- Patient Care & Health Information
- Diseases & Conditions
- Type 1 diabetes
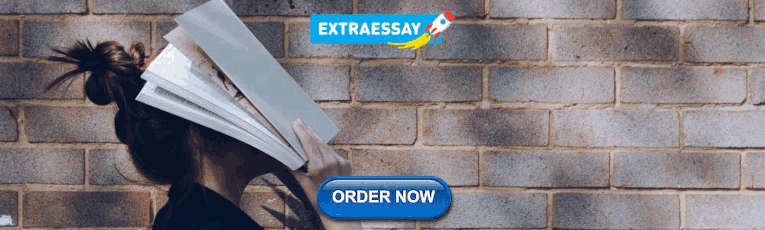
What is type 1 diabetes? A Mayo Clinic expert explains
Learn more about type 1 diabetes from endocrinologist Yogish Kudva, M.B.B.S.
I'm Dr. Yogish C. Kudva an endocrinologist at Mayo Clinic. In this video, we'll cover the basics of type 1 diabetes. What is it? Who gets it? The symptoms, diagnosis, and treatment. Whether you're looking for answers for yourself or someone you love. We are here to give you the best information available. Type 1 diabetes is a chronic condition that affects the insulin making cells of the pancreas. It's estimated that about 1.25 million Americans live with it. People with type 1 diabetes don't make enough insulin. An important hormone produced by the pancreas. Insulin allows your cells to store sugar or glucose and fat and produce energy. Unfortunately, there is no known cure. But treatment can prevent complications and also improve everyday life for patients with type 1 diabetes. Lots of people with type 1 diabetes live a full life. And the more we learn and develop treatment for the disorder, the better the outcome.
We don't know what exactly causes type 1 diabetes. We believe that it is an auto-immune disorder where the body mistakenly destroys insulin producing cells in the pancreas. Typically, the pancreas secretes insulin into the bloodstream. The insulin circulates, letting sugar enter your cells. This sugar or glucose, is the main source of energy for cells in the brain, muscle cells, and other tissues. However, once most insulin producing cells are destroyed, the pancreas can't produce enough insulin, meaning the glucose can't enter the cells, resulting in an excess of blood sugar floating in the bloodstream. This can cause life-threatening complications. And this condition is called diabetic ketoacidosis. Although we don't know what causes it, we do know certain factors can contribute to the onset of type 1 diabetes. Family history. Anyone with a parent or sibling with type 1 diabetes has a slightly increased risk of developing it. Genetics. The presence of certain genes can also indicate an increased risk. Geography. Type 1 diabetes becomes more common as you travel away from the equator. Age, although it can occur at any age there are two noticeable peaks. The first occurs in children between four and seven years of age and the second is between 10 and 14 years old.
Signs and symptoms of type 1 diabetes can appear rather suddenly, especially in children. They may include increased thirst, frequent urination, bed wetting in children who previously didn't wet the bed. Extreme hunger, unintended weight loss, fatigue and weakness, blurred vision, irritability, and other mood changes. If you or your child are experiencing any of these symptoms, you should talk to your doctor.
The best way to determine if you have type 1 diabetes is a blood test. There are different methods such as an A1C test, a random blood sugar test, or a fasting blood sugar test. They are all effective and your doctor can help determine what's appropriate for you. If you are diagnosed with diabetes, your doctor may order additional tests to check for antibodies that are common in type 1 diabetes in the test called C-peptide, which measures the amount of insulin produced when checked simultaneously with a fasting glucose. These tests can help distinguish between type 1 and type 2 diabetes when a diagnosis is uncertain.
If you have been diagnosed with type 1 diabetes, you may be wondering what treatment looks like. It could mean taking insulin, counting carbohydrates, fat protein, and monitoring your glucose frequently, eating healthy foods, and exercising regularly to maintain a healthy weight. Generally, those with type 1 diabetes will need lifelong insulin therapy. There are many different types of insulin and more are being developed that are more efficient. And what you may take may change. Again, your doctor will help you navigate what's right for you. A significant advance in treatment from the last several years has been the development and availability of continuous glucose monitoring and insulin pumps that automatically adjust insulin working with the continuous glucose monitor. This type of treatment is the best treatment at this time for type 1 diabetes. This is an exciting time for patients and for physicians that are keen to develop, prescribe such therapies. Surgery is another option. A successful pancreas transplant can erase the need for additional insulin. However, transplants aren't always available, not successful and the procedure can pose serious risks. Sometimes it may outweigh the dangers of diabetes itself. So transplants are often reserved for those with very difficult to manage conditions. A successful transplant can bring life transforming results. However, surgery is always a serious endeavor and requires ample research and concentration from you, your family, and your medical team.
The fact that we don't know what causes type 1 diabetes can be alarming. The fact that we don't have a cure for it even more so. But with the right doctor, medical team and treatment, type 1 diabetes can be managed. So those who live with it can get on living. If you would like to learn even more about type 1 diabetes, watch our other related videos or visit mayoclinic.org. We wish you well.
Type 1 diabetes, once known as juvenile diabetes or insulin-dependent diabetes, is a chronic condition. In this condition, the pancreas makes little or no insulin. Insulin is a hormone the body uses to allow sugar (glucose) to enter cells to produce energy.
Different factors, such as genetics and some viruses, may cause type 1 diabetes. Although type 1 diabetes usually appears during childhood or adolescence, it can develop in adults.
Even after a lot of research, type 1 diabetes has no cure. Treatment is directed toward managing the amount of sugar in the blood using insulin, diet and lifestyle to prevent complications.
Products & Services
- A Book: The Essential Diabetes Book
Type 1 diabetes symptoms can appear suddenly and may include:
- Feeling more thirsty than usual
- Urinating a lot
- Bed-wetting in children who have never wet the bed during the night
- Feeling very hungry
- Losing weight without trying
- Feeling irritable or having other mood changes
- Feeling tired and weak
- Having blurry vision
When to see a doctor
Talk to your health care provider if you notice any of the above symptoms in you or your child.
The exact cause of type 1 diabetes is unknown. Usually, the body's own immune system — which normally fights harmful bacteria and viruses — destroys the insulin-producing (islet) cells in the pancreas. Other possible causes include:
- Exposure to viruses and other environmental factors
The role of insulin
Once a large number of islet cells are destroyed, the body will produce little or no insulin. Insulin is a hormone that comes from a gland behind and below the stomach (pancreas).
- The pancreas puts insulin into the bloodstream.
- Insulin travels through the body, allowing sugar to enter the cells.
- Insulin lowers the amount of sugar in the bloodstream.
- As the blood sugar level drops, the pancreas puts less insulin into the bloodstream.
The role of glucose
Glucose — a sugar — is a main source of energy for the cells that make up muscles and other tissues.
- Glucose comes from two major sources: food and the liver.
- Sugar is absorbed into the bloodstream, where it enters cells with the help of insulin.
- The liver stores glucose in the form of glycogen.
- When glucose levels are low, such as when you haven't eaten in a while, the liver breaks down the stored glycogen into glucose. This keeps glucose levels within a typical range.
In type 1 diabetes, there's no insulin to let glucose into the cells. Because of this, sugar builds up in the bloodstream. This can cause life-threatening complications.
Risk factors
Some factors that can raise your risk for type 1 diabetes include:
- Family history. Anyone with a parent or sibling with type 1 diabetes has a slightly higher risk of developing the condition.
- Genetics. Having certain genes increases the risk of developing type 1 diabetes.
- Geography. The number of people who have type 1 diabetes tends to be higher as you travel away from the equator.
- Age. Type 1 diabetes can appear at any age, but it appears at two noticeable peaks. The first peak occurs in children between 4 and 7 years old. The second is in children between 10 and 14 years old.
Complications
Over time, type 1 diabetes complications can affect major organs in the body. These organs include the heart, blood vessels, nerves, eyes and kidneys. Having a normal blood sugar level can lower the risk of many complications.
Diabetes complications can lead to disabilities or even threaten your life.
- Heart and blood vessel disease. Diabetes increases the risk of some problems with the heart and blood vessels. These include coronary artery disease with chest pain (angina), heart attack, stroke, narrowing of the arteries (atherosclerosis) and high blood pressure.
Nerve damage (neuropathy). Too much sugar in the blood can injure the walls of the tiny blood vessels (capillaries) that feed the nerves. This is especially true in the legs. This can cause tingling, numbness, burning or pain. This usually begins at the tips of the toes or fingers and spreads upward. Poorly controlled blood sugar could cause you to lose all sense of feeling in the affected limbs over time.
Damage to the nerves that affect the digestive system can cause problems with nausea, vomiting, diarrhea or constipation. For men, erectile dysfunction may be an issue.
- Kidney damage (nephropathy). The kidneys have millions of tiny blood vessels that keep waste from entering the blood. Diabetes can damage this system. Severe damage can lead to kidney failure or end-stage kidney disease that can't be reversed. End-stage kidney disease needs to be treated with mechanical filtering of the kidneys (dialysis) or a kidney transplant.
- Eye damage. Diabetes can damage the blood vessels in the retina (part of the eye that senses light) (diabetic retinopathy). This could cause blindness. Diabetes also increases the risk of other serious vision conditions, such as cataracts and glaucoma.
- Foot damage. Nerve damage in the feet or poor blood flow to the feet increases the risk of some foot complications. Left untreated, cuts and blisters can become serious infections. These infections may need to be treated with toe, foot or leg removal (amputation).
- Skin and mouth conditions. Diabetes may leave you more prone to infections of the skin and mouth. These include bacterial and fungal infections. Gum disease and dry mouth also are more likely.
- Pregnancy complications. High blood sugar levels can be dangerous for both the parent and the baby. The risk of miscarriage, stillbirth and birth defects increases when diabetes isn't well-controlled. For the parent, diabetes increases the risk of diabetic ketoacidosis, diabetic eye problems (retinopathy), pregnancy-induced high blood pressure and preeclampsia.
There's no known way to prevent type 1 diabetes. But researchers are working on preventing the disease or further damage of the islet cells in people who are newly diagnosed.
Ask your provider if you might be eligible for one of these clinical trials. It is important to carefully weigh the risks and benefits of any treatment available in a trial.
- Summary of revisions: Standards of medical care in diabetes — 2022. Diabetes Care. 2022; doi:10.2337/dc22-Srev.
- Papadakis MA, et al., eds. Diabetes mellitus. In: Current Medical Diagnosis & Treatment 2022. 61st ed. McGraw Hill; 2022. https://accessmedicine.mhmedical.com. Accessed May 4, 2022.
- What is diabetes? National Institute of Diabetes and Digestive and Kidney Diseases. https://www.niddk.nih.gov/health-information/diabetes/overview/what-is-diabetes. Accessed May 4, 2022.
- Levitsky LL, et al. Epidemiology, presentation, and diagnosis of type 1 diabetes mellitus in children and adolescents. https://www.uptodate.com/contents/search. Accessed May 4, 2022.
- Diabetes mellitus (DM). Merck Manual Professional Version. https://www.merckmanuals.com/professional/endocrine-and-metabolic-disorders/diabetes-mellitus-and-disorders-of-carbohydrate-metabolism/diabetes-mellitus-dm. Accessed May 4, 2022.
- AskMayoExpert. Type 1 diabetes mellitus. Mayo Clinic; 2021.
- Robertson RP. Pancreas and islet transplantation in diabetes mellitus. https://www.uptodate.com/contents/search. Accessed May 4, 2022.
- Levitsky LL, et al. Management of type 1 diabetes mellitus in children during illness, procedures, school, or travel. https://www.uptodate.com/contents/search. Accessed May 4, 2022.
- Hyperglycemia (high blood glucose). American Diabetes Association. https://www.diabetes.org/healthy-living/medication-treatments/blood-glucose-testing-and-control/hyperglycemia. Accessed May 4, 2022.
- Diabetes and DKA (ketoacidosis). American Diabetes Association. https://www.diabetes.org/diabetes/dka-ketoacidosis-ketones. Accessed May 4, 2022.
- Insulin resistance & prediabetes. National Institute of Diabetes and Digestive and Kidney Diseases. https://www.niddk.nih.gov/health-information/diabetes/overview/what-is-diabetes/prediabetes-insulin-resistance. Accessed May 4, 2022.
- Blood sugar and insulin at work. American Diabetes Association. https://www.diabetes.org/tools-support/diabetes-prevention/high-blood-sugar. Accessed May 4, 2022.
- Inzucchi SE, et al. Glycemic control and vascular complications in type 1 diabetes. https://www.uptodate.com/contents/search. Accessed May 4, 2022.
- Diabetes and oral health. American Diabetes Association. https://www.diabetes.org/diabetes/keeping-your-mouth-healthy. Accessed May 4, 2022.
- Drug treatment of diabetes mellitus. Merck Manual Professional Version. https://www.merckmanuals.com/professional/endocrine-and-metabolic-disorders/diabetes-mellitus-and-disorders-of-carbohydrate-metabolism/drug-treatment-of-diabetes-mellitus. Accessed May 4, 2022.
- Weinstock DK, et al. Management of blood glucose in adults with type 1 diabetes mellitus. https://www.uptodate.com/contents/search. Accessed May 7, 2022.
- FDA proves first automated insulin delivery device for type 1 diabetes. U.S. Food and Drug Administration. https://www.fda.gov/news-events/press-announcements/fda-approves-first-automated-insulin-delivery-device-type-1-diabetes. Accessed May 4, 2022.
- Boughton CK, et al. Advances in artificial pancreas systems. Science Translational Medicine. 2019; doi:10.1126/scitranslmed.aaw4949.
- Hypoglycemia (low blood sugar). American Diabetes Association. https://www.diabetes.org/healthy-living/medication-treatments/blood-glucose-testing-and-control/hypoglycemia. Accessed May 4, 2022.
- Diabetes in the workplace and the ADA. U.S. Equal Opportunity Employment Commission. https://www.eeoc.gov/laws/guidance/diabetes-workplace-and-ada. Accessed May 4, 2022.
- Cardiovascular disease and risk management: Standards of medical care in diabetes — 2022. Diabetes Care. 2022; doi:10.2337/dc22-S010.
- Diabetes technology. Standards of Medical Care in Diabetes — 2022. 2022; doi:10.2337/dc22-S007.
- FDA authorizes a second artificial pancreas system. JDRF. https://www.jdrf.org/blog/2019/12/13/jdrf-reports-fda-authorizes-second-artificial-pancreas-system/. Accessed May 4, 2022.
- Classification and diagnosis of diabetes: Standards of medical care in diabetes — 2022. Diabetes Care. 2022; doi:10.2337/dc22-S002.
- Retinopathy, neuropathy, and foot care: Standards of medical care in diabetes — 2022. Diabetes Care. 2022; doi:10.2337/dc22-S012.
- Glycemic targets: Standards of medical care in diabetes — 2022. Diabetes Care. 2022; doi:10.2337/dc22-S012.
- Pharmacologic approaches to glycemic treatment: Standards of medical care in diabetes — 2022. Diabetes Care. 2022; doi:10.2337/dc22-S009.
- Facilitating behavior change and well-being to improve health outcomes: Standards of medical care in diabetes — 2022. Diabetes Care. 2022; doi:10.2337/dc22-S005.
- Centers for Disease Control and Prevention. Use of hepatitis B vaccination for adults with diabetes mellitus: Recommendations of the Advisory Committee on Immunization Practices (ACIP). Morbidity and Mortality Weekly Report. 2011;60:1709.
- Management of diabetes in pregnancy: Standards of medical care in diabetes — 2022. Diabetes Care. 2022; doi:10.2337/dc22-S015.
- Older adults: Standards of medical care in diabetes — 2022. Diabetes Care. 2022; doi:10.2337/dc22-S013.
- FDA approves first-of-its-kind automated insulin delivery and monitoring system for use in young pediatric patients. U.S. Food and Drug Administration. https://www.fda.gov/news-events/press-announcements/fda-approves-first-its-kind-automated-insulin-delivery-and-monitoring-system-use-young-pediatric#:~:text=Today, the U.S. Food and,by individuals aged 2 to. Accessed May 8, 2022.
- What you need to know: Getting a COVID-19 vaccine. American Diabetes Association. https://www.diabetes.org/coronavirus-covid-19/vaccination-guide. Accessed June 1, 2022.
News from Mayo Clinic
- Driven by family, fueled by hope: Mayo Clinic researcher fights against Type 1 diabetes Aug. 06, 2023, 11:00 a.m. CDT
- Symptoms & causes
- Diagnosis & treatment
- Doctors & departments
Mayo Clinic does not endorse companies or products. Advertising revenue supports our not-for-profit mission.
- Opportunities
Mayo Clinic Press
Check out these best-sellers and special offers on books and newsletters from Mayo Clinic Press .
- Mayo Clinic on Incontinence - Mayo Clinic Press Mayo Clinic on Incontinence
- The Essential Diabetes Book - Mayo Clinic Press The Essential Diabetes Book
- Mayo Clinic on Hearing and Balance - Mayo Clinic Press Mayo Clinic on Hearing and Balance
- FREE Mayo Clinic Diet Assessment - Mayo Clinic Press FREE Mayo Clinic Diet Assessment
- Mayo Clinic Health Letter - FREE book - Mayo Clinic Press Mayo Clinic Health Letter - FREE book
Your gift holds great power – donate today!
Make your tax-deductible gift and be a part of the cutting-edge research and care that's changing medicine.
What Is Type 1 Diabetes?
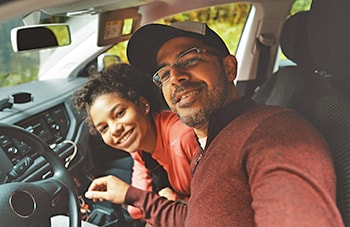
People of all ages can develop type 1 diabetes.
If you have type 1 diabetes, your pancreas doesn’t make insulin or makes very little insulin. Insulin helps blood sugar enter the cells in your body for use as energy. Without insulin, blood sugar can’t get into cells and builds up in the bloodstream. High blood sugar is damaging to the body and causes many of the symptoms and complications of diabetes.
Type 1 diabetes was once called insulin-dependent or juvenile diabetes, but it can develop at any age.
Type 1 diabetes is less common than type 2 —about 5-10% of people with diabetes have type 1. Currently, no one knows how to prevent type 1 diabetes, but it can be treated successfully by:
- Following your doctor’s recommendations for living a healthy lifestyle.
- Managing your blood sugar.
- Getting regular health checkups.
- Getting diabetes self-management education and support .
For Parents
If your child has type 1 diabetes—especially a young child—you’ll handle diabetes care on a day-to-day basis. Daily care will include serving healthy foods, giving insulin injections, and watching for and treating hypoglycemia (low blood sugar). You’ll also need to stay in close contact with your child’s health care team. They will help you understand the treatment plan and how to help your child stay healthy.
Much of the information that follows applies to children as well as adults. You can also visit JDRF’s T1D Resources for more information on managing your child’s type 1 diabetes.
What Causes Type 1 Diabetes?
Type 1 diabetes is thought to be caused by an autoimmune reaction (the body attacks itself by mistake). This reaction destroys the cells in the pancreas that make insulin, called beta cells. This process can go on for months or years before any symptoms appear.
Some people have certain genes (traits passed on from parent to child) that make them more likely to develop type 1 diabetes. However, many of them won’t go on to have type 1 diabetes even if they have the genes. A trigger in the environment, such as a virus, may also play a part in developing type 1 diabetes. Diet and lifestyle habits don’t cause type 1 diabetes.
Symptoms and Risk Factors
It can take months or years before symptoms of type 1 diabetes are noticed. Type 1 diabetes symptoms can develop in just a few weeks or months. Once symptoms appear, they can be severe.
Some type 1 diabetes symptoms are similar to symptoms of other health conditions. Don’t guess! If you think you could have type 1 diabetes, see your doctor to get your blood sugar tested. Untreated diabetes can lead to very serious—even fatal—health problems.
Risk factors for type 1 diabetes are not as clear as for prediabetes and type 2 diabetes. However, studies show that family history plays a part.
Testing for Type 1 Diabetes
A simple blood test will let you know if you have diabetes. If you were tested at a health fair or pharmacy, follow up at a clinic or doctor’s office. That way you’ll be sure the results are accurate.
If your doctor thinks you have type 1 diabetes, your blood may also be tested for autoantibodies. These substances indicate your body is attacking itself and are often found with type 1 diabetes but not with type 2. You may have your urine tested for ketones too. Ketones are produced when your body burns fat for energy. Having ketones in your urine indicates you have type 1 diabetes instead of type 2.
Managing Diabetes
Unlike many health conditions, diabetes is managed mostly by you, with support from your health care team:
- Primary care doctor
- Foot doctor
- Registered dietitian nutritionist
- Diabetes educator
Also ask your family, teachers, and other important people in your life for help and support. Managing diabetes can be challenging, but everything you do to improve your health is worth it!
If you have type 1 diabetes, you’ll need to take insulin shots (or wear an insulin pump) every day. Insulin is needed to manage your blood sugar levels and give your body energy. You can’t take insulin as a pill. That’s because the acid in your stomach would destroy it before it could get into your bloodstream. Your doctor will work with you to figure out the most effective type and dosage of insulin for you.
You’ll also need to do regular blood sugar checks . Ask your doctor how often you should check it and what your target blood sugar levels should be. Keeping your blood sugar levels as close to target as possible will help you prevent or delay diabetes-related complications .
Stress is a part of life, but it can make managing diabetes harder. Both managing your blood sugar levels and dealing with daily diabetes care can be tougher to do. Regular physical activity, getting enough sleep, and exercises to relax can help. Talk to your doctor and diabetes educator about these and other ways you can manage stress.
Healthy lifestyle habits are really important too:
- Making healthy food choices
- Being physically active
- Controlling your blood pressure
- Controlling your cholesterol
Make regular appointments with your health care team. They’ll help you stay on track with your treatment plan and offer new ideas and strategies if needed.
Hypoglycemia and Diabetic Ketoacidosis
These 2 conditions are common complications of diabetes, and you’ll need to know how to handle them. Meet with your doctor for step-by-step instructions. You may want to bring a family member with you to the appointment so they learn the steps too.
Hypoglycemia (low blood sugar) can happen quickly and needs to be treated quickly. It’s most often caused by:
- Too much insulin.
- Waiting too long for a meal or snack.
- Not eating enough.
- Getting extra physical activity.
Talk to your doctor if you have low blood sugar several times a week. Your treatment plan may need to be changed.
Diabetic ketoacidosis (DKA) is a serious complication of diabetes that can be life-threatening. DKA develops when you don’t have enough insulin to let blood sugar into your cells. Very high blood sugar and low insulin levels lead to DKA. The two most common causes are illness and missing insulin shots. Talk with your doctor and make sure you understand how you can prevent and treat DKA.
Get Diabetes Education
Meeting with a diabetes educator is a great way to get support and guidance, including how to:
- Develop and stick to a healthy eating and activity plan
- Test your blood sugar and keep a record of the results
- Recognize the signs of high or low blood sugar and what to do about it
- Give yourself insulin by syringe, pen, or pump
- Monitor your feet, skin, and eyes to catch problems early
- Buy diabetes supplies and store them properly
- Manage stress and deal with daily diabetes care
Ask your doctor about diabetes self-management education and support services and to recommend a diabetes educator. You can also search this nationwide directory for a list of programs in your community.
Get Support
Tap into online diabetes communities for encouragement, insights, and support. Check out the American Diabetes Association’s Community page and JDRF’s TypeOneNation . Both are great ways to connect with others who share your experience.
- Type 1 Diabetes Resources and Support from JDRF
- Living With Diabetes
- Just Diagnosed With Type 1 Diabetes
- Learn About Diabetic Ketoacidosis
- 4 Ways To Take Insulin
- Making the Leap From Type 1 Teen to Adult
To receive updates about diabetes topics, enter your email address:
- Diabetes Home
- State, Local, and National Partner Diabetes Programs
- National Diabetes Prevention Program
- Native Diabetes Wellness Program
- Chronic Kidney Disease
- Vision Health Initiative
- Heart Disease and Stroke
- Overweight & Obesity
Exit Notification / Disclaimer Policy
- The Centers for Disease Control and Prevention (CDC) cannot attest to the accuracy of a non-federal website.
- Linking to a non-federal website does not constitute an endorsement by CDC or any of its employees of the sponsors or the information and products presented on the website.
- You will be subject to the destination website's privacy policy when you follow the link.
- CDC is not responsible for Section 508 compliance (accessibility) on other federal or private website.
Ohio State nav bar
The Ohio State University
- BuckeyeLink
- Find People
- Search Ohio State
Pathophysiology and Clinical Presentation
Pathophysiology:
Type 1 Diabetes Mellitus is a syndrome characterized by hyperglycemia and insulin deficiency resulting from the loss of beta cells in pancreatic islets (Mapes & Faulds, 2014). Nonimmune (type 1B diabetes), occurs secondary to other diseases and is much less common than autoimmune (type 1A). The destruction of beta cells in Type 1A diabetes results from the interaction of both genetic and environmental factors. Although the genetic susceptibility is not well understood, type 1 diabetes is most strongly associated with major histocompatibility complex (MHC), specifically histocompatibility leukocyte antigen (HLA) class II alleles (HLA-DQ and HLA-DR) (McCance & Heuther, 2014). Type 1 diabetes is less hereditary than type 2 but 7-13% of patients also have a first degree relative with type 1 diabetes (Mapes & Faulds, 2014). Environmental factors include viral infections (especially enteroviruses), exposure to infectious microorganisms (such as Helicobacter pylori ), exposure to cow’s milk proteins and a lack of vitamin D (McCance & Heuther, 2014).
The destruction of insulin-producing beta cells in the pancreas starts with the formation of autoantigens. These autoantigens are ingested by antigen-presenting cells which activate T helper 1 (Th1) and T helper 2 (Th2) lmphocytes. Activated Th1 lymphocytes secrete interluekin-2 (IL-2) and interferon. IL-2 activates autoantigen-specific T cytotoxic lymphocytes which destroy islet cells through the secretion of toxic perforins and granzymes. Interferon activates macrophages and stimulates the release of inflammatory cytokines (including IL-1 and tumor necrosis factor [TNF]) which further destroy beta cells (McCance & Heuther, 2014). Activated Th2 lymphocytes produce IL-4 which stimulates B lymphocytes to proliferate and produce islet cell autoantibodies (ICAs) and anti-glutamic acid decarboxylase (antiGAD65) antibodies. AntiGAD65 is an enzyme that helps control the release of insulin from beta cells and can be used to determine the cause of diabetes (McCance & Heuther, 2014). Insulin autoantibodies [IAAs]) and zinc transporter 8 (Znt8) protein are also associated with type 1 diabetes mellitus. Despite it’s complicated pathophysiology, it is important to understand the destruction of beta cells in type 1 diabetes because it leads to a lack of insulin and amylin. Without insulin or amylin the body cannot promote glucose disappearance or limit glucose appearance from the bloodstream, respectively, resulting in hyperglycemia (Mapes & Faulds, 2014).
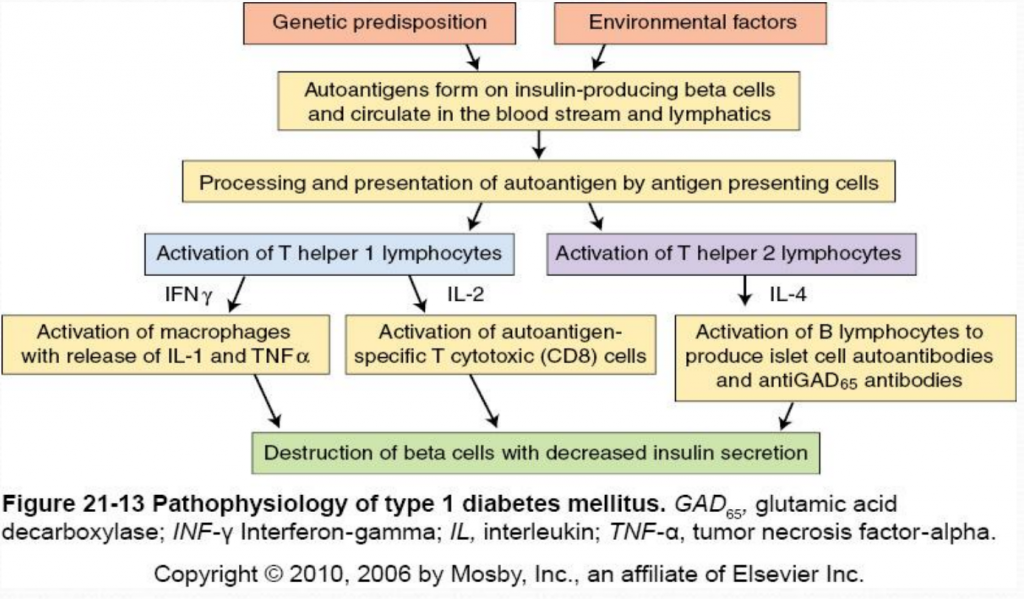
Clinical Presentation:
Type 1 diabetes does not present clinically until 80-90% of the beta cells have been destroyed (McCance & Heuther, 2014). Because insulin stimulates glucose uptake into tissues, stores glycose as glycogen, inhibits glucagon secretion and inhibits glucose production from the liver, the destruction of insulin-producing beta cells causes hyperglycemia (Mapes & Faulds, 2014). Type 1 diabetics may present with abrupt onset of diabetic ketoacidosis, polyuria, polyphagia, polydipsia, or rapid weight loss with marked hyperglycemia (Mapes & Faulds, 2014). To diagnose diabetes, patients must have an A1C level greater than 6.5% percent on two separate tests; the presence of ketones in the urine and/or autoantibodies in the blood can distinguish type 1 from type 2 diabetes (Mayo Clinic, 2014).

2 thoughts on “ Pathophysiology and Clinical Presentation ”
none for now
Leave a Reply Cancel reply
Your email address will not be published. Required fields are marked *
Save my name, email, and website in this browser for the next time I comment.
Learn how UpToDate can help you.
Select the option that best describes you
- Medical Professional
- Resident, Fellow, or Student
- Hospital or Institution
- Group Practice
- Patient or Caregiver
- Find in topic
RELATED PATHWAYS
Related topics.
INTRODUCTION
This topic will review the clinical presentation, diagnosis, and initial evaluation of diabetes in nonpregnant adults. Screening for and prevention of diabetes, the etiologic classification of diabetes mellitus, the treatment of diabetes, as well as diabetes during pregnancy are discussed separately.
● (See "Screening for type 2 diabetes mellitus" .)
● (See "Prevention of type 2 diabetes mellitus" and "Type 1 diabetes mellitus: Prevention and disease-modifying therapy" .)
● (See "Classification of diabetes mellitus and genetic diabetic syndromes" .)
Understanding Type 1 Diabetes
The following symptoms of diabetes are typical. However, some people with diabetes have symptoms so mild that they go unnoticed.
Common symptoms of diabetes:
- Urinating often
- Feeling very thirsty
- Feeling very hungry—even though you are eating
- Extreme fatigue
- Blurry vision
- Cuts/bruises that are slow to heal
- Weight loss—even though you are eating more
Early detection and treatment of diabetes can decrease the risk of developing the complications of diabetes .
Although there are many similarities between type 1 and type 2 diabetes, the cause of each is very different. And the treatment is usually quite different, too. Some people, especially adults who are newly diagnosed with type 1 diabetes, may have symptoms similar to type 2 diabetes and this overlap between types can be confusing. Take our 60-Second Type 2 Diabetes Risk Test to find out if you are at increased risk for having type 2 diabetes.
Can symptoms appear suddenly?
In people with type 1 diabetes, the onset of symptoms can be very sudden, while in type 2 diabetes, they tend to come about more gradually, and sometimes there are no signs at all.
Symptoms sometimes occur after a viral illness. In some cases, a person may reach the point of diabetic ketoacidosis (DKA) before a type 1 diagnosis is made. DKA occurs when blood glucose (blood sugar) is dangerously high and the body can't get nutrients into the cells because of the absence of insulin. The body then breaks down muscle and fat for energy, causing an accumulation of ketones in the blood and urine. Symptoms of DKA include a fruity odor on the breath, heavy, taxed breathing and vomiting. If left untreated, DKA can result in stupor, unconsciousness, and even death.
People who have symptoms—of type 1 or of DKA—should contact their health care provider immediately for an accurate diagnosis. Keep in mind that these symptoms could signal other problems, too.
Some people with type 1 have a "honeymoon" period, a brief remission of symptoms while the pancreas is still secreting some insulin. The honeymoon phase usually occurs after someone has started taking insulin. A honeymoon can last as little as a week or even up to a year. But it’s important to know that the absence of symptoms doesn't mean the diabetes is gone. The pancreas will eventually be unable to secrete insulin, and, if untreated, the symptoms will return.
Symptoms of Type 1 Diabetes Onset in an Infant or Child
The young child who is urinating frequently, drinking large quantities, losing weight, and becoming more and more tired and ill is the classic picture of a child with new-onset type 1 diabetes. If a child who is potty-trained and dry at night starts having accidents and wetting the bed again, diabetes might be the culprit.
Although it is easy to make the diagnosis diabetes in a child by checking blood glucose (blood sugar) at the doctor’s office or emergency room, the tricky part is recognizing the symptoms and knowing to take the child to get checked. Raising the awareness that young children, including infants, can get type 1 diabetes can help parents know when to check for type 1 diabetes.
Sometimes children can be in diabetic ketoacidosis (DKA) when they are diagnosed with diabetes. When there is a lack of insulin in the body, the body can build up high levels of an acid called ketones. DKA is a medical emergency that usually requires hospitalization and immediate care with insulin and IV fluids. After diagnosis and early in treatment, some children may go through a phase where they seem to be making enough insulin again. This is commonly called the “honeymoon phase." It may seem like diabetes has been cured, but over time they will require appropriate doses of insulin to keep their blood glucose levels in the normal range.
Symptoms of Type 1 Diabetes Onset in Adults
When an adult is diagnosed with diabetes, they are often mistakenly told that they have type 2 diabetes. This is because there may be a lack of understanding by some doctors that type 1 diabetes can start at any age, and in people of every race, shape, and size. People with type 1 diabetes who have elevated blood glucose (blood sugar) and classic risk factors for type 2 diabetes, such as being overweight or physically inactive, are often misdiagnosed. It can also be tricky because some adults with new-onset type 1 diabetes are not sick at first. Their doctor finds an elevated blood glucose level at a routine visit and starts them on diet, exercise, and an oral medication.
Maybe it's a Different Type
If you or someone you know is diagnosed with type 2 diabetes but isn’t responding well to the typical treatments for type 2 diabetes, it may be worth a visit to an endocrinologist to determine what type of diabetes is happening. Generally, this requires antibody tests and possibly the measurement of a C-peptide level.
Gestational Diabetes
Women with gestational diabetes often have no symptoms , which is why it's important for at-risk women to be tested at the proper time during pregnancy.
Symptoms of Diabetes Complications
Have you already been diagnosed with diabetes but are concerned about symptoms that may be the result of complications related to diabetes?
Find Out More
- UBC Directories
- UBC Quick Links
- The University of British Columbia
- a place of mind
- Learn Pediatrics
- Diabetes: Approach to First Presentation
Click for pdf: Diabetes
General presentation
Diabetes mellitus (DM) is an important endocrine disorder that presents commonly in children and adolescents. There are two types of diabetes mellitus: type 1 and type 2. Type 1 DM is one of the most common chronic diseases in children and is characterized by insulin deficiency as a result of autoimmune destruction of pancreatic beta islet cells; whereas type 2 DM is the presence of high blood glucose with insulin resistance and relative insulin deficiency. Diabetes mellitus is a chronic condition that requires long-term follow-up and adequate patient (and parent) education to maintain good glycemic control to prevent long-term complications.
Epidemiology
Approximately 2/3 of all new diabetes diagnoses in patients less than 19 years of age in the United States are type 1 DM. Over 300,000 Canadians have type 1 DM, with a 3-5% increase each year; especially in children aged 5-9. Typically, the age of onset has a bimodal distribution, with the first peak in children 4-6 years old, and the second peak in children 10-14 years old (early puberty). Unlike other autoimmune diseases, the overall incidence appears to be equal in both genders. There is a higher risk of developing this condition in children with close relatives who have type 1 DM.
The incidence of type 2 DM has increased 10 fold in the last decade. There is an estimated 3600/100,000 cases of type 2 DM in Canadian adolescents and 1100/100,000 cases in Canadian children. This value may be as high as 1% in Canadian aboriginal youths and children. There is a strong association between increasing rates of obesity in the pediatric population and the development of type 2 DM.
Basic Physiology
In type 1 DM, there is autoimmune-mediated destruction of insulin-producing pancreatic beta cells that results in insulin deficiency. It is a progressive condition that occurs in genetically susceptible individuals. The autoimmune destruction can be triggered by various environmental agents. Some proposed environmental factors include pregnancy-related and perinatal influences, viruses, cow’s milk and cereals. There is a long latency period (where the patient is asymptomatic and euglycemic) between the onset of beta cell destruction and clinical presentation of diabetes mellitus. A large number of functional beta cells must be lost before clinical symptoms like hyperglycemia occurs.
Genetic polymorphisms in six genes have been shown to be associated with type 1 DM. Major Histocompatibility Complex genes and elsewhere in the genome all contribute to the risk, but only the HLA alleles seem to have a large effect.
The natural history has four stages:
- Preclinical autoimmune destruction of pancreatic beta cells
- Onset of clinical symptoms
- Transient remission
- Established diabetes with acute and chronic complications
Type 2 DM is a complex, multifactorial disease characterized by both relative insulin deficiency and insulin resistance with various environmental and behavioural risk factors. Increased hepatic glucose production, insulin resistance and progressive loss of glucose-stimulated insulin release all contribute to the development of hyperglycemia. In Type 2 DM, pancreatic beta cells retain the ability to produce insulin, but levels are not adequate to counteract the developing insulin resistance. The current theory is that insulin resistance develops first, followed and complicated by gradual destruction of beta cells. Insulin resistance worsens with obesity and physical inactivity; and improves with weight loss and increased physical exercise.
Puberty also plays a role in the development of type 2 DM in adolescents. During this period, insulin sensitivity is approximately 30% lower than that of preadolescents or adults, which results in hyperinsulinemia as a compensatory mechanism. In adolescents with both genetic predisposition and negative environmental contributors, this period of relative insulin resistance may result in a decompensated state (inadequate insulin secretion and glucose intolerance). The resulting hyperglycemic state may cause worsening abnormalities of insulin secretion and action, starting a vicious cycle that progress beyond the adolescent years.
Clinical Presentation
Childhood type 1 DM can present in the following ways:
Classic new onset:
Hyperglycemia without acidosis
Symptoms include:
- Can present as nocturia, bedwetting, daytime incontinence in a previously continent child
- Polydipsia – due to increased serum osmolality and hypovolemia
- Impaired glucose utilization in skeletal muscle and increased fat and muscle breakdown
Diabetic ketoacidosis
Similar symptoms but are usually more severe
- Clinical: polydipsia, polyuria, dehydration, hypotension, ketosis, etc.
- Metabolic: hyperglycemia, glycosuria, metabolic acidosis, ketonemia, etc.
Reported frequency varies between 15-67%
- Young children (<6) from low socioeconomic backgrounds are more likely to present with diabetic ketoacidosis
Silent Presentation
Diagnosis before onset of clinical symptoms
Typically occurs in children with a family member with type 1 DM (close monitoring)
Childhood type 2 DM can present in the following ways:
- Hyperglycemia, ketonuria, acidosis
- Frequency varies between 5-25%
Hyperosmolar hyperglycemic state
- Marked hyperglycemia (>33.3 mmol/L) and severe dehydration but no ketonuria
- Less common in adolescents
Symptomatic
- Due to hyperglycemia and include: polyuria, polydipsia, and nocturia
- Recent weight loss is less frequent
- Adolescent girls: vaginal discharge due to candida infection may be initial presentation
Asymptomatic
- Identified based on screening (for type 2 DM or urinalysis as part of a regular physical exam)
Questions to ask
Historical Investigation
Presenting condition:
- Have you been very thirsty? Do you drink a lot?
- Have you been urinating more than usual?
- Has the child had any bedwetting episodes?
- Has there been any recent weight loss?
- Have you been feeling tired lately?
- Have you noticed an increased appetite lately?
- Has the child had more frequent minor skin infections?
Predisposing factors:
- Have you had any viral infections recently?
- What kinds of exercise do you participate in on a regular basis? How frequent do you exercise? How long do you exercise each time?
- How many hours a day do you spend watching TV, using the computer, and play video games?
- What do you normally eat? What is the portion size? How many meals do you have per day? Do you normally eat out or home cooked meals? Do you eat as a family? Do you eat at the table or in front of the tv?
Family history:
- Are there any family members with insulin-dependent diabetes mellitus?
- Are there any family members with autoimmune conditions?
- Does your mother or father have diabetes?
- Are there any other family members with diabetes? (grandparents, aunts, uncles, brothers, sisters, etc.)
Physical Examination
Do a complete physical exam with particular attention to the following:
- Assess hydration status
- Assess circulation: HR, BP, capillary refill
- Temperature: coexisting infection
- Use growth chart to check for weight loss
- Neck examination: look for thyroid abnormalities
- Respiratory: respiratory rate (hyperventilation – DKA), auscultation (respiratory infection), ketones on breath (DKA)
- Measure body weight and height, calculate BMI
- Measure lying and standing BP
- Inspect skin for acanthosisnigricans
- Examine feet to look for decreased sensation and circulation (pulses)
- Measure visual acuity
Differential diagnosis
- DM types 1 and 2
- Diabetes insipidus
- Urinary tract infection
- Malabsorption (e.g. Celiac disease)
- Secondary diabetes
- Maturity-onset diabetes of the young
Procedures for investigation
Diagnostic Criteria
- Fasting plasma glucose >7 mmol/L (no caloric intake for at least 8 hours)
- Symptoms of hyperglycemia, random venous plasma glucose >11.1 mmol/L
- Abnormal oral glucose tolerance test – plasma glucose >11.1 mmol/L measured 2 hours after a glucose load of 1.75 g/kg (max 75g)
- Glycated hemoglobin (A1C) ≥ 6.5%
Other Investigations
- Urinalysis for glucosuria and ketonuria
- Urinalysis for microalbuminemia
- Alemzadeh R, Wyatt DT. Section 6 – Diabetes mellitus in children. In: Kliegman: Nelson Textbook of Pediatrics. 18 th ed. Saunders, Pennsylvania. 2007
- Eisenbarth GS, McCulloch DK. Pathogenesis of type 1 diabetes mellitus. In: UpToDate, Basow, DS (Ed), UpToDate, Waltham, MA, 2011
- Laffel L, Svoren B. Epidemiology, presentation, and diagnosis of type 2 diabetes mellitus in children and adolescents. In: UpToDate, Basow, DS (Ed), UpToDate, Waltham, MA, 2011
- Levinson P, Nelson BA, Scherger JE. Diabetes mellitus type 1 in children. [Online]. 2007. Availabe from: FirstConsult, MDConsult. [cited 2011 Jan 15]
- Levitsky LL, Misra M. Epidemiology, presentation, and diagnosis of type 1 diabetes mellitus in children and adolescents. In: UpToDate, Basow, DS (Ed), UpToDate, Waltham, MA, 2011
- McCulloch DK, Robertson RP. Pathogenesis of type 2 diabetes mellitus. In: UpToDate, Basow, DS (Ed), UpToDate, Waltham, MA, 2011
- Panagiotopoulos C. Type 2 diabetes in children and adolescents. BCMJ. 2004;46(9): 461-466
- Scherger JE, McIntire SDC, Escobar O, Heinzman DM. Diabetes mellitus type 2 in children. [Online]. 2007. Availabe from: FirstConsult, MDConsult. [cited 2011 Jan 15]
Acknowledgements
Written by: Ying Yao
Edited by: Dianna Louie
Last updated on November 10, 2011 @5:01 pm
Feedback: How useful was the above information?
Post comment click here to cancel reply..
You must be logged in to post a comment.
- Approach to the Child with a fever and rash
- Approach to Bradycardia
- Basics of cardiac pharmacology
- Approach to cardiac history taking
- Congestive heart failure in children
- Approach to Pediatric Hypertension
- Approach to Cyanotic Congenital Heart Disease in the Newborn
- Approach to Syncope: Is it Cardiac or Not?
- Approach to Pediatric Tachycardia
- Approach to Pediatric Chest Pain
- Approach to Cardiac Murmurs
- Normal Cardiac Physiology – Transition From Fetal to Neonatal
- Basic Physiology and Approach to Heart Sounds
- Approach to Pediatric ECGs
- Care of a Child with Turner Syndrome
- Approach to the Underweight Child
- Normal sexual maturity rating
- Hypothyroidism
- Approach to the Short Child
- Approach to Vomiting
- Suspected Foreign Body Ingestion
- Approach to a Mediastinal Mass
- Toxic Ingestion
- Nutritional Deficiencies
- Pharmacology of Common Agents Used in Gastrointestinal Conditions
- Approach to Pediatric Abdominal Pain
- Pediatric Gastrointestinal History Taking
- Approach to diarrhea
- Approach to a picky eater
- Constipation
- Hepatomegaly
- Splenomegaly
- Approach to Abdominal Mass
- Failure to Thrive
- Approach to Skin Lesions
- Common Paediatric Skin Conditions & Birthmarks
- Approach to the child with mental health concerns
- Child with a sore ear (Otalgia)
- To vaccinate or not to vaccinate
- Approach to a the Child with a Fever and Rash
- Approach to a Routine Adolescent Interview
- Sore Throat in Children – Clinical Considerations and Evaluation
- Approach to Strabismus
- Approach to Adolescent Substance Use
- Approach to the Child Who is Dry
- Conjunctivitis: Approach to the Child with a Red Eye
- Acne In Teens
- Pediatric Neck Mass
- Fetal Alcohol Spectrum Disorder
- To Circumcise or Not to Circumcise
- Approach to inborn errors of metabolism
- Infants of Diabetic Mothers
- Fever in the Newborn Period
- Neonatal Thrombocytopenia
- Consequences of Prematurity
- Respiratory Distress in the Newborn
- Approach to Neonatal Cyanosis
- Approach to the Child with IUGR/SGA
- Neonatal Jaundice
- Breastfeeding Problems
- Diaper Rash: Clinical Considerations and Evaluation
- The Pediatric Neurological History
- Evaluation of Pediatric Development (Normal)
- Closed Head Injury in Pediatrics
- Approach to the Ataxic Child
- Approach to the Child with a Seizure
- Basics to the Approach of Developmental Delay
- Lumbar Puncture in Pediatrics
- The Basics of Cerebral Palsy
- Approach to a Child with a Headache
- Febrile Seizures
- Approach to the Comatose Child
- Basics of Spina Bifida
- Principles of Pharmacotherapy in Neurology
- Easy Bleeding
- Non-Neonatal Jaundice
- Approach to Lymphadenopathy
- Tumor Lysis Syndrome
- Febrile Neutropenia
- Pediatric Neutropenia
- Blood Transfusion Reactions
- Approach to Sickle Cell Disease
- Iron-deficiency and Health Consequences in Children
- Easy Clotting
- Approach to Thrombocytopenia
- Approach to Pediatric Leukemias and Lymphomas
- Approach to Thalassemia
- Approach to Non-Accidental Injuries
- Common Pediatric Bone Diseases-Approach to Pathological Fractures
- Infant with an Abnormal Hip Exam
- Knock Kneed Children
- Elbow Injuries
- Approach to the Child with a Limp
- Pediatric Fractures
- Clearing the C - Spine
- Approach to a Child With a Cough
- Approach to Pediatric Hemoptysis
- Approach to Pediatric Dyspnea
- Introduction
- Systemic Exam
- Kidneys and Bladder
- Male Infants
- Female Infants
- Acute Assessment
- General exam
- Peripheral palpation
- Precordium palpation
- Auscultation
- General Inspection
- Genito-Urinary
- Musculoskeletal
- Neurological
- Mental Status Exam
- Cranial Nerve Exam
- Sensory Exam
- Cerebellar Exam
- Other Resources
- Physical Exam Setup
- Screening MSK Exam
- Focused MSK Exams
Emergency Procedures | Accessibility | Contact UBC | © Copyright The University of British Columbia

How to Talk to Your Classroom About Type 1 Diabetes
Whether kicking off a new school year or returning to school after a new diagnosis, one of the best ways to ensure your child is safe and supported at school is to ensure the people around them understand type 1. Classmates and teachers may not be familiar with type 1 diabetes (T1D) and may be confused by your child’s routine. Some parents like to educate the class about T1D in coordination with the teacher. Here are our tips on giving a presentation on type 1 diabetes for parents and T1D kids in preschool, kindergarten and elementary/primary school.
Presentation tips
- Ask ahead of time if you can present to the school or class on type 1 diabetes.
- Pick a date and time, place and format for the presentation. Decide whether the child with T1D will give the presentation, or if a parent or guardian will. Ask if they would like to help you present, if they would like to present themselves or if they would prefer to be part of the audience.
- Discuss what topics to present with your child. (Your child may want to help choose specific topics to be discussed.)
- Keep the presentation short—about 15-20 minutes, including a Q & A. Children are more likely to absorb information that is presented!
- Provide only simple, basic facts to begin with. Offer more detailed information only if the children ask for it, and remember that more information is not necessarily better. The language and amount of info shared will slightly vary based on the age group you are presenting to.
- Props are fun! Bring a diabetes “play” kit to demonstrate items used every day. It could include needle-less lancing devices, an empty insulin bottle, alcohol wipes, blood glucose (BG) meter, test strips, glucose tabs, or needle-less syringes or pump supplies, depending on what your child uses. These can be passed around during the presentation, or used to demonstrate on a doll or toy.
- Prepare for the Q & A ahead of time to avoid any mishaps!
Topics to cover
- What type 1 diabetes is
- How you get type 1 diabetes
- How you care for type 1 diabetes
Questions to ask
- Does treatment (like injections and finger pricks) hurt?
- Is type 1 diabetes contagious?
- Can you die from it?
- Why do you have to test your blood?
- Will it ever go away?
- Do you know someone who has it?
- Can you eat sugar?
- When might you need sugar?
For more help, check out our Education Presentations —Beyond Type 1 will send you everything you need to teach others about type 1!
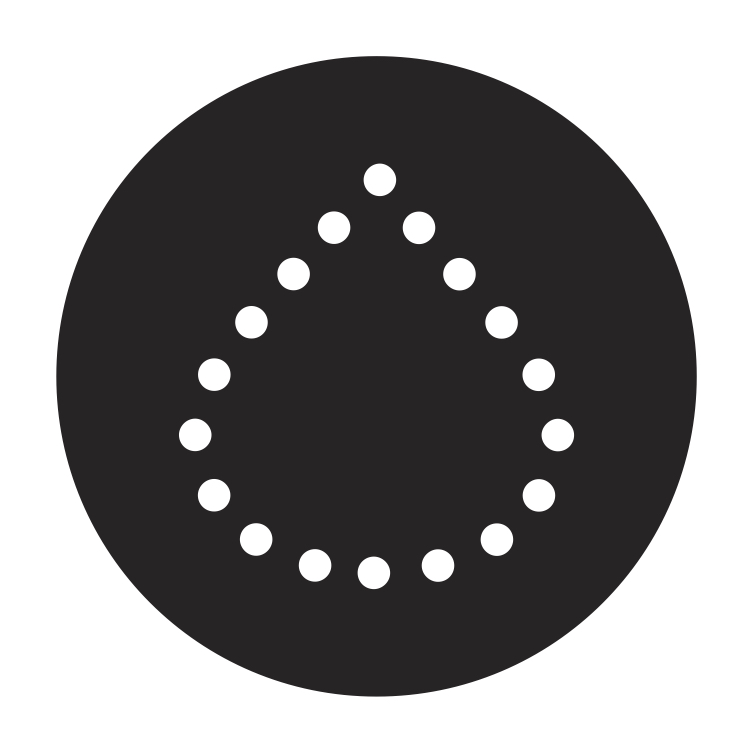
WRITTEN BY BT1 Editorial Team, POSTED 01/30/17, UPDATED 12/27/22
The isolated caregiver—a mom’s story -, ketones — the 6 must-knows -, one day in the life of type 1 diabetes -, understanding your a1c -, signs of type 1 diabetes -.
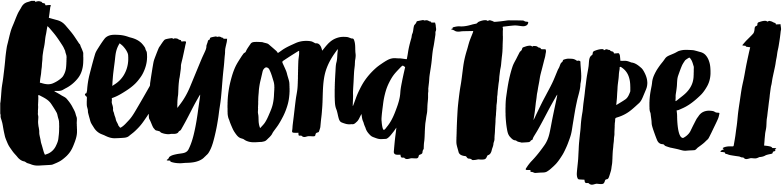
- TERMS OF USE
- 400 CONCAR DR.
- SAN MATEO, CA 94402

- BEYOND TYPE 1 BEYOND TYPE 1 EN ESPAÑOL
- IN ENGLISH EN ESPANOL BEYOND TYPE 1 AUF DEUTSCH BEYOND TYPE 1 IN ITALIANO BEYOND TYPE 1 EN FRANÇAIS BEYOND TYPE 1 IN HET NEDERLANDS BEYOND TYPE 1 PÅ SVENSKA BEYOND TYPE 1 EM PORTUGUÊS BEYOND TYPE 1 بالعربية
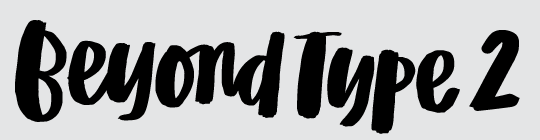
- BEYOND TYPE 2 BEYOND TYPE 2 EN ESPAÑOL IN ENGLISH EN ESPANOL BEYOND TYPE 2 AUF DEUTSCH BEYOND TYPE 2 en Français BEYOND TYPE 2 in Italiano BEYOND TYPE 2 Canada (French) BEYOND TYPE 2 Canada (English)
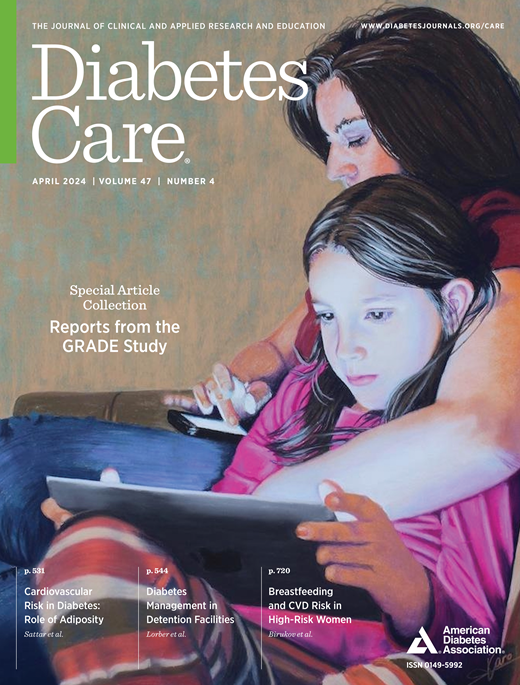
- Previous Article
- Next Article
Article Information
Prompt recognition of new-onset type 1 diabetes is everyone’s responsibility—even on weekends.

- Split-Screen
- Article contents
- Figures & tables
- Supplementary Data
- Peer Review
- Open the PDF for in another window
- Cite Icon Cite
- Get Permissions
Laura M. Jacobsen; Prompt Recognition of New-Onset Type 1 Diabetes Is Everyone’s Responsibility—Even on Weekends. Diabetes Care 25 March 2024; 47 (4): 646–648. https://doi.org/10.2337/dci23-0096
Download citation file:
- Ris (Zotero)
- Reference Manager
While the complex etiopathogenesis and heterogeneity of type 1 diabetes (T1D) creates many challenges regarding disease-modifying interventions, there are ways to meaningfully reduce the morbidity ( 1 , 2 ), and mortality, associated with new-onset T1D when it presents with life-threatening diabetic ketoacidosis (DKA). Early diagnosis and prompt initiation of insulin can prevent DKA, as has been shown in research programs that monitor children at risk for T1D.
In theory, early recognition of T1D to prevent severe metabolic decompensation consists of a simple exchange of knowledge regarding the signs and symptoms with all stakeholders and access to specialized health care. However, we have not been able to reduce the frequency of DKA in the population despite awareness and educational campaigns. Individual efforts have reduced DKA rates within a study setting, such as in Italy through an educational program in clinics, homes, and schools ( 3 ); however, the incidence of DKA at onset continues to rise in Italy and other regions ( 4 – 6 ). The rate of DKA at T1D diagnosis in children and adolescents remains unacceptably high and variable across countries (25–77%) ( 7 – 9 ).
In a study published in this issue of Diabetes Care , Kamrath et al. ( 9 ) analyzed the German Prospective Diabetes Registry (DPV) to assess for differences in when (e.g., the day of the week) individuals were diagnosed with, and started treatment for, new-onset T1D. In addition, over the 10-year period analyzed they assessed the rate of DKA, DKA severity, and effect of the coronavirus disease 2019 (COVID-19) pandemic. These are straightforward but previously unanswered questions that have implications for families, health care systems, and payors.
In this study of the DPV registry, DKA that occurred at diagnosis in children was 1 ) highest (in absolute counts) on Mondays and Tuesdays , but 2 ) highest proportional to the number of new cases of T1D each day on Saturday and Sunday. Similarly, looking at public holidays in Germany and school vacations by region, T1D (and DKA) was less likely to be diagnosed on weekends compared with weekdays.
In 9.1% of cases, insulin was not started on the day of diagnosis. Of note, DKA occurred in 21.2% of cases where treatment initiation was delayed. While the rate of DKA was higher (28%) in children without a treatment delay, if someone were near DKA, often tell-tale signs are evident that should alert a medical provider to the urgent need for treatment, although presentations vary and laboratory studies may not be readily available on weekends. This is especially apparent with the high rate of diabetes diagnosed on public holidays (21.8%), when laboratories may be closed, for example, as opposed to weekends and school vacations (14.4% and 12.4%, respectively). In comparison to 2013–2019, during the COVID-19 pandemic (2020–2022), the proportions of DKA and severe DKA at diagnosis did rise, as seen in other reports. However, there were no significant differences when comparing the type of day (workday versus other) between these time periods.
The analysis by Kamrath et al. ( 9 ) is well designed and includes a large number of children and adolescents. The DPV receives data from the vast majority (93%) of T1D diagnoses throughout the country, increasing its generalizability. While the type of health care system varies between countries, I would still expect these differences between workdays and nonworkdays to be present in many countries. In support of this assumption, in Colorado it was found that while DKA rates in children increased from 2010 to 2017, the increase was driven by insured children ( 5 ), meaning other variables, as Kamrath et al. explore, may play a role. Direct evidence of what information families may have received from acute care clinics, general providers, and on-call answering services is not available.
This study should provide renewed motivation for specialized diabetes-specific trainings for all who care for children. In addition, we can advocate for updates to health care policy to require that all patients have access to pediatric/specialized health care services 24 h a day, 7 days a week, 365 days a year. An influx of monetary support in this area could reduce DKA and intensive care unit hospitalizations for children which may actually save health care dollars. In addition, many children requiring critical care often must be transferred to another hospital that may be far from the family’s home and support system. We can focus on the stakeholders involved in primary prevention of DKA, which include the person with T1D and their family, primary care providers, acute care providers, and on-call services. The latter two especially are used more on weekends and holidays. For children who are likely to have a more rapid metabolic decompensation, parents are a vital stakeholder, as the child may not be able to adequately express their symptoms. Each of these stakeholders interacts with a child developing T1D before a specialist does. Kamrath et al. ( 9 ) bring up valuable points about differences in care that children receive outside standard weekdays. Might we take a multipronged approach to reach these stakeholders, as depicted in Fig. 1 ?
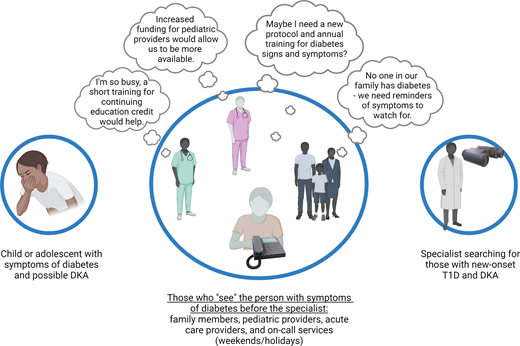
Key stakeholders can help identify T1D before the development of DKA. The specialist, on the right, is on the lookout for cases of T1D and DKA. The specialist, though, is not the first person to interact with a child developing symptoms of diabetes (shown on the left). These people, or stakeholders (in the middle circle), include family members, pediatric or primary care providers, acute care providers, and on-call answering services. Each stakeholder group proposes a potential solution for prompt identification of T1D. Figure created with BioRender.com.
Identifying T1D early, before the development of DKA, is possible, as has been shown in the U.S., Germany, Finland, Sweden, Australia, and New Zealand, where rates of DKA were ≤6% when children at risk for T1D (multiple islet autoantibodies present) were involved in monitoring ( 5 , 10 – 13 ). We, as readers of Diabetes Care , should be taking lessons from these groups as we work toward the goal of zero cases of DKA. We can affect change regarding early recognition of T1D.
See accompanying article, p. 649 .
Funding. L.M.J. is funded by the National Institutes of Health.
Duality of Interest. No potential conflicts of interest relevant to this article were reported.
Email alerts
- Association of Diabetic Ketoacidosis in Childhood New-Onset Type 1 Diabetes With Day of Presentation in Germany
- Online ISSN 1935-5548
- Print ISSN 0149-5992
- Diabetes Care
- Clinical Diabetes
- Diabetes Spectrum
- Standards of Medical Care in Diabetes
- Scientific Sessions Abstracts
- BMJ Open Diabetes Research & Care
- ShopDiabetes.org
- ADA Professional Books
Clinical Compendia
- Clinical Compendia Home
- Latest News
- DiabetesPro SmartBrief
- Special Collections
- DiabetesPro®
- Diabetes Food Hub™
- Insulin Affordability
- Know Diabetes By Heart™
- About the ADA
- Journal Policies
- For Reviewers
- Advertising in ADA Journals
- Reprints and Permission for Reuse
- Copyright Notice/Public Access Policy
- ADA Professional Membership
- ADA Member Directory
- Diabetes.org
- X (Twitter)
- Cookie Policy
- Accessibility
- Terms & Conditions
- Get Adobe Acrobat Reader
- © Copyright American Diabetes Association
This Feature Is Available To Subscribers Only
Sign In or Create an Account
Log in using your username and password
- Search More Search for this keyword Advanced search
- Latest content
- For authors
- Browse by collection
- BMJ Journals More You are viewing from: Google Indexer
You are here
- Volume 5, Issue 3
- The pathway to diagnosis of type 1 diabetes in children: a questionnaire study
- Article Text
- Article info
- Citation Tools
- Rapid Responses
- Article metrics

- http://orcid.org/0000-0002-8501-2531 Juliet A Usher-Smith 1 ,
- Matthew J Thompson 2 ,
- Hannah Zhu 3 ,
- Stephen J Sharp 4 ,
- Fiona M Walter 1
- 1 The Primary Care Unit , University of Cambridge , Cambridge , UK
- 2 Department of Family Medicine , University of Washington , Seattle, Washington , USA
- 3 Cambridge University Hospitals NHS Foundation Trust , Cambridge , UK
- 4 MRC Epidemiology Unit , University of Cambridge, Institute of Metabolic Science , Cambridge , UK
- Correspondence to Dr Juliet A Usher-Smith; jau20{at}medschl.cam.ac.uk
Objective To explore the pathway to diagnosis of type 1 diabetes (T1D) in children.
Design Questionnaire completed by parents.
Participants Parents of children aged 1 month to 16 years diagnosed with T1D within the previous 3 months.
Setting Children and parents from 11 hospitals within the East of England.
Results 88/164 (54%) invited families returned the questionnaire. Children had mean±SD age of 9.41±4.5 years. 35 (39.8%) presented with diabetic ketoacidosis at diagnosis. The most common symptoms were polydipsia (97.7%), polyuria (83.9%), tiredness (75.9%), nocturia (73.6%) and weight loss (64.4%) and all children presented with at least one of those symptoms. The time from symptom onset to diagnosis ranged from 2 to 315 days (median 25 days). Most of this was the appraisal interval from symptom onset until perceiving the need to seek medical advice. Access to healthcare was good but one in five children presenting to primary care were not diagnosed at first encounter, most commonly due to waiting for fasting blood tests or alternative diagnoses. Children diagnosed at first consultation had a shorter duration of symptoms (p=0.022) and children whose parents suspected the diagnosis were 1.3 times more likely (relative risk (RR) 1.3, 95% CI 1.02 to 1.67) to be diagnosed at first consultation.
Conclusions Children present with the known symptoms of T1D but there is considerable scope to improve the diagnostic pathway. Future interventions targeted at parents need to address the tendency of parents to find alternative explanations for symptoms and the perceived barriers to access, in addition to symptom awareness.
- PRIMARY CARE
This is an Open Access article distributed in accordance with the terms of the Creative Commons Attribution (CC BY 4.0) license, which permits others to distribute, remix, adapt and build upon this work, for commercial use, provided the original work is properly cited. See: http://creativecommons.org/licenses/by/4.0/
https://doi.org/10.1136/bmjopen-2014-006470
Statistics from Altmetric.com
Request permissions.
If you wish to reuse any or all of this article please use the link below which will take you to the Copyright Clearance Center’s RightsLink service. You will be able to get a quick price and instant permission to reuse the content in many different ways.
Strengths and limitations of this study
This study uses a questionnaire developed from a previous interview study to explore the diagnostic pathway of children with newly diagnosed type 1 diabetes.
It uses the Model of Pathway to Treatment as a framework to allow analysis of the factors acting at different stages in the pathway.
The inclusion of a calendar with key events in the questionnaires and use of free text responses for internal validation and checking of prompted responses reduced bias but the data was necessarily collected retrospectively and so are subject to recall and framing bias.
Introduction
Approximately 65 000 children are diagnosed with type 1 diabetes (T1D) each year and the incidence is continuing to increase at a rate of approximately 3% per year. 1 , 2 The most common symptoms are well described and include polyuria, polydipsia, weight loss and tiredness. At the early stages of the disease, however, these symptoms are often non-specific and distinguishing the children with T1D from the large number with similar symptoms and minor undifferentiated illness can therefore be difficult. This is reflected in studies which have shown that the mean duration of symptoms prior to diagnosis is over 2 weeks with a significant number of children experiencing delay in diagnosis or misdiagnosis 3 and only one in five diagnosed at first encounter. 4–8 Up to 80% of children additionally present in diabetic ketoacidosis (DKA), 9 which has immediate life-threatening complications and is associated with poorer long-term diabetic control. 10–12
While several studies have highlighted these difficulties in making the diagnosis and the features associated with diabetic ketoacidosis at diagnosis, 3–8 , 13 few have explored the period between symptom onset and diagnosis. Our recent qualitative interview study of parents and general practitioners (GPs) of children newly diagnosed with T1D suggested that the longest component in the diagnostic pathway is the time between onset of symptoms and the decision to seek medical help (known as the appraisal interval). 14 The early symptoms are subtle, and even with some knowledge of T1D it took many parents several weeks of a complex decision-making process and often a physical trigger, such as weight loss or vomiting, to decide to consult a healthcare professional. Once the decision to seek help had been made almost all children were seen immediately and diagnoses were mostly prompt and managed appropriately. Parents continued to play a key role during the diagnostic interval however, with many having already made or suspected the diagnosis themselves, and several feeling that their GP did not take their concerns seriously.
This study builds on this earlier work by using a questionnaire developed from the interview findings to further explore the pathway to diagnosis of T1D in children. By using a structured questionnaire to survey a larger number of families we aimed to quantify the symptoms and their time course prior to diagnosis, the triggers and barriers to seeking help, the influence of parental prior knowledge of diabetes and the role of healthcare services.
A questionnaire about the pathway from first symptom(s) to diagnosis was completed by the parent(s)/guardian(s)/step-parents (hereafter referred to as parents) of children aged 1 month to 16 years diagnosed with T1D within the previous 3 months.
Recruitment
Children and parents were identified and recruited via the paediatric diabetes specialist nurses and research nurses at 11 hospitals within the East of England Diabetes Children and Young People's Network. Parents of all children aged 1 month to 16 years who were diagnosed with T1D within the previous 3 months at participating hospitals were eligible for inclusion unless their clinical team felt that this was not appropriate. Parents who failed to respond within 1 month were sent a reminder letter with a further copy of the questionnaire. Recruitment began at each site between February 2013 and April 2013, and continued across all sites until January 2014.
The clinical or research teams at all sites collected data on the age and gender of each child diagnosed during the study period and whether they had DKA at diagnosis. Each hospital used a slightly different definition of DKA but all included either pH <7.3 or bicarbonate <15 mmol/L (see online appendix table 1).
The questionnaire
The questionnaire was developed from the findings of our previous qualitative study of parents and children recently diagnosed with T1D. 14 It was first reviewed by an expert panel comprising paediatric diabetes consultants, a paediatric diabetes research nurse and primary care researchers, and then piloted with parents of four children recently diagnosed with T1D. In addition to their specific feedback, parents were asked to talk aloud while completing the questionnaire and then interviewed after completion to ensure face validity. Based on feedback from the parents, the questionnaire was revised.
The final questionnaire included five sections (see online supplementary file). The first included questions about the child's age, gender, postcode, ethnic background, family history of diabetes, any medically trained family members and the number of children in the household. Parents were also asked if they knew what the symptoms of diabetes in children were before their child was diagnosed, and if so, to give details of those symptoms they were aware of. The second section asked about the symptoms the children had experienced with yes/no responses for 14 symptoms and space to add the date they noticed the symptoms, what they thought the symptoms were due to at the time and how much they concerned them. The third section focused on help-seeking and asked where parents had looked for information, who they spoke to and then details on when and how they had sought medical advice. It also asked them to describe their main concern at their first appointment and whether they had considered diabetes. Parents were also asked in this section about factors contributing to their decision to seek medical advice sooner or later. The fourth section asked about the diagnosis, including whether it was made at their first appointment with a healthcare professional and, if not, how many subsequent consultations they had, and the investigations that were performed before diagnosis. The final section then asked parents if they felt there was anything that prolonged them finding out their child had diabetes and had further space for free text comments.
Data from the questionnaires were entered into a database and then double checked by a second researcher. Socioeconomic status was computed using postcode and the English indices of deprivation 2010 available online. 15 The presence of DKA at diagnosis was obtained from hospital records rather than self-report. Walter et al 's Model of Pathways to Treatment 16 , 17 provided a theoretic model of the intervals that occur prior to a diagnosis. This model divides the pathway to diagnosis into two intervals prior to presentation to healthcare about a symptom (the appraisal interval from the onset of symptoms to perceiving a reason to discuss symptoms with a healthcare professional, and the help-seeking interval from that decision until presentation to a healthcare professional), and then the diagnostic interval from first presentation to a healthcare professional until diagnosis. The help-seeking interval was further subdivided into the behavioural interval (the time between perceiving the reason to discuss the symptoms with a healthcare professional to making the decision to seek help) and the scheduling interval (the time between making the decision to seek help and the first consultation). 18 Intervals were calculated from responses to the questionnaire. Where dates were incomplete we applied midpoint rules to estimate the actual date. 19 In cases where the responses in free text differed from the dates entered as numbers, the free text was assumed to be correct, and where there was uncertainty the researchers met to agree consensus.
Characteristics (age, gender, presence of DKA) were compared between children whose parents had and had not returned a questionnaire using a t test for age and χ 2 test for gender and presence of DKA. All further analyses used only data from returned questionnaires. The frequency of the 14 symptoms was compared between those with and without DKA using a χ 2 test. Cox regression was used to estimate the association between various factors and the hazard of diagnosis; if a factor was associated with an increased hazard (ie, HR greater than 1), this implied that that factor was associated with a shorter time to diagnosis, and vice versa. Time to diagnosis was from the date of the earliest symptom to the date of diagnosis, and the factors assessed were age, gender, family history of T1D, index of multiple deprivation, prior knowledge of symptoms of T1D, whether the parents suspected T1D, whether the diagnosis was made at the first consultation, whether the first consultation was with primary or secondary care and whether the child had DKA at diagnosis. A similar approach was used to assess factors associated with the length of the appraisal and help-seeking intervals (with the end of the interval being defined as the ‘event’ in the Cox model), but only the first six variables in the list above were considered, as the others do not relate to those time intervals. The Schoenfeld residuals test was used to assess the proportional hazards (PH) assumption for each covariate in each model. Whether parents suspected the diagnosis of T1D did not meet the PH assumption for the total diagnostic interval and so the Cox regression model was stratified by that variable. Logistic regression was used to estimate the association between the same factors and presence of DKA at diagnosis. All analyses were performed using STATA V.12.
Free text responses were grouped into similar categories and coded. Where individual free text responses contained several comments, these were each coded individually.
A total of 172 children were diagnosed with T1D in the 11 hospitals during the study period. Of those, eight families were not invited to take part in the study: five lived outside the hospital catchment area; one emigrated the week after diagnosis; and the clinical team felt it was not appropriate to include two. From the remaining 164 families invited to take part in the study, 88 (54%) completed and returned the questionnaire. There were no significant differences in the proportion presenting in DKA (p=0.27), mean age (p=0.77) or gender (p=0.77) between children of responders and non-responders.
One child was excluded from the analysis as they had no symptoms and the diagnosis was made on a random blood glucose test that the parents were doing at home on an intermittent basis as they had an older child with T1D. Children whose parents checked blood glucose at home after noticing symptoms remain in the analysis. Eighty-seven children are therefore included in the analysis that follows.
Table 1 shows the characteristics of the 87 children and families included in the study. The mean age was 9.34±4.5 years, 49 (56.3%) were male and 35 (40.2%) presented with DKA at diagnosis. The majority (90.8%) were white and as a group they were generally from less deprived areas of England, with 49.4% from the least deprived tertile of English Indices of Deprivation and only 10.3% from the most deprived.
- View inline
Child and family characteristics for those included in the study
Table 2 shows the frequency and duration of the 14 symptoms that were specifically asked about in the questionnaire. The most common symptoms were polydipsia (97.7%), polyuria (83.9%), tiredness (75.9%), nocturia (73.6%) and weight loss (64.4%). Most symptoms were present for a median of between 13 and 17 days. Faster breathing and vomiting both had much shorter median (IQR) durations of 0.5 (0–7.5) and 2.5 (1.5–5.5) days respectively than the other symptoms. Weight loss, vomiting and faster breathing were significantly more frequent in those children who presented in DKA (p=0.014, <0.0005 and 0.001, respectively). All the children had at least one of the four main symptoms (polydipsia, polyuria or nocturia, weight loss, or tiredness), 97.7% had two or more, 79.3% three or more and over half (50.6%) had all four symptoms.
Frequency of symptoms among all children and those with and without diabetic ketoacidosis (DKA) and duration of individual symptoms
A small number of parents mentioned symptoms other than those listed in the questionnaire, these included constipation (9), headaches (3), thrush (3), blurred vision (2), dry skin (2) and different smelling urine (1).
Diagnostic intervals
Table 3 shows the mean±SD and median (IQR) for the diagnostic intervals. Additional details on the diagnostic intervals for different subgroups are shown in online supplementary appendix table 2. The total diagnostic interval ranged from 2 to 315 days with a median (IQR) of 25 days (14–50). In unadjusted Cox regression analysis (data not shown) the time to diagnosis was significantly shorter for children diagnosed at first appointment compared with a subsequent appointment (p=0.046) and for those seen in secondary care rather than primary care (p=0.01). No evidence of associations with time to diagnosis was found for age, gender, family history of T1D, deprivation, prior knowledge of symptoms or DKA at diagnosis. In multivariable cox regression including age, gender, family history of T1D, index of multiple deprivation, prior knowledge of symptoms of T1D, whether the diagnosis was made at the first consultation, whether the first consultation was with primary or secondary care and whether the child had DKA at diagnosis ( figure 1 A), the association between whether the diagnosis of T1D was made at the first or subsequent appointments and total diagnostic interval remained statistically significant (p=0.022).
Duration of diagnostic intervals
- Download figure
- Open in new tab
- Download powerpoint
Associations between parent/child characteristics and (A) the total diagnostic interval, (B) the appraisal interval and (C) the help-seeking interval. HRs adjusted for all variables in each figure. Cox model in (A) stratified by whether parents suspected the diagnosis or not. DKA, diabetic ketoacidosis; IMD, index of multiple deprivation.
The appraisal interval
The appraisal interval was the longest of all the intervals in the pathway for all but three of the families with a mean±SD of 41.0±51.7 days and median (IQR) 20 (9–40) days. During this period nearly two-thirds (64%) of parents discussed the symptoms with family members, 40% with friends and 41% looked on the internet. Only 16% spoke to the child's nursery, school or playgroup and very few (6%) looked for information in books. Over half of parents (49, 56%) reported being aware of some symptoms of T1D in children prior to their child's diagnosis: 40 (45%) were aware of increased thirst, 24 (27%) of polyuria, 17 (19%) of weight loss and 13 (15%) tiredness.
Cox-regression analysis ( figure 1 B) showed no significant associations between parent/child characteristics and the appraisal interval.
Analysis of the free text showed that most parents found explanations for their child's symptoms ( table 4 ). For example, polydipsia was attributed most commonly to hot weather (19/58, 33%) or infection (13/58, 22%), polyuria and nocturia were frequently explained by drinking more (29/47, 62% and 26/40, 65%) and tiredness was thought to be school related (12/44, 27%) or secondary to infection (5/44, 12%) or nocturia (4/44, 10%).
Parents’ explanations for the 10 most common symptoms
The majority of parents (61/87, 70%) additionally reported that they had suspected diabetes before their first consultation with a healthcare professional. When asked what had made them suspect diabetes, the most common reason given was that they knew the symptoms (22/59, 37%), especially thirst (12/59, 20%). Others cited information from the internet (12/59, 20%) or having a family history of diabetes (11/59, 19%).
The help-seeking interval
Twenty-four (28%) children were seen on the same day their parents first thought about seeking medical advice and 64 (74%) within 5 days. Most of this time was the behavioural interval (mean±SD 2.1±3.7 days, median (IQR) 0 (0–3) days) rather than the scheduling interval (mean±SD 1.1±2.6 days, median (IQR) 0 (0–1) days).
Cox-regression analysis ( figure 1 C) showed no significant associations between parent/child characteristics and the help-seeking interval.
The most common reasons that parents cited for seeking medical advice sooner rather than later ( table 5 ) were that the symptoms were not getting better or were getting worse, wanting reassurance or concern something serious was wrong. This was also reflected in the free text responses where 22% of parents noted that worsening or persistent symptoms was the reason they decided to seek help. In general, fewer parents reported factors that led to them seeking medical advice later. Of those that did, the most common reason for waiting was hope that the symptoms would go away (51.6%) but 29.8% felt difficulty getting an appointment contributed and 27.6% and 25.2% were worried about wasting the GPs time or that the GP would not take them seriously, respectively.
Factors influencing parents’ decisions to seek medical advice sooner or later
The diagnostic interval
The diagnostic interval was the shortest of the intervals with a mean±SD of 5 days±34.8 and median 0 (IQR; 0–0) days. Sixty-nine (78%) children were diagnosed at first consultation. Cox regression was not possible given the high number of children with a diagnostic interval of zero. However, children whose parents suspected the diagnosis (n=61, 70.1%) were more likely (unadjusted relative risk (RR) 1.30, 1.02 to 1.67, p=0.046) to be diagnosed at first consultation (n=52, 85.2%) than those in whom there was no suspicion (n=26, 29.9% with 17 (65.4%) diagnosed at first consultation). All children (10) who were seen first in secondary care were diagnosed at first consultation compared with 76.6% (59/77) of those seen first in primary care, but this difference was not statistically significant (p=0.114). None of the variables considered were significantly associated with risk of DKA ( figure 2 ).
Associations between parent/child characteristics and presence/absence of DKA. ORs adjusted for all variables in the figure. DKA, diabetic ketoacidosis; IMD, index of multiple deprivation.
Further details from the questionnaires were available from 14 of the 18 children who were not diagnosed at first encounter with primary care. Of these, six had fasting glucose blood tests arranged by the GP and four were given alternative diagnoses (urine infection, viral infection, tonsillitis, puberty) and diagnosed at a second appointment. Two children were diagnosed with psychological problems: In one case the child's mother had seen the GP alone to discuss her child's ‘obsessive drinking’ and was advised to see the school counsellor, and in the second the GP apparently felt the symptoms were psychological and the child was diagnosed in the emergency department four consultations later. One other family had already performed a finger prick glucose test at home which was high but the GP did not trust the result and asked the child to come back later in the day with a urine sample. In the final case, the child's mother had spoken to a health visitor and suggested diabetes but was told “no, not unless the child is lifeless”. The mother took the child to the GP 12 days later and the diagnosis was made at that consultation.
Principal findings
This study shows that all children with new onset T1D present with one, and 98% present with two, of the four main symptoms of diabetes (polydipsia, polyuria, weight loss and tiredness). Moreover, over half have had symptoms for over 3 weeks before diagnosis. Most of that time is the appraisal interval during which parents found alternative explanations for the symptoms, discussed the symptoms with family and friends and looked on the internet for information. Once they made the decision to seek advice, access to healthcare was generally not difficult with 28% consulting with a healthcare professional on the same day. However, when asked about factors contributing to their decision to seek help, nearly a third of parents felt that difficulty getting an appointment contributed to them waiting to seek help and over a quarter felt that worry about wasting the doctor's time influenced their decision. This suggests that even if access is not difficult, it is perceived as such.
Once parents had sought help, one in five children were then not diagnosed at their first consultation with a healthcare professional, mainly due to being given an alternative diagnosis, most commonly infection, or waiting for further investigations. Diagnosis at first consultation was associated with a shorter total diagnostic interval and children were more likely to be diagnosed at first consultation when their parents suspected the diagnosis of T1D. The association between diagnosis at first consultation and total diagnostic interval may simply reflect the additional time between consultations, or it may be due to biological differences causing some children to develop symptoms more slowly which are then more difficult for parents as well as primary care physicians to recognise.
Strengths and weaknesses
By using a questionnaire developed from a previous interview study 14 and the Model of Pathway to Treatment 16 , 17 as a framework for analysis, this study provides in-depth insights into the diagnostic pathway of children with newly diagnosed T1D and allows factors acting at different stages in the pathway to be explored.
The main weakness is that the data were necessarily collected retrospectively and so are subject to recall and framing bias. Parents have multiple contacts with different healthcare professionals in the period immediately following diagnosis and so their responses to the questionnaire reflect a post hoc rationalisation of events framed by those subsequent encounters and increased knowledge since the diagnosis. The inclusion of a calendar with key events in the questionnaires minimised the error in recall of dates, and the free text responses allowed internal validation and checking of prompted responses. Despite these efforts, we still only have the parents’ perspective on the pathway and were not able to confirm the number of healthcare contacts, diagnostic tests or the parental reports of missed opportunities for diagnosis. We were, however, able to confirm the diagnosis of DKA from clinical records and, although there was variation in the definition of DKA used across the 11 sites, all included a biochemical measurement of either pH or bicarbonate.
Our results are also based on the views of 88 parents. Although not a large number, they were recruited from 11 sites across a large region of the UK and the response rate was over 50% with no significant differences in gender, age or DKA status between the children whose parents responded and those who did not. The fact that they were a predominantly white group from less deprived areas of England limits the generalisability of the results outside the East of England but the main findings are likely to be relevant across the UK and other countries with similar primary care healthcare provision. The questionnaire also did not include questions specifically for the children to complete and so we are unable to comment on the views of the children during this time.
Comparison with existing literature
The median duration of symptoms prior to diagnosis was 13–17 days for the nine most frequent symptoms, with a mean of 30–50 days. This is longer than previous studies relying on retrospective review of medical records 20–23 but similar to studies which have used a checklist to identify subtle symptoms 24 or asked parents soon after diagnosis. 13 , 14 The wide range (a few days to over 6 months) has been described previously 14 , 21 , 23 and highlights the heterogeneous nature of the disease.
The frequency of individual symptoms we report is also similar to previous studies. 13 , 14 , 20 , 22 , 25 Additionally, we showed that all the children had at least one of four symptoms (polydipsia, polyuria, weight loss and fatigue) and over half (50.6%) had all four. Consistent with the known course of the disease and previous studies, vomiting, 4 , 22 , 24 weight loss 13 , 25 , 26 and dyspnoea 22 were more common in those children who presented with DKA.
This is the first quantitative study to compare the time periods during the pathway to diagnosis of T1D in children. The finding that most of the total diagnostic interval was the appraisal interval is consistent with a previous qualitative study 14 and the free text analysis confirms that during that time the parents find alternative explanations for the symptoms initially and make use of a social network of extended family, friends and work colleagues, or the internet. 14 , 27 , 28 The findings that children were more likely to be diagnosed at their first encounter with a healthcare professional when their parents suspected diabetes prior to that consultation may also reflect the findings of previous qualitative work in which a number of parents prompted the GP to consider T1D and pushed for investigations. 14 However, while parental suspicion of T1D has also been shown to be associated with a reduced risk of DKA in a parental survey, 13 in that study the incidence of DKA at presentation was no different whether or not the parents discussed their concerns with the healthcare professional, suggesting other factors may be contributing. The absence of an effect of parental prior knowledge of diabetes either on the total diagnostic interval or the risk of DKA further highlights the complexities around the role of knowledge on help-seeking behaviour.
The finding that parents worry about wasting the doctor's time has also been shown in previous qualitative studies in children 29 , 30 and in studies of help-seeking behaviour for adults with symptoms of cancer in the UK 31 , 32 and so it may reflect a particular British trait rather than be specific to T1D or children.
Implications for clinicians and policymakers
Clinicians should remain alert to the possibility of T1D in all children presenting with one or more symptoms of polyuria, polydipsia, weight loss and tiredness—as almost all children have at least two of these. Interventions targeted at increasing public awareness, such as the 4 T's campaign launched by Diabetes UK to raise awareness of the four most common symptoms of T1D (Toilet, Thirsty, Tired and Thinner), 33 should continue to focus on these established symptoms.
As most of the time between symptom onset and diagnosis is the appraisal interval, the greatest benefit is likely to be seen from interventions directed towards parents and their social network, probably via the internet. Despite ongoing government pressure for better access to primary care, improving access is unlikely to have much impact on the pathway. Instead efforts should be made to address the perception that access is difficult and the general concern in the UK about wasting healthcare professional time, particularly for children with acute or sub-acute health concerns.
Additionally, although the diagnostic interval itself was generally short, one in five children presenting to primary care were not diagnosed at first consultation. Similar numbers have been reported in a recent survey in the UK which found that 24% were not diagnosed at first contact with a healthcare professional, 13 and studies in the USA, Canada and Poland noted between 14% and 35% of children had more than one consultation before diagnosis. 6 , 7 , 34–36 As in those studies, the most common reasons for not being diagnosed at first encounter was either being given an alternative diagnosis, most commonly infection, or waiting for further investigations. In this study 33% of those not diagnosed at first consultation were waiting for fasting glucose tests and in other studies the number waiting for further investigations is as high as 46%. 6 , 13 This suggests that healthcare professionals may have considered a diagnosis of T1D but either lack ready access to rapid tests to confirm or exclude the diagnosis, or are reluctant to use existing tests in children. 14 Access to point of care urine and finger-prick testing and the use of those tests should be routine management for all children presenting with one or more of the four main symptoms of diabetes. The increased use of point of care testing in emergency departments may also explain why all children seen in secondary care were diagnosed at their first consultation. While educational interventions aimed at primary care physicians may help a small number of children not currently diagnosed at first encounter, finding ways to overcome barriers to point-of-care tests in primary care may be more effective and this approach may also improve the diagnosis of other serious illnesses in children and adults.
Unanswered questions and future research
While this study contributes to our understanding of the pathway to diagnosis and the stages at which this may be improved, the findings are unable to explain the large variability in the overall duration of the pathway to diagnosis and why some children develop DKA within a few weeks while others can be symptomatic for up to 6 months before requiring treatment. Further studies are, therefore, needed into the natural course and biology of the disease to better understand these variations. The findings also highlight the need for continuing research into the presentation of serious but rare conditions in primary care and the best ways to improve diagnosis of these conditions.
Acknowledgments
The authors thank all the parents who kindly gave up their time and shared their personal stories with us to either help develop the questionnaire or take part in the study. They also thank the Paediatric diabetes clinical teams at the 11 hospitals, particularly Jon Hassler-Hurst (Ipswich), Holly Roper (Norfolk and Norwich), Elissa Harwood (Cambridgeshire community services), Nazia Bhatti and Claire Pesterfield (Addenbrooke's hospital NHS foundation trust), Julia Harding and Jacky Plumb (West Suffolk), Philippa Corbishley and Laura Ardrey (QE2 and Lister), Suzie Williams (SEPT), Mandy Stevenson (Harlow), Claire Gibbs and Allyson Davison (James Paget) and Meeta Patel (Luton and Dunstable). They are also grateful to the Diabetes Research Network and the East of England Children and Young People's Diabetes Network and network manager Kate Wilson for support, James Brimicombe for advice on data management, Dr Carlo Acerini for comments on the questionnaire and final manuscript and support through his role as Chair of the East of England Children and Young People's Diabetes Network, Professor Jon Emery for comments on the questionnaire, and Professor David Dunger for his support and encouragement throughout the study and comments on the final manuscript.
- Lawrence JM ,
- Imperatore G ,
- Dabelea D , et al
- Fazeli Farsani S ,
- Souverein PC ,
- van der Vorst MMJ , et al
- Usher-Smith JA ,
- Thompson MJ ,
- Sharp SJ , et al
- Lucidarme N ,
- Tubiana-Rufi N
- Hamilton DV ,
- Mundia SS ,
- Mallare JT ,
- Cordice CC ,
- Ryan BA , et al
- Pawłowicz M ,
- Birkholz D ,
- Niedźwiecki M , et al
- Soliman AT ,
- ElZalabany MM ,
- Bappal B , et al
- Thompson M ,
- Ercole A , et al
- Abdul-Rasoul M ,
- Al-Khouly M
- Bowden SA ,
- Fredheim S ,
- Johannesen J ,
- Johansen A , et al
- Lokulo-Sodipe K ,
- Edge J a , et al
- ↵ English Indices of Deprivation 2010 . https://www.gov.uk/government/publications/english-indices-of-deprivation-2010 (accessed 7 Feb 2014 ).
- Webster A ,
- Scott S , et al
- Walter FM ,
- Webster A , et al
- Andersen BL ,
- Cacioppo JT
- Allgar VL ,
- Willasch A ,
- Ehehalt S , et al
- Gill D , et al
- Chen XJ , et al
- Ehehalt S ,
- Willasch A , et al
- Lo FS , et al
- Kapellen TM ,
- Nietzschmann U , et al
- Lévy-Marchal C ,
- Patterson CC ,
- Dixon-Woods M ,
- Findlay M ,
- Young B , et al
- Francis Na ,
- Crocker JC ,
- Gamper A , et al
- Forbes LJL ,
- Thurnham A , et al
- Warburton F , et al
- Diabetes UK
- Stein R , et al
- Fleischman A ,
- Rosner B , et al
- Pawlowicz M ,
- Niedzwiecki M , et al
Supplementary materials
Supplementary data.
This web only file has been produced by the BMJ Publishing Group from an electronic file supplied by the author(s) and has not been edited for content.
Files in this Data Supplement:
- Data supplement 1 - Online Questionnaire
- Data supplement 2 - Online table 1
- Data supplement 3 - Online table 2
Contributors JAU-S, MJT, FMW and SJS were involved in the design of the study and all authors were involved in analysis of the data. JAU-S and FMW developed the questionnaire. JAU-S wrote the first draft of the manuscript and all authors reviewed and edited the manuscript.
Funding The study was funded by the Royal College of General Practitioners Scientific Foundation Board (SFB-2011–15). JAU-S was supported by a National Institute of Health Research (NIHR) Academic Clinical Fellowship and subsequently Clinical Lectureship, and FMW by an NIHR Clinician Scientist award. SJS was supported by the Medical Research Council http://www.mrc.ac.uk (Unit Programme number MC_UU_12015/1). The views expressed in this publication are those of the author(s) and not necessarily those of the NHS, the NIHR or the Department of Health. All researchers were independent of the funding body (although FMW subsequently became a member of the Royal College of General Practitioners Scientific Foundation Board), and the study sponsors and funder had no role in study design; data collection, analysis and interpretation of data; in the writing of the report; or decision to submit the article for publication.
Competing interests None.
Ethics approval The study obtained ethical approval from the East of England Hertfordshire REC (reference number 12/EE/0390).
Provenance and peer review Not commissioned; externally peer reviewed.
Data sharing statement The questionnaire is available from the corresponding author on request.
Read the full text or download the PDF:
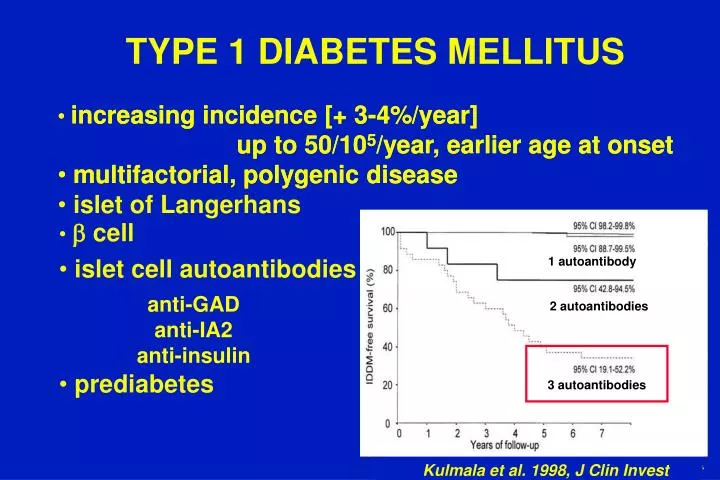
TYPE 1 DIABETES MELLITUS
Jan 23, 2013
250 likes | 508 Views
increasing incidence [ + 3-4%/year ] up to 50/10 5 /year, earlier age at onset multifactorial, polygenic disease islet of Langerhans. 1 autoantibody. 2 autoantibodies. b cell. 3 autoantibodies. islet cell autoantibodies. prediabetes.
Share Presentation
- lancet kulmala et al
- cytokines antigen
- positivity threshold
- embryonic adult stem cells
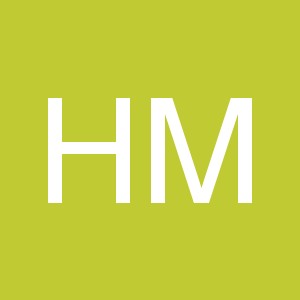
Presentation Transcript
increasing incidence [+ 3-4%/year] • up to 50/105/year, earlier age at onset • multifactorial, polygenic disease • islet of Langerhans 1 autoantibody 2 autoantibodies • b cell 3 autoantibodies • islet cell autoantibodies • prediabetes Bottazzo & Doniach 1974, Lancet Kulmala et al. 1998, J Clin Invest TYPE 1 DIABETES MELLITUS • increasing incidence [+ 3-4%/year] • up to 50/105/year, earlier age at onset • multifactorial, polygenic disease anti-GAD anti-IA2 anti-insulin
MAIN ADVANCES 2003-2006 1- prediction autoantibodies genetic puzzle 2- proinsulin: the primary autoantigen 3- the importance of immune regulation 4- immunotherapy: the end of immunosuppression 5- the goal: b cell restoration + induction of immune tolerance 1- prediction autoantibodies genetic puzzle
STRATIFICATION OF RISK anti-IA2 & anti-insulin IgG2/IgG3/IgG4 anti-IA2b & IA2 multiepitopes anti-IA2 & anti-insulin autoantibody titers Achenbach et al, Diabetes, 2006
POSITIVITY THRESHOLD % individuals 0 50 90 95 97 99 autoantibody titer (percentiles) AUTOANTIBODY DETECTION: LIMITS predictive positive value in atypical forms of type 1 delay to diabetes onset in prediabetes other assays ?
T T CD3 CD3 CYTOKINES ANTIGEN RECOGNITION BY T CELLS T cell receptor MHC class II
Patient A2/A3 B18/B38 34-42 tetramet nb spots/106 cells 34-42 tetramet CD8 Pinske et al. PNAS, 2005 CD8+ T CELL RESPONSE [ELISPOT assay] Toma et al. PNAS, 2005
MAIN ADVANCES 2003-2006 1- prediction autoantibodies genetic puzzle 2- proinsulin: the primary autoantigen 3- the importance of immune regulation 4- immunotherapy: the end of immunosuppression 5- the goal: b cell restoration + induction of immune tolerance
ROLE OF PROINSULIN IN TYPE 1 DIABETES • PROINS 1-/-NOD MICE: • absence of diabetes PROINS 2-/-NOD MICE acceleration of diabetes anti-insulin Abs ~ anti-GAD Abs ~ sialitis Ins1-/- Moriyama H et et al. Proc Natl Acad Sci USA 2003 Thebault-Baumont, Tardivel et al. 2003, 2005
AUTOANTIBODY IN OFFSPRING OF DIABETIC PARENTS anti-insulin autoantibodies Ziegler et al, Diabetes, 1999
IMMUNE REGULATION IN TYPE 1 DIABETES EFFECTOR T CELL REGULATORY CELLS EFFECTOR T CELL REGULATORY CELLS T CD4+ CD25+ T CD4+ CD62L+ T CD8+ Th2 IL-4,5 Th3 IL-10 b CELL - Th3 TGFb T CD4+ Th1 cytokines T NK ISLET INFa TLRs
DETECTION IN HUMAN EXPANSION NOD • number ? • function ? protection Krukeja et al. 2002, J Clin Invest Tang Q et al. 2004, J Exp Med Kretschmer K et al. Nat Immunol 2005 Lindley S et al. 2005, Diabetes 10 2 small size CD25 FoxP3 GITR CTLA4 10 1 10 0 10 1 10 0 10 2 CD4 Shimon Sakaguchi 1995
TREATMENT OF DIABETES WITH ANTI-CD3 months months months n = 40 (placebo) n = 40 (anti-CD3) Keymeulen B et al. 2005, N Engl J Med
GENERATION OF NEW BETA CELLS • transdifferenciation from • ductal cells • acinar cells • hepatic precursor cells [through a dedifferenciation stage] differenciation from • embryonic adult stem cells • embryonic stem cells
DIFFERENCIATION OF b CELLS FROM ES CELLS pancreatic endoderm primitive gut tube insulin cells definitive endoderm posterior foregut D’Amour et al. Nat Biotechnol, 2006
DIFFERENCIATION OF b CELLS FROM ES CELLS C-PEPTIDE HUMAN ISLETS ESC-DERIVED CELLS D’Amour et al. 2006, Nart Biotech
- More by User
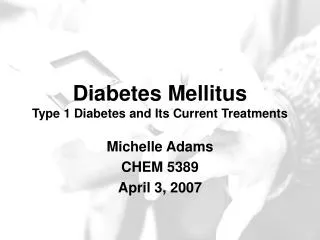
Diabetes Mellitus Type 1 Diabetes and Its Current Treatments
Diabetes Mellitus Type 1 Diabetes and Its Current Treatments. Michelle Adams CHEM 5389 April 3, 2007. Presentation Outline. Diabetes What is Diabetes? How do people get diabetes? What are the signs and symptoms? Importance of Control – Complications of Diabetes Control Being In Control
925 views • 26 slides
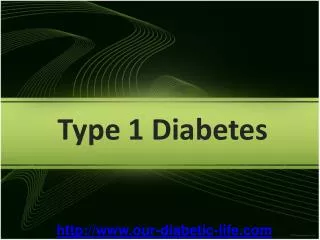
type 1 diabetes mellitus
http://www.our-diabetic-life.com Here are information on Type 1 Diabetes Mellitus
684 views • 5 slides
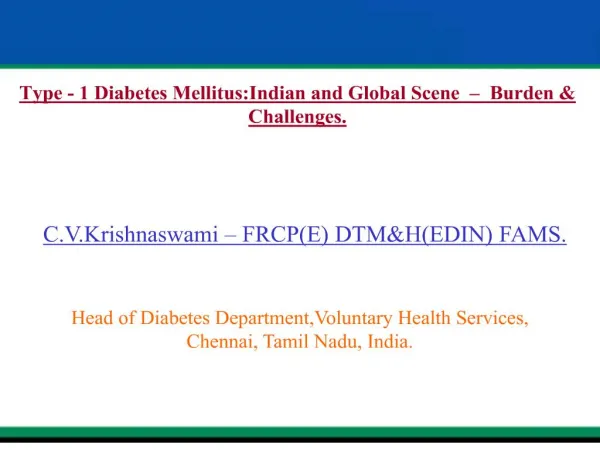
Type - 1 Diabetes Mellitus :Indian and Global Scene
694 views • 43 slides
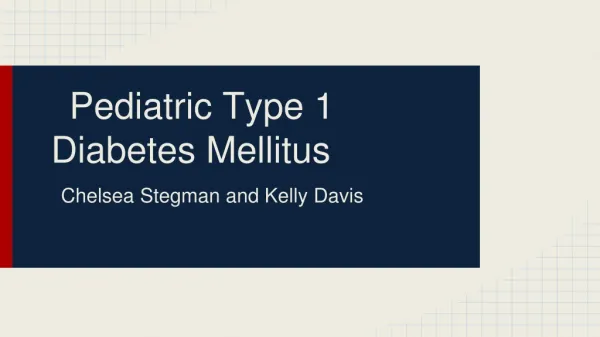
Pediatric Type 1 Diabetes Mellitus
Pediatric Type 1 Diabetes Mellitus. Chelsea Stegman and Kelly Davis. Test your Knowledge. Type 1 Diabetes Mellitus is a/an _____ disease. a. Thyroid b. Bacterial c. Viral d. Autoimmune. Test your Knowledge. Type 1 Diabetes is most commonly diagnosed amongst: A. Overweight adults
896 views • 21 slides
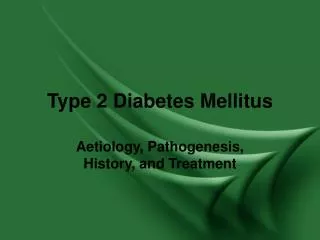
Type 2 Diabetes Mellitus
Type 2 Diabetes Mellitus. Aetiology, Pathogenesis, History, and Treatment. The Diabetes Mellitus epidemic. Estimated 180 million people in the world have DM. That’s roughly 6% of the world population. These numbers are estimated to double by 2030.
738 views • 19 slides
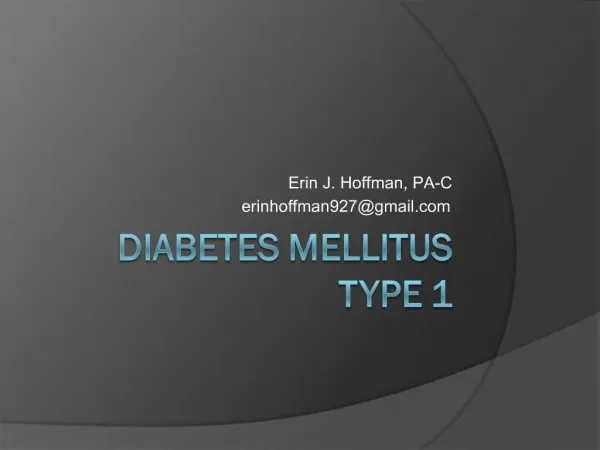
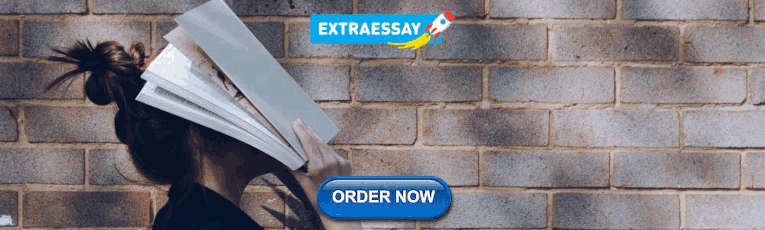
Diabetes Mellitus Type 1
Objectives. Recognize the difference between Type 1 and Type 2 DMIdentify the etoiology, epidemiology, pathophysiology, and common clinical manifestations of DM1.Know the pathophysiology, common presentation, labs, complications and proper treatment of DKABe familiar with different types of insul
753 views • 38 slides
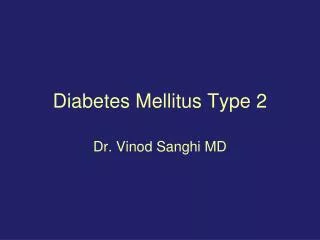
Diabetes Mellitus Type 2
Diabetes Mellitus Type 2. Dr. Vinod Sanghi MD. Overview of Diagnosed and Undiagnosed Diabetes in the United States—2000. Diabetes in India. People with Diabetes : 40 million In 2025 : About 70 million people with diabetes DIABETES CAPITAL OF THE WORLD.
752 views • 37 slides
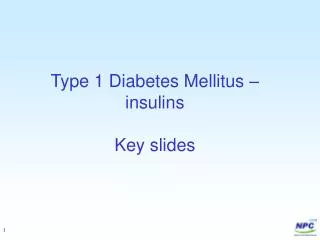
Type 1 Diabetes Mellitus – insulins Key slides
Type 1 Diabetes Mellitus – insulins Key slides. Type 1 vs. type 2 diabetes Lambert P, et al. Medicine 2006; 34(2): 47-51 Nolan JJ. Medicine 2006; 34(2): 52-56. Features of type 2 diabetes Usually presents in over-30s (but also seen increasingly in younger people)
310 views • 9 slides
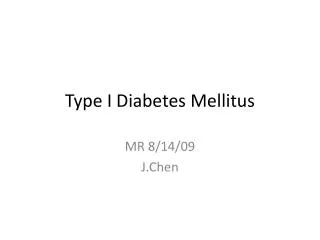
Type I Diabetes Mellitus
Type I Diabetes Mellitus. MR 8/14/09 J.Chen. Management. Insulin Monitoring Nutrition Exercise Education. Insulin. Insulin Requirements. Preadolescent-0.5-1 unit/kg/day Adolescents-0.8-1.2 units/kg/day During honeymoon period-<0.5 units/kg/day. Starting an Insulin Regimen.
313 views • 15 slides
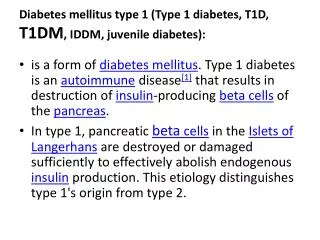
Diabetes mellitus type 1 (Type 1 diabetes, T1D, T1DM , IDDM, juvenile diabetes):
Diabetes mellitus type 1 (Type 1 diabetes, T1D, T1DM , IDDM, juvenile diabetes):. is a form of diabetes mellitus . Type 1 diabetes is an autoimmune disease [1] that results in destruction of insulin -producing beta cells of the pancreas .
1.31k views • 54 slides
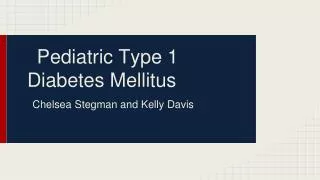
Pediatric Type 1 Diabetes Mellitus. Chelsea Stegman and Kelly Davis. Test your Knowledge . Type 1 Diabetes Mellitus is a/an _____ disease. a. Thyroid b. Bacterial c. Viral d. Autoimmune. Test your Knowledge. Type 1 Diabetes is most commonly diagnosed amongst: A. Overweight adults
674 views • 21 slides
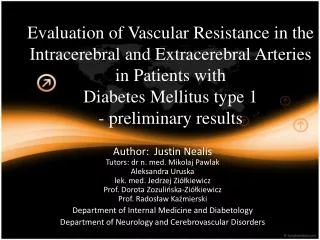
Diabetes Mellitus type 1 & Cerebrovascular disease
Evaluation of Vascular Resistance in the Intracerebral and Extracerebral Arteries in Patients with Diabetes Mellitus type 1 - preliminary results.
382 views • 14 slides
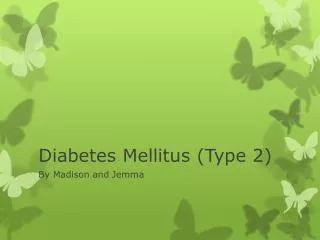
Diabetes Mellitus (Type 2)
Diabetes Mellitus (Type 2). By Madison and Jemma. What is it? Description. Diabetes Mellitus is a chronic condition in which the sufferer is unable to utilise blood glucose correctly. There are three types of this NHPA: type 1 diabetes, type 2 diabetes, gestational diabetes. Different types:.
518 views • 10 slides
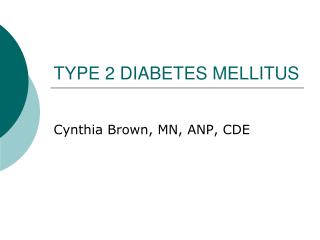
TYPE 2 DIABETES MELLITUS
TYPE 2 DIABETES MELLITUS. Cynthia Brown, MN, ANP, CDE. Type 2 Diabetes Mellitus. Epidemiology: 25 million Americans or 8.3% 7 million undiagnosed 1.9 million older than 20 diagnosed in 2010 7 th leading cause of death In 2007, cost of treating $174 billion
455 views • 27 slides
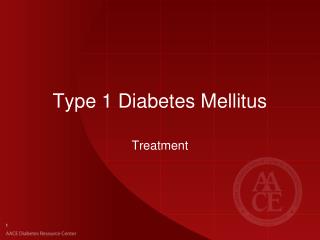
Type 1 Diabetes Mellitus
Type 1 Diabetes Mellitus. Treatment. Goals of T1DM Management. Utilize intensive therapy aimed at near-normal BG and A1C levels Prevent diabetic ketoacidosis and severe hypoglycemia Achieve the highest quality of life compatible with the daily demands of diabetes management
1.27k views • 77 slides
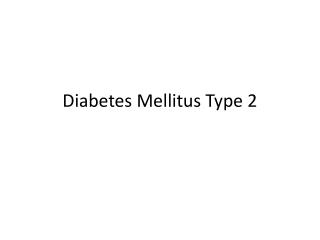
Diabetes Mellitus Type 2. Diabetes Mellitus. Diabetes mellitus is “a group of common metabolic disorders that share the phenotype of hyperglycemia.” (HPIM 17 th ed.)
532 views • 13 slides
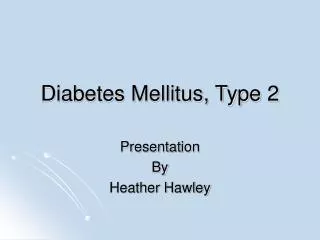
Diabetes Mellitus, Type 2
Diabetes Mellitus, Type 2. Presentation By Heather Hawley. Epidemiology.
831 views • 20 slides
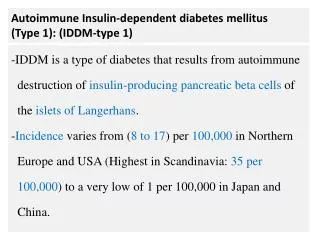
Autoimmune Insulin-dependent diabetes mellitus (Type 1): (IDDM-type 1)
Autoimmune Insulin-dependent diabetes mellitus (Type 1): (IDDM-type 1). -IDDM is a type of diabetes that results from autoimmune destruction of insulin-producing pancreatic beta cells of the islets of Langerhans . - Incidence varies from ( 8 to 17 ) per 100,000 in Northern
378 views • 17 slides
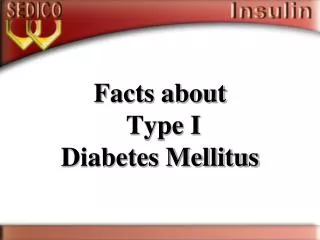
Facts about Type I Diabetes Mellitus
Facts about Type I Diabetes Mellitus. “ Diabetes was long thought to be a kidney disease (Greek & Arabic Methodology). “ Thomas Willis (1621 - 1679), discovered the sweetness of urine, hence, the name Diabetes Mellitus arised”. “Mathew Dobson (1776), identified glycosuria.
535 views • 36 slides
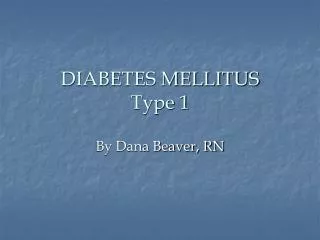
DIABETES MELLITUS Type 1
DIABETES MELLITUS Type 1. By Dana Beaver, RN. Diabetes Mellitus. Is one of the oldest conditions known to man, having been identified in 1500 B.C. (Selekman, J., 2006). What is Diabetes?. Diabetes is a disease in which the body does not produce or properly use insulin
1.19k views • 28 slides
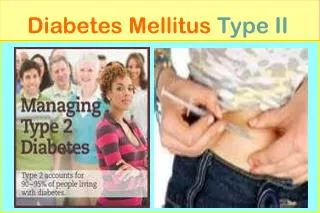
Diabetes Mellitus Type II
Diabetes Mellitus Type II. Beta Cell Failure in DM T2. signaling pathways implicated in β -cell failure. Controls organismal growth and differentiation. Wnt Signaling Pathway. Wnt signalling Pathway and DM T2.
615 views • 40 slides
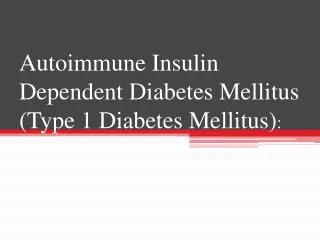
Autoimmune Insulin Dependent Diabetes Mellitus (Type 1 Diabetes Mellitus) :
Autoimmune Insulin Dependent Diabetes Mellitus (Type 1 Diabetes Mellitus) :. Major immunologic Features: HLA-DR3 and DR4 haplotype expression on the beta cells of the islets of Langerhans. Presence of reactive Autoantibodies directed against multiple antigens of islets beta cells.
798 views • 27 slides
- Open access
- Published: 27 March 2024
Systematic immune cell dysregulation and molecular subtypes revealed by single-cell RNA-seq of subjects with type 1 diabetes
- Mohammad Amin Honardoost 1 , 2 , 3 ,
- Andreas Adinatha 1 ,
- Florian Schmidt 1 ,
- Bobby Ranjan 1 ,
- Maryam Ghaeidamini 1 ,
- Nirmala Arul Rayan 1 ,
- Michelle Gek Liang Lim 1 ,
- Ignasius Joanito 1 ,
- Quy Xiao Xuan Lin 1 ,
- Deepa Rajagopalan 1 ,
- Shi Qi Mok 4 ,
- You Yi Hwang 5 ,
- Anis Larbi 5 ,
- Chiea Chuen Khor 4 ,
- Roger Foo 2 , 6 ,
- Bernhard Otto Boehm 7 , 8 , 9 &
- Shyam Prabhakar 1 , 8 , 10
Genome Medicine volume 16 , Article number: 45 ( 2024 ) Cite this article
377 Accesses
2 Altmetric
Metrics details
Type 1 diabetes mellitus (T1DM) is a prototypic endocrine autoimmune disease resulting from an immune-mediated destruction of pancreatic insulin-secreting \(\beta\) cells. A comprehensive immune cell phenotype evaluation in T1DM has not been performed thus far at the single-cell level.
In this cross-sectional analysis, we generated a single-cell transcriptomic dataset of peripheral blood mononuclear cells (PBMCs) from 46 manifest T1DM (stage 3) cases and 31 matched controls.
We surprisingly detected profound alterations in circulatory immune cells (1784 dysregulated genes in 13 immune cell types), far exceeding the count in the comparator systemic autoimmune disease SLE. Genes upregulated in T1DM were involved in WNT signaling, interferon signaling and migration of T/NK cells, antigen presentation by B cells, and monocyte activation. A significant fraction of these differentially expressed genes were also altered in T1DM pancreatic islets. We used the single-cell data to construct a T1DM metagene z-score (TMZ score) that distinguished cases and controls and classified patients into molecular subtypes. This score correlated with known prognostic immune markers of T1DM, as well as with drug response in clinical trials.
Conclusions
Our study reveals a surprisingly strong systemic dimension at the level of immune cell network in T1DM, defines disease-relevant molecular subtypes, and has the potential to guide non-invasive test development and patient stratification.
Type 1 diabetes mellitus (T1DM) is a prototypic endocrine autoimmune disease driven by chronic inflammatory responses against insulin-secreting pancreatic \(\beta\) cells, leading to insulin deficiency with life-long dependence on exogenous insulin treatment [ 1 ]. The disease is characterized by chronic inflammation with impaired homeostasis at the level of innate and adaptive immune cells. The existing therapies for T1DM are still limited in terms of long-term efficacy and addressing the underlying pathophysiology [ 2 , 3 ]. Patient heterogeneity has been considered a key factor in treatment response [ 2 ]. Moreover, data suggest that diverse immune cell types and signaling pathways contribute to T1DM pathology [ 4 ]. Thus, a comprehensive analysis of immune cell phenotypes, cell type-specific molecular traits and their potential variation across affected individuals is called for [ 5 ].
A related challenge is that T1DM-related molecular mechanisms are poorly understood in humans. Mechanistic studies are hindered by the fact that pancreatic islets, which are the primary target of autoimmune attack, are difficult to access in living individuals. However, multiple lines of evidence indicate that systemic immune cell dysregulation, including Treg and NK cells, precedes T1DM diagnosis, and is implicated in disease pathogenesis and progression [ 6 , 7 , 8 , 9 , 10 , 11 , 12 ]. Furthermore, fine-mapping of genetic risk factors indicates that T1DM-associated risk variants exert a significant impact on the transcriptomes of circulating immune cells [ 13 , 14 , 15 , 16 ]. Consequently, non-invasive approaches using peripheral blood samples have become an essential strategy for identification of markers related to the progression of the autoimmune disease.
Along this line, immunological studies of T1DM have greatly advanced the field, for example by highlighting the role of specific, disease-predisposing human leukocyte antigen (HLA) haplotypes [ 17 , 18 ], by revealing insulin, GAD-65, and IA-2A as well as \(\beta\) cells proteins as common autoantigens [ 19 , 20 , 21 ], and by identifying common aberrations in cell composition and gene expression in peripheral immune cells [ 6 , 7 , 22 , 23 , 24 , 25 ]. Of note, most studies have examined the pre-diabetic molecular immune signature of first-degree relatives (FDR) of T1DM subjects [ 26 ]. In contrast to T1DM cases in the general population (GP), FDR T1DM subjects exhibit a lower age at diagnosis [ 27 ] and are enriched for monogenic variants of significant impact [ 28 ]. Moreover, the vast majority of existing molecular analyses are based on analysis of bulk samples, which obscures the heterogeneity of immune cell types and cell states. Consequently, our understanding of molecular and cellular abnormalities in GP T1DM is still limited, as is our ability to identify patient subtypes.
Recently, single-cell RNA-seq (scRNA-seq) profiling of ex vivo (cultured) human pancreatic islets shed light on cellular-resolution gene expression signatures in T1DM [ 29 ]. However, since immune cells comprised only a minor fraction of cells identified, this study focused primarily on endocrine and exocrine cell types. Another study of T1DM-related immune cell phenotypes analyzed scRNA-seq data from PBMCs of 4 T1DM subjects and 4 controls and identified IL32 -expressing cells as a signature of T1DM [ 24 ]. In view of the current situation, there is an unmet need for a more comprehensive analysis of immune cells in T1DM.
We hypothesized that T1DM, an organ specific autoimmune disease, may involve systemic immune perturbation in cell type abundance as well as cell type-specific gene expression. In this cross-sectional study, we used the technique of scRNA-seq to characterize PBMCs from 46 islet-cell autoantibody-positive manifest T1DM cases (stage 3) and 31 healthy controls matched for age, gender, and presence of T1DM-associated risk HLA haplotypes. Our analysis revealed shifts in cellular composition as well as profound molecular aberrations in 12/14 peripheral immune cell types from T1DM subjects. Notably, we identified widespread upregulation of genes associated with immune cell activation, though regulon analysis indicated that this process may be driven by distinct transcription factors (TFs) in T, B, and myeloid cells. The upregulated and downregulated genes we identified in PBMC cell types showed significant overlap with transcriptome changes in T1DM pancreas, suggesting molecular links between systemic and organ-specific immune responses [ 18 , 29 ]. Our differentially expressed genes (DEGs) also showed significant overlap with markers that predict seroconversion (appearance of autoantibodies in peripheral blood) or T1DM onset in high-risk individuals. We also used the single-cell data to define cell type-specific metagene expression scores segregating cases and controls. Importantly, these metagene scores were highly correlated across cell types, even though the DEG sets were largely non-overlapping. To characterize immune variation across the cohort, we therefore aggregated scores across cell types to define a single composite score for each sample, which we defined as the T1DM metagene Z -score (TMZ score). The TMZ score for each individual correlated with GAD autoantibody (GADA) titer and the number of high-risk HLA haplotypes as well as with drug response in interventional clinical trials. Finally, we found that DEGs in effector T cells and B cells were enriched for T1DM genetic risk.
In summary, our case-control study shows unexpectedly strong systemic immune cell dysregulation in T1DM, defines disease-relevant T1DM molecular subtypes, and has the potential for non-invasive test development in T1DM.
Sample collection and cohort details
European stage 3 T1DM subjects were selected from a cohort of 1634 T1D patients from Baden-Wuerttemberg in Germany. The healthy controls matched for age, gender, and T1DM risk HLA haplotypes were selected from a population-based cohort of more than 8367 subjects, again from Baden-Wuerttemberg. To rule out the presence of an infection or any other inflammatory process, we selected subjects with CRP below 1 mg/L (high sensitivity C-reactive protein (hs-CRP); Roche Cobas 6000 analytical system). BMI was restricted within the range from 18.5 to 24.9 kg/m 2 , i.e., the WHO recommendation of a normal BMI for Europeans. Additional inclusion criteria were as follows: normal aspartate transaminase (AST) levels (below 33 units per liter (U/L) and alanine transaminase (ALT) levels (below 40 units per liter (U/L), normal creatine serum values (below 110 µmol/L for men, below 95 µmol/L for women) resulting in normal values of the estimated glomerular filtration rate (eGFR), normal TSH level (range 0.3–4 mU/l) indicative of normal thyroid function, TPO antibody negative, normal vitamin B 12 serum levels (range between 350 and 900 pg/ml), normal 25-OH Vit D levels (range between 30 and 70 µg/l), normal levels of plasma ferritin (ranges between 50 and 330 ng/mL) as a marker of a normal iron status as well as a surrogate marker of inflammation, and normal serum zinc levels (range between 70 and 120 µg/dL) to show an adequate nutritional status and to rule out problems absorbing nutrients.
All control subjects were negative for ICA, GADA, IA-2A, and ZnT8 antibodies. The T1D subjects were positive for at least 2 islet cell autoantibodies. All T1D subjects selected were GADA positive [ 30 , 31 ]. The Roche Elecsys C-Peptide assay was used to quantify fasting C-peptide levels in T1D subjects. All T1D subjects revealed fasting C-peptide levels below 0.01 ng/mL and were therefore considered as “C-peptide negative.” In addition, all T1D subjects were requiring insulin doses above 0.65 units of insulin per kg body weight per day. None of the T1D subjects had evidence of microalbuminuria as a marker of diabetic nephropathy or evidence of diabetic retinopathy. In subjects with T1DM, blood glucose during the blood collection had to be within the range of a predefined target, i.e., 80–140 mg/dl to exclude a potential impact of elevated glucose levels, altered plasma cortisol and catecholamine levels on immune cell counts. Also, HbA1c levels had to label a well-controlled diabetes status with a level below 7.5% (DCCT/NGSP; corresponding to a level below 58 mmol/mol (IFCC; SI unit) [ 32 , 33 , 34 ]. Since the counts of lymphocytes as well as lymphocyte subsets are known to be influenced by hormonal factors and temperature, to achieve pre-analytical consistency, the blood collection was only performed in the morning (8:00–10:00 am) at a fasting state during the seasonal period of spring until autumn. All technical procedures and protocols for the recruitment, blood collection, and PBMC isolation were reviewed and approved by the Institutional Review Board (IRB) at the Division of Endocrinology and Diabetes in Ulm University, Germany (IRB no. 299604393 R ) [ 35 ]. Accordingly, PBMCs were isolated from approximately 10 ml of heparinised whole blood using standard Ficoll-Hypaque density gradient centrifugation method. Isolated PBMCs were frozen in Fetal bovine serum (FBS) containing 10% dimethylsulfoxide (DMSO) and shipped to Genome Institute of Singapore for downstream experiments. The sequence-specific oligonucleotide DNA amplification method was utilized for HLA class II genotyping using HLA-DRB1 , HLA-DQA1 , and HLADQB1 specific primers [ 36 , 37 ]. Autoantibody assay for detection of two main autoantibodies in T1DM (i.e., GADA and IA-2A) was conducted based on previously established fluid-phase-antigen-binding assay [ 38 ]. The clinical information of all samples included in current study are summarized in the Additional file 2 : Table S1-S2.
Sample preparation and scRNA-seq
Frozen PBMCs samples were thawed in 10 ml of pre-warmed thawing media (RPMI 1640 supplemented with 5% human serum, 1% penicillin-streptomycin, and 1% glutamine). After centrifugation at 300g, the supernatant was removed and cells were resuspended in 10 ml of washing media (RPMI 1640 supplemented with 10% fetal bovine serum (FBS), 1% penicillin-streptomycin, 1% glutamine). Cells were washed two times with phosphate-buffered saline (PBS) containing 0.04% bovine serum albumin (BSA) and filtered through a 5-µm cell strainer. The cell concentration and viability were measured in trypan blue using the Bio Rad TC20 TM Automated Cell Counter (Bio-Rad). Droplet-based scRNA-seq was applied to the cohort samples using the single-cell 3′ reagent kit (V2, 10x Genomics), according to the manufacturer’s protocol. Single-cell suspensions from four samples (two cases and two healthy controls) were mixed at the final concentration of \(1 \times 10^{6}\) cells/ml. Before mixing samples, an aliquot of 2 million cells from each sample was centrifuged and kept at − 80 o C and used for DNA extraction and genotyping. Pooled cell suspensions were loaded onto the ChromiumTM Controller instrument at the recommended volume required for capturing 16,000 cells per pool. The cDNA amplification and library preparation were performed using the library construction kit (10x Genomics). Quality, size distribution, and quantity of generated cDNA and sequencing libraries were confirmed using the High Sensitivity DNA kit (Agilent) and Kapa kit (Illumina). After quality control, pooled libraries were diluted in final concentration of 10 nM and were sequenced on Illumina HiSeq 4000 to get on average read-depth of 80,000 reads/cell using following chemistry settings: read 1: 26 cycles; i7 index: 8 cycles; i5 index: 0 cycles; and read 2: 98 cycles.
SNP-array genotyping
Genomic DNA was extracted from 2 to 3 million PBMCs using QIAamp® DNA blood Mini Kit according to the manufacturer’s protocol, and samples were genotyped using the Illumina Infinium® HTS Assay. The intensity data files collected by the Illumina HiScan system were analyzed with the Illumina GenomeStudio software, and PLINK binary genotyping data were extracted for downstream analysis.
Multi-parametric FACS symphony
An antibody staining panel consisting of 23 markers was designed for immunophenotyping of PBMCs populations using a variety of fluorochromes with minimum spectral cross-talk. This panel includes 16 lineage markers (e.g., CD45, CCR7, CD19, IgD, IgM, IgG, IgE, IgA, CD5, CD3, TCRab, TCRgd, CD4, CD8, CD14, and CD16) for detecting different populations of B cells (e.g., naïve IgD + /IgM + B cells or switched IgG + /IgE + /IgA + memory B cells), T cells (TCRab + or TCRgd + T cells), and monocytes (e.g., classical and non-classical monocytes). In addition, seven markers (e.g., CD20, HLA-DR, CD37, CD83, and LAPTM5, CD69, CD78) were selected based on the single-cell data for gene expression validations. The list of all antibodies used in this experiment as well as their corresponding clone, company, and catalogue number are listed in Additional file 2 : Table S10. Frozen PBMCs were thawed as described, and aliquots of \(2 \times 10^{6}\) cells were used for antibody staining. In order to optimize the concentration of each antibody in the panel, a single staining titration experiment with different concentration of each antibody (1 µl, 2 µl, and 4 µl) was performed using aliquots of \(1 \times 10^{6}\) PBMCs derived from a healthy donor. For each antibody, the concentration at which the signal intensity gained the saturation point was selected as the optimal antibody concentration per million cells (Additional file 2 : Table S10). Except for CCR7 antibody which required a pre-incubation step at 37 °C for 10 min, all other antibodies were incubated with cells for 30 min at 4 °C. In order to avoid any cross binding between TCR and CD3 antibodies, a sequential staining strategy was applied for these antibodies. After incubation with fluorescence-conjugated antibodies, the cells were washed and re-suspended in buffer solution (PBS containing 5% FBS and 2 mM EDTA). The stained cells were kept on ice before loading into the FACS symphony machine and data acquisition. The single stained files from the optimal antibody concentration were used to establish the compensation matrix. Finally, immunophenotyping was performed using the BD Symphony® machine for 10 T1DM and 9 healthy samples (independent validation cohort) and a minimum of 50,000 events were acquired per sample (Additional file 1 : Fig. S4a).
Processing and clustering of FACS symphony data
The compensated flow cytometry data were gated in the FlowJo (V 10.6) software using the standard gating strategy for selecting cell population, singlets, live, and CD45 + immune cells. The compensated channel values of the final population (i.e., live, single, CD45 + cells) from case and control samples were exported as CSV files. Next, these files were imported into R, and after down-sampling of each sample into 10,000 single cells, they were concatenated into a Seurat object (Seurat package V3) containing 190,000 cells. The Seurat package functionalities for dimensional reduction using the Uniform Manifold Approximation and Projection (UMAP) method and the unsupervised graph based clustering using the Louvain algorithm were utilized to calculate UMAP embeddings and perform unsupervised clustering based on 12 lineage makers including CD3, TCRab, TCRgd, CD19, IgD, IgM, IgG, IgE, IgA, CD14, CD16, and HLADR (Additional file 1 : Fig. S4b-c).
Processing of genotyping data
The binary PLINK genotyping files were exported from the Illumina GenomeStudio software and converted into the VCF format using the PLINK toolkit [ 39 ]. The vcftool program [ 40 ] was used to filter out insertions/deletions (indels), multiallelic SNPs, and sex chromosome variants. The hg19 human reference genome was used as a template in vcftools to correct the reference and alternative alleles labels in the VCF file and to phase all genotypes. The Beagle toolkit [ 41 ] was utilized to perform the genomic imputation according to the human 1000 genome reference. The imputed variants with low confidence ( R 2 \(< 0.9\) ) were excluded, and the confidently imputed variants were concatenated into the genotyped variants. The final VCF file comprising genotyped variations (750k variants) and confidently imputed variants (3.2 million variants) was sorted and used for the demultiplexing of scRNA-seq data at sample level.
Demultiplexing and quality control of scRNA-seq data
The overall steps for data processing, quality control, and reference-based clustering of PBMCs ( n = 186,671) are represented in Additional file 1 : Fig. S1. The Cell Ranger (V2.0) (with hg19 as reference genome) and demuxlet tool kits [ 42 ] were used to demultiplex paired-end sequencing FASTQ files at the cell and sample level, respectively. As expected, a total of 28,498 cells (average doublet rate of 15.2%) were detected as cross-sample doublets by the demuxlet tool and were removed from the dataset (Additional file 1 : Fig. S1b, d). To check the fidelity of sample assignment by the Demuxlet, sum of average natural logarithm ( nlog ) expression of Y and X chromosome genes for cells of each sample were calculated and shown in a scatter plot, where each dot represents a sample colored based on the gender status obtained from the clinical data. As expected, the cells from female donors were clearly separated from cells from male donors as the former showed negligible (noise-floor) expression of Y chromosome genes (Additional file 1 : Fig. S1c). Genes with low coverage (expressed in less than 0.1% of cells) and cells showing higher than 7% mitochondrial gene expression were filtered out. Read counts were normalized and log transformed using the SCTransform normalization method implemented in Seurat package (V3.1) [ 43 ]. The RCA2 package [ 44 ] was used to perform reference-based clustering of cells into major immune cell types and apply cluster-specific cell filtering based on the distribution of number of detected genes (NODGs) across immune cell types. Accordingly, cells with \(750<\) NODG \(< 2500\) , \(750<\) NODG < 2750, and \(125<\) NODG \(< 1500\) were kept within clusters of pDCs, HSCs, and platelets, respectively. For rest of RCA clusters, cells with \(500<\) NODG \(< 2500\) were included. A total of 19,508 cells were excluded as noisy cells (dead cells, debris, potential undetected doublets) (Additional file 1 : Fig. S1a; Methods ). After removing potential doublets and low quality compromised cells, the DoubletFinder package [ 45 ] was used to detect and exclude 9498 cells as within-sample doublets (average within-sample doublet rate of 6.86%), resulting in a final clean QC-passed dataset of 129,167 single cells used in the downstream re-clustering, cell type annotation, and cleaning.
scRNA-seq clustering and cell type annotation
Reference-based RCA clustering method supervised by a combination of different immune panels from RCA2 (V2) package [ 44 ] was used for clustering of QC-passed single-cell PBMCs. The details of reference panels used in this study are described as follows: (1) Novershtern Panel : including 966 feature genes across 15 PBMC cell types (i.e., CD4 + T naive, CD4 + TCM, CD4 + TEM, CD8 + T naive, CD8 + TCM, CD8 + TEM, NK, B-naive, B-SM, C-monocyte, NC-monocyte, cDCs, pDCs, platelets, HSCs) from the Novershtern et al. study [ 46 ]; (2) Novershtern T Cell Panel : including 420 feature genes across 6 T sub-populations (i.e., CD4 + T naive, CD4 + CM, CD4 + EM, CD8 + T naive, CD8 + CM, CD8 + EM) from the Novershtern et al. study [ 46 ]; (3) Monaco TCell Panel : including 551 feature genes across 15 T and NK sub-populations [i.e., CD4 + naive, CD8 + naive, CD8 + CM, CD8 + EM, CD8 + T effector (TE), MAIT, NK, T follicular helper (TFH), T helper 1 (Th1), Th17, Th1/Th17, Th2, T-reg, VD2 negative, VD2 positive] from the Monaco, G., et al. study [ 47 ]; (4) Monaco B Cell Panel : including 436 feature genes across 5 B sub-populations (i.e., B-naive, B non-switch memory (BNSM), BSM, plasmablasts, B exhausted (Bex)] from the Monaco, G., et al. study [ 47 ]; (5) Monaco Myeloid Panel : including 775 feature genes across 5 myeloid sub-populations (i.e., C-monocyte, I-monocyte, NC-monocyte, pDC, cDC) from the Monaco, G., et al. study [ 47 ]. To perform reference-based clustering supervised by the reference panels, the dataProject function from the RCA2 package was used to project log transformed corrected UMI count of each single cell into each cell type in the immune reference panels by calculating the Pearson correlation coefficient between the nlog transformed (SCTransformed normalized corrected UMI counts) vector from scRNA-seq and log 10 expression vector from the reference bulk transcriptome across feature genes of the reference panel. This function generates the correlation coefficient matrix where each row is a cell type in the panel and each column is a single cell. After raising to the fourth power and row-wised z-transformation, the correlation matrix was used to calculate the correlation-based distance matrix. Next, the dataClust function from RCA2 (V2) package, which uses the internal dataClust function from fastcluster package, was used to apply the correlation-based distance matrix into average-linkage hierarchical clustering. It also takes advantage of the cutreeDynamic function from the WGCNA package to define cell clusters within the resulting dendrogram. To visualize the correlation-based distance matrix in a two-dimensional space, the UMAP dimensional reduction technique [ 48 ] was applied to the correlation-based distance matrix using the computeUMAP function from the RCA2 package. RCA clusters were annotated based on their correlation with immune cell types in each immune panels and expression of the canonical markers. Ambiguous RCA clusters with no correlation or strong correlation with more than one cell type in the panel were excluded from the dataset. First, in order to identify the major immune cell types, the QC-passed cells were projected into the Novershtern Panel resulting in 9 RCA clusters annotated as CD4 + T, CD8 + T, NK, B cell, monocyte, pDCs, platelets, HSCs, and erythrocytes (Fig. 1 b, middle panel; Additional file 1 : Fig. S2a). T/NK cells were further clustered into 11 T cell sub-populations by sequential projection into two T cell specific panels. First, T/NK cells were projected into the Monaco T Cell Panel where the cells with strong correlation with Treg, MAIT, gamma-delta T (VD2P), and NK cells were annotated accordingly and the remaining cells were projected into the Novershtern T Cell Panel resulting in six T sub-populations annotated as CD4 + T naive, CD4 + CM, CD4 + EM, CD8 + naive, CD8 + CM, CD8 + EM (Fig. 1 b, upper-right panel; Additional file 1 : Fig. S2c). B cells were projected into the Monaco B Cell Panel to identify and annotate three B sub-populations including B naive, BSM, and B plasma accordingly (Fig. 1 b, upper left panel; Additional file 1 : Fig. S2d). Likewise, projection of the monocyte/pDC cells into the Monaco Myeloid Panel revealed five myeloid sub-populations including C-monocyte, I-monocyte, NC-monocyte, cDC, and pDC (Fig. 1 b, lower right panel; Additional file 1 : Fig. S2e).
Cell composition enrichment analysis at gene expression space
To evaluate the enrichment of cells from T1DM patients in gene expression space across immune cell types, the nearest 50 neighbor cells around each single cell in the gene expression principal component analysis (PCA) space were collected, and the log 2 (fold-change ratio) of cells from T1DM samples relative to the cells from healthy subjects within nearest 50 neighbor cells was measured. These values were visualized for each single cell at the UMAP space.
Identifying marker genes of PBMCs’ cell types
To define the marker genes for each immune cell type compared with rest of the cells, the negative binomial generalized linear model implemented in FindAllmarker function from seurat package was used, while the sequencing library IDs (batch) and the disease status (healthy/T1DM) were added as variables to regress out. Only genes which were detected in at least 25% of the cells in any of the two testing groups were included in the model. Genes with average nlog (fold-change) higher than 0.25 and FDR q -value less than 0.05 were selected as the marker genes for each immune cell type.
Differential cell composition analysis
To identify cell composition aberrations with taking into account the effect of independent variables, a multiple regression analysis based on the general additive model (GAM) was applied using the gam function from the mgcv R package [ 49 ]. This model benefits from properties of both generalized linear (GLM) models and additive models [ 50 ]. Various independent variables, including disease status (T1DM = 1, healthy = 0), sex (male = 1, female = 0), number of T1DM risk HLA haplotypes, and the GADA titer were included into the model to estimate their effect on predicting the relative proportion of immune cell types (% of PBMC). The regression coefficients were estimated by the model and the F test statistics was applied to compute p -values. By default, the relative proportion is considered as Gaussian response. In all analysis, the p -value less than 0.05 was considered statistically significant.
Pseudo-bulk differential gene expression analysis
The DESeq2 (v.1.30.1) package was used to perform differential gene expression analysis on pseudo-bulk transcriptome profiles of T1DM and healthy samples. First, the pseudo-bulk expression matrices were calculated by summing UMI counts across cells as suggested by DESeq2. The shrunken log2 (fold-change) and standard error were measured by the ashr algorithm. Pseudo-bulk samples resulting from summing-up of less than 5 cells as well as genes that were detected in less than 5% of individuals were excluded from the analysis. Finally, genes with an absolute log 2 (fold-change) \(\ge log2(1.3)\) and FDR q- value (Benjamini-Hochberg) \(\le 0.05\) were defined as DEGs.
Pathway enrichment analysis of T1DM-associated DEGs
Both over-representation and gene set enrichment analysis (GSEA) were performed using the ClusterProfiler R package [ 51 ]. The enrichGO function was used to perform the over-representation analysis on the list of DEGs from each k-mean cluster with the following settings: OrgDb = org.Hs.eg.db, ont = “BP,” pAdjustMethod = “BH,” pvalueCutoff = 0.05, qvalueCutoff = 0.05, minGSSize = 10, maxGSSize = 500. To perform GSEA analysis for each cell type, the gseGO function was applied on the list of DEGs ranked by the differential expression score (DES) [ \(|log2 (Fold-Change)\vert \times -log10 (FDR \textit{q-value})\) ] using the following settings: OrgDb = org.Hs.eg.db, ont = “BP,” keyType = “ENTREZID,” pAdjustMethod = “BH,” pvalueCutoff = 0.05, exponent = 0, nPerm = 10, 000, minGSSize = 15, and maxGSSize = 500. The simplify function was used to simplify the enriched terms. The top 3 significantly enriched terms (ranked by NES) and the cell type-specific terms of each cell type were selected for visualization.
Single-cell regulon activity inference and differential regulon activity (DAR) analysis
The well-established Single-Cell rEgulatory Network Inference and Clustering (SCENIC) algorithm [ 52 ] was utilized to infer the regulon activity in single-cell PBMCs. The SCENIC pipeline with default settings was applied on the whole scRNAseq PBMC dataset and the final matrix of 260 inferred regulons (rows) with their corresponding regulon activity score (RAS) across 117,737 single cells (columns) was extracted as a Seurat object for the downstream analysis. Next, the unpaired Wilcoxon test, implemented in the Seurat FindAllmarker function, was used to identify the list of marker regulons within each immune cell type compared with the rest of the immune cells. For each cell type, the significant upregulated regulons [nlog (fold-change) > 0 and FDR q-value \(< 0.05\) )] were ranked based on their FDR q -values, and the top 5 regulons were selected as the marker regulons of that cell type. The same approach was used to identify the DARs between T1DM and healthy samples across immune cell types. Accordingly, within each cell type, all significant upregulated [nlog (fold-change) > 0] and downregulated [nlog (fold-change) \(< 0\) ] regulons with FDR q- value \(\le 0.05\) were selected as DARs in that cell type and were ranked based on their FDR q -value.
Gene overlap analysis
The GeneOverlap R package [ 53 ] was used to compare the level and significance of overlap between upregulated and downregulated DEGs of T/NK cells, B cells, and monocytes from the current study with reported DEGs from previous RNA-seq profiling studies in T1DM. To do so, first, the list of up and down regulated DEGs was fetched from three studies including (1) longitudinal bulk RNA-seq study of FACS-sorted T cells and PBMCs from seven genetically at-risk case-control pairs at 3, 6, 12, 18, 24, and 36 months of age [ 24 ]; (2) bulk RNA-seq of human pancreatic \(\beta\) cells isolated from 4 T1DM and 12 healthy donors [ 18 ]; and (3) scRNA-seq of macrophages within human cultured islets isolated from 5 T1DM and 11 healthy donors [ 29 ]. The newGOM function from the GeneOverlap package was used to apply the Fisher exact test and calculate the statistical FDR q -value (Benjamini-Hochberg) of intersections.
TMZ score analysis
For each cell type, sample-level pseudo-bulk expression of DEGs were z -transformed across samples, and TMZ score was calculated by the following equation:
where i is the cell type i . The \(z_{ij}\) and \(z_{ik}\) represent the expression z -score for genes j and k in cell type i , respectively. Finally, \(U_i\) and \(D_i\) indicate the set of upregulated and downregulated DEGs in cell type i , respectively. The norm notation denotes the size of the set (i.e., the number of upregulated or downregulated genes in that cell type). Lastly, the average TMZ score is simply the mean of TMZ score across all cell types.
TMZ score validation method
After TMZ score is calculated as explained, to accommodate calculation of TMZ score for bulk RNA-seq from other dataset, DEGs coming from each cell types were grouped into 3 major cell types namely, T/NK, B, and monocytes and 3 TMZ scores were created. The set of upregulated and downregulated DEGs from each groups were used to measure the TMZ score for bulk RNA-seq data like in Fig. 5 e and Additional file 1 : Fig. S7c. Using the predetermined set of genes for each group, the TMZ scores are calculated on the bulk expression data. Subsequently, the retrieved TMZ scores are then quantile transformed per sample to scale the data to the same distribution as in the previously calculated TMZ scores in our single-cell dataset while preserving its gene expression ranking. This method of calculation allows for any new genetic information coming from only as few as 1 sample to have its own TMZ score referenced to our T1D single-cell expression data.
T1DM GWAS enrichment analysis
T1DM GWAS summary statistics fetched from the most recent comprehensive study covering 61,947,369 genetic variants with 37,652,754 single-nucleotide polymorphism (SNPs) passed the general QC in 520,580 European samples (T1DM = 18,942; healthy = 501,638) [ 54 ]. T1D-associated DEGs were ranked based on differential expression score (DES) [ \(|log2(Fold-Change) \vert \times -log10 (FDR \textit{q-value})\) ]. Genes with q-value \(< 0.1\) were filtered out and DES of remaining genes were normalized using min-max normalization method. Next, for each cell type, the ranked normalized DES values were inputted into CELLECT tool [ 55 ] to quantify their association with T1DM polygenetic GWAS signal (T1DM heritability) using MAGMA covariate analysis [ 56 ] with MAGMA window size = 100 kb. Thereby, CELLECT identified cell types whose DEGs were significantly correlated with T1DM heritability and genes that were in the top 200 MAGMA-inferred T1DM GWAS genes and top 30 percentile of normalized DES were set to be the definition of effector genes listed in Fig. 6 b.
Reference-based multi-resolution clustering of PBMCs reveals altered cell populations in T1DM
We leveraged the demuxlet protocol for pooled scRNA-seq [ 42 ] to profile gene expression in single PBMCs from stage 3 46 T1DM and 33 healthy samples matched for age, gender, and T1DM-associated HLA haplotype (Fig. 1 a). After strict QC to discard low-quality cells and doublets ( Methods ), we obtained transcriptomic data on 117,737 PBMCs. All samples were collected from European donors at Ulm University Medical Centre [ 35 ]( Methods ). In brief, PBMC samples from fasting blood taken from well-controlled insulin-dependent subjects with autoimmune diabetes were used in this study. Disease duration was consistent across the majority of the cohort, ranging from 1 to 4 years in 70 percent of subjects. All T1DM cases were negative for C-peptide and were undergoing insulin treatment. Thus, none of the subjects met the criteria for the partial remission (see Methods for details of inclusion and exclusion criteria). Detailed clinical information including gender, age at blood collection, age at disease onset, GADA titer, and T1DM-associated HLA haplotypes is provided in Additional file 2 : Table S1.
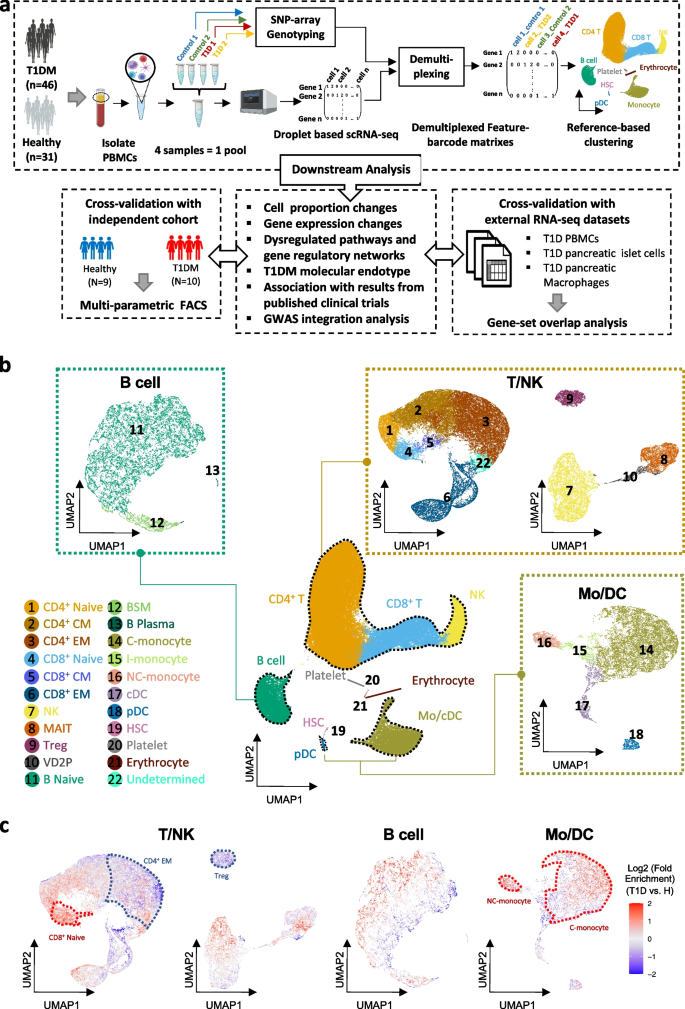
Multi-resolution clustering of PBMCs in T1DM. a Schematic representation of the study design for scRNA-seq and multi-parametric FACS profiling and data analysis of T1DM patients and healthy controls. b UMAP visualization of single cells in gene expression space. Central UMAP plot: low-resolution reference-based clustering to identify the three major immune compartments (T/NK, B, and Mo/DC). Each major cell type was then clustered individually at higher resolution, again using reference panels, to define 22 PBMC cell types. CM, central memory; EM, effector memory; Treg, regulatory T; MAIT, mucosal-associated invariant T; VD2P, gamma-delta T; NK, natural killer; BSM, B switched memory; C-monocyte, I-monocyte, and NC-monocyte: classical, intermediate, and non-classical monocyte; cDC, pDC, conventional, plasmacytoid dendritic cell; HSC, hematopoietic stem cell. c UMAP plot showing fold enrichment of T1DM vs healthy cells at each location in gene expression space. Red, blue: enriched, depleted in T1DM
To minimize batch effects in jointly clustering and annotating cells, we applied a reference-based clustering method, Reference Component Analysis version 2 (RCA2, Methods ) [ 44 , 57 ]. First, using a reference panel of transcriptomes of major immune cell types [ 46 ], we identified and annotated nine high-level cell clusters that were well separated in gene expression space (Fig. 1 b, middle panel, Additional file 1 : Fig. S2a): CD4 + T, CD8 + T, NK, B, monocyte/conventional dendritic cells (Mo/cDC), plasmacytoid dendritic cells (pDCs), hematopoietic stem cells (HSCs), erythrocytes, and platelets. We excluded the three least abundant clusters (HSC, platelet and erythrocyte) and sought to identify sub-populations within the abundant cell types by first merging them into three immune compartments: T/NK, B, and Mo/DC (Mo/cDC plus pDC). Then, in a second, high-resolution round of cell type annotation, we clustered cells from each of the three compartments separately using additional reference panels ( Methods ), resulting in a total of 22 cell types, 21 of which could be annotated using canonical markers (Fig. 1 b; Supp. Fig. Additional file 1 : Fig. S2b-e, i; Methods ). All clusters contained cells from all samples and pooled batches, suggesting that the clustering was mainly driven by biological differences between cell types rather than batch effects or sample specificity (Additional file 1 : Fig. S2f, g).
Next, we investigated the enrichment of immune cells from T1DM patients relative to healthy controls at each location in gene expression space (Fig. 1 c; Additional file 1 : Fig. S3a, e, i; Methods ). We observed depletion of Treg and CD4 + EM cells, as well as enrichment of CD8 + naive T cells, C-monocytes, and NC-monocytes in T1DM (Fig. 1 c, Additional file 1 : Fig. S2h). These results suggest that there could be systematic compositional changes in circulating immune cells in T1DM, particularly at the level of cell sub-populations.
Cell composition changes of peripheral immune cells in T1DM
To systematically investigate cellular composition aberrations of peripheral immune cells in T1DM, we used linear multiple regression analysis with sex [ 58 ] and number of T1DM risk HLA haplotypes as confounders and cell type proportion (% of total PBMCs) as the dependent variable ( Methods ).
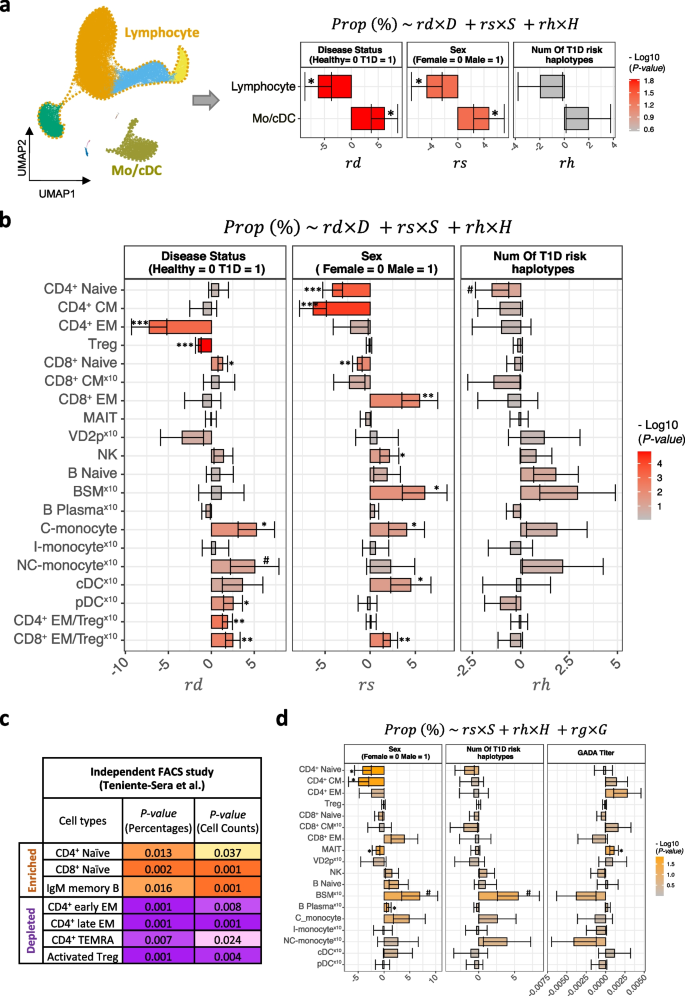
Cell composition alterations of peripheral immune cells in T1DM. a Bar plot of coefficients from multiple regression analysis of lymphocyte and Mo/cDC cell proportion (fraction of total PBMCs) against disease status, sex, and number of T1DM risk HLA haplotypes: 46 T1DM and 31 healthy samples. Error bars: standard error. b Same as a , for the 18 most abundant PBMC sub-populations, as well as the proportion ratios CD4 + EM/Treg and CD8 + EM/Treg. For easier visualization, the smaller values are multiplied by 10 (indicated with a superscript above the cell type label). c Cell composition changes from an independent multi-parametric FACS study of lymphoid cell types in T1DM [ 25 ]. Cell types that showed significant changes in both relative percentage and absolute cell count are shown. P -values from the original study: Mann-Whitney test. d Similar to b , on T1DM subjects alone, with GADA titer included as an additional independent variable. # p \(\le 0.09\) , * p \(\le 0.05\) , ** p \(\le 0.01\) , *** p \(\le 0.001\)
First, we examined compositional changes at the lowest level of resolution, by focusing on two broad compartments: lymphoid and Mo/cDC (Fig. 2 a). Interestingly, the proportion of Mo/cDC cells increased significantly in T1DM, while the proportion of lymphoid cells decreased. Note that these two compartments together account for the vast majority of PBMCs, and thus one would expect that their relative proportions would shift in opposite directions. The Mo/cDC proportion was also significantly higher in male subjects, independent of T1DM status [ 58 ].
Next, we examined T1DM-associated composition changes at higher resolution, for the 18 most abundant PBMC sub-populations (Fig. 2 b). Compared to controls, we noticed significant aberrations in the proportion of 5 immune cell types. CD4 + EM cells were strongly depleted in T1DM PBMCs, perhaps due to their recruitment to the para-pancreatic lymph nodes and pancreatic islets where they are shown to establish immunological synapses with antigen presenting cells (i.e., pDCs, B cells, and \(\beta\) cells) and mediate the inflammatory responses against \(\beta\) cells autoantigens [ 59 , 60 , 61 ]. Defects in immune suppressing function of Tregs have been reported in T1DM and other autoimmune disease [ 62 , 63 , 64 ]. Consistently, our scRNA-seq data revealed significant decrease in relative proportion of Tregs in PBMCs of T1DM patients (Fig. 2 b), suggesting that, in addition to functional changes in Tregs, a reduction in their abundance could potentially contribute to the loss of peripheral tolerance in T1DM. Interestingly, in line with previous studies reporting higher ratio of naive/effector T cells in peripheral blood of T1DM patients [ 25 , 61 ], we also identified enrichment of CD8 + naive T cells in PBMCs of T1DM patients (Fig. 2 b). This observation supports the extensive literature showing a crucial role for CD8 + T cells in destructing of \(\beta\) cells during T1DM pathogenesis [ 61 , 65 , 66 , 67 ]. Finally, we observed a higher proportion of C-monocytes and pDCs in peripheral blood of T1DM patients (Fig. 2 b). We note that some of these T1DM-associated cell population changes may overlap those associated with aging [ 68 ].
We also examined the ratio of CD4 + and CD8 + EM cells to Treg cells, as a measure of T cell activation. Interestingly, both ratios were significantly elevated in T1DM (Fig. 2 b). Consistent with previous studies [ 58 ], sex was again a significant confounding factor for multiple PBMC sub-populations. However, the number of HLA risk haplotypes had no significant effect.
Consistently, our multi-parametric FACS profiling of 190,000 cells from of 10 stage 3 T1DM and 9 healthy samples (10,000 cells per sample) (Additional file 1 : Fig. S4a-c; Methods ) validated the depletion of CD4 + T cells in T1DM ( p- value = 0.037, Additional file 1 : Fig. S4d-e). Likewise, it showed a trend towards higher proportions of CD8 + T and C-monocyte cells (Additional file 1 : Fig. S4d-e). The cell composition shifts observed in our analysis were also supported by an independent multi-parametric FACS study [ 25 ] of B and T lymphocytes in T1DM. Consistently again, this study reported significant depletion of CD4 + early, late, and terminally differentiated EM (TEMRA) T cells as well as activated Tregs (Fig. 2 c). Enrichment of CD8 + naive T cells in T1DM was also supported. These results from FACS profiling validate and support our conclusions from scRNA-seq that specific peripheral cell proportions are significantly altered in T1DM. Nevertheless, our scRNA-seq results encompass a far larger set of markers than can be analyzed using low-plex methods such as FACS.
We then examined the consistency of our results from single-cell analysis of peripheral immune cells with previously reported cell composition changes in relevant tissues, namely T1DM pancreas and para-pancreatic lymph nodes. In line with our own finding that Treg cells were depleted in T1DM peripheral blood, depletion of this cell type was reported as a key feature of para-pancreatic lymph nodes in stage 3 T1DM [ 63 ]. Furthermore, two independent imaging mass cytometry studies revealed an excess of macrophages in stage 3 T1DM pancreatic islets, which may relate to the excess of circulating C-monocytes and NC-monocytes observed in our study. In contrast to the depletion of CD4 + EM T cells in circulation, the overlapping CD4 + helper T cell population was enriched in T1DM islets, suggesting recruitment of these cells to pancreas in the disease state [ 69 , 70 ].
We also investigated concordant signatures between PBMC cell composition changes in organ-specific (manifest T1DM, our study) and systemic autoimmune disease (established systemic lupus erythematosus (SLE)) [ 71 ]. In both T1DM and SLE, the ratio of lymphocytes to Mo/cDC cells was reduced relative to controls. Along the same lines, C-monocytes were enriched in both autoimmune diseases (Additional file 1 : Fig. S5a). However, there were also notable differences. For example, CD4 + naive T cells were depleted in SLE, whereas we observed depletion of Treg and CD4 + EM T cells in T1DM. Similarly, we observed enrichment of CD8 + naive T cells and pDCs in T1DM, but no corresponding shifts were observed in SLE. Thus, the systemic shifts in cell composition we observed in the organ-specific autoimmune disease T1DM were similar in some respects to, but also substantially different from, those seen in SLE.
Next, we analyzed the association of cell composition in T1DM with sex and two clinical features: number of HLA risk haplotypes and GADA titer. We found a positive correlation between GADA titer and the proportion of MAIT ( p = 0.026) and CD4 TEM cells ( p = 0.086), suggesting a possible role for these cell types in GAD autoimmunity (Fig. 2 d).
Cell type-specific gene expression changes of peripheral immune cells in T1DM
To identify transcriptome aberrations in peripheral immune cell types in T1DM, we performed pseudo-bulk differential gene expression analysis between the 46 cases and 31 control samples using DEseq2 [ 72 ] ( Methods ). Since we observed high overlap between DEGs of naive, CM, and EM T sub-populations within CD4 + T cells (data not shown), we merged these three sub-populations into a single CD4 + T cluster. The same approach was used to collapse cell sub-populations within CD8 + T cells. Remarkably, 1784 genes were identified as differentially expressed in at least one cell type ( \(|log2(Fold-Change) \vert \ge log2(1.3)\) ; FDR q- value \(\le 0.1\) ), comprising 1093 upregulated and 691 downregulated DEGs (Fig. 3 a; Additional file 1 : Fig. S3b, f, j; Additional file 1 : Fig. S6a; Additional file 2 : Table S3). Our FACS panel for validating cell composition shifts included 8 of these DEGs, all of which showed protein expression changes in a direction consistent with transcriptome analysis, with 3/8 also showing statistical significance (Additional file 1 : Fig. S4f). Interestingly, NK, Treg, CD8 + T cells comprised the top three cell types in terms of number of DEGs in T1DM (Fig. 3 b), and \(> 300\) genes were upregulated in each of these cell types. This indicates long-lasting systemic immune aberrations after type 1 disease manifestation, most prominently (though not exclusively) in cytotoxic cells and Treg cells that modulate their activity.
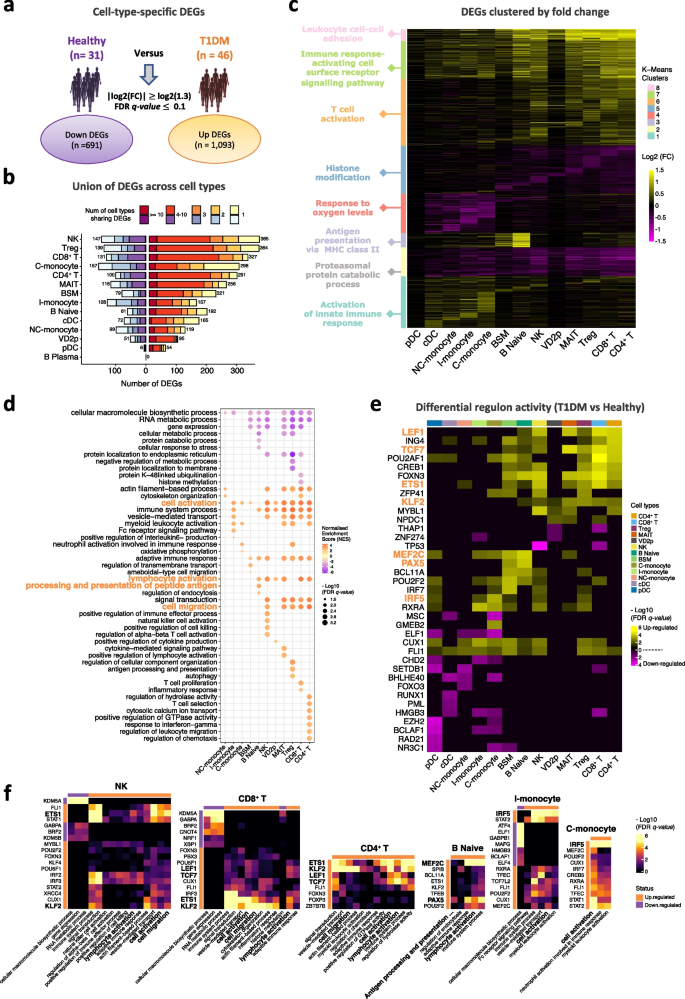
Cell type-specific gene expression changes in T1DM. a Total number of unique upregulated and downregulated DEGs in 14 peripheral immune cell types: 46 T1DM samples vs. 31 healthy. b Bar plot representing the number of DEGs within each cell type, with colored segments indicating the number of cell types that share the same DEG. c Heatmap of T1DM vs healthy log 2 (fold-change) of 1784 DEGs ( \(691+1093\) ) across all immune cell types. Genes are clustered by their fold-change vectors using k-means. Each gene cluster is annotated by the most significantly enriched Gene Ontology (GO) term. d Gene set enrichment analysis (GSEA): dot plot of top shared and cell type-specific biological processes enriched in DEGs of each PBMC cell type, colored by normalized enrichment score (NES). Dot size: \(-log10\) (FDR q- value). e Differential regulon activity: heatmap of transcription factor regulons with increased or decreased activity in T1DM (union of top 5 regulons in each of the 13 peripheral immune cell types). Color indicates \(-log10\) (FDR q- value) of regulons upregulated (yellow) and downregulated (purple) in T1DM. f Overlap between GSEA leading-edge genes and significantly differential regulons in 6 peripheral cell types. Color indicates \(-log10\) (FDR q- value) of overlap (Fisher’s exact test)
To identify gene modules among T1DM-associated DEGs, we clustered them by their cell type-specific log 2 (fold-change) profile using k-means. This yielded 8 major DEG modules, most of which were preferentially altered in specific cell types. We annotated DEG clusters based on their most significantly enriched GO terms (Fig. 3 c, Additional file 2 : Table S4) ( Methods ). Notably, all three major cell lineages showed upregulation of the corresponding activation markers: “T cell activation” in T cells (cluster 6), “antigen presentation via MHC class II” in B cells (cluster 3), and “activation of innate immune responses” in myeloid cells (cluster 1). Consistently, gene set enrichment analyses (GSEA) of DEGs in each cell type ( Methods ) highlighted the enrichment of several overlapping biological terms including “cell activation” enriched in upregulated DEGs of most immune cell types and “processing and presentation of peptide antigens” enriched in up-DEGs of B cells. Furthermore, we observed significant association of T/NK up-DEGs with the term “cell migration” (Fig. 3 d, Additional file 2 : Table S5-S6). These results imply broad immune activation responses across PBMC cell lineages. However, the corresponding sets of DEGs were not entirely the same in T/NK, B, and monocyte populations (Additional file 2 : Table S5-S6). These observations may suggest higher migratory potential of T/NK cells in T1DM, which may explain their active recruitment to the pancreatic islets and parapancreatic lymph nodes [ 63 , 69 , 70 ].
Next, we compared systemic transcriptomic aberrations in organ-specific (T1DM) and systemic (SLE) autoimmune diseases [ 71 ]. Interestingly, peripheral lymphoid and myeloid DEGs in T1DM significantly overlapped with the corresponding SLE DEGs (Additional file 1 : Fig. S5b). These overlapping DEGs imply a shared loss of immune cell homeostasis in the two autoimmune diseases. For example, interferon gamma response genes were enriched in shared up-DEGs of T/NK cells, and myeloid cell activation genes were enriched in shared monocyte up-DEGs (Additional file 1 : Fig. S5c, d, Additional file 2 : Table S7). However, some functional perturbations appeared to be specific to T1DM up-DEGs, such as antigen processing and presentation capacity by B cells, driven by over-expression of a MHC class II cluster including HLA-DM , HLA-DO , HLA-DRB1 , HLA-DQB1 / HLA-DQA1 , CD74 , and CTSS [Fig. 3 d; Additional file 1 : Fig. S6b; [ 71 ]]. Thus, these results suggest significant similarities in the systemic immune response in T1DM and SLE, but also notable differences.
We then leveraged the SCENIC algorithm [ 52 ] ( Methods ) to identify transcription factors (TFs) that may contribute to gene and pathway dysregulation in T1DM. As a positive control, we first confirmed that the regulons (target genes) of canonical cell type marker TFs were upregulated in their corresponding cell types (Additional file 1 : Fig. S6c; Methods ). We then identified differentially active regulons (DARs) in T1DM versus healthy controls across the 13 immune cell types ( Methods ). We detected 123 significantly altered regulons (60 up, 63 down; FDR q- value \(\le 0.05\) ) in at least one immune cell type (Additional file 1 : Fig. S6d; Additional file 2 : Table S9). Notably, we identified several instances of cell type-specific regulon activation in T1DM: TCF7 , LEF1 , ETS1 , and KLF2 in T/NK cells, MEF2C and PCX5 in B cells, and IRF5 in monocytes (Fig. 3 e, orange; Additional file 1 : Fig. S3c, g, k). To identify the TFs contributing to dysregulation of specific functional categories, we intersected the corresponding regulons with leading-edge DEGs of enriched GSEA terms in each immune cell type ( Methods ). This analysis revealed that distinct TFs may drive cell activation in each cell type: TCF7 , LEF1 , and ETS1 in T/NK cells, IRF5 in monocytes, and PAX5 and MEF2C in B cells (Fig. 3 f; Additional file 1 : Fig. S3d, h, i). Furthermore, we identified KLF2 as the master TF driving upregulation of cell migration genes in T/NK cells (Fig. 3 f; Additional file 1 : Fig. S3d) [ 73 , 74 ]. Analysis of the signaling pathways upstream of these TFs that orchestrate T1DM-associated immune cell phenotypes may reveal new insights into the pathobiology of this autoimmune disease.
Overlap with prognostic expression signatures and transcriptome changes in T1DM pancreas
We hypothesized that some of the systemically altered immune cell genes in long-term T1DM could be perturbed even before disease onset. We therefore examined prognostic markers of seroconversion and T1DM onset previously identified in PBMCs of genetically at-risk infants [ 24 ] ( Methods ). Remarkably, these prognostic markers were significantly enriched for overlap with our T/NK up-DEGs (Fig. 4 a, b). The overlapping genes were prominently associated with cytokine production and cytokine-mediated signaling ( TXK , HLA-F , ANXA1 , IL32 , FCER1G , IL7R , CARD8 , CPNE1 , BTN3A2 , and CD8B ), suggesting that they may be involved in production and secretion of inflammatory cytokines by T/NK cells in the pre-disease state (Fig. 4 c; Additional file 2 : Table S8). In summary, numerous transcriptome aberrations of circulating immune cells in young adults with T1DM reflect continuation of pathogenic immune activation processes present at earlier stages of disease development.
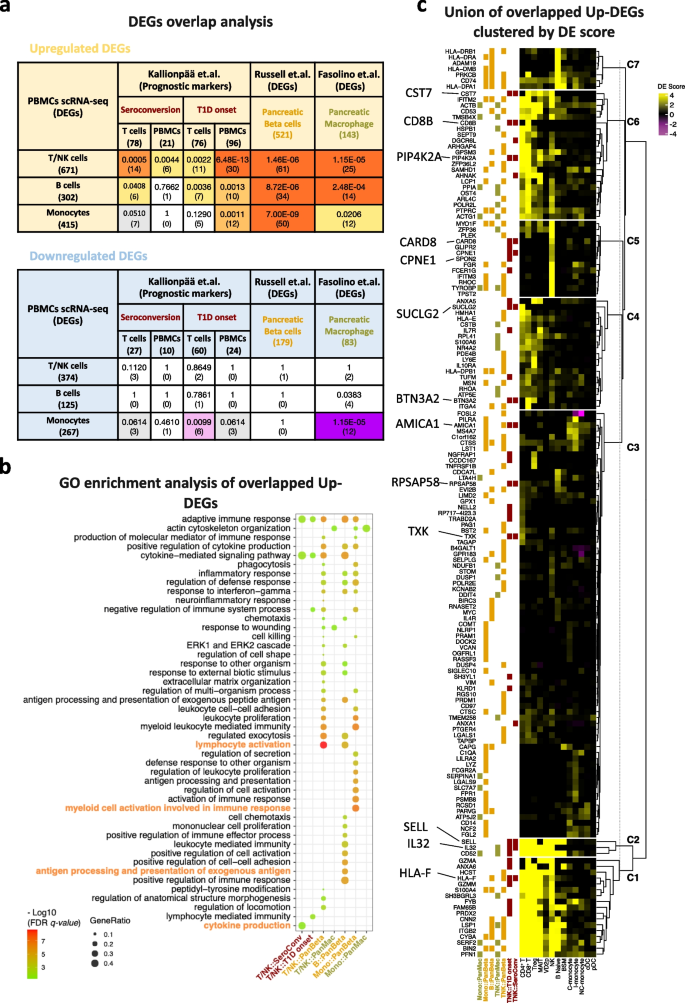
Overlap with prognostic expression signature and transcriptome changes in T1DM pancreas. a Overlap between T1DM versus healthy DEGs in PBMC cell types (this study) and prognostic markers and DEGs identified in other T1DM studies. Kallionpaa et al.: prognostic markers of seroconversion and T1DM onset. Russell et al., Fasolino et al.: T1DM versus healthy DEGs in primary pancreatic \(\beta\) cells, cultured pancreatic macrophages. Statistical significance of overlap [Fisher’s exact test, \(-log10\) (FDR q-value )] is indicated, with number of overlapping genes in parentheses. b Gene Ontology (GO) enrichment analysis: dot plot of biological processes enriched in overlapping up-DEGs in a colored by \(-log10\) (FDR q-value ). T/NK::SeroConv: T/NK DEGs that overlap prognostic markers of seroconversion in T cells or PBMCs ( n = 20); T1DM onset: union of prognostic markers of T1DM onset in T cell and PBMCs; PanBeta: DEGs of pancreatic \(\beta\) cells; PanMac: DEGs of pancreatic macrophages. Dot size: percentage of total DEGs in the given GO term. c Heatmap of differential expression (DE) scores [ \(|log2(Fold-Change) \vert \times -log10 (FDR \textit{q-value})\) ] for union of overlapping up-DEGs in a . DEGs are clustered by k-means into seven clusters (C1-7)
Next, we asked whether the peripheral gene expression changes in T1DM were also reflected in the affected tissue, namely pancreatic islet cells [ 18 , 29 ]. Indeed, T1DM versus healthy DEGs in pancreatic \(\beta\) cells and pancreatic macrophages were highly enriched in peripheral up-DEGs of all three PBMC lineages (Fig. 4 a, b). Consistently, the DEGs shared by pancreatic \(\beta\) cells and PBMC were enriched for roles in lymphocyte (e.g., ZFP36L2 , HLA-E , ITGB2 , LGALS1 , TNFRSF1B ) and myeloid (e.g., CD14 , CTSC , LYZ , FGR , LGALS9 ) cell activation and antigen presentation by B cells (e.g., CD74 and several HLA genes) (Fig. 4 c, orange; Additional file 2 : Table S8). Importantly, 9 of the overlapping genes have genetic associations with T1DM risk: GPSM3 , HLA-DMB , HLA-DPA1 , HLA-DPB1 , HLA-DRA , HLA-DRB1 , HLA-F , LST1 , RPL41 . These results suggest significant similarity between dysregulated genes and pathways in circulatory immune cells and pancreatic islets in T1DM and support the relevance of systemic immune changes to the pathophysiology of T1DM pancreas.
Transcriptome aberrations of peripheral immune cells define clinically relevant T1DM subtypes
We then asked if the expression of T1DM-associated DEGs could be used to define patient subtypes. First, in each cell type of each sample, we summarized the pseudo-bulk expression levels of the corresponding DEGs into a single TMZ score ( Methods ). As expected, this score largely segregated cases and controls. Remarkably, despite substantial differences between the 13 cell type-specific DEG sets, the corresponding 13 TMZ score were highly correlated (Pearson correlation coefficient \(> 0.9\) ; Additional file 1 : Fig. S7a). This result suggests that, in any individual sample, the 13 T1DM-associated molecular programs are activated to approximately the same extent (after z -score normalization). Thus, all 13 cell types are equally indicative of the strength of the systemic immune alteration in T1DM. We then clustered samples by their 13-dimensional TMZ score vectors into three groups to define molecular subtypes of T1DM representing high, intermediate, and low systemic immune response, respectively (Fig. 5 a).
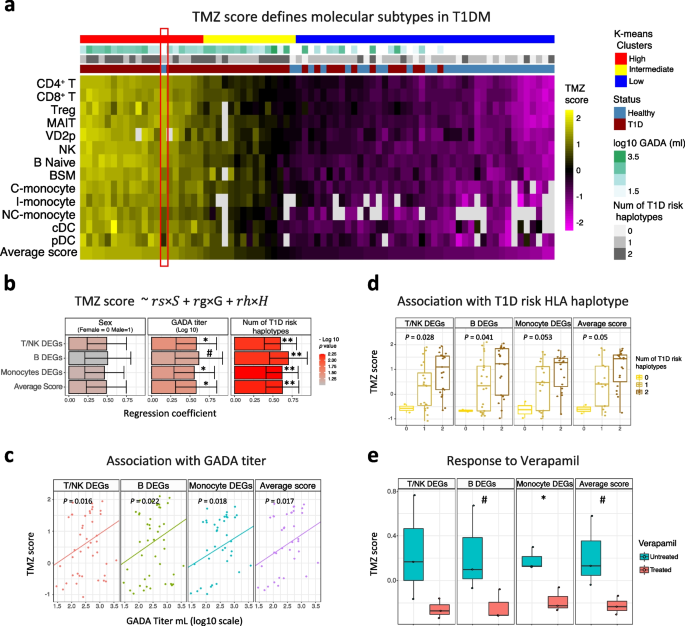
Transcriptome aberrations of peripheral immune cells define T1DM endotype. a TMZ score summarizing pseudo-bulk expression of T1DM-associated DEGs in each of 13 immune cell types: 46 T1DM and 31 Healthy. Samples were clustered by their TMZ score profiles using k-means. Missing values indicate insufficient cell count. b Bar plot of coefficients from multiple regression analysis of TMZ score against gender, GADA titer, and number of T1DM risk HLA haplotypes: 46 T1DM samples. Cell types avg: average of z -score across all cell types. Error bars: standard error. c Scatterplot showing the association of TMZ score with GADA titer across 46 T1DM samples. p- value: Spearman rank correlation. d Box plot representing TMZ score variation within each T1DM risk haplotype category. p- value: Kruskal-Wallis one-way ANOVA. e Effect of verapamil treatment [ 75 ] for 24 h versus untreated controls on TMZ score of human cultured islets. p- value: paired t -test. b , e # p \(\le 0.1\) , * p \(\le 0.05\) , ** p \(\le 0.01\)
As expected, almost all healthy controls (29/31) belonged to the low-response T1DM subtype. Intriguingly, 13/46 T1DM cases were also assigned to the same subtype suggesting that a subset of diagnosed individuals may have relatively low systemic immune response, towards the upper end of healthy range (Fig. 5 a). On the other hand, the intermediate- and high-responding subtypes were almost exclusively T1DM cases (33/35). Of note, the high-response group included one healthy control participant (Fig. 5 a, red box). Remarkably, this participant was subsequently diagnosed with T1DM, 4 years after blood collection. Although anecdotal, this observation raises the possibility that systemic immune alterations may precede the development of insulin-dependence. Overall, these results suggest that T1DM associated DEGs of peripheral immune cells could be used to define molecular subtypes of T1DM.
Next, we asked if the observed variation in TMZ score across patients could be associated with two widely used clinical parameters: the number of T1DM risk haplotypes and GADA titer. Multiple linear regression analysis ( Methods ) revealed significant association of GADA titer as well as T1DM risk HLA haplotypes with TMZ score (Fig. 5 b–d; Additional file 1 : Fig. S7b). However, when we included age at diagnosis as an additional independent variable in the regression model for TMZ score, we did not find any significant association with this covariate (Additional file 1 : Fig. S7b). This result could potentially be attributable to the fact that subjects with early onset T1DM (onset below 13 years) represented only a minority of our cohort (14/46, \(30\%\) ).
Encouraged by the correspondence to clinical parameters, we asked if the TMZ score could be perturbed by treatment with immunomodulatory drugs in a non-antigen-specific manner. Specifically, we hypothesized that these drugs may reduce the TMZ score, i.e., they could move the immune profile of afflicted individuals closer to that of healthy controls. To test this hypothesis, we calculated TMZ score using bulk PBMCs transcriptome data from clinical trials for three drugs, namely teplizumab (anti-CD3) [ 76 ], abatacept (CTLA4 ig) [ 77 ], and rituximab (anti-CD20) [ 78 ] ( Methods ). In addition, we examined bulk transcriptome data from cultured pancreatic islets treated with verapamil [ 75 , 79 ], relative to untreated controls. Verapamil, a drug that improved mixed-meal-stimulated C-peptide area under the curve and reduced the insulin requirement in a phase II trial [ 79 ], also reduced the TMZ score based on monocyte DEGs and had a marginally significant effect on the overall TMZ score (Fig. 5 e; Additional file 1 : Fig. S7c-e). While teplizumab had no detectable influence on the TMZ score, long-term treatment with abatacept, a drug designed to suppress T cell response, reduced the T/NK cell TMZ score. Rituximab, a drug targeting B cells, strongly reduced the B cell TMZ score on day 26, 4 days after the last dose was administered. These results suggest that the TMZ score could potentially be used to monitor drug response in pre-clinical and clinical T1DM studies and also as a readout in high-throughput screens.
Cell type-specific DEGs enriched for T1DM genetic risk
It is possible that some of the systemic immune DEGs detected in our single-cell analysis could be a consequence of chronic hyperglycemia, rather than linked to stage 3 T1DM. To prioritize cell types whose differential expression associates with disease causation, we used the CELLECT tool [ 55 ] to correlate the T1DM risk scores of genes (inferred from GWAS summary statistics [ 52 ]) with their cell type specific differential expression in T1DM versus healthy ( Methods ). This analysis highlighted DEGs in adaptive immune cell types as significantly enriched for T1DM genetic risk. DEGs in B naive cells showed the highest association with T1DM heritability, driven almost exclusively by multiple risk variants of strong effect in the HLA locus (Fig. 6 a upper panel, b). This result indicates the crucial role of antigen presentation by B cells in T1DM pathogenesis [ 80 ].
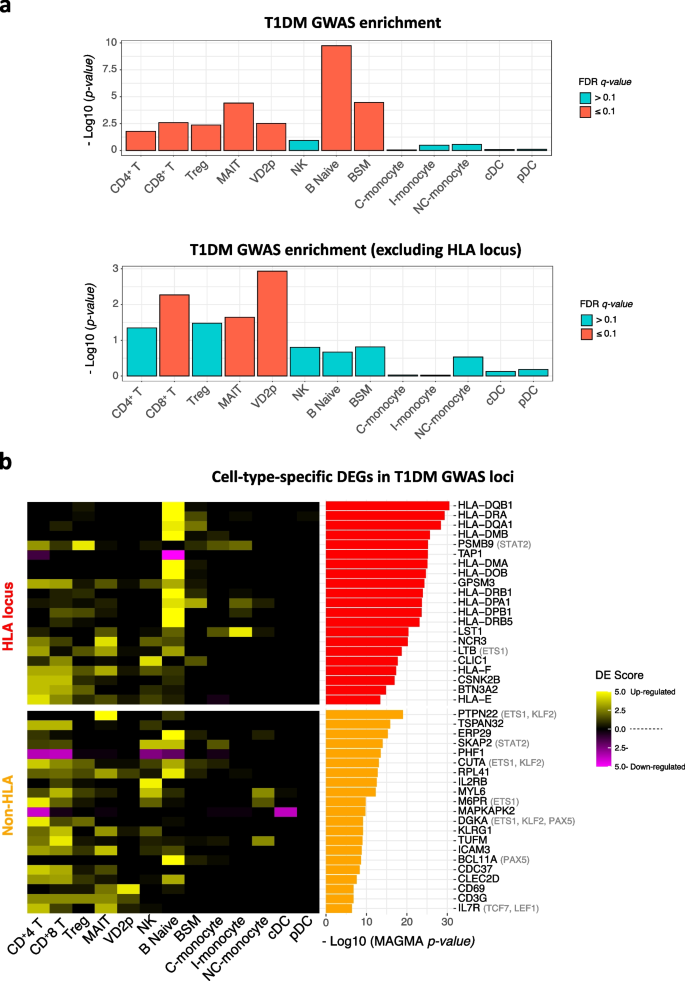
Cell type-specific DEGs enriched for T1DM genetic risk. a Significance of association between cell type-specific DEGs and T1DM risk loci. Upper panel: entire genome, lower panel: non-HLA loci. FDR q- values: Benjamini-Hochberg correction. b Heatmap of cell type-specific differential expression score (DES) of genes in T1DM GWAS loci. Gene within the upper 30th percentile of DES that also rank within top 200 in the genome by T1DM genetic risk score are shown. Genetic risk scores [ \(-log10\) (MAGMA p-value )] of individual genes are shown by the bar plot on the right. Black: DEGs, gray: upstream transcription factors inferred by regulon analysis
To identify cell types associated with T1DM genetic risk in the rest of genome, we excluded the HLA locus and repeated the CELLECT analysis. In this case, DEGs in effector T cells (CD8 + T, MAIT, and VD2p) emerged as significantly enriched for T1DM heritability: PTPN22 , KLRG1 , ICAM3 , CLECC2D , CDC37 , CD69 , CD3G , and IL7R (Fig. 6 a lower panel, b). This result strengthens the evidence for the pathogenic role of effector T cells in T1DM [ 10 , 60 , 65 , 66 , 67 , 81 ].
Also, we observed that 9 of the cell type-specific DEGs in T1DM GWAS loci belong to cell type-specific regulons driving upregulation of cell activation or cell migration genes displayed in Fig. 3 f (Fig. 6 b). These connections suggest that some of the genes influenced by T1DM-causing genetic variants may also be perturbed at the regulon level. In other words, these genes may represent convergence of cis- and trans-regulatory alterations at certain gene loci in T1DM.
Taken together, our GWAS integrative analysis highlights candidate cell types and corresponding DEGs that may mediate T1DM genetic risk in HLA and non-HLA loci. These genes could be targeted for functional assays in the corresponding cell types to investigate molecular mechanisms of T1DM pathogenesis.
We have constructed a cohort-scale single data set of peripheral immune cell states in T1DM as a resource to characterize systemic immune dysregulation in this prototypic endocrine organ-specific autoimmune disease. A key strength of our study is the use of single-cell transcriptome profiling of an autoimmune disease cohort, which provided sufficient resolution to detect cell type-specific molecular traits associated with T1DM. This approach also facilitated cross-validation of our results with T1DM markers found in diseased pancreatic tissue as well as circulating markers from single-cell analysis of SLE. Nevertheless, our study has some limitations. Firstly, this has been a cross-sectional study covering Stage 3 T1DM only. However, we note that the cross-sectional markers we identified from single-cell analysis showed significant overlap with previously detected prognostic markers. Secondly, we did not address signatures of auto-reactive T and B cells in T1DM.
In this study we detected a large number of DEGs between T1DM and control PBMCs (1784 DEGs across 13 cell types), indicating profound systemic immune aberrations in this organ-specific autoimmune disease (Fig. 3 a, b). Remarkably, this was almost six-fold larger than the DEG set detected by scRNA-seq in the systemic autoimmune disease SLE (302 DEGs across 11 immune cell types, [ 71 ]). This increase could reflect an unexpectedly large systemic dimension to T1DM. We also identified substantial overlap in transcriptome changes between T1DM and SLE, including shared upregulation of cell activation genes in lymphoid and myeloid lineages [ 82 , 83 , 84 ]. This may further support the notion of a major systemic dimension to T1DM. These findings are in line with the observation that individuals diagnosed with T1DM are at greater risk of subsequently developing other autoimmune diseases such as celiac disease (small intestine) [ 85 , 86 ], autoimmune gastritis [ 87 , 88 ], autoimmune adrenalitis (Addison’s disease) [ 89 ], and quite common autoimmune thyroiditis (thyroid) [ 90 , 91 ]. The negative clinical impact of the persistence of such immune cell changes is also evident as recurrent autoimmunity after pancreas or islet cell transplantation [ 92 ]. Immunosuppression is thus required to control immediate (re)activation of autoreactivity. Along the same lines, a large population-scale analysis showed that persistent immune activation and/or low-grade inflammation in T1DM appears to substantially increase the risk of cardiovascular disease in autoimmune diabetes subjects (hazard ratio:2.36), to a similar extent as SLE (hazard ratio:2.82) [ 93 ]. Our results, taken together with these epidemiological findings, make a case for expanding the scope of mechanistic studies of T1DM by examining systemic immunopathology and functional impairment in additional organs besides the pancreas.
Despite substantial overlaps between peripheral immune changes in T1DM and SLE, perturbation of antigen presentation by B cells (upregulation of MHC class II antigen/peptide presentation genes including HLA-DR and -DQ ), was specific to T1DM (Fig. 3 d; Additional file 1 : Fig. S6b; [ 71 ]). In type 1 diabetes, it is well known that autoantigen presentation to CD4(+) T cells via MHC class II molecules plays a key role in the disease process [ 94 , 95 , 96 , 97 ]. This finding adds to the evidence from non-obese diabetic (NOD) mice [ 80 , 98 ] supporting a key role for B cells as antigen presenting cells (APCs) in T1DM. Similarly, upregulation of cell migration genes in T/NK cells appears to be specific to T1DM (Fig. 3 d; [ 71 ]). These results indicate qualitative differences between the systemic components of T1DM and SLE, and suggest that broader characterization of the peripheral immune aberrations of diverse autoimmune diseases could lead to new molecular assays for diagnosis and clinical monitoring. For instance, our T1DM-associated DEGs included a significant number of known prognostic markers of seroconversion and T1DM disease onset (Fig. 4 a, c).
Remarkably, we observed significant overlap between the transcriptome aberrations of peripheral immune cells and those observed in pancreatic \(\beta\) cells and macrophages in T1DM (Fig. 4 a, c). This result suggests significant sharing of molecular mechanisms between peripheral immune cells and the relevant target tissue(s) [ 99 ]. Thus, our results provide further motivation for developing therapeutic strategies aimed at reversing the pathology of both pancreatic beta cells and circulating immune cells in T1DM [ 99 , 100 , 101 ].
Regulon analysis highlighted candidate master TFs driving cell type-specific changes in key functional programs in T1DM (Fig. 3 e, f). Multiple lines of evidence support a role for these TFs in the pathophysiology of the corresponding cell types. For example, the WNT-pathway mediators TCF7 and LEF1 , which we identified as drivers of T/NK cell activation, are known to play a key role in maintenance of a Th17 stem-like population which can either give rise to effector Th17 cells or differentiate into highly tissue destructive Th17/Th1-like cells [ 10 , 81 , 102 ]. In addition, we identified IRF5 as a potential driver of monocyte hyperactivation in T1DM. Consistently, genetic variants in this locus are associated with hyper-activation and functional aberrations of monocytes in T1DM and other systemic autoimmune disease such as SLE and Sjögren’s syndrome [ 103 , 104 , 105 , 106 ]. Lastly, our results indicate that MEF2C , a TF necessary for B cell survival and proliferation upon stimulation of antigen receptor [ 107 ] may also drive the abovementioned upregulation of antigen presentation genes in B cells. Targeting WNT [ 108 , 109 ], interferon [ 110 , 111 ], or other signaling pathways mediated by the identified master TFs could represent a promising avenue for therapeutics development in T1DM.
A key observation from our data is substantial heterogeneity across patients in the degree of T1DM-associated transcriptomic change (TMZ score). The clinical relevance of this heterogeneity is supported by the fact that the TMZ score showed significant correlation with GADA titer and number of HLA risk haplotypes, both of which are commonly used to infer disease risk [ 17 , 19 , 21 ] (Fig. 5 ). We therefore used the TMZ score to classify participants into high, intermediate, and low immune-responding molecular subtypes. As evidence for the relevance of this classification, the lone healthy subject assigned to the “high” subtype developed T1DM subsequently, 4 years after blood sampling. Lastly, analysis of transcriptomic response to drug treatment in vitro and in clinical trials revealed reductions in the TMZ score that were consistent with the mechanism of action and clinical response (Fig. 5 e; Additional file 1 : Fig. S7c-e). These results lay a foundation for further studies of the TMZ score as a readout in high-throughput screens, as a tool for T1DM patient stratification, and as an indicator of treatment response.
It should be noted that our dataset does not encompass the entire spectrum of autoimmune diabetes subtypes or endotypes (young-onset T1DM, adult-onset T1DM, as well as latent autoimmune diabetes of adults and other potential variants). Recognizing this limitation is crucial for a nuanced understanding of autoimmune diabetes and its various manifestations, emphasizing the need for future studies in a larger cohort, across a range of disease durations, to examine the temporal dynamics of this signature.
An additional constraint inherent in our study pertains to the absence of quantification of stimulated C-peptide levels in patients, thereby precluding the definitive exclusion of individuals identified as micro-secretors with retained C-peptide. We did, however, exclude T1D subjects with a “positive” fasting C-peptide level. We note that the sensitivity of the C-peptide assay does not compromise the substantive findings and primary conclusions derived from our investigation.
In summary, our study provides a comprehensive characterization of systemic immune dysregulation in type 1 diabetes mellitus (T1DM) by constructing a large-scale single-cell dataset of peripheral immune cell states. Our findings reveal profound systemic immune aberrations in T1DM, suggesting an unexpectedly large systemic dimension to the disease, with a remarkable six-fold increase in the number of differentially expressed genes (DEGs) compared to systemic lupus erythematosus (SLE). The observed transcriptome changes in peripheral immune cells also exhibit significant overlap with those in pancreatic \(\beta\) cells and macrophages, underscoring shared molecular mechanisms and offering insights for therapeutic strategies targeting both tissues. Moreover, our study introduces the T1DM-associated transcriptomic change (TMZ) score as a potential readout for high-throughput screens and an indicator of treatment response. We demonstrate substantial heterogeneity in T1DM-associated transcriptomic changes across patients, as reflected in the TMZ score, which correlates with disease risk indicators and provides a basis for patient stratification.
While our dataset significantly contributes to understanding systemic immunopathology in T1DM, we acknowledge its limitations, particularly the exclusion of the entire spectrum of autoimmune diabetes subtypes and the absence of stimulated C-peptide quantification. Future studies addressing these limitations in larger and more diverse cohorts will enhance our understanding of autoimmune diabetes dynamics over time.
Availability of data and materials
The raw scRNA-seq and snp-array genotyping data are available in the European Genome-phenome Archive (EGA) database and will be accessible upon reasonable request and approval of all authors (accession code EGAS50000000231) [ https://ega-archive.org/studies/EGAS50000000231 ]. The processed single cell count expression matrix is publicly available through Synapse under the accession code syn53641849 [ https://www.synapse.org/#!Synapse:syn53641849 ].
No custom code was used for any aspect of data processing or analysis in this study. All tools and packages used for data analysis in this study were published and are cited either in the main text or “ Methods ” section. Detailed data analysis procedures are described in the Methods section.
Abbreviations
Antigen presenting cells
Butyrophilin subfamily 3 member A2
Caspase recruitment domain family member 8
CD8 subunit beta
Cell division cycle 37
CD3 gamma subunit
C-type lectin domain family 2 member D
Cathepsin C
Cathepsin S
Differentially expressed gene
Differentially active regulons
ETS proto-oncogene 1
Fc epsilon receptor Ig
First-degree relatives
FGR proto-oncogene, Src family tyrosine kinase
Glutamic acid decarboxylase 65-kilodalton isoform
GAD autoantibody
General population
G protein signaling modulator 3
Gene set enrichment analysis
Human leukocyte antigen
Insulinoma-associated-2 autoantibodies
Intercellular adhesion molecule 3
Interleukin 32
Interleukin 7 receptor
Interferon regulatory factor 5
Integrin subunit beta 2
Krüppel-like factor 2
Killer cell lectin like receptor G1
Lymphoid enhancer-binding factor 1
Myocyte enhancer factor 2C
Non-obese diabetic
Paired box 5
Peripheral blood mononuclear cells
Protein tyrosine phosphatase non-receptor type 22
Quality control
Single-cell RNA sequencing
Systemic lupus erythematosus
Transcription factor 7
Type 1 diabetes mellitus
T1DM metagene z -score
TNF receptor superfamily member 1B
Transcription factor
TXK tyrosine kinase
ZFP36 ring finger protein like 2
Katsarou A, Gudbjörnsdottir S, Rawshani A, Dabelea D, Bonifacio E, Anderson BJ, et al. Type 1 diabetes mellitus. Nat Reviews Dis Primers. 2017;3(1):1–7.
Battaglia M, Ahmed S, Anderson MS, Atkinson MA, Becker D, Bingley PJ, et al. Introducing the endotype concept to address the challenge of disease heterogeneity in type 1 diabetes. Diabetes Care. 2020;43(1):5–12.
Article PubMed Google Scholar
Greenbaum C, VanBuecken D, Lord S. Disease-modifying therapies in type 1 diabetes: a look into the future of diabetes practice. Drugs. 2019;79(1):43–61.
Article CAS PubMed PubMed Central Google Scholar
Lehuen A, Diana J, Zaccone P, Cooke A. Immune cell crosstalk in type 1 diabetes. Nat Rev Immunol. 2010;10(7):501–13.
Article CAS PubMed Google Scholar
Eizirik DL, Szymczak F, Alvelos MI, Martin F. From pancreatic \(\beta\) -cell gene networks to novel therapies for type 1 diabetes. Diabetes. 2021;70(9):1915–25.
Mehdi AM, Hamilton-Williams EE, Cristino A, Ziegler A, Bonifacio E, Le Cao KA, et al. A peripheral blood transcriptomic signature predicts autoantibody development in infants at risk of type 1 diabetes. JCI Insight. 2018;3(5):e98212.
Kallionpää H, Elo LL, Laajala E, Mykkänen J, Ricaño-Ponce I, Vaarma M, et al. Innate immune activity is detected prior to seroconversion in children with HLA-conferred type 1 diabetes susceptibility. Diabetes. 2014;63(7):2402–14.
Cabrera SM, Chen YG, Hagopian WA, Hessner MJ. Blood-based signatures in type 1 diabetes. Diabetologia. 2016;59(3):414–25.
Xhonneux LP, Knight O, Lernmark Å, Bonifacio E, Hagopian WA, Rewers MJ, et al. Transcriptional networks in at-risk individuals identify signatures of type 1 diabetes progression. Sci Transl Med. 2021;13(587):eabd5666.
Walker LS, von Herrath M. CD4 T cell differentiation in type 1 diabetes. Clin Exp Immunol. 2016;183(1):16–29.
Danke NA, Koelle DM, Yee C, Beheray S, Kwok WW. Autoreactive T cells in healthy individuals. J Immunol. 2004;172(10):5967–72.
Kurien M, Mollazadegan K, Sanders DS, Ludvigsson JF. Celiac disease increases risk of thyroid disease in patients with type 1 diabetes: a nationwide cohort study. Diabetes Care. 2016;39(3):371–5.
Farh KKH, Marson A, Zhu J, Kleinewietfeld M, Housley WJ, Beik S, et al. Genetic and epigenetic fine mapping of causal autoimmune disease variants. Nature. 2015;518(7539):337–43.
Parker SC, Stitzel ML, Taylor DL, Orozco JM, Erdos MR, Akiyama JA, et al. Chromatin stretch enhancer states drive cell-specific gene regulation and harbor human disease risk variants. Proc Natl Acad Sci. 2013;110(44):17921–6.
Watanabe K, Mirkov MU, de Leeuw CA, van den Heuvel MP, Posthuma D. Genetic mapping of cell type specificity for complex traits. Nat Commun. 2019;10(1):1–13.
Article Google Scholar
Zhu Z, Zhang F, Hu H, Bakshi A, Robinson MR, Powell JE, et al. Integration of summary data from GWAS and eQTL studies predicts complex trait gene targets. Nat Genet. 2016;48(5):481–7.
Noble JA, Valdes AM. Genetics of the HLA region in the prediction of type 1 diabetes. Curr Diabetes Rep. 2011;11(6):533–42.
Article CAS Google Scholar
Russell MA, Redick SD, Blodgett DM, Richardson SJ, Leete P, Krogvold L, et al. HLA class II antigen processing and presentation pathway components demonstrated by transcriptome and protein analyses of islet \(\beta\) -cells from donors with type 1 diabetes. Diabetes. 2019;68(5):988–1001.
Bonifacio E, Yu L, Williams AK, Eisenbarth GS, Bingley PJ, Marcovina SM, et al. Harmonization of glutamic acid decarboxylase and islet antigen-2 autoantibody assays for national institute of diabetes and digestive and kidney diseases consortia. J Clin Endocrinol Metab. 2010;95(7):3360–7.
Vehik K, Beam CA, Mahon JL, Schatz DA, Haller MJ, Sosenko JM, et al. Development of autoantibodies in the TrialNet natural history study. Diabetes Care. 2011;34(9):1897–901.
Article PubMed PubMed Central Google Scholar
Sosenko JM, Skyler JS, Palmer JP, Krischer JP, Yu L, Mahon J, et al. The prediction of type 1 diabetes by multiple autoantibody levels and their incorporation into an autoantibody risk score in relatives of type 1 diabetic patients. Diabetes Care. 2013;36(9):2615–20.
Rahman AH, Homann D. Mass cytometry and type 1 diabetes research in the age of single-cell data science. Curr Opin Endocrinol Diabetes Obes. 2020;27(4):231–9.
Chen YG, Cabrera SM, Jia S, Kaldunski ML, Kramer J, Cheong S, et al. Molecular signatures differentiate immune states in type 1 diabetic families. Diabetes. 2014;63(11):3960–73.
Kallionpää H, Somani J, Tuomela S, Ullah U, De Albuquerque R, Lönnberg T, et al. Early detection of peripheral blood cell signature in children developing \(\beta\) -cell autoimmunity at a young age. Diabetes. 2019;68(10):2024–34.
Teniente-Serra A, Pizarro E, Quirant-Sánchez B, Fernández MA, Vives-Pi M, Martinez-Caceres EM. Identifying changes in peripheral lymphocyte subpopulations in adult onset type 1 diabetes. Front Immunol. 2021;12:784110.
Hippich M, Beyerlein A, Hagopian WA, Krischer JP, Vehik K, Knoop J, et al. Genetic contribution to the divergence in type 1 diabetes risk between children from the general population and children from affected families. Diabetes. 2019;68(4):847–57.
Inshaw JR, Cutler AJ, Crouch DJ, Wicker LS, Todd JA. Genetic variants predisposing most strongly to type 1 diabetes diagnosed under age 7 years lie near candidate genes that function in the immune system and in pancreatic \(\beta\) -cells. Diabetes Care. 2020;43(1):169–77.
Harsunen M, Kettunen JL, Härkönen T, Dwivedi O, Lehtovirta M, Vähäsalo P, et al. Identification of monogenic variants in more than ten per cent of children without type 1 diabetes-related autoantibodies at diagnosis in the Finnish Pediatric Diabetes Register. Diabetologia. 2023;66(3):438–49.
Fasolino M, Schwartz GW, Patil AR, Mongia A, Golson ML, Wang YJ, et al. Single-cell multi-omics analysis of human pancreatic islets reveals novel cellular states in type 1 diabetes. Nat Metab. 2022;4(2):284–99.
Howson JM, Rosinger S, Smyth DJ, Boehm BO, study group AE, Todd JA. Genetic analysis of adult-onset autoimmune diabetes. Diabetes. 2011;60(10):2645–53.
Ong Y, Koh W, Ng M, Tam ZY, Lim S, Boehm BO, et al. Glutamic acid decarboxylase and islet antigen 2 antibody profiles in people with adult-onset diabetes mellitus: a comparison between mixed ethnic populations in Singapore and Germany. Diabet Med. 2017;34(8):1145–53.
Dimitrov S, Benedict C, Heutling D, Westermann J, Born J, Lange T. Cortisol and epinephrine control opposing circadian rhythms in T cell subsets. Blood J Am Soc Hematol. 2009;113(21):5134–43.
CAS Google Scholar
Dimitrov S, Lange T, Nohroudi K, Born J. Number and function of circulating human antigen presenting cells regulated by sleep. Sleep. 2007;30(4):401–11.
Kronfol Z, Nair M, Zhang Q, Hill EE, Brown MB. Circadian immune measures in healthy volunteers: relationship to hypothalamic-pituitary-adrenal axis hormones and sympathetic neurotransmitters. Psychosom Med. 1997;59(1):42–50.
Rosinger S, Nutland S, Mickelson E, Varney MD, Boehm BO, Olsem GJ, et al. Collection and processing of whole blood for transformation of peripheral blood mononuclear cells and extraction of DNA: the Type 1 Diabetes Genetics Consortium. Clin Trials. 2010;7(1_suppl):S65–74.
Kimura A, Dong RP, Harada H, Sasazuki T. DNA typing of HLA class II genes in B-lymphoblastoid cell lines homozygous for HLA. Tissue Antigens. 1992;40(1):5–12.
Endl J, Rosinger S, Schwarz B, Friedrich SO, Rothe G, Karges W, et al. Coexpression of CD25 and OX40 (CD134) receptors delineates autoreactive T-cells in type 1 diabetes. Diabetes. 2006;55(1):50–60.
Strebelow M, Schlosser M, Ziegler B, Rjasanowski I, Ziegler M. Karlsburg Type I diabetes risk study of a general population: frequencies and interactions of the four major type I diabetes-associated autoantibodies studied in 9419 schoolchildren. Diabetologia. 1999;42(6):661–70.
Purcell S, Neale B, Todd-Brown K, Thomas L, Ferreira MA, Bender D, et al. PLINK: a tool set for whole-genome association and population-based linkage analyses. Am J Hum Genet. 2007;81(3):559–75.
Danecek P, Auton A, Abecasis G, Albers CA, Banks E, DePristo MA, et al. The variant call format and VCFtools. Bioinformatics. 2011;27(15):2156–8.
Browning BL, Zhou Y, Browning SR. A one-penny imputed genome from next-generation reference panels. Am J Hum Genet. 2018;103(3):338–48.
Kang HM, Subramaniam M, Targ S, Nguyen M, Maliskova L, McCarthy E, et al. Multiplexed droplet single-cell RNA-sequencing using natural genetic variation. Nat Biotechnol. 2018;36(1):89–94.
Butler A, Hoffman P, Smibert P, Papalexi E, Satija R. Integrating single-cell transcriptomic data across different conditions, technologies, and species. Nat Biotechnol. 2018;36(5):411–20.
Schmidt F, Ranjan B, Lin QXX, Krishnan V, Joanito I, Honardoost MA, et al. RCA2: a scalable supervised clustering algorithm that reduces batch effects in scRNA-seq data. Nucleic Acids Res. 2021;49(15):8505–19.
McGinnis CS, Murrow LM, Gartner ZJ. DoubletFinder: doublet detection in single-cell RNA sequencing data using artificial nearest neighbors. Cell Syst. 2019;8(4):329–37.
Novershtern N, Subramanian A, Lawton LN, Mak RH, Haining WN, McConkey ME, et al. Densely interconnected transcriptional circuits control cell states in human hematopoiesis. Cell. 2011;144(2):296–309.
Monaco G, Lee B, Xu W, Mustafah S, Hwang YY, Carre C, et al. RNA-Seq signatures normalized by mRNA abundance allow absolute deconvolution of human immune cell types. Cell Rep. 2019;26(6):1627–40.
Becht E, McInnes L, Healy J, Dutertre CA, Kwok IW, Ng LG, et al. Dimensionality reduction for visualizing single-cell data using UMAP. Nat Biotechnol. 2019;37(1):38–44.
Wood SN. Fast stable restricted maximum likelihood and marginal likelihood estimation of semiparametric generalized linear models. J R Stat Soc Ser B Stat Methodol. 2011;73(1):3–36.
Wood SN. Generalized additive models: an introduction with R. Chapman and hall/CRC; 2006.
Yu G, Wang LG, Han Y, He QY. clusterProfiler: an R package for comparing biological themes among gene clusters. Omics: J Integr Biol. 2012;16(5):284–7.
Van de Sande B, Flerin C, Davie K, De Waegeneer M, Hulselmans G, Aibar S, et al. A scalable SCENIC workflow for single-cell gene regulatory network analysis. Nat Protoc. 2020;15(7):2247–76.
Shen L, Sinai M. GeneOverlap: test and visualize gene overlaps. R package version. 2013;1(0):444.
Chiou J, Geusz RJ, Okino ML, Han JY, Miller M, Melton R, et al. Interpreting type 1 diabetes risk with genetics and single-cell epigenomics. Nature. 2021;594(7863):398–402.
Timshel PN, Thompson JJ, Pers TH. Genetic mapping of etiologic brain cell types for obesity. Elife. 2020;9:e55851.
Skene NG, Bryois J, Bakken TE, Breen G, Crowley JJ, Gaspar HA, et al. Genetic identification of brain cell types underlying schizophrenia. Nat Genet. 2018;50(6):825–33.
Li H, Courtois ET, Sengupta D, Tan Y, Chen KH, Goh JJL, et al. Reference component analysis of single-cell transcriptomes elucidates cellular heterogeneity in human colorectal tumors. Nat Genet. 2017;49(5):708–18.
Klein SL, Flanagan KL. Sex differences in immune responses. Nat Rev Immunol. 2016;16(10):626–38.
Sandor A, Jacobelli J, Friedman R. Immune cell trafficking to the islets during type 1 diabetes. Clin Exp Immunol. 2019;198(3):314–25.
Michels AW, Landry LG, McDaniel KA, Yu L, Campbell-Thompson M, Kwok WW, et al. Islet-derived CD4 T cells targeting proinsulin in human autoimmune diabetes. Diabetes. 2017;66(3):722–34.
Velthuis J, Unger W, Van der Slik A, Duinkerken G, Engelse M, Schaapherder A, et al. Accumulation of autoreactive effector T cells and allo-specific regulatory T cells in the pancreas allograft of a type 1 diabetic recipient. Diabetologia. 2009;52(3):494–503.
Lindley S, Dayan CM, Bishop A, Roep BO, Peakman M, Tree TI. Defective suppressor function in CD4+ CD25+ T-cells from patients with type 1 diabetes. Diabetes. 2005;54(1):92–9.
Ferraro A, Socci C, Stabilini A, Valle A, Monti P, Piemonti L, et al. Expansion of Th17 cells and functional defects in T regulatory cells are key features of the pancreatic lymph nodes in patients with type 1 diabetes. Diabetes. 2011;60(11):2903–13.
Buckner JH. Mechanisms of impaired regulation by CD4+ CD25+ FOXP3+ regulatory T cells in human autoimmune diseases. Nat Rev Immunol. 2010;10(12):849–59.
Kuric E, Seiron P, Krogvold L, Edwin B, Buanes T, Hanssen KF, et al. Demonstration of tissue resident memory CD8 T cells in insulitic lesions in adult patients with recent-onset type 1 diabetes. Am J Pathol. 2017;187(3):581–8.
Bulek AM, Cole DK, Skowera A, Dolton G, Gras S, Madura F, et al. Structural basis for the killing of human beta cells by CD8+ T cells in type 1 diabetes. Nat Immunol. 2012;13(3):283–9.
Pinkse GG, Tysma OH, Bergen CA, Kester MG, Ossendorp F, Van Veelen PA, et al. Autoreactive CD8 T cells associated with \(\beta\) cell destruction in type 1 diabetes. Proc Natl Acad Sci. 2005;102(51):18425–30.
Shapiro MR, Dong X, Perry DJ, McNichols JM, Thirawatananond P, Posgai AL, et al. Human immune phenotyping reveals accelerated aging in type 1 diabetes. JCI Insight. 2023;8(17):e170767.
Damond N, Engler S, Zanotelli VR, Schapiro D, Wasserfall CH, Kusmartseva I, et al. A map of human type 1 diabetes progression by imaging mass cytometry. Cell Metab. 2019;29(3):755–68.
Wang YJ, Traum D, Schug J, Gao L, Liu C, Atkinson MA, et al. Multiplexed in situ imaging mass cytometry analysis of the human endocrine pancreas and immune system in type 1 diabetes. Cell Metab. 2019;29(3):769–83.
Perez RK, Gordon MG, Subramaniam M, Kim MC, Hartoularos GC, Targ S, et al. Single-cell RNA-seq reveals cell type–specific molecular and genetic associations to lupus. Science. 2022;376(6589):eabf1970.
Love MI, Huber W, Anders S. Moderated estimation of fold change and dispersion for RNA-seq data with DESeq2. Genome Biol. 2014;15(12):1–21.
Wittner J, Schuh W. Krüppel-like factor 2 (KLF2) in immune cell migration. Vaccines. 2021;9(10):1171.
Sebzda E, Zou Z, Lee JS, Wang T, Kahn ML. Transcription factor KLF2 regulates the migration of naive T cells by restricting chemokine receptor expression patterns. Nat Immunol. 2008;9(3):292–300.
Xu G, Grimes TD, Grayson TB, Chen J, Thielen LA, Tse HM, et al. Exploratory study reveals far reaching systemic and cellular effects of verapamil treatment in subjects with type 1 diabetes. Nat Commun. 2022;13(1):1–9.
Google Scholar
Long SA, Thorpe J, DeBerg HA, Gersuk V, Eddy JA, Harris KM, et al. Partial exhaustion of CD8 T cells and clinical response to teplizumab in new-onset type 1 diabetes. Sci Immunol. 2016;1(5):eaai7793.
Linsley PS, Greenbaum CJ, Speake C, Long SA, Dufort MJ. B lymphocyte alterations accompany abatacept resistance in new-onset type 1 diabetes. JCI Insight. 2019;4(4):e126136.
Linsley PS, Greenbaum CJ, Rosasco M, Presnell S, Herold KC, Dufort MJ. Elevated T cell levels in peripheral blood predict poor clinical response following rituximab treatment in new-onset type 1 diabetes. Genes Immun. 2019;20(4):293–307.
Ovalle F, Grimes T, Xu G, Patel AJ, Grayson TB, Thielen LA, et al. Verapamil and beta cell function in adults with recent-onset type 1 diabetes. Nat Med. 2018;24(8):1108–12.
Greeley SAW, Moore DJ, Noorchashm H, Noto LE, Rostami SY, Schlachterman A, et al. Impaired activation of islet-reactive CD4 T cells in pancreatic lymph nodes of B cell-deficient nonobese diabetic mice. J Immunol. 2001;167(8):4351–7.
Heninger AK, Eugster A, Kuehn D, Buettner F, Kuhn M, Lindner A, et al. A divergent population of autoantigen-responsive CD4+ T cells in infants prior to \(\beta\) cell autoimmunity. Sci Transl Med. 2017;9(378):eaaf8848.
Devaraj S, Glaser N, Griffen S, Wang-Polagruto J, Miguelino E, Jialal I. Increased monocytic activity and biomarkers of inflammation in patients with type 1 diabetes. Diabetes. 2006;55(3):774–9.
Toldi G, Vásárhelyi B, Kaposi A, Mészáros G, Pánczél P, Hosszufalusi N, et al. Lymphocyte activation in type 1 diabetes mellitus: the increased significance of Kv1. 3 potassium channels. Immunol Lett. 2010;133(1):35–41.
Josefsen K, Nielsen H, Lorentzen S, Damsbo P, Buschard K. Circulating monocytes are activated in newly diagnosed type 1 diabetes mellitus patients. Clin Exp Immunol. 1994;98(3):489–93.
Sanchez-Albisua I, Wolf J, Neu A, Geiger H, Wäscher I, Stern M. Coeliac disease in children with type 1 diabetes mellitus: the effect of the gluten-free diet. Diabet Med. 2005;22(8):1079–82.
Holmes G. Coeliac disease and type 1 diabetes mellitus-the case for screening. Diabet Med. 2001;18(3):169–77.
De Block CE, De Leeuw IH, Bogers JJ, Pelckmans PA, Ieven MM, Van Marck EA, et al. Autoimmune gastropathy in type 1 diabetic patients with parietal cell antibodies: histological and clinical findings. Diabetes Care. 2003;26(1):82–8.
De Block CE, De Leeuw IH, Van Gaal LF, Registry BD. High prevalence of manifestations of gastric autoimmunity in parietal cell antibody-positive type 1 (insulin-dependent) diabetic patients. J Clin Endocrinol Metab. 1999;84(11):4062–7.
PubMed Google Scholar
Thomas JB, Petrovsky N, Ambler GR. Addison’s disease presenting in four adolescents with type 1 diabetes. Pediatr Diabetes. 2004;5(4):207–11.
Maclaren NK, Riley WJ. Thyroid, gastric, and adrenal autoimmunities associated with insulin-dependent diabetes mellitus. Diabetes Care. 1985;8(Supplement_1):34–8.
De Block C, De Leeuw I, Vertommen J, Rooman R, Du Caju M, Van Campenhout C, et al. Beta-cell, thyroid, gastric, adrenal and coeliac autoimmunity and HLA-DQ types in type 1 diabetes. Clin Exp Immunol. 2001;126(2):236–41.
Pugliese A, Reijonen HK, Nepom J, Burke GW III. Recurrence of autoimmunity in pancreas transplant patients: research update. Diabetes Manag (London, England). 2011;1(2):229.
Article PubMed Central Google Scholar
Conrad N, Verbeke G, Molenberghs G, Goetschalckx L, Callender T, Cambridge G, et al. Autoimmune diseases and cardiovascular risk: a population-based study on 19 autoimmune diseases and 12 cardiovascular diseases in 22 million individuals in the UK. Lancet. 2022;400(10354):733–43.
Burster T, Boehm BO. Processing and presentation of (pro)-insulin in the MHC class II pathway: the generation of antigen-based immunomodulators in the context of type 1 diabetes mellitus. Diabetes Metab Res Rev. 2010;26(4):227–38.
Brown JH, Jardetzky TS, Gorga JC, Stern LJ, Urban RG, Strominger JL, et al. Three-dimensional structure of the human class II histocompatibility antigen HLA-DR1. Nature. 1993;364:33–9.
Durinovic-Belló I, Rosinger S, Olson JA, Congia M, Ahmad RC, Rickert M, et al. DRB1* 0401-restricted human T cell clone specific for the major proinsulin73-90 epitope expresses a down-regulatory T helper 2 phenotype. Proc Natl Acad Sci. 2006;103(31):11683–8.
Friese MA, Jones EY, Fugger L. MHC II molecules in inflammatory diseases: interplay of qualities and quantities. Trends Immunol. 2005;26(11):559–61.
Serreze DV, Fleming SA, Chapman HD, Richard SD, Leiter EH, Tisch RM. B lymphocytes are critical antigen-presenting cells for the initiation of T cell-mediated autoimmune diabetes in nonobese diabetic mice. J Immunol. 1998;161(8):3912–8.
Szymczak F, Colli ML, Mamula M, Evans-Molina C, Eizirik DL. Gene expression signatures of target tissues in type 1 diabetes, lupus erythematosus, multiple sclerosis, and rheumatoid arthritis. Sci Adv. 2021;7(2):eabd7600.
von Herrath M, Bain SC, Bode B, Clausen JO, Coppieters K, Gaysina L, et al. Anti-interleukin-21 antibody and liraglutide for the preservation of \(\beta\) -cell function in adults with recent-onset type 1 diabetes: a randomised, double-blind, placebo-controlled, phase 2 trial. Lancet Diabetes Endocrinol. 2021;9(4):212–24.
Roep BO, Thomaidou S, van Tienhoven R, Zaldumbide A. Type 1 diabetes mellitus as a disease of the \(\beta\) -cell (do not blame the immune system?). Nat Rev Endocrinol. 2021;17(3):150–61.
Escobar G, Mangani D, Anderson AC. T cell factor 1: A master regulator of the T cell response in disease. Sci Immunol. 2020;5(53):eabb9726.
Juhas U, Ryba-Stanisławowska M, Brandt-Varma A, Myśliwiec M, Myśliwska J. Monocytes of newly diagnosed juvenile DM1 patients are prone to differentiate into regulatory IL-10+ M2 macrophages. Immunol Res. 2019;67(1):58–69.
Nordmark G, Kristjansdottir G, Theander E, Eriksson P, Brun J, Wang C, et al. Additive effects of the major risk alleles of IRF5 and STAT4 in primary Sjögren’s syndrome. Genes Immun. 2009;10(1):68–76.
Stone RC, Feng D, Deng J, Singh S, Yang L, Fitzgerald-Bocarsly P, et al. IRF5 activation in monocytes of SLE patients is triggered by circulating autoantigens independent of type I IFN. Arthritis Rheum. 2012;64(3):788.
Li D, Matta B, Song S, Nelson V, Diggins K, Simpfendorfer KR, et al. IRF5 genetic risk variants drive myeloid-specific IRF5 hyperactivation and presymptomatic SLE. JCI Insight. 2020;5(2):e124020.
Wilker PR, Kohyama M, Sandau MM, Albring JC, Nakagawa O, Schwarz JJ, et al. Transcription factor Mef2c is required for B cell proliferation and survival after antigen receptor stimulation. Nat Immunol. 2008;9(6):603–12.
Shi J, Chi S, Xue J, Yang J, Li F, Liu X. Emerging role and therapeutic implication of Wnt signaling pathways in autoimmune diseases. J Immunol Res. 2016;2016:18.
Erlich H, Valdes A, Julier C, Mirel D, Noble J. Evidence for association of the TCF7 locus with type I diabetes. Genes Immun. 2009;10(1):S54–9.
Marwaha AK, Tan S, Dutz JP. Targeting the IL-17/IFN- \(\gamma\) axis as a potential new clinical therapy for type 1 diabetes. Clin Immunol. 2014;154(1):84–9.
Qaisar N, Jurczyk A, Wang JP. Potential role of type I interferon in the pathogenic process leading to type 1 diabetes. Curr Opin Endocrinol Diabetes Obes. 2018;25(2):94.
Download references
Acknowledgements
We are deeply grateful to the participants of the Ulm T1DM Biobank study for their contribution to our work.
This work was funded and supported by the following grants: Grant No. H18/01/a0/020 from Agency for Science, Technology and Research, Singapore; Grant No. 573312 from Simons Foundation Autism Research Initiative (SFARI); and Grant No. OFIRG21jun-0090 from National Medical Research Council, Singapore.
Author information
Authors and affiliations.
Laboratory of Systems Biology and Data Analytics, Genome Institute of Singapore (GIS), A*STAR (Agency for Science, Technology and Research), Singapore, 138672, Singapore
Mohammad Amin Honardoost, Andreas Adinatha, Florian Schmidt, Bobby Ranjan, Maryam Ghaeidamini, Nirmala Arul Rayan, Michelle Gek Liang Lim, Ignasius Joanito, Quy Xiao Xuan Lin, Deepa Rajagopalan & Shyam Prabhakar
Cardiovascular Diseases Translational Research Program, Yong Loo Lin School of Medicine, National University of Singapore, Singapore, 117599, Singapore
Mohammad Amin Honardoost & Roger Foo
School of Public Health and Preventive Medicine, Monash University, Melbourne, Australia
Mohammad Amin Honardoost
Integrated genomics platform, Genome Institute of Singapore (GIS), A*STAR (Agency for Science, Technology and Research), Singapore, 138672, Singapore
Shi Qi Mok & Chiea Chuen Khor
Singapore Immunology Network (SIgN), A*STAR (Agency for Science, Technology and Research), Singapore, 138648, Singapore
You Yi Hwang & Anis Larbi
Institute of Molecular and Cell Biology (IMCB), A*STAR (Agency for Science, Technology and Research), Singapore, 138673, Singapore
Genome Institute of Singapore (GIS), A*STAR (Agency for Science, Technology and Research), Singapore, 138672, Singapore
Bernhard Otto Boehm
Lee Kong Chian School of Medicine, Nanyang Technological University, Singapore, 308232, Singapore
Bernhard Otto Boehm & Shyam Prabhakar
Faculty of Life Sciences and Medicine, King’s College London, London, WC2R 2LS, UK
Cancer Science Institute of Singapore, National University of Singapore, Singapore, Republic of Singapore
Shyam Prabhakar
You can also search for this author in PubMed Google Scholar
Contributions
MAH conceived the project including the study design, generation of scRNA-seq data, experiments and validation, data analysis, and writing of the original draft; AA, FS, BR, IJ, and QXXL assisted with the data analysis; MG, NAR, MGL, and DR assisted with the experiments and validation; SQM and CCK contributed to the generation and management of genotyping data; YYH and AL contributed to the generation and management of FACS data; BB assisted with the management of clinical data and specimens; SP provided resources and consultation; RF, BB, and SP jointly supervised the project. All authors read and approved the final manuscript.
Corresponding authors
Correspondence to Bernhard Otto Boehm or Shyam Prabhakar .
Ethics declarations
Ethics approval and consent to participate.
This research project has undergone review and has been granted ethical approval by the Institutional Review Board (IRB) at the Division of Endocrinology and Diabetes in Ulm University, Germany (Reference no. 299604393 R ). The research conformed to the principles of the Helsinki Declaration. As part of our commitment to protecting the rights and welfare of human participants involved in this study, informed consent was obtained from each participant prior to their participation. To ensure the confidentiality of participants’ data, all collected information were treated with the utmost confidentiality and stored securely. Personal identifiers were removed or replaced with unique codes to protect the participants’ identities.
Consent for publication
Informed consent was obtained from each participant prior to their participation to publish all patient-related information that could potentially identify the individuals involved in this study.
Competing interests
The authors declare no competing interests.
Additional information
Publisher’s note.
Springer Nature remains neutral with regard to jurisdictional claims in published maps and institutional affiliations.
Supplementary information
Additional file 1: figure s1..
Computational workflow for data analysis and demuxlet quality control. Figure S2. RCA Clustering and annotation of 21 immune cell types. Figure S3. Illustration of T1DM and healthy cells, up/down-DEGs, and corresponding regulons/pathways in the UMAP representation of gene expression space. Figure S4. Multi-parametric FACS symphony validated the cellular and molecular aberrations in T1DM patients. Figure S5. Comparison of cellular and molecular changes in peripheral immune cells of SLE and T1DM versus healthy. Figure S6. Differential gene/regulon expression analysis in T1DM patients compared with healthy controls across 13 immune cell types. Figure S7. Association of TMZ score of peripheral immune cells with clinical features and response to immunotherapy in T1DM patients.
Additional file 2: Table S1.
Clinical information of samples used for scRNA-seq. Table S2. Clinical information of samples used for FACS symphony. Table S3. Cell type-specific T1D DEGs. Table S4. Gene Ontology (GO) over-representaion results of T1D DEGs modules. Table S5. GSEA results of T1D DEGs across 13 immune cell types. Table S6. Filtered GSEA results (cell type-specific and top three GO terms in each cell types). Table S7. GO over-representaion results of T1D and SLE overlaping PBMC DEGs. Table S8. GO over-representaion results of overlaping T1D up-DEGs with prognostic expression signatures and transcriptome changes in T1D pancreas. Table S9. Cell type-specific T1D DARs. Table S10. List of antibodies used in this study.
Rights and permissions
Open Access This article is licensed under a Creative Commons Attribution 4.0 International License, which permits use, sharing, adaptation, distribution and reproduction in any medium or format, as long as you give appropriate credit to the original author(s) and the source, provide a link to the Creative Commons licence, and indicate if changes were made. The images or other third party material in this article are included in the article's Creative Commons licence, unless indicated otherwise in a credit line to the material. If material is not included in the article's Creative Commons licence and your intended use is not permitted by statutory regulation or exceeds the permitted use, you will need to obtain permission directly from the copyright holder. To view a copy of this licence, visit http://creativecommons.org/licenses/by/4.0/ . The Creative Commons Public Domain Dedication waiver ( http://creativecommons.org/publicdomain/zero/1.0/ ) applies to the data made available in this article, unless otherwise stated in a credit line to the data.
Reprints and permissions
About this article
Cite this article.
Honardoost, M.A., Adinatha, A., Schmidt, F. et al. Systematic immune cell dysregulation and molecular subtypes revealed by single-cell RNA-seq of subjects with type 1 diabetes. Genome Med 16 , 45 (2024). https://doi.org/10.1186/s13073-024-01300-z
Download citation
Received : 05 August 2023
Accepted : 30 January 2024
Published : 27 March 2024
DOI : https://doi.org/10.1186/s13073-024-01300-z
Share this article
Anyone you share the following link with will be able to read this content:
Sorry, a shareable link is not currently available for this article.
Provided by the Springer Nature SharedIt content-sharing initiative
- Type 1 diabetes mellitus (T1DM)
- Peripheral blood mononuclear cells (PBMCs)
- Single-cell RNA sequencing (scRNA-seq)
Genome Medicine
ISSN: 1756-994X
- Submission enquiries: [email protected]
- General enquiries: [email protected]
How do you get diabetes? Causes of Type 1 and Type 2, according to an expert.
D iabetes affects 37 million people in the U.S. The Centers for Disease Control and Prevention estimates another 96 million people – or 1 in 3 adults – have prediabetes , a condition where blood sugar levels are higher than normal but not high enough to be diagnosed with Type 2.
Most people know of Type 1 and Type 2 diabetes, but not all know how their causes differ. We asked Dr. Rodica Busui, the president of Medicine and Science at the American Diabetes Association. Here’s what you need to know.
What causes diabetes?
Start the day smarter. Get all the news you need in your inbox each morning.
Diabetes is a chronic health condition. With Type 1 diabetes, your body can’t produce enough insulin and with Type 2 diabetes, it doesn’t use it properly.
But even beyond the two types, the effects and treatment associated with diabetes are different from person to person. It can also have serious complications if left untreated. This is why educating the public about diabetes is so crucial, says Busui.
“It can affect every single part of one body, and that’s important to understand – it cannot be taken lightly,” Busui says.
How to prevent diabetes: Here are tips to control the condition, according to a doctor
What causes Type 1 diabetes?
Type 1 diabetes is an autoimmune disease where beta cells, a hormone located in the pancreas that creates insulin, are destroyed, Busui says. This destruction may happen quickly or over some time until a “critical mass” of beta cells is lost and the individual cannot survive without insulin from external sources. This is why many Type 1 diabetics undergo regular insulin injections .
“It’s like your own body creates antibodies against your own structures, in this case, the beta cells,” Busui says.
Type 1 diabetes is more commonly diagnosed in children than Type 2. It can occur at any age – Busui says she’s diagnosed patients in their mid-60s with Type 1 and recent data suggests more than half of new Type 1 cases occur in adulthood.
Anyone can get Type 1 diabetes, Busui says. Type 1 symptoms , which include increased thirst and hunger, frequent urination, fatigue, blurry vision, slow-healing cuts and bruises and weight loss, often come on quicker than Type 2 diabetes.
What causes Type 2 diabetes?
Type 2 diabetes is the more common form of diabetes. In Type 2, beta cell dysfunction has multiple, complex causes, including weight gain, lifestyle changes and lack of exercise. Family history, ethnicity and age can also play a role.
These changes cause your body to stop using the insulin it makes properly. This is called insulin resistance – it’s harder for your body to bring blood glucose levels down.
“The more we are insulin resistant … the more insulin is needed to take the same amount of glucose from the blood inside the cell to produce energy,” Busui says. “And because of that, the beta cells have to work overtime constantly, working nonstop. Eventually, they get exhausted and they cannot produce as much insulin.”
This can cause changes in the brain and warp your sense of satiety, or how full you are. Busui says this causes a “vicious cycle,” for those who dealing with obesity and diabetes.
Doctors are diagnosing more children with Type 2 diabetes than ever, an impact of the obesity epidemic in the U.S. and food insecurity, where many children don’t have access to fresh, healthy food. Children can also develop complications from Type 2 diabetes, Busui says.
The Diabetes Dilemma: American can prevent (and control) Type 2 diabetes. So why aren’t we doing it?
Not everyone who has Type 2 diabetes needs insulin from an external source – many Type 2 diabetics’ bodies can still produce insulin. This is why early diagnosis is key and can help patients make lifestyle changes or start on medication to prevent further complications. But because Type 2 diabetes symptoms are much more subtle than Type 1, they may not be taken seriously enough to seek care.
“If people ignore (high blood glucose levels) saying ‘Well, I don’t have a pain, I don’t want to do anything,’ then there is a progressive decrease,” Busui says. “The higher blood glucose then generates some changes in the body metabolism that will lead to all these toxic radicals that actually have an additional effect on the beta cells to make them less and less functional.”
The Diabetes Dilemma: Managing Type 2 diabetes is complicated.
Can eating too much sugar cause diabetes?
Consumption of sugary drinks is associated with a higher risk of Type 2 diabetes , studies show. But there are other risk factors including family history, age and ethnicity. Sugar-sweetened beverages are the largest source of added sugar in American diets.
The American Diabetes Association recommends avoiding sugar-sweetened beverages and switching to water. The Dietary Guidelines for Americans advise no more than 10% of daily caloric intake be from added sugar.
Diabetes is one of the leading causes of death and disability in the country, but Busui says she has a “glass half full” outlook on where diabetes care and research are today. Part of that is providing greater education and access to care, especially for high-risk, historically underserved populations .
“It’s truly remarkable how much progress we have made in discovering effective strategies, medications, technologies,” Busui says.
The Diabetes Dilemma: Solutions exist to end the Type 2 diabetes dilemma but too few get the help they need
Diabetes treatment can be costly: The biggest cost is (surprisingly) not insulin
Just Curious for more? We've got you covered
USA TODAY is exploring the questions you and others ask every day. From "What is the healthiest bread?" to "How to treat dog flu" to "How much do nurses make?" , we're striving to find answers to the most common questions you ask every day. Head to our Just Curious section to see what else we can answer for you.
Read more on diabetes in America
Diabetes runs deep in rural Mississippi: Locals have taken to growing their own solutions.
A diabetes disparity: Why Colorado's healthy lifestyle brand isn't shared by all
The steep cost of Type 2: When diabetes dragged her down, she chose to fight
More: America can prevent (and control) Type 2 diabetes. So why aren’t we doing it?
More: Solutions exist to end the Type 2 diabetes dilemma but too few get the help they need
This article originally appeared on USA TODAY: How do you get diabetes? Causes of Type 1 and Type 2, according to an expert.
Associations of Dietary Intake with the Intestinal Microbiota and Short-Chain Fatty Acids Among Young Adults with Type 1 Diabetes and Overweight or Obesity
Add to collection, downloadable content.
- Affiliation: Gillings School of Global Public Health, Department of Nutrition
- Affiliation: Gillings School of Global Public Health, Department of Biostatistics
- Other Affiliation: AdventHealth Translational Research Institute
- Other Affiliation: Stanford University
- Affiliation: Gillings School of Global Public Health, Department of Epidemiology
- Background: Diet, a key component of type 1 diabetes (T1D) management, modulates the intestinal microbiota and its metabolically active byproducts—including SCFA—through fermentation of dietary carbohydrates such as fiber. However, the diet–microbiome relationship remains largely unexplored in longstanding T1D. Objectives: We evaluated whether increased carbohydrate intake, including fiber, is associated with increased SCFA-producing gut microbes, SCFA, and intestinal microbial diversity among young adults with longstanding T1D and overweight or obesity. Methods: Young adult men and women with T1D for ≥1 y, aged 19–30 y, and BMI of 27.0–39.9 kg/m2 at baseline provided stool samples at baseline and 3, 6, and 9 mo of a randomized dietary weight loss trial. Diet was assessed by 1–2 24-h recalls. The abundance of SCFA-producing microbes was measured using 16S rRNA gene sequencing. GC-MS measured fecal SCFA (acetate, butyrate, propionate, and total) concentrations. Adjusted and Bonferroni-corrected generalized estimating equations modeled associations of dietary fiber (total, soluble, and pectins) and carbohydrate (available carbohydrate, and fructose) with microbiome-related outcomes. Primary analyses were restricted to data collected before COVID-19 interruptions. Results: Fiber (total and soluble) and carbohydrates (available and fructose) were positively associated with total SCFA and acetate concentrations (n = 40 participants, 52 visits). Each 10 g/d of total and soluble fiber intake was associated with an additional 8.8 μmol/g (95% CI: 4.5, 12.8 μmol/g; P = 0.006) and 24.0 μmol/g (95% CI: 12.9, 35.1 μmol/g; P = 0.003) of fecal acetate, respectively. Available carbohydrate intake was positively associated with SCFA producers Roseburia and Ruminococcus gnavus. All diet variables except pectin were inversely associated with normalized abundance of Bacteroides and Alistipes. Fructose was inversely associated with Akkermansia abundance. Conclusions: In young adults with longstanding T1D, fiber and carbohydrate intake were associated positively with fecal SCFA but had variable associations with SCFA-producing gut microbes. Controlled feeding studies should determine whether gut microbes and SCFA can be directly manipulated in T1D.
- gut microbiome
- short-chain fatty acids
- type 1 diabetes
- https://doi.org/10.17615/1z30-p506
- https://doi.org/10.1016/j.tjnut.2022.12.017
- Journal of Nutrition
- University of North Carolina, UNC
- National Institutes of Health, NIH
- National Institute of Diabetes and Digestive and Kidney Diseases, NIDDK: 1DP3DK113358-01, P30 DK034987, PNC DK056350
- Elsevier B.V.
This work has no parents.
Select type of work
Master's papers.
Deposit your masters paper, project or other capstone work. Theses will be sent to the CDR automatically via ProQuest and do not need to be deposited.
Scholarly Articles and Book Chapters
Deposit a peer-reviewed article or book chapter. If you would like to deposit a poster, presentation, conference paper or white paper, use the “Scholarly Works” deposit form.
Undergraduate Honors Theses
Deposit your senior honors thesis.
Scholarly Journal, Newsletter or Book
Deposit a complete issue of a scholarly journal, newsletter or book. If you would like to deposit an article or book chapter, use the “Scholarly Articles and Book Chapters” deposit option.
Deposit your dataset. Datasets may be associated with an article or deposited separately.
Deposit your 3D objects, audio, images or video.
Poster, Presentation, Protocol or Paper
Deposit scholarly works such as posters, presentations, research protocols, conference papers or white papers. If you would like to deposit a peer-reviewed article or book chapter, use the “Scholarly Articles and Book Chapters” deposit option.

An official website of the United States government
The .gov means it’s official. Federal government websites often end in .gov or .mil. Before sharing sensitive information, make sure you’re on a federal government site.
The site is secure. The https:// ensures that you are connecting to the official website and that any information you provide is encrypted and transmitted securely.
- Publications
- Account settings
Preview improvements coming to the PMC website in October 2024. Learn More or Try it out now .
- Advanced Search
- Journal List
- Ann Saudi Med
- v.31(3); May-Jun 2011

Pattern of presentation in type 1 diabetic patients at the diabetes center of a university hospital
Abdulaziz m. al rashed.
From the Department of Pediatrics, King Abdulaziz University Hospital, College of Medicine, King Saud University, Riyadh, Saudi Arabia
BACKGROUND AND OBJECTIVES:
Diabetes mellitus (DM) is a major health problem worldwide. This study aimed to investigate the pattern of presentation and complications of pediatric diabetes.
DESIGN AND SETTING:
Retrospective study of children treated at a diabetes clinic at a university hospitalfor diabetes over 12-year period.
PATIENTS AND METHODS:
We collected data on the age at onset, sex, clinical presentation, duration of symptoms before diagnosis, and partial remission rate that were obtained from the hospital medical records, the National Diabetes Registry, and the statistics department.
Of 369 diabetic children, most (n=321) children had polyuria (92%) 321/369=87% as the presenting symptom; other symptoms included polydipsia (310 patients, 88.8% 310/369=84%), weight loss (292 patients, 83.9%), nocturia (240 patients, 68.8% 240/369=65%), diabetic ketoacidosis (DKA) (174 patients, 49.9% 174/369=47.20%), and abdominal pain (172 patients, 49.3% 174/369=46.6%). Presenting symptoms were missing in 20 files, so the percentages were calculated among 349 patients. Most patients had acute diabetic complications such as hypoglycemia (222 patients, 62%) and DKA (88 patients, 38.1%, but none had severe complications such as coma and cerebral edema. Chronic complications included retinopathy (4 patients, 1.3%), neuropathy (2 patients, 0.6%), coronary heart disease (2 patients, 0.6%), and nephropathy (1 patient, 0.4%).
CONCLUSION:
The pattern of presentation of type 1 diabetes has changed as the incidence of DKA has decreased; unlike in previous studies, DKA was not the most common presenting symptom in this study. Chronic complications of diabetes, such as retinopathy, neuropathy, coronary heart disease, and nephropathy are mostly rare but still present. These complications might be prevented by achieving better awareness of the need for glycemic control.
Diabetes mellitus (DM) is a major health problem worldwide. Current studies have revealed a definite global increase in the incidence and prevalence of diabetes, with the World Health Organization (WHO) projecting that there will be almost 221 million cases in the year 2010 and up to 285 million cases in the year 2025. 1 It is the fourth or fifth leading cause of death in most developed countries. 1 , 2 Although this increase is mainly expected in type 2 diabetes, a parallel increase in childhood diabetes, including type 1 and 2 diabetes, has been reported. 3 DM in children has previously been considered rare in African and Asian populations. 4 – 8 The WHO Diabetes Mondiale (WHO DIAMOND) project group has reported a worldwide increase in the incidence and variation (over 400-fold) of type 1 diabetes, with the highest occurring in Finland (over 45 per 100 000 children under the age of 15 years) and the lowest in parts of China and Fiji. 9
DM in children in Saudi Arabia has not been studied well and further studies are needed. 10 Little local information on the disease is available, and most cases reported have been of type 2 DM. 11 The epidemiology and characteristics of DM, particularly insulin-dependent DM, are not known in the Saudi community, and only a small amount of data is available. 11 Moreover, the data confirm the need to develop a national registry and the need for further epidemiological research. 12 Furthermore, adolescents are not examined in pediatric clinics, and they do not receive adequate attention in adult clinics. 13
Saudi Arabia is a unique country among developing nations in view of its excellent economic status and relatively low literacy rate, particularly among mothers, in addition to the cultural and religious background, which might influence the management of diabetes. 14 The presentation of type 1 diabetes in Saudi children seems to differ from that in children from Western countries. 15 The most common clinical sign is diabetic ketoacidosis (DKA), which is observed in 67.2% of the patients. 11 DKA is the most serious presenting symptom of type 1 DM. The frequency and severity of DKA at presentation vary significantly worldwide. 16 In Saudi Arabia, studies have revealed that DKA is present in 55% to 77% of the DM cases. 15 , 17 Ketoacidosis is the most common presenting symptom of childhood DM in this region. 18
This study presents some of the epidemiological and clinical features and complications of childhood DM as recorded in the Diabetes Center at King Abdulaziz University Hospital, Riyadh, Saudi Arabia. The Diabetes Center receives patients from Riyadh District and suburban areas; in addition, it is a tertiary care center that receives referred patients from different cities in the country. The objective of this study was to investigate the pattern of presentation of pediatric diabetes in patients enrolled in the diabetes center of a university hospital and to review the complications of diabetes in the study group.
PATIENTS AND METHODS
All diabetic children who were enrolled in the study from among those treated at the King Abdulaziz University Hospital over a 12-year period from 1993 to 2005. Vital data for the study were extracted from several sources, including hospital medical records, the National Diabetes Registry, and the statistics department. The data were extracted by an experienced physician under the strict supervision of the author, who also checked for the consistency and completeness of the extracted data. The recorded information included the age at onset, sex, nationality, consanguinity, clinical presentation, duration of symptoms before diagnosis, and partial remission rate (which was defined according to the criteria of the International Study Group of Diabetes in Children and Adolescents as a period of freedom from clinical symptoms of diabetes with insulin requirements of <0.5 units/kg/day and absent or minimal glycosuria for more than 4 weeks). During this study, type 1 diabetes was predominantly diagnosed on the basis of the clinical and biological features. Polyuria, polydipsia, weight loss and fatigability were the principal clinical features for diagnosis. Significant hyperglycemia was taken into account as a biological feature according to the National Diabetes Data Group criteria of fasting blood glucose of >140 mg/dL (>7.7 mmol/L), 2-hour postprandial blood glucose level of >200 mg/dL (>11.1 mmol/L), and glycosuria with or without ketonuria.
Both clinical and biological features were included in the diagnosis of DKA. Clinical features such as vomiting, abdominal pain, moderate-to-severe dehydration, and stupor, in addition to hyperglycemia with blood glucose levels exceeding 15 mmol/L, ketonuria and metabolic acidosis with a bicarbonate level of <15 mmol/L, played significant roles in determining DKA. The chronic complications such as retinopathy, nephropathy and neuropathy were identified by ophthalmic findings indicative of retinopathy, persistent microalbuminuria, and abnormal nerve conductions, respectively. Data analyses (chi square tests, Fischer exact test) were performed using the statistical packages STATA, R, and Minitab.
Of the 369 diabetic patients, 159 (43.1%) patients were between 11 and 15 years of age. The age groups 6-10 years and >15 years consisted of a similar number of patients—100 (27.1%) and 97 (26.3%) patients, respectively. Only 13 (3.5%) patients were less than 5 years old. The mean (standard deviation) age was 12.3 (4.0) years with a range of 2-18 years ( Table 1 ). Of the enrolled patients, 175 (47.4%) were male and 194 (52.6%) were female. The study group included 324 (87.8%) Saudi patients and 45 (12.2%) patients of different Arab nationalities. A positive family history of DM was recorded in 260 (73.7%) patients, including both type 1 and type 2 diabetes patients. The overall mean (SD) duration of diabetes was 4.6 (3.7) years. There were two major peaks of age at diagnosis, one at the age of 7 years and the other at 11 years, with a sharp drop after the age of 11 years; the curve almost reached a plateau at the age of 18 years ( Figure 1 ). Most patients (134 patients, 58.5%) had a less than 15 days duration of symptoms before diagnosis. The duration of symptoms before diagnosis ranged from 1 to 365 days, with a median of 14 days ( Table 1 ). The mean total insulin intake was 36.0 units/d, with a range of 2-106 units/d and a median of 37 units/d. Partial remission was observed in 21 (9.1%) patients ( Table 1 ). Numbers of patients by age group, duration of diabetes, family history, diabetic complications, and were above 17 years of age at the time of diagnosis, and these were the oldest patients in this study. Two peaks [peaks of age at time of diagnosis?] were observed, one as early as at 12 days of age in a case that was diagnosed in another hospital and referred to the Diabetes Center of King Abdulaziz University Hospital. Three patients duration of partial remission by the other variables are presented in Tables Tables2a, 2a , ,2b, 2b , and and2c 2c .
Characteristics of pediatric diabetic patients attending the diabetes center at a university hospital (1993-2005) (n=369).

Distribution curve of age of diagnosis pediatric patients attending the diabetes center at a university hospital (1993-2005).
Sex of pediatric diabetic patients by age group

Sex and and age group by duration of diabetes

Sex, age group and duration by family history of diabetes, diabetic complications and duration of partial remission

The most frequent presenting symptoms were polyuria, polydipsia, weight loss, nocturia ( Table 3 ) while DKA was present in about half ( Table 3 ). Because of missing data, not all information on all parients was available. The data on fatigability was available for 231 patients; fatigability was observed in 179 of these 231 patients. The less frequent symptoms included fever, obesity, delayed wound healing, vomiting, loss of consciousness, and diarrhea; a history of preceding illness was also less frequent. Ten (4.3%) patients of the studied cohort were asymptomatic. Most patients had acute diabetic complications such as hypoglycemia, and DKA ( Table 4 ). None of the patients had severe complications such as coma and cerebral edema. Chronic complications included retinopathy, neuropathy, coronary heart disease and nephropathy.
Symptoms of pediatric diabetic patients on presentation at the diabetes center according sex, age group, and duration of symptoms

Diabetic complications (acute and chronic)

Among the patients in the study, diabetes was diagnosed as early as at 12 days of age in a case that was diagnosed in another hospital and referred to the Diabetes Center of King Abdulaziz University Hospital. Three patients were above 17 years of age at the time of diagnosis, and these were the oldest patients in this study. Two peaks in age at time of diagnosis were observed, one at 7 and the other at 11 years of age. In a study by Salman et al, the age at onset ranged from 7.5 months to 12 years, with a peak at around 5-7 years and 11-14 years, respectively. The second peak in this study was observed to occur in the age range similar to that reported by Abdullah (10-13 years), while the first peak was observed to occur slightly earlier (4-6 years). 19 , 20 In the study by Abdullah, the youngest patient was 6 months old at diagnosis.
The present study showed a female preponderance, with 194 (52.6%) females versus 175 (47.4%) males; such a female preponderance was also observed in the series conducted by Salman et al, wherein 53.6% of patients were female. On the contrary, the series conducted by Abdullah showed a male preponderance, with a male-to-female ratio of 1.3:1; this ratio is similar to the ratios observed in the UK, Denmark and India. 19 , 20 In this study, the duration of symptoms before diagnosis was 1-35 days with a median of 14 days as compared to a duration of 2-60 days with a mean of 18.2 days in the series conducted by Salman et al.
The most common clinical presentations in the present study were polyuria (92%) and polydipsia (88.8%). In the study by Salman et al, DKA was the most common clinical presentation and was observed in 74 (67.3%) patients; while in the present study, DKA was observed in 49.9% of the patients. In the study by Abdullah, 55% of the patients presented with DKA. Studies in Malaysia revealed a figure (48%) similar to that in the present study, while studies in Philippines and India revealed figures of 63% and 20%-40%, respectively. 6 , 21 , 22 DKA is considered uncommon in Japan and Indonesia. 23 , 24 DKA was observed in 49.9% of the patients in this series; thus DKA was less common in this study than in other local studies, such as those by Salman et al (DKA was observed in 67.2% of the patients) and Abdullah (DKA observed in 55% of the patients). This difference may be explained by a higher level of awareness among parents and improvement in health services with early diagnosis.
The partial remission rate in this study was only 9.1%, which is lower than the rates observed in the studies by Abdullah (32%) and Salman et al. (30.9%). It correlates to those studies in relation to age group; none of the patients below 5 years of age had any episode. Partial remission is considered more common when diabetes is diagnosed in older children and teenagers, and most patients in the present study were diagnosed when they were less than 11 years of age ( Figure 1 ); this might explain the low rate of partial remission observed in this study. The lower incidence of DKA may further explain the low rate of partial remission.
A positive family history of both types (1 and 2) of diabetes was observed in 73.7% of the patients in this study; this figure is higher than that reported in the study by Abdullah (56.7%). DM occurs significantly more frequently in the parents and siblings of diabetics than in those of the control population. 25 , 26 In the study by Salman et al,, both the consanguinity rate and family history of type 1 and 2 diabetes were higher than those reported in the literature and also in a similar local study. 25 – 29
The treatment of DM in children requires the provision of a comprehensive, well-coordinated and continuous service. This is best achieved by teamwork. Adolescents or “young adults” in Saudi Arabia and in some other non-Western countries are not examined at pediatric clinics and do not receive adequate attention at adult clinics. Studies of the microvascular complications in non-insulin-dependent DM patients suggest that the onset of these complications occurs at least 4-6 years before clinical diagnosis. Evidence shows that strict glycemic control prevents microvascular complications. 30
In summary, the incidence of DKA was lower than that reported in previous studies; in addition, unlike in previous studies, DKA was not the most common clinical presentation. This difference is due to better awareness and early diagnosis. Additionally, the partial remission rate was lower, which indicates early diagnosis. Although chronic complications are uncommon in children, retinopathy, neuropathy, coronary heart disease and nephropathy have been observed; this necessitates an awareness among physicians, caretakers and patients about the importance of early diagnosis and strict control of DM. The incidence of family history was higher than that reported previously, which can be explained by the higher rate of consanguinity in the Saudi community. This observation indicates the need for further genetic studies of DM in the Saudi population.
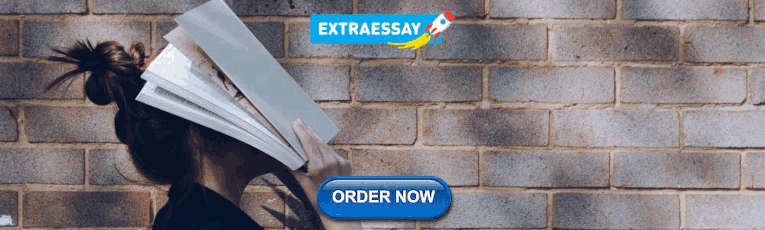
IMAGES
VIDEO
COMMENTS
Type 1 diabetes, once known as juvenile diabetes or insulin-dependent diabetes, is a chronic condition. In this condition, the pancreas makes little or no insulin. Insulin is a hormone the body uses to allow sugar (glucose) to enter cells to produce energy. Different factors, such as genetics and some viruses, may cause type 1 diabetes.
Type 1 diabetes is a chronic illness characterized by the body's inability to produce insulin due to the autoimmune destruction of the beta cells in the pancreas. Onset most often occurs in childhood, but the disease can also develop in adults in their late 30s and early 40s. ... Symptoms at the time of the first clinical presentation can ...
Type 1 diabetes is thought to be caused by an autoimmune reaction (the body attacks itself by mistake). This reaction destroys the cells in the pancreas that make insulin, called beta cells. This process can go on for months or years before any symptoms appear. Some people have certain genes (traits passed on from parent to child) that make ...
Type 1 diabetes is a challenging condition to manage properly, especially consistently throughout your lifetime. Because of this, T1D is associated with several complications. Close to 50% of people with Type 1 diabetes will develop a serious complication over their lifetime. Some may lose eyesight while others may develop end-stage kidney disease.
Type 1 diabetes mellitus is a chronic medical condition that occurs when the pancreas, an organ in the abdomen, produces very little or no insulin . Insulin is a hormone that helps the body to use glucose for energy. ... Clinical presentation, diagnosis, and initial evaluation of diabetes mellitus in adults
Type 1 diabetes mellitus (T1D) is an autoimmune disease that leads to the destruction of insulin-producing pancreatic beta cells. There is heterogeneity in the metabolic, genetic, and immunogenetic characteristics of T1D and age-related differences, requiring a personalized approach for each individual. Loss of insulin secretion can occur quickly or gradually.
Pathophysiology and Clinical Presentation. Pathophysiology: Type 1 Diabetes Mellitus is a syndrome characterized by hyperglycemia and insulin deficiency resulting from the loss of beta cells in pancreatic islets (Mapes & Faulds, 2014). Nonimmune (type 1B diabetes), occurs secondary to other diseases and is much less common than autoimmune (type ...
This topic will review the clinical presentation, diagnosis, and initial evaluation of diabetes in nonpregnant adults. Screening for and prevention of diabetes, the etiologic classification of diabetes mellitus, the treatment of diabetes, as well as diabetes during pregnancy are discussed separately. (See "Screening for type 2 diabetes mellitus" .)
People with type 1 diabetes who have elevated blood glucose (blood sugar) and classic risk factors for type 2 diabetes, such as being overweight or physically inactive, are often misdiagnosed. It can also be tricky because some adults with new-onset type 1 diabetes are not sick at first. Their doctor finds an elevated blood glucose level at a ...
Diabetes mellitus is a chronic heterogeneous metabolic disorder with complex pathogenesis. It is characterized by elevated blood glucose levels or hyperglycemia, which results from abnormalities in either insulin secretion or insulin action or both. Hyperglycemia manifests in various forms with a varied presentation and results in carbohydrate ...
Definition and Description. Type 1 diabetes (T1D) is a T-cell mediated autoimmune disease in which destruction of pancreatic β-cells causes insulin deficiency which leads to hyperglycemia and a tendency to ketoacidosis. 1 Excesses glucose levels must be managed by exogenous insulin injections several times a day. 2 Patients with T1D constitute ...
Type 2 Diabetes Warning Signs. Warning Signs and Symptoms - Can occur slowly over time. Blurred vision. Tingling or numbness in legs, feet or fingers. Recurring skin, gum or urinary tract infections. Drowsiness. Slow healing of cuts and bruises. Any symptoms that occur with Type 1 diabetes.
General presentation. Diabetes mellitus (DM) is an important endocrine disorder that presents commonly in children and adolescents. There are two types of diabetes mellitus: type 1 and type 2. Type 1 DM is one of the most common chronic diseases in children and is characterized by insulin deficiency as a result of autoimmune destruction of ...
Presentation tips. Ask ahead of time if you can present to the school or class on type 1 diabetes. Pick a date and time, place and format for the presentation. Decide whether the child with T1D will give the presentation, or if a parent or guardian will. Ask if they would like to help you present, if they would like to present themselves or if ...
This article reviews our current understanding of the etiology, presentation, and management of type 1 diabetes. The discussion includes a review of the natural history of diabetes, the complex relationship between genetic and environmental risk for type 1 diabetes, and current methods for prediction of type 1 diabetes. The article also reviews the current management of children who have new ...
Type 1 Diabetes: Cellular, Molecular & Clinical Immunology Edited by George S. Eisenbarth Online Edition Version 3.0. ... ADA Update June 2008.ppt Powerpoint slide set (added 7/08) 13. Theoretical Essays Kevin J. Lafferty and George S. Eisenbarth, Editors A. The Development of Autoimmune Diabetes
The clinical determination of type 1 vs. 2 diabetes is made with consideration of factors including the patient's age, body composition, symptom progression and clinical presentation. Type 1 diabetes typically manifests in young patients, often before the age of 14, who frequently appear thin and have a sudden onset of symptoms, with diabetic ...
While the complex etiopathogenesis and heterogeneity of type 1 diabetes (T1D) creates many challenges regarding disease-modifying interventions, there are ways to meaningfully reduce the morbidity (1,2), and mortality, associated with new-onset T1D when it presents with life-threatening diabetic ketoacidosis (DKA).Early diagnosis and prompt initiation of insulin can prevent DKA, as has been ...
Introduction. Approximately 65 000 children are diagnosed with type 1 diabetes (T1D) each year and the incidence is continuing to increase at a rate of approximately 3% per year.1, 2 The most common symptoms are well described and include polyuria, polydipsia, weight loss and tiredness. At the early stages of the disease, however, these symptoms are often non-specific and distinguishing the ...
Pediatric Type 1 Diabetes Mellitus. Pediatric Type 1 Diabetes Mellitus. Chelsea Stegman and Kelly Davis. Test your Knowledge. Type 1 Diabetes Mellitus is a/an _____ disease. a. Thyroid b. Bacterial c. Viral d. Autoimmune. Test your Knowledge. Type 1 Diabetes is most commonly diagnosed amongst: A. Overweight adults. 891 views • 21 slides
Type 1 diabetes mellitus (T1DM) is a prototypic endocrine autoimmune disease resulting from an immune-mediated destruction of pancreatic insulin-secreting $$\beta$$ cells. A comprehensive immune cell phenotype evaluation in T1DM has not been performed thus far at the single-cell level. In this cross-sectional analysis, we generated a single-cell transcriptomic dataset of peripheral blood ...
Type 1 diabetes is a chronic autoimmune disease characterised by insulin deficiency and resultant hyperglycaemia. ... is slightly more common in men and boys than in women and girls. 23 Two HLA class 2 haplotypes involved in anti gen presentation, HLA DRB1*0301-DQA1*0501-DQ* B10201 (DR3) and HLA DRB1*0401-DQA1*0301-DQB1*0301 (DR4-DQ8), are ...
Diabetes is a chronic health condition. With Type 1 diabetes, your body can't produce enough insulin and with Type 2 diabetes, it doesn't use it properly. But even beyond the two types, the ...
Background: Diet, a key component of type 1 diabetes (T1D) management, modulates the intestinal microbiota and its metabolically active byproducts—including SCFA—through fermentation of dietary carbohydrates such as fiber. ... Deposit scholarly works such as posters, presentations, research protocols, conference papers or white papers. If ...
The pattern of presentation of type 1 diabetes has changed as the incidence of DKA has decreased; unlike in previous studies, DKA was not the most common presenting symptom in this study. Chronic complications of diabetes, such as retinopathy, neuropathy, coronary heart disease, and nephropathy are mostly rare but still present. ...