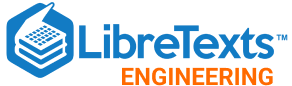
- school Campus Bookshelves
- menu_book Bookshelves
- perm_media Learning Objects
- login Login
- how_to_reg Request Instructor Account
- hub Instructor Commons
- Download Page (PDF)
- Download Full Book (PDF)
- Periodic Table
- Physics Constants
- Scientific Calculator
- Reference & Cite
- Tools expand_more
- Readability
selected template will load here
This action is not available.
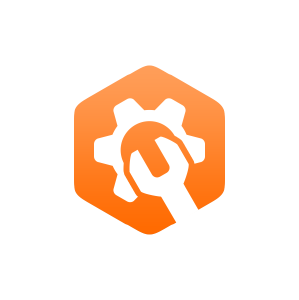
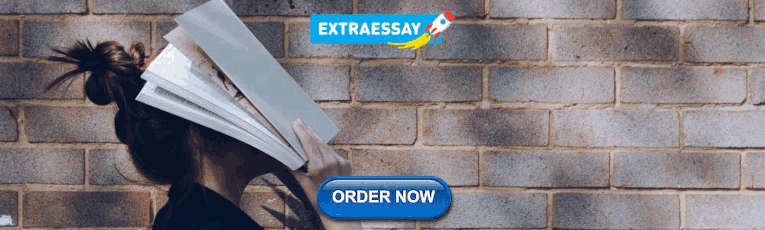
3.6: 3-6 Route Choice
- Last updated
- Save as PDF
- Page ID 48085
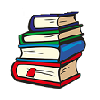
- David Levinson et al.
- Associate Professor (Engineering) via Wikipedia
Route assignment , route choice , or traffic assignment concerns the selection of routes (alternative called paths) between origins and destinations in transportation networks. It is the fourth step in the conventional transportation forecasting model, following Trip Generation, Destination Choice, and Mode Choice. The zonal interchange analysis of trip distribution provides origin-destination trip tables. Mode choice analysis tells which travelers will use which mode. To determine facility needs and costs and benefits, we need to know the number of travelers on each route and link of the network (a route is simply a chain of links between an origin and destination). We need to undertake traffic (or trip) assignment. Suppose there is a network of highways and transit systems and a proposed addition. We first want to know the present pattern of travel times and flows and then what would happen if the addition were made.
Link Performance Function
The cost that a driver imposes on others is called the marginal cost. However, when making decisions, a driver only faces his own cost (the average cost) and ignores any costs imposed on others (the marginal cost).
- \[AverageCost=\dfrac{S_T}{Q}\]
- \[MarginalCost=\dfrac{\delta S_T}{\delta Q}\]
where \(S_T\) is the total cost, and \(Q\) is the flow.
BPR Link Performance Function
Suppose we are considering a highway network. For each link there is a function stating the relationship between resistance and volume of traffic. The Bureau of Public Roads (BPR) developed a link (arc) congestion (or volume-delay, or link performance) function, which we will term S a (Q a )
\[S_a(Q_a)=t_a(1+0.15\dfrac ({Q_a}{c_a})^4)\]
t a = free-flow travel time on link a per unit of time
Q a = flow (or volume) of traffic on link a per unit of time (somewhat more accurately: flow attempting to use link a )
c a = capacity of link a per unit of time
S a (Q a ) is the average travel time for a vehicle on link a
There are other congestion functions. The CATS has long used a function different from that used by the BPR, but there seems to be little difference between results when the CATS and BPR functions are compared.
Can Flow Exceed Capacity?
On a link, the capacity is thought of as “outflow.” Demand is inflow.
If inflow > outflow for a period of time, there is queueing (and delay).
For Example, for a 1 hour period, if 2100 cars arrive and 2000 depart, 100 are still there. The link performance function tries to represent that phenomenon in a simple way.
Wardrop's Principles of Equilibrium
User Equilibrium
Each user acts to minimize his/her own cost, subject to every other user doing the same. Travel times are equal on all used routes and lower than on any unused route.
- System optimal
Each user acts to minimize the total travel time on the system.
Price of Anarchy
The reason we have congestion is that people are selfish. The cost of that selfishness (when people behave according to their own interest rather than society's) is the price of anarchy .
The ratio of system-wide travel time under User Equilibrium and System Optimal conditions.
For a two-link network with linear link performance functions (latency functions), Price of Anarchy is < 4/3.
Is this too much? Should something be done, or is 33% waste acceptable? [The loss may be larger/smaller in other cases, under different assumptions, etc.]
Conservation of Flow
An important factor in road assignment is the conservation of flow. This means that the number of vehicles entering the intersection (link segment) equals the number of vehicles exiting the intersection for a given period of time (except for sources and sinks).
Similarly, the number of vehicles entering the back of the link equals the number exiting the front (over a long period of time).
Auto assignment
Long-standing techniques.
The above examples are adequate for a problem of two links, however real networks are much more complicated. The problem of estimating how many users are on each route is long standing. Planners started looking hard at it as freeways and expressways (motorways) began to be developed. The freeway offered a superior level of service over the local street system and diverted traffic from the local system. At first, diversion was the technique. Ratios of travel time were used, tempered by considerations of costs, comfort, and level of service.
The Chicago Area Transportation Study (CATS) researchers developed diversion curves for freeways versus local streets. There was much work in California also, for California had early experiences with freeway planning. In addition to work of a diversion sort, the CATS attacked some technical problems that arise when one works with complex networks. One result was the Moore algorithm for finding shortest paths on networks.
The issue the diversion approach didn’t handle was the feedback from the quantity of traffic on links and routes. If a lot of vehicles try to use a facility, the facility becomes congested and travel time increases. Absent some way to consider feedback, early planning studies (actually, most in the period 1960-1975) ignored feedback. They used the Moore algorithm to determine shortest paths and assigned all traffic to shortest paths. That’s called all or nothing assignment because either all of the traffic from i to j moves along a route or it does not.
The all-or-nothing or shortest path assignment is not trivial from a technical-computational view. Each traffic zone is connected to n - 1 zones, so there are numerous paths to be considered. In addition, we are ultimately interested in traffic on links. A link may be a part of several paths, and traffic along paths has to be summed link by link.
An argument can be made favoring the all-or-nothing approach. It goes this way: The planning study is to support investments so that a good level of service is available on all links. Using the travel times associated with the planned level of service, calculations indicate how traffic will flow once improvements are in place. Knowing the quantities of traffic on links, the capacity to be supplied to meet the desired level of service can be calculated.
Heuristic procedures
To take account of the affect of traffic loading on travel times and traffic equilibria, several heuristic calculation procedures were developed. One heuristic proceeds incrementally. The traffic to be assigned is divided into parts (usually 4). Assign the first part of the traffic. Compute new travel times and assign the next part of the traffic. The last step is repeated until all the traffic is assigned. The CATS used a variation on this; it assigned row by row in the O-D table.
The heuristic included in the FHWA collection of computer programs proceeds another way.
- Step 0: Start by loading all traffic using an all or nothing procedure.
- Step 1: Compute the resulting travel times and reassign traffic.
- Step 2: Now, begin to reassign using weights. Compute the weighted travel times in the previous two loadings and use those for the next assignment. The latest iteration gets a weight of 0.25 and the previous gets a weight of 0.75.
- Step 3. Continue.
These procedures seem to work “pretty well,” but they are not exact.
Frank-Wolfe algorithm
Dafermos (1968) applied the Frank-Wolfe algorithm (1956, Florian 1976), which can be used to deal with the traffic equilibrium problem.
Equilibrium Assignment
To assign traffic to paths and links we have to have rules, and there are the well-known Wardrop equilibrium (1952) conditions. The essence of these is that travelers will strive to find the shortest (least resistance) path from origin to destination, and network equilibrium occurs when no traveler can decrease travel effort by shifting to a new path. These are termed user optimal conditions, for no user will gain from changing travel paths once the system is in equilibrium.
The user optimum equilibrium can be found by solving the following nonlinear programming problem
\[min \displaystyle \sum_{a} \displaystyle\int\limits_{0}^{v_a}S_a(Q_a)\, dx\]
subject to:
\[Q_a=\displaystyle\sum_{i}\displaystyle\sum_{j}\displaystyle\sum_{r}\alpha_{ij}^{ar}Q_{ij}^r\]
\[sum_{r}Q_{ij}^r=Q_{ij}\]
\[Q_a\ge 0, Q_{ij}^r\ge 0\]
where \(Q_{ij}^r\) is the number of vehicles on path r from origin i to destination j . So constraint (2) says that all travel must take place: i = 1 ... n; j = 1 ... n
\(\alpha_{ij}^{ar}\)= 1 if link a is on path r from i to j ; zero otherwise.
So constraint (1) sums traffic on each link. There is a constraint for each link on the network. Constraint (3) assures no negative traffic.
Transit assignment
There are also methods that have been developed to assign passengers to transit vehicles. In an effort to increase the accuracy of transit assignment estimates, a number of assumptions are generally made. Examples of these include the following:
- All transit trips are run on a set and predefined schedule that is known or readily available to the users.
- There is a fixed capacity associated with the transit service (car/trolley/bus capacity).

Solve for the flows on Links a and b in the Simple Network of two parallel links just shown if the link performance function on link a :
\(S_a=5+2*Q_a\)
and the function on link b :
\(S_b=10+Q_b\)
where total flow between the origin and destination is 1000 trips.
Time (Cost) is equal on all used routes so \(S_a=S_b\)
And we have Conservation of flow so, \(Q_a+Q_b=Q_o=Q_d=1000\)
\(5+2*(1000-Q_b)=10+Q_b\)
\(1995=3Q_b\)
\(Q_b=665;Q_a=335\)
An example from Eash, Janson, and Boyce (1979) will illustrate the solution to the nonlinear program problem. There are two links from node 1 to node 2, and there is a resistance function for each link (see Figure 1). Areas under the curves in Figure 2 correspond to the integration from 0 to a in equation 1, they sum to 220,674. Note that the function for link b is plotted in the reverse direction.
\(S_a=15(1+0.15(\dfrac{Q_a}{1000})^4)\)
\(S_b=20(1+0.15(\dfrac{Q_a}{3000})^4)\)
\(Q_a+Q_b=8000\)
Show graphically the equilibrium result.

At equilibrium there are 2,152 vehicles on link a and 5,847 on link b . Travel time is the same on each route: about 63.
Figure 3 illustrates an allocation of vehicles that is not consistent with the equilibrium solution. The curves are unchanged, but with the new allocation of vehicles to routes the shaded area has to be included in the solution, so the Figure 3 solution is larger than the solution in Figure 2 by the area of the shaded area.
Assume the traffic flow from Milwaukee to Chicago, is 15000 vehicles per hour. The flow is divided between two parallel facilities, a freeway and an arterial. Flow on the freeway is denoted \(Q_f\), and flow on the two-lane arterial is denoted \(Q_a\).
The travel time (in minutes) on the freeway (\(C_f\)) is given by:
\(C_f=10+Q_f/1500\)
\(C_a=15+Q_a/1000\)
Apply Wardrop's User Equilibrium Principle, and determine the flow and travel time on both routes.
The travel times are set equal to one another
\(C_f=C_a\)
\(10+Q_f/1500=15+Q_a/1000\)
The total traffic flow is equal to 15000
\(Q_f+Q_a=15000\)
\(Q_a=15000-Q_f\)
\(10+Q_f/1500=15+(15000-Q_f)/1000\)
Solve for \(Q_f\)
\(Q_f=60000/5=12000\)
\(Q_a=15000-Q_f=3000\)
Thought Questions
- How can we get drivers to consider their marginal cost?
- Alternatively: How can we get drivers to behave in a “System Optimal” way?
Sample Problems
Given a flow of six (6) units from origin “o” to destination “r”. Flow on each route ab is designated with Qab in the Time Function. Apply Wardrop's Network Equilibrium Principle (Users Equalize Travel Times on all used routes)
A. What is the flow and travel time on each link? (complete the table below) for Network A
Link Attributes
B. What is the system optimal assignment?
C. What is the Price of Anarchy?
What is the flow and travel time on each link? Complete the table below for Network A:
These four links are really 2 links O-P-R and O-Q-R, because by conservation of flow Qop = Qpr and Qoq = Qqr.
By Wardrop's Equilibrium Principle, the travel time (cost) on each used route must be equal. Therefore \(C_{opr}=C_{oqr}\)
OR \(25+6*Q_{opr}=20+7*Q_{oqr}\)
\(5+6*Q_{opr}=7*Q_{oqr}\)
\(Q_{oqr}=5/7+6*Q_{opr}/7\)
By the conservation of flow principle
\(Q_{oqr}+Q_{opr}=6\)
\(Q_{opr}=6-Q_{oqr}\)
By substitution
\Q_{oqr}=5/7+6/7(6-Q_{oqr})=41/7-6*Q_{oqr}/7\)
\(13*Q_{oqr}=41\)
\(Q_{oqr}=41/13=3.15\)
\(Q_{opr}=2.84\)
\(42.01=25+6(2.84)\)
\(42.05=20+7(3.15)\)
Check (within rounding error)
or expanding back to the original table:
User Equilibrium: Total Delay = 42.01 * 6 = 252.06
What is the system optimal assignment?
Conservation of Flow:
\(Q_{opr}+Q_{oqr}=6\)
\(TotalDelay=Q_{opr}(25+6*Q_{oqr})+Q_{oqr}(20+7*Q_{oqr})\)
\(25Q_{opr}+6Q_{opr}^2+(6_Q_{opr})(20+7(6-Q_{opr}))\)
\(25Q_{opr}+6Q_{opr}^2+(6_Q_{opr})(62-7Q_{opr}))\)
\(25Q_{opr}+6Q_{opr}^2+372-62Q_{opr}-42Q_{opr}+7Q_{opr}^2\)
\(13Q_{opr}^2-79Q_{opr}+372\)
Analytic Solution requires minimizing total delay
\(\deltaC/\deltaQ=26Q_{opr}-79=0\)
\(Q_{opr}=79/26-3.04\)
\(Q_{oqr}=6-Q_{opr}=2.96\)
And we can compute the SO travel times on each path
\(C_{opr,SO}=25+6*3.04=43.24\)
\(C_{opr,SO}=20+7*2.96=40.72\)
Note that unlike the UE solution, \(C_{opr,SO}\g C_{oqr,SO}\)
Total Delay = 3.04(25+ 6*3.04) + 2.96(20+7*2.96) = 131.45+120.53= 251.98
Note: one could also use software such as a "Solver" algorithm to find this solution.
What is the Price of Anarchy?
User Equilibrium: Total Delay =252.06 System Optimal: Total Delay = 251.98
Price of Anarchy = 252.06/251.98 = 1.0003 < 4/3
The Marcytown - Rivertown corridor was served by 3 bridges, according to the attached map. The bridge over the River on the route directly connecting Marcytown and Citytown collapsed, leaving two alternatives, via Donkeytown and a direct. Assume the travel time functions Cij in minutes, Qij in vehicles/hour, on the five links routes are as given.
Marcytown - Rivertown Cmr = 5 + Qmr/1000
Marcytown - Citytown (prior to collapse) Cmc = 5 + Qmc/1000
Marcytown - Citytown (after collapse) Cmr = ∞
Citytown - Rivertown Ccr = 1 + Qcr/500
Marcytown - Donkeytown Cmd = 7 + Qmd/500
Donkeytown - Rivertown Cdr = 9 + Qdr/1000
Also assume there are 10000 vehicles per hour that want to make the trip. If travelers behave according to Wardrops user equilibrium principle.
A) Prior to the collapse, how many vehicles used each route?
Route A (Marcytown-Rivertown) = Ca = 5 + Qa/1000
Route B (Marcytown-Citytown-Rivertown) = Cb = 5 + Qb/1000 + 1 + Qb/500 = 6 + 3Qb/1000
Route C (Marcytown-Donkeytown-Rivertown)= Cc = 7 + Qc/500 + 9 + Qc/1000 = 16 + 3Qc/1000
At equilibrium the travel time on all three used routes will be the same: Ca = Cb = Cc
We also know that Qa + Qb + Qc = 10000
Solving the above set of equations will provide the following results:
Qa = 8467;Qb = 2267;Qc = −867
We know that flow cannot be negative. By looking at the travel time equations we can see a pattern.
Even with a flow of 0 vehicles the travel time on route C(16 minutes) is higher than A or B. This indicates that vehicles will choose route A or B and we can ignore Route C.
Solving the following equations:
Route A (Marcytown-Rivertown) = Ca = 5 + Qa /1000
Route B (Marcytown-Citytown-Rivertown) = Cb = 6 + 3Qb /1000
Qa + Qb = 10000
We can the following values:
Qa = 7750; Qb = 2250; Qc = 0
B) After the collapse, how many vehicles used each route?
We now have only two routes, route A and C since Route B is no longer possible. We could solve the following equations:
Route C (Marcytown- Donkeytown-Rivertown) = Cc = 16 + 3Qc /1000
Qa+ Qc= 10000
But we know from above table that Route C is going to be more expensive in terms of travel time even with zero vehicles using that route. We can therefore assume that Route A is the only option and allocate all the 10,000 vehicles to Route A.
If we actually solve the problem using the above set of equations, you will get the following results:
Qa = 10250; Qc = -250
which again indicates that route C is not an option since flow cannot be negative.
C) After the collapse, public officials want to reduce inefficiencies in the system, how many vehicles would have to be shifted between routes? What is the “price of anarchy” in this case?
TotalDelayUE =(15)(10,000)=150,000
System Optimal
TotalDelaySO =(Qa)(5+Qa/1000)+(Qc)(16+3Qc/1000)
Using Qa + Qc = 10,000
TotalDelaySO =(Qa2)/250−71Qa+460000
Minimize total delay ∂((Qa2)/250 − 71Qa + 460000)/∂Qa = 0
Qa/125−7 → Qa = 8875 Qc = 1125 Ca = 13,875 Cc = 19,375
TotalDelaySO =144938
Price of Anarchy = 150,000/144,938 = 1.035
- \(C_T\) - total cost
- \(C_k\) - travel cost on link \(k\)
- \(Q_k\) - flow (volume) on link \(k\)
Abbreviations
- VDF - Volume Delay Function
- LPF - Link Performance Function
- BPR - Bureau of Public Roads
- UE - User Equilbrium
- SO - System Optimal
- DTA - Dynamic Traffic Assignment
- DUE - Deterministic User Equilibrium
- SUE - Stochastic User Equilibrium
- AC - Average Cost
- MC - Marginal Cost
- Route assignment, route choice, auto assignment
- Volume-delay function, link performance function
- User equilibrium
- Conservation of flow
- Average cost
- Marginal cost
External Exercises
Use the ADAM software at the STREET website and try Assignment #3 to learn how changes in network characteristics impact route choice.
Additional Questions
1. If trip distribution depends on travel times, and travel times depend on the trip table (resulting from trip distribution) that is assigned to the road network, how do we solve this problem (conceptually)?
2. Do drivers behave in a system optimal or a user optimal way? How can you get them to move from one to the other.
3. Identify a mechanism that can ensure the system optimal outcome is achieved in route assignment, rather than the user equilibrium. Why would we want such an outcome? What are the drawbacks to the mechanism you identified?
4. Assume the flow from Dakotopolis to New Fargo, is 5300 vehicles per hour. The flow is divided between two parallel facilities, a freeway and an arterial. Flow on the freeway is denoted \(Q_f\), and flow on the two-lane arterial is denoted \(Q_r\). The travel time on the freeway \(C_f\) is given by:
\(C_f=5+Q_f/1000\)
The travel time on the arterial (Cr) is given by
\(C_r=7+Q_r/500\)
(a) Apply Wardrop's User Equilibrium Principle, and determine the flow and travel time on both routes from Dakotopolis to New Fargo.
(b) Solve for the System Optimal Solution and determine the flow and travel time on both routes.
5. Given a flow of 10,000 vehicles from origin to destination traveling on three parallel routes. Flow on each route A, B, or C is designated with \(Q_a\), \(Q_b\), \(Q_c\) in the Time Function Respectively. Apply Wardrop's Network Equilibrium Principle (Users Equalize Travel Times on all used routes), and determine the flow on each route.
\(T_A=500+20Q_A\)
\(T_B=1000+10Q_B\)
\(T_C=2000+30Q_C\)
- How does average cost differ from marginal cost?
- How do System Optimal and User Equilibrium travel time differ?
- Why do we want people to behave in an SO way?
- How can you get people to behave in an SO way?
- Who was John Glen Wardrop?
- What are Wardrop’s Two Principles?
- What does conservation of flow require in route assignment?
- Can Variable Message Signs be used to encourage System Optimal behavior?
- What is freeflow travel time?
- If a problem has more than two routes, where does the extra equation come from?
- How can you determine if a route is unused?
- What is the difference between capacity and flow
- Draw a typical volume-delay function for a deterministic, static user equilibrium assignment.
- Can Q be negative?
- What is route assignment?
- Is it important that the output travel times from route choice be consistent with the input travel times for destination choice and mode choice? Why?
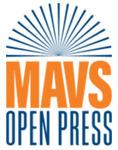
Want to create or adapt books like this? Learn more about how Pressbooks supports open publishing practices.
Part III: Travel Demand Modeling
13 Chapter 13: Last Step of Four Step Modeling (Trip Assignment Models)
Chapter 13 is the last chapter of the book unpacking the last step of four-step travel demand modeling, i.e., trip assignment. This step determines which paths travelers choose for moving between each pair of zones. Additionally, this step can yield numerous results such as traffic volumes in different transportation corridors, the patterns of vehicular movements, total VMTs and VTTs in the network, and zone-to-zone travel costs. The identification of the heavily congested links is crucial for transportation planning and engineering practitioners. This chapter begins with some fundamental concepts, such as the link cost functions. Next, it presents some common and useful trip assignment methods with relevant examples. The methods covered in this chapter include all-or-nothing (AON), user equilibrium (UE), system optimum (SO), feedback loop between distribution and assignment (LDA), incremental increase assignment, capacity restrained assignment, and stochastic user equilibrium assignment.
Learning Objectives
Student Learning Outcomes
- Describe the reasons for performing trip assignment models in FSM and relate these models’ foundation through the cost-function concept.
- Compare static and dynamic trip assignment models and infer the appropriateness of each model for different situations.
- Explain Wardrop principles and relate them to traffic assignment algorithms.
- Complete simple network traffic assignment models using static models such as the all-or-nothing and user equilibrium models.
- Solve modal split analyses manually for small samples using the discrete choice modeling framework and multinominal logit models.
Prep/quiz/assessments
- Explain what the link performance function is in trip assignment models and how it is related to link capacity.
- Name a few static and dynamic traffic assignment models and discuss how different their rules or algorithms are.
- How does stochastic decision-making on route choice affect the transportation level of service, and how it is incorporated into traffic assignment problems?
- Name one extension of the all-or-nothing assignment model and explain how this extension improves the model results.
13.1 Introduction
in this chapter, we continue the discussion about FSM and elaborate on different methods of traffic assignment, which is the last step in the FSM model after trip generation, trip distribution, and modal split. The traffic assignment step, which is also called route assignment or route choice , simulates the choice of route selection from a set of alternatives between origin zone and the destination zone (Levinson et al., 2014). After the first three steps, we know the number of trips produced between each pair of zones and what portion of these trips are completed by different transportation modes. As the final step, we would be also interested in determining what routes or links within our study areas will likely be used. For instance, in a Regional Transportation Plan (RTP), we would be interested in determining how much shift or diversion in daily traffic happens if we introduce an additional transit line or extent a highway corridor (Levinson et al., 2014). Similar to trip distribution, the impedance function has an important role in route choice for travelers. Normally, the impedance function is related to travel cost or travel time. The longer the trip or the higher the cost, the larger the impedance for the trip along that path (Wang & Hofe, 2008).
The output from the last step of the FSM model can provide modelers with numerous valuable results. Such results can yield the planner an insight of good and bad characteristics of various preconceived plans. The results of trip assignment analysis can be:
- The traffic flows in the transportation system andthe pattern of vehicular movements
- Volume of traffic on network links
- Travel costs between trip origins and destinations
- Aggregated network measures, e.g. total flows of vehicles, total distance travelled by vehicles (VMT) , total vehicle travel time (VTT)
- Zone-to-zone travel costs (travel time) for a given demand level
- Obtaining modeled link flows and highlighting congested corridors
- Analysis of turning movements for future intersection design
- Finding out which O-D pairs have taken a particular link or path
- Simulation of the individual’s choice for each pair of origins and destinations (Mathew & Rao, 2006)
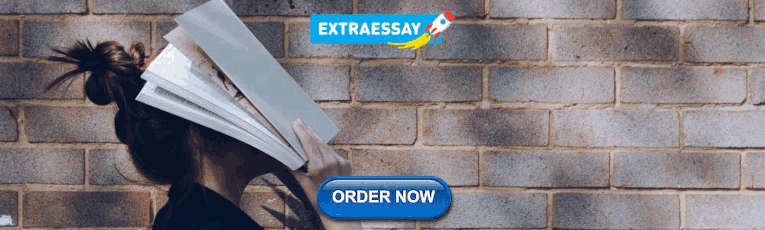
13.2 Link Performance Function
One of the most important and fundamental concepts of the traffic assignment process is to build a link performance function . This function is usually used for estimating travel time, travel cost, and speed on the network based on the relationship between speed and travel flow. While this function can take different forms such as linear, polynomial , exponential , and hyperbolic , there are different equations that represent the above-mentioned relationship. One of the most common functions is the link cost function that represents generalized travel costs (United States Bureau of Public Roads, 1964). This equation estimates travel time on a free-flow road (travel with speed limit) adding a function that increases travel time exponentially as the road gets more congested. The congestion can be represented by the road volume to capacity ratio (Meyer, 2016). However, throughout recent years transportation planners have realized that in many cases, the delay on the links is caused by delays on the intersection, an observation that is not encompassed by the link cost function. Nevertheless, in the following sections we will resort to the traditional function. Equation (1) is the most common and general formula for link performance function.
![Rendered by QuickLaTeX.com t=t_o[1+\alpha\left(\frac{x}{k}\right)\beta]](https://uta.pressbooks.pub/app/uploads/quicklatex/quicklatex.com-b0069e9cc96ab2ee3dd2a513568bed91_l3.png)
- t and x are the travel time and vehicle flow;
- t 0 is the link free flow travel time;
- k is the link capacity;
- α and β are parameters for specific type of links and calibrated using the field data. In the absence of any field data, it is usually assumed = 0.15, and β= 4.0.
α and β are the coefficient for this formula and can take different values (model parameters). However, most studies and planning practices use the same value for them. That said, these values can be locally calibrated for the most efficient results. Also, Figure 13.1 shows the relationship between capacity and travel time. In this plot, the travel time remains constant by increase in vehicle volumes until the turning point which indicates the volume on the link is beginning to exceed the capacity.
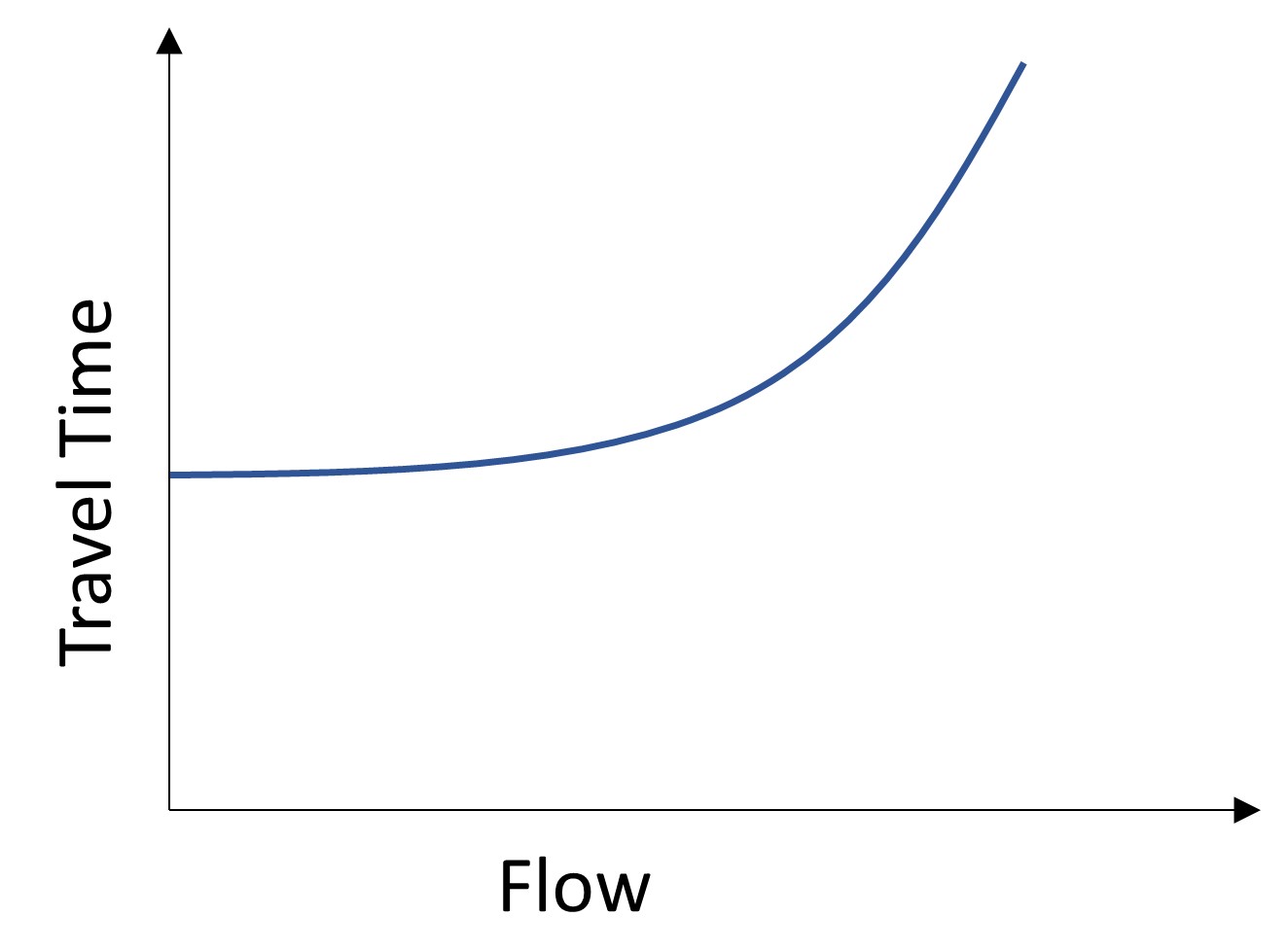
Figure 13.1 Link Flow and Travel Time Relationship
The following example shows how the link performance function helps us to determine the travel time according to flow and capacity.
13.2.1 Example 1
Assume the traffic volume on a path between zone i and j was 525. The travel time recorded on this path is 15 minutes. If the capacity of this path would be 550, then calculate the new travel time for future iteration of the model.
Based on the link performance function, we have:
Now we have to plug in the numbers into the formula to determine the new travel time:
![Rendered by QuickLaTeX.com t=15[1+\0.15\left(\frac{525}{550}\right)\4]=16.86](https://uta.pressbooks.pub/app/uploads/quicklatex/quicklatex.com-4815be46eaf8d0307c939444a157a3ea_l3.png)
13.3 Traffic Assignment Models
Through the rest of this chapter, we are going to discuss different traffic assignment models. In general, the process of traffic assignment is usually done separately for private cars and transit systems. As we specified in previous chapters, the transit impedance function is different from private auto; thus, simulating a utility maximization behavior for a driver and rider should be different. For public transit assignment, variables such as fare, stop or transfer, waiting time, and trip times define the utility (equilibrium) (Sheffi, 1985). However, in some cases the two mentioned networks are related when public buses share highways with cars, and congestion can also affect the performance of public transit (Rojo, 2020). Typically, private car traffic assignment models the path choice of trip makers using:
- algorithms like all-or-nothing
- incremental
- capacity-restrained
- user equilibrium
- system optimum assignment
User equilibrium is based on the principle assumption that travelers try to minimize their travel costs. In this algorithm, the equilibrium occurs when there is no user able to reduce their travel time or cost by changing path. This is the most popular algorithms employed for simulation in the U.S. (Meyer, 2016). Moreover, more recent trip assignment models use approaches such as:
- static user-equilibrium assignment algorithm
- “multiple-time-period assignment for multiple classes (for example, drive-alone, rideshare, and bike/walk)
- an iterative feedback loop mechanism between, at a minimum, the network assignment step and the trip distribution step
- separate specification of facilities like HOV and high-occupancy toll (HOT) lanes
- independent transit assignment using congested highway travel times to estimate a bus ridership assignment” (Meyer, 2016, p.226).
13.3.1 All-or-nothing Model
Through the all-or-nothing (AON) assignment, we assume that the impedance of a road or path between each origin and destination is constant and is equal to free-flow level of service, meaning that the traffic time is not affected by the traffic flow on the path. The only logic behind this model is that each traveler simply uses the shortest path from his or her origin to the destination and no vehicle is assigned to other paths (Hui, 2014). This method is called the all-or-nothing assignment model and is the simplest one among all assignment models. This method is also called the 0-1 assignment model, and its advantage is its simple procedure and calculation. The assumptions of this method are:
- Congestion does not affect travel time or cost, meaning that no matter how much traffic is loaded on the route, congestion does not take place.
- Since the method assigns one route to any travel between each pair of OD, travelers traveling from particular zone to another particular zone all choose the same route (Hui, 2014).
To run the AON model, the following process can be followed:
- Step 0: Initialization. Use free flow travel costs Ca=Ca(0) , for each link a on the empty network. Ɐ
- Step 1: Path finding. Find the shortest path P for each zonal pair.
- Step 2: Path flows assigning. Assign both passenger trips (hppod) and freight trips (hfpod) in PCEs from zonal o to d to path P.
- Step 3: Link flows computing. Sum the flows on all paths going through a link as total flows of this link.
Example 2 illustrates the above-mentioned process for the AON model.
13.3.2 Example 2
Table 13.1 shows a trip distribution matrix with 4 zones. Using the travel costs between each pair of them shown in Figure 13.2, assign the traffic to the network.
Load the vehicle trips from the trip distribution table shown below using the AON technique. After assigning the traffic, illustrate the links and the traffic volume on each on them.
Table 13.1 Trip Distribution Results
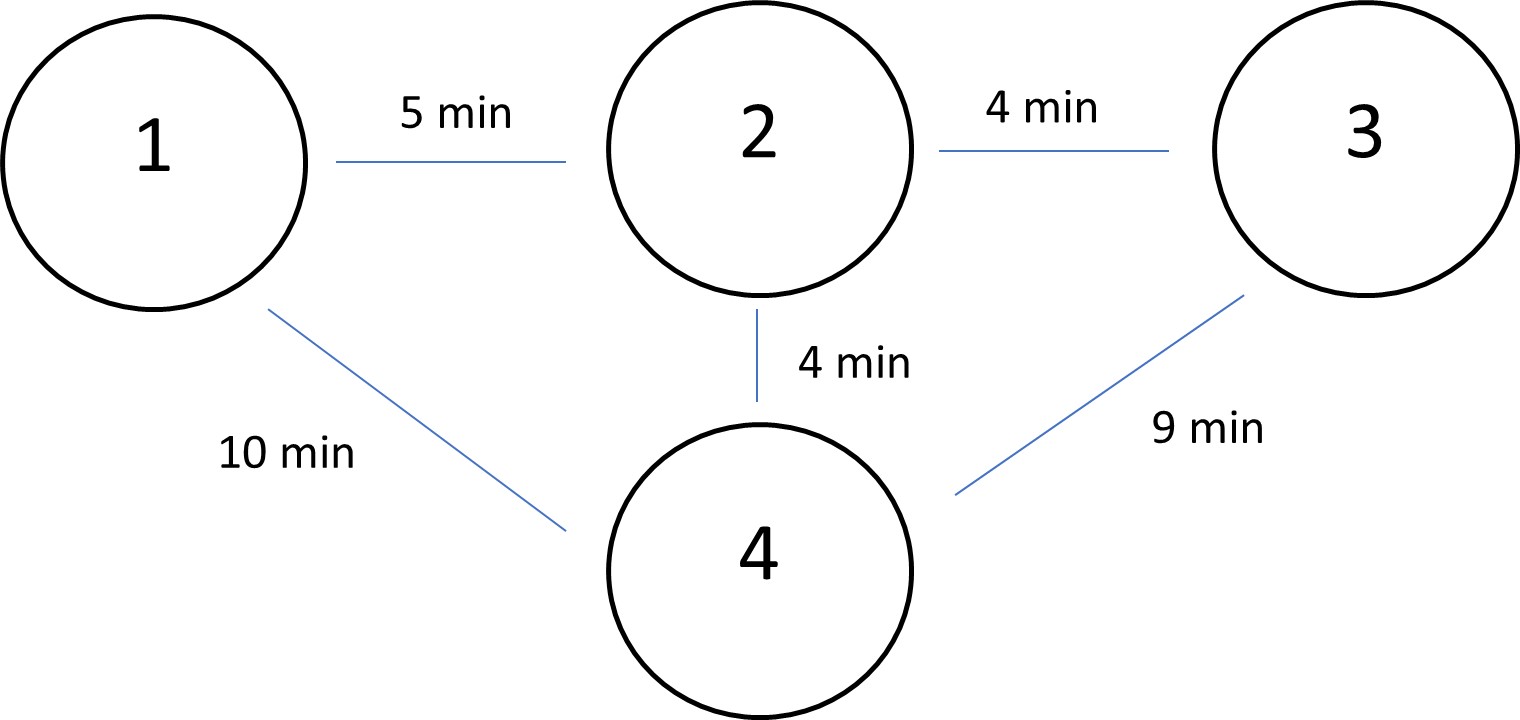
Figure 13.2 Transportation Network
To solve this problem, we need to find the shortest path among all alternatives for each pair of zones. The result of this procedure would be 10 routes in total, each of which bears a specific amount of travels. For instance, the shortest path between zone 1 and 2 is the straight line with 5 min travel time. All other routes like 1 to 4 to 2 or 1 to 4 to 3 to 2 would be empty from travelers going from zone 1 to zone 2. The results are shown in Table 13.2.
Table 13.2 Traffic Volumes for Each Route
As you can see, some of the routes remained unused. This is because in all-or-nothing if a route has longer travel time or higher costs, then it is assumed it would not be used at all.
13.3.4 User Equilibrium
The next method for traffic assignment is called user equilibrium (UE). The rule or algorithm is adopted from the well- known Wardrop equilibrium (19 52) conditions (Correa & Stier-Moses, 2011). In this algorithm, it is assumed that travelers will always choose the shortest path and equilibrium condition would be realized when no traveler is able to decrease their travel impedance by changing paths (Levinson et al., 2014).
As we discussed, the UE method is based on the first principle of Wardrop : “for each origin-destination (OD) pair, with UE, the travel time on all used paths is equal and less than or equally to the travel time that would be experienced by a single vehicle on any unused path”(Jeihani Koohbanani, 2004). The mathematical format of this principle is shown in equation (3):
T 1 =T 2 (2)
For a given OD pair, the UE condition can be expressed in equation (3):

This means that all paths will have the same travel time. Also, for this model we have the following general assumptions:
- The users possess all the knowledge needed about different paths.
- The users have perfect knowledge of the path cost.
- Travel time in a route is subject to change only by the cost flow function of that route.
- Travel times increases as we load travel into the network (Mathew & Rao, 2006).
Hence, the UE assignment comes to an optimization problem that can be formulated using equation (4):

k is the path x a equilibrium flow in link a t a travel time on link a f k rs flow on path connecting OD pairs q rs trip rate between and δ a, k rs is constraint function defined as 1 if link a belongs to path k and 0 otherwise
Example 3 shows how the UE method can be applied for the traffic assignment step. This example is a very simple network consisting of two zones with two possible paths between them.
13.3.5 Example 3
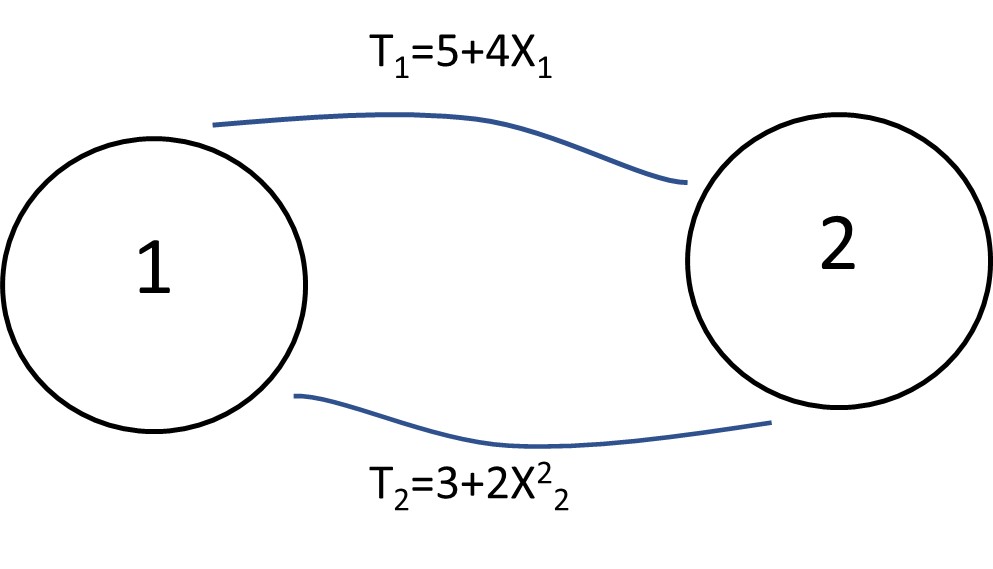
Figure 13.3 A Simple Two-Zone System with Cost Function
In this example, t 1 and t 2 are travel times measured by min on each route, and x 1 and x 2 are traffic flows on each route measured by (Veh/Hour).
Using the UE method, assign 4,500 Veh/Hour to the network and calculate travel time on each route after assignment, traffic volume, and system total travel time.
According to the information provided, total flow (X 1 +X 2 ) is equal to 4,500 (4.5).
First, we need to check, with all traffic assigned to one route, whether that route is still the shortest path. Thus we have:
T 1 (4.5)=23min
T 2 (0)=3min
if all traffic is assigned to route 2:
T 1 (0)=3min
T 2 (4.5)=43.5 min
Step 2: Wardrope equilibrium rule: t 1 =t 2 5+4x 1 =3+ 2x 2 2 and we have x 1 =4.5-x 2
Now the equilibrium equation can be written as: 6 + 4(4.5 − x2)=4+ x222
x 1 = 4.5 − x 2 = 1.58
Now the updated average travel times are: t 1 =5+4(1.58)=11.3min and T 2 =3+2(2.92)2=20.05min
Now the total system travel time is:
Z(x)=X 1 T 1 (X 1 )+X 2 T 2 (X 2 )=2920 veh/hr(11.32)+1585 veh/hr(20.05)=33054+31779=64833 min
13.3.6 System Optimum Assignment
One other traffic assignment model similar to the previous one is called system optimum (SO) in which the second principle of the Wardrop defines the logic of the model. Based on this principle, drivers’ rationale for choosing a path is to minimize total system costs with one another in order to minimize total system travel time (Mathew & Rao, 2006). Using the SO traffic assignment, problems like optimizing departure time for a single commuting route, minimizing total travels from multiple origins to one destination, or minimizing travel time in stochastic time-dependent OD flows from several origins to a single destination can be solved (Jeihani Koohbanani, 2004).
The basic mathematical formula for this model that satisfies the principle of the model is shown in equation (5):

In example 4, we will use the same network we described in the UE example in order to compare the results for the two models.
13.3.7 Example 4
In that simple two-zone network, we had:
T 1 =5+4X 1 T2=3+2X 2 2
Now, based on the principle of the model we have:
Z(x)=x 1 t 1 (x 1 )+x 2 t 2 (x 2 )
Z(x)=x 1 (5+4x 1 )+x 2 (3+2x 2 2 )
Z(x)=5x 1 +4x 1 2 +3x 2 +2x 2 3
From the flow conservation. we have: x 1 +x 2 =4.5 x 1 =4.5-x 2
Z(x)=5(4.5-x 2 )+4(4.5-x 2 )2+4x 2 +x 2 3
Z(x)=x 3 2 +4x 2 2 -27x 2 +103.5
In order to minimize the above equation, we have to take derivatives and equate it to zero. After doing the calculations, we have:
Based on our finding, the system travel time would be:
T 1 =5+4*1.94=12.76min T 2 =3+ 2(2.56)2=10.52 min
And the total travel time of the system would be:
Z(x)=X 1 T 1 (X 1 )+X 2 T 2 (X 2 )=1940 veh/hr(12.76)+2560 veh/hr(10.52)=24754+26931=51685 min
13.3.8 Feedback Loop Model (Combined Traffic Assignment and Trip Distribution)
In the feedback loop model , an interaction between the trip distribution route choice step with several iterations is defined. The essence of this model is that in case a route between an origin and destination gets too congested, then the traveler may replace their destination, for instance choosing between several shopping malls available in a region. In other words, in a real-world situation, travelers usually make decisions about their travel characteristics simultaneously (Qasim, 2012).
The chart below shows how the combination of these two modes can take place:
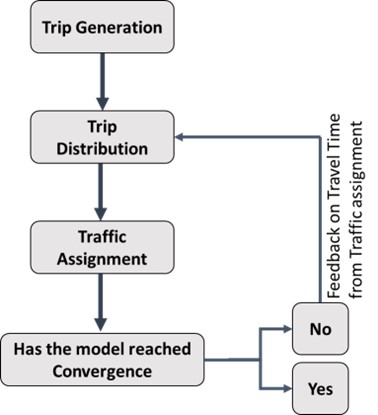
Figure 13.4 The Feedback Loop between the Second and the Fourth Step
Equation (6), shown below for this model, ensures convergence at the end of the model is:

where C a (t) is the same as previous
P a , is total personal trip flows on link a,
f a ; is total freight trip flows on link a,
T od is the total flow from node o to node d,
p od is personal trip from node o to node d,
F od is freight trip from node o to node d,
ζ is a parameter estimated from empirical data,
K is a parameter depending on the type of gravity model used to calculate T od , Evans (1976) proved that K’ equals to 1 for distribution using doubly constrained gravity model and it equals to 1 plus attractiveness for distribution using singly constrained model. Florian et al. (1975) ignored K for distribution using a doubly constrained gravity model because it is a constant.
13.3.9 Incremental Increase model
Another model of traffic assignment we are going to elaborate on here is called incremental increase . In this model, which is based on the logic of the AON model, a process is designed with multiple steps. In each step or level, a fraction of the total traffic volume is assigned, and travel time is calculated based on the allocated traffic volume. Through this incremental addition of traffic, the travel time of each route in step (n) is the updated travel time from the previous step (n-1)(Rojo, 2020).
The steps for the incremental increase traffic assignment model are:
- Finding the shortest path between each pair of O-Ds
- Assigning a portion of the trips according to the matrix (usually 40, 30, 20 and 10 percent to the shortest path)
- Updating the travel time after each iteration (each incremental increase)
- Continuing until all trips are assigned
- Summing the results
Example 4 illustrates the process of this method’s implementation.
13.3.10 Example 4
A hypothetical network accommodates two zones with three possible links between them. Perform an incremental increase traffic assignment model for assigning 200 trips between the two zones with increments of: 30%, 30%, 20%, 20%. (The capacity is 50 trips.)
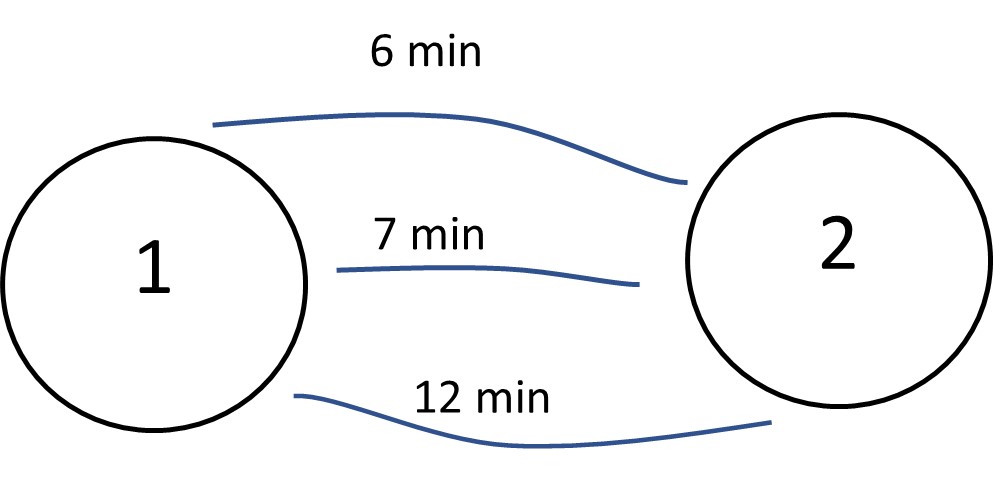
Figure 13.5 A Two-Zone Network with Three Possible Routes
Step 1 (first iteration): Using the method of AON, we now assign the flow to the network using the function below:
![Rendered by QuickLaTeX.com t=to[1+\alpha\left(\frac{x}{k}\right)\beta]](https://uta.pressbooks.pub/app/uploads/quicklatex/quicklatex.com-e6ae32391e4ce2864e22b32f4ce8036d_l3.png)
Since the first route has the shortest travel time, the first 30% of the trips will be assigned to route 1. The updated travel time for this path would be:
![Rendered by QuickLaTeX.com t=6\left[1+0.15\left(\frac{60}{50}\right)4\right]=7.86](https://uta.pressbooks.pub/app/uploads/quicklatex/quicklatex.com-0efdc05f3641753417dd22056d786d27_l3.png)
And the remaining route will be empty, and thus their travel times are unchanged.
Step 2 (second iteration): Now, we can see that the second route has the shortest travel time, with 30% of the trips being assigned to this route, and the new travel time would be:
![Rendered by QuickLaTeX.com t=7\left[1+0.15\left(\frac{60}{50}\right)4\right]=9.17](https://uta.pressbooks.pub/app/uploads/quicklatex/quicklatex.com-ee2cb7dd6e67408c0fd206917565f183_l3.png)
Step 3 (third iteration): In the third step, the 20% of the remaining trips will be assigned to the shortest path, which in this case is the first route again. The updated travel time for this route is:
![Rendered by QuickLaTeX.com t=7.86\left[1+0.15\left(\frac{40}{50}\right)4\right]=8.34](https://uta.pressbooks.pub/app/uploads/quicklatex/quicklatex.com-edaab16adde9967db55782f11d92a0e6_l3.png)
Step 4 (fourth iteration): In the last iteration, the remaining 10% would be assigned to first route, and the time is:
![Rendered by QuickLaTeX.com t=8.34\left[1+0.15\left(\frac{40}{50}\right)4\right]=8.85](https://uta.pressbooks.pub/app/uploads/quicklatex/quicklatex.com-95a27b24b4ec1169c1b611960683dbfe_l3.png)
Finally, we can see that route 1 has a total of 140 trips with a 8.85 travel time, the second route has a total of 60 trips with a 9.17 travel time, and the third route was never used.
13.3.11 Capacity Restraint Assignment
Thus far, in all the algorithms or rules presented, the capacity of the link was incorporated into the model, and travel time was the only factor for assigning the flow to a link. In this model, after each iteration, the total number of trips are compared with the capacity to observe how much increase in travel time was realized by the added volume. In this model, the iteration stops if the added volume in step (n) does not change the travel time updated in step (n-1). With the incorporation of such a constraint, the cost or performance function would be different from cost functions discussed in previous algorithms (Mathew & Rao, 2006). Figure 13.6 visualizes the relationship between flow and travel time with a capacity constraint.
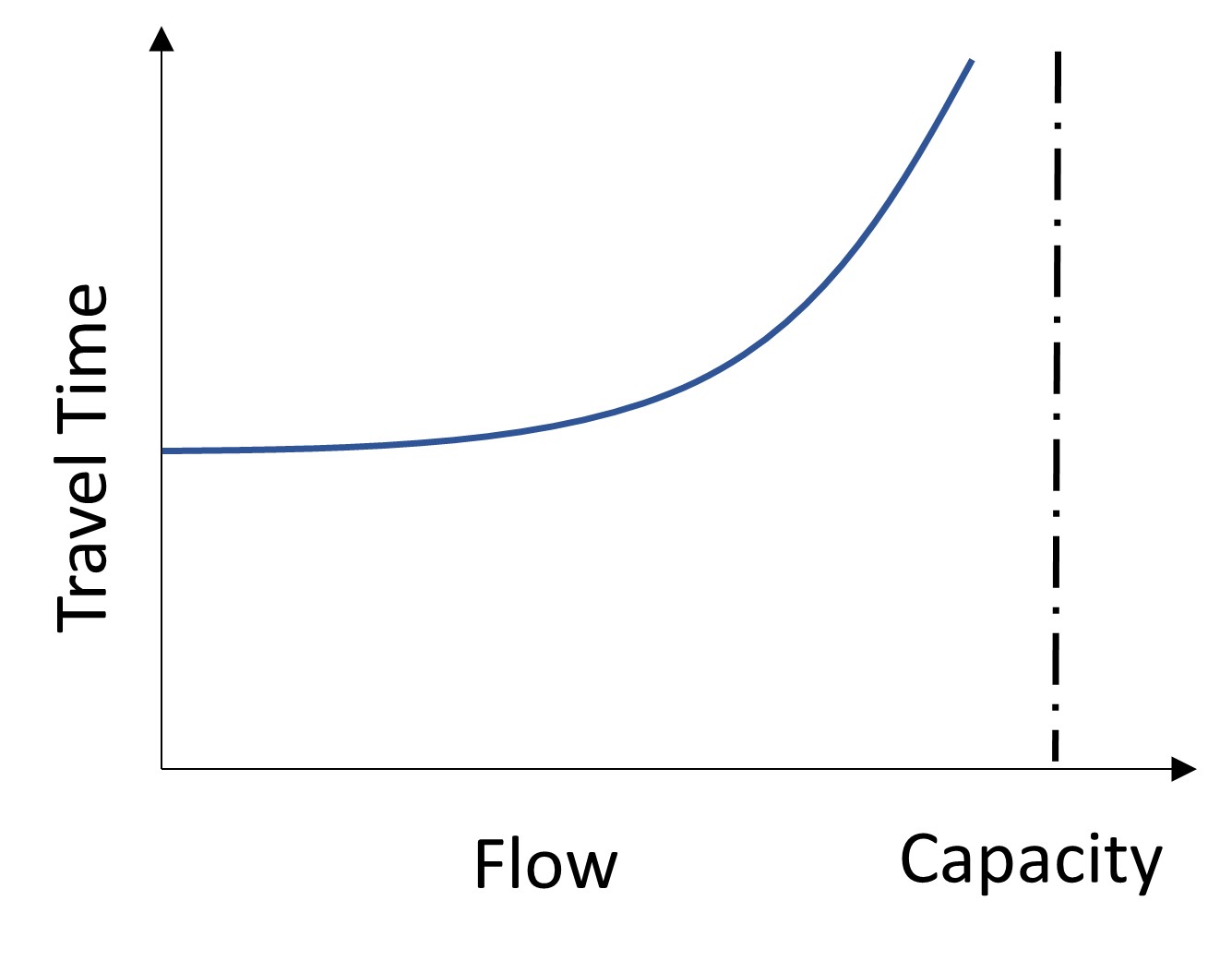
Figure 13.6 Link Flow and Travel Time Relationship
Based on this capacity constraint specific to each link, the α, β can be readjusted for different links such as highways, freeways, and other roads.
13.3.12 Stochastic User Equilibrium Traffic Assignment
Stochastic user equilibrium traffic assignment is a sophisticated and more realistic model, in which the level of uncertainty in regard to which link should be used based on a measurement of utility function is introduced. This model performs a discrete choice analysis through a logistic model. In this model, it is assumed that based on the first Wardrop principle, all drivers perceive the costs of traveling in each link identically and choose the route with minimum cost. In stochastic UE, however, the model allows different individuals to have different perceptions about the costs, and thus, they may choose non-minimum cost routes as well( Mathew & Rao, 2006). In this model, the flow is assigned to almost all links from the beginning (unlike previous models) which is closer to reality. The probability of using each path is calculated with the following logit formula shown in equation (7):
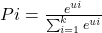
P i is the probability of using path i
U i is the utility function for path i
In the following, an example of a simple network is presented.
13.3.13 Example 6
There is a flow of 200 trips between two points and their possible path, each of which has a travel time specified in Figure 13.7.
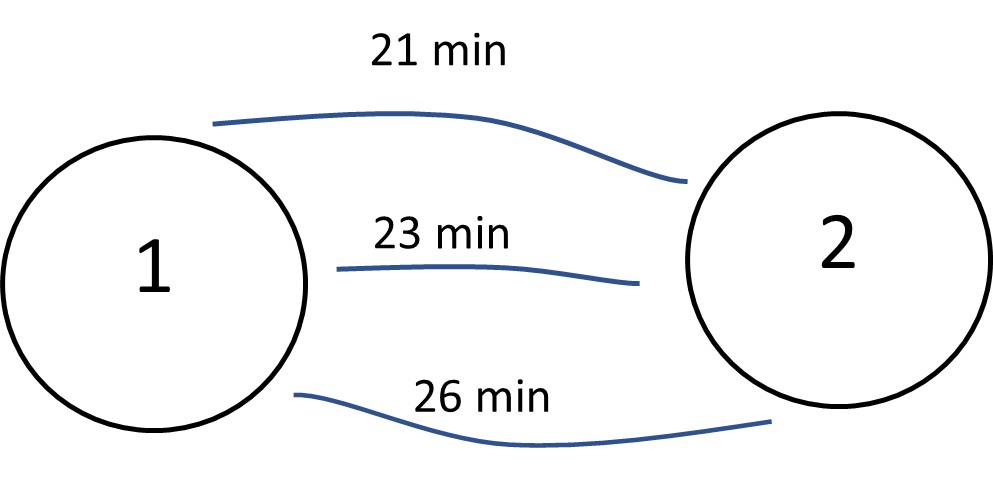
Figure 13.7 A Simple Two-Zone Network with Three Links
Using the mentioned logit formula for these paths, we have:

Based on the calculated probabilities, the distribution of the traffic flow would be:
Q 1 =175 trips
Q 2 =24 trips
Q 3 =1 trips
13.3.14 Dynamic Traffic Assignment
Recall the first Wardrop principle, in which travelers are believed to choose their routes with the minimum cost. Dynamic traffic assignment is based on the same rule, but the difference is that delays resulted from congestion. In this way, not only travelers’ route choice affects the network’s level of service, but also the network’s level of service affects travelers’ choice as well. However, it is not theoretically proven that an equilibrium would result under such conditions (Mathew & Rao, 2006).
Today, various algorithms are developed to solve traffic assignment problems. In any urban transportation system, travelers’ route choice and different links’ level of service have a dynamic feedback loop and affect each other simultaneously. However, a lot of these rules are not present in the models presented here. In real world cases, there can be more than thousands of nodes and links in the network, and therefore more sensitivity to dynamic changes is required for a realistic traffic assignment (Meyer, 2016). Also, the travel demand model applies a linear sequence of the four steps, in which case it is also unlike reality. In fact, travelers may have narrow knowledge about all possible paths, modes, and opportunities and may not make rational decisions.
Route choice is the process of choosing a certain path for a trip from a very large choice sets.
Regional Transportation Plan is long term planning document for a region’s transportation usually updated every five years.
Vehicles (VMT) is the aggregate number of miles deriven from in an area in particular time of day.
Total vehicle travel time is the aggregate amount of time spent in transportation usually in minutes.
Link performance function is function used for estimating travel time, travel cost, and speed on the network based on the relationship between speed and travel flow.
Hyperbolic function is a function used for linear differential equations like calculating distances and angels in hyperbolic geometry.
Free-flow road is situation where vehicles can travel with the maximum allowed travel speed.
- Algorithms like all-or-nothing an assignment model where we assume that the impedance of a road or path between each origin and destination is constant and is equal to free-flow level of service, meaning that the traffic time is not affected by the traffic flow on the path.
Capacity-restrained is a model which takes into account the capacity of a road compared to volume and updates travel times.
User equilibrium is a traffic assignment model where we assume that travelers will always choose the shortest path and equilibrium condition would be realized when no traveler is able to decrease their travel impedance by changing paths.
System optimum assignment is an assignment model based on the principle that drivers’ rationale for choosing a path is to minimize total system costs with one another in order to minimize total system travel time.
Static user-equilibrium assignment algorithm is an iterative traffic assignment process which assumes that travelers chooses the travel path with minimum travel time subject to constraints.
- Iterative feedback loop is a model that iterates between trip distribution and route choice step based on the rational that if a path gets too congested, the travel may alter travel destination.
- First principle of Wardrop is the assumption that for each origin-destination (OD) pair, with UE, the travel time on all used paths is equal and less than or equally to the travel time that would be experienced by a single vehicle on any unused path.
- System optimum (SO) is a condition in trip assignment model where total travel time for the whole area is at a minimum.
- Stochastic time-dependent OD is a modeling framework where generation and distribution of trips are randomly assigned to the area.
- Incremental increase is AON-based model with multiple steps in each of which, a fraction of the total traffic volume is assigned, and travel time is calculated based on the allocated traffic volume.
- Stochastic user equilibrium traffic assignment employs a probability distribution function that controls for uncertainties when drivers compare alternative routes and make decisions.
- Dynamic traffic assignment is a model based on Wardrop first principle in which delays resulted from congestion is incorporated in the algorithm.
Key Takeaways
In this chapter, we covered:
- Traffic assignment is the last step of FSM, and the link cost function is a fundamental concept for traffic assignment.
- Different static and dynamic assignments and how to perform them using a simplistic transportation network.
- Incorporating stochastic decision-making about route choice and how to solve assignment problems with regard to this feature.
Correa, J.R., & Stier-Moses, N.E.(2010).Wardrope equilibria. In J.J. Cochran( Ed.), Wiley encyclopedia of operations research and management science (pp.1–12). Hoboken, NJ: John Wiley & Sons. http://dii.uchile.cl/~jcorrea/papers/Chapters/CS2010.pdf
Hui, C. (2014). Application study of all-or-nothing assignment method for determination of logistic transport route in urban planning. Computer Modelling & New Technologies , 18 , 932–937. http://www.cmnt.lv/upload-files/ns_25crt_170vr.pdf
Jeihani Koohbanani, M. (2004). Enhancements to transportation analysis and simulation systems (Unpublished Doctoral dissertation, Virginia Tech). https://vtechworks.lib.vt.edu/bitstream/handle/10919/30092/dissertation-final.pdf?sequence=1&isAllowed=y
Levinson, D., Liu, H., Garrison, W., Hickman, M., Danczyk, A., Corbett, M., & Dixon, K. (2014). Fundamentals of transportation . Wikimedia. https://upload.wikimedia.org/wikipedia/commons/7/79/Fundamentals_of_Transportation.pdf
Mathew, T. V., & Rao, K. K. (2006). Introduction to transportation engineering. Civil engineering–Transportation engineering. IIT Bombay, NPTEL ONLINE, Http://Www. Cdeep. Iitb. Ac. in/Nptel/Civil% 20Engineering .
Meyer, M. D. (2016). Transportation planning handbook . John Wiley & Sons.
Qasim, G. (2015). Travel demand modeling: AL-Amarah city as a case study . [Unpublished Doctoral dissertation , the Engineering College University of Baghdad]
Rojo, M. (2020). Evaluation of traffic assignment models through simulation. Sustainability , 12 (14), 5536. https://doi.org/10.3390/su12145536
Sheffi, Y. (1985). Urban transportation networks: Equilibrium analysis with mathematical programming method . Prentice-Hall. http://web.mit.edu/sheffi/www/selectedMedia/sheffi_urban_trans_networks.pdf
US Bureau of Public Roads. (1964). Traffic assignment manual for application with a large, high speed computer . U.S. Department of Commerce, Bureau of Public Roads, Office of Planning, Urban Planning Division.
https://books.google.com/books/about/Traffic_Assignment_Manual_for_Applicatio.html?id=gkNZAAAAMAAJ
Wang, X., & Hofe, R. (2008). Research methods in urban and regional planning . Springer Science & Business Media.
Polynomial is distribution that involves the non-negative integer powers of a variable.
Hyperbolic function is a function that the uses the variable values as the power to the constant of e.
A point on the curve where the derivation of the function becomes either maximum or minimum.
all-or-nothing is an assignment model where we assume that the impedance of a road or path between each origin and destination is constant and is equal to free-flow level
Incremental model is a model that the predictions or estimates or fed into the model for forecasting incrementally to account for changes that may occur during each increment.
Iterative feedback loop is a model that iterates between trip distribution and route choice step based on the rational that if a path gets too congested, the travel may alter travel destination
feedback loop model is type of dynamic traffic assignment model where an iteration between route choice and traffic assignment step is peformed, based on the assumption that if a particular route gets heavily congested, the travel may change the destination (like another shopping center).
Transportation Land-Use Modeling & Policy Copyright © by Mavs Open Press. All Rights Reserved.
Share This Book
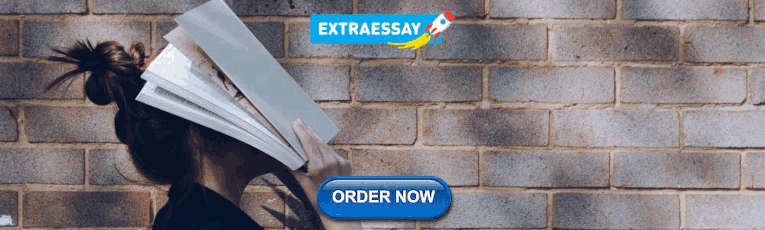
IMAGES
VIDEO
COMMENTS
Route assignment, route choice, or traffic assignment concerns the selection of routes (alternative called paths) between origins and destinations in transportation networks. It is the fourth step in the conventional transportation forecasting model, following Trip Generation, Destination Choice, and Mode Choice.
The traffic assignment step, which is also called route assignment or route choice, simulates the choice of route selection from a set of alternatives between origin zone and the destination zone (Levinson et al., 2014). After the first three steps, we know the number of trips produced between each pair of zones and what portion of these trips ...
The route choice model is integrated in an agent-based transport assignment framework. The model allows combined journey with fixed and flexible service as well as a journey that consists exclusively of fixed or flexible transport; with endogenous demand.