- Privacy Policy
Buy Me a Coffee

Home » Discourse Analysis – Methods, Types and Examples
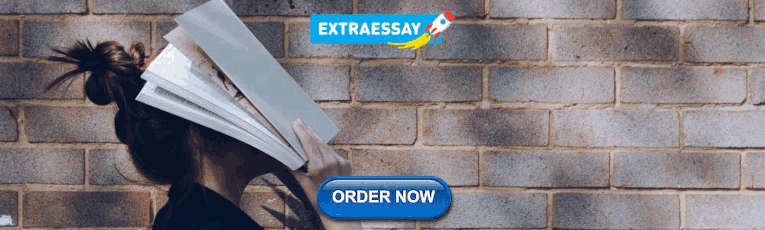
Discourse Analysis – Methods, Types and Examples
Table of Contents

Discourse Analysis
Definition:
Discourse Analysis is a method of studying how people use language in different situations to understand what they really mean and what messages they are sending. It helps us understand how language is used to create social relationships and cultural norms.
It examines language use in various forms of communication such as spoken, written, visual or multi-modal texts, and focuses on how language is used to construct social meaning and relationships, and how it reflects and reinforces power dynamics, ideologies, and cultural norms.
Types of Discourse Analysis
Some of the most common types of discourse analysis are:
Conversation Analysis
This type of discourse analysis focuses on analyzing the structure of talk and how participants in a conversation make meaning through their interaction. It is often used to study face-to-face interactions, such as interviews or everyday conversations.
Critical discourse Analysis
This approach focuses on the ways in which language use reflects and reinforces power relations, social hierarchies, and ideologies. It is often used to analyze media texts or political speeches, with the aim of uncovering the hidden meanings and assumptions that are embedded in these texts.
Discursive Psychology
This type of discourse analysis focuses on the ways in which language use is related to psychological processes such as identity construction and attribution of motives. It is often used to study narratives or personal accounts, with the aim of understanding how individuals make sense of their experiences.
Multimodal Discourse Analysis
This approach focuses on analyzing not only language use, but also other modes of communication, such as images, gestures, and layout. It is often used to study digital or visual media, with the aim of understanding how different modes of communication work together to create meaning.
Corpus-based Discourse Analysis
This type of discourse analysis uses large collections of texts, or corpora, to analyze patterns of language use across different genres or contexts. It is often used to study language use in specific domains, such as academic writing or legal discourse.
Descriptive Discourse
This type of discourse analysis aims to describe the features and characteristics of language use, without making any value judgments or interpretations. It is often used in linguistic studies to describe grammatical structures or phonetic features of language.
Narrative Discourse
This approach focuses on analyzing the structure and content of stories or narratives, with the aim of understanding how they are constructed and how they shape our understanding of the world. It is often used to study personal narratives or cultural myths.
Expository Discourse
This type of discourse analysis is used to study texts that explain or describe a concept, process, or idea. It aims to understand how information is organized and presented in such texts and how it influences the reader’s understanding of the topic.
Argumentative Discourse
This approach focuses on analyzing texts that present an argument or attempt to persuade the reader or listener. It aims to understand how the argument is constructed, what strategies are used to persuade, and how the audience is likely to respond to the argument.
Discourse Analysis Conducting Guide
Here is a step-by-step guide for conducting discourse analysis:
- What are you trying to understand about the language use in a particular context?
- What are the key concepts or themes that you want to explore?
- Select the data: Decide on the type of data that you will analyze, such as written texts, spoken conversations, or media content. Consider the source of the data, such as news articles, interviews, or social media posts, and how this might affect your analysis.
- Transcribe or collect the data: If you are analyzing spoken language, you will need to transcribe the data into written form. If you are using written texts, make sure that you have access to the full text and that it is in a format that can be easily analyzed.
- Read and re-read the data: Read through the data carefully, paying attention to key themes, patterns, and discursive features. Take notes on what stands out to you and make preliminary observations about the language use.
- Develop a coding scheme : Develop a coding scheme that will allow you to categorize and organize different types of language use. This might include categories such as metaphors, narratives, or persuasive strategies, depending on your research question.
- Code the data: Use your coding scheme to analyze the data, coding different sections of text or spoken language according to the categories that you have developed. This can be a time-consuming process, so consider using software tools to assist with coding and analysis.
- Analyze the data: Once you have coded the data, analyze it to identify patterns and themes that emerge. Look for similarities and differences across different parts of the data, and consider how different categories of language use are related to your research question.
- Interpret the findings: Draw conclusions from your analysis and interpret the findings in relation to your research question. Consider how the language use in your data sheds light on broader cultural or social issues, and what implications it might have for understanding language use in other contexts.
- Write up the results: Write up your findings in a clear and concise way, using examples from the data to support your arguments. Consider how your research contributes to the broader field of discourse analysis and what implications it might have for future research.
Applications of Discourse Analysis
Here are some of the key areas where discourse analysis is commonly used:
- Political discourse: Discourse analysis can be used to analyze political speeches, debates, and media coverage of political events. By examining the language used in these contexts, researchers can gain insight into the political ideologies, values, and agendas that underpin different political positions.
- Media analysis: Discourse analysis is frequently used to analyze media content, including news reports, television shows, and social media posts. By examining the language used in media content, researchers can understand how media narratives are constructed and how they influence public opinion.
- Education : Discourse analysis can be used to examine classroom discourse, student-teacher interactions, and educational policies. By analyzing the language used in these contexts, researchers can gain insight into the social and cultural factors that shape educational outcomes.
- Healthcare : Discourse analysis is used in healthcare to examine the language used by healthcare professionals and patients in medical consultations. This can help to identify communication barriers, cultural differences, and other factors that may impact the quality of healthcare.
- Marketing and advertising: Discourse analysis can be used to analyze marketing and advertising messages, including the language used in product descriptions, slogans, and commercials. By examining these messages, researchers can gain insight into the cultural values and beliefs that underpin consumer behavior.
When to use Discourse Analysis
Discourse analysis is a valuable research methodology that can be used in a variety of contexts. Here are some situations where discourse analysis may be particularly useful:
- When studying language use in a particular context: Discourse analysis can be used to examine how language is used in a specific context, such as political speeches, media coverage, or healthcare interactions. By analyzing language use in these contexts, researchers can gain insight into the social and cultural factors that shape communication.
- When exploring the meaning of language: Discourse analysis can be used to examine how language is used to construct meaning and shape social reality. This can be particularly useful in fields such as sociology, anthropology, and cultural studies.
- When examining power relations: Discourse analysis can be used to examine how language is used to reinforce or challenge power relations in society. By analyzing language use in contexts such as political discourse, media coverage, or workplace interactions, researchers can gain insight into how power is negotiated and maintained.
- When conducting qualitative research: Discourse analysis can be used as a qualitative research method, allowing researchers to explore complex social phenomena in depth. By analyzing language use in a particular context, researchers can gain rich and nuanced insights into the social and cultural factors that shape communication.
Examples of Discourse Analysis
Here are some examples of discourse analysis in action:
- A study of media coverage of climate change: This study analyzed media coverage of climate change to examine how language was used to construct the issue. The researchers found that media coverage tended to frame climate change as a matter of scientific debate rather than a pressing environmental issue, thereby undermining public support for action on climate change.
- A study of political speeches: This study analyzed political speeches to examine how language was used to construct political identity. The researchers found that politicians used language strategically to construct themselves as trustworthy and competent leaders, while painting their opponents as untrustworthy and incompetent.
- A study of medical consultations: This study analyzed medical consultations to examine how language was used to negotiate power and authority between doctors and patients. The researchers found that doctors used language to assert their authority and control over medical decisions, while patients used language to negotiate their own preferences and concerns.
- A study of workplace interactions: This study analyzed workplace interactions to examine how language was used to construct social identity and maintain power relations. The researchers found that language was used to construct a hierarchy of power and status within the workplace, with those in positions of authority using language to assert their dominance over subordinates.
Purpose of Discourse Analysis
The purpose of discourse analysis is to examine the ways in which language is used to construct social meaning, relationships, and power relations. By analyzing language use in a systematic and rigorous way, discourse analysis can provide valuable insights into the social and cultural factors that shape communication and interaction.
The specific purposes of discourse analysis may vary depending on the research context, but some common goals include:
- To understand how language constructs social reality: Discourse analysis can help researchers understand how language is used to construct meaning and shape social reality. By analyzing language use in a particular context, researchers can gain insight into the cultural and social factors that shape communication.
- To identify power relations: Discourse analysis can be used to examine how language use reinforces or challenges power relations in society. By analyzing language use in contexts such as political discourse, media coverage, or workplace interactions, researchers can gain insight into how power is negotiated and maintained.
- To explore social and cultural norms: Discourse analysis can help researchers understand how social and cultural norms are constructed and maintained through language use. By analyzing language use in different contexts, researchers can gain insight into how social and cultural norms are reproduced and challenged.
- To provide insights for social change: Discourse analysis can provide insights that can be used to promote social change. By identifying problematic language use or power imbalances, researchers can provide insights that can be used to challenge social norms and promote more equitable and inclusive communication.
Characteristics of Discourse Analysis
Here are some key characteristics of discourse analysis:
- Focus on language use: Discourse analysis is centered on language use and how it constructs social meaning, relationships, and power relations.
- Multidisciplinary approach: Discourse analysis draws on theories and methodologies from a range of disciplines, including linguistics, anthropology, sociology, and psychology.
- Systematic and rigorous methodology: Discourse analysis employs a systematic and rigorous methodology, often involving transcription and coding of language data, in order to identify patterns and themes in language use.
- Contextual analysis : Discourse analysis emphasizes the importance of context in shaping language use, and takes into account the social and cultural factors that shape communication.
- Focus on power relations: Discourse analysis often examines power relations and how language use reinforces or challenges power imbalances in society.
- Interpretive approach: Discourse analysis is an interpretive approach, meaning that it seeks to understand the meaning and significance of language use from the perspective of the participants in a particular discourse.
- Emphasis on reflexivity: Discourse analysis emphasizes the importance of reflexivity, or self-awareness, in the research process. Researchers are encouraged to reflect on their own positionality and how it may shape their interpretation of language use.
Advantages of Discourse Analysis
Discourse analysis has several advantages as a methodological approach. Here are some of the main advantages:
- Provides a detailed understanding of language use: Discourse analysis allows for a detailed and nuanced understanding of language use in specific social contexts. It enables researchers to identify patterns and themes in language use, and to understand how language constructs social reality.
- Emphasizes the importance of context : Discourse analysis emphasizes the importance of context in shaping language use. By taking into account the social and cultural factors that shape communication, discourse analysis provides a more complete understanding of language use than other approaches.
- Allows for an examination of power relations: Discourse analysis enables researchers to examine power relations and how language use reinforces or challenges power imbalances in society. By identifying problematic language use, discourse analysis can contribute to efforts to promote social justice and equality.
- Provides insights for social change: Discourse analysis can provide insights that can be used to promote social change. By identifying problematic language use or power imbalances, researchers can provide insights that can be used to challenge social norms and promote more equitable and inclusive communication.
- Multidisciplinary approach: Discourse analysis draws on theories and methodologies from a range of disciplines, including linguistics, anthropology, sociology, and psychology. This multidisciplinary approach allows for a more holistic understanding of language use in social contexts.
Limitations of Discourse Analysis
Some Limitations of Discourse Analysis are as follows:
- Time-consuming and resource-intensive: Discourse analysis can be a time-consuming and resource-intensive process. Collecting and transcribing language data can be a time-consuming task, and analyzing the data requires careful attention to detail and a significant investment of time and resources.
- Limited generalizability: Discourse analysis is often focused on a particular social context or community, and therefore the findings may not be easily generalized to other contexts or populations. This means that the insights gained from discourse analysis may have limited applicability beyond the specific context being studied.
- Interpretive nature: Discourse analysis is an interpretive approach, meaning that it relies on the interpretation of the researcher to identify patterns and themes in language use. This subjectivity can be a limitation, as different researchers may interpret language data differently.
- Limited quantitative analysis: Discourse analysis tends to focus on qualitative analysis of language data, which can limit the ability to draw statistical conclusions or make quantitative comparisons across different language uses or contexts.
- Ethical considerations: Discourse analysis may involve the collection and analysis of sensitive language data, such as language related to trauma or marginalization. Researchers must carefully consider the ethical implications of collecting and analyzing this type of data, and ensure that the privacy and confidentiality of participants is protected.
About the author
Muhammad Hassan
Researcher, Academic Writer, Web developer
You may also like

Cluster Analysis – Types, Methods and Examples

Discriminant Analysis – Methods, Types and...

MANOVA (Multivariate Analysis of Variance) –...

Documentary Analysis – Methods, Applications and...

ANOVA (Analysis of variance) – Formulas, Types...

Graphical Methods – Types, Examples and Guide
Have a language expert improve your writing
Run a free plagiarism check in 10 minutes, generate accurate citations for free.
- Knowledge Base
Methodology
- Critical Discourse Analysis | Definition, Guide & Examples
Critical Discourse Analysis | Definition, Guide & Examples
Published on August 23, 2019 by Amy Luo . Revised on June 22, 2023.
Critical discourse analysis (or discourse analysis) is a research method for studying written or spoken language in relation to its social context. It aims to understand how language is used in real life situations.
When you conduct discourse analysis, you might focus on:
- The purposes and effects of different types of language
- Cultural rules and conventions in communication
- How values, beliefs and assumptions are communicated
- How language use relates to its social, political and historical context
Discourse analysis is a common qualitative research method in many humanities and social science disciplines, including linguistics, sociology, anthropology, psychology and cultural studies.
Table of contents
What is discourse analysis used for, how is discourse analysis different from other methods, how to conduct discourse analysis, other interesting articles.
Conducting discourse analysis means examining how language functions and how meaning is created in different social contexts. It can be applied to any instance of written or oral language, as well as non-verbal aspects of communication such as tone and gestures.
Materials that are suitable for discourse analysis include:
- Books, newspapers and periodicals
- Marketing material, such as brochures and advertisements
- Business and government documents
- Websites, forums, social media posts and comments
- Interviews and conversations
By analyzing these types of discourse, researchers aim to gain an understanding of social groups and how they communicate.
Prevent plagiarism. Run a free check.
Unlike linguistic approaches that focus only on the rules of language use, discourse analysis emphasizes the contextual meaning of language.
It focuses on the social aspects of communication and the ways people use language to achieve specific effects (e.g. to build trust, to create doubt, to evoke emotions, or to manage conflict).
Instead of focusing on smaller units of language, such as sounds, words or phrases, discourse analysis is used to study larger chunks of language, such as entire conversations, texts, or collections of texts. The selected sources can be analyzed on multiple levels.
Discourse analysis is a qualitative and interpretive method of analyzing texts (in contrast to more systematic methods like content analysis ). You make interpretations based on both the details of the material itself and on contextual knowledge.
There are many different approaches and techniques you can use to conduct discourse analysis, but the steps below outline the basic structure you need to follow. Following these steps can help you avoid pitfalls of confirmation bias that can cloud your analysis.
Step 1: Define the research question and select the content of analysis
To do discourse analysis, you begin with a clearly defined research question . Once you have developed your question, select a range of material that is appropriate to answer it.
Discourse analysis is a method that can be applied both to large volumes of material and to smaller samples, depending on the aims and timescale of your research.
Step 2: Gather information and theory on the context
Next, you must establish the social and historical context in which the material was produced and intended to be received. Gather factual details of when and where the content was created, who the author is, who published it, and whom it was disseminated to.
As well as understanding the real-life context of the discourse, you can also conduct a literature review on the topic and construct a theoretical framework to guide your analysis.
Step 3: Analyze the content for themes and patterns
This step involves closely examining various elements of the material – such as words, sentences, paragraphs, and overall structure – and relating them to attributes, themes, and patterns relevant to your research question.
Step 4: Review your results and draw conclusions
Once you have assigned particular attributes to elements of the material, reflect on your results to examine the function and meaning of the language used. Here, you will consider your analysis in relation to the broader context that you established earlier to draw conclusions that answer your research question.
If you want to know more about statistics , methodology , or research bias , make sure to check out some of our other articles with explanations and examples.
- Normal distribution
- Measures of central tendency
- Chi square tests
- Confidence interval
- Quartiles & Quantiles
- Cluster sampling
- Stratified sampling
- Thematic analysis
- Cohort study
- Peer review
- Ethnography
Research bias
- Implicit bias
- Cognitive bias
- Conformity bias
- Hawthorne effect
- Availability heuristic
- Attrition bias
- Social desirability bias
Cite this Scribbr article
If you want to cite this source, you can copy and paste the citation or click the “Cite this Scribbr article” button to automatically add the citation to our free Citation Generator.
Luo, A. (2023, June 22). Critical Discourse Analysis | Definition, Guide & Examples. Scribbr. Retrieved March 27, 2024, from https://www.scribbr.com/methodology/discourse-analysis/
Is this article helpful?
Other students also liked
What is qualitative research | methods & examples, what is a case study | definition, examples & methods, how to do thematic analysis | step-by-step guide & examples, "i thought ai proofreading was useless but..".
I've been using Scribbr for years now and I know it's a service that won't disappoint. It does a good job spotting mistakes”

What (Exactly) Is Discourse Analysis? A Plain-Language Explanation & Definition (With Examples)
By: Jenna Crosley (PhD). Expert Reviewed By: Dr Eunice Rautenbach | June 2021
Discourse analysis is one of the most popular qualitative analysis techniques we encounter at Grad Coach. If you’ve landed on this post, you’re probably interested in discourse analysis, but you’re not sure whether it’s the right fit for your project, or you don’t know where to start. If so, you’ve come to the right place.
Overview: Discourse Analysis Basics
In this post, we’ll explain in plain, straightforward language :
- What discourse analysis is
- When to use discourse analysis
- The main approaches to discourse analysis
- How to conduct discourse analysis
What is discourse analysis?
Let’s start with the word “discourse”.
In its simplest form, discourse is verbal or written communication between people that goes beyond a single sentence . Importantly, discourse is more than just language. The term “language” can include all forms of linguistic and symbolic units (even things such as road signs), and language studies can focus on the individual meanings of words. Discourse goes beyond this and looks at the overall meanings conveyed by language in context . “Context” here refers to the social, cultural, political, and historical background of the discourse, and it is important to take this into account to understand underlying meanings expressed through language.
A popular way of viewing discourse is as language used in specific social contexts, and as such language serves as a means of prompting some form of social change or meeting some form of goal.
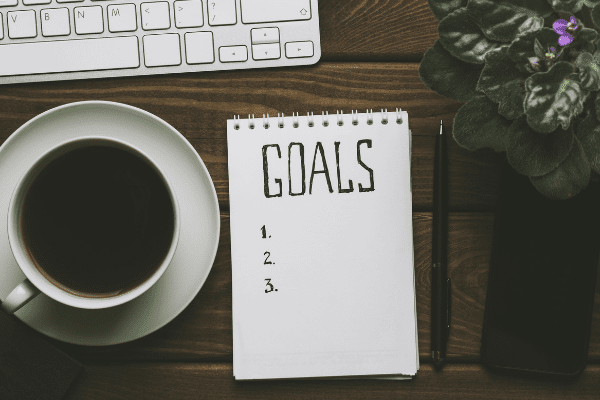
Now that we’ve defined discourse, let’s look at discourse analysis .
Discourse analysis uses the language presented in a corpus or body of data to draw meaning . This body of data could include a set of interviews or focus group discussion transcripts. While some forms of discourse analysis center in on the specifics of language (such as sounds or grammar), other forms focus on how this language is used to achieve its aims. We’ll dig deeper into these two above-mentioned approaches later.
As Wodak and Krzyżanowski (2008) put it: “discourse analysis provides a general framework to problem-oriented social research”. Basically, discourse analysis is used to conduct research on the use of language in context in a wide variety of social problems (i.e., issues in society that affect individuals negatively).
For example, discourse analysis could be used to assess how language is used to express differing viewpoints on financial inequality and would look at how the topic should or shouldn’t be addressed or resolved, and whether this so-called inequality is perceived as such by participants.
What makes discourse analysis unique is that it posits that social reality is socially constructed , or that our experience of the world is understood from a subjective standpoint. Discourse analysis goes beyond the literal meaning of words and languages
For example, people in countries that make use of a lot of censorship will likely have their knowledge, and thus views, limited by this, and will thus have a different subjective reality to those within countries with more lax laws on censorship.
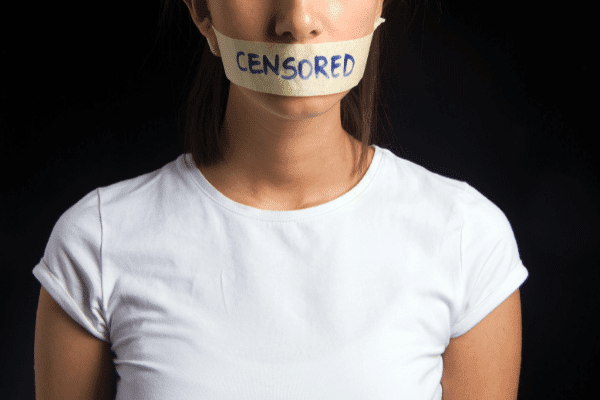
When should you use discourse analysis?
There are many ways to analyze qualitative data (such as content analysis , narrative analysis , and thematic analysis ), so why should you choose discourse analysis? Well, as with all analysis methods, the nature of your research aims, objectives and research questions (i.e. the purpose of your research) will heavily influence the right choice of analysis method.
The purpose of discourse analysis is to investigate the functions of language (i.e., what language is used for) and how meaning is constructed in different contexts, which, to recap, include the social, cultural, political, and historical backgrounds of the discourse.
For example, if you were to study a politician’s speeches, you would need to situate these speeches in their context, which would involve looking at the politician’s background and views, the reasons for presenting the speech, the history or context of the audience, and the country’s social and political history (just to name a few – there are always multiple contextual factors).
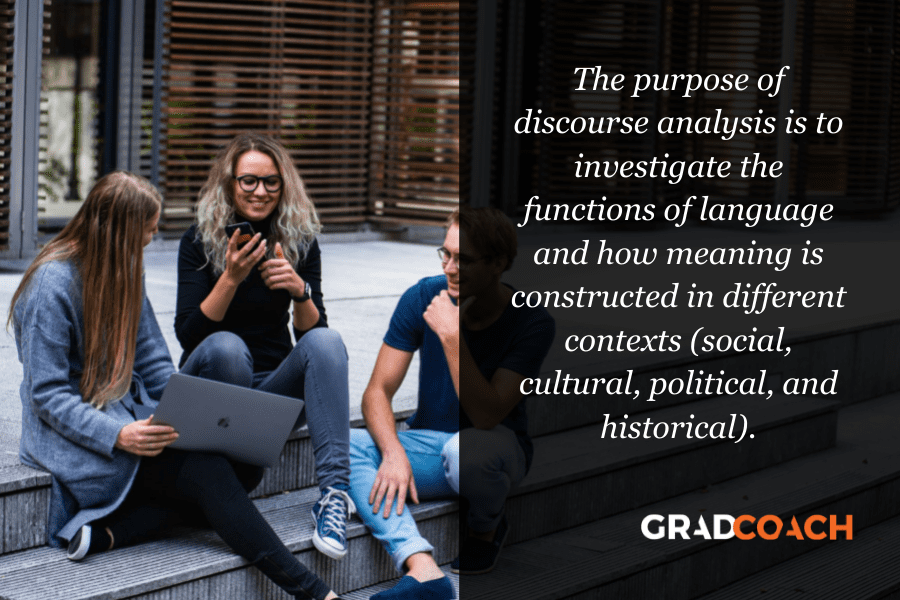
Discourse analysis can also tell you a lot about power and power imbalances , including how this is developed and maintained, how this plays out in real life (for example, inequalities because of this power), and how language can be used to maintain it. For example, you could look at the way that someone with more power (for example, a CEO) speaks to someone with less power (for example, a lower-level employee).
Therefore, you may consider discourse analysis if you are researching:
- Some form of power or inequality (for example, how affluent individuals interact with those who are less wealthy
- How people communicate in a specific context (such as in a social situation with colleagues versus a board meeting)
- Ideology and how ideas (such as values and beliefs) are shared using language (like in political speeches)
- How communication is used to achieve social goals (such as maintaining a friendship or navigating conflict)
As you can see, discourse analysis can be a powerful tool for assessing social issues , as well as power and power imbalances . So, if your research aims and objectives are oriented around these types of issues, discourse analysis could be a good fit for you.
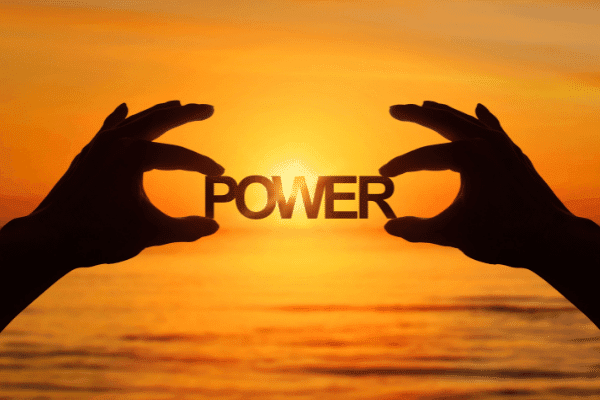
Discourse Analysis: The main approaches
There are two main approaches to discourse analysis. These are the language-in-use (also referred to as socially situated text and talk ) approaches and the socio-political approaches (most commonly Critical Discourse Analysis ). Let’s take a look at each of these.
Approach #1: Language-in-use
Language-in-use approaches focus on the finer details of language used within discourse, such as sentence structures (grammar) and phonology (sounds). This approach is very descriptive and is seldom seen outside of studies focusing on literature and/or linguistics.
Because of its formalist roots, language-in-use pays attention to different rules of communication, such as grammaticality (i.e., when something “sounds okay” to a native speaker of a language). Analyzing discourse through a language-in-use framework involves identifying key technicalities of language used in discourse and investigating how the features are used within a particular social context.
For example, English makes use of affixes (for example, “un” in “unbelievable”) and suffixes (“able” in “unbelievable”) but doesn’t typically make use of infixes (units that can be placed within other words to alter their meaning). However, an English speaker may say something along the lines of, “that’s un-flipping-believable”. From a language-in-use perspective, the infix “flipping” could be investigated by assessing how rare the phenomenon is in English, and then answering questions such as, “What role does the infix play?” or “What is the goal of using such an infix?”
Need a helping hand?
Approach #2: Socio-political
Socio-political approaches to discourse analysis look beyond the technicalities of language and instead focus on the influence that language has in social context , and vice versa. One of the main socio-political approaches is Critical Discourse Analysis , which focuses on power structures (for example, the power dynamic between a teacher and a student) and how discourse is influenced by society and culture. Critical Discourse Analysis is born out of Michel Foucault’s early work on power, which focuses on power structures through the analysis of normalized power .
Normalized power is ingrained and relatively allusive. It’s what makes us exist within society (and within the underlying norms of society, as accepted in a specific social context) and do the things that we need to do. Contrasted to this, a more obvious form of power is repressive power , which is power that is actively asserted.
Sounds a bit fluffy? Let’s look at an example.
Consider a situation where a teacher threatens a student with detention if they don’t stop speaking in class. This would be an example of repressive power (i.e. it was actively asserted).
Normalized power, on the other hand, is what makes us not want to talk in class . It’s the subtle clues we’re given from our environment that tell us how to behave, and this form of power is so normal to us that we don’t even realize that our beliefs, desires, and decisions are being shaped by it.
In the view of Critical Discourse Analysis, language is power and, if we want to understand power dynamics and structures in society, we must look to language for answers. In other words, analyzing the use of language can help us understand the social context, especially the power dynamics.
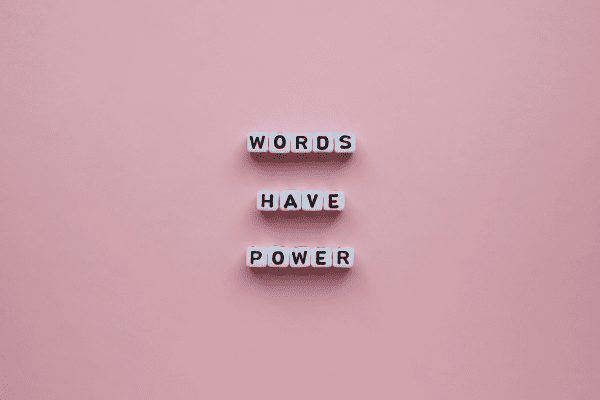
While the above-mentioned approaches are the two most popular approaches to discourse analysis, other forms of analysis exist. For example, ethnography-based discourse analysis and multimodal analysis. Ethnography-based discourse analysis aims to gain an insider understanding of culture , customs, and habits through participant observation (i.e. directly observing participants, rather than focusing on pre-existing texts).
On the other hand, multimodal analysis focuses on a variety of texts that are both verbal and nonverbal (such as a combination of political speeches and written press releases). So, if you’re considering using discourse analysis, familiarize yourself with the various approaches available so that you can make a well-informed decision.
How to “do” discourse analysis
As every study is different, it’s challenging to outline exactly what steps need to be taken to complete your research. However, the following steps can be used as a guideline if you choose to adopt discourse analysis for your research.
Step 1: Decide on your discourse analysis approach
The first step of the process is to decide on which approach you will take in terms. For example, the language in use approach or a socio-political approach such as critical discourse analysis. To do this, you need to consider your research aims, objectives and research questions . Of course, this means that you need to have these components clearly defined. If you’re still a bit uncertain about these, check out our video post covering topic development here.
While discourse analysis can be exploratory (as in, used to find out about a topic that hasn’t really been touched on yet), it is still vital to have a set of clearly defined research questions to guide your analysis. Without these, you may find that you lack direction when you get to your analysis. Since discourse analysis places such a focus on context, it is also vital that your research questions are linked to studying language within context.
Based on your research aims, objectives and research questions, you need to assess which discourse analysis would best suit your needs. Importantly, you need to adopt an approach that aligns with your study’s purpose . So, think carefully about what you are investigating and what you want to achieve, and then consider the various options available within discourse analysis.
It’s vital to determine your discourse analysis approach from the get-go , so that you don’t waste time randomly analyzing your data without any specific plan.
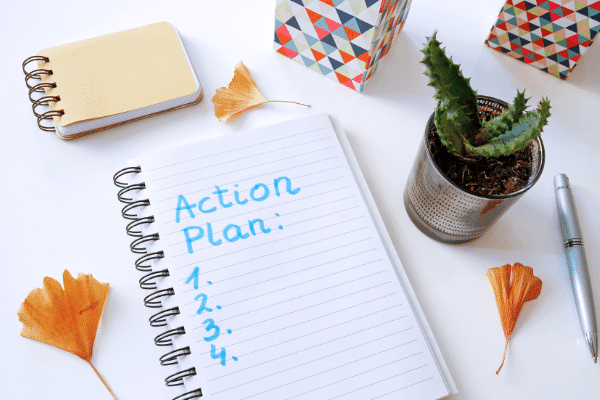
Step 2: Design your collection method and gather your data
Once you’ve got determined your overarching approach, you can start looking at how to collect your data. Data in discourse analysis is drawn from different forms of “talk” and “text” , which means that it can consist of interviews , ethnographies, discussions, case studies, blog posts.
The type of data you collect will largely depend on your research questions (and broader research aims and objectives). So, when you’re gathering your data, make sure that you keep in mind the “what”, “who” and “why” of your study, so that you don’t end up with a corpus full of irrelevant data. Discourse analysis can be very time-consuming, so you want to ensure that you’re not wasting time on information that doesn’t directly pertain to your research questions.
When considering potential collection methods, you should also consider the practicalities . What type of data can you access in reality? How many participants do you have access to and how much time do you have available to collect data and make sense of it? These are important factors, as you’ll run into problems if your chosen methods are impractical in light of your constraints.
Once you’ve determined your data collection method, you can get to work with the collection.
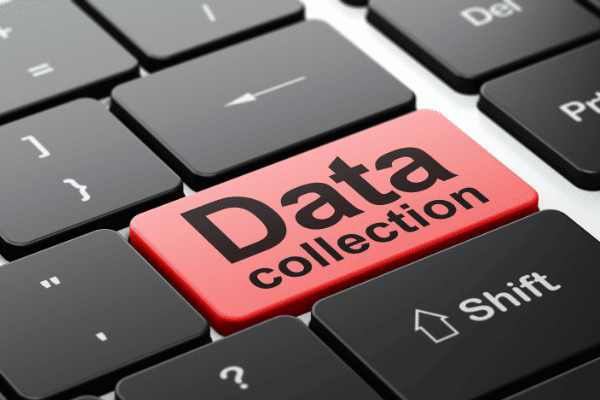
Step 3: Investigate the context
A key part of discourse analysis is context and understanding meaning in context. For this reason, it is vital that you thoroughly and systematically investigate the context of your discourse. Make sure that you can answer (at least the majority) of the following questions:
- What is the discourse?
- Why does the discourse exist? What is the purpose and what are the aims of the discourse?
- When did the discourse take place?
- Where did it happen?
- Who participated in the discourse? Who created it and who consumed it?
- What does the discourse say about society in general?
- How is meaning being conveyed in the context of the discourse?
Make sure that you include all aspects of the discourse context in your analysis to eliminate any confounding factors. For example, are there any social, political, or historical reasons as to why the discourse would exist as it does? What other factors could contribute to the existence of the discourse? Discourse can be influenced by many factors, so it is vital that you take as many of them into account as possible.
Once you’ve investigated the context of your data, you’ll have a much better idea of what you’re working with, and you’ll be far more familiar with your content. It’s then time to begin your analysis.
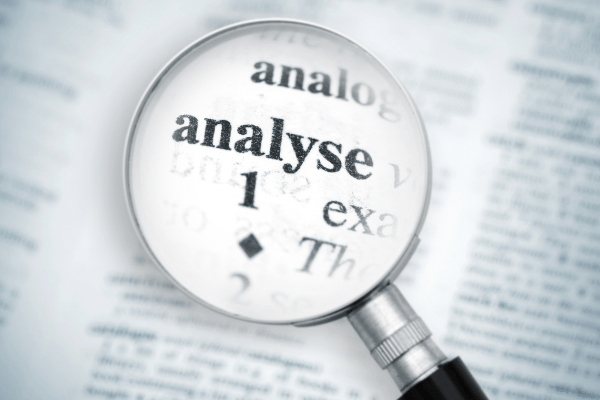
Step 4: Analyze your data
When performing a discourse analysis, you’ll need to look for themes and patterns . To do this, you’ll start by looking at codes , which are specific topics within your data. You can find more information about the qualitative data coding process here.
Next, you’ll take these codes and identify themes. Themes are patterns of language (such as specific words or sentences) that pop up repeatedly in your data, and that can tell you something about the discourse. For example, if you’re wanting to know about women’s perspectives of living in a certain area, potential themes may be “safety” or “convenience”.
In discourse analysis, it is important to reach what is called data saturation . This refers to when you’ve investigated your topic and analyzed your data to the point where no new information can be found. To achieve this, you need to work your way through your data set multiple times, developing greater depth and insight each time. This can be quite time consuming and even a bit boring at times, but it’s essential.
Once you’ve reached the point of saturation, you should have an almost-complete analysis and you’re ready to move onto the next step – final review.
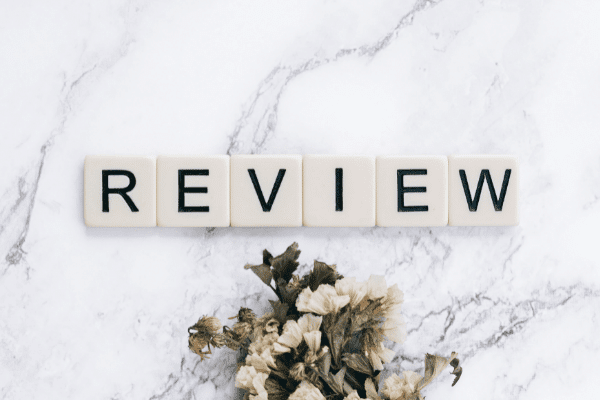
Step 5: Review your work
Hey, you’re nearly there. Good job! Now it’s time to review your work.
This final step requires you to return to your research questions and compile your answers to them, based on the analysis. Make sure that you can answer your research questions thoroughly, and also substantiate your responses with evidence from your data.
Usually, discourse analysis studies make use of appendices, which are referenced within your thesis or dissertation. This makes it easier for reviewers or markers to jump between your analysis (and findings) and your corpus (your evidence) so that it’s easier for them to assess your work.
When answering your research questions, make you should also revisit your research aims and objectives , and assess your answers against these. This process will help you zoom out a little and give you a bigger picture view. With your newfound insights from the analysis, you may find, for example, that it makes sense to expand the research question set a little to achieve a more comprehensive view of the topic.
Let’s recap…
In this article, we’ve covered quite a bit of ground. The key takeaways are:
- Discourse analysis is a qualitative analysis method used to draw meaning from language in context.
- You should consider using discourse analysis when you wish to analyze the functions and underlying meanings of language in context.
- The two overarching approaches to discourse analysis are language-in-use and socio-political approaches .
- The main steps involved in undertaking discourse analysis are deciding on your analysis approach (based on your research questions), choosing a data collection method, collecting your data, investigating the context of your data, analyzing your data, and reviewing your work.
If you have any questions about discourse analysis, feel free to leave a comment below. If you’d like 1-on-1 help with your analysis, book an initial consultation with a friendly Grad Coach to see how we can help.
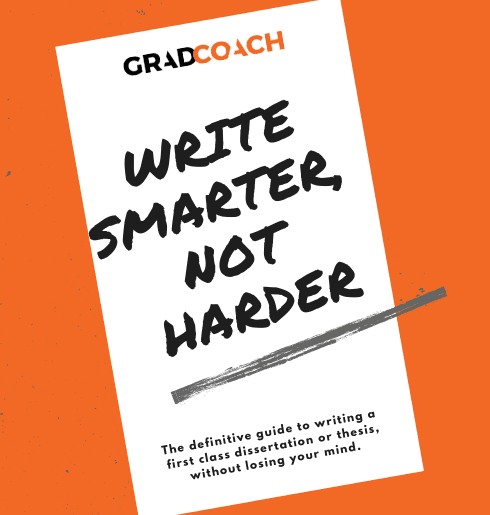
Psst… there’s more (for free)
This post is part of our dissertation mini-course, which covers everything you need to get started with your dissertation, thesis or research project.
You Might Also Like:
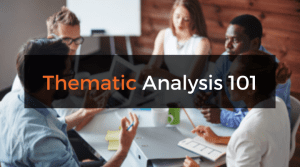
30 Comments
This was really helpful to me
I would like to know the importance of discourse analysis analysis to academic writing
In academic writing coherence and cohesion are very important. DA will assist us to decide cohesiveness of the continuum of discourse that are used in it. We can judge it well.
Thank you so much for this piece, can you please direct how I can use Discourse Analysis to investigate politics of ethnicity in a particular society
Fantastically helpful! Could you write on how discourse analysis can be done using computer aided technique? Many thanks
I would like to know if I can use discourse analysis to research on electoral integrity deviation and when election are considered free & fair
I also to know the importance of discourse analysis and it’s purpose and characteristics
Thanks, we are doing discourse analysis as a subject this year and this helped a lot!
Please can you help explain and answer this question? With illustrations,Hymes’ Acronym SPEAKING, as a feature of Discourse Analysis.
What are the three objectives of discourse analysis especially on the topic how people communicate between doctor and patient
Very useful Thank you for your work and information
thank you so much , I wanna know more about discourse analysis tools , such as , latent analysis , active powers analysis, proof paths analysis, image analysis, rhetorical analysis, propositions analysis, and so on, I wish I can get references about it , thanks in advance
Its beyond my expectations. It made me clear everything which I was struggling since last 4 months. 👏 👏 👏 👏
Thank you so much … It is clear and helpful
Thanks for sharing this material. My question is related to the online newspaper articles on COVID -19 pandemic the way this new normal is constructed as a social reality. How discourse analysis is an appropriate approach to examine theese articles?
This very helpful and interesting information
This was incredible! And massively helpful.
I’m seeking further assistance if you don’t mind.
Found it worth consuming!
What are the four types of discourse analysis?
very helpful. And I’d like to know more about Ethnography-based discourse analysis as I’m studying arts and humanities, I’d like to know how can I use it in my study.
Amazing info. Very happy to read this helpful piece of documentation. Thank you.
is discourse analysis can take data from medias like TV, Radio…?
I need to know what is general discourse analysis
Direct to the point, simple and deep explanation. this is helpful indeed.
Thank you so much was really helpful
really impressive
Thank you very much, for the clear explanations and examples.
It is really awesome. Anybody within just in 5 minutes understand this critical topic so easily. Thank you so much.
Thank you for enriching my knowledge on Discourse Analysis . Very helpful thanks again
This was extremely helpful. I feel less anxious now. Thank you so much.
Submit a Comment Cancel reply
Your email address will not be published. Required fields are marked *
Save my name, email, and website in this browser for the next time I comment.
- Print Friendly
Have a language expert improve your writing
Run a free plagiarism check in 10 minutes, automatically generate references for free.
- Knowledge Base
- Methodology
- Critical Discourse Analysis | Definition, Guide & Examples
Critical Discourse Analysis | Definition, Guide & Examples
Published on 5 May 2022 by Amy Luo . Revised on 5 December 2022.
Discourse analysis is a research method for studying written or spoken language in relation to its social context. It aims to understand how language is used in real-life situations.
When you do discourse analysis, you might focus on:
- The purposes and effects of different types of language
- Cultural rules and conventions in communication
- How values, beliefs, and assumptions are communicated
- How language use relates to its social, political, and historical context
Discourse analysis is a common qualitative research method in many humanities and social science disciplines, including linguistics, sociology, anthropology, psychology, and cultural studies. It is also called critical discourse analysis.
Table of contents
What is discourse analysis used for, how is discourse analysis different from other methods, how to conduct discourse analysis.
Conducting discourse analysis means examining how language functions and how meaning is created in different social contexts. It can be applied to any instance of written or oral language, as well as non-verbal aspects of communication, such as tone and gestures.
Materials that are suitable for discourse analysis include:
- Books, newspapers, and periodicals
- Marketing material, such as brochures and advertisements
- Business and government documents
- Websites, forums, social media posts, and comments
- Interviews and conversations
By analysing these types of discourse, researchers aim to gain an understanding of social groups and how they communicate.
Prevent plagiarism, run a free check.
Unlike linguistic approaches that focus only on the rules of language use, discourse analysis emphasises the contextual meaning of language.
It focuses on the social aspects of communication and the ways people use language to achieve specific effects (e.g., to build trust, to create doubt, to evoke emotions, or to manage conflict).
Instead of focusing on smaller units of language, such as sounds, words, or phrases, discourse analysis is used to study larger chunks of language, such as entire conversations, texts, or collections of texts. The selected sources can be analysed on multiple levels.
Discourse analysis is a qualitative and interpretive method of analysing texts (in contrast to more systematic methods like content analysis ). You make interpretations based on both the details of the material itself and on contextual knowledge.
There are many different approaches and techniques you can use to conduct discourse analysis, but the steps below outline the basic structure you need to follow.
Step 1: Define the research question and select the content of analysis
To do discourse analysis, you begin with a clearly defined research question . Once you have developed your question, select a range of material that is appropriate to answer it.
Discourse analysis is a method that can be applied both to large volumes of material and to smaller samples, depending on the aims and timescale of your research.
Step 2: Gather information and theory on the context
Next, you must establish the social and historical context in which the material was produced and intended to be received. Gather factual details of when and where the content was created, who the author is, who published it, and whom it was disseminated to.
As well as understanding the real-life context of the discourse, you can also conduct a literature review on the topic and construct a theoretical framework to guide your analysis.
Step 3: Analyse the content for themes and patterns
This step involves closely examining various elements of the material – such as words, sentences, paragraphs, and overall structure – and relating them to attributes, themes, and patterns relevant to your research question.
Step 4: Review your results and draw conclusions
Once you have assigned particular attributes to elements of the material, reflect on your results to examine the function and meaning of the language used. Here, you will consider your analysis in relation to the broader context that you established earlier to draw conclusions that answer your research question.
Cite this Scribbr article
If you want to cite this source, you can copy and paste the citation or click the ‘Cite this Scribbr article’ button to automatically add the citation to our free Reference Generator.
Luo, A. (2022, December 05). Critical Discourse Analysis | Definition, Guide & Examples. Scribbr. Retrieved 25 March 2024, from https://www.scribbr.co.uk/research-methods/discourse-analysis-explained/
Is this article helpful?
Other students also liked
Case study | definition, examples & methods, how to do thematic analysis | guide & examples, content analysis | a step-by-step guide with examples.

An official website of the United States government
The .gov means it’s official. Federal government websites often end in .gov or .mil. Before sharing sensitive information, make sure you’re on a federal government site.
The site is secure. The https:// ensures that you are connecting to the official website and that any information you provide is encrypted and transmitted securely.
- Publications
- Account settings
Preview improvements coming to the PMC website in October 2024. Learn More or Try it out now .
- Advanced Search
- Journal List
- J Educ Health Promot
Discourse analysis: A useful methodology for health-care system researches
Ahmadreza yazdannik.
Nursing and Midwifery Care Research Center, Nursing and Midwifery Faculty, Isfahan University of Medical Sciences, Isfahan, Iran
Alireza Yousefy
1 Department of Medical Education, Medical Education Research Center, Isfahan University of Medical Sciences, Isfahan, Iran
Sepideh Mohammadi
2 Department of Nursing and Midwifery Ramsar, Babol University of Medical Sciences, Ramsar, I.R Iran
Discourse analysis (DA) is an interdisciplinary field of inquiry and becoming an increasingly popular research strategy for researchers in various disciplines which has been little employed by health-care researchers. The methodology involves a focus on the sociocultural and political context in which text and talk occur. DA adds a linguistic approach to an understanding of the relationship between language and ideology, exploring the way in which theories of reality and relations of power are encoded in such aspects as the syntax, style, and rhetorical devices used in texts. DA is a useful and productive qualitative methodology but has been underutilized within health-care system research. Without a clear understanding of discourse theory and DA it is difficult to comprehend important research findings and impossible to use DA as a research strategy. To redress this deficiency, in this article, represents an introduction to concepts of discourse and DA, DA history, Philosophical background, DA types and analysis strategy. Finally, we discuss how affect to the ideological dimension of such phenomena discourse in health-care system, health beliefs and intra-disciplinary relationship in health-care system.
Introduction
For at least then years now, “discourse” and “discourse analysis (DA)” has been the fashionable term. Usually, in scientific research and debates, it is used indiscriminately, without being defined. Without a clear understanding of discourse theory and DA, it is difficult to comprehend important research findings and impossible to use DA as a research strategy. Hence, this paper aims to help health-care practitioner employ DA as an effective research strategy.
Materials and Methods
This study was a narrative review. Electronic databases such as PubMed, Medline, ProQuest, and science direct were searched using the keywords discourse analysis, methodology, and health-care system. A manual search of various journals and books was also carried out. Not only all the searched articles and books were included, but also highly relevant articles from English literature were considered for the present review.
Discourse analysis description
There are many explanations and definitions of discourse and DA.[ 1 ] Discourse has been defined as “a group of ideas or patterned way of thinking which can be identified in textual and verbal communications, and can also be located in wider social structures.”[ 2 ] In other definition “discourse is a belief, practice or knowledge that constructs reality and provides a shared way of understanding the world.” In a broad sense, discourses are defined as systems of meaning that are related to the interactional and wider sociocultural context and operate regardless of the speakers’ intentions. DA is a broad and diverse field, including a variety of approaches to the study of language, which derive from different scientific disciplines and utilize various analytical. DA examines language in use.[ 3 ] As suggested, by Fairclough, “discourse is the use of language as a form of social practice and DA is an analysis of how texts work within the sociocultural practice.”[ 4 ] DA focuses on the ways that language and symbols shape interpretations of negotiators’ identities, instrumental activity, and relationships.[ 5 ]
Discourse analysis history background
DA is both an old and a new discipline. Historically, DA path a way from linguistic approaches to socialistic approaches. Its origins can be traced back to the study of language, public speech, and literature more than 2000 years ago. One major historical source is undoubtedly classical rhetoric, the art of good speaking. Whereas the grammatica, the historical antecedent of linguistics, was concerned with the normative rules of correct language use, its sister discipline of rhetorical dealt with the precepts for the planning, organization, specific operations, and performance of public speech in political and legal settings.[ 6 ] The term of DA first came into general use following the publication of a series of papers by ZelligHarris beginning in 1952 and reporting on work from which he developed transformational grammar in the late 1930s.[ 7 ] DA in this decade concerned with such microelements of discourse as the use of grammar, rhetorical devices, syntax, sound forms and the overt meaning and content matter of words and sentences of a text or talk, and such macro structures as topics and themes. After two decades, a new form of DA emerged in the middle decades of the 60s and 70s, following the development of knowledge in the social sciences and humanities. Formal sentence grammars had been challenged from several sides and were at least complemented with new ideas about language use, linguistic variation, speech acts, conversation, other dialogs, text structures, communicative events, and their cognitive and social contexts. Much formal rigor and theoretical sophistication had to be temporarily bracketed out to formulate completely new approaches.[ 6 ]
A new cross-discipline of DA began to develop in most of the humanities and social sciences concurrently with and related to, other disciplines, such as anthropology, semiology, psycholinguistic, sociolinguistics, and pragmatics. Many of these approaches, especially those influenced by the social sciences, favor a more dynamic study of oral talk-in-interaction. In this view, DA concerned with how an individual's experience is socially and historically constructed by language and DA assumes that language constructs how we think about and experience ourselves and our relationships with others.[ 6 ] In Europe, Michel Foucault and Jacques Derrida became the key theorists of the subject, especially of discourse. In this context, the term “discourse” no longer refers to formal linguistic aspects, but to institutionalized patterns of knowledge that become manifest in disciplinary structures and operate by the connection of knowledge, community and power. Since the 1970s, Foucault's works have had an increasing impact, especially on DA in the social sciences. Now DA as qualitative methods apply in various fields such as anthropology, ethnography, sociology, intellect, cognitive and social psychology, politic science, communication, and critical linguistics, and health-care system.
Philosophical background
Mainly DA philosophical base is a social constructionist approach.[ 8 ] Social constructionism is an umbrella term for a range of new theories about culture and society.[ 9 ] DA is just one among several social constructionist approaches, but it is one of the most widely used approaches within social constructionism.[ 10 ]
Discourse analytical approaches take as their starting point the claim of structuralist and poststructuralist linguistic philosophy, which our access to reality is always through language. With language, we create representations of reality that are never mere reflections of a preexisting reality but contribute to constructing reality. That does not mean that reality itself does not exist. Meanings and representations are real. Physical objects also exist, but they only gain meaning through discourse. Language, then, is not merely a channel through which information about underlying mental states and behavior or facts about the world are communicated. On the contrary, language is a “machine” that generates, and as a result constitutes the social world. This also extends to the constitution of social identities and social relations. It means that changes in discourse are a means by which the social world is changed.[ 9 ] In other words “individuals are not intentional agents of their own words, creatively and privately converting thoughts to sounds or inscriptions. Rather they gain their status as selves by taking a position within a preexisting form of language.”[ 11 ]
In terms of epistemology, many discourse theorists adopt a relativist view; they assume that there exist no objective grounds on which the truth of claims can be proven and propose that the value of knowledge should be evaluated according to other criteria, such as its applicability, usefulness and clarity.[ 12 ] Others, however, claim that relativism does not allow for a position from which social critique and action can be developed and adopt a critical realist position; they acknowledge that knowledge is always mediated by social processes but propose that underlying enduring structures do exist and that these can be known through their effects.[ 8 ]
Burr provided an outline of the general philosophical assumptions that underpin most discourse analytical approaches, drawing on the accounts of social constructionism.
They are as follows:
- A critical approach to taken-for-granted knowledge - Our knowledge of the world should not be treated as objective truth. Reality is only accessible to us through categories, so our knowledge and representations of the world are not reflections of the reality “out there,” but rather are products of our ways of categorizing the world, or, in discursive analytical terms, products of discourse[ 10 ]
- Historical and cultural specificity; We are fundamentally historical and cultural beings and our views of, and knowledge about, the world are the products of historically situated interchanges among people.[ 13 ] Consequently, the ways in which we understand and represent the world are historically and culturally specific and contingent: our worldviews and our identities could have been different, and they can change over time. This view match by this view that all knowledge is contingent is an anti-foundationalism and anti-essentialist[ 9 ]
- The link between knowledge and social processes – Our ways of understanding the world are created and maintained by social processes.[ 10 , 13 ] Knowledge is created through social interaction in which we construct common truths and compete about what is true and false.[ 9 ]
The link between knowledge and social action - Within a particular worldview, some forms of action become natural, others unthinkable. Different social understandings of the world lead to different social actions, and therefore, the social construction of knowledge and truth has social consequences.[ 10 , 13 ]
Discourse analysis approaches
DA is not only one approach but also a series of interdisciplinary approaches that have been applied in varying ways, from purely linguistic research into a conversation on a “micro” level to the broadly historic philosophical, and societal context.[ 2 ] DA Different perspectives offer their own suggestions and to some extent, compete to appropriate the terms “discourse” and “DA” for their own definitions.[ 9 ] One major difference between the various types of DA is in their methods of analysis.[ 14 ]
DA is composed of two main dimensions, textual, and contextual. Textual dimensions are those which account for the structure of discourses, while contextual dimensions relate these structural descriptions to various properties of the social, political, or cultural context in which they take place.[ 6 ] The DA that is rooted in linguistics and in textual form is concerned with such microelements of discourse as the use of grammar, rhetorical devices, syntax, sound forms and the overt meaning and content matter of words and sentences of a text or talk, and such macro-structures as topics and themes. The contextual form examines the production and reception processes of discourse, with particular attention to the reproduction of ideology and hegemony in such processes, and the links between discourse structures and social interaction and situations.[ 2 , 6 ]
Some DA mixes one or more of these approaches; for example, one kind of critical DA (CDA) combines linguistic analysis and ideological critique.[ 4 ]
There are various categorizations of discourse analytical research.[ 15 ] Phillips describes four main styles of discourse analytical research [ Figure 1 ]. The styles are categorized along two axes: (1) between text and context, and (2) between constructivist and critical approaches.[ 16 ]

Four perspectives of discourse analysis
The first axis is about the degree to which research focuses on individual texts or on the surrounding texts.[ 15 ] Phillips distinguishes between a proximal and a distal context. The proximal context is the local context, for example, a discipline or science. The distal context is a broader social context, for example ecological, regional, or cultural settings.[ 16 ]
The second axis describes the degree to which the research focuses on ideology and power, as opposed to processes of social construction. The axes are seen as continua, not as dichotomies. Thus, combinations of elements of both axes are possible and usual.[ 16 ]
Jansen in his article summarized the four perspectives of DA that described by Phillips; they are as follows.
Social linguistic analysis
A social linguistic analysis is constructivist and focuses on individual texts. It gives insight into the organization and construction of these texts and how they work to construct and organize other phenomena. The focus is not on the exploration of the power dynamics in which the texts are implicated.
Interpretive structuralism
Similar to social linguistic analysis, these discourse analyses are interested in the way in which broader discursive contexts come into being. They are not directly concerned with power. Individual texts are more important as background material.
Critical linguistic analysis
Critical linguistic analysis shares with social linguistic analysis its focus on individual texts, but its main concern is with the dynamics of power that surround the text. The examination of individual texts is for understanding how the structures of domination of the proximal context are implicated in the text.
Critical discourse analysis
The main interest of CDA is in the discursive activity to construct and maintain unequal power relations. The distal context is of interest, that is, the ecological, cultural, or regional setting that surrounds individual texts.[ 15 , 16 ] The CDA process derived from the work of Fairclough and is a study of language as a social and cultural practice. It is based on the premise that texts have a constructive effect in shaping how we experience ourselves and others and how we act in relation to this, example, the ability to prescribe medication.[ 4 ]
In addition to the above DA types, there are other classifications for DA theoretical approaches.
Discursive psychology
Discursive psychology is part of the general movement of critical psychology, which has been reacting against mainstream social psychology, especially the sort of experimental psychology.[ 17 ] The aim of discursive psychologists is not so much to analyze the changes in society's “large-scale discourses,” which concrete language use can bring about, as to investigate how people use the available discourses flexibly in creating and negotiating representations of the world and identities in talk-in-interaction and to analyze the social consequences of this. Despite the choice of label for this approach “discursive psychology” its main focus is not internal psychological conditions. Discursive psychology is an approach to social psychology that has developed a type of DA to explore the ways in which people's selves, thoughts, and emotions are formed and transformed through social interaction and to cast light on the role of these processes in social and cultural reproduction and change.[ 9 ]
Historical discourse analysis
Historical DA is a poststructuralist approach to reading and writing history; a mode of conceptualizing history through a theorized lens of critique. Historical DA works against the objectivist fallacy of traditional positivist historical methods in decentering the authority of the historian as a neutral recorder of facts and the claim of historical writings as objective reconstructions of past events. In line with its intent to disrupt taken for granted ways of conceptualizing history, the task of historical DA is not to find truths about past events or to identify the origins or causes of past events, but to expose history as a genre contingent, ambiguous, and interpretive. Historical DA is, therefore, less a set methodology than a set of postmethodological methodologies.[ 18 ]
Foucaultian discourse analysis
Today the theoretical work of Michel Foucault is widely considered as being part of the theoretical body of social sciences such as sociology, social history, political sciences, and social psychology.[ 19 ] Discourse, as defined by Foucault, refers to: ways of constituting knowledge, together with the social practices, forms of subjectivity, and power relations which inhere in such knowledge's and relations between them. Discourses are more than ways of thinking and producing meaning. They constitute the “nature” of the body, unconscious and conscious mind and emotional life of the subjects they seek to govern.[ 20 ] Foucault's focus is on questions of how some discourses have shaped and created meaning systems that have gained the status and currency of “truth,” and dominate how we define and organize both ourselves and our social world while other alternative discourses are marginalized and subjugated, yet potentially “offer” sites where hegemonic practices can be contested, challenged, and “resisted”. In Foucault's view, social context in which certain knowledge's and practices emerged as permissible and desirable or changed. In his view knowledge is inextricably connected to power. Power has an important role in Foucault's view, and power is a process that operates in continuous struggles and confrontations that change, strengthen, or reverse the polarity of the force relations between power and resistance. This means that power is described as a relational process that is embodied in context-specific situations and is partially identifiable through its ideological effects on the lives of people. Power is productive of truth, rights, and the conceptualization of individuals, through the processes, or discursive practices of the human sciences and other major discourses such as social sciences, bureaucracy, medicine, law, and education.[ 21 ] Discourse analysts in this way need to be aware of the conceptualizations of power and resistance to be able to recognize them within a discourse. Emancipatory of the marginalized group is an important goal of recognizing power in Foucault's approach.
Analytical strategies
The concrete representation of discourses is texts or discursive “units.” They make have a variety of forms: formal written records, such as news information, company statements and reports, academic papers; spoken words, pictures, symbols, artifacts, transcripts of social interactions such as conversations, focus group discussions, and individual interviews; or involve media such as TV programs, advertisements, magazines, novels, etc. In fact, texts are depositories of discourses, they “store” complex social meanings produced in a particular historical situation that involved individual producer of a text unit, and social surrounds that is appealed to the play.[ 1 ] If we are to understand discourse, we should also understand the context, in which they arise.[ 6 ] Researchers usually distinguish two types of context: broad and local. There is also a more detailed classification of the degree of a context, involved in a study: micro-discourse (specific study of language), meso-discourse (still study of a language but with a broader perspective), grand discourse (study of a system of discourses that are integrated in a particular theme such as culture), and mega discourse (referring to a certain phenomenon like globalization).[ 22 ]
DA is a process rather than a step-by-step research method and can be employed within different epistemological paradigms.[ 23 ] Crowe described the most important questions in data analysis; how it is structured as particular type of text; what politeness strategies are used; how subject positions are constructed; the types and functions of the language used and the identification of keywords; the thematic structure; how social relations are constructed; and how reality is represented.
The content of discourses can be investigated using many different tools. In a specific analysis, it may be a problem where to begin and which tools to select. In this section, we will present four strategies expressed by Jørgensen and Phillips which can be used across all the approaches to provide an overall understanding of the material and identify analytical focus points for further investigation.
The simplest way of building an impression of the nature of a text is to compare it with other texts. The strategy of comparison is based theoretically on the structuralist point that a statement always gains its meaning through being different from something else which has been said or could have been said. In applying this strategy, the researcher asks the following questions: In what ways is the text under study different from other texts and what are the consequences? Which understanding of the world is taken for granted and which understandings are not recognized?
Substitution
Substitution is a form of comparison in which the analyst herself creates the text for comparison. Substitution involves substituting a word with a different word, resulting in two versions of the text which can be compared with one another; in this way, the meaning of the original word can be pinned down. Through such comparisons, a picture can gradually be formed of how the text establishes her identity in relation to the world around her including the decisions she constructs as within her control and the ones that she constructs as out with her control. In common with the strategy of comparison, substitution draws on the structuralist point that words acquire their meaning by being different from other words. In the case of a long text, a single word can be substituted throughout the text to see how it changes the meaning of the text as a whole. However, textual aspects other than single words can also be subject to substitution.
Exaggeration of detail
The exaggeration of detail involves blowing up a particular textual detail out of proportion. The analyst may have identified a textual feature which appears odd or significant, but, as it is just one isolated feature, does not know what its significance is or how it relates to the text as a whole. To explore the significance of the feature, one can overexaggerate it, and then ask what conditions would be necessary in order for the feature to make sense and into what overall interpretation of the text the feature would fit.
Multivocality
The strategy of multivocality consists of the delineation of different voices or discursive logics in the text. The strategy is based on the discourse analytical premise concerning intertextuality– that is, the premise that all utterances inevitably draw on, incorporate or challenge earlier utterances. The aim of the strategy is to use the multivocality to generate new questions to pose to the text: what characterizes the different voices of the text? When does each voice speak? What meanings do the different voices contribute to producing?
Validation and rigor
DA is a highly interpretative process that acknowledges that multiple interpretations can emerge from the data.[ 4 ] DA is an interpretative process that can result in different researchers examining the same data yet arriving at different findings. The reliability and validity of findings, therefore, rely on the strength and logic of the researcher's argument in reports and presentations pertaining to study findings.[ 6 ] Crowe offers several key questions to consider when establishing rigor in DA studies:
Methodological rigor
- Does the research question “fit” the DA
- Do the texts under analysis “fit” the research question
- Have sufficient resources, including historical, political, and clinical resources, been sampled
- Has the interpretative paradigm been described clearly
- Are the data-gathering and analysis congruent with the interpretative paradigm
- Is there a detailed description of the data gathering and analytic processes
- Is the description of the methods detailed enough to enable readers to follow and understand context?
Interpretative rigor
- Have the linkages between the discourse and findings been adequately described
- Is there inclusion of verbatim text to support the findings
- Are the linkages between the discourse and the interpretation plausible
- Have these linkages been described and supported adequately
- How are these findings related to existing knowledge in the subject?
DA application in health-care system
The nature of the knowledge fundamental to health care and the power it wields during its practice, is of continuing interest to philosophers, social scientists and anthropologists, as well as to those individuals who directly use it in administering health care, namely, doctors, nurses, and allied health professionals. The development of sociopolitical critique has centered on the nature of the foundations of knowledge and how this influences our present understanding of the human condition. With the advent of the modern world, there have been continuing controversies about the essential characteristics of rationality.[ 21 ]
In Foucault's view, social context in which certain knowledge's and practices emerged as permissible and desirable or changed. In his view knowledge is inextricably connected to power. Power has an important role in Foucault's view, and power is a process that operates in continuous struggles and confrontations that change, strengthen, or reverse the polarity of the force relations between power and resistance. This means that power is described as a relational process that is embodied in context-specific situations and is partially identifiable through its ideological effects on the lives of people. Power is productive of truth, rights, and the conceptualization of individuals, through the processes, or discursive practices of the human sciences and other major discourses such as social sciences, bureaucracy, medicine, law, and education.[ 22 , 24 ]
DA has the potential to reveal valuable insights into the social and political contexts in which varied discourses about health take place. Areas of research which are relevant to healthcare concerns include the discourses of: the interpersonal communication processes between doctors or nurse and patients, interprofessional conversation, in-depth interviews about lay health beliefs, conversations between lay people about health risks and issues, government-sponsored health promotion messages, health information in the mass entertainment and news media, service protocols, information/education pamphlets for patients; texts describing particular understandings of health and illness or clinical approaches to treatment medical and health-care journals and official texts, textbooks in health-care specialties, health care's system communication about such disease, paternalistic manners in health-care system.
Human is one of the most important concepts in health-care system. Crowe believes that “Individuals can be considered as particular individuals, The meaning and value preexists the identification of these characteristics in an individual, and thus language does not reflect an external reality but expresses cultural conventions.”[ 20 ] Hence, we can say emancipatory of the oppressed group, marginalized patient (cause of race, ethnicity or disease types, such as HIV patient) and giving the voice is the one of the most important uses of DA in health care system.
In this part of article to learn more about the DA application in health care system, we expressed summary an article in this area.
DA as a qualitative approach has an important role in health-care system because health-care system needs to be knowledgeable across the multiple paradigms and perspectives that inform an understanding of the biological, psychological, social, cultural, ethical, and political dimensions of human lives.”[ 25 ] Practice in this area is a political, cultural, and social practice and needs to be understood as such to improve the quality of care provided. Effective clinical reasoning relies on employing several different kinds of knowledge and research[ 26 ] that draw on different perspectives, methodologies, and techniques to generate the breadth of knowledge and depth of understanding of clinical practices and patients’ experiences of those practices. DA can make a contribution to the development of this knowledge.
Financial support and sponsorship
This study was financially supported by Isfahan University of Medical Sciences.
Conflicts of interest
There are no conflicts of interest.
- - Google Chrome
Intended for healthcare professionals
- Access provided by Google Indexer
- My email alerts
- BMA member login
- Username * Password * Forgot your log in details? Need to activate BMA Member Log In Log in via OpenAthens Log in via your institution

Search form
- Advanced search
- Search responses
- Search blogs
Discourse analysis
- Related content
- Peer review
- Brian David Hodges , associate professor, vice chair (education), and director 1 ,
- Ayelet Kuper , assistant professor 2 ,
- Scott Reeves , associate professor 3
- 1 Department of Psychiatry, Wilson Centre for Research in Education, University of Toronto, 200 Elizabeth Street, Eaton South 1-565, Toronto, ON, Canada M5G 2C4
- 2 Department of Medicine, Sunnybrook Health Sciences Centre, and Wilson Centre for Research in Education, University of Toronto, 2075 Bayview Avenue, Room HG 08, Toronto, ON, Canada M4N 3M5
- 3 Department of Psychiatry, Li Ka Shing Knowledge Institute, Centre for Faculty Development, and Wilson Centre for Research in Education, University of Toronto, 200 Elizabeth Street, Eaton South 1-565, Toronto, ON, Canada M5G 2C4
- Correspondence to: B D Hodges brian.hodges{at}utoronto.ca
This articles explores how discourse analysis is useful for a wide range of research questions in health care and the health professions
Previous articles in this series discussed several methodological approaches used by qualitative researchers in the health professions. This article focuses on discourse analysis. It provides background information for those who will encounter this approach in their reading, rather than instructions for conducting such research.
What is discourse analysis?
Discourse analysis is about studying and analysing the uses of language. Because the term is used in many different ways, we have simplified approaches to discourse analysis into three clusters (table 1 ⇓ ) and illustrated how each of these approaches might be used to study a single domain: doctor-patient communication about diabetes management (table 2 ⇓ ). Regardless of approach, a vast array of data sources is available to the discourse analyst, including transcripts from interviews, focus groups, samples of conversations, published literature, media, and web based materials.
Three approaches to discourse analysis
- View inline
Three approaches to a specific research question: example of doctor-patient communications about diabetes management
What is formal linguistic discourse analysis?
The first approach, formal linguistic discourse analysis, involves a structured analysis of text in order to find general underlying rules of linguistic or communicative function behind the text. 4 For example, Lacson and colleagues compared human-human and machine-human dialogues in order to study the possibility of using computers to compress human conversations about patients in a dialysis unit into a form that physicians could use to make clinical decisions. 5 They transcribed phone conversations between nurses and 25 adult dialysis patients over a three month period and coded all 17 385 words by semantic type (categories of meaning) and structure (for example, sentence length, word position). They presented their work as a “first step towards an automatic analysis of spoken medical dialogue” that would allow physicians to “answer questions related to patient care by looking at [computer generated] summaries alone.” 5
What is empirical discourse analysis?
Researchers using empirical discourse analysis 4 do not use highly structured methods to code individual words and utterances in detail. Rather, they look for broad themes and functions of language in action using approaches called conversation analysis (the study of “talk-in-interaction”) 6 and genre analysis (the study of recurrent patterns, or genres of language that share similar structure and context—such as the case report, the scientific article). 7
Conversation analysis and genre analysis give more prominence to sociological uses of language than to grammatical or linguistic structures of words and sentences and are used to study human conversations or other forms of communication in order to elucidate the ways in which meaning and action are created by individuals producing the language. 4 Lingard and colleagues, for example, studied communication between nurses and surgeons during 128 hours of observing 35 different procedures in the operating room and categorised recurrent patterns of communication. They then used their findings to draw links between interpersonal tensions, the use of language, and the occurrence of errors in the operating room. 8 Genre analysis is presented in detail in box 1.
Box 1 An empirical discourse analysis (genre analysis) of case presentations by medical students*
This study took place at a tertiary care teaching hospital in Canada. It was conducted in the context of a medical student rotation in paediatrics. The aim of the study was to gain understanding of how the formal linguistic structure of the case presentation is used in academic medical settings.
The researchers conducted 21 in-depth interviews with medical students and faculty members. Pairs of researchers also observed 16 oral case presentations as well as the teaching exchanges that surrounded them. All of these encounters were tape recorded and transcribed (for a total of 555 pages of text); the transcriptions were iteratively analysed. The analysis was structured to allow themes to emerge from the data (that is, as indicated by multiple examples of such themes throughout the data). However, it particularly focused on themes that helped to illuminate the rules around certain modes of case presentation and on the role of these rules in teaching and learning.
The study showed a pronounced tension between the educational (“schooling”) uses and clinical (“workplace”) functions of case presentations. For example, students saw the case presentation as a school mode and emphasised that they wanted to get through their presentations without being asked any questions. Faculty, on the other hand, understood the case presentation as a way for professionals to jointly create shared knowledge. Their cross-purposes affected the effectiveness of faculty feedback to the students about their case presentations.
*Description based on study by Lingard et al 9
What is critical discourse analysis?
Researchers in cultural studies, sociology, and philosophy use the term critical discourse analysis to encompass an even wider sphere that includes all of the social practices, individuals, and institutions that make it possible or legitimate to understand phenomena in a particular way, and to make certain statements about what is “true.” Critical discourse analysis is particularly concerned with power and is rooted in “constructivism.” Thus the discourse analyses of Michel Foucault, for example, illustrated how particular discourses “systematically construct versions of the social world.” 4 Discourse analysis at this level involves not only the examination of text and the social uses of language but also the study of the ways in which the very existence of specific institutions and of roles for individuals to play are made possible by ways of thinking and speaking.
Foucault’s study of madness, for example, uncovered three distinct discourses that have constructed what madness is in different historical periods and in different places: madness as spiritual possession, madness as social deviancy, and madness as mental illness. 10 In a similarly oriented study, Speed showed how different discourses about mental health service in use today construct individuals’ identities as “patients,” “consumers,” or “survivors” and are made possible by specific institutional practices and ways for individuals to “be.” 11
In a different context, Stone contrasted the specific discourses used in the education literature for diabetes patients (“patient self care” and “autonomy”) with the medical literature’s use of doctor centred discourses (“compliance” and “adherence”). Stone related the resulting tension (and the important implications for patients’ behaviours) to the ways in which the roles that physicians and patients play are historically determined by different and conflicting models of what disease and healing are. 12
Finally, Shaw and colleagues used a discourse analysis to illustrate the many ways in which research itself can be defined (for example, by a lay person, a medical editor, the World Medical Association, a hospital, the taxman) and how these various definitions are linked to the power and objectives of particular institutions. 13
In these examples of critical discourse analysis, the language and practices of healthcare professionals and institutions are examined with the aim of understanding how these practices shape and limit the ways that individuals and institutions can think, speak, and conduct themselves. Table 2 ⇑ illustrates how a critical discourse approach to diabetes education would compare with discourse analyses using other linguistic and empirical approaches to research.
Although our categorisation (tables 1 ⇑ and 2 ⇑ ) emphasises the distinctions between these approaches to discourse analysis, in practice researchers often use more than one of the approaches together in a study. For example, genre analysts may invoke critical theorists in order to study the origins of the sanctioned methods of communication, asking, for example, “What historical and contextual factors led to the adoption of the scientific journal article as a legitimate form of expression of medical ‘truth’ rather than the adoption of another format?”
What should we be looking for in a discourse analysis?
Given the wide variety of approaches to discourse analysis, the elements that constitute a high quality study vary. Rogers has argued that some discourse analysis research suffers from scanty explanation of the analytical method used. 14 Thus one should expect clear documentation of the sources of information used and delimitation of data sources 3 (including a description of decisions made with regard to selection of groups or individuals for interviews, focus groups, or observation) and, importantly, a description of the context of the study. The method of analysis should be clearly explained, including assumptions made and methods used to code and synthesise data. Finally, given that the goal of critical discourse analysis is to illuminate and critique structures of power, it is especially important that researchers describe the ways in which their own individual sociocultural roles may influence their perspectives.
Discourse analysis is an effective method to approach a wide range of research questions in health care and the health professions. What underpins all variants of discourse analysis is the idea of examining segments, or frames of communication, and using this to understand meaning at a “meta” level, rather than simply at the level of actual semantic meaning. In this way, all of the various methods of discourse analysis provide rigorous and powerful approaches to understanding complex phenomena, ranging from the nature of on-the-ground human communication to the inner workings of systems of power that construct what is “true” about health and health care. While these methods are gaining popularity, much remains to be done to develop a widespread appreciation for the use, funding, and publication of discourse analyses. As a start, we hope this article will help readers who encounter these approaches to understand the basic premises of discourse analysis. Box 2 offers further reading for those interested in learning more or undertaking discourse analytical research.
Box 2 Further reading
Fairclough N. Language and power . London: Longman, 1989.
Foucault. The archaeology of knowledge and the discourse on language . New York: Random House, 1972.
Jaworski A, Coupland N, eds. The discourse reader . London: Routledge, 1999.
Kendall G, Wickham G. Using Foucault’s method . London: Sage, 2003.
Mills S. Discourse . London: Routledge, 2004.
Journal articles
Barnes R. Conversation analysis: a practical resource in the health care setting. Med Educ 2005;39:113-5.
Ford-Sumner S. Genre analysis: a means of learning more about the language of health care. Nurse Researcher 2006;14(1):7-17.
Roberts C, Sarangi S. Theme-oriented discourse analysis of medical encounters. Med Educ 2005;39:632-40.
Summary points
Discourse analysis is an effective method for approaching a wide range of research questions in health care and the health professions
Discourse analysis is about studying and analysing the uses of language
A vast array of data sources is available to the discourse analyst
The various methods of discourse analysis provide rigorous and powerful approaches to understanding complex phenomena, ranging from the nature of on-the-ground human communication to the inner workings of systems of power that construct what is “true” about health and health care
Cite this as: BMJ 2008;337:a879
- Related to doi: , 10.1136/bmj.a288
- doi: , 10.1136/bmj.39602.690162.47
- doi: , 10.1136/bmj.a1020
- doi: , 10.1136/bmj.a949
- doi: 10.1136/bmj.a1035
This is the fourth in a series of six articles that aim to help readers to critically appraise the increasing number of qualitative research articles in clinical journals. The series editors are Ayelet Kuper and Scott Reeves.
For a definition of general terms relating to qualitative research, see the first article in this series.
Contributors: All authors contributed to the conception and drafting of the article and its revisions, and all approved the final version.
Competing interests: None declared.
Provenance and peer review: Commissioned; externally peer reviewed.
- Harris ZS. Methods in structural linguistics . Chicago: University Press, 1951 .
- Sacks H. Lectures on conversation . Jefferson G, ed. Cambridge, MA: Blackwell, 1995 .
- ↵ Foucault M. The archaeology of knowledge and the discourse on language . New York: Random House, 1972 .
- ↵ McHoul A, Grace W. A Foucault primer: discourse, power and the subject . New York: New York University Press, 1993 .
- ↵ Lacson RC, Barzilay R, Long WJ. Automatic analysis of medical dialogue in the home hemodialysis domain: structure induction and summarization. J Biomed Informatics 2006 ; 39 : 541 -55. OpenUrl CrossRef PubMed Web of Science
- ↵ Ten Have P. Medical ethnomethodology: an overview. Human Studies 1995 ; 18 : 245 -261. OpenUrl CrossRef Web of Science
- ↵ Ford-Sumner S. Genre analysis: a means of learning more about the language of health care. Nurse Researcher 2006 ; 14 (1): 7 -17. OpenUrl PubMed
- ↵ Lingard L, Espin S, Whyte S, Regehr G, Baker GR, Reznick R, et al. Communication failures in the operating room: an observational classification of recurrent types and effects. Qual Saf Health Care 2004 ; 13 : 330 -4. OpenUrl Abstract / FREE Full Text
- ↵ Lingard L, Schryer C, Garwood K, Spafford M. “Talking the talk”: school and workplace genre tensions in clerkship case presentations. Med Educ 2003 ; 37 : 612 -20. OpenUrl CrossRef PubMed Web of Science
- ↵ Foucault M. Madness and civilization; a history of insanity in the age of reason [Howard R, translation]. New York: Vintage Books, 1988. (Original work published in 1961 .)
- ↵ Speed E. Patients, consumers and survivors: a case study of mental health service user discourses. Soc Sci Med 2006 ; 62 (1): 28 -38. OpenUrl CrossRef PubMed Web of Science
- ↵ Stone MS. In search of patient agency in the rhetoric of diabetes care. Technical Communication Quarterly 1997 :6:201-17.
- ↵ Shaw S, Boynton PM, Greenhalgh T. Research governance: where did it come from, what does it mean? J R Soc Med 2005 ; 98 : 496 -502. OpenUrl Abstract / FREE Full Text
- ↵ Rogers R, Malancharuvil-Berkes R, Mosley M, Hui D, O’Garro JG. Critical discourse analysis in education: a review of the literature. Rev Educ Res 2005 ; 75 : 365 -416. OpenUrl CrossRef
No internet connection.
All search filters on the page have been cleared., your search has been saved..
- All content
- Dictionaries
- Encyclopedias
- Expert Insights
- Foundations
- How-to Guides
- Journal Articles
- Little Blue Books
- Little Green Books
- Project Planner
- Tools Directory
- Sign in to my profile My Profile
- Sign in Signed in
- My profile My Profile
- FOUNDATION ENTRY Critical Discourse Analysis
- FOUNDATION ENTRY Linguistic Relativity
- FOUNDATION ENTRY Transcription
- FOUNDATION ENTRY Cazden, Courtney
- FOUNDATION ENTRY Conversation Analysis
- FOUNDATION ENTRY Prosopography
- FOUNDATION ENTRY Sensitizing Concepts
- FOUNDATION ENTRY Indexicality
- FOUNDATION ENTRY Translation in Qualitative Methods
- FOUNDATION ENTRY Belonging
- FOUNDATION ENTRY Whorf, Benjamin Lee
- FOUNDATION ENTRY Humour and Jokes
- FOUNDATION ENTRY Labov, William
- FOUNDATION ENTRY Hymes, Dell Hathaway
- FOUNDATION ENTRY Ethnostatistics
- FOUNDATION ENTRY Smith, Dorothy
- FOUNDATION ENTRY Garfinkel, Harold
- FOUNDATION ENTRY Unique Adequacy
- FOUNDATION ENTRY Cicourel, Aaron
- FOUNDATION ENTRY Harvey, David
- FOUNDATION ENTRY Membership Categorisation Analysis
- FOUNDATION ENTRY Ethnomethodological Analysis
- FOUNDATION ENTRY Douglas, Jack D.
- FOUNDATION ENTRY Jefferson, Gail
- FOUNDATION ENTRY Goodwin, Charles, and Marjorie Harness Goodwin
- FOUNDATION ENTRY Phenomenology
- FOUNDATION ENTRY Discourse Research
- FOUNDATION ENTRY Ethnoscience
Discover method in the Methods Map
Critical discourse analysis.
- By: Michael Farrelly | Edited by: Paul Atkinson, Sara Delamont, Alexandru Cernat, Joseph W. Sakshaug & Richard A.Williams
- Publisher: SAGE Publications Ltd
- Publication year: 2019
- Online pub date: September 17, 2019
- Discipline: Anthropology , Business and Management , Communication and Media Studies , Computer Science , Counseling and Psychotherapy , Criminology and Criminal Justice , Economics , Education , Engineering , Geography , Health , History , Marketing , Mathematics , Medicine , Nursing , Political Science and International Relations , Psychology , Social Policy and Public Policy , Science , Social Work , Sociology , Technology
- Methods: Critical discourse analysis
- Length: 10k+ Words
- DOI: https:// doi. org/10.4135/9781526421036815631
- Online ISBN: 9781529746983 More information Less information
- What's Next
Critical discourse analysis (CDA) is a social scientific theory and method for analyzing and critiquing the use of language and its contribution to forming and sustaining social practice and for analysis of how language can contribute to reproducing or transforming social problems. CDA adopts the position that the analysis of how language is used can be a way in to, or complementary to, an interdisciplinary critique of events or social practices. The use of language may be an indicator of a more extensive problem, or it might be implicated in reproducing a problematic social practice. This entry examines some of the core concepts which inform the methods of CDA and describe analytical methods associated with each concept. It begins with an overview of the concepts which underpin the critical and analytical methods of CDA: critical analysis, social practices, social and historical context, dialectical relations, power and ideology, and the conceptual distinction of text, discourse, and language. It goes on to describe the major analytical concepts of CDA with subsections on text, discourse, and orders of discourse. The final section discusses further implications of the CDA method for engaging in full research projects: data collection, tools for handling data analysis, interpretation, and interdisciplinary working.
Introduction
Critical discourse analysis (CDA) is a social scientific theory and method for analyzing and critiquing the use of language and its contribution to forming and sustaining social practices. Of prime concern to CDA is the way that the use of language can contribute to reproducing or transforming social problems. CDA has roots in linguistics and language studies, but it recognizes that social practices are only partly constituted by discourse. There are nondiscursive elements of social practices, and the character of the relation between the discursive and nondiscursive elements of a social practice is an important question for CDA research. CDA, therefore, adopts the position that the analysis of how language is used can be an entry point, or complement, to an interdisciplinary critique of events or social practices. The concept of discourse, or the way in which language is used, is an aid to understanding the tendencies by which societies organize and understand themselves and how parts of society become organized by forms of language use.
CDA situates itself in the broader tradition of critical social science. It takes the position that contemporary social structures and practices are not inevitable nor are they always necessary. Where social structures and practices lead to social problems, social science has a place in analyzing the causes and seeking solutions to those problems, including ways of transforming them for the better. The use of language may be an indicator of a more extensive problem or be implicated in reproducing a problematic social practice. As such, CDA is not confined to descriptions of language use but seeks to use the analysis of language, as it is used in society, as a route towards addressing social problems.
Theoretical Background
A prime concern for CDA is the contribution of language use to the reproduction or transformation of social problems. The “critical” orientation of this form of discourse analysis often pushes research to problematize discourses and ideologies that are in the service of powerful groups of people who benefit from the social problem at stake. CDA recognizes discourses and ideologies as having a great effect in perpetuating social problems and constraining human flourishing. An orientation towards critical analysis does not imply that CDA can provide complete solutions to social problems. Rather, CDA is one social scientific route towards identifying and clarifying the causes of, and context for, social problems. As Lilie Chouliaraki and Norman Fairclough (1999) have put it, CDA seeks to clarify “obstacles to change and possibilities for change” (p. 35). Ideally, it puts these insights in the service of those beyond the academy who can push for social change. CDA, then, is an orientation to research which seeks to identify discursive aspects of social practices which contribute to the creation or perpetuation of social problems.
The concept of “social practices” is essential to CDA. Social practices are the “habitualised ways, tied to particular times and places, in which people apply resources (material or symbolic) to act together in the world” (Chouliaraki & Fairclough, 1999, p. 21; see also Gee, 2014, p. 86). They include types of activity, materials and objects, times and places, social relations and roles, types of semantic (or meaning-making) systems, including the use of language. Social practices are important for CDA because they enable researchers to conceptualize the conditions of possibility for social problems: A persistent social problem may have origins in the habits and conventions of a social practice. This conceptualization gives rise to questions over the extent to which features of an instance of language use are unique and which are indicative or typical of the habits and conventions of the broader social practice. A CDA analysis may seek to establish how language habits relate to the nondiscursive elements of a social practice and how those language habits reveal or contribute to a social problem.
The social and historical context in which texts are produced and discourses are formed is important for CDA. The discourse historic approach to CDA, associated with Ruth Wodak, puts emphasis on context. It seeks to account for the influence of social and historical context on the use of language but also sees that influence as being essential for our interpretation of texts and discourses. Understanding these contexts is likely to enable a richer interpretation. The aim is to identify salient aspects of social and historical context and to show how they affect one’s interpretation of a text. This approach has been used in Wodak’s own work (2015) to highlight “dog whistle” rhetoric in which certain words or phrases may seem innocuous but which, given their history, carry a clear message to audiences who are in the know. This approach, as with all approaches to CDA, invites interdisciplinary research in which textual and discourse analysis is brought together with other social science approaches to social and historical analysis.
For CDA, the nature of the relationship between discourse and other aspects of social practices is an important one. Fairclough (2003) argues that discourse is in a dialectical relationship with other elements of social practices: It is a two-way relationship in which discourse influences a social practice and in which those other elements of a social practice also influence discourse. On the one hand, discursive elements of a social practice can contribute to the reproduction or transformation of a social practice; on the other, elements of a social practice can reproduce or transform the discursive elements. This lays down an interpretive and methodological challenge for critical discourse analysts. A text may reproduce effects from the wider social practice, or it may be an attempt, or precursor, to change in the social practice, or it may be a combination of the two. A critical discourse analytical approach may seek to analyze, interpret, and critique the relationship between discourse and other elements of a social practice; the challenge is, to some extent, determining which of these applies to their own case.
Ideology can be an important reference point or focus of critique in CDA. There has been disagreement over the nature of ideology amongst scholars of CDA. On the one hand are scholars who see ideology, in a neutral way, as referring to a system of thought (e.g., van Dijk, 1998). Ideology, in this view, is neither good nor bad, it is simply a way of conceptualizing a system of thought. On the other hand, there are scholars who use “ideology” to refer to those systems of thought which help to exert social control. In this sense, ideologies are “constructions of practices from particular perspectives (and in that sense “one-sided”) which “iron out” the contradictions, dilemmas, and antagonisms of practices in ways which accord with the interests and projects of domination” (Chouliaraki & Fairclough, 1999, p. 26). Fairclough (1989) argued that:
There are in gross terms two ways in which those who have power can exercise and keep it: through coercing others to go along with them, with the ultimate sanction of physical violence or death; or through winning others’ consent to, or at least acquiescence in, their possession and exercise of power … . Ideology is the key mechanism of rule by consent, and because it is the favoured vehicle of ideology, discourse is of considerable social significance in this connection. (pp. 33, 34)
In each case, ideologies are expressed, shaped, and transmitted through discourse. The analysis of discourse, therefore, can show the details of ideologies and can be a way of critiquing them.
As a theory of discourse, CDA distinguishes three general aspects of language: language, discourse, and text. Each refers to language in some sense, but each has distinguishing characteristics that are important methodologically. “Language” is the meaning potential of the semiotic system of speaking and writing. A “text” is the language that is produced during social events, events which are finite and unique. A text, spoken or written, is produced in a unique context. However, language in use has a tendency towards repetition and elements of language appear and reappear in texts that are produced at different times and in different contexts. In CDA, the language patterns and conventions associated with a social practice is “discourse.” The distinction between text and discourse gives CDA a way of conceptualizing and interpreting language in use. It gives rise to analytical questions over the extent to which the properties and features of a text are unique to the event in which the text has been produced and the extent to which they are the product of the habits and conventions of a social practice. The theoretical distinction between text and discourse—not unique to CDA, but crucial to it nonetheless—is, therefore, an important basis for the analytical and interpretive method of CDA.
Analytical Concepts
A prime theoretical distinction for CDA is the separation of text and discourse: A text is the language produced as part of a social event. Texts can take many forms—spoken or written, short or long. A text can be mainly composed of language, or it can be multimodal (include image, colour, sound, or movement). A defining factor is that it is bounded in some way—either temporally or spatially—to make it distinct from other texts. This distinction is not an absolute one—texts can be in dialogue with one another, responding and replying to other texts, and conversations can take place over days through short message services (SMS), for example, but even here one can distinguish boundaries and identify individual SMS messages as “texts” which may or may not combine to make an identifiable conversation or “chat.” Drawing on the body of CDA literature, one can see texts as embodying numerous processes, CDA has been largely concerned with three processes: the presentation of identities, the representation of aspects of the world which are extraneous to those identities, and the organization of the elements of the text, both in form and in the meaningful ordering of those elements.
Typically, texts are analyzed in a “qualitative” way. Analytical focus on texts also has the advantage of being open to seeing contradictions or new hybrid discursive formations within texts—both of which can be important for a critical engagement with social issues and social change. The advantage is that one can see how something is represented and not just that it is present in a text. Since the analyst cannot know in advance which linguistic features will have a potentially problematic effect, CDA studies have been eclectic and diverse in the textual features it focuses on when analyzing a text. As demonstrated in the following sections, analysis of texts in CDA tends to approach texts as modes of identification, representation, or action and interaction.
Texts as Identification
Texts convey something about the people involved in their production. CDA refers to a process of “identification” that runs through a text—process by which the producer(s) of a text perform or emphasize some aspects of identity and not others. The producer of a text may range from a single author to multiple producers—from the people who physically write or speak the words, to the people who control the tone and content of a text, and to those who edit and amend a text. Some of these people will be visible to the consumer of a text and this visibility, or lack of it, may be an important feature of a text that an analyst may wish to point out. Each of the producers of the texts will have multiple aspects to their identity, complex histories, and multifaceted circumstances. From an analytical point of view, much of this is not present in a text.
This textual process is very closely linked to the concept of discursive styles. At the level of a text, though, one can conduct an analysis from the perspective of recovering the results of this process of identification. Here, one can look for the wording or vocabulary and for grammatical structures which point towards personal and social identities. The most obvious are the personal identities that are overtly stated in a text: “I am a …,” “as an …. ,” and “being a ….” Personal identities can also be seen in formulations that show attitude, evaluation, and orientation towards the topic that is being discussed. In both cases, one can ask which aspects are included but also which are excluded. Finally, social identities can also be present in texts. Studies in sociolinguistics reveal that there can be differences in the way that social groups tend to use language. Gender, sexuality, social class, age, regional identity all tend to have language features associated with them. A textual analysis of these features can show the extent to which these are present in a text. Furthermore, institutional roles may require, or tend towards, the presentation of a particular identity.
Fairclough (2003, pp. 161, 162) suggests the following as elements of texts which can be analyzed for the perspective of identification:
- phonological features (the sounds or accent of a spoken text)
- vocabulary and metaphor
- commitments in modality and evaluation
Identities can be talked about and performed or embodied. There is a strong research tradition in CDA which critically analyses the construction of identities through discourse which construct differences between groups or which construct sameness within groups. Rudolf De Cillia and colleagues (1999), for example, developed a method for analyzing the discursive construction of sameness through national identity in the case of Austria. The method distinguishes “three interrelated dimensions” of discursive identity construction: “contents/topics, strategies and linguistic means and forms of realization” (De Cillia et al., 1999, p. 157) which the analyst looks for and identifies in the data. This approach works well for data in which speakers or writers reflect on their identity.
Jane Mulderrig (2012), on the other hand, analyses the performance of identity of the UK Labour Party, in the “New Labour” era, showing how the use of the pronoun “we” in prominent party speeches and documents performed an “inclusive identity” as part of its “shift towards a more ‘personalized’ form of self-representation.” This study used corpus linguistics to show “that the extent and manner of (pronoun) use under New Labour” were “unprecedented in education policy discourse” (Mulderrig, 2012, p. 702).
Texts as Representation
Texts are also engaged in a process of representation: the text shows—or represents—things that are beyond the identity of the text producer. These could be abstract concepts or concrete descriptions. For CDA, key questions are over what a text represents and how it represents it. Almost all texts—of more than a few words—will represent an aspect of the world. Since a text cannot represent every aspect of a thing, and since the meaning potential of language is vast, alternative representations are almost always possible. This provides grounds for critically analyzing which representations were made and which alternatives were not. Drawing on Fairclough (2003, p. 134), one can take the following as starting points for analyzing representations in texts:
- Exclusion or inclusion
- Representation of social action
- Representation of social actors
- Representation of time and space
There may well be overlap between these categories and some ambiguity; often it is the overlap or ambiguity which is of note in critical analysis.
Exclusion and Inclusion
The analysis of exclusion and inclusion can extend to any of the possible types of entity that may be represented in a text: people, objects, places, ideas. As discussed in detail later in this entry, this can include the representation of social actions (processes), social actors (people), and times and places. Yet it is worth considering the analysis of inclusion and exclusion here on a general level. The research design for a project may not call for detailed analysis of how social actors, actions, time, and space are represented and a general analysis of inclusion and exclusion may suffice. In any case, contextual knowledge would be useful for identifying those things that could have been included, but were not. Similarly, contextual knowledge is useful for identifying the significance of details that have been included in a text—it may be that the inclusion of a detail carries implicit meaning that is not obvious from the text itself. In addition, comparative analysis with other texts which refer to the same event or topic is likely to reveal differences between what is included in one text but excluded from the other. Critically, inclusion and exclusion may point to the orientation of a text producer towards an issue and may affect the understanding of an issue amongst consumers of the text.
Representation of Social Action
Analyzing the representation of social action can be a useful way of uncovering or highlighting aspects of the representational function of a text that is not immediately clear from even a close reading of a text. All texts of any length include representations of social processes. Theo Van Leeuwen (1995) gives a set of categories for the analysis of the representation of social action from which one can derive a set of general research questions:
- Is the action material or semiotic?
- Is the action activated or deactivated? That is, represented as a dynamic process or as if it were an object.
- Is the action transactive? That is, action that takes place between two or more people with one “doing” and the other “receiving” the action.
- Is the action instrumental? An action that has an effect on something.
- Is the action agentalized or deagentalized? That is, brought about by human agency, or not.
- Is the action abstracted or generalized? If so, relevant details may be missing.
Critically, the representation may emphasize some kinds of action over others, and this could influence the overall representation of an event or topic—a text may emphasize material action over semiotic action, or vice versa. A text may imply that some people are active “doers,” whilst others are passive receivers of action. A process of human agency may be represented as though it were a natural phenomenon over which one has no control.
Representation of Social Actors
Analyzing the representation of social actors, the people involved in events or topics, can also be a useful way of uncovering or highlighting aspects of the representational function of a text. Again, from van Leeuwen (1996), one can derive a set of generalized analytical questions with which to classify the representation of social actors in text. Is the representation of the social actor:
- active or passive?
- personal or impersonal?
- named or classified?
- specific or generic?
- functional (referring to what they do) or identification (referring to what they are)?
Critically, the representation may treat social actors, or groups of social actors, differently, and this will affect the overall sense-meaning of a text. Without using derogatory or overt claims, a text may present a group of people in a way that deemphasizes some of their human qualities whilst emphasizing others. Conversely, a text may valorize a group of people in an indirect way. A comparison of how a text represents the people to whom it refers differently from each other is crucial for this kind of analysis.
Representation of Time and Space
The analysis of time and space can also be important in textual analysis. The representation of time and space can come in several forms:
- vocabulary of space (nouns referring to places or types of place)
- vocabulary of time (nouns referring to time or period, adverbs of time)
- grammar of time (verb tenses)
Significant issues of time and space that the analyst might focus on could include:
- the times which are objectified and referred to
- the scales of time which are referred to
- the places that are objectified and referred to
- the scales of place and space referred to
- how they are represented as relating to each other
A text may refer to specific towns and cities or refer to towns and cities in general, for example. Critically, representations of space may contribute to a discourse in which the concerns and issues of one part of an entity are subsumed by those of a more extensive entity. Conversely, the concerns and issues of a small entity may come to predominate those of a larger entity. Similarly, with representations of time, the concerns of a previous period may come to dominate the discourse of the present or future, or come to be effaced.
In this example, an extract from the UK Labour Party’s General Election manifesto (see Farrelly, 2015, p. 60), one can see instances of the representation of social actors, action, and time and space as part of a representation of “democracy”:
The Conservatives seem opposed to the very idea of democracy. They support hereditary peers, unaccountable quangos and secretive government. They have debased democracy through their MPs who have taken cash for asking questions in the House of Commons. They are opposed to the development of decentralised government. The party which once opposed universal suffrage and votes for women now says our constitution is so perfect that it cannot be improved. (Labour Party, 1997)
The representation of social action in this context is interesting in that the social action (opposing, supporting, debasing) is largely generalized and nontransactive. The more specific action of “taking cash for questions,” which would be a transaction between people, is represented here in a nontransactive, nonpersonalized way. This kind of representation may be typical of UK election manifestos in which parties seek to generalize their critique of opponents to a whole political party but, in so doing, it also represents democracy in a generalized and static way. The only specifically (potential) democratic actions here deactivated, that is, presented as things rather than processes (e.g., votes, suffrage).
Further, in the representation of social actors it is politicians who are emphasized and who occupy the active roles in this example. Crucially, these politicians are represented as having the ability to “debase democracy.” The reference to “votes for women” implies that the attitude towards democracy described here has a long history and the reference to “the House of Commons” puts the location of democracy in one of the legislative chambers of Parliament. One might summarize this as showing democracy as a static kind of thing which largely involves professional politicians in the legislature, rather than an active relation in which people seek to influence their government.
Texts as Ways of Acting and Interacting
Texts are, according to CDA, also ways of acing and interacting. On a fundamental level, they are ways of organizing the various functions of language and discourse. The organization of ideas, descriptions, and arguments into a coherent and comprehensible text is a key process for the producer of a text. Beyond this fundamental process, there are ways of acting through texts that are even more finessed. One may want to report, recount, argue, explain, or persuade; each of these may be achieved successfully, or not, depending on how the language of a text is organized for the accomplishment of these actions. Further, depending on the social practice in which one is involved, there may be well-established “ways of doing” these things or of combining various discursive actions in a single text. In other words, there are likely to be conventions for how to organize the elements of a text which may depend on the social practice one is engaged in when producing a text. These ways of acting with texts are closely linked to the CDA account of genre and by looking at features of genre, it is possible to begin analyzing texts on the basis of how they perform actions.
First, one can identify “text-types” or “situated genres.” These are names one can give to texts that define or describe the text. This could be a name that the analyst themselves uses to describe a text, but, often, the discourse of a social practice will already have names ascribed to the common or important text-types that are used in that practice. For example, traditional newspapers may name various text-types in their publications as “news report,” “leader column,” “feature column,” amongst others.
These text-types are likely to have conventional features. Fairclough’s (2003) description of the features of text that are shaped by genre (p. 67) includes the structure of a text and the semantic relations between clauses. What one is looking for here is:
- an indication of what the text-type is
- in the overall structure of its constituent parts
- in the semantic relation between clauses
One can begin to analyze these by looking at the structure and semantic relations of a text (see Fairclough, 2003, p. 67 for other text features shaped by genre the formal relations between sentences and clauses; types of exchange, speech function, and mood; the mode of intertextuality).
The Structure of a Text
One can look for identifiable stages of a text. For some text-types, these stages may have readily identifiable names and conventions. An academic essay in social science, for example, is likely to follow a structure such as: title, introduction, background, theory, (possibly) methods, analysis or results, discussion and conclusion, reference list. For some text-types, the analyst may need to develop a classificatory scheme for identifying the stages of a text.
The Semantic Relations Between Clauses
One can also look for the kinds of semantic relations present in a text, or at points in a text. “Semantic relations” refer to the meaning that is given to the relationship between clauses and, therefore, to the relationship between the content of those clauses. Fairclough (2003, p. 89) identifies the following types of semantic relation and the conjunctions that can mark them:
- giving reasons (because)
- giving consequences (was, so)
- giving purposes (in order to, to, so that)
- conditional (if, then)
- temporal (when)
- additive (and)
- elaborative (no conjunction, typically)
- contrastive (but)
Elaborative semantic relations tend to be unmarked by conjunctions, instead they rely on lexical cohesion. For example, when reading a text, one typically infers that a pronoun refers to a preceding noun and that phrases that are related semantically to a previous phrase refer to that preceding phrase. In Fairclough’s (2003) example: “the train was delayed—it was due at 7.30 and arrived at 9.00” (p. 89), one infers that “it” refers to “the train” and that “due at 7.30 and arrived at 9.00” is an elaboration on “delayed.”
At a more abstract level than texts, CDA refers to “discourse.” Discourse is the pattern of language use across a social practice. At this level, the concern is language that is typical, conventional, or which tends to appear at certain points in a social practice rather than the language that is used in a specific text. Discourse emerges from the repeated action and interaction of social practices. Through engaging in social practices, people come to shared understandings about the kinds of identities, representations, and ways of acting that are appropriate for that practice. For CDA, though, discourse does not just emerge from social practices, it also influences, and helps to define, the social practice. It becomes a template for how people might be expected to use language when engaged in that practice. Discourse is seen as “real,” in this sense, and as having real effects on other elements of the world. CDA conceives of discourse (written in the singular) as having three major aspects or functions which it calls styles, discourses (written in the plural to distinguish this aspect of discourse from the overarching concept of discourse), and genres.
As previously noted, CDA can focus on the presentation of identities—it can analyze texts for the “identification” that is carried out through the production and circulation of texts. Beyond individual texts, however, CDA suggests that social practices—the discourse of social practices—have a range of identities that are associated with them. The discursive markers of these identities are, in CDA, called styles. Styles are discursive conventions which enable and constrain the performance of a range of roles and dispositions for a social practice. The types of identities and styles that are associated with social practices and the way in which identities and styles are valued or marginalized can be a focus for a CDA of a social practice.
Styles can be analyzed in a similar way to identities. At the level of social practices, though, one is looking for more general patterns:
- of identification markers associated with a social practice,
- associated with different stages of a social practice,
- associated with institutions or organizations engaged in a social practice,
- identification markers associated with the allocation of roles,
- and adoption of dispositions in a social practice.
The point at which an analyst can make claims about the styles associated with social practices is a methodological issue. There is a distinction to be made between claims that an analyst can make about identities and a collection of texts produced around a defined series of events, and claims that can be made about the patterns of identification which tend to appear as a general feature of a social practice. The point at which this distinguishing point lies will vary according to the social practice under investigation, and the analyst will have to be clear about how and when they have reached this point in discussion of their own case. Once reached satisfactorily, though, an analysis of styles in a social practice can pose questions over the points at which a social practice:
- shows the presence of personal style,
- shows the presence of general social identities (of gender, sexuality, social class, regional identity, age, for example),
- shows the presence of styles that are indicative of institutional roles.
Discourses (plural) are the ways of representing aspects of the world, beyond identities, that are typical of a social practice. Importantly, though, they represent the world, or elements of it, from a point of view. These perspectives can, sometimes, be recognized by those involved in a social practice and, sometimes, not recognized. Pointing out that a discourse comes from a particular perspective can be an instructive critique; pointing out the efficacy (or lack of it) of a particular discourse for our theoretical or practical understanding may be another focus of critique; contrasting a discourse with more exacting measures of describing the world might be further focus for a critique of discourses. In any case, as with styles, discourses can become a kind of social resource for participants of a social practice.
An important conceptual point is that a specific text might represent an aspect of the world which is not typical of the social practice within which that text was produced. When the researcher identifies discourses , then, he or she is dealing with tendencies, patterns, and conventions. As noted, discourses are closely associated with the ways of representing that this entry previously discussed in relation to texts. Indeed, discourses need to be given voice in actual texts to have an effect.
The idea that discourses represent things in the world can be expanded by elaborating on what kinds of things that can reasonably be expected to be represented, Fairclough (2003, p. 135) suggests that, in broad terms, social events include “forms of activity, persons (with beliefs/desires/values … histories), social relations, objects, means (technologies …), times and places, language (and other types of semiosis).” The discourse of social practices can be analyzed for patterns in the ways these tend to be included, given prominence, or excluded. A CDA study might also analyze the discourse of groups or factions within a social practice. Discourses can be analyzed using similar concepts to those previously discussed for analyzing representations in texts but now at a more general level of abstraction:
- of discourses associated with a social practice,
- discourses associated with roles,
- discourses associated with dispositions.
As already noted, in the case of styles, the point at which an analyst can make claims about the discourses associated with social practices is a methodological issue and identifying this point will vary according to the social practice under investigation.
An analysis of discourses in a social practice can pose questions over the relative role or position of discourses in influencing patterns of material or mental action in a social practice. An analysis may be able to trace the characteristics of discourses which are apparently successful in influencing action. Such characteristics could include:
- the conceptual metaphors associated with a discourse,
- the field with which the vocabulary of a discourse is primarily associated,
- the classification system implied in word choice,
- the system of values implied by a discourse.
Such an analysis may reveal insight into why it is that some discourses persist in being influential whilst others do not.
Genres are patterns for acting through texts that are associated with social practices. Typically, a social practice will have a set of text-types associated with it as well as a pattern of pregenres and disembedded genres that are drawn upon in that practice. As previously discussed in relation to styles and discourses, genres are the discursive actions that are typical of a social practice. There is, of course, leeway for innovation and departure from these conventions in some social practices, or some parts of social practices. Such innovation might lead to permanent change in the genres of a social practice, but, for CDA, such a change would be indicative of, even a mechanism for, a fundamental shift in the character of that social practice. Genres can be associated directly with institutionalized power relations—institutional context can be significant for certain text-types because they are only valid if they are produced in the correct institutional context. Institutional contexts can restrict the production of these text-types to a limited number of individuals or types of individual.
An analysis of genre can begin by cataloguing the text-types that are typical of a social practice and the characteristics of these text-types can be analyzed in a similar vein to that previously discussed but with an emphasis on the more extensive social practice rather than on a small number of specific texts. Specifically, one can look for:
- the text-types typical of a social practice,
- which text-types appear at which stages of a social practice,
- the text-types associated with institutions or organizations engaged in a social practice,
- the text-types associated the social roles present in a social practice.
However, how more abstract discursive actions are utilized in a social practice can also be considered. There are, according to CDA, types of discursive action that can be recognized across many social practices. These can be called pregenres (Swales, 1990) and are the primary actions that can be undertaken in the use of language. These are highly abstract categories and “transcend” networks of social practice (Fairclough, 2003, p. 68). They include the discursive actions of argumentation, description, narration, conversation. One can ask where these pregenres are present and how they are used.
Orders of Discourse
At a broader level, one can analyze the way in which styles, discourses,, and genres are combined in social practices. A social practice is likely to have a range of styles, discourses, and genres. This gives rise to questions over the relationships between:
- the different styles associated with a social practice
- the different discourses associated with a social practice
- the different genres associated with a social practice
Further, there are analytical questions over how different styles, discourses, and genres tend to be configured with each other. The analysis may, for example, look to identify which styles tend to appear with which discourses and with which genres. For CDA, the configuration of styles, discourses, and genres is not random but “ordered” and, borrowing a term from Michel Foucault, refers to this ordering as the “order of discourse.”
Orders of discourse, as understood from a CDA point of view, are the patterns of language use that correspond to social practices but also to networks of social practices. The existence of links between social practices, or the absence of links between social practices, is essential feature or characteristic of societies. One task for critical analysis, therefore, is to map out the presence and absence of discursive links. Mapping the presence and absence of discursive links between practices can be a way of opening a critique of the network of social practices. Further, the nature of the links between social practices might be significant—one set of social practices might come to predominate and alter other social practices. A social practice may come to hold an elevated position in a society, and the texts produced in that social practice may come to be referred to throughout many of the other social practices of the society. Similarly, its styles, discourses, and genres may come to be adopted in a range of related but distinct social practices. Analysis of intertextuality is, therefore, key to mapping out the character of discursive links within and between social practices. A CDA analysis of the discursive links between social practices may open a critique of the quality of the relation between social practices.
For example, the practices and discourses of managerialism developed in some parts of the commercial business world may be adopted in other sectors of an economy. Managerialism might be seen to colonize those sectors. At one level, these colonizing or appropriated discourses and genres may exert very little influence: If one adopts a managerialist type of discourse to appear “modern” but continues to act in the same way as before, then the potential ideology of managerialism has not taken hold but remains as a potential. If one, however, also begins to act in a managerialist way, by employing certain genres, and if this new way of acting smooths over contradictions to the greater benefit of the powerful, then the ideology has taken hold because it has altered practice, whether the practitioners believe in managerialism or not. In this case, one could say that the flow between orders of discourse has begun to have a real ideological effect. Yet there would still be room for resistance to the ideology: People might employ contradictory discourses and genres, yet act out these genres ironically or reluctantly. There is, then, a cline on which ideology works through orders of discourse; once the order of discourse has been filled with other genres and discourses and people take on the associated styles, then one might say that the ideological effect is complete, although it is unlikely that this stage would ever be reached as one would expect competing interests to be present.
In the following sections, it will become apparent that the concept of “intertextuality”—the effect of texts on one another—is key for an analysis of orders of discourse. First, an overview of intertextuality is presented, followed by an examination of two forms of intertextuality: manifest intertextuality, in which a text makes direct reference to another; and interdiscursivity, in which texts adopt the conventions of style, discourse, and genre of other texts. Finally, this section looks at “recontextualisation,” which refers to the process by which nondiscursive elements of social practices are brought into orders of discourse.
Intertextuality
Intertextuality is the presence, use, and effect of one text in another. The term was coined by Julia Kristeva (1986) in her work on literary theory but has been taken up and developed in CDA as an important, if underused, theoretical and methodological concept for working on the critical analysis of orders of discourse. Fairclough (1992) has argued that: “an intertextual perspective is helpful in exploring relatively stable networks of which texts move along” (p. 84).
Conceptually, the analysis of intertextuality in both of its forms, manifest and constitutive, is the key to a CDA analysis of the discursive relations of networks of social practices. Discursive relations may appear as patterns in the source for manifest intertextuality or in the patterns of similarity in discourses, genres, and styles in networks of social practices. An analysis can identify features of texts that are produced in related social practices and then go on to map and assess the patterns of intertextuality that these features show. The mapping and analysis of the discursive relations between networks of social practices is not a small undertaking and is likely to require analytical tools and aids that enable the analyst to keep track of relations across large numbers of texts. Corpus linguistics may be useful for assessing vocabulary, grammatical patterns, and collocations (words that tend to appear near other words). Qualitative data analytical software can be useful in analyzing texts for a range of representation, action, and style features and then visualizing and naming discursive links between these features in texts from a range of social practices. For example, an analyst can identify and code (mark) instances of manifest intertextuality in a text in the way described earlier. “Mapping” intertextual links can be one way of exploring orders of discourse and analyzing the intertextual relations that can show how an order of discourse is configured and how it changes over time. Further, such an analysis can give grounds for interpreting and theorizing power relations:
the structuring of discourse practices in particular ways within orders of discourse can be seen, where it comes to be naturalized and win widespread acceptance, as itself a form of (specifically cultural) hegemony. (Fairclough, 1992, p. 10)
Manifest Intertextuality
Manifest intertextuality refers to other texts being “explicitly present in the text under analysis,” constitutive intertextuality is “the configuration of discourse conventions that go into its production” (Fairclough, 1992, p. 104). The first analytical task when focusing on manifest intertextuality is identification of the texts that are incorporated—brought into an intertextual relation with a text under analysis. For the presence of other texts, one can look for indicators such as:
- quotation marks,
- reporting verbs,
- the names of texts or documents,
- generic reference to document types.
These give an indication of the way a text is brought into another text and it may well be worth analyzing and interpreting the significance of the manner of incorporation—is there direct or indirect representation of speech or thought? If indirect, how congruent with the original is the indirect representation? What aspects of the original does the intertextual reference emphasize or deemphasize? Once identified, one can categorize the intertextual reference according to:
- text-type (what type of text is drawn on? News reports rather than scientific papers, for example)
- text origin (where does the source text originate? A university or a think-tank, for example)
- attitudinal orientation towards the source text (is source text framed as trustworthy or not, for example)
As an example, the following three excerpts are from a speech on UK energy policy given in a speech by the UK’s Energy and Climate Change Secretary on November 18, 2018:
- 1. This could provide up to 30% of the low carbon electricity which we’re likely to need through the 2030s and create 30,000 new jobs.
- 2. As the former Chief Scientist at DECC, David Mackay, said: “If everyone does a little, we’ll achieve only a little. We must do a lot. What’s required are big changes.”
- 3. The industry tells us they can meet that challenge and assumes people will hold them to it.
Excerpt 1 is an example of specific information which appears to be the result of some expert analysis on the projected consequences of a set of actions. The intertextuality, though, is not marked at all: There is no indication of quotation or reporting verb, no name of a document from which this information came, and no generic reference to the type of document in which this information could be found. The speech giver appears to value and trust this information, but from where the information originated is not known. Excerpt 2 shows marked intertextuality: There are quotation marks, a reporting verb, and the audience is told who it was that said these words: the former Chief Scientist at DECC, David Mackay. However, his quoted words do not appear to be particularly scientific in nature, nor is the context in which they were said apparent. Excerpt 3 has a reporting verb, “tells,” but the words of the industry are paraphrased, and it is not known if this was a commitment given on a specific occasion or one which has been repeatedly given, indeed one cannot be sure how firm the commitment given by industry is. In these examples, it appears that specific information is given through unmarked or unspecified intertextual reference whilst specific intertextual reference is given to motivational phrases. A CDA research project on intertextuality may go on to establish whether this is a pattern typical of UK political speeches.
Interdiscursivity
Interdiscursivity, in CDA, is a form of intertextuality that is equally as important as manifest intertextuality but which appears in texts in a different way and which requires a different analytical and interpretive orientation. Interdiscursivity refers to “the configuration of discourse conventions” that go into the production of texts (Fairclough, 1992, p. 104). The kinds of conventions that one might find could entail any of the elements of a text which follow patterns that have been already established as being constitutive of a discourse. As far as interdiscursivity goes, one can conclude that a textual feature may be “typical” of a discourse. So, for example, a university library might send an email in which it refers to “customers” when it means students or staff who are members of the university and who may have borrowed items and use the facilities of the library. In terms of interdiscursivity, one could say that “customer” is typical of the discourse of the practices of commodity exchange such as, perhaps, in this case of a retailer and people who buy goods and services from the retailer. Yet the same email might also refer to payment of fines for late return of items which is not typical of retailer practices.
This distinction shows how the analysis of interdiscursivity may show up a heterogeneous set of sources for the discourse conventions that constitute texts. An analysis of this kind of interdiscursivity requires identification of features of the text, identification of those features as being typical of a discourse and identification of the social practices with which that discourse is associated. This can be some undertaking—analysts may have an intuition over the source discourses of textual features—but that intuition can only be a starting point for an analysis. An analysis must be able to show that the feature is present in text that are taken from the source practice. In some cases, corpus linguistics may be a useful supplement to the analysis of interdiscursivity if the feature is one of semantic prosody, vocabulary, or phrasing. A corpus search may indicate that a word is often associated with a domain of practice, for example.
An analysis, then, looks for source discourse and practice and can focus upon:
- circumstances
- orientation
- emphasizing
Interdiscursivity may indicate a legacy of social change or an attempt to effect social change through the adoption of the conventions and habits of one practice in another.
Recontextualization
One implication of orders of discourse is that texts are produced and consumed in different places and at different times but can be linked by being part of a social practice or a network of social practices. They also report or refer to events that take place as part of that social practice. In this case, the nondiscourse elements are “recontextualised” from their original context into the context of another social practice with its own habits and conventions for how it produces texts. So, for example, the events of a professional sport contest may be recontextualized into a media report, from the practice of professional sport to the practices of media production.
CDA holds that recontextualisation follows principles and practices by which the representation of events moves from one context to another. When looking at recontextualization, one can analyze the apparent rules that determine the form that recontextualization takes and interpret reasons for these apparent rules. The concept of recontextualization also gives a way in to analysis of the relationship between the nondiscursive and the discursive elements of a social practice. Van Leeuwen (1993) has noted:
The practical knowledge of a social practice, the knowledge of how to perform as a participant of that practice, is knowledge in an “unrepresented” state. As soon as the practice is represented (taught, described, discussed etc.) it is recontextualized. (p. 204)
Recontextualization may rearrange sequences of events, remove elements that are not seen as relevant to the new context or add elements to the representation such as explanation, legitimation, and evaluation, or it may generalize from an event. What one is looking for, here, then is a pattern which indicates a set of principles which operate when one kind of event is regularly recontextualized into the texts of another part of a social practice or into another social practice altogether.
Further Methodological Issues
The theoretical and methodological stance of CDA in each of the areas described thus far gives rise to further practical questions when conducting a CDA study or research project. Four of these are outlined in the following sections:
- The method of data collection—towards which CDA is generally agnostic;
- Consideration of how, practically, the analyst will handle the data analysis and it includes;
- The process and systematic approach to be taken in interpreting results of analysis;
- The interdisciplinary work or interdisciplinary dialogue through which an understanding of social and historical context and the theoretical positions towards a specific social practice can be brought to bear in a CDA study.
There remain many practical and theoretical issues for a CDA analysts to work out methodologically for the specific research project that they undertake but these are some of the issues that they might face for each one. It is important that they are considered for each case in point.
Data Collection
CDA is not a method of data collection but a theory and method for the analysis, interpretation, and critique of language use as an element of social events and practices. In many ways, CDA is agnostic about the methods by which data are collected. CDA studies include analysis that has been collected through social scientific interviews, from the collection of public and private documents (e.g., media, government, business, charity) and from transcripts of naturally occurring speech. The understanding that language use is an element of social practices and that social practices are more than language means that CDA is often used in research that combines forms of textual data with nontextual data. Further, though CDA often focuses on the written or spoken language, there is also a strand in CDA that has recognized other semiotic systems as important sites for critical analysis (Hodge & Kress, 1988). There are also approaches to critical analytical approaches to multimodal analysis, which finds ways to analyze the combination of different modes of language use. For example, analysis of web pages which combine written words, visual images of various types (including pictures and video), and sound (see Machin & van Leeuwen, 2009; van Leeuwen, 2005).
Computer-Assisted Qualitative Data Analysis Software (CAQDAS)
CAQDAS has been available to social science for some time and has been used in CDA studies (see Wodak, 2006, for example). Yet, there has been little explicit methodological engagement in the literature with how CAQDAS might extend the capacities of CDA in textual analysis (see Paulus & Lester, 2015, for one exception which argues a strong case for the use of CAQDAS in discourse analysis). There are now multiple CAQDAS packages (e.g., ATLAS.ti, NVivo, and MAXQDA) with various advantages and functions. The potential of CAQDAS for CDA is that it can be used in the analysis of the full range of analytical tools that have been traditionally used in CDA, but it is much easier to apply these tools across a more substantial body of texts than pen-and-paper analysis. This is because searches and filters can help to keep track of quotations and codes across many texts. In addition, this software can facilitate analysis and mapping of networks of texts; one can label and visualize connections between elements of texts and between different texts.
Analyzing Corpora of Texts
From around 2010, there was a marked uptake in the use of corpus linguistics in CDA studies. The rationale for this uptake is 2-fold. First, the aim, for some, is to extend the amount of data that can be analyzed in a critical discourse analytical study. The grounds for such a move are the claim, in CDA, that texts draw on discourse rather than containing a discourse. A discourse is always beyond texts and cannot be reduced to the contents of a single text. Discourses must have qualities that make them identifiable as one discourse rather than another and so must have a patterned set of features which differentiates it from other discourses. If this is the case, then, according to advocates of corpus linguistic approaches to discourse analysis, analysis of large bodies of texts, corpora, ought to give up clear indicators of the patterns that occur beyond individual and small bodies of texts and which occur more abstractly across large bodies of texts. Potentially, corpus linguistics can be used to analyze a considerable number of texts from across a social practice and provide a comparative analysis of change within a social practice across time or of differences between social practices.
Interpretation
As already noted, there are a range of analytical concepts and techniques and a range of theoretical positions associated with CDA. The analysis can involve a degree of interpretation—when there is a phrase which is ambiguously either a social actor or a geographical location (or both), for example, and these instances of ambiguity are often telling when it comes to critical analysis because they can indicate a difficulty or contradiction in the way that something is being represented. Often, though, the identification of discursive or textual features is straightforward. In these cases, the interpretation of what the presence of these features means for one’s understanding or critique of the text, discourse, or order of discourse comes after analytical identification. In this interpretive phase, the theoretical positions of CDA can point researchers to a range on possible interpretations of their analysis. However, an interpretation of which of these possibilities is most likely, or an interpretation which is beyond the scope of CDA, would likely depend on interdisciplinary work. The theories, concepts, and knowledge accrued about the topic in question from other disciplinary research, in dialogue with CDA, are key to interpretation of our analytical findings.
Interdisciplinarity
The requirement to refer to context when interpreting, explaining, or theorizing the results of analysis is best fulfilled through interdisciplinary dialogue. This dialogue is not a simple importation of insight from other disciplines. It is a genuine reworking of understanding both text or discourse analysis and of the contextual perspective of the other discipline. CDA offers a general system for interpreting the relation of text and event and for interpreting the relation of discourse and social practice. The other disciplines offer a system for interpreting those relations considering some of the specifics of that event or practice. CDA studies have been concerned with a significant number of topics and appear in many social scientific studies. An examination of the four-volume Critical Discourse Analysis , edited by Ruth Wodak (2013), shows some of the areas of social life to which CDA has been applied:
- Human rights
- Asylum seekers
- National identity and identity in colonial withdrawal
- Political practices
- Economic practices
- Business practices
- Climate change
- Ethnic and racial discrimination
Interdisciplinarity requires work; CDA cannot be bolted-on to studies with which it does not have a commensurable take on critical social science and the position of discourse within social practices. Interdisciplinary working requires a shared understanding of fundamental concepts, and for CDA, these include those discussed earlier: critical analysis, social practices, social and historical context, dialectical relations, power, and ideology. If a study does not link its analysis back to the understanding that there is a distinction to be made between social structure, practice, and event, then it is likely to fail as CDA. Without the sharing or development of these fundamental concepts about society then a “CDA” contribution is likely to be shorn of its critical edge and be reduced to discourse analysis or textual analysis. These may turn up interesting findings, but they would not be CDA.
Final Thoughts
CDA is then a social scientific theory and method for analyzing and critiquing the use of language and its contribution to forming and sustaining social practices. A CDA study can focus on a small number of texts and analyze the way that texts are a way of presenting or performing identities, a way of representing aspects of the world, and ways of acting and interacting. At the level of textual analysis, the critique is aimed at the contribution of the texts under analysis to problems in a specific set of events. A more extensive analysis of texts across a social practice can show the patterns and conventions of the discursive aspect of social practices and networks of practices. At this level of critique, the focus is on the way that social practices can be altered but which also shape the production of texts. In short, CDA endeavours to work towards interdisciplinary critique of social practices and that a primary aim of CDA is to contribute a principled analysis of how the use of language may reproduce or transform social problems.
Sign in to access this content
Get a 30 day free trial, more like this, sage recommends.
We found other relevant content for you on other Sage platforms.
Have you created a personal profile? Login or create a profile so that you can save clips, playlists and searches
- Sign in/register
Navigating away from this page will delete your results
Please save your results to "My Self-Assessments" in your profile before navigating away from this page.
Sign in to my profile
Sign up for a free trial and experience all Sage Learning Resources have to offer.
You must have a valid academic email address to sign up.
Get off-campus access
- View or download all content my institution has access to.
Sign up for a free trial and experience all Sage Research Methods has to offer.
- view my profile
- view my lists
- How it works
Discourse Analysis – A Definitive Guide With Steps & Types
Published by Alvin Nicolas at August 14th, 2021 , Revised On August 29, 2023
What is Discourse Analysis?
Discourse analysis is an essential aspect of studying a language and its uses in day-to-day life.
It aims to gain in-depth knowledge about the language and identify its association with society, culture, and people’s perception.
It is used in various social science and humanities disciplines, such as linguistic, sociolinguistics, and psycholinguistics.
Aims of Discourse Analysis
It focuses on
- The clear, in-depth meaning of the language.
- The uses of language and its effects.
- The association of the language with cultures, interpersonal relationships, and communication.
- Various components of the language like vocabulary, grammar, pronunciation, tone of voice, fonts, and written form.
Uses of Discourse Analysis
Discourse analysis is
- Used to study the language and its applications in texts and contexts.
- It focuses on the entire conversation and real text instead of constructed or artificial text.
- It helps linguists to know the role of language in improving the understanding of people.
- It enables teachers to learn many language strategies to teach students writing/speaking skills better.
Materials Used in Discourse Analysis
The material includes
Types of Discourse
What to analyse, does your research methodology have the following.
- Great Research/Sources
- Perfect Language
- Accurate Sources
If not, we can help. Our panel of experts makes sure to keep the 3 pillars of Research Methodology strong.
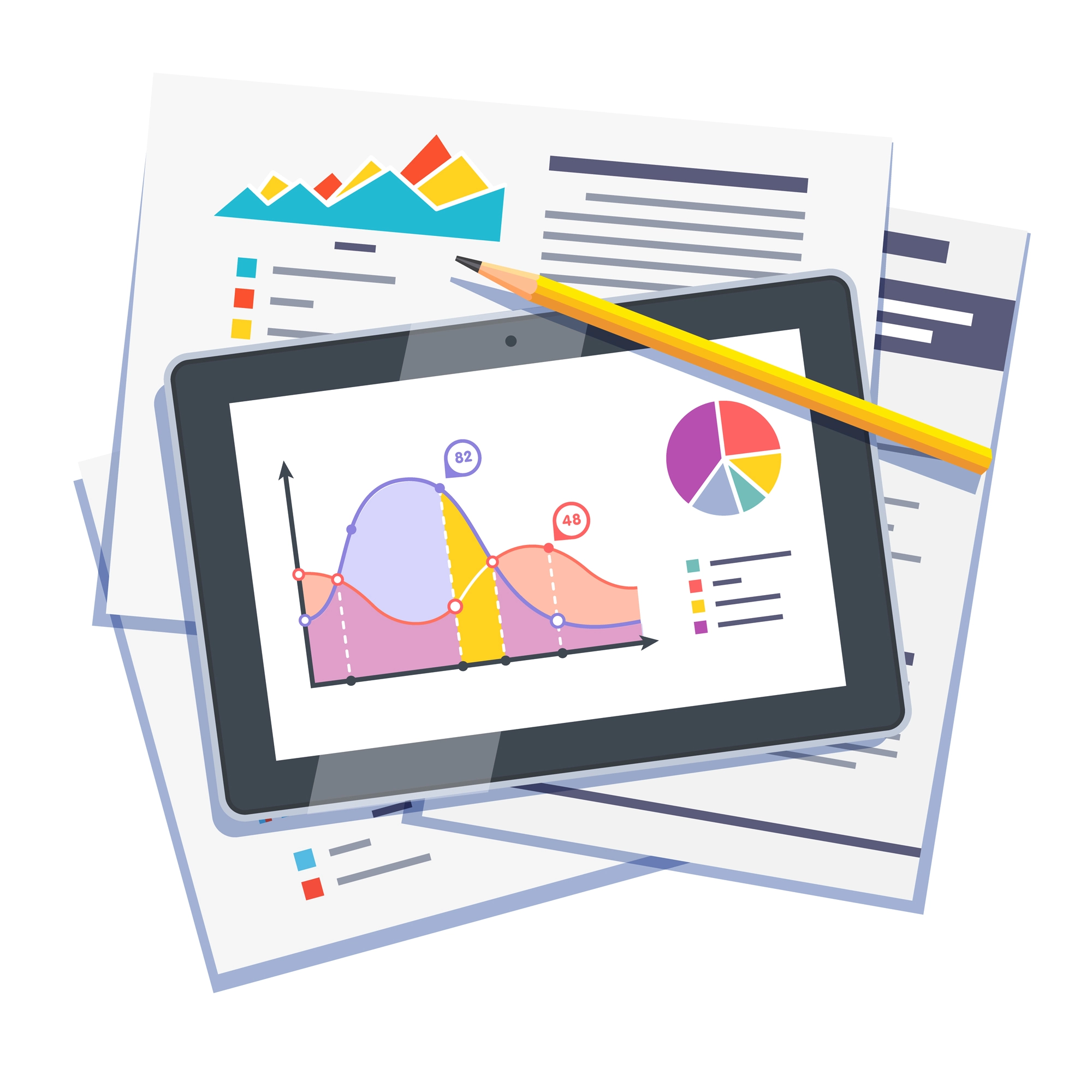
How to Conduct Discourse Analysis?
While conducting discourse analysis, you need to focus on the following points.
- Purpose of the writer
- The context of the speech/passage
- Type of the language used.
- The organisation of the text
You need to interpret the meaning and context of the discourse based on the available material and resources. There are various methods to conduct discourse analysis, but we are discussing the most basic method below.
Step1: Develop a Research Question
Like any other research in discourse analysis, it’s essential to have a research question to proceed with your study. After selecting your research question, you need to find out the relevant resources to find the answer to it. Discourse analysis can be applied to smaller or larger samples depending on your research’s aims and requirements.
Example : If you want to find out the impact of plagiarism on the credibility of the authors. You can examine the relevant materials available on the topic from the internet, newspapers, and books published during the past 5-10 years.
Step 2: Collect Information and Establish the Context
After formulating a research question, you can review the literature and find out the details about the source material, such as:
- Who is the author?
- What is the year and date of publication?
- What’s the name of the publication?
- What country and place is it from?
- What language is used?
- How and where did you find it?
- How can others get access to the same source?
- What kind of impact did it make on its audience?
- What’s the association between discourse material and real life?
These questions enable you to construct a strong evidence-based theory about your study.
Example: While investigating the history and origin of a particular religion. You also have to research the political events, culture, language of the people, and their association with society.
Generally, details about the publication and production of the material are available in the about section on their online websites. If you don’t find the relevant information online, don’t hesitate to contact the editor or publication via email, phone calls, etc.
Step 3: Analyse the Content
In this step, you should analyse various aspects of the materials such as:
- Sentence structure
- Inter-relationship between the text
- Layout and Page quality (if you are using offline materials)
- Links, comments, technical excellence, readability, multimedia content (if you are using online material)
- The genre of the source (a news item, political speech, a report, interview, biography, commentary, etc.)
The analysis of these elements gives you a clear understanding, and you can present your findings more accurately. Once you have analysed the above features, you should analyse the following aspects:
- The structure of the argument
- The role of the introduction and conclusion of the material
- The context of the material
- Patterns and themes
- Discursive statements (arguments, perspective, thoughts of the writer/speaker
- Grammatical features (use of pronouns, adjectives, phrases, active or passive voice, and their meaning)
- Literary figures (idioms, similes, metaphors, allegories, proverbs)
Step 4: Interpret the Data
Now you have all the information, but the question that arises here is:
What does it all mean?
To answer this question, compile all your findings to explain the meaning and context of the discourse.
Step 5: Present your Findings
It’s time to present your results. Throughout the process, you gathered detailed notes of the discourse, building a strong presentation or thesis. You can use the references of other relevant sources as evidence to support your discussion. Always try to make your paper interesting to grab the attention of the reader.
Advantages and Disadvantages of Discourse Analysis
- It provides a way of thinking and analysing the problem.
- It enables us to understand the context and perception of the speaker.
- It can be applied at any given time, place, and people.
- It helps to learn any language its origin and association with society and culture.
Disadvantages
- There are many options available as each tradition has its own concepts, procedures, and a specific understanding of discourse and its analysis.
- Discourse analysis doesn’t help to find out the answer to scientific problems.
Frequently Asked Questions
How to describe the discourse analysis.
Discourse analysis examines language use in context. It studies how communication shapes and reflects social meaning, power dynamics, and cultural norms. By analyzing spoken, written, or visual language, it unveils hidden ideologies, identities, and social structures within various contexts.
You May Also Like
In correlational research, a researcher measures the relationship between two or more variables or sets of scores without having control over the variables.
What are the different types of research you can use in your dissertation? Here are some guidelines to help you choose a research strategy that would make your research more credible.
A case study is a detailed analysis of a situation concerning organizations, industries, and markets. The case study generally aims at identifying the weak areas.
USEFUL LINKS
LEARNING RESOURCES
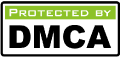
COMPANY DETAILS
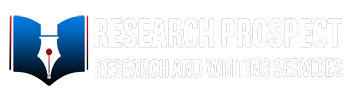
- How It Works
Introducing Discourse Analysis for Qualitative Research
Qualitative researchers often try to understand the world by listening to how people talk, but it can be really revealing to look at not just what people say, but how. This is how discourse analysis (DA) can be used to examine qualitative data.
Daniel Turner
Qualitative research often focuses on what people say: be that in interviews , focus-groups , diaries , social media or documents . Qualitative researchers often try to understand the world by listening to how people talk, but it can be really revealing to look at not just what people say, but how. Essentially this is the how discourse analysis (DA) can be used to examine qualitative data. Discourse is the complete system by which people communicate, it’s the widest interpretation of what we call ‘language’. It includes both written, verbal and non-verbal communication, as well as the wider social concepts that underpin what language means, and how it changes. For example, it can be revealing to look at how some people use a particular word, or terms from a particular local dialect. This can show their upbringing and life history, or influences from other people and workplace culture. It can also be interesting to look at non-verbal communication: people’s facial expressions and hand movements are an important part of the context of what people say. But language is also a dynamic part of culture, and the meanings behind terms change over time. How we understand terms like ‘fake news’ or ‘immigration’ or ‘freedom’ tells us a lot, not just about the times we live in or the people using those terms, but groups that have power to change the discourse on such issues. We will look at all these as separate types of discourse analysis. But first it’s important to understand why language is so important; it is much more than just a method of communication.
“Language allows us to do things. It allows us to engage in actions and activities. We promise people things, we open committee meetings, we propose to our lovers, we argue over politics, and we “talk to God”…
Language allows us to be things. It allows us to take on different socially significant identities. We can speak as experts—as doctors, lawyers, anime aficionados, or carpenters—or as ‘everyday people’. To take on any identity at a given time and place we have to ‘talk the talk’…” - Gee 2011
Language is more than a neutral way of communicating, it’s deeply connected with actions and personal identity, and can even shape the way we think about and understand the world. Who we are, what we do, and our beliefs are all shaped by the language we use. This makes it a very rich avenue for analysis.
Types of discourse analysis Just like so many blanket qualitative terms , there are a lot of different practices and types of analysis called ‘discourse’ analysis, and many different ways of applying them. Hodges et al. (2008) identify 3 meta-types, broadly going from more face-value to conceptual analysis: • Formal linguistic (basically looking at words/phrases, grammar or semantics) • Empirical (social practice constructed through text) • Critical (language constructing and limiting thought)
Tannen et al., 2015 categorise three similar broad types of analysis, again becoming increasingly socially conceptual:
• language use
• anything beyond the sentence
• a broader range of social practice that includes non-linguistic and non-specific instances of language
However Gee (2011) only recognises two main categories, essentially those that look at the use of words, and ‘critical discourse analysis’: like the latter of both groupings above, this is analysis of how language is situated in cultural and contextual power dynamics. But before we get there, let’s start with an example of some more obvious linguistic level discourse analysis.
Example Imagine the following scenario from your favourite fictional medical drama. A patient is wheeled into the ER/casualty unit, conscious but suffering from burns. The doctor attending says three things:
To Patient: “We’re just going to give you a little injection to help with the pain.”
To Nurse: “10cc’s of sodium pentothal, stat!”
To Surgeon: “We’ve got severe second-degree chemical burns, GA administered”
In this situation, the doctor has said essentially the same thing 3 times, but each time using a different response for each recipient. Firstly, when talking to the patient, the doctor doesn’t use any medical terminology, and uses calming and minimising language to comfort the patient. This is a classic type of discourse we are familiar with from medical TV dramas, the ‘good bed-side manner’.
To the nurse, the doctor has a different tone, more commanding and even condescending. It’s a barked command, finished with the term ‘stat!’ - a commonly used medial slang word (actually from the Latin word ‘statum’ meaning immediately, that’s your linguistic analysis!). This is interesting, because it’s not a term you’d hear used in other professional places like a busy kitchen. It shows there is a specific discourse for the setting (a hospital) and for different people in the setting. The ‘10cc of sodium pentothal’ is a commonly used anaesthetic: the same ‘something to help with the pain’ but now with a (trademarked) pharmacological name and dose.
Finally, to the surgeon the same prescription is described by the doctor as an abbreviation (GA for General Anaesthetic). Between senior health professionals, abbreviations might be used more often, in this case actually hiding the specific drug given, perhaps on the basis that the surgeon doesn’t need to know. It could also imply that since only that basic first step has been made, there has been little assessment or intervention so far, telling to an experienced ear what stage of the proceedings they are walking in on. The use of the term ‘we’ might imply the doctor and surgeon are on the same level, as part of the team, a term not used when addressing the nurse.
Even in this small example, there are a lot of different aspects of discourse to unpack. It is very contextually dependent, none of the phrases or manners are likely to be adopted by the doctor in the supermarket or at home. This shows how the identity and performativity of the doctor is connected to their job (and shaped by it, and contextual norms). It also shows differences in discourse between different actors, and power dynamics which are expressed and created through discursive norms.
At a very basic level, we could probably do an interesting study on TV shows and the use of the term ‘stat!’. We could look at how often the term was used, how often it was used by doctors to nurses (often) and by nurses to doctors (rarely). This would probably be more like a basic linguistic analysis, possibly even quantitative. It’s one of the few occasions that a keyword search in a qualitative corpus can be useful – because you are looking at the use of a single, non-replaceable word. If someone says ‘now please’ or ‘as soon as you can’ it has a very different meaning and power dynamic, so we are not interested in synonyms here. However, we probably still want to trawl through the whole text to look at different phrases that are used, and why ‘stat!’ was not the command in all situations. This would be close to the ‘formal linguistic’ approach listed above.
But a more detailed, critical and contextual examination of the discourse might show that nurses struggle with out-moded power dynamics in hospitals (eg Fealy and McNamara 2007 , Turner et al 2007 ). Both of these papers are described as ‘critical’ discourse analysis. However, this term is used in many different ways.
Critical discourse analysis is probably the most often cited, but often used in the most literal sense – that it looks at discourse critically, and takes a comparative and critical analytic stance. It’s another term like ‘grounded theory’ that is used as a catch-all for many different nuanced approaches. But there is another ‘level’ of critical discourse analysis, influenced by Foucault (1972, 1980) and others, that goes beyond reasons for use and local context, to examine how thought processes in society influenced by the control of language and meanings.
Critical discourse analysis (hardcore mode)
“What we commonly accept as objective or obviously true is only so because of negotiated agreement among people” – Gee (2011)
Language and discourse are not absolute. Gee (2011) notes at least three different ways that the positionality of discourse can be shown to be constructed and non-universal: meanings and reality can change over time, between cultures, and finally with ‘discursive construction’ – due to power dynamics in setting language that controls how we understand concepts. Gee uses the term ‘deconstruction’ in the Derridian sense of the word, advocating for the critical examining and dismantling of unquestioned assumptions about what words mean and where they come from.
But ‘deep’ critical discourse analysis also draws heavily from Foucault and an examination of how language is a result of power dynamics, and that the discourse of society heavily regulates what words are understood to mean, as well as who can use them. It also implies that because of these systems of control, discourse is used to actually change and reshape thought and expression. But the key jump is to understand and explain that “what we take to be the truth about the world importantly depends on the social relationships of which we are a part” (Gergen 2015). This is social construction, and a key part of the philosophy behind much critical discourse analysis.
Think of the use of the term ‘freedom’ in mainstream and political discourse in the United States. It is one of the most powerful words used by politicians, and has been for centuries (eg Chanley and Chanley 2015 ) However, it’s use and meaning have changed over time, and what different people from different parts of the political spectrum understand to be enshrined under this concept can be radically different, and even exclusionary. Those in powerful political and media positions are able to change the rhetoric around words like freedom, and sub-terms like ‘freedom of speech’ and ‘freedom of religion’ are both being shifted in public discourse, even on a daily basis, and taking our own internal concepts and ideas with them. It may be that there has never been an age when so much power to manipulate discourse is concentrated in so few places, and able to shift it so rapidly.
Doing Discourse
So do we ‘do’ discourse analysis? How can we start examining complex qualitative data from many voices from a point of view of discourse? Like so many qualitative analytical techniques , researchers will usually adopt a blend of approaches: doing some elements of linguistic analysis, as well as critical discourse analysis for some parts or research questions. They may also draw on narrative and thematic analysis . But discourse analysis is often comparative, it lends itself to differences in the use of language between individuals, professionals and contexts.
From a practical point of view, it can be started by a close reading of key words and terms, especially if it is not clear from the outset what the important and illustrative ones are going to be. For building a complete picture of discourse, a line-by-line approach can be adopted, but it’s also useful to use ‘codes’ or ‘themes’ to tag every use of some terms, or just significant ones. A qualitative software tool like Quirkos can help you do this.

For critical discourse analysis, examination of primary data is rarely enough – it needs to be deeply contextualised within the wider societal or environmental norms that govern a particular subset of discourse. So policy and document analysis are often entwined and can be analysed in the same project. From here, it’s difficult to describe a single technique further, as it will greatly vary by type of source. It is possible in discourse analysis for a single sentence or word to be the major focus of the study, or it may look widely across many different people and data sources.
The textbooks below are all classic works on discourse analysis, each a rabbit hole in itself to digest (especially the new edition of Gergen (2015) which goes much wider into social construction). However, Hodges et al. (2008) is a nice short, practical overview to start your journey.
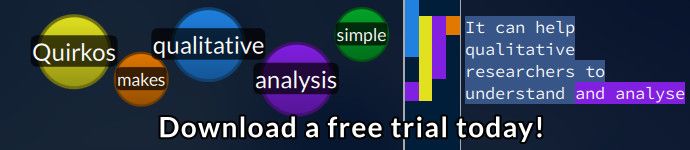
If you are looking for a tool to help your qualitative discourse analysis, why not give Quirkos a try? It was designed by qualitative researchers to be the software they wanted to use, and is flexible enough for a whole number of analytical approaches, including discourse analysis. Download a free trial , or read more about it here .
Gee, J., P., 2011. An Introduction to Discourse Analysis . Routledge, London.
Gergen, K. J., 2015, An invitation to Social Construction . Sage, London.
Hodges, B. D., Kuper, A., Reeves, S. 2008. Discourse Analysis. BMJ , a879.
Johnstone, B., 2017. Discourse Analysis . Wiley, London.
Paltridge, B., 2012. Discourse Analysis: An Introduction . Bloomsbury.
Tannen, D., Hamilton, H., Schiffrin, D. 2015. The Handbook of Discourse Analysis . Wiley, Chichester.
Sign up for more like this.

21 Great Examples of Discourse Analysis
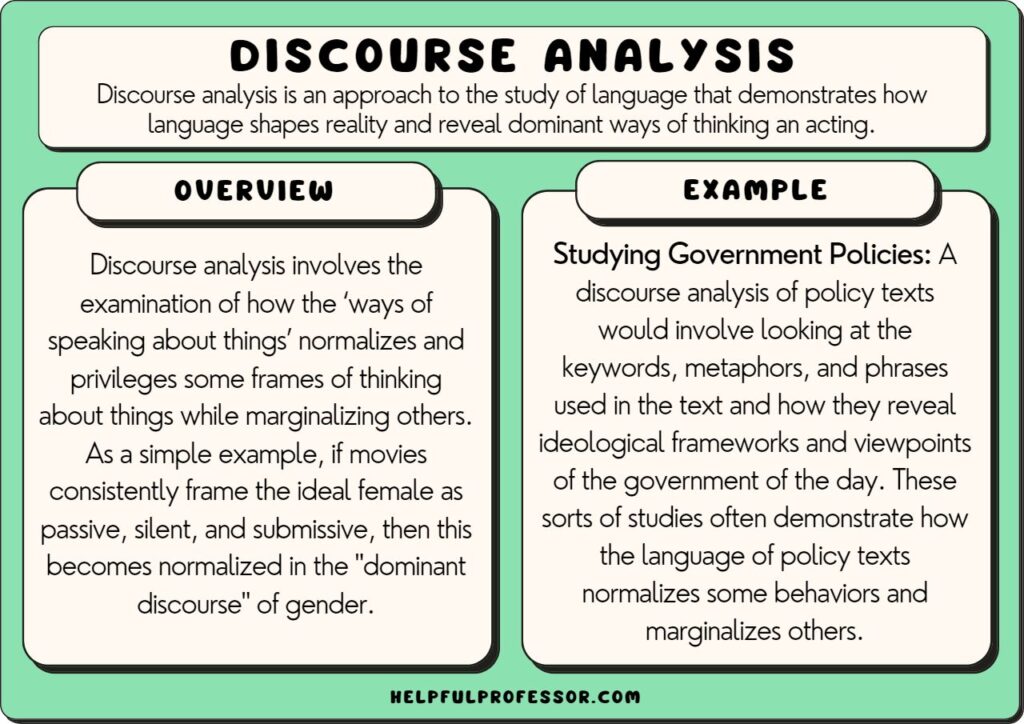
Discourse analysis is an approach to the study of language that demonstrates how language shapes reality. It usually takes the form of a textual or content analysis .
Discourse is understood as a way of perceiving, framing, and viewing the world.
For example:
- A dominant discourse of gender often positions women as gentle and men as active heroes.
- A dominant discourse of race often positions whiteness as the norm and colored bodies as ‘others’ (see: social construction of race )
Through discourse analysis, scholars look at texts and examine how those texts shape discourse.
In other words, it involves the examination of how the ‘ways of speaking about things’ normalizes and privileges some frames of thinking about things while marginalizing others.
As a simple example, if movies consistently frame the ideal female as passive, silent, and submissive, then society comes to think that this is how women should behave and makes us think that this is normal , so women who don’t fit this mold are abnormal .
Instead of seeing this as just the way things are, discourse analysts know that norms are produced in language and are not necessarily as natural as we may have assumed.
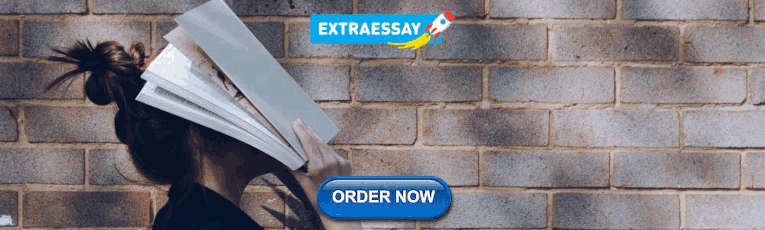
Examples of Discourse Analysis
1. language choice in policy texts.
A study of policy texts can reveal ideological frameworks and viewpoints of the writers of the policy. These sorts of studies often demonstrate how policy texts often categorize people in ways that construct social hierarchies and restrict people’s agency .
Examples include:
2. Newspaper Bias
Conducting a critical discourse analysis of newspapers involves gathering together a quorum of newspaper articles based on a pre-defined range and scope (e.g. newspapers from a particular set of publishers within a set date range).
Then, the researcher conducts a close examination of the texts to examine how they frame subjects (i.e. people, groups of people, etc.) from a particular ideological, political, or cultural perspective.
3. Language in Interviews
Discourse analysis can also be utilized to analyze interview transcripts. While coding methods to identify themes are the most common methods for analyzing interviews, discourse analysis is a valuable approach when looking at power relations and the framing of subjects through speech.
4. Television Analysis
Discourse analysis is commonly used to explore ideologies and framing devices in television shows and advertisements.
Due to the fact advertising is not just textual but rather multimodal , scholars often mix a discourse analytic methodology (i.e. exploring how television constructs dominant ways of thinking) with semiotic methods (i.e. exploration of how color, movement, font choice, and so on create meaning).
I did this, for example, in my PhD (listed below).
5. Film Critique
Scholars can explore discourse in film in a very similar way to how they study discourse in television shows. This can include the framing of sexuality gender, race, nationalism, and social class in films.
A common example is the study of Disney films and how they construct idealized feminine and masculine identities that children should aspire toward.
6. Analysis of Political Speech
Political speeches have also been subject to a significant amount of discourse analysis. These studies generally explore how influential politicians indicate a shift in policy and frame those policy shifts in the context of underlying ideological assumptions.
9. Examining Marketing Texts
Advertising is more present than ever in the context of neoliberal capitalism. As a result, it has an outsized role in shaping public discourse. Critical discourse analyses of advertising texts tend to explore how advertisements, and the capitalist context that underpins their proliferation, normalize gendered, racialized, and class-based discourses.
11. Analyzing Lesson Plans
As written texts, lesson plans can be analyzed for how they construct discourses around education as well as student and teacher identities. These texts tend to examine how teachers and governing bodies in education prioritize certain ideologies around what and how to learn. These texts can enter into discussions around the ‘history wars’ (what and whose history should be taught) as well as ideological approaches to religious and language learning.
12. Looking at Graffiti
One of my favorite creative uses of discourse analysis is in the study of graffiti. By looking at graffiti, researchers can identify how youth countercultures and counter discourses are spread through subversive means. These counterdiscourses offer ruptures where dominant discourses can be unsettled and displaced.
Get a Pdf of this article for class
Enjoy subscriber-only access to this article’s pdf
The Origins of Discourse Analysis
1. foucault.
French philosopher Michel Foucault is a central thinker who shaped discourse analysis. His work in studies like Madness and Civilization and The History of Sexuality demonstrate how our ideas about insanity and sexuality have been shaped through language.
The ways the church speaks about sex, for example, shapes people’s thoughts and feelings about it.
The church didn’t simply make sex a silent taboo. Rather, it actively worked to teach people that desire was a thing of evil, forcing them to suppress their desires.
Over time, society at large developed a suppressed normative approach to the concept of sex that is not necessarily normal except for the fact that the church reiterates that this is the only acceptable way of thinking about the topic.
Similarly, in Madness and Civilization , a discourse around insanity was examined. Medical discourse pathologized behaviors that were ‘abnormal’ as signs of insanity. Were the dominant medical discourse to change, it’s possible that abnormal people would no longer be seen as insane.
One clear example of this is homosexuality. Up until the 1990s, being gay was seen in medical discourse as an illness. Today, most of Western society sees that this way of looking at homosexuality was extremely damaging and exclusionary, and yet at the time, because it was the dominant discourse, people didn’t question it.
2. Norman Fairclough
Fairclough (2013), inspired by Foucault, created some key methodological frameworks for conducting discourse analysis.
Fairclough was one of the first scholars to articulate some frameworks around exploring ‘text as discourse’ and provided key tools for scholars to conduct analyses of newspaper and policy texts.
Today, most methodology chapters in dissertations that use discourse analysis will have extensive discussions of Fairclough’s methods.
Discourse analysis is a popular primary research method in media studies, cultural studies, education studies, and communication studies. It helps scholars to show how texts and language have the power to shape people’s perceptions of reality and, over time, shift dominant ways of framing thought. It also helps us to see how power flows thought texts, creating ‘in-groups’ and ‘out-groups’ in society.
Key examples of discourse analysis include the study of television, film, newspaper, advertising, political speeches, and interviews.
Al Kharusi, R. (2017). Ideologies of Arab media and politics: a CDA of Al Jazeera debates on the Yemeni revolution. PhD Dissertation: University of Hertfordshire.
Alaazi, D. A., Ahola, A. N., Okeke-Ihejirika, P., Yohani, S., Vallianatos, H., & Salami, B. (2021). Immigrants and the Western media: a CDA of newspaper framings of African immigrant parenting in Canada. Journal of Ethnic and Migration Studies , 47 (19), 4478-4496. Doi: https://doi.org/10.1080/1369183X.2020.1798746
Al-Khawaldeh, N. N., Khawaldeh, I., Bani-Khair, B., & Al-Khawaldeh, A. (2017). An exploration of graffiti on university’s walls: A corpus-based discourse analysis study. Indonesian Journal of Applied Linguistics , 7 (1), 29-42. Doi: https://doi.org/10.17509/ijal.v7i1.6856
Alsaraireh, M. Y., Singh, M. K. S., & Hajimia, H. (2020). Critical DA of gender representation of male and female characters in the animation movie, Frozen. Linguistica Antverpiensia , 104-121.
Baig, F. Z., Khan, K., & Aslam, M. J. (2021). Child Rearing and Gender Socialisation: A Feminist CDA of Kids’ Popular Fictional Movies. Journal of Educational Research and Social Sciences Review (JERSSR) , 1 (3), 36-46.
Barker, M. E. (2021). Exploring Canadian Integration through CDA of English Language Lesson Plans for Immigrant Learners. Canadian Journal of Applied Linguistics/Revue canadienne de linguistique appliquée , 24 (1), 75-91. Doi: https://doi.org/10.37213/cjal.2021.28959
Coleman, B. (2017). An Ideological Unveiling: Using Critical Narrative and Discourse Analysis to Examine Discursive White Teacher Identity. AERA Online Paper Repository .
Drew, C. (2013). Soak up the goodness: Discourses of Australian childhoods on television advertisements, 2006-2012. PhD Dissertation: Australian Catholic University. Doi: https://doi.org/10.4226/66/5a9780223babd
Fairclough, N. (2013). Critical discourse analysis: The critical study of language . London: Routledge.
Foucault, M. (1990). The history of sexuality: An introduction . London: Vintage.
Foucault, M. (2003). Madness and civilization . New York: Routledge.
Hahn, A. D. (2018). Uncovering the ideologies of internationalization in lesson plans through CDA. The New English Teacher , 12 (1), 121-121.
Isti’anah, A. (2018). Rohingya in media: CDA of Myanmar and Bangladesh newspaper headlines. Language in the Online and Offline World , 6 , 18-23. Doi: http://repository.usd.ac.id/id/eprint/25962
Khan, M. H., Adnan, H. M., Kaur, S., Qazalbash, F., & Ismail, I. N. (2020). A CDA of anti-Muslim rhetoric in Donald Trump’s historic 2016 AIPAC policy speech. Journal of Muslim Minority Affairs , 40 (4), 543-558. Doi: https://doi.org/10.1080/13602004.2020.1828507
Louise Cooper, K., Luck, L., Chang, E., & Dixon, K. (2021). What is the practice of spiritual care? A CDA of registered nurses’ understanding of spirituality. Nursing Inquiry , 28 (2), e12385. Doi: https://doi.org/10.1111/nin.12385
Mohammadi, D., Momeni, S., & Labafi, S. (2021). Representation of Iranians family’s life style in TV advertising (Case study: food ads). Religion & Communication , 27 (58), 333-379.
Munro, M. (2018) House price inflation in the news: a CDA of newspaper coverage in the UK. Housing Studies, 33(7), pp. 1085-1105. doi: 10.1080/02673037.2017.1421911
Ravn, I. M., Frederiksen, K., & Beedholm, K. (2016). The chronic responsibility: a CDA of Danish chronic care policies. Qualitative Health Research , 26 (4), 545-554. Doi: https://doi.org/10.1177%2F1049732315570133
Sengul, K. (2019). Critical discourse analysis in political communication research: a case study of right-wing populist discourse in Australia. Communication Research and Practice , 5 (4), 376-392. Doi: https://doi.org/10.1080/22041451.2019.1695082
Serafis, D., Kitis, E. D., & Archakis, A. (2018). Graffiti slogans and the construction of collective identity: evidence from the anti-austerity protests in Greece. Text & Talk , 38 (6), 775-797. Doi: https://doi.org/10.1515/text-2018-0023
Suphaborwornrat, W., & Punkasirikul, P. (2022). A Multimodal CDA of Online Soft Drink Advertisements. LEARN Journal: Language Education and Acquisition Research Network , 15 (1), 627-653.
Symes, C., & Drew, C. (2017). Education on the rails: a textual ethnography of university advertising in mobile contexts. Critical Studies in Education , 58 (2), 205-223. Doi: https://doi.org/10.1080/17508487.2016.1252783
Thomas, S. (2005). The construction of teacher identities in educational policy documents: A critical discourse analysis. Critical Studies in Education , 46 (2), 25-44. Doi: https://doi.org/10.1080/17508480509556423
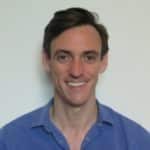
Chris Drew (PhD)
Dr. Chris Drew is the founder of the Helpful Professor. He holds a PhD in education and has published over 20 articles in scholarly journals. He is the former editor of the Journal of Learning Development in Higher Education. [Image Descriptor: Photo of Chris]
- Chris Drew (PhD) https://helpfulprofessor.com/author/chris-drew-phd/ 5 Top Tips for Succeeding at University
- Chris Drew (PhD) https://helpfulprofessor.com/author/chris-drew-phd/ 50 Durable Goods Examples
- Chris Drew (PhD) https://helpfulprofessor.com/author/chris-drew-phd/ 100 Consumer Goods Examples
- Chris Drew (PhD) https://helpfulprofessor.com/author/chris-drew-phd/ 30 Globalization Pros and Cons
Leave a Comment Cancel Reply
Your email address will not be published. Required fields are marked *
Explore our publications and services.
University of michigan press.
Publishes award-winning books that advance humanities and social science fields, as well as English language teaching and regional resources.
Michigan Publishing Services
Assists the U-M community of faculty, staff, and students in achieving their publishing ambitions.
Deep Blue Repositories
Share and access research data, articles, chapters, dissertations and more produced by the U-M community.
A community-based, open source publishing platform that helps publishers present the full richness of their authors' research outputs in a durable, discoverable, accessible and flexible form. Developed by Michigan Publishing and University of Michigan Library.

- shopping_cart Cart
Browse Our Books
- See All Books
- Distributed Clients
Feature Selections
- New Releases
- Forthcoming
- Bestsellers
- Great Lakes
English Language Teaching
- Companion Websites
- Subject Index
- Resources for Teachers and Students
By Skill Area
- Academic Skills/EAP
- Teacher Training
For Authors
Prospective authors.
- Why Publish with Michigan?
- Open Access
- Our Publishing Program
- Submission Guidelines
Author's Guide
- Introduction
- Final Manuscript Preparation
- Production Process
- Marketing and Sales
- Guidelines for Indexing
For Instructors
- Exam Copies
- Desk Copies
For Librarians and Booksellers
- Our Ebook Collection
- Ordering Information for Booksellers
- Review Copies
Background and Contacts
- About the Press
- Customer Service
- Staff Directory
News and Information
- Conferences and Events
Policies and Requests
- Rights and Permissions
- Accessibility
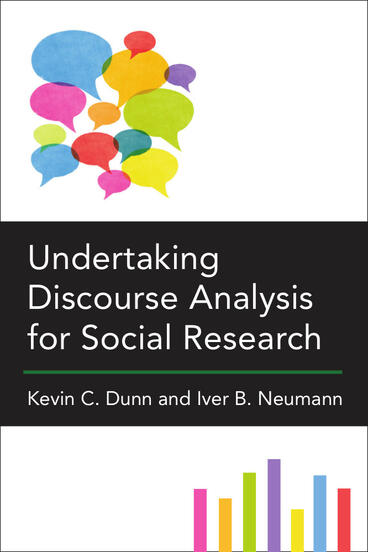
Undertaking Discourse Analysis for Social Research
An indispensable guide to the theory, concept, and application of discourse analysis in the social sciences
Look Inside
- Table of Contents
Description
Kevin C. Dunn and Iver B. Neumann offer a concise, accessible introduction to discourse analysis in the social sciences. A vital resource for students and scholars alike, Undertaking Discourse Analysis for Social Research combines a theoretical and conceptual review with a “how-to” guide for using the method. In the first part of the book, the authors discuss the development of discourse analysis as a research method and identify the main theoretical elements and epistemological assumptions that have led to its emergence as one of the primary qualitative methods of analysis in contemporary scholarship. Then, drawing from a wide-range of examples of social science scholarship, Dunn and Neumann provide an indispensable guide to the variety of ways discourse analysis has been used. They delve into what is gained by using this approach and demonstrate how one actually applies it. They cover such important issues as research prerequisites, how one conceives of a research question, what “counts” as evidence, how one “reads” the data, and some common obstacles and pitfalls. The result is a clear and accessible manual for successfully implementing discourse analysis in social research.
Kevin C. Dunn is Professor of Political Science at Hobart and William Smith Colleges. Iver B. Neumann is the Montague Burton Professor of International Relations at the London School of Economics and Political Science and a Research Professor at the Norwegian Institute of International Affairs.
“This is quite simply the best introductory overview of how to actually ‘do’ discourse analysis I know of. Spanning the distance between analytical and critical methodology, poststructural theory, and concrete steps for gathering and evaluating relevant data, Dunn and Neumann have filled an important gap in the literature for students of discourse analysis at every level.” — Patrick Thaddeus Jackson, American University
“In this concise, well-written, impressively researched text, Dunn and Neumann provide a badly needed resource for the social sciences: a discourse analysis methods book with instructions, examples, and substantive analysis. This text makes discourse analysis in the field accessible, and provides a guide not only to doing it but also to understanding it. An impressive accomplishment!” — Laura Sjoberg, University of Florida
“An appropriate and useful source for both graduate and postgraduate courses on qualitative research methodology as well as for introduction to specialized courses on discourse analysis.” — Milena Komarova, Queen’s University Belfast “Whether it is undergraduate, postgraduate, or doctoral research, this book will be an invaluable guide to the conduct of discourse-theoretical analysis in the social sciences.” — Laura J. Shepherd, UNSW Australia
Thank you for visiting nature.com. You are using a browser version with limited support for CSS. To obtain the best experience, we recommend you use a more up to date browser (or turn off compatibility mode in Internet Explorer). In the meantime, to ensure continued support, we are displaying the site without styles and JavaScript.
- View all journals
- My Account Login
- Explore content
- About the journal
- Publish with us
- Sign up for alerts
- Open access
- Published: 20 March 2024
Persistent interaction patterns across social media platforms and over time
- Michele Avalle ORCID: orcid.org/0009-0007-4934-2326 1 na1 ,
- Niccolò Di Marco 1 na1 ,
- Gabriele Etta 1 na1 ,
- Emanuele Sangiorgio ORCID: orcid.org/0009-0003-1024-3735 2 ,
- Shayan Alipour 1 ,
- Anita Bonetti 3 ,
- Lorenzo Alvisi 1 ,
- Antonio Scala 4 ,
- Andrea Baronchelli 5 , 6 ,
- Matteo Cinelli ORCID: orcid.org/0000-0003-3899-4592 1 &
- Walter Quattrociocchi ORCID: orcid.org/0000-0002-4374-9324 1
Nature ( 2024 ) Cite this article
11k Accesses
215 Altmetric
Metrics details
- Mathematics and computing
- Social sciences
Growing concern surrounds the impact of social media platforms on public discourse 1 , 2 , 3 , 4 and their influence on social dynamics 5 , 6 , 7 , 8 , 9 , especially in the context of toxicity 10 , 11 , 12 . Here, to better understand these phenomena, we use a comparative approach to isolate human behavioural patterns across multiple social media platforms. In particular, we analyse conversations in different online communities, focusing on identifying consistent patterns of toxic content. Drawing from an extensive dataset that spans eight platforms over 34 years—from Usenet to contemporary social media—our findings show consistent conversation patterns and user behaviour, irrespective of the platform, topic or time. Notably, although long conversations consistently exhibit higher toxicity, toxic language does not invariably discourage people from participating in a conversation, and toxicity does not necessarily escalate as discussions evolve. Our analysis suggests that debates and contrasting sentiments among users significantly contribute to more intense and hostile discussions. Moreover, the persistence of these patterns across three decades, despite changes in platforms and societal norms, underscores the pivotal role of human behaviour in shaping online discourse.
Similar content being viewed by others
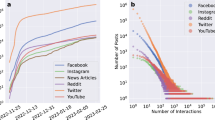
Cross-platform social dynamics: an analysis of ChatGPT and COVID-19 vaccine conversations
Shayan Alipour, Alessandro Galeazzi, … Walter Quattrociocchi
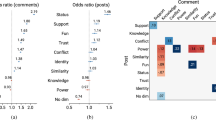
The language of opinion change on social media under the lens of communicative action
Corrado Monti, Luca Maria Aiello, … Francesco Bonchi
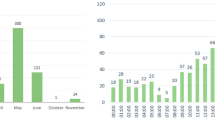
A social media network analysis of trypophobia communication
Xanat Vargas Meza & Shinichi Koyama
The advent and proliferation of social media platforms have not only transformed the landscape of online participation 2 but have also become integral to our daily lives, serving as primary sources for information, entertainment and personal communication 13 , 14 . Although these platforms offer unprecedented connectivity and information exchange opportunities, they also present challenges by entangling their business models with complex social dynamics, raising substantial concerns about their broader impact on society. Previous research has extensively addressed issues such as polarization, misinformation and antisocial behaviours in online spaces 5 , 7 , 12 , 15 , 16 , 17 , revealing the multifaceted nature of social media’s influence on public discourse. However, a considerable challenge in understanding how these platforms might influence inherent human behaviours lies in the general lack of accessible data 18 . Even when researchers obtain data through special agreements with companies like Meta, it may not be enough to clearly distinguish between inherent human behaviours and the effects of the platform’s design 3 , 4 , 8 , 9 . This difficulty arises because the data, deeply embedded in platform interactions, complicate separating intrinsic human behaviour from the influences exerted by the platform’s design and algorithms.
Here we address this challenge by focusing on toxicity, one of the most prominent aspects of concern in online conversations. We use a comparative analysis to uncover consistent patterns across diverse social media platforms and timeframes, aiming to shed light on toxicity dynamics across various digital environments. In particular, our goal is to gain insights into inherently invariant human patterns of online conversations.
The lack of non-verbal cues and physical presence on the web can contribute to increased incivility in online discussions compared with face-to-face interactions 19 . This trend is especially pronounced in online arenas such as newspaper comment sections and political discussions, where exchanges may degenerate into offensive comments or mockery, undermining the potential for productive and democratic debate 20 , 21 . When exposed to such uncivil language, users are more likely to interpret these messages as hostile, influencing their judgement and leading them to form opinions based on their beliefs rather than the information presented and may foster polarized perspectives, especially among groups with differing values 22 . Indeed, there is a natural tendency for online users to seek out and align with information that echoes their pre-existing beliefs, often ignoring contrasting views 6 , 23 . This behaviour may result in the creation of echo chambers, in which like-minded individuals congregate and mutually reinforce shared narratives 5 , 24 , 25 . These echo chambers, along with increased polarization, vary in their prevalence and intensity across different social media platforms 1 , suggesting that the design and algorithms of these platforms, intended to maximize user engagement, can substantially shape online social dynamics. This focus on engagement can inadvertently highlight certain behaviours, making it challenging to differentiate between organic user interaction and the influence of the platform’s design. A substantial portion of current research is devoted to examining harmful language on social media and its wider effects, online and offline 10 , 26 . This examination is crucial, as it reveals how social media may reflect and amplify societal issues, including the deterioration of public discourse. The growing interest in analysing online toxicity through massive data analysis coincides with advancements in machine learning capable of detecting toxic language 27 . Although numerous studies have focused on online toxicity, most concentrate on specific platforms and topics 28 , 29 . Broader, multiplatform studies are still limited in scale and reach 12 , 30 . Research fragmentation complicates understanding whether perceptions about online toxicity are accurate or misconceptions 31 . Key questions include whether online discussions are inherently toxic and how toxic and non-toxic conversations differ. Clarifying these dynamics and how they have evolved over time is crucial for developing effective strategies and policies to mitigate online toxicity.
Our study involves a comparative analysis of online conversations, focusing on three dimensions: time, platform and topic. We examine conversations from eight different platforms, totalling about 500 million comments. For our analysis, we adopt the toxicity definition provided by the Perspective API, a state-of-the-art classifier for the automatic detection of toxic speech. This API considers toxicity as “a rude, disrespectful or unreasonable comment likely to make someone leave a discussion”. We further validate this definition by confirming its consistency with outcomes from other detection tools, ensuring the reliability and comparability of our results. The concept of toxicity in online discourse varies widely in the literature, reflecting its complexity, as seen in various studies 32 , 33 , 34 . The efficacy and constraints of current machine-learning-based automated toxicity detection systems have recently been debated 11 , 35 . Despite these discussions, automated systems are still the most practical means for large-scale analyses.
Here we analyse online conversations, challenging common assumptions about their dynamics. Our findings reveal consistent patterns across various platforms and different times, such as the heavy-tailed nature of engagement dynamics, a decrease in user participation and an increase in toxic speech in lengthier conversations. Our analysis indicates that, although toxicity and user participation in debates are independent variables, the diversity of opinions and sentiments among users may have a substantial role in escalating conversation toxicity.
To obtain a comprehensive picture of online social media conversations, we analysed a dataset of about 500 million comments from Facebook, Gab, Reddit, Telegram, Twitter, Usenet, Voat and YouTube, covering diverse topics and spanning over three decades (a dataset breakdown is shown in Table 1 and Supplementary Table 1 ; for details regarding the data collection, see the ‘Data collection’ section of the Methods ).
Our analysis aims to comprehensively compare the dynamics of diverse social media accounting for human behaviours and how they evolved. In particular, we first characterize conversations at a macroscopic level by means of their engagement and participation, and we then analyse the toxicity of conversations both after and during their unfolding. We conclude the paper by examining potential drivers for the emergence of toxic speech.
Conversations on different platforms
This section provides an overview of online conversations by considering user activity and thread size metrics. We define a conversation (or a thread) as a sequence of comments that follow chronologically from an initial post. In Fig. 1a and Extended Data Fig. 1 , we observe that, across all platforms, both user activity (defined as the number of comments posted by the user) and thread length (defined as the number of comments in a thread) exhibit heavy-tailed distributions. The summary statistics about these distributions are reported in Supplementary Tables 1 and 2 .
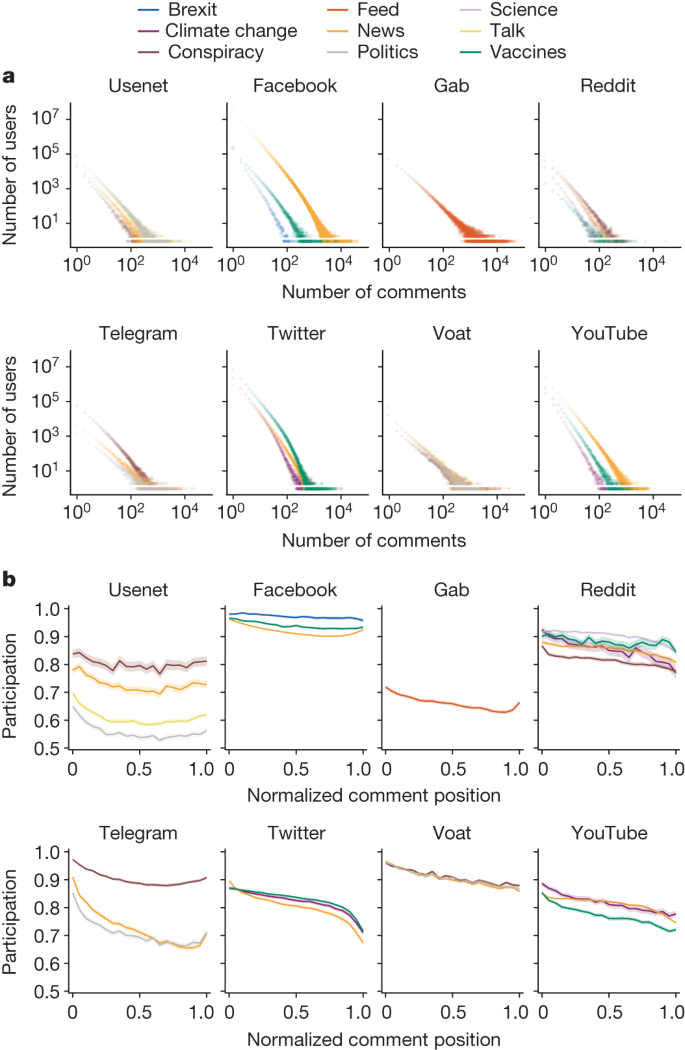
a , The distributions of user activity in terms of comments posted for each platform and each topic. b , The mean user participation as conversations evolve. For each dataset, participation is computed for the threads belonging to the size interval [0.7–1] (Supplementary Table 2 ). Trends are reported with their 95% confidence intervals. The x axis represents the normalized position of comment intervals in the threads.
Consistent with previous studies 36 , 37 our analysis shows that the macroscopic patterns of online conversations, such as the distribution of users/threads activity and lifetime, are consistent across all datasets and topics (Supplementary Tables 1 – 4 ). This observation holds regardless of the specific features of the diverse platforms, such as recommendation algorithms and moderation policies (described in the ‘Content moderation policies’ of the Methods ), as well as other factors, including the user base and the conversation topics. We extend our analysis by examining another aspect of user activity within conversations across all platforms. To do this, we introduce a metric for the participation of users as a thread evolves. In this analysis, threads are filtered to ensure sufficient length as explained in the ‘Logarithmic binning and conversation size’ section of the Methods .
The participation metric, defined over different conversation intervals (that is, 0–5% of the thread arranged in chronological order, 5–10%, and so on), is the ratio of the number of unique users to the number of comments in the interval. Considering a fixed number of comments c , smaller values of participation indicate that fewer unique users are producing c comments in a segment of the conversation. In turn, a value of participation equal to 1 means that each user is producing one of the c comments, therefore obtaining the maximal homogeneity of user participation. Our findings show that, across all datasets, the participation of users in the evolution of conversations, averaged over almost all considered threads, is decreasing, as indicated by the results of Mann–Kendall test—a nonparametric test assessing the presence of a monotonic upward or downward tendency—shown in Extended Data Table 1 . This indicates that fewer users tend to take part in a conversation as it evolves, but those who do are more active (Fig. 1b ). Regarding patterns and values, the trends in user participation for various topics are consistent across each platform. According to the Mann–Kendall test, the only exceptions were Usenet Conspiracy and Talk, for which an ambiguous trend was detected. However, we note that their regression slopes are negative, suggesting a decreasing trend, even if with a weaker effect. Overall, our first set of findings highlights the shared nature of certain online interactions, revealing a decrease in user participation over time but an increase in activity among participants. This insight, consistent across most platforms, underscores the dynamic interplay between conversation length, user engagement and topic-driven participation.
Conversation size and toxicity
To detect the presence of toxic language, we used Google’s Perspective API 34 , a state-of-the-art toxicity classifier that has been used extensively in recent literature 29 , 38 . Perspective API defines a toxic comment as “A rude, disrespectful, or unreasonable comment that is likely to make people leave a discussion”. On the basis of this definition, the classifier assigns a toxicity score in the [0,1] range to a piece of text that can be interpreted as an estimate of the likelihood that a reader would perceive the comment as toxic ( https://developers.perspectiveapi.com/s/about-the-api-score ). To define an appropriate classification threshold, we draw from the existing literature 39 , which uses 0.6 as the threshold for considering a comment as toxic. A robustness check of our results using different threshold and classification tools is reported in the ‘Toxicity detection and validation of employed models’ section of the Methods , together with a discussion regarding potential shortcomings deriving from automatic classifiers. To further investigate the interplay between toxicity and conversation features across various platforms, our study first examines the prevalence of toxic speech in each dataset. We then analyse the occurrence of highly toxic users and conversations. Lastly, we investigate how the length of conversations correlates with the probability of encountering toxic comments. First of all, we define the toxicity of a user as the fraction of toxic comments that she/he left. Similarly, the toxicity of a thread is the fraction of toxic comments it contains. We begin by observing that, although some toxic datasets exist on unmoderated platforms such as Gab, Usenet and Voat, the prevalence of toxic speech is generally low. Indeed, the percentage of toxic comments in each dataset is mostly below 10% (Table 1 ). Moreover, the complementary cumulative distribution functions illustrated in Extended Data Fig. 2 show that the fraction of extremely toxic users is very low for each dataset (in the range between 10 −3 and 10 −4 ), and the majority of active users wrote at least one toxic comment, as reported in Supplementary Table 5 , therefore suggesting that the overall volume of toxicity is not a phenomenon limited to the activity of very few users and localized in few conversations. Indeed, the number of users versus their toxicity decreases sharply following an exponential trend. The toxicity of threads follows a similar pattern. To understand the association between the size and toxicity of a conversation, we start by grouping conversations according to their length to analyse their structural differences 40 . The grouping is implemented by means of logarithmic binning (see the ‘Logarithmic binning and conversation size’ section of the Methods ) and the evolution of the average fraction of toxic comments in threads versus the thread size intervals is reported in Fig. 2 . Notably, the resulting trends are almost all increasing, showing that, independently of the platform and topic, the longer the conversation, the more toxic it tends to be.
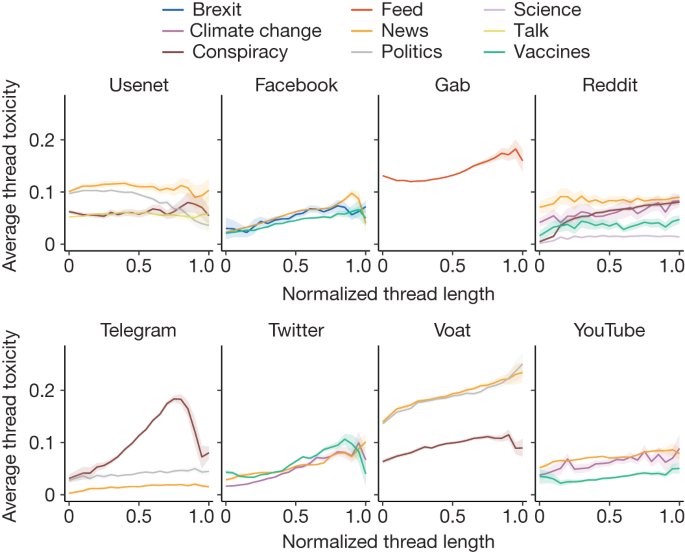
The mean fraction of toxic comments in conversations versus conversation size for each dataset. Trends represent the mean toxicity over each size interval and their 95% confidence interval. Size ranges are normalized to enable visual comparison of the different trends.
We assessed the increase in the trends by both performing linear regression and applying the Mann–Kendall test to ensure the statistical significance of our results (Extended Data Table 2 ). To further validate these outcomes, we shuffled the toxicity labels of comments, finding that trends are almost always non-increasing when data are randomized. Furthermore, the z -scores of the regression slopes indicate that the observed trends deviate from the mean of the distributions resulting from randomizations, being at least 2 s.d. greater in almost all cases. This provides additional evidence of a remarkable difference from randomness. The only decreasing trend is Usenet Politics. Moreover, we verified that our results are not influenced by the specific number of bins as, after estimating the same trends again with different intervals, we found that the qualitative nature of the results remains unchanged. These findings are summarized in Extended Data Table 2 . These analyses have been validated on the same data using a different threshold for identifying toxic comments and on a new dataset labelled with three different classifiers, obtaining similar results (Extended Data Fig. 5 , Extended Data Table 5 , Supplementary Fig. 1 and Supplementary Table 8 ). Finally, using a similar approach, we studied the toxicity content of conversations versus their lifetime—that is, the time elapsed between the first and last comment. In this case, most trends are flat, and there is no indication that toxicity is generally associated either with the duration of a conversation or the lifetime of user interactions (Extended Data Fig. 4 ).
Conversation evolution and toxicity
In the previous sections, we analysed the toxicity level of online conversations after their conclusion. We next focus on how toxicity evolves during a conversation and its effect on the dynamics of the discussion. The common beliefs that (1) online interactions inevitably devolve into toxic exchanges over time and (2) once a conversation reaches a certain toxicity threshold, it would naturally conclude, are not modern notions but they were also prevalent in the early days of the World Wide Web 41 . Assumption 2 aligns with the Perspective API’s definition of toxic language, suggesting that increased toxicity reduces the likelihood of continued participation in a conversation. However, this observation should be reconsidered, as it is not only the peak levels of toxicity that might influence a conversation but, for example, also a consistent rate of toxic content. To test these common assumptions, we used a method similar to that used for measuring participation; we select sufficiently long threads, divide each of them into a fixed number of equal intervals, compute the fraction of toxic comments for each of these intervals, average it over all threads and plot the toxicity trend through the unfolding of the conversations. We find that the average toxicity level remains mostly stable throughout, without showing a distinctive increase around the final part of threads (Fig. 3a (bottom) and Extended Data Fig. 3 ). Note that a similar observation was made previously 41 , but referring only to Reddit. Our findings challenge the assumption that toxicity discourages people from participating in a conversation, even though this notion is part of the definition of toxicity used by the detection tool. This can be seen by checking the relationship between trends in user participation, a quantity related to the number of users in a discussion at some point, and toxicity. The fact that the former typically decreases while the latter remains stable during conversations indicates that toxicity is not associated with participation in conversations (an example is shown in Fig. 3a ; box plots of the slopes of participation and toxicity for the whole dataset are shown in Fig. 3b ). This suggests that, on average, people may leave discussions regardless of the toxicity of the exchanges. We calculated the Pearson’s correlation between user participation and toxicity trends for each dataset to support this hypothesis. As shown in Fig. 3d , the resulting correlation coefficients are very heterogeneous, indicating no consistent pattern across different datasets. To further validate this analysis, we tested the differences in the participation of users commenting on either toxic or non-toxic conversations. To split such conversations into two disjoint sets, we first compute the toxicity distribution T i of long threads in each dataset i , and we then label a conversation j in dataset i as toxic if it has toxicity t ij ≥ µ ( T i ) + σ ( T i ), with µ ( T i ) being mean and σ ( T i ) the standard deviation of T i ; all of the other conversations are considered to be non-toxic. After splitting the threads, for each dataset, we compute the Pearson’s correlation of user participation between sets to find strongly positive values of the coefficient in all cases (Fig. 3c,e ). This result is also confirmed by a different analysis of which the results are reported in Supplementary Table 8 , in which no significant difference between slopes in toxic and non-toxic threads can be found. Thus, user behaviour in toxic and non-toxic conversations shows almost identical patterns in terms of participation. This reinforces our finding that toxicity, on average, does not appear to affect the likelihood of people participating in a conversation. These analyses were repeated with a lower toxicity classification threshold (Extended Data Fig. 5 ) and on additional datasets (Supplementary Fig. 2 and Supplementary Table 11 ), finding consistent results.
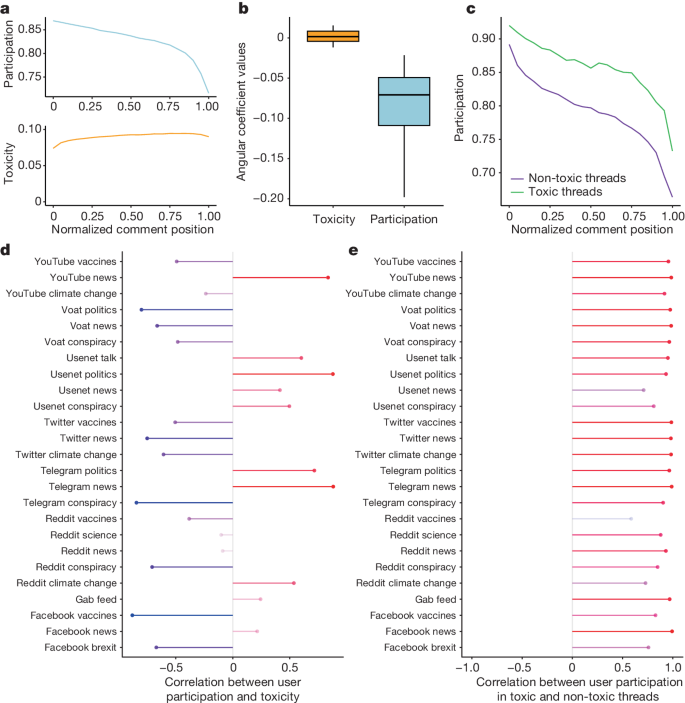
a , Examples of a typical trend in averaged user participation (top) and toxicity (bottom) versus the normalized position of comment intervals in the threads (Twitter news dataset). b , Box plot distributions of toxicity ( n = 25, minimum = −0.012, maximum = 0.015, lower whisker = −0.012, quartile 1 (Q1) = − 0.004, Q2 = 0.002, Q3 = 0.008, upper whisker = 0.015) and participation ( n = 25, minimum = −0.198, maximum = −0.022, lower whisker = −0.198, Q1 = − 0.109, Q2 = − 0.071, Q3 = − 0.049, upper whisker = −0.022) trend slopes for all datasets, as resulting from linear regression. c , An example of user participation in toxic and non-toxic thread sets (Twitter news dataset). d , Pearson’s correlation coefficients between user participation and toxicity trends for each dataset. e , Pearson’s correlation coefficients between user participation in toxic and non-toxic threads for each dataset.
Controversy and toxicity
In this section, we aim to explore why people participate in toxic online conversations and why longer discussions tend to be more toxic. Several factors could be the subject matter. First, controversial topics might lead to longer, more heated debates with increased toxicity. Second, the endorsement of toxic content by other users may act as an incentive to increase the discussion’s toxicity. Third, engagement peaks, due to factors such as reduced discussion focus or the intervention of trolls, may bring a higher share of toxic exchanges. Pursuing this line of inquiry, we identified proxies to measure the level of controversy in conversations and examined how these relate to toxicity and conversation size. Concurrently, we investigated the relationship between toxicity, endorsement and engagement.
As shown previously 24 , 42 , controversy is likely to emerge when people with opposing views engage in the same debate. Thus, the presence of users with diverse political leanings within a conversation could be a valid proxy for measuring controversy. We operationalize this definition as follows. Exploiting the peculiarities of our data, we can infer the political leaning of a subset of users in the Facebook News, Twitter News, Twitter Vaccines and Gab Feed datasets. This is achieved by examining the endorsement, for example, in the form of likes, expressed towards news outlets of which the political inclinations have been independently assessed by news rating agencies (see the ‘Polarization and user leaning attribution’ section of the Methods ). Extended Data Table 3 shows a breakdown of the datasets. As a result, we label users with a leaning score l ∈ [−1, 1], −1 being left leaning and +1 being right leaning. We then select threads with at least ten different labelled users, in which at least 10% of comments (with a minimum of 20) are produced by such users and assign to each of these comments the same leaning score of those who posted them. In this setting, the level of controversy within a conversation is assumed to be captured by the spread of the political leaning of the participants in the conversation. A natural way for measuring such a spread is the s.d. σ ( l ) of the distribution of comments possessing a leaning score: the higher the σ ( l ), the greater the level of ideological disagreement and therefore controversy in a thread. We analysed the relationship between controversy and toxicity in online conversations of different sizes. Figure 4a shows that controversy increases with the size of conversations in all datasets, and its trends are positively correlated with the corresponding trends in toxicity (Extended Data Table 3 ). This supports our hypothesis that controversy and toxicity are closely related in online discussions.
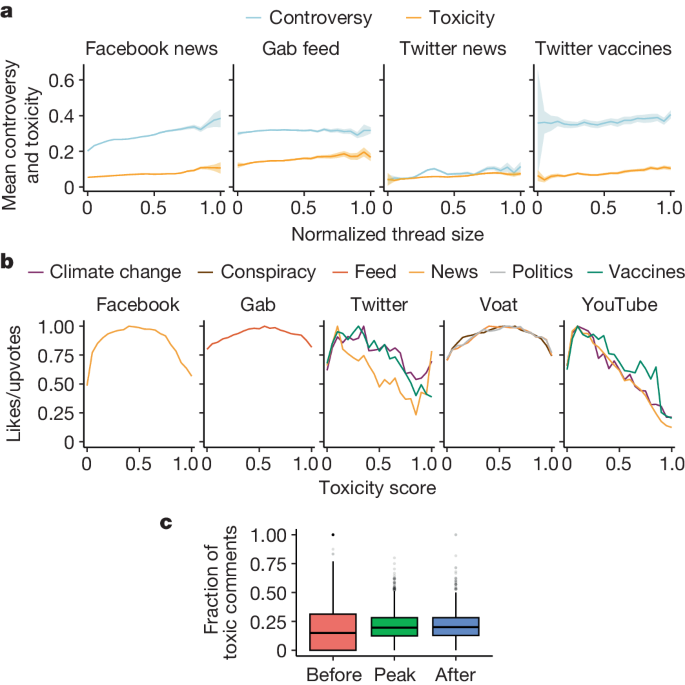
a , The mean controversy ( σ ( l )) and mean toxicity versus thread size (log-binned and normalized) for the Facebook news, Twitter news, Twitter vaccines and Gab feed datasets. Here toxicity is calculated in the same conversations in which controversy could be computed (Extended Data Table 3 ); the relative Pearson’s, Spearman’s and Kendall’s correlation coefficients are also provided in Extended Data Table 3 . Trends are reported with their 95% confidence interval. b , Likes/upvotes versus toxicity (linearly binned). c , An example (Voat politics dataset) of the distributions of the frequency of toxic comments in threads before ( n = 2,201, minimum = 0, maximum = 1, lower whisker = 0, Q1 = 0, Q2 = 0.15, Q3 = 0.313, upper whisker = 0.769) at the peak ( n = 2,798, minimum = 0, maximum = 0.8, lower whisker = 0, Q1 = 0.125, Q2 = 0.196, Q3 = 0.282, upper whisker = 0.513) and after the peak ( n = 2,791, minimum = 0, maximum = 1, lower whisker = 0, Q1 = 0.129, Q2 = 0.200, Q3 = 0.282, upper whisker = 0.500) of activity, as detected by Kleinberg’s burst detection algorithm.
As a complementary analysis, we draw on previous results 43 . In that study, using a definition of controversy operationally different but conceptually related to ours, a link was found between a greater degree of controversy of a discussion topic and a wider distribution of sentiment scores attributed to the set of its posts and comments. We quantified the sentiment of comments using a pretrained BERT model available from Hugging Face 44 , used also in previous studies 45 . The model predicts the sentiment of a sentence through a scoring system ranging from 1 (negative) to 5 (positive). We define the sentiment attributed to a comment c as its weighted mean \(s(c)=\sum _{i=1.5}{x}_{i}{p}_{i}\) , where x i ∈ [1, 5] is the output score from the model and p i is the probability associated to that value. Moreover, we normalize the sentiment score s for each dataset between 0 and 1. We observe the trends of the mean s.d. of sentiment in conversations, \(\bar{\sigma }(s)\) , and toxicity are positively correlated for moderated platforms such as Facebook and Twitter but are negatively correlated on Gab (Extended Data Table 3 ). The positive correlation observed in Facebook and Twitter indicates that greater discrepancies in sentiment of the conversations can, in general, be linked to toxic conversations and vice versa. Instead, on unregulated platforms such as Gab, highly conflicting sentiments seem to be more likely to emerge in less toxic conversations.
As anticipated, another factor that may be associated with the emergence of toxic comments is the endorsement they receive. Indeed, such positive reactions may motivate posting even more comments of the same kind. Using the mean number of likes/upvotes as a proxy of endorsement, we have an indication that this may not be the case. Figure 4b shows that the trend in likes/upvotes versus comments toxicity is never increasing past the toxicity score threshold (0.6).
Finally, to complement our analysis, we inspect the relationship between toxicity and user engagement within conversations, measured as the intensity of the number of comments over time. To do so, we used a method for burst detection 46 that, after reconstructing the density profile of a temporal stream of elements, separates the stream into different levels of intensity and assigns each element to the level to which it belongs (see the ‘Burst analysis’ section of the Methods ). We computed the fraction of toxic comments at the highest intensity level of each conversation and for the levels right before and after it. By comparing the distributions of the fraction of toxic comments for the three intervals, we find that these distributions are statistically different in almost all cases (Fig. 4c and Extended Data Table 4 ). In all datasets but one, distributions are consistently shifted towards higher toxicity at the peak of engagement, compared with the previous phase. Likewise, in most cases, the peak shows higher toxicity even if compared to the following phase, which in turn is mainly more toxic than the phase before the peak. These results suggest that toxicity is likely to increase together with user engagement.
Here we examine one of the most prominent and persistent characteristics online discussions—toxic behaviour, defined here as rude, disrespectful or unreasonable conduct. Our analysis suggests that toxicity is neither a deterrent to user involvement nor an engagement amplifier; rather, it tends to emerge when exchanges become more frequent and may be a product of opinion polarization. Our findings suggest that the polarization of user opinions—intended as the degree of opposed partisanship of users in a conversation—may have a more crucial role than toxicity in shaping the evolution of online discussions. Thus, monitoring polarization could indicate early interventions in online discussions. However, it is important to acknowledge that the dynamics at play in shaping online discourse are probably multifaceted and require a nuanced approach for effective moderation. Other factors may influence toxicity and engagement, such as the specific subject of the conversation, the presence of influential users or ‘trolls’, the time and day of posting, as well as cultural or demographic aspects, such as user average age or geographical location. Furthermore, even though extremely toxic users are rare (Extended Data Fig. 2 ), the relationship between participation and toxicity of a discussion may in principle be affected also by small groups of highly toxic and engaged users driving the conversation dynamics. Although the analysis of such subtler aspects is beyond the scope of this Article, they are certainly worth investigating in future research.
However, when people encounter views that contradict their own, they may react with hostility and contempt, consistent with previous research 47 . In turn, it may create a cycle of negative emotions and behaviours that fuels toxicity. We also show that some online conversation features have remained consistent over the past three decades despite the evolution of platforms and social norms.
Our study has some limitations that we acknowledge and discuss. First, we use political leaning as a proxy for general leaning, which may capture only some of the nuances of online opinions. However, political leaning represents a broad spectrum of opinions across different topics, and it correlates well with other dimensions of leaning, such as news preferences, vaccine attitudes and stance on climate change 48 , 49 . We could not assign a political leaning to users to analyse controversies on all platforms. Still, those considered—Facebook, Gab and Twitter—represent different populations and moderation policies, and the combined data account for nearly 90% of the content in our entire dataset. Our analysis approach is based on breadth and heterogeneity. As such, it may raise concerns about potential reductionism due to the comparison of different datasets from different sources and time periods. We acknowledge that each discussion thread, platform and context has unique characteristics and complexities that might be diminished when homogenizing data. However, we aim not to capture the full depth of every discussion but to identify and highlight general patterns and trends in online toxicity across platforms and time. The quantitative approach used in our study is similar to numerous other studies 15 and enables us to uncover these overarching principles and patterns that may otherwise remain hidden. Of course, it is not possible to account for the behaviours of passive users. This entails, for example, that even if toxicity does not seem to make people leave conversations, it could still be a factor that discourages them from joining them. Our study leverages an extensive dataset to examine the intricate relationship between persistent online human behaviours and the characteristics of different social media platforms. Our findings challenge the prevailing assumption by demonstrating that toxic content, as traditionally defined, does not necessarily reduce user engagement, thereby questioning the assumed direct correlation between toxic content and negative discourse dynamics. This highlights the necessity for a detailed examination of the effect of toxic interactions on user behaviour and the quality of discussions across various platforms. Our results, showing user resilience to toxic content, indicate the potential for creating advanced, context-aware moderation tools that can accurately navigate the complex influence of antagonistic interactions on community engagement and discussion quality. Moreover, our study sets the stage for further exploration into the complexities of toxicity and its effect on engagement within online communities. Advancing our grasp of online discourse necessitates refining content moderation techniques grounded in a thorough understanding of human behaviour. Thus, our research adds to the dialogue on creating more constructive online spaces, promoting moderation approaches that are effective yet nuanced, facilitating engaging exchanges and reducing the tangible negative effects of toxic behaviour.
Through the extensive dataset presented here, critical aspects of the online platform ecosystem and fundamental dynamics of user interactions can be explored. Moreover, we provide insights that a comparative approach such as the one followed here can prove invaluable in discerning human behaviour from platform-specific features. This may be used to investigate further sensitive issues, such as the formation of polarization and misinformation. The resulting outcomes have multiple potential impacts. Our findings reveal consistent toxicity patterns across platforms, topics and time, suggesting that future research in this field should prioritize the concept of invariance. Recognizing that toxic behaviour is a widespread phenomenon that is not limited by platform-specific features underscores the need for a broader, unified approach to understanding online discourse. Furthermore, the participation of users in toxic conversations suggests that a simple approach to removing toxic comments may not be sufficient to prevent user exposure to such phenomena. This indicates a need for more sophisticated moderation techniques to manage conversation dynamics, including early interventions in discussions that show warnings of becoming toxic. Furthermore, our findings support the idea that examining content pieces in connection with others could enhance the effectiveness of automatic toxicity detection models. The observed homogeneity suggests that models trained using data from one platform may also have applicability to other platforms. Future research could explore further into the role of controversy and its interaction with other elements contributing to toxicity. Moreover, comparing platforms could enhance our understanding of invariant human factors related to polarization, disinformation and content consumption. Such studies would be instrumental in capturing the drivers of the effect of social media platforms on human behaviour, offering valuable insights into the underlying dynamics of online interactions.
Data collection
In our study, data collection from various social media platforms was strategically designed to encompass various topics, ensuring maximal heterogeneity in the discussion themes. For each platform, where feasible, we focus on gathering posts related to diverse areas such as politics, news, environment and vaccinations. This approach aims to capture a broad spectrum of discourse, providing a comprehensive view of conversation dynamics across different content categories.
We use datasets from previous studies that covered discussions about vaccines 50 , news 51 and brexit 52 . For the vaccines topic, the resulting dataset contains around 2 million comments retrieved from public groups and pages in a period that ranges from 2 January 2010 to 17 July 2017. For the news topic, we selected a list of pages from the Europe Media Monitor that reported the news in English. As a result, the obtained dataset contains around 362 million comments between 9 September 2009 and 18 August 2016. Furthermore, we collect a total of about 4.5 billion likes that the users put on posts and comments concerning these pages. Finally, for the brexit topic, the dataset contains around 460,000 comments from 31 December 2015 to 29 July 2016.
We collect data from the Pushshift.io archive ( https://files.pushshift.io/gab/ ) concerning discussions taking place from 10 August 2016, when the platform was launched, to 29 October 2018, when Gab went temporarily offline due to the Pittsburgh shooting 53 . As a result, we collect a total of around 14 million comments.
Data were collected from the Pushshift.io archive ( https://pushshift.io/ ) for the period ranging from 1 January 2018 to 31 December 2022. For each topic, whenever possible, we manually identified and selected subreddits that best represented the targeted topics. As a result of this operation, we obtained about 800,000 comments from the r/conspiracy subreddit for the conspiracy topic. For the vaccines topic, we collected about 70,000 comments from the r/VaccineDebate subreddit, focusing on the COVID-19 vaccine debate. We collected around 400,000 comments from the r/News subreddit for the news topic. We collected about 70,000 comments from the r/environment subreddit for the climate change topic. Finally, we collected around 550,000 comments from the r/science subreddit for the science topic.
We created a list of 14 channels, associating each with one of the topics considered in the study. For each channel, we manually collected messages and their related comments. As a result, from the four channels associated with the news topic (news notiziae, news ultimora, news edizionestraordinaria, news covidultimora), we obtained around 724,000 comments from posts between 9 April 2018 and 20 December 2022. For the politics topic, instead, the corresponding two channels (politics besttimeline, politics polmemes) produced a total of around 490,000 comments between 4 August 2017 and 19 December 2022. Finally, the eight channels assigned to the conspiracy topic (conspiracy bennyjhonson, conspiracy tommyrobinsonnews, conspiracy britainsfirst, conspiracy loomeredofficial, conspiracy thetrumpistgroup, conspiracy trumpjr, conspiracy pauljwatson, conspiracy iononmivaccino) produced a total of about 1.4 million comments between 30 August 2019 and 20 December 2022.
We used a list of datasets from previous studies that includes discussions about vaccines 54 , climate change 49 and news 55 topics. For the vaccines topic, we collected around 50 million comments from 23 January 2010 to 25 January 2023. For the news topic, we extend the dataset used previously 55 by collecting all threads composed of less than 20 comments, obtaining a total of about 9.5 million comments for a period ranging from 1 January 2020 to 29 November 2022. Finally, for the climate change topic, we collected around 9.7 million comments between 1 January 2020 and 10 January 2023.
We collected data for the Usenet discussion system by querying the Usenet Archive ( https://archive.org/details/usenet?tab=about ). We selected a list of topics considered adequate to contain a large, broad and heterogeneous number of discussions involving active and populated newsgroups. As a result of this selection, we selected conspiracy, politics, news and talk as topic candidates for our analysis. For the conspiracy topic, we collected around 280,000 comments between 1 September 1994 and 30 December 2005 from the alt.conspiracy newsgroup. For the politics topics, we collected around 2.6 million comments between 29 June 1992 and 31 December 2005 from the alt.politics newsgroup. For the news topic, we collected about 620,000 comments between 5 December 1992 and 31 December 2005 from the alt.news newsgroup. Finally, for the talk topic, we collected all of the conversations from the homonym newsgroup on a period that ranges from 13 February 1989 to 31 December 2005 for around 2.1 million contents.
We used a dataset presented previously 56 that covers the entire lifetime of the platform, from 9 January 2018 to 25 December 2020, including a total of around 16.2 million posts and comments shared by around 113,000 users in about 7,100 subverses (the equivalent of a subreddit for Voat). Similarly to previous platforms, we associated the topics to specific subverses. As a result of this operation, for the conspiracy topic, we collected about 1 million comments from the greatawakening subverse between 9 January 2018 and 25 December 2020. For the politics topic, we collected around 1 million comments from the politics subverse between 16 June 2014 and 25 December 2020. Finally, for the news topic, we collected about 1.4 million comments from the news subverse between 21 November 2013 and 25 December 2020.
We used a dataset proposed in previous studies that collected conversations about the climate change topic 49 , which is extended, coherently with previous platforms, by including conversations about vaccines and news topics. The data collection process for YouTube is performed using the YouTube Data API ( https://developers.google.com/youtube/v3 ). For the climate change topic, we collected around 840,000 comments between 16 March 2014 and 28 February 2022. For the vaccines topic, we collected conversations between 31 January 2020 and 24 October 2021 containing keywords about COVID-19 vaccines, namely Sinopharm, CanSino, Janssen, Johnson&Johnson, Novavax, CureVac, Pfizer, BioNTech, AstraZeneca and Moderna. As a result of this operation, we gathered a total of around 2.6 million comments to videos. Finally, for the news topic, we collected about 20 million comments between 13 February 2006 and 8 February 2022, including videos and comments from a list of news outlets, limited to the UK and provided by Newsguard (see the ‘Polarization and user leaning attribution’ section).
Content moderation policies
Content moderation policies are guidelines that online platforms use to monitor the content that users post on their sites. Platforms have different goals and audiences, and their moderation policies may vary greatly, with some placing more emphasis on free expression and others prioritizing safety and community guidelines.
Facebook and YouTube have strict moderation policies prohibiting hate speech, violence and harassment 57 . To address harmful content, Facebook follows a ‘remove, reduce, inform’ strategy and uses a combination of human reviewers and artificial intelligence to enforce its policies 58 . Similarly, YouTube has a similar set of community guidelines regarding hate speech policy, covering a wide range of behaviours such as vulgar language 59 , harassment 60 and, in general, does not allow the presence of hate speech and violence against individuals or groups based on various attributes 61 . To ensure that these guidelines are respected, the platform uses a mix of artificial intelligence algorithms and human reviewers 62 .
Twitter also has a comprehensive content moderation policy and specific rules against hateful conduct 63 , 64 . They use automation 65 and human review in the moderation process 66 . At the date of submission, Twitter’s content policies have remained unchanged since Elon Musk’s takeover, except that they ceased enforcing their COVID-19 misleading information policy on 23 November 2022. Their policy enforcement has faced criticism for inconsistency 67 .
Reddit falls somewhere in between regarding how strict its moderation policy is. Reddit’s content policy has eight rules, including prohibiting violence, harassment and promoting hate based on identity or vulnerability 68 , 69 . Reddit relies heavily on user reports and volunteer moderators. Thus, it could be considered more lenient than Facebook, YouTube and Twitter regarding enforcing rules. In October 2022, Reddit announced that they intend to update their enforcement practices to apply automation in content moderation 70 .
By contrast, Telegram, Gab and Voat take a more hands-off approach with fewer restrictions on content. Telegram has ambiguity in its guidelines, which arises from broad or subjective terms and can lead to different interpretations 71 . Although they mentioned they may use automated algorithms to analyse messages, Telegram relies mainly on users to report a range of content, such as violence, child abuse, spam, illegal drugs, personal details and pornography 72 . According to Telegram’s privacy policy, reported content may be checked by moderators and, if it is confirmed to violate their terms, temporary or permanent restrictions may be imposed on the account 73 . Gab’s Terms of Service allow all speech protected under the First Amendment to the US Constitution, and unlawful content is removed. They state that they do not review material before it is posted on their website and cannot guarantee prompt removal of illegal content after it has been posted 74 . Voat was once known as a ‘free-speech’ alternative to Reddit and allowed content even if it may be considered offensive or controversial 56 .
Usenet is a decentralized online discussion system created in 1979. Owing to its decentralized nature, Usenet has been difficult to moderate effectively, and it has a reputation for being a place where controversial and even illegal content can be posted without consequence. Each individual group on Usenet can have its own moderators, who are responsible for monitoring and enforcing their group’s rules, and there is no single set of rules that applies to the entire platform 75 .
Logarithmic binning and conversation size
Owing to the heavy-tailed distributions of conversation length (Extended Data Fig. 1 ), to plot the figures and perform the analyses, we used logarithmic binning. Thus, according to its length, each thread of each dataset is assigned to 1 out of 21 bins. To ensure a minimal number of points in each bin, we iteratively change the left bound of the last bin so that it contains at least N = 50 elements (we set N = 100 in the case of Facebook news, due to its larger size). Specifically, considering threads ordered in increasing length, the size of the largest thread is changed to that of the second last largest one, and the binning is recalculated accordingly until the last bin contains at least N points.
For visualization purposes, we provide a normalization of the logarithmic binning outcome that consists of mapping discrete points into coordinates of the x axis such that the bins correspond to {0, 0.05, 0.1, ..., 0.95, 1}.
To perform the part of the analysis, we select conversations belonging to the [0.7, 1] interval of the normalized logarithmic binning of thread length. This interval ensures that the conversations are sufficiently long and that we have a substantial number of threads. Participation and toxicity trends are obtained by applying to such conversations a linear binning of 21 elements to a chronologically ordered sequence of comments, that is, threads. A breakdown of the resulting datasets is provided in Supplementary Table 2 .
Finally, to assess the equality of the growth rates of participation values in toxic and non-toxic threads (see the ‘Conversation evolution and toxicity’ section), we implemented the following linear regression model:
where the term β 2 accounts for the effect that being a toxic conversation has on the growth of participation. Our results show that β 2 is not significantly different from 0 in most original and validation datasets (Supplementary Tables 8 and 11 )
Toxicity detection and validation of the models used
The problem of detecting toxicity is highly debated, to the point that there is currently no agreement on the very definition of toxic speech 64 , 76 . A toxic comment can be regarded as one that includes obscene or derogatory language 32 , that uses harsh, abusive language and personal attacks 33 , or contains extremism, violence and harassment 11 , just to give a few examples. Even though toxic speech should, in principle, be distinguished from hate speech, which is commonly more related to targeted attacks that denigrate a person or a group on the basis of attributes such as race, religion, gender, sex, sexual orientation and so on 77 , it sometimes may also be used as an umbrella term 78 , 79 . This lack of agreement directly reflects the challenging and inherent subjective nature of the concept of toxicity. The complexity of the topic makes it particularly difficult to assess the reliability of natural language processing models for automatic toxicity detection despite the impressive improvements in the field. Modern natural language processing models, such as Perspective API, are deep learning models that leverage word-embedding techniques to build representations of words as vectors in a high-dimensional space, in which a metric distance should reflect the conceptual distance among words, therefore providing linguistic context. A primary concern regarding toxicity detection models is their limited ability to contextualize conversations 11 , 80 . These models often struggle to incorporate factors beyond the text itself, such as the participant’s personal characteristics, motivations, relationships, group memberships and the overall tone of the discussion 11 . Consequently, what is considered to be toxic content can vary significantly among different groups, such as ethnicities or age groups 81 , leading to potential biases. These biases may stem from the annotators’ backgrounds and the datasets used for training, which might not adequately represent cultural heterogeneity. Moreover, subtle forms of toxic content, like indirect allusions, memes and inside jokes targeted at specific groups, can be particularly challenging to detect. Word embeddings equip current classifiers with a rich linguistic context, enhancing their ability to recognize a wide range of patterns characteristic of toxic expression. However, the requirements for understanding the broader context of a conversation, such as personal characteristics, motivations and group dynamics, remain beyond the scope of automatic detection models. We acknowledge these inherent limitations in our approach. Nonetheless, reliance on automatic detection models is essential for large-scale analyses of online toxicity like the one conducted in this study. We specifically resort to the Perspective API for this task, as it represents state-of-the-art automatic toxicity detection, offering a balance between linguistic nuance and scalable analysis capabilities. To define an appropriate classification threshold, we draw from the existing literature 64 , which uses 0.6 as the threshold for considering a comment to be toxic. This threshold can also be considered a reasonable one as, according to the developer guidelines offered by Perspective, it would indicate that the majority of the sample of readers, namely 6 out of 10, would perceive that comment as toxic. Due to the limitations mentioned above (for a criticism of Perspective API, see ref. 82 ), we validate our results by performing a comparative analysis using two other toxicity detectors: Detoxify ( https://github.com/unitaryai/detoxify ), which is similar to Perspective, and IMSYPP, a classifier developed for a European Project on hate speech 16 ( https://huggingface.co/IMSyPP ). In Supplementary Table 14 , the percentages of agreement among the three models in classifying 100,000 comments taken randomly from each of our datasets are reported. For Detoxify we used the same binary toxicity threshold (0.6) as used with Perspective. Although IMSYPP operates on a distinct definition of toxicity as outlined previously 16 , our comparative analysis shows a general agreement in the results. This alignment, despite the differences in underlying definitions and methodologies, underscores the robustness of our findings across various toxicity detection frameworks. Moreover, we perform the core analyses of this study using all classifiers on a further, vast and heterogeneous dataset. As shown in Supplementary Figs. 1 and 2 , the results regarding toxicity increase with conversation size and user participation and toxicity are quantitatively very similar. Furthermore, we verify the stability of our findings under different toxicity thresholds. Although the main analyses in this paper use the threshold value recommended by the Perspective API, set at 0.6, to minimize false positives, our results remain consistent even when applying a less conservative threshold of 0.5. This is demonstrated in Extended Data Fig. 5 , confirming the robustness of our observations across varying toxicity levels. For this study, we used the API support for languages prevalent in the European and American continents, including English, Spanish, French, Portuguese, German, Italian, Dutch, Polish, Swedish and Russian. Detoxify also offers multilingual support. However, IMSYPP is limited to English and Italian text, a factor considered in our comparative analysis.
Polarization and user leaning attribution
Our approach to measuring controversy in a conversation is based on estimating the degree of political partisanship among the participants. This measure is closely related to the political science concept of political polarization. Political polarization is the process by which political attitudes diverge from moderate positions and gravitate towards ideological extremes, as described previously 83 . By quantifying the level of partisanship within discussions, we aim to provide insights into the extent and nature of polarization in online debates. In this context, it is important to distinguish between ‘ideological polarization’ and ‘affective polarization’. Ideological polarization refers to divisions based on political viewpoints. By contrast, affective polarization is characterized by positive emotions towards members of one’s group and hostility towards those of opposing groups 84 , 85 . Here we focus specifically on ideological polarization. The subsequent description of our procedure for attributing user political leanings will further clarify this focus. On online social media, the individual leaning of a user toward a topic can be inferred through the content produced or the endorsement shown toward specific content. In this study, we consider the endorsement of users to news outlets of which the political leaning has been evaluated by trustworthy external sources. Although not without limitations—which we address below—this is a standard approach that has been used in several studies, and has become a common and established practice in the field of social media analysis due to its practicality and effectiveness in providing a broad understanding of political dynamics on these online platforms 1 , 43 , 86 , 87 , 88 . We label news outlets with a political score based on the information reported by Media Bias/Fact Check (MBFC) ( https://mediabiasfactcheck.com ), integrating with the equivalent information from Newsguard ( https://www.newsguardtech.com/ ). MBFC is an independent fact-checking organization that rates news outlets on the basis of the reliability and the political bias of the content that they produce and share. Similarly, Newsguard is a tool created by an international team of journalists that provides news outlet trust and political bias scores. Following standard methods used in the literature 1 , 43 , we calculated the individual leaning of a user l ∈ [−1, 1] as the average of the leaning scores l c ∈ [−1, 1] attributed to each of the content it produced/shared, where l c results from a mapping of the news organizations political scores provided by MBFC and Newsguard, respectively: [left, centre-left, centre, centre-right, right] to [−1, − 0.5, 0, 0.5, 1], and [far left, left, right, far right] to [−1, −0.5, 0.5, 1]). Our datasets have different structures, so we have to evaluate user leanings in different ways. For Facebook News, we assign a leaning score to users who posted a like at least three times and commented at least three times under news outlet pages that have a political score. For Twitter News, a leaning is assigned to users who posted at least 15 comments under scored news outlet pages. For Twitter Vaccines and Gab, we consider users who shared content produced by scored news outlet pages at least three times. A limitation of our approach is that engaging with politically aligned content does not always imply agreement; users may interact with opposing viewpoints for critical discussion. However, research indicates that users predominantly share content aligning with their own views, especially in politically charged contexts 87 , 89 , 90 . Moreover, our method captures users who actively express their political leanings, omitting the ‘passive’ ones. This is due to the lack of available data on users who do not explicitly state their opinions. Nevertheless, analysing active users offers valuable insights into the discourse of those most engaged and influential on social media platforms.
Burst analysis
We used the Kleinberg burst detection algorithm 46 (see the ‘Controversy and toxicity’ section) to all conversations with at least 50 comments in a dataset. In our analysis, we randomly sample up to 5,000 conversations, each containing a specific number of comments. To ensure the reliability of our data, we exclude conversations with an excessive number of double timestamps—defined as more than 10 consecutive or over 100 within the first 24 h. This criterion helps to mitigate the influence of bots, which could distort the patterns of human activity. Furthermore, we focus on the first 24 h of each thread to analyse streams of comments during their peak activity period. Consequently, Usenet was excluded from our study. The unique usage characteristics of Usenet render such a time-constrained analysis inappropriate, as its activity patterns do not align with those of the other platforms under consideration. By reconstructing the density profile of the comment stream, the algorithm divides the entire stream’s interval into subintervals on the basis of their level of intensity. Labelled as discrete positive values, higher levels of burstiness represent higher activity segments. To avoid considering flat-density phases, threads with a maximum burst level equal to 2 are excluded from this analysis. To assess whether a higher intensity of comments results in a higher comment toxicity, we perform a Mann–Whitney U -test 91 with Bonferroni correction for multiple testing between the distributions of the fraction of toxic comments t i in three intensity phases: during the peak of engagement and at the highest levels before and after. Extended Data Table 4 shows the corrected P values of each test, at a 0.99 confidence level, with H1 indicated in the column header. An example of the distribution of the frequency of toxic comments in threads at the three phases of a conversation considered (pre-peak, peak and post-peak) is reported in Fig. 4c .
Toxicity detection on Usenet
As discussed in the section on toxicity detection and the Perspective API above, automatic detectors derive their understanding of toxicity from the annotated datasets that they are trained on. The Perspective API is predominantly trained on recent texts, and its human labellers conform to contemporary cultural norms. Thus, although our dataset dates back to no more than the early 1990s, we provide a discussion on the viability of the application of Perspective API to Usenet and validation analysis. Contemporary society, especially in Western contexts, is more sensitive to issues of toxicity, including gender, race and sexual orientation, compared with a few decades ago. This means that some comments identified as toxic today, including those from older platforms like Usenet, might not have been considered as such in the past. However, this discrepancy does not significantly affect our analysis, which is centred on current standards of toxicity. On the other hand, changes in linguistic features may have some repercussions: there may be words and locutions that were frequently used in the 1990s that instead appear sparsely in today’s language, making Perspective potentially less effective in classifying short texts that contain them. We therefore proceeded to evaluate the impact that such a possible scenario could have on our results. In light of the above considerations, we consider texts labelled as toxic as correctly classified; instead, we assume that there is a fixed probability p that a comment may be incorrectly labelled as non-toxic. Consequently, we randomly designate a proportion p of non-toxic comments, relabel them as toxic and compute the toxicity versus conversation size trend (Fig. 2 ) on the altered dataset across various p . Specifically, for each value, we simulate 500 different trends, collecting their regression slopes to obtain a null distribution for them. To assess if the probability of error could lead to significant differences in the observed trend, we compute the fraction f of slopes lying outside the interval (−| s |,| s |), where s is the slope of the observed trend. We report the result in Supplementary Table 9 for different values of p . In agreement with our previous analysis, we assume that the slope differs significantly from the ones obtained from randomized data if f is less than 0.05.
We observed that only the Usenet Talk dataset shows sensitivity to small error probabilities, and the others do not show a significant difference. Consequently, our results indicate that Perspective API is suitable for application to Usenet data in our analyses, notwithstanding the potential linguistic and cultural shifts that might affect the classifier’s reliability with older texts.
Toxicity of short conversations
Our study focuses on the relationship between user participation and the toxicity of conversations, particularly in engaged or prolonged discussions. A potential concern is that concentrating on longer threads overlooks conversations that terminate quickly due to early toxicity, therefore potentially biasing our analysis. To address this, we analysed shorter conversations, comprising 6 to 20 comments, in each dataset. In particular, we computed the distributions of toxicity scores of the first and last three comments in each thread. This approach helps to ensure that our analysis accounts for a range of conversation lengths and patterns of toxicity development, providing a more comprehensive understanding of the dynamics at play. As shown in Supplementary Fig. 3 , for each dataset, the distributions of the toxicity scores display high similarity, meaning that, in short conversations, the last comments are not significantly more toxic than the initial ones, indicating that the potential effects mentioned above do not undermine our conclusions. Regarding our analysis of longer threads, we notice here that the participation quantity can give rise to similar trends in various cases. For example, high participation can be achieved because many users take part in the conversation, but also with small groups of users in which everyone is equally contributing over time. Or, in very large discussions, the contributions of individual outliers may remain hidden. By measuring participation, these and other borderline cases may not be distinct from the statistically highly likely discussion dynamics but, ultimately, this lack of discriminatory power does not have any implications on our findings nor on the validity of the conclusions that we draw.
Reporting summary
Further information on research design is available in the Nature Portfolio Reporting Summary linked to this article.
Data availability
Facebook, Twitter and YouTube data are made available in accordance with their respective terms of use. IDs of comments used in this work are provided at Open Science Framework ( https://doi.org/10.17605/osf.io/fq5dy ). For the remaining platforms (Gab, Reddit, Telegram, Usenet and Voat), all of the necessary information to recreate the datasets used in this study can be found in the ‘Data collection’ section.
Code availability
The code used for the analyses presented in the Article is available at Open Science Framework ( https://doi.org/10.17605/osf.io/fq5dy ). The repository includes dummy datasets to illustrate the required data format and make the code run.
Cinelli, M., Morales, G. D. F., Galeazzi, A., Quattrociocchi, W. & Starnini, M. The echo chamber effect on social media. Proc. Natl Acad. Sci. USA 118 , e2023301118 (2021).
Article CAS PubMed PubMed Central Google Scholar
Tucker, J. A. et al. Social media, political polarization, and political disinformation: a review of the scientific literature. Preprint at SSRN https://doi.org/10.2139/ssrn.3144139 (2018).
González-Bailón, S. et al. Asymmetric ideological segregation in exposure to political news on Facebook. Science 381 , 392–398 (2023).
Article PubMed ADS Google Scholar
Guess, A. et al. How do social media feed algorithms affect attitudes and behavior in an election campaign? Science 381 , 398–404 (2023).
Article CAS PubMed ADS Google Scholar
Del Vicario, M. et al. The spreading of misinformation online. Proc. Natl Acad. Sci. USA 113 , 554–559 (2016).
Article PubMed PubMed Central ADS Google Scholar
Bakshy, E., Messing, S. & Adamic, L. A. Exposure to ideologically diverse news and opinion on Facebook. Science 348 , 1130–1132 (2015).
Article MathSciNet CAS PubMed ADS Google Scholar
Bail, C. A. et al. Exposure to opposing views on social media can increase political polarization. Proc. Natl Acad. Sci. USA 115 , 9216–9221 (2018).
Article CAS PubMed PubMed Central ADS Google Scholar
Nyhan, B. et al. Like-minded sources on Facebook are prevalent but not polarizing. Nature 620 , 137–144 (2023).
Guess, A. et al. Reshares on social media amplify political news but do not detectably affect beliefs or opinions. Science 381 , 404–408 (2023).
Castaño-Pulgaŕın, S. A., Suárez-Betancur, N., Vega, L. M. T. & López, H. M. H. Internet, social media and online hate speech. Systematic review. Aggress. Viol. Behav. 58 , 101608 (2021).
Article Google Scholar
Sheth, A., Shalin, V. L. & Kursuncu, U. Defining and detecting toxicity on social media: context and knowledge are key. Neurocomputing 490 , 312–318 (2022).
Lupu, Y. et al. Offline events and online hate. PLoS ONE 18 , e0278511 (2023).
Gentzkow, M. & Shapiro, J. M. Ideological segregation online and offline. Q. J. Econ. 126 , 1799–1839 (2011).
Aichner, T., Grünfelder, M., Maurer, O. & Jegeni, D. Twenty-five years of social media: a review of social media applications and definitions from 1994 to 2019. Cyberpsychol. Behav. Social Netw. 24 , 215–222 (2021).
Lazer, D. M. et al. The science of fake news. Science 359 , 1094–1096 (2018).
Cinelli, M. et al. Dynamics of online hate and misinformation. Sci. Rep. 11 , 22083 (2021).
González-Bailón, S. & Lelkes, Y. Do social media undermine social cohesion? A critical review. Soc. Issues Pol. Rev. 17 , 155–180 (2023).
Roozenbeek, J. & Zollo, F. Democratize social-media research—with access and funding. Nature 612 , 404–404 (2022).
Article CAS PubMed Google Scholar
Dutton, W. H. Network rules of order: regulating speech in public electronic fora. Media Cult. Soc. 18 , 269–290 (1996).
Papacharissi, Z. Democracy online: civility, politeness, and the democratic potential of online political discussion groups. N. Media Soc. 6 , 259–283 (2004).
Coe, K., Kenski, K. & Rains, S. A. Online and uncivil? Patterns and determinants of incivility in newspaper website comments. J. Commun. 64 , 658–679 (2014).
Anderson, A. A., Brossard, D., Scheufele, D. A., Xenos, M. A. & Ladwig, P. The “nasty effect:” online incivility and risk perceptions of emerging technologies. J. Comput. Med. Commun. 19 , 373–387 (2014).
Garrett, R. K. Echo chambers online?: Politically motivated selective exposure among internet news users. J. Comput. Med. Commun. 14 , 265–285 (2009).
Del Vicario, M. et al. Echo chambers: emotional contagion and group polarization on Facebook. Sci. Rep. 6 , 37825 (2016).
Garimella, K., De Francisci Morales, G., Gionis, A. & Mathioudakis, M. Echo chambers, gatekeepers, and the price of bipartisanship. In Proc. 2018 World Wide Web Conference , 913–922 (International World Wide Web Conferences Steering Committee, 2018).
Johnson, N. et al. Hidden resilience and adaptive dynamics of the global online hate ecology. Nature 573 , 261–265 (2019).
Fortuna, P. & Nunes, S. A survey on automatic detection of hate speech in text. ACM Comput. Surv. 51 , 85 (2018).
Phadke, S. & Mitra, T. Many faced hate: a cross platform study of content framing and information sharing by online hate groups. In Proceedings of the 2020 CHI Conference on Human Factors in Computing Systems 1–13 (Association for Computing Machinery, 2020).
Xia, Y., Zhu, H., Lu, T., Zhang, P. & Gu, N. Exploring antecedents and consequences of toxicity in online discussions: a case study on Reddit. Proc. ACM Hum. Comput. Interact. 4 , 108 (2020).
Sipka, A., Hannak, A. & Urman, A. Comparing the language of qanon-related content on Parler, GAB, and Twitter. In Proc. 14th ACM Web Science Conference 2022 411–421 (Association for Computing Machinery, 2022).
Fortuna, P., Soler, J. & Wanner, L. Toxic, hateful, offensive or abusive? What are we really classifying? An empirical analysis of hate speech datasets. In Proc. 12th Language Resources and Evaluation Conference (eds Calzolari, E. et al.) 6786–6794 (European Language Resources Association, 2020).
Davidson, T., Warmsley, D., Macy, M. & Weber, I. Automated hate speech detection and the problem of offensive language. In Proc. International AAAI Conference on Web and Social Media 11 (Association for the Advancement of Artificial Intelligence, 2017).
Kolhatkar, V. et al. The SFU opinion and comments corpus: a corpus for the analysis of online news comments. Corpus Pragmat. 4 , 155–190 (2020).
Article PubMed Google Scholar
Lees, A. et al. A new generation of perspective API: efficient multilingual character-level transformers. In KDD'22: The 28th ACM SIGKDD Conference on Knowledge Discovery and Data Mining 3197–3207 (Association for Computing Machinery, 2022).
Vidgen, B. & Derczynski, L. Directions in abusive language training data, a systematic review: garbage in, garbage out. PLoS ONE 15 , e0243300 (2020).
Ross, G. J. & Jones, T. Understanding the heavy-tailed dynamics in human behavior. Phys. Rev. E 91 , 062809 (2015).
Article MathSciNet ADS Google Scholar
Choi, D., Chun, S., Oh, H., Han, J. & Kwon, T. T. Rumor propagation is amplified by echo chambers in social media. Sci. Rep. 10 , 310 (2020).
Beel, J., Xiang, T., Soni, S. & Yang, D. Linguistic characterization of divisive topics online: case studies on contentiousness in abortion, climate change, and gun control. In Proc. International AAAI Conference on Web and Social Media Vol. 16, 32–42 (Association for the Advancement of Artificial Intelligence, 2022).
Saveski, M., Roy, B. & Roy, D. The structure of toxic conversations on Twitter. In Proc. Web Conference 2021 (eds Leskovec, J. et al.) 1086–1097 (Association for Computing Machinery, 2021).
Juul, J. L. & Ugander, J. Comparing information diffusion mechanisms by matching on cascade size. Proc. Natl Acad. Sci. USA 118 , e2100786118 (2021).
Fariello, G., Jemielniak, D. & Sulkowski, A. Does Godwin’s law (rule of Nazi analogies) apply in observable reality? An empirical study of selected words in 199 million Reddit posts. N. Media Soc. 26 , 14614448211062070 (2021).
Qiu, J., Lin, Z. & Shuai, Q. Investigating the opinions distribution in the controversy on social media. Inf. Sci. 489 , 274–288 (2019).
Garimella, K., Morales, G. D. F., Gionis, A. & Mathioudakis, M. Quantifying controversy on social media. ACM Trans. Soc. Comput. 1 , 3 (2018).
NLPTown. bert-base-multilingual-uncased-sentiment, huggingface.co/nlptown/bert-base-multilingual-uncased-sentiment (2023).
Ta, H. T., Rahman, A. B. S., Najjar, L. & Gelbukh, A. Transfer Learning from Multilingual DeBERTa for Sexism Identification CEUR Workshop Proceedings Vol. 3202 (CEUR-WS, 2022).
Kleinberg, J. Bursty and hierarchical structure in streams. Data Min. Knowl. Discov. 7 , 373–397 (2003).
Article MathSciNet Google Scholar
Zollo, F. et al. Debunking in a world of tribes. PLoS ONE 12 , e0181821 (2017).
Article PubMed PubMed Central Google Scholar
Albrecht, D. Vaccination, politics and COVID-19 impacts. BMC Publ. Health 22 , 96 (2022).
Article CAS Google Scholar
Falkenberg, M. et al. Growing polarization around climate change on social media. Nat. Clim. Change 12 , 1114–1121 (2022).
Schmidt, A. L., Zollo, F., Scala, A., Betsch, C. & Quattrociocchi, W. Polarization of the vaccination debate on Facebook. Vaccine 36 , 3606–3612 (2018).
Schmidt, A. L. et al. Anatomy of news consumption on Facebook. Proc. Natl Acad. Sci. USA 114 , 3035–3039 (2017).
Del Vicario, M., Zollo, F., Caldarelli, G., Scala, A. & Quattrociocchi, W. Mapping social dynamics on Facebook: the brexit debate. Soc. Netw. 50 , 6–16 (2017).
Hunnicutt, T. & Dave, P. Gab.com goes offline after Pittsburgh synagogue shooting. Reuters , www.reuters.com/article/uk-pennsylvania-shooting-gab-idUKKCN1N20QN (29 October 2018).
Valensise, C. M. et al. Lack of evidence for correlation between COVID-19 infodemic and vaccine acceptance. Preprint at arxiv.org/abs/2107.07946 (2021).
Quattrociocchi, A., Etta, G., Avalle, M., Cinelli, M. & Quattrociocchi, W. in Social Informatics (eds Hopfgartner, F. et al.) 245–256 (Springer, 2022).
Mekacher, A. & Papasavva, A. “I can’t keep it up” a dataset from the defunct voat.co news aggregator. In Proc. International AAAI Conference on Web and Social Media Vol. 16, 1302–1311 (AAAI, 2022).
Facebook Community Standards , transparency.fb.com/policies/community-standards/hate-speech/ (Facebook, 2023).
Rosen, G. & Lyons, T. Remove, reduce, inform: new steps to manage problematic content. Meta , about.fb.com/news/2019/04/remove-reduce-inform-new-steps/ (10 April 2019).
Vulgar Language Policy , support.google.com/youtube/answer/10072685? (YouTube, 2023).
Harassment & Cyberbullying Policies , support.google.com/youtube/answer/2802268 (YouTube, 2023).
Hate Speech Policy , support.google.com/youtube/answer/2801939 (YouTube, 2023).
How Does YouTube Enforce Its Community Guidelines? , www.youtube.com/intl/enus/howyoutubeworks/policies/community-guidelines/enforcing-community-guidelines (YouTube, 2023).
The Twitter Rules , help.twitter.com/en/rules-and-policies/twitter-rules (Twitter, 2023).
Hateful Conduct , help.twitter.com/en/rules-and-policies/hateful-conduct-policy (Twitter, 2023).
Gorwa, R., Binns, R. & Katzenbach, C. Algorithmic content moderation: technical and political challenges in the automation of platform governance. Big Data Soc. 7 , 2053951719897945 (2020).
Our Range of Enforcement Options , help.twitter.com/en/rules-and-policies/enforcement-options (Twitter, 2023).
Elliott, V. & Stokel-Walker, C. Twitter’s moderation system is in tatters. WIRED (17 November 2022).
Reddit Content Policy , www.redditinc.com/policies/content-policy (Reddit, 2023).
Promoting Hate Based on Identity or Vulnerability , www.reddithelp.com/hc/en-us/articles/360045715951 (Reddit, 2023).
Malik, A. Reddit acqui-hires team from ML content moderation startup Oterlu. TechCrunch , tcrn.ch/3yeS2Kd (4 October 2022).
Terms of Service , telegram.org/tos (Telegram, 2023).
Durov, P. The rules of @telegram prohibit calls for violence and hate speech. We rely on our users to report public content that violates this rule. Twitter , twitter.com/durov/status/917076707055751168?lang=en (8 October 2017).
Telegram Privacy Policy , telegram.org/privacy (Telegram, 2023).
Terms of Service , gab.com/about/tos (Gab, 2023).
Salzenberg, C. & Spafford, G. What is Usenet? , www0.mi.infn.it/ ∼ calcolo/Wis usenet.html (1995).
Castelle, M. The linguistic ideologies of deep abusive language classification. In Proc. 2nd Workshop on Abusive Language Online (ALW2) (eds Fišer, D. et al.) 160–170, aclanthology.org/W18-5120 (Association for Computational Linguistics, 2018).
Tontodimamma, A., Nissi, E. & Sarra, A. E. A. Thirty years of research into hate speech: topics of interest and their evolution. Scientometrics 126 , 157–179 (2021).
Sap, M. et al. Annotators with attitudes: how annotator beliefs and identities bias toxic language detection. In Proc. 2022 Conference of the North American Chapter of the Association for Computational Linguistics: Human Language Technologies (eds. Carpuat, M. et al.) 5884–5906 (Association for Computational Linguistics, 2022).
Pavlopoulos, J., Sorensen, J., Dixon, L., Thain, N. & Androutsopoulos, I. Toxicity detection: does context really matter? In Proc. 58th Annual Meeting of the Association for Computational Linguistics (eds Jurafsky, D. et al.) 4296–4305 (Association for Computational Linguistics, 2020).
Yin, W. & Zubiaga, A. Hidden behind the obvious: misleading keywords and implicitly abusive language on social media. Online Soc. Netw. Media 30 , 100210 (2022).
Sap, M., Card, D., Gabriel, S., Choi, Y. & Smith, N. A. The risk of racial bias in hate speech detection. In Proc. 57th Annual Meeting of the Association for Computational Linguistics (eds Kohonen, A. et al.) 1668–1678 (Association for Computational Linguistics, 2019).
Rosenblatt, L., Piedras, L. & Wilkins, J. Critical perspectives: a benchmark revealing pitfalls in PerspectiveAPI. In Proc. Second Workshop on NLP for Positive Impact (NLP4PI) (eds Biester, L. et al.) 15–24 (Association for Computational Linguistics, 2022).
DiMaggio, P., Evans, J. & Bryson, B. Have American’s social attitudes become more polarized? Am. J. Sociol. 102 , 690–755 (1996).
Fiorina, M. P. & Abrams, S. J. Political polarization in the American public. Annu. Rev. Polit. Sci. 11 , 563–588 (2008).
Iyengar, S., Gaurav, S. & Lelkes, Y. Affect, not ideology: a social identity perspective on polarization. Publ. Opin. Q. 76 , 405–431 (2012).
Cota, W., Ferreira, S. & Pastor-Satorras, R. E. A. Quantifying echo chamber effects in information spreading over political communication networks. EPJ Data Sci. 8 , 38 (2019).
Bessi, A. et al. Users polarization on Facebook and Youtube. PLoS ONE 11 , e0159641 (2016).
Bessi, A. et al. Science vs conspiracy: collective narratives in the age of misinformation. PLoS ONE 10 , e0118093 (2015).
Himelboim, I., McCreery, S. & Smith, M. Birds of a feather tweet together: integrating network and content analyses to examine cross-ideology exposure on Twitter. J. Comput. Med. Commun. 18 , 40–60 (2013).
An, J., Quercia, D. & Crowcroft, J. Partisan sharing: Facebook evidence and societal consequences. In Proc. Second ACM Conference on Online Social Networks, COSN ′ 14 13–24 (Association for Computing Machinery, 2014).
Mann, H. B. & Whitney, D. R. On a test of whether one of two random variables is stochastically larger than the other. Ann. Math. Stat. 18 , 50–60 (1947).
Download references
Acknowledgements
We thank M. Samory for discussions; T. Quandt and Z. Zhang for suggestions during the review process; and Geronimo Stilton and the Hypnotoad for inspiring the data analysis and result interpretation. The work is supported by IRIS Infodemic Coalition (UK government, grant no. SCH-00001-3391), SERICS (PE00000014) under the NRRP MUR program funded by the EU NextGenerationEU project CRESP from the Italian Ministry of Health under the program CCM 2022, PON project ‘Ricerca e Innovazione’ 2014-2020, and PRIN Project MUSMA for Italian Ministry of University and Research (MUR) through the PRIN 2022CUP G53D23002930006 and EU Next-Generation EU, M4 C2 I1.1.
Author information
These authors contributed equally: Michele Avalle, Niccolò Di Marco, Gabriele Etta
Authors and Affiliations
Department of Computer Science, Sapienza University of Rome, Rome, Italy
Michele Avalle, Niccolò Di Marco, Gabriele Etta, Shayan Alipour, Lorenzo Alvisi, Matteo Cinelli & Walter Quattrociocchi
Department of Social Sciences and Economics, Sapienza University of Rome, Rome, Italy
Emanuele Sangiorgio
Department of Communication and Social Research, Sapienza University of Rome, Rome, Italy
Anita Bonetti
Institute of Complex Systems, CNR, Rome, Italy
Antonio Scala
Department of Mathematics, City University of London, London, UK
Andrea Baronchelli
The Alan Turing Institute, London, UK
You can also search for this author in PubMed Google Scholar
Contributions
Conception and design: W.Q., M.A., M.C., G.E. and N.D.M. Data collection: G.E. and N.D.M. with collaboration from M.C., M.A. and S.A. Data analysis: G.E., N.D.M., M.A., M.C., W.Q., E.S., A. Bonetti, A. Baronchelli and A.S. Code writing: G.E. and N.D.M. with collaboration from M.A., E.S., S.A. and M.C. All of the authors provided critical feedback and helped to shape the research, analysis and manuscript, and contributed to the preparation of the manuscript.
Corresponding authors
Correspondence to Matteo Cinelli or Walter Quattrociocchi .
Ethics declarations
Competing interests.
The authors declare no competing interests.
Peer review
Peer review information.
Nature thanks Thorsten Quandt, Ziqi Zhang and the other, anonymous, reviewer(s) for their contribution to the peer review of this work.
Additional information
Publisher’s note Springer Nature remains neutral with regard to jurisdictional claims in published maps and institutional affiliations.
Extended data figures and tables
Extended data fig. 1 general characteristics of online conversations..
a . Distributions of conversation length (number of comments in a thread). b . Distributions of the time duration (days) of user activity on a platform for each platform and each topic. c . Time duration (days) distributions of threads. Colour-coded legend on the side.
Extended Data Fig. 2 Extremely toxic authors and conversations are rare.
a . Complementary cumulative distribution functions (CCDFs) of the toxicity of authors who posted more than 10 comments. Toxicity is defined as usual as the fraction of toxic comments over the total of comments posted by a user. b . CCDFs of the toxicity of conversations containing more than 10 comments. Colour-coded legend on the side.
Extended Data Fig. 3 User toxicity as conversations evolve.
Mean fraction of toxic comments as conversations progress. The x-axis represents the normalized position of comment intervals in the threads. For each dataset, toxicity is computed in the thread size interval [0.7−1] (see main text and Tab. S 2 in SI). Trends are reported with their 95% confidence interval. Colour-coded legend on the side.
Extended Data Fig. 4 Toxicity is not associated with conversation lifetime.
Mean toxicity of a . users versus their time of permanence in the dataset and b . threads versus their time duration. Trends are reported with their 95% confidence interval and they are reported using a normalized log-binning. Colour-coded legend on the side.
Extended Data Fig. 5 Results hold for a different toxicity threshold.
Core analyses presented in the paper repeated employing a lower (0.5) toxicity binary classification threshold. a . Mean fraction of toxic comments in conversations versus conversation size, for each dataset (see Fig. 2 ). Trends are reported with their 95% confidence interval. b . Pearson’s correlation coefficients between user participation and toxicity trends for each dataset. c . Pearson’s correlation coefficients between users’ participation in toxic and non-toxic thread sets, for each dataset. d . Boxplot of the distribution of toxicity ( n = 25, min = −0.016, max = 0.020, lower whisker = −0.005, Q 1 = − 0.005, Q 2 = 0.004, Q 3 = 0.012, upper whisker = 0.020) and participation ( n = 25, min = −0.198, max = −0.022, lower whisker = −0.198, Q 1 = − 0.109, Q 2 = − 0.070, Q 3 = − 0.049, upper whisker = −0.022) trend slopes for all datasets, as resulting from linear regression. The results of the relative Mann-Kendall tests for trend assessment are shown in Extended Data Table 5 .
Supplementary information
Supplementary information.
Supplementary Information 1–4, including details regarding data collection for validation dataset, Supplementary Figs. 1–3, Supplementary Tables 1–17 and software and coding specifications.
Reporting Summary
Rights and permissions.
Open Access This article is licensed under a Creative Commons Attribution 4.0 International License, which permits use, sharing, adaptation, distribution and reproduction in any medium or format, as long as you give appropriate credit to the original author(s) and the source, provide a link to the Creative Commons licence, and indicate if changes were made. The images or other third party material in this article are included in the article’s Creative Commons licence, unless indicated otherwise in a credit line to the material. If material is not included in the article’s Creative Commons licence and your intended use is not permitted by statutory regulation or exceeds the permitted use, you will need to obtain permission directly from the copyright holder. To view a copy of this licence, visit http://creativecommons.org/licenses/by/4.0/ .
Reprints and permissions
About this article
Cite this article.
Avalle, M., Di Marco, N., Etta, G. et al. Persistent interaction patterns across social media platforms and over time. Nature (2024). https://doi.org/10.1038/s41586-024-07229-y
Download citation
Received : 30 April 2023
Accepted : 22 February 2024
Published : 20 March 2024
DOI : https://doi.org/10.1038/s41586-024-07229-y
Share this article
Anyone you share the following link with will be able to read this content:
Sorry, a shareable link is not currently available for this article.
Provided by the Springer Nature SharedIt content-sharing initiative
By submitting a comment you agree to abide by our Terms and Community Guidelines . If you find something abusive or that does not comply with our terms or guidelines please flag it as inappropriate.
Quick links
- Explore articles by subject
- Guide to authors
- Editorial policies
Sign up for the Nature Briefing newsletter — what matters in science, free to your inbox daily.

- Reference Manager
- Simple TEXT file
People also looked at
Original research article, the materiality key: how work on empirical data can improve analytical models and theoretical frameworks for multimodal discourse analysis.
- Communication and Media Department, School of Social Sciences and Humanities, Loughborough University, Loughborough, United Kingdom
This article is a critical reflection on the way the notion of materiality informed the project and the development of The Kinesemiotic Body project carried out by a UK and German research teams and of the model of analysis it adopted, the Functional Grammar of Dance. It starts with an excursus of some of the most interesting developments in other discipline that turned to the investigation of materiality as an epistemological perspective, and it shows how the same type of focus has impacted on multimodal discourse analysis focusing on movement-based communication. The overarching theme that characterises this multidisciplinary attention to materiality is its anchoring function to the temporal and spatial coordinates in which social phenomena are contextualised, which is taken as the fundamental condition for shaping our perception and understanding of the world in all areas of experience and knowledge. A more specific example of how the notion of materiality impacted on the development of movement-based discourse analysis will be provided by an example of analysis of rich movement data captured live from professional dancers from the English National Ballet.
Introduction
This paper provides a critical reflection on the role played by the notion of materiality in the development of movement-based discourse analysis within the wider area of Multimodality studies. It is positioned within an even wider area of multidisciplinary research that focused on this notion in the last few decades and that foregrounded some very interesting points for reflection and development across disciplines. Through examples drawn from a recent research project in movement-based communication, it will demonstrate how in order to incorporate effectively the awareness and understanding of materiality in a communicative environment, it is essential to turn to the analysis of empirical data, which in turn provides solid evidence to strengthen and/or advance theoretical frameworks. The project in question is The Kinesemiotic Body, funded by the Arts and Humanities Research Council (AHRC) in the UK and the German Research Foundation (DFG) in Germany. The fact that the project focused on movement-based analysis (specifically on dance choreography) carried out by scholars from very different disciplines, where the importance of incorporating the materiality of the human body in interaction with a performance environment was considered through different approaches (Multimodal Discourse Analysis, Engineering, Computer Science, etc.) makes the examples of empirical data analysis proposed here particularly appropriate to the consideration of the notion of materiality as an interdisciplinary one and provides a clear connection with John Bateman’s discussion of materiality in relation to the development of Multimodality as a practice that encompasses borders between disciplines and research areas ( Bateman et al., 2017 ; Bateman, 2019 , 2022 ). This article will also show how the consideration of the materiality of dance allowed in primis for the further development of the Functional Grammar of Dance ( Maiorani, 2021 ; Maiorani et al., 2022 ; Maiorani and Liu, 2023 ), which is now a more comprehensive and even more flexible tool that scholars have started to use for analysing movement-based communication in dance performances other than ballet or even outside the domain of dance altogether (see Mouard Ruiz, 2021 ; Bolens, 2022 ; Meissl et al., 2022 ; Prové, 2022 ; Sindoni, 2022 ; Vidal Claramonte, 2022 ; Wu, 2022 ; Elyamany, 2023 ). The examples of empirical data analysis will be preceded by a presentation of how the Functional Grammar of Dance is implemented in ELAN, a widespread commercial, free-to-use software traditionally used for annotating conversations or verbal interactions, for which we created a completely set of interdependent tiers and controlled vocabulary. By including spatial annotation categories and the distinction of internal discourse structures, our annotation offers quite innovative insights into the way movement-based communication can be annotated and analysed.
In order to describe the impact of the concept of materiality on The Kinesemiotic Body project—and especially the way materiality was foregrounded by Bateman’s work in multimodal discourse analysis—I need to take a series of steps backwards, from the time when Kinesemiotics, a new interdisciplinary research area, developed at Loughborough University. Kinesemiotics started with an interdisciplinary team of researchers created at Loughborugh University in 2016, where we covered Linguistics, Semiotics, Multimodality, sensor Engineering, and Computer Science. After receiving funding from the Loughborough University CALIBRE programme in 2017 to work in collaboration with the English National on the investigation of movement-based discourse analysis by capturing a small amount of dance movement data, we joined forces with John Bateman for a joint grant application to the Arts and Humanities Research Council (AHRC) and the German Research Foundation (Deutsche Forschungsgemeinschaft, DFG) and we were funded for the collaborative international project called The Kinesemiotic Body. 1 The project aim was to advance the understanding of movement-based communication starting from choreographed movement in a worldwide renown movement-based form of performance: ballet. This choice was driven by the team’s specific expertise as well as by the pre-existing collaboration with the English National Ballet and the status of ballet as a form of performance based on movement with a tradition long recognised and established at international level, a tradition that has had an enormous impact on the elaboration and the development of many other forms of movement-based performances. Our intention was to evaluate whether and how we could apply a linguistically-motivated model for the analysis of verbal discourse to the study of how the body communicates by interacting with the space within the context of a performance setup. This would not only allow to deepen our understanding of the specific form of performance on which we were focusing, but also to develop a new approach to non-verbal communication with a more finely elaborated notion of movement-based discourse structure.
To contextualise the results of the project within a much more comprehensive scientific overview, this article will start by considering how the same notion has been approached by different disciplines in recent years looking at some significant examples of literature, thus showing how this concept actually taps into the very foundations of a multidisciplinary idea of knowledge.
Materiality across research areas and disciplines
In several research areas, materiality seems to be a considered as a contextualised configuration of the spatial and temporal location of multimodal communication, an architecture that anchors theory to real-life situations and allows for the encounter and cross-fertilisation of diverse fields of study. Contemporary ontological philosophy puts materiality at the centre of social life and interaction ( Schatzki, 2010 ), positing social phenomena as configurations of practices and material arrangements, thus recognising materiality itself as a component of social phenomena that combines with technology and practices. In this way, the relationship between practices (including meaning-making practices) and the material arrangements in which these practices take place spatially and temporally -the fundamental socio-cultural coordinates – becomes the focus of contemporary social ontology. Schatzki (2010 , p. 125) also points out that materiality is not merely physicality: it is rather to be defined as ‘composition’, the ‘stuff’ of which social life is made. The issue at stake is therefore to find a way to describe it systematically. Schatzki (2010 , p. 129) also defines practices as ‘organized spatial–temporal manifolds of human activity. Examples are cooking practices, political practices, manufacturing practices, football practices, dating practices, and horse breeding practices’. The material arrangements that form nexi with practices to generate social phenomena are ‘sets of interconnected material entities’ ( Schatzki, 2010 ) that can be human beings, artifacts, organisms, and other natural items. The materiality of a social phenomenon can therefore be extremely complex, and the problem is finding a systematic way to pick up the elements that compose it.
In the area of semiotic studies, and essentially drawing on Peirce, the complexity and centrality of the notion of materiality has already been foregrounded by Petrilli (2008) through the specification of two types of materiality that inhere the sign itself: ‘In a global semiotic perspective, it would seem that the first claim to be made is that the existence of biological material is the initial condition for sign material or semiosic material to exist. It goes without saying that no less necessary for the existence of biological material is the existence of chemicophysical material. Therefore, we could begin by stating that the materiality of signs presents itself on various levels, upon which basis we may propose a typology of semiosic materiality’ ( Petrilli, 2008 , p. 139). Understanding semiosic materiality in this respect involves the recognition of a clear distinction between physical and biological materiality, the latter generating a further distinction between living and non-living organic materiality. Materiality is therefore seen as being at the origin of human experience of communication.
One of the most interesting examples of the analysis and use of materiality as a foundational epistemological concept, comes from energy studies, where energy is conceptualised in its materiality to understand how its perception impacts on daily practices and transactions worldwide. A whole trend of energy studies has been working for decades on the reconceptualisation of the very notion of ‘energy’ by drawing on multidisciplinary and interdisciplinary approaches that include also theories from geography, politics, history, anthropology, etc. All these approaches focus on the effort to define the materiality of energy. Balmaceda et al. (2019) pose four fundamental questions to open a fruitful dialogue and exchange amongst different research areas; their queries are about the location of energy materiality, its users and the way they use it, its relational characteristics with context in terms of spatial and temporal scales, and the analytical role of energy materiality in the different epistemological areas. These questions are meant to anchor a theoretical enquiry on this fundamental notion to specific contexts in real life, such as the way energy materiality determines constraints in agency that will then impact on infrastructures and politics (i.e., energy consumption, supply chains, etc.). These questions also highlight the historical relationship between energy materiality and the evolution of technology ( Leonardi and Barley, 2010 ), which is also a factor that impacts in a fundamental way social semiotics practices across time and space. Dance discourse—the meaning produced and shaped by choreographed movement in dance performances—is movement-based and movement involves the flow of kinetic energy. The way we experience and capture this flow for various purposes (archiving, documenting, visualising, etc.) is also impacted by the development of technology and of the devices that allow us to anchor to a specific time and place performances that would otherwise be lost once they have taken place. To understand how these questions may be of considerable relevance even when studying the development and perception of dance discourse, it suffices to think of the way an audience perception of live dance performances has changed considerably during and after COVID 19 lockdowns, when the perception of temporal and spatial location of performances worldwide was dramatically changed by the impossibility of actually attending a live performance in theatres. It was the audience’s perception of these coordinates that technological affordances successfully managed to change when a number of theatre and ballet companies survived thanks to the broadcast of performances originally recorded for live streaming in cinemas and then turned into ‘live pre-recorded events’ packaged for home entertainment ( Maiorani, 2020 ).
The importance of how the flow of movement is anchored to a spatially and temporally located context also emerges in trans-contextual analysis, a branch of social semiotics that looks at how materiality is perceived in different contexts through mobility. Kell (2015 , p. 425) proposes the concept of ‘meaning-making trajectories which are made up of recontextualizing and resemiotising moves’. This concept is meant to incorporate the flow of meaning movement and transformation within contextualised communication through language, and it is linked to the materiality of communicative contexts moving across time and space; it also resonates with that of trajectory in Minimal Ballet Sequences, a unit of dance discourse analysis that I will explain below and that provides the description of dance discourse with a connective thread that incorporates the flow of movement and allows for the understanding of the different functions of orientation and direction in movement-based discourse. Meaning-making practices in trans-contextual analysis do not only take into consideration movement across contexts but also the role of material objects that interact with the ‘text-artefacts’ ( Kell, 2015 , p. 426), thus advocating for multimodality as a more comprehensive approach to the analysis of communication.
The connection between materiality, flow of experience and energy and embodiment is also at the centre of several cutting-edge theoretical approaches to knowledge understanding in the humanities. Whilst creative writing practices and cultural anthropology interrogate the relationship between identity and the materiality of the semiotic forms ( Wilf, 2011 ), experimental literature focuses on the notion of materiality when trying to provide a flow of multimodal experiences to its readers ( Lee, 2014 ). The consideration of materiality becomes particularly crucial in translation practices, where the materiality of the text emerges in all its complexity, ranging from its physical features to the way the written word conveys auditory, tactile, visual, and other sensorially-perceived (in other words, multimodal) meanings. In this case and drawing on Gibbons’s (2012) idea of reading as an activity involving multisensory perception, the embodiment of a text materiality is once more conceived as the anchoring of the reader’s meaning perception of multimodal, multisensorial meaning to a specifically located spatial and temporal context. In this way, the reader’s body and its physical environment, its way of perceiving the world through the senses, becomes the nexus , the filter through which the very act of reading, of perceiving the materiality of a text turns into its embodiment.
Whilst experimental literature focuses on the nexus between narrated spaces and topographies and the way these are perceived through reading, recent studies on national mobility and infrastructures also pay attention to the materiality of the environment and how it influences the emergence and understanding of nationalisms and national identities ( Merriman and Jones, 2017 ). Also in this case, materiality is theorised as the constellation of materials of diverse nature that anchors the flow of multimodal discourse—one of nation and identity—to specific temporal and spatial locations or to the process of crossing them. This perspective was generated by a wider context of studies on the relationship between discourse and materiality and its impact on management and organisational theory ( Putnam, 2014 ), which has at its centre the dimensions of time and space and sees communication as the locus of the interplay between human agency and discourse.
Educational contexts have also turned to the study of the materiality in the context of traditional teaching and learning activities to develop more updated and effective pedagogical strategies within the perspective of multimodality. The materiality of multimodal forms of feedback has been studied to improve and update current forms of teaching and learning practices ( Tyrer, 2021 ), whilst lectures have been considered as a form of ‘multimodal, sociomaterial performance’ ( Lacković and Popova, 2021 ) that has the human body and movement-based communication at its centre. This reconceptualisation of lectures as a multimodal, movement-based practice draws on the concept of sociomateriality ( Gherardi, 2017 ), which is grounded in post-humanist studies and essentially describes the interplay between social structures and material contexts made of bodies and items interacting in space in which every day meaning-making practices are habitually carried out. This new epistemological approach to knowledge shuns from human-centred approaches to learning and considers human experiences through materially contextualised phenomena. One of its central areas of research is embodied work practices, which posits the human body as epistemological focus.
In the more specific area of science education, the educational environment is seen as a synthesis of semiotic agents that interact to produce meaning ( Pantidos et al., 2010 ). The teaching of physics is particularly seen as an activity that involves creating connections amongst different signifying items and anchoring them to specific spatial and temporal contexts to explain theories. This activity generates narratives that make use of verbal language, gestures, objects, graphs, body movement, etc., a specific teaching practice whose general features can be observed in all types of science teaching. In this respect, science teaching is very similar to theatre practice, and its materiality is very similar to the materiality of theatre, where narrative spaces are characterised by referents whose meanings define a specific semiotic landscape anchored to a specific time and space (which is more or less what happens with the set-up of a dance performance space). The materiality of these narrative spaces is also similar to those used to teach robots when providing them with exemplary situations: thus, concepts are taught and learnt by anchoring them to the spatial and temporal materiality of a real-life context, to the materiality of everyday semiosis that is shaped into meaning through discourse ( Björkvall and Karlsson, 2011 ). Björkvall and Karlsson draw strongly on social semiotics and anchor the specificity of contextual materiality into culture: according to them, materiality offers a meaning potential ( Kress, 2010 ) that is then shaped through meaning making practices grounded in specific cultures. The shaping activity of cultures also involves choices amongst affordances that will be selected to become semiotic resources for communication. As a matter of fact, in a specific context within a specific culture, not all material affordances will become semiotic affordances. It is therefore the regularity and recurrence of configurations of semiotic affordances that allows us to identify modes ( Bateman et al., 2017 ) within specific temporally and spatially located cultures: ‘for an affordance to be turned into a semiotic resource, it needs to be picked up by a culture or by a social group and be continuously worked upon in activity types of various kinds. In other words, the affordance needs to be shaped by culture to become what we call a semiotic resource. From this it follows that even if affordances are material resources for humans to perceive when they act in their environment, they are not necessarily semiotic resources. However, also affordances that are not defined as semiotic resources can have meaning potential’. ( Björkvall and Karlsson, 2011 , p. 147).
As it will be demonstrated below, the challenge of understanding which affordances in dance are regularly and consistently used as semiotic resources was one that was faced by The Kinesemiotic Body project and one that benefited from the consideration of the notion of materiality as a an external language for description, a language that applies to the analysis of rich, live-captured movement data by taking into consideration the specific configurations of materials that are shaped into semiotic resources in the meaning-making practice of dance performances.
A theoretical framework to anchor the flow of dance to its materiality
The theoretical framework of The Kinesemiotic Body project was strongly based in linguistic theory and multimodal analysis; besides the Functional Grammar of Dance (FGD, Maiorani, 2017 , 2021 ), our work also drew on segmented discourse representation theory ( Asher and Lascarides, 2003 ; Bateman and Wildfeuer, 2014 ) as well as on recent developments in corpora analysis, live movement data collection and data visualisation. Working both on video materials and on movement data collected live from professional dancers of the English National Ballet (ENB)—who performed whole ballet sequences both as single performers and in couple—we developed a method of multimodal annotation using ELAN software that allowed us to annotate and analyse not just how movement is structurally carried out along a temporal line and within a specific space, but also how through structured movement sequences, dancers communicate by projecting their body parts towards meaningful portions of space, thus creating semantic connections that guide the audience’s interpretation. In this way, we created a method for annotating dance sequences that incorporates both movement structures and meaning structures in a flow of data. To show the effect of the research carried out through the analysis of empirical data within The Kinesemiotic Body project, I will first describe the original version of the Functional Grammar of Dance model and then I will introduced the updated version with all the relevant additions.
The first model of the Functional Grammar of Dance
The first model of the Functional Grammar of Dance was published in 2017 and it clearly drew on Halliday’s Functional Grammar for verbal language. The model was already completely different from traditional dance notation systems as it was created and used for the manual analysis of dance discourse (movement structures and corresponding meaning), not for the notation of deconstructed movements and their physical qualities. With respect to the current and updated FGD model, it was simpler but it already incorporated first and foremost the dancer’s point of view as the starting point of movement, even if at the time it had only been applied to manual analysis of video clips. The FGD posits that movement-based communication, like verbal communication, always happens in a specific Context of Situation whose variables, Field (what is happening), Tenor (who is taking part), and Mode (how communication is being carried out by the participants to the communicative event), activate as many meanings (respectively Experiential, Interpersonal, and Textual) that will then be realised by different linguistic structures. In movement-based communication, these structures are also movement-based and they are called Choreographic Affordances, namely all possible body-structure combinations performed by dancers whilst moving, structures that vary according to the dance style that is being adopted and the possibilities and limitations of human bodies. Figure 1 shows the first version of the FGD.
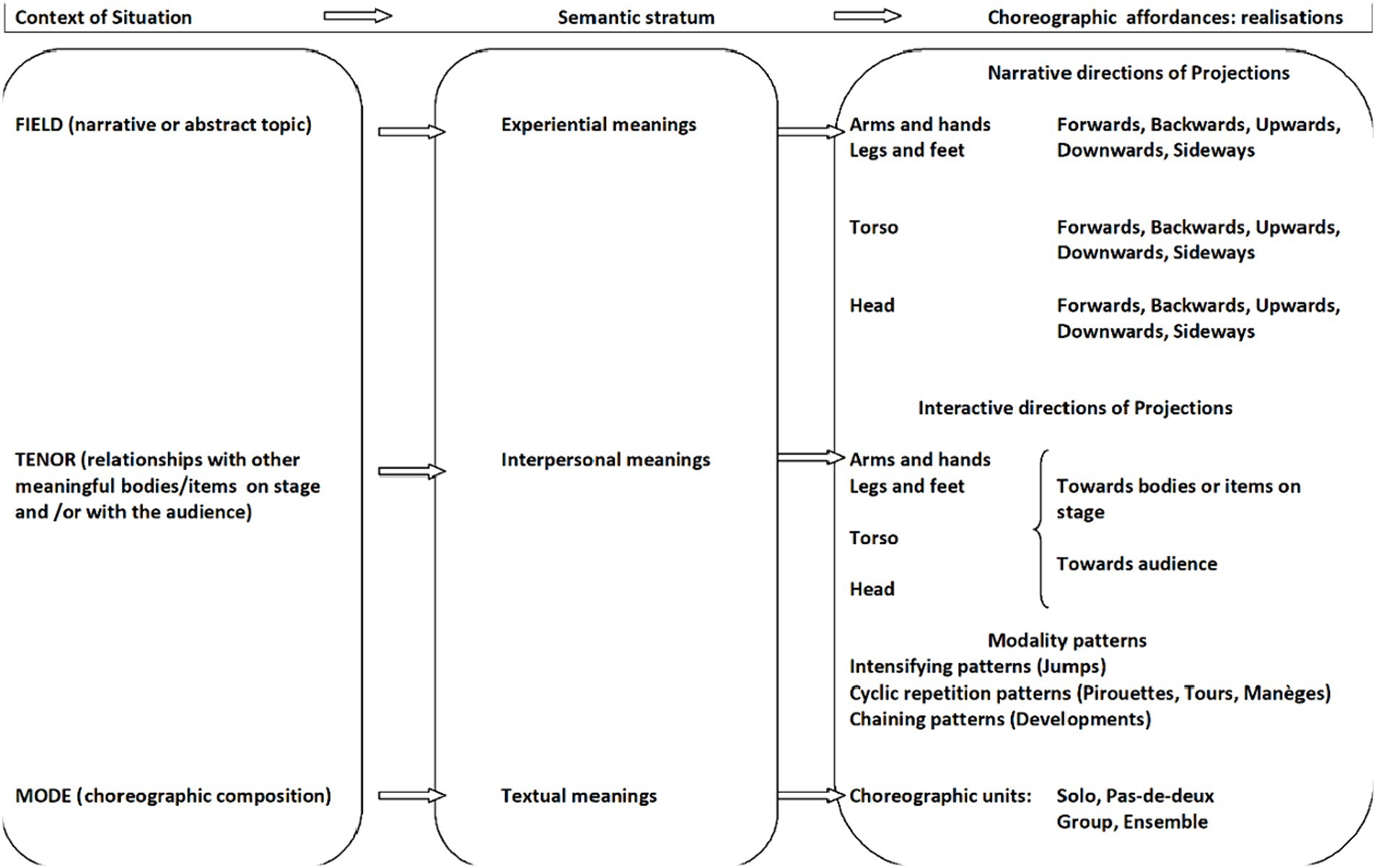
Figure 1 . The 2017 version of the Functional Grammar of Dance.
In order to generate meaning, choreographic affordances allow for the creation of structured projections of body parts towards meaningful portions of the performance space. Th first fundamental difference between the Hallidayan Functional Grammar and the FGD is the distinction between physical space and contextual space. Space is a fundamental dimension of the FGD: “A body is a spatial construct. It exists and functions through its relationship with space. Space itself is defined by the presence of bodies of any kind: without bodies, we call it ‘void’”( Maiorani, 2021 , p. 1). From its first version, the FGD posited that whereas dance movement can be instantiated during training classes by dancers carrying out choreographic combinations in a studio physical space just for the purpose of training, meaning is only created through the interaction between body structures and the performance space, which is populated by contextually relevant objects, people, props, etc., and it is therefore designed for this purpose. Whilst dancing a choreography, dancers extend in various manners their body parts towards meaningful spots in the performance space, thus creating interactions between their dancing bodies and people, or objects, or props, or light effects, and these interactions will provide the audience with cues to follow a narrative, to understand who is interacting with whom or what, and to enjoy the choreographed sequences as a whole. The visualisations of these interactions are called Projections : the narrative ones indicate action (i.e., going to, coming from, locating, connecting, addressing, engaging, etc.), the interactive ones indicate interaction either with the audience (AU) or with participants on stage (POS). Only in the contextual space—whether actually built or just imagined during rehearsals it does not matter, provided that there is a shared awareness of it—can dance discourse actually be realised.
Interestingly, the elaboration of the FGD also allowed for a more in-depth discussion of the discussion of instantiation as a foundational concept of Systemic Functional Linguistic theory, leading to its definition as a dynamic relationship and to a further elaboration of the theory of Context (see Maiorani and Wegener, 2022 ). However, the first FGD model in Figure 1 shows how some areas of analysis could not be fully developed without the use of a larger amount of data collected live from dancers: the whole model is based on the development of its theoretical foundations and on manual analysis performed on small scale video data and drawing on a solid knowledge of the range offered by choreographic affordances, especially in terms of ballet. The lack of analysis of richer data sourced from different dancers performing different roles shows particularly in the area of Textual meanings, which was still developed on merely theoretical assumptions that needed to be tested empirically. The work carried out through The Kinesemiotic Body project on a corpus of live-dance captured data provided exactly this opportunity to test the FGD application empirically and to develop an analytical method that could be implemented in a widely commercially available software for annotation.
The updated model of the Functional Grammar of Dance and our annotation system
The updated version of the Functional Grammar of Dance was elaborated whilst annotating rich live-captured movement data with the ELAN software. The annotation system we have developed is not an alternative to traditional notation systems like Labanotation or Benesh notation, which involve intensive training in using specific scores and symbols and provide a notation of the physical characteristics and qualities of unstructured movement along the music score. These systems are movement notation systems. The FGD annotation we implemented using ELAN is a dance discourse annotation that always puts the dancer’s point of view at the centre of each movement and provides information both on movement structures and on discursive structures using labels that make no use of specialistic terminology. The FGD annotation is a dance annotation method, which implies that dance is not considered only as physical movement but as a meaningful and contextualised movement-based performance ( Maiorani, 2021 ; Maiorani et al., 2022 ). Our annotation system in ELAN develops on different levels: the lower level of Move, which is the basic unit of analysis of the FGD, and the level of Minimal Ballet Sequence (MBS), which is the smallest discursive unit and comprises two consecutive Moves. The annotation is based on the work of the body articulators: head, torso, arms and hands, legs and feet. The Move marks the minimum movement across space performed by a dancer and is delimited by a starting set of projections and an arrival set of projections. The two consecutive Moves in an MBS are the smallest discursive unit that provides a trajectory in direction: if the Move direction is the same for both consecutive Moves, the MBS trajectory is defined as continuous ; it the direction changes, the MBS trajectory is defined as var ied. When the choreography requires it, we also annotate at the level of Elaborations: these are extra arrival sets of projections that mark a change in position of the body articulators at the end of a Move that does not involve any movement across space.
The use of sensors and the related software to capture live movement data from dancers immediately showed us that we had to deal with a complexity of movement parameters that needed to be ordered and put into clear functional relationships in the annotation. As soon as we started working with ELAN, we realised that we had to make three dimensions of annotation visibly distinguished and integrated at the same time: the level of physical movement, which accounts for the way each body articulator moves in relation to the surrounding space coordinates (i.e., inwards/outwards, up/down, backwards/forwards, etc.), the level of structure, which accounts for the way the different body articulators are positioned with respect to the Move direction, and the level of projections , which accounts for the narrative and interactive values of body parts projections towards meaningful portions of the performance space. These distinctions were necessary to show the complexity of the movement-based discourse enacted by dance, where the meaning created by projections is determined also by the position of articulators with respect to the immediate space references and the direction that a whole Move has taken. This complexity of relationships became visible when we started capturing live-data from dancers and had to take into consideration all the elements of movement we had to measure in order to account for all the factors that determined choreographic choices. The tiers related to each dimension are separated and colour coded but they are all at the same level, thus allowing for the visualisation of the complexity of factors all contributing at the same time to the realisation of projections within the performance space. After segmenting the flow of data into Moves, the first tier we annotate is always that of physical movement, which provides us with a picture of where every articulator is at the moment of annotation with respect to the immediate spatial references as they are perceived by the dancer; then we annotate the structures, which incorporate the direction the dancer takes when moving and the respective positioning in space of all the articulators with respect to movement; finally, we incorporate the discursive dimension by annotating narrative and interactive projections, which shows what type of actions and interactions the choices made in terms of physical movement and structure determine. The version of the FGD we used within ELAN is the most recent one, which we started developing after a preliminary work of live-movement capture data with the English National Ballet in 2017 and then kept on elaborating during The Kinesemiotic Body project. The impact of this work carried out on empirical data is reflected in a more detailed distinction of units of analysis (Choreoraphic units) specifically devised for empirical data segmentation and in the inclusion of narrathletic enhancers (showcasing dancers’ athletic qualities) and modal values of projections (highlighting concentrations of projections in one direction) that reinforce the integration of physical and semantic description of the collected data. Figure 2 shows the current, updated model of the FGD ( Maiorani, 2021 , p. 30).
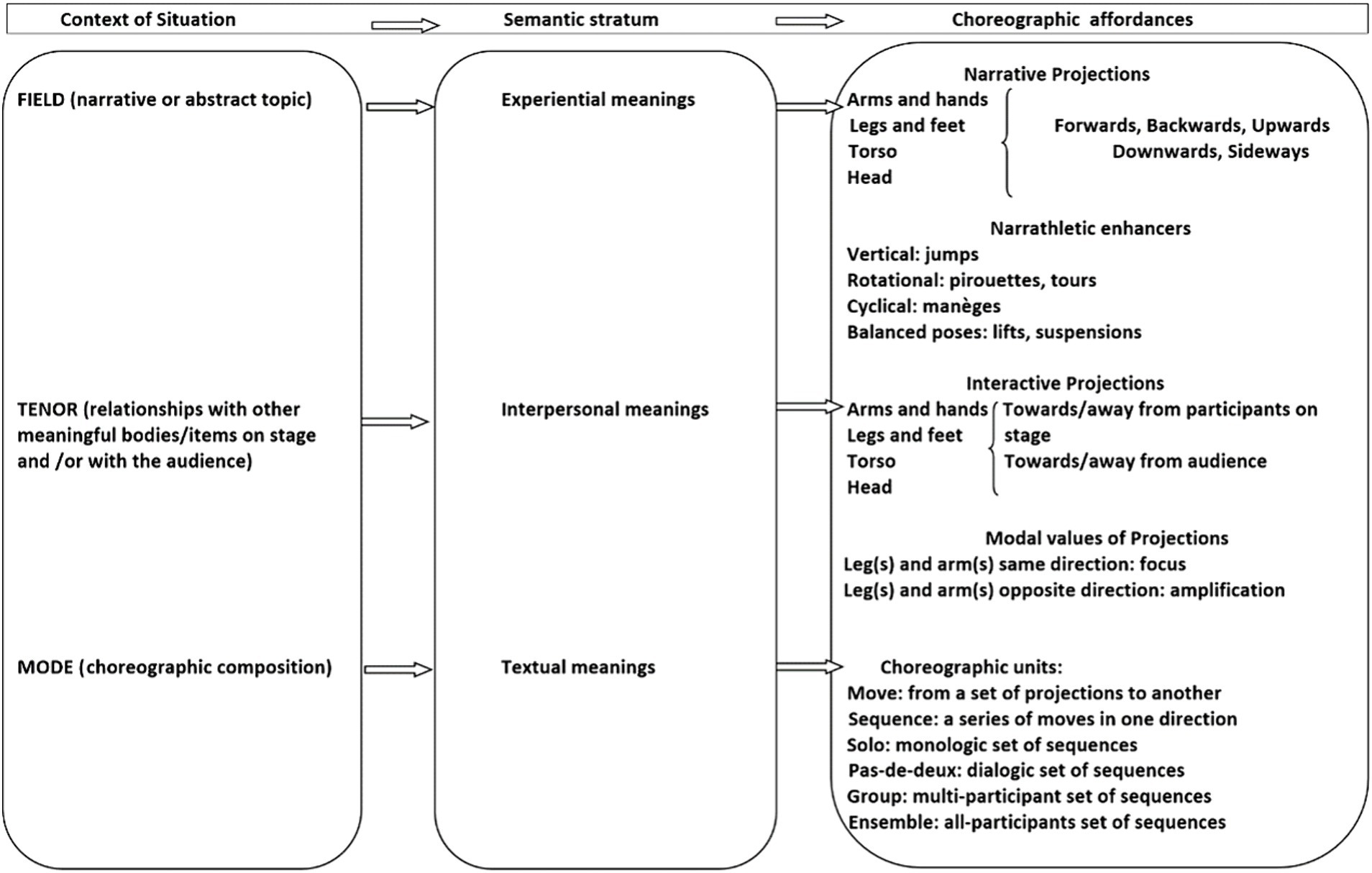
Figure 2 . The updated version of the FGD model ( Maiorani, 2021 , p. 30).
The improved work on the role of Move direction carried out on empirical data, also allowed us to understand the discursive role of trajectories , designed by two consecutive moves, thus highlighting the importance of segmenting MBSs. Figure 3 outlines the annotation framework we have developed.
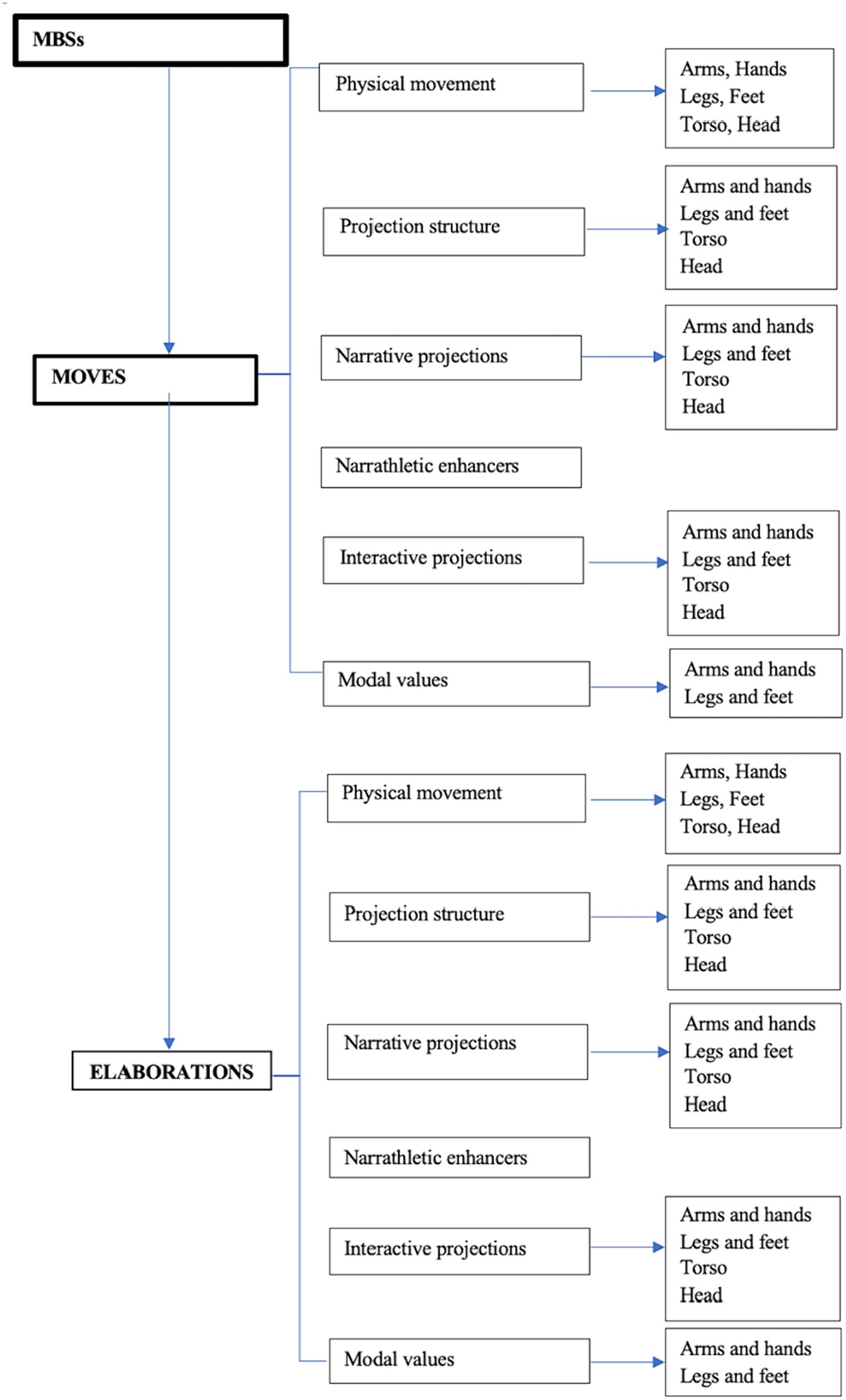
Figure 3 . An FGD-derived framework for the analysis of dance discourse ( Maiorani and Liu, 2023 , p. 9).
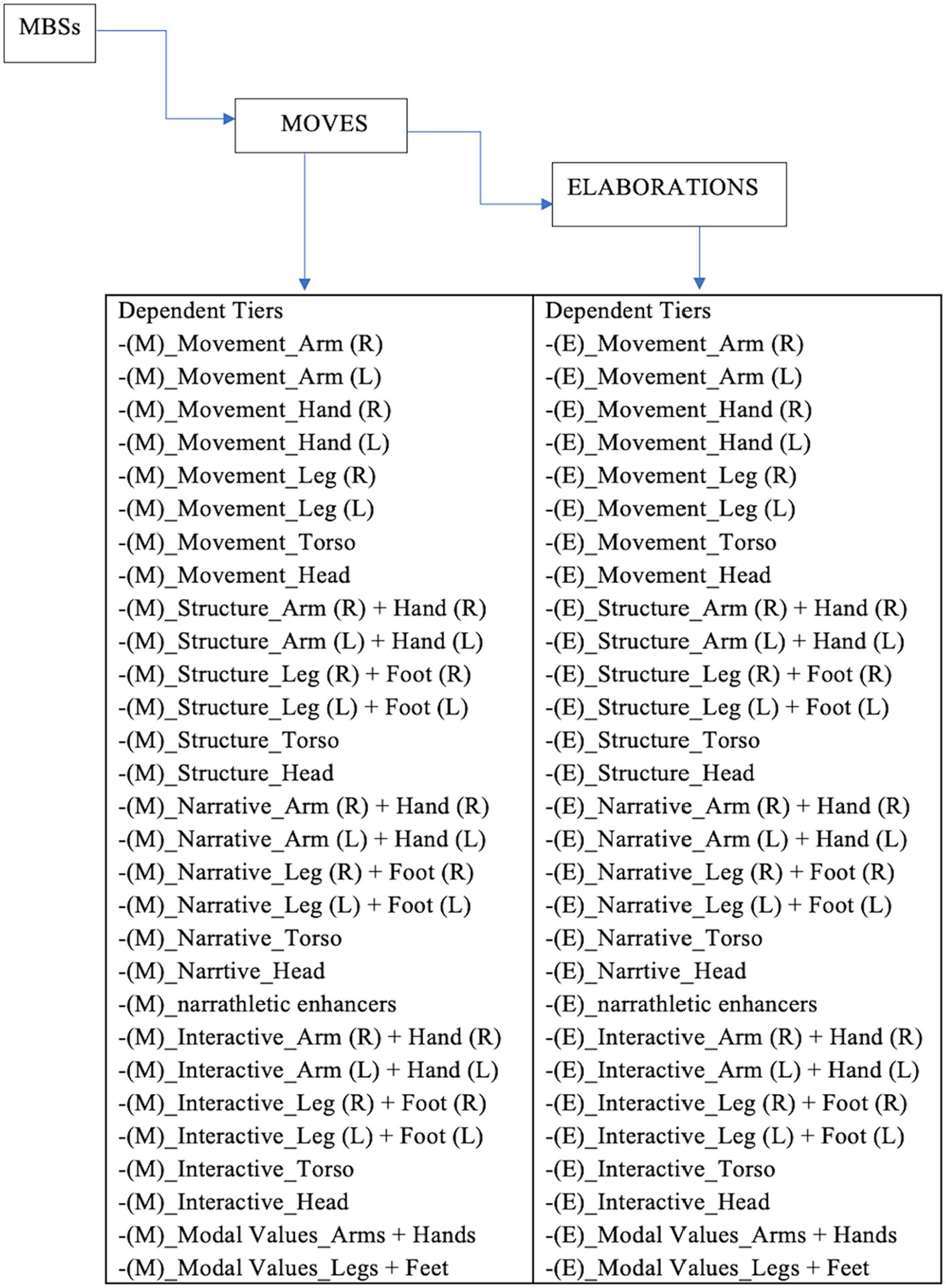
Figure 4 . FGD annotation framework implemented in ELAN ( Maiorani and Liu, 2023 , p. 10).
When we transfer this annotation framework in the ELAN, we create different tiers to annotate the Moves and MBSs. The highest level of description is that of the MBS tier, under which we annotate the Move tier (second description level) and Elaboration tier (third description level). The tiers with the descriptions of physical movement, structures, narrative projection, interactive projections, narrathletic enhancers and modal values of projections are all dependent on the Move tier and on the Elaboration tier when this occurs.
Every dance sequence is segmented according to the three levels of Move and couples of consecutive Moves are then grouped into MBSs. Therefore, the tiers depending on the Move (and Elaboration when present) align with Move (and Elaboration) segmentation. The end point of each Move aligns with the start of the subsequent one, and the same happens with the MBS segmentation, thus incorporating the flow of movement into the annotation. Figure 4 shows the FGD annotation framework implemented in ELAN.
The annotation tiers are linked to a controlled vocabulary divided into menus that provides specific options for each type of information annotated (i.e., physical movement, structures, projections, etc.) and draws on the FGD. The vocabulary is generated to a drop-down list in the ELAN annotation template, from which the annotator can select the most appropriate choice. The vocabulary does not contain any technical term and it is therefore very user friendly and open to non-specialist users. For this reason, it can also be easily adapted to the annotation of movements other than ballet. Figure 5 shows an example of the drop-down controlled vocabulary list with options provided by labels that do not contain specialistic language.
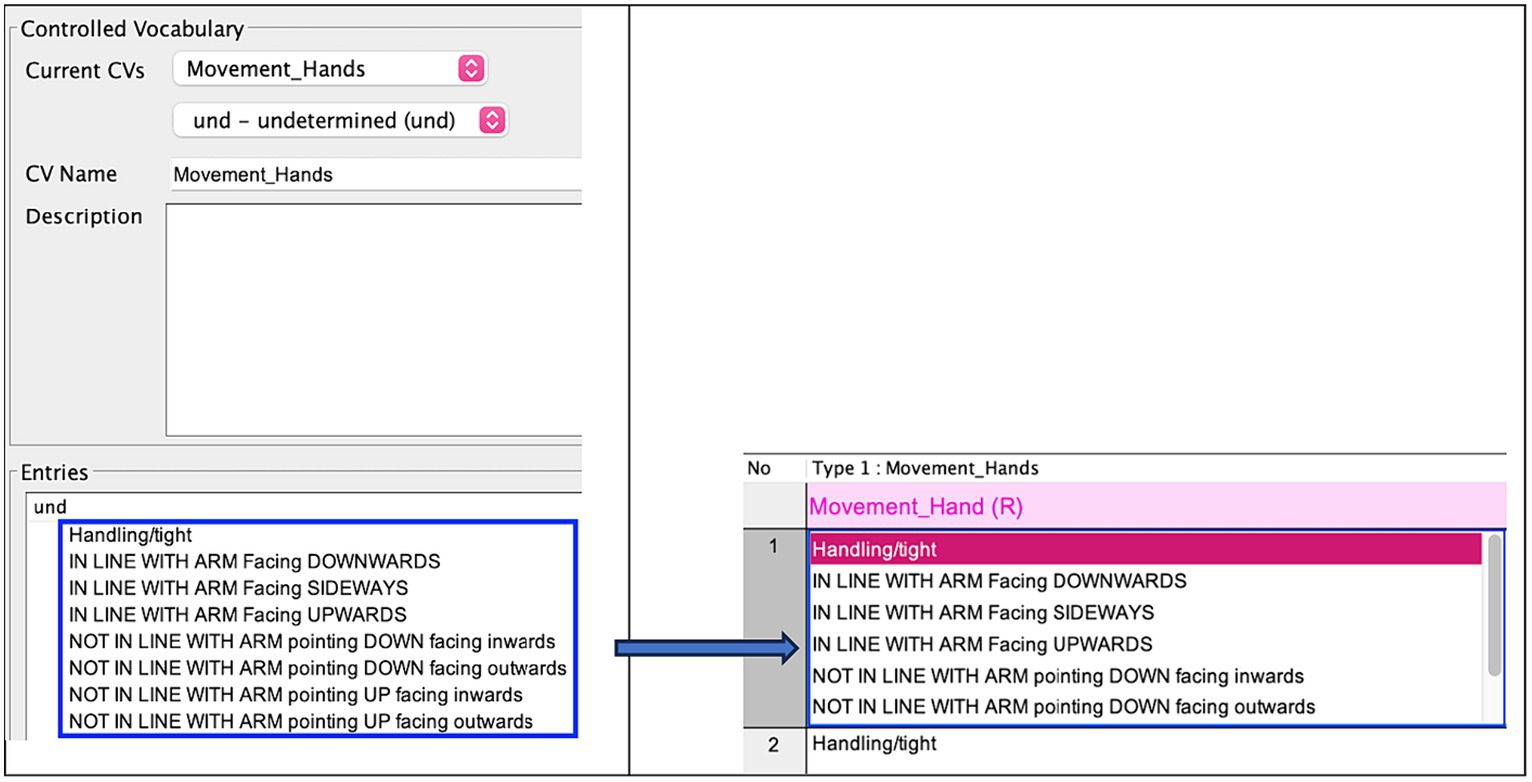
Figure 5 . Example of drop-down controlled vocabulary ( Maiorani and Liu, 2023 , p. 11).
The annotation template includes a window where the dance sequence that is being annotated is visualised and this can be reduced or enlarged in size according to necessity. Figure 6 provides an example of annotation made on data collected live from a dancer from the English National Ballet whose body was synthesised into an avatar. The figure does not offer the whole annotation but just a screenshot of a section as for the whole script it is necessary to scroll the text down. The video window has been reduced in size to provide a larger view of the annotation.
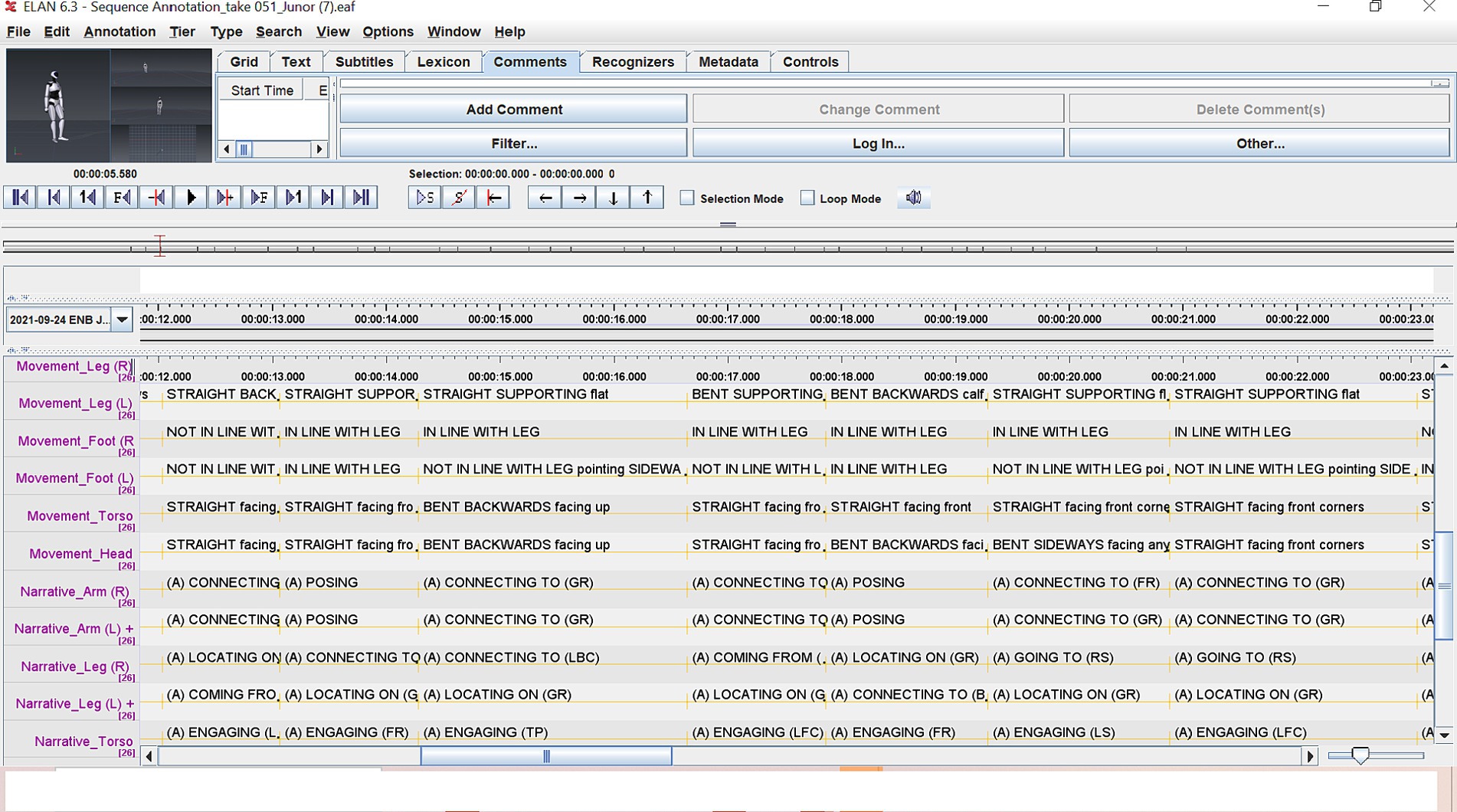
Figure 6 . Example of annotation with visualisation of a dancer’s avatar.
Figure 7 offers an example of annotation that highlights the segmentation into Moves and MBSs. The visualisation of the sequence that is being annotated is a video taken in a rehearsal studio at the English National Ballet headquarters in London.
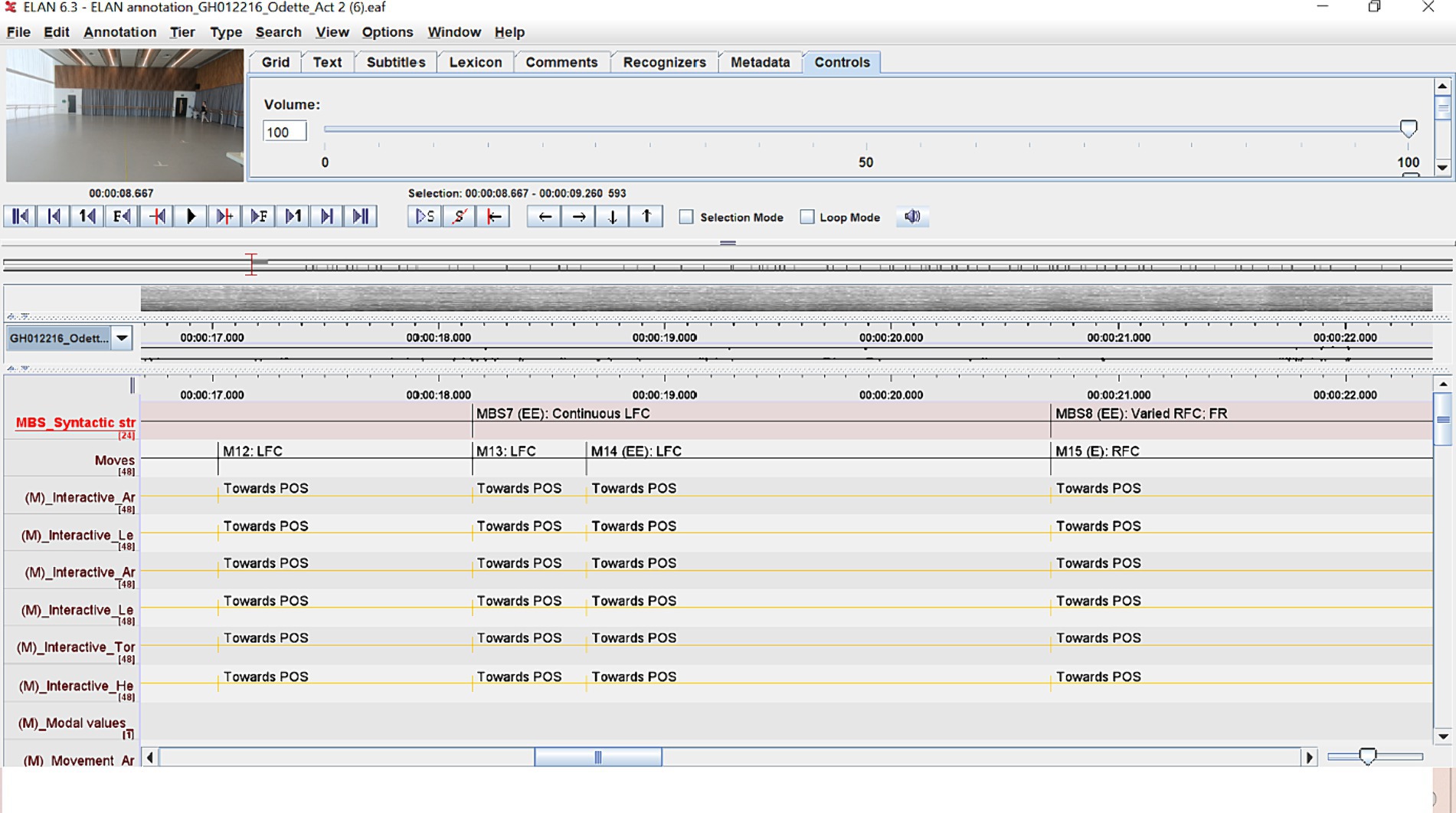
Figure 7 . Example of annotation showing the segmentation into Moves and MBSs.
The various tiers in which the annotation is organised is evidence in itself of the complexity of the materiality of dance that we were capable of capturing when working empirically and with live-captured movement data. The empirical work we carried out within The Kinesemiotic Body project allowed us to capture not only the relationships between movement structures and projections at various levels but it also made us realise that there are different levels of meaning carried out at different levels of discourse segmentation, and that direction and orientation have different and complementary roles in the perception of dance discourse, as will be discussed in the following section.
Results of working with empirical data of a ballet sequence corpus
The FGD model was elaborated further when we started capturing live data from the dancers in a real rehearsal studio in preliminary work carried out in 2017 and then implemented in the analysis of the dance data corpus carried out with The Kinesemiotic Body project. When having to organise and annotate the data we recorded from dancers in rehearsal studios, the research activity based on data analysis had to face two main challenges. The first challenge was posed by the complexity of data which involved not only the dancers’ movements but also the space set-up and the use of direction and orientation. Unlike what we had to take into consideration in the first examples of analysis performed manually with the FGD, where select movement structures and projections were analysed on the basis of the systemic functional theoretical framework, a much greater amount of features and levels of communication deployment were suddenly available for analysis through the corpus of dance sequences collected with the English National Ballet. The second, consequential challenge was that the organisation of all these new features and levels that had not yet been captured or addressed by manual analysis had to be systematised in a consistent and replicable framework for annotation to be used for all items of the corpus. The initial manual analysis with the FGD had paved the road for a systematic investigation of dance discourse as movement-based communication in context but had not yet benefited from the amount of information provided by live-captured data. It lacked empirical application and was therefore limited in its scope and capability. Bateman (2022 , p. 42) highlights this problem with reference to work carried out before empirical data collection and analysis by stating that ‘many multimodal analyses were overly impressionistic, and that analyses tended in any case to be restricted to small-scale studies rarely capable of producing the degree of empirical robustness that would be necessary to improve on impressionistic categories; even when the intuitions underlying such categories are generally sound, it is unlikely that they offer the last word on the precise treatments required’. The FGD application was therefore still restricted to a small case study, a first step that needed to be developed through more empirical work and larger, more complex data analysis.
The first development we achieved when we started working on the rich data provided by the live movement-capture sessions with the English National Ballet was the distinction between more local levels of annotation and more discursive ones. By implementing the FGD in ELAN for annotating our data, we found out that Moves and MBSs create different types of meaning at different levels that are then integrated through the movement flow: the same features that at the more local level of the Move have a specific structural function, at the level of MBS acquire a more discursive one. The analysis carried out on Moves through our annotation method reveals that at this level meaning is created more locally. The annotation of Moves provides three important sets of information that this minimal semantic unit delivers: two sets (starting and arrival) of narrative and interactive projections that the dancer realises for the viewer to interpret within the context of the performance; the positions in which the dancer moves their articulators in relation to each other across space; the flow of relationships between direction and orientation , which not only determines the possible values to be attached to projections for the viewer (i.e., moving towards VS moving away from, going forwards VS going backwards, etc.) but also connects the more local meanings realised at Move level to the syntactic choices observable at MBS level, where the direction of two consecutive Moves determines the type of MBS trajectory . These findings allowed us to gain an important insight into the mechanism of movement-based communication realised through choreography that the manual analysis simply based on the application of theory and tools did not allow us to uncover; the annotation of the rich data we collected through live movement-capture sessions put us in front of a multi-level discursive complexity that we would not have captured otherwise. The meanings expressed at the more local level of Move acquire a discursive flow in a more complex relation with the performance space at the level of MBS, which provides them with trajectories and highlights discursive patterns where bodies and space are integrated. Thus, whereas in previous manual analyses the focus had been predominantly on reading narrative and interactive projections on the basis of the theoretical framework underlying the FGD, thanks to empirical work our annotation had to take into consideration the analysis of physical movement as a separate but integrated part of the analysis, foregrounding the importance of annotating the positions of the articulators with respect to each other and with respect to the physical space and to the dimensions of direction and orientation. The result was a systematic integration of the physical and the semantic data that are described in integration through the annotation within the same model and according to the same theoretical principles. The consideration of the materiality of dance, which involves also the integration of spatial features, direction, and orientation, led us to a discourse description that anchors the theoretically grounded visualisations of projections to the time and space of the physical dance performance phenomenon.
I will illustrate this point with a simple example of annotation taken from the English National Ballet most recent production of Raymonda , a very traditional ballet from the classical repertoire first choreographed by Marius Petipa in 1987. Even in the most recent version, this traditional piece is based on an intricate and equally traditional love-triangle story against a romanticised historical setting, and it is therefore not too difficult for the audience to follow the flow of its scenes and the relationships amongst the characters based on the synopsis presented in the programme, which is supported by a very classical choreography. However, the extracts we annotated, reserved us some surprises. Amongst the extracts we chose, there is one from a solo danced by Abdur, one of the three protagonists: in love with Raymonda, who is engaged already with his best friend, in the extract we analysed he finds himself alone with her and declares his feelings by dancing a solo variation. The annotation we carried out is exemplified in Figure 8 , where a particular pattern is showing. The annotation includes four Moves and two MBSs and the figure shows annotations both of some physical movement structures and of some narrative and interactive projections. The more local annotation of the four Moves shows a considerable amount of repetition of specific physical movements that corresponds to an equally repetitive series of meanings: through narrative projections, the dancer interpreting Abdur forms repeated connections with Raymonda, who is sitting in the corner in the stage setup with which he is also repeatedly addressing and engaging. Interactive projections show that his interactions are entirely devoted to her and the stage space around her. However, the physical data annotation shows that the same types of narrative and interactive projections are being repeated alternatively in opposite directions and maintaining the same orientation, thus indicating that Abdur is moving back and forth, towards and away from Raymonda, which impacts on the way narrative and interactive projections are perceived by the audience. The annotation itself offers a visualisation of this discursive pattern that develops across two MBSs, which we named ‘mirrored pattern’. It also shows how the more local meanings at Move level are incorporated and shaped into a discursive strategy at the higher level of MBS. These patterns also made us realise that whereas movement orientation is important to capture the local value of narrative and interactive projections within Moves because it determines the perception of the narrative and interactive meanings realised by each set of movement structures, movement direction has a more discursive value because it incorporates those more locally determined meanings within a discursive flow that shows how those meanings can change in relation to the perception of the whole performance space surrounding the dancer. Whereas Abdur’s unchanging Move orientation repetitively shows his focus towards Raymonda, his alternatively changing direction at MBS level shows the conflictual situation in which that focus is experienced by the character.
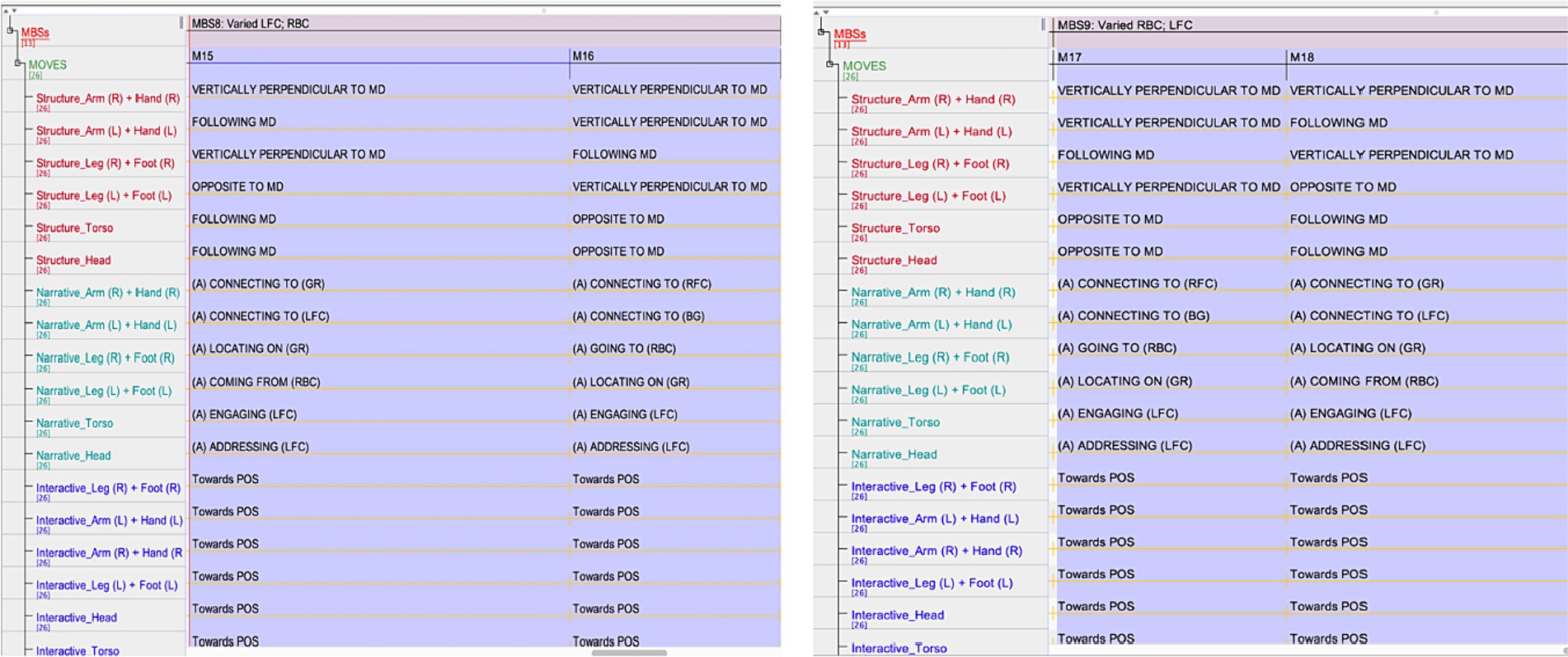
Figure 8 . Example of annotation from Abdur’s sequence in ENB’s version of Raymonda.
Projecting conclusions
I have started this article with an overview of the way the notion of materiality is understood and used as a nexus for connecting the different components of complex phenomena approached by a variety of disciplines and research areas. The pattern that emerged from such an overview highlights how materiality is actually used, as foregrounded by Bateman (2022) , as an external language for description that can be applied to several contexts where human experience manifests itself in and is carried out through multimodal meaning-making socio-semiotic practices. The overview also showed that the notion of materiality helps anchoring theoretical advances to phenomenological studies, thus highlighting the importance of empirical data in any analytical activity across disciplines. The developments observed in other research areas were echoed by the developments evidenced in multimodal discourse analysis through an excursus of the work carried out within The Kinesemiotic Body project, which focused on movement-based discourse. In this specific case, the application of the Functional Grammar of Dance in the annotation and testing of empirical data led not only to the further development of this analytical model and its theoretical framework of reference, but also to a much better understanding of the complex structures that underlie movement-based discourse and their interaction with the contextual space in which communication happens, thus providing much stronger foundations for the extension of this type of analysis to forms of movement-based communication other than ballet and dance in general.
Focusing on materiality really means looking at the complexity of human experience and the processes through which it is shaped into semiotic constellations where configurations of modes work in interplay. Stage performances offer great examples of this complexity, involving music, dance, sung or recited text, movement, lighting, settings, costumes, all in need of more empirical investigation. When working on movement-based communication and in particular on movement-based performance, the ‘materiality key’ has opened the door to the integrated work of linguists, computer scientists, semioticians and engineers as it has provided a common ground for collecting, processing and analysing movement data under mutually understood and shared theoretical principles, and also for creating a common language for defining fundamental concepts. It has also highlighted the complex relationships occurring amongst the different factors that enable this type of communication where human bodies interact with space and its perception. Eventually, the project led to the creation of more effective ways of collecting, annotating and understanding movement data. Our work is still ongoing: one of the project’s results was the creation of short videos where live-captured movement data is turned into avatars which can be inserted in virtual stage set-ups that can be modelled ad-hoc for experiments on perception of how the body interacts with contextual space in communication. The avatars represent both female and male dancers and can now be visualised as carrying out projections when dancing across the virtual stage as the software is now capable of reading automatically the FGD annotations in ELAN. We can even select which types of projections to visualise and how to distinguish one type from the other. These visualisations are still undergoing some level of refinement but there is great potential for future applications in dance education for both dance students, professionals and general audience and for different forms of performance studies and movement-based communication analysis. The same principles of visualisation are currently being applied to the study of potential gender bias in the representation of avatars’ movement in popular fighting games, thus extending the work started with The Kinesemiotic Body project to EDI issues related to the gaming world and relevant communities. These extensions of the work carried out by The Kinesemiotic Body project are possible precisely because the advances we made both in analysis and theory benefited from the focus on materiality as a descriptive language for unpacking and understanding the complexity of semiotic resources that work in interplay to produce dance discourse.
Data availability statement
The datasets presented in this study can be found in online repositories. The names of the repository/repositories and accession number(s) can be found at: https://doi.org/10.17028/rd.lboro.c.6230502.v1 , Loughborough University Research Repository.
Ethics statement
The studies involving humans were approved by Loughborough University Ethics Review Sub-Committee. The studies were conducted in accordance with the local legislation and institutional requirements. The participants provided their written informed consent to participate in this study.
Author contributions
AM: Funding acquisition, Methodology, Writing – original draft, Writing – review & editing.
The author(s) declare that financial support was received for the research, authorship, and/or publication of this article. Funding received from Loughborough University through the UKRI.
Conflict of interest
The author declares that the research was conducted in the absence of any commercial or financial relationships that could be construed as a potential conflict of interest.
Publisher’s note
All claims expressed in this article are solely those of the authors and do not necessarily represent those of their affiliated organizations, or those of the publisher, the editors and the reviewers. Any product that may be evaluated in this article, or claim that may be made by its manufacturer, is not guaranteed or endorsed by the publisher.
1. ^ The collaboration with John Bateman and the University of Bremen had actually started with John’s fellowship at Loughborough University funded by the Institute of Advanced Studies and aimed at fostering our interdisciplinary collaboration in 2017. The rest of the research team was made by Massimiliano Zecca, Russell Lock, Chun Liu from Loughborough University and Dayana Markhabayeva from the University of Bremen.
Asher, N., and Lascarides, A. (2003). Logics of conversation . Cambridge: Cambridge University Press.
Google Scholar
Balmaceda, M., Högselius, P., Johnson, C., Pleines, H., Rogers, D., and Tynkkynen, V. P. (2019). Energy materiality: a conceptual review of multi-disciplinary approaches. Energy Res. Soc. Sci. 56:101220. doi: 10.1016/j.erss.2019.101220
Crossref Full Text | Google Scholar
Bateman, J. A. (2019). Multimodality and materiality: the interplay of textuality and Texturality in the aesthetics of film. Poetics Today 40, 235–268. doi: 10.1215/03335372-7298536
Bateman, J. A. (2022). Multimodality, where next? Some meta-methodological considerations. Multimodal Soc 2, 41–63. doi: 10.1177/26349795211073043
Bateman, J. A., and Wildfeuer, J. (2014). A multimodal discourse theory of visual narrative. J. Pragmat. 74, 180–208. doi: 10.1016/j.pragma.2014.10.001
Bateman, J., Wildfeuer, J., and Hiippala, T. (2017). Multimodality: foundations, research and analysis. A problem-orented introduction. Berlin, Boston: De Gruyter.
Björkvall, A., and Karlsson, A. M. (2011). The materiality of discourses and the semiotics of materials: a social perspective on the meaning potentials of written texts and furniture. Semiotica 2011, 187–1/4. doi: 10.1515/semi.2011.068
Bolens, G. (2022). Embodied cognition, Kinaesthetic knowledge, and Kinesic imagination in literature and visual arts. Front Commun 7:232. doi: 10.3389/fcomm.2022.926232
Elyamany, N. (2023). A chronotopic approach to identity performance in musical numbers: a choreo-musical case study of ‘rewrite the stars’ and ‘this is me’. Vis. Commun. 22, 278–296. doi: 10.1177/1470357220974069
Gherardi, S. (2017). “Sociomateriality in posthuman practice theory” in The Nexus of practices: Connections, constellations, and practitioners . eds. S. Hui, E. Shove, and T. Schatzki (London-New York: Routledge), 38–51.
Gibbons, A. (2012). Multimodality, cognition, and experimental literature . London: Routledge.
Kell, C. (2015). “Making people happen”: materiality and movement in meaning-making trajectories. Soc. Semiot. 25, 423–445. doi: 10.1080/10350330.2015.1060666
Kress, G. (2010). Multimodality: A social semiotic approach to contemporary communication . London-New York: Routledge.
Lacković, N., and Popova, B. (2021). Multimodality and sociomateriality of lectures in global universities’ media: accounting for bodies and things. Learn. Media Technol. 46, 531–549. doi: 10.1080/17439884.2021.1928694
Lee, T.-K. (2014). Translation, materiality, intersemioticity: excursions in experimental literature. Semiotica 202, 345–364. doi: 10.1515/sem-2014-0044
Leonardi, P. M., and Barley, S. R. (2010). What’s under construction Here? Social action, materiality, and power in constructivist studies of technology and organizing. Acad. Manag. Ann. 4, 1–51. doi: 10.5465/19416521003654160
Maiorani, A. (2017). “Making meaning through movement: a functional grammar of dance movement” in Mapping multimodal performance studies . eds. M. G. Sindoni, J. Wildfeuer, and K. L. O'Halloran (London/New York: Routledge), 39–60.
Maiorani, A. (2020). Selling the past and the present alike: streaming ballet for live audiences during lockdown. J Int Cult Arts 1, 1–9. doi: 10.46506/jica.2020.1.2.001
Maiorani, A. (2021). Kinesemiotics: Modelling how choreographed movement means in space . London-New York: Routledge.
Maiorani, A., Bateman, J. A., Liu, C., Markhabayeva, D., Lock, R., and Zecca, M. (2022). Towards semiotically driven empirical studies of ballet as a communicative form. Human Soc Sci Commun 9:429. doi: 10.1057/s41599-022-01399-8
Maiorani, A., and Liu, C. (2023). The functional grammar of dance applied to ELAN annotation: meaning beyond the naked eye. J World Lang. doi: 10.1515/jwl-2023-0050
Maiorani, A., and Wegener, R. (2022). “Challenging instantiation in modelling movement-based multimodal communication” in Empirical evidences and theoretical assumptions in functional linguistics . eds. E. Asp and M. Aldridge (London – New York: Routledge), 151–169.
Meissl, K., Sambre, P., and Feyaerts, K. (2022). Mapping musical dynamics in space. A qualitative analysis of conductors' movements in orchestra rehearsals. Front Commun 7:733. doi: 10.3389/fcomm.2022.986733
Merriman, P., and Jones, R. (2017). Nations, materialities and affects. Prog. Hum. Geogr. 41, 600–617. doi: 10.1177/0309132516649453
Mouard Ruiz, E. (2021). En movimiento: audiodescripción del acuario del arrecife de coral. Universidad de Granada. Departamento de Traducción e Interpretación. Available at: http://hdl.handle.net/10481/69420
Pantidos, P., Valakas, K., Vitoratos, E., and Ravanis, K. (2010). The materiality of narrative spaces: a theatre semiotics perspective into the teaching of physics. Semiotica 2010, 305–325. doi: 10.1515/semi.2010.062
Petrilli, S. (2008). Bodies and signs: for a typology of semiosic materiality. Am J Semiotics 24, 137–158. doi: 10.5840/ajs200824422
Prové, V. (2022). Measuring embodied conceptualizations of pitch in singing performances: insights from an OpenPose study. Front Commun 7:987. doi: 10.3389/fcomm.2022.957987
Putnam, L. (2014). Unpacking the dialectic: alternative views on the discourse–materiality relationship. J. Manag. Stud. 52:115. doi: 10.1111/joms.12115
Schatzki, T. (2010). Materiality and social life. Nat Cult 5, 123–149. doi: 10.3167/nc.2010.050202
Sindoni, M. G. (2022). Traiettorie della multimodalità: gli snodi teorici e i modelli applicativi. Ital LinguaDue 14:3597. doi: 10.54103/2037-3597/2
Tyrer, C. (2021). The voice, text, and the visual as semiotic companions: an analysis of the materiality and meaning potential of multimodal screen feedback. Educ. Inf. Technol. 26, 4241–4260. doi: 10.1007/s10639-021-10455-w
Vidal Claramonte, M. Á. (2022). Translation and contemporary art: transdisciplinary encounters. New York: Routledge.
Wilf, E. (2011). Sincerity versus self-expression: modern creative agency and the materiality of semiotic forms. Cult. Anthropol. 26, 462–484. doi: 10.1111/j.1548-1360.2011.01107.x
Wu, X. (2022). Space and practice: a multifaceted understanding of the designs and uses of “active learning classrooms” . UNSW: Sydney.
ELAN (Version 6.2) [Computer software] . (2021). Nijmegen: Max Planck Institute for Psycholinguistics, The Language Archive. Available at: https://archive.mpi.nl/tla/elan .
Keywords: materiality, multimodal discourse analysis, Functional Grammar of Dance, empirical data analysis, movement-based communication
Citation: Maiorani A (2024) The materiality key: how work on empirical data can improve analytical models and theoretical frameworks for multimodal discourse analysis. Front. Commun . 9:1365145. doi: 10.3389/fcomm.2024.1365145
Received: 03 January 2024; Accepted: 19 March 2024; Published: 28 March 2024.
Reviewed by:
Copyright © 2024 Maiorani. This is an open-access article distributed under the terms of the Creative Commons Attribution License (CC BY) . The use, distribution or reproduction in other forums is permitted, provided the original author(s) and the copyright owner(s) are credited and that the original publication in this journal is cited, in accordance with accepted academic practice. No use, distribution or reproduction is permitted which does not comply with these terms.
*Correspondence: Arianna Maiorani, [email protected]
This article is part of the Research Topic
Drawing Multimodality's Bigger Picture: Metalanguages and Corpora for Multimodal Analyses
- Open access
- Published: 21 March 2024
“Anxiety is not cute” analysis of twitter users’ discourses on romanticizing mental illness
- Barikisu Issaka ORCID: orcid.org/0009-0002-6468-9882 1 , 5 ,
- Ebenezer Ato Kwamena Aidoo 2 ,
- Sandra Freda Wood 3 &
- Fatima Mohammed 4
BMC Psychiatry volume 24 , Article number: 221 ( 2024 ) Cite this article
701 Accesses
15 Altmetric
Metrics details
The proliferation of social media platforms has provided a unique space for discourse on mental health, originally intended to destigmatize mental illness. However, recent discourses on these platforms have shown a concerning shift towards the romanticization of mental health issues. This research focuses on Twitter (now called X) users’ authentic discussions on the phenomenon of romanticizing mental health, aiming to uncover unique perspectives, themes, and language used by users when engaging with this complex topic.
A comprehensive content analysis was conducted on 600 relevant tweets, with the application of topic modeling techniques. This methodology allowed for the identification and exploration of six primary themes that emerged from Twitter users’ discussions. Statistical tests were not applied in this qualitative analysis.
The study identified six primary themes resulting from Twitter users’ discussions on the romanticization of mental health. These themes include rejecting/critiquing the glamorization of mental health, monetization of mental health by corporate organizations, societal misconceptions of mental health, the role of traditional media and social media, unfiltered realities of depression, and the emphasis on not romanticizing mental health.
Conclusions
This study provides valuable insights into the multifaceted discourses surrounding the romanticization of mental health on Twitter. It highlights users’ critiques, concerns, and calls for change, emphasizing the potential harm caused by romanticizing mental illness. The findings underscore the importance of fostering responsible and empathetic discussions about mental health on social media platforms. By examining how Twitter users interact with and respond to the romanticization of mental health, this research advances our understanding of emerging perspectives on mental health issues among social media users, particularly young adolescents. The study also underscores the effects of this phenomenon on individuals, society, and the mental health community. Overall, this research emphasizes the need for more responsible and knowledgeable discussions around mental health in the digital age.
Peer Review reports
Mental illness (MI) is characterized by significant disturbances in thoughts and emotions [ 1 ]. The World Health Organization (WHO) reports that approximately one billion individuals, equating to over one-eighth of the global population, grapple with mental disorders, with depression impacting 280 million and anxiety affecting 301 million [ 2 , 3 ]. Despite the prevalence of MI, persistent stigma surrounds it, often driven by historical fears and media portrayals [ 4 ]. Corrigan et al. [ 5 ] identified two forms of stigma associated with mental illness: internalized stigma, where individuals within the stigmatized group develop negative self-perceptions; and public stigma, encompassing society’s adverse attitudes leading to prejudice and discrimination [ 5 ].
The emergence of social media has revolutionized the discourse on mental health, providing a platform for open discussions and education [ 6 , 7 ]. Social media enables individuals to share their experiences, reducing stigma and initiating conversations [ 8 ]. However, social media has also given rise to a concerning trend—the romanticization of mental illness, portraying it as glamorous and desirable [ 9 , 10 , 11 ]. Some users normalize mental illness by treating it as an accessory and characterizing its challenges as a form of victimization [ 12 ]. This phenomenon distorts the true nature of mental illness and may encourage it as an alternative form of self-expression [ 4 ]. While prior research has explored romanticization in traditional media [ 9 , 12 ], a significant gap exists in understanding how social media users perceive and discuss this phenomenon on platforms like Twitter. Existing studies have predominantly examined content analysis and framing in traditional media, overlooking the unique perspectives and language employed by users in the social media context. This study aims to analyze how Twitter users discuss the issue of romanticizing mental health on the platform, addressing the gap in the literature and providing insights into this specific social media context. It seeks to inform policies and interventions addressing the challenges posed by mental health romanticization on Twitter.
Mental health in traditional media
Traditional media, including movies, has a history of inaccurately portraying mental illnesses, perpetuating negative stereotypes across various cultural contexts. Wood et al. [ 13 ] observed that mental illness has frequently featured in mass media, but these representations have consistently fallen short. They tend to depict mental illnesses as violent, unpredictable, overly simplistic, detached from reality, or innocent [ 14 ].
These portrayals often serve as narrative devices, aiding in character development and scene establishment [ 15 ]. By presenting mentally ill individuals as behaving and appearing distinct from “normal” individuals, these depictions reinforce an “us vs. them” narrative [ 16 ]. Hyler and colleagues identified and examined six recurring movie clichés that further stigmatized individuals with mental illnesses, including the narcissistic parasite, seductress, enlightened member of society, rebellious free spirit, and homicidal lunatic [ 17 ]. Numerous studies conducted across diverse cultural contexts have highlighted the prevalence of these inaccurate representations of mental health in traditional media. For example, studies have shown that news coverage in various countries can link mental illness to violence [ 18 ]. News stories often attribute gun violence to “dangerous people “rather than “dangerous weapons,” perpetuating stigmatizing narratives [ 19 ].
Similarly, Corrigan et al. [ 5 ] reported that a substantial portion of newspaper stories in different regions portrayed mental illness as dangerous and violent, with such stories frequently featured prominently. Misrepresentations of mental illness are not limited to newspapers but extend to global literature, including novels. Works like “Liar” (2009) by Justine Larbalestier depict individuals with mental illness as criminal and dehumanized, potentially perpetuating inaccurate perceptions across international audiences [ 20 ]. Films like “All the Bright Places” illustrate how the stigma associated with mental illness exacerbates characters’ mental health challenges, impacting individuals from diverse cultural backgrounds within the narrative [ 21 ].
Apart from stigmatization, mental illnesses like OCD and ADHD are also frequently trivialized. One aspect of trivialization involves the normalization of symptoms associated with mental illness, which may result in the dismissal of these symptoms as commonplace experiences rather than indicators of significant distress or impairment. For example, behaviors related to obsessive-compulsive disorder (OCD), such as frequent handwashing or checking rituals, may be trivialized as mere quirks or personality traits rather than recognized as symptoms of a debilitating mental health condition. Additionally, trivialization can manifest through societal attitudes and media portrayals that depict mental illness in a lighthearted or humorous manner, thereby minimizing the lived experiences of individuals affected by these conditions. This can contribute to a lack of empathy and understanding toward those struggling with mental health challenges.
Research has shown that trivialization of mental illness can have detrimental effects on help-seeking behaviors and treatment adherence among individuals experiencing psychological distress. When symptoms are trivialized or dismissed, individuals may feel invalidated or reluctant to seek professional help, fearing that their concerns will not be taken seriously [ 68 , 69 ]. Furthermore, trivialization intersects with other forms of stigma, such as social rejection and discrimination, exacerbating the challenges faced by individuals with mental health conditions in various social and institutional settings.
Several studies have contributed to our understanding of trivialization in relation to mental illness. For example, Clemente and colleagues [ 68 ] conducted qualitative research to understand meanings and implications of the stigma related to bipolar disorder and found that trivialization of their symptoms by others contributed to feelings of frustration and alienation, it further contributed to cause of treatment refusal. This demonstrates how trivialization represents a significant barrier to the recognition and support of individuals with mental health conditions in society.
The literature discussed above underscores the historical prevalence of unfavorable portrayals of mental health in traditional media, transcending national borders and encompassing various cultural contexts, including TV, movies, novels, and fictional characters. Providing a brief explanation of how mental health has been depicted on social media would offer valuable insights and enhance the contextual understanding of this study.
Social media and mental health
In the past decade, social media has undergone a profound transformation, reshaping the way individuals interact, communicate, and address mental health concerns [ 22 ]. It has evolved into a dynamic platform for self-expression, enabling people to openly share their thoughts, life experiences, and memories. Moreover, it has become a virtual space where individuals grappling with mental health challenges can connect, find solace, and extend their support to like-minded peers [ 23 ]. Research has illuminated the prevalence of constructive and positive discussions surrounding mental health on social media. For instance, it’s worth noting that tweets conveying inspirational messages tend to receive more retweets (an average of 4.17) than stigmatizing content (with an average of 3.66), underscoring the pervasive presence of uplifting messages in the digital sphere [ 24 ]. The discourse encompassing mental illnesses like depression and anxiety on social media overwhelmingly leans toward supportiveness and encouragement, creating a sense of solidarity among individuals facing similar struggles [ 25 ]. Young people, particularly, utilize platforms such as.
Facebook and Twitter as digital sanctuaries to escape external pressures that can adversely affect their mental well-being [ 26 ]. In essence, social media platforms serve as open canvases for unfiltered expressions and candid discussions about mental health, cultivating a sense of online community [ 27 , 28 ]. Studies reveal that discussions on social media related to depression primarily revolve around offering support and sharing personal experiences, rather than perpetuating stigma or shame [ 29 ]. Similarly, research conducted on Sina Weibo, a prominent microblogging platform in China, illustrates that influential users tend to generate more supportive and helpful posts than negative or stigmatizing ones [ 30 ].
Information concerning recovery and treatment plays a particularly pivotal role in nurturing a positive discourse on mental health. These findings not only underscore the essential role of social media in dismantling the stigma associated with mental health issues but also emphasize its capacity to foster a compassionate and supportive digital community. In this light, social media emerges as a potent tool for advancing mental health awareness and advocacy [ 19 ]. These findings emphasize the profound potential of social media to reshape how we approach mental health, offering not only a space for candid conversations but also a powerful means to combat stigma and promote understanding and support among individuals facing mental health challenges.
Suicide contagion and responsible media reporting guidelines
The issue of suicide contagion, particularly in contexts where romanticization occurs, has garnered significant attention in academic literature due to its potentially dangerous implications. Gould and Shaffer [ 70 ] and subsequent studies have shown that media portrayals of suicide, particularly those glamorizing or sensationalizing the act, can lead to copycat suicides, especially among vulnerable populations like adolescents. Efforts to address this issue include guidelines from the World Health Organization (WHO), Samaritans, the American Foundation for Suicide Prevention, and other mental health and prevention organizations. These guidelines seeks to streamline and deglamorize media reporting of suicide in a responsible manner more specifically news and media information. Among these guidelines is the avoidance of glorification of suicide to help prevent the Werther effect and possible imitation or contagion effects [ 71 , 72 ]. However, despite these guidelines, research by Stack [ 74 ] and others suggests that sensationalized or glamorized media reporting on suicide persists, contributing to increased suicide rates, as evidenced by studies such as Fu and Yip [ 75 ] in Asia.
In recent years, attention has turned to the role of social media platforms like Twitter in influencing suicidal behavior. While social media offers real-time engagement and information dissemination, it also presents challenges in monitoring and regulating content related to suicide. Research by Markman [ 76 ] and Jashinshy et al. [ 77 ] has shown associations between Twitter activity related to suicide and actual suicide rates, prompting platforms like Twitter to update their policies on harmful content [ 78 ]. However, the implementation and enforcement of these policies remain a concern, as studies suggest limited uptake of recommendations. Therefore, despite efforts to address suicide contagion through media guidelines and social media policies, further research and action are needed to effectively mitigate the risks associated with romanticized depictions of suicide in digital environments.
Romanticizing mental health on social media
While discourses on social media were initially aimed at destigmatizing mental health, there has been a notable shift towards romanticizing mental illness, including the creation of memes and content glamorizing conditions such as anxiety [ 21 ]. Users, in their attempt to make conversations about mental illness empathetic, often inadvertently end up romanticizing it, portraying it as an accessory, and those experiencing it as martyrs [ 31 ]. This romanticization can manifest in various forms, from seemingly harmless expressions to more troubling instances such as the glorification of suicide [ 4 ]. Chen [ 32 ] shared her experience of encountering romanticized mental illness content online, highlighting how depression and anxiety were portrayed as fashionable. This phenomenon extends to the proliferation of melancholic content, black-and-white imagery, and narratives of low self-esteem on platforms like Tumblr.
Similar concerns are observed on platforms like Facebook and blogs, where mental health disorders, including anorexia nervosa, self-harm, depression, and anxiety, are increasingly romanticized [ 1 ]. For instance, pro-anorexia (pro-ana) websites have gained notoriety for promoting dangerous behaviors linked to anorexia nervosa, such as extreme dieting practices and unhealthy weight loss methods [ 79 ]. These platforms often perpetuate unrealistic body ideals and encourage individuals to pursue thinness at any cost, disregarding the severe physical and psychological consequences of eating disorders [ 80 ].
Pro-ana websites, for instance, promote dangerous behaviors related to anorexia, perpetuating unrealistic dieting tips and methods [ 33 ]. Furthermore, the influence of celebrities like Lana Del Rey, who has incorporated themes of depression into her music and videos, contributes to the normalization of mental health struggles [ 1 ]. This trend has serious consequences, including the normalization of suicidal behaviors among young people, a rise in feigned mental illness, and self-diagnosis [ 9 , 31 ]. It distorts societal perceptions of mental health and hinders genuine expressions of mental health concerns [ 12 ].
However, the use of pro-ana communities extends beyond the promotion of harmful behaviors, as they also serve as complex online environments where individuals with eating disorders find social support and understanding. Research suggests that individuals who engage with pro-ana content may do so as a means of coping with the challenges of their disorder, seeking validation from peers who share similar struggles [ 80 , 81 , 82 , 83 ,]. Within these communities, individuals often engage in open and candid discussions about their experiences with disordered eating, sharing personal stories, struggles, and successes. For many, the sense of belonging and camaraderie found in these online spaces provides a form of support that may be lacking in their offline lives [ 72 ].
Moreover, some individuals perceive pro-ana websites as empowering spaces where they can exercise agency over their bodies and identities, challenging societal norms and expectations [ 73 ]. This perception underscores the complexity of motivations underlying engagement with pro-ana content and highlights the need for a nuanced understanding of these online communities. Despite the potential benefits of social support within pro-ana communities, it is essential to acknowledge the inherent risks associated with these platforms. Exposure to pro-ana content has been linked to increased body dissatisfaction, disordered eating behaviors, and decreased motivation to seek professional help [ 81 , 84 ]. It is important to acknowledge that pro-ana websites represent multifaceted online environments where individuals with eating disorders navigate between the promotion of harmful behaviors and the search for social support and understanding.
Romanticization of mental illness can be viewed as a contemporary form of stigma, akin to historical media stereotypes [ 4 ]. While prior research has explored various aspects of this phenomenon, including its framing and negative effects, these studies have mostly focused on traditional media. There is a noticeable gap in understanding how social media users engage in discussions about and articulate the romanticization of mental health in their own words on the platform. Furthermore, while studies have examined this issue on other social media platforms, Twitter has received limited attention despite its significant role in mental health discourse [ 34 , 35 ]. Accordingly, there is a critical need to directly explore authentic discourses initiated by Twitter users. Twitter users frequently adopt pseudonyms and tend to interact with strangers, which allows them to communicate anonymously. Analyzing discourse on Twitter could offer a less biased representation of people’s experiences, as it is more naturalistic, includes a diverse population, and is often anonymous, thereby mitigating some of the constraints of conventional data collection approaches [ 36 ].
Research question
How do Twitter users discuss the phenomenon of romanticizing mental health in their own words on the platform, and what themes and perspectives emerge from their conversations?
This research question aims to uncover the unique perspectives and language employed by Twitter users as they actively engage with the topic of romanticizing mental health, providing an in-depth exploration of their authentic discussions and viewpoints. By focusing on Twitter, it addresses the existing gap in the literature, emphasizing the importance of understanding the specific dynamics of this platform in shaping conversations about mental health romanticization.
Methodology
Content analysis.
Our research methodology involved conducting content analysis on Twitter data using topic modeling. Content analysis is a commonly employed qualitative research method [ 37 ] that allows for a comprehensive examination of individuals’ experiences by extracting relevant topics from textual documents [ 38 ].
Research setting and ethics
Twitter, with 166 million active users worldwide [ 39 ], was the research platform chosen. It is a prominent social networking site (SNS) for sharing daily activities, expressing opinions, and delivering news [ 40 ]. This unique platform enables the investigation of health and illness narratives [ 41 ], traditionally studied through methods like interviews and autobiographies [ 42 ]. Unlike conventional human subject research, analyzing public tweets does not require informed consent because such tweets are public [ 43 , 44 ,, 45 ]. However, this pertains solely to public tweets, as private profiles are excluded. Adhering to ethical guidelines [ 45 ], the study omitted user-identifying information and respected the privacy of individuals with private profiles [ 36 ].
Data collection
Researchers collected data from Twitter using the 4CAT, a reference and analysis tool developed by researchers at the University of Amsterdam [ 46 ]. We inductively identified words associated with romanticizing mental health through earlier research [ 4 , 21 ]. This resulted in a list of specific keywords which were used to scrape tweets using the following query:
#Mental health month #Romanticizing mental health# Mental health aesthetics #mental health is not cute #Mental health #glamourizing mental health # Mental health in the US #Romanticizing mental health in the US
To ensure the inclusion of mental health-related tweets, data analysis focused on a specific period, spanning from May 1st, 2022, to May 30th, 2023, corresponding to the global mental health month [ 47 ]. Researchers retrieved 1000 tweets. The observations gathered for analysis included key information such as username, followers, following, tweet ID, text, date, and time tweeted [ 36 ]. To maintain relevance, only tweets that specifically addressed the topic of mental health and romanticization were included, while tweets with irrelevant content or media attachments were excluded [ 48 ]. The final dataset was limited to English-language tweets, and after removing spam and public service announcements, a comprehensive set of relevant tweets (600 in number) was obtained.
Data analysis
Latent Dirichlet Allocation (LDA) was employed as the topic modeling algorithm in this study. The primary goal was to discover latent topics within the text corpus and examine the distribution of topics across the documents. The model used is a powerful technique for extracting hidden themes or topics from a collection of text documents which is Latent Dirichlet Allocation (LDA). LDA was selected due to its ability to uncover hidden themes or topics within a corpus of text. This makes it a valuable tool for exploring various types of text data, such as customer reviews and news articles.
LDA is a hierarchical Bayesian model with three layers that is sought to investigate latent schemes in document corpora. Each document is represented as a random mixture of subjects, with each topic characterized by a distribution across words [ 49 ]. LDA is built on top of the premise that each document can be described by the probabilistic distribution of topics, and each topic can be described by the probabilistic distribution of words. This can provide a much clearer vision of how topics are connected [ 50 ].
Prior to applying LDA, the text data underwent a series of preprocessing steps such as tokenization, bag-of-words. The preprocessing is aimed to enhance the modeling accuracy and avoid the influence of search-related terms on the discovered topics. The model was trained using an optimum number of 5 topics. To determine the optimal number of topics (K), we used coherence and perplexity scores.
We experimented with different values of K, ranging from 2 to 11, and evaluated the coherence and perplexity interpretability of the resulting topics. A moderate range, such as 2 to 11 was chosen because training LDA with a very large number of topics may not lead to meaningful insights. If the number of topics selected is too small, the meaning under each topic will be too broad; if the number of topics selected is too large, it will lead to an over-clustering of data, producing useless topics or topics with too much similarity [ 51 ]. It also facilitates basic understanding of the topic’s structure. Seeing the same key words being repeated in multiple topics is a sign that ‘k’ is too large. Topic coherence can be defined as how interpretable a topic is based on the degree of relevance between the words within the topic itself [ 52 ]. Higher coherence scores indicate better-defined topics.
Perplexity is a measure of how well our LDA model predicts the held-out or unseen data. It quantifies how surprised the model is when it encounters new documents. Lower perplexity scores indicate that the model is better at predicting unseen text. Basically, the lower the perplexity, the more predictable it is. This indicates better generalization and performance [ 53 ]. Having the right balance between quantitative metrics like perplexity and qualitative assessments of topic quality is key to a successful LDA analysis. After careful consideration of the coherence perplexity scores, an optimal number of 5 topics was selected. The 5 Topics have a coherence score of 0.43 and perplexity score of -6.077. To finish with, we generated word clouds for each category of topic to visually represent the most important terms in each topic, which will be discussed in the analysis.
To strengthen the credibility and provide context for the LDA model findings, which include the five topics in Table 2 the word frequencies in Table 3 , and the visual representations of the word cloud in Figure 1 – 5 , representing topics 1–5 respectively, we conducted a qualitative analysis to delve deeper into the underlying themes.
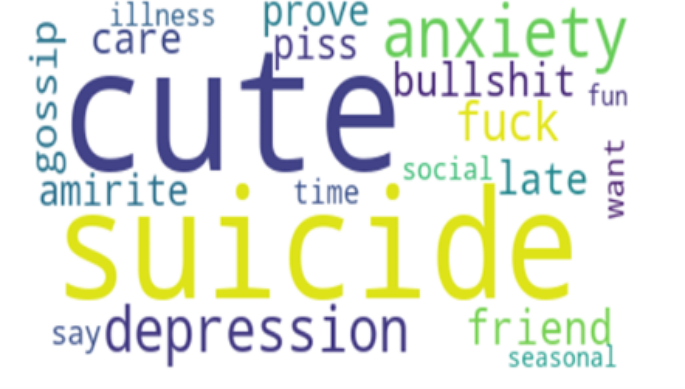
Word Cloud for Topic 1
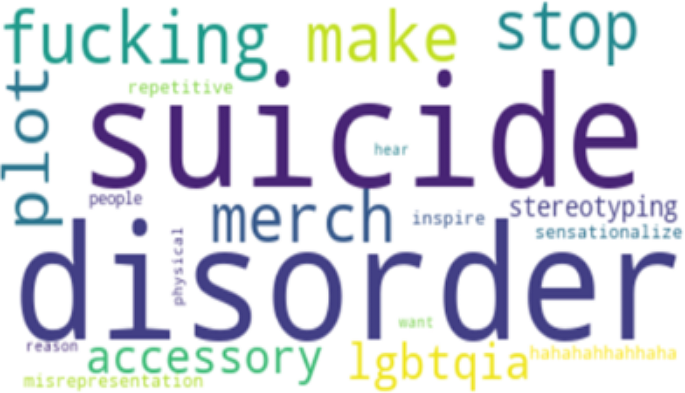
Word Cloud for Topic 2
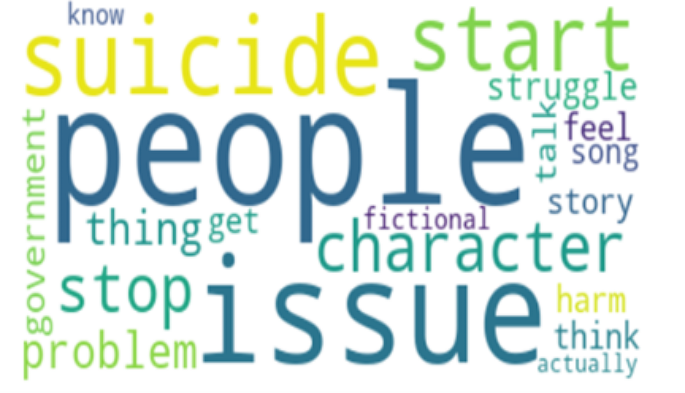
Word Cloud for Topic 3
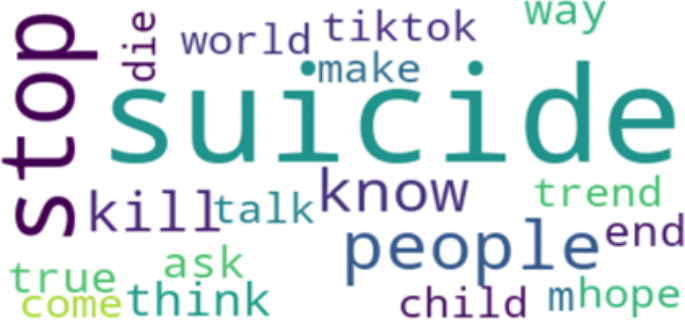
Word Cloud for Topic 4
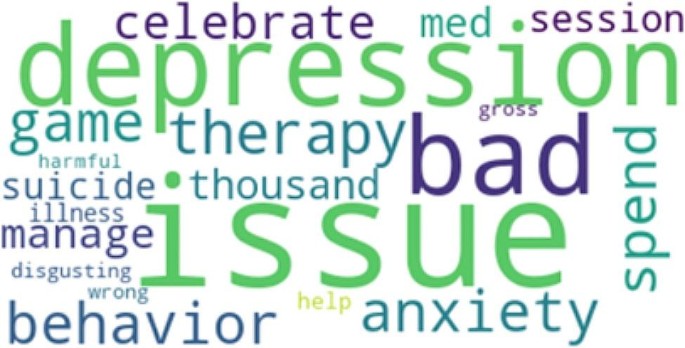
Word Cloud for Topic 5
In Table 3 you will find data related to the top-ranked words and their frequencies based on the LDA analysis. This table indicates the top-ranked words identified by LDA analysis and the number of times each of these words appears in the dataset.
Consistent with prior research, we applied Braun and Clarke’s six-step thematic analysis: (i). familiarizing ourselves with the keyword data, (ii) creating initial codes, (iii) identifying themes, (iv) reviewing potential themes, (v) defining the themes, and (vi) reporting the results [ 53 ]. Our approach was iterative and reflective, allowing us to move back and forth through these phases.
To minimize biases, each of the team members independently went through this process and then cross-compared their findings. We reviewed all identified codes to ensure alignment with the identified topics. Six themes were categorized and named, aligning with the overarching meaning of the five identified topics. These six themes and their corresponding tweets can be found in Table 1 .
Below, we will explain each theme and how they were expressed on Twitter. The corresponding tweets associated with each topic are shown in Table 1 .
Rejecting/critiquing the glamorization of mental health
Users’ responses conveyed strong critiques and concerns regarding the way mental health issues are portrayed on the platform. Many users expressed their vehement disapproval of the glamorization of mental health issues. They employed explicit language and terminology associated with specific mental health concerns to underscore their critique. Notably, one user remarked:
Anxiety is not cute at all for y’all to make stupid TikToks like that
Users also called attention to the glamorization of particular mental health conditions, highlighting their specific concerns. For instance:
Need to get out of this bed and get cute cause this depression is not cute” (Depression)
Within this theme, some users also expressed their strong desire to seek help and transform the way sensitive mental health topics are discussed on the platform. An illustrative example includes:
One day I’ll get the help I need instead of romanticizing suicide on Twitter, not today, but one day
Additionally, several users advocated for a fundamental shift in the portrayal and discourse surrounding mental health disorders. A notable tweet within this context is:
We need to stop romanticizing mental health disorders like adhd and autism
These tweets collectively shed light on users’ critiques and expressions within Theme 1.
Monetization of mental health by corporate organization/confronting the commercialization of mental health (CMH)
Twitter users expressed their concerns and critiques regarding the commercialization of mental health issues through merchandise. In response to the repetitive use of certain themes, one user provided a critique:
They’re using repetitive plots, eh haha! sensationalizing and romanticizing suicide and making a fucking merch and accessories out of it.
Some users also highlighted the significance of businesses having a comprehensive understanding of what they are selling, particularly when it involves merchandise related to mental health:
This is why businesses should know the background of what they’re selling, especially when it involves merchandise. You’re selling something you don’t know that you ended up unintentionally romanticizing suicide. It is honestly triggering.
Users also expressed their concerns regarding specific phrases used on merchandise items:
Finally! I have always been bothered by merch with the phrase ‘to be one with the stars’ like fls huhu stop just stop romanticizing suicide.
These tweets collectively reflect users’ concerns about how phrases used on merchandise sold for profit can intentionally or unintentionally promote romanticization of mental health.
Societal misconception of mental health
Within the theme of “Societal Misconception of Mental Health,” users shared their perspectives on common societal misunderstandings and misconceptions surrounding mental health. Many users expressed their concerns about the societal misconception of romanticizing suicide and its harmful consequences:
The amount of people romanticizing suicide in the comments is sickening. The firefighter saved someone’s life after they made an irrational decision. This is a real person with real family and friends, and a future ahead of them. Their life matters.
When and how cultural contagion happens is an interesting topic. The only analogy I have is about suicide. Talking about suicide does not increase rates of suicide (people think it does). But ROMANTICIZING suicide has caused contagion of the behavior.
Some users took the opportunity to challenge common misconceptions about mental health, aiming to correct these misunderstandings:
More common than I think? I have read up on OCD last year. A lot of you assume perfectionists have OCD, which is very, very wrong. I have a friend with diagnosed OCD, and I sure asl know how bad it is.
Users also criticized the societal misconception of romanticizing mental health issues, particularly among young people:
This era of youths and romanticizing mental health issues. God abeg oh.
Finally, users encouraged a more balanced perspective on mental health, emphasizing the importance of self-improvement rather than romanticizing mental health issues:
Don’t romanticize your mental issues and romanticize working on yourself.
Role of Traditional Media and Social Media
Users voiced their observations and concerns regarding the portrayal of mental health on these platforms. Many users denounced the tendency to romanticize mental health disorders on platforms like TikTok, emphasizing the need to address this issue:
TikTok is like if you breathe through your nose you have adhd, autism and bipolar. CAN we stop romanticizing mental health disorders? You have bpd because the clock app told you so?
It’s crazy how many TikTok’s I’ve seen of people romanticizing mental health issues…like girl it ain’t cute/trendy to have them…
Between therapy becoming a trend and TikTok romanticizing mental health y’all have all the language but none of the tools.
Some users observed that the romanticization of mental health issues on social media has been a long-term trend:
Yes they are. Social media has been romanticizing mental health issues of all sorts since I was in middle school. I’m in my 30s…
Additionally, a user pointed out inconsistencies in media criticism related to the romanticization of mental health:
I’ve seen a few Yandere creators criticize people for romanticizing suicide in fiction when they’re okay with people romanticizing MURDERS and abusive relationships in fiction? Like dude you’re making Yandere focused content, you really can’t be talking.
Unfiltered realities of depression
Within the theme of “Unfiltered Realities of Depression,” users candidly shared their personal experiences and unfiltered perspectives on living with depression.
Some users provided raw and unfiltered insights into their personal experiences with depression:
My depression was bad. I wouldn’t shower, brush my teeth or hair, clean my room, nothing. I would legit work, come home, and sit on my computer all night and think about just driving till my car was empty and then killing myself.
Others expressed a strong desire to break free from the grip of depression and regain a sense of joy in their lives:
I need to seek out fun again and not give a fuck if ppl get weird, butt hurt or jealous bc I want to have a good time. How long do I need to be a miserable adult for?
Depression is not cute but I have to learn to push back before it consumes me.
Users also critiqued the way Twitter seems to celebrate bad behavior while romanticizing mental health issues:
I spend thousands on therapy and meds just to manage my depression and anxiety….Twitter they celebrate bad behavior by romanticizing mental health issues? Baket?
Another user pointed out the inconsistencies in how mental health issues are perceived, especially when the “bad” symptoms are revealed:
Ppl love romanticizing mental health issues until someone shows the ‘bad’ symptoms.
These tweets collectively reflect users’ unfiltered experiences and perspectives within the theme of “Unfiltered Realities of Depression,” emphasizing the harsh realities of living with depression.
We are not romanticizing
Within the theme of “We are not romanticizing,” users defended themselves and others against accusations of romanticizing suicide or mental health struggles. Many users took a stand against being labeled as romanticizing suicide or mental health issues and emphasized that discussing these topics is crucial:
I don’t think that I’m the only one! BUT again, it really doesn’t help things to call ppl ‘crazy’ or ‘batshit’ or say that they’re romanticizing suicide when they’re literally talking about a current event.
ALSO, the suicide taboo is empirically bad at reducing suicides!!
Some users also shared their frustration with being accused of romanticizing suicide or seeking attention”
I often vent about my depression and my suicidal thoughts and a few weeks ago, some chick literally said I was romanticizing suicide and was just whining for attention… Seriously, gfy!
These tweets collectively reflect users’ strong reactions and defenses within the theme of “We are not romanticizing
With romanticization of mental health issues, discourses on Twitter are complicating the stigmatization and destigmatization dichotomy of mental health issues. The theme of rejecting the glamorization of mental health in the tweets analyzed is indicative of the findings from several studies that have considered the negative effects of glamorizing mental health on social media [ 12 , 54 ]. Similar to previous studies, society is now moving mental illness a step further from being stigmatized to glorified [ 21 ]. Such a shift masks the attention that needs to be paid to mental illness. The harmful effect of this trend of glamorization of health issues has been emphasized by researchers, medical (psychiatry) experts, and institutions [ 1 , 55 ]. Unique to our study, we highlight the outcry for people in their tweet to end this glorification act. Interestingly, Twitter users are calling for legal actions to be taken against this act because it is not “cute”. People believed that engaging in these glamorization acts shift attention from the “actual” or “real” symptoms that people experiencing mental illness have to deal with such as depression anxiety, personality disorder, and schizophrenia. Embedded in this call is the misformation and disformation that is spread within these glamorized tweets on mental illness.
In addition, our findings on monetization of mental health by corporate organizations/confronting the commercialization of mental health (CMH) explains the frustration Twitter users expressed towards businesses’ inhumane acts of promoting romanticization of mental illness through their advertisement, brands, logo, slogans or even products. Businesses over the years have been blamed for their insatiable drive to make money even out of most illnesses including mental illness. Some influencers and celebrities used this opportunity to increase their followers or likes/comments whereas others created various forms of clubs. In a story published on invisible illness medium, several bloggers explored some of these businesses created and how they are making money out of mental health. For instance, Corinna Kopf’s anxiety merch developed through a clothing line were criticized as well as appreciated by others. This suggests businesses are co-opting mental health issues into trends and fashion statements to gain profit. Given that research has also shown how people are influenced mostly by influencers or celebrities in several ways [ 56 ] commercializing mental health issues through the use of symbols and phrases in sale of merchandise for profit downplays the severity of mental illness particularly in relation to suicide. From our findings, Twitter users emphasized in their discussion the societal misconceptions of mental health that are propagated and the need for these to be corrected. Data from the Substance Abuse and Mental Health Services Administration (SAMHSA) explained several myths that have been associated with people living with mental conditions, which the participants in this study confirmed. Due to these misconceptions, people are deprived of opportunities. Yu et al. [ 28 ] confirmed the lack of knowledge exhibited about depression on social media ranging from quality of life, access to good jobs, social networks, healthcare satisfaction and general care from people [ 5 ].
Being a stereotype group, Twitter users projected their dismay about the misinformation/disinformation and lack of knowledge that is out there about mental health. These myths and misconception have been disclosed to consequently reinforce the already existing discrimination public and self-stigma that people with mental conditions are faced with [ 57 , 58 , 59 ]. Romanticizing mental illness on social media has worsened these effects and caused people to engage in self harm, wrong diagnosis, and death.
We found that the role of traditional and social media is pivotal in shaping public understanding of mental health. The influence of media platforms, both traditional and social, in shaping public understanding of mental health has become a subject of growing concern [ 60 , 61 ]. This is because they play a very important role in framing the discussion on mental health [ 62 ]. Certain media platforms have played a constructive role in addressing mental health concerns by disseminating messages aimed at educating and enlightening individuals about mental well-being, as well as promoting the importance of seeking support [ 63 ]. Several researchers argue that some platforms have failed to fulfill their responsibility in educating and enlightening the public about mental health, instead, potentially glamorizing, and idealizing it [ 9 , 11 , 64 ].
In our findings, participants expressed apprehensions regarding potential glorification and oversimplification of mental health issues on social media e.g. Tik Tok and other traditional platforms. These findings align with the observation made by Jadayel et al. [ 1 ] that young individuals tend to romanticize mental health disorders on social media platforms. This raises pertinent questions about the ethical responsibility of media platforms in portraying mental health accurately and responsibly. This may give credence to Frost and Casey’s [ 65 ] argument that people seeking online support might be susceptible to increased levels of exposure to suicidal ideation, which ratifies the need for online posting monitoring.
On the contrary, other findings example tweets like:
My depression was bad I wouldn’t shower, brush my teeth or hair, clean my room nothing. I would legit work, come home, and sit on my computer all night and think about just driving till my car was empty and then killing my self Depression is not cute stop it.
, portrays Twitter as a significant platform for individuals to share unfiltered, real-life experiences of grappling with mental health, particularly depression. These narratives shed light on the raw and often challenging aspects of living with this condition. In doing so, they provide a counterbalance to potentially romanticized portrayals, emphasizing the importance of authenticity in mental health discussions. This finding aligns with research by Berry et al. [ 66 ] that found that Twitter provides an online community that allows communication on mental health to flourish, awareness to be raised and space for authentic mental health related discussions and self- disclosure. Moreover, the narratives draw attention to practical challenges faced by individuals, such as the financial burden of accessing therapy. This aspect underscores the urgent need for increased accessibility to mental health resources, ensuring that individuals have the support they require. Although these may be seen as romanticizing mental health, it might be important for policy makers and public health agencies to use as a feedback platform to gain valuable feedback from members of the society having mental health challenges.
Along the same line, our final finding “We are not romanticizing” indicates how some users vehemently reject the tag on them that portrayed them as glorifying mental health. Users passionately advocate for authentic, unvarnished discussions surrounding mental health, while vehemently asserting that their intentions are far from romanticizing the very real struggles individuals face. Nevertheless, this outcome contradicts the conclusions drawn by Chan and Sireling [ 67 ], who argue that the recent positive media coverage and romanticization of mental health challenges of bipolar disorder might contribute to its growing popularity as a self-diagnosed condition.
In all these contradictions, an important aspect of these findings is the active challenge against societal taboos surrounding discussions of suicide. Users courageously step into a realm that has long been shrouded in silence and stigma. By doing so, they contribute to a broader movement that seeks to deconstruct harmful narratives and replace them with open, empathetic conversations. The importance of this cannot be overstated, as these conversations are instrumental in reducing the isolation felt by individuals struggling with suicidal thoughts. They pave the way for increased understanding, support, and access to resources for those who need it the most. Additionally, this finding also underscores a fundamental human right: the right to vent about mental health challenges without facing stigmatization as a romanticizer. This reaffirms the validity of individual experiences and emphasizes that venting is not an endorsement of romanticization, but a crucial aspect of processing and coping [ 66 ]. By upholding this right, users foster an environment where other individuals can express themselves without fear, and where their experiences are acknowledged and respected.
In conclusion, this study explored the complex terrain of Twitter conversations about mental health, uncovering a range of issues and viewpoints related to the phenomena of romanticizing mental health. We discovered six major themes through content analysis and topic modeling: we are not romanticizing; we reject/critique the glamorization of mental health; corporate organizations monetize of mental health; societal misconceptions about mental health; the role of traditional media and social media; and the glamorization of mental health.
Our research highlighted how critical it is to address the complicated problem of social media romanticization of mental health. Users voiced their worries, criticized the glamorization of mental health issues, and demanded more appropriate representations of diseases associated with mental health. These Twitter conversations show how the conversation surrounding mental health is changing, moving from stigmatization to a growing concern about the romanticization of mental illness.
Research and practical implications
This study significantly contributes to the growing body of research on mental health discussions in the digital era. Our study underscores the importance of conducting platform-specific analyses to understand how users engage in discussions about sensitive topics like mental health. Our study calls for further research on the romanticization of mental health in the context of social media. Future studies can explore how these conversations have changed over time, considering how different events and trends may impact the conversation. Additionally, examining other social media platforms and their unique dynamics is essential for a comprehensive understanding of this issue.
Understanding the motivations and demographics of users engaging in these discussions can provide valuable insights. Research could delve deeper into user intentions, whether they aim to raise awareness, seek support, or engage in performative behavior. Traditional and social media platforms must recognize their role in shaping mental health perceptions responsibly. Mental health organizations and advocates can leverage Twitter as a platform to engage with users actively discussing these issues. By providing accurate information and support, mental health experts can contribute to a more informed and empathetic online community.
Promoting digital literacy and responsible online behavior is crucial. Users should be educated about the potential harms of glamorizing mental health and encouraged to engage in meaningful, empathetic conversations. Online support groups and communities can benefit from our findings by emphasizing the importance of authenticity. Encouraging members to share unfiltered experiences can foster a sense of belonging and understanding.
Limitations
The study primarily relied on data from Twitter, offering a diverse dataset, but it’s important to acknowledge its limitations. Firstly, the dataset collected on Twitter, while informative, may not encompass the entirety of discussions regarding the romanticization of mental health, potentially overlooking conversations occurring on other social media platforms or in private settings.
Additionally, the study’s use of specific keywords for data collection could have unintentionally excluded relevant discussions that didn’t explicitly employ those keywords, potentially leading to the omission of valuable insights. While the findings provide valuable insights into discussions among Twitter users, they may not be entirely representative of the broader Twitter user population. Additionally, it’s important to note that the dataset collected from Twitter, although informative, was not extensive in size and was limited to a relatively short time frame. This temporal constraint may have restricted the study’s ability to capture the full spectrum of discussions and developments related to the romanticization of mental health on the platform over a more extended period.
Furthermore, due to privacy concerns and the public nature of Twitter data, the study was unable to access detailed demographic information about users, which could have offered valuable contextual information to enhance the analysis. Additionally, while the study employed topic modeling to identify key themes, it’s essential to acknowledge that this approach might not capture the full depth of user intentions and emotions in their discussions.
Future studies should take these limitations into account in order to offer a broader understanding of the phenomenon.
Table 1 above displays the six themes that were manually categorized and named based on the identified topics from the topic modeling. It also highlights words related to each theme, as well as corresponding tweets specific to each theme.
Table 2 above represents the five main topics from the LDA topic modeling of our Twitter dataset, including the top ten words for each topic and example tweets that support the findings.
Data availability
The datasets generated and/or analyzed will be made available from the corresponding author upon request.
Jadayel R, Medlej K, Jadayel JJ. Mental disorders: a glamorous attraction on social media. J Teach Educ. 2017;7(1):465–76.
Google Scholar
Freeman M. The World Mental Health Report: transforming mental health for all. World Psychiatry [Internet]. 2022;21(3):391–2. https://doi.org/10.1002/wps.21018 .
Cuijpers P, Miguel C, Ciharova M, Harrer M, Moir F, Roskvist R et al. Psychological treatment of adult depression in primary care compared with outpatient mental health care: A meta-analysis. J Affect Disord [Internet]. 2023;339:660–75. https://doi.org/10.1016/j.jad.2023.07.011 .
Shrestha ECHO, Echo. The romanticization of mental illness on Tumblr. 69 Echo: the Romanticization of Mental Illness on Tumblr| Anima Shrestha. 2018.
Corrigan PW, Watson AC. Understanding the impact of stigma on people with mental illness. World Psychiatry. 2002;1(1):16–20.
PubMed PubMed Central Google Scholar
Chivilgina O, Elger BS, Jotterand F. Digital technologies for schizophrenia management: A descriptive review. Sci Eng Ethics [Internet]. 2021;27(2):25. https://doi.org/10.1007/s11948-021-00302-z .
Naslund JA, Deng D. Addressing mental health stigma in low-income and middle-income countries: A new frontier for digital mental health. Ethics Med Public Health [Internet]. 2021;19(100719):100719. https://doi.org/10.1016/j.jemep.2021.100719 .
Gallagher L. Welcome to AnxietyTok: An empirical review of peer support for individuals living with mental illness on social networking site TikTok. VVRJ [Internet]. 2021 [cited 2023 Oct 10];3(1):24–32. Available from: https://jbh.journals.villanova.edu/index.php/veritas/article/view/2641 .
Vidamaly S, Lee SL. Young adults’ mental illness aesthetics on social media. Int J Cyber Behav Psychol Learn [Internet]. 2021;11(2):13–32. https://doi.org/10.4018/ijcbpl.2021040102 .
Castillo EG, Ijadi-Maghsoodi R, Shadravan S, Moore E, Mensah MO III, Docherty M et al. Community interventions to promote mental health and social equity. Curr Psychiatry Rep [Internet]. 2019;21(5):35. https://doi.org/10.1007/s11920-019-1017-0 .
Shankar S, Simão AMV, Matos A, Widjaja AE, Chen JV, Hiele TM. The romanticization of Mental illness and adolescent identity. Psychol Learn Int J. 2016;6(3).
Dunn HK, Clark MA, Pearlman DN. The relationship between sexual history, bullying victimization, and poor mental health outcomes among heterosexual and sexual minority high school students: A feminist perspective. J Interpers Violence [Internet]. 2017;32(22):3497–519. https://doi.org/10.1177/0886260515599658 .
Wood AL, Wahl OF. Evaluating the effectiveness of a consumer-provided mental health recovery education presentation. Psychiatr Rehabil J. 2006.
Wedding D, Boyd MA, Niemiec RM. Movies and mental illness: Using films to understand psychopathology. 1999.
Zhang X, Liu Q. Aestheticization of illness in A Dream of the Red mansions. Comp Literature: East West. 2015;23(1):57–70.
Cross S. Visualizing madness: Mental illness and public representation. Telev New Media [Internet]. 2004;5(3):197–216. https://doi.org/10.1177/1527476403254001 .
Hyler SE, Gabbard GO, Schneider I. Homicidal maniacs and narcissisfic parasites: Stigmatization of mentally ill persons in the movies. Psychiatr Serv [Internet]. 1991;42(10):1044–8. https://doi.org/10.1176/ps.42.10.1044 .
McGinty EE, Webster DW, Jarlenski M, Barry CL. News media framing of serious mental illness and gun violence in the United States, 1997–2012. Am J Public Health [Internet]. 2014;104(3):406–13. https://doi.org/10.2105/ajph.2013.301557 .
Ma Z. How the media cover mental illnesses: a review. Health Educ (Lond) [Internet]. 2017;117(1):90–109. https://doi.org/10.1108/he-01-2016-0004 .
Thaller S. Troubled teens and monstrous others: Problematic depictions of characters with mental illness in young adult literature. Study Scrut Res Young Adult Lit [Internet]. 2015;1(1):215. https://doi.org/10.15763/issn.2376-5275.2015.1.1.215-253 .
Oumeddour Chiraz DG. Romanticizing Mental Illness in Jennifer Niven’s All the Bright Places. 2021.
Pantic I. Online social networking and mental health. Cyberpsychol Behav Soc Netw [Internet]. 2014;17(10):652–7. https://doi.org/10.1089/cyber.2014.0070 .
Robinson J, Rodrigues M, Fisher S, Herrman H, Suicide. and social media findings from the literature review [Internet]. Psu.edu. [cited 2023 Oct 10]. Available from: https://citeseerx.ist.psu.edu/document?repid=rep1&type=pdf&doi=8bdc1674d0fed4a74ea79efb7c5d0c7521773573
Saha K, Torous J, Ernala SK, Rizuto C, Stafford A, De Choudhury M. A computational study of mental health awareness campaigns on social media. Transl Behav Med [Internet]. 2019;9(6):1197–207. https://doi.org/10.1093/tbm/ibz028 .
Huh J. Clinical questions in online health communities: the case of See your doctor threads. In: Proceedings of the 18th ACM Conference on Computer Supported Cooperative Work & Social Computing. 2015. p. 1488–99.
Boyd K, Nugent C, Donnelly M, Bond R, Sterritt R, Gibson L. Investigating methods for increasing the adoption of social media amongst carers for the elderly. In: IFMBE Proceedings. Cham: Springer International Publishing; 2014. p. 1439–42.
Choudhury D. Can social media help us reason about mental health? In: Proceedings of the 23rd International Conference on World Wide Web. 2014.
Yu L, Jiang W, Ren Z, Xu S, Zhang L, Hu X. Detecting changes in attitudes toward depression on Chinese social media: A text analysis. J Affect Disord [Internet]. 2021;280:354–63. https://doi.org/10.1016/j.jad.2020.11.040 .
Cavazos-Rehg PA, Krauss MJ, Sowles S, Connolly S, Rosas C, Bharadwaj M et al. A content analysis of depression-related tweets. Comput Human Behav [Internet]. 2016;54:351–7. https://doi.org/10.1016/j.chb.2015.08.023 .
Wang W, Liu Y. Communication message cues and opinions about people with depression: an investigation of discussion on Weibo. Asian J Commun [Internet]. 2015;25(1):33–47. https://doi.org/10.1080/01292986.2014.989238 .
Brown M, Brown RS, editors. Emancipatory perspectives on madness: psychological, social, and spiritual dimensions. London, England: Routledge; 2020.
Chen JA, Courtwright A, Wu KC-C. The role of stigma and denormalization in suicide-prevention laws in East Asia: A sociocultural, historical, and ethical perspective. Harv Rev Psychiatry [Internet]. 2017;25(5):229–40. https://doi.org/10.1097/hrp.0000000000000160 .
Tanner E, Girls. Instagram, and the glamorization of self-loathing. 2015 [cited 2023 Oct 10]; Available from: https://soar.suny.edu/handle/20.500.12648/2718 .
Valdez D, ten Thij M, Bathina K, Rutter LA, Bollen J. Social media insights into US mental health during the COVID-19 pandemic: Longitudinal analysis of Twitter data. J Med Internet Res [Internet]. 2020;22(12):e21418. https://doi.org/10.2196/21418 .
Pavlova A, Berkers P. Mental health discourse and social media: Which mechanisms of cultural power drive discourse on Twitter. Soc Sci Med [Internet]. 2020;263(113250):113250. https://doi.org/10.1016/j.socscimed.2020.113250 .
Lachmar EM, Wittenborn AK, Bogen KW, Mccauley HL. # MyDepressionLooksLike: examining public discourse about depression on Twitter. JMIR Mental Health. 2017;4(4).
Hsieh H-F, Shannon SE. Three approaches to qualitative content analysis. Qual Health Res [Internet]. 2005;15(9):1277–88. https://doi.org/10.1177/1049732305276687 .
Robert D, Haghighi P, Burstein F, Urquhart D, Cicuttini F. Investigating individuals’ perceptions regarding the context around the low back pain experience: Topic modeling analysis of twitter data. J Med Internet Res [Internet]. 2021;23(12):e26093. Available from: https://pubmed.ncbi.nlm.nih.gov/36260398/ .
X/Twitter global mDAU. 2022 [Internet]. Statista. [cited 2023 Oct 10]. Available from: https://www.statista.com/statistics/970920/monetizable-daily-active-twitter-users-worldwide/ .
Java A, Song X, Finin T, Tseng B. Why we twitter: understanding microblogging usage and communities. In: Proceedings of the 9th WebKDD and 1st SNA-KDD 2007 workshop on Web mining and social network analysis. 2007. p. 56–65.
Makita M, Mas-Bleda A, Morris S, Thelwall M. Mental health discourses on twitter during mental health awareness week. Issues Ment Health Nurs [Internet]. 2021;42(5):437–50. https://doi.org/10.1080/01612840.2020.1814914 .
Charmaz K. The self as habit: The reconstruction of self in chronic illness. OTJR (Thorofare N J) [Internet]. 2002;22(1_suppl):31S-41S. https://doi.org/10.1177/15394492020220s105 .
Bruckman A. Studying the amateur artist: A perspective on disguising data collected in human subjects research on the Internet. Ethics Inf Technol [Internet]. 2002;4(3):217–31. https://doi.org/10.1023/a:1021316409277 .
Williams ML, Burnap P, Sloan L. Towards an ethical framework for publishing Twitter data in social research: Taking into account users’ views, online context and algorithmic estimation. Sociology [Internet]. 2017;51(6):1149–68. https://doi.org/10.1177/0038038517708140 .
Franzke AS, Bechmann A, Zimmer M, Ess C, Internet Research. Ethical Guidelines 3.0 [Internet]. Association of Internet Researchers; 2020. Available from: https://aoir.org/reports/ethics3.pdf .
Willaert T, Van Raemdonck N, Peeters S, Seijbel J. Mapping the dutchophone fringe on Telegram. In: Digital Methods Summer School 2021: Fake everything: Social media’s struggles with inauthentic activities. 2021.
Ghosh T, Banna MHA, Nahian MJA, Taher KA, Kaiser MS, Mahmud M. A hybrid deep learning model to predict the impact of COVID-19 on mental health form social media big data [Internet]. Preprints. 2021. https://doi.org/10.20944/preprints202106.0654.v1 .
Cravens JD, Whiting JB, Aamar RO. Why I stayed/left: An analysis of voices of intimate partner violence on social media. Contemp Fam Ther [Internet]. 2015;37(4):372–85. https://doi.org/10.1007/s10591-015-9360-8 .
Karras C, Karras A, Tsolis D, Giotopoulos KC, Sioutas S. Distributed Gibbs sampling and LDA modelling for large scale big data management on PySpark. In: 2022 7th South-East Europe Design Automation, Computer Engineering, Computer Networks and Social Media Conference (SEEDA-CECNSM). IEEE; 2022.
Onan A, Korukoglu S, Bulut H. LDA-based topic modelling in text sentiment classification: an empirical analysis. Int J Comput Linguistics Appl. 2016;7(1):101–19.
Gan J, Qi Y. Selection of the optimal number of topics for LDA topic model—taking patent policy analysis as an example. Entropy (Basel) [Internet]. 2021;23(10):1301. https://doi.org/10.3390/e23101301 .
Blair SJ, Bi Y, Mulvenna MD. Aggregated topic models for increasing social media topic coherence. Appl Intell [Internet]. 2020;50(1):138–56. https://doi.org/10.1007/s10489-019-01438-z .
Matthews TJ. Recreating literary works using ChatGPT3 and evaluating results using natural language processing analysis (Doctoral dissertation). 2023;30486638.
Braun V, Clarke V. Using thematic analysis in psychology. Qual Res Psychol [Internet]. 2006;3(2):77–101. https://doi.org/10.1191/1478088706qp063oa .
Acuna K. Netflix adds a new message to viewers at the start of its controversial show 13 Reasons Why. Business Insider [Internet]. 2017 May 9 [cited 2023 Oct 10]; Available from: https://www.businessinsider.com/13-reasons-why-adds-message-at-start-of-show-2017-5 .
New WHO/Europe report calls for. urgent action to protect children from digital marketing of food [Internet]. Who.int. [cited 2023 Oct 10]. Available from: http://www.euro.who.int/en/media-centre/sections/press-releases/2016/11/new-whoeurope-report-calls-for-urgent-action-to-protect-children-from-digital-marketing-of-food .
Jenkins EL, Ilicic J, Barklamb AM, McCaffrey TA. Assessing the credibility and authenticity of social media content for applications in health communication: Scoping review. J Med Internet Res [Internet]. 2020;22(7):e17296. https://doi.org/10.2196/17296 .
Fabrega H Jr. Psychiatric stigma in non-Western societies. Compr Psychiatry [Internet]. 1991;32(6):534–51. https://doi.org/10.1016/0010-440x(91)90033-9 .
Martin JK, Pescosolido BA, Tuch SA. Of fear and loathing: the role of’disturbing behavior,’labels, and causal attributions in shaping public attitudes toward people with mental illness. J Health Soc Behav. 2000;208–23.
Socall DW, Holtgraves T. Attitudes toward the mentally ill: The effects of label and beliefs. Sociol Q [Internet]. 1992;33(3):435–45. https://doi.org/10.1111/j.1533-8525.1992.tb00383.x .
Happer C, Philo G. The role of the media in the construction of public belief and social change. J Soc Polit Psychol [Internet]. 2013;1(1):321–36. https://doi.org/10.5964/jspp.v1i1.96 .
Zhang T, Yang K, Ji S, Ananiadou S. Emotion fusion for mental illness detection from social media: A survey. Inf Fusion [Internet]. 2023;92:231–46. https://doi.org/10.1016/j.inffus.2022.11.031 .
Quintero Johnson JM, Yilmaz G, Najarian K. Optimizing the presentation of mental health information in social media: The effects of health testimonials and platform on source perceptions, message processing, and health outcomes. Health Commun [Internet]. 2017;32(9):1121–32. https://doi.org/10.1080/10410236.2016.1214218 .
Ju R, Jia M, Cheng J. Promoting mental health on social media: A content analysis of organizational tweets. Health Commun [Internet]. 2023;38(8):1540–9. https://doi.org/10.1080/10410236.2021.2018834 .
Wang W, Liu Y. Discussing mental illness in Chinese social media: the impact of influential sources on stigmatization and support among their followers. Health Commun [Internet]. 2016;31(3):355–63. https://doi.org/10.1080/10410236.2014.957376 .
Frost M, Casey L. Who seeks help online for self-injury? Arch Suicide Res [Internet]. 2016;20(1):69–79. https://doi.org/10.1080/13811118.2015.1004470 .
Berry N, Lobban F, Belousov M, Emsley R, Nenadic G, Bucci S, #WhyWeTweetMH. Understanding why people use twitter to discuss mental health problems. J Med Internet Res [Internet]. 2017;19(4):e107. https://doi.org/10.2196/jmir.6173 .
Chan D, Sireling L. ‘I want to be bipolar’… a new phenomenon. Psychiatrist [Internet]. 2010;34(3):103–5. https://doi.org/10.1192/pb.bp.108.022129 .
Clemente AS, Santos WJ, Nicolato R, Firmo JO. Stigma related to bipolar disorder in the perception of psychiatrists from Belo Horizonte, Minas Gerais State, Brazil. Cadernos De Saude Publica. 2017;33:e00050016.
Article PubMed Google Scholar
Pavelko RL, Myrick JG. Measuring trivialization of mental illness: developing a scale of perceptions that mental illness symptoms are beneficial. Health Commun. 2019 Feb 5.
Gould MS, Shaffer D. The impact of suicide in television movies: evidence of imitation. N Engl J Med. 2003;279(10):1095–100.
Phillips DP. The influence of suggestion on suicide: substantive and theoretical implications of the Werther effect. American sociological review. Jun. 1974;1:340–54.
World Health Organization (WHO). Preventing suicide: a global imperative. World Health Organ, 2014. 16.
World Health Organization (WHO). Preventing suicide: a resource for media professionals- update 2017. World Health Organization; 2017.
Stack S. Suicide in the media: a quantitative review of studies based on nonfictional stories. Suicide Life-Threatening Behav. 2005;35(2):121–33.
Article Google Scholar
Fu KW, Yip PS. Estimating the risk for suicide following the suicide deaths of 3 Asian entertainment celebrities: a meta-analytic approach. J Clin Psychiatry. 2009;70(6):869.
Markman R. Hip-hop artist Freddy E dead in apparent suicide.2013.
Jashinsky J, Burton SH, Hanson CL, West J, Giraud-Carrier C, Barnes MD, Argyle T. Tracking suicide risk factors through Twitter in the US. Crisis. 2014.
Suicide Prevention Resource Center (SPRC). INTERNATIONAL: Twitter Updates Its Policy on Tweets that Encourage Self-Harm and Suicide 2018 March.
Mitchison D, Hay P. The epidemiology of eating disorders: genetic, environmental, and societal factors. Clin Epidemiol. 2014;6:89–97.
Juarascio AS, Shoaib A, Timko CA. Pro-eating disorder communities on social networking sites: a content analysis. Eat Disord. 2010;18(5):393–407.
Yeshua-Katz D, Martins N. Communicating stigma: the pro-ana paradox. Health Commun. 2013;28(5):499–508. https://doi.org/10.1080/10410236.2012.699889 . Epub 2012 Aug 8. PMID: 22873763.
Arseniev-Koehler A, Lee H, McCormick T, Moreno MA. # Proana: pro-eating disorder socialization on Twitter. J Adolesc Health. 2016;58(6):659–64.
Crowe N, Watts M. We’re just like Gok, but in reverse’: Ana girls–empowerment and resistance in digital communities. Int J Adolescence Youth. 2016;21(3):379–90.
Borzekowski DL, Schenk S, Wilson JL, Peebles R. e-Ana and e-Mia: a content analysis of pro–eating disorder websites. Am J Public Health. 2010;100(8):1526–34.
Article PubMed PubMed Central Google Scholar
Download references
Acknowledgements
We would like to acknowledge and express our gratitude to Huu Dat Tran for assisting in collecting the tweets from Twitter used in this study, and also to Sofiyyah Akolade for providing additional insights for the LDA analysis.
Not applicable. The authors did not receive any funding for this research.
Author information
Authors and affiliations.
Department of Advertising and Public Relations, Michigan State University, East Lansing, USA
Barikisu Issaka
Communication Studies Department, University of Iowa, Iowa City, USA
Ebenezer Ato Kwamena Aidoo
Hugh Downs School of Human Communication, Arizona State University, Tempe, USA
Sandra Freda Wood
Department of Information Systems , University of Nevada, Reno, USA, Reno
Fatima Mohammed
Michigan State University, Lansing, USA
You can also search for this author in PubMed Google Scholar
Contributions
Author BI roles included writing the introduction, literature review, methods, conclusion, qualitative analysis, and results sections of the paper. Author FM conducted the LDA analysis for the data. Author EA contributed to the results, qualitative analysis, and discussion sections of the paper, and Author SW also contributed to the results, qualitative analysis, and discussion sections. All authors made additional contributions to various sections of the paper and collectively reviewed and approved the final manuscript.
Corresponding author
Correspondence to Barikisu Issaka .
Ethics declarations
Ethics approval and consent to participate.
Not applicable as publicly available data was used.
Consent for publication
Not applicable.
Competing interests
The authors declare no competing interests.
Additional information
Publisher’s note.
Springer Nature remains neutral with regard to jurisdictional claims in published maps and institutional affiliations.
Rights and permissions
Open Access This article is licensed under a Creative Commons Attribution 4.0 International License, which permits use, sharing, adaptation, distribution and reproduction in any medium or format, as long as you give appropriate credit to the original author(s) and the source, provide a link to the Creative Commons licence, and indicate if changes were made. The images or other third party material in this article are included in the article’s Creative Commons licence, unless indicated otherwise in a credit line to the material. If material is not included in the article’s Creative Commons licence and your intended use is not permitted by statutory regulation or exceeds the permitted use, you will need to obtain permission directly from the copyright holder. To view a copy of this licence, visit http://creativecommons.org/licenses/by/4.0/ . The Creative Commons Public Domain Dedication waiver ( http://creativecommons.org/publicdomain/zero/1.0/ ) applies to the data made available in this article, unless otherwise stated in a credit line to the data.
Reprints and permissions
About this article
Cite this article.
Issaka, B., Aidoo, E.A.K., Wood, S.F. et al. “Anxiety is not cute” analysis of twitter users’ discourses on romanticizing mental illness. BMC Psychiatry 24 , 221 (2024). https://doi.org/10.1186/s12888-024-05663-w
Download citation
Received : 15 October 2023
Accepted : 06 March 2024
Published : 21 March 2024
DOI : https://doi.org/10.1186/s12888-024-05663-w
Share this article
Anyone you share the following link with will be able to read this content:
Sorry, a shareable link is not currently available for this article.
Provided by the Springer Nature SharedIt content-sharing initiative
- Social media
- Mental health
- Romanticization
- Authentic discussions
- Destigmatization
- Topic modeling
- Responsible discussions
BMC Psychiatry
ISSN: 1471-244X
- Submission enquiries: [email protected]
- General enquiries: [email protected]
The crisis communication of the COVID-19 pandemic in media discourse: text mining for infectious disease frames and environmental pollution
- Article contents
- Figures & tables
- Supplementary Data
- Open the PDF for in another window
- Guest Access
- Cite Icon Cite
- Permissions
- Search Site
Yuhang Li , Zhifa Zeng , Lisai Yu; The crisis communication of the COVID-19 pandemic in media discourse: text mining for infectious disease frames and environmental pollution. AQUA - Water Infrastructure, Ecosystems and Society 2024; jws2024063. doi: https://doi.org/10.2166/aqua.2024.063
Download citation file:
- Ris (Zotero)
- Reference Manager
Presented here is a study on the semantic analysis of mainstream media news related to the COVID-19 outbreak in China that occurred at the end of 2019. Examining the most frequently used keywords and their co-occurrences, researchers can infer a semantic network that represents the major frames used in a large amount of text. Frames are cognitive structures that people use to understand and communicate about issues. Through framing, media and individuals choose to highlight certain aspects of the crisis while downplaying other aspects. This study demonstrates that Chinese mainstream media users applied 12 frames, including basic information, vaccines, politics, economy, and war metaphors, to analyze the public health crisis related to the COVID-19 outbreak. The study also explores how the use of these frameworks changed in different stages of the COVID-19 pandemic, providing new perspectives and content for research on crisis and emergency risk communication. Methodologically, this study demonstrates the feasibility of identifying frames in Chinese media news through text mining and semantic network analysis. From a practical perspective, the findings provide valuable insights for public health professionals in understanding Chinese media perception and formulating crisis communication strategies for future public health emergencies .
This study is to investigate the text semantic analysis of mainstream media news related to the COVID-19 outbreak in China that occurred at the end of 2019.
Examining the most frequently used keywords and their co-occurrences, researchers can infer a semantic network that represents the major frames.
Frames are cognitive structures that people use to understand and communicate about issues.
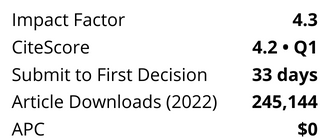
Affiliations
- ISSN 2709-8028 EISSN 2709-8036
- Open Access
- Collections
- Subscriptions
- Subscribe to Open
- Editorial Services
- Rights and Permissions
- Sign Up for Our Mailing List
- IWA Publishing
- Republic – Export Building, Units 1.04 & 1.05
- 1 Clove Crescent
- London, E14 2BA, UK
- Telephone: +44 208 054 8208
- Fax: +44 207 654 5555
- IWAPublishing.com
- IWA-network.org
- IWA-connect.org
- Cookie Policy
- Terms & Conditions
- Get Adobe Acrobat Reader
- ©Copyright 2021 IWA Publishing
This Feature Is Available To Subscribers Only
Sign In or Create an Account
This paper is in the following e-collection/theme issue:
Published on 26.3.2024 in Vol 8 (2024)
This is a member publication of University College London (Jisc)
A Snapshot of COVID-19 Vaccine Discourse Related to Ethnic Minority Communities in the United Kingdom Between January and April 2022: Mixed Methods Analysis
Authors of this article:

Original Paper
- Nazifa Ullah 1 , MBBS, BSc ;
- Sam Martin 2 , LLB, MSc, PhD ;
- Shoba Poduval 3 , MBBS, PhD
1 Research Department of Primary Care & Population Health, University College London, London, United Kingdom
2 Vaccines and Society Unit, Oxford Vaccine Group, University of Oxford, Oxford, United Kingdom
3 Institute of Health Informatics, University College London, London, United Kingdom
Corresponding Author:
Shoba Poduval, MBBS, PhD
Institute of Health Informatics
University College London
222 Euston Road
London, NW1 2DA
United Kingdom
Phone: 44 (0)20 3549 5969
Email: [email protected]
Background: Existing literature highlights the role of social media as a key source of information for the public during the COVID-19 pandemic and its influence on vaccination attempts. Yet there is little research exploring its role in the public discourse specifically among ethnic minority communities, who have the highest rates of vaccine hesitancy (delay or refusal of vaccination despite availability of services).
Objective: This study aims to understand the discourse related to minority communities on social media platforms Twitter and YouTube.
Methods: Social media data from the United Kingdom was extracted from Twitter and YouTube using the software Netlytics and YouTube Data Tools to provide a “snapshot” of the discourse between January and April 2022. A mixed method approach was used where qualitative data were contextualized into codes. Network analysis was applied to provide insight into the most frequent and weighted keywords and topics of conversations.
Results: A total of 260 tweets and 156 comments from 4 YouTube videos were included in our analysis. Our data suggests that the most popular topics of conversation during the period sampled were related to communication strategies adopted during the booster vaccine rollout. These were noted to be divisive in nature and linked to wider conversations around racism and historical mistrust toward institutions.
Conclusions: Our study suggests a shift in narrative from concerns about the COVID-19 vaccine itself, toward the strategies used in vaccination implementation, in particular the targeting of ethnic minority groups through vaccination campaigns. The implications for public health communication during crisis management in a pandemic context include acknowledging wider experiences of discrimination when addressing ethnic minority communities.
Introduction
In December 2020, the United Kingdom began its COVID-19 vaccination program [ 1 ]. Population-level studies have demonstrated persistently lower levels of uptake of the first dose of the COVID-19 vaccine among all minority groups compared with the White British ethnic group, and these differences widened over time [ 2 ]. Vaccine hesitancy, that is, the delay or refusal of vaccinations despite the availability of services [ 3 ], has been recognized as a significant contributing factor to the unequal uptake of COVID-19 vaccines across different ethnic groups [ 4 ]. This contrasts with anti-vaxxers, defined as a group of individuals who may refuse most if not all vaccinations [ 5 ].
Several studies have investigated the causes of hesitancy within the context of the COVID-19 vaccine [ 6 - 8 ]. A summary provided by the World Health Organization 3 C’s model identifies the following 3 core areas that influence hesitancy: confidence (toward the vaccine or its provider), complacency (perceived risk toward vaccine-preventable diseases), and convenience (access to vaccines) [ 8 ].
Historical mistrust toward governments, racism within the health care system, and lack of diversity in medical research are further points in the literature noted as contributing factors to hesitancy specifically in relation to ethnic minority groups [ 5 , 9 - 11 ].
Among the many factors noted, the rising use of social media as an interactive health ecosystem fuelling vaccine hesitancy remains a recurrent concern contributing to vaccine confidence, as identified in the literature [ 12 ]. During the COVID-19 pandemic, there was noted to be the issue of an “infodemic,” that is, the spread of large volumes of information including false or misleading information [ 13 , 14 ]. Social media played a significant role in this infodemic via the rapid dissemination of information. A total of 49% (n=980) of respondents to an online survey in the United Kingdom used social media as a source of information about COVID-19 [ 15 ] and Twitter reported COVID-19–related posts every 45 milliseconds in 2020 [ 16 - 18 ].
Social media was a prominent source of misinformation during the pandemic, particularly in relation to vaccinations. Misinformation refers to false or inaccurate information shared unknowingly and without the intention to cause harm [ 19 ]. Studies have highlighted the correlation between exposure to misinformation on social media and both vaccine hesitancy and reduced compliance to public health measures and disease prevention activities such as vaccinations [ 20 - 22 ]. Loomba et al [ 21 ] conducted a randomized control trial in the United Kingdom and the United States quantifying exposure to misinformation related to COVID-19 vaccines on social media platforms and vaccination intent and found that exposure to misinformation resulted in a 6.2% decline in the number of respondents who would “definitely” take the vaccine in comparison to control groups. Betsch et al [ 20 ] found that even brief exposure to vaccine-critical websites would increase an individual’s overall perception of vaccine risk in comparison to exposure to control websites. Furthermore, negative information related to vaccinations tends to encourage greater user engagement in comparison to positive content [ 23 , 24 ]. This may be due to the persuasive narratives and powerful imagery often used in antivaccination content [ 22 ]. Another important note is the creation of “echo chambers” on social media, that is, the network of users in which each user encounters beliefs supporting pre-existing opinions without being exposed to opposing viewpoints [ 12 ]. Such effects further reinforce antivaccine perspectives by connecting like-minded individuals thereby amplifying antivaccine narratives and potentially dampening vaccination attempts [ 7 ].
Despite the clear role of social media as a source of information during a pandemic and its impact on hesitancy and consequently vaccination attempts, there is little published data on its role in the public discourse on COVID-19 vaccines among ethnic minority groups in the United Kingdom. For ethnic minority communities, there may be a greater reliance on social media as a source of information because of barriers in accessing health care and health information due to, for example, language barriers or poor health literacy [ 25 ]. Dickson et al [ 26 ] study looking at culturally and linguistically diverse communities found that individuals would often receive information by “word of mouth,” that is, from peers from the same cultural groups as these would be seen as a trusted source of information. Those peers themselves would receive information from social media platforms such as Facebook and WhatsApp [ 26 ]. It is for these reasons that understanding hesitancy in minority communities through the lens of social media is of paramount importance.
Our article aims to address the following research question by obtaining data from social platforms Twitter and YouTube between January and April 2022:
- What was the discourse related to and within ethnic minority communities on social media platforms?
- What were the general sentiments and stances of social media posts analyzed?
- What were the most frequent topics of conversation seen on social media?
Study Design
The study analyzed Twitter and YouTube comments using a mixed method approach. This approach is often used when analyzing social media posts due to the large quantity of data that allows for both quantitative exploration as well as qualitative analysis of the contents, further enhancing the understanding of a research topic [ 27 ].
Data Source
We obtained publicly available data from Twitter and YouTube comments in the United Kingdom between January 17, 2022, and April 7, 2022, using the software Netlytics (for Twitter posts) and YouTube Data Tools (for YouTube data) [ 28 , 29 ]. The platforms Twitter and YouTube were selected because they provided the most openly available application programming interfaces (APIs) [ 16 ]. APIs are mechanisms that enable 2 software components to communicate with one another and allow third-party developers to access data such as tweets and YouTube comments [ 30 ].
Drawing on prior research studies on social media and the COVID-19 vaccine [ 6 - 8 ], a pilot Boolean search strategy was created ( Multimedia Appendix 1 ) and applied to Netlytics and YouTube Data Tools in order to understand recurrent topics of discussion around the vaccine. As of April 7, 2022, which was the end date for our data retrieval, the UK government was in the midst of its booster vaccination rollout and proposed that all eligible adults older than 18 years would be offered the booster vaccine [ 31 ]. The government had also proposed mandatory vaccinations for National Health Service workers during this period, a proposal that was later reversed by January 31 [ 31 , 32 ].
Consequently, our preliminary search of the data informed the development of a second more focused Boolean search ( Multimedia Appendix 1 ) containing keywords and relevant hashtags such as those related to booster vaccination campaigns. All posts were screened by NU according to our inclusion and exclusion criteria ( Textbox 1 ) and duplicates were removed. To note, the platform Netlytics provided geo-coded social media data, enabling us to ensure that tweets analyzed are from those based in the United Kingdom [ 28 ]. Geo-coded data may be provided through the Netlytics filtering system that allows users to include or exclude tweets based on a given radius and latitude [ 28 ]. The YouTube videos selected were checked by NU to ensure the content was related to the United Kingdom.
A total of 260 tweets (that were retweeted 15,331 times) and 156 comments from 4 YouTube videos remained after duplications were removed and went through the inclusion and exclusion processes.
Data were managed on Microsoft Excel [ 33 ]. To meet ethics and European Union General Data Protection Regulation guidelines [ 34 , 35 ], usernames were anonymized using a Python script [ 36 ] to replace all usernames and links with an encrypted tag code.
Inclusion criteria
- Posts where ethnic minority groups are discussed or mentioned (all ethnic groups except White British groups). For example use of the terms: (“BAME” OR “BME” OR “Black*” OR “Asian*” OR “minority ethnic” OR “ethnic minority” OR “minority” OR “non-white” OR “raci*” OR “MinorityHealth” OR “Minority” OR “race”; see Multimedia Appendix 1 for full list).
- Posts referring specifically to COVID-19 vaccination. For example use of the terms: (“vaccin*” OR “immunis*” OR “vax*” OR “jab*” OR “covidvaccin*” OR “COVIDVaccine” OR “COVID19” OR “COVID19Vaccine” OR “CovidVaccine” OR “covid-19” OR “covid vaccine,” OR “covid vax”).
- Example of a hypothetical post to include: “Racism and historical injustices fuelled low COVID vaccine uptake by minorities.” We would include this as it refers specifically to COVID-19 vaccinations in relation to ethnic minority groups.
Exclusion criteria
- Posts that discussed the COVID-19 vaccine without mention of ethnic minority groups.
- Posts that do not mention the COVID-19 vaccine.
- Posts by individuals not based in the United Kingdom.
- Example of hypothetical post to exclude: “COVID has caused many deaths.” This would be excluded as there is no mention of the COVID-19 vaccines and minority groups.
Measures of Variables
In order to identify key themes of topics in our social media posts, we developed an initial analytic coding framework ( Multimedia Appendix 1 ) that was informed by previous literature investigating causes of hesitancy as discussed previously [ 6 - 11 ]. We added the coding framework to a Microsoft Excel spreadsheet, with codes in the columns and social media posts entered as individual comments in the rows. The framework was refined during team discussions and all authors applied the same framework to the data.
Sentiment and Stance Coding
In order to appreciate the nuanced opinions on social media and how they may be contributing to positive or negative attitudes toward COVID-19 vaccines [ 37 ], individual posts were manually coded into sentiment and stance categories ( Multimedia Appendix 1 ). Sentiment refers to the overall tone of a post and the categorization of opinions toward a subject expressed by the author (being positive, negative, neutral, or ambiguous) [ 37 , 38 ]. Whereas stance refers to the process of determining the author’s attitude or stance toward a target [ 37 ]. For example, a post may have a positive sentiment toward anti-vaxxers but a negative stance toward vaccines.
Our stance framework ( Multimedia Appendix 1 ) was informed by the Leask et al [ 39 ] categorization of vaccine intention. Hypothetical examples derived from our social media data and the sentiment and stance assigned to each text can be found in Multimedia Appendix 1 . We coded the sentiment and stance of the data and added both to our Microsoft Excel spreadsheet. For example, a positive sentiment post would be coded as P and a positive stance post would be coded PS (codes clarified in Multimedia Appendix 1 ). Any disagreements regarding the classification of the social media posts were discussed during team meetings where a unanimous decision was made regarding their categorization.
Data Analysis Procedure
The percentage of Twitter and YouTube posts assigned to each sentiment and stance category was calculated and posts coded according to themes derived were quantified for further analysis. Where a post was assigned more than 1 theme, they were calculated as separate entities, for example, if a post was assigned to themes 1 and 2, they would be included separately when calculating overall percentages.
Taking an inductive approach, we collated the results from our analysis and a random sample of posts (30 tweets and 20 YouTube comments) were selected for a more detailed analysis and qualitative interrogation of the data to address our overall aims [ 40 , 41 ]. Our thematic analysis framework was updated accordingly to ensure themes identified were grounded in the data obtained.
Network Analysis and Visualization
Network analysis was also carried out to highlight the most prevalent topics of discussion on social media. Text network analysis software Infranodus (Nodus Labs) was used [ 42 ]. Data were imported into Infrandous and semantic networks were generated with data organized by specific topics and subtopics. Word clusters were derived using modularity measurement tools to highlight the most common topics of conversations within a group of tweets or YouTube comments. Keywords obtained using betweenness centrality, an analysis of connections between subtopics or words that link different clusters of conversations together, helped deepen our understanding of influential words or topics that may be linking clusters of conversations together. Word frequency analysis was then performed to highlight the most frequent and weighted subtopics of conversations.
Ethical Considerations
Ethics and data protection approval were obtained from University College London’s (UCL’s) Research Ethics Committee (ID 21773/001) and UCL Data Protection Officer (registration number: Z6364106/2021/10/60). All data obtained were publicly available via YouTube’s and Twitter’s APIs.
Using our search strategy, a total of 44,144 Twitter posts and 9 YouTube videos were identified. After the deduplication process and screening according to inclusion and exclusion criteria, a total of 416 deduplicated social media posts were identified. This included 260 tweets (that were retweeted 15,331 times) and 156 comments from 4 YouTube videos that were included in our analysis. Video 1 was a documentary exploring whether ethnic minorities and in particular minority health care workers, should be prioritized in having the COVID-19 vaccine. Video 2 was a debate on a news channel looking at the causes of vaccine hesitancy specifically in minority communities. Videos 3 and 4 were documentaries that focused on similar topics and explored the causes of vaccine hesitancy within ethnic minority communities. Out of the 4 videos analyzed, video 2 had the highest number of total comments of 1273.
The following 3 themes were identified in the discourse on both Twitter and YouTube: concerns related to vaccination implementation, questions over vaccine development and safety, and wider systemic issues such as institutional racism and historical abuse of power.
Concerns related to vaccine implementation were mostly related to mandatory vaccinations for health care workers and the wider public and public health messaging, in particular the use of the phrase “BAME.” Most of the topics connected to vaccine development were related to the speed of development of the vaccine and its side effects. Comments alluding to conspiracy theories suggesting the vaccine to be gene-altering, experimental in nature, and causing infertility as a side effect were particularly seen on YouTube. Moreover, systemic racism, that is, a form of racism that is embedded within the laws and regulations of a society [ 43 ], was a prevalent topic noted under the theme of wider systems. The topic of racism was particularly prevalent when discussing the strategies of vaccine rollout such as mandatory vaccinations for health care workers and the focus on vaccinating ethnic minorities. Table 1 summarizes the themes noted in our discourse analysis with hypothetical examples of tweets and YouTube comments derived from our data. Hypothetical examples are given in order to abide by ethics guidelines preventing any identifiable information from being published.
Sentiment and Stance
Table 2 demonstrates the sentiment and stance of tweets and YouTube comments as a percentage. In general, YouTube depicted a higher percentage of negative sentiment and stance posts over Twitter.
Network Analysis
Network analysis identified the following 4 key most frequent topics of conversations on Twitter, portrayed as clusters of tweets: booster vaccinations (cluster 1), mistrust (cluster 2), vaccination risk (cluster 3), and racism (cluster 4). Cluster 1 was the most popular group of conversations, with 23% (n=60) of tweets containing the keywords “vaccine,” “covid,” and “uptake.” Most tweets were related to encouraging booster vaccinations in minority communities. Some comments were noted addressing issues around misinformation and hesitancy in an endeavor to encourage booster vaccinations. Cluster 2 of tweets contained keywords, “trust,” “pandemic,” and “work.” There were discussions related to vaccinations for health workers as well as topics related to health inequalities in minority communities and historical abuses of power. Cluster 3 contained the keywords “jab,” “risk,” and “explain,” with conversations focused on the side effects of vaccines. Cluster 4 contained keywords “black,” “people,” and “race,” paying particular attention to the uptake of vaccines within the Black African and Caribbean community.
The word “vaccine” had the greatest betweenness centrality of 0.71, meaning that it was this phrase that predominantly linked the different clusters of conversations in the network, that is, the distribution of tweets, together. This is followed by “minority,” “covid,” and then “black.” The network structure, that is, the network of comments that people have made around a specific topic and their relation to one another, had a focused influence distribution of 80% with a modularity measure of 0.28. This illustrates that although there were several conversations related to the vaccine, the conversations were focused on a small number of key themes discussed above. Further statistics discussing the average degree and weighted betweenness between nodes can be found in the Multimedia Appendix 2 . Further images of individual clusters can be found in Multimedia Appendix 3 .
Regarding YouTube, video 1 keywords found in the most popular cluster of conversations included “race,” “worker,” and “baiting.” “Worker,” in this context was related to debates on whether ethnic minority health care workers should be prioritized in having the vaccine. Most conversations were hence related to racial tension and worries that vaccine campaigns prioritizing specific ethnic minority communities would seed further division. Video 2 had a similar focus in that the main topic of conversation was related to critiquing the vaccination program and the focus on ethnic minorities. Both videos 1 and 2 had a focused network structure reflecting that opinions expressed were similar in nature, hence a focused structure.
Video 3 had the most popular cluster of conversations containing the keywords “time,” “treatment,” and “American.” This video had multiple mentions of the Tuskegee trial, an experiment from 1932 to 1972 that involved the unethical testing of over 400 Black men who were falsely led to believe that they were being treated for syphilis [ 44 ]. Video 4 contained similar clusters of conversations related to mistrust. Both videos had a diverse network structure due to the various causes of vaccine hesitancy discussed in the comments sections. Multimedia Appendix 4 provides examples of conversation clusters in Videos 1 and 3 related to themes of racism and mistrust.
Principal Findings
Our study aimed to provide an overview of the discourse related to and within ethnic minority communities on the social media platforms Twitter and YouTube. This is one of the few studies to our knowledge that has explored the use of social media in this manner specifically related to ethnic minority communities. The summary of our results can be found in Table 3 . The themes identified were intrinsically linked with one another. For example, concerns raised around vaccination strategy such as the use of the term “BAME” were associated with themes related to wider systemic issues such as racism. The higher percentage of negative sentiment and stance seen on YouTube may be attributed to the fact that on YouTube, there is less of a focus on the individual profile page giving rise to the perception of greater anonymity when posting negative statements [ 45 ]. However, the nature of the YouTube videos may have also contributed to the higher levels of negative posts. For example, video 1 explored the potential public health policy of prioritizing minority groups for vaccination, a controversial debate that was not mentioned in the Twitter data we had collected. Naturally, with such contentious issues, greater negative comments could be seen as users shared their opinions via the comment section.
a Where a post was assigned more than 1 theme, they were calculated as separate entities, for example, if a post was assigned to themes 1 and 2, they would be included separately when calculating overall percentages.
Our paper contributes to the existing literature by highlighting that one of the predominant and most popular topics of conversations within ethnic minority communities during the 3 months of data analyzed, was related to the implementation of the vaccination program, particularly communication strategies adopted during part of the booster vaccine rollout. The focus on ethnic minority communities and alienation of these groups through terms such as “BAME,” in vaccination campaigns, has been highlighted from our data as being divisive and causing further racial division. Policies such as mandatory vaccinations for health workers were deemed as particularly discriminatory toward minority workers. In fact, it seemed to have further propelled discussions related to trust or mistrust toward institutions on social media. Few papers explicitly highlight this connection between vaccination implementation strategies propelling concerns related to racism and fuelling further mistrust toward institutions specifically within minority communities.
There is an acknowledgment in the literature about the importance of adopting appropriate communication strategies in public health messaging. The Commission on Race and Ethnic Disparities has acknowledged the generalization that terms such as “BAME,” can cause [ 46 ]. Consequently, recent government guidelines have recommended using the terms “ethnic minorities” or “people from ethnic minority backgrounds” in communication [ 46 ]. Coccia’s [ 47 ] study looking at the maximum level of COVID-19 vaccinations that could be achieved across 150 countries without social impositions, for example, restrictions on public gatherings and government lockdowns, found the maximum level to be 70% based on normal hesitancy in society. In order to increase the number of vaccinated above this threshold, communicating effectively using “humble inquiry, compassionate listening and storytelling,” is a more effective approach than implementing strict health policies that impact individual freedoms [ 47 ].
Our study depicted how opinions toward vaccination strategies were linked to concepts related to trust and mistrust toward institutions. Mistrust toward institutions contributing to hesitancy in minority communities has already been noted by previous studies [ 5 , 7 ]. A systematic review looking at factors influencing vaccination uptake in minority communities found 6 studies that attributed lower uptake to “mistrust including pre-existing lower scientific or medical trust, conspiracy suspicions and attitudes” [ 48 ].
Moreover, our paper suggested that mistrust was mostly directed toward governments and pharmaceutical companies. These findings are echoed in a study looking at survey data from 100 countries and the roles of different forms of trust in predicting vaccine hesitancy [ 49 ]. Trust in political institutions was a consistent predictor of vaccine hesitancy [ 49 ]. The impact of a lack of trust in governments in vaccination attempts is exemplified in Romania’s COVID-19 vaccination strategies. Romania has one of the lowest confidence rates in their national government and this played a significant role in the low COVID-19 vaccination rates seen [ 50 ]. Analysis of comments from Romania’s #storiesfromvaccination campaign, found politically independent sources, for example, from health experts and laypersons, were deemed as being legitimate sources of information and consequently more trustworthy [ 50 ].
The continued concerns over vaccine development and side effects identified in our data are consistent with other studies investigating the causes of hesitancy in ethnic minority communities [ 11 ]. Fertility was a side effect most often noted to be of concern on YouTube and this is further supported by existing literature. A qualitative study of 12 participants done in 2021 exploring vaccine hesitancy in ethnic minority communities found infertility as a prevalent theme, as well as concerns related to period irregularities and breastfeeding [ 4 ]. Conspiracy theories suggesting the vaccine to be gene-altering or experimental in nature were also reported in our results. The spread of such theories could have a detrimental effect on vaccination efforts as studies have suggested that vaccination conspiracy belief was the most relevant predictor in willingness to get vaccinated (ie, a negative association) [ 51 , 52 ].
Strengths and Limitations
The findings of our paper must be considered in light of the limitations of our study. First, our data extraction was limited by Twitter and YouTube API policies that restrict the amount of data that can be extracted over a certain period of time [ 28 , 29 ]. Moreover, our data set was small with a total of 416 social media posts analyzed. However, a rigorous qualitative analysis was conducted with multiple steps taken to validate our analysis as described in the methodology. Furthermore, the demographic of individuals using Twitter and YouTube tends to represent a younger and more politically engaged population [ 53 ]. Consequently, the data obtained may be biased toward this population. However, there are few studies looking at social media as data and by capturing the opinions of a younger population, a greater understanding may be gained of the viewpoints behind a traditionally low uptake group [ 7 ]. Moreover, it is difficult to be conclusive about our sentiment and stance analysis since we were limited by looking at each individual tweet and YouTube comment as isolated posts rather than in the context in which the comment was made (ie, as part of a thread of posts). This was to ensure our methodology of analysis remained consistent and prevented us from making assumptions about the users’ beliefs or opinions.
Much of the literature looking at social media data tends to focus on Twitter, although few on YouTube, due to the ease of accessing Twitter data when compared with other social media platforms [ 37 ]. Hence, we acknowledge that this study is not representative of all social media activity on this topic. Data from other social media sites such as Facebook, Instagram, and TikTok, remain underexplored, and more research is needed looking at such platforms and their contribution to vaccine hesitancy.
Implications and Future Directions
Our findings suggest that when planning communication strategies for future public health interventions such as vaccination, policymakers and health practitioners must acknowledge the wider experiences of individuals, particularly in minority groups. Public health messaging around the COVID-19 vaccine has placed the emphasis on ethnic minorities to become less hesitant and more trusting rather than acknowledging the systemic racism and experiences of discrimination raised by individuals [ 54 ]. Future vaccination strategies and public health messages targeted toward minority groups must make greater concerted efforts to acknowledge the historical abuses of power and contextualize hesitancy accordingly [ 43 , 54 ].
The sources in which information is disseminated should also be considered.
Minority communities may have a greater trust in information obtained from peers from similar cultural groups [ 26 ] and trusted community sources [ 55 ]. Hence, it is critical that communication strategies are not only culturally sensitive and tailored toward individual groups but also use trusted sources of information.
Using existing social networks would also be useful in framing vaccinations as a social norm. Descriptive norms, aka what other people do, say, and believe, have an impact on an individual’s intentions to accept a vaccine [ 56 ]. People often underestimate vaccine acceptance by others making hesitancy more noticeable and influencing their decision-making [ 56 ]. However, presenting vaccinations as a descriptive norm and correcting people’s overestimation of the prevalence of vaccine hesitancy, may have a positive impact on improving uptake [ 56 ].
When deciding what messages to communicate, having an understanding of the discourse on social media in “real time” may help guide communication strategies. By identifying trends in opinions, changes in sentiments, and stances toward a particular intervention such as vaccinations, behaviors can be anticipated [ 57 ]. Policymakers may then be able to intervene in a timely manner to encourage and sustain support [ 58 ].
What is clear to see is the importance of effective communication that explains the reasons for targeted approaches toward vaccinations in the context of a pandemic in a manner that is sensitive and addresses misconceptions from minority communities [ 59 ]. Underlying this is the need for strong governance led by effective leadership that engages communities and adjusts to a population’s needs [ 60 , 61 ]. Good governance not only allows for timely and effective vaccination campaigns [ 60 - 62 ] but also greater investment in research and development and higher public spending [ 60 ]. Greater public spending may address some of the wider socioeconomic factors that contribute to reduced vaccine uptake in minority communities [ 7 ]. More investment in research may allow governments to better address and implement nonpharmaceutical methods of control during a pandemic such as effective contract systems and stronger early warning systems [ 63 ]. Such steps will help improve governments’ prevention and preparedness for future pandemic threats.
Conclusions
Our study highlighted the concerns minority groups had in relation to the vaccine implementation, specifically the targeting of minority groups through vaccination campaigns. The shortfalls in the communication strategies adopted to relay public health information to minority groups during the pandemic must be acknowledged if any meaningful improvement is to be made moving forward to strengthen future interventions targeted toward these groups.
Acknowledgments
The authors are appreciative of ongoing collaborations at University College London (UCL) and the University of Oxford that have enabled this social media research on COVID-19. NU was funded by the National Institute for Health and Care Research School for Primary Care Research Undergraduate Student Internship Programme. The views expressed are those of the authors and not necessarily those of the National Institute for Health and Care Research or the Department of Health and Social Care.
Data Availability
The data sets generated or analyzed during this study are not publicly available due to restrictions from the UCL Research Ethics Council that prohibit the publication of any identifiable information from social media platforms.
Conflicts of Interest
None declared.
Initial Boolean search term, final Boolean search term, thematic analysis framework, sentiment analysis coding, stance analysis coding, hypothetical examples of social media posts, and their sentiment and stance.
Statistics from network analysis.
Most frequent clusters of tweets and key themes noted in relation to the COVID-19 vaccines between Jan 2022 to April 2022 in the UK obtained from network analysis.
Conversation clusters in YouTube videos 1 and 3 related to themes of racism and mistrust in the United Kingdom.
- Baird B, Timmins N. The Covid-19 vaccination programme: trials, tribulations and successes. The Kings Fund. 2022. URL: https://www.kingsfund.org.uk/publications/covid-19-vaccination-programme [accessed 2022-04-03]
- Gaughan CH, Razieh C, Khunti K, Banerjee A, Chudasama YV, Davies MJ, et al. COVID-19 vaccination uptake amongst ethnic minority communities in England: a linked study exploring the drivers of differential vaccination rates. J Public Health (Oxf). 2023;45(1):e65-e74. [ FREE Full text ] [ CrossRef ] [ Medline ]
- Ten threats to global health in 2019. World Health Organisation. 2021. URL: https://www.who.int/news-room/spotlight/ten-threats-to-global-health-in-2019 [accessed 2021-08-08]
- Naqvi M, Li L, Woodrow M, Yadav P, Kostkova P. Understanding COVID-19 vaccine hesitancy in ethnic minorities groups in the UK. Front Public Health. 2022;10:917242. [ FREE Full text ] [ CrossRef ] [ Medline ]
- Burgess RA, Osborne RH, Yongabi KA, Greenhalgh T, Gurdasani D, Kang G, et al. The COVID-19 vaccines rush: participatory community engagement matters more than ever. Lancet. 2021;397(10268):8-10. [ FREE Full text ] [ CrossRef ] [ Medline ]
- Cascini F, Pantovic A, Al-Ajlouni Y, Failla G, Ricciardi W. Attitudes, acceptance and hesitancy among the general population worldwide to receive the COVID-19 vaccines and their contributing factors: a systematic review. EClinicalMedicine. 2021;40:101113. [ FREE Full text ] [ CrossRef ] [ Medline ]
- Al-Jayyousi GF, Sherbash MAM, Ali LAM, El-Heneidy A, Alhussaini NWZ, Elhassan MEA, et al. Factors influencing public attitudes towards COVID-19 vaccination: a scoping review informed by the socio-ecological model. Vaccines (Basel). 2021;9(6):548. [ FREE Full text ] [ CrossRef ] [ Medline ]
- Report of the SAGE working group on vaccine hesitancy. World Health Organisation. 2014. URL: https://www.who.int/immunization/sage/meetings/2014/october/SAGE_working_group_revised_report_vaccine_hesitancy.pdf [accessed 2021-02-21]
- Public attitudes to a Covid-19 vaccine, and their variations across ethnic and socioeconomic groups. Royal Society for Public Health. 2020. URL: https://www.rsph.org.uk/our-work/policy/vaccinations/public-attitudes-to-a-covid-19-vaccine.html [accessed 2022-04-04]
- Scientific Advisory Group for Emergencies. Factors influencing COVID-19 vaccine uptake among minority ethnic groups. GOV.UK. 2020. URL: https://tinyurl.com/26mu6tht [accessed 2022-04-04]
- Razai MS, Osama T, McKechnie DGJ, Majeed A. Covid-19 vaccine hesitancy among ethnic minority groups. BMJ. 2021;372:n513. [ CrossRef ] [ Medline ]
- Cascini F, Pantovic A, Al-Ajlouni YA, Failla G, Puleo V, Melnyk A, et al. Social media and attitudes towards a COVID-19 vaccination: a systematic review of the literature. EClinicalMedicine. 2022;48:101454. [ FREE Full text ] [ CrossRef ] [ Medline ]
- Park MS, Park J, Kim H, Lee JH, Park H. Measuring the impacts of quantity and trustworthiness of information on COVID-19 vaccination intent. J Assoc Inf Sci Technol. 2023;74(7):846-865. [ FREE Full text ] [ CrossRef ]
- Buonomo B. Effects of information-dependent vaccination behavior on coronavirus outbreak: insights from a SIRI model. Ricerche mat. 2020;69(2):483-499. [ FREE Full text ] [ CrossRef ]
- Covid-19 news and information: consumption and attitudes. Ofcom. 2020. URL: https://www.ofcom.org.uk/__data/assets/pdf_file/0031/193747/covid-19-news-consumption-week-one-findings.pdf [accessed 2024-02-23]
- Karafillakis E, Martin S, Simas C, Olsson K, Takacs J, Dada S, et al. Methods for social media monitoring related to vaccination: systematic scoping review. JMIR Public Health Surveill. 2021;7(2):e17149. [ FREE Full text ] [ CrossRef ] [ Medline ]
- Cinelli M, Quattrociocchi W, Galeazzi A, Valensise CM, Brugnoli E, Schmidt AL, et al. The COVID-19 social media infodemic. Sci Rep. 2020;10(1):16598. [ FREE Full text ] [ CrossRef ] [ Medline ]
- Stahl JP, Cohen R, Denis F, Gaudelus J, Martinot A, Lery T, et al. The impact of the web and social networks on vaccination. new challenges and opportunities offered to fight against vaccine hesitancy. Med Mal Infect. 2016;46(3):117-122. [ FREE Full text ] [ CrossRef ] [ Medline ]
- Ngai CSB, Singh RG, Yao L. Impact of COVID-19 vaccine misinformation on social media virality: content analysis of message themes and writing strategies. J Med Internet Res. 2022;24(7):e37806. [ FREE Full text ] [ CrossRef ] [ Medline ]
- Betsch C, Renkewitz F, Betsch T, Ulshöfer C. The influence of vaccine-critical websites on perceiving vaccination risks. J Health Psychol. 2010;15(3):446-455. [ FREE Full text ] [ CrossRef ] [ Medline ]
- Loomba S, de Figueiredo A, Piatek SJ, de Graaf K, Larson HJ. Measuring the impact of COVID-19 vaccine misinformation on vaccination intent in the UK and USA. Nat Hum Behav. 2021;5(3):337-348. [ FREE Full text ] [ CrossRef ] [ Medline ]
- Puri N, Coomes EA, Haghbayan H, Gunaratne K. Social media and vaccine hesitancy: new updates for the era of COVID-19 and globalized infectious diseases. Hum Vaccin Immunother. 2020;16(11):2586-2593. [ FREE Full text ] [ CrossRef ] [ Medline ]
- Basch CH, MacLean SA. A content analysis of HPV related posts on instagram. Hum Vaccin Immunother. 2019;15(7-8):1476-1478. [ FREE Full text ] [ CrossRef ] [ Medline ]
- Blankenship EB, Goff ME, Yin J, Tse ZTH, Fu KW, Liang H, et al. Sentiment, contents, and retweets: a study of two vaccine-related Twitter datasets. Perm J. 2018;22:17-138. [ FREE Full text ] [ CrossRef ] [ Medline ]
- Goldsmith LP, Rowland-Pomp M, Hanson K, Deal A, Crawshaw AF, Hayward SE, et al. Use of social media platforms by migrant and ethnic minority populations during the COVID-19 pandemic: a systematic review. BMJ Open. 2022;12(11):e061896. [ FREE Full text ] [ CrossRef ] [ Medline ]
- Dickson K, Aboltins C, Pelly J, Jessup RL. Effective communication of COVID-19 vaccine information to recently-arrived culturally and linguistically diverse communities from the perspective of community engagement and partnership organisations: a qualitative study. BMC Health Serv Res. 2023;23(1):877. [ FREE Full text ] [ CrossRef ] [ Medline ]
- Dobbs PD, Boykin AA, Ezike N, Myers AJ, Colditz JB, Primack BA. Twitter sentiment about the US federal tobacco 21 law: mixed methods analysis. JMIR Form Res. 2023;7:e50346. [ FREE Full text ] [ CrossRef ] [ Medline ]
- Netlytics. 2021. URL: https://netlytic.org/home/?page_id=10834 [accessed 2021-08-08]
- YouTube Data Tools. 2021. URL: https://tools.digitalmethods.net/netvizz/youtube/faq.php [accessed 2021-09-01]
- Qiu Y. The openness of open application programming interfaces. Inf Commun Soc. 2016;20(11):1720-1736. [ FREE Full text ] [ CrossRef ]
- The rollout of the COVID-19 vaccine programme in England. House of Commons Committee of Public Accounts. 2022. URL: https://committees.parliament.uk/publications/23019/documents/168825/default/ [accessed 2022-12-01]
- Iacobucci G. Covid-19: government abandons mandatory vaccination of NHS staff. BMJ. 2022;376:o269. [ CrossRef ] [ Medline ]
- Microsoft Excel. Microsoft Corporation. 2018. URL: https://office.microsoft.com/excel [accessed 2024-03-07]
- UK general data protection regulation. UK GDPR. 2022. URL: https://uk-gdpr.org/ [accessed 2022-10-13]
- Guide to the UK general data protection regulation. Information Commissioner's Office. 2022. URL: https://ico.org.uk/for-organisations/guide-to-data-protection/guide-to-the-general-data-protection-regulation-gdpr/ [accessed 2022-10-13]
- VACMA GitHub repository. GitHub. URL: https://github.com/peregilk/VACMA-PUBLIC [accessed 2022-08-26]
- Martin S, Kilich E, Dada S, Kummervold PE, Denny C, Paterson P, et al. "Vaccines for pregnant women…?! Absurd" - mapping maternal vaccination discourse and stance on social media over six months. Vaccine. 2020;38(42):6627-6637. [ FREE Full text ] [ CrossRef ] [ Medline ]
- Kummervold PE, Martin S, Dada S, Kilich E, Denny C, Paterson P, et al. Categorizing vaccine confidence with a transformer-based machine learning model: analysis of nuances of vaccine sentiment in Twitter discourse. JMIR Med Inform. 2021;9(10):e29584. [ FREE Full text ] [ CrossRef ] [ Medline ]
- Leask J, Kinnersley P, Jackson C, Cheater F, Bedford H, Rowles G. Communicating with parents about vaccination: a framework for health professionals. BMC Pediatr. 2012;12(1):154. [ FREE Full text ] [ CrossRef ] [ Medline ]
- Chawla N, Kazienko P, editors. Applications of Social Media and Social Network Analysis. Cham. Springer International Publishing; 2015.
- Fürsich E. In defense of textual analysis. Journal Stud. 2009;10(2):238-252. [ FREE Full text ] [ CrossRef ]
- Paranyushkin D. InfraNodus: generating insight using text network analysis. 2022. Presented at: The World Wide Web Conference; San Francisco, USA; May 13, 2019. URL: https://noduslabs.com/wp-content/uploads/2019/06/InfraNodus-Paranyushkin-WWW19-Conference.pdf [ CrossRef ]
- Braveman PA, Arkin E, Proctor D, Kauh T, Holm N. Systemic and structural racism: definitions, examples, health damages, and approaches to dismantling. Health Aff (Millwood). 2022;41(2):171-178. [ FREE Full text ] [ CrossRef ] [ Medline ]
- Corbie-Smith G. The continuing legacy of the tuskegee syphilis study: considerations for clinical investigation. Am J Med Sci. 1999;317(1):5-8. [ CrossRef ] [ Medline ]
- Murthy D, Sharma S. Visualizing YouTube’s comment space: online hostility as a networked phenomena. New Media Soc. 2018;21(1):191-213. [ FREE Full text ] [ CrossRef ]
- Laux R, Nisar S. Why we’ve stopped using the term 'BAME' in government. GOV.UK. 2022. URL: https://civilservice.blog.gov.uk/2022/05/19/why-weve-stopped-using-the-term-bame-in-government/ [accessed 2022-09-11]
- Coccia M. Improving preparedness for next pandemics: max level of COVID-19 vaccinations without social impositions to design effective health policy and avoid flawed democracies. Environ Res. 2022;213:113566. [ FREE Full text ] [ CrossRef ] [ Medline ]
- Kamal A, Hodson A, Pearce JM. A rapid systematic review of factors influencing COVID-19 vaccination uptake in minority ethnic groups in the UK. Vaccines (Basel). 2021;9(10):1121. [ FREE Full text ] [ CrossRef ] [ Medline ]
- Jennings W, Valgarðsson V, McKay L, Stoker G, Mello E, Baniamin HM. Trust and vaccine hesitancy during the COVID-19 pandemic: a cross-national analysis. Vaccine X. 2023;14:100299. [ FREE Full text ] [ CrossRef ] [ Medline ]
- Cmeciu C. (De)legitimation of COVID-19 vaccination narratives on Facebook comments in Romania: beyond the co-occurrence patterns of discursive strategies. Discourse Soc. 2023;4(5-29):09579265231174793. [ FREE Full text ] [ CrossRef ] [ Medline ]
- Lincoln TM, Schlier B, Strakeljahn F, Gaudiano BA, So SH, Kingston J, et al. Taking a machine learning approach to optimize prediction of vaccine hesitancy in high income countries. Sci Rep. 2022;12(1):2055. [ FREE Full text ] [ CrossRef ] [ Medline ]
- Soleimanpour H, Sarbazi E, Esmaeili ED, Mehri A, Fam SG, Nikbakht HA, et al. Predictors of receiving COVID-19 vaccine among adult population in Iran: an observational study. BMC Public Health. 2023;23(1):490. [ FREE Full text ] [ CrossRef ] [ Medline ]
- Xu P, Dredze M, Broniatowski DA. The Twitter social mobility index: measuring social distancing practices with geolocated tweets. J Med Internet Res. 2020;22(12):e21499. [ FREE Full text ] [ CrossRef ] [ Medline ]
- Nellums LB, Latif A, Nkhoma K, Timmons S, Pareek M, Almidani S, et al. COVID-19 boosters and building trust among UK minority ethnic communities. Lancet. 2022;400(10353):643-644. [ FREE Full text ] [ CrossRef ] [ Medline ]
- Pierz AJ, Rauh L, Masoud D, Cruz AK, Palmedo PC, Ratzan SC, et al. Supporting US healthcare providers for successful vaccine communication. BMC Health Serv Res. 2023;23(1):423. [ FREE Full text ] [ CrossRef ] [ Medline ]
- Moehring A, Collis A, Garimella K, Rahimian MA, Aral S, Eckles D. Providing normative information increases intentions to accept a COVID-19 vaccine. Nat Commun. 2023;14(1):126. [ FREE Full text ] [ CrossRef ] [ Medline ]
- Verger P, Peretti-Watel P. Understanding the determinants of acceptance of COVID-19 vaccines: a challenge in a fast-moving situation. Lancet Public Health. 2021;6(4):e195-e196. [ FREE Full text ] [ CrossRef ] [ Medline ]
- de Figueiredo A, Simas C, Larson HJ. COVID-19 vaccine acceptance and its socio-demographic and emotional determinants: a multi-country cross-sectional study. Vaccine. 2023;41(2):354-364. [ FREE Full text ] [ CrossRef ] [ Medline ]
- Coccia M. Sources, diffusion and prediction in COVID-19 pandemic: lessons learned to face next health emergency. AIMS Public Health. 2023;10(1):145-168. [ FREE Full text ] [ CrossRef ] [ Medline ]
- Coccia M. Optimal levels of vaccination to reduce COVID-19 infected individuals and deaths: a global analysis. Environ Res. 2022;204(Pt C):112314. [ FREE Full text ] [ CrossRef ] [ Medline ]
- Benati I, Coccia M. Global analysis of timely COVID-19 vaccinations: improving governance to reinforce response policies for pandemic crises. Int J Health Gov. 2022;27(3):240-253. [ CrossRef ]
- Coccia M. Preparedness of countries to face COVID-19 pandemic crisis: strategic positioning and factors supporting effective strategies of prevention of pandemic threats. Environ Res. 2022;203:111678. [ FREE Full text ] [ CrossRef ] [ Medline ]
- Coccia M. COVID-19 vaccination is not a sufficient public policy to face crisis management of next pandemic threats. Public Organiz Rev. 2022;23(4):1353-1367. [ FREE Full text ] [ CrossRef ]
Abbreviations
Edited by A Mavragani; submitted 22.07.23; peer-reviewed by H Mondal, M Coccia; comments to author 07.02.24; revised version received 27.02.24; accepted 29.02.24; published 26.03.24.
©Nazifa Ullah, Sam Martin, Shoba Poduval. Originally published in JMIR Formative Research (https://formative.jmir.org), 26.03.2024.
This is an open-access article distributed under the terms of the Creative Commons Attribution License (https://creativecommons.org/licenses/by/4.0/), which permits unrestricted use, distribution, and reproduction in any medium, provided the original work, first published in JMIR Formative Research, is properly cited. The complete bibliographic information, a link to the original publication on https://formative.jmir.org, as well as this copyright and license information must be included.

- LIVE DISCOURSE
- BLOG / OPINION
- SUBMIT PRESS RELEASE
- Advertisement
- Knowledge Partnership
- Media Partnership
- Science & Environment
Eating some chocolate really might be good for you - here’s what the research says
It contains many different active compounds which can evoke pharmacological effects within the body, like medicines or drugs.compounds that lead to neurological effects in the brain have to be able to cross the blood-brain barrier, the protective shield which prevents harmful substances like toxins and bacteria entering the delicate nervous tissue.one of these is the compound theobromine, which is also found in tea and contributes towards its bitter taste..
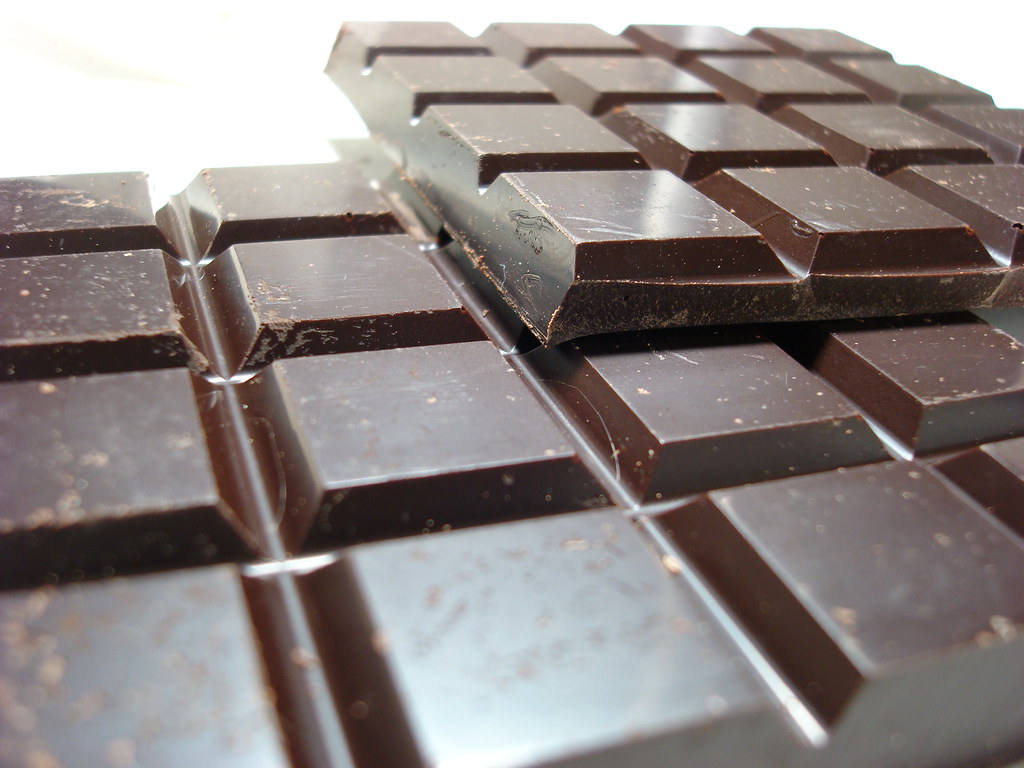
- United Kingdom
Although it always makes me scoff slightly to see Easter eggs making their first appearance in supermarkets at the end of December, few people aren’t delighted to receive a bit of chocolate every year.
It makes sense that too much chocolate would be bad for you because of the high fat and sugar content in most products. But what should we make of common claims that eating some chocolate is actually good for you? Happily, there is a fair amount of evidence that shows, in the right circumstances, chocolate may be both beneficial for your heart and good for your mental state.
In fact, chocolate – or more specifically cacao, the raw, unrefined bean – is a medicinal wonder. It contains many different active compounds which can evoke pharmacological effects within the body, like medicines or drugs.
Compounds that lead to neurological effects in the brain have to be able to cross the blood-brain barrier, the protective shield which prevents harmful substances – like toxins and bacteria – entering the delicate nervous tissue.
One of these is the compound theobromine, which is also found in tea and contributes towards its bitter taste. Tea and chocolate also contain caffeine, which theobromine is related to as part of the purine family of chemicals.
These chemicals, among others, contribute to chocolate’s addictive nature. They have the ability to cross the blood-brain barrier, where they can influence the nervous system. They are therefore known as psychoactive chemicals.
What effects can chocolate have on mood? Well, a systematic review looked at a group of studies which examined the feelings and emotions associated with consuming chocolate. Most demonstrated improvements in mood, anxiety, energy and states of arousal.
Some noted the feeling of guilt, which is perhaps something we’ve all felt after one too many Dairy Milks.
Health benefits of cocoa There are other organs, aside from the brain, that might benefit from the medicinal effects of cocoa. For centuries, chocolate has been used as a medicine to treat a long list of diseases including anaemia, tuberculosis, gout and even low libido.
These might be spurious claims but there is evidence to suggest that eating cacao has a positive effect on the cardiovascular system. First, it can prevent endothelial dysfunction. This is the process through which arteries harden and get laden down with fatty plaques, which can in turn lead to heart attacks and strokes.
Eating dark chocolate may also reduce blood pressure, which is another risk factor for developing arterial disease, and prevent formation of clots which block up blood vessels.
Some studies have suggested that dark chocolate might be useful in adjusting ratios of high-density lipoprotein cholesterol, which can help protect the heart.
Others have examined insulin resistance, the phenomenon associated with Type 2 diabetes and weight gain. They suggest that the polyphenols – chemical compounds present in plants – found in foodstuffs like chocolate may also lead to improved control of blood sugars.
Chocolate toxicity As much as chocolate might be considered a medicine for some, it can be a poison for others.
It’s well documented that the ingestion of caffeine and theobromine is highly toxic for domestic animals. Dogs are particularly affected because of their often voracious appetites and generally unfussy natures.
The culprit is often dark chocolate, which can provoke symptoms of agitation, rigid muscles and even seizures. In certain cases, if ingested in high enough quantities, it can lead to comas and abnormal, even fatal heart rhythms.
Some of the compounds found in chocolate have also been found to have potentially negative effects in humans. Chocolate is a source of oxalate which, along with calcium, is one of the main components of kidney stones.
Some clinical groups have advised against consuming oxalate rich foods, such as spinach and rhubarb – and chocolate, for those who suffer from recurrent kidney stones.
So, what should all this mean for our chocolate consumption habits? Science points in the direction of chocolate that has as high a cocoa solid content as possible, and the minimum of extras. The potentially harmful effects of chocolate are more related to fat and sugar, and may counteract any possible benefits.
A daily dose of 20g-30g of plain or dark chocolate with cocoa solids above 70 per cent – rather than milk chocolate, which contains fewer solids and white chocolate, which contains none – could lead to a greater health benefit, as well as a greater high.
But whatever chocolate you go for, please don’t share it with the dog.

Quantum computing just got hotter: 1 degree above absolute zero

Health News Roundup: Weight-loss drug deals to drive around 4% of Gerresheim...

Samsung's Bespoke home appliances earn top IoT security rating from UL Solut...

Reuters US Domestic News Summary
Latest news, special court dismisses regular bail plea of pfi delhi president perwez ahmed in ed case, pope francis to take part in easter vigil, vatican says, rs 2000 banknotes: exchange, deposit at rbi offices won't be available on april 1.

OPINION / BLOG / INTERVIEW
The impact of climate change on renewable energy production and reliability, ai's revolutionary role in shaping investment futures, natural language processing (nlp): beyond translation - understanding context and emotion, role of artificial intelligence in energy management and optimization, connect us on.
- ADVERTISEMENT
- KNOWLEDGE PARTNERSHIP
- MEDIA PARTNERSHIP
- Agro-Forestry
- Art & Culture
- Economy & Business
- Energy & Extractives
- Law & Governance
- Science & Environment
- Social & Gender
- Urban Development
- East and South East Asia
- Europe and Central Asia
- Central Africa
- East Africa
- Southern Africa
- West Africa
- Middle East and North Africa
- North America
- Latin America and Caribbean
OTHER LINKS
- Write for us
- Submit Press Release
- Opinion / Blog / Analysis
- Business News
- Entertainment News
- Technology News
- Law-order News
- Lifestyle News
- National News
- International News
OTHER PRODUCTS
Email: [email protected] Phone: +91-720-6444012, +91-7027739813, 14, 15
© Copyright 2024
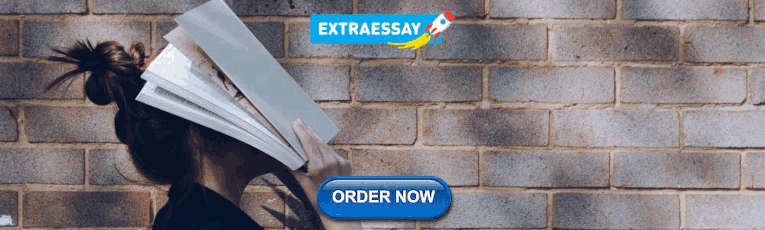
IMAGES
VIDEO
COMMENTS
Interpretive approach: Discourse analysis is an interpretive approach, meaning that it seeks to understand the meaning and significance of language use from the perspective of the participants in a particular discourse. Emphasis on reflexivity: Discourse analysis emphasizes the importance of reflexivity, or self-awareness, in the research process.
Critical discourse analysis (or discourse analysis) is a research method for studying written or spoken language in relation to its social context. It aims to understand how language is used in real life situations. How language use relates to its social, political and historical context. Discourse analysis is a common qualitative research ...
However, the following steps can be used as a guideline if you choose to adopt discourse analysis for your research. Step 1: Decide on your discourse analysis approach . The first step of the process is to decide on which approach you will take in terms. For example, the language in use approach or a socio-political approach such as critical ...
Discourse analysis is a research method for studying written or spoken language in relation to its social context. It aims to understand how language is used in real-life situations. When you do discourse analysis, you might focus on: The purposes and effects of different types of language.
Research. Discourse analysis ( DA ), or discourse studies, is an approach to the analysis of written, spoken, or sign language, including any significant semiotic event. The objects of discourse analysis ( discourse, writing, conversation, communicative event) are variously defined in terms of coherent sequences of sentences, propositions ...
Discourse analysis is an effective method to approach a wide range of research questions in health care and the. health professions. What underpins all variants of. discourse analysis is the idea of examining segments, or frames of communication, and using this to understand.
Abstract. Discourse analysis (DA) is an interdisciplinary field of inquiry and becoming an increasingly popular research strategy for researchers in various disciplines which has been little employed by health-care researchers. The methodology involves a focus on the sociocultural and political context in which text and talk occur.
Critical discourse analysis (CDA) is a qualitative analytical approach for critically describing, interpreting, and explaining the ways in which discourses construct, maintain, and legitimize social inequalities. CDA rests on the notion that the way we use language is purposeful, regardless of whether discursive choices are conscious or ...
This articles explores how discourse analysis is useful for a wide range of research questions in health care and the health professions Previous articles in this series discussed several methodological approaches used by qualitative researchers in the health professions. This article focuses on discourse analysis. It provides background information for those who will encounter this approach ...
Abstract. Critical discourse analysis (CDA) is a social scientific theory and method for analyzing and critiquing the use of language and its contribution to forming and sustaining social practice and for analysis of how language can contribute to reproducing or transforming social problems. CDA adopts the position that the analysis of how ...
Discourse analysis is defined as 'the study of language use above and beyond the sentence'. 12 Discourse analysis examines the language functions and sees how the language is used in different ...
Discourse analysis is a qualitative research method for studying "language in context."[1] The process goes beyond analyzing words and sentences, establishing a deeper context about how language is used to engage in actions and form social identity.
Step 5: Present your Findings. It's time to present your results. Throughout the process, you gathered detailed notes of the discourse, building a strong presentation or thesis. You can use the references of other relevant sources as evidence to support your discussion.
This book seeks to be a concise, accessible introduction to discourse analysis in the social sciences. It is aimed at being a guide for graduate students, advanced undergraduates, and new scholars interested in expanding their research methods. In the chapters that follow, we will provide an overview of the development of discourse analysis in ...
critical discourse analysis, education research, social inequality, qualitative research, analytical framework. Critical discourse analysis (CDA) is a qualitative analytical approach for critically describing, interpreting, and explaining the ways in which discourses construct, main-tain, and legitimize social inequalities (Wodak & Meyer, 2009).
Qualitative research often focuses on what people say: be that in interviews, focus-groups, diaries, social media or documents. Qualitative researchers often try to understand the world by listening to how people talk, but it can be really revealing to look at not just what people say, but how. Essentially this is the how discourse analysis (DA ...
Today, most methodology chapters in dissertations that use discourse analysis will have extensive discussions of Fairclough's methods. Conclusion. Discourse analysis is a popular primary research method in media studies, cultural studies, education studies, and communication studies. It helps scholars to show how texts and language have the ...
What can discourse analysis contribute to research? The big advantage of discourse analysis is that it challenges "the taken-for-granted nature of language" (Sitz, 2008). Thus it can probe the way in which organisational language displays subtle shifts in values and priorities, disclose how documents may appear to present a positive agenda to ...
Kevin C. Dunn and Iver B. Neumann offer a concise, accessible introduction to discourse analysis in the social sciences. A vital resource for students and scholars alike, Undertaking Discourse Analysis for Social Research combines a theoretical and conceptual review with a "how-to" guide for using the method.In the first part of the book, the authors discuss the development of discourse ...
approach to discourse analysis (a version of 'critical discourse analysis') is based upon the assumption that language is an irreducible part of social life, dialectically interconnected with other elements of social life, so that social analysis and research always has to take account of language. ('Dialectical' relations will be ...
Abstract. Discourse analysis (DA) is a broad field of study that draws some of its theories and methods of analysis from disciplines such as linguistics, sociology, philosophy and psychology. More ...
Norman Fairclough, Analyzing discourse: Textual analysis for social research.London: Routledge, 2003. Pp. vii + 270. Hb $135.00, Pb $31.95. This latest book by Norman Fairclough is an extension of his earlier work on critical discourse analysis (CDA) (e.g., Fairclough 1989, 1995, 2001).Relying on systemic functional linguistics (SFL) as his linguistic theoretical standpoint on one hand, and on ...
The analysis follows the methodological template of critical discourse. analysis, by identifying the nomination, predication, and argumentation strategies employed. in the text. In conclusion, we ...
Growing concern surrounds the impact of social media platforms on public discourse 1,2,3,4 and their influence on social dynamics 5,6,7,8,9, especially in the context of toxicity 10,11,12.Here, to ...
This article is a critical reflection on the way the notion of materiality informed the project and the development of The Kinesemiotic Body project carried out by a UK and German research teams and of the model of analysis it adopted, the Functional Grammar of Dance. It starts with an excursus of some of the most interesting developments in other discipline that turned to the investigation of ...
The proliferation of social media platforms has provided a unique space for discourse on mental health, originally intended to destigmatize mental illness. However, recent discourses on these platforms have shown a concerning shift towards the romanticization of mental health issues. This research focuses on Twitter (now called X) users' authentic discussions on the phenomenon of ...
Presented here is a study on the semantic analysis of mainstream media news related to the COVID-19 outbreak in China that occurred at the end of 2019. Examining the most frequently used keywords and their co-occurrences, researchers can infer a semantic network that represents the major frames used in a large amount of text.
Background: Existing literature highlights the role of social media as a key source of information for the public during the COVID-19 pandemic and its influence on vaccination attempts. Yet there is little research exploring its role in the public discourse specifically among ethnic minority communities, who have the highest rates of vaccine hesitancy (delay or refusal of vaccination despite ...
Based on discourse analysis, combined with the current situation of junior high school English writing teaching, this study adopts the teaching model of "reading to write", and uses discourse analysis to assist in the structure, content, and syntax of writing, aiming to cultivate students to acquire the required discourse knowledge and use it to improve the quality of writing.
Eating some chocolate really might be good for you - here's what the research says It contains many different active compounds which can evoke pharmacological effects within the body, like medicines or drugs.Compounds that lead to neurological effects in the brain have to be able to cross the blood-brain barrier, the protective shield which prevents harmful substances like toxins and ...