (MLLMs). In particular, we study the importance of various architecture components and data choices. Through careful and comprehensive ablations of the image encoder, the vision language connector, and various pre-training data choices, we identified several crucial design lessons. For example, we demonstrate that ...
Qualitative research involves collecting and analyzing non-numerical data (e.g., text, video, or audio) to understand concepts, opinions, or experiences. It can be used to gather in-depth insights into a problem or generate new ideas for research. Qualitative research is the opposite of quantitative research, which involves collecting and ...
Classical multivariate statistics and machine learning methods have been used to predict flavor of specific compounds by, for example, linking structural properties of a compound to its potential ...
The 100 categories they identified can be divided into six top-level themes, which give an immediate sense of what generative AI is being used for: Technical Assistance & Troubleshooting (23% ...