Text Analyzer
Text Analyzer is a simple free online tool for SEO web content analysis that helps you find most frequent phrases and words, number of characters, words, sentences and paragraphs, and estimated read and speak time of your content.
Remove Stop Words
Click "Analyze Passage" button to analyze text.
..download as image
..try "word-cloud-generator" for more data visualization
Click "Analyze Passage" button to visualize text.
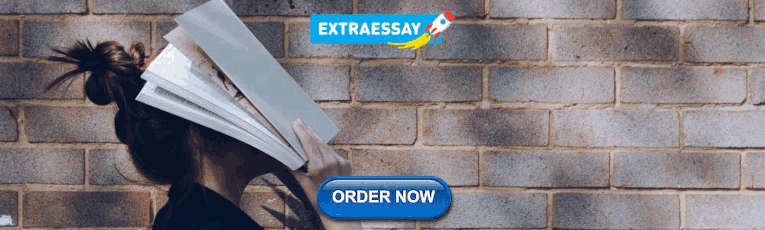
Online Text Analyzer
Text Analyzer is a SEO web content analysis tool that provides extensive statistic about your content like count of characters, words, sentences, paragraphs, spaces, punctuations, the frequency of phrases and words, measure of estimated read and speak time.
Text Analyzer is useful for writers and editors of blogs, forums, websites, product reviews, and anyone who wants to ensure their content stays within limits, wants to avoid over-usage of certain phrases and words, and check for best distribution of words in their writings.
To use Text Analyzer, copy/paste or type your content in the above textarea. Counters like count of characters, words, sentences, paragraphs, spaces, punctuations, the estimated reading and speaking time will update instantly. After typing your content press "Analyze Passage" button to generate count and density of phrases and words.
Link This Tool
You can link this tool by copying and pasting below HTML code in your page:
<a href="https://www.webtools.services/text-analyzer">Text Analyzer Tool</a>
Found an issue, or have feedback?
Help us make "Text Analyzer" tool better by submitting an issue or a feedback today.
- Keyword Density Tool
Textalyzer: Keyword Density + Word Count Tool
Go beyond keyword density.
- Forget 1990s SEO techniques. Take a modern approach to keyword analysis and content optimization with our state of the art topic research reports
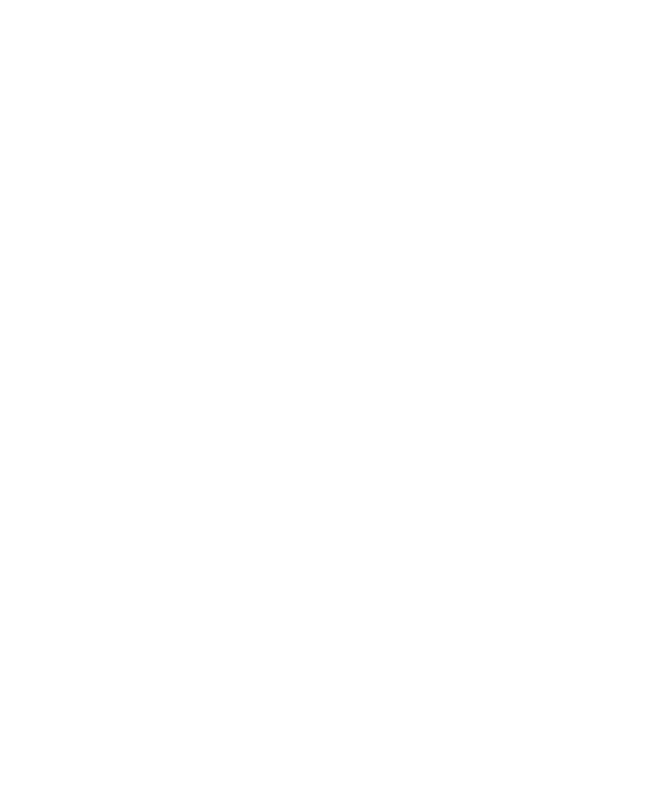
Analyze your copy for SEO with our text analytics tools
Word count and frequency checker, how is the word count calculated by this tool, how many words is the average sentence, when is a sentence too long, whatâs the ideal word count for a blog post, keyword density analysis tool, what is keyword density, how is keyword density calculated, so what is a perfect keyword density when writing online, using a keyword density analysis tool to optimize your content, if itâs so out of date, why did you create a free keyword density tool, how to perform an seo keyword analysis in 2020 using entity and topic analysis, improve your writing using readability scores, check your readability score and grade level using our free tool.
Our free software helps you understand how machines and humans might interpret your text, which can help you improve your writing for SEO. By mining the text for data on readability, word count and frequency, and keyword density, you can understand how a search engine may see your text.
Make sure youâre hitting your targets when writing, with an accurate analysis of the word count youâre using. Explore our keyword density reports to analyze the frequency of keyword usage, and tweak your content to ensure it is highly readable and your content is not over-optimized for specific terms.
We strip all punctuation, from periods and colons through to hyphens and commas. Once this is done, we remove all the excess spaces and then count the gaps between the words. This gives us an accurate count of the words, letters and other characters in your text.
On average we expect a sentence to contain 20 words. But average writing is boring. Good writing should vary in pace and length, to keep the reader engaged and hold their attention for longer. This is especially important online, where people tend to skim read.
Sometimes long sentences are neccessary. But it can make it hard for your reader to digest if you cram too many words into one sentance, as we are deliberately doing here - as it gets longer the reading difficulty increases and you might find you..
So we suggest keeping your sentences shorter if you can. You want your writing to be enjoyable, so donât overcomplicate things. Just use fewer words.
Thereâs really no set answer for the ideal length of the perfect blog post. Your article should try and cover a topic in sufficient depth to keep it interesting and informative for readers. Depending on the topic this may be as little as 300 or 500 words, or it may required a longer essay, such as 1,000, 2,000 or even 5,000 words.
Unless youâre being paid by the word, you should really be aiming to write clearly and succinctly. Thereâs no point us writing 1,000 words on the perfect word count, and you wouldnât enjoy it either. But if we were to talk about how to write for SEO we could probably manage 10,000 words and still have things to say.
Keyword density is a measure of how frequently a keyword or phrase (for example, âcheap sofasâ) occurs in a page. It used to be used by SEOs to determine how well âoptimizedâ their content was. At one point search engines were fairly simple machines, and you could appear higher by simply repeating your main keyword more often than the competition.
This doesnât work anymore.
Calculating keyword density uses a really simple formula. All you need to do is count the number of times a keyword or phrase appears in the text, and divide by the total number of words on the page. For example, if I mention âkeywordâ 100 times in this article, and my article is 1,000 times long, my keyword density would be 10%. And my article would be practically unreadable!
There is no ideal keyword density, no magic number that will make you rank higher in Google. Occasionally people give a figure of 1-2%, which is not unreasonable - and certainly anything over 2% might be deemed a little spammy. But this defeats the point.
Search engines have been part of our life for decades, and they have some of the smartest people in the world working for them. Their approach to understand your article is so far beyond counting words on a page.
Instead of whipping out a calculator and measuring tape, they now use advanced machine learning techniques. The field of natural language understanding has come on so far that it is now simple for a computer to extract the meaning of an article using AI. This means rather than counting word frequency, they seek to analyze whether your article answers the question a searcher is asking.
Using keyword density is a fairly outdated approach to optimizing your content for SEO - in most cases you will want to be writing your text for humans rather than obsession over the precise frequency of keyword usage. It is unlikely you can find an âidealâ keyword density to rank your pages for your target keywords, as Googleâs relevance measures have advanced so much in recent years.
That said, we still feel analyzing keyword density has some value in avoiding over-use of specific terms and phrases in your text. By avoiding âkeyword stuffingâ or over-use of specific phrases you can ensure you donât trip any algorithmic filters or penalties.
You got me. Itâs a trap!
I really want to help people succeed online with SEO, and understand how to correctly optimize their website content for better rankings. By creating a tool and article explaining the pros (and many cons!) of keyword density, I can help direct people towards more effective techniques.
Frankly I think we all spend too long behind screens. If I can help someone out there waste less time optimizing their keyword density ratios, and make their SEO strategies more efficient, we can all go home and play sooner.
The last thing to remember is that Google is not the only search engine. There are some engines and platforms outside of organic search that are much more naive in their approach to information retrieval.. it may be worth testing the impact of keyword density on other 'listing' sites that are important to your business..
A modern approach to keyword analysis goes far beyond simply counting word frequencies, instead we need to look at what ranks in Google, what kind of content Google wants and how we can create something even greater. A far better approach than keyword density is to analyze your content in the context of the search results you are targeting. By mimicing Googleâs use of Natural Language Processing , (a kind of artificial intelligence devoted to understanding the wording we use) and considering your use of entities and topics in comparison to your competitionâs pages you can develop an understanding of how to make your content more topically relevant.
Google's informational retrieval technology has moved on a long way from simple word frequency analysis and keyword density scores, and its time our SEO strategies did too. Their use of machine learning has dramatically increased their ability to understand natural language. By using the same processes they've developed to extract meaning and knowlege from content, we can draw comparisons and see patterns in the sources they choose to promote in their search results. We can use this knowlege to improve the semantic relevance of our work, and better communicate the subject and context of our text to our customers and search engines alike.
You should cover the same topics and entities in your text as other pages in your niche to ensure your content doesnât miss any vital information that searchers are looking for. Tools such as SEO Scout use named entity recognition to analyze competing pages and suggest ways in which your own content may be lacking in depth and context.
When you analyze the top results for your keywords, SEO Scout's service will generate a range of insights based on the content found ranking in the top thirty results for your keyword. Using information extracted from the article found in the top position, in combination with n atural language understanding algorithms, it is possible to predict which topics and linked entities Google expect to find in your text. Writing in greater depth on these topics within your article is a great technique to improve the overall relevance of your piece, that has helped many SEOs improve their placement in the search engines. It can also dramatically improve the number of long tail keywords your page can rank for
Whether writing for SEO or for the web in general, itâs vital you can get your ideas across in your text clearly. Attention spans are limited, and complex sentences can kill conversions.
We use the Flesh Kinkaid reading ease score to assess your contentâs readability. This can be presented as a percentage, where a high score means it is easier to read, or as a grade level. The grade level signifies how many of years of school you would need to read the text. The lower the level, the better for all readers , no matter their level of education!
The score is calculated by looking at factors such as the number of words - or syllables - per sentence. By counting words or syllables per sentence we get a measure of how difficult a text is to read.
To improve your readability score you need to write shorter sentences. You should avoid the use of complex phrases. Shorter, simpler language helps get your point across more easily. The less a reader needs to think, the more likely they are to read your writing. If you need to use longer words, try to keep the sentences short.
As well as analyzing your keyword density, the word count and other text analytics, we automatically check how easy your content is to read. Simply paste your article in the box above and weâll check for difficult words and hard to read sentences. Readability scores are calculated using the Flesch Kincaid reading east algorithm, and supplemented with similar metrics such as the SMOG index, Automated Readability Index, Gunning Fog and Coleman Liau scores.
Do you really need all of those? Probably not - any reading ease score should help you understand where your content is getting too complex. But we like to be complete đ
- Google SERP Simulator
- Schema Generator
- Keyword Combiner
- Keyword Grouping Tool
- Keyword Intent Categorizer
- SEO Content Analysis
How To Guides
- How to Fine-tune Your Content by Adding Missing Long-tail Keywords
- How to Check a Site and See What Keywords it Ranks for: Competitor Research for SEO
- Discover question keywords and other low hanging fruit in your Search Console data to rank for easily
- How To Find and Rank for Long Tail Keywords: SEO Guide
- Find and Rank for Long Tail Keywords: SEO Guide
- How to optimise your content for topical relevance using SEO Scout's content grader
- How to Find New SEO Content Opportunities, Consistently
- How to steal your competitor's top keywords using the competitive research tool
- Content Pruning: How To Consolidate Pages with Little to no Search Visibility
- How to find the FAQ keywords you can easily rank for
Related Blogs
- The 13 Best SEO Tools for Content Optimization & Analysis
- Long Tail Keywords: How to find, target and rank for low competition terms in SEO
- 12 Best Content Brief Generators & Planning Tools for Content Marketers
- How to Write a Blog Post Outline
- How a Content Brief Template Can Level-up Your SEO Content Marketing Strategy
- 10 Simple Tips & Techniques For Effective Keyword Research
- How to Refresh Your Content in 2021 & Beat Content Decay
- The Best Keyword Research Tools For SEO in 2020
- Is Your Content Working? - An Analytical Guide
- Understanding Google Monthly Keyword Search Volume
A guide to text analysis techniques with use cases
Last updated
Reviewed by
Text analysis is a powerful tool for understanding the meaning behind written language. It can be used to uncover hidden insights and gain actionable data from large amounts of text.
In this guide, discover how to use text analysis to aid customer services and marketing communications. Find out how it can help you gain insights into customer sentiment, leading to data-driven decisions. Learn the steps to take when analyzing text and the tools available to help you get started.
- What is text analysis?
Text analysis is a technique for uncovering insights from data sources such as text documents, web pages, emails, and social media posts. It enables companies to gain actionable data and better understand the sentiment of their customer or potential customer.
The technique combines linguistics and computer science to derive meaning from text sources. Itâs sometimes referred to as text mining or text analytics, but these terms have slightly different meanings. Text mining focuses on extracting key information from large amounts of text, while text analytics involves analyzing text data to extract meaningful insights.
- Why is text analysis important?
Text analysis is essential for businesses that are looking to uncover insights, understand customer sentiment, and gain actionable data. The technique can accurately locate patterns, trends, and relationships in large amounts of text data that may otherwise be hard to detect.
With text analysis, businesses can
Better understand customer needs and preferences.
Optimize customer service experiences.
Uncover potential customer segments and market opportunities, enabling them to stay ahead of competitors.
Develop strategies that drive business growth, such as making informed pricing strategies and product development decisions.
Create targeted marketing campaigns.
Identify potential risks.
Improve customer loyalty and retention.
Letâs look at three key uses for text analysis in more detail.
Sentiment analysis â analyzing customer sentiment with text analysis helps businesses understand how customers feel about their product or service. Suppose a customer leaves a review on an online store and mentions they like the product. Sentiment analysis can detect the positive comment and note that the customer is satisfied.
Record management âtext analysis is used to identify potential records in unstructured text data. This allows businesses to easily locate and organize records. Text analysis can process customer feedback from an online survey and detect key topics. Then, it will store the results in an organized way.
Personalizing customer experience âtext analysis is also used to personalize customer experiences by understanding customer needs and preferences.
Text analysis is becoming crucial for businesses as it helps them gain crucial insights. The tools and techniques used in text analysis are constantly evolving, making it an essential tool for any company that wants to stay ahead of its competition.
- Text analysis for customer service teams
Text analysis tools can enable customer service teams to effectively analyze customer conversations and gain actionable data. These tools provide analytics capabilities such as sentiment analysis, topic modeling, and clustering.
The process derives insights from unstructured text data using algorithms, machine learning, and natural language processing (NLP). This can help customer service teams gain valuable feedback through voice of customer (VoC) reporting. Text analysis also enables customer service teams to create and maintain a knowledge base for frequently asked questions with ease.
By harnessing this technique, customer service teams can uncover customer insights that empower them to deliver personalized and improved experiences. In practice, this might involve detecting high-value customers, anticipating their problems, and implementing automated ticket routing, prioritization, and tagging.
- Text analysis for marketing teams
Marketing teams can use text analytics to help them develop more productive campaigns and target audiences more effectively.
Monitoring customer conversations unlocks vital insights into customersâ needs, wants, and expectations. Marketers can gain a better understanding of customer loyalty, predict customer lifetime value , and identify potential customer churn .
The knowledge that text analysis uncovers enables marketers to detect early customer trends and potential lead-to-customer opportunities. They can also determine which strategies are working and which need improvement. Campaigns can be adjusted quickly to capitalize on opportunities as they arise.
- Text analysis techniques
Text analysis is an umbrella term for several methods used to interpret, classify, and extract meaning from text data. In this section, we will look at the four most commonly used text analysis techniques:
Text classification is the process of assigning a label or category to a piece of text either manually or through automated algorithms. For example, you could use text classification to group customer feedback into âpositiveâ or ânegativeâ categories.
Text extraction involves extracting specific pieces of information from unstructured text either manually or with an automated tool. For example, text extraction could automatically find customer names and addresses from emails or customer feedback forms.
Topic modeling is a type of text analysis that uses natural language processing to identify topics or themes. The goal is to identify the most critical themes in a piece of text and then analyze how the topics relate to each other.
Personally identifiable information (PII) redaction removes sensitive information from text before it is shared publicly. This could include social security, bank account, and credit card details.
- Stages of text analysis
Text analysis uses computers to understand large amounts of text. It uses natural language processing techniques, machine learning, and data science to analyze unstructured text and extract valuable insights.
The four main stages of text analysis are:
1. Data gathering
The first step is to collect data from various sources such as online reviews, social media conversations, website feedback, and emails.
The data needs to be formatted to make it easier to analyze. For example, if youâre analyzing customer reviews, you would have to structure the data into a format that includes the customer name, product type, review rating, and review content.
2. Data preparation
Once the data is gathered, it needs to be prepared for analysis. This involves cleaning the data to remove any irrelevant words or punctuation. Youâll also need to categorize the data based on sentiment, topic, or other criteria.
3. Text analysis
Now that the data is clean and categorized, itâs time to use text analysis tools to uncover insights. Text analysis tools use algorithms to detect patterns and sentiments in the text. Some of these tools can detect topics of conversation or even identify individual people from their comments.
4. Visualization
The last step of text analysis is to visualize the data. This helps make the insights easier to understand and can help provide context.
Visualization tools like charts and graphs can be used to show the relationships between different categories of data or show trends over time.
- Text analysis vs. natural language processing
Natural language processing is a branch of artificial intelligence that focuses on understanding human language using computers. NLP enables machines to interpret and analyze natural language, such as spoken or written words, to extract useful information.
On the other hand, text analysis involves analyzing unstructured or semi-structured text data to extract meaningful insights. Businesses can use it to extract facts, figures, and other important information from text that can be used for analysis.
Unlike NLP, text analysis
Focuses on analyzing the text to uncover insights , whereas NLP focuses on understanding the meaning behind the text.
Is concerned with extracting facts and figures from the text, while natural language processing is more concerned with understanding the textâs underlying context and sentiment.
Relies heavily on structured data , while natural language processing requires unstructured data.
Doesnât require the use of advanced algorithms like those used in natural language processing.
Is used for tasks like summarizing and classifying documents, while NLP is used for more complex tasks like machine translation, answering questions, and conversational agents.
- Essential tools for text analysis
Basic text analysis tools:
A customer relationship management (CRM) system is a digital platform that allows businesses to manage customer data, including customer interaction and purchase history. It also helps in tracking customer trends and buying patterns. Salesforce is a popular CRM system used by many companies.
Microsoft Excel is a popular spreadsheet program data analysts use to store, organize, and analyze data. It can help perform various types of text analysis, such as sentiment analysis , topic modeling , and keyword extraction.
Social listening tools are used to monitor and analyze conversations on social media networks like Twitter, Facebook, and Instagram. They allow you to track customer sentiment , measure your contentâs reach, and identify influencers. Hootsuite is an example of a popular social listening tool that many companies use.
Advanced text analysis tools:
 Google NLP products are a suite of natural language processing services that make it easy to analyze text for its sentiment, entities, syntax, and more. These tools allow you to take unstructured text data and create structured insights. For example, Googleâs Natural Language API can detect the sentiment of a customer review and automatically assign it a score based on how positive or negative it is.
Amazon Comprehend is an NLP service designed to help customers quickly and accurately extract meaningful insights from large volumes of text data. It provides sentiment recognition, sentiment analysis, language detection, and keyphrase extraction features. For example, businesses can use Amazon Comprehend to extract customer feedback from online reviews to better understand what customers think about their products or services.
Watson Natural Language Understanding is a cloud-based NLP service that allows users to analyze text data and gain insights. It offers sentiment analysis, entity extraction, keyword extraction, concept tagging, and sentiment scoring. Businesses can use Watson Natural Language Understanding to extract customer sentiment from tweets about their product or service, allowing them to identify areas of improvement quickly.
Azure Text Analysis API is a cloud-based NLP service that provides features such as sentiment analysis, keyphrase extraction, language detection, and entity extraction. It allows businesses to analyze unstructured text and extract insights from it quickly and cost-effectively. For example, Azure Text Analysis API can generate a sentiment score for customer feedback to quickly assess customer satisfaction levels.
- What is the best language for text analytics?
When it comes to text analytics, the language you choose greatly impacts the results you can get. Different languages are best suited to different tasks, so itâs important to know which one will work best for your analysis.
Some of the most popular languages for text analytics include Python, R, and SQL. Itâs helpful to understand the strengths and weaknesses of each language before selecting the one best suited to your needs.
Python is an excellent choice because of its versatility and ability to create advanced models. It is well suited to NLP tasks like sentiment analysis and entity extraction.
Extracting customer feedback from social media posts is an example of a task Python can help with.
R is an ideal language for statistical analysis and data visualization. It is used for text mining tasks such as classification, clustering, and topic modeling.
An example of a task R can help with is creating visualizations of customer sentiment across product categories.Â
SQL is a powerful language for querying data in databases. It can be used for text analytics tasks such as text classification and search.
For example, SQL can help with performing keyword searches of customer support emails to find relevant topics quickly.
Text analysis is a powerful tool that enables businesses to gain insights, understand customer sentiment, design more targeted campaigns, and generally make more informed decisions.
As an essential part of data-driven decision-making, the technique has become increasingly important as more information is available online. It can help companies stay competitive by giving them an advantage in the ever-changing digital landscape.
The demand for text analysis is only increasing, and businesses that leverage this technology will be well-positioned to remain competitive in their respective markets.
Businesses need to identify the right tools for their specific needs and build processes around them to get the most out of text analysis. While it requires some effort up front, investing time into text analysis now will pay dividends in the future.
Get started today
Go from raw data to valuable insights with a flexible research platform
Editorâs picks
Last updated: 22 April 2023
Last updated: 8 May 2023
Last updated: 16 August 2023
Last updated: 18 July 2023
Last updated: 9 July 2023
Last updated: 20 March 2024
Last updated: 21 March 2024
Last updated: 16 March 2024
Last updated: 5 April 2023
Last updated: 22 May 2023
Last updated: 29 May 2023
Last updated: 23 March 2024
Last updated: 25 June 2023
Latest articles
Related topics, log in or sign up.
Get started for free
Analyzing Text Data
An introduction to text analysis and text mining, an overview of text analysis methods, additional resources.
- Text Analysis Methods
- Library Databases
- Social Media
- Open Source
- Language Corpora
- Web Scraping
- Software for Text Analysis
- Text Data Citation
Library Data Services
What is text analysis.
Text analysis is a broad term that encompasses the examination and interpretation of textual data. It involves various techniques to understand, organize, and derive insights from text, including methods from linguistics, statistics, and machine learning. Text analysis often includes processes like text categorization, sentiment analysis, and entity recognition, to gain valuable insights from textual data.
What is text mining?
Text mining , also known as text data mining, is a process of using computer programs and algorithms to dig through large amounts of text, like books, articles, websites, or social media posts, to find valuable and hidden information. This information could be patterns, trends, insights, or specific pieces of knowledge that are not immediately obvious when you read the texts on your own. Text data mining helps people make sense of vast amounts of text data quickly and efficiently, making it easier to discover useful information and gain new perspectives from written content.
This video is an introduction to text mining and how it can be used in research.
There are many different methods for text analysis, such as:
- word frequency analysis
- natural language processing
- sentiment analysis
These text analysis techniques serve various purposes, from organizing and understanding text data to making predictions, extracting knowledge, and automating tasks.
Before beginning your text analysis project, it is important to specify your goals and then choose the method that will allow you to meet those goals. Then, consider how much data you need, and identify a sampling plan , before beginning data collection.
- Examples of Text and Data Mining Research Using Copyrighted Materials By Sean Flynn and Lokesh Vyas, an exploration of text and data mining across disciplines, from medicine to literature. Published December 5, 2022.
- Next: Text Analysis Methods >>
- Last Updated: Feb 7, 2024 10:26 AM
- URL: https://libguides.gwu.edu/textanalysis
Free high-quality Text Analysis Tools
powered by Prose Analyzer
Text Structure Analysis
A versatile solution for SEO optimization, readability improvement, and comprehensive linguistic insights. Our all-in-one tool!
Keyword Analysis
Identifies and evaluates the significance and usage of specific keywords and phrases in your text, enhancing the focus and relevance of your writing.
Sentiment Analysis
Analyzes text to determine the underlying emotional tone, providing insights into the overall sentiment conveyed by the writing.
Paragraph Analysis
Evaluates paragraph structure and composition, ensuring each section contributes effectively to the overall message and readability of the text.
Sentence Analysis
Focuses on analyzing individual sentences for grammatical accuracy and clarity, aiding in the creation of concise and impactful writing.
Word Analysis
Examines word choice and frequency, offering insights into the effectiveness and diversity of language used in the text.
Elevate Your Writing with Prose Analyzer
Welcome to Prose Analyzer â your advanced online text analysis tool.
Whether you are a student polishing an essay, an educator guiding learners, a researcher handling vast amounts of data, or a content creator shaping impactful prose, Prose Analyzer is your comprehensive solution. Tailor-made for users with a diversity of needs, our tool brings unprecedented insights into the fabric of your written content.
When to use Prose Analyzer?
Unlock the full potential of Prose Analyzer across a spectrum of applications:
Word and Character Count:
Swiftly assess the length of your content with our powerful word counter and character counter, capable of handling text volumes from 100k to 1 million words.
Keyword Analysis:
Fine-tune your content with detailed keyword analytics, ensuring your message resonates effectively with your audience.
Paragraph and Sentence Averages:
Gain insights into your writing structure with averages for characters, words, and sentences per paragraph, as well as characters and words per sentence. Explore these features now to elevate your text analysis experience and boost your understanding of written content.
Reading and Speaking Time:
Estimate engagement and presentation durations at 230 words per minute and 150 words per minute, respectively.
Sentiment Analysis:
Dive into the emotional tone of your text with our intuitive pie chart, providing nuanced insights into global sentiment.
Who needs Prose Analyzer?
Prose Analyzer caters to a diverse audience, making it an indispensable tool for various purposes:
Elevate your academic writing with Prose Analyzer. Ensure your essays and assignments meet word count requirements while refining the structure for maximum impact. Prose Analyzer empowers you to submit polished, well-structured work, impressing both instructors and peers.
Researchers:
Efficiently navigate vast amounts of textual data with Prose Analyzer. Save time in the research process by gaining quick insights into word and character counts. Leverage features like paragraph and sentence averages, enhancing your ability to analyze and comprehend large volumes of text, ultimately boosting your research output.
Job-seekers:
Craft impactful resumes and cover letters with Prose Analyzer. Tailor your application materials to meet optimal word count standards while ensuring clarity and precision. Prose Analyzer empowers job-seekers to present themselves compellingly in writing, making a lasting impression on potential employers.
Digital Marketers:
Maximize the impact of your content strategy with Prose Analyzer. Analyze and refine your written material to resonate effectively with your target audience. Prose Analyzer's insights into word and keyword usage provide digital marketers with the tools to enhance the effectiveness of their campaigns and messaging.
Guide your students toward improved writing practices with Prose Analyzer. Assess their work with detailed text analysis, providing constructive feedback on word usage, structure, and overall composition. Prose Analyzer becomes an invaluable tool in fostering better writing habits and facilitating impactful learning experiences.
Refine your craft with Prose Analyzer's detailed insights. Gain a nuanced understanding of your writing structure, word usage, and overall composition. Whether you are a seasoned author or aspiring wordsmith, Prose Analyzer equips you with the tools to enhance the effectiveness and impact of your prose.
Social Media Users:
Optimize your social media posts for maximum engagement with Prose Analyzer. Understand the analytics behind your text, ensuring clarity and resonance with your audience. Whether you are a casual user or a social media influencer, Prose Analyzer helps you fine-tune your content for optimal impact.
Bloggers and Online Business Owners:
Improve the visibility and impact of your online content with Prose Analyzer. Understand the structure and keyword usage in your articles, blog posts, or product descriptions. Prose Analyzer is an essential tool for bloggers and online business owners looking to optimize their content for search engines and audience engagement.
How to use Prose Analyzer?
Prose Analyzer is user-friendly and efficient:
1. Paste or Type: Begin typing or paste your text effortlessly.
2. Live Analysis: Prose Analyzer provides real-time analysis as you type, offering instant insights into your content.
3. Review Results: Explore paragraph, sentence, word, and character counts, and delve into advanced analytics for a thorough understanding of your text.
How Prose Analyzer differs from others?
Prose Analyzer sets itself apart by offering more than just basic text analysis. Dive into the emotional tone of your content with our unique sentiment analysis feature. Visualize sentiments through an intuitive pie chart, providing nuanced insights into the global emotional context expressed in your text.
Versatile Analytics:
Prose Analyzer's power lies in its versatility, making it an indispensable tool for a diverse audience. From writers and students to professionals and content creators, our tool caters to various needs. Tailor your analysis to meet specific requirements, whether it is refining prose, meeting academic standards, or optimizing content for different platforms.
Detailed Averages:
Gain a deeper understanding of your writing structure with Prose Analyzer's detailed averages. Explore per-paragraph, per-sentence, and per-word averages, allowing you to scrutinize and refine your content with precision. This level of granularity empowers you to craft content that is not only well-structured but also impactful.
Intuitive Interface:
Prose Analyzer prioritizes user experience with its intuitive interface. Whether you are a novice or an experienced user, our tool ensures accessibility for all. Seamlessly navigate through its features, making text analysis a straightforward and enjoyable process.
This comprehensive feature set, coupled with an accessible and user-friendly design, positions Prose Analyzer as a standout tool for individuals seeking detailed insights into their written content.
Start Analyzing Your Prose Now!
Embark on a journey of text analysis with Prose Analyzer
Gain profound insights into your written content. Perfect for writers, students, professionals, and anyone seeking in-depth understanding of the nuances within their prose.
- Bahasa Indonesia
- Sign out of AWS Builder ID
- AWS Management Console
- Account Settings
- Billing & Cost Management
- Security Credentials
- AWS Personal Health Dashboard
- Support Center
- Expert Help
- Knowledge Center
- AWS Support Overview
- AWS re:Post
- What is Cloud Computing?
- Cloud Computing Concepts Hub
- Machine Learning & AI
What is Text Analysis?

What is text analysis?
Text analysis is the process of using computer systems to read and understand human-written text for business insights. Text analysis software can independently classify, sort, and extract information from text to identify patterns, relationships, sentiments, and other actionable knowledge. You can use text analysis to efficiently and accurately process multiple text-based sources such as emails, documents, social media content, and product reviews, like a human would.
Why is text analysis important?
Businesses use text analysis to extract actionable insights from various unstructured data sources. They depend on feedback from sources like emails, social media, and customer survey responses to aid decision making. However, the immense volume of text from such sources proves to be overwhelming without text analytics software.
With text analysis, you can get accurate information from the sources more quickly. The process is fully automated and consistent, and it displays data you can act on. For example, using text analysis software allows you to immediately detect negative sentiment on social media posts so you can work to solve the problem
Sentiment analysis
Sentiment analysis or opinion mining uses text analysis methods to understand the opinion conveyed in a piece of text. You can use sentiment analysis of reviews, blogs, forums, and other online media to determine if your customers are happy with their purchases. Sentiment analysis helps you spot new trends, track sentiment changes, and tackle PR issues. By using sentiment analysis and identifying specific keywords, you can track changes in customer opinion and identify the root cause of the problem.
Record management
Text analysis leads to efficient management, categorization, and searches of documents. This includes automating patient record management, monitoring brand mentions, and detecting insurance fraud. For example, LexisNexis Legal & Professional uses text extraction to identify specific records among 200 million documents.
Personalizing customer experience
You can use text analysis software to process emails, reviews, chats, and other text-based correspondence. With insights about customersâ preferences, buying habits, and overall brand perception, you can tailor personalized experiences for different customer segments.
How does text analysis work?
The core of text analysis is training computer software to associate words with specific meanings and to understand the semantic context of unstructured data. This is similar to how humans learn a new language by associating words with objects, actions, and emotions.
Text analysis software works on the principles of deep learning and natural language processing.
Deep learning
Artificial intelligence is the field of data science that teaches computers to think like humans. Machine learning is a technique within artificial intelligence that uses specific methods to teach or train computers. Deep learning is a highly specialized machine learning method that uses neural networks or software structures that mimic the human brain. Deep learning technology powers text analysis software so these networks can read text in a similar way to the human brain.
Natural language processing
Natural language processing (NLP) is a branch of artificial intelligence that gives computers the ability to automatically derive meaning from natural, human-created text. It uses linguistic models and statistics to train the deep learning technology to process and analyze text data, including handwritten text images. NLP methods such as optical character recognition (OCR) convert text images into text documents by finding and understanding the words in the images.
.655cd7c9dbef3880a6317df2ca98b06ae664f4a1.png)
What are the types of text analysis techniques?
The text analysis software uses these common techniques.
Text classification
In text classification, the text analysis software learns how to associate certain keywords with specific topics, usersâ intentions, or sentiments. It does so by using the following methods:
- Rule-based classification assigns tags to the text based on predefined rules for semantic components or syntactic patterns.
- Machine learning-based systems work by training the text analysis software with examples and increasing their accuracy in tagging the text. They use linguistic models like Naive Bayes, Support Vector Machines, and Deep Learning to process structured data, categorize words, and develop a semantic understanding between them.
For example, a favorable review often contains words like good, fast, and great. However, negative reviews might contain words like unhappy, slow, and bad . Data scientists train the text analysis software to look for such specific terms and categorize the reviews as positive or negative. This way, the customer support team can easily monitor customer sentiments from the reviews.
Text extraction
Text extraction scans the text and pulls out key information. It can identify keywords, product attributes, brand names, names of places, and more in a piece of text. The extraction software applies the following methods:
- Regular expression (REGEX): This is a formatted array of symbols that serves as a precondition of what needs to be extracted.
- Conditional random fields (CRFs): This is a machine learning method that extracts text by evaluating specific patterns or phrases. It is more refined and flexible than REGEX.
For example, you can use text extraction to monitor brand mentions on social media. Manually tracking every occurrence of your brand on social media is impossible. Text extraction will alert you to mentions of your brand in real time.
Topic modeling
Topic modeling methods identify and group related keywords that occur in an unstructured text into a topic or theme. These methods can read multiple text documents and sort them into themes based on the frequency of various words in the document. Topic modeling methods give context for further analysis of the documents.
For example, you can use topic modeling methods to read through your scanned document archive and classify documents into invoices, legal documents, and customer agreements. Then you can run different analysis methods on invoices to gain financial insights or on customer agreements to gain customer insights.
PII redaction
PII redaction automatically detects and removes personally identifiable information (PII) such as names, addresses, or account numbers from a document. PII redaction helps protect privacy and comply with local laws and regulations.
For example, you can analyze support tickets and knowledge articles to detect and redact PII before you index the documents in the search solution. After that, search solutions are free of PII in documents.
What are the stages in text analysis?
To implement text analysis, you need to follow a systematic process that goes through four stages.
Stage 1âData gathering
In this stage, you gather text data from internal or external sources.
Internal data
Internal data is text content that is internal to your business and is readily availableâfor example, emails, chats, invoices, and employee surveys.
External data
You can find external data in sources such as social media posts, online reviews, news articles, and online forums. It is harder to acquire external data because it is beyond your control. You might need to use web scraping tools or integrate with third-party solutions to extract external data.
Stage 2âData preparation
Data preparation is an essential part of text analysis. It involves structuring raw text data in an acceptable format for analysis. The text analysis software automates the process and involves the following common natural language processing (NLP) methods.
Tokenization
Tokenization is segregating the raw text into multiple parts that make semantic sense. For example, the phrase text analytics benefits businesses tokenizes to the words text , analytics , benefits , and businesses .
Part-of-speech tagging
Part-of-speech tagging assigns grammatical tags to the tokenized text. For example, applying this step to the previously mentioned tokens results in text: Noun; analytics: Noun; benefits: Verb; businesses: Noun .
Parsing establishes meaningful connections between the tokenized words with English grammar. It helps the text analysis software visualize the relationship between words.
Lemmatization
Lemmatization is a linguistic process that simplifies words into their dictionary form, or lemma. For example, the dictionary form of visualizing is visualize .
Stop words removal
Stop words are words that offer little or no semantic context to a sentence, such as and , or , and for . Depending on the use case, the software might remove them from the structured text.
Stage 3âText analysis
Text analysis is the core part of the process, in which text analysis software processes the text by using different methods.
Classification is the process of assigning tags to the text data that are based on rules or machine learning-based systems.
Extraction involves identifying the presence of specific keywords in the text and associating them with tags. The software uses methods such as regular expressions and conditional random fields (CRFs) to do this.
Stage 4âVisualization
Visualization is about turning the text analysis results into an easily understandable format. You will find text analytics results in graphs, charts, and tables. The visualized results help you identify patterns and trends and build action plans. For example, suppose youâre getting a spike in product returns, but you have trouble finding the causes. With visualization, you look for words such as defects , wrong size , or not a good fit in the feedback and tabulate them into a chart. Then youâll know which is the major issue that takes top priority.
What is text analytics?
Text analytics is the quantitative data that you can obtain by analyzing patterns in multiple samples of text. It is presented in charts, tables, or graphs.
Text analysis vs. text analytics
Text analytics helps you determine if thereâs a particular trend or pattern from the results of analyzing thousands of pieces of feedback. Meanwhile, you can use text analysis to determine whether a customerâs feedback is positive or negative.
What is text mining?
Text mining is the process of obtaining qualitative insights by analyzing unstructured text.
Text analysis vs. text mining
There is no difference between text analysis and text mining. Both terms refer to the same process of gaining valuable insights from sources such as email, survey responses, and social media feeds.
How can Amazon Comprehend help?
Amazon Comprehend is a natural language processing service that uses machine learning to uncover valuable insights and connections in text. You can use it to simplify document processing workflows by automatically classifying and extracting information from them. For example, you can use Amazon Comprehend to do the following tasks:
- Perform sentiment analysis on customer support tickets, product reviews, social media feeds, and more.
- Integrate Amazon Comprehend with Amazon Lex to develop an intelligent, conversational chatbot.
- Extract medical terms from documents and identify the relationship between them with Amazon Comprehend Medical .
Get started by creating an AWS account today.
Next steps on AWS
Ending Support for Internet Explorer
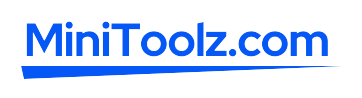
Free Online Text Analyzer: Count Characters, Words, Sentences, Paragraphs, and Frequency
With our free online Text Analyzer, you can effortlessly count the number of characters, words, sentences, paragraphs, and frequency in your text. This powerful tool provides quick and accurate analysis, helping you understand and optimize your written content.
Text Analyzer
Count characters, words, sentences, and paragraphs.
Our intuitive and efficient Text Analyzer simplifies the process of text analysis. It provides accurate counts of characters, words, sentences, and paragraphs in your text, allowing you to easily monitor the length and structure of your content.
Analyze Word Frequency and Occurrences
By analyzing word frequency, you can gain deeper insights into your writing patterns and identify commonly used words or phrases. This information is incredibly useful for content optimization, as you can eliminate repetitive or overused words, enhance clarity, and engage your audience with fresh and varied vocabulary.
Benefits of Using the Text Analyzer
- Save time and effort by automating the process of text analysis.
- Gain valuable insights into the length, and frequency of your text.
- Optimize your writing by identifying repetitive or overused words.
Similar Tools
Convert images to webp online, convert images to png online, convert images to jpg/jpeg online.
Text Analytics
What is text analytics.
Text analytics is the process of transforming unstructured text documents into usable, structured data. Text analysis works by breaking apart sentences and phrases into their components, and then evaluating each partâs role and meaning using complex software rules and machine learning algorithms.
Text analytics forms the foundation of numerous natural language processing (NLP) features, including named entity recognition, categorization, and sentiment analysis. In broad terms, these NLP features aim to answer four questions:
- Who is talking?
- What are they talking about?
- What are they saying about those subjects?
- How do they feel?
Data analysts and other professionals use text mining tools to derive useful information and context-rich insights from large volumes of raw text, such as social media comments, online reviews, and news articles. In this way, text analytics software forms the backbone of business intelligence programs, including voice of customer/customer experience management, social listening and media monitoring, and voice of employee/workforce analytics.
This article will cover the basics of text analytics, starting with the difference between text analytics, text mining, and natural language processing. Then weâll explain the seven functions of text analytics and explore some basic applications of text mining. Finally, weâll tell you where you can try text analytics for free and share some resources for further reading.
Table of contents:
Text analytics
Text mining
- Natural language processing
- Language identification
- Tokenization
- Sentence breaking
- Part of speech tagging
- Syntax parsing
- Sentence chaining
- Voice of customer
- Social media monitoring
- Voice of employee
Try text analytics and text mining for free
Learn more about text analytics, what is the difference between text mining, text analytics and natural language processing.
describes the general act of gathering useful information from text documents.
refers to the actual computational processes of breaking down unstructured text documents, such as tweets, articles, reviews and comments, so they can be analyzed further.
Natural language processing (NLP)
is how a computer understands the underlying meaning of those text documents: whoâs talking, what theyâre talking about, and how they feel about those subjects.
As a term, text mining is often used interchangeably with text analytics . In most cases, thatâs fine. But there is a difference. If text mining refers to collecting useful information from text documents, text analytics is how a computer actually transforms those raw words into information. Meanwhile, the low-level computational functions of text analytics form the foundation of natural language processing features, such as sentiment analysis, named entity recognition, categorization, and theme analysis.
How does text analytics work?
Text analytics starts by breaking down each sentence and phrase into its basic parts. Each of these components, including parts of speech, tokens, and chunks, serve a vital role in accomplishing deeper natural language processing and contextual analysis.
There are seven computational steps involved in preparing an unstructured text document for deeper analysis:
- Language Identification
- Part of Speech tagging
Some text analytics functions are accomplished exclusively through rules-based software systems. Other functions require machine learning models (including deep learning algorithms) to achieve.
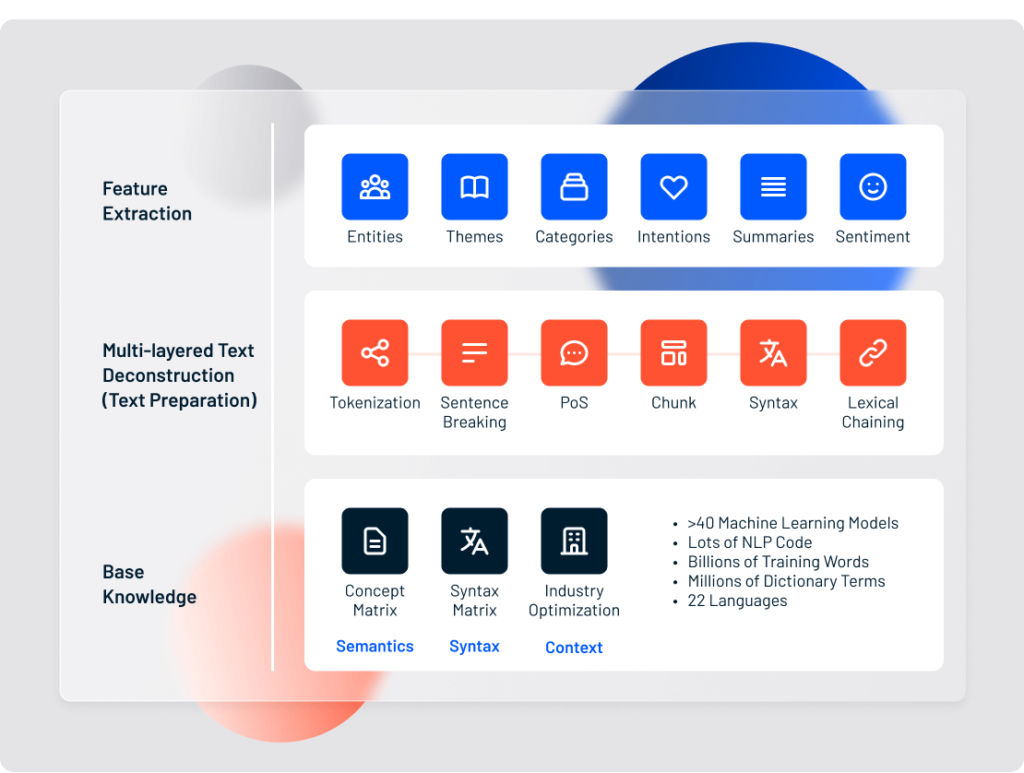
1. Language identification
The first step in text analytics is identifying what language the text is written in. Spanish? Russian? Arabic? Chinese?
Lexalytics supports text analytics for more than 30 languages and dialects . Together, these languages include a complex tangle of alphabets, abjads and logographies. Each language has its own idiosyncrasies and unique rules of grammar. So, as basic as it might seem, language identification determines the whole process for every other text analytics function.
2. Tokenization
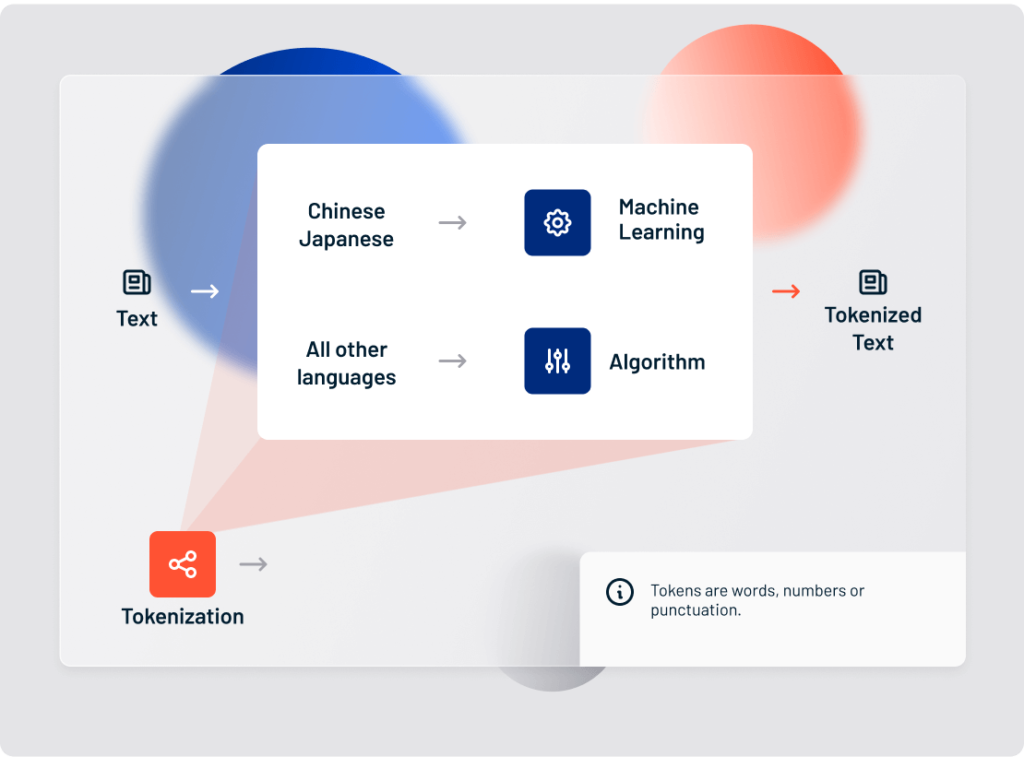
Tokenization is the process of breaking apart a sentence or phrase into its component pieces. Tokens are usually words or numbers. Depending on the type of unstructured text youâre processing, however, tokens can also be:
- Punctuation (exclamation points amplify sentiment )
- Hyperlinks (https://âŠ)
- Possessive markers (apostrophes)
Tokenization is language-specific, so itâs important to know which language youâre analyzing. Most alphabetic languages use whitespace and punctuation to denote tokens within a phrase or sentence. Logographic (character-based) languages such as Chinese, however, use other systems.
Lexalytics uses rules-based algorithms to tokenize alphabetic languages, but logographic languages require the use of complex machine learning algorithms.
3. Sentence breaking
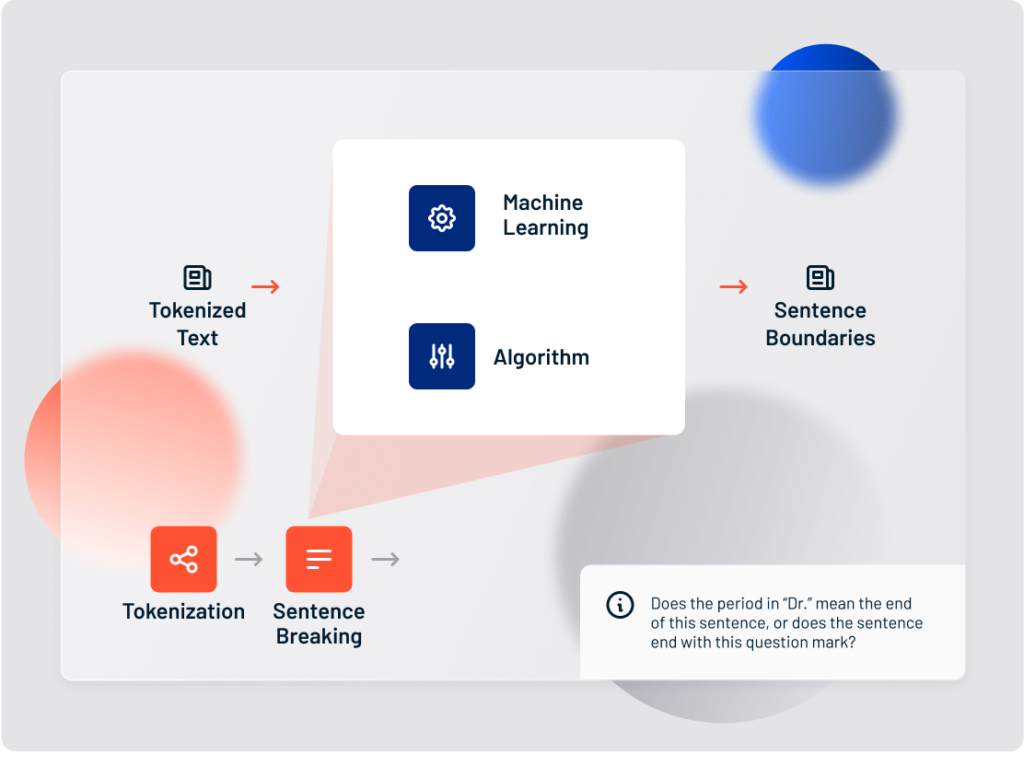
Small text documents, such as tweets, usually contain a single sentence. But longer documents require sentence breaking to separate each unique statement. In some documents, each sentence is separated by a punctuation mark. But some sentences contain punctuation marks that donât mean the end of the statement (like the period in âDr.â)
4. Part of Speech tagging
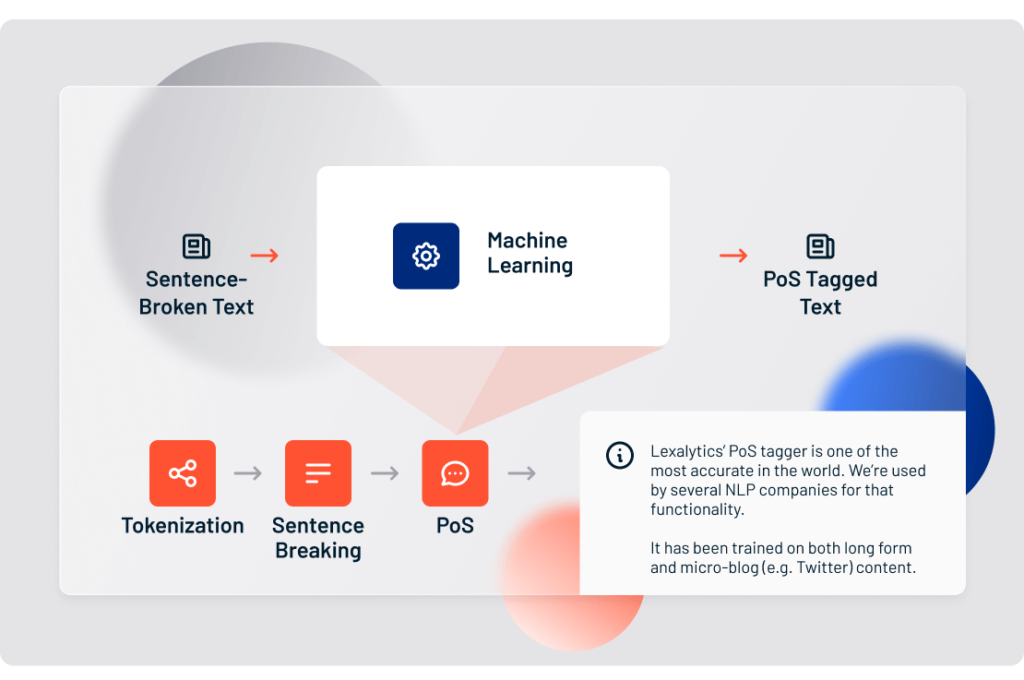
Part of Speech tagging (or PoS tagging) is the process of determining the part of speech of every token in a document, and then tagging it as such. Most languages follow some basic rules and patterns that can be written into a basic Part of Speech tagger. When shown a text document, the tagger figures out whether a given token represents a proper noun or a common noun, or if itâs a verb, an adjective, or something else entirely.
Accurate part of speech tagging is critical for reliable sentiment analysis. Through identifying adjective-noun combinations, a sentiment analysis system gains its first clue that itâs looking at a sentiment-bearing phrase. At Lexalytics, due to our breadth of language coverage, weâve had to train our systems to understand 93 unique Part of Speech tags.
5. Chunking
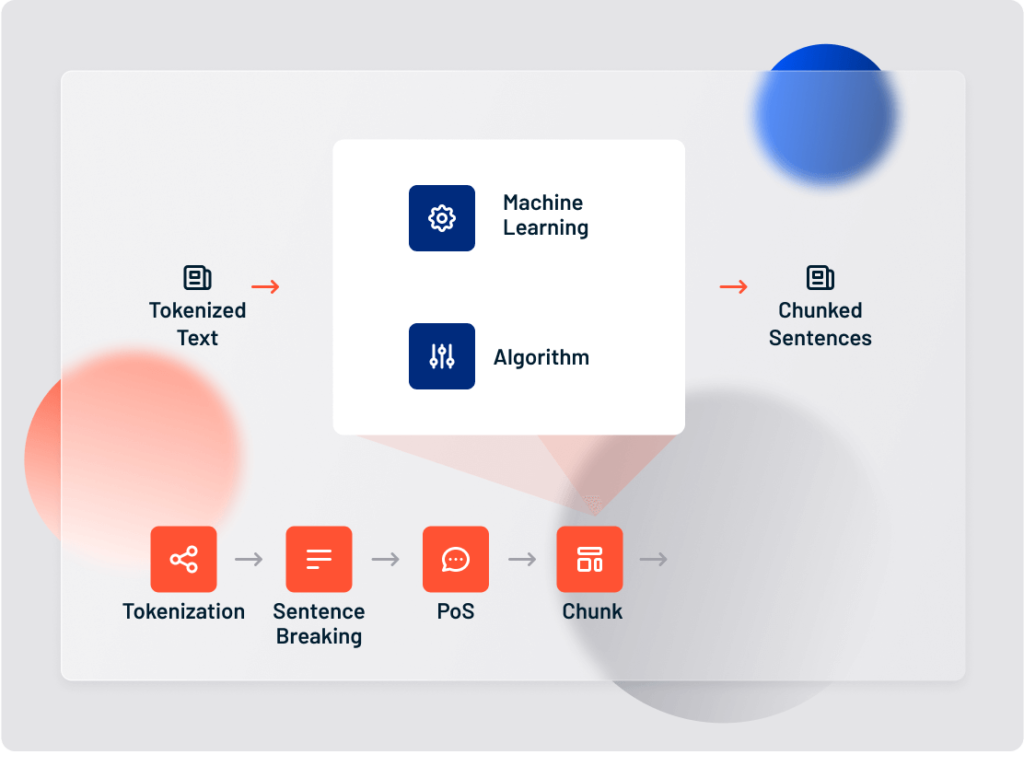
Chunking refers to a range of sentence-breaking systems that splinter a sentence into its component phrases (noun phrases, verb phrases, and so on).
Chunking in text analytics is different than Part of Speech tagging:
- PoS tagging means assigning parts of speech to tokens
- Chunking means assigning PoS-tagged tokens to phrases
For example, take the sentence: The tall man is going to quickly walk under the ladder.
PoS tagging will identify man and ladder as nouns and walk as a verb.
Chunking will return: [the tall man]_np [is going to quickly walk]_vp [under the ladder]_pp
( np stands for ânoun phrase,â vp stands for âverb phrase,â and pp stands for âprepositional phrase.â)
6. Syntax parsing
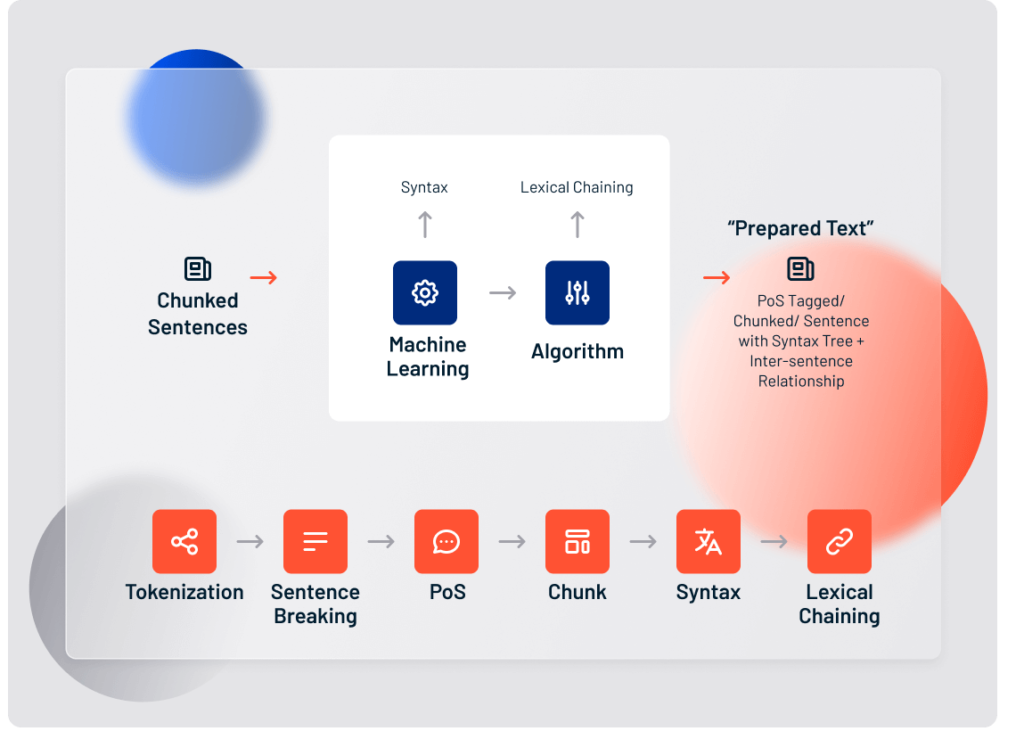
Syntax parsing is the analysis of how a sentence is formed. Syntax parsing is a critical preparatory step in sentiment analysis and other natural language processing features.
The same sentence can have multiple meanings depending on how itâs structured:
- Apple was doing poorly until Steve Jobs âŠ
- Because Apple was doing poorly, Steve Jobs âŠ
- Apple was doing poorly because Steve Jobs âŠ
In the first sentence, Apple is negative, whereas Steve Jobs is positive.
In the second, Apple is still negative, but Steve Jobs is now neutral.
In the final example, both Apple and Steve Jobs are negative.
Syntax parsing is one of the most computationally-intensive steps in text analytics. At Lexalytics, we use special unsupervised machine learning models, based on billions of input words and complex matrix factorization, to help us understand syntax just like a human would.
7. Sentence chaining
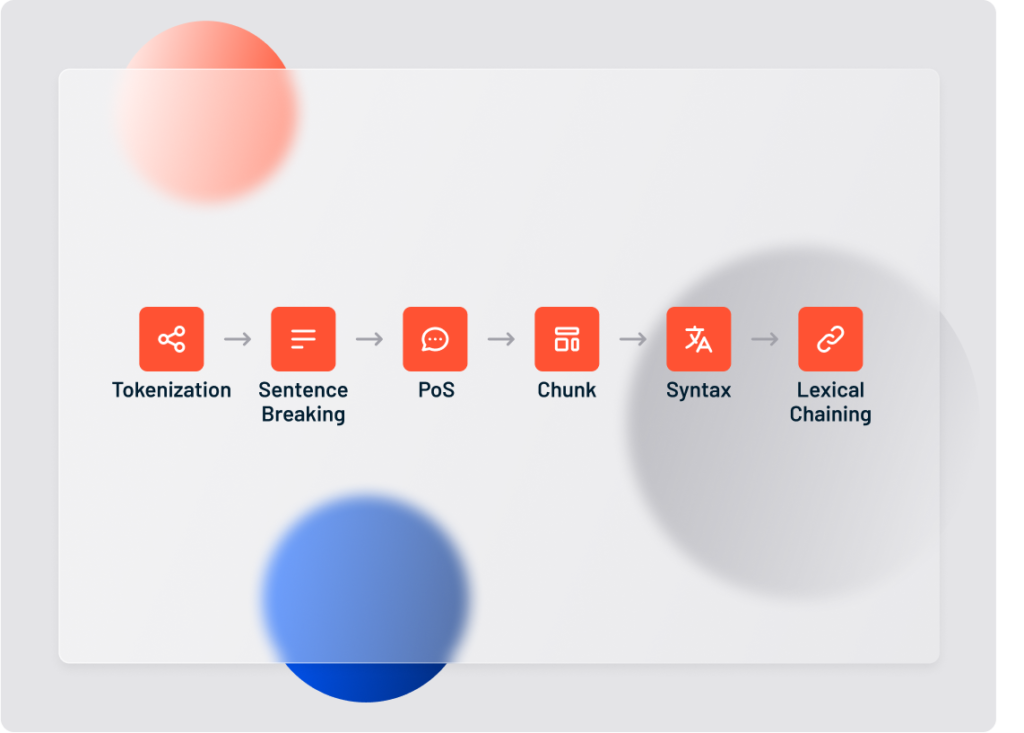
The final step in preparing unstructured text for deeper analysis is sentence chaining . Sentence chaining uses a technique called lexical chaining to connect individual sentences based on their association to a larger topic . Take the sentences:
- I like beer.
- Miller just launched a new pilsner.
- But I only drink Belgian ale.
Even if these sentences donât appear near each other in a body of text, they are still connected to each other through the topics of beer->pilsner->ale . Lexical chaining allows us to make these kinds of connections. The âscoreâ of a lexical chain is directly related to the length of the chain and the relationships between the chaining nouns (same words, antonyms, synonyms, homonyms, meronyms, hypernyms or holonyms).
Lexical chains flow through the document and help a machine detect over-arching topics and quantify the overall âfeelâ. Lexalytics uses sentence chaining to weight individual themes, compare sentiment scores and summarize long documents.
Basic applications of text mining and natural language processing
Text mining and natural language processing technologies add powerful historical and predictive analytics capabilities to business intelligence and data analytics platforms. The flexibility and customizability of these systems make them applicable across a wide range of industries, such as hospitality, financial services, pharmaceuticals, and retail.
Broadly speaking, applications of text mining and NLP fall into three categories:
Voice of Customer
Customer Experience Management and Market Research
It can take years to gain a customer, but only minutes to lose them. Business analysts use text mining tools to understand what consumers are saying about their brands, products and services on social media, in open-ended experience surveys, and around the web. Through sentiment analysis, categorization and other natural language processing features, text mining tools form the backbone of data-driven Voice of Customer programs.
Read more about text analytics for Voice of Customer
Social Media Monitoring
Social Listening and Brand Management
Social media users generate a goldmine of natural-language content for brands to mine. But social comments are usually riddled with spelling errors, and laden with abbreviations, acronyms, and emoticons. The sheer volume poses a problem, too. On your own, analyzing all this data would be impossible. Business Intelligence tools like the Lexalytics Intelligence Platform use text analytics and natural language processing to quickly transform these mountains of hashtags, slang, and poor grammar into useful data and insights into how people feel, in their own words.
Read more about text analytics for Social Media Monitoring
Voice of Employee
Workforce Analytics and Employee Satisfaction
The cost of replacing a single employee can range from 20-30% of salary. But companies struggle to attract and retain good talent. Structured employee satisfaction surveys rarely give people the chance to voice their true opinions. And by the time youâve identified the causes of the factors that reduce productivity and drive employees to leave, itâs too late. Text analytics tools help human resources professionals uncover and act on these issues faster and more effectively, cutting off employee churn at the source.
Read more about text analytics for Voice of Employee
Further reading
Text analytics and nlp in action:.
Try our web demo for a quick sample of Lexalyticsâ own text analytics and NLP features
Contact us for a live demo with your data, or to discuss our on-premise and cloud APIs
Build your own text analytics system:
Start working with the Stanford NLP or Natural Language Toolkit (NLTK) open source distributions
Browse this Predictive Analytics list of 27 free text analytics toolkits
Take a Coursera course on Text Mining and Analytics
Read about sentiment analysis and other natural language processing features
Explore the difference between machine learning and natural language processing
Dive deep with this practitionerâs guide to NLP on KDnuggets
Quick links
- Case Studies
- White Papers
Send Inquiry

What Is Text Analytics? Tools, Examples, and Applications
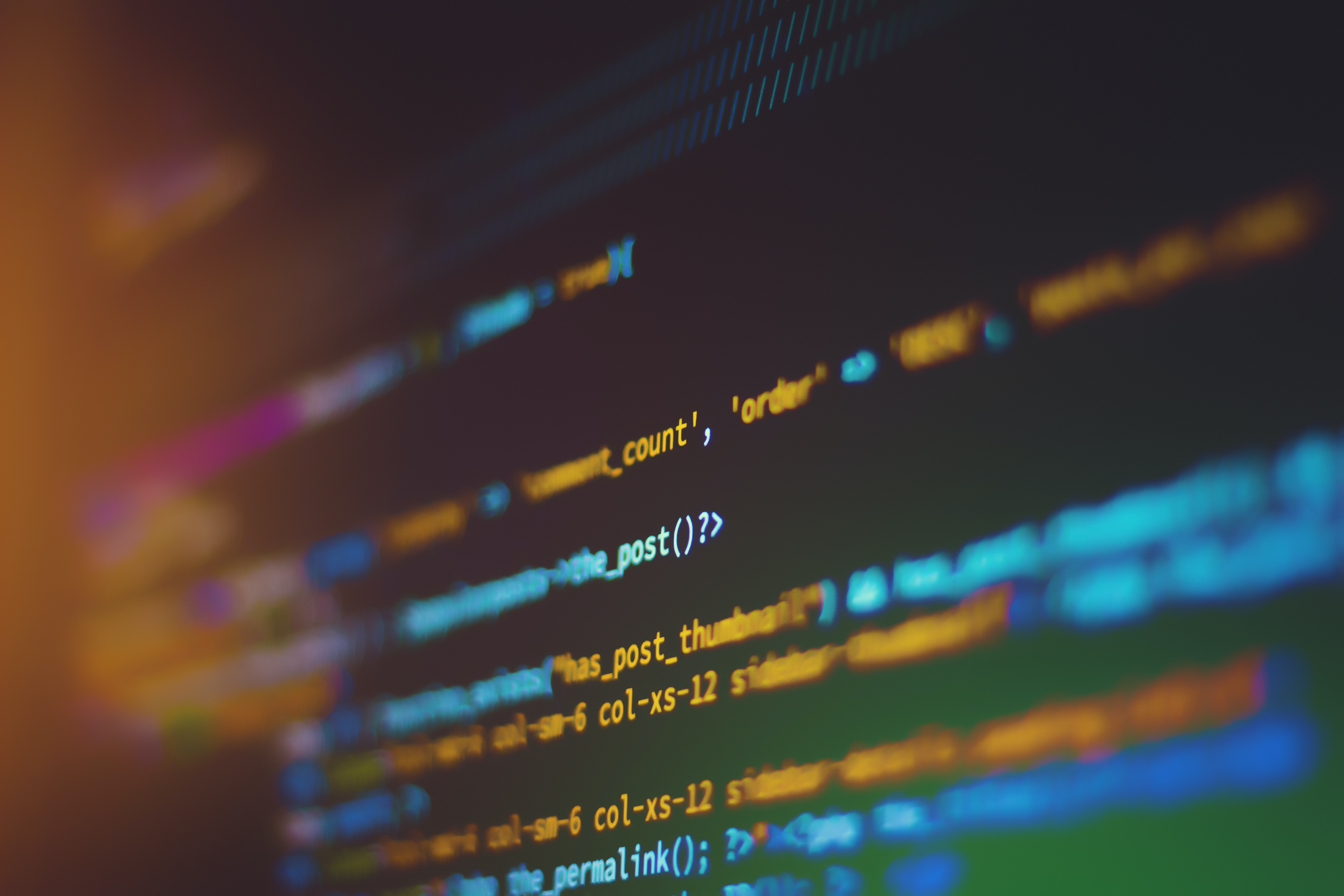
Published on Aug 04, 2021
With the advent of emergent technologies, businesses are having to hustle to sustain momentum. While it may seem a little overwhelming to start with, businesses that are reinventing and adopting such technologies stand to benefit greatly in terms of sharpening their competitive edge by being able to understand their customers and their needs in an unprecedented fashion.
Like data analytics , text analysis is one such advancement that promises to take businesses to the next level with the assistance of NLP and AI .
So, What is Text Analytics?
Text analytics is a sub-set of Natural Language Processing (NLP) that aims to automate the extraction and classification of actionable insights from unstructured text disguised as emails, tweets, chats, tickets, reviews, and survey responses scattered all over the internet.
Text analytics or text mining is multi-faceted and anchors NLP to gather and process text and other language data to deliver meaningful insights.
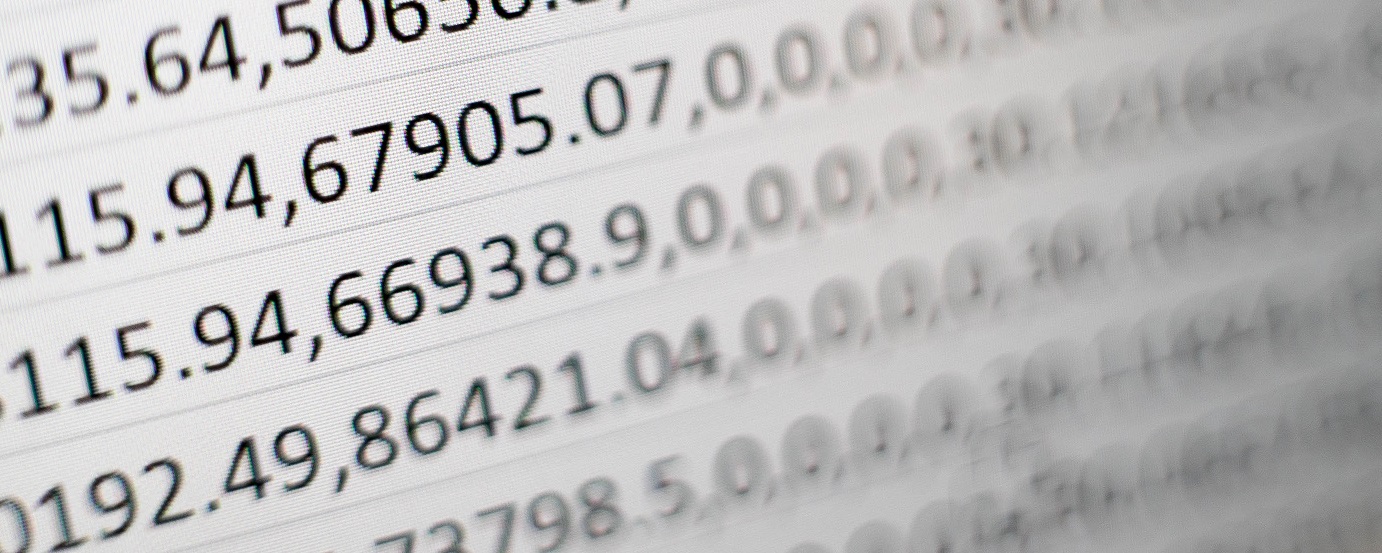
Why Text Analytics is Needed?
Maintain Consistency: Manual tasks are repetitive and tiring. Humans tend to make errors while performing such tasks – and, on top of everything else, performing such tasks is time-consuming. Cognitive biasing is another factor that hinders consistency in data analysis. Leveraging advanced algorithms like text analytics techniques enables performing quick and collective analysis rationally and provides reliable and consistent data.
Scalability: With text analytics techniques, enormous data across social media , emails, chats, websites, and documents can be structured and processed without difficulty, helping businesses improve efficiency with more information.
Real-time Analysis: Real-time data in today’s world is a game-changer. Evaluating this information with text analytics allows businesses to detect and attend to urgent matters without delay. Applications of Text analytics enable monitoring and automated flagging of tweets, shares, likes, and spotting expressions and sentiments that convey urgency or negativity.
Text Analytics Techniques
Word / Term Frequency
This nifty text mining technique captures frequently occurring words across a data set and rates their importance accordingly. To give a context: consider a customer feedback data set on a recently launched product. If the word ‘good’ appears more frequently, this may mean that the customers greatly like the product.
The biggest challenge with word/term frequency is that different but related terms aren’t checked. Different words may be applied to mean the same thought, but this can be reckoned as two different topics and rated accordingly, leading to disparities in coming to a conclusion.
Collocation
Collocation focuses on identifying commonly co-occurred words. In other words, bigrams and trigrams. Bigrams are two adjacent words, e.g., customer service, while Trigrams are three adjacent words, e.g., near the hotel. The understanding can help underpin semantic structures and improve the granularity of insights.
Sentiment Analysis
As the name suggests, sentiment analysis helps understand the impact of a product/service on the customer’s sentiments. Also, it helps compare the customer’s sentiment towards one product/service against that of a competitor.
There are three steps to performing sentiment analysis extensively:
Polarity Analysis: Measuring the tone of the data, i.e., positive or negative.
Categorization: Ranking the data in a specific metric, i.e., positive – happy, excited, pleased; negative – sad, angry, confused, frustrated.
Scaling: Scaling the emotions on a scale of 0 to 10.
Although sentiment analysis aims to glean valuable insights by contextualizing data, the biggest challenge with sentiment analysis is to spot sarcasm or irony and software is currently being developed to overcome this challenge.
Text Classification
The most advantageous NLP ( Natural Processing Language ) technique, it is language-agnostic. It can order, structure, and segment pretty much any data. Text classification helps assign predefined tags or categories to unstructured data. Sentiment Analysis, topic modelling, language, and intent detection all come under the text classification umbrella.
Topic Modeling
Topic modelling helps categorize documents based on specific topics. Topic modelling helps process different documents and abstract recurring themes and is less individualized. Topic modelling classifies and gives a percentage or count of words of each document assigned to a specified topic.
Named Entity Recognition
Named Entity recognition helps identify nouns with data sets. Consider numbers accompanied by ‘INR’ as being monetary; likewise, “Ms.” or “Mr.” or “Mrs.” followed by one or more capital words is probably a person’s name.
The major challenge is that, although some nouns define important categories like geographic location, name, or monetary values, some lack abbreviations, leading to a lot of confusion.
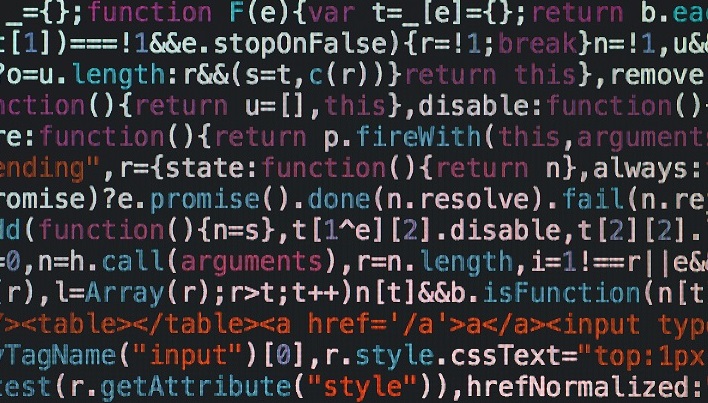
Applications of Text Analytics
Regardless of the industry, applications of text analytics can be of substantial support for businesses for understanding as well as reaching out to customers comfortably. Check below some of the use cases/benefits of text analysis for businesses.
#1. Social Media Listening
Apart from being a medium for staying connected, social media has also become a platform for branding and marketing. Customers talk about their favorite brands and share their experiences all across social media. Leveraging sentiment analysis of the data available on social media with the help of text analytics tools helps to understand the positive or negative sentiments of users towards products/services and the impact and relations of brands with their customers. Furthermore, social media listening can enable brands to build trust with customers.
#2. Sales & Marketing
Prospecting is a nightmare for a sales team. Sales teams make every effort to improve sales and performance. According to a MonkeyLearn study, 27 % of sales agents spend more than an hour a day on data entry work instead of selling, signifying critical time is lost in administrative work and not closing deals.
Text analytics techniques help reduce this menial work with automation while providing valuable and specific insights to nurture the marketing funnel.
To give you an idea: Chatbots are employed to cater to customer queries in real-time. Analyzing this data helps the sales team predict the likelihood of a customer buying a product, perform target marketing and advertising, and make product adjustments.
#3. Brand Monitoring
Businesses fight tooth and nail to establish and brand supremacy. Today, professionals are paid to write false or hype reviews across the internet and social media. Also, customers often write angry reviews in the spur of a moment. Such reviews often spread across the internet like wildfire and do unmitigated harm to a company’s brand image.
Negative reviews often drive away customers. Studies show, 40% of consumers are put off from buying a product/service if there is a negative review.
Visual web scrapers and web scraping frameworks in text analytics empower brand monitoring, comprehending one’s brand evolution, and pinpointing aspects affecting one’s brand in real-time, thus, enabling businesses to take necessary action immediately.
#4. Customer Service
Businesses constantly endeavor to facilitate seamless customer service. Much of the customer churn factors occur due to customer service flaws. With text analytics tools, you can scrape together customer concerns/queries and feedback to streamline customer service processes. Aside from improving responsiveness, this can also help automatically route tickets to reduce manual work and errors. To give an example: the algorithm draws a point ‘My order isn’t delivered yet’ out of customer queries – this will be compared and matched with a Delivery Issues tag automatically with the assistance of text analytics tools.
Additionally, text analytics tools will also help establish personalized customer services, employ the right person for the job, and set priorities efficiently.
#5. Business Intelligence
Although businesses can glean “what is happening?” with data analysis, they struggle to figure out “why this is happening?”. Applications of text analytics help businesses obtain context out of the numeric data and reason out why a situation has happened or is happening or what may happen in the future. Case in point, a large number of factors contribute to sales performance. While data analysis will provide one with numerical statistics, text analytics techniques will help determine why there is a drop or rise in the performance.
#6. Product Analytics
Text analytics not only helps in understanding customer needs but also helps in improving the product. Analyzing customer reviews gives a clear picture of what exactly the customer is looking for vis-à-vis a product and what they think about the competitor’s product. It enables businesses and brands to build quality products that meet customer requirements.
#7. Knowledge Management
We suffer from an overabundance of data today. Processing this behemoth data to draw actionable insights in less time is hardly possible without sophisticated technology advancements. This puts time-sensitive professions like healthcare in dire straits. However, text mining or text analytics techniques can help sort through surplus data in a short time and provide valuable insights for real-time solutions and efficiency.
#8. Email Filtering
Text mining can help detect junk, spam, or malicious emails, preventing fraud and other kinds of cyberattacks.
What Are the Tools for Text Analytics?
The top brands often have a robust consent-gathering system and privacy policy to meet specific countries’ privacy regulations. Nevertheless, they must explore, compare, and try the available text analytics tools and services to benefit from their multi-disciplinary applications. Here are the text analytics providers that global corporations leverage for business operations.
Text Analytics Technologies Businesses Must Know About
1. advanced text analytics by sg analytics (sga) .
SG Analytics offers sentiment detection, consumer opinion discovery, and trend identification across unstructured datasets. Therefore, client organizations can employ SGA’s NLP-powered insights for customer journey personalization, enhancing retention and repeat purchase rate.
The analysts will increase the reliability of your chatbots and similar conversational marketing technologies. So, customer engagement will improve. Besides, their trend reporting will help ensure that your market research methodologies align with your business development strategies. They also customize NLP models, including multilingual text analytics, according to clients’ requirements.
2. NLP by Lexalytics, Inc.
Lexalytics offers natural language processing APIs or application programming interfaces. It includes sentiment analysis and content categorization. Clients have three APIs: Salience, Semantria, and Spotlight.
Salience is for NLP-related data science and architecture. Meanwhile, Semantria adds graphical user interface (GUI) elements on top of Lexalytics’ core APIs. Finally, Spotlight focuses on unstructured text document visualization and dashboards.
Future Trends in Text Analytics
Novel tools for text analytics and sentiment-based content evaluation increase the effectiveness of the applications discussed above. Simultaneously, visionary investors empower academic and corporate research projects exploring more creative NLP integration pathways. Let us understand which innovations are already driving industry growth and what are other text analysis ideas in the making.
Emerging Technologies and Advancements in Text Analytics
Multilingual nlp.
YouTube, Twitter (X), and Google Translate enabled netizens to interact with each other irrespective of language barriers. Today’s brands also want to develop identical text analytics technologies to increase their market share and expand beyond borders. Multilingual natural language processing models facilitate these solutions empowering global companies.
Customers reviewing your offerings use their first language when discussing the pros and cons of your products or services online. Therefore, integrate multilingual NLP-based text analytics tools across all your market research (MR) and sentiment detection processes. Doing so will help reduce the time, effort, and resources.
Contextual Brand Mentions Tracking in News
One of the remarkable text analytics examples is how the analysts can monitor whether news publications and industry magazines mention your brand with a positive value association. For instance, imagine business leaders concerned about controversial coverage or rumors related to their company. They can leverage text analysis, NLP, and sentiment attribution to study what the press has said about their policies and decisions.
Visualizations Based on Unstructured Data
Text analytics have evolved, highlighting different parts of a sentence of an entire article according to topic relevance, correctness, and emotions. Specific tools also demonstrate when the readers will lose interest in your marketing copy, work resumes, news piece, or technical proposals. The most popular visualizations for unstructured data, like consumer reviews, utilize color-coded geometric areas.
Accordingly, the examples or applications of text analytics visualizations encompass word cloud, tag cloud, Sankey chart, and slope graph.
Word Cloud:âŻIt describes frequently utilized phrases in distinct sizes and colors according to how often they appear in a text document.
Tag Cloud:âŻIt shows the actual number of each word’s presence in a copy.
Sankey Chart:âŻIt uses a custom flow chart to break down complex, technical literature at a sub-topic level. Sankey charts have helped engineers and product designers explain as well as learn energy transfer, resource consumption, and project management mechanisms. Thanks to modern text analytics tools, you can use them to streamline skill development and consumer education material.
Slope Graph:âŻIt outlines whether a word’s popularity or relevance has fluctuated over a finite period. As such, it helps inspect the ever-changing consumer interests and search engine optimization (SEO) opportunities.
Predictions for the Future of Text Analytics Applications
The following text analytics innovations are still under development or require more research.
1| Universal Accessibility Enhancements
Screen reading software allows individuals with unique audio-visual conditions to enjoy multimedia content. However, several websites, streaming platforms, and e-books lack the necessary encoding to help accessibility applications understand what the screen contains.
Text analytics examples in user accessibility will rely on machine learning (ML) models that can describe screen elements irrespective of the format. Although a few mobility devices include “experimental” accessibility options to deliver identical experiences, they offer a robotic feel.
An adequately-optimized ML and text analytics integration can overcome the challenge of “sounding artificial” or describing insignificant screen elements. Therefore, organizations must heavily invest in this space, extending it to converting videos and audio into more accessible formats.
2| Data Localization and Privacy Regulation
Cloud computing has assisted text analytics providers in accelerating sentiment, context, and consumer intent recognition. While it might streamline market research and social listening, the rising regulatory requirements concerning consumer data protection affect its future.
Therefore, data localization without losing efficiency is a significant hurdle to text analytics’ effectiveness. The mobile and computing devices that users possess cannot mimic the capabilities of remote servers powered by enterprise-grade central processing units (CPUs) and memory modules.
Similarly, this situation raises these questions:
How will text analytics services comply with regional data and privacy protection laws?
How do we decentralize cloud-related processes to respect data localization norms?
Does the decentralization threaten multilingual NLP models’ training dataset quality?
Who will be legally liable if text analytics applications accidentally or deliberately process a user’s personally identifiable information (PII)?
Text Analytics in the Future
Text mining or text analysis is useless without NLP. It intends to deliver practical, persistent, and credible insights with machine learning. The key objective is to arm businesses with real-time insights that help them drive innovation as well as rack up customer service and profits. The rapid growth in the requirement of understanding the customer will result in an increase in text analytics tools.
Conclusion
Text mining or text analysis is useless without NLP.âŻItâŻintends to deliver practical, persistent,âŻand credible insights with machine learning. The key objective is to arm businessesâŻwith real-time insights that help them drive innovation as well as rack up customer service and profits.âŻThe rapid growth in the requirement of understanding theâŻcustomer willâŻresult in an increase inâŻtext analytics tools.
Our Services
Investment Insights
Market Research
Data Analytics
ESG Services
Data Solutions
ESG Data Services
Technology Services
Investment Banking
Private Equity/VC
ESG Data and Research
Marketing Analytics
Advanced Analytics
Customer Analytics
Hedge Fund Services
Market Intelligence
Equity Research
Recent Blogs
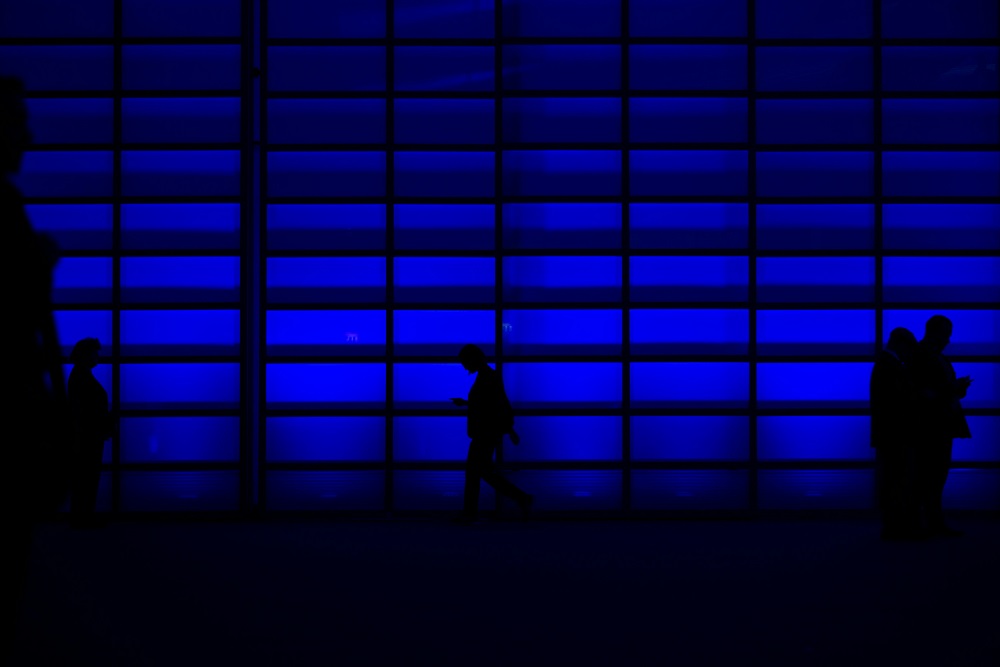
Harnessing the Power of Data to Transform Business Models and Drive Growth
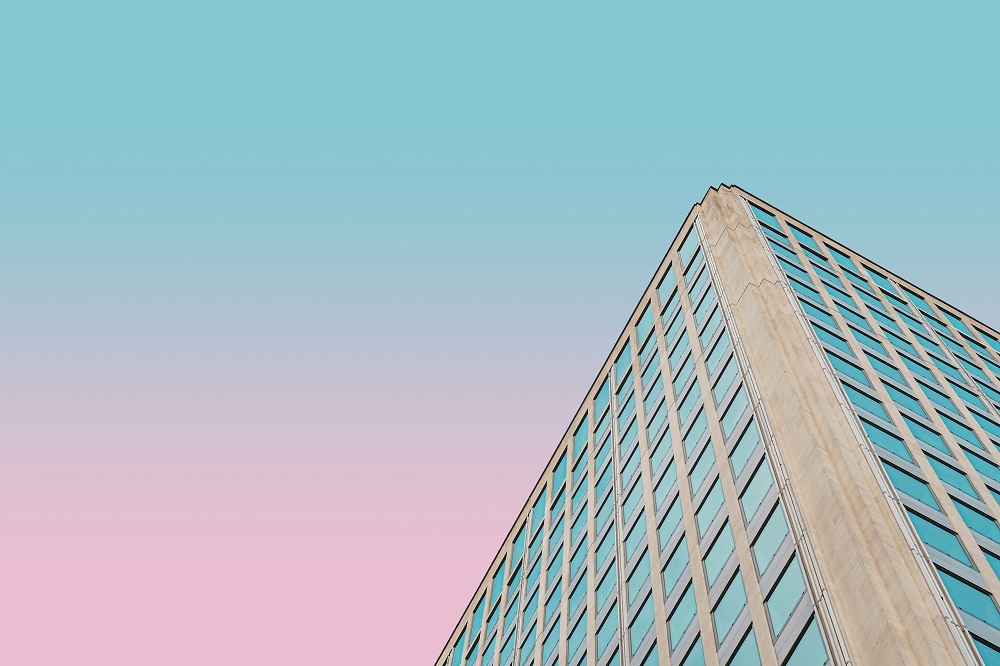
Integrating Generative AI In Banking: Risks and Benefits
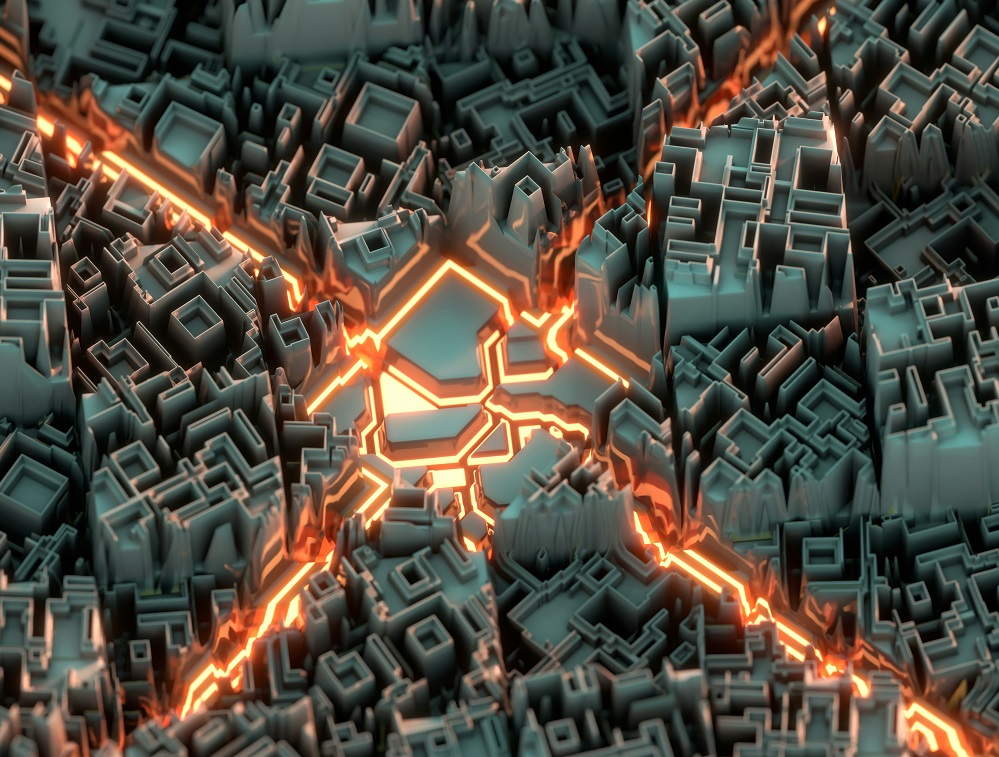
Data Revolution: Empowering Businesses for a Data-driven Future
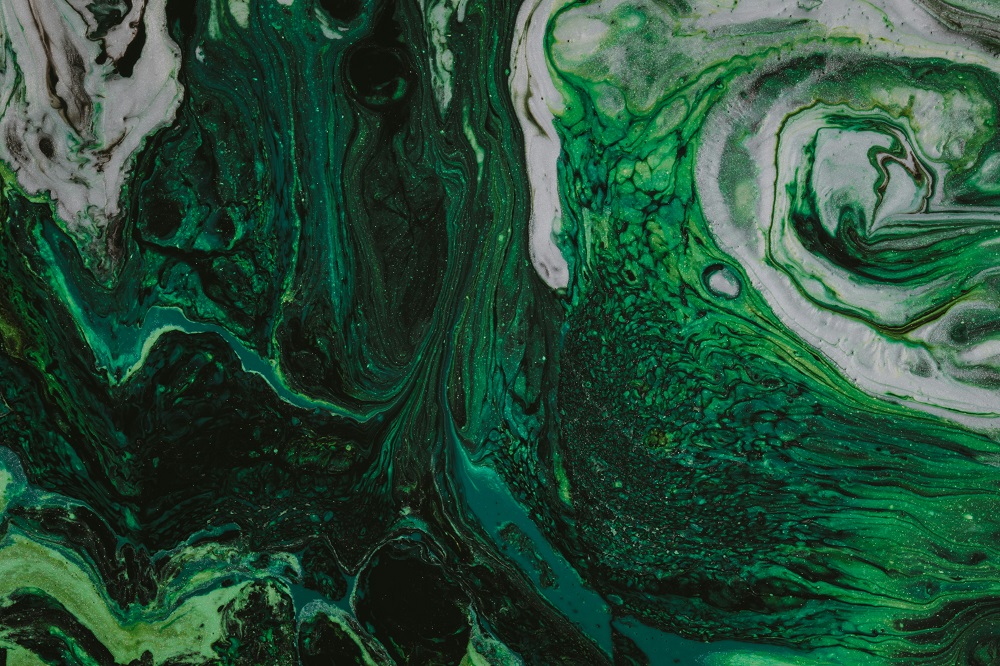
A Simple Guide on How to Avoid Greenwashing Traps
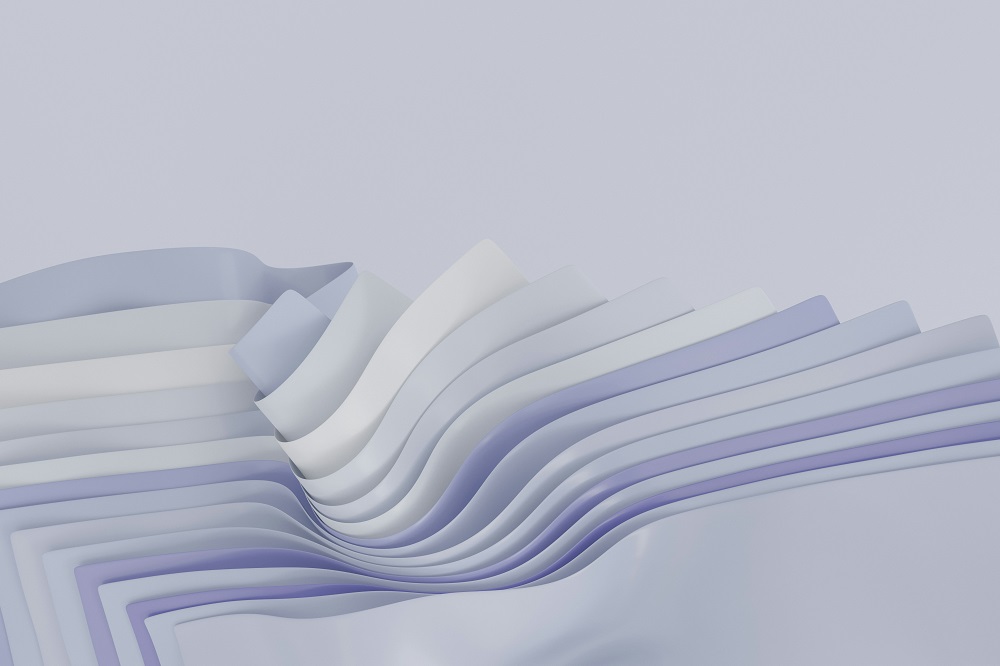
Data-Driven Decision-Making: The Key to Thriving in the Digital Age
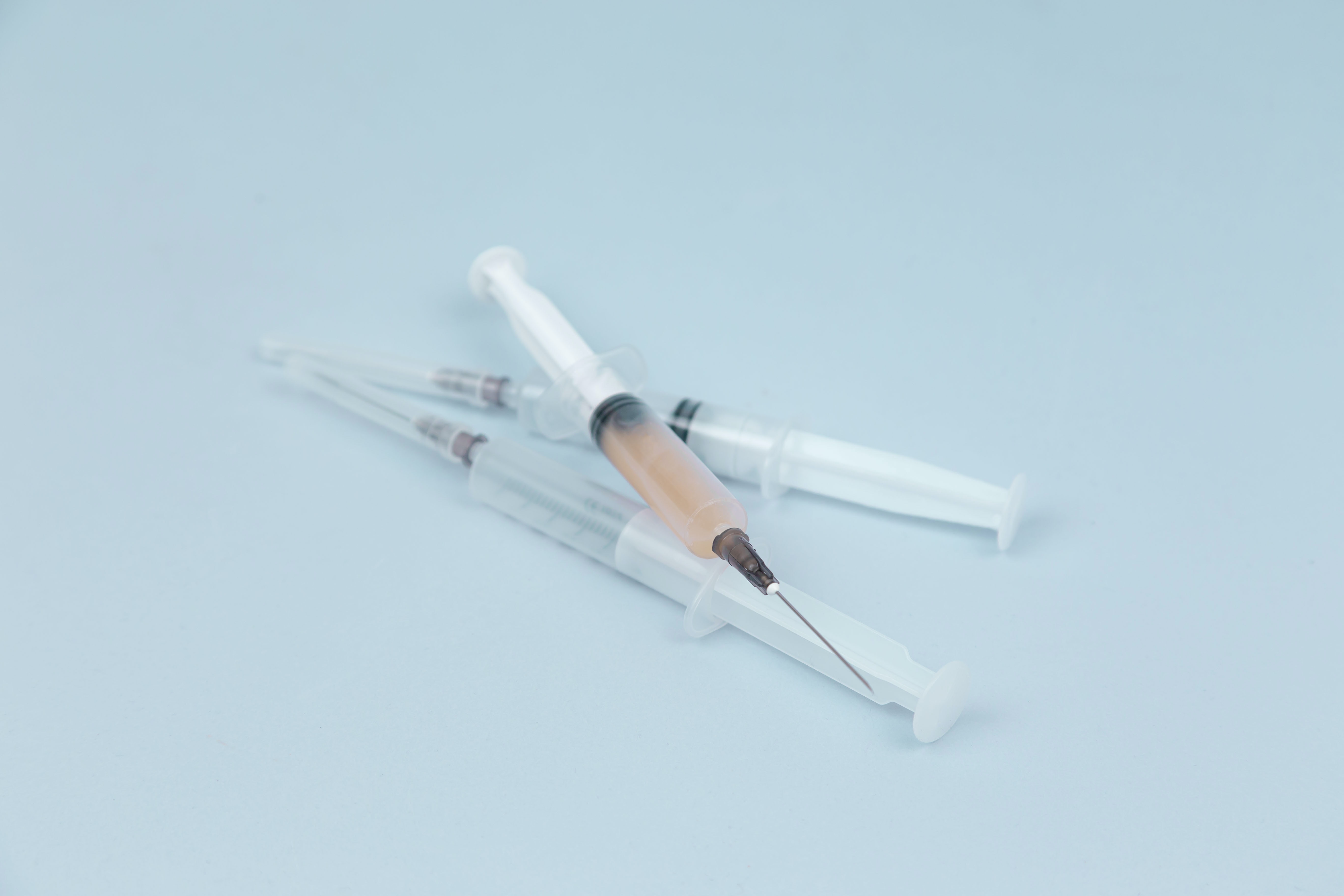
Future Trends and Technological Advances in Vaccine Treatment and Disease Prevention
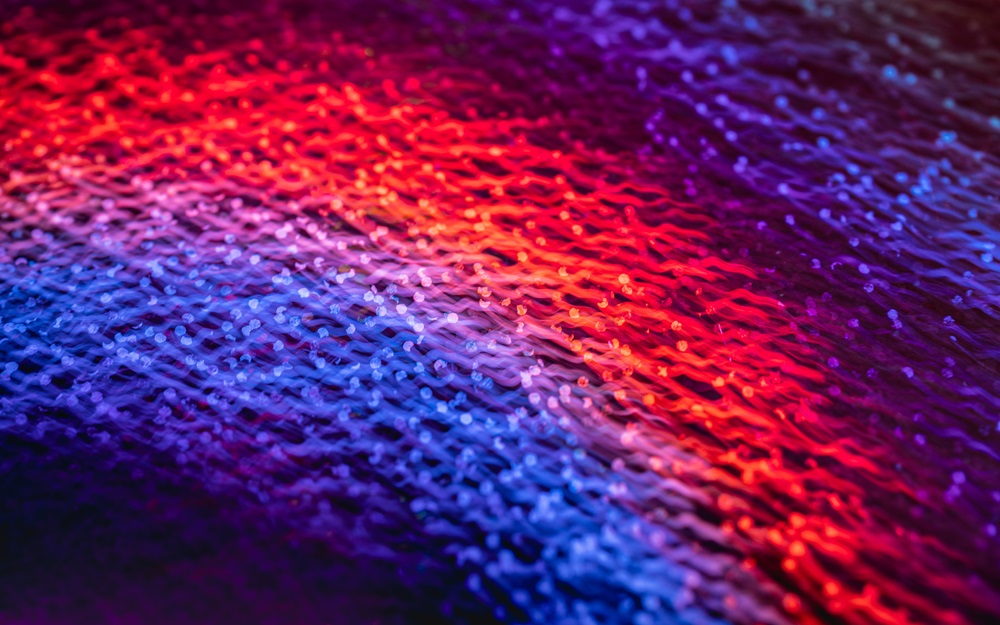
Emergence of Novel Immune - Mediated Therapies
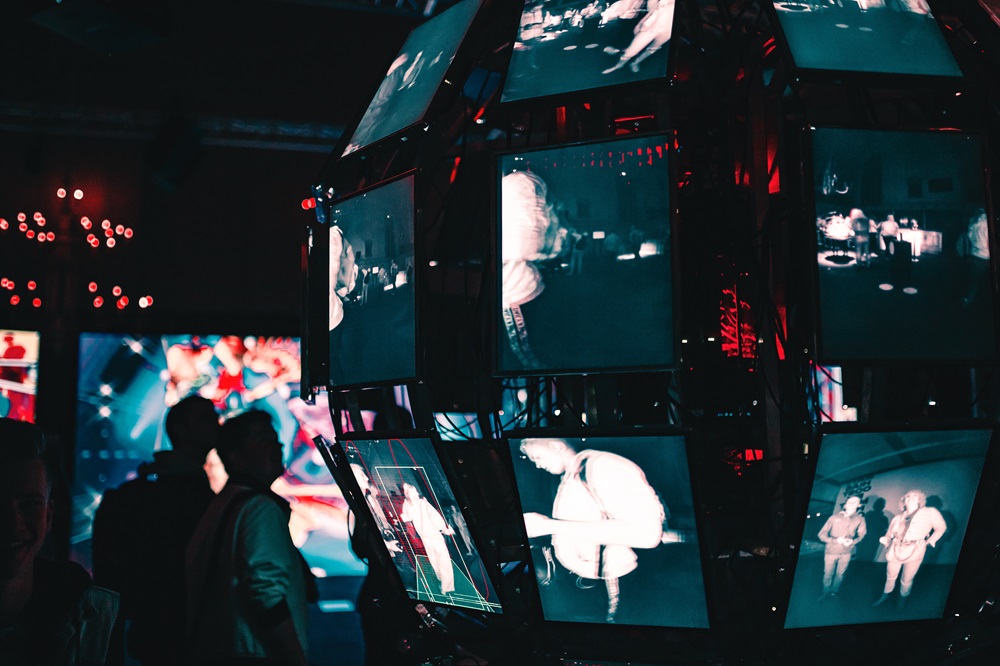
2024 Trends to Watch -TV & Video Technology
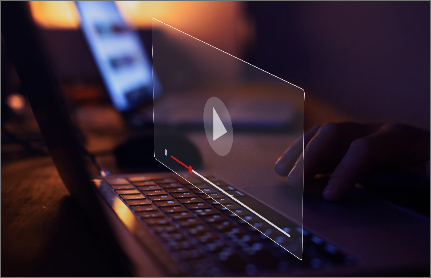
US OTT (SVOD) - The New Frontier

SGA Knowledge Team
We are a dynamic team of subject matter experts who create informative, relevant and...
Sprinklr Service
Sprinklr Social
Works Best With
Sprinklr Insights
Sprinklr Marketing
Marketing Teams
Customer Service Teams
- Unified-CXM
- Customers Customer Stories Sprinklr Champions
- Company Our Story Leadership Newsroom Partners Careers Culture & Talent Investor Relations Security & Data Privacy
- Resources Learn Services Support CX-WISE Podcast Analyst Reports Product Demo Days eBooks & Reports Events & Webinars Blog Unified-CXM Guide Our Services Training For Agencies Help Center Release Notes Contact Us
.css-14us1xl{box-sizing:border-box;margin:0;min-width:0;white-space:pre-wrap;} Text Analysis
An introduction to text analysis and related terms, the business applications and implications, and important tools for getting started.
Share this Topic
.css-1qemmfx{font-weight:700;} What is text analysis?
Text analysis involves analyzing and processing text data to extract meaningful information. It can be done manually, by reading and interpreting the text, or it can be done computationally using various algorithms. Text analysis, when carried out computationally is referred to as text mining or text analytics.
Text analysis can be applied to all kinds of documents, including written documents, social media posts, customer reviews, and other forms of digital communication. It can be used to identify the sentiment, emotion, and meaning of text, as well as to classify and categorize it, extract key phrases and terms, and summarize and translate it. It is often used in a variety of business and research applications, such as customer sentiment analysis, content classification, and market research.
What's the difference between text analysis, text mining and natural language processing?
Text analysis, mining, and natural language processing (NLP) are terms that are often used interchangeably. However, there are some key differences:
Text analysis is the process of examining and interpreting text in order to extract meaningful insights. It can involve both manual and computational methods.
Text miningor text analytics is a subfield of text analysis that specifically involves computational algorithms to extract and analyze the data.
Natural language processing (NLP) is a subfield of AI that involves the development of algorithms to understand, interpret, and generate human language. NLP algorithms are used for text analysis, but also have applicationsin language translation, and voice recognition.
The importance of text analysis
Text analysis has gained prominence over the previous years due to changing dynamics in industry:
Unstructured text data is increasingly prevalent: text data is generated in large quantities through sources such as social media, customer reviews, and online articles.
Text analysis can unravel patterns: algorithms can identify patterns in large datasets that might not be immediately obvious to humans. This is useful for understanding customer sentiment, identifying trends, and making data-backed decisions.
Text analysis can improve efficiency: manually analyzing large volumes of data can be time-consuming and error-prone. Algorithms can automate the process, allowing organizations to extract insights more efficiently.
Text analysis use cases
Text analysis has a wide range of business applications, including:
Market research : Text analysis can be used to analyse online reviews, social media posts, and other forms of customer feedback. This can help businesses understand consumer wants and attitude towards different products and services.
Customer segmentation: Text analysis can be used to identify groups of customers with similar needs or preferences. This allows businesses to tailor their efforts to specific groups of customers. Techniques like clustering can be used to divide the text data into groups based on similarities. The business can then use the characteristics of each group to tailor their marketing or support efforts.
Language & location identification: NLP techniques can be used to identify the language and location of text. This can improve content accuracy and relevance for applications like translation, geotargeting, and content recommendation.
Content classification: Large volume of text data can be classified and categorized using text analysis. This can be useful for organizing and managing datasets and for identifying relevant information.
Text summarization: Algorithms can summarize large datasets making it easier for businesses to quickly extract key insights and ideas.
Trend identification: Large amount of text data can be analyzed to identify patterns and trends over time. This can be useful for identifying changes in public opinion, emerging topics, and seasonal trends.
Intent identification: Conversations can be analyzed to identify the underlying reason or goal that a customer has when interacting with a business. This can be useful for improving customer experience, customer support, and identifying new product or service opportunities.
Fraud detection: Algorithms can be trained to identify fraudulent activity by analyzing the language and content of digital communication.
HR and talent management: Text analysis can be used to analyze resumes, job descriptions, and other text data to identify the right candidates. It can also be used to analyze employee feedback and performance reviews.
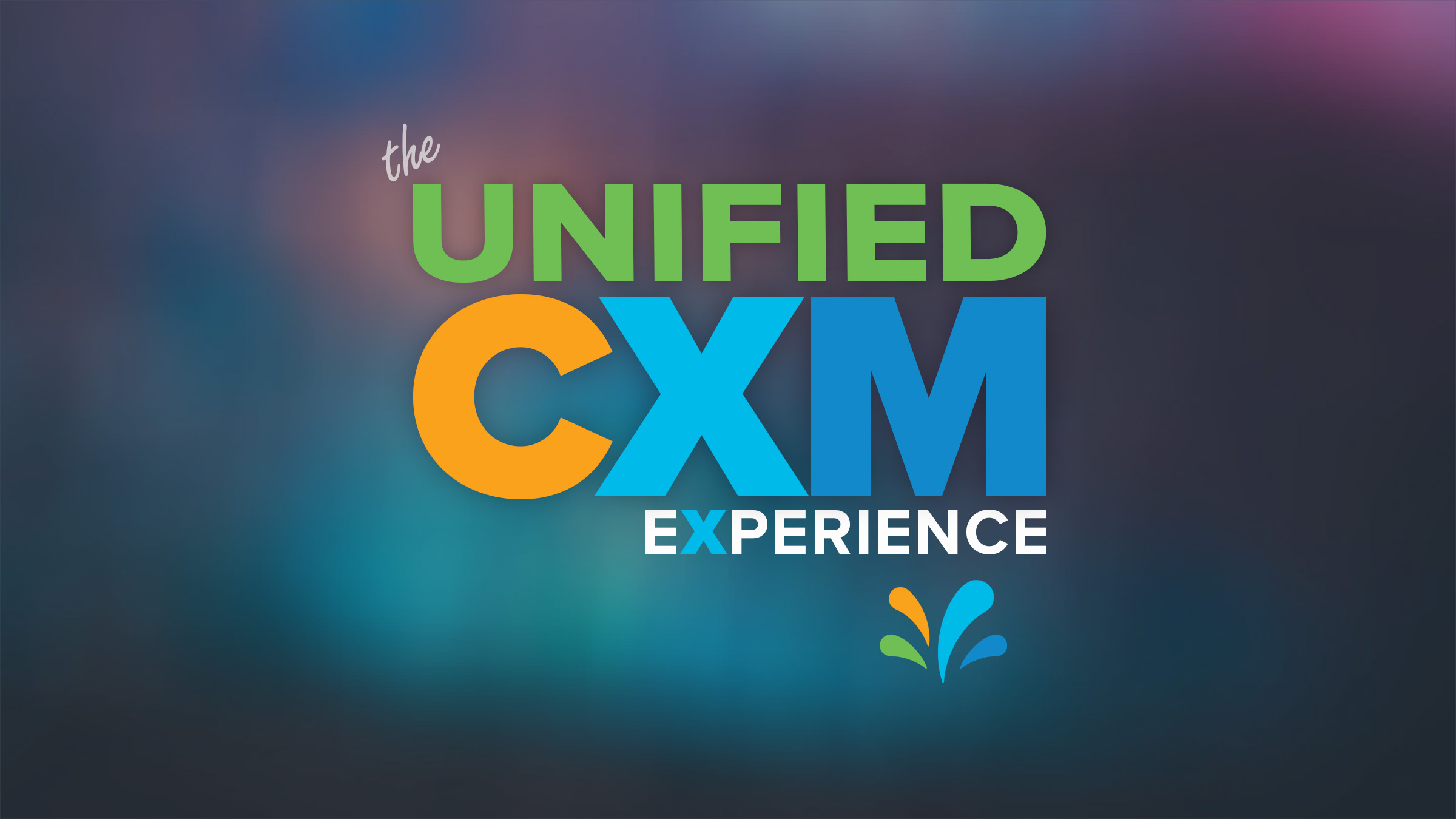
Episode #14: The Complete Guide to AI-Powered Intent Analysis
The best text analysis method - Topic modeling
Topic modeling is used to identify the main topics or themes in a large dataset. It involves identifying patterns in the text data and grouping similar data together. Topic modeling is often used in combination with other techniques, such as text summarization and content classification, to extract insights and identify trends.
Topic modeling can be used to identify key terms that are most representative of each topic, and to generate a list of the most relevant text for each topic. This can be useful for organizing large datasets such as customer reviews, and for identifying the main topics of discussion.
Key elements of topic modeling in text analysis
There are several key elements of topic modeling in text analysis. They are used to identify the main topics or themes in a large dataset of text documents and to extract insights and trends from the data.
Text corpus: The collection of documents that will be used for topic modeling. This could include customer reviews, online articles, or social media posts.
Preprocessing: Topic modeling cannot be applied directly to text data, it requires preprocessing to remove unnecessary information and format the data for analysis. This can include tasks such as tokenization, stemming, and stop word removal.
Term frequency-inverse document frequency (TF-IDF): TF-IDF is a statistical measure that calculates the importance of a term in a document, relative to the entire dictionary. It is commonly used in topic modeling to identify the most important terms and phrases that describe each topic.
Latent Dirichlet allocation (LDA): LDA is a well-known algorithm that is used to find the main topics in a collection of text documents. It works by assuming that each document is a mixture of a small number of topics, and it employs statistical methods to determine the likelihood of each topic is present in the corpus.
Topic representation: Once the topics have been identified, they can be represented in a variety of ways, such as as a list of key terms or as a visual representation of the most relevant documents for each topic.
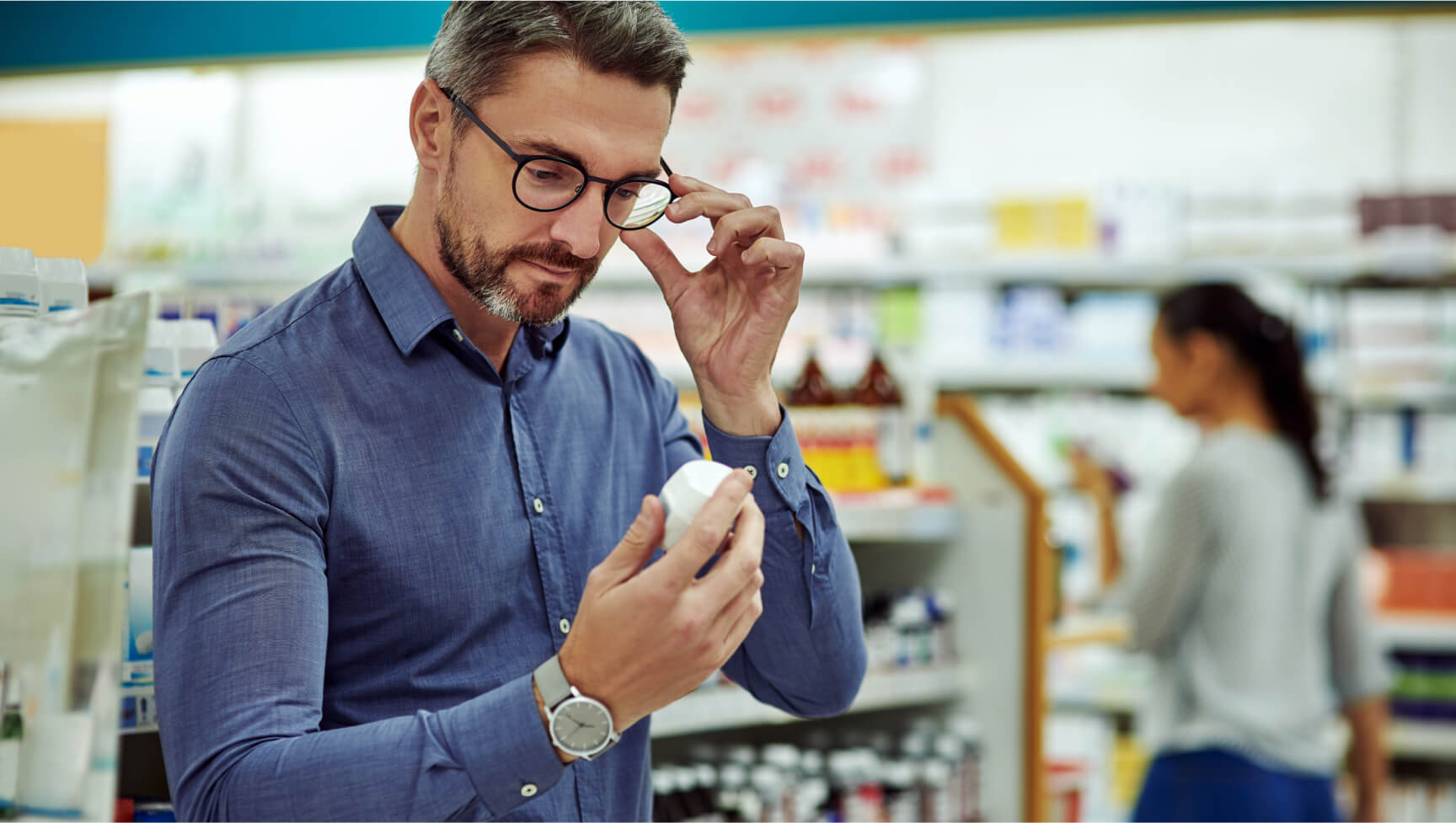
5 questions to answer about customer insights
How to model topics for text analysis
There are several steps involved in modeling topics for text analysis:
Preprocessing: This step is used to remove noise and prepare the dataset for analysis. It includes tasks such as tokenization, stemming, and stop word removal.
Term frequency-inverse document frequency (TF-IDF): TF-IDF is a measure of the importance of a term within a document in relation to the entire dictionary. It is used to identify the key terms and phrases that are most representative of each topic.
Algorithm selection: A wide variety of algorithms can be used for topic modeling, including LDA and non-negative matrix factorization (NMF). The appropriate choice is one that works for the size and complexity of the dataset and that meets the specific goals and objectives of the analysis.
Model training: This process involves fitting the model to the data and adjusting the model parameters to optimize model performance.
Topic identification: The trained model can be used to identify the key themes in the text data. This can be achieved by extracting the most significant terms and phrases that best describe each topic, and by clustering similar documents.
Visualization and interpretation: The output of topic modeling can be represented in different formats, such as a list of essential terms or a visualisation of the most related documents. Interpreting the results is crucial to identify their significance in relation to the intended goals and objectives of the analysis.
Bottom-up topic modeling in text analysis
Bottom-up topic modelling identifies topics by grouping individual terms into larger, abstract concepts or topics, in contrast to the top-down approach, which starts with pre-defined topics and associates documents or terms.
Algorithms such as LSA, LDA, identify patterns and trends by analyzing relationships between individual terms. They can be used to identify the main topics in a dataset of text documents and to group similar documents together based on their content.
Bottom-up topic modeling can be useful for identifying hidden patterns and trends in large datasets of unstructured text data and for extracting insights and trends from the data. It is often used in combination with other text analysis techniques, such as text summarization and content classification, to extract insights and identify trends in large datasets of text data.
Top-down topic modeling in text analysis
Top-down topic modeling is an approach to identifying topics that involve starting with a pre-defined set of topics and identifying documents or terms that are most closely associated with each topic.
Algorithms such as hierarchical clustering and latent class analysis work by analyzing the relationships between individual terms and identifying patterns and trends in the data. They can be used to identify the main topics in a dataset of text documents and to group similar documents together based on their content.
Top-down topic modeling is handy for identifying patterns and trends in large, unstructured datasets and for extracting insights from the data. It is used in combination with text summarization and content classification to extract insights and identify trends in large datasets.
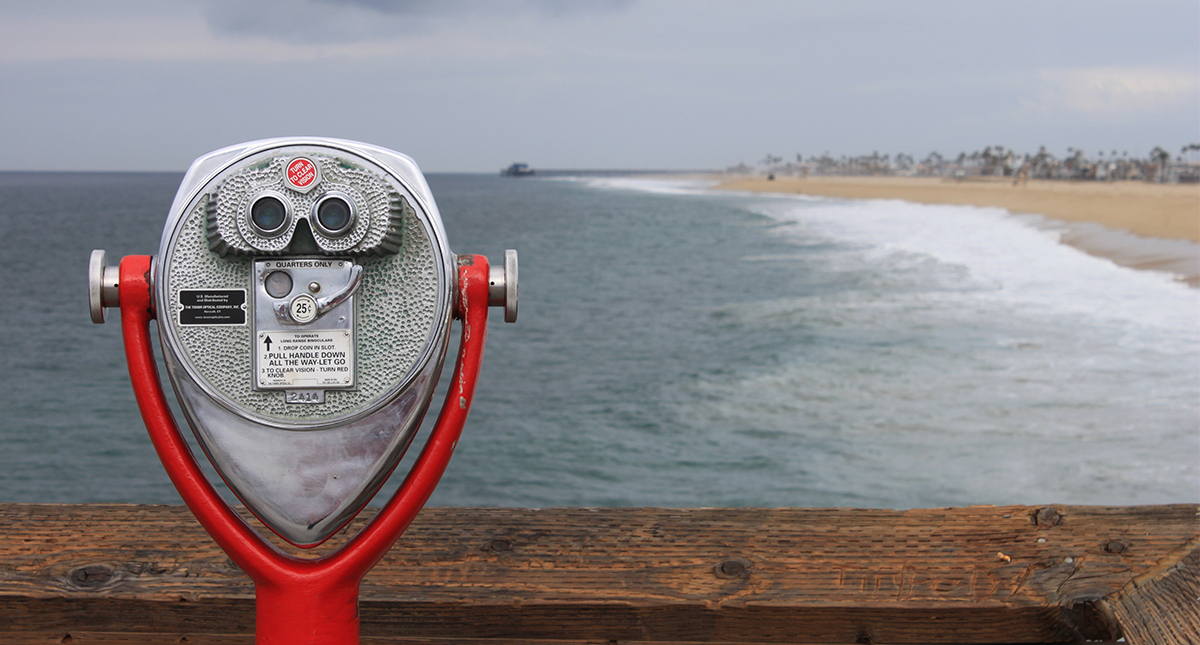
Best practices of modeling topics for text analysis
Here are some best practices for modeling topics for text analysis:
Preprocessing: It is important to preprocess the data to remove noise.
Choice of dataset: The text corpus should be chosen to make sure that it is relevant and contains enough information to generate meaningful topics.
Choice of algorithm: There are various algorithms that can be used for topic modeling. It is important to select the algorithm that is appropriate for the size and complexity of the dataset and meets the specific goals and objectives of the analysis.
Interpreting the results: The analysis results need to be interpreted and considered in relation to the objectives of the analysis.
Visualizing the results: The results of the analysis can be visualized in many ways - a list of key terms or a visual representation of the most relevant documents for each topic.
Validation: Topic modeling is an unsupervised algorithm, and results can be subjective, hence the need for validating the results with human experts.
Fine-tuning: Continuously monitoring and fine-tuning the model based on the results and feedback obtained is necessary for its continued success.
How accurate does your text analysis need to be?
The required accuracy for text analysis algorithms depends on the objectives of the analysis and the consequences of errors. In some cases, where the results of the analysis will be used to make important decisions, high accuracy may be critical. In other cases, lower accuracy may be acceptable, as long as the results are still useful and provide meaningful customer insights .
The accuracy can also vary depending on the complexity of the data and the quality of the training data available. Some common tasks, such as text classification and sentiment analysis, can have relatively high accuracy levels, while others, such as language translation and text generation, can be more challenging and may have lower accuracy levels.
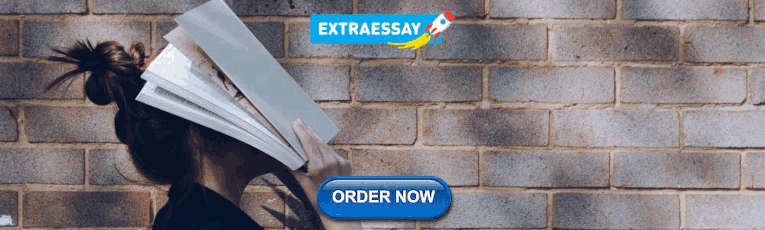
Text analysis in multiple languages
Text analysis can be carried out across different languages using a variety of approaches:
Translation: The simplest approach is to translate the text data into a single language, e.g., English, and then analyze the translated data.
Multilingual text analysis: Another approach is to use algorithms that are trained to handle multiple languages. These algorithms may have language-specific models or may be designed to handle multiple languages in a single model.
Cross-lingual text analysis: Cross-lingual models identify patterns and trends in text across different languages. This is achieved using translation algorithms or multilingual models, or a combination of the two.
Tools for text analysis
A variety of software can be useful for text analysis, depending on the objectives of the analysis and the characteristics of the data. Some essential, general-purpose software may include:
Programming language: A programming language, such as Python or R, is essential for developing custom text analysis algorithms. The also provide access to a wide range of readymade libraries and frameworks.
Spreadsheet software: Spreadsheet software, such as Excel or Google Sheets, can be useful for basic preprocessing tasks.
Data visualization tools: Tools such as Tableau can be useful for creating interactive visualizations of data, such as word clouds and sentiment analysis charts.
NLP library: An NLP framework, such as NLTK or spaCy, can be useful for setting up tasks such as tokenization, stemming, and part-of-speech tagging.
ML library: A machine learning framework, such as scikit-learn or TensorFlow, can be useful for developing custom algorithms and for accessing a wide range of pre-made algorithms.
Apart from the above-mentioned general purpose software, a large number of software companies provide text analysis software suites catering to various use cases through a simplified user experience.
Why you should use text analytics in customer experience
Text analysis is important for CXM because it can help businesses understand how customers feel about their products and services, and to identify strengths and weaknesses. By analyzing customer feedback, businesses can get a better understanding of customer sentiment around their products. This information can be leveraged to improve customer experience.
Text analysis can also be used to identify common themes or issues that customers are concerned about. This can help businesses identify opportunities for improvement and to make data-driven business decisions.
Businesses can use text analysis to identify groups of customers with similar needs and preferences, allowing them to tailor their efforts to specific groups. Clustering techniques can be used to divide text data, and the characteristics of each group can inform targeted marketing or support efforts.
Lastly, text analysis techniques can be used for identifying and tracking patterns and trends over time, which can be useful for identifying potential problems before they become significant.
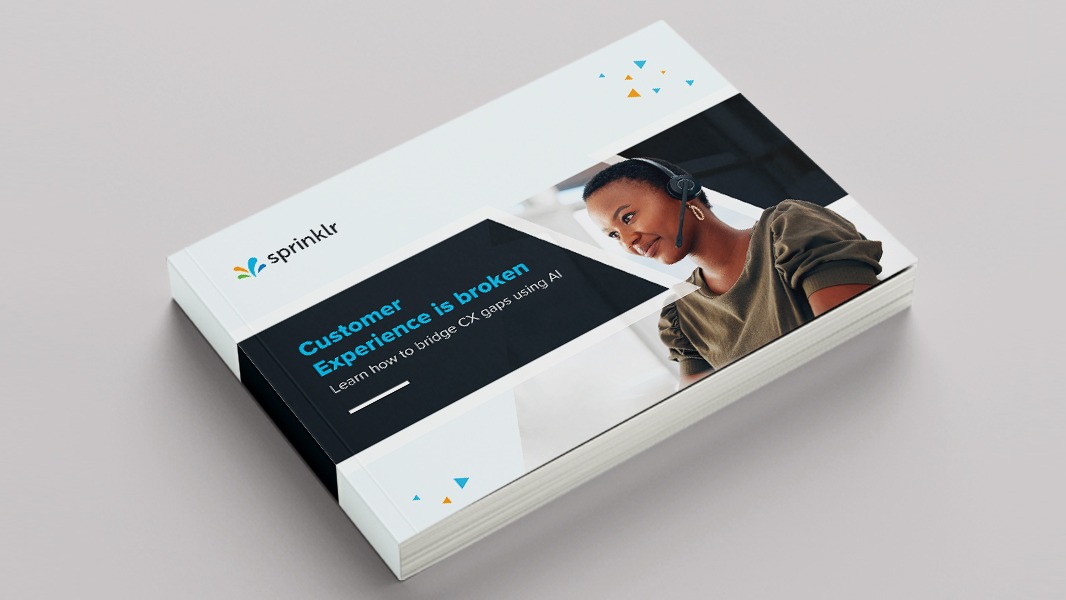
Customer Experience is broken. Learn how to bridge CX gaps using AI and automation
Why Sprinklr is the best text analysis software
Sprinklr offers a variety of products aligned to different aspects of the text analysis value chain. Through its product suites, Sprinklr is able to support the following:
Widest source coverage: Sprinklr's customers have access to the widest source coverage, including popular social media platforms (Facebook, X, formerly Twitter, Reddit, Instagram, YouTube), as well as over 100 million+ web sources (blogs, forums, and news websites). Additionally, through Sprinklr's Product Insights , customers get access to 600+ premium review sources with full historical coverage. Customers can also easily connect internal data sources for analysis within Sprinklr. This allows businesses to get a holistic view of what is being said about their brand and industry, and to identify trends and insights that can inform their business strategies.
Best-in-class sentiment analysis: Sprinklr's sentiment analysis capabilities allow businesses to track and monitor the sentiment of customer conversations over time and to identify changes in customer sentiment. Sprinklrâs sentiment analysis model supports 100+ languages to provide a well-rounded global view of conversations.
Granular insights through Product Insights: Sprinklrâs Product Insights provides advanced analytics capabilities at the phrase-level, including sentiment analysis, category classification, and entity detection.
Verticalized AI models: All Product Insights AI models are verticalized - specialized for a specific industry/ product segment - enabling customers to dig deeper into consumer conversations and identify actionable insights.
Simplified reporting: Sprinklr provides access to a wide variety of standard dashboard and reporting templates that cater to the most common use cases. Users can also create custom dashboards using Sprinklrâs library of 45+ chart types to create visualisations for their unique needs.
Dedicated data science team: Sprinklrâs products are developed using a staff of over 200+ ML engineers and data scientists. This deep domain expertise enables Sprinklr to create best-in-class AI models, leading to improved model performance.
SaaS solution: Sprinklr is a true SaaS solution that is deployed on the cloud and available to customers through a web-based interface. This provides Sprinklr users a high degree of flexibility and scalability.
Advanced capabilities: Sprinklr offers advanced AI and NLP capabilities, such as trend detection, audience insights, text summarization, and descriptive and prescriptive insight identification, as part of its product suite, enabling customers to gather deeper business insights from their text data.
Sprinklr's text analysis capabilities can be used to identify trends and patterns in customer feedback and to understand how customers feel about a company's products or services. This can be useful for identifying opportunities for improvement and for making data-driven decisions about how to enhance the customer experience.
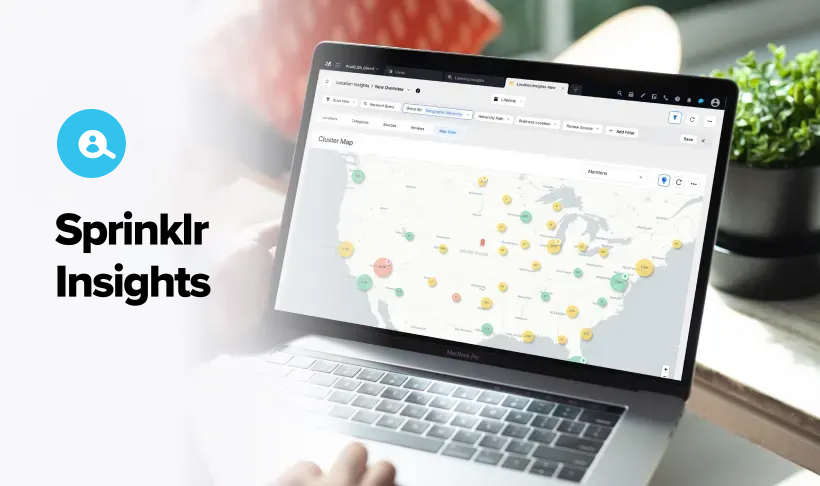
Learn How to Harness AI for Product Insights
Frequently Asked Questions
Text analysis involves collecting the text data, preprocessing the data, extracting relevant features or variables from the data, and applying statistical or machine learning techniques to analyze and interpret the data. The specific steps will depend on the goals and techniques being used for the analysis.
Text analysis can be used by businesses to gain insights from customer feedback, identify trends and patterns in market research data, identify customer segments for targeted marketing, and automate processes such as customer service inquiries.
Some common types of text analytics include sentiment analysis, topic modeling, keyword extraction, and natural language processing (NLP). These techniques can be used to understand customer sentiment, identify trends and patterns in data, extract key information from documents, etc.
related products
Related Topics
Thank you for contacting us.
A Sprinklr representative will be in touch with you shortly.
Contact us today, and we'll create a customized proposal that addresses your unique business needs.
Request a Demo
Welcome Back,
No need to fill out any forms â you're all set.
Related Content
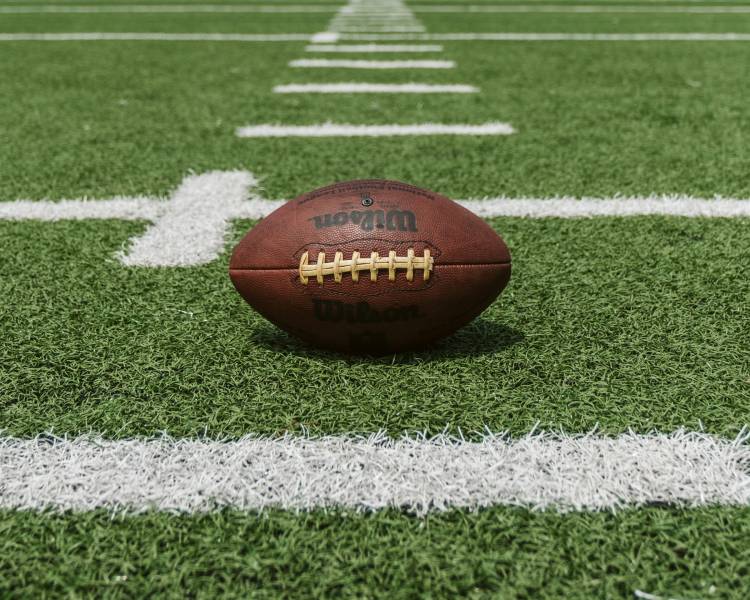
Super Bowl 2024 wasnât just a showdown between football teams, it was also the arena where top brands competed to capture the attention of millions of viewers through their memorable commercials.
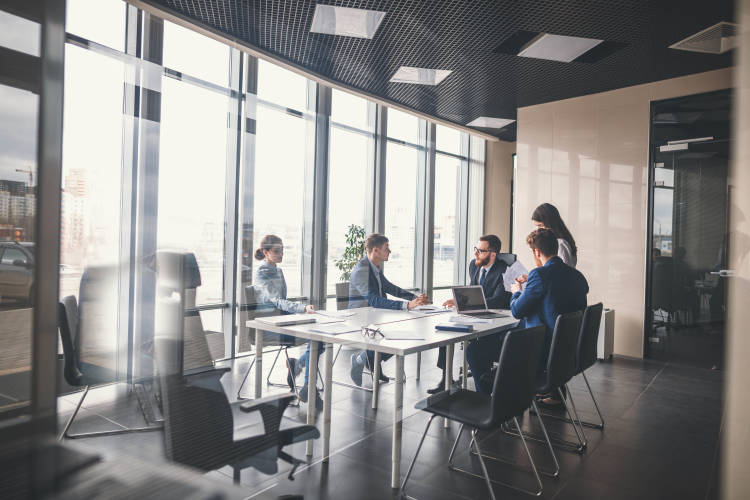
How to manage crisis communication in 2024 (+ checklist)
Improve your brand image with crisis communication management. Nine out of 10 brands trust Sprinklr to manage crises. Learn more about Sprinklr.
Vincent Washington , Priyanka Malik February 2, 2024   âąÂ  9 min read
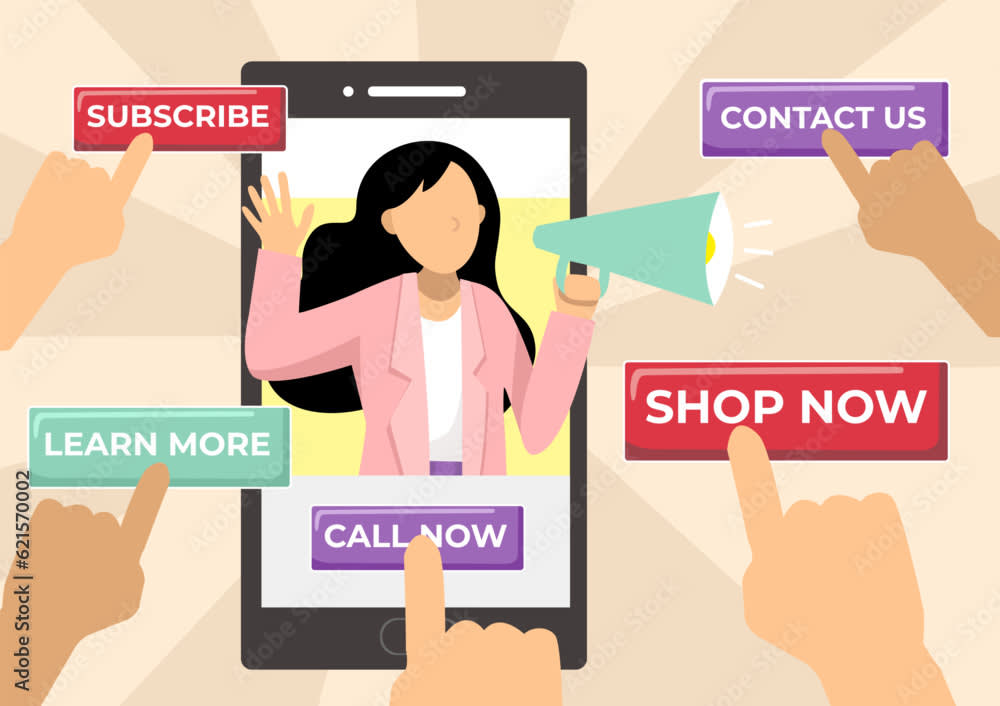
Social media conversion: A guide for beginners to experts
Unlock the potential of social media conversions and learn how to transform online interactions into meaningful results.
Aishwarya Suresh December 20, 2023   âąÂ  11 min read
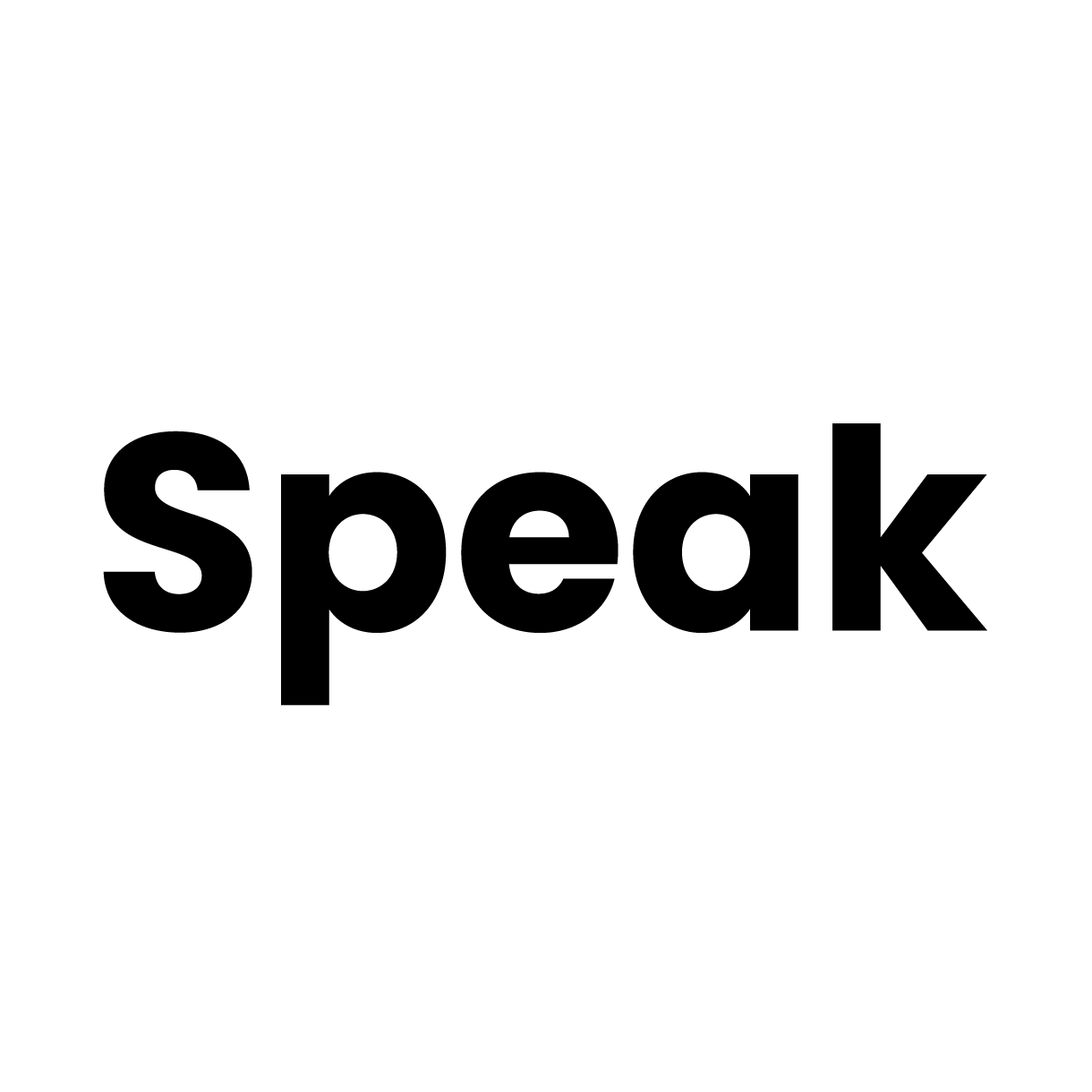
Text Analysis Tools
Analyze your text files to identify sentiment, brands, topics and trends with speak ai's text analysis tools..
With the large amounts of text data that businesses process every day, many companies are sitting on large untapped goldmines in the form of unstructured text documents. Using text analysis to break these massive amounts of data into sentences, phrases, keywords and sentiment, can allow you to better understand trends and topics present across all your files - regardless of the source.Â
You can try out Speak Ai's Named Entity Recognition and Sentiment Analysis below. These are just samples of the type of analysis our platform can help you with, though you can explore the system and analyze multiple files at once with our 7-day trial .
Named Entity Recognition
Sentiment analysis.
Get a 7-day fully-featured trial.
Free Text Analysis Tools Value Proposition
Whether you are trying to do text sentiment analysis online, find the best free text mining software or perform data mining on unstructured text data, we believe Speak is a great option to start your journey.
The best text analytics tools are simple to use and enable you to do text analysis with having to do a text mining software free download.Â
Try a demo of our text mining software right on page to see what kind of output you can expect when analyzing unstructured text data!Â
What is the difference between text analysis, text mining and text analytics?
To start off, there is no difference between text mining and text analysis which are often used interchangeably to talk about the process of grabbing data through statistical pattern learning. As a result, you can use either to talk about this process, but we'll be using text analysis for our explanations.Â
Now, when it comes to text analysis vs text analytics the main difference is that text analysis refers to qualitative results while text analytics is the process of identifying trends and stats - aka quantitative results from your text data.Â
When it comes to machine learning, software that identifies important information within your data such as names, sentiment and brands are conducting text analysis while software that identifies patterns and trends related to the text data are conducting text analytics.Â
Tl;dr Text analysis = decoding human language; Text analytics = finding patterns and trends that are statistically significant
Why you should integrate text analysis into your workflow?
The power of machine learning and AI is the ability to compile and analyze massive amounts of data in a fraction of the time that's possible for a human.Â
As a result, you are able to experiment and uncover important insights at a much quicker rate which comes with a large number of benefits.Â
Using AI for text analysis is scalable
Using an AI text analysis tool allows you to greatly increase the amount of information capture and analysis that can be done to speed up insight extraction. With many systems this is purely limited to parsing through text documents such as emails, customer chats, support tickets and surveys to name a few. However, with the Speak platform, you are able to analyze your text data across audio, video and text files due to our in-built transcription and deep search. Â
Create custom data sets and analysis
If you work with industry-specific vocabulary or are looking to identify uncommon terms or topics, it's easy to set up our system to do that for you. Simply create custom categories and populate them with the terms you would be looking to identify, and our system will do the rest across all your media files.Â
Easily identify key information and repurpose captured content
Finding important information across your media files is made easy with a good text analysis tool. This allows businesses or creators to use their media library as an idea safe, with the ability to resurface and repurpose previously captured information.
What are some use cases of text analysis and natural language processing (NLP)?
Adding text analysis and NLP to your technology stack can be an incredible boost to the business intelligence and data analysis work done for your business. Whether it's through APIs or a user-friendly software, it's possible to build out applications of this across many different industries including research, healthcare, filmmaking, retail and SAAS to name a few.
Some examples of text analysis being applied include:
Conducting qualitative research
Qualitative researchers can use text analysis to identify important keywords, topics and trends based on their interviews. With line-by-line sentiment analysis as well, it's possible to code every project accordingly and end up with a final result that is easy to extract insights from.
Voice of customer
Identifying how customers feel about your product as well as gaining a deeper understanding of how they interact with your support team is an integral business function. With customer success growing as its own discipline, practitioners are looking for ways to better understand all the language data that their teams have to work with.Â
Knowledge management
An uncommon but equally important use case of text analysis and NLP can be for knowledge management and recall. This is particularly true among organizations or individuals who parse through hundreds of hours of transcripts, interviews and other language data to find relevant information. With a system like Speak, you can easily find mentions of topics and keywords as well as the specific parts of different files that they appear in. This is all achieved through text analysis and NLP, with a bit of engineering magic to boot.Â
How To Use Speak As ChatGPT For Text Analysis
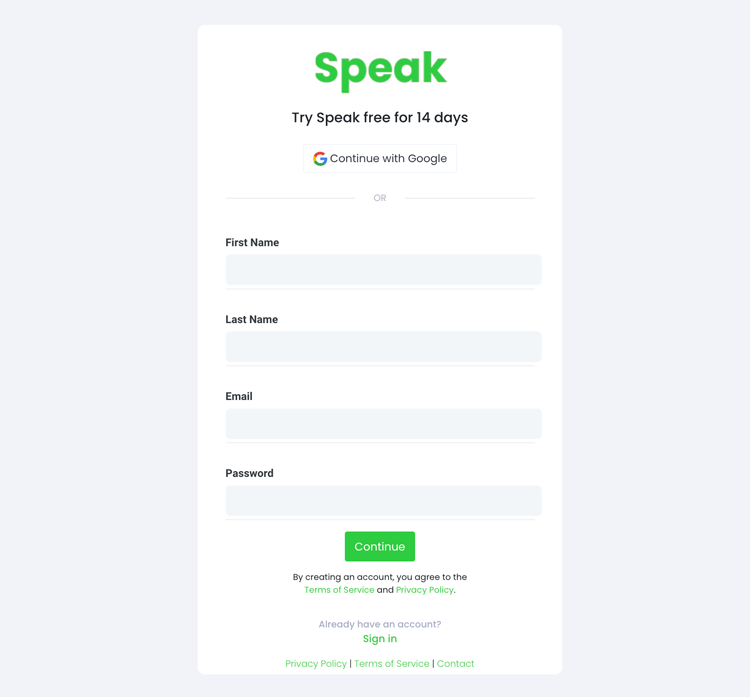
Step 1: Create Your Speak Account
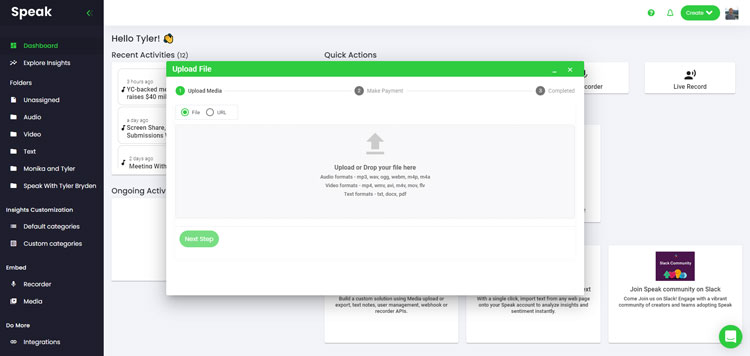
Step 2: Upload Your Text Analysis
Accepted audio file types, accepted video file types, accepted text file types, csv imports, publicly available urls, youtube urls, speak integrations.
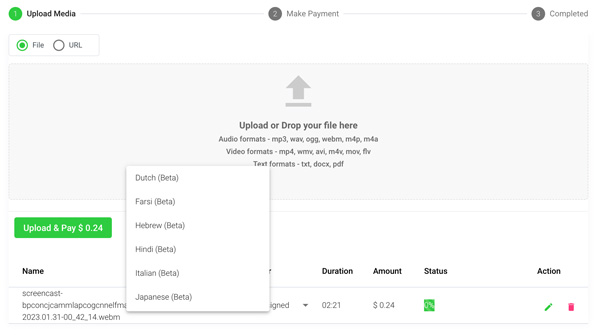
Step 3: Calculate and Pay the Total Automatically
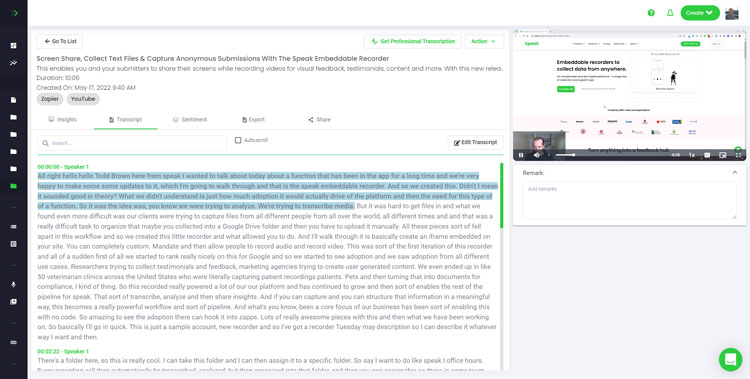
Step 4: Wait for Speak to Analyze Your Text Analysis

Step 5: Visit Your File Or Folder

Step 6: Select Speak Magic Prompts To Analyze Your Data
What are magic prompts.
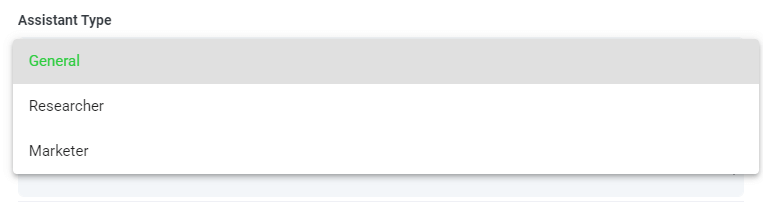
Step 7: Select Your Assistant Type

Step 8: Create Or Select Your Desired Prompt
- Create a SWOT Analysis
- Give me the top action items
- Create a bullet point list summary
- Tell me the key issues that were left unresolved
- Tell me what questions were asked
- Create Your Own Custom Prompts
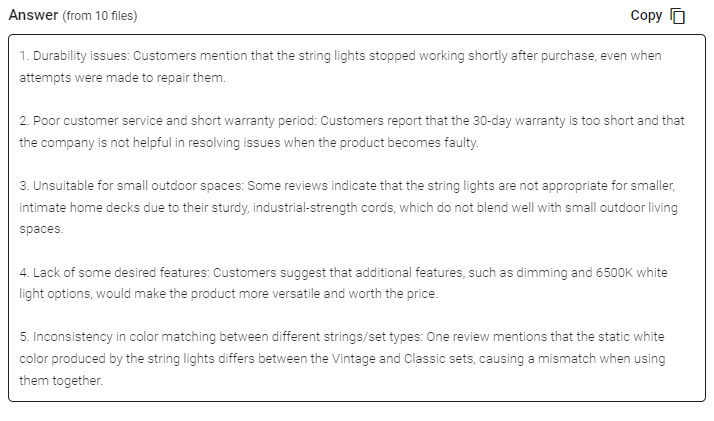
Step 9: Review & Share Responses
Speak magic prompts as chatgpt for text analysis pricing, completely personalize your plan đ, claim your special offer đ.
- Premium Export Options (Word, CSV & More)
- Custom Categories & Insights
- Bulk Editing & Data Organization
- Recorder Customization (Branding, Input & More)
- Media Player Customization
- Shareable Media Libraries
Refer Others & Earn Real Money đž
Check out our dedicated resourcesđ.
- Speak Ai YouTube Channel
- Guide To Building Your Perfect Speak Plan
Book A Free Implementation Session đ€
Our customers love us.
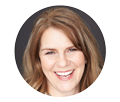
Other free tools
Word cloud generator.
Create eye catching word clouds using important topics and words from your transcripts and text.
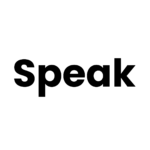
Transcribe and analyze your media like never before.
Automatically generate transcripts, captions, insights and reports with intuitive software and APIs.
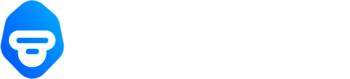
- NPS Analysis
- Review Analysis
- CSAT Analysis
- Support Analysis
- Survey Analysis
- VoC Analysis
- Support Ticket Routing
- How it Works
- Text Classifiers
- Text Extractors
- Integrations
Sentiment Analyzer
Use sentiment analysis to quickly detect emotions in text data.
Play around with our sentiment analyzer, below:
Test with your own text
Get sentiment insights like these:
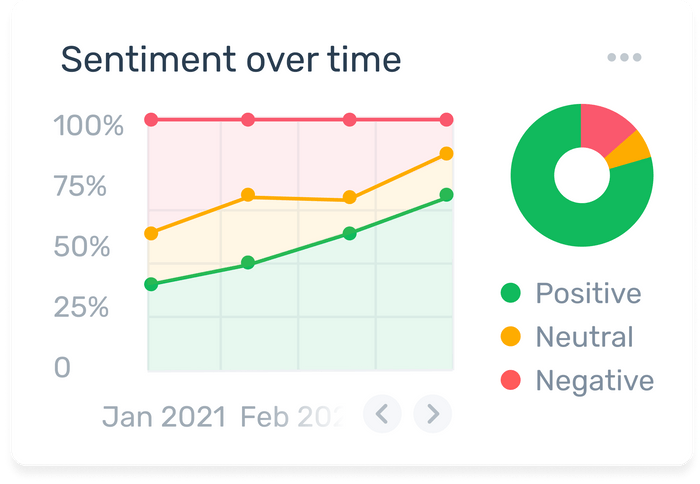
Sentiment analysis benefits:
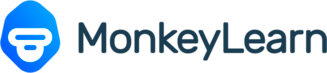
MonkeyLearn Inc. All rights reserved 2024
Text Analyzer
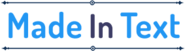
Text Analyzer
Text analyzer is an online tool for complete text analysis. check the number of characters, spaces, words, sentences, paragraphs, shortest and longest words used in your text or article., online text analyzer tool.
Sometimes we all need to analyze whatever we have written, especially if you work as a writer. You need to check and count characters , spaces, words , sentences , and other things in your text to fulfill all guidelines.
Well, with our Text Analyzer tool, this has never been easier. All you need to do is paste your text into the toolbox, and it will analyze the text for you. You will then get to see many things about your text such as:
And much more. In short, it is your one-stop-solution to all your text analysis needs. You can then modify your content accordingly and check again through our tool. It is a free and extremely powerful tool in analyzing the text in no time. No more worrying about not getting your work done on time or missing any guidelines. With this tool, you will know everything you need to about your content. What are you waiting for then? Paste some text now and see for yourself how easy and amazing our tool is. Once you use it, you will never look back.Â
Text Inspector Helps You
Analyse and adapt your text.
Get an overall difficulty score for your English content based on over 200 metrics

Guest are limited to 250 words per document. To process these documents upgrade your subscription. If you are already a subscriber, go to analyse.
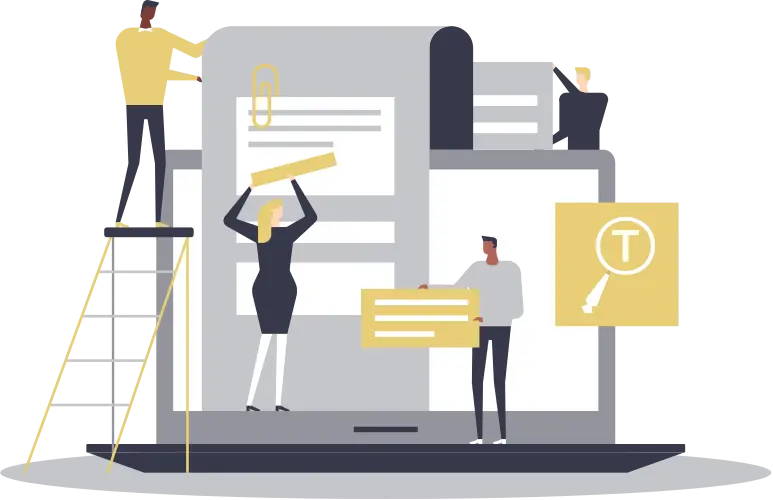
– What is Text Inspector? –
Text Inspector is the most widely used text analyser for preparing teaching materials, tests and evaluating studentsâ work.
Created by renowned Professor Applied Linguistics, Stephen Bax, Text Inspector won the 2017 British Council ELTons Digital Innovation Award. Text Inspector is also supported by its academic partners, the Centre of Research in English Language Learning and Assessment (CRELLA) at the University of Bedforshire.
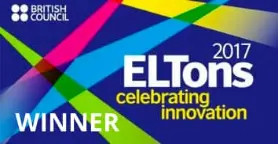
Winner of the British Council Award for Digital Innovation.

Documents Analysed

Unique Users

Using the latest research into Applied Linguistics, our tool is trusted by universities, colleges and organisations across the world, including:
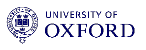
-How we can help you-
Quickly analyse the vocabulary of your texts and get instant feedback on their overall impression, lexical diversity, complexity, and CEFR level.

Teach Confidently
Text Inspector will help you to create materials in-line with the international CEFR grading standard and verify the appropriate difficulty, reading and vocabulary level of your students.

Write high quality articles
Text Inspector quickly analyses your research paper, academic article or textbook and gives you a quick feedback with key linguistic statistics.

Improve your texts
Text Inspector helps you to get a greater understanding of the parts of your texts providing you a precise CEFR level for each word such as common/rare words, difficulty level, and more.
Discover how each feature of Text Inspector can help you below:

Statistics And Readability
Get a detailed overview of the language level and complexity of a text

Lexical Diversity
Discover how diverse the language used in the text is.

British National Corpus
Understand real-world usage in the British English- speaking world

Corpus of Contemporary American English
Analyse texts according to use in the American English-speaking world

Academic Word List & Phrases
Learn the vocabulary you need for academic success and improve teaching materials for TOEFL and IELTS

English Vocabulary Profile
Understand texts in terms of the CEFR level of individual words.

Get a Lexical Profile © Score and detailed information of key language metrics used in the text

Knowledge-Based Vocabulary List

Parts of Speech Tagger
Analyse the text and label the role it plays in a sentence

Metadiscourse Markers
Discover the transition words that add extra information to a text
Monthly / Yearly
Perfect for occasional users

Automatically deletes data from the server after 6hrs.
ÂŁ9.96 / ÂŁ59.76
For frequent users who want to analyse longer texts, use the EVP and download their data.
10,000 words
Can export data. Access to the English Vocabulary Profile, Academic Word List and Scorecard.
Automatically deletes data from the server after 6hrs. Manual data clearing.
Organisation
ÂŁ59.76 / ÂŁ358.56.
For organisations of up to 10 people who want to analyse longer texts, download their data and use the EVP.
13,000 words
For Developers
Full access to our API to use in your own applications. Access from ÂŁ360 per year.
Bespoke Analysis
For researchers or students analysing large texts or corpora. Contact us for more information.
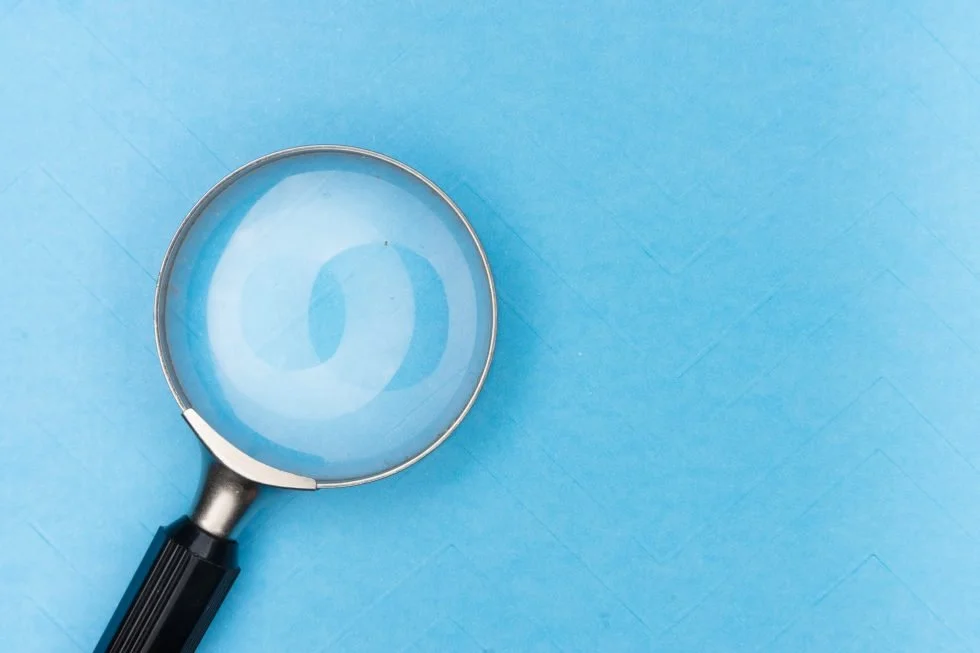
Welcome to the Text Inspector Blog
Announcements
June 24, 2022
Text Inspector is the web-based language analysis tool created by renowned Professor of Applied Linguistics, Stephen Bax.
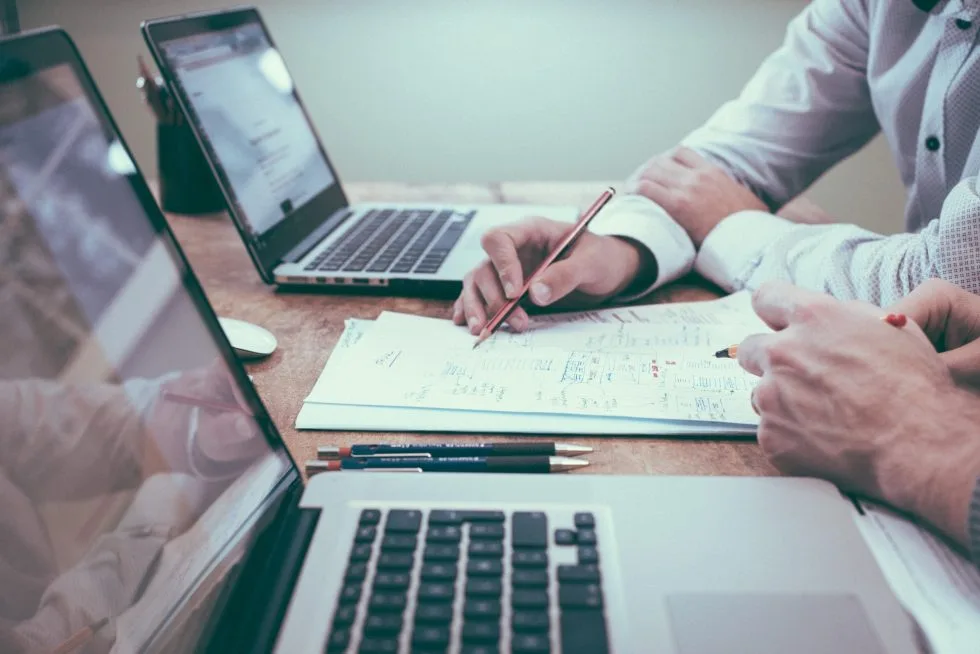
What Are Discourse & Metadiscourse Markers?
Metadiscourse markers are words or phrases that help connect and organise text, express attitude, provide evidence, to connect the reader to the writer and to ensure that the text âflowsâ from one idea to the next.Â
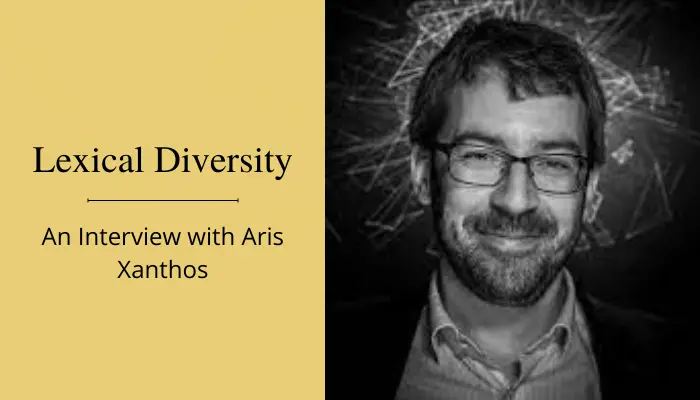
Lexical Diversity: An Interview with Aris Xanthos
Aris Xanthos is a lecturer and researcher in Humanities Computing at the University of Lausanne, CEO at LangTech Sà rl. and creator of the open-source software Textable.
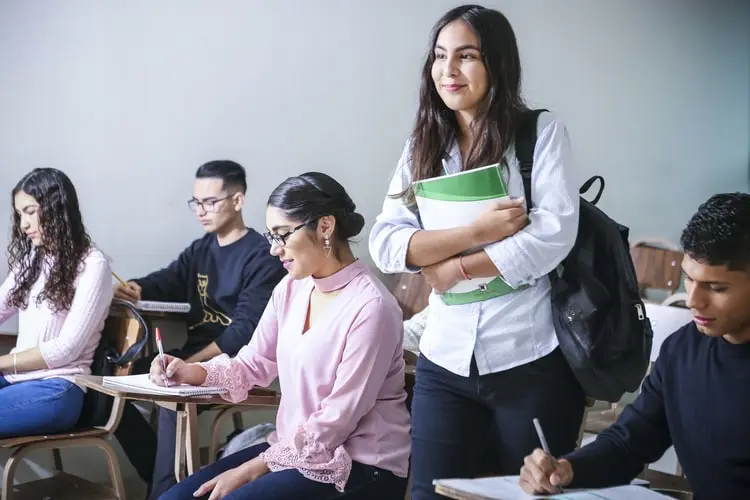
Lexical Profiles According to the CEFR: What Does Research Say?
One of our most widely used tools, the Text Inspector Scorecard uses widely respected metrics to explain the level of text according to the CEFR (the Common European Framework of Reference).
-Testimonials-
Teacher and ma student in spain.

I analysed three main textbooks used in secondary schools in the region where I live to see how much key vocabulary they shared. Thanks to Text Inspector I discovered that the vocabulary they had was from a disproportionately wide range of levels, from A1 to C2. I also found a high proportion of vocabulary not listed. Thanks! Text Inspector formed an important part of the investigation.

Williams, Sarah
Text inspector [online]. english australia journal, vol. 33, no. 2, 2018: 80-83..
This system is ideal for language professionals, academics and students conducting linguistic research. Overall, I found Text Inspector to be a fascinating tool to explore. It is fast and comprehensive, taking a matter of seconds to produce pages of data, and it allows users to analyse reading, writing and listening texts with great accuracy and reliability. I recommend all English language institutions and teaching professionals wishing to ensure that their materials are pitched at the right level utilise Text Inspector.
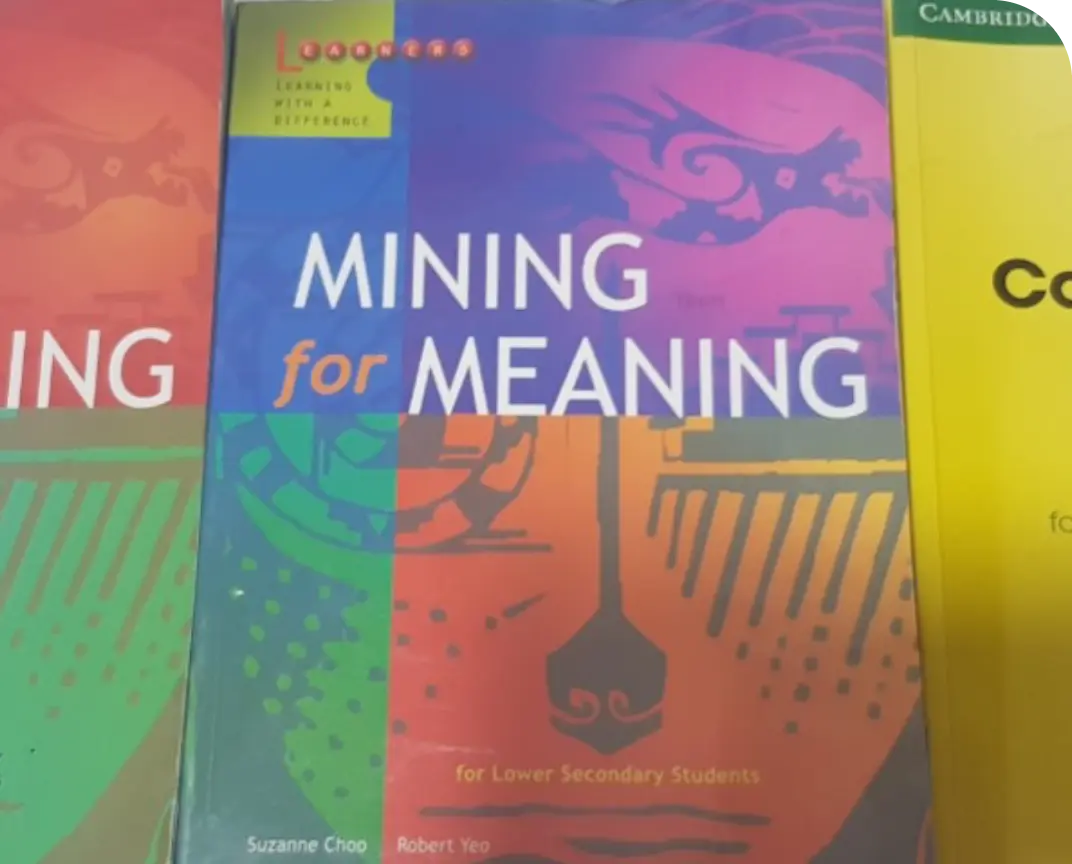
Analyse texts quickly
Get a detailed feedback of your English texts with more than 200 metrics benchmarked against the CEFR.
University Library, University of Illinois at Urbana-Champaign

Text Mining Tools and Methods
- Introduction
- Acquiring Text This link opens in a new window
- Text Analysis Methods
- Additional Downloadable Tools
About Web-Based Tools
- Analysis Tools
Visualization Tools
- More resources
Scholarly Commons
CITL Data Analytics
Web-based tools provide a variety of easy to use and manage visual ization and analysis tools. Some of the possible tools available include word clouds, charts, graphics, and other analysis tools that create visual images and statistically interpret your text.
Analysis Tools
- Lexos âLexos is a great resource for visualizing large text sets through a web-based platform. The site has capabilities to upload multiple files, prepare, visualize, and analyze your data. The visualization tools encompassed in this tool include word clouds, multicloud, bubbleviz, and rollingwindow graph. The analysis tools included are statistical analysis, clustering, similarity query, and topword.â
- Textalyser Textalyser is an online text analysis tool that generates statistics about your text. This analysis tool provides instant results for analyzing word groups, keyword density, the prominence of word or expression, and word count.
- Voyant Voyant is a web-based reading and analysis tool for digital texts. The tool allows you to type in multiple URLs, paste in full text, or upload your own files for analysis. The site is a collaborative project by Stefan Sinclair and Geoffrey Rockwell specifically built for digital humanities projects. The site also provides helpful instruction guides for getting started and additional information about other Voyant tools.
- Word and Phrase Word and Phrase is an online text analysis tool that has a variety of capabilities for analyzing text. Text can be copied and pasted into a text box or take advantage of the data from the Corpus of Contemporary American English (COCA). The tool will first highlight all the medium and lower-frequency words in the text and create lists of the words. Secondly, the words can be clicked upon to create a "word sketch" of any of the words--this will show their definitions and detailed information from from the COCA. Finally, the tool has the capability to conduct powerful searches on select phrases and show related phrases in the COCA.
- Infogram Infogram is a great tool for graphically visualizing your data. This web-based tool has the capability to create over 30+ charts and graphs, including maps, to visualize data for research. When your project is created through the site, you can publish your visualization and/or generate an embed code to post onto a blog or website.
- Tagxedo Tagxedo is a web-based graphic word cloud generator that creates word clouds from famous speeches, news articles, slogans, tweets, etc. This resource is particularly valuable for creating word clouds from websites, news, web searches, RSS feeds, and Twitter IDs. While this resource does not have the capability of creating word clouds from copied and pasted text, it does provide great capabilities for creating word clouds from other data.
- Wordle Wordle is a web-based word cloud tool that creates word clouds from text you provide. The tool has the capability to tweak images, fonts, layouts, and color schemes that allow you to customize your Wordle for your particular project. You can print or create a PDF of your project to share your work. Wordle is a very accessible resource and is incredibly easy to use. Its a great tool for getting started with creating word clouds and for small visualization projects.
- << Previous: Additional Downloadable Tools
- Next: More resources >>
- Last Updated: Nov 8, 2023 3:52 PM
- URL: https://guides.library.illinois.edu/textmining
Exploring the Power of Text Analysis with Gale Digital Scholar Lab
by Rahil Virani
In a recent event by Gale for UW-Madison, participants explored investigative journalism and digital humanities using the Gale Digital Scholar Lab . The event titled “Going Hands-on with the Gale Digital Scholar Lab: A Closer Look at the âFemale Stunt Reporterâ Dataset,” offered a chance to learn new skills and knowledge and to get ready to apply text analysis techniques in their projects/research.
Overview of the Dataset The Female Stunt Reporters Dataset comprises 91 documents sourced from various archives including the Women’s Studies Archive, Nineteenth Century U.S. Newspapers, American Historical Periodicals, and others, covering the period from 1885 to 1925. These documents, compiled using Gale Primary Sources and Gale Digital Scholar Lab, offer a rich resource for exploring the roles and contributions of female journalists during the late 19th and early 20th centuries. The dataset represents an array of publications and perspectives, providing insight into the challenges, achievements, and evolving narratives surrounding women in journalism during this period. Researchers utilizing this dataset can delve into the experiences and impacts of female reporters, shedding light on their historical significance within the field.
Getting Started Using Google or Microsoft Office 365 accounts via NetID, users could easily access the lab and save their research securely on the Gale Digital Scholar Lab. Each project or workspace could be created using different datasets, allowing for organized management. Gale Digital Scholar Lab features 16 preinstalled datasets covering historical events and social movements, providing valuable resources for exploration and research. However, users should be cautious of potential data incompleteness, biases, and the necessity for cleaning before analysis. To learn how to create and set up an account, please click on this link .
Building Your Dataset Building a Content Set in Gale Digital Scholar Lab involves two main steps: searching for documents and curating the results.
- Search : Search Gale Primary Sources, accessible through your institution’s library. Use keywords or terms relevant to your research interests to find documents. You can search based on words within documents or by utilizing metadata fields.
- Review and Curate Results : After searching, review the information of each document to assess its suitability for your Content Set. Consider factors such as relevance to your research questions, quality of content, and metadata associated with the document.
Once you’ve identified and curated the documents you want to analyze, add them to your Content Set for further exploration and analysis in Gale Digital Scholar Lab.
Preparing Your Dataset Preparing your dataset for analysis is crucial in text analysis, and Gale Digital Scholar Lab offers a comprehensive cleaning feature to ensure your documents are formatted appropriately. By creating multiple cleaning configurations, users can tailor the cleaning process to suit specific analysis needs. This involves removing unwanted words or characters that could impact analysis results. The process includes creating a cleaning configuration, testing it on a subset of documents to ensure effectiveness, and then applying it during analysis. This ensures consistency and accuracy in data preparation, allowing researchers to focus on extracting insights rather than dealing with data inconsistencies.
Analyzing Your Dataset This phase empowers you to interrogate hundreds or thousands of documents using digital tools, which would have been too time-consuming without computational algorithms. It guides you through selecting the right tool by asking pertinent questions about your analysis goals. It also covers setting up and running tools effectively to refine results. The available tools include Document Clustering for grouping documents based on similarity, Named Entity Recognition for extracting proper and common nouns, Ngrams for analyzing term frequencies, Parts of Speech for identifying grammatical components, Sentiment Analysis for gauging overall sentiment, and Topic Modeling for grouping frequently co-occurring terms into topics. Each tool offers unique insights, facilitating a comprehensive analysis of your dataset.
Overview – Gale Digital Scholar lab Overall, the event helped in understand how Gale’s platform facilitates research and academic endeavors by integrating its extensive Primary Sources collections with open-source text mining and natural language processing tools. This Single Platform Text and Data Mining Environment streamlines content set creation, cleaning, parsing, and analysis, while optimized cloud-hosted data ensures accessibility and ease of use. To find out about future text analysis related events, please visit UW-Madison Libraries’ Data Services page .Â
Here are some other laws Arizona had on the books in 1864

Arizona sprang into existence in February 1863, just under halfway through the Civil War. President Abraham Lincoln, having signed the act that created the new territory, appointed judges to administer it. Among them was a native New Yorker, William T. Howell .
The appointed governor, John Goodwin, soon determined that the laws established at the territoryâs founding (imported from New Mexico) didnât work. He tasked Howell with writing Arizonaâs first set of laws and procedures, a job Howell began with the help of a former Wisconsin governor, Coles Bashford. In late 1864, the Howell Code , Arizonaâs first set of laws, was born.
And on Tuesday, its original ban on abortion again became Arizonaâs legal standard.
Sign up for the How to Read This Chart newsletter
The decision by Arizonaâs Supreme Court to revive that initial ban triggered an enormous backlash and elevated difficult political questions for Republicans nationally and in the state. It also spurred no small amount of scoffing about how archaic the law must be, given the year of its provenance. California Gov. Gavin Newsom (D), for example, pointed out that Arizona relied on dirt roads when the territorial legislature passed the Howell Code.
Those particular complaints, though, miss the point. It isnât that the law is old that makes it a dubious fit for the moment. After all, the Bill of Rights is old and it contains rules and guidelines that deserve to be maintained. Instead, the point is that the Howell Code was a product of its time and its timeâs morality , a point that is made more obvious when considering other elements of the law that clearly do not conform to 2024 beliefs.
The most obvious difference between now and then that is reflected in the Howell Code is that the United States was, at the time, at war with Southern secessionists desperate to maintain the institution of slavery. (Coincidentally, Tuesday also marked the anniversary of the Southâs final surrender.) Slavery was not allowed in Arizona, but the Howell Code does recognize it as a legal issue. Section 55 of Chapter 10 (âOf Crimes and Punishmentsâ) makes it illegal to entice Black people to leave Arizona so that they can be sold into slavery.
The prohibition on abortion comes a bit before that, just after the section banning duels. Section 45, focused initially on making intentional poisoning a crime, also establishes the ban on abortion:
â[E]very person who shall administer or cause to be administered or taken, any medicinal substances, or shall use or cause to be used any instruments whatever, with the intention· to procure the miscarriage of any woman then being with child, and shall be thereof duly convicted, shall be punished by imprisonment in the Territorial prison for a term not less than two years nor more than five years: Provided, that no physician shall be affected by the last clause of this section, who in the discharge of his professional duties deems it necessary to produce the miscarriage of any woman in order to save her life.â
This is the origin of the law that remains on the books .
Consider, though, the other prohibitions that surround the initial Howell language. A bit before that, for example, the code establishes what constitutes a murder or a manslaughter. In Section 34, it also creates the category of âexcusable homicides.â Those include situations such as when âa man is at work with an axe, and the head flies off and kills a bystanderâ or âa parent is moderately correcting his child, or a master his servant or scholar.â Only when that correction is âmoderate,â mind you. Exceed the bounds of moderation correction, and youâre subject to more severe charges.
Section 38 offers another assessment of pregnancy.
âIf any woman shall endeavor, privately, either by herself or the procurement of others, to conceal the death of any issue of her body, male or female, which, if born alive, would be a bastard, so that it may not come to light, whether it shall have been murdered or not,â it reads, âevery such mother being convicted thereof shall suffer imprisonment in the county jail for a term not exceeding one year.â
In other words, if you were to become pregnant out of wedlock and have a miscarriage â and then conceal it â you could go to jail for a year. If you disagreed with this rule, of course, you had little recourse. Only âwhite male citizensâ of the United States or Mexico whoâd lived in the territory for six months were allowed to vote.
In Section 47, the Howell Code addresses rape, which is defined as âthe carnal knowledge of a female, forcibly and against her will.â It also specifies penalties for having âcarnal knowledge of any female child under the age of ten years, either with or without her consent.â To put a fine point on it, the âconsentâ at issue there is purportedly coming from a 9-year-old.
Many of the punishments identified for criminal actions result in execution. Thereâs even a stipulation that those who âby willful and corrupt perjury or subornation of perjuryâ get someone convicted and executed for a crime might themselves be subject to capital punishment.
Here, again, we encounter a specific rule centered on women. If there was âgood reasonâ to believe that a condemned woman was pregnant, the Code states, the sheriff could ask a panel of three doctors to determine her status to the best of her ability. If she wasnât pregnant, she was executed. If she was, she was allowed to live â until she gave birth, at which point the governor could once again move the execution forward.
There are other reminders that this is a legal framework centered around the frontier, from sanctions for refusing to join a posse or the rules governing citizenâs arrests. Thereâs also an interesting process established for holding accountable elected officials. The Howell Code establishes that third parties could level accusations of misconduct against sitting officials, forcing the official to appear at a hearing. Barring admission, a jury trial ensued and could leave to removal from office. Safe to say, some prominent elected officials would rather not have that be the national standard.
The Arizona Supreme Court decision Tuesday did not immediately revert the state law on abortion to the standard established in 1864, allowing two weeks for challenges. Should those challenges fail, though, women in Arizona could face criminal punishment for seeking an abortion, in keeping with the mores of a 19th-century society in which parents were allowed to accidentally beat their children to death and 9-year-olds were considered capable of giving consent to sexual encounters.


An official website of the United States government
Official websites use .gov A .gov website belongs to an official government organization in the United States.
National Weather Service launches new website for water prediction and products
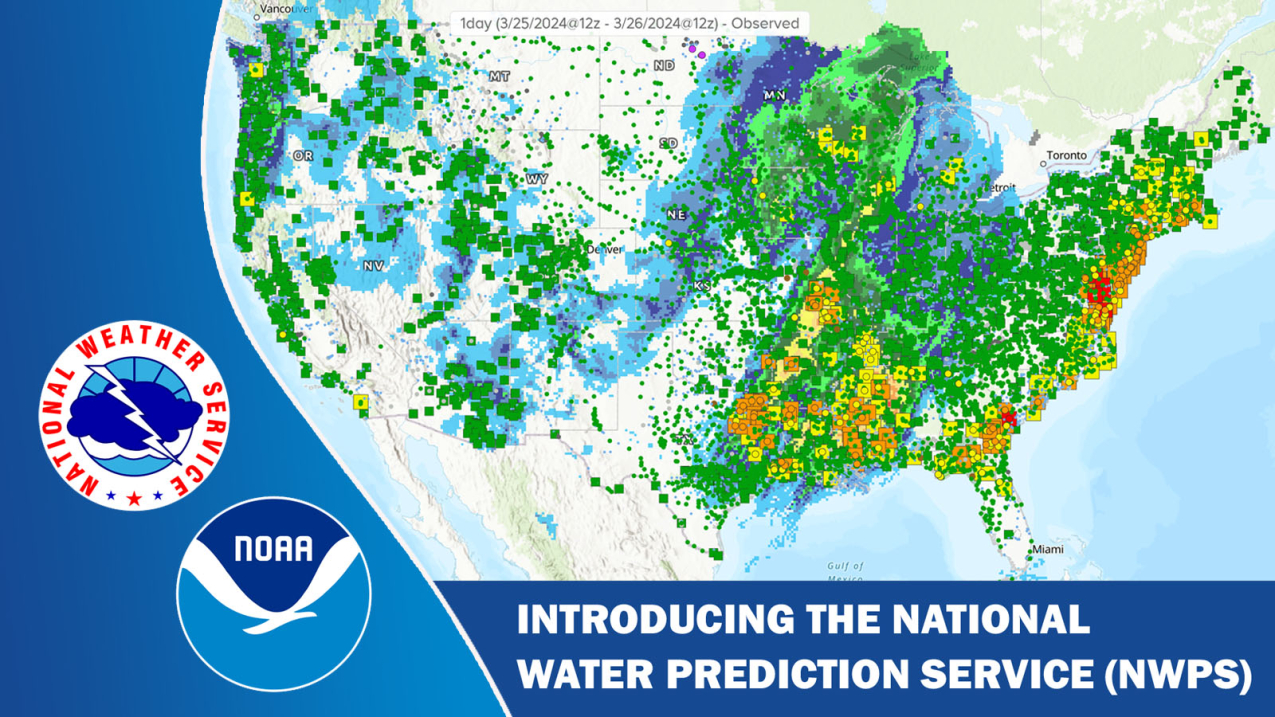
The National Weather Service (NWS) is introducing the new National Water Prediction Service (NWPS), transforming how water resources information and services are delivered, and providing a greatly improved user experience through enhanced displays. (Image credit: NOAA)
NOAAâs National Weather Service launched a new website today: The National Water Prediction Service . This new hub for water data, products and services combines local and regional forecasts with water data and new national level capabilities, such as flood inundation maps and the National Water Model.
"This online water hub is modern and flexible â providing information to help our partners and the public make sound decisions for water safety and management," said Ed Clark, director of NOAAâs National Water Center. âThe new site leverages modern software, geospatial technology and cloud infrastructure, vastly improving the customer experience before, during and after extreme water events such as floods and droughts.â
Key features integrated into the National Water Prediction Service website
- A new, dynamic and seamless national map with flexible options and expansive layers available to help analyze water conditions anywhere in the country.
- Improved hydrographs that are frequently updated, depict water level observations over the past 30 days and provide river flood forecasts up to 10 days in advance.
- The National Water Model , which provides 24 hour coverage, seven days a week hydrologic forecast guidance along 3.4 million river miles across the U.S., including river segments, streams and creeks that have no river gauges.
- Real-time, comprehensive Flood Inundation Maps, which are being implemented in phases, will cover nearly 100% of the U.S. by October 2026.
- An Application Programming Interface (API) has been added to the traditional geographic information system (GIS) data, which will allow customers to flow water information into their own applications and services.
NWS continues to improve the essential support services it provides to communities locally and nationwide as part of its transformation into a more nimble, flexible and mobile agency that works hand-in-hand with decision makers. The National Water Prediction Service website provides tools that deliver actionable hydrologic information across all time scales to address the growing risk of flooding, drought and water availability, and enables partners and the American public to make smart water decisions.
Weather.gov Webstory - January 12, 2024
NWPS Product and Users Guide
Flood Inundation Mapping news release
Biden-Harris Administration announces $80 million to improve flood prediction capabilities
National Weather Service - Flood Safety Tips and Resources
Media Contact
Michael Musher, michael.musher@noaa.gov , (771) 233-1304
Related Features //
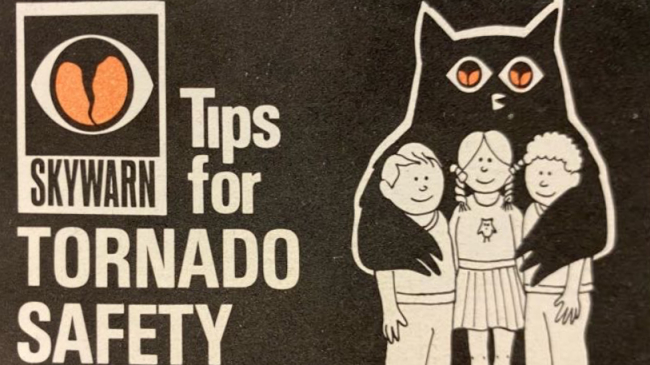
An official website of the United States government
Hereâs how you know
Official websites use .gov A .gov website belongs to an official government organization in the United States.
Secure .gov websites use HTTPS A lock ( Lock Locked padlock icon ) or https:// means youâve safely connected to the .gov website. Share sensitive information only on official, secure websites.
- Entire Site
- Research & Funding
- Health Information
- About NIDDK
- Diabetes Overview
Healthy Living with Diabetes
- Español
On this page:
How can I plan what to eat or drink when I have diabetes?
How can physical activity help manage my diabetes, what can i do to reach or maintain a healthy weight, should i quit smoking, how can i take care of my mental health, clinical trials for healthy living with diabetes.
Healthy living is a way to manage diabetes . To have a healthy lifestyle, take steps now to plan healthy meals and snacks, do physical activities, get enough sleep, and quit smoking or using tobacco products.
Healthy living may help keep your bodyâs blood pressure , cholesterol , and blood glucose level, also called blood sugar level, in the range your primary health care professional recommends. Your primary health care professional may be a doctor, a physician assistant, or a nurse practitioner. Healthy living may also help prevent or delay health problems from diabetes that can affect your heart, kidneys, eyes, brain, and other parts of your body.
Making lifestyle changes can be hard, but starting with small changes and building from there may benefit your health. You may want to get help from family, loved ones, friends, and other trusted people in your community. You can also get information from your health care professionals.
What you choose to eat, how much you eat, and when you eat are parts of a meal plan. Having healthy foods and drinks can help keep your blood glucose, blood pressure, and cholesterol levels in the ranges your health care professional recommends. If you have overweight or obesity, a healthy meal planâalong with regular physical activity, getting enough sleep, and other healthy behaviorsâmay help you reach and maintain a healthy weight. In some cases, health care professionals may also recommend diabetes medicines that may help you lose weight, or weight-loss surgery, also called metabolic and bariatric surgery.
Choose healthy foods and drinks
There is no right or wrong way to choose healthy foods and drinks that may help manage your diabetes. Healthy meal plans for people who have diabetes may include
- dairy or plant-based dairy products
- nonstarchy vegetables
- protein foods
- whole grains
Try to choose foods that include nutrients such as vitamins, calcium , fiber , and healthy fats . Also try to choose drinks with little or no added sugar , such as tap or bottled water, low-fat or non-fat milk, and unsweetened tea, coffee, or sparkling water.
Try to plan meals and snacks that have fewer
- foods high in saturated fat
- foods high in sodium, a mineral found in salt
- sugary foods , such as cookies and cakes, and sweet drinks, such as soda, juice, flavored coffee, and sports drinks
Your body turns carbohydrates , or carbs, from food into glucose, which can raise your blood glucose level. Some fruits, beans, and starchy vegetablesâsuch as potatoes and cornâhave more carbs than other foods. Keep carbs in mind when planning your meals.
You should also limit how much alcohol you drink. If you take insulin or certain diabetes medicines , drinking alcohol can make your blood glucose level drop too low, which is called hypoglycemia . If you do drink alcohol, be sure to eat food when you drink and remember to check your blood glucose level after drinking. Talk with your health care team about your alcohol-drinking habits.
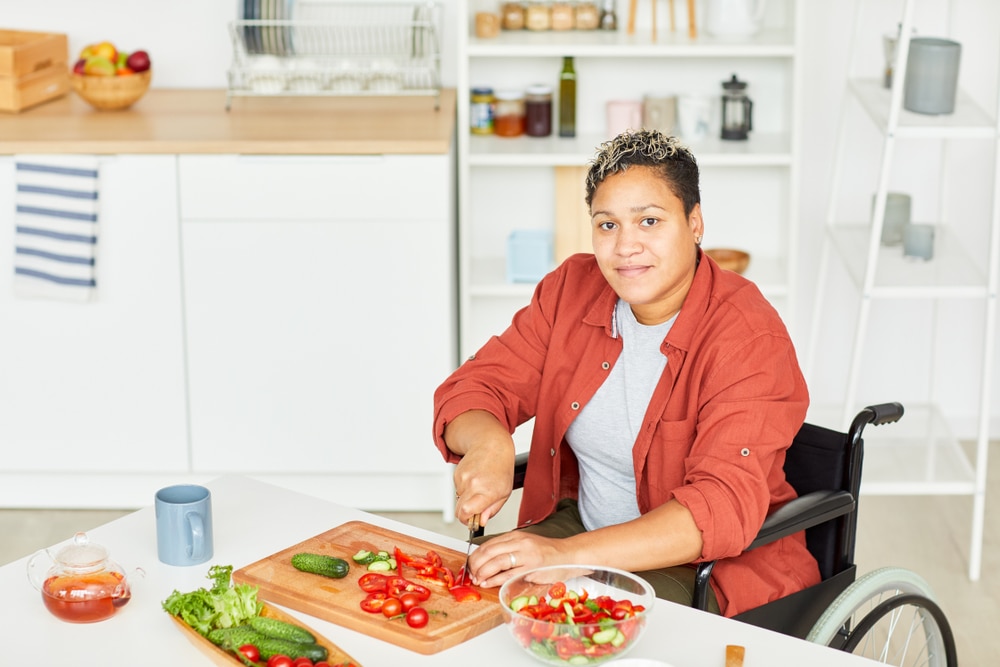
Find the best times to eat or drink
Talk with your health care professional or health care team about when you should eat or drink. The best time to have meals and snacks may depend on
- what medicines you take for diabetes
- what your level of physical activity or your work schedule is
- whether you have other health conditions or diseases
Ask your health care team if you should eat before, during, or after physical activity. Some diabetes medicines, such as sulfonylureas or insulin, may make your blood glucose level drop too low during exercise or if you skip or delay a meal.
Plan how much to eat or drink
You may worry that having diabetes means giving up foods and drinks you enjoy. The good news is you can still have your favorite foods and drinks, but you might need to have them in smaller portions or enjoy them less often.
For people who have diabetes, carb counting and the plate method are two common ways to plan how much to eat or drink. Talk with your health care professional or health care team to find a method that works for you.
Carb counting
Carbohydrate counting , or carb counting, means planning and keeping track of the amount of carbs you eat and drink in each meal or snack. Not all people with diabetes need to count carbs. However, if you take insulin, counting carbs can help you know how much insulin to take.
Plate method
The plate method helps you control portion sizes without counting and measuring. This method divides a 9-inch plate into the following three sections to help you choose the types and amounts of foods to eat for each meal.
- Nonstarchy vegetablesâsuch as leafy greens, peppers, carrots, or green beansâshould make up half of your plate.
- Carb foods that are high in fiberâsuch as brown rice, whole grains, beans, or fruitsâshould make up one-quarter of your plate.
- Protein foodsâsuch as lean meats, fish, dairy, or tofu or other soy productsâshould make up one quarter of your plate.
If you are not taking insulin, you may not need to count carbs when using the plate method.
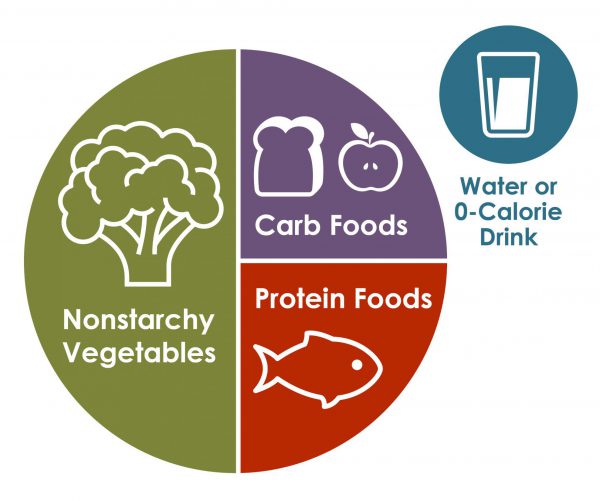
Work with your health care team to create a meal plan that works for you. You may want to have a diabetes educator or a registered dietitian on your team. A registered dietitian can provide medical nutrition therapy , which includes counseling to help you create and follow a meal plan. Your health care team may be able to recommend other resources, such as a healthy lifestyle coach, to help you with making changes. Ask your health care team or your insurance company if your benefits include medical nutrition therapy or other diabetes care resources.
Talk with your health care professional before taking dietary supplements
There is no clear proof that specific foods, herbs, spices, or dietary supplements âsuch as vitamins or mineralsâcan help manage diabetes. Your health care professional may ask you to take vitamins or minerals if you canât get enough from foods. Talk with your health care professional before you take any supplements, because some may cause side effects or affect how well your diabetes medicines work.
Research shows that regular physical activity helps people manage their diabetes and stay healthy. Benefits of physical activity may include
- lower blood glucose, blood pressure, and cholesterol levels
- better heart health
- healthier weight
- better mood and sleep
- better balance and memory
Talk with your health care professional before starting a new physical activity or changing how much physical activity you do. They may suggest types of activities based on your ability, schedule, meal plan, interests, and diabetes medicines. Your health care professional may also tell you the best times of day to be active or what to do if your blood glucose level goes out of the range recommended for you.
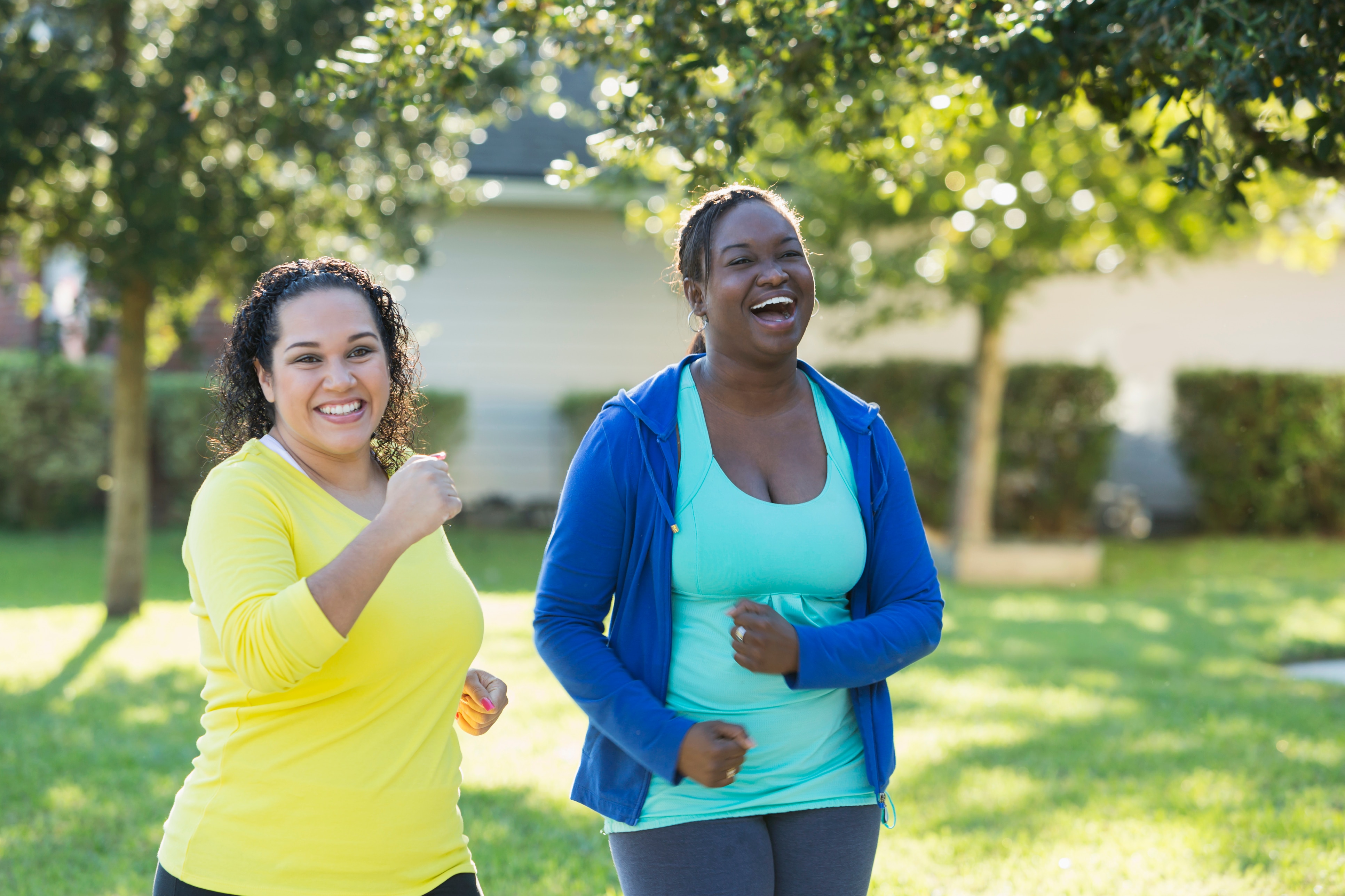
Do different types of physical activity
People with diabetes can be active, even if they take insulin or use technology such as insulin pumps .
Try to do different kinds of activities . While being more active may have more health benefits, any physical activity is better than none. Start slowly with activities you enjoy. You may be able to change your level of effort and try other activities over time. Having a friend or family member join you may help you stick to your routine.
The physical activities you do may need to be different if you are age 65 or older , are pregnant , or have a disability or health condition . Physical activities may also need to be different for children and teens . Ask your health care professional or health care team about activities that are safe for you.
Aerobic activities
Aerobic activities make you breathe harder and make your heart beat faster. You can try walking, dancing, wheelchair rolling, or swimming. Most adults should try to get at least 150 minutes of moderate-intensity physical activity each week. Aim to do 30 minutes a day on most days of the week. You donât have to do all 30 minutes at one time. You can break up physical activity into small amounts during your day and still get the benefit. 1
Strength training or resistance training
Strength training or resistance training may make your muscles and bones stronger. You can try lifting weights or doing other exercises such as wall pushups or arm raises. Try to do this kind of training two times a week. 1
Balance and stretching activities
Balance and stretching activities may help you move better and have stronger muscles and bones. You may want to try standing on one leg or stretching your legs when sitting on the floor. Try to do these kinds of activities two or three times a week. 1
Some activities that need balance may be unsafe for people with nerve damage or vision problems caused by diabetes. Ask your health care professional or health care team about activities that are safe for you.
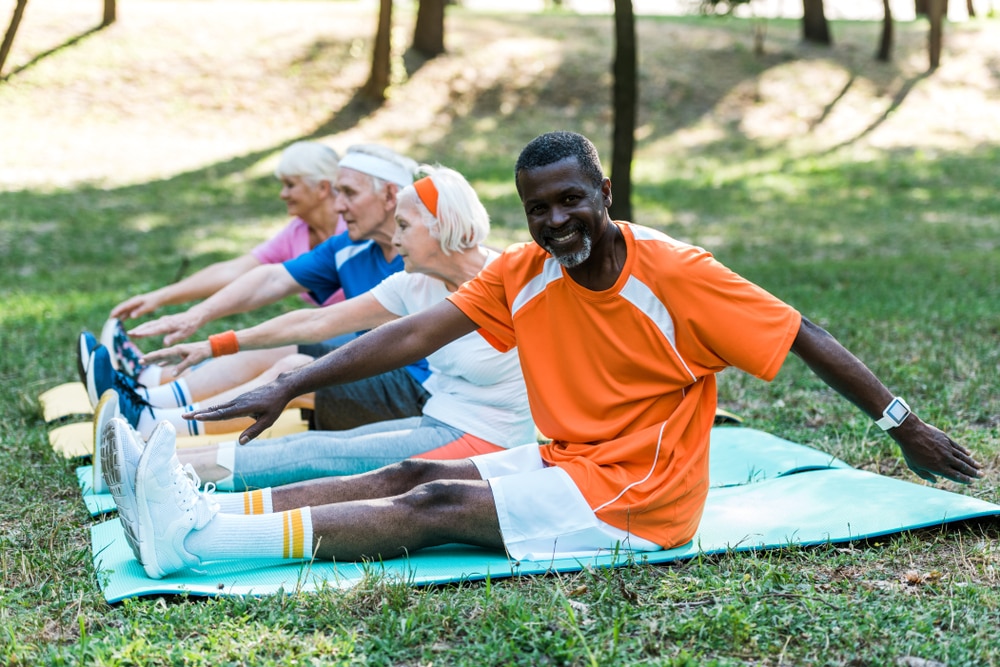
Stay safe during physical activity
Staying safe during physical activity is important. Here are some tips to keep in mind.
Drink liquids
Drinking liquids helps prevent dehydration , or the loss of too much water in your body. Drinking water is a way to stay hydrated. Sports drinks often have a lot of sugar and calories , and you donât need them for most moderate physical activities.
Avoid low blood glucose
Check your blood glucose level before, during, and right after physical activity. Physical activity often lowers the level of glucose in your blood. Low blood glucose levels may last for hours or days after physical activity. You are most likely to have low blood glucose if you take insulin or some other diabetes medicines, such as sulfonylureas.
Ask your health care professional if you should take less insulin or eat carbs before, during, or after physical activity. Low blood glucose can be a serious medical emergency that must be treated right away. Take steps to protect yourself. You can learn how to treat low blood glucose , let other people know what to do if you need help, and use a medical alert bracelet.
Avoid high blood glucose and ketoacidosis
Taking less insulin before physical activity may help prevent low blood glucose, but it may also make you more likely to have high blood glucose. If your body does not have enough insulin, it canât use glucose as a source of energy and will use fat instead. When your body uses fat for energy, your body makes chemicals called ketones .
High levels of ketones in your blood can lead to a condition called diabetic ketoacidosis (DKA) . DKA is a medical emergency that should be treated right away. DKA is most common in people with type 1 diabetes . Occasionally, DKA may affect people with type 2 diabetes who have lost their ability to produce insulin. Ask your health care professional how much insulin you should take before physical activity, whether you need to test your urine for ketones, and what level of ketones is dangerous for you.
Take care of your feet
People with diabetes may have problems with their feet because high blood glucose levels can damage blood vessels and nerves. To help prevent foot problems, wear comfortable and supportive shoes and take care of your feet before, during, and after physical activity.
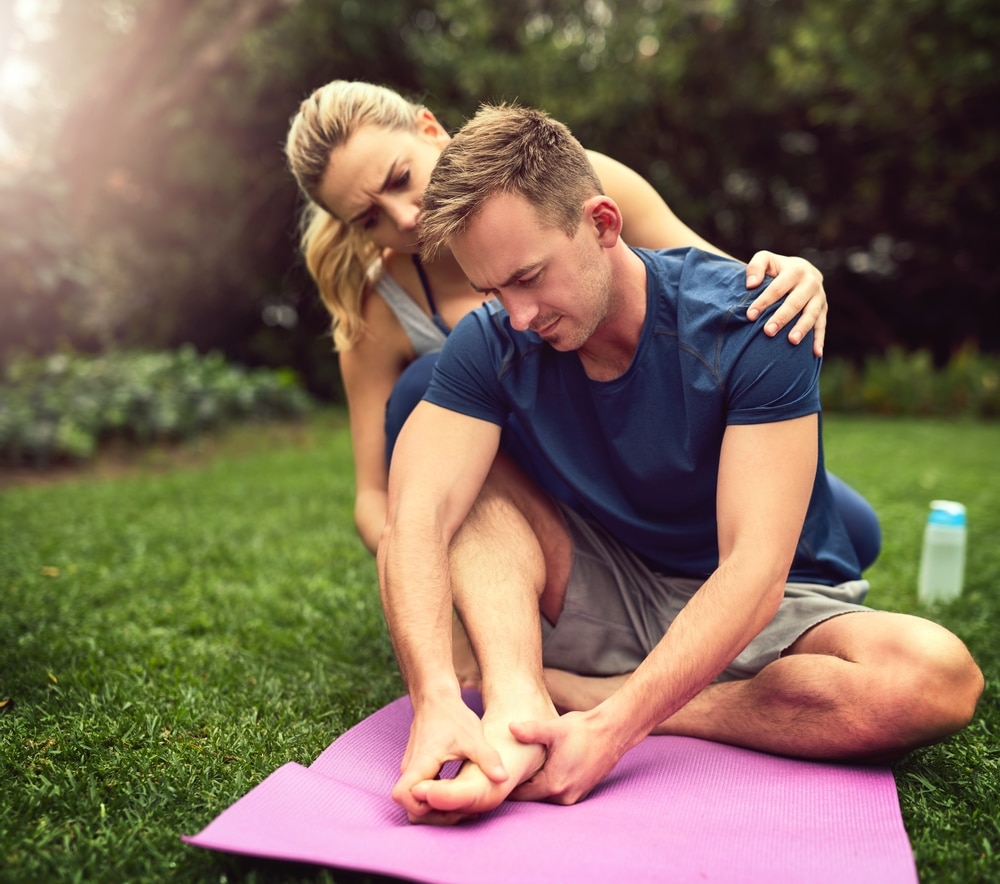
If you have diabetes, managing your weight may bring you several health benefits. Ask your health care professional or health care team if you are at a healthy weight or if you should try to lose weight.
If you are an adult with overweight or obesity, work with your health care team to create a weight-loss plan. Losing 5% to 7% of your current weight may help you prevent or improve some health problems and manage your blood glucose, cholesterol, and blood pressure levels. 2 If you are worried about your childâs weight and they have diabetes, talk with their health care professional before your child starts a new weight-loss plan.
You may be able to reach and maintain a healthy weight by
- following a healthy meal plan
- consuming fewer calories
- being physically active
- getting 7 to 8 hours of sleep each night 3
If you have type 2 diabetes, your health care professional may recommend diabetes medicines that may help you lose weight.
Online tools such as the Body Weight Planner may help you create eating and physical activity plans. You may want to talk with your health care professional about other options for managing your weight, including joining a weight-loss program that can provide helpful information, support, and behavioral or lifestyle counseling. These options may have a cost, so make sure to check the details of the programs.
Your health care professional may recommend weight-loss surgery if you arenât able to reach a healthy weight with meal planning, physical activity, and taking diabetes medicines that help with weight loss.
If you are pregnant , trying to lose weight may not be healthy. However, you should ask your health care professional whether it makes sense to monitor or limit your weight gain during pregnancy.
Both diabetes and smoking âincluding using tobacco products and e-cigarettesâcause your blood vessels to narrow. Both diabetes and smoking increase your risk of having a heart attack or stroke , nerve damage , kidney disease , eye disease , or amputation . Secondhand smoke can also affect the health of your family or others who live with you.
If you smoke or use other tobacco products, stop. Ask for help . You donât have to do it alone.
Feeling stressed, sad, or angry can be common for people with diabetes. Managing diabetes or learning to cope with new information about your health can be hard. People with chronic illnesses such as diabetes may develop anxiety or other mental health conditions .
Learn healthy ways to lower your stress , and ask for help from your health care team or a mental health professional. While it may be uncomfortable to talk about your feelings, finding a health care professional whom you trust and want to talk with may help you
- lower your feelings of stress, depression, or anxiety
- manage problems sleeping or remembering things
- see how diabetes affects your family, school, work, or financial situation
Ask your health care team for mental health resources for people with diabetes.
Sleeping too much or too little may raise your blood glucose levels. Your sleep habits may also affect your mental health and vice versa. People with diabetes and overweight or obesity can also have other health conditions that affect sleep, such as sleep apnea , which can raise your blood pressure and risk of heart disease.
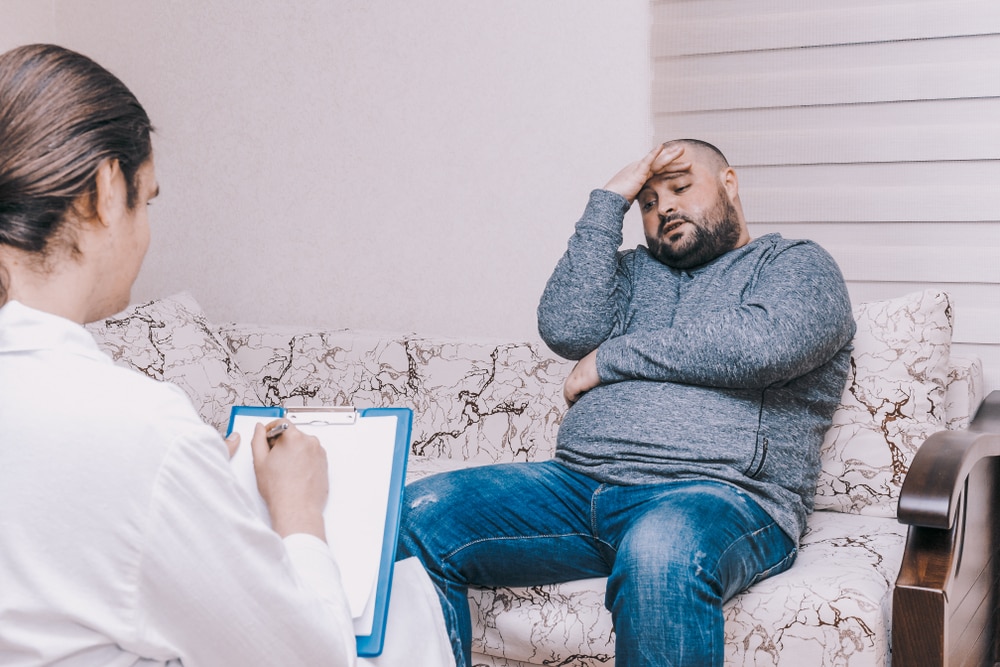
NIDDK conducts and supports clinical trials in many diseases and conditions, including diabetes. The trials look to find new ways to prevent, detect, or treat disease and improve quality of life.
What are clinical trials for healthy living with diabetes?
Clinical trialsâand other types of clinical studies âare part of medical research and involve people like you. When you volunteer to take part in a clinical study, you help health care professionals and researchers learn more about disease and improve health care for people in the future.
Researchers are studying many aspects of healthy living for people with diabetes, such as
- how changing when you eat may affect body weight and metabolism
- how less access to healthy foods may affect diabetes management, other health problems, and risk of dying
- whether low-carbohydrate meal plans can help lower blood glucose levels
- which diabetes medicines are more likely to help people lose weight
Find out if clinical trials are right for you .
Watch a video of NIDDK Director Dr. Griffin P. Rodgers explaining the importance of participating in clinical trials.
What clinical trials for healthy living with diabetes are looking for participants?
You can view a filtered list of clinical studies on healthy living with diabetes that are federally funded, open, and recruiting at www.ClinicalTrials.gov . You can expand or narrow the list to include clinical studies from industry, universities, and individuals; however, the National Institutes of Health does not review these studies and cannot ensure they are safe for you. Always talk with your primary health care professional before you participate in a clinical study.
This content is provided as a service of the National Institute of Diabetes and Digestive and Kidney Diseases (NIDDK), part of the National Institutes of Health. NIDDK translates and disseminates research findings to increase knowledge and understanding about health and disease among patients, health professionals, and the public. Content produced by NIDDK is carefully reviewed by NIDDK scientists and other experts.
NIDDK would like to thank: Elizabeth M. Venditti, Ph.D., University of Pittsburgh School of Medicine.
- Open access
- Published: 12 April 2024
Role of radiomics in staging liver fibrosis: a meta-analysis
- Xiao-min Wang 1 &
- Xiao-jing Zhang 2 Â
BMC Medical Imaging volume  24 , Article number: 87 ( 2024 ) Cite this article
Metrics details
Fibrosis has important pathoetiological and prognostic roles in chronic liver disease. This study evaluates the role of radiomics in staging liver fibrosis.
After literature search in electronic databases (Embase, Ovid, Science Direct, Springer, and Web of Science), studies were selected by following precise eligibility criteria. The quality of included studies was assessed, and meta-analyses were performed to achieve pooled estimates of area under receiver-operator curve (AUROC), accuracy, sensitivity, and specificity of radiomics in staging liver fibrosis compared to histopathology.
Fifteen studies (3718 patients; age 47 years [95% confidence interval (CI): 42, 53]; 69% [95% CI: 65, 73] males) were included. AUROC values of radiomics for detecting significant fibrosis (F2-4), advanced fibrosis (F3-4), and cirrhosis (F4) were 0.91 [95%CI: 0.89, 0.94], 0.92 [95%CI: 0.90, 0.95], and 0.94 [95%CI: 0.93, 0.96] in training cohorts and 0.89 [95%CI: 0.83, 0.91], 0.89 [95%CI: 0.83, 0.94], and 0.93 [95%CI: 0.91, 0.95] in validation cohorts, respectively. For diagnosing significant fibrosis, advanced fibrosis, and cirrhosis the sensitivity of radiomics was 84.0% [95%CI: 76.1, 91.9], 86.9% [95%CI: 76.8, 97.0], and 92.7% [95%CI: 89.7, 95.7] in training cohorts, and 75.6% [95%CI: 67.7, 83.5], 80.0% [95%CI: 70.7, 89.3], and 92.0% [95%CI: 87.8, 96.1] in validation cohorts, respectively. Respective specificity was 88.6% [95% CI: 83.0, 94.2], 88.4% [95% CI: 81.9, 94.8], and 91.1% [95% CI: 86.8, 95.5] in training cohorts, and 86.8% [95% CI: 83.3, 90.3], 94.0% [95% CI: 89.5, 98.4], and 88.3% [95% CI: 84.4, 92.2] in validation cohorts. Limitations included use of several methods for feature selection and classification, less availability of studies evaluating a particular radiological modality, lack of a direct comparison between radiology and radiomics, and lack of external validation.
Although radiomics offers good diagnostic accuracy in detecting liver fibrosis, its role in clinical practice is not as clear at present due to comparability and validation constraints.
Peer Review reports
Introduction
Chronic liver disease is an important health concern due to high prevalence of metabolic dysfunction associated fatty liver disease (MAFLD), hepatitis B/C, and alcoholic liver disease. Whereas mortality due to liver disease has declined in some countries like the USA and China, some countries such as India and Mongolia still have higher mortality rates. Increasing trends in the prevalence are also noted in the United Kingdom and Russia [ 1 ]. In the USA, 1.8% of the population has a liver disease diagnosis [ 2 ]. In China, although the mortality rates have decreased, the prevalence of liver disease is increasing [ 3 ]. Globally, the incidence of non-alcoholic steatohepatitis-caused cirrhosis is increasing by 1.35% each year [ 4 ].
Liver fibrosis is a modifiable factor that is associated with worse health outcomes, transplants, and mortality [ 5 ]. Liver fibrosis may develop due to chronic viral infection, long-term alcohol use, or steatohepatitis. It is estimated that liver fibrosis affects 7.7% of individuals in the general population of the United States of America and obese individuals are at much higher risk [ 6 ]. The latest stage of fibrosis, cirrhosis, is the eleventh leading cause of mortality [ 1 ]. An increasing trend in mortality due to cirrhosis has been observed globally from 1990 to 2017 [ 7 ]. Several methods are available to measure liver fibrosis. The liver biopsy is considered diagnostic âgold standardâ for staging liver fibrosis. The biopsy is a highly valuable tool but can be associated with complications, sampling errors, and between-observer variations in judgments. Fibrosis can be patchy so that not all parts of the liver contain fibrosis evenly, therefore, a biopsy may fail to capture some samples [ 8 ]. Among other methods, serum biomarkers may also help in detecting fibrosis. Radiological methods including magnetic resonance imaging (MRI) magnetic resonance elastography (MRE), computed tomography (CT), ultrasonography, and elastography also provide non-invasive means of measuring fibrosis [ 9 ].
Radiomics is a post-radiology process of high-throughput extraction of features from radiological images for conversion into mineable data involving complex processes of artificial intelligence such as machine learning, deep learning, and convolutional neural networks to maximize predictability. It is developed on the premise that pathophysiological tissues and organs contain much information that can be quantified and differentiated from normal tissues and organs. Extraction of a large number of features to form a database and then mining the data for analyses aids decision support leading to improved diagnostic accuracy and prognostic capability [ 10 , 11 , 12 ]. Radiomics takes texture as a spatial arrangement of predefined voxels through which complicated features of the image can be read and mathematical calculations of these arrangement characteristics differentiate normal from abnormal. The heterogeneity in the selected features reflects the heterogeneity of histopathological changes [ 13 ]. Radiomics features can be morphological, histogram, textural, and high-order features. Morphological features include the shape, size, and volume of the region of interest. Histogram is the plotting of pixel values against pixel frequency and can be used to describe many features such as magnitude, dispersion, asymmetry, peakedness, flatness, randomness, uniformity, etc. Textural features provide spatial relationships between neighboring pixels. High-order features are those acquired after applying filters to images [ 12 , 14 ].
Several reviews have described the role of radiomics in the diagnosis and staging of various types of cancers [ 15 , 16 ]. Among other clinical applications, radiomics has been found to be a valuable aid in cardiomyopathy [ 17 , 18 ], musculoskeletal diseases [ 19 , 20 ], neurological and psychiatric disorders [ 21 , 22 , 23 , 24 ], and liver diseases [ 25 ]. Several studies have reported the diagnostic accuracy indices of radiomics in diagnosing and staging liver fibrosis. However, there is no synthesis of these outcomes which are sometimes variable and even inconsistent. The present study aimed to evaluate the role of radiomics in diagnosing and staging liver fibrosis by conducting a systematic review of relevant studies and performing meta-analyses of statistical indices.
The present study was conducted by following PRISMA guidelines.
Inclusion and exclusion criteria
A study was included in the meta-analysis if a) it prospectively or retrospectively recruited patients with chronic liver disease who had histologically confirmed fibrosis in the liver; b) performed radiomic analyses based on any radiological modality to diagnose and/or differentiate fibrosis; and c) reported diagnostic accuracy indices of radiomics in diagnosing and differentiating liver fibrosis stages by in comparison with histopathology. Studies were excluded based on the following criteria: a study a) reported diagnostic performance of radiomics for liver fibrosis without adequate statistical data; b) reported the outcomes of pediatric patients; and c) reported the diagnostic accuracy of a combined clinical-radiological radiomics model.
Literature search
Electronic scientific databases (Embase, Ovid, Science Direct, Springer, and Web of Science) were searched for the identification of relevant studies using area-specific keywords. The primary search strategy was âRadiomics AND liver fibrosis OR cirrhosis AND diagnostic accuracyâ. Secondary keywords were used in several other combinations with this primary string. The detailed literature search strategy is given in Appendix S1. After the identification of studies, reference lists of related articles were also screened for additional studies. The literature search encompassed peer-reviewed research articles published in English from the date of database inception till May 2023.
Data analysis
Data on the design and conduct of studies, patient demographics, clinical characteristics, fibrosis stage, radiomics design and analyses, and diagnostic accuracy outcome data were extracted from the research articles of respective studies and organized in data sheets. The quality of the included studies was assessed with the Quality Assessment of Diagnostic Accuracy Studies (QUADAS-2) scale. This scale assesses the quality of studies under the domains of risk of bias and applicability concerns by evaluating patient selection, index test, reference standard, and flow and timing. Diagnostic accuracy endpoints (Accuracy, area under receiver operator curve (AUROC), sensitivity, and specificity of various radiomics models compared to histologically proven fibrosis were extracted from the research articles of respective studies and pooled under random effects model using the point estimates and their 95% confidence intervals of these indices. Subgroup analyses were performed with respect to fibrosis stage (significant fibrosis; stages F2-4, advanced fibrosis; stages F3-4, and cirrhosis; stage F4) and with respect to the study cohort (training, test/validation). Statistical analyses were performed with Stata software (Stata Corporation, College Station, Texas, USA).
Fifteen studies [ 13 , 26 , 27 , 28 , 29 , 30 , 31 , 32 , 33 , 34 , 35 , 36 , 37 , 38 , 39 ] were included that were published between 2018 and 2023 (Fig. 1 ). In these studies, radiomic analyses were performed involving 3718 patients with chronic liver diseases including hepatitis and MAFLD. The age of these patients was 47.3 years [95% confidence interval (CI): 42.0, 52.5]. The proportion of males was 69% [95% CI: 65, 73]. Histologically (biopsy/surgical) confirmed fibrosis stage was F0 in 13% [95% CI: 7,20], F1 in 17% [95% CI: 13, 23], F2 in 21% [95% CI: 17,26], F3 in 16% [95% CI: 13,19], and F4 in 27% [95% CI: 23, 33] of the patients. Radiological modalities used in these studies were: MRI (5), CT (3), ultrasonography (2), positron emission tomography (1), shear-wave elastography (2), and MRE (2).
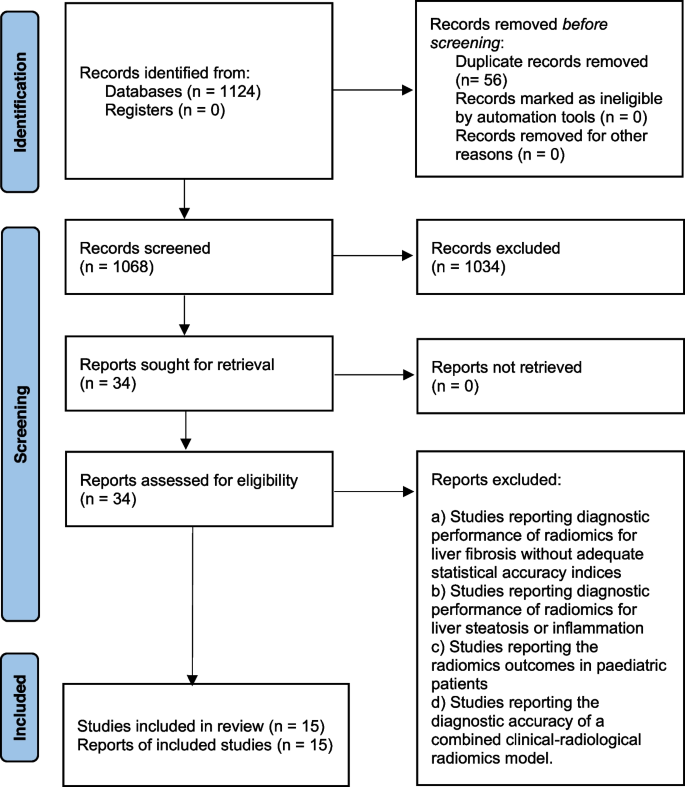
A flowchart of study screening and selection process
Important characteristics of the included studies are given in Table S 1 . The quality of the included studies was moderate in general according to the QUADAS-2 scale (Table S 2 ). The risk of bias was limited to the retrospective design of studies which could have introduced patient selection bias. Moreover, the interval between the index test and reference test was up to six months which might have impacted adequate flow and timing. Included studies also varied with regards to validation ranging from no validation [ 26 ], Five/Ten-fold cross-validation [ 27 , 30 , 32 , 36 , 39 ], and leave-one-out cross-validation [ 13 ] to internal validation [ 28 , 30 , 31 , 33 , 34 , 37 , 38 ], and internal and external validation [ 29 ]. There was no significant publication bias according to Eggerâs test (Bias coefficient 0.114 [-11.80, 12.03]; p =0.984) or Beggâs test (Adjusted Kendallâs score -29±18; p =0.125) (Figure S 1 a and b).
The pooled AUROC value of radiomics for the diagnosis of any liver fibrosis was 0.878 [95% CI: 0.850, 0.906]. The AUROC values for the detection of significant fibrosis, advanced fibrosis, and cirrhosis were 0.914 [95% CI: 0.889, 0.938], 0.924 [95% CI: 0.901, 0.946], and 0.944 [95% CI: 0.929, 0.963] respectively in training cohorts, and 0.886 [95% CI: 0.826, 0.905], 0.887 [95% CI: 0.834, 0.939], and 0.930 [95% CI: 0.905, 0.954] respectively in test/validation cohorts (Figs. 2 and 3 ).
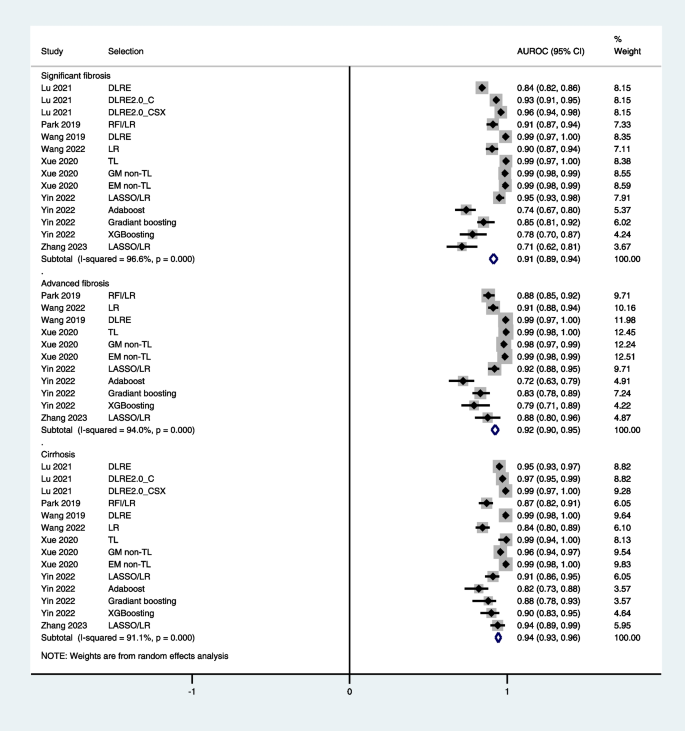
Forest graphs showing the outcomes of meta-analysis of AUROC values of radiomics in diagnosing fibrosis stages in training cohorts
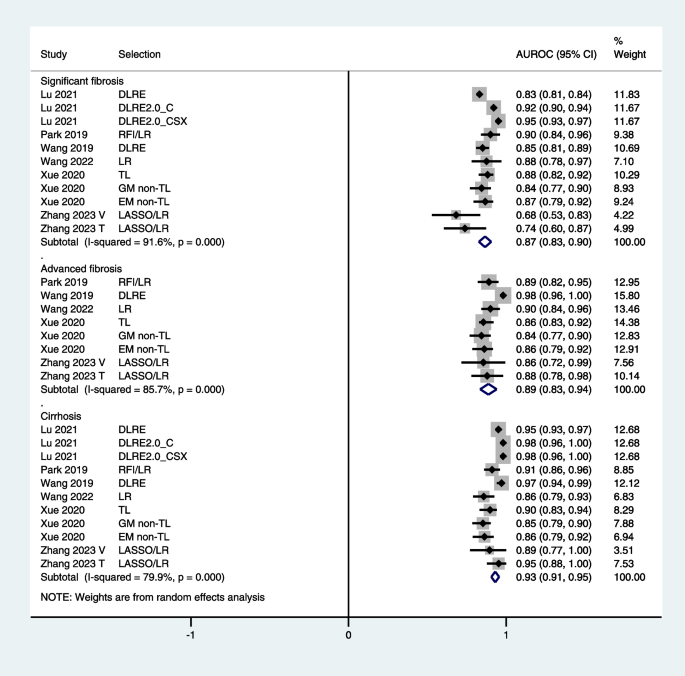
Forest graphs showing the outcomes of meta-analysis of AUROC values of radiomics in diagnosing fibrosis stages in test/validation cohorts
The pooled accuracy of radiomics in diagnosing any liver fibrosis was 83.5% [95% CI: 81.7, 85.4]. The accuracy values of radiomics in the detection of significant fibrosis, advanced fibrosis, and cirrhosis were 80.6% [95% CI: 76.2, 85.1], 83.5% [95% CI: 81.3, 85.8], and 81.6% [95% CI: 78.7, 84.5] respectively in training cohorts, and 77.0% [95% CI: 68.6, 85.5], 84.3% [95% CI: 79.8, 88.8], and 81.3% [95% CI: 77.0, 85.7] respectively in test/validation cohorts (Figures S 2 a and b).
In training cohorts, the sensitivity of radiomics in diagnosing significant fibrosis, advanced fibrosis, and cirrhosis was 84.0% [95% CI: 76.1, 91.9], 86.9% [95% CI: 76.8, 97.0], and 92.7% [95% CI: 89.7, 95.7] respectively (Fig. 4 ). In test/validation cohorts, the sensitivity of radiomics in diagnosing significant fibrosis, advanced fibrosis, and cirrhosis was 75.6% [95% CI: 67.7, 83.5], 80.0% [95% CI: 70.8, 89.3], and 92.0% [95% CI: 87.8, 96.1] respectively (Fig. 5 ).
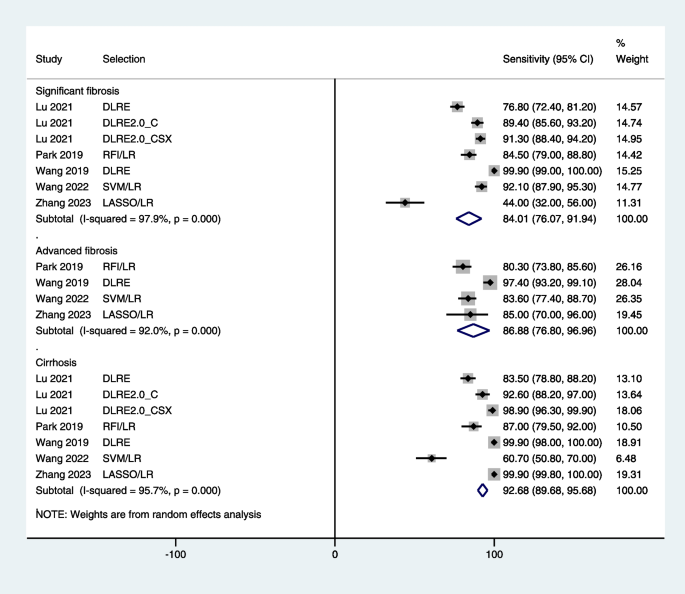
A forest graph showing the outcomes of meta-analysis of the sensitivity of radiomics in diagnosing fibrosis stages in training cohort
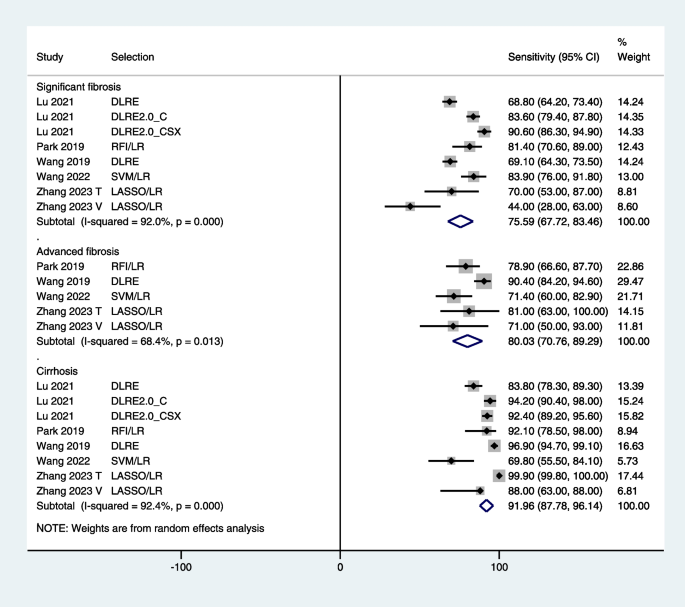
A forest graph showing the outcomes of meta-analysis of the sensitivity of radiomics in diagnosing fibrosis stages in test/validation cohorts
In training cohorts, the specificity of radiomics in diagnosing significant fibrosis, advanced fibrosis, and cirrhosis was 88.6% [95% CI: 83.0, 94.2], 88.4% [95% CI: 81.9, 94.8], and 91.1% [95% CI: 86.8, 95.5], whereas in test/validation cohorts, the specificity was 86.8% [95% CI: 83.3, 90.3], 94.0% [95% CI: 89.5, 98.4], and 88.3% [95% CI: 84.4, 92.2] respectively (Figure S 3 a and b).
Among the included studies, Hu et al. [ 27 ] who found thin-sliced CT images to yield better performance of radiomics than thick-sliced CT images reported AUROC values of 0.90 [95% CI: 0.84, 0.96] for F1 vs F2-4, 0.85 [95% CI: 0.78, 0.92] for F1-2 vs F3-4, and 0.94 [95% CI: 0.89, 0.97] for F1-3 vs F4 stages. Lan et al. [ 13 ] who studied MRE radiomics found AUROC values of 0.89 [95% CI: 0.84, 0.94] for F0 vs F1-4, 0.93 [95% CI: 0.89, 0.98] for F0-1 vs F2-4, 0.92 [95% CI: 0.88, 0.97] for F0-2 vs F3-4, and 0.95 [95% CI: 0.90, 0.997] for F0-3 vs F4 stages in their echo-planar images. They observed AUROC values of 0.89 [95% CI: 0.83, 0.94], 0.87 [95% CI: 0.81, 0.93], 0.89 [95% CI: 0.84, 0.95], and 0.94 [95% CI: 0.89, 0.997] for F0 vs F1-4, F0-1 vs F2-4, F0-2 vs F3-4 and F0-3 vs F4 stages respectively in their gradient recalled echo images.
This meta-analysis found that radiomics exhibits high accuracy in diagnosing and staging liver fibrosis. The AUROC values for the detection of significant fibrosis, advanced fibrosis, and cirrhosis were approximately 0.91, 0.92, and 0.94 in training cohorts and 0.89, 0.89, and 0.93 in validation cohorts, respectively. However, despite the good efficiency of radiomics in diagnosis and staging liver fibrosis observed herein, several factors make these findings inconclusive and dependent on future studies to refine this evidence. For example, several types of radiological modalities were used for radiomics in individual studies and a direct comparison of diagnostic performance between radiomics and radiology was mostly lacking. Moreover, various models were utilized for radiomics, and these studies lacked external validation.
Some studies that could not be included in this meta-analysis have also reported variable diagnostic performance of radiomics in staging liver fibrosis. Cui et al. [ 40 ] utilized multiphase CT-based radiomics to stage liver fibrosis and found the sensitivity of diagnosing significant fibrosis, advanced fibrosis, and cirrhosis to be 30-41%, 59-71%, and 84-87%, and the specificities being 84-90%, 71-79%, and 50-58% respectively. Duan et al. [ 41 ] observed better performance of ultrasound-based radiomics in diagnosing cirrhosis (AUROC 0.86) than advanced fibrosis (AUROC 0.77). Zhou et al. [ 42 ], also reported higher efficieny of ultrasound-based radiomics in diagnosing cirrhosis (AUROC 0.83-0.86) than significant fibrosis (AUROC 0.69-0.71) or advanced fibrosis (AUROC 0.67-0.72).
Many studies have shown that the diagnostic accuracy of radiomics is better than serological biomarkers [ 30 , 42 , 43 , 44 ]. Sim et al. [ 32 ] found better diagnostic performance of MRE radiomics (AUROC 0.97 [95% CI: 0.93, 1]) than clinical features (AUROC 0.91 [95% CI: 0.81, 0.97]) in diagnosing significant fibrosis. Wang et al. [ 34 ] also reported that the AUROC values for significant fibrosis, advanced fibrosis, and cirrhosis were higher with CT-based radiomics (AUROC 0.88, 0.90, and 0.86) than with either aspartate transaminase-to-platelet ratio (AUROC 0.69, 0.67, and 0.65) or Fibrosis-4 index (AUROC 0.71, 0.71, and 0.7) respectively. Xue et al. [ 35 ] also found better diagnostic performance of multimodal ultrasound radiomics (AUROC 0.9-0.93) than either aspartate transaminase-to-platelet ratio (AUROC 0.72-0.78) or Fibrosis-4 index (AUROC 0.69-0.75) in staging liver fibrosis. Zhao et al. [ 38 ] found better diagnostic efficiency of MRI radiomics (accuracy 0.8) than clinical markers (accuracy 0.68) in differentiating non-significant fibrosis from clinically significant fibrosis in the test cohort. Some authors have suggested that a model combining radiomics and clinical biomarkers may further improve the diagnostic accuracy of fibrosis [ 38 , 45 ].
Despite good diagnostic accuracy values observed for radiomics in liver fibrosis assessment in the present study, inconsistencies were observed in the outcomes of individual studies. Whereas Wang et al. [ 34 ] and Lan et al. [ 13 ] found radiomics better than radiological elastography in diagnosing advanced fibrosis and cirrhosis, Sim et al. [ 32 ] did not find a statistically significant difference. Lu et al. [ 29 ] and Sim et al. [ 32 ] found that radiomics distinguished well between significant fibrosis (F2-4) and non-significant fibrosis (F0-1). However, Zhang et al. [ 37 ] reported that radiomics was unable to distinguish between non-significant fibrosis and significant fibrosis. Zhao et al. [ 38 ] reported that a combined use of radiomics and clinical biomarkers performed better than radiomics alone, whereas Sim et al. [ 32 ] did not find a significant difference in performance between radiomics and combined use of radiomics, radiology, and clinical biomarkers.
We have observed that some diagnostic accuracy indices were slightly higher in training cohorts than in validation cohorts. Wang et al. [ 33 ] who found that the accuracy of deep learning radiomics of elastography for the diagnosis of significant fibrosis was lower in the validation cohort in comparison with the training cohort suggested that this could be because of the lower heterogeneity in F0 and F1 groups and can be overcome possibly by adapting multiple strategies for fibrosis classification. Lu et al. [ 29 ] found that AUROC values increased for their deep learning radiomics of elastography when datasets had a higher prevalence of patients with F0 and F1 stages. They suggested that the inclusion of higher proportions of patients with F0 and F1 stages can yield better accuracy because about 80% of patients with chronic hepatitis B have F0 or F1 stage in the general population. In the present study, the pooled percentages of patients with F0 and F1 stages were 13% and 17% respectively.
Fibrosis develops by the excessive deposition of extracellular matrix in the liver as a response to wound healing after which satellite cells activate, higher levels of alpha smooth muscle actins are produced, and collagen I/II are synthesized. Such processes increase the stiffness of the liver progressively and may lead to cirrhosis. Fibrosis is usually associated with the accumulation of collagen fibers, not well-defined portal vein walls, and irregular hepatic vein margins. Gray-scale ultrasound images capture such information to reflect the scattering of fine structures. Moreover, coarse echotexture and a mild increase in echogenicity of hepatic parenchyma are usually observed in cirrhosis [ 35 ].
So far, studies addressing radiomics lack robust validation in larger and clinically diverse settings which present reproducibility challenges. In the present review, we found that all except one study involved internal validation, and therefore, the synthesis of these outcomes remains inconclusive and dependent on future studies with larger sample sizes and better designs with special focus on external validation. Radiomics models without external validation are at increased risk of being specialized in specific radiographs that hamper generalizability due to overfitting [ 46 ].
Overfitting and multi-collinearity may affect radiomics models. During training, high-dimensional features may overfit and thus may yield optimistic outcomes. Moreover, traditional statistical models may not work adequately to deal with multicollinearity among textural features. To avoid this, it is suggested that the removal of unreliable or irrelevant features and the reduction of dimensions of predictors may yield better outcomes [ 30 , 31 ]. The sensitivity of AdaBoost to noisy data or outliers makes it more suitable for cases facing overfitting problems. A frequently used classifier, the Support Vector Machine, uses preselected nonlinear mapping to map input parameters in a high-dimensional feature space to optimize feature classification. Random forest unifies several weak predictor classifiers to make an accurate and stable predictor [ 28 ].
The AUROC is a performance metric to quantify the power of a model in discriminating cases from non-cases. An AUROC value can lie between 0 and 1. It combines the sensitivity and specificity of a marker/modality for the diagnosis of a precisely defined stage of fibrosis. Sensitivity is usually evaluated in patients with advanced fibrosis and specificity in non-advanced fibrosis [ 47 ]. However, the AUROC values can be biased if the fibrosis distribution in the study population differs from that of the whole population to which it is being applied [ 48 ]. Although biopsy is considered a gold standard for the diagnosis of fibrosis in the liver, it has a high rate of false positives and false negatives in comparison with the whole liver due to sampling error. An AUROC value of 0.82 for distinguishing between F2 and F1 when the entire liver was used as the reference index will inform approximately 20% error rate of the biopsy (false positive and false negative rates) compared with the entire liver. Thus, discordance in the staging of liver fibrosis between a modality such as radiomics and biopsy can be due to an error of the modality as well as due to an error of the biopsy [ 47 ].
Currently, the evidence regarding the role of radiomics in diagnosis of liver fibrosis and staging is constrained with several caveats. Quality of medical images acquired through different modalities may vary depending on several factors such as scanners, protocols, and personnel that can affect the reproducibility of radiomics output. A lack of standardization of image acquisition, preprocessing steps, extraction of features, and analyses also makes it difficult to compare radiomics outcomes of various studies performed under different settings. The etiology of fibrosis, progression, and the presence of comorbidities may also affect the accuracy of radiomics outcomes. Moreover, biological interpretation of radiomic features is lacking due to which it is difficult to associate radiomic features with histopathological characteristics.
Several limitations of the present study need to be considered while interpreting the outcomes of this review. An important limitation of the present study was the presence of high statistical heterogeneity in the meta-analyses. Although sources of heterogeneity could not be traced statistically, it is reasonable to assume that clinical and methodological heterogeneity might have played an influencing role. Authors utilized different methods for feature selection and classification, worked with a variety of software, and analyzed a highly variable number of features. Radiomics analyses were based on several radiological modalities and fewer studies were available to evaluate a particular modality in a pooled design. Most studies were retrospective in design due to which several types of biases could have been introduced. Inclusion and exclusion criteria differed substantially across the included studies that recruited several conditions of chronic liver disease including hepatitis B/C, autoimmune hepatitis, liver failure, early-stage cirrhosis, nonalcoholic fatty liver disease, and primary sclerosing cholangitis. Some studies could not be included because of the lack of variance data for diagnostic accuracy indices.
In this meta-analysis of 15 studies, the use of radiomics in staging liver fibrosis has been found to be associated with good diagnostic accuracy. However, the present-day outcome data are inconclusive regarding the use of radiomics in clinical practice owing to heterogeneity in methodology and outcomes of reviewed studies in which the radiomic evaluations were based on several radiological modalities subjected to a variety of analytical models yielding varying outcomes and lacking external validation. Non-invasiveness and the involvement of machine learning make radiomics an attractive option for decision support. The outcomes reported so far are promising and need to be validated in multicenter studies having larger datasets and better comparability and validation aspects in designs.
Availability of data and materials
The datasets used or analyzed during the current study are available from the corresponding author on reasonable request.
Cheemerla S, Balakrishnan M. Global epidemiology of chronic liver disease. Clin Liver Dis (Hoboken). 2021;17(5):365â70.
Article  PubMed  PubMed Central  Google Scholar Â
Centers for disease control and prevention. national center for health statistics. fast stats homepage. Chronic liver disease. Available at: https://www.cdc.gov/nchs/fastats/liver-disease.htm . Last accessed on March 16, 2024.
Li M, Wang ZQ, Zhang L, Zheng H, Liu DW, Zhou MG. Burden of cirrhosis and other chronic liver diseases caused by specific etiologies in China, 1990â2016: Findings from the Global Burden of Disease Study 2016. Biomed Environ Sci. 2020;33(1):1â10.
PubMed  Google Scholar Â
Zhai M, Liu Z, Long J, Zhou Q, Yang L, Zhou Q, et al. The incidence trends of liver cirrhosis caused by nonalcoholic steatohepatitis via the GBD study 2017. Sci Rep. 2021;11:5195. https://doi.org/10.1038/s41598-021-84577-z .
Article  CAS  PubMed  PubMed Central  Google Scholar Â
Angulo P, Kleiner DE, Dam-Larsen S, Adams LA, Bjornsson ES, Charatcharoenwitthaya P, et al. liver fibrosis, but no other histologic features, is associated with long-term outcomes of patients with nonalcoholic fatty liver disease. Gastroenterology. 2015;149(2):389â97.
Article  PubMed  Google Scholar Â
Choi YS, Beltran TA, Calder SA, Padilla CR, Berry-Caban CS, Salyer KR. Prevalence of hepatic steatosis and fibrosis in the United States. Metab Syndr Relat Disord. 2022;20(3):141â7.
GBD 2017 Cirrhosis Collaborators. The global, regional, and national burden of cirrhosis by cause in 195 countries and territories, 1990â2017: a systematic analysis for the Global Burden of Disease Study 2017. Lancet Gastroenterol Hepatol. 2020;5(3):245â66.
Article  Google Scholar Â
Chu LC, Park S, Kawamoto S, Yuille AL, Hruban RH, Fishman EK. Current status of radiomics and deep learning in liver imaging. J Comput Assist Tomogr. 2021;45:343â51.
Manning DS, Afdhal NH. Diagnosis and quantitation of fibrosis. Gastroenterology. 2008;134(6):1670â81.
Article  CAS  PubMed  Google Scholar Â
Gillies RJ, Kinahan PE, Hricak H. Radiomics: images are more than pictures, they are data. Radiology. 2016;278:563â77.
Lambin P, Leijenaar RTH, Deist TM, Peerlings J, de Jong EEC, van Timmeren J, et al. Radiomics: the bridge between medical imaging and personalized medicine. Nat Rev Clin Oncol. 2017;14(12):749â62. https://doi.org/10.1038/nrclinonc.2017.141 .
Scapicchio C, Gabelloni M, Barucci A, Cioni D, Saba L, Neri E. A deep look into radiomics. Radiol Med. 2021;126(10):1296â311.
Lan GY, Guo Y, Zhang XY, Cai XL, Shi Y. Value of radiomic analysis of data from magnetic resonance elastography for diagnosing fibrosis stages in patients with hepatitis B/C. Chin J Acad Radiol. 2019;1:74â84.
Park HJ, Park B, Lee SS. Radiomics and deep learning: hepatic applications. Korean J Radiol. 2020;21:387â401.
Liu Z, Wang S, Dong D, Wei J, Fang C, Zhou X, et al. The applications of radiomics in precision diagnosis and treatment of oncology: Opportunities and challenges. Theranostics. 2019;9(5):1303â22.
Zhang YP, Zhang XY, Cheng YT, Li B, Teng XZ, Zhang J, et al. Artificial intelligence-driven radiomics study in cancer: the role of feature engineering and modeling. Mil Med Res. 2023;10(1):22. https://doi.org/10.1186/s40779-023-00458-8 .
Neisius U, El-Rewaidy H, Nakamori S, Rodriguez J, Manning WJ, Nezafat R. Radiomic analysis of myocardial native T1 imaging discriminates between hypertensive heart disease and hypertrophic cardiomyopathy. JACC Cardiovasc Imaging. 2019;12(10):1946â54.
Neisius U, El-Rewaidy H, Kucukseymen S, Tsao CW, Mancio J, Nakamori S, et al. Texture signatures of native myocardial T1 as novel imaging markers for identification of hypertrophic cardiomyopathy patients without scar. J Magn Reson Imaging. 2020;52(3):906â19.
Cuadra MB, Favre J, Omoumi P. Quantification in musculoskeletal imaging using computational analysis and machine learning: Segmentation and radiomics. Semin Musculoskelet Radiol. 2020;24(1):50â64. https://doi.org/10.1055/s-0039-3400268 .
Chea P, Mandell JC. Current applications and future directions of deep learning in musculoskeletal radiology. Skeletal Radiol. 2020;49(2):183â97.
Park YW, Choi D, Lee J, Ahn SS, Lee SK, Lee SH, et al. Differentiating patients with schizophrenia from healthy controls by hippocampal subfields using radiomics. Schizophr Res. 2020;223:337â44.
Park YW, Choi YS, Kim SE, Choi D, Han K, Kim H, et al. Radiomics features of hippocampal regions in magnetic resonance imaging can differentiate medial temporal lobe epilepsy patients from healthy controls. Sci Rep. 2020;10:19567.
Bang M, Park YW, Eom J, Ahn SS, Kim J, Lee SK, et al. An interpretable radiomics model for the diagnosis of panic disorder with or without agoraphobia using magnetic resonance imaging. J Affect Disord. 2022;305:47â54.
Zhao K, Ding Y, Han Y, Fan Y, Alexander-Bloch AF, Han T, et al. Independent and reproducible hippocampal radiomic biomarkers for multisite Alzheimerâs disease: diagnosis, longitudinal progress and biological basis. Sci Bull. 2020;65:1103â13.
Article  CAS  Google Scholar Â
Liu F, Ning Z, Liu Y, Liu D, Tian J, Luo H, et al. Development and validation of a radiomics signature for clinically significant portal hypertension in cirrhosis (CHESS1701): a prospective multicenter study. EBioMedicine. 2018;36:151â8.
Chen ZW, Tang K, Zhao YF, Chen YZ, Tang LJ, Li G, et al. Radiomics based on fluoro-deoxyglucose positron emission tomography predicts liver fibrosis in biopsy-proven MAFLD: a pilot study. Int J Med Sci. 2021;18:3624â30.
Hu P, Chen L, Zhong Y, Lin Y, Yu X, Hu X, et al. Effects of slice thickness on CT radiomics features and models for staging liver fibrosis caused by chronic liver disease. Japanese J Radiol. 2022;40:1061â8.
Li W, Huang Y, Zhuang BW, Liu GJ, Hu HT, Li X, et al. Multiparametric ultrasomics of significant liver fibrosis: A machine learning-based analysis. Eur Radiol. 2019;29:1496â506.
Lu X, Zhou H, Wang K, Jin J, Meng F, Mu X, et al. Comparing radiomics models with different inputs for accurate diagnosis of significant fibrosis in chronic liver disease. Eur Radiol. 2021;31:8743â54.
Park HJ, Lee SS, Park B, Yun J, Sung YS, Shim WH, et al. Radiomics analysis of Gadoxetic Acidâenhanced MRI for staging liver fibrosis. Radiology. 2019;290:380â7.
Qiu QT, Zhang J, Duan JH, Wu SZ, Ding JL, Yin Y. Development and validation of radiomics model built by incorporating machine learning for identifying liver fibrosis and early-stage cirrhosis. Chin Med J. 2020;133(22):2653â9.
Sim KC, Kim MJ, Cho Y, Kim HJ, Park BJ, Sung DJ, et al. Diagnostic feasibility of magnetic resonance elastography radiomics analysis for the assessment of hepatic fibrosis in patients with nonalcoholic fatty liver disease. J Comput Assist Tomogr. 2022;46:505â13.
Wang K, Lu X, Zhou H, Gao Y, Zheng J, Tong M, et al. Deep learning Radiomics of shear wave elastography significantly improved diagnostic performance for assessing liver fibrosis in chronic hepatitis B: a prospective multicentre study. Gut. 2019;68:729â41.
Wang J, Tang S, Mao Y, Wu J, Xu S, Yue Q, et al. Radiomics analysis of contrast-enhanced CT for staging liver fibrosis: an update for image biomarker. Hepatol Int. 2022;16:627â39.
Xue LY, Jiang ZY, Fu TT, Wang QM, Zhu YL, Dai M, et al. Transfer learning radiomics based on multimodal ultrasound imaging for staging liver fibrosis. Eur Radiol. 2020;30:2973â83.
Yin Y, Yakar D, Dierckx RAJO, Mouridsen KB, Kwee TC, de Haas RJ. Combining hepatic and splenic CT radiomic features improves radiomic analysis performance for liver fibrosis staging. Diagnostics. 2022;12:550. https://doi.org/10.3390/diagnostics12020550 .
Zhang D, Cao Y, Sun Y, Xia Zhao X, Peng C, Zhao J, et al. Radiomics nomograms based on R2* mapping and clinical biomarkers for staging of liver fibrosis in patients with chronic hepatitis B: a single-center retrospective study. Eur Radiol. 2023;33:1653â67.
Zhao R, Zhao H, Ge YQ, Zhou FF, Wang LS, Yu HZ, et al. Usefulness of noncontrast MRI-based radiomics combined clinic biomarkers in stratification of liver fibrosis. Canadian J Gastroenterol Hepatol. 2022;2022:2249447. Article ID 2249447.
Zheng R, Shi C, Wang C, Shi N, Qiu T, Chen W, et al. Imaging-based staging of hepatic fibrosis in patients with hepatitis B: A dynamic radiomics model based on Gd-EOB-DTPA-enhanced MRI. Biomolecules. 2021;11:307.
Cui E, Long W, Wu J, Li Q, Ma C, Lei Y, et al. Predicting the stages of liver fibrosis with multiphase CT radiomics based on volumetric features. Abdom Radiol. 2021;46:3866â76.
Duan YY, Qin J, Qiu WQ, Li SY, Li C, Liu AS, et al. Performance of a generative adversarial network using ultrasound images to stage liver fibrosis and predict cirrhosis based on a deep-learning radiomics nomogram. Clin Radiol. 2022;77(10):e723â31.
Zhou Z, Zhang Z, Gao A, Tai DI, Wu S, Tsui PH. Liver fibrosis assessment using radiomics of ultrasound homodyned-K imaging based on the artificial neural network estimator. Ultrasonic Imaging. 2022;44(5â6):229â41.
Yasaka K, Akai H, Kunimatsu A, Abe O, Kiryu S. Liver fibrosis: deep convolutional neural network for staging by using gadoxetic acidâenhanced hepatobiliary phase MR images. Radiology. 2018;287:146â55.
Choi KJ, Jang JK, Lee SS, Sung YS, Shim WH, Kim HS, et al. Development and validation of a deep learning system for staging liver fibrosis by using contrast agentâenhanced CT images in the liver. Radiology. 2018;289:688â97.
Zheng W, Guo W, Xiong M, Chen X, Gao L, Song Y, et al. Clinic-radiological features and radiomics signatures based on Gd-BOPTA-enhanced MRI for predicting advanced liver fibrosis. European Radiology. 2023;33:633â44.
Yamada A, Kamagata K, Hirata K, Ito R, Nakaura T, Ueda D, et al. Clinical applications of artificial intelligence in liver imaging. Radiol Med. 2023;128(6):655â67.
Poynard T, Halfon P, Castera L, Charlotte F, Le Bail B, Munteanu M, et al. Variability of the area under the receiver operating characteristic curves in the diagnostic evaluation of liver fibrosis markers: impact of biopsy length and fragmentation. Aliment Pharmacol Ther. 2007;25(6):733â9.
Guha IN, Myers RP, Patel K, Talwalkar JA. Biomarkers of liver fibrosis: What lies beneath the receiver operating characteristic curve? Hepatology. 2011;54:1454â62.
Download references
Acknowledgements
Not applicable.
Author information
Authors and affiliations.
School of Medical Imaging, Tianjin Medical University, No.1, Guangdong Road, Hexi District, Tianjin, 300203, China
Xiao-min Wang
Department of Radiology, The First Medical Center, Chinese PLA General Hospital, Beijing, 100853, China
Xiao-jing Zhang
You can also search for this author in PubMed  Google Scholar
Contributions
XMW: Literature search, data analysis, manuscript writing; manuscript review; XJZ: Literature search; manuscript editing.
Corresponding author
Correspondence to Xiao-min Wang .
Ethics declarations
Ethics approval and consent to participate, consent for publication, competing interests.
The authors declare no competing interests.
Additional information
Publisherâs note.
Springer Nature remains neutral with regard to jurisdictional claims in published maps and institutional affiliations.
Supplementary Information
Supplementary material 1., rights and permissions.
Open Access This article is licensed under a Creative Commons Attribution 4.0 International License, which permits use, sharing, adaptation, distribution and reproduction in any medium or format, as long as you give appropriate credit to the original author(s) and the source, provide a link to the Creative Commons licence, and indicate if changes were made. The images or other third party material in this article are included in the article's Creative Commons licence, unless indicated otherwise in a credit line to the material. If material is not included in the article's Creative Commons licence and your intended use is not permitted by statutory regulation or exceeds the permitted use, you will need to obtain permission directly from the copyright holder. To view a copy of this licence, visit http://creativecommons.org/licenses/by/4.0/ . The Creative Commons Public Domain Dedication waiver ( http://creativecommons.org/publicdomain/zero/1.0/ ) applies to the data made available in this article, unless otherwise stated in a credit line to the data.
Reprints and permissions
About this article
Cite this article.
Wang, Xm., Zhang, Xj. Role of radiomics in staging liver fibrosis: a meta-analysis. BMC Med Imaging 24 , 87 (2024). https://doi.org/10.1186/s12880-024-01272-x
Download citation
Received : 13 June 2023
Accepted : 10 April 2024
Published : 12 April 2024
DOI : https://doi.org/10.1186/s12880-024-01272-x
Share this article
Anyone you share the following link with will be able to read this content:
Sorry, a shareable link is not currently available for this article.
Provided by the Springer Nature SharedIt content-sharing initiative
BMC Medical Imaging
ISSN: 1471-2342
- General enquiries: [email protected]
Europe stands firm against US-driven ESG backlash
- Medium Text
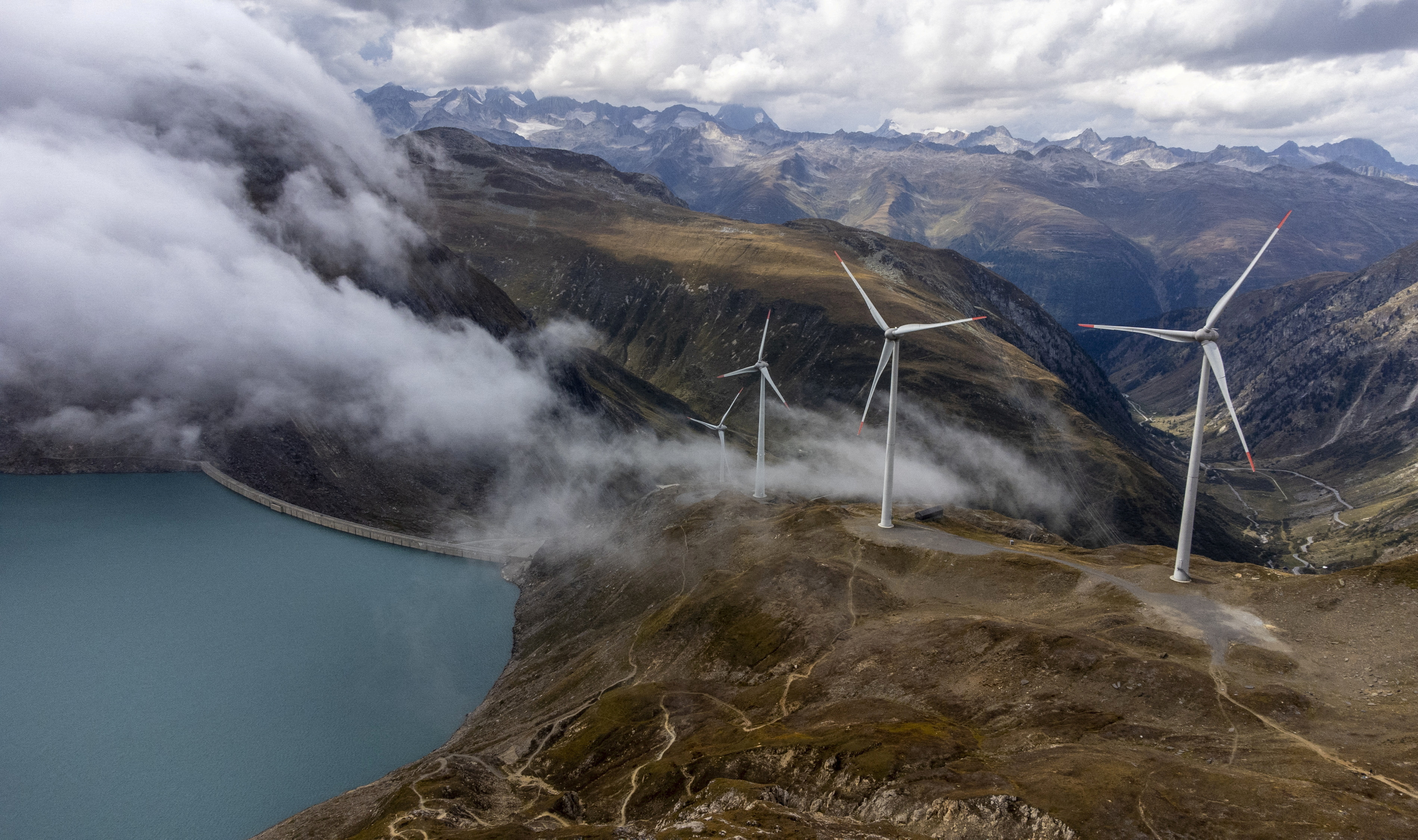
- Regulations, public support greater in Europe
- Europe ESG fund flows stay strong vs U.S. flows
- Climate coalitions dominated by European firms
REGULATORY SUPPORT

The Reuters Daily Briefing newsletter provides all the news you need to start your day. Sign up here.
Reporting by Simon Jessop in London, Ross Kerber in London and Isla Binnie in New York Editing by Greg Roumeliotis and Jane Merriman
Our Standards: The Thomson Reuters Trust Principles. New Tab , opens new tab

Thomson Reuters
Simon leads a team tracking how the financial system and companies more broadly are responding to the challenges posed by climate change, nature loss and other environmental, social and governance (ESG) issues including diversity and inclusion.
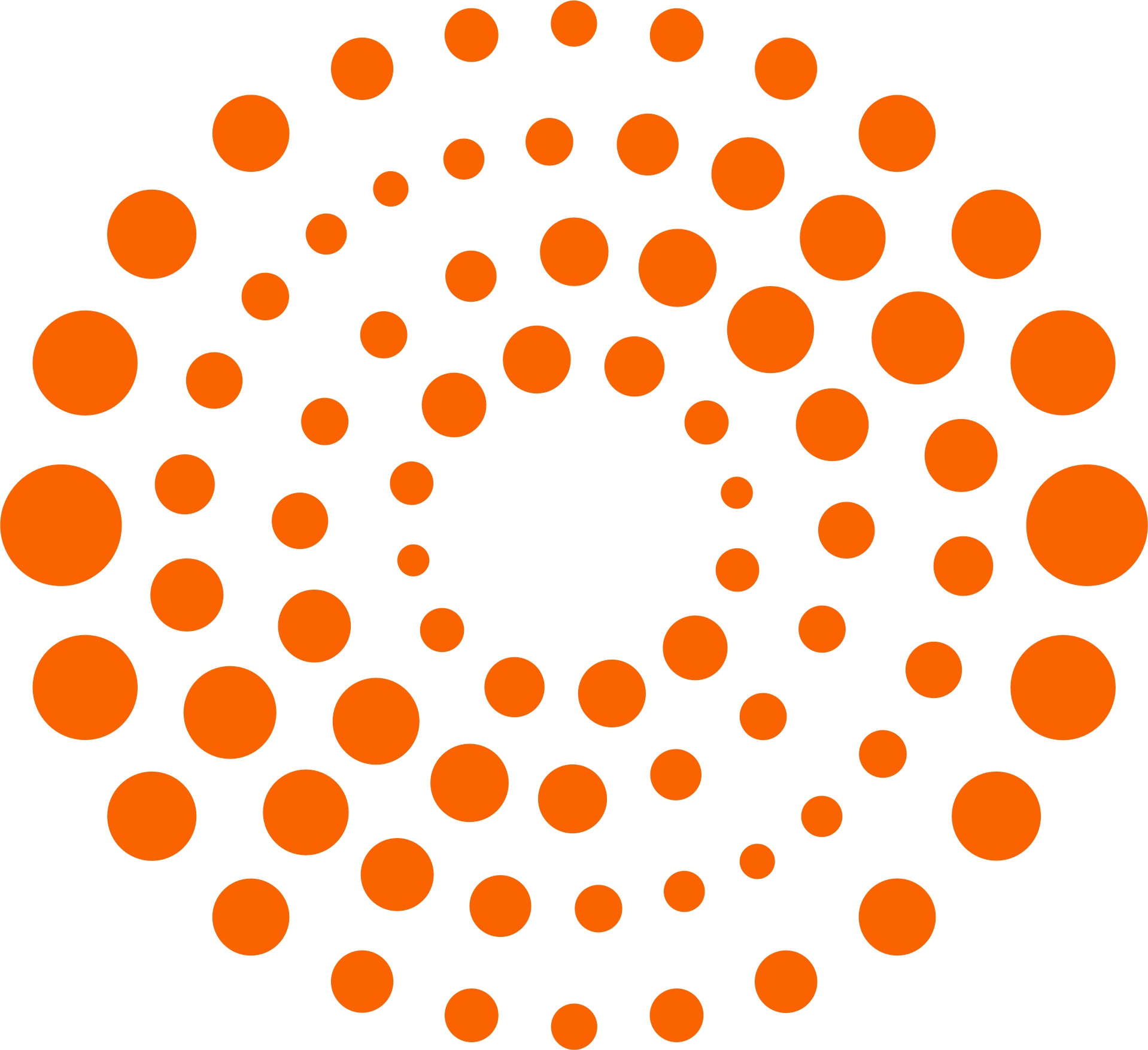
Ross Kerber is U.S. Sustainable Business Correspondent for Reuters News, a beat he created to cover investorsâ growing concern for environmental, social and governance (ESG) issues, and the response from executives and policymakers. Ross joined Reuters in 2009 after a decade at The Boston Globe and has written on topics including proxy voting by the largest asset managers, the corporate response to social movements like Black Lives Matter, and the backlash to ESG efforts by conservatives. He writes the weekly Reuters Sustainable Finance Newsletter.

Isla Binnie is Reuters's New York-based Sustainable Finance Correspondent, reporting on how the worlds of business and finance are affected by and respond to environmental, social and governance issues. Isla previously covered a broad range of business, politics and general news in Spain and Italy, where she also moderated events. She trained with Reuters in London and covered emerging markets debt for the International Financing Review (IFR).
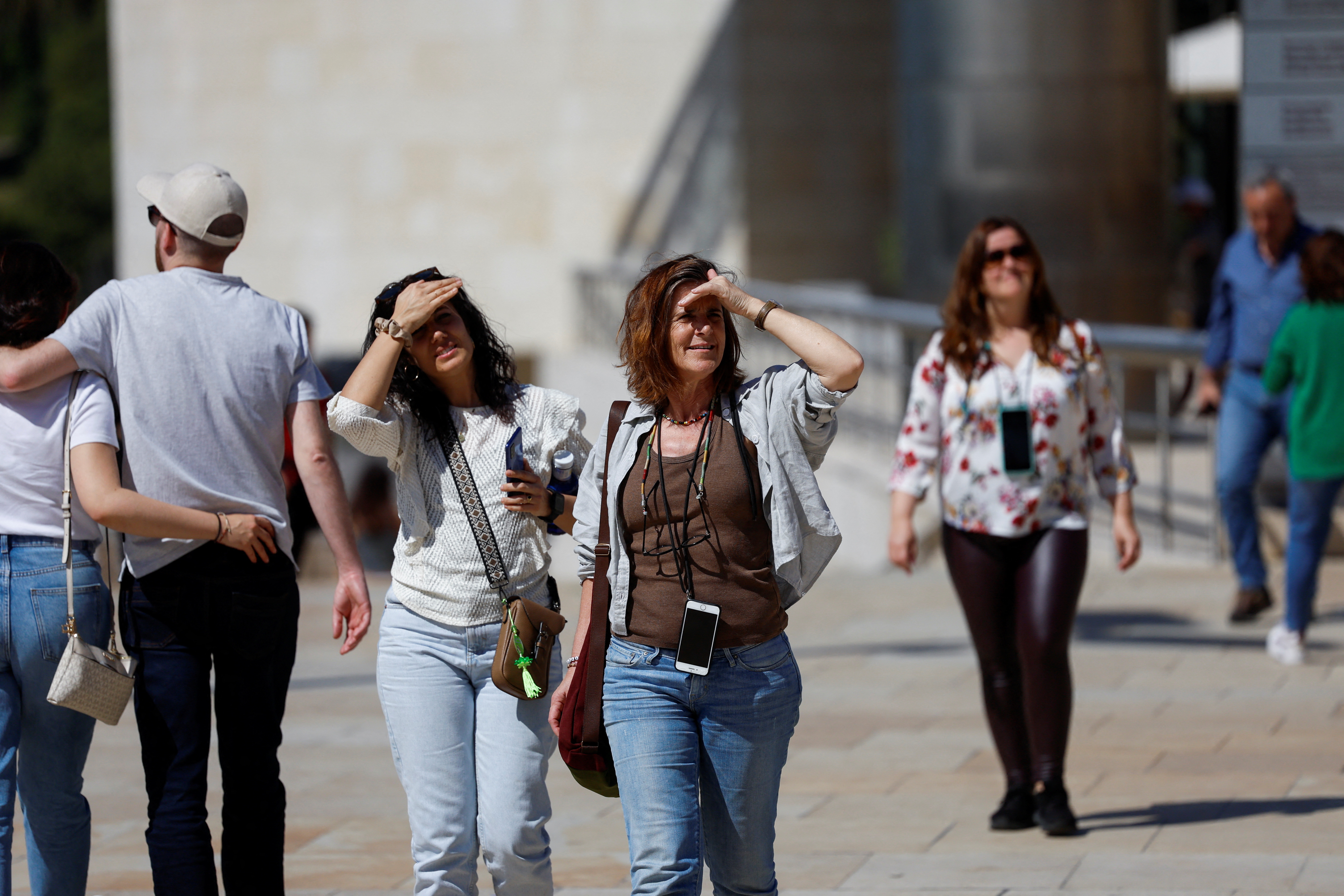
Johnson Controls said on Friday its subsidiary Tyco Fire Products had agreed to a $750 million settlement with some U.S. public water systems that claimed toxic "forever chemicals" in firefighting foam made by the company had contaminated their water supplies.
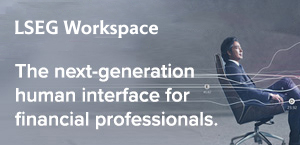
Sustainability Chevron
Iberians hit the beaches as temperatures rise 10c above normal.
People in Bilbao in northwestern Spain normally spend April dodging the showers but on Saturday many hit the beach as temperatures were up to 10 degrees Celsius (18 degrees Fahrenheit) above normal.
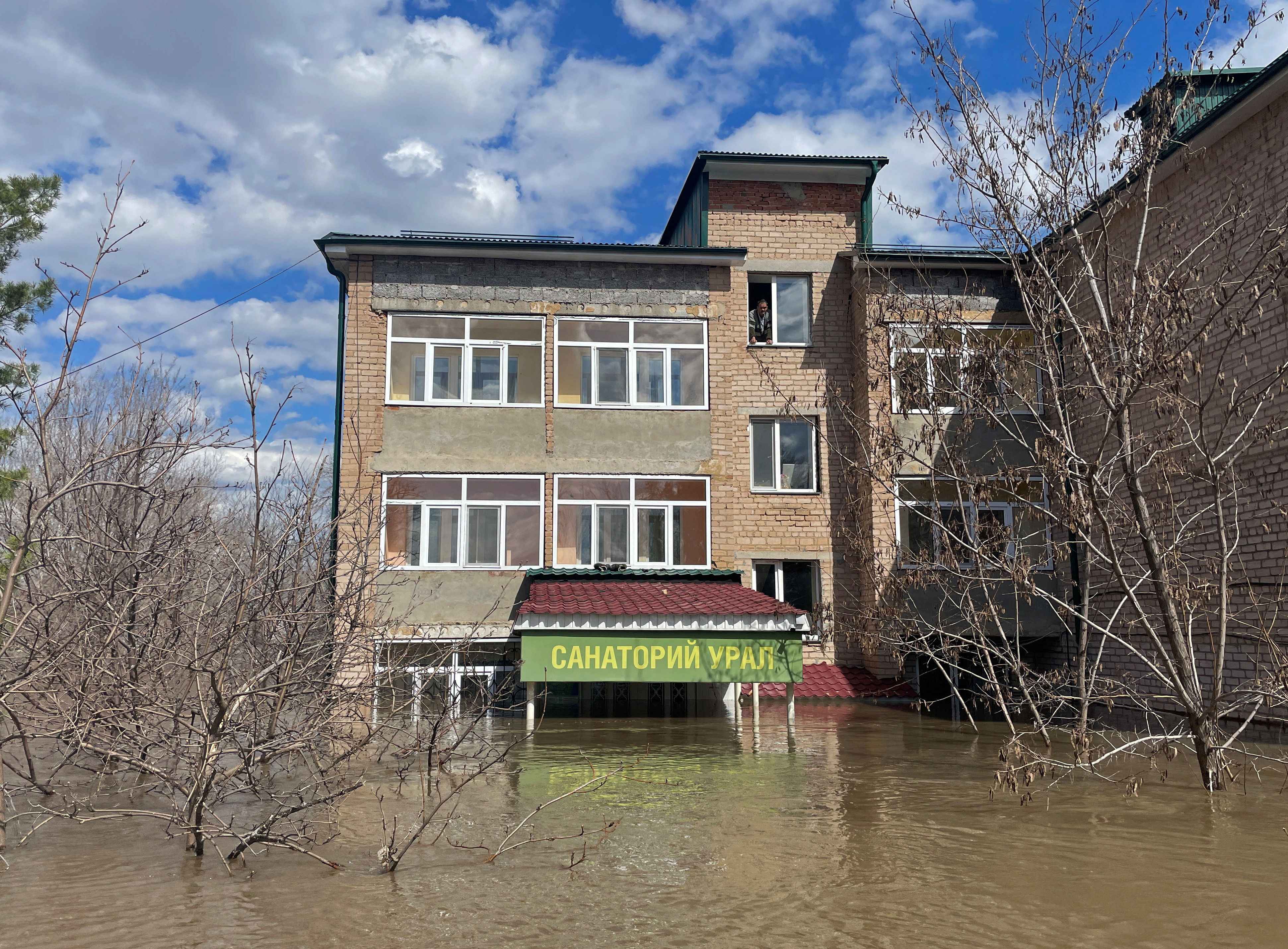
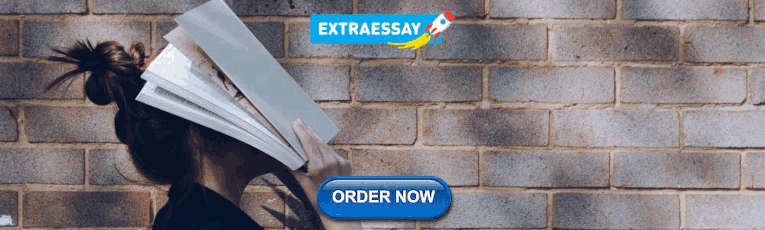
IMAGES
VIDEO
COMMENTS
The advanced version of our text analyser gives a much more detailed analysis of text with many more statistics, analysis by word length, by word frequency and by common phrases. Advanced Features Include: Save up to 20 texts for future reference and comparison; Analyse longer texts of up to 100,000 characters; A Graded Text Analysis tool
Text analysis (TA) is a machine learning technique used to automatically extract valuable insights from unstructured text data. Companies use text analysis tools to quickly digest online data and documents, and transform them into actionable insights. You can us text analysis to extract specific information, like keywords, names, or company ...
Text Analyzer is a SEO web content analysis tool that provides extensive statistic about your content like count of characters, words, sentences, paragraphs, spaces, punctuations, the frequency of phrases and words, measure of estimated read and speak time. Text Analyzer is useful for writers and editors of blogs, forums, websites, product ...
By mining the text for data on readability, word count and frequency, and keyword density, you can understand how a search engine may see your text. Word count and frequency checker. Make sure you're hitting your targets when writing, with an accurate analysis of the word count you're using.
Text analysis is a technique for uncovering insights from data sources such as text documents, web pages, emails, and social media posts. It enables companies to gain actionable data and better understand the sentiment of their customer or potential customer. The technique combines linguistics and computer science to derive meaning from text ...
Text analysis aims to derive quality insights from solely the text or words itself. Semantics in the text is not considered. It answers questions like frequency of words, length of sentence, and presence or absence of words. On the other hand, NLP aims to understand the linguistic use and context behind the text.
Text analysis is a broad term that encompasses the examination and interpretation of textual data. It involves various techniques to understand, organize, and derive insights from text, including methods from linguistics, statistics, and machine learning. Text analysis often includes processes like text categorization, sentiment analysis, and ...
Text Analysis is a complex and specialized task. Textalytic makes it super simple to extract insight from textual content. Use our text builder to preprocess your text. Copy and paste text into the editor or upload a file from your computer or Dropbox. You can view your results in a table, graphs, or export them to csv and pdf format.
Welcome to Prose Analyzer - your advanced online text analysis tool. Whether you are a student polishing an essay, an educator guiding learners, a researcher handling vast amounts of data, or a content creator shaping impactful prose, Prose Analyzer is your comprehensive solution. Tailor-made for users with a diversity of needs, our tool ...
Text Analysis. Choose between multiple language features to analyze the structure of texts. Gain insight into the patterns of text such as word usage, grammar, and occurrences of words and clauses. Even if you do not have any text ready, you can choose from our wide range of texts in our library or bring in messages from Slack or tweets from ...
Text analysis is the process of using computer systems to read and understand human-written text for business insights. Text analysis software can independently classify, sort, and extract information from text to identify patterns, relationships, sentiments, and other actionable knowledge. You can use text analysis to efficiently and ...
Automate how you analyze huge amounts of text. Free text analysis tool that finds the most frequent keywords and phrases. Try MonkeyLearn's text analyzer for free.
With our free online Text Analyzer, you can effortlessly count the number of characters, words, sentences, paragraphs, and frequency in your text. This powerful tool provides quick and accurate analysis, helping you understand and optimize your written content.
Text analytics is the process of transforming unstructured text documents into usable, structured data. Text analysis works by breaking apart sentences and phrases into their components, and then evaluating each part's role and meaning using complex software rules and machine learning algorithms. Text analytics forms the foundation of ...
Scalability: With text analytics techniques, enormous data across social media, emails, chats, websites, and documents can be structured and processed without difficulty, helping businesses improve efficiency with more information. Real-time Analysis: Real-time data in today's world is a game-changer. Evaluating this information with text ...
Text analysis is the process of examining and interpreting text in order to extract meaningful insights. It can involve both manual and computational methods. Text miningor text analytics is a subfield of text analysis that specifically involves computational algorithms to extract and analyze the data. Natural language processing (NLP) is a ...
Whether you are trying to do text sentiment analysis online, find the best free text mining software or perform data mining on unstructured text data, we believe Speak is a great option to start your journey. The best text analytics tools are simple to use and enable you to do text analysis with having to do a text mining software free download.
Use sentiment analysis to quickly detect emotions in text data. Play around with our sentiment analyzer, below: Test with your own text. Classify Text. Results. Tag Confidence. Positive 99.1%. Get sentiment insights like these: Sentiment analysis benefits: đ ...
Text Analysis Online Program. Finds most frequent phrases and words, gives overview about text style, number of words, characters, sentences and syllables.
Well, with our Text Analyzer tool, this has never been easier. All you need to do is paste your text into the toolbox, and it will analyze the text for you. And much more. In short, it is your one-stop-solution to all your text analysis needs. You can then modify your content accordingly and check again through our tool.
Get an overall difficulty score for your English content based on over 200 metrics. Try the tool out with this paragraph of text here, by pressing Analyse below. Or you can replace this whole text with some text of your choosing. Simply paste any text into this box, or else type it in yourself! Text Inspector gives you different scores for ...
perform. text. analysis with scholarly and primary. source content from JSTOR, Portico, and partners. Constellate enables you to easily and confidently incorporate text analysis into your curriculum. Whether you're new to the practice or a seasoned pro, our user-friendly software and pedagogical approach will meet your educational needs.
About Web-Based Tools. Web-based tools provide a variety of easy to use and manage visual ization and analysis tools. Some of the possible tools available include word clouds, charts, graphics, and other analysis tools that create visual images and statistically interpret your text.
by Rahil Virani. In a recent event by Gale for UW-Madison, participants explored investigative journalism and digital humanities using the Gale Digital Scholar Lab.The event titled "Going Hands-on with the Gale Digital Scholar Lab: A Closer Look at the 'Female Stunt Reporter' Dataset," offered a chance to learn new skills and knowledge and to get ready to apply text analysis techniques ...
Here are some other laws Arizona had on the books in 1864. A person in Tucson on Tuesday protests after the Arizona Supreme Court revived a near-total ban on abortion. (Rebecca Noble/Reuters ...
Key features integrated into the National Water Prediction Service website. A new, dynamic and seamless national map with flexible options and expansive layers available to help analyze water conditions anywhere in the country. Improved hydrographs that are frequently updated, depict water level observations over the past 30 days and provide ...
Healthy living is a way to manage diabetes. To have a healthy lifestyle, take steps now to plan healthy meals and snacks, do physical activities, get enough sleep, and quit smoking or using tobacco products. Healthy living may help keep your body's blood pressure, cholesterol, and blood glucose level, also called blood sugar level, in the ...
After assessing the market and holding talks with rival teams - Fernando Alonso decided the lucrative multi-year offer to stay at Aston Martin was too irresistible to turn down. So, what does that mean for the rest of the driver market?
The present study was conducted by following PRISMA guidelines. Inclusion and exclusion criteria. A study was included in the meta-analysis if a) it prospectively or retrospectively recruited patients with chronic liver disease who had histologically confirmed fibrosis in the liver; b) performed radiomic analyses based on any radiological modality to diagnose and/or differentiate fibrosis; and ...
New ESG fund launches fell 10% in Europe in 2023, but the slide in the United States was even more pronounced, down 75%, according to Morningstar. Reuters Graphics. U.S. outflows from sustainable ...