Comunicação Pública
Início Números Vol.2 nº4 / nº3 Nº3 Artigos Drivers of shopping online: a lit...
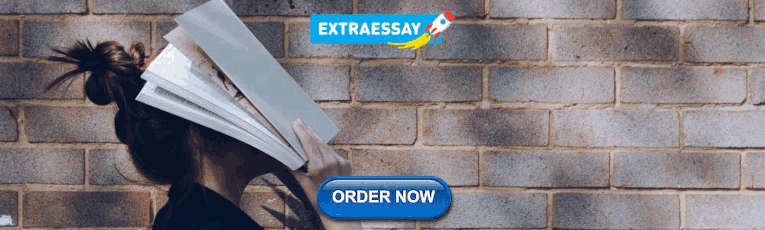
Drivers of shopping online: a literature review
Consumers are increasingly adopting electronic channels for purchasing. Explaining online consumer behavior is still a major issue as studies available focus on a multiple set of variables and relied on different approaches and theoretical foundations. Based on previous research two main drivers of online behavior are identified: perceived benefits of online shopping related to utilitarian and hedonic characteristics and perceived risk. Additionally, exogenous factors are presented as moderating variables of the relationship between perceived advantages and disadvantages of internet shopping and online consumer behavior.
Entradas no índice
Keywords: , texto integral, 1. introduction.
1 The increasing dependence of firms on e-commerce activities and the recent failure of a large number of dot-com companies stresses the challenges of operating through virtual channels and also highlights the need to better understand consumer behavior in online market channels in order to attract and retain consumers.
2 While performing all the functions of a traditional consumer, in Internet shopping the consumer is simultaneously a computer user as he or she interacts with a system, i.e., a commercial Web site. On the other hand, the physical store has been transformed into Web-based stores that use networks and Internet technology for communications and transactions.
3 In this sense, there seems to be an understanding that online shopping behavior is fundamentally different from that in conventional retail environment, (Peterson et al ., 1997) as e-commerce relies on hypertext Computer Mediated Environments (CMEs) and the interaction customer-supplier is ruled by totally different principles.
4 Understanding the factors that explain how consumers interact with technology, their purchase behavior in electronic channels and their preferences to transact with an electronic vendor on a repeated basis is crucial to identify the main drivers of consumer behavior in online market channels.
5 Online consumer behavior research is a young and dynamic academic domain that is characterized by a diverse set of variables studied from multiple theoretical perspectives.
6 Researchers have relied on the Technology Acceptance Model (Davis, 1989: Davis et al ., 1989), the Theory of Reasoned Action (Fisbein and Ajzen, 1975), the Theory of Planned Behavior (Ajzen, 1991), Innovation Diffusion Theory (Rogers, 1995), Flow Theory (Czikszentmihalyi, 1998), Marketing, Information Systems and Human Computer Interaction Literature in investigating consumer’s adoption and use of electronic commerce.
7 While these studies individually provide meaningful insights on online consumer behavior, the empirical research in this area is sparse and the lack of a comprehensive understanding of online consumer behavior is still a major issue (Saeed et al ., 2003).
8 Previous research on consumer adoption of Internet shopping (Childers et al ., 2001; Dabholkar and Bagozzi, 2002; Doolin et al ., 2005; Monsuwé et al .; 2004; O´Cass and Fenech, 2002) suggests that consumers’ attitude toward Internet shopping and intention to shop online depends primarily on the perceived features of online shopping and on the perceived risk associated with online purchase. These relationships are moderated by exogenous factors like “consumer traits”, “situational factors”, “product characteristics” and “previous online shopping experiences”.
9 The outline of this paper is as follow. In the next section an assessment of the basic determinants that positively affect consumers’ intention to buy on the Internet is carried out. Second, the main perceived risks of shopping online are identified as factors that have a negative impact on the intention to buy from Internet vendors. Third, since it has been argued that the relationship between consumers’ attitude and intentions to buy online is moderated by independent factors, an examination of the influence of these factors is presented. Finally, the main findings, the importance to professionals and researchers and limitations are summarized.
2. Perceived benefits in online shopping
10 According to several authors (Childers et al ., 2001; Mathwick et al ., 2001; Menon and Kahn, 2002;) online shopping features can be either consumers’ perceptions of functional or utilitarian dimensions, or their perceptions of emotional and hedonic dimensions.
11 Functional or utilitarian perceptions relate to how effective shopping on the Internet is in helping consumers to accomplish their task, and how easy the Internet as a shopping medium is to use. Implicit to these perceptions is the perceived convenience offered by Internet vendor whereas convenience includes the time and effort saved by consumers when engaging in online shopping (Doolin, 2005; Monsuwé, 2004).
12 Emotional or hedonic dimensions reflect consumers’ perceptions regarding the potential enjoyment or entertainment of Internet shopping (Doolin, 2005; Monsuwé, 2004).
13 Venkatesh (2000) reported that perceived convenience offered by Internet Vendors has a positive impact on consumers’ attitude towards online shopping, as they perceive Internet as a medium that enhances the outcome of their shopping experience in an easy way.
14 Childers et al . (2001) found “enjoyment” to be a consistent and strong predictor of attitude toward online shopping. If consumers enjoy their online shopping experience, they have a more positive attitude toward online shop ping, and are more likely to adopt the Internet as a shopping medium.
15 Vijayasarathy and Jones (2000) showed that Internet shopping convenience, lifestyle compatibility and fun positively influence attitude towards Internet shopping and intention to shop online.
16 Despite the perceived benefits in online shopping mainly associated with convenience and enjoyment, there are a number of possible negative factors associated with the Internet shopping experience. These include the loss of sensory shopping or the loss of social benefits associated with shopping (Vijayasarathy and Jones, 2000).
17 In their research, Swaminathan et al . (1999) found that the lack of social interaction in Internet shopping deterred consumers from online purchase who preferred dealing with people or who treated shopping as a social ex perience.
3. Perceived risk in online shopping
18 Although most of the purchase decisions are perceived with some degree of risk, Internet shopping is associated with higher ri sk by consumers due to its newness and intrinsic characteristics associated to virtual stores where there is no human contact and consumers cannot physically check the quality of a product or monitor the safety and security of sending sensitive personal and financial information while shopping on the Internet (Lee and Turban, 2001).
19 Several studies reported similar findings that perceived risk negatively influenced consumers’ attitude or intention to purchase online (Doolin, 2005; Liu and Wei, 2003; Van der Heidjen et al ., 2003).
20 Opposing results were reported in two studies (Corbitt et al ., 2003; Jar venpaa et al ., 1999). The authors found that perceived risk of Internet shopping did not affect willingness to buy from an online store. One of the reasons for this contradictory conclusion might be due to the countries analyzed, respectively New Zealand and Australia, where individuals could be more risk- taken or more Internet heavy-users.
21 In examining the influences on the perceived risk of purchasing online, Pires at al. (2004) stated that no association was found between the fre quency of online purchasing and perceived risk, although satisfaction with prior Internet purchases was negatively associated with the perceived risk of intended purchases, but only for low-involvement products. Differences in perceived risk were associated with whether the intended purchase was a good or service and whether it was a high or low-involvement product. The perceived risk of purchasing goods through the Internet is higher than for services. Perceived risk was found to be higher for high-involvement than for low-involvement-products, be they goods or services.
22 Various types of risk are perceived in purchase decisions, including prod uct risk, security risk and privacy risk.
23 Product risk is the risk of making a poor or inappropriate purchase deci sion. Aspects involving product risk can be an inability to compare prices, being unable to return a product, not receiving a product paid for and product not performing as expected (Bhatnagar et al ., 2000; Jarvenpaa and Todd, 1997; Tan, 1999; Vijayasarathy and Jones, 2000).
24 Bhatnagar et al . (2000) suggest that the likelihood of purchasing on the Internet decreases with increases in product risk.
25 Other dimensions of perceived risk related to consumers’ perceptions on the Internet as a trustworthy shopping medium. For example, a common perception among consumers is that communicating credit card information over the Internet is inherently risky, due to the possibility of credit card fraud (Bhatnagar et al ., 2000; George, 2002; Hoffman et al ., (1999); Jarvenpaa and Todd, 1997; Liebermann and Stashevsky, 2002).
26 Previous studies found that beliefs about trustworthiness of the Internet were associated with positive attitudes toward Internet purchasing (George, 2002; Hoffman et al ., (1999); Liebermann and Stashevsky, 2002).
27 Privacy risk includes the unauthorized acquisition of personal information during Internet use or the provision of personal information collected by companies to third parties.
28 Perceived privacy risk causes consumers to be reluctant in exchanging personal information with Web providers (Hoffman et al ., 1999). The same authors suggest that with increasing privacy concerns, the likelihood of purchasing online decreases. Similarly, George (2002) found that a belief in the privacy of personal information was associated with negative attitudes toward Internet purchasing.
4. Exogenous factors
29 Based on the previous literature review, four exogenous factors were reported to be key drivers in moving consumers to ultim ately adopt the Internet as a shopping medium.
4.1. Consumer traits
30 Studies on online shopping behavior have focus mainly on demographic, psychographics and personality characteristics.
31 Bellman et al . (1999) cautioned that demographic variables alone explain a very low percentage of variance in the purchase decision.
32 According to Burke (2002) four relevant demographic factors – age, gen der, education, and income have a significant moderating effect on consum ers’ attitude toward online shopping.
33 In studying these variables several studies arrived to some contradictory results.
34 Concerning age, it was found that younger people are more interested in using new technologies, like the Internet, to search for comparative information on products (Wood, 2002). Older consumers avoid shopping online as the potential benefits from shopping online are offset by the perceived cost in skill needed to do it (Ratchford et al ., 2001).
35 On the other hand as younger people are associated with less income it was found that the higher a person’s income and age, the higher the propen sity to buy online (Bellman et al ., 1999; Liao and Cheung, 2001).
36 Gender differences are also related to different attitudes towards online shopping. Although men are more positive about using Internet as a shop ping medium, female shoppers that prefer to shop online, do it more frequently than male (Burke, 2002; Li et al ., 1999).
37 Furthermore Slyke et al . (2002) reported that as women view shopping as a social activity they were found to be less oriented to shop online than men.
38 Regarding education, higher educated consumers have a higher propen sity to use no-store channels, like the Internet to shop (Burke, 2002). This fact can be justified as education has been positively associated with individ ual’s level of Internet literacy (Li et al ., 1999).
39 Higher household income is often positively correlated with possession of computers, Internet access and higher education levels of consumers and consequently with a higher intention to shop online (Lohse et al ., 2000).
40 In terms of psychographics characteristics, Bellman et al . (1999) stated that consumers that are more likely to buy on the Internet have a “wired life” and are “starving of time”. Such consumers use the Internet for a long time for a multiple of purposes such as communicating through e-mail, reading news and search for information.
41 A personality characteristic closely associated with Internet adoption for shopping is innovativeness defined as the relative willingness of a person to try a new product or service (Goldsmith and Hokafer, 1991).
42 Innovativeness seems to influence more than frequency of online purchasing. It relates to the variety of product classes bought online, both in regard to purchasing and to visiting Web sites seeking information. (Blake et al ., 2003). In this sense innovativeness might be a fundamental factor determining the quantity and quality of online shopping.
4.2. Situational factors
43 Situational factors are found to be factors that affect significantly the choice between different retail store formats when consumers are faced with a shopping decision (Gehrt and Yan, 2004). According to this study, the time pressure and purpose of the shopping (for a gift or for themselves) can change the consumers’ shopping habits. Results showed that traditional stores were preferred for self-purchase situations rather than for gift occasions as in this case other store formats (catalog and Internet) performed better in terms of expedition. As for time pressure it was found that it was not a significantly predictor of online shopping as consumers when faced with scarcity of time responded to temporal issues related to whether there is a lag of time between the purchase transaction and receipt of goods rather than whether shopping can take place anytime.
44 Contradictory results were reported by Wolfinbarger and Gilly (2001). According to this study important attributes of online shopping are convenience and accessibility. When faced with time pressure situations, consumers engaged in online shopping but no conclusions should be taken on the effect of this factor on the attitude toward Internet shopping.
45 Lack of mobility and geographical distance has also been addressed has drivers of online shopping as Internet medium offers a viable solution to overcome these barriers (Monsuwé et al ., 2004). According to the same au thors the physical proximity of a traditional store that sells the same prod ucts available online, can lead consumers to shop in the “brick and mortar” alternative due to its perceived attractiveness despite consumers’ positive attitude toward shopping on the Internet.
46 The need for special items difficult to find in traditional retail stores has been reported a situational factor that attenuates the relationship between attitude and consumers’ intention to shop online (Wolfinbarger and Gilly, 2001).
4.3. Product characteristics
47 Consumers' decisions whether or not to shop online are also influenced by the type of product or service under consideration.
48 The lack of physical contact and assistance as well as the need to “feel” somehow the product differentiates products according to their suitability for online shopping.
49 Relying on product categories conceptualized by information economists, Gehrt and Yan (2004), reported that it is more likely that search goods (i.e. books) can be adequately assessed within a Web than experience goods (i.e. clothing), which usually require closer scrutiny.
50 Grewal et al . (2002) and Reibstein (1999) referred to standardized and fa miliar products as those in which quality uncertainty is almost absent and do not need physical assistance or pre-trial. These products such as groceries, books, CDs, videotapes have a high potential to be considered when shopping online.
51 Furthermore in case of certain sensitive products there is high potential to shop online to ensure adequate levels of privacy and anonymity (Grewal et al ., 2002). Some of these products like medicine and pornographic movies are raising legal and ethical issues among international community.
52 On the other hand, personal-care products like perfume or products that required personal knowledge and experience like cars or computers, are less likely to be considered when shopping online (Elliot and Fowell, 2000).
4.4. Previous online shopping experiences
53 Past research suggests that prior online shopping experiences have a direct impact on Internet shopping intentions. Satisfactory previous experiences decreases consumers’ perceived risk levels associated with online shopping but only across low-involvement goods and services (Monsuwé et al ., 2004).
54 Consumers that evaluate positively the previous online experience are motivated to continue shopping on the Internet (Eastlick and Lotz, 1999; Shim et al ., 2001; Weber and Roehl, 1999).
5. Conclusion
55 Relying on an extensive literature review, this paper aims to identify the main drivers of online shopping and thus to give further insights in explaining consumer behavior when adopting the Internet for buying as this issue is still in its infancy stage despite its major importance for academic and professionals.
56 This literature review shows that attitude toward online shopping and in- tention to shop online are not only affected by perceived benefits and perceived risks, but also by exogenous factors like consumer traits, situations factors, product characteristics, previous online shopping experiences.
57 Understanding consumers’ motivations and limitations to shop online is of major importance in e-business for making adequate strategic options and guiding technological and marketing decisions in order to increase customer satisfaction. As reported before consumers´ attitude toward online shopping is influenced by both utilitarian and hedonic factors. Therefore, e-marketers should emphasize the enjoyable feature of their sites as they promote the convenience of shopping online. As personal characteristics also affect buyers´ attitudes and intentions to engage in Internet shopping e-tailers should customize customers´ treatment. Furthermore, the e-vendor should assure a trust-building relationship with its customers to minimize perceived risk associated to online shopping. Adopting and communicating a clear privacy policy, using a third party seal and offering guarantees are mechanisms that can help in creating a reliable environment.
58 Some limitations of this paper must be pointed out as avenues for future. The factors identified as main drives of shopping online are the result of a literature review and there can always be factors of influence on consumers´ intentions to shop on the Internet that are not included because they are addressed in other studies not included in this review. However there are methodological reasons to believe that the most relevant factors were identified in this context. A second limitation is that this paper is the result of a literature review and has never been tested in its entirety using empirical evidence. This implies that some caution should be taken in applying the findings that can be derived from this paper Further research is also needed to determine which of the factors have the most significant effect on behavioral intention to shop on the Internet.
Bibliografia
Ajzen, I. (1991) The theory of planned behavior: some unresolved issues. Organizational Behavior and Human Decisions Processes , 50 (2), pp. 179-211.
Bellman, S., Lohse, G., and Johnson, E. (1999) Predictors of online buying behavior. Communica tions of the Association for the Comptuting Machinery , 42 (12), pp. 32-38.
Bhatnagar, A., Misra, S., and Rao, H. R. (2000) On risk, convenience and internet shopping behavior. Communications of the Association for Computing Machinery , pp. 43 (11), 98-105.
Blake, B. F., Kimberly, A. N., and Colin, M. V. (2003) Innovativeness and variety of internet shopping. Internet Research , 13 (3), pp. 156-169.
Burke, R. R. (2002) Technology and the customer interface: what consumers want in the physical and virtual store. Journal of the Academy of Marketing Science , 30 (4), pp. 411-432.
Childers, T. L., Carr, C. L., Peck, J., and Carson, S. (2001) Hedonic and utilitarian motivations for online retail shopping behavior. Journal of Retailing , 77 (4), pp. 511-535.
Corbitt, B. J., Thanasanki, T., and Yi, H. (2003) Trust and e-commerce: a study of consumer perceptions. Electronic Commerce Research and Applications , 2, pp. 203-215.
Csikszentmihalyi, M. (1988) Optimal experience: psychological studies of flow in cousciousness . U.K, Cambridge University Press.
Dabholkar, P. A. and Bagozzi R. P. (2002) An attitudinal model of technology-based self-service: moderating effects of consumer traits and situational factors. Journal of the Academy of Marketing Science , 30 (3), pp. 184-201.
Davis, F. D. (1989) Perceived usefulness, perceived ease of use and user acceptance of information techonology. MIS Quaterly , 13 (4), pp. 319-340.
Davis, F. D., Bagozzi, R. P., and Warshaw, P. R. (1989) User acceptance of computer technology: a comparation of two theoretical models. Management Science , 35 (8), pp. 982-1002.
Doolin, B., Dillon, S., Thompson, F., and Corner, J. L. (2005) Perceived risk, the internet shopping experience and online purchasing behavior: a New Zeland perspective. Journal of Global Information Management , 13 (2), pp. 66-88.
Eastlick, M. A. and Lotz, S. L. (1999) Profiling potential adopters of an interactive shopping medium. International Journal of Retail and Distribution Management, pp. 27 (6/7), 209-223.
Elliot, S. and Fowell, S. (2000) Expectations versus reality: a snapshot of consumer experiences with internet retailing. International Journal of Information Management, 20 (5), pp. 323- 336.
Fishbein, M., and Ajzen, I. (1975) Belief, attitude, intention and behavior: an introduction to theory and research . Reading, MA, Addison-Wesley.
Gehrt, K. C. and Yan, R-N. (2004) Situational, consumer, and retail factors affecting internet, catalog, and store shopping. International Journal of Retail and Distribution Management , 32 (1), pp. 5-18.
George, J. F. (2002) Influences on the intent to make internet purchases. Internet Research , 12 (2), pp. 165-180.
Goldsmith, R. E. and Hofacker, C. F. (1991) Measuring consumer innovativeness. Journal of the Academy of Marketing Science , 19 (3), pp. 209-221.
Grewal, D., Iyer, G. R., and Levy, M. (2002) Internet retailing: enablers, limiters and market con sequences. Journal of Business Research .
Hoffman, D. L., Novak, T. P., and Peralta, M. (1999) Building consumer trust online. Communica tion of the Association of Computing Machinery , 42 (4), pp. 80-85.
Jarvenpaa, S. and Todd, P. (1997) Consumer reactions to electronic shopping on the world wide web. International Journal of Electronic Commerce , 1 (2), pp. 59-88.
Jarvenpaa, S., Tractinsky, N., and Vitale, M. (1999) Consumer trust in an internet store. Informa tion Technology and Managemet , 1 (1/2), pp. 45-72.
Lee, M. K.-O. and Turban, E. (2001). A trust model for consumer internet shopping. International Journal of Electronic Commerce , 6 (1), 75-91.
Li, H., Kuo, C., and Russel, M. G. (1999) The impact of perceived channel utilities, shopping orientations, and demographics on the consumer’s online buying behavior. Journal of Com- puter-Mediated Communications , 5 (2).
Liao, Z. and Cheung, M. T. (2001) Internet based e-shopping and consumer attitudes: an empirical study. Information and Management , 38 (5), pp. 299-306.
Liebermann, Y. and Stashevsky, S. (2002) Perceived risks as barriers to internet and e-commerce usage. Qualitative Market Research , 5 (4), pp. 291-300.
Liu, X. and Wei, K. K. (2003) An empirical study of product differences in consumers’ e-commerce adoption behavior. Electronic Commerce Research and Applications , 2, pp. 229-239.
Lohse, G. L., Bellman, S., and Johnson, E. J. (2000) Consumer buying behavior on the internet: findings from panel data. Journal of Interactive Marketing , 14 (1), pp. 15-29.
Mathwick, C., Malhotra, N. K. and Rigdon, E. (2001) Experiential value: conceptualisation, measurement and application in the catalog and internet shopping environment. Journal of Re- tailing , 77 (1), pp. 39-56.
Menon, S. and Kahn, P. (2002) Cross-category effects of induced arousal and pleasure on the internet shopping experience. Journal of Retailing , 78 (1), pp. 31-40.
Monsuwé, T. P., Dellaert, G. C.and de Ruyter, K. (2004) What drives consumers to shop online? A literature review. International Journal of Service Industry Management , 15 (1), pp. 102-121.
O’Cass, A. and Fenech, T. (2002) Web retailing adotion: exploring the nature of Internet users web retailing behavior. Journal of Retailing and Consumer Services , 13 (2), pp. 151-167.
Peterson, R. A., Balasubramaniam, S., and Bronnenberg, B. J. (1997) Exploring the implications of the internet for consumer marketing. Journal of the Academy of Marketing Science , 25 (4), pp. 329-346.
Pires, G., Staton, J., and Eckford, A. (2004) Influences of the perceived risk of purchasing online. Journal of Consumer Behavior , 4 (2), pp. 118-131.
Ranganathan, C. and Ganapathy, S. (2002) Key dimensions of business-to-consumer web sites. Information and Management , 39 (6), pp. 457-465.
Ratchford, B. T., Talukdar, D., and Lee, M.-S. (2001) A model of consumer choice of the internet as an information source. International Journal of Electronic Commerce , 5 (3), pp. 7-21.
Reibstein, D. J. (1999) Who is buying on the Internet, 1999? Working Paper, The Wharton School, University of Philadelphia, PA.
Rogers, E. M. (1985) Diffusion of innovations . New York: Free Press.
Saeed, K. A., Hwang, Y., and Yi, M. Y. (2003) Toward an integrative framework for online con sumer behavior research: a meta-analysis approach. Journal of End User Computing , 15 (4), pp. 1-26.
Shim, S., Eastlick, M. A., Lotz, S. L., and Warrington, P. (2001) An online prepurchase intentions model: the role of intention to saerch. Journal of Retailing , 77 (3), pp. 397-416.
Slyke, C. V., Comunale, C. L., and Belanger, F. (2002). Gender differences in perceptions of web-based shoping. Communications of the Association for Computing Machinery , 45 (7), 82-86.
Swaminathan, V., Lepkowska-White, E., and Rao, B. P. (1999) Browsers or buyers in cyberspace? An investigation of factors influencing electronic exchanges. Journal of Computer-Mediated Communication , 5 (2).
Tan, S. J. (1999) Strategies for reducing consumers’ risk aversion in internet shopping. Journal of Consumer Marketing , 16 (2), pp. 163-180.
van der Heidjen, H., Verhagen, T., and Creemers, M. (2003) Understanding online purchase intentions: Contributions from technology and trust perspectives. European Journal of Infor- mation Systems , 12, pp. 41-48.
Vijayasarathy, L. R. and Jones, J. M. (2000) Print and internet catalog shopping: assessing atti tudes and intentions. Internet Research , 10 (3), pp. 191-202.
Weber, K. and Roehl, W. S. (1999). Profiling people searching for and purchasing travel products on the world wide web. Journal of Travel Research , 37, 291-298.
Wolfinbarger, M. and Gilly, M. C. (2001) Shopping online for freedom, control, and fun. California Management Review , 43 (2), pp. 34-55.
Wood, S. L. (2002) Future fantasies: a social change perspective of retailing in the 21 st century. Journal of Retailing , 78 (1), pp. 77-83.
Para citar este artigo
Referência do documento impresso.
Ana Teresa Machado , «Drivers of shopping online: a literature review» , Comunicação Pública , Vol.2 nº4 / nº3 | 2006, 39-50.
Referência eletrónica
Ana Teresa Machado , «Drivers of shopping online: a literature review» , Comunicação Pública [Online], Vol.2 nº4 / nº3 | 2006, posto online no dia 30 outubro 2020 , consultado o 08 abril 2024 . URL : http://journals.openedition.org/cp/8402; DOI : https://doi.org/10.4000/cp.8402
Ana Teresa Machado
Escola Superior de Comunicação Social Instituto Politécnico de Lisboa
Artigos do mesmo autor
- Música na publicidade: compondo a relação entre marca e consumidor [Texto integral] Music in advertising: composing the relationship between brand and consumer Publicado em Comunicação Pública , Vol.11 nº 20 | 2016
- Patrocínio e influência na atitude relativamente à marca e intenção de compra: caso Nike e Selecção Portuguesa de Futebol [Texto integral] Sponsorship and its influence on attitude towards the brand and purchase intention: Nike and Portuguese Soccer Team case study Publicado em Comunicação Pública , Vol.9 n15 | 2014
Direitos de autor

Apenas o texto pode ser utilizado sob licença CC BY-NC 4.0 . Outros elementos (ilustrações, anexos importados) são "Todos os direitos reservados", à exceção de indicação em contrário.
- Palavras-chave
Números em texto integral
- 2021 Vol.16 nº 30
- 2020 Vol.15 nº 28 | Vol.15 nº 29
- 2019 Vol.14 nº 26 | Vol.14 nº 27
- 2018 Vol.13 nº 24 | Vol.13 nº 25
- 2017 Vol.12 nº 22 | Vol.12 nº 23
- 2016 Vol.11 nº 20 | Vol.11 nº 21
- 2015 Vol.10 nº17 | vol.10 nº 18 | Vol.10 nº 19
- 2014 Vol.9 n15 | Vol.9 nº16
- 2013 vol.8 n13 | vol.8 n14
- 2012 vol.7 n11 | vol.7 n12
- 2011 vol.6 n10 | Especial 01E
- 2010 Vol.5 nº 9
- 2009 Vol.4 nº8 / nº7
- 2008 Vol.3 nº 6
- 2007 vol.3 nº5
- 2006 Vol.2 nº4 / nº3
- 2005 Vol.1 nº1 | Vol.1 nº2
Todos os números
Apresentação.
- Projeto Editorial
- Equipa Editorial
- Comissão Editorial
- Comissão Científica
- Revisores 2009-2020
- Normas de publicação
- Declaração de normas éticas de publicação e boas-práticas editoriais
Informações
- Menções legais e creditos
Chamadas para contribuições
- Chamadas fechadas
- Chamadas abertas

Newsletter informativa
- Newsletter da OpenEdition
Filiações/parceiros

ISSN electrónico 2183-2269
Consultar a ficha no catálogo OpenEdition
Mapa do site – Feed RSS
Privacy Policy – About Cookies – Assinalar um problema
Subscrevemos OpenEdition – Editado com Lodel – Acesso reservado
Você sera redirecionado para OpenEdition Search
Academia.edu no longer supports Internet Explorer.
To browse Academia.edu and the wider internet faster and more securely, please take a few seconds to upgrade your browser .
Enter the email address you signed up with and we'll email you a reset link.
- We're Hiring!
- Help Center
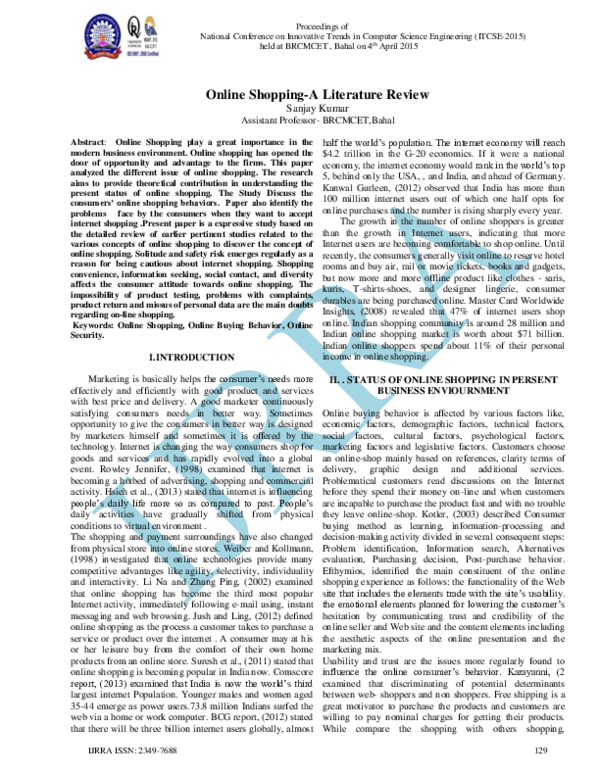
Online Shopping-A Literature Review

Online Shopping play a great importance in the modern business environment. Online shopping has opened the door of opportunity and advantage to the firms. This paper analyzed the different issue of online shopping. The research aims to provide theoretical contribution in understanding the present status of online shopping. The Study Discuss the consumers' online shopping behaviors. Paper also identify the problems face by the consumers when they want to accept internet shopping .Present paper is a expressive study based on the detailed review of earlier pertinent studies related to the various concepts of online shopping to discover the concept of online shopping. Solitude and safety risk emerges regularly as a reason for being cautious about internet shopping. Shopping convenience, information seeking, social contact, and diversity affects the consumer attitude towards online shopping. The impossibility of product testing, problems with complaints, product return and missus of personal data are the main doubts regarding on-line shopping.
- We're Hiring!
- Help Center
- Find new research papers in:
- Health Sciences
- Earth Sciences
- Cognitive Science
- Mathematics
- Computer Science
- Academia ©2024
To read this content please select one of the options below:
Please note you do not have access to teaching notes, what drives consumers to shop online a literature review.
International Journal of Service Industry Management
ISSN : 0956-4233
Article publication date: 1 February 2004
While a large number of consumers in the US and Europe frequently shop on the Internet, research on what drives consumers to shop online has typically been fragmented. This paper therefore proposes a framework to increase researchers’ understanding of consumers’ attitudes toward online shopping and their intention to shop on the Internet. The framework uses the constructs of the Technology Acceptance Model (TAM) as a basis, extended by exogenous factors and applies it to the online shopping context. The review shows that attitudes toward online shopping and intention to shop online are not only affected by ease of use, usefulness, and enjoyment, but also by exogenous factors like consumer traits, situational factors, product characteristics, previous online shopping experiences, and trust in online shopping.
- Information media
Perea y Monsuwé, T. , Dellaert, B.G.C. and de Ruyter, K. (2004), "What drives consumers to shop online? A literature review", International Journal of Service Industry Management , Vol. 15 No. 1, pp. 102-121. https://doi.org/10.1108/09564230410523358
Emerald Group Publishing Limited
Copyright © 2004, Emerald Group Publishing Limited
Related articles
We’re listening — tell us what you think, something didn’t work….
Report bugs here
All feedback is valuable
Please share your general feedback
Join us on our journey
Platform update page.
Visit emeraldpublishing.com/platformupdate to discover the latest news and updates
Questions & More Information
Answers to the most commonly asked questions here
Online shopping behavior model: A literature review and proposed model
Ieee account.
- Change Username/Password
- Update Address
Purchase Details
- Payment Options
- Order History
- View Purchased Documents
Profile Information
- Communications Preferences
- Profession and Education
- Technical Interests
- US & Canada: +1 800 678 4333
- Worldwide: +1 732 981 0060
- Contact & Support
- About IEEE Xplore
- Accessibility
- Terms of Use
- Nondiscrimination Policy
- Privacy & Opting Out of Cookies
A not-for-profit organization, IEEE is the world's largest technical professional organization dedicated to advancing technology for the benefit of humanity. © Copyright 2024 IEEE - All rights reserved. Use of this web site signifies your agreement to the terms and conditions.
Advertisement
E-Commerce and Consumer Protection in India: The Emerging Trend
- Original Paper
- Published: 09 July 2021
- Volume 180 , pages 581–604, ( 2022 )
Cite this article
- Neelam Chawla ORCID: orcid.org/0000-0003-2161-1102 1 &
- Basanta Kumar ORCID: orcid.org/0000-0003-3339-7481 2
70k Accesses
34 Citations
8 Altmetric
Explore all metrics
Given the rapid growth and emerging trend of e-commerce have changed consumer preferences to buy online, this study analyzes the current Indian legal framework that protects online consumers ’ interests. A thorough analysis of the two newly enacted laws, i.e., the Consumer Protection Act, 2019 and Consumer Protection (E-commerce) Rules, 2020 and literature review support analysis of 290 online consumers answering the research questions and achieving research objectives. The significant findings are that a secure and reliable system is essential for e-business firms to work successfully; cash on delivery is the priority option for online shopping; website information and effective customer care services build a customer's trust. The new regulations are arguably strong enough to protect and safeguard online consumers' rights and boost India’s e-commerce growth. Besides factors such as s ecurity, privacy, warranty, customer service, and website information, laws governing consumer rights protection in e-commerce influence customers’ trust. Growing e-commerce looks promising with a robust legal framework and consumer protection measures. The findings contribute to the body of knowledge on e-commerce and consumer rights protection by elucidating the key factors that affect customer trust and loyalty and offering an informative perspective on e-consumer protection in the Indian context with broader implications.
Similar content being viewed by others
Consumer Protection in e-Commerce and Online Services
Service Issues Affecting Consumer Behaviour Towards E-commerce Purchases in India
Protection of Consumer Rights in E-Commerce in India
Avoid common mistakes on your manuscript.
Study Background
The study context, which discusses two key aspects, namely the rationale for consumer protection in e-commerce and its growth, is presented hereunder:
The Rationale for Consumer Protection in E-commerce
Consumer protection is a burning issue in e-commerce throughout the globe. E-Commerce refers to a mechanism that mediates transactions to sell goods and services through electronic exchange. E-commerce increases productivity and widens choice through cost savings, competitiveness and a better production process organisation Footnote 1 (Vancauteren et al., 2011 ). According to the guidelines-1999 of the Organisation for Economic Cooperation and Development (OECD), e-commerce is online business activities-both communications, including advertising and marketing, and transactions comprising ordering, invoicing and payments (OECD, 2000 ). OCED-1999 guidelines recognised, among others, three essential dimensions of consumer protection in e-commerce. All consumers need to have access to e-commerce. Second, to build consumer trust/confidence in e-commerce, the continued development of transparent and effective consumer protection mechanisms is required to check fraudulent, misleading, and unfair practices online. Third, all stakeholders-government, businesses, consumers, and their representatives- must pay close attention to creating effective redress systems. These guidelines are primarily for cross-border transactions (OECD, 2000 ).
Considering the technological advances, internet penetration, massive use of smartphones and social media penetration led e-commerce growth, the OECD revised its 1999 recommendations for consumer protection in 2016. The 2016-guidelines aim to address the growing challenges of e-consumers’ protection by stimulating innovation and competition, including non-monetary transactions, digital content products, consumers-to-consumers (C2C) transactions, mobile devices, privacy and security risks, payment protection and product safety. Furthermore, it emphasises the importance of consumer protection authorities in ensuring their ability to protect e-commerce consumers and cooperate in cross-border matters (OECD, 2016 ). The United Nations Conference on Trade and Development (UNCTAD), in its notes-2017, also recognises similar consumer protection challenges in e-commerce. The notes look into policy measures covering relevant laws and their enforcement, consumer education, fair business practices and international cooperation to build consumer trust (UNCTAD, 2017 ).
E-commerce takes either the domestic (intra-border) route or cross-border (International) transactions. Invariably, six e-commerce models, i.e. Business-to-Consumer (B2C), Business-to-Business (B2B), Consumer-to-Business (C2B), Consumer-to-Consumer (C2C), Business-to-Administration (B2A) and Consumer-to-Administration (C2A) operate across countries (UNESAP and ADB, 2019 ; Kumar & Chandrasekar, 2016 ). Irrespective of the model, the consumer is the King in the marketplace and needs to protect his interest. However, the focus of this paper is the major e-commerce activities covering B2B and B2C.
The OECD and UNCTAD are two global consumer protection agencies that promote healthy and competitive international trade. Founded in 1960, Consumer International Footnote 2 (CI) is a group of around 250 consumer organisations in over 100 countries representing and defending consumer rights in international policy forums and the global marketplace. The other leading international agencies promoting healthy competition in national and international trade are European Consumer Cooperation Network, ECC-Net (European Consumer Center Network), APEC Electronic Consumer Directing Group (APECSG), Iberoamerikanische Forum der Konsumer Protection Agenturen (FIAGC), International Consumer Protection and Enforcement Agencies (Durovic, 2020 ).
ICPEN, in the new form, started functioning in 2002 and is now a global membership organisation of consumer protection authorities from 64 countries, including India joining in 2019 and six observing authorities (COMESA, EU, GPEN, FIAGC, OECD and UNCTAD). While it addresses coordination and cooperation on consumer protection enforcement issues, disseminates information on consumer protection trends and shares best practices on consumer protection laws, it does not regulate financial services or product safety. Through econsumer.gov Footnote 3 enduring initiative, ICPEN, in association with the Federal Trade Commission (FTC), redresses international online fraud. Footnote 4 Econsumer.gov, a collaboration of consumer protection agencies from 41 countries around the world, investigates the following types of international online fraud:
Online shopping/internet services/computer equipment
Credit and debit
Telemarketing & spam
Jobs & making money
Imposters scam: family, friend, government, business or romance
Lottery or sweepstake or prize scams
Travel & vacations
Phones/mobile devices & phone services
Something else
Online criminals target personal and financial information. Online trading issues involve scammers targeting customers who buy/sell/trade online. Table 1 on online cross-border complaints of fraud reported by econsumer.gov reveals that international scams are rising. Total cross-border fraud during 2020 (till 30 June) was 33,968 with a reported loss of US$91.95 million as against 40,432 cases with a loss of US$ 151.3 million and 14,797 complaints with the loss of US$40.83 million 5 years back. Among others, these complaints included online shopping fraud, misrepresented products, products that did not arrive, and refund issues. Figure 1 shows that the United States ranked first among the ten countries where consumers lodged online fraud complaints based on consumer and business locations. India was the third country next to France for online fraud reporting in consumer locations, while it was the fifth nation for company location-based reporting. Besides the USA and India, Poland, Australia, the United Kingdom, Canada, Turkey, Spain, and Mexico reported many consumer complaints. Companies in China, the United Kingdom, France, Hong Kong, Spain, Canada, Poland and Turkey received the most complaints. The trend is a serious global concern, with a magnitude of reported loss of above 60%.
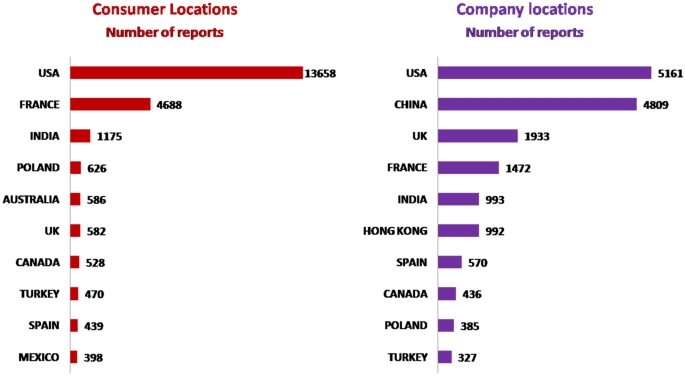
Source: Data compiled from https://public.tableau.com/profile/federal.trade.commission#!/vizhome/eConsumer/Infographic , Accessed 7 October 2020
Online shopping-top consumer locations and company locations.
The international scenario and views on consumer protection in e-commerce provide impetus to discuss consumer protection in e-business in a regional context-India. The reason for this is that India has become a leading country for online consumer fraud, putting a spotlight on electronic governance systems-which may have an impact on India's ease of doing business ranking. However, to check fraud and ensure consumer protection in e-commerce, the government has replaced the earlier Consumer Protection Act, 1986, with the new Act-2019 and E-Commerce Rule-2020 is in place now.
E-commerce Growth
E-commerce has been booming since the advent of the worldwide web (internet) in 1991, but its root is traced back to the Berlin Blockade for ordering and airlifting goods via telex between 24 June 1948 and 12 May 1949. Since then, new technological developments, improvements in internet connectivity, and widespread consumer and business adoption, e-commerce has helped countless companies grow. The first e-commerce transaction took place with the Boston Computer Exchange that launched its first e-commerce platform way back in 1982 (Azamat et al., 2011 ; Boateng et al., 2008 ). E-commerce growth potential is directly associated with internet penetration (Nielsen, 2018 ). The increase in the worldwide use of mobile devices/smartphones has primarily led to the growth of e-commerce. With mobile devices, individuals are more versatile and passive in buying and selling over the internet (Harrisson et al., 2017 ; Išoraitė & Miniotienė, 2018 ; Milan et al., ( 2020 ); Nielsen, 2018 ; Singh, 2019 ; UNCTAD, 2019a , 2019b ). The growth of the millennial digital-savvy workforce, mobile ubiquity and continuous optimisation of e-commerce technology is pressing the hand and speed of the historically slow-moving B2B market. The nearly US$1 Billion B2B e-commerce industry is about to hit the perfect storm that is driving the growth of B2C businesses (Harrisson et al., 2017 ). Now, e-commerce has reshaped the global retail market (Nielsen, 2019 ). The observation is that e-commerce is vibrant and an ever-expanding business model; its future is even more competitive than ever, with the increasing purchasing power of global buyers, the proliferation of social media users, and the increasingly advancing infrastructure and technology (McKinsey Global Institute, 2019 ; UNCTAD, 2019a , 2019b ).
The analysis of the growth trend in e-commerce, especially since 2015, explains that online consumers continue to place a premium on both flexibility and scope of shopping online. With the convenience of buying and returning items locally, online retailers will increase their footprint (Harrisson et al., 2017 ). Today, e-commerce is growing across countries with a compound annual growth rate (CAGR) of 15% between 2014 and 2020; it is likely to grow at 25% between 2020 and 2025. Further analysis of e-commerce business reveals that internet penetration will be nearly 60% of the population in 2020, and Smartphone penetration has reached almost 42%. Among the users, 31% are in the age group of 25–34 years old, followed by 24% among the 35–44 years bracket and 22% in 18–24 years. Such a vast infrastructure and networking have ensured over 70% of the global e-commerce activities in the Asia–Pacific region. While China alone accounts for US$740 billion, the USA accounts for over US$$560 billion (Kerick, 2019 ). A review of global shoppers making online purchases (Fig. 2 ) shows that consumers look beyond their borders-cross-border purchases in all regions. While 90% of consumers visited an online retail site by July 2020, 74% purchased a product online, and 52% used a mobile device.
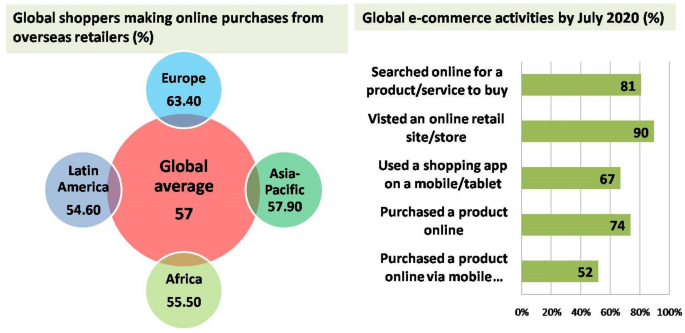
Source: Data compiled from https://datareportal.com/global-dig ital-overview#: ~ :text = There%20are%205.15%20billion%20unique,of%202.4%20percent%20per%20 year and , Accessed 12 October 2020
Global e-commerce activities and overseas online purchase.
The e-commerce uprising in Asia and the Pacific presents vast economic potential. The region holds the largest share of the B2C e-commerce market (UNCTAD, 2017 ). The size of e-commerce relative to the gross domestic product was 4.5% in the region by 2015. E-commerce enables small and medium-sized enterprises to reach global markets and compete on an international scale. It has improved economic efficiency and created many new jobs in developing economies and least developed countries, offering them a chance to narrow development gaps and increase inclusiveness—whether demographic, economic, geographic, cultural, or linguistic. It also helps narrow the rural–urban divide.
Nevertheless, Asia’s e-commerce market remains highly heterogeneous. In terms of e-commerce readiness—based on the UNCTAD e-commerce index 2017, the Republic of Korea ranks fifth globally (score 95.5) while Afghanistan, with 17 points, ranks 132 (UNCTAD, 2017 ). According to a joint study (2018) by the United Nations Economic and Social Commission for Asia and the Pacific (UNESCAP) and Asian Development Bank (ADB), Asia is the fastest-growing region in the global e-commerce marketplace. The region accounted for the largest share of the world’s business-to-consumer e-commerce market (UNESCAP and ADB, 2019). World Retail Congress (2019) brought out the Global E-Commerce Market Ranking 2019 assessing the top 30 ranking e-commerce markets on various parameters-USA, UK, China, Japan and Germany were the first top countries. India figured at 15 with a CAGR of 19.8% between 2018 and 2022. The report suggests that companies need to enhance every aspect of online buying, focusing on localised payment mode and duty-free return. Footnote 5 The observation of this trend implies online consumers’ safety and security.
Figure 3 explains that global cross-border e-commerce (B2C) shopping is growing significantly and is estimated to cross US$1 Trillion in 2020. Adobe Digital Economic Index Survey-2020 Footnote 6 in March 2020 reported that a remarkable fact to note is about steadily accelerated growth in global e-commerce because of COVID-19. While virus protection-related goods increased by 807%, toilet paper spiked by 231%. Online consumers worldwide prefer the eWallet payment system. The survey also revealed an exciting constellation that COVID-19 is further pushing overall online inflation down.
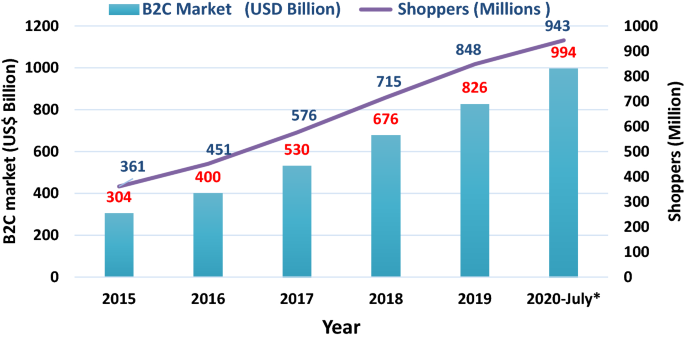
Source: Authors’ compilation from https://www.invespcro.com/blog/cross-border-shopping/ , Accessed on 15 October 2020
Global cross-border e-commerce (B2C) market. *Estimated to cross US$ 1 Trillion in 2020.
According to UNCTD’s B2C E-Commerce Index 2019 survey measuring an economy’s preparedness to support online shopping, India ranks 73rd with 57 index values, seven times better than the 80th rank index report 2018 (UNCTAD, 2019a , 2019b ). The E-commerce industry has emerged as a front-runner in the Indian economy with an internet penetration rate of about 50% now, nearly 37% of smartphone internet users, launching the 4G network, internet content in the local language, and increasing consumer wealth. Massive infrastructure and policy support propelled the e-commerce industry to reach US$ 64 billion in 2020, up by 39% from 2017 and will touch US$ 200 by 2026 with a CAGR of 21%. Footnote 7 Now, India envisions a five trillion dollar economy Footnote 8 by 2024. It would be difficult with the present growth rate, but not impossible, pushing for robust e-governance and a digitally empowered society. The proliferation of smartphones, growing internet access and booming digital payments and policy reforms are accelerating the growth of the e-commerce sector vis-a-vis the economy.
Analysis of different studies on the growth of e-commerce in India shows that while retail spending has grown by a CAGR of 22.52% during 2015–2020, online buyers have climbed by a CAGR of 35.44% during the same period (Fig. 4 ). The government’s Digital India drive beginning 1 July 2015-surge using mobile wallets like Paytm, Ola Money, Mobiwik, BHIM etc., and the declaration of demonetisation on 9 November 2016 appears to be the prime reasons for such a vast growth in the country’s e-commerce industry. The Times of India (2020 October 12), a daily leading Indian newspaper, reported that India's increase in digital payments was at a CAGR of 55.1% from March 2016 to March 2020, jumping from US$ 73,90 million to 470.40, reflecting the country's positive policy environment and preparedness for the digital economy. The government's policy objective is to promote a safe, secure, sound and efficient payment system; hence, the Reserve Bank of India (RBI), the national financial and fiscal regulating authority, attempts to ensure security and increase customer trust in digital payments (RBI, 2020 ).
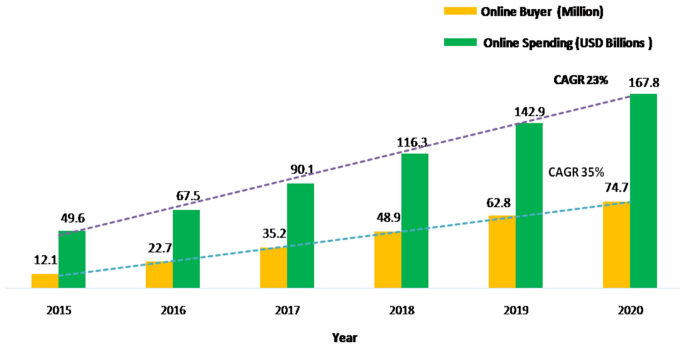
Source: Data compiled from https://www.ibef.org/news/vision-of-a-new-india-US$-5-trillion-economy , http://www.ficci.in/ficci-in-news-page.asp?nid=19630 , https://www.pwc.in/research-insights/2018/propelling-india-towards-global-leadership-in-e-commerce.html , https://www.forrester.com/data/forecastview/reports# , Accessed 12 October 2020
E-Commerce growth in India during 2015–2020.
The massive growth of e-commerce in countries worldwide, especially in India, has prompted an examination of the legal structure regulating online consumer protection.
Literature Review and Research Gap
Theoretical framework.
Generally speaking, customers, as treated inferior to their contracting partners, need protection (Daniel, 2005 ). Therefore, due to low bargaining power, it is agreed that their interests need to be secured. The ‘inequality of negotiating power’ theory emphasises the consumer's economically weaker status than suppliers (Haupt, 2003 ; Liyang, 2019 ; Porter, 1979 ). The ‘inequality in bargaining power’ principle emphasises the customer's economically inferior position to suppliers (Haupt, 2003 ). The ‘exploitation theory’ also supports a similar view to the ‘weaker party’ argument. According to this theory, for two reasons, consumers need protection: first, consumers have little choice but to buy and contract on the terms set by increasingly large and powerful businesses; second, companies can manipulate significant discrepancies in knowledge and complexity in their favour (Cockshott & Dieterich, 2011 ). However, a researcher such as Ruhl ( 2011 ) believed that this conventional theoretical claim about defining the customer as the weaker party is no longer valid in modern times. The logic was that the exploitation theory did not take into account competition between firms. Through competition from other businesses, any negotiating power that companies have vis-a-vis clients is minimal. The study, therefore, considers that the ‘economic theory’ is the suitable theoretical rationale for consumer protection today.
The principle of ‘economic philosophy’ focuses primarily on promoting economic productivity and preserving wealth as a benefit (Siciliani et al., 2019 ). As such, the contract law had to change a great deal to deal with modern-age consumer transactions where there is no delay between agreement and outcomes (McCoubrey & White, 1999 ). Thus, the ‘economic theory’ justifies the flow of goods and services through electronic transactions since online markets' versatility and rewards are greater than those of face-to-face transactions. The further argument suggests that a robust consumer protection framework can provide an impetus for the growth of reliability and trust in electronic commerce. The ‘incentive theory’ works based on that argument to describe consumer protection in electronic transactions (McCoubrey & White, 1999 ).
Online shopping needs greater trust than purchasing offline (Nielsen, 2018 ). From the viewpoint of ‘behavioural economics, trust (faith/confidence) has long been considered a trigger for buyer–seller transactions that can provide high standards of fulfilling trade relationships for customers (Pavlou, 2003 ). Pavlou ( 2003 ) supports the logical reasoning of Lee and Turban ( 2001 ) that the role of trust is of fundamental importance in adequately capturing e-commerce customer behaviour. The study by O'Hara ( 2005 ) also suggests a relationship between law and trust (belief/faith), referred to as ‘safety net evaluation’, suggesting that law may play a role in building trust between two parties. However, with cross-border transactions, the constraint of establishing adequate online trust increases, especially if one of the parties to the transaction comes from another jurisdiction with a high incidence of counterfeits or a weak rule of law (Loannis et al., 2019 ). Thus, the law promotes the parties' ability to enter into a contractual obligation to the extent that it works to reduce a contractual relationship's insecurity. The present research uses the idea of trust (faith/belief/confidence) as another theoretical context in line with ‘behavioural economics’.
As a focal point in e-commerce, trust refers to a party's ability to be vulnerable to another party's actions; the trustor, with its involvement in networking, sees trust in the form of risk-taking activity (Mayer et al., 1995 ; Helge et al., 2020 ). Lack of confidence could result in weak contracts, expensive legal protections, sales loss and business failure. Therefore, trust plays a crucial role in serving customers transcend the perceived risk of doing business online and in helping them become susceptible, actual or imaginary, to those inherent e-business risks. While mutual benefit is usually the reason behind a dealing/transaction, trust is the insurance or chance that the customer can receive that profit (Cazier, 2007 ). The level of trust can be low or high. Low risk-taking behaviour leads to lower trustor engagement, whereas high risk-taking participation leads to higher trustor engagement (Helge et al., 2020 ). The theory of trust propounded by (Mayer et al., 1995 ) suggests that trust formation depends on three components, viz. ability, benevolence, and integrity (ABI model). From the analysis of the previous studies (Mayer et al., 1995 ; Cazier, 2007 ; Helge et al., 2020 ), the following dimensions of the ABI model emerge:
Precisely, ability, benevolence and integrity have a direct influence on the trust of e-commerce customers.
Gaining the trust of consumers and developing a relationship has become more challenging for e-businesses. The primary reasons are weak online security, lack of effectiveness of the electronic payment system, lack of effective marketing program, delay in delivery, low quality of goods and services, and ineffective return policy (Kamari & Kamari, 2012 ; Mangiaracina & Perego, 2009 ). These weaknesses adversely impact business operations profoundly later. Among the challenges that are the reasons for the distrust of customers and downsides of e-commerce is that the online payment mechanism is widely insecure. The lack of trust in electronic payment is the one that impacts negatively on the e-commerce industry, and this issue is still prevalent (Mangiaracina & Perego, 2009 ). The revelation of a recent study (Orendorff, 2019 ) and survey results Footnote 9 on trust-building, particularly about the method of payment, preferred language and data protection, is fascinating. The mode of payment is another matter of trust-building. Today’s customers wish to shop in their local currency seamlessly. In an online shoppers’ survey of 30,000 respondents in 2019, about 92% of customers preferred to purchase in their local currency, and 33% abandoned a buy if pricing was listed in US$ only (Orendorff, 2019 ). Airbnb, an online accommodation booking e-business that began operations in 2009, has expanded and spread its wings globally as of September 2020-over 220 countries and 100 k + cities serving 7 + billion customers (guests) with local currency payment options. Footnote 10
Common Sense Advisory Survey Footnote 11 -Nov. 2019-Feb. 2020 with 8709 online shoppers (B2C) in 29 countries, reported that 75% of them preferred to purchase products if the information was in their native language. About 60% confirmed that they rarely/never bought from an English-only website because they can’t read. Similarly, its survey of 956 business people (B2B) moved in a similar direction. Whether it is B2B or B2C customers, they wanted to go beyond Google translator-this is about language being a front-line issue making or breaking global sales. Leading Indian e-commerce companies like Amazon Footnote 12 and Flipkart Footnote 13 have started capturing the subsequent 100 million users by providing text and voice-based consumer support in vernacular languages. These observations suggest trust in information that the customers can rely upon for a successful transaction.
Data protection is probably the most severe risk of e-commerce. The marketplaces witness so many violations that it often seems that everyone gets hacked, which makes it a real challenge to guarantee that your store is safe and secure. For e-commerce firms, preserving the data is a considerable expense; it points a finger to maintaining the safety and security of the e-commerce consumers’ data privacy in compliance with General Data Protection Regulations (GDPR) across countries. Footnote 14
PwC’s Global Consumer Insight Survey 2020 reports that while customers’ buying habits would become more volatile post-COVID 19, consumers’ experience requires safety, accessibility, and digital engagement would be robust and diversified. Footnote 15 The report reveals that the COVID-19 outbreak pushed the popularity of mobile shopping. Online grocery shopping (including phone use) has increased by nearly 63% post-COVID than before social distancing execution and is likely to increase to 86% until its removal. Knowing the speed of market change will place companies in a position to handle the disruption-74% of the work is from home, at least for the time being. Again, the trend applies to consumers’ and businesses’ confidence/trust-building. The safety and security of customers or consumer protection are of paramount importance.
Given the rationale above, the doctrine of low bargaining power, exploitation theory and the economic approach provides the theoretical justification for consumer protection. Economic theory also justifies electronic transactions and e-commerce operations as instruments for optimising income. The trust theory based on behavioural economic conception also builds up the relationship between the law and customer trust and thus increases confidence in the online market. These premises form the basis for this research.
Need and Instruments for Online Consumer Protection
The law of the land guides people and the living society. Prevailing rules and regulations, when followed, provide peace of mind and security in all spheres, including business activities (Bolton et al., 2004 ). Previous research by Young & Wilkinson ( 1989 ) suggested that those who have more legally strict contracts face more legal problems in contrast to trust-related issues (Young & Wilkinson, 1989 ). Time has changed; people going for online transactions go with the legal framework and feel safe and secured (Bolton et al., 2004 ). An online agreement is a valid contract. Most UNCTAD member countries, including India, have adopted various laws concerning e-governance/e-business/e-society, such as e-transaction laws, consumer protection laws, cyber-crime laws, and data privacy and protection laws. The trend indicates that the law is vital in establishing trust in online transactions.
A review of literature on e-commerce and consumer protection suggests that over the years, consumer protection in e-commerce has received significant attention, particularly from the regulatory authorities-government agencies, trade associations and other associated actors (Belwal et al., 2020 ; Cortés, 2010 ; Dhanya, 2015 ; Emma et al., 2017 ; Ibidapo-Obe, 2011 ; ITU, 2018 ; Jaipuriar et al., 2020 ; Rothchild, 1999 ; Saif, 2018 ). The OECD ( 2016 ), UNCTAD ( 2017 ), and World Economic Forum ( 2019 ) guidelines on e-commerce have facilitated countries to have regulations/laws to provide online customers with data privacy, safe transaction and build trust. Table 2 explains policy guidelines on consumer protection based on a summary of online consumer challenges and possible remedies at different purchases stages.
Research Issue and Objective
The research gap identification involves reviewing the literature on various aspects of e-commerce and consumer rights protection issues spanning two decades. An objective review of 36 highly rated (Scopus/Web Services/ABDC Ranking or the like) e-commerce related publications from over 100 articles published in the last 20 years (2000–2020) suggests that the vast majority of earlier studies in this field have been conceptual/theoretical and generic. Regarding the legal framework of e-commerce and consumers’ rights protection, six current papers exclusively in the Indian context were available for analysis and review. The observations are that while the focus on consumer privacy and rights protection concerns is too general, the legal framework's scrutiny has limited its scope. A review of selected studies on trust and consumer rights protection in e-commerce, as shown in Table 3 , reveals that application aspects, particularly legal issues, are lacking. Indian experience in e-commerce consumer rights protection through jurisprudence is nascent. Review studies show the research of a combination of management and law-related analysis in e-commerce and consumer rights protection is lacking. This scenario showed a gap in exploring a more comprehensive research opportunity in the Indian context.
While e-commerce and electronic transactions have evolved as a global trend, it is noteworthy that Indian customers are still reluctant to place complete confidence and trust in commercial online transactions. Compared to conventional offline customers, online customers face greater risk in cyberspace because they negotiate with unknown vendors and suppliers. Footnote 16 The common issues Footnote 17 related to e-commerce are data privacy and security, product quality, uncertain delivery, no/low scope of replacement, the jurisdiction of filing complaints, and inconceivable terms and conditions (Lahiri, 2018 ). “Country of origin” of the product is a significant issue in e-commerce, particularly in cross-border transactions (Bhattacharya et al., 2020 ). The inadequacy of the Consumer Protection Act, 1986 and other associated laws has surged the insecurity and lack of trust among online customers. The significance of digital payments pursued by the Government of India's essential demonetisation policy-2016 has pushed for online transaction security and consumer protection in e-commerce activities. Therefore, the Consumer Protection Act, 2019 Footnote 18 replaced the Consumer Protection Act 1986 and became effective with effect from 20 July 2020, Footnote 19 while on 7 July 2020, the Consumer Protection (E-commerce) Rules, 2020 Footnote 20 came into force to address the e-commerce challenges. Nevertheless, it was evident that to attract additional investment and to engage with the global market, India, as an emerging country, had to gain the confidence of e-consumers.
These two legislations primarily govern domestic e-commerce businesses. Therefore, the research focuses on these two legal infrastructure strands-new laws enacted during 2019 and 2020 and discusses their implications for online consumer security to increase customers' interest and trust in India's electronic transactions. Like the ABI model , the study also examines the factors influencing e-commerce customers' confidence in the present research context.
Methodology
The research initially depended on the rigorous review of the consumer protection guidelines released from time to time by various bodies, such as the OECD and UNCATD, accompanied by an analysis of the Indian consumer protection legal structure. The Indian Consumer Protection Act, 2019 and the Consumer Protection (E-commerce) Rules, 2020 were the review and analysis subjects. The study used e-commerce driver data collected from secondary sources-published material; the survey reported e-commerce growth and trends and consumer protection and conducted an online survey of 432 online consumers during August and September 2020.
Analysing the arguments of Zikmund ( 2000 ), Bryman ( 2004 ), Saumure & Given ( 2008 ), Bill et al., ( 2010 ) and Bornstein et al. ( 2013 ) about the representative of convenience sampling and bias, we consider it is similar to that of the population, and there is no harm with due care. Regarding inherent bias in convenience sampling, data collection from different sources with different respondents’ inclusion provides more data variability and considerably reduces prejudice (Sousa et al., 2004 ; Edgar and Manz, 2017 ). Therefore, the respondents included in the research were students, professors, advocates, doctors, professionals, and homemakers, avoiding excluding family, relatives and friends to ensure bias-free. Their contact details sources were various channels, including public institution websites, social networking sites, and the authors’ email box. Assuming that more respondents feel fun filling out online questionnaires and providing truthful answers (Chen & Barnes, 2007 ; Saunders et al., 2007 ), the study used an online survey. Furthermore, because people in the digital age are more computer/smartphone savvy, they are more likely to follow a similar trend. Besides, such a technique was convenient during the COVID-19 pandemic condition because of its timeliness, inexpensive methods, ease of research, low cost (no support for this research), readily available, and fewer rules to follow. The respondents' contact details sources were various channels, including public institution websites, social networking sites, and the authors' email box.
The study used a structured questionnaire comprising seven questions with sub-questions except the 7th one being open-ended, consuming about 8–10 min, designed based on the insights gained from responding to customer surveys of different e-commerce companies last year. Pretesting the questionnaire with 17 responses from the target group supported modifying the final questionnaire partially. The first four questions were background questions-gender, age, respondent's attitude towards internet purchasing. Question number five with sub-questions, being the focused question, provided the answer to some trust-building factors found in the literature review. Following previous research (McKnight et al., 2002 ; Corbit et al., 2003 ; Pavlou, 2003 ) tested the Likert-scale, this question's solicited response relied on a five-point Likert-rating scale (1 = Not important at all, 2 = Less important, 3 = Somewhat important, 4 = Important, 5 = Very important). The query six asked was about the consumer protection issues in e-commerce/online transaction-scam/fraud and grievance settlement. The final question seven was open-ended for any remark the respondent wanted to make. The questionnaire was reliable on a reasonable basis with greater internal consistency on overall internal reliability (Cronbach's alpha = 0.829) at a 1% level of significance. The Zoho Survey technique was used to solicit required information. The response rate was 76% (327) of the total emails sent (432). The retained responses were 290, i.e. 88.69% of the replies received, completed in all respects and satisfying the research requirement. The research applied statistical instruments like percentage, weighted mean and multiple regression analysis using SPSS-26 for analysis and interpretation.
Figure 5 highlights the research framework and process.
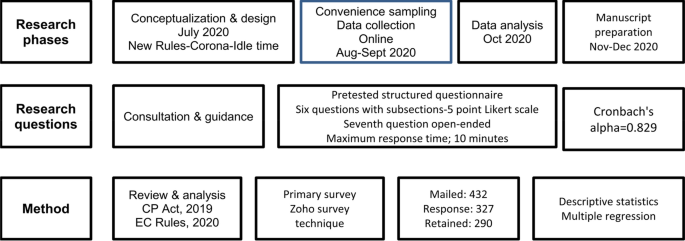
Research framework and process
Deficiency in Act, 1986 and Key Feature of the New Act Governing E-Commerce Consumer Protection
The rapid development of e-commerce has led to new delivery systems for goods and services and has provided new opportunities for consumers. Simultaneously, this has also exposed the consumer vulnerable to new forms of unfair trade and unethical business. The old Act, 1986, has severe limitations regarding its applicability and adjudication processes in consumer rights protection in e-commerce. The new Act, 2020 brings fundamental changes regarding its scope of application, penalty and governance; and envisages CCPA and vests regulating and controlling powers. Table 4 explains the comparative picture between the old Act, 1986 and the new Act, 2019.
The Act, 2019 applies to buying or selling goods or services over the digital or electronic network, including digital products [s.2 (16)] and to a person who provides technologies enabling a product seller to engage in advertising/selling goods/services to a consumer. The Act also covers online market places or online auction sites [s.2 (17)].
Necessary definition/explanation connected to e-commerce provided by the Act are:
Consumer: Meaning
If a person buys any goods and hires or avails any service online through electronic means, the person would be a consumer of the Act [Explanation b to s.2 (7)].
Product Seller: Electronic Service Providers
The electronic service providers are the product sellers under the Act and have the same duties, responsibilities, and liabilities as a product seller [s.2 (37)].
Unfair Trade Practice: Disclosing Personal Information
Unfair trade practice under the Act [s.2 (47) (ix)] refers to electronic service providers disclosing to another person any personal information given in confidence by the consumer.
Authorities: Central Consumer Protection Authority (CCPA)
The Act, 2019 provides, in addition to the existing three-tier grievance redress structure, the establishment of the Central Consumer Protection Authority [CCPA] [s.10 & 18] to provide regulatory, investigative or adjudicatory services to protect consumers’ rights. The CCPA has the powers to regulate/inquire/investigate into consumer rights violations and/unfair trade practice suo motu or on a complaint received from an aggrieved consumer or on a directive from the government. The specific actions it can take include:
Execute inquiries into infringements of customer rights and initiate lawsuits.
Order for the recall of dangerous/hazardous/unsafe products and services.
Order the suspension of unethical commercial practises and false ads.
Impose fines on suppliers or endorsers or publishers of false advertising.
The power of CCPA is categorical regarding dangerous/hazardous/unsafe goods and false/misleading advertisements. The CCPA has the authority to impose a fine ranging from Rs 100 k to Rs 5 million and/imprisonment up to life term for the violators depending on the type of offences committed by them (Table 5 ).
Redress Mechanism
The provisions laid down in Sect. 28 through Sect. 73 deal with various aspects of the consumer dispute redress system. The new Act has changed the District Consumer Dispute Redressal Forum terminology to the District Consumer Dispute Redressal Commission. The pecuniary jurisdiction of filling complaints in the three-tier consumer courts at the District, State and National level has increased (Table 5 ). For better understanding, Fig. 6 shows a diagrammatic picture of the judicial system of dispute settlement.
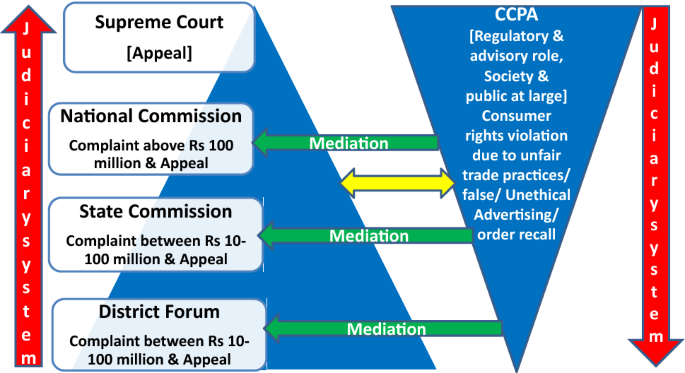
Grievance redress mechanism
The Act, 2019 provides a dispute settlement mechanism through the mediation process in case of compromise at the acceptance point of the complaint or some future date on mutual consent (Sec 37). A mediation cell would operate in each city, state, national commission, and regional bench to expedite redress. Section 74 through 81 of the Act lays down the detailed procedure. Section 81(1) maintains that no appeal lies against the order passed by Mediation, implying that the redress process at the initial stage would be speedy, impacting both the consumers and service providers.
Consumer Protection (E-Commerce) Rules, 2020
The Consumer Protection (E-Commerce) Rules, 2020, notified under the Consumer Protection Act, 2019 on 23 July 2020, aims to prevent unfair trade practices and protect consumers' interests and rights in e-commerce.
Applicability (Rule 2)
The Rules apply to:
Both products and services acquired or sold through automated or electronic networks;
All models of e-commerce retail;
All the e-commerce entities, whether they have inventory or market place model. The inventory-based model includes an inventory of goods and services owned by an e-commerce entity and directly sold to consumers [Rule 3(1) f]. In the marketplace model, an e-commerce entity has an information infrastructure platform on a digital and electronic network that facilitates the consumer and the seller. [Rule 3(1)g];
All aspects of unfair trading practise in all models of e-commerce; and
An e-commerce entity is offering goods or services to consumers in India but not established in India.
General Duties of E-commerce Entities (Rule 4)
The duties of e-commerce entities are:
An e-commerce entity must be a company incorporated under the Companies Act.
Entities must appoint a point of contact to ensure compliance with the Act.
They have to establish an adequate grievance redress mechanism; they would appoint a grievance officer for this purpose and display his name, contact details, and designation of their platform. He would acknowledge the complaint's receipt within 48 h and resolve the complaint within a month from receipt of the complaint.
If they are offering imported goods, the importers’ names and details from whom the imported goods are purchased, and the sellers’ names are to be mentioned on the platform.
They cannot impose cancellation charges on consumers unless they bear similar costs.
They have to affect all payments towards accepted refund requests of the consumers within a reasonable period.
They cannot manipulate the goods' prices to gain unreasonable profit by imposing unjustified costs and discriminating against the same class of consumers.
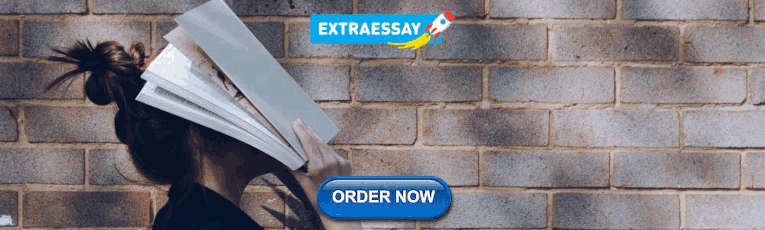
Liabilities of Marketplace E-commerce Entities (Rule 5)
The liabilities of marketplace e-commerce entities include the following:
The marketplace e-commerce entity would require sellers to ensure that information about goods on their platform is accurate and corresponds with the appearance, nature, quality, purpose of goods.
They would display the following information prominently to its users at the appropriate place on its platform:
Details about the sellers offering goods-principal geographic address of its headquarters and all branches and name and details of its website for effective dispute resolution.
Separate ticket/docket/complaint number for each complaint lodged through which the user can monitor the status of the complaint.
Information about return/refund/exchange, warranty and guarantee, delivery and shipment, payment modes and dispute/grievance redress mechanism.
Information on the methods of payment available, the protection of such forms of payment, any fees or charges payable by users.
They would make reasonable efforts to maintain a record of relevant information allowing for the identification of all sellers who have repeatedly offered goods that were previously removed under the Copyright Act/Trademarks Act/Information Technology Act.
Sellers’ Duties on the Marketplace (Rule 6)
The duties of sellers on the market encompass:
The seller would not adopt any unfair trade practice while offering goods.
He should not falsely represent himself as a consumer and post-product review or misrepresent any products' essence or features.
He could not refuse to take back goods purchased or to refund consideration of goods or services that were defective/deficient/spurious.
He would have a prior written contract with the e-commerce entity to undertake sale.
He would appoint a grievance officer for consumer grievance redressal.
He would ensure that the advertisements for the marketing of goods or services are consistent with the actual characteristics, access and usage conditions of goods.
He will provide the e-commerce company with its legal name, the primary geographic address of its headquarters and all subsidiaries/branches, the name and details of the website, e-mail address, customer contact details such as faxes, landlines and mobile numbers, etc.
Duties and Liabilities of Inventory E-commerce Entities (Rule 7)
As in the inventory-based model, inventory of goods and services is owned and sold directly to consumers by e-commerce entities, so inventory e-commerce entities have the same liabilities as marketplace e-commerce entities and the same duties as marketplace sellers.
The Act 2019 has several provisions for regulating e-commerce transactions with safety and trust. Since the Act is new, it would be premature to comment on its operational aspects and effectiveness. In a recent judgement in Consumer Complaint No 883 of 2020 ( M/s Pyaridevi Chabiraj Steels Pvt. Ltd vs National Insurance Company Ltd , the NCDRC Footnote 21 has proved the Act's operational effectiveness by deciding the maintainability of a claim's jurisdiction based on the new Act's provisions. However, it is inevitable that "beware buyer" will be replaced by "beware seller/manufacturer"; the consumer will be the real king. The Rules 2020 strike a balance between the responsibilities of e-commerce business owners and on-the-platform vendors. Contravention, if any, of the new regulation/rules would invite the provisions of the Act 2019. The observation is that limited liability partnerships are missing from the e-commerce entities. However, with the Act and Rules' operational experience, the judiciary or legislature will address this issue sooner or later.
Nevertheless, the Rules 2020 provide a robust legal framework to build consumers' trust in e-commerce transactions and protect their rights and interests, thereby proving the notion, "consumer is the king". The COVID-19 impact has pushed the government to adopt and encourage online compliant filling procedures through the National Consumer Helpline. Using various APPs is likely to expedite the adjudication process and benefit the aggrieved consumer and build trust in the governance system.
Reading the Rules, 2020, with the Act, 2019, the observation is that by making smartphones the primary target of the new legislation, the Act, 2019 is hailed as an all-inclusive regulatory regime that would raise customer interest investment in e-commerce. To safeguard consumers' rights in all modern-day retail commerce models, the Act, 2019 attempts to turn the jurisprudence pervading consumer protectionism from a caveat emptor to a caveat seller. In addition, the Act formally incorporated e-commerce within its limits and entered the realm of B2C e-commerce. One crucial takeaway benefit for consumers is simplifying the complaint filing process, enabling consumers to file complaints online and redress grievances.
E-commerce has become a gift to all customers in the COVID-19 pandemic's aftermath. The E-Commerce Rules, 2020 follow the stringent consumer protection regime under the new Act, 2019. In the raging pandemic, the timing of the E-Commerce Rules, 2020 is beneficial considering the current limitations on customers' freedom of travel and increased reliance on e-commerce. The grievances redress mechanism as provided in the Rules, 2020 is indubitably a calibrated step ensuring neutrality in the e-commerce market place, greater transparency, stringent penalties and a striking balance between the commitments of e-commerce firms and vendors in the marketplace. The mandatory provisions of appointing a consumer grievance redress officer and a nodal contact person or an alternative senior appointed official (resident in India) with contact details, acknowledging consumer complaints within 48 h of receipt with a ticket number, and resolving complaints within 1 month of receipt are unquestionably beneficial to consumers. Although each e-commerce company has its refund policy, all refund claims must have a timely settlement. However, anxiety abounds as daily online fraud and unethical trading practices have made consumers fearful of exposing themselves to unscrupulous vendors and service providers. Moreover, the regulations' effective enforcement would dissuade unethical retailers and service providers, thereby building consumer trust, which time will see.
Practical Contributions
The practical contributions of the paper emerge from survey findings. Concerning the primary survey, the male–female ratio is nearly 1:1, with an average age of 36 years in the age range of 20–65. As regards profession, 67% were working professionals, and 22% were students. While all of the respondents were computer/tablet/mobile-savvy, 96% had at least a five-time online shopping experience during the last 7 months between January–July 2020. The desktop with 61% response is still the preferred device for online shopping. The pricing with cash on delivery, shipping convenience, and quality reviews determined online shopping factors. About 57% of them agreed that COVID-19 impacted their online purchase habits and pushed for online transactions even though they feared insecurity about online shopping. The primary concerns were low-quality products at a high price, a refund for defective products, and a delay in settlement of wrong/excess payments. The top five leading e-commerce platforms reported were Amazon, Flipkart, Alibaba, Myntra, and IndiaMart. Netmeds was also a leading e-commerce business platform in the pharmaceutical sector. During the COVID-19 pandemic, JioMart was very popular for home-delivery food products, groceries and vegetables in the metro locality. The customer feedback system was found robust on Amazon.
The respondents' trust in online shopping reveals that a secure and reliable system was essential for 93% of the respondents. For nearly the same proportion, information about how e-business firms work provided security solutions was a priority factor. Choosing a payment option, 76% of the respondents prioritised “cash on delivery-online transfer at the doorstep. Regarding the privacy of personal information shared by online shoppers, 52% said that they cared about this aspect. Factors like warranty and guarantee (67%) and customer service (69%) were important factors of trust-building with the e-entities. Information on the websites (easy navigation/user friendly and reviews) was either important or very important, with 77% of the respondents’ confidence building to buy online. Information about the product features and its manufacturer/supplier was essential to 86% of the respondents for trust-building on the product and the supplier (manufacture) and e-commerce entity. Along with the ABI model discussed above, the presumption is that security, privacy, warranty/guarantee, customer service, and website information factors positively influence e-commerce customers' trust.
Multiple regression analysis suggests that as the P = value of every independent variable is below 0.05% level of significance, the independent variables security, privacy, warranty, customer service, and website information are all significant. Alternatively, the overall P value of 0.032 with R 2 0.82 supports the presumption that security, privacy, warranty/guarantee, customer service, website information factors have a combined influence on e-commerce customers' trust.
Given this backdrop, Table 6 summarises the micro findings on respondents' online shopping behaviour, their trust and safety aspects, and understanding of the provisions of the new Act, 2019 and Rules, 2020. The higher mean value for a sub-factor implies higher importance attached to the factor by the respondents. P value at a 5% level of significance explains an individual element's contribution to trust-building behaviour for online buying.
Managerial Insights
The first observation from the data analysis is that, comparatively, the younger generation is prone to online shopping; it goes along with Xiaodong and Min ( 2020 ). Secondly, the respondents of all age groups have online buying experience even in a pandemic situation forced by COVID-19, compromising their safety and security concerns. The third observation is that factors like “cash on the delivery option (COD)”, adequate information on the e-commerce entity corporate website, and effective grievance/complaint redress mechanism are the three crucial factors that build consumers’ trust in e-commerce transactions. The reason probably is that this Act and Rules are new and significant dispute (s) could yet be reported seeking invoking the relevant provisions of the Act and Rules in an appropriate legal forum.
Further, the logical observation of the COD option being a perceived influential factor in trust-building emanates from the fact that protection and security are the essential elements that make customers hesitant toward utilizing other e-payment options. The studies by Mekovec and Hutinski ( 2012 ), Maqableh ( 2015 ) and Ponte et al.( 2015 ); have similar views. However, post-demonetization (2016), India is growing with more digital payments. In this context, we value Harvard researchers Bandi et al. ( 2017 ) contention that customers who switch to digital payments maintain their purchasing recurrence but spend more and are less likely to restore their purchases. The firms in emerging markets may appreciate gains from customer interest, notwithstanding operational increases from payment digitalization. The coherent perception about the impact of website information on trust-building is in line with the findings of Brian et al. ( 2019 ) that the online information source creates a spill-over effect on satisfaction and trust toward the retailer. The implication of the need for an effective grievance redress mechanism is that trust-building would be a tricky proposition if the company cannot ensure dedicated and tailored customer service and support. Kamari and Kamari ( 2012 ) and Mangiaracina and Perego ( 2009 ) had comparative perspectives likewise.
The final observation is that the level of trust required to engage in online shopping/transaction varies among the respondents depending on their trust perception level. The younger generation, less than 35 years old, is more risk-taking when it comes to pre-purchase online payment, but women over 45 years old are a little hesitant and prefer to do their online shopping with payment at the time of placing an order. This is ostensibly because the younger generation is more tuned to network connectivity via smartphone/tablet, and they perceive online transactions as less dangerous. The present research findings on the influence of security, privacy, warranty/guarantee, customer service, and website information on e-commerce customers' confidence-building support the earlier discussed ABI model proposition (Mayer et al., 1995 ; Cazier, 2007 ; Helge et al., 2020 ). The R 2 -value of 0.82 implies that there are other factors beyond what is studied. The other probable factor (s) that might have influenced trust is the new Act and Rules' effectiveness in protecting online consumers' interests. The new regulations need a couple of years (at least 2 years) of operational experience for proper assessment. The Act 2019 appears robust to protect consumer rights and interests of e-commerce customers with specific regulations (i.e. Consumer Protection (E-Commerce) Rules, 2020) in force, helping the country's economic growth.
The study variably supports Nehf ( 2007 ) view that consumers make decisions about distributing their data in exchange for different benefits like, e.g., information on web sites and access to databases. Trust, credibility, privacy issues, security concerns, the nature of the information on the website, and the e-commerce firm's reputation directly influence consumers' internet trust (Kim et al., 2008 ). Trust is the focal point of online consumers' decision-making; the observation endorses Larose and Rifon ( 2007 ) creation of privacy alerts as part of consumer privacy self-regulation initiatives and the use of a social cognitive model to consider consumer privacy behaviours. Besides, data privacy and trust breaches adversely affect the firm's market value (Tripathi & Mukhopadhyay, 2020 ) also hold good in the present context. Figure 7 demonstrates a diagrammatic model of trust of the consumer on e-commerce transactions leading to his decision-making.
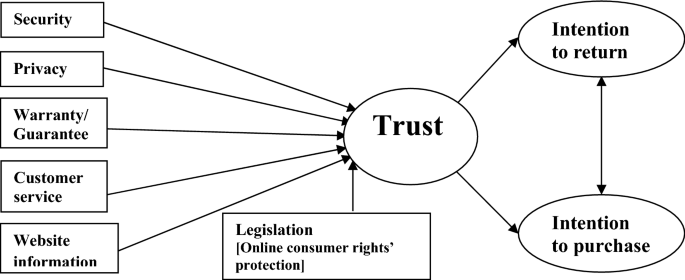
Model for consumers’ trust on e-commerce transactions
Limitations
Every research has more or less some limitations; this one has too. The main impediment was the non-availability of adequate literature defining the impact assessment of the legal framework of consumer protection measures in e-commerce. The probable reasoning is that the Acts/Laws governing e-commerce and online consumer rights protection under consideration are new; ethical dispute resolution and judicial interventions have only recently begun. Sample size limitation is also a hindering factor in the generalisation of the findings. The observations and managerial insights are likely to change with a few more years of implementation experience of the Acts.
Conclusions, Implications and Future Research
Conclusions.
Lack of trust in goods and their suppliers/manufacturers was one of the primary reasons for people not buying online. The widespread internet penetration and the growing use of computer/tablets/smartphones have pushed e-commerce growth across countries, including India. The rapid e-commerce development has brought about new distribution methods. It has provided new opportunities for consumers, forcing consumers vulnerable to new forms of unfair trade and unethical business. Further, the government's measures to protect consumer rights, particularly online consumers, are inadequate. Hence, the government enacted the Consumer Protection Act, 2019 and the Consumer Protection (E-commerce) Rules, 2020 and made them effective from July 2020. The new Act and Rules have less than 6 months of operational experience, implying premature comment on its effectiveness in providing safety and security to online consumers. However, online consumers' positive responses suggest that people gain confidence in online shopping with safety and security. Because consumer rights protection is paramount in the growth of e-commerce, the new regulations strengthen the grievance redress mechanism of online consumers, ensuring their trust-building ability, safety, and security. The "Consumer is the King with power" now. The new reform, i.e., enactment of the two laws, aids in doing business too. Some legal complications may arise with more operational experience in the future. Still, with judiciary intervention and directives, the online consumer's safety and security will pave the growth of e-commerce in India.
Implications
Some stakeholders have apprehension about the new Act and Rules' effectiveness because of the slow judiciary process, inadequate infrastructure support, and corrupt practices. The findings provide some practical implications for consumer activists, policymakers, and research communities to explore how to strengthen trust-building among online consumers. Regarding theoretical implications, the research improves the scientific community's understanding of the existing body of knowledge about online trust and e-consumer protection. The article further contributes to the body of literature on e-commerce and consumer protection, understanding the crucial factors impacting customer trust and loyalty and provides an insightful perspective on e-consumer protection in the Indian context on the eve of the new legislation enacted in 2019–2020.
Future Research
Given the presumption that e-commerce and trust are areas of constant change, trust in e-commerce will change, and it will be more challenging to integrate e-commerce into people's lives. The scope for further research to test the effectiveness of the Act, 2019, and Rule, 2020 in redressing e-commerce consumers' grievances and protecting their rights is wider only after a couple of years of operational experience. The government's policy drive for accelerating online transactions also poses challenges considering the importance of trust-building and consumer rights protection in e-commerce. Future research would shed more light on these issues.
See The United Nations Economic Commission for Europe (UNECE) guidelines on e-commerce https://www.unece.org/fileadmin/DAM/stats/groups/wggna/GuideByChapters/Chapter_13.pdf , pp 249–263, Accessed 7 October 2020.
Consumer International is a champion in the sustainable consumer movement for the last 60 years. Its vision for the future of 2030 is to address three issues-sustainability, digitalization and inclusion. See for more details https://www.consumersinternational.org/who-we-are/ .
econsumer.gov came into being in April 2001, addresses international scams and guides its members to combat fraud worldwide; see for details https://econsumer.gov/#crnt .
For more details, refer to https://icpen.org/consumer-protection-around-world .
For a detailed report, see https://www.worldretailcongress.com/__media/Global_ecommerce_Market_Ranking_2019_001.pdf , Accessed 10 October 2020.
Complete report available at https://business.adobe.com/resources/digital-economy-index.html , Accessed 10 October 2020.
https://www.ibef.org/news/vision-of-a-new-india-US$-5-trillion-economy , Accessed 7 October 2020.
Government of India’s press release, see https://pib.gov.in/PressReleseDetailm.aspx?PRID=1603982 , Accessed 7 October 2020.
See CUTS International survey at https://cuts-citee.org/pdf/Discussion_Paper_E-Commerce_in_the_Context_of_Trade_Consumer_Protection_and_Competition_in_India.pdf , CSA Research at https://insights.csa-research.com/reportaction/305013126/Marketing , https://insights.csa-research.com/reportaction/305013125/Marketing , and UNTAD study at https://unctad.org/page/data-protection-and-privacy-legislation-worldwide , Accessed 12 December 2020.
https://innovationtactics.com/business-model-canvas-airbnb/ , Accessed 12 December 2020.
Detailed findings at https://insights.csa-research.com/reportaction/305013125/Marketing , Accessed 19 October 2020.
Amazon India began testing a Hindi for its mobile website, marking its first foray into vernacular languages in August 2018.
Flipkart started voice assist in multiple languages - Hindi and English to make shopping easier in June 2020 .
See cross-border shopping statistics and trends at https://www.invespcro.com/blog/cross-border-shopping/ , Accessed 15 October 2020.
https://www.pwc.com/gx/en/consumer-markets/consumer-insights-survey/2020/pwc-consumer-insights-survey-2020.pdf , Accessed 15 October 2020.
For global trend-access, explore, and personalized insights, see details at https://www.forrester.com/data/forecastview/reports# , Accessed 15 October 2020.
For defined common issues, see Government’s e-gazette at http://egazette.nic.in/WriteReadData/2020/220661.pdf , Accessed 15 October 2020.
https://consumeraffairs.nic.in/sites/default/files/Act%20into%20force.pdf , Accessed 30 October 2020.
See Government’s e-gazette notification http://egazette.nic.in/WriteReadData/2019/210422.pdf , Accessed 30 October 2020.
Government of India’s press release see https://pib.gov.in/Pressreleaseshare.aspx?PRID=1656161 and for detailed Rules see https://consumeraffairs.nic.in/theconsumerprotection/consumer-protection-e-commerce-rules-2020 .
Full reported case details are available at https://indiankanoon.org/doc/49459460/ , Accessed November 22, 2020.
Agag, G. (2019). E-commerce ethics and its impact on buyer repurchase intentions and loyalty: An empirical study of small and medium Egyptian businesses. Journal of Business Ethics, 154 , 389–410. https://doi.org/10.1007/s10551-017-3452-3 .
Article Google Scholar
Agag, G., & El-Masry, A. (2016). Understanding consumer intention to participate in online travel community and effects on consumer intention to purchase travel online and WOM: An integration of innovation diffusion theory and TAM with trust. Computers in Human Behavior, 60 , 97–111. https://doi.org/10.1016/j.chb.2016.02.038 .
Ayilyath, M. (2020). Consumer protection in E-commerce transactions in India—need for reforms, SSRN . https://doi.org/10.2139/ssrn.3571069 .
Azamat, N., Rashad, Y., Shahriar, M., Behrang, S., & Menon, M. (2011). The evolution and development of E-commerce market and E-cash. SSRN Electronic Journal . https://doi.org/10.1115/1.859858 .
Bandi, C., Ngwe D., Moreno, A., & Xu Z. (2017). The effect of payment choices on online retail: Evidence from the 2016 Indian demonetization, https://www.hbs.edu/faculty/Publication%20Files/19-123_ea5e9c88-8207-4aef-acb5-b206333b70dc.pdf . Accessed 15 Oct 2020.
Belanche, D., Flavián, M., & Pérez-Rueda, A. (2020). Mobile apps use and WOM in the food delivery sector: The role of planned behavior, perceived security and customer lifestyle compatibility. Sustainability, 12 (10), 4275. https://doi.org/10.3390/su12104275 .
Belwal, R., Al Shibli, R., & Belwal, S. (2020). Consumer protection and electronic commerce in the Sultanate of Oman. Journal of Information, Communication and Ethics in Society . https://doi.org/10.1108/JICES-09-2019-0110 .
Bhattacharya, S., Sanghvi, K., & Chaturvedi, A. (2020). India: E-Commerce Rules, https://www.mondaq.com/india/dodd-frank-consumer-protection-Act/976876/e-commerce-rules-2020#:~:text=The%20Rules%20restrict%20the%20sellers,that%20represent%20an%20inaccurate%20picture . Accessed 12 Oct 2020.
Bill, A., Tom T., & Donna, T. (2010). Chapter 2-Planning the study. In Beyond the Usability Lab, Conducting Large-scale Online User Experience Studies (pp. 17–47). ScienceDirect. https://doi.org/10.1016/B978-0-12-374892-8.00002-8
Boateng, R., Heeks, R., Molla, A., & Hinson, R. (2008). E-commerce and socio-economic development: Conceptualizing the link. Internet Research, 18 , 562–594. https://doi.org/10.1108/10662240810912783 .
Bolton, G., Katok, E., & Ockenfels, A. (2004). How effective are electronic reputation mechanisms? An experimental investigation. Management Science, 50 (11), 1587–1602. http://www.jstor.org/stable/30047967 . Accessed 10 Dec 2020.
Bornstein, M. H., Jager, J., & Putnick, D. L. (2013). Sampling in developmental science: Situations, shortcomings, solutions, and standards. Developmental Review, 33 , 357–370. https://doi.org/10.1016/j.dr.2013.08.003 .
Brian, I. S., O’Neill, B. S., & Terence, T. O. (2019). The upside of showrooming: How online information creates positive spill-over for the brick-and-mortar retailer. Journal of Organizational Computing and Electronic Commerce, 29 (4), 294–315. https://doi.org/10.1080/10919392.2019.1671738 .
Bryman, A. (2004). Social research methods . 2nd Edition, Oxford University Press, New York, 592.
Cazier, J. A. (2007). A framework and guide for understanding the creation of consumer trust, Journal of International Technology and Information Management , 16 (2). https://scholarworks.lib.csusb.edu/jitim/vol16/iss2/4 . Accessed 14 Oct 2020.
Chandni, G. (2017). Carving the map for a protected consumer: Establishing the need of a separate legislation for E-commerce, 6.1 NULJ, 123–138. SCC Online Web Edition. file:///E:/Downloads/Need%20for%20separate%20legislation.pdf. Accessed 22 Mar 2021.
Chen, Y-.H., & Barnes, S. (2007). Initial trust and online buyer behavior. Industrial Management and Data Systems, 107 (1), 21–36. https://doi.org/10.1108/02635570710719034
Chia, T. S. (2014). E-Business; The New Strategies Ande-Business Ethics, that Leads Organizations to Success, Global Journal of Management and Business Research: A Administration and Management , 14 (8), Version 1.0. https://globaljournals.org/GJMBR_Volume14/2-E-Business-The-New-Strategies.pdf . Accessed 15 Nov 2020.
Cockshott, P., & Dieterich, H. (2011). The contemporary relevance of exploitation theory. MARXISM 21 (8), 206–236. https://doi.org/10.26587/marx.8.1.201102.009 .
Corbitt, B., Thanasankit, T., & Yi, H. (2003). Trust and e-commerce: a study of consumer perceptions. Electronic Commerce Research and Applications, 2 , 203–215. https://doi.org/10.1016/S1567-4223(03)00024-3
Cortés, P. (2010). Online dispute resolution for consumers in the European Union. In Routledge Research in IT and E-commerce Law , Routledge, London. https://www.econstor.eu/bitstream/10419/181972/1/391038.pdf .
Daniel, D.B. (2005). Inequality of bargaining power, 76 U. Colo. L. Rev . 139. https://digitalcommons.law.msu.edu/facpubs/107/ . Accessed 17 Aug 2020.
Dhanya, K.A. (2015). Consumer protection in the E-commerce Era. International Journal of Legal Research , 3 (4):1. https://ssrn.com/abstrAct=3489753 . Accessed 6 July 2020.
Durovic, M. (2020). International consumer law: What is it all about? Journal of Consumer Policy, 43 (125–143), 2020. https://doi.org/10.1007/s10603-019-09438-9 .
Eastlick, M., Lotz, S.L., & Warrington, P. (2006). Understanding online B-to-C relationships: An integrated model of privacy concerns, trust, and commitment. Journal of Business Research, 59 , 877–886. https://doi.org/10.1016/j.jbusres.2006.02.006 .
Edgar, T. W., & Manz, D. O. (2017). Research Methods for Cyber Security . Elsevier. ISBN:9780128129302.
Elbeltagi, I., & Agag, G. (2016). E-retailing ethics and its impact on customer satisfaction and repurchase intention: A cultural and commitment-trust theory perspective. Internet Research, 26 (1), 288–310. https://doi.org/10.1108/IntR-10-2014-0244 .
Emma, P., Sara, F., Marta, S., & Ana, G. (2017). Exploratory study of consumer issues in online peer-to-peer platform markets. European Commission. file:///C:/Users/BASANT~1/AppData/Local/Temp/Annex5_Task5_ReportMay2017pdf.pdf. Accessed 6 July 2020.
Fang, Y., Chiu, C., & Wang, E. T. G. (2011). Understanding customers’ satisfaction and repurchase intentions: An integration of IS success model, trust, and justice. Internet Research, 21 (4), 479–503. https://doi.org/10.1108/10662241111158335 .
Freestone, O. & V. W. Mitchell. (2004) Generation Y attitudes towards E-ethics and Internet-related Misbehaviours. Journal of Business Ethics, 54 , 121–128, http://www.jstor.org/stable/25123331 . Accessed 27 Nov 2020.
Grabner-Kraeuter, S. (2002). The role of consumers’ trust in online-shopping. Journal of Business Ethics, 39 , 43–50. https://doi.org/10.1023/A:1016323815802 .
Harrigan, M., Feddema, K., Wang, S., Harrigan, P., & Diot, E. (2021). How trust leads to online purchase intention founded in perceived usefulness and peer communication. Journal of Consumer Behaviour: An International Research Review , 1-16. https://doi.org/10.1002/cb.1936 .
Harrisson, B., Jean, P., & Dahl, B. (2017). 10 eCOMMERCE TRENDS FOR 2018 . Project: Growth Strategies in an Omnichannel Retail Context. https://doi.org/10.13140/RG.2.2.34264.19205 .
Haupt, S. (2003). An economic analysis of consumer protection in Con-trAct law. German Law Review , 4 (11), 1137–1164. https://static1.squarespace.com/static/56330ad3e4b0733dcc0c8495/t/56b96e2f22482e110fab1f78/1454992944362/GLJ_Vol_04_No_11_Haupt.pdf . Accessed 6 Oct 2020.
Helge, S., Anne, H., & Guido, M. (2020). The function of ability, benevolence, and integrity-based trust in innovation networks. Industry and Innovation, 27 (6), 585–604. https://doi.org/10.1080/13662716.2019.1632695 .
Husted, B. W., & Allen, D. B. (2008). Toward a model of cross-cultural business ethics: The impact of individualism and collectivism on the ethical decision-making process. Journal of Business Ethics, 82 (2), 293–305. https://doi.org/10.1007/s10551-008-9888-8 .
Ibidapo-Obe, T. (2011). Online consumer protection in E-commerce transactions in Nigeria: An analysis. SSRN Electronic Journal . https://doi.org/10.2139/ssrn.2683927 .
Išoraitė, M., & Miniotienė, N. (2018). Electronic commerce: Theory and practice. Integrated Journal of Business and Economics . https://doi.org/10.33019/ijbe.v2i2.78 .
ITU. (2018). Big data, machine learning, consumer protection and privacy. https://www.itu.int/en/ITU-T/extcoop/figisymposium/2019/Documents/Presentations/Big%20data,%20Machine%20learning,%20Consumer%20protection%20and%20Privacy.pdf . Accessed 6 July 2020.
Jain, S., & Jain, S. (2018). E-commerce and competition law: Challenges and the way ahead. Indian Competition Law Review, 3 , 7–32.
Google Scholar
Jaipuriar, A., Khera, A., & Ganguly, S. (2020). Consumer protection (e-commerce) rules, 2020: A compliance framework in the digital market. https://www.mondaq.com/india/dodd-frank-consumer-protection-act/977768/consumer-protection-e-commerce-rules-2020-a-compliance-framework-in-the-digital-market . Accessed 22 Sept 2020.
Kamari, F., & Kamari, S. (2012). Trust in electronic commerce: A new model for building online trust in B2C. European Journal of Business and Management, 4 (10), 126–133.
Kerick, F. (2019). The growth of ecommerce. https://medium.com/swlh/the-growth-of-ecommerce-2220cf2851f3 . Accessed 4 July 2020.
Khandelwal, P. (2018). Ease of doing E-commerce business in India : The FDI policy relating to Ecommerce and its impact on the Indian economy. RGNUL Financial and Mercantile Law Review , 20–37. https://cf9d2836-9a17-4889-b084-bc78a1bb74ee.filesusr.com/ugd/0fa0b3_d7453802d99a4dd7a20ff4b3886b1d04.pdf . Accessed 12 Dec 2020.
Kim, D. J., Ferrin, D. L., & Rao, H. R. (2008). A trust-based consumer decision-making model in electronic commerce: The role of trust, perceived risk, and their antecedents. Decision Support Systems, 44 (2), 544–564. https://doi.org/10.1016/j.dss.2007.07.001 .
Kracher, B., Corritore, C. L., & Wiedenbeck, S. (2005). A foundation for understanding online trust in electronic commerce. Journal of Information, Communication and Ethics in Society, 3 (3), 131–141. https://doi.org/10.1108/14779960580000267 .
Kumar, P., & Chandrasekar, S. (2016). E-Commerce trends and future analytics tools. Indian Journal of Science and Technology . https://doi.org/10.17485/ijst/2016/v9i32/98653 .
Lahiri, A. (2018). Consumer protection in E-commerce in India. https://amielegal.com/consumer-protection-in-e-commerce-in-india/ . Accessed 12 Oct 2020.
Larose, R., & Rifon, Nora, J. (2007). Promoting i-safety: Effects of privacy warnings and privacy seals on risk assessment and online privacy behavior. Journal of Consumer Affairs . https://doi.org/10.1111/j.1745-6606.2006.00071.x .
Lăzăroiu, G., Neguriţă, O., Grecu, I., Grecu, G., & Mitran, P. C. (2020). Consumers’ decision-making process on social commerce platforms: Online trust, perceived risk, and purchase intentions. Frontiers in Psychology, 11 , 890. https://doi.org/10.3389/fpsyg.2020.00890 .
Lee, O. M. K., & Turban, E. (2001). A trust model for consumer internet shopping. International Journal of Electronic Commerce . https://doi.org/10.1080/10864415.2001.11044227 .
Leonidou, L. C., Kvasova, O., Leonidou, C. N., & Chari, S. (2013). Business unethicality as an impediment to consumer trust: The moderating role of demographic and cultural characteristics. Journal of Business Ethics, 112 (3), 397–415. https://doi.org/10.1007/s10551-012-1267-9 .
Lin, C., & Lekhawipat, W. (2014). Factors affecting online repurchase intention. Industrial Management & Data Systems, 114 (4), 597–611. https://doi.org/10.1108/IMDS-10-2013-0432 .
Liyang, H. (2019). Superior bargaining power: The good, the bad and the ugly. Asia Pacific Law Review, 27 (1), 39–61. https://doi.org/10.1080/10192557.2019.1661589 .
Loannis, L., Despoina, M., Gracia, M. D., Amber, D., & d Azza R. (2019). The global governance of online consumer protection and E-commerce-building trust, http://www3.weforum.org/docs/WEF_consumer_protection.pdf . Accessed 9 Oct 2020.
Majithia, V. (2019). The changing landscape of intermediary liability for E-commerce platforms : Emergence of a new regime. International Journal of Law and Technology, 15 , 470–493..
Mangiaracina, R., & Perego, A. (2009). Payment systems in the B2C eCommerce: Are they a barrier for the online customer? Journal of Internet Banking and Commerce, 14 (3), 1–16..
MaqablehMasa’deh, M. R., Shannak, R., & Nahar, K. (2015). Perceived trust and payment methods: An empirical study of MarkaVIP company. International Journal of Communications Network and System Sciences, 8 (11), 409–427. https://doi.org/10.4236/ijcns.2015.811038 .
Mayer, R. C., Davis, J. H., & Schoorman, F. D. (1995). An integrative model of organizational trust . Academy of Management Review, 20 , 709–734. http://www.makinggood.ac.nz/media/1270/mayeretal_1995_organizationaltrust.pdf . Accessed 14 Oct 2020.
McCoubrey, H., & White, N. D. (1999). Textbook on jurisprudence (3rd ed.). Blackstone Press Limited.
Mcknight, D., Choudhury, V., & Kacmar, C. (2002). The impact of initial consumer trust on intentions to transact with a web site: A trust building model. The Journal of Strategic Information Systems, 11 , 297–323. https://doi.org/10.1016/S0963-8687(02)00020-3 .
McKinsey global institute. (2019). Digital India: Technology to transform a connected nation, https://www.mckinsey.com/~/media/McKinsey/Business%20Functions/McKinsey%20Digital/Our%20Insights/Digital%20India%20Technology%20to%20transform%20a%20connected%20nation/MGI-Digital-India-Report-April-2019.pdf . Accessed 10 Oct 2020.
Mekovec, R., & Hutinski, Ž. (2012). The role of perceived privacy and perceived security in online market. In: Proceedings of the 35th international convention MIPRO (pp. 1549–1554). Institute of Electrical and Electronics Engineers. https://ieeexplore.ieee.org/document/6240857 . Accessed 25 Oct 2020.
Milan, J., Antonio, G., & Niklas, A. (2020). Exploring the growth challenge of mobile payment platforms: A business model perspective. Electronic Commerce Research and Applications . https://doi.org/10.1016/j.elerap.2019.100908 .
Miyazaki, A., & Fernandez, A. N. A. (2001). Consumer perceptions of privacy and security risks for online shopping. Journal of Consumer Affairs, 35 , 27–44. https://doi.org/10.1111/j.1745-6606.2001.tb00101.x .
Nandini, C. P. (2018). B2C E-commerce and consumer protection with special reference to India—ADR a best possible solution. International Journal of Consumer Law and Practice, 6 , 74–87.
Nardal, S., & Sahin, A. (2011). Ethical issues in E-commerce on the basis of online retailing. Journal of Social Sciences, 7 (2), 190–198. https://doi.org/10.3844/jssp.2011.190.198 .
Nehf, J. (2007). Shopping for privacy on the internet. Journal of Consumer Affairs . https://doi.org/10.1111/j.1745-6606.2007.00085_1.x .
Nielsen. (2018). Future opportunities in FMCG E-commerce: Market drivers and five-year forecast. https://www.nielsen.com/wp-content/uploads/sites/3/2019/04/fmcg-eCommerce-report.pdf . Accessed 14 Oct 2020.
Nielsen. (2019). Total consumer report 2019. https://www.nielsen.com/us/en/insights/report/2019/total-consumer-report-2019/ . Accessed 14 Oct 2020.
OECD. (2000). Guidelines for consumer protection in the context of electronic commerce. https://www.oecd.org/sti/consumer/34023811.pdf . Accessed 5 Oct 2020.
OECD. (2016). Consumer protection in E-commerce: OECD recommendation . OECD Publishing. https://doi.org/10.1787/9789264255258-en .
Book Google Scholar
O’Hara, E. A. (2005). Choice of law for internet transactions: The uneasy case for online consumer protection. University of Pennsylvania Law Review, 153 , 1883–1950.
Orendorff, A. (2019). Global ecommerce statistics and trends to launch your business beyond borders, https://www.shopify.com/enterprise/global-ecommerce-statistics#8 . Accessed 5 Oct 2020.
Pavlou, P. A. (2003). Consumer acceptance of electronic commerce: Integrating trust and risk with the technology acceptance model. International Journal of Electronic Commerce, 7 (3), 101–134. https://doi.org/10.1080/10864415.2003.11044275 .
Ponte, E. B., Carvajal-Trujillo, E., & Escobar-Rodríguez, T. (2015). Influence of trust and perceived value on the intention to purchase travel online: Integrating the effects of assurance on trust antecedents. Tourism Management, 47 , 286–302. https://doi.org/10.1016/j.tourman.2014.10.009 .
Porter, M. (1979). How competitive forces shape strategy. https://hbr.org/1979/03/how-competitive-forces-shape-strategy . Accessed 9 Oct 2020.
RBI. (2020). Consumer protection measures by leading e-commerce retailers: Digital payments rise manifold in 5 yrs (p. 11). The Times of India.
Roman, S. (2007). The ethics of online retailing: A scale development and validation from the consumers’ perspective. Journal of Business Ethics, 72 , 131–148. https://doi.org/10.1007/s10551-006-9161-y .
Roman, S., & Cuestas, P. J. (2008). The perception of consumers regarding online retailers’ ethics and their relationship with the consumers’ general internet expertise and word of mouth: A preliminary analysis. Journal of Business Ethics, 83 (4), 641–656. https://doi.org/10.1007/s10551-007-9645-4 .
Rothchild, J. (1999). Protecting the digital consumer: The limits of cyberspace utopianism. Indiana Law Journal , 74 (3). https://www.repository.law.indiana.edu/ilj/vol74/iss3/5 . Accessed 8 Oct 2020.
Ruhl, G. (2011). Consumer protection in choice of law. Cornell International Law Journal, 44 (3), 569–601. https://www.lawschool.cornell.edu . Accessed 7 July 2020.
Rybak, A. (2019). Consumer trust in E-commerce: The case of Poland. Folia Oeconomica Stetinensia, 18 (2), 59–71. https://doi.org/10.2478/foli-2018-0019 .
Saif, N. A. AL M. (2018). General principles of consumer protection in E-commerce trade: A comparative study between Sharia law and EU laws. Unpublished Ph.D thesis. University of Exeter. https://ore.exeter.ac.uk/repository/bitstream/handle/10871/36623/Al-MamariS.pdf?sequence=1&isAllowed=y . Accessed 10 Oct 2020.
Saunders, M., Lewis, P., & Thornhill, A. (2007). Research Methods for Business Students . 4th Edition, Financial Times Prentice Hall, Edinburgh Gate, Harlow.
Saumure, K., & Given, L. M. (2008). Convenience sample. In L. M. Given (Ed.), The sage encyclopedia of qualitative research methods (pp. 124–125). Sage Publications.
Sharma, G., & Lijuan, W. (2014). Ethical perspectives on e-commerce: An empirical investigation. Internet Research: Electronic Networking Applications and Policy . https://doi.org/10.1108/IntR-07-2013-0162 .
Shirazi, F., Adam, N. A., Shanmugam, M., & Schultz, C. D. (2020). The importance of trust for electronic commerce satisfaction: An entrepreneurial perspective. British Food Journal, 123 (2), 789–802. https://doi.org/10.1108/BFJ-07-2020-0626 .
Siciliani, P., Riefa, C., & Gamper, H. (2019). Consumer theories of harm: An economic approach to consumer law enforcement and policy making . Hart Publishing. https://doi.org/10.5040/9781509916887 .
Singh, R. K. (2019). E-commerce in India: Opportunities and challenges, In: Proceedings of 10th international conference on digital strategies for organizational success. SSRN Electronic Journal. https://doi.org/10.2139/ssrn.3315048 . Accessed 27 Nov 2020.
Sousa, V. D., Zauszniewski, J., & Musil, C. (2004). How to determine whether a convenience sample represents the population. Applied Nursing Research, 17 , 130–133. https://doi.org/10.1016/j.apnr.2004.03.003 .
Stead, B., & Gilbert, J. (2001). Ethical issues in electronic commerce. Journal of Business Ethics, 34 (2), 75–85. https://doi.org/10.1023/A:1012266020988 .
Suh, B., & Han, I. (2003). The impact of customer trust and perception of security control on the acceptance of electronic commerce. International Journal of Electronic Commerce, 7 , 135–161. https://doi.org/10.1080/10864415.2003.11044270 .
Thakur, A., Shabnam, & Kaur, R. (2017). An empirical study on consumer trust in online shopping in Punjab. Indian Journal of Marketing . https://doi.org/10.17010/ijom/2017/v47/i2/110027 .
Tripathi, M., & Mukhopadhyay, A. (2020). Financial loss due to a data privacy breach: An empirical analysis. Journal of Organizational Computing and Electronic Commerce, 30 (4), 381–400. https://doi.org/10.1080/10919392.2020.1818521 .
UNCTAD. (2017). Consumer protection in electronic commerce, https://unctad.org/meetings/en/SessionalDocuments/cicplpd7_en.pdf . Accessed 7 October 2020.
UNCTAD. (2019). Digital economy report 2019, value creation and capture: Implications for developing countries, https://unctad.org/system/files/official-document/der2019_en.pdf . Accessed 7 November 2020.
UNCTAD. (2019). Measuring E-commerce and the digital economy, https://unctad.org/topic/ecommerce-and-digital-economy/measuring-ecommerce-digital-economy . Accessed 11 July 2020.
UNESAP & ADB. (2019). Embracing the E-commerce revolution in Asia and the Pacific, https://www.adb.org/sites/default/files/publication/430401/embracing-e-commerce-revolution.pdf . Accessed 9 October 2020.
Van Dyke, T. P., Midha, V., & Nemati, H. (2007). The effect of consumer privacy empowerment on trust and privacy concerns in E-commerce. Electronic Markets, 17 (1), 68–81. https://doi.org/10.1080/10196780601136997 .
Vancauteren, M., Reinsdorf, M., Veldhuizen, E., Eugene van der, P., Carsten, B., & Airaksinen, A. (2011). E-commerce. In United Nations Economic Commission for Europe (Eds.), The Impact of Globalization on National Accounts (pp 249–261). https://www.unece.org/fileadmin/DAM/stats/groups/wggna/GuideByChapters/Chapter_13.pdf . Accessed 7 Oct 2020.
Wajeeha, A., Annas, H., & Kashif, F. (2019). Underlying factors influencing consumers’ trust and loyalty in E-commerce, Business Perspectives and Research, 8 (2), 186–204. https://doi.org/10.1177/2278533719887451 .
Wang, X., Tajvidi, M., Lin, X., & Hajli, N. (2019). Towards an ethical and trustworthy social commerce community for brand value Co-creation: A trust-commitment perspective. Journal of Business Ethics Issue, 167 , 137–152. https://doi.org/10.1007/s10551-019-04182-z .
World Economic Forum. (2019). The global governance of online consumer protection and e-commerce building trust, http://www3.weforum.org/docs/WEF_consumer_protection.pdf . Accessed 9 Oct 2020.
Xiaodong, Q., & Min, L. (2020). E-commerce user type recognition based on access sequence similarity, Journal of Organizational Computing and Electronic Commerce , 30 (3), 209–223. https://doi.org/10.1080/10919392.2020.1742552 .
Yang, Z., Van Ngo, Q., Chen, Y., Nguyen, C. X. -T., & Hoang, H. T. (2019). Does ethics perception foster consumer repurchase intention? Role of trust, perceived uncertainty, and shopping habit. SAGE Open . https://doi.org/10.1177/2158244019848844 .
Young, L. C., & Wilkinson, I. F. (1989). The role of trust and co-operation in marketing channels: A preliminary study. European Journal of Marketing, 23 (2), 109–122. https://doi.org/10.1108/EUM0000000000550 .
Zikmund, W. G. (2000). Business Research Methods . 6th Edition, The Dryden Press, Fort Worth.
Zuhal, H. (2019). The gamut of E-commerce: Between online fraud and trust towards technology among generation Y. International Journal of Mechanical Engineering and Technology, 10 (01), 830–836. http://www.iaeme.com/IJMET/issues.asp?JType=IJMET&VType=10&Type=01 . Accessed 27 Nov 2020.
Download references
Acknowledgements
The authors express their appreciation to the section editor and two anonymous reviewers for providing insightful comments and constructive suggestions to improve the paper's quality.
This research did not receive any specific grant from funding agencies in the public, commercial, or not-for-profit sectors.
Author information
Authors and affiliations.
Vivekananda Institute of Professional Studies, Guru Govind Singh Indraprastha University, New Delhi, 110027, India
Neelam Chawla
Academic, Research and Legal Consultant, C-202/Satyam Tower, Bomikhal, Bhubaneswar, 751010, India
Basanta Kumar
You can also search for this author in PubMed Google Scholar
Corresponding author
Correspondence to Neelam Chawla .
Ethics declarations
Conflict of interest.
The authors declare that they have no known competing financial interests or personal relationships that could have appeared to influence the work reported in this paper.
Ethical Approval
This article does not contain any studies with human participants or animals performed by any of the authors.
Additional information
Publisher's note.
Springer Nature remains neutral with regard to jurisdictional claims in published maps and institutional affiliations.
Rights and permissions
Reprints and permissions
About this article
Chawla, N., Kumar, B. E-Commerce and Consumer Protection in India: The Emerging Trend. J Bus Ethics 180 , 581–604 (2022). https://doi.org/10.1007/s10551-021-04884-3
Download citation
Received : 20 December 2020
Accepted : 24 June 2021
Published : 09 July 2021
Issue Date : October 2022
DOI : https://doi.org/10.1007/s10551-021-04884-3
Share this article
Anyone you share the following link with will be able to read this content:
Sorry, a shareable link is not currently available for this article.
Provided by the Springer Nature SharedIt content-sharing initiative
- Consumer protection
- Online protection
- Find a journal
- Publish with us
- Track your research
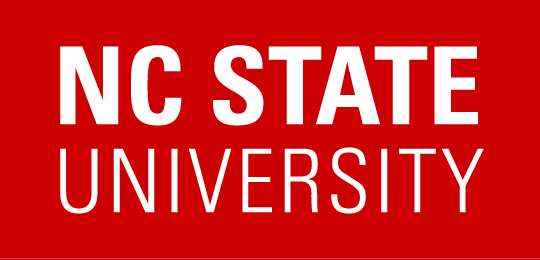
BioResources
- About the Journal
- Authors & Reviewers
- How to Self-Register
- Full Site Navigation
- Editorial Board
- Meet the Staff
- Editorial Policies
- General Instructions
- Ethics & Responsibilities
- Article Preparation
- Submission Instructions
- Acknowledgment of your Peer-Reviewing
- Writing Style Suggestions
- Reviewer Guidelines
- Back and Current Issues
- Scholarly Reviews
- Special Conference Collection Issues
- Competition Print Edition
- FRC: Pulp and Paper Fundamental Research Symposia Proceedings
- Paper Manufacturing Chemistry
- BioResources Early Career Investigator Award
- Distance Education: Online Masters Degree & Individual Courses
- Upcoming Conferences
- Hands-On Courses
- Affiliate Journal
Furniture online consumer experience: A literature review
In recent years, people’s acceptance of online shopping has increased markedly with the gradual maturing of e-commerce. The furniture industry in China, along with many other countries, is paying increased attention to the online retail business. The furniture online consumption experience has attracted attention both in academic and industrial fields. The purpose of this paper is to provide a literature review of the furniture online consumption with an aim to extend the concept of consumer experience to the context of online furniture consumption. The paper offers three important contributions for both academics and practitioners. First, it analyzes the main influencing factors of the consumer experience concerning wood furniture online consumption in China. And secondly, it proposes a conceptual framework of furniture online consumer experience (FOCE), which divides online consumption experience into three dimensions: perceived risk experience, emotional experience, and new technology interactive experience. Finally, from a managerial perspective, the authors put forward constructive strategies in terms of furniture online sales. The findings of this study afford practical implications for the improvement of the online shopping experience of consumers for furniture companies.
Full Article
Furniture Online Consumer Experience: A Literature Review
Shuangjie Zhang, Jiangang Zhu,* Guokun Wang, Shuangyue Reng,and Huan Yan
DOI: 10.15376/biores.17.1.1627-1642
Keywords: Furniture consumption; Online shopping; Consumer experience; Interactive experience
Contact information: College of Furnishings and Industrial Design, Nanjing Forestry University, Nanjing 210037, China; *Corresponding author: [email protected]
INTRODUCTION
Since the advent of e-commerce, China’s online retail industry has developed rapidly, and consumers have a greater interest in online shopping. The “Internet Plus” policy announced by Chinese government in 2015, encourages people to use network information technology (IT) to promote innovations in e-commerce and online shopping (Xiong et al. 2017). The proportion of furniture companies that choose to sell furniture online is higher than before; as of 2020, the market share of online Chinese brands in the furniture industry has reached more than 80% (Alibaba Research Institute 2020). According to the China National Bureau of Statistics, the number of furniture markets in China has gradually decreased, from 179 in 2016 to 149 in 2020 (China National Bureau of Statistics). The scale of furniture e-commerce is becoming increasingly larger (Qianzhan Industry Research Institute 2018), from 49.47 billion in 2011 to 165.06 billion in 2017. The scale of Chinese furniture e-commerce market is shown in Fig. 1. E-commerce in China’s furniture industry is mainly based on the business-to-customer (B2C) model. The following three main approaches have emerged in the online retail market under this model.
The first approach is the furniture brands’ self-built electronic mall and self-developed mobile application. In this mode, consumers can complete the transaction by browsing the product information on the website or application, selecting the product and the delivery address, and completing the payment. In the context of sustainability and customers’ demand on green consumption, the transition towards green manufacturing of furniture industry increasingly urgent than ever. (Xiong et al. 2020 and Wang et al. 2020). This way makes the process of furniture retailing and purchasing informatized and networked, saves a certain amount of manpower and material costs, and brings consumers a unique and complete consumer experience. But the subsequent development and post-maintenance of the website and application costs also discourage most small businesses, since there is a need for continuous investment in labor and material costs related to networking.
Fig. 1. China Furniture E-Commerce Market Scale
The second approach is to integrate sales on e-commerce platforms such as Taobao and JD.com. Alibaba and JD account for more than 90% of China’s online retail market share (China Internet Information Center 2015). E-commerce platforms provide commodity information a display space and transaction methods for the settled stores. At the same time, the e-commerce platform has gradually standardized the management of furniture stores, which has made the service offering by various furniture brands online stores become more comprehensive and standardized, reducing the psychological risks of consumers buying furniture online. However, it has also caused the service promised by the retailers on the product display page to become more uniform with higher homogeneity.
The third approach is mobile commerce platform sales. With the vigorous development of new media technology, new media platforms, such as Weibo, WeChat Mini Programs, and TikTok, have launched commodity retail functions. In particular, the more intuitive way of displaying goods, such as live broadcast selling, which is an emerging marketing method that uses Internet platforms to introduce and display products to be sold in real time (Liu and Shi 2020). Furniture companies in China are actively participating in this new marketing method. According to Xiao and Yu (2017), although there are various forms of new media, it is found that the most suitable for information spreading in the furniture industry is still graphic, live broadcast, followed by short video.
There have been many studies that have demonstrated the influence of consumer experience on consumer satisfaction and repurchase intentions (George 2002; Rose et al . 2011). In a saturated and highly competitive market, improving the online shopping experience is important for companies to provide more special and differentiated products and services (Rose et al . 2011). The research of Janda and Ybarra (2005) purports that excellent consumer experience has a positive impact on consumer satisfaction. Khalifa and Liu (2007) defined repurchase intention as “using online channels to buy goods from specific retailers”. After investigation, it was found that positive online consumer satisfaction is positively correlated with repurchase willingness (Khalifa and Liu 2007). In the context of the fierce competition in the Chinese furniture industry and the highly saturated market, the current article analyzes the components of online consumer experience and their impact and provides some suggestions for furniture companies to optimize customer service.
The aim of this paper is to provide a comprehensive review of contemporary literature that informs our understanding of the antecedents and consequences of consumer experience (CE) in the furniture online consumption context. The review is undertaken in hope of highlighting the importance of this emerging area of interest. This paper expects to carry out several explorations. The first is adding the knowledge of the subject of CE in the furniture online consumption context. The paper extends the understanding of the factors related to online consumer experience from the perspective of the furniture consumption. The second is to propose a theoretical framework for furniture online consumer experience (FOCE) through a summary of the literature on furniture online consumption. Third, the theoretical framework proposed in the thesis puts forward many suggestions to improve furniture online consumption experience for retailers in the Chinese furniture industry.
The structure of the paper is as follows. The first section presents the research method used for this literature review. A literature review of the concept of FOCE is then provided, and the proposed conceptual framework is presented. The following section provides the substantive literature review, structured according to the framework. Finally, the paper ends with a summary of the conclusions that can be drawn from the review and makes proposals for further research.
LITERATURE REVIEW
A systematic review of the literature was undertaken using the following method. A review question was identified by the research team: What is the role of consumer experience in the furniture online consumption environment? Then search keywords were drawn up by the team, which included: furniture consumption; furniture industry; furniture online consumption; consumer experience; online consumer experience; interactive experience; and augmented reality. References came from Google Scholar, Web of Science, and China National Knowledge Infrastructure (CNKI).
The articles were screened according to the topics expressed in the title and abstract of the paper. The content analysis of selected papers was done manually, and data extraction forms were used to summarize key data, such as key findings and methodological characteristics. This enables researchers to identify the overall nature, epistemological assumptions, and methodological characteristics of existing research. Furthermore, the team created a list to categorize the content of the forms according to the research direction.
This paper focuses on the consumer experience in furniture online purchase environment and the features of furniture consumption in China. Therefore, the authors paid equal attention on the concept of consumer experience, OCE, and furniture retail in China to propose a theoretical framework to systematically answer the review questions mentioned above.
The Concept of FOCE
There have been many studies on the concept of consumer experience (CE) in the fields of consumer marketing, service delivery, tourism, and retail before the advent of the Internet. With the rapid development of e-commerce, research on retail consumer experience has gradually shifted from offline to online. However, people’s attitudes towards the online shopping experience are often related to the types of goods, i.e. , people are more likely to perceive the shape and weight of electronic products, and they have a higher tactile demand for clothes (Li et al . 2001). Because of the large volume and price of furniture products, people rely much on consumer experience (Lin et al . 2019) to perceive the spatial and tactile elements of furniture during the consumption process.
Many studies have recognized the importance of CE in the retail market (Grewal et al . 2009). Furniture products are suitable for China’s e-commerce platform, as large-scale durable products, under certain conditions (Li et al . 2020). However, CE is still an unfamiliar concept for online furniture retail, which is not conducive to the establishment of a theoretical framework. Next in this paper, a certain literature review and summary of the concepts of CE and OCE is given, and the theoretical framework of FOCE is proposed.
Consumer Experience
Meyer and Schwager (2007) defined consumer experience as the customer’s internal and subjective response to any direct or indirect contact with the company. Similarly, Gentile et al . (2007) argued that consumer experience comes from a series of interactions between consumers and services, products, companies, or their organizations. The above literature analyzes the source of consumer experience from the perspective of the consumption process.
In terms of the dimensions of consumer experience, Babin et al . (1994) proposed that consumer experience is composed of utilitarian elements and hedonic elements. They further demonstrated the rational combination of the two elements of the consumer experience under specific circumstances. Berry et al. (2006) made important contributions. According to Berry et al . (2006), consumers often evaluate their consumption experience through functional clues (meeting customer expectations), mechanic clues (influencing first impressions, expectations, and value creation), and humanic clues (exceeding customer expectations) in the process of interacting with organizations. The importance of this classification is to classify the consumer experience as different aspects of the contact between consumers and organizations.
Based on the above literature review, the overall consumer experience can be defined as: customer experience is the internal and subjective reaction of any direct or indirect contact between customers and the company. Direct contact usually occurs during purchase, use, and maintenance, a process usually initiated by the customer. The most common way of indirect contact is contact with the company’s products, services, or brands, in the form of word-of-mouth recommendations or advertisements, news reports, reviews, etc . The process of generating consumer experience includes the first impression generated by indirect contact, consumer expectations, to functional satisfaction generated by direct contact, and finally the consumer’s evaluation of the consumer behavior after using the product and service (whether it meets or exceeds the consumer’s expectations).
Online Consumer Experience
With the rapid development of commerce online, increased attention is being paid to the research of consumers’ online experience. Consumer experience in the context of e-commerce is an important factor restricting the development of Chinese furniture e-commerce (Niu and Liu 2017). Therefore, online consumer experience has become an important concept, especially in the context of online shopping. It is believed that the offline context can provide a richer information display method than online. The online environment can only display the brand through an audio-visual way, but a range of visible devices can be used to present the brand in an offline environment.
Long (2004) believes that the online consumer experience is mainly affected by the trustworthiness of the website, convenience, customer autonomy, and the relationship between the website and the customer. Constantinides and Geurts (2005) believe that online consumer experience is related to website interactivity, aesthetics, trust, convenience, and marketing. Although the above authors put forward the factors affecting online consumer experience, these works did not propose a reliable conceptual model of online consumer experience. Frow and Payne (2007) proposed that the rational, cognitive process, and the perceptual and emotional process are all part of the formation of consumer experience. Similarly, Hansen (2005) argued that cognitive and emotional identification, as well as the interaction of these, is an appropriate method to understand consumer experience. With the recent rapid development of the internet, more authors have shifted their research focus from offline to online. Many authors have proposed their own online consumer experience models with reference to the Frow and Payne (2007) model. The table below summarizes some of them.
Table 1. Dimensions of Online Consumer Experience
Compared with the offline environment, the online consumer experience places more emphasis on considering the enterprise’s application of data and technology to the consumer level, such as convenience, interactivity, process experience, technical experience, etc . (Tan 2019).
A CONCEPTUAL FRAMEWORK OF FOCE
Previous reviews of the literature mainly have focused on consumer experience and online consumer experience, while the focus of this article is on the particularity of online consumer experience in furniture consumption. Furniture products have the following characteristics due to their high prices: low standardization, high transportation costs, and many additional services.
The online consumption of furniture has the following characteristics. First, consumers are more cautious when buying furniture products online, hoping to obtain more product information. Online consumers want to check the material, workmanship, style, and shape of furniture in offline physical stores (Cao et al . 2014).
Secondly, delivery restricts online sales of furniture. This is because furniture products have the characteristics of larger size, heavier weight, and relatively higher value. Moreover, furniture e-commerce companies in China are mainly small and medium-sized enterprises. Most of them complete the logistics process through third-party logistics companies or joint distribution. The logistics links are prone to differences in reliability and response. It is difficult for consumers to obtain the fast and convenient logistics services they want (Zhang and Xu 2019).
Finally, spiritual connotation of furniture consumption can bring users a consumption experience similar to luxury goods. It is believed that furniture consumption tends to become symbolic (Dou and Chen 2014). As China enters the consumer society, more consumers are purchasing furniture to show their social status and lifestyle, and furniture consumption is increasingly showing a trend of pursuing spirit demand rather than material demand.
The importance of consumption experience lies in the fact that in a highly competitive and saturated market, enterprises hope to provide differentiated products by enriching basic products and services (Rose et al . 2011). It is useful to understand the dimension of consumption experience from the perspective of the relationship between enterprises and consumers, because the concept of consumption experience comes from this. De Keyser et al . (2015) think that consumers are active participants in the shopping experience, and they pursue cognitive and emotional goals in online and offline shopping (Kawaf and Tagg 2017).
The consumer’s cognitive experience is related to the perceived value of consumers. Wang (2008) define the consumer’s perceived value as the overall evaluation of the trade-off between the perceived quality of the received product or service and the total cost of obtaining the product or service. Many scholars define perceived value separately as perceived advantage and perceived risk. Forsythe et al . (2006) define perceived advantage as the sum of consumer needs or desires and online shopping advantage or satisfaction. Perceived advantages include advantages related to online shopping experience, including convenience, price comparison, time saving, return policies, shopping convenience, entertainment, and enhanced consumer-retailer relations (Elwalda et al . 2016). With regard to the elements of perceived risk, Wang et al . (2006) pointed out that the quality of products or services, personal privacy, and security are frequent risk elements. The basic concerns of consumers are those of conducting online financial transactions. When the authors discuss online furniture consumption, the boundary between the two concepts does not seem to exist. Online furniture consumption is different from other products; consumers pay more attention to the cognitive experience, such as the shape, size, and quality of the furniture (Dai 2013). These factors are often the premise for consumers to determine whether goods are available or not to avoid economic losses and the failure of return services. Therefore, this paper refines the cognitive experience in online furniture consumption into a low-risk experience perceived by consumers.
The emotional experience is more personal and subjective, and shopping experience during shopping relies on entertainment and escapism (De Keyser et al . 2015). These aspects bring more entertainment to customers. Jeong et al. (2009) tested four experience areas (entertainment, educational, escapist, and aesthetic experiences) of Pine and Gilmore (1999) in the online shopping environment. In the online sales of furniture in China, the best-selling of various styles of furniture demonstrates the consumers’ demand for the aesthetic experience of furniture. The major e-commerce platforms hold a variety of furniture purchase experience activities every year to meet consumers’ demand for shopping and entertainment.
Most previous research divided online consumption experience into cognitive experience and emotional experience. This paper argues that the experience brought by new technology cannot be ignored; the application of high-end technology is the inevitable way to meet the needs of consumers (Chen and Wu 2018). According to the degree of interaction between consumers and goods, Li et al . (2001) divides consumer experience into direct experience, indirect experience, and virtual experience, in which the experience brought to consumers by virtual experience often combines cognitive experience and emotional experience. The virtual fitting technology defined as VTO (virtual try-on) has both use value and hedonic value (Zhang et al . 2019), which means that the virtual experience involves both cognitive and emotional experience. Similarly, another virtual technology defined as AR (augmented reality) has similar characteristics. Poushneh and Vasquez-Parraga (2017) pointed out that AR can reflect four product characteristics: aesthetic quality, pragmatic quality, stimulating hedonistic quality, and identifying hedonistic quality. As augmented reality technology becomes more affordable, many retailers, such as IKEA, have implemented augmented reality in their experiential retail channels. Therefore, this paper takes the new technology interactive experience as the third part of the online furniture consumption experience.
Based on the above literature review on the characteristics of online furniture consumption, this paper proposes the theoretical framework of furniture online consumption experience in Fig. 2 based on the theoretical structure of Frow and Payne (2007). This framework divides the furniture online consumption experience into three parts: perceived low-risk experience, emotional experience, and new technology interactive experience. There are several influencing elements in each experience.
Fig. 2. A conceptual framework of FOCE
Perceived Low-risk Experience
Bhatnagar et al. (2000) and Lim (2003) put forward the concept of risk perception when shopping online. They believe that it has an important influence on consumers’ willingness to interact online. Perceived risk of online shopping is primarily the uncertainty of product information, followed by the severity of the consequences of purchase (Cases 2002). In China, online furniture consumers are mainly concerned about the product inconsistency with the description and quality issues (Li et al. 2016). This paper combines various viewpoints in the literature and divides the perceived risk experience into three aspects: the reliability of product information, logistics and delivery, and after-sales service (repair and return). The reasons for selecting these three factors will be discussed separately below.
When shopping online, the reliability of furniture product information is important, because furniture products have larger size, their materials are highly relevant to people’s daily life, and it is important that the product matches the home environment when placed in the domestic environment after purchase. Patro and Katta (2020) found that among perceived risks, the greatest impact on consumers is psychological risk, and an important part of psychological risk is whether the retailer’s commitment is consistent with the facts (Zhang et al . 2011). Therefore, for online furniture consumption, the reliability of product information provided by online retailers is crucial.
Another factor that can bring lower perceived risk to consumers is on-time delivery. Delivery has the greatest impact on consumers’ perceived benefits online (Patro and Katta 2020), which means that excellent delivery can reduce consumer perceptions risk. On the contrary, any failure or delay in delivery will leave a bad evaluation for online consumers and reduce repurchases (Reichheld and Schefter 2000). Similarly, Rao et al . (2011) identified that the delay in the delivery of goods will reduce the frequency of online purchases by consumers in the future. In other words, online retailers can improve the consumer experience and repurchase rate by promising a shorter delivery time on the website and fulfilling these promises.
The last factor that affects consumers’ perceived risks is the after-sales experience, including returns and installation, maintenance services, etc . Furniture products (such as beds, wardrobes, etc .) often require professionals to complete the installation work. After consumers purchase furniture online, if retailers can provide door-to-door installation services, it will increase consumer satisfaction and repurchase rates (Zhang and Xu 2019). In addition, after purchasing furniture online, if consumers are not satisfied with the product after receiving the goods, they often will not choose to return the goods because of the inconvenience of returning the goods (Dai 2013). This also leads to a bad online consumer experience.
The above discussion of perceived risk experience mainly involves the cognitive part of online consumer experience, and the research of Barari et al . (2020) shows that consumers tend to pursue emotional experience when sufficient cognitive value has been obtained during their consumption experience. Next the emotional experience part of the furniture online consumer experience is discussed.
Emotional Experience
Emotional experience depends on entertainment and escapism in the shopping process (De Keyser et al . 2015). The emotional experience is personalized and subjective, bringing fun and pleasure to customers (Holbrook and Hirschman 1982). Users’ different preferences for furniture products are related to the positive, neutral, or negative state of their emotions (Yang et al . 2019). When people are engaged in entertainment activities, such as the popular online celebrity live broadcasts in China, it is easy for this to become an incentive for consumers to buy goods.
Another factor related to emotional experience is online trust. Many studies have pointed out that due to more unknowns, compared with face-to-face retail, the online environment requires a higher degree of trust (Corbitt et al . 2003; Van der Heijden et al. 2003). Van der Heijden et al . (2003) shows that a high degree of trust can reduce consumers’ concerns about product performance and retailer policies.
There have been many academic studies on the source of online trust. Lv et al . (2016) argues that real-time communication between buyers and sellers through online chat services reduces the uncertainty of buyers in making purchasing decisions. Similarly, Mero (2018) proved the positive impact of two-way communication on trust through empirical research.
Perceived interaction is the premise of trust based on interpersonal relationships. Online consumers form online trust in websites through the three aspects of perceptual interaction (perceived interactivity, perceptual reactivity, and perceptual personalization) (Wu et al . 2010). The rise of the internet has brought the need for interactivity in digital media and digital technology researching background. A review of the new contributions of some new interactive technologies to the online consumer experience will be discussed next.
New Technology Interactive Experience
Liu and Shrum (2002) define interactivity as “the degree to which two or more communicating parties can interact, their role on the communication medium, the role of messages, and the degree to which this influence is synchronized.” They also proposed three aspects of interaction: active control (user’s ability to voluntarily participate in and influence communication with tools); two-way communication (two-way information flow); and synchronization (the speed of interaction). Chinese customers’ cognitions around products have changed from store + facade to reality + VR, which gives consumers a different experience via VR (Xiong et al. 2017). Traditional product information acquisition is often passive and single (only through sound and video acquisition), but the augmented reality technology that has gradually attracted the attention of academia recently seems to be suitable for online sales: shopping in the context of enhanced interactive technology can arouse consumers’ greater purchase intentions than passively accepting product information display (Kim and Forsythe 2008).
To understand more concretely, the current authors will use AR as an example to describe the innovation of new technologies in online consumer experience. Poushneh and Vasquez-Parraga (2017) proposed that AR is an interactive technology that can overlay virtual 3D models into the real environment. Users can rotate, move, and zoom in and out in the 3D model to change its state in the real environment.
On the one hand, AR does have some similarities with the risk perception experience and emotional experience. In terms of risk perception experience, the virtual try-on application can provide clues about the physical properties of the product to help consumers evaluate the product more completely (Dennis et al . 2010). Some previous studies have found evidence that virtual try-on may reduce the likelihood that consumers may perceive clothes purchased online as ill-fitting (Shim and Lee 2011; Kim 2016). In terms of emotional experience, Watson et al . (2020) argue that AR seems to provide a happier experience rather than a utilitarian experience. It is also believed that AR creates a rich sensory experience and thus closer emotional responses. Both those with strong or weak hedonistic motives can have fun from AR shopping applications, and those with strong hedonistic motives are more obvious.
In contrast, AR technology seems to explore some new forms of experience, and virtual try-on applications can further enhance the shopping experience. Users can share the results of fittings with friends and family easily (Dennis et al . 2010). Therefore, it can become a social channel, especially when combined with social media applications, to provide online consumers a pleasant shopping experience by meeting their social needs.
In the furniture industry, the furniture brand IKEA has developed the AR application IKEA Place, which can automatically scan the floor in the scene through the mobile phone camera and place the desired product model in the real scene captured by the camera, effectively enhancing the reality sense. The goods added to the scene can be directly clicked to view the product information and purchase, which greatly reduces the process of collecting information, evaluating purchase risks, purchasing and installing, and evaluating purchase results in the consumer process. It brings consumers a new and fast experience. When using the IKEA Place application, users will feel greater confidence and greater purchasing convenience (Alves and Luís Reis 2020).
CONCLUSIONS
- A review of key literature is presented with an aim to give insight and direction to an understanding of the online furniture purchases and online consumption experience in China. The three main influencing factors of the consumer experience on Chinese furniture product line can be drawn: (1) Consumers hope to access more furniture information such as materials, style, and size before buying furniture; (2) Consumers want to get a better delivery service and after-sales return service; and (3) Consumers have gradually enhanced demand for entertaining furniture products. This paper addresses the adaptability of the online consumer experience model in the Chinese furniture industry by expanding the theoretical structure of Frow and Payne (2007).
- A conceptual model of furniture online customer experience (FOCE) has been proposed in this work. According to FOCE, the furniture online consumer experience can be divided into three parts: (1) perceived low-risk experience, (2) emotional experience, and (3) new technology interactive experience. In the context of the fast-growing Chinese furniture e-commerce market, consumers have a strong demand for new consumer experiences. The new technology interactive experience is considered as a new consumption experience type due to its scarcity in online consumption experience in research, although the new technologies interactive experience often combines a perceived experience and emotional experience, and these are often difficult to segment.
- Furniture companies in China should take solid measures in online product display, size customization, logistics, door-to-door installation, after-sales, and other online value-added services. The offline store appointment experiences also are helpful to minimize consumers’ psychological expectations of risks associated with online shopping. Furthermore, surveying consumers’ furniture style preferences and organizing brand-related entertainment activities can be more conducive to meeting consumers’ emotional needs. Finally, the new technologies, such as AR, have revolutionary advantages in improving the high-level experience brought by the existing services and brands of e-commerce platforms. Furniture companies in China should therefore pay more attention to improvement of the user consumption experience brought by related new technologies.
- This article has attempted to discuss the essence of consumer experience in furniture online consumption by proposing a theoretical framework. There is no denying that the proposed framework is only conceptual; thus, subsequent development and empirical tests are required to establish more systemic understanding.
ACKNOWLEDGMENTS
The authors are grateful the support of the Joint Research program of Nanjing Forestry University, Jiangsu Co-Innovation Center of Efficient Processing and Utilization of Forest Resources, and Sino-foreign Cooperation in Running Schools of Jiangsu Province, Jiangsu, China.
REFERENCES CITED
Alibaba Research Institute (2020). “2020 China consumer brand development report,” (https://file.01caijing.com/attachment/202005/1215D966F6CC4F3.pdf), Accessed 31 May 2020.
Alves, C., and Luís Reis, J. (2020). “The intention to use e-commerce using augmented reality – The case of IKEA Place,” in: Information Technology and Systems . ICITS 2020. Advances in Intelligent Systems and Computing (Vol. 1137), Á. Rocha, C. Ferrás, C. Montenegro Marin, and V. Medina García (eds.), Springer, Cham, Switzerland, pp. 114-123. DOI: 10.1007/978-3-030-40690-5_12
Babin, B. J., Darden, W. R., and Griffin, M. (1994). “Work and/or fun: Measuring hedonic and utilitarian shopping value,” Journal of Consumer Research 20(4), 644-656. DOI: 10.1086/209376
Barari, M., Ross, M., and Surachartkumtonkun, J. (2020). “Negative and positive customer shopping experience in an online context,” Journal of Retailing and Consumer Services 53(1), Article ID 101985. DOI: 10.1016/j.jretconser.2019.101985
Berry, L. L., Wall, E. A., and Carbone, L. P. (2006). “Service clues and customer assessment of the service experience: Lessons from marketing,” Academy of Management Perspectives 20(2), 43-57. DOI: 10.5465/amp.2006.20591004
Bhatnagar, A., Misra, S., and Rao, R. (2000). “On risk, convenience, and internet shopping behaviour,” Communications of the ACM 43(11), 98-105. DOI: 10.1145/353360.353371
Bi, D., and Qiu, C. (2014). “Research on the influence mechanism of e-commerce business-customer interaction on customer experience,” China Software Science 2014(12), 124-135. DOI: 10.3969/j.issn.1002-9753.2014.12.012
Cao, Y., Wu, Z., Yang, B., Li, X., and Li, X. (2014). “Research of status quo and promotion programs of Hongmu furniture e-commerce,” Furniture 35(5), 8-14. DOI: 10.16610/j.cnki.jiaju.2014.05.014
Cases, A. (2002). “Perceived risk and risk-reduction strategies in internet shopping,” International Review of Retail Distribution and Consumer Research 12(4), 375-394. DOI: 10.1080/09593960210151162
Chen, Y., and Wu, Z. (2018). “Study on the application of particle swarm optimization in the virtual reality of the modified wood furniture,” Journal of Intelligent and Fuzzy Systems 35(3), 2741-2747. DOI: 10.3233/JIFS-169626
China National Bureau of Statistics(2021). “The number of China’s furniture market in the past five years,” (https://data.stats.gov.cn/easyquery.htm?cn= C01&zb=A0I0901&sj=2020), Accessed 19 December 2021.
Constantinides, E., and Geurts, P. (2005). “The impacts of web experience on virtual buying behavior: An empirical study,” Journal of Customer Behavior 4(3), 307-336. DOI: 10.1362/147539205775181249
Corbitt, B. J., Thanasankit, T., and Yi, H. (2003). “Trust and e-commerce: A study of consumer perceptions,” Electronic Commerce Research and Applications 2(3), 203-215. DOI: 10.1016/S1567-4223(03)00024-3
Dai, L. (2013). “The present and the future of the electronic commerce of Chinese furniture industry,” Furniture 34(1), 68-78. DOI: 10.16610/j.cnki.jiaju.2013.01.007
De Keyser, A., Lemon, K. N., Klaus, P., and Keiningham, T. L. (2015). A Framework for Understanding and Managing the Customer Experience (Report No. 15-121), Marketing Science Institute, Cambridge, MA, USA.
Dennis, C., Morgan, A., Wright, L. T., and Jayawardhena, C. (2010). “The influences of social e-shopping in enhancing young women’s online shopping behaviour,” Journal of Customer Behaviour 9(2), 151-174. DOI: 10.1362/147539210X511353
Dou, L., and Chen, Y. (2014). “Review on Chinese furniture’s symbolic consumer behavior,” Furniture and Interior Design 2014(1), 82-83. DOI: 10.16771/j.cnki.cn43-1247/ts.2014.01.029
Elwalda, A., Lü, K., and Ali, M. (2016). “Perceived derived attributes of online consumer reviews,” Computers in Human Behavior 56, 306-319. DOI: 10.1016/j.chb.2015.11.051
Forsythe, S., Liu, C., Shannon, D., and Gardner, L. C. (2006). “Development of a scale to measure the perceived benefits and risks of online shopping,” Journal of Interactive Marketing 20(2), 55-75. DOI: 10.1002/dir.20061
Frow, P., and Payne, A. (2007). “Towards the ‘perfect’ customer experience,” Journal of Brand Management 15(2), 89-101. DOI: 10.1057/palgrave.bm.2550120
Gentile, C., Spiller, N., and Noci, G. (2007). “How to sustain the customer experience: An overview of experience components that co-create value with the customer,” European Management Journal 25(5), 395-410. DOI: 10.1016/j.emj.2007.08.005
George, J. F. (2002). “Influences on the intent to make internet purchases,” Internet Research 12(2), 165-180. DOI: 10.1108/10662240210422521
Grewal, D., Levy, M., and Kumar, V. (2009). “Customer experience management in retailing: An organizing framework,” Journal of Retailing 85(1), 1-14. DOI: 10.1016/j.jretai.2009.01.001
Guo, H., and Wang, J. (2013). “The study of B2C customer experience model based on Tam model,” Science and Technology Management Research 33(19)184-188. DOI: 10.3969/j.issn.1000-7695.2013.19.042
Hansen, T. (2005). “Perspectives on consumer decision-making: An integrated approach,” Journal of Consumer Behaviour 4(6), 420-437. DOI: 10.1002/cb.33
Holbrook, M. B., and Hirschman, E. C. (1982). “The experiential aspects of consumption: Consumer fantasies, feelings, and fun,” Journal of Consumer Research 9(2), 132-140. DOI: 10.1086/208906
Janda, S., and Ybarra, A. (2005). “Do product and consumer characteristics affect the relationship between online experience and customer satisfaction?,” Journal of Internet Commerce 4(4), 133-151. DOI: 10.1300/J179v04n04_09
Jeong, S. W., Fiore, A. M., Niehm, L. S., and Lorenz, F. O. (2009). “The role of experiential value in online shopping: The impacts of product presentation on consumer responses towards an apparel web site,” Internet Research 19(1), 105-124. DOI: 10.1108/10662240910927858
Long, K. (2004). “Customer loyalty and experience design in e-business,” Design Management Review 22(16), 60-67. DOI: 10.1111/j.1948-7169.2004.tb00163.x
Kawaf, F., and Tagg, S. (2017). “The construction of online shopping experience: A repertory grid approach,” Computers in Human Behavior 72, 222-232. DOI: 10.1016/j.chb.2017.02.055
Khalifa, M., and Liu, V. (2007). “Online consumer retention: Contingent effects of online shopping habit and online shopping experience,” European Journal of Information Systems 16(6), 780-792. DOI: 10.1057/palgrave.ejis.3000711
Kim, D. (2016). “Psychophysical testing of garment size variation using three-dimensional virtual try-on technology,” Textile Research Journal 86(4), 365-379. DOI: 10.1177/0040517515591782
Kim, J., and Forsythe, S. (2008). “Adoption of virtual try-on technology for online apparel shopping,” Journal of Interactive Marketing 22(2), 45-59. DOI: 10.1002/dir.20113
Li, H., Daugherty, T., and Biocca, T. (2001). “Characteristics of virtual experience in electronic commerce: A protocol analysis,” Journal of Interactive Marketing 15(3), 13-30. DOI: 10.1002/dir.1013
Li, Y, Gong, M., Li, X., and Zhao, S. (2016). “Research on the Development Strategy of Electronic Commerce of Furniture – The comparative analysis of the furniture buying of entity shop and online shop,” Issues of Forestry Economics 36(03), 268-275 DOI:10.16832/j.cnki.1005-9709.2016.03.014
Li, Y., Li, X., Zhang, Z., Zhang, G., and Gong, M. (2020). “Understanding consumers online furniture purchase behavior: An updated UTAUT perspective,” Journal of Forest Economics 35(4), 267-303. DOI: 10.1561/112.00000516
Lim, N. (2003). “Consumers’ perceived risk: Sources versus consequences,” Electronic Commerce Research and Applications 2(3), 216-228. DOI: 10.1016/s1567-4223(03)00025-5
Lin, M., Wang, Z., Zhang, Z., and Cao, Y. (2019). “Research on consumers’ attitudes in china about using online-to-offline mode for purchasing wooden furniture,” Forest Products Journal 69(2), 159-172. DOI: 10.13073/FPJ-D-18-00039
Liu, P., and Shi, Y. (2020). “Research on the influencing mechanism of live broadcasting marketing pattern on consumers’ purchase decision,” China Business and Market (10), 38-47. DOI:10.14089/j.cnki.cn11-3664/f.2020.10.004.
Liu, Y., and Shrum, L. J. (2002). “What is interactivity and is it always such a good thing? Implications of definition, person, and situation for the influence of interactivity on advertising effectiveness,” Journal of Advertising 31(4), 53-64. DOI: 10.1080/00913367.2002.10673685
Luo, G. (2011). “Comprehensive evaluation of B2C E-commerce website service quality based on user experience,” Market Modernization 2011(1), 100-102. DOI: 10.3969/j.issn.1006-3102.2011.01.063
Mero, J. (2018). “The effects of two-way communication and chat service usage on consumer attitudes in the e-commerce retailing sector,” Electronic Markets 28(2), 205-217. DOI: 10.1007/s12525-017-0281-2
Meyer, C., and Schwager, A. (2007). “Understanding customer experience,” Harvard Business Review 85(2), 116-126.
Niu, Q., and Liu, H. (2017). “Research progress of e-commerce in furniture industry,” Issues of Forestry Economics 37(01), 68-73+108. DOI: 10.16832/j.cnki.1005-9709.2017.01.012.
Patro, C. S., and Katta, R. M. R. (2020). “Consumers’ perceived value in internet shopping: An empirical study,” International Journal of Customer Relationship Marketing and Management 11(2), 17-36. DOI: 10.4018/IJCRMM.2020040102
Poushneh, A., and Vasquez-Parraga, A. Z. (2017). “Discernible impact of augmented reality on retail customer’s experience, satisfaction and willingness to buy,” Journal and Consumer Services 34, 229-234. DOI: 10.1016/j.jretconser.2016.10.005
Qianzhan Industry Research Institute (2018). “Report of forward and investment strategic planning analysis on China furniture manufacturing industry,” (https://bg.qianzhan.com/report/detail/459/181129-adfe1b7d.html), Accessed 23 May 2021.
Rao, S., Griffis, S. E., and Goldsby, T. J. (2011). “Failure to deliver? Linking online order fulfillment glitches with future purchase behavior,” Journal of Operations Management 29(7-8), 692-703. DOI: 10.1016/j.jom.2011.04.001
Reichheld, F. F., and Schefter, P. (2000). “E-loyalty: Your secret weapon on the web,” Harvard Business Review 78(4), 105-113.
Rose, S., Hair, N., and Clark, M. (2011). “Online customer experience: A review of the business-to-consumer online purchase context,” International Journal of Management Reviews 13(1), 24-39. DOI: 10.1111/j.1468-2370.2010.00280.x
Shim, S. I., and Lee, Y. (2011). “Consumer’s perceived risk reduction by 3D virtual model,” International Journal of Retail & Distribution Management 39(12), 945-959. DOI: 10.1108/09590551111183326
Song, W. (2012). A Study of Customer Experience’s Effect on Customer Loyalty of B2C Electronic Commerce , Master’s Thesis, Shandong University, Shandong, China.
Tan, H. (2019). Research on the Influence of E-commerce Offline Store Experience on Consumers’ Online Purchase Intention , Master’s Thesis, Chongqing University of Posts and Telecommunications, Chongqing, China.
Van der Heijden, H., Verhagen, T., and Creemers, M. (2003). “Understanding online purchase intentions: Contributions from technology and trust perspectives,” European Journal of Information Systems 12(1), 41-48. DOI: 10.1057/palgrave.ejis.3000445
Wang, G., Zhu, J., Cai, W., Liu, B., Tian, Y., and Meng, F. (2021). “Research on packaging optimization in customized panel furniture enterprises,” Bioresources , 16(1), 1186-1206. DOI: 10.15376/biores.16.1.1186-1206
Wang, J., Ashleigh, M., and Meyer, E. (2006). “Knowledge sharing and team trustworthiness: It’s all about social ties!,” Knowledge Management Research & Practice 4(3), 175-186. DOI: 10.1057/palgrave.kmrp.8500098
Wang, Y. (2008). “Assessing e-commerce systems success: A respecification and validation of the DeLone and McLean model of IS success,” Information Systems Journal 18(5), 529-557. DOI: 10.1111/j.1365-2575.2007.00268.x
Watson, A., Alexander, B., and Salavati, L. (2018). “The impact of experiential augmented reality applications on fashion purchase intention,” International Journal of Retail & Distribution Management 48(5), 433-451. DOI: 10.1108/IJRDM-06-2017-0117
Wu, G., Hu, X., and Wu, Y. (2010). “Effects of perceived interactivity, perceived web assurance and disposition to trust on initial online trust,” Journal of Computer-Mediated Communication 16(1), 1-26. DOI: 10.1111/j.1083-6101.2010.01528.x
Xiao, C., and Yu, S. (2017). “Status survey of furniture information dissemination in new medium enviroment,” Furniture 38(3), 13-16, +49. DOI: 10.16610/j.cnki.jiaju.2017.03.003
Xiong, X., Guo, W., Fang, L., Zhang, M., Wu, Z., Lu, R., and Miyakoshi, T. (2017). “Current state and development trend of Chinese furniture industry,” Journal of Wood Science 63(5), 433-444. DOI: 10.1007/s10086-017-1643-2
Xiong, X., Ma, Q., Yuan, Y, Wu, Z., and Zhang, M. (2020). “Current situation and key
manufacturing considerations of green furniture in China: A review,” Journal of Cleaner Production , DOI:10.1016/j.jclepro.2020.121957.
Yang, Y., Liu, W., and Li, G. (2019). “Research on users’cognition of different preferences based on ERPs,” Journal of Forestry Engineering 4(05), 152-158. DOI:10.13360/j.issn.2096-1359.2019.05.022
Zhang, L., Tan, W., Xu, Y., and Tan, G. (2011). “Dimensions of perceived risk and their influence on consumers’ purchasing behavior in the overall process of B2C,” Engineering Education and Management 111, 1-10. DOI: 10.1007/978-3-642-24823-8_1
Zhang, T., Wang, W. Y. C., Cao, L., and Wang, Y. (2019). “The role of virtual try-on technology in online purchase decision from consumers’ aspect,” Internet Research 29(3), 529-551. DOI: 10.1108/IntR-12-2017-0540
Zhang, Z., and Xu, B. (2019). “Research on e-commerce logistics service quality problem based on electronic word-of-mouth data mining,” China Business and Market 2019(1), 43-55. DOI: 10.14089/j.cnki.cn11-3664/f.2019.01.005
Article submitted: August 16, 2021; Peer review completed: November 14, 2021; Revised version received and accepted: January 3, 2022; Published: January 18, 2022.
TechRepublic
Status and scope of online shopping: an interactive analysis through literature review.
Online shopping is a current phenomenon which has developed a great importance in the modern business environment. The evolution of online shopping has opened the door of opportunity to exploit and provide a competitive advantage over firms. This paper analyzed the different issue of online shopping. The paper aims to provide theoretical contribution in understanding the present status of online shopping and explores the factors that affecting the online shopping. The paper provides insights into consumers’ online shopping behaviors and preferences. Moreover, paper also identify the hurdles that customers’ face when they want to adopt internet shopping as their main shopping medium.
Subscribe to the Cybersecurity Insider Newsletter
Strengthen your organization's IT security defenses by keeping abreast of the latest cybersecurity news, solutions, and best practices. Delivered Tuesdays and Thursdays
Resource Details
Create a techrepublic account.
Get the web's best business technology news, tutorials, reviews, trends, and analysis—in your inbox. Let's start with the basics.
* - indicates required fields
Sign in to TechRepublic
Lost your password? Request a new password
Reset Password
Please enter your email adress. You will receive an email message with instructions on how to reset your password.
Check your email for a password reset link. If you didn't receive an email don't forgot to check your spam folder, otherwise contact support .
Welcome. Tell us a little bit about you.
This will help us provide you with customized content.
Want to receive more TechRepublic news?
You're all set.
Thanks for signing up! Keep an eye out for a confirmation email from our team. To ensure any newsletters you subscribed to hit your inbox, make sure to add [email protected] to your contacts list.

An official website of the United States government
The .gov means it’s official. Federal government websites often end in .gov or .mil. Before sharing sensitive information, make sure you’re on a federal government site.
The site is secure. The https:// ensures that you are connecting to the official website and that any information you provide is encrypted and transmitted securely.
- Publications
- Account settings
Preview improvements coming to the PMC website in October 2024. Learn More or Try it out now .
- Advanced Search
- Journal List
- Springer Nature - PMC COVID-19 Collection

Impacts of teleworking and online shopping on travel: a tour-based analysis
1 Department of Civil, Environmental, and Geodetic Engineering, The Ohio State University, Columbus, OH USA
Andre L. Carrel
2 Knowlton School of Architecture, City and Regional Planning Section, The Ohio State University, Columbus, OH USA
Huyen T. K. Le
3 Department of Geography, The Ohio State University, Columbus, OH USA
Large-scale adoption of telemobility, such as teleworking and online shopping, has affected travel patterns significantly. The impacts of teleworking and online shopping on travel have been studied separately and with trip-level analyses, thereby ignoring tour complexity, trip chaining, and activity scheduling. We aim to address this gap by investigating the interactions between online shopping, teleworking, and travel at a tour level, considering trip chaining and the importance of the activities involved. We classify tours into mandatory (e.g., travel for work, school), maintenance (e.g., travel for grocery shopping, appointments, errands), and discretionary (e.g., travel for non-grocery shopping, leisure, religious activities) tours according to the primary activity purpose. We then estimate a structural equation model using a one-week activity-travel diary from the 2019 Puget Sound Regional Travel Study. The results indicate that teleworking reduced mandatory and maintenance tours while increasing online shopping. Mandatory tours were negatively associated with both maintenance tours and online shopping, whereas the number of maintenance tours was positively associated with the number of discretionary tours. We did not find a statistically significant relationship between online shopping, maintenance tours, and discretionary tours. Overall, this study offers new insights into the effect of teleworking and online shopping on travel, with potential implications for travel demand modeling and management, as well as for the design of travel surveys that take such virtual activities into account.
Introduction
Telemobility eases spatial and temporal constraints on socio-economic activity participation, as activities that previously required traveling, such as working, shopping, healthcare, and schooling, can instead be performed virtually. Even before the COVID-19 pandemic, large-scale adoption of information and communication technologies (ICT) resulted in some types of telemobility, such as teleworking and online shopping ( or e-shopping ), having a considerable impact on mobility patterns and travel behavior (Choo and Mokhtarian 2007 ; Le et al. 2022 ; Rotem-Mindali and Weltevreden 2013 ). Hence, to gain a complete understanding of activity patterns, one cannot separate physical and virtual activities as they are intertwined.
Physical travel is largely a derived demand that depends on the locations of successive scheduled activities. Attributes such as activity type (i.e., trip purpose), duration, destination, time of day, and travel mode directly affect activity-travel planning. Based on these attributes, activities can be classified as “ mandatory ” (i.e., non-negotiable activities such as work, school, picking up/dropping off someone), “ maintenance ” (i.e., less-negotiable activities: grocery shopping, personal business, appointments, errands), and “ discretionary ” activities (i.e., negotiable activities: entertainment, social, recreational, religious, non-grocery shopping) (Ortúzar and Willumsen, 2011 ).
While most prior studies on ICT and travel assessed either the impact of teleworking on travel or the impact of online shopping on travel, they did not consider these impacts simultaneously. Simultaneous assessments of the relationship between online shopping, teleworking, and travel are becoming increasingly important as rapidly evolving technology and increased Internet access are making teleworking and online shopping more accessible and transforming daily travel-activity patterns. Yet to date, only few studies have explored this interrelationship. Özbilen et al. ( 2021 ) studied the relationship between ICT use (online shopping and teleworking) and travel time expenditures by different travel modes. Furthermore, most prior studies explored the impact of ICT use on travel without considering interdependencies between activities conducted during the same tour. This ignores the fact that many trip attributes, such as destination, time of travel, and transportation mode, depend on the tour within which this trip is being chained (Bowman and Ben-Akiva 2001 ; Ye et al. 2007 ). As a result, research has generally ignored space–time constraints due to activity scheduling and complex tour forming behavior. This leaves open the question of how various types of telemobility interact with physical mobility to form complex activity-travel patterns.
Our study addresses these gaps by analyzing home-to-home tours that we classify according to the purpose of the primary activity, i.e., as mandatory, maintenance, and discretionary tours. We employ the 2019 Puget Sound Regional Travel Study dataset, a household travel survey with a one-week activity-travel diary that allows us to comprehensively test the intricate relationship between teleworking, online shopping, and travel. Our study extends the literature in ICT and travel as well as tour complexity by considering trip chaining and hierarchy of activity importance. Insights from this study may support practitioners and researchers in the modeling and forecasting of travel behavior and travel demand considering the interactions of physical mobility with telemobility.
This paper is organized as follows: Section Literature review discusses the literature on teleworking, online shopping, and their relationship with travel. Sections Objectives and Methods present the study objectives and methodology. The last two sections discuss the model results, key conclusions, and implications.
Literature review
The relationship between ICT use and travel has been studied extensively (Cao 2009 ; Gössling 2018 ; Le et al. 2022 ). These studies mainly focused on the trip level and relate to either online shopping or teleworking, but not both. Moreover, ICT use in the form of social media has also been found to affect travel for social activities, such as face-to-face social interaction or leisure activities (Calastri et al. 2017 ; Delbosc and Mokhtarian 2018 ; van der Waerden et al. 2019 ). Given the focus of this study, in this section, we review the literature examining the impact of online shopping and teleworking on travel as well as literature on tour-based travel.
Relationship between ICT and travel: the four effects
Numerous studies have found that ICT use significantly alters the way people fulfill their activity and travel needs (Cao 2009 ; Gössling 2018 ; Le et al. 2022 ; Suel and Polak 2018 ). The literature has identified four possible types of effects of ICT use on travel, namely, complementarity, substitution, modification, and neutrality (Mokhtarian 2002 ). Substitution refers to a net reduction in physical travel due to the use of ICT, whereas complementarity implies a generation of physical travel due to ICT use. Salomon ( 1986 , 1985 ) and Mokhtarian ( 1990 , 1988 ) were the first to describe these effects. Salomon also introduced the modification effect, which refers to changes in one or more travel variables, such as destination, travel mode, or travel distance due to the use of ICT (Salomon 1985 ). Lastly, the neutrality effect occurs when there is no relationship between ICT use and travel, as discussed by Mokhtarian ( 1990 ) and Salomon ( 1985 ). Mokhtarian et al. ( 2006 ) noted the possible coexistence of these four types of effects. For instance, the complementarity and substitution effects of ICT use on travel can coexist at an individual level (Konrad and Wittowsky 2018 ; Zhang et al. 2007 ). Other than these four effects, a few studies have identified the presence of a so-called rebound effect (Kim 2017 ; Kim et al. 2015 ), referring to additional demand for travel generated due to travel time savings from ICT use for unrelated purposes, as further discussed in Sect. Relationship between teleworking and travel .
Relationship between online shopping and travel
The literature on the relationship between online shopping and travel builds on the more general literature covering ICT use and travel. In exploring the effects of online shopping on in-store shopping trips, many researchers have reported one or more of the effects introduced above, with the substitution effect and complementarity effect being the most common ones (Le et al. 2022 ). The type of effect found tends to vary by study design and measurements of travel outcomes. For instance, several studies in China and Europe using longitudinal or quasi-longitudinal surveys found the presence of substitution effects (Suel et al. 2018 ; Suel and Polak 2017 ; Weltevreden and Rietbergen 2009 ; Xi et al. 2020b ), while other studies that reported complementarity effects mostly used cross-sectional data (Cao 2012 ; Lee et al. 2017 ). One study (Bhat et al. 2003 ) with longitudinal data also found a combination of the two effects (i.e., substitution and complementarity). A few studies found modification effects (Shi et al. 2020a , 2020b ) when measuring changes in travel distances of online shoppers. Some studies also indicated the co-presence of multiple effects, such as complementarity and substitution (Etminani-Ghasrodashti and Hamidi 2020 ; Farag et al. 2007 ). Despite a large number of studies, there is currently no consensus regarding the dominant type of effect of online shopping on shopping travel.
Most studies considered individual shopping trips and did not explore the trip chaining aspect of travel. Le et al. ( 2022 ) noted that trip characteristics, Internet experience and access, household structure, delivery characteristics, and socio-demographics are primary determinants of the choice between shopping in-store and shopping online. Furthermore, they also concluded that past literature had extensively relied on one-day travel survey data to analyze the impact of ICT use on travel. However, as shopping activities do not occur on a daily basis, using one-day travel diary data and cross-sectional surveys might not provide a full picture of shoppers’ behavior. Thus, the findings of many studies may have been impacted by data limitations.
Relationship between teleworking and travel
Teleworking, or telecommuting , allows workers to work from a location of their choice rather than a central workplace. In the 1970s, researchers began considering teleworking a possible net substitution to the work commute (Nilles et al. 1976 ) and a means to reduce travel and air pollution (Handy and Mokhtarian 1996 ; Salomon 1998 ). Recent studies provided empirical evidence for a substitution effect of teleworking on commute trips (Elldér, 2020 ; Helminen and Ristimäki 2007 ).
However, teleworking may not result in a net reduction in travel in all cases. The ability to telecommute on some days and work at the office other days may lead individuals to live farther away from their workplace, thereby potentially increasing the total distance traveled for commuting purposes. Furthermore, the presence of a rebound effect with teleworking has also been detected, where a teleworker reduces their work-related commute travel but instead engages in additional travel for other purposes, e.g., for leisure purposes (Kim 2017 ; Kim et al. 2015 ). In some cases, this additional travel may be due to reallocation of time saved from commuting to other activities. Asgari et al. ( 2016 ) found an increase in both nonmandatory activity duration and total daily trip rates for telecommuters. Thus, the total distance traveled per week by teleworkers in comparison to non-teleworkers may be greater, and some studies have shown a (net) complementarity effect between teleworking and overall travel (Choo and Mokhtarian 2007 ; Silva and Melo 2018 ; Zhu et al. 2018 ). Özbilen et al. ( 2021 ) found that teleworking reduced the use of motorized modes of travel, i.e., car and transit. Overall, the rebound effect can be considered as a special case of the modification effect (Le et al. 2022 ) and in the case of some outcome variables can also involve a complementarity effect.
Previous studies mostly analyzed the effects of teleworking and online shopping on travel separately. However, some individuals may engage in both online shopping and teleworking, especially with rapidly evolving technology and increased Internet access. Hence, simultaneous assessments of the relationship between online shopping, teleworking, and travel are extremely important, but such assessments have hardly been conducted to date (Özbilen et al. 2021 ). Analyzing the relationship between online shopping and teleworking simultaneously can provide a more comprehensive picture of the interactions between ICT use and travel.
Tour-based activity-travel behavior
The literature has classified tour-forming behavior by the complexity of a tour (Daisy et al. 2018 ; Schneider et al. 2021 ; Ye et al. 2007 ) or the purpose of a primary activity of a tour (Bowman and Ben-Akiva 2001 ; Golob 2000 ; Ortúzar and Willumsen, 2011). For instance, Bowman and Ben-Akiva ( 2001 ) presented an activity-based travel demand modeling system to capture space–time constraints of activities in a tour in which they defined tour complexity as the number of trips in each tour while also classifying tours based on the primary activity.
One of the reasons that studies classified tours based on their complexity was to detect relationships between mode choice and trip chaining (Daisy et al. 2018 ; Schneider et al. 2021 ; Ye et al. 2007 ). For example, Ye et al. ( 2007 ) found that mode choice depends on the tour complexity and trip purpose. In a study in Norway, Vågane ( 2012 ) found that individuals are likely to chain non-work trips with work travel during weekdays, and that the complexity of tours depends on family structure. Lee and McNally ( 2006 ) found that individuals plan their daily tours based on the importance level of each activity. Moreover, if an opportunity presents itself, they might chain trips with lower priority to previously planned anchored trips (i.e., the most important or prioritized trip with an extended activity duration).
While researchers have found that tour complexity and activity scheduling are important variables when analyzing daily travel, as noted in the literature review, most studies on the impact of online shopping and teleworking on travel were conducted as trip-level analyses, thereby ignoring these complexities. Moreover, there is a lack of consensus regarding the impact of online shopping and teleworking on travel in the literature, likely due to different survey designs, including but not limited to aspects such as sampling methods, the cross-sectional or longitudinal nature of the survey, or the presence and length of a travel diary (Le et al. 2022 ; Xi et al. 2020a ). Our study seeks to address some of these issues by (1) simultaneously quantifying the relationship between online shopping, teleworking, and tour-forming behavior while considering the importance of the activities involved; and (2) analyzing the impact of the length of the survey period on the relationship between ICT use and travel in the Puget Sound data set. The second analysis serves to understand the sensitivity of our results to the tracking period and to support future researchers seeking to determine an appropriate tracking period for a study on ICT use and travel.
We used data from the 2019 Puget Sound Regional Household Travel Survey, which collected an activity-travel diary, socio-demographic information, and socio-economic information at both the individual and the household level (Puget Sound Regional Council 2020 ). The survey was conducted in four counties of the central Puget Sound region in the US state of Washington, namely, King, Pierce, Kitsap, and Snohomish counties, between April and June 2019. Traditional address-based sampling was used to select households and mail invitations to participate in the study.
Invited households completed an initial recruitment screening through the study website or by phone. Based on their responses regarding smartphone ownership in the recruitment screening, all individuals from selected households were invited to complete either a one-day or a one-week activity-travel diary. That is, if all individuals in the household owned a smartphone less than four years old and agreed to participate in the one-week travel diary collection, they were invited to do so. Households that completed the one-day activity-travel diary were assigned to a Tuesday, Wednesday, or Thursday as the day on which they completed the diary (the “travel day”), whereas households completing the one-week activity-travel diary were assigned Tuesday as first day of the diary. Households reported their activity-travel diaries through the rMove smartphone app or, if not all household members owned smartphones less than four years old, a web-based survey. The study collected telemobility information, such as the amount of telework time, online shopping time, and information about deliveries of goods purchased online on each day surveyed, separately for all members of household. Compared to other regional or national travel surveys in the US, the Puget Sound data set is unique in its detailed information on telemobility as well as the long tracking duration for all individuals in the households selected for the one-week travel-activity diary. This allows us to capture behavior that cannot be observed in a one-day diary (Le et al. 2022 ). In this study, we use the one-week activity-travel diary data to explore the relationship between telemobility and travel.
Data processing
The original sample includes 801 individuals from 595 households who completed the one-week activity-travel diary. We removed data from participants who did not provide information on their online shopping, teleworking duration, or online delivery (n = 113 people). Moreover, as we focus on the interactions between online shopping, teleworking, and travel, we also removed individuals who reported that they spent no time shopping online and no time teleworking during the survey week (n = 143 people). As individuals within the second group may have been subject to unobserved constraints that did not allow them to perform online activities (e.g., not allowed to work from home, no access to a credit card), this prevented the introduction of a bias due to the unobserved constraints. Therefore, the final data set includes one-week activity-travel diaries of 545 individuals from 470 households. We performed the analysis at the individual level and did not account for the interactions between individuals of the same household.
We defined a tour as a combination of trips that originated from home and ended at home. We discarded tours that did not begin or end at home, consistent with the tour definition proposed by Primerano et al. ( 2008 ). As a result, we removed 393 trips out of a total of 22,818 trips that were not part of tours that started and ended at home during the survey period. Out of the 393 discarded trips, 99 were for mandatory purposes, 31 for maintenance purposes, 204 for discretionary purposes, and 59 were trips to home. We did not remove any non-home-based sub-tours that were embedded into home-based tours. We classified tours into three categories, namely mandatory tours, maintenance tours, and discretionary tours, based on the type of primary activity undertaken during the tour. A mandatory tour contains at least one mandatory activity (e.g., work, school, pick up/drop off someone) and might contain additional maintenance and discretionary activities. A maintenance tour contains a maintenance activity as the primary purpose (e.g., grocery shopping, personal business, appointment, errands) and does not contain any mandatory activity. It may also include discretionary activities. Lastly, a discretionary tour only contains one or more discretionary activities (e.g., entertainment, social, recreational, religious activity, shopping in a mall), and does not include any mandatory or maintenance activities. For example, a tour composed of “Home → Work → Errands → Social activities → Home” would be classified as a mandatory tour since work is the primary activity. A tour composed of “Home → Personal business → Religious activity → Home” would be classified as a maintenance tour since personal business and the religious activity are the primary activities, whereas a tour composed of “Home → Entertainment → Eating out → Home” would be classified as a discretionary tour.
We performed the analysis at an individual level and aggregated all variables from the travel-activity diary over the full week to capture the impact across all travel reported in the seven-day activity-travel diary. For each type of tour, we calculated the total number of trips, total travel time, and percentage of chained vehicle miles traveled (VMT). For example, for a given individual’s mandatory tours, the percentage of chained mandatory VMT is calculated by summing the VMT of all chained mandatory tours (tours with more than one activity) and dividing that by the total VMT of all mandatory tours. We aggregated online shopping time over the week and counted the number of days on which a person received package deliveries and other deliveries (i.e., groceries and food). The Puget Sound data set does not include the total number of individual deliveries, only the number of days on which a delivery was received. Given the data aggregation over the week, our study is cross-sectional.
We motivated this study by observing that trip-level analyses ignore the complexities of tours and may yield different results than tour-level analyses. Recognizing that a tour-based analysis is complex, and its results may depend on the tour classification and travel outcome variables, we took further steps to address these issues. First, we chose a classification based on the primary activity conducted in the tour, following a hierarchy of activity purposes. By doing so we can capture the scheduling of tours based on the importance of the activities involved. Second, our analysis accounts for multiple outcome variables simultaneously by using a latent variable model, thus capturing a more holistic view of the interactions between ICT use and travel. This helps overcome the limitations of prior studies that reached contradictory conclusions due to their reliance on single travel outcomes.
Model specification and analysis approach
We used structural equation modeling (SEM) to analyze the relationship between telework, online shopping, and travel behavior. A full-scale SEM model has two components, the structural model and the measurement model. It can include both latent and observed variables as dependent (endogenous) and independent (exogenous) variables. The structural model depicts the relationships between exogenous and endogenous variables and between different endogenous variables. Since latent variables are unobserved, a set of observed variables (indicators) is used in the measurement model to identify a latent variable. Equations ( 1 , 2 and 3 ) show the basic structure of an SEM model in standard matrix notation (Kline 2015 ). Equations ( 1 and 2 ) are measurement models for latent exogenous and endogenous variables, and Eq. ( 3 ) represents the structural model.
where: x : (m × 1) vector of observed exogeneous variables, y : (n × 1) vector of observed endogenous variables, ξ : (p × 1) vector of latent exogenous variables, η : (q × 1) vector of latent endogenous variables, Λ x : (m x p) matrix of the effect of latent exogenous variables on the observed exogenous variables, Λ y : (n x q) matrix of the effect of latent endogenous variables on the observed endogenous variables, B , Γ : (q x q) matrix (for latent endogenous variables) and (q x p) matrix (for latent exogenous variables) of coefficients, δ , ε , ζ : (m × 1) , (n × 1) , and (q × 1) vectors of errors.
In addition to direct effects, SEM can also measure indirect effects between variables. The sum of the direct and indirect effects between a pair of variables is known as the total effect between them. In an SEM model, if variable X affects variable Y, then the path (X→Y) represents the direct effect of X on Y. Furthermore, if variable Y affects variable Z, then the path (X→Y→Z) is the indirect effect of X on Z.
Figure 1 shows the hypothesized relationships between the endogenous variables representing travel, teleworking, and online shopping. Based on prior literature, we hypothesize that (i) teleworking duration affects all three tour types and online shopping behavior, (ii) online shopping behavior affects maintenance and discretionary tours, (iii) mandatory tours affect maintenance and online shopping behavior, (iv) maintenance tours affect discretionary tours, and (v) socio-demographic variables (exogenous variables) affect all five endogenous variables. The hypothesized relationships between tour types, shown here as arrows, are derived from the literature (Bowman and Ben-Akiva 2001 ; Ortúzar and Willumsen 2011 ; Singleton 2013 ) and represent the way travelers are assumed to plan their daily activities (Bowman and Ben-Akiva 2001 ): individuals first plan mandatory tours, which are more constrained than the other two types, before scheduling maintenance and discretionary tours. Given that mandatory tours have the least flexibility, we hypothesize that they also affect online shopping behavior, which is often a maintenance activity and is more negotiable than mandatory activities. Furthermore, online shopping may affect maintenance and discretionary tours that contain shopping trips since such tours can also contain other non-shopping activities. Given the interactions of online and in-store shopping activities, the scheduling of other non-mandatory activities may be modified. A large body of literature has found a substitution effect of online shopping on shopping trips (Le et al. 2022 ; Suel et al. 2018 ; Suel and Polak 2017 ; Weltevreden and Rietbergen 2009 ; Xi et al. 2020b ), therefore a reduction in shopping travel would impact other maintenance or discretionary activities that could have been chained with shopping trips.

Conceptual relationships between online shopping, telework, and travel (endogenous variables)
Online shopping behavior, mandatory tours, maintenance tours, and discretionary tours were defined as latent endogenous variables that were measured using observed indicator variables in the measurement model. For each tour-related latent variable, the indicators were the total number of trips in all respective tours, total travel time, and percent of chained VMT. The latent variables capture the respondent’s tour-based travel behavior, measured along several dimensions, and classified according to the primary activity conducted during the tour. Online shopping was measured using total time spent shopping online, the number of days on which package deliveries were received, and the number of days on which other deliveries (i.e., grocery, and food) were received. Teleworking was measured by the total time spent teleworking during the week.
We estimated the SEM model using the general analysis and maximum likelihood robust (MLR) estimators in Mplus 8.4 (Muthén and Muthén, 2017 ). As some of the continuous variables were non-normal and some dummy variables were included in the structural part, we selected MLR to generate parameter estimates and standard errors that are robust to non-normality. We first ensured that the relationship between the latent variables and their observed indicators in the measurement model fit properly using confirmatory factor analysis (CFA) (Jöreskog et al. 2016 ). After that, we identified significant demographic variables in the structural part of the SEM model. We employed the nonparametric bootstrapping method (Preacher and Hayes 2008 ), which does not assume normality, to estimate the standard errors (S.E.) and perform significance testing for all indirect effects (mediation effects) and total effects. We used 10,000 bootstrap draws to estimate the standard errors and p-values of indirect and total effects.
To better understand the impact of the tour-based analysis and the use of multiple outcome variables, we also conducted a comparative analysis, where we estimated additional models with the same structure and addressing the same research question as the model shown in Fig. 1 , but using different outcome variables. Namely, we specified a trip-based latent variable model as well as two sets (one tour-based and one trip-based) of three single-indicator models, each using one of the three indicator variables as the travel outcome instead of a latent variable. For example, one of the models used the number of trips by purpose in lieu of the latent variables. As the comparative analysis is not the core focus of this paper, details are provided in the appendix.
Assessing the impact of travel survey design on the ICT–travel relationship
To analyze the impact of the length of the survey period on the relationship between ICT use and travel, we created two pseudo one-day travel-activity diaries from the one-week diary data with 545 individuals from 470 households. In the first pseudo one-day diary (Sample I), all 470 households were randomly assigned to either Tuesday, Wednesday, or Thursday as the travel day, with equal probability of travel day assignment. This assignment method mimics the one-day travel diary collection method used in the Puget Sound survey. In the second pseudo diary (Sample II), households were randomly assigned to one of the seven days of the week as their travel day, with equal probability of assignment. This assignment mimics the one-day travel diary collection method used in the 2017 National Household Travel Survey (Federal Highway Administration 2017 ). For both samples, we extracted the activity and travel information on the assigned travel day, thus creating pseudo one-day travel-activity diaries of the same 545 individuals as we had in the one-week travel-activity diary.
We estimated SEM models using the specification introduced in Sect. Model specification and analysis approach for both pseudo one-day travel diaries. That is, we followed the same data aggregation and modeling approach as with the one-week data, albeit using the one-day activity and travel data.
This section discusses the descriptive statistics of the final sample. We present the results for the relationship between online shopping, teleworking, and tour complexity modeled using the one-week travel data set. Then, we assess the results for the one-day travel data to demonstrate the potential pitfalls of using one-day travel diaries in studying ICT use and travel.
Descriptive statistics
Table Table1 1 shows the descriptive statistics of the final sample of 545 individuals from 470 households. Statistics on travel and ICT use are reported based on the one-week activity-travel diary. The modes were respondents 18–34 years of age, working full time, having a bachelor’s degree, and earning more than $100,000 at the household level. The mean household size was 2.1, with an average of 1.7 adults and 1.4 workers in the household. One-adult households accounted for 30.9% of the sample, and households without a motor vehicle accounted for 22.8%. On average, individuals spent 534 min per week teleworking and 117 min shopping online, and received package deliveries and other deliveries (i.e., groceries and food) on 1.64 days and 0.35 days of the week, respectively.
* ACS 2016–2020 reported educational attainment only for the population aged 25 years and over
# ACS 2016–2020 did not report values for the “vocational/technical training” and “associate degree” categories, thus we assumed that the “some college” category included these two categories
Also shown in Table Table1 1 are the descriptive statistics for the original sample, including all individuals who completed the one-week activity-travel diary in the Puget Sound Regional Household Travel Survey, and for the population of the central Puget Sound region, based on the American Community Survey (ACS) 2016–2020 5-year estimates (U.S. Census Bureau 2020 ). A comparison between the final sample after data cleaning (see Sect. Data processing ) and the original sample indicates that the data processing had minimal impact on the distribution of demographics. A comparison between the original sample and the ACS 2016–2020 data shows a similar distribution of the household income, household size, and gender variables, but there are considerable differences for vehicle ownership and age. These may be due to differences in the survey design and sampling method.
Modeling the relationship between ICT use and tour complexity
Table Table2 2 shows the goodness-of-fit statistics of the SEM model based on the one-week travel and ICT use data and with the model structure presented in Sect. Model specification and analysis approach . All indicators suggest that the model is a good fit according to the guidelines of Hu and Bentler ( 1999 ). The CFI values range from 0 to 1, where 1 is the best model fit. The CFI estimates the deviation of the researcher’s model from a perfect fit while comparing it against a null model (Kline 2015 ). The RMSEA and SRMR are absolute metrics that indicate how far the model is from a perfect fit, which is at 0. An RMSEA value below 0.05 indicates a good fit in terms of the (co)variance in the data explained by the model, whereas an SRMR value below 0.05 indicates that not much (co)variance is left unexplained in the model (Kline 2015 ).
Model goodness-of-fit statistics
a χ 2 is not a good measure of goodness of fit, as it increases with sample size
Table Table3 3 show the standardized coefficients of indicators for the latent variables (from the measurement model) and Table Table4 4 show the direct and total effects of both endogenous and exogenous variables on endogenous variables. At a significance level of 0.05, all indicator estimates in the measurement model (Table (Table3) 3 ) are greater than the threshold value of 0.3 (Xi et al. 2020b ).
Measurement model with standardized coefficient estimates (n = 545)
Structural model with standardized coefficient estimates ( n = 545 )
(1) A direct effect of 0.00 in exogeneous variables means that the estimate of the direct effect between variables is insignificant at a significance level of 0.1 and was replaced manually with 0.00
(2) All categories of age and household income variables (shown in Table Table1) 1 ) were included in the model, however, only significant variable categories are reported here
(3) The total effect of teleworking on online shopping doesn’t include the direct effect, as the direct effect was insignificant (p-value: 0.8)
(4) Significance level codes: *0.05 ≤ p < 0.1; ** p < 0.05
The structural model results represent the relationships between teleworking, online shopping, and travel behavior. Teleworking time reduced the tendency to make mandatory tours ( β ^ = − 0.201) and maintenance tours ( β ^ = -0.068), controlling for socio-demographics. However, teleworking time indirectly increased online shopping ( β ^ = 0.055). If teleworking duration increased by one standard deviation, the number of mandatory tours would decrease by 0.201 standard deviations and online shopping behavior would increase by 0.055 standard deviations through the total effects. Making mandatory tours also reduced the tendency to make maintenance tours and to shop online. Conversely, making maintenance tours increased the tendency to make discretionary tours. The model does not show any statistically significant effects of online shopping on maintenance and discretionary tours, or of teleworking on discretionary tours. Figure 2 depicts the relationships (direct effects) between the endogenous variables.

SEM model results: Direct effects between online shopping, teleworking, and travel
We found several socio-demographic factors that affect telemobility. Specifically, households without a vehicle made fewer maintenance and discretionary tours ( p < 0.05). Households with more children made more mandatory tours and did less teleworking. Individuals with graduate/post-graduate degrees spent more time teleworking and performed fewer mandatory tours. People from households in the highest income brackets (i.e., more than $100,000 per year) tended to telework more than households with incomes between $25,000 and $74,999. Individuals from single-adult households shopped online less than other those from other types of households. Compared to self-employed individuals, full-time employees teleworked and traveled more for mandatory tours, but they made fewer maintenance and discretionary tours and also performed less online shopping. Working part-time also to an increase in mandatory tours and hence indirectly reduced maintenance tours and online shopping behavior. Adults in the 18–54 age group teleworked more than individuals of age 55 or more. Moreover, individuals in the 18–54 age group performed more online shopping than individuals of age 55 or more.
As discussed in Sect. Model specification and analysis approach , we estimated several additional models with the same structure and addressing the same research questions as the model shown in Fig. 2 , but using different outcome variables and different analysis levels (i.e., trip-based and tour-based analysis). A trip-based latent variable model similar to the tour-based latent variable model shown in Fig. 2 failed to converge. The other two sets of models (one tour-based and one trip-based set) that each contained three single-indicator converged successfully. However, their goodness-of-fit statistics were unsatisfactory, and the tour-based latent variable model remained the best model in terms of fit. Detailed results are presented in the appendix.
Assessment of the impact of travel survey design on the ICT–travel relationship
As discussed in Sect. Assessing the impact of travel survey design on the ICT – travel relationship , we created two pseudo one-day travel diaries from the one-week sample used in our tour-based one-week model. In Sample I, households were randomly assigned with equal probability to either Tuesday, Wednesday, or Thursday as their travel day (i.e., excluding weekend). In Sample II, households were randomly assigned with equal probability to one of the seven days (i.e., including weekend). As these samples were extracted from the one-week activity-travel diary, both have the same socio-demographic characteristics as shown in Table Table1. 1 . The descriptive statistics of variables related to travel and ICT use can be found in Table Table5. 5 . On average, Sample I includes more mandatory tours, more teleworking time, and fewer maintenance and discretionary tours than Sample II. This was expected, as Sample I only consisted of weekdays whereas Sample II included all seven days of the week. As Sample I and Sample II were one-day diaries, the two online shopping variables, package received on travel day and other delivery received on a travel day, effectively became binary variables.
Additional descriptive statistics for pseudo one-day travel diaries (n = 545)
For the analysis of Sample I and Sample II, we used the same model specification and the same individuals as we did with the one-week travel diary data, thus the results are directly comparable (Table (Table3). 3 ). Both models converged, however they produced considerably different results than the model based on the one-week travel diary, the results of which are shown in Table Table3 3 . The model results based on Sample I and Sample II also differ substantially. We observed three notable differences between the tour-based, one-day models and the tour-based, one-week model. First, in both one-day models, some of the indicator estimates in the measurement model did not pass the threshold value of 0.3 (Xi et al. 2020b ) at a 0.05 level of significance. Specifically, the online shopping behavior latent variable was poorly quantified by the “package delivery” and “other delivery” indicator variables, as both had very low factor loadings (on average, β ^ package _ delivery = 0.13 and β ^ other _ delivery = 0.28, p < 0.05) . In the one-week travel diary model, all indicators of all four latent variables (i.e., online shopping behavior, mandatory tours, maintenance tours, and discretionary tours) were significant with factor loadings greater than 0.3. Second, the relationship between maintenance tours and discretionary tours was not significant in the one-day models despite being significant in the tour-based latent variable model. Third, many socio-demographic variables that significantly explained ICT use (i.e., online shopping behavior and teleworking) and discretionary tours in the tour-based, one-week model (Fig. 2 ) were no longer significant with the one-day diary data.
Effects of ICT use on tour complexity and activity scheduling
The tour-level analysis model results show that teleworking is negatively associated with mandatory and maintenance tours and there is no significant effect on discretionary tours. This suggests an overall modification effect, where the specific effect on travel depends on the type of tour. Our observation is consistent with other literature based on trip-level analyses that found the presence of both substitution and neutrality effects of ICT use on travel (Calderwood and Freathy 2014 ; Zhai et al. 2017 ). Our findings also indicate that telework indirectly increases maintenance tours via its effect on mandatory tours. In other words, if the teleworker makes fewer mandatory tours, they will likely make additional separate maintenance tours due to the lack of opportunities to chain maintenance trips with mandatory trips. These results are consistent with prior literature showing that ICT use reduces the number of work trips (i.e., has a substitution effect) (Elldér, 2020 ; Helminen and Ristimäki 2007 ) and has a modification effect on non-commute trips (Asgari et al. 2016 ). Hence, our findings suggest that previously observed effects of teleworking on individual trips are also observable at a tour level.
To the best of our knowledge, this is the first study to establish that teleworking has a positive relationship with online shopping, albeit via an indirect path. We hypothesize that due to reduced travel as a result of teleworking, individuals have fewer opportunities to chain non-mandatory trips with their mandatory trips, which may induce more online shopping. Furthermore, the literature suggests that access to computers and experience with the Internet are significant variables in explaining online shopping behavior (Le et al. 2022 ). Since these are generally also prerequisites for teleworking, they may catalyze more online shopping.
This study also finds that more mandatory tours result in fewer maintenance tours, whereas maintenance tours generate additional discretionary tours. These results are indicative of complex activity scheduling and tour-forming behavior and suggest that individuals tend to chain non-work trips with work trips. The existence of such chaining behavior is supported by prior literature that also observed a negative association between work tours and non-work tours (Golob 2000 ; Vågane 2012 ). The positive association between maintenance tours and discretionary tours suggests that there are further discretionary activities that are not chained with maintenance activities. Although this requires further research, a possible explanation may be that some maintenance activities are primarily performed during business hours (e.g., bank visits, appointments) whereas many discretionary activities are conducted after business hours. The disconnect between the timing of these activities may result in separate discretionary tours.
We further found that more mandatory tours lead to less online shopping. This finding may suggest that individuals commuting for work trips may prefer in-store shopping over online shopping if they can chain their shopping trips with work trips or shop during their lunch break. However, we did not find any significant relationship between online shopping and maintenance tours nor between online shopping and discretionary tours. In part, this may be due to the aggregation of both shopping travel and non-shopping travel (e.g., personal business, appointment, errands) in the “maintenance tours” variable. Non-shopping activities might not have a direct relationship with online shopping, and as a result, the model did not show a significant relationship.
Mokhtarian et al. ( 2006 ) noted that ICT use may have mixed effects on leisure-related travel, as in some cases ICT use can promote new leisure travel whereas in other cases, the adoption of ICT is can reduce leisure travel. These contradictory effects of ICT use on discretionary travel might have caused the statistically insignificant relationships between ICT use (both teleworking and online shopping) and discretionary travel in our model.
Impact of socio-demographics on teleworking, online shopping, and travel behavior
The effects of exogenous variables, notably the socio-demographics of individuals, on the endogenous variables are also of interest. Our results show that having a graduate/post-graduate degree, working full-time, being 18–54 years of age, living in a household with income greater than $100,000 per year, and having fewer children than average are associated with an increased likelihood of teleworking. Gender, vehicle ownership, and being in a single-adult household had no significant impact on teleworking. Online shopping, on the other hand, was performed less by older adults (aged more than 55), and single-adult households shopped online less than households with multiple adults. The latter result may be due to a combination of lower consumption levels in smaller households and policies of online shops that make shopping online for small quantities more expensive, such as minimum order quantities. Being employed (full-time or part-time) and having children present in the household also reduced online shopping behavior.
Regarding travel behavior, not owning a vehicle reduces maintenance and discretionary tours, whereas the number of mandatory tours tends to increase with the number of children in the household. The latter may in part be driven by travel to pick up or drop off children. Being employed full-time or part-time results in more mandatory tours and fewer non-mandatory tours, and overall, male travelers are less likely to make separate maintenance and discretionary tours.
Comparative analysis of tour-based and trip-based models
An in-depth comparative analysis, which is presented in detail in the appendix, Sections A2 and A3, shows that the direct effects between teleworking, online shopping, and the travel outcome variables depend upon the outcome variable used. This observation holds for both the trip-level and tour-level models. While the results for the individual outcome variables may be valid, the diverging findings make it difficult to answer the question, “what is the overall effect of teleworking and online shopping on travel?” This corroborates the need for a holistic view of the impact of ICT use on travel, using multiple indicators as the tour-based latent variable model does (Fig. 2 ). Overall, comparing the tour-based latent variable model to tour-based single-indicator models, we observe that the former found a similar or greater number of significant effects of ICT use on travel than the single-indicator models, and the direction and magnitudes of the relationships were consistent. This underlines the fact that using multiple travel indicators provides a more holistic view of the impact of ICT use on travel.
A comparison between the trip-level single-indicator models and the tour-level single-indicator models shows that the impacts of ICT use on travel identified by the models depend on whether trip purposes are assigned based on the individual trip purpose or based on the tour that a trip is part of. Some of the effects that were significant in the single-indicator trip-level models were found to be insignificant in the tour-level single-indicator models and vice-versa. This is discussed in further detail in the appendix. The results in Section A2 indicate that a tour-level analysis that accounts for interdependencies within tours can provide insights that are missed by the trip-level analysis. Furthermore, they show that some effects that are detected at the trip level are not of the same relevance at the tour level, possibly because additional trips are added to tours that were going to be made anyway or because reductions in individual trips do not necessarily result in a reduction in tours.
Implications for travel survey design on the ICT–travel relationship
To understand the sensitivity of our results to the length of the travel diary and to support future research on ICT use and travel, this paper also analyzes the impact of survey methods on the model results regarding the relationship between ICT use and travel. The results indicate that one-day activity-travel diaries may not be effective in capturing the effects of ICT use on travel that can be detected with a one-week activity-travel diary. By comparing the estimation results of the various models, we found that the models based on the one-day diaries faced three issues. First, the delivery variables failed to capture the frequency of online shopping and led to poor performance of the measurement models. This is likely because shopping does not occur daily. Moreover, individuals might concentrate their online shopping on certain days, such as weekends, resulting in delivery patterns that are not evenly distributed throughout the week.
Second and relatedly, the one-day diary fails to capture lagged modification effects. For instance, if a person shops online during the week and consequently modifies their shopping travel on the weekend, a one-day travel diary would fail to measure this modification of travel. This issue can be remedied by collecting longer-duration activity-travel diaries, e.g., by extending the tracking period to one week or more. Alternatively, researchers could measure travel patterns before and after individuals increase their ICT use, or ask survey participants to report their past and current ICT use and travel behavior, i.e., conduct a quasi-longitudinal survey (Le et al. 2022 ; Xi et al. 2020a ).
Third, considering the two different approaches to creating one-day diaries, the difference in assigned travel day (i.e., either including weekdays only or both weekdays and weekends) resulted in differences in observed travel patterns, which in turn affected the coefficients of the latent variables in the structural parts of the models. This suggests that one-day travel diaries struggle to capture daily variations in travel and ICT use and therefore may yield biased results. Moreover, one-day travel diaries have a strict cutoff at midnight, such that tours that start in the evening and finish after mid-night are not fully recorded. Similarly, one-day travel diaries also have trouble capturing commute travel by night shift workers, which may cause bias in the data, in particular since there is also an association between night shift work and socio-demographics.
Our results confirm that the length of the activity-travel diary may affect the observed impact of ICT use on travel. Thus, a shorter travel diary duration may be less effective at capturing the actual effects of ICT use on travel.
Strengths and limitations of this study
This study contributes to the literature by innovating upon prior studies that considered telework and online shopping separately and modeled travel at a trip level. We address these shortcomings by accounting for trip chaining and the complexity of travel. First, this study simultaneously captures both teleworking and online shopping behavior and quantifies their impact on travel behavior as well as the relationship between them. Second, modeling travel at the tour level enables us to incorporate tour complexity and trip chaining and thus capture individual activity-travel behavior more realistically than trip-based analyses. Third, we relied on a one-week travel survey, which enables us to capture modification effects on travel that may not occur on the same day on which a person shops online or performs telework. Fourth, this study is among the few studies to analyze how the length of the travel-activity diary affects the detected relationships between teleworking, online shopping, and travel, thereby contributing to the literature on travel survey methods.
This study has several limitations. First, we classified tours based on a primary activity performed during the tour, thereby omitting information about trips for non-primary purposes (i.e., trips for a purpose of lower priority than the primary purpose of the tour) that were embedded in mandatory and maintenance tours. Second, shopping trips were not considered on their own and were grouped with other non-mandatory trips. This may have limited our ability to specifically investigate the relationship between online shopping and shopping travel. Third, the Puget Sound travel-activity diary measured the number of days when individuals received deliveries from online orders, but it did not measure the total number of deliveries during the travel day(s) or week. Doing so could have enabled a more precise measurement of the online shopping behavior latent variable. Moreover, data on package deliveries will represent a lagged effect of online shopping, though the consideration of deliveries and travel over the course of one week reduces the impact of this issue. Fourth, our model did not control for the effect of land use patterns, which may affect teleworking duration and trip chaining behavior. For instance, people living in suburban areas may telework more frequently. Lastly, the model did not account for intra-household interactions or the clustering effect (i.e., correlation between members of the same household), even though the final dataset included individuals who belonged to the same households (Shah et al. 2021 ).
Conclusions
The purpose of this study was to simultaneously quantify the relationship between teleworking, online shopping, and tour-based travel behavior at an individual level. It accounts for tour-forming by including measures capturing trip chaining and tour complexity and emphasizing the importance of the hierarchy of activities involved in a tour. We found modification effects where teleworking indirectly increases online shopping, reduces mandatory and maintenance tours, but has no significant impact on discretionary tours. Mandatory tours reduce maintenance tours and online shopping, whereas maintenance tours have a positive impact on discretionary tours. We did not find a statistically significant relationship between online shopping and maintenance tours and discretionary tours, nor between teleworking and discretionary tours. In an additional analysis concerning the duration of travel-activity diaries, we found that one-day travel-activity diaries may not provide a full picture of the impact of ICT use. One-day travel diaries also suffer from other shortcomings, such as an inability to capture tours that go past midnight, which may disproportionately exclude specific groups of individuals such as night shift workers.
Our study findings have several implications for transportation practice. First, while past studies focused separately on the effects of online shopping and teleworking, our results show that these two activities may have additional interactions between them. This also suggests that other components of telemobility, such as teleconferencing, telehealth, or e-learning, should not be ignored in future research on the effects of ICT use on travel. Second, our results show that virtual and physical activity and travel are intertwined and should be considered in the same model. The interactions between physical mobility and telemobility may complicate the modeling and forecasting of travel behavior and travel demand, and ignoring them can lead modelers to overlook important effects.
Acquiring a complete picture of the effects of telemobility in all its forms on travel is particularly important due to the ongoing changes to mobility patterns following the COVID-19 pandemic and in light of constant improvements in telemobility technologies and services. Some virtual forms of engagement that were introduced due to constraints during the pandemic may become a permanent part of life in the future. Therefore, there is a need for further studies on the effects of ICT use on travel behavior that consider the many different types and aspects of ICT and travel behavior and that do so simultaneously to capture possible interaction effects. As an example, investigating the impact of the level of teleworking, such as part-time or full-time status, on travel would be an important future research avenue. A further research need is on the topic of telehealth, to understand which types of healthcare visits are being replaced by virtual means and the respective impact on travel behavior.
Lastly, the findings of this paper concerning the duration of travel-activity diaries are of importance to both practitioners and researchers who conduct travel surveys. They show that longer diaries can capture ICT use more accurately and reveal relationships between ICT use and travel that are not visible or adequately captured in one-day diary data. Importantly, travel-activity diary data covering longer time frames may also allow researchers to better understand modification effects of ICT use on travel, as such effects are very difficult to detect without suitable data.
Our study provides several directions for future research. To gain a more detailed picture of tour forming (or trip chaining) behavior, tour complexity, and their interactions with activity scheduling, future research should develop an approach that does not conceal secondary activities behind the primary activities of a tour. More studies are needed to explicitly measure and incorporate maintenance and discretionary trips that are embedded in tours for higher-priority activities, and to better quantify trip chaining behavior and tour complexity. Future studies should also consider household-level models that capture intra-household activity scheduling and travel interactions. This would support the understanding of the effect of various types of telemobility on household travel patterns rather than individual travel patterns. With regard to the interaction between online shopping and in-store shopping, future studies should test the effects of maintenance tours and discretionary tours on online shopping behavior. Likewise, future studies can examine whether mandatory tours influence teleworking.
Biographies
is a Ph.D. Candidate in the Department of Civil, Environmental, and Geodetic Engineering at the Ohio State University. His research interests include the relationship between travel and telecommunication technology, sustainable transportation, travel survey methods, and travel demand modeling. His recent research has focused on the impacts of information and communication technology (ICT) on travel behavior and mobility patterns.
is an Assistant Professor in the Department of Civil, Environmental, and Geodetic Engineering and the Knowlton School of Architecture, City and Regional Planning Section, at the Ohio State University. His research focuses on travel demand analysis, sustainable mobility behavior, interactions between information and communication technologies and travel behavior, and public transportation planning and operations.
is an Assistant Professor in the Department of Geography at the Ohio State University. Her research includes sustainable transportation, travel behavior, information and communication technologies, and the environmental health impacts of travel.
Appendix: Comparative analysis of tour-based and trip-based models
A1. methodology.
Following the approach described in Sects. Data processing and Model specification and analysis approach , we first aggregated the data at the trip level, with each trip classified based on the activity purpose at its destination. Since a trip-level latent variable model with the structure shown in Fig. 1 failed to converge using the same sample of 545 individuals that underlies the model shown in Sect. Modeling the relationship between ICT and tour complexity , the comparative analysis was performed with path models. Instead of latent variables capturing travel, each path model used one of the three indicators of the travel-related latent variables, i.e., travel time, number of trips, and percent chained VMT. In three of the models (labeled M1-M3 hereafter), the aggregation of the variables was based on the trip purpose, and in a further three models (labeled M4-M6), it was based on the tour purpose. As an example, in Model M1, the three travel-related latent variables were replaced with the respective travel time indicators, as shown in Fig. 3 . In Model M1, to determine an individual’s travel time for mandatory trips, the total travel time of all trips for mandatory purposes was calculated. Models M2 and M3 used the number of trips and percent of chained VMT instead of the travel times. In Models M4-M6, the purpose assigned to each trip was the primary purpose of the tour that the trip was part of.

Trip-level model: Direct effects considering Travel time indicator (M1)
A2. Results
Table 6 shows the goodness-of-fit statistics for the single-indicator models M1-M6 as well as the tour-based latent variable model. As can be seen, the tour-based latent variable model has a better fit compared than the six single indicator models, and the CFI values of all six single-indicator models suggest that these models have a comparatively poor fit. The estimation results of Models M1-M6 are shown in Figs. Figs.3, 3 , (M2)4, 4 , (M3)5, 5 , (M4)6, 6 , (M5)7 7 and (M6)8 8 .

Trip-level model: Direct effects considering Number of trips indicator (M2)

Trip-level model: Direct effects considering Percent of chained VMT indicator (M3)

Tour-level model: Direct effects considering Travel time indicator (M4)

Tour-level model: Direct effects considering Number of trips indicator (M5)

Tour-level model: Direct effects considering Percent of chained VMT indicator (M6)
Comparison of Goodness-of-fit statistics: Simpler path models
A3. Discussion
A comparison between the tour-based latent variable model and Models M4-M6 shows that the various single-indicator models were only able to identify subsets of the relationships found by the tour-based latent variable model. Additionally, a comparison between the trip-level models (M1-M3) and the tour-level models (M4-M6) shows that the results regarding the impacts of ICT use on travel differ depending on whether trip purposes are assigned individually or based on the tour they are part of. For instance, in the trip-level models, the relationship between teleworking and the number of mandatory trips is significant, whereas the relationship between teleworking and travel time for mandatory trips is insignificant. In the tour-level models, both relationships are significant. Furthermore, models M2 and M3 found that both online shopping and teleworking significantly reduce the number of discretionary trips and the percent of chained VMT for discretionary travel, but the counterparts of these models with the tour-level aggregation did not find this relationship to be significant. Taking Model M2 and M5 as an example, this indicates that while online shopping and teleworking may reduce the number of trips made for discretionary purposes, they do not significantly affect the number of tours for discretionary purposes.
However, given that teleworking does appear to significantly reduce maintenance and mandatory tours in Model M5, the results suggest that discretionary travel that was previously weaved into those tours is not resulting in a significant increase in discretionary tours to compensate. As discretionary activities are the most negotiable, people might just chain them with other non-negotiable activities once the original primary activities are lost in order to avoid separate tours for those discretionary activities. To the extent that new discretionary tours are formed, multiple discretionary trips may be aggregated into a small set of tours that does not correspond to a statistically significant increase. This is an example of the additional types of insights that a tour-level analysis that accounts for interdependencies within tours can provide above and beyond the insights that can be gained by focusing only on the trip level.
Author contributions
The authors confirm contribution to the paper as follows: study conception and design: HS, ALC, HTKL; Data and Software: HS; analysis and interpretation of results: HS, ALC, HTKL; draft manuscript preparation: HS, ALC, HTKL.
Declarations
On behalf of all authors, the corresponding author states that there is no conflict of interest.
An earlier version of this paper was presented at the 101st Transportation Research Board Annual Meeting, Washington DC, 2022.
Publisher's Note
Springer Nature remains neutral with regard to jurisdictional claims in published maps and institutional affiliations.
- Asgari H, Jin X, Du Y. Examination of the impacts of telecommuting on the time use of nonmandatory activities. Transp. Res. Rec. 2016; 2566 :83–92. doi: 10.3141/2566-09. [ CrossRef ] [ Google Scholar ]
- Bhat CR, Sivakumar A, Axhausen KW. An analysis of the impact of information and communication technologies on non-maintenance shopping activities. Transp. Res. Part B Methodol. 2003; 37 :857–881. doi: 10.1016/S0191-2615(02)00062-0. [ CrossRef ] [ Google Scholar ]
- Bowman JL, Ben-Akiva ME. Activity-based disaggregate travel demand model system with activity schedules. Transp. Res. Part A Policy Pract. 2001; 35 :1–28. doi: 10.1016/S0965-8564(99)00043-9. [ CrossRef ] [ Google Scholar ]
- Calastri C, Hess S, Daly A, Maness M, Kowald M, Axhausen K. Modelling contact mode and frequency of interactions with social network members using the multiple discrete–continuous extreme value model. Transp. Res. Part C Emerg. Technol. 2017; 76 :16–34. doi: 10.1016/J.TRC.2016.12.012. [ CrossRef ] [ Google Scholar ]
- Calderwood E, Freathy P. Consumer mobility in the Scottish isles: the impact of internet adoption upon retail travel patterns. Transp. Res. Part A Policy Pract. 2014; 59 :192–203. doi: 10.1016/j.tra.2013.11.012. [ CrossRef ] [ Google Scholar ]
- Cao XJ. E-shopping, spatial attributes, and personal travel: a review of empirical studies. Transp. Res. Rec. 2009 doi: 10.3141/2135-19. [ CrossRef ] [ Google Scholar ]
- Cao XJ. The relationships between e-shopping and store shopping in the shopping process of search goods. Transp. Res. Part A Policy Pract. 2012; 46 :993–1002. doi: 10.1016/j.tra.2012.04.007. [ CrossRef ] [ Google Scholar ]
- U.S. Census Bureau, 2020. 2016–2020 American Community Survey (5-Year Estimates)
- Choo S, Mokhtarian PL. Telecommunications and travel demand and supply: aggregate structural equation models for the US. Transp. Res. Part A Policy Pract. 2007; 41 :4–18. doi: 10.1016/j.tra.2006.01.001. [ CrossRef ] [ Google Scholar ]
- Daisy NS, Millward H, Liu L. Trip chaining and tour mode choice of non-workers grouped by daily activity patterns. J. Transp. Geogr. 2018; 69 :150–162. doi: 10.1016/j.jtrangeo.2018.04.016. [ CrossRef ] [ Google Scholar ]
- de Ortúzar JD, Willumsen LG. Modelling Transport. UK: Wiley; 2011. [ Google Scholar ]
- Delbosc A, Mokhtarian P. Face to Facebook: the relationship between social media and social travel. Transp. Policy. 2018; 68 :20–27. doi: 10.1016/J.TRANPOL.2018.04.005. [ CrossRef ] [ Google Scholar ]
- Elldér E. Telework and daily travel: new evidence from Sweden. J. Transp. Geogr. 2020; 86 :102777. doi: 10.1016/j.jtrangeo.2020.102777. [ PMC free article ] [ PubMed ] [ CrossRef ] [ Google Scholar ]
- Etminani-Ghasrodashti R, Hamidi S. Online shopping as a substitute or complement to in-store shopping trips in Iran? Cities. 2020; 103 :102768. doi: 10.1016/j.cities.2020.102768. [ CrossRef ] [ Google Scholar ]
- Farag S, Schwanen T, Dijst M, Faber J. Shopping online and/or in-store? A structural equation model of the relationships between e-shopping and in-store shopping. Transp. Res. Part A Policy Pract. 2007; 41 :125–141. doi: 10.1016/j.tra.2006.02.003. [ CrossRef ] [ Google Scholar ]
- Federal Highway Administration, 2017. 2017 National Household Travel Survey. U.S. Department of Transportation, Washington, DC.
- Golob TF. A simultaneous model of household activity participation and trip chain generation. Transp. Res. Part B Methodol. 2000; 34 :355–376. doi: 10.1016/S0191-2615(99)00028-4. [ CrossRef ] [ Google Scholar ]
- Gössling S. ICT and transport behavior: a conceptual review. Int. J. Sustain. Transp. 2018; 12 :153–164. doi: 10.1080/15568318.2017.1338318. [ CrossRef ] [ Google Scholar ]
- Handy S, Mokhtarian PL. Forecasting telecommuting. Transportation (amst). 1996; 23 :163–190. doi: 10.1007/bf00170034. [ CrossRef ] [ Google Scholar ]
- Helminen V, Ristimäki M. Relationships between commuting distance, frequency and telework in Finland. J. Transp. Geogr. 2007; 15 :331–342. doi: 10.1016/j.jtrangeo.2006.12.004. [ CrossRef ] [ Google Scholar ]
- Hu L, Bentler PM. Cutoff criteria for fit indexes in covariance structure analysis: conventional criteria versus new alternatives. Struct. Equ. Model. 1999; 6 :1–55. doi: 10.1080/10705519909540118. [ CrossRef ] [ Google Scholar ]
- Jöreskog, K.G., Olsson, U.H., Wallentin, F.Y.: Confirmatory Factor Analysis (CFA). Springer, Cham, pp. 283–339. (2016) 10.1007/978-3-319-33153-9_7
- Kim SN. Is telecommuting sustainable? an alternative approach to estimating the impact of home-based telecommuting on household travel. Int. J. Sustain. Transp. 2017; 11 :72–85. doi: 10.1080/15568318.2016.1193779. [ CrossRef ] [ Google Scholar ]
- Kim SN, Choo S, Mokhtarian PL. Home-based telecommuting and intra-household interactions in work and non-work travel: a seemingly unrelated censored regression approach. Transp. Res. Part A Policy Pract. 2015; 80 :197–214. doi: 10.1016/j.tra.2015.07.018. [ CrossRef ] [ Google Scholar ]
- Kline RB. Principles and Practice of Structural Equation Modeling. 4. New York: Guilford Press; 2015. [ Google Scholar ]
- Konrad K, Wittowsky D. Virtual mobility and travel behavior of young people–connections of two dimensions of mobility. Res. Transp. Econ. 2018; 68 :11–17. doi: 10.1016/J.RETREC.2017.11.002. [ CrossRef ] [ Google Scholar ]
- Le HTK, Carrel AL, Shah H. Impacts of online shopping on travel demand: a systematic review. Transp. Rev. 2022; 42 :273–295. doi: 10.1080/01441647.2021.1961917. [ CrossRef ] [ Google Scholar ]
- Lee M, McNally MG. An empirical investigation on the dynamic processes of activity scheduling and trip chaining. Transportation (amst). 2006; 33 :553–565. doi: 10.1007/s11116-006-7728-1. [ CrossRef ] [ Google Scholar ]
- Lee RJ, Sener IN, Mokhtarian PL, Handy SL. Relationships between the online and in-store shopping frequency of Davis, California residents. Transp. Res. Part A Policy Pract. 2017; 100 :40–52. doi: 10.1016/j.tra.2017.03.001. [ CrossRef ] [ Google Scholar ]
- Mokhtarian PL. An empirical evaluation of the travel impacts of teleconferencing. Transp. Res. Part A Gen. 1988; 22 :283–289. doi: 10.1016/0191-2607(88)90006-4. [ CrossRef ] [ Google Scholar ]
- Mokhtarian PL. A typology of relationships between telecommunications and transportation. Transp. Res. Part A Gen. 1990; 24 :231–242. doi: 10.1016/0191-2607(90)90060-J. [ CrossRef ] [ Google Scholar ]
- Mokhtarian PL. Telecommunications and travel: The case for complementarity. J. Ind. Ecol. 2002; 6 :43–57. doi: 10.1162/108819802763471771. [ CrossRef ] [ Google Scholar ]
- Mokhtarian PL, Salomon I, Handy SL. The impacts of ict on leisure activities and travel: A conceptual exploration. Transportation (amst). 2006; 33 :263–289. doi: 10.1007/s11116-005-2305-6. [ CrossRef ] [ Google Scholar ]
- Muthén LK, Muthén BO. Mplus User’s Guide. 8. CA: Los Angeles; 2017. [ Google Scholar ]
- Nilles JM, Carlson FR, Gray P, Hanneman G. Telecommuting—an alternative to urban transportation congestion. IEEE Trans. Syst. Man Cybern. 1976; SMC-6 :77–84. doi: 10.1109/TSMC.1976.5409177. [ CrossRef ] [ Google Scholar ]
- Özbilen B, Wang K, Akar G. Revisiting the impacts of virtual mobility on travel behavior: an exploration of daily travel time expenditures. Transp. Res. Part A Policy Pract. 2021; 145 :49–62. doi: 10.1016/j.tra.2021.01.002. [ CrossRef ] [ Google Scholar ]
- Preacher KJ, Hayes AF. Asymptotic and resampling strategies for assessing and comparing indirect effects in multiple mediator models. Behav. Res. Methods. 2008 doi: 10.3758/BRM.40.3.879. [ PubMed ] [ CrossRef ] [ Google Scholar ]
- Primerano F, Taylor MAPAP, Pitaksringkarn L, Tisato P. Defining and understanding trip chaining behaviour. Transportation (Amst). 2008; 35 :55–72. doi: 10.1007/s11116-007-9134-8. [ CrossRef ] [ Google Scholar ]
- Puget Sound Regional Council, 2020. 2019 Puget Sound Regional Travel Study.
- Rotem-Mindali O, Weltevreden JWJ. Transport effects of e-commerce: what can be learned after years of research? Transportation (amst). 2013; 40 :867–885. doi: 10.1007/s11116-013-9457-6. [ CrossRef ] [ Google Scholar ]
- Salomon I. Telecommunications and travel: substitution or modified mobility? J. Transp. Econ. Policy. 1985; 19 (3):219–235. [ Google Scholar ]
- Salomon I. Telecommunications and Travel Relationships: A Review. Transp. Res. Part A Gen. 1986; 20 :223–238. doi: 10.1016/0191-2607(86)90096-8. [ CrossRef ] [ Google Scholar ]
- Salomon I. Technological change and social forecasting: the case of telecommuting as a travel substitute. Transp. Res. Part C Emerg. Technol. 1998; 6C :17–45. doi: 10.1016/S0968-090X(98)00006-0. [ CrossRef ] [ Google Scholar ]
- Schneider F, Ton D, Zomer LB, Daamen W, Duives D, Hoogendoorn-Lanser S, Hoogendoorn S. Trip chain complexity: a comparison among latent classes of daily mobility patterns. Transportation (amst). 2021; 48 :953–975. doi: 10.1007/s11116-020-10084-1. [ CrossRef ] [ Google Scholar ]
- Shah H, Carrel AL, Le HTK. What is your shopping travel style? heterogeneity in US households’ online shopping and travel. Transp. Res. Part A Policy Pract. 2021; 153 :83–98. doi: 10.1016/J.TRA.2021.08.013. [ CrossRef ] [ Google Scholar ]
- Shi K, Cheng L, De Vos J, Yang Y, Cao W, Witlox F. How does purchasing intangible services online influence the travel to consume these services? A Focus on a Chinese Context. Transportation (amst). 2020 doi: 10.1007/s11116-020-10141-9. [ CrossRef ] [ Google Scholar ]
- Shi K, De Vos J, Yang Y, Li E, Witlox F. Does e-shopping for intangible services attenuate the effect of spatial attributes on travel distance and duration? Transp. Res. Part A Policy Pract. 2020; 141 :86–97. doi: 10.1016/j.tra.2020.09.004. [ CrossRef ] [ Google Scholar ]
- Silva e JDA, Melo PC. Home telework, travel behavior, and land-use patterns: a path analysis of British single-worker households. J. Transp. Land Use. 2018; 11 :419–441. doi: 10.5198/jtlu.2018.1134. [ CrossRef ] [ Google Scholar ]
- Singleton PA. A Theory of Travel Decision-Making with Applications for Modeling Active Travel Demand. Oregon: Portland State University; 2013. [ Google Scholar ]
- Suel E, Polak JW. Development of joint models for channel, store, and travel mode choice: Grocery shopping in London. Transp. Res. Part A Policy Pract. 2017; 99 :147–162. doi: 10.1016/j.tra.2017.03.009. [ CrossRef ] [ Google Scholar ]
- Suel E, Polak JW. Incorporating online shopping into travel demand modelling: challenges, progress, and opportunities. Transp. Rev. 2018; 38 :576–601. doi: 10.1080/01441647.2017.1381864. [ CrossRef ] [ Google Scholar ]
- Suel E, Daina N, Polak JW. A hazard-based approach to modelling the effects of online shopping on intershopping duration. Transportation (amst). 2018; 45 :415–428. doi: 10.1007/s11116-017-9838-3. [ PMC free article ] [ PubMed ] [ CrossRef ] [ Google Scholar ]
- Vågane, L.: The complexity of travel: Trip chaining in Norway. Eur. Transp. Conf. 1–11. (2012)
- van der Waerden P, Bérénos M, Wets G. Communication and its relationship with digital and physical mobility patterns—a review. Adv. Transp. Policy Plan. 2019; 3 :3–27. doi: 10.1016/BS.ATPP.2019.05.001. [ CrossRef ] [ Google Scholar ]
- Weltevreden JWJ, Rietbergen TV. The implications of e-shopping for in-store shopping at various shopping locations in the Netherlands. Environ. Plan. B Plan. Des. 2009; 36 :279–299. doi: 10.1068/b34011t. [ CrossRef ] [ Google Scholar ]
- Xi G, Cao X, Zhen F. The impacts of same day delivery online shopping on local store shopping in Nanjing, China. Transp. Res. Part A Policy Pract. 2020; 136 :35–47. doi: 10.1016/j.tra.2020.03.030. [ CrossRef ] [ Google Scholar ]
- Xi G, Zhen F, Cao Jason X, Xu F. The interaction between e-shopping and store shopping: empirical evidence from Nanjing China. Transp Lett. 2020; 12 :157–165. doi: 10.1080/19427867.2018.1546797. [ CrossRef ] [ Google Scholar ]
- Ye X, Pendyala RM, Gottardi G. An exploration of the relationship between mode choice and complexity of trip chaining patterns. Transp. Res. Part B Methodol. 2007; 41 :96–113. doi: 10.1016/j.trb.2006.03.004. [ CrossRef ] [ Google Scholar ]
- Zhai Q, Cao X, Mokhtarian PL, Zhen F. The interactions between e-shopping and store shopping in the shopping process for search goods and experience goods. Transportation (amst). 2017; 44 :885–904. doi: 10.1007/s11116-016-9683-9. [ CrossRef ] [ Google Scholar ]
- Zhang, F., Clifton, K.J., Shen, Q.: Reexamining ICT impact on travel using the 2001 NHTS data for baltimore metropolitan area, in: GeoJournal Library. pp. 153–166. (2007) 10.1007/1-4020-5427-0_10
- Zhu P, Wang L, Jiang Y, Zhou J. Metropolitan size and the impacts of telecommuting on personal travel. Transportation (amst). 2018; 45 :385–414. doi: 10.1007/S11116-017-9846-3/TABLES/8. [ CrossRef ] [ Google Scholar ]
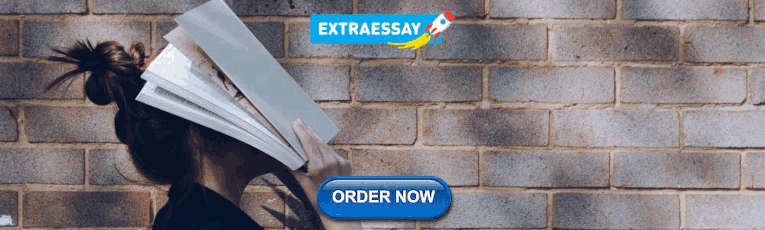
IMAGES
VIDEO
COMMENTS
Conclusion. 55 Relying on an extensive literature review, this paper aims to identify the main drivers of online shopping and thus to give further insights in explaining consumer behavior when adopting the Internet for buying as this issue is still in its infancy stage despite its major importance for academic and professionals.
The current SLR gathers and synthesizes research records of the last 13 years (2007-May2020) on consumer perceived risks, trust and concerning behavioral intention in online shopping in sport domain.
The impact of online shopping attributes on customer satisfaction and loyalty: Moderating effects of e-commerce experience ... 2. Literature review. In South Africa, total retail spending increased from 1.2% in 2016 to 1.8% in 2017, with retail sales reaching the R1 trillion mark. ... during their interaction with the online system, and that ...
Umesh Jaiswal. Online Shopping play a great importance in the modern business environment. Online shopping has opened the door of opportunity and advantage to the firms. This paper analyzed the different issue of online shopping. The research aims to provide theoretical contribution in understanding the present status of online shopping.
Searching the literature. The search was performed in both SCOPUS and Web of Science (WOS) databases, in favour of high-quality, peer-reviewed articles (Booth, Papaioannou, and Sutton Citation 2012; Gusenbauer and Haddaway Citation 2020).The search covers articles until the end of 2021 without limits on the publication date (beyond those already inherent in the databases used) considering an ...
This study conducts a systematic literature review to synthesize the extant literature primarily on "online shopping consumer behavior" and to gain insight into "What drives consumers toward online shopping".,The authors followed guidelines for systematic literature reviews with stringent inclusion and exclusion criteria. The review is ...
The academic interest in Online Grocery Shopping (OGS) has proliferated in retailing and business management over the past two decades. Previous research on OGS was primarily focused on consumer-level consequences such as purchase intention, purchase decision, and post-purchase behavior. However, there is a lack of literature integrating intrinsic and extrinsic factors that influence the ...
Jérôme Lacoeuilhe. Dorsaf Fehri. ... According to recent studies, online grocery shopping (OGS) will increasingly establish itself as a new (complementary) sales channel for manufacturers and ...
shopping, online shopping significantly reduces transaction cost by eliminating several monetary and non-monetary costs (Yang, Zha o &Wan, 2010). Customer satisfaction in an online shopping context
The framework uses the constructs of the Technology Acceptance Model (TAM) as a basis, extended by exogenous factors and applies it to the online shopping context. The review shows that attitudes toward online shopping and intention to shop online are not only affected by ease of use, usefulness, and enjoyment, but also by exogenous factors ...
Due to the first and second waves of COVID-19, blockades were imposed in many countries. The literature points out that from 2020 onwards, many people who were not fans of online shopping actually switched to the online platform during this period of COVID-19 (Chaudhary, 2020) and this caused an increase in online sales (Kim, 2020).
Abstract: In this study, we conducted extensive reviews of online shopping literatures and proposed a hierarchy model of online shopping behavior. We collected 47 studies and classified them by variables used. Some critical points were found that research framework, methodology, and lack of cross-cultural comparison, etc So we developed a cross-cultural model of online shopping including ...
A Systematic Literature Review or SLR of the emerging 'e-grocery adoption' research scope is presented, investigating the antecedents of grocery application adoption and identifying the promising research streams for the near future. This article presents a Systematic Literature Review or SLR of the emerging 'e-grocery adoption' research scope. It proposes grocery application adoption ...
Literature review. Online shopping indicates electronic commerce to buy products or services directly from the seller through the Internet. Internet-based or Click and Order business model has replaced the traditional Brick and Mortar business model. ... Most of the consumers believe that the payment system for online shopping is not secured ...
Given the rapid growth and emerging trend of e-commerce have changed consumer preferences to buy online, this study analyzes the current Indian legal framework that protects online consumers' interests. A thorough analysis of the two newly enacted laws, i.e., the Consumer Protection Act, 2019 and Consumer Protection (E-commerce) Rules, 2020 and literature review support analysis of 290 ...
Review of literature on offline and online consumer buying behavi or. This paper includes the findings and methodologies used in 9 doctorial thesis and 64 research papers. The. following t able ...
costly shops but online shopping has changed this scenario,Dennis et al(2007) B. Online Shopping Resistant Factors Major inhibitors of online shopping for this research adopted are lack of trust on online site, enjoyment of traditional shopping , complex websites, lack of touch and feel experience.
The purpose of this paper is to provide a literature review of the furniture online consumption with an aim to extend the concept of consumer experience to the context of online furniture consumption. The paper offers three important contributions for both academics and practitioners. First, it analyzes the main influencing factors of the ...
In the study by Lian and Yen ( 2014 ), authors tested the two dimensions (drivers and barriers) that might affect intention to purchase online. Drivers consisted of performance expectation, effort expectation, social influence and facilitating conditions. Usage, value, risk, tradition and image were all among barriers.
Online shopping is a current phenomenon which has developed a great importance in the modern business environment. The evolution of online shopping has opened the door of opportunity to exploit ...
Ordered products are directly delivered to the door is the greatest interest to many consumers because online shopping does not requires us to leave the hours or office (Chen and Chang, 2003). According to Monsuwe, Delleart and Ruyter (2004), the main drive of online shopping is that the internet is time saving and accessible 24 hours a day.
The purpose of this study is to elaborate the previous research on theories, methods, analysis and variables involve in the study of online shopping. A study based on previous research on a topic ...
This paper is organized as follows: Section Literature review discusses the literature on teleworking, online shopping, and their relationship with travel. Sections Objectives and Methods present the study objectives and methodology. The last two sections discuss the model results, key conclusions, and implications.