Experimental Design: Types, Examples & Methods
Saul Mcleod, PhD
Editor-in-Chief for Simply Psychology
BSc (Hons) Psychology, MRes, PhD, University of Manchester
Saul Mcleod, PhD., is a qualified psychology teacher with over 18 years of experience in further and higher education. He has been published in peer-reviewed journals, including the Journal of Clinical Psychology.
Learn about our Editorial Process
Olivia Guy-Evans, MSc
Associate Editor for Simply Psychology
BSc (Hons) Psychology, MSc Psychology of Education
Olivia Guy-Evans is a writer and associate editor for Simply Psychology. She has previously worked in healthcare and educational sectors.
On This Page:
Experimental design refers to how participants are allocated to different groups in an experiment. Types of design include repeated measures, independent groups, and matched pairs designs.
Probably the most common way to design an experiment in psychology is to divide the participants into two groups, the experimental group and the control group, and then introduce a change to the experimental group, not the control group.
The researcher must decide how he/she will allocate their sample to the different experimental groups. For example, if there are 10 participants, will all 10 participants participate in both groups (e.g., repeated measures), or will the participants be split in half and take part in only one group each?
Three types of experimental designs are commonly used:
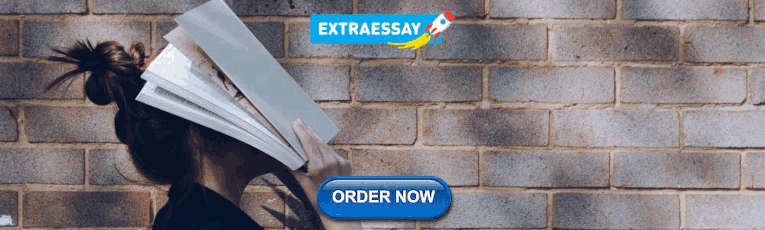
1. Independent Measures
Independent measures design, also known as between-groups , is an experimental design where different participants are used in each condition of the independent variable. This means that each condition of the experiment includes a different group of participants.
This should be done by random allocation, ensuring that each participant has an equal chance of being assigned to one group.
Independent measures involve using two separate groups of participants, one in each condition. For example:
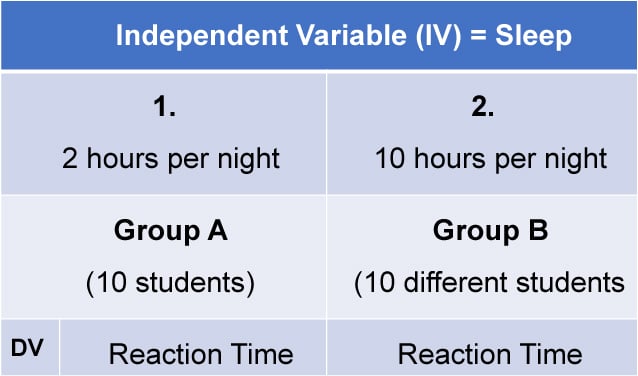
- Con : More people are needed than with the repeated measures design (i.e., more time-consuming).
- Pro : Avoids order effects (such as practice or fatigue) as people participate in one condition only. If a person is involved in several conditions, they may become bored, tired, and fed up by the time they come to the second condition or become wise to the requirements of the experiment!
- Con : Differences between participants in the groups may affect results, for example, variations in age, gender, or social background. These differences are known as participant variables (i.e., a type of extraneous variable ).
- Control : After the participants have been recruited, they should be randomly assigned to their groups. This should ensure the groups are similar, on average (reducing participant variables).
2. Repeated Measures Design
Repeated Measures design is an experimental design where the same participants participate in each independent variable condition. This means that each experiment condition includes the same group of participants.
Repeated Measures design is also known as within-groups or within-subjects design .
- Pro : As the same participants are used in each condition, participant variables (i.e., individual differences) are reduced.
- Con : There may be order effects. Order effects refer to the order of the conditions affecting the participants’ behavior. Performance in the second condition may be better because the participants know what to do (i.e., practice effect). Or their performance might be worse in the second condition because they are tired (i.e., fatigue effect). This limitation can be controlled using counterbalancing.
- Pro : Fewer people are needed as they participate in all conditions (i.e., saves time).
- Control : To combat order effects, the researcher counter-balances the order of the conditions for the participants. Alternating the order in which participants perform in different conditions of an experiment.
Counterbalancing
Suppose we used a repeated measures design in which all of the participants first learned words in “loud noise” and then learned them in “no noise.”
We expect the participants to learn better in “no noise” because of order effects, such as practice. However, a researcher can control for order effects using counterbalancing.
The sample would be split into two groups: experimental (A) and control (B). For example, group 1 does ‘A’ then ‘B,’ and group 2 does ‘B’ then ‘A.’ This is to eliminate order effects.
Although order effects occur for each participant, they balance each other out in the results because they occur equally in both groups.
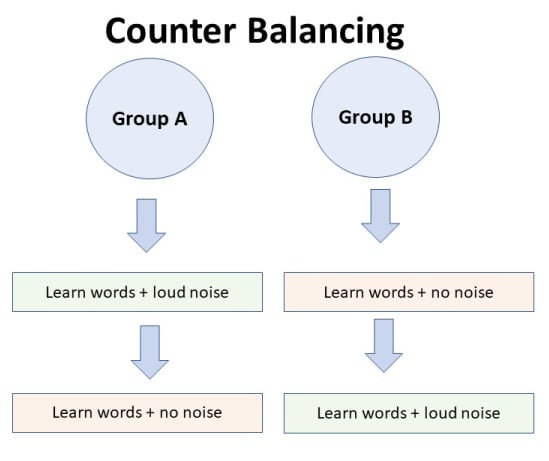
3. Matched Pairs Design
A matched pairs design is an experimental design where pairs of participants are matched in terms of key variables, such as age or socioeconomic status. One member of each pair is then placed into the experimental group and the other member into the control group .
One member of each matched pair must be randomly assigned to the experimental group and the other to the control group.
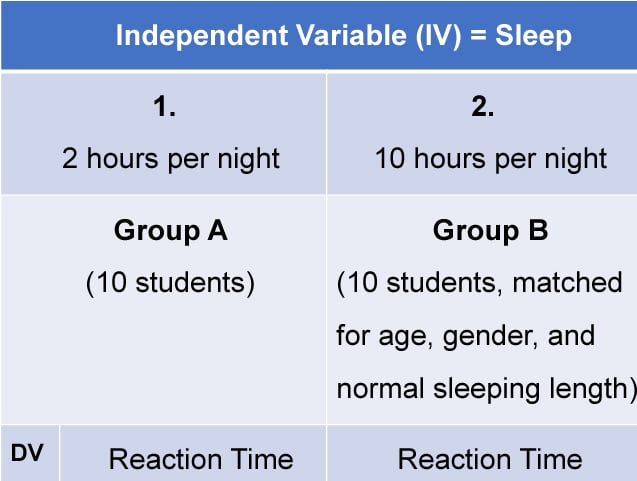
- Con : If one participant drops out, you lose 2 PPs’ data.
- Pro : Reduces participant variables because the researcher has tried to pair up the participants so that each condition has people with similar abilities and characteristics.
- Con : Very time-consuming trying to find closely matched pairs.
- Pro : It avoids order effects, so counterbalancing is not necessary.
- Con : Impossible to match people exactly unless they are identical twins!
- Control : Members of each pair should be randomly assigned to conditions. However, this does not solve all these problems.
Experimental design refers to how participants are allocated to an experiment’s different conditions (or IV levels). There are three types:
1. Independent measures / between-groups : Different participants are used in each condition of the independent variable.
2. Repeated measures /within groups : The same participants take part in each condition of the independent variable.
3. Matched pairs : Each condition uses different participants, but they are matched in terms of important characteristics, e.g., gender, age, intelligence, etc.
Learning Check
Read about each of the experiments below. For each experiment, identify (1) which experimental design was used; and (2) why the researcher might have used that design.
1 . To compare the effectiveness of two different types of therapy for depression, depressed patients were assigned to receive either cognitive therapy or behavior therapy for a 12-week period.
The researchers attempted to ensure that the patients in the two groups had similar severity of depressed symptoms by administering a standardized test of depression to each participant, then pairing them according to the severity of their symptoms.
2 . To assess the difference in reading comprehension between 7 and 9-year-olds, a researcher recruited each group from a local primary school. They were given the same passage of text to read and then asked a series of questions to assess their understanding.
3 . To assess the effectiveness of two different ways of teaching reading, a group of 5-year-olds was recruited from a primary school. Their level of reading ability was assessed, and then they were taught using scheme one for 20 weeks.
At the end of this period, their reading was reassessed, and a reading improvement score was calculated. They were then taught using scheme two for a further 20 weeks, and another reading improvement score for this period was calculated. The reading improvement scores for each child were then compared.
4 . To assess the effect of the organization on recall, a researcher randomly assigned student volunteers to two conditions.
Condition one attempted to recall a list of words that were organized into meaningful categories; condition two attempted to recall the same words, randomly grouped on the page.
Experiment Terminology
Ecological validity.
The degree to which an investigation represents real-life experiences.
Experimenter effects
These are the ways that the experimenter can accidentally influence the participant through their appearance or behavior.
Demand characteristics
The clues in an experiment lead the participants to think they know what the researcher is looking for (e.g., the experimenter’s body language).
Independent variable (IV)
The variable the experimenter manipulates (i.e., changes) is assumed to have a direct effect on the dependent variable.
Dependent variable (DV)
Variable the experimenter measures. This is the outcome (i.e., the result) of a study.
Extraneous variables (EV)
All variables which are not independent variables but could affect the results (DV) of the experiment. Extraneous variables should be controlled where possible.
Confounding variables
Variable(s) that have affected the results (DV), apart from the IV. A confounding variable could be an extraneous variable that has not been controlled.
Random Allocation
Randomly allocating participants to independent variable conditions means that all participants should have an equal chance of taking part in each condition.
The principle of random allocation is to avoid bias in how the experiment is carried out and limit the effects of participant variables.
Order effects
Changes in participants’ performance due to their repeating the same or similar test more than once. Examples of order effects include:
(i) practice effect: an improvement in performance on a task due to repetition, for example, because of familiarity with the task;
(ii) fatigue effect: a decrease in performance of a task due to repetition, for example, because of boredom or tiredness.

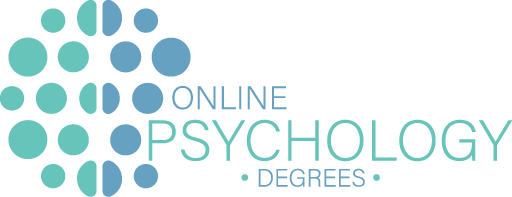
5 Classic Psychology Research Designs
- By Cliff Stamp, BS Psychology, MS Rehabilitation Counseling
- Published November 10, 2019
- Last Updated November 17, 2023
- Read Time 6 mins
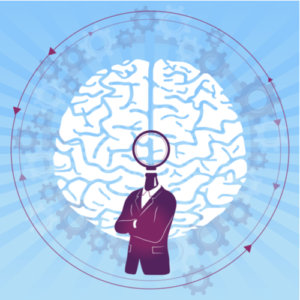
Posted November 2019 by Clifton Stamp, B.S. Psychology; M.A. Rehabilitation Counseling, M.A. English; 10 updates since. Reading time: 5 min. Reading level: Grade 11+. Questions on psychology research designs? Email Toni at: [email protected] .
Psychology research is carried out by a variety of methods, all of which are intended to increase the fund of knowledge we have concerning human behavior. Research is a formalized, systematic way of deriving accurate and reproducible results. Research designs are the particular methods and procedures used to generate, collect and analyze information.
Research can be carried out in many different ways, but can broadly be defined as qualitative or quantitative. Quantitative psychological research refers to research that yields outcomes that derive from statistics or mathematical modeling. Quantitative research is centered around testing objective hypotheses . It is based on empiricism and attempts to show the accuracy of a hypothesis.
Qualitative psychological research attempts to understand behavior within its natural context and setting. Qualitative psychological research uses observation, interviews, focus groups and participant observation as its most common methods.
Classic Psychology Research Designs
Research is typically focused on finding a particular answer or answers to a question or problem, logically enough called the research question. A research design is a formalized means of finding answers to a research question. Research designs create a framework for gathering and collecting information in a structured, orderly way. Five of the most common psychology research designs include descriptive, correlational, semi-experimental, experimental, review and meta-analytic designs.
Descriptive Research Designs
- Case study . Case study research involves researchers conduction a close-up look at an individual, a phenomenon, or a group in its real-world naturalistic environment. Case studies are more intrusive than naturalistic observational studies.
- Naturalistic observation . Naturalistic observation , a kind of field research, involves observing research subjects in their own environment, without any introduced external factors. Naturalistic observation has a high degree of external validity .
- Surveys . Everyone has taken a survey at one time or another. Surveys sample a group of individuals that are chosen to be representative of a larger population. Surveys naturally cannot research every individual in a population, thus a great deal of study is conducted to ensure that samples truly represent the populations they’re supposed to describe. Polls about public opinion, market-research surveys, public-health surveys, and government surveys are examples of mass spectrum surveys.
Correlational Research Designs
In correlational research designs, groups are studied and compared, but researchers cannot introduce variables or manipulate independent variables.
- Case-control study . A case-control study is a comparison between two groups, one of which experienced a condition while the other group did not . Case-control studies are retrospective; that is, they observe a situation that has already happened. Two groups exist that are as similar as possible, save that a hypothesized agent affected the case group. This hypothesized agent, condition or singular difference between groups is said to correlate with differences in outcomes.
- Observational study . Observational studies allow researchers to make some inferences from a group sample to an overall population. In an observational study, the independent variable cannot be controlled or modified directly. Consider a study that compares the outcomes of fetal alcohol exposure on the development of psychological disorders. It would be unethical to cause a group of fetuses to be exposed to alcohol in vivo. Thus, two groups of individuals, as alike as possible are compared. The difference is that one group has been selected due to their exposure to alcohol during their fetal development. Researchers are not manipulating the measure of the independent variable, but they are attempting to measure its effect by group to group comparison .
Semi-Experimental Research Design
- Field experiment . A field experiment occurs in the everyday environment of the research subjects. In a field experiment, researchers manipulate an independent variable and measure changes in the tested, dependent variable. Although field experiments generalize extremely well, it’s not possible to eliminate extraneous variables. This can limit the usefulness of any conclusions.
Experimental Research Design
Experimental research is a major component of experimental psychology. In experimental psychology, researchers perform tightly controlled laboratory experiments that eliminate external, erroneous variables. This high level of control allows experimental results to have a high degree of internal validity. Internal validity refers to the degree to which an experiment’s outcomes come from manipulations of the independent variable. On the other hand, highly controlled lab experiments may not generalize to the natural environment, precisely due to the presence of many external variables.
Review Designs and Meta-Analysis
- Literature review . A literature review is a paper examining other experiments or research into a particular subject. Literature reviews examine research published in academic and other scholarly journals. All research starts with a search for research similar, or at least fundamentally similar, to the research question in question.
- Systematic review . A systematic review examines as much published, verified research that matches the researchers’ guidelines for a particular line of research. Systematic review involves multiple and exhaustive literature reviews. After conducting a systematic review of all other research on a topic that meets criteria, psychology researchers conduct a meta-analysis.
- Meta-analyses. Meta-analyses involve complex statistical analysis of former research to answer an overall research question.
Literature reviews and systematic reviews and meta-analyses all work together to provide psychology researchers with a big-picture view of the body of study they are investigating.
Descriptive, Correlational and Experimental Designs
All research may be thought of as having descriptive or inferential value, although there are usually aspects of both present in all research projects. Descriptive research often comes before experimental research, as examining what’s been discovered about a research topic helps guide and refine experimental research, which has a high inferential value.
Descriptive research designs include literature reviews, systematic reviews and meta-analyses. They’re able to assess and evaluate what the state of a body of knowledge is, but no experimentation is conducted. Correlational designs investigate the strength of the relationship between or among variables. Correlational studies are good for pointing out possible relationships but cannot establish causation, or a cause-and-effect relationship among variables. This leaves experimental designs. which do allow inferences to be made about cause-and-effect. Experimental designs are the most scientifically, mathematically rigorous, but that fine level of control doesn’t always extrapolate well to the world outside the lab.
More Articles of Interest:
- How Do Psychology Researchers Find Funding?
- What Makes Psychology Research Ethical?
- How is the Field of Psychology Changing?
- The Human Connectome Project
- How Is Technology Changing The Study Of Psychology?
- What are the Best TV Shows About Psychology?
Trending now
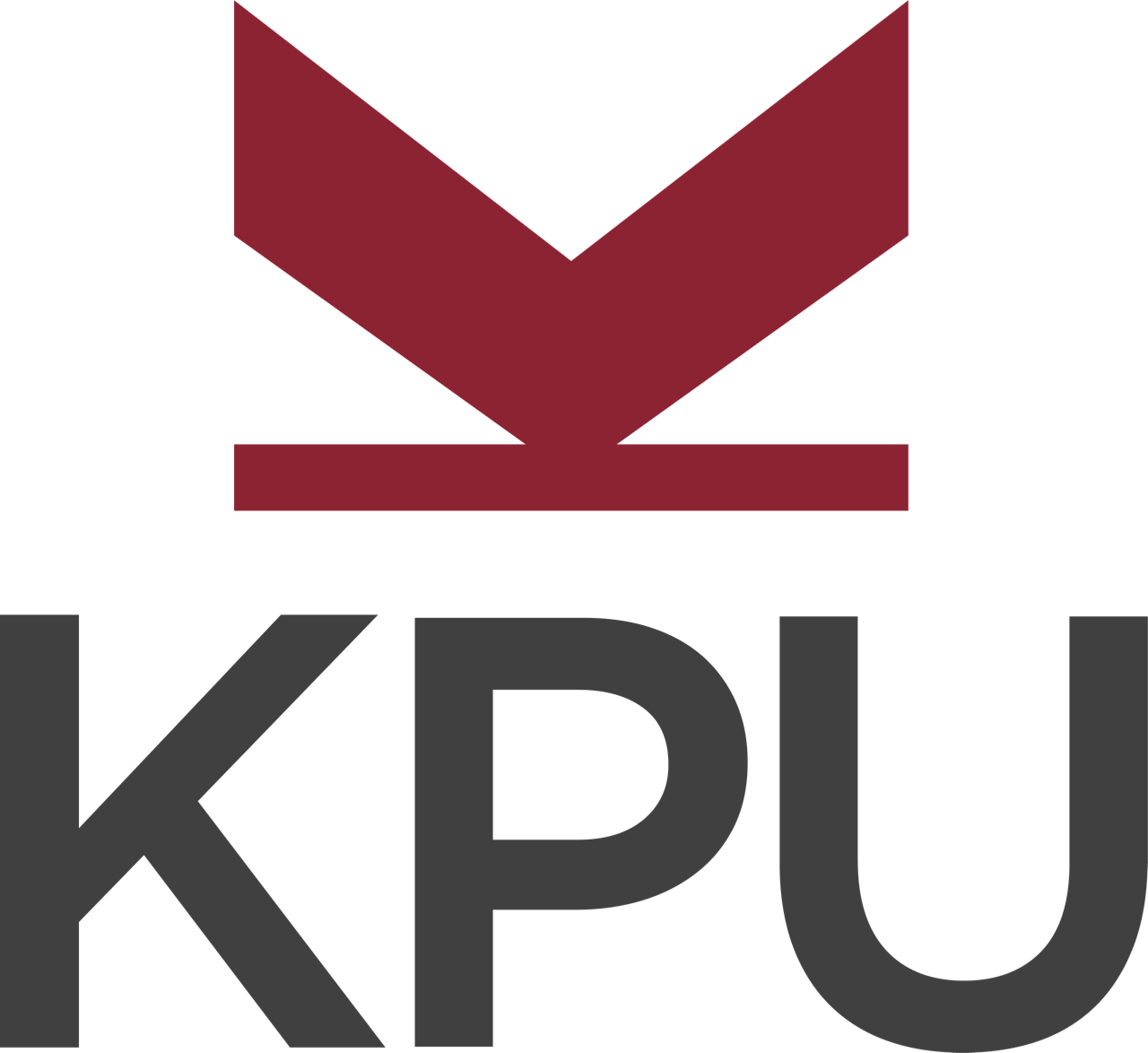
Want to create or adapt books like this? Learn more about how Pressbooks supports open publishing practices.
Overview of the Scientific Method
11 Designing a Research Study
Learning objectives.
- Define the concept of a variable, distinguish quantitative from categorical variables, and give examples of variables that might be of interest to psychologists.
- Explain the difference between a population and a sample.
- Distinguish between experimental and non-experimental research.
- Distinguish between lab studies, field studies, and field experiments.
Identifying and Defining the Variables and Population
Variables and operational definitions.
Part of generating a hypothesis involves identifying the variables that you want to study and operationally defining those variables so that they can be measured. Research questions in psychology are about variables. A variable is a quantity or quality that varies across people or situations. For example, the height of the students enrolled in a university course is a variable because it varies from student to student. The chosen major of the students is also a variable as long as not everyone in the class has declared the same major. Almost everything in our world varies and as such thinking of examples of constants (things that don’t vary) is far more difficult. A rare example of a constant is the speed of light. Variables can be either quantitative or categorical. A quantitative variable is a quantity, such as height, that is typically measured by assigning a number to each individual. Other examples of quantitative variables include people’s level of talkativeness, how depressed they are, and the number of siblings they have. A categorical variable is a quality, such as chosen major, and is typically measured by assigning a category label to each individual (e.g., Psychology, English, Nursing, etc.). Other examples include people’s nationality, their occupation, and whether they are receiving psychotherapy.
After the researcher generates their hypothesis and selects the variables they want to manipulate and measure, the researcher needs to find ways to actually measure the variables of interest. This requires an operational definition —a definition of the variable in terms of precisely how it is to be measured. Most variables that researchers are interested in studying cannot be directly observed or measured and this poses a problem because empiricism (observation) is at the heart of the scientific method. Operationally defining a variable involves taking an abstract construct like depression that cannot be directly observed and transforming it into something that can be directly observed and measured. Most variables can be operationally defined in many different ways. For example, depression can be operationally defined as people’s scores on a paper-and-pencil depression scale such as the Beck Depression Inventory, the number of depressive symptoms they are experiencing, or whether they have been diagnosed with major depressive disorder. Researchers are wise to choose an operational definition that has been used extensively in the research literature.
Sampling and Measurement
In addition to identifying which variables to manipulate and measure, and operationally defining those variables, researchers need to identify the population of interest. Researchers in psychology are usually interested in drawing conclusions about some very large group of people. This is called the population . It could be all American teenagers, children with autism, professional athletes, or even just human beings—depending on the interests and goals of the researcher. But they usually study only a small subset or sample of the population. For example, a researcher might measure the talkativeness of a few hundred university students with the intention of drawing conclusions about the talkativeness of men and women in general. It is important, therefore, for researchers to use a representative sample—one that is similar to the population in important respects.
One method of obtaining a sample is simple random sampling , in which every member of the population has an equal chance of being selected for the sample. For example, a pollster could start with a list of all the registered voters in a city (the population), randomly select 100 of them from the list (the sample), and ask those 100 whom they intend to vote for. Unfortunately, random sampling is difficult or impossible in most psychological research because the populations are less clearly defined than the registered voters in a city. How could a researcher give all American teenagers or all children with autism an equal chance of being selected for a sample? The most common alternative to random sampling is convenience sampling , in which the sample consists of individuals who happen to be nearby and willing to participate (such as introductory psychology students). Of course, the obvious problem with convenience sampling is that the sample might not be representative of the population and therefore it may be less appropriate to generalize the results from the sample to that population.
Experimental vs. Non-Experimental Research
The next step a researcher must take is to decide which type of approach they will use to collect the data. As you will learn in your research methods course there are many different approaches to research that can be divided in many different ways. One of the most fundamental distinctions is between experimental and non-experimental research.
Experimental Research
Researchers who want to test hypotheses about causal relationships between variables (i.e., their goal is to explain) need to use an experimental method. This is because the experimental method is the only method that allows us to determine causal relationships. Using the experimental approach, researchers first manipulate one or more variables while attempting to control extraneous variables, and then they measure how the manipulated variables affect participants’ responses.
The terms independent variable and dependent variable are used in the context of experimental research. The independent variable is the variable the experimenter manipulates (it is the presumed cause) and the dependent variable is the variable the experimenter measures (it is the presumed effect).
Extraneous variables are any variable other than the dependent variable. Confounds are a specific type of extraneous variable that systematically varies along with the variables under investigation and therefore provides an alternative explanation for the results. When researchers design an experiment they need to ensure that they control for confounds; they need to ensure that extraneous variables don’t become confounding variables because in order to make a causal conclusion they need to make sure alternative explanations for the results have been ruled out.
As an example, if we manipulate the lighting in the room and examine the effects of that manipulation on workers’ productivity, then the lighting conditions (bright lights vs. dim lights) would be considered the independent variable and the workers’ productivity would be considered the dependent variable. If the bright lights are noisy then that noise would be a confound since the noise would be present whenever the lights are bright and the noise would be absent when the lights are dim. If noise is varying systematically with light then we wouldn’t know if a difference in worker productivity across the two lighting conditions is due to noise or light. So confounds are bad, they disrupt our ability to make causal conclusions about the nature of the relationship between variables. However, if there is noise in the room both when the lights are on and when the lights are off then noise is merely an extraneous variable (it is a variable other than the independent or dependent variable) and we don’t worry much about extraneous variables. This is because unless a variable varies systematically with the manipulated independent variable it cannot be a competing explanation for the results.
Non-Experimental Research
Researchers who are simply interested in describing characteristics of people, describing relationships between variables, and using those relationships to make predictions can use non-experimental research. Using the non-experimental approach, the researcher simply measures variables as they naturally occur, but they do not manipulate them. For instance, if I just measured the number of traffic fatalities in America last year that involved the use of a cell phone but I did not actually manipulate cell phone use then this would be categorized as non-experimental research. Alternatively, if I stood at a busy intersection and recorded drivers’ genders and whether or not they were using a cell phone when they passed through the intersection to see whether men or women are more likely to use a cell phone when driving, then this would be non-experimental research. It is important to point out that non-experimental does not mean nonscientific. Non-experimental research is scientific in nature. It can be used to fulfill two of the three goals of science (to describe and to predict). However, unlike with experimental research, we cannot make causal conclusions using this method; we cannot say that one variable causes another variable using this method.
Laboratory vs. Field Research
The next major distinction between research methods is between laboratory and field studies. A laboratory study is a study that is conducted in the laboratory environment. In contrast, a field study is a study that is conducted in the real-world, in a natural environment.
Laboratory experiments typically have high internal validity . Internal validity refers to the degree to which we can confidently infer a causal relationship between variables. When we conduct an experimental study in a laboratory environment we have very high internal validity because we manipulate one variable while controlling all other outside extraneous variables. When we manipulate an independent variable and observe an effect on a dependent variable and we control for everything else so that the only difference between our experimental groups or conditions is the one manipulated variable then we can be quite confident that it is the independent variable that is causing the change in the dependent variable. In contrast, because field studies are conducted in the real-world, the experimenter typically has less control over the environment and potential extraneous variables, and this decreases internal validity, making it less appropriate to arrive at causal conclusions.
But there is typically a trade-off between internal and external validity. External validity simply refers to the degree to which we can generalize the findings to other circumstances or settings, like the real-world environment. When internal validity is high, external validity tends to be low; and when internal validity is low, external validity tends to be high. So laboratory studies are typically low in external validity, while field studies are typically high in external validity. Since field studies are conducted in the real-world environment it is far more appropriate to generalize the findings to that real-world environment than when the research is conducted in the more artificial sterile laboratory.
Finally, there are field studies which are non-experimental in nature because nothing is manipulated. But there are also field experiment s where an independent variable is manipulated in a natural setting and extraneous variables are controlled. Depending on their overall quality and the level of control of extraneous variables, such field experiments can have high external and high internal validity.
A quantity or quality that varies across people or situations.
A quantity, such as height, that is typically measured by assigning a number to each individual.
A variable that represents a characteristic of an individual, such as chosen major, and is typically measured by assigning each individual's response to one of several categories (e.g., Psychology, English, Nursing, Engineering, etc.).
A definition of the variable in terms of precisely how it is to be measured.
A large group of people about whom researchers in psychology are usually interested in drawing conclusions, and from whom the sample is drawn.
A smaller portion of the population the researcher would like to study.
A common method of non-probability sampling in which the sample consists of individuals who happen to be easily available and willing to participate (such as introductory psychology students).
The variable the experimenter manipulates.
The variable the experimenter measures (it is the presumed effect).
Any variable other than the dependent and independent variable.
A specific type of extraneous variable that systematically varies along with the variables under investigation and therefore provides an alternative explanation for the results.
A study that is conducted in the laboratory environment.
A study that is conducted in a "real world" environment outside the laboratory.
Refers to the degree to which we can confidently infer a causal relationship between variables.
Refers to the degree to which we can generalize the findings to other circumstances or settings, like the real-world environment.
A type of field study where an independent variable is manipulated in a natural setting and extraneous variables are controlled as much as possible.
Research Methods in Psychology Copyright © 2019 by Rajiv S. Jhangiani, I-Chant A. Chiang, Carrie Cuttler, & Dana C. Leighton is licensed under a Creative Commons Attribution-NonCommercial-ShareAlike 4.0 International License , except where otherwise noted.
Share This Book
Have a language expert improve your writing
Run a free plagiarism check in 10 minutes, automatically generate references for free.
- Knowledge Base
- Methodology
Research Design | Step-by-Step Guide with Examples
Published on 5 May 2022 by Shona McCombes . Revised on 20 March 2023.
A research design is a strategy for answering your research question using empirical data. Creating a research design means making decisions about:
- Your overall aims and approach
- The type of research design you’ll use
- Your sampling methods or criteria for selecting subjects
- Your data collection methods
- The procedures you’ll follow to collect data
- Your data analysis methods
A well-planned research design helps ensure that your methods match your research aims and that you use the right kind of analysis for your data.
Table of contents
Step 1: consider your aims and approach, step 2: choose a type of research design, step 3: identify your population and sampling method, step 4: choose your data collection methods, step 5: plan your data collection procedures, step 6: decide on your data analysis strategies, frequently asked questions.
- Introduction
Before you can start designing your research, you should already have a clear idea of the research question you want to investigate.
There are many different ways you could go about answering this question. Your research design choices should be driven by your aims and priorities – start by thinking carefully about what you want to achieve.
The first choice you need to make is whether you’ll take a qualitative or quantitative approach.
Qualitative research designs tend to be more flexible and inductive , allowing you to adjust your approach based on what you find throughout the research process.
Quantitative research designs tend to be more fixed and deductive , with variables and hypotheses clearly defined in advance of data collection.
It’s also possible to use a mixed methods design that integrates aspects of both approaches. By combining qualitative and quantitative insights, you can gain a more complete picture of the problem you’re studying and strengthen the credibility of your conclusions.
Practical and ethical considerations when designing research
As well as scientific considerations, you need to think practically when designing your research. If your research involves people or animals, you also need to consider research ethics .
- How much time do you have to collect data and write up the research?
- Will you be able to gain access to the data you need (e.g., by travelling to a specific location or contacting specific people)?
- Do you have the necessary research skills (e.g., statistical analysis or interview techniques)?
- Will you need ethical approval ?
At each stage of the research design process, make sure that your choices are practically feasible.
Prevent plagiarism, run a free check.
Within both qualitative and quantitative approaches, there are several types of research design to choose from. Each type provides a framework for the overall shape of your research.
Types of quantitative research designs
Quantitative designs can be split into four main types. Experimental and quasi-experimental designs allow you to test cause-and-effect relationships, while descriptive and correlational designs allow you to measure variables and describe relationships between them.
With descriptive and correlational designs, you can get a clear picture of characteristics, trends, and relationships as they exist in the real world. However, you can’t draw conclusions about cause and effect (because correlation doesn’t imply causation ).
Experiments are the strongest way to test cause-and-effect relationships without the risk of other variables influencing the results. However, their controlled conditions may not always reflect how things work in the real world. They’re often also more difficult and expensive to implement.
Types of qualitative research designs
Qualitative designs are less strictly defined. This approach is about gaining a rich, detailed understanding of a specific context or phenomenon, and you can often be more creative and flexible in designing your research.
The table below shows some common types of qualitative design. They often have similar approaches in terms of data collection, but focus on different aspects when analysing the data.
Your research design should clearly define who or what your research will focus on, and how you’ll go about choosing your participants or subjects.
In research, a population is the entire group that you want to draw conclusions about, while a sample is the smaller group of individuals you’ll actually collect data from.
Defining the population
A population can be made up of anything you want to study – plants, animals, organisations, texts, countries, etc. In the social sciences, it most often refers to a group of people.
For example, will you focus on people from a specific demographic, region, or background? Are you interested in people with a certain job or medical condition, or users of a particular product?
The more precisely you define your population, the easier it will be to gather a representative sample.
Sampling methods
Even with a narrowly defined population, it’s rarely possible to collect data from every individual. Instead, you’ll collect data from a sample.
To select a sample, there are two main approaches: probability sampling and non-probability sampling . The sampling method you use affects how confidently you can generalise your results to the population as a whole.
Probability sampling is the most statistically valid option, but it’s often difficult to achieve unless you’re dealing with a very small and accessible population.
For practical reasons, many studies use non-probability sampling, but it’s important to be aware of the limitations and carefully consider potential biases. You should always make an effort to gather a sample that’s as representative as possible of the population.
Case selection in qualitative research
In some types of qualitative designs, sampling may not be relevant.
For example, in an ethnography or a case study, your aim is to deeply understand a specific context, not to generalise to a population. Instead of sampling, you may simply aim to collect as much data as possible about the context you are studying.
In these types of design, you still have to carefully consider your choice of case or community. You should have a clear rationale for why this particular case is suitable for answering your research question.
For example, you might choose a case study that reveals an unusual or neglected aspect of your research problem, or you might choose several very similar or very different cases in order to compare them.
Data collection methods are ways of directly measuring variables and gathering information. They allow you to gain first-hand knowledge and original insights into your research problem.
You can choose just one data collection method, or use several methods in the same study.
Survey methods
Surveys allow you to collect data about opinions, behaviours, experiences, and characteristics by asking people directly. There are two main survey methods to choose from: questionnaires and interviews.
Observation methods
Observations allow you to collect data unobtrusively, observing characteristics, behaviours, or social interactions without relying on self-reporting.
Observations may be conducted in real time, taking notes as you observe, or you might make audiovisual recordings for later analysis. They can be qualitative or quantitative.
Other methods of data collection
There are many other ways you might collect data depending on your field and topic.
If you’re not sure which methods will work best for your research design, try reading some papers in your field to see what data collection methods they used.
Secondary data
If you don’t have the time or resources to collect data from the population you’re interested in, you can also choose to use secondary data that other researchers already collected – for example, datasets from government surveys or previous studies on your topic.
With this raw data, you can do your own analysis to answer new research questions that weren’t addressed by the original study.
Using secondary data can expand the scope of your research, as you may be able to access much larger and more varied samples than you could collect yourself.
However, it also means you don’t have any control over which variables to measure or how to measure them, so the conclusions you can draw may be limited.
As well as deciding on your methods, you need to plan exactly how you’ll use these methods to collect data that’s consistent, accurate, and unbiased.
Planning systematic procedures is especially important in quantitative research, where you need to precisely define your variables and ensure your measurements are reliable and valid.
Operationalisation
Some variables, like height or age, are easily measured. But often you’ll be dealing with more abstract concepts, like satisfaction, anxiety, or competence. Operationalisation means turning these fuzzy ideas into measurable indicators.
If you’re using observations , which events or actions will you count?
If you’re using surveys , which questions will you ask and what range of responses will be offered?
You may also choose to use or adapt existing materials designed to measure the concept you’re interested in – for example, questionnaires or inventories whose reliability and validity has already been established.
Reliability and validity
Reliability means your results can be consistently reproduced , while validity means that you’re actually measuring the concept you’re interested in.
For valid and reliable results, your measurement materials should be thoroughly researched and carefully designed. Plan your procedures to make sure you carry out the same steps in the same way for each participant.
If you’re developing a new questionnaire or other instrument to measure a specific concept, running a pilot study allows you to check its validity and reliability in advance.
Sampling procedures
As well as choosing an appropriate sampling method, you need a concrete plan for how you’ll actually contact and recruit your selected sample.
That means making decisions about things like:
- How many participants do you need for an adequate sample size?
- What inclusion and exclusion criteria will you use to identify eligible participants?
- How will you contact your sample – by mail, online, by phone, or in person?
If you’re using a probability sampling method, it’s important that everyone who is randomly selected actually participates in the study. How will you ensure a high response rate?
If you’re using a non-probability method, how will you avoid bias and ensure a representative sample?
Data management
It’s also important to create a data management plan for organising and storing your data.
Will you need to transcribe interviews or perform data entry for observations? You should anonymise and safeguard any sensitive data, and make sure it’s backed up regularly.
Keeping your data well organised will save time when it comes to analysing them. It can also help other researchers validate and add to your findings.
On their own, raw data can’t answer your research question. The last step of designing your research is planning how you’ll analyse the data.
Quantitative data analysis
In quantitative research, you’ll most likely use some form of statistical analysis . With statistics, you can summarise your sample data, make estimates, and test hypotheses.
Using descriptive statistics , you can summarise your sample data in terms of:
- The distribution of the data (e.g., the frequency of each score on a test)
- The central tendency of the data (e.g., the mean to describe the average score)
- The variability of the data (e.g., the standard deviation to describe how spread out the scores are)
The specific calculations you can do depend on the level of measurement of your variables.
Using inferential statistics , you can:
- Make estimates about the population based on your sample data.
- Test hypotheses about a relationship between variables.
Regression and correlation tests look for associations between two or more variables, while comparison tests (such as t tests and ANOVAs ) look for differences in the outcomes of different groups.
Your choice of statistical test depends on various aspects of your research design, including the types of variables you’re dealing with and the distribution of your data.
Qualitative data analysis
In qualitative research, your data will usually be very dense with information and ideas. Instead of summing it up in numbers, you’ll need to comb through the data in detail, interpret its meanings, identify patterns, and extract the parts that are most relevant to your research question.
Two of the most common approaches to doing this are thematic analysis and discourse analysis .
There are many other ways of analysing qualitative data depending on the aims of your research. To get a sense of potential approaches, try reading some qualitative research papers in your field.
A sample is a subset of individuals from a larger population. Sampling means selecting the group that you will actually collect data from in your research.
For example, if you are researching the opinions of students in your university, you could survey a sample of 100 students.
Statistical sampling allows you to test a hypothesis about the characteristics of a population. There are various sampling methods you can use to ensure that your sample is representative of the population as a whole.
Operationalisation means turning abstract conceptual ideas into measurable observations.
For example, the concept of social anxiety isn’t directly observable, but it can be operationally defined in terms of self-rating scores, behavioural avoidance of crowded places, or physical anxiety symptoms in social situations.
Before collecting data , it’s important to consider how you will operationalise the variables that you want to measure.
The research methods you use depend on the type of data you need to answer your research question .
- If you want to measure something or test a hypothesis , use quantitative methods . If you want to explore ideas, thoughts, and meanings, use qualitative methods .
- If you want to analyse a large amount of readily available data, use secondary data. If you want data specific to your purposes with control over how they are generated, collect primary data.
- If you want to establish cause-and-effect relationships between variables , use experimental methods. If you want to understand the characteristics of a research subject, use descriptive methods.
Cite this Scribbr article
If you want to cite this source, you can copy and paste the citation or click the ‘Cite this Scribbr article’ button to automatically add the citation to our free Reference Generator.
McCombes, S. (2023, March 20). Research Design | Step-by-Step Guide with Examples. Scribbr. Retrieved 29 April 2024, from https://www.scribbr.co.uk/research-methods/research-design/
Is this article helpful?
Shona McCombes
- Bipolar Disorder
- Therapy Center
- When To See a Therapist
- Types of Therapy
- Best Online Therapy
- Best Couples Therapy
- Best Family Therapy
- Managing Stress
- Sleep and Dreaming
- Understanding Emotions
- Self-Improvement
- Healthy Relationships
- Student Resources
- Personality Types
- Guided Meditations
- Verywell Mind Insights
- 2024 Verywell Mind 25
- Mental Health in the Classroom
- Editorial Process
- Meet Our Review Board
- Crisis Support
Understanding Methods for Research in Psychology
A Psychology Research Methods Study Guide
Kendra Cherry, MS, is a psychosocial rehabilitation specialist, psychology educator, and author of the "Everything Psychology Book."
:max_bytes(150000):strip_icc():format(webp)/IMG_9791-89504ab694d54b66bbd72cb84ffb860e.jpg)
Emily is a board-certified science editor who has worked with top digital publishing brands like Voices for Biodiversity, Study.com, GoodTherapy, Vox, and Verywell.
:max_bytes(150000):strip_icc():format(webp)/Emily-Swaim-1000-0f3197de18f74329aeffb690a177160c.jpg)
Types of Research in Psychology
- Cross-Sectional vs. Longitudinal Research
- Reliability and Validity
Glossary of Terms
Research in psychology focuses on a variety of topics , ranging from the development of infants to the behavior of social groups. Psychologists use the scientific method to investigate questions both systematically and empirically.
Research in psychology is important because it provides us with valuable information that helps to improve human lives. By learning more about the brain, cognition, behavior, and mental health conditions, researchers are able to solve real-world problems that affect our day-to-day lives.
At a Glance
Knowing more about how research in psychology is conducted can give you a better understanding of what those findings might mean to you. Psychology experiments can range from simple to complex, but there are some basic terms and concepts that all psychology students should understand.
Start your studies by learning more about the different types of research, the basics of experimental design, and the relationships between variables.
Research in Psychology: The Basics
The first step in your review should include a basic introduction to psychology research methods . Psychology research can have a variety of goals. What researchers learn can be used to describe, explain, predict, or change human behavior.
Psychologists use the scientific method to conduct studies and research in psychology. The basic process of conducting psychology research involves asking a question, designing a study, collecting data, analyzing results, reaching conclusions, and sharing the findings.
The Scientific Method in Psychology Research
The steps of the scientific method in psychology research are:
- Make an observation
- Ask a research question and make predictions about what you expect to find
- Test your hypothesis and gather data
- Examine the results and form conclusions
- Report your findings
Research in psychology can take several different forms. It can describe a phenomenon, explore the causes of a phenomenon, or look at relationships between one or more variables. Three of the main types of psychological research focus on:
Descriptive Studies
This type of research can tell us more about what is happening in a specific population. It relies on techniques such as observation, surveys, and case studies.
Correlational Studies
Correlational research is frequently used in psychology to look for relationships between variables. While research look at how variables are related, they do not manipulate any of the variables.
While correlational studies can suggest a relationship between two variables, finding a correlation does not prove that one variable causes a change in another. In other words, correlation does not equal causation.
Experimental Research Methods
Experiments are a research method that can look at whether changes in one variable cause changes in another. The simple experiment is one of the most basic methods of determining if there is a cause-and-effect relationship between two variables.
A simple experiment utilizes a control group of participants who receive no treatment and an experimental group of participants who receive the treatment.
Experimenters then compare the results of the two groups to determine if the treatment had an effect.
Cross-Sectional vs. Longitudinal Research in Psychology
Research in psychology can also involve collecting data at a single point in time, or gathering information at several points over a period of time.
Cross-Sectional Research
In a cross-sectional study , researchers collect data from participants at a single point in time. These are descriptive type of research and cannot be used to determine cause and effect because researchers do not manipulate the independent variables.
However, cross-sectional research does allow researchers to look at the characteristics of the population and explore relationships between different variables at a single point in time.
Longitudinal Research
A longitudinal study is a type of research in psychology that involves looking at the same group of participants over a period of time. Researchers start by collecting initial data that serves as a baseline, and then collect follow-up data at certain intervals. These studies can last days, months, or years.
The longest longitudinal study in psychology was started in 1921 and the study is planned to continue until the last participant dies or withdraws. As of 2003, more than 200 of the partipants were still alive.
The Reliability and Validity of Research in Psychology
Reliability and validity are two concepts that are also critical in psychology research. In order to trust the results, we need to know if the findings are consistent (reliability) and that we are actually measuring what we think we are measuring (validity).
Reliability
Reliability is a vital component of a valid psychological test. What is reliability? How do we measure it? Simply put, reliability refers to the consistency of a measure. A test is considered reliable if we get the same result repeatedly.
When determining the merits of a psychological test, validity is one of the most important factors to consider. What exactly is validity? One of the greatest concerns when creating a psychological test is whether or not it actually measures what we think it is measuring.
For example, a test might be designed to measure a stable personality trait but instead measures transitory emotions generated by situational or environmental conditions. A valid test ensures that the results accurately reflect the dimension undergoing assessment.
Review some of the key terms that you should know and understand about psychology research methods. Spend some time studying these terms and definitions before your exam. Some key terms that you should know include:
- Correlation
- Demand characteristic
- Dependent variable
- Hawthorne effect
- Independent variable
- Naturalistic observation
- Placebo effect
- Random assignment
- Replication
- Selective attrition
Erol A. How to conduct scientific research ? Noro Psikiyatr Ars . 2017;54(2):97-98. doi:10.5152/npa.2017.0120102
Aggarwal R, Ranganathan P. Study designs: Part 2 - Descriptive studies . Perspect Clin Res . 2019;10(1):34-36. doi:10.4103/picr.PICR_154_18
Curtis EA, Comiskey C, Dempsey O. Importance and use of correlational research . Nurse Res . 2016;23(6):20-25. doi:10.7748/nr.2016.e1382
Wang X, Cheng Z. Cross-sectional studies: Strengths, weaknesses, and recommendations . Chest . 2020;158(1S):S65-S71. doi:10.1016/j.chest.2020.03.012
Caruana EJ, Roman M, Hernández-Sánchez J, Solli P. Longitudinal studies . J Thorac Dis . 2015;7(11):E537-E540. doi:10.3978/j.issn.2072-1439.2015.10.63
Stanford Magazine. The vexing legacy of Lewis Terman .
By Kendra Cherry, MSEd Kendra Cherry, MS, is a psychosocial rehabilitation specialist, psychology educator, and author of the "Everything Psychology Book."
- Technical Support
- Find My Rep
You are here
Research Methods and Design in Psychology
- Paul Richardson - Sheffield Hallam University, UK
- Allen Goodwin - Sheffield Hallam University, UK
- Emma Vine - Sheffield Hallam University, UK
- Description
Activities help readers build the underpinning generic critical thinking and transferable skills they need in order to become independent learners, and to meet the relevant requirements of their programme of study.
The text provides core information on designing psychology research studies with key chapters on both quantitative and qualitative designs. Other chapters look at ethics, common problems, and advances and innovations.
See what’s new to this edition by selecting the Features tab on this page. Should you need additional information or have questions regarding the HEOA information provided for this title, including what is new to this edition, please email [email protected] . Please include your name, contact information, and the name of the title for which you would like more information. For information on the HEOA, please go to http://ed.gov/policy/highered/leg/hea08/index.html .
For assistance with your order: Please email us at [email protected] or connect with your SAGE representative.
SAGE 2455 Teller Road Thousand Oaks, CA 91320 www.sagepub.com
Excellent book that will be recommended for my students preparing a research project.
Great resource for those new to research
Thanks. Very interesting overview with good examples.
What a perfect text book for level 4? Absolutely what i was looking for.
This is a great starting point for students who want a clear and basic introduction to \research methods. Each chapter takes you through an understanding of the various components you will need to consider in a Undergraduate Reseach project.
A thorough grounding in the key areas of research design and the application of various research methods. well structured and very easy to follow. A good text for social work students to see the range of methods and how they can be used.
Complete, accessible, very well structured
A powerpack for starting researchers. Really informative introductions to a couple of different resarch designs and into data analysis.
Not a suitable scholarly level for our students
Our existing texts had this material covered.
Preview this book
For instructors, select a purchasing option, related products.


Want to create or adapt books like this? Learn more about how Pressbooks supports open publishing practices.
6.2 Experimental Design
Learning objectives.
- Explain the difference between between-subjects and within-subjects experiments, list some of the pros and cons of each approach, and decide which approach to use to answer a particular research question.
- Define random assignment, distinguish it from random sampling, explain its purpose in experimental research, and use some simple strategies to implement it.
- Define what a control condition is, explain its purpose in research on treatment effectiveness, and describe some alternative types of control conditions.
- Define several types of carryover effect, give examples of each, and explain how counterbalancing helps to deal with them.
In this section, we look at some different ways to design an experiment. The primary distinction we will make is between approaches in which each participant experiences one level of the independent variable and approaches in which each participant experiences all levels of the independent variable. The former are called between-subjects experiments and the latter are called within-subjects experiments.
Between-Subjects Experiments
In a between-subjects experiment , each participant is tested in only one condition. For example, a researcher with a sample of 100 college students might assign half of them to write about a traumatic event and the other half write about a neutral event. Or a researcher with a sample of 60 people with severe agoraphobia (fear of open spaces) might assign 20 of them to receive each of three different treatments for that disorder. It is essential in a between-subjects experiment that the researcher assign participants to conditions so that the different groups are, on average, highly similar to each other. Those in a trauma condition and a neutral condition, for example, should include a similar proportion of men and women, and they should have similar average intelligence quotients (IQs), similar average levels of motivation, similar average numbers of health problems, and so on. This is a matter of controlling these extraneous participant variables across conditions so that they do not become confounding variables.
Random Assignment
The primary way that researchers accomplish this kind of control of extraneous variables across conditions is called random assignment , which means using a random process to decide which participants are tested in which conditions. Do not confuse random assignment with random sampling. Random sampling is a method for selecting a sample from a population, and it is rarely used in psychological research. Random assignment is a method for assigning participants in a sample to the different conditions, and it is an important element of all experimental research in psychology and other fields too.
In its strictest sense, random assignment should meet two criteria. One is that each participant has an equal chance of being assigned to each condition (e.g., a 50% chance of being assigned to each of two conditions). The second is that each participant is assigned to a condition independently of other participants. Thus one way to assign participants to two conditions would be to flip a coin for each one. If the coin lands heads, the participant is assigned to Condition A, and if it lands tails, the participant is assigned to Condition B. For three conditions, one could use a computer to generate a random integer from 1 to 3 for each participant. If the integer is 1, the participant is assigned to Condition A; if it is 2, the participant is assigned to Condition B; and if it is 3, the participant is assigned to Condition C. In practice, a full sequence of conditions—one for each participant expected to be in the experiment—is usually created ahead of time, and each new participant is assigned to the next condition in the sequence as he or she is tested. When the procedure is computerized, the computer program often handles the random assignment.
One problem with coin flipping and other strict procedures for random assignment is that they are likely to result in unequal sample sizes in the different conditions. Unequal sample sizes are generally not a serious problem, and you should never throw away data you have already collected to achieve equal sample sizes. However, for a fixed number of participants, it is statistically most efficient to divide them into equal-sized groups. It is standard practice, therefore, to use a kind of modified random assignment that keeps the number of participants in each group as similar as possible. One approach is block randomization . In block randomization, all the conditions occur once in the sequence before any of them is repeated. Then they all occur again before any of them is repeated again. Within each of these “blocks,” the conditions occur in a random order. Again, the sequence of conditions is usually generated before any participants are tested, and each new participant is assigned to the next condition in the sequence. Table 6.2 “Block Randomization Sequence for Assigning Nine Participants to Three Conditions” shows such a sequence for assigning nine participants to three conditions. The Research Randomizer website ( http://www.randomizer.org ) will generate block randomization sequences for any number of participants and conditions. Again, when the procedure is computerized, the computer program often handles the block randomization.
Table 6.2 Block Randomization Sequence for Assigning Nine Participants to Three Conditions
Random assignment is not guaranteed to control all extraneous variables across conditions. It is always possible that just by chance, the participants in one condition might turn out to be substantially older, less tired, more motivated, or less depressed on average than the participants in another condition. However, there are some reasons that this is not a major concern. One is that random assignment works better than one might expect, especially for large samples. Another is that the inferential statistics that researchers use to decide whether a difference between groups reflects a difference in the population takes the “fallibility” of random assignment into account. Yet another reason is that even if random assignment does result in a confounding variable and therefore produces misleading results, this is likely to be detected when the experiment is replicated. The upshot is that random assignment to conditions—although not infallible in terms of controlling extraneous variables—is always considered a strength of a research design.
Treatment and Control Conditions
Between-subjects experiments are often used to determine whether a treatment works. In psychological research, a treatment is any intervention meant to change people’s behavior for the better. This includes psychotherapies and medical treatments for psychological disorders but also interventions designed to improve learning, promote conservation, reduce prejudice, and so on. To determine whether a treatment works, participants are randomly assigned to either a treatment condition , in which they receive the treatment, or a control condition , in which they do not receive the treatment. If participants in the treatment condition end up better off than participants in the control condition—for example, they are less depressed, learn faster, conserve more, express less prejudice—then the researcher can conclude that the treatment works. In research on the effectiveness of psychotherapies and medical treatments, this type of experiment is often called a randomized clinical trial .
There are different types of control conditions. In a no-treatment control condition , participants receive no treatment whatsoever. One problem with this approach, however, is the existence of placebo effects. A placebo is a simulated treatment that lacks any active ingredient or element that should make it effective, and a placebo effect is a positive effect of such a treatment. Many folk remedies that seem to work—such as eating chicken soup for a cold or placing soap under the bedsheets to stop nighttime leg cramps—are probably nothing more than placebos. Although placebo effects are not well understood, they are probably driven primarily by people’s expectations that they will improve. Having the expectation to improve can result in reduced stress, anxiety, and depression, which can alter perceptions and even improve immune system functioning (Price, Finniss, & Benedetti, 2008).
Placebo effects are interesting in their own right (see Note 6.28 “The Powerful Placebo” ), but they also pose a serious problem for researchers who want to determine whether a treatment works. Figure 6.2 “Hypothetical Results From a Study Including Treatment, No-Treatment, and Placebo Conditions” shows some hypothetical results in which participants in a treatment condition improved more on average than participants in a no-treatment control condition. If these conditions (the two leftmost bars in Figure 6.2 “Hypothetical Results From a Study Including Treatment, No-Treatment, and Placebo Conditions” ) were the only conditions in this experiment, however, one could not conclude that the treatment worked. It could be instead that participants in the treatment group improved more because they expected to improve, while those in the no-treatment control condition did not.
Figure 6.2 Hypothetical Results From a Study Including Treatment, No-Treatment, and Placebo Conditions

Fortunately, there are several solutions to this problem. One is to include a placebo control condition , in which participants receive a placebo that looks much like the treatment but lacks the active ingredient or element thought to be responsible for the treatment’s effectiveness. When participants in a treatment condition take a pill, for example, then those in a placebo control condition would take an identical-looking pill that lacks the active ingredient in the treatment (a “sugar pill”). In research on psychotherapy effectiveness, the placebo might involve going to a psychotherapist and talking in an unstructured way about one’s problems. The idea is that if participants in both the treatment and the placebo control groups expect to improve, then any improvement in the treatment group over and above that in the placebo control group must have been caused by the treatment and not by participants’ expectations. This is what is shown by a comparison of the two outer bars in Figure 6.2 “Hypothetical Results From a Study Including Treatment, No-Treatment, and Placebo Conditions” .
Of course, the principle of informed consent requires that participants be told that they will be assigned to either a treatment or a placebo control condition—even though they cannot be told which until the experiment ends. In many cases the participants who had been in the control condition are then offered an opportunity to have the real treatment. An alternative approach is to use a waitlist control condition , in which participants are told that they will receive the treatment but must wait until the participants in the treatment condition have already received it. This allows researchers to compare participants who have received the treatment with participants who are not currently receiving it but who still expect to improve (eventually). A final solution to the problem of placebo effects is to leave out the control condition completely and compare any new treatment with the best available alternative treatment. For example, a new treatment for simple phobia could be compared with standard exposure therapy. Because participants in both conditions receive a treatment, their expectations about improvement should be similar. This approach also makes sense because once there is an effective treatment, the interesting question about a new treatment is not simply “Does it work?” but “Does it work better than what is already available?”
The Powerful Placebo
Many people are not surprised that placebos can have a positive effect on disorders that seem fundamentally psychological, including depression, anxiety, and insomnia. However, placebos can also have a positive effect on disorders that most people think of as fundamentally physiological. These include asthma, ulcers, and warts (Shapiro & Shapiro, 1999). There is even evidence that placebo surgery—also called “sham surgery”—can be as effective as actual surgery.
Medical researcher J. Bruce Moseley and his colleagues conducted a study on the effectiveness of two arthroscopic surgery procedures for osteoarthritis of the knee (Moseley et al., 2002). The control participants in this study were prepped for surgery, received a tranquilizer, and even received three small incisions in their knees. But they did not receive the actual arthroscopic surgical procedure. The surprising result was that all participants improved in terms of both knee pain and function, and the sham surgery group improved just as much as the treatment groups. According to the researchers, “This study provides strong evidence that arthroscopic lavage with or without débridement [the surgical procedures used] is not better than and appears to be equivalent to a placebo procedure in improving knee pain and self-reported function” (p. 85).

Research has shown that patients with osteoarthritis of the knee who receive a “sham surgery” experience reductions in pain and improvement in knee function similar to those of patients who receive a real surgery.
Army Medicine – Surgery – CC BY 2.0.
Within-Subjects Experiments
In a within-subjects experiment , each participant is tested under all conditions. Consider an experiment on the effect of a defendant’s physical attractiveness on judgments of his guilt. Again, in a between-subjects experiment, one group of participants would be shown an attractive defendant and asked to judge his guilt, and another group of participants would be shown an unattractive defendant and asked to judge his guilt. In a within-subjects experiment, however, the same group of participants would judge the guilt of both an attractive and an unattractive defendant.
The primary advantage of this approach is that it provides maximum control of extraneous participant variables. Participants in all conditions have the same mean IQ, same socioeconomic status, same number of siblings, and so on—because they are the very same people. Within-subjects experiments also make it possible to use statistical procedures that remove the effect of these extraneous participant variables on the dependent variable and therefore make the data less “noisy” and the effect of the independent variable easier to detect. We will look more closely at this idea later in the book.
Carryover Effects and Counterbalancing
The primary disadvantage of within-subjects designs is that they can result in carryover effects. A carryover effect is an effect of being tested in one condition on participants’ behavior in later conditions. One type of carryover effect is a practice effect , where participants perform a task better in later conditions because they have had a chance to practice it. Another type is a fatigue effect , where participants perform a task worse in later conditions because they become tired or bored. Being tested in one condition can also change how participants perceive stimuli or interpret their task in later conditions. This is called a context effect . For example, an average-looking defendant might be judged more harshly when participants have just judged an attractive defendant than when they have just judged an unattractive defendant. Within-subjects experiments also make it easier for participants to guess the hypothesis. For example, a participant who is asked to judge the guilt of an attractive defendant and then is asked to judge the guilt of an unattractive defendant is likely to guess that the hypothesis is that defendant attractiveness affects judgments of guilt. This could lead the participant to judge the unattractive defendant more harshly because he thinks this is what he is expected to do. Or it could make participants judge the two defendants similarly in an effort to be “fair.”
Carryover effects can be interesting in their own right. (Does the attractiveness of one person depend on the attractiveness of other people that we have seen recently?) But when they are not the focus of the research, carryover effects can be problematic. Imagine, for example, that participants judge the guilt of an attractive defendant and then judge the guilt of an unattractive defendant. If they judge the unattractive defendant more harshly, this might be because of his unattractiveness. But it could be instead that they judge him more harshly because they are becoming bored or tired. In other words, the order of the conditions is a confounding variable. The attractive condition is always the first condition and the unattractive condition the second. Thus any difference between the conditions in terms of the dependent variable could be caused by the order of the conditions and not the independent variable itself.
There is a solution to the problem of order effects, however, that can be used in many situations. It is counterbalancing , which means testing different participants in different orders. For example, some participants would be tested in the attractive defendant condition followed by the unattractive defendant condition, and others would be tested in the unattractive condition followed by the attractive condition. With three conditions, there would be six different orders (ABC, ACB, BAC, BCA, CAB, and CBA), so some participants would be tested in each of the six orders. With counterbalancing, participants are assigned to orders randomly, using the techniques we have already discussed. Thus random assignment plays an important role in within-subjects designs just as in between-subjects designs. Here, instead of randomly assigning to conditions, they are randomly assigned to different orders of conditions. In fact, it can safely be said that if a study does not involve random assignment in one form or another, it is not an experiment.
There are two ways to think about what counterbalancing accomplishes. One is that it controls the order of conditions so that it is no longer a confounding variable. Instead of the attractive condition always being first and the unattractive condition always being second, the attractive condition comes first for some participants and second for others. Likewise, the unattractive condition comes first for some participants and second for others. Thus any overall difference in the dependent variable between the two conditions cannot have been caused by the order of conditions. A second way to think about what counterbalancing accomplishes is that if there are carryover effects, it makes it possible to detect them. One can analyze the data separately for each order to see whether it had an effect.
When 9 Is “Larger” Than 221
Researcher Michael Birnbaum has argued that the lack of context provided by between-subjects designs is often a bigger problem than the context effects created by within-subjects designs. To demonstrate this, he asked one group of participants to rate how large the number 9 was on a 1-to-10 rating scale and another group to rate how large the number 221 was on the same 1-to-10 rating scale (Birnbaum, 1999). Participants in this between-subjects design gave the number 9 a mean rating of 5.13 and the number 221 a mean rating of 3.10. In other words, they rated 9 as larger than 221! According to Birnbaum, this is because participants spontaneously compared 9 with other one-digit numbers (in which case it is relatively large) and compared 221 with other three-digit numbers (in which case it is relatively small).
Simultaneous Within-Subjects Designs
So far, we have discussed an approach to within-subjects designs in which participants are tested in one condition at a time. There is another approach, however, that is often used when participants make multiple responses in each condition. Imagine, for example, that participants judge the guilt of 10 attractive defendants and 10 unattractive defendants. Instead of having people make judgments about all 10 defendants of one type followed by all 10 defendants of the other type, the researcher could present all 20 defendants in a sequence that mixed the two types. The researcher could then compute each participant’s mean rating for each type of defendant. Or imagine an experiment designed to see whether people with social anxiety disorder remember negative adjectives (e.g., “stupid,” “incompetent”) better than positive ones (e.g., “happy,” “productive”). The researcher could have participants study a single list that includes both kinds of words and then have them try to recall as many words as possible. The researcher could then count the number of each type of word that was recalled. There are many ways to determine the order in which the stimuli are presented, but one common way is to generate a different random order for each participant.
Between-Subjects or Within-Subjects?
Almost every experiment can be conducted using either a between-subjects design or a within-subjects design. This means that researchers must choose between the two approaches based on their relative merits for the particular situation.
Between-subjects experiments have the advantage of being conceptually simpler and requiring less testing time per participant. They also avoid carryover effects without the need for counterbalancing. Within-subjects experiments have the advantage of controlling extraneous participant variables, which generally reduces noise in the data and makes it easier to detect a relationship between the independent and dependent variables.
A good rule of thumb, then, is that if it is possible to conduct a within-subjects experiment (with proper counterbalancing) in the time that is available per participant—and you have no serious concerns about carryover effects—this is probably the best option. If a within-subjects design would be difficult or impossible to carry out, then you should consider a between-subjects design instead. For example, if you were testing participants in a doctor’s waiting room or shoppers in line at a grocery store, you might not have enough time to test each participant in all conditions and therefore would opt for a between-subjects design. Or imagine you were trying to reduce people’s level of prejudice by having them interact with someone of another race. A within-subjects design with counterbalancing would require testing some participants in the treatment condition first and then in a control condition. But if the treatment works and reduces people’s level of prejudice, then they would no longer be suitable for testing in the control condition. This is true for many designs that involve a treatment meant to produce long-term change in participants’ behavior (e.g., studies testing the effectiveness of psychotherapy). Clearly, a between-subjects design would be necessary here.
Remember also that using one type of design does not preclude using the other type in a different study. There is no reason that a researcher could not use both a between-subjects design and a within-subjects design to answer the same research question. In fact, professional researchers often do exactly this.
Key Takeaways
- Experiments can be conducted using either between-subjects or within-subjects designs. Deciding which to use in a particular situation requires careful consideration of the pros and cons of each approach.
- Random assignment to conditions in between-subjects experiments or to orders of conditions in within-subjects experiments is a fundamental element of experimental research. Its purpose is to control extraneous variables so that they do not become confounding variables.
- Experimental research on the effectiveness of a treatment requires both a treatment condition and a control condition, which can be a no-treatment control condition, a placebo control condition, or a waitlist control condition. Experimental treatments can also be compared with the best available alternative.
Discussion: For each of the following topics, list the pros and cons of a between-subjects and within-subjects design and decide which would be better.
- You want to test the relative effectiveness of two training programs for running a marathon.
- Using photographs of people as stimuli, you want to see if smiling people are perceived as more intelligent than people who are not smiling.
- In a field experiment, you want to see if the way a panhandler is dressed (neatly vs. sloppily) affects whether or not passersby give him any money.
- You want to see if concrete nouns (e.g., dog ) are recalled better than abstract nouns (e.g., truth ).
- Discussion: Imagine that an experiment shows that participants who receive psychodynamic therapy for a dog phobia improve more than participants in a no-treatment control group. Explain a fundamental problem with this research design and at least two ways that it might be corrected.
Birnbaum, M. H. (1999). How to show that 9 > 221: Collect judgments in a between-subjects design. Psychological Methods, 4 , 243–249.
Moseley, J. B., O’Malley, K., Petersen, N. J., Menke, T. J., Brody, B. A., Kuykendall, D. H., … Wray, N. P. (2002). A controlled trial of arthroscopic surgery for osteoarthritis of the knee. The New England Journal of Medicine, 347 , 81–88.
Price, D. D., Finniss, D. G., & Benedetti, F. (2008). A comprehensive review of the placebo effect: Recent advances and current thought. Annual Review of Psychology, 59 , 565–590.
Shapiro, A. K., & Shapiro, E. (1999). The powerful placebo: From ancient priest to modern physician . Baltimore, MD: Johns Hopkins University Press.
Research Methods in Psychology Copyright © 2016 by University of Minnesota is licensed under a Creative Commons Attribution-NonCommercial-ShareAlike 4.0 International License , except where otherwise noted.
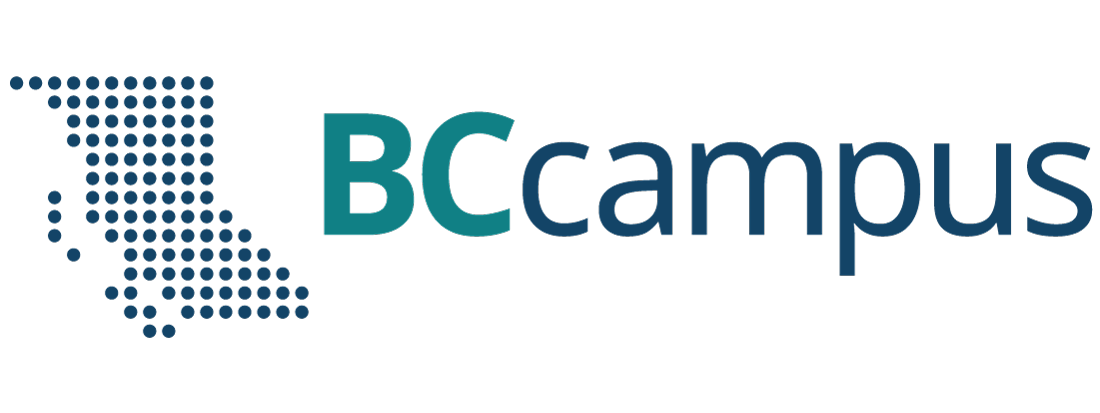
Want to create or adapt books like this? Learn more about how Pressbooks supports open publishing practices.
Chapter 3. Psychological Science
3.2 Psychologists Use Descriptive, Correlational, and Experimental Research Designs to Understand Behaviour
Learning objectives.
- Differentiate the goals of descriptive, correlational, and experimental research designs and explain the advantages and disadvantages of each.
- Explain the goals of descriptive research and the statistical techniques used to interpret it.
- Summarize the uses of correlational research and describe why correlational research cannot be used to infer causality.
- Review the procedures of experimental research and explain how it can be used to draw causal inferences.
Psychologists agree that if their ideas and theories about human behaviour are to be taken seriously, they must be backed up by data. However, the research of different psychologists is designed with different goals in mind, and the different goals require different approaches. These varying approaches, summarized in Table 3.2, are known as research designs . A research design is the specific method a researcher uses to collect, analyze, and interpret data . Psychologists use three major types of research designs in their research, and each provides an essential avenue for scientific investigation. Descriptive research is research designed to provide a snapshot of the current state of affairs . Correlational research is research designed to discover relationships among variables and to allow the prediction of future events from present knowledge . Experimental research is research in which initial equivalence among research participants in more than one group is created, followed by a manipulation of a given experience for these groups and a measurement of the influence of the manipulation . Each of the three research designs varies according to its strengths and limitations, and it is important to understand how each differs.
Descriptive Research: Assessing the Current State of Affairs
Descriptive research is designed to create a snapshot of the current thoughts, feelings, or behaviour of individuals. This section reviews three types of descriptive research : case studies , surveys , and naturalistic observation (Figure 3.4).
Sometimes the data in a descriptive research project are based on only a small set of individuals, often only one person or a single small group. These research designs are known as case studies — descriptive records of one or more individual’s experiences and behaviour . Sometimes case studies involve ordinary individuals, as when developmental psychologist Jean Piaget used his observation of his own children to develop his stage theory of cognitive development. More frequently, case studies are conducted on individuals who have unusual or abnormal experiences or characteristics or who find themselves in particularly difficult or stressful situations. The assumption is that by carefully studying individuals who are socially marginal, who are experiencing unusual situations, or who are going through a difficult phase in their lives, we can learn something about human nature.
Sigmund Freud was a master of using the psychological difficulties of individuals to draw conclusions about basic psychological processes. Freud wrote case studies of some of his most interesting patients and used these careful examinations to develop his important theories of personality. One classic example is Freud’s description of “Little Hans,” a child whose fear of horses the psychoanalyst interpreted in terms of repressed sexual impulses and the Oedipus complex (Freud, 1909/1964).
Another well-known case study is Phineas Gage, a man whose thoughts and emotions were extensively studied by cognitive psychologists after a railroad spike was blasted through his skull in an accident. Although there are questions about the interpretation of this case study (Kotowicz, 2007), it did provide early evidence that the brain’s frontal lobe is involved in emotion and morality (Damasio et al., 2005). An interesting example of a case study in clinical psychology is described by Rokeach (1964), who investigated in detail the beliefs of and interactions among three patients with schizophrenia, all of whom were convinced they were Jesus Christ.
In other cases the data from descriptive research projects come in the form of a survey — a measure administered through either an interview or a written questionnaire to get a picture of the beliefs or behaviours of a sample of people of interest . The people chosen to participate in the research (known as the sample) are selected to be representative of all the people that the researcher wishes to know about (the population). In election polls, for instance, a sample is taken from the population of all “likely voters” in the upcoming elections.
The results of surveys may sometimes be rather mundane, such as “Nine out of 10 doctors prefer Tymenocin” or “The median income in the city of Hamilton is $46,712.” Yet other times (particularly in discussions of social behaviour), the results can be shocking: “More than 40,000 people are killed by gunfire in the United States every year” or “More than 60% of women between the ages of 50 and 60 suffer from depression.” Descriptive research is frequently used by psychologists to get an estimate of the prevalence (or incidence ) of psychological disorders.
A final type of descriptive research — known as naturalistic observation — is research based on the observation of everyday events . For instance, a developmental psychologist who watches children on a playground and describes what they say to each other while they play is conducting descriptive research, as is a biopsychologist who observes animals in their natural habitats. One example of observational research involves a systematic procedure known as the strange situation , used to get a picture of how adults and young children interact. The data that are collected in the strange situation are systematically coded in a coding sheet such as that shown in Table 3.3.
The results of descriptive research projects are analyzed using descriptive statistics — numbers that summarize the distribution of scores on a measured variable . Most variables have distributions similar to that shown in Figure 3.5 where most of the scores are located near the centre of the distribution, and the distribution is symmetrical and bell-shaped. A data distribution that is shaped like a bell is known as a normal distribution .
A distribution can be described in terms of its central tendency — that is, the point in the distribution around which the data are centred — and its dispersion, or spread . The arithmetic average, or arithmetic mean , symbolized by the letter M , is the most commonly used measure of central tendency . It is computed by calculating the sum of all the scores of the variable and dividing this sum by the number of participants in the distribution (denoted by the letter N ). In the data presented in Figure 3.5 the mean height of the students is 67.12 inches (170.5 cm). The sample mean is usually indicated by the letter M .
In some cases, however, the data distribution is not symmetrical. This occurs when there are one or more extreme scores (known as outliers ) at one end of the distribution. Consider, for instance, the variable of family income (see Figure 3.6), which includes an outlier (a value of $3,800,000). In this case the mean is not a good measure of central tendency. Although it appears from Figure 3.6 that the central tendency of the family income variable should be around $70,000, the mean family income is actually $223,960. The single very extreme income has a disproportionate impact on the mean, resulting in a value that does not well represent the central tendency.
The median is used as an alternative measure of central tendency when distributions are not symmetrical. The median is the score in the center of the distribution, meaning that 50% of the scores are greater than the median and 50% of the scores are less than the median . In our case, the median household income ($73,000) is a much better indication of central tendency than is the mean household income ($223,960).
A final measure of central tendency, known as the mode , represents the value that occurs most frequently in the distribution . You can see from Figure 3.6 that the mode for the family income variable is $93,000 (it occurs four times).
In addition to summarizing the central tendency of a distribution, descriptive statistics convey information about how the scores of the variable are spread around the central tendency. Dispersion refers to the extent to which the scores are all tightly clustered around the central tendency , as seen in Figure 3.7.
Or they may be more spread out away from it, as seen in Figure 3.8.
One simple measure of dispersion is to find the largest (the maximum ) and the smallest (the minimum ) observed values of the variable and to compute the range of the variable as the maximum observed score minus the minimum observed score. You can check that the range of the height variable in Figure 3.5 is 72 – 62 = 10. The standard deviation , symbolized as s , is the most commonly used measure of dispersion . Distributions with a larger standard deviation have more spread. The standard deviation of the height variable is s = 2.74, and the standard deviation of the family income variable is s = $745,337.
An advantage of descriptive research is that it attempts to capture the complexity of everyday behaviour. Case studies provide detailed information about a single person or a small group of people, surveys capture the thoughts or reported behaviours of a large population of people, and naturalistic observation objectively records the behaviour of people or animals as it occurs naturally. Thus descriptive research is used to provide a relatively complete understanding of what is currently happening.
Despite these advantages, descriptive research has a distinct disadvantage in that, although it allows us to get an idea of what is currently happening, it is usually limited to static pictures. Although descriptions of particular experiences may be interesting, they are not always transferable to other individuals in other situations, nor do they tell us exactly why specific behaviours or events occurred. For instance, descriptions of individuals who have suffered a stressful event, such as a war or an earthquake, can be used to understand the individuals’ reactions to the event but cannot tell us anything about the long-term effects of the stress. And because there is no comparison group that did not experience the stressful situation, we cannot know what these individuals would be like if they hadn’t had the stressful experience.
Correlational Research: Seeking Relationships among Variables
In contrast to descriptive research, which is designed primarily to provide static pictures, correlational research involves the measurement of two or more relevant variables and an assessment of the relationship between or among those variables. For instance, the variables of height and weight are systematically related (correlated) because taller people generally weigh more than shorter people. In the same way, study time and memory errors are also related, because the more time a person is given to study a list of words, the fewer errors he or she will make. When there are two variables in the research design, one of them is called the predictor variable and the other the outcome variable . The research design can be visualized as shown in Figure 3.9, where the curved arrow represents the expected correlation between these two variables.
One way of organizing the data from a correlational study with two variables is to graph the values of each of the measured variables using a scatter plot . As you can see in Figure 3.10 a scatter plot is a visual image of the relationship between two variables . A point is plotted for each individual at the intersection of his or her scores for the two variables. When the association between the variables on the scatter plot can be easily approximated with a straight line , as in parts (a) and (b) of Figure 3.10 the variables are said to have a linear relationship .
When the straight line indicates that individuals who have above-average values for one variable also tend to have above-average values for the other variable , as in part (a), the relationship is said to be positive linear . Examples of positive linear relationships include those between height and weight, between education and income, and between age and mathematical abilities in children. In each case, people who score higher on one of the variables also tend to score higher on the other variable. Negative linear relationships , in contrast, as shown in part (b), occur when above-average values for one variable tend to be associated with below-average values for the other variable. Examples of negative linear relationships include those between the age of a child and the number of diapers the child uses, and between practice on and errors made on a learning task. In these cases, people who score higher on one of the variables tend to score lower on the other variable.
Relationships between variables that cannot be described with a straight line are known as nonlinear relationships . Part (c) of Figure 3.10 shows a common pattern in which the distribution of the points is essentially random. In this case there is no relationship at all between the two variables, and they are said to be independent . Parts (d) and (e) of Figure 3.10 show patterns of association in which, although there is an association, the points are not well described by a single straight line. For instance, part (d) shows the type of relationship that frequently occurs between anxiety and performance. Increases in anxiety from low to moderate levels are associated with performance increases, whereas increases in anxiety from moderate to high levels are associated with decreases in performance. Relationships that change in direction and thus are not described by a single straight line are called curvilinear relationships .
The most common statistical measure of the strength of linear relationships among variables is the Pearson correlation coefficient , which is symbolized by the letter r . The value of the correlation coefficient ranges from r = –1.00 to r = +1.00. The direction of the linear relationship is indicated by the sign of the correlation coefficient. Positive values of r (such as r = .54 or r = .67) indicate that the relationship is positive linear (i.e., the pattern of the dots on the scatter plot runs from the lower left to the upper right), whereas negative values of r (such as r = –.30 or r = –.72) indicate negative linear relationships (i.e., the dots run from the upper left to the lower right). The strength of the linear relationship is indexed by the distance of the correlation coefficient from zero (its absolute value). For instance, r = –.54 is a stronger relationship than r = .30, and r = .72 is a stronger relationship than r = –.57. Because the Pearson correlation coefficient only measures linear relationships, variables that have curvilinear relationships are not well described by r , and the observed correlation will be close to zero.
It is also possible to study relationships among more than two measures at the same time. A research design in which more than one predictor variable is used to predict a single outcome variable is analyzed through multiple regression (Aiken & West, 1991). Multiple regression is a statistical technique, based on correlation coefficients among variables, that allows predicting a single outcome variable from more than one predictor variable . For instance, Figure 3.11 shows a multiple regression analysis in which three predictor variables (Salary, job satisfaction, and years employed) are used to predict a single outcome (job performance). The use of multiple regression analysis shows an important advantage of correlational research designs — they can be used to make predictions about a person’s likely score on an outcome variable (e.g., job performance) based on knowledge of other variables.
An important limitation of correlational research designs is that they cannot be used to draw conclusions about the causal relationships among the measured variables. Consider, for instance, a researcher who has hypothesized that viewing violent behaviour will cause increased aggressive play in children. He has collected, from a sample of Grade 4 children, a measure of how many violent television shows each child views during the week, as well as a measure of how aggressively each child plays on the school playground. From his collected data, the researcher discovers a positive correlation between the two measured variables.
Although this positive correlation appears to support the researcher’s hypothesis, it cannot be taken to indicate that viewing violent television causes aggressive behaviour. Although the researcher is tempted to assume that viewing violent television causes aggressive play, there are other possibilities. One alternative possibility is that the causal direction is exactly opposite from what has been hypothesized. Perhaps children who have behaved aggressively at school develop residual excitement that leads them to want to watch violent television shows at home (Figure 3.13):
Although this possibility may seem less likely, there is no way to rule out the possibility of such reverse causation on the basis of this observed correlation. It is also possible that both causal directions are operating and that the two variables cause each other (Figure 3.14).
Still another possible explanation for the observed correlation is that it has been produced by the presence of a common-causal variable (also known as a third variable ). A common-causal variable is a variable that is not part of the research hypothesis but that causes both the predictor and the outcome variable and thus produces the observed correlation between them . In our example, a potential common-causal variable is the discipline style of the children’s parents. Parents who use a harsh and punitive discipline style may produce children who like to watch violent television and who also behave aggressively in comparison to children whose parents use less harsh discipline (Figure 3.15)
In this case, television viewing and aggressive play would be positively correlated (as indicated by the curved arrow between them), even though neither one caused the other but they were both caused by the discipline style of the parents (the straight arrows). When the predictor and outcome variables are both caused by a common-causal variable, the observed relationship between them is said to be spurious . A spurious relationship is a relationship between two variables in which a common-causal variable produces and “explains away” the relationship . If effects of the common-causal variable were taken away, or controlled for, the relationship between the predictor and outcome variables would disappear. In the example, the relationship between aggression and television viewing might be spurious because by controlling for the effect of the parents’ disciplining style, the relationship between television viewing and aggressive behaviour might go away.
Common-causal variables in correlational research designs can be thought of as mystery variables because, as they have not been measured, their presence and identity are usually unknown to the researcher. Since it is not possible to measure every variable that could cause both the predictor and outcome variables, the existence of an unknown common-causal variable is always a possibility. For this reason, we are left with the basic limitation of correlational research: correlation does not demonstrate causation. It is important that when you read about correlational research projects, you keep in mind the possibility of spurious relationships, and be sure to interpret the findings appropriately. Although correlational research is sometimes reported as demonstrating causality without any mention being made of the possibility of reverse causation or common-causal variables, informed consumers of research, like you, are aware of these interpretational problems.
In sum, correlational research designs have both strengths and limitations. One strength is that they can be used when experimental research is not possible because the predictor variables cannot be manipulated. Correlational designs also have the advantage of allowing the researcher to study behaviour as it occurs in everyday life. And we can also use correlational designs to make predictions — for instance, to predict from the scores on their battery of tests the success of job trainees during a training session. But we cannot use such correlational information to determine whether the training caused better job performance. For that, researchers rely on experiments.
Experimental Research: Understanding the Causes of Behaviour
The goal of experimental research design is to provide more definitive conclusions about the causal relationships among the variables in the research hypothesis than is available from correlational designs. In an experimental research design, the variables of interest are called the independent variable (or variables ) and the dependent variable . The independent variable in an experiment is the causing variable that is created (manipulated) by the experimenter . The dependent variable in an experiment is a measured variable that is expected to be influenced by the experimental manipulation . The research hypothesis suggests that the manipulated independent variable or variables will cause changes in the measured dependent variables. We can diagram the research hypothesis by using an arrow that points in one direction. This demonstrates the expected direction of causality (Figure 3.16):
Research Focus: Video Games and Aggression
Consider an experiment conducted by Anderson and Dill (2000). The study was designed to test the hypothesis that viewing violent video games would increase aggressive behaviour. In this research, male and female undergraduates from Iowa State University were given a chance to play with either a violent video game (Wolfenstein 3D) or a nonviolent video game (Myst). During the experimental session, the participants played their assigned video games for 15 minutes. Then, after the play, each participant played a competitive game with an opponent in which the participant could deliver blasts of white noise through the earphones of the opponent. The operational definition of the dependent variable (aggressive behaviour) was the level and duration of noise delivered to the opponent. The design of the experiment is shown in Figure 3.17
Two advantages of the experimental research design are (a) the assurance that the independent variable (also known as the experimental manipulation ) occurs prior to the measured dependent variable, and (b) the creation of initial equivalence between the conditions of the experiment (in this case by using random assignment to conditions).
Experimental designs have two very nice features. For one, they guarantee that the independent variable occurs prior to the measurement of the dependent variable. This eliminates the possibility of reverse causation. Second, the influence of common-causal variables is controlled, and thus eliminated, by creating initial equivalence among the participants in each of the experimental conditions before the manipulation occurs.
The most common method of creating equivalence among the experimental conditions is through random assignment to conditions, a procedure in which the condition that each participant is assigned to is determined through a random process, such as drawing numbers out of an envelope or using a random number table . Anderson and Dill first randomly assigned about 100 participants to each of their two groups (Group A and Group B). Because they used random assignment to conditions, they could be confident that, before the experimental manipulation occurred, the students in Group A were, on average, equivalent to the students in Group B on every possible variable, including variables that are likely to be related to aggression, such as parental discipline style, peer relationships, hormone levels, diet — and in fact everything else.
Then, after they had created initial equivalence, Anderson and Dill created the experimental manipulation — they had the participants in Group A play the violent game and the participants in Group B play the nonviolent game. Then they compared the dependent variable (the white noise blasts) between the two groups, finding that the students who had viewed the violent video game gave significantly longer noise blasts than did the students who had played the nonviolent game.
Anderson and Dill had from the outset created initial equivalence between the groups. This initial equivalence allowed them to observe differences in the white noise levels between the two groups after the experimental manipulation, leading to the conclusion that it was the independent variable (and not some other variable) that caused these differences. The idea is that the only thing that was different between the students in the two groups was the video game they had played.
Despite the advantage of determining causation, experiments do have limitations. One is that they are often conducted in laboratory situations rather than in the everyday lives of people. Therefore, we do not know whether results that we find in a laboratory setting will necessarily hold up in everyday life. Second, and more important, is that some of the most interesting and key social variables cannot be experimentally manipulated. If we want to study the influence of the size of a mob on the destructiveness of its behaviour, or to compare the personality characteristics of people who join suicide cults with those of people who do not join such cults, these relationships must be assessed using correlational designs, because it is simply not possible to experimentally manipulate these variables.
Key Takeaways
- Descriptive, correlational, and experimental research designs are used to collect and analyze data.
- Descriptive designs include case studies, surveys, and naturalistic observation. The goal of these designs is to get a picture of the current thoughts, feelings, or behaviours in a given group of people. Descriptive research is summarized using descriptive statistics.
- Correlational research designs measure two or more relevant variables and assess a relationship between or among them. The variables may be presented on a scatter plot to visually show the relationships. The Pearson Correlation Coefficient ( r ) is a measure of the strength of linear relationship between two variables.
- Common-causal variables may cause both the predictor and outcome variable in a correlational design, producing a spurious relationship. The possibility of common-causal variables makes it impossible to draw causal conclusions from correlational research designs.
- Experimental research involves the manipulation of an independent variable and the measurement of a dependent variable. Random assignment to conditions is normally used to create initial equivalence between the groups, allowing researchers to draw causal conclusions.
Exercises and Critical Thinking
- There is a negative correlation between the row that a student sits in in a large class (when the rows are numbered from front to back) and his or her final grade in the class. Do you think this represents a causal relationship or a spurious relationship, and why?
- Think of two variables (other than those mentioned in this book) that are likely to be correlated, but in which the correlation is probably spurious. What is the likely common-causal variable that is producing the relationship?
- Imagine a researcher wants to test the hypothesis that participating in psychotherapy will cause a decrease in reported anxiety. Describe the type of research design the investigator might use to draw this conclusion. What would be the independent and dependent variables in the research?
Image Attributions
Figure 3.4: “ Reading newspaper ” by Alaskan Dude (http://commons.wikimedia.org/wiki/File:Reading_newspaper.jpg) is licensed under CC BY 2.0
Aiken, L., & West, S. (1991). Multiple regression: Testing and interpreting interactions . Newbury Park, CA: Sage.
Ainsworth, M. S., Blehar, M. C., Waters, E., & Wall, S. (1978). Patterns of attachment: A psychological study of the strange situation . Hillsdale, NJ: Lawrence Erlbaum Associates.
Anderson, C. A., & Dill, K. E. (2000). Video games and aggressive thoughts, feelings, and behavior in the laboratory and in life. Journal of Personality and Social Psychology, 78 (4), 772–790.
Damasio, H., Grabowski, T., Frank, R., Galaburda, A. M., Damasio, A. R., Cacioppo, J. T., & Berntson, G. G. (2005). The return of Phineas Gage: Clues about the brain from the skull of a famous patient. In Social neuroscience: Key readings. (pp. 21–28). New York, NY: Psychology Press.
Freud, S. (1909/1964). Analysis of phobia in a five-year-old boy. In E. A. Southwell & M. Merbaum (Eds.), Personality: Readings in theory and research (pp. 3–32). Belmont, CA: Wadsworth. (Original work published 1909).
Kotowicz, Z. (2007). The strange case of Phineas Gage. History of the Human Sciences, 20 (1), 115–131.
Rokeach, M. (1964). The three Christs of Ypsilanti: A psychological study . New York, NY: Knopf.
Stangor, C. (2011). Research methods for the behavioural sciences (4th ed.). Mountain View, CA: Cengage.
Long Descriptions
Figure 3.6 long description: There are 25 families. 24 families have an income between $44,000 and $111,000 and one family has an income of $3,800,000. The mean income is $223,960 while the median income is $73,000. [Return to Figure 3.6]
Figure 3.10 long description: Types of scatter plots.
- Positive linear, r=positive .82. The plots on the graph form a rough line that runs from lower left to upper right.
- Negative linear, r=negative .70. The plots on the graph form a rough line that runs from upper left to lower right.
- Independent, r=0.00. The plots on the graph are spread out around the centre.
- Curvilinear, r=0.00. The plots of the graph form a rough line that goes up and then down like a hill.
- Curvilinear, r=0.00. The plots on the graph for a rough line that goes down and then up like a ditch.
[Return to Figure 3.10]
Introduction to Psychology - 1st Canadian Edition Copyright © 2014 by Jennifer Walinga and Charles Stangor is licensed under a Creative Commons Attribution-NonCommercial-ShareAlike 4.0 International License , except where otherwise noted.
Share This Book
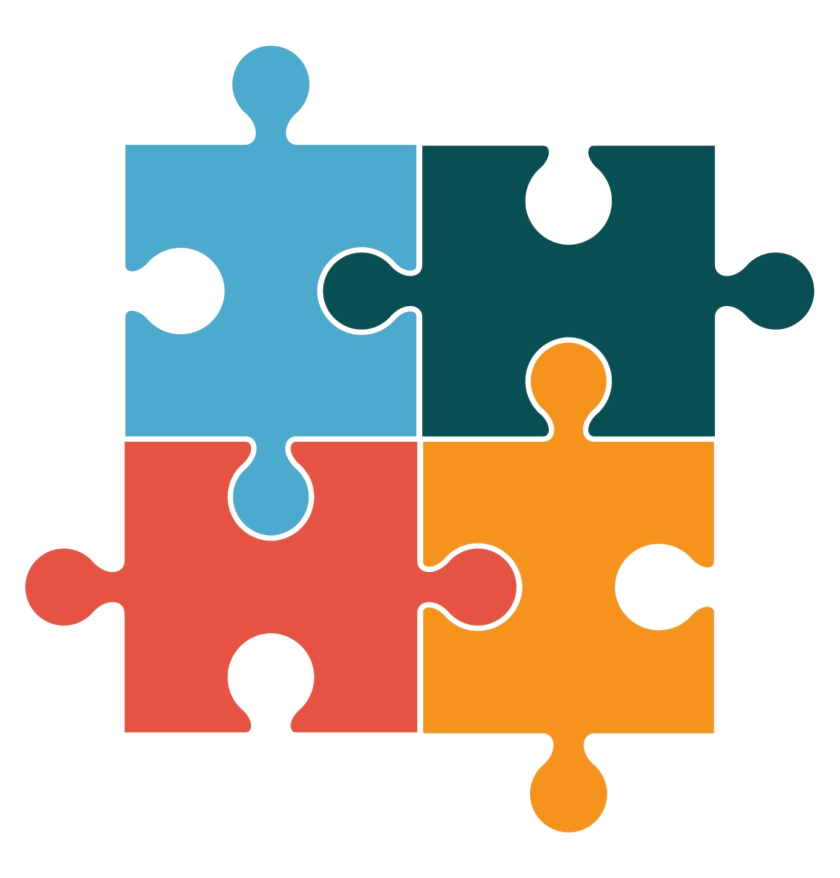
Research Methods in Psychology
5. factorial designs ¶.
We have usually no knowledge that any one factor will exert its effects independently of all others that can be varied, or that its effects are particularly simply related to variations in these other factors. —Ronald Fisher
In Chapter 1 we briefly described a study conducted by Simone Schnall and her colleagues, in which they found that washing one’s hands leads people to view moral transgressions as less wrong [SBH08] . In a different but related study, Schnall and her colleagues investigated whether feeling physically disgusted causes people to make harsher moral judgments [SHCJ08] . In this experiment, they manipulated participants’ feelings of disgust by testing them in either a clean room or a messy room that contained dirty dishes, an overflowing wastebasket, and a chewed-up pen. They also used a self-report questionnaire to measure the amount of attention that people pay to their own bodily sensations. They called this “private body consciousness”. They measured their primary dependent variable, the harshness of people’s moral judgments, by describing different behaviors (e.g., eating one’s dead dog, failing to return a found wallet) and having participants rate the moral acceptability of each one on a scale of 1 to 7. They also measured some other dependent variables, including participants’ willingness to eat at a new restaurant. Finally, the researchers asked participants to rate their current level of disgust and other emotions. The primary results of this study were that participants in the messy room were in fact more disgusted and made harsher moral judgments than participants in the clean room—but only if they scored relatively high in private body consciousness.
The research designs we have considered so far have been simple—focusing on a question about one variable or about a statistical relationship between two variables. But in many ways, the complex design of this experiment undertaken by Schnall and her colleagues is more typical of research in psychology. Fortunately, we have already covered the basic elements of such designs in previous chapters. In this chapter, we look closely at how and why researchers combine these basic elements into more complex designs. We start with complex experiments—considering first the inclusion of multiple dependent variables and then the inclusion of multiple independent variables. Finally, we look at complex correlational designs.
5.1. Multiple Dependent Variables ¶
5.1.1. learning objectives ¶.
Explain why researchers often include multiple dependent variables in their studies.
Explain what a manipulation check is and when it would be included in an experiment.
Imagine that you have made the effort to find a research topic, review the research literature, formulate a question, design an experiment, obtain approval from teh relevant institutional review board (IRB), recruit research participants, and manipulate an independent variable. It would seem almost wasteful to measure a single dependent variable. Even if you are primarily interested in the relationship between an independent variable and one primary dependent variable, there are usually several more questions that you can answer easily by including multiple dependent variables.
5.1.2. Measures of Different Constructs ¶
Often a researcher wants to know how an independent variable affects several distinct dependent variables. For example, Schnall and her colleagues were interested in how feeling disgusted affects the harshness of people’s moral judgments, but they were also curious about how disgust affects other variables, such as people’s willingness to eat in a restaurant. As another example, researcher Susan Knasko was interested in how different odors affect people’s behavior [Kna92] . She conducted an experiment in which the independent variable was whether participants were tested in a room with no odor or in one scented with lemon, lavender, or dimethyl sulfide (which has a cabbage-like smell). Although she was primarily interested in how the odors affected people’s creativity, she was also curious about how they affected people’s moods and perceived health—and it was a simple enough matter to measure these dependent variables too. Although she found that creativity was unaffected by the ambient odor, she found that people’s moods were lower in the dimethyl sulfide condition, and that their perceived health was greater in the lemon condition.
When an experiment includes multiple dependent variables, there is again a possibility of carryover effects. For example, it is possible that measuring participants’ moods before measuring their perceived health could affect their perceived health or that measuring their perceived health before their moods could affect their moods. So the order in which multiple dependent variables are measured becomes an issue. One approach is to measure them in the same order for all participants—usually with the most important one first so that it cannot be affected by measuring the others. Another approach is to counterbalance, or systematically vary, the order in which the dependent variables are measured.
5.1.3. Manipulation Checks ¶
When the independent variable is a construct that can only be manipulated indirectly—such as emotions and other internal states—an additional measure of that independent variable is often included as a manipulation check. This is done to confirm that the independent variable was, in fact, successfully manipulated. For example, Schnall and her colleagues had their participants rate their level of disgust to be sure that those in the messy room actually felt more disgusted than those in the clean room.
Manipulation checks are usually done at the end of the procedure to be sure that the effect of the manipulation lasted throughout the entire procedure and to avoid calling unnecessary attention to the manipulation. Manipulation checks become especially important when the manipulation of the independent variable turns out to have no effect on the dependent variable. Imagine, for example, that you exposed participants to happy or sad movie music—intending to put them in happy or sad moods—but you found that this had no effect on the number of happy or sad childhood events they recalled. This could be because being in a happy or sad mood has no effect on memories for childhood events. But it could also be that the music was ineffective at putting participants in happy or sad moods. A manipulation check, in this case, a measure of participants’ moods, would help resolve this uncertainty. If it showed that you had successfully manipulated participants’ moods, then it would appear that there is indeed no effect of mood on memory for childhood events. But if it showed that you did not successfully manipulate participants’ moods, then it would appear that you need a more effective manipulation to answer your research question.
5.1.4. Measures of the Same Construct ¶
Another common approach to including multiple dependent variables is to operationalize and measure the same construct, or closely related ones, in different ways. Imagine, for example, that a researcher conducts an experiment on the effect of daily exercise on stress. The dependent variable, stress, is a construct that can be operationalized in different ways. For this reason, the researcher might have participants complete the paper-and-pencil Perceived Stress Scale and also measure their levels of the stress hormone cortisol. This is an example of the use of converging operations. If the researcher finds that the different measures are affected by exercise in the same way, then he or she can be confident in the conclusion that exercise affects the more general construct of stress.
When multiple dependent variables are different measures of the same construct - especially if they are measured on the same scale - researchers have the option of combining them into a single measure of that construct. Recall that Schnall and her colleagues were interested in the harshness of people’s moral judgments. To measure this construct, they presented their participants with seven different scenarios describing morally questionable behaviors and asked them to rate the moral acceptability of each one. Although the researchers could have treated each of the seven ratings as a separate dependent variable, these researchers combined them into a single dependent variable by computing their mean.
When researchers combine dependent variables in this way, they are treating them collectively as a multiple-response measure of a single construct. The advantage of this is that multiple-response measures are generally more reliable than single-response measures. However, it is important to make sure the individual dependent variables are correlated with each other by computing an internal consistency measure such as Cronbach’s \(\alpha\) . If they are not correlated with each other, then it does not make sense to combine them into a measure of a single construct. If they have poor internal consistency, then they should be treated as separate dependent variables.
5.1.5. Key Takeaways ¶
Researchers in psychology often include multiple dependent variables in their studies. The primary reason is that this easily allows them to answer more research questions with minimal additional effort.
When an independent variable is a construct that is manipulated indirectly, it is a good idea to include a manipulation check. This is a measure of the independent variable typically given at the end of the procedure to confirm that it was successfully manipulated.
Multiple measures of the same construct can be analyzed separately or combined to produce a single multiple-item measure of that construct. The latter approach requires that the measures taken together have good internal consistency.
5.1.6. Exercises ¶
Practice: List three independent variables for which it would be good to include a manipulation check. List three others for which a manipulation check would be unnecessary. Hint: Consider whether there is any ambiguity concerning whether the manipulation will have its intended effect.
Practice: Imagine a study in which the independent variable is whether the room where participants are tested is warm (30°) or cool (12°). List three dependent variables that you might treat as measures of separate variables. List three more that you might combine and treat as measures of the same underlying construct.
5.2. Multiple Independent Variables ¶
5.2.1. learning objectives ¶.
Explain why researchers often include multiple independent variables in their studies.
Define factorial design, and use a factorial design table to represent and interpret simple factorial designs.
Distinguish between main effects and interactions, and recognize and give examples of each.
Sketch and interpret bar graphs and line graphs showing the results of studies with simple factorial designs.
Just as it is common for studies in psychology to include multiple dependent variables, it is also common for them to include multiple independent variables. Schnall and her colleagues studied the effect of both disgust and private body consciousness in the same study. The tendency to include multiple independent variables in one experiment is further illustrated by the following titles of actual research articles published in professional journals:
The Effects of Temporal Delay and Orientation on Haptic Object Recognition
Opening Closed Minds: The Combined Effects of Intergroup Contact and Need for Closure on Prejudice
Effects of Expectancies and Coping on Pain-Induced Intentions to Smoke
The Effect of Age and Divided Attention on Spontaneous Recognition
The Effects of Reduced Food Size and Package Size on the Consumption Behavior of Restrained and Unrestrained Eaters
Just as including multiple dependent variables in the same experiment allows one to answer more research questions, so too does including multiple independent variables in the same experiment. For example, instead of conducting one study on the effect of disgust on moral judgment and another on the effect of private body consciousness on moral judgment, Schnall and colleagues were able to conduct one study that addressed both variables. But including multiple independent variables also allows the researcher to answer questions about whether the effect of one independent variable depends on the level of another. This is referred to as an interaction between the independent variables. Schnall and her colleagues, for example, observed an interaction between disgust and private body consciousness because the effect of disgust depended on whether participants were high or low in private body consciousness. As we will see, interactions are often among the most interesting results in psychological research.
5.2.2. Factorial Designs ¶
By far the most common approach to including multiple independent variables in an experiment is the factorial design. In a factorial design, each level of one independent variable (which can also be called a factor) is combined with each level of the others to produce all possible combinations. Each combination, then, becomes a condition in the experiment. Imagine, for example, an experiment on the effect of cell phone use (yes vs. no) and time of day (day vs. night) on driving ability. This is shown in the factorial design table in Figure 5.1 . The columns of the table represent cell phone use, and the rows represent time of day. The four cells of the table represent the four possible combinations or conditions: using a cell phone during the day, not using a cell phone during the day, using a cell phone at night, and not using a cell phone at night. This particular design is referred to as a 2 x 2 (read “two-by- two”) factorial design because it combines two variables, each of which has two levels. If one of the independent variables had a third level (e.g., using a hand-held cell phone, using a hands-free cell phone, and not using a cell phone), then it would be a 3 x 2 factorial design, and there would be six distinct conditions. Notice that the number of possible conditions is the product of the numbers of levels. A 2 x 2 factorial design has four conditions, a 3 x 2 factorial design has six conditions, a 4 x 5 factorial design would have 20 conditions, and so on.
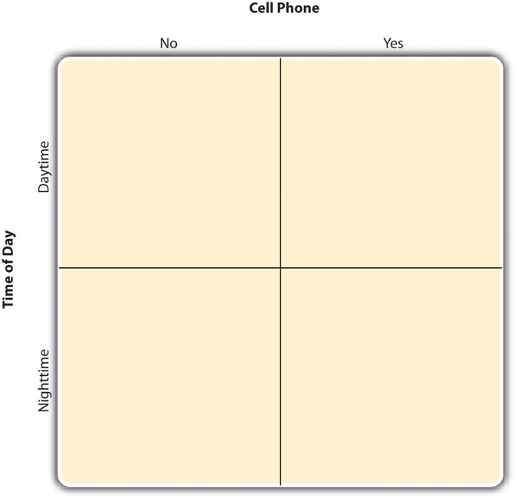
Fig. 5.1 Factorial Design Table Representing a 2 x 2 Factorial Design ¶
In principle, factorial designs can include any number of independent variables with any number of levels. For example, an experiment could include the type of psychotherapy (cognitive vs. behavioral), the length of the psychotherapy (2 weeks vs. 2 months), and the sex of the psychotherapist (female vs. male). This would be a 2 x 2 x 2 factorial design and would have eight conditions. Figure 5.2 shows one way to represent this design. In practice, it is unusual for there to be more than three independent variables with more than two or three levels each.
This is for at least two reasons: For one, the number of conditions can quickly become unmanageable. For example, adding a fourth independent variable with three levels (e.g., therapist experience: low vs. medium vs. high) to the current example would make it a 2 x 2 x 2 x 3 factorial design with 24 distinct conditions. Second, the number of participants required to populate all of these conditions (while maintaining a reasonable ability to detect a real underlying effect) can render the design unfeasible (for more information, see the discussion about the importance of adequate statistical power in Chapter 13 ). As a result, in the remainder of this section we will focus on designs with two independent variables. The general principles discussed here extend in a straightforward way to more complex factorial designs.
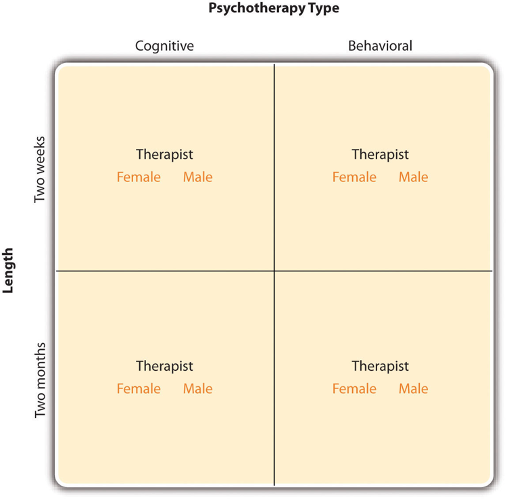
Fig. 5.2 Factorial Design Table Representing a 2 x 2 x 2 Factorial Design ¶
5.2.3. Assigning Participants to Conditions ¶
Recall that in a simple between-subjects design, each participant is tested in only one condition. In a simple within-subjects design, each participant is tested in all conditions. In a factorial experiment, the decision to take the between-subjects or within-subjects approach must be made separately for each independent variable. In a between-subjects factorial design, all of the independent variables are manipulated between subjects. For example, all participants could be tested either while using a cell phone or while not using a cell phone and either during the day or during the night. This would mean that each participant was tested in one and only one condition. In a within-subjects factorial design, all of the independent variables are manipulated within subjects. All participants could be tested both while using a cell phone and while not using a cell phone and both during the day and during the night. This would mean that each participant was tested in all conditions. The advantages and disadvantages of these two approaches are the same as those discussed in Chapter 4 ). The between-subjects design is conceptually simpler, avoids carryover effects, and minimizes the time and effort of each participant. The within-subjects design is more efficient for the researcher and help to control extraneous variables.
It is also possible to manipulate one independent variable between subjects and another within subjects. This is called a mixed factorial design. For example, a researcher might choose to treat cell phone use as a within-subjects factor by testing the same participants both while using a cell phone and while not using a cell phone (while counterbalancing the order of these two conditions). But he or she might choose to treat time of day as a between-subjects factor by testing each participant either during the day or during the night (perhaps because this only requires them to come in for testing once). Thus each participant in this mixed design would be tested in two of the four conditions.
Regardless of whether the design is between subjects, within subjects, or mixed, the actual assignment of participants to conditions or orders of conditions is typically done randomly.
5.2.4. Non-manipulated Independent Variables ¶
In many factorial designs, one of the independent variables is a non-manipulated independent variable. The researcher measures it but does not manipulate it. The study by Schnall and colleagues is a good example. One independent variable was disgust, which the researchers manipulated by testing participants in a clean room or a messy room. The other was private body consciousness, a variable which the researchers simply measured. Another example is a study by Halle Brown and colleagues in which participants were exposed to several words that they were later asked to recall [BKD+99] . The manipulated independent variable was the type of word. Some were negative, health-related words (e.g., tumor, coronary), and others were not health related (e.g., election, geometry). The non-manipulated independent variable was whether participants were high or low in hypochondriasis (excessive concern with ordinary bodily symptoms). Results from this study suggested that participants high in hypochondriasis were better than those low in hypochondriasis at recalling the health-related words, but that they were no better at recalling the non-health-related words.
Such studies are extremely common, and there are several points worth making about them. First, non-manipulated independent variables are usually participant characteristics (private body consciousness, hypochondriasis, self-esteem, and so on), and as such they are, by definition, between-subject factors. For example, people are either low in hypochondriasis or high in hypochondriasis; they cannot be in both of these conditions. Second, such studies are generally considered to be experiments as long as at least one independent variable is manipulated, regardless of how many non-manipulated independent variables are included. Third, it is important to remember that causal conclusions can only be drawn about the manipulated independent variable. For example, Schnall and her colleagues were justified in concluding that disgust affected the harshness of their participants’ moral judgments because they manipulated that variable and randomly assigned participants to the clean or messy room. But they would not have been justified in concluding that participants’ private body consciousness affected the harshness of their participants’ moral judgments because they did not manipulate that variable. It could be, for example, that having a strict moral code and a heightened awareness of one’s body are both caused by some third variable (e.g., neuroticism). Thus it is important to be aware of which variables in a study are manipulated and which are not.
5.2.5. Graphing the Results of Factorial Experiments ¶
The results of factorial experiments with two independent variables can be graphed by representing one independent variable on the x-axis and representing the other by using different kinds of bars or lines. (The y-axis is always reserved for the dependent variable.)
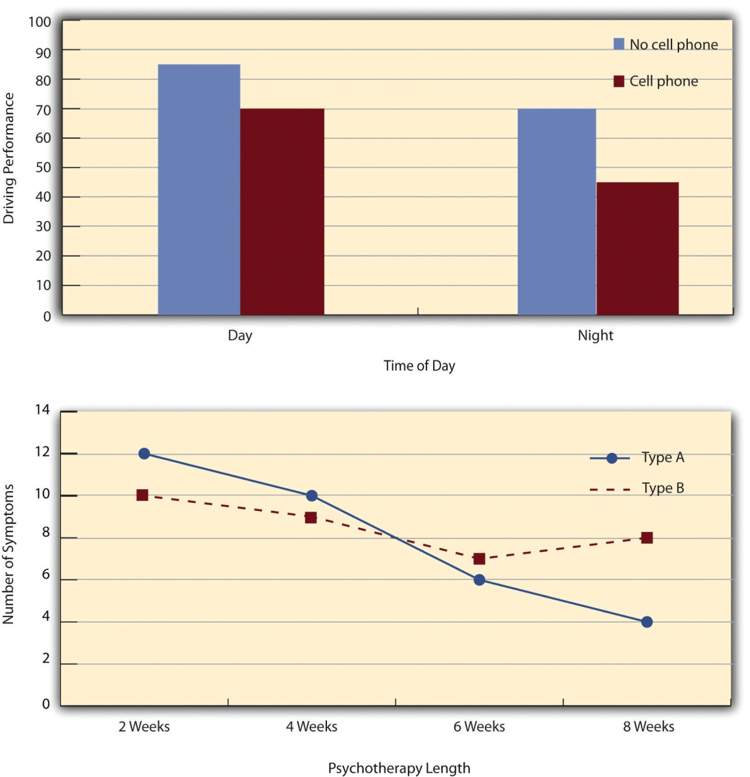
Fig. 5.3 Two ways to plot the results of a factorial experiment with two independent variables ¶
Figure 5.3 shows results for two hypothetical factorial experiments. The top panel shows the results of a 2 x 2 design. Time of day (day vs. night) is represented by different locations on the x-axis, and cell phone use (no vs. yes) is represented by different-colored bars. It would also be possible to represent cell phone use on the x-axis and time of day as different-colored bars. The choice comes down to which way seems to communicate the results most clearly. The bottom panel of Figure 5.3 shows the results of a 4 x 2 design in which one of the variables is quantitative. This variable, psychotherapy length, is represented along the x-axis, and the other variable (psychotherapy type) is represented by differently formatted lines. This is a line graph rather than a bar graph because the variable on the x-axis is quantitative with a small number of distinct levels. Line graphs are also appropriate when representing measurements made over a time interval (also referred to as time series information) on the x-axis.
5.2.6. Main Effects and Interactions ¶
In factorial designs, there are two kinds of results that are of interest: main effects and interactions. A main effect is the statistical relationship between one independent variable and a dependent variable-averaging across the levels of the other independent variable(s). Thus there is one main effect to consider for each independent variable in the study. The top panel of Figure 5.4 shows a main effect of cell phone use because driving performance was better, on average, when participants were not using cell phones than when they were. The blue bars are, on average, higher than the red bars. It also shows a main effect of time of day because driving performance was better during the day than during the night-both when participants were using cell phones and when they were not. Main effects are independent of each other in the sense that whether or not there is a main effect of one independent variable says nothing about whether or not there is a main effect of the other. The bottom panel of Figure 5.4 , for example, shows a clear main effect of psychotherapy length. The longer the psychotherapy, the better it worked.
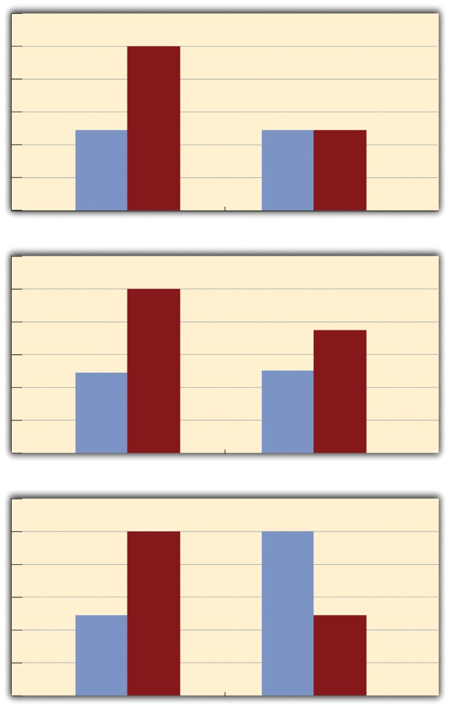
Fig. 5.4 Bar graphs showing three types of interactions. In the top panel, one independent variable has an effect at one level of the second independent variable but not at the other. In the middle panel, one independent variable has a stronger effect at one level of the second independent variable than at the other. In the bottom panel, one independent variable has the opposite effect at one level of the second independent variable than at the other. ¶
There is an interaction effect (or just “interaction”) when the effect of one independent variable depends on the level of another. Although this might seem complicated, you already have an intuitive understanding of interactions. It probably would not surprise you, for example, to hear that the effect of receiving psychotherapy is stronger among people who are highly motivated to change than among people who are not motivated to change. This is an interaction because the effect of one independent variable (whether or not one receives psychotherapy) depends on the level of another (motivation to change). Schnall and her colleagues also demonstrated an interaction because the effect of whether the room was clean or messy on participants’ moral judgments depended on whether the participants were low or high in private body consciousness. If they were high in private body consciousness, then those in the messy room made harsher judgments. If they were low in private body consciousness, then whether the room was clean or messy did not matter.
The effect of one independent variable can depend on the level of the other in several different ways. This is shown in Figure 5.5 .
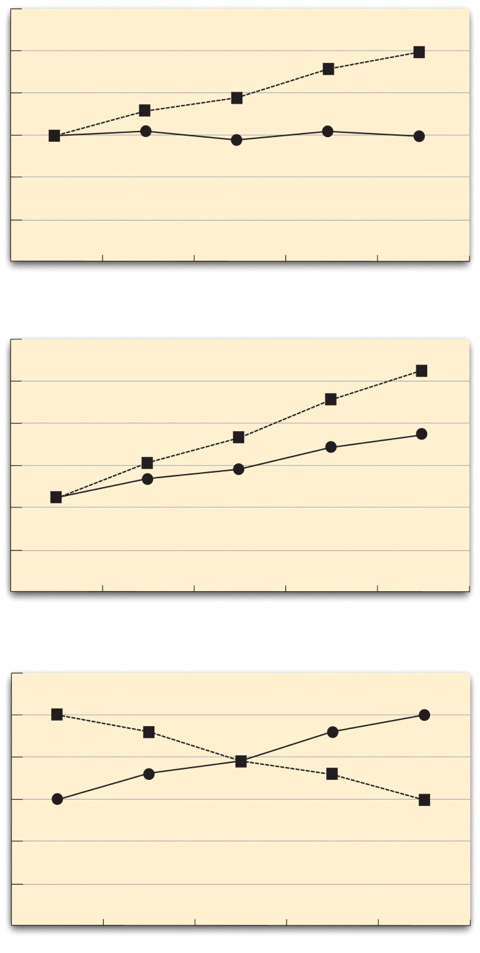
Fig. 5.5 Line Graphs Showing Three Types of Interactions. In the top panel, one independent variable has an effect at one level of the second independent variable but not at the other. In the middle panel, one independent variable has a stronger effect at one level of the second independent variable than at the other. In the bottom panel, one independent variable has the opposite effect at one level of the second independent variable than at the other. ¶
In the top panel, independent variable “B” has an effect at level 1 of independent variable “A” but no effect at level 2 of independent variable “A” (much like the study of Schnall in which there was an effect of disgust for those high in private body consciousness but not for those low in private body consciousness). In the middle panel, independent variable “B” has a stronger effect at level 1 of independent variable “A” than at level 2. This is like the hypothetical driving example where there was a stronger effect of using a cell phone at night than during the day. In the bottom panel, independent variable “B” again has an effect at both levels of independent variable “A”, but the effects are in opposite directions. This is what is called called a crossover interaction. One example of a crossover interaction comes from a study by Kathy Gilliland on the effect of caffeine on the verbal test scores of introverts and extraverts [Gil80] . Introverts perform better than extraverts when they have not ingested any caffeine. But extraverts perform better than introverts when they have ingested 4 mg of caffeine per kilogram of body weight.
In many studies, the primary research question is about an interaction. The study by Brown and her colleagues was inspired by the idea that people with hypochondriasis are especially attentive to any negative health-related information. This led to the hypothesis that people high in hypochondriasis would recall negative health-related words more accurately than people low in hypochondriasis but recall non-health-related words about the same as people low in hypochondriasis. And this is exactly what happened in this study.
5.2.7. Key Takeaways ¶
Researchers often include multiple independent variables in their experiments. The most common approach is the factorial design, in which each level of one independent variable is combined with each level of the others to create all possible conditions.
In a factorial design, the main effect of an independent variable is its overall effect averaged across all other independent variables. There is one main effect for each independent variable.
There is an interaction between two independent variables when the effect of one depends on the level of the other. Some of the most interesting research questions and results in psychology are specifically about interactions.
5.2.8. Exercises ¶
Practice: Return to the five article titles presented at the beginning of this section. For each one, identify the independent variables and the dependent variable.
Practice: Create a factorial design table for an experiment on the effects of room temperature and noise level on performance on the MCAT. Be sure to indicate whether each independent variable will be manipulated between-subjects or within-subjects and explain why.
Practice: Sketch 8 different bar graphs to depict each of the following possible results in a 2 x 2 factorial experiment:
No main effect of A; no main effect of B; no interaction
Main effect of A; no main effect of B; no interaction
No main effect of A; main effect of B; no interaction
Main effect of A; main effect of B; no interaction
Main effect of A; main effect of B; interaction
Main effect of A; no main effect of B; interaction
No main effect of A; main effect of B; interaction
No main effect of A; no main effect of B; interaction
5.3. Factorial designs: Round 2 ¶
Factorial designs require the experimenter to manipulate at least two independent variables. Consider the light-switch example from earlier. Imagine you are trying to figure out which of two light switches turns on a light. The dependent variable is the light (we measure whether it is on or off). The first independent variable is light switch #1, and it has two levels, up or down. The second independent variable is light switch #2, and it also has two levels, up or down. When there are two independent variables, each with two levels, there are four total conditions that can be tested. We can describe these four conditions in a 2x2 table.
This kind of design has a special property that makes it a factorial design. That is, the levels of each independent variable are each manipulated across the levels of the other indpendent variable. In other words, we manipulate whether switch #1 is up or down when switch #2 is up, and when switch numebr #2 is down. Another term for this property of factorial designs is “fully-crossed”.
It is possible to conduct experiments with more than independent variable that are not fully-crossed, or factorial designs. This would mean that each of the levels of one independent variable are not necessarilly manipulated for each of the levels of the other independent variables. These kinds of designs are sometimes called unbalanced designs, and they are not as common as fully-factorial designs. An example, of an unbalanced design would be the following design with only 3 conditions:
Factorial designs are often described using notation such as AXB, where A indicates the number of levels for the first independent variable, and B indicates the number of levels for the second independent variable. The fully-crossed version of the 2-light switch experiment would be called a 2x2 factorial design. This notation is convenient because by multiplying the numbers in the equation we can find the number of conditions in the design. For example 2x2 = 4 conditions.
More complicated factorial designs have more indepdent variables and more levels. We use the same notation describe these designs. Each number represents the number of levels for one of the independent variables, and the number of numbers represents the number of variables. So, a 2x2x2 design has three independent variables, and each one has 2 levels, for a total of 2x2x2=6 conditions. A 3x3 design has two independent variables, each with three levels, for a total of 9 conditions. Designs can get very complicated, such as a 5x3x6x2x7 experiment, with five independent variables, each with differing numbers of levels, for a total of 1260 conditions. If you are considering a complicated design like that one, you might want to consider how to simplify it.
5.3.1. 2x2 Factorial designs ¶
For simplicity, we will focus mainly on 2x2 factorial designs. As with simple designs with only one independent variable, factorial designs have the same basic empirical question. Did manipulation of the independent variables cause changes in the dependent variables? However, 2x2 designs have more than one manipulation, so there is more than one way that the dependent variable can change. So, we end up asking the basic empirical question more than once.
More specifically, the analysis of factorial designs is split into two parts: main effects and interactions. Main effects occur when the manipulation of one independent variable cause a change in the dependent variable. In a 2x2 design, there are two independent variables, so there are two possible main effects: the main effect of independent variable 1, and the main effect of independent variable 2. An interaction occurs when the effect of one independent variable depends on the levels of the other independent variable. My experience in teaching the concept of main effects and interactions is that they are confusing. So, I expect that these definitions will not be very helpful, and although they are clear and precise, they only become helpful as definitions after you understand the concepts…so they are not useful for explaining the concepts. To explain the concepts we will go through several different kinds of examples.
To briefly add to the confusion, or perhaps to illustrate why these two concepts can be confusing, we will look at the eight possible outcomes that could occur in a 2x2 factorial experiment.
In the table, a yes means that there was statistically significant difference for one of the main effects or interaction, and a no means that there was not a statisically significant difference. As you can see, just by adding one more independent variable, the number of possible outcomes quickly become more complicated. When you conduct a 2x2 design, the task for analysis is to determine which of the 8 possibilites occured, and then explain the patterns for each of the effects that occurred. That’s a lot of explaining to do.
5.3.2. Main effects ¶
Main effects occur when the levels of an independent variable cause change in the measurement or dependent variable. There is one possible main effect for each independent variable in the design. When we find that independent variable did influence the dependent variable, then we say there was a main effect. When we find that the independent variable did not influence the dependent variable, then we say there was no main effect.
The simplest way to understand a main effect is to pretend that the other independent variables do not exist. If you do this, then you simply have a single-factor design, and you are asking whether that single factor caused change in the measurement. For a 2x2 experiment, you do this twice, once for each independent variable.
Let’s consider a silly example to illustrate an important property of main effects. In this experiment the dependent variable will be height in inches. The independent variables will be shoes and hats. The shoes independent variable will have two levels: wearing shoes vs. no shoes. The hats independent variable will have two levels: wearing a hat vs. not wearing a hat. The experimenter will provide the shoes and hats. The shoes add 1 inch to a person’s height, and the hats add 6 inches to a person’s height. Further imagine that we conduct a within-subjects design, so we measure each person’s height in each of the fours conditions. Before we look at some example data, the findings from this experiment should be pretty obvious. People will be 1 inch taller when they wear shoes, and 6 inches taller when they where a hat. We see this in the example data from 10 subjects presented below:
The mean heights in each condition are:
To find the main effect of the shoes manipulation we want to find the mean height in the no shoes condition, and compare it to the mean height of the shoes condition. To do this, we collapse , or average over the observations in the hat conditions. For example, looking only at the no shoes vs. shoes conditions we see the following averages for each subject.
The group means are:
As expected, we see that the average height is 1 inch taller when subjects wear shoes vs. do not wear shoes. So, the main effect of wearing shoes is to add 1 inch to a person’s height.
We can do the very same thing to find the main effect of hats. Except in this case, we find the average heights in the no hat vs. hat conditions by averaging over the shoe variable.
As expected, we the average height is 6 inches taller when the subjects wear a hat vs. do not wear a hat. So, the main effect of wearing hats is to add 1 inch to a person’s height.
Instead of using tables to show the data, let’s use some bar graphs. First, we will plot the average heights in all four conditions.
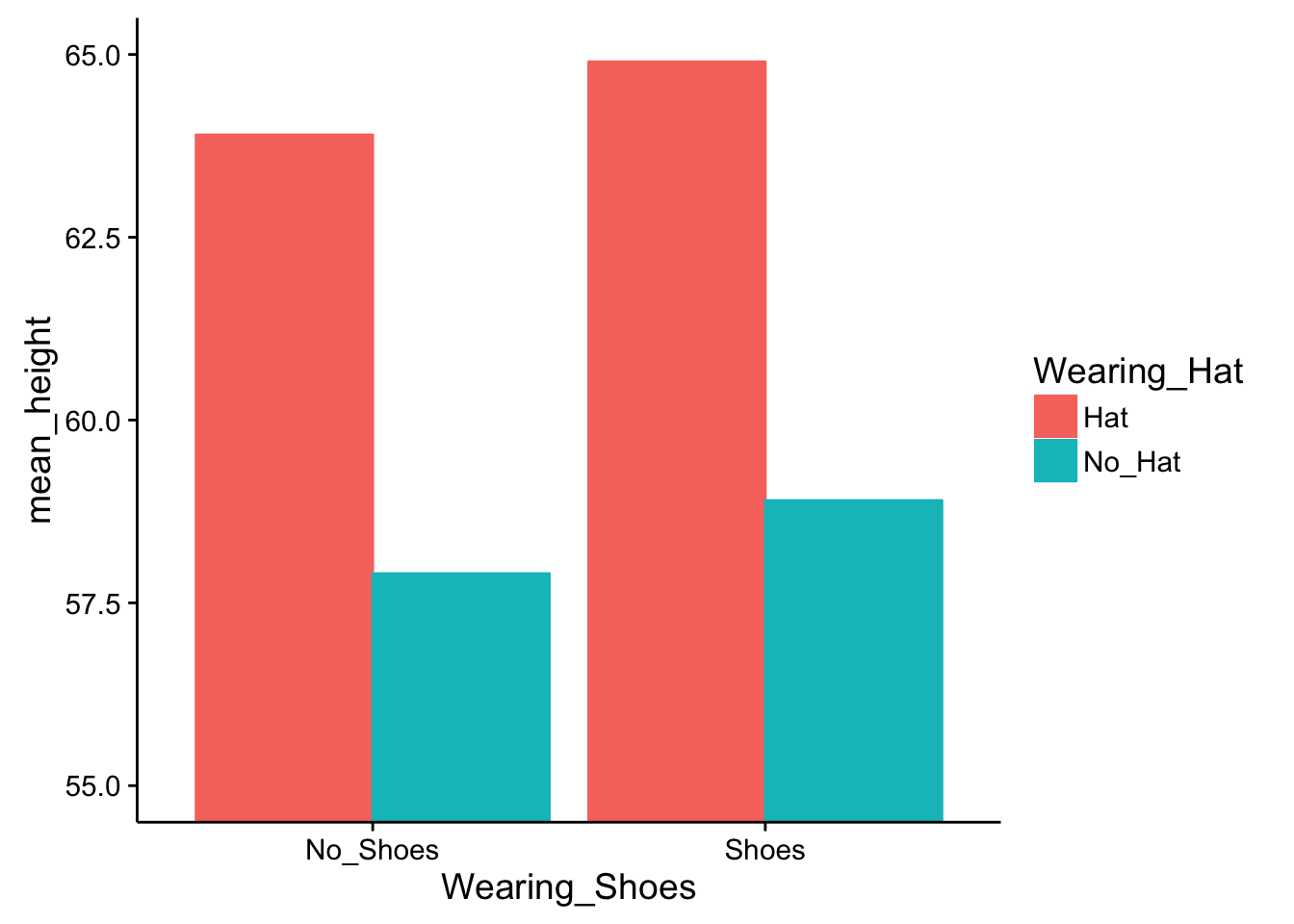
Fig. 5.6 Means from our experiment involving hats and shoes. ¶
Some questions to ask yourself are 1) can you identify the main effect of wearing shoes in the figure, and 2) can you identify the main effet of wearing hats in the figure. Both of these main effects can be seen in the figure, but they aren’t fully clear. You have to do some visual averaging.
Perhaps the most clear is the main effect of wearing a hat. The red bars show the conditions where people wear hats, and the green bars show the conditions where people do not wear hats. For both levels of the wearing shoes variable, the red bars are higher than the green bars. That is easy enough to see. More specifically, in both cases, wearing a hat adds exactly 6 inches to the height, no more no less.
Less clear is the main effect of wearing shoes. This is less clear because the effect is smaller so it is harder to see. How to find it? You can look at the red bars first and see that the red bar for no-shoes is slightly smaller than the red bar for shoes. The same is true for the green bars. The green bar for no-shoes is slightly smaller than the green bar for shoes.
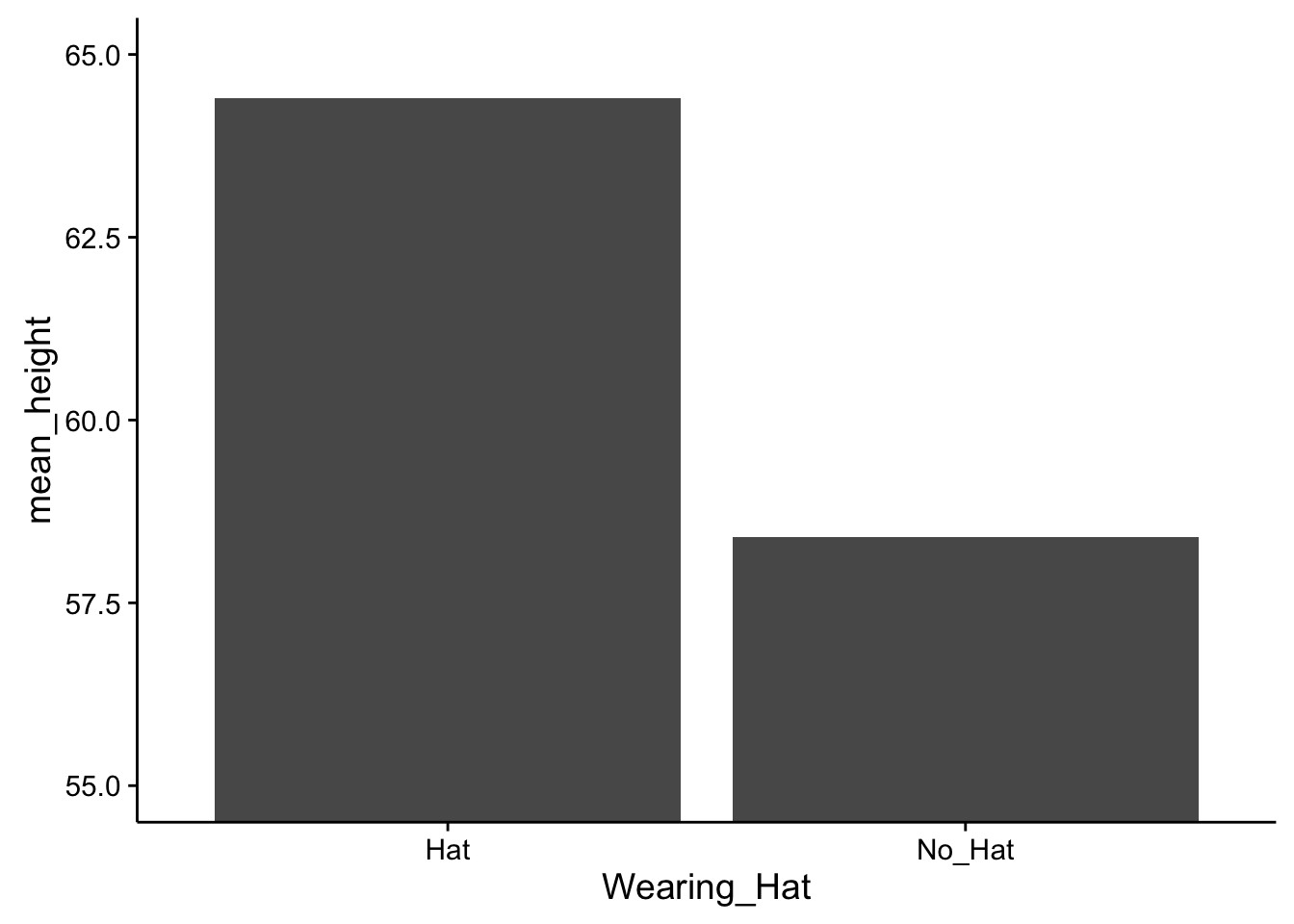
Fig. 5.7 Means of our Hat and No-Hat conditions (averaging over the shoe condition). ¶
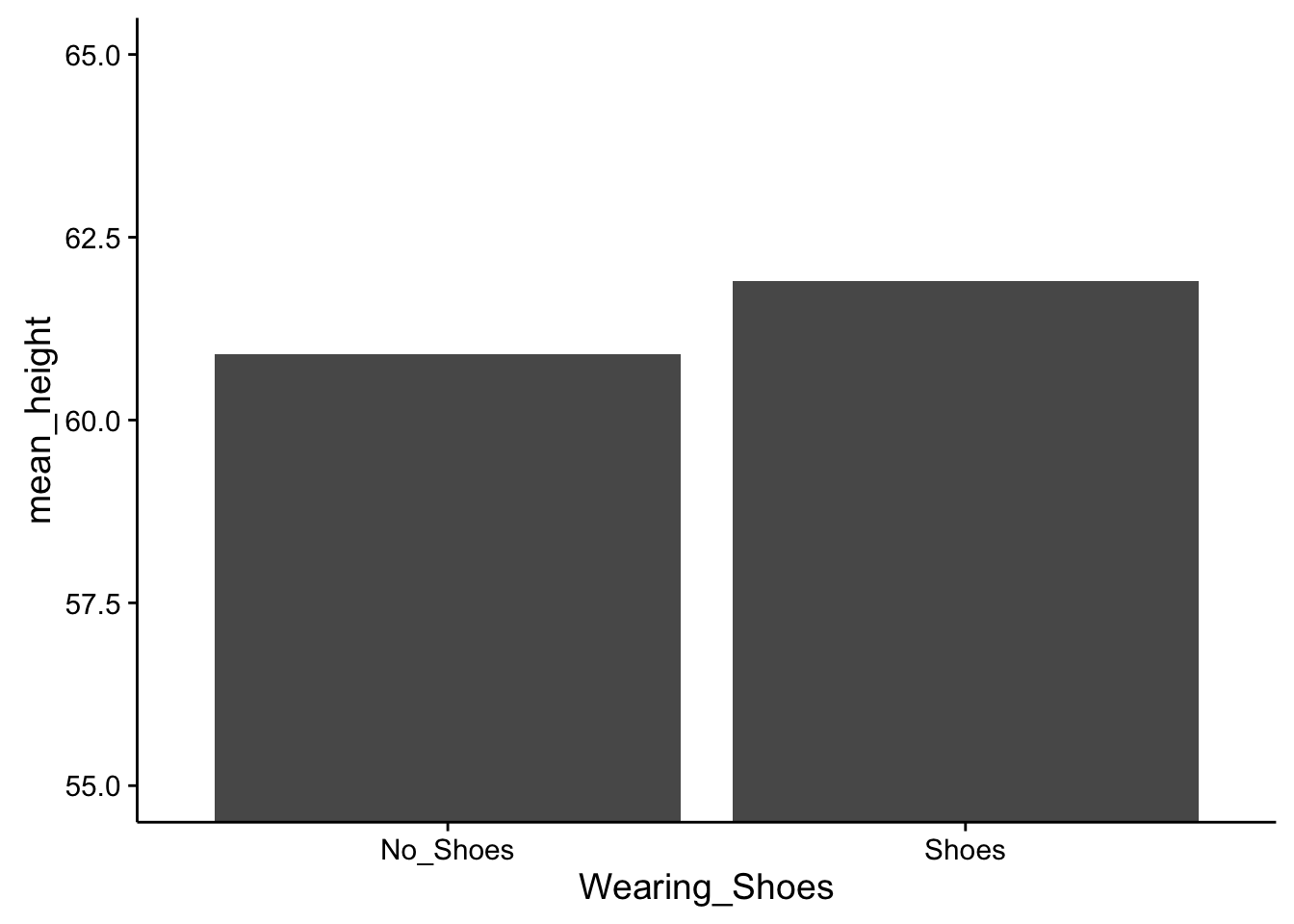
Fig. 5.8 Means of our Shoe and No-Shoe conditions (averaging over the hat condition). ¶
Data from 2x2 designs is often present in graphs like the one above. An advantage of these graphs is that they display means in all four conditions of the design. However, they do not clearly show the two main effects. Someone looking at this graph alone would have to guesstimate the main effects. Or, in addition to the main effects, a researcher could present two more graphs, one for each main effect (however, in practice this is not commonly done because it takes up space in a journal article, and with practice it becomes second nature to “see” the presence or absence of main effects in graphs showing all of the conditions). If we made a separate graph for the main effect of shoes we should see a difference of 1 inch between conditions. Similarly, if we made a separate graph for the main effect of hats then we should see a difference of 6 between conditions. Examples of both of those graphs appear in the margin.
Why have we been talking about shoes and hats? These independent variables are good examples of variables that are truly independent from one another. Neither one influences the other. For example, shoes with a 1 inch sole will always add 1 inch to a person’s height. This will be true no matter whether they wear a hat or not, and no matter how tall the hat is. In other words, the effect of wearing a shoe does not depend on wearing a hat. More formally, this means that the shoe and hat independent variables do not interact. It would be very strange if they did interact. It would mean that the effect of wearing a shoe on height would depend on wearing a hat. This does not happen in our universe. But in some other imaginary universe, it could mean, for example, that wearing a shoe adds 1 to your height when you do not wear a hat, but adds more than 1 inch (or less than 1 inch) when you do wear a hat. This thought experiment will be our entry point into discussing interactions. A take-home message before we begin is that some independent variables (like shoes and hats) do not interact; however, there are many other independent variables that do.
5.3.3. Interactions ¶
Interactions occur when the effect of an independent variable depends on the levels of the other independent variable. As we discussed above, some independent variables are independent from one another and will not produce interactions. However, other combinations of independent variables are not independent from one another and they produce interactions. Remember, independent variables are always manipulated independently from the measured variable (see margin note), but they are not necessarilly independent from each other.
Independence
These ideas can be confusing if you think that the word “independent” refers to the relationship between independent variables. However, the term “independent variable” refers to the relationship between the manipulated variable and the measured variable. Remember, “independent variables” are manipulated independently from the measured variable. Specifically, the levels of any independent variable do not change because we take measurements. Instead, the experimenter changes the levels of the independent variable and then observes possible changes in the measures.
There are many simple examples of two independent variables being dependent on one another to produce an outcome. Consider driving a car. The dependent variable (outcome that is measured) could be how far the car can drive in 1 minute. Independent variable 1 could be gas (has gas vs. no gas). Independent variable 2 could be keys (has keys vs. no keys). This is a 2x2 design, with four conditions.
Importantly, the effect of the gas variable on driving depends on the levels of having a key. Or, to state it in reverse, the effect of the key variable on driving depends on the levesl of the gas variable. Finally, in plain english. You need the keys and gas to drive. Otherwise, there is no driving.
5.3.4. What makes a people hangry? ¶
To continue with more examples, let’s consider an imaginary experiment examining what makes people hangry. You may have been hangry before. It’s when you become highly irritated and angry because you are very hungry…hangry. I will propose an experiment to measure conditions that are required to produce hangriness. The pretend experiment will measure hangriness (we ask people how hangry they are on a scale from 1-10, with 10 being most hangry, and 0 being not hangry at all). The first independent variable will be time since last meal (1 hour vs. 5 hours), and the second independent variable will be how tired someone is (not tired vs very tired). I imagine the data could look something the following bar graph.
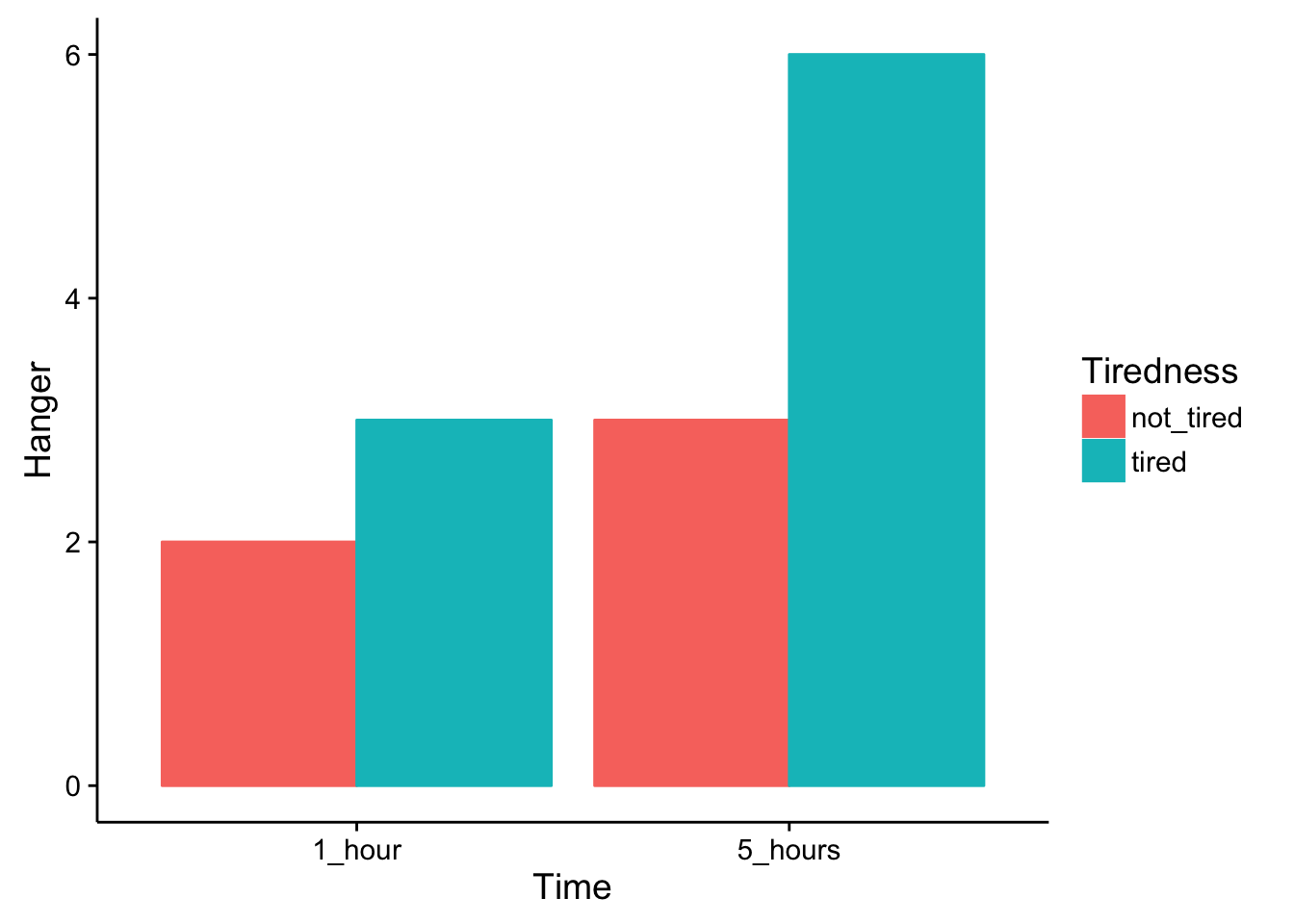
Fig. 5.9 Means from our study of hangriness. ¶
The graph shows clear evidence of two main effects, and an interaction . There is a main effect of time since last meal. Both the bars in the 1 hour conditions have smaller hanger ratings than both of the bars in the 5 hour conditions. There is a main effect of being tired. Both of the bars in the “not tired” conditions are smaller than than both of the bars in the “tired” conditions. What about the interaction?
Remember, an interaction occurs when the effect of one independent variable depends on the level of the other independent variable. We can look at this two ways, and either way shows the presence of the very same interaction. First, does the effect of being tired depend on the levels of the time since last meal? Yes. Look first at the effect of being tired only for the “1 hour condition”. We see the red bar (tired) is 1 unit lower than the green bar (not tired). So, there is an effect of 1 unit of being tired in the 1 hour condition. Next, look at the effect of being tired only for the “5 hour” condition. We see the red bar (tired) is 3 units lower than the green bar (not tired). So, there is an effect of 3 units for being tired in the 5 hour condition. Clearly, the size of the effect for being tired depends on the levels of the time since last meal variable. We call this an interaction.
The second way of looking at the interaction is to start by looking at the other variable. For example, does the effect of time since last meal depend on the levels of the tired variable? The answer again is yes. Look first at the effect of time since last meal only for the red bars in the “not tired” condition. The red bar in the 1 hour condition is 1 unit smaller than the red bar in the 5 hour condition. Next, look at the effect of time since last meal only for the green bars in the “tired” condition. The green bar in the 1 hour condition is 3 units smaller than the green bar in the 5 hour condition. Again, the size of the effect of time since last meal depends on the levels of the tired variable.No matter which way you look at the interaction, we get the same numbers for the size of the interaction effect, which is 2 units (i.e., the difference between 3 and 1). The interaction suggests that something special happens when people are tired and haven’t eaten in 5 hours. In this condition, they can become very hangry. Whereas, in the other conditions, there are only small increases in being hangry.
5.3.5. Identifying main effects and interactions ¶
Research findings are often presented to readers using graphs or tables. For example, the very same pattern of data can be displayed in a bar graph, line graph, or table of means. These different formats can make the data look different, even though the pattern in the data is the same. An important skill to develop is the ability to identify the patterns in the data, regardless of the format they are presented in. Some examples of bar and line graphs are presented in the margin, and two example tables are presented below. Each format displays the same pattern of data.
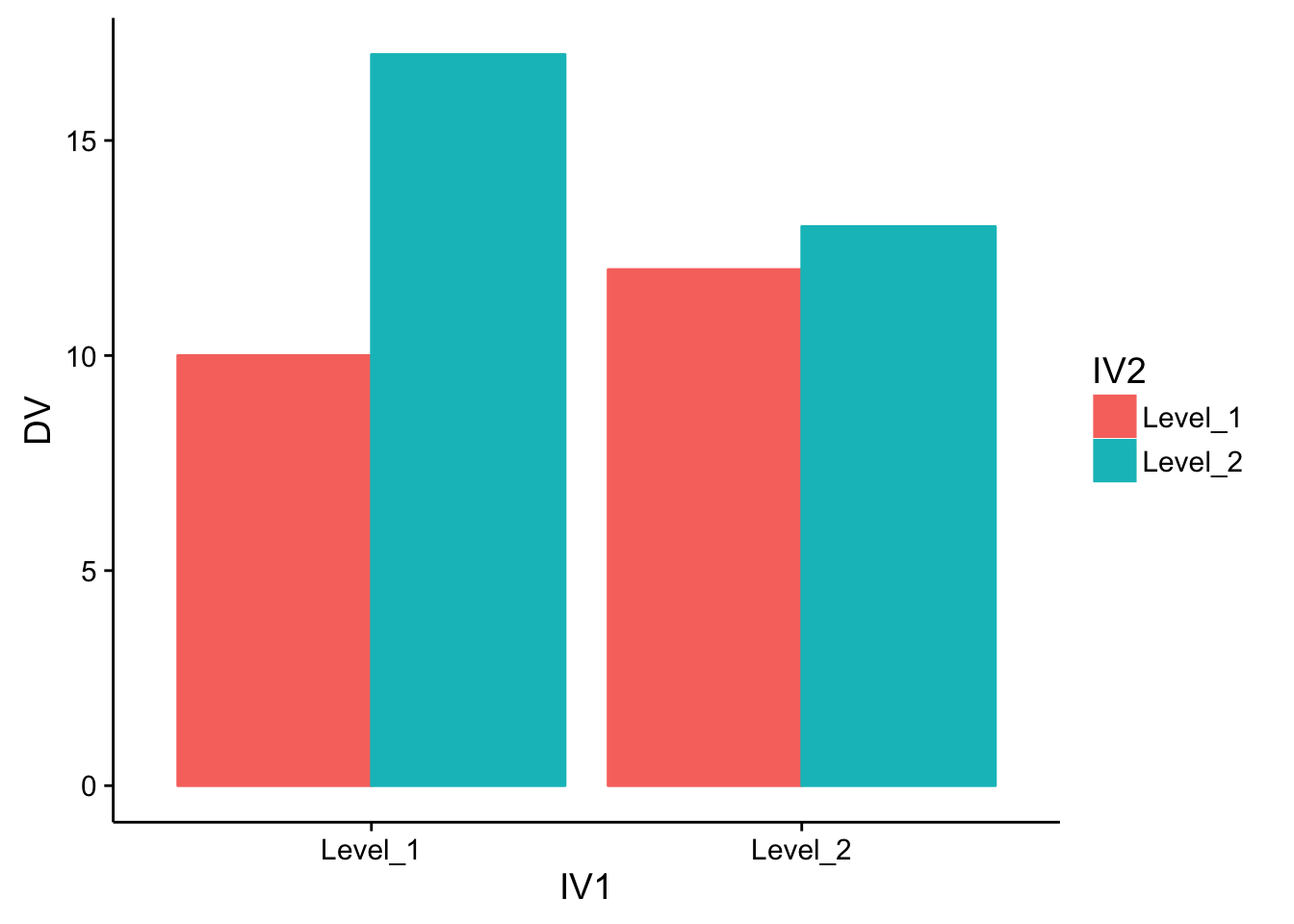
Fig. 5.10 Data from a 2x2 factorial design summarized in a bar plot. ¶
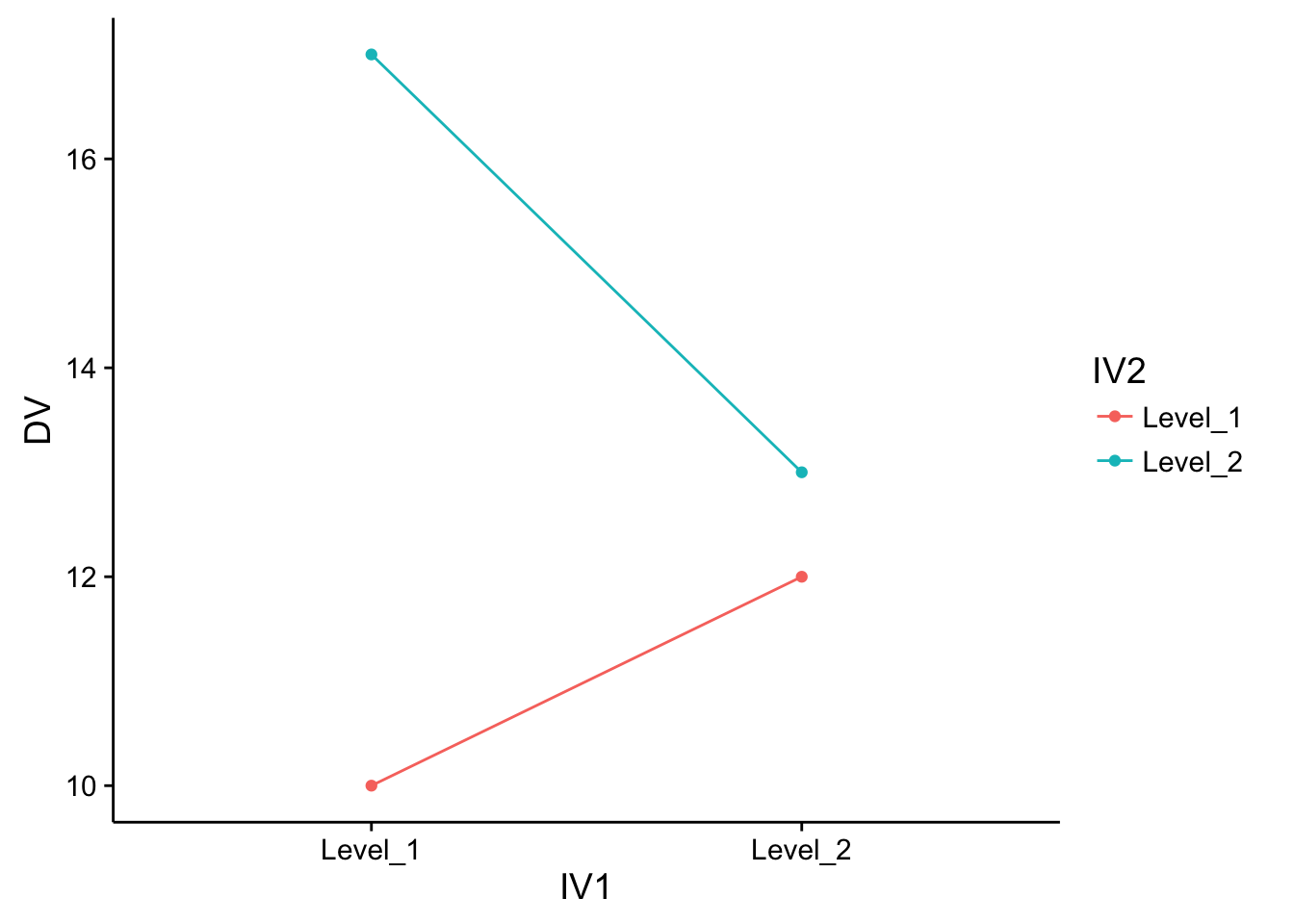
Fig. 5.11 The same data from above, but instead summarized in a line plot. ¶
After you become comfortable with interpreting data in these different formats, you should be able to quickly identify the pattern of main effects and interactions. For example, you would be able to notice that all of these graphs and tables show evidence for two main effects and one interaction.
As an exercise toward this goal, we will first take a closer look at extracting main effects and interactions from tables. This exercise will how the condition means are used to calculate the main effects and interactions. Consider the table of condition means below.
5.3.6. Main effects ¶
Main effects are the differences between the means of single independent variable. Notice, this table only shows the condition means for each level of all independent variables. So, the means for each IV must be calculated. The main effect for IV1 is the comparison between level A and level B, which involves calculating the two column means. The mean for IV1 Level A is (4+3)/2 = 3.5. The mean for IV1 Level B is (5+8)/2 = 6.5. So the main effect is 3 (6.5 - 3.5). The main effect for IV2 is the comparison between level 1 and level 2, which involves calculating the two row means. The mean for IV2 Level 1 is (4+5)/2 = 4.5. The mean for IV2 Level 2 is (3+8)/2 = 5.5. So the main effect is 1 (5.5 - 4.5). The process of computing the average for each level of a single independent variable, always involves collapsing, or averaging over, all of the other conditions from other variables that also occured in that condition
5.3.7. Interactions ¶
Interactions ask whether the effect of one independent variable depends on the levels of the other independent variables. This question is answered by computing difference scores between the condition means. For example, we look the effect of IV1 (A vs. B) for both levels of of IV2. Focus first on the condition means in the first row for IV2 level 1. We see that A=4 and B=5, so the effect IV1 here was 5-4 = 1. Next, look at the condition in the second row for IV2 level 2. We see that A=3 and B=8, so the effect of IV1 here was 8-3 = 5. We have just calculated two differences (5-4=1, and 8-3=5). These difference scores show that the size of the IV1 effect was different across the levels of IV2. To calculate the interaction effect we simply find the difference between the difference scores, 5-1=4. In general, if the difference between the difference scores is different, then there is an interaction effect.
5.3.8. Example bar graphs ¶
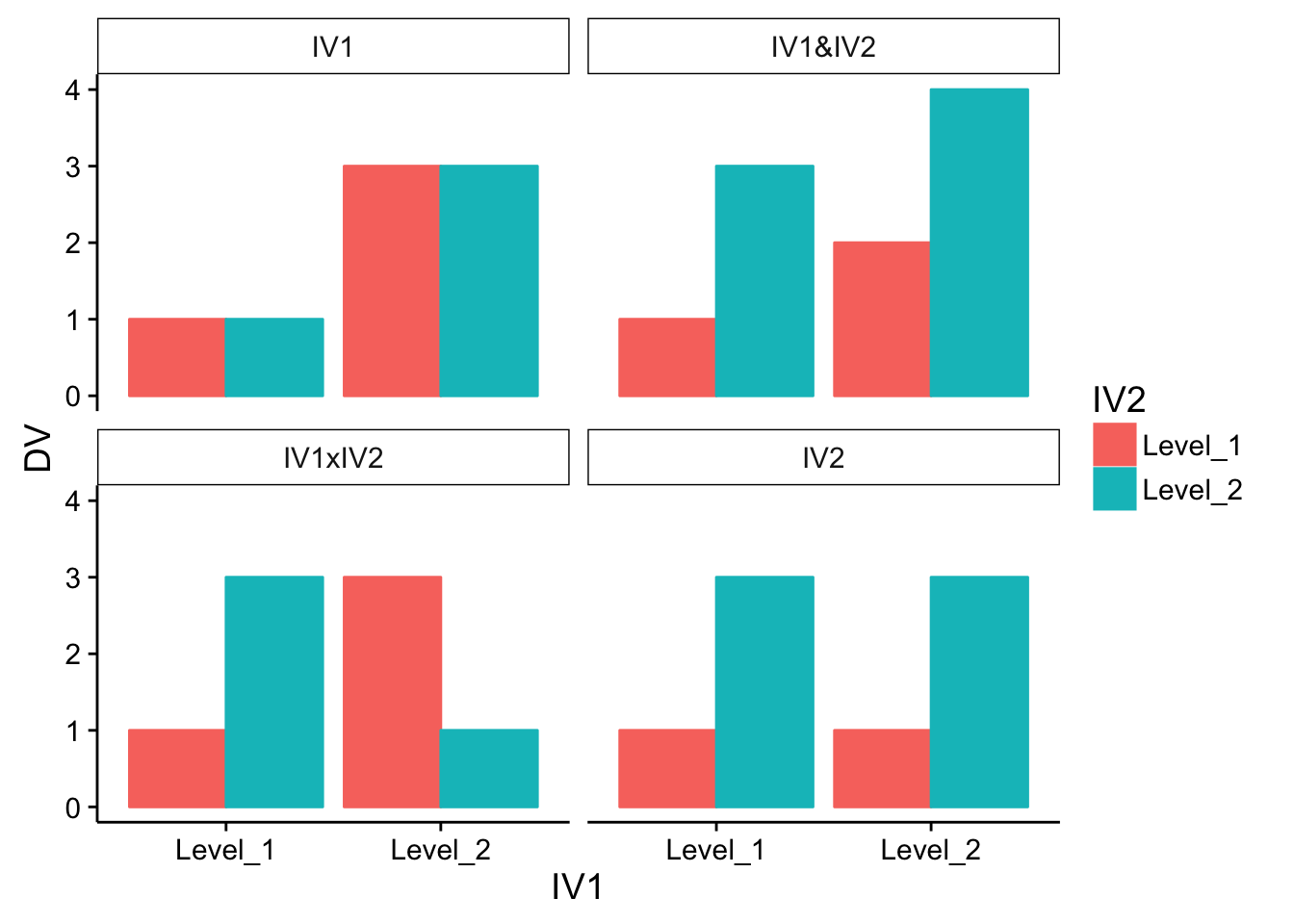
Fig. 5.12 Four patterns that could be observed in a 2x2 factorial design. ¶
The IV1 shows a main effect only for IV1 (both red and green bars are lower for level 1 than level 2). The IV1&IV2 graphs shows main effects for both variables. The two bars on the left are both lower than the two on the right, and the red bars are both lower than the green bars. The IV1xIV2 graph shows an example of a classic cross-over interaction. Here, there are no main effects, just an interaction. There is a difference of 2 between the green and red bar for Level 1 of IV1, and a difference of -2 for Level 2 of IV1. That makes the differences between the differences = 4. Why are their no main effects? Well the average of the red bars would equal the average of the green bars, so there is no main effect for IV2. And, the average of the red and green bars for level 1 of IV1 would equal the average of the red and green bars for level 2 of IV1, so there is no main effect. The bar graph for IV2 shows only a main effect for IV2, as the red bars are both lower than the green bars.
5.3.9. Example line graphs ¶
You may find that the patterns of main effects and interaction looks different depending on the visual format of the graph. The exact same patterns of data plotted up in bar graph format, are plotted as line graphs for your viewing pleasure. Note that for the IV1 graph, the red line does not appear because it is hidden behind the green line (the points for both numbers are identical).
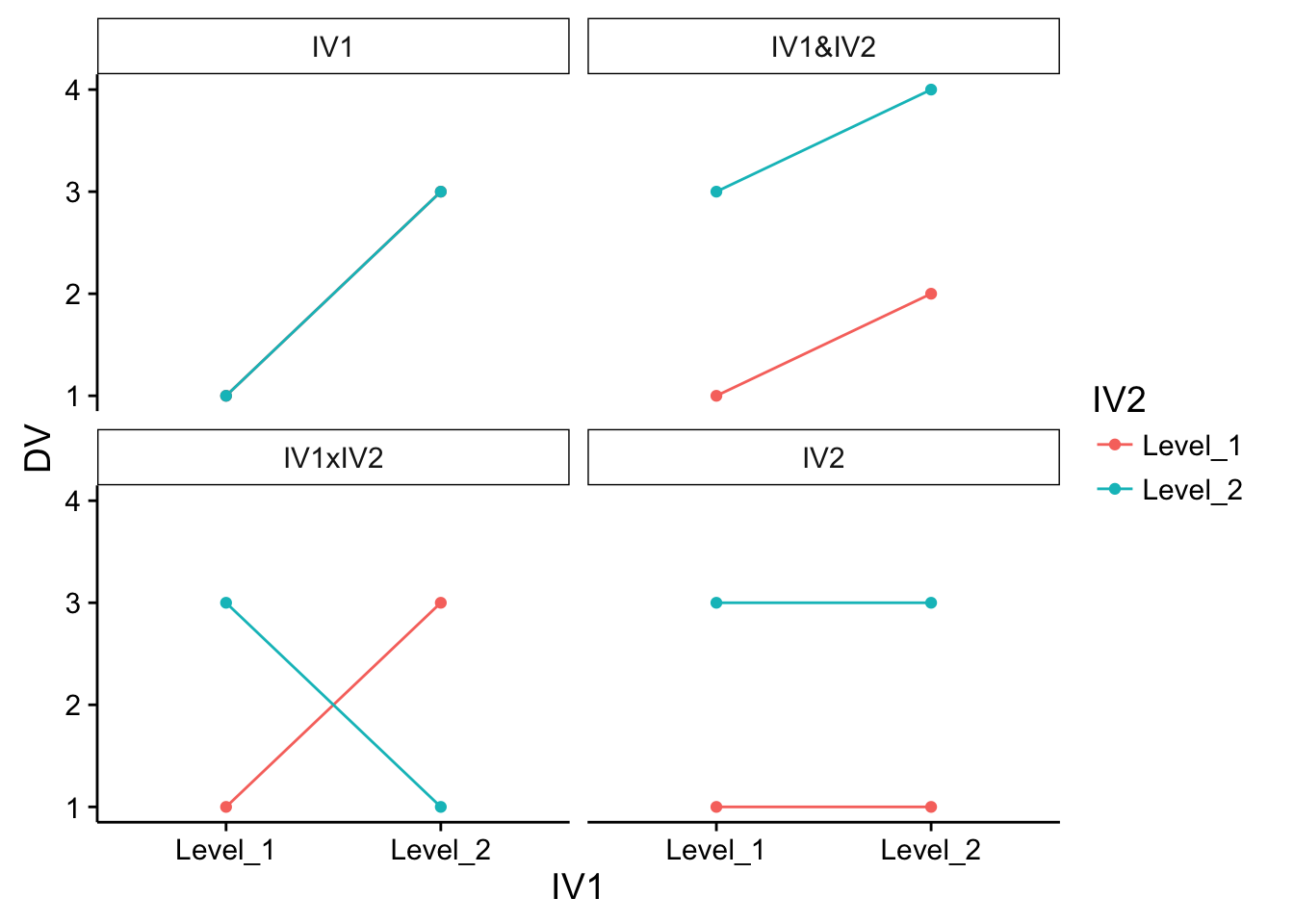
Fig. 5.13 Four patterns that could be observed in a 2x2 factorial design, now depicted using line plots. ¶
5.3.10. Interpreting main effects and interactions ¶
The presence of an interaction, particularly a strong interaction, can sometimes make it challenging to interpet main effects. For example, take a look at Figure 5.14 , which indicates a very strong interaction.
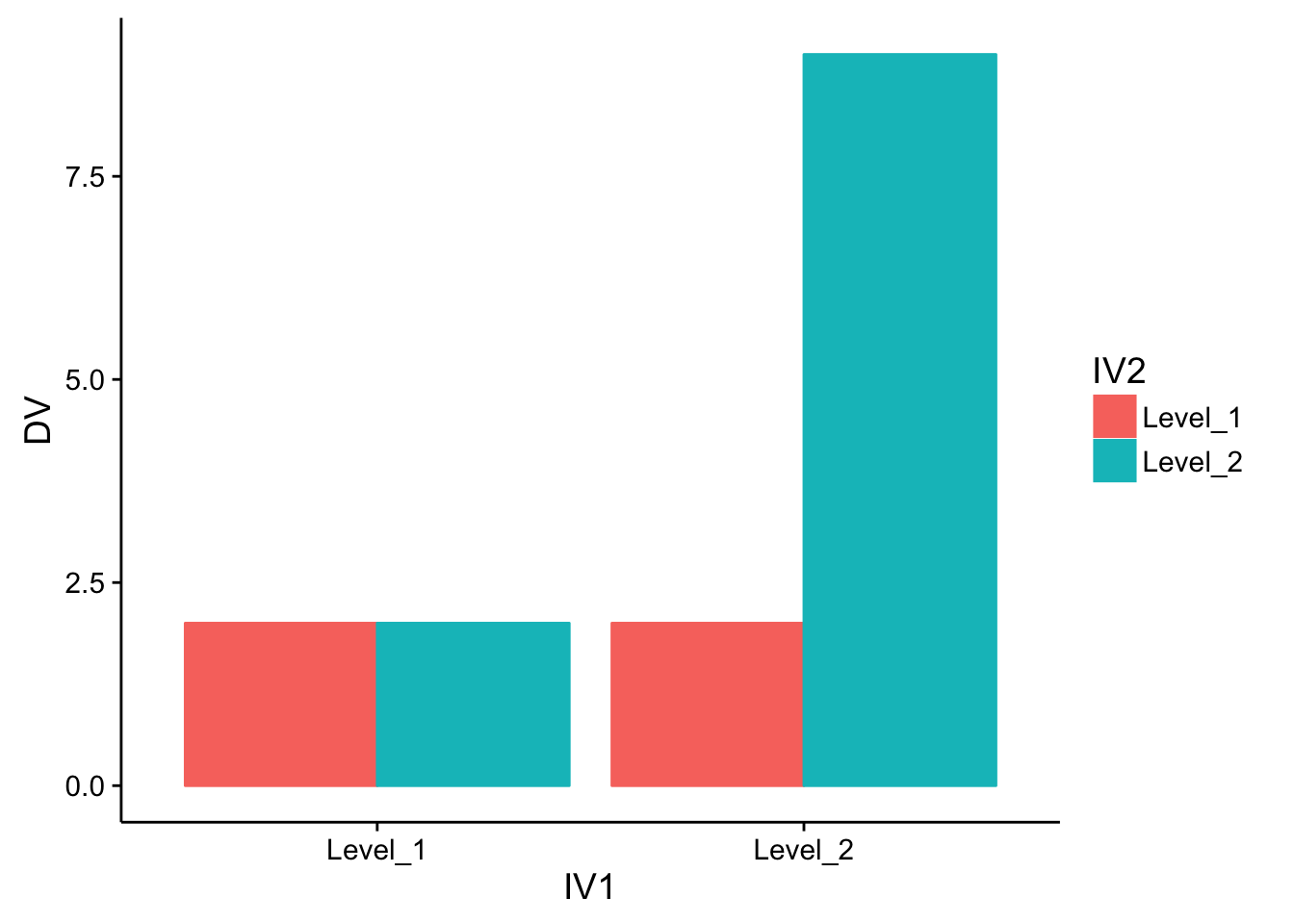
Fig. 5.14 A clear interaction effect. But what about the main effects? ¶
In Figure 5.14 , IV2 has no effect under level 1 of IV1 (e.g., the red and green bars are the same). IV2 has a large effect under level 2 of IV2 (the red bar is 2 and the green bar is 9). So, the interaction effect is a total of 7. Are there any main effects? Yes there are. Consider the main effect for IV1. The mean for level 1 is (2+2)/2 = 2, and the mean for level 2 is (2+9)/2 = 5.5. There is a difference between the means of 3.5, which is consistent with a main effect. Consider, the main effect for IV2. The mean for level 1 is again (2+2)/2 = 2, and the mean for level 2 is again (2+9)/2 = 5.5. Again, there is a difference between the means of 3.5, which is consistent with a main effect. However, it may seem somewhat misleading to say that our manipulation of IV1 influenced the DV. Why? Well, it only seemed to have have this influence half the time. The same is true for our manipulation of IV2. For this reason, we often say that the presence of interactions qualifies our main effects. In other words, there are two main effects here, but they must be interpreting knowing that we also have an interaction.
The example in Figure 5.15 shows a case in which it is probably a bit more straightforward to interpret both the main effects and the interaction.
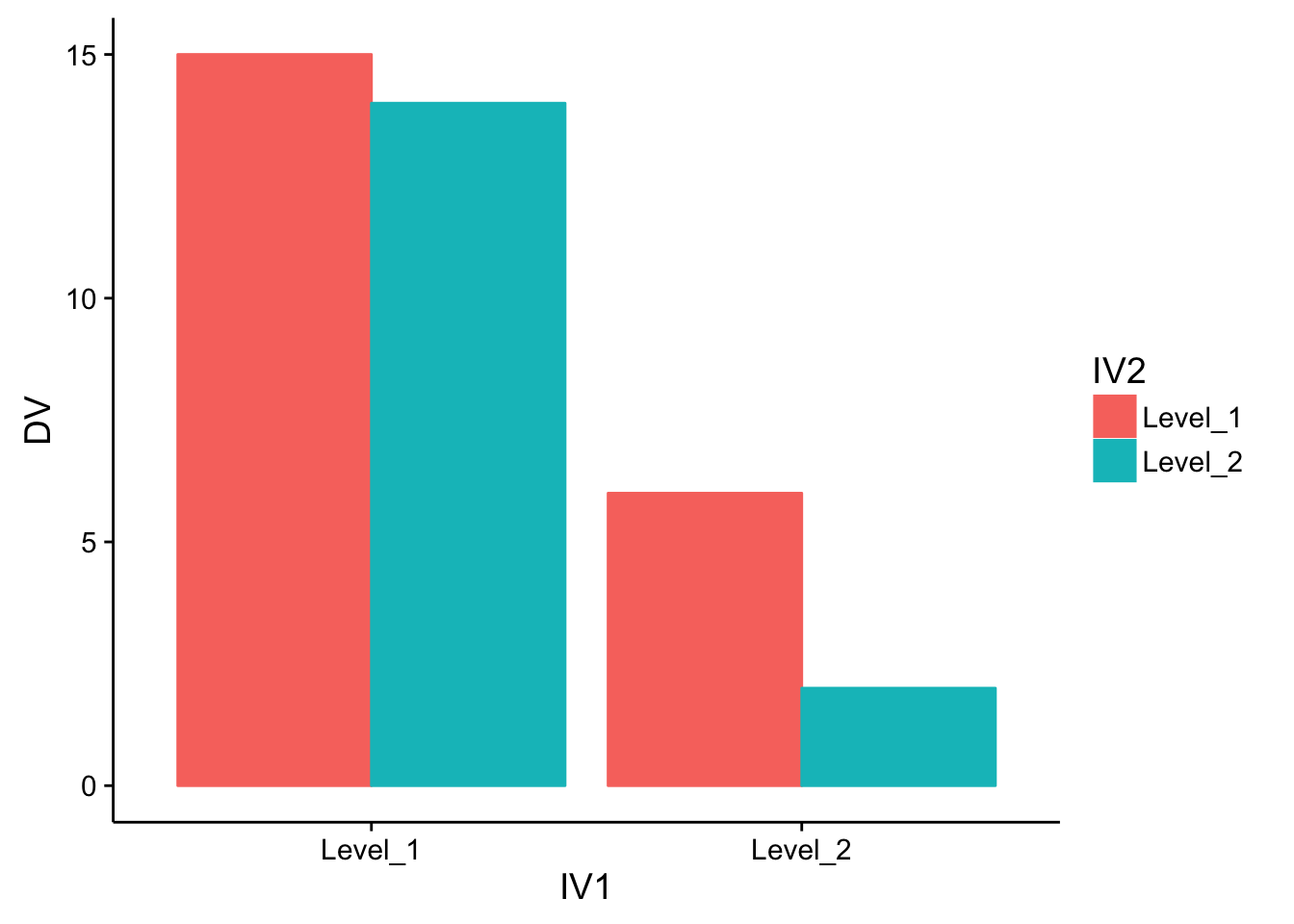
Fig. 5.15 Perhaps the main effects are more straightforward to interpret in this example. ¶
Can you spot the interaction right away? The difference between red and green bars is small for level 1 of IV1, but large for level 2. The differences between the differences are different, so there is an interaction. But, we also see clear evidence of two main effects. For example, both the red and green bars for IV1 level 1 are higher than IV1 Level 2. And, both of the red bars (IV2 level 1) are higher than the green bars (IV2 level 2).
5.4. Complex Correlational Designs ¶
5.5. learning objectives ¶.
Explain why researchers use complex correlational designs.
Create and interpret a correlation matrix.
Describe how researchers can use correlational research to explore causal relationships among variables—including the limits of this approach.
As we have already seen, researchers conduct correlational studies rather than experiments when they are interested in noncausal relationships or when they are interested variables that cannot be manipulated for practical or ethical reasons. In this section, we look at some approaches to complex correlational research that involve measuring several variables and assessing the relationships among them.
5.5.1. Correlational Studies With Factorial Designs ¶
We have already seen that factorial experiments can include manipulated independent variables or a combination of manipulated and non-manipulated independent variables. But factorial designs can also consist exclusively of non-manipulated independent variables, in which case they are no longer experiments but correlational studies. Consider a hypothetical study in which a researcher measures two variables. First, the researcher measures participants’ mood and self-esteem. The research then also measure participants’ willingness to have unprotected sexual intercourse. This study can be conceptualized as a 2 x 2 factorial design with mood (positive vs. negative) and self-esteem (high vs. low) as between-subjects factors. Willingness to have unprotected sex is the dependent variable. This design can be represented in a factorial design table and the results in a bar graph of the sort we have already seen. The researcher would consider the main effect of sex, the main effect of self-esteem, and the interaction between these two independent variables.
Again, because neither independent variable in this example was manipulated, it is a correlational study rather than an experiment (the study by MacDonald and Martineau [MM02] was similar, but was an experiment because they manipulated their participants’ moods). This is important because, as always, one must be cautious about inferring causality from correlational studies because of the directionality and third-variable problems. For example, a main effect of participants’ moods on their willingness to have unprotected sex might be caused by any other variable that happens to be correlated with their moods.
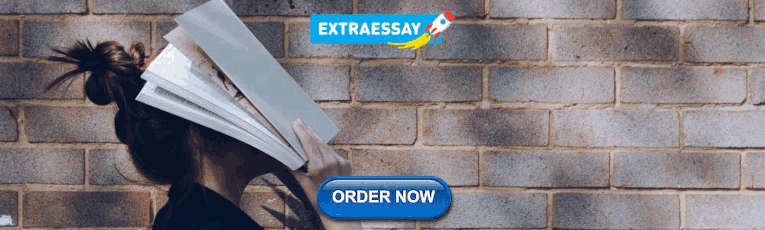
5.5.2. Assessing Relationships Among Multiple Variables ¶
Most complex correlational research, however, does not fit neatly into a factorial design. Instead, it involves measuring several variables, often both categorical and quantitative, and then assessing the statistical relationships among them. For example, researchers Nathan Radcliffe and William Klein studied a sample of middle-aged adults to see how their level of optimism (measured by using a short questionnaire called the Life Orientation Test) was related to several other heart-health-related variables [RK02] . These included health, knowledge of heart attack risk factors, and beliefs about their own risk of having a heart attack. They found that more optimistic participants were healthier (e.g., they exercised more and had lower blood pressure), knew about heart attack risk factors, and correctly believed their own risk to be lower than that of their peers.
This approach is often used to assess the validity of new psychological measures. For example, when John Cacioppo and Richard Petty created their Need for Cognition Scale, a measure of the extent to which people like to think and value thinking, they used it to measure the need for cognition for a large sample of college students along with three other variables: intelligence, socially desirable responding (the tendency to give what one thinks is the “appropriate” response), and dogmatism [CP82] . The results of this study are summarized in Figure 5.16 , which is a correlation matrix showing the correlation (Pearson’s \(r\) ) between every possible pair of variables in the study.
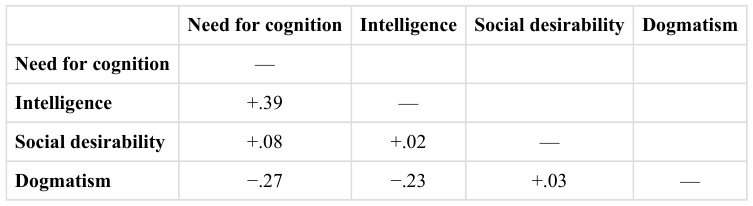
Fig. 5.16 Correlation matrix showing correlations among need for cognition and three other variables based on research by Cacioppo and Petty (1982). Only half the matrix is filled in because the other half would contain exactly the same information. Also, because the correlation between a variable and itself is always \(r=1.0\) , these values are replaced with dashes throughout the matrix. ¶
For example, the correlation between the need for cognition and intelligence was \(r=.39\) , the correlation between intelligence and socially desirable responding was \(r=.02\) , and so on. In this case, the overall pattern of correlations was consistent with the researchers’ ideas about how scores on the need for cognition should be related to these other constructs.
When researchers study relationships among a large number of conceptually similar variables, they often use a complex statistical technique called factor analysis. In essence, factor analysis organizes the variables into a smaller number of clusters, such that they are strongly correlated within each cluster but weakly correlated between clusters. Each cluster is then interpreted as multiple measures of the same underlying construct. These underlying constructs are also called “factors.” For example, when people perform a wide variety of mental tasks, factor analysis typically organizes them into two main factors—one that researchers interpret as mathematical intelligence (arithmetic, quantitative estimation, spatial reasoning, and so on) and another that they interpret as verbal intelligence (grammar, reading comprehension, vocabulary, and so on). The Big Five personality factors have been identified through factor analyses of people’s scores on a large number of more specific traits. For example, measures of warmth, gregariousness, activity level, and positive emotions tend to be highly correlated with each other and are interpreted as representing the construct of extraversion. As a final example, researchers Peter Rentfrow and Samuel Gosling asked more than 1,700 university students to rate how much they liked 14 different popular genres of music [RG03] . They then submitted these 14 variables to a factor analysis, which identified four distinct factors. The researchers called them Reflective and Complex (blues, jazz, classical, and folk), Intense and Rebellious (rock, alternative, and heavy metal), Upbeat and Conventional (country, soundtrack, religious, pop), and Energetic and Rhythmic (rap/hip-hop, soul/funk, and electronica).
Two additional points about factor analysis are worth making here. One is that factors are not categories. Factor analysis does not tell us that people are either extraverted or conscientious or that they like either “reflective and complex” music or “intense and rebellious” music. Instead, factors are constructs that operate independently of each other. So people who are high in extraversion might be high or low in conscientiousness, and people who like reflective and complex music might or might not also like intense and rebellious music. The second point is that factor analysis reveals only the underlying structure of the variables. It is up to researchers to interpret and label the factors and to explain the origin of that particular factor structure. For example, one reason that extraversion and the other Big Five operate as separate factors is that they appear to be controlled by different genes [PDMM08] .
5.5.3. Exploring Causal Relationships ¶
NO NO NO NO NO NO NO NO NO
IGNORE, SECTION UNDER CONSTRUCTION (or destruction)
Another important use of complex correlational research is to explore possible causal relationships among variables. This might seem surprising given that “correlation does not imply causation”. It is true that correlational research cannot unambiguously establish that one variable causes another. Complex correlational research, however, can often be used to rule out other plausible interpretations.
The primary way of doing this is through the statistical control of potential third variables. Instead of controlling these variables by random assignment or by holding them constant as in an experiment, the researcher measures them and includes them in the statistical analysis. Consider some research by Paul Piff and his colleagues, who hypothesized that being lower in socioeconomic status (SES) causes people to be more generous [PKCote+10] . They measured their participants’ SES and had them play the “dictator game.” They told participants that each would be paired with another participant in a different room. (In reality, there was no other participant.) Then they gave each participant 10 points (which could later be converted to money) to split with the “partner” in whatever way he or she decided. Because the participants were the “dictators,” they could even keep all 10 points for themselves if they wanted to.
As these researchers expected, participants who were lower in SES tended to give away more of their points than participants who were higher in SES. This is consistent with the idea that being lower in SES causes people to be more generous. But there are also plausible third variables that could explain this relationship. It could be, for example, that people who are lower in SES tend to be more religious and that it is their greater religiosity that causes them to be more generous. Or it could be that people who are lower in SES tend to come from certain ethnic groups that emphasize generosity more than other ethnic groups. The researchers dealt with these potential third variables, however, by measuring them and including them in their statistical analyses. They found that neither religiosity nor ethnicity was correlated with generosity and were therefore able to rule them out as third variables. This does not prove that SES causes greater generosity because there could still be other third variables that the researchers did not measure. But by ruling out some of the most plausible third variables, the researchers made a stronger case for SES as the cause of the greater generosity.
Many studies of this type use a statistical technique called multiple regression. This involves measuring several independent variables (X1, X2, X3,…Xi), all of which are possible causes of a single dependent variable (Y). The result of a multiple regression analysis is an equation that expresses the dependent variable as an additive combination of the independent variables. This regression equation has the following general form:
\(b1X1+ b2X2+ b3X3+ ... + biXi = Y\)
The quantities b1, b2, and so on are regression weights that indicate how large a contribution an independent variable makes, on average, to the dependent variable. Specifically, they indicate how much the dependent variable changes for each one-unit change in the independent variable.
The advantage of multiple regression is that it can show whether an independent variable makes a contribution to a dependent variable over and above the contributions made by other independent variables. As a hypothetical example, imagine that a researcher wants to know how the independent variables of income and health relate to the dependent variable of happiness. This is tricky because income and health are themselves related to each other. Thus if people with greater incomes tend to be happier, then perhaps this is only because they tend to be healthier. Likewise, if people who are healthier tend to be happier, perhaps this is only because they tend to make more money. But a multiple regression analysis including both income and happiness as independent variables would show whether each one makes a contribution to happiness when the other is taken into account. Research like this, by the way, has shown both income and health make extremely small contributions to happiness except in the case of severe poverty or illness [Die00] .
The examples discussed in this section only scratch the surface of how researchers use complex correlational research to explore possible causal relationships among variables. It is important to keep in mind, however, that purely correlational approaches cannot unambiguously establish that one variable causes another. The best they can do is show patterns of relationships that are consistent with some causal interpretations and inconsistent with others.
5.5.4. Key Takeaways ¶
Researchers often use complex correlational research to explore relationships among several variables in the same study.
Complex correlational research can be used to explore possible causal relationships among variables using techniques such as multiple regression. Such designs can show patterns of relationships that are consistent with some causal interpretations and inconsistent with others, but they cannot unambiguously establish that one variable causes another.
5.5.5. Exercises ¶
Practice: Construct a correlation matrix for a hypothetical study including the variables of depression, anxiety, self-esteem, and happiness. Include the Pearson’s r values that you would expect.
Discussion: Imagine a correlational study that looks at intelligence, the need for cognition, and high school students’ performance in a critical-thinking course. A multiple regression analysis shows that intelligence is not related to performance in the class but that the need for cognition is. Explain what this study has shown in terms of what causes good performance in the critical- thinking course.
Using Science to Inform Educational Practices
Developmental Research Designs
Sometimes, especially in developmental research, the researcher is interested in examining changes over time and will need to consider a research design that will capture these changes. Remember, research methods are tools that are used to collect information, while r esearch design is the strategy or blueprint for deciding how to collect and analyze information. Research design dictates which methods are used and how. There are three types of developmental research designs: cross-sectional, longitudinal, and sequential.
Video 2.9.1. Developmental Research Design summarizes the benefits of challenges of the three developmental design models.
Cross-Sectional Designs
The majority of developmental studies use cross-sectional designs because they are less time-consuming and less expensive than other developmental designs. Cross-sectional research designs are used to examine behavior in participants of different ages who are tested at the same point in time. Let’s suppose that researchers are interested in the relationship between intelligence and aging. They might have a hypothesis that intelligence declines as people get older. The researchers might choose to give a particular intelligence test to individuals who are 20 years old, individuals who are 50 years old, and individuals who are 80 years old at the same time and compare the data from each age group. This research is cross-sectional in design because the researchers plan to examine the intelligence scores of individuals of different ages within the same study at the same time; they are taking a “cross-section” of people at one point in time. Let’s say that the comparisons find that the 80-year-old adults score lower on the intelligence test than the 50-year-old adults, and the 50-year-old adults score lower on the intelligence test than the 20-year-old adults. Based on these data, the researchers might conclude that individuals become less intelligent as they get older. Would that be a valid (accurate) interpretation of the results?
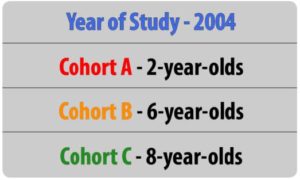
Figure 2.9.1. Example of cross-sectional research design
No, that would not be a valid conclusion because the researchers did not follow individuals as they aged from 20 to 50 to 80 years old. One of the primary limitations of cross-sectional research is that the results yield information about age differences not necessarily changes over time. That is, although the study described above can show that the 80-year-olds scored lower on the intelligence test than the 50-year-olds, and the 50-year-olds scored lower than the 20-year-olds, the data used for this conclusion were collected from different individuals (or groups). It could be, for instance, that when these 20-year-olds get older, they will still score just as high on the intelligence test as they did at age 20. Similarly, maybe the 80-year-olds would have scored relatively low on the intelligence test when they were young; the researchers don’t know for certain because they did not follow the same individuals as they got older.
With each cohort being members of a different generation, it is also possible that the differences found between the groups are not due to age, per se, but due to cohort effects. Differences between these cohorts’ IQ results could be due to differences in life experiences specific to their generation, such as differences in education, economic conditions, advances in technology, or changes in health and nutrition standards, and not due to age-related changes.
Another disadvantage of cross-sectional research is that it is limited to one time of measurement. Data are collected at one point in time, and it’s possible that something could have happened in that year in history that affected all of the participants, although possibly each cohort may have been affected differently.
Longitudinal Research Designs
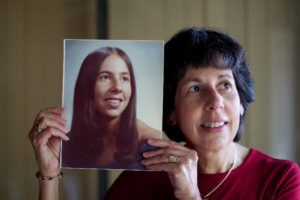
Longitudinal research designs are used to examine behavior in the same individuals over time. For instance, with our example of studying intelligence and aging, a researcher might conduct a longitudinal study to examine whether 20-year-olds become less intelligent with age over time. To this end, a researcher might give an intelligence test to individuals when they are 20 years old, again when they are 50 years old, and then again when they are 80 years old. This study is longitudinal in nature because the researcher plans to study the same individuals as they age. Based on these data, the pattern of intelligence and age might look different than from the cross-sectional research; it might be found that participants’ intelligence scores are higher at age 50 than at age 20 and then remain stable or decline a little by age 80. How can that be when cross-sectional research revealed declines in intelligence with age?

Figure 2.9.2. Example of a longitudinal research design
Since longitudinal research happens over a period of time (which could be short-term, as in months, but is often longer, as in years), there is a risk of attrition. Attrition occurs when participants fail to complete all portions of a study. Participants may move, change their phone numbers, die, or simply become disinterested in participating over time. Researchers should account for the possibility of attrition by enrolling a larger sample into their study initially, as some participants will likely drop out over time. There is also something known as selective attrition— this means that certain groups of individuals may tend to drop out. It is often the least healthy, least educated, and lower socioeconomic participants who tend to drop out over time. That means that the remaining participants may no longer be representative of the whole population, as they are, in general, healthier, better educated, and have more money. This could be a factor in why our hypothetical research found a more optimistic picture of intelligence and aging as the years went by. What can researchers do about selective attrition? At each time of testing, they could randomly recruit more participants from the same cohort as the original members to replace those who have dropped out.
The results from longitudinal studies may also be impacted by repeated assessments. Consider how well you would do on a math test if you were given the exact same exam every day for a week. Your performance would likely improve over time, not necessarily because you developed better math abilities, but because you were continuously practicing the same math problems. This phenomenon is known as a practice effect. Practice effects occur when participants become better at a task over time because they have done it again and again (not due to natural psychological development). So our participants may have become familiar with the intelligence test each time (and with the computerized testing administration).
Another limitation of longitudinal research is that the data are limited to only one cohort. As an example, think about how comfortable the participants in the 2010 cohort of 20-year-olds are with computers. Since only one cohort is being studied, there is no way to know if findings would be different from other cohorts. In addition, changes that are found as individuals age over time could be due to age or to time of measurement effects. That is, the participants are tested at different periods in history, so the variables of age and time of measurement could be confounded (mixed up). For example, what if there is a major shift in workplace training and education between 2020 and 2040, and many of the participants experience a lot more formal education in adulthood, which positively impacts their intelligence scores in 2040? Researchers wouldn’t know if the intelligence scores increased due to growing older or due to a more educated workforce over time between measurements.
Sequential Research Designs
Sequential research designs include elements of both longitudinal and cross-sectional research designs. Similar to longitudinal designs, sequential research features participants who are followed over time; similar to cross-sectional designs, sequential research includes participants of different ages. This research design is also distinct from those that have been discussed previously in that individuals of different ages are enrolled into a study at various points in time to examine age-related changes, development within the same individuals as they age, and to account for the possibility of cohort and/or time of measurement effects
Consider, once again, our example of intelligence and aging. In a study with a sequential design, a researcher might recruit three separate groups of participants (Groups A, B, and C). Group A would be recruited when they are 20 years old in 2010 and would be tested again when they are 50 and 80 years old in 2040 and 2070, respectively (similar in design to the longitudinal study described previously). Group B would be recruited when they are 20 years old in 2040 and would be tested again when they are 50 years old in 2070. Group C would be recruited when they are 20 years old in 2070, and so on.
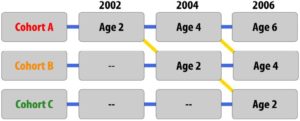
Figure 2.9.3. Example of sequential research design
Studies with sequential designs are powerful because they allow for both longitudinal and cross-sectional comparisons—changes and/or stability with age over time can be measured and compared with differences between age and cohort groups. This research design also allows for the examination of cohort and time of measurement effects. For example, the researcher could examine the intelligence scores of 20-year-olds at different times in history and different cohorts (follow the yellow diagonal lines in figure 2.9.3). This might be examined by researchers who are interested in sociocultural and historical changes (because we know that lifespan development is multidisciplinary). One way of looking at the usefulness of the various developmental research designs was described by Schaie and Baltes (1975): cross-sectional and longitudinal designs might reveal change patterns while sequential designs might identify developmental origins for the observed change patterns.
Since they include elements of longitudinal and cross-sectional designs, sequential research has many of the same strengths and limitations as these other approaches. For example, sequential work may require less time and effort than longitudinal research (if data are collected more frequently than over the 30-year spans in our example) but more time and effort than cross-sectional research. Although practice effects may be an issue if participants are asked to complete the same tasks or assessments over time, attrition may be less problematic than what is commonly experienced in longitudinal research since participants may not have to remain involved in the study for such a long period of time.
Comparing Developmental Research Designs
When considering the best research design to use in their research, scientists think about their main research question and the best way to come up with an answer. A table of advantages and disadvantages for each of the described research designs is provided here to help you as you consider what sorts of studies would be best conducted using each of these different approaches.
Table 2.9.1. Advantages and disadvantages of different research designs
Candela Citations
- Developmental Research Design. Authored by : Nicole Arduini-Van Hoose. Provided by : Hudson Valley Community College. Retrieved from : https://courses.lumenlearning.com/edpsy/chapter/developmental-research-designs/. License : CC BY-NC-SA: Attribution-NonCommercial-ShareAlike
- Developmental Research Designs. Authored by : Nicole Arduini-Van Hoose. Provided by : Hudson Valley Community College. Retrieved from : https://courses.lumenlearning.com/adolescent/chapter/developmental-research-design/. License : CC BY-NC-SA: Attribution-NonCommercial-ShareAlike
Educational Psychology Copyright © 2020 by Nicole Arduini-Van Hoose is licensed under a Creative Commons Attribution-NonCommercial-ShareAlike 4.0 International License , except where otherwise noted.
Share This Book
ORIGINAL RESEARCH article
A short-term longitudinal study on the development of moral disengagement among schoolchildren: the role of collective moral disengagement, authoritative teaching, and student-teacher relationship quality.
- 1 Department of Primary Teacher Education, Kristianstad University, Kristianstad, Sweden
- 2 Department of Behavioural Sciences and Learning, Linköping University, Linköping, Sweden
- 3 Department of Developmental Psychology and Socialisation, University of Padua, Padua, Italy
The aim of this study was to examine whether collective moral disengagement and authoritative teaching at the classroom level, and student-teacher relationship quality at the individual level, predicted individual moral disengagement among pre-adolescent students 1 year later. In this short-term longitudinal study, 1,373 students from 108 classrooms answered a web-based questionnaire on tablets during school, once in fifth grade (T1) and once in sixth grade (T2). The results showed, after controlling for T1 moral disengagement, gender, and immigrant background, that students with better student-teacher relationship quality at T1 were more inclined to score lower on moral disengagement at T2, whereas students in classrooms with higher levels of collective moral disengagement at T1 were more inclined to score higher on moral disengagement at T2. In addition, both collective moral disengagement and authoritative teaching were found to moderate the associations between student-teacher relationship quality at T1 and moral disengagement at T2. These findings underscore the importance of fostering positive relationships between students and teachers, as well as minimizing collective moral disengagement in classrooms. These measures may prevent the potential escalation of moral disengagement in a negative direction.
Introduction
Although there exist different theories and research traditions of children’s moral development, most of them focus on how children progress in and increase their morality ( Jensen, 2020 ; Killen and Smetana, 2022 ). However, as Bussey (2020) notes, there is a lack of research regarding children’s adherence to moral standards, including the moral standards–behavior gap. For instance, already by age three, children typically recognize moral transgressions, such as being mean to others, independently of authority figures and rules (for a meta-analysis, see Yoo and Smetana, 2022 ), yet schoolchildren still engage in immoral actions, such as bullying. Considering the pervasive moral socialization of children taking place at home and in school, and despite their own progress and advancement in moral development, including the understanding by the time they are of preschool age that bullying is wrong, why do schoolchildren engage in such immoral behavior?
Bandura’s social cognitive theory provides insights into this possible moral standard–behavior gap, highlighting the role of moral disengagement in justifying immoral behaviors ( Bandura, 1999 , 2002 , 2016 ). Moral disengagement is a social cognitive process allowing individuals to justify harmful behaviors towards others, enabling them to act immorally without experiencing typical moral self-sanctions like remorse, guilt, or shame ( Bandura, 2002 , 2016 ). Previous research has established a clear link between moral disengagement and bullying ( Killer et al., 2019 ; Thornberg, 2023 ), a pervasive issue affecting children and adolescents worldwide in school settings ( Bradshaw et al., 2017 ; Cosma et al., 2020 ). Consequently, the development of moral disengagement emerges as a critical concern in the field of child development and education. Recognizing the pivotal role of moral development within educational contexts and the significant influence of school environments on students’ behavior ( Eccles and Roeser, 2015 ), longitudinal research on predictors of moral disengagement within a school context is needed. However, existing longitudinal studies often treat moral disengagement as a predictor rather than investigating its antecedents ( Thornberg, 2023 ). To address this gap, the aim of our study is to examine whether students’ moral disengagement in peer aggression is predicted by students’ perceptions of student-teacher relationships, the degree of authoritative teaching and collective moral disengagement within the classroom over the course of 1 year.
In Sweden, compulsory schooling comprises four stages: a pre-school class (age 6), lower elementary school (grades 1–3, ages 7–9), upper elementary school (grades 4–6, ages 10–12), and lower secondary school (grades 7–9, ages 13–15). In elementary school, students typically remain in a single classroom (homeroom) with the same classmates for most subjects, where one or two primary class teachers teach the majority of subjects, with only a few additional teachers (e.g., physical education, arts). Additionally, it is common for class teachers to stay with the same group of students from first to third grade (lower elementary teachers) and then from fourth to sixth grade (upper elementary teachers). Thus, the classroom, defined as the social setting in which students and teachers interact and influence each other’s attitudes and behaviors ( Farmer et al., 2011 ; Hendricks et al., 2016 ), emerges as an important unit of analysis when examining factors influencing the development of moral disengagement in Swedish children.
In the current study, we focus on early adolescence and the last 2 years of the Swedish elementary school, from age 11 to age 12. Focusing on this age group is particularly important because bullying seems to be most prevalent during these years, both in Sweden ( Friends, 2022 ) and elsewhere ( Due et al., 2005 ). Furthermore, studying moral disengagement development at the beginning of adolescence is crucial because it’s a phase where individuals start to actively shape their sense of identity and values ( Sawyer et al., 2012 ). This period offers a vital window for investigating the foundational aspects, precursors, and implications of morality, given its role in shaping lifelong ethical attitudes and behaviors ( Malti et al., 2021 ). Further, our focus on the role of teachers and peers in the present study aligns with Bronfenbrenner’s (1979) conceptualization of the microsystem , and thus aims to explore how specific factors—on their own and in interaction—within a student’s immediate and direct environment influence the development of moral disengagement. Relationships with teachers and peers play a significant role in shaping a students’ development, influencing their beliefs, behaviors, psychological health, and social relationships ( Hamre and Pianta, 2001 ; Cornelius-White, 2007 ; Farmer et al., 2019 ; Troop-Gordon et al., 2019 ).
Moral disengagement theory
Within the framework of moral disengagement theory, Bandura (2016) described eight mechanisms through which moral disengagement occurs: through moral justification, using euphemistic language to soften destructive actions, comparing one’s actions favorably to others’, avoiding personal responsibility by shifting blame elsewhere, diffusing responsibility within a group, distorting or minimizing the consequences of one’s actions, dehumanizing others to justify mistreatment, and attributing blame to victims. By selectively using these moral disengagement mechanisms, individuals can avoid negative self-sanction, thereby increasing the likelihood of engaging in harmful behaviors.
A fundamental principle in the social cognitive theory is viewing humans as active agents who can intentionally influence their functioning and life circumstances ( Bandura, 1986 ). Moral agency – the ability to refrain from immoral actions and act humanely - results from the interactions among personal factors (e.g., cognition, emotions), social and environmental factors (e.g., family, peer group reactions), and behaviors (e.g., aggression). In other words, behavior and cognition - such as moral disengagement - are partly the result of socialization processes within different socio-cultural contexts ( Bandura, 1999 ). Indeed, previous research has shown that poor parental supervision and monitoring ( Campaert et al., 2018 ), rejecting parenting, and neighborhood impoverishment ( Hyde et al., 2010 ) positively predict moral disengagement over time. Regarding the peer context, Fontaine et al. (2014) revealed that peer rejection predicted subsequent moral disengagement, while Caravita et al. (2014) found that in early adolescence (but not in late childhood), Italian students became more like their friends in terms of level of moral disengagement over time. In a recent study, Korean elementary school students were also found to become more like their friends concerning moral disengagement over time ( Kim et al., 2024 ).
Collective moral disengagement
Social cognitive theory does not only include personal agency but also collective agency as a central part of the self-regulatory process ( Bandura, 2002 ). Therefore, moral disengagement can also be considered a group characteristic ( White et al., 2009 ; Bandura, 2016 ). Specifically, collective moral disengagement is “an emergent group-level property arising from the interactive, coordinative, and synergistic group dynamics” ( White et al., 2009 , p. 43). In schools, collective moral disengagement may emerge at the school or classroom level, whereas it may operate more broadly in other contexts (e.g., the community; Bussey, 2020 ). At the classroom level, it captures the shared beliefs of students about the extent to which moral disengagement mechanisms are common among classmates ( Gini et al., 2014b ). Previous research from Sweden ( Thornberg et al., 2021 ; Bjärehed, 2022 ) and other European countries ( Gini et al., 2014b ; Kollerová et al., 2018 ) has demonstrated that students in classrooms characterized by higher levels of collective moral disengagement are more likely to engage in peer aggression and bullying. With the particular interest of the current study, a meta-analytical review ( Luo and Bussey, 2023 ) identified collective moral disengagement as one of the environmental correlates of moral disengagement, suggesting a positive association between the two constructs. In other words, in classrooms with higher levels of collective moral disengagement, students are also more prone to enlist moral disengagement mechanisms ( Gini et al., 2022 ).
Furthermore, collective moral disengagement has been found to moderate the association between individual moral disengagement and aggressive behaviors. For instance, in one Swedish study, Sjögren et al. (2021a) found that students more often reinforced or assisted in bullying situations if they belonged to classrooms with higher levels of collective moral disengagement and, at the same time, scored higher on individual moral disengagement. This aligns with the social cognitive theory, which suggests that collective processes interact with and influence individual behavior. Whether or not collective moral disengagement influences the development of individual students’ tendency to moral disengagement is still not well known. To the best of our knowledge, the present study is the first to examine whether or not collective moral disengagement at the classroom level predicts students’ moral disengagement over time.
Authoritative teaching
The concept of authoritative parenting was introduced by Baumrind (1966) , in her influential work on parenting styles, and has been negatively linked with moral disengagement in young adults ( Di Pentima et al., 2023 ). Although this theory primarily addresses parents and child-rearing, scholars have highlighted the parallels between parents and teachers in their shared objective of fostering positive development and learning in their children and students ( Wentzel, 2002 ; Walker, 2009 ; Ertesvåg, 2011 ). Teachers play a significant role in shaping the growth of young individuals and can serve as influential socialization agents in their students’ personal and social development ( Farmer et al., 2011 , 2019 ). Authoritative teaching refers to high levels of support (responsiveness) and structure (demandingness). While support includes warmth, care, responsiveness, and open communication, structure is about high expectations, demandingness, and strict but fair enforcement of school rules and classroom order ( Walker, 2009 ; Gregory et al., 2010 ; Thornberg et al., 2018 ). Finne et al. (2018) have argued that an authoritative teaching style can counteract a destructive classroom power structure and foster moral engagement among students. Accordingly, authoritative schools and teaching have been linked to greater academic achievement in the United States ( Dever and Karabenick, 2011 ) and less bullying and victimization among Swedish children ( Thornberg et al., 2018 ; Kloo et al., 2023 ), Chinese adolescents ( Wang et al., 2022 ), and American adolescents ( Gregory et al., 2010 ; Cornell et al., 2015 ; Lau et al., 2018 ). However, less is known about the role of authoritative teaching for the development of students’ moral disengagement. Thus, the current study is the first to investigate whether authoritative teaching at the classroom level predicts students’ moral disengagement over time.
Student-teacher relationship quality
A warm, caring, and supportive student-teacher relationship is a vital part of authoritative teaching. However, the quality of the relationship between an individual student and the teacher does not necessarily align with the overall teaching style at the classroom level. Previous research has shown that a higher quality relationship between a student and teacher (warmer, more caring and supportive, more respectful interaction patterns) is associated with less bullying and peer victimization (for a meta-analysis, see ten Bokkel et al., 2023 ). Although it is our understanding that no studies have examined the predictive role of student-teacher relationship quality on student’s moral disengagement within a longitudinal design, a few cross-sectional studies on bullying and peer victimization have included both moral disengagement and student-teacher relationship quality (or aspects of the latter). For instance, one Swedish study showed that students demonstrating higher moral disengagement in conjunction with poorer student-teacher relationship quality were more prone to reinforce in bullying situations ( Sjögren et al., 2021b ). Another study on ethnic bullying in Italy showed that closeness to teachers might restrain morally disengaged children from bullying ( Iannello et al., 2021 ).
Furthermore, moral disengagement has been tested as a mediator between different school factors (e.g., school climate, teachers’ responses to bullying, student–student relationships) and bullying and aggression (e.g., Campaert et al., 2017 ; Ivaniushina and Alexandrov, 2022 ; Gao et al., 2023 ), suggesting that school factors may influence students’ moral disengagement. Concerning student-teacher relationships, one recent cross-sectional study examined whether moral disengagement mediated the association between student-teacher relationship quality and classroom incivility ( Gao et al., 2024 ). Classroom incivility here refers to student behaviors that negatively impact the learning environment, encompassing everything from minor disturbances to physical violence. The findings from this study showed that students with poorer student-teacher relationship quality scored also higher in moral disengagement, which in turn was linked to more classroom incivility behaviors. The authors suggest that a warm, caring, and supportive teacher may model positive communication patterns and behaviors, possibly helping students to “respect and understand others, strengthen their moral and rule constraints, and thereby reduce their levels of moral disengagement” ( Gao et al., 2024 , p. 508). Nevertheless, the cross-sectional design did not allow conclusions about the directionalities of these associations. The present study is the first to examine whether student-teacher relationship quality at the individual level predicts students’ moral disengagement over time.
The present study
The present study aimed to examine whether collective moral disengagement and authoritative teaching at the classroom level and student-teacher relationship quality at the individual level predicted individual moral disengagement among pre-adolescent students 1 year later. Given that previous studies suggest there are gender differences in moral disengagement ( Caravita et al., 2012 ; Thornberg et al., 2023 ; Gao et al., 2024 ), and considering that a student’s tendency to morally disengage may vary due to differing socialization practices across cultures ( Bussey, 2020 ), we included gender and immigrant background as control variables, along with the initial level of moral disengagement. Because Bandura (2016) argues that moral disengagement is “manifested differently depending on the sphere of activity” (p. 26), we delimited classroom collective moral disengagement and students’ moral disengagement in this study to the activity of peer aggression, including bullying and other forms of mean, unwanted or harmful behaviors toward peers. Thus, we did not study students’ proneness to morally disengage in general but how inclined they were to morally disengage when considering peer aggression.
Drawing on social cognitive theory, which posits that behaviors and cognitions are, in part, the result of socialization processes within different socio-cultural contexts ( Bandura, 1999 ), and empirical evidence from cross-sectional studies that collective moral disengagement at the classroom level is negatively associated with defending behavior ( Gini et al., 2015 ; Kollerová et al., 2018 ), and positively linked with peer aggression ( Gini et al., 2015 ), bullying perpetration ( Kollerová et al., 2018 ; Bjärehed et al., 2021 ; Thornberg et al., 2021 ), siding with peer aggressors ( Sjögren et al., 2021a ), and individual moral disengagement ( Luo and Bussey, 2023 ), we hypothesized that greater collective moral disengagement at the classroom level would predict greater individual moral disengagement 1 year later ( Hypothesis 1 ).
Based on previous cross-sectional findings showing that authoritative parenting is negatively linked with moral disengagement ( Di Pentima et al., 2023 ), that higher school structure (a dimension of an authoritative school construct) is associated with less moral disengagement ( Ivaniushina and Alexandrov, 2022 ), that authoritative teaching is negatively linked with bullying and pro-bullying behaviors ( Lau et al., 2018 ; Thornberg et al., 2018 ; Kloo et al., 2023 ), and that individually perceived authoritative school climate is negatively linked with moral disengagement ( Teng et al., 2020 ), we hypothesized that greater authoritative teaching at the classroom level would predict less moral disengagement 1 year later ( Hypothesis 2 ).
With reference to research showing that greater student-teacher relationship quality decreases the risk of bullying perpetration ( ten Bokkel et al., 2023 ) and cross-sectional studies showing a negative correlation between student-teacher relationship quality and moral disengagement ( Sjögren et al., 2021b ; Gao et al., 2024 ), we hypothesized that greater student-teacher relationship quality at the student level would predict less moral disengagement 1 year later ( Hypothesis 3 ).
Given that social cognitive theory ( Bandura, 1986 ) emphasizes the interplay between personal, behavioral, and environmental factors, and that previous research has not yet studied whether collective moral disengagement or authoritative teaching at the classroom level interacts with student-teacher relationship quality at the individual level to predict moral disengagement, we examined potential cross-level interaction effects in an exploratory manner.
Participants and procedure
Data were collected within a four-year longitudinal project that examined individual and classroom social and moral correlates of school bullying among Swedish school children from upper elementary school to the second year of lower secondary school. The overarching project started in the academic year 2015/2016. A total of 2,448 fifth-grade students in 64 schools were invited to participate in the current study. We used a strategic selection methodology which meant that the sample included students from different socio-geographical areas in Sweden (e.g., rural areas, small towns, and cities). Out of the original sample, 1,623 students filled in the questionnaire in grade 5 (51% girls). Reasons for non-participation included failure to submit parental consent (785 students) or absence on the day of data collection (40 students).
The students answered a web-based questionnaire on tablets in their regular classroom setting on two occasions: the first time in grade 5 and the second 1 year later in grade 6. Of those who answered the questionnaire in both grade 5 and grade 6, the final sample consisted of data from 1,373 students (grade 5, M = 11.5 and SD = 0.3), nested in 108 classrooms. For attrition analyses, we assessed whether students who continued their participation ( n = 1,373) from fifth to sixth grade differed from those who only participated in fifth grade ( n = 250) in terms of their levels of individual moral disengagement, student-teacher relationship quality, and perceptions of authoritative teaching and collective moral disengagement in their classroom in fifth grade. Independent t tests showed that there were no group differences in any of these variables in fifth grade. Written informed parental consent and student assent were obtained from all participants, and no incentives were provided for participation. During each session, either a member of the research team or a teacher was present to explain the study procedures and aid participants as needed. This assistance included providing reading support and clarifying specific items or words on the questionnaire. Participants were also informed of their right to withdraw from the study at any time, with assurance that their individual responses would remain inaccessible to both parents and school personnel. Additionally, participants were instructed to sit at a distance from one another to prevent viewing each other’s responses. On average, participants took approximately 30 min to complete the questionnaire.
Moral disengagement
Moral disengagement at the individual level was measured with an 18-item self-report scale in grade 5 (T1) and grade 6 (T2). This scale was specifically developed in Swedish for the overarching longitudinal project to capture moral disengagement in peer aggression. Previous scales have commonly addressed either moral disengagement in more general antisocial behavior ( Bandura et al., 1996 ), or specifically in bullying situations ( Hymel et al., 2005 ; Thornberg and Jungert, 2014 ). The scale used in the current study has previously demonstrated adequate psychometric properties among Swedish school children ( Thornberg et al., 2019 ; Bjärehed et al., 2021 ; Sjögren et al., 2021a ; Bjärehed, 2022 ).
The students were asked to rate the extent to which they agreed (1 = Strongly disagree to 7 = Strongly agree ) with each of the 18 items (e.g., “If you cannot be like everybody else, it is your fault if you get bullied or frozen out.” or “If my friends begin to tease a classmate, I cannot be blamed for being with them and teasing that person too.”) The scale captured all eight moral disengagement mechanisms described by Bandura (2016) . CFAs with the MLM estimator displayed adequate fit: (grade 5: χ 2 (135) = 392.018, p < 0.001, CFI = 0.90, RMSEA = 0.067; 90% CI [0.059, 0.074], SRMR = 0.055; grade 6: χ 2 (135) = 405.179, p < 0.001, CFI = 0.91, RMSEA = 0.063; 90% CI [0.056, 0.070], SRMR = 0.046). For the current sample, Cronbach’s α was 0.87 in the fifth and 0.89 in the sixth grades. Therefore, the mean score of all items was computed as an index for moral disengagement. This measured the students’ overall tendency to morally disengage in peer aggression situations.
Student–teacher relationship quality
Student-teacher relationship quality (STRQ) was measured in grade 5 (T1) with a 13-item self-report scale, specifically developed in Swedish for the overarching project. The scale has demonstrated adequate psychometric properties among Swedish school children ( Forsberg et al., 2023 ). The students were asked to rate the extent to which they agreed (1 = Strongly disagree to 7 = Strongly agree ) with each of the 13 items. Seven items were positively worded to capture positive student–teacher relationship qualities, and six were negatively worded to capture negative student–teacher relationship qualities (as perceived by the student). Some example items are: “My teachers really care about me” (positive STRQ) and “My teachers do not like me” (negative STRQ). The negatively worded items were reversed and the mean score of all thirteen items was computed as an index variable. Thus, higher values on the index variable represent a positive relationship. CFA with the MLM estimator (two factors and accounting for the nested structure) displayed adequate fit: χ 2 (64) = 364.825, p < 0.001, CFI = 0.95, RMSEA = 0.083; 90% CI [0.074, 0.091], SRMR = 0.048. Cronbach’s α for the whole scale (with reversed items) was 0.94.
Collective moral disengagement (CMD) was measured in grade 5 (T1) with an 18-item self-report scale, specifically developed in Swedish for the overarching project, that has demonstrated adequate psychometric properties among Swedish school children ( Alsaadi et al., 2018 ; Bjärehed et al., 2021 ; Sjögren et al., 2021a ; Bjärehed, 2022 ). The scale consisted of the same items as those measuring individual moral disengagement. However, to capture the collective dimension, this scale used the same procedure as in Gini et al.’s (2014b) classroom CMD scale and asked: “In your class, how many students think that…?” which the students then answered by selecting one of the following response categories: “None,” “About one quarter,” “About half,” “About three quarters,” and “Everyone.” At the individual level, the scale represented the individual’s perception of the degree to which moral disengagement was shared among peers in their classroom (referred to as student-perceived collective moral disengagement ; Gini et al., 2014a ). CFA with the MLM estimator (accounting for the nested structure) displayed adequate fit: χ 2 (135) = 558.574, p < 0.001, CFI = 0.91, RMSEA = 0.070; 90% CI [0.064, 0.077], SRMR = 0.043. Cronbach’s α for the current sample was 0.92. Collective moral disengagement as a classroom-level construct was obtained by calculating the average score of all classroom members’ mean scores.
To measure authoritative teaching in grade 5 (T1), we used two subscales from the 15-item Authoritative Classroom Climate Scale ( Thornberg et al., 2018 ). This scale was specifically developed in Swedish for the overarching project and has demonstrated adequate psychometric properties among Swedish school children ( Thornberg et al., 2018 ). The scale consists of two subscales of authoritative teaching: teacher support (4 items, e.g., “our teachers really care about the students,” “our teachers really give the students good help and support”) and teacher structure (4 items, e.g., “our teachers bring order and undisturbed working atmosphere in the classroom,” “our teachers make clear demands on students”). The students were asked to rate the extent to which they agreed (1 = Strongly disagree to 7 = Strongly agree ) with the eight statements. CFA with the MLM estimator (two factors and accounting for the nested structure) displayed adequate fit: χ 2 (19) = 68.751, p < 0.001, CFI = 0.97, RMSEA = 0.044; 90% CI [0.036, 0.052], SRMR = 0.021. Cronbach’s α for the current sample was 0.91. As with the scale measuring collective moral disengagement, we calculated each student’s mean and then averaged the scores for each classroom.
Control variables
Gender and immigrant background were included as control variables at the individual level. The students were asked whether they identified as a girl or a boy (girl = 0, boy = 1). For immigrant background, defined as not being born in Sweden or having two foreign-born parents, the students were asked whether they and their parents were born in Sweden. From these answers, a dummy variable was created indicating whether the students had a Swedish or immigrant background (0 = Swedish background, 1 = Immigrant background).
The students ( N = 1,373) were nested within classrooms ( M = 108). Thus, multilevel modeling techniques were used to analyze the data. This allowed us to disentangle individual-level and classroom-level effects on grade 6 moral disengagement (MD6). The individual-level variables were student-teacher relationship quality (STRQ5), immigrant background, gender, and moral disengagement (MD5), all reported in grade 5. The classroom-level variables examined were authoritative teaching (AUTH5) and collective moral disengagement (CMD5), also reported in grade 5.
First, we estimated an unconditional model with a random intercept. In this model, we estimated the overall classroom-level variance in moral disengagement (MD6). To test whether greater student-teacher relationship quality (STRQ5) predicted greater moral disengagement (MD6) 1 year later ( Hypothesis 3 ), we added the individual-level variables as fixed effects (Model 1). Thus, this model examined the influence of student-teacher relationship quality in grade 5 on grade 6 moral disengagement while controlling for grade 5 moral disengagement, gender, and immigrant background.
To test whether greater authoritative teaching ( Hypothesis 2 ) and less collective moral disengagement ( Hypothesis 1 ) at the classroom level would predict less moral disengagement 1 year later, the two grade 5 classroom variables (AUTH5 and CMD5) were added as fixed effects. That is, in model 2, we examined the contribution of authoritative teaching and collective moral disengagement on grade 6 moral disengagement, over and above the individual’s student-teacher relationship quality (STRQ5), and the control variables. Lastly, in Model 3, we added the four cross-level interaction effects between moral disengagement, student-teacher relationship quality, authoritative teaching, and collective moral disengagement (MD5 × AUTH5, STRQ5 × AUTH5, IMD5 × CMD5, and STRQ5 × CMD5). This final model examined whether the effects of the individual-level variables differed depending on different classroom levels of authoritative teaching and collective moral disengagement.
We examined model fit improvement for each new model to assess the added variables’ explanatory value to the overall model. To help interpretation, the individual-level variables (except immigrant background and gender) were grand-mean centered, whereas the classroom-level variables were centered around the mean of all classrooms. All multilevel regression analyses were conducted in RStudio (version 2023.06.2) with the package lme4 and the restricted maximum likelihood estimator (REML). All models were refitted with the maximum likelihood estimator (ML) to examine model improvement.
Descriptive statistics and correlations
Descriptive statistics and correlations of the individual-level and classroom-level variables are presented in Tables 1 , 2 . Moral disengagement in grade 5 was positively correlated with grade 6 moral disengagement ( r = 0.54, p < 0.001). In contrast, student-teacher relationship quality in grade 5 was negatively correlated with moral disengagement in grade 5 ( r = −0.42, p < 0.001) and grade 6 ( r = −0.38, p < 0.001). In other words, students with better student-teacher relationship quality in the fifth grade were more likely to score lower on moral disengagement in both grade 5 and grade 6. At the classroom level, collective moral disengagement in grade 5 was positively associated with the class mean of moral disengagement in grade 6 ( r = 0.61, p < 0.001), whereas authoritative teaching in grade 5 was negatively associated with the class mean of moral disengagement in grade 6 ( r = −0.50, p < 0.001). Thus, classrooms with greater authoritative teaching and classrooms with less collective moral disengagement were more likely to have a lower class mean of moral disengagement than other classrooms 1 year later. In addition, there was a negative correlation at the classroom level between authoritative teaching and collective moral disengagement in grade 5 ( r = −0.71, p < 0.001), which means that collective moral disengagement tended to be lower in classrooms where teachers displayed greater authoritative teaching.

Table 1 . Correlations, means, and standard deviations for individual-level variables.

Table 2 . Correlations, means, and standard deviations for classroom-level variables.
Multilevel regression analyses
Calculations of the intraclass coefficient (ICC) revealed variation at the classroom level, accounting for about 9% of the total variance in moral disengagement in the sixth grade. Therefore, the use of multilevel modeling was justified. As described earlier, Model 1 examined the influence of student-teacher relationship quality in grade 5 on grade 6 moral disengagement while controlling for grade 5 moral disengagement, gender, and immigrant background ( Hypothesis 3 ). In Model 2, Hypotheses 1 and 2 were examined. In the third model, interaction effects were added and examined in an exploratory manner. Reported coefficients, as presented in Table 3 and in the text, are unstandardized and thus indicate the expected change in moral disengagement for each unit of change in the independent variable. The results of the multilevel modeling are summarized in Table 3 .
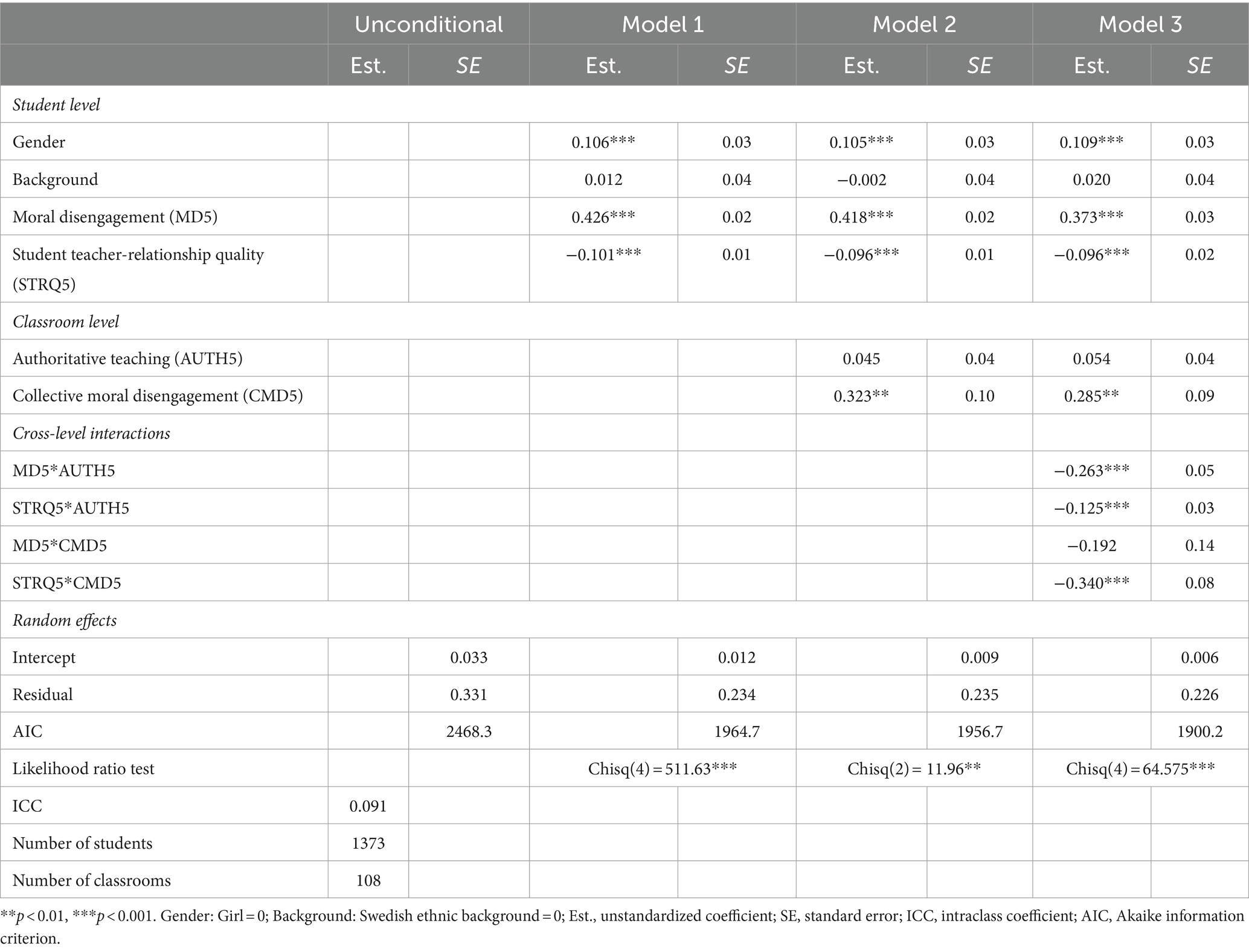
Table 3 . Multilevel estimates for models predicting student moral disengagement in grade 6.
At the individual level, our results revealed that girls ( b = 0.109, SE = 0.03, p < 0.001) and students who reported less moral disengagement ( b = 0.373, SE = 0.03, p < 0.001) and greater student-teacher relationship quality in grade 5 ( b = −0.096, SE = 0.02, p < 0.001) were inclined to score lower on moral disengagement in grade 6 (see Table 3 , Model 3). In other words, we found support for our third hypothesis, as the quality of student-teacher relationship at the individual level negatively predicted students’ moral disengagement 1 year later, even when controlling for their moral disengagement in grade 5 and other variables in the model.
Additionally, we found support for our first hypothesis, as classroom levels of collective moral disengagement in grade 5 positively predicted individual students’ moral disengagement in grade 6 ( b = 0.285, SE = 0.09, p < 0.01). Thus, students in classrooms characterized by higher levels of collective moral disengagement were more likely to score higher on moral disengagement 1 year later compared to students in classrooms with lower levels of collective moral disengagement in grade 5. Contrary to Hypothesis 2 , authoritative teaching in grade 5 did not significantly predict moral disengagement in grade 6. However, interaction effects were found for three of the four interactions tested (see Figures 1 – 3 ).
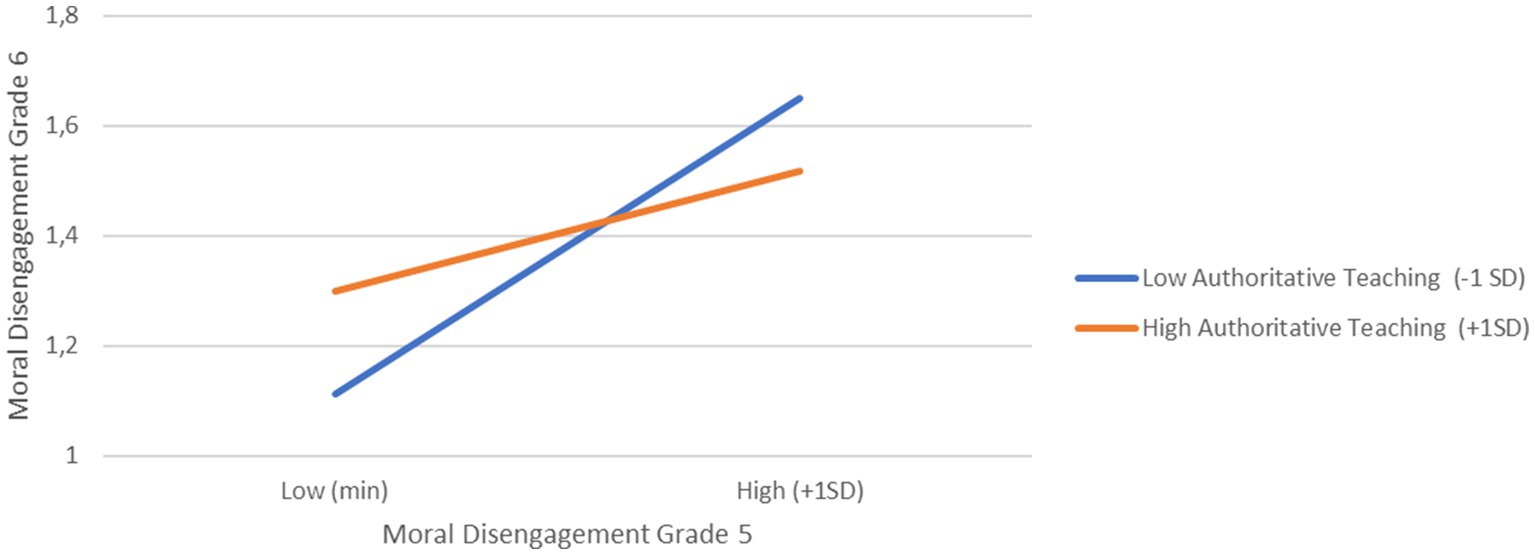
Figure 1 . Cross-level interaction between moral disengagement and authoritative teaching predicting moral disengagement in grade 6.
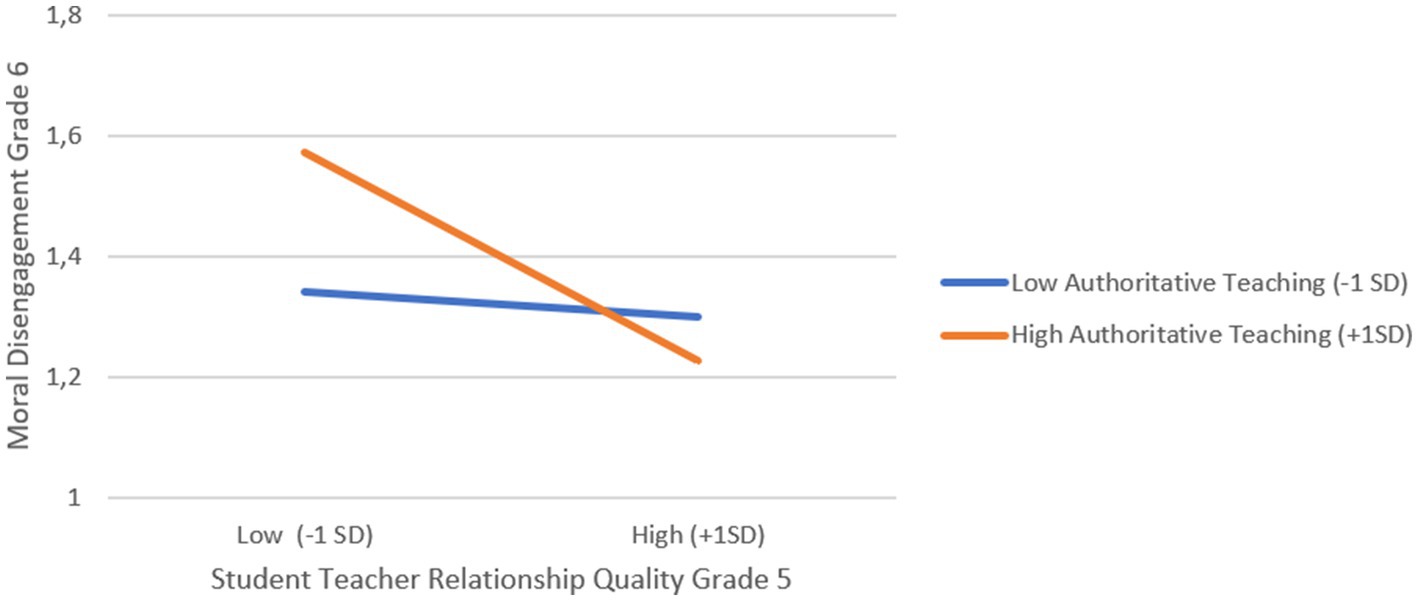
Figure 2 . Cross-level interaction between student-teacher relationship quality and authoritative teaching predicting moral disengagement in grade 6.
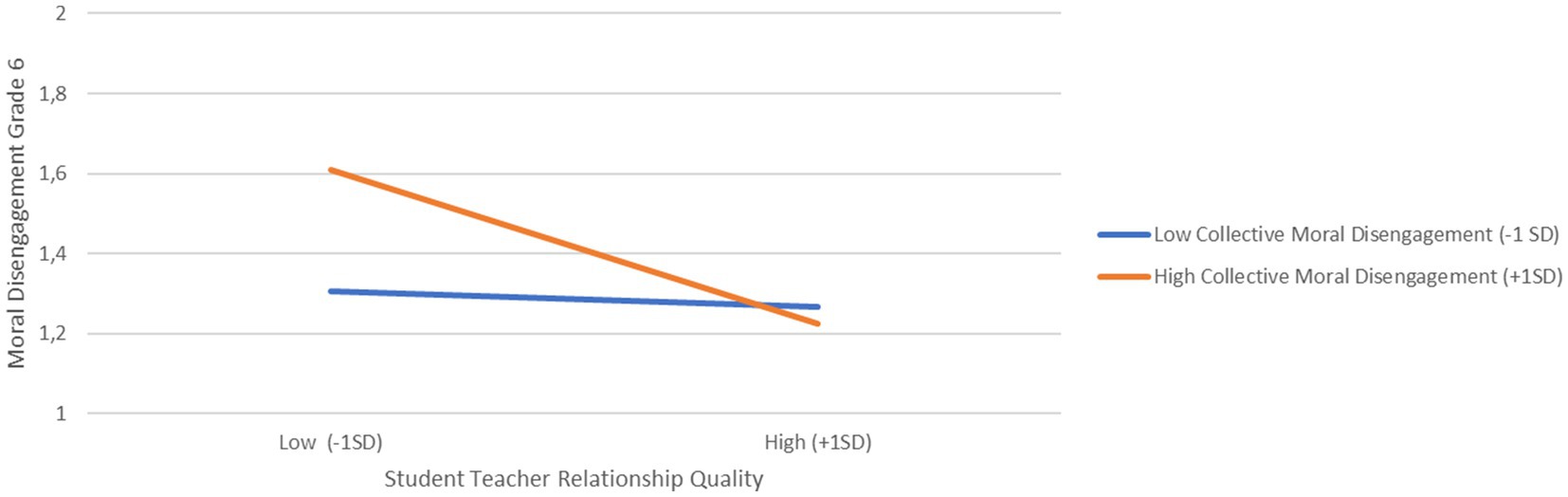
Figure 3 . Cross-level interaction between student-teacher relationship quality and collective moral disengagement predicting moral disengagement in grade 6.
First, there was a significant interaction between students’ moral disengagement and authoritative teaching in grade 5 ( b = −0.26, SE = 0.05, p < 0.001). To interpret these significant interaction effects, we computed simple slopes (see Preacher et al., 2006 ) for low (–1SD) and high (+1SD) levels of authoritative teaching. As shown in Figure 1 , the effect of moral disengagement in grade 5 on moral disengagement in grade 6 was stronger in classrooms characterized by low authoritative teaching ( b low = 0.53, p < 0.001) compared with classrooms characterized as high in authoritative teaching ( b high = 0.21, p < 0.001). Second, and as shown in Figure 2 , we found a significant interaction between student-teacher relationship quality and authoritative teaching in grade 5 ( b = −0.13, SE = 0.03, p < 0.001). Simple slope analysis for low (−1SD) and high classroom levels (+1SD) of authoritative teaching showed that in classrooms with high authoritative teaching, better student-teacher relationship quality was associated with less moral disengagement in the sixth grade ( b high = −0.17, p < 0.001), whereas in classrooms with low levels of authoritative teaching, grade 6 moral disengagement did not vary as a function of student-teacher relationship quality ( b low = −0.02, p = 0 .31). Lastly, and as illustrated in Figure 3 , there was a significant interaction between student-teacher relationship quality and collective moral disengagement in grade 5 ( b = −0.340, SE = 0.09, p < 0.001). The simple slope for low (–1SD) collective moral disengagement was not significant ( b low = −0.02, p = 0 .42), whereas the simple slope for high (+1SD) collective moral disengagement was negative and significant ( b high = −0.17, p < 0.001). That is, in classrooms with high levels of collective moral disengagement, better student-teacher relationship quality was associated with lower levels of moral disengagement 1 year later.
With reference to social cognitive theory ( Bandura, 2016 ), children can develop their moral standards, self-regulatory processes, and behaviors but can also learn to use moral disengagement mechanisms from socialization agents such as teachers and peers in school. Understanding the antecedents of moral disengagement is crucial, given its well-established link to school bullying ( Killer et al., 2019 ; Luo and Bussey, 2023 ; Thornberg, 2023 ) and peer aggression more generally ( Gini et al., 2014a ; Luo and Bussey, 2023 ). In alignment with social cognitive theory and reciprocal determinism ( Bandura, 2016 ), this study offers insights into the complex interplay between personal factors, including cognitions, and social factors, that are transmitted through the influence of peers and teachers. It examines the impact of specific school microsystem factors ( Bronfenbrenner, 1979 ) on the development of moral disengagement in pre-adolescence, specifically focusing on collective moral disengagement, authoritative teaching, and the quality of student-teacher relationships. To our knowledge, the present study has been the first to examine how these factors, uniquely and interactively, predict moral disengagement within a longitudinal design.
As hypothesized, our study revealed that students in classrooms with higher levels of collective moral disengagement in fifth grade were more prone to endorse morally disengaged beliefs about peer aggression 1 year later ( Hypothesis 1 ). Thus, collective moral disengagement is a contextual factor that affects not only behaviors such as bullying ( Gini et al., 2014b ; Kollerová et al., 2018 ; Thornberg et al., 2021 ; Bjärehed, 2022 ) but also socio-cognitive processes, such as an individual’s moral disengagement. This underscores the significant influence of the peer group on the development of moral disengagement among pre-adolescents. Our finding adds to previous research by suggesting that not only do friends tend to become more similar in moral disengagement levels over time in early adolescence ( Caravita et al., 2014 ; Kim et al., 2024 ), but pre-adolescents who belong to a classroom with higher levels of collective moral disengagement are at an increased risk of developing greater individual moral disengagement.
While students’ moral disengagement is in itself a risk factor for peer aggression and bullying ( Gini et al., 2014a ; Luo and Bussey, 2023 ), and a predictor of later bullying perpetration (for a review see Thornberg, 2023 ), collective moral disengagement at the classroom level has also been shown to be associated with bullying behaviors, over and above individual moral disengagement ( Bjärehed et al., 2021 ; Sjögren et al., 2021a ). In addition to this existing research, and a recent longitudinal study showing that classroom collective moral disengagement explains between-classroom variability in later aggression ( Gini et al., 2022 ), the current findings contribute to the literature by demonstrating that classroom collective moral disengagement also predicts students’ moral disengagement over time. In other words, collective moral disengagement at the classroom level can be linked to a negative development of students’ moral cognition and behavior.
Although authoritative teaching was negatively associated with classroom levels of moral disengagement 1 year later in the bivariate analysis, there was no significant direct effect of authoritative teaching in fifth grade on moral disengagement in the sixth grade in the final model ( Hypothesis 2 ). One study has found that students perceiving school rules as transparent and fair are less likely to activate mechanisms of moral disengagement, resulting in decreased engagement in negative behaviors ( Ivaniushina and Alexandrov, 2022 ). The inconsistency with our findings may be explained by the focus of our study on students’ collective perceptions of their teacher’s teaching style rather than individual students’ perceptions. Ivaniushina and Alexandrov’s study also included a slightly older sample (ages 12–15). Further, they focused on structure as a school characteristic, while our measure included both structure and support and specifically examined the teacher and classroom setting. It is also plausible that support and structure are differentially associated with moral disengagement. In a Swedish study with grade 4 students, Kloo et al. (2023) distinguished between these two dimensions of authoritative teaching; their results suggest that teacher support drives the negative association between authoritative teaching and bullying perpetration. For example, high teacher structure might impact bullying through reduced moral disengagement, as proposed by Ivaniushina and Alexandrov (2022) , while a classroom characterized by support (i.e., warmth, open communication, and caring) might be directly linked to lower levels of bullying and victimization ( Lau et al., 2018 ; Thornberg et al., 2018 ; Kloo et al., 2023 ). In addition to the impact of collective moral disengagement at the classroom level found in the present study, another reasonable explanation could be that students’ perception of their relationship quality with the teacher ( Gao et al., 2024 ) is a more influential factor for their development and changes in moral disengagement over time than the overall teaching style at the classroom level. This suggestion is supported by the finding in our study that more positive, warm, and supportive student-teacher relationships predicted lower levels of moral disengagement.
The current results suggest that a higher quality of student-teacher relationships may protect against subsequent increases in moral disengagement ( Hypothesis 3 ). This indicates that how teachers establish and maintain relationships with their students, and how this relationship quality varies across their student cohort, may play an essential role in their students’ development of moral disengagement. This finding holds significance, particularly in light of the well-established connection between moral disengagement and later involvement in school bullying, as identified in other studies ( Thornberg, 2023 ). In our study, students with more negative, less supportive, and less caring student-teacher relationships were more likely to exhibit elevated levels of moral disengagement in the sixth grade, regardless of their initial level of moral disengagement. This result expands upon previous cross-sectional findings demonstrating a positive link between student-teacher relationships and moral disengagement ( Gao et al., 2024 ) that also incorporate two time points within a longitudinal design.
In Gao et al.’s study, the association between student-teacher relationships and moral disengagement was stronger in early adolescence (11–14 years) compared to middle adolescence (15–17 years). From a developmental standpoint, adolescents progressively gain independence, thus suggesting that the influence of adults, including teachers, on their socialization might diminish later in adolescence. Consistent with this notion, prior longitudinal studies have indicated that moral disengagement is influenced by factors in home environments until late childhood ( Hyde et al., 2010 ), after which it becomes more influenced by peers ( Caravita et al., 2014 ). Our study adds to this literature by suggesting that teachers are influential socialization agents impacting students’ tendency to morally disengage in pre-adolescence. Not only can higher student-teacher relationship quality be associated with less bullying ( ten Bokkel et al., 2023 ) and peer aggression ( Krause and Smith, 2023 ). According to our study it can also be associated with less moral disengagement. The literature, in turn, has linked low moral disengagement with less bullying ( Killer et al., 2019 ; Thornberg, 2023 ) and peer aggression ( Gini et al., 2014a ; Luo and Bussey, 2023 ). Altogether, this could be interpreted in terms of what Bandura (2016) calls triadic codetermination, which means that “human functioning is a product of the interplay of personal influences, the behavior individuals engage in, and the environmental forces that impinge on them” (p. 6). Personal influences would here be moral disengagement, behavior would refer to bullying or peer aggression, and environmental forces the student-teacher relationship quality. Our study contributes to the literature by showing that student-teacher relationship quality negatively predicted moral disengagement 1 year later. Since our study focused on changes between the ages of 11 and 12, it would be desirable to include a more extended period in future studies, both with younger school children and those in later adolescence. This could provide insights into the potentially changing influence of student-teacher relationship quality on moral disengagement across different developmental stages.
Cross-level interaction effects
In our study, three out of four tested cross-level interactions significantly predicted moral disengagement 1 year later: the interaction between moral disengagement and authoritative teaching, the interaction between student-teacher relationship quality and authoritative teaching, and the interaction between student-teacher relationship quality and collective moral disengagement.
Although we did not find that authoritative teaching at the classroom level predicted subsequent moral disengagement (when controlling for collective moral disengagement at the classroom level and student-teacher relationship quality, gender, immigrant background, and previous moral disengagement at the individual level), authoritative teaching was found to moderate the effects of moral disengagement and student-teacher relationship quality in grade 5 on subsequent moral disengagement. In classrooms with less authoritative teaching, the impact of moral disengagement on subsequent moral disengagement was more pronounced. This finding suggests that an authoritative teaching style can act as a buffer against a negative spiral of escalating moral disengagement over time, especially for students who already exhibit high levels of moral disengagement in grade 5.
Our findings further indicate that lower student-teacher relationship quality in grade 5 is associated with higher moral disengagement in grade 6. However, this association was only significant in classrooms with high levels of authoritative teaching. In other words, this result suggests that having poor student-teacher relationships when belonging to classrooms where teachers are high in authoritative teaching is a risk factor of moral disengagement. With reference to the self-categorization theory ( Turner and Oakes, 1989 ; Abrams and Hogg, 1990 ), a possible explanation for our findings might be that the few students who had poor relationships with their teachers compared themselves with the majority of their classmates who had more positive relationships with their teachers due to the authoritative teaching style (teachers showed greater warmth, care, support, and responsiveness to students in general). It is plausible to assume that these everyday social comparisons in school increase the risk of developing a sense of non-belonging in the classroom context together with a more deviant social identity. As a part of their self-categorization process ( Turner and Oakes, 1989 ), students with poor teacher relationships would be prone to develop a self-serving bias of favoring the social category they identify themselves with (e.g., anti-school, antisocial, or rejected in-group) while devaluating the majority group of others in the classroom and other peers whom they perceive belong to the same well-adjusted outgroup. These “upward comparisons” ( Laninga-Wijnen et al., 2023 ) might, in this case, contribute to an antisocial trajectory and a greater need for moral disengagement to maintain positive self-esteem and avoid self-sanctions.
In the final regression model, collective moral disengagement at the classroom level and the quality of the student-teacher relationship at the individual level were uniquely linked to moral disengagement in sixth grade. Nevertheless, the extent to which student-teacher relationship quality predicted moral disengagement also depended on the levels of classroom collective moral disengagement. Previous studies have demonstrated that collective moral disengagement can act as a protective group property within the classroom, reducing the risk of bullying perpetration ( Gini et al., 2014b ; Kollerová et al., 2018 ; Thornberg et al., 2019 ; Thornberg et al., 2021 ; Bjärehed, 2022 ). Our study contributes to this body of literature by suggesting that students with high student-teacher relationship quality score low on subsequent moral disengagement independently of classroom levels of collective moral disengagement. In contrast, students with low student-teacher relationship quality tend to score higher on subsequent moral disengagement in classrooms with high levels of collective moral disengagement compared to students in classrooms with low collective moral disengagement. Thus, having a positive student-teacher relationship appears to be an important protective factor against moral disengagement. The interaction effect in our results suggest that students who had positive, warm, and supportive relationships with their teachers were also better equipped to resist the bad influence of classroom collective moral disengagement on their moral development. One year later they still showed low levels of moral disengagement despite belonging to a classroom with high collective moral disengagement.
Limitations
The current study fills an important gap in the literature by examining the role played by specific school microsystem factors ( Bronfenbrenner, 1979 ) on the development of moral disengagement in pre-adolescence. Nevertheless, some methodological limitations need to be addressed. First, all the studied variables were assessed using self-report measures. Self-reports are vulnerable to social desirability biases, and there may be a risk that students underreport their moral disengagement while exaggerating how common it is among classmates. Nevertheless, as both moral disengagement and collective moral disengagement relate to students’ perceptions and beliefs, self-reports may be the best way to capture these constructs. Further, some controversy exists about whether students should rate their teachers’ behaviors ( den Brok et al., 2006 ), like authoritative teaching. Thus, future studies could examine whether the current findings hold when teacher reports and/or direct observations are used to capture authoritative teaching.
Although our study implemented a longitudinal design, only two time points over a relatively short period of 1 year were included. A more extensive longitudinal approach with additional time points could be employed to enhance the robustness of our findings. This would provide a more comprehensive understanding of the observed associations and enable us to capture potential developmental trends or variations over an extended period. In addition, changes in moral disengagement may also be influenced by several factors not included in the current study.
Furthermore, no classroom mean score of authoritative teaching was below 3.9 (max 7); for collective moral disengagement, no classroom score exceeded 2.6 (max 5). This suggests that our study compared classrooms characterized as relatively authoritative and with relatively low levels of collective moral disengagement. Consequently, caution is advised when generalizing findings to classrooms characterized by a lower degree of authoritative teaching and higher levels of collective moral disengagement. Finally, our study exclusively involved students in Swedish schools. Future studies should investigate the transferability of these findings to other countries and cultural contexts.
Practical implications
The current findings have implications for teacher educators and school personnel. First, fostering a positive and supportive relationship between students and teachers seems crucial in minimizing a negative developmental spiral of moral disengagement in pre-adolescence. Teacher educators should, therefore, focus on equipping pre-service teachers with the skills and knowledge to build positive and supportive relationships with their students ( Bouchard and Smith, 2017 ). Teachers who engage in authoritative teaching as a part of their bullying prevention strategy (see Lau et al., 2018 ; Thornberg et al., 2018 ; Kloo et al., 2023 ) need to make the effort to build warm, caring, and responsive relationships with all of the students in their classroom and include targeted actions for students they fail to reach and with whom they fail to develop supportive relationships. To prevent an adverse moral climate, pre-service teachers and teachers can also benefit from professional training on influencing group dynamics ( Hymel et al., 2015 ). This training should include strategies that proactively prevent collective moral disengagement from emerging in the first place and promote a moral climate defined by active engagement and social responsibility. In line with the democratic mission ( Swedish Education Act, SFS 2010:800, 2010 ), teachers could integrate discussions about ethics and moral decision-making into their ordinary lessons by making students aware of moral disengagement mechanisms and how these can contribute to explaining a range of negative, inhumane and aggressive behaviors, including bullying and peer aggression, delinquency/criminality, political oppression, terrorism, genocide, and war ( Bandura, 2016 ). When students are encouraged to reflect on ethical considerations and to identify moral disengagement mechanisms, they might be more likely to develop a moral compass and resist moral disengagement.
Data availability statement
The datasets generated for this study are available on request to the corresponding author.
Ethics statement
The studies involving humans were approved by Regional Ethical Review Board at Linköping. The studies were conducted in accordance with the local legislation and institutional requirements. Written informed consent for participation in this study was provided by the participants’ legal guardians/next of kin.
Author contributions
MB: Writing – original draft, Writing – review & editing. BS: Writing – original draft, Writing – review & editing. RT: Writing – original draft, Writing – review & editing. GG: Writing – review & editing. TP: Writing – review & editing.
The author(s) declare financial support was received for the research, authorship, and/or publication of this article. This research was supported by a grant awarded to RT from the Swedish Research Council (grant number D0775301).
Conflict of interest
The authors declare that the research was conducted in the absence of any commercial or financial relationships that could be construed as a potential conflict of interest.
Publisher’s note
All claims expressed in this article are solely those of the authors and do not necessarily represent those of their affiliated organizations, or those of the publisher, the editors and the reviewers. Any product that may be evaluated in this article, or claim that may be made by its manufacturer, is not guaranteed or endorsed by the publisher.
Abrams, D., and Hogg, M. A. (1990). Social identification, self-categorization, and social influence. Eur. Rev. Soc. Psychol. 1, 195–228. doi: 10.1080/14792779108401862
Crossref Full Text | Google Scholar
Alsaadi, S., Wänström, L., Thornberg, R., Sjögren, B., Bjärehed, M., and Forsberg, C. (2018) Paper presented at the 2018 annual meeting of the American Educational Research Association. Retrieved from the AERA Online Paper Repository. (Accessed April 16, 2024).
Google Scholar
Bandura, A. (1986). Social Foundations of Thought and Action: A Social Cognitive Theory . New Jersey: Prentice-Hall.
Bandura, A., Barbaranelli, C., Caprara, G. V., and Pastorelli, C. (1996). Mechanisms of moral disengagement in the exercise of moral agency. J. Pers. Soc. Psychol. 71, 364–374. doi: 10.1037/0022-3514.71.2.364
Bandura, A. (1999). Moral disengagement in the perpetration of inhumanities. Personal. Soc. Psychol. Rev. 3, 193–209. doi: 10.1207/s15327957pspr0303_3
PubMed Abstract | Crossref Full Text | Google Scholar
Bandura, A. (2002). Selective moral disengagement in the exercise of moral agency. J. Moral Educ. 31, 101–119. doi: 10.1080/0305724022014322
Bandura, A. (2016). Moral Disengagement: How People Do Harm and Live with Themselves . New York: Worth.
Baumrind, D. (1966). Effects of authoritative parental control on child behavior. Child Dev. 37, 887–907. doi: 10.2307/1126611
Bjärehed, M. (2022). Individual and classroom collective moral disengagement in offline and online bullying: a short-term multilevel growth model study. Psychol. Sch. 59, 356–375. doi: 10.1002/pits.22612
Bjärehed, M., Thornberg, R., Wänström, L., and Gini, G. (2021). Individual moral disengagement and bullying among Swedish fifth graders: the role of collective moral disengagement and pro-bullying behavior within classrooms. J. Interpers. Violence 36, NP9576–NP9600. doi: 10.1177/0886260519860889
Bouchard, K. L., and Smith, J. D. (2017). Teacher–student relationship quality and children’s bullying experiences with peers: reflecting on the mesosystem. Educ. Forum 81, 108–125. doi: 10.1080/00131725.2016.1243182
Bradshaw, J., Crous, G., Rees, G., and Turner, N. (2017). Comparing children's experiences of schools-based bullying across countries. Child Youth Serv. Rev. 80, 171–180. doi: 10.1016/j.childyouth.2017.06.060
Bronfenbrenner, U. (1979). The Ecology of Human Development: Experiments by Nature and Design . Cambridge, MA: Harvard University Press.
Bussey, K. (2020). “Development of moral disengagement” in The Oxford Handbook of Moral Development . ed. L. A. Jensen (Oxford: Oxford University Press), 305–326.
Campaert, K., Nocentini, A., and Menesini, E. (2017). The efficacy of teachers’ responses to incidents of bullying and victimization: the mediational role of moral disengagement for bullying. Aggress. Behav. 43, 483–492. doi: 10.1002/ab.21706
Campaert, K., Nocentini, A., and Menesini, E. (2018). The role of poor parenting and parental approval for children’s moral disengagement. J. Child Fam. Stud. 27, 2656–2667. doi: 10.1007/s10826-018-1097-1
Caravita, S. C., Gini, G., and Pozzoli, T. (2012). Main and moderated effects of moral cognition and status on bullying and defending. Aggress. Behav. 38, 456–468. doi: 10.1002/ab.21447
Caravita, S. C., Sijtsema, J. J., Rambaran, J. A., and Gini, G. (2014). Peer influences on moral disengagement in late childhood and early adolescence. J. Youth Adolesc. 43, 193–207. doi: 10.1007/s10964-013-9953-1
Cornelius-White, J. (2007). Learner-centered teacher-student relationships are effective: a meta-analysis. Rev. Educ. Res. 77, 113–143. doi: 10.3102/003465430298563
Cornell, D., Shukla, K., and Konold, T. (2015). Peer victimization and authoritative school climate: a multilevel approach. J. Educ. Psychol. 107, 1186–1201. doi: 10.1037/edu0000038
Cosma, A., Walsh, S. D., Chester, K. L., Callaghan, M., Molcho, M., Craig, W., et al. (2020). Bullying victimization: time trends and the overlap between traditional and cyberbullying across countries in Europe and North America. Int. J. Public Health 65, 75–85. doi: 10.1007/s00038-019-01320-2
den Brok, P. D., Bergen, T., and Brekelmans, M. (2006). “Convergence and divergence between students’ and teachers’ perceptions of instructional behaviour in Dutch secondary education” in Contemporary Approaches to Research on Learning Environments: Worldviews . eds. D. F. Fisher and M. S. Khine (Singapore: World Scientific Publishing), 125–160.
Dever, B. V., and Karabenick, S. A. (2011). Is authoritative teaching beneficial for all students? A multi-level model of the effects of teaching style on interest and achievement. Sch. Psychol. Q. 26, 131–144. doi: 10.1037/a0022985
Di Pentima, L., Toni, A., and Roazzi, A. (2023). Parenting styles and moral disengagement in young adults: the mediating role of attachment experiences. J. Genet. Psychol. 184, 322–338. doi: 10.1080/00221325.2023.2205451
Due, P., Holstein, B. E., Lynch, J., Diderichsen, F., Gabhain, S. N., Scheidt, P., et al. (2005). Bullying and symptoms among school-aged children: international comparative cross sectional study in 28 countries. Eur. J. Pub. Health 15, 128–132. doi: 10.1093/eurpub/cki105
Eccles, J. S., and Roeser, R. W. (2015). “School and community influences on human development” in Developmental Science: An Advanced Textbook . eds. M. H. Bornstein and M. E. Lamb. 7th ed (New York: Psychology Press), 645–728.
Ertesvåg, S. K. (2011). Measuring authoritative teaching. Teach. Teach. Educ. 27, 51–61. doi: 10.1016/j.tate.2010.07.002
Farmer, T. W., Hamm, J. V., Dawes, M., Barko-Alva, K., and Cross, J. R. (2019). Promoting inclusive communities in diverse classrooms: teacher attunement and social dynamics management. Educ. Psychol. 54, 286–305. doi: 10.1080/00461520.2019.1635020
Farmer, T. W., McAuliffe Lines, M., and Hamm, J. V. (2011). Revealing the invisible hand: the role of teachers in children’s peer experiences. J. Appl. Dev. Psychol. 32, 247–256. doi: 10.1016/j.appdev.2011.04.006
Finne, J., Roland, E., and Svartdal, F. (2018). Relational rehabilitation: reducing harmful effects of bullying. Nordic Stud. Educ. 38, 352–367. doi: 10.18261/issn.1891-2018-04-05
Fontaine, R. G., Fida, R., Paciello, M., Tisak, M. S., and Caprara, G. V. (2014). The mediating role of moral disengagement in the developmental course from peer rejection in adolescence to crime in early adulthood. Psychol. Crime Law 20, 1–19. doi: 10.1080/1068316X.2012.719622
Forsberg, C., Sjögren, B., Thornberg, R., Hong, J. S., and Longobardi, C. (2023). Longitudinal reciprocal associations between, student-teacher relationship quality and verbal and relational bullying victimization. Soc. Psychol. Educ. 27, 151–173. doi: 10.1007/s11218-023-09821-y
Friends . (2022). Friendsrapporten 2022. 10 år av Barns Röster! [The Friend’s Report 2022. 10 Years of Children’s Voices!]. Available at: https://friends.se/uploads/2022/08/Friendsrapporten-2022_WEBB.pdf (Accessed April 16, 2024).
Gao, L., Kong, F., Cui, L., Feng, N., and Wang, X. (2024). Teacher–student relationships and adolescents’ classroom incivility: a moderated mediation model of moral disengagement and negative coping style. Psychol. Sch. 61, 496–513. doi: 10.1002/pits.23064
Gao, L., Li, X., Wu, X., and Wang, X. (2023). Longitudinal associations among student-student relationship, moral disengagement, and adolescents' bullying perpetration. Sch. Psychol. 38, 337–347. doi: 10.1037/spq0000534
Gini, G., Pozzoli, T., and Bussey, K. (2014b). Collective moral disengagement: initial validation of a scale for adolescents. Eur. J. Dev. Psychol. 11, 386–395. doi: 10.1080/17405629.2013.851024
Gini, G., Pozzoli, T., and Bussey, K. (2015). The role of individual and collective moral disengagement in peer aggression and by standing: a multilevel analysis. J. Abnorm. Child Psychol. 43, 441–452. doi: 10.1007/s10802-014-992
Gini, G., Pozzoli, T., and Hymel, S. (2014a). Moral disengagement among children and youth: a meta-analytic review of links to aggressive behavior. Aggress. Behav. 40, 56–68. doi: 10.1002/ab.21502
Gini, G., Thornberg, R., Bussey, K., Angelini, F., and Pozzoli, T. (2022). Longitudinal links of individual and collective morality with adolescents’ peer aggression. J. Youth Adolesc. 51, 524–539. doi: 10.1007/s10964-021-01518-9
Gregory, A., Cornell, D., Fan, X., Sheras, P., Shih, T.-H., and Huang, F. (2010). Authoritative school discipline: high school practices associated with lower bullying and victimization. J. Educ. Psychol. 102, 483–496. doi: 10.1037/a0018562
Hamre, B. K., and Pianta, R. C. (2001). Early teacher–child relationships and the trajectory of children's school outcomes through eighth grade. Child Dev. 72, 625–638. doi: 10.1111/1467-8624.00301
Hendricks, M. M. H. G., Mainhard, M. T., Boor-Klip, H. J., Cillessen, A. H. M., and Brekelmans, M. (2016). Social dynamics in the classroom: teacher support and conflict and the peer ecology. Teach. Teach. Educ. 53, 30–40. doi: 10.1016/j.tate.2015.10.004
Hyde, L. W., Shaw, D. S., and Moilanen, K. L. (2010). Developmental precursors of moral disengagement and the role of moral disengagement in the development of antisocial behavior. J. Abnorm. Child Psychol. 38, 197–209. doi: 10.1007/s10802-009-9358-5
Hymel, S., McClure, R., Miller, M., Shumka, E., and Trach, J. (2015). Addressing school bullying: insights from theories of group processes. J. Appl. Dev. Psychol. 37, 16–24. doi: 10.1016/j.appdev.2014.11.008
Hymel, S., Rocke-Hendersson, N., and Bonanno, R. A. (2005). Moral disengagement: a framework for understanding bullying among adolescents. J. Soc. Sci. 8, 1–11.
Iannello, N. M., Camodeca, M., Gelati, C., and Papotti, N. (2021). Prejudice and ethnic bullying among children: the role of moral disengagement and student-teacher relationship. Front. Psychol. 12:713081. doi: 10.3389/fpsyg.2021.713081
Ivaniushina, V., and Alexandrov, D. (2022). School structure, bullying by teachers, moral disengagement, and students' aggression: a mediation model. Front. Psychol. 13:883750. doi: 10.3389/fpsyg.2022.883750
Jensen, L. A. (Ed.) (2020). The Oxford Handbook of Moral Development: An Interdisciplinary Perspective . Oxford: Oxford University Press.
Killen, M., and Smetana, J. G. (Eds.) (2022). Handbook of Moral Development . 3rd Edn. New York: Routledge.
Killer, B., Bussey, K., Hawes, D. J., and Hunt, C. (2019). A meta-analysis of the relationship between moral disengagement and bullying roles in youth. Aggress. Behav. 45, 450–462. doi: 10.1002/ab.21833
Kim, J., Sijtsema, J. J., Thornberg, R., Caravita, S. C. S., and Hong, J. S. (2024). Shaping citizenship in the classroom: peer influences on moral disengagement, social goals, and a sense of peer community. J. Youth Adolesc. 53, 732–743. doi: 10.1007/s10964-023-01916-1
Kloo, M., Thornberg, R., and Wänström, L. (2023). Classroom-level authoritative teaching and its associations with bullying perpetration and victimization. J. Sch. Violence 22, 276–289. doi: 10.1080/15388220.2023.2180746
Kollerová, L., Soukup, P., and Gini, G. (2018). Classroom collective moral disengagement scale: validation in Czech adolescents. Eur. J. Dev. Psychol. 15, 184–191. doi: 10.1080/17405629.2017.1292907
Krause, A., and Smith, J. D. (2023). The interconnected school context: Meta-analyses of the associations between peer aggression involvement and teacher-student relationship closeness. Sch. Psychol. Int. 44, 396–446. doi: 10.1177/01430343221138038
Laninga-Wijnen, L., Yanagida, T., Garandeau, C. F., Malamut, S. T., Veenstra, R., and Salmivalli, C. (2023). Is there really a healthy context paradox for victims of bullying? A longitudinal test of bidirectional within-and between-person associations between victimization and psychological problems. Dev. Psychopathol. , 1–15. doi: 10.1017/S0954579423001384
Lau, C., Wong, M., and Dudovitz, R. (2018). School disciplinary style and adolescent health. J. Adolesc. Health 62, 136–142. doi: 10.1016/j.jadohealth.2017.08.011
Luo, A., and Bussey, K. (2023). Moral disengagement in youth: a meta-analytic review. Dev. Rev. 70:101101. doi: 10.1016/j.dr.2023.101101
Malti, T., Galarneau, E., and Peplak, J. (2021). Moral development in adolescence. J. Res. Adolesc. 31, 1097–1113. doi: 10.1111/jora.12639
Preacher, K. J., Curran, P. J., and Bauer, D. J. (2006). Computational tools for probing interactions in multiple linear regression, multilevel modeling, and latent curve analysis. J. Educ. Behav. Stat. 31, 437–448. doi: 10.3102/10769986031004437
Sawyer, S. M., Afifi, R. A., Bearinger, L. H., Blakemore, S. J., Dick, B., Ezeh, A. C., et al. (2012). Adolescence: a foundation for future health. Lancet 379, 1630–1640. doi: 10.1016/S0140-6736(12)60072-5
Sjögren, B., Thornberg, R., Wänström, L., and Gini, G. (2021a). Associations between students’ bystander behavior and individual and classroom collective moral disengagement. Educ. Psychol. 41, 264–281. doi: 10.1080/01443410.2020.1828832
Sjögren, B., Thornberg, R., Wänström, L., and Gini, G. (2021b). Bystander behaviour in peer victimisation: moral disengagement, defender self-efficacy and student-teacher relationship quality. Res. Pap. Educ. 36, 588–610. doi: 10.1080/02671522.2020.1723679
Swedish Education Act, SFS 2010:800 . (2010). Available at: https://rkrattsbaser.gov.se/sfst?bet=2010:800 (Accessed April 16, 2024).
ten Bokkel, I. M., Roorda, D. L., Maes, M., Verschueren, K., and Colpin, H. (2023). The role of affective teacher–student relationships in bullying and peer victimization: a multilevel meta-analysis. Sch. Psychol. Rev. 52, 110–129. doi: 10.1080/2372966X.2022.2029218
Teng, Z., Bear, G. G., Yang, C., Nie, Q., and Guo, C. (2020). Moral disengagement and bullying perpetration: a longitudinal study of the moderating effect of school climate. Sch. Psychol. 35, 99–109. doi: 10.1037/spq0000348
Thornberg, R. (2023). Longitudinal link between moral disengagement and bullying among children and adolescents: a systematic review. Eur. J. Dev. Psychol. 20, 1099–1129. doi: 10.1080/17405629.2023.2191945
Thornberg, R., and Jungert, T. (2014). School bullying and the mechanisms of moral disengagement. Aggress. Behav. 40, 99–108. doi: 10.1002/ab.21509
Thornberg, R., Jungert, T., and Hong, J. S. (2023). The indirect association between moral disengagement and bystander behaviors in school bullying through motivation: structural equation modelling and mediation analysis. Soc. Psychol. Educ. 26, 533–556. doi: 10.1007/s11218-022-09754-y
Thornberg, R., Wänström, L., Gini, G., Varjas, K., Meyers, J., Elmelid, R., et al. (2021). Collective moral disengagement and its associations with bullying perpetration and victimization in students. Educ. Psychol. 41, 952–966. doi: 10.1080/01443410.2020.1843005
Thornberg, R., Wänström, L., and Hymel, S. (2019). Individual and classroom social-cognitive processes in bullying: a short-term longitudinal multilevel study. Front. Psychol. 10:1752. doi: 10.3389/fpsyg.2019.01752
Thornberg, R., Wänström, L., and Jungert, T. (2018). Authoritative classroom climate and its relations to bullying victimization and bystander behaviors. Sch. Psychol. Int. 39, 663–680. doi: 10.1177/0143034318809762
Troop-Gordon, W., MacDonald, A. P., and Corbitt-Hall, D. J. (2019). Children’s peer beliefs, friendlessness, and friendship quality: reciprocal influences and contributions to internalizing symptoms. Dev. Psychol. 55, 2428–2439. doi: 10.1037/dev0000812
Turner, J. C., and Oakes, P. J. (1989). “Self-categorization theory and social influence” in Psychology of Group Influence . ed. P. B. Paulus . 2nd ed (Hillsdale, NJ: Erlbaum), 233–275.
Walker, J. M. T. (2009). Authoritative classroom management: how control and nurturance work together. Theory Pract. 48, 122–129. doi: 10.1080/00405840902776392
Wang, C., Li, B., Zhang, L., Liu, Y., and Xu, P. (2022). Prosocial behavior and teachers’ attitudes towards bullying on peer victimization among middle school students: examining the cross-level moderating effect of classroom climate. Sch. Psychol. Rev. , 1–14. doi: 10.1080/2372966X.2021.2009313
Wentzel, K. R. (2002). Are effective teachers like good parents? Teaching styles and student adjustment in early adolescence. Child Dev. 73, 287–301. doi: 10.1111/1467-8624.00406
White, J., Bandura, A., and Bero, L. A. (2009). Moral disengagement in the corporate world. Accountability in Research 16, 41–74. doi: 10.1080/08989620802689847
Yoo, H. N., and Smetana, J. G. (2022). Distinctions between moral and conventional judgments from early to middle childhood: a meta-analysis of social domain theory research. Dev. Psychol. 58, 874–889. doi: 10.1037/dev0001330
Keywords: moral disengagement, collective moral disengagement, authoritative teaching, student-teacher relationship quality, bullying, peer aggression
Citation: Bjärehed M, Sjögren B, Thornberg R, Gini G and Pozzoli T (2024) A short-term longitudinal study on the development of moral disengagement among schoolchildren: the role of collective moral disengagement, authoritative teaching, and student-teacher relationship quality. Front. Psychol . 15:1381015. doi: 10.3389/fpsyg.2024.1381015
Received: 02 February 2024; Accepted: 09 April 2024; Published: 01 May 2024.
Reviewed by:
Copyright © 2024 Bjärehed, Sjögren, Thornberg, Gini and Pozzoli. This is an open-access article distributed under the terms of the Creative Commons Attribution License (CC BY) . The use, distribution or reproduction in other forums is permitted, provided the original author(s) and the copyright owner(s) are credited and that the original publication in this journal is cited, in accordance with accepted academic practice. No use, distribution or reproduction is permitted which does not comply with these terms.
*Correspondence: Marlene Bjärehed, [email protected]
Disclaimer: All claims expressed in this article are solely those of the authors and do not necessarily represent those of their affiliated organizations, or those of the publisher, the editors and the reviewers. Any product that may be evaluated in this article or claim that may be made by its manufacturer is not guaranteed or endorsed by the publisher.
The relationship between T7-Fz alpha coherence and peak performance in self-paced sports: a meta-analytical review
- Published: 01 May 2024
Cite this article
- Dhruv Raman ORCID: orcid.org/0000-0002-2692-4047 1 &
- Edson Filho ORCID: orcid.org/0000-0002-8548-4651 1
We examined whether the alpha-band coherence between the T7-Fz (verbal analytical-motor planning) brain areas were related to superior performance in sports. We searched for related papers across eight databases: ProQuest Central, ProQuest Psychology Journals, PsycARTICLES, PsycINFO, SPORTDiscus, MEDLINE, Scopus, and Web of Science using relevant keywords (i.e., EEG AND sports AND coherence). Seven studies, with a total of 194 participants, met our inclusion criteria and were shortlisted for statistical analysis. We compared EEG coherence data for both within-subject and between-subject experimental designs. Our analysis revealed that athletes had lower coherence in the T7-Fz brain pathway for alpha- band activation (Hedges’ g = − 0.54; p = 0.03) when performing better. Theoretically, these results corroborate the notion that athletes become more “neurally efficient” as the verbal and motor areas of their brains function more independently, i.e., the neural efficiency hypothesis. Accordingly, athletes who can limit verbal interference are more likely to perform a sporting task successfully.
This is a preview of subscription content, log in via an institution to check access.
Access this article
Price includes VAT (Russian Federation)
Instant access to the full article PDF.
Rent this article via DeepDyve
Institutional subscriptions
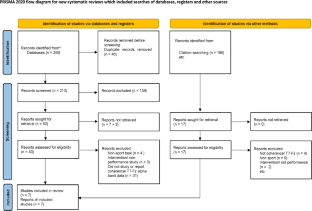
Data availability
The data used to conduct analysis is publicly available and the process used for data extraction is explained in the manuscript.
Acharya JN, Hani AJ, Cheek J, Thirumala P, Tsuchida TN (2016) American clinical neurophysiology society guideline 2: guidelines for standard electrode position nomenclature. Neurodiag J 56(4):245–252. https://doi.org/10.1080/21646821.2016.1245558
Article Google Scholar
Aminoff MJ (2012) Electroencephalography: general principles and clinical applications. In: Aminoff MJ (ed) Aminoff’s electrodiagnosis in clinical neurology. WB Saunders, pp 37–84
Arredondo MM, Hu XS, Satterfield T, Riobóo AT, Gelman SA, Kovelman I (2019) Bilingual effects on lexical selection: a neurodevelopmental perspective. Brain Lang 195:104640. https://doi.org/10.1016/j.bandl.2019.104640
Article PubMed PubMed Central Google Scholar
Bertollo M, di Fronso S, Conforto S, Schmid M, Bortoli L, Comani S, Robazza C (2016) Proficient brain for optimal performance: the MAP model perspective. PeerJ 4:e2082. https://doi.org/10.7717/peerj.2082
Bertollo, M., Doppelmayr, M., and Robazza, C. (2020) Using brain technologies in practice. In G. Tenenbaum and R. C. Eklund (Eds), Handbook of sport psychology. Wiley, 1st edn, pp. 666–693 https://doi.org/10.1002/9781119568124.ch32
Borenstein M, Hedges LV, Higgins JP, Rothstein HR (2010) A basic introduction to fixed-effect and random-effects models for meta-analysis. Res Synth Method 1(2):97–111. https://doi.org/10.1002/jrsm.12
Borenstein M, Hedges LV, Higgins JP, Rothstein HR (2021) Introduction to meta-analysis (2nd edn). Wiley
Book Google Scholar
Busk J, Galbraith GC (1975) EEG correlates of visual-motor practice in man. Electroencephalogr Clin Neurophysiol 38(4):415–422. https://doi.org/10.1016/0013-4694(75)90265-5
Article CAS PubMed Google Scholar
Cheng MY, Wang KP, Hung CL, Tu YL, Huang CJ, Koester D, Schack T, Hung TM (2017) Higher power of sensorimotor rhythm is associated with better performance in skilled air-pistol shooters. Psychol Sport Exerc 32:47–53. https://doi.org/10.1016/j.psychsport.2017.05.007
Cheng MY, Wang KP, Doppelmayr M, Steinberg F, Hung TM, Lu C, Tan YY, Hatfield B (2023) QEEG markers of superior shooting performance in skilled marksmen: an investigation of cortical activity on psychomotor efficiency hypothesis. Psychol Sport Exerc 65:102320. https://doi.org/10.1016/j.psychsport.2022.102320
Article PubMed Google Scholar
Cheron G, Petit G, Cheron J, Leroy A, Cebolla A, Cevallos C, Petieau M, Hoellinger T, Zarka D, Clarinval AM, Dan B (2016) Brain oscillations in sport: toward EEG biomarkers of performance. Front Psychol 7:246. https://doi.org/10.3389/fpsyg.2016.00246
Cheron G, Petit G, Cheron J, Leroy A, Cebolla A, Cevallos C, Petieau M, Hoellinger T, Zarka D, Clarinval A-M, Dan B (2016) Brain oscillations in sport: toward EEG biomarkers of performance. Front Psychol 7:246. https://doi.org/10.3389/fpsyg.2016.00246
Coben R, Wright EK, Decker SL, Morgan T (2015) The impact of coherence neurofeedback on reading delays in learning disabled children: a randomized controlled study. NeuroRegulation 2(4):168–178. https://doi.org/10.15540/nr.2.4.168
Cohen MX (2017) Where does EEG come from and what does it mean? Trends Neurosci 40(4):208–218. https://doi.org/10.1016/j.tins.2017.02.004
Deeny SP, Hillman CH, Janelle CM, Hatfield BD (2003) Cortico-cortical communication and superior performance in skilled marksmen: an EEG coherence analysis. J Sport Exerc Psychol 25(2):188–204. https://doi.org/10.1123/jsep.25.2.188
Deeny SP, Haufler AJ, Saffer M, Hatfield BD (2009) Electroencephalographic coherence during visuomotor performance: a comparison of cortico-cortical communication in experts and novices. J Mot Behav 41(2):106–116. https://doi.org/10.3200/JMBR.41.2.106-116
Ellmers TJ, Machado G, Wong TWL, Zhu F, Williams AM, Young WR (2016) A validation of neural co-activation as a measure of attentional focus in a postural task. Gait Posture 50:229–231. https://doi.org/10.1016/j.gaitpost.2016.09.001
Filho E, Di Fronso S, Mazzoni C, Robazza C, Bortoli L, Bertollo M (2015) My heart is racing! Psychophysiological dynamics of skilled racecar drivers. J Sports Sci 33(9):945–959
Filho E, Dobersek U, Husselman T (2021) The role of neural efficiency, transient hypofrontality and neural proficiency in optimal performance in self-paced sports: a meta-analytic review. Exp Brain Res 239:1381–1393. https://doi.org/10.1007/s00221-021-06078-9
Gallicchio G, Cooke A, Ring C (2016) Lower left temporal-frontal connectivity characterizes expert and accurate performance: high-alpha T7-Fz connectivity as a marker of conscious processing during movement. Sport Exerc Perform Psychol 5(1):14–24. https://doi.org/10.1037/spy0000055
Gazzaley, A., Lee, T., & D'Esposito, M. (2018). The frontal lobes and cognitive control. In B.L. Miller, and J.L., Cummings (Eds), The human frontal lobes: Functions and disorders (3rd ed., pp. 103–123). The Guilford Press
Hatfield BD (2018) Brain dynamics and motor behavior: a case for efficiency and refinement for superior performance. Kinesiol Rev 7(1):42–50. https://doi.org/10.1123/kr.2017-0056
Hatfield BD, Landers DM, Ray WJ (1984) Cognitive processes during self-paced motor performance: an electroencephalographic profile of skilled marksmen. J Sport Exerc Psychol 6(1):42–59. https://doi.org/10.1123/jsp.6.1.42
Hatfield BD, Landers DM, Ray WJ (1987) Cardiovascuiar-CNS interactions during a self-paced, intentional attentive state: elite marksmanship performance. Psychophysiology 24(5):542–549. https://doi.org/10.1111/j.1469-8986.1987.tb00335.x
Hatfield, B. D., Jaquess, K. J., Lo, L. C., and Oh, H. (2020). The cognitive and affective neuroscience of superior athletic performance. In G. Tenenbaum and R. C. Eklund (Eds.), Handbook of sport psychology (pp. 487–512). John Wiley and Sons. https://doi.org/10.1002/9781119568124.ch23
He M, Qi C, Lu Y, Song A, Hayat SZ, Xu X (2018) The sport expert’s attention superiority on skill-related scene dynamic by the activation of left medial frontal Gyrus: an ERP and LORETA study. Neuroscience 379:93–102. https://doi.org/10.1016/j.neuroscience.2018.02.043
Hedges LV, Olkin I (1985) Statistical methods for meta-analysis. Academic Press
Google Scholar
Holmes PS, Wright DJ (2017) Motor cognition and neuroscience in sport psychology. Curr Opin Psychol 16:43–47. https://doi.org/10.1016/j.copsyc.2017.03.009
Jackson AF, Bolger DJ (2014) The neurophysiological bases of EEG and EEG measurement: a review for the rest of us. Psychophysiology 51(11):1061–1071. https://doi.org/10.1111/psyp.12283
Jackson SR, Jackson GM, Roberts M (1999) The selection and suppression of action: ERP correlates of executive control in humans. NeuroReport 10(4):861–865. https://doi.org/10.1097/00001756-199903170-00035
Konttinen N, Lyytinen H, Viitasalo J (1998) Rifle-balancing in precision shooting:behavioral aspects and psychophysiological implication. Scand J Med Sci Sports 8(2):78–83. https://doi.org/10.1111/j.1600-0838.1998.tb00172.x
Kerick SE, McDowell K, Hung TM, Santa Maria DL, Spalding TW, Hatfield BD (2001) The role of the left temporal region under the cognitive motor demands of shooting in skilled marksmen. Biol Psychol 58(3):263–277. https://doi.org/10.1016/S0301-0511(01)00116-8
Lambert, M. I., Viljoen, W., Bosch, A., Pearce, A. J., and Sayers, M. (2008). General principles of training. Olympic textbook of medicine in sport, 1–48. https://doi.org/10.1002/9781444300635.ch1
Landers DM, Han M, Salazar W, Petruzzello SJ et al (1994) Effects of learning on electroencephalographic and electrocardiographic patterns in novice archers. Int J Sport Psychol 25(3):313–330
Li L, Smith DM (2021) Neural efficiency in athletes: a systematic review. Front Behav Neurosci 15:698555. https://doi.org/10.3389/fnbeh.2021.698555
Lo LC, Hatfield BD, Wu CT, Chang CC, Hung TM (2019) Elevated state anxiety alters cerebral cortical dynamics and degrades precision cognitive-motor performance. Sport Exerc Perform Psychol 8(1):21–37. https://doi.org/10.1037/spy0000155
Masic I, Jankovic SM (2021) Inflated co-authorship introduces bias to current scientometric indices. Medical Archives 75(4):248–255. https://doi.org/10.5455/medarh.2021.75.248-255
Masters R, Maxwell J (2008) The theory of reinvestment. Int Rev Sport Exerc Psychol 1(2):160–183. https://doi.org/10.1080/17509840802287218
Mesagno C, Beckmann J (2017) Choking under pressure: theoretical models and interventions. Curr Opin Psychol 16:170–175. https://doi.org/10.1016/j.copsyc.2017.05.015
Mesagno C, Hill DM (2013) Definition of choking in sport: re-conceptualization and debate. Int J Sport Psychol 44(4):267–277. https://doi.org/10.7352/IJSP2013.44.267
Mottaz A, Solcà M, Magnin C, Corbet T, Schnider A, Guggisberg AG (2015) Neurofeedback training of alpha-band coherence enhances motor performance. Clin Neurophysiol 126(9):1754–1760. https://doi.org/10.1016/j.clinph.2014.11.023
Newson JJ, Thiagarajan TC (2019) EEG frequency bands in psychiatric disorders: A review of resting state studies. Frontiers in human neuroscience 12, Article 521. https://doi.org/10.3389/fnhum.2018.00521
Pacheco NC (2016) Neurofeedback for peak performance training. J Mental Health Counse 38(2):116–123
Park JL, Fairweather MM, Donaldson DI (2015) Making the case for mobile cognition: EEG and sports performance. Neurosci Biobehav Rev 52:117–130. https://doi.org/10.1016/j.neubiorev.2015.02.014
Parr JV, Gallicchio G, Wood G (2021) EEG correlates of verbal and conscious processing of motor control in sport and human movement: a systematic review. Int Rev Sport Exerc Psychol. https://doi.org/10.1080/1750984X.2021.1878548
Rohatgi, A. (2022). WebPlotDigitizer (Version 4.6) [Computer software]. https://automeris.io/WebPlotDigitizer
Saikley A, Haroush K (2021) Toward a neurobiological model of human performance under pressure. Proc Natl Acad Sci USA 118(36):e2113777118. https://doi.org/10.1073/pnas.2113777118
Article CAS PubMed PubMed Central Google Scholar
Tenenbaum G, Filho E (2015) Measurement considerations in performance psychology. In: Raab M, Lobinger B, Hoffmann S, Pizzera A, Laborde S (eds) Performance psychology: perception, action, cognition, and emotion. Elsevier, pp 31–44
Tenenbaum G, Basevitch I, Gershgoren L, Filho E (2013) Emotions—decision-making in sport: theoretical conceptualization and experimental evidence. Int J Sport Exercise Psychol 11(2):151–168. https://doi.org/10.1080/1612197X.2013.773687
van Duijn T, Hoskens MC, Masters RS (2019) Analogy instructions promote efficiency of cognitive processes during hockey push-pass performance. Sport Exerc Perform Psychol 8(1):7–20. https://doi.org/10.1037/spy0000142
Von Stein A, Sarnthein J (2000) Different frequencies for different scales of cortical integration: from local gamma to long range alpha/theta synchronization. Int J Psychophysiol 38(3):301–313
Voss MW, Prakash RS, Erickson KI, Basak C, Chaddock L, Kim JS, Kramer AF (2010) Plasticity of brain networks in a randomized intervention trial of exercise training in older adults. Front Aging Neurosci 2:1803. https://doi.org/10.3389/fnagi.2010.00032
Wang KP, Cheng MY, Chen TT, Huang CJ, Schack T, Hung TM (2020) Elite golfers are characterized by psychomotor refinement in cognitive-motor processes. Psychol Sport Exerc 50:101739. https://doi.org/10.1016/j.psychsport.2020.101739
Wang KP, Cheng MY, Chen TT, Lin KH, Huang CJ, Schack T, Hung TM (2022) Successful motor performance of a difficult task: reduced cognitive-motor coupling. Sport Exerc Perform Psychol 11(2):174. https://doi.org/10.1037/spy0000279
Article CAS Google Scholar
Wolf S, Brölz E, Keune PM, Wesa B, Hautzinger M, Birbaumer N, Strehl U (2015) Motor skill failure or flow-experience? Functional brain asymmetry and brain connectivity in elite and amateur table tennis players. Biol Psychol 105:95–105. https://doi.org/10.1016/j.biopsycho.2015.01.007
Woo M, Kim Y (2017) Inter-and intrahemispheric EEG coherence and visuomotor performance during shooting competition and practice. Percept Mot Skills 124(4):830–845. https://doi.org/10.1177/0031512517709150
Xiang MQ, Hou XH, Liao BG, Liao JW, Hu M (2018) The effect of neurofeedback training for sport performance in athletes: a meta-analysis. Psychol Sport Exerc 36:114–122. https://doi.org/10.1016/j.psychsport.2018.02.004
Yarrow K, Brown P, Krakauer JW (2009) Inside the brain of an elite athlete: the neural processes that support high achievement in sports. Nat Rev Neurosci 10(8):585–596. https://doi.org/10.1038/nrn2672
Yu R (2015) Choking under pressure: the neuropsychological mechanisms of incentive-induced performance decrements. Front Behav Neurosci 9:19. https://doi.org/10.3389/fnbeh.2015.00019
Zach S, Dobersek U, Inglis V, Tenenbaum G (2018) A meta-analysis of mental imagery effects on post-injury functional mobility, perceived pain, and self-efficacy. Psychol Sport Exerc 34:79–87. https://doi.org/10.1016/j.psychsport.2017.09.011
Zhu FF, Poolton JM, Wilson MR, Maxwell JP, Masters RSW (2011) Neural co-activation as a yardstick of implicit motor learning and the propensity for conscious control of movement. Biol Psychol 87(1):66–73. https://doi.org/10.1016/j.biopsycho.2011.02.004
Download references
Acknowledgements
Author information, authors and affiliations.
Performance Recovery and Optimization Lab, Wheelock College of Education and Human Development, Boston University, 2 Silber Way, Boston, MA, 02215, USA
Dhruv Raman & Edson Filho
You can also search for this author in PubMed Google Scholar
Contributions
Dhruv Raman (first author) conducted primary data search, extraction and manuscript writing; Edson Filho (lastauthor) supervised project, conducted data analysis, manuscript writing and editing.
Corresponding author
Correspondence to Edson Filho .
Ethics declarations
Conflict of interest.
We have no conflict of interest to disclose.
Ethics approval and consent to participate
Not applicable.
Consent for publication
I have consent to publish from the co-author.
Additional information
Communicated by Melvyn A. Goodale.
Publisher's Note
Springer Nature remains neutral with regard to jurisdictional claims in published maps and institutional affiliations.
Rights and permissions
Springer Nature or its licensor (e.g. a society or other partner) holds exclusive rights to this article under a publishing agreement with the author(s) or other rightsholder(s); author self-archiving of the accepted manuscript version of this article is solely governed by the terms of such publishing agreement and applicable law.
Reprints and permissions
About this article
Raman, D., Filho, E. The relationship between T7-Fz alpha coherence and peak performance in self-paced sports: a meta-analytical review. Exp Brain Res (2024). https://doi.org/10.1007/s00221-024-06833-8
Download citation
Received : 07 May 2023
Accepted : 08 April 2024
Published : 01 May 2024
DOI : https://doi.org/10.1007/s00221-024-06833-8
Share this article
Anyone you share the following link with will be able to read this content:
Sorry, a shareable link is not currently available for this article.
Provided by the Springer Nature SharedIt content-sharing initiative
- Sports performance
- Neurofeedback
- Neural efficiency
- Find a journal
- Publish with us
- Track your research

Quick links
- Find a course
- Request a prospectus
- Postgraduate
Psychology Conversion MSc
Search the website.
Study a BPS accredited Psychology Conversion master's at Middlesex University and learn from our highly respected team
Register your interest
- Book an open day
/199x0:1702x1100/prod01/channel_3/media/middlesex-university/courses-images/ug-courses/Psychology.jpg)
Viewing course details for 2024 year of entry
- Course overview
About your course
- Teaching and learning
Facilities and support
Entry requirements.
- Download prospectus
Why choose a Psychology Conversion masters degree at Middlesex University?
This course is accredited by the British Psychological Society (BPS) which means you'll gain Graduate Basis of Chartered Membership once you successfully complete this master's course.
If you want to start a career in psychology and/or acquire the Graduate basis for Chartered Membership of the British Psychological Society, this course is for you.
You may have a degree in another subject but wish to change career or pursue your interest in psychology in more depth. We will teach you a broad range of theoretical and methodological approaches and encourage you to develop a critical approach to studies, evaluating theory and evidence.
We have been teaching Psychology for over 50 years and are one of the largest providers of Psychology education in the UK.
What you will gain
By the end of this course, you will be able to perform quantitative and qualitative data analyses and use statistical software to analyse complex data. You will design, implement, analyse and communicate independent and group project work to an advanced level culminating in a dissertation on an agreed topic. You will also be able to design and run experiments to gather and interpret data. You will have gained knowledge in the core areas in psychology and be able to apply research skills across domains.
You will learn using cutting-edge facilities including fully equipped lecture theatres and tutorial spaces, a psychophysiology laboratory, social observation laboratories, an auditory cognition laboratory, and testing cubicles.
Accreditations
Our course is accredited by the British Psychological Society (BPS) . This accreditation means that successfully completing the graduate course with an average of 50% or better confers the Graduate Basis for Chartered (GBC) Membership with the BPS.
The BPS is a professional body of graduates, many of whom work as psychologists and the main aim of the association is to maintain high standards in the discipline. Some of the benefits of being eligible to become a member of the BPS include being able to join special interest sections and divisions of the BPS (e.g. Developmental Section, Cognitive Section, etc.) receiving The Psychologist house journal and appointments memorandum, as well as being able to order books and journals at special reduced rates.
After completion of our course, many students go on to study in a more specialised area in psychology. A BPS-recognised degree is often a necessary first step for those who wish to pursue further training and careers in particular areas of psychology such as Clinical, Counselling, Educational, Forensic, Health & Occupational Psychology and for those wanting to obtain chartered status as a practicing psychologist.
3 great reasons to pick this course
Use specialist facilities and equipment.
Use our state-of-the-art facilities and specialist equipment include a psychophysiology laboratory, social observation laboratories, an auditory cognition laboratory, and testing cubicles
BPS accredited course
This course is accredited by the British Psychological Society (BPS), so you’ll gain Graduate Basis for Chartered (GBC) membership
International Research
Our academics are regularly and widely published, and 95% of our research is internationally recognised.
You will learn cognitive skills through class discussion, guided independent study and lecturer feedback, as well as learning practical skills through presenting your ideas in written and oral form along with participation in practical exercises.
You will gain an in-depth understanding of your subject through participation in teaching sessions and independent research, with tutor support. Your learning will also be supported with online self-study materials.
Here is a brief overview of what you will study each year
Social, Personality & Developmental (30 credits)
This BPS core module aims to develop your expertise and mastery of primary and contemporary theory and research in three essential areas: social psychology, developmental psychology and individual differences.
The subject matter is introduced and investigated through topic-focused lectures and augmented with advanced research workshops. A focus on developmental psychology will see you study the biological, social, emotional and cognitive processes and changes of infancy and childhood as these are the periods during an individual's lifespan when the most change occurs.
In social psychology, interpersonal and intergroup processes will be studied with the objective of developing your understanding of the social dimension to human psychology. Throughout the module, several aspects of psychological approaches to understanding individual differences will be considered such as humanistic theories of development and social learning theory.
Research: Practice and Reporting (60 credits)
This module aim to provide you with an opportunity for an in-depth, advanced study in a specific area of applied psychology, pertinent to the degree for which you are registered, guided by, but largely independent of, tutor support. You will apply appropriate principles of empirical research, and present your research study in the form of a written journal article, using appropriate styles and conventions.
Research Methods (30 credits)
This module conforms with the statistics and methodology requirements of the British Psychological Society’s Graduate Basis for Chartered Membership (GBC). The module enables you to understand & critically evaluate psychological research & to understand how research design relates to research questions. It provides you with advanced skills in a variety of statistical analyses and enables them to conduct ethical psychological research at masters level utilising quantitative and qualitative methods. It provides skills in interpretation & critical discussion of published psychological research. It prepares you for your dissertation and enables you to choose appropriate methodologies and analyses for research.
Research and Issues in Applied Psychology (30 credits)
The aim of the module is to provide an intellectual setting within which you can both develop a fuller appreciation of substantive areas of applied psychology and improve their practical and analytical skills. The main aim is to direct you to develop an appreciation of the controversies and issues related to traditional and contemporary research, ethical issues, and up-to-date knowledge in the domain of applied psychology such as neuropsychology, infertility, sports and exercise and literacy acquisition.
Brain, Body and Mind (30 credits)
The module will give you an overview of biological and cognitive psychology as well as an introduction to the biological basis of heritable traits. The anatomy and physiology of the nervous system is considered; attention is focused on aspects of behaviour that have a clear biological component, with an emphasis on individual differences in biology. Through a series of psychophysiology lab sessions you will investigate specific central and peripheral nervous system variables and their relation to behaviour and individual differences. The cognitivist approach to psychology will be outlined and key theories relating to major cognitive faculties explored. Understanding of these is enhanced through a series of interactive seminars, consisting of group problem-solving activities. Both cognitive and biological approaches will explore pathology and neuropsychological case studies.
To find out more about this course, please download the Psychology Conversion MSc specification (PDF) .
Sign up now to receive more information about studying at Middlesex University London.
You'll be taught by an experienced teaching team with a wide range of expertise and professional experience.
How we'll teach you
Coursework and assessments.
You will participate in practical sessions, lectures, seminars and workshops, where you will take part in class discussions, and work on research projects, group assignments and critical analyses. Teaching is in person, on campus and supplemented with bespoke online resources. You will also be expected to engage in independent study and tutorial support is available for all modules.
The MSc can be studied over 12 months full-time or 24 months part-time. Part-time students take 60 taught credits and start their dissertation in year 1 and 60 taught credits and complete their dissertation in year 2.
The course is assessed at 180 credit points. Each 30-credit module represents approximately 300 hours of student study time, with two-four hours of contact time per week. The postgraduate dissertation module is 60 credits and encourages students to work independently, develop expertise in a particular area of psychology and demonstrate advanced research skills.
For two of the taught modules, some lecture content will be taught along with our undergraduate students. All modules include small group teaching sessions which are specifically designed and exclusive for the MSc Psychology conversion students. Staff are also available for individual tutorials. You will supplement all this with your own independent study and will submit a dissertation. There is a specific module on research methods for conversion students and the course also aims to improve your analytical, statistical and IT skills.
Where will I study?
You'll be based at our Hendon campus, north London.
Whether you are studying full or part-time – your course timetable will balance your study commitments on campus with time for work, life commitments and independent study.
We aim to make timetables available to students at least 2 weeks before the start of term. Some weeks are different due to how we schedule classes and arrange on-campus sessions.
Teaching vs independent study
In a typical year, you’ll spend about 1200 hours on your course.
Outside of teaching hours, you’ll learn independently through reading articles and books, working on projects, undertaking research, and preparing for assessments including coursework and presentations.
Typical weekly breakdown
The table below gives you an idea of what learning looks like across a typical week. Some weeks are different due to how we schedule classes and arrange on-campus sessions.
You'll receive full information on your teaching before you start your course.
A typical week looks like this:
Learning terms
On-campus: This includes tutor-led sessions such as seminars, lab sessions and demonstrations as well as student-led sessions for work in small groups.
Online learning: This is teaching that is delivered online using tools like Skype or Zoom, as well as work that you do yourself using online teaching resources.
Independent study: This is the work you do in your own time including reading and research.
Part-time study
You can also study this course part-time.
Academic support
We have a strong support network online and on campus to help you develop your academic skills. We offer one-to-one and group sessions to develop your learning skills together with academic support from our library, IT teams and learning experts.
A wide variety of assessment methods are employed on the course including laboratory reports, essays, poster presentations, other written assignments and practical tests.
A major part of your assessment will be your 7,000 to 10,000-word report in the style of a journal article. We will be looking, among other things, at your ability to look critically at both theory and empirical research, and material from different sources.
You will receive regular feedback on your work.
/0x0:1050x1407/prod01/channel_3/media/middlesex-university/courses-images/ug-courses/facilities/north-london-campus-banner.jpg)
North London campus
Our north London campus is 23 minutes away by underground train, travelling from London Kings Cross.
Our Sheppard Library provides a wide range of resources and support to help you to succeed in your studies.
/159x0:1342x1000/prod01/channel_3/media/middlesex-university/courses-images/ug-courses/business-and-law/007---Students-in-the-Sheppard-Library.jpg)
Over 1,000 study spaces including rooms for group study and over 500 computers available.
/101x0:851x634/prod01/channel_3/media/middlesex-university/courses-images/ug-courses/facilities/Sheppard-Library-Facilities-2014-40.jpg)
We have one of London's biggest and best university campuses with everything you need in one place.
Student support.
We offer lots of support to help you while you're studying including financial advice, wellbeing, mental health, and disability support.
Additional needs
We'll support you if you have additional needs such as sensory impairment or dyslexia. And if you want to find out whether Middlesex is the right place for you before you apply, get in touch with our Disability and Dyslexia team .
Our specialist teams will support your mental health. We have free individual counselling sessions, workshops, support groups and useful guides.
Work while you study
Our Middlesex Unitemps branch will help you find work that fits around uni and your other commitments. We have hundreds of student jobs on campus that pay the London Living Wage and above. Visit the Middlesex Unitemps page.
- International entry
- How to apply
We welcome your application regardless of your background or experience.
For this course, we are looking for these minimum qualifications:
- 2:1. but applicants who have a 2:2 with high module grades may be considered
- Other relevant qualifications equivalent to an honours degree
- Three years' relevant work experience and the ability to study at postgraduate level.
If you have relevant qualifications , we may be able to count this towards your entry requirements.
We welcome students from the UK, EU and all over the world. Join students from over 122 countries and discover why so many international students call our campus home:
Quality teaching with top facilities plus flexible online learning
Welcoming north London campus that's only 30 minutes from central London
Work placements and networking with top London employers
Career support to get you where you want to go after university.
Qualifications
Here are our requirements for this course:
If you have relevant qualifications or work experience , we may be able to count this towards your entry requirements.
English language
You'll need good English language skills to study with us. Find out more about our English language requirements. And, don’t worry If you don't meet our minimum English language requirements, as we offer an intensive pre-sessional English course .
To study with us in the UK, you will need a Student Route visa .
Apply as early as possible to make sure you get a place. You can submit your application before you receive your final qualification.
Personal statements
Make sure that you highlight your best qualities in your personal statement that are relevant to this course, such as your ability to be forward-thinking, creative and collaborative.
We'll invite you to attend an online interview. Visit our interview tips page for advice.
Find out how to apply for postgraduate taught courses and watch our step-by-step video.
How can the Psychological Therapies and Interventions MSc/PGDip support your career?
Our postgraduate courses have been recognised for their ability to support your career.
95% of our postgraduate students go on to work or further study – Graduate Outcomes, 2022
Career paths
There are excellent employment prospects for Psychology graduates. The range of professional skills that you will develop ensures that you are highly valued across the economy. You could progress into a successful career as a counsellor, social worker, teacher or academic, or pursue careers in areas such as law, business management or education.
Many of our graduates pursue further education in more specialised areas in psychology including clinical, forensic, health, educational and occupational psychology and our BPS-recognised degree facilitates this. Others have pursued careers in research and academia.
MDXworks, our employability service, will help you make the most of your experience and connections to achieve your career goals. You'll have unlimited access to one-to-one advice and support from specialists in your sector plus 24/7 online support. You can also make the most of events and networking opportunities, on and off campus.
Global alumni network
You’ll be studying with students from 122 countries who’ll become part of your personal global network. You'll learn how to work with a global mindset and make invaluable connections on your course for your chosen industry.
Fees and funding
The fees below are for the 2024/25 academic year.
UK students 1
Full-time students: £10,500
Part-time students: £70 per credit
Part-time students: £35 per dissertation credit
International students 2
Full-time students: £16,800
Part-time students: £112 per credit
Part-time students: £56 per dissertation credit
Additional costs
We cover your costs for the day-to-day things that you need to do well in your studies.
- Free – laptop loans, Wi-Fi and printing
- Free specialist software for your course
- Free online training with LinkedIn Learning
The following course-related costs are not included in the fees, and you will need to budget for these:
- The cost of course-related books you may wish to purchase
Financial support
We offer lots of support to help you with fees and living costs. Check out our guide to student life on a budget and find out more about postgraduate funding .
Postgraduate scholarships
You may be eligible for one of our scholarships including:
- The Alumni Postgraduate Award – for all UK/EU Middlesex alumni a 20% fee reduction
- The Commonwealth Scholarship – full course fees, airfares and a living allowance
- The Chevening Scholarship – full course fees
- The European Academic Awards – £1000 to £7000 for students showing academic excellence
- Regional or International Merit Award – up to £2,000 towards course fees
For international students, we also have a limited number of other awards specific to certain regions, and work in partnership with funding providers in your country to help support you financially with your study.
Find out more about our postgraduate scholarships .
Help from your employer
Your employer can contribute towards the cost of your postgraduate study as part of their staff development programme.
Fees disclaimers
1. UK fees: The university reserves the right to increase postgraduate tuition fees in line with changes to legislation, regulation and any government guidance or decisions. The tuition fees for part-time UK study are subject to annual review and we reserve the right to increase the fees each academic year by no more than the level of inflation.
2. International fees: Tuition fees are subject to annual review and we reserve the right to increase the fees each academic year by no more than the level of inflation.
Any annual increase in tuition fees as provided for above will be notified to students at the earliest opportunity in advance of the academic year to which any applicable inflationary rise may apply.
Get answers from our Unibuddy student ambassadors
Related courses for you.
/49x0:617x385/prod01/channel_3/media/middlesex-university/courses-images/foundation-courses/Foundation-Year-in-Visual-Arts.jpg)
- How to apply arrow_forward_ios
- Get in touch arrow_forward_ios
- Book an open day arrow_forward_ios
Explore your prospectus
Take your first step to the future you want with our guide to Middlesex
We’ll carefully manage any future changes to courses, or the support and other services available to you, if these are necessary because of things like changes to government health and safety advice, or any changes to the law.
Any decisions will be taken in line with both external advice and the University’s Regulations which include information on this.
Our priority will always be to maintain academic standards and quality so that your learning outcomes are not affected by any adjustments that we may have to make.
At all times we’ll aim to keep you well informed of how we may need to respond to changing circumstances, and about support that we’ll provide to you.
Why not explore
Join us on campus to discover more about our range of courses, and get a feel for life at Middlesex for yourself
The university promotes research and encourages students to participate in research projects
Accommodation
We’ll help you find your new home in London while you study at Middlesex
Student Life
Find out more about what life is like on Campus
Modal video
- Open access
- Published: 30 April 2024
Designing, implementation and evaluation of story reading: a solution to increase general empathy in medical students
- Masoumeh Mahmoudi 1 ,
- Ali Asghar Ghorbani 2 ,
- Mehdi Pourasghar 3 ,
- Azita Balaghafari 4 ,
- Jamshid Yazdani Charati 5 ,
- Nassim Ghahrani 6 &
- Farzaneh Amini 7
BMC Medical Education volume 24 , Article number: 477 ( 2024 ) Cite this article
Metrics details
Communication and mutual understanding among healthcare providers is a significant concern within the healthcare system, and enhancing empathy is one way to foster effective communication and mutual understanding. The aim of this research is to evaluate and compare the impact of story reading on the level of empathy in medical students at Mazandaran University of Medical Sciences.
The study employed an intervention educational design (a quasi-experimental), with a convenience sample of 51 medical students selected as the statistical population. The process of story reading was conducted over six two-hour virtual sessions in the students' classroom, spanning six weeks. Selected stories were discussed in an online virtual class under the supervision of an instructor, focusing on story elements. To assess students' empathy in this educational program, the Davis General Empathy Questionnaire was administered before each of the six sessions, after, and one week later at the end of the course. Statistical analysis of the collected data was performed using repeated measures analysis of variance and Bonferroni's post hoc test through SPSS version 28 software, with a significance level set at 0.05.
The findings revealed that 27 participants (58.7%) were female students, with the remaining being male students, having an average age of 19.5 ± 0.86 years. The level of general empathy among the students significantly increased after the intervention compared to before the intervention ( P <0.001). Furthermore, the analysis of variance with repeated measures indicated a significant effect of the story reading program on enhancing empathy in terms of emotional and cognitive transfer among students in the intervention group ( P <0.001).
Conclusions
The research findings suggest that the story reading program effectively enhances the overall sense of empathy among medical students at the University of Medical Sciences. Therefore, implementing this method in universities, higher education centers, libraries, and psychology centers for teaching empathy can be valuable in fostering empathy skills and improving healthcare.
Peer Review reports
Introduction
In the realm of medicine, even with remarkable technological progress, it remains imperative to maintain effective communication and personal interactions when interacting with and providing care for patients. Research outcomes underscore the importance of the doctor-patient relationship in comprehending and addressing the suffering caused by illnesses, thereby emphasizing the necessity to enhance this relationship [ 1 ]. Many researchers acknowledge the importance of empathy among healthcare professionals and the value of training in developing this skill [ 2 , 3 ]. Furthermore, investigations into complaints related to doctors confirm that many of these grievances are not linked to the doctors' scientific skills or efficiency but rather arise from their communication approach with patients [ 4 ]. In simple terms, it can be said that communication errors are the primary cause of most medical complaints and violations. While information and communication technology (ICT) have made obtaining health and medical information easier, medical technology cannot validate the doctor-patient relationship, which is built upon their mutual attitudes towards one another. As a result, a therapist's behavior may vary depending on their perception of and attitude towards their patient since people's behavior is influenced by their orientation towards others and their perceptions.
Carl Rogers, a renowned humanist in the early 20th century, believed that empathy was a crucial and effective process for facilitating psychological changes within the doctor-patient relationship [ 5 ]. Furthermore, doctor-patient empathy holds a significant ethical importance within the medical community [ 6 ], which has led to discussions on this topic during medical ethics congresses. The need for well-designed educational plans to enhance empathetic abilities is highlighted by research that indicates a lack of satisfaction in this area [ 7 ]. Empathy training can strengthen this moral virtue and improve the mental and spiritual well-being of patients by incorporating it into the medical curriculum [ 8 ]. Empathy skills will help healthcare providers better understand and address their patients’ emotional needs, thereby fostering more positive therapeutic outcomes.
We are narrative creatures and we grow by telling stories and listening to stories [ 9 ]. Many believe that storytelling has many benefits and as an educational tool can improve the ability to retain words, improve their vocabulary, encourage children to learn English. , increasing moral value in them and providing cheap media in teaching rich language experience and increasing students' interest in reading and improving listening and writing abilities [ 10 ]. Also some narration and storytelling are considered useful for treatment, intervention and as a tool and technique for collecting qualitative data about treatment processes [ 11 ]. There is a view that how to read a story and get to know the structure of a story text in literature has similarities with narrative medicine. In fact, just as literature deals with the sufferings and problems of humans, narrative medicine mainly deals with the sufferings and problems of patients, patients' families or health care doctors. Therefore, the narrative reading techniques used in the literature study can be used in the study of narrative medicine to better understand the suffering of patients and thus help medical students and health care professionals to communicate with patients. And this can be effective in creating empathy [ 12 ]. To apply the narrative approach in teaching, we must answer the key questions that are necessary to understand fiction; Questions like who, what, where, when and why? What is the storyline? How will it begin, develop and end? [ 13 ]. Indeed, the field of narrative medicine has provided a useful way to open up our understanding of clinical reasoning, as we realize that the task of arriving at a diagnosis and treatment plan is largely narrative in nature [ 14 ].
In addition, many researchers in the field of medical humanities [ 15 ], medical professionalism [ 16 ] and the study of literary fiction in the creation and development of clinical abilities for compassionate and professional interaction with the patient has been highlighted by the importance and significance of phenomenological research and the lived experience of illness [ 17 ]. They have used experimental methods such as FMRI to track the effect of reading a story text on brain activities and its positive effect [ 18 ]. Since reading literary fiction is a cheap and effective way to develop empathy, story-reading and discussion sessions about the plot of tales has been held for years for hospital staff [ 19 ].
Of course, many of these studies are dedicated to reading a text or a part of a literary text and that too in one session, and the research community in them consists of people who have a tendency to read a story text, so it is possible that the influence of the text is due to the tendency and preparation of them to learn more empathy. Another important aspect is that while these studies highlight the impact of reading literary stories on readers, improving their communication skills and fostering empathy, none of them have been specifically designed to incorporate educational lessons centered on text analysis and teaching of story elements as this current study does. Although we have not discovered any evidence of a similar process being implemented in educational institutions nationwide, internal studies have suggested that reading stories can have an impact on reducing behavioral inconsistencies or enhancing learning abilities [ 20 , 21 ].
Considering the researches that have raised the need for training and creating empathy among therapists (8, 40, 41 and 42), we decided to include reading the text of fiction and familiarizing with the elements of story structure in the curriculum of medical students. Our research has several features: using Persian stories, reading stories along with explaining and discussing their story elements, and placing these training sessions in the students' curriculum and implementing it in 6 two-hour training sessions.
These four theories (cognitive Learning theory, behaviorism Learning theory, Constructivism Learning and Connectivism Learning) can be effective in storytelling. According to the cognitive theory, internal and external factors can affect the learners, and in the behaviorist learning theory, the concept is emphasized that the behavior of the learners depends on how they interact with their environment. In constructivism learning theory, the learner designs its learning based on previous experiences, and Connectivism learning theory focuses on the concept that people learn and grow when creating relationships [ 10 ].
Therefore, it seems that storytelling and active exposure to story can be considered by each of these theories, and considering the relationship between the understanding of the text structure of fictional story and the story of patients and diseases, storytelling and knowing the elements and structure of the story can be effective in creating narrative skills in learners.
Currently, although the significance of empathy skills is acknowledged in courses such as medical ethics, the teaching of this skill is not officially sanctioned in medical courses. Given the impact of accurately understanding a story in cultivating empathy, it is worth considering the inclusion of indirect instruction on this skill in the Persian literature course. This course is a three-unit general course. Medical students are mandated to successfully complete three units of Persian literature as part of their curriculum. The course content has been authorized by the Ministry of Science, although the Ministry of Health's educational planning office has yet to designate an official curriculum for it. Despite being approved by the Ministry of Science with the aim of providing comprehensive education in the Persian language and developing proficient reading and writing skills, critics argue that the general Persian course falls short in adhering to the approved curriculum. They claim that the lecturers, disregarding the audience's needs, tend to design educational content based on their personal preferences and areas of expertise [ 22 , 23 ]. In recent years, the importance of focusing on empathy skills among medical staff and the need for their training has consistently been emphasized in medical ethics meetings and conferences. Therefore, the authors of this article recognize the significance of enabling medical students to enhance their empathy skills. They also acknowledge the potential impact that reading literary stories can have on improving this skill. Consequently, they have decided to focus on teaching the art of accurately interpreting stories. Therefore, this study aims to evaluate and compare the impact of story reading on the level of empathy in medical students at Mazandaran University of Medical Sciences.
We designed an intervention educational study (a quasi-experimental) with utilizing the ADDIE model, which comprises of five primary elements: Analysis, Design, Development, Implementation, and Evaluation. Out of the 51 medical students (Second year) enrolled at medical school of Mazandaran University of Medical Sciences in Sari, 46 students participated in this research study during the first semester of the academic year 2021. During the design phase, the researchers developed the stories, execution method, and test method. Before each session, the students read the selected stories specific to that session. Then, they were required to participate in an exam based on the content of the stories and the session. To assess the students' empathy levels (Design phase), we utilized the Davis empathy questionnaires prior to and following the sessions. Three subscales of the IRI (Interpersonal Reactivity Index) (Davis, 1980) were used to assess empathic concern (EC), perspective-taking (PT) and Fantasy Scale (FS). Consisted of seven items answered on a 5-point scale (describes me very well to does not describe me at all). Which each of subscales included seven items that were answered on a 5-point scale (very agree to very disagree).
The EC subscale measures the feelings of compassion and concern for others, also known as emotional empathy. For example, "I often become emotionally affected by witnessing events". The PT subscale measures the inclination to consider the psychological viewpoint of others, also known as cognitive empathy. For example, "I make an effort to pay attention to the opposing perspective of each person before engaging in a debate". Also, the FS subscale is a measure of an individual's tendency to identify with fictitious characters from books, films, or video games. (e.g., “When I am reading an interesting story or novel, I imagine how I would feel if the events in the story were happening to me”).
During the developmental phase, we gathered the stories using research methods that have established the impact of reading stories on empathy. For this study, we selected stories from prestigious literary festivals or renowned authors who have caught the attention of literary criticism theorists and were carefully chosen by literature experts. Additionally, this aspect is encompassed in the selection of stories that are deemed appropriate for teaching story elements during the assigned sessions. During the implementation phase, students who expressed disinterest in participating in the research were instructed to study the material covered in the in-person classes and respond to tailored questions in the final examination. Please note that the offline content lacks criticism and analysis of the story, and it consists of typical educational texts found in this course. Additionally, it includes ancient Persian texts that have been translated into modern Persian poetry and prose.
In this study, the reading of literary texts was conducted virtually and online in the classroom of medical students for a total of six 2-hour sessions over a period of six weeks. This arrangement was necessary due to the Covid-19 pandemic and the resulting limitations on in-person educational activities. The reason for allocating 2 hours of time for this training over 6 sessions is to equate it to 1 lesson unit. This unit will be included in the proposed subject of a 3-unit Persian literature course for medical students. In order to assess the students' empathy during this educational course, the Davis General Empathy Questionnaire was administered before each of the six sessions, after, and one week later at the end of the course.
Another important aspect is that during these sessions, the teacher offered explanations on story elements by referring to relevant specialized books. The stories selected for this study were based on the author's esteemed reputation and literary recognition, as supported by research in this field [ 24 , 25 , 26 , 27 , 28 , 29 ]. The stories were selected by the literature expert (MM) who taught the training sessions. These stories are known in Persian literature and have famous authors. As mentioned in the Additional file 1 : Appendix 1, some of these stories have won awards from festivals, and the rest are famous writers of Persian literature, and have been selected from the book "Short Story in Iran" by Dr. Hossein Payandeh, a well-known Iranian critic, holder of a chair of literary criticism and Theorizing in Allameh Tabatabai University.
After every session, in order for the professor to make sure that the students' attention, listening and active presence in the class, a test was administered, which focused on the topics covered during that session. This was done in support of the measured empathy resulting from reading the story. Additionally, the level of student satisfaction with the educational material and the manner in which the sessions were conducted was gauged using an interview and self-expression by students. To assess the durability of empathy, students were required to complete the Davis General Empathy Questionnaire one week later at the end of the course.
We evaluated 46 students, including 27 (58.7%) female students and the rest male students with an average age of 19.5 ± 0.8. The highest age of the intervention subjects was 22 years and the youngest was 18 years. In this study, empathy was evaluated in three time periods before the intervention, after the intervention, and then 7 days after the end of the intervention, and the results listed below are related to the overall empathy scores (Table 1 ).
To compare overall empathy, analysis of variance with repeated measurement was used because Mauchly's Test of Sphericity showed significant results ( P <0.001), therefore Greenhouse- Geisser test was used and a significant difference was observed in three periods of overall empathy measurement ( P <0.001).
For comparisons in three times of measuring empathy, the results listed in the following table were also obtained: the type of paired t-test and the significance level of 5%, which was considered to be 0.015 with Bonferroni's correction based on the three times of measurement (Table 2 ).
The results showed that the average empathy score increased by 18.2 ± 0.97 after the intervention ( P < 0.001). And in the third time compared to the second time, it increased by 16.25 ± 0.95 ( P <0.001). In the third time, it decreased by 1.94 ± 0.54 compared to the second time ( P =0.003), whose graph is as follows (Fig. 1 ).
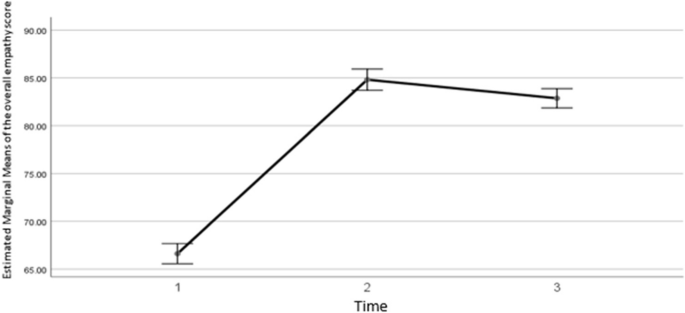
The changing trend of the overall average empathy score in three time periods
The results showed that the overall average score of empathy increased in the third time compared to the first time. In the first dimension of empathy (EC=empathic concern), the results were calculated as follows (Table 3 ).
The above results show the description of scores with a 95% confidence interval in the first dimension of empathy. To compare the first component of empathy, analysis of variance with repeated measurements was used, Mauchly's Test of Sphericity showed significant results ( P <0.001), therefore Greenhouse-Geisser test was used and a significant difference was observed in the first three measurement periods of the first component of empathy ( P <0.001). For comparisons, the results listed in the following table were obtained these three times by measuring the first dimension of empathy: the type of paired t-test and a significance level of 5%, which was considered to be 0.015 with Bonferroni's correction based on the three times of measurement (Table 4 ).
The results showed that the average score of the first dimension of empathy increased by 5.92 ± 0.4997 after the intervention ( P < 0.001). And in the third time compared to the second time, it increased by 5.07 ± 0.56 ( P <0.001). Also, the third time, it decreased by 0.85 ± 0.28 compared to the second time ( P =0.013) whose diagram is as follows (Fig. 2 ).
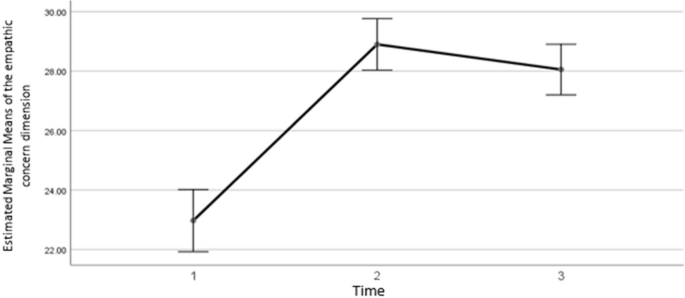
The changing trend of the average empathy score in the dimension of empathic concern in three time periods
The results showed that the average score of empathy in the dimension of empathic concern increased in the third time compared to the first time. In the second dimension of empathy (PT=perspective-taking), the results were calculated as follows (Table 5 ).
The above results show the description of scores with a 95% confidence interval in the second dimension of empathy. To compare the second component of empathy, analysis of variance with repeated measurements was used, Mauchly's Test of Sphericity showed significant results ( P <0.001), the Greenhouse-Geisser test was used and a significant difference was observed in the three measurement periods of the second component of empathy ( P <0.001). For comparisons in these three times of measuring the second dimension of empathy, the results listed in the following table were also obtained: the type of paired t-test and the significance level of 5%, which was considered to be 0.015 with Bonferroni's correction according to the three times of measurement (Table 6 ).
The results showed that the average score of the second dimension of empathy increased by 6.37 ± 0.46 after the intervention ( P <0.001), and in the third time compared to the second time it increased by an average of 5.94±0.48 ( P <0.001). Also, in the third time, no significant difference was observed compared to the second time ( P =0.61), whose graph is as follows (Fig. 3 ).
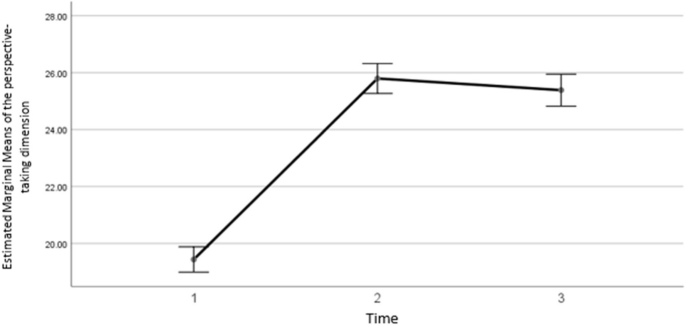
The changing trend of the average empathy score in the dimension of perspective-taking in three time periods
The results showed that the average score of empathy in the dimension of e perspective-taking increased in the third time compared to the first time. In the third dimension of empathy (FS= Fantasy Scale), the results were calculated as follows (Table 7 ).
The above results show the description of scores with a 95% confidence interval in the third dimension of empathy. To compare the third component of empathy, analysis of variance with repeated measurements was used, Mauchly's Test of Sphericity showed significant results ( P <0.001), the Greenhouse-Geisser test was used and a significant difference was observed in the three measurement periods of the third component of empathy ( P <0.001). For comparisons in these three times of measuring the third dimension of empathy, the results listed in the following table were also obtained: the type of paired t-test and the significance level of 5%, which was considered to be 0.015 with Bonferroni's correction according to the three times of measurement (Table 8 ).
The results showed that the average score of the third dimension of empathy increased by 5.92 ± 0.43 after the intervention ( P <0.001), and in the third time compared to the second time it increased by an average of 5.23±0.47 (P < 0.001). Also, in the third time, no significant difference was observed compared to the second time ( P =0.028), whose graph is as follows (Fig. 4 ). The results showed that the average score of empathy in the dimension of Fantasy Scale increased in the third time compared to the first time.
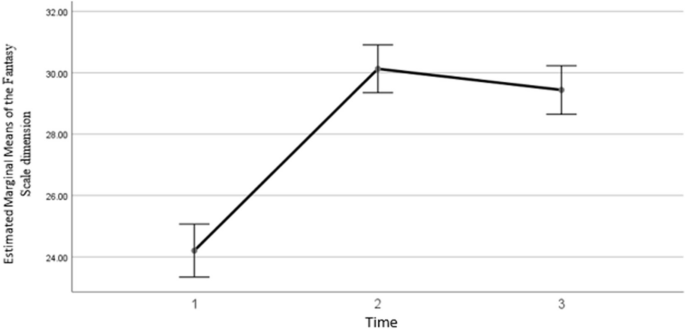
The changing trend of the average empathy score in the dimension of Fantasy Scale in three time periods
This study was conducted with the aim of investigating the effectiveness of reading stories in the Persian literature course on increasing empathy in medical students. The results of the present study indicated that reading stories had an effect on increasing empathy in the fields of emotional transfer (FS), cognitive and emotional empathy of students in the intervention group. In line with the current research, Maria et al.(2016) found that reading stories increases empathy among students [ 30 ]. Also, Matis et al. (2013), and Maja et al. (2013) showed the effectiveness of reading stories on empathy [ 31 , 32 ].
Also, the findings of the present study indicated that the story reading program had an effect on increasing empathy in the emotional transfer dimension of medical students in the intervention group, and the effectiveness of the intervention was stable in the follow-up phase. In line with the findings of the present study, Mumper and Gerrig (2017) concluded that reading stories can improve empathy [ 33 ]. In the Netherlands (2013), during a university research, it was found that reading the story carefully and conveying it emotionally to the reader can increase their empathy [ 31 ]. The research of Djikic et al. (2013) also confirmed the same result [ 32 ].
Also, the findings showed that the story reading program had a significant effect on increasing empathy in the cognitive dimension of students in the intervention group. In line with this finding, Panro et al. (2016) concluded that reading stories can affect the cognitive dimension and improve empathy [ 34 ]. In the explanation of the mentioned findings, it can be said that since reading the story emphasizes the mental and cognitive abilities of humans, it can lead to the development of cognitive skills in people [ 35 ] and increase people’s aware of others cognitive, emotional and sense states [ 36 ]. The research of Kidd et al. (2016) also showed that reading stories in the long term can increase our understanding of the cognitive and emotional states of others [ 37 ]. Also, the findings showed that reading stories had a significant impact on emotional empathy. In line with these findings, Koopman et al. and Dickij et al. also reached these results [ 32 , 38 ]. This finding is due to the fact that empathy is an important concept in the field of psychology and interpersonal communication. As a personal characteristic and also as an ability, it makes a person show desirable emotional reactions by perceiving the emotional reactions of others. In order to establish empathetic relationships, a person must be able to put himself in the place of others. Empathetic people are kind and caring towards others. They worry about others when they get hurt, on the other hand, their sensitivity to the behavior of others and trying to understand the nature of the behavior of the people around them makes them feel close and empathetic with the person to whom an incident has happened [ 39 , 40 ].
The findings of Rafati et al. (2015) also showed that the level of empathy of medical students with patients varies during the academic years. In such a way that the level of empathy in medical students decreases with increasing age and educational level, which is in line with the findings of the present study. These researchers also believe that due to the importance of empathy as a moral virtue, it is necessary to plan to strengthen empathy and include this concept in the curriculum of medical students to improve the mental and spiritual health of patients [ 8 ]. Khairabadi et al.'s research also informs about the weakness in the field of empathy and the importance of its training [ 41 ]. Shariat and Kikhaoni (2009) in a study that measured the level of empathy in clinical assistants of Iranian medical sciences universities, reported the decrease of this skill in students during their studies [ 42 ]. Considering this shortcoming, although in order to reduce this dissatisfaction, empathy training workshops have been held for the treatment staff in some universities, but there is still a need for planning in this regard [ 43 ]. One of the important limitations of the present study is the involvement of intervening factors in the effect of medical students' empathy, which are uncontrollable. Another limitation of this study is the small number of female participants compared to male participants. This issue does not allow us to draw conclusions about gender differences. The next limitation was that giving the right to choose to participate in the research for students made only students who are interested in fiction to participate in it, and there is a possibility that these people are more prepared to teach empathy and be influenced by texts. Finally, considering the relationship between reading fiction and general empathy, it is suggested for future researches to examine the ranking of general empathy with real empathy and also how empathy research evolves over time.
The findings of this research showed that story reading increased the general empathy of medical students in the fields of transfer of empathy, cognitive and emotional empathy in the post-intervention phase significantly more than the pre-intervention phase. This problem implicitly confirms the positive effect of reading stories in improving the sense of empathy. These results show the necessity of planning to include the study of stories and teaching them to read in the curriculum of medical students as a low-cost way to increase empathy. There is a literature course as a general course in the students' curriculum, but it does not have a specific educational framework. Considering the importance of storytelling in narrative medicine and the role of stories in creating empathy, it is suggested that more importance be given to reading stories in the curriculum of this course, especially during Interns and Stagers .
Availability of data and materials
The datasets used and/or analysed during the current study are available from the corresponding author on reasonable request.
Samuel CA, Mbah O, Schaal J, Eng E, Black KZ, Baker S, et al. The role of patient-physician relationship on health-related quality of life and pain in cancer patients. Supportive Care Cancer. 2020;28:2615–26.
Article Google Scholar
Halpern J. Empathy and patient–physician conflicts. J Gen Intern Med. 2007;22(5):696–700.
Yune SJ, Kang SH, Park K. Medical students' perceptions of patient-doctor relationship in South Korea: concept mapping analysis. Front Public Health. 2021:1606.
Van Der Merwe J. Physician-patient communication using ancestral spirits to achieve holistic healing. Am J Obstet Gynecol. 1995;172(4):1080–7.
Rogers CR. Significant learning in therapy and in education. Educ Leader. 1959;16(4):232–42.
Google Scholar
Khodabakhsh MR, Mansoori P. Empathy and its impact on promoting physician-patients relationship. Iran J Med Ethics History Med. 2011;4(3):38–46.
Kazemipoor M, Sattar BS, Hakimian R. Patient empathy and related factors in undergraduate and postgraduate dental students. 2018.
Shiva R, Nahid R, Ali D, Forotani F. Empathic perspective of medical students based on Jefferson Empathy Scale. J Med Sci Res Ethics. 2016;10(36):25–34.
Loftus S, Greenhalgh T. Towards a narrative mode of practice. Education for future practice: Brill; 2010. p. 85-94.
Linda NC, Clement M. The Application of Storytelling in Teaching and Learning: Implication on Pupil’s Performance and Enrolment in Schools. 2023.
Cersosimo G. Storytelling in medical education programs. Ital J Sociol Educ. 2019;11(Italian Journal of Sociology of Education 11/3):212–25.
Liao H-C, Wang Y-H. Storytelling in medical education: narrative medicine as a resource for interdisciplinary collaboration. Int J Environ Res Public Health. 2020;17(4):1135.
Kamel-ElSayed S, Loftus S. Using and combining learning theories in medical education. Med Sci Educ. 2018;28:255–8.
Loftus S. The language of clinical reasoning. Clinical Reasoning in the Health Professions E-Book. 2018:129.
Jones AH. Why teach literature and medicine? Answers from three decades. J Med Humanit. 2013;34:415–28.
Shapiro J, Nixon LL, Wear SE, Doukas DJ. Medical professionalism: what the study of literature can contribute to the conversation. Philos Ethics Humanit Med. 2015;10:1–8.
Sklar DP. Health humanities and medical education: joined by a common purpose. Acad Med. 2017;92(12):1647–9.
Tamir DI, Bricker AB, Dodell-Feder D, Mitchell JP. Reading fiction and reading minds: the role of simulation in the default network. Soc Cogn Affect Neurosci. 2016;11(2):215–24.
Bonebakker V. Literature & medicine: Humanities at the heart of health care: a hospital-based reading and discussion program developed by the Maine Humanities Council. Acad Med. 2003;78(10):963–7.
Panahifar S, Nouriani JM. The Effectiveness of Narrative therapy on Behavioral Maladaptation and Psychological Health of Children with ADHD in Kerman. 2021.
Shahabizadeh F, Khageaminiyan F. The effectiveness of narrative therapy based on cognitive-behavioral perspective on symptoms depression and dysthymic disorders in children. J Psychol Achieve. 2019;26(1):39–58.
Azmi hjsa. farsi omomi, ahamiyat wa asibshenasi 1 th hamayesh amozeshe zabane farsi. 2016. p. 195-200.
Kiyani barforoshi hR, ghodsiye. Pathological Criticism in farsi ye omomi; Critical Studies in Texts and Programs of Human Sciences 19. 2019;3:181-205.
Boulter A. Writing fiction: creative and critical approaches: Bloomsbury Publishing; 2007.
Damrosch D. How to read world literature: John Wiley & Sons; 2017.
Foster TC. How to Read Literature Like a Professor. Revised. New York: HarperCollins; 2014.
Rasley A. The Power of Point of View: Make Your Story Come to Life: Penguin. 2008.
Robert S. Elements of Fiction: An Anthology. Oxford University Press; 1981.
Truby J. The anatomy of story: 22 steps to becoming a master storyteller: Farrar, Straus and Giroux; 2008.
Pino MC, Mazza M. The use of “literary fiction” to promote mentalizing ability. PloS one. 2016;11(8):e0160254.
Bal PM, Veltkamp M. How does fiction reading influence empathy? An experimental investigation on the role of emotional transportation. PloS one. 2013;8(1):e55341.
Djikic M, Oatley K, Moldoveanu MC. Reading other minds: effects of literature on empathy. Sci Study Lit. 2013;3(1):28–47.
Mumper ML, Gerrig RJ. Leisure reading and social cognition: a meta-analysis. Psychol Aesthetics Creativity Arts. 2017;11(1):109.
Panero ME, Weisberg DS, Black J, Goldstein TR, Barnes JL, Brownell H, et al. Does reading a single passage of literary fiction really improve theory of mind? An attempt at replication. J Personal Soc Psychol. 2016;111(5):e46.
Dodell-Feder D, Lincoln SH, Coulson JP, Hooker CI. Using fiction to assess mental state understanding: a new task for assessing theory of mind in adults. PloS one. 2013;8(11):e81279.
Beaudoin C, Leblanc É, Gagner C, Beauchamp MH. Systematic review and inventory of theory of mind measures for young children. Front Psychol. 2020;10:2905.
Kidd D, Ongis M, Castano E. On literary fiction and its effects on theory of mind. Sci Study Lit. 2016;6(1):42–58.
Koopman EME. Effects of “literariness” on emotions and on empathy and reflection after reading. Psychol Aesthetics Creativity Arts. 2016;10(1):82.
Engbretson AM, Poehlmann-Tynan JA, Zahn-Waxler CJ, Vigna AJ, Gerstein ED, Raison CL. Effects of cognitively-based compassion training on parenting interactions and children’s empathy. Mindfulness. 2020;11:2841–52.
Stansfield J, Bunce L. The relationship between empathy and reading fiction: separate roles for cognitive and affective components. J Eur Psychol Students. 2014;5(3).
Kheirabadi G H-RM, Mahki B, Masaiely N, Yahaei M, Golshani L,Kheirabadi D. Empathy with patients in medical sciences faculty physicians at Isfahan University of Medical Sciences, Iran. J Res Behav Sci. 2016;14(2):154-60.
Shariat SV, Kaykhavoni A. Empathy in medical residents at Iran University of Medical Sciences. Iran J Psychiatry Clin Psychol. 2010;16(3):248–56.
Managheb E, Bagheri S. The impact of empathy training workshops on empathic practice of family physicians of Jahrom University of Medical Sciences. Iran J Med Educ. 2013;13(2):114–22.
Download references
Acknowledgements
The authors would like to express our gratitude to all those who helped us in this research and participated in the intervention. This research has been done with the financial support of the National Center for Strategic Research in Medical Education, Tehran, Iran (project number 1400130).
This research has been done with the financial support of the National Center for Strategic Research in Medical Education, Tehran, Iran (project number 1400130).
Author information
Authors and affiliations.
Department of General Education, School of Paramedical Sciences, Mazandaran University of Medical Sciences, Sari, Iran
Masoumeh Mahmoudi
School of Paramedical Sciences, Mazandaran University of Medical Sciences, Sari, Iran
Ali Asghar Ghorbani
Psychiatry and Behavioral Sciences Research Center, Addiction Institute, Mazandaran University of Medical Sciences, Sari, Iran
Mehdi Pourasghar
Department of Health Information Technology, School of Paramedical Sciences, Mazandaran University of Medical Sciences, Sari, Iran
Azita Balaghafari
Department of Biostatistics and Epidemiology, School of Health, Health Sciences Research Center, Mazandaran University of Medical Sciences, Sari, Iran
Jamshid Yazdani Charati
Mazandaran University of Medical Sciences, Sari, Iran
Nassim Ghahrani
Department of Biostatistics and Epidemiology, Student Research Committee, School of Health, Mazandaran University of Medical Sciences, Sari, Iran
Farzaneh Amini
You can also search for this author in PubMed Google Scholar
Contributions
MM, MP: developed the stories, AB, NGH and AAGH: Study design, intervention, implementation and interpretation of the results. JY and FA: Data analysis, and interpretation of the results. All authors confirmed the final version for submission. We confirm that instruction for authors of this journal has been read carefully, and all points are compiled for the whole manuscript.
Corresponding author
Correspondence to Ali Asghar Ghorbani .
Ethics declarations
Ethics approval and consent to participate.
In this study, after coordination with the relevant authorities and obtaining the consent and approval of the participants, the intervention was conducted. Confidentiality was also observed.
Also, written informed consent was obtained from all participants.
All authors confirm that all experiments were performed in accordance with relevant guidelines and regulations. Also, they confirm that all methods were carried out in accordance with relevant guidelines and regulations.
The authors confirm that the experimental protocols were approved by the Ethics Committee of the National Center for Strategic Research in Medical Education, Tehran, Iran (Ethical Code: 1400130).
Consent for publication
Not applicable.
Competing interests
The authors declare no competing interests.
Additional information
Publisher’s note.
Springer Nature remains neutral with regard to jurisdictional claims in published maps and institutional affiliations.
Supplementary Information
Additional file 1: appendix 1..
The Stories.
Rights and permissions
Open Access This article is licensed under a Creative Commons Attribution 4.0 International License, which permits use, sharing, adaptation, distribution and reproduction in any medium or format, as long as you give appropriate credit to the original author(s) and the source, provide a link to the Creative Commons licence, and indicate if changes were made. The images or other third party material in this article are included in the article's Creative Commons licence, unless indicated otherwise in a credit line to the material. If material is not included in the article's Creative Commons licence and your intended use is not permitted by statutory regulation or exceeds the permitted use, you will need to obtain permission directly from the copyright holder. To view a copy of this licence, visit http://creativecommons.org/licenses/by/4.0/ . The Creative Commons Public Domain Dedication waiver ( http://creativecommons.org/publicdomain/zero/1.0/ ) applies to the data made available in this article, unless otherwise stated in a credit line to the data.
Reprints and permissions
About this article
Cite this article.
Mahmoudi, M., Ghorbani, A.A., Pourasghar, M. et al. Designing, implementation and evaluation of story reading: a solution to increase general empathy in medical students. BMC Med Educ 24 , 477 (2024). https://doi.org/10.1186/s12909-024-05384-4
Download citation
Received : 07 September 2023
Accepted : 03 April 2024
Published : 30 April 2024
DOI : https://doi.org/10.1186/s12909-024-05384-4
Share this article
Anyone you share the following link with will be able to read this content:
Sorry, a shareable link is not currently available for this article.
Provided by the Springer Nature SharedIt content-sharing initiative
- Story reading
- General empathy
- Medical students
- Interventional education
BMC Medical Education
ISSN: 1472-6920
- Submission enquiries: [email protected]
- General enquiries: [email protected]
To read this content please select one of the options below:
Please note you do not have access to teaching notes, construction of a competency evaluation index system for postgraduates of educational technology.
Education + Training
ISSN : 0040-0912
Article publication date: 2 May 2024
The purpose of this study is to determine the competency characteristics required for the employment of master’s degree students in educational technology.
Design/methodology/approach
A combined qualitative and quantitative method was used to consult multiple experts through a modified Delphi method. Competency characteristics were extracted from Chinese recruitment apps, national recruitment websites and university training programs. Ten senior teacher experts who teach educational technology master’s students were consulted through a questionnaire consultation to validate the proposed competency model. The weights of competency characteristics were determined through a combination of the analytic hierarchy process and entropy method.
The results show that when recruiting educational technology master’s students, more emphasis is placed on operational skills. The majority of companies tend to assess practical abilities rather than theoretical knowledge. Relevant knowledge of educational technology, psychology, computer science and education is considered to be the basic knowledge components of educational technology master’s students, while professional skills are the core skills required for their positions. Therefore, universities need to focus on training, educational technology graduate students in these areas of competence. The study also found that professional qualities (such as physical and mental fitness) and personality traits (interpersonal communication and interaction) receive more attention from companies and are essential competencies for educational technology master’s students.
Originality/value
A competence model for educational technology master’s students is proposed, which includes aspects such as knowledge, personal skills/abilities, professional qualities and personality traits. The competence elements included in this model can serve as reference indicators for universities to cultivate the competence of educational technology master’s students, as well as reference points for recruiting units to help them select talents. This represents a new dimension in research related to the employment of educational technology master’s students. The study enriches the research objects and competence dictionary in the field of competence research.
- Competency model
- Master’s degree in educational technology
- Analytic hierarchy process
Acknowledgements
The authors thank two anonymous reviewers who provided helpful and constructive comments that improved the manuscript substantially. This work was supported by the Chongqing Normal University [grant numbers xyjg20014]; the Chongqing Science and Technology Bureau [grant number cstc2019jscx-mbdxX0061]; and the 13th Five-Year Plan project of Education and Science Planning of Chongqing [grant number 2019-GX-105].
Luo, L. , Ji, H. , Chen, S.-N. and Chen, X. (2024), "Construction of a competency evaluation index system for postgraduates of educational technology", Education + Training , Vol. ahead-of-print No. ahead-of-print. https://doi.org/10.1108/ET-03-2023-0075
Emerald Publishing Limited
Copyright © 2024, Emerald Publishing Limited
Related articles
We’re listening — tell us what you think, something didn’t work….
Report bugs here
All feedback is valuable
Please share your general feedback
Join us on our journey
Platform update page.
Visit emeraldpublishing.com/platformupdate to discover the latest news and updates
Questions & More Information
Answers to the most commonly asked questions here
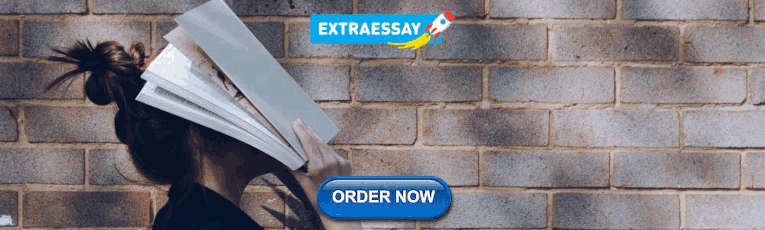
IMAGES
VIDEO
COMMENTS
Three types of experimental designs are commonly used: 1. Independent Measures. Independent measures design, also known as between-groups, is an experimental design where different participants are used in each condition of the independent variable. This means that each condition of the experiment includes a different group of participants.
Research Methods in Psychology AP A Han dbook s in Psychology VOLUME Research Designs: Quantitative, Qualitative, Neuropsychological, and Biological SECOND EDITION Harris Cooper, Editor-in-Chief Marc N. Coutanche, Linda M. McMullen, A. T. Panter, sychological Association. Not for further distribution.
Correlational Research Designs. In correlational research designs, groups are studied and compared, but researchers cannot introduce variables or manipulate independent variables. Case-control study. A case-control study is a comparison between two groups, one of which experienced a condition while the other group did not. Case-control studies ...
The study design used to answer a particular research question depends on the nature of the question and the availability of resources. In this article, which is the first part of a series on "study designs," we provide an overview of research study designs and their classification. The subsequent articles will focus on individual designs.
Quantitative Research Designs Involving Single Participants or Units ... PhD, is an associate professor of psychology and research scientist in the Learning Research and Development Center at the University of Pittsburgh. Dr. ... At the University of Missouri he directed the predoctoral and postdoctoral training program in alcohol studies, and ...
Part of generating a hypothesis involves identifying the variables that you want to study and operationally defining those variables so that they can be measured. Research questions in psychology are about variables. A variable is a quantity or quality that varies across people or situations. For example, the height of the students enrolled in ...
In the pages of this handbook, you will find descriptions of many techniques that psychologists and others have developed to help them pursue a shared understanding of why humans think, feel, and behave the way they do. These are the tools that we use to conduct our rational analyses. At the broadest level, when choosing a method you make decisions about (a) what data or measurement techniques ...
Specifically, Part III presents issues in sampling for quantitative studies; Part IV focuses on designs that build and test the implications of causal models; Part V focuses on research with experimental manipulations, in which participants are deliberately treated differently; Part VI introduces designs that focus on theory-testing questions ...
A research design is a strategy for answering your research question using empirical data. Creating a research design means making decisions about: ... Type of design Purpose and characteristics; Case study: Detailed study of a specific subject (e.g., a place, event, organisation) ... Psychology: Using technologies like neuroimaging, eye ...
Research in psychology focuses on a variety of topics, ranging from the development of infants to the behavior of social groups. Psychologists use the scientific method to investigate questions both systematically and empirically. Research in psychology is important because it provides us with valuable information that helps to improve human lives.
A research design is a strategy for answering your research question using empirical data. Creating a research design means making decisions about: Your overall research objectives and approach. Whether you'll rely on primary research or secondary research. Your sampling methods or criteria for selecting subjects. Your data collection methods.
The three common types of descriptive research designs in psychology are observation, case studies and surveys. Observational research is a detailed recording of behaviors that occur in a specific ...
'Dr. Kazdin's Research Design in Clinical Psychology (6th edition) is the perfect textbook to help students develop skills in and appreciation for sound research design. Research Methods is often the course that students dread, finding the material dry and inaccessible, but Dr. Kazdin's approach to balancing scientific rigor with humor ...
Research Methods and Design in Psychology. This accessible introductory text addresses the core knowledge domain of research methods. It provides concise coverage of the central concepts, techniques, problems and debates in this key area, while encouraging a critical approach and developing students' higher level skills.
Research design is an overall action plan in conducting research, and experimental design provides the guidelines of allocating treatments to the subjects in the experiment. There are several designs which the researcher can choose depending upon the nature of the study and experimental material.
Random assignment is a method for assigning participants in a sample to the different conditions, and it is an important element of all experimental research in psychology and other fields too. In its strictest sense, random assignment should meet two criteria. One is that each participant has an equal chance of being assigned to each condition ...
A research design is the specific method a researcher uses to collect, analyze, ... An interesting example of a case study in clinical psychology is described by Rokeach (1964), who investigated in detail the beliefs of and interactions among three patients with schizophrenia, all of whom were convinced they were Jesus Christ. ...
In Volume 2, interpretive research designs that emphasize a qualitative approach are detailed in Part I. Volume 2, Parts II through VI, introduces designs that emphasize an etic (or theory-specified), more quantitative approach to research. ... rely heavily on quantification, and are used to study change in individual units. The designs in ...
5.2.6. Main Effects and Interactions. In factorial designs, there are two kinds of results that are of interest: main effects and interactions. A main effect is the statistical relationship between one independent variable and a dependent variable-averaging across the levels of the other independent variable (s).
Remember, research methods are tools that are used to collect information, while r esearch design is the strategy or blueprint for deciding how to collect and analyze information. Research design dictates which methods are used and how. There are three types of developmental research designs: cross-sectional, longitudinal, and sequential.
When Kyle Palmberg set out to design a research study as the capstone project for his psychology major at St. Mary's University of M i nnesota in Winona, he knew he wanted his focus to be ...
Introduction. Although there exist different theories and research traditions of children's moral development, most of them focus on how children progress in and increase their morality (Jensen, 2020; Killen and Smetana, 2022).However, as Bussey (2020) notes, there is a lack of research regarding children's adherence to moral standards, including the moral standards-behavior gap.
Quality assessment. The quality of each study was assessed using a scale which has been used in sport psychology meta-analyses (see Zach et al. 2018).We rated the study on seven different criteria: (1) statement of purpose and hypothesis, (2) population, (3) description of EEG and results, (4) study design, (5) statistical analyses, (6) results, and (7) overall quality.
Study a BPS accredited Psychology Conversion master's at Middlesex University and learn from our highly respected team. ... The module enables you to understand & critically evaluate psychological research & to understand how research design relates to research questions. It provides you with advanced skills in a variety of statistical analyses ...
The aim of this research is to evaluate and compare the impact of story reading on the level of empathy in medical students at Mazandaran University of Medical Sciences. Methods. The study employed an intervention educational design (a quasi-experimental), with a convenience sample of 51 medical students selected as the statistical population.
The study enriches the research objects and competence dictionary in the field of competence research. ... Design/methodology/approach. ... Relevant knowledge of educational technology, psychology, computer science and education is considered to be the basic knowledge components of educational technology master's students, while professional ...