
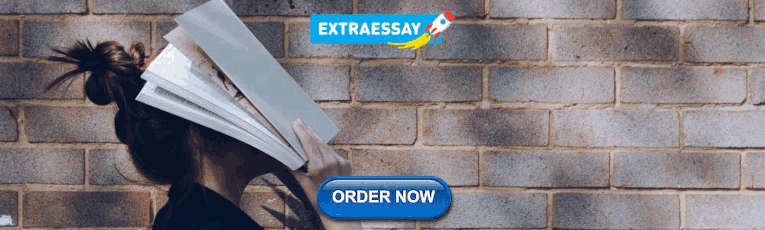
Free Mathematics Tutorials
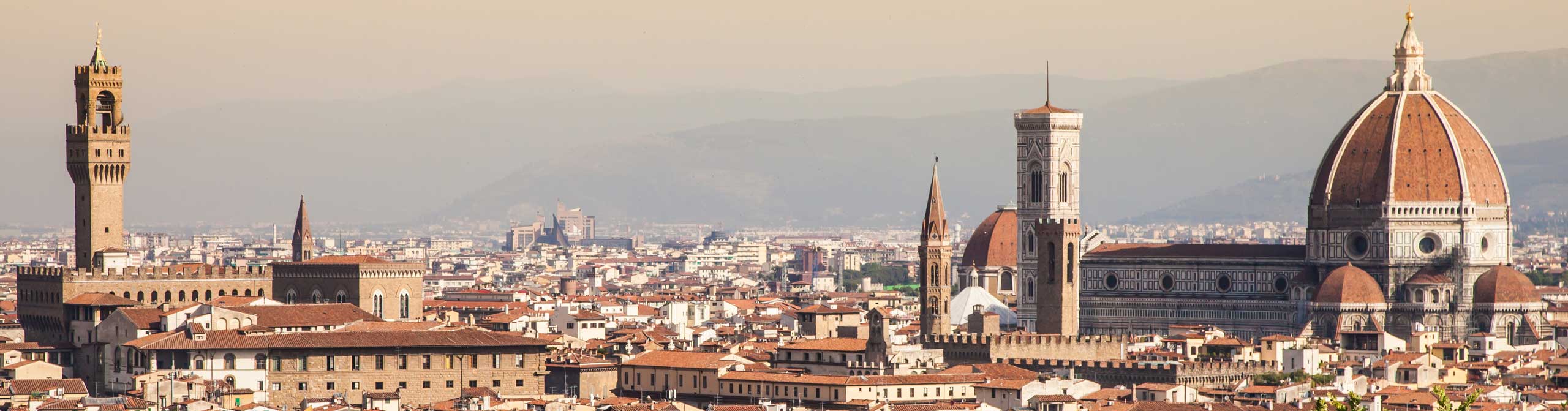
Normal Distribution Problems with Solutions
Problems and applications on normal distributions are presented. The solutions to these problems are at the bottom of the page. An online normal probability calculator and an inverse normal probability calculator may be useful to check your answers.
Problems with Solutions
- X is a normally distributed variable with mean μ = 30 and standard deviation σ = 4. Find the probabilities a) P(X < 40) b) P(X > 21) c) P(30 < X < 35)
- A radar unit is used to measure the speeds of cars on a motorway. The speeds are normally distributed with a mean of 90 km/hr and a standard deviation of 10 km/hr. What is the probability that a car picked at random is traveling at more than 100 km/hr?
- For certain types of computers, the length of time between charges of the battery is normally distributed with a mean of 50 hours and a standard deviation of 15 hours. John owns one of these computers and wants to know the probability that the length of time will be between 50 and 70 hours.
- Entry to a certain University is determined by a national test. The scores on this test are normally distributed with a mean of 500 and a standard deviation of 100. Tom wants to be admitted to this university and he knows that he must score better than at least 70% of the students who took the test. Tom takes the test and scores 585. Will he be admitted to this university?
- The length of similar components produced by a company is approximated by a normal distribution model with a mean of 5 cm and a standard deviation of 0.02 cm. If a component is chosen at random a) what is the probability that the length of this component is between 4.98 and 5.02 cm? b) What is the probability that the length of this component is between 4.96 and 5.04 cm?
- The length of life of an instrument produced by a machine has a normal distribution with a mean of 12 months and a standard deviation of 2 months. Find the probability that an instrument produced by this machine will last a) less than 7 months. b) between 7 and 12 months.
- The time taken to assemble a car in a certain plant is a random variable having a normal distribution of 20 hours and a standard deviation of 2 hours. What is the probability that a car can be assembled at this plant in a period of time a) less than 19.5 hours? b) between 20 and 22 hours?
- A large group of students took a test in Physics and the final grades have a mean of 70 and a standard deviation of 10. If we can approximate the distribution of these grades by a normal distribution, what percent of the students a) scored higher than 80? b) Should pass the test (grades≥60)? c) Should fail the test (grades<60)?
- The annual salaries of employees in a large company are approximately normally distributed with a mean of $50,000 and a standard deviation of $20,000. a) What percent of people earn less than $40,000? b) What percent of people earn between $45,000 and $65,000? c) What percent of people earn more than $70,000?
Solutions to the Above Problems
- Note: What is meant here by area is the area under the standard normal curve. a) For x = 40, the z-value z = (40 - 30) / 4 = 2.5 Hence P(x < 40) = P(z < 2.5) = [area to the left of 2.5] = 0.9938 b) For x = 21, z = (21 - 30) / 4 = -2.25 Hence P(x > 21) = P(z > -2.25) = [total area] - [area to the left of -2.25] = 1 - 0.0122 = 0.9878 c) For x = 30 , z = (30 - 30) / 4 = 0 and for x = 35, z = (35 - 30) / 4 = 1.25 Hence P(30 < x < 35) = P(0 < z < 1.25) = [area to the left of z = 1.25] - [area to the left of 0] = 0.8944 - 0.5 = 0.3944
- Let x be the random variable that represents the speed of cars. x has μ = 90 and σ = 10. We have to find the probability that x is higher than 100 or P(x > 100) For x = 100 , z = (100 - 90) / 10 = 1 P(x > 90) = P(z > 1) = [total area] - [area to the left of z = 1] = 1 - 0.8413 = 0.1587 The probability that a car selected at random has a speed greater than 100 km/hr is equal to 0.1587
- Let x be the random variable that represents the length of time. It has a mean of 50 and a standard deviation of 15. We have to find the probability that x is between 50 and 70 or P( 50< x < 70) For x = 50 , z = (50 - 50) / 15 = 0 For x = 70 , z = (70 - 50) / 15 = 1.33 (rounded to 2 decimal places) P( 50< x < 70) = P( 0< z < 1.33) = [area to the left of z = 1.33] - [area to the left of z = 0] = 0.9082 - 0.5 = 0.4082 The probability that John's computer has a length of time between 50 and 70 hours is equal to 0.4082.
- Let x be the random variable that represents the scores. x is normally distributed with a mean of 500 and a standard deviation of 100. The total area under the normal curve represents the total number of students who took the test. If we multiply the values of the areas under the curve by 100, we obtain percentages. For x = 585 , z = (585 - 500) / 100 = 0.85 The proportion P of students who scored below 585 is given by P = [area to the left of z = 0.85] = 0.8023 = 80.23% Tom scored better than 80.23% of the students who took the test and he will be admitted to this University.
- a) P(4.98 < x < 5.02) = P(-1 < z < 1) = 0.6826 b) P(4.96 < x < 5.04) = P(-2 < z < 2) = 0.9544
- a) P(x < 7) = P(z < -2.5) = 0.0062 b) P(7 < x < 12) = P(-2.5 < z < 0) = 0.4938
- a) P(x < 19.5) = P(z < -0.25) = 0.4013 b) P(20 < x < 22) = P(0 < z < 1) = 0.3413
- a) For x = 80, z = 1 Area to the right (higher than) z = 1 is equal to 0.1586 = 15.87% scored more that 80. b) For x = 60, z = -1 The area to the right of z = -1 is equal to 0.8413 = 84.13% should pass the test. c) 100% - 84.13% = 15.87% should fail the test.
- a) For x = 40000, z = -0.5 The area to the left (less than) of z = -0.5 is equal to 0.3085 = 30.85% earn less than $40,000. b) For x = 45000 , z = -0.25 and for x = 65000, z = 0.75 The area between z = -0.25 and z = 0.75 is equal to 0.3720 = 37.20 earning between $45,000 and $65,000. c) For x = 70000, z = 1 The area to the right (higher) of z = 1 is equal to 0.1586 = 15.86% earning more than $70,000.
More References and links
- Normal Distribution Definition
- Elementary statistics and probabilities
Popular Pages
- Normal Distribution Probability Calculator
- Probability Questions with Solutions
- Elementary Statistics and Probability Tutorials and Problems
- Introduction to Probability
- Mean and Standard deviation - Problems with Solutions
Stay In Touch
- Privacy Policy
Normal Distribution
In these lessons, we learn the characteristics of the normal distribution and its applications.
Related Pages Normal Distribution Normal Distribution: Probability Standard Deviation More Lessons for Statistics Math Worksheets
What is the Normal Distribution? Probably the most widely known and used of all distributions is the normal distribution. It fits many human characteristics, such as height, weight, speed etc. Many living things in nature, such as trees, animals and insects have many characteristics that are normally distributed. Many variables in business and industry are also normally distributed.
Discovery of the normal curve is generally credited to Karl Gauss (1777 – 1855), who recognized that the errors of repeated measurement of objects are often normally distributed. Sometimes, the normal distribution is also called the Gaussian distribution.
The normal distribution has the following characteristics:
- It is a continuous distribution
- It is symmetrical about the mean. Each half of the distribution is a mirror image of the other half.
- It is asymptotic to the horizontal axis. That is, it does not touch the x -axis and it goes on forever in each direction.
- It is unimodal. The normal curve is sometimes called a bell-shaped curve. All the values are “bunched up” in only one portion of the graph – the center of the curve.
- It is a family of curves. Every unique value of the mean and every unique value of the standard deviation result in a different normal curve.
- The area under the curve is 1. The area under the curve yields the probabilities, so the total of all probabilities for a normal distribution is 1. Since the distribution is symmetric, the area of the distribution on each side of the mean is 0.5.
What is the Probability density function of the normal distribution? The normal distribution is described by two parameters: the mean, μ, and the standard deviation, σ. We write X - N(μ, σ 2 ).
The following diagram shows the formula for Normal Distribution. Scroll down the page for more examples and solutions on using the normal distribution formula.
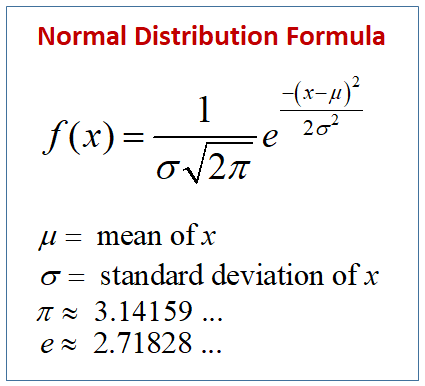
Since the formula is so complex, using it to determine area under the curve is cumbersome and time consuming. Instead, tables and software are used to find the probabilities for the normal distribution. This will be discussed in the lesson on Z-Score .
Normal Distribution The 68-95-99.7 Rule
- Approximately 68% of the data falls ±1 standard deviation from the mean.
- Approximately 95% of the data falls ±2 standard deviation from the mean.
- Approximately 99.7% of the data falls ±3 standard deviation from the mean.
In a call center, the distribution of the number of phone calls answered each day by each of the 12 receptionists is bell-shaped and has a mean of 63 and a standard deviation of 3. Use the empirical rule, what is the approximate percentage of daily phone calls numbering between 60 and 66?
The scores of a midterm are normally distributed with a mean of 85% and a standard deviation of 6%. Find the percentage of the class that score above and below the given score. Use the 68-95-99.7 rule from the text. a) Score: 91% b) Score: 73%
The following video explores the normal distribution
Presentation on spreadsheet to show that the normal distribution approximates the binomial distribution for a large number of trials.
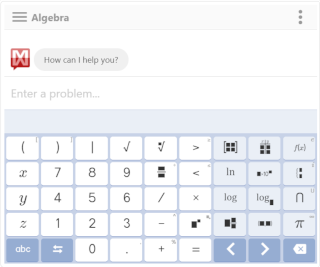
We welcome your feedback, comments and questions about this site or page. Please submit your feedback or enquiries via our Feedback page.
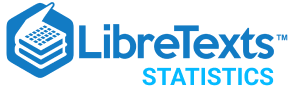
- school Campus Bookshelves
- menu_book Bookshelves
- perm_media Learning Objects
- login Login
- how_to_reg Request Instructor Account
- hub Instructor Commons
- Download Page (PDF)
- Download Full Book (PDF)
- Periodic Table
- Physics Constants
- Scientific Calculator
- Reference & Cite
- Tools expand_more
- Readability
selected template will load here
This action is not available.
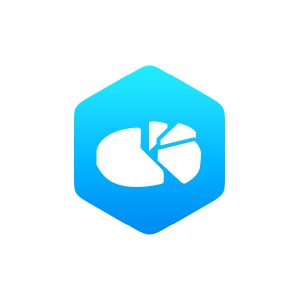
6.3: Finding Probabilities for the Normal Distribution
- Last updated
- Save as PDF
- Page ID 16356
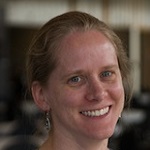
- Kathryn Kozak
- Coconino Community College
The Empirical Rule is just an approximation and only works for certain values. What if you want to find the probability for x values that are not integer multiples of the standard deviation? The probability is the area under the curve. To find areas under the curve, you need calculus. Before technology, you needed to convert every x value to a standardized number, called the z-score or z-value or simply just z . The z-score is a measure of how many standard deviations an x value is from the mean. To convert from a normally distributed x value to a z-score, you use the following formula.
Definition \(\PageIndex{1}\): z-score
\[z=\dfrac{x-\mu}{\sigma} \label{z-score}\]
where \(\mu\)= mean of the population of the x value and \(\sigma\)= standard deviation for the population of the x value
The z-score is normally distributed, with a mean of 0 and a standard deviation of 1. It is known as the standard normal curve. Once you have the z-score, you can look up the z-score in the standard normal distribution table.
Definition \(\PageIndex{2}\): standard normal distribution
The standard normal distribution , z, has a mean of \(\mu =0\) and a standard deviation of \(\sigma =1\).
.png?revision=1)
Luckily, these days technology can find probabilities for you without converting to the zscore and looking the probabilities up in a table. There are many programs available that will calculate the probability for a normal curve including Excel and the TI-83/84. There are also online sites available. The following examples show how to do the calculation on the TI-83/84 and with R. The command on the TI-83/84 is in the DISTR menu and is normalcdf(. You then type in the lower limit, upper limit, mean, standard deviation in that order and including the commas.
Example \(\PageIndex{1}\) general normal distribution
The length of a human pregnancy is normally distributed with a mean of 272 days with a standard deviation of 9 days (Bhat & Kushtagi, 2006).
- State the random variable.
- Find the probability of a pregnancy lasting more than 280 days.
- Find the probability of a pregnancy lasting less than 250 days.
- Find the probability that a pregnancy lasts between 265 and 280 days.
- Find the length of pregnancy that 10% of all pregnancies last less than.
- Suppose you meet a woman who says that she was pregnant for less than 250 days. Would this be unusual and what might you think?
a. x = length of a human pregnancy
b. First translate the statement into a mathematical statement.
P (x>280)
Now, draw a picture. Remember the center of this normal curve is 272.
.png?revision=1)
To find the probability on the TI-83/84, looking at the picture you realize the lower limit is 280. The upper limit is infinity. The calculator doesn’t have infinity on it, so you need to put in a really big number. Some people like to put in 1000, but if you are working with numbers that are bigger than 1000, then you would have to remember to change the upper limit. The safest number to use is \(1 \times 10^{99}\), which you put in the calculator as 1E99 (where E is the EE button on the calculator). The command looks like:
\(\text{normalcdf}(280,1 E 99,272,9)\)
.png?revision=1)
Thus 18.7% of all pregnancies last more than 280 days. This is not unusual since the probability is greater than 5%.
c. First translate the statement into a mathematical statement.
P (x<250)
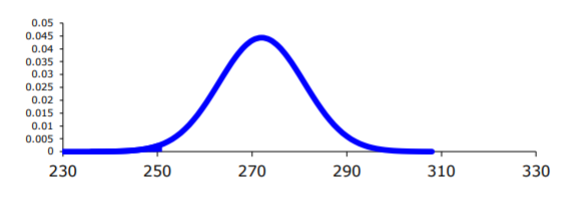.png?revision=1)
To find the probability on the TI-83/84, looking at the picture, though it is hard to see in this case, the lower limit is negative infinity. Again, the calculator doesn’t have this on it, put in a really small number, such as \(-1 \times 10^{99}=-1 E 99\) on the calculator.
.png?revision=1)
\(P(x<250)=\text { normalcdf }(-1 E 99,250,272,9)=0.0073\)
Thus 0.73% of all pregnancies last less than 250 days. This is unusual since the probability is less than 5%.
d. First translate the statement into a mathematical statement.
\(P(265<x<280)\)
.png?revision=1)
In this case, the lower limit is 265 and the upper limit is 280.
Using the calculator
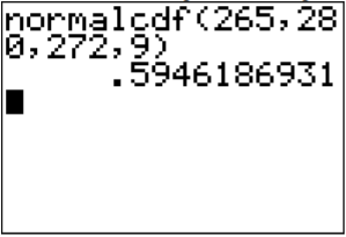.png?revision=1)
\(P(265<x<280)=\text { normalcdf }(265,280,272,9)=0.595\)
Many software programs always give you the area to the left. So \(P(x<280)\) is the area to the left of 280 and \(P(x<265)\) is the area to the left of 265. So the area is between the two would be the bigger one minus the smaller one. So, \(P(265<x<280)= P(x<280) - P(x<265) =0.595\). Thus 59.5% of all pregnancies last between 265 and 280 days.
e. This problem is asking you to find an x value from a probability. You want to find the x value that has 10% of the length of pregnancies to the left of it. On the TI-83/84, the command is in the DISTR menu and is called invNorm(. The invNorm( command needs the area to the left. In this case, that is the area you are given. For the command on the calculator, once you have invNorm( on the main screen you type in the probability to the left, mean, standard deviation, in that order with the commas.
.png?revision=1)
Thus 10% of all pregnancies last less than approximately 260 days.
f. From part (c) you found the probability that a pregnancy lasts less than 250 days is 0.73%. Since this is less than 5%, it is very unusual. You would think that either the woman had a premature baby, or that she may be wrong about when she actually became pregnant.
Example \(\PageIndex{2}\) general normal distribution
The mean mathematics SAT score in 2012 was 514 with a standard deviation of 117 ("Total group profile," 2012). Assume the mathematics SAT score is normally distributed.
- Find the probability that a person has a mathematics SAT score over 700.
- Find the probability that a person has a mathematics SAT score of less than 400.
- Find the probability that a person has a mathematics SAT score between a 500 and a 650.
- Find the mathematics SAT score that represents the top 1% of all scores.
a. x = mathematics SAT score
P (x>700)
Now, draw a picture. Remember the center of this normal curve is 514.
.png?revision=1)
On TI-83/84: \(P(x>700)=\text { normalcdf }(700,1 E 99,514,117) \approx 0.056\)
On R: \(P(x>700)=1-\text { pnorm }(700,514,117) \approx 0.056\)
There is a 5.6% chance that a person scored above a 700 on the mathematics SAT test. This is not unusual.
P (x<400)
.png?revision=1)
On TI-83/84: \(P(x<400)=\text { normalcdf }(-1 E 99,400,514,117) \approx 0.165\)
On R: \(P(x<400)=\operatorname{pnorm}(400,514,117) \approx 0.165\)
So, there is a 16.5% chance that a person scores less than a 400 on the mathematics part of the SAT.
P (500<x<650)
.png?revision=1)
On TI-83/84: \(P(500<x<650)=\text { normalcdf }(500,650,514,117) \approx 0.425\)
On R: \(P(500<x<650)=\text { pnorm }(650,514,117)-\text { pnorm }(500,514,117) \approx 0.425\)
So, there is a 42.5% chance that a person has a mathematical SAT score between 500 and 650.
e. This problem is asking you to find an x value from a probability. You want to find the x value that has 1% of the mathematics SAT scores to the right of it. Remember, the calculator and R always need the area to the left, you need to find the area to the left by 1 - 0.01 = 0.99.
On TI-83/84: \(\text{invNorm}(.99,514,117) \approx 786\)
On R: \(\text{qnorm}(.99,514,117) \approx 786\)
So, 1% of all people who took the SAT scored over about 786 points on the mathematics SAT.
Exercise \(\PageIndex{1}\)
- P (z<2.36)
- P (z>0.67)
- P (0<z<2.11)
- P (-2.78<z<1.97)
- The area to the left of z is 15%.
- The area to the right of z is 65%.
- The area to the left of z is 10%.
- The area to the right of z is 5%.
- The area between -z and z is 95%. (Hint draw a picture and figure out the area to the left of the -z.)
- The area between -z and z is 99%.
- Find the probability that a person in China has blood pressure of 135 mmHg or more.
- Find the probability that a person in China has blood pressure of 141 mmHg or less.
- Find the probability that a person in China has blood pressure between 120 and 125 mmHg.
- Is it unusual for a person in China to have a blood pressure of 135 mmHg? Why or why not?
- What blood pressure do 90% of all people in China have less than?
- Find the probability that an Atlantic cod has a length less than 52 cm.
- Find the probability that an Atlantic cod has a length of more than 74 cm.
- Find the probability that an Atlantic cod has a length between 40.5 and 57.5 cm.
- If you found an Atlantic cod to have a length of more than 74 cm, what could you conclude?
- What length are 15% of all Atlantic cod longer than?
- Find the probability that a woman age 45-59 in Ghana, Nigeria, or Seychelles has a cholesterol level above 6.2 mmol/l (considered a high level).
- Find the probability that a woman age 45-59 in Ghana, Nigeria, or Seychelles has a cholesterol level below 5.2 mmol/l (considered a normal level).
- Find the probability that a woman age 45-59 in Ghana, Nigeria, or Seychelles has a cholesterol level between 5.2 and 6.2 mmol/l (considered borderline high).
- If you found a woman age 45-59 in Ghana, Nigeria, or Seychelles having a cholesterol level above 6.2 mmol/l, what could you conclude?
- What value do 5% of all woman ages 45-59 in Ghana, Nigeria, or Seychelles have a cholesterol level less than?
- Find the probability that a man age 40-49 in the U.S. eats more than 110 g of fat every day.
- Find the probability that a man age 40-49 in the U.S. eats less than 93 g of fat every day.
- Find the probability that a man age 40-49 in the U.S. eats less than 65 g of fat every day.
- If you found a man age 40-49 in the U.S. who says he eats less than 65 g of fat every day, would you believe him? Why or why not?
- What daily fat level do 5% of all men age 40-49 in the U.S. eat more than?
- Find the probability that a dishwasher will last more than 15 years.
- Find the probability that a dishwasher will last less than 6 years.
- Find the probability that a dishwasher will last between 8 and 10 years.
- If you found a dishwasher that lasted less than 6 years, would you think that you have a problem with the manufacturing process? Why or why not?
- A manufacturer of dishwashers only wants to replace free of charge 5% of all dishwashers. How long should the manufacturer make the warranty period?
- Find the probability that a starting nurse will make more than $80,000.
- Find the probability that a starting nurse will make less than $60,000.
- Find the probability that a starting nurse will make between $55,000 and $72,000.
- If a nurse made less than $50,000, would you think the nurse was under paid? Why or why not?
- What salary do 30% of all nurses make more than?
- Find the probability that the yearly rainfall is less than 100 mm.
- Find the probability that the yearly rainfall is more than 240 mm.
- Find the probability that the yearly rainfall is between 140 and 250 mm.
- If a year has a rainfall less than 100mm, does that mean it is an unusually dry year? Why or why not?
- What rainfall amount are 90% of all yearly rainfalls more than?
1. a. \(P(z<2.36)=0.9909\), b. \(P(z>0.67)=0.2514\), c. \(P(0<z<2.11)=0.4826\), d. \(P(-2.78<z<1.97)=0.9729\)
3. a. -0.6667, b. -2.6667, c. -2, d. 6.6667
5. a. See solutions, b. \(P(x<52 \mathrm{cm})=0.7128\), c. \(P(x>74 \mathrm{cm})=5.852 \times 10^{-11}\), d. \(P(40.5 \mathrm{cm}<x<57.5 \mathrm{cm})=0.9729\), e. See solutions, f. 53.8 cm
7. a. See solutions, b. \(P(x>110 \mathrm{g})=0.0551\) c. \(P(x<93 \mathrm{g})=0.0097\), d. \(P(x<65 \mathrm{g}) \approx 0\) or \(5.57 \times 10^{-19}\), e. See solutions, f. 110.2 g
9. a. See solutions, b. \(P(x>\$ 80,000)=0.1168\), c. \(P(x>\$ 80,000)=0.2283\), d. \(P(\$ 55,000<x<\$ 72,000)=0.5519\), e. See solutions, f. $73,112
Have a language expert improve your writing
Run a free plagiarism check in 10 minutes, generate accurate citations for free.
- Knowledge Base
- The Standard Normal Distribution | Calculator, Examples & Uses
The Standard Normal Distribution | Calculator, Examples & Uses
Published on November 5, 2020 by Pritha Bhandari . Revised on June 21, 2023.
The standard normal distribution , also called the z -distribution , is a special normal distribution where the mean is 0 and the standard deviation is 1.
Any normal distribution can be standardized by converting its values into z scores. Z scores tell you how many standard deviations from the mean each value lies.
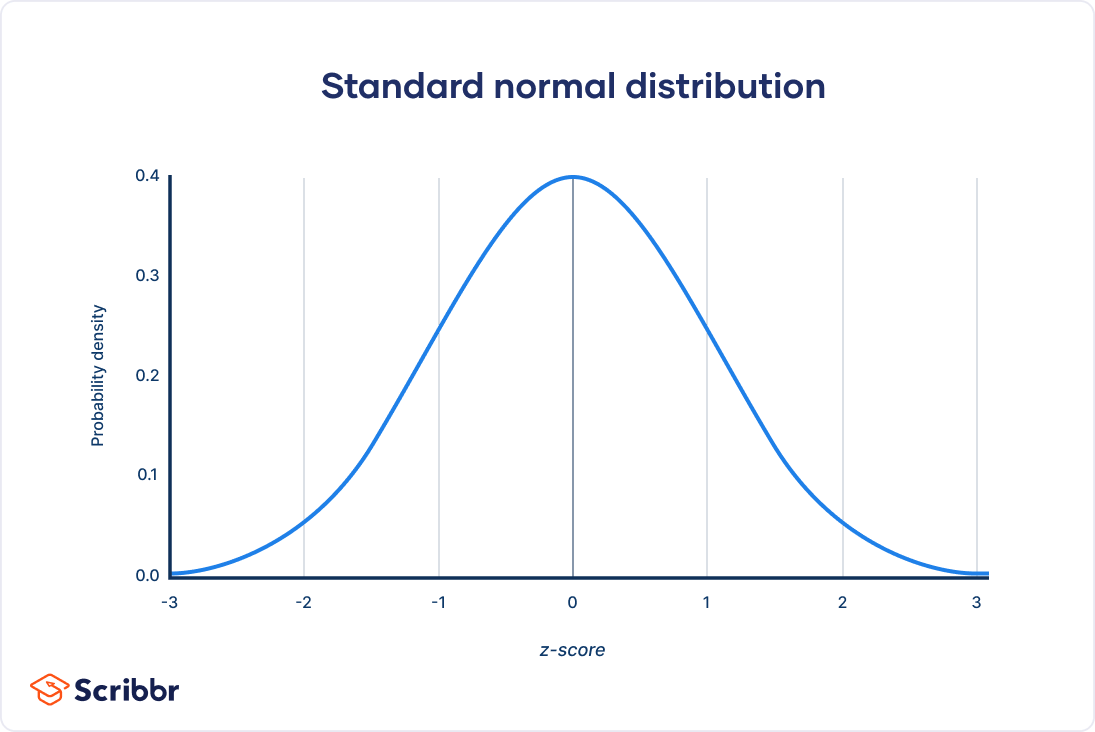
Converting a normal distribution into a z -distribution allows you to calculate the probability of certain values occurring and to compare different data sets.
Download the z table
Table of contents
Standard normal distribution calculator, normal distribution vs the standard normal distribution, standardizing a normal distribution, use the standard normal distribution to find probability, step-by-step example of using the z distribution, other interesting articles, frequently asked questions about the standard normal distribution, receive feedback on language, structure, and formatting.
Professional editors proofread and edit your paper by focusing on:
- Academic style
- Vague sentences
- Style consistency
See an example
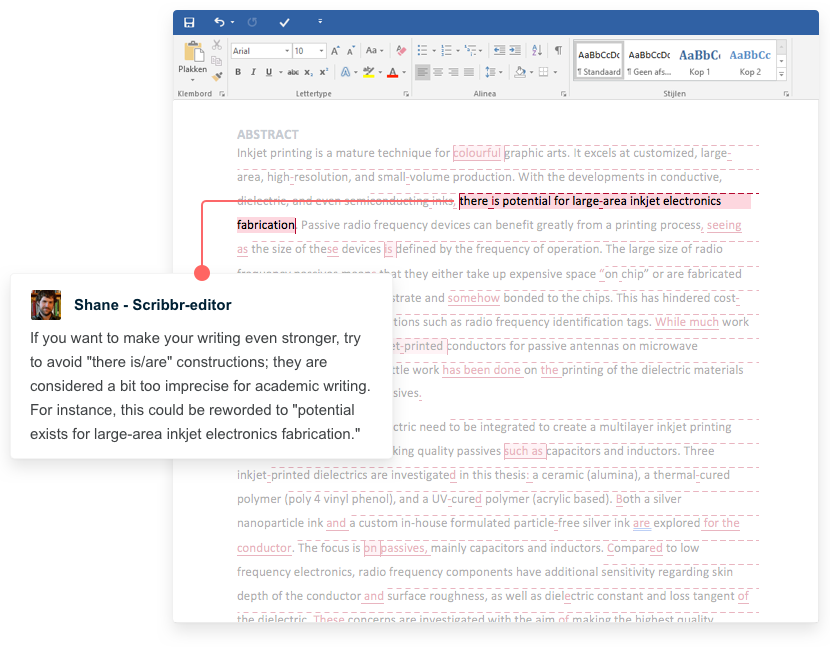
All normal distributions , like the standard normal distribution, are unimodal and symmetrically distributed with a bell-shaped curve. However, a normal distribution can take on any value as its mean and standard deviation. In the standard normal distribution, the mean and standard deviation are always fixed.
Every normal distribution is a version of the standard normal distribution that’s been stretched or squeezed and moved horizontally right or left.
The mean determines where the curve is centered. Increasing the mean moves the curve right, while decreasing it moves the curve left.
The standard deviation stretches or squeezes the curve. A small standard deviation results in a narrow curve, while a large standard deviation leads to a wide curve.
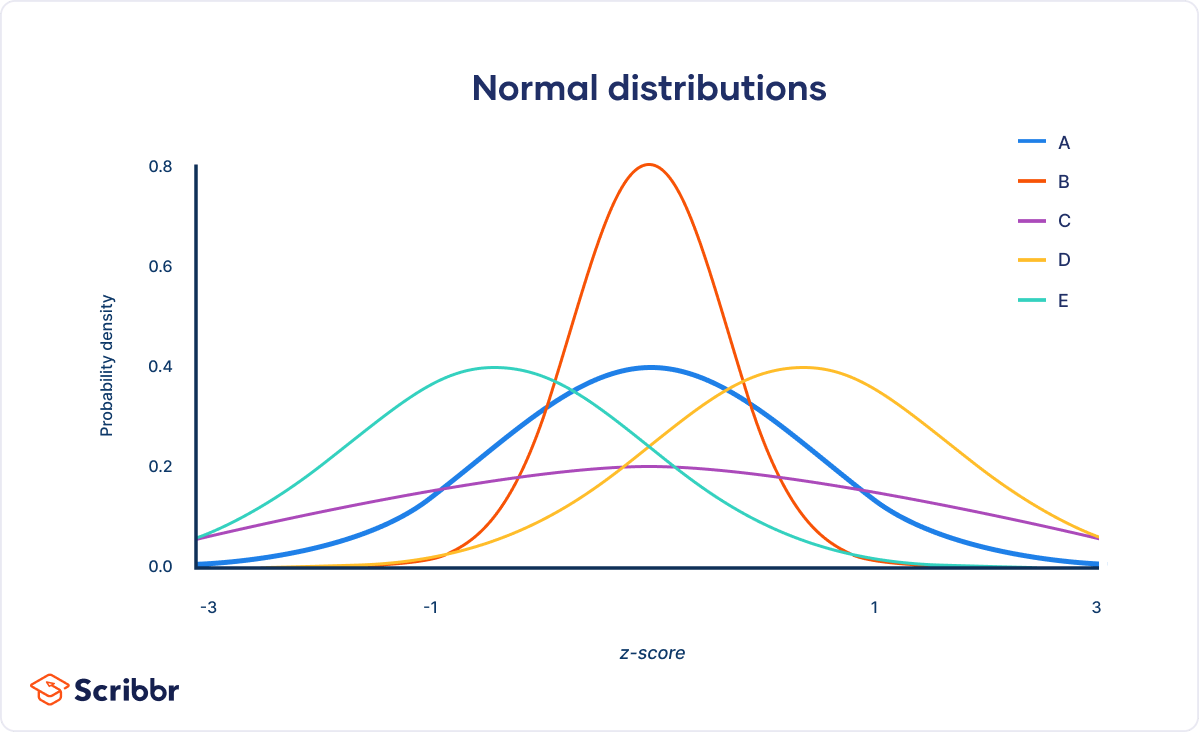
When you standardize a normal distribution, the mean becomes 0 and the standard deviation becomes 1. This allows you to easily calculate the probability of certain values occurring in your distribution, or to compare data sets with different means and standard deviations.
While data points are referred to as x in a normal distribution, they are called z or z scores in the z distribution. A z score is a standard score that tells you how many standard deviations away from the mean an individual value ( x ) lies:
- A positive z score means that your x value is greater than the mean.
- A negative z score means that your x value is less than the mean.
- A z score of zero means that your x value is equal to the mean.
Converting a normal distribution into the standard normal distribution allows you to:
- Compare scores on different distributions with different means and standard deviations.
- Normalize scores for statistical decision-making (e.g., grading on a curve).
- Find the probability of observations in a distribution falling above or below a given value.
- Find the probability that a sample mean significantly differs from a known population mean.
How to calculate a z score
To standardize a value from a normal distribution, convert the individual value into a z -score:
- Subtract the mean from your individual value.
- Divide the difference by the standard deviation.
To standardize your data, you first find the z score for 1380. The z score tells you how many standard deviations away 1380 is from the mean.
The z score for a value of 1380 is 1.53 . That means 1380 is 1.53 standard deviations from the mean of your distribution.
The standard normal distribution is a probability distribution , so the area under the curve between two points tells you the probability of variables taking on a range of values. The total area under the curve is 1 or 100%.
Every z score has an associated p value that tells you the probability of all values below or above that z score occuring. This is the area under the curve left or right of that z score.
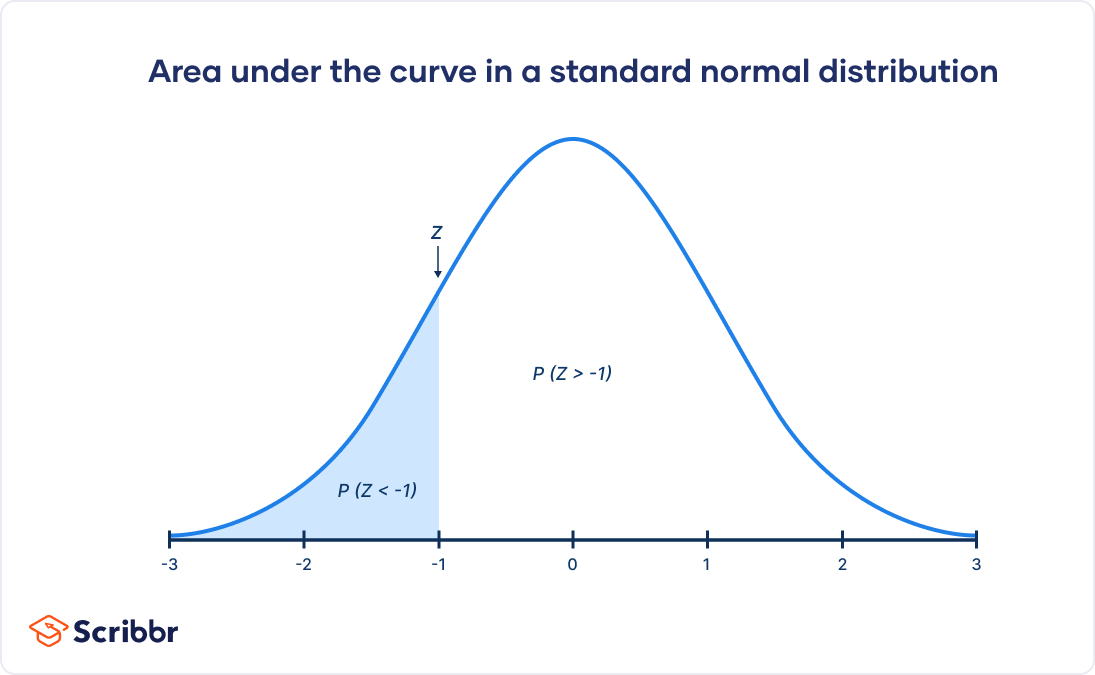
Z tests and p values
The z score is the test statistic used in a z test . The z test is used to compare the means of two groups, or to compare the mean of a group to a set value. Its null hypothesis typically assumes no difference between groups.
The area under the curve to the right of a z score is the p value, and it’s the likelihood of your observation occurring if the null hypothesis is true.
Usually, a p value of 0.05 or less means that your results are unlikely to have arisen by chance; it indicates a statistically significant effect.
By converting a value in a normal distribution into a z score, you can easily find the p value for a z test.
How to use a z table
Once you have a z score, you can look up the corresponding probability in a z table .
In a z table, the area under the curve is reported for every z value between -4 and 4 at intervals of 0.01.
There are a few different formats for the z table. Here, we use a portion of the cumulative table. This table tells you the total area under the curve up to a given z score—this area is equal to the probability of values below that z score occurring.
The first column of a z table contains the z score up to the first decimal place. The top row of the table gives the second decimal place.
To find the corresponding area under the curve (probability) for a z score:
- Go down to the row with the first two digits of your z score.
- Go across to the column with the same third digit as your z score.
- Find the value at the intersection of the row and column from the previous steps.
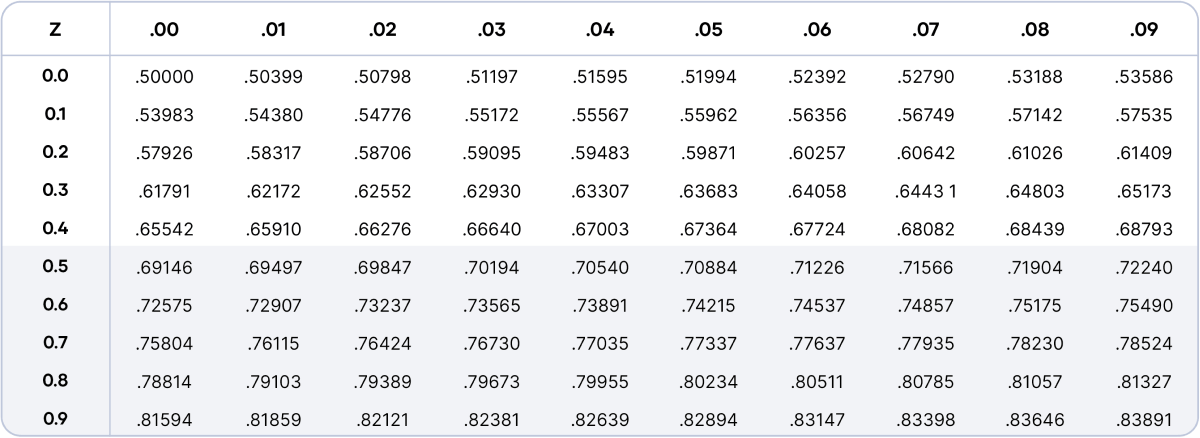
To find the shaded area, you take away 0.937 from 1, which is the total area under the curve.
Probability of x > 1380 = 1 − 0.937 = 0.063
Here's why students love Scribbr's proofreading services
Discover proofreading & editing
Let’s walk through an invented research example to better understand how the standard normal distribution works.
As a sleep researcher, you’re curious about how sleep habits changed during COVID-19 lockdowns. You collect sleep duration data from a sample during a full lockdown.
Before the lockdown, the population mean was 6.5 hours of sleep. The lockdown sample mean is 7.62.
To assess whether your sample mean significantly differs from the pre-lockdown population mean, you perform a z test :
- First, you calculate a z score for the sample mean value.
- Then, you find the p value for your z score using a z table.
Step 1: Calculate a z -score
To compare sleep duration during and before the lockdown, you convert your lockdown sample mean into a z score using the pre-lockdown population mean and standard deviation.
A z score of 2.24 means that your sample mean is 2.24 standard deviations greater than the population mean.
Step 2: Find the p value
To find the probability of your sample mean z score of 2.24 or less occurring, you use the z table to find the value at the intersection of row 2.2 and column +0.04.
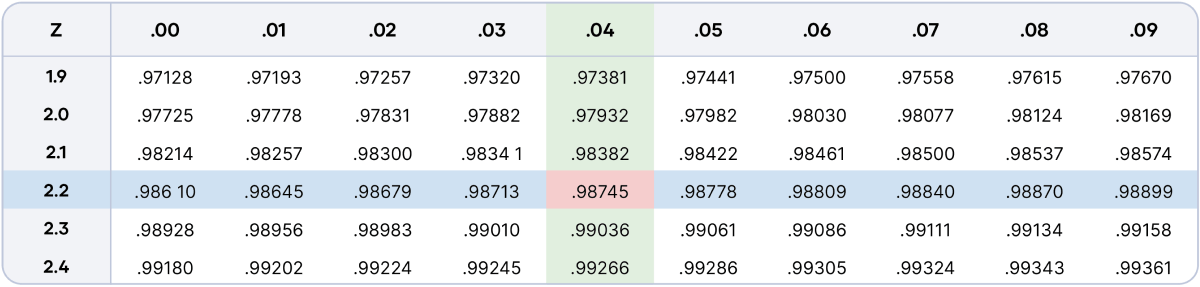
The table tells you that the area under the curve up to or below your z score is 0.9874. This means that your sample’s mean sleep duration is higher than about 98.74% of the population’s mean sleep duration pre-lockdown.
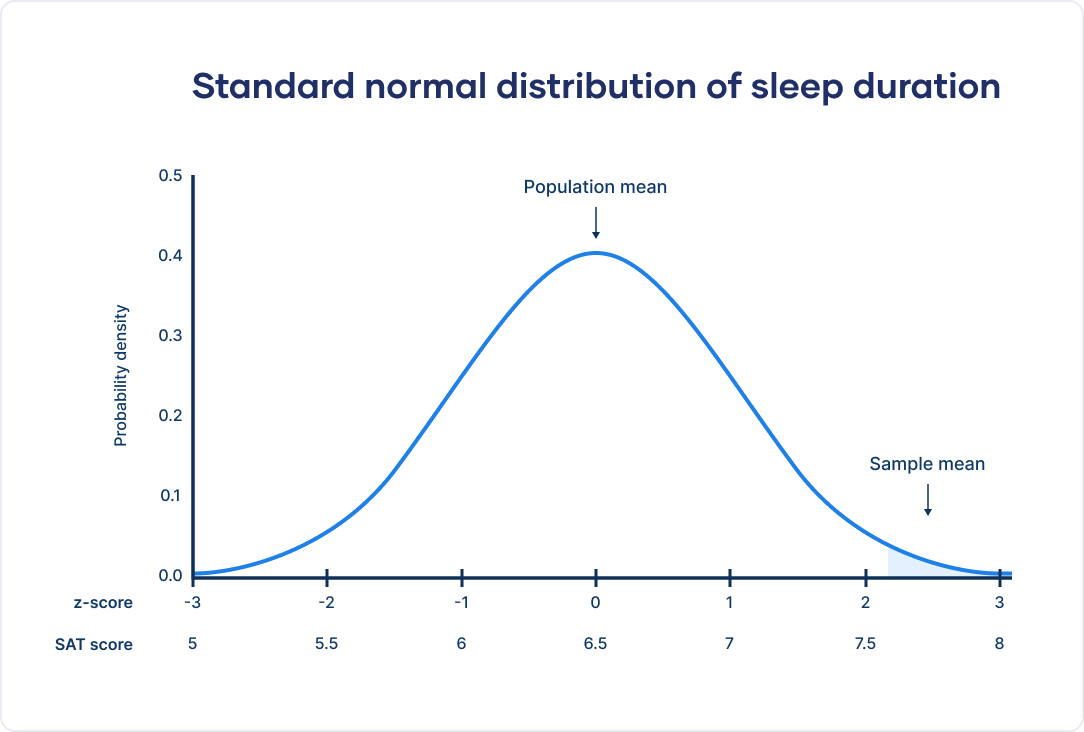
To find the p value to assess whether the sample differs from the population, you calculate the area under the curve above or to the right of your z score. Since the total area under the curve is 1, you subtract the area under the curve below your z score from 1.
A p value of less than 0.05 or 5% means that the sample significantly differs from the population.
Probability of z > 2.24 = 1 − 0.9874 = 0.0126 or 1.26%
With a p value of less than 0.05, you can conclude that average sleep duration in the COVID-19 lockdown was significantly higher than the pre-lockdown average.
If you want to know more about statistics , methodology , or research bias , make sure to check out some of our other articles with explanations and examples.
- Student’s t table
- Student’s t distribution
- Descriptive statistics
- Measures of central tendency
- Correlation coefficient
Methodology
- Cluster sampling
- Stratified sampling
- Types of interviews
- Cohort study
- Thematic analysis
Research bias
- Implicit bias
- Cognitive bias
- Survivorship bias
- Availability heuristic
- Nonresponse bias
- Regression to the mean
In a normal distribution , data are symmetrically distributed with no skew. Most values cluster around a central region, with values tapering off as they go further away from the center.
The measures of central tendency (mean, mode, and median) are exactly the same in a normal distribution.
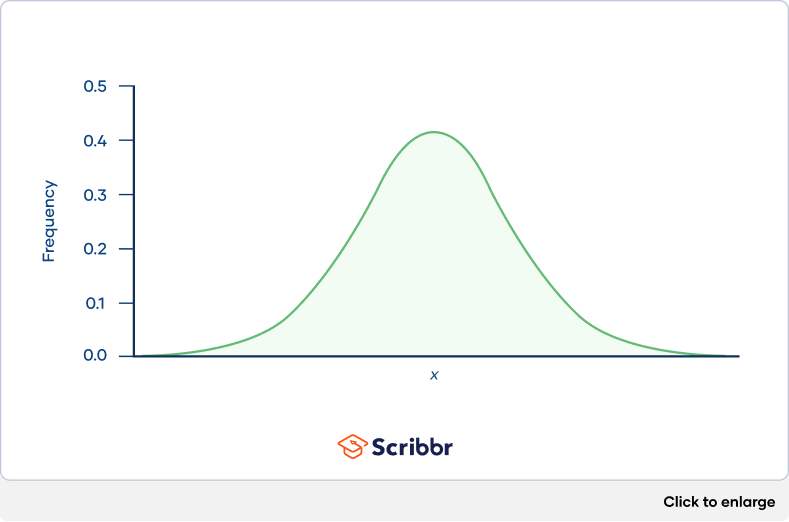
The standard normal distribution , also called the z -distribution, is a special normal distribution where the mean is 0 and the standard deviation is 1.
Any normal distribution can be converted into the standard normal distribution by turning the individual values into z -scores. In a z -distribution, z -scores tell you how many standard deviations away from the mean each value lies.
The empirical rule, or the 68-95-99.7 rule, tells you where most of the values lie in a normal distribution :
- Around 68% of values are within 1 standard deviation of the mean.
- Around 95% of values are within 2 standard deviations of the mean.
- Around 99.7% of values are within 3 standard deviations of the mean.
The empirical rule is a quick way to get an overview of your data and check for any outliers or extreme values that don’t follow this pattern.
The t -distribution gives more probability to observations in the tails of the distribution than the standard normal distribution (a.k.a. the z -distribution).
In this way, the t -distribution is more conservative than the standard normal distribution: to reach the same level of confidence or statistical significance , you will need to include a wider range of the data.
Cite this Scribbr article
If you want to cite this source, you can copy and paste the citation or click the “Cite this Scribbr article” button to automatically add the citation to our free Citation Generator.
Bhandari, P. (2023, June 21). The Standard Normal Distribution | Calculator, Examples & Uses. Scribbr. Retrieved March 25, 2024, from https://www.scribbr.com/statistics/standard-normal-distribution/
Is this article helpful?
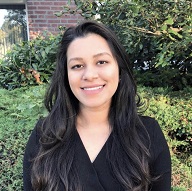
Pritha Bhandari
Other students also liked, normal distribution | examples, formulas, & uses, t-distribution: what it is and how to use it, understanding p values | definition and examples, what is your plagiarism score.
Normal Distribution
Data can be "distributed" (spread out) in different ways.
But there are many cases where the data tends to be around a central value with no bias left or right, and it gets close to a "Normal Distribution" like this:
The blue curve is a Normal Distribution. The yellow histogram shows some data that follows it closely, but not perfectly (which is usual).
Many things closely follow a Normal Distribution:
- heights of people
- size of things produced by machines
- errors in measurements
- blood pressure
- marks on a test
We say the data is "normally distributed":
The Normal Distribution has:
- mean = median = mode
- symmetry about the center
- 50% of values less than the mean and 50% greater than the mean
Standard Deviations
The Standard Deviation is a measure of how spread out numbers are (read that page for details on how to calculate it).
When we calculate the standard deviation we find that generally :
Example: 95% of students at school are between 1.1m and 1.7m tall.
Assuming this data is normally distributed can you calculate the mean and standard deviation?
The mean is halfway between 1.1m and 1.7m:
Mean = (1.1m + 1.7m) / 2 = 1.4m
95% is 2 standard deviations either side of the mean (a total of 4 standard deviations) so:
It is good to know the standard deviation, because we can say that any value is:
- likely to be within 1 standard deviation (68 out of 100 should be)
- very likely to be within 2 standard deviations (95 out of 100 should be)
- almost certainly within 3 standard deviations (997 out of 1000 should be)
Standard Scores
The number of standard deviations from the mean is also called the "Standard Score", "sigma" or "z-score". Get used to those words!
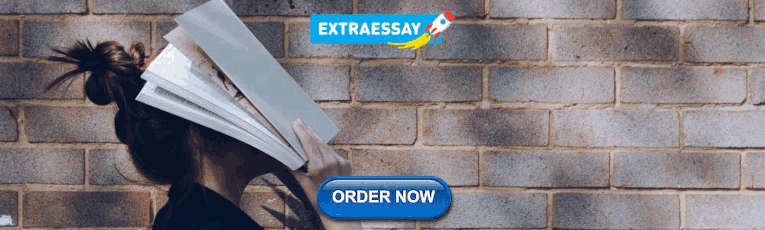
Example: In that same school one of your friends is 1.85m tall
You can see on the bell curve that 1.85m is 3 standard deviations from the mean of 1.4, so:
Your friend's height has a "z-score" of 3.0
It is also possible to calculate how many standard deviations 1.85 is from the mean
How far is 1.85 from the mean?
It is 1.85 - 1.4 = 0.45m from the mean
How many standard deviations is that? The standard deviation is 0.15m, so:
0.45m / 0.15m = 3 standard deviations
So to convert a value to a Standard Score ("z-score"):
- first subtract the mean,
- then divide by the Standard Deviation
And doing that is called "Standardizing":
We can take any Normal Distribution and convert it to The Standard Normal Distribution.
Example: Travel Time
A survey of daily travel time had these results (in minutes):
26, 33, 65, 28, 34, 55, 25, 44, 50, 36, 26, 37, 43, 62, 35, 38, 45, 32, 28, 34
The Mean is 38.8 minutes , and the Standard Deviation is 11.4 minutes (you can copy and paste the values into the Standard Deviation Calculator if you want).
Convert the values to z-scores ("standard scores").
To convert 26 :
So 26 is −1.12 Standard Deviations from the Mean
Here are the first three conversions
And here they are graphically:
You can calculate the rest of the z-scores yourself!
The z-score formula that we have been using is:
z = x − μ σ
- z is the "z-score" (Standard Score)
- x is the value to be standardized
- μ ('mu") is the mean
- σ ("sigma") is the standard deviation
And this is how to use it:
Example: Travel Time (continued)
Here are the first three conversions using the "z-score formula":
The exact calculations we did before, just following the formula.
Why Standardize ... ?
It can help us make decisions about our data.
Example: Professor Willoughby is marking a test.
Here are the students' results (out of 60 points):
20, 15, 26, 32, 18, 28, 35, 14, 26, 22, 17
Most students didn't even get 30 out of 60, and most will fail .
The test must have been really hard, so the Prof decides to Standardize all the scores and only fail people more than 1 standard deviation below the mean.
The Mean is 23 , and the Standard Deviation is 6.6 , and these are the Standard Scores:
-0.45, -1.21 , 0.45, 1.36, -0.76, 0.76, 1.82, -1.36 , 0.45, -0.15, -0.91
Now only 2 students will fail (the ones lower than −1 standard deviation)
Much fairer!
It also makes life easier because we only need one table (the Standard Normal Distribution Table ), rather than doing calculations individually for each value of mean and standard deviation.
In More Detail
Here is the Standard Normal Distribution with percentages for every half of a standard deviation , and cumulative percentages:
Example: Your score in a recent test was 0.5 standard deviations above the average, how many people scored lower than you did?
- Between 0 and 0.5 is 19.1%
- Less than 0 is 50% (left half of the curve)
So the total less than you is:
50% + 19.1% = 69.1%
In theory 69.1% scored less than you did (but with real data the percentage may be different)
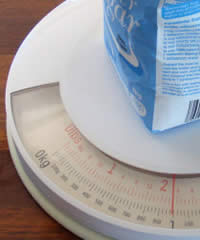
A Practical Example: Your company packages sugar in 1 kg bags.
When you weigh a sample of bags you get these results:
- 1007g, 1032g, 1002g, 983g, 1004g, ... (a hundred measurements)
- Mean = 1010g
- Standard Deviation = 20g
Some values are less than 1000g ... can you fix that?
The normal distribution of your measurements looks like this:
31% of the bags are less than 1000g, which is cheating the customer!
It is a random thing, so we can't stop bags having less than 1000g, but we can try to reduce it a lot.
Let's adjust the machine so that 1000g is:
- at −3 standard deviations:
- at −2.5 standard deviations:
So let us adjust the machine to have 1000g at −2.5 standard deviations from the mean.
Now, we can adjust it to:
- increase the amount of sugar in each bag (which changes the mean), or
- make it more accurate (which reduces the standard deviation)
Let us try both.
Adjust the mean amount in each bag
The standard deviation is 20g, and we need 2.5 of them:
2.5 × 20g = 50g
So the machine should average 1050g , like this:
Adjust the accuracy of the machine
Or we can keep the same mean (of 1010g), but then we need 2.5 standard deviations to be equal to 10g:
10g / 2.5 = 4g
So the standard deviation should be 4g , like this:
(We hope the machine is that accurate!)
Or perhaps we could have some combination of better accuracy and slightly larger average size, I will leave that up to you!
More Accurate Values ...
Use the Standard Normal Distribution Table when you want more accurate values.

- school Campus Bookshelves
- menu_book Bookshelves
- perm_media Learning Objects
- login Login
- how_to_reg Request Instructor Account
- hub Instructor Commons
- Download Page (PDF)
- Download Full Book (PDF)
- Periodic Table
- Physics Constants
- Scientific Calculator
- Reference & Cite
- Tools expand_more
- Readability
selected template will load here
This action is not available.
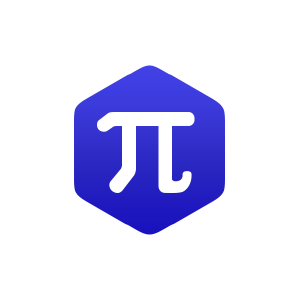
11.8: Understanding Normal Distribution
- Last updated
- Save as PDF
- Page ID 51642
Here you will learn about the Normal Distribution. You will learn what it is and why it is important, and you will begin to develop an intuition for the rarity of a value in a set by comparing it to the mean and standard deviation of the data.
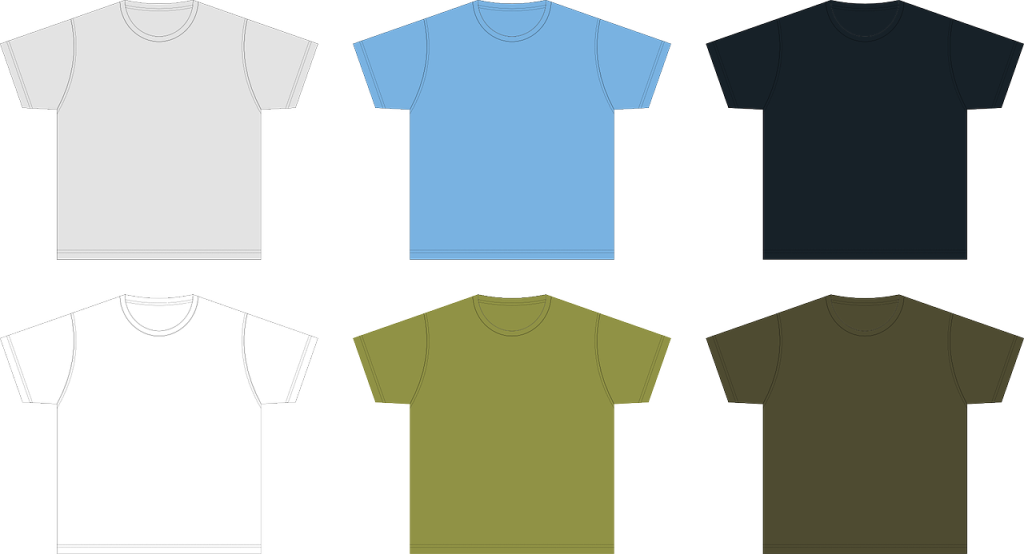
Watch This: Spread of a Normal Distribution
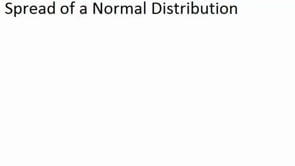
A Vimeo element has been excluded from this version of the text. You can view it online here: http://pb.libretexts.org/mala/?p=154
There are a number of reasons that it is important to become familiar with the normal distribution, as you will discover throughout this chapter. Examples of values associated with normal distribution:
- Physical characteristics such as height, weight, arm or leg length, etc.
- The percentile rankings of standardized testing such as the ACT and SAT
- The volume of water produced by a river on a monthly or yearly basis
- The velocity of molecules in an ideal gas
Knowing that the values in a set are exactly or approximately normally distributed allows you to get a feel for how common a particular value might be in that set. Because the values of a normal distribution are predictably clustered around the mean, you can estimate in short order the rarity of a given value in the set. In our upcoming lesson on the Empirical Rule, you will see that it is worth memorizing that normally distributed data has the characteristics mentioned above:
- 50% of all data points are above the mean and 50% are below
- Apx 68% of all data points are within 1 standard deviation of the mean
- Apx 95% of all data points are within 2 standard deviations of the mean
- Apx 99.7% of all data points are within 3 standard deviations of the mean
In this lesson, we will be practicing a ’rough estimate’ of the probability that a value within a given range will occur in a particular set of data, just to develop an intuition of the use of a normal distribution. In subsequent lessons, we will become more specific with our estimates. The image below will be used in greater detail in the lesson on the Empirical Rule, but you may use it as a reference for this lesson also.
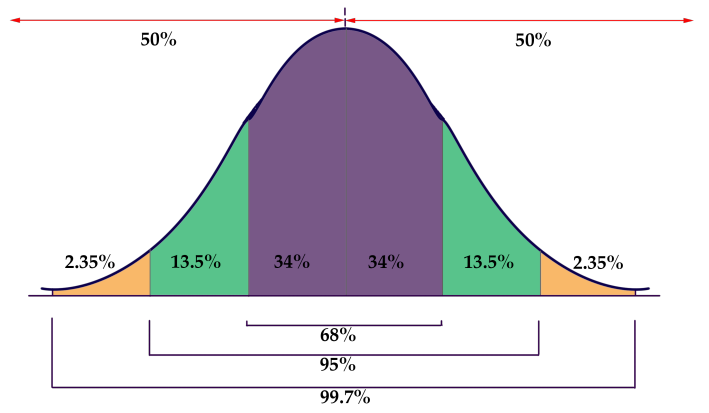
Human height is commonly considered an approximately normally distributed measure. If the mean height of a male adult in the United States is 5′ 1″, with a standard deviation of 1.5″, how common are men with heights greater than 6′ 2″?
Since each standard deviation of this normally distributed data is 1.5″, and 6′ 2″ is 400 above the mean for the population, 6′ 2″ is nearly 3 standard deviations above the mean. That tells us that men taller than 6′ 2″ are quite rare in this population.
If the fuel mileage of a particular model of car is normally distributed, with a mean of 26 mpg and a standard deviation of 2 mpg, how common are cars with a fuel efficiency of 24 to 25 mpg?

We know that apx 68% of the cars in the population have an efficiency of between 24 and 28 mpg, since that would be 1 SD below and 1 SD above the mean. That suggests that apx 34% have an efficiency of 24 to 26 mpg, so we can say that it is uncommon to see a car with an efficiency between 24 and 25 mpg, but not extremely so.
If the maximum jumping height of US high school high jumpers is normally distributed with a mean of 5′ 11.5″ and a SD of 2.2″, how unusual is it to see a high school jumper clear 6′ 3″?
If the mean is 5′ 11.5″, then 1 SD above is 6′ 1.7″ and 2 SDs is 6′ 3.9″. That means that less than 2.5% of jumpers 6′ 3.9″, so it would be pretty uncommon to see a high-school competitor exceed 6′ 3″.
Intro Problem Revisited
If you knew that the prices of t-shirts sold in an online shopping site were normally distributed , and had a mean cost of $10, with a standard deviation of $1.50, how could that information benefit you as you are looking at various t-shirt styles and designs on the site? How could you use what you know if you were looking to make a profit by purchasing unusually inexpensive shirts to resell at prices that are more common?
By knowing the mean and SD of the shirt prices, and knowing that they are normally distributed, you can estimate right away if a shirt is priced at a point significantly below the norm. For instance, with this data, we can estimate that a shirt priced at $7.00 is less expensive than apx 97.5% of all shirts on the site, and could likely be resold at a profit (assuming there is not something wrong the shirt that is not obvious from the listing).
Distribution: an arrangement of values of a variable showing their observed or theoretical frequency of occurrence.
Range of values of a distribution: is the difference between the least and greatest values.
Normal distribution: a very specific distribution that is symmetric about its mean. Half the values of the random variable are below the mean and half are above the mean. Approximately 68% of the data is within 1 standard deviation of the mean; aproximately 96% is within 2 SDs, and 99.7% within 3 SDs.
Standard deviation: a measure of how spread out the data is from the mean. To determine if a data value is far from the mean, determine how many standard deviations it is from the mean. The SD is calculated as the square root of the variance.
Guided Practice
Assume the data to be normally distributed, and describe the rarity of an event using the following scale:
- 0% to < 1% probability = very rare
- 1% to < 5% = rare
- 5% to < 34% = uncommon
- 34% to < 50% = common
- 50% to 100% = likely
- If the mean (µ) of the data is 75, and the standard deviation (σ) is 5, how common is a value between 70 and 75?
- If the µ is .02 and the σ is .005, how common is a value between .005 and .01?
- If the µ is 1280 and the σ is 70, how common is a value between 1210 and 1350?
- If the mean defect rate at a cellphone production plant is .1%, with a standard deviation of .03%, would it seem reasonable for a quality assurance manager to be concerned about 3 defective phones in a single 1000 unit run?
- A value of 70 is only 1 standard deviation below the mean, so a value between 70 and 75 would be expected approximately 34% of the time, so it would be common.
- A value of .01 is 2 SDs below the mean, and .005 is 3 SDs below, so we would expect there to be about a 2.5% probability of a value occurring in that range. A value between 0.005 and 0.01 would be rare.
- 1210 is 1 SD below the mean, and 1350 is 1 SD above the mean, so we would expect approximately 68% of the data to be in that range, meaning that it is likely that a value in that range would occur.
- .1% translates into 1 per thousand, with a standard deviation of 3 per ten thousand. That means that 3 defects in the same thousand is nearly 7 SDs above the mean, well into the very rare category. While it is not impossible for random chance to result in such a value, it would certainly be prudent for the manager to investigate.
Practice Questions
Assume all sets/populations to be approximately normally distributed, and describe the rarity of an event using the following scale:
- 0% to< 1% probability = very rare
- 1% to < 5% = rare
- 5% to < 34% = uncommon
- 34% to < 50% = common
- 50% to 100% = likely.
You may reference the image below:
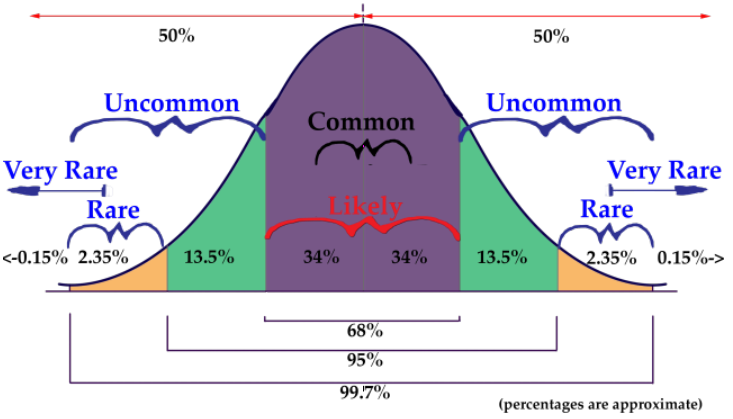
- Scores on a certain standardized test have a mean of 500, and a standard deviation of 100. How common is a score between 600 and 700?
- Considering a full-grown show-quality male Siberian Husky has a mean weight of 52.5 lbs, with SD of 7.5 lbs, how common are male huskies in the 37.5–45 lbs range?
- A population µ = 125, and σ = 25, how common are values in the 100 – 150 range?
- Population µ = 0.0025 and σ = 0.0005, how common are values between 0.0025 and 0.0030?
- A 12 oz can of soda has a mean volume of 12 oz, with a standard deviation of .25 oz. How common are cans with between 11 and 11.5 oz of soda?
- µ = 0.0025 and σ = 0.0005, how common are values between 0.0045 and 0.005?
- If a population µ = 1130 and σ = 5, how common are values between 0 and 1100?
- Assuming population µ = 1130 and σ = 5, how common are values between 1125 and 1135?
- The American Robin Redbreast has a mean weight of 77 g, with a standard deviation of 6 g. How common are Robins in the 59 g−71 g range?
- Population µ = 0.25% and σ = 0.05%, how common are values between 0.35% and 0.45%?
- Population µ = 156.5 and σ = 0.25, how common are values between 155 and 156?
- The Normal Distribution. Authored by : Karen Walters. Provided by : CK-12 Foundation. Located at : http://www.ck12.org/ . License : CC BY-NC: Attribution-NonCommercial
- Chapter6SpreadofaNormalDistributionA. Authored by : CK-12 Foundation. Located at : https://vimeo.com/47099905 . License : CC BY-NC-SA: Attribution-NonCommercial-ShareAlike
- T-Shirts. Authored by : OpenClipArtVectors. Located at : https://pixabay.com/en/t-shirts-shirts-clothing-apparel-154312/?oq=shirt . License : Public Domain: No Known Copyright
- Gas prices. Authored by : stux. Located at : https://pixabay.com/en/gasoline-prices-petrol-fuel-206098/?oq=gas%20price . License : Public Domain: No Known Copyright
- Math Article
Normal Distribution
In probability theory and statistics, the Normal Distribution , also called the Gaussian Distribution , is the most significant continuous probability distribution. Sometimes it is also called a bell curve. A large number of random variables are either nearly or exactly represented by the normal distribution, in every physical science and economics. Furthermore, it can be used to approximate other probability distributions , therefore supporting the usage of the word ‘normal ‘as in about the one, mostly used.
Table of Contents:
Normal Distribution Standard Deviation
- Problems and Solutions
Applications
Normal distribution definition.
The Normal Distribution is defined by the probability density function for a continuous random variable in a system. Let us say, f(x) is the probability density function and X is the random variable. Hence, it defines a function which is integrated between the range or interval (x to x + dx), giving the probability of random variable X, by considering the values between x and x+dx.
f(x) ≥ 0 ∀ x ϵ (−∞,+∞)
And -∞ ∫ +∞ f(x) = 1
Normal Distribution Formula
The probability density function of normal or gaussian distribution is given by;
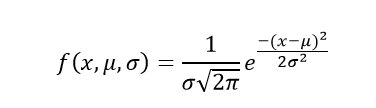
- x is the variable
- μ is the mean
- σ is the standard deviation
Also, read:
Normal Distribution Curve
The random variables following the normal distribution are those whose values can find any unknown value in a given range. For example, finding the height of the students in the school. Here, the distribution can consider any value, but it will be bounded in the range say, 0 to 6ft. This limitation is forced physically in our query.
Whereas, the normal distribution doesn’t even bother about the range. The range can also extend to –∞ to + ∞ and still we can find a smooth curve. These random variables are called Continuous Variables, and the Normal Distribution then provides here probability of the value lying in a particular range for a given experiment. Also, use the normal distribution calculator to find the probability density function by just providing the mean and standard deviation value.
Generally, the normal distribution has any positive standard deviation. We know that the mean helps to determine the line of symmetry of a graph, whereas the standard deviation helps to know how far the data are spread out. If the standard deviation is smaller, the data are somewhat close to each other and the graph becomes narrower. If the standard deviation is larger, the data are dispersed more, and the graph becomes wider. The standard deviations are used to subdivide the area under the normal curve. Each subdivided section defines the percentage of data, which falls into the specific region of a graph.
Using 1 standard deviation, the Empirical Rule states that,
- Approximately 68% of the data falls within one standard deviation of the mean. (i.e., Between Mean- one Standard Deviation and Mean + one standard deviation)
- Approximately 95% of the data falls within two standard deviations of the mean. (i.e., Between Mean- two Standard Deviation and Mean + two standard deviations)
- Approximately 99.7% of the data fall within three standard deviations of the mean. (i.e., Between Mean- three Standard Deviation and Mean + three standard deviations)
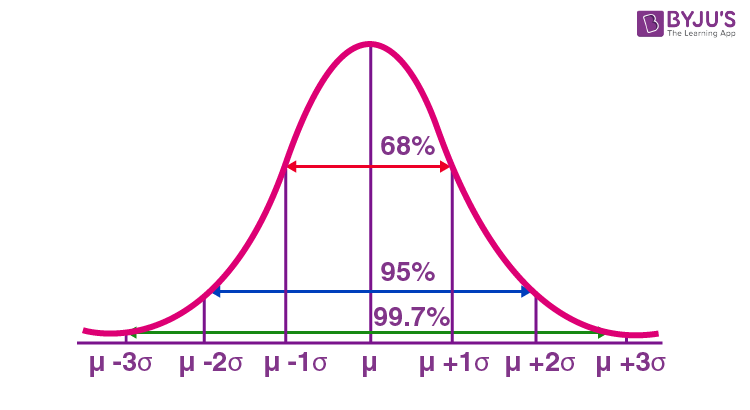
Thus, the empirical rule is also called the 68 – 95 – 99.7 rule.
Normal Distribution Table
The table here shows the area from 0 to Z-value.
Normal Distribution Problems and Solutions
Question 1: Calculate the probability density function of normal distribution using the following data. x = 3, μ = 4 and σ = 2.
Solution: Given, variable, x = 3
Mean = 4 and
Standard deviation = 2
By the formula of the probability density of normal distribution, we can write;
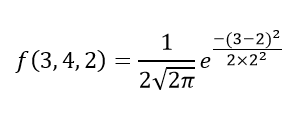
Hence, f(3,4,2) = 1.106.
Question 2: If the value of random variable is 2, mean is 5 and the standard deviation is 4, then find the probability density function of the gaussian distribution.
Solution: Given,
Variable, x = 2
Mean = 5 and
Standard deviation = 4
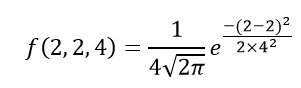
f(2,2,4) = 1/(4√2π) e 0
f(2,2,4) = 0.0997
There are two main parameters of normal distribution in statistics namely mean and standard deviation. The location and scale parameters of the given normal distribution can be estimated using these two parameters.
Normal Distribution Properties
Some of the important properties of the normal distribution are listed below:
- In a normal distribution, the mean, median and mode are equal.(i.e., Mean = Median= Mode).
- The total area under the curve should be equal to 1.
- The normally distributed curve should be symmetric at the centre.
- There should be exactly half of the values are to the right of the centre and exactly half of the values are to the left of the centre.
- The normal distribution should be defined by the mean and standard deviation.
- The normal distribution curve must have only one peak. (i.e., Unimodal)
- The curve approaches the x-axis, but it never touches, and it extends farther away from the mean.
The normal distributions are closely associated with many things such as:
- Marks scored on the test
- Heights of different persons
- Size of objects produced by the machine
- Blood pressure and so on.
Frequently Asked Questions on Normal Distribution – FAQs
What is a normal distribution in statistics, what does normal distribution mean, what is a normal distribution used for, what are the characteristics of a normal distribution, how do you know if data is normally distributed, how do you use a normal distribution table.

- Share Share
Register with BYJU'S & Download Free PDFs
Register with byju's & watch live videos.

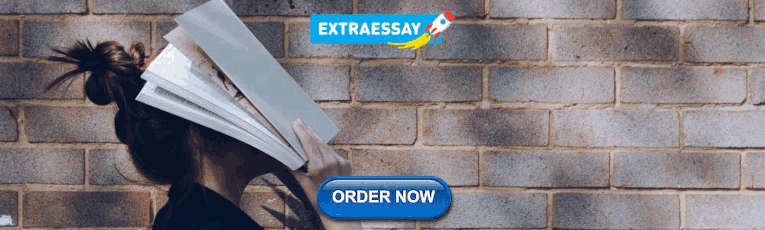
IMAGES
VIDEO
COMMENTS
Problems with Solutions. X is a normally distributed variable with mean μ = 30 and standard deviation σ = 4. Find the probabilities. a) P (X < 40) b) P (X > 21) c) P (30 < X < 35) A radar unit is used to measure the speeds of cars on a motorway. The speeds are normally distributed with a mean of 90 km/hr and a standard deviation of 10 km/hr.
Multiply the sample size (found in step 1) by the z-value you found in step 4. For example, 0.300 * 100 = 30. That's it! 2. "More Than" or "Above". This how-to covers solving normal distribution problems that contain the phrase " more than " (or a phrase like "above"). Step 1:Break up the word problem into parts.
The distribution is normal with a mean of 25 25, and a standard deviation of 4 4. Everyone who scores in the top 30% 30 % of the distribution gets a certificate. What is the lowest score someone can get and still earn a certificate? The top 5% 5 % of the scores get to compete in a statewide history contest. What is the lowest score someone can ...
Height, birth weight, reading ability, job satisfaction, or SAT scores are just a few examples of such variables. Because normally distributed variables are so common, many statistical tests are designed for normally distributed populations. Understanding the properties of normal distributions means you can use inferential statistics to compare ...
Solution: Step 1: Sketch a normal distribution with a mean of μ = 150 cm and a standard deviation of σ = 30 cm . Step 2: The diameter of 120 cm is one standard deviation below the mean. Shade below that point. Step 3: Add the percentages in the shaded area: 0.15 % + 2.35 % + 13.5 % = 16 %.
The normal distribution is described by two parameters: the mean, μ, and the standard deviation, σ. We write X - N (μ, σ 2 ). The following diagram shows the formula for Normal Distribution. Scroll down the page for more examples and solutions on using the normal distribution formula.
For example, suppose we have a set of data that follows the normal distribution with mean 400 and standard deviation 100. This means 68% of the data would fall between the values of 300 (one standard deviation below the mean: 400 - 100 = 300 400 - 100 = 300 ) and 500 (one standard deviation above the mean: 400 + 100 = 500 400 + 100 = 500 ).
Learn how to solve any Normal Probability Distribution problem. This tutorial first explains the concept behind the normal distribution, then it discusses h...
This calculus video tutorial provides a basic introduction into normal distribution and probability. It explains how to solve normal distribution problems u...
The probability is given by the area under that curve. It be given by this area. For those of you who know calculus, if p of x is our probability density function -- doesn't have to be a normal distribution although it often is a normal distribution -- the way you actually figure out the probability, let's say between 4 and a half and 5 and half.
Introduction. This section will cover some of the types of questions that can be answered using the properties of a normal distribution. The first examples deal with more theoretical questions that will help you master basic understandings and computational skills, while the later problems will provide examples with real data, or at least a real context.
For example, in a normal distribution, 68% of the observations fall within +/- 1 standard deviation from the mean. This property is part of the Empirical Rule, which describes the percentage of the data that fall within specific numbers of standard deviations from the mean for bell-shaped curves. ... My problem in interpreting normal and ...
The normal distribution, which is continuous, is the most important of all the probability distributions. Its graph is bell-shaped. This bell-shaped curve is used in almost all disciplines. Since it is a continuous distribution, the total area under the curve is one. The parameters of the normal are the mean \(\mu\) and the standard deviation σ.
Solving Problems Involving Using Normal Distribution. Problem 1. : Suppose that the data concerning the first-year salaries of Baruch graduates is normally distributed with the population mean µ = $60000 and the population standard deviation σ = $15000. Find the probability of a randomly selected Baruch graduate earning less than $45000 annually.
Example \(\PageIndex{1}\) general normal distribution. The length of a human pregnancy is normally distributed with a mean of 272 days with a standard deviation of 9 days (Bhat & Kushtagi, 2006). State the random variable. Find the probability of a pregnancy lasting more than 280 days. Find the probability of a pregnancy lasting less than 250 days.
x - M = 1380 − 1150 = 230. Step 2: Divide the difference by the standard deviation. SD = 150. z = 230 ÷ 150 = 1.53. The z score for a value of 1380 is 1.53. That means 1380 is 1.53 standard deviations from the mean of your distribution. Next, we can find the probability of this score using a z table.
These numerical values (68 - 95 - 99.7) come from the cumulative distribution function (CDF) of the normal distribution. For example, F (2) = 0.9772, or Pr (x ≤ μ + 2σ) = 0.9772. Note that this is not a symmetrical interval - this is merely the probability that an observation is less than μ + 2σ.
Example 11.3.2 11.3. 2. Suppose that the distribution of the masses of female marine iguanas in Puerto Villamil in the Galapagos Islands is approximately normal, with a mean mass of 950 g and a standard deviation of 325 g. There are very few young marine iguanas in the populated areas of the islands, because feral cats tend to kill them.
The Standard Deviation is a measure of how spread out numbers are (read that page for details on how to calculate it). When we calculate the standard deviation we find that generally: 68% of values are within. 1 standard deviation of the mean. 95% of values are within. 2 standard deviations of the mean.
Normal distribution: a very specific distribution that is symmetric about its mean. Half the values of the random variable are below the mean and half are above the mean. Approximately 68% of the data is within 1 standard deviation of the mean; aproximately 96% is within 2 SDs, and 99.7% within 3 SDs. Standard deviation: a measure of how spread ...
The grades on a statistics midterm for a high school are normally distributed with a mean of 81 and a standard deviation of 6.3. All right. Calculate the z-scores for each of the following exam grades. Draw and label a sketch for each example. We can probably do it all on the same example.
For example, finding the height of the students in the school. Here, the distribution can consider any value, but it will be bounded in the range say, 0 to 6ft. This limitation is forced physically in our query. ... Normal Distribution Problems and Solutions. Question 1: Calculate the probability density function of normal distribution using ...