Information Processing Theory: Definition and Examples
Yuichiro Chino / Getty Images
- Archaeology
:max_bytes(150000):strip_icc():format(webp)/CVinney_Headshot-1-5b6ced71c9e77c00508aedfd.jpg)
- Ph.D., Psychology, Fielding Graduate University
- M.A., Psychology, Fielding Graduate University
- B.A., Film Studies, Cornell University
Information processing theory is a cognitive theory that uses computer processing as a metaphor for the workings of the human brain. Initially proposed by George A. Miller and other American psychologists in the 1950s, the theory describes how people focus on information and encode it into their memories.
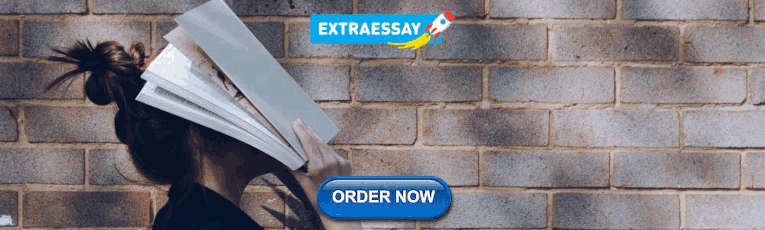
Key Takeaways: Information Processing Model
- Information processing theory is a cornerstone of cognitive psychology that uses computers as a metaphor for the way the human mind works.
- It was initially proposed in the mid-50s by American psychologists including George Miller to explain how people process information into memory.
- The most important theory in information processing is the stage theory originated by Atkinson and Shiffrin, which specifies a sequence of three stages information goes through to become encoded into long-term memory: sensory memory, short-term or working memory, and long-term memory.
Origins of Information Processing Theory
During the first half of the twentieth century, American psychology was dominated by behaviorism . Behaviorists only studied behaviors that could be directly observed. This made the inner-workings of the mind seem like an unknowable “black box.” Around the 1950s, however, computers came into existence, giving psychologists a metaphor to explain how the human mind functioned. The metaphor helped psychologists explain the different processes the brain engages in, including attention and perception, which could be compared to inputting information into a computer, and memory, which could be compared to a computer’s storage space.
This was referred to as the information processing approach and is still fundamental to cognitive psychology today. Information processing is especially interested in how people select, store and retrieve memories. In 1956, psychologist George A. Miller developed the theory and also contributed the idea that one can only hold a limited number of pieces of information in short-term memory. Miller specified this number as seven plus or minus two (or five to nine chunks of information), but more recently other scholars have suggested the number may be smaller .
Significant Models
The development of the information processing framework has continued through the years and has been broadened. Below are four models that are especially important to the approach:
Atkinson and Shiffrin’s Stage Theory
In 1968, Atkinson and Shiffrin developed the stage theory model. The model was later modified by other researchers but the basic outline of stage theory continues to be a cornerstone of information processing theory. The model concerns how information is stored in memory and presents a sequence of three stages, as follows:
Sensory Memory — Sensory memory involves whatever we take in through our senses. This kind of memory is exceedingly brief, only lasting up to 3 seconds. In order for something to enter sensory memory, the individual has to pay attention to it. Sensory memory can’t attend to every piece of information in the environment, so it filters out what it deems irrelevant and only sends what seems important to the next stage, short-term memory. The information that’s most likely to reach the next stage is either interesting or familiar.
Short-Term Memory/Working Memory — Once information reaches short-term memory , which is also called working memory, it is filtered further. Once again, this kind of memory doesn’t last long, only about 15 to 20 seconds. However, if information is repeated, which is referred to as maintenance rehearsal, it can be stored for up to 20 minutes. As observed by Miller, working memory’s capacity is limited so it can only process a certain number of pieces of information at a time. How many pieces is not agreed on, although many still point to Miller to identify the number as five to nine.
There are several factors that will impact what and how much information will be processed in working memory. Cognitive load capacity varies from person to person and from moment to moment based on an individual’s cognitive abilities, the amount of information being processed, and one's ability to focus and pay attention. Also, information that is familiar and has often been repeated doesn’t require as much cognitive capacity and, therefore, will be easier to process. For example, riding a bike or driving a car take minimal cognitive load if you’ve performed these tasks numerous times. Finally, people will pay more attention to information they believe is important, so that information is more likely to be processed. For example, if a student is preparing for a test, they are more likely to attend to information that will be on the test and forget about information they don’t believe they will be asked about.
Long-Term Memory — Although short-term memory has a limited capacity, the capacity of long-term memory is thought to be limitless. Several different types of information are encoded and organized in long-term memory: declarative information, which is information that can be discussed such as facts, concepts, and ideas (semantic memory) and personal experiences (episodic memory); procedural information, which is information about how to do something like drive a car or brush your teeth; and imagery, which are mental pictures.
Craik and Lockhart’s Level of Processing Model
Although Atkinson and Shiffrin’s stage theory is still highly influential and is the basic outline on which many later models are built, its sequential nature over-simplified how memories are stored. As a result, additional models were created to expand upon it. The first of these was created by Craik and Lockhart in 1973. Their levels of processing theory states that the ability to access information in long-term memory will be affected by how much it was elaborated upon. Elaboration is the process of making information meaningful so it is more likely to be remembered.
People process information with different levels of elaboration that will make the information more or less likely to be retrieved later. Craik and Lockhart specified a continuum of elaboration that starts with perception, continues through attention and labeling, and ends at meaning. Regardless of the level of elaboration, all information is likely to be stored in long-term memory, but higher levels of elaboration make it more likely that the information will be able to be retrieved. In other words, we can recall far less information that we’ve actually stored in long-term memory.
Parallel-Distributed Processing Model and Connectionist Model
The parallel-distributed processing model and connectionist model contrast to the linear three-step process specified by the stage theory. The parallel-distributed processing model was a precursor to connectionism that proposed that information is processed by multiple parts of the memory system at the same time.
This was extended by Rumelhart and McClelland’s connectionist model in 1986, which said that information is stored in various locations throughout the brain that is connected through a network. Information that has more connections will be easier for an individual to retrieve.
Limitations
While the information processing theory’s use of a computer as a metaphor for the human mind has proven to be potent, it’s also limited . Computers aren’t influenced by things like emotions or motivations in their ability to learn and remember information, but these things can have a powerful impact on people. In addition, while computers tend to process things sequentially, evidence shows humans are capable of parallel processing.
- Anderson, John R. Cognitive Psychology and Its Implications . 7th ed., Worth Publishers, 2010.
- Carlston, Don. “Social Cognition.” Advanced Social Psychology: The State of the Science , edited by Roy F. Baumeister and Eli J. Finkel, Oxford University Press, 2010, pp. 63-99.
- David L. "Information Processing Theory." Learning Theories. 2015 December 5. https://www.learning-theories.com/information-processing-theory.html
- Huitt, William G. "The Information Processing Approach to Cognition." Educational Psychology Interactive. 2003. http://www.edpsycinteractive.org/topics/cognition/infoproc.html
- Instructional Design. "Information Processing Theory (G. Miller)." https://www.instructionaldesign.org/theories/information-processing/
- McLeod, Saul. “Information Processing.” Simply Psychology , 24 October 2018. https://www.simplypsychology.org/information-processing.html
- Psychology Research and Reference. "Information Processing Theory." iResearchnet.com. https://psychology.iresearchnet.com/developmental-psychology/cognitive-development/information-processing-theory/
- Proactive and Retroactive Interference: Definition and Examples
- Hippocampus and Memory
- What Is a Schema in Psychology? Definition and Examples
- Definition and Examples of Conceptual Blending
- The Value of Analogies in Writing and Speech
- Social Cognitive Theory: How We Learn From the Behavior of Others
- Cognitive Linguistics
- What Is Relevance Theory in Terms of Communication?
- Chunk (Language Acquisition)
- Information Content (Language)
- What Is the Recency Effect in Psychology?
- Fluid Versus Crystallized Intelligence: What’s the Difference?
- An Introduction to Queuing Theory
- What Is the Elaboration Likelihood Model in Psychology?
- What Is Psycholinguistics?
- The Limbic System of the Brain
- Bipolar Disorder
- Therapy Center
- When To See a Therapist
- Types of Therapy
- Best Online Therapy
- Best Couples Therapy
- Best Family Therapy
- Managing Stress
- Sleep and Dreaming
- Understanding Emotions
- Self-Improvement
- Healthy Relationships
- Student Resources
- Personality Types
- Guided Meditations
- Verywell Mind Insights
- 2023 Verywell Mind 25
- Mental Health in the Classroom
- Editorial Process
- Meet Our Review Board
- Crisis Support
Information Processing Theory in Psychology
Arlin Cuncic, MA, is the author of The Anxiety Workbook and founder of the website About Social Anxiety. She has a Master's degree in clinical psychology.
:max_bytes(150000):strip_icc():format(webp)/ArlinCuncic_1000-21af8749d2144aa0b0491c29319591c4.jpg)
Daniel B. Block, MD, is an award-winning, board-certified psychiatrist who operates a private practice in Pennsylvania.
:max_bytes(150000):strip_icc():format(webp)/block-8924ca72ff94426d940e8f7e639e3942.jpg)
Willie B. Thomas / Getty Images
- Real-Life Examples
- Limitations
- Application
- Improving Your Skills
Information processing theory is a cognitive psychology theory that studies mental processes involved in acquiring, storing, and using knowledge. It focuses on the flow of information as it is passed from one stage to another within a person’s mind.
By examining different types of information processing as well as real-world examples of them in action, we can better understand how our minds work and why certain tasks require more effort than others.
According to this theory, the human mind is seen as an information processor similar to that of a computer. This model suggests that events comprise individual pieces of information that are processed in stages such as encoding, storage, and retrieval.
Read ahead to learn more about why this theory is important and how it applies to daily life.
What Are the Origins of the Information Processing Theory?
The origins of information processing theory can be traced back to George Miller , a cognitive psychologist and computer scientist.
He is known for his seminal paper “The Magical Number Seven, Plus or Minus Two” which proposed that human short-term memory has a limited capacity of seven items plus or minus two.
This theory has been expanded upon by other psychologists, such as Ulric Neisser who suggested that information is initially encoded in the sensory register before it moves on through different processing stages.
What Are the 3 Types of Information Processing Theory?
Let's take at the three types of information processing theory:
- Serial Processing Theory: This theory proposes that information is processed one step at a time in the order received. Each piece of information is held in short-term memory until the next piece of information comes in, and then it is transferred to long-term memory or discarded if it is no longer relevant.
- Parallel Processing Theory: This theory suggests that multiple pieces of information can be processed simultaneously instead of sequentially. It argues against the concept of a limited capacity for short-term memory since various pieces of information can be stored and processed simultaneously.
- Hierarchical Processing Theory: This model suggests that different levels of complexity exist within cognitive processes, with higher-level tasks requiring more complex mental operations than lower-level tasks. This theory developed as a result of Miller's work on the limited capacity of short-term memory and has been used to explain cognitive phenomena such as learning, problem-solving, and decision-making .
What Are Some Examples of Information Processing in Daily Life?
Information processing theory has been a handy tool for understanding the cognitive processes involved in acquiring, storing, and using knowledge.
By studying different types of information processing as well as examining real-world examples of them in action, we can better understand how our minds work and why certain tasks require more effort than others.
This knowledge is invaluable for improving educational practices and designing better problem-solving techniques.
- Driving: When driving , you must be constantly aware of your surroundings and use information from all of your senses to respond quickly and accurately to any potential dangers. This requires processing multiple pieces of sensory information in parallel to make safe decisions when behind the wheel.
- Learning a Language: When learning a new language , you must first encode new words in short-term memory before transferring them into long-term memory through rehearsal and repetition. This process involves both serial and parallel processing as you are encoding new words while also rehearsing previously learned words at the same time.
- Problem-Solving: Problem-solving often involves hierarchical processing as different levels of complexity are required for different tasks. For example, when solving a complex math problem , you may need to break it down into smaller components in order to understand each part and how they all fit together.
What Are the Four Stages of Information Processing Theory?
Information processing theory proposes that cognitive processes happen in four distinct stages, each with its own purpose and functionality.
- Encoding: This is the process of taking in information from the environment and assigning meaning to it. It involves perceiving or attending to stimuli before they can be stored in short-term memory.
- Storage: This is when information is held temporarily in short-term memory while it is processed or transferred into long-term memory for more permanent storage.
- Retrieval: This stage focuses on accessing previously stored information from either short-term or long-term memory in order to utilize it for a current task.
- Transformation: The final stage involves transforming information into a more useful form, such as organizing it in different ways or using reasoning and problem-solving skills to come up with a solution.
What This Means For You
By understanding these four stages of information processing, we can gain valuable insight into how our minds work and make use of this knowledge to improve educational practices and increase learning efficiency.
Why Is Information Processing Theory Important?
Information processing theory is an important tool for understanding cognitive processes and the way humans acquire , store, and use knowledge.
It Can Help Improve Education Overall
Furthermore, this theory has been used to explain a variety of complex phenomena such as learning, decision-making, and information recall. In short, it provides us with valuable insight into the inner workings of our minds that can be used to improve education in many different areas.
Limitations of Information Processing Theory
It is important to note that information processing theory is not without its limitations and criticisms.
- The theory ignores emotion and creativity: One of the main issues with this theory is that it relies too heavily on a computer-like model of how our brains work, which fails to take into account certain aspects such as emotion and creativity .
- The theory may be too simple: Additionally, some argue that the four stages are too simplistic and do not accurately represent the complex processes involved in acquiring knowledge.
Despite these critiques, however, information processing theory remains an invaluable tool for understanding cognitive processes and improving educational practices.
How Can We Apply Information Processing Theory?
Information processing theory can be applied in a variety of different ways. For example, it can help provide insight into how students learn and how best to structure educational material for maximum comprehension.
Additionally, it can be used to improve problem-solving techniques, as well as understand why certain tasks are more difficult than others and develop strategies that make them easier to complete.
How Can I Improve My Information Processing Skills?
Improving your information processing skills requires practice and dedication. Here are a few tips that can help you improve your ability to process information:
- Utilize memory techniques such as mnemonic devices and chunking in order to better remember the material.
- Take notes while studying or reading in order to reinforce important concepts.
- Break up complex tasks into smaller, more manageable chunks instead of trying to tackle them all at once.
- Make use of various visual aids such as charts, diagrams, and illustrations; these can make complex topics easier to understand.
- Stay focused on the task at hand by eliminating distractions and setting aside enough time for adequate concentration .
By following these tips and consistently practicing your information processing skills, you can significantly improve your ability to acquire, store, and use knowledge.
In conclusion, understanding the principles of information processing theory is essential for gaining insight into how our minds work and making use of this knowledge to improve educational practices and increase learning efficiency.
Cowan N. George Miller's magical number of immediate memory in retrospect: Observations on the faltering progression of science . Psychol Rev . 2015;122(3):536-541. doi:10.1037/a0039035
Association for Psychological Science. Remembering the Father of Cognitive Psychology .
Li K, Kadohisa M, Kusunoki M, Duncan J, Bundesen C, Ditlevsen S. Distinguishing between parallel and serial processing in visual attention from neurobiological data . R Soc Open Sci . 2020;7(1):191553. Published 2020 Jan 29. doi:10.1098/rsos.191553
Agliari E, Barra A, Galluzzi A, Guerra F, Tantari D, Tavani F. Hierarchical neural networks perform both serial and parallel processing . Neural Netw . 2015;66:22-35. doi:10.1016/j.neunet.2015.02.010
Campos TF, Barroso MT, de Lara Menezes AA. Encoding, storage and retrieval processes of the memory and the implications for motor practice in stroke patients . NeuroRehabilitation . 2010;26(2):135-142. doi:10.3233/NRE-2010-0545
Liu J, Zhang H, Yu T, Ren L, Ni D, Yang Q, Lu B, Zhang L, Axmacher N, Xue G. Transformative neural representations support long-term episodic memory . Sci Adv. 2021 Oct 8;7(41):eabg9715. doi: 10.1126/sciadv.abg9715. Epub 2021 Oct 8. PMID: 34623910; PMCID: PMC8500506.
By Arlin Cuncic, MA Arlin Cuncic, MA, is the author of The Anxiety Workbook and founder of the website About Social Anxiety. She has a Master's degree in clinical psychology.
Information Processing Theory In Psychology
Saul Mcleod, PhD
Editor-in-Chief for Simply Psychology
BSc (Hons) Psychology, MRes, PhD, University of Manchester
Saul Mcleod, PhD., is a qualified psychology teacher with over 18 years of experience in further and higher education. He has been published in peer-reviewed journals, including the Journal of Clinical Psychology.
Learn about our Editorial Process
Olivia Guy-Evans, MSc
Associate Editor for Simply Psychology
BSc (Hons) Psychology, MSc Psychology of Education
Olivia Guy-Evans is a writer and associate editor for Simply Psychology. She has previously worked in healthcare and educational sectors.
On This Page:
At the very heart of cognitive psychology is the idea of information processing.
Cognitive psychology sees the individual as a processor of information, in much the same way that a computer takes in information and follows a program to produce an output.
Basic Assumptions
The information processing approach is based on several assumptions, including:
- Information made available by the environment is processed by a series of processing systems (e.g., attention, perception, short-term memory);
- These processing systems transform or alter the information in systematic ways;
- The research aims to specify the processes and structures that underlie cognitive performance;
- Information processing in humans resembles that in computers.
Computer-Mind Analogy
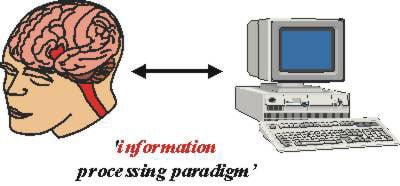
The development of the computer in the 1950s and 1960s had an important influence on psychology. It was, in part, responsible for the cognitive approach becoming the dominant approach in modern psychology (taking over from Behaviorism).
The computer gave cognitive psychologists a metaphor, or analogy, to which they could compare human mental processing. The use of the computer as a tool for thinking about how the human mind handles information is known as the computer analogy.
Essentially, a computer codes (i.e., changes) information, stores information, uses information and produces an output (retrieves info).
The idea of information processing was adopted by cognitive psychologists as a model of how human thought works.
For example, the eye receives visual information and codes information into electric neural activity, which is fed back to the brain where it is “stored” and “coded.”
This information can be used by other parts of the brain relating to mental activities such as memory, perception, and attention. The output (i.e., behavior) might be, for example, to read what you can see on a printed page.
Hence the information processing approach characterizes thinking as the environment providing input of data, which is then transformed by our senses.
The information can be stored, retrieved, and transformed using “mental programs,” with the results being behavioral responses.
Cognitive psychology has influenced and integrated with many other approaches and areas of study to produce, for example, social learning theory, cognitive neuropsychology, and artificial intelligence (AI).
Information Processing Model of Memory
When we selectively attend to one activity, we tend to ignore other stimulation. However, our attention can be distracted by something else, like the telephone ringing or someone using our name.
Psychologists are interested in what makes us attend to one thing rather than another ( selective attention ), why we sometimes switch our attention to something that was previously unattended (e.g., Cocktail Party Syndrome), and how many things we can attend to at the same time (attentional capacity).
One way of conceptualizing attention is to think of humans as information processors who can only process a limited amount of information at a time without becoming overloaded.
Broadbent and others in the 1950s adopted a model of the brain as a limited capacity information processing system through which external input is transmitted.

The information processing model of memory consists of a series of stages, or boxes, representing stages of processing. Arrows indicate the flow of information from one stage to the next.
- Input processes are concerned with the analysis of the stimuli.
- Storage processes cover everything that happens to stimuli internally in the brain and can include coding and manipulation of the stimuli.
- Output processes are responsible for preparing an appropriate response to a stimulus.
Critical Evaluation
A number of models of attention within the Information Processing framework have been proposed, including:
Broadbent’s Filter Model (1958), Treisman’s Attenuation Model (1964), and Deutsch and Deutsch’s Late Selection Model (1963).
However, there are a number of evaluative points to bear in mind when studying these models and the information processing approach in general. These include:
1. The information processing models assume serial processing of stimulus inputs.
- Serial processing effectively means one process has to be completed before the next starts.
- Parallel processing assumes some or all processes involved in a cognitive task(s) occur at the same time.
There is evidence from dual-task experiments that parallel processing is possible. It is difficult to determine whether a particular task is processed in a serial or parallel fashion as it probably depends (a) on the processes required to solve a task and (b) the amount of practice on a task.
Parallel processing is probably more frequent when someone is highly skilled; for example, a skilled typist thinks several letters ahead, while a novice focuses on just one letter at a time.
2. The analogy between human cognition and computer functioning adopted by the information processing approach is limited.
Computers can be regarded as information processing systems insofar as they:
- Combine information presented with the stored information to provide solutions to a variety of problems, and
- Most computers have a central processor of limited capacity, and it is usually assumed that capacity limitations affect the human attentional system.
BUT –
- The human brain has the capacity for extensive parallel processing, and computers often rely on serial processing;
- Humans are influenced in their cognitions by a number of conflicting emotional and motivational factors.
3. The evidence for the theories/models of attention that come under the information processing approach is largely based on experiments under controlled, scientific conditions.
Most laboratory studies are artificial and could be said to lack ecological validity.
In everyday life, cognitive processes are often linked to a goal (e.g., you pay attention in class because you want to pass the examination), whereas in the laboratory, the experiments are carried out in isolation from other cognitive and motivational factors.
Although these laboratory experiments are easy to interpret, the data may not apply to the real world outside the laboratory. More recently, ecologically valid approaches to cognition have been proposed (e.g., the Perceptual Cycle, Neisser, 1976).
Attention has been studied largely in isolation from other cognitive processes, although clearly, it operates as an interdependent system with the related cognitive processes of perception and memory.
The more successful we become at examining part of the cognitive system in isolation, the less our data are likely to tell us about cognition in everyday life.
4. Although it is agreed that stimulus-driven (bottom-up) information in cognition is important, what the individual brings to the task regarding expectations/past experiences is also important.
These influences are known as “top-down” or “conceptually-driven” processes. For example, read the triangle below:
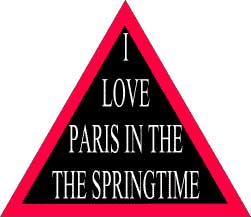
Expectation ( top-down processing ) often overrides information actually available in the stimulus ( bottom-up ), which we are, supposedly, attending to. How did you read the text in the triangle above?
Broadbent, D. (1958). Perception and Communication. London: Pergamon Press.
Deutsch, J. A., & Deutsch, D. (1963). Attention: Some Theoretical Considerations. Psychological Review , 70, 80–90
Neisser, U. (1967). Cognitive Psychology . New York: Appleton-Century-Crofts.
Treisman, A. (1964). Selective attention in man. British Medical Bulletin , 20, 12-16.
Further information
- Why Your Brain is Not a Computer
- BBC Radio: Donald Broadbent and the Cocktail Party.
- Attention Journal Article
- Attention Essay


An official website of the United States government
The .gov means it’s official. Federal government websites often end in .gov or .mil. Before sharing sensitive information, make sure you’re on a federal government site.
The site is secure. The https:// ensures that you are connecting to the official website and that any information you provide is encrypted and transmitted securely.
- Publications
- Account settings
Preview improvements coming to the PMC website in October 2024. Learn More or Try it out now .
- Advanced Search
- Journal List
- Front Psychol
Information Processing: The Language and Analytical Tools for Cognitive Psychology in the Information Age
The information age can be dated to the work of Norbert Wiener and Claude Shannon in the 1940s. Their work on cybernetics and information theory, and many subsequent developments, had a profound influence on reshaping the field of psychology from what it was prior to the 1950s. Contemporaneously, advances also occurred in experimental design and inferential statistical testing stemming from the work of Ronald Fisher, Jerzy Neyman, and Egon Pearson. These interdisciplinary advances from outside of psychology provided the conceptual and methodological tools for what is often called the cognitive revolution but is more accurately described as the information-processing revolution. Cybernetics set the stage with the idea that everything ranging from neurophysiological mechanisms to societal activities can be modeled as structured control systems with feedforward and feedback loops. Information theory offered a way to quantify entropy and information, and promoted theorizing in terms of information flow. Statistical theory provided means for making scientific inferences from the results of controlled experiments and for conceptualizing human decision making. With those three pillars, a cognitive psychology adapted to the information age evolved. The growth of technology in the information age has resulted in human lives being increasingly interweaved with the cyber environment, making cognitive psychology an essential part of interdisciplinary research on such interweaving. Continued engagement in interdisciplinary research at the forefront of technology development provides a chance for psychologists not only to refine their theories but also to play a major role in the advent of a new age of science.
Information is information, not matter or energy Wiener (1952 , p. 132)
Introduction
The period of human history in which we live is frequently called the information age , and it is often dated to the work of Wiener (1894–1964) and Shannon (1916–2001) on cybernetics and information theory. Each of these individuals has been dubbed the “father of the information age” ( Conway and Siegelman, 2005 ; Nahin, 2013 ). Wiener’s and Shannon’s work quantitatively described the fundamental phenomena of communication, and subsequent developments linked to that work had a profound influence on re-shaping many fields, including cognitive psychology from what it was prior to the 1950s ( Cherry, 1957 ; Edwards, 1997 , p. 222). Another closely related influence during that same period is the statistical hypothesis testing of Fisher (1890–1962), the father of modern statistics and experimental design ( Dawkins, 2010 ), and Jerzy Neyman (1894–1981), and Egon Pearson (1895–1980). In the U.S., during the first half of the 20th century, the behaviorist approach dominated psychology ( Mandler, 2007 ). In the 1950s, though, based mainly on the progress made in communication system engineering, as well as statistics, the human information-processing approach emerged in what is often called the cognitive revolution ( Gardner, 1985 ; Miller, 2003 ).
The information age has had, and continues to have, a great impact on psychology and society at large. Since the 1950s, science and technology have progressed with each passing day. The promise of the information-processing approach was to bring knowledge of human mind to a level in which cognitive mechanisms could be modeled to explain the processes between people’s perception and action. This promise, though far from completely fulfilled, has been increasingly realized. However, as any period in human history, the information age will come to an end at some future time and be replaced by another age. We are not claiming that information will become obsolete in the new age, just that it will become necessary but not sufficient for understanding people and society in the new era. Comprehending how and why the information-processing revolution in psychology occurred should prepare psychologists to deal with the changes that accompany the new era.
In the present paper, we consider the information age from a historical viewpoint and examine its impact on the emergence of contemporary cognitive psychology. Our analysis of the historical origins of cognitive psychology reveals that applied research incorporating multiple disciplines provided conceptual and methodological tools that advanced the field. An implication, which we explore briefly, is that interdisciplinary research oriented toward solving applied problems is likely to be the source of the next advance in conceptual and methodological tools that will enable a new age of psychology. In the following sections, we examine milestones of the information age and link them to the specific language and methodology for conducting psychological studies. We illustrate how the research methods and theory evolved over time and provide hints for developing research tools in the next age for cognitive psychology.
Cybernetics and Information Theory
Wiener and cybernetics.
Norbert Wiener is an individual whose impact on the field of psychology has not been acknowledged adequately. Wiener, a mathematician and philosopher, was a child prodigy who earned his Ph.D. from Harvard University at age 18 years. He is best-known for establishing what he labeled Cybernetics ( Wiener, 1948b ), which is also known as control theory, although he made many other contributions of note. A key feature of Wiener’s intellectual development and scientific work is its interdisciplinary nature ( Montagnini, 2017b ).
Prior to college, Wiener was influenced by Harvard physiologist Walter B. Cannon ( Conway and Siegelman, 2005 ), who later, in 1926, devised the term homeostasis , “the tendency of an organism or a cell to regulate its internal conditions, usually by a system of feedback controls…” ( Biology Online Dictionary, 2018 ). During his undergraduate and graduate education, Wiener was inspired by several Harvard philosophers ( Montagnini, 2017a ), including William James (pragmatism), George Santayana (positivistic idealism), and Josiah Royce (idealism and the scientific method). Motivated by Royce, Wiener made his commitment to study logic and completed his dissertation on mathematic logic. Following graduate school, Wiener traveled on a postdoctoral fellowship to pursue his study of mathematics and logic, working with philosopher/logician Bertrand Russell and mathematician/geneticist Godfrey H. Hardy in England, mathematicians David Hilbert and Edmund Landau in Europe, and philosopher/psychologist John Dewey in the U.S.
Wiener’s career was characterized by a commitment to apply mathematics and logic to real-world problems, which was sparked by his working for the U.S. Army. According to Hulbert ( 2018 , p. 50),
- simple He returned to the United States in 1915 to figure out what he might do next, at 21 jumping among jobs… His stint in 1918 at the U.S. Army’s Aberdeen Proving Ground was especially rewarding…. Busy doing invaluable work on antiaircraft targeting with fellow mathematicians, he found the camaraderie and the independence he yearned for. Soon, in a now-flourishing postwar academic market for the brainiacs needed in a science-guided era, Norbert found his niche. At MIT, social graces and pedigrees didn’t count for much, and wartime technical experience like his did. He got hired. The latest mathematical tools were much in demand as electronic communication technology took off in the 1920s.
Wiener began his early research in applied mathematics on stochastic noise processes (i.e., Brownian motion; Wiener, 1921 ). The Wiener process named in honor of him has been widely used in engineering, finance, physical sciences, and, as described later, psychology. From the mid 1930s until 1953, Wiener also was actively involved in a series of interdisciplinary seminars and conferences with a group of researchers that included mathematicians (John von Neumann, Walter Pitts), engineers (Julian Bigelow, Claude Shannon), physiologists (Warren McCulloch, Arturo Rosenblueth), and psychologists (Wolfgang Köhler, Joseph C. R. Licklider, Duncan Luce). “Models of the human brain” is one topic discussed in those meetings, and concepts proposed during those conferences had significant influence on the research in information technologies and the human sciences ( Heims, 1991 ).
One of Wiener’s major contributions was in World War II, when he applied mathematics to electronics problems and developed a statistical prediction method for fire control theory. This method predicted the position in space where an enemy aircraft would be located in the future so that an artillery shell fired from a distance would hit the aircraft ( Conway and Siegelman, 2005 ). As told by Conway and Siegelman, “Wiener’s focus on a practical real-world problem had led him into that paradoxical realm of nature where there was no certainty, only probabilities, compromises, and statistical conclusions…” (p. 113). Advances in probability and statistics provided a tool for Wiener and others to investigate this paradoxial realm. Early in 1942 Wiener wrote a classified report for the National Defense Research Committee (NRDC), “The Extrapolation, Interpolating, and Smoothing of Stationary Time Series,” which was published as a book in 1949. This report is credited as the founding work in communications engineering, in which Wiener concluded that communication in all fields is in terms of information. In his words,
- simple The proper field of communication engineering is far wider than that generally assigned to it. Communication engineering concerns itself with the transmission of messages. For the existence of a message, it is indeed essential that variable information be transmitted. The transmission of a single fixed item of information is of no communicative value. We must have a repertory of possible messages, and over this repertory a measure determining the probability of these messages ( Wiener, 1949 , p. 2).
Wiener went on to say “such information will generally be of a statistical nature” (p. 10).
From 1942 onward, Wiener developed his ideas of control theory more broadly in Cybernetics , as described in a Scientific American article ( Wiener, 1948a ):
- simple It combines under one heading the study of what in a human context is sometimes loosely described as thinking and in engineering is known as control and communication. In other words, cybernetics attempts to find the common elements in the functioning of automatic machines and of the human nervous system, and to develop a theory which will cover the entire field of control and communication in machines and in living organisms (p. 14).
Wiener (1948a) made apparent in that article that the term cybernetics was chosen to emphasize the concept of feedback mechanism. The example he used was one of human action:
- simple Suppose that I pick up a pencil. To do this I have to move certain muscles. Only an expert anatomist knows what all these muscles are, and even an anatomist could hardly perform the act by a conscious exertion of the will to contract each muscle concerned in succession. Actually, what we will is not to move individual muscles but to pick up the pencil. Once we have determined on this, the motion of the arm and hand proceeds in such a way that we may say that the amount by which the pencil is not yet picked up is decreased at each stage. This part of the action is not in full conscious (p. 14; see also p. 7 of Wiener, 1961 ).
Note that in this example, Wiener hits on the central idea behind contemporary theorizing in action selection – the choice of action is with reference to a distal goal ( Hommel et al., 2001 ; Dignath et al., 2014 ). Wiener went on to say,
- simple To perform an action in such a manner, there must be a report to the nervous system, conscious or unconscious, of the amount by which we have failed to pick up the pencil at each instant. The report may be visual, at least in part, but it is more generally kinesthetic, or, to use a term now in vogue, proprioceptive (p. 14; see also p. 7 of Wiener, 1961 ).
That is, Wiener emphasizes the role of negative feedback in control of the motor system, as in theories of motor control ( Adams, 1971 ; Schmidt, 1975 ).
Wiener (1948b) developed his views more thoroughly and mathematically in his master work, Cybernetics or Control and Communication in the Animal and in the Machine , which was extended in a second edition published in 1961. In this book, Wiener devoted considerable coverage to psychological and sociological phenomena, emphasizing a systems view that takes into account feedback mechanisms. Although he was interested in sensory physiology and neural functioning, he later noted, “The need of including psychologists had indeed been obvious from the beginning. He who studies the nervous system cannot forget the mind, and he who studies the mind cannot forget the nervous system” ( Wiener, 1961 , p. 18).
Later in the Cybernetics book, Wiener indicated the value of viewing society as a control system, stating “Of all of these anti-homeostatic factors in society, the control of the means of communication is the most effective and most important” (p. 160). This statement is followed immediately by a focus on information processing of the individual: “One of the lessons of the present book is that any organism is held together in this action by the possession of means for the acquisition, use, retention, and transmission of information” ( Wiener, 1961 , p. 160).
Cybernetics, or the study of control and communication in machines and living things, is a general approach to understanding self-regulating systems. The basic unit of cybernetic control is the negative feedback loop, whose function is to reduce the sensed deviations from an expected outcome to maintain a steady state. Specifically, a present condition is perceived by the input function and then compared against a point of reference through a mechanism called a comparator. If there is a discrepancy between the present state and the reference value, an action is taken. This arrangement thus constitutes a closed loop of control, the overall purpose of which is to minimize deviations from the standard of comparison (reference point). Reference values are typically provided by superordinate systems, which output behaviors that constitute the setting of standards for the next lower level.
Cybernetics thus illustrates one of the most valuable characteristics of mathematics: to identify a common feature (feedback) across many domains and then study it abstracted from those domains. This abstracted study draws the domains closer together and often enables results from one domain to be extended to the other. From its birth, Wiener conceived of cybernetics as an interdisciplinary field, and control theory has had a major impact on diverse areas of work, such as biology, psychology, engineering, and computer science. Besides the mathematical nature, cybernetics has also been claimed as the science of complex probabilistic systems ( Beer, 1959 ). In other words, cybernetics is a science of combined constant flows of communication and self-regulating systems.
Shannon and Information Theory
With backgrounds in electrical engineering and mathematics, Claude Shannon obtained his Ph.D. in electrical engineering at MIT in 1940. Shannon is known within psychology primarily for information theory, but prior to his contribution on that topic, in his Master’s thesis, he showed how to design switch circuits according to Boole’s symbolic logic. Use of combinations of switches that represent binary values provides the foundation of modern computers and telecommunication systems ( O’Regan, 2012 ). In the 1940s, Shannon’s work on digit circuit theory opened the doors for him and allowed him to make connections with great scientists of the day, including von Neumann, Albert Einstein, and Alan Turing. These connections, along with his work on cryptography, affected his thoughts about communication theory.
With regard to information theory, or what he called communication theory, Shannon (1948a) stated the essential problem of communication in the first page of his classic article:
- simple The fundamental problem of communication is that of reproducing at one point either exactly or approximately a message selected at another point… The significant aspect is that the actual message is one selected from a set of possible messages. The system must be designed to operate for each possible selection, not just the one which will actually be chosen since this is unknown at the time of design. If the number of messages in the set is finite then this number or any monotonic function of this number can be regarded as a measure of the information produced when one message is chosen from the set, all choices being equally likely. As was pointed out by Hartley the most natural choice is the logarithmic function (p. 379).
Shannon (1948a) characterized an information system as having five elements: (1) an information source; (2) a transmitter; (3) a channel; (4) a receiver; (5) a destination. Note the similarity of Figure Figure1 1 , taken from his article, to the human information-processing models of cognitive psychology. Shannon provided mathematical analyses of each element for three categories of communication systems: discrete, continuous, and mixed. A key measure in information theory is entropy , which Shannon defined as the amount of uncertainty involved in the value of a random variable or the outcome of a random process. Shannon also introduced the concepts of encoding and decoding for the transmitter and receiver, respectively. His main concern was to find explicit methods, also called codes , to increase the efficiency and reduce the error rate during data communication over noisy channels to near the channel capacity.

Shannon’s schematic diagram of a general communication system ( Shannon, 1948a ).
Shannon explicitly acknowledged Wiener’s influence on the development of information theory:
- simple Communication theory is heavily indebted to Wiener for much of its basic philosophy and theory. His classic NDRC report, The Interpolation, Extrapolation, and Smoothing of Stationary Time Series ( Wiener, 1949 ), contains the first clear-cut formulation of communication theory as a statistical problem, the study of operations on time series. This work, although chiefly concerned with the linear prediction and filtering problem, is an important collateral reference in connection with the present paper. We may also refer here to Wiener’s Cybernetics ( Wiener, 1948b ), dealing with the general problems of communication and control ( Shannon and Weaver, 1949 , p. 85).
Although Shannon and Weaver (1949) developed similar measures of information independently from Wiener, they approached the same problem from different angles. Wiener developed the statistical theory of communication, equated information with negative entropy and related it to solve the problems of prediction and filtering while he worked on designing anti-aircraft fire-control systems ( Galison, 1994 ). Shannon, working primarily on cryptography at Bell Labs, drew an analogy between a secrecy system and a noisy communication system through coding messages into signals to transmit information in the presence of noise ( Shannon, 1945 ). According to Shannon, the amount of information and channel capacity were expressed in terms of positive entropy. With regard to the difference in sign for entropy in his and Wiener’s formulations, Shannon wrote to Wiener:
- simple I do not believe this difference has any real significance but is due to our taking somewhat complementary views of information. I consider how much information is produced when a choice is made from a set – the larger the set the more the information. You consider the larger uncertainty in the case of a larger set to mean less knowledge of the situation and hence less information ( Shannon, 1948b ).
A key element of the mathematical theory of communication developed by Shannon is that it omits “the question of interpretation” ( Shannon and Weaver, 1949 ). In other words, it separates information from the “psychological factors” involved in the ordinary use of information and establishes a neutral or non-specific human meaning of the information content ( Luce, 2003 ). In such sense, consistent with cybernetics, information theory also confirmed this neutral meaning common to systems of machines, human beings, or combinations of them. The view that information refers not to “what” you send but what you “can” send, based on probability and statistics, opened a new science that used the same methods to study machines, humans, and their interactions.
Inference Revolution
Although it is often overlooked, a related impact on psychological research during roughly the same period was that of using statistical thinking and methodology for small sample experiments. The two approaches that have been most influential in psychology, the null hypothesis significance testing of Ronald Fisher and the more general hypothesis testing view of Jerzy Neyman and Egon Pearson, resulted in what Gigerenzer and Murray (1987) called the inference revolution .
Fisher, Information, Inferential Statistics, and Experiment Design
Ronald Fisher got his degree in mathematics from Cambridge University where he spent another year studying statistical mechanics and quantum theory ( Yates and Mather, 1963 ). He has been described as “a genius who almost single-handedly created the foundations for modern statistical science” ( Hald, 2008 , p. 147) and “the single most important figure of 20th century statistics” ( Efron, 1998 , p. 95). Fisher is also “rightly regarded as the founder of the modern methods of design and analysis of experiments” ( Yates, 1964 , p. 307). In addition to his work on statistics and experimental design, Fisher made significant scientic contributions to genetics and evolutionary biology. Indeed, Dawkins (2010) , the famous biologist, called Fisher the greatest biologist since Darwin, saying:
- simple Not only was he the most original and constructive of the architects of the neo-Darwinian synthesis. Fisher also was the father of modern statistics and experimental design. He therefore could be said to have provided researchers in biology and medicine with their most important research tools.
Our interest in this paper is, of course, with the research tools and logic that Fisher provided, along with their application to scientific content.
Fisher began his early research as a statistician at Rothamsted Experimental Station in Harpenden, England (1919–1933). There, he was hired to develop statistical methods that could be applied to interpret the cumulative results of agriculture experiments ( Russell, 1966 , p. 326). Besides dealing with the past data, he became involved with ongoing experiments and developing methods to improve them ( Lehmann, 2011 ). Fisher’s hands-on work with experiments is a necessary feature of his background for understanding his positions regarding statistics and experimental design. Fisher (1962 , p. 529) essentially said as much in an address published posthumously:
- simple There is, frankly, no easy substitute for the educational discipline of whole time personal responsibility for the planning and conduct of experiments, designed for the ascertainment of fact, or the improvement of Natural Knowledge. I say “educational discipline” because such experience trains the mind and deepens the judgment for innumerable ancillary decisions, on which the value or cogency of an experimental program depends.
The analysis of variance (ANOVA, Fisher, 1925 ) and an emphasis on experimental design ( Fisher, 1937 ) were both outcomes of Fisher’s work in response to the experimental problems posed by the agricultural research performed at Rothamsted ( Parolini, 2015 ).
Fisher’s work synthesized mathematics with practicality and reshaped the scientific tools and practice for conducting and analyzing experiments. In the preface to the first edition of his textbook Statistical Methods for Research Workers , Fisher (1925 , p. vii) made clear that his main concern was application:
- simple Daily contact with the statistical problems which present themselves to the laboratory worker has stimulated the purely mathematical researches upon which are based the methods here presented. Little experience is sufficient to show that the traditional machinery of statistical processes is wholly unsuited to the needs of practical research.
Although prior statisticians developed probabilistic methods to estimate errors of experimental data [e.g., Student’s, 1908 (Gosset’s) t -test], Fisher carried the work a step further, developing the concept of null hypothesis testing using ANOVA ( Fisher, 1925 , 1935 ). Fisher demonstrated that by proposing a null hypothesis (usually no effect of an independent variable over a population), a researcher could evaluate whether a difference between conditions was sufficiently unlikely to occur due to chance to allow rejection of the null hypothesis. Fisher proposed that tests of significance with a low p -value can be taken as evidence against the null hypothesis. The following quote from Fisher (1937 , pp. 15–16), captures his position well:
- simple It is usual and convenient for experimenters to take 5 per cent. as a standard level of significance, in the sense that they are prepared to ignore all results which fail to reach this standard, and, by this means, to eliminate from further discussion the greater part of the fluctuations which chance causes have introduced into their experimental results.
While Fisher recommended using the 0.05 probability level as a criterion to decide whether to reject the null hypothesis, his general position was that researchers should set the critical level of significance at sufficiently low probability so as to limit the chance of concluding that an independent variable has an effect when the null hypothesis is true. Therefore, the criterion of significance does not necessarily have to be 0.05 (see also Lehmann, 1993 ), and Fisher’s main point was that failing to reject the null hypothesis regardless of what criterion is used does not warrant accepting it (see Fisher, 1956 , pp. 4 and 42).
Fisher (1925 , 1935 ) also showed how different sources of variance can be partitioned to allow tests of separate and combined effects of two or more independent variables. Prior to this work, much experimental research in psychology, though not all, used designs in which a single independent variable was manipulated. Fisher made a case that designs with two or more independent variables were more informative than multiple experiments using different single independent variables because they allowed determination of whether the variables interacted. Fisher’s application of the ANOVA to data from factorial experimental designs, coupled with his treatment of extracting always the maximum amount of information (likelihood) conveyed by a statistic (see later), apparently influenced both Wiener and Shannon ( Wiener, 1948a , p. 10).
In “The Place of Design of Experiments in the Logic of Scientific Inference,” Fisher (1962) linked experimental design, the application of correct statistical methods, and the subsequent extraction of a valid conclusion through the concept of information (known as Fisher information ). Fisher information measures the amount of information that an obtained random sample of data has about the variable of interest ( Ly et al., 2017 ). It is the expected value of the second moment of the log-likelihood function, which is the probability density function for obtained data conditional on the variable. In other words, the variance is defined to be the Fisher information, which measures the sensitivity of the likelihood function to the changes of a manipulated variable on the obtained results. Furthermore, Fisher argued that experimenters should be interested in not only minimizing loss of information in the process of statistical reduction (e.g., use ANOVA to summarize evidence that preserves the relevant information from data, Fisher, 1925 , pp. 1 and 7) but also the deliberate study of experimental design, for example, by introducing randomization or control, to maximize the amount of information provided by estimates derived from the resulting experimental data (see Fisher, 1947 ). Therefore, Fisher unfied experimental design and statistical analysis through information ( Seidenfeld, 1992 ; Aldrich, 2007 ), an approach that resonates with the system view of cybernetics.
Neyman-Pearson Approach
Jerzy Neyman obtained his doctorate from the University of Warsaw with a thesis based on his statistical work at the Agricultural Institute in Bydgoszcz, Poland, in 1924. Egon Pearson received his undergraduate degree in mathematics and continued his graduate study in astronomy at Cambridge University until 1921. In 1926, Neyman and Pearson started their collaboration and raised a question with regard to Fisher’s method, why test only the null hypothesis? They proposed a solution in which not only the null hypothesis but also a class of possible alternatives are considered, and the decision is one of accepting or rejecting the null hypothesis. This decision yields probabilities of two kinds of error: false rejection of the null hypothesis (Type I or Alpha) or false acceptance of the alternative hypothesis (Type II or Beta; Neyman and Pearson, 1928 , 1933 ). They suggested that the best test was the one that minimized the Type II error subject to a bound on the Type I error, i.e., the significance level of the test. Thus, instead of classifying the null hypothesis as rejected or not, the central consideration of the Neyman-Pearson approach was that one must specify not only the null hypothesis but also the alternative hypotheses against which it is tested. With this symmetric decision approach, statistical power (1 – Type II error) becomes an issue. Fisher (1947) also realized the importance and necessity of power but argued that it is a qualitative concern addressed during the experimental design to increase the sensitivity of the experiment and not part of the statistical decision process. In other words, Fisher thought that researchers should “conduct experimental and observational inquiries so as to maximize the information obtained for a given expenditure” ( Fisher, 1951 , p. 54), but did not see that as being part of statistics.
To reject or accept the null hypothesis, rather than disregarding results that do not allow rejection of the null hypothesis, was the rule of behavior for Neyman-Pearson hypothesis testing. Thus, their approach assumed that a decision made from the statistical analysis was sufficient to draw a conclusion as to whether the null or alternative hypothesis was most likely and did not put emphasis on the need for non-statistical inductive inference to understand the problems. In other words, tests of significance were interpreted as means to make decisions in an acceptance procedure (also see Wald, 1950 ) but not specifically for research workers to gain a better understanding of the experimental material. Also, the Neyman-Pearson approach interpreted probability (or the value of a significance level) as a realization of long-run frequency in a sequence of repetitions under constant conditions. Their view was that if a sequence of independent events was obtained with probability p of success, then the long-run success frequency will be close to p (this is known as a frequentist probability, Neyman, 1977 ). Fisher (1956) vehemently disagreed with this frequentist position.
Fisherian vs. Frequentist Approaches
In a nutshell, the differences between Fisherians and frequentists are mostly about research philosophy and how to interpret the results ( Fisher, 1956 ). In particular, Fisher emphasized that in scientific research, failure to reject the null hypothesis should not be interpreted as acceptance of it, whereas Neyman and Pearson portrayed the process as a decision between accepting or rejecting the null hypothesis. Nevertheless, the usual practice for statistical testing in psychology is based on a hybrid of Fisher’s and Neyman-Pearson’s approaches ( Gigerenzer and Murray, 1987 ). In practice, when behavioral researchers speak of the results of research, they are primarily referring to the statistically significant results and less often to null effects and the effect size estimates associated with those p -values.
The reliance on the results of significance testing has been explained from two perspectives: (1) Neither experienced behavioral researchers nor experienced statisticians have a good intuitive feel for the practical meaning of effect size estimation (e.g., Rosenthal and Rubin, 1982 ); (2) The reliance on a reject-or-accept dichotomous decision procedure, in which the differences between p levels are taken to be trivial relative to the difference between exceeding or failing to exceed a 0.05 or some other accepted level of significance ( Nelson et al., 1986 ). The reject-accept procedure follows the Neyman-Pearson approach and is compatible with the view that information is binary ( Shannon, 1948a ). Nevertheless, even if an accurate statistical power analysis is conducted, a replication study properly powered can produce results that are consistent with the effect size of interest or consistent with absolutely no effect ( Nelson et al., 1986 ; Maxwell et al., 2015 ). Therefore, instead of solely relying on hypothesis testing, or whether an effect is true or false, a report of actual p level obtained along with a statement of the effect size estimation, should be considered.
Fisher emphasized in his writings that an essential ingredient in the research process is the judgment of the researcher, who must decide by how much the obtained results have advanced a particular theoretical proposition (that is, how meaningful the results are). This decision is based in large part on decisions made during experimental design. The statistical significance test is just a useful tool to inform such decisions during the process to allow the researcher to be confident that the results are likely not due to chance. Moreover, he wanted this statistical decision in scientific research to be independent of a priori probabilities or estimates because he did not think these could be made accurately. Consequently, Fisher considered that only a statistically significant effect in an exact test for which the null hypothesis can be rejected should be open to subsequent interpretation by the researcher.
Acree (1978 , pp. 397–398) conducted a thorough evaluation of statistical inference in psychological research that for the most part captures why Fisher’s views had greater impact on the practice of psychological researchers than those of Neyman and Pearson (emphasis ours):
- simple On logical grounds, Neyman and Pearson had decidedly the better theory; but Fisher’s claims were closer to the ostensible needs of psychological research . The upshot is that psychologists have mostly followed Fisher in their thinking and practice: in the use of the hypothetical infinite population to justify probabilistic statements about a single data set; in treating the significance level evidentially; in setting it after the experiment is performed; in never accepting the null hypothesis; in disregarding power…. Yet the rationale for all our statistical methods, insofar as it is presented, is that of Neyman and Pearson, rather than Fisher.
Although Neyman and Pearson may have had “decidedly the better theory” for statistical decisions in general, Fisher’s approach provides a better theory for scientific inferences from controlled experiments.
Interim Summary
The work we described in Sections “Cybernetics and Information Theory” and “Inference Revolution” identifies three crucial pillars of research that were developed mainly in the period from 1940 to 1955: cybernetics/control theory, information/communication theory, and inferential statistical theory. Moreover, our analysis revealed a correspondence among those pillars. Specifically, the cybernetics/control theory corresponds to the experimental design, both of which provide the framework for cognitive psychology. The information theory corresponds to the statistical test, both of which provide quantitative evidence for the qualitative assumption.
These pillars were identified as early as 1952 in the preface to the proceedings of a conference called Information Theory in Biology , in which the editor, Quastler (1953 , p. 1), said:
- simple The “new movement” [what we would call information-processing theory] is based on evaluative concepts (R. A. Fisher’s experimental design, A. Wald’s statistical decision function, J. von Neumann’s theory of games), on the development of a measure of information (R. Hartley, D. Gabor, N. Wiener, C. Shannon), on studies of control mechanisms, and the analysis and design of large systems (W. S. McCulloch and W. Pitt’s “neurons,” J. von Neumann’s theory of complicated automata, N. Wiener’s cybernetics).
The pillars undergirded not only the new movement in biology but also the new movement in psychology. The concepts introduced in the dawning information age of 1940–1955 had tremendous impact on applied and basic research in experimental psychology that transformed psychological research into a form that has developed to the present.
Human Information Processing
As noted in earlier sections, psychologists and neurophysiologists were involved in the cybernetics, information theory, and inferential statistics movements from the earliest days. Each of these movements was crucial to the ascension of the information-processing approach in psychology and the emergence of cognitive science, which are often dated to 1956. In this section, we review developments in cognitive psychology linked to each of the three pillars, starting with the most fundamental one, cybernetics.
The Systems Viewpoint of Cybernetics
George A. Miller explicitly credited cybernetics as being seminal in 1979, stating, “I have picked September 11, 1956 [the date of the second MIT symposium on Information Theory] as the birthday of cognitive science – the day that cognitive science burst from the womb of cybernetics and became a recognizable interdisciplinary adventure in its own right” (quoted by Elias, 1994 , p. 24; emphasis ours). With regard to the development of human factors (ergonomics) in the United Kingdom, Waterson (2011 , pp. 1126–1127) remarks similarly:
- simple During the 1960s, the ‘systems approach’ within ergonomics took on a precedence which has lasted until the present day, and a lot of research was informed from cybernetics and general systems theory. In many respects, a concern in applying a systemic approach to ergonomic issues could be said to be one of the factors which ‘glues’ together all of the elements and sub-disciplines within ergonomics.
This seminal role for cybernetics is due to its fundamental idea that various levels of processing in humans and non-humans can be viewed as control systems with interconnected stages and feedback loops. It should be apparent that the human information-processing approach, which emphasizes the human as a processing system with feedback loops, stems directly from cybernetics, and the human-machine system view that underlies contemporary human factors and ergonomics, can be traced directly to cybernetics.
We will provide a few more specific examples of the impact of cybernetics. McCulloch and Pitts (1943) , members of the cybernetics movement, are given credit for developing “the first conceptual model of an artificial neural network” ( Shiffman, 2012 ) and “the first modern computational theory of mind and brain” ( Piccinini, 2004 ). The McCulloch and Pitts model identified the neurons as logical decision elements by on and off states, which are the basis of building brain-like machines. Since then, Boolean function, together with feedback through neurons, has been used extensively to quantify theorizing in relation to both neural and artificial intelligent systems ( Piccinini, 2004 ). Thus, computational modeling of brain processes was part of the cybernetics movement from the outset.
Franklin Taylor a noted engineering psychologist, reviewed Wiener’ (1948b) Cybernetics book, calling it “a curious and provocative book” ( Taylor, 1949 , p. 236). Taylor noted, “The author’s most important assertion for psychology is his suggestion, often repeated, that computers, servos, and other machines may profitably be used as models of human and animal behavior” (p. 236), and “It seems that Dr. Wiener is suggesting that psychologists should borrow the theory and mathematics worked out for machines and apply them to the behavior of men” (p. 237). Psychologists have clearly followed this suggestion, making ample use of the theory and mathematics of control systems. Craik (1947 , 1948 ) in the UK had in fact already started to take a control theory approach to human tracking performance, stating that his analysis “puts the human operator in the class of ‘intermittent definite correction servos’ ” ( Craik, 1948 , p. 148).
Wiener’s work seemingly had considerable impact on Taylor, as reflected in the opening paragraphs of a famous article by Birmingham and Taylor (1954) on human performance of tracking tasks and design of manual control systems:
- simple The cardinal purpose of this report is to discuss a principle of control system design based upon considerations of engineering psychology. This principle will be found to advocate design practices for man-operated systems similar to those customarily employed by engineers with fully automatic systems…. In many control systems the human acts as the error detector… During the last decade it has become clear that, in order to develop control systems with maximum precision and stability, human response characteristics have to be taken into account. Accordingly, the new discipline of engineering psychology was created to undertake the study of man from an engineering point of view (p. 1748).
Control theory continues to provide a quantitative means for modeling basic and applied human performance ( Jagacinski and Flach, 2003 ; Flach et al., 2015 ).
Colin Cherry, who performed the formative study on auditory selective attention, studied with Wiener and Jerome Wiesner at MIT in 1952. It was during this time that he conducted his classic experiments on the cocktail party problem – the question of how we identify what one person is saying when others are speaking at the same time ( Cherry, 1953 ). His detailed investigations of selective listening, including attention switching, provided the basis for much research on the topic in the next decade that laid the foundation for contemporary studies of attention. The initial models explored the features and locus of a “limited-capacity processing channel” ( Broadbent, 1958 ; Deutsch and Deutsch, 1963 ). Subsequent landmark studies of attention include the attentuation theory of Treisman (1960 ; also see Moray, 1959 ); capacity models that conceive of attention as a resource to be flexibly allocated to various stages of human information processing ( Kahneman, 1973 ; Posner, 1978 ); the distinction between controlled and automatic processing ( Shiffrin and Schneider, 1977 ); the feature-integration theory of visual search ( Treisman and Gelade, 1980 ).
As noted, Miller (2003) and others identified the year 1956 as a critical one in the development of contemporary psychology ( Newell and Simon, 1972 ; Mandler, 2007 ). Mandler lists two events that year that ignited the field, in both of which Allan Newell and Herbert Simon participated. The first is the meeting of the Special Group on Information Theory of the Institute of Electrical and Electronics Engineers, which included papers by linguist Noam Chomsky (who argued against an information theory approach to language for his transformational-generative grammer) and psychologist Miller (on avoiding the short-term memory bottleneck), in addition to Newell and Simon (on their Logic Theorist “thinking machine”) and others ( Miller, 2003 ). The other event is the Dartmouth Summer Seminar on Artificial Intelligence (AI), which was organized by John McCarthy, who had coined the term AI the previous year. It included Shannon, Oliver Selfridge (who discussed initial ideas that led to his Pandemonium model of human pattern recognition, described in the next paragraph), and Marvin Minsky (a pioneer of AI, who turned to symbolic AI after earlier work on neural net; Moor, 2006 ), among others. A presentation by Newell and Simon at that seminar is regarded as essential in the birth of AI, and their work on human problem solving exploited concepts from work on AI.
Newell applied a combination of experimental and theoretical research during his work in RAND Corporation from 1950 ( Simon, 1997 ). For example, in 1952, he and his colleagues designed and conducted laboratory experiments on a full-scale simulation of an Air-Force Early Warning Station to study the decision-making and information-handling processes of the station crews. Central to the research was the recording and analyzing the crew’s interaction with their radar screens, with interception aircraft, and with each other. From these studies, Newell became to believe that information processing is the central activity in organizations (systems).
Selfridge (1959) laid the foundation for a cognitive theory of letter perception with his Pandemonium model, in which the letter identification is achieved by way of hierarchically organized layers of features and letter detectors. Inspired by Selfridge’s work on Pandemonium, Newell started to converge on the idea that systems can be created that contain intelligence and have the ability to adapt. Based on his understanding of computers, heuristics, information processing in organizations (systems), and cybernetics, Newell (1955) delineated the design of a computer program to play chess in “The Chess Machine: An Example of Dealing with a Complex Task by Adaptation.” After that, for Newell, the investigation of organizations (systems) became the examination of the mind, and he committed himself to understand human learning and thinking through computer simulations.
In the study of problem solving, think-aloud protocols in laboratory settings revealed that means-end analysis is a key heuristic mechanism. Specifically, the current situation is compared to the desired goal state and mental or physical actions are taken to reduce the gap. Newell, Simon, and Jeff Shaw developed the General Problem Solver, a computer program that could solve problems in various domains if given a problem space (domain representation), possible actions to move between space states, and information about which actions would reduce the gap between the current and goal states (see Ernst and Newell, 1969 , for a detailed treatment, and Newell and Simon, 1972 , for an overview). The program built into the system underlined the importance of control structure for solving the problems, revealing a combination of cybernetics and information theory.
Besides using cybernetics, neuroscientists further developed it to explain anticipation in biological systems. Although closed-loop feedback can perform online corrections in a determinate machine, it does not give any direction ( Ashby, 1956 , pp. 224–225). Therefore, a feedforward loop was proposed in cybernetics that could improve control over systems through anticipation of future actions ( Ashby, 1956 ; MacKay, 1956 ). Generally, the feedforward mechansim is constructed as another input pathway parallel to the actual input, which enables comparison between the actual and anticipated inputs before they are processed by the system ( Ashby, 1960 ; Pribram, 1976 , p. 309). In other words, a self-organized system is not only capable of self-adjusting its own behavior (feedback), but is also able to change its own internal organization in such a way as to select the response that eliminates a disturbance from the outside among the random responses that it attempts ( Ashby, 1960 ). Therefore, the feedforward loop “nudges” the inputs based on predefined parameters in an automatic manner to account for cognitive adaptation, indicating a higher level action planning. Moreover, different from error-based feedback control, the knowledge-based feedforward control cannot be further adjusted once the feedforward input has been processed. The feedforward control from cybernetics has been used by psychologists to understand human action control at behavioral, motoric, and neural levels (for a review, see Basso and Olivetti Belardinelli, 2006 ).
Therefore, both feedback and feedforward are critical to a control system, in which feedforward control is valuable and could improve the performance when feedback control is not sufficient. A control system with feedforward and feedback loops allows the interaction between top-down and bottom-up information processing. Consequently, the main function of a control system is not to create “behavior” but to create and maintain the anticipation of a specific desired condition, which constitutes its reference value or standard of comparison.
Cognitive psychology and neuroscience suggest that the combination of anticipatory and hierarchical structures are involved for human action learning and control ( Herbort et al., 2005 ). Specifically, anticipatory mechanisms lead to direct action selections in inverse model and effective filtering mechanisms in forward models, both of which are based on sensorimotor contingencies through people’s interaction with the environment (ideomotor principle, Greenwald, 1970 ; James, 1890 ). Therefore, the feedback loop included in cybernetics as well as the feedforward loop are essential to the learning processes.
We conclude this section with mention of one of the milestone books in cognitive psychology, Plans and the Structure of Behavior , by Miller et al. (1960) . In the prolog to the book, the authors indicate that they worked on it together for a year at the Center for Advanced Study in the Behavioral Sciences in California. As indicated by the title, the central idea motivating the book was that of a plan, or program, that guides behavior. But, the authors said:
- simple Our fundamental concern, however, was to discover whether the cybernetic ideas have any relevance for psychology…. There must be some way to phrase the new ideas [of cybernetics] so that they can contribute to and profit from the science of behavior that psychologists have created. It was the search for that favorable intersection that directed the course of our year-long debate (p. 3, emphasis ours).
In developing the central concept of the test-operate-test (TOTE) unit in the book, Miller et al. (1960) stated, “The interpretation to which the argument builds is one that has been called the ‘cybernetic hypothesis,’ namely that the fundamental building block of the nervous system is the feedback loop” (pp. 26–27). As noted by Edwards (1997) , the TOTE concept “is the same principle upon which Weiner, Rosenblueth, and Bigelow had based ‘Behavior, Purpose, and Teleology”’ (p. 231). Thus, although Miller later gave 1956 as the date that cognitive science “burst from the womb of cybernetics,” even after the birth of cognitive science, the genes inherited from cybernetics continued to influence its development.
Information and Uncertainty
Information theory, a useful way to quantify psychological and behavior concepts, had possibly a more direct impact than cybernetics on psychological research. No articles were retrieved from the PsycINFO database prior to 1950 when we entered “information theory” as an unrestricted field search term on May 3, 2018. But, from 1950 to 1956 there were 37 entries with “information theory” in the title and 153 entries with the term in some field. Two articles applying information theory to speech communication appeared in 1950, a general theoretical article by Fano (1950) of the Research Laboratory in Electronics at MIT, and an empirical article by Licklider (1950) of the Acoustics Laboratory, also at MIT. Licklider (1950) presented two methods of reducing the frequncies of speech without destoying the intelligibility by using the Shannon-Weaver information formula based on first-order probability.
Given Licklider’s background in cybernetics and information theory, it is not too surprising that he played a major role in establishing the ARPAnet, which was later replaced by the Internet:
- simple His 1968 paper called “The Computer as a Communication Device” illustrated his vision of network applications and predicted the use of computer networks for communications. Until then, computers had generally been thought of as mathematical devices for speeding up computations ( Internet Hall of Fame, 2016 ).
Licklider worked from 1943–1950 at the Psyco-Acoustics Laboratory (PAL) of Harvard University University, headed by Edwards ( 1997 , p. 212) noted, “The PAL played a crucial role in the genesis of postwar information processing psychologies.” He pointed out, “A large number of those who worked at the lab… helped to develop computer models and metaphors and to introduce information theory into human experimental psychology” (p. 212). Among those were George Miller and Wendell Garner, who did much to promulgate information theory in psychology ( Garner and Hake, 1951 ; Miller, 1953 ), as well as Licklider, Galanter and Pribram. Much of PAL’s research was based in solving engineering problems for the military and industry.
The exploration of human information-processing limitations using information theory that led to one of the most influential applications was that of Hick (1952) and Hyman (1953) to explain increases in reaction time as a function of uncertainty regarding the potential stimulus-response alternatives. Their analyses showed that reaction time increased as a logarithmic function of the number of equally likely alternatives and as a function of the amount of information as computed from differential probabilities of occurrence and sequential effects. This relation, call Hick’s law or the Hick-Hyman law has continued to be a source of research to the present and is considered to be a fundamental law of human-computer interaction ( Proctor and Schneider, 2018 ). Fitts and Seeger (1953) showed that uncertainty was not the only factor influencing reaction time. They examined performance of eight-choice task for all combinations of three spatial-location stimulus and response arrays. Responses were faster and more accurate when the response array corresponded to that of the stimulus array than when it did not, which Fitts and Seeger called a stimulus-response compatibility effect. The main point of their demonstration was that correspondence of the spatial codes for the stimulus and response alternatives was crucial, and this led to detailed investigation of compatibility effects that continue to the present ( Proctor and Vu, 2006 , 2016 ).
Even more influential has been Fitts’s law, which describes movement time in tasks where people make discrete aimed movements to targets or series of repetitive movements between two targets. Fitts (1954) developed the index of difficulty as –log 2 W/2A bits/response, where W , is the target width and A is the amplitude (or distance) of the movement. The resulting movement time is a linear function of the index of difficulty, with the slope differing for different movement types. Fitts’s law continues to be the subject of basic and applied research to the present ( Glazebrook et al., 2015 ; Velasco et al., 2017 ).
Information theory was applied to a range of other topics during the 1950s, including intelligence tests ( Hick, 1951 ), memory ( Aborn and Rubenstein, 1952 ; Miller, 1956 ), perception ( Attneave, 1959 ), skilled performance ( Kay, 1957 ), music ( Meyer, 1957 ), and psychiatry ( Brosin, 1953 ). However, the key concept of information theory, entropy, or uncertainty, was found not to provide an adequate basis for theories of human performance (e.g., Ambler et al., 1977 ; Proctor and Schneider, 2018 ).
Shannon’ (1948a) advocacy of information theory for electronic communication was mainly built on there being a mature understanding of the structured pattern of information transmission within electromagnetic systems at that time. In spite of cognitive research having greatly expanded our knowledge about how humans select, store, manipulate, recover, and output information, the fundamental mechanisms of those information processes remained under further investigation ( Neisser, 1967 , p. 8). Thus, although information theory provided a useful mathematic metric, it did not provide a comprehensive account of events between the stimulus and response, which is what most psychologists were interested in ( Broadbent, 1959 ). With the requirement that information be applicable to a vast array of psychological issues, “information” has been expanded from a measure of informativeness of stimuli and responses, to a framework for describing the mental or neural events between stimuli and responses in cognitive psychology ( Collins, 2007 ). Therefore, the more enduring impact of information theory was through getting cognitive psychologists to focus on the nature of human information processing, such that by Lachman et al. (1979) titled their introduction to the field, Cognitive Psychology and Information Processing .
Along with the information theory, the arrival of the computer provided one of the most viable models to help researchers understand the human mind. Computers grew from a desire to make machines smart ( Laird et al., 1987 ), which assumes that stored knowledge inside of a machine can be applied to the world similar to the way that people do, constituting intelligence (e.g., intelligent machine, Turing, 1937 ; AI, Minsky, 1968 ; McCarthy et al., 2006 ). The core idea of the computer metaphor is that the mind functions like a digital computer, in which mental states are computational states and mental processes are computational processes. The use of the computer as a tool for thinking about how the mind handles information has been highly influential in cognitive psychology. For example, the PsycINFO database returned no articles prior to 1950 when “encoding” was entered as an unrestricted field search term on May 3, 2018. From 1950 to 1956 there was 1 entry with “encoding” in the title and 4 entries with the term in some field. But, from 1956 to 1973, there were 214 entries with “encoding” in the title and 578 entries with term in some field, including the famous encoding specificity principle of Tulving and Thomson (1973) . Some models in cognitive psychology were directly inspired by how the memory system of a computer works, for example, the multi-store memory ( Atkinson and Shiffrin, 1968 ) and working memory ( Baddeley and Hitch, 1974 ) models.
Although cybernetics is the origin of early AI ( Kline, 2011 ) and the computer metaphor and cybernetics share similar concepts (e.g., representation), they are fundamentally different at the conceptual level. The computer metaphor represents a genuine simplification: Terms like “encoding” and “retrieving” can be used to describe human behavior analogously to machine operation but without specifying a precise mapping between the analogical “computer” and the target “human” domain ( Gentner and Grudin, 1985 ). In contrast, cybernetics provides a powerful framework to help people understand the human mind, which holds that, regardless of human or machine, it is necessary and possible to achieve goals through correcting action using feedback and adapting to the external environment using feedforward. Recent breakthroughs in AI (e.g., AlphaGo beating professional Go players) rely on training the machine to learn how to perform tasks at a level not seen before using a large number of examples and an artificial neural network (ANN) without human guidance. This unsupervised learning allows the machine to determine on its own whether a certain function should be executed. The development of ANN has been greatly influenced by consideration of dynamic properties of cybernetics ( Cruse, 2009 ), to achieve the goal of self-organization or self-regulation.
Statistical Inference and Decisions
Statistical decision theory also had substantial impact. Engineering psychologists were among the leaders in promulgating use of the ANOVA, with Chapanis and Schachter (1945 ; Schachter and Chapanis, 1945 ) using it in research on depth perception through distorted glass, conducted in the latter part of World War II and presented in Technical Reports. As noted by Rucci and Tweney (1980) , “Following the war, these [engineering] psychologists entered the academic world and began to publish in regular journals, using ANOVA” (p. 180).
Factorial experiments and use of the ANOVA were slow to take hold in psychology. Rucci and Tweney (1980) counted the frequency with which the t -test and ANOVA were used in major psychology journals from 1935 to 1952. They described the relation as, “Use of both t and ANOVA increased gradually prior to World War II, declined during the war, and increased immediately thereafter” (p. 172). Rucci and Tweney concluded, “By 1952 it [ANOVA] was fully established as the most frequently used technique in experimental research” (p. 166). They emphasized that this increased use of ANOVA reflected a radical change in experimental design, and emphasized that although one could argue that the statistical technique caused the change in psychological research, “It is just as plausible that the discipline had developed in such a way that the time was ripe for adoption of the technique” (p. 167). Note that the rise in use of null hypothesis testing and ANOVA paralleled that of cybernetics and information theory, which suggests that the time was indeed ripe for the use of probability theory, multiple independent variables, and formal scientific decision making through hypothesis testing that is embodied in the factorial design and ANOVA.
The first half of the 1950s also saw the introduction of signal detection theory, a variant of statistical decision theory, for analyzing human perception and performance. Initial articles by Peterson et al. (1954) and Van Meter and Middleton (1954) were published in a journal of the Institute of Electrical and Electronics Engineers (IEEE), but psychologists were quick to realize the importance of the approach. This point is evident in the first sentence of Swets et al.’s (1961) article describing signal detection theory in detail:
- simple About 5 years ago, the theory of statistical decision was translated into a theory of signal detection. Although the translation was motivated by problems in radar, the detection theory that resulted is a general theory… The generality of the theory suggested to us that it might also be relevant to the detection of signals by human observers… The detection theory seemed to provide a framework for a realistic description of the behavior of the human observer in a variety of perceptual tasks (p. 301).
Signal detection theory has proved to be an invaluable tool because it dissociates influences of the evidence on which decisions are based from the criteria applied to that evidence. This way of conceiving decisions is useful not only for perceptual tasks but for a variety of tasks in which choices on the basis of noisy information are required, including recognition memory ( Kellen et al., 2012 ). Indeed, Wixted (2014) states, “Signal-detection theory is one of psychology’s most notable achievements, but it is not a theory about typical psychological phenomena such as memory, attention, vision or psychopathology (even though it applies to all of those areas and more). Instead, it is a theory about how we use evidence to make decisions.”
In the 1960s, Sternberg (1969) formalized the additive factors method of analyzing reaction-time data to identify different information-processing stages. Specifically, a factorial experiment is conducted, and if two independent variables affect different processing stages, the two variables do not interact. If, on the other hand, there is a significant interaction, then the variables can be assumed to affect at least one processing stage in common. Note that the subtitle of Sternberg’s article is “Extension of Donders’ Method,” which is reference to the research reported by F. C. Donders 100 years earlier in which he estimated the time for various processing stages by subtracting the reaction time obtained for a task that did not have an additional processing stage inserted from one that did. A limitation of Donders’s (1868/1969) subtraction method is that the stages had to be assumed and could not be identified. Sternberg’s extension that provided a means for identifying the stages did not occur until both the language of information processing and the factorial ANOVA were available as tools for analyzing reaction-time data. Additive factors method formed a cornerstone for much research in cognitive psychology for the following couple of decades, and the logic is still often applied to interpret empirical results, often without explicit acknowledgment.
In psychology, how people make decisions in perceptual and cognitive tasks has often been proposed on the basis of sequential sampling to explain the pattern of obtained reaction time (RT) and percentage error. The study of such mechanisms addresses one of the fundamental question in psychology, namely, how the central nervous system translates perception into action and how this translation depends on the interaction and expectation of individuals. Like signal detection theory, the theory of sequential sampling starts from the premise that perceptual and cognitive decisions are statistical in nature. It also follws the widely accepted assumption that sensory and cognitive systems are inherently noise and time-varying. In practice, the study of a given sequential model reduce to the study of a stochastic process, which represents the accumulative information avaliable to the decision at a given time. A Wiener process forms the basis of Ratcliff’ (1978) influential diffusion model of reaction times, in which noisy information accumulates continuously over time from a starting point to response thresholds ( Ratcliff and Smith, 2004 ). Recently, Srivastava et al. (2017) extended this diffusion model to make the Wiener process time dependent. More generally, Shalizi (2007 , p. 126) makes the point, “The fully general theory of stochastic calculus considers integration with respect to a very broad range of stochastic processes, but the original case, which is still the most important, is integration with respect to the Wiener process.”
In parallel to use of the computer metaphor to understand human mind, use of the laws of probability as metaphors of the mind also has had a profound influence on physiology and psychology ( Gigerenzer, 1991 ). Gregory (1968) regarded seeing an object from an image as an inference from a hypothesis (also see “unconscious inference” of Helmholtz, 1866/1925 ). According to Gregory (1980) , in spite of differences between perception and science, the cognitive procedures carried out by perceptual neural processes are essentially the same as the processes of predictive hypotheses of science. Especially, Gregory emphasized the importance and distinction between bottom–up and top–down procedures in perception. For normal perception and the perceptual illusions, the bottom–up procedures filter and structure the input and the top–down procedures refer to stored knowledge or assumptions that can work downwards to parcel signals and data into object.
A recent development of the statistics metaphor is the Bayesian brain hypothesis, which has been used to model perception and decision making since the 1990s ( Friston, 2012 ). Rao and Ballard (1997) described a hierarchical neural network model of visual recognition, in which both input-driven bottom–up signals and expectation-driven top–down signals were used to predict the current recognition state. They showed that feedback from a higher layer to the input later carries predictions of expected inputs, and the feedforward connections convey the errors in prediction which are used to correct the estimation. Rao (2004) illustrated how the Bayesian model could be implemented with neural networks by feedforward and recurrent connections, showing that for both perception and decision-making tasks the resulting network exhibits direction selectivity and computes posterior error corrections.
We would like to highlight that, different from the analogy to computers, the cybernetics view is essential for the Bayesian brain hypothesis. This reliance on cybernetics is because the Bayesian brain hypothesis models the interaction between prior knowledge (top–down) and sensory evidence (bottom–up) quantitatively. Therefore, the success of Bayesian brain modeling is due to both the framework from cybernetics and the computation of probability. Seth (2015) explicitly acknowledges this relation in his article, The Cybernetic Bayesian Brain .
Meanwhile, the external information format (or representation) on which the Bayesian inferences and statistical reasoning operate has been investigated. For example, Gigerenzer and Hoffrage (1995) varied mathematically equivalent representation of information in percentage or frequency for various problems (e.g., the mammography problem, the cab problem) and found that frequency formats enabled participants’ inferences to conform to Bayes’ theorem without any teaching or instruction.
The Information Age of humans follows on periods that are called the Stone Age , Bronze Age , Iron Age , and Industrial Age . These labels indicate that the advancement and time period of a specific human history are often represented by the type of tool material used by humans. The formation of the Information Age is inseparable from the interdisciplinary work of cybernetics, information theory, and statistical inference, which together generated a cognitive psychology adapted to the age. Each of these three pillars has been acknowledged separately by other authors, and contemporary scientifical approaches to motor control and cognitive processing have been continuously inspired by cybernetics, information theory, and statistical inference.
Kline (2015 , p. 1) aptly summarized the importance of cybernetics in the founding of the information age:
- simple During contentious meetings filled with brilliant arguments, rambling digressions, and disciplinary posturing, the cybernetics group shaped a language of feedback, control, and information that transformed the idiom of the biological and social sciences, sparked the invention of information technologies, and set the intellectual foundation for what came to be called the information age. The premise of cybernetics was a powerful analogy: that the principles of information-feedback machines, which explained how a thermostat controlled a household furnace, for example, could also explain how all living things—from the level of the cell to that of society—behaved as they interacted with their environment.
Pezzulo and Cisek (2016) extended the cybernetic principles of feedback and forward control for understanding cognition. In particular, they proposed hierachical feedback control, indicating that adaptive action selection is influenced not only by prediction of immediate outcomes but also prediction of new opportunites afforded by the outcomes. Scott (2016) highlighted the use of sensory feedback, after a person becomes familiar with performing a perceptual-motor task, to drive goal-directed motor control, reducing the role of top–down control through utilizing bottom–up sensory feedback.
Although less expansive than Kline (2015) , Fan (2014 , p. 2) emphasized the role of information theory. He stated, “Information theory has a long and distinguished role in cognitive science and neuroscience. The ‘cognitive revolution’ of the 1950s, as spearheaded by Miller (1956) and Broadbent (1958) , was highly influenced by information theory.” Likewise, Gigerenzer (1991 , p. 255) said, “Inferential statistics… provided a large part of the new concepts for mental processes that have fueled the so called cognitive revolution since the 1960s.” The separate treatment of the three pillars by various authors indicates that the pillars have distinct emphases, which are sometimes treated as in opposition ( Verschure, 2016 ). However, we have highlighted the convergent aspects of the three that were critical to the founding of cognitive psychology and its continued development to the present. An example of a contemporary approach utilizing information theory and statistics in computational and cognitive neuroscience is the study of activity of neuronal populations to understand how the brain processes information. Quian Quiroga and Panzeri (2009) reviewed methods based on statistical decoding and information theory, and concluded, “Decoding and information theory describe complementary aspects of knowledge extraction… A more systematic joint application of both methodologies may offer additional insights” (p. 183).
Leahey (1992) claimed that it is incorrect to say that there was a “cognitive revolution” in the 1950s, but he acknowledged “that information-processing psychology has had world-wide influence…” (p. 315). Likewise, Mandler (2007) pointed out that the term “cognitive revolution” for the changes that occurred in the 1950s is a misnomer because, although behaviorism was dominant in the United States, much psychology outside of the United States prior to that time could be classified as “cognitive.” However, he also said, after reviewing the 1956 meetings and ones in 1958, that “the 1950s surely were ready for the emergence of the new information-processing psychology—the new cognitive psychology” (p. 187). Ironically, although both Leahey and Mandler identified the change as being one of information processing, neither author acknowledged the implication of their analyses, which is that there was a transformation that is more aptly labeled the information-processing revolution rather than the cognitive revolution. The concepts provided by the advances in cybernetics, information theory, and inferential statistical theory together provided the language and methodological tools that enabled a significant leap forward in theorizing.
Wootton (2015) , says of his book The Invention of Science: A New History of the Scientific Revolution , “We can state one of its core premises quite simply: a revolution in ideas requires a revolution in language” (p. 48). That language is what the concepts of communications systems engineering and inferential statistical theory provided for psychological research. Assessing the early influence of cybernetics and information theory on cognitive psychology, Broadbent (1959) stated, “It is in fact, the cybernetic approach above all others which has provided a clear language for discussing those various internal complexities which make the nervous system differ from a simple channel” (p. 113). He also identified a central feature of information theory as being crucial: The information conveyed by a stimulus is dependent on the stimuli that might have occurred but did not.
Posner (1986) , in his introduction to the Information Processing section of the Handbook of Perception and Human Performance , highlights more generally that the language of information processing affords many benefits to cognitive psychologists. He states, “Information processing language provides an alternative way of discussing internal mental operations intermediate between subjective experience and activity of neurons” (p. V-3). Later in the chapter, he elaborates:
- simple The view of the nervous system in terms of information flow provided a common language in which both conscious and unconscious events might be discussed. Computers could be programmed to simulate exciting tasks heretofore only performed by human beings without requiring any discussion of consciousness. By analogies with computing systems, one could deal with the format (code) in which information is presented to the senses and the computations required to change code (recodings) and for storage and overt responses. These concepts brought a new unity to areas of psychology and a way of translating between psychological and physiological processes. The presence of the new information processing metaphor reawakened interest in internal mental processes beyond that of simple sensory and motor events and brought cognition back to a position of centrality in psychology (p. V-7).
Posner also notes, “The information processing approach has a long and respected relationship with applications of experimental psychology to industrial and military settings” (V-7). The reason, as emphasized years earlier by Wiener, is that it allows descriptions of humans to be integrated with those of the nonhuman parts of the system. Again, from our perspective, there was a revolution, but it was specifically an information-processing revolution.
Our main points can be summarized as follows:
- simple (1) The information age originated in interdisciplinary research of an applied nature.
- simple (2) Cybernetics and information theory played pivotal roles, with the former being more fundamental than the latter through its emphasis on a systems approach.
- simple (3) These roles of communication systems theory were closely linked to developments in inferential statistical theory and applications.
- simple (4) The three pillars of cybernetics, information theory, and inferential statistical theory undergirded the so-called cognitive revolution in psychology, which is more appropriately called the information-processing revolution.
- simple (5) Those pillars, rooted in solving real-world problems, provided the language and methodological tools that enabled growth of the basic and applied fields of psychology.
- simple (6) The experimental design and inferential statistics adopted in cognitive psychology, with emphasis on rejecting null hypotheses, originated in the applied statistical analyses of the scientist Ronald Fisher and were influential because of their compatibility with scientific research conducted using controlled experiments.
Simon (1969) , in an article entitled “Designing Organizations for an Information-Rich World,” pointed out the problems created by the wealth of information:
- simple Now, when we speak of an information-rich world, we may expect, analogically, that the wealth of information means a dearth of something else – a scarcity of whatever it is that information consumes. What information consumes is rather obvious: it consumes the attention of its recipients. Hence a wealth of information creates a poverty of attention, and a need to allocate that attention efficiently among the overabundance of information sources that might consume it (manuscript pp. 6-7).
What Simon described is valid and even more evident in the current smart device and Internet era, where the amount of information is overwhelming. Phenomena as disparate as accidents caused by talking on a cellphone while driving ( Banducci et al., 2016 ) and difficulty assessing the credibility of information reported on the Internet or other media ( Chen et al., 2015 ) can be attributed to the overload. Moreover, the rapid rate at which information is encountered may have a negative impact on maintaining a prolonged focus of attention ( Microsoft Canada, 2015 ). Therefore, knowing how people process information and allocate attention is increasingly essential in the current explosion of information.
As noted, the predominant method of cognitive psychology in the information age has been that of drawing theoretical inferences from the statistical results of small-scale sets of data collected in controlled experimental settings (e.g., laboratory). The progress in psychology is tied to progress in statistics as well as technological developments that improve our ability to measure and analyze human behavior. Outside of the lab, with the continuing development of the Internet of Things (IoT), especially the implementation of AI, human physical lives are becoming increasingly interweaved into the cyber world. Ubiquitous records of human behavior, or “big data,” offer the potential to examine cognitive mechanisms at an escalated scale and level of ecological validity that cannot be achieved in the lab. This opportunity seems to require another significant transformation of cognitive psychology to use those data effectively to push forward understanding of the human mind and ensure seamless integration with cyber physical systems.
In a posthumous article, Brunswik (1956) noted that psychology should have the goal of broadening perception and learning by including interactions with a probabilistic environment. He insisted that psychology “must link behavior and environment statistically in bivariate or multivariate correlation rather than with the predominant emphasis on strict law…” (p. 158). As part of this proposal, Brunswik indicated a need to relate psychology more closely to disciplines that “use autocorrelation and intercorrelation, as theoretically stressed especially by Wiener (1949) , for probability prediction” (p. 160). With the ubiquitous data being collected within cyber physical systems, more extensive use of sophisticated correlational methods to extract the information embedded within the data will likely be necessary.
Using Stokes (1997) two dimensions of scientific research (considerations of use; quest for fundamental understanding), the work of pioneers of the Information Age, including Wiener, Shannon, and Fisher, falls within Pasteur’s Quadrant of use-inspired basic research. They were motivated by the need to solve immediate applied problems and through their research advanced human’s fundamental understanding of nature. Likewise, in seizing the opportunity to use big data to inform cognitive psychology, psychologists need to increase their involvement in interdisciplinary research targeted at real-world problems. In seeking to mine the information from big data, a new age is likely to emerge for cognitive psychology and related disciplines.
Although we reviewed the history of the information-processing revolution and subsequent developments in this paper, our ultimate concern is with the future of cognitive psychology. So, it is fitting to end as we began with a quote from Wiener (1951 , p. 68):
To respect the future, we must be aware of the past.
Author Contributions
AX and RP contributed jointly and equally to the paper.
Conflict of Interest Statement
The authors declare that the research was conducted in the absence of any commercial or financial relationships that could be construed as a potential conflict of interest.
- Aborn M., Rubenstein H. (1952). Information theory and immediate recall. J. Exp. Psychol. 44 260–266. 10.1037/h0061660 [ PubMed ] [ CrossRef ] [ Google Scholar ]
- Acree M. C. (1978). Theories of Statistical Inference in Psychological Research: A Historico-Critical Study. Doctoral dissertation, Clark University, Worcester, MS. [ Google Scholar ]
- Adams J. A. (1971). A closed-loop theory of motor learning. J. Mot. Behav. 3 111–150. 10.1080/00222895.1971.10734898 [ PubMed ] [ CrossRef ] [ Google Scholar ]
- Aldrich J. (2007). Information and economics in Fisher’s design of experiments. Int. Stat. Rev. 75 131–149. 10.1111/j.1751-5823.2007.00020.x [ CrossRef ] [ Google Scholar ]
- Ambler B. A., Fisicaro S. A., Proctor R. W. (1977). Information reduction, internal transformations, and task difficulty. Bull. Psychon. Soc. 10 463–466. 10.3758/BF03337698 [ CrossRef ] [ Google Scholar ]
- Ashby W. R. (1956). An Introduction to Cybernetics. London: Chapman & Hall; 10.5962/bhl.title.5851 [ CrossRef ] [ Google Scholar ]
- Ashby W. R. (1960). Design for a Brain: The Origin of Adaptive Behavior. New York, NY: Wiley & Sons; 10.1037/11592-000 [ CrossRef ] [ Google Scholar ]
- Atkinson R. C., Shiffrin R. M. (1968). “Human memory: a proposed system and its control,” in The Psychology of Learning and Motivation Vol. 2 eds Spence K. W., Spence J. T. (New York, NY: Academic Press; ), 89–195. [ Google Scholar ]
- Attneave F. (1959). Applications of Information theory to Psychology: A Summary of Basic Concepts, Methods, and Results. New York, NY: Henry Holt. [ Google Scholar ]
- Baddeley A. D., Hitch G. (1974). “Working memory,” in The Psychology of Learning and Motivation Vol. 8 ed. Bower G. A. (New York, NY: Academic press; ), 47–89. [ Google Scholar ]
- Banducci S. E., Ward N., Gaspar J. G., Schab K. R., Crowell J. A., Kaczmarski H., et al. (2016). The effects of cell phone and text message conversations on simulated street crossing. Hum. Factors 58 150–162. 10.1177/0018720815609501 [ PubMed ] [ CrossRef ] [ Google Scholar ]
- Basso D., Olivetti Belardinelli M. (2006). The role of the feedforward paradigm in cognitive psychology. Cogn. Process. 7 73–88. 10.1007/s10339-006-0034-1 [ PubMed ] [ CrossRef ] [ Google Scholar ]
- Beer S. (1959). Cybernetics and Management. New York, NY: John Wiley & Sons. [ Google Scholar ]
- Biology Online Dictionary (2018). Homeostasis. Available at: https://www.biology-online.org/dictionary/Homeostasis [ Google Scholar ]
- Birmingham H. P., Taylor F. V. (1954). A design philosophy for man-machine control systems. Proc. Inst. Radio Eng. 42 1748–1758. 10.1109/JRPROC.1954.274775 [ CrossRef ] [ Google Scholar ]
- Broadbent D. E. (1958). Perception and Communication. London: Pergamon Press; 10.1037/10037-000 [ CrossRef ] [ Google Scholar ]
- Broadbent D. E. (1959). Information theory and older approaches in psychology. Acta Psychol. 15 111–115. 10.1016/S0001-6918(59)80030-5 [ CrossRef ] [ Google Scholar ]
- Brosin H. W. (1953). “Information theory and clinical medicine (psychiatry),” in Current Trends in Information theory , ed. Patton R. A. (Pittsburgh, PA: University of Pittsburgh Press; ), 140–188. [ Google Scholar ]
- Brunswik E. (1956). Historical and thematic relations of psychology to other sciences. Sci. Mon. 83 151–161. [ Google Scholar ]
- Chapanis A., Schachter S. (1945). Depth Perception through a P-80 Canopy and through Distorted Glass. Memorandum Rep. TSEAL-69S-48N. Dayton, OH: Aero Medical Laboratory. [ Google Scholar ]
- Chen Y., Conroy N. J., Rubin V. L. (2015). News in an online world: the need for an “automatic crap detector”. Proc. Assoc. Inform. Sci. Technol. 52 1–4. 10.1002/pra2.2015.145052010081 [ CrossRef ] [ Google Scholar ]
- Cherry E. C. (1953). Some experiments on the recognition of speech, with one and with two ears. J. Acoust. Soc. Am. 25 975–979. 10.1121/1.1907229 [ CrossRef ] [ Google Scholar ]
- Cherry E. C. (1957). On Human Communication. New York, NY: John Wiley. [ Google Scholar ]
- Collins A. (2007). From H = log s n to conceptual framework: a short history of information. Hist. Psychol. 10 44–72. 10.1037/1093-4510.10.1.44 [ PubMed ] [ CrossRef ] [ Google Scholar ]
- Conway F., Siegelman J. (2005). Dark Hero of the Information Age. New York, NY: Basic Books. [ Google Scholar ]
- Craik K. J. (1947). Theory of the human operator in control systems. I. The operator as an engineering system. Br. J. Psychol. 38 56–61. [ PubMed ] [ Google Scholar ]
- Craik K. J. (1948). Theory of the human operator in control systems. II. Man as an element in a control system. Br. J. Psychol. 38 142–148. [ PubMed ] [ Google Scholar ]
- Cruse H. (2009). Neural Networks as Cybernetic Systems , 3rd Edn. Bielefeld: Brain, Minds, and Media. [ Google Scholar ]
- Dawkins R. (2010). Who is the Greatest Biologist Since Darwin? Why? Available at: https://www.edge.org/3rd_culture/leroi11/leroi11_index.html#dawkins [ Google Scholar ]
- Deutsch J. A., Deutsch D. (1963). Attention: some theoretical considerations. Psychol. Rev. 70 80–90. 10.1037/h0039515 [ PubMed ] [ CrossRef ] [ Google Scholar ]
- Dignath D., Pfister R., Eder A. B., Kiesel A., Kunde W. (2014). Representing the hyphen in action–effect associations: automatic acquisition and bidirectional retrieval of action–effect intervals. J. Exp. Psychol. Learn. Mem. Cogn. 40 1701–1712. 10.1037/xlm0000022 [ PubMed ] [ CrossRef ] [ Google Scholar ]
- Donders F. C. (1868/1969). “On the speed of mental processes,” in Attention and Performance II , ed. Koster W. G. (Amsterdam: North Holland Publishing Company; ), 412–431. [ Google Scholar ]
- Edwards P. N. (1997). The Closed World: Computers and the Politics of Discourse in Cold War America. Cambridge, MA: MIT Press. [ Google Scholar ]
- Efron B. (1998). R. A. Fisher in the 21st century: invited paper presented at the 1996 R. A. Fisher lecture. Stat. Sci. 13 95–122. [ Google Scholar ]
- Elias P. (1994). “The rise and fall of cybernetics in the US and USSR,” in The Legacy of Norbert Wiener: A Centennial Symposium , eds D. Jerison I, Singer M., Stroock D. W. (Providence, RI: American Mathematical Society; ), 21–30. [ Google Scholar ]
- Ernst G. W., Newell A. (1969). GPS: A Case Study in Generality and Problem Solving. New York, NY: Academic Press. [ Google Scholar ]
- Fan J. (2014). An information theory account of cognitive control. Front. Hum. Neurosci. 8 : 680 . 10.3389/fnhum.2014.00680 [ PMC free article ] [ PubMed ] [ CrossRef ] [ Google Scholar ]
- Fano R. M. (1950). The information theory point of view in speech communication. J. Acoust. Soc. Am. 22 691–696. 10.1121/1.1906671 [ CrossRef ] [ Google Scholar ]
- Fisher R. A. (1925). Statistical Methods for Research Workers. London: Oliver & Boyd. [ Google Scholar ]
- Fisher R. A. (1935). The Design of Experiments. London: Oliver & Boyd. [ Google Scholar ]
- Fisher R. A. (1937). The Design of Experiments , 2nd Edn. London: Oliver & Boyd. [ Google Scholar ]
- Fisher R. A. (1947). “Development of the theory of experimental design,” in Proceedings of the International Statistical Conferences Vol. 3 Poznań, 434–439. [ Google Scholar ]
- Fisher R. A. (1951). “Statistics,” in Scientific thought in the Twentieth century , ed. Heath A. E. (London: Watts; ). [ Google Scholar ]
- Fisher R. A. (1956). Statistical Methods and Scientific Inference. Edinburgh: Oliver & Boyd. [ Google Scholar ]
- Fisher R. A. (ed.). (1962). “The place of the design of experiments in the logic of scientific inference,” in Fisher: Collected Papers Relating to Statistical and Mathematical theory and Applications Vol. 110 (Paris: Centre National de la Recherche Scientifique), 528–532. [ Google Scholar ]
- Fitts P. M. (1954). The information capacity of the human motor system in controlling the amplitude of movement. J. Exp. Psychol. 47 381–391. 10.1037/h0055392 [ PubMed ] [ CrossRef ] [ Google Scholar ]
- Fitts P. M., Seeger C. M. (1953). S-R compatibility: spatial characteristics of stimulus and response codes. J. Exp. Psychol. 46 199–210. 10.1037/h0062827 [ PubMed ] [ CrossRef ] [ Google Scholar ]
- Flach J. M., Bennett K. B., Woods D. D., Jagacinski R. J. (2015). “Interface design: a control theoretic context for a triadic meaning processing approach,” in The Cambridge Handbook of Applied Perception Research , Vol. II , eds Hoffman R. R., Hancock P. A., Scerbo M. W., Parasuraman R., Szalma J. L. (New York, NY: Cambridge University Press; ), 647–668. [ Google Scholar ]
- Friston K. (2012). The history of the future of the Bayesian brain. Neuroimage 62 1230–1233. 10.1016/j.neuroimage.2011.10.004 [ PMC free article ] [ PubMed ] [ CrossRef ] [ Google Scholar ]
- Galison P. (1994). The ontology of the enemy: norbert Wiener and the cybernetic vision. Crit. Inq. 21 228–266. 10.1086/448747 [ CrossRef ] [ Google Scholar ]
- Gardner H. E. (1985). The Mind’s New Science: A History of the Cognitive Revolution. New York, NY: Basic Books. [ Google Scholar ]
- Garner W. R., Hake H. W. (1951). The amount of information in absolute judgments. Psychol. Rev. 58 446–459. 10.1037/h0054482 [ PubMed ] [ CrossRef ] [ Google Scholar ]
- Gentner D., Grudin J. (1985). The evolution of mental metaphors in psychology: a 90-year retrospective. Am. Psychol. 40 181–192. 10.1037/0003-066X.40.2.181 [ CrossRef ] [ Google Scholar ]
- Gigerenzer G. (1991). From tools to theories: a heuristic of discovery in Cognitive Psychology. Psychol. Rev. 98 254–267. 10.1037/0033-295X.98.2.254 [ CrossRef ] [ Google Scholar ]
- Gigerenzer G., Hoffrage U. (1995). How to improve Bayesian reasoning without instruction: frequency formats. Psychol. Rev. 102 684–704. 10.1037/0033-295X.102.4.684 [ CrossRef ] [ Google Scholar ]
- Gigerenzer G., Murray D. J. (1987). Cognition as Intuitive Statistics. Mahwah, NJ: Lawrence Erlbaum. [ Google Scholar ]
- Glazebrook C. M., Kiernan D., Welsh T. N., Tremblay L. (2015). How one breaks Fitts’s Law and gets away with it: moving further and faster involves more efficient online control. Hum. Mov. Sci. 39 163–176. 10.1016/j.humov.2014.11.005 [ PubMed ] [ CrossRef ] [ Google Scholar ]
- Greenwald A. G. (1970). Sensory feedback mechanisms in performance control: with special reference to the ideo-motor mechanism. Psychol. Rev. 77 73–99. 10.1037/h0028689 [ PubMed ] [ CrossRef ] [ Google Scholar ]
- Gregory R. L. (1968). Perceptual illusions and brain models. Proc. R. Soc. Lond. B Biol. Sci. 171 279–296. 10.1098/rspb.1968.0071 [ PubMed ] [ CrossRef ] [ Google Scholar ]
- Gregory R. L. (1980). Perceptions as hypotheses. Philos. Trans. R. Soc. Lond. B 290 181–197. 10.1098/rstb.1980.0090 [ PubMed ] [ CrossRef ] [ Google Scholar ]
- Hald A. (2008). A History of Parametric Statistical Inference from Bernoulli to Fisher. Copenhagen: Springer Science & Business Media, 1713–1935. [ Google Scholar ]
- Heims S. J. (1991). The Cybernetics Group. Cambridge, MA: Massachusetts Institute of Technology. [ Google Scholar ]
- Helmholtz H. (1866/1925). Handbuch der Physiologischen Optik [Treatise on Physiological Optics] Vol. 3 ed. Southall J. (Rochester, NY: Optical Society of America; ). [ Google Scholar ]
- Herbort O., Butz M. V., Hoffmann J. (2005). “Towards an adaptive hierarchical anticipatory behavioral control system,” in From Reactive to Anticipatory Cognitive Embodied Systems: Papers from the AAAI Fall Symposium , eds Castelfranchi C., Balkenius C. Butz M. V. Ortony A. (Menlo Park, CA: AAAI Press; ), 83–90. [ Google Scholar ]
- Hick W. E. (1951). Information theory and intelligence tests. Br. J. Math. Stat. Psychol. 4 157–164. 10.1111/j.2044-8317.1951.tb00317.x [ CrossRef ] [ Google Scholar ]
- Hick W. E. (1952). On the rate of gain of information. Q. J. Exp. Psychol. 4 11–26. 10.1080/17470215208416600 [ CrossRef ] [ Google Scholar ]
- Hommel B., Müsseler J., Aschersleben G., Prinz W. (2001). The theory of event coding (TEC): a framework for perception and action planning. Behav. Brain Sci. 24 849–878. 10.1017/S0140525X01000103 [ PubMed ] [ CrossRef ] [ Google Scholar ]
- Hulbert A. (2018). Prodigies’ Progress: Parents and Superkids, then and Now. Cambridge, MA: Harvard Magazine, 46–51. [ Google Scholar ]
- Hyman R. (1953). Stimulus information as a determinant of reaction time. J. Exp. Psychol. 53 188–196. 10.1037/h0056940 [ PubMed ] [ CrossRef ] [ Google Scholar ]
- Internet Hall of Fame (2016). Internet Hall of Fame Pioneer J.C.R. Licklider: Posthumous Recipient. Available at: https://www.internethalloffame.org/inductees/jcr-licklider [ Google Scholar ]
- Jagacinski R. J., Flach J. M. (2003). Control theory for Humans: Quantitative Approaches to Modeling Performance. Mahwah, NJ: Lawrence Erlbaum. [ Google Scholar ]
- James W. (1890). The Principles of Psychology. New York, NY: Dover. [ Google Scholar ]
- Kahneman D. (1973). Attention and Effort. Englewood Cliffs, NJ: Prentice Hall. [ Google Scholar ]
- Kay H. (1957). Information theory in the understanding of skills. Occup. Psychol. 31 218–224. [ Google Scholar ]
- Kellen D., Klauer K. C., Singmann H. (2012). On the measurement of criterion noise in signal detection theory: the case of recognition memory. Psychol. Rev. 119 457–479. 10.1037/a0027727 [ PubMed ] [ CrossRef ] [ Google Scholar ]
- Kline R. R. (2011). Cybernetics, automata studies, and the Dartmouth conference on artificial intelligence. IEEE Ann. His. Comput. 33 5–16. 10.1109/MAHC.2010.44 [ CrossRef ] [ Google Scholar ]
- Kline R. R. (2015). The Cybernetics Moment: Or why We Call our Age the Information Age. Baltimore, MD: John Hopkins University Press. [ Google Scholar ]
- Lachman R., Lachman J. L., Butterfield E. C. (1979). Cognitive Psychology and Information Processing: An Introduction. Hillsdale, NJ: Lawrence Erlbaum. [ Google Scholar ]
- Laird J., Newell A., Rosenbloom P. (1987). SOAR: an architecture for general intelligence. Artif. Intell. 33 1–64. 10.1016/0004-3702(87)90050-6 [ CrossRef ] [ Google Scholar ]
- Leahey T. H. (1992). The mythical revolutions of American psychology. Am. Psychol. 47 308–318. 10.1037/0003-066X.47.2.308 [ CrossRef ] [ Google Scholar ]
- Lehmann E. L. (1993). The Fisher, Neyman-Pearson theories of testing hypotheses: one theory or two? J. Am. Stat. Assoc. 88 1242–1249. 10.1080/01621459.1993.10476404 [ CrossRef ] [ Google Scholar ]
- Lehmann E. L. (2011). Fisher, Neyman, and the Creation of Classical Statistics. New York, NY: Springer Science & Business Media; 10.1007/978-1-4419-9500-1 [ CrossRef ] [ Google Scholar ]
- Licklider J. R. (1950). The intelligibility of amplitude-dichotomized, time-quantized speech waves. J. Acoust. Soc. Am. 22 820–823. 10.1121/1.1906695 [ CrossRef ] [ Google Scholar ]
- Luce R. D. (2003). Whatever happened to information theory in psychology? Rev. Gen. Psychol. 7 183–188. 10.1037/1089-2680.7.2.183 [ CrossRef ] [ Google Scholar ]
- Ly A., Marsman M., Verhagen J., Grasman R. P., Wagenmakers E. J. (2017). A tutorial on Fisher information. J. Math. Psychol. 80 40–55. 10.1016/j.jmp.2017.05.006 [ PMC free article ] [ PubMed ] [ CrossRef ] [ Google Scholar ]
- MacKay D. M. (1956). Towards an information-flow model of human behaviour. Br. J. Psychol. 47 30–43. 10.1111/j.2044-8295.1956.tb00559.x [ PubMed ] [ CrossRef ] [ Google Scholar ]
- Mandler G. (2007). A History of Modern Experimental Psychology: From James and Wundt to Cognitive Science. Cambridge, MA: MIT Press. [ Google Scholar ]
- Maxwell S. E., Lau M. Y., Howard G. S. (2015). Is psychology suffering from a replication crisis? What does “failure to replicate” really mean? Am. Psychol. 70 487–498. 10.1037/a0039400 [ PubMed ] [ CrossRef ] [ Google Scholar ]
- McCarthy J., Minsky M. L., Rochester N., Shannon C. E. (2006). A proposal for the Dartmouth summer research project on artificial intelligence, August 31 1955. AI Mag. 27 12–14. [ Google Scholar ]
- McCulloch W., Pitts W. (1943). A logical calculus of ideas immanent in nervous activity. Bull. Math. Biophys. 5 115–133. 10.1007/BF02478259 [ PubMed ] [ CrossRef ] [ Google Scholar ]
- Meyer L. B. (1957). Meaning in music and information theory. J. Aesthet. Art Crit. 15 412–424. 10.1016/j.plrev.2013.05.008 [ PubMed ] [ CrossRef ] [ Google Scholar ]
- Microsoft Canada (2015). Attention Spans Research Report. Available at: https://www.scribd.com/document/317442018/microsoft-attention-spans-research-report-pdf [ Google Scholar ]
- Miller G. A. (1953). What is information measurement? Am. Psychol. 8 3–11. 10.1037/h0057808 [ CrossRef ] [ Google Scholar ]
- Miller G. A. (1956). The magical number seven, plus or minus two: some limits on our capacity for processing information. Psychol. Rev. 63 81–97. 10.1037/h0043158 [ PubMed ] [ CrossRef ] [ Google Scholar ]
- Miller G. A. (2003). The cognitive revolution: a historical perspective. Trends Cogn. Sci. 7 141–144. 10.1016/S1364-6613(03)00029-9 [ PubMed ] [ CrossRef ] [ Google Scholar ]
- Miller G. A., Galanter E., Pribram K. H. (1960). Plans and the Structure of Behavior. New York, NY: Holt; 10.1037/10039-000 [ CrossRef ] [ Google Scholar ]
- Minsky M. (ed.). (1968). Semantic Information Processing. Cambridge, MA: The MIT Press. [ Google Scholar ]
- Montagnini L. (2017a). Harmonies of Disorder: Norbert Wiener: A Mathematician-Philosopher of our Time. Roma: Springer. [ PubMed ] [ Google Scholar ]
- Montagnini L. (2017b). Interdisciplinarity in Norbert Wiener, a mathematician-philosopher of our time. Biophys. Chem. 229 173–180. 10.1016/j.bpc.2017.06.009 [ PubMed ] [ CrossRef ] [ Google Scholar ]
- Moor J. (2006). The Dartmouth College artificial intelligence conference: the next fifty years. AI Mag. 27 87–91. [ Google Scholar ]
- Moray N. (1959). Attention in dichotic listening: affective cues and the influence of instructions. Q. J. Exp. Psychol. 11 56–60. 10.1080/17470215908416289 [ CrossRef ] [ Google Scholar ]
- Nahin P. J. (2013). The Logician and the Engineer: How George Boole and Claude Shannon Created the Information Age. Princeton, NJ: Princeton University Press. [ Google Scholar ]
- Neisser U. (1967). Cognitive Psychology. New York, NY: Apple Century Crofts. [ Google Scholar ]
- Nelson N., Rosenthal R., Rosnow R. L. (1986). Interpretation of significance levels and effect sizes by psychological researchers. Am. Psychol. 41 1299–1301. 10.1037/0003-066X.41.11.1299 [ CrossRef ] [ Google Scholar ]
- Newell A. (1955). “The chess machine: an example of dealing with a complex task by adaptation,” in Proceedings of the March 1-3 1955 Western Joint Computer Conference , (New York, NY: ACM; ), 101–108. 10.1145/1455292.1455312 [ CrossRef ] [ Google Scholar ]
- Newell A., Simon H. A. (1972). Human Problem Solving. Englewood Cliffs, NJ: Prentice-Hall. [ Google Scholar ]
- Neyman J. (1977). Frequentist probability and frequentist statistics. Synthese 36 97–131. 10.1007/BF00485695 [ CrossRef ] [ Google Scholar ]
- Neyman J., Pearson E. S. (1928). On the use and interpretation of certain test criteria for purposes of statistical inference: Part I. Biometrika 20A , 175–240. [ Google Scholar ]
- Neyman J., Pearson E. S. (1933). IX. On the problem of the most efficient tests of statistical hypotheses. Philos. Trans. R. Soc. Lond. A 231 289–337. 10.1098/rsta.1933.0009 [ CrossRef ] [ Google Scholar ]
- O’Regan G. (2012). A Brief History of Computing , 2nd Edn. London: Springer; 10.1007/978-1-4471-2359-0 [ CrossRef ] [ Google Scholar ]
- Parolini G. (2015). The emergence of modern statistics in agricultural science: analysis of variance, experimental design and the reshaping of research at Rothamsted Experimental Station, 1919–1933. J. His. Biol. 48 301–335. 10.1007/s10739-014-9394-z [ PubMed ] [ CrossRef ] [ Google Scholar ]
- Peterson W. W. T. G., Birdsall T., Fox W. (1954). The theory of signal detectability. Trans. IRE Prof. Group Inform. Theory 4 171–212. 10.1109/TIT.1954.1057460 [ CrossRef ] [ Google Scholar ]
- Pezzulo G., Cisek P. (2016). Navigating the affordance landscape: feedback control as a process model of behavior and cognition. Trends Cogn. Sci. 20 414–424. 10.1016/j.tics.2016.03.013 [ PubMed ] [ CrossRef ] [ Google Scholar ]
- Piccinini G. (2004). The First computational theory of mind and brain: a close look at McCulloch and Pitts’s “Logical calculus of ideas immanent in nervous activity”. Synthese 141 175–215. 10.1023/B:SYNT.0000043018.52445.3e [ CrossRef ] [ Google Scholar ]
- Posner M. I. (1978). Chronometric Explorations of Mind. Hillsdale, NJ: Lawrence Erlbaum. [ Google Scholar ]
- Posner M. I. (1986). “Overview,” in Handbook of Perception and Human Performance: Cognitive Processes and Performance Vol. 2 eds Boff K. R., Kaufman L. I., Thomas J. P. (New York, NY: John Wiley; ), V.1—-V.10 . [ Google Scholar ]
- Pribram K. H. (1976). “Problems concerning the structure of consciousness,” in Consciousness and the Brain: A Scientific and Philosophical Inquiry , eds Globus G. G., Maxwell G., Savodnik I. (New York, NY: Plenum; ), 297–313. [ Google Scholar ]
- Proctor R. W., Schneider D. W. (2018). Hick’s law for choice reaction time: a review. Q. J. Exp. Psychol. 71 1281–1299. 10.1080/17470218.2017.1322622 [ PubMed ] [ CrossRef ] [ Google Scholar ]
- Proctor R. W., Vu K. P. L. (2006). Stimulus-Response Compatibility Principles: Data, theory, and Application. Boca Raton, FL: CRC Press. [ Google Scholar ]
- Proctor R. W., Vu K. P. L. (2016). Principles for designing interfaces compatible with human information processing. Int. J. Hum. Comput. Interact. 32 2–22. 10.1080/10447318.2016.1105009 [ CrossRef ] [ Google Scholar ]
- Quastler H. (ed.). (1953). Information theory in Biology. Urbana, IL: University of Illinois Press. [ Google Scholar ]
- Quian Quiroga R., Panzeri E. (2009). Extracting information from neuronal populations: information theory and decoding approaches. Nat. Rev. Neurosci. 10 173–185. 10.1038/nrn2578 [ PubMed ] [ CrossRef ] [ Google Scholar ]
- Rao R. P. (2004). Bayesian computation in recurrent neural circuits. Neural Comput. 16 1–38. 10.1162/08997660460733976 [ PubMed ] [ CrossRef ] [ Google Scholar ]
- Rao R. P., Ballard D. H. (1997). Dynamic model of visual recognition predicts neural response properties in the visual cortex. Neural Comput. 9 721–763. 10.1162/neco.1997.9.4.721 [ PubMed ] [ CrossRef ] [ Google Scholar ]
- Ratcliff R. (1978). A theory of memory retrieval. Psychol. Rev. 85 59–108. 10.1037/0033-295X.85.2.59 [ CrossRef ] [ Google Scholar ]
- Ratcliff R., Smith P. L. (2004). A comparison of sequential sampling models for two-choice reaction time. Psychol. Rev. 111 333–367. 10.1037/0033-295X.111.2.333 [ PMC free article ] [ PubMed ] [ CrossRef ] [ Google Scholar ]
- Rosenthal R., Rubin D. B. (1982). A simple, general purpose display of magnitude of experimental effect. J. Educ. Psychol. 74 166–169. 10.1037/0022-0663.74.2.166 [ CrossRef ] [ Google Scholar ]
- Rucci A. J., Tweney R. D. (1980). Analysis of variance and the “second discipline” of scientific psychology: a historical account. Psychol. Bull. 87 166–184. 10.1037/0033-2909.87.1.166 [ CrossRef ] [ Google Scholar ]
- Russell E. J. (1966). A History of Agricultural Science in Great Britain. London: George Allen and Unwin, 1620–1954. [ Google Scholar ]
- Schachter S., Chapanis A. (1945). Distortion in Glass and Its Effect on Depth Perception. Memorandum Report No. TSEAL-695-48B. Dayton, OH: Aero Medical Laboratory. [ Google Scholar ]
- Schmidt R. A. (1975). A schema theory of discrete motor skill learning. Psychol. Rev. 82 225–260. 10.1037/h0076770 [ CrossRef ] [ Google Scholar ]
- Scott S. H. (2016). A functional taxonomy of bottom-up sensory feedback processing for motor actions. Trends Neurosci. 39 512–526. 10.1016/j.tins.2016.06.001 [ PubMed ] [ CrossRef ] [ Google Scholar ]
- Seidenfeld T. (1992). “R. A. Fisher on the design of experiments and statistical estimation,” in The Founders of Evolutionary Genetics , ed. Sarkar S. (Dordrecht: Springer; ), 23–36. [ Google Scholar ]
- Selfridge O. G. (1959). “Pandemonium: a paradigm for learning,” in Proceedings of the Symposium on Mechanisation of thought Processes , (London: Her Majesty’s Stationery Office; ), 511–529. [ Google Scholar ]
- Seth A. K. (2015). “The cybernetic Bayesian brain - From interoceptive inference to sensorimotor contingencies,” in Open MIND: 35(T) , eds Metzinger T., Windt J. M. (Frankfurt: MIND Group; ). [ Google Scholar ]
- Shalizi C. (2007). Advanced Probability II or Almost None of the theory of Stochastic Processes. Available at: http://www.stat.cmu.edu/cshalizi/754/notes/all.pdf [ Google Scholar ]
- Shannon C. E. (1945). A Mathematical theory of Cryptography. Technical Report Memoranda 45-110-02 . Murray Hill, NJ: Bell; Labs. [ Google Scholar ]
- Shannon C. E. (1948a). A mathematical theory of communication. Bell Syst. Tech. J. 27 379–423. 10.1002/j.1538-7305.1948.tb01338.x [ CrossRef ] [ Google Scholar ]
- Shannon C. E. (1948b). Letter to Norbert Wiener, October 13. In box 5-85 Norbert Wiener Papers. Cambridge, MA: MIT Archives. [ Google Scholar ]
- Shannon C. E., Weaver W. (1949). The Mathematical theory of Communication. Urbana, IL: University of Illinois Press. [ Google Scholar ]
- Shiffman D. (2012). The Nature of Code. Available at: http://natureofcode.com/book/ [ Google Scholar ]
- Shiffrin R. M., Schneider W. (1977). Controlled and automatic human information processing: II. Perceptual learning, automatic attending and a general theory. Psychol. Rev. 84 127–190. 10.1037/0033-295X.84.2.127 [ CrossRef ] [ Google Scholar ]
- Simon H. A. (1969). Designing organizations for an information-rich world. Int. Libr. Crit. Writ. Econ. 70 187–202. [ Google Scholar ]
- Simon H. A. (1997). Allen Newell (1927-1992). Biographical Memoir. Washington DC: National Academics Press. [ Google Scholar ]
- Srivastava V., Feng S. F., Cohen J. D., Leonard N. E., Shenhav A. (2017). A martingale analysis of first passage times of time-dependent Wiener diffusion models. J. Math. Psychol. 77 94–110. 10.1016/j.jmp.2016.10.001 [ PMC free article ] [ PubMed ] [ CrossRef ] [ Google Scholar ]
- Sternberg S. (1969). The discovery of processing stages: extensions of Donders’ method. Acta Psychol. 30 276–315. 10.1016/0001-6918(69)90055-9 [ CrossRef ] [ Google Scholar ]
- Stokes D. E. (1997). Pasteur’s Quadrant – Basic Science and Technological Innovation. Washington DC: Brookings Institution Press. [ Google Scholar ]
- Student’s (1908). The probable error of a mean. Biometrika 6 1–25. [ Google Scholar ]
- Swets J. A., Tanner W. P., Jr., Birdsall T. G. (1961). Decision processes in perception. Psychol. Rev. 68 301–340. 10.1037/h0040547 [ PubMed ] [ CrossRef ] [ Google Scholar ]
- Taylor F. V. (1949). Review of Cybernetics (or control and communication in the animal and the machine). Psychol. Bull. 46 236–237. 10.1037/h0051026 [ CrossRef ] [ Google Scholar ]
- Treisman A. M. (1960). Contextual cues in selective listening. Q. J. Exp. Psychol. 12 242–248. 10.1080/17470216008416732 [ CrossRef ] [ Google Scholar ]
- Treisman A. M., Gelade G. (1980). A feature-integration theory of attention. Cogn. Psychol. 12 97–136. 10.1016/0010-0285(80)90005-5 [ PubMed ] [ CrossRef ] [ Google Scholar ]
- Tulving E., Thomson D. M. (1973). Encoding specificity and retrieval processes in episodic memory. Psychol. Rev. 80 352–373. 10.1037/h0020071 [ CrossRef ] [ Google Scholar ]
- Turing A. M. (1937). On computable numbers, with an application to the Entscheidungsproblem. Proc. Lond. Math. Soc. 2 230–265. 10.1112/plms/s2-42.1.230 [ CrossRef ] [ Google Scholar ]
- Van Meter D., Middleton D. (1954). Modern statistical approaches to reception in communication theory. Trans. IRE Prof. Group Inform. Theory 4 119–145. 10.1109/TIT.1954.1057471 [ CrossRef ] [ Google Scholar ]
- Velasco M. A., Clemotte A., Raya R., Ceres R., Rocon E. (2017). Human-computer interaction for users with cerebral palsy based on head orientation. Can cursor’s movement be modeled by Fitts’s law? Int. J. Hum. Comput. Stud. 106 1–9. 10.1016/j.ijhcs.2017.05.002 [ CrossRef ] [ Google Scholar ]
- Verschure P. F. M. J. (2016). “Consciousness in action: the unconscious parallel present optimized by the conscious sequential projected future,” in The Pragmatic Turn: Toward Action-Oriented Views in Cognitive Science , eds Engel A. K., Friston K. J., Kragic D. (Cambridge, MA: MIT Press; ). [ Google Scholar ]
- Wald A. (1950). Statistical Decision Functions. New York, NY: John Wiley. [ Google Scholar ]
- Waterson P. (2011). World War II and other historical influences on the formation of the Ergonomics Research Society. Ergonomics 54 1111–1129. 10.1080/00140139.2011.622796 [ PubMed ] [ CrossRef ] [ Google Scholar ]
- Wiener N. (1921). The average of an analytic functional and the Brownian movement. Proc. Natl. Acad. Sci. U.S.A. 7 294–298. 10.1073/pnas.7.10.294 [ PMC free article ] [ PubMed ] [ CrossRef ] [ Google Scholar ]
- Wiener N. (1948a). Cybernetics. Sci. Am. 179 14–19. 10.1038/scientificamerican1148-14 [ PubMed ] [ CrossRef ] [ Google Scholar ]
- Wiener N. (1948b). Cybernetics or Control and Communication in the Animal and the Machine. New York, NY: John Wiley. [ Google Scholar ]
- Wiener N. (1949). Extrapolation, Interpolation, and Smoothing of Stationary Time Series, with Engineering Applications. Cambridge, MA: Technology Press of the Massachusetts Institute of Technology. [ Google Scholar ]
- Wiener N. (1951). Homeostasis in the individual and society. J. Franklin Inst. 251 65–68. 10.1016/0016-0032(51)90897-6 [ CrossRef ] [ Google Scholar ]
- Wiener N. (1952). Cybernetics or Control and Communication in the Animal and the Machine. Cambridge, MA: The MIT Press. [ Google Scholar ]
- Wiener N. (1961). Cybernetics or Control and Communication in the Animal and the Machine , 2nd Edn. Cambridge MA: MIT Press; 10.1037/13140-000 [ CrossRef ] [ Google Scholar ]
- Wixted J. T. (2014). Signal Detection theory. Hoboken, NJ: Wiley; 10.1002/9781118445112.stat06743 [ CrossRef ] [ Google Scholar ]
- Wootton D. (2015). The Invention of Science: A new History of the Scientific Revolution. New York, NY: Harper. [ Google Scholar ]
- Yates F. (1964). Sir Ronald Fisher and the design of experiments. Biometrics 20 307–321. 10.1080/03639045.2017.1291672 [ PubMed ] [ CrossRef ] [ Google Scholar ]
- Yates F., Mather K. (1963). Ronald Aylmer fisher. Biogr. Mem. Fellows R. Soc. Lond. 9 91–120. 10.1098/rsbm.1963.0006 [ CrossRef ] [ Google Scholar ]
Theory and Implications of Information Processing
- First Online: 22 October 2020
Cite this chapter
- G. Sucharitha 2 ,
- Anjanna Matta 2 ,
- Kanagala Dwarakamai 2 &
- Bodepu Tannmayee 2
751 Accesses
2 Citations
- The original version of this chapter was revised. The correction to this chapter is available at https://doi.org/10.1007/978-3-030-48849-9_14
This chapter talks about the theories connected with information processing and memory. It comprises the descriptions and definitions of important stipulations and models that have been used to represent memory types and workstations (PC, processors). Information processing and communications are investigated in various disciplines: technology, psychology, mathematics, sociology, social science, and plenty of others. Nowadays, human learning has become one of the research interests. This is due to how one acquires new information and retrieve the information, collection of long-term and short-term learning goals, and methods of useful instruction. Therefore, the study of cognition as a psychological area reaches its height, just not in the captivating and recovering information. It has become a wide area of research devoted to the study of mind holistically. Many significant researchers in cognition defined that it is the study of how individuals encode, organize, store, recover, employ, or else learn knowledge. Cognitive psychologists imagine a dominant variable or set of variables between environment and behavior, which shows the difference in behavioral theories.
This is a preview of subscription content, log in via an institution to check access.
Access this chapter
- Available as PDF
- Read on any device
- Instant download
- Own it forever
- Available as EPUB and PDF
- Compact, lightweight edition
- Dispatched in 3 to 5 business days
- Free shipping worldwide - see info
- Durable hardcover edition
Tax calculation will be finalised at checkout
Purchases are for personal use only
Institutional subscriptions
Change history
01 december 2020.
This book was inadvertently published without updating the following error.
Abbot, B. (2002). Human memory . Fort Wayne, IN: Indiana University-Purdue University at Fort Wayne, Psychology Department. Retrieved June 22, 2002.
Google Scholar
Anderson, M. (1998). Jerome Bruner. “Educational psychology” . Portland, OR: Cortland College. Retrieved June 12, 2002.
Atkinson, R., & Shiffrin, R. (1968). Human memory. A proposed system and its control processes. In K. W. Spence & J. T. Spence (Eds.), The psychology of learning and motivation (Vol. 2, pp. 89–195). New York, NY: Academic Press.
Clark, J. M., & Paivio, A. (1991). Dual coding theory and education. Educational Psychology Review, 3 (3), 149–170.
Article Google Scholar
Craik, F. I. M., & Lockhart, R. S. (1972). Levels of processing: A framework for memory research 1. Journal of Verbal Learning and Verbal Behavior, 11 , 671–684.
Driscoll, M. (2001). Psychology of learning for assessment (2nd ed.). Boston, MA: Allyn & Bacon.
Eliasmith, C. (Ed.). (2001). Memory. Dictionary of philosophy of mind . Pullman, WA: Washington State University. Retrieved June 19, 2002.
Huitt, W. (2000). The information processing approach. Educational psychology interactive . Valdosta, GA: Valdosta State University. Retrieved June 12, 2002.
Kearsley, G. (2001a). Dual coding theory. In A. Paivio (Ed.), Theory into practice . Jacksonville, FL: Jacksonville State University. Retrieved June 14, 2002.
Kearsley, G. (2001b). Levels of processing. Theory into practice . Jacksonville, FL: Jacksonville State University. Retrieved June 14, 2002.
McClelland, J., & Rumelhart, D. (1981). An interactive activation model of context effects in letter perception. Part I: An account of basic findings. Psychological Review, 88 , 375–407.
Mohanty, S. N., & Suar, D. (2013a). Decision-making in positive and negative prospects: Influence of certainty and affectivity. International Journal of Advances in Psychology , 2 (1),19–28.
Mohanty, S. N. & Suar, D. (2013b). Influence of mood states, group discussion, and interpersonal comparison on change in decision-making and information processing. International Journal of Decision Science, Risk and Management, 5(2) ,101–123.
O’reilly, T. (2005). Web 2.0: compact definition, 137–158.
Porter, A. L., & Read W. H., eds. (1998). The information revolution: Current and future consequences. Greenwood Publishing Group.
Rumelhart, D. (1980). Schemata: The building blocks of cognition . Hillsdale, NJ: Erlbaum.
Rumelhart, D. E., & McClelland J. L. (1986). On learning the past tenses of English verbs, 216–271.
Suthers, D. (1996). Attention and automaticity . Pittsburgh, PA: University of Pittsburg, Learning Research and Development Center. Retrieved June 21, 2002.
Wiener, N. (1948). Cybernetics or control and communication in the animal and the machine. Technology Press.
Winn, W., & Snyder, D. (2001). Mental representation. The handbook of research for educational communications and technology (Chap. 5) . Bloomington, IN: The Association of Educational Communications and Technology. Retrieved June 12, 2002.
Download references
Author information
Authors and affiliations.
Faculty of Science and Technology, ICFAI Foundation for Higher Education, Hyderabad, India
G. Sucharitha, Anjanna Matta, Kanagala Dwarakamai & Bodepu Tannmayee
You can also search for this author in PubMed Google Scholar
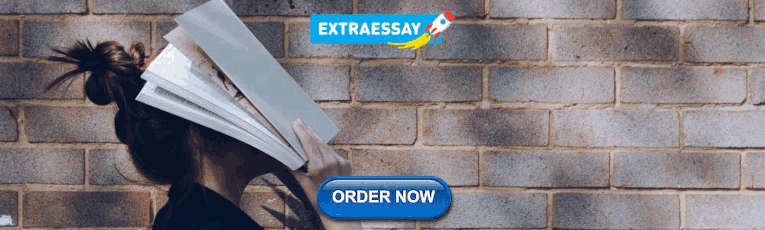
Corresponding author
Correspondence to G. Sucharitha .
Editor information
Editors and affiliations.
Department of Computer Science & Engineering, ICFAI Foundation for Higher Education, Hyderabad, India
Sachi Nandan Mohanty
Rights and permissions
Reprints and permissions
Copyright information
© 2020 The Editor(s) (if applicable) and The Author(s), under exclusive license to Springer Nature Switzerland AG
About this chapter
Sucharitha, G., Matta, A., Dwarakamai, K., Tannmayee, B. (2020). Theory and Implications of Information Processing. In: Mohanty, S.N. (eds) Emotion and Information Processing. Springer, Cham. https://doi.org/10.1007/978-3-030-48849-9_4
Download citation
DOI : https://doi.org/10.1007/978-3-030-48849-9_4
Published : 22 October 2020
Publisher Name : Springer, Cham
Print ISBN : 978-3-030-48848-2
Online ISBN : 978-3-030-48849-9
eBook Packages : Behavioral Science and Psychology Behavioral Science and Psychology (R0)
Share this chapter
Anyone you share the following link with will be able to read this content:
Sorry, a shareable link is not currently available for this article.
Provided by the Springer Nature SharedIt content-sharing initiative
- Publish with us
Policies and ethics
- Find a journal
- Track your research
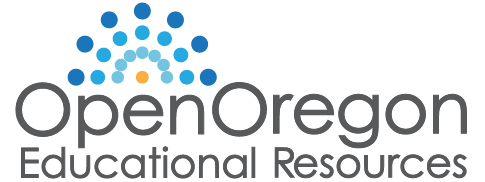
12 Information Processing Theory
At the end of this chapter, you will be able to:
- Identify key elements of information processing theory
- Explain strategies utilized to implement information processing theory
- Summarize the criticisms of and educational implications of information processing theory
- Explain how equity is impacted by information processing theory
- Identify classroom strategies to support the use of information processing theory
- Select strategies to support student success utilizing information processing theory
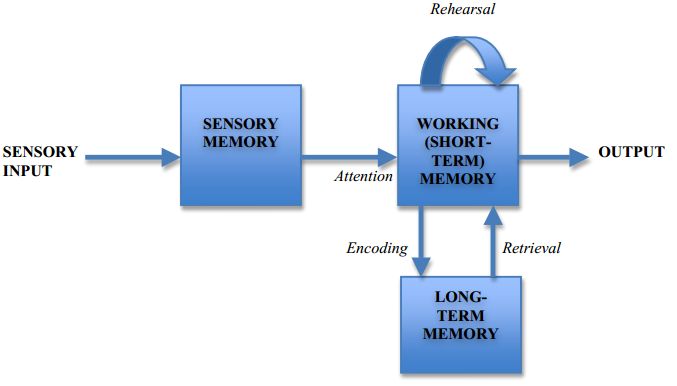
INTRODUCTION
Information Processing (IP) Theory is concerned with how people view their environment, how they put that information into memory, and how they retrieve that information later on. The Information Processing Theory approach is based on the idea that humans process information they receive instead of simply responding to external stimuli. According to the Information Processing Theory model , the mind is often compared to a computer. The computer, like minds, analyzes information and determines how the information will be stored. There are three components of the Information Processing Theory:
- sensory memory
- short-term memory
- long-term memory
Sensory memory is all of the things that you experience through your five senses-hearing, vision, taste, smell, and touch. The capacity of sensory memory is about four items and the duration is limited to .5 to 3 seconds.
Short-term memory (STM) , also called working memory, is the temporary storage, lasts about 15-30 seconds, holds about 7 items of information, and includes the thinking part of applying what comes out of the sensory memory.
Long-term memory (LTM) is memory that can be accessed at a later time, is long lasting, and can hold infinite information. The Information Processing Theory addresses how people respond to the information they receive through their senses and how they further process that information with steps of attention, forgetting, and retention. Unlike other cognitive developmental theories, the information processing theory includes a continuous pattern of development instead of development in stages.
The information processing (IP) theory is a cognitive approach to understanding how the human mind transforms sensory information. The model (Figure 12.1) assumes that information that comes from the environment is subject to mental processes beyond a simple stimulus-response pattern. “Input” from the environment goes through the cognitive systems which is then measured by the “output.” Information that is received can take several paths depending on attention, encoding, recognition, and storage. The central executive feature controls how much information is being processed, though more primitive sensory areas of the brain first accept environmental input. The theory looks at real time responses to presented stimuli and how the mind transforms that information.
Figure 12.1
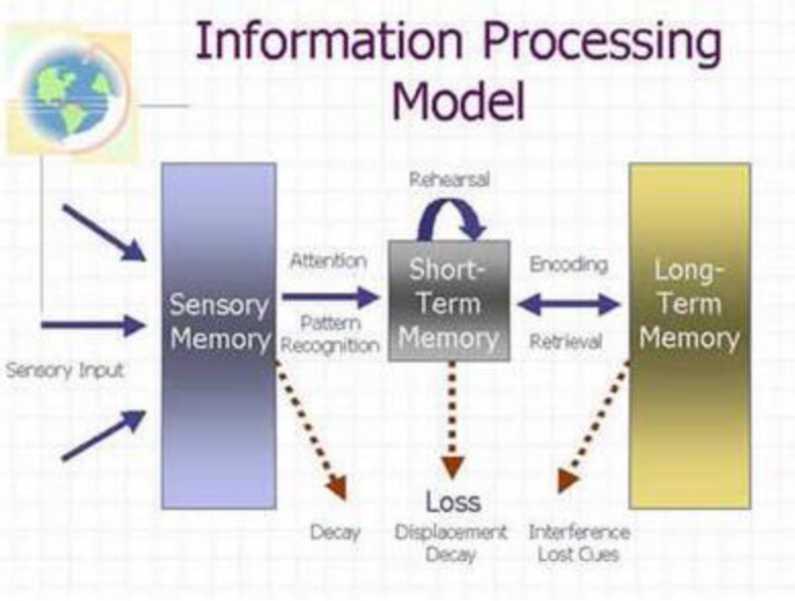
Video 3.8.1. Attention explains the ways in which we may attend or fail to attend to stimuli.
Selective Attention
The ability with selective attention tasks improves through childhood and into adolescence. While children’s selective attention may be inconsistent during middle childhood, adolescents demonstrate the ability to select and prioritize stimuli for attention reliably. The development of this ability is influenced by the child’s temperament (Rothbart & Rueda, 2005), the complexity of the stimulus or task (Porporino, Shore, Iarocci & Burack, 2004), and may be dependent on whether the stimuli are visual or auditory (Guy, Rogers & Cornish, 2013). Guy et al. (2013) found that children’s ability to attend to visual information selectively outpaced that of auditory stimuli. This change may explain why young children are not able to hear the voice of the teacher over the cacophony of sounds in the typical preschool classroom (Jones, Moore & Amitay, 2015). Jones and his colleagues found that 4 to 7-year-olds could not filter out background noise, especially when its frequencies were close in sound to the target sound. In comparison, teens often performed similarly to adults.
Video 3.8.2. Theories of Selective Attention explains how and why we attend to some stimuli and not others.
Sustained Attention
Most measures of sustained attention typically ask individuals to spend several minutes focusing on one task, while waiting for an infrequent event, while there are multiple distractors for several minutes. Young children can retain their visual and auditory attention for approximately 5 minutes if they are 5 years old, 6 minutes if they are 6 years old, 7 minutes if they are 7 years old, and so on. If a task is interesting or novel, the child may sustain attention substantially longer. Sustained attention improves to around age 10, then plateaus with only small improvements to adulthood. Common estimates of the attention span of healthy teenagers and adults range from 10 to 20 minutes. There is some debate as to whether attention is consistently sustained or whether people repeatedly choose to re-focus on the same thing (Raichle, 1999) This ability to renew attention permits people to ‘ pay attention’ to things that last for more than a few minutes.
For time-on-task measurements, the type of activity used in the test affects the results, as people are generally capable of a longer attention span when they are doing something that they find enjoyable or intrinsically motivating (Raichle,1999). Attention is also increased if the person can perform the task fluently, compared to a person who has difficulty performing the task, or to the same person when he or she is just learning the task. Fatigue, hunger, noise, and emotional stress reduce the time focused on the task. After losing attention from a topic, a person may restore it by resting, doing a different kind of activity, changing mental focus, or deliberately choosing to re-focus on the first topic.
Divided Attention
Divided attention can be thought of in a couple of ways. We may look at how well people can multitask, performing two or more tasks simultaneously, or how people can alternate attention between two or more tasks. For example, walking and talking to a friend at the same time is multitasking, where trying to text while driving requires us to alternate attention between two tasks quickly.
Young children (age 3-4) have considerable difficulties in dividing their attention between two tasks and often perform at levels equivalent to our closest relative, the chimpanzee. However, by age five, they have surpassed the chimp (Hermann, Misch, Hernandez-Lloreda & Tomasello, 2015; Hermann & Tomasello, 2015). Despite these improvements, 5-year-olds continue to perform below the level of school-age children, adolescents, and adults. These skills continue to develop into adolescence.
Regardless of age, we have a limited capacity for attention, and the division of attention is confined to that limitation. Our ability to effectively multitask or alternate attention is dependent on the automaticity or complexity of the task but is also influenced by conditions like anxiety, arousal, task difficulty, and skills (Sternberg & Sternberg, 2012). Research shows that when dividing attention, people are more apt to make mistakes or perform their tasks more slowly (Matlin, 2013). Attention must be divided among all of the component tasks to perform them.
Classical research on divided attention involved people performing simultaneous tasks, like reading stories while listening and writing something else, or listening to two separate messages through different ears. Subjects were often tested on their ability to learn new information while engaged in multiple tasks. More current research examines the performance of doing two tasks simultaneously (Matlin, 2013), such as driving while performing another task. This research reveals that the human attentional system has limits on what it can process. For example, driving performance is worse while engaged in other tasks; drivers make more mistakes, brake harder and later, get into more accidents, veer into other lanes, and/or are less aware of their surroundings when engaged in the previously discussed tasks (Collet et al., 2009; Salvucci & Taatgen, 2008; Strayer & Drews, 2007).
Video 3.8.3. The Spotlight Model of Attention and Our Ability to Multitask explains how we divide our attention to attend to different tasks or information.
Memory is an information processing system; therefore, we often compare it to a computer. Memory is the set of processes used to encode, store, and retrieve information over different periods of time.
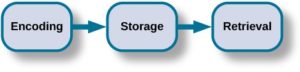
Figure 3.8.1 . Encoding involves the input of information into the memory system. Storage is the retention of encoded information. Retrieval, or getting the information out of memory and back into awareness, is the third function.
We get information into our brains through a process called encoding , which is the input of information into the memory system. Once we receive sensory information from the environment, our brains label or code it. We organize the information with other similar information and connect new concepts to existing concepts. Encoding information occurs through automatic processing and effortful processing.
If someone asks you what you ate for lunch today, more than likely, you could recall this information quite easily. This is known as automatic processing , or the encoding of details like time, space, frequency, and the meaning of words. Automatic processing is usually done without any conscious awareness. Recalling the last time you studied for a test is another example of automatic processing. However, what about the actual test material that you studied? It probably required a lot of work and attention on your part in order to encode that information. This is known as effortful processing .
There are three types of encoding. The encoding of words and their meaning is known as semantic encoding . It was first demonstrated by William Bousfield (1935) in an experiment in which he asked people to memorize words. The 60 words were divided into 4 categories of meaning, although the participants did not know this because the words were randomly presented. When they were asked to remember the words, they tended to recall them in categories, showing that they paid attention to the meanings of the words as they learned them.
Visual encoding is the encoding of images, and acoustic encoding is the encoding of sounds, words in particular. To see how visual encoding works, read over this list of words: car, level, dog, truth, book, value . If you were asked later to recall the words from this list, which ones do you think you’d most likely remember? You would probably have an easier time recalling the words car, dog, and book , and a more difficult time recalling the words level, truth, and value . Why is this? Because you can recall images (mental pictures) more easily than words alone. When you read the words car, dog, and book, you created images of these things in your mind. These are concrete, high-imagery words. On the other hand, abstract words like level, truth, and value are low-imagery words. High-imagery words are encoded both visually and semantically (Paivio, 1986), thus building a more reliable memory.
Now let us turn our attention to acoustic encoding. You are driving in your car, and a song comes on the radio that you have not heard in at least ten years, but you sing along, recalling every word. In the United States, children often learn the alphabet through song, and they learn the number of days in each month through rhyme: “ Thirty days hath September, / April, June, and November; / All the rest have thirty-one, / Save February, with twenty-eight days clear, / And twenty-nine each leap year.” These lessons are easy to remember because of acoustic encoding. We encode the sounds the words make. This is one of the reasons why much of what we teach young children is done through song, rhyme, and rhythm.
Which of the three types of encoding do you think would give you the best memory of verbal information? Some years ago, psychologists Fergus Craik and Endel Tulving (1975) conducted a series of experiments to find out. Participants were given words along with questions about them. The questions required the participants to process the words at one of the three levels. The visual processing questions included such things as asking the participants about the font of the letters. The acoustic processing questions asked the participants about the sound or rhyming of the words, and the semantic processing questions asked the participants about the meaning of the words. After participants were presented with the words and questions, they were given an unexpected recall or recognition task.
Words that had been encoded semantically were better remembered than those encoded visually or acoustically. Semantic encoding involves a deeper level of processing than shallower visual or acoustic encoding. Craik and Tulving concluded that we process verbal information best through semantic encoding, especially if we apply what is called the self-reference effect. The self-reference effect is the tendency for an individual to have a better memory for information that relates to oneself in comparison to material that has less personal relevance (Rogers, Kuiper & Kirker, 1977). Could semantic encoding be beneficial to you as you attempt to memorize the concepts in this chapter?
Video 3.8.4. Encoding Strategies discusses various encoding techniques that help us store information in memory.
Once the information has been encoded, we have to retain it somehow. Our brains take the encoded information and place it in storage. Storage is the creation of a permanent record of information.
In order for a memory to go into storage (i.e., long-term memory), it has to pass through three distinct stages: Sensory Memory, Short-Term Memory, and finally, Long-Term Memory. These stages were first proposed by Richard Atkinson and Richard Shiffrin (1968). Their model of human memory, called Atkinson-Shiffrin (A-S) or three-box model, is based on the belief that we process memories in the same way that a computer processes information.
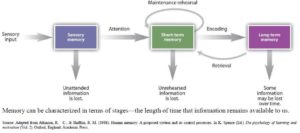
Figure 3 .8.2. According to the Atkinson-Shiffrin model of memory, information passes through three distinct stages in order for it to be stored in long-term memory.
The three-box is just one model of memory. Others, such as Baddeley and Hitch (1974), have proposed a model where short-term memory itself has different forms. In this model, storing memories in short-term memory is like opening different files on a computer and adding information. The type of short-term memory (or computer file) depends on the type of information received. There are memories in visual-spatial form, as well as memories of spoken or written material, and they are stored in three short-term systems: a visuospatial sketchpad, an episodic buffer, and a phonological loop. According to Baddeley and Hitch, a central executive part of memory supervises or controls the flow of information to and from the three short-term systems.
Video 3.8.5. Information Processing Model: Sensory, Working, and Long Term Memory explains the three-box model of memory.
Sensory Memory
In the Atkinson-Shiffrin model, stimuli from the environment are processed first in sensory memory : storage of brief sensory events, such as sights, sounds, and tastes. It is very brief storage, essentially long enough for the brain to register and start processing the information . Sensory memory can hold visual information for about half second and auditory information for a few seconds. Unlike other cognitive processes, it seems that sensory memory does not change from infancy (Siegler, 1998). However, without the ability to encode the information, it fades from sensory memory quickly (Papalia et al., 2008). As children and adolescents become more capable of encoding, they can take more advantage of the information available to them in their sensory memory.
We are constantly bombarded with sensory information. We cannot absorb all of it, or even most of it. Moreover, most of it has no impact on our lives. For example, what was your professor wearing during the last class period? As long as the professor was dressed appropriately, it does not matter what she was wearing. Sensory information about sights, sounds, smells, and even textures, which we do not view as valuable information, we discard. If we view something as valuable, the information will move into our short-term memory system.
One study of sensory memory researched the significance of valuable information on short-term memory storage. J. R. Stroop discovered a memory phenomenon in the 1930s: you will name a color more easily if it appears printed in that color, which is called the Stroop effect. In other words, the word “red” will be named more quickly, regardless of the color, the word appears in, than any word that is colored red. Try an experiment: name the colors of the words you are given in Figure 3.8.3. Do not read the words, but say the color the word is printed in. For example, upon seeing the word “yellow” in green print, you should say “green,” not “yellow.” This experiment is fun, but it is not as easy as it seems.
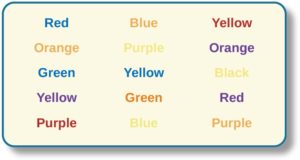
Figure 3.8.3 . The Stroop effect describes why it is difficult for us to name a color when the word and the color of the word are different.
Short-Term (Working) Memory
Short-term memory (STM) , also called working memory , is a temporary storage system that processes incoming sensory memory. Short-term memory is the bridge between information taken in through sensory memory and the more permanent storage of information in long-term memory. Information that is not moved along from short-term memory to long-term memory will be forgotten. Short-term memory is also called working memory because this is the system where the “work” of memory happens. If you are retrieving information from your long-term memory, you are moving it into your working memory, where you can think about that information.
Think of working memory as the information you have displayed on your computer screen—a document, a spreadsheet, or a web page. Then, the information in this memory system goes to long-term memory (you save it to your hard drive), or it is discarded (you delete a document or close a web browser). This step of rehearsal , the conscious repetition of information to be remembered, to move STM into long-term memory is called memory consolidation .
You may find yourself asking, “How much information can our memory handle at once?” To explore the capacity and duration of your short-term memory, have a partner read the strings of random numbers (Figure 3.8.4) out loud to you, beginning each string by saying, “Ready?” and ending each by saying, “Recall,” at which point you should try to write down the string of numbers from memory.

Figure 3.8.4 . Work through this series of numbers using the recall exercise explained above to determine the longest string of digits that you can store.
Note the longest string at which you got the series correct. For most people, this will be close to 7. Recall is somewhat better for random numbers than for random letters (Jacobs, 1887), and also often slightly better for information we hear (acoustic encoding) rather than see (visual encoding) (Anderson, 1969).
Short-term or working memory often requires conscious effort and adequate use of attention to function effectively. As you read earlier, children struggle with many aspects of attention, and this greatly diminishes their ability to juggle several pieces of information in memory consciously. The capacity of working memory is the amount of information someone can hold in consciousness is smaller in young children than in older children and adults. The typical 5-year-old can hold only a four-digit number active. The typical adult and teenager can hold a seven-digit number active in their short-term memory. The capacity of working memory expands during middle and late childhood, and research has suggested that both an increase in processing speed and the ability to inhibit irrelevant information from entering memory are contributing to the greater efficiency of working memory during this age (de Ribaupierre, 2002). Changes in myelination and synaptic pruning in the cortex are likely behind the increase in processing speed and ability to filter out irrelevant stimuli (Kail, McBride-chang, Ferrer, Cho, & Shu, 2013).
Short-term memory can only hold information for a short period of time, without rehearsal. For a typical adolescent or adult, storage lasts about 20-30 seconds. Older children and adults use mental strategies to aid their memory performance. For instance, simple rote rehearsal may be used to commit information to memory. Young children often do not rehearse unless reminded to do so, and when they do rehearse, they often fail to use clustering rehearsal. In clustering rehearsal, the person rehearses previous material while adding additional information. If a list of words is read out loud to you, you are likely to rehearse each word as you hear it along with any previous words you were given. Young children will repeat each word they hear, but often fail to repeat the prior words in the list. In Schneider, Kron-Sperl, and Hunnerkopf’s (2009) longitudinal study of 102 kindergarten children, the majority of children used no strategy to remember information, a finding that was consistent with previous research. As a result, their memory performance was reduced when compared to their abilities as they aged and started to use more effective memory strategies.
Executive Functions
Changes in attention and the working memory system also involve changes in executive function. Executive function (ef) refers to self-regulatory processes, such as the ability to inhibit behavior or cognitive flexibility, that enable adaptive responses to new situations or to reach a specific goal. Executive function skills gradually emerge during early childhood and continue to develop throughout childhood and adolescence. Like many cognitive changes, brain maturation, especially the prefrontal cortex, along with experience, influence the development of executive function skills. A child, whose parents are more warm and responsive, uses scaffolding when the child is trying to solve a problem, and who provides cognitively stimulating environments for the child to show higher executive function skills (Fay-Stammbach, Hawes & Meredith, 2014). For instance, scaffolding was positively correlated with greater cognitive flexibility at age two and inhibitory control at age four (Bibok, Carpendale & Müller, 2009).
STM and Learning
Individuals differ in their memory abilities, and these differences predict academic performance (Prebler, Krajewski, & Hasselhorn, 2013). Children with learning disabilities in math and reading often have difficulties with working memory (Alloway, 2009). They may struggle with following the directions of an assignment. When a task calls for multiple steps, children with poor working memory may miss steps because they may lose track of where they are in the task. Adults working with such children may need to communicate: using more familiar vocabulary, using shorter sentences, repeating task instructions more frequently, and breaking more complex tasks into smaller, more manageable steps. Some studies have also shown that more intensive training in working memory strategies, such as chunking, aids in improving the capacity of working memory in children with poor working memory (Alloway, Bibile, & Lau, 2013).
Long-term Memory
Long-term memory (LTM) is the continuous storage of information. Unlike short-term memory, the storage capacity of LTM has no real limits. It encompasses all the things you can remember what happened more than just a few minutes ago to all of the things that you can remember what happened days, weeks, and years ago. In keeping with the computer analogy, the information in your LTM would be like the information you have saved on the hard drive. It is not there on your desktop (your short-term memory), but you can pull up this information when you want it, at least most of the time. Not all long-term memories are strong memories. Some memories can only be recalled through prompts. For example, you might easily recall a fact— “What is the capital of the United States?”—or a procedure—”How do you ride a bike?”—but you might struggle to recall the name of the restaurant you had dinner when you were on vacation in France last summer. A prompt, such as that the restaurant was named after its owner, who spoke to you about your shared interest in soccer, may help you recall the name of the restaurant.
Long-term memory is divided into two types: explicit and implicit (Figure 3.8.5). Understanding the different types is important because a person’s age or particular types of brain trauma or disorders can leave certain types of LTM intact while having disastrous consequences for other types. Explicit memories, also called declarative memories, are those we consciously try to remember and recall. For example, if you are studying for your chemistry exam, the material you are learning will be part of your explicit memory. (Note: Sometimes, but not always, the terms explicit memory and declarative memory are used interchangeably.)
Implicit memories, also called non-declarative memories, are memories that are not part of our consciousness. They are memories formed from behaviors. Implicit memory is also called non-declarative memory.
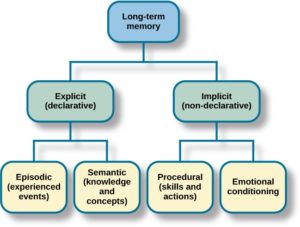
Figure 3.8.5 . There are two components of long-term memory: explicit and implicit. Explicit memory includes episodic and semantic memory. Implicit memory includes procedural memory and things learned through conditioning.
Procedural memory is a type of implicit memory: it stores information about how to do things. It is the memory for skilled actions, such as how to brush your teeth, how to drive a car, how to swim the crawl (freestyle) stroke. If you are learning how to swim freestyle, you practice the stroke: how to move your arms, how to turn your head to alternate breathing from side to side, and how to kick your legs. You would practice this many times until you become good at it. Once you learn how to swim freestyle and your body knows how to move through the water, you will never forget how to swim freestyle, even if you do not swim for a couple of decades. Similarly, if you present an accomplished guitarist with a guitar, even if he has not played in a long time, he will still be able to play quite well.
Explicit or declarative memory has to do with the storage of facts and events we personally experienced. Explicit (declarative) memory has two parts: semantic memory and episodic memory. Semantic means having to do with language and knowledge about language. An example would be the question, “what does argumentative mean?” Stored in our semantic memory is knowledge about words, concepts, and language-based knowledge and facts. For example, answers to the following questions are stored in your semantic memory:
- Who was the first President of the United States?
- What is democracy?
- What is the longest river in the world?
Episodic memory is information about events we have personally experienced. The concept of episodic memory was first proposed about 40 years ago (Tulving, 1972). Since then, Tulving and others have looked at the scientific evidence and reformulated the theory. Currently, scientists believe that episodic memory is memory about happenings in particular places at particular times, the what, where, and when of an event (Tulving, 2002). It involves recollection of visual imagery as well as the feeling of familiarity (Hassabis & Maguire, 2007).
A component of episodic memory is autobiographical memory, or our personal narrative. Adolescents and adults rarely remember events from the first few years of life. We refer to this normal experience as infantile amnesia. In other words, we lack autobiographical memories from our experiences as an infant, toddler, and very young preschooler. Several factors contribute to the emergence of autobiographical memory, including brain maturation, improvements in language, opportunities to talk about experiences with parents and others, the development of the theory of mind, and a representation of “self” (Nelson & Fivush, 2004). Two-year-olds do remember fragments of personal experiences, but these are rarely coherent accounts of past events (Nelson & Ross, 1980). Between 2 and 2 ½ years of age, children can provide more information about past experiences. However, these recollections require considerable prodding by adults (Nelson & Fivush, 2004). Over the next few years, children will form more detailed autobiographical memories and engage in more reflection on the past.
So you have worked hard to encode (via effortful processing) and store some important information for your upcoming final exam. How do you get that information back out of storage when you need it? The act of getting information out of memory storage and back into conscious awareness is known as retrieval . This process would be similar to finding and opening a paper you had previously saved on your computer’s hard drive. Now it is back on your desktop, and you can work with it again. Our ability to retrieve information from long-term memory is vital to our everyday functioning. You must be able to retrieve information from memory in order to do everything from knowing how to brush your hair and teeth, to driving to work, to knowing how to perform your job once you get there.
Video 3.8.6. Retrieval Cues discusses how cues prompt memory retrieval.
There are three ways to retrieve information from long-term memory storage systems: recall, recognition, and relearning. Recall is what we most often think about when we talk about memory retrieval: it means you can access information without cues. For example, you would use recall for an essay test. Recognition happens when you identify information that you have previously learned after re-encountering it. It involves a process of comparison. When you take a multiple-choice test, you are relying on recognition to help you choose the correct answer. Here is another example. Let us say you graduated from high school ten years ago, and you have returned to your hometown for your 10-year reunion. You may not be able to recall all of your classmates, but you recognize many of them based on their yearbook photos.
Video 3.8.7. Free Recall, Cued Recall, and Recognition discusses the various ways in which information can be retrieved from long term memory.
The third form of retrieval is relearning , and it is just as it sounds. It involves learning information that you previously learned. Whitney took Spanish in high school, but after high school, she did not have the opportunity to speak Spanish. Whitney is now 31, and her company has offered her an opportunity to work in their Mexico City office. In order to prepare herself, she enrolls in a Spanish course at the local community center. She is surprised at how quickly she can pick up the language after not speaking it for 13 years; this is an example of relearning.
Organization of Thinking
During middle childhood and adolescence, young people can learn and remember more due to improvements in the way they attend to and store information. As people learn more about the world, they develop more categories for concepts and learn more efficient strategies for storing and retrieving information. One significant reason is that they continue to have more experiences on which to tie new information. In other words, their knowledge base , knowledge in particular areas that makes learning new information easier , expands (Berger, 2014).
Cognitive control
As noted earlier, executive functions, such as attention, increases in working memory, and cognitive flexibility, have been steadily improving since early childhood. Studies have found that executive function is very competent in adolescence. However, self-regulation , or the ability to control impulses, may still fail. A failure in self-regulation is especially true when there is high stress or high demand on mental functions (Luciano & Collins, 2012). While high stress or demand may tax even an adult’s self-regulatory abilities, neurological changes in the adolescent brain may make teens particularly prone to more risky decision-making under these conditions.
Inductive and Deductive Reasoning
Inductive reasoning emerges in childhood and is a type of reasoning that is sometimes characterized as “bottom-up- processing” in which specific observations, or specific comments from those in authority, may be used to draw general conclusions. However, in inductive reasoning, the veracity of the information that created the general conclusion does not guarantee the accuracy of that conclusion. For instance, a child who has only observed thunder on summer days may conclude that it only thunders in the summer. In contrast, deductive reasoning , sometimes called “top-down-processing,” emerges in adolescence. This type of reasoning starts with some overarching principle and, based on this, propose specific conclusions. Deductive reasoning guarantees an accurate conclusion if the premises on which it is based are accurate.
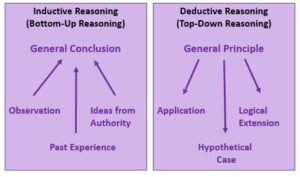
Figure 3.8.6. Models of inductive and deductive reasoning.
Intuitive versus Analytic Thinking
Cognitive psychologists often refer to intuitive and analytic thought as the Dual-Process Model, the notion that humans have two distinct networks for processing information (Albert & Steinberg, 2011). Intuitive thought is automatic, unconscious, and fast (Kahneman, 2011), and it is more experiential and emotional. In contrast, Analytic thought is deliberate, conscious, and rational. While these systems interact, they are distinct (Kuhn, 2013). Intuitive thought is easier and more commonly used in everyday life. It is also more commonly used by children and teens than by adults (Klaczynski, 2001). The quickness of adolescent thought, along with the maturation of the limbic system, may make teens more prone to emotional, intuitive thinking than adults.
Critical Thinking
According to Bruning et al. (2004), there is a debate in U.S. education as to whether schools should teach students what to think or how to think. Critical thinking , or a detailed examination of beliefs, courses of action, and evidence, involves teaching children how to think. The purpose of critical thinking is to evaluate information in ways that help us make informed decisions. Critical thinking involves better understanding a problem through gathering, evaluating, and selecting information, and also by considering many possible solutions. Ennis (1987) identified several skills useful in critical thinking. These include: Analyzing arguments, clarifying information, judging the credibility of a source, making value judgments, and deciding on an action. Metacognition is essential to critical thinking because it allows us to reflect on the information as we make decisions.
Metacognition
As children mature through middle and late childhood and into adolescence, they have a better understanding of how well they are performing a task and the level of difficulty of a task. As they become more realistic about their abilities, they can adapt studying strategies to meet those needs. Young children spend as much time on an unimportant aspect of a problem as they do on the main point, while older children start to learn to prioritize and gauge what is significant and what is not. As a result, they develop metacognition. Metacognition refers to the knowledge we have about our thinking and our ability to use this awareness to regulate our cognitive processes (Bruning, Schraw, Norby, & Ronning, 2004).
Bjorklund (2005) describes a developmental progression in the acquisition and use of memory strategies. Such strategies are often lacking in younger children but increase in frequency as children progress through elementary school. Examples of memory strategies include rehearsing information you wish to recall, visualizing and organizing information, creating rhymes, such as “i” before “e” except after “c,” or inventing acronyms, such as “ROYGBIV” to remember the colors of the rainbow. Schneider, Kron-Sperl, and hünnerkopf (2009) reported a steady increase in the use of memory strategies from ages six to ten in their longitudinal study (see table 3.8.1). Moreover, by age ten, many children were using two or more memory strategies to help them recall information. Schneider and colleagues found that there were considerable individual differences at each age in the use of strategies and that children who utilized more strategies had better memory performance than their same-aged peers.
Table 3.8.1. Percentage of children who did not use any memory strategies by age.
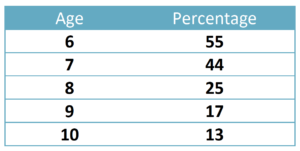
A person may experience three deficiencies in their use of memory strategies. A mediation deficiency occurs when a person does not grasp the strategy being taught, and thus, does not benefit from its use. If you do not understand why using an acronym might be helpful, or how to create an acronym, the strategy is not likely to help you. In a production deficiency, the person does not spontaneously use a memory strategy and has to be prompted to do so. In this case, the person knows the strategy and is more than capable of using it, but they fail to “produce” the strategy on their own. For example, a child might know how to make a list but may fail to do this to help them remember what to bring on a family vacation. A utilization deficiency refers to a person using an appropriate strategy, but it fails to aid their performance. Utilization deficiency is common in the early stages of learning a new memory strategy (Schneider & Pressley, 1997; miller, 2000). Until the use of the strategy becomes automatic, it may slow down the learning process, as space is taken up in memory by the strategy itself. Initially, children may get frustrated because their memory performance may seem worse when they try to use the new strategy. Once children become more adept at using the strategy, their memory performance will improve. Sodian and Schneider (1999) found that new memory strategies acquired before age 8 often show utilization deficiencies, with there being a gradual improvement in the child’s use of the strategy. In contrast, strategies acquired after this age often followed an “all-or-nothing” principle in which improvement was not gradual, but abrupt.
Candela Citations
- Information Processing Theory. Authored by : Nicole Arduini-Van Hoose. Provided by : Hudson Valley Community College. Retrieved from : https://courses.lumenlearning.com/edpsy/chapter/information-processing-theories/. License : CC BY-NC-SA: Attribution-NonCommercial-ShareAlike
- Adolescent Psychology. Authored by : Nicole Arduini-Van Hoose. Provided by : Hudson Valley Community College. Retrieved from : https://courses.lumenlearning.com/adolescent. License : CC BY-NC-SA: Attribution-NonCommercial-ShareAlike
- Divided Attention, Selective Attention, Inattentional Blindness, & Change Blindness. Authored by : Carole Yue. Provided by : Khan Academy. Retrieved from : https://youtu.be/s4JBqLoY3tY. License : CC BY-NC-SA: Attribution-NonCommercial-ShareAlike
- Theories of Selective Attention. Authored by : Carole Yue. Provided by : Khan Academy . Retrieved from : https://youtu.be/qpsaHE_uZic. License : CC BY-NC-SA: Attribution-NonCommercial-ShareAlike
- The Spotlight Model of Attention and Our Ability to Multitask. Provided by : Khan Academy. Retrieved from : https://youtu.be/THJgaznSBu8. License : CC BY-NC-SA: Attribution-NonCommercial-ShareAlike
- Information Processing Model: Sensory, Working, and Long Term Memory. Authored by : Carole Yue. Provided by : Khan Academy. Retrieved from : https://youtu.be/pMMRE4Q2FGk. License : CC BY-NC-SA: Attribution-NonCommercial-ShareAlike
- Free Recall, Cued Recall, and Recognition. Authored by : Carole Yue. Provided by : Khan Academy. Retrieved from : https://youtu.be/Uhyk2bRTguI. License : CC BY-NC-SA: Attribution-NonCommercial-ShareAlike
Educational Psychology Copyright © 2020 by Nicole Arduini-Van Hoose is licensed under a Creative Commons Attribution-NonCommercial-ShareAlike 4.0 International License , except where otherwise noted.
Share This Book
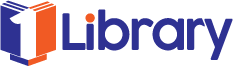
- No results found
Newell and Simon's problem-space theory
Review of the literature, 2.4. information-processing theory and the problem understanding task, 2.4.1. newell and simon's problem-space theory.
Newell and Simon's research into human problem solving, especially their seminal work, "Human problem solving" (1972), still remains a much quoted reference in contemporary information-processing theory and research (Dawson, 1998). Despite the age o f this theory, it was found with a few modifications to be a useful way of structuring thinking
in the area o f interviewer reasoning. The current debate between the classical and the connectionist views of information processing is beyond the scope of this thesis, however readers are referred to Dawson (1998) who provides an excellent coverage o f the issues involved.
Newell and Simon (1972) conducted a wide range of experiments under controlled laboratory conditions into how people (usually undergraduates), approached a range of three-dimensional puzzles, and in particular the Tower o f Hanoi problem. In the Tower o f Hanoi problem participants were presented with three vertical pegs in a row, the first of which had three disks piled on it in order of size; that is the largest disk was at the bottom, the next on top, and so on. The goal o f the problem was to have all the disks piled in the same order on the last peg. However, disks could only be moved in certain ways. Only one disk could be moved at a time, and a larger disk could not be placed on top o f a smaller disk (Eysenck & Keane, 1995, p.363).
Figure 2.2 presents the problem space o f legal moves for the Tower o f Hanoi problem which will be referred to in the discussions below. From Newell and Simon's perspective people went about solving problems by first exploring a range o f possible ways (paths) o f finding a solution. Puzzles, such as the Tower of Hanoi, begin with a point outside the problem space (or maze), and then progress through a series o f moves to the centre - the solution. To achieve the goal o f getting to the centre, the person comes across many junctions where they have to make decisions (e.g., go straight, turn left, and so on). Each of these alternative paths may branch again and again, with some leading to the centre and the solution, and some not. Different strategies can be employed to find one's way inside the problem space (e.g., mark where you have come from, initially take left turns, and so on). These strategies provide the person with a systematic way of searching the problem space, and selecting one path firom a range o f alternative paths to get closer to the solution.
Their findings suggested that the stmcture of a problem could be characterised by a set o f states, beginning with an initial problem state (e.g., standing outside the problem space), involving many intermediate states (e.g., moving through the problem space), and ending with a goal state (e.g., being at the centre of the problem space). People in their studies began with an initial state and "searched" through a space o f alternative mental
States until they reached a goal state. Each of these alternative states can also have alternatives. The number o f these alternatives increases greatly as one moves away from the initial state to the goal state.
In order for people to solve the Tower of Hanoi problem they have to employ a range of cognitive strategies to reduce the number of states which they have to pass through to reach the goal state. Newell and Simon describe such strategies as heuristics. A heuristic strategy is in essence a nonrigorous way of achieving a solution to a problem. While heuristic procedures often lead to solutions, they offer no guarantee o f doing so (Bruner, 1996). Heuristics are contrasted with algorithms, which are methods that produce a definite solution. For example, in the Tower of Hanoi problem, a person could check every state, by starting at the beginning and systematically checking every alternative state until the goal state were achieved. This procedure would take far too long to be efficient, but is guaranteed to solve the problem. Heuristics, on the other hand are "rules of thumb", that may not guarantee a solution to a given problem every time, but most o f the time, thus saving time and effort.
One of the most important heuristic principles proposed by Newell and Simon was means-ends analysis. It consists of three main steps: first, the person notes the difference between the initial state and the goal state, second, they create subgoals to reduce this observed difference, and third, they select an operator that will solve this subgoal. Moves from one state to another are achieved by the application o f "mental operators". As problems may have a large number o f alternative paths, people use strategies to move from the initial state to the goal state efficiently. Thus, people's conception of a problem (i.e. the nature of the initial state), and the knowledge they bring to it (the operators and strategies available to them), make contributions to their problem solving behaviour (Newell & Simon, 1972).
Newell and Simon's problem-space theory identifies the various hypothetical states, processes and strategies that people may use to go about solving problems, at least puzzle based problems. The theory also predicts the types o f constraints that will make solving problems difficult, for example, the constraint o f human working memory and the interaction between this and the types o f strategies people use to search it. From a theoretical perspective it provides a normative theory o f human problem solving. The
theory allows for the structure of the problem to be specified and the best solution to the problem to be defined. In puzzle-based research from the 1950s to the present day, it is possible to elaborate the problem space and identify the correct or best solution to the problem by tracing the shortest sequence of moves from the initial state to the goal state. It provides a normative model o f what an "expert" problem solver would do, and how and why people's behaviour diverges from that o f the "expert".
2.4.2. Sum m ary of Newell and Simon's problem-space theory
Newell and Simon's (1972) information-processing theory o f problem solving suggests that when people move from an initial problem state towards a solution state they form a mental representation of the problem, which in this thesis is called a problem map. Research on expert problem solvers (outlined in section 2.7 o f this chapter) shows that they acquire through experience mechanisms for internally representing the problem space. This internal model acts as a precondition for planning, reasoning, anticipating and controlling subsequent cognitive behaviour (Ericsson & Lehmann, 1996).
The problem map undergoes a series of transformations as the problem solver tries to move from the initial problem state to a solution state. These transitions are achieved by the problem solver employing a series of cognitive operations, or strategies, such as means-ends analysis (this is the strategy whereby the problem solver evaluates the difference between the initial problem and the solution state). In summary, Newell and Simon's theory suggests that:
1. Problems have a large number o f alternative paths from the initial problem state to the solution state.
2. The total number o f such states, as generated by mental operators, is called the 'basic problem' space.
3. People's problem-solving behaviour is seen as the production of knowledge states by using mental operators to move from the initial knowledge state to a goal knowledge state.
4. People use their knowledge and various heuristic methods (i.e. means-ends analysis) to search through the problem space to find an efficient path from the initial state to the goal state.
5. All o f these processes occur within the limitations o f the individual's cognitive system, that is their working memory and information processing limitations (i.e. sorting and retrieving information from long-term memory).
6. The contents of people's short-term memory are open to conscious reporting by the individual. This assumption guided much of their work in which they used think-aloud protocols. This is a method whereby individuals say out loud what is going through their minds as they solve problems. The researcher records responses which are analysed later (Eysenck & Keane, 1995, p.363).
- Context-constraints
- Newell and Simon's problem-space theory (You are here)
- THE HYPOTHESIS-TESTING FRAMEWORK TO GUIDE PROBLEM UNDERSTANDING
- THE CHARACTERISTICS OF EXPERT AND NOVICE PROBLEM SOLVERS
- ARGYRIS AND SCHON'S THEORY OF INTERPERSONAL EFFECTIVENESS
- ROBINSON AND HALLIDAY’S RESEARCH ON ACCESSIBLE REASONING
- Critique of model
- Section One (Interviewing)
- Interviewing
- LIMITATIONS OF STUDY ONE
- CONCLUSIONS
- RATIONALE FOR STUDY TWO
- General design and interviewing tasks
- Use o f accessible reasoning statements
- RATIONALE FOR STUDY TWO (A)
- Research questions and hypotheses for study two (a)
Related documents
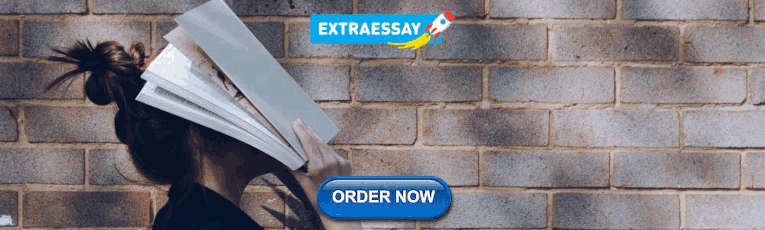
IMAGES
VIDEO
COMMENTS
Abstract. Sets forth the general theory of human problem solving that has emerged from research in the past 2 decades and examines recent research on (a) the role of perceptual processes in problem solving, (b) the processes for generating problem representations, and (c) research aimed at extending the theory to new domains. Issues involved in ...
Problem Solving. R.E. Mayer, in Encyclopedia of Human Behavior (Second Edition), 2012 Problem Space and Search Processes. Information-processing theories of problem solving focus on constructing a problem space and finding a path through the problem space (Newell and Simon, 1972; Novick and Bassok, 2005).A problem space consists of a representation of the initial state, goal state, and all ...
Published on March 21, 2020. Information processing theory is a cognitive theory that uses computer processing as a metaphor for the workings of the human brain. Initially proposed by George A. Miller and other American psychologists in the 1950s, the theory describes how people focus on information and encode it into their memories.
Information processing theory is a cognitive psychology theory that studies mental processes involved in acquiring, storing, and using knowledge. It focuses on the flow of information as it is passed from one stage to another within a person's mind. By examining different types of information processing as well as real-world examples of them ...
Information processing theory is the approach to the study of cognitive development evolved out of the American experimental tradition in psychology. ... or obtaining problem-solving rules (Miller, 2011). Increases in the knowledge base or the ability to remember more items in working memory are examples of quantitative changes, as well as ...
Hence the information processing approach characterizes thinking as the environment providing input of data, which is then transformed by our senses. The information can be stored, retrieved, and transformed using "mental programs," with the results being behavioral responses. Cognitive psychology has influenced and integrated with many ...
Originally known as the cognitive information processing approach to career problem solving and decision making (Peterson, Sampson, & Reardon, 1991; Sampson, Reardon, Peterson, & Lenz, 2004; & Sampson, 2008), the theory's name has evolved over time to simply "CIP theory" and includes both a theory of vocational behavior and a theory
Information processing theory is a cognitive developmental approach that lays a structural foundation for the human mind. The information processing theory assumes that the human mind contains mental structures that process information received by the senses. ... fluid intelligence, problem-solving, and language comprehension. Working memory is ...
The present chapter, adopting the same information-processing point of view as the previous one, undertakes three tasks. The first part of the chapter sets forth the general theory of human problem solving thai has emerged from research in the past two decades, especially research that has employed the m~thods of computer simulation and ...
The "new movement" [what we would call information-processing theory] is based on evaluative concepts (R. A. Fisher's experimental design, A. Wald's statistical decision function, J. von Neumann's theory of games), on the development of a measure of information (R. Hartley, D. Gabor, N. Wiener, C. Shannon), on studies of control ...
Information processing is a model for human thinking and learning, and it is a part of the resurgence of cognitive perspectives of learning. The cognitive perspective asserts that complex mental states affect human learning and behavior, that such mental states can be scientifically investigated. Computers, which process information, include ...
This chapter sets forth the general theory of human problem Solving that has emerged from research in the past two decades, especially research that has employed the methods of computer simulation and analysis of thinking-aloud protocols. In the preceding chapter a taxonomy of pr oblem types was proposed, and some hypotheses set forth about the general kin ds of skills and knowledge needed to ...
Information Processing Theory model, the mind is often compared to a computer. The computer, like minds, analyzes information and determines how the information will be stored. ... or obtaining problem-solving rules (Miller, 2011). Increases in the knowledge base or the ability to remember more items in short-term (working) memory are examples ...
Basically, information processing theory attempts to explain how humans think. Prior to the evolution of information processing theory, the field of psychology was dominated by behaviorism, a school of thought in which emphasis was placed solely on externally observable behaviors. ... was essentially a theory of human problem solving stated in ...
Summary. Information processing is a cognitive learning theory that helps explain how individuals acquire, process, store, and retrieve information from memory. The cognitive architecture that facilitates the processing of information consists of three components: memory stores, cognitive processes, and metacognition.
An information-processing view of personal problem solving is presented, involving the way people take in information, process that information into plans for solutions to personal problems and carry out those plans. An abbreviated view of how we view the effect of some important individual differences is presented.
Abstract. This article compares the information processing and dynamical systems perspectives on problem solving. Key theoretical constructs of the information-processing perspective include "searching" a "problem space" by using "heuristics" that produce "incremental" changes such as reaching a "subgoal" to solve a puzzle.
Video 3.8.5. Information Processing Model: Sensory, Working, and Long Term Memory explains the three-box model of memory. Sensory Memory. In the Atkinson-Shiffrin model, stimuli from the environment are processed first in sensory memory: storage of brief sensory events, such as sights, sounds, and tastes.It is very brief storage, essentially long enough for the brain to register and start ...
Sum m ary of Newell and Simon's problem-space theory. Newell and Simon's (1972) information-processing theory o f problem solving suggests that when people move from an initial problem state towards a solution state they form a mental representation of the problem, which in this thesis is called a problem map. Research on expert problem solvers ...
2. Information processing systems: Simon and Newell An analysis follows what happens from the beginning of a task, such as being given a problem to solve to the end with the problem solved. The basic theory is that much of the sequence of events can be thought of as the movement, storage and transformation of information. Major components.
Abstract. Using the Newell, Shaw, and Simon postulates for an information processing theory of human problem solving, decision net models were constructed for two individual consumers' choices of grocery products. The models were tested against actual data, and the resulting predictions were highly accurate.
An overview is presented of information processing analyses of knowledge and performance in three areas of the elementary mathematics curriculum. These include basic addition and subtraction facts, complex procedures such as multicolumn subtraction, and the solution of word problems. Theories are presented of the knowledge associated with ...
4.2 The Emergence of Information Processing. Information processing is a model for human thinking and learning, and it is a part. of the resurgence of cognitive perspecti ves of learning. The ...