Standard Score
The standard score (more commonly referred to as a z-score) is a very useful statistic because it (a) allows us to calculate the probability of a score occurring within our normal distribution and (b) enables us to compare two scores that are from different normal distributions. The standard score does this by converting (in other words, standardizing) scores in a normal distribution to z-scores in what becomes a standard normal distribution. To explain what this means in simple terms, let's use an example (if needed, see our statistical guide, Normal Distribution Calculations , for background information on normal distribution calculations).
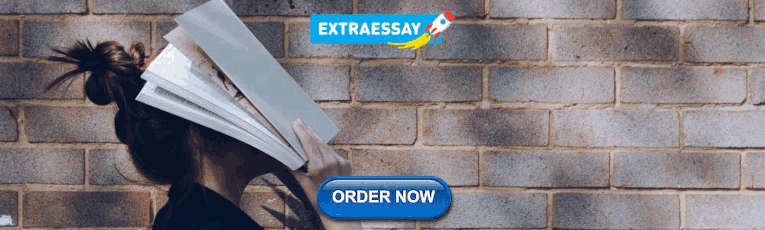
Setting the scene: Part 1
A tutor sets a piece of English Literature coursework for the 50 students in his class. We make the assumption that when the scores are presented on a histogram, the data is found to be normally distributed. The mean score is 60 out of 100 and the standard deviation (in other words, the variation in the scores) is 15 marks (see our statistical guides, Measures of Central Tendency and Standard Deviation , for more information about the mean and standard deviation).
Having looked at the performance of the tutor's class, one student, Sarah, has asked the tutor if, by scoring 70 out of 100, she has done well. Bearing in mind that the mean score was 60 out of 100 and that Sarah scored 70, then at first sight it may appear that since Sarah has scored 10 marks above the 'average' mark, she has achieved one of the best marks. However, this does not take into consideration the variation in scores amongst the 50 students (in other words, the standard deviation). After all, if the standard deviation is 15, then there is a reasonable amount of variation amongst the scores when compared with the mean.
Whilst Sarah has still scored much higher than the mean score, she has not necessarily achieved one of the best marks in her class. The question arises: How well did Sarah perform in her English Literature coursework compared to the other 50 students? Before answering this question, let us look at another problem.
The tutor has a dilemma. In the next academic year, he must choose which of his students have performed well enough to be entered into an advanced English Literature class. He decides to use the coursework scores as an indicator of the performance of his students. As such, he feels that only those students that are in the top 10% of the class should be entered into the advanced English Literature class. The question arises: Which students came in the top 10% of the class?
Therefore, we are left with two questions to answer. First, how well did Sarah perform in her English Literature coursework compared to the other 50 students? Second, which students came in the top 10% of the class?
Whilst it is possible to calculate the answer to both of these questions using the existing mean score and standard deviation, this is very complex. Therefore, statisticians have come up with probability distributions , which are ways of calculating the probability of a score occurring for a number of common distributions, such as the normal distribution. In our case, we make the assumption that the students' scores are normally distributed. As such, we can use something called the standard normal distribution and its related z-scores to answer these questions much more easily.
Standard Normal Distribution and Standard Score (z-score)
When a frequency distribution is normally distributed, we can find out the probability of a score occurring by standardising the scores, known as standard scores (or z scores). The standard normal distribution simply converts the group of data in our frequency distribution such that the mean is 0 and the standard deviation is 1 (see below).
Z-Score: Definition, Formula, Calculation & Interpretation
Saul Mcleod, PhD
Editor-in-Chief for Simply Psychology
BSc (Hons) Psychology, MRes, PhD, University of Manchester
Saul Mcleod, PhD., is a qualified psychology teacher with over 18 years of experience in further and higher education. He has been published in peer-reviewed journals, including the Journal of Clinical Psychology.
Learn about our Editorial Process
Olivia Guy-Evans, MSc
Associate Editor for Simply Psychology
BSc (Hons) Psychology, MSc Psychology of Education
Olivia Guy-Evans is a writer and associate editor for Simply Psychology. She has previously worked in healthcare and educational sectors.
On This Page:
A z-score describes the position of a raw score in terms of its distance from the mean when measured in standard deviation units. The z-score is positive if the value lies above the mean and negative if it lies below the mean.
It is also known as a standard score because it allows the comparison of scores on different kinds of variables by standardizing the distribution. A standard normal distribution (SND) is a normally shaped distribution with a mean of 0 and a standard deviation (SD) of 1 (see Fig. 1).
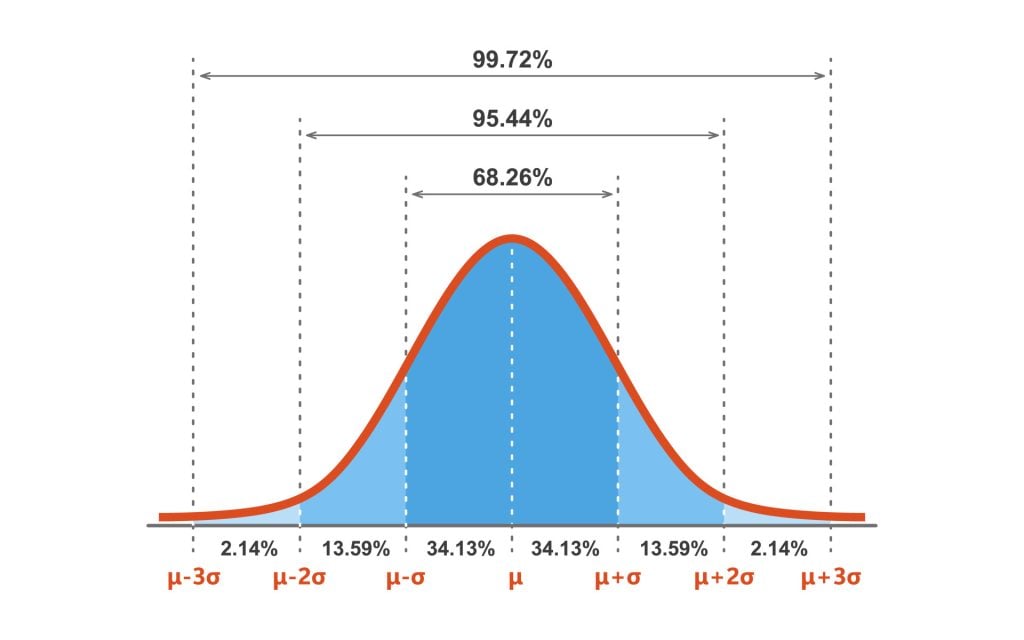
Why Are Z-Scores Important?
It is useful to standardize the values (raw scores) of a normal distribution by converting them into z-scores because:
- It allows researchers to calculate the probability of a score occurring within a standard normal distribution;
- It enables us to compare two scores from different samples (which may have different means and standard deviations).
How To Calculate
The formula for calculating a z-score is z = (x-μ)/σ, where x is the raw score, μ is the population mean, and σ is the population standard deviation.
As the formula shows, the z-score is simply the raw score minus the population mean, divided by the population standard deviation.
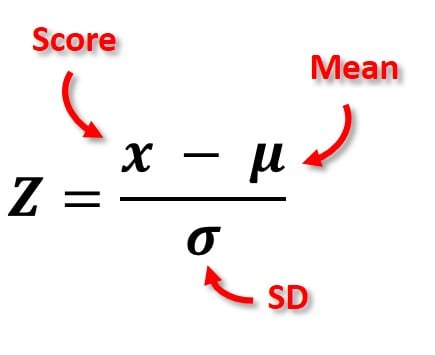
When the population mean and the population standard deviation are unknown, the standard score may be calculated using the sample mean (x̄) and sample standard deviation (s) as estimates of the population values.
Interpretation
The value of the z-score tells you how many standard deviations you are away from the mean. If a z-score is equal to 0, it is on the mean.
- A positive z-score indicates the raw score is higher than the mean average. For example, if a z-score is equal to +1, it is 1 standard deviation above the mean.
- A negative z-score reveals the raw score is below the mean average. For example, if a z-score is equal to -2, it is two standard deviations below the mean.
Another way to interpret z-scores is by creating a standard normal distribution, also known as the z-score distribution, or probability distribution (see Fig. 3).
Standard Normal Distribution (SND)
- The SND (i.e., z-distribution) is always the same shape as the raw score distribution. For example, if the distribution of raw scores is normally distributed, so is the distribution of z-scores.
- The mean of any SND always = 0.
- The standard deviation of any SND always = 1. Therefore, one standard deviation of the raw score (whatever raw value this is) converts into 1 z-score unit.
The SND allows researchers to calculate the probability of randomly obtaining a score from the distribution (i.e., sample). For example, there is a 68% probability of randomly selecting a score between -1 and +1 standard deviations from the mean (see Fig. 3).
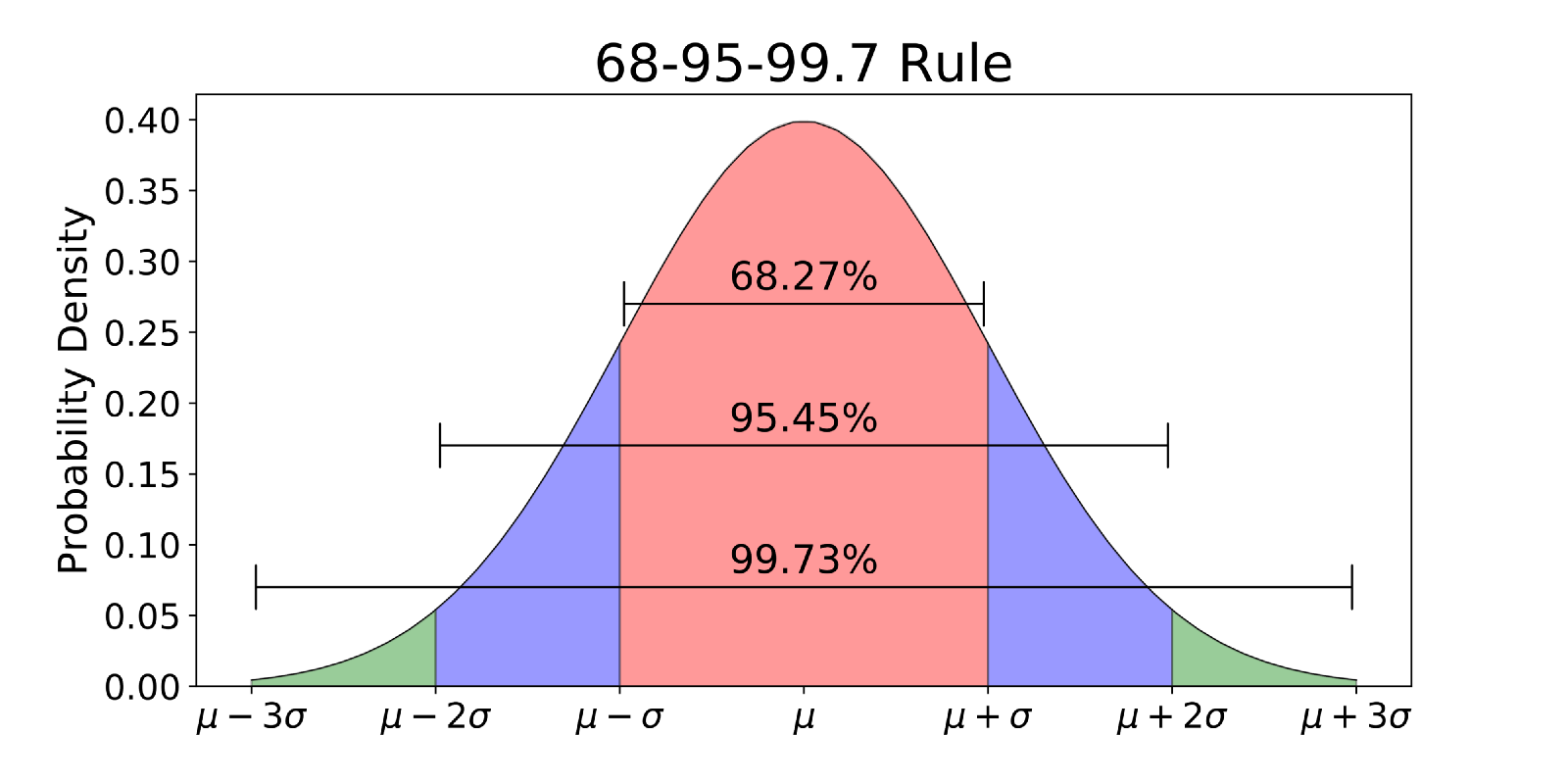
The probability of randomly selecting a score between -1.96 and +1.96 standard deviations from the mean is 95% (see Fig. 3).
If there is less than a 5% chance of a raw score being selected randomly, then this is a statistically significant result.
Learn how to use a z-score table
Practice Problems for Z-Scores
Calculate the z-scores for the following:
Sample Questions
- Scores on a psychological well-being scale range from 1 to 10, with an average score of 6 and a standard deviation of 2. What is the z-score for a person who scored 4?
- On a measure of anxiety, a group of participants show a mean score of 35 with a standard deviation of 5. What is the z-score corresponding to a score of 30?
- A depression inventory has an average score of 50 with a standard deviation of 10. What is the z-score corresponding to a score of 70?
- In a study on sleep, participants report an average of 7 hours of sleep per night, with a standard deviation of 1 hour. What is the z-score for a person reporting 5 hours of sleep?
- On a memory test, the average score is 100, with a standard deviation of 15. What is the z-score corresponding to a score of 85?
- A happiness scale has an average score of 75 with a standard deviation of 10. What is the z-score corresponding to a score of 95?
- An intelligence test has a mean score of 100 with a standard deviation of 15. What is the z-score that corresponds to a score of 130?
Answers for Sample Questions
Double-check your answers with these solutions. Remember, for each problem, you subtract the average from your value, then divide by how much values typically vary (the standard deviation).
- Z-score = (4 – 6)/2 = -1
- Z-score = (30 – 35)/5 = -1
- Z-score = (70 – 50)/10 = 2
- Z-score = (5 – 7)/1 = -2
- Z-score = (85 – 100)/15 = -1
- Z-score = (95 – 75)/10 = 2
- Z-score = (130 – 100)/15 = 2
Calculating a Raw Score
Sometimes we know a z-score and want to find the corresponding raw score. The formula for calculating a z-score in a sample into a raw score is given below:
X = (z)(SD) + mean
As the formula shows, the z-score and standard deviation are multiplied together, and this figure is added to the mean.
Check your answer makes sense: If we have a negative z-score, the corresponding raw score should be less than the mean, and a positive z-score must correspond to a raw score higher than the mean.
Calculating a Z-Score using Excel
To calculate the z-score of a specific value, x, first, you must calculate the mean of the sample by using the AVERAGE formula.
For example, if the range of scores in your sample begins at cell A1 and ends at cell A20, the formula =AVERAGE(A1:A20) returns the average of those numbers.
Next, you must calculate the standard deviation of the sample by using the STDEV.S formula. For example, if the range of scores in your sample begins at cell A1 and ends at cell A20, the formula = STDEV.S (A1:A20) returns the standard deviation of those numbers.
Now to calculate the z-score, type the following formula in an empty cell: = (x – mean) / [standard deviation].
To make things easier, instead of writing the mean and SD values in the formula, you could use the cell values corresponding to these values. For example, = (A12 – B1) / [C1].
Then, to calculate the probability for a SMALLER z-score, which is the probability of observing a value less than x (the area under the curve to the LEFT of x), type the following into a blank cell: = NORMSDIST( and input the z-score you calculated).
To find the probability of LARGER z-score, which is the probability of observing a value greater than x (the area under the curve to the RIGHT of x), type: =1 – NORMSDIST (and input the z-score you calculated).
Frequently Asked Questions
Can z-scores be used with any type of data, regardless of distribution.
Z-scores are commonly used to standardize and compare data across different distributions. They are most appropriate for data that follows a roughly symmetric and bell-shaped distribution.
However, they can still provide useful insights for other types of data, as long as certain assumptions are met. Yet, for highly skewed or non-normal distributions, alternative methods may be more appropriate.
It’s important to consider the characteristics of the data and the goals of the analysis when determining whether z-scores are suitable or if other approaches should be considered.
How can understanding z-scores contribute to better research and statistical analysis in psychology?
Understanding z-scores enhances research and statistical analysis in psychology. Z-scores standardize data for meaningful comparisons, identify outliers, and assess likelihood.
They aid in interpreting practical significance, applying statistical tests, and making accurate conclusions. Z-scores provide a common metric, facilitating communication of findings.
By using z-scores, researchers improve rigor, objectivity, and clarity in their work, leading to better understanding and knowledge in psychology.
Can a z-score be used to determine the likelihood of an event occurring?
No, a z-score itself cannot directly determine the likelihood of an event occurring. However, it provides information about the relative position of a data point within a distribution.
By converting data to z-scores, researchers can assess how unusual or extreme a value is compared to the rest of the distribution. This can help estimate the probability or likelihood of obtaining a particular score or more extreme values.
So, while z-scores provide insights into the relative rarity of an event, they do not directly determine the likelihood of the event occurring on their own.
Further Information
How to Use a Z-Table (Standard Normal Table) to Calculate the Percentage of Scores Above or Below the Z-Score
Z-Score Table (for positive or negative scores)
Statistics for Psychology Book Download

Advantages And Pitfalls Of Using The Z Score
There’s no question that using the z score can be very helpful in certain situations. However, its use may also lead to pitfalls that you need to know about. However, before we start, we believe that it is important to remind you about what actually ar z scores.
Discover the best statistics calculators.
What Are Z Scores?
Simply put, z scores are a way that you have that allows you to standardize a score in respect to other scores in the group. As you already know, in order to determine the z score, you need to know both the mean and the standard deviation of the group.
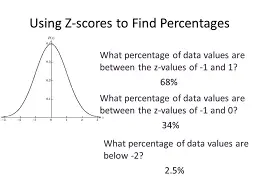
So, we can then state that a z score expresses a specific score in terms of how many standard deviations it is away from the mean.
Looking to know more about the z score table?
Notice that when you convert a raw score into a z score, you are ultimately expressing that score on a z score scale which always has a mean of zero and a standard deviation of one. So, to sum up, when you calculate the z score you are redefining each raw score in terms of how far away it is from the group mean.
Now that you are already reminded about what a score is, it’s time to check out the advantages and pitfalls of using the z score.
Advantages Of Using The Z Score
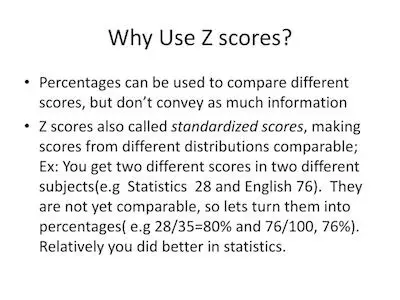
As you know, you can’t always use the z score. However, when it is possible, using it can bring different advantages:
#1: Clarity:
One of the main advantages of using the z score is the fact that you can see and understand the relationship between the raw score and the distribution of scores much clearer. So, this means that it is possible to get an idea of how good or bad a score is relative to the entire group.
Discover more about the z table.
#2: Comparison:
Another great advantage of using the z score is related to the fact that you can easily compare scores that are measured on different scales.
#3: The Area Under The Curve:
If you think about it, you already know many different properties of the normal distribution. So, by converting to a normal distribution of z scores, you will be able to discover how many scores actually fall between certain limits. So, this means that you can then calculate the probability of a specific score occur.
#4: The Area Between The Mean And Z:
Ultimately, this specific area of the table tells you the proportion of scores that are between the mean and a specific z score. Notice that this proportion is the area under the curve between those points.
#5: Area Beyond Z:
This part of the table tells you the proportion of scores that are greater than a specific z score.
Pitfalls Of Using The Z Score
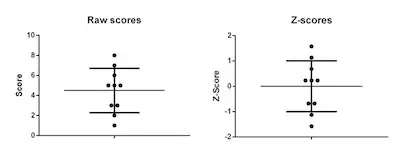
#1: When you calculate the z score from raw scores, you may end up losing the meaningfulness of these raw scores.
Understanding the z score table normal distribution.
#2: Since you need to know the standard deviations to calculate the z score, you may also lose the meaning standard scores.
#3: When you are using the z score, you may end up magnifying small differences.
#4: Linear transformations require interval data and some of the data that you use may bot be interval level.

An official website of the United States government
The .gov means it’s official. Federal government websites often end in .gov or .mil. Before sharing sensitive information, make sure you’re on a federal government site.
The site is secure. The https:// ensures that you are connecting to the official website and that any information you provide is encrypted and transmitted securely.
- Publications
- Account settings
Preview improvements coming to the PMC website in October 2024. Learn More or Try it out now .
- Advanced Search
- Journal List
- Indian J Psychol Med
- v.43(6); 2021 Nov
Z Scores, Standard Scores, and Composite Test Scores Explained
Chittaranjan andrade.
1 Dept. of Clinical Psychopharmacology and Neurotoxicology, National Institute of Mental Health and Neurosciences, Bengaluru, Karnataka, India.
Patients may be assessed using a battery of tests where different tests yield scores in different units, where different tests have different minimum and maximum scores, and where higher or lower scores mean different things in different tests. Therefore, a composite test score cannot be obtained by simple addition or averaging of scores in the individual tests. However, if performances in individual tests are converted to Z scores, the Z scores can be added or averaged to yield a composite score that can be interpreted or processed using conventional statistical methods. This article explains in simple ways how Z scores are calculated, what the properties of Z scores are, how Z scores can be interpreted, and how Z scores can be converted into other standard scores.
In a hypothetical study, I randomize schizophrenia patients to computer-based cognitive remediation (CR) or television viewing (TV) thrice weekly for three months. I administer five cognitive tasks at the study baseline and, again, at the study endpoint. I wish to determine whether CR improves cognitive task scores more than TV does. One way of doing this is to use statistical tests to compare CR and TV groups, task by task; however, there are several problems associated with this approach. For example, performing five separate statistical tests, one for each cognitive task, increases the risk of a Type 1 (false positive) error. 1 Or, patients in one group may perform better in some tasks and worse in other tasks relative to patients in the other group; so, what should the overall conclusion be? Or, patients in one group may perform better than patients in the other group in all tasks without the results reaching statistical significance for any task; again, what should the overall conclusion be?
Need for Composite Scores
One way to get an overall perspective is to create a composite score. This is easily done in some circumstances; for example, one may reasonably add or average language, science, math, geography, and history marks to get a single composite score in school examinations. Simple addition or averaging of marks is possible because all subjects are treated equally, all subjects are marked from 0 to 100, and all subjects have higher marks indicating better performance. Simple addition or averaging is not possible for cognitive tasks because different tasks have different everyday importance, because different tasks have different minimum and maximum scores, because in some tasks lower scores indicate better performance, and in other tasks, higher scores indicate better performance, and because some tasks yield scores measured in units of time, others yield scores measured as the number of correct responses, and so on. As examples, verbal memory may be more important than visual memory because of its application to everyday life; tests of processing speed are measured in units of time and lower scores indicate better performance; and tests of memory are measured in the number of units correctly recalled and higher scores indicate better performance. So, simple addition or averaging of scores is not possible.
Computing Z Scores
One solution is to first convert the original (raw) scores for each cognitive task into a new score that is described in the same unit for all tasks. The new scores can then be added or averaged to form a composite score. Conversion of the raw scores into Z scores is one such approach. To do this (for example) for the verbal memory test in the cognitive battery, I would need to perform the following actions with the verbal memory raw scores.
- Calculate the mean (M) and standard deviation (SD) verbal memory score for the CR and TV groups combined into a single group ; that is, for the pooled sample .
- Calculate the Z score for each patient; the formula is Z = (x – M)/SD, where x is the patient’s verbal memory raw score and M and SD are the estimates from the previous step. Positive Z values indicate scores that are greater than the mean of the pooled sample, and negative values indicate scores that are less than the pooled mean. 2
Z scores are similarly calculated for each patient for each of the remaining four cognitive tasks. This is done separately for the baseline and endpoint data.
Understanding Z Scores
If we look at the formula for the Z score, we will immediately realize that the Z score tells us how far above or below the mean an individual’s score is, expressed in units of SD . So, if the M(SD) is 18(4) for the verbal memory scores in the pooled sample, a patient with a verbal memory score of 20 has a Z score of (20−18)/4, or 0.5. That is, the patient’s verbal memory score is half an SD above the mean of the sample. Another patient whose raw score is 12 would have a Z score of (12−18)/4, or −1.5; that is, one and a half SDs below the sample mean.
Interpreting and Using the Z Scores
The raw scores were in different units in the different cognitive tasks. Z scores are all in the same unit, that is, SD. The Z score distribution has a mean of 0 and an SD of 1. Z scores are useful because they allow data to be interpreted or used in many ways, as the following examples show:
- The Z score tells us at a glance how the patient has performed relative to the rest of the sample, something that is not evident from the inspection of a raw score.
- Because we understand the relationship between M and SD in the normal distribution, and because the Z score is an SD unit, we know that Z scores of 2 and above (either positive or negative) are quite far from the mean and that Z scores of 3 and above (either positive or negative) are so far from the mean as to represent outliers. So, an inspection of Z scores can identify outliers in the sample.
- Using published tables, such as a table of the area under the normal curve, we can read off the probability of obtaining any individual Z value.
- If a patient has a Z score of, for example, 1 for verbal memory and a Z score of −0.3 for processing speed, because the unit of Z is the same for both tasks, we can conclude that this patient performed better in the verbal memory task than in the processing speed task. This conclusion would not have been possible from an inspection of the raw scores.
- For tests where lower scores indicate better performance, Z scores should be multiplied by −1 so that when the Z scores are added, the composite score will correctly indicate the direction of change.
- Whereas the individual Z scores are in units of SD, the composite score, created by adding the Z scores for the five tests, is no longer in units of SD. However, if the composite score is divided by the number of tests, we get a composite (average) score that is again a unit of SD.
Z Scores and Composite Scores
When Z scores are added or averaged as described above, each cognitive task receives equal weightage. It is possible to create composite scores in which some tasks are given higher weightage than others, based on preset values for weights. For example, it can a priori be decided that, because verbal tasks are more relevant in everyday life than visual tasks, the verbal memory task should receive twice the weight that the visual memory task receives when computing the composite score. Weights can also be determined and assigned through statistical methods. 3 , 4
Once the composite score has been calculated for each patient, the M(SD) composite score can be calculated for CR and TV groups separately at the study baseline and at the study endpoint and then processed using usual statistical methods; this can be done whether the composite score is a total or an average of the Z scores of the individual cognitive tasks.
Standard Scores
Some people find it hard to understand Z scores, especially when values are negative (readers are reminded that Z scores have a mean of 0, and that Z values that are negative indicate scores that are below the mean of the sample). This difficulty can be resolved by converting Z scores into other standard scores. The Z score is one example of a standard score; using simple formulae, Z scores can be converted to other standard scores that have only positive values and other specific properties. An example is the T score which has M=50 and SD=10. Stanine and sten scores are based on the same principle. Stanine (standard nine) scores range from 1 to 9, with a mean of 5 and an SD of 2; sten (standard ten) scores range from 1 to 10, with a mean of 5.5 and an SD of 2. Stanine and sten scores are used in some psychological tests. IQ scores are also standardized; they have a mean of 100 and an SD of 15. Readers may note that Z transformation and other methods of standardization do not change the ranking of the original data.
Computing Z Scores: Reprise
The Z score for an individual measurement can be calculated using the mean and standard deviation of a sample, or of a pooled sample, or of the population, depending on the context in which the Z score requires to be derived and used. If the sample comprises a single group, such as a class of students, the Z scores are based on the M(SD) of that group. If there are two groups, such as in the study described in this article, Z scores should be calculated based on the M(SD) of the pooled sample. However, when Z scores are interpreted for a single individual on a test for which population norms are available, the population mean and standard deviation are used rather than the sample M(SD).
As an aside, for the study described in this article, why are the Z scores computed for the pooled sample; why cannot the Z scores be computed for each group separately, and then M(SD) Z scores compared between groups? The answer ought to be obvious. Z scores may create new values but do not change the ranks (order) of the raw scores within a group; and these new values have an M(SD) of 0(1) because, as stated earlier, this is a property of Z scores. So, if Z scores are computed separately for CR and TV groups, the M(SD) of the z scores for each group will be 0(1), making comparisons between groups illogical. However, if the CR and TV groups are pooled, the order of the raw scores will change; whereas the M(SD) of the Z scores for the pooled group will be 0(1), the Z scores for the CR and TV group patients will depend on the new order, and the M(SD) of the Z scores for the CR and TV groups will no longer each be 0(1). So, the M(SD) Z scores thus created can now be validly compared between the CR and TV groups. Pooling of groups is done in certain nonparametric tests, as well. For example, in the Mann–Whitney and Kruskal–Wallis tests, the groups are pooled, individual values are ranked, and then the ranks are compared across groups.
Parting Notes
Knowledgeable readers may recognize that the standardized mean difference that is a measure of pooled effect size in meta-analysis and the (standardized) beta coefficient in regression analysis are both based on principles similar to those discussed in this article. A discussion on these, however, is out of the scope of the present article.
Declaration of Conflicting Interests: The author declared no potential conflicts of interest with respect to the research, authorship, and/or publication of this article.
Funding: The author received no financial support for the research, authorship, and/or publication of this article.
- Bipolar Disorder
- Therapy Center
- When To See a Therapist
- Types of Therapy
- Best Online Therapy
- Best Couples Therapy
- Best Family Therapy
- Managing Stress
- Sleep and Dreaming
- Understanding Emotions
- Self-Improvement
- Healthy Relationships
- Student Resources
- Personality Types
- Guided Meditations
- Verywell Mind Insights
- 2023 Verywell Mind 25
- Mental Health in the Classroom
- Editorial Process
- Meet Our Review Board
- Crisis Support
How a Z-Score Can Help You Compare Data—Here's How to Calculate It
LaKeisha Fleming is a prolific writer with over 20 years of experience writing for a variety of formats, from film and television scripts to magazines articles and digital content. She is passionate about parenting and family, as well as destigmatizing mental health issues. Her book, There Is No Heartbeat: From Miscarriage to Depression to Hope , is authentic, transparent, and provides hope to many.
:max_bytes(150000):strip_icc():format(webp)/LaKeishaFlemingPhoto1-584c31b6e5684e6d84a7d37cd33752c7.jpg)
Steven Gans, MD is board-certified in psychiatry and is an active supervisor, teacher, and mentor at Massachusetts General Hospital.
:max_bytes(150000):strip_icc():format(webp)/steven-gans-1000-51582b7f23b6462f8713961deb74959f.jpg)
Carol Yepes / Getty Images
What Does a Z-Score Tell You?
Z-score vs. standard deviation, how to calculate the z-score, z-scores and iq testing, z-score and other psychological tests.
"You can’t compare apples to oranges." Although the saying is cliché, the message behind it is clear. Comparing two things that are completely different is hard to do. You need a way to make the objects equal so it can give you a fair comparison.
Similarly, when analyzing numbers, experts have to find a way to make the numbers comparable. This allows them to draw parallels between the information. Finding the Z-score can help make sense of different types of data.
“Z-scores are a handy way to determine how a specific value compares to the average,” explains Nathan Brunner, CEO of Salarship . “Researchers find the Z-score very useful because it is standardized, which allows them to perform standardized statistical tests and compute prediction intervals,” he notes.
Finding the Z-score can help make sense of different types of data.
We’ll look at what the Z-score is, when having a Z-score is helpful, and how you can calculate the score.
A Z-score gives you a composite number for a specific set of data. It helps provide standardized numbers for data that may otherwise be hard to compare. The American Psychological Association notes that it is “the standardized score that results from applying a Z-score transformation to raw data.” By using the formula to get a standardized number, you can compare that finding to other sets of data.
In a recent study, researchers wanted to find out what activity helped improve cognitive function in schizophrenia patients more—watching TV or cognitive remediation on a computer. Because different scores and insights were used to measure responses to the input, researchers had to find a common way to measure both sets of data. Using the raw information to find the Z-score gave them the comparison tool that they needed.
You may be thinking that since the Z-score finds the average of several sets of data as a part of its formula, it is like finding the standard deviation. That’s not exactly the case.
“The Z-score and standard deviation are related but different concepts. The standard deviation measures the spread or variability of the whole dataset. A larger standard deviation indicates greater variability, while a smaller standard deviation indicates less variability,” Brunner notes.
The Z-score gives a way to compare information. The standard deviation tells you the difference between the two sets of information.
Calculating the Z-score starts with you having the raw data that you want a composite number for. With that data, you will need to find the mean or average number, as well as the standard deviation value for all the data.
You can find out the mean by adding all the numbers together, then dividing them by the total amount of values that you have. For example, if you have 4 + 5 + 6, you add those numbers together, giving you 15. You then divide 15 by the number 3, because you have 3 separate values. That gives you the number 5, which is the average of those numbers.
The standard deviation is a bit more detailed.
“To get the standard deviation … you need to take the square root of the sum of squared differences from the mean divided by the size of the data set,” Brunner tells. “Once you have both values, you can easily compute the z-score.”
You would use the following formula: Z-score = (the initial data point – mean)/standard deviation.
Brunner uses an example of finding out how a student’s test score of 90 compared with the scores his peers received, which are 75, 80, 85, 90, and 95. First, we find out the mean of this data set, which is 85. Then you calculate the standard deviation, which is approximately 7.9. Plugging the numbers into the formula, the Z-score is (90-85)/7.9, which equals 0.63.
If following the math is confusing, a Z-score calculator can help you find your answer.
Interesting information, but where is it applicable?
IQ test scores are highly prized for admission to certain clubs and organizations. Researchers note that Z-scores help you to understand the actual value of the IQ test, as well as where the test score falls in comparison to other IQ scores .
How to Convert a Z-Score to IQ
To compute the IQ score from a z-score, you multiply the Z-score by 15 for most tests, then multiply that number by 100.
For some tests, you multiply the Z-score by 16 initially. With a basic example, if you have a Z-score of 0.10, and you multiply that number by 15, you’ll get 1.5. You then multiply 1.5 by 100, for an IQ score of 150.
Which Z-Score Indicates a Genius-Level IQ?
While this number can fluctuate, Brunner provides insight on a high-level z-score.
“Some psychologists consider an IQ of 145 or above to be genius-level. The z-score is (145 - 100) / 15 = 3 for this value. This is extremely restrictive as only 0.135% of the population has an IQ of 145 or above,” he states.
Z-scores and the information they provide can also be beneficial in the mental health arena.
Studies have shown that when comparing the impact of different levels of stress on subjects’ mood , hormones, and cognition, Z-scores were extremely useful.
The scores helped to create a uniform way to compare the information.
Another study found that using Z-scores to provide neurofeedback and biofeedback in older adults with memory issues helped to target methods of treatment.
Experts say Z-scores can also help with tracking changes in patient's depression and anxiety levels .
Ultimately, being able to take raw data and put it into a form that is useful for understanding and processing information is valuable. Z-scores serve as that beneficial tool on a variety of platforms.
American Psychological Assocation. APA Dictionary of Psychology.
Andrade C. Z scores, standard scores, and composite test scores explained . Indian Journal of Psychological Medicine. 2021;43(6):555-557.
Caldwell JA, Niro PJ, Farina EK, McClung JP, Caron GR, Lieberman HR. A Z-score based method for comparing the relative sensitivity of behavioral and physiological metrics including cognitive performance, mood, and hormone levels. PLoS One. 2019;14(8):e0220749.
Meeuwsen KD, Groeneveld KM, Walker LA, Mennenga AM, Tittle RK, White EK. Z-score neurofeedback, heart rate variability biofeedback, and brain coaching for older adults with memory concerns. Restor Neurol Neurosci. 39(1):9-37.
- Statistics Tutorials
- Calculators
- Book Recommendations
Advantages and Disadvantages of Z Scores
Distributions differ in measures of their central tendency and variability. When conducting or reading about research, we will find ourselves working with distributions that are indeed different, yet we will be required to compare them with one another.
And to do such a comparison, we need some kind of a standard. One such kind of standardized score is a Z score. We now list some of the advantages and disadvantages of using Z scores in statistical applications.
Advantages of Z Scores:
1) The Z scores tell us how far away the raw data value is from the mean, in units of standard deviations . For example, a Z score of 2 means the value is at a distance of 2 standard deviations to the right of the mean.
A Z score of -1 means that the value is at a distance of 1 standard deviation to the left of the mean.
2) Since the Z scores are standardized scores they allow us to make comparisons between two different data sets that may have different means and standard deviations .
They help to level the playing field in comparing performances from one group to another. For example, suppose that two students give two different tests and obtain absolute scores of 200 and 300 marks respectively.
This is not helpful for comparison since we do not know how the students performed compared to the other exam takers. The level of difficulty of the two exams might also not be the same.
But suppose that we are told that their Z scores are +2 and +3 respectively. Then we can conclude that the second student showed a better performance.
3) The Z scores serve as the test statistic when conducting many of the usual tests of hypothesis such as the Z test for equality of means .
We can also easily compute the p-value corresponding to the Z score by looking at the standard Z probability table.
4) The Z scores allow us to calculate the probability and relative position of a particular value since we already understand the standard normal distribution .
For example, suppose that we are given data about the exam scores of 100 students in terms of Z scores. If a particular student has a Z score of +2 we can conclude that the student is in the top 5% among the 100 students.
Disadvantages of Z Scores:
1) We cannot compute or assign meaning to Z scores for the nominal or ordinal type of data .
2) The original data values cannot be recovered from the Z score unless we know the mean and the standard deviation of the distribution .
3) Although the Z score takes into account the mean and standard deviation of the distribution, it does not take into account the skewness and kurtosis of the distribution .
If the distribution is not symmetric then using the Z scores can lead to erroneous conclusions.
4) The computation of Z scores is based on the assumption that the data is roughly approximated by the normal distribution . The assumption of normality is not true under all circumstances.
I have always been passionate about statistics and mathematics education.
I created this website to explain mathematical and statistical concepts in the simplest possible manner.
If you've found value from reading my content, feel free to support me in even the smallest way you can.
Share this article
Recent posts, what is a frequency distribution table (with examples), what is a two way table (with examples), what is a relative frequency histogram (with examples), what is joint relative frequency (with examples), what is split half reliability (with examples), popular categories.
Mode Formula for Class 10 (Grouped Data)
Examples of manipulated variables (definition + examples), recent comments.
About Privacy Policy © Copyright - All Things Statistics

Z-Scores – What and Why?
Z-scores are scores that have mean = 0 and standard deviation = 1. Z-scores are also known as standardized scores; they are scores (or data values) that have been given a common standard . This standard is a mean of zero and a standard deviation of 1. Contrary to what many people believe, z-scores are not necessarily normally distributed .
Z-Scores - Example
A group of 100 people took some IQ test. My score was 5. So is that good or bad? At this point, there's no way of telling because we don't know what people typically score on this test. However, if my score of 5 corresponds to a z-score of 0.91, you'll know it was pretty good: it's roughly a standard deviation higher than the average (which is always zero for z-scores). What we see here is that standardizing scores facilitates the interpretation of a single test score. Let's see how that works.
Scores - Histogram
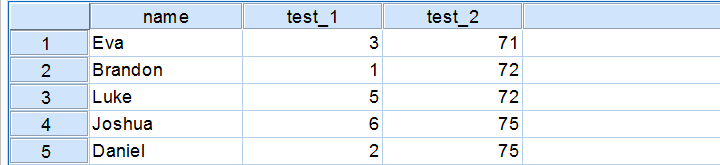
A quick peek at some of our 100 scores on our first IQ test shows a minimum of 1 and a maximum of 6. However, we'll gain much more insight into these scores by inspecting their histogram as shown below.
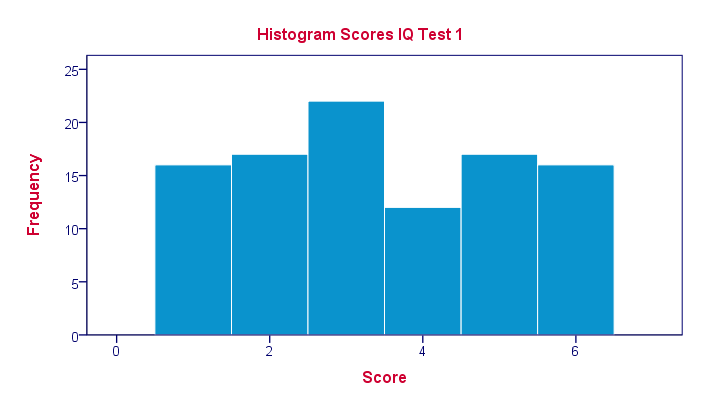
The histogram confirms that scores range from 1 through 6 and each of these scores occurs about equally frequently. This pattern is known as a uniform distribution and we typically see this when we roll a die a lot of times: numbers 1 through 6 are equally likely to come up. Note that these scores are clearly not normally distributed .
Z-Scores - Standardization
We suggested earlier on that giving scores a common standard of zero mean and unity standard deviation facilitates their interpretation. We can do just that by
- first subtracting the mean over all scores from each individual score and
- then dividing each remainder by the standard deviation over all scores.

Z-Scores - Histogram
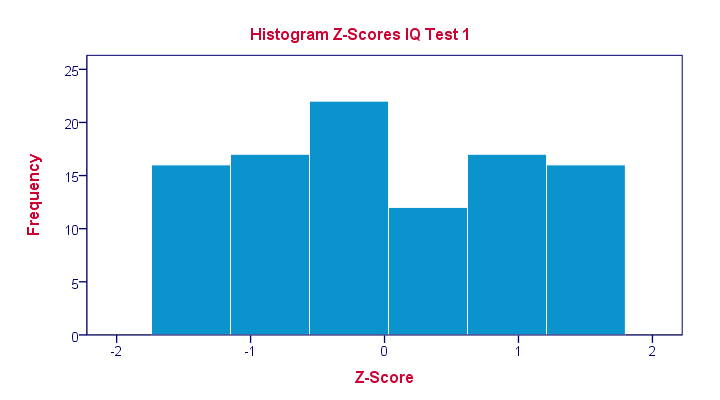
What's a Linear Transformation?
Z-scores are linearly transformed scores. What we mean by this, is that if we run a scatterplot of scores versus z-scores, all dots will be exactly on a straight line (hence, “linear”). The scatterplot below illustrates this. It contains 100 points but many end up right on top of each other.
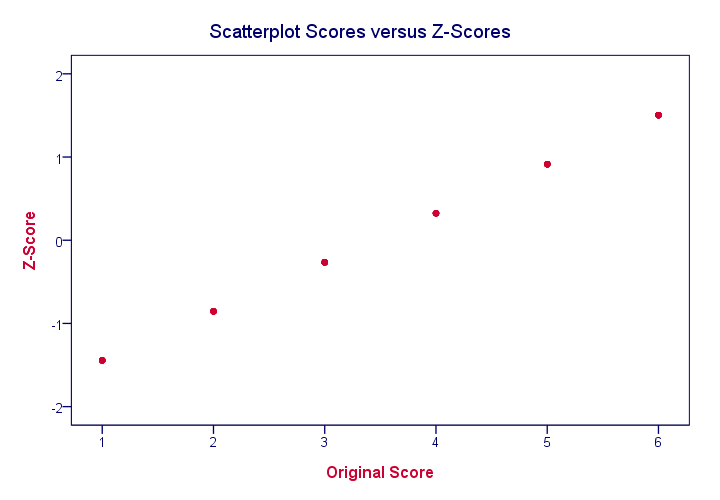
In a similar vein, if we had plotted scores versus squared scores, our line would have been curved; in contrast to standardizing, taking squares is a non linear transformation.
Z-Scores and the Normal Distribution
We saw earlier that standardizing scores doesn't change the shape of their distribution in any way; distribution don't become any more or less “normal”. So why do people relate z-scores to normal distributions?
The reason may be that many variables actually do follow normal distributions. Due to the central limit theorem , this holds especially for test statistics. If a normally distributed variable is standardized, it will follow a standard normal distribution.
This is a common procedure in statistics because values that (roughly) follow a standard normal distribution are easily interpretable. For instance, it's well known that some 2.5% of values are larger than two and some 68% of values are between -1 and 1.
The histogram below illustrates this: if a variable is roughly normally distributed, z-scores will roughly follow a standard normal distribution. For z-scores, it always holds (by definition) that a score of 1.5 means “1.5 standard deviations higher than average”. However, if a variable also follows a standard normal distribution, then we also know that 1.5 roughly corresponds to the 95th percentile .
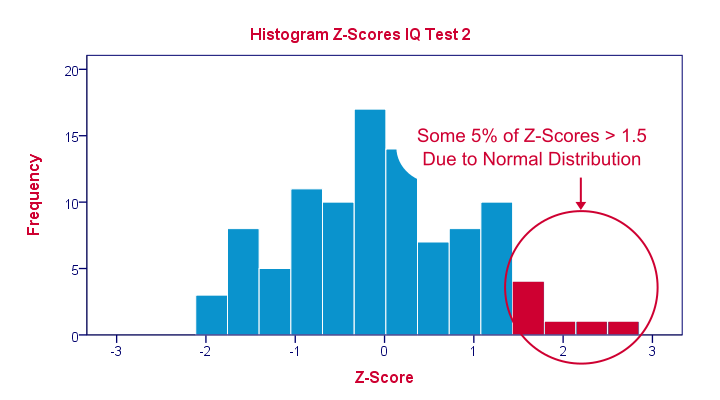
Z-Scores in SPSS
SPSS users can easily add z-scores to their data by using a DESCRIPTIVES command as in descriptives test_1 test_2/save. in which “save” means “save z-scores as new variables in my data”. For more details, see z-scores in SPSS .
Tell us what you think!
This tutorial has 8 comments:.
By christina on April 21st, 2020
very excellent explaination
By Davidson on June 15th, 2021
It was useful! Thanks.
By DG Shankar on October 13th, 2021
Privacy overview.
- Field Exchange

The practical implications of using z-scores: Concern's experience in Angola
by Beth Matthews, Maureen Billiet (Concern field staff Angola) Annalies Borrell (Concern chief nutritionist Dublin).
In children the three most commonly used anthropometric indices are weight for height, height for age and weight for age. These indices can be used to assess wasting, stunting and underweight respectively. For the purpose of this paper we are discussing wasting (weight / height), and where the term malnutrition is used it refers to this index.
When a child's "weight for height" is assessed, the child's weight is being compared to a reference weight for a child of the same height. The reference weights for each height are known as the NCHS/WHO reference values and are derived from the combination of two distinct data sets, which cover two age groups:
- 2 to 18 years, based on nation-wide data from the US National Centre for Health Statistics (NCHS), and
- birth to 3 years from a more geographically and socio-economically restricted sample from the Fels Research Institute.
Though NCHS/WHO reference values are currently deemed the most appropriate for assessing the nutritional status of infants and young children, it is also recognised that they are not ideal. The WHO expert committee has recently recommended the development of a new reference conceming weight and height of infants and children.
- percentage of median: the ratio of a child's weight to the median weight of a child of the same height in the reference data, expressed as a percentage, e.g., if the median weight of the reference data for a particular height is I Okgs then to say that a child is 80% weight for height means that the child is 8kgs
- percentiles: the position of an individuals weight of a particular height in the reference values in terms of the percentage of values ex ceeded or equalled, e.g., if 10% of the refer ence population weigh less than the child being considered, then the child is in the 10th per centile.
- z-scores: by describing how far in (units called standard deviations) a child's weight is from the median weight of a child at the same height in the reference data.
While 'percentage of median' is the most commonly used index for selection criteria for feeding programmes it has not been deemed an appropriate index for use with the NCHS/WHO reference values. It has the advantage of being easy to use and, more influential perhaps, of being easy to understand. But this ease of use is paid for in loss of accuracy. The method cannot take into account the fact that the variation in weight found in normal children at one height will be different to the variation found at a different height.
Percentiles are considered appropriate descriptors for malnutrition, using the NCHS/WHO reference values, but do not distinguish well between children who are in the 3rd percentile. It is difficult therefore to classify further these children into moderately and severely malnourished. Z-scores and percentage of the median can be used to further distinguish between severely and moderately malnourished.
Z-scores use an application of statistical theory to describe how far a child's weight is from the average (the median for the NCHS/WHO values) weight of a child of the same height in the reference data. This "distance" is called a z-score. It is expressed in multiples of the standard deviation and is derived as follows:
where both the median weight and the standard deviation (how the different values are distributed about the mean) are those taken from the normalised growth curves derived from the NCHS /WHO reference values for the given height.
The z-score is a more sensitive descriptor than either percentiles' or 'percentage of the median'. The use of z scores has the advantage that it recognises that the spread of(or variation in) weights at one height may be different than that at a different height. Since the percentage of median' does not do this, its use may mean that children who are actually malnourished are not identified.
The table below illustrates how, as a child grows taller (older), s/he will be more likely tobe classified as malnourished by the use of z-score than by the use of percentage of the median.
Z-scores are useful in practice because they can:
- be applied to the individual or population;
- pinpoint any given weight and height, noting improvement or deterioration over time in relation to the reference values; and
- classify children of all ages and sizes equally.
Although there is substantial recognition that the z score is the most appropriate descriptor of malnutrition, health and nutrition facilities have been, in practice, reluctant to adopt its use as a means for individual assessment.
In programme use the practical requirements that a descriptor should fulfil are several. The descriptor should ensure the correct identification of malnourished children. It should allow estimation of absolute numbers of malnourished children through nutrition surveys. This would then lead to informed estimation of programme size and eventual programme coverage. Finally, the use of a particular descriptor should provide information on the relative seriousness of the nutritional situation, thus helping to identify the most appropriate type of intervention needed.
The use of z-scores in nutrition surveys
Concern, like many intemational agencies, analyses and reports nutrition survey results using z-scores. A comparison of the reported prevalence of malnutrition according to z-scores and 'percentage of the median' show that the two descriptors provide quite different results. See figure 1 below.
On average the use of the z-score selected an undemourished group which was 1.6 times greater than that selected by the use of the '80% of the median' method (range: 1.3 - 1.8). Similar differences were obtained from nutrition surveys carried out in other locations in Angola and in Sierra Leone.
Again, the use of z-scores selected a group which was between 1.4 and 1.8 times greater then that selected by the use of the 'percentage of the median' method.
The difference in the results obtained from z-scores and percentage of the median may become larger when proportionally more older stunted children are included in the survey; for example, when the selection criteria for measurement is 'less than 110cm' as opposed to 'less than 5 years' and the former includes more older stunted children.
Z-scores as selection criteria
Z-scores were introduced as admission criteria in May and June 1996 in five Concem nutrition centres in the provinces of Huambo and Bie in Angola. The programmes were all dry supplementary feeding programmes.
The rationale for implementing z-scores was:
- its greater compatibility with the NCHS/WHO reference values and suitability for selecting malnourished children irrespective of height;
- the children being classified as malnourished in the nutrition surveys would be the same children being admitted into the nutrition centres thus ensuring compatibility between the programmes and the nutrition survey results;
- the Ministry of Heath in Angola together with WHO and UNICEF recognised and supported the use of z-scores;
- there had been no practical evaluation of the use of z-scores and its use in Angolan nutrition centres provided the opportunity for this sort of evaluation.
- Following the introduction of z-scores, a significant increase in admissions was reported. The increase was considered a direct consequence of the change in criteria since the methodology for case detection and the nutritional situation remained constant. These increases are described in Figure 2 and 3 .
- There was an increase of 55 - 60% and 40-45% in the numbers of children being admitted to the nutrition programmes in Kuito and Huambo respectively.
- Once established, the nutrition centres would only have operated at 65% of their capacity had z-scores not been introduced.
- In November 1996, when programme closure was being considered, one fifth (20%) of those registered in the nutrition centres would have been discharged according to weight-for-height percentage of the median.
Since the use of z-scores was a new concept, co ordination difficulties periodically arose between Concern and other agencies over issues arising from its use.
WFP co-operated with Concern during the initial period that z-scores were implemented and provided the necessary additional increase in food requirements. However, problems arose during food shortages later on during the year since numbers of children registered in the programme remained unusually high. The concept of z-scores was difficult for WFP field level food-monitors and staff at headquarters in Luanda.
At field level, staff experienced difficulties during the referral procedure to therapeutic centre with those children falling below -3 z-scores but not less than 70% weight-for-height. These children remained in the Concern supplementary feeding programme.
The implementation of z-scores was supported by the nutrition department at the Ministry of Health and also by UNICEF and WHO. However, MoH nurses at field level had difficulties with understanding z-scores.
Using z-scores... what are the practical implications?
Z-score tables.
The availability of z-score tables is essential for enabling staff to use the index in the field. Z-score cards are available and the methodology for using these cards is similar to that of the percentage median.
- Concern has produced their own z-score cards with values for - 1.5 z-score and a lower standard deviation included (this is a value necessary for calculating exact z-scores).
- WHO has produced z score cards in target countries.
- Other agencies are producing z-scores tables e.g. MSF and Oxfam
The calculation of individual z-scores
Sometimes rather than saying a child is between -2 and -3 z-scores we may wish to calculate the exact z-score value. This calculation involves three figures (see formula above); an observed weight, an expected weight and an associated standard deviation. In the calculation of z-scores based on the NCHS/WHO reference values there are two relevant standard deviations, a lower standard deviation which is used in the calculation of z-scores for children less than the median weight at a specific height and an upper standard deviation used to calculate z-scores for children greater than the median weight at a given height. It follows, that for selection purposes to feeding centres only the lower standard deviation is necessary. However, in situations where all children (those greater and less then the median weight at particular heights) require classification e.g. in the analysis of nutrition surveys, both lower and upper standard deviations should be provided for each height. Generally, field staff can learn the mechanical calculation but experience difficulties with the concept of z-scores.
Measurement of Child Progress
Nutritional deterioration and improvement of individuals can be monitored using z-scores as long as the median and the standard deviation for a given height are known. In circumstances where this is difficult alternative means for monitoring progress can be used, for example expected weight for a 5kg boy. This would be 15g/kg/day i.e. a total of 15g x 5kg x 7days = 525 grams per week.
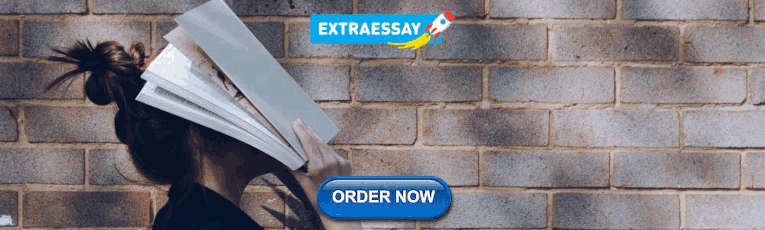
Discharge criteria
The admission criteria are similar to those used in defining moderate and severe malnutrition in nutrition surveys (<-3 z-score for severe malnutrition etc.). The discharge criteria from therapeutic feeding programmes is >-2 z-score and from supplementary feeding programmes would normally be > - 1.5 z score. Staff who have been used to working with percentage of median' cut-offs may consider discharge too early at -1.5 z-score since this is almost equivalent to less than 85% for younger children. However, discharge at - 1 z-score may be considered inappropriate as it may prolong the length of stay in the nutrition programme. Using z-scores, selective feeding programmes will tend to include proportionally more older (stunted) children, therefore targeting those who are suffering acute or chronic malnutrition.
Compatibility between Surveys and Feeding programmes
Children can be referred during nutrition surveys since z-scores can be calculated by hand using a pocket calculator and z-score tables during the nutrition surveys. Coverage of the target group, as identified by the survey assessment, is more feasible since there is compatibility between surveys and programrnes.
Appropriate technology
Accurate results from nutrition surveys can be obtained from analysis by field staff without computers provided that the lower and upper standard deviations are given and calculations are sex-specific.
Programme assessment
The indicators for assessing the effectiveness of a nutrition centre, as established by MSF and other NGOs and based on 'percentage of the median' may be inappropriate for programmes using z-scores. Target weight gains and length of stay may be inappropriate, particularly where discharge is at -1 z-score.
Teaching z-scores is undoubtedly challenging. There is a need to address imaginative and interesting ways to teach z-scores using graphs, colours and scales.
Resource implications
A change in criteria to z-scores may have significant implications for food requirements for supplementary feeding programmes. Predicted estimates for food requirements based on survey findings may be one and a half times previous estimates. Furthermore, the actual food consumption could be more than double if length of stay increases (x 1.5) and the number of admissions increases (x 1.5). In many circumstances where food resources are limited, it may be necessary to alter the criteria for selection accordingly i.e. from -2 z-scoreto -1.8 or-l.5 etc.
Conclusions
- This study provides some evidence to suggest there is an increase in the size of the target population for supplementary programmes when using z-scores.
- A single descriptor of nutritional status compared to the NCHS/WHO reference values that can be used for individuals (selection) and populations (surveys) will be useful for field practitioners operating nutrition programmes.
- In circumstances where nutrition centres are taken over by or assimilated into MoH structures and supported by the WHO, the use of z-scores ensures compatibility in selection criteria.
Questions and Challenges
- More field studies to evaluate the use of z-scores in- practice are required. These should include the identification and follow up of children excluded by either method.
- Z-scores should be included into all nutrition in emergencies training courses for practitioners and management staff, using imaginative and simple tools.
- There is a need for population based research focusing on the relationship between the various indices and outcomes such as mortality, illness, child development, etc.
Show footnotes
1 Gorstein J, Sullivan K, Yip R, de Onis Metal. Issues in assessment of nutritional status using anthropometry. Bulletin of the World Health Organisation 1994, 72:273 - 283 2 de Onis M Habicht JP. Anthropometric reference data for international use. recommendations from a World Health Organisation expert Committee. Am. Journal of din. Nutrition. 1996, 64:650-658. 3 WHO Technical Report No. 854. Physical Status. The use and interpretation ofAnthropometry pp.7-9 and pp. 219-224.
More like this
En-net: who growth standards.
Why does the WHO Growth Standards have median in their z-score tables instead of mean? If they already have mean, why don't they have the percentage of median? It is easy to...
FEX: Letter on WHO 2006 Growth Standards, by Marko Kerac and Andrew Seal
This new 2006 WHO Growth standards: What will they mean for emergency nutrition programmes? Dear Editor Whilst welcoming the principles which have driven the development of...
en-net: Converting WHO reference median to z-scores
I sometimes see exclusion criteria in papers expressed as weight for length (WFL) < 75% or <80% of WHO reference median. I can't figure out how to convert this to...
FEX: Impact of WHO Growth Standards on programme admissions in Niger
Summary of research1 Severely malnourished children managed in the MSF-run Centre de Récupération Nutritionnelle Intensive (CRENI) in Maradi, Niger A recent study by...
FEX: WHO growth reference for children and adolescents
Summary of published research1 The need for a widely applicable growth reference for older children and adolescents has increasingly been recognised by countries attempting to...
FEX: Comparison of Weight-for-Height Based Indices for Assessing the Risk of Death
Summary of Published Paper Mortality rates among children with severe malnutrition vary considerably between different treatment centres. This variation is due to differences...
en-net: Urgent: Weight for Height Z-scores: Unisex Z-score table used in West African countries vs. calculated Z score values
In the 2011 Guidelines for THE INTEGRATED MANAGEMENT OF SEVERE ACUTE MALNUTRITION published by ACF, Annex 4 depicts a Weight-for-Height table. This same table has been adopted...
en-net: Why do I need to adjust for age and sex of children again if z score calculation already involved gender and sex in its calculation?
Hello, I have already z score using WHO anthropometric survey analyzer. One question arised was that why do I need to still control for gender and age in my regression again...
FEX: Impact of WHO Growth Standards on SAM response to treatment
Summary of published research1 More younger admissions to therapeutic feeding programmes are one of the implications of moving to the new WHO Growth Standards. A recent study...
en-net: Automatic WHZ calculation in excel database
I would like to introduce a automatic calculation of WHZ in a CMAM program patient database where I have the needed variables (gender, length/height and weight). Does anyone...
FEX: Letter on practically using z scores in Angola, by Saskia van der Kam
Dear Field Exchange, I have the following comments to make about the article "The practical implications of using Z-scores: CONCERN's experience in Angola" (Field...
FEX: Including infants in nutrition surveys
Experiences of ACF in Kabul city Claudine Prudhon is the head of the nutrition department in ACF HQ. Claudine is interested in improving the nutritional management of the under...
Resource: Changing sex differences in undernutrition of African children: findings from Demographic and Health Surveys
Abstract The study investigates sex differences in the prevalence of undernutrition in sub-Saharan Africa. Undernutrition was defined by Z-scores using the CDC-2000 growth...
FEX: Effect of nutrition survey ‘cleaning criteria’ on estimates of malnutrition prevalence and disease burden: secondary data analysis
Summary of research1 Location: Global What we know: Standardised methods for collection and reporting malnutrition prevalence data in nutrition surveys are used. What this...
FEX: A proposed new method of estimating weight deficits in children
Summary of paper presented at the Intenational Child Health Meeting on 'Managing Childhood Malnutrition' held in Birmingham At the recent Birmingham meeting reported on in...
en-net: What is the lowest WAZ score that would be "compatible with human life
Hello, I am passing on a question from a colleague. I am keeping his wording: "What is the lowest WAZ score that would be "compatible with human life"? I know that Epi-Info...
en-net: Anthropometric data of children 6-24 from Africa using Stata
Hi, I am using Stata for analysis my data from Africa focusing on children aged from 6 to 24 months and need to calculate MUAC-for-age z-score and WAZ. I found there are...
en-net: Are the children considered heavier or overweight if Weight for Height Z is +5 even after taking out flagged ones.
Hello, World Health Organization Anthro survey analyzer already flags implausible z score. After not including the implausible z score, the maximum Weight for Height z score...
FEX: Supplementary Feeding Programme - Current Guidelines
Circumstances leading to the setting up of SFPs (from WFP guidelines) Blanket SFP Targeted SFP A generalised SFP for prevention purposes maybe implemented in the absence of a...
FEX: MUAC Versus Weight-for-Height in Assessing Severe Malnutrition
Summary of published paper1 An infant having MUAC measured during the study in Kenya Current WHO guidelines for the management of severe malnutrition in children recommend...
Download and bookmark
- PDF version
- Preferred Citation
- Citation Tools
Tags for this page
About this article.
- Date: May 1997
- Issue: Field Exchange 1
- Page number: 5
- Location: Angola
- Article Type: Field Articles
About this page
- Updated on 1 May 1997
- 33,339 views
Reference this page
Beth Matthews, Maureen Billiet, Annalies Borrell (). The practical implications of using z-scores: Concern's experience in Angola. Field Exchange 1, May 1997. p5. www.ennonline.net/fex/1/practical
Download to a citation manager
The below files can be imported into your preferred reference management tool, most tools will allow you to manually import the RIS file. Endnote may required a specific filter file to be used.
ENN Strategy Annual reports & accounts Our funding
Subscribe Update your details Who you are Support the ENN
IFE Core group Global Nutrition Cluster Technical Alliance Global Nutrition Cluster MAMI Global Network
Email the ENN Office +44 (0)1865 372340 Map and address

Statistics Made Easy
5 Examples of Using Z-Scores in Real Life
In statistics, a z-score tells us how many standard deviations away a given value lies from a population mean.
We use the following formula to calculate a z-score for a given value:
- z = (x – μ) / σ
- x : Individual data value
- μ : Mean of population
- σ : Standard deviation of population
The following examples show how z-scores are used in real life in different scenarios.
Example 1: Exam Scores
Z-scores are often used in academic settings to analyze how well a student’s score compares to the mean score on a given exam.
For example, suppose the scores on a certain college entrance exam are roughly normally distributed with a mean of 82 and a standard deviation of 5.
If a certain student received a 90 on the exam, we would calculate their z-score to be:
- z = (90 – 82) / 5
This means that this student received a score that was 1.6 standard deviations above the mean.
We could use the Area To The Left of Z-Score Calculator to find that a z-score of 1.6 represents a value that is greater than 94.52% of all exam scores.
Example 2: Newborn Weights
Z-scores are often used in a medical setting to analyze how a certain newborn’s weight compares to the mean weight of all babies.
For example, it’s well-documented that the weights of newborns are normally distributed with a mean of about 7.5 pounds and a standard deviation of 0.5 pounds.
If a certain newborn weights 7.7 pounds, we would calculate their z-score to be:
- z = (7.7 – 7.5) / 0.5
This means that this baby weighs 0.4 standard deviations above the mean.
We could use the Area To The Left of Z-Score Calculator to find that a z-score of 0.4 represents a weight that is greater than 65.54% of all baby weights.
Example 3: Giraffe Heights
Z-scores are often used in a biology to assess how the height of a certain animal compares to the mean population height of that particular animal.
For example, suppose the heights of a certain species of giraffe is normally distributed with a mean of 16 feet and a standard deviation of 2 feet.
If a certain giraffe from this species is 15 feet tall, we would calculate their z-score to be:
- z = (15 – 16) / 2
This means that this giraffe has a height that is 0.5 standard deviations below the mean.
We could use the Area To The Left of Z-Score Calculator to find that a z-score of -0.5 represents a height that is greater than just 30.85% of all giraffes.
Example 4: Shoe Size
Z-scores can be used to determine how a certain shoe size compares to the mean population size.
For example, it’s known that shoe sizes for males in the U.S. is roughly normally distributed with a mean of size 10 and a standard deviation of 1.
If a certain man has a shoe size of 10, we would calculate their z-score to be:
- z = (10 – 10) / 1
This means that this man has a shoe size that is 0 standard deviations away from the mean.
We could use the Area To The Left of Z-Score Calculator to find that a z-score of 0 represents a shoe size that is greater than exactly 50% of all males.
Example 5: Blood Pressure
Z-scores are often used in medical settings to assess how an individual’s blood pressure compares to the mean population blood pressure.
For example, the distribution of diastolic blood pressure for men is normally distributed with a mean of about 80 and a standard deviation of 20.
If a certain man has a diastolic blood pressure of 100, we would calculate their z-score to be:
- z = (100 – 80) / 20
This means that this man has a diastolic blood pressure that is 1 standard deviation above the mean.
We could use the Area To The Left of Z-Score Calculator to find that a z-score of 1 represents a blood pressure size that is greater than 84.13% of all males.
Additional Resources
The following tutorials provide additional information about z-scores:
How to Interpret Z-Scores How to Find Area to the Right of Z-Scores How to Find Area to the Left of Z-Scores What is Considered a Good Z-Score?

Published by Zach
Leave a reply cancel reply.
Your email address will not be published. Required fields are marked *
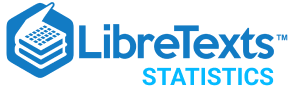
- school Campus Bookshelves
- menu_book Bookshelves
- perm_media Learning Objects
- login Login
- how_to_reg Request Instructor Account
- hub Instructor Commons
- Download Page (PDF)
- Download Full Book (PDF)
- Periodic Table
- Physics Constants
- Scientific Calculator
- Reference & Cite
- Tools expand_more
- Readability
selected template will load here
This action is not available.
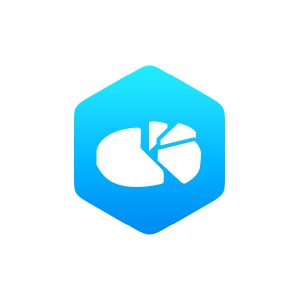
5.5: Summary of z Scores
- Last updated
- Save as PDF
- Page ID 22054
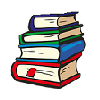
- Danielle Navarro
- University of New South Wales
Although this section has some math and the z-score formula, it's really to hit the main points of z-scores.
Summary of z-scores
Suppose Dr. Navarro's friend is putting together a new questionnaire intended to measure “grumpiness”. The survey has 50 questions, which you can answer in a grumpy way or not. Across a big sample (hypothetically, let’s imagine a million people or so!) the data are fairly normally distributed, with the mean grumpiness score being 17 out of 50 questions answered in a grumpy way, and the standard deviation is 5. In contrast, when Dr. Navarro takes the questionnaire, she answer 35 out of 50 questions in a grumpy way. So, how grumpy is she? One way to think about would be to say that I have grumpiness of 35/50, so you might say that she's 70% grumpy. But that’s a bit weird, when you think about it. If Dr. Navarro's friend had phrased her questions a bit differently, people might have answered them in a different way, so the overall distribution of answers could easily move up or down depending on the precise way in which the questions were asked. So, I’m only 70% grumpy with respect to this set of survey questions . Even if it’s a very good questionnaire, this isn’t very a informative statement.
Can we standardize?
A simpler way around this is to describe Dr. Navarro's grumpiness by comparing me to other people. Shockingly, out of the friend’s sample of 1,000,000 people, only 159 people were as grumpy as Dr. Navarro (which Dr. Navarro believes is realistic), suggesting that she's in the top \(0.016\%\) of people for grumpiness. This makes much more sense than trying to interpret the raw data. This idea – that we should describe a person's grumpiness in terms of the overall distribution of the grumpiness of humans – is the idea that standardization attempts to get at. One way to do this is to do exactly what I just did, and describe everything in terms of percentiles. However, the problem with doing this is that “it’s lonely at the top”. Suppose that the friend had only collected a sample of 1000 people (still a pretty big sample for the purposes of testing a new questionnaire, I’d like to add), and this time gotten a mean of 16 out of 50 with a standard deviation of 5, let’s say. The problem is that almost certainly, not a single person in that sample would be as grumpy as me.
However, all is not lost. A different approach is to convert my grumpiness score into a standard score , also referred to as a z-score. The standard score is defined as the number of standard deviations above the mean that my grumpiness score lies. To phrase it in “pseudo-math” the standard score is calculated like this:
standard score \(=\frac{\text { raw score }-\text { mean }}{\text { standard deviation }}\)
In actual math, the equation for the z-score is:
\(z=\frac{X-\bar{X}}{s}\)
So, going back to the grumpiness data, we can now transform Dr. Navarro's raw grumpiness into a standardized grumpiness score. If the mean is 17 and the standard deviation is 5 then her standardized grumpiness score would be
\(z=\dfrac{X-\bar{X}}{s} = \dfrac{35-17}{5} = \dfrac{18}{5} = 3.6 \)
To interpret this value, recall that 99.7% of values are expected to lie within 3 standard deviations of the mean. So the fact that my grumpiness corresponds to a z score of 3.6 (3.6 standard deviations above the mean) indicates that I’m very grumpy indeed.
So now that we know that we can compare one person's individual raw score to the whole distribution of scores, what else can the z-score do?
Compare Across Distributions
In addition to allowing you to interpret a raw score in relation to a larger population (and thereby allowing you to make sense of variables that lie on arbitrary scales), standard scores serve a second useful function. Standard scores can be compared to one another in situations where the raw scores can’t. Suppose, for instance, my friend also had another questionnaire that measured extraversion using a 24 items questionnaire. The overall mean for this measure turns out to be 13 with standard deviation 4; and I scored a 2. As you can imagine, it doesn’t make a lot of sense to try to compare my raw score of 2 on the extraversion questionnaire to my raw score of 35 on the grumpiness questionnaire. The raw scores for the two variables are about fundamentally different things, so this would be like comparing apples to oranges. BUT, if we calculate the z-scores, we get \(z=\dfrac{(35−17)}{5}=3.6 \) for grumpiness and \( z=\dfrac{(2−13)}{4}=−2.75 \) for extraversion. These two numbers can be compared to each other. Dr. Navarro is much less extraverted than most people (\(z=−2.75\)) (the negative sign means that Dr. Navarro is 2.75 standard deviations below the average extraversion of the sample) and much grumpier than most people (z=3.6): but the extent of my unusualness is much more extreme for grumpiness (since 3.6 is a bigger number than 2.75). Because each standardized score is a statement about where an observation falls relative to its own population , it is possible to compare standardized scores across completely different variables.
This ability to compare scores across different distributions is the foundation of statistical analyses. It allows us to compare the sample that we have to a probability sample, and then make predictions!
Proportions & Amounts
But that's not all! Using this z-score formula to turn a raw score into a standardized z-score, we can then use what we know about the proportions of scores in standard normal curves to predict how likely that score was. And if we know the sample size, we can even predict how many people from the sample will score above (or below) that initial raw score. Amazing!
This all is pretty cool, although you probably don't think so yet. Or maybe never will. But the way that the Standard Normal Distribution and these standardized scores (z-scores) function allows much of the rest of statistics to exist.
Next: Write-Ups
You are in a social science class, not a statistics class, so finding the number is almost never the end of your task. The next section will describe the important components of a good concluding sentence or concluding paragraph.
Contributors and Attributions
- Danielle Navarro ( University of New South Wales )
Dr. MO ( Taft College )
Use of Percentiles and Z -Scores in Anthropometry
- First Online: 01 January 2012
Cite this chapter
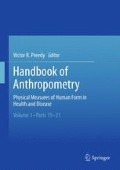
- Youfa Wang 2 &
- Hsin-Jen Chen
2085 Accesses
98 Citations
Percentiles and Z -scores are often used to assess anthropometric measures to help evaluate children’s growth and nutritional status. In this chapter, we first compare the concepts and applications of percentiles and Z -scores and their strengths and limitations. Compared to percentiles, Z -scores have a number of advantages: first, they are calculated based on the distribution of the reference population (mean and standard deviation), and thus reflect the reference distribution; second, as standardized quantities, they are comparable across ages, sexes, and anthropometric measures; third, Z -scores can be analyzed as a continuous variable in studies. In addition, they can quantify extreme growth status at both ends of the distribution. However, Z -scores are not straightforward to explain to the public and are hard to use in clinical settings. In recent years, there has been growing support to the use of percentiles in some growth and obesity references. We also discuss the issues related to cut point selections and outline the fitting/smoothing techniques for developing reference curves. Finally, several important growth references and standards including the previous and new WHO growth reference/standards and the US 2000 CDC Growth Charts, are presented and compared. They have been developed based on different principles and data sets and have provided different cut points for the same anthropometric measures; they could, thus, provide different results. This chapter will guide readers to understand and use percentiles and Z -scores based on recent growth references and standards.
- Growth Standard
- Growth Chart
- Growth Reference
International Obesity Task Force
- Percentile Curve
These keywords were added by machine and not by the authors. This process is experimental and the keywords may be updated as the learning algorithm improves.
This is a preview of subscription content, log in via an institution to check access.
Access this chapter
- Available as PDF
- Read on any device
- Instant download
- Own it forever
- Available as EPUB and PDF
- Compact, lightweight edition
- Dispatched in 3 to 5 business days
- Free shipping worldwide - see info
- Durable hardcover edition
Tax calculation will be finalised at checkout
Purchases are for personal use only
Institutional subscriptions
Abbreviations
Body mass index
Centers for Disease Control and Prevention
Height- or length-for-age Z -score
Multicentre Growth Reference Study
National Center for Health Statistics
National Health and Nutrition Examination Survey
Standard deviation
World Health Organization
Weight-for-age Z -score
Centers For Disease Control and Prevention. CDC growth charts [Online]. 2000. http://www.cdc.gov/growthcharts/Default.htm . Accessed 31 Jan 2010.
Cole TJ, Green PJ. Smoothing Reference Centile Curves - the LMS Method and Penalized Likelihood. Stat Med. 1992;11:1305–19.
Article PubMed CAS Google Scholar
Cole TJ, Bellizzi MC, Flegal KM, Dietz WH. Establishing a standard definition for child overweight and obesity worldwide: international survey. BMJ. 2000;320:1240–3.
Cole TJ, Flegal KM, Nicholls D, Jackson AA. Body mass index cut offs to define thinness in children and adolescents: international survey. BMJ. 2007;335:194–7.
Article PubMed Google Scholar
de Onis M, Onyango AW. The Centers for Disease Control and Prevention 2000 growth charts and the growth of breastfed infants. Acta Paediatr. 2003;92:413–9.
de Onis M, Onyango AW, Borghi E, Garza C, Yang H; WHO Multicentre Growth Reference Study Group. Comparison of the World Health Organization (WHO) Child Growth Standards and the National Center for Health Statistics/WHO international growth reference: implications for child health programmes. Public Health Nutr. 2006;9:942–7.
de Onis M, Onyango AW, Borghi E, Siyam A, Nishida C, Siekmann J. Development of a WHO growth reference for school-aged children and adolescents. Bull World Health Organ. 2007;85:660–7.
Hamill PV, Drizd TA, Johnson CL, Reed RB, Roche AF, Moore WM. Physical growth: National Center for Health Statistics percentiles. Am J Clin Nutr. 1979;32:607–29.
PubMed CAS Google Scholar
Hammer LD, Kraemer HC, Wilson DM, Ritter PL, Dornbusch SM. Standardized percentile curves of body-mass index for children and adolescents. Am J Dis Child. 1991;145:259–63.
Inoue S, Zimmet P, Caterson I, Chen C, Ikeda Y, Khalid AK, Kim YS, Bassett J. The Asia-Pacific perspective: redefining obesity and its treatment. 2000. http://iotf.org/
Kuczmarski RJ, Ogden CL, Guo SS, Grummer-Strawn LM, Flegal KM, Mei Z, Wei R, Curtin LR, Roche AF, Johnson CL. 2000 CDC Growth Charts for the United States: methods and development. Vital Health Stat. 2002;11:1–190.
Google Scholar
Mei Z, Ogden CL, Flegal KM, Grummer-Strawn LM. Comparison of the prevalence of shortness, underweight, and overweight among US children aged 0 to 59 months by using the CDC 2000 and the WHO 2006 growth charts. J Pediatr. 2008;153:622–8.
Must A, Dallal GE, Dietz WH. Reference data for obesity: 85th and 95th percentiles of body mass index (wt/ht2) and triceps skinfold thickness. Am J Clin Nutr. 1991;53:839–46.
National High Blood Pressure Education Program. Update on the 1987 Task Force Report on High Blood Pressure in Children and Adolescents: A working group report from the National High Blood Pressure Education Program. National High Blood Pressure Education Program Working Group on Hypertension Control in Children and Adolescents. Pediatrics. 1996;98:649–58.
NHLBI. Blood pressure tables for children and adolescents. http://www.nhlbi.nih.gov/guidelines/hypertension/child_tbl.htm . Accessed 10 Jan 2009.
Pelletier D. Theoretical considerations related to cutoff points. Food Nutr Bull. 2006;27:S224–36.
PubMed Google Scholar
Poskitt EME. Defining Childhood Obesity - the Relative Body-Mass Index (BMI). Acta Paediatr. 1995;84:961–3.
Roberfroid D, Lerude MP, Pérez-Cueto A, Kolsteren P. Is the 2000 CDC growth reference appropriate for developing countries? Public Health Nutr. 2006;9:266–8.
Rolland-Cachera MF, Cole TJ, Sempé M, Tichet J, Rossignol C, Charraud A. Body-Mass Index Variations - Centiles from Birth to 87 Years. Eur J Clin Nutr. 1991;45:13–21.
Schwarz NG, Grobusch MP, Decker ML, Goesch J, Poetschke M, Oyakhirome S, Kombila D, Fortin J, Lell B, Issifou S, Kremsner PG, Klipstein-Grobusch K. WHO 2006 child growth standards: implications for the prevalence of stunting and underweight-for-age in a birth cohort of Gabonese children in comparison to the Centers for Disease Control and Prevention 2000 growth charts and the National Center for Health Statistics 1978 growth references. Public Health Nutr. 2008;11:714–9.
Shan X, Xi B, Chen H, Hou D, Wang Y, Mi J. Prevalence and behavioral risk factors of overweight and obesity among children aged 2–18 in Beijing, China. Int J Ped Obe. 2010;5:383–9.
Victora CG, Morris SS, Barros FC, de Onis M, Yip R. The NCHS reference and the growth of breastand bottle-fed infants. J Nutr. 1998;128:1134–8.
Wang Y. Epidemiology of childhood obesity - methodological aspects and guidelines: what is new? Int J Obes. 2004;28:S21–8.
Article Google Scholar
Wang Y, Moreno LA, Caballero B, Cole TJ. Limitations of the current world health organization growth references for children and adolescents. Food Nutr Bull. 2006;27:S175–88.
WHO. Physical status: the use and interpretation of anthropometry. Report of a WHO Expert Committee. World Health Organ Tech Rep Ser. Geneva; 1995.
WHO. The WHO child growth standards website [Online]. 2006. http://www.who.int/childgrowth/en/ . Accessed 31 Jan 2010.
WHO Expert Consultation. Appropriate body-mass index for Asian populations and its implications for policy and intervention strategies. Lancet. 2004;363:157–63.
WHO Multicentre Growth Reference Study Group. WHO child growth standards: length/height-for-age, weight-forage, weight-for-length, weight-for-height and body mass index-for-age: Methods and development. Geneva: World Health Organization; 2006a.
WHO Multicentre Growth Reference Study Group. Assessment of differences in linear growth among populations in the WHO Multicentre Growth Reference Study. Acta Paediatr Suppl. 2006b;450:56–65.
WHO Training Course. Training course on child growth assessment: investigating causes of undernutrition and of overweight. November 2006. Geneva: WHO. http://www.who.int/childgrowth/training/jobaid_investigating_causes.pdf . Accessed 30 Jan 2010.
Download references
Acknowledgments
This work was supported in part by research grants from the NIH/NIDDK (R01DK81335-01A1, 1R03HD058077-01A1, R03HD058077-01A1S1) and the Nestle Foundation. We also thank Irwin Shorr for his comments on an earlier draft of this chapter.
Author information
Authors and affiliations.
Department of International Health, Johns Hopkins Bloomberg School of Public Health, Center for Human Nutrition, 615 N Wolfe St. E2546, Baltimore, MD, 21205, USA
You can also search for this author in PubMed Google Scholar
Corresponding author
Correspondence to Youfa Wang .
Editor information
Editors and affiliations.
Dept. Nutrition & Dietetics, King's College, Stamford St. 150, London, SE1 9NH, United Kingdom
Victor R. Preedy
Rights and permissions
Reprints and permissions
Copyright information
© 2012 Springer Science+Business Media, LLC
About this chapter
Wang, Y., Chen, HJ. (2012). Use of Percentiles and Z -Scores in Anthropometry. In: Preedy, V. (eds) Handbook of Anthropometry. Springer, New York, NY. https://doi.org/10.1007/978-1-4419-1788-1_2
Download citation
DOI : https://doi.org/10.1007/978-1-4419-1788-1_2
Published : 12 January 2012
Publisher Name : Springer, New York, NY
Print ISBN : 978-1-4419-1787-4
Online ISBN : 978-1-4419-1788-1
eBook Packages : Medicine Medicine (R0)
Share this chapter
Anyone you share the following link with will be able to read this content:
Sorry, a shareable link is not currently available for this article.
Provided by the Springer Nature SharedIt content-sharing initiative
- Publish with us
Policies and ethics
- Find a journal
- Track your research
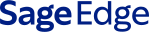
- Request new password
- Create a new account
Essentials of Social Statistics for a Diverse Society, 3e
Student resources, discussion questions.
1. Describe the key features of the normal curve and explain why the normal curve in real-life distributions never matches the model perfectly.
2. Imagine that you recently took a statistics exam and your instructor just returned your graded exam. The instructor announces that 75% of students scored below the mean. How do you reconcile this with the fact that, in a normal distribution, half of the scores should fall below the mean and half of the scores should fall above the mean?
3. Compare and contrast an empirical distribution and a normal distribution. Describe the value of each distribution. Discuss the terminology that is invoked to denote the former and the terminology that is invoked to denote the latter.
4. According to the material presented in chapter 6, why do researchers use Z scores? What are the advantages of using Z scores? Describe some research questions that would require the use of Z scores. Are there any research questions that would not be appropriate for the use of Z scores?
5. Why can we NOT assume that for any scale variable, 50% of cases are above the mean and 50% are below the mean?
6. Why do we use a bell curve to assess the normality of a variable as opposed to a square, triangle, or some other symmetrical shape?
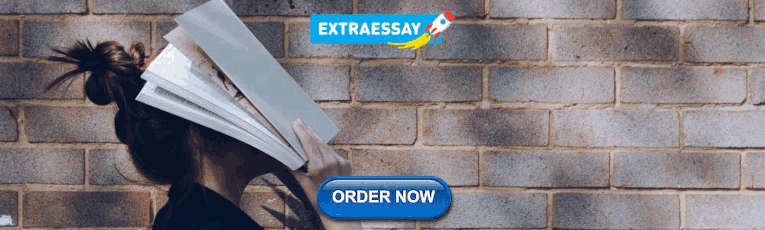
IMAGES
VIDEO
COMMENTS
Standard Score. The standard score (more commonly referred to as a z-score) is a very useful statistic because it (a) allows us to calculate the probability of a score occurring within our normal distribution and (b) enables us to compare two scores that are from different normal distributions. The standard score does this by converting (in ...
A z-score measures the distance between a data point and the mean using standard deviations. Z-scores can be positive or negative. The sign tells you whether the observation is above or below the mean. For example, a z-score of +2 indicates that the data point falls two standard deviations above the mean, while a -2 signifies it is two standard ...
The value of the z-score tells you how many standard deviations you are away from the mean. If a z-score is equal to 0, it is on the mean. A positive z-score indicates the raw score is higher than the mean average. For example, if a z-score is equal to +1, it is 1 standard deviation above the mean. A negative z-score reveals the raw score is ...
Understanding the z score table normal distribution. #2: Since you need to know the standard deviations to calculate the z score, you may also lose the meaning standard scores. #3: When you are using the z score, you may end up magnifying small differences. #4: Linear transformations require interval data and some of the data that you use may ...
Stanine (standard nine) scores range from 1 to 9, with a mean of 5 and an SD of 2; sten (standard ten) scores range from 1 to 10, with a mean of 5.5 and an SD of 2. Stanine and sten scores are used in some psychological tests. IQ scores are also standardized; they have a mean of 100 and an SD of 15. Readers may note that Z transformation and ...
Use a Z test when you need to compare group means. Use the 1-sample analysis to determine whether a population mean is different from a hypothesized value. Or use the 2-sample version to determine whether two population means differ. A Z test is a form of inferential statistics. It uses samples to draw conclusions about populations.
Z score formula for sample. How to interpret the results? z-score = 0, the score (x) is exactly average; z-score is positive, the score (x) is above average; z-score is negative, the score (x) is below average. Z-scores can be used either to compare scores between the same distribution, or between different distributions. Let's look now at an ...
4.1: Normal Distributions. The normal distribution is the most important and most widely used distribution in statistics. It is sometimes called the "bell curve," although the tonal qualities of such a bell would be less than pleasing. It is also called the "Gaussian curve" of Gaussian distribution after the mathematician Karl Friedrich ...
Example: Calculate and Interpret Z-Scores. Suppose the scores for a certain exam are normally distributed with a mean of 80 and a standard deviation of 4. Question 1: Find the z-score for an exam score of 87. We can use the following steps to calculate the z-score: Thus, z = (X - μ) / σ = (87 - 80) /4 = 1.75.
How to Convert a Z-Score to IQ. To compute the IQ score from a z-score, you multiply the Z-score by 15 for most tests, then multiply that number by 100. For some tests, you multiply the Z-score by 16 initially. With a basic example, if you have a Z-score of 0.10, and you multiply that number by 15, you'll get 1.5.
1) We cannot compute or assign meaning to Z scores for the nominal or ordinal type of data. 2) The original data values cannot be recovered from the Z score unless we know the mean and the standard deviation of the distribution. 3) Although the Z score takes into account the mean and standard deviation of the distribution, it does not take into ...
These two steps are the same as the following formula: Zx = Xi − X¯¯¯¯ Sx Z x = X i − X ¯ S x. As shown by the table below, our 100 scores have a mean of 3.45 and a standard deviation of 1.70. By entering these numbers into the formula, we see why a score of 5 corresponds to a z-score of 0.91: Zx = 5 − 3.45 1.70 = 0.91 Z x = 5 − 3. ...
Statistical analysis of research results is similarly facilitated and indeed enhanced by using Z scores. The analytic power added through the use of Z scores is often underappreciated and can be illustrated through an example. A common study design is to attempt to control for the effects of age and body size by selecting controls matched for age and body size and then performing a paired ...
Nov. '96. 810. 450. On average the use of the z-score selected an undemourished group which was 1.6 times greater than that selected by the use of the '80% of the median' method (range: 1.3 - 1.8). Similar differences were obtained from nutrition surveys carried out in other locations in Angola and in Sierra Leone.
Example 1: Exam Scores. Z-scores are often used in academic settings to analyze how well a student's score compares to the mean score on a given exam. For example, suppose the scores on a certain college entrance exam are roughly normally distributed with a mean of 82 and a standard deviation of 5. If a certain student received a 90 on the ...
Stanine (standard nine) scores range from 1 to 9, with a mean of 5 and an SD of 2; sten (standard ten) scores range from 1 to 10, with a mean of 5.5 and an SD of 2. Stanine and sten scores are used in some psychological tests. IQ scores are also standardized; they have a mean of 100 and an SD of 15. Readers may note that Z transformation and ...
The raw scores for the two variables are about fundamentally different things, so this would be like comparing apples to oranges. BUT, if we calculate the z-scores, we get z = (35 − 17) 5 = 3.6 z = ( 35 − 17) 5 = 3.6 for grumpiness and z = (2 − 13) 4 = −2.75 z = ( 2 − 13) 4 = − 2.75 for extraversion. These two numbers can be ...
The use of Z-scores is recommended for several reasons (Table 2.1).First, Z-scores are calculated based on the distribution of the reference population (both the mean and the standard deviation [SD]); thus, they reflect the reference distribution.Second, as standardized measures, Z-scores are comparable across age, sex and measure (as a measure of "dimensionless quantity").
According to the material presented in Chapter 5, why do researchers use Z scores? What are the advantages of using Z scores? Describe some research questions that would require the use of Z scores. ... Why do we use a bell curve to assess the normality of a variable as opposed to a square, triangle, or some other symmetrical shape? Chapter 6.
Discussion Questions. 1. Describe the key features of the normal curve and explain why the normal curve in real-life distributions never matches the model perfectly. 2. Imagine that you recently took a statistics exam and your instructor just returned your graded exam. The instructor announces that 75% of students scored below the mean.
This might be related to the same letter z being frequently used for the Normal distribution, but, again, I have no idea why the letter z was chosen here. Does it stand for anything? The connection with the frequent use of Z Z for normal random variables is clear. Then the question becomes, why the use of Z Z for normal random variables.
Z-scores. A z-score (also known as standard score) shows the relationship a value and the mean of the group's values have. When z-score is equals to 0, it means that the mean and the score of data points are similar. Formula: Z = (X - mean)/ standard deviation. Answer and Explanation: 1
This problem has been solved! You'll get a detailed solution from a subject matter expert that helps you learn core concepts. Question: According to the material presented in chapter 5 of Social Statistics for a Diverse Society ed. 8, why do researchers use z scores? What are the advantages of using Z scores? Describe some research questions ...