- Our Mission
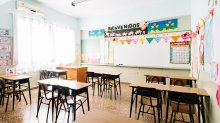
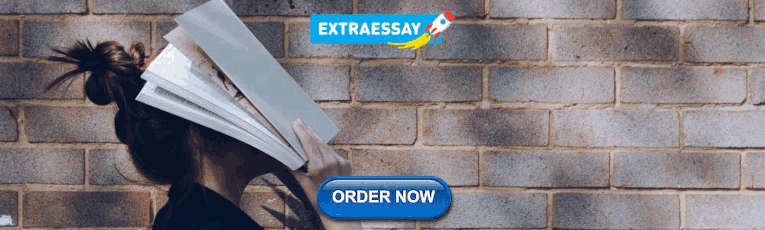
The Science of Classroom Design
Our comprehensive, all-in, research-based look at the design of effective learning spaces.
When a team of researchers led by University of Salford professor Peter Barrett analyzed the design of 153 classrooms across 27 elementary schools in the United Kingdom, they went all in and kept it real, taking measurements and making observations of seating arrangements, wall decorations, and often-overlooked ambient factors such as lighting, temperature, acoustics, and air quality—all inside real classrooms.
Good classrooms should be “designed to make attending school an interesting and pleasurable experience,” the researchers enthused, balancing visual stimulation with comfort and a sense of ownership. Combined, these classroom design elements accounted for 16 percent of the variation in students’ academic progress.
We tried to take a similar approach, getting beyond the more obvious classroom design factors to survey the science of learning environments more comprehensively. Not everything in this list is within a teacher’s purview—you can’t very well open your walls and let in more sunlight, at least not without a good saw and the district’s permission—but we tried to identify factors that might be addressed within classrooms immediately, or within school or district budgets over a longer term.
“Lighting is one of the most critical physical characteristics in a learning space,” researchers explain in a 2020 review of 130 studies. Poor lighting not only makes it harder to see materials clearly but also can dampen engagement, especially for students with developmental disabilities. Good lighting, on the other hand, has a significant impact across many dimensions of successful learning, including “attention rates, working speed, productivity, and accuracy, among other reported effects.”
If you have outside-facing windows, try to allow as much natural sunlight into your room as possible, researchers suggest in a 2021 study . After analyzing over two dozen variables, from lighting type (artificial, sunlight, or a mix) to window size and window shades in 53 European schools, they concluded that lighting is “a strong enabler of performance, which is crucial for child development.”
There was one notable wrinkle: Too much direct sunlight impaired test scores, the researchers found. You might consider using shades or window decorations to prevent glare from entering the classroom, especially when students are focused on screens or paper for longer periods of time.
VENTILATION AND AIR QUALITY
When three coal-fired power plants closed at about the same time in Chicago, Illinois, the downwind effects in schools were significant. “For the typical elementary school in our sample,” researchers studying the shutdowns reported, there was a 7 percent reduction in student absences, translating “into around 372 fewer absence-days per year.” Students in classrooms with poor air-conditioning saw outsized improvements after the plants closed, indicating that school ventilation and air-purifying systems had been keeping kids healthier and in school more often. Two years later, a study analyzing pollution data from over 10,000 U.S. school districts found a direct link to academic performance, concluding that elevated levels of particulate matter in the air—dust or soot, for instance—lead to reduced test scores.
Installing air-conditioning, better HVAC systems, or new windows is the responsibility of administrators and district leaders, but there are simpler measures teachers can take. Researchers, for example, found that carbon dioxide, a by-product of human breathing, steadily accumulated in classrooms over the course of the day—readings were often six times higher than the level that a 2015 Harvard study linked to substantial declines in higher-order thinking.
“If air quality is OK at the start of the class, it won’t be by the end unless you do something,” according to Barrett, whose team recorded the gas levels, when we interviewed him in 2018 . The fix is easy enough: “You have to open a window or a door,” he suggested.
COMPLEXITY AND COLOR
There’s a difference between cluttered walls and visually stimulating ones. In a landmark 2015 study that was largely confirmed by two studies we recently reviewed ( here and here ), researchers found that students are more frequently off task when visual clutter overwhelms “their still-developing and fragile ability to actively maintain task goals and ignore distractions.”
The good news, as we recently reported , is that the studies tend to point to a commonsensical middle ground, where classrooms are neither too cluttered nor too austere: “Classroom decoration can alter academic trajectories, the research suggests, but the task shouldn’t stress teachers out,” we wrote. “The rules appear to be relatively straightforward: Hang academically relevant work on the walls, and avoid the extremes—working within the broad constraints suggested by common sense and moderation.”
Color palettes make a difference, too, according to a 2022 study . The same principle of moderation applies: Avoid extreme wall colors such as black or neon green, and opt for a pleasant mix of color across your walls, floor, and wall displays. Use a simple scheme, such as a single neutral color that’s accompanied by splashes of brightness, for example.
DATA WALLS
Some schools believe that data walls motivate students to try harder. The research casts doubt on that conclusion, especially at the margins—where struggling students need the most help. Students in “the red zone” of public data walls are “often mocked or derided by other students for their poor performance,” researchers who reviewed 30 empirical studies on the topic explain in a 2020 review , dampening enthusiasm and confidence for kids who need it the most. Data walls can trigger “positive emotions such as pride, hopefulness, and joy, as well as negative feelings of stress, anxiety, or disappointment,” depending on where a student ranks in the list, the researchers asserted.
“The idea behind ‘data-driven decision-making’ is a good one,” explains assessment expert Lorrie Shepard. But even data walls that use an “anonymous ID number” are harmful to many students because kids “know what it means if they see themselves as a red or a yellow learner.”
The takeaway: Using data to inform instruction is good practice, but public displays or public ranking are neutral or beneficial for only a subset of higher-performing students.
NATURE, PLANTS, AND GREENERY
A classroom space that is conducive to learning should feel natural and fresh, not cramped and stuffy, researchers explain in a 2021 study . Views of nature and green spaces from windows appear to make a difference: “Students reported less stress and were more focused on a task in classrooms with more natural window views.” If you don’t have open spaces outside your window, you can bring in plants and other natural decorations—“students displayed stronger feelings of friendliness and comfort in the presence of these plants,” the researchers note.
When researchers added potted plants to high school classrooms, the older students also expressed more satisfaction in their surroundings, paid more attention in class, and rated the lessons and their teachers higher, a 2020 study found. “Incorporating indoor nature can thus improve students’ satisfaction with their study environment, which may positively influence retention and students’ beliefs about their academic performance,” the researchers concluded, though you can expect improvements to be modest.
REPRESENTATION
Students can experience representation in classrooms by seeing their own or peers’ artifacts on walls and in shared virtual spaces, or by being exposed to images and references that mirror their interests, passions, and backgrounds.
In a 2015 study , researchers explain that “intimate and personalized spaces are better for absorbing, memorizing and recalling information.” To help students see the classroom as a space they belong in, “you can use your walls to showcase your students’ nonacademic talents and activities,” writes special education math teacher Rachel Fuhrman. “It’s incredibly empowering for students… to see something they did or something they created on display in the space.”
Exposure to resonant cultural imagery on walls and in materials—what researchers often call the “symbolic classroom”—also appears to improve a sense of belonging and has positive effects on engagement and academic outcomes. In a 2014 review and a 2019 study , for example, researchers discovered that making culturally relevant adjustments to lessons—and displaying inspiring, inclusive posters and other visuals that mirrored student interests—helped students feel a greater sense of connection to their classroom learning and could boost final course performance by nearly a full letter grade.
FLEXIBILITY
Teachers sometimes chafe at so-called flexible classrooms that look like they were designed by the House of Dior. Beautiful classrooms, the teachers argue persuasively, are not necessarily successful learning environments, and flexibility as a standard of classroom design should be judged by factors like versatility (they support multiple uses) and modifiability (they allow for “active manipulation and appropriation”), according to one review of modern classrooms. The research on flexible classroom design, meanwhile, is scarce but promising , we reported a few years back, with Peter Barrett’s team concluding that flexible classrooms were about as important as air quality, light, and temperature in boosting academic outcomes. Taken together, flexibility and a student’s “sense of ownership” account for just over a quarter of the academic improvements attributed to classroom design.
In the end, flexible classroom design contributes modestly to daily academic outcomes but has the benefit of durability: It works behind the scenes to improve learning for the entirety of the school year. If you’re interested in trying the approach, consider cheap options like rugs, standing desks, and reading nooks with pillows, and use the full range of seating alternatives to support independent and group learning, as the educational tasks demand.
Learning Differences and Neurodivergence
Out of the 7.3 million students with a disability in the U.S., about one in three has a learning disability or neurodevelopmental condition such as autism. For these students, who often have sensory or executive function issues, colorful, richly decorated environments may be perceived as a cacophony of visual noise, according to a 2021 study .
The study provides several research-based recommendations to guide teachers, which actually align nicely with the best research on general education environments:
- Align wall displays with the current topic so that “if students focus on the visual displays rather than the teacher, they will still be focusing on relevant information.”
- Use the front wall for daily materials such as a calendar and word walls.
- Create distinct activity areas—circle time, reading space, and desk work, for example—to help students transition between tasks.
- Be mindful of excessive brightness and glare. A partially shaded window can still allow natural light to enter the room, and carpets can block the glare from vinyl floors.
Sweltering classrooms can have profound academic effects. In a 2018 study , researchers analyzed 10 million PSAT scores and found that a one-degree Fahrenheit rise in local temperature resulted in a corresponding 1 percent drop in test scores. While air-conditioning helped mitigate the impact, the researchers observed stark differences in the school infrastructure and funding: “We estimate that between three and seven percent of the gap in PSAT scores between White students and Black and Hispanic students can be explained by differences in the temperature environment,” the researchers concluded.
In a popular Reddit thread , teachers share their own ideas for dealing with hot classrooms. While it may seem counterintuitive, you can use fans to direct air into the hallway to regulate the air pressure in your own classroom—pulling cooler air from outside into your room, suggests a physics teacher. If you’re looking for fun art project ideas, you can replace some of your window shades with stained glass art to soften sun glare. Other teachers suggest turning off heat-generating electronic devices, such as computers and projectors.
ACOUSTICS/NOISE
Five decades ago, progressive schools began experimenting with open classrooms. In an attempt to create “a less authoritarian environment,” the classroom walls came down, ushering in an era that promised “a greater range of learning methodologies and group sizes,” according to a 2023 study . But the results fell far short. Students in the open classrooms read half as fast as their peers in the traditional classrooms, largely because the open layouts were acoustical chaos and probably “require a significantly higher degree of listening effort.”
When deep focus is needed for independent learning, there’s really no substitute for quiet spaces—and conveying information orally only works when kids can discern who is speaking and what they’re saying. “Effective listening is a linchpin of school learning,” researchers assert in a 2013 study .
SEATING ARRANGEMENTS
Should you assign seats or let kids choose? Several recent studies arrived at what appear, superficially, to be conflicting conclusions. One 2021 study found that assigned seating can forge new friendships between students who would otherwise never bond. Another, published in 2013 , suggests that intentionally separating close friends can reduce classroom disruptions by as much as 70 percent.
The contradiction is easily reconciled in light of other research that finds that it’s best to think of classroom seating arrangements within the context of learning goals. While there’s some evidence that elementary school children are most attentive when they are arranged in semicircles—as opposed to rows or clusters—the best arrangements should match the learning task. For more collaborative work, small clusters of desks or standing arrangements are best, the researchers suggest, while for independent work the oft-demonized row arrangements work well. Likewise, friends can be seated together or apart, depending on the teacher’s immediate objectives.
Finally, consider the space between desks and configurations carefully, or find other ways to allow for movement during the day. Dozens of studies reveal that brain breaks and movement breaks are underestimated as methods to improve engagement, behavior, and learning outcomes.
LEARNING ZONES
In a 2019 study , researchers made the case for creating multiple learning zones in your classroom—a main space for teacher-centered instruction and several smaller areas that can be rotated throughout the school year that involve “students actively working on learning tasks and reflecting on their work, apart from watching, listening, and taking notes.”
Such classrooms often resulted in “improved measurable student learning outcomes, whether those outcomes are traditional quantitative measures such as exams and course grades or measures of ‘21st century skills.’”
Using learning zones can be a low-cost approach to tailoring instruction to meet the needs of students, according to a 2020 study that encompassed 610 elementary and middle school classrooms. The researchers point to a growing body of research suggesting that learning zones have a wide range of benefits, allowing teachers to improve transitions, facilitate differentiated instruction, and motivate and engage students more effectively.
“Don’t let a small classroom be your kryptonite,” writes former teacher and principal Veronica Lopez. “You can set up a learning zone in a bookcase, on a shelf, on one bulletin board, or on a small desk or table.”
Advertisement
Conducting classroom design research with teachers
- Original Article
- Published: 02 December 2014
- Volume 47 , pages 905–917, ( 2015 )
Cite this article
- Michelle. L. Stephan 1
2025 Accesses
5 Citations
Explore all metrics
Design research is usually motivated by university members with experience and interest in building theory and instructional designs in collaboration with one teacher. Typically, the teacher is considered as a member of the research team, with the primary responsibility of implementing instruction. However, in this chapter, I describe a Classroom Design Research project that was conducted by a team comprised mostly of classroom teachers. Their goal was to create a stable instructional unit for integer addition and subtraction that they could use to help students learn the topic with meaning. In this paper, I outline the basic tenets of Classroom Design Research, including the instructional theory of Realistic Mathematics Education and how it guided them in designing instruction. I introduce the construct of a classroom learning trajectory and elaborate on it with the integer project. Finally, I argue that Design Research is mutually beneficial for researchers and teachers. The team of teachers that participated in Design Research embarked on a unique professional development experience, one in which they engaged in practices that supported a new way of preparing their instruction. Reciprocally, the teachers’ unique craft and pedagogical content knowledge shaped the integer instruction theory in unique ways that do not occur in typical Design Research.
This is a preview of subscription content, log in via an institution to check access.
Access this article
Price includes VAT (Russian Federation)
Instant access to the full article PDF.
Rent this article via DeepDyve
Institutional subscriptions
Similar content being viewed by others
Educational Design Research to Support System-Wide Instructional Improvement
Impact of classroom design on teacher pedagogy and student engagement and performance in mathematics.
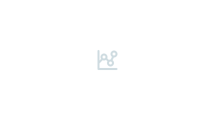
There is more variation within than across domains: an interview with Paul A. Kirschner about applying cognitive psychology-based instructional design principles in mathematics teaching and learning
Battista, M. T. (1983). A complete model for operations on integers. Arithmetic Teacher, 30 (9), 26–31.
Google Scholar
Bofferding, L. (2010). Addition and subtraction with negatives: acknowledging the multiple meanings of the minus sign. In P. Brosnan, D. B. Erchick, & L. Flevares (Eds.), Proceedings of the 32nd annual meeting of the North American Chapter of the International Group for the Psychology of Mathematics Education (pp. 703–710). Columbus: The Ohio State University.
Burkhardt, H. & Swan, M. (2013). Task design for systemic improvement: principles and frameworks (pp. 431–440). Proceedings of the International Commission on Mathematical Instruction , Oxford.
Clements, D., & Sarama, J. (2004). Learning trajectories in mathematics education. Mathematical Thinking and Learning, 6 (2), 81–89.
Article Google Scholar
Cobb, P. (2000). Conducting teaching experiments in collaboration with teachers. In A. E. Kelly & R. A. Lesh (Eds.), Handbook of research design in mathematics and science education (pp. 307–334). Mahwah: Erlbaum.
Cobb, P. (2003). Investigating students’ reasoning about linear measurement as a paradigm case of design research. In N. Pateman (Series Ed.), M. Stephan, J. Bowers, & P. Cobb (with K. Gravemeijer) (Vol. Eds.), Journal for Research in Mathematics Education Monograph Series: Vol. 12. Supporting students’ development of measuring conceptions: analyzing students’ learning in social context (pp. 1–16). Reston: National Council of Teachers of Mathematics.
Cobb, P., Confrey, J., diSessa, A., Lehrer, R., & Schauble, L. (2003). Design experiments in educational research. Educational Researcher, 32 (1), 9–13. doi: 10.3102/0013189X032001009 .
Cobb, P., Gravemeijer, K., Yackel, E., McClain, K., & Whitenack, J. (1997). Mathematizing and symbolizing: the emergence of chains of signification in one first-grade classroom. In D. Kirshner & J. A. Whitson (Eds.), Situated cognition: social, semiotic, and psychological perspectives (pp. 151–233). Mahwah: Erlbaum.
Cobb, P., & Jackson, K. (2011). Towards an empirically grounded theory of action for improving the quality of mathematics teaching at scale. Mathematics Teacher Education and Development, 13 (1), 6–33.
Cobb, P., & Jackson, K. (2015). Supporting teachers’ use of research-based instructional sequences. ZDM —The International Journal on Mathematics Education, 47 (6) (this issue).
Cobb, P., & Yackel, E. (1996). Constructivist, emergent, and sociocultural perspectives in the context of developmental research. Educational Psychologist, 31 , 175–190.
Confrey, J., & Lachance, A. (2000). Transformative reading experiments through conjecture-driven research design. In A. E. Kelly & A. Lesh (Eds.), Handbook of research design in mathematics and science education (pp. 231–266). Mahwah: Erlbaum.
Confrey, J., Maloney, A., Nguyen, K., Mojica, G., & Myers, M. (2009). Equipartitioning/splitting as a foundation of rational number reasoning. In M. Tzekaki, M. Kaldrimidou, & C. Sakonidis (Eds.), Proceedings of the 33rd conference of the International Group for the Psychology of Mathematics Education (Vol. 2, pp. 345–352). Thessaloniki: PME.
Corcoran, T., Mosher, F., & Rogat, A. (2009). Learning progressions in science: an evidence-based approach to reform . Philadelphia: Consortium for Policy Research in Education.
Daro, P., Mosher, F., & Cocoran, T. (2011). Learning trajectories in mathematics: A foundation for standards, curriculum, assessment, and instruction . Philadelphia: Consortium for Policy Research in Education.
De Beer, H., Gravemeijer, K., & van Eijck, M. (2015). Discrete and continuous reasoning about change in primary school classrooms. ZDM—The International Journal on Mathematics Education , 47 (6) (this issue).
Doorman, M., & Gravemeijer, K. (2008). Emergent modeling: discrete graphs to support the understanding of change and velocity. ZDM—The International Journal on Mathematics Education, 41 , 199–211. doi: 10.1007/s11858-008-0130-z .
Fosnot, C. T., & Dolk, M. (2005). “Mathematics” or “mathematizing”? In C. T. Fosnot (Ed.), Constructivism: theory, perspectives and practice (2nd ed., pp. 175–191). New York: Teachers College Press.
Freudenthal, H. (1973). Mathematics as an educational task . Dordrecht: D. Reidel.
Gallardo, A. (2002). The extension of the natural-number domain to the integers in the transition from arithmetic to algebra. Educational Studies in Mathematics, 49 , 171–192. doi: 10.1023/A:1016210906658 .
Garcia, J. & Ruiz-Higueras, L (2013). Task design within the anthropological theory of the didactics: study and research courses for pre-school (pp. 421–430). In Proceedings of the International Commission on Mathematical Instruction , Oxford.
Glaeser, G. (1981). Epistémologie des nombres relatifs. Recherches en Didactique des Mathématiques, 2 , 303–346.
Glaser, B., & Strauss, A. (1967). The discovery of grounded research: strategies for qualitative research . New York: Aldine De Gruyter.
Gravemeijer, K. (1994). Developing realistic mathematics education . Utrecht: CD Press.
Gravemeijer, K. (2004). Local instruction theories as a means of support for teachers in reform mathematics education. Mathematical Thinking and Learning, 6 (2), 105–128.
Gravemeijer, K., & Stephan, M. (2002). Emergent models as an instructional design heuristic. In K. Gravemeijer, R. Lehrer, B. van Oers, & L. Verschaffel (Eds.), Symbolizing, modeling and tool use in mathematics education (pp. 145–169). Dordrecht: Kluwer.
Chapter Google Scholar
Gravemeijer, K., & van Eerde, D. (2009). Design research as a means for building a knowledge vase for teachers and teaching in mathematics education. The Elementary School Journal, 109 (5), 510–524.
Koichu, B., Zaslavsky, O., & Dolev, L. (2013). Effects of variations in task design using different representations of mathematical objects on learning: a case of a sorting task. In C. Margolinas (Ed.), Task design in mathematics education (pp. 461–470). Oxford: Proceedings of the International Commission on Mathematical Instruction.
Lampert, M., Beasley, H., Ghousseini, H., Kazemi, E., & Franke, M. (2010). Using designed instructional activities to enable novices to manage ambitious mathematics teaching. In M.K. Stein, & L. Kucan (Eds.), Instructional explanations in the disciplines. doi: 10.1007/978-1-4419-0594-9_9 . New York: Springer, LLC.
Lampert, M., Franke, M., Kazemi, E., Ghousseini, H., Turrou, A., Beasley, H., et al. (2013). Keeping it complex: using rehearsals to support novice teacher learning of ambitious teaching. Journal of Teacher Education, 64 (3), 226–243. doi: 10.1177/0022487112473837 .
Lappan, G., Fey, J., Fitzgerald, W., Friel, S., & Phillips, E. (2002). Connected mathematics . Upper Saddle River: Prentice Hall.
Linchevski, L., & Williams, J. (1999). Using intuition from everyday life in “filling” the gap in children’s extension of their number concept to include the negative numbers. Educational Studies in Mathematics, 39 , 131–147. doi: 10.1023/A:1003726317920 .
Lytle, P. A. (1994). Investigation of a model based on the neutralization of opposites to teach integer addition and subtraction. In J. P. da Ponte & J. F. Matos (Eds.), Proceedings of the 18th international conference for the psychology of mathematics education (Vol. III, pp. 192–199). Lisbon: University of Lisbon.
Peled, I., Mukhopadhyay, S., & Resnick, L. B. (1989). Formal and informal sources of mental models for negative numbers. In G. Vergnaud, J. Rogalski, & M. Artigue (Eds.), Proceedings of the 13th international conference for the psychology of mathematics education (Vol. III, pp. 106–110). Paris: PME.
Prediger, S. & Krägeloh, K. (2015). Low achievers learning to crack algebraic word problems—a design research project for aligning a strategic scaffolding tool to students’ mental processes. ZDM—The International Journal on Mathematics Education , 47 (6) (this issue).
Rasmussen, C., Stephan, M., & Whitehead, K. (2004). Classroom mathematical practices and gesturing. Journal of Mathematical Behavior, 23 , 301–323.
Schoenfeld, A. (2000). Models of the teaching process. Journal of Mathematical Behavior, 18 (3), 243–261.
Schwarz, B. B., Kohn, A. S., & Resnick, L. B. (1993/1994). Positives about negatives: a case study of an intermediate model for signed numbers. The Journal of the Learning Sciences , 3 , 37–92. doi: 10.1207/s15327809jls0301_2 .
Shulman, L. S. (1986). Those who understand: knowledge growth in teaching. Educational Researcher, 75 (2), 4–14.
Simon, M. A. (1995). Reconstructing mathematics pedagogy from a constructivist perspective. Journal for Research in Mathematics Education, 26 , 114–145. doi: 10.2307/749205 .
Smith, J. P. (1995). The effects of a computer microworld on middle school students’ use and understanding of integers (unpublished doctoral dissertation). The Ohio State University, Columbus. http://rave.ohiolink.edu/etdc/view?acc_num=osu1248798217 .
Steffe, L., & Olive, J. (2010). Children’s fractional knowledge . New York: Springer.
Book Google Scholar
Steffe, L. P., von Glasersfeld, E., Richards, J., & Cobb, P. (1983). Children’s counting types: philosophy, theory, and application . New York: Praeger.
Stephan, M., & Akyuz, D. (2012). A proposed instructional theory for integer addition and subtraction. Journal for Research in Mathematics Education, 43 (4), 428–464.
Stephan, M., Akyuz, D., McManus, G., & Smith, J. (2012). Conditions that support the creation of a middle-school mathematics communities of learners. NCSM Journal of Mathematics Education Leadership, 14 (1), 19–27.
Stephan, M., Bowers, J., Cobb, P., & Gravemeijer K. (Eds.). (2003). Journal for Research in Mathematics Education Monograph Series: Vol. 12. Supporting students’ development of measuring conceptions: analyzing students’ learning in social context (N. Pateman, Series Ed.). Reston: National Council of Teachers of Mathematics.
Stephan, M. & Cobb, P. (2013). Teachers engaging in integer design research. In T. Plomp & N. Nieveen (Eds.), Educational design research: introduction and illustrative cases (pp. 277–298). Enschede: SLO (Netherlands institute for curriculum development).
Streefland, L. (1996). Negative numbers: reflections of a learning researcher. Journal of Mathematical Behavior, 15 , 57–79. doi: 10.1016/S0732-3123(96)90040-1 .
Thompson, P. W., & Dreyfus, T. (1988). Integers as transformations. Journal for Research in Mathematics Education, 19 , 115–133. doi: 10.2307/749406 .
Van den Heuvel-Panhuizen, M., & Drijvers, P. (in press). Realistic mathematics education. In S. Lerman (Ed.), Encyclopedia of mathematics education . Dordrecht: Springer.
Vlassis, J. (2008). The role of mathematical symbols in the development of number conceptualization: the case of the minus sign. Philosophical Psychology, 21 , 555–570. doi: 10.1080/09515080802285552 .
Download references
Author information
Authors and affiliations.
College of Education-MDSK, University of North Carolina at Charlotte, 9201 University City Boulevard, Charlotte, NC, 28223, USA
Michelle. L. Stephan
You can also search for this author in PubMed Google Scholar
Corresponding author
Correspondence to Michelle. L. Stephan .
Rights and permissions
Reprints and permissions
About this article
Stephan, M.L. Conducting classroom design research with teachers. ZDM Mathematics Education 47 , 905–917 (2015). https://doi.org/10.1007/s11858-014-0651-6
Download citation
Accepted : 15 November 2014
Published : 02 December 2014
Issue Date : October 2015
DOI : https://doi.org/10.1007/s11858-014-0651-6
Share this article
Anyone you share the following link with will be able to read this content:
Sorry, a shareable link is not currently available for this article.
Provided by the Springer Nature SharedIt content-sharing initiative
- Instructional Design
- Pedagogical Content Knowledge
- Number Line
- Lesson Study
- Learning Trajectory
- Find a journal
- Publish with us
- Track your research
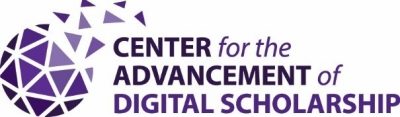
Want to create or adapt books like this? Learn more about how Pressbooks supports open publishing practices.
1 What is Action Research for Classroom Teachers?
ESSENTIAL QUESTIONS
- What is the nature of action research?
- How does action research develop in the classroom?
- What models of action research work best for your classroom?
- What are the epistemological, ontological, theoretical underpinnings of action research?
Educational research provides a vast landscape of knowledge on topics related to teaching and learning, curriculum and assessment, students’ cognitive and affective needs, cultural and socio-economic factors of schools, and many other factors considered viable to improving schools. Educational stakeholders rely on research to make informed decisions that ultimately affect the quality of schooling for their students. Accordingly, the purpose of educational research is to engage in disciplined inquiry to generate knowledge on topics significant to the students, teachers, administrators, schools, and other educational stakeholders. Just as the topics of educational research vary, so do the approaches to conducting educational research in the classroom. Your approach to research will be shaped by your context, your professional identity, and paradigm (set of beliefs and assumptions that guide your inquiry). These will all be key factors in how you generate knowledge related to your work as an educator.
Action research is an approach to educational research that is commonly used by educational practitioners and professionals to examine, and ultimately improve, their pedagogy and practice. In this way, action research represents an extension of the reflection and critical self-reflection that an educator employs on a daily basis in their classroom. When students are actively engaged in learning, the classroom can be dynamic and uncertain, demanding the constant attention of the educator. Considering these demands, educators are often only able to engage in reflection that is fleeting, and for the purpose of accommodation, modification, or formative assessment. Action research offers one path to more deliberate, substantial, and critical reflection that can be documented and analyzed to improve an educator’s practice.
Purpose of Action Research
As one of many approaches to educational research, it is important to distinguish the potential purposes of action research in the classroom. This book focuses on action research as a method to enable and support educators in pursuing effective pedagogical practices by transforming the quality of teaching decisions and actions, to subsequently enhance student engagement and learning. Being mindful of this purpose, the following aspects of action research are important to consider as you contemplate and engage with action research methodology in your classroom:
- Action research is a process for improving educational practice. Its methods involve action, evaluation, and reflection. It is a process to gather evidence to implement change in practices.
- Action research is participative and collaborative. It is undertaken by individuals with a common purpose.
- Action research is situation and context-based.
- Action research develops reflection practices based on the interpretations made by participants.
- Knowledge is created through action and application.
- Action research can be based in problem-solving, if the solution to the problem results in the improvement of practice.
- Action research is iterative; plans are created, implemented, revised, then implemented, lending itself to an ongoing process of reflection and revision.
- In action research, findings emerge as action develops and takes place; however, they are not conclusive or absolute, but ongoing (Koshy, 2010, pgs. 1-2).
In thinking about the purpose of action research, it is helpful to situate action research as a distinct paradigm of educational research. I like to think about action research as part of the larger concept of living knowledge. Living knowledge has been characterized as “a quest for life, to understand life and to create… knowledge which is valid for the people with whom I work and for myself” (Swantz, in Reason & Bradbury, 2001, pg. 1). Why should educators care about living knowledge as part of educational research? As mentioned above, action research is meant “to produce practical knowledge that is useful to people in the everyday conduct of their lives and to see that action research is about working towards practical outcomes” (Koshy, 2010, pg. 2). However, it is also about:
creating new forms of understanding, since action without reflection and understanding is blind, just as theory without action is meaningless. The participatory nature of action research makes it only possible with, for and by persons and communities, ideally involving all stakeholders both in the questioning and sense making that informs the research, and in the action, which is its focus. (Reason & Bradbury, 2001, pg. 2)
In an effort to further situate action research as living knowledge, Jean McNiff reminds us that “there is no such ‘thing’ as ‘action research’” (2013, pg. 24). In other words, action research is not static or finished, it defines itself as it proceeds. McNiff’s reminder characterizes action research as action-oriented, and a process that individuals go through to make their learning public to explain how it informs their practice. Action research does not derive its meaning from an abstract idea, or a self-contained discovery – action research’s meaning stems from the way educators negotiate the problems and successes of living and working in the classroom, school, and community.
While we can debate the idea of action research, there are people who are action researchers, and they use the idea of action research to develop principles and theories to guide their practice. Action research, then, refers to an organization of principles that guide action researchers as they act on shared beliefs, commitments, and expectations in their inquiry.
Reflection and the Process of Action Research
When an individual engages in reflection on their actions or experiences, it is typically for the purpose of better understanding those experiences, or the consequences of those actions to improve related action and experiences in the future. Reflection in this way develops knowledge around these actions and experiences to help us better regulate those actions in the future. The reflective process generates new knowledge regularly for classroom teachers and informs their classroom actions.
Unfortunately, the knowledge generated by educators through the reflective process is not always prioritized among the other sources of knowledge educators are expected to utilize in the classroom. Educators are expected to draw upon formal types of knowledge, such as textbooks, content standards, teaching standards, district curriculum and behavioral programs, etc., to gain new knowledge and make decisions in the classroom. While these forms of knowledge are important, the reflective knowledge that educators generate through their pedagogy is the amalgamation of these types of knowledge enacted in the classroom. Therefore, reflective knowledge is uniquely developed based on the action and implementation of an educator’s pedagogy in the classroom. Action research offers a way to formalize the knowledge generated by educators so that it can be utilized and disseminated throughout the teaching profession.
Research is concerned with the generation of knowledge, and typically creating knowledge related to a concept, idea, phenomenon, or topic. Action research generates knowledge around inquiry in practical educational contexts. Action research allows educators to learn through their actions with the purpose of developing personally or professionally. Due to its participatory nature, the process of action research is also distinct in educational research. There are many models for how the action research process takes shape. I will share a few of those here. Each model utilizes the following processes to some extent:
- Plan a change;
- Take action to enact the change;
- Observe the process and consequences of the change;
- Reflect on the process and consequences;
- Act, observe, & reflect again and so on.
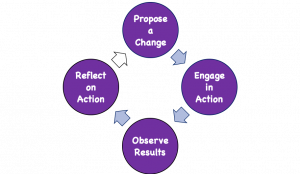
Figure 1.1 Basic action research cycle
There are many other models that supplement the basic process of action research with other aspects of the research process to consider. For example, figure 1.2 illustrates a spiral model of action research proposed by Kemmis and McTaggart (2004). The spiral model emphasizes the cyclical process that moves beyond the initial plan for change. The spiral model also emphasizes revisiting the initial plan and revising based on the initial cycle of research:
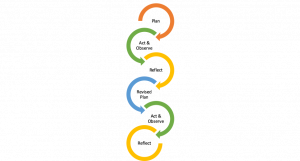
Figure 1.2 Interpretation of action research spiral, Kemmis and McTaggart (2004, p. 595)
Other models of action research reorganize the process to emphasize the distinct ways knowledge takes shape in the reflection process. O’Leary’s (2004, p. 141) model, for example, recognizes that the research may take shape in the classroom as knowledge emerges from the teacher’s observations. O’Leary highlights the need for action research to be focused on situational understanding and implementation of action, initiated organically from real-time issues:
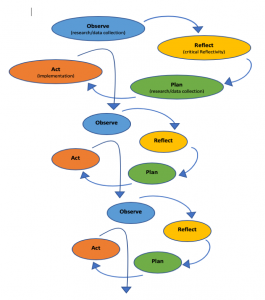
Figure 1.3 Interpretation of O’Leary’s cycles of research, O’Leary (2000, p. 141)
Lastly, Macintyre’s (2000, p. 1) model, offers a different characterization of the action research process. Macintyre emphasizes a messier process of research with the initial reflections and conclusions as the benchmarks for guiding the research process. Macintyre emphasizes the flexibility in planning, acting, and observing stages to allow the process to be naturalistic. Our interpretation of Macintyre process is below:
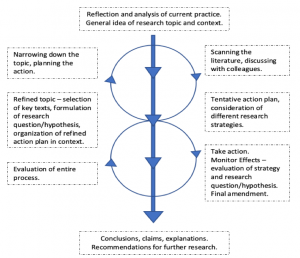
Figure 1.4 Interpretation of the action research cycle, Macintyre (2000, p. 1)
We believe it is important to prioritize the flexibility of the process, and encourage you to only use these models as basic guides for your process. Your process may look similar, or you may diverge from these models as you better understand your students, context, and data.
Definitions of Action Research and Examples
At this point, it may be helpful for readers to have a working definition of action research and some examples to illustrate the methodology in the classroom. Bassey (1998, p. 93) offers a very practical definition and describes “action research as an inquiry which is carried out in order to understand, to evaluate and then to change, in order to improve educational practice.” Cohen and Manion (1994, p. 192) situate action research differently, and describe action research as emergent, writing:
essentially an on-the-spot procedure designed to deal with a concrete problem located in an immediate situation. This means that ideally, the step-by-step process is constantly monitored over varying periods of time and by a variety of mechanisms (questionnaires, diaries, interviews and case studies, for example) so that the ensuing feedback may be translated into modifications, adjustment, directional changes, redefinitions, as necessary, so as to bring about lasting benefit to the ongoing process itself rather than to some future occasion.
Lastly, Koshy (2010, p. 9) describes action research as:
a constructive inquiry, during which the researcher constructs his or her knowledge of specific issues through planning, acting, evaluating, refining and learning from the experience. It is a continuous learning process in which the researcher learns and also shares the newly generated knowledge with those who may benefit from it.
These definitions highlight the distinct features of action research and emphasize the purposeful intent of action researchers to improve, refine, reform, and problem-solve issues in their educational context. To better understand the distinctness of action research, these are some examples of action research topics:
Examples of Action Research Topics
- Flexible seating in 4th grade classroom to increase effective collaborative learning.
- Structured homework protocols for increasing student achievement.
- Developing a system of formative feedback for 8th grade writing.
- Using music to stimulate creative writing.
- Weekly brown bag lunch sessions to improve responses to PD from staff.
- Using exercise balls as chairs for better classroom management.
Action Research in Theory
Action research-based inquiry in educational contexts and classrooms involves distinct participants – students, teachers, and other educational stakeholders within the system. All of these participants are engaged in activities to benefit the students, and subsequently society as a whole. Action research contributes to these activities and potentially enhances the participants’ roles in the education system. Participants’ roles are enhanced based on two underlying principles:
- communities, schools, and classrooms are sites of socially mediated actions, and action research provides a greater understanding of self and new knowledge of how to negotiate these socially mediated environments;
- communities, schools, and classrooms are part of social systems in which humans interact with many cultural tools, and action research provides a basis to construct and analyze these interactions.
In our quest for knowledge and understanding, we have consistently analyzed human experience over time and have distinguished between types of reality. Humans have constantly sought “facts” and “truth” about reality that can be empirically demonstrated or observed.
Social systems are based on beliefs, and generally, beliefs about what will benefit the greatest amount of people in that society. Beliefs, and more specifically the rationale or support for beliefs, are not always easy to demonstrate or observe as part of our reality. Take the example of an English Language Arts teacher who prioritizes argumentative writing in her class. She believes that argumentative writing demonstrates the mechanics of writing best among types of writing, while also providing students a skill they will need as citizens and professionals. While we can observe the students writing, and we can assess their ability to develop a written argument, it is difficult to observe the students’ understanding of argumentative writing and its purpose in their future. This relates to the teacher’s beliefs about argumentative writing; we cannot observe the real value of the teaching of argumentative writing. The teacher’s rationale and beliefs about teaching argumentative writing are bound to the social system and the skills their students will need to be active parts of that system. Therefore, our goal through action research is to demonstrate the best ways to teach argumentative writing to help all participants understand its value as part of a social system.
The knowledge that is conveyed in a classroom is bound to, and justified by, a social system. A postmodernist approach to understanding our world seeks knowledge within a social system, which is directly opposed to the empirical or positivist approach which demands evidence based on logic or science as rationale for beliefs. Action research does not rely on a positivist viewpoint to develop evidence and conclusions as part of the research process. Action research offers a postmodernist stance to epistemology (theory of knowledge) and supports developing questions and new inquiries during the research process. In this way action research is an emergent process that allows beliefs and decisions to be negotiated as reality and meaning are being constructed in the socially mediated space of the classroom.
Theorizing Action Research for the Classroom
All research, at its core, is for the purpose of generating new knowledge and contributing to the knowledge base of educational research. Action researchers in the classroom want to explore methods of improving their pedagogy and practice. The starting place of their inquiry stems from their pedagogy and practice, so by nature the knowledge created from their inquiry is often contextually specific to their classroom, school, or community. Therefore, we should examine the theoretical underpinnings of action research for the classroom. It is important to connect action research conceptually to experience; for example, Levin and Greenwood (2001, p. 105) make these connections:
- Action research is context bound and addresses real life problems.
- Action research is inquiry where participants and researchers cogenerate knowledge through collaborative communicative processes in which all participants’ contributions are taken seriously.
- The meanings constructed in the inquiry process lead to social action or these reflections and action lead to the construction of new meanings.
- The credibility/validity of action research knowledge is measured according to whether the actions that arise from it solve problems (workability) and increase participants’ control over their own situation.
Educators who engage in action research will generate new knowledge and beliefs based on their experiences in the classroom. Let us emphasize that these are all important to you and your work, as both an educator and researcher. It is these experiences, beliefs, and theories that are often discounted when more official forms of knowledge (e.g., textbooks, curriculum standards, districts standards) are prioritized. These beliefs and theories based on experiences should be valued and explored further, and this is one of the primary purposes of action research in the classroom. These beliefs and theories should be valued because they were meaningful aspects of knowledge constructed from teachers’ experiences. Developing meaning and knowledge in this way forms the basis of constructivist ideology, just as teachers often try to get their students to construct their own meanings and understandings when experiencing new ideas.
Classroom Teachers Constructing their Own Knowledge
Most of you are probably at least minimally familiar with constructivism, or the process of constructing knowledge. However, what is constructivism precisely, for the purposes of action research? Many scholars have theorized constructivism and have identified two key attributes (Koshy, 2010; von Glasersfeld, 1987):
- Knowledge is not passively received, but actively developed through an individual’s cognition;
- Human cognition is adaptive and finds purpose in organizing the new experiences of the world, instead of settling for absolute or objective truth.
Considering these two attributes, constructivism is distinct from conventional knowledge formation because people can develop a theory of knowledge that orders and organizes the world based on their experiences, instead of an objective or neutral reality. When individuals construct knowledge, there are interactions between an individual and their environment where communication, negotiation and meaning-making are collectively developing knowledge. For most educators, constructivism may be a natural inclination of their pedagogy. Action researchers have a similar relationship to constructivism because they are actively engaged in a process of constructing knowledge. However, their constructions may be more formal and based on the data they collect in the research process. Action researchers also are engaged in the meaning making process, making interpretations from their data. These aspects of the action research process situate them in the constructivist ideology. Just like constructivist educators, action researchers’ constructions of knowledge will be affected by their individual and professional ideas and values, as well as the ecological context in which they work (Biesta & Tedder, 2006). The relations between constructivist inquiry and action research is important, as Lincoln (2001, p. 130) states:
much of the epistemological, ontological, and axiological belief systems are the same or similar, and methodologically, constructivists and action researchers work in similar ways, relying on qualitative methods in face-to-face work, while buttressing information, data and background with quantitative method work when necessary or useful.
While there are many links between action research and educators in the classroom, constructivism offers the most familiar and practical threads to bind the beliefs of educators and action researchers.
Epistemology, Ontology, and Action Research
It is also important for educators to consider the philosophical stances related to action research to better situate it with their beliefs and reality. When researchers make decisions about the methodology they intend to use, they will consider their ontological and epistemological stances. It is vital that researchers clearly distinguish their philosophical stances and understand the implications of their stance in the research process, especially when collecting and analyzing their data. In what follows, we will discuss ontological and epistemological stances in relation to action research methodology.
Ontology, or the theory of being, is concerned with the claims or assumptions we make about ourselves within our social reality – what do we think exists, what does it look like, what entities are involved and how do these entities interact with each other (Blaikie, 2007). In relation to the discussion of constructivism, generally action researchers would consider their educational reality as socially constructed. Social construction of reality happens when individuals interact in a social system. Meaningful construction of concepts and representations of reality develop through an individual’s interpretations of others’ actions. These interpretations become agreed upon by members of a social system and become part of social fabric, reproduced as knowledge and beliefs to develop assumptions about reality. Researchers develop meaningful constructions based on their experiences and through communication. Educators as action researchers will be examining the socially constructed reality of schools. In the United States, many of our concepts, knowledge, and beliefs about schooling have been socially constructed over the last hundred years. For example, a group of teachers may look at why fewer female students enroll in upper-level science courses at their school. This question deals directly with the social construction of gender and specifically what careers females have been conditioned to pursue. We know this is a social construction in some school social systems because in other parts of the world, or even the United States, there are schools that have more females enrolled in upper level science courses than male students. Therefore, the educators conducting the research have to recognize the socially constructed reality of their school and consider this reality throughout the research process. Action researchers will use methods of data collection that support their ontological stance and clarify their theoretical stance throughout the research process.
Koshy (2010, p. 23-24) offers another example of addressing the ontological challenges in the classroom:
A teacher who was concerned with increasing her pupils’ motivation and enthusiasm for learning decided to introduce learning diaries which the children could take home. They were invited to record their reactions to the day’s lessons and what they had learnt. The teacher reported in her field diary that the learning diaries stimulated the children’s interest in her lessons, increased their capacity to learn, and generally improved their level of participation in lessons. The challenge for the teacher here is in the analysis and interpretation of the multiplicity of factors accompanying the use of diaries. The diaries were taken home so the entries may have been influenced by discussions with parents. Another possibility is that children felt the need to please their teacher. Another possible influence was that their increased motivation was as a result of the difference in style of teaching which included more discussions in the classroom based on the entries in the dairies.
Here you can see the challenge for the action researcher is working in a social context with multiple factors, values, and experiences that were outside of the teacher’s control. The teacher was only responsible for introducing the diaries as a new style of learning. The students’ engagement and interactions with this new style of learning were all based upon their socially constructed notions of learning inside and outside of the classroom. A researcher with a positivist ontological stance would not consider these factors, and instead might simply conclude that the dairies increased motivation and interest in the topic, as a result of introducing the diaries as a learning strategy.
Epistemology, or the theory of knowledge, signifies a philosophical view of what counts as knowledge – it justifies what is possible to be known and what criteria distinguishes knowledge from beliefs (Blaikie, 1993). Positivist researchers, for example, consider knowledge to be certain and discovered through scientific processes. Action researchers collect data that is more subjective and examine personal experience, insights, and beliefs.
Action researchers utilize interpretation as a means for knowledge creation. Action researchers have many epistemologies to choose from as means of situating the types of knowledge they will generate by interpreting the data from their research. For example, Koro-Ljungberg et al., (2009) identified several common epistemologies in their article that examined epistemological awareness in qualitative educational research, such as: objectivism, subjectivism, constructionism, contextualism, social epistemology, feminist epistemology, idealism, naturalized epistemology, externalism, relativism, skepticism, and pluralism. All of these epistemological stances have implications for the research process, especially data collection and analysis. Please see the table on pages 689-90, linked below for a sketch of these potential implications:
Again, Koshy (2010, p. 24) provides an excellent example to illustrate the epistemological challenges within action research:
A teacher of 11-year-old children decided to carry out an action research project which involved a change in style in teaching mathematics. Instead of giving children mathematical tasks displaying the subject as abstract principles, she made links with other subjects which she believed would encourage children to see mathematics as a discipline that could improve their understanding of the environment and historic events. At the conclusion of the project, the teacher reported that applicable mathematics generated greater enthusiasm and understanding of the subject.
The educator/researcher engaged in action research-based inquiry to improve an aspect of her pedagogy. She generated knowledge that indicated she had improved her students’ understanding of mathematics by integrating it with other subjects – specifically in the social and ecological context of her classroom, school, and community. She valued constructivism and students generating their own understanding of mathematics based on related topics in other subjects. Action researchers working in a social context do not generate certain knowledge, but knowledge that emerges and can be observed and researched again, building upon their knowledge each time.
Researcher Positionality in Action Research
In this first chapter, we have discussed a lot about the role of experiences in sparking the research process in the classroom. Your experiences as an educator will shape how you approach action research in your classroom. Your experiences as a person in general will also shape how you create knowledge from your research process. In particular, your experiences will shape how you make meaning from your findings. It is important to be clear about your experiences when developing your methodology too. This is referred to as researcher positionality. Maher and Tetreault (1993, p. 118) define positionality as:
Gender, race, class, and other aspects of our identities are markers of relational positions rather than essential qualities. Knowledge is valid when it includes an acknowledgment of the knower’s specific position in any context, because changing contextual and relational factors are crucial for defining identities and our knowledge in any given situation.
By presenting your positionality in the research process, you are signifying the type of socially constructed, and other types of, knowledge you will be using to make sense of the data. As Maher and Tetreault explain, this increases the trustworthiness of your conclusions about the data. This would not be possible with a positivist ontology. We will discuss positionality more in chapter 6, but we wanted to connect it to the overall theoretical underpinnings of action research.
Advantages of Engaging in Action Research in the Classroom
In the following chapters, we will discuss how action research takes shape in your classroom, and we wanted to briefly summarize the key advantages to action research methodology over other types of research methodology. As Koshy (2010, p. 25) notes, action research provides useful methodology for school and classroom research because:
Advantages of Action Research for the Classroom
- research can be set within a specific context or situation;
- researchers can be participants – they don’t have to be distant and detached from the situation;
- it involves continuous evaluation and modifications can be made easily as the project progresses;
- there are opportunities for theory to emerge from the research rather than always follow a previously formulated theory;
- the study can lead to open-ended outcomes;
- through action research, a researcher can bring a story to life.
Action Research Copyright © by J. Spencer Clark; Suzanne Porath; Julie Thiele; and Morgan Jobe is licensed under a Creative Commons Attribution-NonCommercial 4.0 International License , except where otherwise noted.
Share This Book

Classrooms by design
Research-based primary classroom design.
However often you have set up a new classroom, according to Kate McCallam it’s worth pausing to consider new research about the impact of classroom design on learning first.
It’s classroom set-up time
Love it or loathe it, as we start this new school year, if you are a primary or Early Years teacher, you’ve probably spent the latter part of your summer holiday on the Great Interior Design Challenge that is your classroom. Whether you favour the traditional palette of bright, primary colours or you’ve joined the revolution and gone for muted, neutral tones and swathes of hessian, you probably know what you like and have designed it that way.
Impact of physical spaces on learning – the research
You might be interested to know, however, that there is now research to show that the way you design your classroom has a significant impact on learning. Before you get too busy with that staple-gun again, it’s worth considering what this research says about what impacts learning positively.
‘ Clever Classrooms ’ a study by Emeritus Professor Peter Barrett of the University of Salford [1] found that ‘there is clear evidence that the physical characteristics of primary schools do impact on pupils’ learning in reading, writing and mathematics, explaining 16% of the variation in the overall progress over a year of the 3,766 pupils in 27 UK primary schools who took part in the study in the study.’
Ten classroom design tips
Professor Barrett’s paper is an interesting read that I highly recommend, but he has also, and very helpfully helpfully produced a fantastic summary with 10 research-backed tips for time-poor teachers considering classroom design. Very briefly, this is what he advises:
- Maximise daylight
- Ensure adequate ventilation
- Control the temperature
- Choose the right level of flexibility
- Engender ownership
- Manage the visual complexity
- Use colour carefully
- Attack on all fronts!
- Don’t assume a ‘good school’ means a ‘good classroom’
- Remember to see the classroom as another teaching tool
I think given Covid restrictions in the past 12 months we can all safely say that we’ve well and truly done Tip 2!
Voice, Choice, Agency
Although all the tips are equally important, I am going to focus on Tip 5, Engendering Ownership , as this is something I really focused on at the beginning of the last school year. I learnt a lot about it from a brilliant PYP course – Making the PYP Happen. As many of you will know, much of the PYP centres around Voice, Choice and Agency with a particular focus on Learning Environments:
‘Teachers co-construct learning spaces with students, providing voice, choice and a sense of ownership. This supports well-being, a sense of familiarity and belonging, and pleasure in inhabiting those spaces, for teachers and students alike.’ [2]
Less is more
So, in terms of the ‘co-construct’, the first thing I did was just less. I deliberately left areas blank, was mindful of colour and took into account the 20-50% of clear wall space that Professor Barrett advocates. There are some teachers out there who hate displays and challenge their effectiveness in supporting learning at all, so having the evidence that 50% blank wall space ‘works’ will no doubt be music to their ears. As primary school teachers we tend to want to engage and excite with our rooms, but if we take a step back and think about the spaces in which we like to learn as adults, I’d bet the vast majority of us favour a more minimalist and open environment. It therefore makes sense to apply this to the learning environments of our children.
First week back
I like to dedicate quite a bit of time in the first week back discussing with my Year 6 (Grade 5) class that their opinion matters and that together we will create a learning environment that suits us all. They are normally brimming with ideas.
Last year, I admit, I couldn’t quite get on board with the disco ball or find the money to finance the mini-fridge they were after, but there are normally plenty of ideas we can agree on as a class. Here are some suggestions from last year’s cohort that we agreed on and implemented in our collaborative classroom.
- Cinnamon air freshener – (Marks and Spencer – they loved this and very helpful throughout the year for accidental smells!))
- History Ti meline so they could see what came when (this is good idea to link to prior learning)
- Treat Box (a positive attribute decided on each week.Vote anonymously for person most displaying attribute. Prize for winner) They loved this.
- A cactus named Spike!
- House Posters of Prominent People – we have Romans, Saxons, Vikings and Normans and willing members of the class designed a poster of a famous person from their House with a little biography on them for our House Points board
- Welcome Sign for classroom door
- Uplifting Quotes
- Worry box/jar/questions
- Post-its in trays ‘so we don’t have to write on hands to remember things’
This might not seem like a lot, but it is a really powerful exercise and did so much at the beginning of the school year to identify ‘us’ as a class. From this point, we were able to consider class rules and routines.

Door Sign Poster created in Canva by a pupil in my class
IT skills and posters
The posters and signage are a great way for pupils using their IT skills for purpose and getting creative. Even something as simple as asking them to design classroom banners for Google Classroom is a positive step in encouraging ownership and collaboration.
Designing your room together really is a great exercise, but however often you have done it, taking a little time to look at the research and to tweak your approach accordingly can and does make a difference to learning in the course of the new year.

Her website https://www.lovewriting4kids.com / provides KS2 teachers with free modelled texts and lesson ideas.
Get in touch or follow Kate on Twitter @ChattyStaffroom
[1] https://www.cleverclassroomsdesign.co.uk
[2] IB PYP – Making the PYP Happen – Implementing Agency
FEATURE IMAGE: by klimkin from Pixabay
You may also like

Ask the author

British and International Schools Library Network
Academia.edu no longer supports Internet Explorer.
To browse Academia.edu and the wider internet faster and more securely, please take a few seconds to upgrade your browser .
Enter the email address you signed up with and we'll email you a reset link.
- We're Hiring!
- Help Center
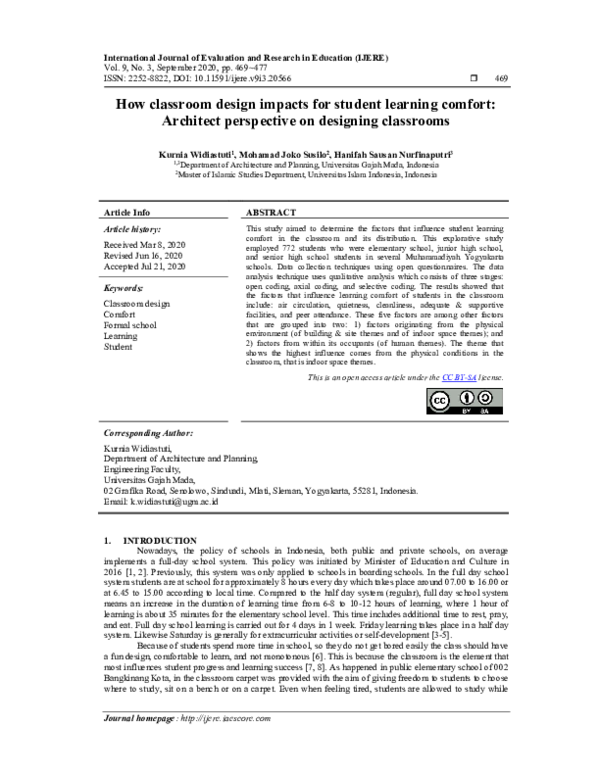
How classroom design impacts for student learning comfort: Architect perspective on designing classrooms

International Journal of Evaluation and Research in Education (IJERE)
This study aimed to determine the factors that influence student learning comfort in the classroom and its distribution. This explorative study employed 772 students who were elementary school, junior high school, and senior high school students in several Muhammadiyah Yogyakarta schools. Data collection techniques using open questionnaires. The data analysis technique uses qualitative analysis which consists of three stages: open coding, axial coding, and selective coding. The results showed that the factors that influence learning comfort of students in the classroom include: air circulation, quietness, cleanliness, adequate & supportive facilities, and peer attendance. These five factors are among other factors that are grouped into two: 1) factors originating from the physical environment (of building & site themes and of indoor space themes); and 2) factors from within its occupants (of human themes). The theme that shows the highest influence comes from the physical conditions...
Related Papers
School space plays an essential role in creating a pleasurable learning atmosphere. The tendency of everyone to choose a school space also varies. By knowing this trend pattern, schools can be designed to improve student learning effectiveness. The purpose of this study was to find out which school spaces students choose to study, what kind of room criteria are needed, and distribution patterns of students' preference choices. This research used both the qualitative exploratory and quantitative methods using an open-ended question questionnaire for data collection. Data analysis techniques used qualitative analysis methods consisting of open coding, axial coding, and selective coding. The results showed that the library, mosque, and multimedia laboratory were the most preferred space for students to study at school. Some factors that influence the selection include thermal comfort, completeness of supporting facilities, and acoustic comfort.
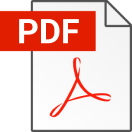
SCRI Research Report Series
Peter Barrett
Applied Sciences
Khairul Nizam Abdul Maulud
The indoor environmental aspects of classrooms in secondary school buildings need to be determined to ensure that they meet the users’ basic requirements. Students’ efficiency and learning productivity can be affected if the classroom’s indoor environment is of poor quality. The question raised here: how can we ensure that the comfort level provided to building users in terms of indoor aspects is up to their satisfaction? Post Occupancy Evaluation (POE) is an instrument to examine the success of building design and performance after occupancy. It indicates users’ satisfaction and comfort level related with the indoor environment. Considering users as a benchmark, there is a large potential for improvement in buildings’ indoor environmental aspects. As reflected by the title, the study’s main purpose is to evaluate students’ satisfaction and perception of their classrooms’ comfort level along with recommendations to enhance the quality of their indoor environment. The survey method a...
romeo puyat
Axel rome Puyat
Introduction The 1997 renovation of the Charles Young Hill Top Academy in the District of Columbia is a classic illustration of how an improved school environment contributes to higher levels of educational performance. This case illustrates the connection between environmental quality, comfort, health and well-being, positive attitudes and behavior, and higher levels of educational performance. This case shows that aging city schools do not have to be abandoned; they can be successfully revitalized and made contribute effectively to the process of education. Regardless of where a school is located, a healthy school environment is comfortable and secure from danger radiates a "sense of wellbeing" and a sends a caring message. These healthy school environments are the key to a high performance educational institution. Successfully managing a school environment is a necessary and essential educational investment. Research increasingly shows that there is a clear link between environmental quality of schools and educational performance: • Facility management systems determine environmental quality in schools. • The quality of the school environment shapes attitudes of students, teachers and staff. • Attitudes affect teaching and learning behavior. • Behavior affects performance. • Educational performance determines future outcomes of individuals and society as a whole. In preparing this case, a variety of information and data were examined that were provided by an extensive review of educational facility publications, the Charles Young Elementary School, the University of North Carolina Environmental Studies Program, the US Environmental Protection Agency, the District of Columbia, and the Carpet and Rug Institute. The key findings of the work start with the identifiable and measurable environmental conditions required of all high performance schools and the basic finding that an academically successful school must radiate a sense of well-being which is the essence of health. The information gathered for this case study clearly indicates there must be a serious, if not passionate, desire accompanied by positive action, to restore non-performing schools to a constantly healthy state. Effective restoration is achieved through good design that addresses total environmental quality to include general sanitation, good air quality, noise control, lighting and glare reduction,
mohamed matboully
Jeffrey Edwards
The various environmental factors that occur within a Sunday School classroom setting has a direct relationship with the ability of the adult student to learn biblical truths.
Lisa Heschong
Lorato Motsatsi
Ihab Elzeyadi
RELATED PAPERS
Hadil Al Bustami
Advances in Civil Engineering
Dr. Nishant Raj Kapoor
Energy Procedia
Mounir Asmar
Building and Environment
DR. EMMA MARINIE AHMAD ZAWAWI
Dominique Hes
INTED2017 Proceedings
Prof.Eziyi Ibem
Journal of Physics: Conference Series
Siswo Wardoyo
Journal of Civil Engineering and Architecture
Journal of Civil Engineering and Architecture DPC
Nextgen Research Publication
Nur Samancioglu
Stella Koci
Sustainability
Rokhshid Ghaziani
IPTEK Journal of Proceedings Series
Rani Prihatmanti
Dr Jeremy Gibberd
Rotraut Walden , Henry Sanoff
Jill Loughlin
International Journal of Academic Research in Progressive Education and Development
Mohd Hazwan Mohd Puad
Halil Alibaba
Garcia Aubrey John
Hikari Kahyee
Haşim Altan
Journal of Education and Practice
Christiana Kudawe
Publisher ijmra.us UGC Approved
MATEC Web of Conferences
Naziah salleh
IJEFMS An Open Access Journal
Sustainable Built Environments
Charlene Bayer
Procedia - Social and Behavioral Sciences
Prima Vitasari
Anisah Madden
Eva Latipah Latipah
EPI International Journal of Engineering
Nimah Natsir
Claire Wolstenholme
Godfrey Udo
Journal of Construction Engineering, Management & Innovation
Gökçe Nihan Taşkın
Retrofitting Passive Cooling Strategies to a Brisbane School: A Case Study of the Physical and Social Aspects
RELATED TOPICS
- We're Hiring!
- Help Center
- Find new research papers in:
- Health Sciences
- Earth Sciences
- Cognitive Science
- Mathematics
- Computer Science
- Academia ©2024

Scientific Research in Education (2002)
Chapter: 5 designs for the conduct of scientific research in education, 5 designs for the conduct of scientific research in education.
The salient features of education delineated in Chapter 4 and the guiding principles of scientific research laid out in Chapter 3 set boundaries for the design and conduct of scientific education research. Thus, the design of a study (e.g., randomized experiment, ethnography, multiwave survey) does not itself make it scientific. However, if the design directly addresses a question that can be addressed empirically, is linked to prior research and relevant theory, is competently implemented in context, logically links the findings to interpretation ruling out counterinterpretations, and is made accessible to scientific scrutiny, it could then be considered scientific. That is: Is there a clear set of questions underlying the design? Are the methods appropriate to answer the questions and rule out competing answers? Does the study take previous research into account? Is there a conceptual basis? Are data collected in light of local conditions and analyzed systematically? Is the study clearly described and made available for criticism? The more closely aligned it is with these principles, the higher the quality of the scientific study. And the particular features of education require that the research process be explicitly designed to anticipate the implications of these features and to model and plan accordingly.
RESEARCH DESIGN
Our scientific principles include research design—the subject of this chapter—as but one aspect of a larger process of rigorous inquiry. How-
ever, research design (and corresponding scientific methods) is a crucial aspect of science. It is also the subject of much debate in many fields, including education. In this chapter, we describe some of the most frequently used and trusted designs for scientifically addressing broad classes of research questions in education.
In doing so, we develop three related themes. First, as we posit earlier, a variety of legitimate scientific approaches exist in education research. Therefore, the description of methods discussed in this chapter is illustrative of a range of trusted approaches; it should not be taken as an authoritative list of tools to the exclusion of any others. 1 As we stress in earlier chapters, the history of science has shown that research designs evolve, as do the questions they address, the theories they inform, and the overall state of knowledge.
Second, we extend the argument we make in Chapter 3 that designs and methods must be carefully selected and implemented to best address the question at hand. Some methods are better than others for particular purposes, and scientific inferences are constrained by the type of design employed. Methods that may be appropriate for estimating the effect of an educational intervention, for example, would rarely be appropriate for use in estimating dropout rates. While researchers—in education or any other field—may overstate the conclusions from an inquiry, the strength of scientific inference must be judged in terms of the design used to address the question under investigation. A comprehensive explication of a hierarchy of appropriate designs and analytic approaches under various conditions would require a depth of treatment found in research methods textbooks. This is not our objective. Rather, our goal is to illustrate that among available techniques, certain designs are better suited to address particular kinds of questions under particular conditions than others.
Third, in order to generate a rich source of scientific knowledge in education that is refined and revised over time, different types of inquiries and methods are required. At any time, the types of questions and methods depend in large part on an accurate assessment of the overall state of knowl-
edge and professional judgment about how a particular line of inquiry could advance understanding. In areas with little prior knowledge, for example, research will generally need to involve careful description to formulate initial ideas. In such situations, descriptive studies might be undertaken to help bring education problems or trends into sharper relief or to generate plausible theories about the underlying structure of behavior or learning. If the effects of education programs that have been implemented on a large scale are to be understood, however, investigations must be designed to test a set of causal hypotheses. Thus, while we treat the topic of design in this chapter as applying to individual studies, research design has a broader quality as it relates to lines of inquiry that develop over time.
While a full development of these notions goes considerably beyond our charge, we offer this brief overview to place the discussion of methods that follows into perspective. Also, in the concluding section of this chapter, we make a few targeted suggestions for the kinds of work we believe are most needed in education research to make further progress toward robust knowledge.
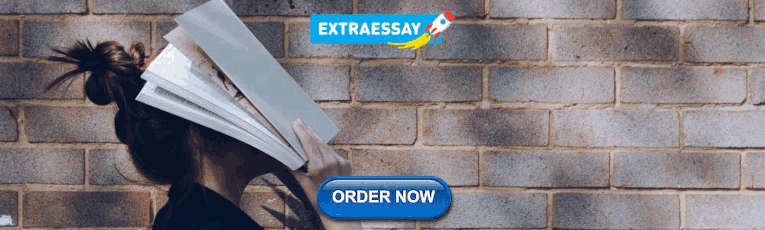
TYPES OF RESEARCH QUESTIONS
In discussing design, we have to be true to our admonition that the research question drives the design, not vice versa. To simplify matters, the committee recognized that a great number of education research questions fall into three (interrelated) types: description—What is happening? cause—Is there a systematic effect? and process or mechanism—Why or how is it happening?
The first question—What is happening?—invites description of various kinds, so as to properly characterize a population of students, understand the scope and severity of a problem, develop a theory or conjecture, or identify changes over time among different educational indicators—for example, achievement, spending, or teacher qualifications. Description also can include associations among variables, such as the characteristics of schools (e.g., size, location, economic base) that are related to (say) the provision of music and art instruction. The second question is focused on establishing causal effects: Does x cause y ? The search for cause, for example,
can include seeking to understand the effect of teaching strategies on student learning or state policy changes on district resource decisions. The third question confronts the need to understand the mechanism or process by which x causes y . Studies that seek to model how various parts of a complex system—like U.S. education—fit together help explain the conditions that facilitate or impede change in teaching, learning, and schooling. Within each type of question, we separate the discussion into subsections that show the use of different methods given more fine-grained goals and conditions of an inquiry.
Although for ease of discussion we treat these types of questions separately, in practice they are closely related. As our examples show, within particular studies, several kinds of queries can be addressed. Furthermore, various genres of scientific education research often address more than one of these types of questions. Evaluation research—the rigorous and systematic evaluation of an education program or policy—exemplifies the use of multiple questions and corresponding designs. As applied in education, this type of scientific research is distinguished from other scientific research by its purpose: to contribute to program improvement (Weiss, 1998a). Evaluation often entails an assessment of whether the program caused improvements in the outcome or outcomes of interest (Is there a systematic effect?). It also can involve detailed descriptions of the way the program is implemented in practice and in what contexts ( What is happening? ) and the ways that program services influence outcomes (How is it happening?).
Throughout the discussion, we provide several examples of scientific education research, connecting them to scientific principles ( Chapter 3 ) and the features of education ( Chapter 4 ). We have chosen these studies because they align closely with several of the scientific principles. These examples include studies that generate hypotheses or conjectures as well as those that test them. Both tasks are essential to science, but as a general rule they cannot be accomplished simultaneously.
Moreover, just as we argue that the design of a study does not itself make it scientific, an investigation that seeks to address one of these questions is not necessarily scientific either. For example, many descriptive studies—however useful they may be—bear little resemblance to careful scientific study. They might record observations without any clear conceptual viewpoint, without reproducible protocols for recording data, and so
forth. Again, studies may be considered scientific by assessing the rigor with which they meet scientific principles and are designed to account for the context of the study.
Finally, we have tended to speak of research in terms of a simple dichotomy— scientific or not scientific—but the reality is more complicated. Individual research projects may adhere to each of the principles in varying degrees, and the extent to which they meet these goals goes a long way toward defining the scientific quality of a study. For example, while all scientific studies must pose clear questions that can be investigated empirically and be grounded in existing knowledge, more rigorous studies will begin with more precise statements of the underlying theory driving the inquiry and will generally have a well-specified hypothesis before the data collection and testing phase is begun. Studies that do not start with clear conceptual frameworks and hypotheses may still be scientific, although they are obviously at a more rudimentary level and will generally require follow-on study to contribute significantly to scientific knowledge.
Similarly, lines of research encompassing collections of studies may be more or less productive and useful in advancing knowledge. An area of research that, for example, does not advance beyond the descriptive phase toward more precise scientific investigation of causal effects and mechanisms for a long period of time is clearly not contributing as much to knowledge as one that builds on prior work and moves toward more complete understanding of the causal structure. This is not to say that descriptive work cannot generate important breakthroughs. However, the rate of progress should—as we discuss at the end of this chapter—enter into consideration of the support for advanced lines of inquiry. The three classes of questions we discuss in the remainder of this chapter are ordered in a way that reflects the sequence that research studies tend to follow as well as their interconnected nature.
WHAT IS HAPPENING?
Answers to “What is happening?” questions can be found by following Yogi Berra’s counsel in a systematic way: if you want to know what’s going on, you have to go out and look at what is going on. Such inquiries are descriptive. They are intended to provide a range of information from
documenting trends and issues in a range of geopolitical jurisdictions, populations, and institutions to rich descriptions of the complexities of educational practice in a particular locality, to relationships among such elements as socioeconomic status, teacher qualifications, and achievement.
Estimates of Population Characteristics
Descriptive scientific research in education can make generalizable statements about the national scope of a problem, student achievement levels across the states, or the demographics of children, teachers, or schools. Methods that enable the collection of data from a randomly selected sample of the population provide the best way of addressing such questions. Questionnaires and telephone interviews are common survey instruments developed to gather information from a representative sample of some population of interest. Policy makers at the national, state, and sometimes district levels depend on this method to paint a picture of the educational landscape. Aggregate estimates of the academic achievement level of children at the national level (e.g., National Center for Education Statistics [NCES], National Assessment of Educational Progress [NAEP]), the supply, demand, and turnover of teachers (e.g., NCES Schools and Staffing Survey), the nation’s dropout rates (e.g., NCES Common Core of Data), how U.S. children fare on tests of mathematics and science achievement relative to children in other nations (e.g., Third International Mathematics and Science Study) and the distribution of doctorate degrees across the nation (e.g., National Science Foundation’s Science and Engineering Indicators) are all based on surveys from populations of school children, teachers, and schools.
To yield credible results, such data collection usually depends on a random sample (alternatively called a probability sample) of the target population. If every observation (e.g., person, school) has a known chance of being selected into the study, researchers can make estimates of the larger population of interest based on statistical technology and theory. The validity of inferences about population characteristics based on sample data depends heavily on response rates, that is, the percentage of those randomly selected for whom data are collected. The measures used must have known reliability—that is, the extent to which they reproduce results. Finally, the value of a data collection instrument hinges not only on the
sampling method, participation rate, and reliability, but also on their validity: that the questionnaire or survey items measure what they are supposed to measure.
The NAEP survey tracks national trends in student achievement across several subject domains and collects a range of data on school, student, and teacher characteristics (see Box 5-1 ). This rich source of information enables several kinds of descriptive work. For example, researchers can estimate the average score of eighth graders on the mathematics assessment (i.e., measures of central tendency) and compare that performance to prior years. Part of the study we feature (see below) about college women’s career choices featured a similar estimation of population characteristics. In that study, the researchers developed a survey to collect data from a representative sample of women at the two universities to aid them in assessing the generalizability of their findings from the in-depth studies of the 23 women.
Simple Relationships
The NAEP survey also illustrates how researchers can describe patterns of relationships between variables. For example, NCES reports that in 2000, eighth graders whose teachers majored in mathematics or mathematics education scored higher, on average, than did students whose teachers did not major in these fields (U.S. Department of Education, 2000). This finding is the result of descriptive work that explores the correlation between variables: in this case, the relationship between student mathematics performance and their teachers’ undergraduate major.
Such associations cannot be used to infer cause. However, there is a common tendency to make unsubstantiated jumps from establishing a relationship to concluding cause. As committee member Paul Holland quipped during the committee’s deliberations, “Casual comparisons inevitably invite careless causal conclusions.” To illustrate the problem with drawing causal inferences from simple correlations, we use an example from work that compares Catholic schools to public schools. We feature this study later in the chapter as one that competently examines causal mechanisms. Before addressing questions of mechanism, foundational work involved simple correlational results that compared the performance of Catholic high school students on standardized mathematics tests with their
counterparts in public schools. These simple correlations revealed that average mathematics achievement was considerably higher for Catholic school students than for public school students (Bryk, Lee, and Holland, 1993). However, the researchers were careful not to conclude from this analysis that attending a Catholic school causes better student outcomes, because there are a host of potential explanations (other than attending a Catholic school) for this relationship between school type and achievement. For example, since Catholic schools can screen children for aptitude, they may have a more able student population than public schools at the outset. (This is an example of the classic selectivity bias that commonly threatens the validity of causal claims in nonrandomized studies; we return to this issue in the next section.) In short, there are other hypotheses that could explain the observed differences in achievement between students in different sectors that must be considered systematically in assessing the potential causal relationship between Catholic schooling and student outcomes.
Descriptions of Localized Educational Settings
In some cases, scientists are interested in the fine details (rather than the distribution or central tendency) of what is happening in a particular organization, group of people, or setting. This type of work is especially important when good information about the group or setting is non-existent or scant. In this type of research, then, it is important to obtain first-hand, in-depth information from the particular focal group or site. For such purposes, selecting a random sample from the population of interest may not be the proper method of choice; rather, samples may be purposively selected to illuminate phenomena in depth. 2 For example, to better understand a high-achieving school in an urban setting with children of predominantly low socioeconomic status, a researcher might conduct a detailed case study or an ethnographic study (a case study with a focus on culture) of such a school (Yin and White, 1986; Miles and Huberman,
1994). This type of scientific description can provide rich depictions of the policies, procedures, and contexts in which the school operates and generate plausible hypotheses about what might account for its success. Researchers often spend long periods of time in the setting or group in order to understand what decisions are made, what beliefs and attitudes are formed, what relationships are developed, and what forms of success are celebrated. These descriptions, when used in conjunction with causal methods, are often critical to understand such educational outcomes as student achievement because they illuminate key contextual factors.
Box 5-2 provides an example of a study that described in detail (and also modeled several possible mechanisms; see later discussion) a small group of women, half who began their college careers in science and half in what were considered more traditional majors for women. This descriptive part of the inquiry involved an ethnographic study of the lives of 23 first-year women enrolled in two large universities.
Scientific description of this type can generate systematic observations about the focal group or site, and patterns in results may be generalizable to other similar groups or sites or for the future. As with any other method, a scientifically rigorous case study has to be designed to address the research question it addresses. That is, the investigator has to choose sites, occasions, respondents, and times with a clear research purpose in mind and be sensitive to his or her own expectations and biases (Maxwell, 1996; Silverman, 1993). Data should typically be collected from varied sources, by varied methods, and corroborated by other investigators. Furthermore, the account of the case needs to draw on original evidence and provide enough detail so that the reader can make judgments about the validity of the conclusions (Yin, 2000).
Results may also be used as the basis for new theoretical developments, new experiments, or improved measures on surveys that indicate the extent of generalizability. In the work done by Holland and Eisenhart (1990), for example (see Box 5-2 ), a number of theoretical models were developed and tested to explain how women decide to pursue or abandon nontraditional careers in the fields they had studied in college. Their finding that commitment to college life—not fear of competing with men or other hypotheses that had previously been set forth—best explained these decisions was new knowledge. It has been shown in subsequent studies to
generalize somewhat to similar schools, though additional models seem to exist at some schools (Seymour and Hewitt, 1997).
Although such purposively selected samples may not be scientifically generalizable to other locations or people, these vivid descriptions often appeal to practitioners. Scientifically rigorous case studies have strengths and weaknesses for such use. They can, for example, help local decision makers by providing them with ideas and strategies that have promise in their educational setting. They cannot (unless combined with other methods) provide estimates of the likelihood that an educational approach might work under other conditions or that they have identified the right underlying causes. As we argue throughout this volume, research designs can often be strengthened considerably by using multiple methods— integrating the use of both quantitative estimates of population characteristics and qualitative studies of localized context.
Other descriptive designs may involve interviews with respondents or document reviews in a fairly large number of cases, such as 30 school districts or 60 colleges. Cases are often selected to represent a variety of conditions (e.g., urban/rural; east/west; affluent/poor). Such descriptive studies can be longitudinal, returning to the same cases over several years to see how conditions change.
These examples of descriptive work meet the principles of science, and have clearly contributed important insights to the base of scientific knowledge. If research is to be used to answer questions about “what works,” however, it must advance to other levels of scientific investigation such as those considered next.
IS THERE A SYSTEMATIC EFFECT?
Research designs that attempt to identify systematic effects have at their root an intent to establish a cause-and-effect relationship. Causal work is built on both theory and descriptive studies. In other words, the search for causal effects cannot be conducted in a vacuum: ideally, a strong theoretical base as well as extensive descriptive information are in place to provide the intellectual foundation for understanding causal relationships.
The simple question of “does x cause y ?” typically involves several different kinds of studies undertaken sequentially (Holland, 1993). In basic
terms, several conditions must be met to establish cause. Usually, a relationship or correlation between the variables is first identified. 3 Researchers also confirm that x preceded y in time (temporal sequence) and, crucially, that all presently conceivable rival explanations for the observed relationship have been “ruled out.” As alternative explanations are eliminated, confidence increases that it was indeed x that caused y . “Ruling out” competing explanations is a central metaphor in medical research, diagnosis, and other fields, including education, and it is the key element of causal queries (Campbell and Stanley 1963; Cook and Campbell 1979, 1986).
The use of multiple qualitative methods, especially in conjunction with a comparative study of the kind we describe in this section, can be particularly helpful in ruling out alternative explanations for the results observed (Yin, 2000; Weiss, in press). Such investigative tools can enable stronger causal inferences by enhancing the analysis of whether competing explanations can account for patterns in the data (e.g., unreliable measures or contamination of the comparison group). Similarly, qualitative methods can examine possible explanations for observed effects that arise outside of the purview of the study. For example, while an intervention was in progress, another program or policy may have offered participants opportunities similar to, and reinforcing of, those that the intervention provided. Thus, the “effects” that the study observed may have been due to the other program (“history” as the counterinterpretation; see Chapter 3 ). When all plausible rival explanations are identified and various forms of data can be used as evidence to rule them out, the causal claim that the intervention caused the observed effects is strengthened. In education, research that explores students’ and teachers’ in-depth experiences, observes their actions, and documents the constraints that affect their day-to-day activities provides a key source of generating plausible causal hypotheses.
We have organized the remainder of this section into two parts. The first treats randomized field trials, an ideal method when entities being examined can be randomly assigned to groups. Experiments are especially well-suited to situations in which the causal hypothesis is relatively simple. The second describes situations in which randomized field trials are not
feasible or desirable, and showcases a study that employed causal modeling techniques to address a complex causal question. We have distinguished randomized studies from others primarily to signal the difference in the strength with which causal claims can typically be made from them. The key difference between randomized field trials and other methods with respect to making causal claims is the extent to which the assumptions that underlie them are testable. By this simple criterion, nonrandomized studies are weaker in their ability to establish causation than randomized field trials, in large part because the role of other factors in influencing the outcome of interest is more difficult to gauge in nonrandomized studies. Other conditions that affect the choice of method are discussed in the course of the section.
Causal Relationships When Randomization Is Feasible
A fundamental scientific concept in making causal claims—that is, inferring that x caused y —is comparison. Comparing outcomes (e.g., student achievement) between two groups that are similar except for the causal variable (e.g., the educational intervention) helps to isolate the effect of that causal agent on the outcome of interest. 4 As we discuss in Chapter 4 , it is sometimes difficult to retain the sharpness of a comparison in education due to proximity (e.g., a design that features students in one classroom assigned to different interventions is subject to “spillover” effects) or human volition (e.g., teacher, parent, or student decisions to switch to another condition threaten the integrity of the randomly formed groups). Yet, from a scientific perspective, randomized trials (we also use the term “experiment” to refer to causal studies that feature random assignment) are the ideal for establishing whether one or more factors caused change in an outcome because of their strong ability to enable fair comparisons (Campbell and Stanley, 1963; Boruch, 1997; Cook and Payne, in press). Random allocation of students, classrooms, schools—whatever the unit of comparison may be—to different treatment groups assures that these comparison groups are, roughly speaking, equivalent at the time an intervention is introduced (that is, they do not differ systematically on account of hidden
influences) and chance differences between the groups can be taken into account statistically. As a result, the independent effect of the intervention on the outcome of interest can be isolated. In addition, these studies enable legitimate statistical statements of confidence in the results.
The Tennessee STAR experiment (see Chapter 3 ) on class-size reduction is a good example of the use of randomization to assess cause in an education study; in particular, this tool was used to gauge the effectiveness of an intervention. Some policy makers and scientists were unwilling to accept earlier, largely nonexperimental studies on class-size reduction as a basis for major policy decisions in the state. Those studies could not guarantee a fair comparison of children in small versus large classes because the comparisons relied on statistical adjustment rather than on actual construction of statistically equivalent groups. In Tennessee, statistical equivalence was achieved by randomly assigning eligible children and teachers to classrooms of different size. If the trial was properly carried out, 5 this randomization would lead to an unbiased estimate of the relative effect of class-size reduction and a statistical statement of confidence in the results.
Randomized trials are used frequently in the medical sciences and certain areas of the behavioral and social sciences, including prevention studies of mental health disorders (e.g., Beardslee, Wright, Salt, and Drezner, 1997), behavioral approaches to smoking cessation (e.g., Pieterse, Seydel, DeVries, Mudde, and Kok, 2001), and drug abuse prevention (e.g., Cook, Lawrence, Morse, and Roehl, 1984). It would not be ethical to assign individuals randomly to smoke and drink, and thus much of the evidence regarding the harmful effects of nicotine and alcohol comes from descriptive and correlational studies. However, randomized trials that show reductions in health detriments and improved social and behavioral functioning strengthen the causal links that have been established between drug use and adverse health and behavioral outcomes (Moses, 1995; Mosteller, Gilbert, and McPeek, 1980). In medical research, the relative effectiveness of the Salk vaccine (see Lambert and Markel, 2000) and streptomycin (Medical Research Council, 1948) was demonstrated through such trials. We have also learned about which drugs and surgical treatments are useless by depending on randomized controlled experiments (e.g., Schulte et al.,
2001; Gorman et al., 2001; Paradise et al., 1999). Randomized controlled trials are also used in industrial, market, and agricultural research.
Such trials are not frequently conducted in education research (Boruch, De Moya, and Snyder, in press). Nonetheless, it is not difficult to identify good examples in a variety of education areas that demonstrate their feasibility (see Boruch, 1997; Orr, 1999; and Cook and Payne, in press). For example, among the education programs whose effectiveness have been evaluated in randomized trials are the Sesame Street television series (Bogatz and Ball, 1972), peer-assisted learning and tutoring for young children with reading problems (Fuchs, Fuchs, and Kazdan, 1999), and Upward Bound (Myers and Schirm, 1999). And many of these trials have been successfully implemented on a large scale, randomizing entire classrooms or schools to intervention conditions. For numerous examples of trials in which schools, work places, and other entities are the units of random allocation and analysis, see Murray (1998), Donner and Klar (2000), Boruch and Foley (2000), and the Campbell Collaboration register of trials at http://campbell.gse.upenn.edu .
Causal Relationships When Randomization Is Not Feasible
In this section we discuss the conditions under which randomization is not feasible nor desirable, highlight alternative methods for addressing causal questions, and provide an illustrative example. Many nonexperimental methods and analytic approaches are commonly classified under the blanket rubric “quasi-experiment” because they attempt to approximate the underlying logic of the experiment without random assignment (Campbell and Stanley, 1963; Caporaso and Roos, 1973). These designs were developed because social science researchers recognized that in some social contexts (e.g., schools), researchers do not have the control afforded in laboratory settings and thus cannot always randomly assign units (e.g., classrooms).
Quasi-experiments (alternatively called observational studies), 6 for example, sometimes compare groups of interest that exist naturally (e.g.,
existing classes varying in size) rather than assigning them randomly to different conditions (e.g., assigning students to small, medium, or large class size). These studies must attempt to ensure fair comparisons through means other than randomization, such as by using statistical techniques to adjust for background variables that may account for differences in the outcome of interest. For example, researchers might come across schools that vary in the size of their classes and compare the achievement of students in large and small classes, adjusting for other differences among schools and children. If the class size conjecture holds after this adjustment is made, the researchers would expect students in smaller classes to have higher achievement scores than students in larger size classes. If indeed this difference is observed, the causal effect is more plausible.
The plausibility of the researchers’ causal interpretation, however, depends on some strong assumptions. They must assume that their attempts to equate schools and children were, indeed, successful. Yet, there is always the possibility that some unmeasured, prior existing difference among schools and children caused the effect, not the reduced class size. Or, there is the possibility that teachers with reduced classes were actively involved in school reform and that their increased effort and motivation (which might wane over time) caused the effect, not the smaller classes themselves. In short, these designs are less effective at eliminating competing plausible hypotheses with the same authority as a true experiment.
The major weakness of nonrandomized designs is selectivity bias—the counter-interpretation that the treatment did not cause the difference in outcomes but, rather, unmeasured prior existing differences (differential selectivity) between the groups did. 7 For example, a comparison of early literacy skills among low-income children who participated in a local preschool program and those who did not may be confounded by selectivity bias. That is, the parents of the children who were enrolled in preschool may be more motivated than other parents to provide reading experiences to their children at home, thus making it difficult to disentangle the several potential causes (e.g., preschool program or home reading experiences) for early reading success.
It is critical in such studies, then, to be aware of potential sources of bias and to measure them so their influence can be accounted for in relation to the outcome of interest. 8 It is when these biases are not known that quasi-experiments may yield misleading results. Thus, the scientific principle of making assumptions explicit and carefully attending to ruling out competing hypotheses about what caused a difference takes on heightened importance.
In some settings, well-controlled quasi-experiments may have greater “external validity”—generalizability to other people, times, and settings— than experiments with completely random assignment (Cronbach et al., 1980; Weiss, 1998a). It may be useful to take advantage of the experience and investment of a school with a particular program and try to design a quasi-experiment that compares the school that has a good implementation of the program to a similar school without the program (or with a different program). In such cases, there is less risk of poor implementation, more investment of the implementers in the program, and potentially greater impact. The findings may be more generalizable than in a randomized experiment because the latter may be externally mandated (i.e., by the researcher) and thus may not be feasible to implement in the “real-life” practice of education settings. The results may also have stronger external validity because if a school or district uses a single program, the possible contamination of different programs because teachers or administrators talk and interact will be reduced. Random assignment within a school at the level of the classroom or child often carries the risk of dilution or blending the programs. If assignment is truly random, such threats to internal validity will not bias the comparison of programs—just the estimation of the strength of the effects.
In the section above ( What Is Happening? ), we note that some kinds of correlational work make important contributions to understanding broad patterns of relationships among educational phenomena; here, we highlight a correlational design that allows causal inferences about the relationship between two or more variables. When correlational methods use what are called “model-fitting” techniques based on a theoretically gener-
ated system of variables, they permit stronger, albeit still tentative, causal inferences.
In Chapter 3 , we offer an example that illustrates the use of model-fitting techniques from the geophysical sciences that tested alternative hypotheses about the causes of glaciation. In Box 5-3 , we provide an example of causal modeling that shows the value of such techniques in education. This work examined the potential causal connection between teacher compensation and student dropout rates. Exploring this relationship is quite relevant to education policy, but it cannot be studied through a randomized field trail: teacher salaries, of course, cannot be randomly assigned nor can students be randomly assigned to those teachers. Because important questions like these often cannot be examined experimentally, statisticians have developed sophisticated model-fitting techniques to statistically rule out potential alternative explanations and deal with the problem of selection bias.
The key difference between simple correlational work and model-fitting is that the latter enhances causal attribution. In the study examining teacher compensation and dropout rates, for example, researchers introduced a conceptual model for the relationship between student outcomes and teacher salary, set forth an explicit hypothesis to test about the nature of that relationship, and assessed competing models of interpretation. By empirically rejecting competing theoretical models, confidence is increased in the explanatory power of the remaining model(s) (although other alternative models may also exist that provide a comparable fit to the data).
The study highlighted in Box 5-3 tested different models in this way. Loeb and Page (2000) took a fresh look at a question that had a good bit of history, addressing what appeared to be converging evidence that there was no causal relationship between teacher salaries and student outcomes. They reasoned that one possible explanation for these results was that the usual “production-function” model for the effects of salary on student outcomes was inadequately specified. Specifically, they hypothesized that nonpecuniary job characteristics and alternative wage opportunities that previous models had not accounted for may be relevant in understanding the relationship between teacher compensation and student outcomes. After incorporating these opportunity costs in their model and finding a sophisticated way to control the fact that wealthier parents are likely to send their
children to schools that pay teachers more, Loeb and Page found that raising teacher wages by 10 percent reduced high school dropout rates by 3 to 4 percent.
WHY OR HOW IS IT HAPPENING?
In many situations, finding that a causal agent ( x ) leads to the outcome ( y ) is not sufficient. Important questions remain about how x causes y . Questions about how things work demand attention to the processes and mechanisms by which the causes produce their effects. However, scientific research can also legitimately proceed in the opposite direction: that is, the search for mechanism can come before an effect has been established. For example, if the process by which an intervention influences student outcomes is established, researchers can often predict its effectiveness with known probability. In either case, the processes and mechanisms should be linked to theories so as to form an explanation for the phenomena of interest.
The search for causal mechanisms, especially once a causal effect has garnered strong empirical support, can use all of the designs we have discussed. In Chapter 2 , we trace a sequence of investigations in molecular biology that investigated how genes are turned on and off. Very different techniques, but ones that share the same basic intellectual approach to casual analysis reflected in these genetic studies, have yielded understandings in education. Consider, for example, the Tennessee class-size experiment (see discussion in Chapter 3 ). In addition to examining whether reduced class size produced achievement benefits, especially for minority students, a research team and others in the field asked (see, e.g., Grissmer, 1999) what might explain the Tennessee and other class-size effects. That is, what was the causal mechanism through which reduced class size affected achievement? To this end, researchers (Bohrnstedt and Stecher, 1999) used classroom observations and interviews to compare teaching in different class sizes. They conducted ethnographic studies in search of mechanism. They correlated measures of teaching behavior with student achievement scores. These questions are important because they enhance understanding of the foundational processes at work when class size is reduced and thus
improve the capacity to implement these reforms effectively in different times, places, and contexts.
Exploring Mechanism When Theory Is Fairly Well Established
A well-known study of Catholic schools provides another example of a rigorous attempt to understand mechanism (see Box 5-4 ). Previous and highly controversial work on Catholic schools (e.g., Coleman, Hoffer, and
Kilgore, 1982) had examined the relative benefits to students of Catholic and public schools. Drawing on these studies, as well as a fairly substantial literature related to effective schools, Bryk and his colleagues (Byrk, Lee, and Holland, 1993) focused on the mechanism by which Catholic schools seemed to achieve success relative to public schools. A series of models were developed (sector effects only, compositional effects, and school effects) and tested to explain the mechanism by which Catholic schools successfully achieve an equitable social distribution of academic achievement. The
researchers’ analyses suggested that aspects of school life that enhance a sense of community within Catholic schools most effectively explained the differences in student outcomes between Catholic and public schools.
Exploring Mechanism When Theory Is Weak
When the theoretical basis for addressing questions related to mechanism is weak, contested, or poorly understood, other types of methods may be more appropriate. These queries often have strong descriptive components and derive their strength from in-depth study that can illuminate unforeseen relationships and generate new insights. We provide two examples in this section of such approaches: the first is the ethnographic study of college women (see Box 5-2 ) and the second is a “design study” that resulted in a theoretical model for how young children learn the mathematical concepts of ratio and proportion.
After generating a rich description of women’s lives in their universities based on extensive analysis of ethnographic and survey data, the researchers turned to the question of why women who majored in nontraditional majors typically did not pursue those fields as careers (see Box 5-2 ). Was it because women were not well prepared before college? Were they discriminated against? Did they not want to compete with men? To address these questions, the researchers developed several theoretical models depicting commitment to schoolwork to describe how the women participated in college life. Extrapolating from the models, the researchers predicted what each woman would do after completing college, and in all cases, the models’ predictions were confirmed.
A second example highlights another analytic approach for examining mechanism that begins with theoretical ideas that are tested through the design, implementation, and systematic study of educational tools (curriculum, teaching methods, computer applets) that embody the initial conjectured mechanism. The studies go by different names; perhaps the two most popular names are “design studies” (Brown, 1992) and “teaching experiments” (Lesh and Kelly, 2000; Schoenfeld, in press).
Box 5-5 illustrates a design study whose aim was to develop and elaborate the theoretical mechanism by which ratio reasoning develops in young children and to build and modify appropriate tasks and assessments that
incorporate the models of learning developed through observation and interaction in the classroom. The work was linked to substantial existing literature in the field about the theoretical nature of ratio and proportion as mathematical ideas and teaching approaches to convey them (e.g., Behr, Lesh, Post, and Silver, 1983; Harel and Confrey, 1994; Mack, 1990, 1995). The initial model was tested and refined as careful distinctions and extensions were noted, explained, and considered as alternative explanations as the work progressed over a 3-year period, studying one classroom intensively. The design experiment methodology was selected because, unlike laboratory or other highly controlled approaches, it involved research within the complex interactions of teachers and students and allowed the everyday demands and opportunities of schooling to affect the investigation.
Like many such design studies, there were two main products of this work. First, through a theory-driven process of designing—and a data-driven process of refining—instructional strategies for teaching ratio and proportion, researchers produced an elaborated explanatory model of how young children come to understand these core mathematical concepts. Second, the instructional strategies developed in the course of the work itself hold promise because they were crafted based on a number of relevant research literatures. Through comparisons of achievement outcomes between children who received the new instruction and students in other classrooms and schools, the researchers provided preliminary evidence that the intervention designed to embody this theoretical mechanism is effective. The intervention would require further development, testing, and comparisons of the kind we describe in the previous section before it could be reasonably scaled up for widespread curriculum use.
Steffe and Thompson (2000) are careful to point out that design studies and teaching experiments must be conducted scientifically. In their words:
We use experiment in “teaching experiment” in a scientific sense…. What is important is that the teaching experiments are done to test hypotheses as well as to generate them. One does not embark on the intensive work of a teaching experiment without having major research hypotheses to test (p. 277).
This genre of method and approach is a relative newcomer to the field of education research and is not nearly as accepted as many of the other
methods described in this chapter. We highlight it here as an illustrative example of the creative development of new methods to embed the complex instructional settings that typify U.S. education in the research process. We echo Steffe and Thompson’s (2000) call to ensure a careful application of the scientific principles we describe in this report in the conduct of such research. 9
CONCLUDING COMMENTS
This chapter, building on the scientific principles outlined in Chapter 3 and the features of education that influence their application in education presented in Chapter 4 , illustrates that a wide range of methods can legitimately be employed in scientific education research and that some methods are better than others for particular purposes. As John Dewey put it:
We know that some methods of inquiry are better than others in just the same way in which we know that some methods of surgery, arming, road-making, navigating, or what-not are better than others. It does not follow in any of these cases that the “better” methods are ideally perfect…We ascertain how and why certain means and agencies have provided warrantably assertible conclusions, while others have not and cannot do so (Dewey, 1938, p. 104, italics in original).
The chapter also makes clear that knowledge is generated through a sequence of interrelated descriptive and causal studies, through a constant process of refining theory and knowledge. These lines of inquiry typically require a range of methods and approaches to subject theories and conjectures to scrutiny from several perspectives.
We conclude this chapter with several observations and suggestions about the current state of education research that we believe warrant attention if scientific understanding is to advance beyond its current state. We do not provide a comprehensive agenda for the nation. Rather, we
wish to offer constructive guidance by pointing to issues we have identified throughout our deliberations as key to future improvements.
First, there are a number of areas in education practice and policy in which basic theoretical understanding is weak. For example, very little is known about how young children learn ratio and proportion—mathematical concepts that play a key role in developing mathematical proficiency. The study we highlight in this chapter generated an initial theoretical model that must undergo sustained development and testing. In such areas, we believe priority should be given to descriptive and theory-building studies of the sort we highlight in this chapter. Scientific description is an essential part of any scientific endeavor, and education is no different. These studies are often extremely valuable in themselves, and they also provide the critical theoretical grounding needed to conduct causal studies. We believe that attention to the development and systematic testing of theories and conjectures across multiple studies and using multiple methods—a key scientific principle that threads throughout all of the questions and designs we have discussed—is currently undervalued in education relative to other scientific fields. The physical sciences have made progress by continuously developing and testing theories; something of that nature has not been done systematically in education. And while it is not clear that grand, unifying theories exist in the social world, conceptual understanding forms the foundation for scientific understanding and progresses—as we showed in Chapter 2 —through the systematic assessment and refinement of theory.
Second, while large-scale education policies and programs are constantly undertaken, we reiterate our belief that they are typically launched without an adequate evidentiary base to inform their development, implementation, or refinement over time (Campbell, 1969; President’s Committee of Advisors on Science and Technology, 1997). The “demand” for education research in general, and education program evaluation in particular, is very difficult to quantify, but we believe it tends to be low from educators, policy makers, and the public. There are encouraging signs that public attitudes toward the use of objective evidence to guide decisions is improving (e.g., statutory requirements to set aside a percentage of annual appropriations to conduct evaluations of federal programs, the Government Performance and Results Act, and common rhetoric about “evidence-based” and “research-based” policy and practice). However, we believe stronger
scientific knowledge is needed about educational interventions to promote its use in decision making.
In order to generate a rich store of scientific evidence that could enhance effective decision making about education programs, it will be necessary to strengthen a few related strands of work. First, systematic study is needed about the ways that programs are implemented in diverse educational settings. We view implementation research—the genre of research that examines the ways that the structural elements of school settings interact with efforts to improve instruction—as a critical, underfunded, and underappreciated form of education research. We also believe that understanding how to “scale up” (Elmore, 1996) educational interventions that have promise in a small number of cases will depend critically on a deep understanding of how policies and practices are adopted and sustained (Rogers, 1995) in the complex U.S. education system. 10
In all of this work, more knowledge is needed about causal relationships. In estimating the effects of programs, we urge the expanded use of random assignment. Randomized experiments are not perfect. Indeed, the merits of their use in education have been seriously questioned (Cronbach et al., 1980; Cronbach, 1982; Guba and Lincoln, 1981). For instance, they typically cannot test complex causal hypotheses, they may lack generalizability to other settings, and they can be expensive. However, we believe that these and other issues do not generate a compelling rationale against their use in education research and that issues related to ethical concerns, political obstacles, and other potential barriers often can be resolved. We believe that the credible objections to their use that have been raised have clarified the purposes, strengths, limitations, and uses of randomized experiments as well as other research methods in education. Establishing cause is often exceedingly important—for example, in the large-scale deployment of interventions—and the ambiguity of correlational studies or quasi-experiments can be undesirable for practical purposes.
In keeping with our arguments throughout this report, we also urge that randomized field trials be supplemented with other methods, including in-depth qualitative approaches that can illuminate important nuances,
identify potential counterhypotheses, and provide additional sources of evidence for supporting causal claims in complex educational settings.
In sum, theory building and rigorous studies of implementations and interventions are two broad-based areas that we believe deserve attention. Within the framework of a comprehensive research agenda, targeting these aspects of research will build on the successes of the enterprise we highlight throughout this report.
Researchers, historians, and philosophers of science have debated the nature of scientific research in education for more than 100 years. Recent enthusiasm for "evidence-based" policy and practice in education—now codified in the federal law that authorizes the bulk of elementary and secondary education programs—have brought a new sense of urgency to understanding the ways in which the basic tenets of science manifest in the study of teaching, learning, and schooling.
Scientific Research in Education describes the similarities and differences between scientific inquiry in education and scientific inquiry in other fields and disciplines and provides a number of examples to illustrate these ideas. Its main argument is that all scientific endeavors share a common set of principles, and that each field—including education research—develops a specialization that accounts for the particulars of what is being studied. The book also provides suggestions for how the federal government can best support high-quality scientific research in education.
READ FREE ONLINE
Welcome to OpenBook!
You're looking at OpenBook, NAP.edu's online reading room since 1999. Based on feedback from you, our users, we've made some improvements that make it easier than ever to read thousands of publications on our website.
Do you want to take a quick tour of the OpenBook's features?
Show this book's table of contents , where you can jump to any chapter by name.
...or use these buttons to go back to the previous chapter or skip to the next one.
Jump up to the previous page or down to the next one. Also, you can type in a page number and press Enter to go directly to that page in the book.
Switch between the Original Pages , where you can read the report as it appeared in print, and Text Pages for the web version, where you can highlight and search the text.
To search the entire text of this book, type in your search term here and press Enter .
Share a link to this book page on your preferred social network or via email.
View our suggested citation for this chapter.
Ready to take your reading offline? Click here to buy this book in print or download it as a free PDF, if available.
Get Email Updates
Do you enjoy reading reports from the Academies online for free ? Sign up for email notifications and we'll let you know about new publications in your areas of interest when they're released.
- Reference Manager
- Simple TEXT file
People also looked at
Review article, why comparing matters – on case comparisons in organic chemistry.
- Institute of Chemistry Education, Justus-Liebig-University Giessen, Giessen, Germany
When working with domain-specific representations such as structural molecular representations and reaction mechanisms, learners need to be engaged in multiple cognitive operations, from attending to relevant areas of representations, linking implicit information to structural features, and making meaningful connections between information and reaction processes. For these processes, appropriate instruction, such as a clever task design, becomes a crucial factor for successful learning. Chemistry learning, and especially organic chemistry, merely addressed meaningful task design in classes, often using more reproduction-oriented predict-the-product tasks. In recent years, rethinking task design has become a major focus for instructional design in chemistry education research. Thus, this perspective aims to illustrate the theoretical underpinning of comparing cases from different perspectives, such as the structure-mapping theory, the cognitive load theory, and the variation theory, and outlines, based on the cognitive theory of multimedia learning, how instructors can support their students. Variations of this task design in the chemistry classroom and recommendations for teaching with case comparisons based on current state-of-the-art evidence from research studies in chemistry education research are provided.
1 Introduction
As educators in chemistry, we would unanimously agree that understanding the relationship between the Lewis structure representations of organic molecules and their chemical properties, the molecular architecture, as named by Laszlo (2002) , is essential for explaining or predicting chemical behavior. When learning chemistry, students, thus, encounter various ways of representing structures and processes (i.e., electron-pushing formalism) and must connect this to chemical and physical characteristics and energetic considerations ( Goodwin, 2010 ). As a chemical entity has both a visible structural representation and an underlying conceptual aspect, difficulties in linking these two aspects can lead to a superficial understanding. Studies consistently show that students often focus on surface features or patterns when estimating the reactivity of molecules, overlooking functional or more abstract relational similarities ( cf. Cooper et al., 2013 ; Anzovino and Bretz, 2016 ; Talanquer, 2017 ). They tend to equate visual similarity with chemical similarity, potentially missing out on understanding how different structural environments can lead to property changes, i.e., changes in chemical reactivity ( Bhattacharyya, 2014 ; Graulich et al., 2019 ).
One may now ask, why comparing and contrasting should be an important part of learning in chemistry. The act of comparing is inherent to the discipline because it allows us to understand the properties of substances by comparing their behavior in different conditions ( Goodwin, 2008 ). Chemists often compare different substances to identify similarities and differences of chemical and physical properties. In chemical synthesis, making small changes in functional groups at a target catalyst, for example, allows us to determine which ones are most effective at promoting specific chemical reactions ( Afagh and Yudin, 2010 ). By comparing the behavior of chemical systems, chemists can gain a deeper understanding of the underlying principles of chemical processes to monitor and control chemical reactions or refine computational models. Comparing either experimental, machine learning or computational data allows us to estimate the magnitude of effects ( Keith et al., 2021 ). Comparing, for instance, kinetic data of reactions helps determine the magnitude of reaction speed, for instance, influenced by changes of electronic substituent effects ( Trabert and Schween, 2018 ). In some cases, we have this data at hand in terms of empirical properties, such as electronegativity or p K a values, but in other cases, in which we do not have access to these data, chemists often express qualitatively the properties of a functional group or molecule, e.g., this leaving group or nucleophile is good, or this structure is stable ( Popova and Bretz, 2018 ). However, to estimate what “good” means requires answering the question “Good, compared to what” and essentially answering the question “why is it better?.” This is an inherently comparative process that requires knowledge about implicit properties, electron distribution, strength of effects, and energetic considerations. Purposeful case comparisons may engage learners in meaningful sense-making about organic reactions. This assumption is further supported by studies in psychology that have highlighted the educational value of using case comparisons to assist students in grasping new concepts ( Schwartz and Bransford, 1998 ; Gentner et al., 2003 ). In particular, Gentner et al. (2003) found that comparing two cases simultaneously was more effective for learning than studying five single cases in sequence. By comparing and contrasting different cases, students learn to discern both common and distinctive characteristics that help differentiate and understand key concepts or phenomena. As the instruction continues, such comparisons offer a chance for learners to develop inferences and justifications for the specific features. A meta-analysis by Alfieri et al. (2013) has shown that this method significantly enhances learning. This perspective outlines the theoretical underpinning of case comparisons and highlights how instruction in chemistry can profit from well-designed and orchestrated cases.
2 Why should we learn with case comparisons? Theoretical underpinning
2.1 what does structure mapping theory tell us about comparing.
Learning by comparing cases can be rationalized from a cognitive psychology perspective because it taps into several important cognitive processes, essential for learning and problem-solving. When comparing cases, a learner is engaged in a process called analogical reasoning, which involves finding similarities and differences between cases and using those similarities and differences to make inferences and draw conclusions. This analogical reasoning is a fundamental cognitive process that allows transfer knowledge and skills from one domain to another, or from one context to another ( Gick and Holyoak, 1983 ). The structure mapping theory by Gentner (1989) and Gentner and Markman (1997) explains how this analogical reasoning works. When we compare two situations, objects, or reactions, we look for shared relationships. These relationships could either be similarities in surface features or relational features, such as causal or functional ones. Surface features are always visible features and details of a situation or object and, thus, are easy to discern. While relational structures refer to the abstract relationships between features and implicit information conveyed, they can, but do not necessarily share surface similarities. Comparing a set of correspondences between the surface or relational features of two cases leads to a structural alignment, i.e., discerning the information that two cases share. According to the structure mapping theory, the more shared relational features there are between two situations, the stronger the analogy, the easier to transfer our knowledge about one situation to reason about the other. For example, knowing that an electronegativity difference is needed to make a carbon-heteroatom bond polar, we can use that knowledge to infer that other carbon-heteroatom bonds might be polar as well, when there is a difference in electronegativity, even if the functional group looks different. However, attending to the relational similarity between cases is modulated by expertise. With increasing expertise, we can make use of abstract schemas and use them to categorize tasks based on implicit, conceptual aspects, whereas novice chemistry learners tend to focus on more explicit concrete features ( Graulich et al., 2019 ; Lapierre and Flynn, 2020 ).
2.2 Cognitive load – the gatekeeper for accessibility
The Cognitive Load Theory (CLT) ( Sweller and Chandler, 1994 ; Kalyuga et al., 1998 ) offers substantial insights into the use of case comparisons in learning chemistry, emphasizing how instructional design can manage cognitive resources to enhance learning ( Paas et al., 2003 ). The CLT acknowledges the structure or extraneous load of a task (extraneous cognitive load), as well as the cognitive affordances that come with the content (intrinsic cognitive load) and the cognitive effort that a learner needs to activate for learning (germane cognitive load). When we compare cases, we activate our working memory system. However, the use of working memory and the associated capacity is limited, which is why sufficient available capacity must be accessible for effective learning or application of knowledge ( Baddeley, 2010 ). CLT describes that learning is associated with cognitive load and that learning can be simplified or be more challenging depending on the circumstances. Intrinsic cognitive load is related to the difficulty or complexity of the learning material. Sweller (2003) focuses here on element interactivity. In concrete terms, this means that different elements must be processed simultaneously in the working memory during learning. This can happen sequentially, which causes a lower intrinsic cognitive load, or simultaneously, which results in an increased intrinsic cognitive load. If the elements are processed one after the other, e.g., in learning with single cases, this usually leads to memorization; if they are processed simultaneously, e.g., by comparing cases, links are created, which generates understanding but is also more demanding for the working memory ( Sweller, 2010 ). The more prior knowledge learners have, the more links already exist and the lower the intrinsic cognitive load, even when processing elements simultaneously ( Paas and Sweller, 2014 ). Two assumptions support the use of case comparison in light of the intrinsic cognitive load. On the one hand, as our working memory is limited in capacity, comparing cases instead of single cases helps us to be able to attend easily to differences and similarities and neglect other possibly irrelevant features of a situation or object ( Schwartz and Bransford, 1998 ). Simultaneous processing of multiple and maybe irrelevant aspects can be challenging for learners; thus, the extraneous and intrinsic load can be reduced if cases help learners to focus on a reduced number of relevant aspects, as the one variable that needs to be compared can be focused on. This allows us to save capacity in our working memory. Furthermore, studying multiple cases allows learners to see how the same underlying principles apply to different contexts. This can help learners develop a deeper understanding of those principles and how they relate, which makes it easier to build conceptual chunks instead of memorizing single features ( Schwartz and Bransford, 1998 ; Alfieri et al., 2013 ; Roelle and Berthold, 2015 ). Studying a single case in isolation may not give learners enough context or variation to understand the underlying principles involved fully ( Alfieri et al., 2013 ). However, using case comparisons does not, per se , remediate mediocre ways of teaching. If the cases are not fully understood and the learner struggles to determine the relevant aspects, comparing cases might increase the intrinsic cognitive load compared to a single case, especially when multiple variables are involved ( Schwartz and Bransford, 1998 ).
In contrast to the intrinsic cognitive load, the extraneous cognitive load is about how learning materials are designed ( Sweller, 2010 ). The more superfluous or irrelevant information learners are presented with, the greater the possibility that they will not be able to distinguish between relevant and irrelevant information and will be distracted, which increases extraneous cognitive load. To minimize extraneous cognitive load for learners, it is therefore advisable to use design principles such as Mayer’s, which are evidence-based and conducive to learning ( Mayer, 2021 ). In relation to case comparisons, this means, for example, that in addition to reducing irrelevant information, the relevant information can be emphasized, e.g., by highlighting techniques ( Rodemer et al., 2022 ).
The germane cognitive load describes the load that relates directly to learning as an activity and is considered productive ( Paas and Sweller, 2014 ). The more a learner can focus on the learning itself, the more effectively links can be created. The germane cognitive load thus relates to the intrinsic cognitive load. Currently, there is an assumption “that germane cognitive load has a redistributive function from extraneous to intrinsic aspects of the task rather than imposing a load in its own right” ( Sweller et al., 2019 , p. 264). The lower the extraneous cognitive load is kept, the more space is given to the intrinsic cognitive load, which in turn results in an increased germane cognitive load (which is positive). However, this only becomes important with complex learning material, as the intrinsic cognitive load only becomes noticeable here. The simpler a task is, the lower the intrinsic cognitive load and the lower the germane cognitive load ( Paas and Sweller, 2014 ). In relation to case comparisons, this means that the way in which the learning material is designed should be well considered so that there is more space for the germane cognitive load. Complex tasks can be chosen, whereby the complexity must match the prior knowledge and the capacity of the working memory to be able to generate effective learning and links ( Sweller, 1994 ).
Overall, comparing cases as a task design can offload the working memory and engage multiple cognitive processes that are essential for learning and problem-solving when they match the capability of the learners ( Roelle and Berthold, 2015 ).
2.3 Variation theory – instructional design principles
While Cognitive Load Theory (CLT) focuses on the capacity of working memory and how instructional design can be optimized to avoid cognitive overload, Variation theory is a learning theory that emphasizes the importance of variation in the design of instructional materials and activities and places emphasis on the importance of experiencing variations in the learning material to understand and discern the critical aspects of the content. While CLT is more about managing the quantity and complexity of information, Variation Theory is about the quality and structure of learning experiences. According to this theory, learners need to experience variations in the material they are studying in order to fully understand the underlying concepts, i.e., to abstract the relational connections beside surface similarities. Variation theory is based on the work of Swedish researcher Ference Marton and his colleagues, who developed the theory in the 1970s and 1980s ( Marton, 1981 ). Marton (1981) was interested in understanding how students develop their understanding of complex concepts, and he observed that learners often struggle to transfer knowledge from one context to another.
Lo and Marton (2011) proposed that the key to understanding complex concepts is to focus on the variations in the material. They argued that learners need to experience different examples of a concept in order to fully understand it and develop a flexible understanding that can be applied to new contexts, advocating for a deep understanding of the subject matter instead of surface-level memorization.
Variation Theory of Learning helps further to support the use of case comparisons in chemistry education, as it emphasizes the importance of discerning critical features of a concept being taught. Using case comparisons (like different chemical reactions) helps students notice and understand the essential characteristics of each case; for example, contrasting an acid–base reaction with a redox reaction can help students understand the unique features of each type of reaction. Second, Variation Theory suggests that exposure to a range of examples, prototypical and non-prototypical examples, can help students see beyond single examples and support the ability to discriminate between different entities and recognize the significance of these differences. Certain elements become more salient to the viewer through variation, while other elements are kept invariant ( Lo and Marton, 2011 ; Bussey et al., 2013 ), which allows learners to notice critical features more quickly ( Bussey et al., 2013 ). Using case comparisons helps in achieving this by requiring students to apply principles to different scenarios, thereby promoting a deeper understanding of the underlying concepts ( Roelle and Berthold, 2015 ; Bego et al., 2023 ). By focusing on these variations, variation theory aims to help learners develop a more nuanced and flexible understanding of the concept they are studying, which can be applied to new situations and contexts. The theory highlights the importance of experiencing variations in the material being studied in order to develop a flexible understanding that can be applied to new situations.
3 How good are students in comparing chemical reactions?
Multiple studies in chemistry education in the last decades documented that students when either not taught or not prompted appropriately to compare meaningfully, show a more surface-level-oriented comparison behavior when categorizing molecules or reactions. Moreover, by comparing two or more structures just because of their similar surface features, learners may overlook their properties ( Talanquer, 2008 ; DeFever et al., 2015 ). Considering implicit properties and underlying processes of a reaction mechanism is crucial for higher modes of reasoning ( Weinrich and Sevian, 2017 ) and leads to greater success when solving novel mechanistic problems ( Grove et al., 2012 ). Stains and Talanquer (2007 , 2008) compared the behaviors of undergraduate and graduate students while engaged in classifying different chemical representations and analyzed how often surface and deep-level attributes were used in the classification tasks. They determined that graduate students used more implicit information from the representations given than explicit ones for their classification. The most common approach used by undergraduates was a single attribute decision-making process. In the domain of organic chemistry, Domin et al. (2008) investigated the behavior of undergraduate students and experts while engaged in categorizing different cyclic or acyclic a-chloro derivatives of aldehydes and ketones. Consistent with Stains and Talanquer’s findings, they found that students primarily categorized these compounds dichotomously by choosing a single surface-level attribute, such as aldehyde/ketone, cyclic/acyclic, or halogenated/non-halogenated. In Stains and Talanquer’s study, experts tended to build similar categories as novices, also focusing on functional groups, but made the decision based on more implicit considerations, such as reactivity of the functional group toward the addition of nucleophiles. This increased focus on functional similarity, i.e., focusing on nucleophilicity/electrophilicity as well as reactivity of reactants, has been as well observed in various studies using card sorting activities ( Graulich and Bhattacharyya, 2017 ; Galloway et al., 2018 ). It seems as if experts or advanced students in organic chemistry are able to generate more abstract schemas and store implicit information about molecules and reactions in bigger chunks, mirroring chemical reactivity patterns. Regarding investigating the development of expertise, a study revealed that successfully categorizing organic chemistry reaction cards is, with a large effect, correlated with the students’ academic performance ( r = 0.62). Moreover, the findings that academic performance is correlated with the successful online categorization were confirmed over the years ( Lapierre et al., 2022 ). In a study from Graulich et al. (2019) , learners were prompted to identify, for example, which two out of three nucleophiles would react similarly in a given substitution reaction. Thereby, the explicit properties of the given reactants matched or did match with the correct solutions. The findings revealed that students experienced greater challenges with items in which the structural representations of the correct answer did not share explicit similarity. Therefore, it might be helpful from time to time to use molecules or reactions with similar explicit surface features that are not undergoing similar reaction pathways or reactions that seem to be similar on the surface but undergo different pathways ( Graulich and Schween, 2018 ). This could ideally induce cognitive dissonance in learners and challenge their strong focus on surface similarity. As a result, learners are required to use implicit properties to get to a proper solution and might be open to new explanatory concepts. Moreover, studies revealed that learners experience difficulties in activating the same concept knowledge in different contexts; thus, using a variety of molecules to introduce nucleophilicity might help students not to look only for negative charges and may help learners broaden their concept knowledge ( Anzovino and Bretz, 2015 ; Popova and Bretz, 2018 ).
4 Designing and orchestrating cases
Case comparisons have been widely used as a task design across natural sciences and mathematics to foster students’ ability to derive implicit features and weigh multiple arguments when reasoning. In their meta-analysis, Alfieri et al. (2013) found that case comparisons led to a higher number of identified variables than single cases ( d = 0.60, 95% CI[0.47, 0.72]). Appropriately designed case comparisons offer the possibility to support learners to see how the same underlying principles apply to different chemical systems or to what extent reactions might occur differently ( Graulich and Schween, 2018 ). This offers a chance to foster a deeper understanding of those principles and help students abstract from the explicit and sometimes misleading features of structural representations. Case comparisons seem to be more effective at the beginning rather than the end of an instructional topic, as it can prepare students to be sensitive to important features that need to be properly considered or to key features that must be transferred to new cases ( Schwartz and Bransford, 1998 ; Schwartz et al., 2011 ).
When learners compare different chemical reactions that involve similar reactants and products but occur under different conditions, learners can experience how changes in conditions can affect the reaction rate and yield and relate this observation to the principles of thermodynamics and kinetics ( Pölloth et al., 2022 ). Moreover, by comparing different cases, learners are forced to consider multiple influential factors and have to evaluate the similarities and differences. This can help them develop their ability to recognize patterns, make connections, and draw conclusions, which are essential skills in scientific inquiry and research ( Alfieri et al., 2013 ). Figure 1 illustrates the differences between tasks based on single cases, contrasting cases with one variable and contrasting cases with two (or more) variables. When comparing a simple single case ( Figure 1 , upper part), the prompt is often only answered superficially, for example in stating as to whether reactions take place from a thermodynamic point of view. But when another case is added, such as changing the leaving group, this could be considered the simplest format of a case comparison, as only one variable of two displayed reactions is changed ( Figure 1 , middle part). This requires univariate reasoning and a strong focus on how the leaving group, in this case, the bromide or the chloride ion, is influencing the kinetic outcome of the reaction. Case comparisons can be adapted to more complex ones by changing a second variable, for example, several substituents or positions. The lower part of Figure 1 illustrates a case comparison that requires multivariate reasoning, as not only the leaving group (bromide or chloride-ion) but also the nature of the substrate (e.g., carbonyl vs. double bond) influences the reaction kinetic. Thus, learners have to weigh multiple arguments and justify their decisions based on the strength of implicit properties, in this case, mesomeric and inductive effects ( Lieber and Graulich, 2022 ; Watts et al., 2023 ).
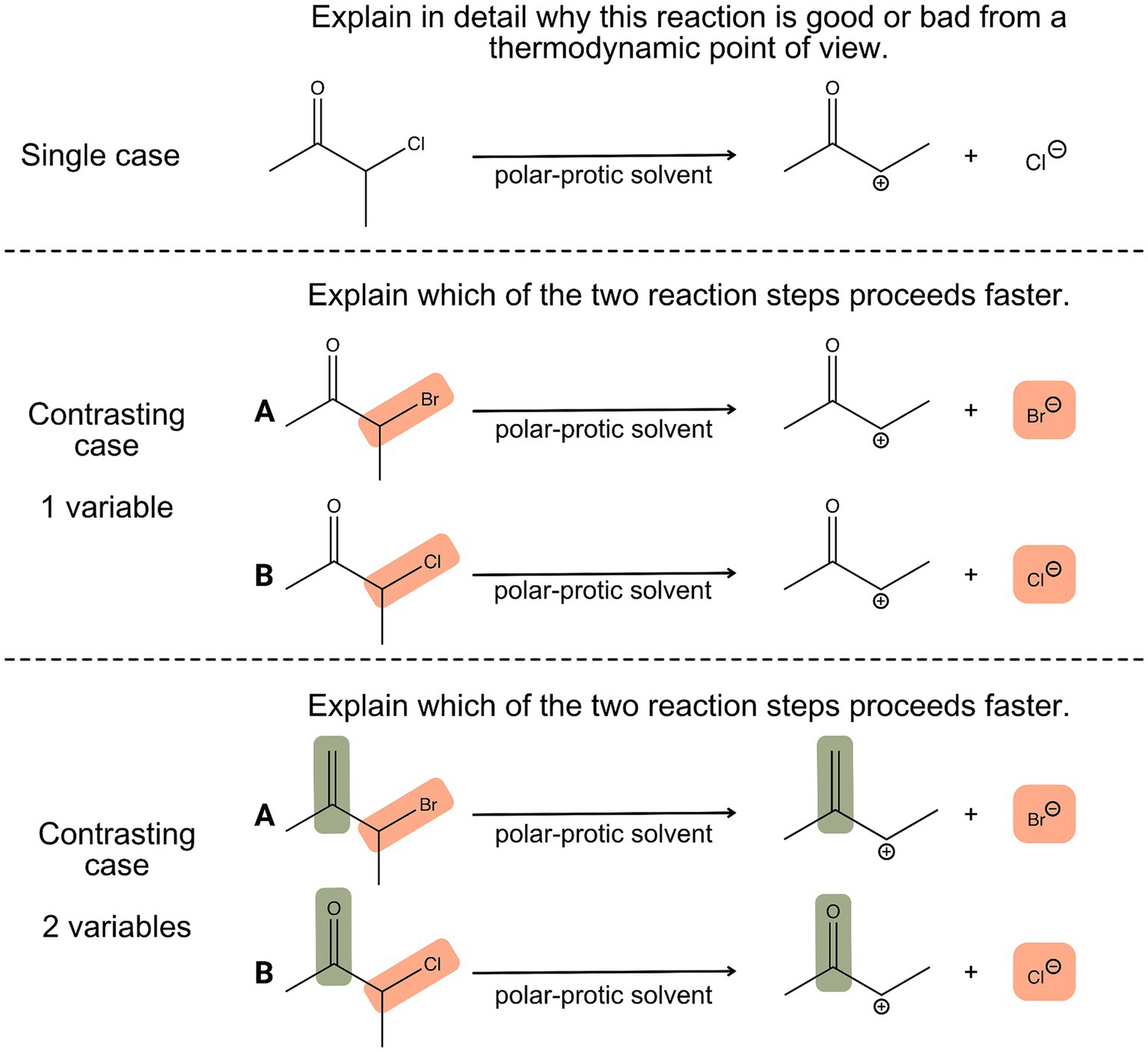
Figure 1 . Example for a single case and case comparisons.
Case comparisons have been widely used in chemistry education studies, but the way in which these case comparisons were used differed (e.g., Bodé et al., 2019 ; Lieber and Graulich, 2022 ; Kranz et al., 2023 ). Figure 2 illustrates three different possibilities for using contrasting cases in argumentation processes. In the simplest case, an argument is divided into three parts: a claim, evidence and reasoning (evidence and reasoning can be combined as justification) ( McNeill and Krajcik, 2012 ). One possibility for a task design involving case comparisons is that students compare two reactions at the beginning of the task to reason deeply about which reaction will proceed more likely. Thereby, the justification process can take place first and is guided by scaffolding which leads to a claim ( Kranz et al., 2023 ) (see Figure 2 , first example). Moreover, after comparing two reaction mechanisms at the beginning, it is also possible that learners first make a claim and justify their claim afterwards ( Bodé et al., 2019 ; Deng and Flynn, 2021 ) (see Figure 2 , second example). Besides comparing reactions at the beginning, it is also possible to build arguments on single reaction products of a reaction but contrast the reaction products at the end of the task. Thereby, students first claim if the respective reaction product is plausible or implausible, which is each justified with evidence and reasoning and compare the plausibilities of the reaction products in the end (see Figure 2 , third example). This can lead to a revision of students’ claims of most plausible reaction products toward a correct claim by weighing key concepts when contrasting them ( Lieber et al., 2022 ; Lieber and Graulich, 2022 ). These studies indicate that the use of case comparison, at the beginning or at the end, has a beneficial effect for building arguments.
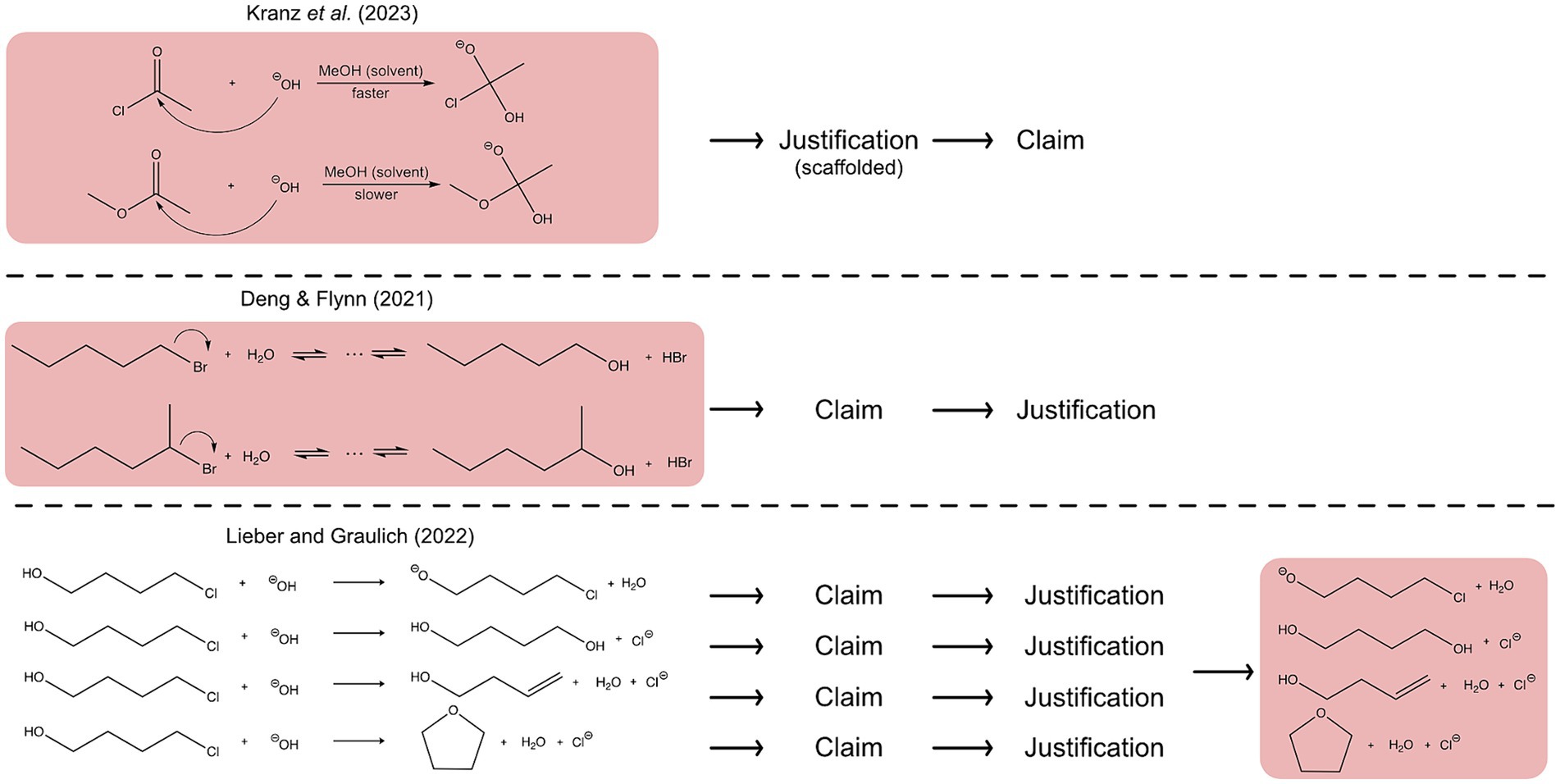
Figure 2 . Illustration of different possibilities for the use of case comparisons in argumentation and reasoning processes. The red background highlights when the case comparison is used during the process.
4.1 CPOE cycle – embedding case comparisons in inquiry processes
One way to combine the use of case comparisons with lab work is to embed these case comparisons in the CPOE cycle ( Graulich and Schween, 2018 ), an adapted form of the Predict-Observe-Explain cycle ( White and Gunstone, 2014 ) with an added “Compare” step. The cycle is based on learners first receiving a case comparison where they need to compare two given reactions (C), to predict (P) by generating a hypothesis which of the two reactions, for example, is faster than the other. This hypothesis can then be tested experimentally. By experimentally testing the hypotheses that have arisen from the case comparison, the outcome of the reactions is observed (O). Once the data has been analyzed, the final step takes place, in which conclusions are drawn about the previously formulated hypothesis based on the experimental results (E). Figure 3 illustrates the theoretical CPOE cycle by giving concrete examples how each step can look like, which is described in more detail in the following section.
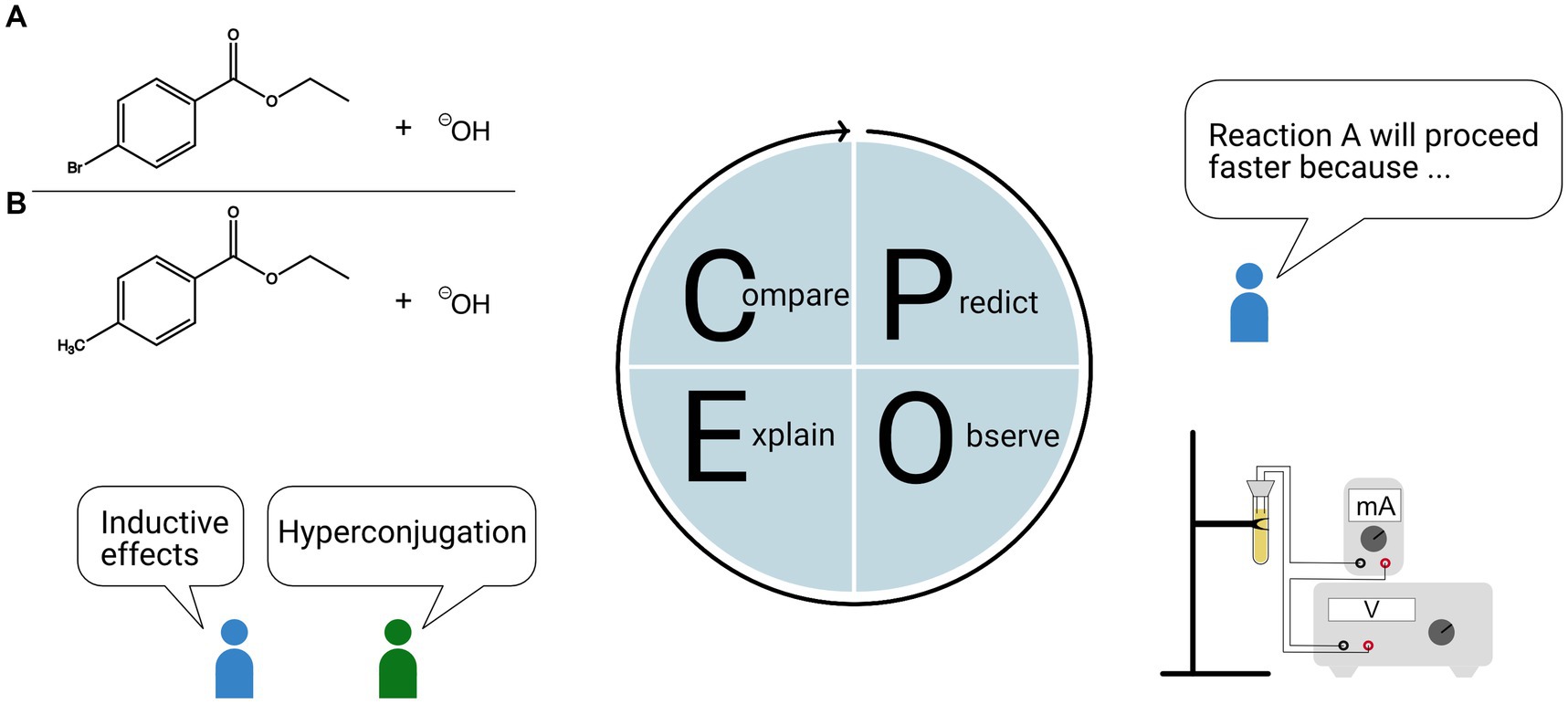
Figure 3 . Embedding case comparisons in the CPOE cycle as illustrated with an example from Trabert and Schween (2020) .
As the name suggests, however, this may not be a linear process with a defined end but a cycle that can be repeated based on new case comparisons. In this way, learners not only become familiar with scientific principles through independent experience, but the targeted choice of contrasting cases and experiments also enables a specific promotion of chemical concepts.
Schween’s group has developed numerous experiments that make intermediate stages “visible,” for example, based on conductivity measurements ( cf. Trabert et al., 2023 for an overview). In each case, two or more reactions are compared with each other and learners are prompted to estimate the reaction with the higher reaction rate. Their work resulted in experimental case comparisons on electrophilic substitution on aromatic compounds, in which the sigma complexes were determined by conductivity measurements ( Vorwerk et al., 2015 ), on the stability of carbenium ions, which makes intermediates directly and indirectly visible through color gradients as well as conductivity measurements ( Schmitt et al., 2013 ), on the competition of primary and secondary haloalkanes in S N 2 reactions ( Schmitt et al., 2018 ), as well as on electronic substituent effects in alkaline ester hydrolysis ( Trabert and Schween, 2018 ). All these experiments can be used in a CPOE cycle. Figure 3 illustrates the linkage of Trabert and Schween’s (2020) case comparisons of an alkaline ester hydrolysis, which is focused on inductive effects and their experimental design to the CPOE cycle. Thereby, students first receive contrasting cases of ester hydrolysis, which differ in their substituents on the phenyl group ( Figure 3 , compare) Based on these two reactions, students have to predict which of the reactions proceed faster including a justification ( Figure 3 , predict). Students test their hypothesis afterwards in the laboratory with conductivity measurements ( Figure 3 , observe). Based on their observations, students are encouraged to explain the phenomenon and refer to their hypothesis ( Figure 3 , explain). When the shown cycle is used in teaching and learning, learners can transfer their knowledge of inductive effects into a second cycle. Therefore, learners can apply their knowledge of inductive effect on new reactions, which focus on the position of substituents. Thereby, learners complete the CPOE cycle a second time by comparing the position of substituents on aromatic compounds, predicting the reaction rate, observing the hypothesis by conducting experiments, and explaining the position dependency of inductive effects. The key aim of these experimental case comparisons is to engage learners in reflection about reaction rate, slowly increasing the sophistication of chemical concepts such as electronic effects that is not only supported by the experimental investigations but can also be advanced to other reactions and contexts. Those cases used in the lab and discussed in lecture might serve as a bridge between these two traditional course formats in organic chemistry.
5 Supporting students to learn meaningfully with case comparisons
When engaged in comparing, meaningful problem-solving requires attending to the relevant features of a representation, as well as linking the necessary implicit information to it ( Mason et al., 2019 ). This may not be an intuitive process for students, as the connection between the feature of a carbonyl group (e.g., C=O) and its electron distribution has to be learned. The first visual selection process when looking at a structure is guided by learners’ perception of saliency, their individual framing of what a given task entails, as well as their prior knowledge and the cognitive resources that a learner is able to activate ( Bodé et al., 2019 ). Just comparing is not a one-size-fits-all solution, especially when implicit or functional information is more important than superficial features and might not result in the intended deeper reasoning about critical features ( Bhattacharyya, 2023 ). For beginners, it might thus be necessary to be supported in attending to the relevant aspects, in order to decrease the extraneous and intrinsic load. The Cognitive Theory of Multimedia Learning (CTML) by Mayer (2021) allows informed instructional design to support students in these aspects. The key assumption of the CTML is that human cognition proceeds by two channels, a visual and a verbal channel, that need to be optimally synchronized in learning. It is thus beneficial to present information both visually, which we typically do with structural representations and verbally (e.g., written or spoken explanations), to engage both channels. Both channels have limited capacity, meaning that learners can only process a limited amount of information at a time. In the context of case comparisons, it is important not to overwhelm students with too much information at once and to guide their attention to the relevant aspect in the visual and verbal channel ( Rodemer et al., 2020 ; Eckhard et al., 2022 ). Thus, both theories, the CLT as well as the CTML, support the same instructional design principles: guiding students visually and conceptually through a task, to make a task accessible for actual learning.
5.1 Visual attention guidance
Guiding learners to attend to the relevant features, i.e., important functional groups involved in a reaction, can be achieved by multiple means, such as simply signaling or highlighting the relevant areas of the representation [i.e., signaling principle as described by Mayer (2021) ], e.g., by zooming in or out, spotlights, coloring, added on-screen text or symbols. Others used experts’ eye gaze as a model for the learner, as used in the context of medicine ( Jarodzka et al., 2012 ; Gegenfurtner et al., 2017 ), whereas transferring this idea to learning organic reaction mechanisms has not yet been convincing ( Graulich et al., 2022 ). By “signaling” (highlighting key structural features in a static or dynamic fashion) students can focus on these key features of the representation and reduce their attentional focus to the rest of the structure, thus, reducing their extraneous cognitive load, if they are not attending to everything all at once ( Richter et al., 2016 ; Schneider et al., 2018 ). It can also allow us to model a certain sequence of comparing by highlighting, for example, a starting point of comparison and then the sequential decoding process. Although attending to the relevant features is a key step. Implicit chemical properties cannot be read out of the functional group but need to be linked to it. When the attention of the learner is on the relevant features of a representation, the respective implicit information needs to be added, either in terms of verbal or written information. This is in line with the dual channel assumption of the CTML, providing highlighting for the visual features and chemical information for the verbal channel, as well as presenting it at the same time, i.e., the contiguity principle ( Mayer and Fiorella, 2014 ). Some instructors might intuitively use highlighting techniques by pointing toward the representational features on the blackboard and explaining simultaneously or by adding conceptual information, such as pK a or partial charges on the board. Redirecting a learner’s attention to the relevant aspects, thus, can be complex, as decisions have to be made that cannot just be guided by the salience of a functional group, and conceptual information needs to be linked to make a purposeful selection.
In a quantitative study, we tested if a highlighting technique actually supports students to attend to relevant areas of organic chemistry case comparisons and solve them more successfully. Thus, we created tutorial videos with case comparisons and used a dynamic moving dot highlighting representational features, which was synchronized with the information given as a verbal explanation in parallel ( Rodemer et al., 2020 ; Eckhard et al., 2022 ). The study could document that all students in the study were profiting from the given verbal explanation, but especially low performing students profited from the highlighting. Following students while watching the videos with highlighting with the help of eye-tracking could show that the attention to relevant areas is focused over the entire time of the video, and the perceived extraneous cognitive load is decreased ( Rodemer et al., 2022 ). These overall results illustrated that beginners need more support in decoding the molecular structures that we use in organic chemistry, and guiding their attention is key for a decreased extraneous cognitive load. Besides using eye-tracking as an analytical lens to track students’ attention, using it in instruction might help students understand their own viewing behavior. In an eye-tracking study conducted by Hansen et al. (2019) , they investigated how students view and critique different animations of redox reactions and precipitation reactions. After their reasoning process, students received visual feedback on their own viewing behavior. Hansen et al. (2019) revealed that viewing this feedback helped the students to be critical about their own viewing behavior and to deepen the critique regarding the animations shown.
5.2 Conceptual guidance
Further breaking down the reasoning process with case comparisons into manageable parts can help students process the information more effectively ( Belland, 2017 ). A simple nucleophilic substitution, taught in an introductory organic chemistry course, for instance, requires the consideration of three main influential factors, i.e., leaving group ability, nucleophilicity, substrate effects, and the cause-effect relationships that determine the reactivity in this type of mechanism. Thus, a lot needs to be considered by the learners. Using case comparison can have positive effects on students’ engagement with the conceptual knowledge, as it shifts the focus onto implicit and influential factors of the organic reaction mechanism ( Watts et al., 2021 ). However, if we expect students to reason in a particular way, i.e., building cause-effect relationships, and connect different concepts and properties, we need to be explicit how students should integrate these multiple pieces of knowledge. Developing mastery requires explicit learning of how to create those mechanistic explanations ( Cooper, 2015 ). Thus, supporting students in solving case comparisons should acknowledge the complexity and reasoning steps required and ideally make these steps transparent through a scaffold ( Caspari et al., 2018 ; Kranz et al., 2023 ). Scaffolding is a known technique widely used as an instruction in science education ( cf. Lin et al., 2012 ; Wilson and Devereux, 2014 ) and helps students to slow down the decision-making process and gives students the opportunity to activate necessary conceptual and procedural knowledge ( Rittle-Johnson and Star, 2007 ; Rittle-Johnson and Star, 2009 ; Shemwell et al., 2015 ; Chin et al., 2016 ). A scaffold for the case comparisons illustrated therein thus can guide the learner through the different considerations necessary to make a claim about the outcome of a case: (1) describing the chemical changes in the given cases; (2) explicitly stating the overall goal of comparison (task prompt); (3) naming the similarities and differences; (4) stating the role of the influential factors (i.e., implicit properties); (5) explaining and contrasting the influences of the implicit properties; (6) stating how the transition state is affected to refer to the energetic account and (7) making a final claim about the reactivity of both reactions ( Bernholt et al., 2023 ).
Various studies already documented the positive effect of using scaffolding with case comparisons on students’ reasoning. In prior studies, we used a scaffold grid, represented by a worksheet with empty boxes, which visually connects the structural differences, changes, and cause-effect relations ( Caspari et al., 2018 ). By utilizing this grid, students can systematically relate each structural difference to each ongoing change, verbalizing the influence of the structural difference on the change. We compared how students are reasoning through contrasting cases with and without a scaffold and could observe that students’ reasoning is more guided and includes the consideration of more implicit properties and influential effects when solving a contrasting case with a scaffold ( Caspari et al., 2018 ). This structured approach helps students avoid jumping to the final answer without considering the underlying reasons. A mixed-methods study could confirm that especially students with a low prior knowledge profited from working with a scaffold and had a higher learning gain, whereas it does also not harm those with higher prior knowledge ( Kranz et al., 2023 ). Lieber et al. (2022) advanced a scaffold further by acknowledging students’ individual needs when arguing about alternative reaction pathways. Those adaptive scaffolds could show that more individualized instruction when using different cases in organic chemistry might be a new avenue to improve teaching.
6 Conclusion
Comparing the outcome of organic reactions, the strength of nucleophiles, or the reaction rate is at the core of organic chemistry. Through asking comparative questions, we gain insight into reaction processes and reactivity patterns, which allow us to predict and explain novel ones. Learning a collection of seemingly unrelated reactions, or even name reactions in organic chemistry, as often the practice in organic chemistry classes, does not allow learners or make it more difficult to understand and derive the underlying principles that govern reactions. Structure mapping theory tells us, that our cognitive structure is barely made to extract with ease a conceptual similarity just by looking at reactions. An explicit surface similarity will always be more salient for an inexperienced learner. The limited capacity of our working memory additionally affects how much effort we can put into learning and understanding. Purposefully comparing and reasoning through case comparisons can help regain the focus on conceptual understanding in organic chemistry but has not yet been fully explored in instructional design as well as assessments. Multiple studies have documented the potential of using case comparisons compared to more traditional task formats, characterized the type of reasoning that can be elicited from learners, and integrated case comparisons into laboratory experiments. We illustrated therein how, based on various theories of cognition and instruction, comparing can serve as a valuable process for selecting attention, limiting the extraneous cognitive load as well as focusing on implicit and explicit properties and cause-effect relationships. This process of comparing can further be supported, following the principles of the Cognitive Theory of Multimedia Learning, by highlighting relevant features of representations through cueing techniques or providing scaffolding by sequentially guiding students through solving a case comparison. This perspective was meant to consolidate the current state of the art around the use of case comparison to provide instructors with a theory-informed basis for changing their practice and exploring comparing.
Author contributions
NG: Conceptualization, Funding acquisition, Visualization, Writing – original draft, Writing – review & editing. LL: Conceptualization, Visualization, Writing – original draft, Writing – review & editing.
The author(s) declare financial support was received for the research, authorship, and/or publication of this article. NG would like to thank the German Research Foundation DFG (Deutsche Forschungsgemeinschaft) for funding (project number: 446349713).
Conflict of interest
The authors declare that the research was conducted in the absence of any commercial or financial relationships that could be construed as a potential conflict of interest.
Publisher’s note
All claims expressed in this article are solely those of the authors and do not necessarily represent those of their affiliated organizations, or those of the publisher, the editors and the reviewers. Any product that may be evaluated in this article, or claim that may be made by its manufacturer, is not guaranteed or endorsed by the publisher.
Afagh, N. A., and Yudin, A. K. (2010). Chemoselectivity and the curious reactivity preferences of functional groups. Angew. Chem. Int. Ed. 49, 262–310. doi: 10.1002/anie.200901317
PubMed Abstract | Crossref Full Text | Google Scholar
Alfieri, L., Nokes-Malach, T. J., and Schunn, C. D. (2013). Learning through case comparisons: a Meta-analytic review. Educ. Psychol. 48, 87–113. doi: 10.1080/00461520.2013.775712
Crossref Full Text | Google Scholar
Anzovino, M. E., and Bretz, S. L. (2015). Organic chemistry students' ideas about nucleophiles and electrophiles: the role of charges and mechanisms. Chem. Educ. Res. Pract. 16, 797–810. doi: 10.1039/C5RP00113G
Anzovino, M. E., and Bretz, S. L. (2016). Organic chemistry students' fragmented ideas about the structure and function of nucleophiles and electrophiles: a concept map analysis. Chem. Educ. Res. Pract. 17, 1019–1029. doi: 10.1039/C6RP00111D
Baddeley, A. (2010). Working memory. Curr. Biol. 20, R136–R140. doi: 10.1016/j.cub.2009.12.014
Bego, C. R., Chastain, R. J., and DeCaro, M. S. (2023). Designing novel activities before instruction: use of contrasting cases and a rich dataset. Br. J. Educ. Psychol. 93, 299–317. doi: 10.1111/bjep.12555
Belland, B. R., (2017), In instructional scaffolding in STEM education: strategies and efficacy evidence , Cham: Springer International Publishing.
Google Scholar
Bernholt, S., Eckhard, J., Rodemer, M., Langner, A., Asmussen, G., and Graulich, N. (2023). In digital learning and teaching in chemistry The Royal Society of Chemistry.
Bhattacharyya, G. (2014). Trials and tribulations: student approaches and difficulties with proposing mechanisms using the electron-pushing formalism. Chem. Educ. Res. Pract. 15, 594–609. doi: 10.1039/C3RP00127J
Bhattacharyya, G. (2023) “Assessment of assessment in organic chemistry - Review and analysis of predominant problem types related to reactions and mechanisms”, in Student reasoning in organic chemistry . eds. N. Graulich and G. Shultz (Cambridge (UK): Roy. Soc. Chemistry), 269–284.
Bodé, N. E., Deng, J. M., and Flynn, A. B. (2019). Getting past the rules and to the WHY: causal mechanistic arguments when judging the plausibility of organic reaction mechanisms. J. Chem. Educ. 96, 1068–1082. doi: 10.1021/acs.jchemed.8b00719
Bussey, T. J., Orgill, M., and Crippen, K. J. (2013). Variation theory: a theory of learning and a useful theoretical framework for chemical education research. Chem. Educ. Res. Pract. 14, 9–22. doi: 10.1039/C2RP20145C
Caspari, I., Kranz, D., and Graulich, N. (2018). Resolving the complexity of organic chemistry students' reasoning through the lens of a mechanistic framework. Chem. Educ. Res. Pract. 19, 1117–1141. doi: 10.1039/C8RP00131F
Chin, D. B., Chi, M., and Schwartz, D. L. (2016). A comparison of two methods of active learning in physics: inventing a general solution versus compare and contrast. Instr. Sci. 44, 177–195. doi: 10.1007/s11251-016-9374-0
Cooper, M. M. (2015). Why ask why? J. Chem. Educ. 92, 1273–1279. doi: 10.1021/acs.jchemed.5b00203
Cooper, M. M., Corley, L. M., and Underwood, S. M. (2013). An investigation of college chemistry students' understanding of structure-property relationships. J. Res. Sci. Teach. 50, 699–721. doi: 10.1002/tea.21093
DeFever, R. S., Bruce, H., and Bhattacharyya, G. (2015). Mental Rolodexing: senior chemistry Majors' understanding of chemical and physical properties. J. Chem. Educ. 92, 415–426. doi: 10.1021/ed500360g
Deng, J. M., and Flynn, A. B. (2021). Reasoning, granularity, and comparisons in students’ arguments on two organic chemistry items. Chem. Educ. Res. Pract. 22, 749–771. doi: 10.1039/D0RP00320D
Domin, D. S., Al-Masum, M., and Mensah, J. (2008). Students' categorizations of organic compounds. Chem. Educ. Res. Pract. 9, 114–121. doi: 10.1039/B806226A
Eckhard, J., Rodemer, M., Bernholt, S., and Graulich, N. (2022). What do University students truly learn when watching tutorial videos in organic chemistry? An exploratory study focusing on mechanistic reasoning. J. Chem. Educ. 99, 2231–2244. doi: 10.1021/acs.jchemed.2c00076
Galloway, K. R., Leung, M. W., and Flynn, A. B. (2018). A comparison of how undergraduates, graduate students, and professors organize organic chemistry reactions. J. Chem. Educ. 95, 355–365. doi: 10.1021/acs.jchemed.7b00743
Gegenfurtner, A., Lehtinen, E., Jarodzka, H., and Säljö, R. (2017). Effects of eye movement modeling examples on adaptive expertise in medical image diagnosis. Comput. Educ. 113, 212–225. doi: 10.1016/j.compedu.2017.06.001
Gentner, D., (1989), Similarity and analogical reasoning , New York: Cambridge University Press.
Gentner, D., Loewenstein, J., and Thompson, L. (2003). Learning and transfer: a general role for analogical encoding. J. Educ. Psychol. 95, 393–408. doi: 10.1037/0022-0663.95.2.393
Gentner, D., and Markman, A. B. (1997). Structure mapping in analogy and similarity. Am. Psychol. 52, 45–56. doi: 10.1037/0003-066X.52.1.45
Gick, M. L., and Holyoak, K. J. (1983). Schema induction and analogical transfer. Cogn. Psychol. 15, 1–38. doi: 10.1016/0010-0285(83)90002-6
Goodwin, W. (2008). Structural formulas and explanation in organic chemistry. Found. Chem. 10, 117–127. doi: 10.1007/s10698-007-9033-2
Goodwin, W. (2010). How do structural formulas embody the theory of organic chemistry? Br. Soc. Philos. Sci. 61, 621–633. doi: 10.1093/bjps/axp052
Graulich, N., and Bhattacharyya, G. (2017). Investigating students' similarity judgments in organic chemistry. Chem. Educ. Res. Pract. 18, 774–784. doi: 10.1039/C7RP00055C
Graulich, N., Hedtrich, S., and Harzenetter, R. (2019). Explicit versus implicit similarity - exploring relational conceptual understanding in organic chemistry. Chem. Educ. Res. Pract. 20, 924–936. doi: 10.1039/C9RP00054B
Graulich, N., Rodemer, M., Eckhard, J., and Bernholt, S., (2022), Eye-Tracking in der Mathematik- und Naturwissenschaftsdidaktik: Forschung und Praxis , Berlin, Heidelberg: Springer Berlin Heidelberg.
Graulich, N., and Schween, M. (2018). Concept-oriented task design: making purposeful case comparisons in organic chemistry. J. Chem. Educ. 95, 376–383. doi: 10.1021/acs.jchemed.7b00672
Grove, N. P., Cooper, M. M., and Cox, E. L. (2012). Does mechanistic thinking improve student success in organic chemistry? J. Chem. Educ. 89, 850–853. doi: 10.1021/ed200394d
Hansen, S., Hu, B., Riedlova, D., Kelly, R., Akaygun, S., and Villalta-Cerdas, A. (2019). Critical consumption of chemistry visuals: eye tracking structured variation and visual feedback of redox and precipitation reactions. Chem. Educ. Res. Pract. 20, 837–850. doi: 10.1039/C9RP00015A
Jarodzka, H., Balslev, T., Holmqvist, K., Nyström, M., Scheiter, K., Gerjets, P., et al. (2012). Conveying clinical reasoning based on visual observation via eye-movement modelling examples. Instr. Sci. 40, 813–827. doi: 10.1007/s11251-012-9218-5
Kalyuga, S., Chandler, P., and Sweller, J. (1998). Levels of expertise and instructional design. Hum. Factors 40, 1–17. doi: 10.1518/001872098779480587
Keith, J. A., Vassilev-Galindo, V., Cheng, B., Chmiela, S., Gastegger, M., Müller, K.-R., et al. (2021). Combining machine learning and computational chemistry for predictive insights into chemical systems. Chem. Rev. 121, 9816–9872. doi: 10.1021/acs.chemrev.1c00107
Kranz, D., Schween, M., and Graulich, N. (2023). Patterns of reasoning - exploring the interplay of students' work with a scaffold and their conceptual knowledge in organic chemistry. Chem. Educ. Res. Pract. 24, 453–477. doi: 10.1039/D2RP00132B
Lapierre, K. R., and Flynn, A. B. (2020). An online categorization task to investigate changes in students' interpretations of organic chemistry reactions. J. Res. Sci. Teach. 57, 87–111. doi: 10.1002/tea.21586
Lapierre, K. R., Streja, N., and Flynn, A. B. (2022). Investigating the role of multiple categorization tasks in a curriculum designed around mechanistic patterns and principles. Chem. Educ. Res. Pract. 23, 545–559. doi: 10.1039/D1RP00267H
Laszlo, P. (2002). Describing reactivity with structural formulas, or when push comes to shove. Chem. Educ. Res. Pract. 3, 113–118. doi: 10.1039/B2RP90009B
Lieber, L., and Graulich, N. (2022). Investigating Students' argumentation when judging the plausibility of alternative reaction pathways in organic chemistry. Chem. Educ. Res. Pract. 23, 38–54. doi: 10.1039/D1RP00145K
Lieber, L., Ibraj, K., Caspari-Gnann, I., and Graulich, N. (2022). Closing the gap of organic chemistry Students' performance with an adaptive scaffold for argumentation patterns. Chem. Educ. Res. Pract. 23, 811–828. doi: 10.1039/D2RP00016D
Lin, T.-C., Hsu, Y.-S., Lin, S.-S., Changlai, M.-L., Yang, K.-Y., and Lai, T.-L. (2012). A review of empirical evidence on scaffolding for science education. Int. J. Sci. Math. Educ. 10, 437–455. doi: 10.1007/s10763-011-9322-z
Lo, M. L., and Marton, F. (2011). Towards a science of the art of teaching: using variation theory as a guiding principle of pedagogical design. Int. J. Lesson Learn. Stud. 1, 7–22. doi: 10.1108/20468251211179678
Marton, F. (1981). Phenomenography—describing conceptions of the world around us. Instr. Sci. 10, 177–200. doi: 10.1007/BF00132516
Mason, B., Rau, M. A., and Nowak, R. (2019). Cognitive task analysis for implicit knowledge about visual representations with similarity learning methods. Cogn. Sci. 43:e12744. doi: 10.1111/cogs.12744
Mayer, R. E. (2021). Multimedia learning. Cambridge: Cambridge University Press.
Mayer, R. E., and Fiorella, L., (2014). The Cambridge handbook of multimedia learning , New York: Cambridge University Press.
McNeill, K. L., and Krajcik, J., (2012), Book study facilitator’s guide: Supporting grade 5–8 students in constructing explanations in science: the claim, evidence and reasoning framework for talk and writing , New York: Pearson Allyn & Bacon.
Paas, F., Renkl, A., and Sweller, J. (2003). Cognitive load theory and instructional design: recent developments. Educ. Psychol. 38, 1–4. doi: 10.1207/S15326985EP3801_1
Paas, F., and Sweller, J., (2014). “Implications of Cognitive Load Theory for Multimedia Learning”, in The Cambridge handbook of multimedia learning, 2nd Edition . Ed. R. Mayer (New York: Cambridge University Press), 27–42.
Pölloth, B., Häfner, M., and Schwarzer, S. (2022). At the same time or one after the other?–exploring reaction paths of nucleophilic substitution reactions using historic insights and experiments. Chemkon 29, 77–83. doi: 10.1002/ckon.202100060
Popova, M., and Bretz, S. L. (2018). Organic chemistry Students' understandings of what makes a good leaving group. J. Chem. Educ. 95, 1094–1101. doi: 10.1021/acs.jchemed.8b00198
Richter, J., Scheiter, K., and Eitel, A. (2016). Signaling text-picture relations in multimedia learning: a comprehensive meta-analysis. Educ. Res. Rev. 17, 19–36. doi: 10.1016/j.edurev.2015.12.003
Rittle-Johnson, B., and Star, J. R. (2007). Does comparing solution methods facilitate conceptual and procedural knowledge? An experimental study on learning to solve equations. J. Educ. Psychol. 99, 561–574. doi: 10.1037/0022-0663.99.3.561
Rittle-Johnson, B., and Star, J. R. (2009). Compared with what? The effects of different comparisons on conceptual knowledge and procedural flexibility for equation solving. J. Chem. Educ. 101:529. doi: 10.1037/a0014224
Rodemer, M., Eckhard, J., Graulich, N., and Bernholt, S. (2020). Decoding case comparisons in organic chemistry: eye-tracking Students' visual behavior. J. Chem. Educ. 97, 3530–3539. doi: 10.1021/acs.jchemed.0c00418
Rodemer, M., Lindner, M. A., Eckhard, J., Graulich, N., and Bernholt, S. (2022). Dynamic signals in instructional videos support students to navigate through complex representations: an eye-tracking study. Appl. Cogn. Psychol. 36, 852–863. doi: 10.1002/acp.3973
Roelle, J., and Berthold, K. (2015). Effects of comparing contrasting cases on learning from subsequent explanations. Cogn. Instr. 33, 199–225. doi: 10.1080/07370008.2015.1063636
Schmitt, C., Bender, M., Trabert, A., and Schween, M. (2018). What's the effect of steric hindrance? Experimental comparison of reaction rates of primary and secondary alkyl halides in competing SN2 reactions. Chemkon 25, 231–237. doi: 10.1002/ckon.201800012
Schmitt, C., Wißner, O., and Schween, M. (2013). Carbenium ions as reactive intermediates – an (experimental) access to a deeper understanding of organic reactions. Chemkon 20, 59–65. doi: 10.1002/ckon.201310195
Schneider, S., Beege, M., Nebel, S., and Rey, G. D. (2018). A meta-analysis of how signaling affects learning with media. Educ. Res. Rev. 23, 1–24. doi: 10.1016/j.edurev.2017.11.001
Schwartz, D. L., and Bransford, J. D. (1998). A time for telling. Cogn. Instr. 16, 475–5223. doi: 10.1207/s1532690xci1604_4
Schwartz, D. L., Chase, C. C., Oppezzo, M. A., and Chin, D. B. (2011). Practicing versus inventing with contrasting cases: the effects of telling first on learning and transfer. J. Educ. Psychol. 103, 759–775. doi: 10.1037/a0025140
Shemwell, J. T., Chase, C. C., and Schwartz, D. L. (2015). Seeking the general explanation: a test of inductive activities for learning and transfer. J. Res. Sci. Teach. 52, 58–83. doi: 10.1002/tea.21185
Stains, M., and Talanquer, V. (2007). Classification of chemical substances using particulate representations of matter: an analysis of student thinking. Int. J. Sci. Educ. 29, 643–661. doi: 10.1080/09500690600931129
Stains, M., and Talanquer, V. (2008). Classification of chemical reactions: stages of expertise. J. Res. Sci. Teach. 45, 771–793. doi: 10.1002/tea.20221
Sweller, J. (1994). Cognitive load theory, learning difficulty, and instructional design. Learn. Instr. 4, 295–312. doi: 10.1016/0959-4752(94)90003-5
Sweller, J., (2003), In psychology of learning and motivation: Advances in research and theory , San Diego, USA: Elsevier Science, pp. 215–266.
Sweller, J. (2010). Element interactivity and intrinsic, extraneous, and germane cognitive load. Educ. Psychol. Rev. 22, 123–138. doi: 10.1007/s10648-010-9128-5
Sweller, J., and Chandler, P. (1994). Why some material is difficult to learn. Cogn. Instr. 12, 185–233. doi: 10.1207/s1532690xci1203_1
Sweller, J., van Merriënboer, J. J., and Paas, F. (2019). Cognitive architecture and instructional design: 20 years later. Educ. Psychol. Rev. 31, 261–292. doi: 10.1007/s10648-019-09465-5
Talanquer, V. (2008). Students' predictions about the sensory properties of chemical compounds: additive versus emergent frameworks. Sci. Educ. 92, 96–114. doi: 10.1002/sce.20235
Talanquer, V. (2017). Concept inventories: predicting the wrong answer may boost performance. J. Chem. Educ. 94, 1805–1810. doi: 10.1021/acs.jchemed.7b00427
Trabert, A., Schmitt, C., and Schween, M. (2023). “Building bridges between tasks and flasks - Design of a coherent experiment-supported learning environment for deep reasoning in organic chemistry”, in Student reasoning in organic chemistry . Eds. N. Graulich and G. Shultz (Cambridge (UK): Roy. Soc. Chemistry), 248–266.
Trabert, A., and Schween, M. (2018). How do electronic substituent effects work?-design of a concept-based approach applying inventing with contrasting cases to the example of alkaline ester hydrolysis. Chemkon 25, 334–342. doi: 10.1002/ckon.201800010
Trabert, A., and Schween, M. (2020). How do electronic substituent effects work?–additional contrasting cases for a differentiated inquiry illustrated by the example of alkaline ester hydrolysis. Chemkon 27, 22–33. doi: 10.1002/ckon.201800076
Vorwerk, N., Schmitt, C., and Schween, M. (2015). Understanding electrophilic aromatic substitutions– sigma-complexes as (experimental) key structures. Chemkon 22, 59–68. doi: 10.1002/ckon.201410237
Watts, F. M., Dood, A. J., Shultz, G. V., and Rodriguez, J.-M. G. (2023). Comparing student and generative artificial intelligence Chatbot responses to organic chemistry writing-to-learn assignments. J. Chem. Educ. 100, 3806–3817. doi: 10.1021/acs.jchemed.3c00664
Watts, F. M., Zaimi, I., Kranz, D., Graulich, N., and Shultz, G. V. (2021). Investigating students' reasoning over time for case comparisons of acyl transfer reaction mechanisms. Chem. Educ. Res. Pract. 22, 364–381. doi: 10.1039/D0RP00298D
Weinrich, M. L., and Sevian, H. (2017). Capturing students’ abstraction while solving organic reaction mechanism problems across a semester. Chem. Educ. Res. Pract. 18, 169–190. doi: 10.1039/C6RP00120C
White, R., and Gunstone, R., (2014), Probing Understanding , New York: Routledge.
Wilson, K., and Devereux, L. (2014). Scaffolding theory: high challenge, high support in academic language and learning (ALL) contexts. J. Acad. Lang. Learn. 8, A91–A100.
Keywords: case comparisons, chemistry education, support, guidance, instruction
Citation: Graulich N and Lieber L (2024) Why comparing matters – on case comparisons in organic chemistry. Front. Educ . 9:1374793. doi: 10.3389/feduc.2024.1374793
Received: 22 January 2024; Accepted: 11 April 2024; Published: 26 April 2024.
Reviewed by:
Copyright © 2024 Graulich and Lieber. This is an open-access article distributed under the terms of the Creative Commons Attribution License (CC BY) . The use, distribution or reproduction in other forums is permitted, provided the original author(s) and the copyright owner(s) are credited and that the original publication in this journal is cited, in accordance with accepted academic practice. No use, distribution or reproduction is permitted which does not comply with these terms.
*Correspondence: Nicole Graulich, [email protected]
This article is part of the Research Topic
Organic Chemistry Education Research into Practice
Voices Blog
Sciences, Mathematics and Biotechnology
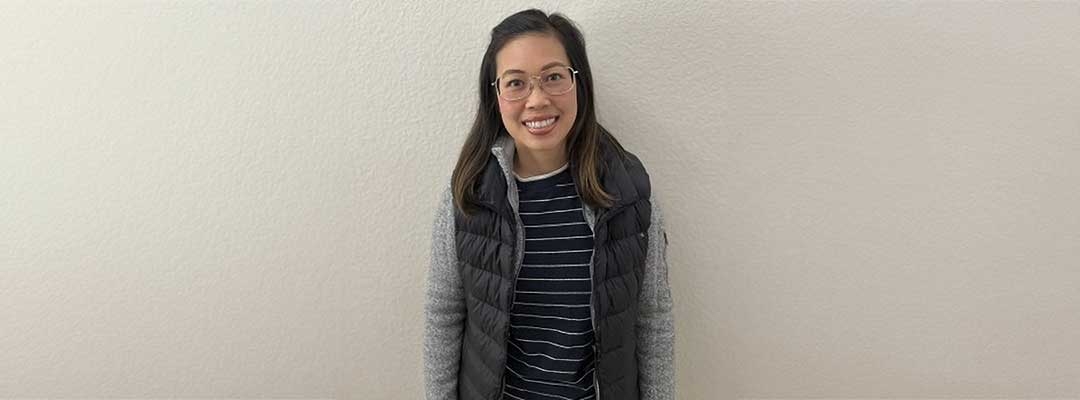
Steeped in Clinical Trial Design Know-How
Certificate graduate vivian dao-tong parlays skills to advance into clinical trial manager role.
For Vivian Dao-Tong, the importance of clinical research and its positive effects on health are personal.
One of her family members participated in a clinical trial for diabetes research and Vivian was taken aback at how much care and attention they received from the trial staff during each monthly visit. “The research team was very knowledgeable and resourceful. They spent a lot of time explaining to us what diabetes was and how to modify lifestyle habits to help improve quality of life,” Vivian explains.
“When the clinical trial was over and the drug went to market, it was neat to see how my family member’s participation in the clinical trial played a huge role in helping other patients have access to a new therapy to help manage their diabetes.”
This experience stuck with her. It’s what led her to earn a B.S. in biological sciences (with a minor in psychology) from Sacramento State and then to continue her education in the field with an M.S. in pharmaceutical sciences from Touro University.
In the field, Vivian has held clinical research coordinator and clinical research associate roles at various biotech companies in the Bay Area. It was during these experiences when Vivian realized that in order to succeed and help others improve their quality of life, she would need the technical know-how to effectively oversee a clinical trial from start to finish.
“The Bay Area is home to a lot of biotech companies and unfortunately not all companies will teach their employees on how to lead and manage a clinical trial,” Vivian explains.
“It was important for me to find a program that would prepare me to be knowledgeable in FDA and ICH Good Clinical Practices regulations and understand best practices on how to start, maintain and close out a clinical trial.”
So Vivian turned to some coworkers for advice, many of whom had excelled in their careers after completing our Certificate Program in Clinical Research Conduct and Management . With those recommendations in mind, Vivian knew that our certificate would be the perfect path toward career success .
“I was also drawn to UC Berkeley Extension’s reputation of upholding rigorous academic standards that would prepare me for my career in clinical trials.”
In August 2019, Vivian took her first step in investing in her future: Enrolling in the Introduction to Clinical Research: Clinical Trial Phases and Design course .
It was important for me to find a program that would prepare me to be knowledgeable in FDA and ICH Good Clinical Practices regulations and understand best practices on how to start, maintain and close out a clinical trial. I was also drawn to UC Berkeley Extension’s reputation of upholding rigorous academic standards that would prepare me for my career in clinical trials.
You took all of the classes online. Tell me about your experience going through the certificate.
I really enjoyed meeting other students and instructors virtually . The teachers really cared for their students and shared their industry experiences with us.
Assignments were applicable to day-to-day operations in the field. I remember we had an assignment where we had to write out what to prepare prior to conducting a monitoring visit .
I really enjoyed the flexibility of taking online classes because I was also working full time as a clinical trials specialist at Exelixis .
The curriculum was very logical. The first course was very high-level and challenging, but as I navigated through the program, it started to get easier. I enjoyed learning about the different life cycles of a clinical trial—such as startup, maintenance , close-out , post-market—different phases of a clinical trial and inspection readiness.
I really enjoyed the flexibility of taking online classes because I was also working full time as a clinical trials specialist at Exelixis.
Since completing the certificate, you’ve moved up from a clinical trials specialist to a clinical trials manager.
Yes, I am working at Alector , a biotechnology company in South San Francisco that focuses on neurodegenerative and immuno-neurology therapies.
Some of my day-to-day responsibilities include:
Ensuring sponsor oversight by managing contract research organizations (CROs) and study vendors
Overseeing regional site management activities
Performing data listing reconciliations and protocol-deviation reviews.
Managing CTA and budget negotiations
Leading study document reviews with cross-functional team members
Overseeing Trial Master File (TMF) as part of inspection readiness
Identifying risks and performing mitigation
Working on clinical-development operations, process, initiatives and improvement
That’s quite a lot of responsibilities! Are you incorporating lessons learned from the certificate to your work?
Absolutely! There was one company that I worked for that was a pre-IPO biotech company. At the time, the company wanted to start a clinical trial but did not know how or what guidelines to follow. I was able to explain what processes must be in place before starting a clinical trial —such as site feasibility, site qualification, site selection, et cetera—and worked very closely with the project manager to set realistic timelines.
This program provided me with a lot of knowledge and gave me the tools to be resourceful so that I can help lead my team to manage a clinical trial successfully.
What does earning this certificate mean to you personally and professionally?
With the constant changes in the world, there are so many new and undiscovered illnesses out there. Being able to research these emerging illnesses and investing the time, effort and money to run these clinical trials is very rewarding. Not everyone can say that they can put a drug out on the market , and for me it is an ultimate career goal to be a part of a team that is able to do this.
What advice would you give to a student who is starting the certificate?
Stay organized and stay disciplined ! Don't be afraid to ask questions if you do not understand the material at first.
Stay up to date with courses and trends in Clinical Research Conduct and Management
Deepen your skills, clinical research conduct and management, related posts.
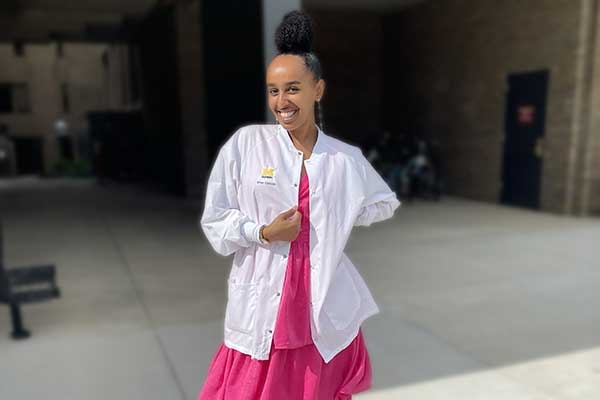
Sticking to Her Roots
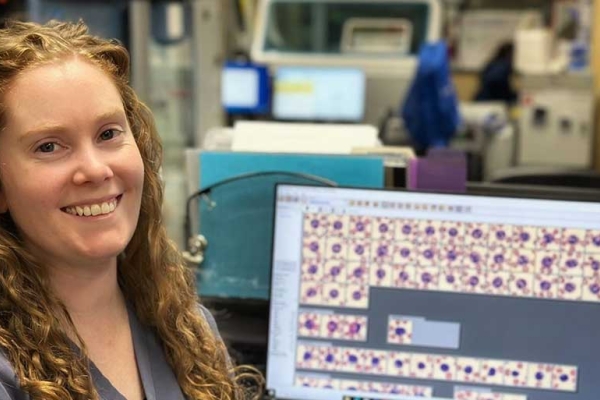
Making a Difference in the Lab
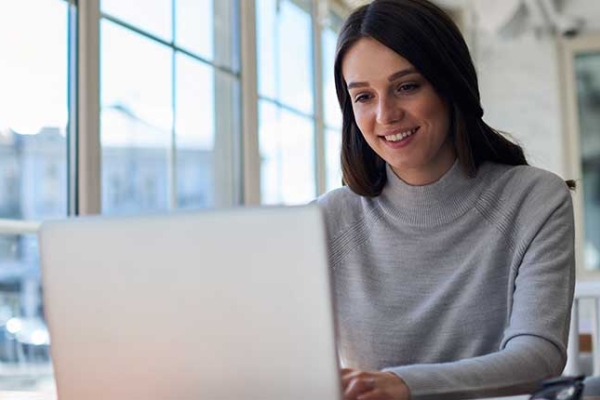
February 20 Online Event: Careers in Biotech
View the discussion thread.
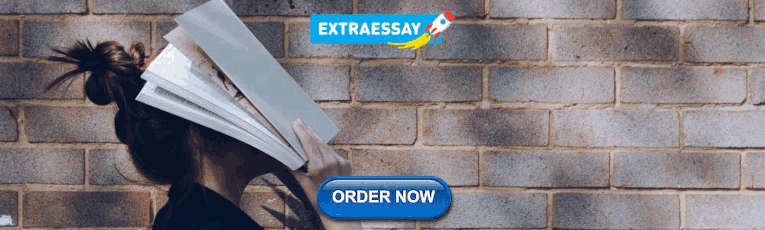
IMAGES
VIDEO
COMMENTS
The purpose of this qualitative case study was to investigate how an active learning classroom (ALC) at ISU influenced student engagement. Using Barkley's (2010) classroom-based model of student engagement, the findings provide insights on how classroom design affords student. Melissa L. Rands is the Assistant Director of Assessment at the ...
The Science of Classroom Design. Our comprehensive, all-in, research-based look at the design of effective learning spaces. By Youki Terada, Stephen Merrill. November 2, 2023. When a team of researchers led by University of Salford professor Peter Barrett analyzed the design of 153 classrooms across 27 elementary schools in the United Kingdom ...
Scientific research shows how the physical classroom environment influences student achievement. Two findings are key: First, the building's structural facilities profoundly influence learning. Inadequate lighting, noise, low air quality, and deficient heating in the classroom are significantly related to worse student achievement.
Overview of HEAD research design (with examples of BE factors). ... by the impact of the most effective classroom design, compared with the least. 5. Discussion. Table 11 takes the findings on the individual parameters and compares them with existing evidence from the literature. Many of the sources used for the latter have been focused on ...
While student‐centered instruction can occur in any style classrooms, active learning classrooms (ALCs) are purposefully designed to promote student engagement in the learning process (Adedokum et al., 2107; Baepler et al., 2016; Freeman et al., 2014; Wiltbank et al., 2019).
The facility's structural features—inadequate light-ing, noise, poor air quality, and deficient heating—can undermine learning. The classroom's symbols, such as objects and décor, also influence student achievement. Evidence-based classroom design can maximize edu-cation outcomes for all students.
Classroom design research is a cyclic process in which a classroom intervention is designed, implemented in classrooms, and analyzed for students' learning (Stephan, 2015). Based upon data ...
Classroom design played a crucial role in shaping the learning environment and had a multidimensional impact on student learning outcomes, creativity, and well-being.
DBR is a promising research approach integrating educational theory and practice. The. Didactical Design in Classroom research group (DDC) strives to establish a research base of. conceptually and ...
A resurgence in interest in classroom and school design has highlighted how little we know about the impact of learning environments on student and teacher performance. This is partly because of a lack of research methods capable of controlling the complex variables inherent to space and education. In a unique study that overcame such difficulties by using a single-subject research design in ...
Design research might be considered to focus more on external than inter- nal validity in that authentic contexts, complex instruction, and social inter- 10. Experimental Research in Classrooms 2 21 action are key features of design research. Design research, however, has not solved the problems of studying complex phenomena in complex settings.
Design research is usually motivated by university members with experience and interest in building theory and instructional designs in collaboration with one teacher. Typically, the teacher is considered as a member of the research team, with the primary responsibility of implementing instruction. However, in this chapter, I describe a Classroom Design Research project that was conducted by a ...
Step 1: Notice. Observe what's going on in your classroom. The important aspect of Step 1 in the classroom research cycle is to be a good observer—to see what you and your students are doing. When observing and noticing your classroom, zero in on something that you struggle with as a teacher and that students struggle with as learners.
This article discusses four key considerations for using research well in the classroom, along with initial resources and practical guides to support teachers to engage with research. 1. Research comes from a variety of sources. The educators in our survey told us about the challenges they face in accessing research.
Introduction. Students are ever more involved in the design of educational practices (e.g. Bovill et al. Citation 2016), which is reflected in the growing body of educational literature about approaches to student participation: design-based research (DBR), participatory design (PD), co-creation, co-design, student voice, student-staff partnership, students as change agents, student ...
Action research is a process for improving educational practice. Its methods involve action, evaluation, and reflection. It is a process to gather evidence to implement change in practices. Action research is participative and collaborative. It is undertaken by individuals with a common purpose.
Research-based primary classroom design. However often you have set up a new classroom, according to Kate McCallam it's worth pausing to consider new research about the impact of classroom design on learning first. It's classroom set-up time. Love it or loathe it, as we start this new school year, if you are a primary or Early Years teacher ...
Classroom design. should be intentional and purposeful, incorporating elements such as natural. light, flexible spaces, comfortable. furniture, strategic use of color, and. technology that ...
International Journal of Evaluation and Research in Education (IJERE) Vol. 9, No. 3, September 2020, pp. 469~477 ISSN: 2252-8822, DOI: 10.11591/ijere.v9i3.20566 469 How classroom design impacts for student learning comfort: Architect perspective on designing classrooms Kurnia Widiastuti1, Mohamad Joko Susilo2, Hanifah Sausan Nurfinaputri3 ...
Research designs that attempt to identify systematic effects have at their root an intent to establish a cause-and-effect relationship. Causal work is built on both theory and descriptive studies. ... a design that features students in one classroom assigned to different interventions is subject to "spillover" effects) or human volition (e ...
The increasing use of quasi-experimental research designs (QEDs) in education, brought into focus following the "credibility revolution" (Angrist & Pischke, 2010) in economics, which sought to use data to empirically test theoretical assertions, has indeed improved causal claims in education (Loeb et al., 2017).However, more recently, scholars, practitioners, and policymakers have ...
Variations of this task design in the chemistry classroom and recommendations for teaching with case comparisons based on current state-of-the-art evidence from research studies in chemistry education research are provided. ... rethinking task design has become a major focus for instructional design in chemistry education research. Thus, this ...
The study explores the influence of classroom design on Grade 8 students' academic performance in English. The sample consisted of teachers and students, with teachers predominantly aged between ...
In August 2019, Vivian took her first step in investing in her future: Enrolling in the Introduction to Clinical Research: Clinical Trial Phases and Design course. It was important for me to find a program that would prepare me to be knowledgeable in FDA and ICH Good Clinical Practices regulations and understand best practices on how to start ...
How classroom design impacts for student learning comfort: Architect perspective on designing classrooms September 2020 International Journal of Evaluation and Research in Education (IJERE) 9(3 ...