- Privacy Policy
Buy Me a Coffee

Home » Quantitative Data – Types, Methods and Examples
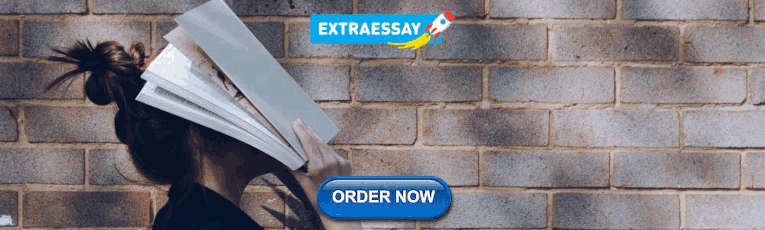
Quantitative Data – Types, Methods and Examples
Table of Contents

Quantitative Data
Definition:
Quantitative data refers to numerical data that can be measured or counted. This type of data is often used in scientific research and is typically collected through methods such as surveys, experiments, and statistical analysis.
Quantitative Data Types
There are two main types of quantitative data: discrete and continuous.
- Discrete data: Discrete data refers to numerical values that can only take on specific, distinct values. This type of data is typically represented as whole numbers and cannot be broken down into smaller units. Examples of discrete data include the number of students in a class, the number of cars in a parking lot, and the number of children in a family.
- Continuous data: Continuous data refers to numerical values that can take on any value within a certain range or interval. This type of data is typically represented as decimal or fractional values and can be broken down into smaller units. Examples of continuous data include measurements of height, weight, temperature, and time.
Quantitative Data Collection Methods
There are several common methods for collecting quantitative data. Some of these methods include:
- Surveys : Surveys involve asking a set of standardized questions to a large number of people. Surveys can be conducted in person, over the phone, via email or online, and can be used to collect data on a wide range of topics.
- Experiments : Experiments involve manipulating one or more variables and observing the effects on a specific outcome. Experiments can be conducted in a controlled laboratory setting or in the real world.
- Observational studies : Observational studies involve observing and collecting data on a specific phenomenon without intervening or manipulating any variables. Observational studies can be conducted in a natural setting or in a laboratory.
- Secondary data analysis : Secondary data analysis involves using existing data that was collected for a different purpose to answer a new research question. This method can be cost-effective and efficient, but it is important to ensure that the data is appropriate for the research question being studied.
- Physiological measures: Physiological measures involve collecting data on biological or physiological processes, such as heart rate, blood pressure, or brain activity.
- Computerized tracking: Computerized tracking involves collecting data automatically from electronic sources, such as social media, online purchases, or website analytics.
Quantitative Data Analysis Methods
There are several methods for analyzing quantitative data, including:
- Descriptive statistics: Descriptive statistics are used to summarize and describe the basic features of the data, such as the mean, median, mode, standard deviation, and range.
- Inferential statistics : Inferential statistics are used to make generalizations about a population based on a sample of data. These methods include hypothesis testing, confidence intervals, and regression analysis.
- Data visualization: Data visualization involves creating charts, graphs, and other visual representations of the data to help identify patterns and trends. Common types of data visualization include histograms, scatterplots, and bar charts.
- Time series analysis: Time series analysis involves analyzing data that is collected over time to identify patterns and trends in the data.
- Multivariate analysis : Multivariate analysis involves analyzing data with multiple variables to identify relationships between the variables.
- Factor analysis : Factor analysis involves identifying underlying factors or dimensions that explain the variation in the data.
- Cluster analysis: Cluster analysis involves identifying groups or clusters of observations that are similar to each other based on multiple variables.
Quantitative Data Formats
Quantitative data can be represented in different formats, depending on the nature of the data and the purpose of the analysis. Here are some common formats:
- Tables : Tables are a common way to present quantitative data, particularly when the data involves multiple variables. Tables can be used to show the frequency or percentage of data in different categories or to display summary statistics.
- Charts and graphs: Charts and graphs are useful for visualizing quantitative data and can be used to highlight patterns and trends in the data. Some common types of charts and graphs include line charts, bar charts, scatterplots, and pie charts.
- Databases : Quantitative data can be stored in databases, which allow for easy sorting, filtering, and analysis of large amounts of data.
- Spreadsheets : Spreadsheets can be used to organize and analyze quantitative data, particularly when the data is relatively small in size. Spreadsheets allow for calculations and data manipulation, as well as the creation of charts and graphs.
- Statistical software : Statistical software, such as SPSS, R, and SAS, can be used to analyze quantitative data. These programs allow for more advanced statistical analyses and data modeling, as well as the creation of charts and graphs.
Quantitative Data Gathering Guide
Here is a basic guide for gathering quantitative data:
- Define the research question: The first step in gathering quantitative data is to clearly define the research question. This will help determine the type of data to be collected, the sample size, and the methods of data analysis.
- Choose the data collection method: Select the appropriate method for collecting data based on the research question and available resources. This could include surveys, experiments, observational studies, or other methods.
- Determine the sample size: Determine the appropriate sample size for the research question. This will depend on the level of precision needed and the variability of the population being studied.
- Develop the data collection instrument: Develop a questionnaire or survey instrument that will be used to collect the data. The instrument should be designed to gather the specific information needed to answer the research question.
- Pilot test the data collection instrument : Before collecting data from the entire sample, pilot test the instrument on a small group to identify any potential problems or issues.
- Collect the data: Collect the data from the selected sample using the chosen data collection method.
- Clean and organize the data : Organize the data into a format that can be easily analyzed. This may involve checking for missing data, outliers, or errors.
- Analyze the data: Analyze the data using appropriate statistical methods. This may involve descriptive statistics, inferential statistics, or other types of analysis.
- Interpret the results: Interpret the results of the analysis in the context of the research question. Identify any patterns, trends, or relationships in the data and draw conclusions based on the findings.
- Communicate the findings: Communicate the findings of the analysis in a clear and concise manner, using appropriate tables, graphs, and other visual aids as necessary. The results should be presented in a way that is accessible to the intended audience.
Examples of Quantitative Data
Here are some examples of quantitative data:
- Height of a person (measured in inches or centimeters)
- Weight of a person (measured in pounds or kilograms)
- Temperature (measured in Fahrenheit or Celsius)
- Age of a person (measured in years)
- Number of cars sold in a month
- Amount of rainfall in a specific area (measured in inches or millimeters)
- Number of hours worked in a week
- GPA (grade point average) of a student
- Sales figures for a product
- Time taken to complete a task.
- Distance traveled (measured in miles or kilometers)
- Speed of an object (measured in miles per hour or kilometers per hour)
- Number of people attending an event
- Price of a product (measured in dollars or other currency)
- Blood pressure (measured in millimeters of mercury)
- Amount of sugar in a food item (measured in grams)
- Test scores (measured on a numerical scale)
- Number of website visitors per day
- Stock prices (measured in dollars)
- Crime rates (measured by the number of crimes per 100,000 people)
Applications of Quantitative Data
Quantitative data has a wide range of applications across various fields, including:
- Scientific research: Quantitative data is used extensively in scientific research to test hypotheses and draw conclusions. For example, in biology, researchers might use quantitative data to measure the growth rate of cells or the effectiveness of a drug treatment.
- Business and economics: Quantitative data is used to analyze business and economic trends, forecast future performance, and make data-driven decisions. For example, a company might use quantitative data to analyze sales figures and customer demographics to determine which products are most popular among which segments of their customer base.
- Education: Quantitative data is used in education to measure student performance, evaluate teaching methods, and identify areas where improvement is needed. For example, a teacher might use quantitative data to track the progress of their students over the course of a semester and adjust their teaching methods accordingly.
- Public policy: Quantitative data is used in public policy to evaluate the effectiveness of policies and programs, identify areas where improvement is needed, and develop evidence-based solutions. For example, a government agency might use quantitative data to evaluate the impact of a social welfare program on poverty rates.
- Healthcare : Quantitative data is used in healthcare to evaluate the effectiveness of medical treatments, track the spread of diseases, and identify risk factors for various health conditions. For example, a doctor might use quantitative data to monitor the blood pressure levels of their patients over time and adjust their treatment plan accordingly.
Purpose of Quantitative Data
The purpose of quantitative data is to provide a numerical representation of a phenomenon or observation. Quantitative data is used to measure and describe the characteristics of a population or sample, and to test hypotheses and draw conclusions based on statistical analysis. Some of the key purposes of quantitative data include:
- Measuring and describing : Quantitative data is used to measure and describe the characteristics of a population or sample, such as age, income, or education level. This allows researchers to better understand the population they are studying.
- Testing hypotheses: Quantitative data is often used to test hypotheses and theories by collecting numerical data and analyzing it using statistical methods. This can help researchers determine whether there is a statistically significant relationship between variables or whether there is support for a particular theory.
- Making predictions : Quantitative data can be used to make predictions about future events or trends based on past data. This is often done through statistical modeling or time series analysis.
- Evaluating programs and policies: Quantitative data is often used to evaluate the effectiveness of programs and policies. This can help policymakers and program managers identify areas where improvements can be made and make evidence-based decisions about future programs and policies.
When to use Quantitative Data
Quantitative data is appropriate to use when you want to collect and analyze numerical data that can be measured and analyzed using statistical methods. Here are some situations where quantitative data is typically used:
- When you want to measure a characteristic or behavior : If you want to measure something like the height or weight of a population or the number of people who smoke, you would use quantitative data to collect this information.
- When you want to compare groups: If you want to compare two or more groups, such as comparing the effectiveness of two different medical treatments, you would use quantitative data to collect and analyze the data.
- When you want to test a hypothesis : If you have a hypothesis or theory that you want to test, you would use quantitative data to collect data that can be analyzed statistically to determine whether your hypothesis is supported by the data.
- When you want to make predictions: If you want to make predictions about future trends or events, such as predicting sales for a new product, you would use quantitative data to collect and analyze data from past trends to make your prediction.
- When you want to evaluate a program or policy : If you want to evaluate the effectiveness of a program or policy, you would use quantitative data to collect data about the program or policy and analyze it statistically to determine whether it has had the intended effect.
Characteristics of Quantitative Data
Quantitative data is characterized by several key features, including:
- Numerical values : Quantitative data consists of numerical values that can be measured and counted. These values are often expressed in terms of units, such as dollars, centimeters, or kilograms.
- Continuous or discrete : Quantitative data can be either continuous or discrete. Continuous data can take on any value within a certain range, while discrete data can only take on certain values.
- Objective: Quantitative data is objective, meaning that it is not influenced by personal biases or opinions. It is based on empirical evidence that can be measured and analyzed using statistical methods.
- Large sample size: Quantitative data is often collected from a large sample size in order to ensure that the results are statistically significant and representative of the population being studied.
- Statistical analysis: Quantitative data is typically analyzed using statistical methods to determine patterns, relationships, and other characteristics of the data. This allows researchers to make more objective conclusions based on empirical evidence.
- Precision : Quantitative data is often very precise, with measurements taken to multiple decimal points or significant figures. This precision allows for more accurate analysis and interpretation of the data.
Advantages of Quantitative Data
Some advantages of quantitative data are:
- Objectivity : Quantitative data is usually objective because it is based on measurable and observable variables. This means that different people who collect the same data will generally get the same results.
- Precision : Quantitative data provides precise measurements of variables. This means that it is easier to make comparisons and draw conclusions from quantitative data.
- Replicability : Since quantitative data is based on objective measurements, it is often easier to replicate research studies using the same or similar data.
- Generalizability : Quantitative data allows researchers to generalize findings to a larger population. This is because quantitative data is often collected using random sampling methods, which help to ensure that the data is representative of the population being studied.
- Statistical analysis : Quantitative data can be analyzed using statistical methods, which allows researchers to test hypotheses and draw conclusions about the relationships between variables.
- Efficiency : Quantitative data can often be collected quickly and efficiently using surveys or other standardized instruments, which makes it a cost-effective way to gather large amounts of data.
Limitations of Quantitative Data
Some Limitations of Quantitative Data are as follows:
- Limited context: Quantitative data does not provide information about the context in which the data was collected. This can make it difficult to understand the meaning behind the numbers.
- Limited depth: Quantitative data is often limited to predetermined variables and questions, which may not capture the complexity of the phenomenon being studied.
- Difficulty in capturing qualitative aspects: Quantitative data is unable to capture the subjective experiences and qualitative aspects of human behavior, such as emotions, attitudes, and motivations.
- Possibility of bias: The collection and interpretation of quantitative data can be influenced by biases, such as sampling bias, measurement bias, or researcher bias.
- Simplification of complex phenomena: Quantitative data may oversimplify complex phenomena by reducing them to numerical measurements and statistical analyses.
- Lack of flexibility: Quantitative data collection methods may not allow for changes or adaptations in the research process, which can limit the ability to respond to unexpected findings or new insights.
About the author
Muhammad Hassan
Researcher, Academic Writer, Web developer
You may also like

Qualitative Data – Types, Methods and Examples

Secondary Data – Types, Methods and Examples

Information in Research – Types and Examples

Primary Data – Types, Methods and Examples

Research Data – Types Methods and Examples
Have a language expert improve your writing
Run a free plagiarism check in 10 minutes, automatically generate references for free.
- Knowledge Base
- Methodology
- Data Collection Methods | Step-by-Step Guide & Examples
Data Collection Methods | Step-by-Step Guide & Examples
Published on 4 May 2022 by Pritha Bhandari .
Data collection is a systematic process of gathering observations or measurements. Whether you are performing research for business, governmental, or academic purposes, data collection allows you to gain first-hand knowledge and original insights into your research problem .
While methods and aims may differ between fields, the overall process of data collection remains largely the same. Before you begin collecting data, you need to consider:
- The aim of the research
- The type of data that you will collect
- The methods and procedures you will use to collect, store, and process the data
To collect high-quality data that is relevant to your purposes, follow these four steps.
Table of contents
Step 1: define the aim of your research, step 2: choose your data collection method, step 3: plan your data collection procedures, step 4: collect the data, frequently asked questions about data collection.
Before you start the process of data collection, you need to identify exactly what you want to achieve. You can start by writing a problem statement : what is the practical or scientific issue that you want to address, and why does it matter?
Next, formulate one or more research questions that precisely define what you want to find out. Depending on your research questions, you might need to collect quantitative or qualitative data :
- Quantitative data is expressed in numbers and graphs and is analysed through statistical methods .
- Qualitative data is expressed in words and analysed through interpretations and categorisations.
If your aim is to test a hypothesis , measure something precisely, or gain large-scale statistical insights, collect quantitative data. If your aim is to explore ideas, understand experiences, or gain detailed insights into a specific context, collect qualitative data.
If you have several aims, you can use a mixed methods approach that collects both types of data.
- Your first aim is to assess whether there are significant differences in perceptions of managers across different departments and office locations.
- Your second aim is to gather meaningful feedback from employees to explore new ideas for how managers can improve.
Prevent plagiarism, run a free check.
Based on the data you want to collect, decide which method is best suited for your research.
- Experimental research is primarily a quantitative method.
- Interviews , focus groups , and ethnographies are qualitative methods.
- Surveys , observations, archival research, and secondary data collection can be quantitative or qualitative methods.
Carefully consider what method you will use to gather data that helps you directly answer your research questions.
When you know which method(s) you are using, you need to plan exactly how you will implement them. What procedures will you follow to make accurate observations or measurements of the variables you are interested in?
For instance, if you’re conducting surveys or interviews, decide what form the questions will take; if you’re conducting an experiment, make decisions about your experimental design .
Operationalisation
Sometimes your variables can be measured directly: for example, you can collect data on the average age of employees simply by asking for dates of birth. However, often you’ll be interested in collecting data on more abstract concepts or variables that can’t be directly observed.
Operationalisation means turning abstract conceptual ideas into measurable observations. When planning how you will collect data, you need to translate the conceptual definition of what you want to study into the operational definition of what you will actually measure.
- You ask managers to rate their own leadership skills on 5-point scales assessing the ability to delegate, decisiveness, and dependability.
- You ask their direct employees to provide anonymous feedback on the managers regarding the same topics.
You may need to develop a sampling plan to obtain data systematically. This involves defining a population , the group you want to draw conclusions about, and a sample, the group you will actually collect data from.
Your sampling method will determine how you recruit participants or obtain measurements for your study. To decide on a sampling method you will need to consider factors like the required sample size, accessibility of the sample, and time frame of the data collection.
Standardising procedures
If multiple researchers are involved, write a detailed manual to standardise data collection procedures in your study.
This means laying out specific step-by-step instructions so that everyone in your research team collects data in a consistent way – for example, by conducting experiments under the same conditions and using objective criteria to record and categorise observations.
This helps ensure the reliability of your data, and you can also use it to replicate the study in the future.
Creating a data management plan
Before beginning data collection, you should also decide how you will organise and store your data.
- If you are collecting data from people, you will likely need to anonymise and safeguard the data to prevent leaks of sensitive information (e.g. names or identity numbers).
- If you are collecting data via interviews or pencil-and-paper formats, you will need to perform transcriptions or data entry in systematic ways to minimise distortion.
- You can prevent loss of data by having an organisation system that is routinely backed up.
Finally, you can implement your chosen methods to measure or observe the variables you are interested in.
The closed-ended questions ask participants to rate their manager’s leadership skills on scales from 1 to 5. The data produced is numerical and can be statistically analysed for averages and patterns.
To ensure that high-quality data is recorded in a systematic way, here are some best practices:
- Record all relevant information as and when you obtain data. For example, note down whether or how lab equipment is recalibrated during an experimental study.
- Double-check manual data entry for errors.
- If you collect quantitative data, you can assess the reliability and validity to get an indication of your data quality.
Data collection is the systematic process by which observations or measurements are gathered in research. It is used in many different contexts by academics, governments, businesses, and other organisations.
When conducting research, collecting original data has significant advantages:
- You can tailor data collection to your specific research aims (e.g., understanding the needs of your consumers or user testing your website).
- You can control and standardise the process for high reliability and validity (e.g., choosing appropriate measurements and sampling methods ).
However, there are also some drawbacks: data collection can be time-consuming, labour-intensive, and expensive. In some cases, it’s more efficient to use secondary data that has already been collected by someone else, but the data might be less reliable.
Quantitative research deals with numbers and statistics, while qualitative research deals with words and meanings.
Quantitative methods allow you to test a hypothesis by systematically collecting and analysing data, while qualitative methods allow you to explore ideas and experiences in depth.
Reliability and validity are both about how well a method measures something:
- Reliability refers to the consistency of a measure (whether the results can be reproduced under the same conditions).
- Validity refers to the accuracy of a measure (whether the results really do represent what they are supposed to measure).
If you are doing experimental research , you also have to consider the internal and external validity of your experiment.
In mixed methods research , you use both qualitative and quantitative data collection and analysis methods to answer your research question .
Operationalisation means turning abstract conceptual ideas into measurable observations.
For example, the concept of social anxiety isn’t directly observable, but it can be operationally defined in terms of self-rating scores, behavioural avoidance of crowded places, or physical anxiety symptoms in social situations.
Before collecting data , it’s important to consider how you will operationalise the variables that you want to measure.
Cite this Scribbr article
If you want to cite this source, you can copy and paste the citation or click the ‘Cite this Scribbr article’ button to automatically add the citation to our free Reference Generator.
Bhandari, P. (2022, May 04). Data Collection Methods | Step-by-Step Guide & Examples. Scribbr. Retrieved 20 March 2024, from https://www.scribbr.co.uk/research-methods/data-collection-guide/
Is this article helpful?
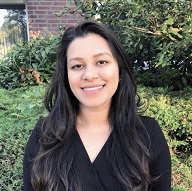
Pritha Bhandari
Other students also liked, qualitative vs quantitative research | examples & methods, triangulation in research | guide, types, examples, what is a conceptual framework | tips & examples.

Want to create or adapt books like this? Learn more about how Pressbooks supports open publishing practices.
3 Gathering and Analyzing Quantitative Data
Although the goal of any research study is to gather information to analyze, this process can be a little daunting. Hopefully, you’ve taken the time to plan your approach so that you have a clear plan for the type of information you’ll be gathering and the process by which you will assign meaning and glean an understanding about what you’ve collected. As was the case with designing your approach to your study, a systematic method by which you plan the analysis of your data will make your life a whole lot easier. This chapter will provide a basic overview of how to gather data and begin the analysis of those data with an overview of quantitative statistical approaches.
Chapter 3: Learning Objectives
As you work to understand how best to approach gathering and analyzing quantitative data you will:
- Describe the methodology of developing measurement instruments to gather and quantify data
- Discuss the factors that influence the coding process
- Describe how relationships between dependent and independent variables influence selection of statistical approach
- Compare questions of difference, association, and description
- Identify both basic and complex statistical approaches specific to a research question
Basic Approaches to Gathering and Analyzing Quantitative Data
Developing or selecting measurement instruments.
There are several methods by which data can be gathered. These methods will be directed by the approach you’ve taken as well as the question you’re investigating. Methods by which data may be gathered include, but are not limited to:
- Case or patient specific information
- Questionnaires or surveys
- Structured interviews
- Observations
- Standardized inventories
The method by which you will gather data is hugely important to the validity of your results. Ideally, a researcher would utilize established measurement tools which have been validated through consistent study. However, this is not always feasible. When you cannot utilize a validated instrument (e.g. a common questionnaire or scale) to measure variables in your specific sample, the instrument you either revise or develop should at least be pilot tested. Pilot testing is a procedure by which measurement tools or instruments are implemented on a small scale to evaluate the feasibility and identify adverse events in design prior to implementing the tool in a larger study.
Collecting Data
Again, the type and amount of data will depend on the approach you’ve selected. Regardless of this, however, all data will need to be checked to ensure that it is ‘clean’. This means removing duplicated responses or entries or other erroneous or inconsistent data that may impact your ability to analyze. Note: You should never change or alter data collected. Rather, if there are inconsistencies in how you expected the data to be collected, you must decide how to deal with those issues before moving forward.
Dealing with Data Collection Issues
You have asked respondents how well the training you provided met the objectives outlined at the beginning of the course using a likert scale (1=strongly disagree; 5= strongly agree). You have several respondents who encircled 4 and 5. You now have to decide what to do with this data. You have a few options:
- Create a new category of scores using the average of 4+5 (9/2=4.5)
- You can exclude all respondents who did this
Note: When designing questionnaires or surveys, it is essential to ensure that the questions are clear and concise by utilizing directive language such as, “Select the option most appropriate…”, or “Which ONE of the following”.
Data must then be transferred into a format where it can be sorted and analyzed. The most common approaches to this is to either input or export data to software such as Microsoft Excel (TM) or specialized statistical software such as Statistical Packages for the Social Sciences (SPSS) (TM) .
Data may be entered either manually or electronically imported to analysis software:
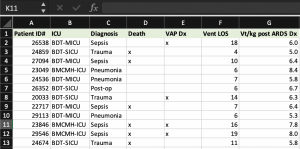
Coding may be thought of as the process of translating the information you’ve gathered such that variables can ‘talk’ to one another through analysis. This is done by numbers to the attributes, or layers, within a variable. There are a few rules governing how this is done:
- Each coded level within a variable must be mutually exclusive: Only one value can be used to code each layer within a variable
Mutually Exclusive Coding
In our dataset example, patients may only be in one location, so you can code the each layer exclusively:
- BDT-MICU (Coded as ‘1’)
- BDT-SICU (Coded as ‘2’)
- BMCMH-ICU (Coded as ‘3’)
Similarly, each diagnosis may be coded independently:
- Sepsis (Coded as ‘1’)
- Trauma (Coded as ‘2’)
- Pneumonia (Coded as ‘3’)
- Post-Op (Coded as ‘4’)
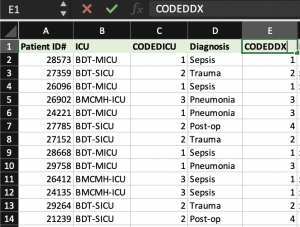
There are instances wherein more than one response is indicated or where there may be a designation within each variable. In these cases, each designation would need to have a separate code (e.g. ‘yes’/’no’). In our example, patients either have ventilator associated pneumonia (VAP), or they did not. Similarly, patients either died, or did not. You will need to code each layer to indicate whether it was experienced:
- VAP (yes= ‘1’, no= ‘0’)
- Death (yes= ‘1’, no= ‘0’)
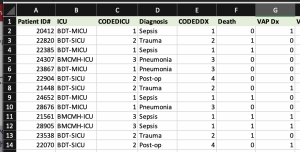
2. For each variable entered, there must be a code assigned: Numeric codes need to be applied to all data entries, except for missing responses. In the case of missing data, leave the cell blank. Leaving the cell blank ensures that the item will be counted as ‘missing’ rather than associated with a different code. Depending on the software being used, designating missing data as ‘N/A’ may result in errors during analysis.
3. Coding needs to be consistent: As you make decisions about how to proceed with your data, it is imperative that your decisions are consistent across the entire data set. For example, if you decide that you will be excluding a participant with missing data, you must exclude all missing data.
4. Data relating to specific cases or responses must be organized: Each variable relating to a participant must be organized in a way which relates to that participant. For our example above, patients are indicated in column ‘A’. Subsequent columns are variables which relate to that patient; however, each piece of data relating to the specific patient is indicated in the row associated with that specific patient. This is important to ensure that data is correctly attributed during analyses.
5. Variables need to be labeled appropriately: It’s common to need to abbreviate the names of variables. Do this in a way which helps you remain organized. If you want to keep the data one one sheet, you can simply add a coded column next to the original variable. Or, you can begin an entirely new spreadsheet of coded variables. Regardless of ;approach, you’ll need to keep a record of how you’ve changed variable names and assigned codes. Creating a codebook will help you recall which codes were assigned to which attributes so that you can correctly interpret analyses.
Creating a Codebook
Creating a codebook is imperative to ensuring you can correctly interpret the analyses you perform on the data
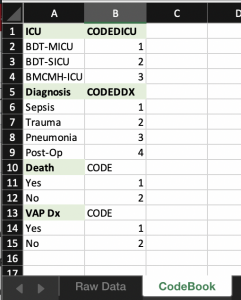
Selecting the Appropriate Statistical Method
In chapter 2, we discussed three types of general statistical approaches:
- Experimental: Has an active independent variable. The purpose of the study is to manipulate the independent variable to evaluate the impact of that variable on the dependent variable; may experimental (randomized sampling) or quasi-experimental (sample not randomized)
- Non-Experimental: Has an attribute independent variable rather than an active independent variable. The purpose of the study is to explore relationships among variables. Survey and observational research fit into this category.
- Descriptive: Does not have an independent variable. The purpose of descriptive research is to describe a selected sample rather than make inferences about that sample to the population.
We also discussed five types of specific approaches:
- Randomized experimental
- Quasi-experimental
- Comparative
- Associational/Correlational
- Descriptive
If you recall, we underscored that the type of question central to your work guides the selection of your research approach. Similarly, the type of question you’re asking will help to guide your selection of appropriate statistical approaches to analyze the data you’ve collected. There are three main types of questions and it’s important to note that a research project may incorporate several different statistical approaches, depending on the type of question(s) asked.
Adding to the chart introduced in chapter two, information relating to the purpose of the research question, we can identify the next step in identifying the most appropriate statistical approach.
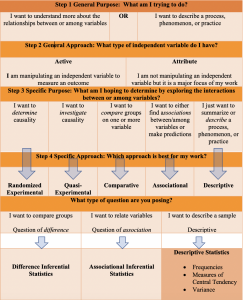
Descriptive questions
Descriptive questions seek to describe a specific sample. Descriptive statistics include measures of central tendency and variability.
Examples of Descriptive Statistics
- This is a description of the number of participants who fit into any one attribute or variable. This number may include the percentage of the sample that this number represents
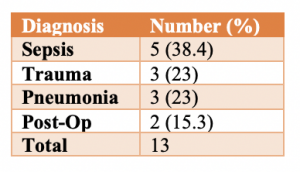
- Mean= Average (sum of the measurements divided by the number of entries) of all numerical data included for a specific variable
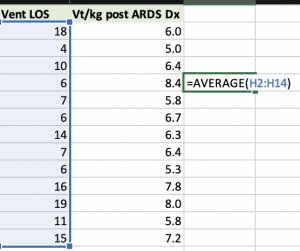
- Median= The middle number when numeric data are arranged in either ascending or descending order
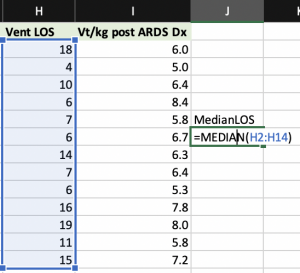
- Mode: The measurement that occurs most frequently in a set of data
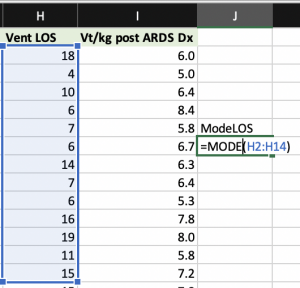
- Standard deviation is the most common when the data is normally distributed
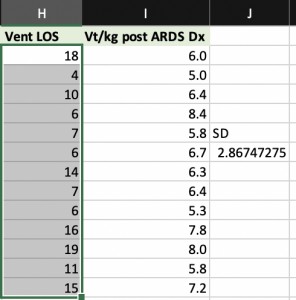
Questions of difference
Answers to these questions center on the comparison of groups and the difference between those groups. Randomized experimental, quasi-experimental, and comparative approaches support questions of difference and therefore, use similar statistical approaches. Questions of difference utilize difference inferential statistics because the goal is to compare groups’ average scores on a dependent variable.
The selection of either basic or complex difference statistics will depend on how many independent and/or independent variables you are comparing:
One dependent and/or independent variable
As we’ve discussed, understanding the relationships between or among your dependent and independent variable(s) is extremely important. If you identify only one dependent and/or independent variable, Gliner, Morgan and Leech (2017) indicate that there are a few considerations you’ll need to investigate:
- Independent t-test : Used to compare means of independent samples or groups with one independent variable with two categories.
- Paired t-test : Used to compare means of repeated measures within the same group with one independent variable with two categories.
- One way ANOVA : Used to compare means of independent samples or groups with one independent variable that has two or more categories.
- Repeated Measures ANOVA : Used to compare means of repeated measures or related samples with one independent variable that has two or more categories.
- Mann-Whitney can be used to compare medians or ranks of groups with one independent variable that has two categories.
- Wilcoxon or Sign test can be used to compare medians or ranks within groups which have one independent variable with two categories
- Kruskal-Wallis can be used to compare medians or ranks of independent samples with one independent variable but that has two or more categories.
- Friedman test can be used to compare medians or ranks for repeated measures or related samples with one independent variable that has two or more categories.
- Chi Square or Fisher’s exact test can be used to compare counts within groups which have one independent variable with two categories. Chi square is a nonparametric test (used when sample is NOT normally distributed)
- Mcnemar can be used to compare counts within groups which have one independent variable with two categories.
- Chi Square can also be used to compare counts of independent samples with one independent variable but with that has two or more categories.
- Cochran Q Test can be used to compare counts for repeated measures or related samples with one independent variable that has two or more categories.
More than one independent and/or dependent variable
The inclusion of more than one independent and/or dependent variable will require the use of fairly complex statistical tests such as:
- Factorial ANOVA can be used to measure two or more independent variables between groups when you have one dependent variable; assuming normal distribution.
- Factorial ANOVA with repeated measures can be used to explore means of groups that are related, have one dependent variable, and two or more independent variables
- Log linear should only be used with a dependent variable is nominal and you are looking at differences between groups with more than two independent variables.
- MANOVA can be used in when looking at differences between groups with several dependent variables and two or more independent variables.
- MANOVA with repeated measures can be used in when looking at differences within a group and has several dependent variables as well as two or more independent variables.
Questions of association
Answers to these questions seek to identify whether there is an association or correlation between at least two variables. Associational inferential statistics can also be used to help predict associations between variables.
The selection of either basic or complex difference statistics will depend on how many independent variables are included in your work (Gliner, Morgan, & Leech, 2017):
Only one independent variable
- Pearson r or Bivariate regression to investigate the relationship between two variables for the same subject
- Spearman (Rho) or Kendall’s Tau can be used to explore the relationship between the ranks of two variables for the same subject
- Phi or Cramer's V can be used to identify relationships between the counts of two variables for the same subject.
Several independent variables
One continuous dependent variable?
- Multiple Regression : Used to predict the value of a variable based on the value of two or more other variables
One dichotomous dependent variable?
- Discriminant analysis : Use with normally distributed independent variables
- Logistic regression : Use when some independent variables are normal and some dichotomous or when ALL independent variables are dichotomous
Making it as easy as possible:
Although there are several factors that influence the selection of a statistical test, there are general questions you can ask to help guide your decision. We’ve covered several of those steps throughout this chapter; however, the figure below, adapted from Salkind and Frey (2020), can be thought of as a quick reference:
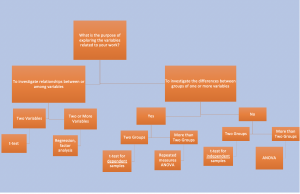
Additional how-to:
Now that you understand the basic concepts of choosing a statistical approach, you will be able to move forward. Although there are several conditions specific to your work which will impact your choices, understanding the general approach and considerations is the focus of this text. Additional information about which statistical tests are most appropriate for your specific design, as well as information about how to perform and interpret specific statistics can be found here: Choosing the correct statistical test
Key Takeaways
- Data must be collected systematically and prepared for analysis
- Mutually exclusive
- Numeric and applied to each entry
- The statistical approach you select will be guided by the study approach you selected and the question you’re asking
- Questions can either be of difference, association, or descriptive
- There are both basic and complex statistical approaches for both questions of difference and association
- You must understand the relationship between your dependent and independent variable(s) to identify the most appropriate statistical test
- The selection of statistical approaches is not ‘one-size-fits all’ and requires specific attention to be paid to the variables specific to the work
Bhattacherjee, A. (2012). Social science research: Principles, methods, and practices. https://open.umn.edu/opentextbooks/textbooks/social-science-research-principles-methods-and-practices
Gliner, J.A., Morgan, G.A., & Leech, N.L. (2017). Research methods in applied settings: An integrated approach to design and analysis . Routledge
Salkind, N.J. & Frey, B.B. (2020). Statistics for people who think they hate statistics . Sage Publications
An initial study performed prior to implementing a large scale study to evaluate the feasibility of the approach on a larger scale
Compares means of at least two different samples or groups with one independent variables with two categories
Compare the means of the same group with two different data points (e.g. pre and post test scores).
Compares the means of different samples with one independent variable which has two or more categories.
Compares means of related or the same group with one independent variable that has two or more categories
Compares medians or ranks within groups which have one independent variable with two categories
Compares medians within a group that has one independent variable with two categories
Compares medians of different samples with one independent variable with two or more categories
Compares counts within groups with have one independent variable with two categories
Can be used when looking at differences either within or between groups with several dependent variables and two or more independent variables
Evaluates the relationship between two variables for the same subject
Explores relationship between ranks of two variables on the same subject
Identifies relationships between the counts of two variables with for the same subject
Used to predict the value of a variable based on the value of two or more other variables
Used to classify observations into non-overlapping groups, based on scores on one or more quantitative predictor variables
Uses a logistic function to model a binary dependent variable
Practical Research: A Basic Guide to Planning, Doing, and Writing Copyright © by megankoster. All Rights Reserved.
Share This Book
How to Research Guide
- What is Research
- Research Terms
- Subject vs. Keyword
- Crafting Searches
- Primary, Secondary, & Tertiary
- Credible Resources
Quantitative Research
What is quantitative research, purpose of quantitative research, how do i know if the study is a quantitative design what type is it, sample quantitative student paper, acknowledgment.
- Qualitative Research
- Qualitative vs. Quantitative
- Scientific Method
- Literature Review
Quantitative methodology is the dominant research framework in the social sciences. It refers to a set of strategies, techniques, and assumptions used to study psychological, social, and economic processes through the exploration of numeric patterns .
- Quantitative research gathers a range of numeric data. Some of the numeric data is intrinsically quantitative (e.g. personal income), while in other cases the numeric structure is imposed (e.g. ‘On a scale from 1 to 10, how depressed did you feel last week?’).
- The collection of quantitative information allows researchers to conduct simple to extremely sophisticated statistical analyses that aggregate the data (e.g. averages, percentages), show relationships among the data (e.g. ‘Students with lower grade point averages tend to score lower on a depression scale’) or compare across aggregated data (e.g. the USA has a higher gross domestic product than Spain).
- Quantitative research includes methodologies such as questionnaires, structured observations, or experiments and stands in contrast to qualitative research. Qualitative research involves the collection and analysis of narratives and/or open-ended observations through methodologies such as interviews, focus groups, or ethnographies.
Source: The SAGE encyclopedia of action research (Vols. 1-2). London, : SAGE Publications Ltd doi: 10.4135/9781446294406
The purpose of quantitative research is to generate knowledge and create an understanding of the social world.
- Quantitative research is used by social scientists, including communication researchers, to observe phenomena or occurrences affecting individuals. Social scientists are concerned with the study of people.
- Quantitative research is a way to learn about a particular group of people, known as a sample population. Using scientific inquiry, quantitative research relies on data that are observed or measured to examine questions about the sample population.
Source: The SAGE encyclopedia of communication research methods;(Vols. 1-4). Thousand Oaks, CA: SAGE Publications, Inc doi: 10.4135/9781483381411<
Quantitative Research Designs: Descriptive non-experimental, Quasi-experimental, or Experimental?
- Studies do not always explicitly state what kind of research design is being used. You will need to know how to decipher which design type is used.
The following video will help you determine the quantitative design type.
- Quantitiative student paper From the American Psychological Association
This resource is adapted from the UTA Libraries, Quantitative and Qualitative Research Guide. Thank you to them for sharing their content, which may be reused and adapted under the Creative Commons Attribution-Share Alike license .

- << Previous: Types of Research
- Next: Qualitative Research >>
- Last Updated: Mar 18, 2024 4:05 PM
- URL: https://davenport.libguides.com/research-how

Quantitative and Qualitative Research
- I NEED TO . . .
What is Quantitative Research?
- What is Qualitative Research?
- Quantitative vs Qualitative
- Step 1: Accessing CINAHL
- Step 2: Create a Keyword Search
- Step 3: Create a Subject Heading Search
- Step 4: Repeat Steps 1-3 for Second Concept
- Step 5: Repeat Steps 1-3 for Quantitative Terms
- Step 6: Combining All Searches
- Step 7: Adding Limiters
- Step 8: Save Your Search!
- What Kind of Article is This?
- More Research Help This link opens in a new window
Quantitative methodology is the dominant research framework in the social sciences. It refers to a set of strategies, techniques and assumptions used to study psychological, social and economic processes through the exploration of numeric patterns . Quantitative research gathers a range of numeric data. Some of the numeric data is intrinsically quantitative (e.g. personal income), while in other cases the numeric structure is imposed (e.g. ‘On a scale from 1 to 10, how depressed did you feel last week?’). The collection of quantitative information allows researchers to conduct simple to extremely sophisticated statistical analyses that aggregate the data (e.g. averages, percentages), show relationships among the data (e.g. ‘Students with lower grade point averages tend to score lower on a depression scale’) or compare across aggregated data (e.g. the USA has a higher gross domestic product than Spain). Quantitative research includes methodologies such as questionnaires, structured observations or experiments and stands in contrast to qualitative research. Qualitative research involves the collection and analysis of narratives and/or open-ended observations through methodologies such as interviews, focus groups or ethnographies.
Coghlan, D., Brydon-Miller, M. (2014). The SAGE encyclopedia of action research (Vols. 1-2). London, : SAGE Publications Ltd doi: 10.4135/9781446294406
What is the purpose of quantitative research?
The purpose of quantitative research is to generate knowledge and create understanding about the social world. Quantitative research is used by social scientists, including communication researchers, to observe phenomena or occurrences affecting individuals. Social scientists are concerned with the study of people. Quantitative research is a way to learn about a particular group of people, known as a sample population. Using scientific inquiry, quantitative research relies on data that are observed or measured to examine questions about the sample population.
Allen, M. (2017). The SAGE encyclopedia of communication research methods (Vols. 1-4). Thousand Oaks, CA: SAGE Publications, Inc doi: 10.4135/9781483381411
How do I know if the study is a quantitative design? What type of quantitative study is it?
Quantitative Research Designs: Descriptive non-experimental, Quasi-experimental or Experimental?
Studies do not always explicitly state what kind of research design is being used. You will need to know how to decipher which design type is used. The following video will help you determine the quantitative design type.
- << Previous: I NEED TO . . .
- Next: What is Qualitative Research? >>
- Last Updated: Dec 8, 2023 10:05 PM
- URL: https://libguides.uta.edu/quantitative_and_qualitative_research
University of Texas Arlington Libraries 702 Planetarium Place · Arlington, TX 76019 · 817-272-3000
- Internet Privacy
- Accessibility
- Problems with a guide? Contact Us.

Health Science
- Find Articles
- Search strategies
- Find Journals
- Web Resources
- Qualitative vs. Quantitative Research
- Evidence Based Practice
- Citation: APA 7
Mixed Methods Research
As its name suggests, mixed methods research involves using elements of both quantitative and qualitative research methods. Using mixed methods, a researcher can more fully explore a research question and provide greater insight.
Need to find quantitative or qualitative research?
The CINAHL and PsycINFO databases both allow for the application of filters that will yield results that are either qualitative or quantitative in nature.
For detailed information about how to do that in CINAHL or PsycINFO, visit the Quantitative and Qualitative LibGuide found here.
What is Qualitative Research?
Quantitative research gathers data that can be measured numerically and analyzed mathematically. Quantitative research attempts to answer research questions through the quantification of data.
Indicators of quantitative research include:
contains statistical analysis
large sample size
objective - little room to argue with the numbers
types of research: descriptive studies, exploratory studies, experimental studies, explanatory studies, predictive studies, clinical trials
What is Quantitative Research?
Qualitative research is based upon data that is gathered by observation. Qualitative research articles will attempt to answer questions that cannot be measured by numbers but rather by perceived meaning. Qualitative research will likely include interviews, case studies, ethnography, or focus groups.
Indicators of qualitative research include:
interviews or focus groups
small sample size
subjective - researchers are often interpreting meaning
methods used: phenomenology, ethnography, grounded theory, historical method, case study
Related Guide
Quantitative and Qualitative Research
- << Previous: Web Resources
- Next: Evidence Based Practice >>
- Last Updated: Mar 19, 2024 1:10 PM
- URL: https://libguides.hofstra.edu/HealthScience

This site is compliant with the W3C-WAI Web Content Accessibility Guidelines HOFSTRA UNIVERSITY Hempstead, NY 11549-1000 (516) 463-6600 © 2000-2009 Hofstra University

Quantitative Research: Examples of Research Questions and Solutions
Are you ready to embark on a journey into the world of quantitative research? Whether you’re a seasoned researcher or just beginning your academic journey, understanding how to formulate effective research questions is essential for conducting meaningful studies. In this blog post, we’ll explore examples of quantitative research questions across various disciplines and discuss how StatsCamp.org courses can provide the tools and support you need to overcome any challenges you may encounter along the way.
Understanding Quantitative Research Questions
Quantitative research involves collecting and analyzing numerical data to answer research questions and test hypotheses. These questions typically seek to understand the relationships between variables, predict outcomes, or compare groups. Let’s explore some examples of quantitative research questions across different fields:
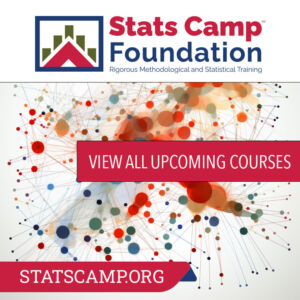
- What is the relationship between class size and student academic performance?
- Does the use of technology in the classroom improve learning outcomes?
- How does parental involvement affect student achievement?
- What is the effect of a new drug treatment on reducing blood pressure?
- Is there a correlation between physical activity levels and the risk of cardiovascular disease?
- How does socioeconomic status influence access to healthcare services?
- What factors influence consumer purchasing behavior?
- Is there a relationship between advertising expenditure and sales revenue?
- How do demographic variables affect brand loyalty?
Stats Camp: Your Solution to Mastering Quantitative Research Methodologies
At StatsCamp.org, we understand that navigating the complexities of quantitative research can be daunting. That’s why we offer a range of courses designed to equip you with the knowledge and skills you need to excel in your research endeavors. Whether you’re interested in learning about regression analysis, experimental design, or structural equation modeling, our experienced instructors are here to guide you every step of the way.
Bringing Your Own Data
One of the unique features of StatsCamp.org is the opportunity to bring your own data to the learning process. Our instructors provide personalized guidance and support to help you analyze your data effectively and overcome any roadblocks you may encounter. Whether you’re struggling with data cleaning, model specification, or interpretation of results, our team is here to help you succeed.
Courses Offered at StatsCamp.org
- Latent Profile Analysis Course : Learn how to identify subgroups, or profiles, within a heterogeneous population based on patterns of responses to multiple observed variables.
- Bayesian Statistics Course : A comprehensive introduction to Bayesian data analysis, a powerful statistical approach for inference and decision-making. Through a series of engaging lectures and hands-on exercises, participants will learn how to apply Bayesian methods to a wide range of research questions and data types.
- Structural Equation Modeling (SEM) Course : Dive into advanced statistical techniques for modeling complex relationships among variables.
- Multilevel Modeling Course : A in-depth exploration of this advanced statistical technique, designed to analyze data with nested structures or hierarchies. Whether you’re studying individuals within groups, schools within districts, or any other nested data structure, multilevel modeling provides the tools to account for the dependencies inherent in such data.
As you embark on your journey into quantitative research, remember that StatsCamp.org is here to support you every step of the way. Whether you’re formulating research questions, analyzing data, or interpreting results, our courses provide the knowledge and expertise you need to succeed. Join us today and unlock the power of quantitative research!
Follow Us On Social! Facebook | Instagram | X
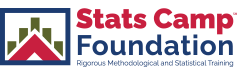
933 San Mateo Blvd NE #500, Albuquerque, NM 87108
3014 23rd Street Lubbock, TX 79410
Monday – Friday: 9:00 AM – 5:00 PM
© Copyright 2003 - 2024 | All Rights Reserved Stats Camp Foundation 501(c)(3) Non-Profit Organization.
- A/B Monadic Test
- A/B Pre-Roll Test
- Key Driver Analysis
- Multiple Implicit
- Penalty Reward
- Price Sensitivity
- Segmentation
- Single Implicit
- Category Exploration
- Competitive Landscape
- Consumer Segmentation
- Innovation & Renovation
- Product Portfolio
- Marketing Creatives
- Advertising
- Shelf Optimization
- Performance Monitoring
- Better Brand Health Tracking
- Ad Tracking
- Trend Tracking
- Satisfaction Tracking
- AI Insights
- Case Studies
quantilope is the Consumer Intelligence Platform for all end-to-end research needs
What Are Quantitative Survey Questions? Types and Examples
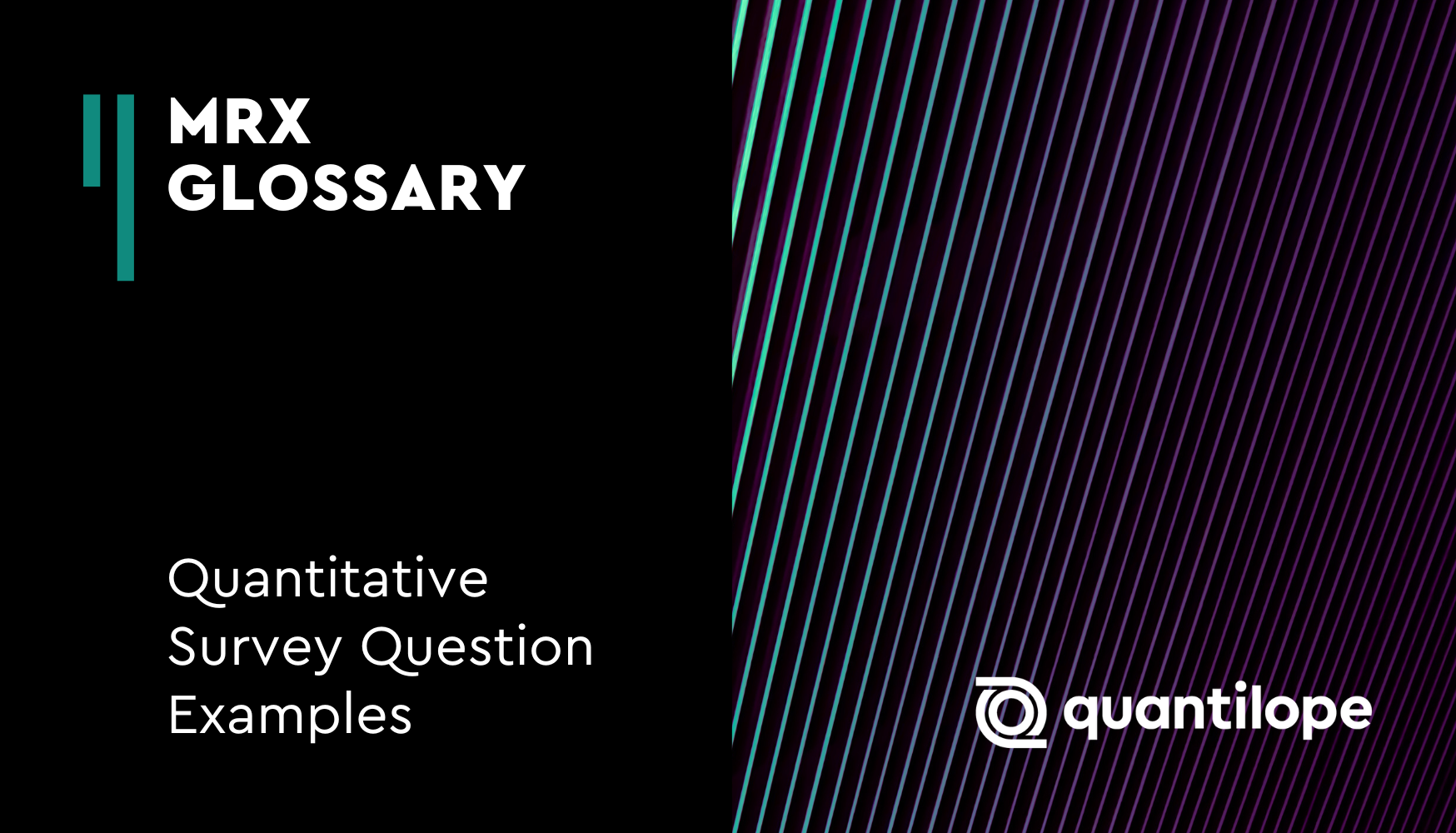
Table of contents:
- Types of quantitative survey questions - with examples
- Quantitative question formats
- How to write quantitative survey questions
- Examples of quantitative survey questions
Leveraging quantilope for your quantitative survey
In a quantitative research study brands will gather numeric data for most of their questions through formats like numerical scale questions or ranking questions. However, brands can also include some non-quantitative questions throughout their quantitative study - like open-ended questions, where respondents will type in their own feedback to a question prompt. Even so, open-ended answers can be numerically coded to sift through feedback easily (e.g. anyone who writes in 'Pepsi' in a soda study would be assigned the number '1', to look at Pepsi feedback as a whole). One of the biggest benefits of using a quantitative research approach is that insights around a research topic can undergo statistical analysis; the same can’t be said for qualitative data like focus group feedback or interviews. Another major difference between quantitative and qualitative research methods is that quantitative surveys require respondents to choose from a limited number of choices in a close-ended question - generating clear, actionable takeaways. However, these distinct quantitative takeaways often pair well with freeform qualitative responses - making quant and qual a great team to use together. The rest of this article focuses on quantitative research, taking a closer look at quantitative survey question types and question formats/layouts.
Back to table of contents
Types of dropdown#toggle" data-dropdown-placement-param="top" data-term-id="281139745">quantitative survey questions - with examples
Quantitative questions come in many forms, each with different benefits depending on dropdown#toggle" data-dropdown-placement-param="top" data-term-id="281139784">your dropdown#toggle" data-dropdown-placement-param="top" data-term-id="281139740">market research objectives. Below we’ll explore some of these dropdown#toggle" data-dropdown-placement-param="top" data-term-id="281139745">quantitative dropdown#toggle" data-dropdown-placement-param="top" data-term-id="281139785">survey question dropdown#toggle" data-dropdown-menu-id-param="menu_term_281139785" data-dropdown-placement-param="top" data-term-id="281139785"> types, which are commonly used together in a single survey to keep things interesting for dropdown#toggle" data-dropdown-placement-param="top" data-term-id="281139737">respondents . The style of questioning used during dropdown#toggle" data-dropdown-placement-param="top" data-term-id="281139739">quantitative dropdown#toggle" data-dropdown-placement-param="top" data-term-id="281139750">data dropdown#toggle" data-dropdown-menu-id-param="menu_term_281139750" data-dropdown-placement-param="top" data-term-id="281139750"> collection is important, as a good mix of the right types of questions will deliver rich data, limit dropdown#toggle" data-dropdown-placement-param="top" data-term-id="281139737">respondent fatigue, and optimize the dropdown#toggle" data-dropdown-placement-param="top" data-term-id="281139757">response rate . dropdown#toggle" data-dropdown-placement-param="top" data-term-id="281139742">Questionnaires should be enjoyable - and varying the dropdown#toggle" data-dropdown-placement-param="top" data-term-id="281139755">types of dropdown#toggle" data-dropdown-menu-id-param="menu_term_281139755" data-dropdown-placement-param="top" data-term-id="281139755">quantitative research dropdown#toggle" data-dropdown-placement-param="top" data-term-id="281139755"> questions used throughout your survey will help achieve that.
Descriptive survey questions
dropdown#toggle" data-dropdown-placement-param="top" data-term-id="281139763">Descriptive research questions (also known as usage and attitude, or, U&A questions) seek a general indication or prediction about how a dropdown#toggle" data-dropdown-placement-param="top" data-term-id="281139773">group of people behaves or will behave, how that group is characterized, or how a group thinks.
For example, a business might want to know what portion of adult men shave, and how often they do so. To find this out, they will survey men (the dropdown#toggle" data-dropdown-placement-param="top" data-term-id="281139743">target audience ) and ask descriptive questions about their frequency of shaving (e.g. daily, a few times a week, once per week, and so on.) Each of these frequencies get assigned a numerical ‘code’ so that it’s simple to chart and analyze the data later on; daily might be assigned ‘5’, a few times a week might be assigned ‘4’, and so on. That way, brands can create charts using the ‘top two’ and ‘bottom two’ values in a descriptive question to view these metrics side by side.
Another business might want to know how important local transit issues are to residents, so dropdown#toggle" data-dropdown-placement-param="top" data-term-id="281139745">quantitative survey questions will allow dropdown#toggle" data-dropdown-placement-param="top" data-term-id="281139737">respondents to indicate the degrees of opinion attached to various transit issues. Perhaps the transit business running this survey would use a sliding numeric scale to see how important a particular issue is.
Comparative survey questions
dropdown#toggle" data-dropdown-placement-param="top" data-term-id="281139782">Comparative research questions are concerned with comparing individuals or groups of people based on one or more variables. These questions might be posed when a business wants to find out which segment of its dropdown#toggle" data-dropdown-placement-param="top" data-term-id="281139743">target audience might be more profitable, or which types of products might appeal to different sets of consumers.
For example, a business might want to know how the popularity of its chocolate bars is spread out across its entire customer base (i.e. do women prefer a certain flavor? Are children drawn to candy bars by certain packaging attributes? etc.). Questions in this case will be designed to profile and ‘compare’ segments of the market.
Other businesses might be looking to compare coffee consumption among older and younger consumers (i.e. dropdown#toggle" data-dropdown-placement-param="top" data-term-id="281139741">demographic segments), the difference in smartphone usage between younger men and women, or how women from different regions differ in their approach to skincare.
Relationship-based survey questions
As the name suggests, relationship-based survey questions are concerned with the relationship between two or more variables within one or more dropdown#toggle" data-dropdown-placement-param="top" data-term-id="281139741">demographic groups. This might be a dropdown#toggle" data-dropdown-placement-param="top" data-term-id="281139759">causal link between one thing and the other - for example, the consumption of caffeine and dropdown#toggle" data-dropdown-placement-param="top" data-term-id="281139737">respondents ’ reported energy levels throughout the day. In this case, a coffee or energy drink brand might be interested in how energy levels differ between those who drink their caffeinated line of beverages and those who drink decaf/non-caffeinated beverages.
Alternatively, it might be a case of two or more factors co-existing, without there necessarily being a dropdown#toggle" data-dropdown-placement-param="top" data-term-id="281139759">causal link - for example, a particular type of air freshener being more popular amongst a certain dropdown#toggle" data-dropdown-placement-param="top" data-term-id="281139741">demographic (maybe one that is controlled wirelessly via Bluetooth is more popular among younger homeowners than one that’s plugged into the wall with no controls). Knowing that millennials favor air fresheners which have options for swapping out scents and setting up schedules would be valuable information for new product development.
Advanced method survey questions
Aside from descriptive, comparative, and relationship-based survey questions, brands can opt to include advanced methodologies in their quantitative dropdown#toggle" data-dropdown-placement-param="top" data-term-id="281139742">questionnaire for richer depth. Though advanced methods are more complex in terms of the insights output, quantilope’s Consumer Intelligence Platform automates the setup and analysis of these methods so that researchers of any background or skillset can leverage them with ease.
With quantilope’s pre-programmed suite of 12 advanced methodologies , including MaxDiff , TURF , Implicit , and more, users can drag and drop any of these into a dropdown#toggle" data-dropdown-placement-param="top" data-term-id="281139742">questionnaire and customize for their own dropdown#toggle" data-dropdown-placement-param="top" data-term-id="281139740">market research objectives.
For example, consider a beverage company that’s looking to expand its flavor profiles. This brand would benefit from a MaxDiff which forces dropdown#toggle" data-dropdown-placement-param="top" data-term-id="281139737">respondents to make tradeoff decisions between a set of flavors. A dropdown#toggle" data-dropdown-placement-param="top" data-term-id="281139737">respondent might say that coconut is their most-preferred flavor, and lime their least (when in a consideration set with strawberry), yet later on in the MaxDiff that same dropdown#toggle" data-dropdown-placement-param="top" data-term-id="281139737">respondent may say Strawberry is their most-preferred flavor (over black cherry and kiwi). While this is just one example of an advanced method, instantly you can see how much richer and more actionable these quantitative metrics become compared to a standard usage and attitude question .
Advanced methods can be used alongside descriptive, comparison, or relationship questions to add a new layer of context wherever a business sees fit. Back to table of contents
Quantitative question formats
So we’ve covered the kinds of dropdown#toggle" data-dropdown-placement-param="top" data-term-id="281139736">quantitative research questions you might want to answer using dropdown#toggle" data-dropdown-placement-param="top" data-term-id="281139740">market research , but how do these translate into the actual format of questions that you might include on your dropdown#toggle" data-dropdown-placement-param="top" data-term-id="281139742">questionnaire ?
Thinking ahead to your reporting process during your dropdown#toggle" data-dropdown-placement-param="top" data-term-id="281139742">questionnaire setup is actually quite important, as the available chart types differ among the types of questions asked; some question data is compatible with bar chart displays, others pie charts, others in trended line graphs, etc. Also consider how well the questions you’re asking will translate onto different devices that your dropdown#toggle" data-dropdown-placement-param="top" data-term-id="281139737">respondents might be using to complete the survey (mobile, PC, or tablet).
Single Select questions
Single select questions are the simplest form of quantitative questioning, as dropdown#toggle" data-dropdown-placement-param="top" data-term-id="281139737">respondents are asked to choose just one answer from a list of items, which tend to be ‘either/or’, ‘yes/no’, or ‘true/false’ questions. These questions are useful when you need to get a clear answer without any qualifying nuances.
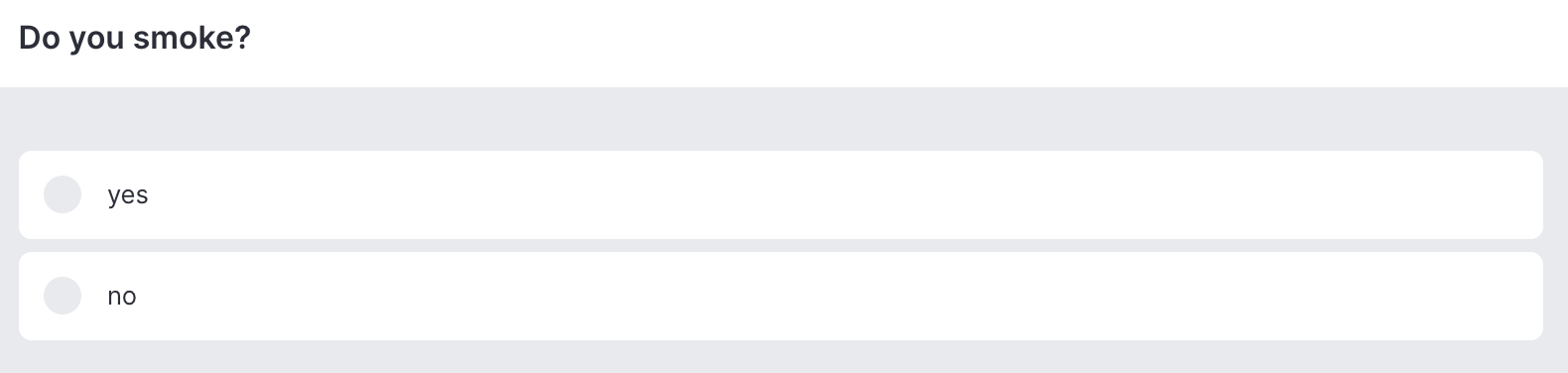
Multi-select questions
Multi-select questions (aka, dropdown#toggle" data-dropdown-placement-param="top" data-term-id="281139767">multiple choice ) offer more flexibility for responses, allowing for a number of responses on a single question. dropdown#toggle" data-dropdown-placement-param="top" data-term-id="281139737">Respondents can be asked to ‘check all that apply’ or a cap can be applied (e.g. ‘select up to 3 choices’).
For example:
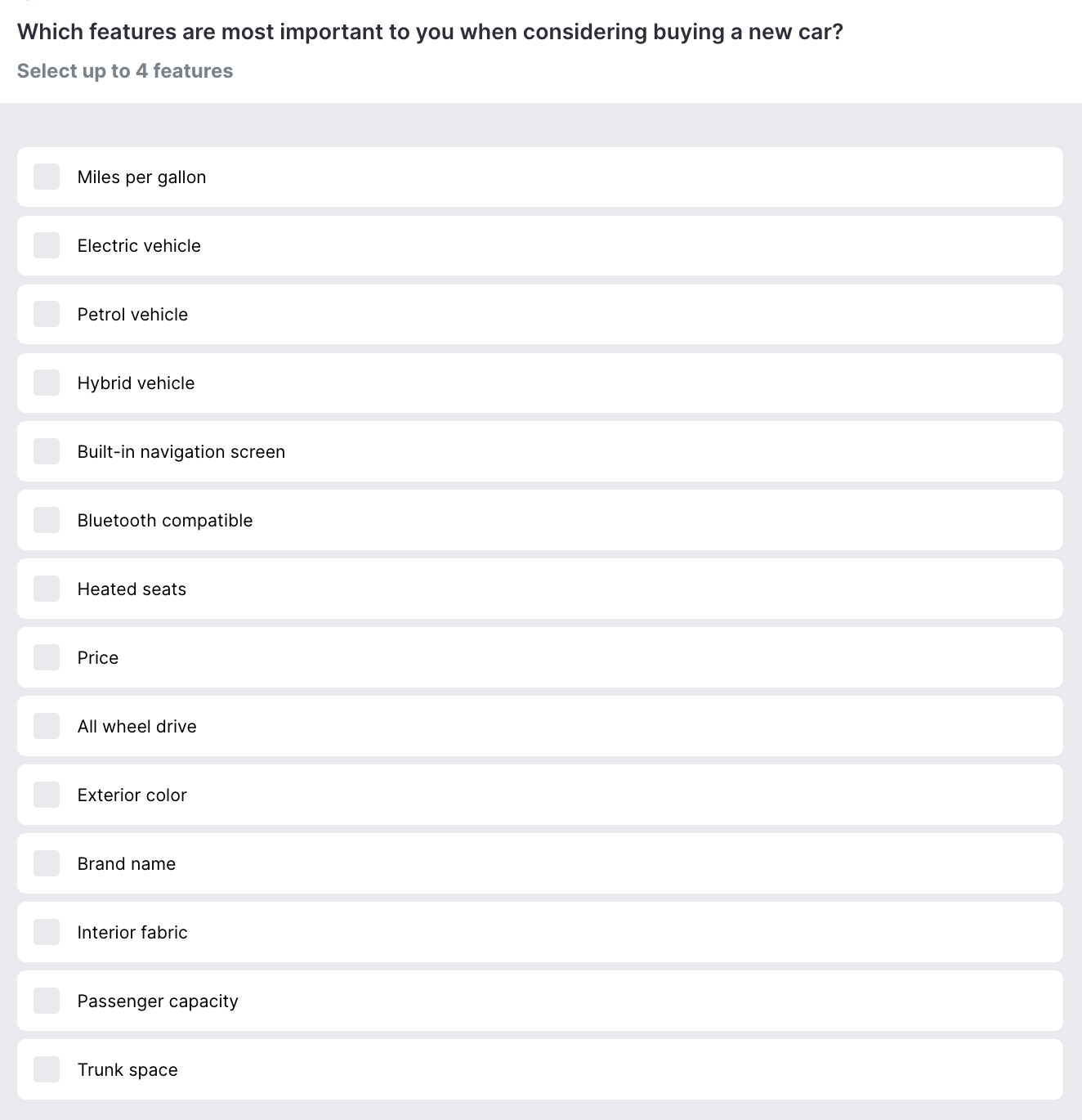
Aside from asking text-based questions like the above examples, a brand could also use a single or multi-select question to ask respondents to select the image they prefer more (like different iterations of a logo design, packaging options, branding colors, etc.).
dropdown#toggle" data-dropdown-placement-param="top" data-term-id="281139749">Likert dropdown#toggle" data-dropdown-placement-param="top" data-term-id="281139766">scale dropdown#toggle" data-dropdown-menu-id-param="menu_term_281139766" data-dropdown-placement-param="top" data-term-id="281139766"> questions
A dropdown#toggle" data-dropdown-placement-param="top" data-term-id="281139749">Likert scale is widely used as a convenient and easy-to-interpret rating method. dropdown#toggle" data-dropdown-placement-param="top" data-term-id="281139737">Respondents find it easy to indicate their degree of feelings by selecting the response they most identify with.
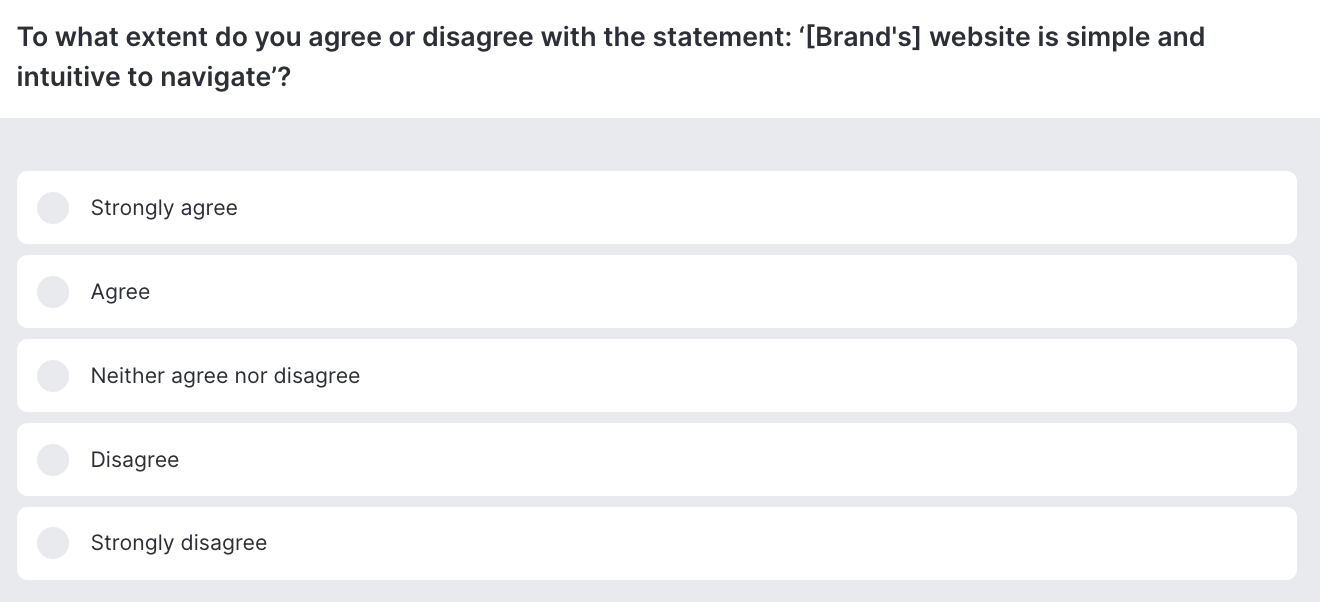
Slider scales
Slider scales are another good interactive way of formatting questions. They allow dropdown#toggle" data-dropdown-placement-param="top" data-term-id="281139737">respondents to customize their level of feeling about a question, with a bit more variance and nuance allowed than a numeric scale:
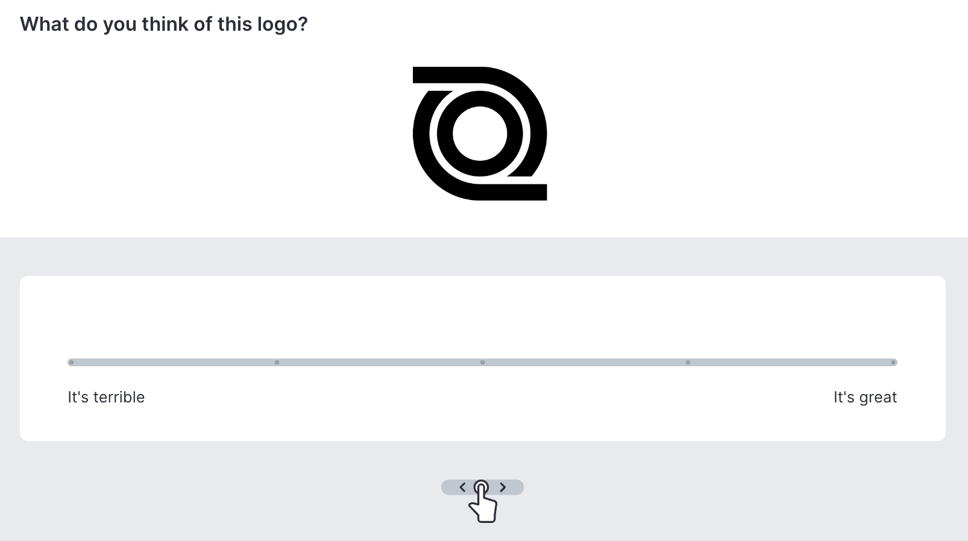
One particularly common use of a slider scale in a dropdown#toggle" data-dropdown-placement-param="top" data-term-id="281139740">market dropdown#toggle" data-dropdown-placement-param="top" data-term-id="281139770">research dropdown#toggle" data-dropdown-menu-id-param="menu_term_281139770" data-dropdown-placement-param="top" data-term-id="281139770"> study is known as a NPS (Net Promoter Score) - a way to measure dropdown#toggle" data-dropdown-placement-param="top" data-term-id="281139775">customer experience and loyalty . A 0-10 scale is used to ask customers how likely they are to recommend a brand’s product or services to others. The NPS score is calculated by subtracting the percentage of ‘detractors’ (those who respond with a 0-6) from the percentage of promoters (those who respond with a 9-10). dropdown#toggle" data-dropdown-placement-param="top" data-term-id="281139737">Respondents who select 7-8 are known as ‘passives’.
For example:

Drag and drop questions
Drag-and-drop question formats are a more ‘gamified’ approach to survey capture as they ask dropdown#toggle" data-dropdown-placement-param="top" data-term-id="281139737">respondents to do more than simply check boxes or slide a scale. Drag-and-drop question formats are great for ranking exercises - asking dropdown#toggle" data-dropdown-placement-param="top" data-term-id="281139737">respondents to place answer options in a certain order by dragging with their mouse. For example, you could ask survey takers to put pizza toppings in order of preference by dragging options from a list of possible answers to a box displaying their personal preferences:
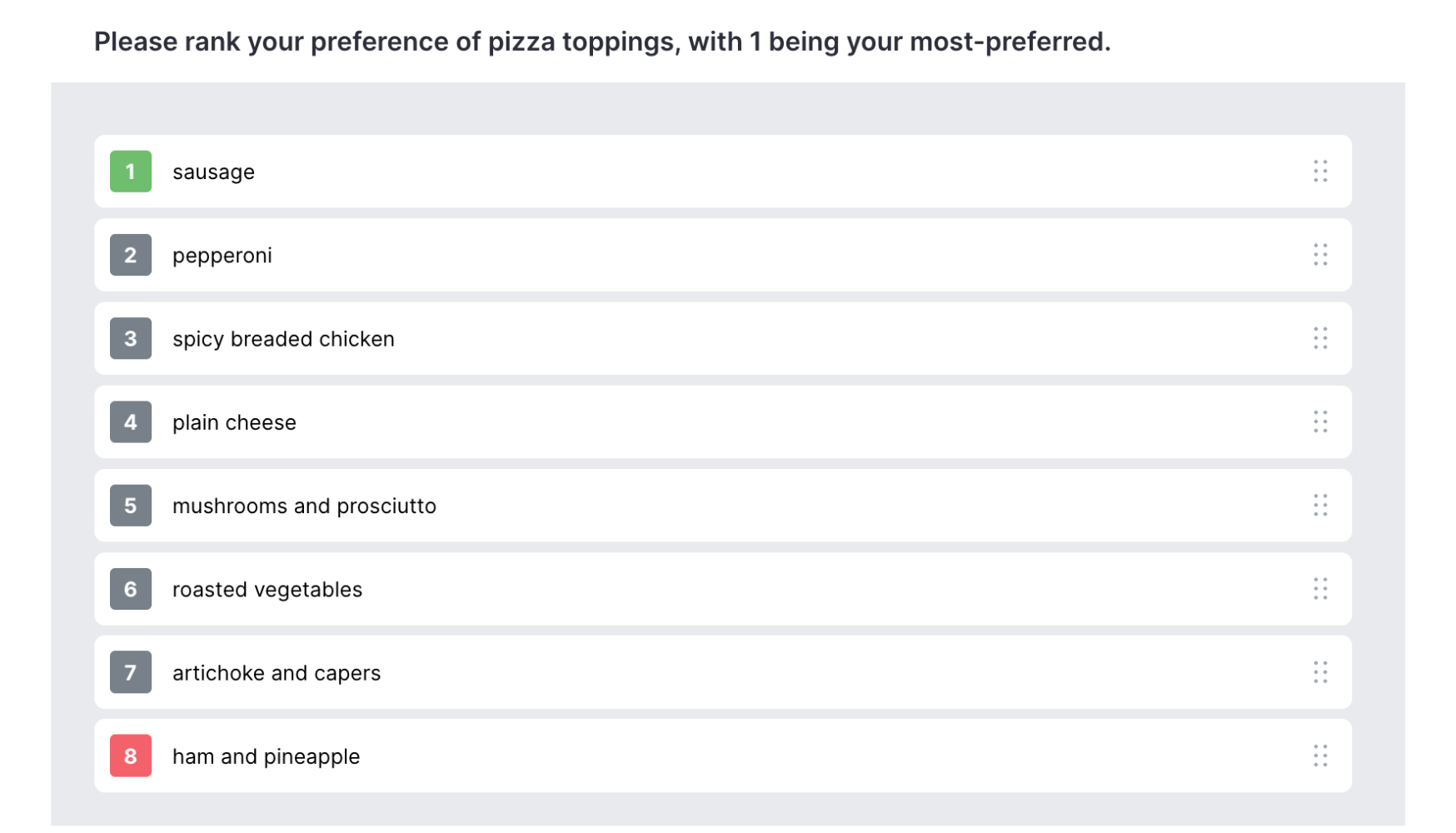
Matrix questions
Matrix questions are a great way to consolidate a number of questions that ask for the same type of response (e.g. single select yes/no, true/false, or multi-select lists). They are mutually beneficial - making a survey look less daunting for the dropdown#toggle" data-dropdown-placement-param="top" data-term-id="281139737">respondent , and easier for a brand to set up than asking multiple separate questions.
Items in a matrix question are presented one by one, as respondents cycle through the pages selecting one answer for each coffee flavor shown.
-1.png?width=1500&height=800&name=Untitled%20design%20(5)-1.png)
While the above example shows a single-matrix question - meaning a respondent can only select one answer per element (in this case, coffee flavors), a matrix setup can also be used for multiple-choice questions - allowing respondents to choose multiple answers per element shown, or for rating questions - allowing respondents to assign a rating (e.g. 1-5) for a list of elements at once. Back to table of contents
How to write dropdown#toggle" data-dropdown-placement-param="top" data-term-id="281139745">quantitative survey questions
We’ve reviewed the types of questions you might ask in a quantitative survey, and how you might format those questions, but now for the actual crafting of the content.
When considering which questions to include in your survey, you’ll first want to establish what your research goals are and how these relate to your business goals. For example, thinking about the three types of dropdown#toggle" data-dropdown-placement-param="top" data-term-id="281139745">quantitative survey questions explained above - descriptive, comparative, and relationship-based - which type (or which combination) will best meet your research needs? The questions you ask dropdown#toggle" data-dropdown-placement-param="top" data-term-id="281139737">respondents may be phrased in similar ways no matter what kind of layout you leverage, but you should have a good idea of how you’ll want to analyze the results as that will make it much easier to correctly set up your survey.
Quantitative questions tend to start with words like ‘how much,’ ‘how often,’ ‘to what degree,’ ‘what do you think of,’ ‘which of the following’ - anything that establishes what consumers do or think and that can be assigned a numerical code or value. Be sure to also include ‘other’ or ‘none of the above’ options in your quant questions, accommodating those who don’t feel the pre-set answers reflect their true opinion. As mentioned earlier, you can always include a small number of dropdown#toggle" data-dropdown-placement-param="top" data-term-id="281139748">open-ended questions in your quant survey to account for any ideas or expanded feedback that the pre-coded questions don’t (or can’t) cover. Back to table of contents
Examples of dropdown#toggle" data-dropdown-placement-param="top" data-term-id="281139745">quantitative survey questions
dropdown#toggle" data-dropdown-placement-param="top" data-term-id="281139745">Quantitative survey questions impose limits on the answers that dropdown#toggle" data-dropdown-placement-param="top" data-term-id="281139737">respondents can choose from, and this is a good thing when it comes to measuring consumer opinions on a large scale and comparing across dropdown#toggle" data-dropdown-placement-param="top" data-term-id="281139737">respondents . A large volume of freeform, open-ended answers is interesting when looking for themes from qualitative studies, but impractical to wade through when dealing with a large dropdown#toggle" data-dropdown-placement-param="top" data-term-id="281139756">sample size , and impossible to subject to dropdown#toggle" data-dropdown-placement-param="top" data-term-id="281139774">statistical analysis .
For example, a quantitative survey might aim to establish consumers' smartphone habits. This could include their frequency of buying a new smartphone, the considerations that drive purchase, which features they use their phone for, and how much they like their smartphone.
Some examples of quantitative survey questions relating to these habits would be:
Q. How often do you buy a new smartphone?
[single select question]
More than once per year
Every 1-2 years
Every 3-5 years
Every 6+ years
Q. Thinking about when you buy a smartphone, please rank the following factors in order of importance:
[drag and drop ranking question]
screen size
storage capacity
Q. How often do you use the following features on your smartphone?
[matrix question]
Q. How do you feel about your current smartphone?
[sliding scale]
I love it <-------> I hate it
Answers from these above questions, and others within the survey, would be analyzed to paint a picture of smartphone usage and attitude trends across a population and its sub-groups. dropdown#toggle" data-dropdown-placement-param="top" data-term-id="281139738">Qualitative research might then be carried out to explore those findings further - for example, people’s detailed attitudes towards their smartphones, how they feel about the amount of time they spend on it, and how features could be improved. Back to table of contents
quantilope’s Consumer Intelligence Platform specializes in automated, advanced survey insights so that researchers of any skill level can benefit from quick, high-quality consumer insights. With 12 advanced methods to choose from and a wide variety of quantitative question formats, quantilope is your one-stop-shop for all things dropdown#toggle" data-dropdown-placement-param="top" data-term-id="281139740">market research (including its dropdown#toggle" data-dropdown-placement-param="top" data-term-id="281139776">in-depth dropdown#toggle" data-dropdown-placement-param="top" data-term-id="281139738">qualitative research solution - inColor ).
When it comes to building your survey, you decide how you want to go about it. You can start with a blank slate and drop questions into your survey from a pre-programmed list, or you can get a head start with a survey dropdown#toggle" data-dropdown-placement-param="top" data-term-id="281139765">template for a particular business use case (like concept testing ) and customize from there. Once your survey is ready to launch, simply specify your dropdown#toggle" data-dropdown-placement-param="top" data-term-id="281139743">target audience , connect any panel (quantilope is panel agnostic), and watch as dropdown#toggle" data-dropdown-placement-param="top" data-term-id="281139737">respondents dropdown#toggle" data-dropdown-placement-param="top" data-term-id="281139783">answer questions in your survey in real-time by monitoring the fieldwork section of your project. AI-driven dropdown#toggle" data-dropdown-placement-param="top" data-term-id="281139764">data analysis takes the raw data and converts it into actionable findings so you never have to worry about manual calculations or statistical testing.
Whether you want to run your quantitative study entirely on your own or with the help of a classically trained research team member, the choice is yours on quantilope’s platform. For more information on how quantilope can help with your next dropdown#toggle" data-dropdown-placement-param="top" data-term-id="281139736">quantitative dropdown#toggle" data-dropdown-placement-param="top" data-term-id="281139768">research dropdown#toggle" data-dropdown-menu-id-param="menu_term_281139768" data-dropdown-placement-param="top" data-term-id="281139768"> project , get in touch below!
Get in touch to learn more about quantitative research with quantilope!
Related posts, quantilope's 5th consecutive year as a 'fastest growing tech company', automated survey setup: how to utilize ai-generated question inputs, quantilope & moneygram: tracking a major f1 sponsorship, quantilope & greenbook showcase: grow with mental availability.
- (855) 776-7763
Training Maker
All Products
Qualaroo Insights
ProProfs.com
- Sign Up Free
Do you want a free Survey Software?
We have the #1 Online Survey Maker Software to get actionable user insights.
How to Write Quantitative Research Questions: Types With Examples
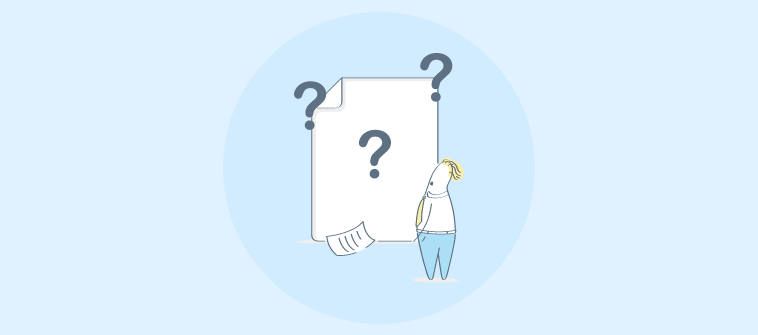
For research to be effective, it becomes crucial to properly formulate the quantitative research questions in a correct way. Otherwise, you will not get the answers you were looking for.
Has it ever happened that you conducted a quantitative research study and found out the results you were expecting are quite different from the actual results?
This could happen due to many factors like the unpredictable nature of respondents, errors in calculation, research bias, etc. However, your quantitative research usually does not provide reliable results when questions are not written correctly.
We get it! Structuring the quantitative research questions can be a difficult task.
Hence, in this blog, we will share a few bits of advice on how to write good quantitative research questions. We will also look at different types of quantitative research questions along with their examples.
Let’s start:
How to Write Quantitative Research Questions?
When you want to obtain actionable insight into the trends and patterns of the research topic to make sense of it, quantitative research questions are your best bet.
Being objective in nature, these questions provide you with detailed information about the research topic and help in collecting quantifiable data that can be easily analyzed. This data can be generalized to the entire population and help make data-driven and sound decisions.
Respondents find it easier to answer quantitative survey questions than qualitative questions . At the same time, researchers can also analyze them quickly using various statistical models.
However, when it comes to writing the quantitative research questions, one can get a little overwhelmed as the entire study depends on the types of questions used.
There is no “one good way” to prepare these questions. However, to design well-structured quantitative research questions, you can follow the 4-steps approach given below:
1. Select the Type of Quantitative Question
The first step is to determine which type of quantitative question you want to add to your study. There are three types of quantitative questions:
- Descriptive
- Comparative
- Relationship-based
This will help you choose the correct words and phrases while constructing the question. At the same time, it will also assist readers in understanding the question correctly.
2. Identify the Type of Variable
The second step involves identifying the type of variable you are trying to measure, manipulate, or control. Basically, there are two types of variables:
- Independent variable (a variable that is being manipulated)
- Dependent variable (outcome variable)
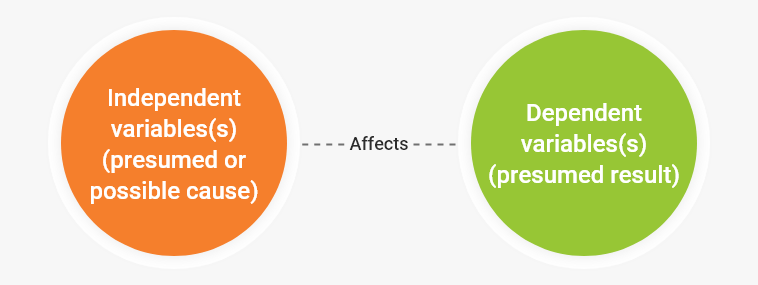
If you plan to use descriptive research questions, you have to deal with a number of dependent variables. However, where you plan to create comparative or relationship research questions, you will deal with both dependent and independent variables.
3. Select the Suitable Structure
The next step is determining the structure of the research question. It involves:
- Identifying the components of the question. It involves the type of dependent or independent variable and a group of interest (the group from which the researcher tries to conclude the population).
- The number of different components used. Like, as to how many variables and groups are being examined.
- Order in which these are presented. For example, the independent variable before the dependent variable or vice versa.
4. Draft the Complete Research Question
The last step involves identifying the problem or issue that you are trying to address in the form of complete quantitative survey questions. Also, make sure to build an exhaustive list of response options to make sure your respondents select the correct response. If you miss adding important answer options, then the ones chosen by respondents may not be entirely true.
Types of Quantitative Research Questions With Examples
Quantitative research questions are generally used to answer the “who” and “what” of the research topic. For quantitative research to be effective, it is crucial that the respondents are able to answer your questions concisely and precisely. With that in mind, let’s look in greater detail at the three types of formats you can use when preparing quantitative market research questions.
1. Descriptive
Descriptive research questions are used to collect participants’ opinions about the variable that you want to quantify. It is the most effortless way to measure the particular variable (single or multiple variables) you are interested in on a large scale. Usually, descriptive research questions begin with “ how much,” “how often,” “what percentage,” “what proportion,” etc.
Examples of descriptive research questions include:
2. Comparative
Comparative research questions help you identify the difference between two or more groups based on one or more variables. In general, a comparative research question is used to quantify one variable; however, you can use two or more variables depending on your market research objectives.
Comparative research questions examples include:
3. Relationship-based
Relationship research questions are used to identify trends, causal relationships, or associations between two or more variables. It is not vital to distinguish between causal relationships, trends, or associations while using these types of questions. These questions begin with “What is the relationship” between independent and dependent variables, amongst or between two or more groups.
Relationship-based quantitative questions examples include:
Ready to Write Your Quantitative Research Questions?
So, there you have it. It was all about quantitative research question types and their examples. By now, you must have figured out a way to write quantitative research questions for your survey to collect actionable customer feedback.
Now, the only thing you need is a good survey maker tool, like ProProfs Survey Maker, that will glide your process of designing and conducting your surveys . You also get access to various survey question types, both qualitative and quantitative, that you can add to any kind of survey along with professionally-designed survey templates .
About the author
Jared cornell.
Jared is a customer support expert. He has been published in CrazyEgg , Foundr , and CXL . As a customer support executive at ProProfs, he has been instrumental in developing a complete customer support system that more than doubled customer satisfaction. You can connect and engage with Jared on Twitter , Facebook , and LinkedIn .
Popular Posts in This Category
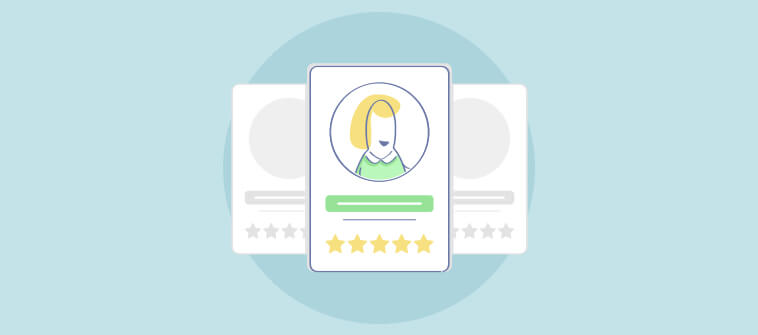
How to Use Real Time Feedback Survey for Business Growth & Development
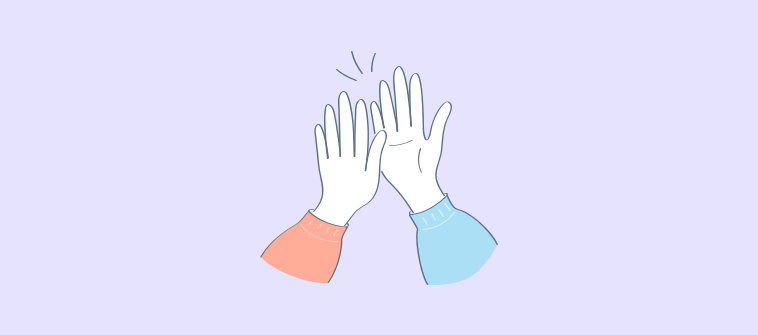
How To Build A Customer Loyalty Program
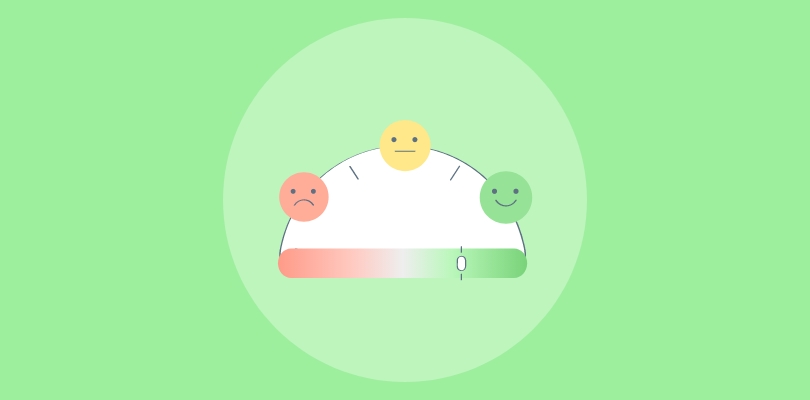
Net Promoter Score: The Ultimate NPS Survey Guide for Growth
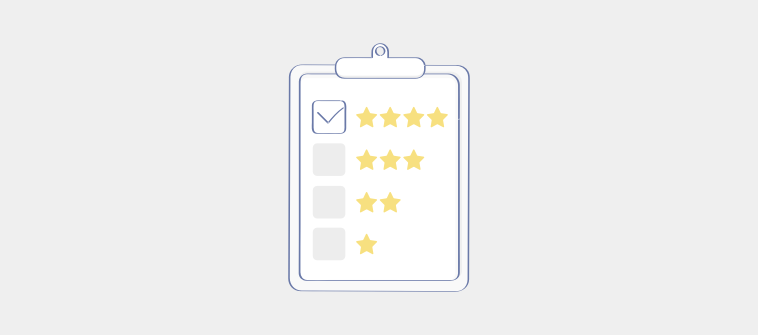
Checklist for Creating an Effective Customer Survey
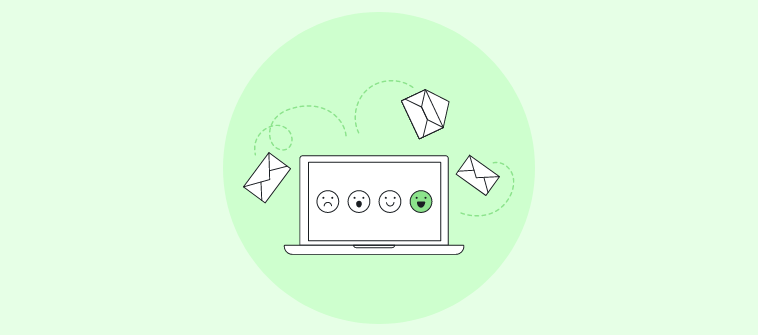
NPS Email: An Ultimate Guide With Tips & Examples
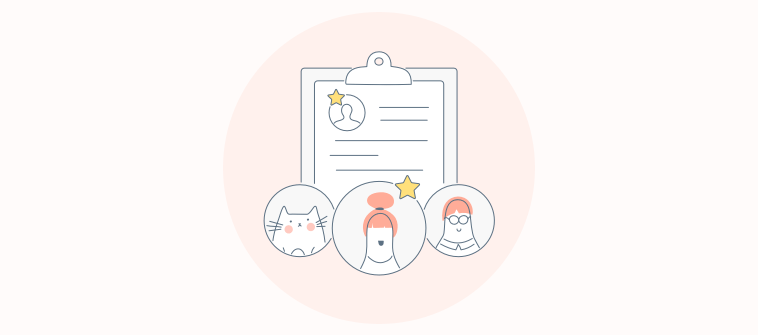
How to Fix Survey Problems: Your Ultimate Guide
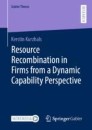
Resource Recombination in Firms from a Dynamic Capability Perspective pp 177–207 Cite as
Quantitative Research: Questionnaire Design and Data Collection
- Kerstin Kurzhals 2
- First Online: 18 November 2021
741 Accesses
1 Citations
Part of the book series: Gabler Theses ((GT))
Following the introduction and discussion of the general research design and the presentation of the results from the qualitative research, this chapter specifies the quantitative research method applied for testing the conceptual model and hypotheses. The chapter starts with a critical examination of the data collection method, a self-administered online survey, chosen for the quantitative research part, and justifies its use. This is followed by a discussion of the questionnaire design, which incorporates the levels of measurement, theory and statistical analysis, an operationalisation of the measurement constructs and scales used, as well as the pre-test of the survey instrument.
This is a preview of subscription content, log in via an institution .
Buying options
- Available as PDF
- Read on any device
- Instant download
- Own it forever
- Available as EPUB and PDF
- Compact, lightweight edition
- Dispatched in 3 to 5 business days
- Free shipping worldwide - see info
Tax calculation will be finalised at checkout
Purchases are for personal use only
In their study Pavlou and El Sawy (2011) focus on the group level, explicitly the NPD unit’s attributes, not the firm’s attributes are addressed.
CINT is a professional panel data provider that was conducted for target sampling as described in 5.4.2 .
Author information
Authors and affiliations.
Department of Strategic and Applied Management, Coventry University Business School, Coventry, UK
Kerstin Kurzhals
You can also search for this author in PubMed Google Scholar
Corresponding author
Correspondence to Kerstin Kurzhals .
5.1 Electronic supplementary material
Below is the link to the electronic supplementary material.
Supplementary file1 (PDF 1802 kb)
Rights and permissions.
Reprints and permissions
Copyright information
© 2021 The Author(s), under exclusive license to Springer Fachmedien Wiesbaden GmbH, part of Springer Nature
About this chapter
Cite this chapter.
Kurzhals, K. (2021). Quantitative Research: Questionnaire Design and Data Collection. In: Resource Recombination in Firms from a Dynamic Capability Perspective. Gabler Theses. Springer Gabler, Wiesbaden. https://doi.org/10.1007/978-3-658-35666-8_5
Download citation
DOI : https://doi.org/10.1007/978-3-658-35666-8_5
Published : 18 November 2021
Publisher Name : Springer Gabler, Wiesbaden
Print ISBN : 978-3-658-35665-1
Online ISBN : 978-3-658-35666-8
eBook Packages : Business and Management Business and Management (R0)
Share this chapter
Anyone you share the following link with will be able to read this content:
Sorry, a shareable link is not currently available for this article.
Provided by the Springer Nature SharedIt content-sharing initiative
- Publish with us
Policies and ethics
- Find a journal
- Track your research
What is Quantitative Data?
Data professionals work with two types of data: quantitative and qualitative. What is quantitative data? What is qualitative data? In simple terms, quantitative data is measurable while qualitative data is descriptive—think numbers versus words.
If you plan on working as a data analyst or a data scientist (or in any field that involves conducting research, like psychology), you’ll need to get to grips with both. In this post, we’ll focus on quantitative data. We’ll explain exactly what quantitative data is, including plenty of useful examples. We’ll also show you what methods you can use to collect and analyze quantitative data.
By the end of this post, you’ll have a clear understanding of quantitative data and how it’s used.
We’ll cover:
- What is quantitative data? (Definition)
- What are some examples of quantitative data?
- What’s the difference between quantitative and qualitative data?
- What are the different types of quantitative data?
- How is quantitative data collected?
- What methods are used to analyze quantitative data?
- What are the advantages and disadvantages of quantitative data?
- Should I use quantitative or qualitative data in my research?
- What are some common quantitative data analysis tools?
- What is quantitative data? FAQs
- Key takeaways
So: what is quantitative data? Let’s find out.
1. What is quantitative data? (Definition)
Quantitative data is, quite simply, information that can be quantified. It can be counted or measured, and given a numerical value—such as length in centimeters or revenue in dollars. Quantitative data tends to be structured in nature and is suitable for statistical analysis. If you have questions such as “How many?”, “How often?” or “How much?”, you’ll find the answers in quantitative data.
2. What are some examples of quantitative data?
Some examples of quantitative data include:
- Revenue in dollars
- Weight in kilograms
- Age in months or years
- Length in centimeters
- Distance in kilometers
- Height in feet or inches
- Number of weeks in a year
3. What is the difference between quantitative and qualitative data?
It’s hard to define quantitative data without comparing it to qualitative data—so what’s the difference between the two?
While quantitative data can be counted and measured, qualitative data is descriptive and, typically, unstructured. It usually takes the form of words and text—for example, a status posted on Facebook or an interview transcript are both forms of qualitative data. You can also think of qualitative data in terms of the “descriptors” you would use to describe certain attributes. For example, if you were to describe someone’s hair color as auburn, or an ice cream flavor as vanilla, these labels count as qualitative data.
Qualitative data cannot be used for statistical analysis; to make sense of such data, researchers and analysts will instead try to identify meaningful groups and themes.
You’ll find a detailed exploration of the differences between qualitative and quantitative data in this post . But, to summarize:
- Quantitative data is countable or measurable, relating to numbers; qualitative data is descriptive, relating to words.
- Quantitative data lends itself to statistical analysis; qualitative data is grouped and categorized according to themes.
- Examples of quantitative data include numerical values such as measurements, cost, and weight; examples of qualitative data include descriptions (or labels) of certain attributes, such as “brown eyes” or “vanilla flavored ice cream”.
Now we know the difference between the two, let’s get back to quantitative data.
4. What are the different types of quantitative data?
There are two main types of quantitative data: discrete and continuous .
Discrete data
Discrete data is quantitative data that can only take on certain numerical values. These values are fixed and cannot be broken down. When you count something, you get discrete data. For example, if a person has three children, this is an example of discrete data. The number of children is fixed—it’s not possible for them to have, say, 3.2 children.
Another example of discrete quantitative data could be the number of visits to your website; you could have 150 visits in one day, but not 150.6 visits. Discrete data is usually visualized using tally charts, bar charts, and pie charts.
Continuous data
Continuous data, on the other hand, can be infinitely broken down into smaller parts. This type of quantitative data can be placed on a measurement scale; for example, the length of a piece of string in centimeters, or the temperature in degrees Celsius. Essentially, continuous data can take any value; it’s not limited to fixed values. What’s more, continuous data can also fluctuate over time—the room temperature will vary throughout the day, for example. Continuous data is usually represented using a line graph.
Continuous data can be further classified depending on whether it’s interval data or ratio data . Let’s take a look at those now.
Interval vs. ratio data
Interval data can be measured along a continuum, where there is an equal distance between each point on the scale. For example: The difference between 30 and 31 degrees C is equal to the difference between 99 and 100 degrees. Another thing to bear in mind is that interval data has no true or meaningful zero value . Temperature is a good example; a temperature of zero degrees does not mean that there is “no temperature”—it just means that it’s extremely cold!
Ratio data is the same as interval data in terms of equally spaced points on a scale, but unlike interval data, ratio data does have a true zero . Weight in grams would be classified as ratio data; the difference between 20 grams and 21 grams is equal to the difference between 8 and 9 grams, and if something weighs zero grams, it truly weighs nothing.
Beyond the distinction between discrete and continuous data, quantitative data can also be broken down into several different types:
- Measurements: This type of data refers to the measurement of physical objects. For example, you might measure the length and width of your living room before ordering new sofas.
- Sensors: A sensor is a device or system which detects changes in the surrounding environment and sends this information to another electronic device, usually a computer. This information is then converted into numbers—that’s your quantitative data. For example, a smart temperature sensor will provide you with a stream of data about the temperature of the room throughout the day.
- Counts: As the name suggests, this is the quantitative data you get when you count things. You might count the number of people who attended an event, or the number of visits to your website in one week.
- Quantification of qualitative data: This is when qualitative data is converted into numbers. Take the example of customer satisfaction. If a customer said “I’m really happy with this product”, that would count as qualitative data. You could turn this into quantitative data by asking them to rate their satisfaction on a scale of 1-10.
- Calculations: This is any quantitative data that results from mathematical calculations, such as calculating your final profit at the end of the month.
- Projections: Analysts may estimate or predict quantities using algorithms, artificial intelligence, or “manual” analysis. For example, you might predict how many sales you expect to make in the next quarter. The figure you come up with is a projection of quantitative data.
Knowing what type of quantitative data you’re working with helps you to apply the correct type of statistical analysis. We’ll look at how quantitative data is analyzed in section five.
5. How is quantitative data collected?
Now we know what quantitative data is, we can start to think about how analysts actually work with it in the real world. Before the data can be analyzed, it first needs to be generated or collected. So how is this done?
Researchers (for example, psychologists or scientists) will often conduct experiments and studies in order to gather quantitative data and test certain hypotheses. A psychologist investigating the relationship between social media usage and self-esteem might devise a questionnaire with various scales—for example, asking participants to rate, on a scale of one to five, the extent to which they agree with certain statements.
If the survey reaches enough people, the psychologist ends up with a large sample of quantitative data (for example, an overall self-esteem score for each participant) which they can then analyze.
Data analysts and data scientists are less likely to conduct experiments, but they may send out questionnaires and surveys—it all depends on the sector they’re working in. Usually, data professionals will work with “naturally occurring” quantitative data, such as the number of sales per quarter, or how often a customer uses a particular service.
Some common methods of data collection include:
- Analytics tools, such as Google Analytics
- Probability sampling
Questionnaires and surveys
- Open-source datasets on the web
Analytics tools
Data analysts and data scientists rely on specialist tools to gather quantitative data from various sources. Google Analytics, for example, will gather data pertaining to your website; at a glance, you can see metrics such as how much traffic you got in one week, how many page views per minute, and average session length—all useful insights if you want to optimize the performance of your site.
Aside from Google Analytics, which tends to be used within the marketing sector, there are loads of tools out there which can be connected to multiple data sources at once. Tools like RapidMiner, Knime, Qlik, and Splunk can be integrated with internal databases, data lakes, cloud storage, business apps, social media, and IoT devices, allowing you to access data from multiple sources all in one place.
You can learn more about the top tools used by data analysts in this guide
Sampling is when, instead of analyzing an entire dataset, you select a sample or “section” of the data. Sampling may be used to save time and money, and in cases where it’s simply not possible to study an entire population. For example, if you wanted to analyze data pertaining to the residents of New York, it’s unlikely that you’d be able to get hold of data for every single person in the state. Instead, you’d analyze a representative sample.
There are two types of sampling: Random probability sampling, where each unit within the overall dataset has the same chance of being selected (i.e. included in the sample), and non-probability sampling, where the sample is actively selected by the researcher or analyst—not at random. Data analysts and scientists may use Python (the popular programming language) and various algorithms to extract samples from large datasets.
Another way to collect quantitative data is through questionnaires and surveys. Nowadays, it’s easy to create a survey and distribute it online—with tools like Typeform , SurveyMonkey , and Qualtrics , practically anyone can collect quantitative data. Surveys are a useful tool for gathering customer or user feedback, and generally finding out how people feel about certain products or services.
To make sure you gather quantitative data from your surveys, it’s important that you ask respondents to quantify their feelings—for example, asking them to rate their satisfaction on a scale of one to ten.
Open-source datasets online
In addition to analyzing data from internal databases, data analysts might also collect quantitative data from external sources. Again, it all depends on the field you’re working in and what kind of data you need. The internet is full of free and open datasets spanning a range of sectors, from government, business and finance, to science, transport, film, and entertainment—pretty much anything you can think of! We’ve put together a list of places where you can find free datasets here .
6. How is quantitative data analyzed?
A defining characteristic of quantitative data is that it’s suitable for statistical analysis. There are many different methods and techniques used for quantitative data analysis, and how you analyze your data depends on what you hope to find out.
Before we go into some specific methods of analysis, it’s important to distinguish between descriptive and inferential analysis .
What’s the difference between descriptive and inferential analysis of quantitative data?
Descriptive analysis does exactly what it says on the tin; it describes the data. This is useful as it allows you to see, at a glance, what the basic qualities of your data are and what you’re working with. Some commonly used descriptive statistics include the range (the difference between the highest and lowest scores), the minimum and maximum (the lowest and highest scores in a dataset), and frequency (how often a certain value appears in the dataset).
You might also calculate various measures of central tendency in order to gauge the general trend of your data. Measures of central tendency include the mean (the sum of all values divided by the number of values, otherwise known as the average), the median (the middle score when all scores are ordered numerically), and the mode (the most frequently occurring score). Another useful calculation is standard deviation . This tells you how representative of the entire dataset the mean value actually is.
While descriptive statistics give you an initial read on your quantitative data, they don’t allow you to draw definitive conclusions. That’s where inferential analysis comes in. With inferential statistics, you can make inferences and predictions. This allows you to test various hypotheses and to predict future outcomes based on probability theory.
Quantitative data analysis methods
When it comes to deriving insights from your quantitative data, there’s a whole host of techniques at your disposal. Some of the most common (and useful) methods of quantitative data analysis include:
- Regression analysis: This is used to estimate the relationship between a set of variables, and to see if there’s any kind of correlation between the two. Regression is especially useful for making predictions and forecasting future trends.
- Monte Carlo simulation : The Monte Carlo method is a computerized technique used to generate models of possible outcomes and their probability distributions based on your dataset. It essentially considers a range of possible outcomes and then calculates how likely it is that each particular outcome will occur. It’s used by data analysts to conduct advanced risk analysis, allowing them to accurately predict what might happen in the future.
- Cohort analysis: A cohort is a group of people who share a common attribute or behavior during a given time period—for example, a cohort of students who all started university in 2020, or a cohort of customers who purchased via your app in the month of February. Cohort analysis essentially divides your dataset into cohorts and analyzes how these cohorts behave over time. This is especially useful for identifying patterns in customer behavior and tailoring your products and services accordingly.
- Cluster analysis : This is an exploratory technique used to identify structures within a dataset. The aim of cluster analysis is to sort different data points into groups that are internally homogenous and externally heterogeneous—in other words, data points within a cluster are similar to each other, but dissimilar to data points in other clusters. Clustering is used to see how data is distributed in a given dataset, or as a preprocessing step for other algorithms.
- Time series analysis : This is used to identify trends and cycles over time. Time series data is a sequence of data points which measure the same variable at different points in time, such as weekly sales figures or monthly email sign-ups. By looking at time-related trends, analysts can forecast how the variable of interest may fluctuate in the future. Extremely handy when it comes to making business decisions!
Above is just a very brief introduction to how you might analyze your quantitative data. For a more in-depth look, check out this comprehensive guide to some of the most useful data analysis techniques .
7. What are the advantages and disadvantages of quantitative data?
As with anything, there are both advantages and disadvantages of using quantitative data. So what are they? Let’s take a look.
Advantages of quantitative data
The main advantages of working with quantitative data are as follows:
- Quantitative data is relatively quick and easy to collect , allowing you to gather a large sample size. And, the larger your sample size, the more accurate your conclusions are likely to be.
- Quantitative data is less susceptible to bias. The use of random sampling helps to ensure that a given dataset is as representative as possible, and protects the sample from bias. This is crucial for drawing reliable conclusions.
- Quantitative data is analyzed objectively. Because quantitative data is suitable for statistical analysis, it can be analyzed according to mathematical rules and principles. This greatly reduces the impact of analyst or researcher bias on how the results are interpreted.
Disadvantages of quantitative data
There are two main drawbacks to be aware of when working with quantitative data, especially within a research context:
- Quantitative data can lack context. In some cases, context is key; for example, if you’re conducting a questionnaire to find out how customers feel about a new product. The quantitative data may tell you that 60% of customers are unhappy with the product, but that figure alone will not tell you why. Sometimes, you’ll need to delve deeper to gain valuable insights beyond the numbers.
- There is a risk of bias when using surveys and questionnaires. Again, this point relates more to a research context, but it’s important to bear in mind when creating surveys and questionnaires. The way in which questions are worded can allow researcher bias to seep in, so it’s important to make sure that surveys are devised carefully. You can learn all about how to reduce survey bias in this post .
8. Should I use quantitative or qualitative data in my research?
Okay—so now we know what the difference between quantitative and qualitative data is, as well as other aspects of quantitative data. But when should you make use of quantitative or qualitative research? This answer to this question will depend on the type of project you’re working on—or client you’re working for—specifically. But use these simple criteria as a guide:
- When to use quantitative research: when you want to confirm or test something, like a theory or hypothesis. When the data can be shown clearly in numbers. Think of a city census that shows the whole number of people living there, as well as their ages, incomes, and other useful information that makes up a city’s demographic.
- When to use qualitative research: when you want to understand something—for example, a concept, experience, or opinions. Maybe you’re testing out a run of experiences for your company, and need to gather reviews for a specific time period. This would be an example of qualitative research.
- When to use both quantitative and qualitative research: when you’re taking on a research project that demands both numerical and non-numerical data.
9. What are some common quantitative analysis tools?
The tools used for quantitative data collection and analysis should come as no surprise to the budding data analyst. You may end up using one tool per project, or a combination of tools:
- Microsoft Power BI
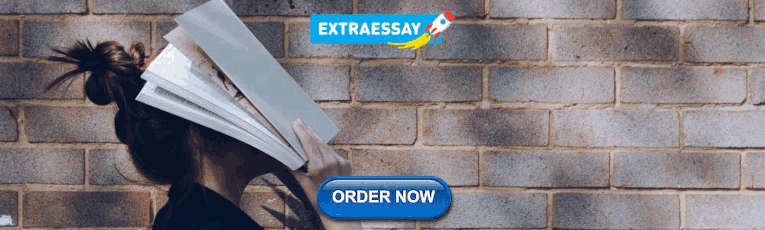
10. What is quantitative data? FAQs
Who uses quantitative data.
Quantitative data is used in many fields—not just data analytics (though, you could argue that all of these fields are at least data-analytics-adjacent)! Those working in the fields of economics, epidemiology, psychology, sociology, and health—to name a few—would make great use of quantitative data in their work. You would be less likely to see quantitative data being used in fields such as anthropology and history.
Is quantitative data better than qualitative data?
It would be hard to make a solid argument of which form of data collection is “better”, as it really depends on the type of project you’re working on. However, quantitative research provides more “hard and fast” information that can be used to make informed, objective decisions.
Where is quantitative data used?
Quantitative data is used when a problem needs to be quantified. That is, to answer the questions that start with “how many…” or “how often…”, for example.
What is quantitative data in statistics?
As statistics is an umbrella term of a discipline concerning the collection, organization and analysis of data, it’s only natural that quantitative data falls under that umbrella—the practice of counting and measuring data sets according to a research question or set of research needs.
Can quantitative data be ordinal?
Ordinal data is a type of statistical data where the variables are sorted into ranges, and the distance between the ranges are not known. Think of the pain scale they sometimes use in the hospital, where you judge the level of pain you have on a scale of 1-10, with 1 being low and 10 being the highest. However, you can’t really quantify the difference between 1-10—it’s a matter of how you feel!
By that logic, ordinal data falls under qualitative data, not quantitative. You can learn more about the data levels of measurement in this post .
Is quantitative data objective?
Due to the nature of how quantitative data is produced—that is, using methods that are verifiable and replicable—it is objective.
11. Key takeaways and further reading
In this post, we answered the question: what is quantitative data? We looked at how it differs from qualitative data, and how it’s collected and analyzed. To recap what we’ve learned:
- Quantitative data is data that can be quantified. It can be counted or measured, and given a numerical value.
- Quantitative data lends itself to statistical analysis, while qualitative data is grouped according to themes.
- Quantitative data can be discrete or continuous. Discrete data takes on fixed values (e.g. a person has three children), while continuous data can be infinitely broken down into smaller parts.
- Quantitative data has several advantages: It is relatively quick and easy to collect, and it is analyzed subjectively.
Collecting and analyzing quantitative data is just one aspect of the data analyst’s work. To learn more about what it’s like to work as a data analyst, check out the following guides. And, if you’d like to dabble in some analytics yourself, why not try our free five-day introductory short course ?
- What is data analytics? A beginner’s guide
- A step-by-step guide to the data analysis process
- Where could a career in data analytics take you?
- Skip to main content
- Skip to primary sidebar
- Skip to footer
- QuestionPro

- Solutions Industries Gaming Automotive Sports and events Education Government Travel & Hospitality Financial Services Healthcare Cannabis Technology Use Case NPS+ Communities Audience Contactless surveys Mobile LivePolls Member Experience GDPR Positive People Science 360 Feedback Surveys
- Resources Blog eBooks Survey Templates Case Studies Training Help center

Home Market Research
Quantitative Data Collection: Best 5 methods
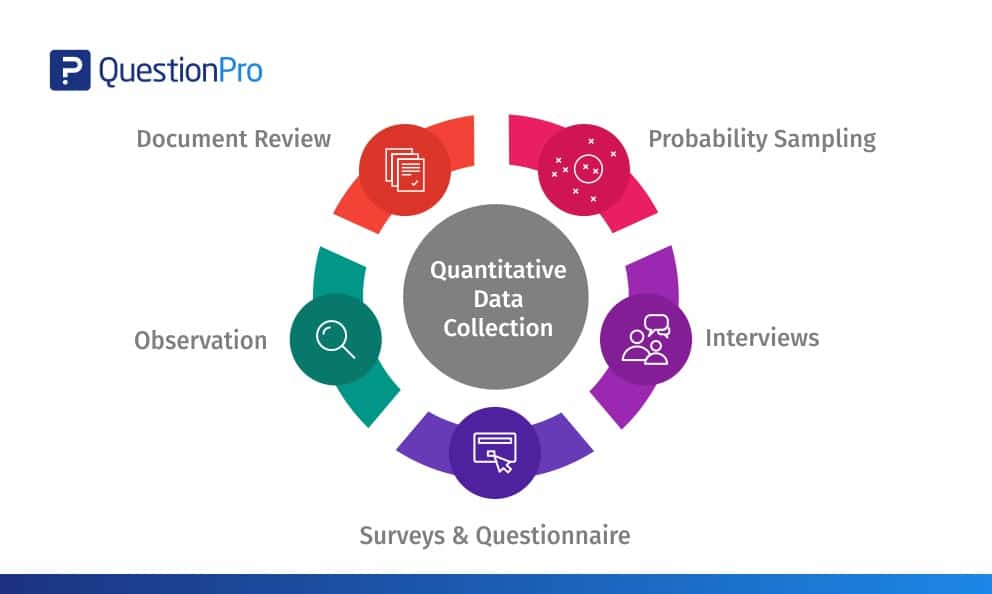
In contrast to qualitative data , quantitative data collection is everything about figures and numbers. Researchers often rely on quantitative data when they intend to quantify attributes, attitudes, behaviors, and other defined variables with a motive to either back or oppose the hypothesis of a specific phenomenon by contextualizing the data obtained via surveying or interviewing the study sample.
Content Index
What is Quantitative Data Collection?
Importance of quantitative data collection, probability sampling, surveys/questionnaires, observations, document review in quantitative data collection.
Quantitative data collection refers to the collection of numerical data that can be analyzed using statistical methods. This type of data collection is often used in surveys, experiments, and other research methods. It measure variables and establish relationships between variables. The data collected through quantitative methods is typically in the form of numbers, such as response frequencies, means, and standard deviations, and can be analyzed using statistical software.
LEARN ABOUT: Research Process Steps
As a researcher, you do have the option to opt either for data collection online or use traditional data collection methods via appropriate research. Quantitative data collection is important for several reasons:
- Objectivity: Quantitative data collection provides objective and verifiable information, as the data is collected in a systematic and standardized manner.
- Generalizability: The results from quantitative data collection can be generalized to a larger population, making it an effective way to study large groups of people.
- Precision: Numerical data allows for precise measurement and unit of analysis , providing more accurate results than other data collection forms.
- Hypothesis testing: Quantitative data collection allows for testing hypotheses and theories, leading to a better understanding of the relationships between variables.
- Comparison: Quantitative data collection allows for data comparison and analysis. It can be useful in making decisions and identifying trends or patterns.
- Replicability: The numerical nature of quantitative data makes it easier to replicate research results. It is essential for building knowledge in a particular field.
LEARN ABOUT: Level of Analysis
Overall, quantitative data collection provides valuable information for understanding complex phenomena and making informed decisions based on empirical evidence.
LEARN ABOUT: Best Data Collection Tools
Methods used for Quantitative Data Collection
A data that can be counted or expressed in numerical’s constitute the quantitative data. It is commonly used to study the events or levels of concurrence. And is collected through a Structured Question & structured questionnaire asking questions starting with “how much” or “how many.” As the quantitative data is numerical, it represents both definitive and objective data. Furthermore, quantitative information is much sorted for statistical analysis and mathematical analysis, making it possible to illustrate it in the form of charts and graphs.
Discrete and continuous are the two major categories of quantitative data where discreet data have finite numbers and the constant data values falling on a continuum possessing the possibility to have fractions or decimals. If research is conducted to find out the number of vehicles owned by the American household, then we get a whole number, which is an excellent example of discrete data. When research is limited to the study of physical measurements of the population like height, weight, age, or distance, then the result is an excellent example of continuous data.
Any traditional or online data collection method that helps in gathering numerical data is a proven method of collecting quantitative data.
LEARN ABOUT: Survey Sampling
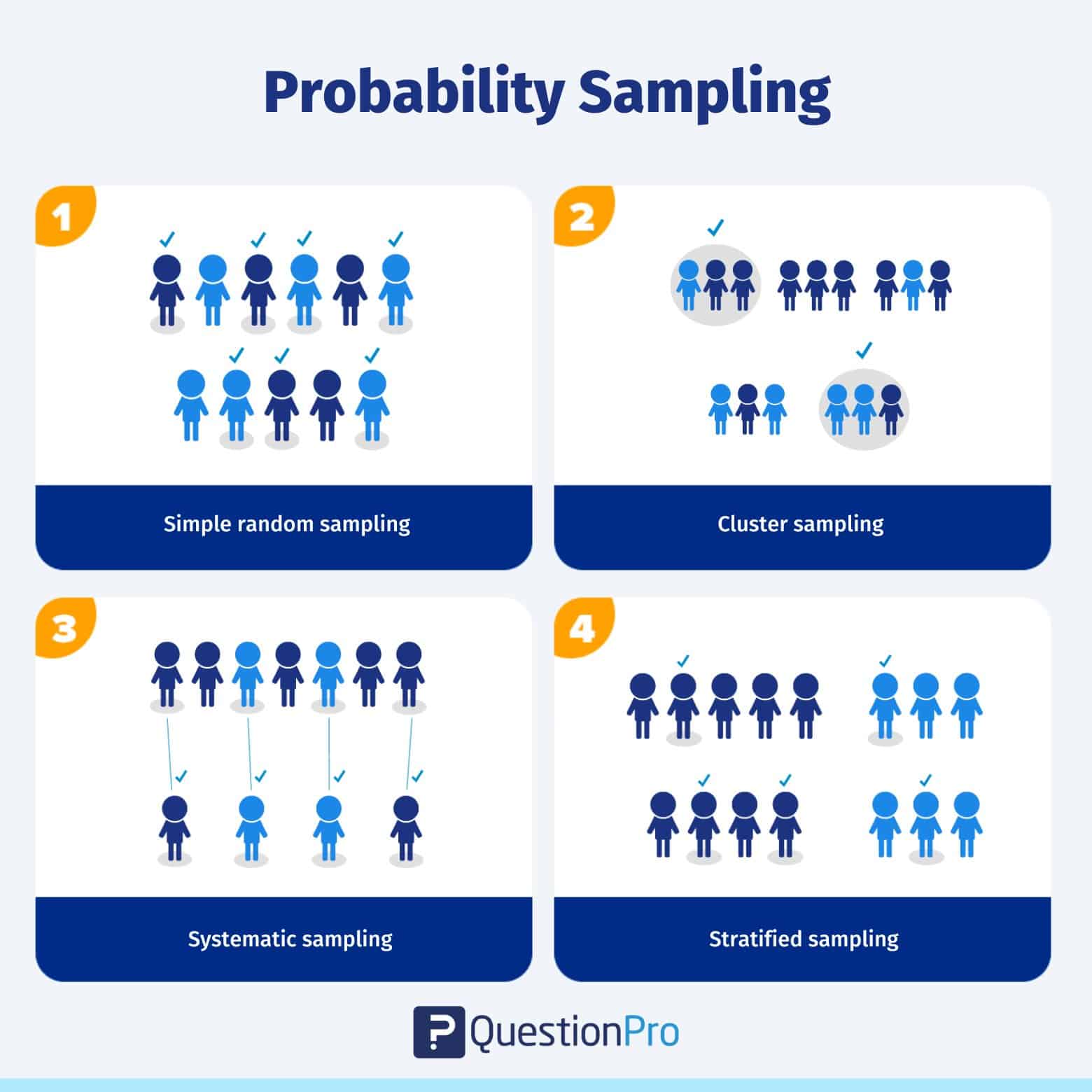
There are four significant types of probability sampling:
- Simple random sampling : More often, the targeted demographic is chosen for inclusion in the sample.
- Cluster sampling : Cluster sampling is a technique in which a population is divided into smaller groups or clusters, and a random sample of these clusters is selected. This method is used when it is impractical or expensive to obtain a random sample from the entire population .
- Systematic sampling : Any of the targeted demographic would be included in the sample, but only the first unit for inclusion in the sample is selected randomly, rest are selected in the ordered fashion as if one out of every ten people on the list .
- Stratified sampling : It allows selecting each unit from a particular group of the targeted audience while creating a sample. It is useful when the researchers are selective about including a specific set of people in the sample, i.e., only males or females, managers or executives, people working within a particular industry.
Interviewing people is a standard method used for data collection . However, the interviews conducted to collect quantitative data are more structured, wherein the researchers ask only a standard set of online questionnaires and nothing more than that.
There are three major types of interviews conducted for data collection
- Telephone interviews: For years, telephone interviews ruled the charts of data collection methods. Nowadays, there is a significant rise in conducting video interviews using the internet, Skype, or similar online video calling platforms.
- Face-to-face interviews: It is a proven technique to collect data directly from the participants. It helps in acquiring quality data as it provides a scope to ask detailed questions and probing further to collect rich and informative data. Literacy requirements of the participant are irrelevant as F2F surveys offer ample opportunities to collect non-verbal data through observation or to explore complex and unknown issues. Although it can be an expensive and time-consuming method, the response rates for F2F interviews are often higher.
- Computer-Assisted Personal Interviewing (CAPI): It is nothing but a similar setup of the face-to-face interview where the interviewer carries a desktop or laptop along with him at the time of interview to upload the data obtained from the interview directly into the database. CAPI saves a lot of time in updating and processing the data and also makes the entire process paperless as the interviewer does not carry a bunch of papers and questionnaires.
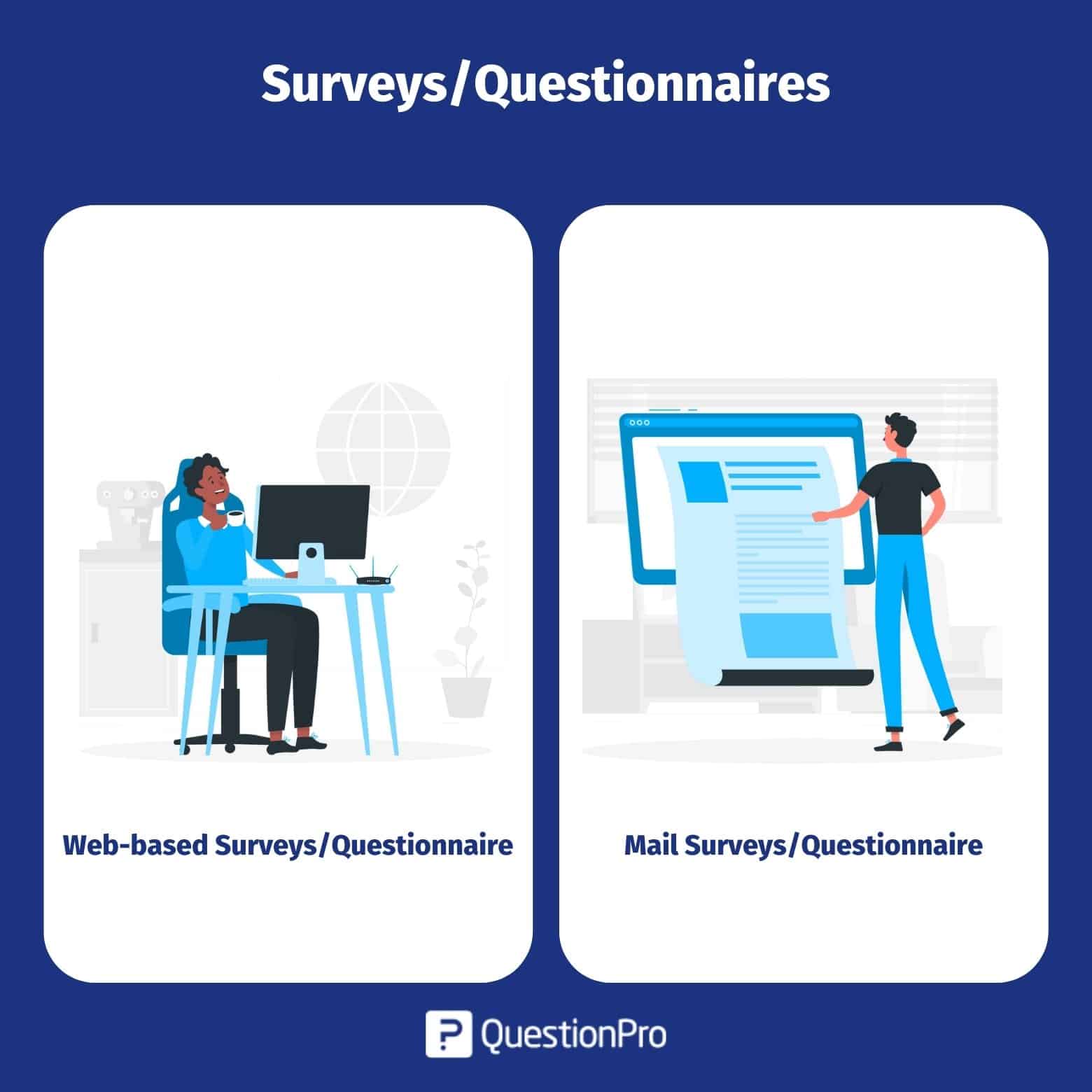
There are two significant types of survey questionnaires used to collect online data for quantitative market research.
- Web-based questionnaire : This is one of the ruling and most trusted methods for internet-based research or online research. In a web-based questionnaire, the receive an email containing the survey link, clicking on which takes the respondent to a secure online survey tool from where he/she can take the survey or fill in the survey questionnaire. Being a cost-efficient, quicker, and having a wider reach, web-based surveys are more preferred by the researchers. The primary benefit of a web-based questionnaire is flexibility. Respondents are free to take the survey in their free time using either a desktop, laptop, tablet, or mobile.
- Mail Questionnaire : In a mail questionnaire, the survey is mailed out to a host of the sample population, enabling the researcher to connect with a wide range of audiences. The mail questionnaire typically consists of a packet containing a cover sheet that introduces the audience about the type of research and reason why it is being conducted along with a prepaid return to collect data online. Although the mail questionnaire has a higher churn rate compared to other quantitative data collection methods, adding certain perks such as reminders and incentives to complete the survey help in drastically improving the churn rate. One of the major benefits of the mail questionnaire is all the responses are anonymous, and respondents are allowed to take as much time as they want to complete the survey and be completely honest about the answer without the fear of prejudice.
LEARN ABOUT: Steps in Qualitative Research
As the name suggests, it is a pretty simple and straightforward method of collecting quantitative data. In this method, researchers collect quantitative data through systematic observations by using techniques like counting the number of people present at the specific event at a particular time and a particular venue or number of people attending the event in a designated place. More often, for quantitative data collection, the researchers have a naturalistic observation approach. It needs keen observation skills and senses for getting the numerical data about the “what” and not about “why” and ”how.”
Naturalistic observation is used to collect both types of data; qualitative and quantitative. However, structured observation is more used to collect quantitative rather than qualitative data collection .
- Structured observation: In this type of observation method, the researcher has to make careful observations of one or more specific behaviors in a more comprehensive or structured setting compared to naturalistic or participant observation . In a structured observation, the researchers, rather than observing everything, focus only on very specific behaviors of interest. It allows them to quantify the behaviors they are observing. When the qualitative observations require a judgment on the part of the observers – it is often described as coding, which requires a clearly defining a set of target behaviors.
Document review is a process used to collect data after reviewing the existing documents. It is an efficient and effective way of gathering data as documents are manageable. Those are the practical resource to get qualified data from the past. Apart from strengthening and supporting the research by providing supplementary research data document review has emerged as one of the beneficial methods to gather quantitative research data.
Three primary document types are being analyzed for collecting supporting quantitative research data.
- Public Records: Under this document review, official, ongoing records of an organization are analyzed for further research. For example, annual reports policy manuals, student activities, game activities in the university, etc.
- Personal Documents: In contrast to public documents, this type of document review deals with individual personal accounts of individuals’ actions, behavior, health, physique, etc. For example, the height and weight of the students, distance students are traveling to attend the school, etc.
- Physical Evidence: Physical evidence or physical documents deal with previous achievements of an individual or of an organization in terms of monetary and scalable growth.
LEARN ABOUT: 12 Best Tools for Researchers
Quantitative data is not about convergent reasoning, but it is about divergent thinking. It deals with the numerical, logic, and an objective stance, by focusing on numeric and unchanging data. More often, data collection methods are used to collect quantitative research data, and the results are dependent on the larger sample sizes that are commonly representing the population researcher intend to study.
Although there are many other methods to collect quantitative data. Those mentioned above probability sampling, interviews, questionnaire observation, and document review are the most common and widely used methods for data collection.
With QuestionPro, you can precise results, and data analysis . QuestionPro provides the opportunity to collect data from a large number of participants. It increases the representativeness of the sample and providing more accurate results.
FREE TRIAL LEARN MORE
MORE LIKE THIS
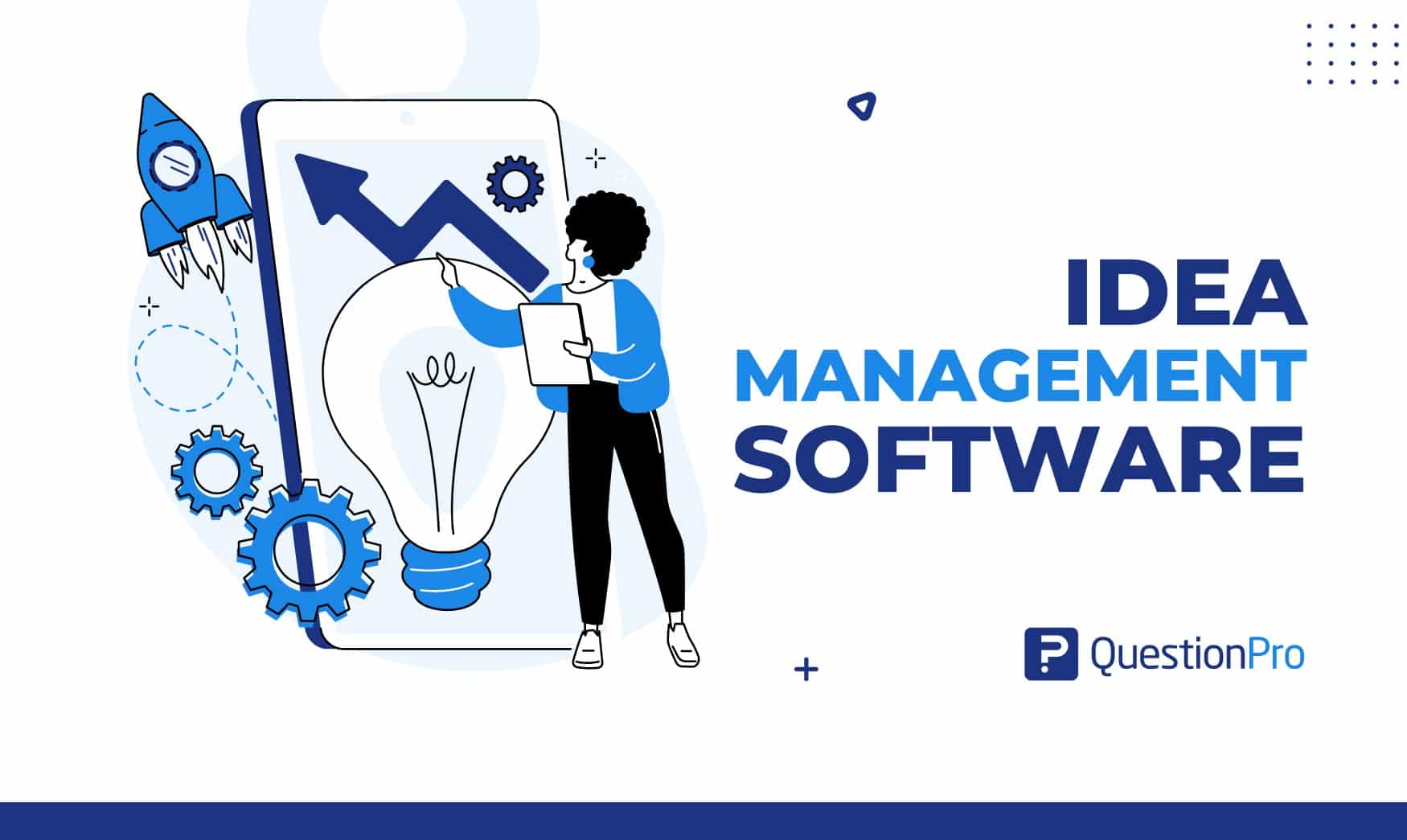
Unlocking Creativity With 10 Top Idea Management Software
Mar 23, 2024
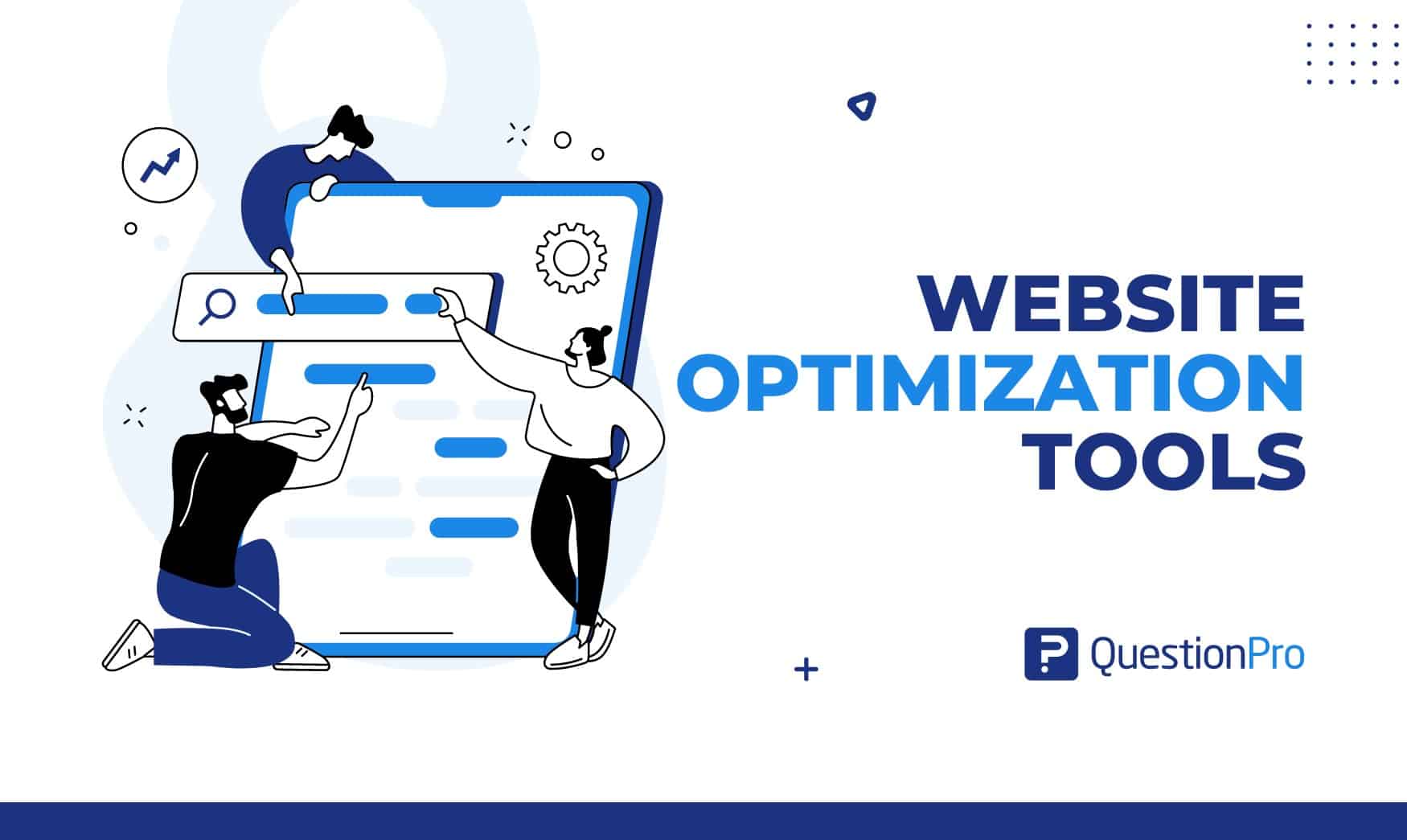
20 Best Website Optimization Tools to Improve Your Website
Mar 22, 2024
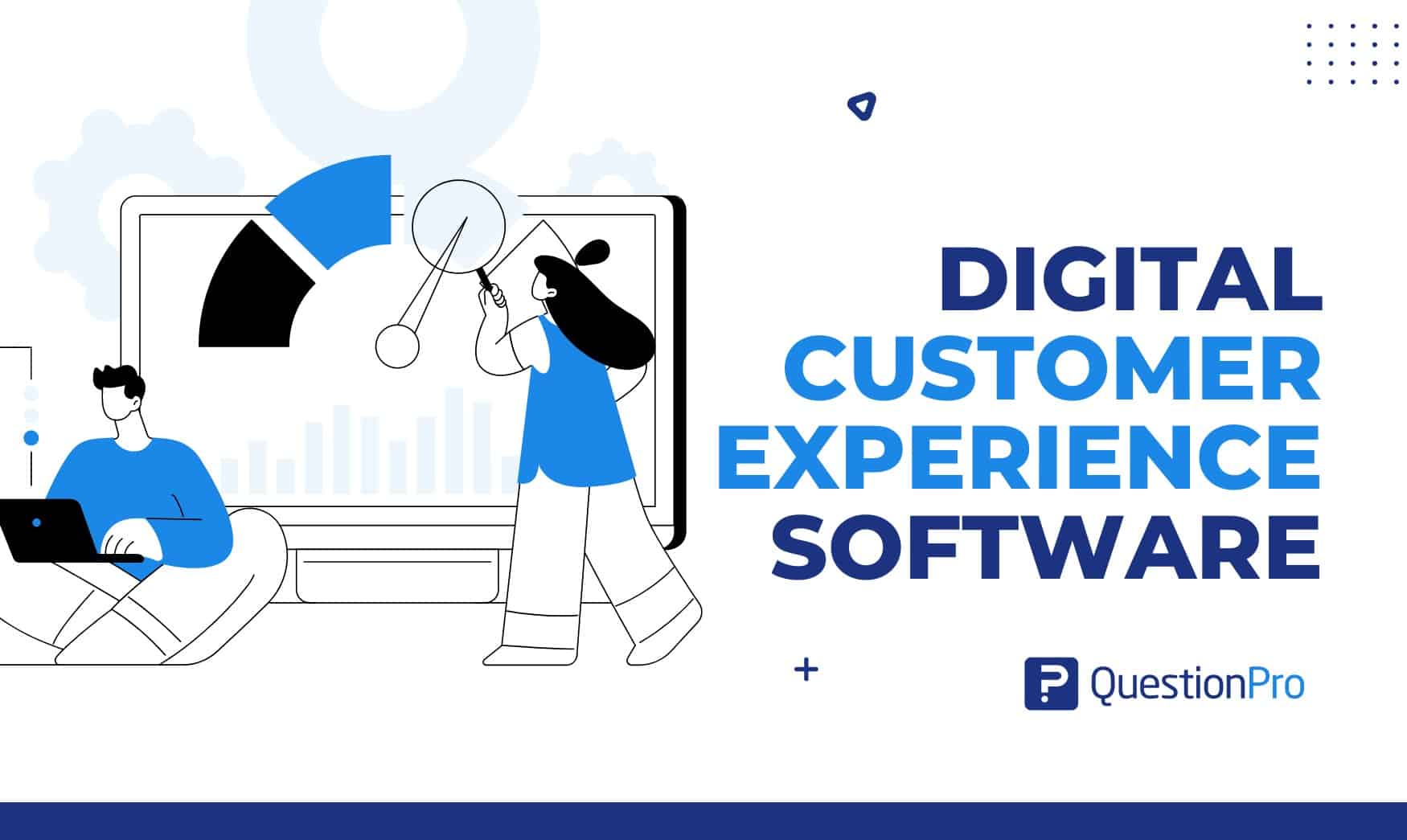
15 Best Digital Customer Experience Software of 2024
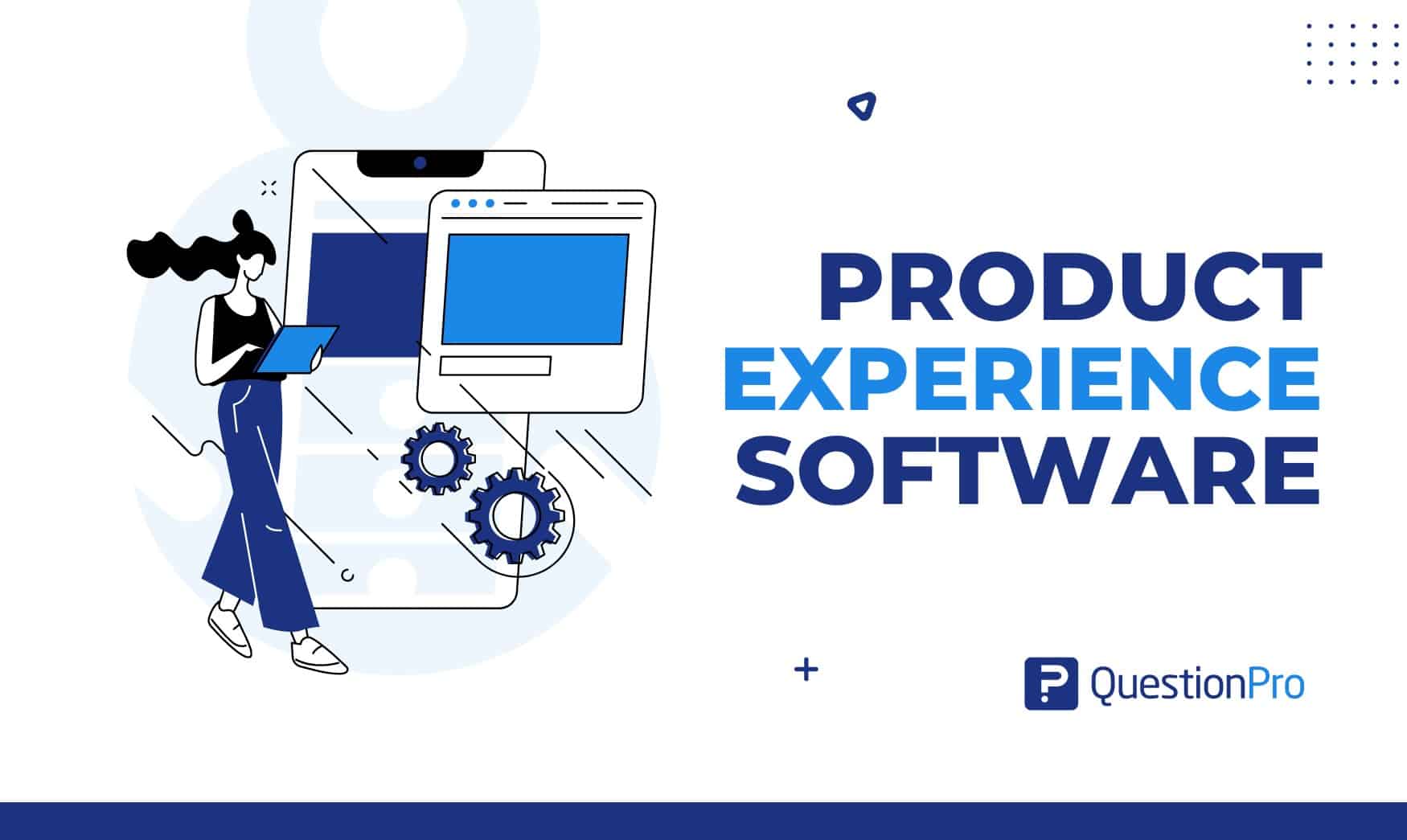
15 Best Product Experience Software of 2024
Other categories.
- Academic Research
- Artificial Intelligence
- Assessments
- Brand Awareness
- Case Studies
- Communities
- Consumer Insights
- Customer effort score
- Customer Engagement
- Customer Experience
- Customer Loyalty
- Customer Research
- Customer Satisfaction
- Employee Benefits
- Employee Engagement
- Employee Retention
- Friday Five
- General Data Protection Regulation
- Insights Hub
- Life@QuestionPro
- Market Research
- Mobile diaries
- Mobile Surveys
- New Features
- Online Communities
- Question Types
- Questionnaire
- QuestionPro Products
- Release Notes
- Research Tools and Apps
- Revenue at Risk
- Survey Templates
- Training Tips
- Uncategorized
- Video Learning Series
- What’s Coming Up
- Workforce Intelligence

Data Module #1: What is Research Data?
- Defining Research Data
- Qualitative vs. Quantitative
- Types of Research Data
- Data and Statistics
- Let's Review...
Quick Navigation
Qualitative vs. quantitative data, research data can be placed into two broad categories: quantitative or qualitative. .

Quantitative data are used when a researcher is trying to quantify a problem, or address the "what" or "how many" aspects of a research question. It is data that can either be counted or compared on a numeric scale. For example, it could be the number of first year students at Macalester, or the ratings on a scale of 1-4 of the quality of food served at Cafe Mac. This data are usually gathered using instruments, such as a questionnaire which includes a ratings scale or a thermometer to collect weather data. Statistical analysis software, such as SPSS, is often used to analyze quantitative data.

Qualitative data describes qualities or characteristics. It is collected using questionnaires, interviews, or observation, and frequently appears in narrative form. For example, it could be notes taken during a focus group on the quality of the food at Cafe Mac, or responses from an open-ended questionnaire. Qualitative data may be difficult to precisely measure and analyze. The data may be in the form of descriptive words that can be examined for patterns or meaning, sometimes through the use of coding. Coding allows the researcher to categorize qualitative data to identify themes that correspond with the research questions and to perform quantitative analysis.
Should I Use Qualitative or Quantitative Data for My Research?
- << Previous: Defining Research Data
- Next: Types of Research Data >>
- Last Updated: Feb 2, 2024 1:41 PM
- URL: https://libguides.macalester.edu/data1

An official website of the United States government
The .gov means it’s official. Federal government websites often end in .gov or .mil. Before sharing sensitive information, make sure you’re on a federal government site.
The site is secure. The https:// ensures that you are connecting to the official website and that any information you provide is encrypted and transmitted securely.
- Publications
- Account settings
Preview improvements coming to the PMC website in October 2024. Learn More or Try it out now .
- Advanced Search
- Journal List
- Perspect Clin Res
- v.14(3); Jul-Sep 2023
- PMC10405529
Designing and validating a research questionnaire - Part 1
Priya ranganathan.
Department of Anaesthesiology, Tata Memorial Centre, Homi Bhabha National Institute, Mumbai, Maharashtra, India
Carlo Caduff
1 Department of Global Health and Social Medicine, King’s College London, London, United Kingdom
Questionnaires are often used as part of research studies to collect data from participants. However, the information obtained through a questionnaire is dependent on how it has been designed, used, and validated. In this article, we look at the types of research questionnaires, their applications and limitations, and how a new questionnaire is developed.
INTRODUCTION
In research studies, questionnaires are commonly used as data collection tools, either as the only source of information or in combination with other techniques in mixed-method studies. However, the quality and accuracy of data collected using a questionnaire depend on how it is designed, used, and validated. In this two-part series, we discuss how to design (part 1) and how to use and validate (part 2) a research questionnaire. It is important to emphasize that questionnaires seek to gather information from other people and therefore entail a social relationship between those who are doing the research and those who are being researched. This social relationship comes with an obligation to learn from others , an obligation that goes beyond the purely instrumental rationality of gathering data. In that sense, we underscore that any research method is not simply a tool but a situation, a relationship, a negotiation, and an encounter. This points to both ethical questions (what is the relationship between the researcher and the researched?) and epistemological ones (what are the conditions under which we can know something?).
At the start of any kind of research project, it is crucial to select the right methodological approach. What is the research question, what is the research object, and what can a questionnaire realistically achieve? Not every research question and not every research object are suitable to the questionnaire as a method. Questionnaires can only provide certain kinds of empirical evidence and it is thus important to be aware of the limitations that are inherent in any kind of methodology.
WHAT IS A RESEARCH QUESTIONNAIRE?
A research questionnaire can be defined as a data collection tool consisting of a series of questions or items that are used to collect information from respondents and thus learn about their knowledge, opinions, attitudes, beliefs, and behavior and informed by a positivist philosophy of the natural sciences that consider methods mainly as a set of rules for the production of knowledge; questionnaires are frequently used instrumentally as a standardized and standardizing tool to ask a set of questions to participants. Outside of such a positivist philosophy, questionnaires can be seen as an encounter between the researcher and the researched, where knowledge is not simply gathered but negotiated through a distinct form of communication that is the questionnaire.
STRENGTHS AND LIMITATIONS OF QUESTIONNAIRES
A questionnaire may not always be the most appropriate way of engaging with research participants and generating knowledge that is needed for a research study. Questionnaires have advantages that have made them very popular, especially in quantitative studies driven by a positivist philosophy: they are a low-cost method for the rapid collection of large amounts of data, even from a wide sample. They are practical, can be standardized, and allow comparison between groups and locations. However, it is important to remember that a questionnaire only captures the information that the method itself (as the structured relationship between the researcher and the researched) allows for and that the respondents are willing to provide. For example, a questionnaire on diet captures what the respondents say they eat and not what they are eating. The problem of social desirability emerges precisely because the research process itself involves a social relationship. This means that respondents may often provide socially acceptable and idealized answers, particularly in relation to sensitive questions, for example, alcohol consumption, drug use, and sexual practices. Questionnaires are most useful for studies investigating knowledge, beliefs, values, self-understandings, and self-perceptions that reflect broader social, cultural, and political norms that may well diverge from actual practices.
TYPES OF RESEARCH QUESTIONNAIRES
Research questionnaires may be classified in several ways:
Depending on mode of administration
Research questionnaires may be self-administered (by the research participant) or researcher administered. Self-administered (also known as self-reported or self-completed) questionnaires are designed to be completed by respondents without assistance from a researcher. Self-reported questionnaires may be administered to participants directly during hospital or clinic visits, mailed through the post or E-mail, or accessed through websites. This technique allows respondents to answer at their own pace and simplifies research costs and logistics. The anonymity offered by self-reporting may facilitate more accurate answers. However, the disadvantages are that there may be misinterpretations of questions and low response rates. Significantly, relevant context information is missing to make sense of the answers provided. Researcher-reported (or interviewer-reported) questionnaires may be administered face-to-face or through remote techniques such as telephone or videoconference and are associated with higher response rates. They allow the researcher to have a better understanding of how the data are collected and how answers are negotiated, but are more resource intensive and require more training from the researchers.
The choice between self-administered and researcher-administered questionnaires depends on various factors such as the characteristics of the target audience (e.g., literacy and comprehension level and ability to use technology), costs involved, and the need for confidentiality/privacy.
Depending on the format of the questions
Research questionnaires can have structured or semi-structured formats. Semi-structured questionnaires allow respondents to answer more freely and on their terms, with no restrictions on their responses. They allow for unusual or surprising responses and are useful to explore and discover a range of answers to determine common themes. Typically, the analysis of responses to open-ended questions is more complex and requires coding and analysis. In contrast, structured questionnaires provide a predefined set of responses for the participant to choose from. The use of standard items makes the questionnaire easier to complete and allows quick aggregation, quantification, and analysis of the data. However, structured questionnaires can be restrictive if the scope of responses is limited and may miss potential answers. They also may suggest answers that respondents may not have considered before. Respondents may be forced to fit their answers into the predetermined format and may not be able to express personal views and say what they really want to say or think. In general, this type of questionnaire can turn the research process into a mechanical, anonymous survey with little incentive for participants to feel engaged, understood, and taken seriously.
STRUCTURED QUESTIONS: FORMATS
Some examples of close-ended questions include:
e.g., Please indicate your marital status:
- Prefer not to say.
e.g., Describe your areas of work (circle or tick all that apply):
- Clinical service
- Administration
- Strongly agree
- Strongly disagree.
- Numerical scales: Please rate your current pain on a scale of 1–10 where 1 is no pain and 10 is the worst imaginable pain
- Symbolic scales: For example, the Wong-Baker FACES scale to rate pain in older children
- Ranking: Rank the following cities as per the quality of public health care, where 1 is the best and 5 is the worst.
A matrix questionnaire consists of a series of rows with items to be answered with a series of columns providing the same answer options. This is an efficient way of getting the respondent to provide answers to multiple questions. The EORTC QLQ-C30 is an example of a matrix questionnaire.[ 1 ]
For a more detailed review of the types of research questions, readers are referred to a paper by Boynton and Greenhalgh.[ 2 ]
USING PRE-EXISTING QUESTIONNAIRES VERSUS DEVELOPING A NEW QUESTIONNAIRE
Before developing a questionnaire for a research study, a researcher can check whether there are any preexisting-validated questionnaires that might be adapted and used for the study. The use of validated questionnaires saves time and resources needed to design a new questionnaire and allows comparability between studies.
However, certain aspects need to be kept in mind: is the population/context/purpose for which the original questionnaire was designed similar to the new study? Is cross-cultural adaptation required? Are there any permission needed to use the questionnaire? In many situations, the development of a new questionnaire may be more appropriate given that any research project entails both methodological and epistemological questions: what is the object of knowledge and what are the conditions under which it can be known? It is important to understand that the standardizing nature of questionnaires contributes to the standardization of objects of knowledge. Thus, the seeming similarity in the object of study across diverse locations may be an artifact of the method. Whatever method one uses, it will always operate as the ground on which the object of study is known.
DESIGNING A NEW RESEARCH QUESTIONNAIRE
Once the researcher has decided to design a new questionnaire, several steps should be considered:
Gathering content
It creates a conceptual framework to identify all relevant areas for which the questionnaire will be used to collect information. This may require a scoping review of the published literature, appraising other questionnaires on similar topics, or the use of focus groups to identify common themes.
Create a list of questions
Questions need to be carefully formulated with attention to language and wording to avoid ambiguity and misinterpretation. Table 1 lists a few examples of poorlyworded questions that could have been phrased in a more appropriate manner. Other important aspects to be noted are:
Examples of poorly phrased questions in a research questionnaire
- Provide a brief introduction to the research study along with instructions on how to complete the questionnaire
- Allow respondents to indicate levels of intensity in their replies, so that they are not forced into “yes” or “no” answers where intensity of feeling may be more appropriate
- Collect specific and detailed data wherever possible – this can be coded into categories. For example, age can be captured in years and later classified as <18 years, 18–45 years, 46 years, and above. The reverse is not possible
- Avoid technical terms, slang, and abbreviations. Tailor the reading level to the expected education level of respondents
- The format of the questionnaire should be attractive with different sections for various subtopics. The font should be large and easy to read, especially if the questionnaire is targeted at the elderly
- Question sequence: questions should be arranged from general to specific, from easy to difficult, from facts to opinions, and sensitive topics should be introduced later in the questionnaire.[ 3 ] Usually, demographic details are captured initially followed by questions on other aspects
- Use contingency questions: these are questions which need to be answered only by a subgroup of the respondents who provide a particular answer to a previous question. This ensures that participants only respond to relevant sections of the questionnaire, for example, Do you smoke? If yes, then how long have you been smoking? If not, then please go to the next section.
TESTING A QUESTIONNAIRE
A questionnaire needs to be valid and reliable, and therefore, any new questionnaire needs to be pilot tested in a small sample of respondents who are representative of the larger population. In addition to validity and reliability, pilot testing provides information on the time taken to complete the questionnaire and whether any questions are confusing or misleading and need to be rephrased. Validity indicates that the questionnaire measures what it claims to measure – this means taking into consideration the limitations that come with any questionnaire-based study. Reliability means that the questionnaire yields consistent responses when administered repeatedly even by different researchers, and any variations in the results are due to actual differences between participants and not because of problems with the interpretation of the questions or their responses. In the next article in this series, we will discuss methods to determine the reliability and validity of a questionnaire.
Financial support and sponsorship
Conflicts of interest.
There are no conflicts of interest.
Have a language expert improve your writing
Run a free plagiarism check in 10 minutes, generate accurate citations for free.
- Knowledge Base
Methodology
- Data Collection | Definition, Methods & Examples
Data Collection | Definition, Methods & Examples
Published on June 5, 2020 by Pritha Bhandari . Revised on June 21, 2023.
Data collection is a systematic process of gathering observations or measurements. Whether you are performing research for business, governmental or academic purposes, data collection allows you to gain first-hand knowledge and original insights into your research problem .
While methods and aims may differ between fields, the overall process of data collection remains largely the same. Before you begin collecting data, you need to consider:
- The aim of the research
- The type of data that you will collect
- The methods and procedures you will use to collect, store, and process the data
To collect high-quality data that is relevant to your purposes, follow these four steps.
Table of contents
Step 1: define the aim of your research, step 2: choose your data collection method, step 3: plan your data collection procedures, step 4: collect the data, other interesting articles, frequently asked questions about data collection.
Before you start the process of data collection, you need to identify exactly what you want to achieve. You can start by writing a problem statement : what is the practical or scientific issue that you want to address and why does it matter?
Next, formulate one or more research questions that precisely define what you want to find out. Depending on your research questions, you might need to collect quantitative or qualitative data :
- Quantitative data is expressed in numbers and graphs and is analyzed through statistical methods .
- Qualitative data is expressed in words and analyzed through interpretations and categorizations.
If your aim is to test a hypothesis , measure something precisely, or gain large-scale statistical insights, collect quantitative data. If your aim is to explore ideas, understand experiences, or gain detailed insights into a specific context, collect qualitative data. If you have several aims, you can use a mixed methods approach that collects both types of data.
- Your first aim is to assess whether there are significant differences in perceptions of managers across different departments and office locations.
- Your second aim is to gather meaningful feedback from employees to explore new ideas for how managers can improve.
Here's why students love Scribbr's proofreading services
Discover proofreading & editing
Based on the data you want to collect, decide which method is best suited for your research.
- Experimental research is primarily a quantitative method.
- Interviews , focus groups , and ethnographies are qualitative methods.
- Surveys , observations, archival research and secondary data collection can be quantitative or qualitative methods.
Carefully consider what method you will use to gather data that helps you directly answer your research questions.
When you know which method(s) you are using, you need to plan exactly how you will implement them. What procedures will you follow to make accurate observations or measurements of the variables you are interested in?
For instance, if you’re conducting surveys or interviews, decide what form the questions will take; if you’re conducting an experiment, make decisions about your experimental design (e.g., determine inclusion and exclusion criteria ).
Operationalization
Sometimes your variables can be measured directly: for example, you can collect data on the average age of employees simply by asking for dates of birth. However, often you’ll be interested in collecting data on more abstract concepts or variables that can’t be directly observed.
Operationalization means turning abstract conceptual ideas into measurable observations. When planning how you will collect data, you need to translate the conceptual definition of what you want to study into the operational definition of what you will actually measure.
- You ask managers to rate their own leadership skills on 5-point scales assessing the ability to delegate, decisiveness and dependability.
- You ask their direct employees to provide anonymous feedback on the managers regarding the same topics.
You may need to develop a sampling plan to obtain data systematically. This involves defining a population , the group you want to draw conclusions about, and a sample, the group you will actually collect data from.
Your sampling method will determine how you recruit participants or obtain measurements for your study. To decide on a sampling method you will need to consider factors like the required sample size, accessibility of the sample, and timeframe of the data collection.
Standardizing procedures
If multiple researchers are involved, write a detailed manual to standardize data collection procedures in your study.
This means laying out specific step-by-step instructions so that everyone in your research team collects data in a consistent way – for example, by conducting experiments under the same conditions and using objective criteria to record and categorize observations. This helps you avoid common research biases like omitted variable bias or information bias .
This helps ensure the reliability of your data, and you can also use it to replicate the study in the future.
Creating a data management plan
Before beginning data collection, you should also decide how you will organize and store your data.
- If you are collecting data from people, you will likely need to anonymize and safeguard the data to prevent leaks of sensitive information (e.g. names or identity numbers).
- If you are collecting data via interviews or pencil-and-paper formats, you will need to perform transcriptions or data entry in systematic ways to minimize distortion.
- You can prevent loss of data by having an organization system that is routinely backed up.
Finally, you can implement your chosen methods to measure or observe the variables you are interested in.
The closed-ended questions ask participants to rate their manager’s leadership skills on scales from 1–5. The data produced is numerical and can be statistically analyzed for averages and patterns.
To ensure that high quality data is recorded in a systematic way, here are some best practices:
- Record all relevant information as and when you obtain data. For example, note down whether or how lab equipment is recalibrated during an experimental study.
- Double-check manual data entry for errors.
- If you collect quantitative data, you can assess the reliability and validity to get an indication of your data quality.
Prevent plagiarism. Run a free check.
If you want to know more about statistics , methodology , or research bias , make sure to check out some of our other articles with explanations and examples.
- Student’s t -distribution
- Normal distribution
- Null and Alternative Hypotheses
- Chi square tests
- Confidence interval
- Cluster sampling
- Stratified sampling
- Data cleansing
- Reproducibility vs Replicability
- Peer review
- Likert scale
Research bias
- Implicit bias
- Framing effect
- Cognitive bias
- Placebo effect
- Hawthorne effect
- Hindsight bias
- Affect heuristic
Data collection is the systematic process by which observations or measurements are gathered in research. It is used in many different contexts by academics, governments, businesses, and other organizations.
When conducting research, collecting original data has significant advantages:
- You can tailor data collection to your specific research aims (e.g. understanding the needs of your consumers or user testing your website)
- You can control and standardize the process for high reliability and validity (e.g. choosing appropriate measurements and sampling methods )
However, there are also some drawbacks: data collection can be time-consuming, labor-intensive and expensive. In some cases, it’s more efficient to use secondary data that has already been collected by someone else, but the data might be less reliable.
Quantitative research deals with numbers and statistics, while qualitative research deals with words and meanings.
Quantitative methods allow you to systematically measure variables and test hypotheses . Qualitative methods allow you to explore concepts and experiences in more detail.
Reliability and validity are both about how well a method measures something:
- Reliability refers to the consistency of a measure (whether the results can be reproduced under the same conditions).
- Validity refers to the accuracy of a measure (whether the results really do represent what they are supposed to measure).
If you are doing experimental research, you also have to consider the internal and external validity of your experiment.
Operationalization means turning abstract conceptual ideas into measurable observations.
For example, the concept of social anxiety isn’t directly observable, but it can be operationally defined in terms of self-rating scores, behavioral avoidance of crowded places, or physical anxiety symptoms in social situations.
Before collecting data , it’s important to consider how you will operationalize the variables that you want to measure.
In mixed methods research , you use both qualitative and quantitative data collection and analysis methods to answer your research question .
Cite this Scribbr article
If you want to cite this source, you can copy and paste the citation or click the “Cite this Scribbr article” button to automatically add the citation to our free Citation Generator.
Bhandari, P. (2023, June 21). Data Collection | Definition, Methods & Examples. Scribbr. Retrieved March 24, 2024, from https://www.scribbr.com/methodology/data-collection/
Is this article helpful?
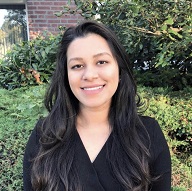
Pritha Bhandari
Other students also liked, qualitative vs. quantitative research | differences, examples & methods, sampling methods | types, techniques & examples, unlimited academic ai-proofreading.
✔ Document error-free in 5minutes ✔ Unlimited document corrections ✔ Specialized in correcting academic texts
- Survey Software The world’s leading omnichannel survey software
- Online Survey Tools Create sophisticated surveys with ease.
- Mobile Offline Conduct efficient field surveys.
- Text Analysis
- Close The Loop
- Automated Translations
- NPS Dashboard
- CATI Manage high volume phone surveys efficiently
- Cloud/On-premise Dialer TCPA compliant Cloud on-premise dialer
- IVR Survey Software Boost productivity with automated call workflows.
- Analytics Analyze survey data with visual dashboards
- Panel Manager Nurture a loyal community of respondents.
- Survey Portal Best-in-class user friendly survey portal.
- Voxco Audience Conduct targeted sample research in hours.
- Predictive Analytics
- Customer 360
- Customer Loyalty
- Fraud & Risk Management
- AI/ML Enablement Services
- Credit Underwriting

Find the best survey software for you! (Along with a checklist to compare platforms)
Get Buyer’s Guide
- 100+ question types
- Drag-and-drop interface
- Skip logic and branching
- Multi-lingual survey
- Text piping
- Question library
- CSS customization
- White-label surveys
- Customizable ‘Thank You’ page
- Customizable survey theme
- Reminder send-outs
- Survey rewards
- Social media
- SMS surveys
- Website surveys
- Correlation analysis
- Cross-tabulation analysis
- Trend analysis
- Real-time dashboard
- Customizable report
- Email address validation
- Recaptcha validation
- SSL security
Take a peek at our powerful survey features to design surveys that scale discoveries.
Download feature sheet.
- Hospitality
- Financial Services
- Academic Research
- Customer Experience
- Employee Experience
- Product Experience
- Market Research
- Social Research
- Data Analysis
- Banking & Financial Services
- Retail Solution
- Risk Management
- Customer Lifecycle Solutions
- Net Promoter Score
- Customer Behaviour Analytics
- Customer Segmentation
- Data Unification
Explore Voxco
Need to map Voxco’s features & offerings? We can help!
Watch a Demo
Download Brochures
Get a Quote
- NPS Calculator
- CES Calculator
- A/B Testing Calculator
- Margin of Error Calculator
- Sample Size Calculator
- CX Strategy & Management Hub
- Market Research Hub
- Patient Experience Hub
- Employee Experience Hub
- Market Research Guide
- Customer Experience Guide
- The Voxco Guide to Customer Experience
- NPS Knowledge Hub
- Survey Research Guides
- Survey Template Library
- Webinars and Events
- Feature Sheets
- Try a sample survey
- Professional services
- Blogs & White papers
- Case Studies
Find the best customer experience platform
Uncover customer pain points, analyze feedback and run successful CX programs with the best CX platform for your team.
Get the Guide Now

We’ve been avid users of the Voxco platform now for over 20 years. It gives us the flexibility to routinely enhance our survey toolkit and provides our clients with a more robust dataset and story to tell their clients.
VP Innovation & Strategic Partnerships, The Logit Group
- Client Stories
- Voxco Reviews
- Why Voxco Research?
- Why Voxco Intelligence?
- Careers at Voxco
- Vulnerabilities and Ethical Hacking
Explore Regional Offices
- Cloud/On-premise Dialer TCPA compliant Cloud & on-premise dialer
- Fraud & Risk Management
Get Buyer’s Guide
- Banking & Financial Services
Explore Voxco
Watch a Demo
Download Brochures
- CX Strategy & Management Hub
- Blogs & White papers
VP Innovation & Strategic Partnerships, The Logit Group
- Our clients
- Client stories
- Featuresheets
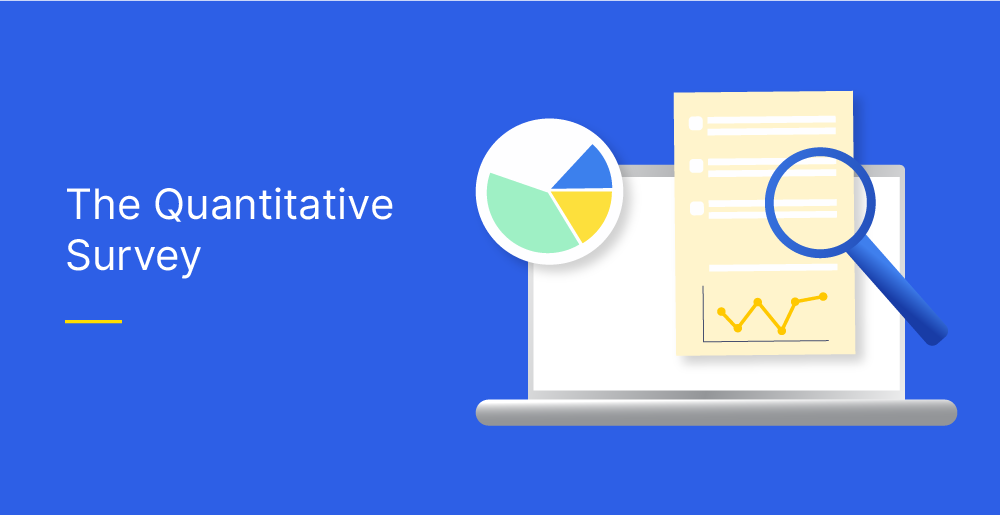
Quantitative Survey Questions: Definition, Types, Examples, and More
SHARE THE ARTICLE ON
Asking the right question to your audience can help you gather accurate and significant data. But what type of questions should you ask your audience – quantitative or qualitative? This depends on the type of data you want to collect.
Quantitative research questions allow respondents to answer concisely. In this article, we’ll go over some popular quantitative research questions and the different types of quantitative research questions.
Definition of quantitative research questions?
Quantitative research questions are objective questions that provide detailed knowledge about a research topic. The data obtained with quantitative research questions are numerical that can be examined statistically.
Quantitative research questions help look into trends and patterns to make logical sense of the research topic. The data gathered can be generalized to the entire population and help make data-driven and sound decisions.
Statistical reports are hard to argue with, making the data more reliable. Quantitative research requires precise information so the data it brings is reliable and valid.
Popular Quantitative Research Questions
Here are some of the most common quantitative research questions you can use in your quantitative surveys:
👉How often do you visit an art gallery?
👉How much do you pay for the hotel’s VIP services?
👉What differences did you notice between our website and app interface?
👉What is the effect of social media on self-confidence?
👉What is the relationship between age and alcohol consumption?
The best way to create a quantitative survey is by using online survey tools that offer a diverse range of question types. This way you can choose which quantitative question would best serve the purpose of your survey.
Launching surveys is easier with a sample
Get a free survey sample from Voxco
Now let’s dive into the different types of quantitative research questions.
What are the different types of quantitative research questions?
There are three types of quantitative research questions .
Each of these type helps you gather unique insights. You can determine which research question types to use based on the type of data you want to gather and the research objective.
- Descriptive Research Questions- These questions are used for gathering customers’ opinions about variables one wants to measure.
- Comparative Research Questions- These questions are used for examining the difference between two or more groups based on one or more dependable variables.
- Relationship-based Research Questions- These questions are used for identifying trends, causal relationships, or associations between two or more variables.
In our next section, we’ll explore the three types of quantitative research questions in detail and look at their examples.
15 quantitative survey question examples
Let’s take a look at some examples of quantitative research questions
1. Descriptive Research Questions
It simply implies that you want to gather information about variables that you are trying to quantify or measure. Descriptive research questions help gather a customer’s opinion about variables you want to measure.
These questions mostly begin with-
- What percentage?
- What proportion?
Descriptive research questions focus mostly on one group and one variable. Rarely do these questions include multiple groups and variables. Here are a few examples of descriptive research questions
We have shared a few questions that you can use as an inspiration to create your own research question. You can use the following quantitative research question examples for your market research.
Let’s look at a few examples of quantitative research question types.
Question 1: How much fast food do Americans consume per week?
- Variable: Fast food intake
- Group: American
Question 2: How often do students between the ages of 15 – 18 use Facebook weekly?
- Variable: Weekly use of Facebook
- Group: Students between ages 15 – 18
Question 3: How often do male and female university students use a food delivery app monthly?
- Variables: Use of food delivery app
- Group: 1. Male University students
- Female University students
Question 4: What is the preferred choice of cuisine of the Italians?
- Variable: Cuisine
- Groups: Italian
Question 5: What are the important factors influencing the career choice of Asian-American University students?
- Variable: Factors influencing career choice
- Group: Asian-American University students
When conducting market research, leverage the best market research software that allows you to reach a global audience across preferred channels.
Also read: What is Descriptive Research?
One-stop-shop to gather, measure, uncover, and act on insightful data.
Curious About The Price? Click Below To Get A Personalized Quote.
2. Comparative Research Question
Comparative research questions help examine the difference between two or more groups based on one or more dependable variables. The number of variables depends on the need of market research.
The question asks the respondents, “what is the difference in” a dependent variable between two or more groups?
Let’s look at some quantitative research question examples for this type that you can use in competitive analysis.
Question 1: What is the difference in time spent on video games between people aged 12 to 17 and 18 to 25?
- Variable: Time spent on video games
- Group 1: People within the age range 12 and 17
- Group 2: People within the age range 18 and 25
Question 2: What is the difference in attitude towards online shopping between millennials adults and Adults born before 1980?
- Variable: Attitude towards online shopping
- Group 1: Millennial adults
- Group 2: Adults born before 1980
Question 3: What is the difference in the attitude towards politics between Mexicans and Americans in America?
- Variable: Attitude towards Politics
- Group 1: Mexicans in America
- Groups 2: Americans in America
Question 4: What is the difference in the usage of Snapchat between Canadian male and female university students?
- Variable: usage of Snapchat
- Group 1: Canadian Male University student
- Group 2: Canadian Female University student
Question 5: What is the difference in attitude toward rock music between Gen-Z and Millennials?
- Variables: Attitude toward rock music
- Group 1: Gen-Z
- Group 2: Millennials
Take a guided tour of Voxco Online.
See how easily you can create, test, distribute and design the surveys.
3. Relationship-based Research Questions
Relationship-based research questions are the best quantitative research question examples when you need to identify trends, causal relationships, or associations between two or more variables.
When using the term relationship in statistics, it is important to remember that it refers to experimental research design.
In this case, examining the cause and effect between two variables is possible. This means it is possible to say which variable was responsible for the effect on the other variable.
However, when you use relationship-based research questions, you do not have to distinguish between causal relationships, trends, or associations.
The question in this type begins with “What is the relationship” between or amongst independent and dependent variables, amongst or between two or more groups?
Question 1: What is the relationship between gender and attitude towards romantic movies amongst Middle-aged people?
- Dependent Variable: Attitude towards romantic movies
- Independent Variables: Gender
- Group: Middle-aged people
Question 2: What is the relationship between age and fast food preference in Australia?
- Dependent Variable: Fast Food preference
- Independent Variable: Age
- Group: Australia
Question 3: What is the relationship between age and lifestyle among women?
- Dependent Variable: Lifestyle
- Group: Women
Question 4: What is the relationship between Salary and Job satisfaction among people working in the corporate business?
- Dependent Variable: Job satisfaction
- Independent Variable: Salary
- Group: People working in corporate business
Question 5: What is the relationship between University degrees and Job positions amongst employees in Multinational Companies?
- Dependent Variable: Job position
- Independent Variable: University Degree
- Group: Employees in a Multinational Company
This quantitative research question example can help you help segment customers based on their shared characteristics. Leverage a data analysis platform that enables you to identify patterns between responses and help you group customers with similar attributes.
This way, you can create unique customer profiles and personalized campaigns that appeal to each customer.
Learn the Key Steps To Conduct Descriptive Research with Voxco’s Guide to Descriptive Research.
400+ Marketers Have Downloaded It & Found It Useful.
How to Write Good Quantitative Survey Questions?
Follow the steps listed below to design quantitative survey questions:
Step 1: Choose the type of quantitative survey question
Select a type that corresponds to the survey’s goal. The kind of answers given by respondents are determined by the type of question posed.
Step 2: Identify the target group as well as dependent and independent variables
The next step is to choose the target audience and the variables to be measured. Variables can be categorized into 4 types: nominal, ordinal, interval, and ratio.
Step 3: Give a proper structure to the survey
After the first 2 points have been taken care of, it is now time to structure the survey such that it is uncomplicated. Simple and unambiguous wordings should be used to frame the questions.
Importance of Quantitative Research Questions
Quantitative research questions play a vital role in the design and implementation of the research method. The questions provide a clear framework to help you understand what data you need to gather and investigate the correlation between quantitative variables.
Here are some key reasons that make quantitative research questions important.
1. Objective analysis
This research question allows you to seek numerical data and focus on measurable variables. It provides a more standardized result and objective measurement.
2. Generalizability
Quantitative data enables you to make generalizations about the target population based on your research sample. By clearly defining variables and the target population, you can draw conclusions that are applicable to the intended population.
3. Statistical analysis
A well-defined quantitative research question gathers statistical data that allows you to perform statistical analysis. This allows you to identify patterns, correlations, trends, and differences within the data, thus providing meaningful insights.
When to use Quantitative Research Questions?
Let’s look at the situations when you should use quantitative research questions.
1. When exploring the relationship between quantitative variables.
What is the relationship between students’ play time and their health?
This is an example of quantitative research question where you intended to identify the relation between the variables of students’ health and their playtime.
2. When comparing different groups.
Is there any significant difference in the satisfaction level between software A and software B?
This is a quantitative research question example where you explore the difference in satisfaction between two variables.
3. When evaluating trends and patterns.
How has the purchase frequency changed over the fourth quarter of the year?
This is an example of quantitative research question where you uncover the trend or changes in purchase frequency over time.
4. When conducting large-scale surveys.
Quantitative questions are valuable when you intend to conduct large-scale surveys targeting a wide range of participants. It allows a standardized and systematic approach to data collection and analysis, enabling you to draw meaningful conclusions.
Also Read: Quantitative Research Vs Qualitative Research .
This sums up our article on quantitative research question examples. You can use these question types to create interactive market research surveys that provide meaningful insights. You can choose to use these questions separately or together in a survey depending on your research goal.
Looking for World’s best Survey Platform?
Voxco Is The Leading Online Survey Tool That Annually Powers More Than 50Mn+ Surveys.
Trusted By 500+ Brands Across 40+ Countries.
See how it works-
Explore all the survey question types possible on Voxco
Explore Voxco Survey Software
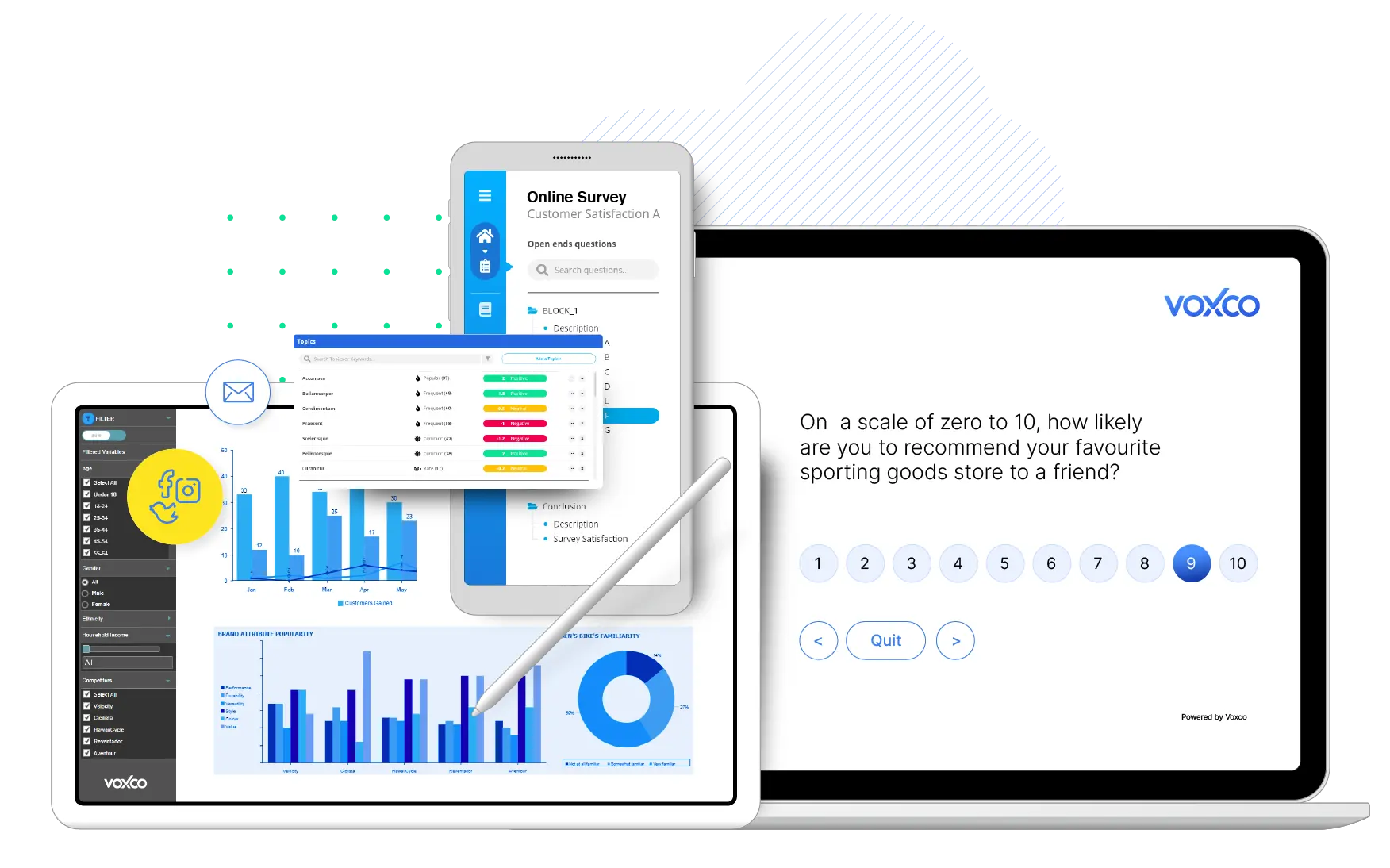
+ Omnichannel Survey Software
+ Online Survey Software
+ CATI Survey Software
+ IVR Survey Software
+ Market Research Tool
+ Customer Experience Tool
+ Product Experience Software
+ Enterprise Survey Software
We use cookies in our website to give you the best browsing experience and to tailor advertising. By continuing to use our website, you give us consent to the use of cookies. Read More
Just one more step to your free trial.
.surveysparrow.com
Already using SurveySparrow? Login
By clicking on "Get Started", I agree to the Privacy Policy and Terms of Service .
This site is protected by reCAPTCHA and the Google Privacy Policy and Terms of Service apply.
Enterprise Survey Software
Enterprise Survey Software to thrive in your business ecosystem
NPS Software
Turn customers into promoters
Offline Survey
Real-time data collection, on the move. Go internet-independent.
360 Assessment
Conduct omnidirectional employee assessments. Increase productivity, grow together.
Reputation Management
Turn your existing customers into raving promoters by monitoring online reviews.
Ticket Management
Build loyalty and advocacy by delivering personalized support experiences that matter.
Chatbot for Website
Collect feedback smartly from your website visitors with the engaging Chatbot for website.
Swift, easy, secure. Scalable for your organization.
Executive Dashboard
Customer journey map, craft beautiful surveys, share surveys, gain rich insights, recurring surveys, white label surveys, embedded surveys, conversational forms, mobile-first surveys, audience management, smart surveys, video surveys, secure surveys, api, webhooks, integrations, survey themes, accept payments, custom workflows, all features, customer experience, employee experience, product experience, marketing experience, sales experience, hospitality & travel, market research, saas startup programs, wall of love, success stories, sparrowcast, nps benchmarks, learning centre, apps & integrations.
Our surveys come with superpowers ⚡
Blog General
Quantitative Data Collection: A Quick Guide for Beginners
Parvathi vijayamohan.
Table Of Contents
- Quantitative Data Collection
- Best Practices
- Common Mistakes
What is quantitative data collection? It’s a research method that gathers and analyzes numerical data. Its purpose is to answer research questions, or test hypotheses. Pretty cool, right?
Moreover, the quantitative data structure allows statistical analysis and objective conclusions. That’s why it’s so popular among researchers.
In this guide, we’re going to explore:
- Data collection methods in quantitative research
- Data collection tools in quantitative research
- 7 best practices for quantitative data collection
- 7 common mistakes to dodge
- The role of quantitative data in decision making
6 Tried-and-True Quantitative Data Collection Methods
Surveys and forms.
Surveys and forms are a common method of collecting quantitative data. They involve asking a large group of people to respond to a set of questions, either in person or online.
Forms are like surveys, but they are usually shorter, and more targeted to a specific group.
The process of conducting a survey involves:
- Designing a set of questions
- Selecting a sample of respondents
- Administering the survey
- Analyzing the results
You can survey people in various ways, including face-to-face, over the phone, by mail, or online. However, online surveys are one of the most popular methods, because they are cost-effective, easy to create and distribute
Need to collect quantitative data for your survey? Start getting insights with 3 active survey projects and custom report filters for FREE. Sign up with SurveySparrow .
Please enter a valid Email ID.
14-Day Free Trial • No Credit Card Required • No Strings Attached
Pros of surveys and forms:
- Their ability to collect data from a large sample of people.
- Flexibility in design, customization and distribution.
- Potential for anonymity, which can encourage respondents to answer truthfully.
- The possibility of low response rates.
- Potential bias in the sample selection.
- The risk of respondents providing inaccurate or incomplete answers.
Interviews involve asking a set of questions to individuals, or groups of people. to collect quantitative data. They can be conducted in person, over the phone, or online.
The process of conducting an interview involves:
- Preparing a set of questions.
- Selecting a sample of participants.
- Conducting the interview.
- Analyzing the results.
Interviews can be structured, semi-structured, or unstructured. It depends on the research question and the level of flexibility needed in the responses.
Pros of interviews:
- Their ability to collect detailed and nuanced data.
- Their potential for follow-up questions and clarifications.
- Ability to build rapport and establish trust with the respondents.
- The potential for interviewer bias.
- Potential for the respondents to provide socially desirable answers.
- The time and resources required to conduct interviews.
Observations
Observations involve observing and recording the behavior of people or objects – in a natural or controlled setting – to collect quantitative data.
This data collection method can be conducted in various ways – including structured or unstructured observations, participant or non-participant observations, and covert or overt observations.
The process of conducting observations involves:
- Selecting a setting or situation.
- Determining the behaviors or events to be observed.
- Recording the data.
Observations can be conducted in real-time or recorded for later analysis.
Pros of observations include:
- Their ability to capture behavior in a natural setting.
- Potential for rich and detailed data.
- Their ability to reveal patterns and trends that may not be evident through other methods.
Cons include:
- The potential for observer bias.
- Potential for participant behavior to be affected by the presence of the observer
- The time and resources required to conduct observations.
Polls involve asking a specific question, or set of questions, to a large group of people to collect quantitative data. Additionally, polls can be conducted in various ways, including over the phone, online, or in person.
The process of conducting a poll involves:
- Preparing a question or set of questions.
- Selecting a sample of respondents.
- Administering the poll.
Polls can be conducted on a one-time or ongoing basis, depending on the research question and the level of data needed.
Pros of polls include:
- Ability to collect data quickly and efficiently
- Their potential for a large sample size
- Ability to track changes in public opinion over time.
- The potential for bias in the sample selection.
- Possibility for leading or ambiguous questions.
- The potential for respondents to provide socially desirable answers.
Experiments
Experiments involve manipulating one or more variables in a controlled setting to collect quantitative data.
In addition, experiments can be conducted in various ways, including laboratory experiments, field experiments, and quasi-experiments.
The process of conducting an experiment involves:
- Selecting the variables to be manipulated.
- Administering the treatment.
Experiments require a high level of control and standardization to ensure accurate results.
Pros of experiments include:
- Their ability to establish cause-and-effect relationships between variables.
- Potential for high internal validity.
- Their ability to replicate and generalize findings.
- The potential for low external validity.
- Possibility of ethical concerns.
- The time and resources required to conduct experiments.
Document/ Archival Reviews
Document reviews involve analyzing existing documents to collect quantitative data. Documents can include official records, reports, publications, or any other written material that is relevant to the research questions.
The process of conducting a document review involves:
- Selecting the documents to be analyzed.
- Developing a coding scheme .
- Collecting and analyzing the data.
- Drawing conclusions based on the findings.
Pros of document reviews include:
- Their ability to collect data from a wide range of sources.
- Potential for high reliability and objectivity.
- Their ability to access historical or hard-to-reach data.
- The possibility of incomplete or inaccurate data.
- Potential for researcher bias in the selection and analysis of documents.
- The time and resources required to conduct a thorough review.
Data Collection Tools in Quantitative Research
Data collection tools are basically instruments that are used to collect data in a standardized and consistent way. So different methods of quantitative data collection need different data collection tools.
- For surveys, data collection tools can include paper questionnaires, online surveys, offline kiosks or phone surveys.
- For interviews, data collection tools can include interview guides or scripts.
- During observations, tools of data collection can include observation checklists or rating scales.
- For polls, data collection tools can include questionnaires or online polling apps.
- For experiments, data collection tools can include treatment protocols or observation protocols.
- In document reviews, data collection tools can include coding schemes or data extraction forms.
7 best practices for collecting quantitative data
If you want to make sure that the data you get is accurate and reliable, there are some best practices you should follow in quantitative data collection.
Here are a few of them:
- First things first, make sure you clearly define your research question and objectives. You need to know what you’re trying to achieve before you start collecting data.
- Next, select an appropriate data collection method and tool that will help you answer your research question.
- It’s also important to develop a sampling plan and select a representative sample. This will help ensure that the data you collect is relevant to your research question.
- Before you start collecting data, it’s a good idea to pilot test your data collection tool to make sure it’s effective.
- Train your data collectors to ensure that everyone is on the same page and knows what to do.
- Monitor the data collection process to ensure quality control. This will help you catch any issues before they become major problems.
- Finally, use appropriate statistical techniques to analyze the data. This will help you make sense of the data you’ve collected and draw accurate conclusions.
7 common mistakes to avoid in quantitative data collection
Gathering quantitative data is no easy feat – it requires careful planning, execution, and analysis.
But even with the best of intentions, researchers can make mistakes that lead to inaccurate or biased data. Here are some of the most common pitfalls to avoid:
Using biased or unrepresentative samples:
It’s important to ensure that the sample you collect is representative of the population you’re interested in. Otherwise you will get results that don’t accurately reflect the population’s characteristics.
To avoid this, researchers should use random sampling techniques that give everyone an equal chance of selection.
Using poorly designed data collection tools
The data collection tool is a key part of quantitative data collection. Messily designed tools can result in incomplete or inaccurate data.
To avoid this, researchers should take the time to design their data collection tools, test them with a small sample of participants, and revise them as needed. You can also take advantage of survey templates online as a lot of thought has already gone into crafting them.
Failing to establish clear research questions and objectives
Having a clear focus is essential for successful data collection. Without a clear research question and objectives, your data collection efforts can become scattered and unfocused, leading to data that’s difficult to interpret and use.
Make sure to establish clear research questions and objectives before you start collecting data.
Failing to pilot test the data collection tool
Testing (or “piloting”) your tool is key to ensuring its effectiveness.
Piloting involves testing the tool with a small sample of participants. Piloting can help researchers identify any issues with its design or implementation, and fix them before they spread.
Skipping the training of data collectors
To ensure consistency and accuracy in data collection, researchers must train their data collectors on the tool and the research objectives.
Data collectors need to understand the protocol and its reasoning. Without adequate training, data collectors may miss important data or record it incorrectly, compromising the data’s validity.
Failing to monitor the data collection process
Researchers need to oversee the data collection process to ensure that:
- The data collectors follow the protocol correctly.
- Data is collected on time.
- The data is recorded accurately.
Without monitoring, data collection efforts can become unfocused and ineffective.
Using inappropriate statistical techniques to analyze the data
The statistical analysis of quantitative data is a vital step in the research process. Using inappropriate statistical techniques can lead to misleading or incorrect conclusions.
Because of this, a lot of researchers use online survey tools, like SurveySparrow or Qualtrics, which have an automatic report generation feature. This cuts short the time spent on statistical techniques and reduces the chance of human error.
In short, dodging these mistakes ensures the accuracy and reliability of your quantitative data collection. By doing so, you’ll be able to gather valuable insights, and make evidence-based decisions.
The role of quantitative data collection in decision making
- Quantitative data collection plays a vital role in decision making in various fields – including business, healthcare, education, and public policy.
- Quantitative data can provide insights into key areas like – consumer behavior, product performance, patient outcomes, student achievement, and social trends.
- By collecting and analyzing quantitative data, decision makers can make informed decisions based on objective evidence rather than intuition or assumptions.
- Quantitative data can help identify patterns, trends, and relationships between variables, which can inform strategies and interventions.
For example:
- In healthcare, quantitative data can help identify risk factors for diseases, evaluate the effectiveness of treatments, and track patient outcomes.
- In business, quantitative data can help identify market trends, assess the performance of products or services, and inform pricing strategies.
- In education, quantitative data can help evaluate the effectiveness of teaching methods, assess student learning outcomes, and inform curriculum development.
Wrapping Up
In conclusion, quantitative data collection is a great example of a deductive research approach. This makes it an essential tool for researchers and decision makers in various fields.
By following best practices and avoiding common mistakes, researchers can collect accurate, reliable and actionable data. Moreover, the right tools can reduce the chances of human error. Get in touch with our team to find out how we can help with your next survey project.
Growth Marketer at SurveySparrow
Fledgling growth marketer. Cloud watcher. Aunty to a naughty beagle.
You Might Also Like
How to launch your product : a complete guide for launching on product hunt, how to conduct a job interview effectively: a pocket guide, how to use lominger competencies to improve key business skills in 2024.
Leave us your email, we wont spam. Promise!
Start your free trial today
No Credit Card Required. 14-Day Free Trial
Request a Demo
Want to learn more about SurveySparrow? We'll be in touch soon!
For all types of insights
Scale up your survey projects with 50+ survey tools. try surveysparrow for free..
14-Day Free Trial • No Credit card required • 40% more completion rate
Hi there, we use cookies to offer you a better browsing experience and to analyze site traffic. By continuing to use our website, you consent to the use of these cookies. Learn More
More From Forbes
How marketers are using qualitative data in the age of big data and ai.

- Share to Facebook
- Share to Twitter
- Share to Linkedin
Lacking a holistic understanding of their target audience limits marketers’ ability to create the most effective strategies. Yet they often prioritize the concrete metrics of quantitative data, such as sales numbers and website traffic that seem easier to measure and analyze, over subjective observations. Consequently, relying solely on quantitative data can lead marketers to overlook the human element of consumer behavior and miss out on valuable insights that only qualitative data can provide.
Qualitative data reveals vital information about customers’ emotions, motivations, preferences and perceptions. Through methods such as interviews and focus groups, marketers can gather rich, nuanced data that goes beyond mere numbers, illuminating a bigger picture that allows them to better tailor strategies, messaging and products to what consumers want. Below, 15 members of Forbes Agency Council explore the role that qualitative data can play in the era of big data and artificial intelligence, sharing how their agencies leverage it to better meet the needs and desires of target audiences.
1. To Get To The Quantitative Data
Without the qualitative data, you can’t get to the quantitative data. All the ad creatives, messaging, campaign structures and so forth that take place behind the scenes are going to feed the big data monster with the engagement, clicks and interactions that can be improved upon. AI helps us get 10 times more iterations and options—but nothing runs without the creative thought that fills the machine. - Bernard May , National Positions
2. To Create A Better User Experience
We use qualitative data to create a better UX for Web design projects. Through user testing, interviews and usability studies, we gain insights into user needs, preferences and pain points. This information guides the design process, ensuring that websites and digital platforms are not only functional, but also enjoyable to use, enhancing user satisfaction and engagement. - Goran Paun , ArtVersion
3. To Understand Sentiment And Brand Perception
Qualitative data adds depth to our understanding of consumer behaviors, emotions and motivations, complementing quantitative insights. Our agency uses it for sentiment analysis and brand perception studies to craft personalized messaging that resonates authentically with target audiences. - Pascal Wilpers , Streamerzone.gg
The Gentlemen Dethroned In Netflix s Top 10 List By A New Show
Ios 17 4 1 update now warning issued to all iphone users, isis claims responsibility for moscow shooting that left 40 dead, 4. to discover unique, actionable insights.
Einstein said, “Not everything that counts can be counted, and not everything that can be counted counts.” In marketing, qualitative data is vital to understanding human emotions and decisions. Despite abundant data, actionable insights require human intuition and experience, especially in hyper-personalized marketing. Global Prairie excels at this by harnessing qualitative data to discover unique insights. - Tom Hileman , Global Prairie
Forbes Agency Council is an invitation-only community for executives in successful public relations, media strategy, creative and advertising agencies. Do I qualify?
5. To Provide Color And Context
Consider qualitative data as the color providing context and sentiment around the rigid boundaries of quantitative data. Often, both types of datasets are needed to derive business value and audience interest. Qualitative data is the “gut check” for the “instincts” you may follow while evaluating datasets. Data cannot always be simplified into a dashboard; sometimes it requires deep analysis. - Tyler Back , Mitosis
6. To Identify Pain Points, Gains And Jobs To Be Done
Customer interviews provide qualitative data that is increasingly vital for identifying pain points, gains and jobs to be done, as well as shaping your communication strategy. However, quantitative data from clients and prospects is also crucial. It informs us about what messages to convey, as well as where and how to communicate them more effectively. - Kate Vasylenko , 42DM Corporation
7. To Connect PR Efforts To Client Goals And Show ROI
Qualitative data is essential to connecting PR efforts to clients’ business goals and showing the ROI of our work. At Next PR, we go beyond reporting on quantitative KPIs, such as number of media placements, and instead focus on qualitative results that support clients’ objectives, such as leveraging Google Analytics to demonstrate how a media placement contributed to increased brand awareness. - Heather Kelly , Next PR
8. To Get Insights That Yield Marketing Recommendations
Qualitative data speaks to the emotion behind customers’ decision making. We use interviews, open-ended surveys, observational studies and focus groups to gather such data. Quantitative techniques capture numerical data (website visits, customer satisfaction scores), revealing trends and correlations in customer behavior. Combining both, we develop insights that yield marketing recommendations. - Robert Finlayson , Bold Marketing and Communications
9. To Understand Our Clients’ Audiences’ Motivations
AI allows us to analyze large sets of data to provide necessary context. The contextual frameworks need to be filled with emotions and insights beyond the numbers—in short, qualitative data. It’s all about storytelling, so leveraging user feedback and social listening helps us understand the motivations and preferences of our clients’ audiences and turn them into meaningful marketing strategies. - Christoph Kastenholz , Pulse Advertising
10. To Get As One-To-One As Possible In Our Messaging
Qualitative data is important, as it allows us to get as one-to-one as possible in our messaging and content. Right now, we’re seeing a lot of junk via email, LinkedIn and content in search engines and other places that, in many cases, is either wrong at worst or okay at best. Higher-quality data allows for brand messaging and content that meets the prospect or potential customer with value, not noise. - Corey Morris , Voltage
11. To Develop Briefs And Leverage Winning Insights
Qualitative data is increasingly precious in an age of data commoditization. Comments on social media are the new briefs, and invariably, the majority of winning insights are the consequence of qualitative inputs from customers. - Aasim Shaikh Zubaer , LPS Brands
12. To Understand Why What Works Is Working
Qualitative data goes deeper than quantitative data. It gives you the “why.” While big data and AI can tell you what works, understanding why it works will help your organization develop a larger story arc to guide customers across the full journey. - Alicia Arnold , AK Arnold
13. To Develop Richer Audience Personas
In the era of big data and AI, qualitative data helps marketers and advertisers gain a deeper understanding of customers’ motivations and preferences. Our agency plans to leverage it by developing richer audience personas, which can help clients get a clearer picture into their customers’ values, inspirations and challenges. - Jordan Edelson , Appetizer Mobile LLC
14. To Inform Campaigns And Evaluate Results
Qualitative data remains a crucial tool for marketers and advertisers. It plays a complementary and valuable role alongside quantitative data, offering deeper insights and richer understanding of consumers. Our platform uses it to conduct research and analysis, develop customer personas for our clients, inform campaign development and measure and evaluate campaign results. - Tanuj Joshi , Eulerity
15. To Accurately Model B2B Audiences
Qualitative data is incredibly important for B2B agencies such as Napier. For example, it’s often not possible to get statistically significant samples to accurately model audiences quantitatively. It’s also important to note that AI’s “pattern matching” capabilities are not quantitative analysis; in fact, I believe AI has introduced a golden age of qualitative data analysis. - Mike Maynard , Napier Partnership Limited
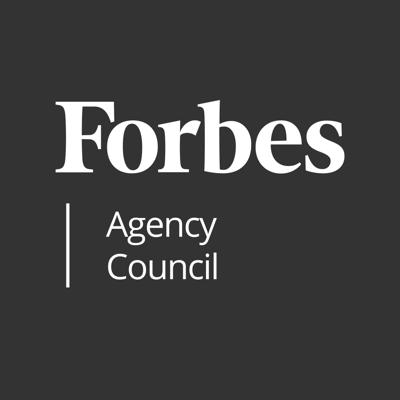
- Editorial Standards
- Reprints & Permissions
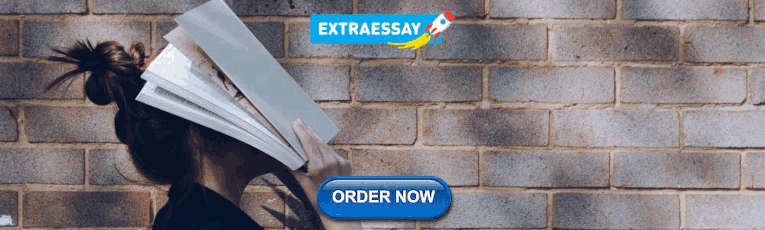
IMAGES
VIDEO
COMMENTS
INTRODUCTION. Scientific research is usually initiated by posing evidenced-based research questions which are then explicitly restated as hypotheses.1,2 The hypotheses provide directions to guide the study, solutions, explanations, and expected results.3,4 Both research questions and hypotheses are essentially formulated based on conventional theories and real-world processes, which allow the ...
Quantitative Research. Quantitative research is a type of research that collects and analyzes numerical data to test hypotheses and answer research questions.This research typically involves a large sample size and uses statistical analysis to make inferences about a population based on the data collected.
Here is a basic guide for gathering quantitative data: Define the research question: The first step in gathering quantitative data is to clearly define the research question. This will help determine the type of data to be collected, the sample size, and the methods of data analysis.
Depending on your research questions, you might need to collect quantitative or qualitative data: Quantitative data is expressed in numbers and graphs and is analysed through statistical methods. Qualitative data is expressed in words and analysed through interpretations and categorisations. If your aim is to test a hypothesis, measure ...
Revised on June 22, 2023. Quantitative research is the process of collecting and analyzing numerical data. It can be used to find patterns and averages, make predictions, test causal relationships, and generalize results to wider populations. Quantitative research is the opposite of qualitative research, which involves collecting and analyzing ...
3 Gathering and Analyzing Quantitative Data . Although the goal of any research study is to gather information to analyze, this process can be a little daunting. Hopefully, you've taken the time to plan your approach so that you have a clear plan for the type of information you'll be gathering and the process by which you will assign meaning and glean an understanding about what you've ...
Quantitative research gathers a range of numeric data. Some of the numeric data is intrinsically quantitative (e.g. personal income), while in other cases the numeric structure is imposed (e.g. ... Using scientific inquiry, quantitative research relies on data that are observed or measured to examine questions about the sample population ...
Social scientists are concerned with the study of people. Quantitative research is a way to learn about a particular group of people, known as a sample population. Using scientific inquiry, quantitative research relies on data that are observed or measured to examine questions about the sample population. Allen, M. (2017). The SAGE encyclopedia ...
What is Quantitative Research? Qualitative research is based upon data that is gathered by observation. Qualitative research articles will attempt to answer questions that cannot be measured by numbers but rather by perceived meaning. Qualitative research will likely include interviews, case studies, ethnography, or focus groups.
Use quantitative research if you want to confirm or test something (a theory or hypothesis) Use qualitative research if you want to understand something (concepts, thoughts, experiences) For most research topics you can choose a qualitative, quantitative or mixed methods approach. Which type you choose depends on, among other things, whether ...
As you embark on your journey into quantitative research, remember that StatsCamp.org is here to support you every step of the way. Whether you're formulating research questions, analyzing data, or interpreting results, our courses provide the knowledge and expertise you need to succeed. Join us today and unlock the power of quantitative ...
Descriptive research questions (also known as usage and attitude, or, U&A questions) seek a general indication or prediction about how a group of people behaves or will behave, how that group is characterized, or how a group thinks. For example, a business might want to know what portion of adult men shave, and how often they do so.
Order in which these are presented. For example, the independent variable before the dependent variable or vice versa. 4. Draft the Complete Research Question. The last step involves identifying the problem or issue that you are trying to address in the form of complete quantitative survey questions.
As briefly introduced in section 4.2.2., a survey was chosen as appropriate data collection method for the quantitative analysis of the model and hypotheses.The decision was made based on the following considerations. Generally, surveys are seen as valuable instrument, commonly used in research practice, when the aim is to collect quantitative data for statistical analysis from a variety of ...
To recap what we've learned: Quantitative data is data that can be quantified. It can be counted or measured, and given a numerical value. Quantitative data lends itself to statistical analysis, while qualitative data is grouped according to themes. Quantitative data can be discrete or continuous.
When conducting qualitative research, scientific researchers raise a question, answer the question by performing a novel study, and propose a new theory to clarify and interpret the obtained results. After which, they should take an inductive approach to writing the formulation of concepts based on collected data.
Those mentioned above probability sampling, interviews, questionnaire observation, and document review are the most common and widely used methods for data collection. With QuestionPro, you can precise results, and data analysis. QuestionPro provides the opportunity to collect data from a large number of participants.
According to Mohajan (2020), quantitative research may be described as a method of data collection that involves administering surveys to a selected group of participants, who provide self ...
Research data can be placed into two broad categories: quantitative or qualitative. Quantitative data are used when a researcher is trying to quantify a problem, or address the "what" or "how many" aspects of a research question.It is data that can either be counted or compared on a numeric scale. For example, it could be the number of first year students at Macalester, or the ratings on a ...
A research questionnaire can be defined as a data collection tool consisting of a series of questions or items that are used to collect information from respondents and thus learn about their knowledge, opinions, attitudes, beliefs, and behavior and informed by a positivist philosophy of the natural sciences that consider methods mainly as a ...
Revised on June 21, 2023. Data collection is a systematic process of gathering observations or measurements. Whether you are performing research for business, governmental or academic purposes, data collection allows you to gain first-hand knowledge and original insights into your research problem. While methods and aims may differ between ...
A well-defined quantitative research question gathers statistical data that allows you to perform statistical analysis. This allows you to identify patterns, correlations, trends, and differences within the data, thus providing meaningful insights.
It's a research method that gathers and analyzes numerical data. Its purpose is to answer research questions, or test hypotheses. ... Data Collection Tools in Quantitative Research. Data collection tools are basically instruments that are used to collect data in a standardized and consistent way. So different methods of quantitative data ...
2. To Create A Better User Experience. We use qualitative data to create a better UX for Web design projects. Through user testing, interviews and usability studies, we gain insights into user ...