- Utility Menu

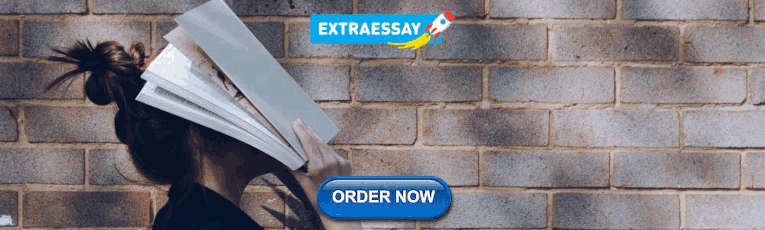
GA4 Tracking Code

fa51e2b1dc8cca8f7467da564e77b5ea
- Make a Gift
- Join Our Email List
- Problem Solving in STEM
Solving problems is a key component of many science, math, and engineering classes. If a goal of a class is for students to emerge with the ability to solve new kinds of problems or to use new problem-solving techniques, then students need numerous opportunities to develop the skills necessary to approach and answer different types of problems. Problem solving during section or class allows students to develop their confidence in these skills under your guidance, better preparing them to succeed on their homework and exams. This page offers advice about strategies for facilitating problem solving during class.
How do I decide which problems to cover in section or class?
In-class problem solving should reinforce the major concepts from the class and provide the opportunity for theoretical concepts to become more concrete. If students have a problem set for homework, then in-class problem solving should prepare students for the types of problems that they will see on their homework. You may wish to include some simpler problems both in the interest of time and to help students gain confidence, but it is ideal if the complexity of at least some of the in-class problems mirrors the level of difficulty of the homework. You may also want to ask your students ahead of time which skills or concepts they find confusing, and include some problems that are directly targeted to their concerns.
You have given your students a problem to solve in class. What are some strategies to work through it?
- Try to give your students a chance to grapple with the problems as much as possible. Offering them the chance to do the problem themselves allows them to learn from their mistakes in the presence of your expertise as their teacher. (If time is limited, they may not be able to get all the way through multi-step problems, in which case it can help to prioritize giving them a chance to tackle the most challenging steps.)
- When you do want to teach by solving the problem yourself at the board, talk through the logic of how you choose to apply certain approaches to solve certain problems. This way you can externalize the type of thinking you hope your students internalize when they solve similar problems themselves.
- Start by setting up the problem on the board (e.g you might write down key variables and equations; draw a figure illustrating the question). Ask students to start solving the problem, either independently or in small groups. As they are working on the problem, walk around to hear what they are saying and see what they are writing down. If several students seem stuck, it might be a good to collect the whole class again to clarify any confusion. After students have made progress, bring the everyone back together and have students guide you as to what to write on the board.
- It can help to first ask students to work on the problem by themselves for a minute, and then get into small groups to work on the problem collaboratively.
- If you have ample board space, have students work in small groups at the board while solving the problem. That way you can monitor their progress by standing back and watching what they put up on the board.
- If you have several problems you would like to have the students practice, but not enough time for everyone to do all of them, you can assign different groups of students to work on different – but related - problems.
When do you want students to work in groups to solve problems?
- Don’t ask students to work in groups for straightforward problems that most students could solve independently in a short amount of time.
- Do have students work in groups for thought-provoking problems, where students will benefit from meaningful collaboration.
- Even in cases where you plan to have students work in groups, it can be useful to give students some time to work on their own before collaborating with others. This ensures that every student engages with the problem and is ready to contribute to a discussion.
What are some benefits of having students work in groups?
- Students bring different strengths, different knowledge, and different ideas for how to solve a problem; collaboration can help students work through problems that are more challenging than they might be able to tackle on their own.
- In working in a group, students might consider multiple ways to approach a problem, thus enriching their repertoire of strategies.
- Students who think they understand the material will gain a deeper understanding by explaining concepts to their peers.
What are some strategies for helping students to form groups?
- Instruct students to work with the person (or people) sitting next to them.
- Count off. (e.g. 1, 2, 3, 4; all the 1’s find each other and form a group, etc)
- Hand out playing cards; students need to find the person with the same number card. (There are many variants to this. For example, you can print pictures of images that go together [rain and umbrella]; each person gets a card and needs to find their partner[s].)
- Based on what you know about the students, assign groups in advance. List the groups on the board.
- Note: Always have students take the time to introduce themselves to each other in a new group.
What should you do while your students are working on problems?
- Walk around and talk to students. Observing their work gives you a sense of what people understand and what they are struggling with. Answer students’ questions, and ask them questions that lead in a productive direction if they are stuck.
- If you discover that many people have the same question—or that someone has a misunderstanding that others might have—you might stop everyone and discuss a key idea with the entire class.
After students work on a problem during class, what are strategies to have them share their answers and their thinking?
- Ask for volunteers to share answers. Depending on the nature of the problem, student might provide answers verbally or by writing on the board. As a variant, for questions where a variety of answers are relevant, ask for at least three volunteers before anyone shares their ideas.
- Use online polling software for students to respond to a multiple-choice question anonymously.
- If students are working in groups, assign reporters ahead of time. For example, the person with the next birthday could be responsible for sharing their group’s work with the class.
- Cold call. To reduce student anxiety about cold calling, it can help to identify students who seem to have the correct answer as you were walking around the class and checking in on their progress solving the assigned problem. You may even want to warn the student ahead of time: "This is a great answer! Do you mind if I call on you when we come back together as a class?"
- Have students write an answer on a notecard that they turn in to you. If your goal is to understand whether students in general solved a problem correctly, the notecards could be submitted anonymously; if you wish to assess individual students’ work, you would want to ask students to put their names on their notecard.
- Use a jigsaw strategy, where you rearrange groups such that each new group is comprised of people who came from different initial groups and had solved different problems. Students now are responsible for teaching the other students in their new group how to solve their problem.
- Have a representative from each group explain their problem to the class.
- Have a representative from each group draw or write the answer on the board.
What happens if a student gives a wrong answer?
- Ask for their reasoning so that you can understand where they went wrong.
- Ask if anyone else has other ideas. You can also ask this sometimes when an answer is right.
- Cultivate an environment where it’s okay to be wrong. Emphasize that you are all learning together, and that you learn through making mistakes.
- Do make sure that you clarify what the correct answer is before moving on.
- Once the correct answer is given, go through some answer-checking techniques that can distinguish between correct and incorrect answers. This can help prepare students to verify their future work.
How can you make your classroom inclusive?
- The goal is that everyone is thinking, talking, and sharing their ideas, and that everyone feels valued and respected. Use a variety of teaching strategies (independent work and group work; allow students to talk to each other before they talk to the class). Create an environment where it is normal to struggle and make mistakes.
- See Kimberly Tanner’s article on strategies to promoste student engagement and cultivate classroom equity.
A few final notes…
- Make sure that you have worked all of the problems and also thought about alternative approaches to solving them.
- Board work matters. You should have a plan beforehand of what you will write on the board, where, when, what needs to be added, and what can be erased when. If students are going to write their answers on the board, you need to also have a plan for making sure that everyone gets to the correct answer. Students will copy what is on the board and use it as their notes for later study, so correct and logical information must be written there.
For more information...
Tipsheet: Problem Solving in STEM Sections
Tanner, K. D. (2013). Structure matters: twenty-one teaching strategies to promote student engagement and cultivate classroom equity . CBE-Life Sciences Education, 12(3), 322-331.
- Designing Your Course
- A Teaching Timeline: From Pre-Term Planning to the Final Exam
- The First Day of Class
- Group Agreements
- Classroom Debate
- Flipped Classrooms
- Leading Discussions
- Polling & Clickers
- Teaching with Cases
- Engaged Scholarship
- Devices in the Classroom
- Beyond the Classroom
- On Professionalism
- Getting Feedback
- Equitable & Inclusive Teaching
- Advising and Mentoring
- Teaching and Your Career
- Teaching Remotely
- Tools and Platforms
- The Science of Learning
- Bok Publications
- Other Resources Around Campus

The Problem Solving Approach in Science Education

Table of Contents
Have you ever wondered how science , with its vast array of facts and figures, becomes so deeply integrated into our understanding of the world? It isn’t just about memorizing data; it’s about engaging with problems and seeking solutions through a systematic approach. This is where the problem\-solving approach in science education takes the spotlight. It transforms passive listeners into active participants, nurturing the next generation of critical thinkers and innovators.
What is the Problem-Solving Approach?
At its core, the problem-solving approach is a student\-centered method that encourages learners to tackle scientific problems with curiosity and rigor. It isn’t just a teaching strategy; it’s a journey that begins with recognizing a problem and ends with reaching a conclusion through investigation and reasoning.
Step 1: Identifying the Problem
Every scientific journey begins with a question. In the classroom, this means fostering an environment where students are prompted to observe phenomena and articulate their curiosities in the form of clear, concise problems. This might look like a teacher demonstrating an unexpected result in an experiment and asking students to ponder why it occurred.
Step 2: Gathering Information
Once the problem is set, the next step is to gather relevant information. Here, students exercise their research skills, looking through textbooks, scientific journals, and credible internet sources to understand the context of their problem. They learn to differentiate between reliable and unreliable information—a skill with far-reaching implications.
Step 3: Formulating Hypotheses
Armed with information, students then formulate hypotheses. A hypothesis is an educated guess that can be tested through experiments. Encouraging learners to come up with their hypotheses promotes creativity and ownership of the learning process.
Step 4: Conducting Experiments
What sets science apart is its reliance on empirical evidence . In this step, students design and conduct experiments to test their hypotheses. They learn about controls, variables, and the importance of replicability. This hands-on experience is invaluable and often the most engaging part of the approach.
Step 5: Analyzing Data
After the experiment, comes the analysis. Students examine their results, often using statistical methods , to see if the data supports or refutes their hypotheses. This is where critical thinking is paramount, as they must interpret the data without bias.
Step 6: Drawing Conclusions
The final step in the process is drawing conclusions. Here, students evaluate the entirety of their work and determine the implications of their findings. Whether their hypotheses were supported or not, they gain insights into the scientific process and develop the ability to argue their conclusions based on evidence.
The Benefits of Problem Solving in Science Education
This methodology goes beyond knowledge acquisition; it’s about instilling a scientific mindset. Let’s explore how this approach benefits learners:
Develops Higher-Order Thinking Skills
By grappling with complex problems, students develop higher\-order thinking skills such as analysis, synthesis, and evaluation. These are not only vital in science but in everyday decision-making as well.
Encourages Active Learning
Active engagement in learning through problem-solving keeps students invested in their education. They’re not passive receivers of information but active participants in their learning journey.
Promotes Autonomy and Confidence
As students navigate through problems on their own, they build autonomy and confidence in their ability to tackle challenges. This self-assurance can translate to various aspects of their lives.
Fosters a Deeper Understanding of Scientific Principles
By connecting theoretical knowledge to practical problems, students develop a more nuanced understanding of scientific principles. It’s one thing to read about a concept; it’s another to see it in action.
Improves Collaboration Skills
Problem-solving often involves teamwork, allowing students to improve their collaborative skills . They learn to communicate ideas, share tasks, and respect different viewpoints.
Enhances Persistence and Resilience
Not every experiment will go as planned, and not every hypothesis will be correct. Navigating these challenges teaches learners persistence and resilience —qualities that are essential in science and in life.
Bringing Problem Solving Into the Classroom
Integrating the problem-solving approach into science education requires careful planning and a shift in mindset. Teachers become facilitators rather than lecturers, guiding students through the process and providing support when needed. Classrooms become active learning environments where mistakes are seen as learning opportunities.
The problem-solving approach in science education is more than a teaching strategy; it’s a blueprint for developing curious, independent, and analytical thinkers. By engaging learners in this manner, we’re not just teaching them science; we’re equipping them with the tools to solve the complex problems of tomorrow.
What do you think? How can we further encourage problem-solving skills in students from an early age? Do you believe that the problem-solving approach should be applied to other subjects beyond science? Share your thoughts and experiences with this dynamic educational strategy.
How useful was this post?
Click on a star to rate it!
Average rating 0 / 5. Vote count: 0
No votes so far! Be the first to rate this post.
We are sorry that this post was not useful for you!
Let us improve this post!
Tell us how we can improve this post?
Submit a Comment Cancel reply
Your email address will not be published. Required fields are marked *
Save my name, email, and website in this browser for the next time I comment.
Submit Comment
Pedagogy of Science
1 Science – Perspectives and Nature
- Understanding Science
- Myths about Nature of Science
- Understanding Nature of Science
- Domains of Science
2 Aims and Objectives of Science Teaching-Learning
- Aims of Science Education
- Objectives of Science Teaching-Learning
- Developing Learning Objectives
- Shift in Pedagogic Approach
3 Process Skills in Science
- Process Skills in Science
- Basic Process Skills in Science
- Developing Scientific Attitude and Scientific Temper
- Nurturing Aesthetic Sense and Curiosity
- Interdependence of Different Aspects of Nature of Science
4 Science in School Curriculum
- Historical Development of Science Education in India
- Teaching of Science as Recommended in National Curriculum Framework-2005
- Correlation of Science with Other Subjects/Disciplines
5 Organizing Teaching – Learning Experiences
- Linking Process Skills with Content
- Formulating Learning Objectives
- Unit Planning in Science
- Lesson Planning in Science
- Using Laboratory for Teaching-Learning
6 Approaches in Science Teaching – Learning
- Science as a Process of Construction of Knowledge
- Inquiry Approach
- Problem Solving Approach
- Cooperative Learning Approach
- Experiential Learning Approach
- Concept Mapping as an Approach for Planning and Transaction
- Adopting Critical Pedagogy in Science Teaching-Learning
7 Methods in Science Teaching – Learning
- Teacher Centric Methods
- Learner Centric Methods
- Cooperative Learning Methods
- Inclusion in Science Classroom
- Adopting Critical Pedagogy
8 Learning Resources in Science
- Identifying Appropriate Learning Resource
- Various Learning Resources
- Classroom Learning Resources
- ICT as Learning Resource
- Developing Learning Resource Centres
- Importance of Various Activities in Science Teaching-Learning
- Innovations in Science Laboratories
- Role of Innovation and Research in Science
- Professional Development of Science Teachers
9 Assessment in Science
- Nature of Assessment in Science
- Assessment Indicators in Science
- Tools and Techniques for Assessment
- Diagnostics Assessment in Science
- Schemes for Promoting Scientific Attitude
- Components of Food
- How to Get Higher Yields
- Animal Husbandry
11 Material
- Classification of Substances
- States of Material
- Mole Valency and Equivalence
- Types of Chemical Reactions
- Basic Metallurgical Processes
12 The Living World
- Diversity in Plants and Animals
- Nomenclature Scientific Names and Hierarchy
- Cell and Cell Organelles
- Life Processes
13 How Things Work
- Electric Current and Electric Circuit
- Electric Potential and Potential Difference
- Combination of Resistors — Series and Parallel
- Electric Power
- Heating Effects of Electric Current
- Magnetic Effects of Electric Current
- Electric Motor
- Electromagnetic Induction
- Electric Generator
- Domestic Electric Circuits
14 Moving Things, People and Ideas
- Newton’s Law of Motion
- Conservation of Momentum
- Kinetic and Potential Energy
15 Natural Phenomenon
- Light as a Natural Phenomenon
- Water Cycle
- Conservation of Water Bodies
- Natural Disasters
- Waste Management
16 Natural Resources
- Physical Resources and their Utilization
- Pollution and Role of Human Being
- Bio-Geo-Chemical Cycles in Nature
- Natural Resource Management
Share on Mastodon
Thank you for visiting nature.com. You are using a browser version with limited support for CSS. To obtain the best experience, we recommend you use a more up to date browser (or turn off compatibility mode in Internet Explorer). In the meantime, to ensure continued support, we are displaying the site without styles and JavaScript.
- View all journals
- My Account Login
- Explore content
- About the journal
- Publish with us
- Sign up for alerts
- Review Article
- Open access
- Published: 11 January 2023
The effectiveness of collaborative problem solving in promoting students’ critical thinking: A meta-analysis based on empirical literature
- Enwei Xu ORCID: orcid.org/0000-0001-6424-8169 1 ,
- Wei Wang 1 &
- Qingxia Wang 1
Humanities and Social Sciences Communications volume 10 , Article number: 16 ( 2023 ) Cite this article
13k Accesses
12 Citations
3 Altmetric
Metrics details
- Science, technology and society
Collaborative problem-solving has been widely embraced in the classroom instruction of critical thinking, which is regarded as the core of curriculum reform based on key competencies in the field of education as well as a key competence for learners in the 21st century. However, the effectiveness of collaborative problem-solving in promoting students’ critical thinking remains uncertain. This current research presents the major findings of a meta-analysis of 36 pieces of the literature revealed in worldwide educational periodicals during the 21st century to identify the effectiveness of collaborative problem-solving in promoting students’ critical thinking and to determine, based on evidence, whether and to what extent collaborative problem solving can result in a rise or decrease in critical thinking. The findings show that (1) collaborative problem solving is an effective teaching approach to foster students’ critical thinking, with a significant overall effect size (ES = 0.82, z = 12.78, P < 0.01, 95% CI [0.69, 0.95]); (2) in respect to the dimensions of critical thinking, collaborative problem solving can significantly and successfully enhance students’ attitudinal tendencies (ES = 1.17, z = 7.62, P < 0.01, 95% CI[0.87, 1.47]); nevertheless, it falls short in terms of improving students’ cognitive skills, having only an upper-middle impact (ES = 0.70, z = 11.55, P < 0.01, 95% CI[0.58, 0.82]); and (3) the teaching type (chi 2 = 7.20, P < 0.05), intervention duration (chi 2 = 12.18, P < 0.01), subject area (chi 2 = 13.36, P < 0.05), group size (chi 2 = 8.77, P < 0.05), and learning scaffold (chi 2 = 9.03, P < 0.01) all have an impact on critical thinking, and they can be viewed as important moderating factors that affect how critical thinking develops. On the basis of these results, recommendations are made for further study and instruction to better support students’ critical thinking in the context of collaborative problem-solving.
Similar content being viewed by others
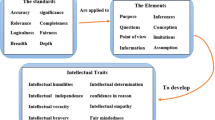
Fostering twenty-first century skills among primary school students through math project-based learning
Nadia Rehman, Wenlan Zhang, … Samia Batool
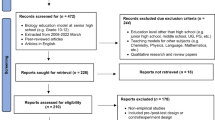
A meta-analysis to gauge the impact of pedagogies employed in mixed-ability high school biology classrooms
Malavika E. Santhosh, Jolly Bhadra, … Noora Al-Thani
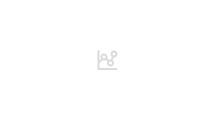
A guide to critical thinking: implications for dental education
Deborah Martin
Introduction
Although critical thinking has a long history in research, the concept of critical thinking, which is regarded as an essential competence for learners in the 21st century, has recently attracted more attention from researchers and teaching practitioners (National Research Council, 2012 ). Critical thinking should be the core of curriculum reform based on key competencies in the field of education (Peng and Deng, 2017 ) because students with critical thinking can not only understand the meaning of knowledge but also effectively solve practical problems in real life even after knowledge is forgotten (Kek and Huijser, 2011 ). The definition of critical thinking is not universal (Ennis, 1989 ; Castle, 2009 ; Niu et al., 2013 ). In general, the definition of critical thinking is a self-aware and self-regulated thought process (Facione, 1990 ; Niu et al., 2013 ). It refers to the cognitive skills needed to interpret, analyze, synthesize, reason, and evaluate information as well as the attitudinal tendency to apply these abilities (Halpern, 2001 ). The view that critical thinking can be taught and learned through curriculum teaching has been widely supported by many researchers (e.g., Kuncel, 2011 ; Leng and Lu, 2020 ), leading to educators’ efforts to foster it among students. In the field of teaching practice, there are three types of courses for teaching critical thinking (Ennis, 1989 ). The first is an independent curriculum in which critical thinking is taught and cultivated without involving the knowledge of specific disciplines; the second is an integrated curriculum in which critical thinking is integrated into the teaching of other disciplines as a clear teaching goal; and the third is a mixed curriculum in which critical thinking is taught in parallel to the teaching of other disciplines for mixed teaching training. Furthermore, numerous measuring tools have been developed by researchers and educators to measure critical thinking in the context of teaching practice. These include standardized measurement tools, such as WGCTA, CCTST, CCTT, and CCTDI, which have been verified by repeated experiments and are considered effective and reliable by international scholars (Facione and Facione, 1992 ). In short, descriptions of critical thinking, including its two dimensions of attitudinal tendency and cognitive skills, different types of teaching courses, and standardized measurement tools provide a complex normative framework for understanding, teaching, and evaluating critical thinking.
Cultivating critical thinking in curriculum teaching can start with a problem, and one of the most popular critical thinking instructional approaches is problem-based learning (Liu et al., 2020 ). Duch et al. ( 2001 ) noted that problem-based learning in group collaboration is progressive active learning, which can improve students’ critical thinking and problem-solving skills. Collaborative problem-solving is the organic integration of collaborative learning and problem-based learning, which takes learners as the center of the learning process and uses problems with poor structure in real-world situations as the starting point for the learning process (Liang et al., 2017 ). Students learn the knowledge needed to solve problems in a collaborative group, reach a consensus on problems in the field, and form solutions through social cooperation methods, such as dialogue, interpretation, questioning, debate, negotiation, and reflection, thus promoting the development of learners’ domain knowledge and critical thinking (Cindy, 2004 ; Liang et al., 2017 ).
Collaborative problem-solving has been widely used in the teaching practice of critical thinking, and several studies have attempted to conduct a systematic review and meta-analysis of the empirical literature on critical thinking from various perspectives. However, little attention has been paid to the impact of collaborative problem-solving on critical thinking. Therefore, the best approach for developing and enhancing critical thinking throughout collaborative problem-solving is to examine how to implement critical thinking instruction; however, this issue is still unexplored, which means that many teachers are incapable of better instructing critical thinking (Leng and Lu, 2020 ; Niu et al., 2013 ). For example, Huber ( 2016 ) provided the meta-analysis findings of 71 publications on gaining critical thinking over various time frames in college with the aim of determining whether critical thinking was truly teachable. These authors found that learners significantly improve their critical thinking while in college and that critical thinking differs with factors such as teaching strategies, intervention duration, subject area, and teaching type. The usefulness of collaborative problem-solving in fostering students’ critical thinking, however, was not determined by this study, nor did it reveal whether there existed significant variations among the different elements. A meta-analysis of 31 pieces of educational literature was conducted by Liu et al. ( 2020 ) to assess the impact of problem-solving on college students’ critical thinking. These authors found that problem-solving could promote the development of critical thinking among college students and proposed establishing a reasonable group structure for problem-solving in a follow-up study to improve students’ critical thinking. Additionally, previous empirical studies have reached inconclusive and even contradictory conclusions about whether and to what extent collaborative problem-solving increases or decreases critical thinking levels. As an illustration, Yang et al. ( 2008 ) carried out an experiment on the integrated curriculum teaching of college students based on a web bulletin board with the goal of fostering participants’ critical thinking in the context of collaborative problem-solving. These authors’ research revealed that through sharing, debating, examining, and reflecting on various experiences and ideas, collaborative problem-solving can considerably enhance students’ critical thinking in real-life problem situations. In contrast, collaborative problem-solving had a positive impact on learners’ interaction and could improve learning interest and motivation but could not significantly improve students’ critical thinking when compared to traditional classroom teaching, according to research by Naber and Wyatt ( 2014 ) and Sendag and Odabasi ( 2009 ) on undergraduate and high school students, respectively.
The above studies show that there is inconsistency regarding the effectiveness of collaborative problem-solving in promoting students’ critical thinking. Therefore, it is essential to conduct a thorough and trustworthy review to detect and decide whether and to what degree collaborative problem-solving can result in a rise or decrease in critical thinking. Meta-analysis is a quantitative analysis approach that is utilized to examine quantitative data from various separate studies that are all focused on the same research topic. This approach characterizes the effectiveness of its impact by averaging the effect sizes of numerous qualitative studies in an effort to reduce the uncertainty brought on by independent research and produce more conclusive findings (Lipsey and Wilson, 2001 ).
This paper used a meta-analytic approach and carried out a meta-analysis to examine the effectiveness of collaborative problem-solving in promoting students’ critical thinking in order to make a contribution to both research and practice. The following research questions were addressed by this meta-analysis:
What is the overall effect size of collaborative problem-solving in promoting students’ critical thinking and its impact on the two dimensions of critical thinking (i.e., attitudinal tendency and cognitive skills)?
How are the disparities between the study conclusions impacted by various moderating variables if the impacts of various experimental designs in the included studies are heterogeneous?
This research followed the strict procedures (e.g., database searching, identification, screening, eligibility, merging, duplicate removal, and analysis of included studies) of Cooper’s ( 2010 ) proposed meta-analysis approach for examining quantitative data from various separate studies that are all focused on the same research topic. The relevant empirical research that appeared in worldwide educational periodicals within the 21st century was subjected to this meta-analysis using Rev-Man 5.4. The consistency of the data extracted separately by two researchers was tested using Cohen’s kappa coefficient, and a publication bias test and a heterogeneity test were run on the sample data to ascertain the quality of this meta-analysis.
Data sources and search strategies
There were three stages to the data collection process for this meta-analysis, as shown in Fig. 1 , which shows the number of articles included and eliminated during the selection process based on the statement and study eligibility criteria.
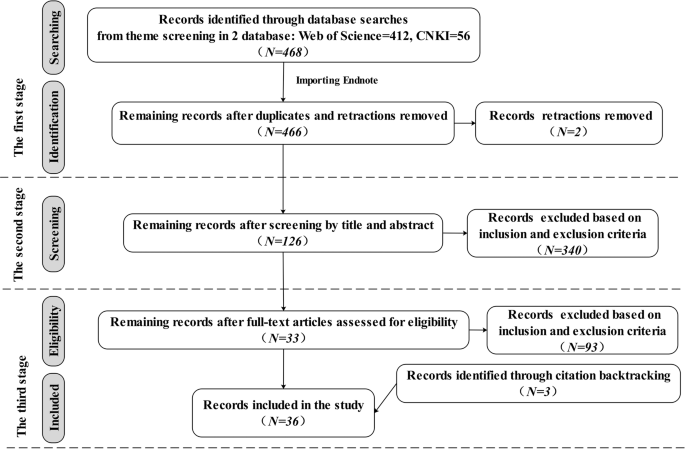
This flowchart shows the number of records identified, included and excluded in the article.
First, the databases used to systematically search for relevant articles were the journal papers of the Web of Science Core Collection and the Chinese Core source journal, as well as the Chinese Social Science Citation Index (CSSCI) source journal papers included in CNKI. These databases were selected because they are credible platforms that are sources of scholarly and peer-reviewed information with advanced search tools and contain literature relevant to the subject of our topic from reliable researchers and experts. The search string with the Boolean operator used in the Web of Science was “TS = (((“critical thinking” or “ct” and “pretest” or “posttest”) or (“critical thinking” or “ct” and “control group” or “quasi experiment” or “experiment”)) and (“collaboration” or “collaborative learning” or “CSCL”) and (“problem solving” or “problem-based learning” or “PBL”))”. The research area was “Education Educational Research”, and the search period was “January 1, 2000, to December 30, 2021”. A total of 412 papers were obtained. The search string with the Boolean operator used in the CNKI was “SU = (‘critical thinking’*‘collaboration’ + ‘critical thinking’*‘collaborative learning’ + ‘critical thinking’*‘CSCL’ + ‘critical thinking’*‘problem solving’ + ‘critical thinking’*‘problem-based learning’ + ‘critical thinking’*‘PBL’ + ‘critical thinking’*‘problem oriented’) AND FT = (‘experiment’ + ‘quasi experiment’ + ‘pretest’ + ‘posttest’ + ‘empirical study’)” (translated into Chinese when searching). A total of 56 studies were found throughout the search period of “January 2000 to December 2021”. From the databases, all duplicates and retractions were eliminated before exporting the references into Endnote, a program for managing bibliographic references. In all, 466 studies were found.
Second, the studies that matched the inclusion and exclusion criteria for the meta-analysis were chosen by two researchers after they had reviewed the abstracts and titles of the gathered articles, yielding a total of 126 studies.
Third, two researchers thoroughly reviewed each included article’s whole text in accordance with the inclusion and exclusion criteria. Meanwhile, a snowball search was performed using the references and citations of the included articles to ensure complete coverage of the articles. Ultimately, 36 articles were kept.
Two researchers worked together to carry out this entire process, and a consensus rate of almost 94.7% was reached after discussion and negotiation to clarify any emerging differences.
Eligibility criteria
Since not all the retrieved studies matched the criteria for this meta-analysis, eligibility criteria for both inclusion and exclusion were developed as follows:
The publication language of the included studies was limited to English and Chinese, and the full text could be obtained. Articles that did not meet the publication language and articles not published between 2000 and 2021 were excluded.
The research design of the included studies must be empirical and quantitative studies that can assess the effect of collaborative problem-solving on the development of critical thinking. Articles that could not identify the causal mechanisms by which collaborative problem-solving affects critical thinking, such as review articles and theoretical articles, were excluded.
The research method of the included studies must feature a randomized control experiment or a quasi-experiment, or a natural experiment, which have a higher degree of internal validity with strong experimental designs and can all plausibly provide evidence that critical thinking and collaborative problem-solving are causally related. Articles with non-experimental research methods, such as purely correlational or observational studies, were excluded.
The participants of the included studies were only students in school, including K-12 students and college students. Articles in which the participants were non-school students, such as social workers or adult learners, were excluded.
The research results of the included studies must mention definite signs that may be utilized to gauge critical thinking’s impact (e.g., sample size, mean value, or standard deviation). Articles that lacked specific measurement indicators for critical thinking and could not calculate the effect size were excluded.
Data coding design
In order to perform a meta-analysis, it is necessary to collect the most important information from the articles, codify that information’s properties, and convert descriptive data into quantitative data. Therefore, this study designed a data coding template (see Table 1 ). Ultimately, 16 coding fields were retained.
The designed data-coding template consisted of three pieces of information. Basic information about the papers was included in the descriptive information: the publishing year, author, serial number, and title of the paper.
The variable information for the experimental design had three variables: the independent variable (instruction method), the dependent variable (critical thinking), and the moderating variable (learning stage, teaching type, intervention duration, learning scaffold, group size, measuring tool, and subject area). Depending on the topic of this study, the intervention strategy, as the independent variable, was coded into collaborative and non-collaborative problem-solving. The dependent variable, critical thinking, was coded as a cognitive skill and an attitudinal tendency. And seven moderating variables were created by grouping and combining the experimental design variables discovered within the 36 studies (see Table 1 ), where learning stages were encoded as higher education, high school, middle school, and primary school or lower; teaching types were encoded as mixed courses, integrated courses, and independent courses; intervention durations were encoded as 0–1 weeks, 1–4 weeks, 4–12 weeks, and more than 12 weeks; group sizes were encoded as 2–3 persons, 4–6 persons, 7–10 persons, and more than 10 persons; learning scaffolds were encoded as teacher-supported learning scaffold, technique-supported learning scaffold, and resource-supported learning scaffold; measuring tools were encoded as standardized measurement tools (e.g., WGCTA, CCTT, CCTST, and CCTDI) and self-adapting measurement tools (e.g., modified or made by researchers); and subject areas were encoded according to the specific subjects used in the 36 included studies.
The data information contained three metrics for measuring critical thinking: sample size, average value, and standard deviation. It is vital to remember that studies with various experimental designs frequently adopt various formulas to determine the effect size. And this paper used Morris’ proposed standardized mean difference (SMD) calculation formula ( 2008 , p. 369; see Supplementary Table S3 ).
Procedure for extracting and coding data
According to the data coding template (see Table 1 ), the 36 papers’ information was retrieved by two researchers, who then entered them into Excel (see Supplementary Table S1 ). The results of each study were extracted separately in the data extraction procedure if an article contained numerous studies on critical thinking, or if a study assessed different critical thinking dimensions. For instance, Tiwari et al. ( 2010 ) used four time points, which were viewed as numerous different studies, to examine the outcomes of critical thinking, and Chen ( 2013 ) included the two outcome variables of attitudinal tendency and cognitive skills, which were regarded as two studies. After discussion and negotiation during data extraction, the two researchers’ consistency test coefficients were roughly 93.27%. Supplementary Table S2 details the key characteristics of the 36 included articles with 79 effect quantities, including descriptive information (e.g., the publishing year, author, serial number, and title of the paper), variable information (e.g., independent variables, dependent variables, and moderating variables), and data information (e.g., mean values, standard deviations, and sample size). Following that, testing for publication bias and heterogeneity was done on the sample data using the Rev-Man 5.4 software, and then the test results were used to conduct a meta-analysis.
Publication bias test
When the sample of studies included in a meta-analysis does not accurately reflect the general status of research on the relevant subject, publication bias is said to be exhibited in this research. The reliability and accuracy of the meta-analysis may be impacted by publication bias. Due to this, the meta-analysis needs to check the sample data for publication bias (Stewart et al., 2006 ). A popular method to check for publication bias is the funnel plot; and it is unlikely that there will be publishing bias when the data are equally dispersed on either side of the average effect size and targeted within the higher region. The data are equally dispersed within the higher portion of the efficient zone, consistent with the funnel plot connected with this analysis (see Fig. 2 ), indicating that publication bias is unlikely in this situation.
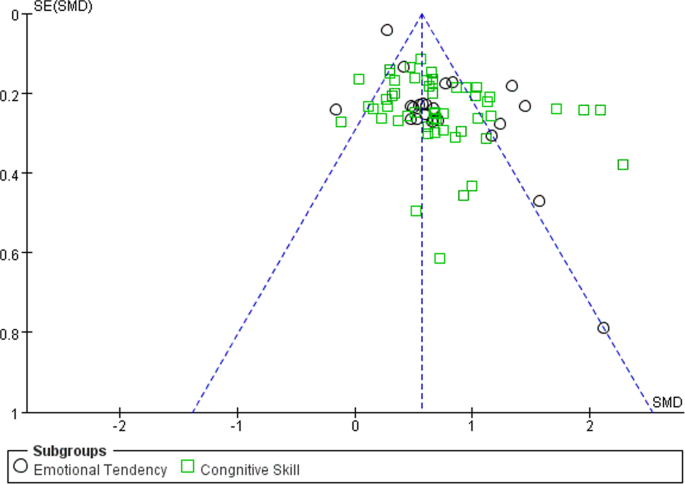
This funnel plot shows the result of publication bias of 79 effect quantities across 36 studies.
Heterogeneity test
To select the appropriate effect models for the meta-analysis, one might use the results of a heterogeneity test on the data effect sizes. In a meta-analysis, it is common practice to gauge the degree of data heterogeneity using the I 2 value, and I 2 ≥ 50% is typically understood to denote medium-high heterogeneity, which calls for the adoption of a random effect model; if not, a fixed effect model ought to be applied (Lipsey and Wilson, 2001 ). The findings of the heterogeneity test in this paper (see Table 2 ) revealed that I 2 was 86% and displayed significant heterogeneity ( P < 0.01). To ensure accuracy and reliability, the overall effect size ought to be calculated utilizing the random effect model.
The analysis of the overall effect size
This meta-analysis utilized a random effect model to examine 79 effect quantities from 36 studies after eliminating heterogeneity. In accordance with Cohen’s criterion (Cohen, 1992 ), it is abundantly clear from the analysis results, which are shown in the forest plot of the overall effect (see Fig. 3 ), that the cumulative impact size of cooperative problem-solving is 0.82, which is statistically significant ( z = 12.78, P < 0.01, 95% CI [0.69, 0.95]), and can encourage learners to practice critical thinking.
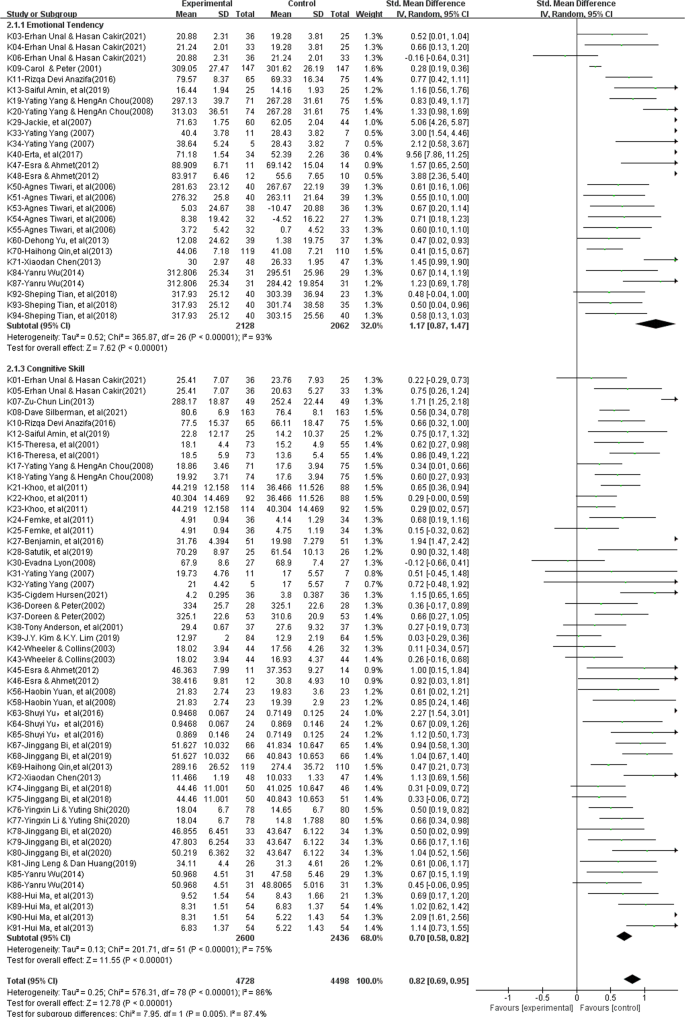
This forest plot shows the analysis result of the overall effect size across 36 studies.
In addition, this study examined two distinct dimensions of critical thinking to better understand the precise contributions that collaborative problem-solving makes to the growth of critical thinking. The findings (see Table 3 ) indicate that collaborative problem-solving improves cognitive skills (ES = 0.70) and attitudinal tendency (ES = 1.17), with significant intergroup differences (chi 2 = 7.95, P < 0.01). Although collaborative problem-solving improves both dimensions of critical thinking, it is essential to point out that the improvements in students’ attitudinal tendency are much more pronounced and have a significant comprehensive effect (ES = 1.17, z = 7.62, P < 0.01, 95% CI [0.87, 1.47]), whereas gains in learners’ cognitive skill are slightly improved and are just above average. (ES = 0.70, z = 11.55, P < 0.01, 95% CI [0.58, 0.82]).
The analysis of moderator effect size
The whole forest plot’s 79 effect quantities underwent a two-tailed test, which revealed significant heterogeneity ( I 2 = 86%, z = 12.78, P < 0.01), indicating differences between various effect sizes that may have been influenced by moderating factors other than sampling error. Therefore, exploring possible moderating factors that might produce considerable heterogeneity was done using subgroup analysis, such as the learning stage, learning scaffold, teaching type, group size, duration of the intervention, measuring tool, and the subject area included in the 36 experimental designs, in order to further explore the key factors that influence critical thinking. The findings (see Table 4 ) indicate that various moderating factors have advantageous effects on critical thinking. In this situation, the subject area (chi 2 = 13.36, P < 0.05), group size (chi 2 = 8.77, P < 0.05), intervention duration (chi 2 = 12.18, P < 0.01), learning scaffold (chi 2 = 9.03, P < 0.01), and teaching type (chi 2 = 7.20, P < 0.05) are all significant moderators that can be applied to support the cultivation of critical thinking. However, since the learning stage and the measuring tools did not significantly differ among intergroup (chi 2 = 3.15, P = 0.21 > 0.05, and chi 2 = 0.08, P = 0.78 > 0.05), we are unable to explain why these two factors are crucial in supporting the cultivation of critical thinking in the context of collaborative problem-solving. These are the precise outcomes, as follows:
Various learning stages influenced critical thinking positively, without significant intergroup differences (chi 2 = 3.15, P = 0.21 > 0.05). High school was first on the list of effect sizes (ES = 1.36, P < 0.01), then higher education (ES = 0.78, P < 0.01), and middle school (ES = 0.73, P < 0.01). These results show that, despite the learning stage’s beneficial influence on cultivating learners’ critical thinking, we are unable to explain why it is essential for cultivating critical thinking in the context of collaborative problem-solving.
Different teaching types had varying degrees of positive impact on critical thinking, with significant intergroup differences (chi 2 = 7.20, P < 0.05). The effect size was ranked as follows: mixed courses (ES = 1.34, P < 0.01), integrated courses (ES = 0.81, P < 0.01), and independent courses (ES = 0.27, P < 0.01). These results indicate that the most effective approach to cultivate critical thinking utilizing collaborative problem solving is through the teaching type of mixed courses.
Various intervention durations significantly improved critical thinking, and there were significant intergroup differences (chi 2 = 12.18, P < 0.01). The effect sizes related to this variable showed a tendency to increase with longer intervention durations. The improvement in critical thinking reached a significant level (ES = 0.85, P < 0.01) after more than 12 weeks of training. These findings indicate that the intervention duration and critical thinking’s impact are positively correlated, with a longer intervention duration having a greater effect.
Different learning scaffolds influenced critical thinking positively, with significant intergroup differences (chi 2 = 9.03, P < 0.01). The resource-supported learning scaffold (ES = 0.69, P < 0.01) acquired a medium-to-higher level of impact, the technique-supported learning scaffold (ES = 0.63, P < 0.01) also attained a medium-to-higher level of impact, and the teacher-supported learning scaffold (ES = 0.92, P < 0.01) displayed a high level of significant impact. These results show that the learning scaffold with teacher support has the greatest impact on cultivating critical thinking.
Various group sizes influenced critical thinking positively, and the intergroup differences were statistically significant (chi 2 = 8.77, P < 0.05). Critical thinking showed a general declining trend with increasing group size. The overall effect size of 2–3 people in this situation was the biggest (ES = 0.99, P < 0.01), and when the group size was greater than 7 people, the improvement in critical thinking was at the lower-middle level (ES < 0.5, P < 0.01). These results show that the impact on critical thinking is positively connected with group size, and as group size grows, so does the overall impact.
Various measuring tools influenced critical thinking positively, with significant intergroup differences (chi 2 = 0.08, P = 0.78 > 0.05). In this situation, the self-adapting measurement tools obtained an upper-medium level of effect (ES = 0.78), whereas the complete effect size of the standardized measurement tools was the largest, achieving a significant level of effect (ES = 0.84, P < 0.01). These results show that, despite the beneficial influence of the measuring tool on cultivating critical thinking, we are unable to explain why it is crucial in fostering the growth of critical thinking by utilizing the approach of collaborative problem-solving.
Different subject areas had a greater impact on critical thinking, and the intergroup differences were statistically significant (chi 2 = 13.36, P < 0.05). Mathematics had the greatest overall impact, achieving a significant level of effect (ES = 1.68, P < 0.01), followed by science (ES = 1.25, P < 0.01) and medical science (ES = 0.87, P < 0.01), both of which also achieved a significant level of effect. Programming technology was the least effective (ES = 0.39, P < 0.01), only having a medium-low degree of effect compared to education (ES = 0.72, P < 0.01) and other fields (such as language, art, and social sciences) (ES = 0.58, P < 0.01). These results suggest that scientific fields (e.g., mathematics, science) may be the most effective subject areas for cultivating critical thinking utilizing the approach of collaborative problem-solving.
The effectiveness of collaborative problem solving with regard to teaching critical thinking
According to this meta-analysis, using collaborative problem-solving as an intervention strategy in critical thinking teaching has a considerable amount of impact on cultivating learners’ critical thinking as a whole and has a favorable promotional effect on the two dimensions of critical thinking. According to certain studies, collaborative problem solving, the most frequently used critical thinking teaching strategy in curriculum instruction can considerably enhance students’ critical thinking (e.g., Liang et al., 2017 ; Liu et al., 2020 ; Cindy, 2004 ). This meta-analysis provides convergent data support for the above research views. Thus, the findings of this meta-analysis not only effectively address the first research query regarding the overall effect of cultivating critical thinking and its impact on the two dimensions of critical thinking (i.e., attitudinal tendency and cognitive skills) utilizing the approach of collaborative problem-solving, but also enhance our confidence in cultivating critical thinking by using collaborative problem-solving intervention approach in the context of classroom teaching.
Furthermore, the associated improvements in attitudinal tendency are much stronger, but the corresponding improvements in cognitive skill are only marginally better. According to certain studies, cognitive skill differs from the attitudinal tendency in classroom instruction; the cultivation and development of the former as a key ability is a process of gradual accumulation, while the latter as an attitude is affected by the context of the teaching situation (e.g., a novel and exciting teaching approach, challenging and rewarding tasks) (Halpern, 2001 ; Wei and Hong, 2022 ). Collaborative problem-solving as a teaching approach is exciting and interesting, as well as rewarding and challenging; because it takes the learners as the focus and examines problems with poor structure in real situations, and it can inspire students to fully realize their potential for problem-solving, which will significantly improve their attitudinal tendency toward solving problems (Liu et al., 2020 ). Similar to how collaborative problem-solving influences attitudinal tendency, attitudinal tendency impacts cognitive skill when attempting to solve a problem (Liu et al., 2020 ; Zhang et al., 2022 ), and stronger attitudinal tendencies are associated with improved learning achievement and cognitive ability in students (Sison, 2008 ; Zhang et al., 2022 ). It can be seen that the two specific dimensions of critical thinking as well as critical thinking as a whole are affected by collaborative problem-solving, and this study illuminates the nuanced links between cognitive skills and attitudinal tendencies with regard to these two dimensions of critical thinking. To fully develop students’ capacity for critical thinking, future empirical research should pay closer attention to cognitive skills.
The moderating effects of collaborative problem solving with regard to teaching critical thinking
In order to further explore the key factors that influence critical thinking, exploring possible moderating effects that might produce considerable heterogeneity was done using subgroup analysis. The findings show that the moderating factors, such as the teaching type, learning stage, group size, learning scaffold, duration of the intervention, measuring tool, and the subject area included in the 36 experimental designs, could all support the cultivation of collaborative problem-solving in critical thinking. Among them, the effect size differences between the learning stage and measuring tool are not significant, which does not explain why these two factors are crucial in supporting the cultivation of critical thinking utilizing the approach of collaborative problem-solving.
In terms of the learning stage, various learning stages influenced critical thinking positively without significant intergroup differences, indicating that we are unable to explain why it is crucial in fostering the growth of critical thinking.
Although high education accounts for 70.89% of all empirical studies performed by researchers, high school may be the appropriate learning stage to foster students’ critical thinking by utilizing the approach of collaborative problem-solving since it has the largest overall effect size. This phenomenon may be related to student’s cognitive development, which needs to be further studied in follow-up research.
With regard to teaching type, mixed course teaching may be the best teaching method to cultivate students’ critical thinking. Relevant studies have shown that in the actual teaching process if students are trained in thinking methods alone, the methods they learn are isolated and divorced from subject knowledge, which is not conducive to their transfer of thinking methods; therefore, if students’ thinking is trained only in subject teaching without systematic method training, it is challenging to apply to real-world circumstances (Ruggiero, 2012 ; Hu and Liu, 2015 ). Teaching critical thinking as mixed course teaching in parallel to other subject teachings can achieve the best effect on learners’ critical thinking, and explicit critical thinking instruction is more effective than less explicit critical thinking instruction (Bensley and Spero, 2014 ).
In terms of the intervention duration, with longer intervention times, the overall effect size shows an upward tendency. Thus, the intervention duration and critical thinking’s impact are positively correlated. Critical thinking, as a key competency for students in the 21st century, is difficult to get a meaningful improvement in a brief intervention duration. Instead, it could be developed over a lengthy period of time through consistent teaching and the progressive accumulation of knowledge (Halpern, 2001 ; Hu and Liu, 2015 ). Therefore, future empirical studies ought to take these restrictions into account throughout a longer period of critical thinking instruction.
With regard to group size, a group size of 2–3 persons has the highest effect size, and the comprehensive effect size decreases with increasing group size in general. This outcome is in line with some research findings; as an example, a group composed of two to four members is most appropriate for collaborative learning (Schellens and Valcke, 2006 ). However, the meta-analysis results also indicate that once the group size exceeds 7 people, small groups cannot produce better interaction and performance than large groups. This may be because the learning scaffolds of technique support, resource support, and teacher support improve the frequency and effectiveness of interaction among group members, and a collaborative group with more members may increase the diversity of views, which is helpful to cultivate critical thinking utilizing the approach of collaborative problem-solving.
With regard to the learning scaffold, the three different kinds of learning scaffolds can all enhance critical thinking. Among them, the teacher-supported learning scaffold has the largest overall effect size, demonstrating the interdependence of effective learning scaffolds and collaborative problem-solving. This outcome is in line with some research findings; as an example, a successful strategy is to encourage learners to collaborate, come up with solutions, and develop critical thinking skills by using learning scaffolds (Reiser, 2004 ; Xu et al., 2022 ); learning scaffolds can lower task complexity and unpleasant feelings while also enticing students to engage in learning activities (Wood et al., 2006 ); learning scaffolds are designed to assist students in using learning approaches more successfully to adapt the collaborative problem-solving process, and the teacher-supported learning scaffolds have the greatest influence on critical thinking in this process because they are more targeted, informative, and timely (Xu et al., 2022 ).
With respect to the measuring tool, despite the fact that standardized measurement tools (such as the WGCTA, CCTT, and CCTST) have been acknowledged as trustworthy and effective by worldwide experts, only 54.43% of the research included in this meta-analysis adopted them for assessment, and the results indicated no intergroup differences. These results suggest that not all teaching circumstances are appropriate for measuring critical thinking using standardized measurement tools. “The measuring tools for measuring thinking ability have limits in assessing learners in educational situations and should be adapted appropriately to accurately assess the changes in learners’ critical thinking.”, according to Simpson and Courtney ( 2002 , p. 91). As a result, in order to more fully and precisely gauge how learners’ critical thinking has evolved, we must properly modify standardized measuring tools based on collaborative problem-solving learning contexts.
With regard to the subject area, the comprehensive effect size of science departments (e.g., mathematics, science, medical science) is larger than that of language arts and social sciences. Some recent international education reforms have noted that critical thinking is a basic part of scientific literacy. Students with scientific literacy can prove the rationality of their judgment according to accurate evidence and reasonable standards when they face challenges or poorly structured problems (Kyndt et al., 2013 ), which makes critical thinking crucial for developing scientific understanding and applying this understanding to practical problem solving for problems related to science, technology, and society (Yore et al., 2007 ).
Suggestions for critical thinking teaching
Other than those stated in the discussion above, the following suggestions are offered for critical thinking instruction utilizing the approach of collaborative problem-solving.
First, teachers should put a special emphasis on the two core elements, which are collaboration and problem-solving, to design real problems based on collaborative situations. This meta-analysis provides evidence to support the view that collaborative problem-solving has a strong synergistic effect on promoting students’ critical thinking. Asking questions about real situations and allowing learners to take part in critical discussions on real problems during class instruction are key ways to teach critical thinking rather than simply reading speculative articles without practice (Mulnix, 2012 ). Furthermore, the improvement of students’ critical thinking is realized through cognitive conflict with other learners in the problem situation (Yang et al., 2008 ). Consequently, it is essential for teachers to put a special emphasis on the two core elements, which are collaboration and problem-solving, and design real problems and encourage students to discuss, negotiate, and argue based on collaborative problem-solving situations.
Second, teachers should design and implement mixed courses to cultivate learners’ critical thinking, utilizing the approach of collaborative problem-solving. Critical thinking can be taught through curriculum instruction (Kuncel, 2011 ; Leng and Lu, 2020 ), with the goal of cultivating learners’ critical thinking for flexible transfer and application in real problem-solving situations. This meta-analysis shows that mixed course teaching has a highly substantial impact on the cultivation and promotion of learners’ critical thinking. Therefore, teachers should design and implement mixed course teaching with real collaborative problem-solving situations in combination with the knowledge content of specific disciplines in conventional teaching, teach methods and strategies of critical thinking based on poorly structured problems to help students master critical thinking, and provide practical activities in which students can interact with each other to develop knowledge construction and critical thinking utilizing the approach of collaborative problem-solving.
Third, teachers should be more trained in critical thinking, particularly preservice teachers, and they also should be conscious of the ways in which teachers’ support for learning scaffolds can promote critical thinking. The learning scaffold supported by teachers had the greatest impact on learners’ critical thinking, in addition to being more directive, targeted, and timely (Wood et al., 2006 ). Critical thinking can only be effectively taught when teachers recognize the significance of critical thinking for students’ growth and use the proper approaches while designing instructional activities (Forawi, 2016 ). Therefore, with the intention of enabling teachers to create learning scaffolds to cultivate learners’ critical thinking utilizing the approach of collaborative problem solving, it is essential to concentrate on the teacher-supported learning scaffolds and enhance the instruction for teaching critical thinking to teachers, especially preservice teachers.
Implications and limitations
There are certain limitations in this meta-analysis, but future research can correct them. First, the search languages were restricted to English and Chinese, so it is possible that pertinent studies that were written in other languages were overlooked, resulting in an inadequate number of articles for review. Second, these data provided by the included studies are partially missing, such as whether teachers were trained in the theory and practice of critical thinking, the average age and gender of learners, and the differences in critical thinking among learners of various ages and genders. Third, as is typical for review articles, more studies were released while this meta-analysis was being done; therefore, it had a time limit. With the development of relevant research, future studies focusing on these issues are highly relevant and needed.
Conclusions
The subject of the magnitude of collaborative problem-solving’s impact on fostering students’ critical thinking, which received scant attention from other studies, was successfully addressed by this study. The question of the effectiveness of collaborative problem-solving in promoting students’ critical thinking was addressed in this study, which addressed a topic that had gotten little attention in earlier research. The following conclusions can be made:
Regarding the results obtained, collaborative problem solving is an effective teaching approach to foster learners’ critical thinking, with a significant overall effect size (ES = 0.82, z = 12.78, P < 0.01, 95% CI [0.69, 0.95]). With respect to the dimensions of critical thinking, collaborative problem-solving can significantly and effectively improve students’ attitudinal tendency, and the comprehensive effect is significant (ES = 1.17, z = 7.62, P < 0.01, 95% CI [0.87, 1.47]); nevertheless, it falls short in terms of improving students’ cognitive skills, having only an upper-middle impact (ES = 0.70, z = 11.55, P < 0.01, 95% CI [0.58, 0.82]).
As demonstrated by both the results and the discussion, there are varying degrees of beneficial effects on students’ critical thinking from all seven moderating factors, which were found across 36 studies. In this context, the teaching type (chi 2 = 7.20, P < 0.05), intervention duration (chi 2 = 12.18, P < 0.01), subject area (chi 2 = 13.36, P < 0.05), group size (chi 2 = 8.77, P < 0.05), and learning scaffold (chi 2 = 9.03, P < 0.01) all have a positive impact on critical thinking, and they can be viewed as important moderating factors that affect how critical thinking develops. Since the learning stage (chi 2 = 3.15, P = 0.21 > 0.05) and measuring tools (chi 2 = 0.08, P = 0.78 > 0.05) did not demonstrate any significant intergroup differences, we are unable to explain why these two factors are crucial in supporting the cultivation of critical thinking in the context of collaborative problem-solving.
Data availability
All data generated or analyzed during this study are included within the article and its supplementary information files, and the supplementary information files are available in the Dataverse repository: https://doi.org/10.7910/DVN/IPFJO6 .
Bensley DA, Spero RA (2014) Improving critical thinking skills and meta-cognitive monitoring through direct infusion. Think Skills Creat 12:55–68. https://doi.org/10.1016/j.tsc.2014.02.001
Article Google Scholar
Castle A (2009) Defining and assessing critical thinking skills for student radiographers. Radiography 15(1):70–76. https://doi.org/10.1016/j.radi.2007.10.007
Chen XD (2013) An empirical study on the influence of PBL teaching model on critical thinking ability of non-English majors. J PLA Foreign Lang College 36 (04):68–72
Google Scholar
Cohen A (1992) Antecedents of organizational commitment across occupational groups: a meta-analysis. J Organ Behav. https://doi.org/10.1002/job.4030130602
Cooper H (2010) Research synthesis and meta-analysis: a step-by-step approach, 4th edn. Sage, London, England
Cindy HS (2004) Problem-based learning: what and how do students learn? Educ Psychol Rev 51(1):31–39
Duch BJ, Gron SD, Allen DE (2001) The power of problem-based learning: a practical “how to” for teaching undergraduate courses in any discipline. Stylus Educ Sci 2:190–198
Ennis RH (1989) Critical thinking and subject specificity: clarification and needed research. Educ Res 18(3):4–10. https://doi.org/10.3102/0013189x018003004
Facione PA (1990) Critical thinking: a statement of expert consensus for purposes of educational assessment and instruction. Research findings and recommendations. Eric document reproduction service. https://eric.ed.gov/?id=ed315423
Facione PA, Facione NC (1992) The California Critical Thinking Dispositions Inventory (CCTDI) and the CCTDI test manual. California Academic Press, Millbrae, CA
Forawi SA (2016) Standard-based science education and critical thinking. Think Skills Creat 20:52–62. https://doi.org/10.1016/j.tsc.2016.02.005
Halpern DF (2001) Assessing the effectiveness of critical thinking instruction. J Gen Educ 50(4):270–286. https://doi.org/10.2307/27797889
Hu WP, Liu J (2015) Cultivation of pupils’ thinking ability: a five-year follow-up study. Psychol Behav Res 13(05):648–654. https://doi.org/10.3969/j.issn.1672-0628.2015.05.010
Huber K (2016) Does college teach critical thinking? A meta-analysis. Rev Educ Res 86(2):431–468. https://doi.org/10.3102/0034654315605917
Kek MYCA, Huijser H (2011) The power of problem-based learning in developing critical thinking skills: preparing students for tomorrow’s digital futures in today’s classrooms. High Educ Res Dev 30(3):329–341. https://doi.org/10.1080/07294360.2010.501074
Kuncel NR (2011) Measurement and meaning of critical thinking (Research report for the NRC 21st Century Skills Workshop). National Research Council, Washington, DC
Kyndt E, Raes E, Lismont B, Timmers F, Cascallar E, Dochy F (2013) A meta-analysis of the effects of face-to-face cooperative learning. Do recent studies falsify or verify earlier findings? Educ Res Rev 10(2):133–149. https://doi.org/10.1016/j.edurev.2013.02.002
Leng J, Lu XX (2020) Is critical thinking really teachable?—A meta-analysis based on 79 experimental or quasi experimental studies. Open Educ Res 26(06):110–118. https://doi.org/10.13966/j.cnki.kfjyyj.2020.06.011
Liang YZ, Zhu K, Zhao CL (2017) An empirical study on the depth of interaction promoted by collaborative problem solving learning activities. J E-educ Res 38(10):87–92. https://doi.org/10.13811/j.cnki.eer.2017.10.014
Lipsey M, Wilson D (2001) Practical meta-analysis. International Educational and Professional, London, pp. 92–160
Liu Z, Wu W, Jiang Q (2020) A study on the influence of problem based learning on college students’ critical thinking-based on a meta-analysis of 31 studies. Explor High Educ 03:43–49
Morris SB (2008) Estimating effect sizes from pretest-posttest-control group designs. Organ Res Methods 11(2):364–386. https://doi.org/10.1177/1094428106291059
Article ADS Google Scholar
Mulnix JW (2012) Thinking critically about critical thinking. Educ Philos Theory 44(5):464–479. https://doi.org/10.1111/j.1469-5812.2010.00673.x
Naber J, Wyatt TH (2014) The effect of reflective writing interventions on the critical thinking skills and dispositions of baccalaureate nursing students. Nurse Educ Today 34(1):67–72. https://doi.org/10.1016/j.nedt.2013.04.002
National Research Council (2012) Education for life and work: developing transferable knowledge and skills in the 21st century. The National Academies Press, Washington, DC
Niu L, Behar HLS, Garvan CW (2013) Do instructional interventions influence college students’ critical thinking skills? A meta-analysis. Educ Res Rev 9(12):114–128. https://doi.org/10.1016/j.edurev.2012.12.002
Peng ZM, Deng L (2017) Towards the core of education reform: cultivating critical thinking skills as the core of skills in the 21st century. Res Educ Dev 24:57–63. https://doi.org/10.14121/j.cnki.1008-3855.2017.24.011
Reiser BJ (2004) Scaffolding complex learning: the mechanisms of structuring and problematizing student work. J Learn Sci 13(3):273–304. https://doi.org/10.1207/s15327809jls1303_2
Ruggiero VR (2012) The art of thinking: a guide to critical and creative thought, 4th edn. Harper Collins College Publishers, New York
Schellens T, Valcke M (2006) Fostering knowledge construction in university students through asynchronous discussion groups. Comput Educ 46(4):349–370. https://doi.org/10.1016/j.compedu.2004.07.010
Sendag S, Odabasi HF (2009) Effects of an online problem based learning course on content knowledge acquisition and critical thinking skills. Comput Educ 53(1):132–141. https://doi.org/10.1016/j.compedu.2009.01.008
Sison R (2008) Investigating Pair Programming in a Software Engineering Course in an Asian Setting. 2008 15th Asia-Pacific Software Engineering Conference, pp. 325–331. https://doi.org/10.1109/APSEC.2008.61
Simpson E, Courtney M (2002) Critical thinking in nursing education: literature review. Mary Courtney 8(2):89–98
Stewart L, Tierney J, Burdett S (2006) Do systematic reviews based on individual patient data offer a means of circumventing biases associated with trial publications? Publication bias in meta-analysis. John Wiley and Sons Inc, New York, pp. 261–286
Tiwari A, Lai P, So M, Yuen K (2010) A comparison of the effects of problem-based learning and lecturing on the development of students’ critical thinking. Med Educ 40(6):547–554. https://doi.org/10.1111/j.1365-2929.2006.02481.x
Wood D, Bruner JS, Ross G (2006) The role of tutoring in problem solving. J Child Psychol Psychiatry 17(2):89–100. https://doi.org/10.1111/j.1469-7610.1976.tb00381.x
Wei T, Hong S (2022) The meaning and realization of teachable critical thinking. Educ Theory Practice 10:51–57
Xu EW, Wang W, Wang QX (2022) A meta-analysis of the effectiveness of programming teaching in promoting K-12 students’ computational thinking. Educ Inf Technol. https://doi.org/10.1007/s10639-022-11445-2
Yang YC, Newby T, Bill R (2008) Facilitating interactions through structured web-based bulletin boards: a quasi-experimental study on promoting learners’ critical thinking skills. Comput Educ 50(4):1572–1585. https://doi.org/10.1016/j.compedu.2007.04.006
Yore LD, Pimm D, Tuan HL (2007) The literacy component of mathematical and scientific literacy. Int J Sci Math Educ 5(4):559–589. https://doi.org/10.1007/s10763-007-9089-4
Zhang T, Zhang S, Gao QQ, Wang JH (2022) Research on the development of learners’ critical thinking in online peer review. Audio Visual Educ Res 6:53–60. https://doi.org/10.13811/j.cnki.eer.2022.06.08
Download references
Acknowledgements
This research was supported by the graduate scientific research and innovation project of Xinjiang Uygur Autonomous Region named “Research on in-depth learning of high school information technology courses for the cultivation of computing thinking” (No. XJ2022G190) and the independent innovation fund project for doctoral students of the College of Educational Science of Xinjiang Normal University named “Research on project-based teaching of high school information technology courses from the perspective of discipline core literacy” (No. XJNUJKYA2003).
Author information
Authors and affiliations.
College of Educational Science, Xinjiang Normal University, 830017, Urumqi, Xinjiang, China
Enwei Xu, Wei Wang & Qingxia Wang
You can also search for this author in PubMed Google Scholar
Corresponding authors
Correspondence to Enwei Xu or Wei Wang .
Ethics declarations
Competing interests.
The authors declare no competing interests.
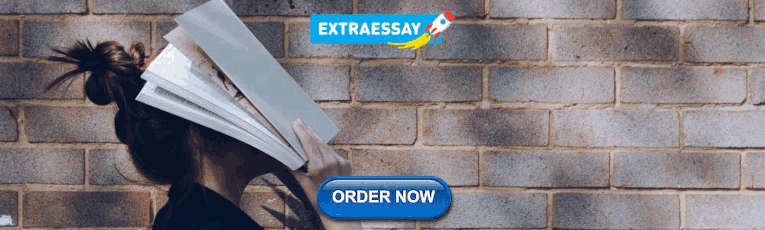
Ethical approval
This article does not contain any studies with human participants performed by any of the authors.
Informed consent
Additional information.
Publisher’s note Springer Nature remains neutral with regard to jurisdictional claims in published maps and institutional affiliations.
Supplementary information
Supplementary tables, rights and permissions.
Open Access This article is licensed under a Creative Commons Attribution 4.0 International License, which permits use, sharing, adaptation, distribution and reproduction in any medium or format, as long as you give appropriate credit to the original author(s) and the source, provide a link to the Creative Commons license, and indicate if changes were made. The images or other third party material in this article are included in the article’s Creative Commons license, unless indicated otherwise in a credit line to the material. If material is not included in the article’s Creative Commons license and your intended use is not permitted by statutory regulation or exceeds the permitted use, you will need to obtain permission directly from the copyright holder. To view a copy of this license, visit http://creativecommons.org/licenses/by/4.0/ .
Reprints and permissions
About this article
Cite this article.
Xu, E., Wang, W. & Wang, Q. The effectiveness of collaborative problem solving in promoting students’ critical thinking: A meta-analysis based on empirical literature. Humanit Soc Sci Commun 10 , 16 (2023). https://doi.org/10.1057/s41599-023-01508-1
Download citation
Received : 07 August 2022
Accepted : 04 January 2023
Published : 11 January 2023
DOI : https://doi.org/10.1057/s41599-023-01508-1
Share this article
Anyone you share the following link with will be able to read this content:
Sorry, a shareable link is not currently available for this article.
Provided by the Springer Nature SharedIt content-sharing initiative
This article is cited by
Impacts of online collaborative learning on students’ intercultural communication apprehension and intercultural communicative competence.
- Hoa Thi Hoang Chau
- Hung Phu Bui
- Quynh Thi Huong Dinh
Education and Information Technologies (2024)
Exploring the effects of digital technology on deep learning: a meta-analysis
Sustainable electricity generation and farm-grid utilization from photovoltaic aquaculture: a bibliometric analysis.
- A. A. Amusa
- M. Alhassan
International Journal of Environmental Science and Technology (2024)
Quick links
- Explore articles by subject
- Guide to authors
- Editorial policies

Teaching Science That Is Inquiry-Based: Practices and Principles
- First Online: 09 February 2023
Cite this chapter
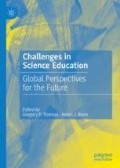
- Robyn M. Gillies 3
477 Accesses
Evidence has emerged in recent years regarding the importance of teaching science through an inquiry-based approach where students are encouraged to be actively involved in investigations that challenge their curiosity, encourage them to ask questions, explore potential solutions, use evidence to help explain different phenomena, and predict outcomes under different conditions. The inquiry process is complex and multifaceted as it involves students reconciling their current understandings of a problem with both the evidence obtained from an inquiry while also being able to demonstrate their understandings in ways that are logical, well-reasoned, and viewed as justifiable. Drawing on current research, this chapter proposes three curriculum-based interventions in cooperative learning, scientific literacy, and scientific discourses that have the potential to promote student understanding during inquiry learning.
This is a preview of subscription content, log in via an institution to check access.
Access this chapter
- Available as PDF
- Read on any device
- Instant download
- Own it forever
- Available as EPUB and PDF
- Compact, lightweight edition
- Dispatched in 3 to 5 business days
- Free shipping worldwide - see info
- Durable hardcover edition
Tax calculation will be finalised at checkout
Purchases are for personal use only
Institutional subscriptions
Alexander, R. (2008). Culture, dialogue and learning: Notes on an emerging pedagogy. In N. Mercer & S. Hodgkinson (Eds.), Exploring talk in school (pp. 91–114). Sage.
Google Scholar
Areepattamannil, S., Cairns, D., & Dickson, M. (2020). Teacher-directed versus inquiry-based science instruction: Investigating links to adolescent students’ science dispositions across 66 countries. Journal of Science Teacher Education, 31 , 675–704. https://doi.org/10.1080/1046560X.2020.1753309
Article Google Scholar
Bathgate, M., Crowell, A., Schunn, C., Cannady, M., & Dorph, R. (2015). The learning benefits of being willing and able to engage in scientific argumentation. International Journal of Science Education, 37 , 1590–1612. https://psycnet.apa.org/doi/10.1080/09500693.2015.1045958
Bybee, R. (2015). The BSCS 5 E Instructional Model: Creating teachable moments (p. 126). National Science Teachers’ Association Press.
Bybee, R. (2019). Using the BSCS 5E Instructional Model to introduce STEM Disciplines. Science and Children, 56 (6), 8–12.
Bybee, R., & Van Scotter, P. (2007). Reinventing the science curriculum. Educational Leadership, 64 (4), 43–47.
Chinn, C., Duncan, R., Dianovsky, M., & Rhinehart, R. (2013). Promoting conceptual change through inquiry. In S. Vosniadou (Ed.), International handbook of research in conceptual change (2nd ed., pp. 339–559). Routledge.
Duschl, R., & Grandy, R. (2008). Reconsidering the character and role of inquiry in school science: Framing the debates. In R. Duschl & R. Grandy (Eds.), Teaching scientific inquiry: Recommendations for research and implementation (pp. 1–37). Sense.
Chapter Google Scholar
Felton, M., Garcia-Mila, M., & Gilabert, S. (2009). Deliberation versus dispute: The impact of argumentative discourse on learning and reasoning in the science classroom. Informal Logic, 29 (417), 446. https://doi.org/10.22329/il.v29i4.2907
Felton, M., Garcia-Mila, M., Villarroel, C., & Gilabert, S. (2015). Arguing collaboratively: Argumentative discourse types and their potential for knowledge building. British Journal of Educational Psychology, 85 , 372–386. https://psycnet.apa.org/doi/10.1111/bjep.12078
Firman, M., Ertikanto, C., & Abdurrahman, A. (2019). Description of meta-analysis of inquiry-based learning of science in improving students’ inquiry skills. International Conference on Mathematics and Science Education , 1–6. Journal of Physics Conference Series. 1157 022018.
Ford, M. J., & Forman, E. A. (2015). Uncertainty and scientific progress in classroom dialogue. In L. B. Resnick, C. S. C. Asterhan, & S. N. Clarke (Eds.), Socializing intelligence through academic talk and dialogue (pp. 143–156). AERA.
Giamellaro, M. (2014). Primary contextualization of science through immersion in content-rich settings. International Journal of Science Education, 36 , 2848–2871. https://doi.org/10.1080/09500693.2014.937787
Gillies, R. M. (2007). Cooperative learning: Integrating theory and practice . Sage. https://doi.org/10.4135/9781483329598
Book Google Scholar
Gillies, R. M. (2016). Enhancing classroom-based talk: Blending practice, research and theory . Routledge.
Gillies, R. M. (2020). Inquiry-based science education . CRC Press. https://doi.org/10.1201/9780429299179
Gillies, R. M., & Nichols, K. (2015). How to support primary teachers’ implementation of inquiry: Teachers’ reflections on teaching cooperative inquiry-based science. Research in Science Education, 45 , 171–191. https://doi.org/10.1007/s11165-014-9418-x
Gillies, R. M., Nichols, K., Burgh, G., & Haynes, M. (2012). The effects of two strategic and meta-cognitive questioning approaches on children’s explanatory behaviour, problem-solving, and learning during cooperative, inquiry-based science. International Journal of Educational Research, 53 , 93–106. https://doi.org/10.1016/j.ijer.2012.02.003
Gillies, R. M., Nichols, K., Burgh, G., & Haynes, M. (2014). Primary students scientific reasoning and discourse during cooperative inquiry-based science activities. International Journal of Educational Research, 63 , 127–140. https://doi.org/10.1016/j.ijer.2013.01.001
Howe, C., & Abedin, M. (2013). Classroom dialogue: A systematic review across four decades of research. Cambridge Journal of Education, 43 , 325–356. https://doi.org/10.1080/0305764X.2013.786024
Huff, K., & Bybee, R. (2013). The practice of critical discourse in science classrooms. Science Scope, 36 (9), 30–34.
Johnson, D., & Johnson, R. (2002). Learning together and alone: Overview and meta-analysis. Asia Pacific Journal of Education, 22 , 95–105. https://doi.org/10.1080/0218879020220110
Johnson, D., & Johnson, F. (2009). Joining together: Group theory and group skills (10th ed.). Allyn and Bacon.
Johnson, D., Johnson, R., Roseth, C., & Shin, T. (2014). The relationship between motivation and achievement in interdependent situations. Journal of Applied Social Psychology, 44 , 622–633. https://doi.org/10.1111/jasp.12280
Kang, J., & Keinonen, T. (2018). The effect of student-centered approaches on students’ interest and achievement in science: Relevant topic-based, open and guided inquiry-based, and discussion-based approaches. Research in Science Education, 48 , 865–885. https://doi.org/10.1007/s11165-016-9590-2
King, A. (1997). Ask to think-tel why: A model of transactive peer tutoring for scaffolding higher level complex learning. Educational Psychologist, 32 , 221–235. https://doi.org/10.1207/s15326985ep3204_3
Krajcik, J., & Sutherland, L. (2010). Supporting students in developing literacy in science. Science, 328 , 456–459. https://www.science.org/doi/10.1126/science.1182593
Lazonder, A., & Harmsen, R. (2016). Meta-analysis of inquiry-based learning: Effects of guidance. Review of Educational Research, 86 , 681–718. https://doi.org/10.3102/0034654315627366
Liou, P. (2020). Students’ attitudes towards science and science achievement: An analysis of the differential effects of science instructional practices. Journal of Research in Science Teaching, 58 , 310–331. https://doi.org/10.1002/tea.21643
Liu, O., Lee, H., & Linn, M. (2010). An investigation of teacher impact on student inquiry science performance using a hierarchical linear model. Journal of Research in Science Teaching, 47 , 807–819. https://doi.org/10.1002/tea.20372
Murphy, C., Smith, G., & Broderick, N. (2019). A starting point: Provide children opportunities to engage with scientific inquiry and nature of science. Research in Science Education, 51 , 1759–1793. https://doi.org/10.1007/s11165-019-9825-0
National Research Council. (1996). National Science Education Standards . National Academy Press.
National Science Teachers Association. (2004). NSTA position statement: Scientific Inquiry . Retrieved from http://www.nsta.org/about/positions/inquiry.aspx/
Rennie, L. (2005). Science awareness and scientific literacy. Teaching Science, 51 (1), 10–14. http://hdl.handle.net/20.500.11937/31481
Resnick, L., Michaels, S., & O’Connor, C. (2010). How (well structured) talk builds the mind. In D. Pressis & R. Sternberg (Eds.), Innovations in educational psychology: Perspectives on learning, teaching and human development (pp. 163–194). Springer.
Salchegger, S., Wallner-Paschon, C., & Bertsch, C. (2021). Explaining Waldorf Students’ high motivation but moderate achievement in science: Is inquiry-based science education the key? Large Scale Assessments in Education, 9 , 14. https://doi.org/10.1186/s40536-021-00107-3
Soysal, Y. (2021). Argument-based inquiry, teachers’ talk moves, and students’ critical thinking in the classroom. Science & Education, 30 , 33–65. https://doi.org/10.1007/s11191-020-00163-1
Tang, K., & Moje, E. (2010). Relating multimodal representations to the literacies of science. Research in Science Education, 40 , 81–85. http://hdl.handle.net/20.500.11937/27263
Treagust, D., Qureshi, S., Vishnumolakala, V., Ojeil, J., Mocerino, M., & Southam, D. (2020). Process-orientated guided learning inquiry (POGIL) as a culturally relevant pedagogy (CRP) in Qatar: A perspective from Grade 10 chemistry classes. Research in Science Education, 50 , 813–831. https://doi.org/10.1007/s11165-018-9712-0
Tseng, C., Tuan, H., & Chin, C. (2013). How to help teachers develop inquiry teaching: Perspectives from experienced science teachers. Research in Science Education, 43 , 809–825. https://doi.org/10.1007/s11165-012-9292-3
Download references
Author information
Authors and affiliations.
The University of Queensland, St Lucia, QLD, Australia
Robyn M. Gillies
You can also search for this author in PubMed Google Scholar
Corresponding author
Correspondence to Robyn M. Gillies .
Editor information
Editors and affiliations.
The University of Alberta, Edmonton, AB, Canada
Gregory P. Thomas
James Cook University, Townsville, QLD, Australia
Helen J. Boon
Rights and permissions
Reprints and permissions
Copyright information
© 2023 The Author(s), under exclusive license to Springer Nature Switzerland AG
About this chapter
Gillies, R.M. (2023). Teaching Science That Is Inquiry-Based: Practices and Principles. In: Thomas, G.P., Boon, H.J. (eds) Challenges in Science Education. Palgrave Macmillan, Cham. https://doi.org/10.1007/978-3-031-18092-7_3
Download citation
DOI : https://doi.org/10.1007/978-3-031-18092-7_3
Published : 09 February 2023
Publisher Name : Palgrave Macmillan, Cham
Print ISBN : 978-3-031-18091-0
Online ISBN : 978-3-031-18092-7
eBook Packages : Education Education (R0)
Share this chapter
Anyone you share the following link with will be able to read this content:
Sorry, a shareable link is not currently available for this article.
Provided by the Springer Nature SharedIt content-sharing initiative
- Publish with us
Policies and ethics
- Find a journal
- Track your research
Change Password
Your password must have 8 characters or more and contain 3 of the following:.
- a lower case character,
- an upper case character,
- a special character
Password Changed Successfully
Your password has been changed
- Sign in / Register
Request Username
Can't sign in? Forgot your username?
Enter your email address below and we will send you your username
If the address matches an existing account you will receive an email with instructions to retrieve your username
Variations in Student Approaches to Problem Solving in Undergraduate Biology Education
- Jeremy L. Hsu
- Rou-Jia Sung
- Su L. Swarat
- Alexandra J. Gore
- Stephanie Kim
- Stanley M. Lo
Schmid College of Science and Technology, Chapman University, Orange, CA 92866
Search for more papers by this author
Department of Biology, Carleton College, Northfield, MN 55057
Office of Institutional Effectiveness and Planning, California State University, Fullerton, CA 92831
Program in Mathematical Methods in Social Sciences, and
Program in Biological Sciences, Northwestern University, Evanston, IL 60201
*Address correspondence to: Stanley M. Lo ( E-mail Address: [email protected] )
Department of Cell and Developmental Biology
Joint Doctoral Program in Mathematics and Science Education, and
Research Ethics Program University of California San Diego, La Jolla, CA 92093
Existing research has investigated student problem-solving strategies across science, technology, engineering, and mathematics; however, there is limited work in undergraduate biology education on how various aspects that influence learning combine to generate holistic approaches to problem solving. Through the lens of situated cognition, we consider problem solving as a learning phenomenon that involves the interactions between internal cognition of the learner and the external learning environment. Using phenomenography as a methodology, we investigated undergraduate student approaches to problem solving in biology through interviews. We identified five aspects of problem solving (including knowledge, strategy, intention, metacognition, and mindset) that define three qualitatively different approaches to problem solving; each approach is distinguishable by variations across the aspects. Variations in the knowledge and strategy aspects largely aligned with previous work on how the use or avoidance of biological knowledge informed both concept-based and nonconcept-based strategies. Variations in the other aspects revealed intentions spanning complete disengagement to deep interest with the course material, different degrees of metacognitive reflections, and a continuum of fixed to growth mindsets. We discuss implications for how these characterizations can improve instruction and efforts to support development of problem-solving skills.
INTRODUCTION
Creating learning opportunities for students to engage with complex, real-world problems is a major goal for undergraduate science, technology, engineering, and math (STEM) education ( Harper, 2006 ; Klegeris and Hurren, 2011 ; Hoskinson et al. , 2013 ; Conana et al. , 2020 ; Avena et al. , 2021 ; Frey et al. , 2022 ). There have been multiple calls to align learning and teaching with real-world problems ( Jacob, 2004 ; American Association for the Advancement of Science, 2011 ). One important component is problem solving, where students apply concepts and think through a series of decisions that allow them to define, interpret, and solve a problem ( Martinez, 1998 ; Carlson and Bloom, 2005 ; Price et al. , 2021 ).
Multiple studies have characterized the knowledge and strategies that students use when solving problems in STEM ( Carlson and Bloom, 2005 ; Jones, 2009 ; Fredlund et al ., 2015 ; Price et al. , 2021 ; Frey et al. , 2022 ). Both domain-general knowledge (which can be applied across any discipline) and domain-specific knowledge (which are skills unique to a given discipline) can influence problem solving, suggesting the existence of discipline-related variations ( Alexander et al. , 1989 ; Jones, 2009 ; Fredlund et al. , 2015 ; Prevost and Lemons, 2016 ). In the context of undergraduate biology education, students utilize a variety of conceptual strategies (that rely on biological reasoning) and nonconceptual strategies (that are algorithmic or heuristic in nature but without connecting to biological principles) to solve problems ( Brumby, 1982 ; Hoskinson et al. , 2013 ; Avena and Knight, 2019 ; Avena et al. , 2021 ; Sung et al. , 2022 ). Similarly, a previous case study on problem solving identified one conceptual strategy grounded in biological understanding and two nonconceptual strategies based on algorithms or patterns ( Sung et al. , 2022 ). While our previous work aligns well with other existing literature, its focus on knowledge and strategies alone limits our understanding of how other aspects of student learning can impact problem solving.
A number of aspects that influence learning are correlated to how students solve problems in STEM. For example, students’ intentions for choosing particular problem-solving strategies inform the specific actions that they perform in engineering ( Case and Marshall, 2004 ). Similarly, the level of metacognitive reflection has been found to correlate with problem-solving outcomes in mathematics and medical sciences ( Safari and Meskini, 2016 ; Izzati and Mahmudi, 2018 ). Students’ mindsets, or their beliefs about their ability to improve, may also influence their problem-solving potential in mathematics ( Callejo and Vila, 2009 ). However, little work has been done to investigate how these aspects of intention, metacognition, and mindset interact with strategies to inform students’ problem-solving approaches, particularly in undergraduate biology education.
To complement existing work in the literature, we identify and characterize student approaches to problem solving in undergraduate biology education, while accounting for multiple aspects that influence learning. This current study significantly extends previous work ( Sung et al. , 2022 ) beyond considering only knowledge and strategies in problem solving, providing an opportunity to develop a more comprehensive model of how multiple aspects of problem solving are integrated in a particular approach. Specifically, our research question is: What are the qualitatively different approaches that undergraduate students use to solve problems in biology?
THEORETICAL FRAMEWORKS
In discipline-based education research across STEM, the term framework or theoretical framework is used inconsistently to convey a combination of conceptual perspectives that inform the overall interpretation of the results, methodological rationale that guides the research process from data collection to analysis, and existing literature on the research topic that situates the novel contributions of the conclusions ( Bussey et al. , 2020 ; Luft et al. , 2022 ). To distinguish these overlapping elements for our current study, we articulate situated cognition as a conceptual framework for understanding problem solving as a learning phenomenon, explain the utility of phenomenography as the methodology, and describe relevant literature on problem solving in STEM.
Situated cognition
Research on problem solving in STEM included cognitive, metacognitive, affective, and contextual dimensions ( Lee et al. , 1996 ; Taconis et al. , 2001 ; Shin et al. , 2003 ; Reigosa and Jiménez‐Aleixandre, 2007 ; Taasoobshirazi and Glynn, 2009 ; Jonassen, 2010 ; Löffler et al. , 2018 ; Akben, 2020 ). To account for these intersecting dimensions, we use situated cognition as the conceptual framework for this study ( Table 1 ). Situated cognition is a sociocultural learning theory positing that knowledge is not simply a product of cognition but is further situated in the activities, contexts, and cultures in which the knowledge is produced and used ( Brown et al. , 1989 ; Cakmakci et al. , 2020 ). Correspondingly, learning is viewed not just as constructing an understanding of disciplinary knowledge but rather more expansively as the interactions between the learner and the environment as learning occurs ( Lave and Wenger, 1991 ; Cakmakci et al. , 2020 ). Past work examining problem solving through the lens of situated cognition has identified that contextual and situational factors can impact students’ problem-solving approaches ( Kirsh, 2008 ; Roth and Jornet, 2013 ). By considering problem solving as a learning phenomenon from the perspective of situated cognition, we expect to identify a coherent way to understand student approaches to problem solving that can likely accommodate the different aspects in the existing literature, as well as additional dimensions related to course and disciplinary contexts that may emerge from the data as we observe students interacting with the problem-solving process.
Developed from situated cognition as a learning theory, cognitive apprenticeship describes six processes that can facilitate learning: modeling, coaching, scaffolding, articulation, reflection, and exploration ( Table 1 ; Hennessy, 1993 ). Modeling, coaching, and scaffolding are processes that involve an expert making explicit their tacit knowledge and thought processes, providing feedback as learners engage in a task, and designing increasingly complex tasks that successively expands on the understanding of a concept or development of a skill, respectively ( Hennessy, 1993 ; Cakmakci et al. , 2020 ). Articulation, reflection, and exploration are processes that involve learners describing their knowledge and reasoning, comparing their own thought processes with those of other people or a model, and entering a mode of learning on their own, respectively ( Hennessy, 1993 ; Cakmakci et al. , 2020 ), all of which relate to metacognition or a student’s ability to reflect on their own thinking ( Tanner, 2012 ). Because cognitive apprenticeship is a pedagogical articulation of situated cognition, fundamental insights about student approaches to problem solving emerging from this study can likely be translated into tangible teaching implications.
Phenomenography
We use phenomenography as the methodological framework to guide our research process ( Table 1 ). Phenomenography is the empirical examination of the variations in how people think about the world, with the goal of identifying and characterizing the qualitatively different ways of experiencing a specific phenomenon ( Figure 1 ; Marton, 1981 , 1986; Hajar, 2021 ). Variation theory in phenomenography further organizes the results of a research study into a two-dimensional outcome space , which articulates the aspects or specific features of a phenomenon that individuals are aware of and pay attention to, as well as the variations or distinctions in how each of these aspects are experienced by different individuals ( Table 1 ; Marton and Tsui, 2004 ; Åkerlind, 2018 ). Together across multiple aspects, the outcome space represents a hypothesis based on a set of logically related descriptions that define the qualitatively different ways of experiencing the phenomenon by different individuals ( Marton and Booth, 1997 ), that is, distinct approaches to problem solving.
FIGURE 1. Phenomenography as methodology. We use phenomenography to investigate the qualitatively different ways that undergraduate students approach problem solving in biology education. Instead of directly studying problem solving as a phenomenon, we are examining how different study participants experience and understand problem solving. The research team interacts empirically with study participants in terms of data collection and conceptually with the phenomenon of problem solving situated as positionality.
Variation theory can be further applied to explain how instructors and students may experience the same learning phenomenon differently ( Bussey et al. , 2013 ). Previously, this application of variation theory was used to articulate why it is important to examine instructors’ definition of understanding in biology education ( Hsu et al. , 2021 ) and students’ strategies in solving biology problems ( Sung et al. , 2022 ). Furthermore, variation theory can be used as a framework to examine how individual students may experience the same learning phenomenon differently. For example, when presented with an exam problem, students could attend to the features of the problem itself, patterns of the problem that are similar to other practice problems, or biological concepts embedded within the problem, thus resulting in a variety of conceptual and nonconceptual problem-solving strategies ( Sung et al. , 2022 ).
Phenomenography is a research methodology developed within higher education research by higher education researchers ( Tight, 2016 ) and has been used extensively to study learning and teaching across disciplines ( Akerlind, 2005 ; Booth, 1997 ; Entwistle, 1997 ), including STEM as well as medical and nursing education ( Swarat et al. , 2011 ; Stenfors-Hayes et al. , 2013 ; Barry et al. , 2017 ; Han and Ellis, 2019 ). Within this literature, an approach to learning was initially defined as having two aspects, including the strategy (plans for doing something) and the intention (motivation for doing it) ( Table 1 ; Marton, 1988 ; Case and Marshall, 2004 ). Three general student approaches to learning have been identified: (1) a surface approach that utilizes rote memorization to reproduce details of the course, (2) a procedural approach that relies on study strategies to achieve course outcomes, and (3) a deep approach that internalizes and assimilates meaning to understand disciplinary content ( Biggs, 1979 ; Entwistle et al. , 1979 ; Case and Marshall, 2009 ). Subsequent research highlighted the importance of affect and metacognition in student approaches to learning ( Case and Gunstone, 2002 ; Pintrich, 2004 ; Case, 2008 ). More recently, a few studies have specifically examined approaches to problem solving in biology, engineering, physics, and physiotherapy using phenomenography, further highlighting the potential connections between different aspects in problem solving across STEM ( Walsh et al. , 2007 ; Lönngren et al. , 2017 ; Dringenberg and Purzer, 2018 ; Dahlgren et al. , 2021 ; Sung et al. , 2022 ).
Problem solving in STEM
Existing literature on problem solving in undergraduate biology education primarily focus on knowledge and strategies. In addition to domain-general knowledge that can be applied across disciplines, students need to utilize declarative knowledge such as disciplinary concepts, procedural knowledge such as problem-solving strategies specific to the discipline, and conditional knowledge such as metacognitive awareness on when to use certain strategies ( Prevost and Lemons, 2016 ). Problem-solving strategies can be algorithmic, when students memorize a pattern or formula and utilize it without recognizing the underlying biological concepts ( Avena et al. , 2021 ; Sung et al. , 2022 ), or can include more complex sets of actions, where students organize biological concepts into a mental framework to solve the problem ( Nehm, 2010 ; Prevost and Lemons, 2016 ). Furthermore, students often rely on domain-general knowledge and nonbiological reasoning, particularly when approaching problems with higher-order cognitive demands in biology ( Prevost and Lemons, 2016 ; Sung et al. , 2022 ).
Beyond knowledge and strategies, approaches to learning and problem solving include intention , or motivation to use a certain strategy ( Table 1 ; Marton, 1988 ; Case and Marshall, 2004 ). Motivation is a complex term encompassing distinct and related constructs from multiple theoretical traditions ( Conradi et al. , 2014 ; Murphy et al. , 2019 ; Richardson et al. , 2020 ). One motivational factor that can influence student learning and persistence across STEM is interest ( Schiefele, 1991 ; Wang, 2013 ), a term that has been applied in many contexts in biology education research ( Rowland et al. , 2019 ). Development of interest as motivation for learning can be serendipitous (e.g., trigged by an unplanned event), promoted by other people (e.g., in response to external demands as imposed by course structure), or self-generated by students (e.g., internally recognizing connections among different concepts; Renninger and Hidi, 2022 ). Interest can drive student learning, leading to more efforts and reflections ( Zimmerman, 2002 ). While we acknowledge that there are entire bodies of research focused on motivation and interest, we use the term intention in this paper, operationalized as a combination of motivation and interest, to be consistent with prior literature in phenomenography that has studied student approaches to learning and problem solving.
Metacognition is another aspect that can influence problem solving and learning in undergraduate biology education ( Table 1 ; Tanner, 2012 ). There are multiple definitions of metacognition ( Schraw and Moshman, 1995 ; Pintrich, 2004 ; Veenman et al. , 2006 ; Dinsmore et al. , 2008 ). Here, we refer to metacognition based on self-regulated learning (SRL), where metacognition in conjunction with motivational and behavioral processes can influence the ability of learners to regulate their own learning ( Zimmerman, 2002 ; Sebasta and Bray Speth, 2017 ). In this definition, the term metacognition encompasses metacognitive knowledge (understanding and awareness of one’s own thinking and learning) and metacognitive processes or regulation (activation and utilization of specific reflective skills such as planning, monitoring, and evaluating to support learning; Pintrich, 2004 ; Dinsmore et al. , 2008 ; Stanton et al. , 2021 ). Past work has identified that increased metacognitive knowledge and processes can drive regulation of learning and improve problem solving ( Swanson, 1990 ; Antonietti et al. , 2000 ; Aşık and Erktin, 2019 ); thus, we hypothesize that different problem-solving approaches may be associated with specific forms of metacognition.
Mindset can refer to several distinct and related constructs that shape student beliefs about the nature of their abilities, which can impact learning; most work in this area has focused specifically on student beliefs about their ability to improve, which is the definition that we use in this study ( Table 1 ; Dweck and Yeager, 2019 ). Students who possess a growth mindset and believe that they are able to improve their skills tend to have more success at developing those skills, compared with students who possess a fixed mindset and believe that abilities are static and unmalleable ( Limeri et al. , 2020 ; Miller and Srougi, 2021 ). The impact of such mindsets on student learning is further influenced the instructor’s mindset as well as the sociocultural and institutional contexts of the learning environment ( Muenks et al. , 2020 ; Muenks et al. , 2021 ; Canning and Limeri, 2023 ). Because of these complex interactions among mindset, student learning, and the learning environment, we believe that this study with situated cognition as the conceptual framework can provide insights into how mindsets may impact student approaches to problem solving.
Taken together, the existing literature suggests that multiple aspects can influence student learning and problem solving. In a previous study, case studies were developed to describe the knowledge and strategies used by three participants in solving problems, with a focus on implications for assessments, and the results were largely aligned with those in the existing literature ( Sung et al. , 2022 ). However, there was additional richness to these participants’ experiences in other aspects such as intention, metacognition, and mindset that were left unexplored. More broadly, there is a need in the literature for studies that examine how these various aspects integrate together to form different approaches to problem solving, specifically in biology. Consequently, the goal of this study is to develop a comprehensive model for how students approach problem solving in undergraduate biology education.
Context and participants
This study took place at a 4-y, private not-for-profit, doctoral university with very high research activity in the United States, with an undergraduate profile described as 4-y, full-time, more selective, lower transfer-in, large, and primarily residential, according to the Carnegie Classification of Institutions of Higher Education ( McCormick and Zhao, 2005 ). We recruited 22 study participants over three academic years from an introductory course on genetics and molecular biology using a purposeful stratified sampling plan that included exam performance and gender ( Sung et al. , 2022 ). The sampling variables were included to maximize the potential number of aspects and variations in the outcome space for student approaches to problem solving ( Han and Ellis, 2019 ) but not to determine whether these variables correlated with the different approaches identified in the study. Additional descriptions on the study context and participants were reported previously ( Sung et al. , 2022 ).
We chose genetics as the disciplinary context because genetics has been identified as a critical component of undergraduate biology education ( Smith and Wood, 2016 ). Furthermore, there has been a long history of other work examining a variety of issues related to problem solving in genetics ( Tolman, 1982 ; Smith and Good, 1984 ; Stewart and Kirk, 1990 ; Cavallo, 1996 ; Avena et al. , 2021 ), providing an existing literature to build upon. Finally, a variety of qualitative and quantitative problems are available in genetics coursework that can be used as interview tasks ( Sung et al. , 2022 ).
Data collection and analysis
We used clinical interview originating from Piagetian constructivist traditions as a method ( Piaget, 2007 ) to explore students’ approaches to problem solving in biology. Additional descriptions on this method were reported previously ( Sung et al. , 2022 ). Here, we highlight that the interview protocol was semistructured, a common feature for phenomenography as a methodology ( Han and Ellis, 2019 ). Participants were asked to explain, elaborate, or confirm their problem solving approaches by verbalizing their thought processes and drawing diagrams ( diSessa, 2007 ) in interview tasks with problems that involved nondisjunction and genetic recombination, with possible follow-up prompts that further explore ideas brought up by participants (Supplemental Material). Participants were also asked if and how the course could be structured differently to support their learning, an interview question that was not analyzed in the previous study ( Sung et al. , 2022 ). The interviewers (S.L.S. and S.M.L.) had no prior contacts with the participants and were not involved with the courses that served as the recruitment site for this study. Interviews were audio-recorded and transcribed semiverbatim to remove verbal nods such as “um” and “ah” by a professional service, and Transcripts were spot-checked. Drawings also were collected as artifacts. Additional descriptions on the data collection process were reported previously ( Sung et al. , 2022 ).
Transcripts were analyzed using qualitative methodologies in three stages ( Saldana, 2021 ) as described previously ( Zuckerman and Lo, 2022 ). First, preliminary codes were developed through iterative close reading of the transcripts to provide a large corpus of thought processes emerging from the data (S.L.S., A.J.G., S.K., and S.M.L.). Second, codes were condensed to identify different aspects of approaches to problem solving, leading to the creation of a preliminary outcome space (J.L.H., R.J.S., and S.M.L.). Using constant comparative method ( Glaser, 1965 ), excerpts that describe each aspect and variations across the different approaches were contrasted with previously analyzed transcripts, allowing for the confirmation or disconfirmation of working conjectures. Third, data for each intersection in the preliminary outcome space were revisited to guard against biases, maintain consistency, and further refine specific variations from one approach to the next within each aspect, resulting in the final outcome space (J.L.H., R.J.S., and S.M.L.). We note that as the outcome space is emergent from the data, the interview protocol was not constructed around the aspects identified through this analysis process, nor were the participants asked to discuss them in the follow-up prompts. Finally, results are reported as excerpts associated with pseudonyms for study participants.
The data analyses in this current study were expanded from the set of interviews conducted previously ( Sung et al. , 2022 ). Revisiting prior data in what is called secondary analysis has emerged as common practice in qualitative methodologies in the past two decades ( Bishop and Kuula-Luumi, 2017 ). In general, data sources for such secondary analyses include formal sharing through public repositories, informal sharing among collaborators, and reuse of previously self-collected data to investigate additional research questions ( Heaton, 2008 ). This study falls under the third category of reusing our own data that were collected previously. Furthermore, there are five purposes to secondary analyses of qualitative data; specifically, this study falls under the category of supra analysis, where the focus of the new study transcends that of the previous work to address novel research questions and to reach new theoretical, empirical, and/or methodological insights ( Heaton, 2008 ).
Compared to the previous study ( Sung et al. , 2022 ), the results reported in this study represent a novel analyses of: (1) study participants who were not previously included, (2) additional data with previously unreported excerpts from the three study participants in the prior study, and (3) additional data from an interview question that had not been analyzed. We are also investigating a supra research question that asks how the previously identified strategies ( Sung et al. , 2022 ) are more comprehensively situated in an outcome space with other aspects of problem solving. Given that the goals of this study are to extend our previous work, we believe that it was of value to reexamine the previous interview data as well. Consequently, the excerpts reported in this study for the previous three case-study participants ( Sung et al. , 2022 ) represent an extension of existing work specific to the new research question outlined in this study.
Reliability, validity, and trustworthiness
In phenomenography, reliability focuses on dialogic agreement “through discussion and mutual critique of the data and of each researcher’s interpretive hypotheses” ( Åkerlind, 2005 ). The research team met regularly to examine the preliminary codes, the emergent aspects and their variations, and iterations of the outcome space, providing checks against personal biases and supporting reliability throughout the process. All disagreements were resolved through dialogic discussions in the form of argumentation ( Schoenfeld, 1992 ) to reach consensus on the structural relationships between the various aspects and approaches of the final outcome space.
Research in phenomenography is also expected to yield an outcome space considered to be appropriate and useful by relevant communities ( Entwistle, 1997 ; Åkerlind, 2005 ; van Rossum and Hamer, 2010 ; Hajar, 2021 ). Throughout data analyses, our results were presented to various communities of biology education researchers and practitioners, including faculty, staff, postdoctoral scholars, and students, for feedback. Validity stems from these repeated cycles of critique and refinement as well as the perceived usefulness of the outcome space, resulting in potentially new understanding of student learning and educational interventions ( Åkerlind, 2005 ).
Theoretical saturation was achieved by collecting and analyzing data through the constant comparative method over a sufficient length of time ( Aldiabat and Le Navenec, 2018 ) as described previously ( Sung et al. , 2022 ). In short, preliminary descriptions were developed from data in the first year and were confirmed by data in the second year. While refinement of the outcome space was supported by data in the third year, the overall structure of the outcome space did not change. Furthermore, the number of interviews conducted ( n = 22) are within the estimated range that would typically result in theoretical saturation ( Hennink et al. , 2017 ).
We recognize that our own experiences can influence the way we collected and analyzed the data, and we include a statement of positionality to situate our identities as researchers and educators in the context of this study to enhance the trustworthiness of our findings ( Bourke, 2014 ). J.L.H. and R.J.S. are pretenure faculty in biology at a comprehensive university and a primarily undergraduate institution, respectively, and both have programs in biology education research. A.J.G. and S.K. were undergraduate students majoring in quantitative methods in social sciences and biological sciences, respectively, when they contributed to the data analyses. S.L.S. has an M.S. in evolutionary biology and Ph.D. in learning sciences and was a senior research associate focused on STEM education at a teaching and learning center at the time of the study. S.M.L. is tenured teaching faculty in biology who primarily works in discipline-based education research at a research-intensive university. Together, our collective professional experiences with complementary expertise and career stages provide enriched interpretations of the data and guard against potential biases.
We identified the following five aspects with variations that distinguish different approaches to problem solving: (1) Knowledge: What is the knowledge base that students are drawing from as they work on problems? (2) Strategies: How are students connecting and applying their knowledge to reach an answer to the problem? (3) Intention: Why are students engaging with problems from this course and/or the discipline? (4) Metacognition: How do students reflect on their experiences when solving problems in biology? (5) Mindset: What are the beliefs that students have in their abilities to solve the problems?
Variations in each of these aspects revealed three hypothesized student approaches to problem solving in biology that aligned with approaches to learning previously identified in higher education ( Biggs, 1979 ; Entwistle et al. , 1979 ; Case and Marshall, 2009 ). To maintain continuity with this existing work, we have named our three approaches as the surface approach, procedural approach, and deep approach. Here, the surface approach to problem solving is primarily characterized by the use of nonbiological concepts in knowledge and strategy, a desire to complete the course as the primary intention, minimal metacognitive reflection, and a fixed mindset about biology. In the procedural approach, students develop pattern-based processes from prior experience with course-specific material but do not rely on a conceptual understanding of biology; the metacognition, intention, and mindset aspects of this approach reflect complex dynamics between interest in biology as a discipline but a fixed mindset with regards to the specific genetics course in the study. The deep approach is characterized by a strong emphasis on biological concepts and pronounced interest in the intellectual challenges afforded by participation in biology and/or genetics.
In the sections below, we detail the variations in each aspect along with the three hypothesized approaches to problem solving in undergraduate biology education. Although most participants displayed variations in all aspects (i.e., knowledge, strategies, intention, metacognition, and mindset) that correlated within one approach (i.e., surface, procedural, or deep), we also identified some participants who displayed hybrid combinations of variations related to distinct approaches in different aspects. Such inconsistencies within an outcome space can occur when individuals are transitioning from one approach to another or are at a liminal state in the learning process ( Cousin, 2006 ; Land et al. , 2014 ). Therefore, as we will further elaborate in the Discussion section, we do not necessarily view these approaches as fixed for a given participant in time and, in fact, propose that the variations we identified may be opportunities for fluidity in supporting how students can shift between approaches and towards the deep approach in the classroom.
Existing studies generally distinguished the knowledge underlying different problem-solving strategies as being conceptual (grounded in disciplinary concepts) or nonconceptual (not utilizing concepts in the discipline; Walsh et al. , 2007 ; Lönngren et al. , 2017 ; Sung et al. , 2022 ). In this study, we find that the binary distinction to be insufficient to capture the observed variations. While the surface and deep approaches are aligned with nonconceptual and conceptual cognitive knowledge, respectively, participants using the procedural approach cannot be fully characterized by either variation and instead demonstrate elements from both conceptual and nonconceptual knowledge. We can only fully capture the range of variations and describe all three approaches by considering two dimensions to knowledge emerging from the data: whether the knowledge involves biological concepts and what the participants consider as their source of the knowledge.
Key features of the surface approach in the knowledge aspect are a lack of biology, both in concept and in the source of the knowledge. For example, William described:
Usually what I do whenever I start a problem [is to] try to look at which ones are bogus answers. I look for the ones that don’t really make much sense and then from there I try to think about it. If I don’t necessarily know it, like with one of the questions, I kind of try to logically figure it out.
The knowledge used in William’s approach is devoid of biological concepts, and the primary source of knowledge is prior experience with the format and/or style of the question. In the excerpt, William focused on identifying self-described “bogus” answers in the question without mentioning any biological concepts for his initial attempts to solve the problem. William also hinted at a shift in his approach to use logic in figuring out the answer, and we will further explore this type of shift in the Discussion section.
The knowledge aspect in the procedural approach, similarly to that in the surface approach, does not rely on conceptual understanding of biology. However, participants characterized by the procedural approach drew on a different source of knowledge. Whereas in the surface approach, participants used knowledge based on the question itself irrespective of the course from which those experiences are drawn, in the procedural approach, students used their prior experiences with information specifically from this genetics course. For example, Martin described:
I worked enough problems that I saw, and also like in the solutions manual, I remember it stated that like a simple way you can tell the order of the genes was if there is only one type of like recombination that occurs, you can look at whatever occurs together.
Although Martin was clearly relying on knowledge sourced from other genetics problems in the course, he made no attempt to meaningfully connect this information to biological concepts. Therefore, even though participants using both the surface and procedural approaches did not incorporate biological concepts in their knowledge base when solving problems, superficial connections to biology may make an appearance in the procedural approach in the form of specific references to the course material. These examples highlight the variation in the knowledge aspect that distinguishes the surface approach from the procedural approach: Participants can draw on knowledge that is unrelated to biology (surface approach) or draw on information related to course material but use it in a way unconnected to biological concepts (procedural approach).
The knowledge aspect in the deep approach is characterized by its grounding in biological concepts, in contrast to that in the surface approach and the procedural approach. For example, Evan described:
Translocation is when a chunk from one chromosome breaks off and joins another chromosome and then the opposite chunk joins. So it’s just when chromosomes switch sections of DNA. I don’t how it’d affect map distance. It gets smaller, so it can’t be it. Yeah, that makes sense. It can’t be a translocation because it should do the opposite. It should be bigger. And there would be a new phenotype. Or usually there’s a new phenotype in translocation, when translocation happens because where the chromosome breaks, a new phenotype is created because the protein that’s encoded for at that breakage point gets messed up. So that’s why it’s not a translocation. It’s an inversion.
Evan used biological concepts and his internal conceptualization of this knowledge. Based on Evan’s logical reasoning, we argue that the source of knowledge went beyond simple recall of concepts from course material, as Evan was drawing inferences and making connections using his conceptual understanding.
Participants using the deep approach similarly drew on knowledge that originated from the course material and was grounded in their own understanding of biological concepts, reflecting a deep engagement between the participants as learners in the course and the course material as part of the learning environment. In contrast, while participants in the procedural approach also draw on knowledge that originated from the course material, this knowledge was grounded in processes that allowed the participants to bypass biological concepts as they solve the problem.
Variations in the knowledge aspect across the three distinct approaches provide a valuable lens with which to situate our current and prior work related to the strategy aspect. Contextualizing the strategy aspect with variations in the knowledge aspect allows us to identify connections between these two aspects within each of the three distinct approaches. Below, we apply this analysis to data from new participants specific to the current study as well as unreported data for Samuel, Kylie, and Michael (2022) from the previous case-study paper.
In the surface approach, the absence of biological concepts in the knowledge aspect likely reinforces a strategy that relies on information from a nonbiological source, such as the format, style, and/or language of the question itself. For example, William shared:
Generally, I don’t usually go with the nonpossible, so I pretty much cross that out from the beginning, because logically, I think there is something that goes on between one of the first couple of choices.
William’s strategy is based on the logic that it is necessary to eliminate nonpossible answers first, and from previous experience, those are typically the first few choices. Consequently, this strategy could potentially be applied to questions of a similar style in different contexts, not necessarily just in the genetics course. Similarly, Samuel explained how he eliminated one of the options in a multiple-choice question based on the structure of the options:
You realize that out of these four [options], like three of the four, like “describe four progeny being created”, so this one is two and two, this one is two and two, and this one is one, one, one, one, one, and this one is only one. So that’s another reason why [Option] A seems wrong, because it’s the odd one out in that sense, which again is not like super good related to biology skills.
The strategy aspect in both the surface and procedural approaches is characterized by patterns of process based on prior experience with similar problems. However, the knowledge base for the procedural approach relies on course-specific information such as practice problems, examples from class, and even (unintentional) instructor cues. Nonetheless, participants characterized by the procedural approach still focused on the nonconceptual features to support a strategy that allowed them to reason through the problems without conceptual understanding. For example, Martin shared:
I just looked at the numbers to see which was a double recombination and which one was a single recombination, and that is the way it was set up, the largest one was the parental and then smallest one was like the most recombinations. I think the most [the instructor] did was two. So I look at these numbers, and the smallest number is usually the double, and the largest is the parental. Again, I don’t really know why. I just know that the higher the frequency, the further away they are from each other, or the more like normal they are.
Martin acknowledged an explicit awareness of how to do the problem without any understanding of the concepts. Similarly, as seen below, Kylie explained her elaborate process for how to solve a recombination problem by identifying pair-wise additions that arithmetically summed to an even round number while also gauging the complexity of the problem based on the point value assigned to it. She further implied toward the end of this excerpt that other students in the course also used this type of strategy:
Also, it really helps because [the instructor] makes the numbers add up really nicely together, and you know if you’re doing it right, if you get an even number. So like here, you get 100. (…) So I knew that like you add 26 and 24, and you add 27 and 23, you get 50 each. So like that helped too. I mean, he said that out loud to us once. He was like, I’m not going to ask you to, it’s not a math test. It’s not like an understanding, but it’s a clue. And also, the point values tell you how difficult the problem is going to, like how difficult the explanation is. So like, sometimes he asks if, are these genes linked, and it’s like two points, then you know that it’s yeah they’re all linked, or they’re not linked. And if it’s more than that, then you know there’s something else going on. And it’s true every time. Don’t tell [the instructor] that, ’cause all the [other students] will hate me!
As opposed to processes based solely on the question itself, and absent any course context as seen in the surface approach, participants using the procedural approach were reliant on information related to the specific course material. The processes ranged in complexity from a simple single-step recall from course material to connecting multiple pieces of information and/or steps to form a more complex set of associations, as respectively exemplified by Martin’s and Kylie’s descriptions above.
The deep approach is also characterized by a range in complexity, from a single-step conceptual connection to an elaborate process involving multiple concepts. Instead of connecting course-based information and perceived rules to generate essentially a heuristic strategy as seen in the procedural approach, the strategy in the deep approach relies on connecting conceptual ideas grounded in biological understanding. For example, in a single-step conceptual connection, Evan described nondisjunction:
[Nondisjunction is] when the, in meiosis whenever all the chromosomes line up, and then the spindles attach, and pull them apart to separate them, haploid gametes. If for some reason, it doesn’t do that correctly, and it doesn’t split the two homologues up then, and say one gamete gets both, and one gets none, that’s a nondisjunction.
While it is possible that Evan could have memorized such a definition from a textbook, there was a certain fluency in how he described the idea using language grounded in biological concepts. In contrast, in the procedural approach, Cara described homologous chromosomes explicitly through references to visualizations provided in class: “In lecture, the visual [the instructor] put up made it seem like they, they’re similar, and so I’m like, and they look according in the visual [the instructor] put up in lecture, it looked like they were similar in size.” Evan further elaborated on how he solved a recombination problem, connecting multiple concepts and processes:
First, what you’d do is just draw the two chromosomes next to each other, and then you have to figure out which genes in what order. You knew from these numbers that these are the two wild-type phenotypes, and so in that worm, you know that the two chromosomes have these alleles, and you know the order, and you know which ones are on it, and it’s asking you what crossover would give rise to a wild-type progeny. You know that you have to get all, you have to get big C, big W, and big D. So the only way that happens is a crossover right there, which would give you all dominant alleles. So the hardest part is just figuring out what the gene order is, and which alleles are on which chromosome. And the way you get that information is from these two parental classes.
Even though Evan seemingly used what appears to be a heuristic process with sequential steps, it was also evident that this strategy involved connecting each step together using his conceptual understanding of the biological system. This aligns with the knowledge base identified for the deep approach, which is centered on the participant’s own understanding of biological concepts. It is interesting to note that Evan shifted to a formula-based strategy only when he did not understand or remember the meaning of a concept, i.e., interference in the excerpt below. We will further explore this type of shift in the Discussion section.
The last part is where applicable calculate a value for interference. And that’s just an equation. It’s one minus d, number of double recombinants, divided by the expected number of double recombinants. (…) That’s just the equation for interference. (…) Just whenever the number of double recombinants you get deviates from what you expect. Then interference is happening. I don’t know exactly why, or I don’t remember.
As we consider the connections between the two cognitive aspects of knowledge and strategy through the lens of situated cognition, we see that each approach begins to capture distinct interactions between the learner and the environment as learning occurs. For example, the near absolute avoidance of conceptual understanding and even in relation to the course material seen in the surface approach is suggestive of a lack of interaction, or disengagement, between the learner and the learning environment. The procedural approach is characterized by an almost transactional interaction; the selective knowledge that is observed and retained from the course is specific information such as formulas and patterns in previous questions that allows the learner to solve the problem without application of concepts. In the deep approach, the strong conceptual connections within both the knowledge and the strategy aspects suggests a deeper engagement in the interaction, in which the learner is internalizing and integrating concepts seen in the learning environment into their own understanding.
We characterize the intention aspect as the self-identified reasons why participants were interested and motivated to engage with problems from this course and/or the discipline. To describe the intention aspect, we consider two dimensions emerging from the data: desire and demand. We describe desire as being representative of the participant’s interest in being intellectually challenged and/or connected to the subject matter, likely correlating with interest in biological concepts and/or problem solving. On the other hand, demand captures the participant’s underlying motivation in the educational enterprise at hand, either specifically in the genetics course from which these problems originated or in the discipline of biology as a whole. Similar to our analysis of the knowledge aspect, the use of two dimensions allows us to more fully describe the variations in the intention aspect among the three distinct approaches.
Broadly, the intention aspect in the surface approach shows a disinterested perspective towards biology as a discipline, suggesting low interest in the underlying biological concepts and/or problem solving. There is a lack of desire to connect with the course material; consistently, the primary demand is motivation to complete the course and move past the content, external structures set forth by other people such as requirements for future careers or the instructor. For example, Samuel described:
I wish I was learning biology, but at some point, I have to square with the fact that I’m not. I hate this class, and I don’t think I like these kinds of premed sciences that much. You just have to get through it and then, ‘cause when you’re a doctor, I’m not going to be dealing with biology. So you just have to get through it, which is why I’m not giving it up just yet.
Similarly, William said:
I think I have never been like a cheat-for-the-test kind of person. I think learning it is probably better, but sometimes I don’t see things coming at all, and if I could be exposed to that during a review session or during class sometimes. We get taught how it works, but I don’t exactly see how that translates to problems at times, so I think getting exposed to problems more would probably help. I was trying to focus on what [the instructor] told us to study on.
In both examples, there was a lack of desire to connect to the material: Samuel did not consider the course learning biology and consequently hated the course, and for William, the perceived disconnection between what was taught and what was tested in the course frustrated him so much that he focused his efforts on what he was told to study. For both Samuel and William, this lack of connection to the course material reinforced the motivation to just finish the course.
The procedural approach is characterized by a combination of desire that is different from that of the surface approach and demand that is similar to that of the surface approach. For example, the demand of focusing on motivation to complete the course was also reflected in Kylie’s experience:
I thought it was kind of ridiculous that I knew all the information, yet I couldn’t apply it to anything, and I just think it’s a really bad way to test. [The instructor] did go through practice problems, but they were always straightforward. Then they threw you a curveball at you on the test. I’m used to tests being challenging and not presenting the information as you see it when you study, and that’s fine, but I feel like this had to do a lot with like logic and just like. I don’t know, I just, it’s not a logic class, you know. It’s biology. You just memorize how it’s done. I’m a little bit bitter about it. I kind of felt really frustrated, and the only reason why I would’ve bothered to play the game is because I’m competing with everyone else to do well, so I knew everyone else would.
Kylie’s response demonstrated her frustration that the genetics course material included on the exam did not reflect her preconceived idea of what biology should be, instead consisting of questions that were more akin to logic questions to her. This frustration underscored her need to play the game, with her primary demand also focused on just finishing the course. However, what distinguishes Kylie’s response from those of Samuel and William is her desire to be challenged on the test and/or see things presented differently in the course, suggesting interest in the underlying biological concepts and/or problem solving. This distinction is particularly striking given that William cited those exact reasons for not intellectually engaging with the class. This variation in desire, in this case a demonstrated interest to be intellectually challenged, is what distinguishes the procedural approach from the surface approach.
Similarly, Martin described:
Genetics was not my favorite. I was just like, I have to get through it to be honest. I just like really tried to cover as many problems as I could until I found a trend in the problems. And I don’t like that it is not really a way of learning, I don’t feel like. In molecular biology, because there were not so many problems per se, you really had to get an understanding of the concepts and like all the basics to really understand that, and I like that a lot better than just working problems to find an answer. Even in math the trend was always there, but you actually had to understand the basics. You couldn’t just memorize a typical pattern in the problems, because you know that wouldn’t help you all the time. These [genetics problems], if you found a pattern nine times out of ten, it would be the same, which is okay, but if the basic understanding of the concept isn’t there, I don’t feel like it is very helpful. I like learning a lot. That is why I am in college, I don’t like just doing problems and finishing it up there. I memorized how to do it, and applied it on the tests, and then pretty much forgot about it, because I wasn’t very interested in it.
Here, Martin expresses their feelings about genetics through comparisons to other courses he has taken, highlighting features that simultaneously underscored his sense of learning in those spaces and his sense of not learning in genetics. Similar to Kylie’s experience, Martin had a strong desire to be intellectually challenged. However, the seeming mismatch between how he expected those intellectual challenges to manifest in the course and how he actually did on the exam resulted in focusing the demand on his motivation to just move past the course.
Both procedural and deep approaches share the desire to be intellectually challenged; what distinguishes between the two is the variation in demand, where the deep approach is characterized by a self-generated motivation to learn both for themselves and in the course. For example, Evan shared:
I mean the test wasn’t, [the instructor] didn’t make it as hard as he could’ve, could’ve made it a lot trickier. But it’s just, there’s a lot of different things that could happen, and you have to really understand what causes those things to happen so that you, ’cause you can’t just memorize every scenario. You just have to logically work your way through it. It’s just a long process, and if you do that, you can get the right answer, but a lot of people struggled with that ’cause they don’t get that initial foundation to build off of.
Similarly, Sean explained:
I also appreciate the fact that there are some more difficult questions, such as these ones, that actually require you to, you know, work out a calculation or think of something in a new way that actually involves some sort of thought process, not just simple memorization of the notes, and then go to the test, and recognize the pattern, and just circle it. So, I appreciate the challenge. The bio class doesn’t really have that rule that you have to get a certain percentage right, it just encourages you, not just to, you know, focus on getting the right answer, and stress about that. It doesn’t matter if I get it right or wrong, as long as I learn.
These responses from Evan and Sean demonstrate that both participants appreciated intellectual challenges, having scenarios that were possibly beyond what had already been shown and citing the questions that required some sort of thought process compared with memorization of the notes. In turn, these participants indicated that their intention supported a demand that is largely driven by an internal motivation to deeply engage with course material. Moreover, the mechanism of this deeper engagement aligns with both the knowledge and strategy aspects already identified for the deep approach, where participants drew on their own conceptual understanding and form connections between these concepts to deduce a solution to the problem.
Similar to how we observed interactions between the knowledge and strategy aspects, it is possible that the intention aspect also aligns with other aspects within each approach. For example, avoiding the use of biological concepts in both knowledge and strategy in the surface approach could reinforce the notion that the participants were not learning biology, and the lack of desire or demand in their intention could in turn reinforce a deliberate choice to avoid the use of biological concepts to solve problems. Participants described above in the procedural approach commented on intellectual challenges when working through the problems; however, these challenges were sources of frustration that ultimately led to intellectual disengagement, reinforcing the use of biological concepts in strategies that supported completing course requirements rather than deeper conceptual learning.
Metacognition
Based on ideas emerging from the data, we focused this aspect on how participants reflected on their experience solving problems based on metacognitive knowledge and metacognitive process or regulation. Specifically, we examined how much participants were aware of their own knowledge and strategies in relation to the problems (metacognitive knowledge) and how the features of the course may influence connections between such awareness and problem solving by participants (metacognitive process or regulation).
The surface approach captures a largely passive engagement with the reflective process used by participants, with limited metacognitive knowledge and metacognitive process in their reflections. For example, Samuel described: “I think you’re gonna expose how superficial my understanding is. I’m not sure if that’s right. So I guess again, in explaining it, I’m realizing that I don’t know it as well as I thought I knew it.” Similarly, William states: “I know the thought process behind it. I think my biggest issue with genetics so far has been like I know what is going on, [but] I don’t know how it [is] going on.”
Both participants were clearly metacognitively aware of the limitations of their biological knowledge, recognizing that they had significant gaps in their conceptual understanding. Samuel named his understanding as superficial, whereas William identified his lack of understanding by distinguishing between his familiarity with the topic of what was going on versus his lack of the conceptual knowledge needed to solve a problem and explain how it was going on. In Samuel’s case, beyond identifying a sense that the course was not biology, there was limited reflection on metacognitive processes, with very little reflection on the additional factors that contributed to his experience. Similarly, as described earlier, William proffered only a superficial reflection on factors that would shape his metacognitive processes: “We get taught how it works, but I don’t exactly see how that translates to problems at times, so I think getting exposed to problems more would probably help.” In both cases, metacognition in the surface approach reflects some level of metacognitive knowledge and almost no metacognitive process, reflection that connects the knowledge and strategy aspects with broader contextual factors that may influence problem solving.
The procedural approach is characterized by a degree of reflection that captures a greater amount of metacognitive knowledge though with still a limited amount of metacognitive process, resulting in disconnections or incompatibilities between their increased metacognitive knowledge and their limited recognition of metacognitive processes. Specifically, participants using the strategic approach can recognize their own content knowledge and a set of expectations of what is necessary to achieve success in problem solving. While participants reflected on expectations of how the course should support them as learners, the contextual factors that shape the limited metacognitive processes that emerged as part of this reflection were often viewed as oppositions to their success. For example, Kylie indicated:
I have a friend that took this over the summer and is really good at logic problems and puzzles. Like he’s the best and he got like 95 [percent] on all his exams because of it. Even though I know for a fact I studied way harder than he did, and I probably knew the information better. It’s just like, you shouldn’t have a test that people do well because they’re good at taking it. It should be because you know the information better, you should do well, better than others, I guess.
Here, Kylie implicitly recognized that she was not as proficient as her friend in logic problems, conveying limited metacognitive processes. She explicitly laid out contextual reasons in her reflection for this limited metacognitive process, suggesting that her performance was due to the tests being a measure of logic rather than knowledge. In doing so, the reflection provided a more complex lens into her experience of problem solving than participants using the surface approach. Similarly, Bailey described:
I couldn’t get some of [the concepts]. I’m not sure. Some of these really screwed me up. I think my problem was that I never actually learned what was happening or like, why it would happen. It was like, okay, I see these numbers and so [the instructor] kinda asked the same questions, so I would try to figure out how to solve them instead of actually learning it. It definitely put me in a robot mode a little bit, maybe practice problems in class or explanation, like step-by-step what conceptually I guess is happening. I know [the instructor] did a little bit of that, but like I feel like a lot of people struggle with this concept.
Here, Bailey demonstrated her metacognitive knowledge by explicitly recognizing that she never learned some of the concepts and instead developed algorithmic strategies to solve the problems. Although she was less direct than Kylie, Bailey also highlighted limited ability for metacognitive processes, instead focusing on contextual reasons that would explain her lack of content knowledge and her reliance on nonconceptual problem-solving strategies. For instance, she cited how being asked similar questions and the lack of opportunity to review the conceptual basis for problems contributed to her not learning the biological concepts.
In contrast, the deep approach is characterized by reflections that recognize and connect metacognitive knowledge related to the expectations necessary to achieve success and a high degree of metacognitive processes that are essential for problem solving. These participants were able to distinguish specific patterns and processes that they used to improve their conceptual understanding and problem-solving capacities. For example, as described earlier, Evan noted that on the tests there were “a lot of different things that could happen, and you have to really understand what causes those things to happen” and as a result “you can’t just memorize every scenario.” Evan reflected on a difference in lower-order cognitive problems that required recall and higher-order problems that required application, and then he provided a strategy for how to solve the latter type of problems, stating that “[y]ou have to break it down, and you have to start from the beginning in terms of like segregation and stuff like that.” Similarly, Sean noted that:
There were questions on the test that I found to be very fair, very straightforward. If you had studied the notes and gone over the material and done the reading you would be in a good position to breeze through them, but I also appreciate the fact that there are some more difficult questions that actually require you to work out a calculation or think of something in a new way that actually involves some sort of thought process, not just simple memorization of the notes and then go to the test and recognize the pattern and just circle it.
Similar to Evan, Sean distinguished between cognitive levels of problems and reflected on his own metacognitive processes on what he needed to do to succeed, such as going over the notes and reading. Both participants indicated that the primary expectation of the course was to provide opportunities for developing problem-solving processes, typically in the form of higher-order cognitive problems. The alignment of metacognitive knowledge and metacognitive processes in these participants reflects a more cooperative relationship between the participants and the learning environment, whereas the reflections in the procedural approach highlight a more combative relationship or tension between these factors. In contrast, the minimal reflection seen in the surface approach represents a more passive engagement with metacognitive knowledge and metacognitive processes for problem solving.
Variations in the metacognition aspect also correlate with those in the aspects described earlier. The surface approach suggests a lack of connections to biological concepts and also a passive relationship to the course, both in intention and metacognition. The procedural approach focuses on a transactional view that uses course-based knowledge and strategies to complete the course requirements, correlating with greater metacognitive knowledge but still limited metacognitive processes. The deep approach emphasizes biological concepts in the knowledge and strategy aspects, an intention to be intellectually challenged and to engage with course material, and a connection between both metacognitive knowledge and processes.
Students can operate on a continuum of attitudes and beliefs between fixed mindset, believing that their intelligence and ability to do well cannot be improved, and growth mindset, believing that such qualities are malleable ( Yeager and Dweck, 2020 ). However, our data indicate that this nearly binary distinction of either a fixed or growth mindset as the two ends of the spectrum is not sufficient to describe the variations within this aspect for the three approaches. Instead, we articulate an additional dimension that further defines an intermediate point in the continuum between fixed and growth mindsets, i.e., whether participants viewed their learning from a deficit-based or asset-based perspective ( Denton et al. , 2020 ; Denton and Borrego, 2021 ).
The surface approach is characterized by attitudes and beliefs that learning cannot be improved as predetermined by certain factors, classic features of a fixed mindset. This variation also adopts a deficit-based perspective. For example, Michael shares:
I think people who have a natural aptitude towards biology maybe can figure [this problem] out. I have a friend who does really well on the tests, because she literally goes to the test and figures out the test. Like, she can read this and understand, think, and figure out what a homologous chromosome is. But I don’t have that kind of natural aptitude towards biology.
Michael then compares himself to another student and explicitly states that he believes that he will not be able to develop the needed aptitude for problem solving in biology, showing a fixed mindset toward his abilities to solve biological problems. He implies from a deficit perspective that he does not have an aptitude for biology, consistent with a fixed mindset that he will not be able to acquire the skills needed to solve the problem. He further characterizes this natural aptitude (or lack thereof) as something that is relevant for the entire field of biology. Michael’s reflections correlate with the idea of brilliance, that success requires innate, natural talent that cannot be taught ( Rattan et al. , 2012 , 2018).
Whereas participants using the surface approach show an alignment with fixed mindset inherently consistent with a deficit-based perspective, we found that the procedural approach can be described by a growth mindset but also coming from a deficit-based perspective. For example, Bailey explains:
I didn’t have the background to understand it in class. I took AP Bio sophomore year in high school, so it wasn’t recent to me anymore. I could’ve done this [problem] five years ago, easily. Trying to learn it in the short time I had, I like, you know, I just didn’t have it down.
Bailey viewed her lack of success as due to her missing some foundational background or ability in terms of a deficit. However, what distinguishes the procedural from the surface approach is the attitude and belief that she could have been able to succeed if she had had more time to learn and/or if the knowledge from her AP Biology experience had been more recent. Bailey also situated this deficit as specific to this genetics course, not necessarily to biology as a discipline, unlike Michael as illustrated in the surface approach. Consequently, this view that there are some specific deficits particular for this one course preventing success implies that these participants believe that they would be able to improve if they were able to rectify the deficit. Bailey further ascribed the path to success and thus improvement as one influenced by external factors, such as time because the prerequisite course was taken, rather than how her work and effort in the course could influence her ability to improve and successfully solve genetics problems. Therefore, participants using the procedural approach share elements of a growth mindset but from a deficit-based perspective.
The deep approach is similar to the procedural approach in that both are described by a growth mindset; however, the deep approach focuses on an asset-based perspective instead of a deficit-based perspective. Participants using the deep approach conveyed the attitudes and beliefs that they and their peers could take specific steps to gain skills and become better at solving problems in biology, focusing on internal factors on what they could do to improve rather than external constraints that limit their success. For example, Evan shares that “[y]ou just have to logically work your way through [problems], and it’s just a long process, and if you do that, you can get the right answer.” Similarly, Sean mentioned that he enjoyed doing practice problems for the following reason: “[i]t just encourages you, not just to focus on getting the right answer and stress about that, and instead it doesn’t matter if I get it right or wrong, as long as, like, I learn.” Both Evan and Sean described that working through problems will aid them in learning, improving, and gaining the ability to become better, aligning with a growth mindset from an asset-based perspective.
As described earlier, the connections between the cognitive aspects of knowledge and strategy suggest that each approach encapsulates distinct degrees of interactions with the learning environment on the basis of what knowledge was used in each strategy. Here, by considering multiple other aspects beyond knowledge and strategy, we can begin to formulate a more coherent understanding of each approach. For example, we can see that the lack of connections to biological concepts in knowledge and strategy correlated with a passive relationship to the course, both in intention and metacognition, and subsequently with a fixed mindset of not being able to improve in learning biology. In contrast, a transactional view as described by the knowledge and strategy aspects for the procedural approach fails to capture the sense of internal conflict between the student and the course environment as reflected in the intention, metacognition, and mindset aspects. On the other extreme, the depth of interactions between the learner and the learning environment seen in the knowledge and strategy aspects for the deep approach also correlate with the depth of engagement and cooperative relationship between internal factors of the participants and contextual factors in the course. By considering multiple dimensions of a learner’s experience with their environment through the lens of situated cognition, we see that individuals characterized by each of the approaches can have complex relationships with the course and course material. As we will discuss later, this creates potential opportunities for the instructor to acknowledge and address features of this interaction to potentially shift individuals’ problem-solving from one approach to another.
Our work provides one of the first examples to examine student approaches to problem solving in a broader and more holistic perspective that can potentially integrate multiple different aspects. The outcome space emerging from our data ( Figure 2 ) represents a hypothesis for how variations in each of the five aspects (i.e., knowledge, strategies, intention, metacognition, and mindset) are connected to one of the three approaches (i.e., surface, procedural, or deep). As we considered problem solving as a learning phenomenon through the lens of situated cognition, it is perhaps not surprising that we identified three approaches that are aligned with the previously characterized student approaches to learning ( Biggs, 1979 ; Entwistle et al. , 1979 ; Case and Marshall, 2009 ), even though we did not set out to constrain ourselves to a specific number of approaches in the outcome space. Classically, approaches as defined in phenomenography consisted only of strategies and intentions ( Marton, 1988 ; Case and Marshall, 2004 ). Here, our outcome space expands the number of aspects to include knowledge, metacognition, and mindset in addition to strategies and intentions.
FIGURE 2. Outcome space for different student approaches to problem solving. This outcome space articulates hypothesized relationships among the three approaches illustrated by specific variations across five aspects: the aspects of knowledge (blue), strategy (red), intention (green), metacognition (yellow), and mindset (purple). Under each aspect, variations are described to distinguish between two neighboring approaches, either surface versus procedural or procedural versus deep.
The three approaches to problem solving span a continuum from deliberate avoidance of using conceptual information to intentional application of biological concepts. The knowledge and strategy aspects align with other studies that have observed both biological and nonbiological strategies for problem solving ( Schoenfeld, 2016 ; Sung et al. , 2022 ). Moreover, we show that the knowledge and strategy aspects may be connected with other aspects including intention, metacognition, and mindset. These results provide an expanded view of factors that may impact how students solve problems beyond considering the domain-specific factors of declarative, procedural, and conditional knowledge ( Prevost and Lemons, 2016 ; Avena et al. , 2021 ).
Previous phenomenographic studies
Our current work is further situated in other phenomenographic studies characterizing problem solving across STEM disciplines ( Walsh et al. , 2007 ; Lönngren et al. , 2017 ; Dringenberg and Purzer, 2018 ; Dahlgren et al. , 2021 ; Sung et al. , 2022 ), which previously identified variations in problem solving in a limited number of aspects ( Figure 3 ). Therefore, our results extend these previous findings by providing an outcome space that incorporates a larger number of aspects and synthesizes the different aspects into a coherent hypothesis as a model for student approaches to problem solving.
FIGURE 3. Outcome space with mapping of prior work characterizing approaches to problem solving in STEM. Our outcome space is shown encompassing different approaches identified in previous phenomenographic studies, which are highlighted by different colors: sustainability in engineering education ( Lönngren et al. , 2017 ) in blue, physics education ( Walsh et al. , 2007 ) in red, collaborative problem solving in engineering education ( Dringenberg and Purzer, 2018 ) in green, physiology education ( Dahlgren et al. , 2021 ) in yellow, and biology education ( Sung et al. , 2022 ) in purple. The highlighted text represents the approaches as named and described in each of the original studies. Some of these approaches span multiple aspects. For example, the cognitive-based clinical reasoning approach in yellow aligns with the knowledge, strategy, and mindset aspects but not the intention and metacognition aspects.
In engineering education focusing on sustainability development, four problem-solving approaches were identified ( Lönngren et al. , 2017 ) that overlap with the variations in the strategy aspect in our outcome space ( Figure 3 , blue). In the simplify-and-avoid approach, students worked on the problems without structure or meaning; in the divide-and-control approach, students did not consider the context of the problem or draw upon knowledge of the field ( Lönngren et al. , 2017 ). This lack of conceptual application in both approaches parallels the strategies seen in the surface approach in our current study. In the isolate-and-succumb approach, students show some understanding of the concepts needed to solve the problem, but their responses remain superficial, disjointed, and lack recognition of the true complexity of engineering systems ( Lönngren et al. , 2017 ). This superficial and limited understanding of the system, despite drawing on information from the course, aligns with strategies in the procedural approach. In the integrate-and-balance approach, students show the most thorough understanding of the problem and apply appropriate engineering concepts to solve the problem ( Lönngren et al. , 2017 ), aligning with strategies in the deep approach.
In physics education, approaches to problem solving were identified ( Walsh et al. , 2007 ) with variations corresponding to the knowledge and strategy aspects in our outcome space ( Figure 3 , red). In the approach named no clear approach, students relied on features of the problem with no conceptual knowledge from the course to solve the problem ( Walsh et al. , 2007 ), aligning with knowledge and strategies in the surface approach in our current study. In two plug-and-chug approaches, students used heuristics that were either devoid of meaning in physics (memory based) or incorporated some conceptual understanding (structured), but this conceptual understanding was applied in a formulaic manner with limited meaning of the complexity of the system ( Walsh et al. , 2007 ). These features correspond to the knowledge and strategies in the procedural approach. In the scientific approach, students drew upon their own understanding and knowledge of physics principles to address each problem, aligning with knowledge and strategies in the deep approach.
Similarly, the previous study on student problem solving in biology ( Sung et al. , 2022 ) identified variations only in the knowledge and strategies aspects in the new outcome space presented in this paper ( Figure 3 , purple). In the nonconceptual approach, students utilized clues identified in the formatting of the question itself or in the language of the question to arrive at their final answer through a process of eliminating seemingly incorrect answers ( Sung et al. , 2022 ). These features correspond to the knowledge and strategies of the surface approach in our current study. In another nonconceptual approach, students relied on algorithmic processes that lacked conceptual understanding and were instead based on patterns identified from either instructor cues or previous problems ( Sung et al. , 2022 ). These features correspond to the knowledge and strategies of the procedural approach in our current study. Lastly, in the conceptual approach, students drew upon their own conceptual knowledge and understanding of biology in order to address the problem ( Sung et al. , 2022 ), aligning with the knowledge and strategy aspects of the deep approach identified in our current study.
Collaborative problem-solving approaches in engineering centered on two aspects ( Dringenberg and Purzer, 2018 ) aligned with variations in the intention and metacognition aspects in our outcome space ( Figure 3 , green). In one extreme, students using a completion approach focused primarily on completing the problem as quickly as possible and did not view their work as authentic engineering ( Dringenberg and Purzer, 2018 ), aligning with our surface approach characterized by a motivation to just complete the course (intention) and limited self-reflection that viewed the coursework as not biology (metacognition). In the middle, students using a transition approach recognized problem solving as a needed and fulfilling process that could lead them to success in future engineering classes but resisted working through the ambiguity inherent in complex engineering systems ( Dringenberg and Purzer, 2018 ), aligning with the conflicting desire and demand (intention) we observed in our procedural approach. In the other extreme, students using a growth approach viewed problem solving as an integral part of personal growth for becoming an engineer ( Dringenberg and Purzer, 2018 ), aligning with our deep approach characterized by sustained interest and motivation in the discipline (intention) and the ability to reflect on the specific concepts and steps needed to solve a problem (metacognition).
Two approaches to problem solving identified in physiotherapy education ( Dahlgren et al. , 2021 ) span the knowledge, strategy, and mindset aspects in our outcome space ( Figure 3 , yellow). In one approach called cognitive-based clinical reasoning, students relied on a step-by-step problem-solving process that was algorithmic in nature and only depended on a superficial understanding of physiotherapy; these students also centered their own experiences rather than the patient experience in physiotherapy, thus limiting how they adapt their learning to respond to new situations ( Dahlgren et al. , 2021 ). These features share similarities with the variations in the knowledge, strategy, and mindset aspects characterized by the procedural approach in our outcome space. In the second approach called relational understanding of clinical reasoning, students used understanding and reasoning in physiotherapy, which provided a more complex view that connected the student and patient; these students also indicated learning was an ongoing process and acknowledged the need to continually adapt to patient needs ( Dahlgren et al. , 2021 ). These features all align with the variations in knowledge, strategy, and mindset in the deep approach.
Our study expands the current literature on student problem-solving approaches by providing a more comprehensive outcome space that include many different aspects of problem solving. The other outcome spaces for problem solving in biology, engineering, physics, and physiotherapy education can be largely correlated within the one from our study, with some minor exceptions only in the strategy aspect ( Figure 3 ). In previous studies, strategies such as simplify-and-avoid versus divide-and-control ( Lönngren et al. , 2017 ) or memory-based versus structured plug-and-chug ( Walsh et al. , 2007 ) were separately identified within those outcome spaces; here, our data do not allow us to distinguish among these different strategies. Nonetheless, the similarities identified across these disciplines and in our work, which focuses on biology, illustrates that the outcome space emerging from this study may be applicable to other disciplines. Finally, by considering problem solving through the lens of situated cognition, our results allowed for an integration of various aspects that influence problem-solving, thus supporting the importance of sociocultural learning theories beyond cognition.
Implications for teaching
We observed some instances of participants switching approaches even within the same problem, such as Evan and William in this study and Michael in the previous study ( Sung et al. , 2022 ). Therefore, we posit that approaches to problem solving are malleable and that students can learn to adopt the deep approach. Prior work has indicated that changes in how a question is framed or how knowledge of biological concepts is activated can lead to differences in problem-solving strategies used by students ( Weston et al. , 2015 ; Avena and Knight, 2019 ). Similarly, student metacognition and mindset can shift based on the use of specific prompts ( Case and Gunstone, 2002 ; Berthold et al. , 2007 ; Tanner, 2012 ; Limeri et al. , 2020 ); However, much of this work has focused within a limited number of aspects, with little to no exploration on how shifts in one aspect may impact other aspects.
By identifying variations in each of the five aspects (i.e., knowledge, strategies, intention, metacognition, and mindset), our results provide additional context for considering interventions that could potentially shift students between approaches (i.e., surface, procedural, or deep), for example, by targeting transitions from one variation to another within one aspect. As demonstrated by other studies in phenomenography on learning in higher education ( Åkerlind, 2005 , 2018), we hypothesize that interventions shifting students from one variation to another within some aspects could also lead to changes in other aspects. In biology education research, interventions promoting metacognition can shift students’ intention from the surface approach towards the variations seen in the procedural or deep approaches while improving cognitive learning outcomes ( Conner, 2007 ; Tanner, 2012 ; Avargil et al. , 2018 ; Dang et al. , 2018 ). Across STEM disciplines, interventions that guide students away from fixed mindset toward growth mindset can positively influence intention and also cognitive learning outcomes ( Fink et al. , 2018 ; Muenks et al. , 2020 , 2021). Similarly, we speculate that providing students with structured guidance on how to become more aware of and to shape the thinking of others ( Halmo et al. , 2022 ) may spark changes in students’ intention from surface to procedural or deep approaches. For example, instructors who rely on small-group activities in class may wish to provide instructions, so that students have guidance on how to evaluate, question, and challenge their group members’ thinking or to elicit greater explanations from their peers ( Halmo et al. , 2022 ). Instructors may also wish to refer to recent evidence-based teaching guides on metacognition ( Stanton et al. , 2021 ) and problem-solving in biology ( Frey et al. , 2022 ).
To further consider implications for teaching, we draw upon the framework of cognitive apprenticeship ( Hennessy, 1993 ). Instructors could utilize interventions based on modeling and coaching that encourage intended and/or actual adoption of knowledge and strategies based on biological concepts and scaffolding in higher-order cognitive questions that promote understanding of biological concepts ( Tanner and Allen, 2005 ; Jensen et al. , 2014 ; Cleveland et al. , 2021 ), all of which are aligned with variations in the knowledge and strategy aspects of the deep approach. For example, when teaching problem solving, instructors can first link various concepts relating to the problem by explaining their connections and then demonstrate the conceptual steps needed to solve the problem (modeling). Next, instructors can provide time for students to work on similar problems and provide feedback during this process (coaching), before iterating through small-group activities with complex problems designed to expand students’ thinking (scaffolding). By directly modeling, coaching, and scaffolding the thinking process, instructors can encourage students to utilize conceptual strategies and draw upon biological knowledge when solving problems.
Similarly, instructors can take steps to promote student learning and metacognition through articulation, reflection, and exploration. Students who are able to internalize concepts and produce a mental model of the new information are more successful at learning ( Bierema et al. , 2017 ). As an example, instructors teaching problem solving in genetics can consider implementing a variant of the jigsaw intervention ( Aronson, 1978 ; Premo et al. , 2018 ; Baken et al. , 2022 ). For example, instructors can provide several scenarios that are related but require distinct solutions; students can work in small groups on one scenario, with different groups solving different scenarios. Next, the class forms new groups that merge students who worked on different scenarios. In these new groups, students can work together to share their solutions and tackle new problems that synthesize ideas from these different scenarios. In such a manner, students describe their knowledge and reasoning to each other (articulation), compare different approaches to related scenarios (reflection), and solve broader problems that require the integration of different concepts and strategies (exploration). Throughout this time, students develop their conceptual understanding of the relevant biological concepts and familiarity with different approaches to problem solving. In addition, structured reflection prompts from instructors throughout this process can be used to spark metacognition.
The relationship among different aspects influencing each other as well as impacting student learning has been empirically demonstrated in other contexts ( Case and Marshall, 2004 ; Limeri et al. , 2020 ). Theoretically, within phenomenography, the outcome space represents descriptions that coherently define the different ways of experiencing a phenomenon across the identified aspects ( Marton and Booth, 1997 ). Therefore, it is reasonable to hypothesize that by developing student metacognition or fostering an asset-based growth mindset in the classroom, we may be able to shift students toward the deep approach to problem solving. Together, we further speculate that there may be a complex, linked relationship among the five aspects of approaches to problem solving, and more work is needed to investigate the interaction among these different aspects.
LIMITATIONS AND FUTURE DIRECTIONS
We acknowledge that our results may not be broadly generalizable to biology education. Our study population includes students at a single institution who were enrolled in one specific course, albeit across several years. Furthermore, we chose genetics to be the disciplinary context for specific methodological reasons, and there may be differences in how students approach problem solving in other subdisciplines of biology. Nonetheless, the contribution of this study is the novel phenomenographic outcome space on student approaches to problem solving that incorporates many aspects previously observed in the education research literature across STEM disciplines.
Within our study sample, only a small number of participants described all five aspects of the outcome space, making it more challenging to draw inferences about the intersections between aspects and approaches. This limitation may be due to the variability in how loquacious participants were when reflecting upon one or more of the aspects. The interview protocol did not directly ask about each aspect, as they emerged from data analysis as part of the methodology in phenomenography ( Åkerlind, 2005 , 2018), Instead, our results illustrate how participants organically provided insights into these aspects when discussing their thought processes. Future work will be able to build upon this study by directly exploring the different aspects identified in the outcome space of approaches to solving problems.
We also did not explore what specific steps or sequential actions that students took to solve problems, as others have studied such heuristics ( Prevost and Lemons, 2016 ; Price et al. , 2021 ). Instead, this study focused on the aspects and variations in how students approach problem solving to generate complementary insights in the existing literature. Future work can explore how variations in these aspects intersect to influence each step of the problem-solving process.
ACKNOWLEDGMENTS
We are grateful to the study participants for their time, and we thank the course instructors for their support in providing the exam problems. We also thank R. Holmgren and G. Light for thoughtful discussions in the early phases of the study. This project was initiated with support by an institutional award from the Howard Hughes Medical Institute for undergraduate biology education under award number 52006934 and the Hewlett Fund for Curricular Innovation from the Weinberg College of Arts and Sciences at Northwestern University. S.K. and A.J.G. were supported by the Undergraduate Research Assistant Program in the Office of Undergraduate Research at Northwestern University. S.M.L. was supported in part by the Faculty Career Development Program at the University of California San Diego.
- Akben, N. ( 2020 ). Effects of the problem-posing approach on students’ problem-solving skills and metacognitive awareness in science education . Research in Science Education , 50 (3), 1143–1165. https://doi.org/10.1007/s11165-018-9726-7 Google Scholar
- Åkerlind, G. S. ( 2005 ). Variation and commonality in phenomenographic research methods . Higher Education Research & Development , 24 (4), 321–334. https://doi.org/10.1080/07294360500284672 Google Scholar
- Åkerlind, G. S. ( 2018 ). What future for phenomenographic research? On continuity and development in the phenomenography and variation theory research tradition . Scandinavian Journal of Educational Research , 62 (6), 949–958. https://doi.org/10.1080/00313831.2017.1324899 Google Scholar
- Aldiabat, K. M., & Le Navenec, C.-L. ( 2018 ). Data saturation: The mysterious step in grounded theory methodology . The Qualitative Report , 23 (1), 245–261. https://doi.org/10.46743/2160-3715/2018.2994 Google Scholar
- Alexander, P. A., Pate, P. E., Kulikowich, J. M., Farrell, D. M., & Wright, N. L. ( 1989 ). Domain-specific and strategic knowledge: Effects of training on students of differing ages or competence levels . Learning and Individual Differences , 1 (3), 283–325. https://doi.org/10.1016/1041-6080(89)90014-9 Google Scholar
- American Association for the Advancement of Science . ( 2011 ). Vision and change in undergraduate biology education: A call to action . Washington, DC: American Association for the Advancement of Science. Google Scholar
- Antonietti, A., Ignazi, S., & Perego, P. ( 2000 ). Metacognitive knowledge about problem-solving methods . British Journal of Educational Psychology , 70 (1), 1–16. https://doi.org/10.1348/000709900157921 Medline , Google Scholar
- Aronson, E. ( 1978 ). The Jigsaw Classroom . Thousand Oaks, CA: SAGE Publications. Google Scholar
- Aşık, G., & Erktin, E. ( 2019 ). Metacognitive experiences: Mediating the relationship between metacognitive knowledge and problem solving . Egitim ve Bilim/Education and Science , 44 (197), 85–103. Google Scholar
- Avargil, S., Lavi, R., & Dori, Y. J. ( 2018 ). Students’ metacognition and metacognitive strategies in science education . In Dori, Y. J.Mevarech, Z. R.Baker, D. R. Eds.), Cognition, Metacognition, and Culture in STEM Education: Learning, Teaching and Assessment (pp. 33–64). Cham, Switzerland: Springer International Publishing. Google Scholar
- Avena, J. S., & Knight, J. K. ( 2019 ). Problem solving in genetics: Content hints can help . CBE—Life Sciences Education , 18 (2), ar23. https://doi.org/10.1187/cbe.18-06-0093 Link , Google Scholar
- Avena, J. S., McIntosh, B. B., Whitney, O. N., Wiens, A., & Knight, J. K. ( 2021 ). Successful problem solving in genetics varies based on question content . CBE—Life Sciences Education , 20 (4), ar51. https://doi.org/10.1187/cbe.21-01-0016 Medline , Google Scholar
- Baken, E. K., Adams, D. C., & Rentz, M. S. ( 2022 ). Jigsaw method improves learning and retention for observation-based undergraduate biology laboratory activities . Journal of Biological Education , 56 (3), 317–322. https://doi.org/10.1080/00219266.2020.1796757 Google Scholar
- Barry, S., Ward, L., & Walter, R. ( 2017 ). Exploring nursing students’ experiences of learning using phenomenography: A literature review . Journal of Nursing Education , 56 (10), 591–598. https://doi.org/10.3928/01484834-20170918-03 Medline , Google Scholar
- Berthold, K., Nückles, M., & Renkl, A. ( 2007 ). Do learning protocols support learning strategies and outcomes? The role of cognitive and metacognitive prompts . Learning and Instruction , 17 (5), 564–577. https://doi.org/10.1016/j.learninstruc.2007.09.007 Google Scholar
- Bierema, A. M.-K., Schwartz, R. S., & Gill, S. A. ( 2017 ). To what extent does current scientific research and textbook content align? A methodology and case study . Journal of Research in Science Teaching , 54 (8), 1097–1118. https://doi.org/10.1002/tea.21399 Google Scholar
- Biggs, J. ( 1979 ). Individual differences in study processes and the quality of learning outcomes . Higher Education , 8 (4), 381–394. https://doi.org/10.1007/BF01680526 Google Scholar
- Bishop, L., & Kuula-Luumi, A. ( 2017 ). Revisiting qualitative data reuse: A decade on . SAGE Open , 7 (1), 2158244016685136. https://doi.org/10.1177/2158244016685136 Google Scholar
- Booth, S. ( 1997 ). On phenomenography, learning, and teaching . Higher Education Research & Development , 16 (2), 135–158. https://doi.org/10.1080/0729436970160203 Google Scholar
- Bourke, B. ( 2014 ). Positionality: Reflecting on the research process . The Qualitative Report , 19 (33), 1–9. https://doi.org/10.46743/2160-3715/2014.1026 Google Scholar
- Brown, J. S., Collins, A., & Duguid, P. ( 1989 ). Situated cognition and the culture of learning . Educational Researcher , 18 (1), 32–42. https://doi.org/10.3102/0013189X018001032 Google Scholar
- Brumby, M. N. ( 1982 ). Consistent differences in cognitive styles shown for qualitative biological problem-solving . British Journal of Educational Psychology , 52 (2), 244–257. https://doi.org/10.1111/j.2044-8279.1982.tb00833.x Google Scholar
- Bussey, T. J., Lo, S. M., & Rasmussen, C. ( 2020 ). Theoretical frameworks for STEM education research . In Johnson, C. C.Mohr-Schroeder, M. J.Moore, T. J.English, L. D. (Eds.), Handbook of Research on STEM Education (pp. 51–62). New York, NY: Routledge. Google Scholar
- Bussey, T. J., Orgill, M., & Crippen, K. J. ( 2013 ). Variation theory: A theory of learning and a useful theoretical framework for chemical education research . Chemistry Education Research and Practice , 14 (1), 9–22. https://doi.org/10.1039/C2RP20145C Google Scholar
- Cakmakci, G., Aydeniz, M., Brown, A., & Makokha, J. M. ( 2020 ). Situated cognition and cognitive apprenticeship learning . In Akpan, B.Kennedy, T. J. (Eds.), Science Education in Theory and Practice: An Introductory Guide to Learning Theory (pp. 293–310). Cham, Switzerland: Springer International Publishing. Google Scholar
- Callejo, M. L., & Vila, A. ( 2009 ). Approach to mathematical problem solving and students’ belief systems: Two case studies . Educational Studies in Mathematics , 72 (1), 111–126. https://doi.org/10.1007/s10649-009-9195-z Google Scholar
- Canning, E. A., & Limeri, L. B. ( 2023 ). Theoretical and methodological directions in mindset intervention research . Social and Personality Psychology Compass , 17 (6), e12758. https://doi.org/10.1111/spc3.12758 Google Scholar
- Carlson, M. P., & Bloom, I. ( 2005 ). The cyclic nature of problem solving: An emergent multidimensional problem-solving framework . Educational Studies in Mathematics , 58 (1), 45–75. https://doi.org/10.1007/s10649-005-0808-x Google Scholar
- Case, J. M. ( 2008 ). Alienation and engagement: Development of an alternative theoretical framework for understanding student learning . Higher Education , 55 (3), 321–332. https://doi.org/10.1007/s10734-007-9057-5 Google Scholar
- Case, J. M., & Marshall, D. ( 2009 ). Approaches to Learning . In Tight, M.Mok, K. H.Huisman, J.Morphew, C. (Eds.), The Routledge International Handbook of Higher Education (pp. 9–22). New York, NY: Routledge. Google Scholar
- Case, J., & Gunstone, R. ( 2002 ). Metacognitive development as a shift in approach to learning: An in-depth study . Studies in Higher Education , 27 (4), 459–470. https://doi.org/10.1080/0307507022000011561 Google Scholar
- Case, J., & Marshall, D. ( 2004 ). Between deep and surface: Procedural approaches to learning in engineering education contexts . Studies in Higher Education , 29 (5), 605–615. https://doi.org/10.1080/0307507042000261571 Google Scholar
- Cavallo, A. M. L. ( 1996 ). Meaningful learning, reasoning ability, and students’ understanding and problem solving of topics in genetics . Journal of Research in Science Teaching , 33 (6), 625–656. https://doi.org/10.1002/(SICI)1098-2736(199608)33:6<625::AID-TEA3>3.0.CO;2-Q Google Scholar
- Cleveland, A., Sezen-Barrie, A., & Marbach-Ad, G. ( 2021 ). The conceptualization of quantitative reasoning among introductory biology faculty . Journal of Microbiology & Biology Education , 22 (3), e00203–21. https://doi.org/10.1128/jmbe.00203-21 Medline , Google Scholar
- Conana, H., Marshall, D., & Case, J. ( 2020 ). A semantics analysis of first-year physics teaching: Developing students’ use of representations in problem-solving . In Winberg, C.McKenna, S.Wilmot, K. (Eds.), Building Knowledge in Higher Education: Enhancing Teaching and Learning with Legitimation Code Theory (pp. 162–179). New York, NY: Routledge. Google Scholar
- Conner, L. N. ( 2007 ). Cueing metacognition to improve researching and essay writing in a final year high school biology class . Research in Science Education , 37 (1), 1–16. https://doi.org/10.1007/s11165-004-3952-x Google Scholar
- Conradi, K., Jang, B. G., & McKenna, M. C. ( 2014 ). Motivation terminology in reading research: A conceptual review . Educational Psychology Review , 26 , 127–164. https://doi.org/10.1007/s10648-013-9245-z Google Scholar
- Cousin, G. ( 2006 ). An introduction to threshold concepts . Planet , 17 (1), 4–5. https://doi.org/10.11120/plan.2006.00170004 Google Scholar
- Dahlgren, M. A., Valeskog, K., Johansson, K., & Edelbring, S. ( 2021 ). Understanding clinical reasoning: A phenomenographic study with entry-level physiotherapy students . Physiotherapy Theory and Practice , 38 (13), 2817–2826. https://doi.org/10.1080/09593985.2021.1976332 Medline , Google Scholar
- Dang, N. V., Chiang, J. C., Brown, H. M., & McDonald, K. K. ( 2018 ). Curricular activities that promote metacognitive skills impact lower-performing students in an introductory biology course . Journal of Microbiology & Biology Education , 19 (1), 19.1.5. https://doi.org/10.1128/jmbe.v19i1.1324 Medline , Google Scholar
- Denton, M., & Borrego, M. ( 2021 ). Funds of knowledge in STEM education: A scoping review . Studies in Engineering Education , 1 (2), 71092. https://doi.org/10.21061/see.19 Google Scholar
- Denton, M., Borrego, M., & Boklage, A. ( 2020 ). Community cultural wealth in science, technology, engineering, and mathematics education: A systematic review . Journal of Engineering Education , 109 (3), 556–580. https://doi.org/10.1002/jee.20322 Google Scholar
- Dinsmore, D. L., Alexander, P. A., & Loughlin, S. M. ( 2008 ). Focusing the conceptual lens on metacognition, self-regulation, and self-regulated learning . Educational Psychology Review , 20 , 391–409. https://doi.org/10.1007/s10648-008-9083-6 Google Scholar
- diSessa, A. A. ( 2007 ). An interactional analysis of clinical interviewing . Cognition and Instruction , 25 (4), 523–565. https://doi.org/10.1080/07370000701632413 Google Scholar
- Dringenberg, E., & Purzer, Ş. ( 2018 ). Experiences of first-year engineering students working on ill-structured problems in teams . Journal of Engineering Education , 107 (3), 442–467. https://doi.org/10.1002/jee.20220 Google Scholar
- Dweck, C. S., & Yeager, D. S. ( 2019 ). Mindsets: A view from two eras . Perspectives on Psychological Science , 14 (3), 481–496. https://doi.org/10.1177/1745691618804166 Medline , Google Scholar
- Entwistle, N. ( 1997 ). Introduction: Phenomenography in higher education . Higher Education Research & Development , 16 (2), 127–134. https://doi.org/10.1080/0729436970160202 Google Scholar
- Entwistle, N., Hanley, M., & Hounsell, D. ( 1979 ). Identifying distinctive approaches to studying . Higher Education , 8 (4), 365–380. https://doi.org/10.1007/BF01680525 Google Scholar
- Fink, A., Cahill, J. M., McDaniel, M. A., Hoffman, A., & Frey, F. R. ( 2018 ). Improving general chemistry performance through a growth mindset intervention: Selective effects on underrepresented minorities . Chemistry Education Research and Practice , 19 (3), 783–806. https://doi.org/10.1039/C7RP00244K Google Scholar
- Fredlund, T., Airey, J., & Linder, C. ( 2015 ). Enhancing the possibilities for learning: Variation of disciplinary-relevant aspects in physics representations . European Journal of Physics , 36 (5), 055001. https://doi.org/10.1088/0143-0807/36/5/055001 Google Scholar
- Frey, R. F., Brame, C. J., Fink, A., & Lemons, P. P. ( 2022 ). Teaching discipline-based problem solving . CBE—Life Sciences Education , 21 (2), fe1. https://doi.org/10.1187/cbe.22-02-0030 Medline , Google Scholar
- Glaser, B. G. ( 1965 ). The constant comparative method of qualitative analysis . Social Problems , 12 (4), 436–445. https://doi.org/10.2307/798843 Google Scholar
- Hajar, A. ( 2021 ). Theoretical foundations of phenomenography: A critical review . Higher Education Research & Development , 40 (7), 1421–1436. https://doi.org/10.1080/07294360.2020.1833844 Google Scholar
- Halmo, S. M., Bremers, E. K., Fuller, S., & Stanton, J. D. ( 2022 ). “Oh, that makes sense”: Social metacognition in small-group problem solving . CBE—Life Sciences Education , 21 (3), ar58. https://doi.org/10.1187/cbe.22-01-0009 Medline , Google Scholar
- Han, F., & Ellis, R. A. ( 2019 ). Using phenomenography to tackle key challenges in science education . Frontiers in Psychology , 10 , 1414. https://doi.org/10.3389/fpsyg.2019.01414 Medline , Google Scholar
- Harper, K. A. ( 2006 ). Student problem-solving behaviors . The Physics Teacher , 44 (4), 250–251. https://doi.org/10.1119/1.2186244 Google Scholar
- Heaton, J. ( 2008 ). Secondary analysis of qualitative data: An overview . Historical Social Research/Historische Sozialforschung , 33 (3125), 33–45. https://doi.org/10.12759/hsr.33.2008.3.33-45 Google Scholar
- Hennessy, S. ( 1993 ). Situated cognition and cognitive apprenticeship: Implications for classroom learning . Studies in Science Education , 22 (1), 1–41. https://doi.org/10.1080/03057269308560019 Google Scholar
- Hennink, M. M., Kaiser, B. N., & Marconi, V. C. ( 2017 ). Code saturation versus meaning saturation: How many interviews are enough? Qualitative Health Research , 27 (4), 591–608. https://doi.org/10.1177/1049732316665344 Medline , Google Scholar
- Hoskinson, A.-M., Caballero, M. D., & Knight, J. K. ( 2013 ). How can we improve problem solving in undergraduate biology? Applying lessons from 30 years of physics education research . CBE—Life Sciences Education , 12 (2), 153–161. https://doi.org/10.1187/cbe.12-09-0149 Link , Google Scholar
- Hsu, J. L., Lo, S. M., & Sato, B. K. ( 2021 ). Defining understanding: Perspectives from biology instructors & biology education researchers . The American Biology Teacher , 83 (6), 372–376. https://doi.org/10.1525/abt.2021.83.6.372 Google Scholar
- Izzati, L. R., & Mahmudi, A. ( 2018 ). The influence of metacognition in mathematical problem solving . Journal of Physics: Conference Series , 1097 , 012107. https://doi.org/10.1088/1742-6596/1097/1/012107 Google Scholar
- Jacob, C. ( 2004 ). Critical thinking in the chemistry classroom and beyond . Journal of Chemical Education , 81 (8), 1216–1223. https://doi.org/10.1021/ed081p1216 Google Scholar
- Jensen, J. L., McDaniel, M. A., Woodard, S. M., & Kummer, T. A. ( 2014 ). Teaching to the test…or testing to teach: Exams requiring higher order thinking skills encourage greater conceptual understanding . Educational Psychology Review , 26 (2), 307–329. https://doi.org/10.1007/s10648-013-9248-9 Google Scholar
- Jonassen, D. H. ( 2010 ). Learning to Solve Problems: A Handbook for Designing Problem-Solving Learning Environments . New York, NY: Routledge. https://doi.org/10.4324/9780203847527 Google Scholar
- Jones, A. ( 2009 ). Redisciplining generic attributes: The disciplinary context in focus . Studies in Higher Education , 34 (1), 85–100. https://doi.org/10.1080/03075070802602018 Google Scholar
- Kirsh, D. ( 2008 ). Problem solving and situated cognition . In Robbins, P.Aydede, M. (Eds.), The Cambridge Handbook of Situated Cognition , (pp. 264–306). Cambridge, United Kingdom: Cambridge University Press. Google Scholar
- Klegeris, A., & Hurren, H. ( 2011 ). Impact of problem-based learning in a large classroom setting: Student perception and problem-solving skills . Advances in Physiology Education , 35 (4), 408–415. https://doi.org/10.1152/advan.00046.2011 Medline , Google Scholar
- Land, R., Rattray, J., & Vivian, P. ( 2014 ). Learning in the liminal space: A semiotic approach to threshold concepts . Higher Education , 67 , 199–217. https://doi.org/10.1007/s10734-013-9705-x Google Scholar
- Lave, J., & Wenger, E. ( 1991 ). Situated Learning: Legitimate Peripheral Participation . Cambridge, United Kingdom: Cambridge University Press. https://doi.org/10.1017/CBO9780511815355 Google Scholar
- Lee, K.-W. L., Goh, N.-K., Chia, L.-S., & Chin, C. ( 1996 ). Cognitive variables in problem solving in chemistry: A revisited study . Science Education , 80 (6), 691–710. https://doi.org/10.1002/(SICI)1098-237X(199611)80:6<691::AID-SCE4>3.0.CO;2-E Google Scholar
- Limeri, L. B., Carter, N. T., Choe, J., Harper, H. G., Martin, H. R., Benton, A., & Dolan, E. L. ( 2020 ). Growing a growth mindset: Characterizing how and why undergraduate students’ mindsets change . International Journal of STEM Education , 7 (1), 35. https://doi.org/10.1186/s40594-020-00227-2 Google Scholar
- Löffler, P., Pozas, M., & Kauertz, A. ( 2018 ). How do students coordinate context-based information and elements of their own knowledge? An analysis of students’ context-based problem-solving in thermodynamics . International Journal of Science Education , 40 (16), 1935–1956. https://doi.org/10.1080/09500693.2018.1514673 Google Scholar
- Lönngren, J., Ingerman, Å., & Svanström, M. ( 2017 ). Avoid, control, succumb, or balance: Engineering students’ approaches to a wicked sustainability problem . Research in Science Education , 47 (4), 805–831. https://doi.org/10.1007/s11165-016-9529-7 Google Scholar
- Luft, J. A., Jeong, S., Idsardi, R., & Gardner, G. ( 2022 ). Literature reviews, theoretical frameworks, and conceptual frameworks: An introduction for new biology education researchers . CBE—Life Sciences Education , 21 (3), rm33. https://doi.org/10.1187/cbe.21-05-0134 Medline , Google Scholar
- Martinez, M. E. ( 1998 ). What is problem solving? The Phi Delta Kappan , 79 (8), 605–609. www.jstor.org/stable/20439287 Google Scholar
- Marton, F. ( 1981 ). Phenomenography: Describing conceptions of the world around us . Instructional Science , 10 (2), 177–200. https://doi.org/10.1007/BF00132516 Google Scholar
- Marton, F. ( 1986 ). Phenomenography: A research approach to investigating different understandings of reality . Journal of Thought , 21 (3), 28–49. www.jstor.org/stable/42589189 Google Scholar
- Marton, F. ( 1988 ). Describing and improving learning . In Schmeck, R. R. (Ed.), Learning Strategies and Learning Styles (pp. 53–82). Boston, MA: Springer. Google Scholar
- Marton, F., & Booth, S. ( 1997 ). Learning and awareness . Hillsdale, NJ: Lawrence Erlbaum. https://doi.org/10.4324/9780203053690 Google Scholar
- Marton, F., & Tsui, A. B. ( 2004 ). Classroom Discourse and the Space of Learning . Hillsdale, NJ: Lawrence Erlbaum. https://doi.org/10.4324/9781410609762 Google Scholar
- McCormick, A. C., & Zhao, C.-M. ( 2005 ). Rethinking and reframing the Carnegie classification . Change: The Magazine of Higher Learning , 37 (5), 51–57. https://doi.org/10.3200/CHNG.37.5.51-57 Google Scholar
- Miller, H. B., & Srougi, M. C. ( 2021 ). Growth mindset interventions improve academic performance but not mindset in biochemistry . Biochemistry and Molecular Biology Education , 49 (5), 748–757. https://doi.org/10.1002/bmb.21556 Medline , Google Scholar
- Muenks, K., Canning, E. A., LaCosse, J., Green, D. J., Zirkel, S., Garcia, J. A., & Murphy, M. C. ( 2020 ). Does my professor think my ability can change? Students’ perceptions of their STEM professors’ mindset beliefs predict their psychological vulnerability, engagement, and performance in class . Journal of Experimental Psychology: General , 149 (11), 2119–2144. https://doi.org/10.1037/xge0000763 Medline , Google Scholar
- Muenks, K., Yan, V. X., Woodward, N. R., & Frey, S. E. ( 2021 ). Elaborative learning practices are associated with perceived faculty growth mindset in undergraduate science classrooms . Learning and Individual Differences , 92 , 102088. https://doi.org/10.1016/j.lindif.2021.102088 Google Scholar
- Murphy, S., MacDonald, A., Wang, C. A., & Danaia, L. ( 2019 ). Towards an understanding of STEM engagement: A review of the literature on motivation and academic emotions . Canadian Journal of Science, Mathematics and Technology Education , 19 , 304–320. https://doi.org/10.1007/s42330-019-00054-w Google Scholar
- Nehm, R. H. ( 2010 ). Understanding undergraduates’ problem-solving processes . Journal of Microbiology & Biology Education , 11 (2), 119–122. https://doi.org/10.1128/jmbe.v11i2.203 Medline , Google Scholar
- Piaget, J. ( 2007 ). The Child’s Conception of the World . Lanham, MD: Rowman & Littlefield. Google Scholar
- Pintrich, P. R. ( 2004 ). A conceptual framework for assessing motivation and self-regulated learning in college students . Educational Psychology Review , 16 (4), 385–407. https:/doi.org/10.1007/s10648-004-0006-x Google Scholar
- Premo, J., Cavagnetto, A., & Davis, W. B. ( 2018 ). Promoting collaborative classrooms: The impacts of interdependent cooperative learning on undergraduate interactions and achievement . CBE—Life Sciences Education , 17 (2), ar32. https://doi.org/10.1187/cbe.17-08-0176 Link , Google Scholar
- Prevost, L. B., & Lemons, P. P. ( 2016 ). Step by step: Biology undergraduates’ problem-solving procedures during multiple-choice assessment . CBE—Life Sciences Education , 15 (4), ar71. https://doi.org/10.1187/cbe.15-12-0255 Link , Google Scholar
- Price, A. M., Kim, C. J., Burkholder, E. W., Fritz, A. V., & Wieman, C. E. ( 2021 ). A detailed characterization of the expert problem-solving process in science and engineering: Guidance for teaching and assessment . CBE—Life Sciences Education , 20 (3), ar43. https://doi.org/10.1187/cbe.20-12-0276 Medline , Google Scholar
- Rattan, A., Savani, K., Komarraju, M., Morrison, M. M., Boggs, C., & Ambady, N. ( 2018 ). Meta-lay theories of scientific potential drive underrepresented students’ sense of belonging to science, technology, engineering, and mathematics (STEM) . Journal of Personality and Social Psychology , 115 (1), 54–75. https://doi.org/10.1037/pspi0000130 Medline , Google Scholar
- Rattan, A., Savani, K., Naidu, N. V. R., & Dweck, C. S. ( 2012 ). Can everyone become highly intelligent? Cultural differences in and societal consequences of beliefs about the universal potential for intelligence . Journal of Personality and Social Psychology , 103 (5), 787–803. https://doi.org/10.1037/a0029263 Medline , Google Scholar
- Reigosa, C., & Jiménez-Aleixandre, M. ( 2007 ). Scaffolded problem-solving in the physics and chemistry laboratory: Difficulties hindering students’ assumption of responsibility . International Journal of Science Education , 29 (3), 307–329. https://doi.org/10.1080/09500690600702454 Google Scholar
- Renninger, K. A., & Hidi, S. E. ( 2022 ). Interest development, self-related information processing, and practice . Theory into Practice , 61 (1), 23–34. https://doi.org/10.1080/00405841.2021.1932159 Google Scholar
- Richardson, D. S., Bledsoe, R. S., & Cortez, Z. ( 2020 ). Mindset, motivation, and teaching practice: Psychology applied to understanding teaching and learning in STEM disciplines . CBE—Life Sciences Education , 19 (3), ar46. https://doi.org/10.1187/cbe.19-11-0238 Link , Google Scholar
- Roth, W.-M., & Jornet, A. ( 2013 ). Situated cognition . WIREs Cognitive Science , 4 (5), 463–478. https://doi.org/10.1002/wcs.1242 Google Scholar
- Rowland, A. A., Knekta, E., Eddy, S., & Corwin, L. A. ( 2019 ). Defining and measuring students’ interest in biology: An analysis of the biology education literature . CBE—Life Sciences Education , 18 (3), ar34. https://doi.org/10.1187/cbe.19-02-0037 Link , Google Scholar
- Safari, Y., & Meskini, H. ( 2016 ). The effect of metacognitive instruction on problem solving skills in Iranian students of health sciences . Global Journal of Health Science , 8 (1), 150–156. https://doi.org/10.5539/gjhs.v8n1p150 Google Scholar
- Saldana, J. ( 2021 ). The Coding Manual for Qualitative Researchers 4th ed., Thousand Oaks, CA: SAGE Publications. Google Scholar
- Schiefele, U. ( 1991 ). Interest, learning, and motivation . Educational Psychologist , 26 (3-4), 299–323. https://doi.org/10.1207/s15326985ep2603&4_5 Google Scholar
- Schoenfeld, A. H. ( 1992 ). On paradigms and methods: What do you do when the ones you know don’t do what you want them to? Issues in the analysis of data in the form of videotapes . Journal of the Learning Sciences , 2 (2), 179–214. https://doi.org/10.1207/s15327809jls0202_3 Google Scholar
- Schoenfeld, A. H. ( 2016 ). Learning to think mathematically: Problem solving, metacognition, and sense making in mathematics . Journal of Education , 196 (2), 1–38. https://doi.org/10.1177/002205741619600202 Google Scholar
- Schraw, G., & Moshman, D. ( 1995 ). Metacognitive theories . Educational Psychology Review , 7 , 351–371. https://doi.org/10.1007/BF02212307 Google Scholar
- Sebasta, A. J., & Bray Speth, E. ( 2017 ). How should I study for the exam? Self-regulated learning strategies and achievement in introductory biology. CBE—Life Sciences Education , 16 (2), ar30. https://doi.org/10.1187/cbe.16-09-0269 Medline , Google Scholar
- Shin, N., Jonassen, D. H., & McGee, S. ( 2003 ). Predictors of well-structured and ill-structured problem solving in an astronomy simulation . Journal of Research in Science Teaching , 40 (1), 6–33. https://doi.org/10.1002/tea.10058 Google Scholar
- Smith, M. K., & Wood, W. B. ( 2016 ). Teaching genetics: Past, present, and future . Genetics , 204 (1), 5–10. https://doi.org/10.1534/genetics.116.187138 Medline , Google Scholar
- Smith, M. U., & Good, R. ( 1984 ). Problem solving and classical genetics: Successful versus unsuccessful performance . Journal of Research in Science Teaching , 21 (9), 895–912. https://doi.org/10.1002/tea.3660210905 Google Scholar
- Stanton, J. D., Sebasta, A. J., & Dunlosky, J. ( 2021 ). Fostering metacognition to support student learning and performance . CBE—Life Sciences Education , 20 (2), fe13. https://doi.org/10.1187/cbe.20-12-0289 Google Scholar
- Stenfors-Hayes, T., Hult, H., & Dahlgren, M. A. ( 2013 ). A phenomenographic approach to research in medical education . Medical Education , 47 (3), 261–270. https://doi.org/10.1111/medu.12101 Medline , Google Scholar
- Stewart, J., & Kirk, J. V. ( 1990 ). Understanding and problem-solving in classical genetics . International Journal of Science Education , 12 (5), 575–588. https://doi.org/10.1080/0950069900120509 Google Scholar
- Sung, R.-J., Swarat, S. L., & Lo, S. M. ( 2022 ). Doing coursework without doing biology: Undergraduate students’ non-conceptual strategies to problem solving . Journal of Biological Education , 56 (3), 271–283. https://doi.org/10.1080/00219266.2020.1785925 Google Scholar
- Swanson, H. L. ( 1990 ). Influence of metacognitive knowledge and aptitude on problem solving . Journal of Educational Psychology , 82 (2), 306. https://doi.org/10.1037/0022-0663.82.2.306 Google Scholar
- Swarat, S., Light, G., Park, E. J., & Drane, D. ( 2011 ). A typology of undergraduate students’ conceptions of size and scale: Identifying and characterizing conceptual variation . Journal of Research in Science Teaching , 48 (5), 512–533. https://doi.org/10.1002/tea.20403 Google Scholar
- Taasoobshirazi, G., & Glynn, S. M. ( 2009 ). College students solving chemistry problems: A theoretical model of expertise . Journal of Research in Science Teaching , 46 (10), 1070–1089. https://doi.org/10.1002/tea.20301 Google Scholar
- Taconis, R., Ferguson-Hessler, M., & Broekkamp, H. ( 2001 ). Teaching science problem solving: An overview of experimental work . Journal of Research in Science Teaching , 38 (4), 442–468. https://doi.org/10.1002/tea.1013 Google Scholar
- Tanner, K. D. ( 2012 ). Promoting student metacognition . CBE—Life Sciences Education , 11 (2), 113–120. https://doi.org/10.1187/cbe.12-03-0033 Link , Google Scholar
- Tanner, K., & Allen, D. ( 2005 ). Approaches to biology teaching and learning: Understanding the wrong answers and teaching toward conceptual change . Cell Biology Education , 4 (2), 112–117. https://doi.org/10.1187/cbe.05-02-0068 Link , Google Scholar
- Tight, M. ( 2016 ). Phenomenography: The development and application of an innovative research design in higher education research . International Journal of Social Research Methodology , 19 (3), 319–338. https://doi.org/10.1080/13645579.2015.1010284 Google Scholar
- Tolman, R. R. ( 1982 ). Difficulties in genetics problem solving . American Biology Teacher , 44 (9), 525–527. https://doi.org/10.2307/4447599 Google Scholar
- van Rossum, E. J., & Hamer, R. ( 2010 ). A pragmatic view on phenomenography and issues of validity and reliability . In van Rossum, E. J.Hamer, R. (Eds.), The Meaning of Learning and Knowing (pp. 33–53). Leiden, Netherlands: Brill. Google Scholar
- Veenman, M. V., Van Hout-Wolters, B. H., & Afflerbach, P. ( 2006 ). Metacognition and learning: Conceptual and methodological considerations . Metacognition and Learning , 1 , 3–14. https://doi.org/10.1007/s11409-006-6893-0 Google Scholar
- Yeager, D. S., & Dweck, C. S. ( 2020 ). What can be learned from growth mindset controversies? American Psychologist , 75 (9), 1269–1284. https://doi.org/10.1037/amp0000794 Medline , Google Scholar
- Walsh, L. N., Howard, R. G., & Bowe, B. ( 2007 ). Phenomenographic study of students’ problem-solving approaches in physics . Physical Review Special Topics-Physics Education Research , 3 (2), 020108. https://doi.org/10.1103/PhysRevSTPER.3.020108 Google Scholar
- Wang, X. ( 2013 ). Why students choose STEM majors: Motivation, high school learning, and postsecondary context of support . American Educational Research Journal , 50 (5), 1081–1121. https://doi.org/10.3102/0002831213488622 Google Scholar
- Weston, M., Haudek, K. C., Prevost, L., Urban-Lurain, M., & Merrill, J. ( 2015 ). Examining the impact of question surface features on students’ answers to constructed-response questions on photosynthesis . CBE—Life Sciences Education , 14 (2), ar19. https://doi.org/10.1187/cbe.14-07-0110 Link , Google Scholar
- Zimmerman, B. J. ( 2002 ). Becoming a self-regulated learner: An overview . Theory Into Practice , 41 (2), 64–70. https://doi.org/10.1207/s15430421tip4102_2 Google Scholar
- Zuckerman, A. L., & Lo, S. M. ( 2022 ). Examining the variations in undergraduate students’ conceptions of successful researchers: A phenomenographic study . CBE—Life Sciences Education , 21 (3), ar55. https://doi.org/10.1187/cbe.21-10-0295 Medline , Google Scholar
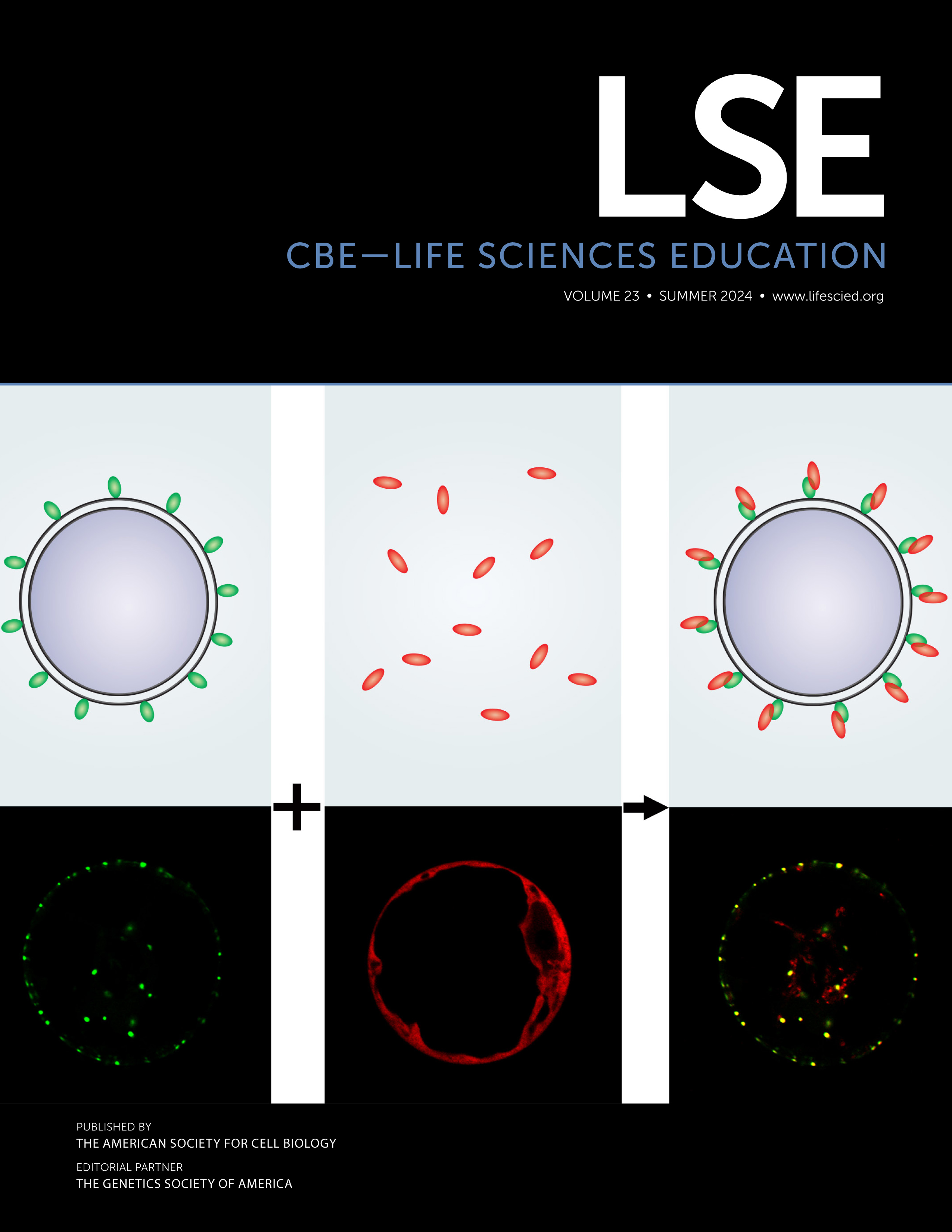
Submitted: 21 February 2023 Revised: 19 January 2024 Accepted: 9 February 2024
© 2024 J. L. Hsu et al. CBE—Life Sciences Education © 2024 The American Society for Cell Biology. This article is distributed by The American Society for Cell Biology under license from the author(s). It is available to the public under an Attribution–Noncommercial–Share Alike 3.0 Unported Creative Commons License (http://creativecommons.org/licenses/by-nc-sa/3.0).

An official website of the United States government
The .gov means it’s official. Federal government websites often end in .gov or .mil. Before sharing sensitive information, make sure you’re on a federal government site.
The site is secure. The https:// ensures that you are connecting to the official website and that any information you provide is encrypted and transmitted securely.
- Publications
- Account settings
Preview improvements coming to the PMC website in October 2024. Learn More or Try it out now .
- Advanced Search
- Journal List
- CBE Life Sci Educ
- v.8(3); Fall 2009
Teaching Creativity and Inventive Problem Solving in Science
Robert l. dehaan.
Division of Educational Studies, Emory University, Atlanta, GA 30322
Engaging learners in the excitement of science, helping them discover the value of evidence-based reasoning and higher-order cognitive skills, and teaching them to become creative problem solvers have long been goals of science education reformers. But the means to achieve these goals, especially methods to promote creative thinking in scientific problem solving, have not become widely known or used. In this essay, I review the evidence that creativity is not a single hard-to-measure property. The creative process can be explained by reference to increasingly well-understood cognitive skills such as cognitive flexibility and inhibitory control that are widely distributed in the population. I explore the relationship between creativity and the higher-order cognitive skills, review assessment methods, and describe several instructional strategies for enhancing creative problem solving in the college classroom. Evidence suggests that instruction to support the development of creativity requires inquiry-based teaching that includes explicit strategies to promote cognitive flexibility. Students need to be repeatedly reminded and shown how to be creative, to integrate material across subject areas, to question their own assumptions, and to imagine other viewpoints and possibilities. Further research is required to determine whether college students' learning will be enhanced by these measures.
INTRODUCTION
Dr. Dunne paces in front of his section of first-year college students, today not as their Bio 110 teacher but in the role of facilitator in their monthly “invention session.” For this meeting, the topic is stem cell therapy in heart disease. Members of each team of four students have primed themselves on the topic by reading selected articles from accessible sources such as Science, Nature, and Scientific American, and searching the World Wide Web, triangulating for up-to-date, accurate, background information. Each team knows that their first goal is to define a set of problems or limitations to overcome within the topic and to begin to think of possible solutions. Dr. Dunne starts the conversation by reminding the group of the few ground rules: one speaker at a time, listen carefully and have respect for others' ideas, question your own and others' assumptions, focus on alternative paths or solutions, maintain an atmosphere of collaboration and mutual support. He then sparks the discussion by asking one of the teams to describe a problem in need of solution.
Science in the United States is widely credited as a major source of discovery and economic development. According to the 2005 TAP Report produced by a prominent group of corporate leaders, “To maintain our country's competitiveness in the twenty-first century, we must cultivate the skilled scientists and engineers needed to create tomorrow's innovations.” ( www.tap2015.org/about/TAP_report2.pdf ). A panel of scientists, engineers, educators, and policy makers convened by the National Research Council (NRC) concurred with this view, reporting that the vitality of the nation “is derived in large part from the productivity of well-trained people and the steady stream of scientific and technical innovations they produce” ( NRC, 2007 ).
For many decades, science education reformers have promoted the idea that learners should be engaged in the excitement of science; they should be helped to discover the value of evidence-based reasoning and higher-order cognitive skills, and be taught to become innovative problem solvers (for reviews, see DeHaan, 2005 ; Hake, 2005 ; Nelson, 2008 ; Perkins and Wieman, 2008 ). But the means to achieve these goals, especially methods to promote creative thinking in scientific problem solving, are not widely known or used. An invention session such as that led by the fictional Dr. Dunne, described above, may seem fanciful as a means of teaching students to think about science as something more than a body of facts and terms to memorize. In recent years, however, models for promoting creative problem solving were developed for classroom use, as detailed by Treffinger and Isaksen (2005) , and such techniques are often used in the real world of high technology. To promote imaginative thinking, the advertising executive Alex F. Osborn invented brainstorming ( Osborn, 1948 , 1979 ), a technique that has since been successful in stimulating inventiveness among engineers and scientists. Could such strategies be transferred to a class for college students? Could they serve as a supplement to a high-quality, scientific teaching curriculum that helps students learn the facts and conceptual frameworks of science and make progress along the novice–expert continuum? Could brainstorming or other instructional strategies that are specifically designed to promote creativity teach students to be more adaptive in their growing expertise, more innovative in their problem-solving abilities? To begin to answer those questions, we first need to understand what is meant by “creativity.”
What Is Creativity? Big-C versus Mini-C Creativity
How to define creativity is an age-old question. Justice Potter Stewart's famous dictum regarding obscenity “I know it when I see it” has also long been an accepted test of creativity. But this is not an adequate criterion for developing an instructional approach. A scientist colleague of mine recently noted that “Many of us [in the scientific community] rarely give the creative process a second thought, imagining one either ‘has it’ or doesn't.” We often think of inventiveness or creativity in scientific fields as the kind of gift associated with a Michelangelo or Einstein. This is what Kaufman and Beghetto (2008) call big-C creativity, borrowing the term that earlier workers applied to the talents of experts in various fields who were identified as particularly creative by their expert colleagues ( MacKinnon, 1978 ). In this sense, creativity is seen as the ability of individuals to generate new ideas that contribute substantially to an intellectual domain. Howard Gardner defined such a creative person as one who “regularly solves problems, fashions products, or defines new questions in a domain in a way that is initially considered novel but that ultimately comes to be accepted in a particular cultural setting” ( Gardner, 1993 , p. 35).
But there is another level of inventiveness termed by various authors as “little-c” ( Craft, 2000 ) or “mini-c” ( Kaufman and Beghetto, 2008 ) creativity that is widespread among all populations. This would be consistent with the workplace definition of creativity offered by Amabile and her coworkers: “coming up with fresh ideas for changing products, services and processes so as to better achieve the organization's goals” ( Amabile et al. , 2005 ). Mini-c creativity is based on what Craft calls “possibility thinking” ( Craft, 2000 , pp. 3–4), as experienced when a worker suddenly has the insight to visualize a new, improved way to accomplish a task; it is represented by the “aha” moment when a student first sees two previously disparate concepts or facts in a new relationship, an example of what Arthur Koestler identified as bisociation: “perceiving a situation or event in two habitually incompatible associative contexts” ( Koestler, 1964 , p. 95).
In this essay, I maintain that mini-c creativity is not a mysterious, innate endowment of rare individuals. Instead, I argue that creative thinking is a multicomponent process, mediated through social interactions, that can be explained by reference to increasingly well-understood mental abilities such as cognitive flexibility and cognitive control that are widely distributed in the population. Moreover, I explore some of the recent research evidence (though with no effort at a comprehensive literature review) showing that these mental abilities are teachable; like other higher-order cognitive skills (HOCS), they can be enhanced by explicit instruction.
Creativity Is a Multicomponent Process
Efforts to define creativity in psychological terms go back to J. P. Guilford ( Guilford, 1950 ) and E. P. Torrance ( Torrance, 1974 ), both of whom recognized that underlying the construct were other cognitive variables such as ideational fluency, originality of ideas, and sensitivity to missing elements. Many authors since then have extended the argument that a creative act is not a singular event but a process, an interplay among several interactive cognitive and affective elements. In this view, the creative act has two phases, a generative and an exploratory or evaluative phase ( Finke et al. , 1996 ). During the generative process, the creative mind pictures a set of novel mental models as potential solutions to a problem. In the exploratory phase, we evaluate the multiple options and select the best one. Early scholars of creativity, such as J. P. Guilford, characterized the two phases as divergent thinking and convergent thinking ( Guilford, 1950 ). Guilford defined divergent thinking as the ability to produce a broad range of associations to a given stimulus or to arrive at many solutions to a problem (for overviews of the field from different perspectives, see Amabile, 1996 ; Banaji et al. , 2006 ; Sawyer, 2006 ). In neurocognitive terms, divergent thinking is referred to as associative richness ( Gabora, 2002 ; Simonton, 2004 ), which is often measured experimentally by comparing the number of words that an individual generates from memory in response to stimulus words on a word association test. In contrast, convergent thinking refers to the capacity to quickly focus on the one best solution to a problem.
The idea that there are two stages to the creative process is consistent with results from cognition research indicating that there are two distinct modes of thought, associative and analytical ( Neisser, 1963 ; Sloman, 1996 ). In the associative mode, thinking is defocused, suggestive, and intuitive, revealing remote or subtle connections between items that may be correlated, or may not, and are usually not causally related ( Burton, 2008 ). In the analytical mode, thought is focused and evaluative, more conducive to analyzing relationships of cause and effect (for a review of other cognitive aspects of creativity, see Runco, 2004 ). Science educators associate the analytical mode with the upper levels (analysis, synthesis, and evaluation) of Bloom's taxonomy (e.g., Crowe et al. , 2008 ), or with “critical thinking,” the process that underlies the “purposeful, self-regulatory judgment that drives problem-solving and decision-making” ( Quitadamo et al. , 2008 , p. 328). These modes of thinking are under cognitive control through the executive functions of the brain. The core executive functions, which are thought to underlie all planning, problem solving, and reasoning, are defined ( Blair and Razza, 2007 ) as working memory control (mentally holding and retrieving information), cognitive flexibility (considering multiple ideas and seeing different perspectives), and inhibitory control (resisting several thoughts or actions to focus on one). Readers wishing to delve further into the neuroscience of the creative process can refer to the cerebrocerebellar theory of creativity ( Vandervert et al. , 2007 ) in which these mental activities are described neurophysiologically as arising through interactions among different parts of the brain.
The main point from all of these works is that creativity is not some single hard-to-measure property or act. There is ample evidence that the creative process requires both divergent and convergent thinking and that it can be explained by reference to increasingly well-understood underlying mental abilities ( Haring-Smith, 2006 ; Kim, 2006 ; Sawyer, 2006 ; Kaufman and Sternberg, 2007 ) and cognitive processes ( Simonton, 2004 ; Diamond et al. , 2007 ; Vandervert et al. , 2007 ).
Creativity Is Widely Distributed and Occurs in a Social Context
Although it is understandable to speak of an aha moment as a creative act by the person who experiences it, authorities in the field have long recognized (e.g., Simonton, 1975 ) that creative thinking is not so much an individual trait but rather a social phenomenon involving interactions among people within their specific group or cultural settings. “Creativity isn't just a property of individuals, it is also a property of social groups” ( Sawyer, 2006 , p. 305). Indeed, Osborn introduced his brainstorming method because he was convinced that group creativity is always superior to individual creativity. He drew evidence for this conclusion from activities that demand collaborative output, for example, the improvisations of a jazz ensemble. Although each musician is individually creative during a performance, the novelty and inventiveness of each performer's playing is clearly influenced, and often enhanced, by “social and interactional processes” among the musicians ( Sawyer, 2006 , p. 120). Recently, Brophy (2006) offered evidence that for problem solving, the situation may be more nuanced. He confirmed that groups of interacting individuals were better at solving complex, multipart problems than single individuals. However, when dealing with certain kinds of single-issue problems, individual problem solvers produced a greater number of solutions than interacting groups, and those solutions were judged to be more original and useful.
Consistent with the findings of Brophy (2006) , many scholars acknowledge that creative discoveries in the real world such as solving the problems of cutting-edge science—which are usually complex and multipart—are influenced or even stimulated by social interaction among experts. The common image of the lone scientist in the laboratory experiencing a flash of creative inspiration is probably a myth from earlier days. As a case in point, the science historian Mara Beller analyzed the social processes that underlay some of the major discoveries of early twentieth-century quantum physics. Close examination of successive drafts of publications by members of the Copenhagen group revealed a remarkable degree of influence and collaboration among 10 or more colleagues, although many of these papers were published under the name of a single author ( Beller, 1999 ). Sociologists Bruno Latour and Steve Woolgar's study ( Latour and Woolgar, 1986 ) of a neuroendocrinology laboratory at the Salk Institute for Biological Studies make the related point that social interactions among the participating scientists determined to a remarkable degree what discoveries were made and how they were interpreted. In the laboratory, researchers studied the chemical structure of substances released by the brain. By analysis of the Salk scientists' verbalizations of concepts, theories, formulas, and results of their investigations, Latour and Woolgar showed that the structures and interpretations that were agreed upon, that is, the discoveries announced by the laboratory, were mediated by social interactions and power relationships among members of the laboratory group. By studying the discovery process in other fields of the natural sciences, sociologists and anthropologists have provided more cases that further illustrate how social and cultural dimensions affect scientific insights (for a thoughtful review, see Knorr Cetina, 1995 ).
In sum, when an individual experiences an aha moment that feels like a singular creative act, it may rather have resulted from a multicomponent process, under the influence of group interactions and social context. The process that led up to what may be sensed as a sudden insight will probably have included at least three diverse, but testable elements: 1) divergent thinking, including ideational fluency or cognitive flexibility, which is the cognitive executive function that underlies the ability to visualize and accept many ideas related to a problem; 2) convergent thinking or the application of inhibitory control to focus and mentally evaluate ideas; and 3) analogical thinking, the ability to understand a novel idea in terms of one that is already familiar.
LITERATURE REVIEW
What do we know about how to teach creativity.
The possibility of teaching for creative problem solving gained credence in the 1960s with the studies of Jerome Bruner, who argued that children should be encouraged to “treat a task as a problem for which one invents an answer, rather than finding one out there in a book or on the blackboard” ( Bruner, 1965 , pp. 1013–1014). Since that time, educators and psychologists have devised programs of instruction designed to promote creativity and inventiveness in virtually every student population: pre–K, elementary, high school, and college, as well as in disadvantaged students, athletes, and students in a variety of specific disciplines (for review, see Scott et al. , 2004 ). Smith (1998) identified 172 instructional approaches that have been applied at one time or another to develop divergent thinking skills.
Some of the most convincing evidence that elements of creativity can be enhanced by instruction comes from work with young children. Bodrova and Leong (2001) developed the Tools of the Mind (Tools) curriculum to improve all of the three core mental executive functions involved in creative problem solving: cognitive flexibility, working memory, and inhibitory control. In a year-long randomized study of 5-yr-olds from low-income families in 21 preschool classrooms, half of the teachers applied the districts' balanced literacy curriculum (literacy), whereas the experimenters trained the other half to teach the same academic content by using the Tools curriculum ( Diamond et al. , 2007 ). At the end of the year, when the children were tested with a battery of neurocognitive tests including a test for cognitive flexibility ( Durston et al. , 2003 ; Davidson et al. , 2006 ), those exposed to the Tools curriculum outperformed the literacy children by as much as 25% ( Diamond et al. , 2007 ). Although the Tools curriculum and literacy program were similar in academic content and in many other ways, they differed primarily in that Tools teachers spent 80% of their time explicitly reminding the children to think of alternative ways to solve a problem and building their executive function skills.
Teaching older students to be innovative also demands instruction that explicitly promotes creativity but is rigorously content-rich as well. A large body of research on the differences between novice and expert cognition indicates that creative thinking requires at least a minimal level of expertise and fluency within a knowledge domain ( Bransford et al. , 2000 ; Crawford and Brophy, 2006 ). What distinguishes experts from novices, in addition to their deeper knowledge of the subject, is their recognition of patterns in information, their ability to see relationships among disparate facts and concepts, and their capacity for organizing content into conceptual frameworks or schemata ( Bransford et al. , 2000 ; Sawyer, 2005 ).
Such expertise is often lacking in the traditional classroom. For students attempting to grapple with new subject matter, many kinds of problems that are presented in high school or college courses or that arise in the real world can be solved merely by applying newly learned algorithms or procedural knowledge. With practice, problem solving of this kind can become routine and is often considered to represent mastery of a subject, producing what Sternberg refers to as “pseudoexperts” ( Sternberg, 2003 ). But beyond such routine use of content knowledge the instructor's goal must be to produce students who have gained the HOCS needed to apply, analyze, synthesize, and evaluate knowledge ( Crowe et al. , 2008 ). The aim is to produce students who know enough about a field to grasp meaningful patterns of information, who can readily retrieve relevant knowledge from memory, and who can apply such knowledge effectively to novel problems. This condition is referred to as adaptive expertise ( Hatano and Ouro, 2003 ; Schwartz et al. , 2005 ). Instead of applying already mastered procedures, adaptive experts are able to draw on their knowledge to invent or adapt strategies for solving unique or novel problems within a knowledge domain. They are also able, ideally, to transfer conceptual frameworks and schemata from one domain to another (e.g., Schwartz et al. , 2005 ). Such flexible, innovative application of knowledge is what results in inventive or creative solutions to problems ( Crawford and Brophy, 2006 ; Crawford, 2007 ).
Promoting Creative Problem Solving in the College Classroom
In most college courses, instructors teach science primarily through lectures and textbooks that are dominated by facts and algorithmic processing rather than by concepts, principles, and evidence-based ways of thinking. This is despite ample evidence that many students gain little new knowledge from traditional lectures ( Hrepic et al. , 2007 ). Moreover, it is well documented that these methods engender passive learning rather than active engagement, boredom instead of intellectual excitement, and linear thinking rather than cognitive flexibility (e.g., Halpern and Hakel, 2003 ; Nelson, 2008 ; Perkins and Wieman, 2008 ). Cognitive flexibility, as noted, is one of the three core mental executive functions involved in creative problem solving ( Ausubel, 1963 , 2000 ). The capacity to apply ideas creatively in new contexts, referred to as the ability to “transfer” knowledge (see Mestre, 2005 ), requires that learners have opportunities to actively develop their own representations of information to convert it to a usable form. Especially when a knowledge domain is complex and fraught with ill-structured information, as in a typical introductory college biology course, instruction that emphasizes active-learning strategies is demonstrably more effective than traditional linear teaching in reducing failure rates and in promoting learning and transfer (e.g., Freeman et al. , 2007 ). Furthermore, there is already some evidence that inclusion of creativity training as part of a college curriculum can have positive effects. Hunsaker (2005) has reviewed a number of such studies. He cites work by McGregor (2001) , for example, showing that various creativity training programs including brainstorming and creative problem solving increase student scores on tests of creative-thinking abilities.
What explicit instructional strategies are available to promote creative problem solving? In addition to brainstorming, McFadzean (2002) discusses several “paradigm-stretching” techniques that can encourage creative ideas. One method, known as heuristic ideation, encourages participants to force together two unrelated concepts to discover novel relationships, a modern version of Koestler's bisociation ( Koestler, 1964 ). On the website of the Center for Development and Learning, Robert Sternberg and Wendy M. Williams offer 24 “tips” for teachers wishing to promote creativity in their students ( Sternberg and Williams, 1998 ). Among them, the following techniques might apply to a science classroom:
- Model creativity—students develop creativity when instructors model creative thinking and inventiveness.
- Repeatedly encourage idea generation—students need to be reminded to generate their own ideas and solutions in an environment free of criticism.
- Cross-fertilize ideas—where possible, avoid teaching in subject-area boxes: a math box, a social studies box, etc; students' creative ideas and insights often result from learning to integrate material across subject areas.
- Build self-efficacy—all students have the capacity to create and to experience the joy of having new ideas, but they must be helped to believe in their own capacity to be creative.
- Constantly question assumptions—make questioning a part of the daily classroom exchange; it is more important for students to learn what questions to ask and how to ask them than to learn the answers.
- Imagine other viewpoints—students broaden their perspectives by learning to reflect upon ideas and concepts from different points of view.
Although these strategies are all consistent with the knowledge about creativity that I have reviewed above, evidence from well-designed investigations to warrant the claim that they can enhance measurable indicators of creativity in college students is only recently beginning to materialize. If creativity most often occurs in “a mental state where attention is defocused, thought is associative, and a large number of mental representations are simultaneously activated” ( Martindale, 1999 , p. 149), the question arises whether instructional strategies designed to enhance the HOCS also foster such a mental state? Do valid tests exist to show that creative problem solving can be enhanced by such instruction?
How Is Creativity Related to Critical Thinking and the Higher-Order Cognitive Skills?
It is not uncommon to associate creativity and ingenuity with scientific reasoning ( Sawyer, 2005 ; 2006 ). When instructors apply scientific teaching strategies ( Handelsman et al. , 2004 ; DeHaan, 2005 ; Wood, 2009 ) by using instructional methods based on learning research, according to Ebert-May and Hodder ( 2008 ), “we see students actively engaged in the thinking, creativity, rigor, and experimentation we associate with the practice of science—in much the same way we see students learn in the field and in laboratories” (p. 2). Perkins and Wieman (2008) note that “To be successful innovators in science and engineering, students must develop a deep conceptual understanding of the underlying science ideas, an ability to apply these ideas and concepts broadly in different contexts, and a vision to see their relevance and usefulness in real-world applications … An innovator is able to perceive and realize potential connections and opportunities better than others” (pp. 181–182). The results of Scott et al. (2004) suggest that nontraditional courses in science that are based on constructivist principles and that use strategies of scientific teaching to promote the HOCS and enhance content mastery and dexterity in scientific thinking ( Handelsman et al. , 2007 ; Nelson, 2008 ) also should be effective in promoting creativity and cognitive flexibility if students are explicitly guided to learn these skills.
Creativity is an essential element of problem solving ( Mumford et al. , 1991 ; Runco, 2004 ) and of critical thinking ( Abrami et al. , 2008 ). As such, it is common to think of applications of creativity such as inventiveness and ingenuity among the HOCS as defined in Bloom's taxonomy ( Crowe et al. , 2008 ). Thus, it should come as no surprise that creativity, like other elements of the HOCS, can be taught most effectively through inquiry-based instruction, informed by constructivist theory ( Ausubel, 1963 , 2000 ; Duch et al. , 2001 ; Nelson, 2008 ). In a survey of 103 instructors who taught college courses that included creativity instruction, Bull et al. (1995) asked respondents to rate the importance of various course characteristics for enhancing student creativity. Items ranking high on the list were: providing a social climate in which students feels safe, an open classroom environment that promotes tolerance for ambiguity and independence, the use of humor, metaphorical thinking, and problem defining. Many of the responses emphasized the same strategies as those advanced to promote creative problem solving (e.g., Mumford et al. , 1991 ; McFadzean, 2002 ; Treffinger and Isaksen, 2005 ) and critical thinking ( Abrami et al. , 2008 ).
In a careful meta-analysis, Scott et al. (2004) examined 70 instructional interventions designed to enhance and measure creative performance. The results were striking. Courses that stressed techniques such as critical thinking, convergent thinking, and constraint identification produced the largest positive effect sizes. More open techniques that provided less guidance in strategic approaches had less impact on the instructional outcomes. A striking finding was the effectiveness of being explicit; approaches that clearly informed students about the nature of creativity and offered clear strategies for creative thinking were most effective. Approaches such as social modeling, cooperative learning, and case-based (project-based) techniques that required the application of newly acquired knowledge were found to be positively correlated to high effect sizes. The most clear-cut result to emerge from the Scott et al. (2004) study was simply to confirm that creativity instruction can be highly successful in enhancing divergent thinking, problem solving, and imaginative performance. Most importantly, of the various cognitive processes examined, those linked to the generation of new ideas such as problem finding, conceptual combination, and idea generation showed the greatest improvement. The success of creativity instruction, the authors concluded, can be attributed to “developing and providing guidance concerning the application of requisite cognitive capacities … [and] a set of heuristics or strategies for working with already available knowledge” (p. 382).
Many of the scientific teaching practices that have been shown by research to foster content mastery and HOCS, and that are coming more widely into use, also would be consistent with promoting creativity. Wood (2009) has recently reviewed examples of such practices and how to apply them. These include relatively small modifications of the traditional lecture to engender more active learning, such as the use of concept tests and peer instruction ( Mazur, 1996 ), Just-in-Time-Teaching techniques ( Novak et al. , 1999 ), and student response systems known as “clickers” ( Knight and Wood, 2005 ; Crossgrove and Curran, 2008 ), all designed to allow the instructor to frequently and effortlessly elicit and respond to student thinking. Other strategies can transform the lecture hall into a workshop or studio classroom ( Gaffney et al. , 2008 ) where the teaching curriculum may emphasize problem-based (also known as project-based or case-based) learning strategies ( Duch et al. , 2001 ; Ebert-May and Hodder, 2008 ) or “community-based inquiry” in which students engage in research that enhances their critical-thinking skills ( Quitadamo et al. , 2008 ).
Another important approach that could readily subserve explicit creativity instruction is the use of computer-based interactive simulations, or “sims” ( Perkins and Wieman, 2008 ) to facilitate inquiry learning and effective, easy self-assessment. An example in the biological sciences would be Neurons in Action ( http://neuronsinaction.com/home/main ). In such educational environments, students gain conceptual understanding of scientific ideas through interactive engagement with materials (real or virtual), with each other, and with instructors. Following the tenets of scientific teaching, students are encouraged to pose and answer their own questions, to make sense of the materials, and to construct their own understanding. The question I pose here is whether an additional focus—guiding students to meet these challenges in a context that explicitly promotes creativity—would enhance learning and advance students' progress toward adaptive expertise?
Assessment of Creativity
To teach creativity, there must be measurable indicators to judge how much students have gained from instruction. Educational programs intended to teach creativity became popular after the Torrance Tests of Creative Thinking (TTCT) was introduced in the 1960s ( Torrance, 1974 ). But it soon became apparent that there were major problems in devising tests for creativity, both because of the difficulty of defining the construct and because of the number and complexity of elements that underlie it. Tests of intelligence and other personality characteristics on creative individuals revealed a host of related traits such as verbal fluency, metaphorical thinking, flexible decision making, tolerance of ambiguity, willingness to take risks, autonomy, divergent thinking, self-confidence, problem finding, ideational fluency, and belief in oneself as being “creative” ( Barron and Harrington, 1981 ; Tardif and Sternberg, 1988 ; Runco and Nemiro, 1994 ; Snyder et al. , 2004 ). Many of these traits have been the focus of extensive research of recent decades, but, as noted above, creativity is not defined by any one trait; there is now reason to believe that it is the interplay among the cognitive and affective processes that underlie inventiveness and the ability to find novel solutions to a problem.
Although the early creativity researchers recognized that assessing divergent thinking as a measure of creativity required tests for other underlying capacities ( Guilford, 1950 ; Torrance, 1974 ), these workers and their colleagues nonetheless believed that a high score for divergent thinking alone would correlate with real creative output. Unfortunately, no such correlation was shown ( Barron and Harrington, 1981 ). Results produced by many of the instruments initially designed to measure various aspects of creative thinking proved to be highly dependent on the test itself. A review of several hundred early studies showed that an individual's creativity score could be affected by simple test variables, for example, how the verbal pretest instructions were worded ( Barron and Harrington, 1981 , pp. 442–443). Most scholars now agree that divergent thinking, as originally defined, was not an adequate measure of creativity. The process of creative thinking requires a complex combination of elements that include cognitive flexibility, memory control, inhibitory control, and analogical thinking, enabling the mind to free-range and analogize, as well as to focus and test.
More recently, numerous psychometric measures have been developed and empirically tested (see Plucker and Renzulli, 1999 ) that allow more reliable and valid assessment of specific aspects of creativity. For example, the creativity quotient devised by Snyder et al. (2004) tests the ability of individuals to link different ideas and different categories of ideas into a novel synthesis. The Wallach–Kogan creativity test ( Wallach and Kogan, 1965 ) explores the uniqueness of ideas associated with a stimulus. For a more complete list and discussion, see the Creativity Tests website ( www.indiana.edu/∼bobweb/Handout/cretv_6.html ).
The most widely used measure of creativity is the TTCT, which has been modified four times since its original version in 1966 to take into account subsequent research. The TTCT-Verbal and the TTCT-Figural are two versions ( Torrance, 1998 ; see http://ststesting.com/2005giftttct.html ). The TTCT-Verbal consists of five tasks; the “stimulus” for each task is a picture to which the test-taker responds briefly in writing. A sample task that can be viewed from the TTCT Demonstrator website asks, “Suppose that people could transport themselves from place to place with just a wink of the eye or a twitch of the nose. What might be some things that would happen as a result? You have 3 min.” ( www.indiana.edu/∼bobweb/Handout/d3.ttct.htm ).
In the TTCT-Figural, participants are asked to construct a picture from a stimulus in the form of a partial line drawing given on the test sheet (see example below; Figure 1 ). Specific instructions are to “Add lines to the incomplete figures below to make pictures out of them. Try to tell complete stories with your pictures. Give your pictures titles. You have 3 min.” In the introductory materials, test-takers are urged to “… think of a picture or object that no one else will think of. Try to make it tell as complete and as interesting a story as you can …” ( Torrance et al. , 2008 , p. 2).

Sample figural test item from the TTCT Demonstrator website ( www.indiana.edu/∼bobweb/Handout/d3.ttct.htm ).
How would an instructor in a biology course judge the creativity of students' responses to such an item? To assist in this task, the TTCT has scoring and norming guides ( Torrance, 1998 ; Torrance et al. , 2008 ) with numerous samples and responses representing different levels of creativity. The guides show sample evaluations based upon specific indicators such as fluency, originality, elaboration (or complexity), unusual visualization, extending or breaking boundaries, humor, and imagery. These examples are easy to use and provide a high degree of validity and generalizability to the tests. The TTCT has been more intensively researched and analyzed than any other creativity instrument, and the norming samples have longitudinal validations and high predictive validity over a wide age range. In addition to global creativity scores, the TTCT is designed to provide outcome measures in various domains and thematic areas to allow for more insightful analysis ( Kaufman and Baer, 2006 ). Kim (2006) has examined the characteristics of the TTCT, including norms, reliability, and validity, and concludes that the test is an accurate measure of creativity. When properly used, it has been shown to be fair in terms of gender, race, community status, and language background. According to Kim (2006) and other authorities in the field ( McIntyre et al. , 2003 ; Scott et al. , 2004 ), Torrance's research and the development of the TTCT have provided groundwork for the idea that creative levels can be measured and then increased through instruction and practice.
SCIENTIFIC TEACHING TO PROMOTE CREATIVITY
How could creativity instruction be integrated into scientific teaching.
Guidelines for designing specific course units that emphasize HOCS by using strategies of scientific teaching are now available from the current literature. As an example, Karen Cloud-Hansen and colleagues ( Cloud-Hansen et al. , 2008 ) describe a course titled, “Ciprofloxacin Resistance in Neisseria gonorrhoeae .” They developed this undergraduate seminar to introduce college freshmen to important concepts in biology within a real-world context and to increase their content knowledge and critical-thinking skills. The centerpiece of the unit is a case study in which teams of students are challenged to take the role of a director of a local public health clinic. One of the county commissioners overseeing the clinic is an epidemiologist who wants to know “how you plan to address the emergence of ciprofloxacin resistance in Neisseria gonorrhoeae ” (p. 304). State budget cuts limit availability of expensive antibiotics and some laboratory tests to patients. Student teams are challenged to 1) develop a plan to address the medical, economic, and political questions such a clinic director would face in dealing with ciprofloxacin-resistant N. gonorrhoeae ; 2) provide scientific data to support their conclusions; and 3) describe their clinic plan in a one- to two-page referenced written report.
Throughout the 3-wk unit, in accordance with the principles of problem-based instruction ( Duch et al. , 2001 ), course instructors encourage students to seek, interpret, and synthesize their own information to the extent possible. Students have access to a variety of instructional formats, and active-learning experiences are incorporated throughout the unit. These activities are interspersed among minilectures and give the students opportunities to apply new information to their existing base of knowledge. The active-learning activities emphasize the key concepts of the minilectures and directly confront common misconceptions about antibiotic resistance, gene expression, and evolution. Weekly classes include question/answer/discussion sessions to address student misconceptions and 20-min minilectures on such topics as antibiotic resistance, evolution, and the central dogma of molecular biology. Students gather information about antibiotic resistance in N. gonorrhoeae , epidemiology of gonorrhea, and treatment options for the disease, and each team is expected to formulate a plan to address ciprofloxacin resistance in N. gonorrhoeae .
In this project, the authors assessed student gains in terms of content knowledge regarding topics covered such as the role of evolution in antibiotic resistance, mechanisms of gene expression, and the role of oncogenes in human disease. They also measured HOCS as gains in problem solving, according to a rubric that assessed self-reported abilities to communicate ideas logically, solve difficult problems about microbiology, propose hypotheses, analyze data, and draw conclusions. Comparing the pre- and posttests, students reported significant learning of scientific content. Among the thinking skill categories, students demonstrated measurable gains in their ability to solve problems about microbiology but the unit seemed to have little impact on their more general perceived problem-solving skills ( Cloud-Hansen et al. , 2008 ).
What would such a class look like with the addition of explicit creativity-promoting approaches? Would the gains in problem-solving abilities have been greater if during the minilectures and other activities, students had been introduced explicitly to elements of creative thinking from the Sternberg and Williams (1998) list described above? Would the students have reported greater gains if their instructors had encouraged idea generation with weekly brainstorming sessions; if they had reminded students to cross-fertilize ideas by integrating material across subject areas; built self-efficacy by helping students believe in their own capacity to be creative; helped students question their own assumptions; and encouraged students to imagine other viewpoints and possibilities? Of most relevance, could the authors have been more explicit in assessing the originality of the student plans? In an experiment that required college students to develop plans of a different, but comparable, type, Osborn and Mumford (2006) created an originality rubric ( Figure 2 ) that could apply equally to assist instructors in judging student plans in any course. With such modifications, would student gains in problem-solving abilities or other HOCS have been greater? Would their plans have been measurably more imaginative?

Originality rubric (adapted from Osburn and Mumford, 2006 , p. 183).
Answers to these questions can only be obtained when a course like that described by Cloud-Hansen et al. (2008) is taught with explicit instruction in creativity of the type I described above. But, such answers could be based upon more than subjective impressions of the course instructors. For example, students could be pretested with items from the TTCT-Verbal or TTCT-Figural like those shown. If, during minilectures and at every contact with instructors, students were repeatedly reminded and shown how to be as creative as possible, to integrate material across subject areas, to question their own assumptions and imagine other viewpoints and possibilities, would their scores on TTCT posttest items improve? Would the plans they formulated to address ciprofloxacin resistance become more imaginative?
Recall that in their meta-analysis, Scott et al. (2004) found that explicitly informing students about the nature of creativity and offering strategies for creative thinking were the most effective components of instruction. From their careful examination of 70 experimental studies, they concluded that approaches such as social modeling, cooperative learning, and case-based (project-based) techniques that required the application of newly acquired knowledge were positively correlated with high effect sizes. The study was clear in confirming that explicit creativity instruction can be successful in enhancing divergent thinking and problem solving. Would the same strategies work for courses in ecology and environmental biology, as detailed by Ebert-May and Hodder (2008) , or for a unit elaborated by Knight and Wood (2005) that applies classroom response clickers?
Finally, I return to my opening question with the fictional Dr. Dunne. Could a weekly brainstorming “invention session” included in a course like those described here serve as the site where students are introduced to concepts and strategies of creative problem solving? As frequently applied in schools of engineering ( Paulus and Nijstad, 2003 ), brainstorming provides an opportunity for the instructor to pose a problem and to ask the students to suggest as many solutions as possible in a brief period, thus enhancing ideational fluency. Here, students can be encouraged explicitly to build on the ideas of others and to think flexibly. Would brainstorming enhance students' divergent thinking or creative abilities as measured by TTCT items or an originality rubric? Many studies have demonstrated that group interactions such as brainstorming, under the right conditions, can indeed enhance creativity ( Paulus and Nijstad, 2003 ; Scott et al. , 2004 ), but there is little information from an undergraduate science classroom setting. Intellectual Ventures, a firm founded by Nathan Myhrvold, the creator of Microsoft's Research Division, has gathered groups of engineers and scientists around a table for day-long sessions to brainstorm about a prearranged topic. Here, the method seems to work. Since it was founded in 2000, Intellectual Ventures has filed hundreds of patent applications in more than 30 technology areas, applying the “invention session” strategy ( Gladwell, 2008 ). Currently, the company ranks among the top 50 worldwide in number of patent applications filed annually. Whether such a technique could be applied successfully in a college science course will only be revealed by future research.
- Abrami P. C., Bernard R. M., Borokhovski E., Wadem A., Surkes M. A., Tamim R., Zhang D. Instructional interventions affecting critical thinking skills and dispositions: a stage 1 meta-analysis. Rev. Educ. Res. 2008; 78 :1102–1134. [ Google Scholar ]
- Amabile T. M. Creativity in Context. Boulder, CO: Westview Press; 1996. [ Google Scholar ]
- Amabile T. M., Barsade S. G., Mueller J. S., Staw B. M. Affect and creativity at work. Admin. Sci. Q. 2005; 50 :367–403. [ Google Scholar ]
- Ausubel D. The Psychology of Meaningful Verbal Learning. New York: Grune and Stratton; 1963. [ Google Scholar ]
- Ausubel B. The Acquisition and Retention of Knowledge: A Cognitive View. Boston, MA: Kluwer Academic Publishers; 2000. [ Google Scholar ]
- Banaji S., Burn A., Buckingham D. The Rhetorics of Creativity: A Review of the Literature. London: Centre for the Study of Children, Youth and Media; 2006. [accessed 29 December 2008]. www.creativepartnerships.com/data/files/rhetorics-of-creativity-12.pdf . [ Google Scholar ]
- Barron F., Harrington D. M. Creativity, intelligence and personality. Ann. Rev. Psychol. 1981; 32 :439–476. [ Google Scholar ]
- Beller M. Quantum Dialogue: The Making of a Revolution. Chicago, IL: University of Chicago Press; 1999. [ Google Scholar ]
- Blair C., Razza R. P. Relating effortful control, executive function, and false belief understanding to emerging math and literacy ability in kindergarten. Child Dev. 2007; 78 :647–663. [ PubMed ] [ Google Scholar ]
- Bodrova E., Leong D. J. American Early Childhood and Primary Classrooms. Geneva, Switzerland: UNESCO International Bureau of Education; 2001. The Tool of the Mind: a case study of implementing the Vygotskian approach. [ Google Scholar ]
- Bransford J. D., Brown A. L., Cocking R. R., editors. How People Learn: Brain, Mind, Experience, and School. Washington, DC: National Academies Press; 2000. [ Google Scholar ]
- Brophy D. R. A comparison of individual and group efforts to creatively solve contrasting types of problems. Creativity Res. J. 2006; 18 :293–315. [ Google Scholar ]
- Bruner J. The growth of mind. Am. Psychol. 1965; 20 :1007–1017. [ PubMed ] [ Google Scholar ]
- Bull K. S., Montgomery D., Baloche L. Teaching creativity at the college level: a synthesis of curricular components perceived as important by instructors. Creativity Res. J. 1995; 8 :83–90. [ Google Scholar ]
- Burton R. On Being Certain: Believing You Are Right Even When You're Not. New York: St. Martin's Press; 2008. [ Google Scholar ]
- Cloud-Hanson K. A., Kuehner J. N., Tong L., Miller S., Handelsman J. Money, sex and drugs: a case study to teach the genetics of antibiotic resistance. CBE Life Sci. Educ. 2008; 7 :302–309. [ PMC free article ] [ PubMed ] [ Google Scholar ]
- Craft A. Teaching Creativity: Philosophy and Practice. New York: Routledge; 2000. [ Google Scholar ]
- Crawford V. M. Adaptive expertise as knowledge building in science teachers' problem solving. Proceedings of the Second European Cognitive Science Conference; Delphi, Greece. 2007. [accessed 1 July 2008]. http://ctl.sri.com/publications/downloads/Crawford_EuroCogSci07Proceedings.pdf . [ Google Scholar ]
- Crawford V. M., Brophy S. Menlo Park, CA: SRI International; 2006. [accessed 1 July 2008]. Adaptive Expertise: Theory, Methods, Findings, and Emerging Issues; September 2006. http://ctl.sri.com/publications/downloads/AESymposiumReportOct06.pdf . [ Google Scholar ]
- Crossgrove K., Curran K. L. Using clickers in nonmajors- and majors-level biology courses: student opinion, learning, and long-term retention of course material. CBE Life Sci. Educ. 2008; 7 :146–154. [ PMC free article ] [ PubMed ] [ Google Scholar ]
- Crowe A., Dirks C., Wenderoth M. P. Biology in bloom: implementing Bloom's taxonomy to enhance student learning in biology. CBE Life Sci. Educ. 2008; 7 :368–381. [ PMC free article ] [ PubMed ] [ Google Scholar ]
- Davidson M. C., Amso D., Anderson L. C., Diamond A. Development of cognitive control and executive functions from 4–13 years: evidence from manipulations of memory, inhibition, and task switching. Neuropsychologia. 2006; 44 :2037–2078. [ PMC free article ] [ PubMed ] [ Google Scholar ]
- DeHaan R. L. The impending revolution in undergraduate science education. J. Sci. Educ. Technol. 2005; 14 :253–270. [ Google Scholar ]
- Diamond A., Barnett W. S., Thomas J., Munro S. Preschool program improves cognitive control. Science. 2007; 318 :1387–1388. [ PMC free article ] [ PubMed ] [ Google Scholar ]
- Duch B. J., Groh S. E., Allen D. E. The Power of Problem-based Learning. Sterling, VA: Stylus Publishers; 2001. [ Google Scholar ]
- Durston S., Davidson M. C., Thomas K. M., Worden M. S., Tottenham N., Martinez A., Watts R., Ulug A. M., Caseya B. J. Parametric manipulation of conflict and response competition using rapid mixed-trial event-related fMRI. Neuroimage. 2003; 20 :2135–2141. [ PubMed ] [ Google Scholar ]
- Ebert-May D., Hodder J. Pathways to Scientific Teaching. Sunderland, MA: Sinauer; 2008. [ Google Scholar ]
- Finke R. A., Ward T. B., Smith S. M. Creative Cognition: Theory, Research and Applications. Boston, MA: MIT Press; 1996. [ Google Scholar ]
- Freeman S., O'Connor E., Parks J. W., Cunningham M., Hurley D., Haak D., Dirks C., Wenderoth M. P. Prescribed active learning increases performance in introductory biology. CBE Life Sci. Educ. 2007; 6 :132–139. [ PMC free article ] [ PubMed ] [ Google Scholar ]
- Gabora L. Cognitive mechanisms underlying the creative process. In: Hewett T., Kavanagh E., editors. Proceedings of the Fourth International Conference on Creativity and Cognition; 2002 October 13–16; Loughborough University, United Kingdom. 2002. pp. 126–133. [ Google Scholar ]
- Gaffney J.D.H., Richards E., Kustusch M. B., Ding L., Beichner R. Scaling up education reform. J. Coll. Sci. Teach. 2008; 37 :48–53. [ Google Scholar ]
- Gardner H. New York: Harper Collins; 1993. Creating Minds: An Anatomy of Creativity Seen through the Lives of Freud, Einstein, Picasso, Stravinsky, Eliot, Graham, and Ghandi. [ Google Scholar ]
- Gladwell M. In the air; who says big ideas are rare? The New Yorker. 2008. [accessed 19 May 2008]. www.newyorker.com/reporting/2008/05/12/080512fa_fact_gladwell .
- Guilford J. P. Creativity. Am. Psychol. 1950; 5 :444–454. [ PubMed ] [ Google Scholar ]
- Hake R. The physics education reform effort: a possible model for higher education. Natl. Teach. Learn. Forum. 2005; 15 :1–6. [ Google Scholar ]
- Halpern D. E., Hakel M. D. Applying the science of learning to the university and beyond. Change. 2003; 35 :36–42. [ Google Scholar ]
- Handelsman J. Scientific teaching. Science. 2004; 304 :521–522. [ PubMed ] [ Google Scholar ]
- Handelsman J, Miller S., Pfund C. Scientific Teaching. New York: W. H. Freeman and Co; 2007. [ PubMed ] [ Google Scholar ]
- Haring-Smith T. Creativity research review: some lessons for higher education. Association of American Colleges and Universities. Peer Rev. 2006; 8 :23–27. [ Google Scholar ]
- Hatano G., Ouro Y. Commentary: reconceptualizing school learning using insight from expertise research. Educ. Res. 2003; 32 :26–29. [ Google Scholar ]
- Hrepic Z., Zollman D. A., Rebello N. S. Comparing students' and experts' understanding of the content of a lecture. J. Sci. Educ. Technol. 2007; 16 :213–224. [ Google Scholar ]
- Hunsaker S. L. Outcomes of creativity training programs. Gifted Child Q. 2005; 49 :292–298. [ Google Scholar ]
- Kaufman J. C., Baer J. Intelligent testing with Torrance. Creativity Res. J. 2006; 18 :99–102. [ Google Scholar ]
- Kaufman J. C., Beghetto R. A. Exploring mini-C: creativity across cultures. In: DeHaan R. L., Narayan K.M.V., editors. Education for Innovation: Implications for India, China and America. Rotterdam, The Netherlands: Sense Publishers; 2008. pp. 165–180. [ Google Scholar ]
- Kaufman J. C., Sternberg R. J. Creativity. Change. 2007; 39 :55–58. [ Google Scholar ]
- Kim K. H. Can we trust creativity tests: a review of the Torrance Tests of Creative Thinking (TTCT) Creativity Res. J. 2006; 18 :3–14. [ Google Scholar ]
- Knight J. K., Wood W. B. Teaching more by lecturing less. Cell Biol. Educ. 2005; 4 :298–310. [ PMC free article ] [ PubMed ] [ Google Scholar ]
- Cetina Knorr K. Laboratory studies: the cultural approach to the study of science. In: Jasanoff S., Markle G., Petersen J., Pinch T., editors. Handbook of Science and Technology Studies. Thousand Oaks, CA: Sage Publications; 1995. pp. 140–166. [ Google Scholar ]
- Koestler A. The Act of Creation. New York: Macmillan; 1964. [ Google Scholar ]
- Latour B., Woolgar S. Laboratory Life: The Construction of Scientific Facts. Princeton, NJ: Princeton University Press; 1986. [ Google Scholar ]
- MacKinnon D. W. What makes a person creative? In: MacKinnon D. W., editor. In Search of Human Effectiveness. New York: Universe Books; 1978. pp. 178–186. [ Google Scholar ]
- Martindale C. Biological basis of creativity. In: Sternberg R. J., editor. Handbook of Creativity. Cambridge, United Kingdom: Cambridge University Press; 1999. pp. 137–152. [ Google Scholar ]
- Mazur E. Peer Instruction: A User's Manual. Upper Saddle River, NJ: Prentice Hall; 1996. [ Google Scholar ]
- McFadzean E. Developing and supporting creative problem-solving teams: Part 1—a conceptual model. Manage. Decis. 2002; 40 :463–475. [ Google Scholar ]
- McGregor G. D., Jr Creative thinking instruction for a college study skills program: a case study. Dissert Abstr. Intl. 2001; 62 :3293A. UMI No. AAT 3027933. [ Google Scholar ]
- McIntyre F. S., Hite R. E., Rickard M. K. Individual characteristics and creativity in the marketing classroom: exploratory insights. J. Mark. Educ. 2003; 25 :143–149. [ Google Scholar ]
- Mestre J. P., editor. Transfer of Learning: From a Modern Multidisciplinary Perspective. Greenwich, CT: Information Age Publishing; 2005. [ Google Scholar ]
- Mumford M. D., Mobley M. I., Uhlman C. E., Reiter-Palmon R., Doares L. M. Process analytic models of creative capacities. Creativity Res. J. 1991; 4 :91–122. [ Google Scholar ]
- National Research Council. Washington, DC: National Academies Press; 2007. Rising Above the Gathering Storm: Energizing and Employing America for a Brighter Economic Future, Committee on Science, Engineering and Public Policy. [ Google Scholar ]
- Neisser U. The multiplicity of thought. Br. J. Psychol. 1963; 54 :1–14. [ PubMed ] [ Google Scholar ]
- Nelson C. E. Teaching evolution (and all of biology) more effectively: strategies for engagement, critical reasoning, and confronting misconceptions. Integrative and Comparative Biology Advance Access. 2008. [accessed 15 September 2008]. http://icb.oxfordjournals.org/cgi/reprint/icn027v1.pdf . [ PubMed ]
- Novak G, Gavrin A., Christian W, Patterson E. Just-in-Time Teaching: Blending Active Learning with Web Technology. San Francisco, CA: Pearson Benjamin Cummings; 1999. [ Google Scholar ]
- Osborn A. F. Your Creative Power. New York: Scribner; 1948. [ Google Scholar ]
- Osborn A. F. Applied Imagination. New York: Scribner; 1979. [ Google Scholar ]
- Osburn H. K., Mumford M. D. Creativity and planning: training interventions to develop creative problem-solving skills. Creativity Res. J. 2006; 18 :173–190. [ Google Scholar ]
- Paulus P. B., Nijstad B. A. Group Creativity: Innovation through Collaboration. New York: Oxford University Press; 2003. [ Google Scholar ]
- Perkins K. K., Wieman C. E. Innovative teaching to promote innovative thinking. In: DeHaan R. L., Narayan K.M.V., editors. Education for Innovation: Implications for India, China and America. Rotterdam, The Netherlands: Sense Publishers; 2008. pp. 181–210. [ Google Scholar ]
- Plucker J. A., Renzulli J. S. Psychometric approaches to the study of human creativity. In: Sternberg R. J., editor. Handbook of Creativity. Cambridge, United Kingdom: Cambridge University Press; 1999. pp. 35–61. [ Google Scholar ]
- Quitadamo I. J., Faiola C. L., Johnson J. E., Kurtz M. J. Community-based inquiry improves critical thinking in general education biology. CBE Life Sci. Educ. 2008; 7 :327–337. [ PMC free article ] [ PubMed ] [ Google Scholar ]
- Runco M. A. Creativity. Annu. Rev. Psychol. 2004; 55 :657–687. [ PubMed ] [ Google Scholar ]
- Runco M. A., Nemiro J. Problem finding, creativity, and giftedness. Roeper Rev. 1994; 16 :235–241. [ Google Scholar ]
- Sawyer R. K. Educating for Innovation. [accessed 13 August 2008]; Thinking Skills Creativity. 2005 1 :41–48. www.artsci.wustl.edu/∼ksawyer/PDFs/Thinkjournal.pdf . [ Google Scholar ]
- Sawyer R. K. Explaining Creativity: The Science of Human Innovation. New York: Oxford University Press; 2006. [ Google Scholar ]
- Schwartz D. L., Bransford J. D., Sears D. Efficiency and innovation in transfer. In: Mestre J. P., editor. Transfer of Learning from a Modern Multidisciplinary Perspective. Greenwich, CT: Information Age Publishing; 2005. pp. 1–51. [ Google Scholar ]
- Scott G., Leritz L. E., Mumford M. D. The effectiveness of creativity training: a quantitative review. Creativity Res. J. 2004; 16 :361–388. [ Google Scholar ]
- Simonton D. K. Sociocultural context of individual creativity: a transhistorical time-series analysis. J. Pers. Soc. Psychol. 1975; 32 :1119–1133. [ PubMed ] [ Google Scholar ]
- Simonton D. K. Creativity in Science: Chance, Logic, Genius, and Zeitgeist. Oxford, United Kingdom: Cambridge University Press; 2004. [ Google Scholar ]
- Sloman S. The empirical case for two systems of reasoning. Psychol. Bull. 1996; 9 :3–22. [ Google Scholar ]
- Smith G. F. Idea generation techniques: a formulary of active ingredients. J. Creative Behav. 1998; 32 :107–134. [ Google Scholar ]
- Snyder A., Mitchell J., Bossomaier T., Pallier G. The creativity quotient: an objective scoring of ideational fluency. Creativity Res. J. 2004; 16 :415–420. [ Google Scholar ]
- Sternberg R. J. What is an “expert student?” Educ. Res. 2003; 32 :5–9. [ Google Scholar ]
- Sternberg R., Williams W. M. Teaching for creativity: two dozen tips. 1998. [accessed 25 March 2008]. www.cdl.org/resource-library/articles/teaching_creativity.php .
- Tardif T. Z., Sternberg R. J. What do we know about creativity? In: Sternberg R. J., editor. The Nature of Creativity. New York: Cambridge University Press; 1988. pp. 429–440. [ Google Scholar ]
- Torrance E. P. Norms and Technical Manual for the Torrance Tests of Creative Thinking. Bensenville, IL: Scholastic Testing Service; 1974. [ Google Scholar ]
- Torrance E. P. The Torrance Tests of Creative Thinking Norms—Technical Manual Figural (Streamlined) Forms A and B. Bensenville, IL: Scholastic Testing Service; 1998. [ Google Scholar ]
- Torrance E. P., Ball O. E., Safter H. T. Torrance Tests of Creative Thinking: Streamlined Scoring Guide for Figural Forms A and B. Bensenville, IL: Scholastic Testing Service; 2008. [ Google Scholar ]
- Treffinger D. J., Isaksen S. G. Creative problem solving: the history, development, and implications for gifted education and talent development. Gifted Child Q. 2005; 49 :342–357. [ Google Scholar ]
- Vandervert L. R., Schimpf P. H., Liu H. How working memory and the cerebellum collaborate to produce creativity and innovation. Creativity Res. J. 2007; 9 :1–18. [ Google Scholar ]
- Wallach M. A., Kogan N. Modes of Thinking in Young Children: A Study of the Creativity-Intelligence Distinction. New York: Holt, Rinehart and Winston; 1965. [ Google Scholar ]
- Wood W. B. Innovations in undergraduate biology teaching and why we need them. Annu. Rev. Cell Dev. Biol. 2009 in press. [ PubMed ] [ Google Scholar ]
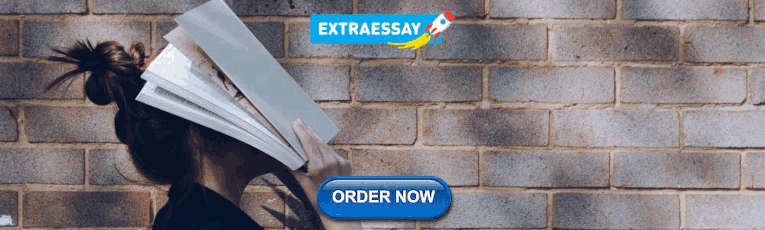
IMAGES
VIDEO
COMMENTS
Problem Solving in STEM. Solving problems is a key component of many science, math, and engineering classes. If a goal of a class is for students to emerge with the ability to solve new kinds of problems or to use new problem-solving techniques, then students need numerous opportunities to develop the skills necessary to approach and answer ...
Problem solving plays an essential role in all scientific disciplines, and solving problems can reveal essential concepts that underlie those disciplines. Thus, problem solving serves both as a common tool and desired outcome in many science classes. Research on teaching problem solving offers principles for instruction that are guided by learning theories. This essay describes an online ...
Details the learner-centered problem-solving approach, outlining steps from problem definition to hypothesis testing and conclusion formulation. It exemplifies how this approach engages learners in active problem solving, enhancing their scientific skills and higher-order thinking through practical experiments and discussions.
Abstract. Engaging learners in the excitement of science, helping them discover the value of evidence-based reasoning and higher-order cognitive skills, and teaching them to become creative problem solvers have long been goals of science education reformers. But the means to achieve these goals, especially methods to promote creative thinking ...
The traditional teaching of science problem solving involves a considerable amount of drill and practice. Research suggests that these practices do not lead to the development of expert-like problem-solving strategies and that there is little correlation between the number of problems solved (exceeding 1,000 problems in one specific study) and the development of a conceptual understanding.
STEM is an effective approach, in which problem solving is an indisputable part of engineering design as described by Kennedy and Odell ... Taconis, R., Ferguson-Hessler, M. G. M., & Broekkamp, H. (2001). Teaching science problem solving: An overview of experimental work. Journal of Research in Science Teaching, 38(4), 442-468.
A systematic approach to solving problems and on designing instruction where students learn this approach. Teaching and learning problem solving in science. Part I: A general strategy | Journal of Chemical Education
The traditional approach to teaching science problem solving is having the students work individually on a large number of problems. This approach has long been overtaken by research suggesting and testing other methods, which are expected to be more effective. To get an overview of the characteristics of good and innovative problem-solving ...
Balancing disciplinary knowledge and practical reasoning in problem solving is needed for meaningful learning. In STEM problem solving, science subject matter with associated practices often appears distant to learners due to its abstract nature. Consequently, learners experience difficulties making meaningful connections between science and their daily experiences. Applying Dewey's idea of ...
Regarding the results obtained, collaborative problem solving is an effective teaching approach to foster learners' critical thinking, with a significant overall effect size (ES = 0.82, z = 12. ...
A primary goal of science and engineering (S&E) education is to produce good problem solvers, but how to best teach and measure the quality of problem solving remains unclear. The process is complex, multifaceted, and not fully characterized. Here, we present a detailed characterization of the S&E problem-solving process as a set of specific interlinked decisions. This framework of decisions ...
In typical classroom problem solving approaches, ... PBL is a curricular and instructional approach which successfully resolves the seemingly contradictory demands of science education reform in a way that is true to the discipline of science, its process, and the larger goals of educating an independent reasoning citizenry. ...
Examines effective and innovative teaching approaches for science problem solving by analyzing experimental research articles published between 1985 and 1995. Authors use a model of the cognitive capacities needed for effective science problem solving, composed of a knowledge base and a skills base. They also analyze learning conditions such as feedback and group work. Researchers identified ...
Higher educational institutions have utilized problem-based learning (PBL) approaches over the last two decades. The approach has been found to enable educators to adopt different teaching and learning strategies. This study examined how teacher educators have adopted technology integrated PBL in teacher education. The study aimed to understand teacher educators perceptions of adopting the ...
The traditional approach to teaching science problem solving is having the students work individually on a large number of problems. This approach has long been overtaken by research suggesting and testing other methods, which are expected to be more effective. To get an overview of the characteristics of good and innovative problem-solving ...
The traditional approach to teaching science problem solving is having the students work individually on a large number of problems. This approach has long been overtaken by research suggesting and testing other methods, which are expected to be more effective. To get an overview of the characteristics of good and innovative problem-solving teaching strategies, we performed an analysis of a ...
Purpose . This theoretical paper builds on the Pedagogy Analysis Framework by integrating it with pedagogical problem-solving theory, illustrating the resultant extended Pedagogy Analysis Framework and Pedagogical Problem Typology using data from a video-based study of one science and one Religious Education (RE) lesson.
One approach to teaching inquiry-based science that has a strong evidence base is the 5E instructional model proposed by Bybee (2015, 2019).This model consists of five phases of learning: engage, explore, explain, elaborate, and evaluate.In the first phase, teachers begin by capturing students' attention and interest by presenting tasks that challenge their curiosity and provoke wonderment.
Abstract. Problem-Based Learning (PBL) is an inquiry approach in teaching involving the use of real-world problems in a student-centered environment and a method to stimulate students ...
Existing research has investigated student problem-solving strategies across science, technology, engineering, and mathematics; however, there is limited work in undergraduate biology education on how various aspects that influence learning combine to generate holistic approaches to problem solving. Through the lens of situated cognition, we consider problem solving as a learning phenomenon ...
A Problem-Solving Approach for Science Learning. Rushikesh Kirtikar. Published 2013. Education. Science education in schools is aimed at providing the knowledge of surrounding science, developing curiosity, scientific attitude and thinking skills. However, in terms of pedagogy, teaching science is limited in achieving the aim of providing ...
Abstract. Engaging learners in the excitement of science, helping them discover the value of evidence-based reasoning and higher-order cognitive skills, and teaching them to become creative problem solvers have long been goals of science education reformers. But the means to achieve these goals, especially methods to promote creative thinking ...
Teach philosophy of science. Much is being made about the erosion of public trust in science. Surveys show a modest decline in the United States from a very high level of trust, but that is seen for other institutions as well. What is apparent from the surveys is that a better explanation of the nature of science—that it is revised as new ...
The traditional approach to teaching science problem solving is having the students work individually on a large number of problems. This approach has long been overtaken by research suggesting and testing other methods, which are expected to be more effective. To get an overview of the characteristics of good and innovative problem-solving ...