
A relational database is a type of database that organizes data into rows and columns, which collectively form a table where the data points are related to each other.
Data is typically structured across multiple tables, which can be joined together via a primary key or a foreign key. These unique identifiers demonstrate the different relationships which exist between tables, and these relationships are usually illustrated through different types of data models . Analysts use SQL queries to combine different data points and summarize business performance, allowing organizations to gain insights, optimize workflows, and identify new opportunities.
For example, imagine your company maintains a database table with customer information, which contains company data at the account level. There may also be a different table, which describes all the individual transactions that align to that account. Together, these tables can provide information about the different industries that purchase a specific software product.
The columns (or fields) for the customer table might be Customer ID , Company Name , Company Address , Industry etc.; the columns for a transaction table might be Transaction Date , Customer ID , Transaction Amount , Payment Method , etc. The tables can be joined together with the common Customer ID field. You can, therefore, query the table to produce valuable reports, such as a sales reports by industry or company, which can inform messaging to prospective clients.
Relational databases are also typically associated with transactional databases, which execute commands, or transactions, collectively. A popular example that is used to illustrate this is a bank transfer. A defined amount is withdrawn from one account, and then it is deposited within another. The total amount of money is withdrawn and deposited, and this transaction cannot occur in any kind of partial sense. Transactions have specific properties. Represented by the acronym, ACID, ACID properties are defined as:
- Atomicity: All changes to data are performed as if they are a single operation. That is, all the changes are performed, or none of them are.
- Consistency: Data remains in a consistent state from state to finish, reinforcing data integrity.
- Isolation: The intermediate state of a transaction is not visible to other transactions, and as a result, transactions that run concurrently appear to be serialized.
- Durability: After the successful completion of a transaction, changes to data persist and are not undone, even in the event of a system failure.
These properties enable reliable transaction processing.
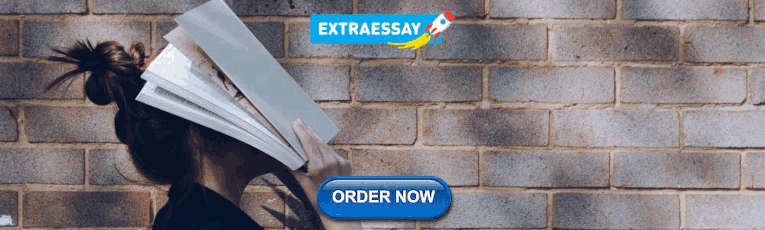
Relational database vs. relational database management system
While a relational database organizes data based off a relational data model, a relational database management system (RDBMS) is a more specific reference to the underlying database software that enables users to maintain it. These programs allow users to create, update, insert, or delete data in the system, and they provide:
- Data structure
- Multi-user access
- Privilege control
- Network access
Examples of popular RDBMS systems include MySQL, PostgreSQL, and IBM DB2. Additionally, a relational database system differs from a basic database management system (DBMS) in that it stores data in tables while a DBMS stores information as files.
Learn key benefits of generative AI and how organizations can incorporate generative AI and machine learning into their business.
Read the guide for data leaders
Invented by Don Chamberlin and Ray Boyce at IBM, Structured Query Language (SQL) is the standard programming language for interacting with relational database management systems, allowing database administrator to add, update, or delete rows of data easily. Originally known as SEQUEL, it was simplified to SQL due to a trademark issue. SQL queries also allows users to retrieve data from databases using only a few lines of code. Given this relationship, it’s easy to see why relational databases are also referred to as “SQL databases” at times.
Using the example from above, you might construct a query to find the top 10 transactions by company for a specific year with the following code:
SELECT COMPANY_NAME, SUM(TRANSACTION_AMOUNT)
FROM TRANSACTION_TABLE A
LEFT JOIN CUSTOMER_TABLE B
ON A.CUSTOMER_ID = B.CUSTOMER_ID
WHERE YEAR(DATE) = 2022
GROUP BY 1
ORDER BY 2 DESC
The ability to join data in this way helps us to reduce redundancy within our data systems, allowing data teams to maintain one master table for customers versus duplicating this information if there was another transaction in the future. To learn more, Don details more of the history of SQL in his paper here (link resides outside ibm.com).
Before relational databases, companies used a hierarchical database system with a tree-like structure for the data tables. These early database management systems (DBMS) enabled users to organize large quantities of data. However, they were complex, often proprietary to a particular application, and limited in the ways in which they could uncover within the data. These limitations eventually led IBM researcher, Edgar F. Codd, to publish a paper (link resides outside ibm.com) in 1970, titled "A Relational Model of Data for Large Shared Data Banks,” which theorized the relational database model. In this proposed model, information could be retrieved without specialized computer knowledge. He proposed arranging data based on meaningful relationships as tuples, or attribute-value pairs. Sets of tuples were referred to as relations, which ultimately enabled the merging of data across tables.
In 1973, the San Jose Research Laboratory—now known as the Almaden Research Center—began a program called System R (R for relational) to prove this relational theory with what it called “an industrial-strength implementation.” It ultimately became a testing ground for SQL as well, enabling it to become more widely adopted in a short period of time. However, Oracle’s adoption of SQL also didn’t hurt its popularity with database administrators.
By 1983, IBM introduced the DB2 family of relational databases, so named because it was IBM’s second family of database management software. Today, it is one of IBM’s most successful products, continuing to handle billions of transactions every day on cloud infrastructure and setting the foundational layer for machine learning applications.
While relational databases structure data into a tabular format, non-relational databases do not have as rigid of a database schema. In fact, non-relational databases organize data differently based on the type of database. Irrespective of the type of non-relational database, they all aim to solve for the flexibility and scalability issues inherent in relational models which are not ideal for unstructured data formats, like text, video, and images. These types of databases include:
- Key-value store: This schema-less data model is organized into a dictionary of key-value pairs, where each item has a key and a value. The key could be like something similar found in a SQL database, like a shopping cart ID, while the value is an array of data, like each individual item in that user’s shopping cart. It’s commonly used for caching and storing user session information, such as shopping carts. However, it's not ideal when you need to pull multiple records at a time. Redis and Memcached are examples of open-source databases with this data model.
- Document store: As suggested by the name, document databases store data as documents. They can be helpful in managing semi-structured data, and data are typically stored in JSON, XML, or BSON formats. This keeps the data together when it is used in applications, reducing the amount of translation needed to use the data. Developers also gain more flexibility since data schemas do not need to match across documents (e.g. name vs. first_name). However, this can be problematic for complex transactions, leading to data corruption. Popular use cases of document databases include content management systems and user profiles. An example of a document-oriented database is MongoDB, the database component of the MEAN stack.
- Wide-column store: These databases store information in columns, enabling users to access only the specific columns they need without allocating additional memory on irrelevant data. This database tries to solve for the shortcomings of key-value and document stores, but since it can be a more complex system to manage, it is not recommended for use for newer teams and projects. Apache HBase and Apache Cassandra are examples of open-source, wide-column databases. Apache HBase is built on top of Hadoop Distributed Files System that provides a way of storing sparse data sets, which is commonly used in many big data applications. Apache Cassandra, on the other hand, has been designed to manage large amounts of data across multiple servers and clustering that spans multiple data centers. It’s been used for a variety of use cases, such as social networking websites and real-time data analytics.
- Graph store: This type of database typically houses data from a knowledge graph. Data elements are stored as nodes, edges and properties. Any object, place, or person can be a node. An edge defines the relationship between the nodes. Graph databases are used for storing and managing a network of connections between elements within the graph. Neo4j (link resides outside IBM), a graph-based database service based on Java with an open-source community edition where users can purchase licenses for online backup and high availability extensions, or pre-package licensed version with backup and extensions included.
NoSQL databases also prioritize availability over consistency.
When computers run over a network , they invariably need to decide to prioritize consistent results (where every answer is always the same) or high uptime, called "availability." This is called the "CAP Theory," which stands for Consistency, Availability, or Partition Tolerance. Relational databases ensure the information is always in-sync and consistent. Some NoSQL databases, like Redis, prefer to always provide a response. That means the information you receive from a query may be incorrect by a few seconds—perhaps up to half a minute. On social media sites, this means seeing an old profile picture when the newest one is only a few moments old. The alternative could be a timeout or error. On the other hand, in banking and financial transactions, an error and resubmit may be better than old, incorrect information.
For a full rundown of the differences between SQL and NoSQL, see " SQL vs. NoSQL Databases: What's the Difference? "
The primary benefit of the relational database approach is the ability to create meaningful information by joining the tables. Joining tables allows you to understand the relations between the data, or how the tables connect. SQL includes the ability to count, add, group, and also combine queries. SQL can perform basic math and subtotal functions and logical transformations. Analysts can order the results by date, name, or any column. These features make the relational approach the single most popular query tool in business today.
Relational databases have several advantages compared to other database formats:
Ease of Use
By virtue of its product lifespan, there is more of a community around relational databases, which partially perpetuates its continued use. SQL also makes it easy to retrieve datasets from multiple tables and perform simple transformations such as filtering and aggregation. The use of indices within relational databases also allows them to locate this information quickly without searching each row in the selected table.
While relational databases have historically been viewed as a more rigid and inflexible data storage option, advances in technology and DBaaS options are changing that perception. While there is still more overhead to develop schemas compared to NoSQL database offerings, relational databases are becoming more flexible as they migrate to cloud environments.
Reduced redundancy
Relational databases can eliminate redundancy in two ways. The relational model itself reduces data redundancy via a process known as normalization. As noted earlier, a customer table should only log unique records of customer information versus duplicating this information for multiple transactions.
Stored procedures also help to reduce repetitive work. For example, if database access is restricted to certain roles, functions or teams, a stored procedure can help to manage access-control. These reusable functions free up coveted application developer time to tackle high impact work.
Ease of backup and disaster recovery
Relational databases are transactional—they guarantee the state of the entire system is consistent at any moment. Most relational databases offer easy export and import options, making backup and restore trivial. These exports can happen even while the database is running, making restore on failure easy. Modern, cloud-based relational databases can do continuous mirroring, making the loss of data on restore measured in seconds or less. Most cloud-managed services allow you to create Read Replicas, like in IBM Cloud® Databases for PostgreSQL . These Read Replicas enable you to store a read-only copy of your data in a cloud data center. Replicas can be promoted to Read/Write instances for disaster recovery as well.
Learn about IBM Db2, the cloud-native database built to power low-latency transactions and real-time analytics at scale.
Discover PostgreSQL as a service, built enterprise-ready with native integration into the IBM Cloud.
Hyper Protect Virtual Servers for Virtual Private Cloud (VPC) is a fully managed confidential compute container runtime that enables the deployment of sensitive containerized workloads in a highly isolated environment with technical assurance.
Develop and run applications on a security-rich, enterprise-class database that's based on open source PostgreSQL.
Look back to the beginning of Db2.
Scale AI workloads for all your data, anywhere, with IBM watsonx.data, a fit-for-purpose data store built on an open data lakehouse architecture.
Two-Bit History
Computing through the ages
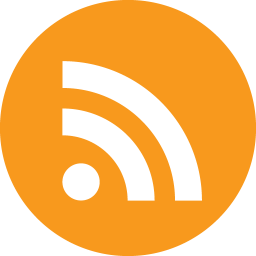
Important Papers: Codd and the Relational Model
29 Dec 2017
It’s hard to believe today, but the relational database was once the cool new kid on the block. In 2017, the relational model competes with all sorts of cutting-edge NoSQL technologies that make relational database systems seem old-fashioned and boring. Yet, 50 years ago, none of the dominant database systems were relational. Nobody had thought to structure their data that way. When the relational model did come along, it was a radical new idea that revolutionized the database world and spawned a multi-billion dollar industry.
The relational model was introduced in 1970. Edgar F. Codd, a researcher at IBM, published a paper called “A Relational Model of Data for Large Shared Data Banks.” The paper was a rewrite of a paper he had circulated internally at IBM a year earlier. The paper is unassuming; Codd does not announce in his abstract that he has discovered a brilliant new approach to storing data. He only claims to have employed a novel tool (the mathematical notion of a “relation”) to address some of the inadequacies of the prevailing database models.
In 1970, there were two schools of thought about how to structure a database: the hierarchical model and the network model. The hierarchical model was used by IBM’s Information Management System (IMS), the dominant database system at the time. The network model had been specified by a standards committee called CODASYL (which also—random tidbit—specified COBOL) and implemented by several other database system vendors. The two models were not really that different; both could be called “navigational” models. They persisted tree or graph data structures to disk using pointers to preserve the links between the data. Retrieving a record stored toward the bottom of the tree would involve first navigating through all of its ancestor records. These databases were fast (IMS is still used by many financial institutions partly for this reason, see this excellent blog post ) but inflexible. Woe unto those database administrators who suddenly found themselves needing to query records from the bottom of the tree without having an obvious place to start at the top.
Codd saw this inflexibility as a symptom of a larger problem. Programs using a hierarchical or network database had to know about how the stored data was structured. Programs had to know this because they were responsible for navigating down this structure to find the information they needed. This was so true that when Charles Bachman, a major pioneer of the network model, received a Turing Award for his work in 1973, he gave a speech titled “ The Programmer as Navigator .” Of course, if programs were saddled with this responsibility, then they would immediately break if the structure of the database ever changed. In the introduction to his 1970 paper, Codd motivates the search for a better model by arguing that we need “data independence,” which he defines as “the independence of application programs and terminal activities from growth in data types and changes in data representation.” The relational model, he argues, “appears to be superior in several respects to the graph or network model presently in vogue,” partly because, among other benefits, the relational model “provides a means of describing data with its natural structure only.” By this he meant that programs could safely ignore any artificial structures (like trees) imposed upon the data for storage and retrieval purposes only.
To further illustrate the problem with the navigational models, Codd devotes the first section of his paper to an example data set involving machine parts and assembly projects. This dataset, he says, could be represented in existing systems in at least five different ways. Any program \(P\) that is developed assuming one of five structures will fail when run against at least three of the other structures. The program \(P\) could instead try to figure out ahead of time which of the structures it might be dealing with, but it would be difficult to do so in this specific case and practically impossible in the general case. So, as long as the program needs to know about how the data is structured, we cannot switch to an alternative structure without breaking the program. This is a real bummer because (and this is from the abstract) “changes in data representation will often be needed as a result of changes in query, update, and report traffic and natural growth in the types of stored information.”
Codd then introduces his relational model. This model would be refined and expanded in subsequent papers: In 1971, Codd wrote about ALPHA, a SQL-like query language he created; in another 1971 paper, he introduced the first three normal forms we know and love today; and in 1972, he further developed relational algebra and relational calculus, the mathematically rigorous underpinnings of the relational model. But Codd’s 1970 paper contains the kernel of the relational idea:
The term relation is used here in its accepted mathematical sense. Given sets \(S_1, S_i, ..., S_n\) (not necessarily distinct), \(R\) is a relation on these \(n\) sets if it is a set of \(n\)-tuples each of which has its first element from \(S_1\), its second element from \(S_2\), and so on. We shall refer to \(S_j\) as the \(j\)th domain of \(R\). As defined above, \(R\) is said to have degree \(n\). Relations of degree 1 are often called unary , degree 2 binary , degree 3 ternary , and degree \(n\) n-ary .
Today, we call a relation a table , and a domain an attribute or a column . The word “table” actually appears nowhere in the paper, though Codd’s visual representations of relations (which he calls “arrays”) do resemble tables. Codd defines several more terms, some of which we continue to use and others we have replaced. He explains primary and foreign keys, as well as what he calls the “active domain,” which is the set of all distinct values that actually appear in a given domain or column. He then spends some time distinguishing between a “simple” and a “nonsimple” domain. A simple domain contains “atomic” or “nondecomposable” values, like integers. A nonsimple domain has relations as elements. The example Codd gives here is that of an employee with a salary history. The salary history is not one salary but a collection of salaries each associated with a date. So a salary history cannot be represented by a single number or string.
It’s not obvious how one could store a nonsimple domain in a multi-dimensional array, AKA a table. The temptation might be to denote the nonsimple relationship using some kind of pointer, but then we would be repeating the mistakes of the navigational models. Instead. Codd introduces normalization, which at least in the 1970 paper involves nothing more than turning nonsimple domains into simple ones. This is done by expanding the child relation so that it includes the primary key of the parent. Each tuple of the child relation references its parent using simple domains, eliminating the need for a nonsimple domain in the parent. Normalization means no pointers, sidestepping all the problems they cause in the navigational models.
At this point, anyone reading Codd’s paper would have several questions, such as “Okay, how would I actually query such a system?” Codd mentions the possibility of creating a universal sublanguage for querying relational databases from other programs, but declines to define such a language in this particular paper. He does explain, in mathematical terms, many of the fundamental operations such a language would have to support, like joins, “projection” ( SELECT in SQL), and “restriction” ( WHERE ). The amazing thing about Codd’s 1970 paper is that, really, all the ideas are there—we’ve been writing SELECT statements and joins for almost half a century now.
Codd wraps up the paper by discussing ways in which a normalized relational database, on top of its other benefits, can reduce redundancy and improve consistency in data storage. Altogether, the paper is only 11 pages long and not that difficult of a read. I encourage you to look through it yourself. It would be another ten years before Codd’s ideas were properly implemented in a functioning system, but, when they finally were, those systems were so obviously better than previous systems that they took the world by storm.
If you enjoyed this post, more like it come out every four weeks! Follow @TwoBitHistory on Twitter or subscribe to the RSS feed to make sure you know when a new post is out.
A Relational Database Management System Approach for Data Integration in Manufacturing Process
Ieee account.
- Change Username/Password
- Update Address
Purchase Details
- Payment Options
- Order History
- View Purchased Documents
Profile Information
- Communications Preferences
- Profession and Education
- Technical Interests
- US & Canada: +1 800 678 4333
- Worldwide: +1 732 981 0060
- Contact & Support
- About IEEE Xplore
- Accessibility
- Terms of Use
- Nondiscrimination Policy
- Privacy & Opting Out of Cookies
A not-for-profit organization, IEEE is the world's largest technical professional organization dedicated to advancing technology for the benefit of humanity. © Copyright 2024 IEEE - All rights reserved. Use of this web site signifies your agreement to the terms and conditions.
Academia.edu no longer supports Internet Explorer.
To browse Academia.edu and the wider internet faster and more securely, please take a few seconds to upgrade your browser .
- We're Hiring!
- Help Center
Relational Database
- Most Cited Papers
- Most Downloaded Papers
- Newest Papers
- Save to Library
- Last »
- Clouds Follow Following
- Free Software Follow Following
- Distributed Systems Follow Following
- Cloud Follow Following
- Database Systems Follow Following
- Database Management Systems Follow Following
- Nomophobia Follow Following
- Risk management and control of ERP projects Follow Following
- Cyber Bullying Follow Following
- Humanities Computing (Digital Humanities) Follow Following
Enter the email address you signed up with and we'll email you a reset link.
- Academia.edu Publishing
- We're Hiring!
- Help Center
- Find new research papers in:
- Health Sciences
- Earth Sciences
- Cognitive Science
- Mathematics
- Computer Science
- Academia ©2024
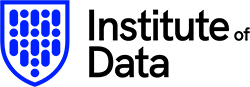
- New Zealand
- United Kingdom
Understanding Databases: Relational and Non-Relational Structures in Data Science

Databases play a fundamental role in data science.
Data scientists rely on databases to store, organize, and analyze vast data.
By understanding databases through their structure and features, data scientists can make informed decisions when choosing the right database for their projects.
Understanding databases: the fundamentals

Databases are repositories of structured data.
They provide a way to store, retrieve, and manipulate data efficiently.
Understanding databases and their central storage capacity for massive datasets in data science is fundamental to analysts accessing and analyzing data quickly and accurately.
The importance of databases in data science
Databases are crucial in data science for several reasons, including:
- Understanding databases provides a structured and standardized way to store data, ensuring its reliability and consistency.
- Understanding databases enables data scientists to perform complex queries and analyses of data.
- Using Structured Query Language (SQL), they can extract specific information from databases, filter data based on certain conditions, and perform advanced calculations.
- Databases support data integrity and security .
- Key terms and concepts in databases
Before delving deeper into relational and non-relational databases, let’s define some key terms and concepts that are commonly used in the realm of databases:
- Entity : A distinct object or concept that is represented in the database. Each entity has attributes that describe its properties or characteristics.
- Primary Key : A unique identifier for each record in a database table. It ensures that each record can be uniquely identified and accessed.
- Query : A request for specific data from a database. Queries are written in SQL and can retrieve, update, or delete records.
- Normalisation : The process of organising data in a database to minimise redundancy and improve efficiency. It involves breaking down tables into smaller, related tables to reduce data duplication.
Diving into relational databases
Relational databases are the most common type of database used in data science.
They organize data into tables with predefined relationships between them.
Each table contains rows, which represent individual records, and columns, which define the records’ attributes or fields.
The structure of relational databases
Relational databases are structured based on Edgar F. Codd’s 1970s proposal of the relational model.
This model organizes data into tables, and the keys establish relationships between tables.
A primary key is a unique identifier for each record in a table. It ensures that each record can be uniquely identified and accessed.
The role of SQL in relational databases
SQL is a powerful language for interacting with relational databases.
With SQL, data scientists can extract specific information from the database using SELECT statements .
SQL provides a standardized and intuitive way to manipulate data in relational databases.
Exploring non-relational databases

Understanding databases means knowing the difference between non-relational and relational databases.
Non-relational databases, also known as NoSQL (Not Only SQL), have gained popularity recently due to their ability to handle large volumes of unstructured and semi-structured data.
Unlike relational databases, non-relational databases do not strictly adhere to a predefined schema.
Understanding the structure of non-relational databases
Non-relational databases are schema-less, meaning they do not require a predefined structure for data.
Key-value stores are the simplest type of non-relational databases.
Graph databases represent relationships between entities.
The role of NoSQL in non-relational databases
NoSQL databases provide several advantages over traditional relational databases, making them suitable for certain data types and applications, including:
- NoSQL databases offer scalability and high-performance capabilities.
- NoSQL databases also excel in handling unstructured and semi-structured data.
- NoSQL databases offer built-in support for horizontal scaling, fault tolerance, and high availability.
Comparing relational and non-relational databases
Relational and non-relational databases have distinct characteristics suited for different use cases.
Performance comparison between the two structures
Regarding performance, relational databases excel in handling complex queries involving multiple joins and aggregations.
They are optimized for structured data and provide strong consistency and data integrity.
However, relational databases may face challenges when dealing with large datasets or high-speed data ingestion.
Suitability for different data types and applications
Understanding databases means choosing between relational and non-relational databases.
What you choose depends on the nature of the data and the application’s specific requirements.
Relational databases are well-suited for structured data that requires strong consistency and complex analysis.
They are commonly used in financial systems, e-commerce platforms, and inventory management applications.
Making the right choice

Understanding databases means choosing the right database structure for your data science project.
Factors to consider when choosing a database structure
- Data requirements : Analyse the characteristics of your data, such as volume, variety, and velocity. Determine whether your data is structured or unstructured, and consider its growth rate and future scalability requirements.
- Query complexity : Assess the types of queries and analysis you need to perform on the data. Determine if your queries involve complex joins and aggregations or require flexibility and adaptability to evolving data requirements.
- Development and maintenance costs : Consider the resources for developing and maintaining the database. Evaluate the skills and expertise required for each database type and the licensing and operational costs associated with each option.
- Integration with existing systems : Evaluate how well the database structure integrates with your existing systems and tools. Consider the database’s compatibility with your data processing and analysis workflows and its ability to support connectivity to other systems.
The impact of database choice on data analysis and results
Understanding databases is knowing the choice of database structure can greatly impact the efficiency and accuracy of data analysis.
Understanding databases also means the outcome will be a well-designed database structure that suits the data and query requirements and can streamline data retrieval and analysis processes, leading to faster insights and better decision-making.
Understanding databases is a pivotal component in the field of data science.
Relational and non-relational databases offer distinct features and advantages, catering to different data types and application needs.
Understanding databases and their structure and capabilities is essential for data scientists, allowing them to make informed decisions and efficiently work with their data.
Are you ready to boost your data science career?
The Institute of Data’s Data Science & AI program offers a real-world, practical curriculum taught by industry-experienced professionals.
We’ll support your learning with extensive resources and flexible learning options to suit your busy schedule.
Ready to learn more about our programs? Contact our local team for a free career consultation .

From Legal Expert to AI Innovator: Charting a New Course with Data Science & AI

From Neuroscience to Data Science & AI: Navigating Rei Masuda’s Career Transition

5 Questions to Ponder Before Switching Careers to Data Science

© Institute of Data. All rights reserved.
Copy Link to Clipboard

An official website of the United States government
The .gov means it’s official. Federal government websites often end in .gov or .mil. Before sharing sensitive information, make sure you’re on a federal government site.
The site is secure. The https:// ensures that you are connecting to the official website and that any information you provide is encrypted and transmitted securely.
- Publications
- Account settings
Preview improvements coming to the PMC website in October 2024. Learn More or Try it out now .
- Advanced Search
- Journal List
- Springer Nature - PMC COVID-19 Collection

Advances in database systems education: Methods, tools, curricula, and way forward
Muhammad ishaq.
1 Department of Computer Science, National University of Computer and Emerging Sciences, Lahore, Pakistan
2 Department of Computer Science, Virtual University of Pakistan, Lahore, Pakistan
3 Department of Computer Science, University of Management and Technology, Lahore, Pakistan
Muhammad Shoaib Farooq
Muhammad faraz manzoor.
4 Department of Computer Science, Lahore Garrison University, Lahore, Pakistan
Uzma Farooq
Kamran abid.
5 Department of Electrical Engineering, University of the Punjab, Lahore, Pakistan
Mamoun Abu Helou
6 Faculty of Information Technology, Al Istiqlal University, Jericho, Palestine
Associated Data
Not Applicable.
Fundamentals of Database Systems is a core course in computing disciplines as almost all small, medium, large, or enterprise systems essentially require data storage component. Database System Education (DSE) provides the foundation as well as advanced concepts in the area of data modeling and its implementation. The first course in DSE holds a pivotal role in developing students’ interest in this area. Over the years, the researchers have devised several different tools and methods to teach this course effectively, and have also been revisiting the curricula for database systems education. In this study a Systematic Literature Review (SLR) is presented that distills the existing literature pertaining to the DSE to discuss these three perspectives for the first course in database systems. Whereby, this SLR also discusses how the developed teaching and learning assistant tools, teaching and assessment methods and database curricula have evolved over the years due to rapid change in database technology. To this end, more than 65 articles related to DSE published between 1995 and 2022 have been shortlisted through a structured mechanism and have been reviewed to find the answers of the aforementioned objectives. The article also provides useful guidelines to the instructors, and discusses ideas to extend this research from several perspectives. To the best of our knowledge, this is the first research work that presents a broader review about the research conducted in the area of DSE.
Introduction
Database systems play a pivotal role in the successful implementation of the information systems to ensure the smooth running of many different organizations and companies (Etemad & Küpçü, 2018 ; Morien, 2006 ). Therefore, at least one course about the fundamentals of database systems is taught in every computing and information systems degree (Nagataki et al., 2013 ). Database System Education (DSE) is concerned with different aspects of data management while developing software (Park et al., 2017 ). The IEEE/ACM computing curricula guidelines endorse 30–50 dedicated hours for teaching fundamentals of design and implementation of database systems so as to build a very strong theoretical and practical understanding of the DSE topics (Cvetanovic et al., 2010 ).
Practically, most of the universities offer one user-oriented course at undergraduate level that covers topics related to the data modeling and design, querying, and a limited number of hours on theory (Conklin & Heinrichs, 2005 ; Robbert & Ricardo, 2003 ), where it is often debatable whether to utilize a design-first or query-first approach. Furthermore, in order to update the course contents, some recent trends, including big data and the notion of NoSQL should also be introduced in this basic course (Dietrich et al., 2008 ; Garcia-Molina, 2008 ). Whereas, the graduate course is more theoretical and includes topics related to DB architecture, transactions, concurrency, reliability, distribution, parallelism, replication, query optimization, along with some specialized classes.
Researchers have designed a variety of tools for making different concepts of introductory database course more interesting and easier to teach and learn interactively (Brusilovsky et al., 2010 ) either using visual support (Nagataki et al., 2013 ), or with the help of gamification (Fisher & Khine, 2006 ). Similarly, the instructors have been improvising different methods to teach (Abid et al., 2015 ; Domínguez & Jaime, 2010 ) and evaluate (Kawash et al., 2020 ) this theoretical and practical course. Also, the emerging and hot topics such as cloud computing and big data has also created the need to revise the curriculum and methods to teach DSE (Manzoor et al., 2020 ).
The research in database systems education has evolved over the years with respect to modern contents influenced by technological advancements, supportive tools to engage the learners for better learning, and improvisations in teaching and assessment methods. Particularly, in recent years there is a shift from self-describing data-driven systems to a problem-driven paradigm that is the bottom-up approach where data exists before being designed. This mainly relies on scientific, quantitative, and empirical methods for building models, while pushing the boundaries of typical data management by involving mathematics, statistics, data mining, and machine learning, thus opening a multidisciplinary perspective. Hence, it is important to devote a few lectures to introducing the relevance of such advance topics.
Researchers have provided useful review articles on other areas including Introductory Programming Language (Mehmood et al., 2020 ), use of gamification (Obaid et al., 2020 ), research trends in the use of enterprise service bus (Aziz et al., 2020 ), and the role of IoT in agriculture (Farooq et al., 2019 , 2020 ) However, to the best of our knowledge, no such study was found in the area of database systems education. Therefore, this study discusses research work published in different areas of database systems education involving curricula, tools, and approaches that have been proposed to teach an introductory course on database systems in an effective manner. The rest of the article has been structured in the following manner: Sect. 2 presents related work and provides a comparison of the related surveys with this study. Section 3 presents the research methodology for this study. Section 4 analyses the major findings of the literature reviewed in this research and categorizes it into different important aspects. Section 5 represents advices for the instructors and future directions. Lastly, Sect. 6 concludes the article.
Related work
Systematic Literature Reviews have been found to be a very useful artifact for covering and understanding a domain. A number of interesting review studies have been found in different fields (Farooq et al., 2021 ; Ishaq et al., 2021 ). Review articles are generally categorized into narrative or traditional reviews (Abid et al., 2016 ; Ramzan et al., 2019 ), systematic literature review (Naeem et al., 2020 ) and meta reviews or mapping study (Aria & Cuccurullo, 2017 ; Cobo et al., 2012 ; Tehseen et al., 2020 ). This study presents a systematic literature review on database system education.
The database systems education has been discussed from many different perspectives which include teaching and learning methods, curriculum development, and the facilitation of instructors and students by developing different tools. For instance, a number of research articles have been published focusing on developing tools for teaching database systems course (Abut & Ozturk, 1997 ; Connolly et al., 2005 ; Pahl et al., 2004 ). Furthermore, few authors have evaluated the DSE tools by conducting surveys and performing empirical experiments so as to gauge the effectiveness of these tools and their degree of acceptance among important stakeholders, teachers and students (Brusilovsky et al., 2010 ; Nelson & Fatimazahra, 2010 ). On the other hand, some case studies have also been discussed to evaluate the effectiveness of the improvised approaches and developed tools. For example, Regueras et al. ( 2007 ) presented a case study using the QUEST system, in which e-learning strategies are used to teach the database course at undergraduate level, while, Myers and Skinner ( 1997 ) identified the conflicts that arise when theories in text books regarding the development of databases do not work on specific applications.
Another important facet of DSE research focuses on the curriculum design and evolution for database systems, whereby (Alrumaih, 2016 ; Bhogal et al., 2012 ; Cvetanovic et al., 2010 ; Sahami et al., 2011 ) have proposed solutions for improvements in database curriculum for the better understanding of DSE among the students, while also keeping the evolving technology into the perspective. Similarly, Mingyu et al. ( 2017 ) have shared their experience in reforming the DSE curriculum by adding topics related to Big Data. A few authors have also developed and evaluated different tools to help the instructors teaching DSE.
There are further studies which focus on different aspects including specialized tools for specific topics in DSE (Mcintyre et al, 1995 ; Nelson & Fatimazahra, 2010 ). For instance, Mcintyre et al. ( 1995 ) conducted a survey about using state of the art software tools to teach advanced relational database design courses at Cleveland State University. However, the authors did not discuss the DSE curricula and pedagogy in their study. Similarly, a review has been conducted by Nelson and Fatimazahra ( 2010 ) to highlight the fact that the understanding of basic knowledge of database is important for students of the computer science domain as well as those belonging to other domains. They highlighted the issues encountered while teaching the database course in universities and suggested the instructors investigate these difficulties so as to make this course more effective for the students. Although authors have discussed and analyzed the tools to teach database, the tools are yet to be categorized according to different methods and research types within DSE. There also exists an interesting systematic mapping study by Taipalus and Seppänen ( 2020 ) that focuses on teaching SQL which is a specific topic of DSE. Whereby, they categorized the selected primary studies into six categories based on their research types. They utilized directed content analysis, such as, student errors in query formulation, characteristics and presentation of the exercise database, specific or non-specific teaching approach suggestions, patterns and visualization, and easing teacher workload.
Another relevant study that focuses on collaborative learning techniques to teach the database course has been conducted by Martin et al. ( 2013 ) This research discusses collaborative learning techniques and adapted it for the introductory database course at the Barcelona School of Informatics. The motive of the authors was to introduce active learning methods to improve learning and encourage the acquisition of competence. However, the focus of the study was only on a few methods for teaching the course of database systems, while other important perspectives, including database curricula, and tools for teaching DSE were not discussed in this study.
The above discussion shows that a considerable amount of research work has been conducted in the field of DSE to propose various teaching methods; develop and test different supportive tools, techniques, and strategies; and to improve the curricula for DSE. However, to the best of our knowledge, there is no study that puts all these relevant and pertinent aspects together while also classifying and discussing the supporting methods, and techniques. This review is considerably different from previous studies. Table Table1 1 highlights the differences between this study and other relevant studies in the field of DSE using ✓ and – symbol reflecting "included" and "not included" respectively. Therefore, this study aims to conduct a systematic mapping study on DSE that focuses on compiling, classifying, and discussing the existing work related to pedagogy, supporting tools, and curricula.
Comparison with other related research articles
Research methodology
In order to preserve the principal aim of this study, which is to review the research conducted in the area of database systems education, a piece of advice has been collected from existing methods described in various studies (Elberzhager et al., 2012 ; Keele et al., 2007 ; Mushtaq et al., 2017 ) to search for the relevant papers. Thus, proper research objectives were formulated, and based on them appropriate research questions and search strategy were formulated as shown in Fig. 1 .

Research objectives
The Following are the research objectives of this study:
- i. To find high quality research work in DSE.
- ii. To categorize different aspects of DSE covered by other researchers in the field.
- iii. To provide a thorough discussion of the existing work in this study to provide useful information in the form of evolution, teaching guidelines, and future research directions of the instructors.
Research questions
In order to fulfill the research objectives, some relevant research questions have been formulated. These questions along with their motivations have been presented in Table Table2 2 .
Study selection results
Search strategy
The Following search string used to find relevant articles to conduct this study. “Database” AND (“System” OR “Management”) AND (“Education*” OR “Train*” OR “Tech*” OR “Learn*” OR “Guide*” OR “Curricul*”).
Articles have been taken from different sources i.e. IEEE, Springer, ACM, Science Direct and other well-known journals and conferences such as Wiley Online Library, PLOS and ArXiv. The planning for search to find the primary study in the field of DSE is a vital task.
Study selection
A total of 29,370 initial studies were found. These articles went through a selection process, and two authors were designated to shortlist the articles based on the defined inclusion criteria as shown in Fig. 2 . Their conflicts were resolved by involving a third author; while the inclusion/exclusion criteria were also refined after resolving the conflicts as shown in Table Table3. 3 . Cohen’s Kappa coefficient 0.89 was observed between the two authors who selected the articles, which reflects almost perfect agreement between them (Landis & Koch, 1977 ). While, the number of papers in different stages of the selection process for all involved portals has been presented in Table Table4 4 .

Selection criteria
Title based search: Papers that are irrelevant based on their title are manually excluded in the first stage. At this stage, there was a large portion of irrelevant papers. Only 609 papers remained after this stage.
Abstract based search: At this stage, abstracts of the selected papers in the previous stage are studied and the papers are categorized for the analysis along with research approach. After this stage only 152 papers were left.
Full text based analysis: Empirical quality of the selected articles in the previous stage is evaluated at this stage. The analysis of full text of the article has been conducted. The total of 70 papers were extracted from 152 papers for primary study. Following questions are defined for the conduction of final data extraction.
Quality assessment criteria
Following are the criteria used to assess the quality of the selected primary studies. This quality assessment was conducted by two authors as explained above.
- The study focuses on curricula, tools, approach, or assessments in DSE, the possible answers were Yes (1), No (0)
- The study presents a solution to the problem in DSE, the possible answers to this question were Yes (1), Partially (0.5), No (0)
- The study focuses on empirical results, Yes (1), No (0)
Score pattern of publication channels
Almost 50.00% of papers had scored more than average and 33.33% of papers had scored between the average range i.e., 2.50–3.50. Some articles with the score below 2.50 have also been included in this study as they present some useful information and were published in education-based journals. Also, these studies discuss important demography and technology based aspects that are directly related to DSE.
Threats to validity
The validity of this study could be influenced by the following factors during the literature of this publication.
Construct validity
In this study this validity identifies the primary study for research (Elberzhager et al., 2012 ). To ensure that many primary studies have been included in this literature two authors have proposed possible search keywords in multiple repetitions. Search string is comprised of different terms related to DS and education. Though, list might be incomplete, count of final papers found can be changed by the alternative terms (Ampatzoglou et al., 2013 ). IEEE digital library, Science direct, ACM digital library, Wiley Online Library, PLOS, ArXiv and Google scholar are the main libraries where search is done. We believe according to the statistics of search engines of literature the most research can be found on these digital libraries (Garousi et al., 2013 ). Researchers also searched related papers in main DS research sites (VLDB, ICDM, EDBT) in order to minimize the risk of missing important publication.
Including the papers that does not belong to top journals or conferences may reduce the quality of primary studies in this research but it indicates that the representativeness of the primary studies is improved. However, certain papers which were not from the top publication sources are included because of their relativeness wisth the literature, even though they reduce the average score for primary studies. It also reduces the possibility of alteration of results which might have caused by the improper handling of duplicate papers. Some cases of duplications were found which were inspected later whether they were the same study or not. The two authors who have conducted the search has taken the final decision to the select the papers. If there is no agreement between then there must be discussion until an agreement is reached.
Internal validity
This validity deals with extraction and data analysis (Elberzhager et al., 2012 ). Two authors carried out the data extraction and primary studies classification. While the conflicts between them were resolved by involving a third author. The Kappa coefficient was 0.89, according to Landis and Koch ( 1977 ), this value indicates almost perfect level of agreement between the authors that reduces this threat significantly.
Conclusion validity
This threat deals with the identification of improper results which may cause the improper conclusions. In this case this threat deals with the factors like missing studies and wrong data extraction (Ampatzoglou et al., 2013 ). The objective of this is to limit these factors so that other authors can perform study and produce the proper conclusions (Elberzhager et al., 2012 ).
Interpretation of results might be affected by the selection and classification of primary studies and analyzing the selected study. Previous section has clearly described each step performed in primary study selection and data extraction activity to minimize this threat. The traceability between the result and data extracted was supported through the different charts. In our point of view, slight difference based on the publication selection and misclassification would not alter the main results.
External validity
This threat deals with the simplification of this research (Mateo et al., 2012 ). The results of this study were only considered that related to the DSE filed and validation of the conclusions extracted from this study only concerns the DSE context. The selected study representativeness was not affected because there was no restriction on time to find the published research. Therefore, this external validity threat is not valid in the context of this research. DS researchers can take search string and the paper classification scheme represented in this study as an initial point and more papers can be searched and categorized according to this scheme.
Analysis of compiled research articles
This section presents the analysis of the compiled research articles carefully selected for this study. It presents the findings with respect to the research questions described in Table Table2 2 .
Selection results
A total of 70 papers were identified and analyzed for the answers of RQs described above. Table Table6 6 represents a list of the nominated papers with detail of the classification results and their quality assessment scores.
Classification and quality assessment of selected articles
RQ1.Categorization of research work in DSE field
The analysis in this study reveals that the literature can be categorized as: Tools: any additional application that helps instructors in teaching and students in learning. Methods: any improvisation aimed at improving pedagogy or cognition. Curriculum: refers to the course content domains and their relative importance in a degree program, as shown in Fig. 3 .

Taxonomy of DSE study types
Most of the articles provide a solution by gathering the data and also prove the novelty of their research through results. These papers are categorized as experiments w.r.t. their research types. Whereas, some of them case study papers which are used to generate an in depth, multifaceted understanding of a complex issue in its real-life context, while few others are review studies analyzing the previously used approaches. On the other hand, a majority of included articles have evaluated their results with the help of experiments, while others conducted reviews to establish an opinion as shown in Fig. 4 .

Cross Mapping of DSE study type and research Types
Educational tools, especially those related to technology, are making their place in market faster than ever before (Calderon et al., 2011 ). The transition to active learning approaches, with the learner more engaged in the process rather than passively taking in information, necessitates a variety of tools to help ensure success. As with most educational initiatives, time should be taken to consider the goals of the activity, the type of learners, and the tools needed to meet the goals. Constant reassessment of tools is important to discover innovation and reforms that improve teaching and learning (Irby & Wilkerson, 2003 ). For this purpose, various type of educational tools such as, interactive, web-based and game based have been introduced to aid the instructors in order to explain the topic in more effective way.
The inclusion of technology into the classroom may help learners to compete in the competitive market when approaching the start of their career. It is important for the instructors to acknowledge that the students are more interested in using technology to learn database course instead of merely being taught traditional theory, project, and practice-based methods of teaching (Adams et al., 2004 ). Keeping these aspects in view many authors have done significant research which includes web-based and interactive tools to help the learners gain better understanding of basic database concepts.
Great research has been conducted with the focus of students learning. In this study we have discussed the students learning supportive with two major finding’s objectives i.e., tools which prove to be more helpful than other tools. Whereas, proposed tools with same outcome as traditional classroom environment. Such as, Abut and Ozturk ( 1997 ) proposed an interactive classroom environment to conduct database classes. The online tools such as electronic “Whiteboard”, electronic textbooks, advance telecommunication networks and few other resources such as Matlab and World Wide Web were the main highlights of their proposed smart classroom. Also, Pahl et al. ( 2004 ) presented an interactive multimedia-based system for the knowledge and skill oriented Web-based education of database course students. The authors had differentiated their proposed classroom environment from traditional classroom-based approach by using tool mediated independent learning and training in an authentic setting. On the other hand, some authors have also evaluated the educational tools based on their usage and impact on students’ learning. For example, Brusilovsky et al. ( 2010 )s evaluated the technical and conceptual difficulties of using several interactive educational tools in the context of a single course. A combined Exploratorium has been presented for database courses and an experimental platform, which delivers modified access to numerous types of interactive learning activities.
Also, Taipalus and Perälä ( 2019 ) investigated the types of errors that are persistent in writing SQL by the students. The authors also contemplated the errors while mapping them onto different query concepts. Moreover, Abelló Gamazo et al. ( 2016 ) presented a software tool for the e-assessment of relational database skills named LearnSQL. The proposed software allows the automatic and efficient e-learning and e-assessment of relational database skills. Apart from these, Yue ( 2013 ) proposed the database tool named Sakila as a unified platform to support instructions and multiple assignments of a graduate database course for five semesters. According to this study, students find this tool more useful and interesting than the highly simplified databases developed by the instructor, or obtained from textbook. On the other hand, authors have proposed tools with the main objective to help the student’s grip on the topic by addressing the pedagogical problems in using the educational tools. Connolly et al. ( 2005 ) discussed some of the pedagogical problems sustaining the development of a constructive learning environment using problem-based learning, a simulation game and interactive visualizations to help teach database analysis and design. Also, Yau and Karim ( 2003 ) proposed smart classroom with prevalent computing technology which will facilitate collaborative learning among the learners. The major aim of this smart classroom is to improve the quality of interaction between the instructors and students during lecture.
Student satisfaction is also an important factor for the educational tools to more effective. While it supports in students learning process it should also be flexible to achieve the student’s confidence by making it as per student’s needs (Brusilovsky et al., 2010 ; Connolly et al., 2005 ; Pahl et al., 2004 ). Also, Cvetanovic et al. ( 2010 ) has proposed a web-based educational system named ADVICE. The proposed solution helps the students to reduce the gap between DBMS, theory and its practice. On the other hand, authors have enhanced the already existing educational tools in the traditional classroom environment to addressed the student’s concerns (Nelson & Fatimazahra, 2010 ; Regueras et al., 2007 ) Table Table7 7 .
Tools: Adopted in DSE and their impacts
Hands on database development is the main concern in most of the institute as well as in industry. However, tools assisting the students in database development and query writing is still major concern especially in SQL (Brusilovsky et al., 2010 ; Nagataki et al., 2013 ).
Student’s grades reflect their conceptual clarity and database development skills. They are also important to secure jobs and scholarships after passing out, which is why it is important to have the educational learning tools to help the students to perform well in the exams (Cvetanovic et al., 2010 ; Taipalus et al., 2018 ). While, few authors (Wang et al., 2010 ) proposed Metube which is a variation of YouTube. Subsequently, existing educational tools needs to be upgraded or replaced by the more suitable assessment oriented interactive tools to attend challenging students needs (Pahl et al., 2004 ; Yuelan et al., 2011 ).
One other objective of developing the educational tools is to increase the interaction between the students and the instructors. In the modern era, almost every institute follows the student centered learning(SCL). In SCL the interaction between students and instructor increases with most of the interaction involves from the students. In order to support SCL the educational based interactive and web-based tools need to assign more roles to students than the instructors (Abbasi et al., 2016 ; Taipalus & Perälä, 2019 ; Yau & Karim, 2003 ).
Theory versus practice is still one of the main issues in DSE teaching methods. The traditional teaching method supports theory first and then the concepts learned in the theoretical lectures implemented in the lab. Whereas, others think that it is better to start by teaching how to write query, which should be followed by teaching the design principles for database, while a limited amount of credit hours are also allocated for the general database theory topics. This part of the article discusses different trends of teaching and learning style along with curriculum and assessments methods discussed in DSE literature.
A variety of teaching methods have been designed, experimented, and evaluated by different researchers (Yuelan et al., 2011 ; Chen et al., 2012 ; Connolly & Begg, 2006 ). Some authors have reformed teaching methods based on the requirements of modern way of delivering lectures such as Yuelan et al. ( 2011 ) reform teaching method by using various approaches e.g. a) Modern ways of education: includes multimedia sound, animation, and simulating the process and working of database systems to motivate and inspire the students. b) Project driven approach: aims to make the students familiar with system operations by implementing a project. c) Strengthening the experimental aspects: to help the students get a strong grip on the basic knowledge of database and also enable them to adopt a self-learning ability. d) Improving the traditional assessment method: the students should turn in their research and development work as the content of the exam, so that they can solve their problem on their own.
The main aim of any teaching method is to make student learn the subject effectively. Student must show interest in order to gain something from the lectures delivered by the instructors. For this, teaching methods should be interactive and interesting enough to develop the interest of the students in the subject. Students can show interest in the subject by asking more relative questions or completing the home task and assignments on time. Authors have proposed few teaching methods to make topic more interesting such as, Chen et al. ( 2012 ) proposed a scaffold concept mapping strategy, which considers a student’s prior knowledge, and provides flexible learning aids (scaffolding and fading) for reading and drawing concept maps. Also, Connolly & Begg (200s6) examined different problems in database analysis and design teaching, and proposed a teaching approach driven by principles found in the constructivist epistemology to overcome these problems. This constructivist approach is based on the cognitive apprenticeship model and project-based learning. Similarly, Domínguez & Jaime ( 2010 ) proposed an active method for database design through practical tasks development in a face-to-face course. They analyzed results of five academic years using quasi experimental. The first three years a traditional strategy was followed and a course management system was used as material repository. On the other hand, Dietrich and Urban ( 1996 ) have described the use of cooperative group learning concepts in support of an undergraduate database management course. They have designed the project deliverables in such a way that students develop skills for database implementation. Similarly, Zhang et al. ( 2018 ) have discussed several effective classroom teaching measures from the aspects of the innovation of teaching content, teaching methods, teaching evaluation and assessment methods. They have practiced the various teaching measures by implementing the database technologies and applications in Qinghai University. Moreover, Hou and Chen ( 2010 ) proposed a new teaching method based on blending learning theory, which merges traditional and constructivist methods. They adopted the method by applying the blending learning theory on Access Database programming course teaching.
Problem solving skills is a key aspect to any type of learning at any age. Student must possess this skill to tackle the hurdles in institute and also in industry. Create mind and innovative students find various and unique ways to solve the daily task which is why they are more likeable to secure good grades and jobs. Authors have been working to introduce teaching methods to develop problem solving skills in the students(Al-Shuaily, 2012 ; Cai & Gao, 2019 ; Martinez-González & Duffing, 2007 ; Gudivada et al., 2007 ). For instance, Al-Shuaily ( 2012 ) has explored four cognitive factors such as i) Novices’ ability in understanding, ii) Novices’ ability to translate, iii) Novice’s ability to write, iv) Novices’ skills that might influence SQL teaching, and learning methods and approaches. Also, Cai and Gao ( 2019 ) have reformed the teaching method in the database course of two higher education institutes in China. Skills and knowledge, innovation ability, and data abstraction were the main objective of their study. Similarly, Martinez-González and Duffing ( 2007 ) analyzed the impact of convergence of European Union (EU) in different universities across Europe. According to their study, these institutes need to restructure their degree program and teaching methodologies. Moreover, Gudivada et al. ( 2007 ) proposed a student’s learning method to work with the large datasets. they have used the Amazon Web Services API and.NET/C# application to extract a subset of the product database to enhance student learning in a relational database course.
On the other hand, authors have also evaluated the traditional teaching methods to enhance the problem-solving skills among the students(Eaglestone & Nunes, 2004 ; Wang & Chen, 2014 ; Efendiouglu & Yelken, 2010 ) Such as, Eaglestone and Nunes ( 2004 ) shared their experiences of delivering a database design course at Sheffield University and discussed some of the issues they faced, regarding teaching, learning and assessments. Likewise, Wang and Chen ( 2014 ) summarized the problems mainly in teaching of the traditional database theory and application. According to the authors the teaching method is outdated and does not focus on the important combination of theory and practice. Moreover, Efendiouglu and Yelken ( 2010 ) investigated the effects of two different methods Programmed Instruction (PI) and Meaningful Learning (ML) on primary school teacher candidates’ academic achievements and attitudes toward computer-based education, and to define their views on these methods. The results show that PI is not favoured for teaching applications because of its behavioural structure Table Table8 8 .
Methods: Teaching approaches adopted in DSE
Students become creative and innovative when the try to study on their own and also from different resources rather than curriculum books only. In the modern era, there are various resources available on both online and offline platforms. Modern teaching methods must emphasize on making the students independent from the curriculum books and educate them to learn independently(Amadio et al., 2003 ; Cai & Gao, 2019 ; Martin et al., 2013 ). Also, in the work of Kawash et al. ( 2020 ) proposed he group study-based learning approach called Graded Group Activities (GGAs). In this method students team up in order to take the exam as a group. On the other hand, few studies have emphasized on course content to prepare students for the final exams such as, Zheng and Dong ( 2011 ) have discussed the issues of computer science teaching with particular focus on database systems, where different characteristics of the course, teaching content and suggestions to teach this course effectively have been presented.
As technology is evolving at rapid speed, so students need to have practical experience from the start. Basic theoretical concepts of database are important but they are of no use without its implementation in real world projects. Most of the students study in the institutes with the aim of only clearing the exams with the help of theoretical knowledge and very few students want to have practical experience(Wang & Chen, 2014 ; Zheng & Dong, 2011 ). To reduce the gap between the theory and its implementation, authors have proposed teaching methods to develop the student’s interest in the real-world projects (Naik & Gajjar, 2021 ; Svahnberg et al., 2008 ; Taipalus et al., 2018 ). Moreover, Juxiang and Zhihong ( 2012 ) have proposed that the teaching organization starts from application scenarios, and associate database theoretical knowledge with the process from analysis, modeling to establishing database application. Also, Svahnberg et al. ( 2008 ) explained that in particular conditions, there is a possibility to use students as subjects for experimental studies in DSE and influencing them by providing responses that are in line with industrial practice.
On the other hand, Nelson et al. ( 2003 ) evaluated the different teaching methods used to teach different modules of database in the School of Computing and Technology at the University of Sunder- land. They outlined suggestions for changes to the database curriculum to further integrate research and state-of-the-art systems in databases.
- III. Curriculum
Database curriculum has been revisited many times in the form of guidelines that not only present the contents but also suggest approximate time to cover different topics. According to the ACM curriculum guidelines (Lunt et al., 2008 ) for the undergraduate programs in computer science, the overall coverage time for this course is 46.50 h distributed in such a way that 11 h is the total coverage time for the core topics such as, Information Models (4 core hours), Database Systems (3 core hours) and Data Modeling (4 course hours). Whereas, the remaining hours are allocated for elective topics such as Indexing, Relational Databases, Query Languages, Relational Database Design, Transaction Processing, Distributed Databases, Physical Database Design, Data Mining, Information Storage and Retrieval, Hypermedia, Multimedia Systems, and Digital Libraries(Marshall, 2012 ). While, according to the ACM curriculum guidelines ( 2013 ) for undergraduate programs in computer science, this course should be completed in 15 weeks with two and half hour lecture per week and lab session of four hours per week on average (Brady et al., 2004 ). Thus, the revised version emphasizes on the practice based learning with the help of lab component. Numerous organizations have exerted efforts in this field to classify DSE (Dietrich et al., 2008 ). DSE model curricula, bodies of knowledge (BOKs), and some standardization aspects in this field are discussed below:
Model curricula
There are standard bodies who set the curriculum guidelines for teaching undergraduate degree programs in computing disciplines. Curricula which include the guidelines to teach database are: Computer Engineering Curricula (CEC) (Meier et al., 2008 ), Information Technology Curricula (ITC) (Alrumaih, 2016 ), Computing Curriculum Software Engineering (CCSE) (Meyer, 2001 ), Cyber Security Curricula (CSC) (Brady et al., 2004 ; Bishop et al., 2017 ).
Bodies of knowledge (BOK)
A BOK includes the set of thoughts and activities related to the professional area, while in model curriculum set of guidelines are given to address the education issues (Sahami et al., 2011 ). Database body of Knowledge comprises of (a) The Data Management Body of Knowledge (DM- BOK), (b) Software Engineering Education Knowledge (SEEK) (Sobel, 2003 ) (Sobel, 2003 ), and (c) The SE body of knowledge (SWEBOK) (Swebok Evolution: IEEE Computer Society n.d. ).
Apart from the model curricula, and bodies of knowledge, there also exist some standards related to the database and its different modules: ISO/IEC 9075–1:2016 (Computing Curricula, 1991 ), ISO/IEC 10,026–1: 1998 (Suryn, 2003 ).
We also utilize advices from some studies (Elberzhager et al., 2012 ; Keele et al., 2007 ) to search for relevant papers. In order to conduct this systematic study, it is essential to formulate the primary research questions (Mushtaq et al., 2017 ). Since the data management techniques and software are evolving rapidly, the database curriculum should also be updated accordingly to meet these new requirements. Some authors have described ways of updating the content of courses to keep pace with specific developments in the field and others have developed new database curricula to keep up with the new data management techniques.
Furthermore, some authors have suggested updates for the database curriculum based on the continuously evolving technology and introduction of big data. For instance Bhogal et al. ( 2012 ) have shown that database curricula need to be updated and modernized, which can be achieved by extending the current database concepts that cover the strategies to handle the ever changing user requirements and how database technology has evolved to meet the requirements. Likewise, Picciano ( 2012 ) examines the evolving world of big data and analytics in American higher education. According to the author, the “data driven” decision making method should be used to help the institutes evaluate strategies that can improve retention and update the curriculum that has big data basic concepts and applications, since data driven decision making has already entered in the big data and learning analytic era. Furthermore, Marshall ( 2011 ) presented the challenges faced when developing a curriculum for a Computer Science degree program in the South African context that is earmarked for international recognition. According to the author, the Curricula needs to adhere both to the policy and content requirements in order to be rated as being of a particular quality.
Similarly, some studies (Abourezq & Idrissi, 2016 ; Mingyu et al., 2017 ) described big data influence from a social perspective and also proceeded with the gaps in database curriculum of computer science, especially, in the big data era and discovers the teaching improvements in practical and theoretical teaching mode, teaching content and teaching practice platform in database curriculum. Also Silva et al. ( 2016 ) propose teaching SQL as a general language that can be used in a wide range of database systems from traditional relational database management systems to big data systems.
On the other hand, different authors have developed a database curriculum based on the different academic background of students. Such as, Dean and Milani ( 1995 ) have recommended changes in computer science curricula based on the practice in United Stated Military Academy (USMA). They emphasized greatly on the practical demonstration of the topic rather than the theoretical explanation. Especially, for the non-computer science major students. Furthermore, Urban and Dietrich ( 2001 ) described the development of a second course on database systems for undergraduates, preparing students for the advanced database concepts that they will exercise in the industry. They also shared their experience with teaching the course, elaborating on the topics and assignments. Also, Andersson et al. ( 2019 ) proposed variations in core topics of database management course for the students with the engineering background. Moreover, Dietrich et al. ( 2014 ) described two animations developed with images and color that visually and dynamically introduce fundamental relational database concepts and querying to students of many majors. The goal is that the educators, in diverse academic disciplines, should be able to incorporate these animations in their existing courses to meet their pedagogical needs.
The information systems have evolved into large scale distributed systems that store and process a huge amount of data across different servers, and process them using different distributed data processing frameworks. This evolution has given birth to new paradigms in database systems domain termed as NoSQL and Big Data systems, which significantly deviate from conventional relational and distributed database management systems. It is pertinent to mention that in order to offer a sustainable and practical CS education, these new paradigms and methodologies as shown in Fig. 5 should be included into database education (Kleiner, 2015 ). Tables Tables9 9 and and10 10 shows the summarized findings of the curriculum based reviewed studies. This section also proposed appropriate text book based on the theory, project, and practice-based teaching methodology as shown in Table Table9. 9 . The proposed books are selected purely on the bases of their usage in top universities around the world such as, Massachusetts Institute of Technology, Stanford University, Harvard University, University of Oxford, University of Cambridge and, University of Singapore and the coverage of core topics mentioned in the database curriculum.

Concepts in Database Systems Education (Kleiner, 2015 )
Recommended text books for DSE
Curriculum: Findings of Reviewed Literature
RQ.2 Evolution of DSE research
This section discusses the evolution of database while focusing the DSE over the past 25 years as shown in Fig. 6 .

Evolution of DSE studies
This study shows that there is significant increase in research in DSE after 2004 with 78% of the selected papers are published after 2004. The main reason of this outcome is that some of the papers are published in well-recognized channels like IEEE Transactions on Education, ACM Transactions on Computing Education, International Conference on Computer Science and Education (ICCSE), and Teaching, Learning and Assessment of Database (TLAD) workshop. It is also evident that several of these papers were published before 2004 and only a few articles were published during late 1990s. This is because of the fact that DSE started to gain interest after the introduction of Body of Knowledge and DSE standards. The data intensive scientific discovery has been discussed as the fourth paradigm (Hey et al., 2009 ): where the first involves empirical science and observations; second contains theoretical science and mathematically driven insights; third considers computational science and simulation driven insights; while the fourth involves data driven insights of modern scientific research.
Over the past few decades, students have gone from attending one-room class to having the world at their fingertips, and it is a great challenge for the instructors to develop the interest of students in learning database. This challenge has led to the development of the different types of interactive tools to help the instructors teach DSE in this technology oriented era. Keeping the importance of interactive tools in DSE in perspective, various authors have proposed different interactive tools over the years, such as during 1995–2003, when different authors proposed various interactive tools. Some studies (Abut & Ozturk, 1997 ; Mcintyre et al., 1995 ) introduced state of the art interactive tools to teach and enhance the collaborative learning among the students. Similarly, during 2004–2005 more interactive tools in the field of DSE were proposed such as Pahl et al. ( 2004 ), Connolly et al. ( 2005 ) introduced multimedia system based interactive model and game based collaborative learning environment.
The Internet has started to become more common in the first decade of the twenty-first century and its positive impact on the education sector was undeniable. Cost effective, student teacher peer interaction, keeping in touch with the latest information were the main reasons which made the instructors employ web-based tools to teach database in the education sector. Due to this spike in the demand of web-based tools, authors also started to introduce new instruments to assist with teaching database. In 2007 Regueras et al. ( 2007 ) proposed an e-learning tool named QUEST with a feedback module to help the students to learn from their mistakes. Similarly, in 2010, multiple authors have proposed and evaluated various web-based tools. Cvetanovic et al. ( 2010 ) proposed ADVICE with the functionality to monitor student’s progress, while, few authors (Wang et al., 2010 ) proposed Metube which is a variation of YouTube. Furthermore, Nelson and Fatimazahra ( 2010 ) evaluated different web-based tools to highlight the complexities of using these web-based instruments.
Technology has changed the teaching methods in the education sector but technology cannot replace teachers, and despite the amount of time most students spend online, virtual learning will never recreate the teacher-student bond. In the modern era, innovation in technology used in educational sectors is not meant to replace the instructors or teaching methods.
During the 1990s some studies (Dietrich & Urban, 1996 ; Urban & Dietrich, 1997 ) proposed learning and teaching methods respectively keeping the evolving technology in view. The highlight of their work was project deliverables and assignments where students progressively advanced to a step-by-step extension, from a tutorial exercise and then attempting more difficult extension of assignment.
During 2002–2007 various authors have discussed a number of teaching and learning methods to keep up the pace with the ever changing database technology, such as Connolly and Begg ( 2006 ) proposing a constructive approach to teach database analysis and design. Similarly, Prince and Felder ( 2006 ) reviewed the effectiveness of inquiry learning, problem based learning, project-based learning, case-based teaching, discovery learning, and just-in-time teaching. Also, McIntyre et al. (Mcintyre et al., 1995 ) brought to light the impact of convergence of European Union (EU) in different universities across Europe. They suggested a reconstruction of teaching and learning methodologies in order to effectively teach database.
During 2008–2013 more work had been done to address the different methods of teaching and learning in the field of DSE, like the work of Dominguez and Jaime ( 2010 ) who proposed an active learning approach. The focus of their study was to develop the interest of students in designing and developing databases. Also, Zheng and Dong ( 2011 ) have highlighted various characteristics of the database course and its teaching content. Similarly, Yuelan et al. ( 2011 ) have reformed database teaching methods. The main focus of their study were the Modern ways of education, project driven approach, strengthening the experimental aspects, and improving the traditional assessment method. Likewise, Al-Shuaily ( 2012 ) has explored 4 cognitive factors that can affect the learning process of database. The main focus of their study was to facilitate the students in learning SQL. Subsequently, Chen et al. ( 2012 ) also proposed scaffolding-based concept mapping strategy. This strategy helps the students to better understand database management courses. Correspondingly, Martin et al. ( 2013 ) discussed various collaborative learning techniques in the field of DSE while keeping database as an introductory course.
In the years between 2014 and 2021, research in the field of DSE increased, which was the main reason that the most of teaching, learning and assessment methods were proposed and discussed during this period. Rashid and Al-Radhy ( 2014 ) discussed the issues of traditional teaching, learning, assessing methods of database courses at different universities in Kurdistan and the main focus of their study being reformation issues, such as absence of teaching determination and contradiction between content and theory. Similarly, Wang and Chen ( 2014 ) summarized the main problems in teaching the traditional database theory and its application. Curriculum assessment mode was the main focus of their study. Eaglestone and Nunes ( 2004 ) shared their experiences of delivering a databases design course at Sheffield University. Their focus of study included was to teach the database design module to a diverse group of students from different backgrounds. Rashid ( 2015 ) discussed some important features of database courses, whereby reforming the conventional teaching, learning, and assessing strategies of database courses at universities were the main focus of this study. Kui et al. ( 2018 ) reformed the teaching mode of database courses based on flipped classroom. Initiative learning of database courses was their main focus in this study. Similarly, Zhang et al. ( 2018 ) discussed several effective classroom teaching measures. The main focus of their study was teaching content, teaching methods, teaching evaluation and assessment methods. Cai and Gao ( 2019 ) also carried out the teaching reforms in the database course of liberal arts. Diversified teaching modes, such as flipping classroom, case oriented teaching and task oriented were the focus of their study. Teaching Kawash et al. ( 2020 ) proposed a learning approach called Graded Group Activities (GGAs). Their main focus of the study was reforming learning and assessment method.
Database course covers several topics that range from data modeling to data implementation and examination. Over the years, various authors have given their suggestions to update these topics in database curriculum to meet the requirements of modern technologies. On the other hand, authors have also proposed a new curriculum for the students of different academic backgrounds and different areas. These reformations in curriculum helped the students in their preparation, practically and theoretically, and enabled them to compete in the competitive market after graduation.
During 2003 and 2006 authors have proposed various suggestions to update and develop computer science curriculum across different universities. Robbert and Ricardo ( 2003 ) evaluated three reviews from 1999 to 2002 that were given to the groups of educators. The focus of their study was to highlight the trends that occurred in database curriculum. Also, Calero et al. ( 2003 ) proposed a first draft for this Database Body of Knowledge (DBBOK). Database (DB), Database Design (DBD), Database Administration (DBAd), Database Application (DBAp) and Advance Databases (ADVDB) were the main focus of their study. Furthermore, Conklin and Heinrichs (Conklin & Heinrichs, 2005 ) compared the content included in 13 database textbooks and the main focus of their study was IS 2002, CC2001, and CC2004 model curricula.
The years from 2007 and 2011, authors managed to developed various database curricula, like Luo et al. ( 2008 ) developed curricula in Zhejiang University City College. The aim of their study to nurture students to be qualified computer scientists. Likewise, Dietrich et al. ( 2008 ) proposed the techniques to assess the development of an advanced database course. The purpose behind the addition of an advanced database course at undergraduate level was to prepare the students to respond to industrial requirements. Also, Marshall ( 2011 ) developed a new database curriculum for Computer Science degree program in the South African context.
During 2012 and 2021 various authors suggested updates for the database curriculum such as Bhogal et al. ( 2012 ) who suggested updating and modernizing the database curriculum. Data management and data analytics were the focus of their study. Similarly, Picciano ( 2012 ) examined the curriculum in the higher level of American education. The focus of their study was big data and analytics. Also, Zhanquan et al. ( 2016 ) proposed the design for the course content and teaching methods in the classroom. Massive Open Online Courses (MOOCs) were the focus of their study. Likewise, Mingyu et al. ( 2017 ) suggested updating the database curriculum while keeping new technology concerning the database in perspective. The focus of their study was big data.
The above discussion clearly shows that the SQL is most discussed topic in the literature where more than 25% of the studies have discussed it in the previous decade as shown in Fig. 7 . It is pertinent to mention that other SQL databases such as Oracle, MS access are discussed under the SQL banner (Chen et al., 2012 ; Hou & Chen, 2010 ; Wang & Chen, 2014 ). It is mainly because of its ability to handle data in a relational database management system and direct implementation of database theoretical concepts. Also, other database topics such as transaction management, application programming etc. are also the main highlights of the topics discussed in the literature.

Evolution of Database topics discussed in literature
Research synthesis, advice for instructors, and way forward
This section presents the synthesized information extracted after reading and analyzing the research articles considered in this study. To this end, it firstly contextualizes the tools and methods to help the instructors find suitable tools and methods for their settings. Similarly, developments in curriculum design have also been discussed. Subsequently, general advice for instructors have been discussed. Lastly, promising future research directions for developing new tools, methods, and for revising the curriculum have also been discussed in this section.
Methods, tools, and curriculum
Methods and tools.
Web-based tools proposed by Cvetanovic et al. ( 2010 ) and Wang et al. ( 2010 ) have been quite useful, as they are growing increasingly pertinent as online mode of education is prevalent all around the globe during COVID-19. On the other hand, interactive tools and smart class room methodology has also been used successfully to develop the interest of students in database class. (Brusilovsky et al., 2010 ; Connolly et al., 2005 ; Pahl et al., 2004 ; Canedo et al., 2021 ; Ko et al., 2021 ).
One of the most promising combination of methodology and tool has been proposed by Cvetanovic et al. ( 2010 ), whereby they developed a tool named ADVICE that helps students learn and implement database concepts while using project centric methodology, while a game based collaborative learning environment was proposed by Connolly et al. ( 2005 ) that involves a methodology comprising of modeling, articulation, feedback, and exploration. As a whole, project centric teaching (Connolly & Begg, 2006 ; Domínguez & Jaime, 2010 ) and teaching database design and problem solving skills Wang and Chen ( 2014 ), are two successful approaches for DSE. Whereas, other studies (Urban & Dietrich, 1997 ) proposed teaching methods that are more inclined towards practicing database concepts. While a topic specific approach has been proposed by Abbasi et al. ( 2016 ), Taipalus et al. ( 2018 ) and Silva et al. ( 2016 ) to teach and learn SQL. On the other hand, Cai and Gao ( 2019 ) developed a teaching method for students who do not have a computer science background. Lastly, some useful ways for defining assessments for DSE have been proposed by Kawash et al. ( 2020 ) and Zhang et al. ( 2018 ).
Curriculum of database adopted by various institutes around the world does not address how to teach the database course to the students who do not have a strong computer science background. Such as Marshall ( 2012 ), Luo et al. ( 2008 ) and Zhanquan et al. ( 2016 ) have proposed the updates in current database curriculum for the students who are not from computer science background. While Abid et al. ( 2015 ) proposed a combined course content and various methodologies that can be used for teaching database systems course. On the other hand, current database curriculum does not include the topics related to latest technologies in database domain. This factor was discussed by many other studies as well (Bhogal et al., 2012 ; Mehmood et al., 2020 ; Picciano, 2012 ).
Guidelines for instructors
The major conclusion of this study are the suggestions based on the impact and importance for instructors who are teaching DSE. Furthermore, an overview of productivity of every method can be provided by the empirical studies. These instructions are for instructors which are the focal audience of this study. These suggestions are subjective opinions after literature analysis in form of guidelines according to the authors and their meaning and purpose were maintained. According to the literature reviewed, various issues have been found in this section. Some other issues were also found, but those were not relevant to DSE. Following are some suggestions that provide interesting information:
Project centric and applied approach
- To inculcate database development skills for the students, basic elements of database development need to be incorporated into teaching and learning at all levels including undergraduate studies (Bakar et al., 2011 ). To fulfill this objective, instructors should also improve the data quality in DSE by assigning the projects and assignments to the students where they can assess, measure and improve the data quality using already deployed databases. They should demonstrate that the quality of data is determined not only by the effective design of a database, but also through the perception of the end user (Mathieu & Khalil, 1997 )
- The gap between the database course theory and industrial practice is big. Fresh graduate students find it difficult to cope up with the industrial pressure because of the contrast between what they have been taught in institutes and its application in industry (Allsopp et al., 2006 ). Involve top performers from classes in industrial projects so that they are able to acquiring sufficient knowledge and practice, especially for post graduate courses. There must be some other activities in which industry practitioners come and present the real projects and also share their industrial experiences with the students. The gap between theoretical and the practical sides of database has been identified by Myers and Skinner ( 1997 ). In order to build practical DS concepts, instructors should provide the students an accurate view of reality and proper tools.
Importance of software development standards and impact of DB in software success
- They should have the strategies, ability and skills that can align the DSE course with the contemporary Global Software Development (GSD) (Akbar & Safdar, 2015 ; Damian et al., 2006 ).
- Enable the students to explain the approaches to problem solving, development tools and methodologies. Also, the DS courses are usually taught in normal lecture format. The result of this method is that students cannot see the influence on the success or failure of projects because they do not realize the importance of DS activities.
Pedagogy and the use of education technology
- Some studies have shown that teaching through play and practical activities helps to improve the knowledge and learning outcome of students (Dicheva et al., 2015 ).
- Interactive classrooms can help the instructors to deliver their lecture in a more effective way by using virtual white board, digital textbooks, and data over network(Abut & Ozturk, 1997 ). We suggest that in order to follow the new concept of smart classroom, instructors should use the experience of Yau and Karim ( 2003 ) which benefits in cooperative learning among students and can also be adopted in DSE.
- The instructors also need to update themselves with full spectrum of technology in education, in general, and for DSE, in particular. This is becoming more imperative as during COVID the world is relying strongly on the use of technology, particularly in education sector.
Periodic Curriculum Revision
- There is also a need to revisit the existing series of courses periodically, so that they are able to offer the following benefits: (a) include the modern day database system concepts; (b) can be offered as a specialization track; (c) a specialized undergraduate degree program may also be designed.
DSE: Way forward
This research combines a significant work done on DSE at one place, thus providing a point to find better ways forward in order to improvise different possible dimensions for improving the teaching process of a database system course in future. This section discusses technology, methods, and modifications in curriculum would most impact the delivery of lectures in coming years.
Several tools have already been developed for effective teaching and learning in database systems. However, there is a great room for developing new tools. Recent rise of the notion of “serious games” is marking its success in several domains. Majority of the research work discussed in this review revolves around web-based tools. The success of serious games invites researchers to explore this new paradigm of developing useful tools for learning and practice database systems concepts.
Likewise, due to COVID-19 the world is setting up new norms, which are expected to affect the methods of teaching as well. This invites the researchers to design, develop, and test flexible tools for online teaching in a more interactive manner. At the same time, it is also imperative to devise new techniques for assessments, especially conducting online exams at massive scale. Moreover, the researchers can implement the idea of instructional design in web-based teaching in which an online classroom can be designed around the learners’ unique backgrounds and effectively delivering the concepts that are considered to be highly important by the instructors.
The teaching, learning and assessment methods discussed in this study can help the instructors to improve their methods in order to teach the database system course in a better way. It is noticed that only 16% of authors have the assessment methods as their focus of study, which clearly highlights that there is still plenty of work needed to be done in this particular domain. Assessment techniques in the database course will help the learners to learn from their mistakes. Also, instructors must realize that there is a massive gap between database theory and practice which can only be reduced with maximum practice and real world database projects.
Similarly, the technology is continuously influencing the development and expansion of modern education, whereas the instructors’ abilities to teach using online platforms are critical to the quality of online education.
In the same way, the ideas like flipped classroom in which students have to prepare the lesson prior to the class can be implemented on web-based teaching. This ensures that the class time can be used for further discussion of the lesson, share ideas and allow students to interact in a dynamic learning environment.
The increasing impact of big data systems, and data science and its anticipated impact on the job market invites the researchers to revisit the fundamental course of database systems as well. There is a need to extend the boundaries of existing contents by including the concepts related to distributed big data systems data storage, processing, and transaction management, with possible glimpse of modern tools and technologies.
As a whole, an interesting and long term extension is to establish a generic and comprehensive framework that engages all the stakeholders with the support of technology to make the teaching, learning, practicing, and assessing easier and more effective.
This SLR presents review on the research work published in the area of database system education, with particular focus on teaching the first course in database systems. The study was carried out by systematically selecting research papers published between 1995 and 2021. Based on the study, a high level categorization presents a taxonomy of the published under the heads of Tools, Methods, and Curriculum. All the selected articles were evaluated on the basis of a quality criteria. Several methods have been developed to effectively teach the database course. These methods focus on improving learning experience, improve student satisfaction, improve students’ course performance, or support the instructors. Similarly, many tools have been developed, whereby some tools are topic based, while others are general purpose tools that apply for whole course. Similarly, the curriculum development activities have also been discussed, where some guidelines provided by ACM/IEEE along with certain standards have been discussed. Apart from this, the evolution in these three areas has also been presented which shows that the researchers have been presenting many different teaching methods throughout the selected period; however, there is a decrease in research articles that address the curriculum and tools in the past five years. Besides, some guidelines for the instructors have also been shared. Also, this SLR proposes a way forward in DSE by emphasizing on the tools: that need to be developed to facilitate instructors and students especially post Covid-19 era, methods: to be adopted by the instructors to close the gap between the theory and practical, Database curricula update after the introduction of emerging technologies such as big data and data science. We also urge that the recognized publication venues for database research including VLDB, ICDM, EDBT should also consider publishing articles related to DSE. The study also highlights the importance of reviving the curricula, tools, and methodologies to cater for recent advancements in the field of database systems.
Data availability
Code availability, declarations.
Publisher's note
Springer Nature remains neutral with regard to jurisdictional claims in published maps and institutional affiliations.
- Abbasi, S., Kazi, H., Khowaja, K., Abelló Gamazo, A., Burgués Illa, X., Casany Guerrero, M. J., Martin Escofet, C., Quer, C., Rodriguez González, M. E., Romero Moral, Ó., Urpi Tubella, A., Abid, A., Farooq, M. S., Raza, I., Farooq, U., Abid, K., Hussain, N., Abid, K., Ahmad, F., …, Yatim, N. F. M. (2016). Research trends in enterprise service bus (ESB) applications: A systematic mapping study. Journal of Informetrics, 27 (1), 217–220.
- Abbasi, S., Kazi, H., & Khowaja, K. (2017). A systematic review of learning object oriented programming through serious games and programming approaches. 2017 4th IEEE International Conference on Engineering Technologies and Applied Sciences (ICETAS) , 1–6.
- Abelló Gamazo A, Burgués Illa X, Casany Guerrero MJ, Martin Escofet C, Quer C, Rodriguez González ME, Romero Moral Ó, Urpi Tubella A. A software tool for E-assessment of relational database skills. International Journal of Engineering Education. 2016; 32 (3A):1289–1312. [ Google Scholar ]
- Abid A, Farooq MS, Raza I, Farooq U, Abid K. Variants of teaching first course in database systems. Bulletin of Education and Research. 2015; 37 (2):9–25. [ Google Scholar ]
- Abid A, Hussain N, Abid K, Ahmad F, Farooq MS, Farooq U, Khan SA, Khan YD, Naeem MA, Sabir N. A survey on search results diversification techniques. Neural Computing and Applications. 2016; 27 (5):1207–1229. [ Google Scholar ]
- Abourezq, M., & Idrissi, A. (2016). Database-as-a-service for big data: An overview. International Journal of Advanced Computer Science and Applications (IJACSA) , 7 (1).
- Abut, H., & Ozturk, Y. (1997). Interactive classroom for DSP/communication courses. 1997 IEEE International Conference on Acoustics, Speech, and Signal Processing , 1 , 15–18.
- Adams ES, Granger M, Goelman D, Ricardo C. Managing the introductory database course: What goes in and what comes out? ACM SIGCSE Bulletin. 2004; 36 (1):497–498. [ Google Scholar ]
- Akbar, R., & Safdar, S. (2015). A short review of global software development (gsd) and latest software development trends. 2015 International Conference on Computer, Communications, and Control Technology (I4CT) , 314–317.
- Allsopp DH, DeMarie D, Alvarez-McHatton P, Doone E. Bridging the gap between theory and practice: Connecting courses with field experiences. Teacher Education Quarterly. 2006; 33 (1):19–35. [ Google Scholar ]
- Alrumaih, H. (2016). ACM/IEEE-CS information technology curriculum 2017: status report. Proceedings of the 1st National Computing Colleges Conference (NC3 2016) .
- Al-Shuaily, H. (2012). Analyzing the influence of SQL teaching and learning methods and approaches. 10 Th International Workshop on the Teaching, Learning and Assessment of Databases , 3.
- Amadio, W., Riyami, B., Mansouri, K., Poirier, F., Ramzan, M., Abid, A., Khan, H. U., Awan, S. M., Ismail, A., Ahmed, M., Ilyas, M., Mahmood, A., Hey, A. J. G., Tansley, S., Tolle, K. M., others, Tehseen, R., Farooq, M. S., Abid, A., …, Fatimazahra, E. (2003). The fourth paradigm: data-intensive scientific discovery. Innovation in Teaching and Learning in Information and Computer Sciences , 1 (1), 823–828. https://www.iso.org/standard/27614.html
- Amadio, W. (2003). The dilemma of Team Learning: An assessment from the SQL programming classroom . 823–828.
- Ampatzoglou A, Charalampidou S, Stamelos I. Research state of the art on GoF design patterns: A mapping study. Journal of Systems and Software. 2013; 86 (7):1945–1964. [ Google Scholar ]
- Andersson C, Kroisandt G, Logofatu D. Including active learning in an online database management course for industrial engineering students. IEEE Global Engineering Education Conference (EDUCON) 2019; 2019 :217–220. [ Google Scholar ]
- Aria M, Cuccurullo C. bibliometrix: An R-tool for comprehensive science mapping analysis. Journal of Informetrics. 2017; 11 (4):959–975. [ Google Scholar ]
- Aziz O, Farooq MS, Abid A, Saher R, Aslam N. Research trends in enterprise service bus (ESB) applications: A systematic mapping study. IEEE Access. 2020; 8 :31180–31197. [ Google Scholar ]
- Bakar MA, Jailani N, Shukur Z, Yatim NFM. Final year supervision management system as a tool for monitoring computer science projects. Procedia-Social and Behavioral Sciences. 2011; 18 :273–281. [ Google Scholar ]
- Beecham S, Baddoo N, Hall T, Robinson H, Sharp H. Motivation in Software Engineering: A systematic literature review. Information and Software Technology. 2008; 50 (9–10):860–878. [ Google Scholar ]
- Bhogal, J. K., Cox, S., & Maitland, K. (2012). Roadmap for Modernizing Database Curricula. 10 Th International Workshop on the Teaching, Learning and Assessment of Databases , 73.
- Bishop, M., Burley, D., Buck, S., Ekstrom, J. J., Futcher, L., Gibson, D., ... & Parrish, A. (2017, May). Cybersecurity curricular guidelines . In IFIP World Conference on Information Security Education (pp. 3–13). Cham: Springer.
- Brady A, Bruce K, Noonan R, Tucker A, Walker H. The 2003 model curriculum for a liberal arts degree in computer science: preliminary report. ACM SIGCSE Bulletin. 2004; 36 (1):282–283. [ Google Scholar ]
- Brusilovsky P, Sosnovsky S, Lee DH, Yudelson M, Zadorozhny V, Zhou X. An open integrated exploratorium for database courses. AcM SIGcSE Bulletin. 2008; 40 (3):22–26. [ Google Scholar ]
- Brusilovsky P, Sosnovsky S, Yudelson MV, Lee DH, Zadorozhny V, Zhou X. Learning SQL programming with interactive tools: From integration to personalization. ACM Transactions on Computing Education (TOCE) 2010; 9 (4):1–15. [ Google Scholar ]
- Cai, Y., & Gao, T. (2019). Teaching Reform in Database Course for Liberal Arts Majors under the Background of" Internet Plus". 2018 6th International Education, Economics, Social Science, Arts, Sports and Management Engineering Conference (IEESASM 2018) , 208–213.
- Calderon KR, Vij RS, Mattana J, Jhaveri KD. Innovative teaching tools in nephrology. Kidney International. 2011; 79 (8):797–799. [ PubMed ] [ Google Scholar ]
- Calero C, Piattini M, Ruiz F. Towards a database body of knowledge: A study from Spain. ACM SIGMOD Record. 2003; 32 (2):48–53. [ Google Scholar ]
- Canedo, E. D., Bandeira, I. N., & Costa, P. H. T. (2021). Challenges of database systems teaching amidst the Covid-19 pandemic. In 2021 IEEE Frontiers in Education Conference (FIE) (pp. 1–9). IEEE.
- Chen H-H, Chen Y-J, Chen K-J. The design and effect of a scaffolded concept mapping strategy on learning performance in an undergraduate database course. IEEE Transactions on Education. 2012; 56 (3):300–307. [ Google Scholar ]
- Cobo MJ, López-Herrera AG, Herrera-Viedma E, Herrera F. SciMAT: A new science mapping analysis software tool. Journal of the American Society for Information Science and Technology. 2012; 63 (8):1609–1630. [ Google Scholar ]
- Conklin M, Heinrichs L. In search of the right database text. Journal of Computing Sciences in Colleges. 2005; 21 (2):305–312. [ Google Scholar ]
- Connolly, T. M., & Begg, C. E. (2006). A constructivist-based approach to teaching database analysis and design. Journal of Information Systems Education , 17 (1).
- Connolly, T. M., Stansfield, M., & McLellan, E. (2005). An online games-based collaborative learning environment to teach database design. Web-Based Education: Proceedings of the Fourth IASTED International Conference(WBE-2005) .
- Curricula Computing. (1991). Report of the ACM/IEEE-CS Joint Curriculum Task Force. Technical Report . New York: Association for Computing Machinery.
- Cvetanovic M, Radivojevic Z, Blagojevic V, Bojovic M. ADVICE—Educational system for teaching database courses. IEEE Transactions on Education. 2010; 54 (3):398–409. [ Google Scholar ]
- Damian, D., Hadwin, A., & Al-Ani, B. (2006). Instructional design and assessment strategies for teaching global software development: a framework. Proceedings of the 28th International Conference on Software Engineering , 685–690.
- Dean, T. J., & Milani, W. G. (1995). Transforming a database systems and design course for non computer science majors. Proceedings Frontiers in Education 1995 25th Annual Conference. Engineering Education for the 21st Century , 2 , 4b2--17.
- Dicheva, D., Dichev, C., Agre, G., & Angelova, G. (2015). Gamification in education: A systematic mapping study. Journal of Educational Technology \& Society , 18 (3), 75–88.
- Dietrich SW, Urban SD, Haag S. Developing advanced courses for undergraduates: A case study in databases. IEEE Transactions on Education. 2008; 51 (1):138–144. [ Google Scholar ]
- Dietrich SW, Goelman D, Borror CM, Crook SM. An animated introduction to relational databases for many majors. IEEE Transactions on Education. 2014; 58 (2):81–89. [ Google Scholar ]
- Dietrich, S. W., & Urban, S. D. (1996). Database theory in practice: learning from cooperative group projects. Proceedings of the Twenty-Seventh SIGCSE Technical Symposium on Computer Science Education , 112–116.
- Dominguez, C., & Jaime, A. (2010). Database design learning: A project-based approach organized through a course management system. Computers \& Education , 55 (3), 1312–1320.
- Eaglestone, B., & Nunes, M. B. (2004). Pragmatics and practicalities of teaching and learning in the quicksand of database syllabuses. Journal of Innovations in Teaching and Learning for Information and Computer Sciences , 3 (1).
- Efendiouglu A, Yelken TY. Programmed instruction versus meaningful learning theory in teaching basic structured query language (SQL) in computer lesson. Computers & Education. 2010; 55 (3):1287–1299. [ Google Scholar ]
- Elberzhager F, Münch J, Nha VTN. A systematic mapping study on the combination of static and dynamic quality assurance techniques. Information and Software Technology. 2012; 54 (1):1–15. [ Google Scholar ]
- Etemad M, Küpçü A. Verifiable database outsourcing supporting join. Journal of Network and Computer Applications. 2018; 115 :1–19. [ Google Scholar ]
- Farooq MS, Riaz S, Abid A, Abid K, Naeem MA. A Survey on the role of IoT in agriculture for the implementation of smart farming. IEEE Access. 2019; 7 :156237–156271. [ Google Scholar ]
- Farooq MS, Riaz S, Abid A, Umer T, Zikria YB. Role of IoT technology in agriculture: A systematic literature review. Electronics. 2020; 9 (2):319. [ Google Scholar ]
- Farooq U, Rahim MSM, Sabir N, Hussain A, Abid A. Advances in machine translation for sign language: Approaches, limitations, and challenges. Neural Computing and Applications. 2021; 33 (21):14357–14399. [ Google Scholar ]
- Fisher, D., & Khine, M. S. (2006). Contemporary approaches to research on learning environments: Worldviews . World Scientific.
- Garcia-Molina, H. (2008). Database systems: the complete book . Pearson Education India.
- Garousi V, Mesbah A, Betin-Can A, Mirshokraie S. A systematic mapping study of web application testing. Information and Software Technology. 2013; 55 (8):1374–1396. [ Google Scholar ]
- Gudivada, V. N., Nandigam, J., & Tao, Y. (2007). Enhancing student learning in database courses with large data sets. 2007 37th Annual Frontiers In Education Conference-Global Engineering: Knowledge Without Borders, Opportunities Without Passports , S2D--13.
- Hey, A. J. G., Tansley, S., Tolle, K. M., & others. (2009). The fourth paradigm: data-intensive scientific discovery (Vol. 1). Microsoft research Redmond, WA.
- Holliday, M. A., & Wang, J. Z. (2009). A multimedia database project and the evolution of the database course. 2009 39th IEEE Frontiers in Education Conference , 1–6.
- Hou, S., & Chen, S. (2010). Research on applying the theory of Blending Learning on Access Database Programming Course teaching. 2010 2nd International Conference on Education Technology and Computer , 3 , V3--396.
- Irby DM, Wilkerson L. Educational innovations in academic medicine and environmental trends. Journal of General Internal Medicine. 2003; 18 (5):370–376. [ PMC free article ] [ PubMed ] [ Google Scholar ]
- Ishaq K, Zin NAM, Rosdi F, Jehanghir M, Ishaq S, Abid A. Mobile-assisted and gamification-based language learning: A systematic literature review. PeerJ Computer Science. 2021; 7 :e496. [ PMC free article ] [ PubMed ] [ Google Scholar ]
- Joint Task Force on Computing Curricula, A. F. C. M. (acm), & Society, I. C. (2013). Computer science curricula 2013: Curriculum guidelines for undergraduate degree programs in computer science . New York, NY, USA: Association for Computing Machinery.
- Juxiang R, Zhihong N. Taking database design as trunk line of database courses. Fourth International Conference on Computational and Information Sciences. 2012; 2012 :767–769. [ Google Scholar ]
- Kawash, J., Jarada, T., & Moshirpour, M. (2020). Group exams as learning tools: Evidence from an undergraduate database course. Proceedings of the 51st ACM Technical Symposium on Computer Science Education , 626–632.
- Keele, S., et al. (2007). Guidelines for performing systematic literature reviews in software engineering .
- Kleiner, C. (2015). New Concepts in Database System Education: Experiences and Ideas. Proceedings of the 46th ACM Technical Symposium on Computer Science Education , 698.
- Ko J, Paek S, Park S, Park J. A news big data analysis of issues in higher education in Korea amid the COVID-19 pandemic. Sustainability. 2021; 13 (13):7347. [ Google Scholar ]
- Kui, X., Du, H., Zhong, P., & Liu, W. (2018). Research and application of flipped classroom in database course. 2018 13th International Conference on Computer Science \& Education (ICCSE) , 1–5.
- Landis, J. R., & Koch, G. G. (1977). The measurement of observer agreement for categorical data. Biometrics , 159–174. [ PubMed ]
- Lunt, B., Ekstrom, J., Gorka, S., Hislop, G., Kamali, R., Lawson, E., ... & Reichgelt, H. (2008). Curriculum guidelines for undergraduate degree programs in information technology . ACM.
- Luo, R., Wu, M., Zhu, Y., & Shen, Y. (2008). Exploration of Curriculum Structures and Educational Models of Database Applications. 2008 The 9th International Conference for Young Computer Scientists , 2664–2668.
- Luxton-Reilly, A., Albluwi, I., Becker, B. A., Giannakos, M., Kumar, A. N., Ott, L., Paterson, J., Scott, M. J., Sheard, J., & Szabo, C. (2018). Introductory programming: a systematic literature review. Proceedings Companion of the 23rd Annual ACM Conference on Innovation and Technology in Computer Science Education , 55–106.
- Manzoor MF, Abid A, Farooq MS, Nawaz NA, Farooq U. Resource allocation techniques in cloud computing: A review and future directions. Elektronika Ir Elektrotechnika. 2020; 26 (6):40–51. doi: 10.5755/j01.eie.26.6.25865. [ CrossRef ] [ Google Scholar ]
- Marshall, L. (2011). Developing a computer science curriculum in the South African context. CSERC , 9–19.
- Marshall, L. (2012). A comparison of the core aspects of the acm/ieee computer science curriculum 2013 strawman report with the specified core of cc2001 and cs2008 review. Proceedings of Second Computer Science Education Research Conference , 29–34.
- Martin C, Urpi T, Casany MJ, Illa XB, Quer C, Rodriguez ME, Abello A. Improving learning in a database course using collaborative learning techniques. The International Journal of Engineering Education. 2013; 29 (4):986–997. [ Google Scholar ]
- Martinez-González MM, Duffing G. Teaching databases in compliance with the European dimension of higher education: Best practices for better competences. Education and Information Technologies. 2007; 12 (4):211–228. [ Google Scholar ]
- Mateo PR, Usaola MP, Alemán JLF. Validating second-order mutation at system level. IEEE Transactions on Software Engineering. 2012; 39 (4):570–587. [ Google Scholar ]
- Mathieu, R. G., & Khalil, O. (1997). Teaching Data Quality in the Undergraduate Database Course. IQ , 249–266.
- Mcintyre, D. R., Pu, H.-C., & Wolff, F. G. (1995). Use of software tools in teaching relational database design. Computers \& Education , 24 (4), 279–286.
- Mehmood E, Abid A, Farooq MS, Nawaz NA. Curriculum, teaching and learning, and assessments for introductory programming course. IEEE Access. 2020; 8 :125961–125981. [ Google Scholar ]
- Meier, R., Barnicki, S. L., Barnekow, W., & Durant, E. (2008). Work in progress-Year 2 results from a balanced, freshman-first computer engineering curriculum. In 38th Annual Frontiers in Education Conference (pp. S1F-17). IEEE.
- Meyer B. Software engineering in the academy. Computer. 2001; 34 (5):28–35. [ Google Scholar ]
- Mingyu, L., Jianping, J., Yi, Z., & Cuili, Z. (2017). Research on the teaching reform of database curriculum major in computer in big data era. 2017 12th International Conference on Computer Science and Education (ICCSE) , 570–573.
- Morien, R. I. (2006). A Critical Evaluation Database Textbooks, Curriculum and Educational Outcomes. Director , 7 .
- Mushtaq Z, Rasool G, Shehzad B. Multilingual source code analysis: A systematic literature review. IEEE Access. 2017; 5 :11307–11336. [ Google Scholar ]
- Myers M, Skinner P. The gap between theory and practice: A database application case study. Journal of International Information Management. 1997; 6 (1):5. [ Google Scholar ]
- Naeem A, Farooq MS, Khelifi A, Abid A. Malignant melanoma classification using deep learning: Datasets, performance measurements, challenges and opportunities. IEEE Access. 2020; 8 :110575–110597. [ Google Scholar ]
- Nagataki, H., Nakano, Y., Nobe, M., Tohyama, T., & Kanemune, S. (2013). A visual learning tool for database operation. Proceedings of the 8th Workshop in Primary and Secondary Computing Education , 39–40.
- Naik, S., & Gajjar, K. (2021). Applying and Evaluating Engagement and Application-Based Learning and Education (ENABLE): A Student-Centered Learning Pedagogy for the Course Database Management System. Journal of Education , 00220574211032319.
- Nelson, D., Stirk, S., Patience, S., & Green, C. (2003). An evaluation of a diverse database teaching curriculum and the impact of research. 1st LTSN Workshop on Teaching, Learning and Assessment of Databases, Coventry .
- Nelson D, Fatimazahra E. Review of Contributions to the Teaching, Learning and Assessment of Databases (TLAD) Workshops. Innovation in Teaching and Learning in Information and Computer Sciences. 2010; 9 (1):78–86. [ Google Scholar ]
- Obaid I, Farooq MS, Abid A. Gamification for recruitment and job training: Model, taxonomy, and challenges. IEEE Access. 2020; 8 :65164–65178. [ Google Scholar ]
- Pahl C, Barrett R, Kenny C. Supporting active database learning and training through interactive multimedia. ACM SIGCSE Bulletin. 2004; 36 (3):27–31. [ Google Scholar ]
- Park, Y., Tajik, A. S., Cafarella, M., & Mozafari, B. (2017). Database learning: Toward a database that becomes smarter every time. Proceedings of the 2017 ACM International Conference on Management of Data , 587–602.
- Picciano AG. The evolution of big data and learning analytics in American higher education. Journal of Asynchronous Learning Networks. 2012; 16 (3):9–20. [ Google Scholar ]
- Prince MJ, Felder RM. Inductive teaching and learning methods: Definitions, comparisons, and research bases. Journal of Engineering Education. 2006; 95 (2):123–138. [ Google Scholar ]
- Ramzan M, Abid A, Khan HU, Awan SM, Ismail A, Ahmed M, Ilyas M, Mahmood A. A review on state-of-the-art violence detection techniques. IEEE Access. 2019; 7 :107560–107575. [ Google Scholar ]
- Rashid, T. A., & Al-Radhy, R. S. (2014). Transformations to issues in teaching, learning, and assessing methods in databases courses. 2014 IEEE International Conference on Teaching, Assessment and Learning for Engineering (TALE) , 252–256.
- Rashid, T. (2015). Investigation of instructing reforms in databases. International Journal of Scientific \& Engineering Research , 6 (8), 64–72.
- Regueras, L. M., Verdú, E., Verdú, M. J., Pérez, M. A., & De Castro, J. P. (2007). E-learning strategies to support databases courses: a case study. First International Conference on Technology, Training and Communication .
- Robbert MA, Ricardo CM. Trends in the evolution of the database curriculum. ACM SIGCSE Bulletin. 2003; 35 (3):139–143. [ Google Scholar ]
- Sahami, M., Guzdial, M., McGettrick, A., & Roach, S. (2011). Setting the stage for computing curricula 2013: computer science--report from the ACM/IEEE-CS joint task force. Proceedings of the 42nd ACM Technical Symposium on Computer Science Education , 161–162.
- Sciore E. SimpleDB: A simple java-based multiuser syst for teaching database internals. ACM SIGCSE Bulletin. 2007; 39 (1):561–565. [ Google Scholar ]
- Shebaro B. Using active learning strategies in teaching introductory database courses. Journal of Computing Sciences in Colleges. 2018; 33 (4):28–36. [ Google Scholar ]
- Sibia, N., & Liut, M. (2022, June). The Positive Effects of using Reflective Prompts in a Database Course. In 1st International Workshop on Data Systems Education (pp. 32–37).
- Silva, Y. N., Almeida, I., & Queiroz, M. (2016). SQL: From traditional databases to big data. Proceedings of the 47th ACM Technical Symposium on Computing Science Education , 413–418.
- Sobel, A. E. K. (2003). Computing Curricula--Software Engineering Volume. Proc. of the Final Draft of the Software Engineering Education Knowledge (SEEK) .
- Suryn, W., Abran, A., & April, A. (2003). ISO/IEC SQuaRE: The second generation of standards for software product quality .
- Svahnberg, M., Aurum, A., & Wohlin, C. (2008). Using students as subjects-an empirical evaluation. Proceedings of the Second ACM-IEEE International Symposium on Empirical Software Engineering and Measurement , 288–290.
- Swebok evolution: IEEE Computer Society. (n.d.). In IEEE Computer Society SWEBOK Evolution Comments . Retrieved March 24, 2021 https://www.computer.org/volunteering/boards-and-committees/professional-educational-activities/software-engineering-committee/swebok-evolution
- Taipalus T, Seppänen V. SQL education: A systematic mapping study and future research agenda. ACM Transactions on Computing Education (TOCE) 2020; 20 (3):1–33. [ Google Scholar ]
- Taipalus T, Siponen M, Vartiainen T. Errors and complications in SQL query formulation. ACM Transactions on Computing Education (TOCE) 2018; 18 (3):1–29. [ Google Scholar ]
- Taipalus, T., & Perälä, P. (2019). What to expect and what to focus on in SQL query teaching. Proceedings of the 50th ACM Technical Symposium on Computer Science Education , 198–203.
- Tehseen R, Farooq MS, Abid A. Earthquake prediction using expert systems: A systematic mapping study. Sustainability. 2020; 12 (6):2420. [ Google Scholar ]
- Urban, S. D., & Dietrich, S. W. (2001). Advanced database concepts for undergraduates: experience with teaching a second course. Proceedings of the Thirty-Second SIGCSE Technical Symposium on Computer Science Education , 357–361.
- Urban SD, Dietrich SW. Integrating the practical use of a database product into a theoretical curriculum. ACM SIGCSE Bulletin. 1997; 29 (1):121–125. [ Google Scholar ]
- Wang, J., & Chen, H. (2014). Research and practice on the teaching reform of database course. International Conference on Education Reform and Modern Management, ERMM .
- Wang, J. Z., Davis, T. A., Westall, J. M., & Srimani, P. K. (2010). Undergraduate database instruction with MeTube. Proceedings of the Fifteenth Annual Conference on Innovation and Technology in Computer Science Education , 279–283.
- Yau, G., & Karim, S. W. (2003). Smart classroom: Enhancing collaborative learning using pervasive computing technology. II American Society… .
- Yue K-B. Using a semi-realistic database to support a database course. Journal of Information Systems Education. 2013; 24 (4):327. [ Google Scholar ]
- Yuelan L, Yiwei L, Yuyan H, Yuefan L. Study on teaching methods of database application courses. Procedia Engineering. 2011; 15 :5425–5428. [ Google Scholar ]
- Zhang, X., Wang, X., Liu, Z., Xue, W., & ZHU, X. (2018). The Exploration and Practice on the Classroom Teaching Reform of the Database Technologies Course in colleges. 2018 3rd International Conference on Modern Management, Education Technology, and Social Science (MMETSS 2018) , 320–323.
- Zhanquan W, Zeping Y, Chunhua G, Fazhi Z, Weibin G. Research of database curriculum construction under the environment of massive open online courses. International Journal of Educational and Pedagogical Sciences. 2016; 10 (12):3873–3877. [ Google Scholar ]
- Zheng, Y., & Dong, J. (2011). Teaching reform and practice of database principles. 2011 6th International Conference on Computer Science \& Education (ICCSE) , 1460–1462.
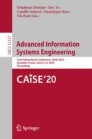
International Conference on Advanced Information Systems Engineering
CAiSE 2020: Advanced Information Systems Engineering pp 498–514 Cite as
Recommendations for Evolving Relational Databases
- Julien Delplanque 13 , 14 ,
- Anne Etien 13 , 14 ,
- Nicolas Anquetil 13 , 14 &
- Stéphane Ducasse 13 , 14
- Conference paper
- First Online: 03 June 2020
4995 Accesses
3 Citations
Part of the book series: Lecture Notes in Computer Science ((LNISA,volume 12127))
Relational databases play a central role in many information systems. Their schemas contain structural and behavioral entity descriptions. Databases must continuously be adapted to new requirements of a world in constant change while: (1) relational database management systems (RDBMS) do not allow inconsistencies in the schema; (2) stored procedure bodies are not meta-described in RDBMS such as PostgreSQL that consider their bodies as plain text. As a consequence, evaluating the impact of an evolution of the database schema is cumbersome, being essentially manual. We present a semi-automatic approach based on recommendations that can be compiled into a SQL patch fulfilling RDBMS constraints. To support recommendations, we designed a meta-model for relational databases easing computation of change impact. We performed an experiment to validate the approach by reproducing a real evolution on a database. The results of our experiment show that our approach can set the database in the same state as the one produced by the manual evolution in 75% less time.
You have full access to this open access chapter, Download conference paper PDF
1 Introduction
Relational Database (DB) schemas contain structural entity descriptions ( e.g., tables and columns), but also sometimes descriptions of behavioral entities such as views ( i.e., named SELECT queries), stored procedures ( i.e., functions written in a programming language), triggers ( i.e., entity listening to events happening on a table and reacting to them), etc. Structural and behavioral entities are referencing each others through foreign keys, function calls, or table/column references in queries.
Continuous evolution happens on databases [ 21 ] to adapt to new requirements of a world in constant change. When databases evolve, problems are twofold:
Issue 1: Relational database management systems (RDBMS) do not allow schema inconsistencies . The consistency of databases is ensured by the RDBMS at any moment . This feature makes the evolution of the database complicated because the database runs during the evolution and continues to ensure its consistency. For other kinds of software, the program is stopped during source code edition. Thus, the program can be temporarily in an inconsistent state.
Issue 2: Stored procedure bodies are not meta-described in RDBMS such as PostgreSQL. Unlike references between tables, columns, constraints or views that are kept and managed through metadata, stored procedures bodies are considered only as text and existing references they make are not known. This second problem slightly alters the first one as inconsistencies can be introduced but only in stored procedures (dangling references).
For example, to remove a column from a table, different cases occur:
If the column is not referenced, the change can be performed.
If the column is a primary key and is referenced by a foreign key in another table, the removal is not allowed.
If the column is referenced in a view, either the change is refused or the view must be dropped. In the latter case, views referencing the one to drop must also be transitively dropped.
If the column is referenced in a function, change can be performed but an error might arise at execution.
Cases (ii) and (iii) result from the first issue whereas the second issue leads to case (iv). This shows that the consequences of even a small change can be complex to handle, particularly cases (iii) and (iv). Such changes need to be anticipated to comply with the constraints imposed by the RDBMS. Meurice et al. studied the history of three applications using databases [ 14 ]. They conclude that the impact of renaming or removing a table or a column on programs using the database is not trivial. To ease the evolutions of the database and the management of their impacts on related programs, the authors provide a tool to detect and prevent program inconsistencies under database schema evolution. Their approach has two major drawbacks: First, only a small number of evolutions of the database are taken into account (removing and renaming table or column); second, internal programs stored in behavioral entities such as views or stored procedures are not studied.
In this paper, we propose a tool automating most modifications required after applying a change on a database, similar to a refactoring browser [ 18 , 19 ]. We do not consider only refactorings [ 5 ], which are by definition behavior preserving, but also deal with other evolutions as illustrated by the above example. Thus, we use the term change rather than refactoring .
We propose an approach based on a meta-model to provide recommendations to database architects. The architects initiate a change and, based on impact analysis, our tool proposes recommendations to the architect. Those recommendations allow the model to reach a consistent state after the change – new changes are induced and new recommendations provided until a stable state is reached. Finally, when the architect accepts the various changes, an analysis is done to generate a patch containing all the SQL queries to perform these changes.
This article is organized as follows. Section 2 sets the context and defines the vocabulary. Section 3 introduces the behavior-aware meta-model used to represent relational databases. Section 4 describes our approach based on impact computation and recommendations to generate SQL evolution patch. It also shows an example of how our approach manages such evolutions illustrated with one evolution operator. Section 5 validates our approach by using our implementation to reproduce an evolution that was performed by an architect on a real database. Section 6 discusses related work. Finally, Sect. 7 concludes this article by summarizing our results and proposing future work.
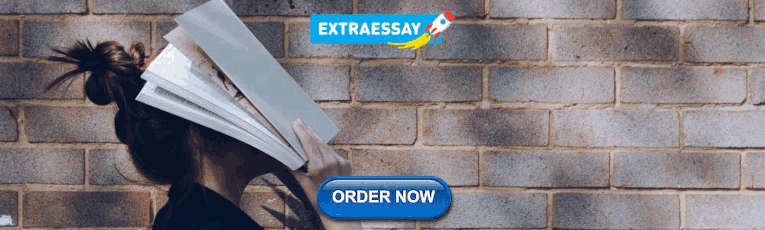
2 Setting the Context
Before getting into the meta-model and approach explanations, let us set the context in which the approach is designed.
Database Schema: The concept of database schema commonly refers to the way data are organized in a database (through tables and referential integrity constraints for relational databases). However, RDBMSs also allows one to define behavior inside the database ( e.g., stored procedure), and this behavior might be used to constrain data ( e.g., triggers or CHECK constraints). Thus, since there is a fine line between the schema as described before and the behavior, in this article when the terms database schema or schema are used, they refer to both structural and behavioral entities.
Impact of a Change: Changing a database will probably affect its structure and behavior. The impact of such a change is defined as the set of database entities that potentially need to be adapted for the change to be applied. For example, RemoveColumn ’s impact set includes constraints applied to the column.
Recommendation: Once the impact of a change has been computed, decisions might need to be taken to handle impacted entities, for example dropping views in cascade in the scenario proposed in the introduction. In the context of this paper, we call each of these potential decisions a recommendation . For example, if one wants to remove a column, we recommend to remove the NOT NULL constraint concerning this column.
Note that Bohnert and Arnold definition of impact [ 1 ] mixes the set of impacted entities and the actions to be done to fix such entities (in the context of this paper, we call these actions “recommendations”): “Identifying the potential consequences of a change, or estimating what needs to be modified to accomplish a change”. To avoid confusion, we decided to use a specific word for each part of the definition.
We identified two kinds of constraints involved in a relational database: (1) data constraints are responsible for data consistency. 5 types of such constraints are available: “primary key”, “foreign key”, “unique”, “not-null” and “check”. (2) schema constraints are responsible for schema consistency and 3 types of such constraints are available: “a table can have a single primary key”, “a column can not have the same constraints applied twice on it” and “foreign key can not reference a column that has no primary key or unique constraint”.
Database Schema Consistency: The RDBMS ensures the consistency of the database schema. This notion of consistency is characterized by the fact that schema constraints are respected and no dangling reference is allowed (except in stored procedure).
Our approach works on a model of the database schema. Using a model allows one to temporarily relax schema constraints and dangling references constraint for the sake of evolution. It allows the developer to focus on changes to be made and not on how to fulfill schema consistency constraints and avoid dangling references at any time.
Operator: An operator represents a change to the database schema. It may impact several entities and require further changes to restore the schema in a consistent state after its application. RemoveColumn is an example of operator.
Entity-Oriented Operator: An entity-oriented operator applies on an element of the model that does not represent a reference. This kind of operator has the particularity to be translatable directly as one or many SQL queries that implement it. An example of such operator is RemoveColumn .
Reference-Oriented Operator: A reference-oriented operator applies on an element of the model representing a reference. RDBMSs do not reify references. Thus, such concepts are implicit and only exist in the source code of DB entities. Because of that, they can not be directly translated as SQL queries. Instead, they need to be converted to entity-oriented operator by interpreting them and generating updated versions of the source code of concerned entities. An example of such operator is ChangeReference Target .
3 A Behavior-Aware Meta-Model for Relational Databases
This section presents our meta-model for relational databases. It takes into account both structural and behavioral entities of the database as well as their relationships.
3.1 Meta-model Objectives
As discussed in the introduction, modifying the structure of a database implies adapting the behavior ( i.e. program) depending on it. Thus, the development of the meta-model is driven by two objectives:
Model the structure and behavior of the database.
Ease the computation of entities impacted by a change.
Objective 1 is fulfilled by modeling tables, columns and constraints. We also model behavioral entities such as CRUD Footnote 1 queries, views ( i.e., named SELECT query stored in the database), stored procedures, and triggers. Objective 2 is fulfilled by reifying references between structural and behavioral entities. The details of these modeling choices are given in Sect. 3.4 .
The implementation of the meta-model is available on github Footnote 2 . The meta-model is instantiated by analysing meta-data provided by the RDBMS and parsing the source code of entities that are not meta-described. The source code of the meta-data reader Footnote 3 and the parser Footnote 4 available on github as well.
3.2 Structural Entities
Figure 1 shows the structural part of the meta-model. To ease reading, for this UML diagram and the following, inheritance links have straight corners while other links are rounded; classes modeling structural entities are red (such as Table ); classes modeling behavioral entities are orange (such as StoredProcedure ); and classes modeling references are white.
A StructuralEntity defines the structure of data held by the database or defining constraints applied on these data ( e.g., Table, Column, Referential integrity constraint, etc.). The containment relation between Table and Column is modeled through ColumnsContainer which is an abstract entity. This entity also has sub-classes in the behavioral part of the meta-model (see Sect. 3.3 ). A Column has a type. This relation is modeled through a TypeReference . A Column can also be subject to Constraint s. Depending on whether a Constraint concerns a single or multiple columns, it inherits from, respectively, ColumnConstraint or TableConstraint . Six concrete constraints inherit from Constraint : PrimaryKey , ForeignKey , Unique , Check (a developer-defined constraint, described by a boolean expression), NotNull , and Default (a default value assigned when no value is explicitly provided, it can be a literal value or an expression to compute). Note that Check and Default constraints also inherit from BehavioralEntity because they contain source code.
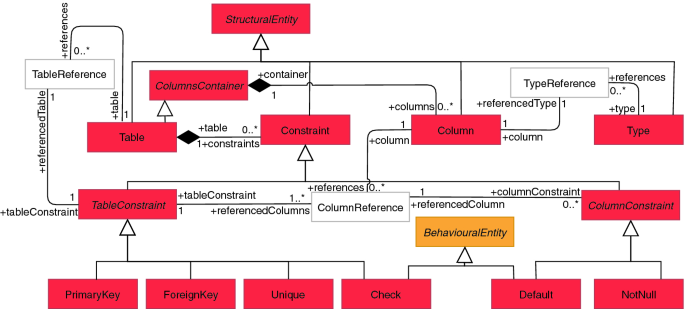
Structural entities of the meta-model.
3.3 Behavioral Entities
A behavioral entity is an entity holding behavior that may interact with StructuralEntities . Figure 2 shows the behavioral part of the meta-model. The main entities are as follows.
View is a named entity holding a SELECT query. StoredProcedure is an entity holding developer-defined behavior which includes queries and calls to other StoredProcedure . A StoredProcedure contains Parameter (s) and LocalVariable (s). These entities can be referenced in clauses of queries that are contained in StoredProcedures or Views . Trigger represents actions happening in response to event on a table ( e.g., row inserted, updated or deleted). CRUDQuery (ies) contain multiple clauses depending on the query. For the sake of readability, we did not include the clause classes in the diagram. In a nutshell, the containment relation between CRUD queries and clauses are: SelectQuery contains With , Select , From , Where , Join , Union , Intersect , Except , GroupBy , OrderBy , Having , Limit , Offset , Fetch clauses. InsertQuery contains With , Into , Returning clauses. UpdateQuery contains With , Update , Set , From , Where , Returning clauses. DeleteQuery contains With , Delete , From , Where , Returning clauses. Each clause holds some Reference s to structural or behavioral entities. The references made to structural or behavioral entities from clauses are detailed in Sect. 3.4 . DerivedTable is an anonymous query usually used in another query but can also appear in the body of a StoredProcedure .
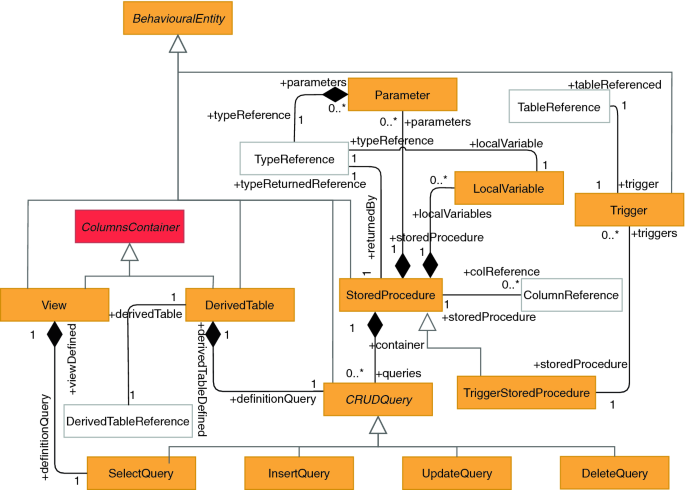
Behavioral entities of the meta-model.
3.4 References
The third and last part of the meta-model represents links between entities. It allows one to track relations between behavioral and structural entities. To simplify the approach, all references have been reified. For example, a column is thus referenced through a ColumnReference , a local variable through a LocalVariableReference and a stored procedure through a StoredProcedureCall .
4 Description of the Approach
To evolve a database, the database architect formulates changes on some of its entities. These changes impact other entities that, in turn, need to evolve to maintain the database in a consistent state. To handle evolutions induced by the initial changes, we developed a 3-step approach. The implementation of this approach is available on github Footnote 5 .
Impact computation : The set of impacted entities is computed from the change. The next step treats impacted entities one by one.
Recommendations selection : Second, depending on the change, and the impacted entity, our approach computes a set of recommendations . These recommendations are presented to the database architect that chooses one when several are proposed. This introduces new changes that will have new impacts. Steps A. and B. are recursively applied until all the impacts have been managed.
Compiling operators as a valid SQL patch : Finally, all operators (the recommendations chosen by the architect) are converted as a set of SQL queries that can be run by the RDBMS. The set of SQL queries is used to migrate the database to a state in which the initial architect ’s change has been applied.
4.1 Impact Computation
To compute the entities potentially affected by a change, one needs to collect all the entities referencing this changed entity. For example, if a Column is subject to a modification, our approach identifies the impacted entities by gathering all the ColumnReference s concerning this column. The impact of the change corresponds to the sources of all ColumnReference s since they can potentially be affected by the modification.
4.2 Recommendations Selection
For each operator, the set of impacted entities is split into disjoint sub-sets called categories . For each of these categories , one or several recommendations are available. We determinated those recommendations by analysing how to handle them according to database schema constraints.
The output of step 4.1 combined with this step (4.2) is a tree of operators where the root is the change initiated by the architect and, each other node corresponds to an operator chosen among recommendations .
4.3 Compiling Operators as a Valid SQL Patch
Once all the impact sets have been considered and recommendations chosen, our approach generates a SQL patch. This patch includes queries belonging to the SQL data definition language (DDL). These queries enable migrating the database from its original state to a state where the initial operator and all induced operators have been applied.
We stress that, during the execution of any operator of the patch, the RDBMS cannot be in inconsistent state. This constraint is fundamentally different from source code refactoring where the state of the program can be temporarily inconsistent. Therefore, each operator must lead the database to a state complying with schema consistency constraints. Else the RDBMS will forbid the execution of the SQL patch. For this purpose, the tree of operators resulting from the previous step has to be transformed into a sequence of SQL queries.
The tree resulting from the step described in Sect. 4.2 is composed of operators on references. However, DDL queries only deal with entities. Thus, reference-oriented operators are transformed into entity-oriented operators. As the RDBMS does not allow inconsistencies, operators concerning a given behavioral entity of the database are aggregated into a single operator per view and per stored procedure. This aggregation is performed in two steps: 1. all reference-oriented operators are grouped according to the entity to which belongs to the source code in which the reference appears, and 2. for each group of reference-oriented operators, we create the new version of the source code for this entity. To do so, we iterate the list of reference-oriented operators and update the part of the source code corresponding to the reference to make it reflect the change implemented by the operator. Once the iteration is complete, a new version of the source code has been built with no more dangling reference.
Those entity-oriented operators are ordered to comply with RDBMS constraints of consistency and serialized as SQL queries. Technical details related to this serialization are not provided in this paper because of space limitation.
4.4 Example
To explain the proposed process, let us take a small example. Consider the simple database shown in Fig. 3 . In this database, there are two tables, t1 with two columns t1.b , t1.c and t2 with column t2.e . Additionally, one stored procedure s() and three views v1 , v2 and v3 are present. On this figure, dependencies between entities are modeled with arrows. These dependencies arrows are a generalization over the various kinds of reference entities of the meta-model. For example, the arrow between s() and t1 is an instance of TableReference and the arrow between s() and b is an instance of ColumnReference. Views and functions have source code displayed inside their box. In this source code, a reference to another entity of the database is underlined.
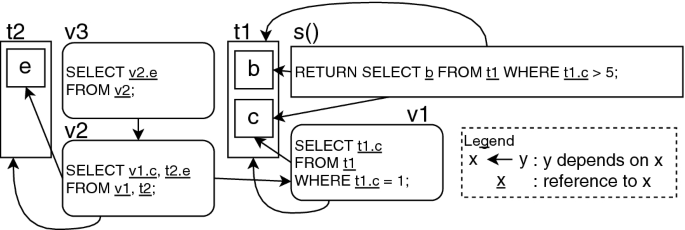
Example database.
The architect wants to rename the column c of table t1 as d .
Impact Computation. First, we compute the impact of this change. Column c of table t1 is referenced three times: (i) in the WHERE clause of the SELECT query of the stored procedure s() ; (ii) in the WHERE clause of the query defining view v1 ; and (iii) in the SELECT clause of the query defining view v1 . Each of these clauses is added in the impact of renaming t1.c as t1.d .
Recommendations Selection. For each of the three impacted entities , recommendations are produced. For the WHERE clause of the stored procedure s() , the recommendation is to replace the reference to column t1.c with a new one corresponding to t1.d . The result of replacing this reference will be the following source code: RETURN SELECT b FROM t1 WHERE t1.d > 5; . From this operator, the impact is computed but is empty which stops the recursive process.
The recommendation concerning the WHERE clause of v1 is the same: replacing the reference to t1.c by a reference to t1.d . Again, there is no further impact for this operator.
For the reference to t1.c in the SELECT clause of view v1 , two recommendations are proposed to the architect: either aliasing the column and replacing the reference ( i.e., replacing SELECT t1.c by SELECT t1.d AS c ) or replacing the reference ( i.e., replacing SELECT t1.c by SELECT t1.d ). In the latter case, the column c in view v1 becomes d ; it is no longer possible to refer to v1.c . Consequently, the second recommendation leads to rename column v1.c . If the architect choose to replace the reference without aliasing, the recursive process continues: new impacts need to be computed and new changes to be performed. The SELECT clause of view v2 is impacted. Two recommendations are again provided: either aliasing the column and replacing the reference or just replacing the reference. In this case, the architect chooses to alias the column and replace the reference. Thus, the rest of the database can continue to refer to column c of view v2 . Figure 4 illustrates this step.

Recommendations selection.
Compiling Operators as a Valid SQL Patch. Figure 5 illustrates the patch generation step. References-oriented operators resulting from the recommendations are transformed into entity-oriented operators. For this purpose, operators concerning the same sourced entity are aggregated. Operators (3) and (4) concern the same sourced entity, v1 . They are thus aggregated into ModifyViewQuery(v1) . At the end, there is a single operator per entity to be modified.
The resulting list of operators is ordered and converted to a SQL patch.
5 Experiment
Our university department uses an information system to manage its members, teams, thematic groups, etc. with 95 tables, 63 views, 109 stored procedures and 20 triggers. This information system is developed by a database architect. Before each migration, he prepares a road map containing, in natural language, the list of operators initially planned for the migration. We observed that these road maps are not complete or accurate [ 4 ]. Following a long manual process, the architect writes a SQL patch ( i.e., a text file containing queries) to migrate from one version of the database to the next one.
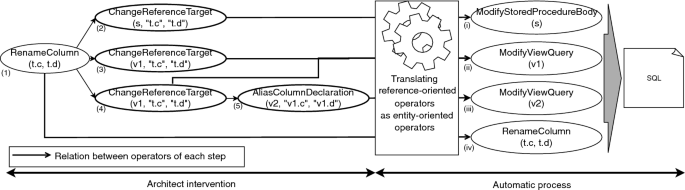
Compiling operators as a valid SQL patch.
The architect gave us access to these patches to do a post-mortem analysis of the DB evolutions. One of the patches implements the renaming of a column belonging to a table that is central to the DB. This is interesting because it is a non-trivial evolution.
We had the opportunity to record the architect ’s screen during this migration [ 4 ]. We observed that the architect used a trial-and-error process to find dependencies between entities of the database. He implements part of the patch and runs it in a transaction that is always rolled back. When the patch fails in between, the architect uses the gained knowledge to correct the SQL patch. Using this methodology, the architect built incrementally the SQL patch implementing the patch during approximately 1 h. The patch is \({\sim }200\) LOC and is composed of 19 SQL statements. To validate our approach, we regenerate this SQL patch with our tool but without the architect ’s expertise. Then, we compare our resulting database with the one obtained by the architect.
5.1 Experimental Protocol
The goals of the experiment are multiple: (i) to illustrate on a concrete case the generation of a SQL patch; (ii) to compare the database resulting from our approach with the one originally written by the architect; and (iii) to estimate the time required to generate a SQL patch as compared to the manual generation.
Based on the road map written by the architect and the comments in the patch we extracted the operators initiated by the architect during this migration. A discussion with the architect allowed us to validate the list of initial operators: RenameColumn(person.uid, login) , RemoveFunction(key_for_uid(varchar)) , RemoveFunction(is_responsible_of(int4)) , RemoveFunction(is_responsible_of(int4,int4)) , Re- nameFunction(uid(integer), login(integer)) , RenameLocalVariable(login.uidperson, login.loginperson) , RemoveView(test_member_view) . Details on these operators can be found at: https://hal.inria.fr/hal-02504949v1.
The experiment consists in choosing these operators in our tool and following the recommendations it proposes. Potentially several recommendations might be proposed, particularly as whether to create aliases in some referencing queries or to rename various columns in cascade (see example in Sect. 4.4 ). The architect told us that, as a rule, he preferred to avoid using aliases and renamed the columns. These were the only decision we had to do during the experiment.
We finished the experiment by executing the SQL patch generated by our tool on an empty (no data) copy of the database. Note that having no data in the database to test the patch might be a problem for operators modifying data ( e.g., changing the type of a column implies converting data to the new type). However, in the case of our experiment no operator modifies data stored in the database. First, we checked whether the generated patch ran without errors. Second, we compared the state of the database after the architect ’s migration and ours. For this, we generated a dump of the SQL schema of both databases and compared these two dumps using a textual diff tool. Third, we also considered the time we spent on our migration and the one used by the architect when he did his.
5.2 Results
We entered the seven operators listed previously in our tool and let it guide us through the decision process to generate the SQL migration patch.
Fifteen decisions were taken to choose among the proposed recommendations. They all concerned the renaming or aliasing of column references. From this process, the tool generated a SQL patch of \({\sim }270\) LOC and 27 SQL statements.
To answer the goals of the experiment listed previously: (i) The generated SQL patch was successfully applied on the database. (ii) The diff of the two databases (one being the result of the hand-written patch and the other being the result of the generated patch) showed a single difference: a comment in one function is modified in the hand-written version. Such changes are not taken into account by our approach. (iii) Encoding the list of changes and taking decisions took approximately 15 min. This corresponds to about \(25\%\) of the time necessary to the architect who has a very good knowledge of his database to obtain the same result.
5.3 Discussion
Validating tools predicting the impact of a software change is not easy. Evidence of that claim can be found in Lehnert’s meta-review [ 10 ]. On the 18 approaches reviewed by Lehnert using either call graphs or program dependency graph techniques, only six have experimental results about the size of the system, time, precision and recall. And only one of these has results on all the metrics together.
Accessing industrial databases with their evolutions is more difficult than accessing source code. Since databases are usually at the core of company business, companies are reluctant to provide their schema. The database schema evolutions are not systematically recorded in tools such as version control systems (VCS) probably because the integration between relational database and VCS is poor. Finding database administrators willing to devote some time to our experiment can also be challenging.
It is also possible to analyze the co-evolution between the source code of a database and the source code of its clients. Analyzing only the behavior inside the database has the advantage that the precision is better as queries are usually not built dynamically. When queries are built dynamically via string concatenation, it is hard to determinate what query is executed in the end. However, it is possible to build query dynamically from inside the database (via PERFORM query). We do not handle these kinds of query at the moment but it would be possible to use an approach similar to Meurice et al. approach [ 14 ].
Note that our approach has been applied on AppSI database but is does not rely on AppSI specificities. DBEvolution relies on the meta-model and operators definitions to provide recommendations for a given change. We can import other databases as model in our tool. For example, we were able to load Liquidfeedback database schema Footnote 6 in our tool and we can use DBEvolution on it to get recommendations.
6 Related Work
Our work needs to be compared to impact analysis and database schema evolution research fields.
Impact Analysis. Since the first paper introducing Impact Analysis by Bohnert and Arnold [ 1 ], the research field has been widely investigated by the scientific community. Meta-analyses on this topic exist, e.g., Lehnert did a review of software change impact analysis in 2011 [ 10 ]. We focus on work adapting impact analysis techniques to relational databases as discussed below.
Karahasanovic and Sjøberg proposed a tool, called SEMT, to find impacts of object-database schema changes on applications [ 9 ]. Their tool allows one to identify and visualize the impact. It uses an improved version of the transitive closure algorithm. It also provides a language to graphically walk the impact graph.
Gardikiotis and Malevris [ 6 ] proposes an approach to estimate the impact of a database schema change on the operability of a web application. To achieve that, they proposed a tool named DaSIAn (Database Schema Impact Analyzer) based on their approach. This tool finds CRUD queries and stored procedures affected by a change on the database schema. The authors also presented an approach assessing impact on client applications from schema changes [ 7 ]. They used this approach to assess both affected source code statements and affected test suites in the application using the database after a change in the database.
Maul et al. [ 13 ] created a static analysis technique to assess the impact of changing a relational database on its object-oriented software clients. They implemented Schema Update Impact Tool Environment (SUITE) which takes the source code of the application using the database and a model of the database schema as input. Then, they queried this model to find out the part of the source code application impacted when modifying an entity of the database.
Nagy et al. [ 15 ] compared two methods for computing dependencies between stored procedures and tables in a database: One using Static Execute After/Before relations [ 8 ] and the other analysing CRUD queries and schema to find database access and propagate this dependency at the stored procedure level. The authors concluded that the two approaches provide different results and should thus be used together to assess dependencies safely.
Liu et al. [ 11 , 12 ], proposed a graph called attribute dependency graph to identify dependencies between columns in a database and parts of client software source code using it. They evaluated their approach on 3 databases and their clients written in PHP. Their tool presents to the architect an overview of a change impact as a graph.
Similarly to approaches covered by Lehnert meta-analysis, the validations for impact analysis on databases are usually quite weak because it is a difficult task. To position our approach, it uses static analysis to determine the impact of a change on an entity. This information is directly available in our model because we reify the references between entities. As explained previously, our approach considers that if you change an entity, all entities referencing it are potentially impacted. That set of impacted entities is decomposed into categories and a recommendation is provided for each of them.
Recommendations for Relational Database Schema Evolution. Sjøberg’s work [ 20 ] quantifies schema evolution. They studied the evolution of a relational database and its application forming a health management system during 18 months. To do so they used “the Thesaurus” tool which analyses how many screens, actions and queries may be affected by a potential schema change. This tool does not propose recommendations to users but rather shows code locations to be manually modified. Their results suggest that change management tools are needed to handle this evolution.
Curino et al. [ 2 , 3 ] proposed PRISM, a tool suite allowing one to predict and evaluate schema modification. PRISM also propose database migration feature through rewriting queries and application to take into account the modification. To do so, they provide a language to express schema modification operators, automatic data migration support and documentation of changes applied on the database. They evaluated their approach and tool on Wikimedia showing it is efficient. In PRISM approach, the operators are limited to modification on structural entities of the database, whereas our approach also deals with change on behavioral entities.
Papastefanatos et al. [ 16 , 17 ] developed Hecataeus, a tool representing the database structural entities, the queries and the views, as a uniform directed graph. Hecataeus allows user to create an arbitrary change and to simulate it to predict its impact. From this perspective, it is close to the aim of our tool. The main difference is that our approach ensures no inconsistency is created at some point during database evolution. It is not clear how Hecataeus addresses this problem in these papers.
Meurice et al. [ 14 ] presented a tool-supported approach that can analyze how the client source code and database schema co-evolved in the past and to simulate a database change to determine client source code locations that would be affected by the change. Additionally, the authors provide strategies (recommendations and warnings) for facing database schema change. Their recommendations describe how to modify client program source code depending on the change performed on the database. The approach presented has been evaluated by comparing historical evolution of a database and its client application with recommendations provided by their approach. From the historical analysis the authors observed that the task of manually propagating database schema change to client software is not trivial. Some schema changes required multiple versions of the software application to be fully propagated. Others were never fully propagated. We argue that, according to what we observed in previous research [ 4 ] and the research made in this article, propagating structural change to behavior entities of the database is a hard task as well.
Compared to previous approaches, DBEvolution brings as a novelty that any entity can be subject to an evolution operator. In particular, stored procedures can be modified and DBEvolution will provide recommandations for the modification. The other way around, modifying a structural entity will provide recommandations to accomodate stored procedures with the change. Such capability is absent from above approaches.
7 Conclusion
We have developed an approach to manage relational database evolution. This approach addresses the two main constraints that a RDBMS sets: 1. no schema inconsistency is allowed during the evolution and 2. stored procedures bodies are not described by meta-data . Addressing these problems allowed us to provide three main contributions: i. a meta-model for relational databases easing the computation of the impact of a change, ii. a semi-automatic approach to evolve a database while managing the impact, and iii. an experiment to assess that our approach can reproduce a change that happened on a database used by a real project with a gain of 75% of the time. These results show that this approach is promising to build the future of relational databases integrated development environments.
Our future works are threefold. First, we would like to extend the set of operators supported by our implementation. More specifically, we want higher-level operators such as: historize column which will modify the database schema to keep track of the history of the values of a column through the database life.
Second, the evolution has been reproduced by us which might bias our results in terms of time to implement a change. Indeed, as we have little knowledge on the DB, it is possible that an expert using our tool would be faster than us. Thus, we would like to do another experiment where we compare the performances of an architect using our tool with the performances of an architect using typical tools to implement an evolution.
Finally, some operators will require to transform or move data stored in the database (for example moving a column from a table to another). We plan to support such operators in our methodology by generating CRUD queries in addition to the DDL queries already generated by the operators.
Create Read Update Delete query in SQL: INSERT, SELECT, UPDATE, DELETE .
https://github.com/juliendelplanque/FAMIXNGSQL .
https://github.com/olivierauverlot/PgMetadata .
https://github.com/juliendelplanque/PostgreSQLParser .
https://github.com/juliendelplanque/DBEvolution .
https://liquidfeedback.org .
Arnold, R.S., Bohnert, S.: Software Change Impact Analysis. IEEE Computer Society Press, Los Alamitos (1996)
Google Scholar
Curino, C., Moon, H.J., Zaniolo, C.: Automating database schema evolution in information system upgrades. In: Proceedings of the 2nd International Workshop on Hot Topics in Software Upgrades, p. 5. ACM (2009)
Curino, C.A., Moon, H.J., Zaniolo, C.: Graceful database schema evolution: the prism workbench. Proc. VLDB Endow. 1 (1), 761–772 (2008)
Article Google Scholar
Delplanque, J., Etien, A., Anquetil, N., Auverlot, O.: Relational database schema evolution: an industrial case study. In: 2018 IEEE International Conference on Software Maintenance and Evolution (ICSME) (2018). https://doi.org/10.1109/ICSME.2018.00073 . http://rmod.inria.fr/archives/papers/Delp18c-ICSME-DatabaseSchemaEvolution.pdf
Fowler, M., Beck, K., Brant, J., Opdyke, W., Roberts, D.: Refactoring: Improving the Design of Existing Code. Addison Wesley, Boston (1999)
Gardikiotis, S.K., Malevris, N.: DaSIAn: a tool for estimating the impact of database schema modifications on web applications. In: 2006 IEEE International Conference on Computer Systems and Applications, pp. 188–195. IEEE (2006)
Gardikiotis, S.K., Malevris, N.: A two-folded impact analysis of schema changes on database applications. Int. J. Autom. Comput. 6 (2), 109–123 (2009)
Jász, J., Beszédes, Á., Gyimóthy, T., Rajlich, V.: Static execute after/before as a replacement of traditional software dependencies. In: 2008 IEEE International Conference on Software Maintenance, pp. 137–146. IEEE (2008)
Karahasanovic, A., Sjoberg, D.I.: Visualizing impacts of database schema changes-a controlled experiment. In: 2001 Proceedings IEEE Symposia on Human-Centric Computing Languages and Environments, pp. 358–365. IEEE (2001)
Lehnert, S.: A review of software change impact analysis, p. 39. Ilmenau University of Technology (2011)
Liu, K., Tan, H.B.K., Chen, X.: Extraction of attribute dependency graph from database applications. In: 2011 18th Asia Pacific Software Engineering Conference (APSEC), pp. 138–145. IEEE (2011)
Liu, K., Tan, H.B.K., Chen, X.: Aiding maintenance of database applications through extracting attribute dependency graph. J. Database Manage. 24 (1), 20–35 (2013)
Maule, A., Emmerich, W., Rosenblum, D.: Impact analysis of database schema changes. In: 2008 ACM/IEEE 30th International Conference on Software Engineering, ICSE 2008, pp. 451–460. IEEE (2008)
Meurice, L., Nagy, C., Cleve, A.: Detecting and preventing program inconsistencies under database schema evolution. In: 2016 IEEE International Conference on Software Quality, Reliability and Security (QRS), pp. 262–273. IEEE (2016)
Nagy, C., Pantos, J., Gergely, T., Besz’edes, A.: Towards a safe method for computing dependencies in database-intensive systems. In: 2010 14th European Conference on Software Maintenance and Reengineering (CSMR), pp. 166–175. IEEE (2010)
Papastefanatos, G., Anagnostou, F., Vassiliou, Y., Vassiliadis, P.: Hecataeus: A what-if analysis tool for database schema evolution. In: 2008 12th European Conference on Software Maintenance and Reengineering, CSMR 2008, pp. 326–328. IEEE (2008)
Papastefanatos, G., Vassiliadis, P., Simitsis, A., Vassiliou, Y.: HECATAEUS: regulating schema evolution. In: 2010 IEEE 26th International Conference on Data Engineering (ICDE), pp. 1181–1184. IEEE (2010)
Roberts, D., Brant, J., Johnson, R.E., Opdyke, B.: An automated refactoring tool. In: Proceedings of ICAST 1996, Chicago, IL, April 1996
Roberts, D.B.: Practical Analysis for Refactoring. Ph.D. thesis, University of Illinois (1999). http://historical.ncstrl.org/tr/pdf/uiuc_cs/UIUCDCS-R-99-2092.pdf
Sjøberg, D.: Quantifying schema evolution. Inf. Softw. Technol. 35 (1), 35–44 (1993)
Skoulis, I., Vassiliadis, P., Zarras, A.: Open-source databases: within, outside, or beyond Lehman’s laws of software evolution? In: Jarke, M., et al. (eds.) CAiSE 2014. LNCS, vol. 8484, pp. 379–393. Springer, Cham (2014). https://doi.org/10.1007/978-3-319-07881-6_26
Chapter Google Scholar
Download references
Author information
Authors and affiliations.
Univ. Lille, CNRS, Centrale Lille, Inria UMR 9189 - CRIStAL, Lille, France
Julien Delplanque, Anne Etien, Nicolas Anquetil & Stéphane Ducasse
INRIA Lille Nord Europe, Villeneuve d’Ascq, France
You can also search for this author in PubMed Google Scholar
Corresponding author
Correspondence to Julien Delplanque .
Editor information
Editors and affiliations.
TU Wien, Vienna, Austria
Schahram Dustdar
University of Toronto, Toronto, ON, Canada
Université Paris 1 Panthéon-Sorbonne, Paris, France
Camille Salinesi
Université Grenoble Alpes, Saint-Martin-d’Hères, France
Dominique Rieu
Rights and permissions
Reprints and permissions
Copyright information
© 2020 Springer Nature Switzerland AG
About this paper
Cite this paper.
Delplanque, J., Etien, A., Anquetil, N., Ducasse, S. (2020). Recommendations for Evolving Relational Databases. In: Dustdar, S., Yu, E., Salinesi, C., Rieu, D., Pant, V. (eds) Advanced Information Systems Engineering. CAiSE 2020. Lecture Notes in Computer Science(), vol 12127. Springer, Cham. https://doi.org/10.1007/978-3-030-49435-3_31
Download citation
DOI : https://doi.org/10.1007/978-3-030-49435-3_31
Published : 03 June 2020
Publisher Name : Springer, Cham
Print ISBN : 978-3-030-49434-6
Online ISBN : 978-3-030-49435-3
eBook Packages : Computer Science Computer Science (R0)
Share this paper
Anyone you share the following link with will be able to read this content:
Sorry, a shareable link is not currently available for this article.
Provided by the Springer Nature SharedIt content-sharing initiative
- Publish with us
Policies and ethics
- Find a journal
- Track your research
This paper is in the following e-collection/theme issue:
Published on 24.4.2024 in Vol 26 (2024)
Behavior Change Approaches in Digital Technology–Based Physical Rehabilitation Interventions Following Stroke: Scoping Review
Authors of this article:

- Helen J Gooch, BSc ;
- Kathryn A Jarvis, PhD ;
- Rachel C Stockley, PhD
Stroke Research Team, School of Nursing and Midwifery, University of Central Lancashire, Preston, United Kingdom
Corresponding Author:
Helen J Gooch, BSc
Stroke Research Team
School of Nursing and Midwifery
University of Central Lancashire
BB247 Brook Building
Victoria Street
Preston, PR1 2HE
United Kingdom
Phone: 44 1772894956
Email: [email protected]
Background: Digital health technologies (DHTs) are increasingly used in physical stroke rehabilitation to support individuals in successfully engaging with the frequent, intensive, and lengthy activities required to optimize recovery. Despite this, little is known about behavior change within these interventions.
Objective: This scoping review aimed to identify if and how behavior change approaches (ie, theories, models, frameworks, and techniques to influence behavior) are incorporated within physical stroke rehabilitation interventions that include a DHT.
Methods: Databases (Embase, MEDLINE, PsycINFO, CINAHL, Cochrane Library, and AMED) were searched using keywords relating to behavior change, DHT, physical rehabilitation, and stroke. The results were independently screened by 2 reviewers. Sources were included if they reported a completed primary research study in which a behavior change approach could be identified within a physical stroke rehabilitation intervention that included a DHT. Data, including the study design, DHT used, and behavior change approaches, were charted. Specific behavior change techniques were coded to the behavior change technique taxonomy version 1 (BCTTv1).
Results: From a total of 1973 identified sources, 103 (5%) studies were included for data charting. The most common reason for exclusion at full-text screening was the absence of an explicit approach to behavior change (165/245, 67%). Almost half (45/103, 44%) of the included studies were described as pilot or feasibility studies. Virtual reality was the most frequently identified DHT type (58/103, 56%), and almost two-thirds (65/103, 63%) of studies focused on upper limb rehabilitation. Only a limited number of studies (18/103, 17%) included a theory, model, or framework for behavior change. The most frequently used BCTTv1 clusters were feedback and monitoring (88/103, 85%), reward and threat (56/103, 54%), goals and planning (33/103, 32%), and shaping knowledge (33/103, 32%). Relationships between feedback and monitoring and reward and threat were identified using a relationship map, with prominent use of both of these clusters in interventions that included virtual reality.
Conclusions: Despite an assumption that DHTs can promote engagement in rehabilitation, this scoping review demonstrates that very few studies of physical stroke rehabilitation that include a DHT overtly used any form of behavior change approach. From those studies that did consider behavior change, most did not report a robust underpinning theory. Future development and research need to explicitly articulate how including DHTs within an intervention may support the behavior change required for optimal engagement in physical rehabilitation following stroke, as well as establish their effectiveness. This understanding is likely to support the realization of the transformative potential of DHTs in stroke rehabilitation.
Introduction
Digital health technologies (DHTs) comprise apps, programs, or software used in the health and social care systems [ 1 ]. They are considered to have almost unlimited potential to transform health care interventions and delivery and empower people to take a greater role in their own care and well-being [ 2 , 3 ].
Stroke is one of the leading causes of acquired disability worldwide, with around 12 million people experiencing a stroke each year [ 4 ]. Rehabilitation is a complex, multifaceted process [ 5 ] that facilitates those with health conditions and disabilities to participate in and gain independence in meaningful life roles [ 6 ]. It is considered an essential aspect of health care provision following a stroke [ 7 ] as a means to address poststroke impairments, which can involve motor, sensory, and cognitive functions. Changes in the ability to move due to impairment of both movement and sensory function are commonly experienced by people following a stroke [ 8 ] and are addressed by physical rehabilitation comprising regular, intensive practice and repetition of movements and tasks [ 9 , 10 ]. Conventional physical rehabilitation often struggles to deliver the intensity required to optimize recovery [ 11 ], and over recent years, there has been significant interest in the use of DHTs, such as virtual reality (VR), telerehabilitation, robotics, and activity monitors [ 12 - 16 ], to enhance and increase the intensity of rehabilitation. DHTs can provide a whole intervention or be used as a component of a wider intervention; the term DHT-based intervention has been used within this review to refer to both situations.
For many people who survive a stroke, rehabilitation requires individuals to engage in regular and frequent rehabilitative activities to achieve improvements in function and realize their optimal recovery. This necessitates adjustments to an individual’s behavior [ 17 ] over a sustained period of time. Changing behavior is a complex process and is underpinned by a variety of different theories, models, and frameworks [ 18 ], such as social cognitive theory [ 19 ] or the behavior change wheel framework [ 20 ]. Individual activities within a complex intervention that are designed to change behavior can be separated into replicable active components widely referred to as behavior change techniques (BCTs) [ 21 ]. Historically, labels applied to BCTs have lacked consensus, resulting in uncertainty and difficulty in comparing interventions. This has been addressed in the behavior change technique taxonomy version 1 (BCTTv1) [ 22 ], a classification system of 93 distinct BCTs clustered into 16 groups, which is a well-recognized tool to provide consistency with BCT reporting in interventions. DHTs provide an emerging opportunity to support the behavior change required within physical stroke rehabilitation interventions through facilitators that are embedded within the technology itself that aim to form, alter, or reinforce behaviors [ 23 ]. Understanding of this area is limited, with most literature exploring the use of DHTs to support behavior change focused on specific health-related behaviors such as physical activity or healthy eating [ 24 ] rather than as a core component of a type of rehabilitation intervention. Motivation is acknowledged to play an integral role in behavior change [ 25 ], and it is often assumed that DHTs provide motivation to engage with rehabilitation [ 26 ]. However, for this assumption to be realized, the DHTs must be able to support and deliver interventions that facilitate the vital changes in behavior needed to promote prolonged and sustained engagement in stroke rehabilitative activities. Imperative to this is understanding the theories, models, and frameworks that underpin interventions and the BCTs (active components) within the interventions [ 27 - 29 ]. The theories, models, and frameworks alongside the BCTs will be referred to hereinafter as approaches. Within the context of DHT-based physical stroke rehabilitation interventions, approaches to behavior change warrant further investigation.
Aim and Objectives
This scoping review aimed to identify if and how behavior change approaches are incorporated within DHT-based physical stroke rehabilitation interventions. Specifically, it sought to:
- Establish if behavior change theories, models, and frameworks, or BCTs, are described when reporting on DHT-based interventions that have been developed or evaluated for use in poststroke physical rehabilitation.
- Identify if behavior change theories, models, or frameworks underpin the interventions and which of these are being used.
- Identify if the BCTTv1 is being used to report BCTs within interventions.
- Determine which BCTs (based on the BCTTv1) can be identified within the interventions.
- Explore whether the type of technology influences the techniques used to change behaviors.
Review Methodology
A scoping review was completed and reported following established guidelines [ 30 , 31 ] and the Preferred Reporting of Systematic Reviews and Meta-Analyses Extension for Scoping Reviews (PRISMA-ScR; Multimedia Appendix 1 ) [ 32 ]. The protocol was registered with the Open Science Framework [ 33 ].
Eligibility Criteria
Any published sources that reported a completed primary research study in which a behavior change approach could be identified within a DHT-based physical stroke rehabilitation intervention were included ( Multimedia Appendix 2 ). Physical rehabilitation comprised interventions that addressed an impairment, or sequela of impairment, of sensory function and pain, neuromusculoskeletal and movement-related functions, or voice and speech, as defined by the International Classification of Functioning, Disability, and Health [ 34 ]. Completed primary research included all types of studies, both quantitative and qualitative, and no minimum sample size or intervention length was set. The BCTTv1 [ 22 ] was used to support the identification of BCTs within the interventions.
Information Sources and Search Strategy
A systematic database search was conducted in Embase, MEDLINE, PsycINFO, CINAHL, Cochrane Database of Systematic Reviews, CENTRAL Register of Controlled Trials, and AMED on March 21, 2023. The search was completed in collaboration with an information specialist who provided support with the development of the free text and thesaurus search terms, created the final search, adjusted the searches for the different databases, and ran the search. It consisted of 4 distinct search streams: behavior change, DHT, physical rehabilitation, and stroke, which were then combined ( Multimedia Appendix 3 ). Searches were restricted to the English language (due to review resources) and by date to search from 2001; the date restriction acknowledges the main time period of DHT growth [ 35 ], captures sources reported in systematic reviews of DHTs in stroke rehabilitation [ 12 - 16 ], and is reflected in other scoping literature exploring DHTs [ 24 ]. Additional sources were identified by hand searching, including scrutiny of the included source reference lists.
Selection of Sources of Evidence
The titles and abstracts of deduplicated sources from database searches and hand searches were independently screened by 2 reviewers, 1 of whom had completed the BCTTv1 web-based training package [ 36 ] to inform decisions made around the use of BCTs. Any conflicts were discussed, and if a consensus was not reached, the source was included for full-text screening. Attempts were made to locate a completed study publication from eligible conference abstracts, protocols, and trial registry entries. Full-text sources were screened independently by 2 reviewers, and disagreements were resolved by a third reviewer. Reasons for full-text exclusion were recorded. EndNote X9 software (Clarivate) and the Rayyan web tool software (Qatar Computing Research Institute) [ 37 ] were used to facilitate the source selection process.
Data Charting Process
A review-specific data charting tool was developed and initially piloted using 3 sources by 3 reviewers, and then further developed iteratively throughout the process [ 30 ]. Data charting was completed collectively by 2 reviewers. When several sources referred to a single study, these sources were grouped together for data charting, and if a source identified additional sources for further detail of the intervention (eg, a protocol or supplementary material), then this information was also used to support data charting.
The data charting tool was developed with reference to the Template for Intervention Description and Replication (TIDieR) checklist [ 27 ] and with a focus on the DHT-based intervention and behavior change approaches ( Multimedia Appendix 4 [ 14 , 38 - 40 ]). In the absence of a recognized predefined taxonomy for DHTs, the DHTs used in the sources were charted iteratively by the type of technology [ 41 ] from the information provided about the intervention. Over time, DHT categories emerged and were defined ( Multimedia Appendix 4 ). Discrete BCTs were identified from the intervention detail provided using the BCTTv1 [ 22 ] ( Multimedia Appendix 5 [ 42 ]). A pragmatic decision was made that the single reviewer who had completed the BCTTv1 web-based training package [ 36 ] would code the interventions to the BCTTv1. Any areas of uncertainty were discussed in detail among the review team.
Synthesis of Results
In accordance with the aims of a scoping review, formal assessments of methodological quality were not completed [ 30 , 31 ]. Findings were synthesized using descriptive statistics facilitated by SPSS Statistics 28.0.0.0 (IBM Corp) and Microsoft Excel (version 2208; Microsoft Corporation) and presented in text, table, and chart formats. The characteristics of the included sources, specifically participant numbers, age, and time since stroke, and intervention details, were summarized to provide contextual information for the review. Time since stroke was based on a published timeline framework [ 43 ], which describes the following phases: acute (1-7 days), early subacute (7 days to 3 months), late subacute (3-6 months), and chronic (greater than 6 months).
The behavior change theories, models, or frameworks underpinning the DHT-based interventions and sources where interventions had already been coded to the BCTTv1 were summarized. The use of individual BCTs, as coded by reviewers from intervention descriptions, was briefly summarized; however, the main focus of the BCT synthesis was completed by grouping the BCTs into the 16 BCTTv1 clusters, in order to provide an overview of their use across the sources and allow comparison with other reviews [ 44 , 45 ]. A cluster was only identified once per source, irrespective of the number of individual BCTs within that cluster. Relationships between BCTTv1 clusters and between DHT type and BCTTv1 clusters were descriptively explored. A relationship map was used to visually represent the strength of the connections between the BCTTv1 clusters, with a thicker line indicating that variables were more frequently reported together. No inferential statistical analysis was used.
From a total of 1973 sources screened, 357 full-text sources were assessed for eligibility, then after grouping sources that referred to a single study, 103 (5%) distinct sources were included in the review [ 46 - 148 ] ( Figure 1 ). Of the 245 sources excluded at full-text screening, 165 (67%) were excluded due to a lack of a behavior change approach.

Characteristics of Sources of Evidence
All sources of evidence were studies and will be referred to as such hereinafter. The number of studies in this field has rapidly increased over time ( Figure 2 ), from a single study in 2004 to 8 in 2022, with a peak of 15 in 2021. The majority (86/103, 83%) [ 47 - 51 , 53 - 56 , 58 , 59 , 61 , 63 - 68 , 71 - 86 , 89 - 95 , 97 - 105 , 107 , 109 , 111 , 112 , 114 , 115 , 117 - 126 , 128 - 136 , 138 - 148 ] were published in the past 10 years. Most studies took place in North America (41/103, 40%) [ 46 - 49 , 52 , 55 , 56 , 60 , 64 - 67 , 69 , 70 , 72 , 74 , 76 - 78 , 80 , 85 - 88 , 92 , 93 , 97 , 99 , 101 , 108 - 110 , 126 - 129 , 137 , 138 , 141 , 142 , 145 ] and Europe (35/103, 34%) [ 51 , 53 , 54 , 57 , 58 , 62 , 63 , 68 , 71 , 79 , 81 - 84 , 89 , 111 , 113 - 125 , 132 , 136 , 140 , 143 , 146 , 147 ], with the remainder in Asia (16/103, 16%) [ 50 , 59 , 61 , 91 , 94 , 95 , 98 , 100 , 102 - 104 , 107 , 135 , 139 , 144 , 148 ], Australasia (9/103, 9%) [ 75 , 96 , 105 , 106 , 112 , 130 , 131 , 133 , 134 ], Africa (1/103, 1%) [ 90 ], and a single multicontinental study (1/103, 1%) [ 73 ]. Almost half (45/103, 44%) the studies are reported as feasibility or pilot studies [ 49 , 56 , 58 , 64 , 66 , 68 , 69 , 72 - 74 , 76 , 77 , 79 , 82 - 84 , 89 , 90 , 92 , 93 , 95 , 97 , 100 - 104 , 106 , 108 , 114 , 116 , 117 , 119 , 122 , 124 - 126 , 131 , 134 , 136 , 138 , 139 , 141 , 143 , 147 ]. Other study designs included randomized controlled trials (20/103, 19%) [ 50 , 51 , 60 , 61 , 65 , 75 , 80 , 85 , 86 , 91 , 107 , 109 , 112 , 128 - 130 , 137 , 144 , 146 , 148 ], single session investigations (19/103, 18%) [ 47 , 52 , 57 , 59 , 71 , 78 , 87 , 88 , 98 , 110 , 115 , 118 , 120 , 123 , 127 , 132 , 133 , 135 , 142 ], nonrandomized experimental designs (13/103, 13%) [ 53 - 55 , 62 , 63 , 67 , 81 , 94 , 96 , 99 , 105 , 113 , 145 ], case studies (4/103, 4%) [ 46 , 48 , 70 , 140 ], and realist evaluations (2/103, 2%) [ 111 , 121 ].

Participants
There were a total of 2825 participants in the 103 included studies. Studies tended to be small, with a median of 16 participants and a range of 1-188. Only half (55/103, 53%) the studies [ 46 - 48 , 50 , 56 , 57 , 59 , 61 , 64 , 67 , 69 - 72 , 78 , 79 , 82 , 87 , 88 , 92 , 93 , 95 - 99 , 101 , 102 , 105 , 106 , 108 , 111 - 121 , 123 - 127 , 134 , 138 - 140 , 142 , 143 , 145 , 147 ] reported the minimum and maximum age of participants, which ranged from 17 to 99 years. Over three-quarters (83/103, 81%; 2508 participants) of studies reported the time since the onset of stroke. Of these 83 studies, 1 (1%; 48 participants) study [ 91 ] was conducted in the acute phase, 14 (17%; 504 participants) studies [ 60 , 61 , 68 , 74 , 79 , 92 , 100 , 102 , 109 , 114 , 133 , 144 , 146 , 148 ] were conducted in the early subacute phase, 11 (13%; 316 participants) studies [ 59 , 65 , 66 , 72 , 75 , 76 , 81 , 104 , 107 , 121 , 134 ] were conducted in the late subacute phase, and 57 (69%; 1640 participants) studies [ 46 , 48 , 49 , 51 , 53 , 54 , 57 , 63 , 64 , 67 , 69 , 70 , 73 , 78 , 80 , 82 , 84 , 85 , 88 , 89 , 93 - 99 , 101 , 103 , 105 , 106 , 108 , 111 - 113 , 117 - 120 , 122 - 125 , 127 - 131 , 136 - 142 , 145 , 147 ] were conducted in the chronic phase [ 43 ].
Study Intervention
An overview of study intervention characteristics is provided ( Table 1 ). Interventions were focused on upper limb rehabilitation in almost two-thirds (65/103, 63%) of the studies [ 46 - 49 , 51 , 54 - 59 , 62 - 65 , 68 , 71 , 72 , 74 , 75 , 77 - 81 , 85 - 88 , 92 , 95 , 96 , 99 , 101 - 103 , 105 - 108 , 110 , 112 , 113 , 116 - 118 , 121 , 123 - 125 , 127 , 128 , 132 , 133 , 135 - 137 , 139 - 142 , 144 - 147 ]. Nearly all interventions (96/103, 93%) [ 46 - 80 , 84 - 94 , 96 - 117 , 119 - 121 , 124 - 148 ] were delivered to individual participants, with over half (62/103, 60%) [ 46 - 50 , 53 - 58 , 60 , 61 , 64 - 70 , 72 , 74 - 77 , 79 , 80 , 82 - 86 , 89 , 90 , 93 , 94 , 96 , 97 , 99 , 101 , 105 , 111 , 112 , 116 , 117 , 119 - 122 , 126 , 129 - 131 , 134 , 136 , 138 , 139 , 141 , 143 - 145 , 147 ] delivered fully or partly in the participant’s homes. Two-thirds (70/103, 68%) of studies [ 46 - 50 , 52 - 54 , 57 , 60 , 62 , 63 , 65 - 74 , 76 - 84 , 86 - 93 , 98 , 100 , 102 , 104 , 108 , 109 , 112 - 115 , 117 , 118 , 120 , 122 - 125 , 129 - 131 , 135 - 138 , 140 - 142 , 144 - 146 , 148 ] included partial or full supervision of the intervention, with this predominately being provided face-to-face (48/70, 69%) [ 46 , 47 , 52 , 57 , 60 , 62 , 63 , 67 , 68 , 71 , 73 , 78 , 81 - 84 , 86 - 89 , 91 , 92 , 98 , 100 , 102 , 104 , 108 , 109 , 112 - 115 , 117 , 118 , 120 , 122 - 125 , 135 - 137 , 140 , 142 , 144 - 146 , 148 ]. Interventions lasted between a single session and 26 weeks.
Of the 103 studies, over half (n=57, 55%) of the studies [ 46 , 47 , 51 - 54 , 57 , 61 , 63 , 67 , 68 , 70 , 71 , 73 , 75 - 78 , 81 , 84 - 86 , 88 - 91 , 93 , 95 , 96 , 98 , 100 , 102 - 104 , 106 , 109 , 112 , 114 , 115 , 123 - 126 , 129 , 130 , 132 , 133 , 135 - 138 , 140 , 143 - 147 ] included 1 type of DHT, 30 (29%) studies [ 48 , 49 , 55 , 56 , 58 - 60 , 62 , 64 , 69 , 83 , 92 , 94 , 97 , 99 , 101 , 105 , 107 , 108 , 110 , 111 , 113 , 116 , 118 , 121 , 122 , 127 , 128 , 139 , 142 ] included 2 types, and 16 (16%) studies [ 50 , 65 , 66 , 72 , 74 , 79 , 80 , 82 , 87 , 117 , 119 , 120 , 131 , 134 , 141 , 148 ] included 3 types. VR was the most frequently used DHT (58/103, 56%) [ 46 - 49 , 51 - 53 , 57 , 59 , 62 , 63 , 65 , 66 , 69 , 71 , 72 , 74 , 77 , 78 , 80 , 81 , 84 - 89 , 92 , 95 , 96 , 98 , 102 - 104 , 106 , 112 , 113 , 115 , 117 - 120 , 123 - 128 , 132 , 135 - 137 , 140 , 142 , 143 , 146 - 148 ] followed by apps (31/103, 30%) [ 50 , 55 , 56 , 58 , 61 , 64 - 66 , 72 , 74 , 75 , 79 , 82 , 83 , 94 , 97 , 99 , 101 , 105 , 108 , 111 , 114 , 116 , 119 - 122 , 131 , 134 , 139 , 141 ]. Further information on intervention characteristics with detail on associated citations is available ( Multimedia Appendix 6 [ 46 - 148 ]).
a F2F: face-to-face.
b DHT: digital health technology.
c VR: virtual reality.
Behavior Change Theories, Models, and Frameworks
Most studies (93/103, 90%) [ 46 - 49 , 51 - 62 , 64 - 73 , 75 - 89 , 91 - 93 , 96 - 106 , 108 - 115 , 117 - 137 , 139 , 140 , 142 - 148 ] endeavored to link the intervention to behavior change; however, in the majority of these studies (75/93, 81%) [ 46 , 51 - 56 , 58 - 62 , 64 - 69 , 71 - 73 , 75 , 77 - 89 , 91 - 93 , 96 , 97 , 99 - 101 , 103 - 106 , 108 , 110 , 112 , 114 , 115 , 117 - 120 , 123 , 124 , 127 , 128 , 131 - 137 , 139 , 140 , 142 - 144 , 146 - 148 ], this explanation was centered on the reporting of the techniques perceived to change behaviors without direct reference to use of the BCTTv1 or on the reporting of a component of the intervention or the whole of the intervention as motivating. These explanations lack detail on how or why this influences behavior change. Examples of this included “the app also provided performance feedback, allowing the user to compare their current performance against their score from the previous session” (Bhattacharjya et al [ 56 ]) and “games motivate patients to engage in enjoyable play behavior” (Cramer et al [ 66 ]). A limited number of studies (18/103, 17%) [ 47 - 49 , 57 , 70 , 76 , 98 , 102 , 109 , 111 , 113 , 121 , 122 , 125 , 126 , 129 , 130 , 145 ] articulated 1 or more theories, models, or frameworks of behavior change. While it is acknowledged that the BCTTv1 is a taxonomy framework rather than a theoretical framework, for the purpose of this review, it has been included as a framework for behavior change. A total of 13 different theories, models, or frameworks were identified within these 18 studies, with social cognitive theory being the most frequently reported (6/18, 33%) [ 76 , 109 , 111 , 121 , 129 , 130 ], followed by the behavior change technique taxonomy (4/18, 22%) [ 48 , 49 , 122 , 129 ], game design theory (3/18, 17%) [ 47 , 57 , 125 ], operant conditioning (3/18, 17%) [ 47 , 98 , 121 ], and self-determination theory (3/18, 17%) [ 48 , 49 , 126 ]. Further information on behavior change theories, models, and frameworks, with details on associated citations, is available ( Multimedia Appendix 7 [ 47 - 49 , 57 , 70 , 76 , 98 , 102 , 109 , 111 , 113 , 121 , 122 , 125 , 126 , 129 , 130 , 145 ]).
Behavior Change Techniques
Despite 4 studies acknowledging the BCTTv1, explicit BCTTv1 codes were only reported in 2 studies (2/103, 2%) [ 48 , 122 ]. However, a third study (1/103, 1%) mapped the techniques used to change behavior directly to the transtheoretical model [ 145 ]. There was a median of 3 (range 1-14) individual BCTs coded per study, with a total of 383 BCTs across the 103 studies. The most frequently identified individual BCTs were feedback on behavior and nonspecific reward ( Multimedia Appendix 8 ).
There was also a median of 3 (range 1-8) BCTTv1 clusters per study, with a total of 288 clusters coded across the 103 studies. The most frequently used of the 16 possible clusters were feedback and monitoring (88/103, 85%) [ 46 - 60 , 62 - 69 , 71 - 74 , 76 , 78 - 80 , 82 - 92 , 94 - 106 , 108 - 113 , 116 , 117 , 119 - 129 , 134 - 146 , 148 ], reward and threat (56/103, 54%) [ 46 - 49 , 51 - 53 , 55 - 57 , 62 , 65 , 69 , 71 , 72 , 74 , 77 , 80 , 81 , 85 , 86 , 88 , 89 , 91 , 92 , 95 , 96 , 98 , 102 , 103 , 106 - 108 , 112 , 113 , 115 , 117 - 119 , 121 - 125 , 128 , 132 , 134 - 137 , 140 , 142 , 143 , 146 - 148 ], goals and planning (33/103, 32%) [ 49 , 58 , 60 , 65 - 68 , 70 , 72 , 74 , 76 , 79 , 80 , 82 , 83 , 90 , 91 , 93 , 94 , 97 , 100 , 109 , 111 , 112 , 121 , 122 , 126 , 129 , 130 , 134 , 138 , 141 , 145 ], and shaping knowledge (33/103, 32%) [ 46 , 48 , 50 , 53 - 56 , 58 , 60 , 61 , 64 - 72 , 74 , 75 , 86 , 94 , 97 , 101 - 103 , 108 , 111 , 113 , 114 , 120 , 129 - 131 , 139 - 141 ]. Other BCTTv1 clusters used were social support (24/103, 23%) [ 48 , 49 , 58 , 60 , 64 , 67 , 70 , 72 , 73 , 79 , 80 , 82 , 84 , 90 , 93 , 101 , 108 , 117 , 119 , 129 - 131 , 134 , 141 ], comparison of behavior (23/103, 22%) [ 46 , 50 , 53 , 54 , 60 , 61 , 64 - 66 , 74 , 75 , 81 , 86 , 101 , 104 , 111 , 114 , 118 , 122 , 123 , 125 , 131 , 139 ], associations (16/103, 15%) [ 58 , 60 , 65 , 66 , 68 , 75 , 80 , 83 , 87 , 90 , 110 , 120 , 131 , 133 , 139 , 144 ], repetition and substitution (6/103, 6%) [ 60 , 82 , 109 , 122 , 129 , 130 ], scheduled consequences (3/103, 3%) [ 47 , 80 , 88 ], natural consequences (2/103, 2%) [ 129 , 138 ], comparison of outcomes (2/103, 2%) [ 47 , 133 ], antecedents (1/103, 1%) [ 60 ], and self-belief (1/103, 1%) [ 70 ]. The clusters of regulation, identity, and covert learning were not identified. Within the context of the review, it was noted that the reward and threat cluster only included reward-based BCTs. A tabulated summary and graphical representation of the BCTTv1 clusters is available ( Multimedia Appendix 9 [ 46 - 148 ]).
The exploration of clusters that were reported together in an intervention ( Figure 3 ) identified the strongest relationship between the clusters of feedback and monitoring and reward and threat. Clear links were also identified between feedback and monitoring and 4 other clusters: goals and planning, shaping knowledge, social support, and comparison of behavior, and between the shaping knowledge and comparison of behavior clusters.

Behavior Change Techniques and Digital Health Technology
The feedback and monitoring cluster was reported most frequently for all types of DHT ( Figure 4 ), with the greatest proportion of this cluster in robotics (11/25, 44%) [ 59 , 62 , 87 , 92 , 110 , 113 , 117 , 127 , 128 , 142 , 148 ], VR (52/148, 35%) [ 46 - 49 , 51 - 53 , 57 , 59 , 62 , 63 , 65 , 66 , 69 , 71 , 72 , 74 , 78 , 80 , 84 - 89 , 92 , 95 , 96 , 98 , 102 - 104 , 106 , 112 , 113 , 117 , 119 , 120 , 123 - 135 - 137 , 140 , 142 , 143 , 146 , 148 ], and sensors (17/48, 35%) [ 50 , 55 , 56 , 87 , 94 , 99 , 101 , 105 , 108 , 110 , 111 , 116 , 119 - 121 , 134 , 141 ]. Robotics and VR also often used the reward and threat cluster (9/25, 36% [ 62 , 92 , 107 , 113 , 117 , 118 , 128 , 142 , 148 ] and 48/148, 32% [ 46 - 49 , 51 - 53 , 57 , 62 , 65 , 69 , 71 , 72 , 74 , 77 , 80 , 81 , 85 , 86 , 88 , 89 , 92 , 95 , 96 , 98 , 102 , 103 , 106 , 112 , 113 , 115 , 117 - 119 , 123 - 125 , 128 , 132 , 135 - 137 , 140 , 142 , 143 , 146 - 148 ], respectively), while the goals and planning cluster was a dominant second cluster in activity monitors (13/53, 25%) [ 67 , 68 , 76 , 79 , 80 , 82 , 91 , 100 , 109 , 122 , 129 , 138 , 145 ].

Summary of Evidence
This scoping review provides a comprehensive overview of approaches used to support changes in behavior in DHT-based physical stroke rehabilitation interventions. Research in this field is in its infancy, with the predominance of studies in this review being described as pilot or feasibility studies with limited participants.
Despite using comprehensive behavior change search terms, only a limited number (103/1973, 6%) of screened sources were included. Over two-thirds of full-text sources were excluded as they did not describe or refer to any behavior change theories, models, or frameworks or BCTs, suggesting that in general, explicit behavior change approaches are not reported as being integral to DHT-based physical stroke rehabilitation.
Only 18 (17%) of the 103 included studies articulated a theory, model, or framework to underpin the intervention, which aimed to change behavior, despite widely published recommendations about the importance of overt use of theory when developing, evaluating, and reporting interventions [ 27 , 29 ], including those related to behavior change [ 28 ]. The proportion of studies articulating a behavior change theory, model, or framework in this work is significantly lower than review findings in non-rehabilitation DHT-based interventions that have sought to influence specific behaviors such as physical activity or weight control [ 24 , 44 ]. These reviews have identified up to two-thirds of sources reporting a theory, model, or framework. However, our findings mirror the relative absence of behavior change theories, models, and frameworks in rehabilitation interventions more generally, irrespective of whether they use digital technology [ 149 ] or not [ 45 ], and it is widely recognized that the complex nature of rehabilitation often results in the essential characteristics of interventions being poorly defined [ 150 ]. Consistent with our findings in these other reviews, a variety of theories, models, and frameworks were found to underpin interventions, with social cognitive theory being the most frequently reported [ 24 , 44 , 45 , 149 ]. The explicit description of BCTs using the BCTTv1 within DHT-based physical stroke rehabilitation interventions is also poorly reported (2%), despite a significant proportion of the sources being dated after the publication of the BCTTv1 in 2013 [ 22 ]. This lack of acknowledgment of behavior change approaches impedes the accumulation of knowledge within this field.
It is important that both the underpinning theory and BCTs are reported so the mechanisms by which the BCTs elicit change can be better understood [ 21 ]. The general assumption that the motivational and captivating aspects of DHTs will promote prolonged and repeated engagement with rehabilitative activities, in particular in those DHTs that incorporate game design [ 151 ], risks suboptimal outcomes for patients and wasted investment of time and money if the mechanisms by which the DHT elicits change are not considered.
When exploring which BCT clusters featured within the reviewed DHT-based interventions, the findings relating to the commonly used clusters of feedback and monitoring, goals and planning, and shaping knowledge are consistent with findings from DHT-based interventions to change a specific behavior [ 44 ] and non-DHT–based rehabilitation [ 45 ]. However, a novel finding in our review was the frequent identification of the reward and threat cluster, although it was noted that all techniques related to reward and none to threat. A large number of studies in this review used VR technology, which frequently incorporates gamified tasks or gameplay. Reward is an integral part of game design theory alongside feedback [ 152 ], and so it is perhaps unsurprising that the feedback and monitoring, and reward and threat clusters dominated and an association between these 2 clusters was seen.
Limitations
Rehabilitation is a process that comprises multiple behaviors and so exploring approaches to change behavior within this context was complicated. There were challenges in searching and screening sources for inclusion as few studies explicitly reported approaches to change behavior, and there is a similarity in the vocabulary used within behavior change and other theoretical approaches (eg, “feedback,” which is used within motor learning). Similarly, only a very small proportion of studies explicitly reported BCTs within interventions. The lack of clear reporting of behavior change introduces the risk that sources may be omitted during both the searching and screening process highlighting the difficulty of comprehensively reviewing this field of work. An inclusive approach to screening reduced the risk of erroneously excluding sources, but it is perhaps inevitable that the sources included reflect those studies that have reported a behavior change approach rather than all studies that have used one.
This lack of clear BCT reporting also posed challenges for intervention coding. The use of the BCTTv1 aimed to ensure the review used a generalizable nomenclature to describe BCTs, and the 1 reviewer who had completed BCTTv1 training coded all the interventions. It is acknowledged that decisions made in the application of the BCTTv1 within the context of the review will have introduced some subjectivity in intervention coding, which will ultimately influence the review findings. Although the coding process could have been made more robust by having a second reviewer trained in the BCTTv1 also code the interventions, regular and extensive discussions between all members of the review team took place with the aim of ensuring consistency with the coding process. Clear documentation as to how the BCTTv1 was used within this review ( Multimedia Appendix 5 ) supports transparency as to the decisions made and the reproducibility of the review.
The absence of a recognized predefined taxonomy for DHTs posed a challenge when categorizing the DHT interventions, acknowledging that the distinction between the categories used to present the results is open to interpretation. A description of how the reviewers interpreted these categories is provided ( Multimedia Appendix 4 ).
Implications for Research
Future studies aimed at developing and evaluating DHT-based rehabilitation interventions, including those relating to physical stroke rehabilitation, need to ensure there is explicit recognition and reporting of the specific approaches used to change behavior, articulating both the theory on which the intervention is based and how the intervention plans to deliver the change in behavior using universally recognized terminology. This should be reported as part of a program theory and potential mechanisms of action, which are key parts of developing and evaluating complex interventions [ 29 ]. This detailed reporting would further support an understanding of how changes in behavior could be best enabled by DHT-based rehabilitation interventions and how this contributes to changes in patient outcomes. It would also enable further evaluation of the optimal behavioral components of interventions, enabling patients to use and clinicians to deliver the most effective DHT-based rehabilitative interventions. More generally, as the use of DHTs expands, there is an urgent need for some form of taxonomy to categorize and clearly define the different types of DHTs to facilitate consistent reporting, replication, and comparison of DHT-based interventions.
This novel and original review is the first to explore if and how approaches to change behavior are incorporated within DHT-based physical stroke rehabilitation. It demonstrates that a minority of studies report using approaches to change behavior within this context, despite these changes in behavior being vital to meet the demands of rehabilitative activities. Those who do report behavior change often lack the underpinning detail as to how the DHT-based intervention will facilitate these changes. In order for DHT-based interventions to realize their potential within rehabilitation and their impact on patient outcomes, approaches to change behavior must be embedded in the intervention and appropriately reported.
Acknowledgments
The authors would like to thank Catherine Harris (Information Specialist, University of Central Lancashire) for her assistance in developing the search strategy and running the searches, and Rebekah Murray (Undergraduate Research Intern, University of Central Lancashire) for her support with aspects of the screening and data charting process.
This work was funded by a UK Research and Innovation Future Leaders Fellowship (grant MR/T022434/1).
Data Availability
All data supporting this study are openly available from the University of Central Lancashire repository [ 153 ].
Authors' Contributions
RCS conceived the review focus and oversaw the work. HJG developed the review design and search strategy. HJG, KAJ, and RCS completed the screening of the identified sources. HJG and KAJ piloted the data charting tool. HJG completed the data charting, data analysis, and the initial manuscript draft. KAJ and RCS reviewed and made substantial contributions to the manuscript. All authors approved the final manuscript.
Conflicts of Interest
None declared.
Preferred Reporting of Systematic Reviews and Meta-Analyses Extension for Scoping Reviews (PRISMA-ScR) checklist.
Inclusion and exclusion criteria.
Full search strategy as used in Medline.
Data charting tool.
Review-specific behavior change technique taxonomy coding decisions.
Intervention characteristics (with associated references).
Behavior change theories, models, and frameworks reported (with associated references).
Individual behavior change techniques coded.
Behavior change technique taxonomy clusters identified (with associated references).
- Evidence standards framework for digital health technologies. National Institute of Clinical Excellence (NICE). 2018. URL: https://tinyurl.com/mpmrwrwx [accessed 2022-01-25]
- Castle-Clark S. The NHS at 70: What will new technology mean for the NHS and its patients? Kings Fund. 2018. URL: https://tinyurl.com/44k9fmat [accessed 2024-04-05]
- Topel E. The Topol review: preparing the healthcare workforce to deliver the digital future. Health Education England, NHS. 2019. URL: https://topol.hee.nhs.uk/ [accessed 2022-01-19]
- GBD 2019 Stroke Collaborators. Global, regional, and national burden of stroke and its risk factors, 1990-2019: a systematic analysis for the Global Burden of Disease Study 2019. Lancet Neurol. 2021;20(10):795-820. [ FREE Full text ] [ CrossRef ] [ Medline ]
- Wade DT. What is rehabilitation? An empirical investigation leading to an evidence-based description. Clin Rehabil. 2020;34(5):571-583. [ FREE Full text ] [ CrossRef ] [ Medline ]
- Rehabilitation. World Health Organisation. 2023. URL: https://www.who.int/news-room/fact-sheets/detail/rehabilitation [accessed 2023-05-04]
- Grefkes C, Fink GR. Recovery from stroke: current concepts and future perspectives. Neurol Res Pract. 2020;2:17. [ FREE Full text ] [ CrossRef ] [ Medline ]
- Lawrence ES, Coshall C, Dundas R, Stewart J, Rudd AG, Howard R, et al. Estimates of the prevalence of acute stroke impairments and disability in a multiethnic population. Stroke. 2001;32(6):1279-1284. [ FREE Full text ] [ CrossRef ] [ Medline ]
- National clinical guideline for stroke for the United Kingdom and Ireland. Intercollegiate Stroke Working Party. 2023. URL: https://tinyurl.com/yxy6b9tp [accessed 2023-05-02]
- Veerbeek JM, van Wegen E, van Peppen R, van der Wees PJ, Hendriks E, Rietberg M, et al. What is the evidence for physical therapy poststroke? a systematic review and meta-analysis. PLoS One. 2014;9(2):e87987. [ FREE Full text ] [ CrossRef ] [ Medline ]
- Lang CE, Macdonald JR, Reisman DS, Boyd L, Kimberley TJ, Schindler-Ivens SM, et al. Observation of amounts of movement practice provided during stroke rehabilitation. Arch Phys Med Rehabil. 2009;90(10):1692-1698. [ FREE Full text ] [ CrossRef ] [ Medline ]
- Laver KE, Lange B, George S, Deutsch JE, Saposnik G, Crotty M. Virtual reality for stroke rehabilitation. Cochrane Database Syst Rev. 2017;11(11):CD008349. [ FREE Full text ] [ CrossRef ] [ Medline ]
- Laver KE, Adey-Wakeling Z, Crotty M, Lannin NA, George S, Sherrington C. Telerehabilitation services for stroke. Cochrane Database Syst Rev. 2020;1(1):CD010255. [ FREE Full text ] [ CrossRef ] [ Medline ]
- Lynch EA, Jones TM, Simpson DB, Fini NA, Kuys SS, Borschmann K, et al. Activity monitors for increasing physical activity in adult stroke survivors. Cochrane Database Syst Rev. 2018;7(7):CD012543. [ FREE Full text ] [ CrossRef ] [ Medline ]
- Mehrholz J, Pohl M, Platz T, Kugler J, Elsner B. Electromechanical and robot-assisted arm training for improving activities of daily living, arm function, and arm muscle strength after stroke. Cochrane Database Syst Rev. 2018;9(9):CD006876. [ FREE Full text ] [ CrossRef ] [ Medline ]
- Mehrholz J, Thomas S, Kugler J, Pohl M, Elsner B. Electromechanical-assisted training for walking after stroke. Cochrane Database Syst Rev. 2020;10(10):CD006185. [ FREE Full text ] [ CrossRef ] [ Medline ]
- Jones F, Riazi A. Self-efficacy and self-management after stroke: a systematic review. Disabil Rehabil. 2011;33(10):797-810. [ CrossRef ] [ Medline ]
- Behaviour change: general approaches. National Institute of Clinical Excellence. UK. National Institute of Clinical Excellence (NICE); 2007. URL: https://tinyurl.com/yfthycch [accessed 2024-04-05]
- Bandura A. Social Foundations of Thought and Action: A Social Cognitive Theory. Englewood Cliffs, NJ. Prentice-Hall; 1986.
- Michie S, van Stralen MM, West R. The behaviour change wheel: a new method for characterising and designing behaviour change interventions. Implement Sci. 2011;6:42. [ FREE Full text ] [ CrossRef ] [ Medline ]
- Carey RN, Connell LE, Johnston M, Rothman AJ, de Bruin M, Kelly MP, et al. Behavior change techniques and their mechanisms of action: a synthesis of links described in published intervention literature. Ann Behav Med. 2019;53(8):693-707. [ FREE Full text ] [ CrossRef ] [ Medline ]
- Michie S, Richardson M, Johnston M, Abraham C, Francis J, Hardeman W, et al. The behavior change technique taxonomy (v1) of 93 hierarchically clustered techniques: building an international consensus for the reporting of behavior change interventions. Ann Behav Med. 2013;46(1):81-95. [ FREE Full text ] [ CrossRef ] [ Medline ]
- Oinas-Kukkonen H. A foundation for the study of behavior change support systems. Pers Ubiquit Comput. 2012;17(6):1223-1235. [ CrossRef ]
- Taj F, Klein MCA, van Halteren A. Digital health behavior change technology: bibliometric and scoping review of two decades of research. JMIR Mhealth Uhealth. 2019;7(12):e13311. [ FREE Full text ] [ CrossRef ] [ Medline ]
- West R, Michie S. A Guide to Development and Evaluation of Digital Behaviour Change Interventions in Healthcare. London. Silverback Publishing; 2016.
- Lewis GN, Rosie JA. Virtual reality games for movement rehabilitation in neurological conditions: how do we meet the needs and expectations of the users? Disabil Rehabil. 2012;34(22):1880-1886. [ CrossRef ] [ Medline ]
- Hoffmann TC, Glasziou PP, Boutron I, Milne R, Perera R, Moher D, et al. Better reporting of interventions: template for intervention description and replication (TIDieR) checklist and guide. BMJ. 2014;348:g1687. [ FREE Full text ] [ CrossRef ] [ Medline ]
- Michie S, Prestwich A. Are interventions theory-based? development of a theory coding scheme. Health Psychol. 2010;29(1):1-8. [ FREE Full text ] [ CrossRef ] [ Medline ]
- Skivington K, Matthews L, Simpson SA, Craig P, Baird J, Blazeby JM, et al. A new framework for developing and evaluating complex interventions: update of Medical Research Council guidance. BMJ. 2021;374:n2061. [ FREE Full text ] [ CrossRef ] [ Medline ]
- Peters MDJ, Godfrey CM, Khalil H, McInerney P, Parker D, Soares CB. Guidance for conducting systematic scoping reviews. Int J Evid Based Healthc. 2015;13(3):141-146. [ FREE Full text ] [ CrossRef ] [ Medline ]
- Peters MDJ, Godfrey CM, McInerney P, Munn Z, Tricco AC, Khalil H. Scoping Reviews (2020 version). In: Aromataris E, Munn Z, editors. JBI Manual for Evidence Synthesis. Adelaide, Australia. JBI; 2020. [ CrossRef ]
- Tricco AC, Lillie E, Zarin W, O'Brien KK, Colquhoun H, Levac D, et al. PRISMA Extension for Scoping Reviews (PRISMA-ScR): checklist and explanation. Ann Intern Med. 2018;169(7):467-473. [ FREE Full text ] [ CrossRef ] [ Medline ]
- Gooch H, Stockley R, Jarvis K. Behaviour change approaches within digital health technology-based interventions in physical rehabilitation following stroke: a scoping review protocol Version 2 081122. OSF Registries. 2022. URL: https://osf.io/yjn5g [accessed 2022-11-15]
- ICF checklist. World Health Organisation. 2003. URL: https://tinyurl.com/5fz6krew [accessed 2022-01-19]
- Hillyer M. How has technology changed—and changed us—in the past 20 years? World Economic Forum. 2020. URL: https://tinyurl.com/5n74wsym [accessed 2023-10-18]
- Online training. BCT Taxonomy v1. 2022. URL: http://www.bct-taxonomy.com/ [accessed 2022-03-08]
- Ouzzani M, Hammady H, Fedorowicz Z, Elmagarmid A. Rayyan-a web and mobile app for systematic reviews. Syst Rev. 2016;5(1):210. [ FREE Full text ] [ CrossRef ] [ Medline ]
- Wadley M, Bradbury M, Stockley R. Virtual reality. The Chartered Society of Physiotherapy. URL: https://www.csp.org.uk/professional-clinical/digital-physiotherapy/virtual-reality [accessed 2024-03-29]
- Cambridge dictionary. Cambridge University Press and Assessment. URL: https://tinyurl.com/2f2mx6kz [accessed 2024-03-29]
- Weber LM, Stein J. The use of robots in stroke rehabilitation: A narrative review. NeuroRehabilitation. 2018;43(1):99-110. [ CrossRef ] [ Medline ]
- English C, Ceravolo MG, Dorsch S, Drummond A, Gandhi DB, Green JH, et al. Telehealth for rehabilitation and recovery after stroke: state of the evidence and future directions. Int J Stroke. 2022;17(5):487-493. [ CrossRef ] [ Medline ]
- Taub E, Crago JE, Burgio LD, Groomes TE, Cook EW, DeLuca SC, et al. An operant approach to rehabilitation medicine: overcoming learned nonuse by shaping. J Exp Anal Behav. 1994;61(2):281-293. [ FREE Full text ] [ CrossRef ] [ Medline ]
- Bernhardt J, Hayward KS, Kwakkel G, Ward NS, Wolf SL, Borschmann K, et al. Agreed definitions and a shared vision for new standards in stroke recovery research: the Stroke Recovery and Rehabilitation Roundtable taskforce. Int J Stroke. 2017;12(5):444-450. [ FREE Full text ] [ CrossRef ] [ Medline ]
- Asbjørnsen RA, Smedsrød ML, Solberg Nes L, Wentzel J, Varsi C, Hjelmesæth J, et al. Persuasive system design principles and behavior change techniques to stimulate motivation and adherence in electronic health interventions to support weight loss maintenance: scoping review. J Med Internet Res. 2019;21(6):e14265. [ FREE Full text ] [ CrossRef ] [ Medline ]
- Bayly J, Wakefield D, Hepgul N, Wilcock A, Higginson IJ, Maddocks M. Changing health behaviour with rehabilitation in thoracic cancer: a systematic review and synthesis. Psychooncology. 2018;27(7):1675-1694. [ CrossRef ] [ Medline ]
- Alankus G, Proffitt R, Kelleher C, Engsberg J. Stroke therapy through motion-based games: a case study. ACM Trans Access Comput. 2011;4(1):1-35. [ CrossRef ]
- Alankus G, Kelleher C. Reducing compensatory motions in motion-based video games for stroke rehabilitation. Hum Comput Interact. 2015;30(3-4):232-262. [ CrossRef ]
- Allegue DR, Kairy D, Higgins J, Archambault PS, Michaud F, Miller W, et al. A personalized home-based rehabilitation program using exergames combined with a telerehabilitation app in a chronic stroke survivor: mixed methods case study. JMIR Serious Games. 2021;9(3):e26153. [ FREE Full text ] [ CrossRef ] [ Medline ]
- Allegue DR, Higgins J, Sweet SN, Archambault PS, Michaud F, Miller W, et al. Rehabilitation of upper extremity by telerehabilitation combined with exergames in survivors of chronic stroke: preliminary findings from a feasibility clinical trial. JMIR Rehabil Assist Technol. 2022;9(2):e33745. [ FREE Full text ] [ CrossRef ] [ Medline ]
- Asano M, Tai BC, Yeo FY, Yen SC, Tay A, Ng YS, et al. Home-based tele-rehabilitation presents comparable positive impact on self-reported functional outcomes as usual care: the Singapore Tele-technology Aided Rehabilitation in Stroke (STARS) randomised controlled trial. J Telemed Telecare. 2021;27(4):231-238. [ CrossRef ] [ Medline ]
- Ballester BR, Maier M, Mozo RMSS, Castañeda V, Duff A, Verschure PFMJ. Counteracting learned non-use in chronic stroke patients with reinforcement-induced movement therapy. J Neuroeng Rehabil. 2016;13(1):74. [ FREE Full text ] [ CrossRef ] [ Medline ]
- Bardack A, Bhandari P, Doggett J, Epstein M, Gagliolo N, Graff S, et al. EMG biofeedback videogame system for the gait rehabilitation of hemiparetic individuals. Digital Repository at the University of Maryland. 2010. URL: https://drum.lib.umd.edu/bitstream/handle/1903/10082/CHIP.pdf?sequence=1&isAllowed=y [accessed 2023-02-27]
- Bellomo RG, Paolucci T, Saggino A, Pezzi L, Bramanti A, Cimino V, et al. The WeReha Project for an innovative home-based exercise training in chronic stroke patients: a clinical study. J Cent Nerv Syst Dis. 2020;12:1179573520979866. [ FREE Full text ] [ CrossRef ] [ Medline ]
- Benvenuti F, Stuart M, Cappena V, Gabella S, Corsi S, Taviani A, et al. Community-based exercise for upper limb paresis: a controlled trial with telerehabilitation. Neurorehabil Neural Repair. 2014;28(7):611-620. [ FREE Full text ] [ CrossRef ] [ Medline ]
- Bhattacharjya S, Stafford MC, Cavuoto LA, Yang Z, Song C, Subryan H, et al. Harnessing smartphone technology and three dimensional printing to create a mobile rehabilitation system, mRehab: assessment of usability and consistency in measurement. J Neuroeng Rehabil. 2019;16(1):127. [ FREE Full text ] [ CrossRef ] [ Medline ]
- Bhattacharjya S, Cavuoto LA, Reilly B, Xu W, Subryan H, Langan J. Usability, usefulness, and acceptance of a novel, portable rehabilitation system (mRehab) using smartphone and 3D printing technology: mixed methods study. JMIR Hum Factors. 2021;8(1):e21312. [ FREE Full text ] [ CrossRef ] [ Medline ]
- Burke JW, McNeill MDJ, Charles DK, Morrow PJ, Crosbie JH, McDonough SM. Optimising engagement for stroke rehabilitation using serious games. Vis Comput. 2009;25(12):1085-1099. [ CrossRef ]
- Burridge JH, Lee ACW, Turk R, Stokes M, Whitall J, Vaidyanathan R, et al. Telehealth, wearable sensors, and the internet: will they improve stroke outcomes through increased intensity of therapy, motivation, and adherence to rehabilitation programs? J Neurol Phys Ther. 2017;41(Suppl 3):S32-S38. [ CrossRef ] [ Medline ]
- Cai S, Wei X, Su E, Wu W, Zheng H, Xie L. Online compensation detecting for real-time reduction of compensatory motions during reaching: a pilot study with stroke survivors. J Neuroeng Rehabil. 2020;17(1):58. [ FREE Full text ] [ CrossRef ] [ Medline ]
- Chumbler NR, Quigley P, Li X, Morey M, Rose D, Sanford J, et al. Effects of telerehabilitation on physical function and disability for stroke patients: a randomized, controlled trial. Stroke. 2012;43(8):2168-2174. [ FREE Full text ] [ CrossRef ] [ Medline ]
- Chung BPH, Chiang WKH, Lau H, Lau TFO, Lai CWK, Sit CSY, et al. Pilot study on comparisons between the effectiveness of mobile video-guided and paper-based home exercise programs on improving exercise adherence, self-efficacy for exercise and functional outcomes of patients with stroke with 3-month follow-up: a single-blind randomized controlled trial. Hong Kong Physiother J. 2020;40(1):63-73. [ FREE Full text ] [ CrossRef ] [ Medline ]
- Colombo R, Pisano F, Mazzone A, Delconte C, Micera S, Carrozza MC, et al. Design strategies to improve patient motivation during robot-aided rehabilitation. J Neuroeng Rehabil. 2007;4:3. [ FREE Full text ] [ CrossRef ] [ Medline ]
- Colomer C, Llorens R, Noé E, Alcañiz M. Effect of a mixed reality-based intervention on arm, hand, and finger function on chronic stroke. J Neuroeng Rehabil. 2016;13(1):45. [ FREE Full text ] [ CrossRef ] [ Medline ]
- Conroy SS, Harcum S, Keldsen L, Bever CT. Novel use of existing technology: a preliminary study of patient portal use for telerehabilitation. J Telemed Telecare. 2022;28(5):380-388. [ CrossRef ] [ Medline ]
- Cramer SC, Dodakian L, Le V, See J, Augsburger R, McKenzie A, et al. Efficacy of home-based telerehabilitation vs in-clinic therapy for adults after stroke: a randomized clinical trial. JAMA Neurol. 2019;76(9):1079-1087. [ FREE Full text ] [ CrossRef ] [ Medline ]
- Cramer SC, Dodakian L, Le V, McKenzie A, See J, Augsburger R, et al. A feasibility study of expanded home-based telerehabilitation after stroke. Front Neurol. 2020;11:611453. [ FREE Full text ] [ CrossRef ] [ Medline ]
- Danks KA, Roos MA, McCoy D, Reisman DS. A step activity monitoring program improves real world walking activity post stroke. Disabil Rehabil. 2014;36(26):2233-2236. [ FREE Full text ] [ CrossRef ] [ Medline ]
- Da-Silva RH, Moore SA, Rodgers H, Shaw L, Sutcliffe L, van Wijck F, et al. Wristband accelerometers to motivate arm exercises after Stroke (WAVES): a pilot randomized controlled trial. Clin Rehabil. 2019;33(8):1391-1403. [ CrossRef ] [ Medline ]
- Deng H, Durfee WK, Nuckley DJ, Rheude BS, Severson AE, Skluzacek KM, et al. Complex versus simple ankle movement training in stroke using telerehabilitation: a randomized controlled trial. Phys Ther. 2012;92(2):197-209. [ FREE Full text ] [ CrossRef ] [ Medline ]
- Deutsch JE, Maidan I, Dickstein R. Patient-centered integrated motor imagery delivered in the home with telerehabilitation to improve walking after stroke. Phys Ther. 2012;92(8):1065-1077. [ FREE Full text ] [ CrossRef ] [ Medline ]
- Dias P, Silva R, Amorim P, Lains J, Roque E, Pereira ISF, et al. Using virtual reality to increase motivation in poststroke rehabilitation. IEEE Comput Graph Appl. 2019;39(1):64-70. [ CrossRef ] [ Medline ]
- Dodakian L, McKenzie AL, Le V, See J, Pearson-Fuhrhop K, Quinlan EB, et al. A home-based telerehabilitation program for patients with stroke. Neurorehabil Neural Repair. 2017;31(10-11):923-933. [ FREE Full text ] [ CrossRef ] [ Medline ]
- Dorsch AK, Thomas S, Xu X, Kaiser W, Dobkin BH, SIRRACT investigators. SIRRACT: an international randomized clinical trial of activity feedback during inpatient stroke rehabilitation enabled by wireless sensing. Neurorehabil Neural Repair. 2015;29(5):407-415. [ FREE Full text ] [ CrossRef ] [ Medline ]
- Edwards D, Kumar S, Brinkman L, Ferreira IC, Esquenazi A, Nguyen T, et al. Telerehabilitation initiated early in post-stroke recovery: a feasibility study. Neurorehabil Neural Repair. 2023;37(2-3):131-141. [ FREE Full text ] [ CrossRef ] [ Medline ]
- Emmerson KB, Harding KE, Taylor NF. Home exercise programmes supported by video and automated reminders compared with standard paper-based home exercise programmes in patients with stroke: a randomized controlled trial. Clin Rehabil. 2017;31(8):1068-1077. [ CrossRef ] [ Medline ]
- Ezeugwu VE, Manns PJ. The feasibility and longitudinal effects of a home-based sedentary behavior change intervention after stroke. Arch Phys Med Rehabil. 2018;99(12):2540-2547. [ CrossRef ] [ Medline ]
- Fluet GG, Qiu Q, Patel J, Cronce A, Merians AS, Adamovich SV. Autonomous use of the home virtual rehabilitation system: a feasibility and pilot study. Games Health J. 2019;8(6):432-438. [ FREE Full text ] [ CrossRef ] [ Medline ]
- Foreman MH, Engsberg JR. A virtual reality tool for measuring and shaping trunk compensation for persons with stroke: design and initial feasibility testing. J Rehabil Assist Technol Eng. 2019;6:2055668318823673. [ FREE Full text ] [ CrossRef ] [ Medline ]
- Fusari G, Gibbs E, Hoskin L, Lawrence-Jones A, Dickens D, Crespo RF, et al. What is the feasibility and patient acceptability of a digital system for arm and hand rehabilitation after stroke? a mixed-methods, single-arm feasibility study of the 'OnTrack' intervention for hospital and home use. BMJ Open. 2022;12(9):e062042. [ FREE Full text ] [ CrossRef ] [ Medline ]
- Gauthier LV, Nichols-Larsen DS, Uswatte G, Strahl N, Simeo M, Proffitt R, et al. Video game rehabilitation for outpatient stroke (VIGoROUS): a multi-site randomized controlled trial of in-home, self-managed, upper-extremity therapy. EClinicalMedicine. 2022;43:101239. [ FREE Full text ] [ CrossRef ] [ Medline ]
- Goršič M, Cikajlo I, Goljar N, Novak D. A multisession evaluation of an adaptive competitive arm rehabilitation game. J Neuroeng Rehabil. 2017;14(1):128. [ FREE Full text ] [ CrossRef ] [ Medline ]
- Grau-Pellicer M, Lalanza JF, Jovell-Fernández E, Capdevila L. Impact of mHealth technology on adherence to healthy PA after stroke: a randomized study. Top Stroke Rehabil. 2020;27(5):354-368. [ CrossRef ] [ Medline ]
- Guidetti S, Gustavsson M, Tham K, Andersson M, Fors U, Ytterberg C. F@ce: a team-based, person-centred intervention for rehabilitation after stroke supported by information and communication technology—a feasibility study. BMC Neurol. 2020;20(1):387. [ FREE Full text ] [ CrossRef ] [ Medline ]
- Held JP, Ferrer B, Mainetti R, Steblin A, Hertler B, Moreno-Conde A, et al. Autonomous rehabilitation at stroke patients home for balance and gait: safety, usability and compliance of a virtual reality system. Eur J Phys Rehabil Med. 2018;54(4):545-553. [ FREE Full text ] [ CrossRef ] [ Medline ]
- Hernandez A, Bubyr L, Archambault PS, Higgins J, Levin MF, Kairy D. Virtual reality-based rehabilitation as a feasible and engaging tool for the management of chronic poststroke upper-extremity function recovery: randomized controlled trial. JMIR Serious Games. 2022;10(3):e37506. [ FREE Full text ] [ CrossRef ] [ Medline ]
- Hung NT, Paul V, Prakash P, Kovach T, Tacy G, Tomic G, et al. Wearable myoelectric interface enables high-dose, home-based training in severely impaired chronic stroke survivors. Ann Clin Transl Neurol. 2021;8(9):1895-1905. [ FREE Full text ] [ CrossRef ] [ Medline ]
- Johnson MJ, Shakya Y, Strachota E, Ahamed SI. Low-cost monitoring of patients during unsupervised robot/computer assisted motivating stroke rehabilitation. Biomed Tech (Berl). 2011;56(1):5-9. [ CrossRef ] [ Medline ]
- Johnson MJ, Van der Loos HFM, Burgar CG, Shor P, Leifer LJ. Experimental results using force-feedback cueing in robot-assisted stroke therapy. IEEE Trans Neural Syst Rehabil Eng. 2005;13(3):335-348. [ CrossRef ] [ Medline ]
- Jonsdottir J, Baglio F, Gindri P, Isernia S, Castiglioni C, Gramigna C, et al. Virtual reality for motor and cognitive rehabilitation from clinic to home: a pilot feasibility and efficacy study for persons with chronic stroke. Front Neurol. 2021;12:601131. [ FREE Full text ] [ CrossRef ] [ Medline ]
- Kamwesiga JT, Eriksson GM, Tham K, Fors U, Ndiwalana A, von Koch L, et al. A feasibility study of a mobile phone supported family-centred ADL intervention, F@ce, after stroke in Uganda. Global Health. 2018;14(1):82. [ FREE Full text ] [ CrossRef ] [ Medline ]
- Kanai M, Izawa KP, Kobayashi M, Onishi A, Kubo H, Nozoe M, et al. Effect of accelerometer-based feedback on physical activity in hospitalized patients with ischemic stroke: a randomized controlled trial. Clin Rehabil. 2018;32(8):1047-1056. [ CrossRef ] [ Medline ]
- Keeling AB, Piitz M, Semrau JA, Hill MD, Scott SH, Dukelow SP. Robot Enhanced Stroke Therapy Optimizes Rehabilitation (RESTORE): a pilot study. J Neuroeng Rehabil. 2021;18(1):10. [ FREE Full text ] [ CrossRef ] [ Medline ]
- Kessler D, Anderson ND, Dawson DR. Occupational performance coaching for stroke survivors delivered via telerehabilitation using a single-case experimental design. Br J Occup Ther. 2021;84(8):488-496. [ FREE Full text ] [ CrossRef ] [ Medline ]
- Kim DY, Kwon H, Nam KW, Lee Y, Kwon HM, Chung YS. Remote management of poststroke patients with a smartphone-based management system integrated in clinical care: prospective, nonrandomized, interventional study. J Med Internet Res. 2020;22(2):e15377. [ FREE Full text ] [ CrossRef ] [ Medline ]
- Kim J, Lee M, Kim Y, Eun SD, Yoon B. Feasibility of an individually tailored virtual reality program for improving upper motor functions and activities of daily living in chronic stroke survivors: a case series. Eur J Integr Med. 2016;8(5):731-737. [ CrossRef ]
- King M, Hijmans JM, Sampson M, Satherley J, Hale L. Home-based stroke rehabilitation using computer gaming. N Z J Physiother. 2012;40(3):128-134. [ FREE Full text ]
- Kringle EA, Setiawan IMA, Golias K, Parmanto B, Skidmore ER. Feasibility of an iterative rehabilitation intervention for stroke delivered remotely using mobile health technology. Disabil Rehabil Assist Technol. 2020;15(8):908-916. [ FREE Full text ] [ CrossRef ] [ Medline ]
- Kumar D, Sinha N, Dutta A, Lahiri U. Virtual reality-based balance training system augmented with operant conditioning paradigm. Biomed Eng Online. 2019;18(1):90. [ FREE Full text ] [ CrossRef ] [ Medline ]
- Langan J, Bhattacharjya S, Subryan H, Xu W, Chen B, Li Z, et al. In-home rehabilitation using a smartphone app coupled with 3D printed functional objects: single-subject design study. JMIR Mhealth Uhealth. 2020;8(7):e19582. [ FREE Full text ] [ CrossRef ] [ Medline ]
- Lawrie S, Dong Y, Steins D, Xia Z, Esser P, Sun S, et al. Evaluation of a smartwatch-based intervention providing feedback of daily activity within a research-naive stroke ward: a pilot randomised controlled trial. Pilot Feasibility Stud. 2018;4:157. [ FREE Full text ] [ CrossRef ] [ Medline ]
- Lawson S, Tang Z, Feng J. Supporting stroke motor recovery through a mobile application: a pilot study. Am J Occup Ther. 2017;71(3):7103350010p1-7103350010p5. [ CrossRef ] [ Medline ]
- Lee M, Pyun SB, Chung J, Kim J, Eun SD, Yoon BC. A further step to develop patient-friendly implementation strategies for virtual reality-based rehabilitation in patients with acute stroke. Phys Ther. 2016;96(10):1554-1564. [ FREE Full text ] [ CrossRef ] [ Medline ]
- Lee M, Son J, Kim J, Pyun SB, Eun SD, Yoon BC. Comparison of individualized virtual reality- and group-based rehabilitation in older adults with chronic stroke in community settings: a pilot randomized controlled trial. Eur J Integr Med. 2016;8(5):738-746. [ CrossRef ]
- Lee MM, Shin DC, Song CH. Canoe game-based virtual reality training to improve trunk postural stability, balance, and upper limb motor function in subacute stroke patients: a randomized controlled pilot study. J Phys Ther Sci. 2016;28(7):2019-2024. [ FREE Full text ] [ CrossRef ] [ Medline ]
- Levy T, Crotty M, Laver K, Lannin N, Killington M. Does the addition of concurrent visual feedback increase adherence to a home exercise program in people with stroke: a single-case series? BMC Res Notes. 2020;13(1):361. [ FREE Full text ] [ CrossRef ] [ Medline ]
- Lewis GN, Woods C, Rosie JA, McPherson KM. Virtual reality games for rehabilitation of people with stroke: perspectives from the users. Disabil Rehabil Assist Technol. 2011;6(5):453-463. [ CrossRef ] [ Medline ]
- Li X, Wang L, Miao S, Yue Z, Tang Z, Su L, et al. Sensorimotor rhythm-brain computer interface with audio-cue, motor observation and multisensory feedback for upper-limb stroke rehabilitation: a controlled study. Front Neurosci. 2022;16:808830. [ FREE Full text ] [ CrossRef ] [ Medline ]
- Lum PS, Taub E, Schwandt D, Postman M, Hardin P, Uswatte G. Automated Constraint-Induced Therapy Extension (AutoCITE) for movement deficits after stroke. J Rehabil Res Dev. 2004;41(3A):249-258. [ FREE Full text ] [ CrossRef ] [ Medline ]
- Mansfield A, Wong JS, Bryce J, Brunton K, Inness EL, Knorr S, et al. Use of accelerometer-based feedback of walking activity for appraising progress with walking-related goals in inpatient stroke rehabilitation: a randomized controlled trial. Neurorehabil Neural Repair. 2015;29(9):847-857. [ FREE Full text ] [ CrossRef ] [ Medline ]
- Matarić MJ, Eriksson J, Feil-Seifer DJ, Winstein CJ. Socially assistive robotics for post-stroke rehabilitation. J Neuroeng Rehabil. 2007;4:5. [ FREE Full text ] [ CrossRef ] [ Medline ]
- Mawson S, Nasr N, Parker J, Davies R, Zheng H, Mountain G. A personalized self-management rehabilitation system with an intelligent shoe for stroke survivors: a realist evaluation. JMIR Rehabil Assist Technol. 2016;3(1):e1. [ FREE Full text ] [ CrossRef ] [ Medline ]
- McNulty PA, Thompson-Butel AG, Faux SG, Lin G, Katrak PH, Harris LR, et al. The efficacy of Wii-based Movement Therapy for upper limb rehabilitation in the chronic poststroke period: a randomized controlled trial. Int J Stroke. 2015;10(8):1253-1260. [ CrossRef ] [ Medline ]
- Mihelj M, Novak D, Milavec M, Ziherl J, Olenšek A, Munih M. Virtual rehabilitation environment using principles of intrinsic motivation and game design. Presence: Teleop Virt Environ. 2012;21(1):1-15. [ CrossRef ]
- Mitchell C, Bowen A, Tyson S, Conroy P. A feasibility randomized controlled trial of ReaDySpeech for people with dysarthria after stroke. Clin Rehabil. 2018;32(8):1037-1046. [ FREE Full text ] [ CrossRef ] [ Medline ]
- Moan ME, Vonstad EK, Su X, Vereijken B, Solbjør M, Skjæret-Maroni N. Experiences of stroke survivors and clinicians with a fully immersive virtual reality treadmill exergame for stroke rehabilitation: a qualitative pilot study. Front Aging Neurosci. 2021;13:735251. [ FREE Full text ] [ CrossRef ] [ Medline ]
- Mountain G, Wilson S, Eccleston C, Mawson S, Hammerton J, Ware T, et al. Developing and testing a telerehabilitation system for people following stroke: issues of usability. J Eng Des. 2010;21(2-3):223-236. [ CrossRef ]
- Nijenhuis SM, Prange GB, Amirabdollahian F, Sale P, Infarinato F, Nasr N, et al. Feasibility study into self-administered training at home using an arm and hand device with motivational gaming environment in chronic stroke. J Neuroeng Rehabil. 2015;12:89. [ FREE Full text ] [ CrossRef ] [ Medline ]
- Novak D, Nagle A, Keller U, Riener R. Increasing motivation in robot-aided arm rehabilitation with competitive and cooperative gameplay. J Neuroeng Rehabil. 2014;11:64. [ FREE Full text ] [ CrossRef ] [ Medline ]
- Olafsdottir SA, Jonsdottir H, Bjartmarz I, Magnusson C, Caltenco H, Kytö M, et al. Feasibility of ActivABLES to promote home-based exercise and physical activity of community-dwelling stroke survivors with support from caregivers: a mixed methods study. BMC Health Serv Res. 2020;20(1):562. [ FREE Full text ] [ CrossRef ] [ Medline ]
- Olafsdottir SA, Jonsdottir H, Magnusson C, Caltenco H, Kytö M, Maye L, et al. Developing ActivABLES for community-dwelling stroke survivors using the Medical Research Council framework for complex interventions. BMC Health Serv Res. 2020;20(1):463. [ FREE Full text ] [ CrossRef ] [ Medline ]
- Parker J, Mawson S, Mountain G, Nasr N, Zheng H. Stroke patients' utilisation of extrinsic feedback from computer-based technology in the home: a multiple case study realistic evaluation. BMC Med Inform Decis Mak. 2014;14:46. [ FREE Full text ] [ CrossRef ] [ Medline ]
- Paul L, Wyke S, Brewster S, Sattar N, Gill JMR, Alexander G, et al. Increasing physical activity in stroke survivors using STARFISH, an interactive mobile phone application: a pilot study. Top Stroke Rehabil. 2016;23(3):170-177. [ CrossRef ] [ Medline ]
- Pereira F, Badia SBI, Jorge C, Cameirão MS. The use of game modes to promote engagement and social involvement in multi-user serious games: a within-person randomized trial with stroke survivors. J Neuroeng Rehabil. 2021;18(1):62. [ FREE Full text ] [ CrossRef ] [ Medline ]
- Perez-Marcos D, Chevalley O, Schmidlin T, Garipelli G, Serino A, Vuadens P, et al. Increasing upper limb training intensity in chronic stroke using embodied virtual reality: a pilot study. J Neuroeng Rehabil. 2017;14(1):119. [ FREE Full text ] [ CrossRef ] [ Medline ]
- Popović MD, Kostić MD, Rodić SZ, Konstantinović LM. Feedback-mediated upper extremities exercise: increasing patient motivation in poststroke rehabilitation. Biomed Res Int. 2014;2014:520374. [ FREE Full text ] [ CrossRef ] [ Medline ]
- Proffitt R, Lange B. Feasibility of a customized, in-home, game-based stroke exercise program using the Microsoft Kinect® sensor. Int J Telerehabil. 2015;7(2):23-34. [ FREE Full text ] [ CrossRef ] [ Medline ]
- Rosati G, Oscari F, Reinkensmeyer DJ, Secoli R, Avanzini F, Spagnol S, et al. Improving robotics for neurorehabilitation: enhancing engagement, performance, and learning with auditory feedback. IEEE Int Conf Rehabil Robot. 2011;2011:5975373. [ CrossRef ] [ Medline ]
- Rowe JB, Chan V, Ingemanson ML, Cramer SC, Wolbrecht ET, Reinkensmeyer DJ. Robotic assistance for training finger movement using a Hebbian model: a randomized controlled trial. Neurorehabil Neural Repair. 2017;31(8):769-780. [ FREE Full text ] [ CrossRef ] [ Medline ]
- Sakakibara BM, Lear SA, Barr SI, Goldsmith CH, Schneeberg A, Silverberg ND, et al. Telehealth coaching to improve self-management for secondary prevention after stroke: a randomized controlled trial of Stroke Coach. Int J Stroke. 2022;17(4):455-464. [ FREE Full text ] [ CrossRef ] [ Medline ]
- Saywell NL, Vandal AC, Mudge S, Hale L, Brown P, Feigin V, et al. Telerehabilitation after stroke using readily available technology: a randomized controlled trial. Neurorehabil Neural Repair. 2021;35(1):88-97. [ FREE Full text ] [ CrossRef ] [ Medline ]
- Scrivener K, Sewastenko J, Bouvier-Farrell A, MacDonald K, Van Rijn T, Tezak J, et al. Feasibility of a self-managed, video-guided exercise program for community-dwelling people with stroke. Stroke Res Treat. 2021;2021:5598100. [ FREE Full text ] [ CrossRef ] [ Medline ]
- Shah N, Amirabdollahian F, Basteris A. Designing motivational games for stroke rehabilitation. New Jersey. IEEE; 2014. Presented at: 7th International Conference on Human System Interactions (HSI); June 16-18, 2014;166-171; Costa da Caparica, Portugal. [ CrossRef ]
- Signal NEJ, McLaren R, Rashid U, Vandal A, King M, Almesfer F, et al. Haptic nudges increase affected upper limb movement during inpatient stroke rehabilitation: multiple-period randomized crossover study. JMIR Mhealth Uhealth. 2020;8(7):e17036. [ FREE Full text ] [ CrossRef ] [ Medline ]
- Simpson DB, Bird ML, English C, Gall SL, Breslin M, Smith S, et al. "Connecting patients and therapists remotely using technology is feasible and facilitates exercise adherence after stroke". Top Stroke Rehabil. 2020;27(2):93-102. [ CrossRef ] [ Medline ]
- Song X, van de Ven SS, Chen S, Kang P, Gao Q, Jia J, et al. Proposal of a wearable multimodal sensing-based serious games approach for hand movement training after stroke. Front Physiol. 2022;13:811950. [ FREE Full text ] [ CrossRef ] [ Medline ]
- Standen PJ, Threapleton K, Richardson A, Connell L, Brown DJ, Battersby S, et al. A low cost virtual reality system for home based rehabilitation of the arm following stroke: a randomised controlled feasibility trial. Clin Rehabil. 2017;31(3):340-350. [ FREE Full text ] [ CrossRef ] [ Medline ]
- Subramanian SK, Lourenço CB, Chilingaryan G, Sveistrup H, Levin MF. Arm motor recovery using a virtual reality intervention in chronic stroke: randomized control trial. Neurorehabil Neural Repair. 2013;27(1):13-23. [ FREE Full text ] [ CrossRef ] [ Medline ]
- Sullivan JE, Espe LE, Kelly AM, Veilbig LE, Kwasny MJ. Feasibility and outcomes of a community-based, pedometer-monitored walking program in chronic stroke: a pilot study. Top Stroke Rehabil. 2014;21(2):101-110. [ CrossRef ] [ Medline ]
- Toh SFM, Gonzalez PC, Fong KNK. Usability of a wearable device for home-based upper limb telerehabilitation in persons with stroke: a mixed-methods study. Digit Health. 2023;9:20552076231153737. [ FREE Full text ] [ CrossRef ] [ Medline ]
- Tsekleves E, Paraskevopoulos IT, Warland A, Kilbride C. Development and preliminary evaluation of a novel low cost VR-based upper limb stroke rehabilitation platform using Wii technology. Disabil Rehabil Assist Technol. 2016;11(5):413-422. [ CrossRef ] [ Medline ]
- Uswatte G, Taub E, Lum P, Brennan D, Barman J, Bowman MH, et al. Tele-rehabilitation of upper-extremity hemiparesis after stroke: proof-of-concept randomized controlled trial of in-home Constraint-Induced Movement therapy. Restor Neurol Neurosci. 2021;39(4):303-318. [ CrossRef ] [ Medline ]
- Valdés BA, Van der Loos HFM. Biofeedback vs. game scores for reducing trunk compensation after stroke: a randomized crossover trial. Top Stroke Rehabil. 2018;25(2):96-113. [ CrossRef ] [ Medline ]
- Wairagkar M, McCrindle R, Robson H, Meteyard L, Sperrin M, Smith A, et al. MaLT—combined motor and language therapy tool for brain injury patients using kinect. Methods Inf Med. 2017;56(2):127-137. [ FREE Full text ] [ CrossRef ] [ Medline ]
- Wei WXJ, Fong KNK, Chung RCK, Cheung HKY, Chow ESL. "Remind-to-Move" for promoting upper extremity recovery using wearable devices in subacute stroke: a multi-center randomized controlled study. IEEE Trans Neural Syst Rehabil Eng. 2019;27(1):51-59. [ CrossRef ] [ Medline ]
- Whitford M, Schearer E, Rowlett M. Effects of in home high dose accelerometer-based feedback on perceived and actual use in participants chronic post-stroke. Physiother Theory Pract. 2020;36(7):799-809. [ CrossRef ] [ Medline ]
- Widmer M, Held JPO, Wittmann F, Valladares B, Lambercy O, Sturzenegger C, et al. Reward during arm training improves impairment and activity after stroke: a randomized controlled trial. Neurorehabil Neural Repair. 2022;36(2):140-150. [ FREE Full text ] [ CrossRef ] [ Medline ]
- Wittmann F, Held JP, Lambercy O, Starkey ML, Curt A, Höver R, et al. Self-directed arm therapy at home after stroke with a sensor-based virtual reality training system. J Neuroeng Rehabil. 2016;13(1):75. [ FREE Full text ] [ CrossRef ] [ Medline ]
- Yuan Z, Peng Y, Wang L, Song S, Chen S, Yang L, et al. Effect of BCI-controlled pedaling training system with multiple modalities of feedback on motor and cognitive function rehabilitation of early subacute stroke patients. IEEE Trans Neural Syst Rehabil Eng. 2021;29:2569-2577. [ FREE Full text ] [ CrossRef ] [ Medline ]
- Chong MS, Sit JWH, Karthikesu K, Chair SY. Effectiveness of technology-assisted cardiac rehabilitation: a systematic review and meta-analysis. Int J Nurs Stud. 2021;124:104087. [ CrossRef ] [ Medline ]
- Hart T, Dijkers MP, Whyte J, Turkstra LS, Zanca JM, Packel A, et al. A theory-driven system for the specification of rehabilitation treatments. Arch Phys Med Rehabil. 2019;100(1):172-180. [ CrossRef ] [ Medline ]
- Deterding S, Dixon D, Khaled R, Nacke L. From game design elements to gamefulness: defining "gamification". New York, NY, US. Association for Computing Machinery; 2011. Presented at: Proceedings of the 15th International Academic MindTrek Conference: Envisioning Future Media Environments; September 28-30, 2011;9-15; Tampere, Finland. [ CrossRef ]
- Barrett N, Swain I, Gatzidis C, Mecheraoui C. The use and effect of video game design theory in the creation of game-based systems for upper limb stroke rehabilitation. J Rehabil Assist Technol Eng. 2016;3:2055668316643644. [ FREE Full text ] [ CrossRef ] [ Medline ]
- Welcome to UCLanData. University of Central Lancashire. URL: https://uclandata.uclan.ac.uk/383/ [accessed 2024-03-29]
Abbreviations
Edited by A Mavragani; submitted 15.05.23; peer-reviewed by M Broderick, G Sweeney, E Crayton, D Pogrebnoy; comments to author 11.10.23; revised version received 14.11.23; accepted 26.12.23; published 24.04.24.
©Helen J Gooch, Kathryn A Jarvis, Rachel C Stockley. Originally published in the Journal of Medical Internet Research (https://www.jmir.org), 24.04.2024.
This is an open-access article distributed under the terms of the Creative Commons Attribution License (https://creativecommons.org/licenses/by/4.0/), which permits unrestricted use, distribution, and reproduction in any medium, provided the original work, first published in the Journal of Medical Internet Research, is properly cited. The complete bibliographic information, a link to the original publication on https://www.jmir.org/, as well as this copyright and license information must be included.
Help | Advanced Search
Computer Science > Computation and Language
Title: phi-3 technical report: a highly capable language model locally on your phone.
Abstract: We introduce phi-3-mini, a 3.8 billion parameter language model trained on 3.3 trillion tokens, whose overall performance, as measured by both academic benchmarks and internal testing, rivals that of models such as Mixtral 8x7B and GPT-3.5 (e.g., phi-3-mini achieves 69% on MMLU and 8.38 on MT-bench), despite being small enough to be deployed on a phone. The innovation lies entirely in our dataset for training, a scaled-up version of the one used for phi-2, composed of heavily filtered web data and synthetic data. The model is also further aligned for robustness, safety, and chat format. We also provide some initial parameter-scaling results with a 7B and 14B models trained for 4.8T tokens, called phi-3-small and phi-3-medium, both significantly more capable than phi-3-mini (e.g., respectively 75% and 78% on MMLU, and 8.7 and 8.9 on MT-bench).
Submission history
Access paper:.
- HTML (experimental)
- Other Formats

References & Citations
- Google Scholar
- Semantic Scholar
BibTeX formatted citation

Bibliographic and Citation Tools
Code, data and media associated with this article, recommenders and search tools.
- Institution
arXivLabs: experimental projects with community collaborators
arXivLabs is a framework that allows collaborators to develop and share new arXiv features directly on our website.
Both individuals and organizations that work with arXivLabs have embraced and accepted our values of openness, community, excellence, and user data privacy. arXiv is committed to these values and only works with partners that adhere to them.
Have an idea for a project that will add value for arXiv's community? Learn more about arXivLabs .
The Federal Register
The daily journal of the united states government, request access.
Due to aggressive automated scraping of FederalRegister.gov and eCFR.gov, programmatic access to these sites is limited to access to our extensive developer APIs.
If you are human user receiving this message, we can add your IP address to a set of IPs that can access FederalRegister.gov & eCFR.gov; complete the CAPTCHA (bot test) below and click "Request Access". This process will be necessary for each IP address you wish to access the site from, requests are valid for approximately one quarter (three months) after which the process may need to be repeated.
An official website of the United States government.
If you want to request a wider IP range, first request access for your current IP, and then use the "Site Feedback" button found in the lower left-hand side to make the request.
Numbers, Facts and Trends Shaping Your World
Read our research on:
Full Topic List
Regions & Countries
- Publications
- Our Methods
- Short Reads
- Tools & Resources
Read Our Research On:
Partisan divides over K-12 education in 8 charts
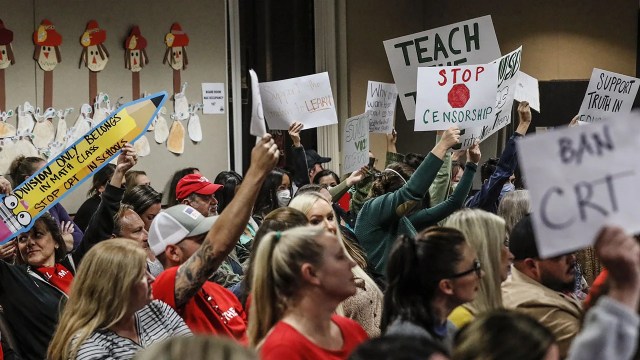
K-12 education is shaping up to be a key issue in the 2024 election cycle. Several prominent Republican leaders, including GOP presidential candidates, have sought to limit discussion of gender identity and race in schools , while the Biden administration has called for expanded protections for transgender students . The coronavirus pandemic also brought out partisan divides on many issues related to K-12 schools .
Today, the public is sharply divided along partisan lines on topics ranging from what should be taught in schools to how much influence parents should have over the curriculum. Here are eight charts that highlight partisan differences over K-12 education, based on recent surveys by Pew Research Center and external data.
Pew Research Center conducted this analysis to provide a snapshot of partisan divides in K-12 education in the run-up to the 2024 election. The analysis is based on data from various Center surveys and analyses conducted from 2021 to 2023, as well as survey data from Education Next, a research journal about education policy. Links to the methodology and questions for each survey or analysis can be found in the text of this analysis.
Most Democrats say K-12 schools are having a positive effect on the country , but a majority of Republicans say schools are having a negative effect, according to a Pew Research Center survey from October 2022. About seven-in-ten Democrats and Democratic-leaning independents (72%) said K-12 public schools were having a positive effect on the way things were going in the United States. About six-in-ten Republicans and GOP leaners (61%) said K-12 schools were having a negative effect.
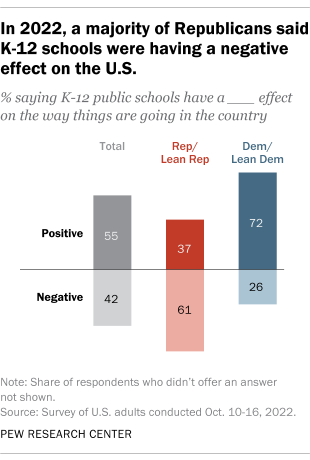
About six-in-ten Democrats (62%) have a favorable opinion of the U.S. Department of Education , while a similar share of Republicans (65%) see it negatively, according to a March 2023 survey by the Center. Democrats and Republicans were more divided over the Department of Education than most of the other 15 federal departments and agencies the Center asked about.
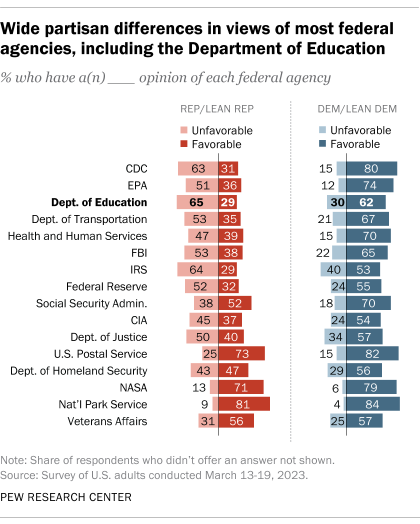
In May 2023, after the survey was conducted, Republican lawmakers scrutinized the Department of Education’s priorities during a House Committee on Education and the Workforce hearing. The lawmakers pressed U.S. Secretary of Education Miguel Cardona on topics including transgender students’ participation in sports and how race-related concepts are taught in schools, while Democratic lawmakers focused on school shootings.
Partisan opinions of K-12 principals have become more divided. In a December 2021 Center survey, about three-quarters of Democrats (76%) expressed a great deal or fair amount of confidence in K-12 principals to act in the best interests of the public. A much smaller share of Republicans (52%) said the same. And nearly half of Republicans (47%) had not too much or no confidence at all in principals, compared with about a quarter of Democrats (24%).
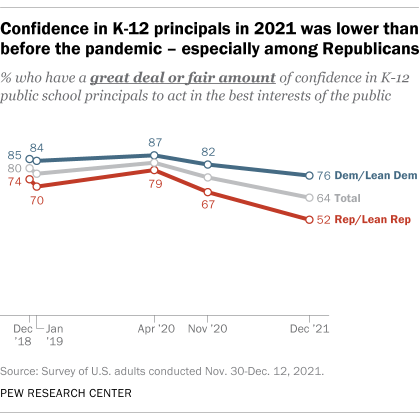
This divide grew between April 2020 and December 2021. While confidence in K-12 principals declined significantly among people in both parties during that span, it fell by 27 percentage points among Republicans, compared with an 11-point decline among Democrats.
Democrats are much more likely than Republicans to say teachers’ unions are having a positive effect on schools. In a May 2022 survey by Education Next , 60% of Democrats said this, compared with 22% of Republicans. Meanwhile, 53% of Republicans and 17% of Democrats said that teachers’ unions were having a negative effect on schools. (In this survey, too, Democrats and Republicans include independents who lean toward each party.)
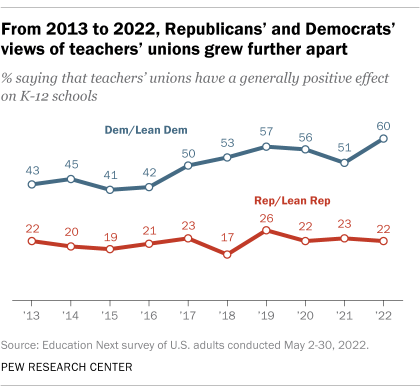
The 38-point difference between Democrats and Republicans on this question was the widest since Education Next first asked it in 2013. However, the gap has exceeded 30 points in four of the last five years for which data is available.
Republican and Democratic parents differ over how much influence they think governments, school boards and others should have on what K-12 schools teach. About half of Republican parents of K-12 students (52%) said in a fall 2022 Center survey that the federal government has too much influence on what their local public schools are teaching, compared with two-in-ten Democratic parents. Republican K-12 parents were also significantly more likely than their Democratic counterparts to say their state government (41% vs. 28%) and their local school board (30% vs. 17%) have too much influence.
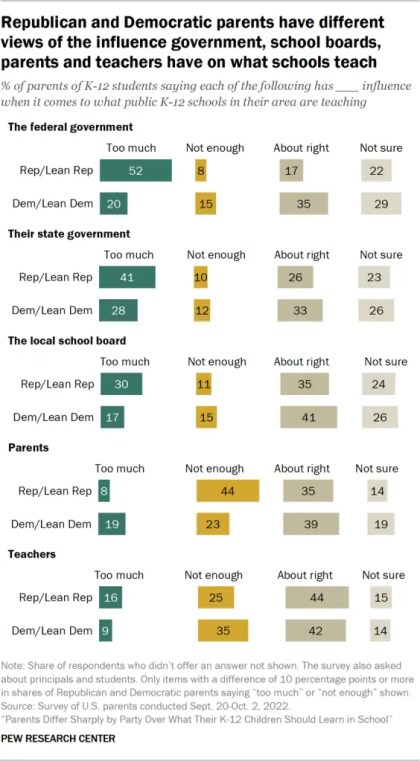
On the other hand, more than four-in-ten Republican parents (44%) said parents themselves don’t have enough influence on what their local K-12 schools teach, compared with roughly a quarter of Democratic parents (23%). A larger share of Democratic parents – about a third (35%) – said teachers don’t have enough influence on what their local schools teach, compared with a quarter of Republican parents who held this view.
Republican and Democratic parents don’t agree on what their children should learn in school about certain topics. Take slavery, for example: While about nine-in-ten parents of K-12 students overall agreed in the fall 2022 survey that their children should learn about it in school, they differed by party over the specifics. About two-thirds of Republican K-12 parents said they would prefer that their children learn that slavery is part of American history but does not affect the position of Black people in American society today. On the other hand, 70% of Democratic parents said they would prefer for their children to learn that the legacy of slavery still affects the position of Black people in American society today.
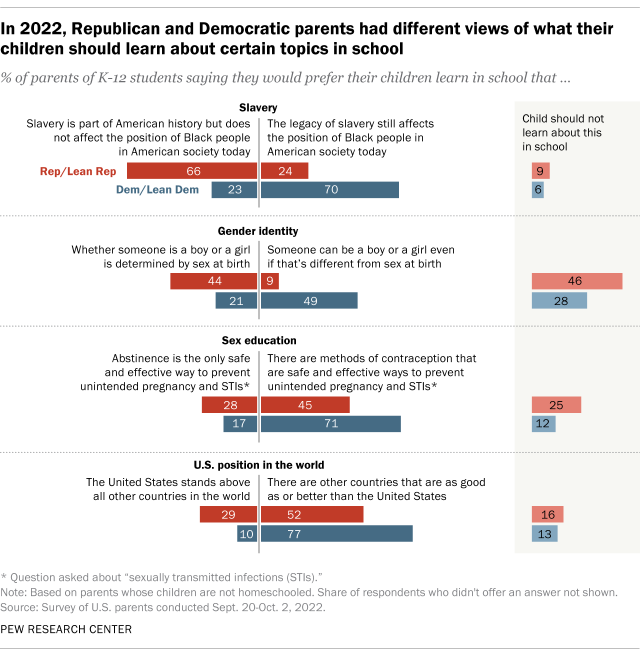
Parents are also divided along partisan lines on the topics of gender identity, sex education and America’s position relative to other countries. Notably, 46% of Republican K-12 parents said their children should not learn about gender identity at all in school, compared with 28% of Democratic parents. Those shares were much larger than the shares of Republican and Democratic parents who said that their children should not learn about the other two topics in school.
Many Republican parents see a place for religion in public schools , whereas a majority of Democratic parents do not. About six-in-ten Republican parents of K-12 students (59%) said in the same survey that public school teachers should be allowed to lead students in Christian prayers, including 29% who said this should be the case even if prayers from other religions are not offered. In contrast, 63% of Democratic parents said that public school teachers should not be allowed to lead students in any type of prayers.

In June 2022, before the Center conducted the survey, the Supreme Court ruled in favor of a football coach at a public high school who had prayed with players at midfield after games. More recently, Texas lawmakers introduced several bills in the 2023 legislative session that would expand the role of religion in K-12 public schools in the state. Those proposals included a bill that would require the Ten Commandments to be displayed in every classroom, a bill that would allow schools to replace guidance counselors with chaplains, and a bill that would allow districts to mandate time during the school day for staff and students to pray and study religious materials.
Mentions of diversity, social-emotional learning and related topics in school mission statements are more common in Democratic areas than in Republican areas. K-12 mission statements from public schools in areas where the majority of residents voted Democratic in the 2020 general election are at least twice as likely as those in Republican-voting areas to include the words “diversity,” “equity” or “inclusion,” according to an April 2023 Pew Research Center analysis .
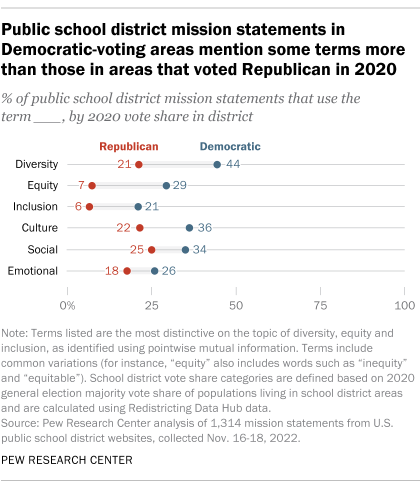
Also, about a third of mission statements in Democratic-voting areas (34%) use the word “social,” compared with a quarter of those in Republican-voting areas, and a similar gap exists for the word “emotional.” Like diversity, equity and inclusion, social-emotional learning is a contentious issue between Democrats and Republicans, even though most K-12 parents think it’s important for their children’s schools to teach these skills . Supporters argue that social-emotional learning helps address mental health needs and student well-being, but some critics consider it emotional manipulation and want it banned.
In contrast, there are broad similarities in school mission statements outside of these hot-button topics. Similar shares of mission statements in Democratic and Republican areas mention students’ future readiness, parent and community involvement, and providing a safe and healthy educational environment for students.
- Education & Politics
- Partisanship & Issues
- Politics & Policy
Jenn Hatfield is a writer/editor at Pew Research Center
Most Americans think U.S. K-12 STEM education isn’t above average, but test results paint a mixed picture
About 1 in 4 u.s. teachers say their school went into a gun-related lockdown in the last school year, about half of americans say public k-12 education is going in the wrong direction, what public k-12 teachers want americans to know about teaching, what’s it like to be a teacher in america today, most popular.
1615 L St. NW, Suite 800 Washington, DC 20036 USA (+1) 202-419-4300 | Main (+1) 202-857-8562 | Fax (+1) 202-419-4372 | Media Inquiries
Research Topics
- Age & Generations
- Coronavirus (COVID-19)
- Economy & Work
- Family & Relationships
- Gender & LGBTQ
- Immigration & Migration
- International Affairs
- Internet & Technology
- Methodological Research
- News Habits & Media
- Non-U.S. Governments
- Other Topics
- Race & Ethnicity
- Email Newsletters
ABOUT PEW RESEARCH CENTER Pew Research Center is a nonpartisan fact tank that informs the public about the issues, attitudes and trends shaping the world. It conducts public opinion polling, demographic research, media content analysis and other empirical social science research. Pew Research Center does not take policy positions. It is a subsidiary of The Pew Charitable Trusts .
Copyright 2024 Pew Research Center
Terms & Conditions
Privacy Policy
Cookie Settings
Reprints, Permissions & Use Policy
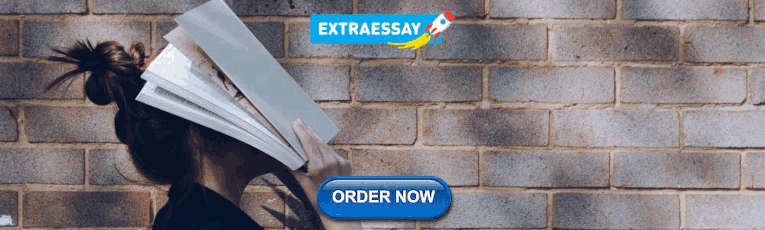
IMAGES
VIDEO
COMMENTS
Thus, despite the relational database's continued dominance in many contexts, modern databases' specific material forms can vary dramatically. For instance, though all relational databases organize data into sets of interlinked tables, the specific file types and querying languages vary depending on the software platform being used.
on relational database systems throughout this paper. At heart, a typical RDBMS has five main components, as illustrated in Figure 1.1. As an introduction to each of these components and the way they fit together, we step through the life of a query in a database system. This also serves as an overview of the remaining sections of the paper.
The relational model was described by E.F. Codd in his 1970 paper, "A Relational Model of Data for Large Shared Data Banks." ... All of these projects resulted in many research papers and projects dealing with issues in the implementation of the relational model, including data representation, data languages, transaction management, user ...
Our objective is to establish deep learning on relational data as a new subfield of machine learning. We hope that this will be a fruitful research direction, with many opportunities for impactful ideas that make much better use of the rich predictive signal in relational data. This paper lays the ground
Logical data models such as the relational model [14] are related to how data is stored and presented, but often without describing how the data is physically stored, e.g., which computing node is responsible for storing the data, where the data is located on a disk, and what types of indices (i.e., redundant data structures
Relational databases play a central role in many information systems. Their schemas contain structural and behavioral entity descriptions. ... Our work needs to be compared to impact analysis and database schema evolution research fields. Impact Analysis. Since the first paper introducing Impact Analysis by Bohnert and Arnold , ...
In 1970, Dr. Edgar F. (Ted) Codd of IBM published a paper entitled "A Relational Model of Data for Large Shared Data Banks" in Communications of the ACM. This paper marked the beginning of the field of relational database. During the 1970s, the relational approach to database progressed from being a technical curiosity to a subject of ...
5 CONCLUSION. Database schema data sets are needed for various ML applications, including to automate and scale the synthesis of databases for use in cyber deception. SchemaDB is intended to enable such research, as well as to provide a standardised example for other potential data set providers.
In this paper we outline research towards a role-concept-enabled relational database system. We describe a definition of this concept based on existing results and discuss open research questions ...
Paper —Design and Analysis of a Relational Database for Behavioral Experiments Data Processing. 3 Design of the relational database. The schema of the designed relational database entitled ...
Origin of Relational Databases. The concept of a RDBS was first described in a seminal article in 1970. 1 The theoretic construct was that all data could be defined or represented as a series of relations with or to other data. The article was quantitative in that it used relational algebra and tuple relational calculus to prove its points. 2 IBM used this theoretic framework to design what ...
A relational database is a type of database that organizes data into rows and columns, which collectively form a table where the data points are related to each other. Data is typically structured across multiple tables, which can be joined together via a primary key or a foreign key. These unique identifiers demonstrate the different ...
The business organization expects that NoSQL database has better performance than a relational database. In This paper, we aim to compare the performance of Redis, which is a key-value database, one kind of NoSQL database, and MariaDB, which is a popular relational database. We designed a set of experiments with a large amount of data and ...
History. The concept of relational database was defined by E. F. Codd at IBM in 1970. Codd introduced the term relational in his research paper "A Relational Model of Data for Large Shared Data Banks". In this paper and later papers, he defined what he meant by relation.One well-known definition of what constitutes a relational database system is composed of Codd's 12 rules.
A relational database management system (RDBMS) is a. program that allows you to create, update, and administer a. relational database. Generally, RDBMS use the SQL language to access the ...
The relational model was introduced in 1970. Edgar F. Codd, a researcher at IBM, published a paper called "A Relational Model of Data for Large Shared Data Banks.". The paper was a rewrite of a paper he had circulated internally at IBM a year earlier. The paper is unassuming; Codd does not announce in his abstract that he has discovered a ...
A Relational Model of Data for Large Shared Data Banks E. F. CODD IBM Research Laboratory, San Jose, California Future users of large data banks must be protected from having to know how the data is organized in the machine (the ... access to large banks of formatted data. Except for a paper by Childs [l], the principal application of relations ...
In this paper we introduce a data integration system by implementing a function into the context of PostgreSQL. The aim of this work is to collect files to process from two different data sources (a platform of Physical Testing Software (PTS) and another one of Physical Simulation Software (PSS)), in order to retrieve specific records through a query and integrate them. Both these platforms ...
Going beyond a simple database table, a relational database fits more complicated systems by relating information from two or more database tables. This paper will use MySQL to develop a basic appreciation of relational databases including user administration, database design, and SQL syntax. It will lead the reader in downloading and ...
In this paper, the performance evaluation of MySQL and MongoDB is performed where MySQL is an example of relational database and MongoDB is an example of non relational databases. A relational database is a data structure that allows you to connect information from different 'tables', or different types of data buckets. Non-relational database ...
The role of SQL in relational databases. SQL is a powerful language for interacting with relational databases. With SQL, data scientists can extract specific information from the database using SELECT statements. SQL provides a standardized and intuitive way to manipulate data in relational databases. Exploring non-relational databases
• Relational database mapping and prototyping, Database system implementation • cooperative group project based learning ... The study was carried out by systematically selecting research papers published between 1995 and 2021. Based on the study, a high level categorization presents a taxonomy of the published under the heads of Tools ...
A Literature Review on Evolving Database. March 2017. International Journal of Computer Applications 162 (9):35-41. DOI: 10.5120/ijca2017913365. Authors: Shagufta Praveen. Glocal University. Umesh ...
Methods in Ecology and Evolution is an open access journal publishing papers across a wide range of subdisciplines, ... Image-based machine learning tools are an ascendant 'big data' research avenue. Citizen science platforms, like iNaturalist, and museum-led initiatives provide researchers with an abundance of data and knowledge to extract
Relational databases play a central role in many information systems. Their schemas contain structural and behavioral entity descriptions. Databases must continuously be adapted to new requirements of a world in constant change while: (1) relational database management systems (RDBMS) do not allow inconsistencies in the schema; (2) stored procedure bodies are not meta-described in RDBMS such ...
The results were independently screened by 2 reviewers. Sources were included if they reported a completed primary research study in which a behavior change approach could be identified within a physical stroke rehabilitation intervention that included a DHT. Data, including the study design, DHT used, and behavior change approaches, were charted.
We introduce phi-3-mini, a 3.8 billion parameter language model trained on 3.3 trillion tokens, whose overall performance, as measured by both academic benchmarks and internal testing, rivals that of models such as Mixtral 8x7B and GPT-3.5 (e.g., phi-3-mini achieves 69% on MMLU and 8.38 on MT-bench), despite being small enough to be deployed on a phone. The innovation lies entirely in our ...
How Pew Research Center will report on generations moving forward. Journalists, researchers and the public often look at society through the lens of generation, using terms like Millennial or Gen Z to describe groups of similarly aged people. This approach can help readers see themselves in the data and assess where we are and where we're ...
In conducting research in these areas, FDA will need to employ the following validation methodology: (1) research to assess knowledge, perceptions, and experiences related to topics in the above-mentioned areas with specific target populations; (2) techniques to evaluate sampling and recruitment methods; and (3) evaluations of the validity and ...
Pew Research Center conducted this analysis to provide a snapshot of partisan divides in K-12 education in the run-up to the 2024 election. The analysis is based on data from various Center surveys and analyses conducted from 2021 to 2023, as well as survey data from Education Next, a research journal about education policy.