Have a language expert improve your writing
Run a free plagiarism check in 10 minutes, generate accurate citations for free.
- Knowledge Base
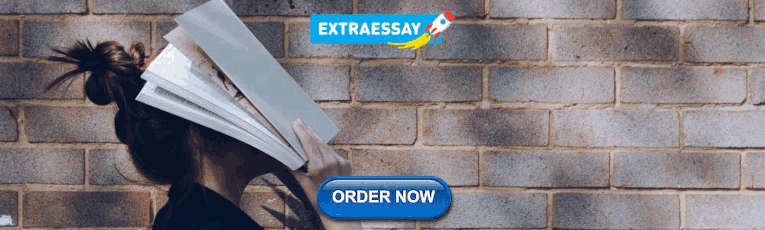
The Beginner's Guide to Statistical Analysis | 5 Steps & Examples
Statistical analysis means investigating trends, patterns, and relationships using quantitative data . It is an important research tool used by scientists, governments, businesses, and other organizations.
To draw valid conclusions, statistical analysis requires careful planning from the very start of the research process . You need to specify your hypotheses and make decisions about your research design, sample size, and sampling procedure.
After collecting data from your sample, you can organize and summarize the data using descriptive statistics . Then, you can use inferential statistics to formally test hypotheses and make estimates about the population. Finally, you can interpret and generalize your findings.
This article is a practical introduction to statistical analysis for students and researchers. We’ll walk you through the steps using two research examples. The first investigates a potential cause-and-effect relationship, while the second investigates a potential correlation between variables.
Table of contents
Step 1: write your hypotheses and plan your research design, step 2: collect data from a sample, step 3: summarize your data with descriptive statistics, step 4: test hypotheses or make estimates with inferential statistics, step 5: interpret your results, other interesting articles.
To collect valid data for statistical analysis, you first need to specify your hypotheses and plan out your research design.
Writing statistical hypotheses
The goal of research is often to investigate a relationship between variables within a population . You start with a prediction, and use statistical analysis to test that prediction.
A statistical hypothesis is a formal way of writing a prediction about a population. Every research prediction is rephrased into null and alternative hypotheses that can be tested using sample data.
While the null hypothesis always predicts no effect or no relationship between variables, the alternative hypothesis states your research prediction of an effect or relationship.
- Null hypothesis: A 5-minute meditation exercise will have no effect on math test scores in teenagers.
- Alternative hypothesis: A 5-minute meditation exercise will improve math test scores in teenagers.
- Null hypothesis: Parental income and GPA have no relationship with each other in college students.
- Alternative hypothesis: Parental income and GPA are positively correlated in college students.
Planning your research design
A research design is your overall strategy for data collection and analysis. It determines the statistical tests you can use to test your hypothesis later on.
First, decide whether your research will use a descriptive, correlational, or experimental design. Experiments directly influence variables, whereas descriptive and correlational studies only measure variables.
- In an experimental design , you can assess a cause-and-effect relationship (e.g., the effect of meditation on test scores) using statistical tests of comparison or regression.
- In a correlational design , you can explore relationships between variables (e.g., parental income and GPA) without any assumption of causality using correlation coefficients and significance tests.
- In a descriptive design , you can study the characteristics of a population or phenomenon (e.g., the prevalence of anxiety in U.S. college students) using statistical tests to draw inferences from sample data.
Your research design also concerns whether you’ll compare participants at the group level or individual level, or both.
- In a between-subjects design , you compare the group-level outcomes of participants who have been exposed to different treatments (e.g., those who performed a meditation exercise vs those who didn’t).
- In a within-subjects design , you compare repeated measures from participants who have participated in all treatments of a study (e.g., scores from before and after performing a meditation exercise).
- In a mixed (factorial) design , one variable is altered between subjects and another is altered within subjects (e.g., pretest and posttest scores from participants who either did or didn’t do a meditation exercise).
- Experimental
- Correlational
First, you’ll take baseline test scores from participants. Then, your participants will undergo a 5-minute meditation exercise. Finally, you’ll record participants’ scores from a second math test.
In this experiment, the independent variable is the 5-minute meditation exercise, and the dependent variable is the math test score from before and after the intervention. Example: Correlational research design In a correlational study, you test whether there is a relationship between parental income and GPA in graduating college students. To collect your data, you will ask participants to fill in a survey and self-report their parents’ incomes and their own GPA.
Measuring variables
When planning a research design, you should operationalize your variables and decide exactly how you will measure them.
For statistical analysis, it’s important to consider the level of measurement of your variables, which tells you what kind of data they contain:
- Categorical data represents groupings. These may be nominal (e.g., gender) or ordinal (e.g. level of language ability).
- Quantitative data represents amounts. These may be on an interval scale (e.g. test score) or a ratio scale (e.g. age).
Many variables can be measured at different levels of precision. For example, age data can be quantitative (8 years old) or categorical (young). If a variable is coded numerically (e.g., level of agreement from 1–5), it doesn’t automatically mean that it’s quantitative instead of categorical.
Identifying the measurement level is important for choosing appropriate statistics and hypothesis tests. For example, you can calculate a mean score with quantitative data, but not with categorical data.
In a research study, along with measures of your variables of interest, you’ll often collect data on relevant participant characteristics.
Prevent plagiarism. Run a free check.
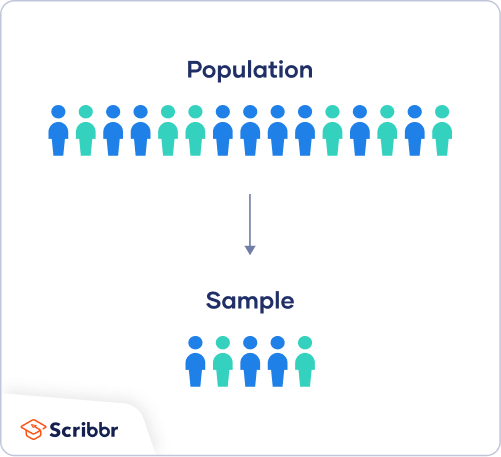
In most cases, it’s too difficult or expensive to collect data from every member of the population you’re interested in studying. Instead, you’ll collect data from a sample.
Statistical analysis allows you to apply your findings beyond your own sample as long as you use appropriate sampling procedures . You should aim for a sample that is representative of the population.
Sampling for statistical analysis
There are two main approaches to selecting a sample.
- Probability sampling: every member of the population has a chance of being selected for the study through random selection.
- Non-probability sampling: some members of the population are more likely than others to be selected for the study because of criteria such as convenience or voluntary self-selection.
In theory, for highly generalizable findings, you should use a probability sampling method. Random selection reduces several types of research bias , like sampling bias , and ensures that data from your sample is actually typical of the population. Parametric tests can be used to make strong statistical inferences when data are collected using probability sampling.
But in practice, it’s rarely possible to gather the ideal sample. While non-probability samples are more likely to at risk for biases like self-selection bias , they are much easier to recruit and collect data from. Non-parametric tests are more appropriate for non-probability samples, but they result in weaker inferences about the population.
If you want to use parametric tests for non-probability samples, you have to make the case that:
- your sample is representative of the population you’re generalizing your findings to.
- your sample lacks systematic bias.
Keep in mind that external validity means that you can only generalize your conclusions to others who share the characteristics of your sample. For instance, results from Western, Educated, Industrialized, Rich and Democratic samples (e.g., college students in the US) aren’t automatically applicable to all non-WEIRD populations.
If you apply parametric tests to data from non-probability samples, be sure to elaborate on the limitations of how far your results can be generalized in your discussion section .
Create an appropriate sampling procedure
Based on the resources available for your research, decide on how you’ll recruit participants.
- Will you have resources to advertise your study widely, including outside of your university setting?
- Will you have the means to recruit a diverse sample that represents a broad population?
- Do you have time to contact and follow up with members of hard-to-reach groups?
Your participants are self-selected by their schools. Although you’re using a non-probability sample, you aim for a diverse and representative sample. Example: Sampling (correlational study) Your main population of interest is male college students in the US. Using social media advertising, you recruit senior-year male college students from a smaller subpopulation: seven universities in the Boston area.
Calculate sufficient sample size
Before recruiting participants, decide on your sample size either by looking at other studies in your field or using statistics. A sample that’s too small may be unrepresentative of the sample, while a sample that’s too large will be more costly than necessary.
There are many sample size calculators online. Different formulas are used depending on whether you have subgroups or how rigorous your study should be (e.g., in clinical research). As a rule of thumb, a minimum of 30 units or more per subgroup is necessary.
To use these calculators, you have to understand and input these key components:
- Significance level (alpha): the risk of rejecting a true null hypothesis that you are willing to take, usually set at 5%.
- Statistical power : the probability of your study detecting an effect of a certain size if there is one, usually 80% or higher.
- Expected effect size : a standardized indication of how large the expected result of your study will be, usually based on other similar studies.
- Population standard deviation: an estimate of the population parameter based on a previous study or a pilot study of your own.
Once you’ve collected all of your data, you can inspect them and calculate descriptive statistics that summarize them.
Inspect your data
There are various ways to inspect your data, including the following:
- Organizing data from each variable in frequency distribution tables .
- Displaying data from a key variable in a bar chart to view the distribution of responses.
- Visualizing the relationship between two variables using a scatter plot .
By visualizing your data in tables and graphs, you can assess whether your data follow a skewed or normal distribution and whether there are any outliers or missing data.
A normal distribution means that your data are symmetrically distributed around a center where most values lie, with the values tapering off at the tail ends.
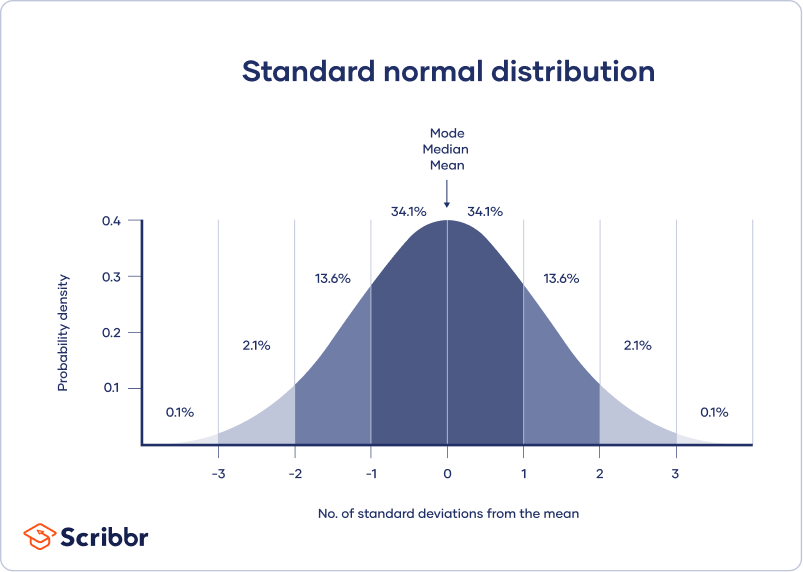
In contrast, a skewed distribution is asymmetric and has more values on one end than the other. The shape of the distribution is important to keep in mind because only some descriptive statistics should be used with skewed distributions.
Extreme outliers can also produce misleading statistics, so you may need a systematic approach to dealing with these values.
Calculate measures of central tendency
Measures of central tendency describe where most of the values in a data set lie. Three main measures of central tendency are often reported:
- Mode : the most popular response or value in the data set.
- Median : the value in the exact middle of the data set when ordered from low to high.
- Mean : the sum of all values divided by the number of values.
However, depending on the shape of the distribution and level of measurement, only one or two of these measures may be appropriate. For example, many demographic characteristics can only be described using the mode or proportions, while a variable like reaction time may not have a mode at all.
Calculate measures of variability
Measures of variability tell you how spread out the values in a data set are. Four main measures of variability are often reported:
- Range : the highest value minus the lowest value of the data set.
- Interquartile range : the range of the middle half of the data set.
- Standard deviation : the average distance between each value in your data set and the mean.
- Variance : the square of the standard deviation.
Once again, the shape of the distribution and level of measurement should guide your choice of variability statistics. The interquartile range is the best measure for skewed distributions, while standard deviation and variance provide the best information for normal distributions.
Using your table, you should check whether the units of the descriptive statistics are comparable for pretest and posttest scores. For example, are the variance levels similar across the groups? Are there any extreme values? If there are, you may need to identify and remove extreme outliers in your data set or transform your data before performing a statistical test.
From this table, we can see that the mean score increased after the meditation exercise, and the variances of the two scores are comparable. Next, we can perform a statistical test to find out if this improvement in test scores is statistically significant in the population. Example: Descriptive statistics (correlational study) After collecting data from 653 students, you tabulate descriptive statistics for annual parental income and GPA.
It’s important to check whether you have a broad range of data points. If you don’t, your data may be skewed towards some groups more than others (e.g., high academic achievers), and only limited inferences can be made about a relationship.
A number that describes a sample is called a statistic , while a number describing a population is called a parameter . Using inferential statistics , you can make conclusions about population parameters based on sample statistics.
Researchers often use two main methods (simultaneously) to make inferences in statistics.
- Estimation: calculating population parameters based on sample statistics.
- Hypothesis testing: a formal process for testing research predictions about the population using samples.
You can make two types of estimates of population parameters from sample statistics:
- A point estimate : a value that represents your best guess of the exact parameter.
- An interval estimate : a range of values that represent your best guess of where the parameter lies.
If your aim is to infer and report population characteristics from sample data, it’s best to use both point and interval estimates in your paper.
You can consider a sample statistic a point estimate for the population parameter when you have a representative sample (e.g., in a wide public opinion poll, the proportion of a sample that supports the current government is taken as the population proportion of government supporters).
There’s always error involved in estimation, so you should also provide a confidence interval as an interval estimate to show the variability around a point estimate.
A confidence interval uses the standard error and the z score from the standard normal distribution to convey where you’d generally expect to find the population parameter most of the time.
Hypothesis testing
Using data from a sample, you can test hypotheses about relationships between variables in the population. Hypothesis testing starts with the assumption that the null hypothesis is true in the population, and you use statistical tests to assess whether the null hypothesis can be rejected or not.
Statistical tests determine where your sample data would lie on an expected distribution of sample data if the null hypothesis were true. These tests give two main outputs:
- A test statistic tells you how much your data differs from the null hypothesis of the test.
- A p value tells you the likelihood of obtaining your results if the null hypothesis is actually true in the population.
Statistical tests come in three main varieties:
- Comparison tests assess group differences in outcomes.
- Regression tests assess cause-and-effect relationships between variables.
- Correlation tests assess relationships between variables without assuming causation.
Your choice of statistical test depends on your research questions, research design, sampling method, and data characteristics.
Parametric tests
Parametric tests make powerful inferences about the population based on sample data. But to use them, some assumptions must be met, and only some types of variables can be used. If your data violate these assumptions, you can perform appropriate data transformations or use alternative non-parametric tests instead.
A regression models the extent to which changes in a predictor variable results in changes in outcome variable(s).
- A simple linear regression includes one predictor variable and one outcome variable.
- A multiple linear regression includes two or more predictor variables and one outcome variable.
Comparison tests usually compare the means of groups. These may be the means of different groups within a sample (e.g., a treatment and control group), the means of one sample group taken at different times (e.g., pretest and posttest scores), or a sample mean and a population mean.
- A t test is for exactly 1 or 2 groups when the sample is small (30 or less).
- A z test is for exactly 1 or 2 groups when the sample is large.
- An ANOVA is for 3 or more groups.
The z and t tests have subtypes based on the number and types of samples and the hypotheses:
- If you have only one sample that you want to compare to a population mean, use a one-sample test .
- If you have paired measurements (within-subjects design), use a dependent (paired) samples test .
- If you have completely separate measurements from two unmatched groups (between-subjects design), use an independent (unpaired) samples test .
- If you expect a difference between groups in a specific direction, use a one-tailed test .
- If you don’t have any expectations for the direction of a difference between groups, use a two-tailed test .
The only parametric correlation test is Pearson’s r . The correlation coefficient ( r ) tells you the strength of a linear relationship between two quantitative variables.
However, to test whether the correlation in the sample is strong enough to be important in the population, you also need to perform a significance test of the correlation coefficient, usually a t test, to obtain a p value. This test uses your sample size to calculate how much the correlation coefficient differs from zero in the population.
You use a dependent-samples, one-tailed t test to assess whether the meditation exercise significantly improved math test scores. The test gives you:
- a t value (test statistic) of 3.00
- a p value of 0.0028
Although Pearson’s r is a test statistic, it doesn’t tell you anything about how significant the correlation is in the population. You also need to test whether this sample correlation coefficient is large enough to demonstrate a correlation in the population.
A t test can also determine how significantly a correlation coefficient differs from zero based on sample size. Since you expect a positive correlation between parental income and GPA, you use a one-sample, one-tailed t test. The t test gives you:
- a t value of 3.08
- a p value of 0.001
The final step of statistical analysis is interpreting your results.
Statistical significance
In hypothesis testing, statistical significance is the main criterion for forming conclusions. You compare your p value to a set significance level (usually 0.05) to decide whether your results are statistically significant or non-significant.
Statistically significant results are considered unlikely to have arisen solely due to chance. There is only a very low chance of such a result occurring if the null hypothesis is true in the population.
This means that you believe the meditation intervention, rather than random factors, directly caused the increase in test scores. Example: Interpret your results (correlational study) You compare your p value of 0.001 to your significance threshold of 0.05. With a p value under this threshold, you can reject the null hypothesis. This indicates a statistically significant correlation between parental income and GPA in male college students.
Note that correlation doesn’t always mean causation, because there are often many underlying factors contributing to a complex variable like GPA. Even if one variable is related to another, this may be because of a third variable influencing both of them, or indirect links between the two variables.
Effect size
A statistically significant result doesn’t necessarily mean that there are important real life applications or clinical outcomes for a finding.
In contrast, the effect size indicates the practical significance of your results. It’s important to report effect sizes along with your inferential statistics for a complete picture of your results. You should also report interval estimates of effect sizes if you’re writing an APA style paper .
With a Cohen’s d of 0.72, there’s medium to high practical significance to your finding that the meditation exercise improved test scores. Example: Effect size (correlational study) To determine the effect size of the correlation coefficient, you compare your Pearson’s r value to Cohen’s effect size criteria.
Decision errors
Type I and Type II errors are mistakes made in research conclusions. A Type I error means rejecting the null hypothesis when it’s actually true, while a Type II error means failing to reject the null hypothesis when it’s false.
You can aim to minimize the risk of these errors by selecting an optimal significance level and ensuring high power . However, there’s a trade-off between the two errors, so a fine balance is necessary.
Frequentist versus Bayesian statistics
Traditionally, frequentist statistics emphasizes null hypothesis significance testing and always starts with the assumption of a true null hypothesis.
However, Bayesian statistics has grown in popularity as an alternative approach in the last few decades. In this approach, you use previous research to continually update your hypotheses based on your expectations and observations.
Bayes factor compares the relative strength of evidence for the null versus the alternative hypothesis rather than making a conclusion about rejecting the null hypothesis or not.
If you want to know more about statistics , methodology , or research bias , make sure to check out some of our other articles with explanations and examples.
- Student’s t -distribution
- Normal distribution
- Null and Alternative Hypotheses
- Chi square tests
- Confidence interval
Methodology
- Cluster sampling
- Stratified sampling
- Data cleansing
- Reproducibility vs Replicability
- Peer review
- Likert scale
Research bias
- Implicit bias
- Framing effect
- Cognitive bias
- Placebo effect
- Hawthorne effect
- Hostile attribution bias
- Affect heuristic
Is this article helpful?
Other students also liked.
- Descriptive Statistics | Definitions, Types, Examples
- Inferential Statistics | An Easy Introduction & Examples
- Choosing the Right Statistical Test | Types & Examples
More interesting articles
- Akaike Information Criterion | When & How to Use It (Example)
- An Easy Introduction to Statistical Significance (With Examples)
- An Introduction to t Tests | Definitions, Formula and Examples
- ANOVA in R | A Complete Step-by-Step Guide with Examples
- Central Limit Theorem | Formula, Definition & Examples
- Central Tendency | Understanding the Mean, Median & Mode
- Chi-Square (Χ²) Distributions | Definition & Examples
- Chi-Square (Χ²) Table | Examples & Downloadable Table
- Chi-Square (Χ²) Tests | Types, Formula & Examples
- Chi-Square Goodness of Fit Test | Formula, Guide & Examples
- Chi-Square Test of Independence | Formula, Guide & Examples
- Coefficient of Determination (R²) | Calculation & Interpretation
- Correlation Coefficient | Types, Formulas & Examples
- Frequency Distribution | Tables, Types & Examples
- How to Calculate Standard Deviation (Guide) | Calculator & Examples
- How to Calculate Variance | Calculator, Analysis & Examples
- How to Find Degrees of Freedom | Definition & Formula
- How to Find Interquartile Range (IQR) | Calculator & Examples
- How to Find Outliers | 4 Ways with Examples & Explanation
- How to Find the Geometric Mean | Calculator & Formula
- How to Find the Mean | Definition, Examples & Calculator
- How to Find the Median | Definition, Examples & Calculator
- How to Find the Mode | Definition, Examples & Calculator
- How to Find the Range of a Data Set | Calculator & Formula
- Hypothesis Testing | A Step-by-Step Guide with Easy Examples
- Interval Data and How to Analyze It | Definitions & Examples
- Levels of Measurement | Nominal, Ordinal, Interval and Ratio
- Linear Regression in R | A Step-by-Step Guide & Examples
- Missing Data | Types, Explanation, & Imputation
- Multiple Linear Regression | A Quick Guide (Examples)
- Nominal Data | Definition, Examples, Data Collection & Analysis
- Normal Distribution | Examples, Formulas, & Uses
- Null and Alternative Hypotheses | Definitions & Examples
- One-way ANOVA | When and How to Use It (With Examples)
- Ordinal Data | Definition, Examples, Data Collection & Analysis
- Parameter vs Statistic | Definitions, Differences & Examples
- Pearson Correlation Coefficient (r) | Guide & Examples
- Poisson Distributions | Definition, Formula & Examples
- Probability Distribution | Formula, Types, & Examples
- Quartiles & Quantiles | Calculation, Definition & Interpretation
- Ratio Scales | Definition, Examples, & Data Analysis
- Simple Linear Regression | An Easy Introduction & Examples
- Skewness | Definition, Examples & Formula
- Statistical Power and Why It Matters | A Simple Introduction
- Student's t Table (Free Download) | Guide & Examples
- T-distribution: What it is and how to use it
- Test statistics | Definition, Interpretation, and Examples
- The Standard Normal Distribution | Calculator, Examples & Uses
- Two-Way ANOVA | Examples & When To Use It
- Type I & Type II Errors | Differences, Examples, Visualizations
- Understanding Confidence Intervals | Easy Examples & Formulas
- Understanding P values | Definition and Examples
- Variability | Calculating Range, IQR, Variance, Standard Deviation
- What is Effect Size and Why Does It Matter? (Examples)
- What Is Kurtosis? | Definition, Examples & Formula
- What Is Standard Error? | How to Calculate (Guide with Examples)
What is your plagiarism score?

An official website of the United States government
The .gov means it’s official. Federal government websites often end in .gov or .mil. Before sharing sensitive information, make sure you’re on a federal government site.
The site is secure. The https:// ensures that you are connecting to the official website and that any information you provide is encrypted and transmitted securely.
- Publications
- Account settings
Preview improvements coming to the PMC website in October 2024. Learn More or Try it out now .
- Advanced Search
- Journal List
- Indian J Anaesth
- v.60(9); 2016 Sep
Basic statistical tools in research and data analysis
Zulfiqar ali.
Department of Anaesthesiology, Division of Neuroanaesthesiology, Sheri Kashmir Institute of Medical Sciences, Soura, Srinagar, Jammu and Kashmir, India
S Bala Bhaskar
1 Department of Anaesthesiology and Critical Care, Vijayanagar Institute of Medical Sciences, Bellary, Karnataka, India
Statistical methods involved in carrying out a study include planning, designing, collecting data, analysing, drawing meaningful interpretation and reporting of the research findings. The statistical analysis gives meaning to the meaningless numbers, thereby breathing life into a lifeless data. The results and inferences are precise only if proper statistical tests are used. This article will try to acquaint the reader with the basic research tools that are utilised while conducting various studies. The article covers a brief outline of the variables, an understanding of quantitative and qualitative variables and the measures of central tendency. An idea of the sample size estimation, power analysis and the statistical errors is given. Finally, there is a summary of parametric and non-parametric tests used for data analysis.
INTRODUCTION
Statistics is a branch of science that deals with the collection, organisation, analysis of data and drawing of inferences from the samples to the whole population.[ 1 ] This requires a proper design of the study, an appropriate selection of the study sample and choice of a suitable statistical test. An adequate knowledge of statistics is necessary for proper designing of an epidemiological study or a clinical trial. Improper statistical methods may result in erroneous conclusions which may lead to unethical practice.[ 2 ]
Variable is a characteristic that varies from one individual member of population to another individual.[ 3 ] Variables such as height and weight are measured by some type of scale, convey quantitative information and are called as quantitative variables. Sex and eye colour give qualitative information and are called as qualitative variables[ 3 ] [ Figure 1 ].

Classification of variables
Quantitative variables
Quantitative or numerical data are subdivided into discrete and continuous measurements. Discrete numerical data are recorded as a whole number such as 0, 1, 2, 3,… (integer), whereas continuous data can assume any value. Observations that can be counted constitute the discrete data and observations that can be measured constitute the continuous data. Examples of discrete data are number of episodes of respiratory arrests or the number of re-intubations in an intensive care unit. Similarly, examples of continuous data are the serial serum glucose levels, partial pressure of oxygen in arterial blood and the oesophageal temperature.
A hierarchical scale of increasing precision can be used for observing and recording the data which is based on categorical, ordinal, interval and ratio scales [ Figure 1 ].
Categorical or nominal variables are unordered. The data are merely classified into categories and cannot be arranged in any particular order. If only two categories exist (as in gender male and female), it is called as a dichotomous (or binary) data. The various causes of re-intubation in an intensive care unit due to upper airway obstruction, impaired clearance of secretions, hypoxemia, hypercapnia, pulmonary oedema and neurological impairment are examples of categorical variables.
Ordinal variables have a clear ordering between the variables. However, the ordered data may not have equal intervals. Examples are the American Society of Anesthesiologists status or Richmond agitation-sedation scale.
Interval variables are similar to an ordinal variable, except that the intervals between the values of the interval variable are equally spaced. A good example of an interval scale is the Fahrenheit degree scale used to measure temperature. With the Fahrenheit scale, the difference between 70° and 75° is equal to the difference between 80° and 85°: The units of measurement are equal throughout the full range of the scale.
Ratio scales are similar to interval scales, in that equal differences between scale values have equal quantitative meaning. However, ratio scales also have a true zero point, which gives them an additional property. For example, the system of centimetres is an example of a ratio scale. There is a true zero point and the value of 0 cm means a complete absence of length. The thyromental distance of 6 cm in an adult may be twice that of a child in whom it may be 3 cm.
STATISTICS: DESCRIPTIVE AND INFERENTIAL STATISTICS
Descriptive statistics[ 4 ] try to describe the relationship between variables in a sample or population. Descriptive statistics provide a summary of data in the form of mean, median and mode. Inferential statistics[ 4 ] use a random sample of data taken from a population to describe and make inferences about the whole population. It is valuable when it is not possible to examine each member of an entire population. The examples if descriptive and inferential statistics are illustrated in Table 1 .
Example of descriptive and inferential statistics

Descriptive statistics
The extent to which the observations cluster around a central location is described by the central tendency and the spread towards the extremes is described by the degree of dispersion.
Measures of central tendency
The measures of central tendency are mean, median and mode.[ 6 ] Mean (or the arithmetic average) is the sum of all the scores divided by the number of scores. Mean may be influenced profoundly by the extreme variables. For example, the average stay of organophosphorus poisoning patients in ICU may be influenced by a single patient who stays in ICU for around 5 months because of septicaemia. The extreme values are called outliers. The formula for the mean is

where x = each observation and n = number of observations. Median[ 6 ] is defined as the middle of a distribution in a ranked data (with half of the variables in the sample above and half below the median value) while mode is the most frequently occurring variable in a distribution. Range defines the spread, or variability, of a sample.[ 7 ] It is described by the minimum and maximum values of the variables. If we rank the data and after ranking, group the observations into percentiles, we can get better information of the pattern of spread of the variables. In percentiles, we rank the observations into 100 equal parts. We can then describe 25%, 50%, 75% or any other percentile amount. The median is the 50 th percentile. The interquartile range will be the observations in the middle 50% of the observations about the median (25 th -75 th percentile). Variance[ 7 ] is a measure of how spread out is the distribution. It gives an indication of how close an individual observation clusters about the mean value. The variance of a population is defined by the following formula:

where σ 2 is the population variance, X is the population mean, X i is the i th element from the population and N is the number of elements in the population. The variance of a sample is defined by slightly different formula:

where s 2 is the sample variance, x is the sample mean, x i is the i th element from the sample and n is the number of elements in the sample. The formula for the variance of a population has the value ‘ n ’ as the denominator. The expression ‘ n −1’ is known as the degrees of freedom and is one less than the number of parameters. Each observation is free to vary, except the last one which must be a defined value. The variance is measured in squared units. To make the interpretation of the data simple and to retain the basic unit of observation, the square root of variance is used. The square root of the variance is the standard deviation (SD).[ 8 ] The SD of a population is defined by the following formula:

where σ is the population SD, X is the population mean, X i is the i th element from the population and N is the number of elements in the population. The SD of a sample is defined by slightly different formula:

where s is the sample SD, x is the sample mean, x i is the i th element from the sample and n is the number of elements in the sample. An example for calculation of variation and SD is illustrated in Table 2 .
Example of mean, variance, standard deviation

Normal distribution or Gaussian distribution
Most of the biological variables usually cluster around a central value, with symmetrical positive and negative deviations about this point.[ 1 ] The standard normal distribution curve is a symmetrical bell-shaped. In a normal distribution curve, about 68% of the scores are within 1 SD of the mean. Around 95% of the scores are within 2 SDs of the mean and 99% within 3 SDs of the mean [ Figure 2 ].

Normal distribution curve
Skewed distribution
It is a distribution with an asymmetry of the variables about its mean. In a negatively skewed distribution [ Figure 3 ], the mass of the distribution is concentrated on the right of Figure 1 . In a positively skewed distribution [ Figure 3 ], the mass of the distribution is concentrated on the left of the figure leading to a longer right tail.

Curves showing negatively skewed and positively skewed distribution
Inferential statistics
In inferential statistics, data are analysed from a sample to make inferences in the larger collection of the population. The purpose is to answer or test the hypotheses. A hypothesis (plural hypotheses) is a proposed explanation for a phenomenon. Hypothesis tests are thus procedures for making rational decisions about the reality of observed effects.
Probability is the measure of the likelihood that an event will occur. Probability is quantified as a number between 0 and 1 (where 0 indicates impossibility and 1 indicates certainty).
In inferential statistics, the term ‘null hypothesis’ ( H 0 ‘ H-naught ,’ ‘ H-null ’) denotes that there is no relationship (difference) between the population variables in question.[ 9 ]
Alternative hypothesis ( H 1 and H a ) denotes that a statement between the variables is expected to be true.[ 9 ]
The P value (or the calculated probability) is the probability of the event occurring by chance if the null hypothesis is true. The P value is a numerical between 0 and 1 and is interpreted by researchers in deciding whether to reject or retain the null hypothesis [ Table 3 ].
P values with interpretation

If P value is less than the arbitrarily chosen value (known as α or the significance level), the null hypothesis (H0) is rejected [ Table 4 ]. However, if null hypotheses (H0) is incorrectly rejected, this is known as a Type I error.[ 11 ] Further details regarding alpha error, beta error and sample size calculation and factors influencing them are dealt with in another section of this issue by Das S et al .[ 12 ]
Illustration for null hypothesis

PARAMETRIC AND NON-PARAMETRIC TESTS
Numerical data (quantitative variables) that are normally distributed are analysed with parametric tests.[ 13 ]
Two most basic prerequisites for parametric statistical analysis are:
- The assumption of normality which specifies that the means of the sample group are normally distributed
- The assumption of equal variance which specifies that the variances of the samples and of their corresponding population are equal.
However, if the distribution of the sample is skewed towards one side or the distribution is unknown due to the small sample size, non-parametric[ 14 ] statistical techniques are used. Non-parametric tests are used to analyse ordinal and categorical data.
Parametric tests
The parametric tests assume that the data are on a quantitative (numerical) scale, with a normal distribution of the underlying population. The samples have the same variance (homogeneity of variances). The samples are randomly drawn from the population, and the observations within a group are independent of each other. The commonly used parametric tests are the Student's t -test, analysis of variance (ANOVA) and repeated measures ANOVA.
Student's t -test
Student's t -test is used to test the null hypothesis that there is no difference between the means of the two groups. It is used in three circumstances:

where X = sample mean, u = population mean and SE = standard error of mean

where X 1 − X 2 is the difference between the means of the two groups and SE denotes the standard error of the difference.
- To test if the population means estimated by two dependent samples differ significantly (the paired t -test). A usual setting for paired t -test is when measurements are made on the same subjects before and after a treatment.
The formula for paired t -test is:

where d is the mean difference and SE denotes the standard error of this difference.
The group variances can be compared using the F -test. The F -test is the ratio of variances (var l/var 2). If F differs significantly from 1.0, then it is concluded that the group variances differ significantly.
Analysis of variance
The Student's t -test cannot be used for comparison of three or more groups. The purpose of ANOVA is to test if there is any significant difference between the means of two or more groups.
In ANOVA, we study two variances – (a) between-group variability and (b) within-group variability. The within-group variability (error variance) is the variation that cannot be accounted for in the study design. It is based on random differences present in our samples.
However, the between-group (or effect variance) is the result of our treatment. These two estimates of variances are compared using the F-test.
A simplified formula for the F statistic is:

where MS b is the mean squares between the groups and MS w is the mean squares within groups.
Repeated measures analysis of variance
As with ANOVA, repeated measures ANOVA analyses the equality of means of three or more groups. However, a repeated measure ANOVA is used when all variables of a sample are measured under different conditions or at different points in time.
As the variables are measured from a sample at different points of time, the measurement of the dependent variable is repeated. Using a standard ANOVA in this case is not appropriate because it fails to model the correlation between the repeated measures: The data violate the ANOVA assumption of independence. Hence, in the measurement of repeated dependent variables, repeated measures ANOVA should be used.
Non-parametric tests
When the assumptions of normality are not met, and the sample means are not normally, distributed parametric tests can lead to erroneous results. Non-parametric tests (distribution-free test) are used in such situation as they do not require the normality assumption.[ 15 ] Non-parametric tests may fail to detect a significant difference when compared with a parametric test. That is, they usually have less power.
As is done for the parametric tests, the test statistic is compared with known values for the sampling distribution of that statistic and the null hypothesis is accepted or rejected. The types of non-parametric analysis techniques and the corresponding parametric analysis techniques are delineated in Table 5 .
Analogue of parametric and non-parametric tests

Median test for one sample: The sign test and Wilcoxon's signed rank test
The sign test and Wilcoxon's signed rank test are used for median tests of one sample. These tests examine whether one instance of sample data is greater or smaller than the median reference value.
This test examines the hypothesis about the median θ0 of a population. It tests the null hypothesis H0 = θ0. When the observed value (Xi) is greater than the reference value (θ0), it is marked as+. If the observed value is smaller than the reference value, it is marked as − sign. If the observed value is equal to the reference value (θ0), it is eliminated from the sample.
If the null hypothesis is true, there will be an equal number of + signs and − signs.
The sign test ignores the actual values of the data and only uses + or − signs. Therefore, it is useful when it is difficult to measure the values.
Wilcoxon's signed rank test
There is a major limitation of sign test as we lose the quantitative information of the given data and merely use the + or – signs. Wilcoxon's signed rank test not only examines the observed values in comparison with θ0 but also takes into consideration the relative sizes, adding more statistical power to the test. As in the sign test, if there is an observed value that is equal to the reference value θ0, this observed value is eliminated from the sample.
Wilcoxon's rank sum test ranks all data points in order, calculates the rank sum of each sample and compares the difference in the rank sums.
Mann-Whitney test
It is used to test the null hypothesis that two samples have the same median or, alternatively, whether observations in one sample tend to be larger than observations in the other.
Mann–Whitney test compares all data (xi) belonging to the X group and all data (yi) belonging to the Y group and calculates the probability of xi being greater than yi: P (xi > yi). The null hypothesis states that P (xi > yi) = P (xi < yi) =1/2 while the alternative hypothesis states that P (xi > yi) ≠1/2.
Kolmogorov-Smirnov test
The two-sample Kolmogorov-Smirnov (KS) test was designed as a generic method to test whether two random samples are drawn from the same distribution. The null hypothesis of the KS test is that both distributions are identical. The statistic of the KS test is a distance between the two empirical distributions, computed as the maximum absolute difference between their cumulative curves.
Kruskal-Wallis test
The Kruskal–Wallis test is a non-parametric test to analyse the variance.[ 14 ] It analyses if there is any difference in the median values of three or more independent samples. The data values are ranked in an increasing order, and the rank sums calculated followed by calculation of the test statistic.
Jonckheere test
In contrast to Kruskal–Wallis test, in Jonckheere test, there is an a priori ordering that gives it a more statistical power than the Kruskal–Wallis test.[ 14 ]
Friedman test
The Friedman test is a non-parametric test for testing the difference between several related samples. The Friedman test is an alternative for repeated measures ANOVAs which is used when the same parameter has been measured under different conditions on the same subjects.[ 13 ]
Tests to analyse the categorical data
Chi-square test, Fischer's exact test and McNemar's test are used to analyse the categorical or nominal variables. The Chi-square test compares the frequencies and tests whether the observed data differ significantly from that of the expected data if there were no differences between groups (i.e., the null hypothesis). It is calculated by the sum of the squared difference between observed ( O ) and the expected ( E ) data (or the deviation, d ) divided by the expected data by the following formula:

A Yates correction factor is used when the sample size is small. Fischer's exact test is used to determine if there are non-random associations between two categorical variables. It does not assume random sampling, and instead of referring a calculated statistic to a sampling distribution, it calculates an exact probability. McNemar's test is used for paired nominal data. It is applied to 2 × 2 table with paired-dependent samples. It is used to determine whether the row and column frequencies are equal (that is, whether there is ‘marginal homogeneity’). The null hypothesis is that the paired proportions are equal. The Mantel-Haenszel Chi-square test is a multivariate test as it analyses multiple grouping variables. It stratifies according to the nominated confounding variables and identifies any that affects the primary outcome variable. If the outcome variable is dichotomous, then logistic regression is used.
SOFTWARES AVAILABLE FOR STATISTICS, SAMPLE SIZE CALCULATION AND POWER ANALYSIS
Numerous statistical software systems are available currently. The commonly used software systems are Statistical Package for the Social Sciences (SPSS – manufactured by IBM corporation), Statistical Analysis System ((SAS – developed by SAS Institute North Carolina, United States of America), R (designed by Ross Ihaka and Robert Gentleman from R core team), Minitab (developed by Minitab Inc), Stata (developed by StataCorp) and the MS Excel (developed by Microsoft).
There are a number of web resources which are related to statistical power analyses. A few are:
- StatPages.net – provides links to a number of online power calculators
- G-Power – provides a downloadable power analysis program that runs under DOS
- Power analysis for ANOVA designs an interactive site that calculates power or sample size needed to attain a given power for one effect in a factorial ANOVA design
- SPSS makes a program called SamplePower. It gives an output of a complete report on the computer screen which can be cut and paste into another document.
It is important that a researcher knows the concepts of the basic statistical methods used for conduct of a research study. This will help to conduct an appropriately well-designed study leading to valid and reliable results. Inappropriate use of statistical techniques may lead to faulty conclusions, inducing errors and undermining the significance of the article. Bad statistics may lead to bad research, and bad research may lead to unethical practice. Hence, an adequate knowledge of statistics and the appropriate use of statistical tests are important. An appropriate knowledge about the basic statistical methods will go a long way in improving the research designs and producing quality medical research which can be utilised for formulating the evidence-based guidelines.
Financial support and sponsorship
Conflicts of interest.
There are no conflicts of interest.
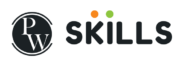
Data Analysis Techniques in Research – Methods, Tools & Examples
By Varun Saharawat | January 22, 2024
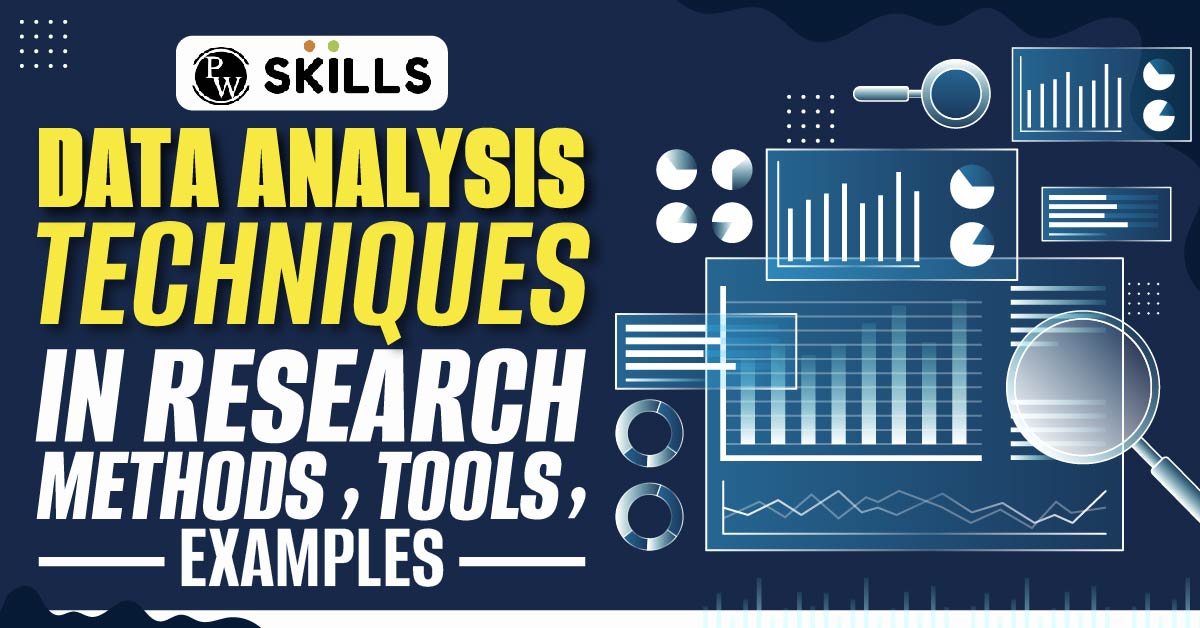
Data analysis techniques in research are essential because they allow researchers to derive meaningful insights from data sets to support their hypotheses or research objectives.
Data Analysis Techniques in Research : While various groups, institutions, and professionals may have diverse approaches to data analysis, a universal definition captures its essence. Data analysis involves refining, transforming, and interpreting raw data to derive actionable insights that guide informed decision-making for businesses.
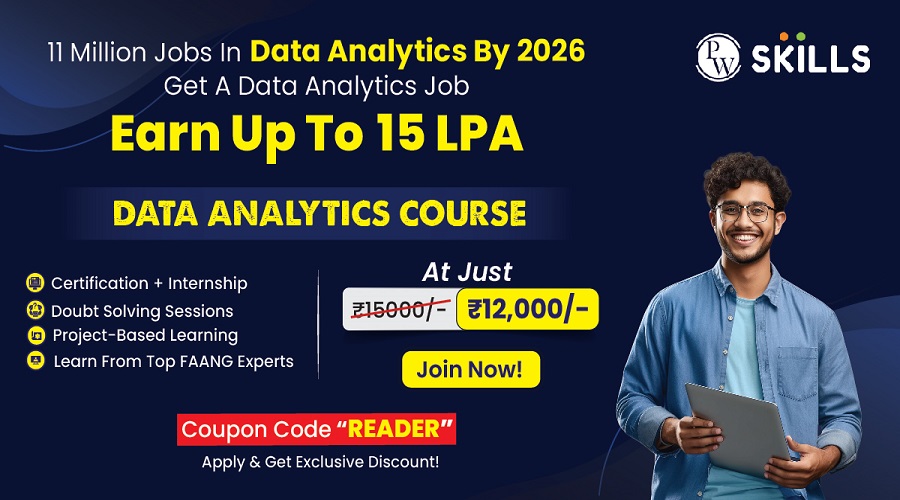
A straightforward illustration of data analysis emerges when we make everyday decisions, basing our choices on past experiences or predictions of potential outcomes.
If you want to learn more about this topic and acquire valuable skills that will set you apart in today’s data-driven world, we highly recommend enrolling in the Data Analytics Course by Physics Wallah . And as a special offer for our readers, use the coupon code “READER” to get a discount on this course.
Table of Contents
What is Data Analysis?
Data analysis is the systematic process of inspecting, cleaning, transforming, and interpreting data with the objective of discovering valuable insights and drawing meaningful conclusions. This process involves several steps:
- Inspecting : Initial examination of data to understand its structure, quality, and completeness.
- Cleaning : Removing errors, inconsistencies, or irrelevant information to ensure accurate analysis.
- Transforming : Converting data into a format suitable for analysis, such as normalization or aggregation.
- Interpreting : Analyzing the transformed data to identify patterns, trends, and relationships.
Types of Data Analysis Techniques in Research
Data analysis techniques in research are categorized into qualitative and quantitative methods, each with its specific approaches and tools. These techniques are instrumental in extracting meaningful insights, patterns, and relationships from data to support informed decision-making, validate hypotheses, and derive actionable recommendations. Below is an in-depth exploration of the various types of data analysis techniques commonly employed in research:
1) Qualitative Analysis:
Definition: Qualitative analysis focuses on understanding non-numerical data, such as opinions, concepts, or experiences, to derive insights into human behavior, attitudes, and perceptions.
- Content Analysis: Examines textual data, such as interview transcripts, articles, or open-ended survey responses, to identify themes, patterns, or trends.
- Narrative Analysis: Analyzes personal stories or narratives to understand individuals’ experiences, emotions, or perspectives.
- Ethnographic Studies: Involves observing and analyzing cultural practices, behaviors, and norms within specific communities or settings.
2) Quantitative Analysis:
Quantitative analysis emphasizes numerical data and employs statistical methods to explore relationships, patterns, and trends. It encompasses several approaches:
Descriptive Analysis:
- Frequency Distribution: Represents the number of occurrences of distinct values within a dataset.
- Central Tendency: Measures such as mean, median, and mode provide insights into the central values of a dataset.
- Dispersion: Techniques like variance and standard deviation indicate the spread or variability of data.
Diagnostic Analysis:
- Regression Analysis: Assesses the relationship between dependent and independent variables, enabling prediction or understanding causality.
- ANOVA (Analysis of Variance): Examines differences between groups to identify significant variations or effects.
Predictive Analysis:
- Time Series Forecasting: Uses historical data points to predict future trends or outcomes.
- Machine Learning Algorithms: Techniques like decision trees, random forests, and neural networks predict outcomes based on patterns in data.
Prescriptive Analysis:
- Optimization Models: Utilizes linear programming, integer programming, or other optimization techniques to identify the best solutions or strategies.
- Simulation: Mimics real-world scenarios to evaluate various strategies or decisions and determine optimal outcomes.
Specific Techniques:
- Monte Carlo Simulation: Models probabilistic outcomes to assess risk and uncertainty.
- Factor Analysis: Reduces the dimensionality of data by identifying underlying factors or components.
- Cohort Analysis: Studies specific groups or cohorts over time to understand trends, behaviors, or patterns within these groups.
- Cluster Analysis: Classifies objects or individuals into homogeneous groups or clusters based on similarities or attributes.
- Sentiment Analysis: Uses natural language processing and machine learning techniques to determine sentiment, emotions, or opinions from textual data.
Also Read: AI and Predictive Analytics: Examples, Tools, Uses, Ai Vs Predictive Analytics
Data Analysis Techniques in Research Examples
To provide a clearer understanding of how data analysis techniques are applied in research, let’s consider a hypothetical research study focused on evaluating the impact of online learning platforms on students’ academic performance.
Research Objective:
Determine if students using online learning platforms achieve higher academic performance compared to those relying solely on traditional classroom instruction.
Data Collection:
- Quantitative Data: Academic scores (grades) of students using online platforms and those using traditional classroom methods.
- Qualitative Data: Feedback from students regarding their learning experiences, challenges faced, and preferences.
Data Analysis Techniques Applied:
1) Descriptive Analysis:
- Calculate the mean, median, and mode of academic scores for both groups.
- Create frequency distributions to represent the distribution of grades in each group.
2) Diagnostic Analysis:
- Conduct an Analysis of Variance (ANOVA) to determine if there’s a statistically significant difference in academic scores between the two groups.
- Perform Regression Analysis to assess the relationship between the time spent on online platforms and academic performance.
3) Predictive Analysis:
- Utilize Time Series Forecasting to predict future academic performance trends based on historical data.
- Implement Machine Learning algorithms to develop a predictive model that identifies factors contributing to academic success on online platforms.
4) Prescriptive Analysis:
- Apply Optimization Models to identify the optimal combination of online learning resources (e.g., video lectures, interactive quizzes) that maximize academic performance.
- Use Simulation Techniques to evaluate different scenarios, such as varying student engagement levels with online resources, to determine the most effective strategies for improving learning outcomes.
5) Specific Techniques:
- Conduct Factor Analysis on qualitative feedback to identify common themes or factors influencing students’ perceptions and experiences with online learning.
- Perform Cluster Analysis to segment students based on their engagement levels, preferences, or academic outcomes, enabling targeted interventions or personalized learning strategies.
- Apply Sentiment Analysis on textual feedback to categorize students’ sentiments as positive, negative, or neutral regarding online learning experiences.
By applying a combination of qualitative and quantitative data analysis techniques, this research example aims to provide comprehensive insights into the effectiveness of online learning platforms.
Also Read: Learning Path to Become a Data Analyst in 2024
Data Analysis Techniques in Quantitative Research
Quantitative research involves collecting numerical data to examine relationships, test hypotheses, and make predictions. Various data analysis techniques are employed to interpret and draw conclusions from quantitative data. Here are some key data analysis techniques commonly used in quantitative research:
1) Descriptive Statistics:
- Description: Descriptive statistics are used to summarize and describe the main aspects of a dataset, such as central tendency (mean, median, mode), variability (range, variance, standard deviation), and distribution (skewness, kurtosis).
- Applications: Summarizing data, identifying patterns, and providing initial insights into the dataset.
2) Inferential Statistics:
- Description: Inferential statistics involve making predictions or inferences about a population based on a sample of data. This technique includes hypothesis testing, confidence intervals, t-tests, chi-square tests, analysis of variance (ANOVA), regression analysis, and correlation analysis.
- Applications: Testing hypotheses, making predictions, and generalizing findings from a sample to a larger population.
3) Regression Analysis:
- Description: Regression analysis is a statistical technique used to model and examine the relationship between a dependent variable and one or more independent variables. Linear regression, multiple regression, logistic regression, and nonlinear regression are common types of regression analysis .
- Applications: Predicting outcomes, identifying relationships between variables, and understanding the impact of independent variables on the dependent variable.
4) Correlation Analysis:
- Description: Correlation analysis is used to measure and assess the strength and direction of the relationship between two or more variables. The Pearson correlation coefficient, Spearman rank correlation coefficient, and Kendall’s tau are commonly used measures of correlation.
- Applications: Identifying associations between variables and assessing the degree and nature of the relationship.
5) Factor Analysis:
- Description: Factor analysis is a multivariate statistical technique used to identify and analyze underlying relationships or factors among a set of observed variables. It helps in reducing the dimensionality of data and identifying latent variables or constructs.
- Applications: Identifying underlying factors or constructs, simplifying data structures, and understanding the underlying relationships among variables.
6) Time Series Analysis:
- Description: Time series analysis involves analyzing data collected or recorded over a specific period at regular intervals to identify patterns, trends, and seasonality. Techniques such as moving averages, exponential smoothing, autoregressive integrated moving average (ARIMA), and Fourier analysis are used.
- Applications: Forecasting future trends, analyzing seasonal patterns, and understanding time-dependent relationships in data.
7) ANOVA (Analysis of Variance):
- Description: Analysis of variance (ANOVA) is a statistical technique used to analyze and compare the means of two or more groups or treatments to determine if they are statistically different from each other. One-way ANOVA, two-way ANOVA, and MANOVA (Multivariate Analysis of Variance) are common types of ANOVA.
- Applications: Comparing group means, testing hypotheses, and determining the effects of categorical independent variables on a continuous dependent variable.
8) Chi-Square Tests:
- Description: Chi-square tests are non-parametric statistical tests used to assess the association between categorical variables in a contingency table. The Chi-square test of independence, goodness-of-fit test, and test of homogeneity are common chi-square tests.
- Applications: Testing relationships between categorical variables, assessing goodness-of-fit, and evaluating independence.
These quantitative data analysis techniques provide researchers with valuable tools and methods to analyze, interpret, and derive meaningful insights from numerical data. The selection of a specific technique often depends on the research objectives, the nature of the data, and the underlying assumptions of the statistical methods being used.
Also Read: Analysis vs. Analytics: How Are They Different?
Data Analysis Methods
Data analysis methods refer to the techniques and procedures used to analyze, interpret, and draw conclusions from data. These methods are essential for transforming raw data into meaningful insights, facilitating decision-making processes, and driving strategies across various fields. Here are some common data analysis methods:
- Description: Descriptive statistics summarize and organize data to provide a clear and concise overview of the dataset. Measures such as mean, median, mode, range, variance, and standard deviation are commonly used.
- Description: Inferential statistics involve making predictions or inferences about a population based on a sample of data. Techniques such as hypothesis testing, confidence intervals, and regression analysis are used.
3) Exploratory Data Analysis (EDA):
- Description: EDA techniques involve visually exploring and analyzing data to discover patterns, relationships, anomalies, and insights. Methods such as scatter plots, histograms, box plots, and correlation matrices are utilized.
- Applications: Identifying trends, patterns, outliers, and relationships within the dataset.
4) Predictive Analytics:
- Description: Predictive analytics use statistical algorithms and machine learning techniques to analyze historical data and make predictions about future events or outcomes. Techniques such as regression analysis, time series forecasting, and machine learning algorithms (e.g., decision trees, random forests, neural networks) are employed.
- Applications: Forecasting future trends, predicting outcomes, and identifying potential risks or opportunities.
5) Prescriptive Analytics:
- Description: Prescriptive analytics involve analyzing data to recommend actions or strategies that optimize specific objectives or outcomes. Optimization techniques, simulation models, and decision-making algorithms are utilized.
- Applications: Recommending optimal strategies, decision-making support, and resource allocation.
6) Qualitative Data Analysis:
- Description: Qualitative data analysis involves analyzing non-numerical data, such as text, images, videos, or audio, to identify themes, patterns, and insights. Methods such as content analysis, thematic analysis, and narrative analysis are used.
- Applications: Understanding human behavior, attitudes, perceptions, and experiences.
7) Big Data Analytics:
- Description: Big data analytics methods are designed to analyze large volumes of structured and unstructured data to extract valuable insights. Technologies such as Hadoop, Spark, and NoSQL databases are used to process and analyze big data.
- Applications: Analyzing large datasets, identifying trends, patterns, and insights from big data sources.
8) Text Analytics:
- Description: Text analytics methods involve analyzing textual data, such as customer reviews, social media posts, emails, and documents, to extract meaningful information and insights. Techniques such as sentiment analysis, text mining, and natural language processing (NLP) are used.
- Applications: Analyzing customer feedback, monitoring brand reputation, and extracting insights from textual data sources.
These data analysis methods are instrumental in transforming data into actionable insights, informing decision-making processes, and driving organizational success across various sectors, including business, healthcare, finance, marketing, and research. The selection of a specific method often depends on the nature of the data, the research objectives, and the analytical requirements of the project or organization.
Also Read: Quantitative Data Analysis: Types, Analysis & Examples
Data Analysis Tools
Data analysis tools are essential instruments that facilitate the process of examining, cleaning, transforming, and modeling data to uncover useful information, make informed decisions, and drive strategies. Here are some prominent data analysis tools widely used across various industries:
1) Microsoft Excel:
- Description: A spreadsheet software that offers basic to advanced data analysis features, including pivot tables, data visualization tools, and statistical functions.
- Applications: Data cleaning, basic statistical analysis, visualization, and reporting.
2) R Programming Language:
- Description: An open-source programming language specifically designed for statistical computing and data visualization.
- Applications: Advanced statistical analysis, data manipulation, visualization, and machine learning.
3) Python (with Libraries like Pandas, NumPy, Matplotlib, and Seaborn):
- Description: A versatile programming language with libraries that support data manipulation, analysis, and visualization.
- Applications: Data cleaning, statistical analysis, machine learning, and data visualization.
4) SPSS (Statistical Package for the Social Sciences):
- Description: A comprehensive statistical software suite used for data analysis, data mining, and predictive analytics.
- Applications: Descriptive statistics, hypothesis testing, regression analysis, and advanced analytics.
5) SAS (Statistical Analysis System):
- Description: A software suite used for advanced analytics, multivariate analysis, and predictive modeling.
- Applications: Data management, statistical analysis, predictive modeling, and business intelligence.
6) Tableau:
- Description: A data visualization tool that allows users to create interactive and shareable dashboards and reports.
- Applications: Data visualization , business intelligence , and interactive dashboard creation.
7) Power BI:
- Description: A business analytics tool developed by Microsoft that provides interactive visualizations and business intelligence capabilities.
- Applications: Data visualization, business intelligence, reporting, and dashboard creation.
8) SQL (Structured Query Language) Databases (e.g., MySQL, PostgreSQL, Microsoft SQL Server):
- Description: Database management systems that support data storage, retrieval, and manipulation using SQL queries.
- Applications: Data retrieval, data cleaning, data transformation, and database management.
9) Apache Spark:
- Description: A fast and general-purpose distributed computing system designed for big data processing and analytics.
- Applications: Big data processing, machine learning, data streaming, and real-time analytics.
10) IBM SPSS Modeler:
- Description: A data mining software application used for building predictive models and conducting advanced analytics.
- Applications: Predictive modeling, data mining, statistical analysis, and decision optimization.
These tools serve various purposes and cater to different data analysis needs, from basic statistical analysis and data visualization to advanced analytics, machine learning, and big data processing. The choice of a specific tool often depends on the nature of the data, the complexity of the analysis, and the specific requirements of the project or organization.
Also Read: How to Analyze Survey Data: Methods & Examples
Importance of Data Analysis in Research
The importance of data analysis in research cannot be overstated; it serves as the backbone of any scientific investigation or study. Here are several key reasons why data analysis is crucial in the research process:
- Data analysis helps ensure that the results obtained are valid and reliable. By systematically examining the data, researchers can identify any inconsistencies or anomalies that may affect the credibility of the findings.
- Effective data analysis provides researchers with the necessary information to make informed decisions. By interpreting the collected data, researchers can draw conclusions, make predictions, or formulate recommendations based on evidence rather than intuition or guesswork.
- Data analysis allows researchers to identify patterns, trends, and relationships within the data. This can lead to a deeper understanding of the research topic, enabling researchers to uncover insights that may not be immediately apparent.
- In empirical research, data analysis plays a critical role in testing hypotheses. Researchers collect data to either support or refute their hypotheses, and data analysis provides the tools and techniques to evaluate these hypotheses rigorously.
- Transparent and well-executed data analysis enhances the credibility of research findings. By clearly documenting the data analysis methods and procedures, researchers allow others to replicate the study, thereby contributing to the reproducibility of research findings.
- In fields such as business or healthcare, data analysis helps organizations allocate resources more efficiently. By analyzing data on consumer behavior, market trends, or patient outcomes, organizations can make strategic decisions about resource allocation, budgeting, and planning.
- In public policy and social sciences, data analysis is instrumental in developing and evaluating policies and interventions. By analyzing data on social, economic, or environmental factors, policymakers can assess the effectiveness of existing policies and inform the development of new ones.
- Data analysis allows for continuous improvement in research methods and practices. By analyzing past research projects, identifying areas for improvement, and implementing changes based on data-driven insights, researchers can refine their approaches and enhance the quality of future research endeavors.
However, it is important to remember that mastering these techniques requires practice and continuous learning. That’s why we highly recommend the Data Analytics Course by Physics Wallah . Not only does it cover all the fundamentals of data analysis, but it also provides hands-on experience with various tools such as Excel, Python, and Tableau. Plus, if you use the “ READER ” coupon code at checkout, you can get a special discount on the course.
For Latest Tech Related Information, Join Our Official Free Telegram Group : PW Skills Telegram Group
Data Analysis Techniques in Research FAQs
What are the 5 techniques for data analysis.
The five techniques for data analysis include: Descriptive Analysis Diagnostic Analysis Predictive Analysis Prescriptive Analysis Qualitative Analysis
What are techniques of data analysis in research?
Techniques of data analysis in research encompass both qualitative and quantitative methods. These techniques involve processes like summarizing raw data, investigating causes of events, forecasting future outcomes, offering recommendations based on predictions, and examining non-numerical data to understand concepts or experiences.
What are the 3 methods of data analysis?
The three primary methods of data analysis are: Qualitative Analysis Quantitative Analysis Mixed-Methods Analysis
What are the four types of data analysis techniques?
The four types of data analysis techniques are: Descriptive Analysis Diagnostic Analysis Predictive Analysis Prescriptive Analysis
Top 20 Big Data Tools Used By Professionals
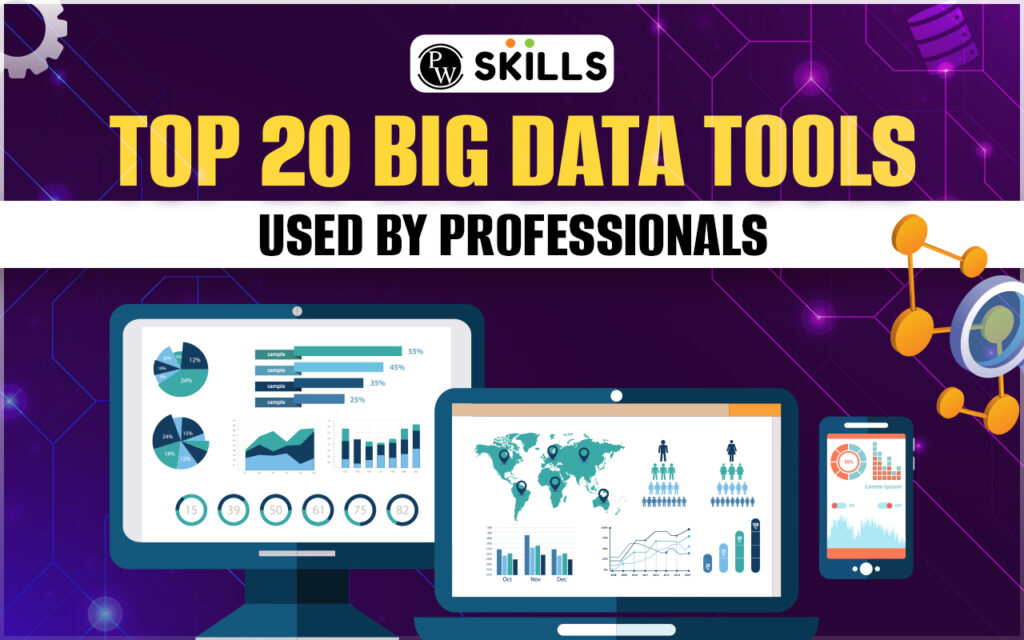
There are plenty of big data tools available online for free. However, some of the handpicked big data tools used…
Top Best Big Data Analytics Classes 2024
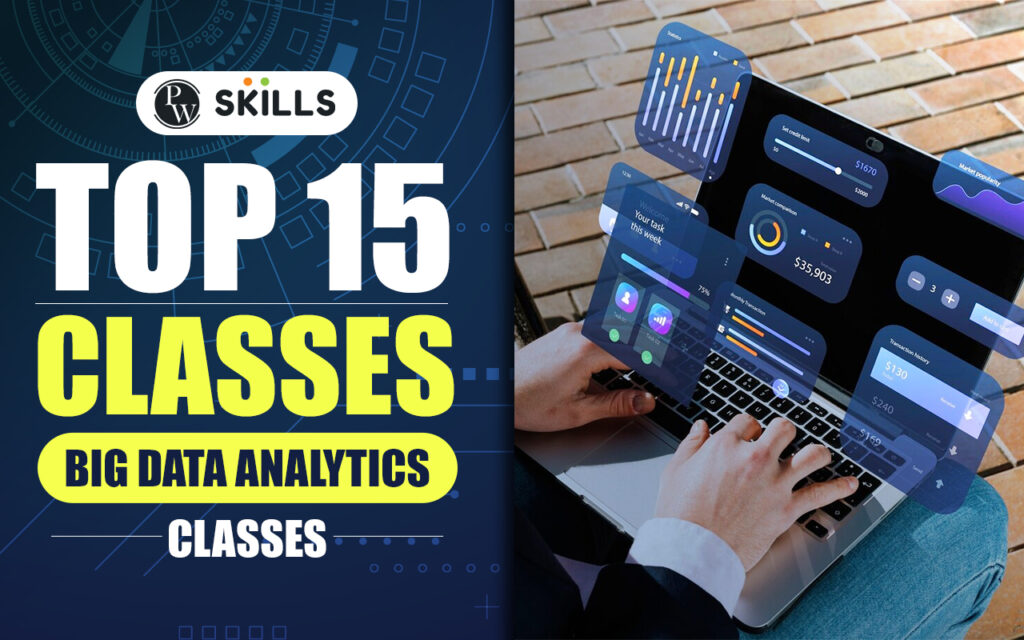
Many websites and institutions provide online remote big data analytics classes to help you learn and also earn certifications for…
Big Data Defined: Examples and Benefits
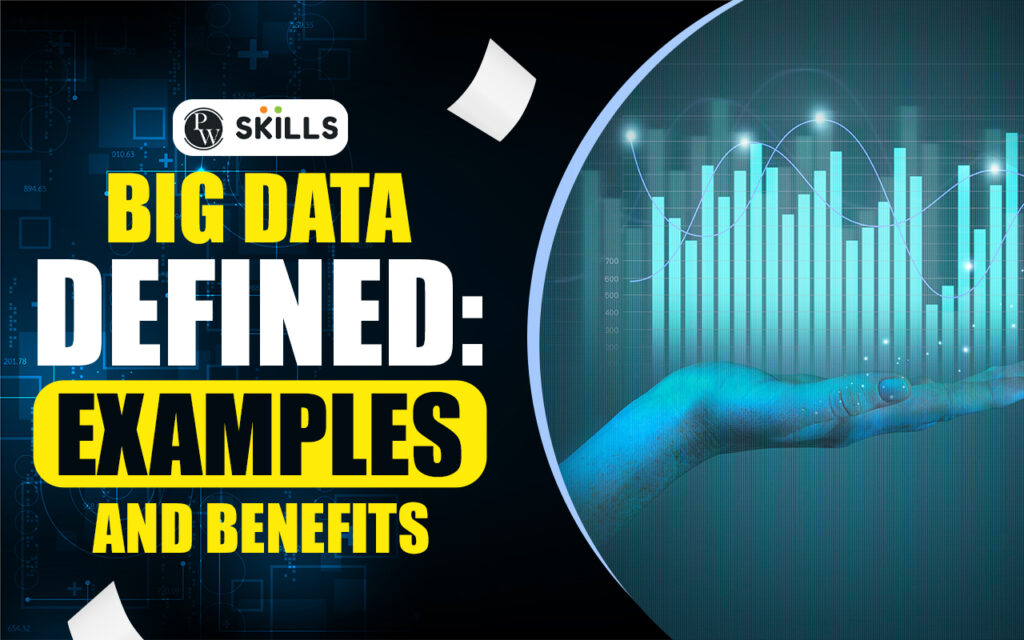
Big data is a tremendous volume of complex data collected from various sources, such as text, videos, audio, email, etc.…
- Privacy Policy
Buy Me a Coffee

Home » Descriptive Analytics – Methods, Tools and Examples
Descriptive Analytics – Methods, Tools and Examples
Table of Contents

Descriptive Analytics
Definition:
Descriptive analytics focused on describing or summarizing raw data and making it interpretable. This type of analytics provides insight into what has happened in the past. It involves the analysis of historical data to identify patterns, trends, and insights. Descriptive analytics often uses visualization tools to represent the data in a way that is easy to interpret.
Descriptive Analytics in Research
Descriptive analytics plays a crucial role in research, helping investigators understand and describe the data collected in their studies. Here’s how descriptive analytics is typically used in a research setting:
- Descriptive Statistics: In research, descriptive analytics often takes the form of descriptive statistics . This includes calculating measures of central tendency (like mean, median, and mode), measures of dispersion (like range, variance, and standard deviation), and measures of frequency (like count, percent, and frequency). These calculations help researchers summarize and understand their data.
- Visualizing Data: Descriptive analytics also involves creating visual representations of data to better understand and communicate research findings . This might involve creating bar graphs, line graphs, pie charts, scatter plots, box plots, and other visualizations.
- Exploratory Data Analysis: Before conducting any formal statistical tests, researchers often conduct an exploratory data analysis, which is a form of descriptive analytics. This might involve looking at distributions of variables, checking for outliers, and exploring relationships between variables.
- Initial Findings: Descriptive analytics are often reported in the results section of a research study to provide readers with an overview of the data. For example, a researcher might report average scores, demographic breakdowns, or the percentage of participants who endorsed each response on a survey.
- Establishing Patterns and Relationships: Descriptive analytics helps in identifying patterns, trends, or relationships in the data, which can guide subsequent analysis or future research. For instance, researchers might look at the correlation between variables as a part of descriptive analytics.
Descriptive Analytics Techniques
Descriptive analytics involves a variety of techniques to summarize, interpret, and visualize historical data. Some commonly used techniques include:
Statistical Analysis
This includes basic statistical methods like mean, median, mode (central tendency), standard deviation, variance (dispersion), correlation, and regression (relationships between variables).
Data Aggregation
It is the process of compiling and summarizing data to obtain a general perspective. It can involve methods like sum, count, average, min, max, etc., often applied to a group of data.
Data Mining
This involves analyzing large volumes of data to discover patterns, trends, and insights. Techniques used in data mining can include clustering (grouping similar data), classification (assigning data into categories), association rules (finding relationships between variables), and anomaly detection (identifying outliers).
Data Visualization
This involves presenting data in a graphical or pictorial format to provide clear and easy understanding of the data patterns, trends, and insights. Common data visualization methods include bar charts, line graphs, pie charts, scatter plots, histograms, and more complex forms like heat maps and interactive dashboards.
This involves organizing data into informational summaries to monitor how different areas of a business are performing. Reports can be generated manually or automatically and can be presented in tables, graphs, or dashboards.
Cross-tabulation (or Pivot Tables)
It involves displaying the relationship between two or more variables in a tabular form. It can provide a deeper understanding of the data by allowing comparisons and revealing patterns and correlations that may not be readily apparent in raw data.
Descriptive Modeling
Some techniques use complex algorithms to interpret data. Examples include decision tree analysis, which provides a graphical representation of decision-making situations, and neural networks, which are used to identify correlations and patterns in large data sets.
Descriptive Analytics Tools
Some common Descriptive Analytics Tools are as follows:
Excel: Microsoft Excel is a widely used tool that can be used for simple descriptive analytics. It has powerful statistical and data visualization capabilities. Pivot tables are a particularly useful feature for summarizing and analyzing large data sets.
Tableau: Tableau is a data visualization tool that is used to represent data in a graphical or pictorial format. It can handle large data sets and allows for real-time data analysis.
Power BI: Power BI, another product from Microsoft, is a business analytics tool that provides interactive visualizations with self-service business intelligence capabilities.
QlikView: QlikView is a data visualization and discovery tool. It allows users to analyze data and use this data to support decision-making.
SAS: SAS is a software suite that can mine, alter, manage and retrieve data from a variety of sources and perform statistical analysis on it.
SPSS: SPSS (Statistical Package for the Social Sciences) is a software package used for statistical analysis. It’s widely used in social sciences research but also in other industries.
Google Analytics: For web data, Google Analytics is a popular tool. It allows businesses to analyze in-depth detail about the visitors on their website, providing valuable insights that can help shape the success strategy of a business.
R and Python: Both are programming languages that have robust capabilities for statistical analysis and data visualization. With packages like pandas, matplotlib, seaborn in Python and ggplot2, dplyr in R, these languages are powerful tools for descriptive analytics.
Looker: Looker is a modern data platform that can take data from any database and let you start exploring and visualizing.
When to use Descriptive Analytics
Descriptive analytics forms the base of the data analysis workflow and is typically the first step in understanding your business or organization’s data. Here are some situations when you might use descriptive analytics:
Understanding Past Behavior: Descriptive analytics is essential for understanding what has happened in the past. If you need to understand past sales trends, customer behavior, or operational performance, descriptive analytics is the tool you’d use.
Reporting Key Metrics: Descriptive analytics is used to establish and report key performance indicators (KPIs). It can help in tracking and presenting these KPIs in dashboards or regular reports.
Identifying Patterns and Trends: If you need to identify patterns or trends in your data, descriptive analytics can provide these insights. This might include identifying seasonality in sales data, understanding peak operational times, or spotting trends in customer behavior.
Informing Business Decisions: The insights provided by descriptive analytics can inform business strategy and decision-making. By understanding what has happened in the past, you can make more informed decisions about what steps to take in the future.
Benchmarking Performance: Descriptive analytics can be used to compare current performance against historical data. This can be used for benchmarking and setting performance goals.
Auditing and Regulatory Compliance: In sectors where compliance and auditing are essential, descriptive analytics can provide the necessary data and trends over specific periods.
Initial Data Exploration: When you first acquire a dataset, descriptive analytics is useful to understand the structure of the data, the relationships between variables, and any apparent anomalies or outliers.
Examples of Descriptive Analytics
Examples of Descriptive Analytics are as follows:
Retail Industry: A retail company might use descriptive analytics to analyze sales data from the past year. They could break down sales by month to identify any seasonality trends. For example, they might find that sales increase in November and December due to holiday shopping. They could also break down sales by product to identify which items are the most popular. This analysis could inform their purchasing and stocking decisions for the next year. Additionally, data on customer demographics could be analyzed to understand who their primary customers are, guiding their marketing strategies.
Healthcare Industry: In healthcare, descriptive analytics could be used to analyze patient data over time. For instance, a hospital might analyze data on patient admissions to identify trends in admission rates. They might find that admissions for certain conditions are higher at certain times of the year. This could help them allocate resources more effectively. Also, analyzing patient outcomes data can help identify the most effective treatments or highlight areas where improvement is needed.
Finance Industry: A financial firm might use descriptive analytics to analyze historical market data. They could look at trends in stock prices, trading volume, or economic indicators to inform their investment decisions. For example, analyzing the price-earnings ratios of stocks in a certain sector over time could reveal patterns that suggest whether the sector is currently overvalued or undervalued. Similarly, credit card companies can analyze transaction data to detect any unusual patterns, which could be signs of fraud.
Advantages of Descriptive Analytics
Descriptive analytics plays a vital role in the world of data analysis, providing numerous advantages:
- Understanding the Past: Descriptive analytics provides an understanding of what has happened in the past, offering valuable context for future decision-making.
- Data Summarization: Descriptive analytics is used to simplify and summarize complex datasets, which can make the information more understandable and accessible.
- Identifying Patterns and Trends: With descriptive analytics, organizations can identify patterns, trends, and correlations in their data, which can provide valuable insights.
- Inform Decision-Making: The insights generated through descriptive analytics can inform strategic decisions and help organizations to react more quickly to events or changes in behavior.
- Basis for Further Analysis: Descriptive analytics lays the groundwork for further analytical activities. It’s the first necessary step before moving on to more advanced forms of analytics like predictive analytics (forecasting future events) or prescriptive analytics (advising on possible outcomes).
- Performance Evaluation: It allows organizations to evaluate their performance by comparing current results with past results, enabling them to see where improvements have been made and where further improvements can be targeted.
- Enhanced Reporting and Dashboards: Through the use of visualization techniques, descriptive analytics can improve the quality of reports and dashboards, making the data more understandable and easier to interpret for stakeholders at all levels of the organization.
- Immediate Value: Unlike some other types of analytics, descriptive analytics can provide immediate insights, as it doesn’t require complex models or deep analytical capabilities to provide value.
Disadvantages of Descriptive Analytics
While descriptive analytics offers numerous benefits, it also has certain limitations or disadvantages. Here are a few to consider:
- Limited to Past Data: Descriptive analytics primarily deals with historical data and provides insights about past events. It does not predict future events or trends and can’t help you understand possible future outcomes on its own.
- Lack of Deep Insights: While descriptive analytics helps in identifying what happened, it does not answer why it happened. For deeper insights, you would need to use diagnostic analytics, which analyzes data to understand the root cause of a particular outcome.
- Can Be Misleading: If not properly executed, descriptive analytics can sometimes lead to incorrect conclusions. For example, correlation does not imply causation, but descriptive analytics might tempt one to make such an inference.
- Data Quality Issues: The accuracy and usefulness of descriptive analytics are heavily reliant on the quality of the underlying data. If the data is incomplete, incorrect, or biased, the results of the descriptive analytics will be too.
- Over-reliance on Descriptive Analytics: Businesses may rely too much on descriptive analytics and not enough on predictive and prescriptive analytics. While understanding past and present data is important, it’s equally vital to forecast future trends and make data-driven decisions based on those predictions.
- Doesn’t Provide Actionable Insights: Descriptive analytics is used to interpret historical data and identify patterns and trends, but it doesn’t provide recommendations or courses of action. For that, prescriptive analytics is needed.
About the author
Muhammad Hassan
Researcher, Academic Writer, Web developer
You may also like

Digital Ethnography – Types, Methods and Examples

Predictive Analytics – Techniques, Tools and...

Big Data Analytics -Types, Tools and Methods

Diagnostic Analytics – Methods, Tools and...

Blockchain Research – Methods, Types and Examples

Social Network Analysis – Types, Tools and...

Effective Use of Statistics in Research – Methods and Tools for Data Analysis
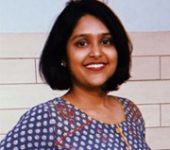
Remember that impending feeling you get when you are asked to analyze your data! Now that you have all the required raw data, you need to statistically prove your hypothesis. Representing your numerical data as part of statistics in research will also help in breaking the stereotype of being a biology student who can’t do math.
Statistical methods are essential for scientific research. In fact, statistical methods dominate the scientific research as they include planning, designing, collecting data, analyzing, drawing meaningful interpretation and reporting of research findings. Furthermore, the results acquired from research project are meaningless raw data unless analyzed with statistical tools. Therefore, determining statistics in research is of utmost necessity to justify research findings. In this article, we will discuss how using statistical methods for biology could help draw meaningful conclusion to analyze biological studies.
Table of Contents
Role of Statistics in Biological Research
Statistics is a branch of science that deals with collection, organization and analysis of data from the sample to the whole population. Moreover, it aids in designing a study more meticulously and also give a logical reasoning in concluding the hypothesis. Furthermore, biology study focuses on study of living organisms and their complex living pathways, which are very dynamic and cannot be explained with logical reasoning. However, statistics is more complex a field of study that defines and explains study patterns based on the sample sizes used. To be precise, statistics provides a trend in the conducted study.
Biological researchers often disregard the use of statistics in their research planning, and mainly use statistical tools at the end of their experiment. Therefore, giving rise to a complicated set of results which are not easily analyzed from statistical tools in research. Statistics in research can help a researcher approach the study in a stepwise manner, wherein the statistical analysis in research follows –
1. Establishing a Sample Size
Usually, a biological experiment starts with choosing samples and selecting the right number of repetitive experiments. Statistics in research deals with basics in statistics that provides statistical randomness and law of using large samples. Statistics teaches how choosing a sample size from a random large pool of sample helps extrapolate statistical findings and reduce experimental bias and errors.
2. Testing of Hypothesis
When conducting a statistical study with large sample pool, biological researchers must make sure that a conclusion is statistically significant. To achieve this, a researcher must create a hypothesis before examining the distribution of data. Furthermore, statistics in research helps interpret the data clustered near the mean of distributed data or spread across the distribution. These trends help analyze the sample and signify the hypothesis.
3. Data Interpretation Through Analysis
When dealing with large data, statistics in research assist in data analysis. This helps researchers to draw an effective conclusion from their experiment and observations. Concluding the study manually or from visual observation may give erroneous results; therefore, thorough statistical analysis will take into consideration all the other statistical measures and variance in the sample to provide a detailed interpretation of the data. Therefore, researchers produce a detailed and important data to support the conclusion.
Types of Statistical Research Methods That Aid in Data Analysis
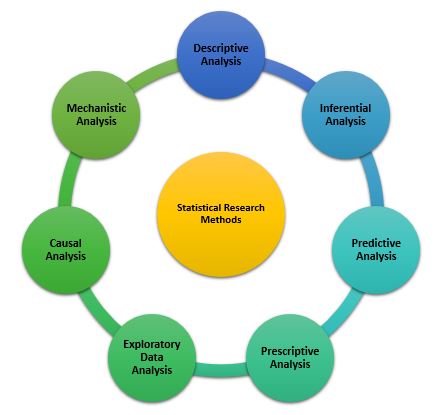
Statistical analysis is the process of analyzing samples of data into patterns or trends that help researchers anticipate situations and make appropriate research conclusions. Based on the type of data, statistical analyses are of the following type:
1. Descriptive Analysis
The descriptive statistical analysis allows organizing and summarizing the large data into graphs and tables . Descriptive analysis involves various processes such as tabulation, measure of central tendency, measure of dispersion or variance, skewness measurements etc.
2. Inferential Analysis
The inferential statistical analysis allows to extrapolate the data acquired from a small sample size to the complete population. This analysis helps draw conclusions and make decisions about the whole population on the basis of sample data. It is a highly recommended statistical method for research projects that work with smaller sample size and meaning to extrapolate conclusion for large population.
3. Predictive Analysis
Predictive analysis is used to make a prediction of future events. This analysis is approached by marketing companies, insurance organizations, online service providers, data-driven marketing, and financial corporations.
4. Prescriptive Analysis
Prescriptive analysis examines data to find out what can be done next. It is widely used in business analysis for finding out the best possible outcome for a situation. It is nearly related to descriptive and predictive analysis. However, prescriptive analysis deals with giving appropriate suggestions among the available preferences.
5. Exploratory Data Analysis
EDA is generally the first step of the data analysis process that is conducted before performing any other statistical analysis technique. It completely focuses on analyzing patterns in the data to recognize potential relationships. EDA is used to discover unknown associations within data, inspect missing data from collected data and obtain maximum insights.
6. Causal Analysis
Causal analysis assists in understanding and determining the reasons behind “why” things happen in a certain way, as they appear. This analysis helps identify root cause of failures or simply find the basic reason why something could happen. For example, causal analysis is used to understand what will happen to the provided variable if another variable changes.
7. Mechanistic Analysis
This is a least common type of statistical analysis. The mechanistic analysis is used in the process of big data analytics and biological science. It uses the concept of understanding individual changes in variables that cause changes in other variables correspondingly while excluding external influences.
Important Statistical Tools In Research
Researchers in the biological field find statistical analysis in research as the scariest aspect of completing research. However, statistical tools in research can help researchers understand what to do with data and how to interpret the results, making this process as easy as possible.
1. Statistical Package for Social Science (SPSS)
It is a widely used software package for human behavior research. SPSS can compile descriptive statistics, as well as graphical depictions of result. Moreover, it includes the option to create scripts that automate analysis or carry out more advanced statistical processing.
2. R Foundation for Statistical Computing
This software package is used among human behavior research and other fields. R is a powerful tool and has a steep learning curve. However, it requires a certain level of coding. Furthermore, it comes with an active community that is engaged in building and enhancing the software and the associated plugins.
3. MATLAB (The Mathworks)
It is an analytical platform and a programming language. Researchers and engineers use this software and create their own code and help answer their research question. While MatLab can be a difficult tool to use for novices, it offers flexibility in terms of what the researcher needs.
4. Microsoft Excel
Not the best solution for statistical analysis in research, but MS Excel offers wide variety of tools for data visualization and simple statistics. It is easy to generate summary and customizable graphs and figures. MS Excel is the most accessible option for those wanting to start with statistics.
5. Statistical Analysis Software (SAS)
It is a statistical platform used in business, healthcare, and human behavior research alike. It can carry out advanced analyzes and produce publication-worthy figures, tables and charts .
6. GraphPad Prism
It is a premium software that is primarily used among biology researchers. But, it offers a range of variety to be used in various other fields. Similar to SPSS, GraphPad gives scripting option to automate analyses to carry out complex statistical calculations.
This software offers basic as well as advanced statistical tools for data analysis. However, similar to GraphPad and SPSS, minitab needs command over coding and can offer automated analyses.
Use of Statistical Tools In Research and Data Analysis
Statistical tools manage the large data. Many biological studies use large data to analyze the trends and patterns in studies. Therefore, using statistical tools becomes essential, as they manage the large data sets, making data processing more convenient.
Following these steps will help biological researchers to showcase the statistics in research in detail, and develop accurate hypothesis and use correct tools for it.
There are a range of statistical tools in research which can help researchers manage their research data and improve the outcome of their research by better interpretation of data. You could use statistics in research by understanding the research question, knowledge of statistics and your personal experience in coding.
Have you faced challenges while using statistics in research? How did you manage it? Did you use any of the statistical tools to help you with your research data? Do write to us or comment below!
Frequently Asked Questions
Statistics in research can help a researcher approach the study in a stepwise manner: 1. Establishing a sample size 2. Testing of hypothesis 3. Data interpretation through analysis
Statistical methods are essential for scientific research. In fact, statistical methods dominate the scientific research as they include planning, designing, collecting data, analyzing, drawing meaningful interpretation and reporting of research findings. Furthermore, the results acquired from research project are meaningless raw data unless analyzed with statistical tools. Therefore, determining statistics in research is of utmost necessity to justify research findings.
Statistical tools in research can help researchers understand what to do with data and how to interpret the results, making this process as easy as possible. They can manage large data sets, making data processing more convenient. A great number of tools are available to carry out statistical analysis of data like SPSS, SAS (Statistical Analysis Software), and Minitab.

nice article to read
Holistic but delineating. A very good read.
Rate this article Cancel Reply
Your email address will not be published.
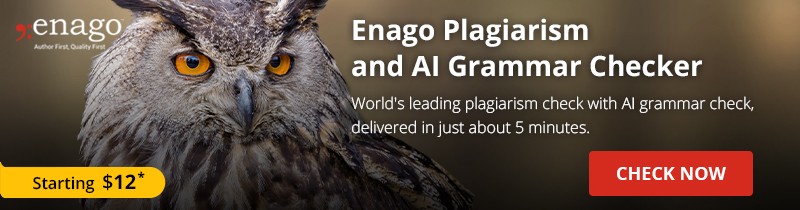
Enago Academy's Most Popular Articles
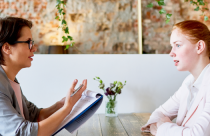
- Reporting Research
Research Interviews: An effective and insightful way of data collection
Research interviews play a pivotal role in collecting data for various academic, scientific, and professional…
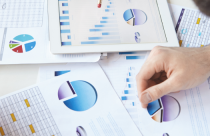
Planning Your Data Collection: Designing methods for effective research
Planning your research is very important to obtain desirable results. In research, the relevance of…
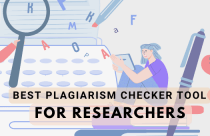
- Language & Grammar
Best Plagiarism Checker Tool for Researchers — Top 4 to choose from!
While common writing issues like language enhancement, punctuation errors, grammatical errors, etc. can be dealt…
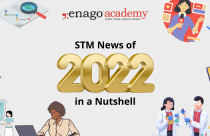
- Industry News
- Publishing News
2022 in a Nutshell — Reminiscing the year when opportunities were seized and feats were achieved!
It’s beginning to look a lot like success! Some of the greatest opportunities to research…
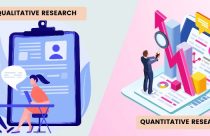
- Manuscript Preparation
- Publishing Research
Qualitative Vs. Quantitative Research — A step-wise guide to conduct research
A research study includes the collection and analysis of data. In quantitative research, the data…
2022 in a Nutshell — Reminiscing the year when opportunities were seized and feats…

Sign-up to read more
Subscribe for free to get unrestricted access to all our resources on research writing and academic publishing including:
- 2000+ blog articles
- 50+ Webinars
- 10+ Expert podcasts
- 50+ Infographics
- 10+ Checklists
- Research Guides
We hate spam too. We promise to protect your privacy and never spam you.
I am looking for Editing/ Proofreading services for my manuscript Tentative date of next journal submission:
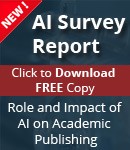
What should universities' stance be on AI tools in research and academic writing?
Join thousands of product people at Insight Out Conf on April 11. Register free.
Insights hub solutions
Analyze data
Uncover deep customer insights with fast, powerful features, store insights, curate and manage insights in one searchable platform, scale research, unlock the potential of customer insights at enterprise scale.
Featured reads
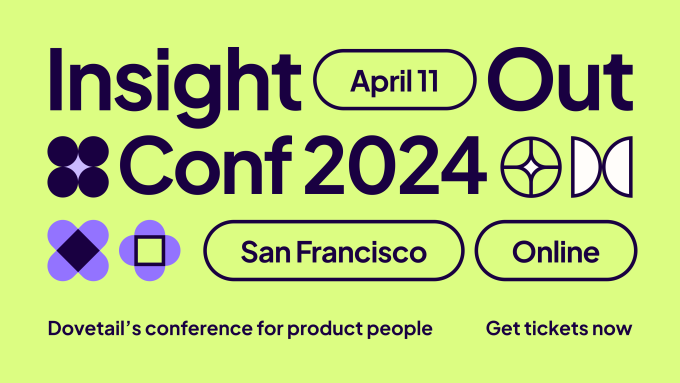
Inspiration
Three things to look forward to at Insight Out
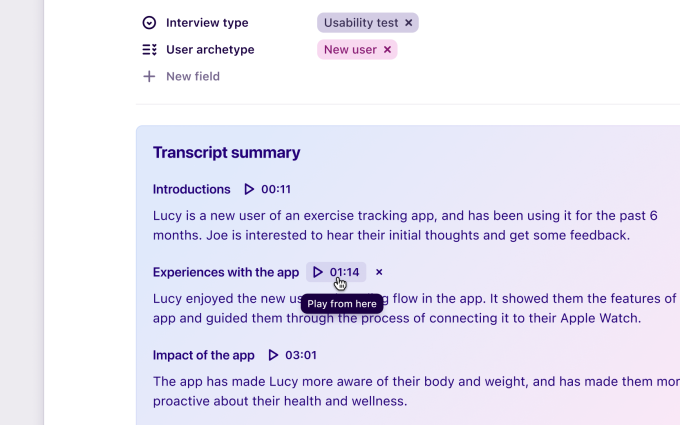
Tips and tricks
Make magic with your customer data in Dovetail
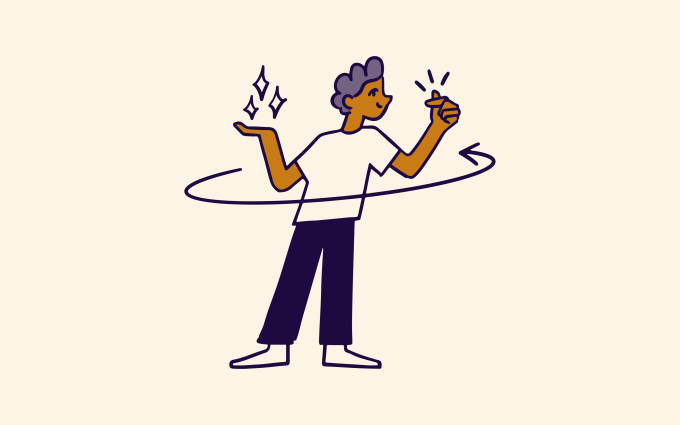
Four ways Dovetail helps Product Managers master continuous product discovery
Events and videos
© Dovetail Research Pty. Ltd.
Top 21 must-have digital tools for researchers
Last updated
12 May 2023
Reviewed by
Jean Kaluza
Research drives many decisions across various industries, including:
Uncovering customer motivations and behaviors to design better products
Assessing whether a market exists for your product or service
Running clinical studies to develop a medical breakthrough
Conducting effective and shareable research can be a painstaking process. Manual processes are sluggish and archaic, and they can also be inaccurate. That’s where advanced online tools can help.
The right tools can enable businesses to lean into research for better forecasting, planning, and more reliable decisions.
- Why do researchers need research tools?
Research is challenging and time-consuming. Analyzing data, running focus groups , reading research papers , and looking for useful insights take plenty of heavy lifting.
These days, researchers can’t just rely on manual processes. Instead, they’re using advanced tools that:
Speed up the research process
Enable new ways of reaching customers
Improve organization and accuracy
Allow better monitoring throughout the process
Enhance collaboration across key stakeholders
- The most important digital tools for researchers
Some tools can help at every stage, making researching simpler and faster.
They ensure accurate and efficient information collection, management, referencing, and analysis.
Some of the most important digital tools for researchers include:
Research management tools
Research management can be a complex and challenging process. Some tools address the various challenges that arise when referencing and managing papers.
.css-32cyld{-webkit-align-items:center;-webkit-box-align:center;-ms-flex-align:center;align-items:center;background:transparent;border:0;color:inherit;cursor:pointer;display:inline-block;-webkit-flex-shrink:0;-ms-flex-negative:0;flex-shrink:0;-webkit-text-decoration:underline;text-decoration:underline;} Zotero
Coined as a personal research assistant, Zotero is a tool that brings efficiency to the research process. Zotero helps researchers collect, organize, annotate, and share research easily.
Zotero integrates with internet browsers, so researchers can easily save an article, publication, or research study on the platform for later.
The tool also has an advanced organizing system to allow users to label, tag, and categorize information for faster insights and a seamless analysis process.
Messy paper stacks––digital or physical––are a thing of the past with Paperpile. This reference management tool integrates with Google Docs, saving users time with citations and paper management.
Referencing, researching, and gaining insights is much cleaner and more productive, as all papers are in the same place. Plus, it’s easier to find a paper when you need it.
Acting as a single source of truth (SSOT), Dovetail houses research from the entire organization in a simple-to-use place. Researchers can use the all-in-one platform to collate and store data from interviews , forms, surveys , focus groups, and more.
Dovetail helps users quickly categorize and analyze data to uncover truly actionable insights . This helps organizations bring customer insights into every decision for better forecasting, planning, and decision-making.
Dovetail integrates with other helpful tools like Slack, Atlassian, Notion, and Zapier for a truly efficient workflow.
Putting together papers and referencing sources can be a huge time consumer. EndNote claims that researchers waste 200,000 hours per year formatting citations.
To address the issue, the tool formats citations automatically––simultaneously creating a bibliography while the user writes.
EndNote is also a cloud-based system that allows remote working, multiple-user interaction and collaboration, and seamless working on different devices.
Information survey tools
Surveys are a common way to gain data from customers. These tools can make the process simpler and more cost-effective.
With ready-made survey templates––to collect NPS data, customer effort scores, five-star surveys, and more––getting going with Delighted is straightforward.
Delighted helps teams collect and analyze survey feedback without needing any technical knowledge. The templates are customizable, so you can align the content with your brand. That way, the survey feels like it’s coming from your company, not a third party.
SurveyMonkey
With millions of customers worldwide, SurveyMonkey is another leader in online surveys. SurveyMonkey offers hundreds of templates that researchers can use to set up and deploy surveys quickly.
Whether your survey is about team performance, hotel feedback, post-event feedback, or an employee exit, SurveyMonkey has a ready-to-use template.
Typeform offers free templates you can quickly embed, which comes with a point of difference: It designs forms and surveys with people in mind, focusing on customer enjoyment.
Typeform employs the ‘one question at a time’ method to keep engagement rates and completions high. It focuses on surveys that feel more like conversations than a list of questions.
Web data analysis tools
Collecting data can take time––especially technical information. Some tools make that process simpler.
For those conducting clinical research, data collection can be incredibly time-consuming. Teamscope provides an online platform to collect and manage data simply and easily.
Researchers and medical professionals often collect clinical data through paper forms or digital means. Those are too easy to lose, tricky to manage, and challenging to collaborate on.
With Teamscope, you can easily collect, store, and electronically analyze data like patient-reported outcomes and surveys.
Heap is a digital insights platform providing context on the entire customer journey . This helps businesses improve customer feedback , conversion rates, and loyalty.
Through Heap, you can seamlessly view and analyze the customer journey across all platforms and touchpoints, whether through the app or website.
Another analytics tool, Smartlook, combines quantitative and qualitative analytics into one platform. This helps organizations understand user behavior and make crucial improvements.
Smartlook is useful for analyzing web pages, purchasing flows, and optimizing conversion rates.
Project management tools
Managing multiple research projects across many teams can be complex and challenging. Project management tools can ease the burden on researchers.
Visual productivity tool Trello helps research teams manage their projects more efficiently. Trello makes product tracking easier with:
A range of workflow options
Unique project board layouts
Advanced descriptions
Integrations
Trello also works as an SSOT to stay on top of projects and collaborate effectively as a team.
To connect research, workflows, and teams, Airtable provides a clean interactive interface.
With Airtable, it’s simple to place research projects in a list view, workstream, or road map to synthesize information and quickly collaborate. The Sync feature makes it easy to link all your research data to one place for faster action.
For product teams, Asana gathers development, copywriting, design, research teams, and product managers in one space.
As a task management platform, Asana offers all the expected features and more, including time-tracking and Jira integration. The platform offers reporting alongside data collection methods, so it’s a favorite for product teams in the tech space.
Grammar checker tools
Grammar tools ensure your research projects are professional and proofed.
No one’s perfect, especially when it comes to spelling, punctuation, and grammar. That’s where Grammarly can help.
Grammarly’s AI-powered platform reviews your content and corrects any mistakes. Through helpful integrations with other platforms––such as Gmail, Google Docs, Twitter, and LinkedIn––it’s simple to spellcheck as you go.
Another helpful grammar tool is Trinka AI. Trinka is specifically for technical and academic styles of writing. It doesn’t just correct mistakes in spelling, punctuation, and grammar; it also offers explanations and additional information when errors show.
Researchers can also use Trinka to enhance their writing and:
Align it with technical and academic styles
Improve areas like syntax and word choice
Discover relevant suggestions based on the content topic
Plagiarism checker tools
Avoiding plagiarism is crucial for the integrity of research. Using checker tools can ensure your work is original.
Plagiarism checker Quetext uses DeepSearch™ technology to quickly sort through online content to search for signs of plagiarism.
With color coding, annotations, and an overall score, it’s easy to identify conflict areas and fix them accordingly.
Duplichecker
Another helpful plagiarism tool is Duplichecker, which scans pieces of content for issues. The service is free for content up to 1000 words, with paid options available after that.
If plagiarism occurs, a percentage identifies how much is duplicate content. However, the interface is relatively basic, offering little additional information.
Journal finder tools
Finding the right journals for your project can be challenging––especially with the plethora of inaccurate or predatory content online. Journal finder tools can solve this issue.
Enago Journal Finder
The Enago Open Access Journal Finder sorts through online journals to verify their legitimacy. Through Engao, you can discover pre-vetted, high-quality journals through a validated journal index.
Enago’s search tool also helps users find relevant journals for their subject matter, speeding up the research process.
JournalFinder
JournalFinder is another journal tool that’s popular with academics and researchers. It makes the process of discovering relevant journals fast by leaning into a machine-learning algorithm.
This is useful for discovering key information and finding the right journals to publish and share your work in.
Social networking for researchers
Collaboration between researchers can improve the accuracy and sharing of information. Promoting research findings can also be essential for public health, safety, and more.
While typical social networks exist, some are specifically designed for academics.
ResearchGate
Networking platform ResearchGate encourages researchers to connect, collaborate, and share within the scientific community. With 20 million researchers on the platform, it's a popular choice.
ResearchGate is founded on an intention to advance research. The platform provides topic pages for easy connection within a field of expertise and access to millions of publications to help users stay up to date.
Academia is another commonly used platform that connects 220 million academics and researchers within their specialties.
The platform aims to accelerate research with discovery tools and grow a researcher’s audience to promote their ideas.
On Academia, users can access 47 million PDFs for free. They cover topics from mechanical engineering to applied economics and child psychology.
- Expedited research with the power of tools
For researchers, finding data and information can be time-consuming and complex to manage. That’s where the power of tools comes in.
Manual processes are slow, outdated, and have a larger potential for inaccuracies.
Leaning into tools can help researchers speed up their processes, conduct efficient research, boost their accuracy, and share their work effectively.
With tools available for project and data management, web data collection, and journal finding, researchers have plenty of assistance at their disposal.
When it comes to connecting with customers, advanced tools boost customer connection while continually bringing their needs and wants into products and services.
What are primary research tools?
Primary research is data and information that you collect firsthand through surveys, customer interviews, or focus groups.
Secondary research is data and information from other sources, such as journals, research bodies, or online content.
Primary researcher tools use methods like surveys and customer interviews. You can use these tools to collect, store, or manage information effectively and uncover more accurate insights.
What is the difference between tools and methods in research?
Research methods relate to how researchers gather information and data.
For example, surveys, focus groups, customer interviews, and A/B testing are research methods that gather information.
On the other hand, tools assist areas of research. Researchers may use tools to more efficiently gather data, store data securely, or uncover insights.
Tools can improve research methods, ensuring efficiency and accuracy while reducing complexity.
Get started today
Go from raw data to valuable insights with a flexible research platform
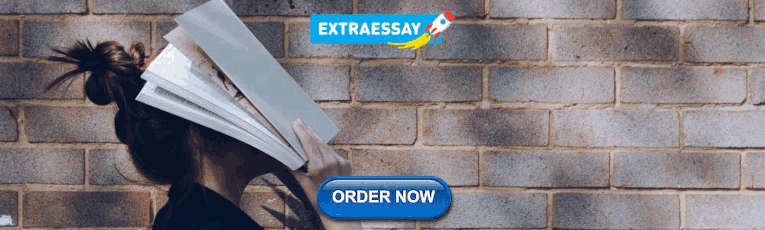
Editor’s picks
Last updated: 21 December 2023
Last updated: 16 December 2023
Last updated: 6 October 2023
Last updated: 17 February 2024
Last updated: 5 March 2024
Last updated: 19 November 2023
Last updated: 15 February 2024
Last updated: 11 March 2024
Last updated: 12 December 2023
Last updated: 6 March 2024
Last updated: 10 April 2023
Last updated: 20 December 2023
Latest articles
Related topics, log in or sign up.
Get started for free
- Skip to main content
- Skip to primary sidebar
- Skip to footer
- QuestionPro

- Solutions Industries Gaming Automotive Sports and events Education Government Travel & Hospitality Financial Services Healthcare Cannabis Technology Use Case NPS+ Communities Audience Contactless surveys Mobile LivePolls Member Experience GDPR Positive People Science 360 Feedback Surveys
- Resources Blog eBooks Survey Templates Case Studies Training Help center

Home Surveys Academic Research
Academic Research Tools: What they are + Top 5 Best
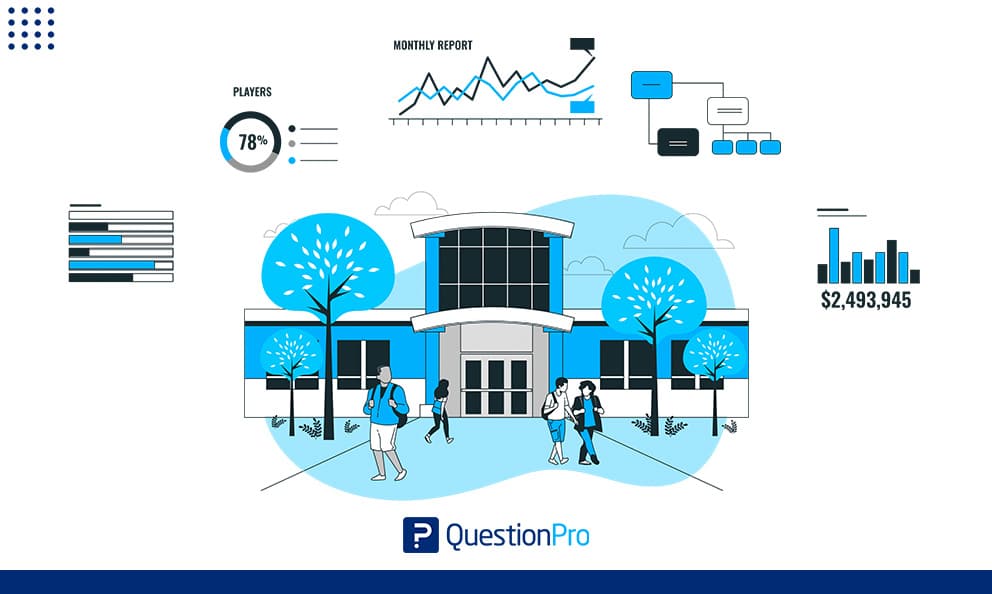
Academic research is a rigorous activity that requires a lot of dedication and diligence to provide great-quality results. Technology has improved how we access information by making access to information faster and more effective, so we carry out research more efficiently.
When conducting research, you must have the best methods and tools to facilitate the process. Every researcher needs a typing assistant to review spelling, grammar, and punctuation mistakes. If your research involves data analysis, you need a statistical research tool. You’ll probably need a virtual library for consulting if it involves psychology or sociology.
What are academic research tools?
An academic research tool is a software or platform that helps researchers organize, analyze, and manage the various components of their research projects. Some examples of academic research tools include reference management software, data visualization software, and survey design tools. These tools are designed to support the various stages of the research process, from literature review and data collection to data analysis and publication.
Reference management software, such as Mendeley or Endnote, allows researchers to organize and manage their bibliographic references and citations. This can be particularly helpful for large literature reviews, as it allows researchers to easily search and access their reference library and format citations and bibliographies in various styles. Additionally, many reference management tools offer features like annotation and collaboration, so researchers can share their reference libraries with colleagues and work on them together.
Data visualization software, like Tableau or R, can help researchers to explore and understand their data. These tools allow researchers to create interactive visualizations from their data, such as charts, graphs, and maps. This can be very useful for identifying patterns and trends that might not be immediately apparent from looking at raw data. These tools also provide a way to communicate the findings from their research clearly and effectively, as visualizations can be more easily understood than raw data.
Data collection software is another important tool that can support the research process. This software can be used to design and administer surveys, collect and store data, and manage participant information. Top data collection software such as QuestionPro offers a variety of question types, such as multiple choice, rating scales, and open-ended questions. They can be used to conduct surveys online or in person. This software also provides features like skip logic, data validation, and data export, which can help to ensure data quality and facilitate analysis. Some data collection software also integrates with data visualization or statistical software, making it easy to analyze and visualize once data is collected.
Top 5 Academic Research Tools
There are endless tools for academic research that can help you in any stage of the research process, from educational search engine software and project management tools to grammar editors and reference managers. Adopting these technologies can improve the quality of academic research, regardless of the field or topic.
From the multiple options in the market, we made a list of the best five academic research tools you can use to level up your academic research:
EndNote gives you the tools you need for searching, organizing, and sharing your research. It allows you to easily create bibliographies while writing your following paper with features like Cite While You Write. Maximize your time with features like finding full text for your references and automatically updating records.
Whether you’re on your desktop, online, or iPad, EndNote’s syncing capabilities let you access all of your references, attachments, and groups from anywhere.
Bit AI is an excellent tool for collaborating on research with your team. It’s essentially like a Google Docs but specifically made for research. You can upload and share different file formats, including PDFs, videos, white papers, etc., and then edit them together with your team.
Typeset is a great tool when it comes to writing your own research papers. You can upload all your references for simple citations and check your work for spelling errors and plagiarism. Typeset also offers features to collaborate with your teammates and get the work done together.
2. Google Scholar
Google Scholar is a classic tool that only some people know about. It’s essentially a version of the traditional Google search but focused on scientific and academic papers, journals, books, and other publications. Instead of using Google, you can use Google Scholar to eliminate the risk of citing non-credible sources.
1. QuestionPro
Most academic research, regardless of field or topic, requires data analysis so the information can have a solid foundation. Online surveys are critical to examine population samples so hypotheses can be proved or disregarded. While methods and techniques may vary, QuestionPro survey software is an excellent academic research tool for conducting online surveys.
QuestionPro’s robust suite of research tools provides you with all you need to derive research results. If someone needs a simple survey tool or a collaborative research solution, this software offers solutions in an intuitive way. The platform is simple to use intuitively, but our certification process can assist you in creating powerful surveys that minimize the risks of information bias. If needed, in the platform, you can also perform Audience Surveys . Audience gives you access to millions of possible respondents so that your segmentation sample for academic research becomes 360°.
QuestionPro also provides easy-to-setup analytical research tools to build dashboards and visualizations for all your research results. Presenting the data collected comprehensively is a crucial factor in research, making it easier for anyone to consult and cite the information.
It’s crucial to decide on the tools for data collection because research is carried out in different ways and for various purposes. Data collection aims to capture quality evidence that allows analysis to formulate convincing and credible answers to the posed questions.
With QuestionPro Education Research Solutions, you gain access to the top survey software in the market. Conduct powerful surveys with a complete set of data analytics tools to gather valuable insights. Join our community of more than 5000+ universities and colleges across the globe that already use our platform to make research of impact.
MORE LIKE THIS
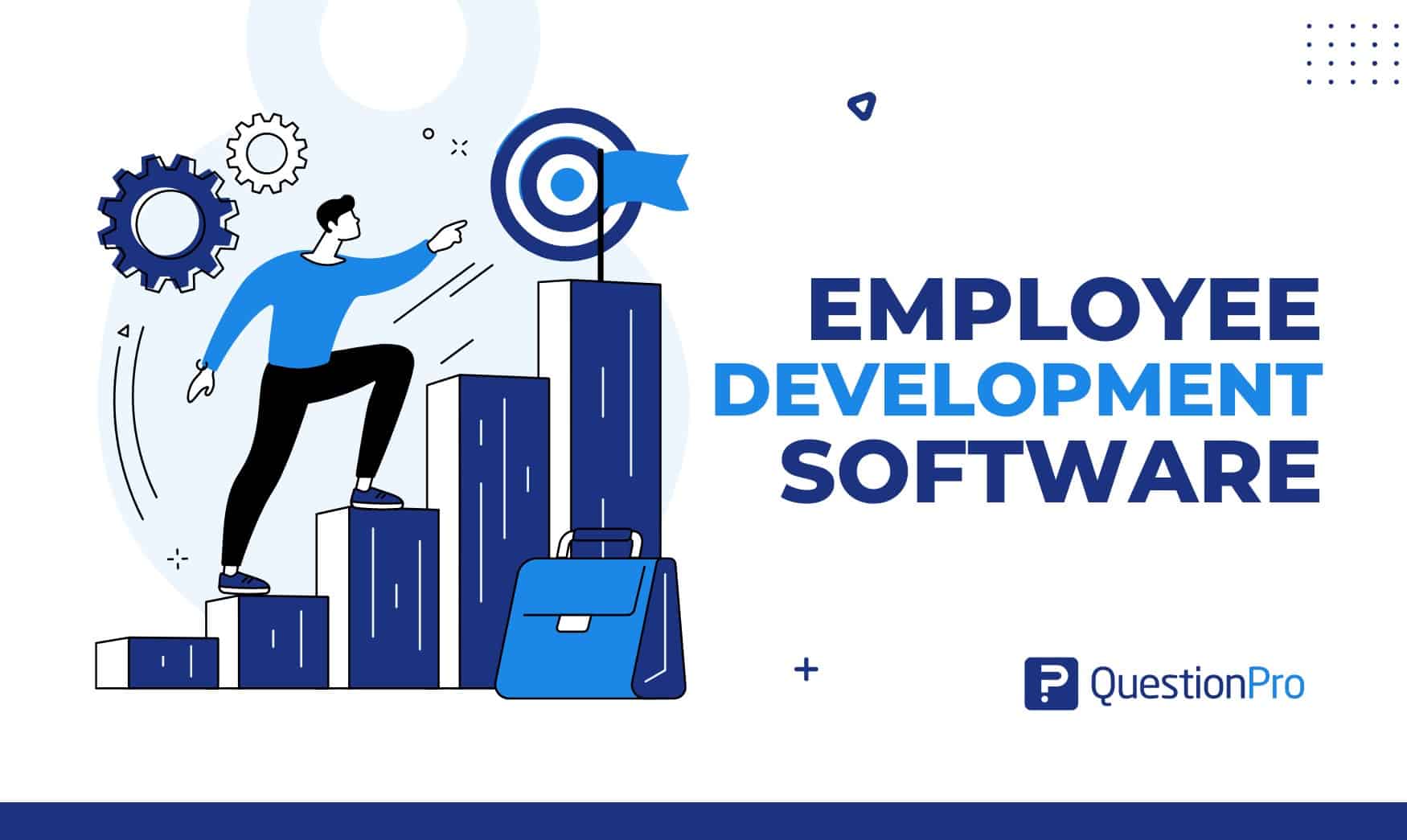
Top 10 Employee Development Software for Talent Growth
Apr 3, 2024
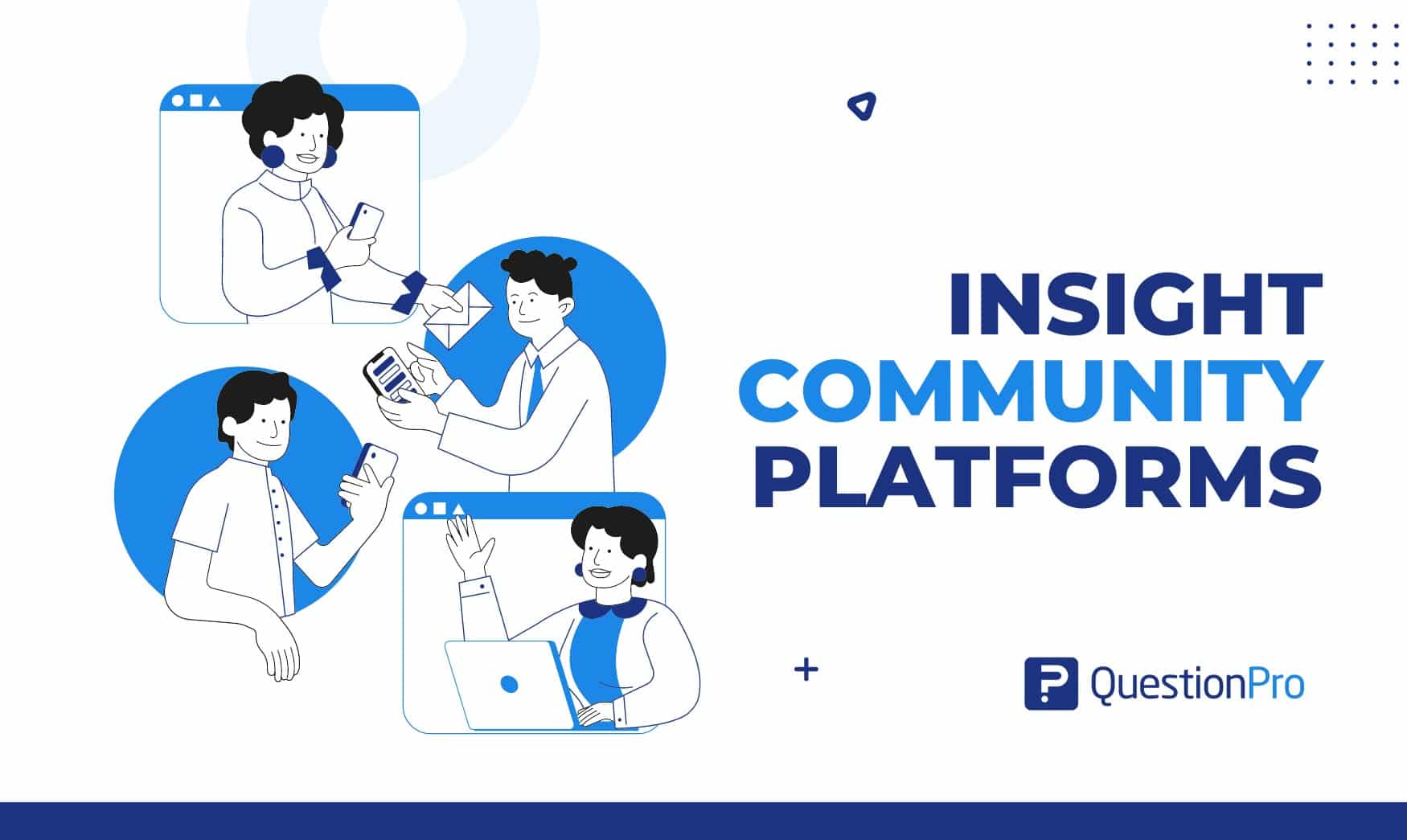
Top 5 Insight Community Platforms to Elevate Your Research
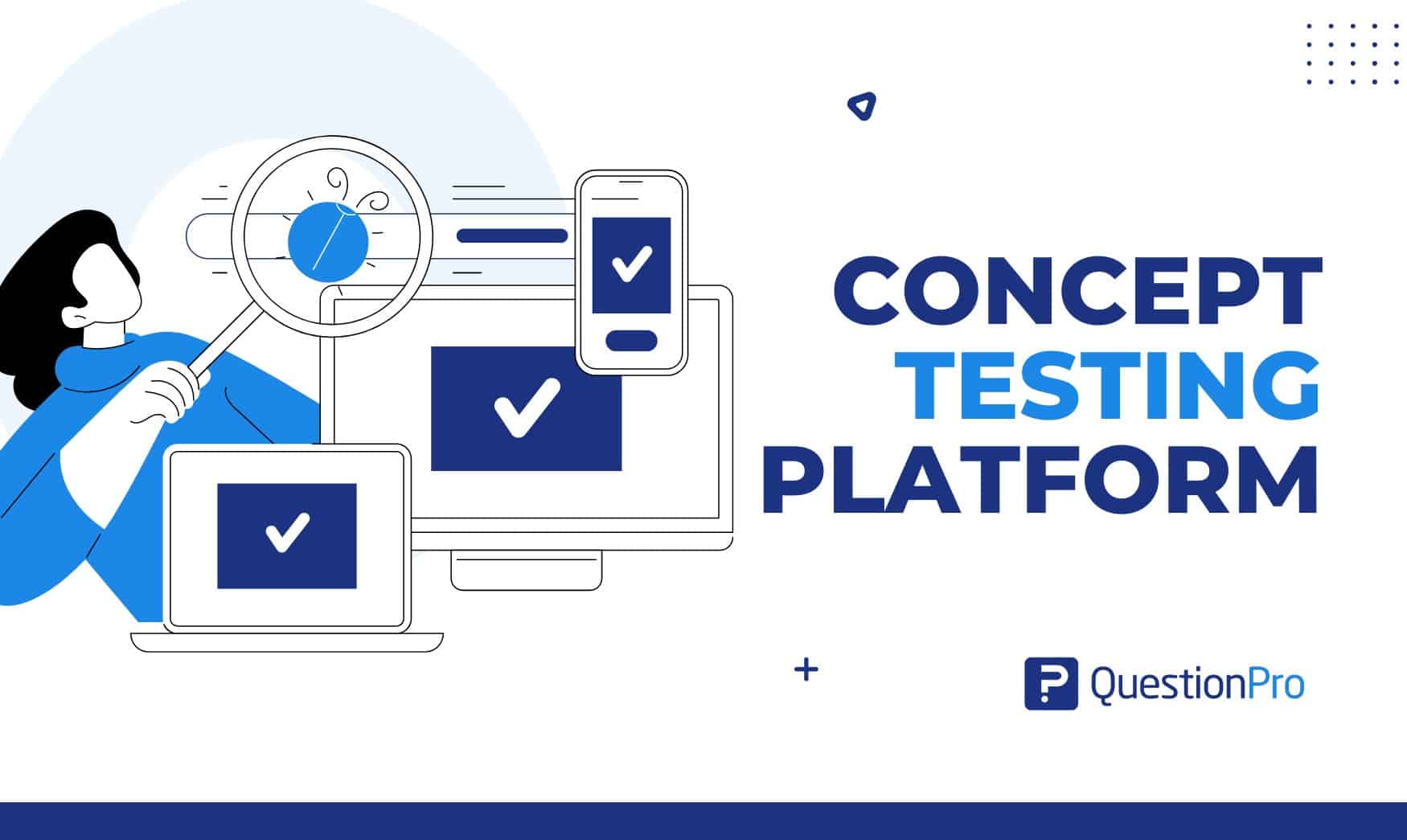
Choose The Right Concept Testing Platform to Boost Your Ideas
Apr 2, 2024
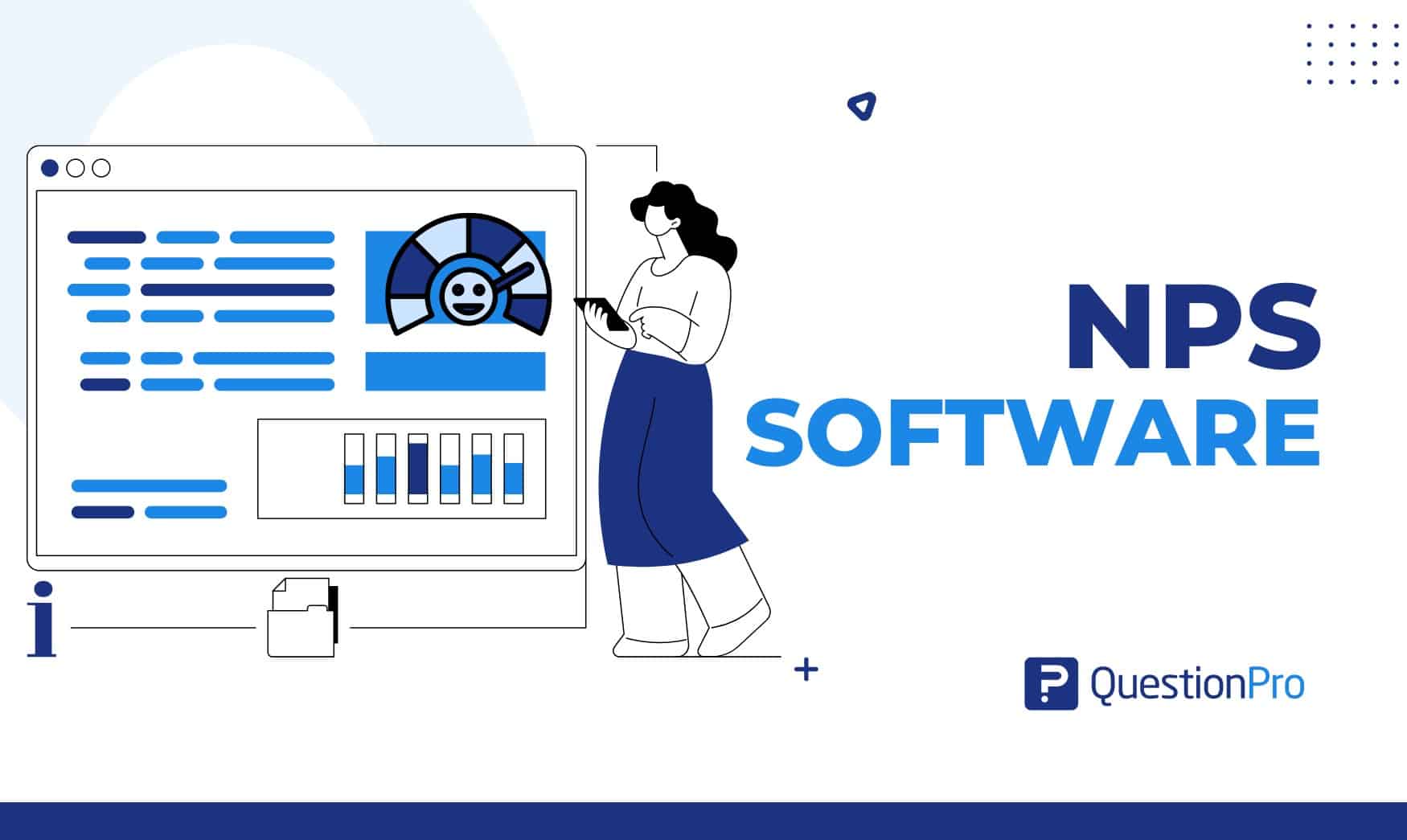
Top 15 NPS Software for Customer Feedback in 2024
Other categories.
- Academic Research
- Artificial Intelligence
- Assessments
- Brand Awareness
- Case Studies
- Communities
- Consumer Insights
- Customer effort score
- Customer Engagement
- Customer Experience
- Customer Loyalty
- Customer Research
- Customer Satisfaction
- Employee Benefits
- Employee Engagement
- Employee Retention
- Friday Five
- General Data Protection Regulation
- Insights Hub
- Life@QuestionPro
- Market Research
- Mobile diaries
- Mobile Surveys
- New Features
- Online Communities
- Question Types
- Questionnaire
- QuestionPro Products
- Release Notes
- Research Tools and Apps
- Revenue at Risk
- Survey Templates
- Training Tips
- Uncategorized
- Video Learning Series
- What’s Coming Up
- Workforce Intelligence
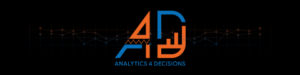
Top 9 Statistical Tools Used in Research
Well-designed research requires a well-chosen study sample and a suitable statistical test selection . To plan an epidemiological study or a clinical trial, you’ll need a solid understanding of the data . Improper inferences from it could lead to false conclusions and unethical behavior . And given the ocean of data available nowadays, it’s often a daunting task for researchers to gauge its credibility and do statistical analysis on it.
With that said, thanks to all the statistical tools available in the market that help researchers make such studies much more manageable. Statistical tools are extensively used in academic and research sectors to study human, animal, and material behaviors and reactions.
Statistical tools aid in the interpretation and use of data. They can be used to evaluate and comprehend any form of data. Some statistical tools can help you see trends, forecast future sales, and create links between causes and effects. When you’re unsure where to go with your study, other tools can assist you in navigating through enormous amounts of data.
In this article, we will discuss some of the best statistical tools and their key features . So, let’s start without any further ado.
What is Statistics? And its Importance in Research
Statistics is the study of collecting, arranging, and interpreting data from samples and inferring it to the total population. Also known as the “Science of Data,” it allows us to derive conclusions from a data set. It may also assist people in all industries in answering research or business queries and forecast outcomes, such as what show you should watch next on your favorite video app.
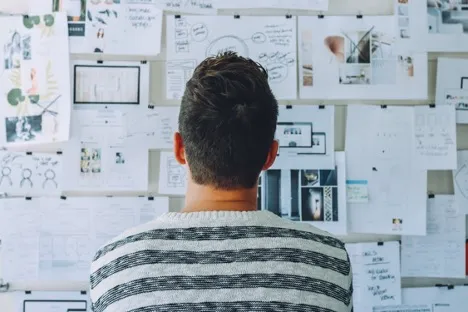
Statistical Tools Used in Research
Researchers often cannot discern a simple truth from a set of data. They can only draw conclusions from data after statistical analysis. On the other hand, creating a statistical analysis is a difficult task. This is when statistical tools come into play. Researchers can use statistical tools to back up their claims, make sense of a vast set of data, graphically show complex data, or help clarify many things in a short period.
Let’s go through the top 9 best statistical tools used in research below:
SPSS (Statistical Package for the Social Sciences) is a collection of software tools compiled as a single package. This program’s primary function is to analyze scientific data in social science. This information can be utilized for market research, surveys, and data mining, among other things. It is mainly used in the following areas like marketing, healthcare, educational research, etc.
SPSS first stores and organizes the data, then compile the data set to generate appropriate output. SPSS is intended to work with a wide range of variable data formats.
Some of the highlights of SPSS :
- It gives you greater tools for analyzing and comprehending your data. With SPSS’s excellent interface, you can easily handle complex commercial and research challenges.
- It assists you in making accurate and high-quality decisions.
- It also comes with a variety of deployment options for managing your software.
- You may also use a point-and-click interface to produce unique visualizations and reports. To start using SPSS, you don’t need prior coding skills.
- It provides the best views of missing data patterns and summarizes variable distributions.
R is a statistical computing and graphics programming language that you may use to clean, analyze and graph your data. It is frequently used to estimate and display results by researchers from various fields and lecturers of statistics and research methodologies. It’s free, making it an appealing option, but it relies upon programming code rather than drop-down menus or buttons.
Some of the highlights of R :
- It offers efficient storage and data handling facility.
- R has the most robust set of operators. They are used for array calculations, namely matrices.
- It has the best data analysis tools.
- It’s a full-featured high-level programming language with conditional loops, decision statements, and various functions.
SAS is a statistical analysis tool that allows users to build scripts for more advanced analyses or use the GUI. It’s a high-end solution frequently used in industries including business, healthcare, and human behavior research. Advanced analysis and publication-worthy figures and charts are conceivable, albeit coding can be a challenging transition for people who aren’t used to this approach.
Many big tech companies are using SAS due to its support and integration for vast teams. Setting up the tool might be a bit time-consuming initially, but once it’s up and running, it’ll surely streamline your statistical processes.
Some of the highlights of SAS are:
- , with a range of tutorials available.
- Its package includes a wide range of statistics tools.
- It has the best technical support available.
- It gives reports of excellent quality and aesthetic appeal
- It provides the best assistance for detecting spelling and grammar issues. As a result, the analysis is more precise.
MATLAB is one of the most well-reputed statistical analysis tools and statistical programming languages. It has a toolbox with several features that make programming languages simple. With MATLAB, you may perform the most complex statistical analysis, such as EEG data analysis . Add-ons for toolboxes can be used to increase the capability of MATLAB.
Moreover, MATLAB provides a multi-paradigm numerical computing environment, which means that the language may be used for both procedural and object-oriented programming. MATLAB is ideal for matrix manipulation, including data function plotting, algorithm implementation, and user interface design, among other things. Last but not least, MATLAB can also run programs written in other programming languages.
Some of the highlights of MATLAB :
- MATLAB toolboxes are meticulously developed and professionally executed. It is also put through its paces by the tester under various settings. Aside from that, MATLAB provides complete documents.
- MATLAB is a production-oriented programming language. As a result, the MATLAB code is ready for production. All that is required is the integration of data sources and business systems with corporate systems.
- It has the ability to convert MATLAB algorithms to C, C++, and CUDA cores.
- For users, MATLAB is the best simulation platform.
- It provides the optimum conditions for performing data analysis procedures.
Some of the highlights of Tableau are:
- It gives the most compelling end-to-end analytics.
- It provides us with a system of high-level security.
- It is compatible with practically all screen resolutions.
Minitab is a data analysis program that includes basic and advanced statistical features. The GUI and written instructions can be used to execute commands, making it accessible to beginners and those wishing to perform more advanced analysis.
Some of the highlights of Minitab are:
- Minitab can be used to perform various sorts of analysis, such as measurement systems analysis, capability analysis, graphical analysis, hypothesis analysis, regression, non-regression, etcetera.
- , such as scatterplots, box plots, dot plots, histograms, time series plots, and so on.
- Minitab also allows you to run a variety of statistical tests, including one-sample Z-tests, one-sample, two-sample t-tests, paired t-tests, and so on.
7. MS EXCEL:
You can apply various formulas and functions to your data in Excel without prior knowledge of statistics. The learning curve is great, and even freshers can achieve great results quickly since everything is just a click away. This makes Excel a great choice not only for amateurs but beginners as well.
Some of the highlights of MS Excel are:
- It has the best GUI for data visualization solutions, allowing you to generate various graphs with it.
- MS Excel has practically every tool needed to undertake any type of data analysis.
- It enables you to do basic to complicated computations.
- Excel has a lot of built-in formulas that make it a good choice for performing extensive data jobs.
8. RAPIDMINER:
RapidMiner is a valuable platform for data preparation, machine learning, and the deployment of predictive models. RapidMiner makes it simple to develop a data model from the beginning to the end. It comes with a complete data science suite. Machine learning, deep learning, text mining, and predictive analytics are all possible with it.
Some of the highlights of RapidMiner are:
- It has outstanding security features.
- It allows for seamless integration with a variety of third-party applications.
- RapidMiner’s primary functionality can be extended with the help of plugins.
- It provides an excellent platform for data processing and visualization of results.
- It has the ability to track and analyze data in real-time.
9. APACHE HADOOP:
Apache Hadoop is an open-source software that is best known for its top-of-the-drawer scaling capabilities. It is capable of resolving the most challenging computational issues and excels at data-intensive activities as well, given its distributed architecture . The primary reason why it outperforms its contenders in terms of computational power and speed is that it does not directly transfer files to the node. It divides enormous files into smaller bits and transmits them to separate nodes with specific instructions using HDFS . More about it here .
So, if you have massive data on your hands and want something that doesn’t slow you down and works in a distributed way, Hadoop is the way to go.
Some of the highlights of Apache Hadoop are:
- It is cost-effective.
- Apache Hadoop offers built-in tools that automatically schedule tasks and manage clusters.
- It can effortlessly integrate with third-party applications and apps.
- Apache Hadoop is also simple to use for beginners. It includes a framework for managing distributed computing with user intervention.
Learn more about Statistics and Key Tools
Elasticity of Demand Explained in Plain Terms
When you think of “elasticity,” you probably think of flexibility or the ability of an object to bounce back to its original conditions after some change. The type of elasticity
Learn More…
An Introduction to Statistical Power And A/B Testing
Statistical power is an integral part of A/B testing. And in this article, you will learn everything you need to know about it and how it is applied in A/B testing. A/B
What Data Analytics Tools Are And How To Use Them
When it comes to improving the quality of your products and services, data analytic tools are the antidotes. Regardless, people often have questions. What are data analytic tools? Why are
There are a variety of software tools available, each of which offers something slightly different to the user – which one you choose will be determined by several things, including your research question, statistical understanding, and coding experience. These factors may indicate that you are on the cutting edge of data analysis, but the quality of the data acquired depends on the study execution, as with any research.
It’s worth noting that even if you have the most powerful statistical software (and the knowledge to utilize it), the results would be meaningless if they weren’t collected properly. Some online statistics tools are an alternative to the above-mentioned statistical tools. However, each of these tools is the finest in its domain. Hence, you really don’t need a second opinion to use any of these tools. But it’s always recommended to get your hands dirty a little and see what works best for your specific use case before choosing it.
Emidio Amadebai
As an IT Engineer, who is passionate about learning and sharing. I have worked and learned quite a bit from Data Engineers, Data Analysts, Business Analysts, and Key Decision Makers almost for the past 5 years. Interested in learning more about Data Science and How to leverage it for better decision-making in my business and hopefully help you do the same in yours.
Recent Posts
Bootstrapping vs. Boosting
Over the past decade, the field of machine learning has witnessed remarkable advancements in predictive techniques and ensemble learning methods. Ensemble techniques are very popular in machine...
Boosting Algorithms vs. Random Forests Explained
Imagine yourself in the position of a marketing analyst for an e-commerce site who has to make a model that will predict if a customer purchases in the next month or not. In such a scenario, you...

The 7 Most Useful Data Analysis Methods and Techniques
Data analytics is the process of analyzing raw data to draw out meaningful insights. These insights are then used to determine the best course of action.
When is the best time to roll out that marketing campaign? Is the current team structure as effective as it could be? Which customer segments are most likely to purchase your new product?
Ultimately, data analytics is a crucial driver of any successful business strategy. But how do data analysts actually turn raw data into something useful? There are a range of methods and techniques that data analysts use depending on the type of data in question and the kinds of insights they want to uncover.
You can get a hands-on introduction to data analytics in this free short course .
In this post, we’ll explore some of the most useful data analysis techniques. By the end, you’ll have a much clearer idea of how you can transform meaningless data into business intelligence. We’ll cover:
- What is data analysis and why is it important?
- What is the difference between qualitative and quantitative data?
- Regression analysis
- Monte Carlo simulation
- Factor analysis
- Cohort analysis
- Cluster analysis
- Time series analysis
- Sentiment analysis
- The data analysis process
- The best tools for data analysis
- Key takeaways
The first six methods listed are used for quantitative data , while the last technique applies to qualitative data. We briefly explain the difference between quantitative and qualitative data in section two, but if you want to skip straight to a particular analysis technique, just use the clickable menu.
1. What is data analysis and why is it important?
Data analysis is, put simply, the process of discovering useful information by evaluating data. This is done through a process of inspecting, cleaning, transforming, and modeling data using analytical and statistical tools, which we will explore in detail further along in this article.
Why is data analysis important? Analyzing data effectively helps organizations make business decisions. Nowadays, data is collected by businesses constantly: through surveys, online tracking, online marketing analytics, collected subscription and registration data (think newsletters), social media monitoring, among other methods.
These data will appear as different structures, including—but not limited to—the following:
The concept of big data —data that is so large, fast, or complex, that it is difficult or impossible to process using traditional methods—gained momentum in the early 2000s. Then, Doug Laney, an industry analyst, articulated what is now known as the mainstream definition of big data as the three Vs: volume, velocity, and variety.
- Volume: As mentioned earlier, organizations are collecting data constantly. In the not-too-distant past it would have been a real issue to store, but nowadays storage is cheap and takes up little space.
- Velocity: Received data needs to be handled in a timely manner. With the growth of the Internet of Things, this can mean these data are coming in constantly, and at an unprecedented speed.
- Variety: The data being collected and stored by organizations comes in many forms, ranging from structured data—that is, more traditional, numerical data—to unstructured data—think emails, videos, audio, and so on. We’ll cover structured and unstructured data a little further on.
This is a form of data that provides information about other data, such as an image. In everyday life you’ll find this by, for example, right-clicking on a file in a folder and selecting “Get Info”, which will show you information such as file size and kind, date of creation, and so on.
Real-time data
This is data that is presented as soon as it is acquired. A good example of this is a stock market ticket, which provides information on the most-active stocks in real time.
Machine data
This is data that is produced wholly by machines, without human instruction. An example of this could be call logs automatically generated by your smartphone.
Quantitative and qualitative data
Quantitative data—otherwise known as structured data— may appear as a “traditional” database—that is, with rows and columns. Qualitative data—otherwise known as unstructured data—are the other types of data that don’t fit into rows and columns, which can include text, images, videos and more. We’ll discuss this further in the next section.
2. What is the difference between quantitative and qualitative data?
How you analyze your data depends on the type of data you’re dealing with— quantitative or qualitative . So what’s the difference?
Quantitative data is anything measurable , comprising specific quantities and numbers. Some examples of quantitative data include sales figures, email click-through rates, number of website visitors, and percentage revenue increase. Quantitative data analysis techniques focus on the statistical, mathematical, or numerical analysis of (usually large) datasets. This includes the manipulation of statistical data using computational techniques and algorithms. Quantitative analysis techniques are often used to explain certain phenomena or to make predictions.
Qualitative data cannot be measured objectively , and is therefore open to more subjective interpretation. Some examples of qualitative data include comments left in response to a survey question, things people have said during interviews, tweets and other social media posts, and the text included in product reviews. With qualitative data analysis, the focus is on making sense of unstructured data (such as written text, or transcripts of spoken conversations). Often, qualitative analysis will organize the data into themes—a process which, fortunately, can be automated.
Data analysts work with both quantitative and qualitative data , so it’s important to be familiar with a variety of analysis methods. Let’s take a look at some of the most useful techniques now.
3. Data analysis techniques
Now we’re familiar with some of the different types of data, let’s focus on the topic at hand: different methods for analyzing data.
a. Regression analysis
Regression analysis is used to estimate the relationship between a set of variables. When conducting any type of regression analysis , you’re looking to see if there’s a correlation between a dependent variable (that’s the variable or outcome you want to measure or predict) and any number of independent variables (factors which may have an impact on the dependent variable). The aim of regression analysis is to estimate how one or more variables might impact the dependent variable, in order to identify trends and patterns. This is especially useful for making predictions and forecasting future trends.
Let’s imagine you work for an ecommerce company and you want to examine the relationship between: (a) how much money is spent on social media marketing, and (b) sales revenue. In this case, sales revenue is your dependent variable—it’s the factor you’re most interested in predicting and boosting. Social media spend is your independent variable; you want to determine whether or not it has an impact on sales and, ultimately, whether it’s worth increasing, decreasing, or keeping the same. Using regression analysis, you’d be able to see if there’s a relationship between the two variables. A positive correlation would imply that the more you spend on social media marketing, the more sales revenue you make. No correlation at all might suggest that social media marketing has no bearing on your sales. Understanding the relationship between these two variables would help you to make informed decisions about the social media budget going forward. However: It’s important to note that, on their own, regressions can only be used to determine whether or not there is a relationship between a set of variables—they don’t tell you anything about cause and effect. So, while a positive correlation between social media spend and sales revenue may suggest that one impacts the other, it’s impossible to draw definitive conclusions based on this analysis alone.
There are many different types of regression analysis, and the model you use depends on the type of data you have for the dependent variable. For example, your dependent variable might be continuous (i.e. something that can be measured on a continuous scale, such as sales revenue in USD), in which case you’d use a different type of regression analysis than if your dependent variable was categorical in nature (i.e. comprising values that can be categorised into a number of distinct groups based on a certain characteristic, such as customer location by continent). You can learn more about different types of dependent variables and how to choose the right regression analysis in this guide .
Regression analysis in action: Investigating the relationship between clothing brand Benetton’s advertising expenditure and sales
b. Monte Carlo simulation
When making decisions or taking certain actions, there are a range of different possible outcomes. If you take the bus, you might get stuck in traffic. If you walk, you might get caught in the rain or bump into your chatty neighbor, potentially delaying your journey. In everyday life, we tend to briefly weigh up the pros and cons before deciding which action to take; however, when the stakes are high, it’s essential to calculate, as thoroughly and accurately as possible, all the potential risks and rewards.
Monte Carlo simulation, otherwise known as the Monte Carlo method, is a computerized technique used to generate models of possible outcomes and their probability distributions. It essentially considers a range of possible outcomes and then calculates how likely it is that each particular outcome will be realized. The Monte Carlo method is used by data analysts to conduct advanced risk analysis, allowing them to better forecast what might happen in the future and make decisions accordingly.
So how does Monte Carlo simulation work, and what can it tell us? To run a Monte Carlo simulation, you’ll start with a mathematical model of your data—such as a spreadsheet. Within your spreadsheet, you’ll have one or several outputs that you’re interested in; profit, for example, or number of sales. You’ll also have a number of inputs; these are variables that may impact your output variable. If you’re looking at profit, relevant inputs might include the number of sales, total marketing spend, and employee salaries. If you knew the exact, definitive values of all your input variables, you’d quite easily be able to calculate what profit you’d be left with at the end. However, when these values are uncertain, a Monte Carlo simulation enables you to calculate all the possible options and their probabilities. What will your profit be if you make 100,000 sales and hire five new employees on a salary of $50,000 each? What is the likelihood of this outcome? What will your profit be if you only make 12,000 sales and hire five new employees? And so on. It does this by replacing all uncertain values with functions which generate random samples from distributions determined by you, and then running a series of calculations and recalculations to produce models of all the possible outcomes and their probability distributions. The Monte Carlo method is one of the most popular techniques for calculating the effect of unpredictable variables on a specific output variable, making it ideal for risk analysis.
Monte Carlo simulation in action: A case study using Monte Carlo simulation for risk analysis
c. Factor analysis
Factor analysis is a technique used to reduce a large number of variables to a smaller number of factors. It works on the basis that multiple separate, observable variables correlate with each other because they are all associated with an underlying construct. This is useful not only because it condenses large datasets into smaller, more manageable samples, but also because it helps to uncover hidden patterns. This allows you to explore concepts that cannot be easily measured or observed—such as wealth, happiness, fitness, or, for a more business-relevant example, customer loyalty and satisfaction.
Let’s imagine you want to get to know your customers better, so you send out a rather long survey comprising one hundred questions. Some of the questions relate to how they feel about your company and product; for example, “Would you recommend us to a friend?” and “How would you rate the overall customer experience?” Other questions ask things like “What is your yearly household income?” and “How much are you willing to spend on skincare each month?”
Once your survey has been sent out and completed by lots of customers, you end up with a large dataset that essentially tells you one hundred different things about each customer (assuming each customer gives one hundred responses). Instead of looking at each of these responses (or variables) individually, you can use factor analysis to group them into factors that belong together—in other words, to relate them to a single underlying construct. In this example, factor analysis works by finding survey items that are strongly correlated. This is known as covariance . So, if there’s a strong positive correlation between household income and how much they’re willing to spend on skincare each month (i.e. as one increases, so does the other), these items may be grouped together. Together with other variables (survey responses), you may find that they can be reduced to a single factor such as “consumer purchasing power”. Likewise, if a customer experience rating of 10/10 correlates strongly with “yes” responses regarding how likely they are to recommend your product to a friend, these items may be reduced to a single factor such as “customer satisfaction”.
In the end, you have a smaller number of factors rather than hundreds of individual variables. These factors are then taken forward for further analysis, allowing you to learn more about your customers (or any other area you’re interested in exploring).
Factor analysis in action: Using factor analysis to explore customer behavior patterns in Tehran
d. Cohort analysis
Cohort analysis is a data analytics technique that groups users based on a shared characteristic , such as the date they signed up for a service or the product they purchased. Once users are grouped into cohorts, analysts can track their behavior over time to identify trends and patterns.
So what does this mean and why is it useful? Let’s break down the above definition further. A cohort is a group of people who share a common characteristic (or action) during a given time period. Students who enrolled at university in 2020 may be referred to as the 2020 cohort. Customers who purchased something from your online store via the app in the month of December may also be considered a cohort.
With cohort analysis, you’re dividing your customers or users into groups and looking at how these groups behave over time. So, rather than looking at a single, isolated snapshot of all your customers at a given moment in time (with each customer at a different point in their journey), you’re examining your customers’ behavior in the context of the customer lifecycle. As a result, you can start to identify patterns of behavior at various points in the customer journey—say, from their first ever visit to your website, through to email newsletter sign-up, to their first purchase, and so on. As such, cohort analysis is dynamic, allowing you to uncover valuable insights about the customer lifecycle.
This is useful because it allows companies to tailor their service to specific customer segments (or cohorts). Let’s imagine you run a 50% discount campaign in order to attract potential new customers to your website. Once you’ve attracted a group of new customers (a cohort), you’ll want to track whether they actually buy anything and, if they do, whether or not (and how frequently) they make a repeat purchase. With these insights, you’ll start to gain a much better understanding of when this particular cohort might benefit from another discount offer or retargeting ads on social media, for example. Ultimately, cohort analysis allows companies to optimize their service offerings (and marketing) to provide a more targeted, personalized experience. You can learn more about how to run cohort analysis using Google Analytics .
Cohort analysis in action: How Ticketmaster used cohort analysis to boost revenue
e. Cluster analysis
Cluster analysis is an exploratory technique that seeks to identify structures within a dataset. The goal of cluster analysis is to sort different data points into groups (or clusters) that are internally homogeneous and externally heterogeneous. This means that data points within a cluster are similar to each other, and dissimilar to data points in another cluster. Clustering is used to gain insight into how data is distributed in a given dataset, or as a preprocessing step for other algorithms.
There are many real-world applications of cluster analysis. In marketing, cluster analysis is commonly used to group a large customer base into distinct segments, allowing for a more targeted approach to advertising and communication. Insurance firms might use cluster analysis to investigate why certain locations are associated with a high number of insurance claims. Another common application is in geology, where experts will use cluster analysis to evaluate which cities are at greatest risk of earthquakes (and thus try to mitigate the risk with protective measures).
It’s important to note that, while cluster analysis may reveal structures within your data, it won’t explain why those structures exist. With that in mind, cluster analysis is a useful starting point for understanding your data and informing further analysis. Clustering algorithms are also used in machine learning—you can learn more about clustering in machine learning in our guide .
Cluster analysis in action: Using cluster analysis for customer segmentation—a telecoms case study example
f. Time series analysis
Time series analysis is a statistical technique used to identify trends and cycles over time. Time series data is a sequence of data points which measure the same variable at different points in time (for example, weekly sales figures or monthly email sign-ups). By looking at time-related trends, analysts are able to forecast how the variable of interest may fluctuate in the future.
When conducting time series analysis, the main patterns you’ll be looking out for in your data are:
- Trends: Stable, linear increases or decreases over an extended time period.
- Seasonality: Predictable fluctuations in the data due to seasonal factors over a short period of time. For example, you might see a peak in swimwear sales in summer around the same time every year.
- Cyclic patterns: Unpredictable cycles where the data fluctuates. Cyclical trends are not due to seasonality, but rather, may occur as a result of economic or industry-related conditions.
As you can imagine, the ability to make informed predictions about the future has immense value for business. Time series analysis and forecasting is used across a variety of industries, most commonly for stock market analysis, economic forecasting, and sales forecasting. There are different types of time series models depending on the data you’re using and the outcomes you want to predict. These models are typically classified into three broad types: the autoregressive (AR) models, the integrated (I) models, and the moving average (MA) models. For an in-depth look at time series analysis, refer to our guide .
Time series analysis in action: Developing a time series model to predict jute yarn demand in Bangladesh
g. Sentiment analysis
When you think of data, your mind probably automatically goes to numbers and spreadsheets.
Many companies overlook the value of qualitative data, but in reality, there are untold insights to be gained from what people (especially customers) write and say about you. So how do you go about analyzing textual data?
One highly useful qualitative technique is sentiment analysis , a technique which belongs to the broader category of text analysis —the (usually automated) process of sorting and understanding textual data.
With sentiment analysis, the goal is to interpret and classify the emotions conveyed within textual data. From a business perspective, this allows you to ascertain how your customers feel about various aspects of your brand, product, or service.
There are several different types of sentiment analysis models, each with a slightly different focus. The three main types include:
Fine-grained sentiment analysis
If you want to focus on opinion polarity (i.e. positive, neutral, or negative) in depth, fine-grained sentiment analysis will allow you to do so.
For example, if you wanted to interpret star ratings given by customers, you might use fine-grained sentiment analysis to categorize the various ratings along a scale ranging from very positive to very negative.
Emotion detection
This model often uses complex machine learning algorithms to pick out various emotions from your textual data.
You might use an emotion detection model to identify words associated with happiness, anger, frustration, and excitement, giving you insight into how your customers feel when writing about you or your product on, say, a product review site.
Aspect-based sentiment analysis
This type of analysis allows you to identify what specific aspects the emotions or opinions relate to, such as a certain product feature or a new ad campaign.
If a customer writes that they “find the new Instagram advert so annoying”, your model should detect not only a negative sentiment, but also the object towards which it’s directed.
In a nutshell, sentiment analysis uses various Natural Language Processing (NLP) algorithms and systems which are trained to associate certain inputs (for example, certain words) with certain outputs.
For example, the input “annoying” would be recognized and tagged as “negative”. Sentiment analysis is crucial to understanding how your customers feel about you and your products, for identifying areas for improvement, and even for averting PR disasters in real-time!
Sentiment analysis in action: 5 Real-world sentiment analysis case studies
4. The data analysis process
In order to gain meaningful insights from data, data analysts will perform a rigorous step-by-step process. We go over this in detail in our step by step guide to the data analysis process —but, to briefly summarize, the data analysis process generally consists of the following phases:
Defining the question
The first step for any data analyst will be to define the objective of the analysis, sometimes called a ‘problem statement’. Essentially, you’re asking a question with regards to a business problem you’re trying to solve. Once you’ve defined this, you’ll then need to determine which data sources will help you answer this question.
Collecting the data
Now that you’ve defined your objective, the next step will be to set up a strategy for collecting and aggregating the appropriate data. Will you be using quantitative (numeric) or qualitative (descriptive) data? Do these data fit into first-party, second-party, or third-party data?
Learn more: Quantitative vs. Qualitative Data: What’s the Difference?
Cleaning the data
Unfortunately, your collected data isn’t automatically ready for analysis—you’ll have to clean it first. As a data analyst, this phase of the process will take up the most time. During the data cleaning process, you will likely be:
- Removing major errors, duplicates, and outliers
- Removing unwanted data points
- Structuring the data—that is, fixing typos, layout issues, etc.
- Filling in major gaps in data
Analyzing the data
Now that we’ve finished cleaning the data, it’s time to analyze it! Many analysis methods have already been described in this article, and it’s up to you to decide which one will best suit the assigned objective. It may fall under one of the following categories:
- Descriptive analysis , which identifies what has already happened
- Diagnostic analysis , which focuses on understanding why something has happened
- Predictive analysis , which identifies future trends based on historical data
- Prescriptive analysis , which allows you to make recommendations for the future
Visualizing and sharing your findings
We’re almost at the end of the road! Analyses have been made, insights have been gleaned—all that remains to be done is to share this information with others. This is usually done with a data visualization tool, such as Google Charts, or Tableau.
Learn more: 13 of the Most Common Types of Data Visualization
To sum up the process, Will’s explained it all excellently in the following video:
5. The best tools for data analysis
As you can imagine, every phase of the data analysis process requires the data analyst to have a variety of tools under their belt that assist in gaining valuable insights from data. We cover these tools in greater detail in this article , but, in summary, here’s our best-of-the-best list, with links to each product:
The top 9 tools for data analysts
- Microsoft Excel
- Jupyter Notebook
- Apache Spark
- Microsoft Power BI
6. Key takeaways and further reading
As you can see, there are many different data analysis techniques at your disposal. In order to turn your raw data into actionable insights, it’s important to consider what kind of data you have (is it qualitative or quantitative?) as well as the kinds of insights that will be useful within the given context. In this post, we’ve introduced seven of the most useful data analysis techniques—but there are many more out there to be discovered!
So what now? If you haven’t already, we recommend reading the case studies for each analysis technique discussed in this post (you’ll find a link at the end of each section). For a more hands-on introduction to the kinds of methods and techniques that data analysts use, try out this free introductory data analytics short course. In the meantime, you might also want to read the following:
- The Best Online Data Analytics Courses for 2024
- What Is Time Series Data and How Is It Analyzed?
- What is Spatial Analysis?
Learn / Guides / Quantitative data analysis guide
Back to guides
The top 9 quantitative data analysis tools you should try in 2024
Quantitative data analysis software helps you track how well your website is doing, understand which marketing channels bring in the most traffic, and increase your chances of turning visitors into customers. But with so many analytics tools to choose from, finding the right solution for your needs can take time and effort.
Last updated
Reading time.
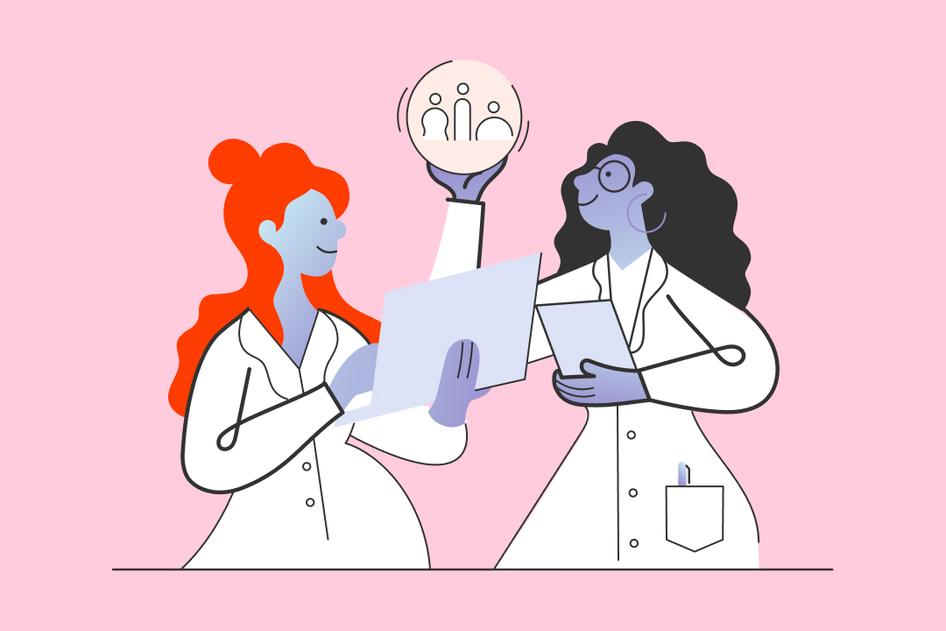
To help you narrow down your options, we’ve compiled a list of the top nine quantitative data analysis tools, along with helpful tips on choosing the best tools for your needs. We also share some practical ways to pair Hotjar with your chosen tool to gain in-depth user insights and increase customer delight .
Gather quantitative user insights with Hotjar
Use Hotjar Funnels, Heatmaps, and Trends to collect user insights, measure customer satisfaction levels, and improve the user experience.
9 quantitative data analysis tools you should consider adding to your tech stack
Quantitative data analysis involves analyzing and interpreting numerical data to identify meaningful patterns and trends in user behavior .
For example, if you want more people to sign up for your product, you can use quantitative data analysis to learn how many people looked at your product or pricing page, how long they stayed on these pages, and how often they signed up.
The true purpose of analytics is to help you make good decisions. If you have an idea, you can use data to check the concept. Is it likely to affect a lot of visitors? Just a few? And once you make the changes, you can use data to see how well it worked. Did it make an impact? Was there a measurable change?
To help you stay on top of your site’s performance, let’s explore the best nine tools for quantitative data analysis, including what each tool does, why you should consider adding it to your tech stack, and how to use it.
1. Google Analytics
Google Analytics is a (very) popular website analytics software for quantitative data analysis and research that lets you track what's happening on your site.
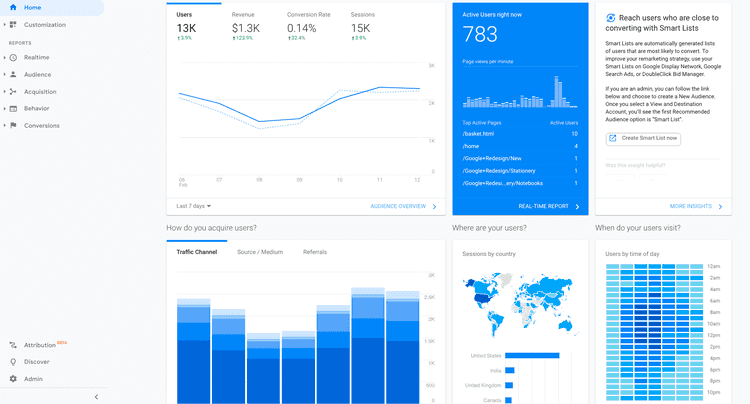
How it helps: use Google Analytics to monitor website user behavior and learn
How many people visit your site
Where they come from (search engines, social media, or referrals)
What devices they use to access your site (desktop or mobile)
Which pages they visit
How long they spend on each page
Which pages are the most popular
Where visitors drop off
How to use it: to start using Google Analytics, create an account and set up a property for your website or app. Once you've set up the software, it automatically collects data on every page view, bounce, drop off, and conversion. You can also configure goals, set up conversion tracking, create custom reports, and explore user behavior in real time.
Note: from July 1, 2023, Google Analytics Universal no longer processes data , but the Hotjar integration remains functional.
💡 Pro tip: use the Hotjar and Google Analytics integration to gain deeper insights into how users interact with your website.
For example, say you want to optimize the checkout process for your ecommerce site to reduce cart abandonment rate . Google Analytics shows you what’s happening on your site, but it doesn't tell you why the drop-offs occur. Hotjar, on the other hand, helps you understand the 'why' behind those numbers.
Take session recordings, for instance:
Session recordings let you see exactly how users navigate through the checkout process and spot potential issues that made them drop off
Recordings let you observe users' mouse movements, clicks, and hesitations, helping you uncover specific areas where they might get confused or frustrated.
Combining quantitative and qualitative data gives you a more complete understanding of user behavior, which empowers you to identify and address specific issues, optimize your site’s UX, and ultimately increase your conversion rates.
The problem is that Google Analytics on its own isn’t enough. It tells me that 100 people have visited a page, whereas Hotjar shows how one specific person engages on a specific part of the page—that’s invaluable!
Hotjar (that’s us 👋) is a product experience insights and behavior analytics platform with quantitative and qualitative tools and features that help you
Observe user behavior with funnels, trends, session recordings, and heatmaps to see what users are doing on your site and where they're dropping off
Ask for direct feedback through feedback widgets and surveys to understand why they're dropping off
Engage with users and customers through one-on-one interviews
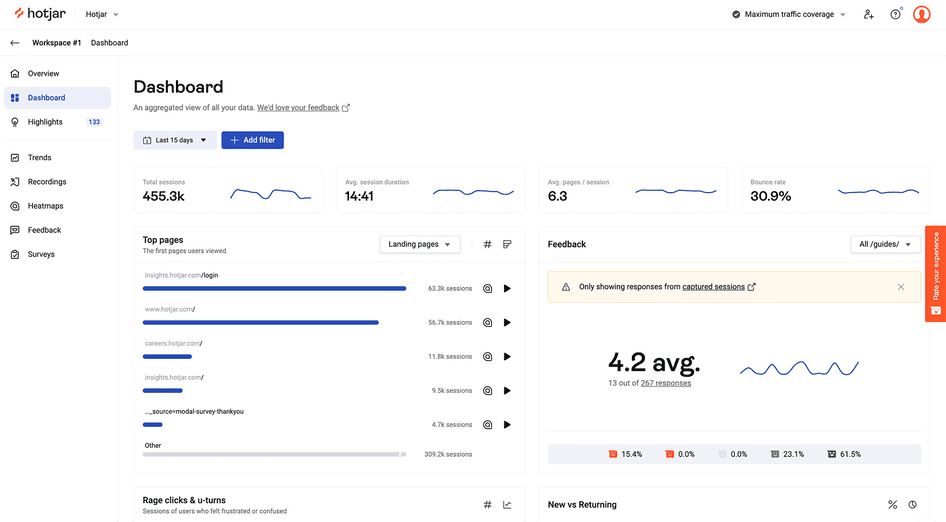
How it helps: Hotjar shows you what your users do on your site and why —you can access visual quantitative and behavior insights, real-time user feedback, and one-to-one interviews, all in one place.
For example, Hotjar Funnels measures how many users converted or dropped off, and Recordings shows you their entire experience while using your site, so you can tell why they dropped off. You can also combine behavior analytics insights from Hotjar with quantitative data from traditional tools like Google Analytics or Mixpanel to
Get a more complete picture of user behavior
Pinpoint specific issues your users might be having
Improve the overall user experience
Ultimately increase conversions
How to use it : if you're looking to use Hotjar for quantitative data analysis, you can approach it in many different ways since our tools and features complement each other. Here are three ideas to help you get started.
1. Use heatmaps to identify where visitors click on your most important pages
When it comes to optimizing your site’s UX, relying on gut feelings or copying competitors won’t take you far, especially since your audiences aren’t exactly the same. You need specific insights about your own users to understand and improve their experience.
Heatmaps show you how users interact with your most important pages—how far they scroll, which elements they ignore, and the buttons they click (or don't click), which helps you identify obstacles that keep them from converting and make informed changes.
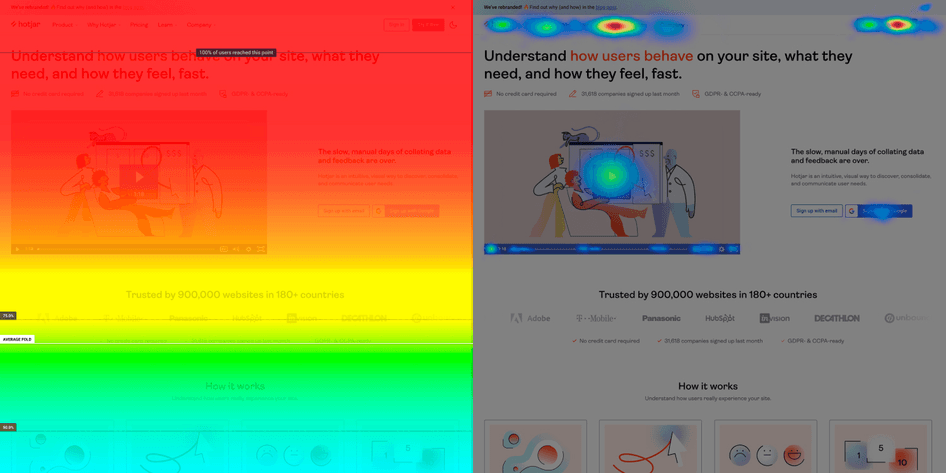
For example, software company TechSmith used Heatmaps to find out where visitors click and how far they scroll on their most important pages. The team found many website visitors clicked on their product icons instead of the CTA buttons. After optimizing those areas, they created a better experience for prospects.
2. Use trends to spot patterns in user behavior
Hotjar Trends gives you the complete picture, so you can visualize your metrics, uncover patterns in user behavior, and understand the real 'why' behind the numbers. Trends let you
See your custom conversion metrics and track their changes over time
Review individual recordings to discover the reasons behind the metrics
Analyze errors spotted in recordings or patterns in rage click maps to determine if the behavior is isolated or widespread
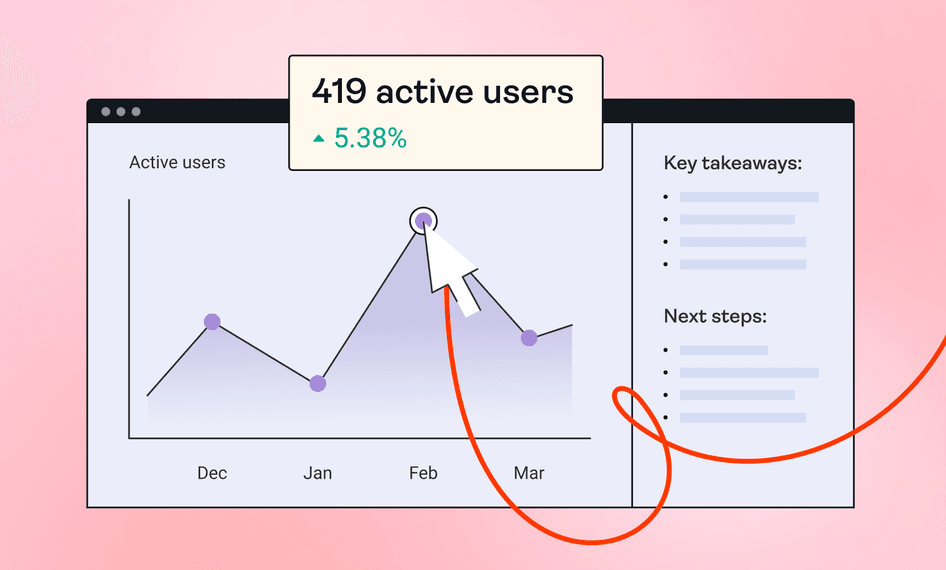
For example, to make your site's checkout process smoother for users, you can use Trends to visualize the checkout conversion rate over time and notice any changes. To better understand their behavior, you can review individual session recordings to see why users abandoned the checkout process and where they struggled.
Trends also lets you segment your data based on user groups, such as new versus returning customers. By comparing their behavior, you may find new users have a higher cart abandonment rate than returning ones, which helps you focus on improving the onboarding process and providing clearer instructions to new users during checkout.
3. Use funnels to identify where in the customer journey users drop off
Hotjar Funnels lets you track how visitors interact with your website and see where visitors convert or drop off. With Funnels, you can
View specific recordings from relevant funnels to see why users churn
See your lowest-converting steps, so you can identify how to improve these areas
Compare conversion performance between different marketing channels and more
Gather rich insights using filters and user attributes
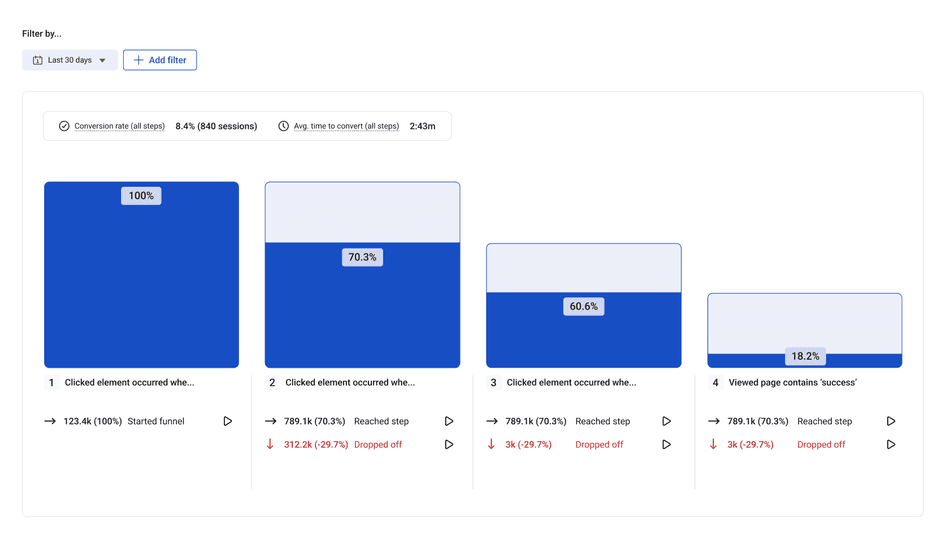
For example, Gogoprint (formerly Zenprint), an online printing service, noticed users were dropping off their site. While Google Analytics provided useful metrics like page time and bounce rate, it didn't reveal how users interacted with the page.
To dig deeper, they combined Hotjar with Google Analytics to identify specific sections of the product page that caused the drop-off and optimized them to improve the customer journey. As a result, Gogoprint saw 7% fewer drop-offs than before.
Hotjar reveals what numbers don’t. Funnels helped me identify where in the customer journey people drop off. Recorded user sessions let me understand what people see when they arrive on our website—what they click and what they don’t click. Heatmaps helped me identify where they spend most of their time and assess if they should be spending time there or not.
3. Mixpanel
Mixpanel is an advanced analytics tool that helps you understand how people use your site, app, or other digital products, so you can make data-backed improvements.
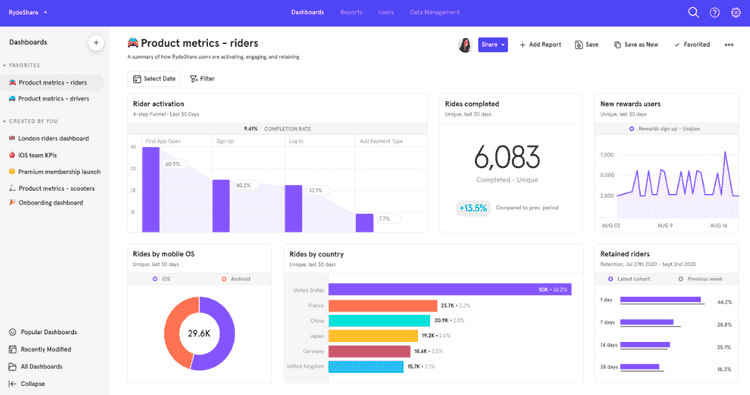
How it helps: use Mixpanel to
See how users go through different steps or actions
Understand which features are working well and which ones need adjustments
Create and analyze reports about how users behave
Group users into categories and track them over time
Make informed changes to increase conversions
How to use it: to start using Mixpanel, you need to integrate it into your product or service by adding tracking code or using its SDKs. Once the integration is complete, Mixpanel starts collecting and analyzing user data.
💡 Pro tip: use Hotjar’s Mixpanel integration to filter and send targeted surveys.
Say you have an ecommerce website that sells clothing, and you want to gather insights about customer preferences to improve the shopping experience.
With Mixpanel, you can track customer actions and segment them based on their behaviors and preferences. Then, with Hotjar, you can send targeted surveys to gather insights about the customer experience and collect suggestions for improvement.
This combination of quantitative and qualitative insights will help you personalize and improve the buying experience for users, ultimately increasing their satisfaction.
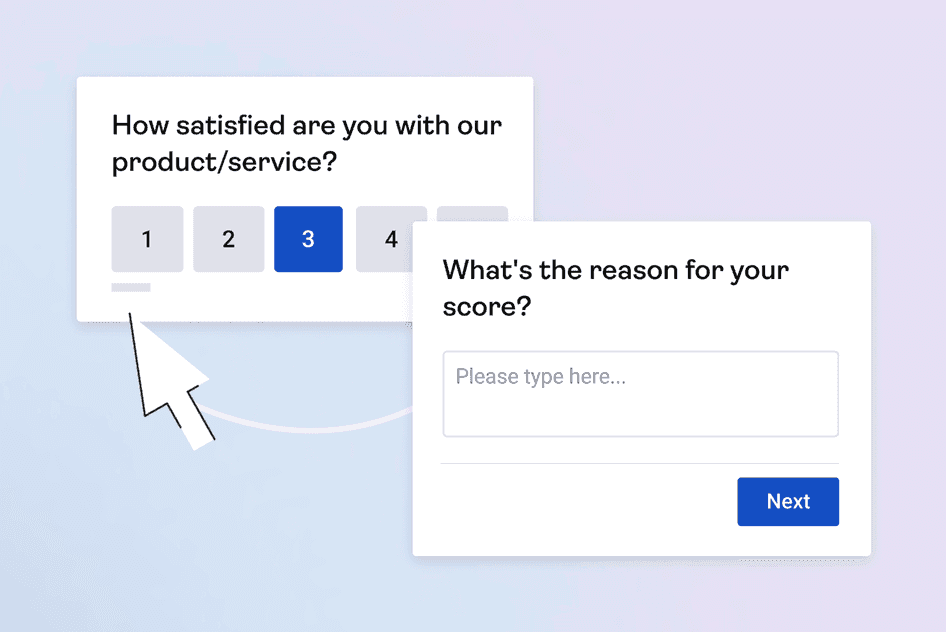
Use a Hotjar survey to learn about customer satisfaction with your site, product, or service
4. Amplitude
Amplitude is a product analytics and event tracking platform that helps you analyze user behavior, track key metrics, and gain insights into product performance.
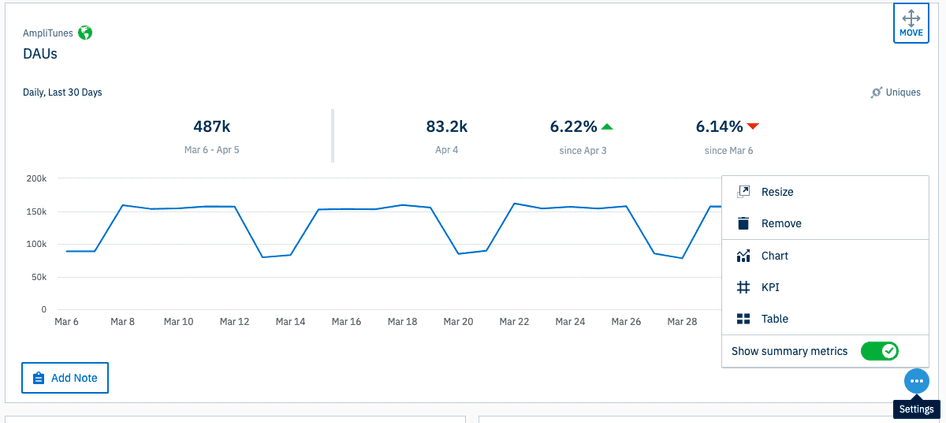
How it helps: Amplitude specializes in behavior analytics—helping you understand how users interact with your product, so you can identify patterns, trends, and opportunities to optimize your site. Amplitude also offers retention tracking and engagement analysis tools that measure user retention over time.
How to use it: to use Amplitude, you need to integrate it into your product or service by adding its tracking code. Once integrated, Amplitude will start collecting and processing user interaction data, so you can see what’s happening on your site.
5. Optimizely
Optimizely is a digital experience platform that lets you A/B test different variations of your site and run experiments to compare their performance and improve the user experience.
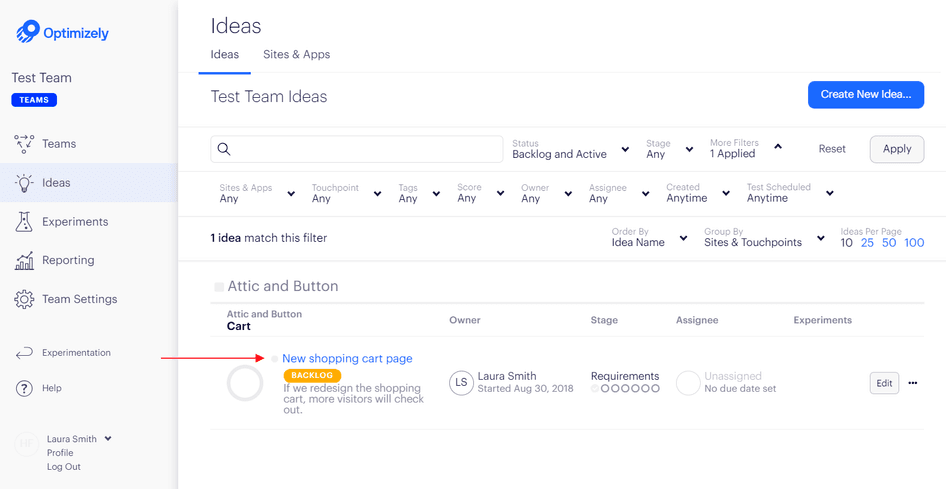
How it helps: Optimizely lets you test variations of your website to understand which version performs better in terms of conversions and click-through rates. Its experiment builder allows you to easily set up A/B tests or multivariate experiments without requiring technical expertise.
How to use it: integrate Optimizely into your website or app by adding its code snippet. After integrating the tool, set up your first experiment by defining the variations, selecting the audience segments, and specifying the goals you want to achieve. Optimizely then tracks user interactions, collects data, and provides analysis and insights to evaluate the experiment results.
💡 Pro tip: combine Optimizely A/B tests with Hotjar Recordings and Surveys to understand how users interact with different versions of your product and learn what they think in their own words.
These tools provide a comprehensive understanding of user behavior, preferences, and opinions, allowing you to optimize your product pages based on real data and deliver a better user experience.
The changes made from the Hotjar survey gave us enough confidence to begin designing the new page template, which we then A/B tested to get to the final version. Ultimately, we saw a 10% boost in conversions.
6. Kissmetrics
Kissmetrics is a software for quantitative data analysis that focuses on customer analytics and helps businesses understand user behavior and customer journeys.
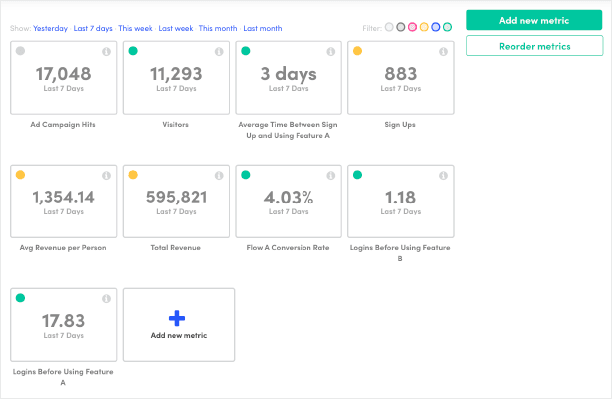
How it helps: Kissmetrics lets you track individual user behavior over time to understand the specific actions users take, engagement levels, and their impact on your business goals. The tool also provides cohort analysis, so you can group users based on their signup or acquisition date and analyze their behavior over time.
How to use it: to use Kissmetrics, integrate their tracking code into your website or application. Then, use the tool to define and track events relevant to your business.
7. Omniconvert
Omniconvert is a website optimization platform with A/B testing, surveys, web personalization, customer segmentation, and behavioral targeting features.
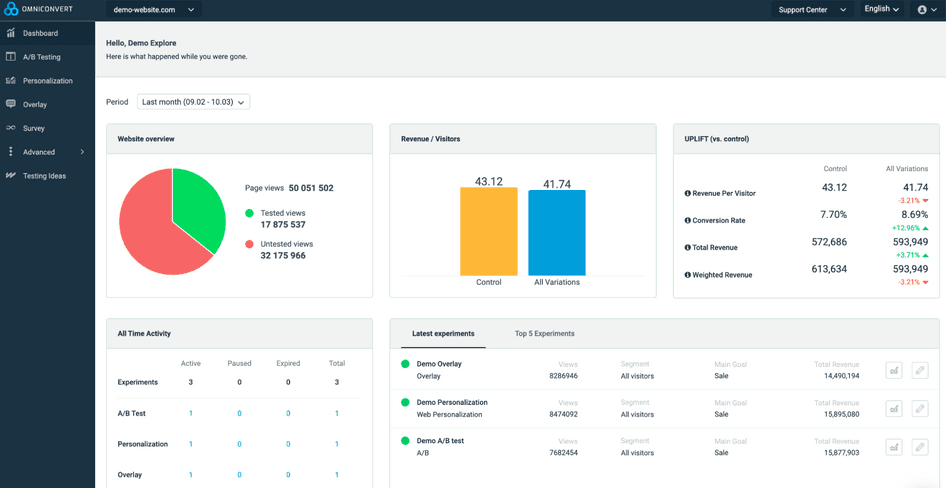
How it helps: Omniconvert focuses on conversion rate optimization , helping you analyze user interactions, identify areas of improvement, and run experiments to increase conversion rates. The tool also provides advanced analytics features to track and measure key user behavior, conversions, and engagement metrics to help you understand the performance of your marketing efforts.
How to use it: to use Omniconvert, integrate its tracking code or use its plugins with your website or app. After integrating, you can set up A/B tests, define segments, and track user interactions.
💡 Pro tip: connect your Omniconvert A/B test experiments to your Hotjar account and start using heatmaps and session recordings to understand user behavior and make changes that ultimately convert more users.
For example, if you want to understand why users aren’t signing up for your product from your site, you can set up a conversion funnel in Omniconvert to track the entire signup process and identify the specific step where users drop off. With Hotjar Recordings, you can watch users experience the signup process to gain insights into their behaviors, struggles, and potential reasons for abandonment.
When you start watching Hotjar recordings, you’ll think, ‘surely this user will complete the action!’ But once you’ve seen 20 people make the same mistake, you know the problem is with your site, not the users.
Heap is a website analytics solution that offers product managers a comprehensive toolkit to understand customer behavior on a large scale.
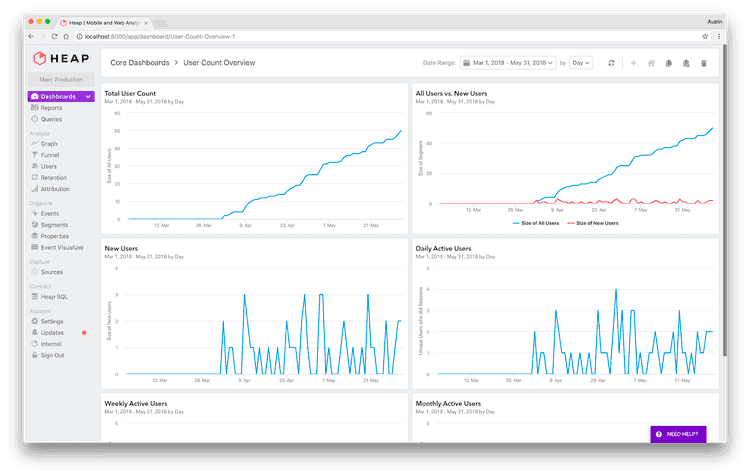
How it helps: Heap's automatic data capture eliminates the need for manual event tracking. The tool also has a retroactive analysis capability that lets you gain insights from past data and make changes without worrying about missed tracking opportunities.
How to use it: to use Heap, integrate its tracking code or install its SDKs in your website or app. You can then log into the Heap platform to explore captured user data, create funnels, segment your audience, and make decisions to improve your site’s UX.
HubSpot is a comprehensive customer relationship management platform that offers a wide range of tools to support your marketing, sales, and customer service efforts.
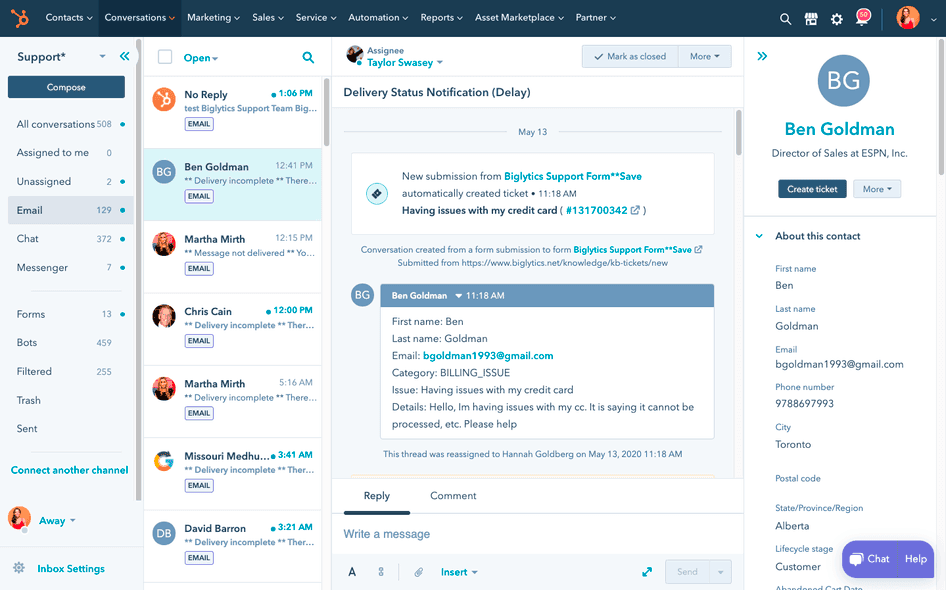
How it helps: with HubSpot, you can track various metrics and data points related to your marketing, sales, and customer service activities, such as website traffic, email open rates, conversion rates, and customer interactions.
Plus, customizable dashboards and reports make it easy to get a quick overview of how things are going—analyze data trends, identify patterns, and gain insights into the performance of your campaigns, content, and channels.
How to use it: to use HubSpot, create an account and set up your marketing, sales, and customer service processes within the platform. Integrate it with your website, CRM, and other relevant tools (including Hotjar!). Then customize your analytics dashboards to track the metrics that matter most to your business, and HubSpot will collect and analyze the data on your behalf.
💡 Pro tip: pair Hubspot with Hotjar to speed up the process of solving customer support issues and make users happier.
Say you have a SaaS product and notice increased support tickets related to a specific feature. By analyzing a Hotjar rage click or Engagement Zone heatmap, you can see where users struggle.
Once you identify the spots where users are having trouble, create a knowledge base article in HubSpot, with clear instructions and helpful screenshots, to guide users through the process smoothly. You can also improve your support email templates in HubSpot, ensuring they direct users to the article for more help.
As you implement these changes, use Hotjar feedback tools to gather continuous feedback, so you can tell if users truly find these resources helpful. That's how you strengthen customer relationships and speed up your support process.
How to pick the best quantitative data analysis software for your business
Choosing the best quantitative data analysis tool for your business will help you gather the most accurate and relevant data for your team to make informed decisions. Here are eight tips for choosing the software that best suits your needs.
Know what you need: before choosing a solution, think about the type of data you work with and the kind of analysis you want to perform
Do your research: look for well-known tools and read reviews from other users on third-party sites to get an idea of their experiences
Check the features: look closely at what each tool offers to ensure it has what you need to make sense of your data
User-friendliness matters: choose a tool that's easy to use and doesn't require a steep learning curve—you want something that your team can quickly adapt to and start using effectively
Consider the cost: think about what you're willing to spend and look for a tool that fits your budget while providing the features your team needs
Give it a test run: many tools offer free trials or demos, so try them out first to determine if it’s the right fit for your business
Ask for recommendations: reach out to other businesses or colleagues who have used any of the data analysis tools you might be considering to hear what they have to say about the product
Support matters, too: consider the level of support the tool provides—look for resources like documentation, tutorials, and responsive customer support
Use a product experience insights platform to gather more holistic, actionable user data
Quantitative data analysis tools focus on the numbers, like how many people visited your website or how much revenue you generated, but they don’t tell you why your customers took a specific action.
A product experience insights platform like Hotjar shows you what's happening and why , giving you a more complete and well-rounded understanding of your users. You can see the big picture while diving deep into the behavior behind the numbers. This combination helps you uncover valuable user insights that create more delight and loyalty.
FAQs about quantitative data analysis software
What is quantitative data analysis software.
Quantitative data analysis software gathers data from various sources to help you understand your online visitors and website performance. Analyzing quantitative website data helps you identify trends and patterns in user behavior.
Why is quantitative data analysis software important?
Quantitative data analysis tools are important because they help you understand your audience, track performance, and make informed decisions about marketing strategies, content creation, and website optimization.
What is the most popular quantitative data analysis software?
When it comes to quantitative data analysis, you have a wide range of software options to choose from. Let's take a look at some of the most popular ones:
Google Analytics lets you track what's happening on your site
Hotjar helps you understand the what and why in one place
Mixpanel helps you understand how people use your site
Amplitude gives you insights into product performance
Optimizely specializes in experimentation and personalization for websites
Kissmetrics helps you understand user behavior and customer journeys
Omniconvert lets you run A/B tests and offers surveys, web personalization, customer segmentation, and behavioral targeting features
Heap helps product managers understand customer behavior at scale
HubSpot helps with your marketing, sales, and customer service efforts
Quantitative data analysis methods
Previous chapter
Guide index

Top 15 Academic Research Tools For Scholars And Tutors
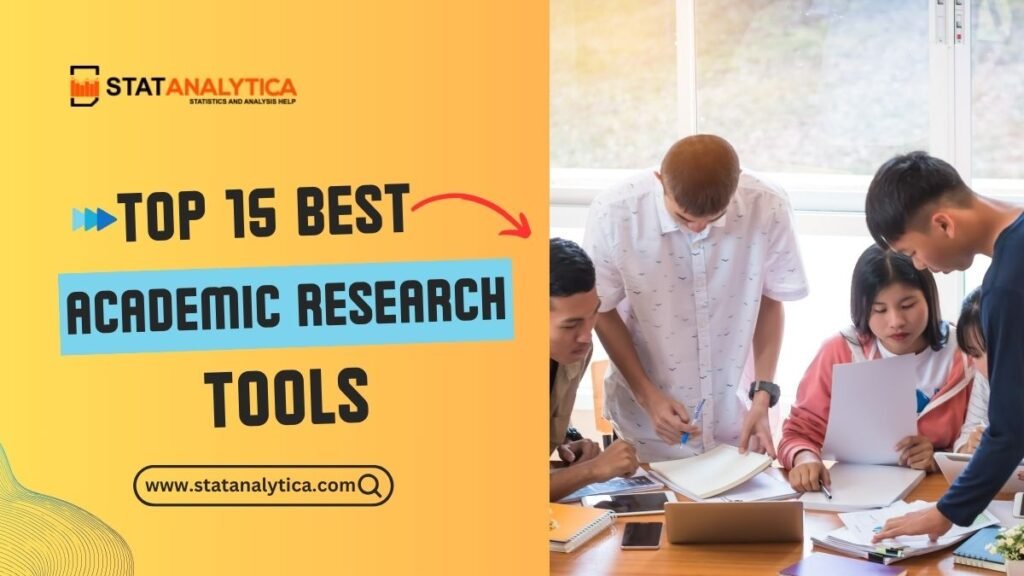
Using specialised tools has become more important in the ever changing field of academic research. These tools reference management, data visualisation, survey design, literature search, writing, and editing, are essential for improving the efficiency of the research process and the quality of scholarly output. Researchers can smoothly collect, assess, and share data using the top 15 academic research tools we are sharing, which will ultimately change the way research is carried out and done.
We will examine the essential resources for successful research in this guide, offering insightful analyses of the top three resources in each category—all discussed in simple language for a general audience. So let us start this discussing the ultimate top 3 for all the categories we have today.
Top 3 Academic Research Tools For Reference Management
Table of Contents
Reference management tools, also known as citation managers, are programs or online services that help researchers collect, organise, and cite references. These tools offer features such as storing references in a searchable database, attaching PDFs and other files, and auto-generating citations and bibliographies in the preferred citation style. They also allow users to share collections of references with others and sync references across multiple devices. Some popular reference management tools include EndNote, Mendeley, Zotero, and RefWorks.
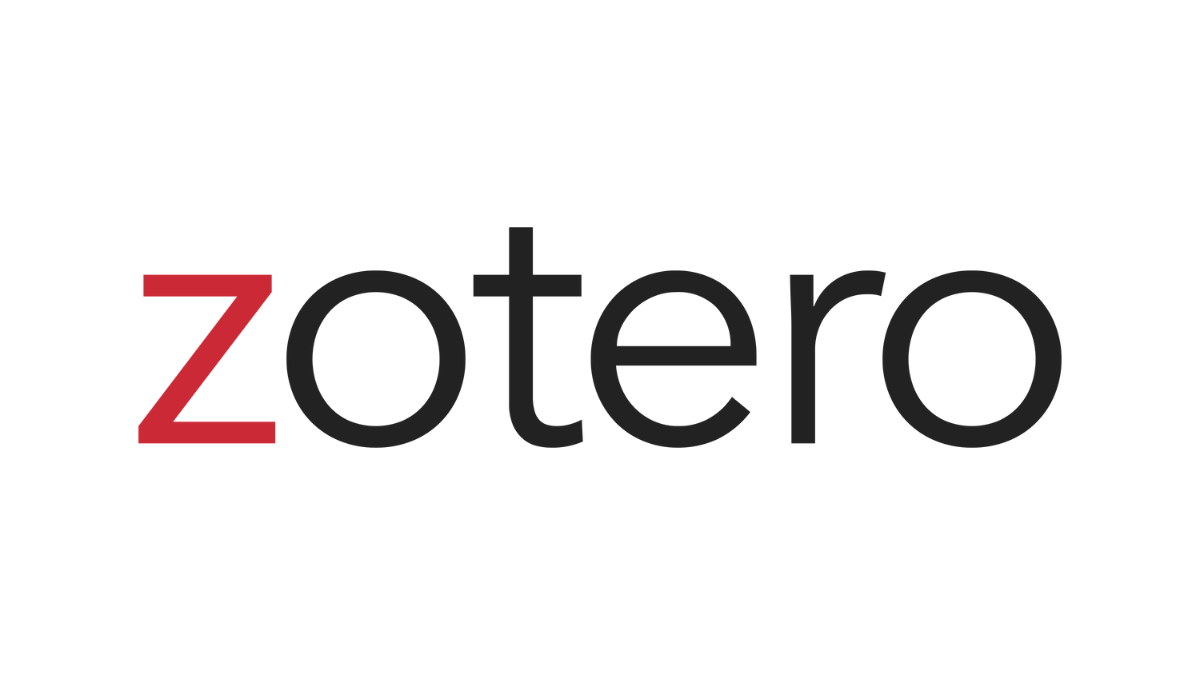
Zotero is a free, open-source research management program that has been a game-changer for many researchers, including myself. Here are some benefits of using Zotero in academic research, drawn from personal experience:
- Time-saving : Zotero’s web browser plugins make it easy to save item information from the web, eliminating the need to manually copy and paste citations. It saves time and effort, especially while dealing with multiple sources.
- Automatic PDF downloading : Zotero is good at capturing and downloading full-text PDFs from databases, which can be particularly useful when working with limited access to certain resources.
- Built-in tools : Zotero has a built-in tool to extract source information from PDFs, making it easier to manage and organise your research materials.
- Citation style flexibility : Zotero supports virtually all citation styles, allowing you to format your citations consistently and according to your institution’s guidelines.
- Word processor integration : Zotero’s word processor plugins let you “cite as you write,” making it easier to insert citations directly into your paper without having to switch between different applications .
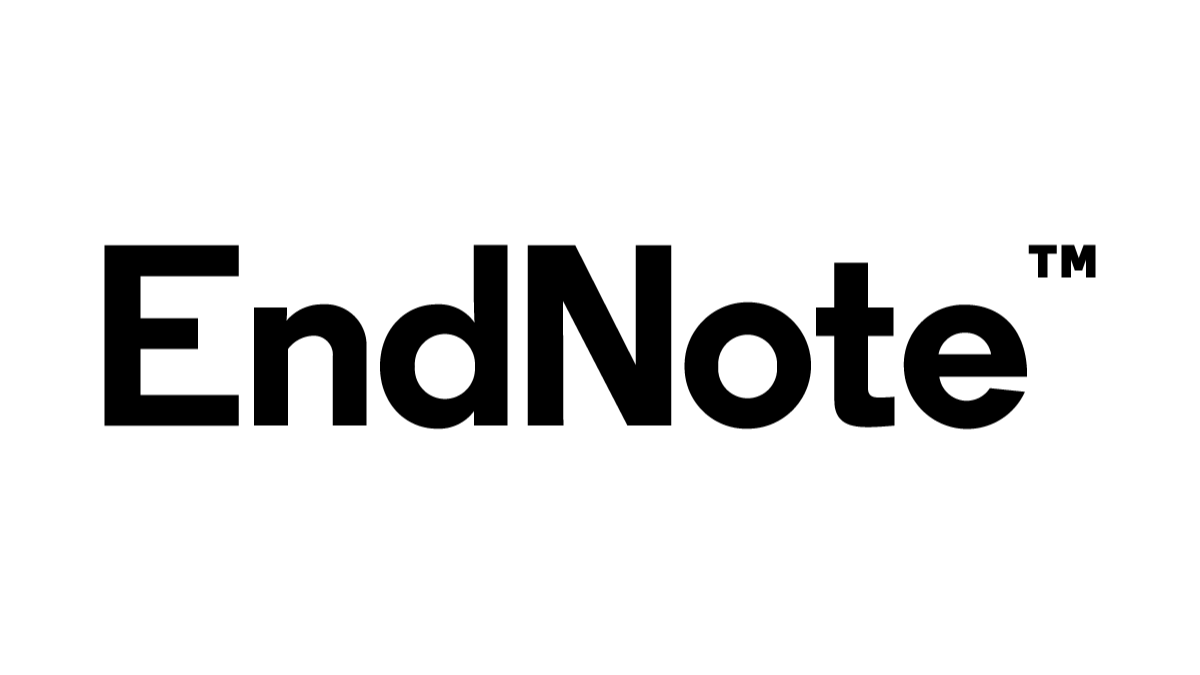
EndNote is a popular citation management tool used by researchers, faculty, and students to store, organise, and cite references. Here are some benefits of using EndNote in academic research:
- Organisation: EndNote allows you to organise your research materials by creating folders, adding notes, and tagging citations, making it easier to locate and manage your sources.
- Better Time Management: By using EndNote, you can manage your research more efficiently, making it easier to meet deadlines and stay organised throughout the research process.
- Full-text PDF downloading: EndNote enables you to automatically attach and download full-text PDFs to saved references, making it easier to read, review, and annotate articles without having to switch between different applications.
- Collaboration: EndNote allows you to share your research with others, facilitating collaboration on research projects and group assignments.
- Bibliography: When it comes time to create a bibliography, EndNote sprinkles some magic. It seamlessly integrates with Word, turning your list of references from a headache-inducing task into a point-and-click breeze.
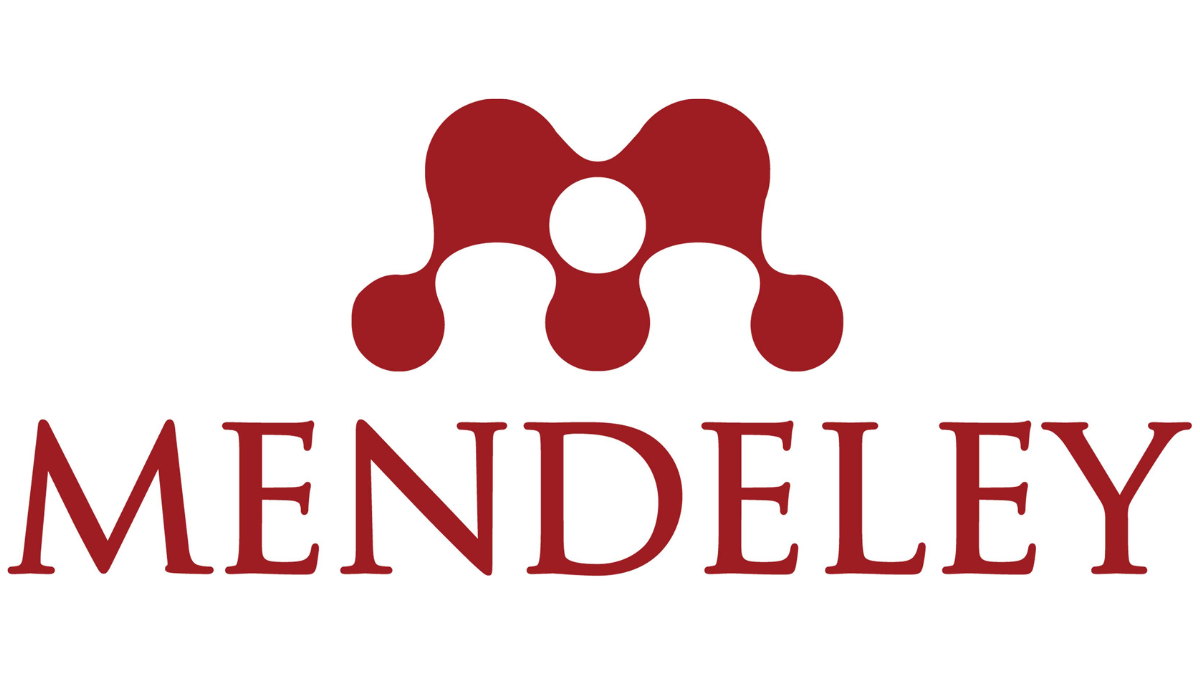
For researchers and journal editors among all the reference management tools Mendley is very popular. Other than its feature of ease of use here are few other features of it:
- Free of Cost: Best of all, Mendeley doesn’t cost anything. It’s free and all of us love these free tools as they are budget friendly and do not cost anything. Library Superpower: Mendeley is like a superhero library for your computer. It keeps all your research papers and articles in one organised place.
- Teamwork Friendly: If you’re working on a project with friends, Mendeley makes it easy to share your library. Teamwork just got a whole lot simpler.
- Highlight and Scribble: If you find something cool in a paper, you can highlight it and write notes on your computer. It’s like doodling on your homework but way more useful.
- Discover New Things: Mendeley even helps you discover new articles based on what you like. It’s like a friend suggesting cool stuff to read.
Top 3 Academic Research Tools For Data Visualisation And Analysis
Data visualisation and analysis tools are software or online platforms that help users create visual representations of data, making it easier to understand and interpret complex information. These tools enable users to generate various types of visualisations, such as charts, graphs, and maps, from their data sets. By presenting data visually, these tools facilitate quicker and more effective decision-making, allowing users to examine trends and information that is not immediately apparent from the raw data. Also it offers features for customising and sharing visualisations, making them valuable for both individual analysis and collaborative work. Some of the popular tools are as:
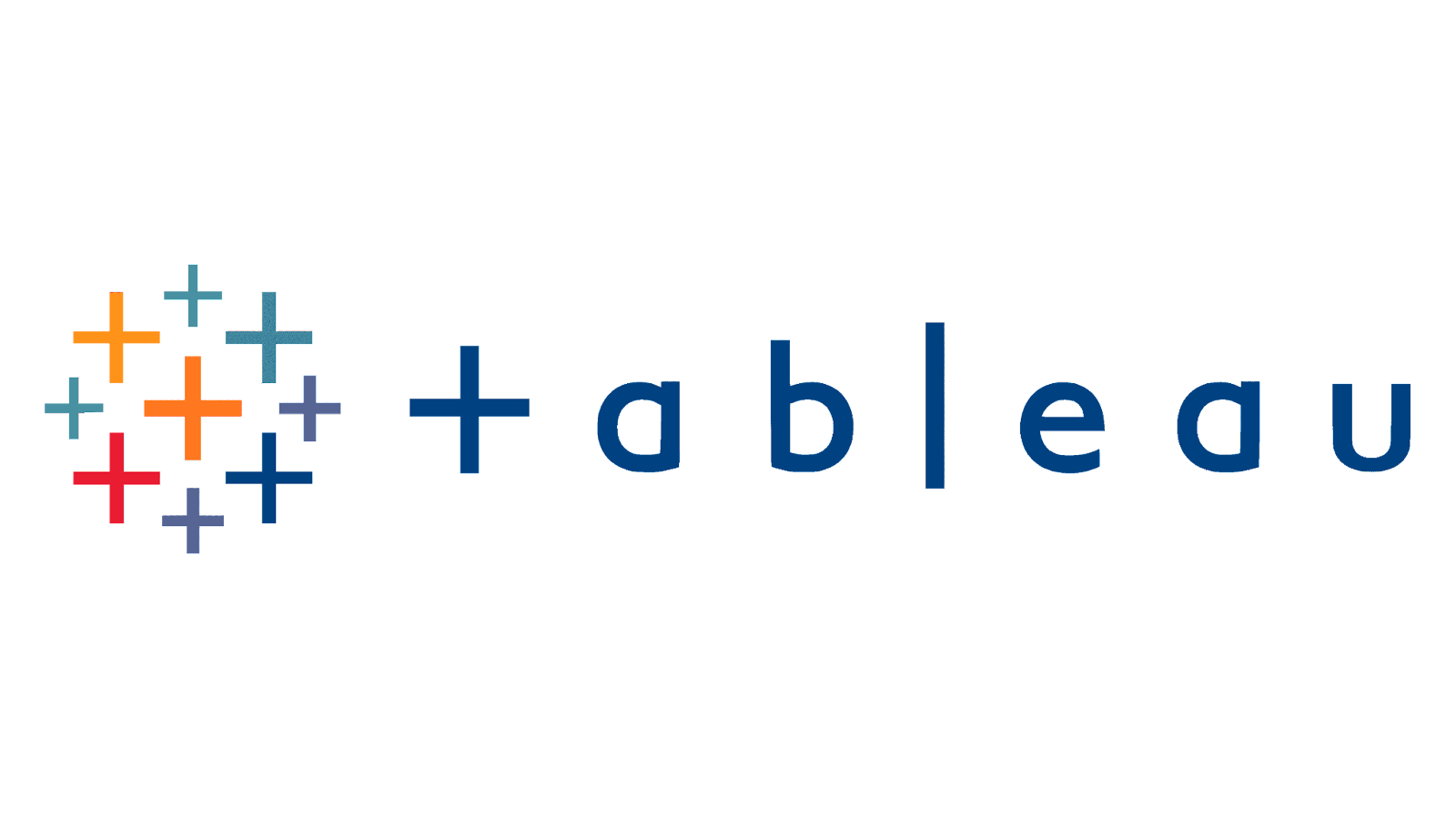
In academic research, Tableau is a data visualisation and analysis application that is becoming more and more popular. Using Tableau in academic research has several advantages, which I have personally experienced:
- Easy to use: Tableau is simple to use and intuitive to understand, making it available to researchers of all experience levels.
- Data visualisation: Tableau makes complex data easier to understand and analyse by enabling you to build dynamic, eye-catching charts, graphs, and maps.
- Integration with different data sources: Tableau facilitates the work with a variety of data types by helping you to access a vast range of data sources.
- Real-time data analysis : With Tableau’s ability to analyse data in real-time, you can act quickly and decisively based on up-to-date knowledge.
- R (Programming Language)
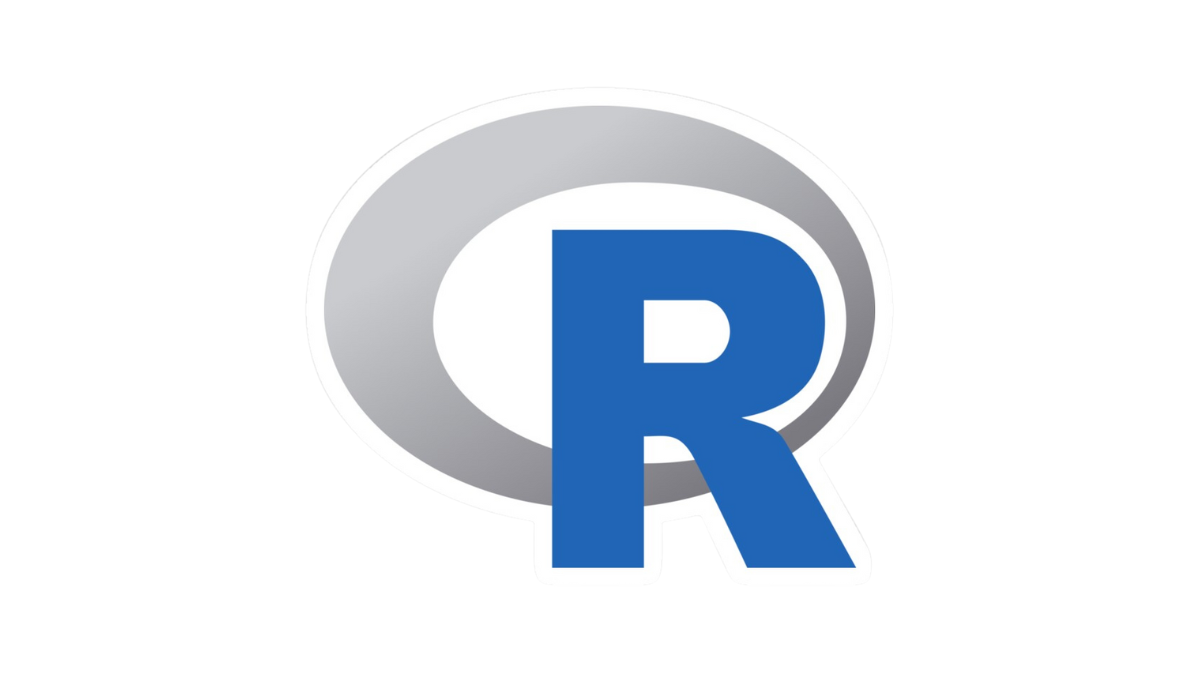
R is a programming language and software database used for statistical computing and graphics. It’s like a super-smart tool for crunching numbers, making cool charts, and handling data in a way that even non-computer wizards can understand. Following are some of the features of it:
- Flexibility and Extensibility : R’s flexible nature allows researchers to create custom functions, tailor analyses to specific needs, and interface with other programming languages like C, Python, and Java, enhancing its extensibility.
- Community Support: The R community is basically my online superhero squad. Whenever I got stuck on some coding conundrum, forums and groups were there with advice and solutions. It’s like having a 24/7 coding help hotline.
- Advanced Visualisations and Quick Implementation : R offers advanced visualisations and allows for the quick implementation of new theoretical approaches, which can be highly beneficial for researchers working with complex data.
- Continuous Evolution: R is always evolving. New packages, updates, and features keep popping up. It’s like your favourite app that keeps getting better with each update.
- Cost-Free Awesomeness: R doesn’t cost a cent. In a world where software can drain your wallet, R is the ultimate budget-friendly genius. It’s like getting a high-end software package without the hefty price tag.
- Python (Having Libraries Like Matplotlib And Seaborn)
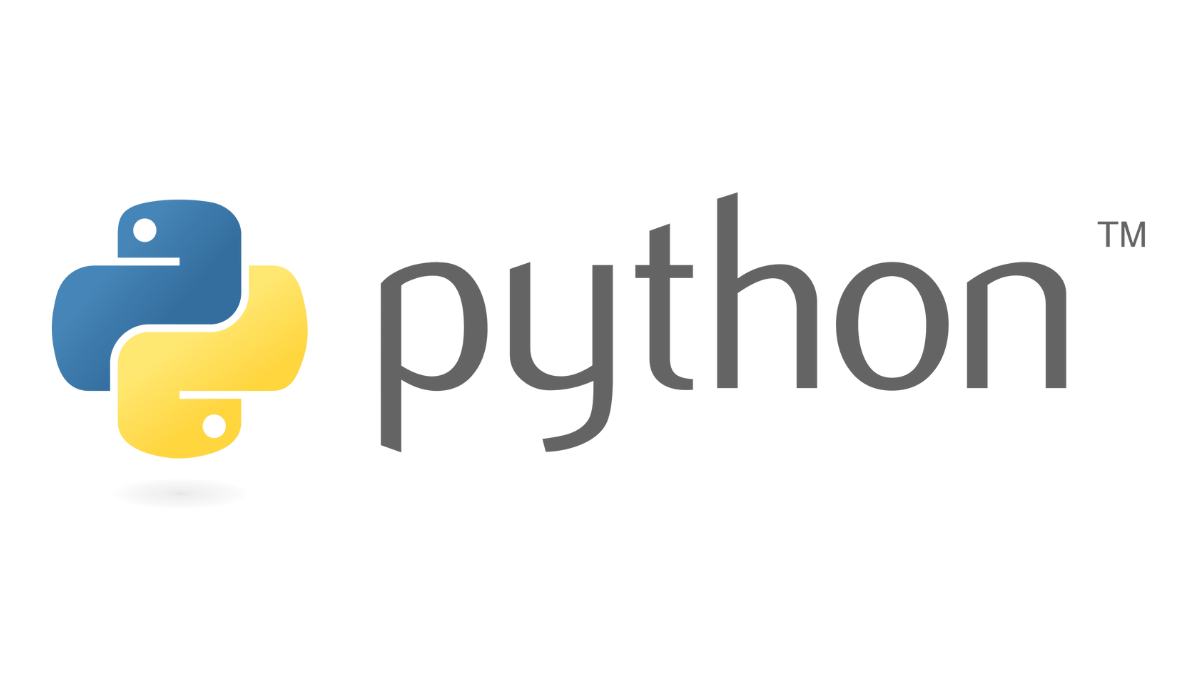
Python is an adaptable and user-friendly programming language. It’s like a friendly guide for beginners, helping them write code effortlessly. With a vast library ecosystem, Python is a go-to language for tasks ranging from simple scripts to complex machine learning projects. Here is a short list of features of Python:
- Coding Zen: Python is like the cool kid in coding class. It’s easy to learn, which is a big relief when you’re juggling research and a gazillion other things.
- Open-Source and Cost-Effective : Python is open-source, platform-independent, and does not require fees or licences, making it a low-risk and cost-effective option for academic research.
- Versatile Toolbox: Python has a toolbox full of libraries. If we need to do something specific there’s probably a library for it. It’s like having a Swiss Army knife for data tasks.
- Machine Learning Marvel: Python is the superhero of machine learning. With libraries like TensorFlow and scikit-learn, it’s like having Iron Man’s suit for training models and making predictions.
- Data Analysis Capabilities : Python offers a large range of statistical tests, models, and capabilities, and it is mainly opted for machine learning and data analysis.
Top 3 Academic Research Tools For Survey Design And Data Collection
Survey design and data collection tools are software or online platforms that help researchers gather information from a specific group of people about their views, interests, or understandings. These tools offer various methods for creating surveys, such as questionnaires, e-surveys, telephone interviews, face-to-face interviews, focus groups, and electronic (e-mail or website) surveys. These tools offer features like customizable templates, question types, and data analysis capabilities, making them essential for conducting effective surveys and collecting valuable data for research purposes. Top 3 survey design and data collections tools are as:
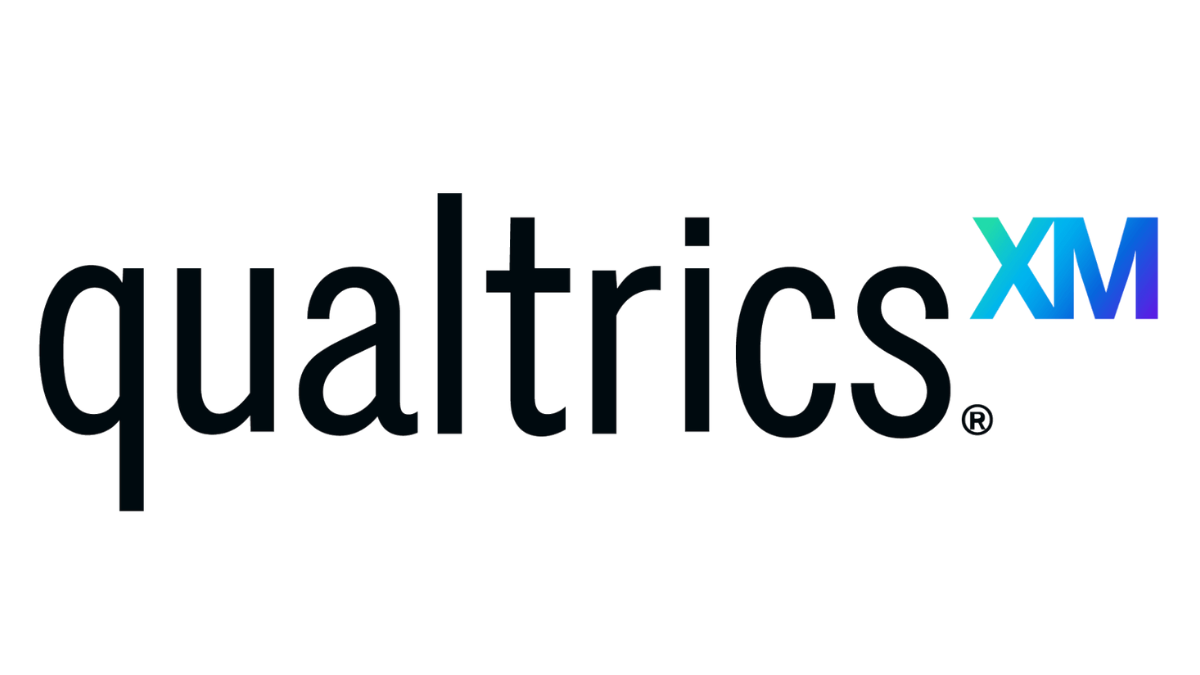
Qualtrics is an online survey platform that helps you create and analyse surveys. It’s like a digital survey wizard, making it easy to collect and understand data. With user-friendly features, Qualtrics simplifies the survey process for everyone.
- Super Easy Surveys: Qualtrics turns survey-making into a piece of cake. You drag, drop, and boom – your survey is ready. No need for a PhD in tech.
- Data Heaven: When the replies start coming in, Qualtrics is a data wizard. It handles calculations and organisation like an expert. No more getting lost in a pile of info.
- Real-Time Feedback : Qualtrics allows researchers to listen to real-time feedback from students, faculty, and staff, making it easier to understand and improve the educational experience
- Student Budget Friendly: Qualtrics won’t cost you a lot. It’s like getting a premium survey experience without losing some weight of your wallet.
- Surveymonkey
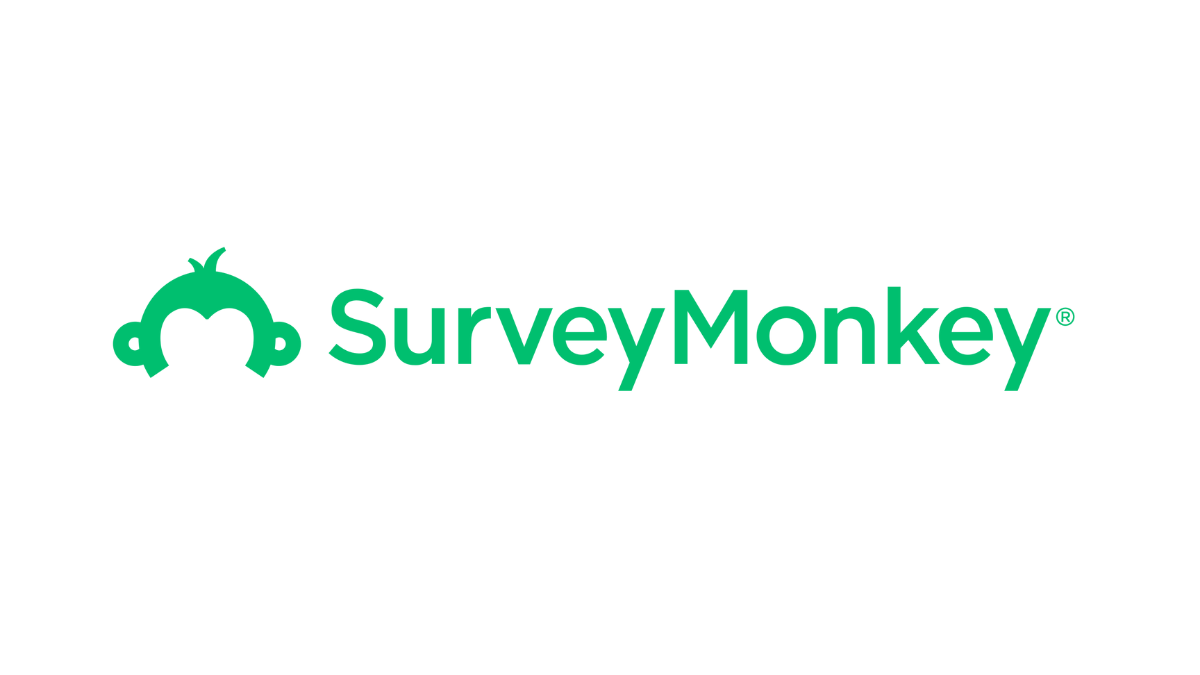
SurveyMonkey is an online platform that creates and analyses surveys. It’s like having a survey guru in your pocket, simplifying the process with a user-friendly interface and tools for gathering insights. Some of the main features are as:
- User Friendly: SurveyMonkey takes the headache out of making surveys. It’s so user-friendly that even a middle school child could create a survey without asking for tech support.
- Mobile Friendly: In a world glued to phones, SurveyMonkey is mobile-friendly. Respondents can tap away on any device, making my surveys accessible and cool.
- Confidentiality: SurveyMonkey provides high-security features and ensures that it is in the right hands and there is no breach of security. SurveyMonkey is like the superhero security guard, ensuring your data stays locked and safe.
- Feedback Buffet: SurveyMonkey isn’t just for surveys; it’s a feedback buffet. From opinions to reviews, it gathers feedback like a pro, turning me into a feedback platter.
- Data Playground: SurveyMonkey transforms data into a playground once the responses start rolling in. Spreadsheet making is a thing of the past; insights are the rollercoaster of a data carnival.
- Redcap (Research Electronic Data Capture)
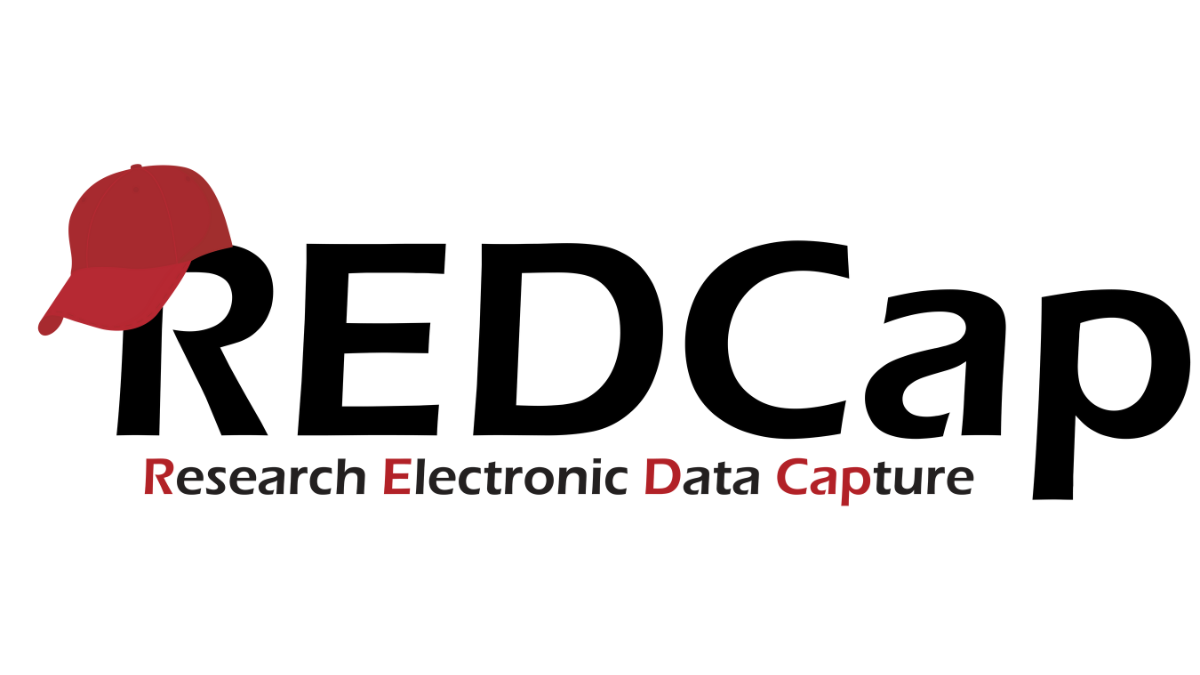
REDCap (Research Electronic Data Capture) is a user-friendly, secure, and web-based application for data collection and management in research. It’s like having a digital assistant that simplifies the process of gathering and organising research data efficiently and safely. Some of the attractive features of Redcap are as:
- User-Friendly Interface : REDCap offers a user-friendly web-based interface that puts researchers in total control of their work, allowing them to manage their own projects whenever and however they wish, through any browser on any device.
- Cost-Effective : REDCap is a cost-effective choice for academic research because it is a free, safe, web-based application that allows data capture for research studies.
- Wide Range of Forms: REDCap offers a versatile solution for creating surveys, whether they are straightforward or intricate. It’s akin to having a variety of forms at your disposal, allowing you to select and customise according to your specific needs, just like choosing items from a buffet.
- Customizable Surveys : REDCap allows researchers to customise their surveys to meet their local security policies and personalise features/functionality to address user needs.
- Secure Data Collection : REDCap provides a secure data collection tool that meets HIPAA compliance standards, making it a reliable and safe option for data collection.
Top 3 Academic Research Tools For Literature Search
Literature search tools are software or online platforms that help researchers find, organise, and analyse relevant information via different sources, such as educational articles, books, and other journals. These tools offer features like search engines for research papers, literature review software based on citation networks, tools for locating open access scientific papers, and more. Some of the best literature search tools are as:
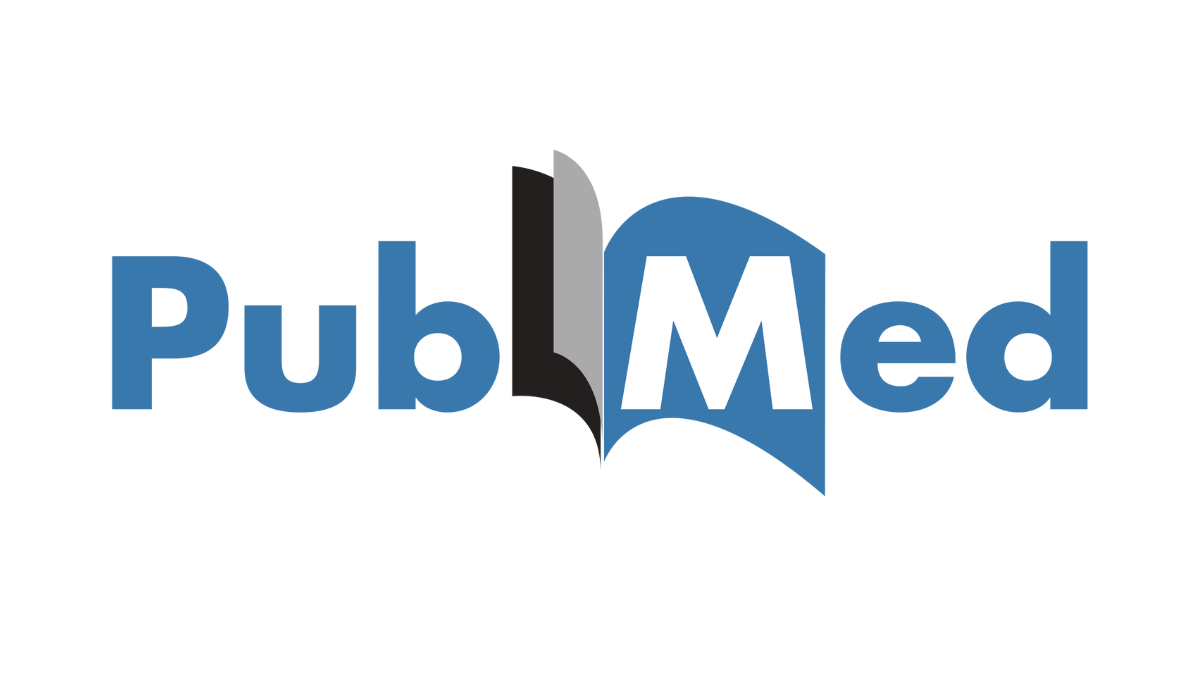
PubMed is a free, easy-to-use search engine that lets you find and read articles on life sciences and biomedical topics. It has more than 35 million citations and abstracts, with links to full-text articles when available. It is managed by the National Library of Medicine and is a reliable source for researchers and students. Some of its main features are as:
- Free Access : PubMed is freely accessible, making it a cost-effective option for academic research.PubMed is like a scholarship for information, supporting my academic journey without draining my student budget.
- Abstract Summaries: Reading full articles can be time-consuming, but PubMed spoils me with abstracts. It’s like having a trailer before committing to the whole movie – efficient and smart.
- Historical Journey: PubMed is a time machine for research. It’s like flipping through the pages of history, seeing how studies evolved over time. Each article tells a story.
- Integration with Other Sources : PubMed can integrate data from other sources, making it easier to analyse and interpret data ensuring that different data sets will react collectively.
- Ease of Use : PubMed offers a user-friendly interface that allows researchers to search for articles using keywords or Medical Subject Headings (MeSH), making it easy to find relevant information.
- Google Scholar
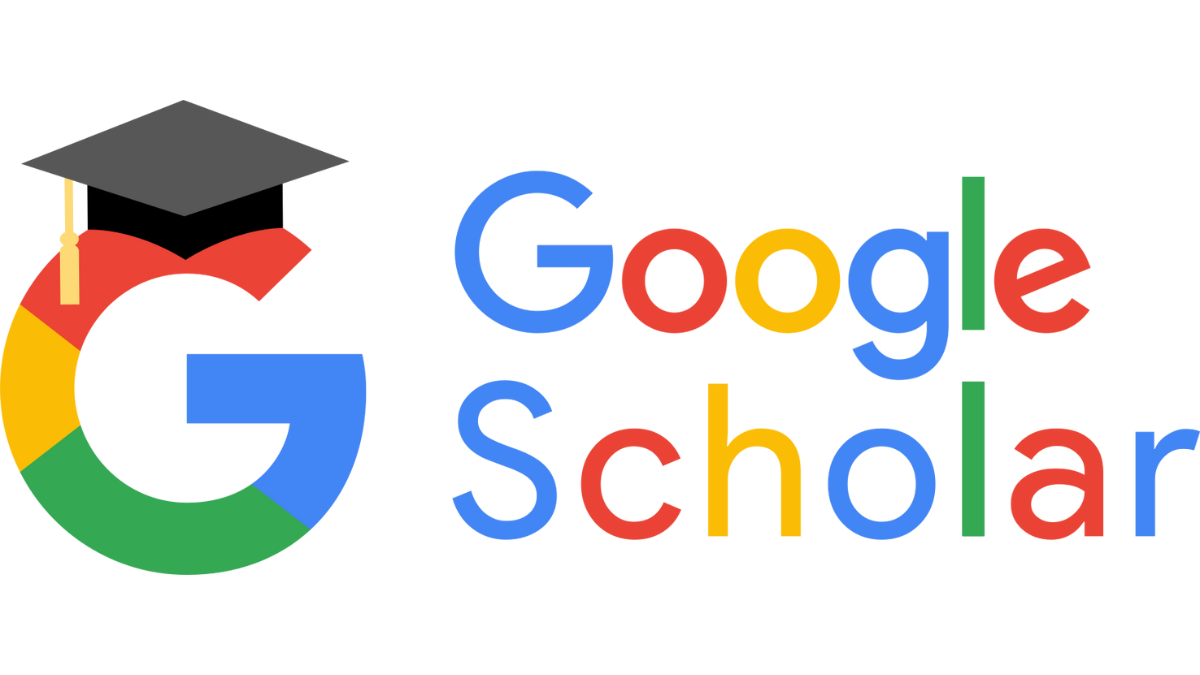
Users can locate academic resources and scholarly literature, such as books, articles, theses, abstracts, and court opinions, using the free Google Scholar search engine. It searches many different sources, such as websites from universities, professional associations, academic publishers, and online repositories. Google Scholar attempts to organise papers according to researcher rankings. Some of its main features are as:
- No Membership Fee: Best of all, it’s free. Google Scholar is like a gift that keeps on giving, supporting my academic journey. It is freely accessible, making it a cost-effective option for academic research.
- Diverse Resource Hub: It’s not just articles; Google Scholar is a hub of diverse resources. It’s like a buffet of knowledge, offering books, theses, and conference papers on my research plate.
- Advanced Search Capabilities : Google Scholar offers advanced search capabilities, including filters and limiters, allowing researchers to refine their search results.
- My Virtual Bookshelf: Google Scholar is my digital bookshelf. It’s like having a tidy shelf where I can collect and revisit my favourite studies. No more going through piles of papers.
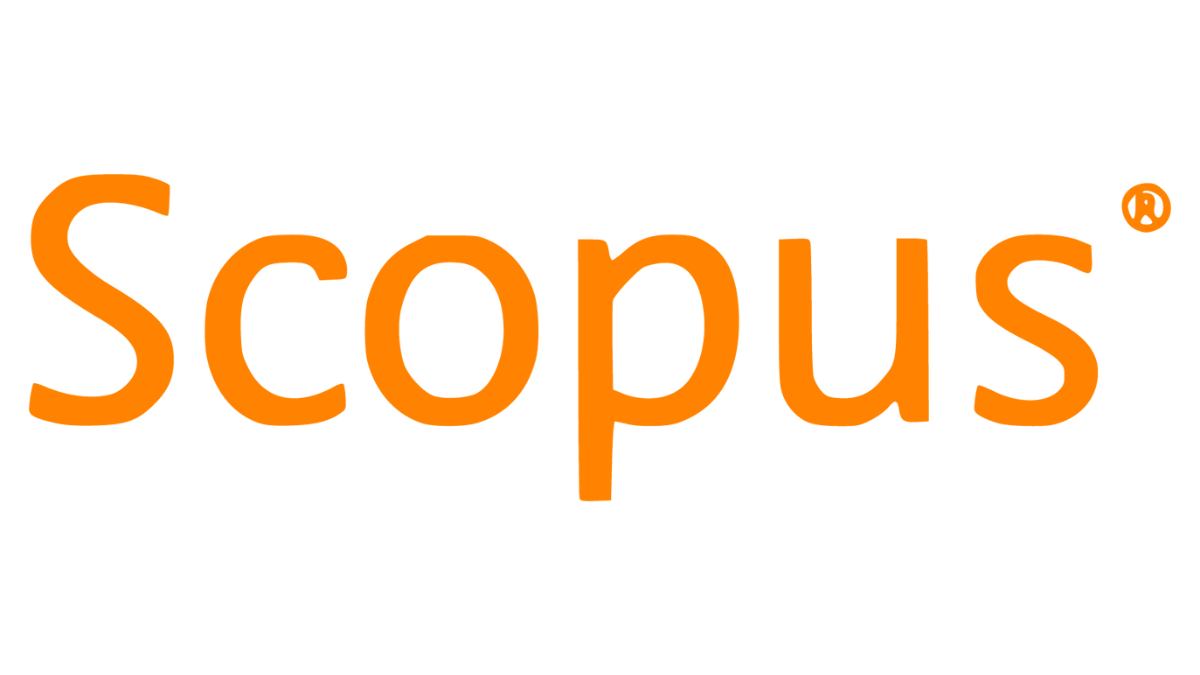
Scopus allows users to search both forward and backward in time for scientific, technical, and medical journal articles and the references included in those articles. Authors, researchers, students, librarians, universities, and others use the database to find, locate, and evaluate research output from around the world. Some of its main features are as:
- Global Coverage : Scopus covers journals from multiple disciplines, like science, technology, medicine, social sciences, arts, and humanities, offering a broad spectrum of research fields and topics.
- Keyword Treasure Hunt: Searching Scopus for particular keywords is similar to going on a scavenger hunt. Similar to looking for hidden treasures, each keyword reveals a wealth of useful information.
- Graphical Journey: Visualizing research connections is like a graphical journey on Scopus. It’s like having a map that shows the academic world’s complex web of ideas and influences.
- Academic Evaluation : Scopus Indexed Journals are often considered in academic evaluation processes, such as tenure and promotion decisions, as a measure of a researcher’s scholarly output and impact.
Top 3 Academic Research Tools For Writing And Editing
Writing and editing tools are software applications that help writers and content creators improve the quality of their work. It offers different features such as grammar and spell-check, style and readability analysis, and plagiarism detection. It can also help you with real-time feedback and suggestions to increase the overall quality of the content. Writing and editing tools are widely used to streamline the writing process, ensure accuracy, and maintain high standards of professionalism in written work. Some popular examples of these tools include Grammarly, Hemingway Editor, and Latex
LaTeX is a document generation system with high-quality and it is commonly used for technical and scientific documents. It is free software and is distributed under the LaTeX Project Public License. LaTeX is not a word processor; instead, it encourages authors to focus on the content of their documents, leaving the typesetting to the system. Some of its main highlights are as:
- Elegant Formatting: LaTeX produces high-quality typesetting, making documents look professional and polished. LaTeX is the fashion designer of documents. It’s like dressing up your research in a sleek suit, making it look elegant and professional .
- Mathematical Expertise: If you are Dealing with equations then LaTeX is like a maths wizard. It weaves equations into your text seamlessly, so your formulas look as good as your arguments.
- Easy Management of References and Citations : LaTeX allows researchers to label any piece of information they would like to use later for citations or as a reference, only requiring them to remember the label, and LaTeX handles everything.
- Portable and Platform-Independent : LaTeX files can be opened and altered with any text editor, and its formatting is consistent and automatically employed once set.
- Hemingway Editor
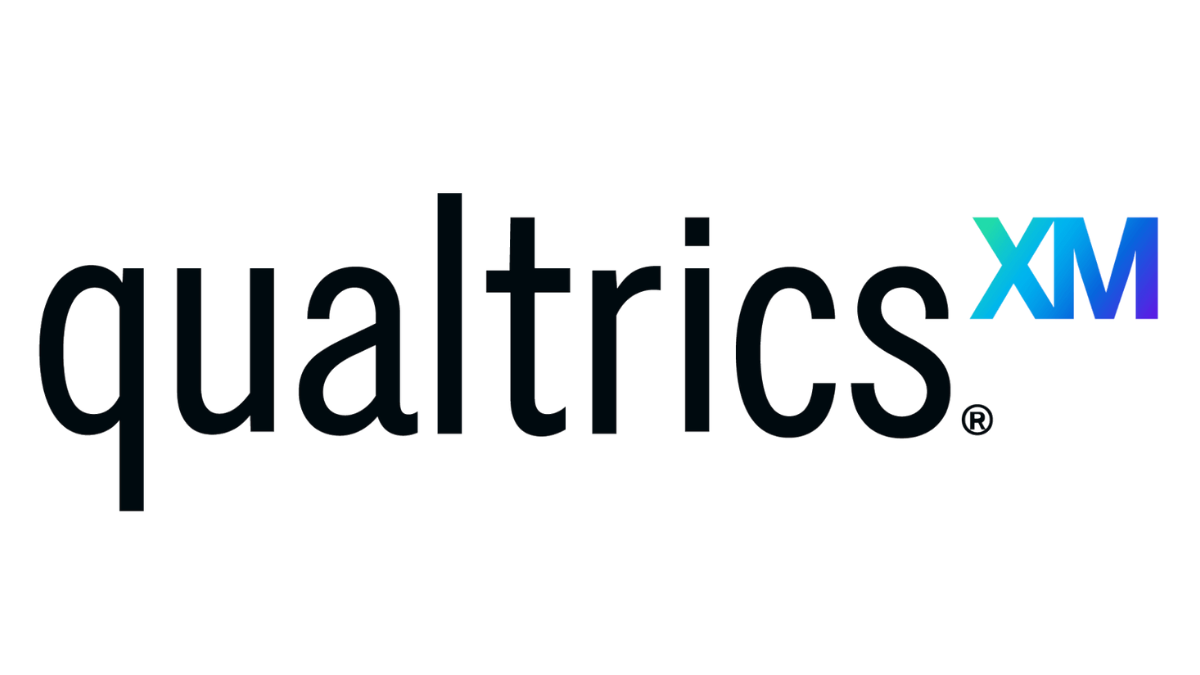
The Hemingway Editor is a user-friendly online tool that helps improve writing by highlighting and correcting common errors. It focuses on enhancing readability by identifying and simplifying complex sentences, passive voice , and adverbs. The tool offers both writing and editing modes, making it easy to create and refine content. Some of its main features are as:
- Simple & Powerful: In the world of writing tools, Hemingway Editor is simple yet powerful. It’s like the minimalist ninja, cutting through complexity and leaving my research polished and potent.
- Active Voice Enthusiast: Hemingway Editor is an active voice cheerleader. It’s like a coach nudging me to ditch passive constructions, making my writing punchier and more engaging.
- Readability Whisperer: Ensuring readability is Hemingway Editor’s secret weapon. It’s like having a readability whisperer, making sure my research doesn’t sound like an ancient manuscript but flows effortlessly.
- Formatting Friend: Hemingway Editor is also a formatting friend. It’s like a design consultant, making sure my text isn’t just clear but visually inviting, making my research a pleasure to read.
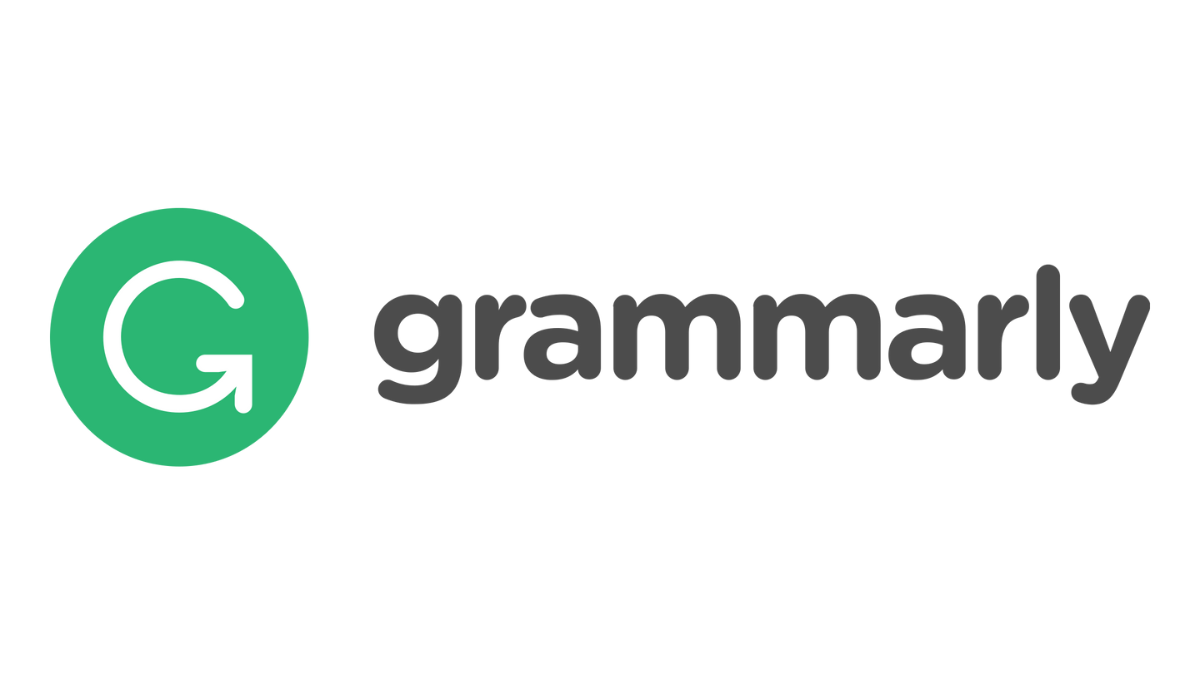
Grammarly is a digital writing tool that acts like a friendly grammar coach. It’s your online proofreader, catching typos, suggesting better words, and ensuring your sentences are clear and error-free. It helps you in making sure that there is no plagiarism in your content. Some its key highlights are as:
- Error Detective: Grammarly is my error detective. It spots typos, punctuation crimes, and grammatical slip-ups like detectives, saving me from embarrassing blunders.
- Plagiarism Protector: If you are worried about your accidental plagiarism then Grammarly is my protector. It’s like a shield, scanning my text to ensure it’s authentically mine and saving me from citation threats.
- Customizable Settings : Grammarly offers customizable settings, allowing researchers to adjust the tool according to their objectives and preferences like type of audience, knowledge level and other.
- Improving Writing Skills : Grammarly helps improve writing skills by providing suggestions and explanations for errors, helping researchers learn from their mistakes.
In conclusion, the top 15 academic research tools offer valuable support for various aspects of the research process. For reference management, tools like Zotero, Mendeley, and EndNote provide efficient organisation and citation capabilities.
In the realm of data visualisation and analysis, Tableau, Power BI, and Google Data Studio offer powerful solutions for interpreting and presenting research findings. Survey design and data collection are facilitated by Qualtrics, REDCap, and SurveyMonkey, which streamline the process of gathering and analysing data.
When it comes to literature search, Google Scholar, PubMed, and Scopus stand out as comprehensive and reliable resources for accessing scholarly literature. Finally, for writing and editing, Grammarly, Hemingway Editor, and LaTex offer indispensable support in enhancing the quality, clarity, and professionalism of academic writing. These tools collectively contribute to the efficiency, accuracy, and impact of academic research endeavours.
Image Reference
Related posts.
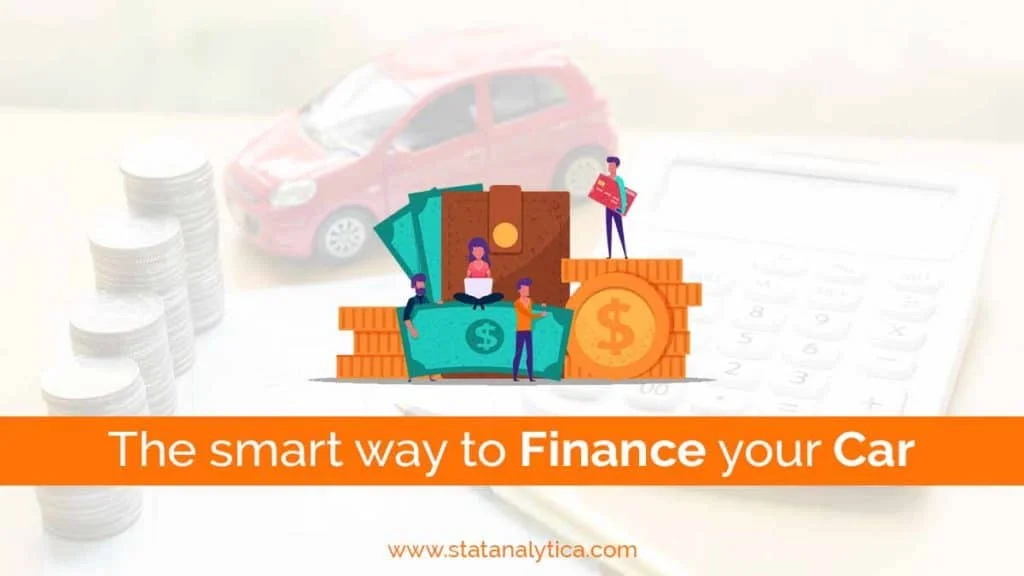
Step by Step Guide on The Best Way to Finance Car
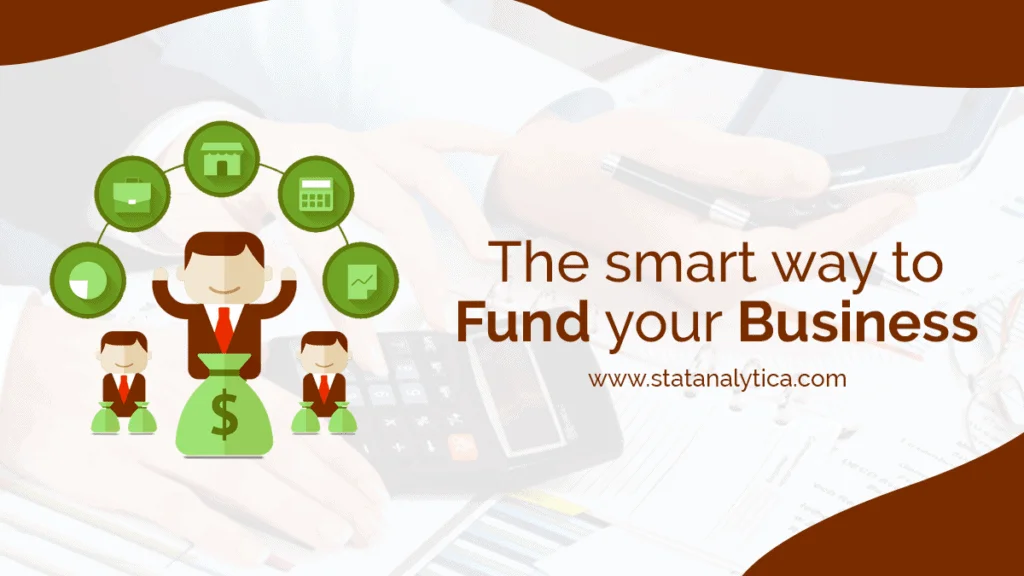
The Best Way on How to Get Fund For Business to Grow it Efficiently
20+ Tools & Resources for Conducting Market Research

Published: September 15, 2023
Finding out if a product will be successful beyond the initial curiosity is just good business. With market research, you determine whether the opportunity exists, how to position the product or service, or what consumers' opinions are after the launch.
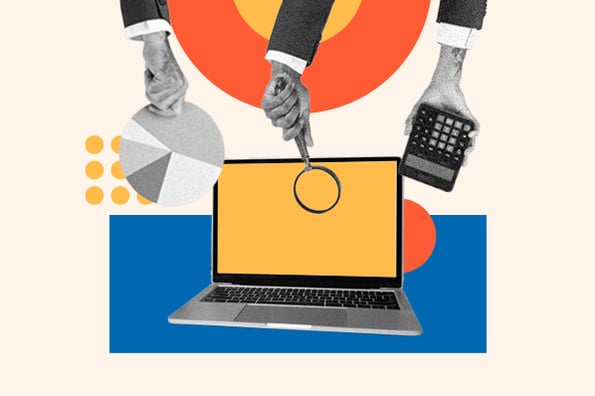
If you're sensitive to the high costs of failure and need to gather facts and opinions to predict whether your new product, feature, or location will be successful, start by investing in market research using these tools and resources.
Here are 21 of the best tools for conducting market research, including a few recommendations directly from HubSpot market researchers. Let's dive in.
Featured Resource: Market Research Kit
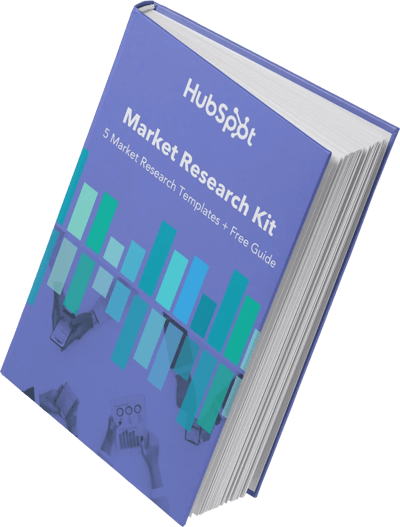
Download the Kit Now
Market Research Tools
- Think With Google Research Tools
- Census Bureau
- Make My Persona
- SurveyMonkey
- Upwave Instant Insights
- Claritas MyBestSegment
- Ubersuggest
- Pew Research Center
- BrandMentions
- Qualtrics Market Research Panels
Helpful Market Research Tools & Resources
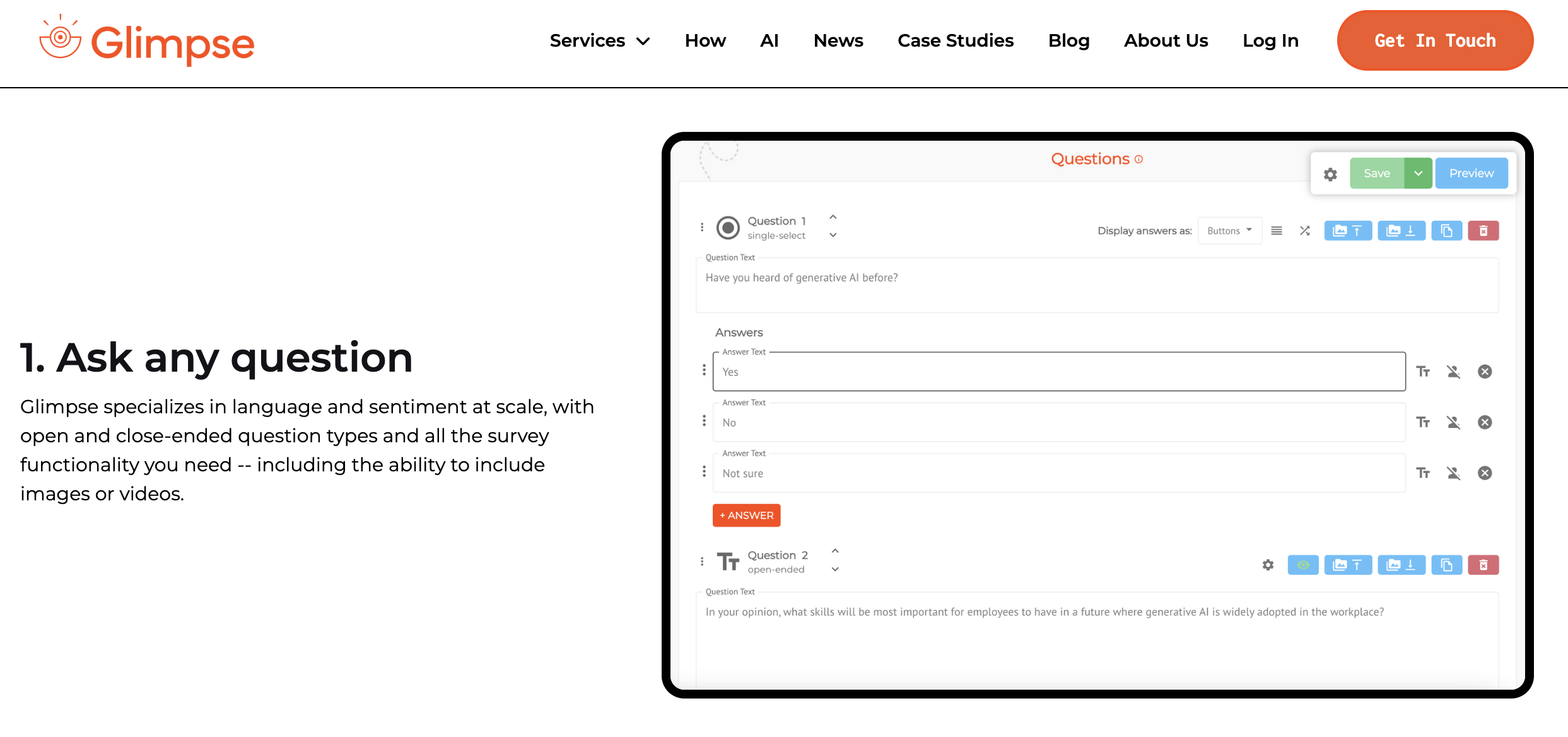
For Max Iskiev , Market Research Analyst at HubSpot, one research tool stands out from the rest, and that's Glimpse .
He told me, "Glimpse is my favorite research tool. It's quick and easy to use, allowing me to design and launch short surveys for real-time insights on trending topics."
As a writer for the HubSpot Marketing Blog, I've also used Glimpse to run short, 100-person surveys for articles (case in point: Are Sales Reps Rushing Back to the Office? ).
Not only is Glimpse valuable for doing quick pulse-checks on the latest trends, but it also leverages the power of AI for even deeper insights.
"Glimpse really shines when it comes to open-ended questions, using natural language processing and AI to analyze emotion and sentiment, saving time and offering invaluable insights," Iskiev shared.
Pricing : $1,000/month (Pro Account)
.png)
Free Market Research Kit
5 Research and Planning Templates + a Free Guide on How to Use Them in Your Market Research
- SWOT Analysis Template
- Survey Template
- Focus Group Template
You're all set!
Click this link to access this resource at any time.
2. Statista
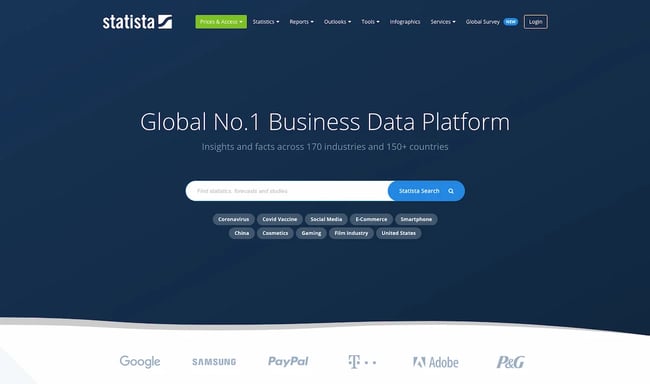
Statista is a data visualization website that takes data from reputable reports across the web and makes them easy and digestible for researchers, marketers, and product creators just like you.
"Statista is like my market research sidekick, giving me all the data I need without the endless search. No more digging through the haystack, with Statista I can spot trends and make informed decisions with ease," Icee Griffin , Market Researcher at HubSpot, told me.
Are you planning on launching a new video game and want to know how many hours people spend playing video games? There’s a chart for that.
One neat aspect of using Statista is that the same chart is updated as the years pass. Say that you want to allude to the value of the beauty market in your proposal. If your investor accesses that same graph a year from now, it will reflect updated numbers, as Statista always finds the most recent research to update their visualizations. (Note that Statista doesn’t carry out original research.)
Pricing : Free; $39/month (billed yearly); $1,950 (one-time 30-day access)
3. Think With Google Research Tools
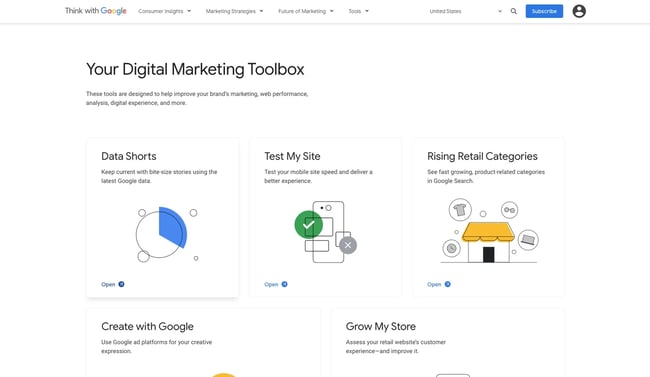
Wish you had information on your product’s likelihood of success? Think With Google's marketing research tools offer interesting insights on whether anyone is looking for your product ( Google Trends ), which markets to launch to ( Market Finder ), and what retail categories rise as the months and seasons pass ( Rising Retail Categories ).
If you’d like to market your product through YouTube, the Find My Audience tool allows you to investigate what your potential viewers are interested in and what you should discuss in your brand’s YouTube channel.
Pricing : Free
4. Census Bureau
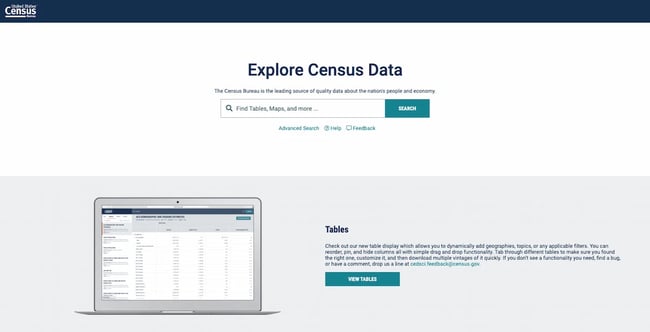
The Census Bureau offers a free resource for searching U.S. census data. You can filter by age, income, year, and location. You can also use some of its shortcuts to access visualizations of the data, allowing you to see potential target markets across the country.
One of the best ways to use this tool is by finding the NAICS code for your business, then accessing the Tables tool, then clicking Filter on the sidebar and searching for your industry. Easily find out where your target industry is most popular — or where the market has been oversaturated. Another helpful tool is the Census Bureau Business and Economy data , where you can also target premade tables depending on your industry.
5. Make My Persona

HubSpot's Make My Persona tool allows you to create a buyer persona for your potential new product. In this tool, you pick a name for the persona, choose their age, identify their career characteristics, and identify their challenges, allowing you to pinpoint both demographic and psychographic information.
This tool is most suited for B2B product launches because you’ll be prompted to document your buyer persona’s career objectives and role-specific challenges. As such, your product would ideally solve a problem for them in the workplace or help their company achieve revenue goals.
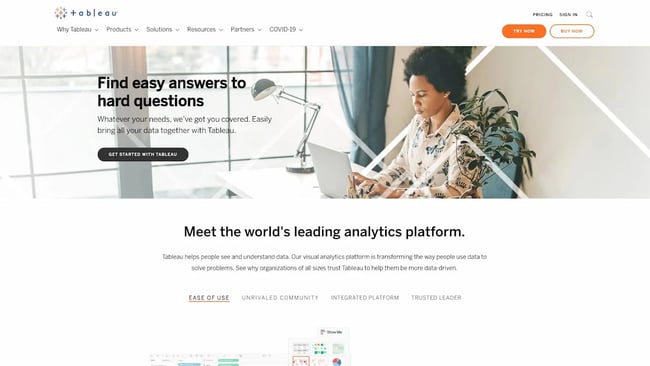
Tableau is a business intelligence suite of products that allows you to “connect to virtually any data source.” But the data isn’t presented in unreadable tables. Rather, Tableau helps you visualize this data in a way that helps you glean insights, appeal to external stakeholders, and communicate the feasibility of your product to potential investors.
You can visualize data on anything from corn production in tropical climate zones to office product sales in North America. With Tableau’s tools, you can take as granular or as general a look you’d like into potential marketplaces and supplier regions. Tableau also integrates well with spreadsheets and databases so that you can export Tableau data to Excel , back up records in Amazon Redshift, and more.
Pricing : $12/user/month (Tableau Viewer); $35/user/month (Tableau Explorer); $70/user/month (Tableau Creator)
7. Paperform
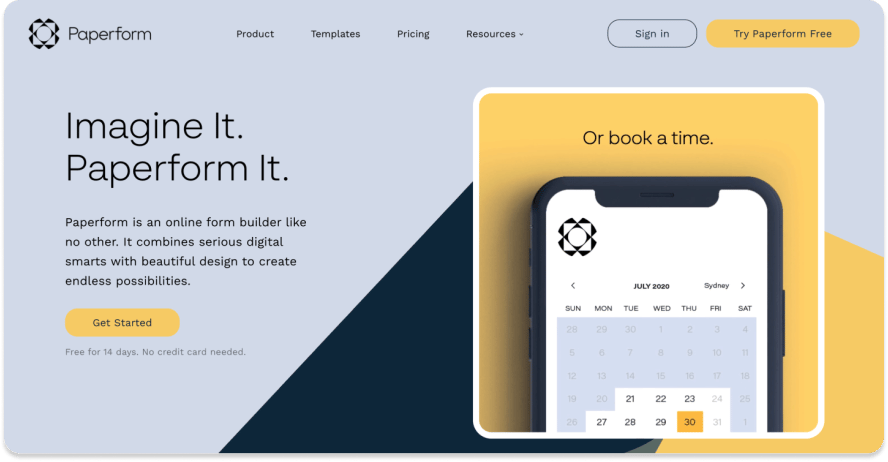
A market research survey is an effective way to understand your target audience and their needs better by asking them directly. Since this step is integral to understanding your dream customer’s problems, you want to ensure the process is as interactive as possible and incites an objective and accurate response.
With its free-text interface, Paperform is as simple as writing a word document. Make your survey stand out by customizing colors, fonts, layouts and themes, and create your unique look and feel. f you’re unsure where to start, you can use one of their expertly made questionnaires or market research survey templates to get you started.
With Paperform, you can add conditional logic to show or hide questions or whole sections of content. Create fully personalized paths for different personas to create more interactive forms that lower drop-off rates and boost customer interaction. Or use any of the 27+ question fields, like the ranking, matrix, or scale fields, to create visually engaging ways to collect information.
Pricing: Free (14-day trial); Essentials ($20/month); Pro ($40/month); and Agency ($135/month)
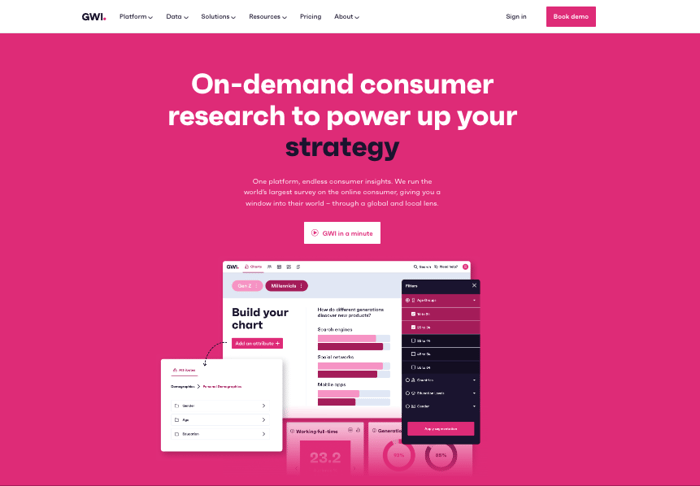
GWI is an on-demand consumer research platform that makes audience research a breeze. Powered by the world’s largest study on the online consumer base, GWI provides insights into the lives of over 2.8 billion consumers, across 50+ markets. Everything you need to know about who they are, what’s on their minds, and what they're up to. With fresh insights at your fingertips in one user-friendly platform, it’s quick and easy to become an expert on your audience and get the answers you need to succeed. Compare markets and create customized, shareable charts and dashboards in seconds.
Pricing : Available upon request
9. SurveyMonkey
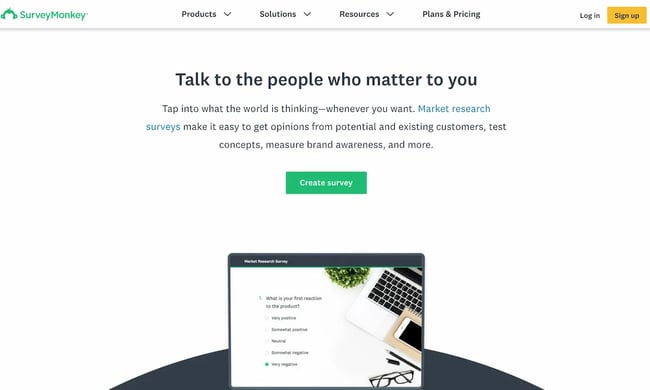
SurveyMonkey is a powerful tool for creating in-depth market research surveys that will help you understand your market and consumer preferences.
With this tool, you can create targeted, uber-specific surveys that help you collect answers that pertain specifically to your product. While using a data source can give you a general overview of your target audience and market, SurveyMonkey can help you get more granular insights from real consumers.
SurveyMonkey offers dedicated market research solutions and services , including a global survey panel, a survey translation service for international research, and a reporting dashboard option that allows you to easily parse through the results.
Pricing : Free, $32/month (Advantage Annual), $99/month (Premier Annual), $99/month (Standard Monthly) ; $25/user/month (Team Advantage, minimum 3 users), $75/user/month (Team Premier, minimum 3 users), Enterprise (Contact for pricing)
10. Typeform

Like SurveyMonkey, Typeform allows you to run research surveys to get direct answers from your target consumers. It’s an easy-to-use, mobile-optimized form-builder that's great for market research.
Typeform’s distinguishing factor is that it shows viewers one form field at a time. In its templates, it encourages a more conversational, casual approach (like in its market research survey template ). This makes it a better fit for product launches that target a younger demographic. If you’re targeting C-suite executives at established firms, consider a more formal option such as SurveyMonkey or keeping your tone more formal in your questions.
You can create a wide range of question types, including multiple choice questions, short-form questions, and rating scale questions. Other features include the ability to recall answers from previous questions and create logic jumps.
In a survey, you’d want to collect both demographic and psychographic information on your customer, seeking to understand their purchasing behaviors and the problems they encounter. The goal is to find out if your product is the solution to one of those problems — and whether, before launching, you should add more features or rethink your product positioning strategy .
Pricing : Free; $35/month (Essentials); $50/month (Professional); $70/month (Premium)
11. Upwave Instant Insights
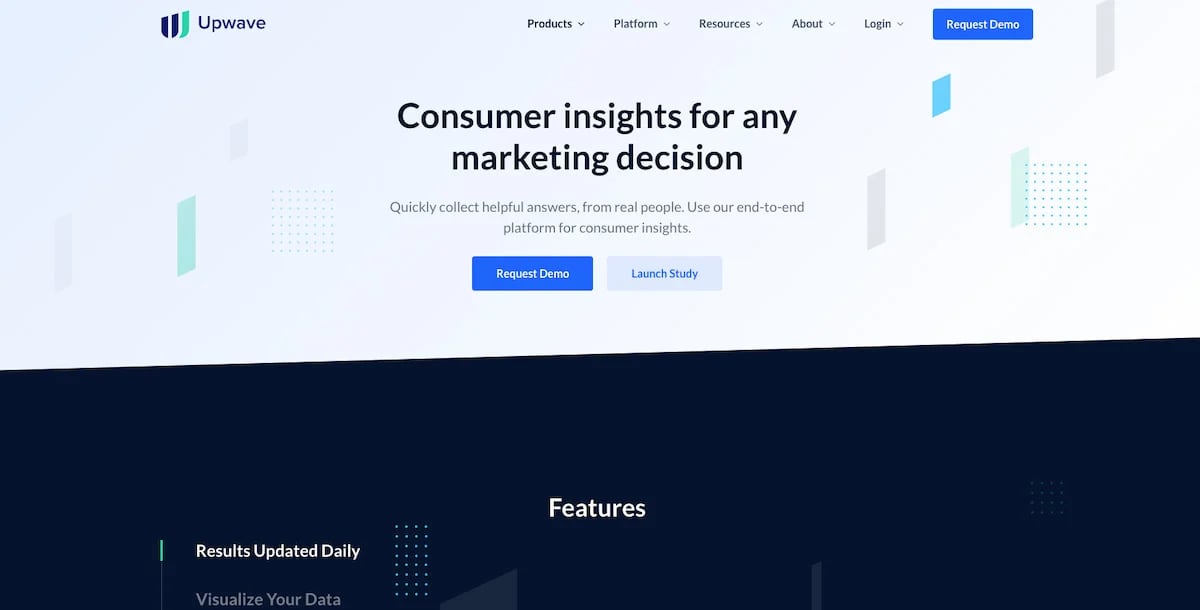
Upwave Instant Insights is a consumer research tool that’s part of the Upwave brand marketing platform. While it isn’t advertised as a survey creation tool, it allows you to launch market research surveys specifically to get consumer insights.
Instant Insights allows you to target audiences on Upwave’s partner ecosystem and visualize the data for easy scanning by key stakeholders and investors. One pro of using this platform is that Upwave distributes your survey to real people — not just people taking surveys for the money, which could skew the results.
To create a survey, you sign up on the Upwave platform , click your name in the upper right-hand corner, and click “My Surveys,” where you can create as many surveys as you want. For the Basic option, you have a 6-question limit, while the Advanced option allows you to include unlimited questions.
Pricing : $2/study participant (Basic); $3/study participant (Extended); $4/study participant (Advanced)
12. Claritas MyBestSegment
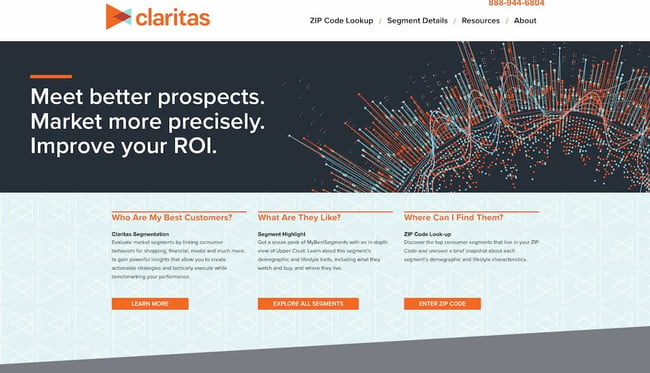
Claritas MyBestSegment provides product researchers with tools to understand an area's demographic information and the area’s inhabitants’ lifestyle habits. By finding out what a segment of the population does — without having to go out and survey them — you can find out which areas would be most receptive to a campaign or launch, which competitors are located nearby, and which lifestyle trends have shifted or are on the rise.
A snapshot of an audience segment gives you basic information on their household income, lifestyle traits, employment levels, and education levels. If you want more specific data relating to these topics, you’ll have to contact Claritas’ sales team to become a customer.
Pricing : Free; Pricing available on request
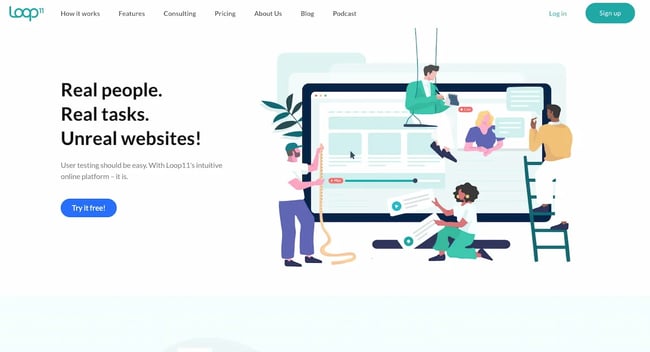
Loop11 is a user experience testing platform that allows you to test the usability of your website, study user intent, test the information architecture of your site, and examine how the user experience changes based on the device they’re using.
This tool is useful for market research because you can find out whether your target consumers find your site easy to navigate. You can also identify snags that prevent conversions.
Loop11 tests your site by making users perform tasks. They then complete a short question about how easy or difficult the task was to complete. Your product may be phenomenal, but unless consumers can buy it through your site, you won’t launch it successfully.
You can use Loop11’s participants or bring in your own.
Pricing : $63/month (Rapid Insights), $239/month (Pro), $399/month (Enterprise)
14. Userlytics
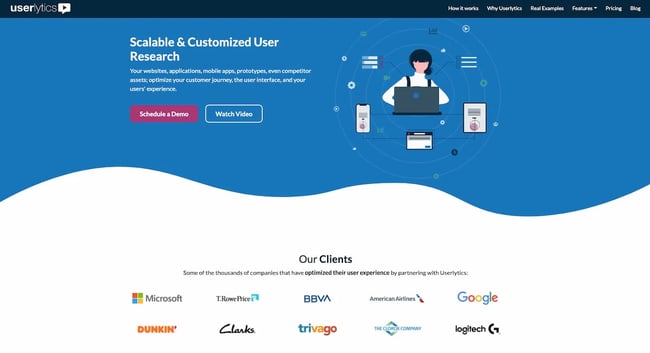
Like Loop11, Userlytics allows you to test the usability of your website, mobile app, and site prototype. You can target different devices, define a buyer persona, and disqualify participants based on screening questions.
Testing is based on tasks that your test-takers carry out. They then answer a simple question about the difficulty of the task. You can structure the question in various ways; you can leave it open-ended, provide multiple choices, or ask for a rating. Other formats you can use include System Usability Scale (SUS) questions, Net Promoter Score (NPS) questions, and Single Ease Questions (SEQ).
Userlytics performs both a webcam and a screen recording. You can compare the user’s answers with their reactions on video to understand how they feel when they’re interacting with your assets.
Pricing : $49/participant (Quick & Easy); $69/participant (Annual Enterprise); Custom pricing available on request
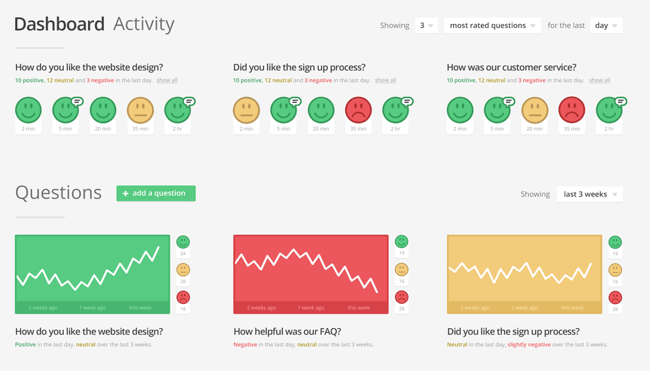
Sometimes you need a no-frills test to take the pulse of consumers. Temper allows you to create a question, grab a snippet of code, and pop it onto your website. The smiley face, "meh" face, and frown face make it easy for viewers to make a snap judgment.
One great way to use this tool is by adding the widget on a blog post announcing the launch of your new product. That way, you can find out general sentiment on the product before launching it. You can also add it to a product page, an email, or a landing page.
When you include the widget, you can change the question to something that’s tailored to your offerings.
Pricing : $12/month (Hobby), $49/month (Pro), $89/month (Business), $199/month (White Label )
16. NielsenIQ
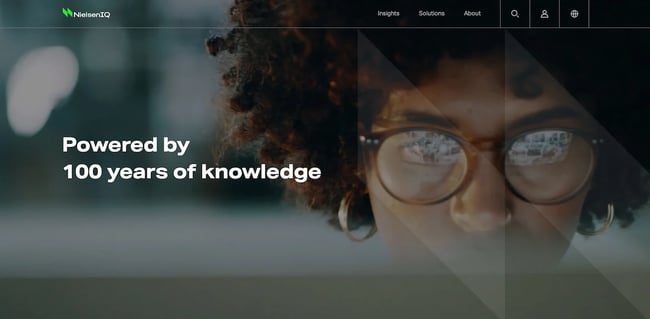
NielsenIQ is a retail and consumer intelligence consultant that works with you to collect consumer insights, identify the best distribution channels for your product, and create a range of products that addresses the needs of your target buyers.
This service helps you look at your product launch from all angles and delivers forecasting data that predicts how your sales will perform upon launch. NielsenIQ can also run consumer insights surveys on their list of panelists and partners.
Because it operates like a consultant and not as a self-service software, NielsenIQ is a better option for established firms with a bigger product launch budget.
Pricing : Pricing available on request
17. Ubersuggest
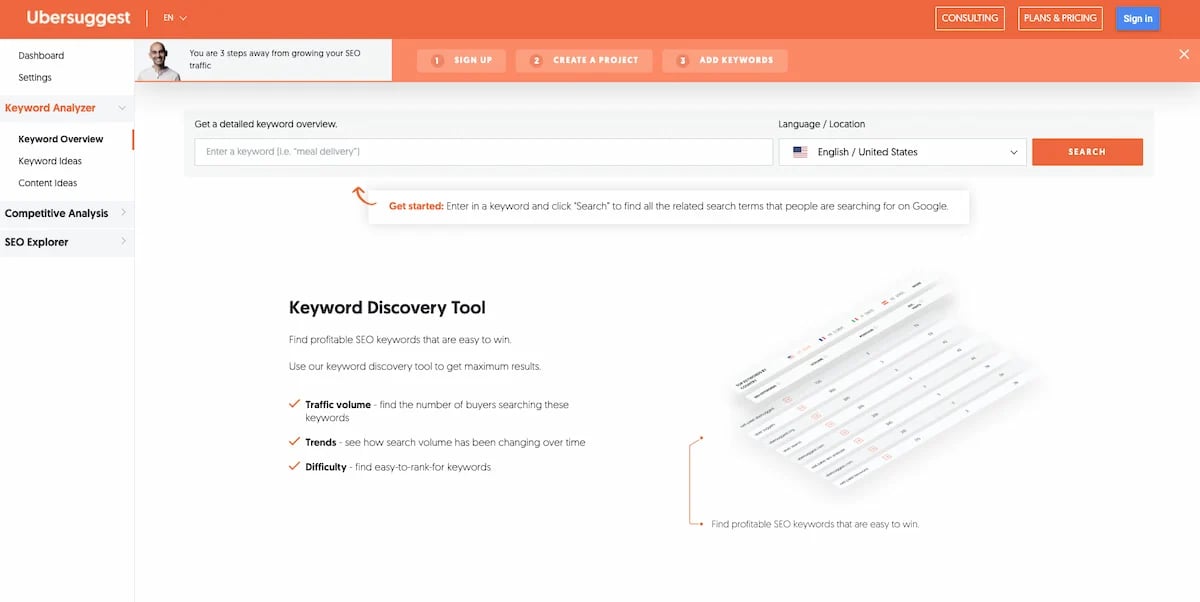
Ubersuggest is a simple tool for doing keyword and content research. You can input a phrase, and it'll create a list of keyword suggestions. You can also see top performing articles and pages so that you get an initial understanding of the type of content that ranks for the keywords.
This tool is useful for market research because you can see who your top competitors are, how often your product is searched for, and whether there’s enough space in the market for the type of product you’re launching. You can also find out the questions your target audience asks in relation to the product. Each of these questions can be turned into a blog post that can inform your audience, increasing your brand authority and driving conversions.
Alternatives to Ubersuggest include Moz , Ahrefs , and SEMRush .
Pricing : Free; $29/month (Individual); $49/month (Business); $99/month (Enterprise / Agency)
18. Pew Research Center
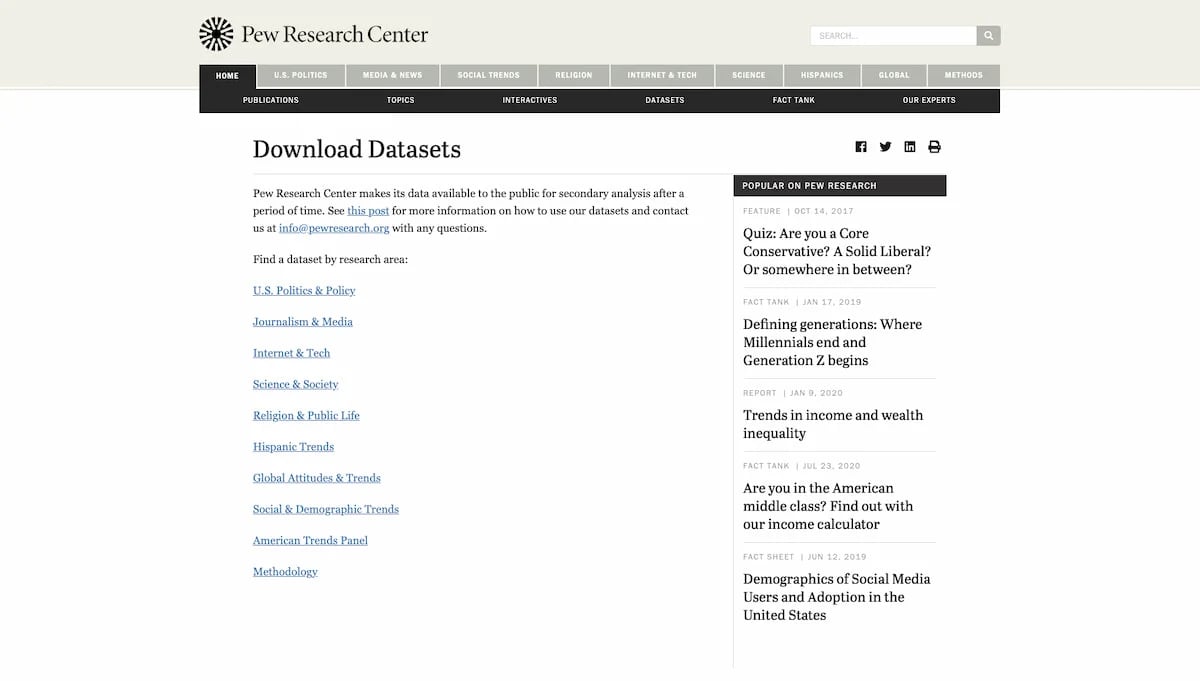
From economic conditions, to political attitudes, to social media usage, the Pew Research Center website has a ton of free research that you can use to better understand your target market. Best of all, the site has interactive articles that allow you to filter and sift through the data for more granular, targeted insights.
Topics include U.S. politics, digital media, social trends, religion, science, and technology.
19. BrandMentions

BrandMentions is a social media monitoring platform that can help you understand what your prospective customers are buzzing about online. Search for a keyword, and BrandMentions will show you recent social posts that contain that keyword, along with the context of its usage. After subscribing to the platform, you can also get sentiment analysis on the keyword.
You’ll also get other metrics such as Reach (how many people view the keyword per day), Performance (how many people engage with the keyword per day), and Mentions by Weekday (when people are mentioning the keyword).
You can use this tool for market research by finding out when people are looking for your product on social media sites. When you start announcing the new product, you can use insights from this tool to post about the launch at exactly the right time. It also allows you to find out how people are generally feeling about the type of product you’re launching. That way, you can better refine the tone of your campaigns.
Pricing : $99/month (Growing Business); $ 299/month (Company); $ 499/month (Enterprise/Agency)
20. Qualtrics Market Research Panels
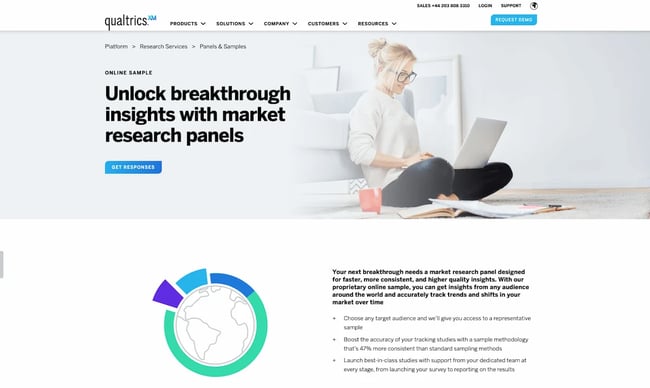
Qualtrics takes the pain of finding respondents for your market research surveys through an online sample service. After identifying your target audience, you can go to Qualtrics for access to a representative sample. You can either use your chosen survey software or use Qualtrics’ built-in platform for insights and feedback.
21. Qualaroo
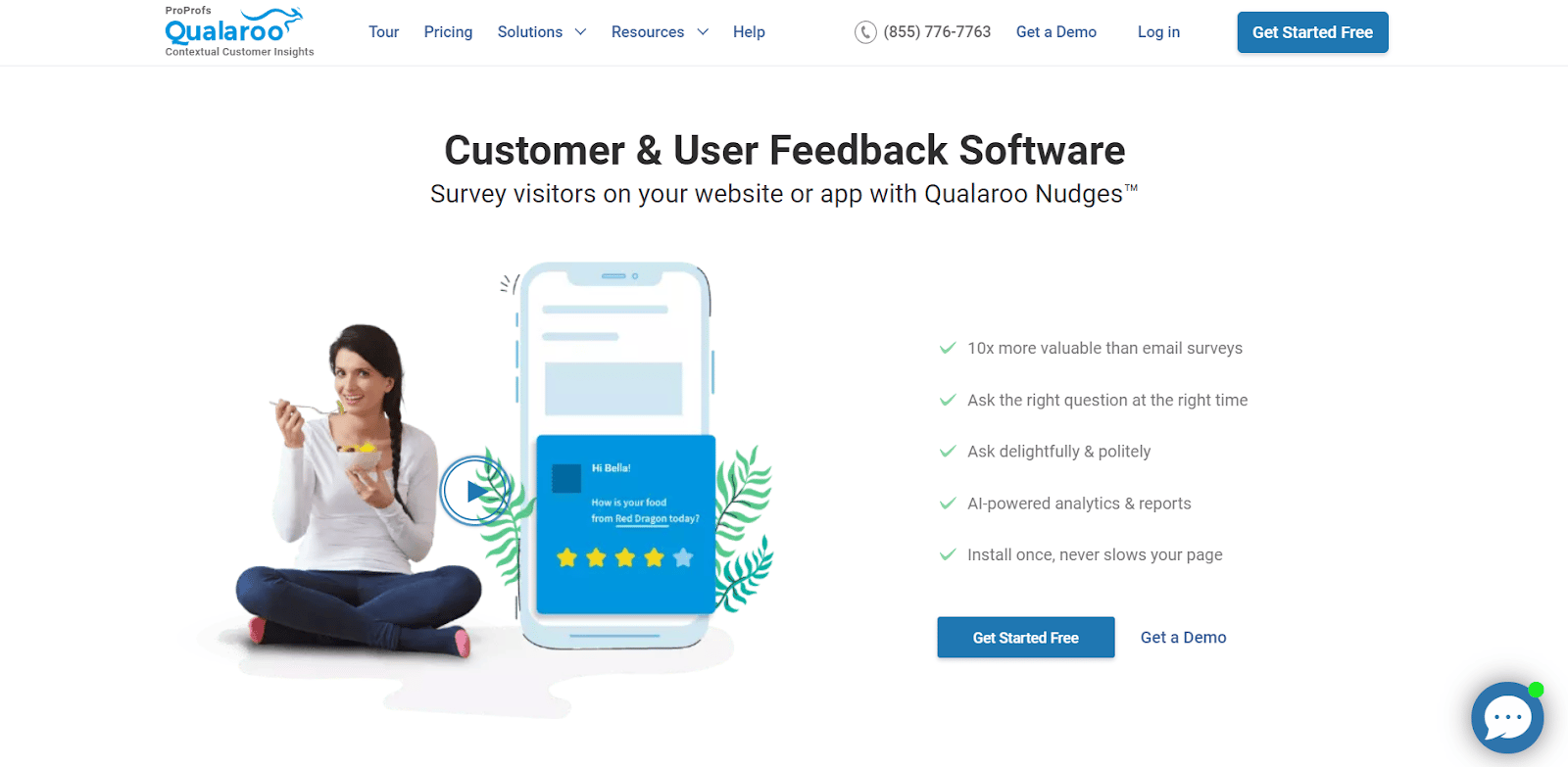
Qualaroo is an advanced user and market research tool that helps you understand your target market with targeted surveys. You can run surveys on over six channels at once (such as website, app, product, social media, and mail,) to get a 360-degree view of your existing and potential customers.
It comes packed with features like question branching, 12+ answer types, automatic survey language translation, in-depth audience targeting, pre-built survey templates, and an extensive repository of professionally designed questions.
You can create various market research surveys in minutes to collect data on the demographic, psychographic, and behavioral traits of your target audience. It can help you map customers' expectations and preferences, create customer personas, and perform audience segmentation.
Qualaroo also promotes quick feedback analysis. Its in-built AI-based sentiment analysis and text analytics engine automatically categorizes the responses based on user moods. It also highlights the key phrases and words in real-time, saving hours of manual work.
Pricing : $80/month billed annually (Essentials); $160/month billed annually (Premium)
Conduct Market Research for a Successful Product Launch
Conducting market research is essential to successfully launch a product to market. With the tools we’ve shared, you can find out who’s looking for your product, why they need it, and how you can better market it upon launch, ensuring that it’s a success.
Editor's note: This post was originally published in April 2016 and has been updated for comprehensiveness.
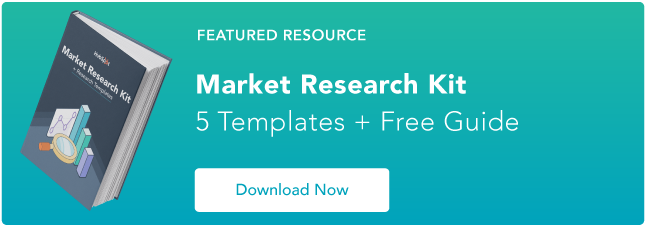
Don't forget to share this post!
Related articles.
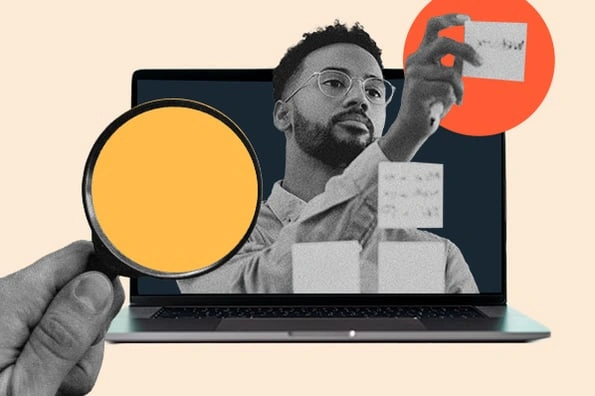
Market Research: A How-To Guide and Template
![research analytical tools SWOT Analysis: How To Do One [With Template & Examples]](https://blog.hubspot.com/hubfs/marketingplan_20.webp)
SWOT Analysis: How To Do One [With Template & Examples]
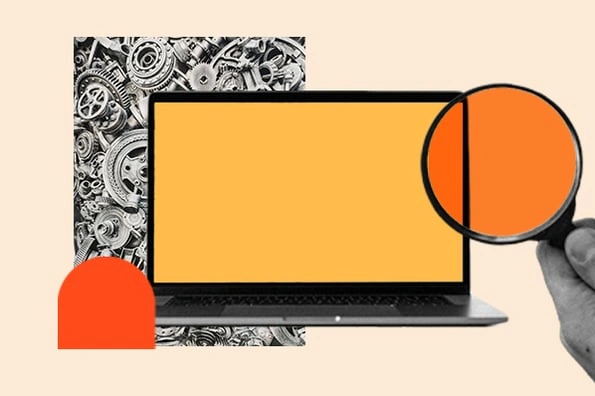
What's a Competitive Analysis & How Do You Conduct One?
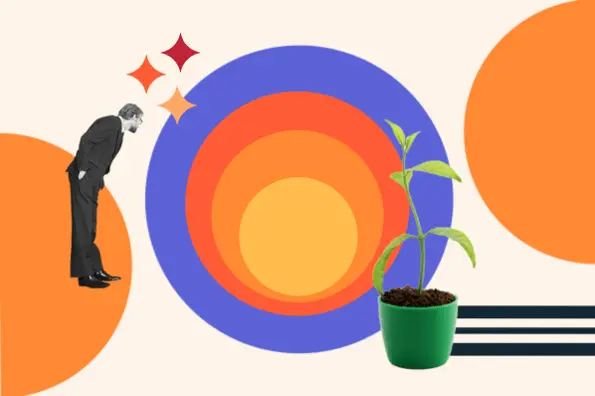
TAM SAM SOM: What Do They Mean & How Do You Calculate Them?
![research analytical tools How to Run a Competitor Analysis [Free Guide]](https://blog.hubspot.com/hubfs/Google%20Drive%20Integration/how%20to%20do%20a%20competitor%20analysis_122022.jpeg)
How to Run a Competitor Analysis [Free Guide]
![research analytical tools 5 Challenges Marketers Face in Understanding Audiences [New Data + Market Researcher Tips]](https://blog.hubspot.com/hubfs/challenges%20marketers%20face%20in%20understanding%20the%20customer%20.png)
5 Challenges Marketers Face in Understanding Audiences [New Data + Market Researcher Tips]
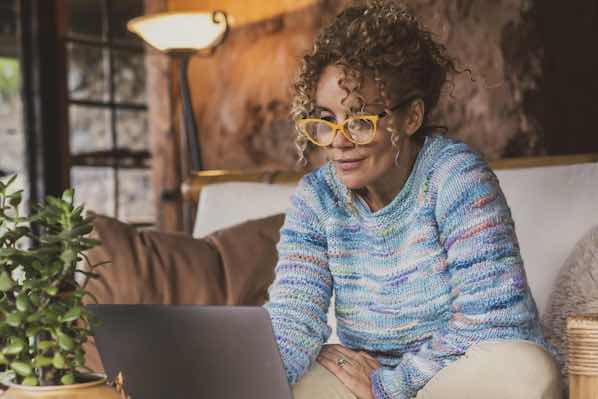
Causal Research: The Complete Guide
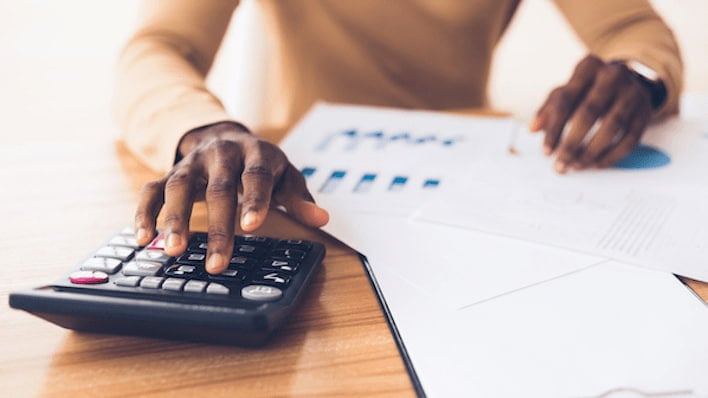
Total Addressable Market (TAM): What It Is & How You Can Calculate It
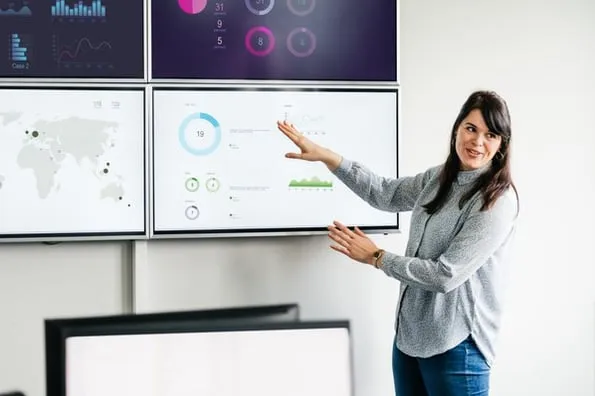
What Is Market Share & How Do You Calculate It?
![research analytical tools 3 Ways Data Privacy Changes Benefit Marketers [New Data]](https://blog.hubspot.com/hubfs/how-data-privacy-benefits-marketers_1.webp)
3 Ways Data Privacy Changes Benefit Marketers [New Data]
Free Guide & Templates to Help Your Market Research
Marketing software that helps you drive revenue, save time and resources, and measure and optimize your investments — all on one easy-to-use platform

- SUGGESTED TOPICS
- The Magazine
- Newsletters
- Managing Yourself
- Managing Teams
- Work-life Balance
- The Big Idea
- Data & Visuals
- Reading Lists
- Case Selections
- HBR Learning
- Topic Feeds
- Account Settings
- Email Preferences
Research: How Different Fields Are Using GenAI to Redefine Roles
- Maryam Alavi
Examples from customer support, management consulting, professional writing, legal analysis, and software and technology.
The interactive, conversational, analytical, and generative features of GenAI offer support for creativity, problem-solving, and processing and digestion of large bodies of information. Therefore, these features can act as cognitive resources for knowledge workers. Moreover, the capabilities of GenAI can mitigate various hindrances to effective performance that knowledge workers may encounter in their jobs, including time pressure, gaps in knowledge and skills, and negative feelings (such as boredom stemming from repetitive tasks or frustration arising from interactions with dissatisfied customers). Empirical research and field observations have already begun to reveal the value of GenAI capabilities and their potential for job crafting.
There is an expectation that implementing new and emerging Generative AI (GenAI) tools enhances the effectiveness and competitiveness of organizations. This belief is evidenced by current and planned investments in GenAI tools, especially by firms in knowledge-intensive industries such as finance, healthcare, and entertainment, among others. According to forecasts, enterprise spending on GenAI will increase by two-fold in 2024 and grow to $151.1 billion by 2027 .
- Maryam Alavi is the Elizabeth D. & Thomas M. Holder Chair & Professor of IT Management, Scheller College of Business, Georgia Institute of Technology .
Partner Center
We've detected unusual activity from your computer network
To continue, please click the box below to let us know you're not a robot.
Why did this happen?
Please make sure your browser supports JavaScript and cookies and that you are not blocking them from loading. For more information you can review our Terms of Service and Cookie Policy .
For inquiries related to this message please contact our support team and provide the reference ID below.
Data & Tools
Data and tools.
The All of Us Research Hub includes powerful data and analysis tools to help researchers with their work. As the program grows, more data types and tools will be available for researchers.
Data Browser
The Data Browser provides interactive views of the publicly available All of Us Research Program aggregate-level participant data, including EHR domains, survey responses, and physical measurements.
Data Snapshots
The Data Snapshots include data visualizations showing the participant cohort size and attributes, including how many participants are from groups underrepresented in biomedical research.
DATA ACCESS TIERS
The Research Hub’s data use policies and tiered-data access model support our commitment to data security and participant privacy.
DATA SOURCES
Participant data available for research include information from EHRs, physical measurements, survey responses, wearables data, and genomic data from biosamples. We will add new participant data sources as the program grows.
DATA METHODS
Participant data have been harmonized and curated to protect participant privacy. We ensure high-quality data for research by standardization methods. Data counts may differ between Research Hub tools and data views. This is because we use different data curation methods for each tool to protect participant privacy.
RESEARCHER WORKBENCH
The Researcher Workbench provides tools to enable powerful analysis of data from All of Us participants. Registered researchers can conduct multiple research projects simultaneously and collaborate within and across teams.
SURVEY EXPLORER
This tool provides source information for the surveys that All of Us asks participants to complete, including each individual question. Aggregate responses to the surveys are available in the Data Browser.
Subscribe to Email Updates
Sign up to receive email news and updates for the All of Us Research Hub.
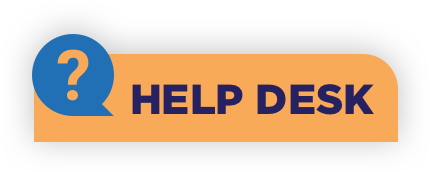
- Open access
- Published: 28 March 2024
Using the consolidated Framework for Implementation Research to integrate innovation recipients’ perspectives into the implementation of a digital version of the spinal cord injury health maintenance tool: a qualitative analysis
- John A Bourke 1 , 2 , 3 ,
- K. Anne Sinnott Jerram 1 , 2 ,
- Mohit Arora 1 , 2 ,
- Ashley Craig 1 , 2 &
- James W Middleton 1 , 2 , 4 , 5
BMC Health Services Research volume 24 , Article number: 390 ( 2024 ) Cite this article
111 Accesses
Metrics details
Despite advances in managing secondary health complications after spinal cord injury (SCI), challenges remain in developing targeted community health strategies. In response, the SCI Health Maintenance Tool (SCI-HMT) was developed between 2018 and 2023 in NSW, Australia to support people with SCI and their general practitioners (GPs) to promote better community self-management. Successful implementation of innovations such as the SCI-HMT are determined by a range of contextual factors, including the perspectives of the innovation recipients for whom the innovation is intended to benefit, who are rarely included in the implementation process. During the digitizing of the booklet version of the SCI-HMT into a website and App, we used the Consolidated Framework for Implementation Research (CFIR) as a tool to guide collection and analysis of qualitative data from a range of innovation recipients to promote equity and to inform actionable findings designed to improve the implementation of the SCI-HMT.
Data from twenty-three innovation recipients in the development phase of the SCI-HMT were coded to the five CFIR domains to inform a semi-structured interview guide. This interview guide was used to prospectively explore the barriers and facilitators to planned implementation of the digital SCI-HMT with six health professionals and four people with SCI. A team including researchers and innovation recipients then interpreted these data to produce a reflective statement matched to each domain. Each reflective statement prefaced an actionable finding, defined as alterations that can be made to a program to improve its adoption into practice.
Five reflective statements synthesizing all participant data and linked to an actionable finding to improve the implementation plan were created. Using the CFIR to guide our research emphasized how partnership is the key theme connecting all implementation facilitators, for example ensuring that the tone, scope, content and presentation of the SCI-HMT balanced the needs of innovation recipients alongside the provision of evidence-based clinical information.
Conclusions
Understanding recipient perspectives is an essential contextual factor to consider when developing implementation strategies for healthcare innovations. The revised CFIR provided an effective, systematic method to understand, integrate and value recipient perspectives in the development of an implementation strategy for the SCI-HMT.
Trial registration
Peer Review reports
Injury to the spinal cord can occur through traumatic causes (e.g., falls or motor vehicle accidents) or from non-traumatic disease or disorder (e.g., tumours or infections) [ 1 ]. The onset of a spinal cord injury (SCI) is often sudden, yet the consequences are lifelong. The impact of a SCI is devastating, with effects on sensory and motor function, bladder and bowel function, sexual function, level of independence, community participation and quality of life [ 2 ]. In order to maintain good health, wellbeing and productivity in society, people with SCI must develop self-management skills and behaviours to manage their newly acquired chronic health condition [ 3 ]. Given the increasing emphasis on primary health care and community management of chronic health conditions, like SCI, there is a growing responsibility on all parties to promote good health practices and minimize the risks of common health complications in their communities.
To address this need, the Spinal Cord Injury Health Maintenance Tool (SCI-HMT) was co-designed between 2018 and 2023 with people living with SCI and their General Practitioners (GPs) in NSW, Australia [ 4 ] The aim of the SCI-HMT is to support self-management of the most common and arguably avoidable potentially life-threatening complications associated with SCI, such as mental health crises, autonomic dysreflexia, kidney infections and pressure injuries. The SCI-HMT provides comprehensible information with resources about the six highest priority health areas related to SCI (as indicated by people with SCI and GPs) and was developed over two phases. Phase 1 focused on developing a booklet version and Phase 2 focused on digitizing this content into a website and smartphone app [ 4 , 5 ].
Enabling the successful implementation of evidence-based innovations such as the SCI-HMT is inevitably influenced by contextual factors: those dynamic and diverse array of forces within real-world settings working for or against implementation efforts [ 6 ]. Contextual factors often include background environmental elements in which an intervention is situated, for example (but not limited to) demographics, clinical environments, organisational culture, legislation, and cultural norms [ 7 ]. Understanding the wider context is necessary to identify and potentially mitigate various challenges to the successful implementation of those innovations. Such work is the focus of determinant frameworks, which focus on categorising or classing groups of contextual determinants that are thought to predict or demonstrate an effect on implementation effectiveness to better understand factors that might influence implementation outcomes [ 8 ].
One of the most highly cited determinant frameworks is the Consolidated Framework for Implementation Research (CFIR) [ 9 ], which is often posited as an ideal framework for pre-implementation preparation. Originally published in 2009, the CFIR has recently been subject to an update by its original authors, which included a literature review, survey of users, and the creation of an outcome addendum [ 10 , 11 ]. A key contribution from this revision was the need for a greater focus on the place of innovation recipients, defined as the constituency for whom the innovation is being designed to benefit; for example, patients receiving treatment, students receiving a learning activity. Traditionally, innovation recipients are rarely positioned as key decision-makers or innovation implementers [ 8 ], and as a consequence, have not often been included in the application of research using frameworks, such as the CFIR [ 11 ].
Such power imbalances within the intersection of healthcare and research, particularly between those receiving and delivering such services and those designing such services, have been widely reported [ 12 , 13 ]. There are concerted efforts within health service development, health research and health research funding, to rectify this power imbalance [ 14 , 15 ]. Importantly, such efforts to promote increased equitable population impact are now being explicitly discussed within the implementation science literature. For example, Damschroder et al. [ 11 ] has recently argued for researchers to use the CFIR to collect data from innovation recipients, and that, ultimately, “equitable population impact is only possible when recipients are integrally involved in implementation and all key constituencies share power and make decisions together” (p. 7). Indeed, increased equity between key constituencies and partnering with innovation recipients promotes the likelihood of sustainable adoption of an innovation [ 4 , 12 , 14 ].
There is a paucity of work using the updated CFIR to include and understand innovation recipients’ perspectives. To address this gap, this paper reports on a process of using the CFIR to guide the collection of qualitative data from a range of innovation recipients within a wider co-design mixed methods study examining the development and implementation of SCI-HMT. The innovation recipients in our research are people living with SCI and GPs. Guided by the CFIR domains (shown in the supplementary material), we used reflexive thematic analysis [ 16 ]to summarize data into reflective summaries, which served to inform actionable findings designed to improve implementation of the SCI-HMT.
The procedure for this research is multi-stepped and is summarized in Fig. 1 . First, we mapped retrospective qualitative data collected during the development of the SCI-HMT [ 4 ] against the five domains of the CFIR in order to create a semi-structured interview guide (Step 1). Then, we used this interview guide to collect prospective data from health professionals and people with SCI during the development of the digital version of the SCI-HMT (Step 2) to identify implementation barriers and facilitators. This enabled us to interpret a reflective summary statement for each CFIR domain. Lastly, we developed an actionable finding for each domain summary. The first (RESP/18/212) and second phase (2019/ETH13961) of the project received ethical approval from The Northern Sydney Local Health District Human Research Ethics Committee. The reporting of this study was conducted in line with the consolidated Criteria for Reporting Qualitative Research (COREQ) guidelines [ 17 ]. All methods were performed in accordance with the relevant guidelines and regulations.
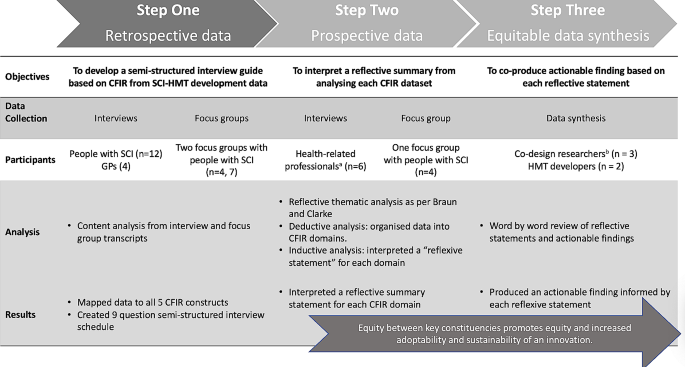
Procedure of synthesising datasets to inform reflective statements and actionable findings. a Two health professionals had a SCI (one being JAB); b Two co-design researchers had a SCI (one being JAB)
Step one: retrospective data collection and analysis
We began by retrospectively analyzing the data set (interview and focus group transcripts) from the previously reported qualitative study from the development phase of the SCI-HMT [ 4 ]. This analysis was undertaken by two team members (KASJ and MA). KASJ has a background in co-design research. Transcript data were uploaded into NVivo software (Version 12: QSR International Pty Ltd) and a directed content analysis approach [ 18 ] was applied to analyze categorized data a priori according to the original 2009 CFIR domains (intervention characteristics, outer setting, inner setting, characteristics of individuals, and process of implementation) described by Damschroder et al. [ 9 ]. This categorized data were summarized and informed the specific questions of a semi-structured interview guide. The final output of step one was an interview guide with context-specific questions arranged according to the CFIR domains (see supplementary file 1). The interview was tested with two people with SCI and one health professional.
Step two: prospective data collection and analysis
In the second step, semi-structured interviews were conducted by KASJ (with MA as observer) with consenting healthcare professionals who had previously contributed to the development of the SCI-HMT. Healthcare professionals included GPs, Nurse Consultants, Specialist Physiotherapists, along with Health Researchers (one being JAB). In addition, a focus group was conducted with consenting individuals with SCI who had contributed to the SCI-HMT design and development phase. The interview schedule designed in step one above guided data collection in all interviews and the focus group.
The focus group and interviews were conducted online, audio recorded, transcribed verbatim and uploaded to NVivo software (Version 12: QSR International Pty Ltd). All data were subject to reflexive, inductive and deductive thematic analysis [ 16 , 19 ] to better understand participants’ perspectives regarding the potential implementation of the SCI-HMT. First, one team member (KASJ) read transcripts and began a deductive analysis whereby data were organized into CFIR domains-specific dataset. Second, KASJ and JAB analyzed this domain-specific dataset to inductively interpret a reflective statement which served to summarise all participant responses to each domain. The final output of step two was a reflective summary statement for each CFIR domain.
Step three: data synthesis
In the third step we aimed to co-create an actionable finding (defined as tangible alteration that can be made to a program, in this case the SCI-HMT [ 20 ]) based on each domain-specific reflective statement. To achieve this, three codesign researchers (KAS and JAB with one person with SCI from Step 2 (deidentified)) focused on operationalising each reflective statement into a recommended modification for the digital version of the SCI-HMT. This was an iterative process guided by the specific CFIR domain and construct definitions, which we deemed salient and relevant to each reflective statement (see Table 2 for example). Data synthesis involved line by line analysis, group discussion, and repeated refinement of actionable findings. A draft synthesis was shared with SCI-HMT developers (JWM and MA) and refinement continued until consensus was agreed on. The final outputs of step three were an actionable finding related to each reflective statement for each CFIR domain.
The characteristics of both the retrospective and prospective study participants are shown in Table 1 . The retrospective data included data from a total of 23 people: 19 people with SCI and four GPs. Of the 19 people with SCI, 12 participated in semi-structured interviews, seven participated in the first focus group, and four returned to the second focus group. In step 2, four people with SCI participated in a focus group and six healthcare professionals participated in one-on-one semi-structured interviews. Two of the healthcare professionals (a GP and a registrar) had lived experience of SCI, as did one researcher (JAB). All interviews and focus groups were conducted either online or in-person and ranged in length between 60 and 120 min.
In our overall synthesis, we actively interpreted five reflective statements based on the updated CFIR domain and construct definitions by Damschroder et al. [ 11 ]. Table 2 provides a summary of how we linked the updated CFIR domain and construct definitions to the reflective statements. We demonstrate this process of co-creation below, including illustrative quotes from participants. Importantly, we guide readers to the actionable findings related to each reflective statement in Table 2 . Each actionable statement represents an alteration that can be made to a program to improve its adoption into practice.
Participants acknowledged that self-management is a major undertaking and very demanding, as one person with SCI said, “ we need to be informed without being terrified and overwhelmed”. Participants felt the HMT could indeed be adapted, tailored, refined, or reinvented to meet local needs. For example, another person with SCI remarked:
“Education needs to be from the get-go but in bite sized pieces from all quarters when readiness is most apparent… at all time points , [not just as a] a newbie tool or for people with [long-term impairment] ” (person with SCI_02).
Therefore, the SCI-HMT had to balance complexity of content while still being accessible and engaging, and required input from both experts in the field and those with lived experience of SCI, for example, a clinical nurse specialist suggested:
“it’s essential [the SCI-HMT] is written by experts in the field as well as with collaboration with people who have had a, you know, the lived experience of SCI” (healthcare professional_03).
Furthermore, the points of contact with healthcare for a person with SCI can be challenging to navigate and the SCI-HMT has the potential to facilitate a smoother engagement process and improve communication between people with SCI and healthcare services. As a GP suggested:
“we need a tool like this to link to that pathway model in primary health care , [the SCI-HMT] it’s a great tool, something that everyone can read and everyone’s reading the same thing” (healthcare professional_05).
Participants highlighted that the ability of the SCI-HMT to facilitate effective communication was very much dependent on the delivery format. The idea of digitizing the SCI-HMT garnered equal support from people with SCI and health care professionals, with one participant with SCI deeming it to be “ essential” ( person with SCI_01) and a health professional suggesting a “digitalized version will be an advantage for most people” (healthcare professional_02).
Outer setting
There was strong interest expressed by both people with SCI and healthcare professionals in using the SCI-HMT. The fundamental premise was that knowledge is power and the SCI-HMT would have strong utility in post-acute rehabilitation services, as well as primary care. As a person with SCI said,
“ we need to leave the [spinal unit] to return to the community with sufficient knowledge, and to know the value of that knowledge and then need to ensure primary healthcare provider [s] are best informed” (person with SCI_04).
The value of the SCI-HMT in facilitating clear and effective communication and shared decision-making between healthcare professionals and people with SCI was also highlighted, as shown by the remarks of an acute nurse specialist:
“I think this tool is really helpful for the consumer and the GP to work together to prioritize particular tests that a patient might need and what the regularity of that is” (healthcare professional_03).
Engaging with SCI peer support networks to promote the SCI-HMT was considered crucial, as one person with SCI emphasized when asked how the SCI-HMT might be best executed in the community, “…peers, peers and peers” (person with SCI_01). Furthermore, the layering of content made possible in the digitalized version will allow for the issue of approachability in terms of readiness for change, as another person with SCI said:
“[putting content into a digital format] is essential and required and there is a need to put summarized content in an App with links to further web-based information… it’s not likely to be accessed otherwise” (person with SCI_02).
Inner setting
Participants acknowledged that self-management of health and well-being is substantial and demanding. It was suggested that the scope, tone, and complexity of the SCI-HMT, while necessary, could potentially be resisted by people with SCI if they felt overwhelmed, as one person with SCI described:
“a manual that is really long and wordy, like, it’s [a] health metric… they maybe lack the health literacy to, to consume the content then yes, it would impede their readiness for [self-management]” (person with SCI_02).
Having support from their GPs was considered essential, and the HMT could enable GP’s, who are under time pressure, to provide more effective health and advice to their patients, as one GP said:
“We GP’s are time poor, if you realize then when you’re time poor you look quickly to say oh this is a patient tool - how can I best use this?” (healthcare professional_05).
Furthermore, health professional skills may be best used with the synthesis of self-reported symptoms, behaviors, or observations. A particular strength of a digitized version would be its ability to facilitate more streamlined communication between a person with SCI and their primary healthcare providers developing healthcare plans, as an acute nurse specialist reflected, “ I think that a digitalized version is essential with links to primary healthcare plans” (healthcare professional_03).
Efficient communication with thorough assessment is essential to ensure serious health issues are not missed, as findings reinforce that the SCI-HMT is an educational tool, not a replacement for healthcare services, as a clinical nurse specialist commented, “ remember, things will go wrong– people end up very sick and in acute care “ (healthcare professional_02).
The SCI-HMT has the potential to provide a pathway to a ‘hope for better than now’ , a hope to ‘remain well’ and a hope to ‘be happy’ , as the informant with SCI (04) declared, “self-management is a long game, if you’re keeping well, you’ve got that possibility of a good life… of happiness”. Participants with SCI felt the tool needed to be genuine and
“acknowledge the huge amount of adjustment required, recognizing that dealing with SCI issues is required to survive and live a good life” (person with SCI_04).
However, there is a risk that an individual is completely overwhelmed by the scale of the SCI-HMT content and the requirement for lifelong vigilance. Careful attention and planning were paid to layering the information accordingly to support self-management as a ‘long game’, which one person with SCI reflected in following:
“the first 2–3 year [period] is probably the toughest to get your head around the learning stuff, because you’ve got to a stage where you’re levelling out, and you’ve kind of made these promises to yourself and then you realize that there’s no quick fix” (person with SCI_01).
It was decided that this could be achieved by providing concrete examples and anecdotes from people with SCI illustrating that a meaningful, healthy life is possible, and that good health is the bedrock of a good life with SCI.
There was universal agreement that the SCI-HMT is aspirational and that it has the potential to improve knowledge and understanding for people with SCI, their families, community workers/carers and primary healthcare professionals, as a GP remarked:
“[different groups] could just read it and realize, ‘Ahh, OK that’s what that means… when you’re doing catheters. That’s what you mean when you’re talking about bladder and bowel function or skin care” (healthcare professional_04).
Despite the SCI-HMT providing an abundance of information and resources to support self-management, participants identified four gaps: (i) the priority issue of sexuality, including pleasure and identity, as one person with SCI remarked:
“ sexuality is one of the biggest issues that people with SCI often might not speak about that often cause you know it’s awkward for them. So yeah, I think that’s a that’s a serious issue” (person with SCI_03).
(ii) consideration of the taboo nature of bladder and bowel topics for indigenous people, (iii) urgent need to ensure links for SCI-HMT care plans are compatible with patient management systems, and (iv) exercise and leisure as a standalone topic taking account of effects of physical activity, including impact on mental health and wellbeing but more especially for fun.
To ensure longevity of the SCI-HMT, maintaining a partnership between people with SCI, SCI community groups and both primary and tertiary health services is required for liaison with the relevant professional bodies, care agencies, funders, policy makers and tertiary care settings to ensure ongoing education and promotion of SCI-HMT is maintained. For example, delivery of ongoing training of healthcare professionals to both increase the knowledge base of primary healthcare providers in relation to SCI, and to promote use of the tools and resources through health communities. As a community nurse specialist suggested:
“ improving knowledge in the health community… would require digital links to clinical/health management platforms” (healthcare professional_02).
In a similar vein, a GP suggested:
“ our common GP body would have continuing education requirements… especially if it’s online, in particular for the rural, rural doctors who you know, might find it hard to get into the city” (healthcare professional_04).
The successful implementation of evidence-based innovations into practice is dependent on a wide array of dynamic and active contextual factors, including the perspectives of the recipients who are destined to use such innovations. Indeed, the recently updated CFIR has called for innovation recipient perspectives to be a priority when considering contextual factors [ 10 , 11 ]. Understanding and including the perspectives of those the innovation is being designed to benefit can promote increased equity and validation of recipient populations, and potentially increase the adoption and sustainability of innovations.
In this paper, we have presented research using the recently updated CFIR to guide the collection of innovation recipients’ perspectives (including people with SCI and GPs working in the community) regarding the potential implementation barriers and facilitators of the digital version of the SCI-HMT. Collected data were synthesized to inform actionable findings– tangible ways in which the SCI-HMT could be modified according of the domains of the CFIR (e.g., see Keith et al. [ 20 ]). It is important to note that we conducted this research using the original domains of the CFIR [ 9 ] prior to Damschroder et al. publishing the updated CFIR [ 11 ]. However, in our analysis we were able to align our findings to the revised CFIR domains and constructs, as Damschroder [ 11 ] suggests, constructs can “be mapped back to the original CFIR to ensure longitudinal consistency” (p. 13).
One of the most poignant findings from our analyses was the need to ensure the content of the SCI-HMT balanced scientific evidence and clinical expertise with lived experience knowledge. This balance of clinical and experiential knowledge demonstrated genuine regard for lived experience knowledge, and created a more accessible, engaging, useable platform. For example, in the innovation and individual domains, the need to include lived experience quotes was immediately apparent once the perspective of people with SCI was included. It was highlighted that while the SCI-HMT will prove useful to many parties at various stages along the continuum of care following onset of SCI, there will be those individuals that are overwhelmed by the scale of the content. That said, the layering of information facilitated by the digitalized version is intended to provide an ease of navigation through the SCI-HMT and enable a far greater sense of control over personal health and wellbeing. Further, despite concerns regarding e-literacy the digitalized version of the SCI-HMT is seen as imperative for accessibility given the wide geographic diversity and recent COVID pandemic [ 21 ]. While there will be people who are challenged by the technology, the universally acceptable use of the internet is seen as less of a barrier than printed material.
The concept of partnership was also apparent within the data analysis focusing on the outer and inner setting domains. In the outer setting domain, our findings emphasized the importance of engaging with SCI community groups, as well as primary and tertiary care providers to maximize uptake at all points in time from the phase of subacute rehabilitation onwards. While the SCI-HMT is intended for use across the continuum of care from post-acute rehabilitation onwards, it may be that certain modules are more relevant at different times, and could serve as key resources during the hand over between acute care, inpatient rehabilitation and community reintegration.
Likewise, findings regarding the inner setting highlighted the necessity of a productive partnership between GPs and individuals with SCI to address the substantial demands of long-term self-management of health and well-being following SCI. Indeed, support is crucial, especially when self-management is the focus. This is particularly so in individuals living with complex disability following survival after illness or injury [ 22 ], where health literacy has been found to be a primary determinant of successful health and wellbeing outcomes [ 23 ]. For people with SCI, this tool potentially holds the most appeal when an individual is ready and has strong partnerships and supportive communication. This can enable potential red flags to be recognized earlier allowing timely intervention to avert health crises, promoting individual well-being, and reducing unnecessary demands on health services.
While the SCI-HMT is an educational tool and not meant to replace health services, findings suggest the current structure would lead nicely to having the conversation with a range of likely support people, including SCI peers, friends and family, GP, community nurses, carers or via on-line support services. The findings within the process domain underscored the importance of ongoing partnership between innovation implementers and a broad array of innovation recipients (e.g., individuals with SCI, healthcare professionals, family, funding agencies and policy-makers). This emphasis on partnership also addresses recent discussions regarding equity and the CFIR. For example, Damschroder et al. [ 11 ] suggests that innovation recipients are too often not included in the CFIR process, as the CFIR is primarily seen as a tool intended “to collect data from individuals who have power and/or influence over implementation outcomes” (p. 5).
Finally, we feel that our inclusion of innovation recipients’ perspectives presented in this article begins to address the notion of equity in implementation, whereby the inclusion of recipient perspectives in research using the CFIR both validates, and increases, the likelihood of sustainable adoption of evidence-based innovations, such as the SCI-HMT. We have used the CFIR in a pragmatic way with an emphasis on meaningful engagement between the innovation recipients and the research team, heeding the call from Damschroder et al. [ 11 ], who recently argued for researchers to use the CFIR to collect data from innovation recipients. Adopting this approach enabled us to give voice to innovation recipient perspectives and subsequently ensure that the tone, scope, content and presentation of the SCI-HMT balanced the needs of innovation recipients alongside the provision of evidence-based clinical information.
Our research is not without limitations. While our study was successful in identifying a number of potential barriers and facilitators to the implementation of the SCI-HMT, we did not test any implementation strategies to impact determinants, mechanisms, or outcomes. This will be the focus of future research on this project, which will investigate the impact of implementation strategies on outcomes. Focus will be given to the context-mechanism configurations which give rise to particular outcomes for different groups in certain circumstances [ 7 , 24 ]. A second potential concern is the relatively small sample size of participants that may not allow for saturation and generalizability of the findings. However, both the significant impact of secondary health complications for people with SCI and the desire for a health maintenance tool have been established in Australia [ 2 , 4 ]. The aim our study reported in this article was to achieve context-specific knowledge of a small sample that shares a particular mutual experience and represents a perspective, rather than a population [ 25 , 26 ]. We feel our findings can stimulate discussion and debate regarding participant-informed approaches to implementation of the SCI-HMT, which can then be subject to larger-sample studies to determine their generalisability, that is, their external validity. Notably, future research could examine the interaction between certain demographic differences (e.g., gender) of people with SCI and potential barriers and facilitators to the implementation of the SCI-HMT. Future research could also include the perspectives of other allied health professionals working in the community, such as occupational therapists. Lastly, while our research gave significant priority to recipient viewpoints, research in this space would benefit for ensuring innovation recipients are engaged as genuine partners throughout the entire research process from conceptualization to implementation.
Employing the CFIR provided an effective, systematic method for identifying recipient perspectives regarding the implementation of a digital health maintenance tool for people living with SCI. Findings emphasized the need to balance clinical and lived experience perspectives when designing an implementation strategy and facilitating strong partnerships with necessary stakeholders to maximise the uptake of SCI-HMT into practice. Ongoing testing will monitor the uptake and implementation of this innovation, specifically focusing on how the SCI-HMT works for different users, in different contexts, at different stages and times of the rehabilitation journey.
Data availability
The datasets supporting the conclusions of this article are available available upon request and with permission gained from the project Steering Committee.
Abbreviations
spinal cord injury
HMT-Spinal Cord Injury Health Maintenance Tool
Consolidated Framework for Implementation Research
Kirshblum S, Vernon WL. Spinal Cord Medicine, Third Edition. New York: Springer Publishing Company; 2018.
Middleton JW, Arora M, Kifley A, Clark J, Borg SJ, Tran Y, et al. Australian arm of the International spinal cord Injury (Aus-InSCI) Community Survey: 2. Understanding the lived experience in people with spinal cord injury. Spinal Cord. 2022;60(12):1069–79.
Article PubMed PubMed Central Google Scholar
Craig A, Nicholson Perry K, Guest R, Tran Y, Middleton J. Adjustment following chronic spinal cord injury: determining factors that contribute to social participation. Br J Health Psychol. 2015;20(4):807–23.
Article PubMed Google Scholar
Middleton JW, Arora M, Jerram KAS, Bourke J, McCormick M, O’Leary D, et al. Co-design of the Spinal Cord Injury Health Maintenance Tool to support Self-Management: a mixed-methods Approach. Top Spinal Cord Injury Rehabilitation. 2024;30(1):59–73.
Article Google Scholar
Middleton JW, Arora M, McCormick M, O’Leary D. Health maintenance Tool: how to stay healthy and well with a spinal cord injury. A tool for consumers by consumers. 1st ed. Sydney, NSW Australia: Royal Rehab and The University of Sydney; 2020.
Google Scholar
Nilsen P, Bernhardsson S. Context matters in implementation science: a scoping review of determinant frameworks that describe contextual determinants for implementation outcomes. BMC Health Serv Res. 2019;19(1):189.
Jagosh J. Realist synthesis for Public Health: building an Ontologically Deep understanding of how Programs Work, for whom, and in which contexts. Annu Rev Public Health. 2019;40(1):361–72.
Nilsen P. Making sense of implementation theories, models and frameworks. Implement Sci. 2015;10(1):53.
Damschroder LJ, Aron DC, Keith RE, Kirsh SR, Alexander JA, Lowery JC. Fostering implementation of health services research findings into practice: a consolidated framework for advancing implementation science. Implement Sci. 2009;4(1):50.
Damschroder LJ, Reardon CM, Opra Widerquist MA, Lowery JC. Conceptualizing outcomes for use with the Consolidated Framework for Implementation Research (CFIR): the CFIR outcomes Addendum. Implement Sci. 2022;17(1):7.
Damschroder LJ, Reardon CM, Widerquist MAO, Lowery JC. The updated Consolidated Framework for Implementation Research based on user feedback. Implement Sci. 2022;17(1):75.
Plamondon K, Ndumbe-Eyoh S, Shahram S. 2.2 Equity, Power, and Transformative Research Coproduction. Research Co-Production in Healthcare2022. p. 34–53.
Verville L, Cancelliere C, Connell G, Lee J, Munce S, Mior S, et al. Exploring clinicians’ experiences and perceptions of end-user roles in knowledge development: a qualitative study. BMC Health Serv Res. 2021;21(1):926.
Gainforth HL, Hoekstra F, McKay R, McBride CB, Sweet SN, Martin Ginis KA, et al. Integrated Knowledge Translation Guiding principles for conducting and Disseminating Spinal Cord Injury Research in Partnership. Arch Phys Med Rehabil. 2021;102(4):656–63.
Langley J, Knowles SE, Ward V. Conducting a Research Coproduction Project. Research Co-Production in Healthcare2022. p. 112– 28.
Braun V, Clarke V. One size fits all? What counts as quality practice in (reflexive) thematic analysis? Qualitative Research in Psychology. 2020:1–25.
Tong A, Sainsbury p, Craig J. Consolidated criteria for reporting qulaitative research (COREQ): a 32-item checklist for interviews and focus groups. Int J Qual Health Care. 2007;19(6):349–57.
Bengtsson M. How to plan and perform a qualitative study using content analysis. NursingPlus Open. 2016;2:8–14.
Braun V, Clarke V. Using thematic analysis in psychology. Qualitative Res Psychol. 2006;3(2):77–101.
Keith RE, Crosson JC, O’Malley AS, Cromp D, Taylor EF. Using the Consolidated Framework for Implementation Research (CFIR) to produce actionable findings: a rapid-cycle evaluation approach to improving implementation. Implement Science: IS. 2017;12(1):15.
Choukou M-A, Sanchez-Ramirez DC, Pol M, Uddin M, Monnin C, Syed-Abdul S. COVID-19 infodemic and digital health literacy in vulnerable populations: a scoping review. Digit HEALTH. 2022;8:20552076221076927.
PubMed PubMed Central Google Scholar
Daniels N. Just Health: Meeting Health needs fairly. Cambridge University Press; 2007. p. 397.
Parker SM, Stocks N, Nutbeam D, Thomas L, Denney-Wilson E, Zwar N, et al. Preventing chronic disease in patients with low health literacy using eHealth and teamwork in primary healthcare: protocol for a cluster randomised controlled trial. BMJ Open. 2018;8(6):e023239–e.
Salter KL, Kothari A. Using realist evaluation to open the black box of knowledge translation: a state-of-the-art review. Implement Sci. 2014;9(1):115.
Sebele-Mpofu FY. The Sampling Conundrum in qualitative research: can Saturation help alleviate the controversy and alleged subjectivity in Sampling? Int’l J Soc Sci Stud. 2021;9:11.
Malterud K, Siersma VD, Guassora AD. Sample size in qualitative interview studies: guided by Information Power. Qual Health Res. 2015;26(13):1753–60.
Download references
Acknowledgements
Authors of this study would like to thank all the consumers with SCI and healthcare professionals for their invaluable contribution to this project. Their participation and insights have been instrumental in shaping the development of the SCI-HMT. The team also acknowledges the support and guidance provided by the members of the Project Steering Committee, as well as the partner organisations, including NSW Agency for Clinical Innovation, and icare NSW. Author would also like to acknowledge the informant group with lived experience, whose perspectives have enriched our understanding and informed the development of SCI-HMT.
The SCI Wellness project was a collaborative project between John Walsh Centre for Rehabilitation Research at The University of Sydney and Royal Rehab. Both organizations provided in-kind support to the project. Additionally, the University of Sydney and Royal Rehab received research funding from Insurance and Care NSW (icare NSW) to undertake the SCI Wellness Project. icare NSW do not take direct responsibility for any of the following: study design, data collection, drafting of the manuscript, or decision to publish.
Author information
Authors and affiliations.
John Walsh Centre for Rehabilitation Research, Northern Sydney Local Health District, St Leonards, NSW, Australia
John A Bourke, K. Anne Sinnott Jerram, Mohit Arora, Ashley Craig & James W Middleton
The Kolling Institute, Faculty of Medicine and Health, The University of Sydney, Sydney, NSW, Australia
Burwood Academy Trust, Burwood Hospital, Christchurch, New Zealand
John A Bourke
Royal Rehab, Ryde, NSW, Australia
James W Middleton
State Spinal Cord Injury Service, NSW Agency for Clinical Innovation, St Leonards, NSW, Australia
You can also search for this author in PubMed Google Scholar
Contributions
Project conceptualization: KASJ, MA, JWM; project methodology: JWM, MA, KASJ, JAB; data collection: KASJ and MA; data analysis: KASJ, JAB, MA, JWM; writing—original draft preparation: JAB; writing—review and editing: JAB, KASJ, JWM, MA, AC; funding acquisition: JWM, MA. All authors contributed to the revision of the paper and approved the final submitted version.
Corresponding author
Correspondence to John A Bourke .
Ethics declarations
Ethics approval and consent to participate.
The first (RESP/18/212) and second phase (2019/ETH13961) of the project received ethical approval from The Northern Sydney Local Health District Human Research Ethics Committee. All participants provided informed, written consent. All data were to be retained for 7 years (23rd May 2030).
Consent for publication
Not applicable.
Competing interests
MA part salary (from Dec 2018 to Dec 2023), KASJ part salary (July 2021 to Dec 2023) and JAB part salary (Jan 2022 to Aug 2022) was paid from the grant monies. Other authors declare no conflicts of interest.
Additional information
Publisher’s note.
Springer Nature remains neutral with regard to jurisdictional claims in published maps and institutional affiliations.
Electronic supplementary material
Below is the link to the electronic supplementary material.
Supplementary Material 1
Rights and permissions.
Open Access This article is licensed under a Creative Commons Attribution 4.0 International License, which permits use, sharing, adaptation, distribution and reproduction in any medium or format, as long as you give appropriate credit to the original author(s) and the source, provide a link to the Creative Commons licence, and indicate if changes were made. The images or other third party material in this article are included in the article’s Creative Commons licence, unless indicated otherwise in a credit line to the material. If material is not included in the article’s Creative Commons licence and your intended use is not permitted by statutory regulation or exceeds the permitted use, you will need to obtain permission directly from the copyright holder. To view a copy of this licence, visit http://creativecommons.org/licenses/by/4.0/ . The Creative Commons Public Domain Dedication waiver ( http://creativecommons.org/publicdomain/zero/1.0/ ) applies to the data made available in this article, unless otherwise stated in a credit line to the data.
Reprints and permissions
About this article
Cite this article.
Bourke, J.A., Jerram, K.A.S., Arora, M. et al. Using the consolidated Framework for Implementation Research to integrate innovation recipients’ perspectives into the implementation of a digital version of the spinal cord injury health maintenance tool: a qualitative analysis. BMC Health Serv Res 24 , 390 (2024). https://doi.org/10.1186/s12913-024-10847-x
Download citation
Received : 14 August 2023
Accepted : 11 March 2024
Published : 28 March 2024
DOI : https://doi.org/10.1186/s12913-024-10847-x
Share this article
Anyone you share the following link with will be able to read this content:
Sorry, a shareable link is not currently available for this article.
Provided by the Springer Nature SharedIt content-sharing initiative
- Spinal Cord injury
- Self-management
- Innovation recipients
- Secondary health conditions
- Primary health care
- Evidence-based innovations
- Actionable findings
- Consolidated Framework for implementation research
BMC Health Services Research
ISSN: 1472-6963
- General enquiries: [email protected]
- University Libraries
- Location Location
- Contact Contact
- Offices and Divisions
- Exhibits, Events and News
Digital Humanities Working Group Offers Research Support for Scholars
From digital mapping to data visualization to tools for text analysis, USC faculty and students in the humanities and humanities-adjacent fields are increasingly using computation to expand both the scope and the reach of their research. But for scholars whose training doesn’t typically include computer science, venturing into the digital space can be daunting.
That’s why the Digital Humanities Working Group , an offshoot of USC’s Humanities Collaborative that’s supported by the Digital Research Services team at University Libraries, has developed a range of resources for faculty who want to explore the ways computational tools can enhance their research.
“Our goal is to make working in digital scholarship more approachable and less intimidating to humanities researchers,” says Scholarly Communications Librarian Amie Freeman. “Many of the scholars we meet with have fabulous ideas but aren’t sure how to get started. We can connect them with collaborators, tools, and even assistants to make their projects a reality.”
In addition to a wide range of software tools, the group can provide support from the Libraries’ Digital Research Services team and from computer science students who can help with everything from digitization to project management to website development.
The Digital Humanities Working Group brings DH-curious and accomplished DH practitioners together to share projects, ideas, and assistance. “We want it to be accessible and casual where people felt comfortable sharing failures and crazy ideas and asking any type of question,” says Kate Boyd, the Libraries’ Director of Digital Research Services and Collections. “Hence, there are no recordings, just the conversation and memories that happened in that moment between those of us connecting online or in person.”
The group, now in its fifth semester, also sponsors a series of talks which showcase the variety and expanse of digital humanities work happening at USC. Recent talks include librarian Greg Wilsbacher’s overview of his NEH-funded project to develop an AI tool that can aid in the description of archival film, music professor Marcelo Hazan’s discussion of his hypertext edition of the songs of a Brazilian composer, and political science professor Joshua Meyer-Gutbrod’s presentation on his work developing a database of state political campaign rhetoric in order to examine partisan agendas and rhetoric.
While many of the presenters have become adept at using digital humanities tools, the working group is not limited to those who already have DH skills, or even those who have clear ideas about how digital tools could enhance their work. The group describes itself as “an open community of digital humanities dabblers, novices, and practitioners who can share new and developing projects, discuss strategies for addressing the challenges of beginning and continuing digital humanities work, and find collaborators and colleagues across campus.”
Senior computer science major Sophia Riley, who has assisted three different faculty members with their projects, says the opportunities for cross-pollination between the humanities and computational sciences that the group affords have been both exciting and professionally enriching: “My work on digital humanities projects has given me the opportunity to learn and practice web development from multiple frameworks. It’s a great exercise in problem solving. All the projects I’ve worked on have been interesting, but what has always stood out to me is the passion my clients have for their projects! It really motivates me to do my best work.”
Boyd says both the list of digital resources available to humanities scholars and the proliferation of DH research across multiple disciplines at USC are impressive. “The work happening across campus includes the creation of digital repositories and online encyclopedias, web scraping, data and text analysis using machine learning, GIS, virtual reality, photogrammetry, and artificial intelligence,” Boyd notes. “The creation of virtual realities by Jason Porter in the School of Journalism, Dr. Sarah Williams’ work on the audible history of medieval music manuscripts in the Singing the Archives project, and the Institute of Southern Studies' Dr. Smith and Dr. Simmons’ work transcribing 18th century probate records are just a few examples of the fascinating projects that have been launched in the last few years.”
For those interested in learning more about DH tools, how they can be deployed in humanities research, and the support that’s available to USC faculty and students as they adopt new digital resources, University Libraries is offering an introductory workshop, Getting Started with Digital Resources , on April 18. The workshop will provide an overview of content management systems like WordPress and Omeka, text analysis tools such as JSTOR’s Constellate, and data cleaning and visualization tools.
“We hope that anyone interested in diving into a digital project or even just dabbling in the digital humanities will attend the workshop,” says Scholarly Communications Librarian Amie Freeman. “This professional training session will equip campus researchers with the skills they need to get started working in the digital humanities realm, and we look forward to building new connections with students and faculty.”
Challenge the conventional. Create the exceptional. No Limits.

New genetic analysis tool tracks risks tied to CRISPR edits
Classification system uses genetic fingerprints to identify unintentional 'bystander' edits linked with new disease therapies.
Since its breakthrough development more than a decade ago, CRISPR has revolutionized DNA editing across a broad range of fields. Now scientists are applying the technology's immense potential to human health and disease, targeting new therapies for an array of disorders spanning cancers, blood conditions and diabetes.
In some designed treatments, patients are injected with CRISPR-treated cells or with packaged CRISPR components with a goal of repairing diseased cells with precision gene edits. Yet, while CRISPR has shown immense promise as a next-generation therapeutic tool, the technology's edits are still imperfect. CRISPR-based gene therapies can cause unintended but harmful "bystander" edits to parts of the genome, at times leading to new cancers or other diseases.
Next-generation solutions are needed to help scientists unravel the complex biological dynamics behind both on- and off-target CRISPR edits. But the landscape for such novel tools is daunting, since intricate bodily tissues feature thousands of different cell types and CRISPR edits can depend on many different biological pathways.
University of California San Diego researchers have developed a new genetic system to test and analyze the underlying mechanisms of CRISPR-based DNA repair outcomes. As described in Nature Communications , Postdoctoral Scholar Zhiqian Li, Professor Ethan Bier and their colleagues developed a sequence analyzer to help track on- and off-target mutational edits and the ways they are inherited from one generation to the next. Based on a concept proposed by former UC San Diego researcher David Kosman, the Integrated Classifier Pipeline (ICP) tool can reveal specific categories of mutations resulting from CRISPR editing.
Developed in flies and mosquitoes, the ICP provides a "fingerprint" of how genetic material is being inherited, which allows scientists to follow the source of mutational edits and related risks emerging from potentially problematic edits.
"The ICP system can cleanly establish whether a given individual insect has inherited specific genetic components of the CRISPR machinery from either their mothers or fathers since maternal versus paternal transmission result in totally different fingerprints," said Bier, a professor in the UC San Diego School of Biological Sciences.
The ICP can help untangle complex biological issues that arise in determining the mechanisms behind CRISPR. While developed in insects, ICP carries vast potential for human applications.
"There are many parallel applications of ICP for analyzing and following CRISPR editing outcomes in humans following gene therapy or during tumor progression," said study first author Li. "This transformative flexible analysis platform has many possible impactful uses to ensure safe application of cutting-edge next-generation health technologies."
ICP also offers help in tracking inheritance across generations in gene drive systems, which are new technologies designed to spread CRISPR edits in applications such as stopping the transmission of malaria and protecting agricultural crops against pest destruction. For example, researchers could select a single mosquito from the field where a gene-drive test is being conducted and use ICP analysis to determine whether that individual had inherited the genetic construct from its mother or its father, and whether it had inherited a defective element lacking the defining visible markers of that genetic element.
"The CRISPR editing system can be more than 90 percent accurate," said Bier, "but since it edits over and over again it will eventually make a mistake. The bottom line is that the ICP system can give you a very high-resolution picture of what can go wrong."
In addition to Li and Bier, coauthors included Lang You and Anita Hermann. Prior Bier lab member Kosman also made important intellectual contributions to this project.
- Gene Therapy
- Personalized Medicine
- Human Biology
- Diseases and Conditions
- Birth Defects
- Medical Topics
- Immune System
- Gene therapy
- Genetically modified organism
- DNA microarray
- Drug discovery
- Biopharmaceutical
Story Source:
Materials provided by University of California - San Diego . Original written by Mario Aguilera. Note: Content may be edited for style and length.
Journal Reference :
- Zhiqian Li, Lang You, Anita Hermann, Ethan Bier. Developmental progression of DNA double-strand break repair deciphered by a single-allele resolution mutation classifier . Nature Communications , 2024; 15 (1) DOI: 10.1038/s41467-024-46479-2
Cite This Page :
Explore More
- 100 Kilometers of Quantum-Encrypted Transfer
- Intelligent Liquid
- New Approach to Searching for Dark Matter
- We've Had Bird Evolution All Wrong
- Physical Activity Protects Against Chronic Pain
- Australia On Track for Decades-Long Megadroughts
- Speed of Visual Perception Ranges Widely
- 3D Printed Replica of an Adult Human Ear
- Extremely Fast Wound Healing: New Treatment
- Micro-Lisa! Novel Nano-Scale Laser Writing
Trending Topics
Strange & offbeat.
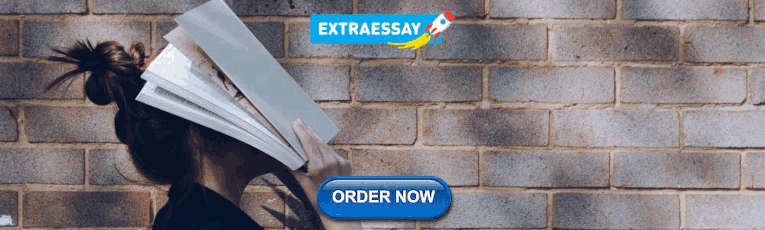
IMAGES
VIDEO
COMMENTS
Research methods are specific procedures for collecting and analyzing data. Developing your research methods is an integral part of your research design. When planning your methods, there are two key decisions you will make. First, decide how you will collect data. Your methods depend on what type of data you need to answer your research question:
Whether you are part of a small or large organization, learning how to effectively utilize data analytics can help you take advantage of the wide range of data-driven benefits. 1. RapidMiner. Primary use: Data mining. RapidMiner is a comprehensive package for data mining and model development.
It is an important research tool used by scientists, governments, businesses, and other organizations. To draw valid conclusions, statistical analysis requires careful planning from the very start of the research process. You need to specify your hypotheses and make decisions about your research design, sample size, and sampling procedure.
Home Market Research Research Tools and Apps. Analytical Research: What is it, Importance + Examples. Finding knowledge is a loose translation of the word "research." It's a systematic and scientific way of researching a particular subject. As a result, research is a form of scientific investigation that seeks to learn more.
Definition of research in data analysis: According to LeCompte and Schensul, research data analysis is a process used by researchers to reduce data to a story and interpret it to derive insights. The data analysis process helps reduce a large chunk of data into smaller fragments, which makes sense. Three essential things occur during the data ...
The statistical analysis gives meaning to the meaningless numbers, thereby breathing life into a lifeless data. The results and inferences are precise only if proper statistical tests are used. This article will try to acquaint the reader with the basic research tools that are utilised while conducting various studies.
Google Cloud AutoML contains a suite of tools across categories from structured data to language translation, image and video classification. As more and more organizations adopt machine learning, there will be a growing demand for data analysts who can use AutoML tools to automate their work easily. 7. SAS.
Data analysis techniques in research are categorized into qualitative and quantitative methods, each with its specific approaches and tools. These techniques are instrumental in extracting meaningful insights, patterns, and relationships from data to support informed decision-making, validate hypotheses, and derive actionable recommendations.
Until the mid-1980s we either had to use pen-and-paper methods (highlighters, whiteboards, scissors, sticky notes, blue tac etc.) or general purpose software (word processors, spreadsheets, etc.). Since they first emerged, dedicated digital tools for qualitative analysis have mushroomed and there are now literally dozens to choose from.
Some common Descriptive Analytics Tools are as follows: Excel: Microsoft Excel is a widely used tool that can be used for simple descriptive analytics. It has powerful statistical and data visualization capabilities. Pivot tables are a particularly useful feature for summarizing and analyzing large data sets.
The descriptive statistical analysis allows organizing and summarizing the large data into graphs and tables. Descriptive analysis involves various processes such as tabulation, measure of central tendency, measure of dispersion or variance, skewness measurements etc. 2. Inferential Analysis.
They ensure accurate and efficient information collection, management, referencing, and analysis. Some of the most important digital tools for researchers include: Research management tools. Research management can be a complex and challenging process. Some tools address the various challenges that arise when referencing and managing papers. Zotero
For many researchers unfamiliar with qualitative research, determining how to conduct qualitative analyses is often quite challenging. Part of this challenge is due to the seemingly limitless approaches that a qualitative researcher might leverage, as well as simply learning to think like a qualitative researcher when analyzing data. From framework analysis (Ritchie & Spencer, 1994) to content ...
14. Excel - Excel is spreadsheet software used for organizing, analyzing, and presenting data. 15. Tableau - Tableau is a data visualization software that allows you to create interactive visualizations and dashboards. 16. NVivo - Nviva is a software tool for qualitative research and data analysis. 17.
Top 5 Academic Research Tools. There are endless tools for academic research that can help you in any stage of the research process, from educational search engine software and project management tools to grammar editors and reference managers. ... QuestionPro also provides easy-to-setup analytical research tools to build dashboards and ...
Let's go through the top 9 best statistical tools used in research below: 1. SPSS: SPSS (Statistical Package for the Social Sciences) is a collection of software tools compiled as a single package. This program's primary function is to analyze scientific data in social science. This information can be utilized for market research, surveys ...
(v) Research demands accurate observation and description. (vi) Research involves gathering new data from primary or first-hand sources or using existing data for a new purpose. (vii) Research is characterized by carefully designed procedures that apply rigorous analysis. (viii) Research involves the quest for answers to un-solved problems.
The best tools for data analysis Key takeaways; The first six methods listed are used for quantitative data, while the last technique applies to qualitative data. We briefly explain the difference between quantitative and qualitative data in section two, but if you want to skip straight to a particular analysis technique, just use the clickable ...
6. Kissmetrics. Kissmetrics is a software for quantitative data analysis that focuses on customer analytics and helps businesses understand user behavior and customer journeys. Kissmetrics lets you track user actions, create funnels to analyze conversion rates, segment your user base, and measure customer lifetime value.
In conclusion, the top 15 academic research tools offer valuable support for various aspects of the research process. For reference management, tools like Zotero, Mendeley, and EndNote provide efficient organisation and citation capabilities. In the realm of data visualisation and analysis, Tableau, Power BI, and Google Data Studio offer ...
However, in general, a research analyst is responsible for the following: Collecting, analyzing, and interpreting data to support company aims. Using statistical modeling to find patterns and trends Understanding and using data analytical tools and research software. Conducting analysis of historical data to highlight trends
21. Qualaroo. Qualaroo is an advanced user and market research tool that helps you understand your target market with targeted surveys. You can run surveys on over six channels at once (such as website, app, product, social media, and mail,) to get a 360-degree view of your existing and potential customers.
2. Facebook Page Insights. Page Insight by Meta is a fantastic free market research option if you use Facebook to market your business. And, if you don't use Facebook for marketing yet, you probably should. With 2.9 billion monthly active users, it's one of the best social media platforms for marketing.
Summary. The interactive, conversational, analytical, and generative features of GenAI offer support for creativity, problem-solving, and processing and digestion of large bodies of information.
Real-time data, unparalleled news and research, powerful analytics, communications tools and world-class execution capabilities. Bloomberg Terminal Overview Research
DATA METHODS. Participant data have been harmonized and curated to protect participant privacy. We ensure high-quality data for research by standardization methods. Data counts may differ between Research Hub tools and data views. This is because we use different data curation methods for each tool to protect participant privacy.
Procedure. The procedure for this research is multi-stepped and is summarized in Fig. 1.First, we mapped retrospective qualitative data collected during the development of the SCI-HMT [] against the five domains of the CFIR in order to create a semi-structured interview guide (Step 1).Then, we used this interview guide to collect prospective data from health professionals and people with SCI ...
From digital mapping to data visualization to tools for text analysis, USC faculty and students in the humanities and humanities-adjacent fields are increasingly using computation to expand both the scope and the reach of their research. But for scholars whose training doesn't typically include computer science, venturing into the digital space can be daunting.
By Shania Kennedy. April 01, 2024 - George Washington University (GW) School of Medicine and Health Sciences (SMHS) and the University of Maryland Eastern Shore (UMES) have been awarded a two-year, $839,000 National Institutes of Health (NIH) grant to advance the development of artificial intelligence (AI) tools to improve health equity.
University of California - San Diego. "New genetic analysis tool tracks risks tied to CRISPR edits." ScienceDaily. ScienceDaily, 26 March 2024. <www.sciencedaily.com / releases / 2024 / 03 ...