- USC Libraries
- Research Guides
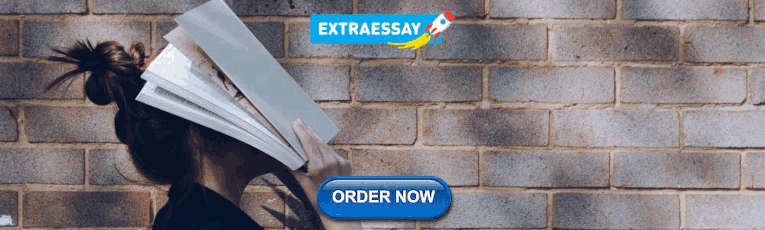
Organizing Your Social Sciences Research Paper
- Independent and Dependent Variables
- Purpose of Guide
- Design Flaws to Avoid
- Glossary of Research Terms
- Reading Research Effectively
- Narrowing a Topic Idea
- Broadening a Topic Idea
- Extending the Timeliness of a Topic Idea
- Academic Writing Style
- Applying Critical Thinking
- Choosing a Title
- Making an Outline
- Paragraph Development
- Research Process Video Series
- Executive Summary
- The C.A.R.S. Model
- Background Information
- The Research Problem/Question
- Theoretical Framework
- Citation Tracking
- Content Alert Services
- Evaluating Sources
- Primary Sources
- Secondary Sources
- Tiertiary Sources
- Scholarly vs. Popular Publications
- Qualitative Methods
- Quantitative Methods
- Insiderness
- Using Non-Textual Elements
- Limitations of the Study
- Common Grammar Mistakes
- Writing Concisely
- Avoiding Plagiarism
- Footnotes or Endnotes?
- Further Readings
- Generative AI and Writing
- USC Libraries Tutorials and Other Guides
- Bibliography
Definitions
Dependent Variable The variable that depends on other factors that are measured. These variables are expected to change as a result of an experimental manipulation of the independent variable or variables. It is the presumed effect.
Independent Variable The variable that is stable and unaffected by the other variables you are trying to measure. It refers to the condition of an experiment that is systematically manipulated by the investigator. It is the presumed cause.
Cramer, Duncan and Dennis Howitt. The SAGE Dictionary of Statistics . London: SAGE, 2004; Penslar, Robin Levin and Joan P. Porter. Institutional Review Board Guidebook: Introduction . Washington, DC: United States Department of Health and Human Services, 2010; "What are Dependent and Independent Variables?" Graphic Tutorial.
Identifying Dependent and Independent Variables
Don't feel bad if you are confused about what is the dependent variable and what is the independent variable in social and behavioral sciences research . However, it's important that you learn the difference because framing a study using these variables is a common approach to organizing the elements of a social sciences research study in order to discover relevant and meaningful results. Specifically, it is important for these two reasons:
- You need to understand and be able to evaluate their application in other people's research.
- You need to apply them correctly in your own research.
A variable in research simply refers to a person, place, thing, or phenomenon that you are trying to measure in some way. The best way to understand the difference between a dependent and independent variable is that the meaning of each is implied by what the words tell us about the variable you are using. You can do this with a simple exercise from the website, Graphic Tutorial. Take the sentence, "The [independent variable] causes a change in [dependent variable] and it is not possible that [dependent variable] could cause a change in [independent variable]." Insert the names of variables you are using in the sentence in the way that makes the most sense. This will help you identify each type of variable. If you're still not sure, consult with your professor before you begin to write.
Fan, Shihe. "Independent Variable." In Encyclopedia of Research Design. Neil J. Salkind, editor. (Thousand Oaks, CA: SAGE, 2010), pp. 592-594; "What are Dependent and Independent Variables?" Graphic Tutorial; Salkind, Neil J. "Dependent Variable." In Encyclopedia of Research Design , Neil J. Salkind, editor. (Thousand Oaks, CA: SAGE, 2010), pp. 348-349;
Structure and Writing Style
The process of examining a research problem in the social and behavioral sciences is often framed around methods of analysis that compare, contrast, correlate, average, or integrate relationships between or among variables . Techniques include associations, sampling, random selection, and blind selection. Designation of the dependent and independent variable involves unpacking the research problem in a way that identifies a general cause and effect and classifying these variables as either independent or dependent.
The variables should be outlined in the introduction of your paper and explained in more detail in the methods section . There are no rules about the structure and style for writing about independent or dependent variables but, as with any academic writing, clarity and being succinct is most important.
After you have described the research problem and its significance in relation to prior research, explain why you have chosen to examine the problem using a method of analysis that investigates the relationships between or among independent and dependent variables . State what it is about the research problem that lends itself to this type of analysis. For example, if you are investigating the relationship between corporate environmental sustainability efforts [the independent variable] and dependent variables associated with measuring employee satisfaction at work using a survey instrument, you would first identify each variable and then provide background information about the variables. What is meant by "environmental sustainability"? Are you looking at a particular company [e.g., General Motors] or are you investigating an industry [e.g., the meat packing industry]? Why is employee satisfaction in the workplace important? How does a company make their employees aware of sustainability efforts and why would a company even care that its employees know about these efforts?
Identify each variable for the reader and define each . In the introduction, this information can be presented in a paragraph or two when you describe how you are going to study the research problem. In the methods section, you build on the literature review of prior studies about the research problem to describe in detail background about each variable, breaking each down for measurement and analysis. For example, what activities do you examine that reflect a company's commitment to environmental sustainability? Levels of employee satisfaction can be measured by a survey that asks about things like volunteerism or a desire to stay at the company for a long time.
The structure and writing style of describing the variables and their application to analyzing the research problem should be stated and unpacked in such a way that the reader obtains a clear understanding of the relationships between the variables and why they are important. This is also important so that the study can be replicated in the future using the same variables but applied in a different way.
Fan, Shihe. "Independent Variable." In Encyclopedia of Research Design. Neil J. Salkind, editor. (Thousand Oaks, CA: SAGE, 2010), pp. 592-594; "What are Dependent and Independent Variables?" Graphic Tutorial; “Case Example for Independent and Dependent Variables.” ORI Curriculum Examples. U.S. Department of Health and Human Services, Office of Research Integrity; Salkind, Neil J. "Dependent Variable." In Encyclopedia of Research Design , Neil J. Salkind, editor. (Thousand Oaks, CA: SAGE, 2010), pp. 348-349; “Independent Variables and Dependent Variables.” Karl L. Wuensch, Department of Psychology, East Carolina University [posted email exchange]; “Variables.” Elements of Research. Dr. Camille Nebeker, San Diego State University.
- << Previous: Design Flaws to Avoid
- Next: Glossary of Research Terms >>
- Last Updated: Apr 9, 2024 1:19 PM
- URL: https://libguides.usc.edu/writingguide

Research Variables 101
Independent variables, dependent variables, control variables and more
By: Derek Jansen (MBA) | Expert Reviewed By: Kerryn Warren (PhD) | January 2023
If you’re new to the world of research, especially scientific research, you’re bound to run into the concept of variables , sooner or later. If you’re feeling a little confused, don’t worry – you’re not the only one! Independent variables, dependent variables, confounding variables – it’s a lot of jargon. In this post, we’ll unpack the terminology surrounding research variables using straightforward language and loads of examples .
Overview: Variables In Research
What (exactly) is a variable.
The simplest way to understand a variable is as any characteristic or attribute that can experience change or vary over time or context – hence the name “variable”. For example, the dosage of a particular medicine could be classified as a variable, as the amount can vary (i.e., a higher dose or a lower dose). Similarly, gender, age or ethnicity could be considered demographic variables, because each person varies in these respects.
Within research, especially scientific research, variables form the foundation of studies, as researchers are often interested in how one variable impacts another, and the relationships between different variables. For example:
- How someone’s age impacts their sleep quality
- How different teaching methods impact learning outcomes
- How diet impacts weight (gain or loss)
As you can see, variables are often used to explain relationships between different elements and phenomena. In scientific studies, especially experimental studies, the objective is often to understand the causal relationships between variables. In other words, the role of cause and effect between variables. This is achieved by manipulating certain variables while controlling others – and then observing the outcome. But, we’ll get into that a little later…
The “Big 3” Variables
Variables can be a little intimidating for new researchers because there are a wide variety of variables, and oftentimes, there are multiple labels for the same thing. To lay a firm foundation, we’ll first look at the three main types of variables, namely:
- Independent variables (IV)
- Dependant variables (DV)
- Control variables
What is an independent variable?
Simply put, the independent variable is the “ cause ” in the relationship between two (or more) variables. In other words, when the independent variable changes, it has an impact on another variable.
For example:
- Increasing the dosage of a medication (Variable A) could result in better (or worse) health outcomes for a patient (Variable B)
- Changing a teaching method (Variable A) could impact the test scores that students earn in a standardised test (Variable B)
- Varying one’s diet (Variable A) could result in weight loss or gain (Variable B).
It’s useful to know that independent variables can go by a few different names, including, explanatory variables (because they explain an event or outcome) and predictor variables (because they predict the value of another variable). Terminology aside though, the most important takeaway is that independent variables are assumed to be the “cause” in any cause-effect relationship. As you can imagine, these types of variables are of major interest to researchers, as many studies seek to understand the causal factors behind a phenomenon.
Need a helping hand?
What is a dependent variable?
While the independent variable is the “ cause ”, the dependent variable is the “ effect ” – or rather, the affected variable . In other words, the dependent variable is the variable that is assumed to change as a result of a change in the independent variable.
Keeping with the previous example, let’s look at some dependent variables in action:
- Health outcomes (DV) could be impacted by dosage changes of a medication (IV)
- Students’ scores (DV) could be impacted by teaching methods (IV)
- Weight gain or loss (DV) could be impacted by diet (IV)
In scientific studies, researchers will typically pay very close attention to the dependent variable (or variables), carefully measuring any changes in response to hypothesised independent variables. This can be tricky in practice, as it’s not always easy to reliably measure specific phenomena or outcomes – or to be certain that the actual cause of the change is in fact the independent variable.
As the adage goes, correlation is not causation . In other words, just because two variables have a relationship doesn’t mean that it’s a causal relationship – they may just happen to vary together. For example, you could find a correlation between the number of people who own a certain brand of car and the number of people who have a certain type of job. Just because the number of people who own that brand of car and the number of people who have that type of job is correlated, it doesn’t mean that owning that brand of car causes someone to have that type of job or vice versa. The correlation could, for example, be caused by another factor such as income level or age group, which would affect both car ownership and job type.
To confidently establish a causal relationship between an independent variable and a dependent variable (i.e., X causes Y), you’ll typically need an experimental design , where you have complete control over the environmen t and the variables of interest. But even so, this doesn’t always translate into the “real world”. Simply put, what happens in the lab sometimes stays in the lab!
As an alternative to pure experimental research, correlational or “ quasi-experimental ” research (where the researcher cannot manipulate or change variables) can be done on a much larger scale more easily, allowing one to understand specific relationships in the real world. These types of studies also assume some causality between independent and dependent variables, but it’s not always clear. So, if you go this route, you need to be cautious in terms of how you describe the impact and causality between variables and be sure to acknowledge any limitations in your own research.
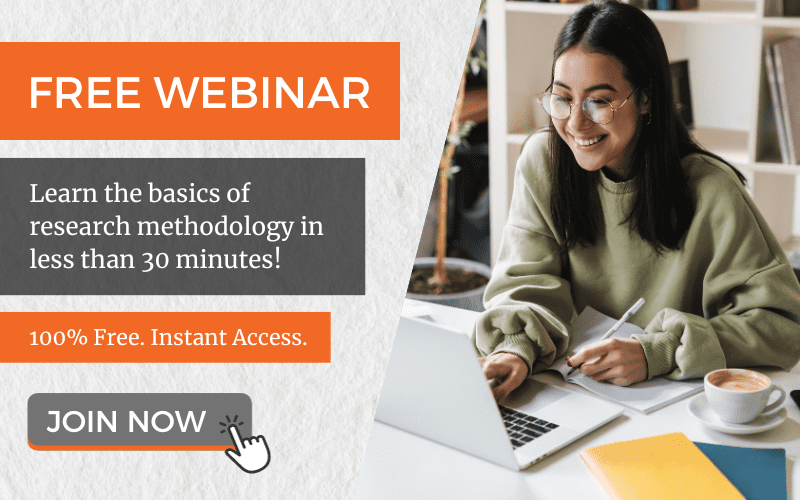
What is a control variable?
In an experimental design, a control variable (or controlled variable) is a variable that is intentionally held constant to ensure it doesn’t have an influence on any other variables. As a result, this variable remains unchanged throughout the course of the study. In other words, it’s a variable that’s not allowed to vary – tough life 🙂
As we mentioned earlier, one of the major challenges in identifying and measuring causal relationships is that it’s difficult to isolate the impact of variables other than the independent variable. Simply put, there’s always a risk that there are factors beyond the ones you’re specifically looking at that might be impacting the results of your study. So, to minimise the risk of this, researchers will attempt (as best possible) to hold other variables constant . These factors are then considered control variables.
Some examples of variables that you may need to control include:
- Temperature
- Time of day
- Noise or distractions
Which specific variables need to be controlled for will vary tremendously depending on the research project at hand, so there’s no generic list of control variables to consult. As a researcher, you’ll need to think carefully about all the factors that could vary within your research context and then consider how you’ll go about controlling them. A good starting point is to look at previous studies similar to yours and pay close attention to which variables they controlled for.
Of course, you won’t always be able to control every possible variable, and so, in many cases, you’ll just have to acknowledge their potential impact and account for them in the conclusions you draw. Every study has its limitations, so don’t get fixated or discouraged by troublesome variables. Nevertheless, always think carefully about the factors beyond what you’re focusing on – don’t make assumptions!
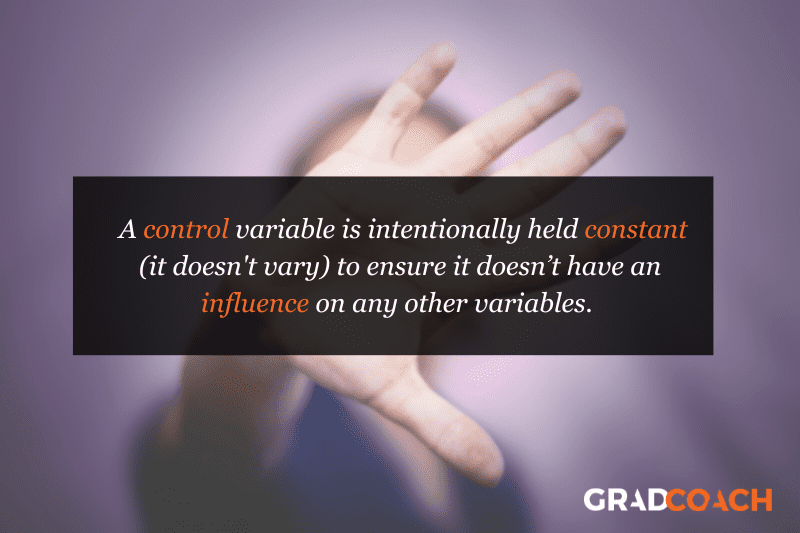
Other types of variables
As we mentioned, independent, dependent and control variables are the most common variables you’ll come across in your research, but they’re certainly not the only ones you need to be aware of. Next, we’ll look at a few “secondary” variables that you need to keep in mind as you design your research.
- Moderating variables
- Mediating variables
- Confounding variables
- Latent variables
Let’s jump into it…
What is a moderating variable?
A moderating variable is a variable that influences the strength or direction of the relationship between an independent variable and a dependent variable. In other words, moderating variables affect how much (or how little) the IV affects the DV, or whether the IV has a positive or negative relationship with the DV (i.e., moves in the same or opposite direction).
For example, in a study about the effects of sleep deprivation on academic performance, gender could be used as a moderating variable to see if there are any differences in how men and women respond to a lack of sleep. In such a case, one may find that gender has an influence on how much students’ scores suffer when they’re deprived of sleep.
It’s important to note that while moderators can have an influence on outcomes , they don’t necessarily cause them ; rather they modify or “moderate” existing relationships between other variables. This means that it’s possible for two different groups with similar characteristics, but different levels of moderation, to experience very different results from the same experiment or study design.
What is a mediating variable?
Mediating variables are often used to explain the relationship between the independent and dependent variable (s). For example, if you were researching the effects of age on job satisfaction, then education level could be considered a mediating variable, as it may explain why older people have higher job satisfaction than younger people – they may have more experience or better qualifications, which lead to greater job satisfaction.
Mediating variables also help researchers understand how different factors interact with each other to influence outcomes. For instance, if you wanted to study the effect of stress on academic performance, then coping strategies might act as a mediating factor by influencing both stress levels and academic performance simultaneously. For example, students who use effective coping strategies might be less stressed but also perform better academically due to their improved mental state.
In addition, mediating variables can provide insight into causal relationships between two variables by helping researchers determine whether changes in one factor directly cause changes in another – or whether there is an indirect relationship between them mediated by some third factor(s). For instance, if you wanted to investigate the impact of parental involvement on student achievement, you would need to consider family dynamics as a potential mediator, since it could influence both parental involvement and student achievement simultaneously.
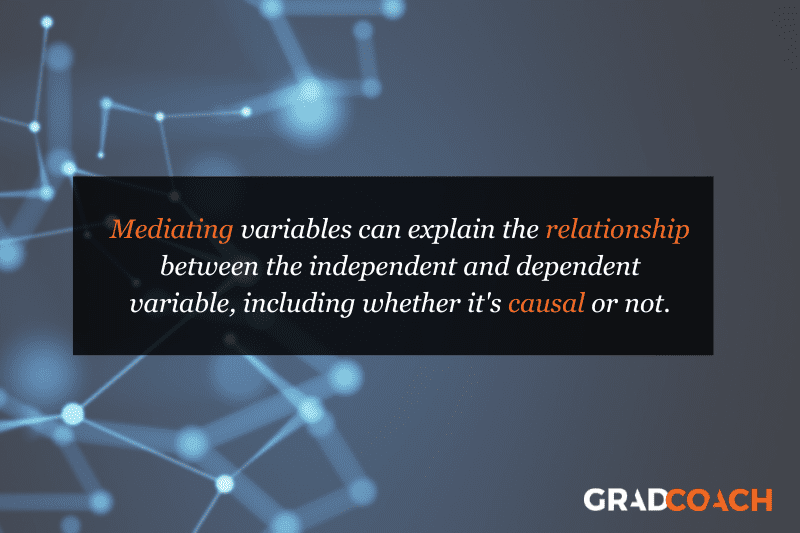
What is a confounding variable?
A confounding variable (also known as a third variable or lurking variable ) is an extraneous factor that can influence the relationship between two variables being studied. Specifically, for a variable to be considered a confounding variable, it needs to meet two criteria:
- It must be correlated with the independent variable (this can be causal or not)
- It must have a causal impact on the dependent variable (i.e., influence the DV)
Some common examples of confounding variables include demographic factors such as gender, ethnicity, socioeconomic status, age, education level, and health status. In addition to these, there are also environmental factors to consider. For example, air pollution could confound the impact of the variables of interest in a study investigating health outcomes.
Naturally, it’s important to identify as many confounding variables as possible when conducting your research, as they can heavily distort the results and lead you to draw incorrect conclusions . So, always think carefully about what factors may have a confounding effect on your variables of interest and try to manage these as best you can.
What is a latent variable?
Latent variables are unobservable factors that can influence the behaviour of individuals and explain certain outcomes within a study. They’re also known as hidden or underlying variables , and what makes them rather tricky is that they can’t be directly observed or measured . Instead, latent variables must be inferred from other observable data points such as responses to surveys or experiments.
For example, in a study of mental health, the variable “resilience” could be considered a latent variable. It can’t be directly measured , but it can be inferred from measures of mental health symptoms, stress, and coping mechanisms. The same applies to a lot of concepts we encounter every day – for example:
- Emotional intelligence
- Quality of life
- Business confidence
- Ease of use
One way in which we overcome the challenge of measuring the immeasurable is latent variable models (LVMs). An LVM is a type of statistical model that describes a relationship between observed variables and one or more unobserved (latent) variables. These models allow researchers to uncover patterns in their data which may not have been visible before, thanks to their complexity and interrelatedness with other variables. Those patterns can then inform hypotheses about cause-and-effect relationships among those same variables which were previously unknown prior to running the LVM. Powerful stuff, we say!
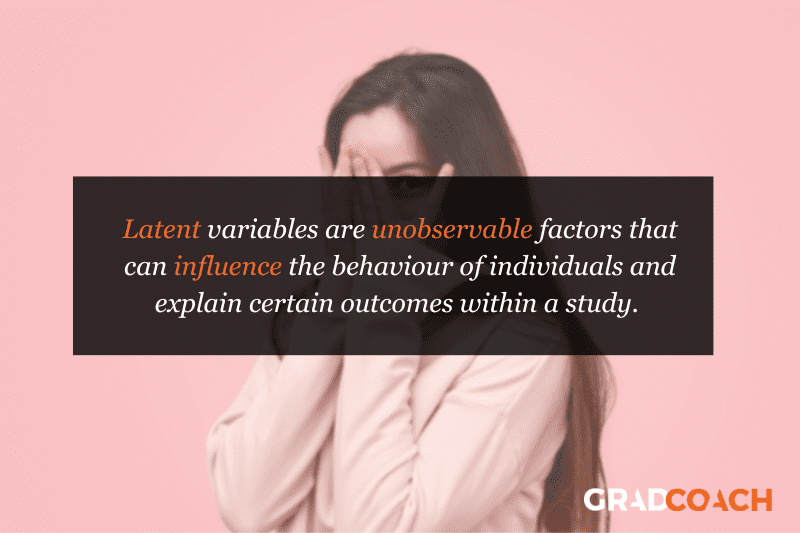
Let’s recap
In the world of scientific research, there’s no shortage of variable types, some of which have multiple names and some of which overlap with each other. In this post, we’ve covered some of the popular ones, but remember that this is not an exhaustive list .
To recap, we’ve explored:
- Independent variables (the “cause”)
- Dependent variables (the “effect”)
- Control variables (the variable that’s not allowed to vary)
If you’re still feeling a bit lost and need a helping hand with your research project, check out our 1-on-1 coaching service , where we guide you through each step of the research journey. Also, be sure to check out our free dissertation writing course and our collection of free, fully-editable chapter templates .
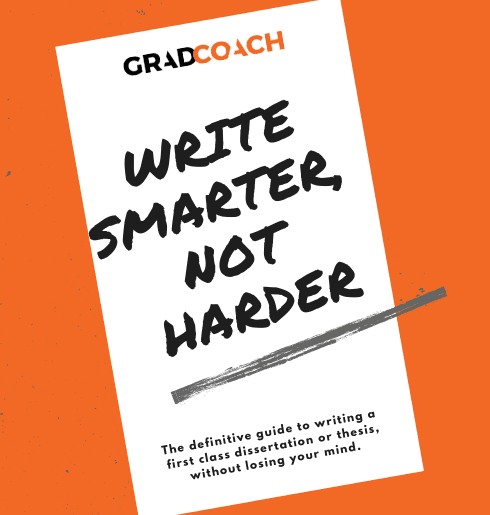
Psst… there’s more (for free)
This post is part of our dissertation mini-course, which covers everything you need to get started with your dissertation, thesis or research project.
You Might Also Like:
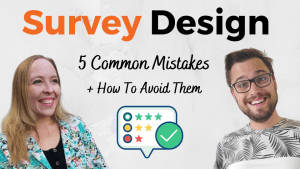
Very informative, concise and helpful. Thank you
Helping information.Thanks
practical and well-demonstrated
Very helpful and insightful
Submit a Comment Cancel reply
Your email address will not be published. Required fields are marked *
Save my name, email, and website in this browser for the next time I comment.
- Print Friendly
Independent and Dependent Variables
Saul Mcleod, PhD
Editor-in-Chief for Simply Psychology
BSc (Hons) Psychology, MRes, PhD, University of Manchester
Saul Mcleod, PhD., is a qualified psychology teacher with over 18 years of experience in further and higher education. He has been published in peer-reviewed journals, including the Journal of Clinical Psychology.
Learn about our Editorial Process
Olivia Guy-Evans, MSc
Associate Editor for Simply Psychology
BSc (Hons) Psychology, MSc Psychology of Education
Olivia Guy-Evans is a writer and associate editor for Simply Psychology. She has previously worked in healthcare and educational sectors.
On This Page:
In research, a variable is any characteristic, number, or quantity that can be measured or counted in experimental investigations . One is called the dependent variable, and the other is the independent variable.
In research, the independent variable is manipulated to observe its effect, while the dependent variable is the measured outcome. Essentially, the independent variable is the presumed cause, and the dependent variable is the observed effect.
Variables provide the foundation for examining relationships, drawing conclusions, and making predictions in research studies.

Independent Variable
In psychology, the independent variable is the variable the experimenter manipulates or changes and is assumed to directly affect the dependent variable.
It’s considered the cause or factor that drives change, allowing psychologists to observe how it influences behavior, emotions, or other dependent variables in an experimental setting. Essentially, it’s the presumed cause in cause-and-effect relationships being studied.
For example, allocating participants to drug or placebo conditions (independent variable) to measure any changes in the intensity of their anxiety (dependent variable).
In a well-designed experimental study , the independent variable is the only important difference between the experimental (e.g., treatment) and control (e.g., placebo) groups.
By changing the independent variable and holding other factors constant, psychologists aim to determine if it causes a change in another variable, called the dependent variable.
For example, in a study investigating the effects of sleep on memory, the amount of sleep (e.g., 4 hours, 8 hours, 12 hours) would be the independent variable, as the researcher might manipulate or categorize it to see its impact on memory recall, which would be the dependent variable.
Dependent Variable
In psychology, the dependent variable is the variable being tested and measured in an experiment and is “dependent” on the independent variable.
In psychology, a dependent variable represents the outcome or results and can change based on the manipulations of the independent variable. Essentially, it’s the presumed effect in a cause-and-effect relationship being studied.
An example of a dependent variable is depression symptoms, which depend on the independent variable (type of therapy).
In an experiment, the researcher looks for the possible effect on the dependent variable that might be caused by changing the independent variable.
For instance, in a study examining the effects of a new study technique on exam performance, the technique would be the independent variable (as it is being introduced or manipulated), while the exam scores would be the dependent variable (as they represent the outcome of interest that’s being measured).
Examples in Research Studies
For example, we might change the type of information (e.g., organized or random) given to participants to see how this might affect the amount of information remembered.
In this example, the type of information is the independent variable (because it changes), and the amount of information remembered is the dependent variable (because this is being measured).
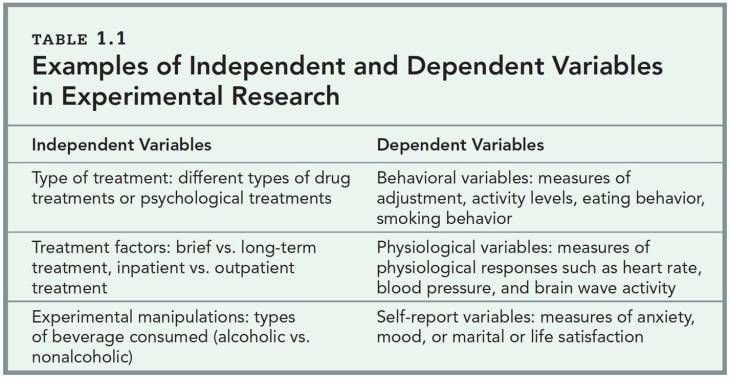
For the following hypotheses, name the IV and the DV.
1. Lack of sleep significantly affects learning in 10-year-old boys.
IV……………………………………………………
DV…………………………………………………..
2. Social class has a significant effect on IQ scores.
DV……………………………………………….…
3. Stressful experiences significantly increase the likelihood of headaches.
4. Time of day has a significant effect on alertness.
Operationalizing Variables
To ensure cause and effect are established, it is important that we identify exactly how the independent and dependent variables will be measured; this is known as operationalizing the variables.
Operational variables (or operationalizing definitions) refer to how you will define and measure a specific variable as it is used in your study. This enables another psychologist to replicate your research and is essential in establishing reliability (achieving consistency in the results).
For example, if we are concerned with the effect of media violence on aggression, then we need to be very clear about what we mean by the different terms. In this case, we must state what we mean by the terms “media violence” and “aggression” as we will study them.
Therefore, you could state that “media violence” is operationally defined (in your experiment) as ‘exposure to a 15-minute film showing scenes of physical assault’; “aggression” is operationally defined as ‘levels of electrical shocks administered to a second ‘participant’ in another room.
In another example, the hypothesis “Young participants will have significantly better memories than older participants” is not operationalized. How do we define “young,” “old,” or “memory”? “Participants aged between 16 – 30 will recall significantly more nouns from a list of twenty than participants aged between 55 – 70” is operationalized.
The key point here is that we have clarified what we mean by the terms as they were studied and measured in our experiment.
If we didn’t do this, it would be very difficult (if not impossible) to compare the findings of different studies to the same behavior.
Operationalization has the advantage of generally providing a clear and objective definition of even complex variables. It also makes it easier for other researchers to replicate a study and check for reliability .
For the following hypotheses, name the IV and the DV and operationalize both variables.
1. Women are more attracted to men without earrings than men with earrings.
I.V._____________________________________________________________
D.V. ____________________________________________________________
Operational definitions:
I.V. ____________________________________________________________
2. People learn more when they study in a quiet versus noisy place.
I.V. _________________________________________________________
D.V. ___________________________________________________________
3. People who exercise regularly sleep better at night.
Can there be more than one independent or dependent variable in a study?
Yes, it is possible to have more than one independent or dependent variable in a study.
In some studies, researchers may want to explore how multiple factors affect the outcome, so they include more than one independent variable.
Similarly, they may measure multiple things to see how they are influenced, resulting in multiple dependent variables. This allows for a more comprehensive understanding of the topic being studied.
What are some ethical considerations related to independent and dependent variables?
Ethical considerations related to independent and dependent variables involve treating participants fairly and protecting their rights.
Researchers must ensure that participants provide informed consent and that their privacy and confidentiality are respected. Additionally, it is important to avoid manipulating independent variables in ways that could cause harm or discomfort to participants.
Researchers should also consider the potential impact of their study on vulnerable populations and ensure that their methods are unbiased and free from discrimination.
Ethical guidelines help ensure that research is conducted responsibly and with respect for the well-being of the participants involved.
Can qualitative data have independent and dependent variables?
Yes, both quantitative and qualitative data can have independent and dependent variables.
In quantitative research, independent variables are usually measured numerically and manipulated to understand their impact on the dependent variable. In qualitative research, independent variables can be qualitative in nature, such as individual experiences, cultural factors, or social contexts, influencing the phenomenon of interest.
The dependent variable, in both cases, is what is being observed or studied to see how it changes in response to the independent variable.
So, regardless of the type of data, researchers analyze the relationship between independent and dependent variables to gain insights into their research questions.
Can the same variable be independent in one study and dependent in another?
Yes, the same variable can be independent in one study and dependent in another.
The classification of a variable as independent or dependent depends on how it is used within a specific study. In one study, a variable might be manipulated or controlled to see its effect on another variable, making it independent.
However, in a different study, that same variable might be the one being measured or observed to understand its relationship with another variable, making it dependent.
The role of a variable as independent or dependent can vary depending on the research question and study design.

Have a language expert improve your writing
Run a free plagiarism check in 10 minutes, generate accurate citations for free.
- Knowledge Base
Methodology
- Types of Variables in Research & Statistics | Examples
Types of Variables in Research & Statistics | Examples
Published on September 19, 2022 by Rebecca Bevans . Revised on June 21, 2023.
In statistical research , a variable is defined as an attribute of an object of study. Choosing which variables to measure is central to good experimental design .
If you want to test whether some plant species are more salt-tolerant than others, some key variables you might measure include the amount of salt you add to the water, the species of plants being studied, and variables related to plant health like growth and wilting .
You need to know which types of variables you are working with in order to choose appropriate statistical tests and interpret the results of your study.
You can usually identify the type of variable by asking two questions:
- What type of data does the variable contain?
- What part of the experiment does the variable represent?
Table of contents
Types of data: quantitative vs categorical variables, parts of the experiment: independent vs dependent variables, other common types of variables, other interesting articles, frequently asked questions about variables.
Data is a specific measurement of a variable – it is the value you record in your data sheet. Data is generally divided into two categories:
- Quantitative data represents amounts
- Categorical data represents groupings
A variable that contains quantitative data is a quantitative variable ; a variable that contains categorical data is a categorical variable . Each of these types of variables can be broken down into further types.
Quantitative variables
When you collect quantitative data, the numbers you record represent real amounts that can be added, subtracted, divided, etc. There are two types of quantitative variables: discrete and continuous .
Categorical variables
Categorical variables represent groupings of some kind. They are sometimes recorded as numbers, but the numbers represent categories rather than actual amounts of things.
There are three types of categorical variables: binary , nominal , and ordinal variables .
*Note that sometimes a variable can work as more than one type! An ordinal variable can also be used as a quantitative variable if the scale is numeric and doesn’t need to be kept as discrete integers. For example, star ratings on product reviews are ordinal (1 to 5 stars), but the average star rating is quantitative.
Example data sheet
To keep track of your salt-tolerance experiment, you make a data sheet where you record information about the variables in the experiment, like salt addition and plant health.
To gather information about plant responses over time, you can fill out the same data sheet every few days until the end of the experiment. This example sheet is color-coded according to the type of variable: nominal , continuous , ordinal , and binary .
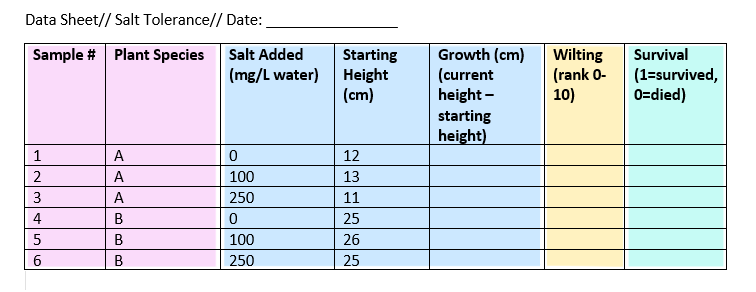
Receive feedback on language, structure, and formatting
Professional editors proofread and edit your paper by focusing on:
- Academic style
- Vague sentences
- Style consistency
See an example
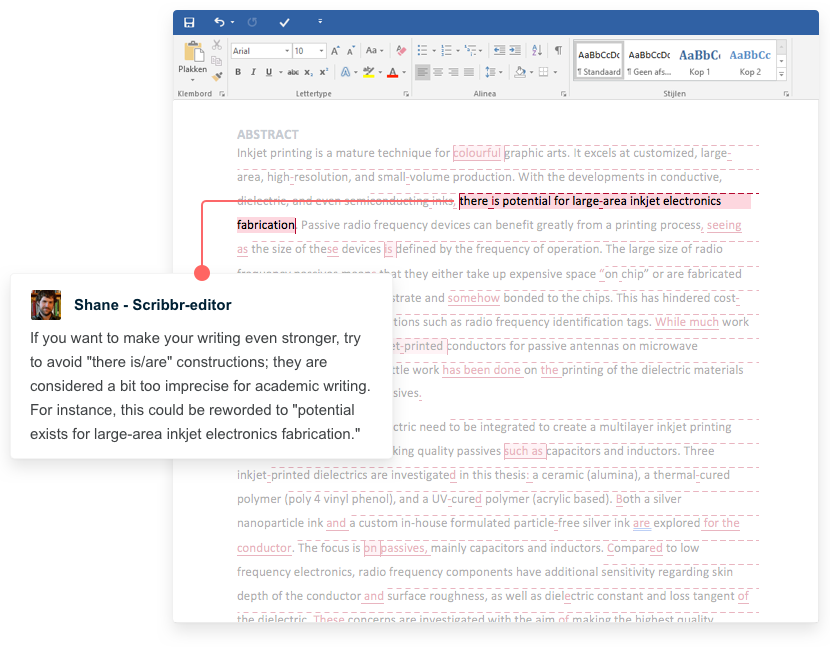
Experiments are usually designed to find out what effect one variable has on another – in our example, the effect of salt addition on plant growth.
You manipulate the independent variable (the one you think might be the cause ) and then measure the dependent variable (the one you think might be the effect ) to find out what this effect might be.
You will probably also have variables that you hold constant ( control variables ) in order to focus on your experimental treatment.
In this experiment, we have one independent and three dependent variables.
The other variables in the sheet can’t be classified as independent or dependent, but they do contain data that you will need in order to interpret your dependent and independent variables.
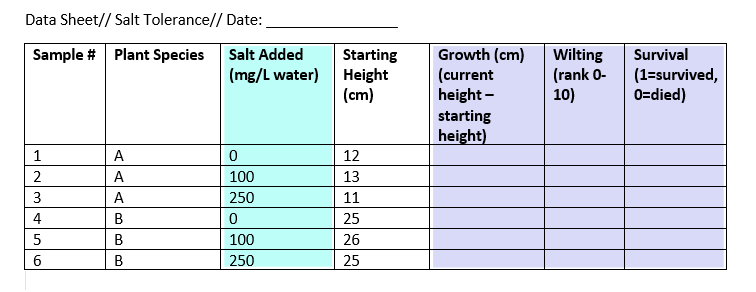
What about correlational research?
When you do correlational research , the terms “dependent” and “independent” don’t apply, because you are not trying to establish a cause and effect relationship ( causation ).
However, there might be cases where one variable clearly precedes the other (for example, rainfall leads to mud, rather than the other way around). In these cases you may call the preceding variable (i.e., the rainfall) the predictor variable and the following variable (i.e. the mud) the outcome variable .
Once you have defined your independent and dependent variables and determined whether they are categorical or quantitative, you will be able to choose the correct statistical test .
But there are many other ways of describing variables that help with interpreting your results. Some useful types of variables are listed below.
If you want to know more about statistics , methodology , or research bias , make sure to check out some of our other articles with explanations and examples.
- Student’s t -distribution
- Normal distribution
- Null and Alternative Hypotheses
- Chi square tests
- Confidence interval
- Cluster sampling
- Stratified sampling
- Data cleansing
- Reproducibility vs Replicability
- Peer review
- Likert scale
Research bias
- Implicit bias
- Framing effect
- Cognitive bias
- Placebo effect
- Hawthorne effect
- Hindsight bias
- Affect heuristic
You can think of independent and dependent variables in terms of cause and effect: an independent variable is the variable you think is the cause , while a dependent variable is the effect .
In an experiment, you manipulate the independent variable and measure the outcome in the dependent variable. For example, in an experiment about the effect of nutrients on crop growth:
- The independent variable is the amount of nutrients added to the crop field.
- The dependent variable is the biomass of the crops at harvest time.
Defining your variables, and deciding how you will manipulate and measure them, is an important part of experimental design .
A confounding variable , also called a confounder or confounding factor, is a third variable in a study examining a potential cause-and-effect relationship.
A confounding variable is related to both the supposed cause and the supposed effect of the study. It can be difficult to separate the true effect of the independent variable from the effect of the confounding variable.
In your research design , it’s important to identify potential confounding variables and plan how you will reduce their impact.
Quantitative variables are any variables where the data represent amounts (e.g. height, weight, or age).
Categorical variables are any variables where the data represent groups. This includes rankings (e.g. finishing places in a race), classifications (e.g. brands of cereal), and binary outcomes (e.g. coin flips).
You need to know what type of variables you are working with to choose the right statistical test for your data and interpret your results .
Discrete and continuous variables are two types of quantitative variables :
- Discrete variables represent counts (e.g. the number of objects in a collection).
- Continuous variables represent measurable amounts (e.g. water volume or weight).
Cite this Scribbr article
If you want to cite this source, you can copy and paste the citation or click the “Cite this Scribbr article” button to automatically add the citation to our free Citation Generator.
Bevans, R. (2023, June 21). Types of Variables in Research & Statistics | Examples. Scribbr. Retrieved April 9, 2024, from https://www.scribbr.com/methodology/types-of-variables/
Is this article helpful?
Rebecca Bevans
Other students also liked, independent vs. dependent variables | definition & examples, confounding variables | definition, examples & controls, control variables | what are they & why do they matter, "i thought ai proofreading was useless but..".
I've been using Scribbr for years now and I know it's a service that won't disappoint. It does a good job spotting mistakes”
- Bipolar Disorder
- Therapy Center
- When To See a Therapist
- Types of Therapy
- Best Online Therapy
- Best Couples Therapy
- Best Family Therapy
- Managing Stress
- Sleep and Dreaming
- Understanding Emotions
- Self-Improvement
- Healthy Relationships
- Student Resources
- Personality Types
- Guided Meditations
- Verywell Mind Insights
- 2023 Verywell Mind 25
- Mental Health in the Classroom
- Editorial Process
- Meet Our Review Board
- Crisis Support
What Is a Dependent Variable?
Kendra Cherry, MS, is a psychosocial rehabilitation specialist, psychology educator, and author of the "Everything Psychology Book."
:max_bytes(150000):strip_icc():format(webp)/IMG_9791-89504ab694d54b66bbd72cb84ffb860e.jpg)
Cara Lustik is a fact-checker and copywriter.
:max_bytes(150000):strip_icc():format(webp)/Cara-Lustik-1000-77abe13cf6c14a34a58c2a0ffb7297da.jpg)
skynesher / Getty Images
- Independent vs. Dependent
- Selection Features
Frequently Asked Questions
The dependent variable is the variable that is being measured or tested in an experiment. For example, in a study looking at how tutoring impacts test scores, the dependent variable would be the participants' test scores since that is what is being measured.
This is different than the independent variable in an experiment, which is a variable that stands on its own. In the example above, the independent variable would be tutoring. The independent variable (tutoring) doesn't change based on other variables, but the dependent variable (test scores) may.
One way to help identify the dependent variable is to remember that it depends on the independent variable. When researchers make changes to the independent variable, they then measure any resulting changes to the dependent variable.
The dependent variable is called "dependent" because it is thought to depend, in some way, on the variations of the independent variable.
Independent vs. Dependent Variable
In a psychology experiment , researchers study how changes in one variable (the independent variable) change another variable (the dependent variable). Manipulating independent variables and measuring the effect on dependent variables allows researchers to draw conclusions about cause-and-effect relationships.
These experiments can range from simple to quite complicated, so it can sometimes be a bit confusing to know how to identify the independent vs. dependent variables. Here are a couple of questions to ask to help you learn which is which.
Which Variable Is the Experimenter Measuring?
Keep in mind that the dependent variable is the one being measured. So, if the experiment is trying to see how one variable affects another, the variable that is being affected is the dependent variable.
In many psychology experiments and studies, the dependent variable is a measure of a certain aspect of a participant's behavior . In an experiment looking at how sleep affects test performance, the dependent variable would be test performance.
Which Variable Does the Experimenter Manipulate?
The independent variable is "independent" because the experimenters are free to vary it as they need. This might mean changing the amount, duration, or type of variable that the participants in the study receive as a treatment or condition.
For example, it's common for treatment-based studies to have some subjects receive a certain treatment while others receive no treatment at all. In this case, the treatment is an independent variable because it is the one being manipulated or changed.
Variable being manipulated
Doesn't change based on other variables
Stands on its own
Variable being measured
May change based on other variables
Depends on other variables
How to Choose a Dependent Variable
How do researchers determine what will be a good dependent variable? There are a few key features that a scientist might consider.
Stability is often a good sign of a higher quality dependent variable. If the experiment is repeated with the same participants, conditions, and experimental manipulations, the effects on the dependent variable should be very close to what they were the first time around.
A researcher might also choose dependent variables based on the complexity of their study. While some studies only have one dependent variable and one independent variable, it is possible to have several of each type.
Researchers might also want to learn how changes in a single independent variable affect several dependent variables. For example, imagine an experiment where a researcher wants to learn how the messiness of a room influences people's creativity levels .
This research might also want to see how the messiness of a room might influence a person's mood. The messiness of a room would be the independent variable and the study would have two dependent variables: level of creativity and mood .
Ability to Operationalize
Operationalization is defined as "translating a construct into its manifestation." In simple terms, it refers to how a variable will be measured. So, a good dependent variable is one that you are able to measure.
If measuring burnout , for instance, researchers might decide to use the Maslach Burnout Inventory. If measuring depression, they could use the Patient Health Questionnaire-9 (PHQ-9).
Dependent Variable Examples
As you are learning to identify the dependent variables in an experiment, it can be helpful to look at examples. Here are just a few dependent variable examples in psychology research .
- How does the amount of time spent studying influence test scores? The test scores would be the dependent variable and the amount of studying would be the independent variable. The researcher could also change the independent variable by instead evaluating how age or gender influences test scores.
- How does stress influence memory? The dependent variable might be scores on a memory test and the independent variable might be exposure to a stressful task.
- How does a specific therapeutic technique influence the symptoms of psychological disorders ? In this case, the dependent variable might be defined as the severity of the symptoms a patient is experiencing, while the independent variable would be the use of a specific therapy method .
- Does listening to classical music help students perform better on a math exam? The scores on the math exams are the dependent variable and classical music is the independent variable.
- How long does it take people to respond to different sounds? The length of time it takes participants to respond to a sound is the dependent variable, while the sounds are the independent variable.
- Do first-born children learn to speak at a younger age than second-born children? In this example, the dependent variable is the age at which the child learns to speak and the independent variable is whether the child is first- or second-born.
- How does alcohol use influence reaction time while driving? The amount of alcohol a participant ingests is the independent variable, while their performance on the driving test is the dependent variable.
A Word From Verywell
Understanding what a dependent variable is and how it is used can be helpful for interpreting different types of research that you encounter in different settings. When you are trying to determine which variables are which, remember that the independent variables are the cause while the dependent variables are the effect.
The dependent variable depends on the independent variable. Thus, if the independent variable changes, the dependent variable would likely change too.
The dependent variable is placed on a graph's y-axis. This is the vertical line or the line that extends upward. The independent variable is placed on the graph's x-axis or the horizontal line.
The dependent variable is the one being measured. If looking at how a lack of sleep affects mental health , for instance, mental health is the dependent variable. In a study that seeks to find the effects of supplements on mood , the participants' mood is the dependent variable.
A controlled variable is a variable that doesn't change during the experiment. This enables researchers to assess the relationship between the dependent and independent variables more accurately. For example, if trying to assess the impact of drinking green tea on memory, researchers might ask subjects to drink it at the same time of day. This would be a controlled variable.
U.S. National Library of Medicine. Dependent and independent variables .
Steingrimsdottir HS, Arntzen E. On the utility of within-participant research design when working with patients with neurocognitive disorders . Clin Interv Aging . 2015;10:1189-1199. doi:10.2147/CIA.S81868
Kaliyadan F, Kulkarni V. Types of variables, descriptive statistics, and sample size . Indian Dermatol Online J . 2019;10(1):82-86. doi:10.4103/idoj.IDOJ_468_18
Flannelly LT, Flannelly KJ, Jankowski KR. Independent, dependent, and other variables in healthcare and chaplaincy research . J Health Care Chaplain . 2014;20(4):161-70. doi:10.1080/08854726.2014.959374
Weiten W. Psychology: Themes and Variations . Cengage Learning.
Roediger HL, Elmes DG, Kantowitz BH. Experimental Psychology . Cengage Learning.
Vassar M, Matthew H. The retrospective chart review: important methodological considerations . J Educ Eval Health Prof . 2013;10:12. doi:10.3352/jeehp.2013.10.12
By Kendra Cherry, MSEd Kendra Cherry, MS, is a psychosocial rehabilitation specialist, psychology educator, and author of the "Everything Psychology Book."
Independent and Dependent Variables
This guide discusses how to identify independent and dependent variables effectively and incorporate their description within the body of a research paper.
A variable can be anything you might aim to measure in your study, whether in the form of numerical data or reflecting complex phenomena such as feelings or reactions. Dependent variables change due to the other factors measured, especially if a study employs an experimental or semi-experimental design. Independent variables are stable: they are both presumed causes and conditions in the environment or milieu being manipulated.
Identifying Independent and Dependent Variables
Even though the definitions of the terms independent and dependent variables may appear to be clear, in the process of analyzing data resulting from actual research, identifying the variables properly might be challenging. Here is a simple rule that you can apply at all times: the independent variable is what a researcher changes, whereas the dependent variable is affected by these changes. To illustrate the difference, a number of examples are provided below.
- The purpose of Study 1 is to measure the impact of different plant fertilizers on how many fruits apple trees bear. Independent variable : plant fertilizers (chosen by researchers) Dependent variable : fruits that the trees bear (affected by choice of fertilizers)
- The purpose of Study 2 is to find an association between living in close vicinity to hydraulic fracturing sites and respiratory diseases. Independent variable: proximity to hydraulic fracturing sites (a presumed cause and a condition of the environment) Dependent variable: the percentage/ likelihood of suffering from respiratory diseases
Confusion is possible in identifying independent and dependent variables in the social sciences. When considering psychological phenomena and human behavior, it can be difficult to distinguish between cause and effect. For example, the purpose of Study 3 is to establish how tactics for coping with stress are linked to the level of stress-resilience in college students. Even though it is feasible to speculate that these variables are interdependent, the following factors should be taken into account in order to clearly define which variable is dependent and which is interdependent.
- The dependent variable is usually the objective of the research. In the study under examination, the levels of stress resilience are being investigated.
- The independent variable precedes the dependent variable. The chosen stress-related coping techniques help to build resilience; thus, they occur earlier.
Writing Style and Structure
Usually, the variables are first described in the introduction of a research paper and then in the method section. No strict guidelines for approaching the subject exist; however, academic writing demands that the researcher make clear and concise statements. It is only reasonable not to leave readers guessing which of the variables is dependent and which is independent. The description should reflect the literature review, where both types of variables are identified in the context of the previous research. For instance, in the case of Study 3, a researcher would have to provide an explanation as to the meaning of stress resilience and coping tactics.
In properly organizing a research paper, it is essential to outline and operationalize the appropriate independent and dependent variables. Moreover, the paper should differentiate clearly between independent and dependent variables. Finding the dependent variable is typically the objective of a study, whereas independent variables reflect influencing factors that can be manipulated. Distinguishing between the two types of variables in social sciences may be somewhat challenging as it can be easy to confuse cause with effect. Academic format calls for the author to mention the variables in the introduction and then provide a detailed description in the method section.
Unfortunately, your browser is too old to work on this site.
For full functionality of this site it is necessary to enable JavaScript.
Have a language expert improve your writing
Run a free plagiarism check in 10 minutes, automatically generate references for free.
- Knowledge Base
- Methodology
- Independent vs Dependent Variables | Definition & Examples
Independent vs Dependent Variables | Definition & Examples
Published on 4 May 2022 by Pritha Bhandari . Revised on 17 October 2022.
In research, variables are any characteristics that can take on different values, such as height, age, temperature, or test scores.
Researchers often manipulate or measure independent and dependent variables in studies to test cause-and-effect relationships.
- The independent variable is the cause. Its value is independent of other variables in your study.
- The dependent variable is the effect. Its value depends on changes in the independent variable.
Your independent variable is the temperature of the room. You vary the room temperature by making it cooler for half the participants, and warmer for the other half.
Table of contents
What is an independent variable, types of independent variables, what is a dependent variable, identifying independent vs dependent variables, independent and dependent variables in research, visualising independent and dependent variables, frequently asked questions about independent and dependent variables.
An independent variable is the variable you manipulate or vary in an experimental study to explore its effects. It’s called ‘independent’ because it’s not influenced by any other variables in the study.
Independent variables are also called:
- Explanatory variables (they explain an event or outcome)
- Predictor variables (they can be used to predict the value of a dependent variable)
- Right-hand-side variables (they appear on the right-hand side of a regression equation).
These terms are especially used in statistics , where you estimate the extent to which an independent variable change can explain or predict changes in the dependent variable.
Prevent plagiarism, run a free check.
There are two main types of independent variables.
- Experimental independent variables can be directly manipulated by researchers.
- Subject variables cannot be manipulated by researchers, but they can be used to group research subjects categorically.
Experimental variables
In experiments, you manipulate independent variables directly to see how they affect your dependent variable. The independent variable is usually applied at different levels to see how the outcomes differ.
You can apply just two levels in order to find out if an independent variable has an effect at all.
You can also apply multiple levels to find out how the independent variable affects the dependent variable.
You have three independent variable levels, and each group gets a different level of treatment.
You randomly assign your patients to one of the three groups:
- A low-dose experimental group
- A high-dose experimental group
- A placebo group
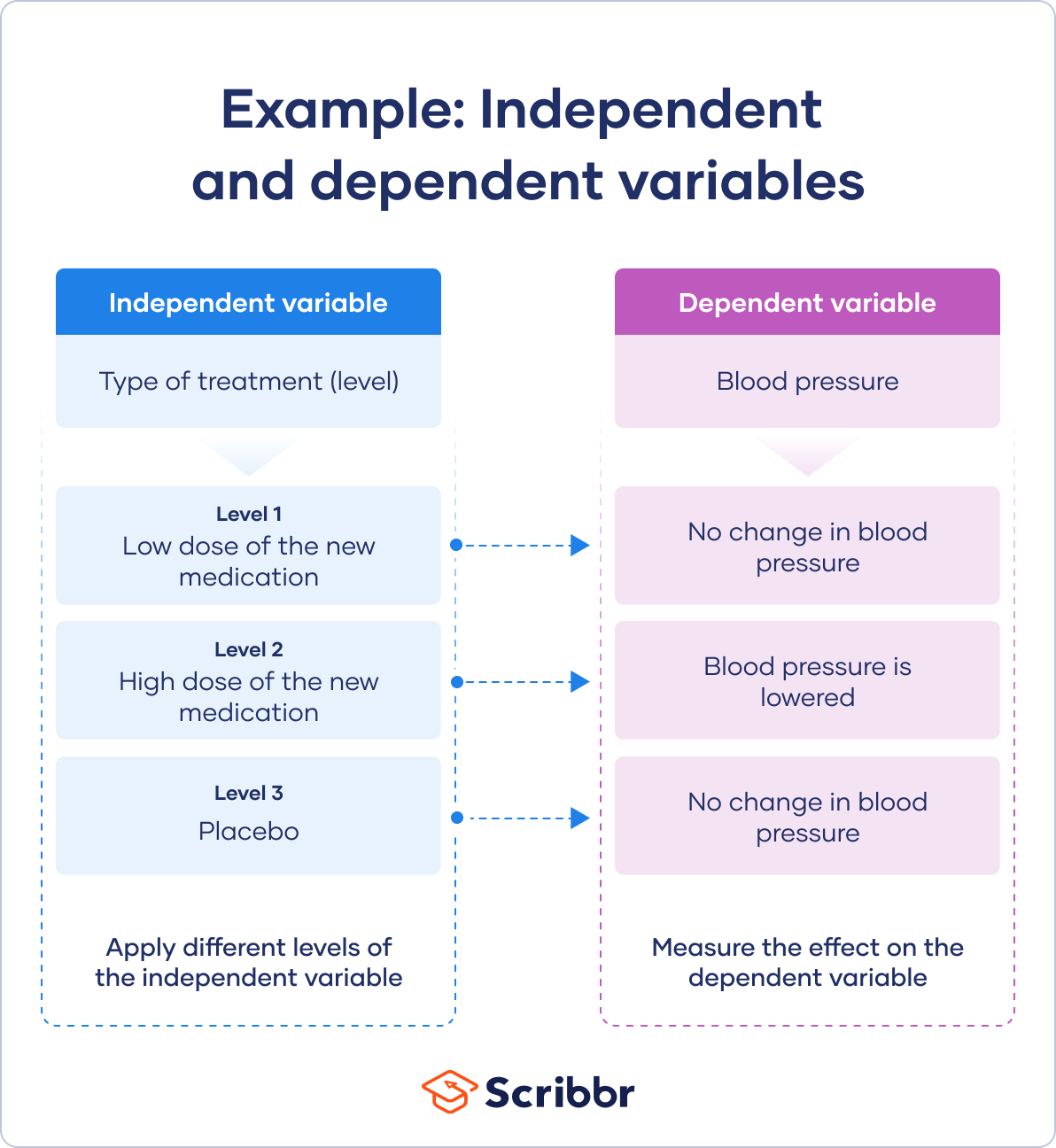
A true experiment requires you to randomly assign different levels of an independent variable to your participants.
Random assignment helps you control participant characteristics, so that they don’t affect your experimental results. This helps you to have confidence that your dependent variable results come solely from the independent variable manipulation.
Subject variables
Subject variables are characteristics that vary across participants, and they can’t be manipulated by researchers. For example, gender identity, ethnicity, race, income, and education are all important subject variables that social researchers treat as independent variables.
It’s not possible to randomly assign these to participants, since these are characteristics of already existing groups. Instead, you can create a research design where you compare the outcomes of groups of participants with characteristics. This is a quasi-experimental design because there’s no random assignment.
Your independent variable is a subject variable, namely the gender identity of the participants. You have three groups: men, women, and other.
Your dependent variable is the brain activity response to hearing infant cries. You record brain activity with fMRI scans when participants hear infant cries without their awareness.
A dependent variable is the variable that changes as a result of the independent variable manipulation. It’s the outcome you’re interested in measuring, and it ‘depends’ on your independent variable.
In statistics , dependent variables are also called:
- Response variables (they respond to a change in another variable)
- Outcome variables (they represent the outcome you want to measure)
- Left-hand-side variables (they appear on the left-hand side of a regression equation)
The dependent variable is what you record after you’ve manipulated the independent variable. You use this measurement data to check whether and to what extent your independent variable influences the dependent variable by conducting statistical analyses.
Based on your findings, you can estimate the degree to which your independent variable variation drives changes in your dependent variable. You can also predict how much your dependent variable will change as a result of variation in the independent variable.
Distinguishing between independent and dependent variables can be tricky when designing a complex study or reading an academic paper.
A dependent variable from one study can be the independent variable in another study, so it’s important to pay attention to research design.
Here are some tips for identifying each variable type.
Recognising independent variables
Use this list of questions to check whether you’re dealing with an independent variable:
- Is the variable manipulated, controlled, or used as a subject grouping method by the researcher?
- Does this variable come before the other variable in time?
- Is the researcher trying to understand whether or how this variable affects another variable?
Recognising dependent variables
Check whether you’re dealing with a dependent variable:
- Is this variable measured as an outcome of the study?
- Is this variable dependent on another variable in the study?
- Does this variable get measured only after other variables are altered?
Independent and dependent variables are generally used in experimental and quasi-experimental research.
Here are some examples of research questions and corresponding independent and dependent variables.
For experimental data, you analyse your results by generating descriptive statistics and visualising your findings. Then, you select an appropriate statistical test to test your hypothesis .
The type of test is determined by:
- Your variable types
- Level of measurement
- Number of independent variable levels
You’ll often use t tests or ANOVAs to analyse your data and answer your research questions.
In quantitative research , it’s good practice to use charts or graphs to visualise the results of studies. Generally, the independent variable goes on the x -axis (horizontal) and the dependent variable on the y -axis (vertical).
The type of visualisation you use depends on the variable types in your research questions:
- A bar chart is ideal when you have a categorical independent variable.
- A scatterplot or line graph is best when your independent and dependent variables are both quantitative.
To inspect your data, you place your independent variable of treatment level on the x -axis and the dependent variable of blood pressure on the y -axis.
You plot bars for each treatment group before and after the treatment to show the difference in blood pressure.
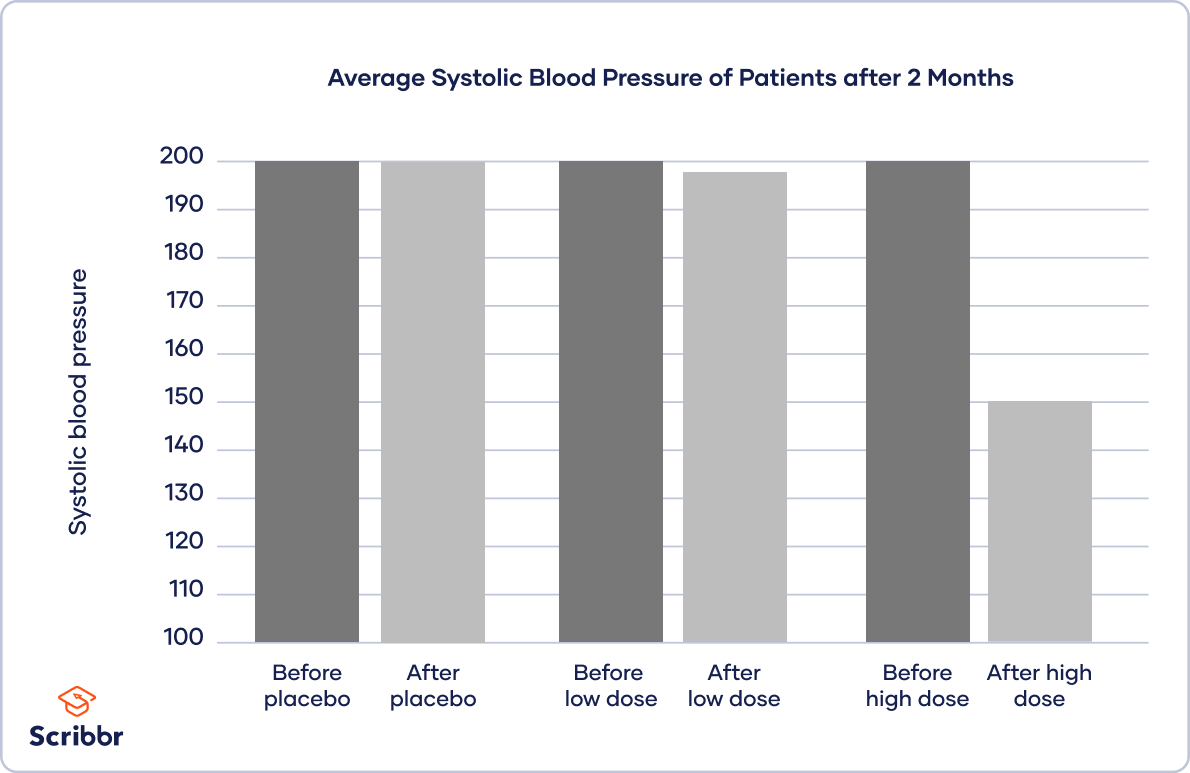
An independent variable is the variable you manipulate, control, or vary in an experimental study to explore its effects. It’s called ‘independent’ because it’s not influenced by any other variables in the study.
- Right-hand-side variables (they appear on the right-hand side of a regression equation)
A dependent variable is what changes as a result of the independent variable manipulation in experiments . It’s what you’re interested in measuring, and it ‘depends’ on your independent variable.
In statistics, dependent variables are also called:
Determining cause and effect is one of the most important parts of scientific research. It’s essential to know which is the cause – the independent variable – and which is the effect – the dependent variable.
You want to find out how blood sugar levels are affected by drinking diet cola and regular cola, so you conduct an experiment .
- The type of cola – diet or regular – is the independent variable .
- The level of blood sugar that you measure is the dependent variable – it changes depending on the type of cola.
Yes, but including more than one of either type requires multiple research questions .
For example, if you are interested in the effect of a diet on health, you can use multiple measures of health: blood sugar, blood pressure, weight, pulse, and many more. Each of these is its own dependent variable with its own research question.
You could also choose to look at the effect of exercise levels as well as diet, or even the additional effect of the two combined. Each of these is a separate independent variable .
To ensure the internal validity of an experiment , you should only change one independent variable at a time.
No. The value of a dependent variable depends on an independent variable, so a variable cannot be both independent and dependent at the same time. It must be either the cause or the effect, not both.
Cite this Scribbr article
If you want to cite this source, you can copy and paste the citation or click the ‘Cite this Scribbr article’ button to automatically add the citation to our free Reference Generator.
Bhandari, P. (2022, October 17). Independent vs Dependent Variables | Definition & Examples. Scribbr. Retrieved 9 April 2024, from https://www.scribbr.co.uk/research-methods/independent-vs-dependent-variables/
Is this article helpful?
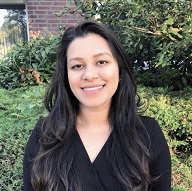
Pritha Bhandari
Other students also liked, a quick guide to experimental design | 5 steps & examples, quasi-experimental design | definition, types & examples, types of variables in research | definitions & examples.
- What is New
- Download Your Software
- Behavioral Research
- Software for Consumer Research
- Software for Human Factors R&D
- Request Live Demo
- Contact Sales
Sensor Hardware
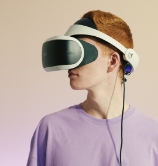
We carry a range of biosensors from the top hardware producers. All compatible with iMotions
iMotions for Higher Education
Imotions for business.
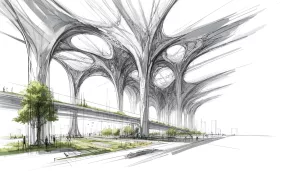
Neuroarchitecture: Designing Spaces with Our Brain in Mind
- Sensory and Perceptual
Morten Pedersen
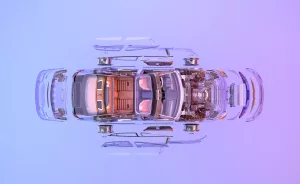
Human Factors in Automotive Human-Machine Interface (HMI) Design
Consumer Insights
News & Events
- iMotions Lab
- iMotions Online
- Eye Tracking
- Eye Tracking Screen Based
- Eye Tracking VR
- Eye Tracking Glasses
- FEA (Facial Expression Analysis)
- Voice Analysis
- EDA/GSR (Electrodermal Activity)
- EEG (Electroencephalography)
- ECG (Electrocardiography)
- EMG (Electromyography)
- Respiration
- iMotions Lab: New features
- iMotions Lab: Developers
- EEG sensors
- Consumer Inights
- Human Factors R&D
- Work Environments, Training and Safety
- Customer Stories
- Published Research Papers
- Document Library
- Customer Support Program
- Help Center
- Release Notes
- Contact Support
- Partnerships
- Mission Statement
- Ownership and Structure
- Executive Management
- Job Opportunities
Best Practice April 5, 2024
Roles of Independent and Dependent Variables in Research
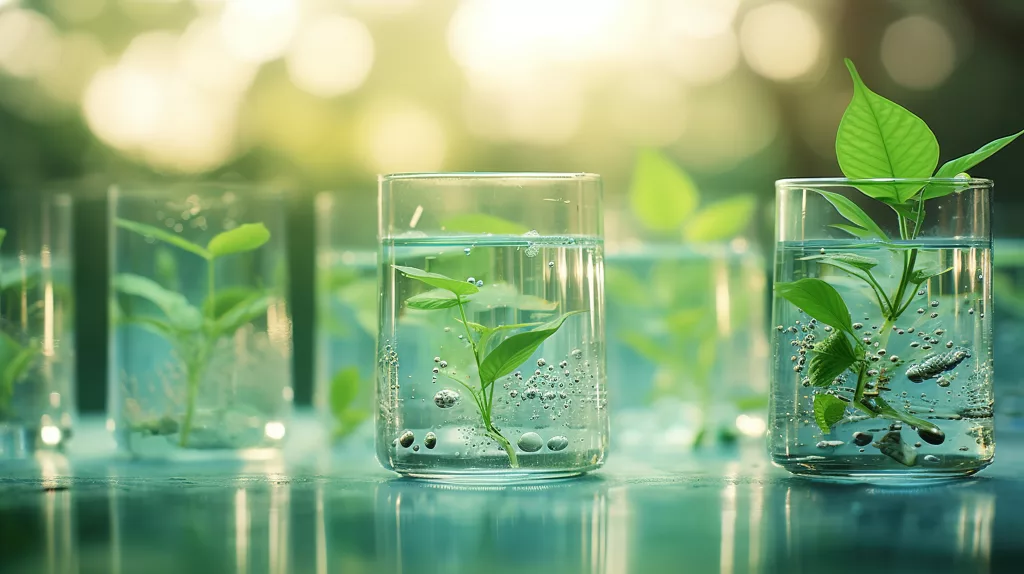
Explore the essential roles of independent and dependent variables in research. This guide delves into their definitions, significance in experiments, and their critical relationship. Learn how these variables are the foundation of research design, influencing hypothesis testing, theory development, and statistical analysis, empowering researchers to understand and predict outcomes of research studies.
Table of Contents
Introduction.
At the very base of scientific inquiry and research design , variables act as the fundamental steps, guiding the rhythm and direction of research. This is particularly true in human behavior research, where the quest to understand the complexities of human actions and reactions hinges on the meticulous manipulation and observation of these variables. At the heart of this endeavor lie two different types of variables, namely: independent and dependent variables, whose roles and interplay are critical in scientific discovery.
Understanding the distinction between independent and dependent variables is not merely an academic exercise; it is essential for anyone venturing into the field of research. This article aims to demystify these concepts, offering clarity on their definitions, roles, and the nuances of their relationship in the study of human behavior, and in science generally. We will cover hypothesis testing and theory development, illuminating how these variables serve as the cornerstone of experimental design and statistical analysis.
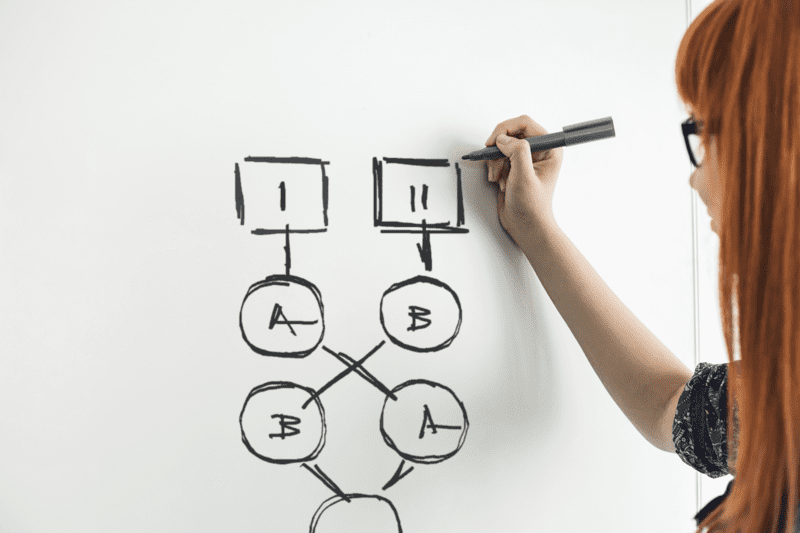
The significance of grasping the difference between independent and dependent variables extends beyond the confines of academia. It empowers researchers to design robust studies, enables critical evaluation of research findings, and fosters an appreciation for the complexity of human behavior research. As we delve into this exploration, our objective is clear: to equip readers with a deep understanding of these fundamental concepts, enhancing their ability to contribute to the ever-evolving field of human behavior research.
Chapter 1: The Role of Independent Variables in Human Behavior Research
In the realm of human behavior research, independent variables are the keystones around which studies are designed and hypotheses are tested. Independent variables are the factors or conditions that researchers manipulate or observe to examine their effects on dependent variables, which typically reflect aspects of human behavior or psychological phenomena. Understanding the role of independent variables is crucial for designing robust research methodologies, ensuring the reliability and validity of findings.
Defining Independent Variables
Independent variables are those variables that are changed or controlled in a scientific experiment to test the effects on dependent variables. In studies focusing on human behavior, these can range from psychological interventions (e.g., cognitive-behavioral therapy), environmental adjustments (e.g., noise levels, lighting, smells, etc), to societal factors (e.g., social media use). For example, in an experiment investigating the impact of sleep on cognitive performance, the amount of sleep participants receive is the independent variable.
Selection and Manipulation
Selecting an independent variable requires careful consideration of the research question and the theoretical framework guiding the study. Researchers must ensure that their chosen variable can be effectively, and consistently manipulated or measured and is ethically and practically feasible, particularly when dealing with human subjects.
Manipulating an independent variable involves creating different conditions (e.g., treatment vs. control groups) to observe how changes in the variable affect outcomes. For instance, researchers studying the effect of educational interventions on learning outcomes might vary the type of instructional material (digital vs. traditional) to assess differences in student performance.
Challenges in Human Behavior Research
Manipulating independent variables in human behavior research presents unique challenges. Ethical considerations are paramount, as interventions must not harm participants. For example, studies involving vulnerable populations or sensitive topics require rigorous ethical oversight to ensure that the manipulation of independent variables does not result in adverse effects.
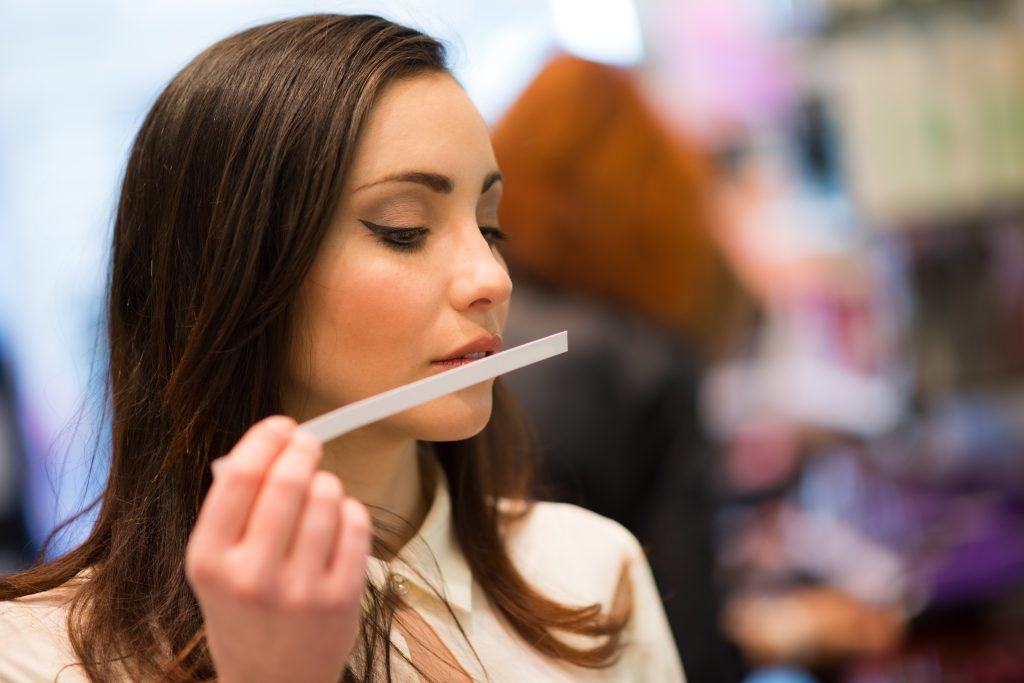
Practical limitations also come into play, such as controlling for extraneous variables that could influence the outcomes. In the aforementioned example of sleep and cognitive performance, factors like caffeine consumption or stress levels could confound the results. Researchers employ various methodological strategies, such as random assignment and controlled environments, to mitigate these influences.
Chapter 2: Dependent Variables: Measuring Human Behavior
The dependent variable in human behavior research acts as a mirror, reflecting the outcomes or effects resulting from variations in the independent variable. It is the aspect of human experience or behavior that researchers aim to understand, predict, or change through their studies. This section explores how dependent variables are measured, the significance of their accurate measurement, and the inherent challenges in capturing the complexities of human behavior.
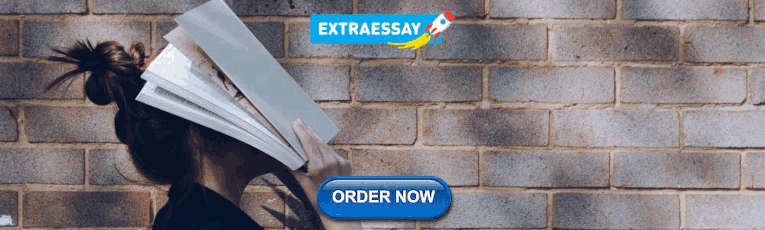
Defining Dependent Variables
Dependent variables are the responses or outcomes that researchers measure in an experiment, expecting them to vary as a direct result of changes in the independent variable. In the context of human behavior research, dependent variables could include measures of emotional well-being, cognitive performance, social interactions, or any other aspect of human behavior influenced by the experimental manipulation. For instance, in a study examining the effect of exercise on stress levels, stress level would be the dependent variable, measured through various psychological assessments or physiological markers.
Measurement Methods and Tools
Measuring dependent variables in human behavior research involves a diverse array of methodologies, ranging from self-reported questionnaires and interviews to physiological measurements and behavioral observations. The choice of measurement tool depends on the nature of the dependent variable and the objectives of the study.
- Self-reported Measures: Often used for assessing psychological states or subjective experiences, such as anxiety, satisfaction, or mood. These measures rely on participants’ introspection and honesty, posing challenges in terms of accuracy and bias.
- Behavioral Observations: Involve the direct observation and recording of participants’ behavior in natural or controlled settings. This method is used for behaviors that can be externally observed and quantified, such as social interactions or task performance.
- Physiological Measurements: Include the use of technology to measure physical responses that indicate psychological states, such as heart rate, cortisol levels, or brain activity. These measures can provide objective data about the physiological aspects of human behavior.
Reliability and Validity
The reliability and validity of the measurement of dependent variables are critical to the integrity of human behavior research.
- Reliability refers to the consistency of a measure; a reliable tool yields similar results under consistent conditions.
- Validity pertains to the accuracy of the measure; a valid tool accurately reflects the concept it aims to measure.
Ensuring reliability and validity often involves the use of established measurement instruments with proven track records, pilot testing new instruments, and applying rigorous statistical analyses to evaluate measurement properties.
Challenges in Measuring Human Behavior
Measuring human behavior presents challenges due to its complexity and the influence of multiple, often interrelated, variables. Researchers must contend with issues such as participant bias, environmental influences, and the subjective nature of many psychological constructs. Additionally, the dynamic nature of human behavior means that it can change over time, necessitating careful consideration of when and how measurements are taken.
Section 3: Relationship between Independent and Dependent Variables
Understanding the relationship between independent and dependent variables is at the core of research in human behavior. This relationship is what researchers aim to elucidate, whether they seek to explain, predict, or influence human actions and psychological states. This section explores the nature of this relationship, the means by which it is analyzed, and common misconceptions that may arise.
The Nature of the Relationship
The relationship between independent and dependent variables can manifest in various forms—direct, indirect, linear, nonlinear, and may be moderated or mediated by other variables. At its most basic, this relationship is often conceptualized as cause and effect: the independent variable (the cause) influences the dependent variable (the effect). For instance, increased physical activity (independent variable) may lead to decreased stress levels (dependent variable).
Analyzing the Relationship
Statistical analyses play a pivotal role in examining the relationship between independent and dependent variables. Techniques vary depending on the nature of the variables and the research design, ranging from simple correlation and regression analyses for quantifying the strength and form of relationships, to complex multivariate analyses for exploring relationships among multiple variables simultaneously.
- Correlation Analysis : Used to determine the degree to which two variables are related. However, it’s crucial to note that correlation does not imply causation.
- Regression Analysis : Goes a step further by not only assessing the strength of the relationship but also predicting the value of the dependent variable based on the independent variable.
- Experimental Design : Provides a more robust framework for inferring causality, where manipulation of the independent variable and control of confounding factors allow researchers to directly observe the impact on the dependent variable.
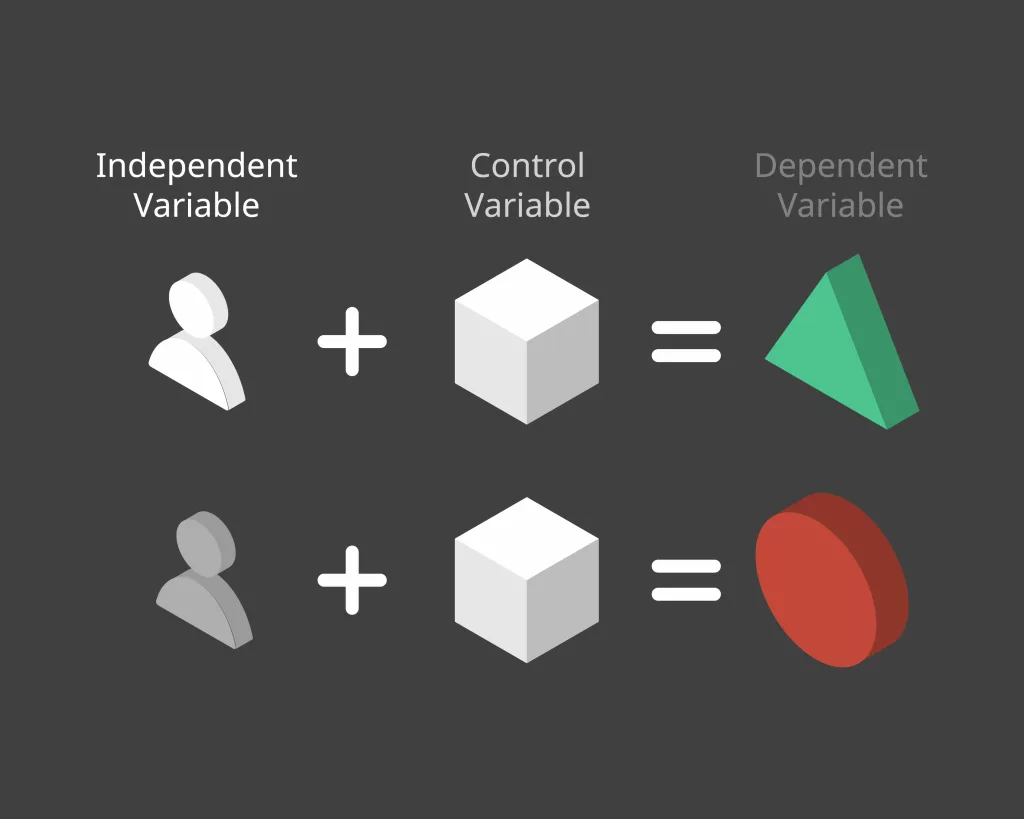
Causality vs. Correlation
A fundamental consideration in human behavior research is the distinction between causality and correlation. Causality implies that changes in the independent variable cause changes in the dependent variable. Correlation, on the other hand, indicates that two variables are related but does not establish a cause-effect relationship. Confounding variables may influence both, creating the appearance of a direct relationship where none exists. Understanding this distinction is crucial for accurate interpretation of research findings.
Common Misinterpretations
The complexity of human behavior and the myriad factors that influence it often lead to challenges in interpreting the relationship between independent and dependent variables. Researchers must be wary of:
- Overestimating the strength of causal relationships based on correlational data.
- Ignoring potential confounding variables that may influence the observed relationship.
- Assuming the directionality of the relationship without adequate evidence.
This exploration highlights the importance of understanding independent and dependent variables in human behavior research. Independent variables act as the initiating factors in experiments, influencing the observed behaviors, while dependent variables reflect the results of these influences, providing insights into human emotions and actions.
Ethical and practical challenges arise, especially in experiments involving human participants, necessitating careful consideration to respect participants’ well-being. The measurement of these variables is critical for testing theories and validating hypotheses, with their relationship offering potential insights into causality and correlation within human behavior.
Rigorous statistical analysis and cautious interpretation of findings are essential to avoid misconceptions. Overall, the study of these variables is fundamental to advancing human behavior research, guiding researchers towards deeper understanding and potential interventions to improve the human condition.
Free 44-page Experimental Design Guide
For Beginners and Intermediates
- Introduction to experimental methods
- Respondent management with groups and populations
- How to set up stimulus selection and arrangement
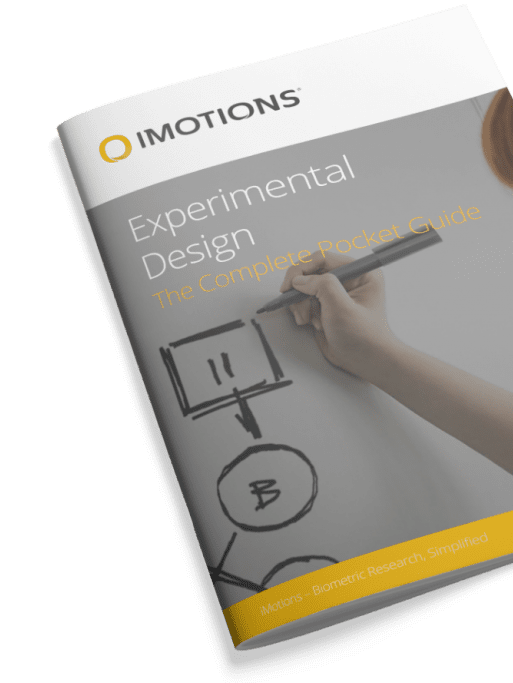
Last edited
About the author
See what is next in human behavior research
Follow our newsletter to get the latest insights and events send to your inbox.
Related Posts
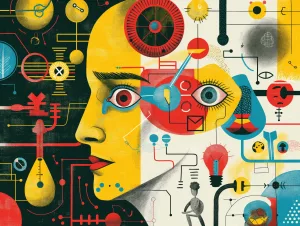
Scientific Method
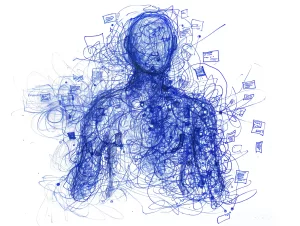
Mixed Methods Research
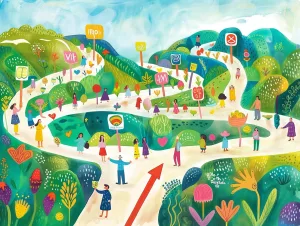
Introduction to Nudge Theory
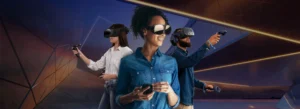
Can you use HTC VIVE Pro Eye for eye tracking research?
Research insights.
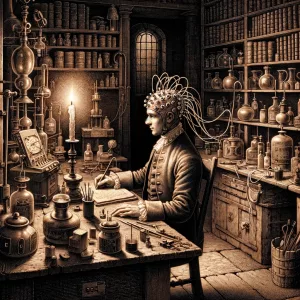
The History of EEG
Bryn Farnsworth
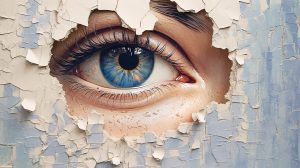
Emotion Detection Software: A Comprehensive Guide by iMotions
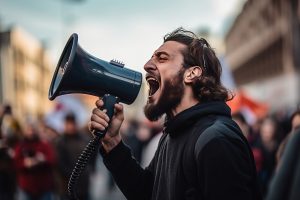
Understanding Emotional Prosody: Unveiling Human Emotion in Speech
Publications.
Case Stories
Explore Blog Categories
Best Practice
Collaboration, product guides, product news, research fundamentals.
Read publications made possible with iMotions
See Publications
Get inspired and learn more from our expert content writers
A monthly close up of latest product and research news
🍪 Use of cookies
We are committed to protecting your privacy and only use cookies to improve the user experience.
Chose which third-party services that you will allow to drop cookies. You can always change your cookie settings via the Cookie Settings link in the footer of the website. For more information read our Privacy Policy.
- gtag This tag is from Google and is used to associate user actions with Google Ad campaigns to measure their effectiveness. Enabling this will load the gtag and allow for the website to share information with Google.
- Livechat Livechat provides you with direct access to the experts in our office. The service tracks visitors to the website but does not store any information unless consent is given. This service is essential and can not be disabled.
- Pardot Collects information such as the IP address, browser type, and referring URL. This information is used to create reports on website traffic and track the effectiveness of marketing campaigns.
- Third-party iFrames Allows you to see thirdparty iFrames.
Dependent Variable Definition and Examples
- Chemical Laws
- Periodic Table
- Projects & Experiments
- Scientific Method
- Biochemistry
- Physical Chemistry
- Medical Chemistry
- Chemistry In Everyday Life
- Famous Chemists
- Activities for Kids
- Abbreviations & Acronyms
- Weather & Climate
- Ph.D., Biomedical Sciences, University of Tennessee at Knoxville
- B.A., Physics and Mathematics, Hastings College
A dependent variable is the variable being tested in a scientific experiment.
The dependent variable is "dependent" on the independent variable . As the experimenter changes the independent variable, the change in the dependent variable is observed and recorded. When you take data in an experiment, the dependent variable is the one being measured.
Common Misspellings: dependant variable
Dependent Variable Examples
- A scientist is testing the effect of light and dark on the behavior of moths by turning a light on and off. The independent variable is the amount of light and the moth's reaction is the dependent variable . A change in the independent variable (amount of light) directly causes a change in the dependent variable (moth behavior).
- You are interested in learning which kind of chicken produces the largest eggs. The size of the eggs depends on the breed of chicken, so breed is the independent variable and egg size is the dependent variable.
- You want to know whether or not stress affects heart rate. Your independent variable is the stress, while the dependent variable would be the heart rate. To perform an experiment, you would provide stress and measure the subject's heartbeat. Note that in a good experiment, you'd want to choose a stress you could control and quantify. Your choice could lead you to perform additional experiments since it might turn out the change in heart rate after exposure to a decrease in temperature 40 degrees (physical stress) might be different from the heart rate after failing a test (psychological stress). Even though your independent variable might be a number that you measure, it's one you control, so it's not "dependent".
Distinguishing Between Dependent and Independent Variables
Sometimes it's easy to tell the two types of variables apart , but if you get confused, here are tips to help keep them straight:
- If you change one variable, which is affected? If you're studying the rate of growth of plants using different fertilizers, can you identify the variables? Start by thinking about what you are controlling and what you will be measuring. The type of fertilizer is the independent variable. The rate of growth is the dependent variable. So, to perform an experiment, you would fertilize plants with one fertilizer and measure the change in height of the plant over time, then switch fertilizers and measure the height of plants over the same span of time. You might be tempted to identify time or height as your variable, not the rate of growth (distance per time). It may help to look at your hypothesis or purpose to remember your goal.
- Write out your variables as a sentence stating cause and effect. The (independent variable) causes a change in the (dependent variable). Usually, the sentence won't make sense if you get them wrong. For example: (Taking vitamins) affects the numbers of (birth defects). = makes sense (Birth defects) affects the number of (vitamins). = probably not so much
Graphing the Dependent Variable
When you graph data, the independent variable is on the x-axis, while the dependent variable is on the y-axis. You can use the DRY MIX acronym to remember this:
D - dependent variable R - responds to change Y - Y-axis
M - manipulated variable (one you change) I - independent variable X - X-axis
- Scientific Variable
- What Are Independent and Dependent Variables?
- What Are the Elements of a Good Hypothesis?
- What Is an Experiment? Definition and Design
- Six Steps of the Scientific Method
- What Is a Control Group?
- Understanding Simple vs Controlled Experiments
- How To Design a Science Fair Experiment
- Scientific Method Vocabulary Terms
- Linear Regression Analysis
- The Significance of Negative Slope
- What Is a Hypothesis? (Science)
- How Intervening Variables Work in Sociology
- Null Hypothesis Definition and Examples
- What Is a Dependent Variable?
College & Research Libraries ( C&RL ) is the official, bi-monthly, online-only scholarly research journal of the Association of College & Research Libraries, a division of the American Library Association.
C&RL is now on Instragram! Follow us today.
William H. Walters is Executive Director of the Library at Manhattan College; email: [email protected] .

C&RL News
ALA JobLIST
Advertising Information
- Research is an Activity and a Subject of Study: A Proposed Metaconcept and Its Practical Application (71142 views)
- Information Code-Switching: A Study of Language Preferences in Academic Libraries (38511 views)
- Three Perspectives on Information Literacy in Academia: Talking to Librarians, Faculty, and Students (27076 views)
Apportioning the Cost of a Full-Text Database Among the Journals in the Database: A Comparison of Six Methods
William H. Walters *
Estimates of the price or value of the individual journals within a full-text database may be useful to librarians engaged in serials reviews or other collection development projects, to scholars investigating the determinants of journal prices, and to publishers seeking to rationalize their pricing strategies. This paper evaluates six methods of apportioning the cost of a full-text database among the individual journals in the database—methods based on variables such as journal size, total citations, Journal Impact Factor (JIF) percentile, and single-journal list price. Each method is evaluated based on how well the resulting prices can be predicted by the determinants of journal prices identified in previous research. Although the six methods yield similar results, the single best option is to use price estimates that account for JIF percentile. If citation data are not available and cannot be estimated, the best alternative is to rely on the equal-value assumption—to split the total price equally among the wanted journals in the database.
Introduction
Although nearly 20 studies have examined the determinants of scholarly journal prices since 1989, virtually all of them have focused exclusively on the prices of single-journal subscriptions. 1 The single-journal approach to price analysis remains common even today, when academic libraries acquire most of their journals through full-text databases. 2
Just a few large-scale price studies have accounted for the journals available through online databases or collections. One approach to evaluating the cost of these journals is to treat each database as an indivisible entity, calculating statistics such as price per article and price per citation for each database. 3 A second approach is to estimate the cost of each individual journal by apportioning the total database price among the journals in the database. 4 The first approach has the advantage of relying on authoritative data; no price estimation is required. However, the second approach may be more useful when the goal is to evaluate journal-specific determinants of price (e.g., subject area and scholarly reputation) or when the prices of individual journals are required for library collection development decisions—when determining whether to bundle or unbundle subscriptions to individual journals and full-text collections, for instance. 5
When estimates of individual journal prices are required, the total cost of each full-text database must be apportioned among the journals in the database. This can be done on the basis of
- The equal-value assumption (total cost split equally among the wanted journals in the database)
- Journal size (articles per year)
- Total citations for the journal as a whole
- Journal Impact Factor (JIF) percentile (average citations per article)
- Single-journal list price, representing the publisher’s own assessment of the journal’s relative value
- A composite indicator that accounts for variables 2–5.
There are other possibilities, of course, but these are the journal-level variables identified in previous research as the most consistent correlates of journal prices. 6
This paper first estimates journal prices based on each of the six criteria. Each price variable is then used as the dependent variable in a regression with independent variables representing resource provider type (scholarly society, university, other non-profit, commercial publisher, or library vendor), subject field (engineering, physical sciences, life sciences, business, social sciences, or education), publisher size, JIF percentile, and journal size. The study evaluates one primary research question: Which method of estimating prices results in a dependent variable that is most fully explained by the combination of independent variables? That is, which method results in the highest R 2 value? The assumption is that an effective method of estimating price is one for which variations in price are (a) systematic rather than random, and (b) closely linked to the variables that might reasonably be expected to contribute to variations in price.
A secondary question is whether the results support or challenge an earlier finding—that for a typical U.S. master’s university, the journals available through commercial publishers’ databases cost substantially less than those available through the databases of non-profit publishers and library vendors. Previous research shows that while commercial databases are especially expensive for the major research universities, they are especially inexpensive for American bachelor’s and master’s universities. 7 This study investigates whether the same finding can be seen when several different methods of price estimation are used.
The data used in this analysis were compiled for a recent Manhattan College serials review. Specifically, we attempted to acquire 2,717 wanted journals —those identified by the faculty as the most important titles for their teaching and research—while minimizing cost per wanted journal. Manhattan College, a 4,000-student university in the Bronx, offers bachelor’s and master’s degrees in engineering, business, arts and sciences, education, health, and professional studies. The college is typical of U.S. universities in the Carnegie master’s—larger category except for the size of its engineering school, which accounts for 30% of undergraduate students.
The price data used here are actual 2019 or 2020 invoice prices (or, in some cases, price quotes) obtained by Manhattan College for the 236 full-text databases considered as possible means of gaining access to the 2,717 wanted journals. Unlike list prices, they represent the amounts actually paid or payable. The details of the data compilation process are described in an earlier study. 8
Journals in the arts and humanities (A&H) were excluded from the study due to data limitations—specifically, because citation data were unavailable for a relatively high proportion of those journals. That is, the A&H journals selected by the faculty include quite a few that are not indexed in Web of Science. Open Access (OA) journals were also excluded from the analysis since they are freely accessible without a subscription. Consequently, number of wanted journals in the database refers to the number of wanted journals that are neither A&H nor OA. Likewise, total database price refers to the total price times the proportion of journals in the database that are neither A&H nor OA.
It is important to keep in mind that any one journal may be acquired through several different subscriptions or databases. Consequently, price is an attribute not of a particular journal, but of a particular acquisition opportunity . 9 To gain current access to Northeastern Naturalist , for instance, a library might choose a single-journal subscription from the publisher or subscribe to any of 13 full-text databases offered by BioOne, EBSCO, or ProQuest. That’s 14 acquisition opportunities with annual prices ranging from $105 to $545. The data file for this investigation has 4,529 cases that correspond to 4,529 acquisition opportunities—4,529 instances in which a particular wanted journal was included in a particular full-text database. For each case, there are 6 dependent variables (price estimates) and 14 independent variables that represent 5 constructs: resource provider type, subject field, publisher size, JIF percentile, and journal size.
Price Estimates (Dependent Variables)
Five of the six price estimates—all but the composite indicator—were calculated using similar methods.
- For price (equal value) , the total database price was split equally among the wanted journals in the database. This calculation is based on the assumption that the value of each journal (relative to that of the other journals in the same database) does not vary systematically on the basis of size, scholarly impact, or list price.
- For price (journal size) , each wanted journal was assigned a value equal to the total database price times the proportion of the wanted-journal articles in the database that appeared in the journal. ( Wanted-journal articles are simply articles that appeared in the wanted journals. No differentiation between wanted and not wanted status was made at the article level.) This calculation is based on the assumption that price is determined mainly by the number of articles in each journal—specifically, the number of Web of Science citable items published in 2019. Citable items include empirical articles, review articles, research notes, and other substantive contributions but not items such as announcements, editorials, and letters to the editor.
- For price (total citations) , each wanted journal was assigned a value equal to the total database price times the proportion of the database’s wanted-journal citation total (number of citing articles) that could be attributed to the journal. With this variable, price is proportional to the number of times the journal (all articles combined) was cited in 2019. 10
- For price (JIF percentile) , each wanted journal’s 2019 Impact Factor was first expressed as the average of the journal’s percentile ranks in all the Web of Science subject categories in which the journal was classified. Each journal was then assigned a value equal to the total database price times the proportion of the database’s wanted-journal percentile-rank total that could be attributed to the journal. Price (JIF percentile) is based on the assumption that price is proportional to the average number of times each article in the journal was cited in 2019. It is therefore different from price (total citations) in two important ways. First, it makes use of data on average citations per article rather than total citations per journal; it is therefore not influenced by the number of articles published in the journal. Second, it is based on percentile ranks rather than raw scores; it represents each journal’s impact relative to that of the other journals in the same subject category. With price (JIF percentile) , a top-tier political science journal is assigned the same price as a top-tier biochemistry journal in the same database. This method disregards the fact that the average citation rate is higher in biochemistry than in political science.
- For price (single-journal price) , each wanted journal was assigned a value equal to the total database price times the proportion of the database’s single-journal list price total (the sum of the single-journal list prices of the wanted journals) that could be attributed to the journal. This calculation assumes that the publishers themselves have a good idea of the value of each of their journals, and that their assessments of value are incorporated into the journals’ list prices. Price (single-journal price) is consistently lower than actual list price, but proportional to it. With just a few exceptions, the single-journal list prices used in this analysis are 2019 or 2020 prices from EBSCO or from the publishers’ web sites.
- A different method was used to arrive at price (composite) , a composite indicator that incorporates dependent variables 2–5, above. First, unweighted least squares extraction—the initial step in factor analysis—was used to calculate communality values, which represent the extent to which each price variable contributes to the shared variance within the set of four variables (i.e., the extent to which each variable can be represented by the other three). 11 Communalities of 0.89, 0.76, 0.67, and 0.72 were obtained for variables 2–5, respectively, revealing that price (journal size) best captures the variance common to the set of four variables. Because the eigenvalues of the extracted factors showed that all four variables could be represented well by a single composite indicator, a composite score for each journal was calculated as the sum of the four ( communality * estimated price ) values. That is, each of the four component variables was weighted in proportion to its contribution to the shared variance. 12 Finally, each wanted journal was assigned an estimated price equal to the total database price times the proportion of the database’s composite-score total that could be attributed to the journal.
Three of the six price estimates require the use of citation data. Because the A&H journals—those most likely to have missing values for the citation variables—were excluded from the analysis, just 5.7% of the remaining 4,529 cases have one or more missing values. For those cases, total citations and JIF percentile were estimated. 13
The correlations among the six price variables are shown in Table 1. As described earlier, each price variable was used as the dependent variable in a regression that included the independent variables identified in earlier research as effective predictors of journal prices. (See below.) The dependent variables were entered in natural log form in order to maintain linearity.
Correlates of Price (Independent Variables)
All six regressions used the same set of independent variables:
- Resource provider type (five categories): scholarly society, university, other non-profit, commercial publisher, or library vendor. The resource provider is almost always the publisher, except for the databases provided by library vendors such as EBSCO and ProQuest. The university category includes both university presses and academic departments/centers.
- Subject field (six categories): engineering, physical sciences, life sciences, business, social sciences, or education, based on the Manhattan College department(s) that identified the journal as a wanted journal. Because some journals were wanted by more than one department, about 10% of the journals have more than one subject designation.
- Publisher size: number of wanted journals published by the publisher (not always the resource provider), including those of subsidiary imprints.
- JIF percentile: 2019 JIF, expressed as a percentile within the relevant Web of Science subject category. If the journal appeared in multiple subject categories, the percentile scores were averaged. Because JIF is independent of journal size, it represents the average citation impact of an article in the journal rather than the impact of the journal as a whole.
- Journal size: number of citable items published in 2019.
Although two of the independent variables were used in the construction of the dependent variables, this is not a problem, since the dependent and independent variables do not represent the same constructs. Moreover, because characteristics not represented within the set of independent variables (e.g., total database price and the number of wanted journals) figure heavily in each price estimate, the correlations between the dependent variables and the independent variables are modest. The correlation between price (journal size) and journal size is 0.27, for instance, indicating that just 7% of the variation in price (journal size) can be explained by journal size (r 2 = 0.07). Likewise, the correlation between price (JIF percentile) and JIF percentile is just 0.17 (r 2 = 0.03).
Results and Discussion
The independent variables, taken together, are more closely associated with some price estimates than with others (Table 2). The highest R 2 value is that for price (JIF percentile) . This indicates that the independent variables are most effective at explaining variations in price when the total database price is allocated among the wanted journals based on the average citation impact of an article in each journal (JIF), expressed as a percentile score (i.e., relative to the other journals in the same Web of Science subject category). If we want the price variable that is most sensitive to the characteristics that might reasonably be expected to influence price, then price (JIF percentile) is the best of the options shown in Table 2.
If price estimates are needed for journals for which citation data are unavailable, then price (equal value) is a good alternative to price (JIF percentile) . As noted earlier, three of the six price estimation methods require actual or estimated citation data for every journal. For journals not included in Web of Science, three options are available: (1) use a price estimation method that does not rely on citation data, such as the equal-value method; (2) use a data source that includes citation data for a broader range of journals (e.g., Scopus rather than Web of Science, and CiteScore rather than JIF); or (3) estimate the citation values for the journals with missing data before calculating price estimates. Fortunately, the regression results suggest that the first of these options is entirely reasonable. Based on the R 2 and SEE values shown in Table 2, the equal-value method is a good alternative to the JIF percentile method. Moreover, the two methods result in price estimates that are very closely related (r = 0.92; see Table 1).
Comparing the Results for Particular Price Variables
The fact that price (JIF percentile) has a higher R 2 value than price (equal value) , price (composite) , and price (total citations) is surprising for at least two reasons. First, we might expect a higher R 2 value for the composite indicator since it incorporates the shared variance common to all four of its component variables. In fact, however, the composite indicator produces less satisfactory results than either price (JIF percentile) or price (equal value) .
Second, we might expect a higher R 2 value for price (total citations ) than for price (JIF percentile) since total citations represents the scholarly impact of the journal as a whole rather than the average impact of a single article in the journal. For instance, if there are two journals with equal JIF percentile scores but one publishes twice as many articles as the other, price (total citations) will account for the difference in journal size while price (JIF percentile) will not. One explanation for the lower R 2 value for price (total citations) is that the price or value of a journal is not closely related to the number of articles it publishes. This first explanation is not unreasonable, especially considering the relatively low R 2 value associated with price (journal size) .
There is a second and perhaps more likely possibility, however; the high R 2 value for price (JIF percentile) may be related to the use of percentile scores. If this is the case, it suggests that the price of a journal is tied to its relative standing within its subject area—not to its actual citation rate—and that we ought to use percentile scores to account for the differences in average citation rates across disciplines. A price variable based on JIF raw scores can be used to test this assertion. If the assertion is valid, then price (JIF raw score) will have a lower R 2 value than price (JIF percentile) —and it does. A regression with price (JIF raw score) as the dependent variable results in a low R 2 value (0.22) and an error (SEE) value of 3.12, far higher than any of the values shown in Table 2. We can therefore conclude that price (JIF percentile) is probably effective due to the use of percentile scores rather than actual JIF values. 14
As Table 2 shows, price (single-journal price) and price (journal size) are associated with the lowest R 2 values. Notably, the price variable with the most shared variance, price (journal size) , has the lowest R 2 value of all. Conversely, the price variable with the least shared variance, price (JIF percentile) , yields the highest R 2 value. The reasons for this are not clear. These results do suggest two related findings, however. First, combining multiple dimensions of price into a single variable (the composite variable) does not increase the extent to which the estimated prices can be explained by the independent variables in the regression. Second, the price estimates that can be predicted most effectively are not necessarily those with the most shared variance.
Correlates of Price
Because the dependent variables were entered in natural log form, the unstandardized regression ( B ) coefficients cannot be interpreted as dollar amounts. Table 3 shows the effect coefficients , which are more intuitively meaningful. Each represents the percentage change in price associated with a one-unit change in the independent variable—or, for categorical variables, the percentage change in price associated with inclusion in the indicated category rather than the reference category. (The complete regression results can be found in the Appendix.)
As Table 3 reveals, the results for resource provider type are similar across all six regressions. Moreover, all six confirm earlier reports that for a typical master’s university, the journals available through commercial publishers’ databases cost less, all else equal, than those available through the databases of library vendors and nonprofit providers. 15 The publisher-type differentials do vary in magnitude, however. All else equal, the journals acquired from scholarly societies may cost from 128% to 319% more than those acquired from commercial publishers, depending on which price variable is used.
Earlier investigations also identified two subject variables, life sciences and physical sciences , as important determinants of journal prices. Those same findings can be seen in Table 3. The very modest effects of publisher size, JIF percentile, and journal size are also consistent with previous research. 16
Because there is no definitive way to determine the correct market price of each journal included in a full-text database, the results presented here cannot be regarded as authoritative. If there is a strong theoretical or methodological reason for estimating prices based on a particular construct, such as journal size or single-journal list price, then that construct should determine the method by which prices are estimated.
In the absence of a strong rationale for a particular price estimation method, however, it seems reasonable to use price estimates that make intuitive sense—estimates that can be explained in terms of the variables most consistently associated with price. By that criterion, the best approach is to use the JIF percentile method described here—to apportion the total database price in accordance with the JIF percentile scores of the wanted journals included in the database. If citation data are unavailable, then price (equal value) is a good alternative to price (JIF percentile) .
The results for all six price variables are consistent with earlier reports that for a typical master’s university, the journals acquired through commercial publishers’ databases cost less than those acquired through the databases of scholarly societies, universities, other non-profits, and library vendors.
Application of These Findings
There are several contexts in which the findings of this investigation may be useful. First, recent studies suggest that the acquisition of full-text journal resources for library collections should involve two separate steps: (1) the selection of individual journals on a title-by-title basis and (2) the identification of the full-text databases that can provide access to those journals in the most cost-effective way. 17 If the serials review or evaluation procedure requires price estimates for every acquisition opportunity—every wanted journal within each full-text database—then a defensible method of apportioning database prices among journals will be needed.
Second, scholarly investigations of the determinants of journal prices are also likely to require the allocation of total database cost among the journals in each database. Some determinants of price (e.g., publisher’s market share and for-profit/non-profit status) are attributes of particular publishers or databases rather than individual journals, while others (e.g., subject area and scholarly reputation) are specific to each journal and therefore require the estimation of prices for individual acquisition opportunities. Recent journal price studies have relied on price (equal value) and price (journal size) , 18 but this investigation shows that at least one indicator, price (JIF percentile) , is likely to be a better choice.
Third, publishers and library vendors may find it useful to disaggregate database prices in order to assess their own pricing strategies, to identify anomalies in the list prices of particular journals, or to demonstrate to libraries that their products are cost-effective—to show, for instance, that their own journals are a good value in comparison with similar titles from other vendors. Because single-journal subscriptions account for relatively few of the titles held by libraries, 19 the most meaningful comparisons involve not single-journal prices, but the prices that would be paid if each journal were acquired through the most cost-effective full-text database offered by the vendor or publisher.
Further Research
Further research using data for a range of institutions might help extend or clarify the findings presented here. Nonetheless, these results, based on Manhattan College price data, are likely to be useful to other universities as well. For one thing, Manhattan College is typical of many U.S. bachelor’s and master’s institutions with regard to its size, mission, reputation, selectivity, student characteristics, teaching/research focus, and library budget. The curriculum is not unusual except for the size of the engineering program, and the wanted journals selected by the faculty include nearly all the high-impact journals in the subjects typically taught at U.S. undergraduate colleges. 20 Moreover, most of the library’s journal budget is devoted to resources acquired through WALDO and LYRASIS, two of the largest library consortia in the United States. The consortial price schedules that apply to Manhattan College also apply to more than 1,400 other member libraries.
Research on journal prices would also benefit from greater transparency and more widespread dissemination of price information. Even today, many investigations rely on list prices, which often bear little relationship to the prices actually paid by libraries. A broader, and perhaps insurmountable, challenge lies in the disconnect between the end user’s desire for particular scholarly works and the publisher’s (and librarian’s) focus on information products . While researchers need access to particular journals—or, more accurately, particular articles—publishers and librarians tend to think of cost or revenue in terms of the journal databases or packages that are marketed and acquired as indivisible units. The main analytical problem stems not from the sale or acquisition of full-text databases, but from the fact that their associated costs cannot be readily disaggregated. As long as this remains true, price estimation methods such as those described here are likely to remain useful despite their limitations.
Each of the six price variables was used as the dependent variable in a separate regression (Tables A1–A6). B is the unstandardized regression coefficient, Beta is the standardized coefficient, n = 4,529, and the significance levels are two-tailed. Each effect coefficient is equal to (exp( B )–1) * 100. Commercial publisher and social sciences are the reference categories for resource provider type and subject field.
1. William H. Walters, “Can Differences in Publisher Size Account for the Relatively Low Prices of the Journals Available to Master’s Universities Through Commercial Publishers’ Databases? The Importance of Price Discrimination and Substitution Effects,” Scientometrics 127 (Feb. 2022): 1065–97, https://doi.org/10.1007/s11192-021-04205-5 .
2. Stephen Bosch, Barbara Albee, and Sion Romaine, “The New Abnormal: Periodicals Price Survey 2021,” Library Journal 146 (Apr. 27, 2021): 20–25, https://www.libraryjournal.com/story/The-New-Abnormal-Periodicals-Price-Survey-2021 ; Oliver T. Coomes, Tim R. Moore, and Sébastien Breau, “The Price of Journals in Geography,” The Professional Geographer 69 (2017): 251–62, https://doi.org/10.1080/00330124.2016.1229624 ; Rob Johnson, Anthony Watkinson, and Michael Mabe, The STM Report: An Overview of Scientific and Scholarly Publishing , 5th ed. (The Hague: STM: International Association of Scientific, Technical, and Medical Publishers, 2018), https://www.stm-assoc.org/2018_10_04_STM_Report_2018.pdf ; Lewis G. Liu and Harold Gee, “Determining Whether Commercial Publishers Overcharge Libraries for Scholarly Journals in the Fields of Science, Technology, and Medicine, with a Semilogarithmic Econometric Model,” Library Quarterly 87 (Apr. 2017): 150–72, https://doi.org/10.1086/690736 ; Karla L. Strieb and Julia C. Blixrud, “Unwrapping the Bundle: An Examination of Research Libraries and the “Big Deal,” Portal: Libraries and the Academy 14 (Oct. 2014): 587–615, https://doi.org/10.1353/pla.2014.0027 .
3. Theodore C. Bergstrom, Paul N. Courant, R. Preston McAfee, and Michael A. Williams, “Evaluating Big Deal Journal Bundles,” PNAS 111 (June 16, 2014): 9425–30, https://doi.org/10.1073/pnas.1403006111 .
4. Walters, “Can Differences in Publisher Size”; William H. Walters and Susanne Markgren, “Comparing the Prices of Commercial and Nonprofit Journals: A Realistic Assessment,” portal: Libraries and the Academy 21 (Apr. 2021): 389–410, http://doi.org/10.1353/pla.2021.0021 .
5. Khue Duong, Carol Perruso, and Hema Ramachandran, “Content Overlap and Replacement Cost Analyses: Tools to Evaluate Abstracting/Indexing (A&I) and Full-Text Databases in Science and Engineering,” Science & Technology Libraries 32 (2013): 84–94, https://doi.org/10.1080/0194262X.2012.758461 ; Asen O. Ivanov, Catherine Anne Johnson, and Samuel Cassady, “Unbundling Practice: The Unbundling of Big Deal Journal Packages as an Information Practice,” Journal of Documentation 76 (2020): 1051–67, https://doi.org/10.1108/JD-09-2019-0187 ; Elizabeth Parang and Jeremy Whitt, “When to Hold Them, When to Fold Them: Reassessing “Big Deals” in 2020,” The Serials Librarian 80 (2021): 147–52, https://doi.org/10.1080/0361526X.2021.1877083 .
6. Walters, “Can Differences in Publisher Size.”
7. See Bergstrom et al., “Evaluating Big Deal Journal Bundles,” appendix table SI 17; Walters, “Can Differences in Publisher Size”; Walters and Markgren, “Comparing the Prices of Commercial and Nonprofit Journals.” Although most journal price studies have reported that commercially published journals are more expensive than those of non-profit publishers, these three papers are more realistic than the others in several respects: (a) unlike other journal price studies, they present data for all types of U.S. colleges and universities rather than just the major research universities; (b) they include only the journals that have met libraries’ selection criteria—the journals that faculty and librarians actually want for their collections; (c) they evaluate not just single-journal subscriptions, but the acquisition opportunities available through full-text databases and other online resources; and (d) they are based not on list prices, but on the prices actually paid by academic libraries—the prices negotiated by library consortia, university systems, and individual institutions.
8. William H. Walters and Susanne Markgren, “Zero-Based Serials Review: An Objective, Comprehensive Method of Selecting Full-Text Journal Resources in Response to Local Needs,” Journal of Academic Librarianship 46 (Sept. 2020), article 102189, https://doi.org/10.1016/j.acalib.2020.102189 ; William H. Walters and Susanne Markgren, “A Two-Stage Approach to Serials Review: Minimizing Journal Costs Through Title-by-Title Selection with Package-Based Acquisition,” Insights: The UKSG Journal 34 (July 21, 2021a): article 18, http://doi.org/10.1629/uksg.550 .
9. Walters and Markgren, “Zero-Based Serials Review.”
10. The data on journal size, total citations, and JIF percentile are all 2019 data from Clarivate Analytics, Journal Citation Reports (London: Clarivate Analytics, 2019).
11. Leandre R. Fabrigar and Duane T. Wegener, Exploratory Factor Analysis (New York: Oxford University Press, 2012); Jae-On Kim and Charles W. Mueller, Factor Analysis: Statistical Methods and Practical Issues (Beverly Hills, CA: SAGE Publications, 1978); Jae-On Kim and Charles W. Mueller, Introduction to Factor Analysis: What It Is and How To Do It (Beverly Hills, CA: SAGE Publications, 1978); Paul Kline, An Easy Guide to Factor Analysis (New York: Routledge, 1994).
12. Although factor scores might have been used in the creation of the composite variable, this procedure seemed more appropriate. Factor scores represent departures from the mean for all the journals in the entire data set, but the goal here is to represent the relative prices of the journals within each particular database.
13. The estimation procedure is based on the assumption that the journals not listed in Web of Science are similar to the lower-impact journals listed in Web of Science. Each missing value was replaced with the average value for the wanted journals in the lowest 20% of the Web of Science distribution within the appropriate subject area: engineering, physical sciences, life sciences, business, social sciences, or education.
14. In the calculation of price (JIF raw score) , each wanted journal was assigned a value equal to the total database price times the proportion of the database’s wanted-journal JIF total that could be attributed to the journal. The regression for price (JIF raw score) used the same independent variables as the other analyses. In all seven regressions, JIF percentile was used as an independent variable because, overall, (a) it was more closely related to the various price variables than either JIF raw score or total citations, and (b) its use resulted in lower levels of multicollinearity among the independent variables.
15. Bergstrom et al., “Evaluating Big Deal Journal Bundles”; Walters, “Can Differences in Publisher Size”; Walters and Markgren, “Comparing the Prices of Commercial and Nonprofit Journals.”
16. Walters, “Can Differences in Publisher Size.”
17. Walters and Markgren, “Zero-Based Serials Review”; Walters and Markgren, “A Two-Stage Approach.”
18. Walters, “Can Differences in Publisher Size”; Walters and Markgren, “Comparing the Prices of Commercial and Nonprofit Journals.”
19. Johnson, Watkinson, and Mabe, The STM Report ; Strieb and Blixrud, “Unwrapping the Bundle.”
20. William H. Walters and Susanne Markgren, “Do Faculty Journal Selections Correspond to Objective Indicators of Citation Impact? Results for 20 Academic Departments at Manhattan College,” Scientometrics 118 (Jan. 2019): 321–37, https://doi.org/10.1007/s11192-018-2972-7 .
* William H. Walters is Executive Director of the Library at Manhattan College; email: [email protected] . Acknowledgements: I am grateful for the advice and assistance of Susanne Markgren, Esther Isabelle Wilder, Brendon Ford, and Helen White. ©2024 William H. Walters, Attribution-NonCommercial ( https://creativecommons.org/licenses/by-nc/4.0/ ) CC BY-NC.

Article Views (Last 12 Months)
Contact ACRL for article usage statistics from 2010-April 2017.
Article Views (By Year/Month)
© 2024 Association of College and Research Libraries , a division of the American Library Association
Print ISSN: 0010-0870 | Online ISSN: 2150-6701
ALA Privacy Policy
ISSN: 2150-6701

An official website of the United States government
The .gov means it’s official. Federal government websites often end in .gov or .mil. Before sharing sensitive information, make sure you’re on a federal government site.
The site is secure. The https:// ensures that you are connecting to the official website and that any information you provide is encrypted and transmitted securely.
- Publications
- Account settings
Preview improvements coming to the PMC website in October 2024. Learn More or Try it out now .
- Advanced Search
- Journal List
- J Korean Med Sci
- v.37(16); 2022 Apr 25

A Practical Guide to Writing Quantitative and Qualitative Research Questions and Hypotheses in Scholarly Articles
Edward barroga.
1 Department of General Education, Graduate School of Nursing Science, St. Luke’s International University, Tokyo, Japan.
Glafera Janet Matanguihan
2 Department of Biological Sciences, Messiah University, Mechanicsburg, PA, USA.
The development of research questions and the subsequent hypotheses are prerequisites to defining the main research purpose and specific objectives of a study. Consequently, these objectives determine the study design and research outcome. The development of research questions is a process based on knowledge of current trends, cutting-edge studies, and technological advances in the research field. Excellent research questions are focused and require a comprehensive literature search and in-depth understanding of the problem being investigated. Initially, research questions may be written as descriptive questions which could be developed into inferential questions. These questions must be specific and concise to provide a clear foundation for developing hypotheses. Hypotheses are more formal predictions about the research outcomes. These specify the possible results that may or may not be expected regarding the relationship between groups. Thus, research questions and hypotheses clarify the main purpose and specific objectives of the study, which in turn dictate the design of the study, its direction, and outcome. Studies developed from good research questions and hypotheses will have trustworthy outcomes with wide-ranging social and health implications.
INTRODUCTION
Scientific research is usually initiated by posing evidenced-based research questions which are then explicitly restated as hypotheses. 1 , 2 The hypotheses provide directions to guide the study, solutions, explanations, and expected results. 3 , 4 Both research questions and hypotheses are essentially formulated based on conventional theories and real-world processes, which allow the inception of novel studies and the ethical testing of ideas. 5 , 6
It is crucial to have knowledge of both quantitative and qualitative research 2 as both types of research involve writing research questions and hypotheses. 7 However, these crucial elements of research are sometimes overlooked; if not overlooked, then framed without the forethought and meticulous attention it needs. Planning and careful consideration are needed when developing quantitative or qualitative research, particularly when conceptualizing research questions and hypotheses. 4
There is a continuing need to support researchers in the creation of innovative research questions and hypotheses, as well as for journal articles that carefully review these elements. 1 When research questions and hypotheses are not carefully thought of, unethical studies and poor outcomes usually ensue. Carefully formulated research questions and hypotheses define well-founded objectives, which in turn determine the appropriate design, course, and outcome of the study. This article then aims to discuss in detail the various aspects of crafting research questions and hypotheses, with the goal of guiding researchers as they develop their own. Examples from the authors and peer-reviewed scientific articles in the healthcare field are provided to illustrate key points.
DEFINITIONS AND RELATIONSHIP OF RESEARCH QUESTIONS AND HYPOTHESES
A research question is what a study aims to answer after data analysis and interpretation. The answer is written in length in the discussion section of the paper. Thus, the research question gives a preview of the different parts and variables of the study meant to address the problem posed in the research question. 1 An excellent research question clarifies the research writing while facilitating understanding of the research topic, objective, scope, and limitations of the study. 5
On the other hand, a research hypothesis is an educated statement of an expected outcome. This statement is based on background research and current knowledge. 8 , 9 The research hypothesis makes a specific prediction about a new phenomenon 10 or a formal statement on the expected relationship between an independent variable and a dependent variable. 3 , 11 It provides a tentative answer to the research question to be tested or explored. 4
Hypotheses employ reasoning to predict a theory-based outcome. 10 These can also be developed from theories by focusing on components of theories that have not yet been observed. 10 The validity of hypotheses is often based on the testability of the prediction made in a reproducible experiment. 8
Conversely, hypotheses can also be rephrased as research questions. Several hypotheses based on existing theories and knowledge may be needed to answer a research question. Developing ethical research questions and hypotheses creates a research design that has logical relationships among variables. These relationships serve as a solid foundation for the conduct of the study. 4 , 11 Haphazardly constructed research questions can result in poorly formulated hypotheses and improper study designs, leading to unreliable results. Thus, the formulations of relevant research questions and verifiable hypotheses are crucial when beginning research. 12
CHARACTERISTICS OF GOOD RESEARCH QUESTIONS AND HYPOTHESES
Excellent research questions are specific and focused. These integrate collective data and observations to confirm or refute the subsequent hypotheses. Well-constructed hypotheses are based on previous reports and verify the research context. These are realistic, in-depth, sufficiently complex, and reproducible. More importantly, these hypotheses can be addressed and tested. 13
There are several characteristics of well-developed hypotheses. Good hypotheses are 1) empirically testable 7 , 10 , 11 , 13 ; 2) backed by preliminary evidence 9 ; 3) testable by ethical research 7 , 9 ; 4) based on original ideas 9 ; 5) have evidenced-based logical reasoning 10 ; and 6) can be predicted. 11 Good hypotheses can infer ethical and positive implications, indicating the presence of a relationship or effect relevant to the research theme. 7 , 11 These are initially developed from a general theory and branch into specific hypotheses by deductive reasoning. In the absence of a theory to base the hypotheses, inductive reasoning based on specific observations or findings form more general hypotheses. 10
TYPES OF RESEARCH QUESTIONS AND HYPOTHESES
Research questions and hypotheses are developed according to the type of research, which can be broadly classified into quantitative and qualitative research. We provide a summary of the types of research questions and hypotheses under quantitative and qualitative research categories in Table 1 .
Research questions in quantitative research
In quantitative research, research questions inquire about the relationships among variables being investigated and are usually framed at the start of the study. These are precise and typically linked to the subject population, dependent and independent variables, and research design. 1 Research questions may also attempt to describe the behavior of a population in relation to one or more variables, or describe the characteristics of variables to be measured ( descriptive research questions ). 1 , 5 , 14 These questions may also aim to discover differences between groups within the context of an outcome variable ( comparative research questions ), 1 , 5 , 14 or elucidate trends and interactions among variables ( relationship research questions ). 1 , 5 We provide examples of descriptive, comparative, and relationship research questions in quantitative research in Table 2 .
Hypotheses in quantitative research
In quantitative research, hypotheses predict the expected relationships among variables. 15 Relationships among variables that can be predicted include 1) between a single dependent variable and a single independent variable ( simple hypothesis ) or 2) between two or more independent and dependent variables ( complex hypothesis ). 4 , 11 Hypotheses may also specify the expected direction to be followed and imply an intellectual commitment to a particular outcome ( directional hypothesis ) 4 . On the other hand, hypotheses may not predict the exact direction and are used in the absence of a theory, or when findings contradict previous studies ( non-directional hypothesis ). 4 In addition, hypotheses can 1) define interdependency between variables ( associative hypothesis ), 4 2) propose an effect on the dependent variable from manipulation of the independent variable ( causal hypothesis ), 4 3) state a negative relationship between two variables ( null hypothesis ), 4 , 11 , 15 4) replace the working hypothesis if rejected ( alternative hypothesis ), 15 explain the relationship of phenomena to possibly generate a theory ( working hypothesis ), 11 5) involve quantifiable variables that can be tested statistically ( statistical hypothesis ), 11 6) or express a relationship whose interlinks can be verified logically ( logical hypothesis ). 11 We provide examples of simple, complex, directional, non-directional, associative, causal, null, alternative, working, statistical, and logical hypotheses in quantitative research, as well as the definition of quantitative hypothesis-testing research in Table 3 .
Research questions in qualitative research
Unlike research questions in quantitative research, research questions in qualitative research are usually continuously reviewed and reformulated. The central question and associated subquestions are stated more than the hypotheses. 15 The central question broadly explores a complex set of factors surrounding the central phenomenon, aiming to present the varied perspectives of participants. 15
There are varied goals for which qualitative research questions are developed. These questions can function in several ways, such as to 1) identify and describe existing conditions ( contextual research question s); 2) describe a phenomenon ( descriptive research questions ); 3) assess the effectiveness of existing methods, protocols, theories, or procedures ( evaluation research questions ); 4) examine a phenomenon or analyze the reasons or relationships between subjects or phenomena ( explanatory research questions ); or 5) focus on unknown aspects of a particular topic ( exploratory research questions ). 5 In addition, some qualitative research questions provide new ideas for the development of theories and actions ( generative research questions ) or advance specific ideologies of a position ( ideological research questions ). 1 Other qualitative research questions may build on a body of existing literature and become working guidelines ( ethnographic research questions ). Research questions may also be broadly stated without specific reference to the existing literature or a typology of questions ( phenomenological research questions ), may be directed towards generating a theory of some process ( grounded theory questions ), or may address a description of the case and the emerging themes ( qualitative case study questions ). 15 We provide examples of contextual, descriptive, evaluation, explanatory, exploratory, generative, ideological, ethnographic, phenomenological, grounded theory, and qualitative case study research questions in qualitative research in Table 4 , and the definition of qualitative hypothesis-generating research in Table 5 .
Qualitative studies usually pose at least one central research question and several subquestions starting with How or What . These research questions use exploratory verbs such as explore or describe . These also focus on one central phenomenon of interest, and may mention the participants and research site. 15
Hypotheses in qualitative research
Hypotheses in qualitative research are stated in the form of a clear statement concerning the problem to be investigated. Unlike in quantitative research where hypotheses are usually developed to be tested, qualitative research can lead to both hypothesis-testing and hypothesis-generating outcomes. 2 When studies require both quantitative and qualitative research questions, this suggests an integrative process between both research methods wherein a single mixed-methods research question can be developed. 1
FRAMEWORKS FOR DEVELOPING RESEARCH QUESTIONS AND HYPOTHESES
Research questions followed by hypotheses should be developed before the start of the study. 1 , 12 , 14 It is crucial to develop feasible research questions on a topic that is interesting to both the researcher and the scientific community. This can be achieved by a meticulous review of previous and current studies to establish a novel topic. Specific areas are subsequently focused on to generate ethical research questions. The relevance of the research questions is evaluated in terms of clarity of the resulting data, specificity of the methodology, objectivity of the outcome, depth of the research, and impact of the study. 1 , 5 These aspects constitute the FINER criteria (i.e., Feasible, Interesting, Novel, Ethical, and Relevant). 1 Clarity and effectiveness are achieved if research questions meet the FINER criteria. In addition to the FINER criteria, Ratan et al. described focus, complexity, novelty, feasibility, and measurability for evaluating the effectiveness of research questions. 14
The PICOT and PEO frameworks are also used when developing research questions. 1 The following elements are addressed in these frameworks, PICOT: P-population/patients/problem, I-intervention or indicator being studied, C-comparison group, O-outcome of interest, and T-timeframe of the study; PEO: P-population being studied, E-exposure to preexisting conditions, and O-outcome of interest. 1 Research questions are also considered good if these meet the “FINERMAPS” framework: Feasible, Interesting, Novel, Ethical, Relevant, Manageable, Appropriate, Potential value/publishable, and Systematic. 14
As we indicated earlier, research questions and hypotheses that are not carefully formulated result in unethical studies or poor outcomes. To illustrate this, we provide some examples of ambiguous research question and hypotheses that result in unclear and weak research objectives in quantitative research ( Table 6 ) 16 and qualitative research ( Table 7 ) 17 , and how to transform these ambiguous research question(s) and hypothesis(es) into clear and good statements.
a These statements were composed for comparison and illustrative purposes only.
b These statements are direct quotes from Higashihara and Horiuchi. 16
a This statement is a direct quote from Shimoda et al. 17
The other statements were composed for comparison and illustrative purposes only.
CONSTRUCTING RESEARCH QUESTIONS AND HYPOTHESES
To construct effective research questions and hypotheses, it is very important to 1) clarify the background and 2) identify the research problem at the outset of the research, within a specific timeframe. 9 Then, 3) review or conduct preliminary research to collect all available knowledge about the possible research questions by studying theories and previous studies. 18 Afterwards, 4) construct research questions to investigate the research problem. Identify variables to be accessed from the research questions 4 and make operational definitions of constructs from the research problem and questions. Thereafter, 5) construct specific deductive or inductive predictions in the form of hypotheses. 4 Finally, 6) state the study aims . This general flow for constructing effective research questions and hypotheses prior to conducting research is shown in Fig. 1 .

Research questions are used more frequently in qualitative research than objectives or hypotheses. 3 These questions seek to discover, understand, explore or describe experiences by asking “What” or “How.” The questions are open-ended to elicit a description rather than to relate variables or compare groups. The questions are continually reviewed, reformulated, and changed during the qualitative study. 3 Research questions are also used more frequently in survey projects than hypotheses in experiments in quantitative research to compare variables and their relationships.
Hypotheses are constructed based on the variables identified and as an if-then statement, following the template, ‘If a specific action is taken, then a certain outcome is expected.’ At this stage, some ideas regarding expectations from the research to be conducted must be drawn. 18 Then, the variables to be manipulated (independent) and influenced (dependent) are defined. 4 Thereafter, the hypothesis is stated and refined, and reproducible data tailored to the hypothesis are identified, collected, and analyzed. 4 The hypotheses must be testable and specific, 18 and should describe the variables and their relationships, the specific group being studied, and the predicted research outcome. 18 Hypotheses construction involves a testable proposition to be deduced from theory, and independent and dependent variables to be separated and measured separately. 3 Therefore, good hypotheses must be based on good research questions constructed at the start of a study or trial. 12
In summary, research questions are constructed after establishing the background of the study. Hypotheses are then developed based on the research questions. Thus, it is crucial to have excellent research questions to generate superior hypotheses. In turn, these would determine the research objectives and the design of the study, and ultimately, the outcome of the research. 12 Algorithms for building research questions and hypotheses are shown in Fig. 2 for quantitative research and in Fig. 3 for qualitative research.

EXAMPLES OF RESEARCH QUESTIONS FROM PUBLISHED ARTICLES
- EXAMPLE 1. Descriptive research question (quantitative research)
- - Presents research variables to be assessed (distinct phenotypes and subphenotypes)
- “BACKGROUND: Since COVID-19 was identified, its clinical and biological heterogeneity has been recognized. Identifying COVID-19 phenotypes might help guide basic, clinical, and translational research efforts.
- RESEARCH QUESTION: Does the clinical spectrum of patients with COVID-19 contain distinct phenotypes and subphenotypes? ” 19
- EXAMPLE 2. Relationship research question (quantitative research)
- - Shows interactions between dependent variable (static postural control) and independent variable (peripheral visual field loss)
- “Background: Integration of visual, vestibular, and proprioceptive sensations contributes to postural control. People with peripheral visual field loss have serious postural instability. However, the directional specificity of postural stability and sensory reweighting caused by gradual peripheral visual field loss remain unclear.
- Research question: What are the effects of peripheral visual field loss on static postural control ?” 20
- EXAMPLE 3. Comparative research question (quantitative research)
- - Clarifies the difference among groups with an outcome variable (patients enrolled in COMPERA with moderate PH or severe PH in COPD) and another group without the outcome variable (patients with idiopathic pulmonary arterial hypertension (IPAH))
- “BACKGROUND: Pulmonary hypertension (PH) in COPD is a poorly investigated clinical condition.
- RESEARCH QUESTION: Which factors determine the outcome of PH in COPD?
- STUDY DESIGN AND METHODS: We analyzed the characteristics and outcome of patients enrolled in the Comparative, Prospective Registry of Newly Initiated Therapies for Pulmonary Hypertension (COMPERA) with moderate or severe PH in COPD as defined during the 6th PH World Symposium who received medical therapy for PH and compared them with patients with idiopathic pulmonary arterial hypertension (IPAH) .” 21
- EXAMPLE 4. Exploratory research question (qualitative research)
- - Explores areas that have not been fully investigated (perspectives of families and children who receive care in clinic-based child obesity treatment) to have a deeper understanding of the research problem
- “Problem: Interventions for children with obesity lead to only modest improvements in BMI and long-term outcomes, and data are limited on the perspectives of families of children with obesity in clinic-based treatment. This scoping review seeks to answer the question: What is known about the perspectives of families and children who receive care in clinic-based child obesity treatment? This review aims to explore the scope of perspectives reported by families of children with obesity who have received individualized outpatient clinic-based obesity treatment.” 22
- EXAMPLE 5. Relationship research question (quantitative research)
- - Defines interactions between dependent variable (use of ankle strategies) and independent variable (changes in muscle tone)
- “Background: To maintain an upright standing posture against external disturbances, the human body mainly employs two types of postural control strategies: “ankle strategy” and “hip strategy.” While it has been reported that the magnitude of the disturbance alters the use of postural control strategies, it has not been elucidated how the level of muscle tone, one of the crucial parameters of bodily function, determines the use of each strategy. We have previously confirmed using forward dynamics simulations of human musculoskeletal models that an increased muscle tone promotes the use of ankle strategies. The objective of the present study was to experimentally evaluate a hypothesis: an increased muscle tone promotes the use of ankle strategies. Research question: Do changes in the muscle tone affect the use of ankle strategies ?” 23
EXAMPLES OF HYPOTHESES IN PUBLISHED ARTICLES
- EXAMPLE 1. Working hypothesis (quantitative research)
- - A hypothesis that is initially accepted for further research to produce a feasible theory
- “As fever may have benefit in shortening the duration of viral illness, it is plausible to hypothesize that the antipyretic efficacy of ibuprofen may be hindering the benefits of a fever response when taken during the early stages of COVID-19 illness .” 24
- “In conclusion, it is plausible to hypothesize that the antipyretic efficacy of ibuprofen may be hindering the benefits of a fever response . The difference in perceived safety of these agents in COVID-19 illness could be related to the more potent efficacy to reduce fever with ibuprofen compared to acetaminophen. Compelling data on the benefit of fever warrant further research and review to determine when to treat or withhold ibuprofen for early stage fever for COVID-19 and other related viral illnesses .” 24
- EXAMPLE 2. Exploratory hypothesis (qualitative research)
- - Explores particular areas deeper to clarify subjective experience and develop a formal hypothesis potentially testable in a future quantitative approach
- “We hypothesized that when thinking about a past experience of help-seeking, a self distancing prompt would cause increased help-seeking intentions and more favorable help-seeking outcome expectations .” 25
- “Conclusion
- Although a priori hypotheses were not supported, further research is warranted as results indicate the potential for using self-distancing approaches to increasing help-seeking among some people with depressive symptomatology.” 25
- EXAMPLE 3. Hypothesis-generating research to establish a framework for hypothesis testing (qualitative research)
- “We hypothesize that compassionate care is beneficial for patients (better outcomes), healthcare systems and payers (lower costs), and healthcare providers (lower burnout). ” 26
- Compassionomics is the branch of knowledge and scientific study of the effects of compassionate healthcare. Our main hypotheses are that compassionate healthcare is beneficial for (1) patients, by improving clinical outcomes, (2) healthcare systems and payers, by supporting financial sustainability, and (3) HCPs, by lowering burnout and promoting resilience and well-being. The purpose of this paper is to establish a scientific framework for testing the hypotheses above . If these hypotheses are confirmed through rigorous research, compassionomics will belong in the science of evidence-based medicine, with major implications for all healthcare domains.” 26
- EXAMPLE 4. Statistical hypothesis (quantitative research)
- - An assumption is made about the relationship among several population characteristics ( gender differences in sociodemographic and clinical characteristics of adults with ADHD ). Validity is tested by statistical experiment or analysis ( chi-square test, Students t-test, and logistic regression analysis)
- “Our research investigated gender differences in sociodemographic and clinical characteristics of adults with ADHD in a Japanese clinical sample. Due to unique Japanese cultural ideals and expectations of women's behavior that are in opposition to ADHD symptoms, we hypothesized that women with ADHD experience more difficulties and present more dysfunctions than men . We tested the following hypotheses: first, women with ADHD have more comorbidities than men with ADHD; second, women with ADHD experience more social hardships than men, such as having less full-time employment and being more likely to be divorced.” 27
- “Statistical Analysis
- ( text omitted ) Between-gender comparisons were made using the chi-squared test for categorical variables and Students t-test for continuous variables…( text omitted ). A logistic regression analysis was performed for employment status, marital status, and comorbidity to evaluate the independent effects of gender on these dependent variables.” 27
EXAMPLES OF HYPOTHESIS AS WRITTEN IN PUBLISHED ARTICLES IN RELATION TO OTHER PARTS
- EXAMPLE 1. Background, hypotheses, and aims are provided
- “Pregnant women need skilled care during pregnancy and childbirth, but that skilled care is often delayed in some countries …( text omitted ). The focused antenatal care (FANC) model of WHO recommends that nurses provide information or counseling to all pregnant women …( text omitted ). Job aids are visual support materials that provide the right kind of information using graphics and words in a simple and yet effective manner. When nurses are not highly trained or have many work details to attend to, these job aids can serve as a content reminder for the nurses and can be used for educating their patients (Jennings, Yebadokpo, Affo, & Agbogbe, 2010) ( text omitted ). Importantly, additional evidence is needed to confirm how job aids can further improve the quality of ANC counseling by health workers in maternal care …( text omitted )” 28
- “ This has led us to hypothesize that the quality of ANC counseling would be better if supported by job aids. Consequently, a better quality of ANC counseling is expected to produce higher levels of awareness concerning the danger signs of pregnancy and a more favorable impression of the caring behavior of nurses .” 28
- “This study aimed to examine the differences in the responses of pregnant women to a job aid-supported intervention during ANC visit in terms of 1) their understanding of the danger signs of pregnancy and 2) their impression of the caring behaviors of nurses to pregnant women in rural Tanzania.” 28
- EXAMPLE 2. Background, hypotheses, and aims are provided
- “We conducted a two-arm randomized controlled trial (RCT) to evaluate and compare changes in salivary cortisol and oxytocin levels of first-time pregnant women between experimental and control groups. The women in the experimental group touched and held an infant for 30 min (experimental intervention protocol), whereas those in the control group watched a DVD movie of an infant (control intervention protocol). The primary outcome was salivary cortisol level and the secondary outcome was salivary oxytocin level.” 29
- “ We hypothesize that at 30 min after touching and holding an infant, the salivary cortisol level will significantly decrease and the salivary oxytocin level will increase in the experimental group compared with the control group .” 29
- EXAMPLE 3. Background, aim, and hypothesis are provided
- “In countries where the maternal mortality ratio remains high, antenatal education to increase Birth Preparedness and Complication Readiness (BPCR) is considered one of the top priorities [1]. BPCR includes birth plans during the antenatal period, such as the birthplace, birth attendant, transportation, health facility for complications, expenses, and birth materials, as well as family coordination to achieve such birth plans. In Tanzania, although increasing, only about half of all pregnant women attend an antenatal clinic more than four times [4]. Moreover, the information provided during antenatal care (ANC) is insufficient. In the resource-poor settings, antenatal group education is a potential approach because of the limited time for individual counseling at antenatal clinics.” 30
- “This study aimed to evaluate an antenatal group education program among pregnant women and their families with respect to birth-preparedness and maternal and infant outcomes in rural villages of Tanzania.” 30
- “ The study hypothesis was if Tanzanian pregnant women and their families received a family-oriented antenatal group education, they would (1) have a higher level of BPCR, (2) attend antenatal clinic four or more times, (3) give birth in a health facility, (4) have less complications of women at birth, and (5) have less complications and deaths of infants than those who did not receive the education .” 30
Research questions and hypotheses are crucial components to any type of research, whether quantitative or qualitative. These questions should be developed at the very beginning of the study. Excellent research questions lead to superior hypotheses, which, like a compass, set the direction of research, and can often determine the successful conduct of the study. Many research studies have floundered because the development of research questions and subsequent hypotheses was not given the thought and meticulous attention needed. The development of research questions and hypotheses is an iterative process based on extensive knowledge of the literature and insightful grasp of the knowledge gap. Focused, concise, and specific research questions provide a strong foundation for constructing hypotheses which serve as formal predictions about the research outcomes. Research questions and hypotheses are crucial elements of research that should not be overlooked. They should be carefully thought of and constructed when planning research. This avoids unethical studies and poor outcomes by defining well-founded objectives that determine the design, course, and outcome of the study.
Disclosure: The authors have no potential conflicts of interest to disclose.
Author Contributions:
- Conceptualization: Barroga E, Matanguihan GJ.
- Methodology: Barroga E, Matanguihan GJ.
- Writing - original draft: Barroga E, Matanguihan GJ.
- Writing - review & editing: Barroga E, Matanguihan GJ.
- Open access
- Published: 08 April 2024
Changes in intention to use an interprofessional approach to decision-making following training: a cluster before-and-after study
- Hajar Taqif 1 , 2 ,
- Lionel Adisso 2 , 3 ,
- Lucas Gomes Souza ORCID: orcid.org/0000-0002-2965-4660 2 , 3 ,
- Suélène Georgina Dofara ORCID: orcid.org/0009-0008-0537-8965 2 ,
- Sergio Cortez Ghio 2 ,
- Louis-Paul Rivest ORCID: orcid.org/0000-0003-4351-4127 1 &
- France Légaré ORCID: orcid.org/0000-0002-2296-6696 2 , 4
BMC Health Services Research volume 24 , Article number: 437 ( 2024 ) Cite this article
Metrics details
Health professionals in home care work in interprofessional teams. Yet most training in decision support assumes a one-on-one relationship with patients. We assessed the impact of an in-person training session in interprofessional shared decision-making (IP-SDM) on home care professionals’ intention to adopt this approach.
We conducted a secondary analysis of a cluster stepped-wedge trial using a before-and-after study design. We collected data among home care professionals from November 2016 to February 2018 in 9 health and social services centers in Quebec, Canada. The intervention was an in-person IP-SDM training session. Intention to engage in IP-SDM pre- and post-session (dependent variable) was compared using a continuing professional development evaluation scale (CPD-Reaction) informed by the Godin’s Integrated Behavioral Model for health professionals. We also assessed socio-demographic and psychosocial variables (beliefs about capabilities, beliefs about consequences, social influence and moral norm). We performed bivariate and multivariate analysis to identify factors influencing post-intervention intention. We used the STROBE reporting guidelines for observational studies to report our results.
Of 134 respondents who provided complete pairs of questionnaires (pre- and post-), most were female (90.9%), mean age was 42 (± 9.3) years and 66.9% were social workers. Mean intention scores decreased from 5.84 (± 1.19) to 5.54 (± 1.35) (Mean difference = -0.30 ± 1.16; p = 0.02). Factors associated with higher intention post-intervention were social influence (ß = 0.34, p = 0.01) and belief about capabilities (ß = 0.49, p < 0.01).
After in-person IP-SDM training, healthcare professionals’ intention to engage in IP-SDM decreased. However, the scope of this decrease is probably not clinically significant. Due to their association with intention, beliefs about capabilities, which translate into having a sense of self-competency in the new clinical behavior, and social influences, which translate into what important others think one should be doing, could be targets for future research aiming to implement IP-SDM in home care settings.
Peer Review reports
Canada’s population is aging, and people aged 65 and over (older adults) are living longer than ever [ 1 , 2 ]. As a society we must ensure that these older adults are supported when they need to make decisions about safe and comfortable accommodation.
Aging is generally associated with a higher risk of developing disabilities that can lead to a loss of autonomy. As they start losing their autonomy, older adults are faced with one of the most difficult decisions: to stay at home or to move somewhere where they will be able to receive appropriate care (e.g., a health care facility or a nursing home) [ 3 ]. This decision is very difficult to make alone. The shared decision-making approach (SDM) represents a middle ground between the traditional paternalistic approach, where the health care professional makes the decision alone, and the consumer approach, where the patient is the sole decision maker [ 4 ]. An interprofessional (IP) approach to SDM is especially relevant to caring for the frail elderly, as chronic illness often means that several different kinds of healthcare professional are involved in their care. The interprofessional approach to SDM (IP-SDM) enables health professionals to collaboratively support patients in facing difficult decisions and reach healthcare choices that are agreed on by the patient, their family members or caregivers, and the interprofessional team [ 3 , 5 ]. Various obstacles hindering SDM have been identified, particularly within multidisciplinary care settings. These barriers include a lack of knowledge of what other disciplines can do, insufficient trust in the expertise of other disciplines, and inadequate communication among the different disciplines [ 6 ]Consequently, training home care teams in SDM through an IP approach that directly addresses these barriers is expected to facilitate the seamless adoption of decision tools in clinical practice. This, in turn, is anticipated to contribute to the widespread implementation of SDM across the health and social care system.
Based on our previous research using the IP-SDM approach [ 7 , 8 ], and considering the potential of an intervention involving task-sharing among diverse kinds of professionals to alleviate the burden of the discussion about transitioning to long term care, we hypothesized that IP-SDM training would increase the intention of healthcare professionals in home care teams to engage in this approach.
To strengthen our analysis, we used Godin's integrated model, which explains healthcare professionals' clinical behavior, as the theoretical framework for our study. Godin et al. conducted a systematic review of 76 studies examining the influence of social cognitive theories on healthcare professionals' adoption of clinical behaviors [ 9 ]. The authors confirmed the key role of intention as a predictor of behavior and identified six psychosocial factors that influence intention: beliefs about capabilities and consequences, moral norms, social influences, role and identity, and individual characteristics. Based on this framework, Legare et al. developed the CPD-Reaction tool for evaluating continuing professional development courses [ 10 ]. The tool evaluates intention (using 2 of the 12 included items) immediately after training and has been shown to be a good predictor of behavior six months after the training [ 11 ]. In addition, it measures the psychosocial factors that influence intention, which suggests elements which could be target in future behavior change interventions [ 10 , 12 ]. Thus this tool was useful for measuring the impact of our intervention and possible psychosocial targets to improve it.
Several studies that have measured behavioral intention used a cross-sectional design [ 13 , 14 , 15 ]. However, intention scores measured at a single point in time do not demonstrate a change in professionals’ intention to adopt a behavior. Therefore, our study aimed to assess the impact of IP-SDM training on healthcare professionals' intention to engage in an IP-SDM approach, both before and after an in-person training session, and to identify factors that influenced this intention.
Study design
We reported this cluster pre-post study according to the STROBE reporting guidelines for observational studies [ 16 ]. We performed a secondary analysis of an existing database collected during a stepped-wedge study [ 17 ]. This primary study aimed to scale up and evaluate the implementation of SDM in interprofessional home care teams caring for older adults or their caregivers facing a decision about staying at home or moving elsewhere. The study was conducted from November 2016 to February 2018 in 9 health and social services centers (HSSCs) in the province of Quebec in Canada. HSSCs are regional health authorities that provide public health and social care for the population of their region. The trial was registered at ClinicalTrials.gov (NCT02592525) on October 30, 2015 and the protocol was published [ 6 ].
In this study, we used a pre-post measurement design with clusters to compare intention and its variables (pre and post-intervention) to engage in an IP-SDM approach among healthcare professionals in home care teams and, post-intervention, we analyzed significant factors of intention.
Participants
Of the 22 HSSCs contacted, 9 participated in the study. Since this was a secondary analysis of a cluster randomized trial, selection criteria applies to the site rather than the individual: all clinicians at the site were invited to participate in the training. Thus healthcare professionals from interprofessional home care teams who were involved in the care of older adults with loss of autonomy, practiced in one of the participating HSSCs, and gave informed consent ( n = 281) were included in the study (Additional file 1 ).
Intervention
Before taking the in-person training program, healthcare professionals in home care teams were invited to complete the Ottawa Decision Support Tutorial (1h30), an online general SDM tutorial (Additional file 2 ) [ 18 ]. The 3.5-h in-person training, based on adult education principles, was designed according to the IP-SDM conceptual model [ 6 ]. In the context of decision-making with older adults about housing, it addressed communication techniques and strategies for engaging frail older adults with cognitive impairment or their caregivers in the decision making. It included the use of a patient decision aid (PtDA), and involved a lecture, a video, and a role play session[ 6 ].
Data collection and variables
Data was collected before and after each of the 9 in-person IP-SDM training sessions from November 2016 to February 2018, using the self-administered CPD-Reaction Questionnaire, which was adapted to the home care context [ 7 ]. Questionnaires were completed upon participants' arrival in the training room and at their departure. The CPD-Reaction questionnaire is a validated theory-based tool [ 10 , 12 ] that followed a strict development procedure. It assesses the impact of training on clinical behavioral intention using items based on Godin’s integrated socio-cognitive model for healthcare professional behavior change [ 10 ]. CPD-Reaction evaluates behavioral intention and its psychosocial variables using 12 questions that are scored with a Likert-type scale from 1 to 7, except for one question (on social influence) which is scored on a scale of 1 to 5. The study questionnaire also collected the sociodemographic characteristics of healthcare professionals in home care teams[ 19 ].
Dependent variable: intention
The dependent variable of this study was the intention of healthcare professionals in home care teams to engage in an IP-SDM approach, defined as the mean of the scores of the 2 CPD-Reaction questions (items) on intention as measured on a 7-point Likert-type scale (1 = Strongly Disagree to 7 = Strongly Agree).
Independent variables (predictor variables)
Our independent variables were beliefs about capabilities (healthcare professional’s perceptions of facilitators and barriers to adopting the behavior, 3 items), beliefs about consequences (the usefulness and the benefits/risks of adopting the behavior, 2 items), social influence (perception of approval or disapproval by significant persons regarding the adoption of the behavior, 3 items), moral norm (feeling of personal obligation to adopt the behavior, 2 items) and sociodemographic characteristics of healthcare professionals in home care teams: number of clients served per week, age, sex, number of years of practice in home care, profession, highest level of education attained, and whether they had completed the online general SDM Tutorial and the in-person IP-SDM training session.
Statistical analysis
Only health professionals in home care teams who completed both questionnaires before and after attending the in-person IP-SDM training were included in the analyzes ( n = 134).
We used descriptive statistics to report on the intention of healthcare professionals in home care teams to engage in IP-SDM and on the other 4 psychosocial variables before and after the intervention, and to describe the sociodemographic characteristics of the participants, using frequencies (n, %) for the categorical variables and mean and standard deviation (SD) for the continuous variables. For the education variable, given that participants could complete multiple response categories, we treated the variable as an ordinal of highest education obtained using the classification of the Quebec educational system.
The data we analyzed herein is from all 9 health and social services centers, making it non-independent (clustering effect).
Using repeated measures models and Wilcoxon signed ranks tests, as the normality assumption was rejected, we compared the levels of intention to engage in IP-SDM as well as the 4 psychosocial variables before and after the intervention, proceeding with the intention-to-treat analysis. This kind of analysis is appropriate for practical clinical scenarios as it makes allowance for non-compliance and protocol deviations [ 20 ]. Then we performed a sensitivity analysis by excluding participants who may or may not have been exposed to the intervention (we have no evidence of their presence). In additional analysis, we compared the pre-intervention intention of healthcare professionals who attended the preliminary online general SDM tutorial before attending the in-person training session to the pre-intervention intention of those who skipped the preliminary online tutorial, in order to see the impact of the online tutorial as well.
To determine which factors influenced participants’ intention to engage in IP-SDM, we then fit mixed linear models specifying a random effect at the health and social service center level. We started with bivariate analyses to examine the relationship between intention and the independent variables of interest (at the 0.20 alpha level). Then we performed multivariate regression analysis using a manual backward stepwise selection of the variables with a significance level (p-value) of 0.05. After obtaining the final model, we reintroduced the variables that had been excluded during the selection process one by one into the model to assess whether their inclusion improved its performance. In the final model, we considered p-values < 0.05 as statistically significant. We performed all the analyzes using R version 4.1.2. All tests were two-sided, and a p-value of < 0.05 was considered statistically significant.
Flow of the trial and participant characteristics
A total of 281 healthcare professionals in home care teams were recruited at the start but 134 provided data before and after the planned intervention and thus were included in these analyses (completed pairs of pre and post questionnaires) (Fig. 1 ). There were 22 health professionals who had completed both questionnaire (pre- and post-) but for whom we were not able to specify for sure if they had been exposed to the intervention as we could not find evidence of their signature on the list of trainees presence at the workshop. We thus conducted sensitivity analyses with and without them and observed no change in our results (data not shown). The characteristics of the 134 who were included in the analyses are described in Table 1 . Mean (± SD) age was 42 (± 9.3) years. Mean patients served per week was 13 (± 7.6) and mean years of practice in home care was 11 (± 7.0).
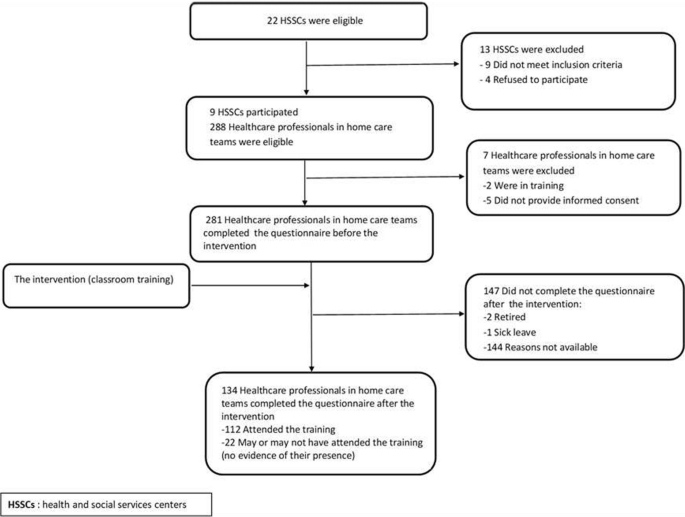
Most of the healthcare professionals in home care teams were female (90.9%). Most were social workers (66.9%), and a bachelor’s degree was most frequently the highest qualification (25.3%).
Intention before and after the intervention
Mean scores of intention to engage in IP-SDM (outcome of interest) ( n = 134) decreased from 5.84 (± 1.19) to 5.54 (± 1.35). Beliefs about consequences also decreased from 5.89 (± 1.12) to 5.65 (± 1.19) (Table 2 ) . Similarly, mean score of social influence and belief about capabilities decreased from 5.50 (± 1.03) to 5.42 (± 1.09) and 5.58 (± 1.10) to 5.51 (± 1.15) respectively. Considering intention-to-treat [ 20 ], mean score of moral norm increased from 6.07 (± 1.02) to 6.15 (± 0.89). Wilcoxon signed ranks tests confirmed these results. We noted a significant difference only for intention and beliefs about consequences (Table 2 ). However, due to the scope of the difference, less than half of a standard deviation, these differences are most probably not clinically significant [ 21 ].
Second, we did a sensitivity analysis: we only analyzed the healthcare professionals in home care teams whom we could be sure were exposed to the intervention based on the list of attendees who signed in (solely the in-person IP-SDM training) ( n = 112), and still noted a significant difference, but only for intention, which decreased from 5.88 (± 1.22) to 5.57 (± 1.35) after the intervention, while there was no significant difference for the other psychosocial variables (Additional file 3 ).
Third, in additional analysis we did not detect a significant difference in the intention of healthcare professionals in home care teams who completed the online general SDM Tutorial before the in-person IP-SDM training ( n = 197) compared to those who did not ( n = 84) (Additional file 4 ).
Factors associated with post-intervention intention
Factors associated with higher intention to engage in IP-SDM post-intervention were perception of approval by colleagues or significant others in the profession ("social influence") (ß = 0.34, p = 0.01) and perceptions of facilitators and barriers to adopting the behavior (“beliefs about capabilities”) (ß = 0.49, p < 0.01) (Table 3 ).
The variance of intention explained by these two factors was 72.1%.
In this study, we assessed healthcare professionals' intention to engage in an IP-SDM approach both before and after receiving in-person training. Additionally, we explored factors associated with this intention after the intervention. Contrary to our main hypothesis, we observed a decrease in intention following the intervention. However, given the scope of the decrease, this is most probably not clinically significant. Beliefs about consequences also decreased, whereas moral norm increased post-intervention. Despite a decline in social influence after the intervention, it remained associated with healthcare professionals' intention to engage in the IP-SDM approach. Similarly, beliefs about capabilities, which also decreased, were also associated with intention. These findings lead us to the make following observations:
First, intention to engage in IP-SDM had a statistically significant decrease after the intervention, but this decrease was most probably not clinically significant as it did not reach half of the standard deviation of the means for the intention to engage in IP-SDM before and after the intervention [ 21 ]. Our main hypothesis that could explain these findings is the fact that this trial was planned before a major reform in the health and social care system, initiated by the Quebec government in March 2015 [ 22 , 23 ]. The reform occurred between the intervention and data collection at exit, which varied from 10 to 32 months [ 7 ]. Briefly, existing healthcare organizations were merged into 22 megastructures which took over the mandates and missions of the previous structures in their areas of jurisdiction. The new organizations gathered a much broader scope of health services under a single governing body per territory. Under this imposed merger, many healthcare teams, including home care teams, faced loss of staff, heavy workloads, low morale, and changes in team composition. This may have been behind the decrease. The ones who remained may have been simply overwhelmed trying to meet the essential basic daily needs of their clients. On the other hand, our finding might underscore a critical point: while training programs targeting behavioral changes among healthcare professionals are valuable, structural and organizational barriers within healthcare systems can impede the successful implementation of evidence-based practices such as SDM. As highlighted by Müller et al., organizational culture, leadership support, and alterations in workflow structures are pivotal factors for the effective integration of SDM in healthcare[ 24 ]. Moreover, there is a dearth of knowledge regarding the influence of system-level characteristics on SDM implementation [ 25 ]. Therefore, future research aimed at implementing IP-SDM in home-care settings should delve into the organizational and system-level characteristics that both facilitate and hinder implementation. Quantifying the varying impacts of these characteristics, understanding their potential interactions, and exploring how the system could operate differently are crucial aspects to consider in advancing SDM implementation efforts [ 25 ] and should be a target of future studies.
Second, following the training, there was a statistically significant decrease in the "beliefs about consequences" construct, which assesses perceptions of positive or negative outcomes linked to specific behaviors. Again, the scope of the decrease is most probably not clinically significant. Existing literature also suggests that some allied health professionals express frustration with engaging in SDM, citing issues such as client characteristics and misalignment with their professional frameworks [ 26 , 27 ]. Our findings confirm this ambivalence among health professionals: despite recognizing the moral importance of an IP-SDM approach, the barriers within their practices and professional frameworks may block their intention to engage in IP-SDM, even after training. Future studies should address these challenges and design interventions tailored to the specific context barriers encountered by these professionals in their daily practices.
Third, factors associated with intention to engage in IP-SDM post-intervention were perception of approval by colleagues or significant others in the profession ("social influence") (ß = 0.34, p = 0.01) and perceptions of facilitators and barriers to adopting the behavior (“beliefs about capabilities”) (ß = 0.49, p < 0.01). The "social influences" construct reflects individuals' perceptions of approval or disapproval from peers regarding a specific behavior. Similarly, a separate review of 19 studies identified the key characteristics of interprofessional teams that influence implementation and that also play a role in social influence, including governance structures, communication power dynamics, and training [ 28 ]. Our intervention addressed only one of these elements – training – and it is possible that integrating these other factors into the IP-SDM model could enhance social influence. This is crucial because our data indicated a statistically significant association between social influence and intention. Consequently, future adaptations of the IP-SDM model may benefit from implementing behavior change techniques specifically targeting social influence. In a separate study with interprofessional teams within a Quebec mental health network, strategies such as providing information about peer approval, promoting trust through social comparison, and fostering social support/change emerged as relevant approaches that could be integrated in future adaptations [ 29 , 30 ]. Moreover, we found a statistically significant association between beliefs about capabilities and intention. This aligns with findings from a systematic review of SDM training programs[ 31 ], which revealed that studies involving allied health professionals or nurses often indicated a desire for more training to enhance their SDM skills [ 32 , 33 ]. Future interventions targeting allied healthcare professionals should consider this and incorporate additional training sessions, practice opportunities or ongoing support programs to build their beliefs about their capabilities and confidence in implementing IP-SDM effectively.
Finally, we did not detect a significant difference in intention, or in any of its variables, among healthcare professionals who attended the online training on general SDM principles meant as a prompt (Additional file 4 ). This suggests that the online portion of the training did not have a great impact on the predisposition of health professionals to attend the IP-SDM in-person training and thus on their intention to engage in an IP-SDM approach. This may be explained by the fact that for health professionals to invest time in training they need to feel that the material is relevant to them from start to finish. Anecdotally, participants told the research team that the online module was too general and not relevant to their work (data not shown). Thus it will be important to develop more targeted online training material in future studies [ 34 ].
The strength of this study lies in its use of a socio-cognitive theory to identify factors associated with professionals' intention to engage in an IP-SDM approach. In keeping with this theory [ 9 ], factors associated with healthcare professionals' intention to adopt a clinical practice or not were identified following a comprehensive and rigorous review. The factors identified on the basis of this theory explained more than two-thirds of the variance in intention, and our results thus confirm the theory’s hypothesis: that modifiable psychosocial factors are more likely to explain healthcare professionals' behavior change than sociodemographic characteristics. Also, a validated tool with acceptable internal consistency of constructs [ 19 ] was used to measure intention as well as its psychosocial determinants.
This study has a number of limitations. First, few healthcare professionals in home care teams completed both the pre and post intervention questionnaires, due to the major healthcare reform that took place during the study. Different information and selection biases could have occurred, as the 134 included participants may have had similar behavioral intentions, and different from the 147 who did not complete the questionnaire after the intervention. Second, because we used a self-reported questionnaire, a social desirability bias may apply. In other words, a healthcare professional may have indicated a higher intention than her actual intention to satisfy a certain social desirability [ 35 ].
We found that the level of intention of healthcare professionals to engage in the IP-SDM approach decreased after a training session on the IP-SDM approach and the use of a PtDA. Based on our results, IP-SDM training should use behavior change techniques that focus on social influence and beliefs about capabilities. Designers of training interventions should also focus on strategies to withstand or mitigate disruptions at the system and organizational levels, thus favoring sustainability. Further research could also explore the effect of more training, as the initial training introduced the IP-SDM concept but then revealed the providers' lack of readiness to engage in SDM.
The results of this study will enable health system jurisdictions to plan more effective training of healthcare professionals to engage in the IP-SDM approach and, to a certain extent, better understand how major healthcare reform can hamper implementation of desirable clinical behaviors.
Availability of data and materials
The datasets used and/or analyzed during the current study are available from the corresponding author on reasonable request.
Abbreviations
Continuing Professional Development-Reaction
Health and social services centers
Patient decision aid
- Interprofessional shared decision-making
He W, Goodkind D, Kowal PR. An aging world: 2015. In.: United States Census Bureau Washington, DC; 2016.
Google Scholar
Statistics Canada. Population Projections for Canada, Provinces and Territories 2009 to 2036; Catalogue no. 91–520-X. https://www150.statcan.gc.ca/n1/pub/91-520-x/91-520-x2010001-eng.pdf . Assessed 2022.06.03.
Lefebvre C. Indspd: Un portrait de la santé des Québécois de 65 ans et plus: Institut national de santé publique du Québec. 2003.
Charles C, Gafni A, Whelan T. Decision-making in the physician-patient encounter: revisiting the shared treatment decision-making model. Soc Sci Med. 1999;49(5):651–61.
Article CAS PubMed Google Scholar
Dagnone T, Review SPF. Health SS: For Patients’ Sake: Patient First Review Commissioner’s Report to the Saskatchewan Minister of Health. Patient First Review Commission; 2010.
Légaré F, Brière N, Stacey D, Lacroix G, Desroches S, Dumont S, Fraser KD, Rivest L-P, Durand PJ, Turcotte S, et al. Implementing shared decision-making in interprofessional home care teams (the IPSDM-SW study): protocol for a stepped wedge cluster randomised trial. BMJ Open. 2016;6(11): e014023.
Article PubMed PubMed Central Google Scholar
Adekpedjou R, Haesebaert J, Stacey D, Brière N, Freitas A, Rivest L-P, Légaré F. Variations in factors associated with healthcare providers’ intention to engage in interprofessional shared decision making in home care: results of two cross-sectional surveys. BMC Health Serv Res. 2020;20(1):1–11.
Article Google Scholar
Légaré F, Stacey D, Brière N, Fraser K, Desroches S, Dumont S, Sales A, Puma C, Aubé D. Healthcare providers’ intentions to engage in an interprofessional approach to shared decision-making in home care programs: a mixed methods study. J Interprof Care. 2013;27(3):214–22.
Godin G, Bélanger-Gravel A, Eccles M, Grimshaw J. Healthcare professionals’ intentions and behaviours: a systematic review of studies based on social cognitive theories. Implement Sci. 2008;3:1–12.
Legare F, Freitas A, Turcotte S, Borduas F, Jacques A, Luconi F, Godin G, Boucher A, Sargeant J, Labrecque M. Responsiveness of a simple tool for assessing change in behavioral intention after continuing professional development activities. PLoS ONE. 2017;12(5): e0176678.
Bakwa Kanyinga F, Gogovor A, Dofara SG, Gadio S, Tremblay M, Daniel SJ, Rivest L-P, Légaré F. Evaluating the impact of continuing professional development courses on physician behavioral intention: a pre-post study with follow-up at six months. BMC Med Educ. 2023;23(1):629.
Legare F, Borduas F, Freitas A, Jacques A, Godin G, Luconi F, Grimshaw J. team C-K: Development of a simple 12-item theory-based instrument to assess the impact of continuing professional development on clinical behavioral intentions. PLoS ONE. 2014;9(3): e91013.
Du X, He Q, Yang T, Wang Y, Xu H, Hao C, Zhou K, Gu J, Hao Y. Intention to start ART after the launch of expanded treatment strategy among people living with HIV in China: a behavioral theory-based cross-sectional study. AIDS Care. 2020;32(9):1182–90.
Article PubMed Google Scholar
Desjardins A, Boulay ME, Gagne M, Simon M, Boulet LP. Family medicine physician teachers and residents’ intentions to prescribe and interpret spirometry: a descriptive cross-sectional study. J Asthma. 2020;57(2):149–59.
Ayivi-Vinz G, Kanyinga FB, Bergeron L, Décary S, Adisso ÉL, Zomahoun HTV, Daniel SJ, Tremblay M, Plourde KV, Guay-Bélanger S. Use of the CPD-REACTION Questionnaire to Evaluate Continuing Professional Development Activities for Health Professionals: Systematic Review. JMIR Medical Education. 2022;8(2): e36948.
Cuschieri S. The STROBE guidelines. Saudi J Anaesth. 2019;13(Suppl 1):S31.
Adisso ÉL, Taljaard M, Stacey D, Brière N, Zomahoun HTV, Durand PJ, Rivest L-P, Légaré F. Shared Decision-Making Training for Home Care Teams to Engage Frail Older Adults and Caregivers in Housing Decisions: Stepped-Wedge Cluster Randomized Trial. JMIR aging. 2022;5(3): e39386.
Stacey D, Legare F, Boland L, Lewis KB, Loiselle M-C, Hoefel L, Garvelink M, O’Connor A. 20th anniversary Ottawa decision support framework: part 3 overview of systematic reviews and updated framework. Med Decis Making. 2020;40(3):379–98.
Légare F, Borduas F, Freitas A, Turcotte S. The Continuing Professional Development (CPD) Reaction Questionnaire User Manual. Quebec: University Laval; 2015.
Gupta SK. Intention-to-treat concept: A review. Perspect Clin Res. 2011;2(3):109–12.
Norman GR, Sloan JA, Wyrwich KW. Interpretation of changes in health-related quality of life: the remarkable universality of half a standard deviation. Med Care. 2003;41(5):582–92.
Wankah P, Guillette M, Dumas S, Couturier Y, Gagnon D, Belzile L, Mosbah Y, Breton M. Reorganising health and social care in Québec: a journey towards integrating care through mergers. London Journal of Primary Care. 2018;10(3):48–53.
Fleury É. Système de santé québécois: défis de taille à relever. In: Le Soleil. 2017.
Müller E, Hahlweg P, Scholl I. What do stakeholders need to implement shared decision making in routine cancer care? A qualitative needs assessment Acta Oncologica. 2016;55(12):1484–91.
Scholl I, LaRussa A, Hahlweg P, Kobrin S, Elwyn G. Organizational- and system-level characteristics that influence implementation of shared decision-making and strategies to address them — a scoping review. Implement Sci. 2018;13(1):40.
Gutman C, Cohen A, Redlich Amirav D. SDM Training Modules for Health and Social Care Professionals in Israel. Front Psychiatry. 2021;12: 679036.
Levin L, Gewirtz S, Cribb A. Shared decision making in Israeli social services: Social workers’ perspectives on policy making and implementation. Br J Soc Work. 2017;47(2):507–23.
Grant A, Kontak J, Jeffers E, Lawson B, MacKenzie A, Burge F, Boulos L, Lackie K, Marshall EG, Mireault A, et al. Barriers and enablers to implementing interprofessional primary care teams: a narrative review of the literature using the consolidated framework for implementation research. BMC Primary Care. 2024;25(1):25.
Ndibu Muntu Keba Kebe N, Chiocchio F, Bamvita J-M, Fleury M-J. Variables associated with interprofessional collaboration:The case of professionals working in Quebec local mental health service networks. J Interprof Care. 2019;33(1):76–84.
Godin G. Les comportements dans le domaine de la santé : comprendre pour mieux intervenir. Montréal: Les Presses de l’Université de Montréal; 2012.
Book Google Scholar
Coates D, Clerke T. Training Interventions to Equip Health Care Professionals With Shared Decision-Making Skills: A Systematic Scoping Review. J Contin Educ Heal Prof. 2020;40(2):100–19.
Boland L, Lawson ML, Graham ID, Légaré F, Dorrance K, Shephard A, Stacey D. Post-training shared decision making barriers and facilitators for pediatric healthcare providers: a mixed-methods study. Acad Pediatr. 2019;19(1):118–29.
Giguere AM, Labrecque M, Haynes RB, Grad R, Pluye P, Légaré F, Cauchon M, Greenway M, Carmichael P-H. Evidence summaries (decision boxes) to prepare clinicians for shared decision-making with patients: a mixed methods implementation study. Implement Sci. 2014;9:1–13.
Herron AP, Agbadje TT, Guay-Bélanger S, Ngueta G, Roch G, Rousseau F, Légaré F. Web-Based Training for Nurses on Using a Decision Aid to Support Shared Decision-making About Prenatal Screening: Parallel Controlled Trial. JMIR nursing. 2022;5(1): e31380.
Elwyn G, Tilburt J, Montori V. The ethical imperative for shared decision-making. Eur J Pers Centered Healthc. 2013;1(1):129–31.
Download references
Acknowledgements
We thank Louisa Blair for revising and editing the manuscript.
The parent study was funded by the Canadian Institutes of Health Research (Grant number: 201403MOP-325236-KTR-CFBA-19158), and also supported by the CIUSSS de la Capitale-Nationale (in kind contribution included in the CIHR grant). No other funding sources were used in this secondary study. The funders had no role in the design and conduct of the study; collection, management, analysis, and interpretation of the data; preparation, review, or approval of the manuscript; or the decision to submit the manuscript for publication.
Author information
Authors and affiliations.
Department of Mathematics and Statistics, Faculty of Science and Engineering, Université Laval, Quebec City, QC, Canada
Hajar Taqif & Louis-Paul Rivest
VITAM - Centre de Recherche en Santé Durable, Centre Intégré Universitaire de Santé Et de Services Sociaux de La Capitale-Nationale, Quebec City, QC, Canada
Hajar Taqif, Lionel Adisso, Lucas Gomes Souza, Suélène Georgina Dofara, Sergio Cortez Ghio & France Légaré
Department of Social and Preventive Medicine, Faculty of Medicine, Université Laval, Quebec City, QC, Canada
Lionel Adisso & Lucas Gomes Souza
Department of Family Medicine and Emergency Medicine, Faculty of Medicine, Université Laval, Quebec City, QC, Canada
France Légaré
You can also search for this author in PubMed Google Scholar
Contributions
This study was secondary analysis of a parent study led by the Chairholder of the Tier 1 Canada Research Chair in Shared Decision Making and Knowledge Translation. FL designed and wrote the parent study. HT designed and wrote this study. HT conceived the statistical analysis plan and performed data cleaning. HT and SCG performed statistical analyses. HT, FL, SCG, LA, SGD, LGS and L-PR interpreted the data. HT and LGS drafted the manuscript. All authors critically reviewed the manuscript for important intellectual content. All authors have read and approved the final version of the manuscript. FL is its guarantor.
Corresponding author
Correspondence to France Légaré .
Ethics declarations
Ethics approval and consent to participate.
Ethics committee review approval was obtained from the Multicenter Ethics Committee of the Centre intégré de santé et de services sociaux de Laval (2015–2016/01–01-E). Throughout the study, all methods followed the approved methodology in accordance with the Declaration of Helsinki and adhered to the relevant guidelines and regulations. Participation was voluntary and anonymous. All participant gave informed consent before completing each questionnaire.
Consent for publication
Not applicable.
Competing interests
The authors declare no competing interests.
Additional information
Publisher’s note.
Springer Nature remains neutral with regard to jurisdictional claims in published maps and institutional affiliations.
Supplementary Information
Supplementary material 1, supplementary material 2, supplementary material 3, supplementary material 4, rights and permissions.
Open Access This article is licensed under a Creative Commons Attribution 4.0 International License, which permits use, sharing, adaptation, distribution and reproduction in any medium or format, as long as you give appropriate credit to the original author(s) and the source, provide a link to the Creative Commons licence, and indicate if changes were made. The images or other third party material in this article are included in the article's Creative Commons licence, unless indicated otherwise in a credit line to the material. If material is not included in the article's Creative Commons licence and your intended use is not permitted by statutory regulation or exceeds the permitted use, you will need to obtain permission directly from the copyright holder. To view a copy of this licence, visit http://creativecommons.org/licenses/by/4.0/ . The Creative Commons Public Domain Dedication waiver ( http://creativecommons.org/publicdomain/zero/1.0/ ) applies to the data made available in this article, unless otherwise stated in a credit line to the data.
Reprints and permissions
About this article
Cite this article.
Taqif, H., Adisso, L., Gomes Souza, L. et al. Changes in intention to use an interprofessional approach to decision-making following training: a cluster before-and-after study. BMC Health Serv Res 24 , 437 (2024). https://doi.org/10.1186/s12913-024-10899-z
Download citation
Received : 17 March 2023
Accepted : 26 March 2024
Published : 08 April 2024
DOI : https://doi.org/10.1186/s12913-024-10899-z
Share this article
Anyone you share the following link with will be able to read this content:
Sorry, a shareable link is not currently available for this article.
Provided by the Springer Nature SharedIt content-sharing initiative
- Health personnel
- Community services
- Shared decision making
BMC Health Services Research
ISSN: 1472-6963
- General enquiries: [email protected]
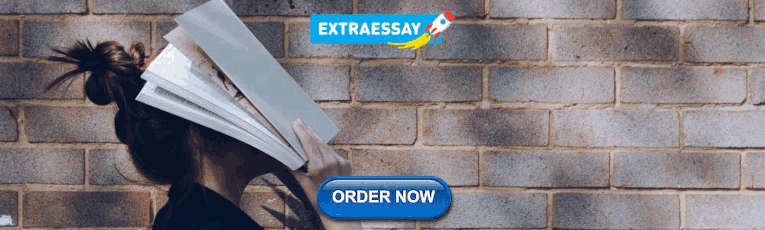
IMAGES
VIDEO
COMMENTS
This article explains the following concepts: Independent variables, dependent variables, confounding variables, operationalization of variables, and construction of hypotheses. In any body of research, the subject of study requires to be described and understood.
The independent variable is the cause. Its value is independent of other variables in your study. The dependent variable is the effect. Its value depends on changes in the independent variable. Example: Independent and dependent variables. You design a study to test whether changes in room temperature have an effect on math test scores.
So, it is usual for research protocols to include many independent variables and many dependent variables in the generation of many hypotheses, as shown in Table 1. Pairing each variable in the "independent variable" column with each variable in the "dependent variable" column would result in the generation of these hypotheses.
Designation of the dependent and independent variable involves unpacking the research problem in a way that identifies a general cause and effect and classifying these variables as either independent or dependent. The variables should be outlined in the introduction of your paper and explained in more detail in the methods section. There are no ...
While the independent variable is the " cause ", the dependent variable is the " effect " - or rather, the affected variable. In other words, the dependent variable is the variable that is assumed to change as a result of a change in the independent variable. Keeping with the previous example, let's look at some dependent variables ...
In research, the independent variable is manipulated to observe its effect, while the dependent variable is the measured outcome. Essentially, the independent variable is the presumed cause, and the dependent variable is the observed effect. Variables provide the foundation for examining relationships, drawing conclusions, and making ...
Independent variables are also labeled explanatory or predictor variables. Dependent variables may be called outcome, response, or criterion variables. Much of the research process investigates the status of "true" independent variables and eliminating false or "spurious" causality. Although it is more difficult to designate independent ...
1. Experimental variables: An experimental independent variable is one that researchers can manipulate to test downstream outcomes. Experimental variables are also called controlled variables due to researchers' ability to control them. 2. Subject variables: Subject variables cannot be controlled by researchers.
A deep understanding of variables is crucial for designing, conducting, and analyzing research effectively. In this article, we break down the complex concept of variables in scientific research, offering insights into their roles and functions, along with examples of independent and dependent variables.
Example (salt tolerance experiment) Independent variables (aka treatment variables) Variables you manipulate in order to affect the outcome of an experiment. The amount of salt added to each plant's water. Dependent variables (aka response variables) Variables that represent the outcome of the experiment.
The dependent variable is the variable that is being measured or tested in an experiment. For example, in a study looking at how tutoring impacts test scores, the dependent variable would be the participants' test scores since that is what is being measured. This is different than the independent variable in an experiment, which is a variable ...
Here is a simple rule that you can apply at all times: the independent variable is what a researcher changes, whereas the dependent variable is affected by these changes. To illustrate the difference, a number of examples are provided below. The purpose of Study 1 is to measure the impact of different plant fertilizers on how many fruits apple ...
The independent variable is the cause. Its value is independent of other variables in your study. The dependent variable is the effect. Its value depends on changes in the independent variable. Example: Independent and dependent variables. You design a study to test whether changes in room temperature have an effect on maths test scores.
Ordinal dependent variable: An ordinal variable is a categorical variable that has a specific order or ranking to its categories. Examples include education level (e.g., high school diploma, college degree, graduate degree), or socioeconomic status (e.g., low, middle, high). Interval dependent variable: An interval variable is a continuous ...
Independent and dependent variables are crucial elements in research. The independent variable is the entity being tested and the dependent variable is the result. Check out this article to learn more about independent and dependent variable types and examples.
Chapter 2: Dependent Variables: Measuring Human Behavior. The dependent variable in human behavior research acts as a mirror, reflecting the outcomes or effects resulting from variations in the independent variable. It is the aspect of human experience or behavior that researchers aim to understand, predict, or change through their studies.
A dependent variable is the variable being tested in a scientific experiment. The dependent variable is "dependent" on the independent variable. As the experimenter changes the independent variable, the change in the dependent variable is observed and recorded. When you take data in an experiment, the dependent variable is the one being measured.
There seems to be a fair amount of research on motivation as an independent variable, but research on motivation as a dependent variable is scarce. This review identifies a gap in the literature on this particular issue, especially because identifying factors influencing motivation could help medical educators incorporate them into design of a ...
As described earlier, each price variable was used as the dependent variable in a regression that included the independent variables identified in earlier research as effective predictors of journal prices. (See below.) The dependent variables were entered in natural log form in order to maintain linearity.
This statement is based on background research and current knowledge.8,9 The research hypothesis makes a specific prediction about a new phenomenon10 or a formal statement on the expected relationship between an independent variable and a dependent variable.3,11 It provides a tentative answer to the research question to be tested or explored.4
Dependent variable: intention. The dependent variable of this study was the intention of healthcare professionals in home care teams to engage in an IP-SDM approach, defined as the mean of the scores of the 2 CPD-Reaction questions (items) on intention as measured on a 7-point Likert-type scale (1 = Strongly Disagree to 7 = Strongly Agree).
In this paper, we deal with the just-in-time job shop scheduling problem with sequence-dependent setup times and release dates. Given a set of jobs characterized by release and due dates, the goal is to execute them by minimizing a weighted sum of their earliness, tardiness, and flow time (i.e., the difference between completion and start time of each job).