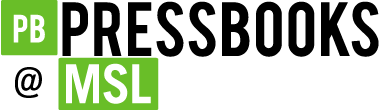
Chapter 6: 21st-century media and issues
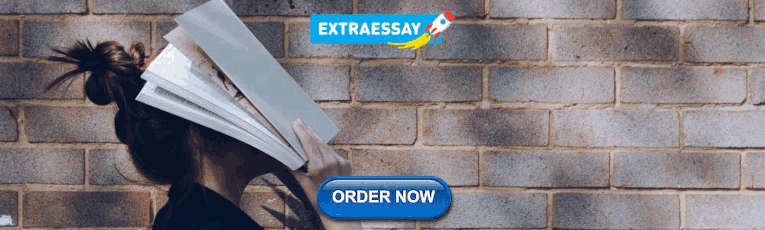
6.10.2 Social media and communication (research essay)
Lindsey Matier
English 102, April 2021
Communication is extremely important in today’s world, whether it be verbal or nonverbal. It can take place through many different forms such as through writing, speaking, listening and physical actions. These forms of communication evolve and continue to improve over time. As humans, we rely on communication for almost everything and it is a way of life. Communication has evolved from talking to writing letters to texting or talking over the phone. Every time a new form of communication is brought up and becomes more popular, we have to adapt and evolve to that new lifestyle. Throughout all the new forms of communication and ways of evolving, social media has been one of the most influential so far. Social media has allowed us to create new ways of communicating, such as texting or posting through different apps. It can connect us with people all over the world and give us a platform to express ourselves in ways that have not been possible before. While social media started off as a small form of technology, it has morphed into aspects of our everyday life. Now there are apps for everything from social media profiles to online shopping. While social media and technology itself has evolved, this has also affected our communication with each other and the world. Social media has created a fast track for information in a matter of seconds. It can give people a platform with millions of followers overnight for doing practically anything. It can help people express themselves in new ways and connect with people who have similar interests. The end goal of social media is to make people happy and ultimately make lives easier.
Introduction
With all this being said, it is evident that social media is in our everyday lives and will continue to change. It has a very strong grip on society as social media usage continues to rise throughout the years. Generalizing social media, we are exposed to forms of media at almost all times of the day. Answering the question of what media is will help give a better understanding of social media as a whole. Media can be defined as a way of mass communication. This could include siting in the car listening to ads on the radio all the way to scrolling on twitter. We are exposed to social media less often than generalized media, but it tends to come in greater quantities when exposed. For example, for people that wake up and check twitter it is an instant flood of information with every scroll. Everything from politics to sports to celebrity news is available at the fingertips. The concern is not all focused on the overwhelming information, but also the overwhelming number of comments and opinions. If we wanted to debate or talk about something before social media it had to be done in person, face to face. Now with social media, we are able to fight with people in comment sections on a backup account with a different name and no connection to who we really are. This new form of communication takes away the vulnerability of speaking to people and having genuine conversation, and makes up for it in internet trolls. Overall, social media is impacting the way we communicate with each other and the real questions are: Is social media impacting us in a positive or negative way? Do the positive aspects outweigh the negative aspects? Is social media hindering the way we communicate in person with each other? Is their more room for improvement when it comes to dealing with communication in the social media spectrum? How is social media impacting younger generation’s communication versus older generation’s communication? How can we help improve our communication skills on social media and in real life?
Personal Research
Along with the other studies that I found from the sources I chose, I also conducted my own study to determine more accurate and recent data. I asked students mostly within high school and college range questions relating to social media and communication. I tried to get a wide range of data dealing with social media apps, screen time, and overall communication as a result of social media. I expected to see almost all negative responses about social media and communication. I figured that most people would respond saying that it has affected them negatively rather than positively, but the results were different compared to what I expected.
The first questions I asked had to do with social media itself. I asked questions about their most used social media apps, screen time, what age they were allowed to start using social media, and whether or not they think social media has had a negative or positive impact on them. As expected, most of the social media apps were some of the most popular ones like Snapchat, Instagram, and TikTok. Overall, the average screen time for all apps was evenly split between 4-6 and 6-8 hours, which I also expected. Something that did surprise me was the amount of time spent on certain social media apps. The data was split pretty evenly three ways and all between 1-4 hours. The next two questions dealt with when they group surveyed started using social media. I asked these questions because a lot of the points I want to discuss later in my paper have to deal with age and whether younger generations are suffering when it comes to communication. More than half the people surveyed said that they wished that they had waited to get social media until they were older. Some said that it is not appropriate for younger kids and that it is just toxic in general. Something that I really like that a couple people mentioned was that in reality, social media at a young age is stupid and useless. A lot of people said they wish they would have enjoyed their childhood more and they would be more extroverted now if they had not been exposed that early. The last question of this section that I asked was if they thought social media has had a more positive or negative impact on them. Overall, the data was split but leaning slightly towards the more positive side. The positive answers mostly dealt with being able to talk to stay in contact with people and meeting new friends. The negative answers all related to mental health and feeling bad about themselves. A lot of people said it is toxic and very controlling and takes up too much of our time.
The next set of questions I asked had to do more with communication and interaction with and without social media. I asked questions like how they feel about social media and how it has impacted their communication, their mental health, and if it has made our lives easier. I decided to ask questions like these because I figured I would get a wide range of responses and a lot of people’s different opinions. I started off by asking if people are an introvert or an extrovert to get an idea of what the responses would be like, and 66% said somewhere in between the two. The response for the next question really shocked me because I received such a one-side response. I asked if they think social media has impacted their communication and the way they interact with others and 75% (18/24 people) said yes. This is the information that I was looking for along with the next two questions. The next question asked if they think social media has negatively impacted their mental health and 50% said yes. I also plan on using this as a research question to show that social media can affect our mental health and therefore affect the way we interact with and around other people. The last two questions are similar but the responses were both very good. Almost everyone answered yes to the question asking if social media has made our lives easier. Everyone that answered yes said they think so because it helps them talk to friends, stay in touch with people they do not see as much, and meet new people that they are comfortable talking to. The people that said no also made good points such as it takes over our lives and it is filled with too much hate and cancel culture. I agree with both sides and am very happy that people can feel a positive response especially when it comes to communicating with other people online. The last question I asked was used to wrap up the whole survey and topic. I asked if they think social media has made our generation’s communication improve or worsen. The data was pretty evenly split, and most people gave a positive and a negative. The people that said improve gave that answer because they said it broadens our communication and allows us to talk to people at a wider range. The people who said it has made it worse all said that it is ruining our face-to-face interaction and causing us to lose emotion. They said that some people do not even know how to have a proper in person conversation and that they are too dependent on their phones. Overall, I agree with both arguments that people made but I do think that the positives outweigh the negatives in most of these situations and questions.
Research Questions
The first question I want to ask has to deal with the overall social media and communication connection and has multiple other questions I would like to cover within it. The main question is: Is social media hindering the way we communicate with each other? I also want to touch on questions like: Is social media impacting us in a positive or negative way? Do the positives outweigh the negatives? The second set of research questions I have is: Is their more room for improvement when it comes to dealing with communication in the social media spectrum? How can we help improve our communication skills on social media and in real life? How is social media impacting younger generation’s communication versus older generation’s communication?
Research Question One
Social media and communication have a direct connection to each other and both have a strong impact on the outcome of the other. My first research question has to do with that. My questions center around how social media has impacted our communication, and whether or not it is positive or negative. First, I think it is important to note the changes and different characteristics that come into play when talking about this. Things like age and problems going on in our world can affect our social media usage and communication. While we connect to people on a deeper level when talking to the in person, social media has also given us a newer and more broad way of communicating. The article “How Social Media Affects Our Ability to Communicate” by Stacey Hanke, talks about different ways social media has impacted our communication. Social media has become so relevant in our day to day lives and Hanke describes it in a couple different ways. She describes it as information binging and the fear of missing out, social graces and conversational boredom. Within these, she explains how social media has become an excuse and escape to talk to people face to face. Hanke also talks about how even though it is limiting our in person communication, it can sometimes make communicating in general easier, by being able to talk to each other in just a few words (Hanke 1). In another article by Ryan J. Fuller titled “The Impact of Social Media Use on Our Social Skills”, he discusses similar topics to Hanke’s article but also brings up more positive attributes of social media. Fuller starts of his article by giving some statistics, stating that 75% of teens own cellphones and 25% of them using it for social media, and also says that they use 7.5 hours a day using it (Fuller 1). I am glad that this was brought up because it is important to know how much time is spent on social media, scrolling through feed. Next, Fuller starts to discuss some of the benefits of social media. He briefly explains how social media is beneficial because we are able to stay in touch with our friends and family, and share important parts of our lives with them. He also explains how it helps people reach out to new friends and provide themselves with more opportunities (Fuller 1). Overall, I really like that he mentioned these because it is important to keep in mind the vast majority of social media and communication. While some use it for more simpler purposes likes just keeping up to date with what is going on in the world, others use it to make new friends, find new job opportunities, and stay in touch with people. Another topic I find important when it comes to answering this research question is how Covid affected everything. With the pandemic, we were left inside with nothing to do but what was at our fingertips. This pandemic increased social media usage drastically. The article “Social Media Insights Into US Mental Health During the COVID-19 Pandemic: Longitudinal Analysis of Twitter Data” by Danny Valdez et al, shows extensive research into determining just how much social media usage in the United States increased during the pandemic. They did experiments and surveys to determine multiple responses to research questions and show how much we rely on social media to communicate with each other. During the pandemic, everyone spent more time on their social media and their phone in general, probably more than they would like to admit. The article helps give more insight into this claim. There is the idea that social media was meant as an addition to our lives. For some people, it has become an addiction and a new piece of their life. The article focuses on how social media could be a toxic place and have a negative effect on our mental health. The time period for this information focuses around the COVID-19 pandemic. Using data from Twitter, Valdez created a study to determine the mood of people during the pandemic and the usage throughout (Valdez et al 2). Collecting tweets with certain hashtags and during time periods, the goal was to determine how much the pandemic affected people’s moods, and how much they put out and shared on social media. They used hashtags, timeline data, and tweets from different periods such as the first lockdown, different stay at home orders, etc. Given the responses to the data, they were able to determine the increase in social media usage. We cannot determine if this had a positive or negative effect on the people who were using Twitter, but we can infer that social media is becoming a key part of our lives. Not being able to talk to people as much in person during the first few months of the pandemic greatly affected communication, in positive and negative ways. Communication over the phone increased due to the amount of free time that people had and were able to spend talking to others. Contrary to that, in person communication also decreased given that people were not really allowed to leave the house. The next article by Tayebi et al, “The Role of Information Systems in Communication Through Social Media” focuses a lot about how we have evolved over time with social media and communication. They start off by talking about how social networks are like social media societies. They explain it by resembling it to a human society, as it is filled with people communicating, regardless of time or place. They also exemplify other aspects such as emotional support, information, emotions (Tayebi 2). Social media is constantly looked at through such a negative light due to some of the major bad events that have taken place. While it can be difficult at times to look past the negatives, it is important to recognize and acknowledge the positives. The growth of scientific research would not be possible without the amount of information received from the media (Tayebi 3). Without social media and media in general, we would not be where we are today as a society. As mentioned earlier, it is so easy to get lost in the negative aspects of social media and discard the positive ones. Positive parts of social media such as widespread communication and unlimited access to information makes it all worth it. Staying on topic with positive aspects of social media and communication, social media in the workplace has also broken down barriers for communication. The article “A Guide to the Successful Use of Social Media in the Workplace” by Clark Boyd gives insight into how social media has improved the workplace, and ultimately communication and interaction as a whole. Companies can use social media as a form of branding and way to communicate their products (Boyd 4). Boyd states, “Harvard Business Review finds that 82% of employees believe social media improves work relationships. Left to their own devices, your teams will connect and communicate on social networks, both inside and outside the office.” This directly relates to the research question asking whether social media hinders our communication with each other. Social media also helps when it comes to dealing with complaints placed online. By seeing these through social media, it can help the company communicate either with the person or their company the concerns that are being stated (Boyd 9). Overall, it is safe to say that social media has directly affected communication throughout different aspects of our lives.
Research Question Two
My second set of research questions has a lot to do with the future and how we can improve. Questions such as: Is their more room for improvement when it comes to dealing with communication in the social media spectrum? How can we help improve our communication skills on social media and in real life? How is social media impacting younger generation’s communication versus older generation’s communication? The article “What is Literacy” by James Paul Gee talks a lot about the basics of communication. I find this an important article to talk about before I go into more detail with this second research question. Gee explains discourse as a socially accepted way of speaking, thinking, and acting (Gee 1). It is important to note this because social media has changed that discourse for us. We no longer communicate and interact the same way in which we use to therefore almost giving us a new discourse. Another thing Gee discusses is identity kits. Gee explains identity kits as “appropriate costumes and instructions on how to act and talk” (Gee 2). This relates to social media because there is a certain way we communicate online that we wouldn’t do in person. For example, we use emojis and abbreviations to communicate on social media or over text, but this is something we would not do when communicating face-to-face. There are also some basic well-known rules of social media that follow along the lines of an identity kit. Such as, for Instagram it is a common idea not to like people’s pictures from too long ago. When you say this aloud it sounds like it is not a big deal and silly almost, but for people that use social media it is something that makes sense. The next article is going to focus more on the question that has to do with room for improvement of communication. The article “The Positive Effect of Not Following Others on Social Media” by Francesca Valsesia, Davide Proserpio, and Joseph C. Nunes involves how we deal with social media and how we react to it. The article has a lot to do with pyramid schemes and marketing schemes on social media, simply due to follower count. Social media has a lot of power over us and the content we see. Influencers have too much impact on what we see every day and this overall effects our communication (Valsesia 1). Social media feeds us information at our fingertips, whether it be true or false. Valsesia is trying to get the point across that social media has no impact on our lives without the phone and therefore, having a smaller follower count is better for our communication and overall wellbeing in the first place. Leading into my next article, social media can have a huge impact on the younger generation. This leads into part of my second research question dealing with the younger generation and their communication. The article “The Impact of Social Media on Youth Mental Health: Challenges and Opportunities” by Jacqueline Nesi shows how social media is a very complex brand of information and makes it complicated for everyone. Younger kids having access to it and multiple devices like computers and phones makes it that much more difficult. There are a lot of positives and negatives for younger kids having access to social media and the internet in general. It has an impact on their mental health and studies show it leads to signs of depression, body dysmorphia, eating disorders (Nesi 2). It can also affect their communication and outward identity due to things such as bullying, internet drama, and behavioral problems. While it does have serious negative risks, social media also can bring a lot of new positive ones. Things like creative ideas, humor and entertainment, and being able to explore their identity are all really great positives that social media gives us (Nesi 4). Most of them using it as a way to connect with friends and family and help them feel a sense of acceptance and belonging (Nesi 4). Similarly to this, social media has given a great outlet for kids and young adults to speak out on issues going on in the world. The article “Building Bridges: Exploring the Communication Trends and Perceived Sociopolitical Benefits of Adolescents Engaging in Online Social Justice Efforts” by Mariah Elsa Kornbluh goes into detail about the racial injustices in the world and how they are communicated through social media. Social media networks can help connect kids to different backgrounds and aspects of their lives (Kornbluh 1). Kornbluh expresses how a society only can flourish under civic engagement and being able to express ourselves, and social media is helping us do that. It is helping the younger generation prepare for the civic role that they will undergo (Kornbluh 2). Social media helps play a major role in participating in political movements and bringing awareness to topics (Kornbluh 3). This all is done by the younger generation and would not be possible without them. So, while it is easy to look at the negative parts of social media and how it effects the younger generation, it also brings great awareness to real life problems in our world. This last article I wanted to go over dealing with this research question has to do with the pandemic. The article “Responses to COVID-19 in Higher Education: Social Media Usage for Sustaining Formal Academic Communication in Developing Countries” by Abu Elnasr E. Sobaih, Ahmed M. Hasanein and Ahmed E. Abu Elnasr briefly talks about communication with social media in higher education systems. Education systems had to switch from in person learning and communication to online learning, which was a struggle for everyone. Throughout the time that this took place, results showed that social media had a positive effect on students dealing with this (Sobaih 1). Students used social media to build a community and help support each other through this rough time. Through these results, proper usage of social media can be shown as a positive result for a new era of learning (Sobaih 1). This is just one more reason why social media can help us improve our future.
After answering my research questions, it has become clear to me that while social media does have negative aspects, the positive aspects outweigh them. Between the articles and my own research, I have enough evidence to prove that social media does effect communication, but in a more positive way. The way we act and present ourselves is heavily influenced by social media and communication between generations are different and can be seen that way. It is important to note the accomplishments we have made as a society with social media and the media in general. It has helped connect families, provide support groups, and provide entertainment in desperate times. Our communication has changed because of social media but has changed and helped us for the better in the long run. Keeping social media a positive place and staying away from the toxic people on it will only help us grow and learn new things about ourselves.
Works Cited
Boyd, Clark. “A Guide to Using Social Media in the Workplace in 2021.” The Blueprint , The Blueprint, 13 May 2020, www.fool.com/the-blueprint/social-media-in-the-workplace/.
https://www.fool.com/the-blueprint/social-media-in-the-workplace/
D, Valdez, et al. “Social Media Insights Into US Mental Health During the Covid-19 Pandemic: Longitudinal Analysis of Twitter Data.” Journal of Medical Internet Research , vol. 22, no. 12, 14 Dec. 2020, pp. 1438–8871.
http://eds.b.ebscohost.com.proxy.ulib.csuohio.edu:2050/eds/detail/detail? vid=8&sid=ff59b04c-b868-44cd-b864-4538e112a2ea%40sessionmgr103&bdata=JnNpdGU9ZWRzLWxpdmUmc2NvcGU9c2l0ZQ%3d%3d#AN=33284783&db=mnh
J, Nesi. “The Impact of Social Media on Youth Health: Challenges and Opportunities.” North Carolina Medical Journal , vol. 81, no. 2, 2020, pp. 116–121.
http://eds.b.ebscohost.com.proxy.ulib.csuohio.edu:2050/eds/detail/detail?vid=10&sid=ff59b04c-b868-44cd-b864-4538e112a2ea%40sessionmgr103&bdata=JnNpdGU9ZWRzLWxpdmUmc2NvcGU9c2l0ZQ%3d%3d#AN=32132255&db=mnh
Gee, James Paul. “What is literacy.” Negotiating academic literacies: Teaching and learning across languages and cultures (1998): 51-59.
https://academic.jamespaulgee.com/pdfs/Gee%20What%20is%20Literacy.pdf
Hanke, Stacey. “How Social Media Affects Our Ability to Communicate.” Thrive Global , 13 Sept. 2018, thriveglobal.com/stories/how-social-media-affects-our-ability-to-communicate/.
https://thriveglobal.com/stories/how-social-media-affects-our-ability-to-communicate/
http://eds.a.ebscohost.com.proxy.ulib.csuohio.edu:2050/eds/pdfviewer/pdfviewer?vid=4&sid=467b825c-34f8-4e47-95df-e5b2b61bbaf4%40sessionmgr4006
Kornbluh, Mariah Elsa. “Building Bridges.” Youth & Society , vol. 51, no. 8, 2017, pp. 1104–1126., doi:10.1177/0044118×17723656.
https://journals-sagepub-com.proxy.ulib.csuohio.edu/doi/pdf/10.1177/0044118X17723656
Retchin, Sarah, et al. “The Impact of Social Media Use on Social Skills.” New York Behavioral Health , 1 Dec. 2020, newyorkbehavioralhealth.com/the-impact-of-social-media-use-on-social-skills/.
https://newyorkbehavioralhealth.com/the-impact-of-social-media-use-on-social-skills/
Sobaih, Abu Elnasr E., et al. “Responses to COVID-19 in Higher Education: Social Media Usage for Sustaining Formal Academic Communication in Developing Countries.” MDPI , Multidisciplinary Digital Publishing Institute, 12 Aug. 2020, www.mdpi.com/2071-1050/12/16/6520/htm.
https://www.mdpi.com/2071-1050/12/16/6520/htm
Tayeb, Seyed Mohammad, et al. “The Role of Information Systems in Communication through Social Media.” International Journal of Data and Network Science , vol. 3, no. 3, 2019, pp. 245–268., doi:10.5267/j.ijdns.2019.2.002.
http://www.growingscience.com/ijds/Vol3/ijdns_2019_15.pdf
Valsesia, Francesca, et al. “The Positive Effect of Not Following Others on Social Media .” Journal of Marketing Research , vol. 57, no. 6, Dec. 2020, pp. 1152–1168.
https://www.francescavalsesia.com/uploads/1/0/5/1/105151509/the_positive_effect_of_not_following_others_on_social_media.pdf
Understanding Literacy in Our Lives by Lindsey Matier is licensed under a Creative Commons Attribution-NonCommercial-NoDerivatives 4.0 International License , except where otherwise noted.
Share This Book
Feb 15, 2023
6 Example Essays on Social Media | Advantages, Effects, and Outlines
Got an essay assignment about the effects of social media we got you covered check out our examples and outlines below.
Social media has become one of our society's most prominent ways of communication and information sharing in a very short time. It has changed how we communicate and has given us a platform to express our views and opinions and connect with others. It keeps us informed about the world around us. Social media platforms such as Facebook, Twitter, Instagram, and LinkedIn have brought individuals from all over the world together, breaking down geographical borders and fostering a genuinely global community.
However, social media comes with its difficulties. With the rise of misinformation, cyberbullying, and privacy problems, it's critical to utilize these platforms properly and be aware of the risks. Students in the academic world are frequently assigned essays about the impact of social media on numerous elements of our lives, such as relationships, politics, and culture. These essays necessitate a thorough comprehension of the subject matter, critical thinking, and the ability to synthesize and convey information clearly and succinctly.
But where do you begin? It can be challenging to know where to start with so much information available. Jenni.ai comes in handy here. Jenni.ai is an AI application built exclusively for students to help them write essays more quickly and easily. Jenni.ai provides students with inspiration and assistance on how to approach their essays with its enormous database of sample essays on a variety of themes, including social media. Jenni.ai is the solution you've been looking for if you're experiencing writer's block or need assistance getting started.
So, whether you're a student looking to better your essay writing skills or want to remain up to date on the latest social media advancements, Jenni.ai is here to help. Jenni.ai is the ideal tool for helping you write your finest essay ever, thanks to its simple design, an extensive database of example essays, and cutting-edge AI technology. So, why delay? Sign up for a free trial of Jenni.ai today and begin exploring the worlds of social networking and essay writing!
Want to learn how to write an argumentative essay? Check out these inspiring examples!
We will provide various examples of social media essays so you may get a feel for the genre.
6 Examples of Social Media Essays
Here are 6 examples of Social Media Essays:
The Impact of Social Media on Relationships and Communication
Introduction:.
The way we share information and build relationships has evolved as a direct result of the prevalence of social media in our daily lives. The influence of social media on interpersonal connections and conversation is a hot topic. Although social media has many positive effects, such as bringing people together regardless of physical proximity and making communication quicker and more accessible, it also has a dark side that can affect interpersonal connections and dialogue.
Positive Effects:
Connecting People Across Distances
One of social media's most significant benefits is its ability to connect individuals across long distances. People can use social media platforms to interact and stay in touch with friends and family far away. People can now maintain intimate relationships with those they care about, even when physically separated.
Improved Communication Speed and Efficiency
Additionally, the proliferation of social media sites has accelerated and simplified communication. Thanks to instant messaging, users can have short, timely conversations rather than lengthy ones via email. Furthermore, social media facilitates group communication, such as with classmates or employees, by providing a unified forum for such activities.
Negative Effects:
Decreased Face-to-Face Communication
The decline in in-person interaction is one of social media's most pernicious consequences on interpersonal connections and dialogue. People's reliance on digital communication over in-person contact has increased along with the popularity of social media. Face-to-face interaction has suffered as a result, which has adverse effects on interpersonal relationships and the development of social skills.
Decreased Emotional Intimacy
Another adverse effect of social media on relationships and communication is decreased emotional intimacy. Digital communication lacks the nonverbal cues and facial expressions critical in building emotional connections with others. This can make it more difficult for people to develop close and meaningful relationships, leading to increased loneliness and isolation.
Increased Conflict and Miscommunication
Finally, social media can also lead to increased conflict and miscommunication. The anonymity and distance provided by digital communication can lead to misunderstandings and hurtful comments that might not have been made face-to-face. Additionally, social media can provide a platform for cyberbullying , which can have severe consequences for the victim's mental health and well-being.
Conclusion:
In conclusion, the impact of social media on relationships and communication is a complex issue with both positive and negative effects. While social media platforms offer many benefits, such as connecting people across distances and enabling faster and more accessible communication, they also have a dark side that can negatively affect relationships and communication. It is up to individuals to use social media responsibly and to prioritize in-person communication in their relationships and interactions with others.
The Role of Social Media in the Spread of Misinformation and Fake News
Social media has revolutionized the way information is shared and disseminated. However, the ease and speed at which data can be spread on social media also make it a powerful tool for spreading misinformation and fake news. Misinformation and fake news can seriously affect public opinion, influence political decisions, and even cause harm to individuals and communities.
The Pervasiveness of Misinformation and Fake News on Social Media
Misinformation and fake news are prevalent on social media platforms, where they can spread quickly and reach a large audience. This is partly due to the way social media algorithms work, which prioritizes content likely to generate engagement, such as sensational or controversial stories. As a result, false information can spread rapidly and be widely shared before it is fact-checked or debunked.
The Influence of Social Media on Public Opinion
Social media can significantly impact public opinion, as people are likelier to believe the information they see shared by their friends and followers. This can lead to a self-reinforcing cycle, where misinformation and fake news are spread and reinforced, even in the face of evidence to the contrary.
The Challenge of Correcting Misinformation and Fake News
Correcting misinformation and fake news on social media can be a challenging task. This is partly due to the speed at which false information can spread and the difficulty of reaching the same audience exposed to the wrong information in the first place. Additionally, some individuals may be resistant to accepting correction, primarily if the incorrect information supports their beliefs or biases.
In conclusion, the function of social media in disseminating misinformation and fake news is complex and urgent. While social media has revolutionized the sharing of information, it has also made it simpler for false information to propagate and be widely believed. Individuals must be accountable for the information they share and consume, and social media firms must take measures to prevent the spread of disinformation and fake news on their platforms.
The Effects of Social Media on Mental Health and Well-Being
Social media has become an integral part of modern life, with billions of people around the world using platforms like Facebook, Instagram, and Twitter to stay connected with others and access information. However, while social media has many benefits, it can also negatively affect mental health and well-being.
Comparison and Low Self-Esteem
One of the key ways that social media can affect mental health is by promoting feelings of comparison and low self-esteem. People often present a curated version of their lives on social media, highlighting their successes and hiding their struggles. This can lead others to compare themselves unfavorably, leading to feelings of inadequacy and low self-esteem.
Cyberbullying and Online Harassment
Another way that social media can negatively impact mental health is through cyberbullying and online harassment. Social media provides a platform for anonymous individuals to harass and abuse others, leading to feelings of anxiety, fear, and depression.
Social Isolation
Despite its name, social media can also contribute to feelings of isolation. At the same time, people may have many online friends but need more meaningful in-person connections and support. This can lead to feelings of loneliness and depression.
Addiction and Overuse
Finally, social media can be addictive, leading to overuse and negatively impacting mental health and well-being. People may spend hours each day scrolling through their feeds, neglecting other important areas of their lives, such as work, family, and self-care.
In sum, social media has positive and negative consequences on one's psychological and emotional well-being. Realizing this, and taking measures like reducing one's social media use, reaching out to loved ones for help, and prioritizing one's well-being, are crucial. In addition, it's vital that social media giants take ownership of their platforms and actively encourage excellent mental health and well-being.
The Use of Social Media in Political Activism and Social Movements
Social media has recently become increasingly crucial in political action and social movements. Platforms such as Twitter, Facebook, and Instagram have given people new ways to express themselves, organize protests, and raise awareness about social and political issues.
Raising Awareness and Mobilizing Action
One of the most important uses of social media in political activity and social movements has been to raise awareness about important issues and mobilize action. Hashtags such as #MeToo and #BlackLivesMatter, for example, have brought attention to sexual harassment and racial injustice, respectively. Similarly, social media has been used to organize protests and other political actions, allowing people to band together and express themselves on a bigger scale.
Connecting with like-minded individuals
A second method in that social media has been utilized in political activity and social movements is to unite like-minded individuals. Through social media, individuals can join online groups, share knowledge and resources, and work with others to accomplish shared objectives. This has been especially significant for geographically scattered individuals or those without access to traditional means of political organizing.
Challenges and Limitations
As a vehicle for political action and social movements, social media has faced many obstacles and restrictions despite its many advantages. For instance, the propagation of misinformation and fake news on social media can impede attempts to disseminate accurate and reliable information. In addition, social media corporations have been condemned for censorship and insufficient protection of user rights.
In conclusion, social media has emerged as a potent instrument for political activism and social movements, giving voice to previously unheard communities and galvanizing support for change. Social media presents many opportunities for communication and collaboration. Still, users and institutions must be conscious of the risks and limitations of these tools to promote their responsible and productive usage.
The Potential Privacy Concerns Raised by Social Media Use and Data Collection Practices
With billions of users each day on sites like Facebook, Twitter, and Instagram, social media has ingrained itself into every aspect of our lives. While these platforms offer a straightforward method to communicate with others and exchange information, they also raise significant concerns over data collecting and privacy. This article will examine the possible privacy issues posed by social media use and data-gathering techniques.
Data Collection and Sharing
The gathering and sharing of personal data are significant privacy issues brought up by social media use. Social networking sites gather user data, including details about their relationships, hobbies, and routines. This information is made available to third-party businesses for various uses, such as marketing and advertising. This can lead to serious concerns about who has access to and uses our personal information.
Lack of Control Over Personal Information
The absence of user control over personal information is a significant privacy issue brought up by social media usage. Social media makes it challenging to limit who has access to and how data is utilized once it has been posted. Sensitive information may end up being extensively disseminated and may be used maliciously as a result.
Personalized Marketing
Social media companies utilize the information they gather about users to target them with adverts relevant to their interests and usage patterns. Although this could be useful, it might also cause consumers to worry about their privacy since they might feel that their personal information is being used without their permission. Furthermore, there are issues with the integrity of the data being used to target users and the possibility of prejudice based on individual traits.
Government Surveillance
Using social media might spark worries about government surveillance. There are significant concerns regarding privacy and free expression when governments in some nations utilize social media platforms to follow and monitor residents.
In conclusion, social media use raises significant concerns regarding data collecting and privacy. While these platforms make it easy to interact with people and exchange information, they also gather a lot of personal information, which raises questions about who may access it and how it will be used. Users should be aware of these privacy issues and take precautions to safeguard their personal information, such as exercising caution when choosing what details to disclose on social media and keeping their information sharing with other firms to a minimum.
The Ethical and Privacy Concerns Surrounding Social Media Use And Data Collection
Our use of social media to communicate with loved ones, acquire information, and even conduct business has become a crucial part of our everyday lives. The extensive use of social media does, however, raise some ethical and privacy issues that must be resolved. The influence of social media use and data collecting on user rights, the accountability of social media businesses, and the need for improved regulation are all topics that will be covered in this article.
Effect on Individual Privacy:
Social networking sites gather tons of personal data from their users, including delicate information like search history, location data, and even health data. Each user's detailed profile may be created with this data and sold to advertising or used for other reasons. Concerns regarding the privacy of personal information might arise because social media businesses can use this data to target users with customized adverts.
Additionally, individuals might need to know how much their personal information is being gathered and exploited. Data breaches or the unauthorized sharing of personal information with other parties may result in instances where sensitive information is exposed. Users should be aware of the privacy rules of social media firms and take precautions to secure their data.
Responsibility of Social Media Companies:
Social media firms should ensure that they responsibly and ethically gather and use user information. This entails establishing strong security measures to safeguard sensitive information and ensuring users are informed of what information is being collected and how it is used.
Many social media businesses, nevertheless, have come under fire for not upholding these obligations. For instance, the Cambridge Analytica incident highlighted how Facebook users' personal information was exploited for political objectives without their knowledge. This demonstrates the necessity of social media corporations being held responsible for their deeds and ensuring that they are safeguarding the security and privacy of their users.
Better Regulation Is Needed
There is a need for tighter regulation in this field, given the effect, social media has on individual privacy as well as the obligations of social media firms. The creation of laws and regulations that ensure social media companies are gathering and using user information ethically and responsibly, as well as making sure users are aware of their rights and have the ability to control the information that is being collected about them, are all part of this.
Additionally, legislation should ensure that social media businesses are held responsible for their behavior, for example, by levying fines for data breaches or the unauthorized use of personal data. This will provide social media businesses with a significant incentive to prioritize their users' privacy and security and ensure they are upholding their obligations.
In conclusion, social media has fundamentally changed how we engage and communicate with one another, but this increased convenience also raises several ethical and privacy issues. Essential concerns that need to be addressed include the effect of social media on individual privacy, the accountability of social media businesses, and the requirement for greater regulation to safeguard user rights. We can make everyone's online experience safer and more secure by looking more closely at these issues.
In conclusion, social media is a complex and multifaceted topic that has recently captured the world's attention. With its ever-growing influence on our lives, it's no surprise that it has become a popular subject for students to explore in their writing. Whether you are writing an argumentative essay on the impact of social media on privacy, a persuasive essay on the role of social media in politics, or a descriptive essay on the changes social media has brought to the way we communicate, there are countless angles to approach this subject.
However, writing a comprehensive and well-researched essay on social media can be daunting. It requires a thorough understanding of the topic and the ability to articulate your ideas clearly and concisely. This is where Jenni.ai comes in. Our AI-powered tool is designed to help students like you save time and energy and focus on what truly matters - your education. With Jenni.ai , you'll have access to a wealth of examples and receive personalized writing suggestions and feedback.
Whether you're a student who's just starting your writing journey or looking to perfect your craft, Jenni.ai has everything you need to succeed. Our tool provides you with the necessary resources to write with confidence and clarity, no matter your experience level. You'll be able to experiment with different styles, explore new ideas , and refine your writing skills.
So why waste your time and energy struggling to write an essay on your own when you can have Jenni.ai by your side? Sign up for our free trial today and experience the difference for yourself! With Jenni.ai, you'll have the resources you need to write confidently, clearly, and creatively. Get started today and see just how easy and efficient writing can be!
Try Jenni for free today
Create your first piece of content with Jenni today and never look back
Academia.edu no longer supports Internet Explorer.
To browse Academia.edu and the wider internet faster and more securely, please take a few seconds to upgrade your browser .
Enter the email address you signed up with and we'll email you a reset link.
- We're Hiring!
- Help Center
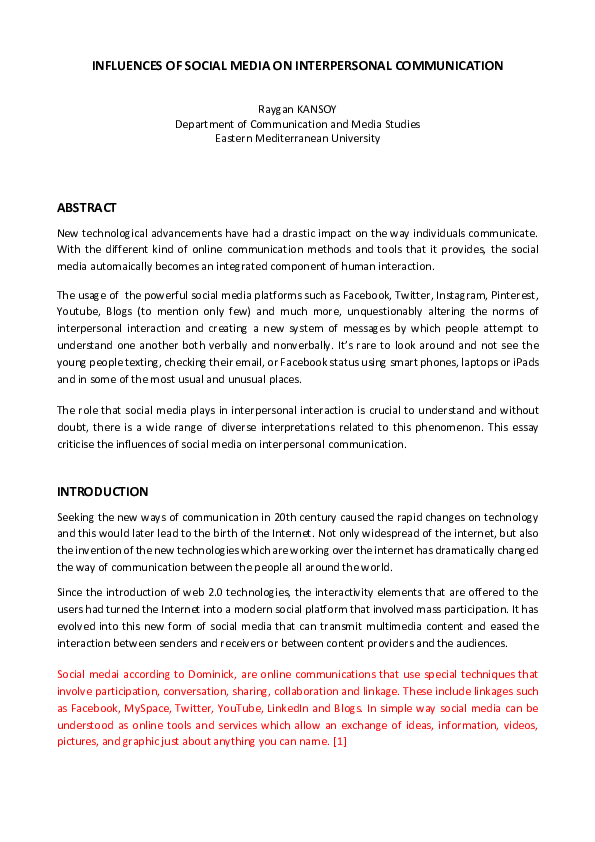
INFLUENCES OF SOCIAL MEDIA ON INTERPERSONAL COMMUNICATION

New technological advancements have had a drastic impact on the way individuals communicate. With the different kind of online communication methods and tools that it provides, the social media automaically becomes an integrated component of human interaction. The usage of the powerful social media platforms such as Facebook, Twitter, Instagram, Pinterest, Youtube, Blogs (to mention only few) and much more, unquestionably altering the norms of interpersonal interaction and creating a new system of messages by which people attempt to understand one another both verbally and nonverbally. It's rare to look around and not see the young people texting, checking their email, or Facebook status using smart phones, laptops or iPads and in some of the most usual and unusual places. The role that social media plays in interpersonal interaction is crucial to understand and without doubt, there is a wide range of diverse interpretations related to this phenomenon. This essay criticise the influences of social media on interpersonal communication.
Related Papers
Review of Management
Srirang Jha , Anju Gulla
Social media has emerged as one of the most potent communication tools in recent times. It has empowered the people to express themselves in an effective manner to reach the fellow human beings with common interests. Social media has also helped significantly in connecting people of extended communities across the globe. However, social media, with all its features, is extremely addictive. Social media creates a unique virtual world where people have hardly any inhibitions in interacting with anyone who are on the same platform like Facebook, Instagram, Twitter, etc. Thus, the people spend more time in the virtual world at the cost of their traditional social interactions in the real world. Such a scenario has remarkably limited their abilities to connect with others in real situations-posing a serious civilizational challenge by undermining interpersonal communication. This article looks at the value of interpersonal communication in contemporary world and examines how we can deal with diminishing social interactions in the wake of phenomenal rise of the social media. INTRODUCTION Berger (2008) has defined interpersonal communication as an interchange of information between two or more people that reflects how humans use verbal and non-verbal cues to realize a number of personal and social goals. However, there are other scholars who believe that interpersonal communication is more than transmission of information entailing how people negotiate meanings, identity and relationships (Braithwaite and Baxter, 2008). Basic components of interpersonal communication include how humans adjust and adapt their verbal communication and non-verbal communication during face to face interaction, how messages are produced, how uncertainty influences behaviour and information-management strategies, deception in interpersonal exchange, social contentions and social interactions mediated by technology (Berger, 2005).
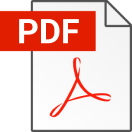
Ken Rhose Waupan
Th e present article deals with the problem of social media communication and its impact on the everyday communicative behavior; namely, on the ways we speak and write. In order to accomplish this task at fi rst we turn to philosophical background of communication, paying a special attention Søren Kierkegaard's media criticism. Th e second chapter of the article is devoted to the explication of the basic notions of social media studies, emphasizing such concepts as new media, new new media, and network society. Th e third part of the article presents the results of students' survey regarding their attitudes towards contemporary communication practices, the main conclusion being that although students recognize the impact of social media on their everyday communication patterns, including the situation and perceive it as a new natural environment of communication.
rasimah yusoff
Nowadays, social media sites have become very popular and provide more convenient lifestyle to the people today. It has modernized the way people connect, interact and socialize with each other. Due to proliferate of social websites and applications, social media has become an indispensable part of life with the increase in complexities of contemporary times. Besides, the variety of social networking platforms delivered by developers gives end user most likely to facilitate communication by sharing through text, video and audio streams. As a matter of fact, the diversity of entertainments in social media sites might cause an individual over spending time on the computer or smartphone. These circumstances show that people might physically become inactive to learn new knowledge and lack of interest to go for outdoor activities. The main purpose of this study is to find out the communication problem among people. This study entails the investigation to discover social media is playing ...
International Journal for Research in Applied Science & Engineering Technology (IJRASET)
IJRASET Publication
Over specific times, the manner human beings speak took numerous paperwork, which includes meeting, traveling, mailing, posting, and calling. Currently, maximum of those communications are changed with digital on line platform which include Facebook, what's app etc. In spite of the truth that of the internet is open for every body and people appears abuse it. It is widely regular that higher procedures of conversation are on hand for us because the internet becomes made. A few Individuals help the concept folk's affiliation thru social media, no matter the truth that others take into account this as something wrong. This studies paper will speak approximately each of those perspectives and could supply my non-public opinion. This studies paper will too delineate the additives which have pushed to the upward push of social media as a success communications medium. You can also additionally in addition to pick up information of ways social media has modified the circulation of conversation. I.
Lia Hernandez
Since the beginnings of time human beings are considered social beings. We live in communities, take care of our own and even though we are based in micro structures, like families, we are supposed to be empathetic to the world that revolves around us with which we interact. We've created social laws such as ethics and morals that reflect as manners and values and even though these exist, these are only things we've put a name to or realize its existence. Every human has a different reality; life is complex and cannot be taught, it can only be lived through experiences. As social beings we have the capacity of understanding the world and relationships through those experiences among the people that surround us. In this essay I present my reflections on the new development of relationships and communication because of the use of social media in the contemporary time. The use of social media has become a basic part of our lives and this dependency has been developed mostly because every aspect of our lives has also taken a place in the virtual world and if it has not, it certainly wants to. But if mankind has created something so advanced that we are able to exist in our reality and at the same time be so connected to other realities, where does the problem rely on? Well, for me, it relays on the importance we give to this new world. The virtual self is a creation of yours that you are free to manage and that out speaks about you. You are able to present yourself as you want and freely talk about your opinions. I believe that is a wonderful opportunity; nonetheless, I separate your virtual self from your real self because its not really you; it is only what you want to say about you. The virtual world is a creation based on the real one, it's a creation that everyday grows into us as something indispensable, as our own reality. We made changes based on technology when it became a part of our everyday life, we started talking everyday instead of seeing each other everyday, we started looking for self validation through likes instead of looking for it outside home being social. We started to care too much about showing how we are instead of actually just being, we started caring about other realities or other places while taking for granted the one we are in. In the late 1990's, which was the beginning of the use of the World Wide Web we never even dreamed of the world, we have today. We had a lot of expectations; we saw machines as something advanced, as something amusing. The Internet was promoted in every show of the early 2000's. It was a revolution. The use of the color white and silver, the minimalism, the idea of everything being computerized, the movies about robots taking the power of earth, the idea of going to space, all this created an urge for more that maybe now we cant control. The creation of social media has developed some unmanageable and increasing wrong wised values in our culture that most of us are free to act on through the web. The virtual world has a lot to do with the ego, because every user is constantly presenting themselves, self-validating themselves.
Jurnal Humanipreneur
Missnoopyy S
The purpose of this study was to find out whether or not the use of social media by youth decreases social interaction with friends. This study used a quantitative method using a simple random sampling technique to take the sample. The subjects of this study were Business English Communication students from batches 2019, 2020, and 2021. This study has two variables, namely social media (X) and interaction with friends (Y). A questionnaire was used as the method for collecting data for this study. Two types of questionnaires: open-ended and closed-ended questionnaires, were used as the instruments and data collection techniques. Data analysis employed the normality test, linearity test, and t-test for testing hypotheses. Based on the questionnaire results, data analysis was conducted, and the results for the two variables ell into the high category. The t-test results showed that when the value of t-count exceeds t-table (7.722> 2.002), H0 is rejected. Thus, it can be said that ...
Bolarinwa E Mowemi
Tolga Sevük
Irudaya Raj
Duvan Vergara
MIT News | Massachusetts Institute of Technology
- Machine learning
- Social justice
- Black holes
- Classes and programs
Departments
- Aeronautics and Astronautics
- Brain and Cognitive Sciences
- Architecture
- Political Science
- Mechanical Engineering
Centers, Labs, & Programs
- Abdul Latif Jameel Poverty Action Lab (J-PAL)
- Picower Institute for Learning and Memory
- Lincoln Laboratory
- School of Architecture + Planning
- School of Engineering
- School of Humanities, Arts, and Social Sciences
- Sloan School of Management
- School of Science
- MIT Schwarzman College of Computing
Why social media has changed the world — and how to fix it
Press contact :, media download.
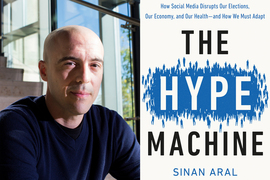
*Terms of Use:
Images for download on the MIT News office website are made available to non-commercial entities, press and the general public under a Creative Commons Attribution Non-Commercial No Derivatives license . You may not alter the images provided, other than to crop them to size. A credit line must be used when reproducing images; if one is not provided below, credit the images to "MIT."
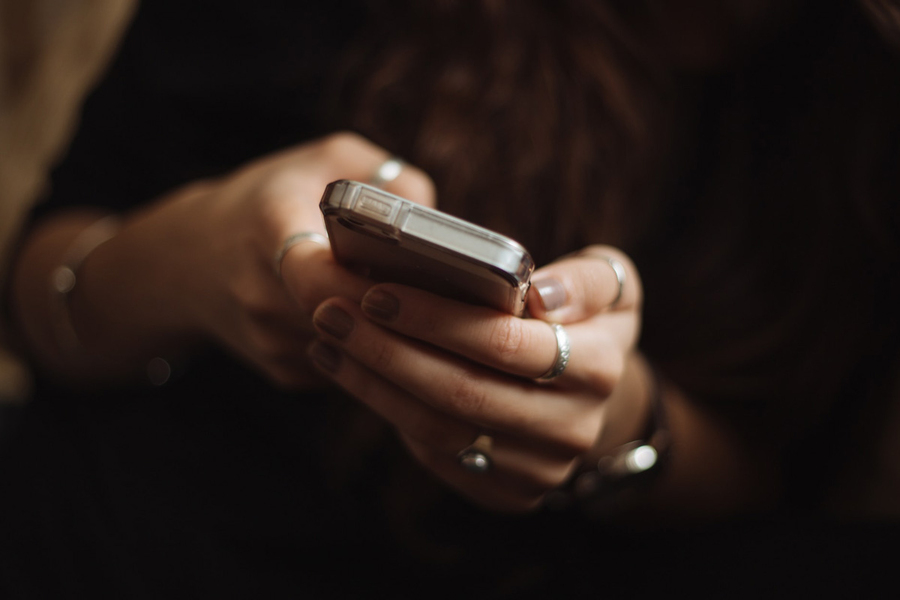
Previous image Next image
Are you on social media a lot? When is the last time you checked Twitter, Facebook, or Instagram? Last night? Before breakfast? Five minutes ago?
If so, you are not alone — which is the point, of course. Humans are highly social creatures. Our brains have become wired to process social information, and we usually feel better when we are connected. Social media taps into this tendency.
“Human brains have essentially evolved because of sociality more than any other thing,” says Sinan Aral, an MIT professor and expert in information technology and marketing. “When you develop a population-scale technology that delivers social signals to the tune of trillions per day in real-time, the rise of social media isn’t unexpected. It’s like tossing a lit match into a pool of gasoline.”
The numbers make this clear. In 2005, about 7 percent of American adults used social media. But by 2017, 80 percent of American adults used Facebook alone. About 3.5 billion people on the planet, out of 7.7 billion, are active social media participants. Globally, during a typical day, people post 500 million tweets, share over 10 billion pieces of Facebook content, and watch over a billion hours of YouTube video.
As social media platforms have grown, though, the once-prevalent, gauzy utopian vision of online community has disappeared. Along with the benefits of easy connectivity and increased information, social media has also become a vehicle for disinformation and political attacks from beyond sovereign borders.
“Social media disrupts our elections, our economy, and our health,” says Aral, who is the David Austin Professor of Management at the MIT Sloan School of Management.
Now Aral has written a book about it. In “The Hype Machine,” published this month by Currency, a Random House imprint, Aral details why social media platforms have become so successful yet so problematic, and suggests ways to improve them.
As Aral notes, the book covers some of the same territory as “The Social Dilemma,” a documentary that is one of the most popular films on Netflix at the moment. But Aral’s book, as he puts it, "starts where ‘The Social Dilemma’ leaves off and goes one step further to ask: What can we do about it?”
“This machine exists in every facet of our lives,” Aral says. “And the question in the book is, what do we do? How do we achieve the promise of this machine and avoid the peril? We’re at a crossroads. What we do next is essential, so I want to equip people, policymakers, and platforms to help us achieve the good outcomes and avoid the bad outcomes.”
When “engagement” equals anger
“The Hype Machine” draws on Aral’s own research about social networks, as well as other findings, from the cognitive sciences, computer science, business, politics, and more. Researchers at the University of California at Los Angeles, for instance, have found that people obtain bigger hits of dopamine — the chemical in our brains highly bound up with motivation and reward — when their social media posts receive more likes.
At the same time, consider a 2018 MIT study by Soroush Vosoughi, an MIT PhD student and now an assistant professor of computer science at Dartmouth College; Deb Roy, MIT professor of media arts and sciences and executive director of the MIT Media Lab; and Aral, who has been studying social networking for 20 years. The three researchers found that on Twitter, from 2006 to 2017, false news stories were 70 percent more likely to be retweeted than true ones. Why? Most likely because false news has greater novelty value compared to the truth, and provokes stronger reactions — especially disgust and surprise.
In this light, the essential tension surrounding social media companies is that their platforms gain audiences and revenue when posts provoke strong emotional responses, often based on dubious content.
“This is a well-designed, well-thought-out machine that has objectives it maximizes,” Aral says. “The business models that run the social-media industrial complex have a lot to do with the outcomes we’re seeing — it’s an attention economy, and businesses want you engaged. How do they get engagement? Well, they give you little dopamine hits, and … get you riled up. That’s why I call it the hype machine. We know strong emotions get us engaged, so [that favors] anger and salacious content.”
From Russia to marketing
“The Hype Machine” explores both the political implications and business dimensions of social media in depth. Certainly social media is fertile terrain for misinformation campaigns. During the 2016 U.S. presidential election, Russia spread false information to at least 126 million people on Facebook and another 20 million people on Instagram (which Facebook owns), and was responsible for 10 million tweets. About 44 percent of adult Americans visited a false news source in the final weeks of the campaign.
“I think we need to be a lot more vigilant than we are,” says Aral.
We do not know if Russia’s efforts altered the outcome of the 2016 election, Aral says, though they may have been fairly effective. Curiously, it is not clear if the same is true of most U.S. corporate engagement efforts.
As Aral examines, digital advertising on most big U.S. online platforms is often wildly ineffective, with academic studies showing that the “lift” generated by ad campaigns — the extent to which they affect consumer action — has been overstated by a factor of hundreds, in some cases. Simply counting clicks on ads is not enough. Instead, online engagement tends to be more effective among new consumers, and when it is targeted well; in that sense, there is a parallel between good marketing and guerilla social media campaigns.
“The two questions I get asked the most these days,” Aral says, “are, one, did Russia succeed in intervening in our democracy? And two, how do I measure the ROI [return on investment] from marketing investments? As I was writing this book, I realized the answer to those two questions is the same.”
Ideas for improvement
“The Hype Machine” has received praise from many commentators. Foster Provost, a professor at New York University’s Stern School of Business, says it is a “masterful integration of science, business, law, and policy.” Duncan Watts, a university professor at the University of Pennsylvania, says the book is “essential reading for anyone who wants to understand how we got here and how we can get somewhere better.”
In that vein, “The Hype Machine” has several detailed suggestions for improving social media. Aral favors automated and user-generated labeling of false news, and limiting revenue-collection that is based on false content. He also calls for firms to help scholars better research the issue of election interference.
Aral believes federal privacy measures could be useful, if we learn from the benefits and missteps of the General Data Protection Regulation (GDPR) in Europe and a new California law that lets consumers stop some data-sharing and allows people to find out what information companies have stored about them. He does not endorse breaking up Facebook, and suggests instead that the social media economy needs structural reform. He calls for data portability and interoperability, so “consumers would own their identities and could freely switch from one network to another.” Aral believes that without such fundamental changes, new platforms will simply replace the old ones, propelled by the network effects that drive the social-media economy.
“I do not advocate any one silver bullet,” says Aral, who emphasizes that changes in four areas together — money, code, norms, and laws — can alter the trajectory of the social media industry.
But if things continue without change, Aral adds, Facebook and the other social media giants risk substantial civic backlash and user burnout.
“If you get me angry and riled up, I might click more in the short term, but I might also grow really tired and annoyed by how this is making my life miserable, and I might turn you off entirely,” Aral observes. “I mean, that’s why we have a Delete Facebook movement, that’s why we have a Stop Hate for Profit movement. People are pushing back against the short-term vision, and I think we need to embrace this longer-term vision of a healthier communications ecosystem.”
Changing the social media giants can seem like a tall order. Still, Aral says, these firms are not necessarily destined for domination.
“I don’t think this technology or any other technology has some deterministic endpoint,” Aral says. “I want to bring us back to a more practical reality, which is that technology is what we make it, and we are abdicating our responsibility to steer technology toward good and away from bad. That is the path I try to illuminate in this book.”
Share this news article on:
Press mentions.
Prof. Sinan Aral’s new book, “The Hype Machine,” has been selected as one of the best books of the year about AI by Wired . Gilad Edelman notes that Aral’s book is “an engagingly written shortcut to expertise on what the likes of Facebook and Twitter are doing to our brains and our society.”
Prof. Sinan Aral speaks with Danny Crichton of TechCrunch about his new book, “The Hype Machine,” which explores the future of social media. Aral notes that he believes a starting point “for solving the social media crisis is creating competition in the social media economy.”
New York Times
Prof. Sinan Aral speaks with New York Times editorial board member Greg Bensinger about how social media platforms can reduce the spread of misinformation. “Human-in-the-loop moderation is the right solution,” says Aral. “It’s not a simple silver bullet, but it would give accountability where these companies have in the past blamed software.”
Prof. Sinan Aral speaks with Kara Miller of GBH’s Innovation Hub about his research examining the impact of social media on everything from business re-openings during the Covid-19 pandemic to politics.
Prof. Sinan Aral speaks with NPR’s Michael Martin about his new book, “The Hype Machine,” which explores the benefits and downfalls posed by social media. “I've been researching social media for 20 years. I've seen its evolution and also the techno utopianism and dystopianism,” says Aral. “I thought it was appropriate to have a book that asks, 'what can we do to really fix the social media morass we find ourselves in?'”
Previous item Next item
Related Links
- MIT Sloan School of Management
Related Topics
- Business and management
- Social media
- Books and authors
- Behavioral economics
Related Articles
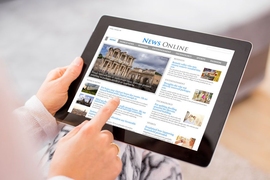
The catch to putting warning labels on fake news
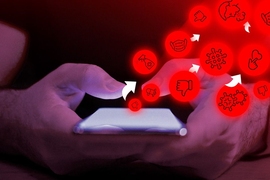
Our itch to share helps spread Covid-19 misinformation
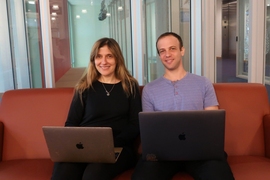
Better fact-checking for fake news
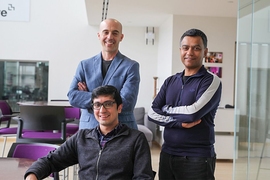
Study: On Twitter, false news travels faster than true stories
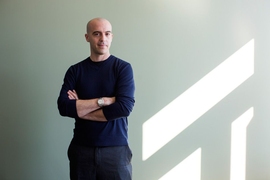
Social networking
More mit news.
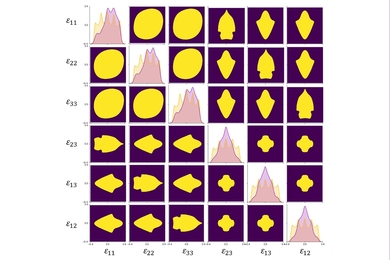
A first-ever complete map for elastic strain engineering
Read full story →
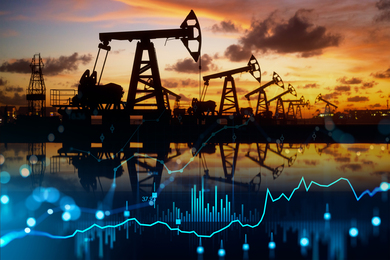
Shining a light on oil fields to make them more sustainable
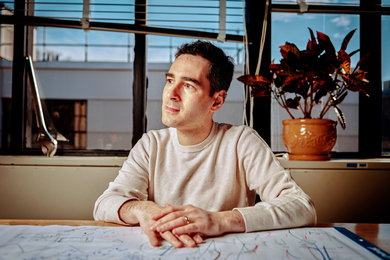
“Life is short, so aim high”
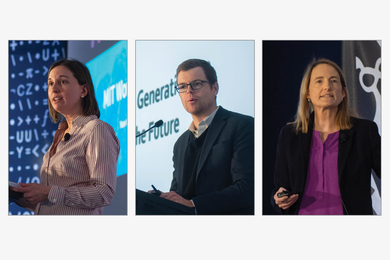
MIT launches Working Group on Generative AI and the Work of the Future
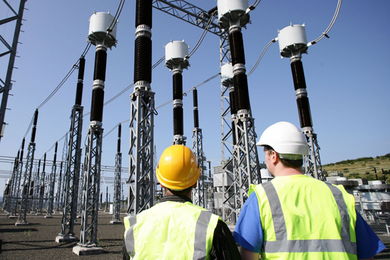
Atmospheric observations in China show rise in emissions of a potent greenhouse gas
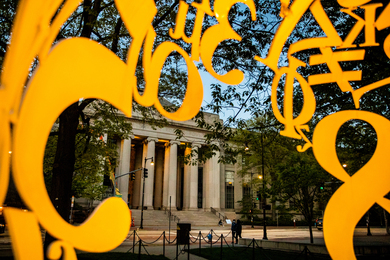
Second round of seed grants awarded to MIT scholars studying the impact and applications of generative AI
- More news on MIT News homepage →
Massachusetts Institute of Technology 77 Massachusetts Avenue, Cambridge, MA, USA
- Map (opens in new window)
- Events (opens in new window)
- People (opens in new window)
- Careers (opens in new window)
- Accessibility
- Social Media Hub
- MIT on Facebook
- MIT on YouTube
- MIT on Instagram
Home — Essay Samples — Sociology — Social Media — The Impact of Social Media: Causes and Effects

The Impact of Social Media: Causes and Effects
- Categories: Effects of Social Media Social Media
About this sample

Words: 1226 |
Published: Feb 7, 2024
Words: 1226 | Pages: 3 | 7 min read
Table of contents
Introduction, cause 1: increased connectivity and communication, cause 2: promotion of self-expression and individuality, cause 3: access to information and awareness, effect 1: impacts on mental health, effect 2: influence on societal norms and values, effect 3: privacy and security concerns.
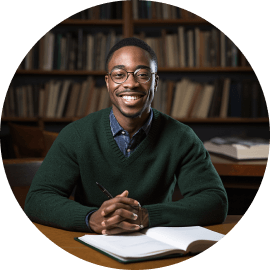
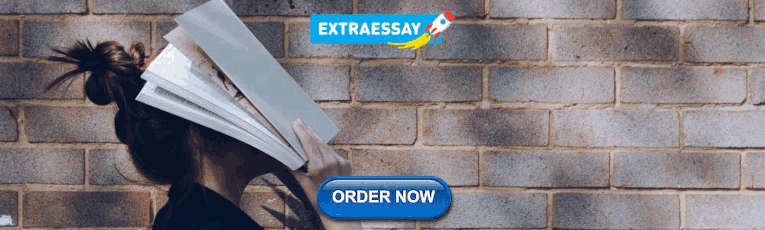
Cite this Essay
Let us write you an essay from scratch
- 450+ experts on 30 subjects ready to help
- Custom essay delivered in as few as 3 hours
Get high-quality help
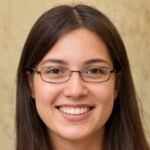
Verified writer
- Expert in: Sociology
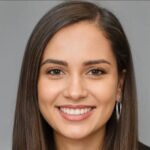
+ 120 experts online
By clicking “Check Writers’ Offers”, you agree to our terms of service and privacy policy . We’ll occasionally send you promo and account related email
No need to pay just yet!
Related Essays
2 pages / 1041 words
6 pages / 2611 words
2 pages / 1121 words
4 pages / 1882 words
Remember! This is just a sample.
You can get your custom paper by one of our expert writers.
121 writers online
Still can’t find what you need?
Browse our vast selection of original essay samples, each expertly formatted and styled
Related Essays on Social Media
The advancements of technology have brought upon many positive and negative effects on society. The most recent technology provides easier access to our everyday needs such as the way we purchase products and the way we [...]
Social media pros and cons are often debated as the influence of these platforms continues to grow. Social media is a term that describes a person’s ability to affect other people’s social thinking in a community. The numerous [...]
Social media has a big role towards today’s life and society as the one who has control towards today’s life has already familiar with social media and the uses of it. Society is a concept used to describe the structured [...]
There are many sites which facilitate the sharing of media throughout a community, but a prominent one is YouTube. YouTube is a free video sharing website that makes it easy to watch online videos. You can even create and [...]
Many of the institutions have embarked on embracing new technological models in order to adapt towards the digital revolution which is why the digital divide is decreasing. The term digital equality is coming to surface because [...]
Are people blind to what’s in front of them because of a screen? The use of social media has grown exponentially to the extent of engaging 3.1 billion users worldwide, with more than half of society linked to a form of social [...]
Related Topics
By clicking “Send”, you agree to our Terms of service and Privacy statement . We will occasionally send you account related emails.
Where do you want us to send this sample?
By clicking “Continue”, you agree to our terms of service and privacy policy.
Be careful. This essay is not unique
This essay was donated by a student and is likely to have been used and submitted before
Download this Sample
Free samples may contain mistakes and not unique parts
Sorry, we could not paraphrase this essay. Our professional writers can rewrite it and get you a unique paper.
Please check your inbox.
We can write you a custom essay that will follow your exact instructions and meet the deadlines. Let's fix your grades together!
Get Your Personalized Essay in 3 Hours or Less!
We use cookies to personalyze your web-site experience. By continuing we’ll assume you board with our cookie policy .
- Instructions Followed To The Letter
- Deadlines Met At Every Stage
- Unique And Plagiarism Free
Social Media: Influences and Impacts on Culture
- Conference paper
- First Online: 04 July 2020
- Cite this conference paper
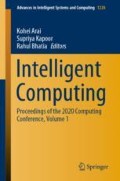
- Mui Joo Tang 17 &
- Eang Teng Chan 17
Part of the book series: Advances in Intelligent Systems and Computing ((AISC,volume 1228))
Included in the following conference series:
- Science and Information Conference
3100 Accesses
5 Citations
Advanced technologies of communication have brought influences and impacts on cultures. There are views that the influences and impacts are brought forward by social media which has been a powerful tool that can affect and form human behaviors as well as culture. Social media may have crossed the boundaries of culture due to the concept of borderless. Facebook may have been the social media that connects people around the world with massive cultural backgrounds to meet at the platform. The media content uploaded may spark the invasion of culture. There are many other social media which come with influencers that may shout about different values and practices around. Local cultures had therefore slowly lost their identities and replaced with a cross-cultural phenomenon. The cultural values invaded may include human behaviors, beliefs, values or even fashion and lifestyle. This research is to examine the factors of cross cultural communication, the reasons of culture being influenced by foreign countries through social media and the possibility of cultural invasion through social media. Social Influence theory is used in this research. Social influence occurs when a person’s emotions, opinions or behaviors are affected by others intentionally or unintentionally. Online survey Google Form is used at the target of 150 respondents aged between 18 to 25 years old. There is a contradicting view that people will lose their own culture after viewing too much of online content. The online activities and actions will slowly lead them to the change without their realization.
- Social media
- Media content
The authors acknowledged the raw material provided by Khoo Rose Lyn and Yong Zheng Wai.
This is a preview of subscription content, log in via an institution to check access.
Access this chapter
- Available as PDF
- Read on any device
- Instant download
- Own it forever
- Available as EPUB and PDF
- Compact, lightweight edition
- Dispatched in 3 to 5 business days
- Free shipping worldwide - see info
Tax calculation will be finalised at checkout
Purchases are for personal use only
Institutional subscriptions
Furedi, F.: http://www.aspeninstitute.cz/en/article/4-2014-how-the-internet-and-social-media-are-changing-culture/ . Accessed 28 Jan 2019
Kirkpatrick, D.: The Facebook Effect: The Inside Story of the Company That is Connecting the World. Simon & Schuster, New York (2010)
Google Scholar
Ta, J.Q.: http://www.business2community.com/social-media/impact-social-media-truly-society-0974685#QClV2qf84zb4iRzf.97 . Accessed 13 July 2016
Glucksman, M.: https://www.elon.edu/u/academics/communications/ . Accessed 1 July 2018
Tapinfluence Research. https://www.tapinfluence.com/tp_resource/future-influencer-marketing/ . Accessed 25 May 2019
Kasteler, J.: http://searchengineland.com/how-social-media-is-influencing-your-behavior-4061 . Accessed 1 July 2018
Mariella, A.: https://www.elitedaily.com/life/culture/x-pro-ii-valencia-instagram-choose-neither/614571 . Accessed 15 July 2018
Barcon, L.: http://monroviainquirer.com/2014/05/30/the-importance-of-culture-and-national-identity-in-the-societythe-sad-story-of-liberia/ . Accessed 15 July 2018
Wikipedia: Culture of Malaysia (2019). https://en.wikipedia.org/wiki/Culture_of_Malaysia . Accessed 13 June 2019
NCBI. http://www.ncbi.nlm.nih.gov/pmc/articles/PMC2792691/ . Accessed 10 Aug 2018
Kevin, H.: Stanford. https://www.business2community.com/brandviews/growing-social-media/social-media-culture-01635249 . Accessed 12 Aug 2018
Cross, T.: http://wallblog.co.uk/2013/11/08/the-culture-of-now-the-rise-of-imagery-in-social-media/ . Accessed 15 Aug 2018
Keller, M.: http://www.socialworktoday.com/archive/051313p10.shtml . Accessed 10 Sept 2018
Kelman, H.: Compliance, identification, and internalization: three processes of attitude change. J. Conflict Resolut. 2 (1), 51–60 (1958). https://doi.org/10.1177/002200275800200106
Article Google Scholar
Kietzmann, J.H., Hermkens, K.: Social media? Get serious! Understanding the functional building blocks of social media. Bus. Horiz. (Submitted manuscript) 54 (3), 241–251 (2011). https://doi.org/10.1016/j.bushor.2011.01.005
Download references
Author information
Authors and affiliations.
Tunku Abdul Rahman University College, Kuala Lumpur, Malaysia
Mui Joo Tang & Eang Teng Chan
You can also search for this author in PubMed Google Scholar
Corresponding author
Correspondence to Mui Joo Tang .
Editor information
Editors and affiliations.
Saga University, Saga, Japan
The Science and Information (SAI) Organization, Bradford, West Yorkshire, UK
Supriya Kapoor
Rahul Bhatia
Please Click (✓) For Your Choice
Student ID: ________________________________________________
Section B – Culture
In your opinion, how has the local culture been influenced?
□ Overseas media/social media content through internet
□ Foreign culture is better than local culture
□ Did not practice own culture well
□ Other than the above: _______________________
Section C – Social Media and Culture
What is your view if local culture is influenced?
(If POSITIVE , proceed to 7a ; NEGATIVE , proceed to 7b )
If positive, why?
□ It is good if we accept other culture
□ It is more educational
□ Other cultures are better
□ Others: ________________________________________________________
If negative, why?
□ Culture makes us who we are in identity
□ Culture is important
Section D – Effects of Social Media on Culture
Please Click (✓) Your Choice
Rights and permissions
Reprints and permissions
Copyright information
© 2020 Springer Nature Switzerland AG
About this paper
Cite this paper.
Tang, M.J., Chan, E.T. (2020). Social Media: Influences and Impacts on Culture. In: Arai, K., Kapoor, S., Bhatia, R. (eds) Intelligent Computing. SAI 2020. Advances in Intelligent Systems and Computing, vol 1228. Springer, Cham. https://doi.org/10.1007/978-3-030-52249-0_33
Download citation
DOI : https://doi.org/10.1007/978-3-030-52249-0_33
Published : 04 July 2020
Publisher Name : Springer, Cham
Print ISBN : 978-3-030-52248-3
Online ISBN : 978-3-030-52249-0
eBook Packages : Intelligent Technologies and Robotics Intelligent Technologies and Robotics (R0)
Share this paper
Anyone you share the following link with will be able to read this content:
Sorry, a shareable link is not currently available for this article.
Provided by the Springer Nature SharedIt content-sharing initiative
- Publish with us
Policies and ethics
- Find a journal
- Track your research

An official website of the United States government
The .gov means it’s official. Federal government websites often end in .gov or .mil. Before sharing sensitive information, make sure you’re on a federal government site.
The site is secure. The https:// ensures that you are connecting to the official website and that any information you provide is encrypted and transmitted securely.
- Publications
- Account settings
Preview improvements coming to the PMC website in October 2024. Learn More or Try it out now .
- Advanced Search
- Journal List
- v.12(6); 2020 Jun

Social Media Use and Its Connection to Mental Health: A Systematic Review
Fazida karim.
1 Psychology, California Institute of Behavioral Neurosciences and Psychology, Fairfield, USA
2 Business & Management, University Sultan Zainal Abidin, Terengganu, MYS
Azeezat A Oyewande
3 Family Medicine, California Institute of Behavioral Neurosciences and Psychology, Fairfield, USA
4 Family Medicine, Lagos State Health Service Commission/Alimosho General Hospital, Lagos, NGA
Lamis F Abdalla
5 Internal Medicine, California Institute of Behavioral Neurosciences and Psychology, Fairfield, USA
Reem Chaudhry Ehsanullah
Safeera khan.
Social media are responsible for aggravating mental health problems. This systematic study summarizes the effects of social network usage on mental health. Fifty papers were shortlisted from google scholar databases, and after the application of various inclusion and exclusion criteria, 16 papers were chosen and all papers were evaluated for quality. Eight papers were cross-sectional studies, three were longitudinal studies, two were qualitative studies, and others were systematic reviews. Findings were classified into two outcomes of mental health: anxiety and depression. Social media activity such as time spent to have a positive effect on the mental health domain. However, due to the cross-sectional design and methodological limitations of sampling, there are considerable differences. The structure of social media influences on mental health needs to be further analyzed through qualitative research and vertical cohort studies.
Introduction and background
Human beings are social creatures that require the companionship of others to make progress in life. Thus, being socially connected with other people can relieve stress, anxiety, and sadness, but lack of social connection can pose serious risks to mental health [ 1 ].
Social media
Social media has recently become part of people's daily activities; many of them spend hours each day on Messenger, Instagram, Facebook, and other popular social media. Thus, many researchers and scholars study the impact of social media and applications on various aspects of people’s lives [ 2 ]. Moreover, the number of social media users worldwide in 2019 is 3.484 billion, up 9% year-on-year [ 3 - 5 ]. A statistic in Figure 1 shows the gender distribution of social media audiences worldwide as of January 2020, sorted by platform. It was found that only 38% of Twitter users were male but 61% were using Snapchat. In contrast, females were more likely to use LinkedIn and Facebook. There is no denying that social media has now become an important part of many people's lives. Social media has many positive and enjoyable benefits, but it can also lead to mental health problems. Previous research found that age did not have an effect but gender did; females were much more likely to experience mental health than males [ 6 , 7 ].

Impact on mental health
Mental health is defined as a state of well-being in which people understand their abilities, solve everyday life problems, work well, and make a significant contribution to the lives of their communities [ 8 ]. There is debated presently going on regarding the benefits and negative impacts of social media on mental health [ 9 , 10 ]. Social networking is a crucial element in protecting our mental health. Both the quantity and quality of social relationships affect mental health, health behavior, physical health, and mortality risk [ 9 ]. The Displaced Behavior Theory may help explain why social media shows a connection with mental health. According to the theory, people who spend more time in sedentary behaviors such as social media use have less time for face-to-face social interaction, both of which have been proven to be protective against mental disorders [ 11 , 12 ]. On the other hand, social theories found how social media use affects mental health by influencing how people view, maintain, and interact with their social network [ 13 ]. A number of studies have been conducted on the impacts of social media, and it has been indicated that the prolonged use of social media platforms such as Facebook may be related to negative signs and symptoms of depression, anxiety, and stress [ 10 - 15 ]. Furthermore, social media can create a lot of pressure to create the stereotype that others want to see and also being as popular as others.
The need for a systematic review
Systematic studies can quantitatively and qualitatively identify, aggregate, and evaluate all accessible data to generate a warm and accurate response to the research questions involved [ 4 ]. In addition, many existing systematic studies related to mental health studies have been conducted worldwide. However, only a limited number of studies are integrated with social media and conducted in the context of social science because the available literature heavily focused on medical science [ 6 ]. Because social media is a relatively new phenomenon, the potential links between their use and mental health have not been widely investigated.
This paper attempt to systematically review all the relevant literature with the aim of filling the gap by examining social media impact on mental health, which is sedentary behavior, which, if in excess, raises the risk of health problems [ 7 , 9 , 12 ]. This study is important because it provides information on the extent of the focus of peer review literature, which can assist the researchers in delivering a prospect with the aim of understanding the future attention related to climate change strategies that require scholarly attention. This study is very useful because it provides information on the extent to which peer review literature can assist researchers in presenting prospects with a view to understanding future concerns related to mental health strategies that require scientific attention. The development of the current systematic review is based on the main research question: how does social media affect mental health?
Research strategy
The research was conducted to identify studies analyzing the role of social media on mental health. Google Scholar was used as our main database to find the relevant articles. Keywords that were used for the search were: (1) “social media”, (2) “mental health”, (3) “social media” AND “mental health”, (4) “social networking” AND “mental health”, and (5) “social networking” OR “social media” AND “mental health” (Table 1 ).
Out of the results in Table 1 , a total of 50 articles relevant to the research question were selected. After applying the inclusion and exclusion criteria, duplicate papers were removed, and, finally, a total of 28 articles were selected for review (Figure 2 ).

PRISMA, Preferred Reporting Items for Systematic Reviews and Meta-Analyses
Inclusion and exclusion criteria
Peer-reviewed, full-text research papers from the past five years were included in the review. All selected articles were in English language and any non-peer-reviewed and duplicate papers were excluded from finally selected articles.
Of the 16 selected research papers, there were a research focus on adults, gender, and preadolescents [ 10 - 19 ]. In the design, there were qualitative and quantitative studies [ 15 , 16 ]. There were three systematic reviews and one thematic analysis that explored the better or worse of using social media among adolescents [ 20 - 23 ]. In addition, eight were cross-sectional studies and only three were longitudinal studies [ 24 - 29 ].The meta-analyses included studies published beyond the last five years in this population. Table 2 presents a selection of studies from the review.
IGU, internet gaming disorder; PSMU, problematic social media use
This study has attempted to systematically analyze the existing literature on the effect of social media use on mental health. Although the results of the study were not completely consistent, this review found a general association between social media use and mental health issues. Although there is positive evidence for a link between social media and mental health, the opposite has been reported.
For example, a previous study found no relationship between the amount of time spent on social media and depression or between social media-related activities, such as the number of online friends and the number of “selfies”, and depression [ 29 ]. Similarly, Neira and Barber found that while higher investment in social media (e.g. active social media use) predicted adolescents’ depressive symptoms, no relationship was found between the frequency of social media use and depressed mood [ 28 ].
In the 16 studies, anxiety and depression were the most commonly measured outcome. The prominent risk factors for anxiety and depression emerging from this study comprised time spent, activity, and addiction to social media. In today's world, anxiety is one of the basic mental health problems. People liked and commented on their uploaded photos and videos. In today's age, everyone is immune to the social media context. Some teens experience anxiety from social media related to fear of loss, which causes teens to try to respond and check all their friends' messages and messages on a regular basis.
On the contrary, depression is one of the unintended significances of unnecessary use of social media. In detail, depression is limited not only to Facebooks but also to other social networking sites, which causes psychological problems. A new study found that individuals who are involved in social media, games, texts, mobile phones, etc. are more likely to experience depression.
The previous study found a 70% increase in self-reported depressive symptoms among the group using social media. The other social media influence that causes depression is sexual fun [ 12 ]. The intimacy fun happens when social media promotes putting on a facade that highlights the fun and excitement but does not tell us much about where we are struggling in our daily lives at a deeper level [ 28 ]. Another study revealed that depression and time spent on Facebook by adolescents are positively correlated [ 22 ]. More importantly, symptoms of major depression have been found among the individuals who spent most of their time in online activities and performing image management on social networking sites [ 14 ].
Another study assessed gender differences in associations between social media use and mental health. Females were found to be more addicted to social media as compared with males [ 26 ]. Passive activity in social media use such as reading posts is more strongly associated with depression than doing active use like making posts [ 23 ]. Other important findings of this review suggest that other factors such as interpersonal trust and family functioning may have a greater influence on the symptoms of depression than the frequency of social media use [ 28 , 29 ].
Limitation and suggestion
The limitations and suggestions were identified by the evidence involved in the study and review process. Previously, 7 of the 16 studies were cross-sectional and slightly failed to determine the causal relationship between the variables of interest. Given the evidence from cross-sectional studies, it is not possible to conclude that the use of social networks causes mental health problems. Only three longitudinal studies examined the causal relationship between social media and mental health, which is hard to examine if the mental health problem appeared more pronounced in those who use social media more compared with those who use it less or do not use at all [ 19 , 20 , 24 ]. Next, despite the fact that the proposed relationship between social media and mental health is complex, a few studies investigated mediating factors that may contribute or exacerbate this relationship. Further investigations are required to clarify the underlying factors that help examine why social media has a negative impact on some peoples’ mental health, whereas it has no or positive effect on others’ mental health.
Conclusions
Social media is a new study that is rapidly growing and gaining popularity. Thus, there are many unexplored and unexpected constructive answers associated with it. Lately, studies have found that using social media platforms can have a detrimental effect on the psychological health of its users. However, the extent to which the use of social media impacts the public is yet to be determined. This systematic review has found that social media envy can affect the level of anxiety and depression in individuals. In addition, other potential causes of anxiety and depression have been identified, which require further exploration.
The importance of such findings is to facilitate further research on social media and mental health. In addition, the information obtained from this study can be helpful not only to medical professionals but also to social science research. The findings of this study suggest that potential causal factors from social media can be considered when cooperating with patients who have been diagnosed with anxiety or depression. Also, if the results from this study were used to explore more relationships with another construct, this could potentially enhance the findings to reduce anxiety and depression rates and prevent suicide rates from occurring.
The content published in Cureus is the result of clinical experience and/or research by independent individuals or organizations. Cureus is not responsible for the scientific accuracy or reliability of data or conclusions published herein. All content published within Cureus is intended only for educational, research and reference purposes. Additionally, articles published within Cureus should not be deemed a suitable substitute for the advice of a qualified health care professional. Do not disregard or avoid professional medical advice due to content published within Cureus.
The authors have declared that no competing interests exist.
Thank you for visiting nature.com. You are using a browser version with limited support for CSS. To obtain the best experience, we recommend you use a more up to date browser (or turn off compatibility mode in Internet Explorer). In the meantime, to ensure continued support, we are displaying the site without styles and JavaScript.
- View all journals
- My Account Login
- Explore content
- About the journal
- Publish with us
- Sign up for alerts
- Open access
- Published: 20 March 2024
Persistent interaction patterns across social media platforms and over time
- Michele Avalle ORCID: orcid.org/0009-0007-4934-2326 1 na1 ,
- Niccolò Di Marco 1 na1 ,
- Gabriele Etta 1 na1 ,
- Emanuele Sangiorgio ORCID: orcid.org/0009-0003-1024-3735 2 ,
- Shayan Alipour 1 ,
- Anita Bonetti 3 ,
- Lorenzo Alvisi 1 ,
- Antonio Scala 4 ,
- Andrea Baronchelli 5 , 6 ,
- Matteo Cinelli ORCID: orcid.org/0000-0003-3899-4592 1 &
- Walter Quattrociocchi ORCID: orcid.org/0000-0002-4374-9324 1
Nature ( 2024 ) Cite this article
12k Accesses
223 Altmetric
Metrics details
- Mathematics and computing
- Social sciences
Growing concern surrounds the impact of social media platforms on public discourse 1 , 2 , 3 , 4 and their influence on social dynamics 5 , 6 , 7 , 8 , 9 , especially in the context of toxicity 10 , 11 , 12 . Here, to better understand these phenomena, we use a comparative approach to isolate human behavioural patterns across multiple social media platforms. In particular, we analyse conversations in different online communities, focusing on identifying consistent patterns of toxic content. Drawing from an extensive dataset that spans eight platforms over 34 years—from Usenet to contemporary social media—our findings show consistent conversation patterns and user behaviour, irrespective of the platform, topic or time. Notably, although long conversations consistently exhibit higher toxicity, toxic language does not invariably discourage people from participating in a conversation, and toxicity does not necessarily escalate as discussions evolve. Our analysis suggests that debates and contrasting sentiments among users significantly contribute to more intense and hostile discussions. Moreover, the persistence of these patterns across three decades, despite changes in platforms and societal norms, underscores the pivotal role of human behaviour in shaping online discourse.
Similar content being viewed by others
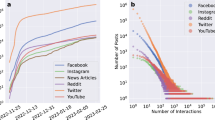
Cross-platform social dynamics: an analysis of ChatGPT and COVID-19 vaccine conversations
Shayan Alipour, Alessandro Galeazzi, … Walter Quattrociocchi
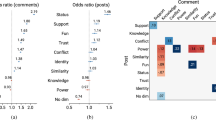
The language of opinion change on social media under the lens of communicative action
Corrado Monti, Luca Maria Aiello, … Francesco Bonchi
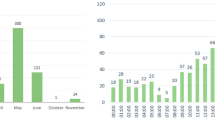
A social media network analysis of trypophobia communication
Xanat Vargas Meza & Shinichi Koyama
The advent and proliferation of social media platforms have not only transformed the landscape of online participation 2 but have also become integral to our daily lives, serving as primary sources for information, entertainment and personal communication 13 , 14 . Although these platforms offer unprecedented connectivity and information exchange opportunities, they also present challenges by entangling their business models with complex social dynamics, raising substantial concerns about their broader impact on society. Previous research has extensively addressed issues such as polarization, misinformation and antisocial behaviours in online spaces 5 , 7 , 12 , 15 , 16 , 17 , revealing the multifaceted nature of social media’s influence on public discourse. However, a considerable challenge in understanding how these platforms might influence inherent human behaviours lies in the general lack of accessible data 18 . Even when researchers obtain data through special agreements with companies like Meta, it may not be enough to clearly distinguish between inherent human behaviours and the effects of the platform’s design 3 , 4 , 8 , 9 . This difficulty arises because the data, deeply embedded in platform interactions, complicate separating intrinsic human behaviour from the influences exerted by the platform’s design and algorithms.
Here we address this challenge by focusing on toxicity, one of the most prominent aspects of concern in online conversations. We use a comparative analysis to uncover consistent patterns across diverse social media platforms and timeframes, aiming to shed light on toxicity dynamics across various digital environments. In particular, our goal is to gain insights into inherently invariant human patterns of online conversations.
The lack of non-verbal cues and physical presence on the web can contribute to increased incivility in online discussions compared with face-to-face interactions 19 . This trend is especially pronounced in online arenas such as newspaper comment sections and political discussions, where exchanges may degenerate into offensive comments or mockery, undermining the potential for productive and democratic debate 20 , 21 . When exposed to such uncivil language, users are more likely to interpret these messages as hostile, influencing their judgement and leading them to form opinions based on their beliefs rather than the information presented and may foster polarized perspectives, especially among groups with differing values 22 . Indeed, there is a natural tendency for online users to seek out and align with information that echoes their pre-existing beliefs, often ignoring contrasting views 6 , 23 . This behaviour may result in the creation of echo chambers, in which like-minded individuals congregate and mutually reinforce shared narratives 5 , 24 , 25 . These echo chambers, along with increased polarization, vary in their prevalence and intensity across different social media platforms 1 , suggesting that the design and algorithms of these platforms, intended to maximize user engagement, can substantially shape online social dynamics. This focus on engagement can inadvertently highlight certain behaviours, making it challenging to differentiate between organic user interaction and the influence of the platform’s design. A substantial portion of current research is devoted to examining harmful language on social media and its wider effects, online and offline 10 , 26 . This examination is crucial, as it reveals how social media may reflect and amplify societal issues, including the deterioration of public discourse. The growing interest in analysing online toxicity through massive data analysis coincides with advancements in machine learning capable of detecting toxic language 27 . Although numerous studies have focused on online toxicity, most concentrate on specific platforms and topics 28 , 29 . Broader, multiplatform studies are still limited in scale and reach 12 , 30 . Research fragmentation complicates understanding whether perceptions about online toxicity are accurate or misconceptions 31 . Key questions include whether online discussions are inherently toxic and how toxic and non-toxic conversations differ. Clarifying these dynamics and how they have evolved over time is crucial for developing effective strategies and policies to mitigate online toxicity.
Our study involves a comparative analysis of online conversations, focusing on three dimensions: time, platform and topic. We examine conversations from eight different platforms, totalling about 500 million comments. For our analysis, we adopt the toxicity definition provided by the Perspective API, a state-of-the-art classifier for the automatic detection of toxic speech. This API considers toxicity as “a rude, disrespectful or unreasonable comment likely to make someone leave a discussion”. We further validate this definition by confirming its consistency with outcomes from other detection tools, ensuring the reliability and comparability of our results. The concept of toxicity in online discourse varies widely in the literature, reflecting its complexity, as seen in various studies 32 , 33 , 34 . The efficacy and constraints of current machine-learning-based automated toxicity detection systems have recently been debated 11 , 35 . Despite these discussions, automated systems are still the most practical means for large-scale analyses.
Here we analyse online conversations, challenging common assumptions about their dynamics. Our findings reveal consistent patterns across various platforms and different times, such as the heavy-tailed nature of engagement dynamics, a decrease in user participation and an increase in toxic speech in lengthier conversations. Our analysis indicates that, although toxicity and user participation in debates are independent variables, the diversity of opinions and sentiments among users may have a substantial role in escalating conversation toxicity.
To obtain a comprehensive picture of online social media conversations, we analysed a dataset of about 500 million comments from Facebook, Gab, Reddit, Telegram, Twitter, Usenet, Voat and YouTube, covering diverse topics and spanning over three decades (a dataset breakdown is shown in Table 1 and Supplementary Table 1 ; for details regarding the data collection, see the ‘Data collection’ section of the Methods ).
Our analysis aims to comprehensively compare the dynamics of diverse social media accounting for human behaviours and how they evolved. In particular, we first characterize conversations at a macroscopic level by means of their engagement and participation, and we then analyse the toxicity of conversations both after and during their unfolding. We conclude the paper by examining potential drivers for the emergence of toxic speech.
Conversations on different platforms
This section provides an overview of online conversations by considering user activity and thread size metrics. We define a conversation (or a thread) as a sequence of comments that follow chronologically from an initial post. In Fig. 1a and Extended Data Fig. 1 , we observe that, across all platforms, both user activity (defined as the number of comments posted by the user) and thread length (defined as the number of comments in a thread) exhibit heavy-tailed distributions. The summary statistics about these distributions are reported in Supplementary Tables 1 and 2 .
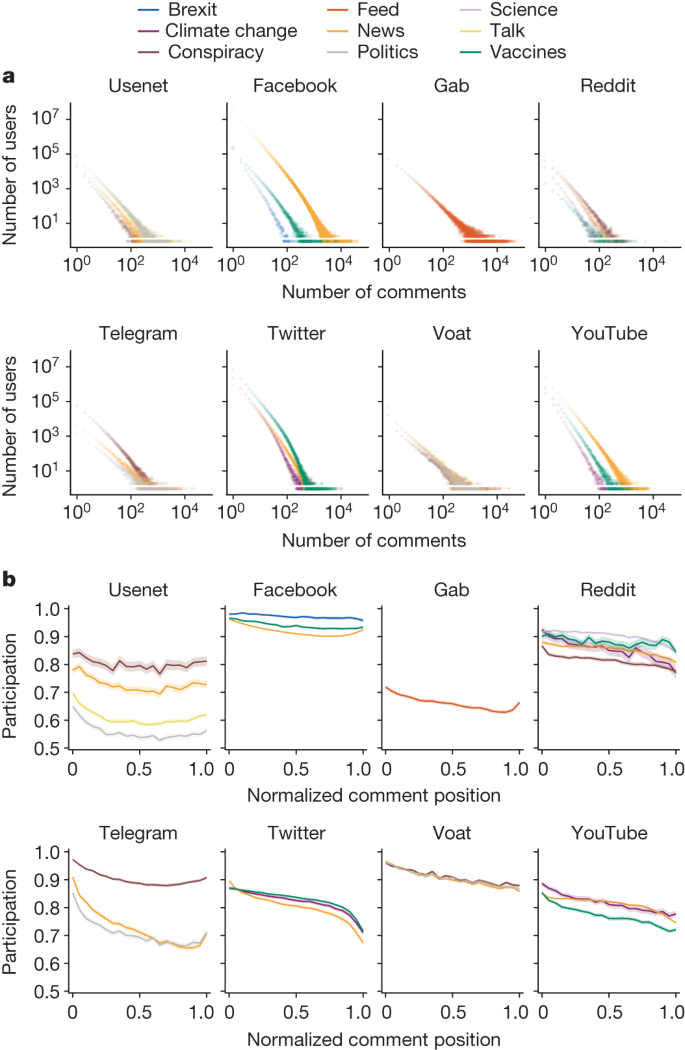
a , The distributions of user activity in terms of comments posted for each platform and each topic. b , The mean user participation as conversations evolve. For each dataset, participation is computed for the threads belonging to the size interval [0.7–1] (Supplementary Table 2 ). Trends are reported with their 95% confidence intervals. The x axis represents the normalized position of comment intervals in the threads.
Consistent with previous studies 36 , 37 our analysis shows that the macroscopic patterns of online conversations, such as the distribution of users/threads activity and lifetime, are consistent across all datasets and topics (Supplementary Tables 1 – 4 ). This observation holds regardless of the specific features of the diverse platforms, such as recommendation algorithms and moderation policies (described in the ‘Content moderation policies’ of the Methods ), as well as other factors, including the user base and the conversation topics. We extend our analysis by examining another aspect of user activity within conversations across all platforms. To do this, we introduce a metric for the participation of users as a thread evolves. In this analysis, threads are filtered to ensure sufficient length as explained in the ‘Logarithmic binning and conversation size’ section of the Methods .
The participation metric, defined over different conversation intervals (that is, 0–5% of the thread arranged in chronological order, 5–10%, and so on), is the ratio of the number of unique users to the number of comments in the interval. Considering a fixed number of comments c , smaller values of participation indicate that fewer unique users are producing c comments in a segment of the conversation. In turn, a value of participation equal to 1 means that each user is producing one of the c comments, therefore obtaining the maximal homogeneity of user participation. Our findings show that, across all datasets, the participation of users in the evolution of conversations, averaged over almost all considered threads, is decreasing, as indicated by the results of Mann–Kendall test—a nonparametric test assessing the presence of a monotonic upward or downward tendency—shown in Extended Data Table 1 . This indicates that fewer users tend to take part in a conversation as it evolves, but those who do are more active (Fig. 1b ). Regarding patterns and values, the trends in user participation for various topics are consistent across each platform. According to the Mann–Kendall test, the only exceptions were Usenet Conspiracy and Talk, for which an ambiguous trend was detected. However, we note that their regression slopes are negative, suggesting a decreasing trend, even if with a weaker effect. Overall, our first set of findings highlights the shared nature of certain online interactions, revealing a decrease in user participation over time but an increase in activity among participants. This insight, consistent across most platforms, underscores the dynamic interplay between conversation length, user engagement and topic-driven participation.
Conversation size and toxicity
To detect the presence of toxic language, we used Google’s Perspective API 34 , a state-of-the-art toxicity classifier that has been used extensively in recent literature 29 , 38 . Perspective API defines a toxic comment as “A rude, disrespectful, or unreasonable comment that is likely to make people leave a discussion”. On the basis of this definition, the classifier assigns a toxicity score in the [0,1] range to a piece of text that can be interpreted as an estimate of the likelihood that a reader would perceive the comment as toxic ( https://developers.perspectiveapi.com/s/about-the-api-score ). To define an appropriate classification threshold, we draw from the existing literature 39 , which uses 0.6 as the threshold for considering a comment as toxic. A robustness check of our results using different threshold and classification tools is reported in the ‘Toxicity detection and validation of employed models’ section of the Methods , together with a discussion regarding potential shortcomings deriving from automatic classifiers. To further investigate the interplay between toxicity and conversation features across various platforms, our study first examines the prevalence of toxic speech in each dataset. We then analyse the occurrence of highly toxic users and conversations. Lastly, we investigate how the length of conversations correlates with the probability of encountering toxic comments. First of all, we define the toxicity of a user as the fraction of toxic comments that she/he left. Similarly, the toxicity of a thread is the fraction of toxic comments it contains. We begin by observing that, although some toxic datasets exist on unmoderated platforms such as Gab, Usenet and Voat, the prevalence of toxic speech is generally low. Indeed, the percentage of toxic comments in each dataset is mostly below 10% (Table 1 ). Moreover, the complementary cumulative distribution functions illustrated in Extended Data Fig. 2 show that the fraction of extremely toxic users is very low for each dataset (in the range between 10 −3 and 10 −4 ), and the majority of active users wrote at least one toxic comment, as reported in Supplementary Table 5 , therefore suggesting that the overall volume of toxicity is not a phenomenon limited to the activity of very few users and localized in few conversations. Indeed, the number of users versus their toxicity decreases sharply following an exponential trend. The toxicity of threads follows a similar pattern. To understand the association between the size and toxicity of a conversation, we start by grouping conversations according to their length to analyse their structural differences 40 . The grouping is implemented by means of logarithmic binning (see the ‘Logarithmic binning and conversation size’ section of the Methods ) and the evolution of the average fraction of toxic comments in threads versus the thread size intervals is reported in Fig. 2 . Notably, the resulting trends are almost all increasing, showing that, independently of the platform and topic, the longer the conversation, the more toxic it tends to be.
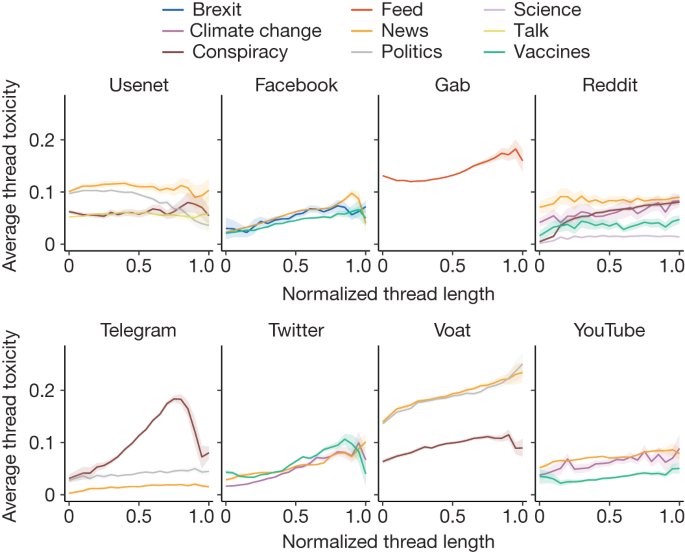
The mean fraction of toxic comments in conversations versus conversation size for each dataset. Trends represent the mean toxicity over each size interval and their 95% confidence interval. Size ranges are normalized to enable visual comparison of the different trends.
We assessed the increase in the trends by both performing linear regression and applying the Mann–Kendall test to ensure the statistical significance of our results (Extended Data Table 2 ). To further validate these outcomes, we shuffled the toxicity labels of comments, finding that trends are almost always non-increasing when data are randomized. Furthermore, the z -scores of the regression slopes indicate that the observed trends deviate from the mean of the distributions resulting from randomizations, being at least 2 s.d. greater in almost all cases. This provides additional evidence of a remarkable difference from randomness. The only decreasing trend is Usenet Politics. Moreover, we verified that our results are not influenced by the specific number of bins as, after estimating the same trends again with different intervals, we found that the qualitative nature of the results remains unchanged. These findings are summarized in Extended Data Table 2 . These analyses have been validated on the same data using a different threshold for identifying toxic comments and on a new dataset labelled with three different classifiers, obtaining similar results (Extended Data Fig. 5 , Extended Data Table 5 , Supplementary Fig. 1 and Supplementary Table 8 ). Finally, using a similar approach, we studied the toxicity content of conversations versus their lifetime—that is, the time elapsed between the first and last comment. In this case, most trends are flat, and there is no indication that toxicity is generally associated either with the duration of a conversation or the lifetime of user interactions (Extended Data Fig. 4 ).
Conversation evolution and toxicity
In the previous sections, we analysed the toxicity level of online conversations after their conclusion. We next focus on how toxicity evolves during a conversation and its effect on the dynamics of the discussion. The common beliefs that (1) online interactions inevitably devolve into toxic exchanges over time and (2) once a conversation reaches a certain toxicity threshold, it would naturally conclude, are not modern notions but they were also prevalent in the early days of the World Wide Web 41 . Assumption 2 aligns with the Perspective API’s definition of toxic language, suggesting that increased toxicity reduces the likelihood of continued participation in a conversation. However, this observation should be reconsidered, as it is not only the peak levels of toxicity that might influence a conversation but, for example, also a consistent rate of toxic content. To test these common assumptions, we used a method similar to that used for measuring participation; we select sufficiently long threads, divide each of them into a fixed number of equal intervals, compute the fraction of toxic comments for each of these intervals, average it over all threads and plot the toxicity trend through the unfolding of the conversations. We find that the average toxicity level remains mostly stable throughout, without showing a distinctive increase around the final part of threads (Fig. 3a (bottom) and Extended Data Fig. 3 ). Note that a similar observation was made previously 41 , but referring only to Reddit. Our findings challenge the assumption that toxicity discourages people from participating in a conversation, even though this notion is part of the definition of toxicity used by the detection tool. This can be seen by checking the relationship between trends in user participation, a quantity related to the number of users in a discussion at some point, and toxicity. The fact that the former typically decreases while the latter remains stable during conversations indicates that toxicity is not associated with participation in conversations (an example is shown in Fig. 3a ; box plots of the slopes of participation and toxicity for the whole dataset are shown in Fig. 3b ). This suggests that, on average, people may leave discussions regardless of the toxicity of the exchanges. We calculated the Pearson’s correlation between user participation and toxicity trends for each dataset to support this hypothesis. As shown in Fig. 3d , the resulting correlation coefficients are very heterogeneous, indicating no consistent pattern across different datasets. To further validate this analysis, we tested the differences in the participation of users commenting on either toxic or non-toxic conversations. To split such conversations into two disjoint sets, we first compute the toxicity distribution T i of long threads in each dataset i , and we then label a conversation j in dataset i as toxic if it has toxicity t ij ≥ µ ( T i ) + σ ( T i ), with µ ( T i ) being mean and σ ( T i ) the standard deviation of T i ; all of the other conversations are considered to be non-toxic. After splitting the threads, for each dataset, we compute the Pearson’s correlation of user participation between sets to find strongly positive values of the coefficient in all cases (Fig. 3c,e ). This result is also confirmed by a different analysis of which the results are reported in Supplementary Table 8 , in which no significant difference between slopes in toxic and non-toxic threads can be found. Thus, user behaviour in toxic and non-toxic conversations shows almost identical patterns in terms of participation. This reinforces our finding that toxicity, on average, does not appear to affect the likelihood of people participating in a conversation. These analyses were repeated with a lower toxicity classification threshold (Extended Data Fig. 5 ) and on additional datasets (Supplementary Fig. 2 and Supplementary Table 11 ), finding consistent results.
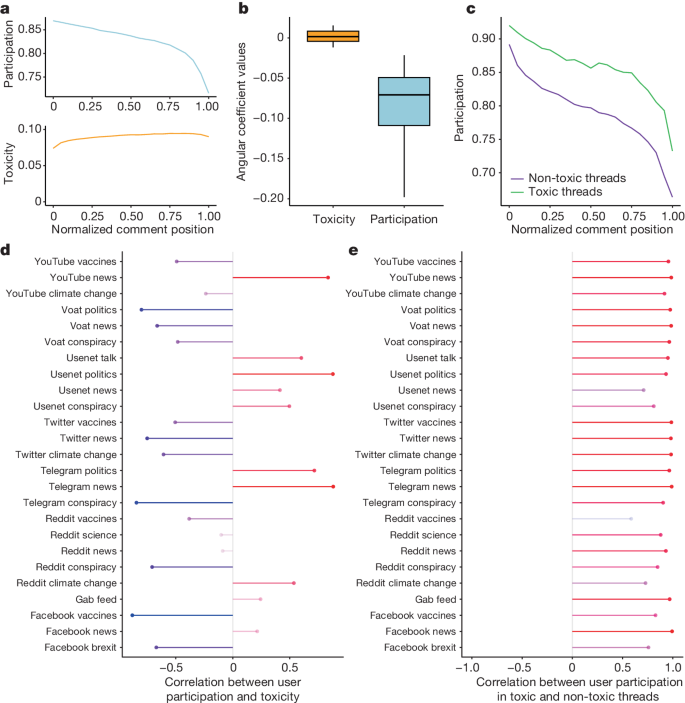
a , Examples of a typical trend in averaged user participation (top) and toxicity (bottom) versus the normalized position of comment intervals in the threads (Twitter news dataset). b , Box plot distributions of toxicity ( n = 25, minimum = −0.012, maximum = 0.015, lower whisker = −0.012, quartile 1 (Q1) = − 0.004, Q2 = 0.002, Q3 = 0.008, upper whisker = 0.015) and participation ( n = 25, minimum = −0.198, maximum = −0.022, lower whisker = −0.198, Q1 = − 0.109, Q2 = − 0.071, Q3 = − 0.049, upper whisker = −0.022) trend slopes for all datasets, as resulting from linear regression. c , An example of user participation in toxic and non-toxic thread sets (Twitter news dataset). d , Pearson’s correlation coefficients between user participation and toxicity trends for each dataset. e , Pearson’s correlation coefficients between user participation in toxic and non-toxic threads for each dataset.
Controversy and toxicity
In this section, we aim to explore why people participate in toxic online conversations and why longer discussions tend to be more toxic. Several factors could be the subject matter. First, controversial topics might lead to longer, more heated debates with increased toxicity. Second, the endorsement of toxic content by other users may act as an incentive to increase the discussion’s toxicity. Third, engagement peaks, due to factors such as reduced discussion focus or the intervention of trolls, may bring a higher share of toxic exchanges. Pursuing this line of inquiry, we identified proxies to measure the level of controversy in conversations and examined how these relate to toxicity and conversation size. Concurrently, we investigated the relationship between toxicity, endorsement and engagement.
As shown previously 24 , 42 , controversy is likely to emerge when people with opposing views engage in the same debate. Thus, the presence of users with diverse political leanings within a conversation could be a valid proxy for measuring controversy. We operationalize this definition as follows. Exploiting the peculiarities of our data, we can infer the political leaning of a subset of users in the Facebook News, Twitter News, Twitter Vaccines and Gab Feed datasets. This is achieved by examining the endorsement, for example, in the form of likes, expressed towards news outlets of which the political inclinations have been independently assessed by news rating agencies (see the ‘Polarization and user leaning attribution’ section of the Methods ). Extended Data Table 3 shows a breakdown of the datasets. As a result, we label users with a leaning score l ∈ [−1, 1], −1 being left leaning and +1 being right leaning. We then select threads with at least ten different labelled users, in which at least 10% of comments (with a minimum of 20) are produced by such users and assign to each of these comments the same leaning score of those who posted them. In this setting, the level of controversy within a conversation is assumed to be captured by the spread of the political leaning of the participants in the conversation. A natural way for measuring such a spread is the s.d. σ ( l ) of the distribution of comments possessing a leaning score: the higher the σ ( l ), the greater the level of ideological disagreement and therefore controversy in a thread. We analysed the relationship between controversy and toxicity in online conversations of different sizes. Figure 4a shows that controversy increases with the size of conversations in all datasets, and its trends are positively correlated with the corresponding trends in toxicity (Extended Data Table 3 ). This supports our hypothesis that controversy and toxicity are closely related in online discussions.
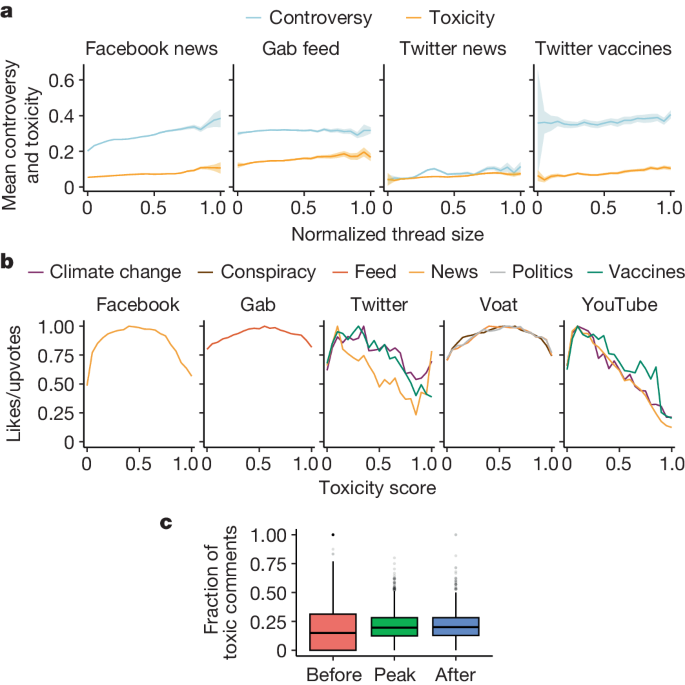
a , The mean controversy ( σ ( l )) and mean toxicity versus thread size (log-binned and normalized) for the Facebook news, Twitter news, Twitter vaccines and Gab feed datasets. Here toxicity is calculated in the same conversations in which controversy could be computed (Extended Data Table 3 ); the relative Pearson’s, Spearman’s and Kendall’s correlation coefficients are also provided in Extended Data Table 3 . Trends are reported with their 95% confidence interval. b , Likes/upvotes versus toxicity (linearly binned). c , An example (Voat politics dataset) of the distributions of the frequency of toxic comments in threads before ( n = 2,201, minimum = 0, maximum = 1, lower whisker = 0, Q1 = 0, Q2 = 0.15, Q3 = 0.313, upper whisker = 0.769) at the peak ( n = 2,798, minimum = 0, maximum = 0.8, lower whisker = 0, Q1 = 0.125, Q2 = 0.196, Q3 = 0.282, upper whisker = 0.513) and after the peak ( n = 2,791, minimum = 0, maximum = 1, lower whisker = 0, Q1 = 0.129, Q2 = 0.200, Q3 = 0.282, upper whisker = 0.500) of activity, as detected by Kleinberg’s burst detection algorithm.
As a complementary analysis, we draw on previous results 43 . In that study, using a definition of controversy operationally different but conceptually related to ours, a link was found between a greater degree of controversy of a discussion topic and a wider distribution of sentiment scores attributed to the set of its posts and comments. We quantified the sentiment of comments using a pretrained BERT model available from Hugging Face 44 , used also in previous studies 45 . The model predicts the sentiment of a sentence through a scoring system ranging from 1 (negative) to 5 (positive). We define the sentiment attributed to a comment c as its weighted mean \(s(c)=\sum _{i=1.5}{x}_{i}{p}_{i}\) , where x i ∈ [1, 5] is the output score from the model and p i is the probability associated to that value. Moreover, we normalize the sentiment score s for each dataset between 0 and 1. We observe the trends of the mean s.d. of sentiment in conversations, \(\bar{\sigma }(s)\) , and toxicity are positively correlated for moderated platforms such as Facebook and Twitter but are negatively correlated on Gab (Extended Data Table 3 ). The positive correlation observed in Facebook and Twitter indicates that greater discrepancies in sentiment of the conversations can, in general, be linked to toxic conversations and vice versa. Instead, on unregulated platforms such as Gab, highly conflicting sentiments seem to be more likely to emerge in less toxic conversations.
As anticipated, another factor that may be associated with the emergence of toxic comments is the endorsement they receive. Indeed, such positive reactions may motivate posting even more comments of the same kind. Using the mean number of likes/upvotes as a proxy of endorsement, we have an indication that this may not be the case. Figure 4b shows that the trend in likes/upvotes versus comments toxicity is never increasing past the toxicity score threshold (0.6).
Finally, to complement our analysis, we inspect the relationship between toxicity and user engagement within conversations, measured as the intensity of the number of comments over time. To do so, we used a method for burst detection 46 that, after reconstructing the density profile of a temporal stream of elements, separates the stream into different levels of intensity and assigns each element to the level to which it belongs (see the ‘Burst analysis’ section of the Methods ). We computed the fraction of toxic comments at the highest intensity level of each conversation and for the levels right before and after it. By comparing the distributions of the fraction of toxic comments for the three intervals, we find that these distributions are statistically different in almost all cases (Fig. 4c and Extended Data Table 4 ). In all datasets but one, distributions are consistently shifted towards higher toxicity at the peak of engagement, compared with the previous phase. Likewise, in most cases, the peak shows higher toxicity even if compared to the following phase, which in turn is mainly more toxic than the phase before the peak. These results suggest that toxicity is likely to increase together with user engagement.
Here we examine one of the most prominent and persistent characteristics online discussions—toxic behaviour, defined here as rude, disrespectful or unreasonable conduct. Our analysis suggests that toxicity is neither a deterrent to user involvement nor an engagement amplifier; rather, it tends to emerge when exchanges become more frequent and may be a product of opinion polarization. Our findings suggest that the polarization of user opinions—intended as the degree of opposed partisanship of users in a conversation—may have a more crucial role than toxicity in shaping the evolution of online discussions. Thus, monitoring polarization could indicate early interventions in online discussions. However, it is important to acknowledge that the dynamics at play in shaping online discourse are probably multifaceted and require a nuanced approach for effective moderation. Other factors may influence toxicity and engagement, such as the specific subject of the conversation, the presence of influential users or ‘trolls’, the time and day of posting, as well as cultural or demographic aspects, such as user average age or geographical location. Furthermore, even though extremely toxic users are rare (Extended Data Fig. 2 ), the relationship between participation and toxicity of a discussion may in principle be affected also by small groups of highly toxic and engaged users driving the conversation dynamics. Although the analysis of such subtler aspects is beyond the scope of this Article, they are certainly worth investigating in future research.
However, when people encounter views that contradict their own, they may react with hostility and contempt, consistent with previous research 47 . In turn, it may create a cycle of negative emotions and behaviours that fuels toxicity. We also show that some online conversation features have remained consistent over the past three decades despite the evolution of platforms and social norms.
Our study has some limitations that we acknowledge and discuss. First, we use political leaning as a proxy for general leaning, which may capture only some of the nuances of online opinions. However, political leaning represents a broad spectrum of opinions across different topics, and it correlates well with other dimensions of leaning, such as news preferences, vaccine attitudes and stance on climate change 48 , 49 . We could not assign a political leaning to users to analyse controversies on all platforms. Still, those considered—Facebook, Gab and Twitter—represent different populations and moderation policies, and the combined data account for nearly 90% of the content in our entire dataset. Our analysis approach is based on breadth and heterogeneity. As such, it may raise concerns about potential reductionism due to the comparison of different datasets from different sources and time periods. We acknowledge that each discussion thread, platform and context has unique characteristics and complexities that might be diminished when homogenizing data. However, we aim not to capture the full depth of every discussion but to identify and highlight general patterns and trends in online toxicity across platforms and time. The quantitative approach used in our study is similar to numerous other studies 15 and enables us to uncover these overarching principles and patterns that may otherwise remain hidden. Of course, it is not possible to account for the behaviours of passive users. This entails, for example, that even if toxicity does not seem to make people leave conversations, it could still be a factor that discourages them from joining them. Our study leverages an extensive dataset to examine the intricate relationship between persistent online human behaviours and the characteristics of different social media platforms. Our findings challenge the prevailing assumption by demonstrating that toxic content, as traditionally defined, does not necessarily reduce user engagement, thereby questioning the assumed direct correlation between toxic content and negative discourse dynamics. This highlights the necessity for a detailed examination of the effect of toxic interactions on user behaviour and the quality of discussions across various platforms. Our results, showing user resilience to toxic content, indicate the potential for creating advanced, context-aware moderation tools that can accurately navigate the complex influence of antagonistic interactions on community engagement and discussion quality. Moreover, our study sets the stage for further exploration into the complexities of toxicity and its effect on engagement within online communities. Advancing our grasp of online discourse necessitates refining content moderation techniques grounded in a thorough understanding of human behaviour. Thus, our research adds to the dialogue on creating more constructive online spaces, promoting moderation approaches that are effective yet nuanced, facilitating engaging exchanges and reducing the tangible negative effects of toxic behaviour.
Through the extensive dataset presented here, critical aspects of the online platform ecosystem and fundamental dynamics of user interactions can be explored. Moreover, we provide insights that a comparative approach such as the one followed here can prove invaluable in discerning human behaviour from platform-specific features. This may be used to investigate further sensitive issues, such as the formation of polarization and misinformation. The resulting outcomes have multiple potential impacts. Our findings reveal consistent toxicity patterns across platforms, topics and time, suggesting that future research in this field should prioritize the concept of invariance. Recognizing that toxic behaviour is a widespread phenomenon that is not limited by platform-specific features underscores the need for a broader, unified approach to understanding online discourse. Furthermore, the participation of users in toxic conversations suggests that a simple approach to removing toxic comments may not be sufficient to prevent user exposure to such phenomena. This indicates a need for more sophisticated moderation techniques to manage conversation dynamics, including early interventions in discussions that show warnings of becoming toxic. Furthermore, our findings support the idea that examining content pieces in connection with others could enhance the effectiveness of automatic toxicity detection models. The observed homogeneity suggests that models trained using data from one platform may also have applicability to other platforms. Future research could explore further into the role of controversy and its interaction with other elements contributing to toxicity. Moreover, comparing platforms could enhance our understanding of invariant human factors related to polarization, disinformation and content consumption. Such studies would be instrumental in capturing the drivers of the effect of social media platforms on human behaviour, offering valuable insights into the underlying dynamics of online interactions.
Data collection
In our study, data collection from various social media platforms was strategically designed to encompass various topics, ensuring maximal heterogeneity in the discussion themes. For each platform, where feasible, we focus on gathering posts related to diverse areas such as politics, news, environment and vaccinations. This approach aims to capture a broad spectrum of discourse, providing a comprehensive view of conversation dynamics across different content categories.
We use datasets from previous studies that covered discussions about vaccines 50 , news 51 and brexit 52 . For the vaccines topic, the resulting dataset contains around 2 million comments retrieved from public groups and pages in a period that ranges from 2 January 2010 to 17 July 2017. For the news topic, we selected a list of pages from the Europe Media Monitor that reported the news in English. As a result, the obtained dataset contains around 362 million comments between 9 September 2009 and 18 August 2016. Furthermore, we collect a total of about 4.5 billion likes that the users put on posts and comments concerning these pages. Finally, for the brexit topic, the dataset contains around 460,000 comments from 31 December 2015 to 29 July 2016.
We collect data from the Pushshift.io archive ( https://files.pushshift.io/gab/ ) concerning discussions taking place from 10 August 2016, when the platform was launched, to 29 October 2018, when Gab went temporarily offline due to the Pittsburgh shooting 53 . As a result, we collect a total of around 14 million comments.
Data were collected from the Pushshift.io archive ( https://pushshift.io/ ) for the period ranging from 1 January 2018 to 31 December 2022. For each topic, whenever possible, we manually identified and selected subreddits that best represented the targeted topics. As a result of this operation, we obtained about 800,000 comments from the r/conspiracy subreddit for the conspiracy topic. For the vaccines topic, we collected about 70,000 comments from the r/VaccineDebate subreddit, focusing on the COVID-19 vaccine debate. We collected around 400,000 comments from the r/News subreddit for the news topic. We collected about 70,000 comments from the r/environment subreddit for the climate change topic. Finally, we collected around 550,000 comments from the r/science subreddit for the science topic.
We created a list of 14 channels, associating each with one of the topics considered in the study. For each channel, we manually collected messages and their related comments. As a result, from the four channels associated with the news topic (news notiziae, news ultimora, news edizionestraordinaria, news covidultimora), we obtained around 724,000 comments from posts between 9 April 2018 and 20 December 2022. For the politics topic, instead, the corresponding two channels (politics besttimeline, politics polmemes) produced a total of around 490,000 comments between 4 August 2017 and 19 December 2022. Finally, the eight channels assigned to the conspiracy topic (conspiracy bennyjhonson, conspiracy tommyrobinsonnews, conspiracy britainsfirst, conspiracy loomeredofficial, conspiracy thetrumpistgroup, conspiracy trumpjr, conspiracy pauljwatson, conspiracy iononmivaccino) produced a total of about 1.4 million comments between 30 August 2019 and 20 December 2022.
We used a list of datasets from previous studies that includes discussions about vaccines 54 , climate change 49 and news 55 topics. For the vaccines topic, we collected around 50 million comments from 23 January 2010 to 25 January 2023. For the news topic, we extend the dataset used previously 55 by collecting all threads composed of less than 20 comments, obtaining a total of about 9.5 million comments for a period ranging from 1 January 2020 to 29 November 2022. Finally, for the climate change topic, we collected around 9.7 million comments between 1 January 2020 and 10 January 2023.
We collected data for the Usenet discussion system by querying the Usenet Archive ( https://archive.org/details/usenet?tab=about ). We selected a list of topics considered adequate to contain a large, broad and heterogeneous number of discussions involving active and populated newsgroups. As a result of this selection, we selected conspiracy, politics, news and talk as topic candidates for our analysis. For the conspiracy topic, we collected around 280,000 comments between 1 September 1994 and 30 December 2005 from the alt.conspiracy newsgroup. For the politics topics, we collected around 2.6 million comments between 29 June 1992 and 31 December 2005 from the alt.politics newsgroup. For the news topic, we collected about 620,000 comments between 5 December 1992 and 31 December 2005 from the alt.news newsgroup. Finally, for the talk topic, we collected all of the conversations from the homonym newsgroup on a period that ranges from 13 February 1989 to 31 December 2005 for around 2.1 million contents.
We used a dataset presented previously 56 that covers the entire lifetime of the platform, from 9 January 2018 to 25 December 2020, including a total of around 16.2 million posts and comments shared by around 113,000 users in about 7,100 subverses (the equivalent of a subreddit for Voat). Similarly to previous platforms, we associated the topics to specific subverses. As a result of this operation, for the conspiracy topic, we collected about 1 million comments from the greatawakening subverse between 9 January 2018 and 25 December 2020. For the politics topic, we collected around 1 million comments from the politics subverse between 16 June 2014 and 25 December 2020. Finally, for the news topic, we collected about 1.4 million comments from the news subverse between 21 November 2013 and 25 December 2020.
We used a dataset proposed in previous studies that collected conversations about the climate change topic 49 , which is extended, coherently with previous platforms, by including conversations about vaccines and news topics. The data collection process for YouTube is performed using the YouTube Data API ( https://developers.google.com/youtube/v3 ). For the climate change topic, we collected around 840,000 comments between 16 March 2014 and 28 February 2022. For the vaccines topic, we collected conversations between 31 January 2020 and 24 October 2021 containing keywords about COVID-19 vaccines, namely Sinopharm, CanSino, Janssen, Johnson&Johnson, Novavax, CureVac, Pfizer, BioNTech, AstraZeneca and Moderna. As a result of this operation, we gathered a total of around 2.6 million comments to videos. Finally, for the news topic, we collected about 20 million comments between 13 February 2006 and 8 February 2022, including videos and comments from a list of news outlets, limited to the UK and provided by Newsguard (see the ‘Polarization and user leaning attribution’ section).
Content moderation policies
Content moderation policies are guidelines that online platforms use to monitor the content that users post on their sites. Platforms have different goals and audiences, and their moderation policies may vary greatly, with some placing more emphasis on free expression and others prioritizing safety and community guidelines.
Facebook and YouTube have strict moderation policies prohibiting hate speech, violence and harassment 57 . To address harmful content, Facebook follows a ‘remove, reduce, inform’ strategy and uses a combination of human reviewers and artificial intelligence to enforce its policies 58 . Similarly, YouTube has a similar set of community guidelines regarding hate speech policy, covering a wide range of behaviours such as vulgar language 59 , harassment 60 and, in general, does not allow the presence of hate speech and violence against individuals or groups based on various attributes 61 . To ensure that these guidelines are respected, the platform uses a mix of artificial intelligence algorithms and human reviewers 62 .
Twitter also has a comprehensive content moderation policy and specific rules against hateful conduct 63 , 64 . They use automation 65 and human review in the moderation process 66 . At the date of submission, Twitter’s content policies have remained unchanged since Elon Musk’s takeover, except that they ceased enforcing their COVID-19 misleading information policy on 23 November 2022. Their policy enforcement has faced criticism for inconsistency 67 .
Reddit falls somewhere in between regarding how strict its moderation policy is. Reddit’s content policy has eight rules, including prohibiting violence, harassment and promoting hate based on identity or vulnerability 68 , 69 . Reddit relies heavily on user reports and volunteer moderators. Thus, it could be considered more lenient than Facebook, YouTube and Twitter regarding enforcing rules. In October 2022, Reddit announced that they intend to update their enforcement practices to apply automation in content moderation 70 .
By contrast, Telegram, Gab and Voat take a more hands-off approach with fewer restrictions on content. Telegram has ambiguity in its guidelines, which arises from broad or subjective terms and can lead to different interpretations 71 . Although they mentioned they may use automated algorithms to analyse messages, Telegram relies mainly on users to report a range of content, such as violence, child abuse, spam, illegal drugs, personal details and pornography 72 . According to Telegram’s privacy policy, reported content may be checked by moderators and, if it is confirmed to violate their terms, temporary or permanent restrictions may be imposed on the account 73 . Gab’s Terms of Service allow all speech protected under the First Amendment to the US Constitution, and unlawful content is removed. They state that they do not review material before it is posted on their website and cannot guarantee prompt removal of illegal content after it has been posted 74 . Voat was once known as a ‘free-speech’ alternative to Reddit and allowed content even if it may be considered offensive or controversial 56 .
Usenet is a decentralized online discussion system created in 1979. Owing to its decentralized nature, Usenet has been difficult to moderate effectively, and it has a reputation for being a place where controversial and even illegal content can be posted without consequence. Each individual group on Usenet can have its own moderators, who are responsible for monitoring and enforcing their group’s rules, and there is no single set of rules that applies to the entire platform 75 .
Logarithmic binning and conversation size
Owing to the heavy-tailed distributions of conversation length (Extended Data Fig. 1 ), to plot the figures and perform the analyses, we used logarithmic binning. Thus, according to its length, each thread of each dataset is assigned to 1 out of 21 bins. To ensure a minimal number of points in each bin, we iteratively change the left bound of the last bin so that it contains at least N = 50 elements (we set N = 100 in the case of Facebook news, due to its larger size). Specifically, considering threads ordered in increasing length, the size of the largest thread is changed to that of the second last largest one, and the binning is recalculated accordingly until the last bin contains at least N points.
For visualization purposes, we provide a normalization of the logarithmic binning outcome that consists of mapping discrete points into coordinates of the x axis such that the bins correspond to {0, 0.05, 0.1, ..., 0.95, 1}.
To perform the part of the analysis, we select conversations belonging to the [0.7, 1] interval of the normalized logarithmic binning of thread length. This interval ensures that the conversations are sufficiently long and that we have a substantial number of threads. Participation and toxicity trends are obtained by applying to such conversations a linear binning of 21 elements to a chronologically ordered sequence of comments, that is, threads. A breakdown of the resulting datasets is provided in Supplementary Table 2 .
Finally, to assess the equality of the growth rates of participation values in toxic and non-toxic threads (see the ‘Conversation evolution and toxicity’ section), we implemented the following linear regression model:
where the term β 2 accounts for the effect that being a toxic conversation has on the growth of participation. Our results show that β 2 is not significantly different from 0 in most original and validation datasets (Supplementary Tables 8 and 11 )
Toxicity detection and validation of the models used
The problem of detecting toxicity is highly debated, to the point that there is currently no agreement on the very definition of toxic speech 64 , 76 . A toxic comment can be regarded as one that includes obscene or derogatory language 32 , that uses harsh, abusive language and personal attacks 33 , or contains extremism, violence and harassment 11 , just to give a few examples. Even though toxic speech should, in principle, be distinguished from hate speech, which is commonly more related to targeted attacks that denigrate a person or a group on the basis of attributes such as race, religion, gender, sex, sexual orientation and so on 77 , it sometimes may also be used as an umbrella term 78 , 79 . This lack of agreement directly reflects the challenging and inherent subjective nature of the concept of toxicity. The complexity of the topic makes it particularly difficult to assess the reliability of natural language processing models for automatic toxicity detection despite the impressive improvements in the field. Modern natural language processing models, such as Perspective API, are deep learning models that leverage word-embedding techniques to build representations of words as vectors in a high-dimensional space, in which a metric distance should reflect the conceptual distance among words, therefore providing linguistic context. A primary concern regarding toxicity detection models is their limited ability to contextualize conversations 11 , 80 . These models often struggle to incorporate factors beyond the text itself, such as the participant’s personal characteristics, motivations, relationships, group memberships and the overall tone of the discussion 11 . Consequently, what is considered to be toxic content can vary significantly among different groups, such as ethnicities or age groups 81 , leading to potential biases. These biases may stem from the annotators’ backgrounds and the datasets used for training, which might not adequately represent cultural heterogeneity. Moreover, subtle forms of toxic content, like indirect allusions, memes and inside jokes targeted at specific groups, can be particularly challenging to detect. Word embeddings equip current classifiers with a rich linguistic context, enhancing their ability to recognize a wide range of patterns characteristic of toxic expression. However, the requirements for understanding the broader context of a conversation, such as personal characteristics, motivations and group dynamics, remain beyond the scope of automatic detection models. We acknowledge these inherent limitations in our approach. Nonetheless, reliance on automatic detection models is essential for large-scale analyses of online toxicity like the one conducted in this study. We specifically resort to the Perspective API for this task, as it represents state-of-the-art automatic toxicity detection, offering a balance between linguistic nuance and scalable analysis capabilities. To define an appropriate classification threshold, we draw from the existing literature 64 , which uses 0.6 as the threshold for considering a comment to be toxic. This threshold can also be considered a reasonable one as, according to the developer guidelines offered by Perspective, it would indicate that the majority of the sample of readers, namely 6 out of 10, would perceive that comment as toxic. Due to the limitations mentioned above (for a criticism of Perspective API, see ref. 82 ), we validate our results by performing a comparative analysis using two other toxicity detectors: Detoxify ( https://github.com/unitaryai/detoxify ), which is similar to Perspective, and IMSYPP, a classifier developed for a European Project on hate speech 16 ( https://huggingface.co/IMSyPP ). In Supplementary Table 14 , the percentages of agreement among the three models in classifying 100,000 comments taken randomly from each of our datasets are reported. For Detoxify we used the same binary toxicity threshold (0.6) as used with Perspective. Although IMSYPP operates on a distinct definition of toxicity as outlined previously 16 , our comparative analysis shows a general agreement in the results. This alignment, despite the differences in underlying definitions and methodologies, underscores the robustness of our findings across various toxicity detection frameworks. Moreover, we perform the core analyses of this study using all classifiers on a further, vast and heterogeneous dataset. As shown in Supplementary Figs. 1 and 2 , the results regarding toxicity increase with conversation size and user participation and toxicity are quantitatively very similar. Furthermore, we verify the stability of our findings under different toxicity thresholds. Although the main analyses in this paper use the threshold value recommended by the Perspective API, set at 0.6, to minimize false positives, our results remain consistent even when applying a less conservative threshold of 0.5. This is demonstrated in Extended Data Fig. 5 , confirming the robustness of our observations across varying toxicity levels. For this study, we used the API support for languages prevalent in the European and American continents, including English, Spanish, French, Portuguese, German, Italian, Dutch, Polish, Swedish and Russian. Detoxify also offers multilingual support. However, IMSYPP is limited to English and Italian text, a factor considered in our comparative analysis.
Polarization and user leaning attribution
Our approach to measuring controversy in a conversation is based on estimating the degree of political partisanship among the participants. This measure is closely related to the political science concept of political polarization. Political polarization is the process by which political attitudes diverge from moderate positions and gravitate towards ideological extremes, as described previously 83 . By quantifying the level of partisanship within discussions, we aim to provide insights into the extent and nature of polarization in online debates. In this context, it is important to distinguish between ‘ideological polarization’ and ‘affective polarization’. Ideological polarization refers to divisions based on political viewpoints. By contrast, affective polarization is characterized by positive emotions towards members of one’s group and hostility towards those of opposing groups 84 , 85 . Here we focus specifically on ideological polarization. The subsequent description of our procedure for attributing user political leanings will further clarify this focus. On online social media, the individual leaning of a user toward a topic can be inferred through the content produced or the endorsement shown toward specific content. In this study, we consider the endorsement of users to news outlets of which the political leaning has been evaluated by trustworthy external sources. Although not without limitations—which we address below—this is a standard approach that has been used in several studies, and has become a common and established practice in the field of social media analysis due to its practicality and effectiveness in providing a broad understanding of political dynamics on these online platforms 1 , 43 , 86 , 87 , 88 . We label news outlets with a political score based on the information reported by Media Bias/Fact Check (MBFC) ( https://mediabiasfactcheck.com ), integrating with the equivalent information from Newsguard ( https://www.newsguardtech.com/ ). MBFC is an independent fact-checking organization that rates news outlets on the basis of the reliability and the political bias of the content that they produce and share. Similarly, Newsguard is a tool created by an international team of journalists that provides news outlet trust and political bias scores. Following standard methods used in the literature 1 , 43 , we calculated the individual leaning of a user l ∈ [−1, 1] as the average of the leaning scores l c ∈ [−1, 1] attributed to each of the content it produced/shared, where l c results from a mapping of the news organizations political scores provided by MBFC and Newsguard, respectively: [left, centre-left, centre, centre-right, right] to [−1, − 0.5, 0, 0.5, 1], and [far left, left, right, far right] to [−1, −0.5, 0.5, 1]). Our datasets have different structures, so we have to evaluate user leanings in different ways. For Facebook News, we assign a leaning score to users who posted a like at least three times and commented at least three times under news outlet pages that have a political score. For Twitter News, a leaning is assigned to users who posted at least 15 comments under scored news outlet pages. For Twitter Vaccines and Gab, we consider users who shared content produced by scored news outlet pages at least three times. A limitation of our approach is that engaging with politically aligned content does not always imply agreement; users may interact with opposing viewpoints for critical discussion. However, research indicates that users predominantly share content aligning with their own views, especially in politically charged contexts 87 , 89 , 90 . Moreover, our method captures users who actively express their political leanings, omitting the ‘passive’ ones. This is due to the lack of available data on users who do not explicitly state their opinions. Nevertheless, analysing active users offers valuable insights into the discourse of those most engaged and influential on social media platforms.
Burst analysis
We used the Kleinberg burst detection algorithm 46 (see the ‘Controversy and toxicity’ section) to all conversations with at least 50 comments in a dataset. In our analysis, we randomly sample up to 5,000 conversations, each containing a specific number of comments. To ensure the reliability of our data, we exclude conversations with an excessive number of double timestamps—defined as more than 10 consecutive or over 100 within the first 24 h. This criterion helps to mitigate the influence of bots, which could distort the patterns of human activity. Furthermore, we focus on the first 24 h of each thread to analyse streams of comments during their peak activity period. Consequently, Usenet was excluded from our study. The unique usage characteristics of Usenet render such a time-constrained analysis inappropriate, as its activity patterns do not align with those of the other platforms under consideration. By reconstructing the density profile of the comment stream, the algorithm divides the entire stream’s interval into subintervals on the basis of their level of intensity. Labelled as discrete positive values, higher levels of burstiness represent higher activity segments. To avoid considering flat-density phases, threads with a maximum burst level equal to 2 are excluded from this analysis. To assess whether a higher intensity of comments results in a higher comment toxicity, we perform a Mann–Whitney U -test 91 with Bonferroni correction for multiple testing between the distributions of the fraction of toxic comments t i in three intensity phases: during the peak of engagement and at the highest levels before and after. Extended Data Table 4 shows the corrected P values of each test, at a 0.99 confidence level, with H1 indicated in the column header. An example of the distribution of the frequency of toxic comments in threads at the three phases of a conversation considered (pre-peak, peak and post-peak) is reported in Fig. 4c .
Toxicity detection on Usenet
As discussed in the section on toxicity detection and the Perspective API above, automatic detectors derive their understanding of toxicity from the annotated datasets that they are trained on. The Perspective API is predominantly trained on recent texts, and its human labellers conform to contemporary cultural norms. Thus, although our dataset dates back to no more than the early 1990s, we provide a discussion on the viability of the application of Perspective API to Usenet and validation analysis. Contemporary society, especially in Western contexts, is more sensitive to issues of toxicity, including gender, race and sexual orientation, compared with a few decades ago. This means that some comments identified as toxic today, including those from older platforms like Usenet, might not have been considered as such in the past. However, this discrepancy does not significantly affect our analysis, which is centred on current standards of toxicity. On the other hand, changes in linguistic features may have some repercussions: there may be words and locutions that were frequently used in the 1990s that instead appear sparsely in today’s language, making Perspective potentially less effective in classifying short texts that contain them. We therefore proceeded to evaluate the impact that such a possible scenario could have on our results. In light of the above considerations, we consider texts labelled as toxic as correctly classified; instead, we assume that there is a fixed probability p that a comment may be incorrectly labelled as non-toxic. Consequently, we randomly designate a proportion p of non-toxic comments, relabel them as toxic and compute the toxicity versus conversation size trend (Fig. 2 ) on the altered dataset across various p . Specifically, for each value, we simulate 500 different trends, collecting their regression slopes to obtain a null distribution for them. To assess if the probability of error could lead to significant differences in the observed trend, we compute the fraction f of slopes lying outside the interval (−| s |,| s |), where s is the slope of the observed trend. We report the result in Supplementary Table 9 for different values of p . In agreement with our previous analysis, we assume that the slope differs significantly from the ones obtained from randomized data if f is less than 0.05.
We observed that only the Usenet Talk dataset shows sensitivity to small error probabilities, and the others do not show a significant difference. Consequently, our results indicate that Perspective API is suitable for application to Usenet data in our analyses, notwithstanding the potential linguistic and cultural shifts that might affect the classifier’s reliability with older texts.
Toxicity of short conversations
Our study focuses on the relationship between user participation and the toxicity of conversations, particularly in engaged or prolonged discussions. A potential concern is that concentrating on longer threads overlooks conversations that terminate quickly due to early toxicity, therefore potentially biasing our analysis. To address this, we analysed shorter conversations, comprising 6 to 20 comments, in each dataset. In particular, we computed the distributions of toxicity scores of the first and last three comments in each thread. This approach helps to ensure that our analysis accounts for a range of conversation lengths and patterns of toxicity development, providing a more comprehensive understanding of the dynamics at play. As shown in Supplementary Fig. 3 , for each dataset, the distributions of the toxicity scores display high similarity, meaning that, in short conversations, the last comments are not significantly more toxic than the initial ones, indicating that the potential effects mentioned above do not undermine our conclusions. Regarding our analysis of longer threads, we notice here that the participation quantity can give rise to similar trends in various cases. For example, high participation can be achieved because many users take part in the conversation, but also with small groups of users in which everyone is equally contributing over time. Or, in very large discussions, the contributions of individual outliers may remain hidden. By measuring participation, these and other borderline cases may not be distinct from the statistically highly likely discussion dynamics but, ultimately, this lack of discriminatory power does not have any implications on our findings nor on the validity of the conclusions that we draw.
Reporting summary
Further information on research design is available in the Nature Portfolio Reporting Summary linked to this article.
Data availability
Facebook, Twitter and YouTube data are made available in accordance with their respective terms of use. IDs of comments used in this work are provided at Open Science Framework ( https://doi.org/10.17605/osf.io/fq5dy ). For the remaining platforms (Gab, Reddit, Telegram, Usenet and Voat), all of the necessary information to recreate the datasets used in this study can be found in the ‘Data collection’ section.
Code availability
The code used for the analyses presented in the Article is available at Open Science Framework ( https://doi.org/10.17605/osf.io/fq5dy ). The repository includes dummy datasets to illustrate the required data format and make the code run.
Cinelli, M., Morales, G. D. F., Galeazzi, A., Quattrociocchi, W. & Starnini, M. The echo chamber effect on social media. Proc. Natl Acad. Sci. USA 118 , e2023301118 (2021).
Article CAS PubMed PubMed Central Google Scholar
Tucker, J. A. et al. Social media, political polarization, and political disinformation: a review of the scientific literature. Preprint at SSRN https://doi.org/10.2139/ssrn.3144139 (2018).
González-Bailón, S. et al. Asymmetric ideological segregation in exposure to political news on Facebook. Science 381 , 392–398 (2023).
Article PubMed ADS Google Scholar
Guess, A. et al. How do social media feed algorithms affect attitudes and behavior in an election campaign? Science 381 , 398–404 (2023).
Article CAS PubMed ADS Google Scholar
Del Vicario, M. et al. The spreading of misinformation online. Proc. Natl Acad. Sci. USA 113 , 554–559 (2016).
Article PubMed PubMed Central ADS Google Scholar
Bakshy, E., Messing, S. & Adamic, L. A. Exposure to ideologically diverse news and opinion on Facebook. Science 348 , 1130–1132 (2015).
Article MathSciNet CAS PubMed ADS Google Scholar
Bail, C. A. et al. Exposure to opposing views on social media can increase political polarization. Proc. Natl Acad. Sci. USA 115 , 9216–9221 (2018).
Article CAS PubMed PubMed Central ADS Google Scholar
Nyhan, B. et al. Like-minded sources on Facebook are prevalent but not polarizing. Nature 620 , 137–144 (2023).
Guess, A. et al. Reshares on social media amplify political news but do not detectably affect beliefs or opinions. Science 381 , 404–408 (2023).
Castaño-Pulgaŕın, S. A., Suárez-Betancur, N., Vega, L. M. T. & López, H. M. H. Internet, social media and online hate speech. Systematic review. Aggress. Viol. Behav. 58 , 101608 (2021).
Article Google Scholar
Sheth, A., Shalin, V. L. & Kursuncu, U. Defining and detecting toxicity on social media: context and knowledge are key. Neurocomputing 490 , 312–318 (2022).
Lupu, Y. et al. Offline events and online hate. PLoS ONE 18 , e0278511 (2023).
Gentzkow, M. & Shapiro, J. M. Ideological segregation online and offline. Q. J. Econ. 126 , 1799–1839 (2011).
Aichner, T., Grünfelder, M., Maurer, O. & Jegeni, D. Twenty-five years of social media: a review of social media applications and definitions from 1994 to 2019. Cyberpsychol. Behav. Social Netw. 24 , 215–222 (2021).
Lazer, D. M. et al. The science of fake news. Science 359 , 1094–1096 (2018).
Cinelli, M. et al. Dynamics of online hate and misinformation. Sci. Rep. 11 , 22083 (2021).
González-Bailón, S. & Lelkes, Y. Do social media undermine social cohesion? A critical review. Soc. Issues Pol. Rev. 17 , 155–180 (2023).
Roozenbeek, J. & Zollo, F. Democratize social-media research—with access and funding. Nature 612 , 404–404 (2022).
Article CAS PubMed Google Scholar
Dutton, W. H. Network rules of order: regulating speech in public electronic fora. Media Cult. Soc. 18 , 269–290 (1996).
Papacharissi, Z. Democracy online: civility, politeness, and the democratic potential of online political discussion groups. N. Media Soc. 6 , 259–283 (2004).
Coe, K., Kenski, K. & Rains, S. A. Online and uncivil? Patterns and determinants of incivility in newspaper website comments. J. Commun. 64 , 658–679 (2014).
Anderson, A. A., Brossard, D., Scheufele, D. A., Xenos, M. A. & Ladwig, P. The “nasty effect:” online incivility and risk perceptions of emerging technologies. J. Comput. Med. Commun. 19 , 373–387 (2014).
Garrett, R. K. Echo chambers online?: Politically motivated selective exposure among internet news users. J. Comput. Med. Commun. 14 , 265–285 (2009).
Del Vicario, M. et al. Echo chambers: emotional contagion and group polarization on Facebook. Sci. Rep. 6 , 37825 (2016).
Garimella, K., De Francisci Morales, G., Gionis, A. & Mathioudakis, M. Echo chambers, gatekeepers, and the price of bipartisanship. In Proc. 2018 World Wide Web Conference , 913–922 (International World Wide Web Conferences Steering Committee, 2018).
Johnson, N. et al. Hidden resilience and adaptive dynamics of the global online hate ecology. Nature 573 , 261–265 (2019).
Fortuna, P. & Nunes, S. A survey on automatic detection of hate speech in text. ACM Comput. Surv. 51 , 85 (2018).
Phadke, S. & Mitra, T. Many faced hate: a cross platform study of content framing and information sharing by online hate groups. In Proceedings of the 2020 CHI Conference on Human Factors in Computing Systems 1–13 (Association for Computing Machinery, 2020).
Xia, Y., Zhu, H., Lu, T., Zhang, P. & Gu, N. Exploring antecedents and consequences of toxicity in online discussions: a case study on Reddit. Proc. ACM Hum. Comput. Interact. 4 , 108 (2020).
Sipka, A., Hannak, A. & Urman, A. Comparing the language of qanon-related content on Parler, GAB, and Twitter. In Proc. 14th ACM Web Science Conference 2022 411–421 (Association for Computing Machinery, 2022).
Fortuna, P., Soler, J. & Wanner, L. Toxic, hateful, offensive or abusive? What are we really classifying? An empirical analysis of hate speech datasets. In Proc. 12th Language Resources and Evaluation Conference (eds Calzolari, E. et al.) 6786–6794 (European Language Resources Association, 2020).
Davidson, T., Warmsley, D., Macy, M. & Weber, I. Automated hate speech detection and the problem of offensive language. In Proc. International AAAI Conference on Web and Social Media 11 (Association for the Advancement of Artificial Intelligence, 2017).
Kolhatkar, V. et al. The SFU opinion and comments corpus: a corpus for the analysis of online news comments. Corpus Pragmat. 4 , 155–190 (2020).
Article PubMed Google Scholar
Lees, A. et al. A new generation of perspective API: efficient multilingual character-level transformers. In KDD'22: The 28th ACM SIGKDD Conference on Knowledge Discovery and Data Mining 3197–3207 (Association for Computing Machinery, 2022).
Vidgen, B. & Derczynski, L. Directions in abusive language training data, a systematic review: garbage in, garbage out. PLoS ONE 15 , e0243300 (2020).
Ross, G. J. & Jones, T. Understanding the heavy-tailed dynamics in human behavior. Phys. Rev. E 91 , 062809 (2015).
Article MathSciNet ADS Google Scholar
Choi, D., Chun, S., Oh, H., Han, J. & Kwon, T. T. Rumor propagation is amplified by echo chambers in social media. Sci. Rep. 10 , 310 (2020).
Beel, J., Xiang, T., Soni, S. & Yang, D. Linguistic characterization of divisive topics online: case studies on contentiousness in abortion, climate change, and gun control. In Proc. International AAAI Conference on Web and Social Media Vol. 16, 32–42 (Association for the Advancement of Artificial Intelligence, 2022).
Saveski, M., Roy, B. & Roy, D. The structure of toxic conversations on Twitter. In Proc. Web Conference 2021 (eds Leskovec, J. et al.) 1086–1097 (Association for Computing Machinery, 2021).
Juul, J. L. & Ugander, J. Comparing information diffusion mechanisms by matching on cascade size. Proc. Natl Acad. Sci. USA 118 , e2100786118 (2021).
Fariello, G., Jemielniak, D. & Sulkowski, A. Does Godwin’s law (rule of Nazi analogies) apply in observable reality? An empirical study of selected words in 199 million Reddit posts. N. Media Soc. 26 , 14614448211062070 (2021).
Qiu, J., Lin, Z. & Shuai, Q. Investigating the opinions distribution in the controversy on social media. Inf. Sci. 489 , 274–288 (2019).
Garimella, K., Morales, G. D. F., Gionis, A. & Mathioudakis, M. Quantifying controversy on social media. ACM Trans. Soc. Comput. 1 , 3 (2018).
NLPTown. bert-base-multilingual-uncased-sentiment, huggingface.co/nlptown/bert-base-multilingual-uncased-sentiment (2023).
Ta, H. T., Rahman, A. B. S., Najjar, L. & Gelbukh, A. Transfer Learning from Multilingual DeBERTa for Sexism Identification CEUR Workshop Proceedings Vol. 3202 (CEUR-WS, 2022).
Kleinberg, J. Bursty and hierarchical structure in streams. Data Min. Knowl. Discov. 7 , 373–397 (2003).
Article MathSciNet Google Scholar
Zollo, F. et al. Debunking in a world of tribes. PLoS ONE 12 , e0181821 (2017).
Article PubMed PubMed Central Google Scholar
Albrecht, D. Vaccination, politics and COVID-19 impacts. BMC Publ. Health 22 , 96 (2022).
Article CAS Google Scholar
Falkenberg, M. et al. Growing polarization around climate change on social media. Nat. Clim. Change 12 , 1114–1121 (2022).
Schmidt, A. L., Zollo, F., Scala, A., Betsch, C. & Quattrociocchi, W. Polarization of the vaccination debate on Facebook. Vaccine 36 , 3606–3612 (2018).
Schmidt, A. L. et al. Anatomy of news consumption on Facebook. Proc. Natl Acad. Sci. USA 114 , 3035–3039 (2017).
Del Vicario, M., Zollo, F., Caldarelli, G., Scala, A. & Quattrociocchi, W. Mapping social dynamics on Facebook: the brexit debate. Soc. Netw. 50 , 6–16 (2017).
Hunnicutt, T. & Dave, P. Gab.com goes offline after Pittsburgh synagogue shooting. Reuters , www.reuters.com/article/uk-pennsylvania-shooting-gab-idUKKCN1N20QN (29 October 2018).
Valensise, C. M. et al. Lack of evidence for correlation between COVID-19 infodemic and vaccine acceptance. Preprint at arxiv.org/abs/2107.07946 (2021).
Quattrociocchi, A., Etta, G., Avalle, M., Cinelli, M. & Quattrociocchi, W. in Social Informatics (eds Hopfgartner, F. et al.) 245–256 (Springer, 2022).
Mekacher, A. & Papasavva, A. “I can’t keep it up” a dataset from the defunct voat.co news aggregator. In Proc. International AAAI Conference on Web and Social Media Vol. 16, 1302–1311 (AAAI, 2022).
Facebook Community Standards , transparency.fb.com/policies/community-standards/hate-speech/ (Facebook, 2023).
Rosen, G. & Lyons, T. Remove, reduce, inform: new steps to manage problematic content. Meta , about.fb.com/news/2019/04/remove-reduce-inform-new-steps/ (10 April 2019).
Vulgar Language Policy , support.google.com/youtube/answer/10072685? (YouTube, 2023).
Harassment & Cyberbullying Policies , support.google.com/youtube/answer/2802268 (YouTube, 2023).
Hate Speech Policy , support.google.com/youtube/answer/2801939 (YouTube, 2023).
How Does YouTube Enforce Its Community Guidelines? , www.youtube.com/intl/enus/howyoutubeworks/policies/community-guidelines/enforcing-community-guidelines (YouTube, 2023).
The Twitter Rules , help.twitter.com/en/rules-and-policies/twitter-rules (Twitter, 2023).
Hateful Conduct , help.twitter.com/en/rules-and-policies/hateful-conduct-policy (Twitter, 2023).
Gorwa, R., Binns, R. & Katzenbach, C. Algorithmic content moderation: technical and political challenges in the automation of platform governance. Big Data Soc. 7 , 2053951719897945 (2020).
Our Range of Enforcement Options , help.twitter.com/en/rules-and-policies/enforcement-options (Twitter, 2023).
Elliott, V. & Stokel-Walker, C. Twitter’s moderation system is in tatters. WIRED (17 November 2022).
Reddit Content Policy , www.redditinc.com/policies/content-policy (Reddit, 2023).
Promoting Hate Based on Identity or Vulnerability , www.reddithelp.com/hc/en-us/articles/360045715951 (Reddit, 2023).
Malik, A. Reddit acqui-hires team from ML content moderation startup Oterlu. TechCrunch , tcrn.ch/3yeS2Kd (4 October 2022).
Terms of Service , telegram.org/tos (Telegram, 2023).
Durov, P. The rules of @telegram prohibit calls for violence and hate speech. We rely on our users to report public content that violates this rule. Twitter , twitter.com/durov/status/917076707055751168?lang=en (8 October 2017).
Telegram Privacy Policy , telegram.org/privacy (Telegram, 2023).
Terms of Service , gab.com/about/tos (Gab, 2023).
Salzenberg, C. & Spafford, G. What is Usenet? , www0.mi.infn.it/ ∼ calcolo/Wis usenet.html (1995).
Castelle, M. The linguistic ideologies of deep abusive language classification. In Proc. 2nd Workshop on Abusive Language Online (ALW2) (eds Fišer, D. et al.) 160–170, aclanthology.org/W18-5120 (Association for Computational Linguistics, 2018).
Tontodimamma, A., Nissi, E. & Sarra, A. E. A. Thirty years of research into hate speech: topics of interest and their evolution. Scientometrics 126 , 157–179 (2021).
Sap, M. et al. Annotators with attitudes: how annotator beliefs and identities bias toxic language detection. In Proc. 2022 Conference of the North American Chapter of the Association for Computational Linguistics: Human Language Technologies (eds. Carpuat, M. et al.) 5884–5906 (Association for Computational Linguistics, 2022).
Pavlopoulos, J., Sorensen, J., Dixon, L., Thain, N. & Androutsopoulos, I. Toxicity detection: does context really matter? In Proc. 58th Annual Meeting of the Association for Computational Linguistics (eds Jurafsky, D. et al.) 4296–4305 (Association for Computational Linguistics, 2020).
Yin, W. & Zubiaga, A. Hidden behind the obvious: misleading keywords and implicitly abusive language on social media. Online Soc. Netw. Media 30 , 100210 (2022).
Sap, M., Card, D., Gabriel, S., Choi, Y. & Smith, N. A. The risk of racial bias in hate speech detection. In Proc. 57th Annual Meeting of the Association for Computational Linguistics (eds Kohonen, A. et al.) 1668–1678 (Association for Computational Linguistics, 2019).
Rosenblatt, L., Piedras, L. & Wilkins, J. Critical perspectives: a benchmark revealing pitfalls in PerspectiveAPI. In Proc. Second Workshop on NLP for Positive Impact (NLP4PI) (eds Biester, L. et al.) 15–24 (Association for Computational Linguistics, 2022).
DiMaggio, P., Evans, J. & Bryson, B. Have American’s social attitudes become more polarized? Am. J. Sociol. 102 , 690–755 (1996).
Fiorina, M. P. & Abrams, S. J. Political polarization in the American public. Annu. Rev. Polit. Sci. 11 , 563–588 (2008).
Iyengar, S., Gaurav, S. & Lelkes, Y. Affect, not ideology: a social identity perspective on polarization. Publ. Opin. Q. 76 , 405–431 (2012).
Cota, W., Ferreira, S. & Pastor-Satorras, R. E. A. Quantifying echo chamber effects in information spreading over political communication networks. EPJ Data Sci. 8 , 38 (2019).
Bessi, A. et al. Users polarization on Facebook and Youtube. PLoS ONE 11 , e0159641 (2016).
Bessi, A. et al. Science vs conspiracy: collective narratives in the age of misinformation. PLoS ONE 10 , e0118093 (2015).
Himelboim, I., McCreery, S. & Smith, M. Birds of a feather tweet together: integrating network and content analyses to examine cross-ideology exposure on Twitter. J. Comput. Med. Commun. 18 , 40–60 (2013).
An, J., Quercia, D. & Crowcroft, J. Partisan sharing: Facebook evidence and societal consequences. In Proc. Second ACM Conference on Online Social Networks, COSN ′ 14 13–24 (Association for Computing Machinery, 2014).
Mann, H. B. & Whitney, D. R. On a test of whether one of two random variables is stochastically larger than the other. Ann. Math. Stat. 18 , 50–60 (1947).
Download references
Acknowledgements
We thank M. Samory for discussions; T. Quandt and Z. Zhang for suggestions during the review process; and Geronimo Stilton and the Hypnotoad for inspiring the data analysis and result interpretation. The work is supported by IRIS Infodemic Coalition (UK government, grant no. SCH-00001-3391), SERICS (PE00000014) under the NRRP MUR program funded by the EU NextGenerationEU project CRESP from the Italian Ministry of Health under the program CCM 2022, PON project ‘Ricerca e Innovazione’ 2014-2020, and PRIN Project MUSMA for Italian Ministry of University and Research (MUR) through the PRIN 2022CUP G53D23002930006 and EU Next-Generation EU, M4 C2 I1.1.
Author information
These authors contributed equally: Michele Avalle, Niccolò Di Marco, Gabriele Etta
Authors and Affiliations
Department of Computer Science, Sapienza University of Rome, Rome, Italy
Michele Avalle, Niccolò Di Marco, Gabriele Etta, Shayan Alipour, Lorenzo Alvisi, Matteo Cinelli & Walter Quattrociocchi
Department of Social Sciences and Economics, Sapienza University of Rome, Rome, Italy
Emanuele Sangiorgio
Department of Communication and Social Research, Sapienza University of Rome, Rome, Italy
Anita Bonetti
Institute of Complex Systems, CNR, Rome, Italy
Antonio Scala
Department of Mathematics, City University of London, London, UK
Andrea Baronchelli
The Alan Turing Institute, London, UK
You can also search for this author in PubMed Google Scholar
Contributions
Conception and design: W.Q., M.A., M.C., G.E. and N.D.M. Data collection: G.E. and N.D.M. with collaboration from M.C., M.A. and S.A. Data analysis: G.E., N.D.M., M.A., M.C., W.Q., E.S., A. Bonetti, A. Baronchelli and A.S. Code writing: G.E. and N.D.M. with collaboration from M.A., E.S., S.A. and M.C. All of the authors provided critical feedback and helped to shape the research, analysis and manuscript, and contributed to the preparation of the manuscript.
Corresponding authors
Correspondence to Matteo Cinelli or Walter Quattrociocchi .
Ethics declarations
Competing interests.
The authors declare no competing interests.
Peer review
Peer review information.
Nature thanks Thorsten Quandt, Ziqi Zhang and the other, anonymous, reviewer(s) for their contribution to the peer review of this work.
Additional information
Publisher’s note Springer Nature remains neutral with regard to jurisdictional claims in published maps and institutional affiliations.
Extended data figures and tables
Extended data fig. 1 general characteristics of online conversations..
a . Distributions of conversation length (number of comments in a thread). b . Distributions of the time duration (days) of user activity on a platform for each platform and each topic. c . Time duration (days) distributions of threads. Colour-coded legend on the side.
Extended Data Fig. 2 Extremely toxic authors and conversations are rare.
a . Complementary cumulative distribution functions (CCDFs) of the toxicity of authors who posted more than 10 comments. Toxicity is defined as usual as the fraction of toxic comments over the total of comments posted by a user. b . CCDFs of the toxicity of conversations containing more than 10 comments. Colour-coded legend on the side.
Extended Data Fig. 3 User toxicity as conversations evolve.
Mean fraction of toxic comments as conversations progress. The x-axis represents the normalized position of comment intervals in the threads. For each dataset, toxicity is computed in the thread size interval [0.7−1] (see main text and Tab. S 2 in SI). Trends are reported with their 95% confidence interval. Colour-coded legend on the side.
Extended Data Fig. 4 Toxicity is not associated with conversation lifetime.
Mean toxicity of a . users versus their time of permanence in the dataset and b . threads versus their time duration. Trends are reported with their 95% confidence interval and they are reported using a normalized log-binning. Colour-coded legend on the side.
Extended Data Fig. 5 Results hold for a different toxicity threshold.
Core analyses presented in the paper repeated employing a lower (0.5) toxicity binary classification threshold. a . Mean fraction of toxic comments in conversations versus conversation size, for each dataset (see Fig. 2 ). Trends are reported with their 95% confidence interval. b . Pearson’s correlation coefficients between user participation and toxicity trends for each dataset. c . Pearson’s correlation coefficients between users’ participation in toxic and non-toxic thread sets, for each dataset. d . Boxplot of the distribution of toxicity ( n = 25, min = −0.016, max = 0.020, lower whisker = −0.005, Q 1 = − 0.005, Q 2 = 0.004, Q 3 = 0.012, upper whisker = 0.020) and participation ( n = 25, min = −0.198, max = −0.022, lower whisker = −0.198, Q 1 = − 0.109, Q 2 = − 0.070, Q 3 = − 0.049, upper whisker = −0.022) trend slopes for all datasets, as resulting from linear regression. The results of the relative Mann-Kendall tests for trend assessment are shown in Extended Data Table 5 .
Supplementary information
Supplementary information.
Supplementary Information 1–4, including details regarding data collection for validation dataset, Supplementary Figs. 1–3, Supplementary Tables 1–17 and software and coding specifications.
Reporting Summary
Rights and permissions.
Open Access This article is licensed under a Creative Commons Attribution 4.0 International License, which permits use, sharing, adaptation, distribution and reproduction in any medium or format, as long as you give appropriate credit to the original author(s) and the source, provide a link to the Creative Commons licence, and indicate if changes were made. The images or other third party material in this article are included in the article’s Creative Commons licence, unless indicated otherwise in a credit line to the material. If material is not included in the article’s Creative Commons licence and your intended use is not permitted by statutory regulation or exceeds the permitted use, you will need to obtain permission directly from the copyright holder. To view a copy of this licence, visit http://creativecommons.org/licenses/by/4.0/ .
Reprints and permissions
About this article
Cite this article.
Avalle, M., Di Marco, N., Etta, G. et al. Persistent interaction patterns across social media platforms and over time. Nature (2024). https://doi.org/10.1038/s41586-024-07229-y
Download citation
Received : 30 April 2023
Accepted : 22 February 2024
Published : 20 March 2024
DOI : https://doi.org/10.1038/s41586-024-07229-y
Share this article
Anyone you share the following link with will be able to read this content:
Sorry, a shareable link is not currently available for this article.
Provided by the Springer Nature SharedIt content-sharing initiative
By submitting a comment you agree to abide by our Terms and Community Guidelines . If you find something abusive or that does not comply with our terms or guidelines please flag it as inappropriate.
Quick links
- Explore articles by subject
- Guide to authors
- Editorial policies
Sign up for the Nature Briefing newsletter — what matters in science, free to your inbox daily.

ComArtSci Presents Fourth Annual Excellence in Communication Scholarship Lecture Series
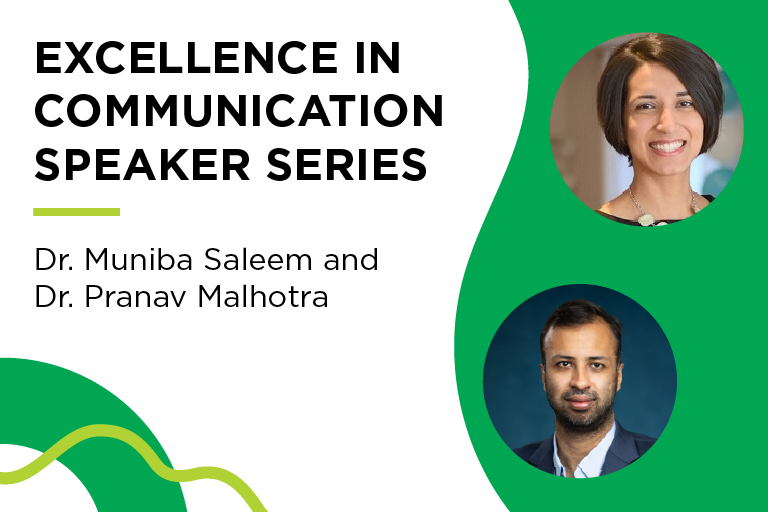
Two communication scholars presented their research on the effects of social and mainstream media on marginalized and international groups during the 4th annual Michigan State University Excellence in Communication Scholarship Speaker Series on March 8.
The Department of Communication hosted Muniba Saleem, Ph.D., Associate Professor at the University of California Santa Barbara and Pranav Malhotra, Ph.D., Assistant Professor at the University of Michigan Department of Communication and Media.
“This speaker series features cutting edge social scientific research on diversity, equity and inclusion topics,” said Dr. Elizabeth Dorrance Hall , director of the Family Communication and Relationships Lab. “Both scholars’ work utilizes communication theory to better understand the relationship between media and the experiences of marginalized groups and / or non-Western populations.”
Media’s Influence on Intergroup Relations
Dr. Saleem led the series with her lecture, “Media’s Influence on Intergroup Relations.” Using social scientific methods, Saleem studies how the media represents marginalized groups in a context of violence, and the detrimental effects that policies enacted towards those groups have on their self-identity.
For example, Saleem noted that most Americans have little to no direct experience with Muslims in their daily lives, and therefore rely on the media for information. She said media narratives of Muslims tend to drive how they’re perceived in the larger society.
“Depicting a group in an aggressive manner is likely to activate aggressive perceptions of that group,” Saleem said. “So, thinking that members of that group are hostile…increases the likelihood of aggressive behaviors against them, including support for public policies that are harming members of those groups.”
Saleem also explained that negative media stereotypes harm minority self-esteem.
“(They have) feelings such as anger, embarrassment, shame…especially when watching content along with majority members,” she said. “So, (it’s) like feeling kind of embarrassed that this is how my group is being depicted. It’s also been associated with psychological stress and anxiety.”
Saleem noted she conducted a recent content analysis that revealed similar findings are relevant to many other racial and ethnic minority groups.
She concluded that having more variation and nuances in media depictions of racial, ethnic and religious minorities would have a powerful effect in reducing negative evaluations of those groups.
“So, it's not necessarily the media that's the problem, it's the content within it that’s really driving our attitudes,” Saleem said.
Internationalization in Communication Research: Experiences Studying Social and Mobile Media Across the Globe
In the second lecture of the series, Dr. Pranav Malhotra focused on interpersonal relationships in his lecture, “Internationalization in Communication Research: Experiences Studying Social and Mobile Media Across the Globe.”
“Very broadly, I focus on how people engage with information and each other through social and mobile media,” Malhotra said. “What I'm really interested in is the relationship between those two aspects. How do our interpersonal relationships impact the ways in which we engage with and evaluate the credibility of information?”
As an example, Malhotra analyzed a Gates Foundation video promoting greater accessibility of mobile phones for the world’s poorest women. Malhotra said the messaging suggests that giving that group a phone begins to break down patriarchal norms and creates a sense of agency and empowerment.
However, Malhotra explains, studies he conducted among Indian women painted a different reality.
“What we found on the ground was that access to a mobile phone does not automatically result in empowerment or even disempowerment,” he said. “What it typically does for marginalized and poor women is that it makes their everyday lives slightly easier. It facilitates coordination. It helps them feel a little safer. It helps them feel a little more connected to their family, especially when they're outside of the house and are working. So, all of those things are great, but it certainly does not come close to challenging existing patriarchal power structures. These women still often lack power and agency within their own families, especially compared to their male counterparts.”
Malhotra used a second example to illustrate how family dynamics in India intersect with social media. He noted the popularity of the messaging platform WhatsApp, describing it as the primary means by which many Indians socially engage with the Internet. Malhotra said WhatsApp has been a vehicle through which misinformation about topics like politics and COVID-19 has been disseminated…particularly in family group chats.
“As I was personally seeing these chats become a real hotbed of misinformation in my own family, I started to see a lot of people, including my friends and family, discuss this issue,” Malhotra said. “And when they did, they talked about the difficulty of correcting misinformation in these chats, especially when misinformation is shared by an older relative.
So, we were interested in the role of ‘face politeness’ and how young Indians react when their older relatives share misinformation, particularly given the salience of Indian cultural norms surrounding showing respect and deference to elders. You’re not supposed to talk back.”
Each lecture demonstrated the importance of considering culture when interpreting the impact of traditional and social media on various groups.
By: Kevin Lavery
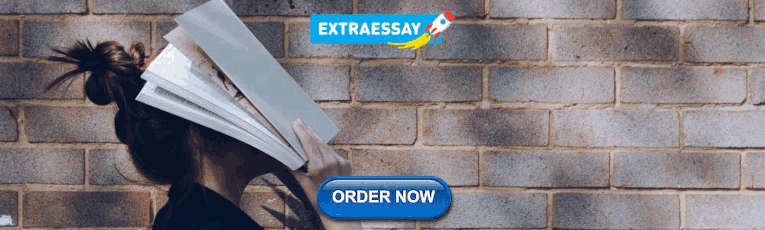
IMAGES
VIDEO
COMMENTS
8.2.3 The overwhelming influence of technology (research essay) 8.3.1 Communication and literacy during COVID (prospectus) ... talks about different ways social media has impacted our communication. Social media has become so relevant in our day to day lives and Hanke describes it in a couple different ways. She describes it as information ...
Social media has revolutionized communication. It allows us to connect with people around the world, share ideas, and express ourselves freely. ... 250 Words Essay on Influence of Social Media The Ubiquity of Social Media. In the digital age, social media platforms have become an integral part of our daily lives. They have transformed the way ...
6 Examples of Social Media Essays. Here are 6 examples of Social Media Essays: The Impact of Social Media on Relationships and Communication; Introduction: The way we share information and build relationships has evolved as a direct result of the prevalence of social media in our daily lives. The influence of social media on interpersonal ...
Abstract. This research paper presents the findings of a research project that investigated how young adult interpersonal communications have changed since using social media. Specifically, the research focused on determining if using social media had a beneficial or an adverse effect on the development of interaction and communication skills ...
3. Unfiltered Interactions. Social media and internet interactions offer a veil between the person sending and the person receiving the message. These interactions are no longer face to face, and this can lead to some unfiltered conversations as people feel they can say anything with no repercussions. 4.
Writing an essay on social media allows for an in-depth examination of its influence on communication, relationships, information sharing, and societal dynamics. It offers an opportunity to analyze the advantages and disadvantages, exploring topics such as privacy, online identities, social activism, and the role of social media in shaping ...
Social media encompass web-based programs and user-generated content that allow people to communicate and collaborate via mobile phones, computers, and other communication technologies. Unlike ...
By examining the various ways social media has influenced language use, linguistic patterns, and communication practices, we can gain a deeper understanding of the. transformative effects of this ...
1 hour! Social media has influenced the society criminally, socially, and economically. Criminally, social media has led to the growth of Internet criminals. Crimes committed over the social media are of different magnitudes and they mainly include impostors, hacking, and hate speeches.
The role that social media plays in interpersonal interaction is crucial to understand and without doubt, there is a wide range of diverse interpretations related to this phenomenon. This essay criticise the influences of social media on interpersonal communication.
"Social media disrupts our elections, our economy, and our health," says Aral, who is the David Austin Professor of Management at the MIT Sloan School of Management. Now Aral has written a book about it. In "The Hype Machine," published this month by Currency, a Random House imprint, Aral details why social media platforms have become ...
A 2018 Common Sense Media report found that 81 percent of teens use social media, and more than a third report using social media sites multiple times an hour. These statistics have risen dramatically over the past six years, likely driven by increased access to mobile devices. Rising along with these stats is a growing interest in the impact ...
Grade: 5. Download. Social media has both a positive and negative influence on our communication within society. Some individuals see social networking as online communities that help to promote increased interaction with their peers. On the other hand, others see social media sites in that they are preventing face-to-face communication from ...
Social media has had a profound impact on society, leading to increased connectivity and communication, promotion of self-expression and individuality, access to information and awareness, negative impacts on mental health, influence on societal norms and values, as well as privacy and security concerns.
The Effects of Social Media on Communication. This essay sample was donated by a student to help the academic community. Papers provided by EduBirdie writers usually outdo students' samples. The enourmous growth in the use of the Internet over the last decade has led to radical changes to the way that people consume and share information. This ...
2.1 Social Media Influences Human Behavior. The internet is shaping the users' culture, whereas social media has strongly influenced our shopping pattern, relationships, and education. This can alter the behaviours, beliefs, and even the basic health of people [ 6 ]. Social media affects lifestyles especially in fashion.
This article focuses on studying the social media influence on an individual through systematic literature review ( Brereton et al., 2007) with respect to TCCM approach (Theory, Context, Characteristics and Methodology). Adopting a framework or lens in literature reviews help in bringing objectivity to the analysis.
Despite these potential drawbacks, social media has undoubtedly had a positive impact on social skills developme nt. Social media can facilitate the exchange. of ideas, experiences, and knowledge ...
Media defines communication and can deliver messages through any number of channels, including text messaging, social media, and, more recently, video conferencing. For better or worse, media is altering our interactions and perceptions of the world around us. Join us as we explore media's effect on communication.
Introduction. Social media has become an integral part of our daily lives, revolutionizing the way we communicate, connect, and share information. This essay delves into my personal journey and explores the profound influence that social media has had on shaping my perspectives, experiences, and relationships.
Abstract. Social media are responsible for aggravating mental health problems. This systematic study summarizes the effects of social network usage on mental health. Fifty papers were shortlisted from google scholar databases, and after the application of various inclusion and exclusion criteria, 16 papers were chosen and all papers were ...
APA's Health Advisory on Social Media Use in Adolescence makes these recommendations based on the scientific evidence to date: Youth using social media should be encouraged to use functions that create opportunities for social support, online companionship, and emotional intimacy that can promote healthy socialization.
Depression, anxiety, catfishing, bullying, terro rism, and. criminal activities are some of the negative side s of social media on societies. Generall y, when peoples use social. media for ...
Growing concern surrounds the impact of social media platforms on public discourse 1,2,3,4 and their influence on social dynamics 5,6,7,8,9, especially in the context of toxicity 10,11,12.Here, to ...
Two communication scholars presented their research on the effects of social and mainstream media on marginalized and international groups during the 4th annual Michigan State University Excellence in Communication Scholarship Speaker Series on March 8.. The Department of Communication hosted Muniba Saleem, Ph.D., Associate Professor at the University of California Santa Barbara and Pranav ...
Murthy v. Missouri concerns whether the federal government's involvement in influencing content moderation choices at private social media companies, such as Facebook, YouTube, and X (formerly Twitter), violated the First Amendment 's Free Speech Clause. 1 Footnote U.S. Const. amend. I (Congress shall make no law . . . abridging the freedom of speech . . . .