- Systematic Review
- Open access
- Published: 27 May 2020
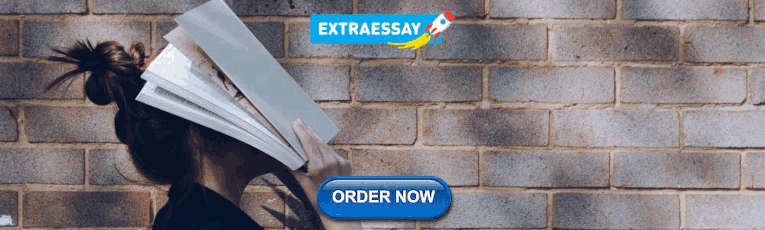
A systematic review on spatial crime forecasting
- Ourania Kounadi 1 ,
- Alina Ristea ORCID: orcid.org/0000-0003-2682-1416 2 , 3 ,
- Adelson Araujo Jr. 4 &
- Michael Leitner 2 , 5
Crime Science volume 9 , Article number: 7 ( 2020 ) Cite this article
16k Accesses
39 Citations
9 Altmetric
Metrics details
Predictive policing and crime analytics with a spatiotemporal focus get increasing attention among a variety of scientific communities and are already being implemented as effective policing tools. The goal of this paper is to provide an overview and evaluation of the state of the art in spatial crime forecasting focusing on study design and technical aspects.
We follow the PRISMA guidelines for reporting this systematic literature review and we analyse 32 papers from 2000 to 2018 that were selected from 786 papers that entered the screening phase and a total of 193 papers that went through the eligibility phase. The eligibility phase included several criteria that were grouped into: (a) the publication type, (b) relevance to research scope, and (c) study characteristics.
The most predominant type of forecasting inference is the hotspots (i.e. binary classification) method. Traditional machine learning methods were mostly used, but also kernel density estimation based approaches, and less frequently point process and deep learning approaches. The top measures of evaluation performance are the Prediction Accuracy, followed by the Prediction Accuracy Index, and the F1-Score. Finally, the most common validation approach was the train-test split while other approaches include the cross-validation, the leave one out, and the rolling horizon.
Limitations
Current studies often lack a clear reporting of study experiments, feature engineering procedures, and are using inconsistent terminology to address similar problems.
Conclusions
There is a remarkable growth in spatial crime forecasting studies as a result of interdisciplinary technical work done by scholars of various backgrounds. These studies address the societal need to understand and combat crime as well as the law enforcement interest in almost real-time prediction.
Implications
Although we identified several opportunities and strengths there are also some weaknesses and threats for which we provide suggestions. Future studies should not neglect the juxtaposition of (existing) algorithms, of which the number is constantly increasing (we enlisted 66). To allow comparison and reproducibility of studies we outline the need for a protocol or standardization of spatial forecasting approaches and suggest the reporting of a study’s key data items.
Environmental criminology provides an important theoretical foundation for exploring and understanding spatial crime distribution (Bruinsma and Johnson 2018 ). The occurrence of crime within an area fluctuates from place to place. Besides, crime occurrences depend on a multitude of factors, and they show an increased strategic complexity and interaction with other networks, such as institutional or community-based. In criminology research, these factors are primarily referred to as crime attractors and crime generators (Kinney et al. 2008 ). Spatial fluctuations and dependencies to attractors and generators suggest that crime is not random in time and in space. A strong foundation for spatial predictive crime analytics is the Crime Pattern Theory (Brantingham and Brantingham 1984 ). It is used to explain why crimes occur in specific areas, suggests that crime is not random, and that it can be organized or opportunistic. In particular, it shows that when the activity space of a victim intersects with the activity space of an offender, there are higher chances for a crime occurrence. The activity perimeter of a person is spatially constrained by locations that are attended (nodes). For example, if one of the personal nodes is in a high-crime neighbourhood, criminals come across new opportunities to offend.
If crime is not random it can be studied, and as such, its patterns, including the spatial component, can be modelled. As a consequence, environmental criminology theories have been tested scientifically and in the past decade various research fields have made much progress in developing methods for (spatiotemporal) crime prediction and evaluation (Caplan et al. 2011 ; Mohler et al. 2011 , 2015 ; Perry 2013 ; Wang and Brown 2011 ; Yu et al. 2011 ).
Most prediction techniques are used for retrospective forecasting, i.e., predicting the future through historical data. Historical crime data are used alone or together with crime attractors and generators (which can be demographic, environmental, etc.) in diverse types of prediction models (Mohler et al. 2011 ; Ohyama and Amemiya 2018 ; Yu et al. 2011 ). Apart from static data, such as demographics or socio-economic variables, as predictors, researchers have recently included dynamic space and time features, thus giving a boost to predicting crime occurrences. These models consist of social media data (Al Boni and Gerber 2016 ; Gerber 2014 ; Kadar et al. 2017 ; Wang et al. 2012 ; Williams and Burnap 2015 ), and taxi pick-up and drop-off data (Kadar and Pletikosa 2018 ; Wang et al. 2016 ; Zhao and Tang 2017 ).
Although current crime prediction models show increasing accuracy, little emphasis has been placed on drawing the empirical and technical landscape to outline strengths and opportunities for future research, but also to identify weaknesses and threats. In this paper, we focus on spatial crime forecasting, which is the spatial forecasting of crime-related information. It has many applications such as the spatial forecast of the number of crimes, the type of criminal activity, the next location of a crime in a series, or other crime-related information. At this point, we should note that we came across papers that claim to do spatial crime forecasting or crime forecasting while extrapolating in space or detecting spatial clusters. Overall, papers in the field of spatial crime analysis use the term prediction synonymous with forecasting and they have a preference for the term prediction (Perry 2013 ). However, there are several spatial prediction types with applications of interpolation or extrapolation. Forecasting is a prediction that extrapolates an estimated variable into a future time. While prediction can be synonymous with forecasting, it is often also used to infer unknown values regardless of the time dimension (e.g., predict the crime in area A using a model derived from area B). Cressie ( 1993 , pp 105–106) refers to spatial prediction as an inference process to predict values at unknown locations from data observed at known locations. His terminology includes the temporal notions of smoothing or interpolation, filtering, and prediction, which traditionally use time units instead of locations. As a result, when searching for forecasting literature you need to add the “prediction” term, which derives a much larger pool of papers, than the ones that actually do “only” forecasting. In this paper, we define the term “Spatial Crime Forecasting” as an inference approach about crime both in time and in space. In the box below, we add definition boundaries by describing variations of forecasting approaches that we consider in our study.
We are driven by the need to harmonize existing concepts and methodologies within and between criminology, sociology, geography, and computer science communities. The goal of this paper is to conduct a systematic literature review in spatial crime predictive analytics, with a focus on crime forecasting, to understand and evaluate the state of the art concerning concepts and methods given the unprecedented pace of published empirical studies. Below, we list the research questions of this study.
What are the types of forecasted information for which space plays a significant role? (“ Overview of selected publications on spatial crime forecasting ” section).
What are the commonly used forecasting methods? (“ Spatial crime forecasting methods ” section).
Which are the technical similarities and differences between spatial crime forecasting models? (“ Spatial crime forecasting methods ” section).
How is predictive performance being measured in spatial crime forecasting? (“ Considerations when analysing forecasting performance ” section).
What are the commonly used model validation strategies? (“ Considerations when analysing forecasting performance ” section).
What are the main dependencies and limitations of crime forecasting performance? (“ Considerations when analysing forecasting performance ” section).
Before presenting the results (“ Results ” section) and discuss them in the form of a SWOT analysis (“ Discussion ” section), we summarize previous literature work on crime prediction and analytics (“ Related work ” section) and then present the methodology to select the papers and ensure the study quality (“ Methods ” section). Last, in “ Conclusion ” section we conclude with the main findings of each research question. With our work, we aim to shed light on future research directions and indicate pitfalls to consider when performing spatial crime forecasting.
Related work
The papers identified as review or related-work studies (a total of 13) date back to 2003 and are connected to the keyword strategy that we used (find further details in “ Study selection ” section). In addition, to review papers (a total of 9), we also include two editorials, one book chapter, and one research paper, because they contain an extensive literature review in the field of crime predictive analytics.
Five papers focus on data mining with a much broader scope than our topics of interest, i.e., prediction, forecasting, or spatial analysis. The oldest one proposes a framework for crime data mining (Chen et al. 2004 ). It groups mining techniques into eight categories, including (a) the entity extraction (usage example: to identify persons), (b) clustering (usage example: to distinguish among groups belonging to different gangs), (c) association rule mining (usage example: to detect network attacks), (d) sequential pattern mining (usage example: same as before), (e) deviation detection (usage example: to identify fraud), (f) classification (usage example: to identify e-mail spamming), (g) string comparator (usage example: to detect deceptive information), and (h) social network analysis (usage example: to construct the criminal’s role in a network). Association rule, clustering, and classification are the ones that have been discussed in other crime data mining reviews, such as for the identification of criminals (i.e., profiling) (Chauhan and Sehgal 2017 ), applications to solve crimes (Thongsatapornwatana 2016 ), and applications of criminal career analysis, investigative profiling, and pattern analysis (with respect to criminal behaviour) (Thongtae and Srisuk 2008 ). Furthermore, Hassani et al. ( 2016 ) conducted a recent in-depth review that looked at over 100 applications of crime data mining. Their taxonomy of applications identifies five types that include those previously described by Chen et al. ( 2004 ) with the exemption of sequential pattern mining, deviation detection, and string comparator. Regarding specific algorithms, the emphasis is put on three types, namely decision trees, neural networks, and support vector machines. Chen et al. ( 2004 ) work covers a broad spectrum of crime analysis and investigation and as such, it identifies a couple of studies related to hotspot detection and forecasting under the mining categories of clustering and classification. These technical review studies gave us examples of the data items that we need to extract and analyse, such as the techniques that are used and the tasks that are performed (Thongsatapornwatana 2016 ) as well as the study purpose and region (Hassani et al. 2016 ).
The oldest, yet still relevant paper to our work is an editorial to six crime forecasting studies (Gorr and Harries 2003 ). The authors refer to crime forecasting as a new application domain, which includes the use of geographical information systems (GIS), performs long- and short-term prediction with univariate and multivariate methods, and fixed boundary versus ad hoc areal units for space and time-series data. More than 15 years later, this application domain is not new but it still involves the same characteristics as described above. Another editorial by Kennedy and Dugato ( 2018 ) introduces a special issue on spatial crime forecasting using the Risk Terrain Modelling (RTM) approach. The focus of most papers is to analyse factors that lead to accurate forecasts because the foundation of the RTM approach is based on the Theory of Risky Places by Kennedy and Caplan ( 2012 ). This theory starts with the proposition that places vary in terms of risk due to the spatial influence of criminogenic factors. Last, a recent review study summarizes past crime forecasting studies of four methods, namely support vector machines, artificial neural networks, fuzzy theory, and multivariate time series (Shamsuddin et al. 2017 ). The authors suggest that researchers propose hybrid methods to produce better results. In our study we group and discuss a much wider number of methods (a list of 66 in Additional file 1 C) and we also identified hybrid approaches (e.g., ensemble methods) one of which dates back to 2011.
In addition, we identified two papers that describe spatial methods for spatial crime prediction per se. The paper by Bernasco and Elffers ( 2010 ) discusses statistical and spatial methods to analyse crime. They interestingly distinguish two types of spatial outcomes for modelling, including spatial distribution and movement. When it comes to spatial distribution, which is relevant to the scope of our paper, the authors describe the following spatial methods, including spatial regression models, spatial filtering, geographically weighted regression, and multilevel regression with spatial dependence. The paper by Chainey et al. ( 2008 ) focuses on hotspot mapping as a basic approach to crime prediction. The techniques they describe and empirically examine are spatial ellipses, thematic mapping of geographic areas, grid thematic mapping, and Kernel Density Estimation (KDE). Among these, KDE yielded the highest prediction accuracy index (PAI) score. Surprisingly, most of the spatial methods (with the exemption of KDE and RTM) have not been used by authors of our selected papers (see methods discussed in “ Spatial crime forecasting methods ” section).
Regarding predictive policing, a recent review explains its definition, how it works, how to evaluate its effectiveness, and it also provides an overview of existing (mostly commercial) applications (Hardyns and Rummens 2018 ). One of the innovative aspects of this review is the section on the evaluation of predictive policing using three criteria, namely the correctness of the prediction, the effect of predictive policing implementations to actual crime rates, and the costs relative to the methods being replaced. The authors of this paper support the definition of predictive policing that originates from Ratcliffe ( 2015 , p. 4), which reads: “ the use of historical data to create a spatiotemporal forecast of areas of criminality or crime hot spots that will be the basis for police resource allocation decisions with the expectation that having officers at the proposed place and time will deter or detect criminal activity ”. In general, spatial crime forecasting has a broader scope and is not synonymous to predictive policing. In addition, the papers that we examine do not aim in assisting policing decisions (although this can be an indirect consequence) but they have an academic and explanatory focus. However, the effectiveness of the predictive analysis- first criterion, as framed by Hardyns and Rummens ( 2018 ), is highly connected to our scope and thus is further analysed, from a technical perspective, in “ Considerations when analysing forecasting performance ” section.
A second predictive policing systematic review by Seele ( 2017 ) examines the potential of big data to promote sustainability and reduce harm and also discusses ethical and legal aspects linked to predictive algorithms. Similarly, Ozkan ( 2018 ) also reviews big data for crime research. This paper provides a critical discussion on the benefits and limitations of data-driven research and draws attention to the imminent threat of eliminating conventional hypothesis testing, which has traditionally been an integral scientific tool for social scientists and criminologists.
Except for Seele ( 2017 ) no other related-work study follows a systematic procedure regarding the methods to identify and select relevant research, and thereafter to collect and analyse data from them. Also, our work focuses only on spatial crime forecasting, which is narrower than crime data mining and broader than predictive policing as discussed above. Last, we aim to contribute with scientific reference for technical issues in future studies. To achieve this, we follow a review protocol (“ Methods ” section), to answer six research questions (mentioned in “ Background ”) that have not been systematically addressed by previous studies.
Study selection
This study follows the reporting guidance “PRISMA” (Preferred Reporting Items for Systematic reviews and Meta-Analyses) (Liberati et al. 2009 ). PRISMA suggests a checklist of 27 items regarding the sections of a systematic literature review and their content, as well as a four-phase flow diagram for the selection of papers. We adopted and modified the PRISMA guidance according to the needs of our study. Our flow diagram contains three phases for the selection of papers. The first phase is “identification” and involves the selection of information sources and a search strategy that yields a set of possible papers. The second phase is “screening” the selected papers from the first phase, and removing the ones that are not relevant to the research scope. The third phase is “eligibility”, in which we applied a more thorough reading of papers and selected the ones that are relevant to our research questions. The count of papers in each phase and their subsequent steps are illustrated in Fig. 1 .
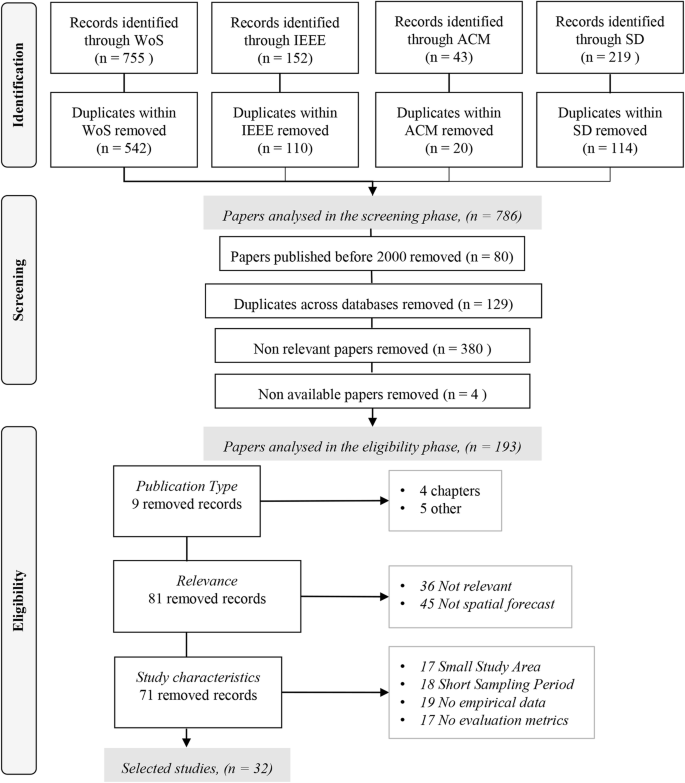
The three phases of the study selection process: identification, screening, and eligibility
The number of papers selected in the Identification phase is based on eleven keywords related to crime prediction (i.e., predict crime, crime predictive, predictive policing, predicting crimes, crime prediction, crime forecasting, crime data mining, crime mining, crime estimation, crime machine learning, crime big data). In addition, we added seven more spatially explicit terms (i.e., crime hotspot, spatial crime prediction, crime risk terrain modelling, spatial crime analysis, spatio-temporal modelling crime, spatiotemporal modelling crime, near-repeat crime). In a subsequent analysis, we have visualized the word frequency of the titles of the selected papers as evidence of the relevance of the keywords used. This can be found in Additional file 1 B: A word cloud of high - frequency words extracted from the titles of the selected papers .
Next, we selected information sources to perform literature searches. Although there is a remarkable number of search engines and academic databases, we focus on scholarly and comprehensive research databases including fields where spatial crime prediction is a representative topic. We considered the following databases, including Web of Science by Clarivate Analytics (WoS), Institute of Electrical and Electronics Engineers (IEEE) Xplore, ScienceDirect by Elsevier (SD), and Association for Computing Machinery (ACM) Digital Library. We consider that an optimal search process should include multiple academic search databases, with searches being carried out at the best level of detail possible. In addition, as also discussed by Bramer et al. ( 2017 ) in an exploratory study for database combinations, if the research question is more interdisciplinary, a broader science database such as Web of Science is likely to add value. With regards to Google Scholar (GS) there are divergent opinions between researchers if GS brings relevant information for an interdisciplinary review or not. Holone ( 2016 ) discusses that some engine searches, specifically GS, have a tendency to selectively expose information by using algorithms that personalize information for the users, calling this the filter bubble effect. Haddaway et al. ( 2015 ) found that when searched for specific papers, the majority of the literature identified using Web of Science was also found using GS. However, their findings showed moderate to poor overlap in results when similar search strings were used in Web of Science and GS (10–67%), and that GS missed some important literature in five of six case studies.
In each database, we used keywords on singular and plural word versions (e.g., crime hotspot/s). For WoS, we used the advanced search option, by looking for papers written in English and matching our keywords with the topic or title. For IEEE, we searched for our keywords in the metadata or papers’ titles. In SD and ACM, we used the advanced search option with Boolean functions that searched our keywords in the title, abstract, or paper’s keywords. The identified papers were integrated directly into the free reference manager Mendeley. Last, we removed duplicates within each database, which resulted in 786 papers for the second phase, the Screening phase. The last search in the Identification phase was run on 5 February 2019.
Whereas, the use of statistical and geostatistical analyses for crime forecasting has been considered for quite some time, during the last two decades there has been an increasing interest in developing tools that use large data sets to make crime predictions (Perry 2013 ). Thus, predictive analytics have been included in law enforcement practices (Brayne 2017 ). This is the main reason that during the Screening phase, we first excluded papers published before 2000. Second, we removed duplicates across the four selected databases (WoS, IEEE, SD, and ACM). Third, we screened all papers to identify the “non-relevant” ones. This decision was made by defining “relevant” papers to contain the following three elements. The first element is that a paper addresses crime events with explicit geographic boundaries. Common examples of excluded papers are the ones dealing with the fear of crime, offenders’ characteristics, offender, victims’ characteristics, geographical profiling, journey to crime, and cyber or financial crime. The second element for a paper to be “relevant” is that it employs a forecasting algorithm and is not limited to exploratory or clustering analysis. The third element is that there is some form of spatial prediction. This means that there are predefined spatial units of analysis, such as inferencing for each census block of the study area. For the relevance elements, our strategy was the following: (a) read title and screen figures and/or maps, (b) if unsure about relevance, read abstract, (c) if still unsure about relevance, search for relevant words (e.g., geo*, location, spatial) within the document. The last step of the Screening phase was to remove relevant papers that authors did not have access to, due to subscription restrictions. The Screening phase resulted in 193 relevant papers to be considered for the third and final phase.
During this final phase, the Eligibility phase, we read the abstract and main body of all 193 papers (e.g., study area, data, methods, and results). The focus was to extract data items that compose the paper’s eligibility criteria. These are grouped into three categories, namely: (a) publication type which is the first data item, (b) relevance: consists of data items relevance and purpose , and (c) study characteristics: consists of data items study area , sampling period , empirical data , evaluation metrics . Next, we discuss each category and the data items it entails.
The first data item is the publication type. Literature reviews sometimes exclude conference papers because their quality is not evaluated like International Scientific Indexing (ISI) papers. However, for some disciplines, such as computer science, many conferences are considered as highly reputable publication outlets. In the Screening phase, we found a large number of papers from computer or information science scholars, hence at this stage we decided not to exclude conference papers (n = 65), but also non-ISI papers (n = 19). In total, we excluded nine papers that are book chapters or belong to other categories (e.g., editorial).
The next two “relevance” criteria (i.e., relevance and purpose) address the fit of the papers’ content to our research scope. Paper relevance was checked again during this phase. For example, some papers that appeared to be relevant in the Screening phase (i.e., a paper is about crime events, space, and forecasting), were actually found not to be relevant after reading the core part of the paper. For example, prediction was mentioned in the abstract, but what the authors implied was that prediction is a future research perspective of the analysis that was actually done in the paper. Also, we added the data item “purpose” to separate methods that model and explore relationships between the dependent and independent variables (e.g., crime attractors to burglaries) from the ones that perform a spatial forecast. The number of papers that were excluded due to these criteria amounted to 81.
Last, there are four more “study characteristics” criteria relevant to the quality and homogeneity of the selected papers. First, the study area should be equal to or greater than a city. Cities are less prone to edge effects compared to smaller administrative units within a city that share boundaries with other units (e.g., districts). In addition, the smaller the study area the more likely it is that conclusions are tailored to the study characteristics and are not scalable. Second, the timeframe of the crime sample should be equal or greater than a year to ensure that seasonality patterns were captured. These two items also increase the homogeneity of the selected studies. Yet, there are significant differences among studies that are discussed further in Results section. The last two criteria are the restriction to analysing empirical data (e.g., proof of concepts or purely methodological papers were excluded) and to use measures that evaluate the models’ performance (e.g., mean square error). The last two criteria ensure that we only analyse studies that are useful to address our research questions. The number of papers that were excluded due to the publication type, the relevance, or the study characteristics were 71. Furthermore, Fig. 1 shows the number of excluded papers for each data item (e.g., 17 papers were excluded due to insufficient size of the study area). Finally, the entire selection processes yielded 32 papers.
Study quality
Two of the four authors of this research performed the selection of the papers to be analysed. Prior to each phase, these two authors discussed and designed the process, tested samples, and divided the workload. Then, results were merged, analysed, and discussed until both authors reached a consensus for the next phase. The same two authors crosschecked several of the results to ensure methodological consistency among them. The reading of the papers during the final phase (i.e., eligibility) was performed two times, by alternating the papers’ samples among the two authors, to ensure all eligible papers were included. In addition, in case some information on the paper’s content was unclear to the two authors, they contacted via email the corresponding authors for clarifications.
Regarding the results subsections that constitute four study stages (“ Study characteristics ”, “ Overview of selected publications on spatial crime forecasting ”, “ Spatial crime forecasting methods ”, and “ Considerations when analysing forecasting performance ” sections), one or two authors performed each and all authors contributed to extracting information and reviewing them. To extract information that is structured as data items we followed a procedure of three steps that was repeated at each stage. First, the papers were read by the authors to extract manually the data items and their values (1—extract). Data items and their values were then discussed and double-checked by the authors (2—discussion/consensus). In case information was still unclear, we contacted via email the corresponding authors for clarifications (3—consultation). This information was structured as a matrix where rows represent the papers and columns are several items of processed information (e.g., a data item is the year of publication). Table 1 shows the data items at the stage at which they were exploited. The attributes (values) of the items are discussed in “ Results ” section.
The risk of bias in individual studies was assessed via the scale of the study. Spatial and temporal constraints were set (already defined in the eligibility phase) to ensure that we analyse medium to large scale studies and that findings are not tied to specific locality or seasonality characteristics. Furthermore, we did not identify duplicate publications (i.e., two or more papers with the same samples and experiments) and did not identify study peculiarities, such as special and uncommon features or research topics.
Last, the risk of bias across studies was assessed via an online survey. We contacted the authors of the publications (in some cases we could not identify contact details) and ask them to respond to a short survey regarding the results of their paper. The introductory email defined the bias across studies as “ Bias across studies may result from non - publication of full studies (publication bias) and selective publication of results (i.e., selective reporting within studies) and is an important risk of bias to a systematic review and meta - analysis” . Then, we explained the content of the survey that is to identify, if there are non-reported results that are considerably different from the ones in their papers. This information was assessed via two questions (for further details we added the questionnaire as a Additional file 1 of this paper). Out of the 32 papers, we received responses for 11 papers ( n = 12, with two authors responding to the same paper). One factor that explains the low response rate is that many authors have changed positions (papers date back to 2001) and for some we could not identify their new contact details, while for others we received several non-delivery email responses.
Regarding the responses’ results, seven authors responded that they never conducted a similar study to the one for which they were contacted for and five responded that they have conducted a similar study to the one for which they were contacted. A similar study was defined as a study in which: (a) the study design, selection of independent variables/predictors, selection of method(s), and parametrization of a method(s) are the same, and (b) data can be different. From those who performed a similar study four responded that their results were not different and one responded that their results were considerably different. However, in a follow-up explanatory answer, this author responded that changing the parametrization yielded different results regarding the performance ranking of three algorithms and that the data and the study area were the same. Based on this small-scale survey there is no indication that there is a risk of bias across studies. However, further investigation of this matter is needed.
Study characteristics
In this section, we discuss generic characteristics of the selected papers. To start with, the type of publication is slightly higher for ISI journal articles (n = 18) than for conference papers (n = 14). The 32 papers were published in a variety of journals and conferences and no preference was observed for a particular publication outlet. In specific, four journals and one conference published two or three of the selected papers each (Table 2 ) and all other papers were published in different journals and conferences. On the other hand, there is little variation regarding the country of the study area. The majority of studies were conducted in the US, which is probably a somewhat biased statistic, considering the large US population size, as well as the used language (e.g., English) of the study selection process. Similarly, institutions that have published more than one paper on spatial crime forecasting are based in the US with the exception of the Federal University of Rio Grande do Norte, Brazil, that has recent publications in this field.
We also collected the discipline associated with each paper. To do so we used the affiliation of the first author and extracted the discipline down to the department level, if this was possible. Then we used as a benchmark reference the 236 categories/disciplines used in Journal Citation Reports (JCR) Footnote 1 by the Web of Science Group. Each affiliation of authors was then matched to one of the categories. In Table 2 , we see the disciplines that appeared more than one time (i.e., computer science, criminology, public administration, and geosciences). Although we collected a variety of disciplines these are the ones that we encountered more than once and account for the majority of the papers ( n = 22). Thus scholars of these disciplines seem to have a greater interest in spatial crime forecasting.
Figure 2 shows the number of eligible and selected articles by year during the study selection period. We included the eligible in addition to the selected papers for two reasons. First, many of the eligible papers looked into spatial crime forecasting but did not meet the criteria defined for this study. Second, other papers may not be relevant to forecasting, but are relevant to the broader topics of prediction or modelling. The graph in Fig. 2 depicts a rapidly increasing trend over the last couple of years. For the eligible papers, the number of articles increased substantially since 2013, whereas for the selected papers, a similar trend is evident in the last 2 years.
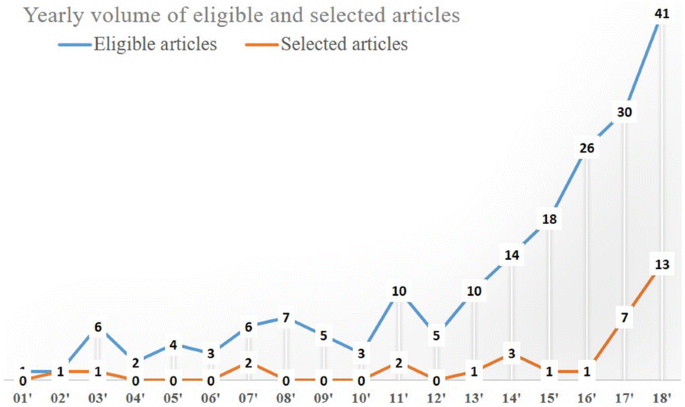
A yearly count of eligible and selected papers from 2001 to 2018
Overview of selected publications on spatial crime forecasting
In Table 3 we enlist each selected paper along with information related to space (i.e., study area and spatial scale), time (i.e., sampling period and period in months), crime data (i.e., crime type and crime sample size), and prediction (i.e., predicted information, task, spatial unit, and temporal unit). In this section, we consider these 10 data items as initial and basic information when reporting a spatial crime forecasting study. A reader who may want to replicate or modify the methodological approach presented in the follow-up research will require the same 10 data items to assess whether such approach is adequate to the author’s follow-up study and research objectives. More importantly, when any of these data items are missing an assessment of the generalizability (or bias) of the conclusions and interpretation of results is limited. Unfortunately, the majority of the 32 selected papers (n = 21) had at least one item with undefined or unclear information for five out of the 10 data items (Fig. 3 ). From these, 52% (n = 11) were conference papers and 48% (n = 10) were ISI articles. On the other hand, 73% (n = 8) of the papers with no undefined or no unclear information were ISI papers and 27% (n = 3) were conference papers.
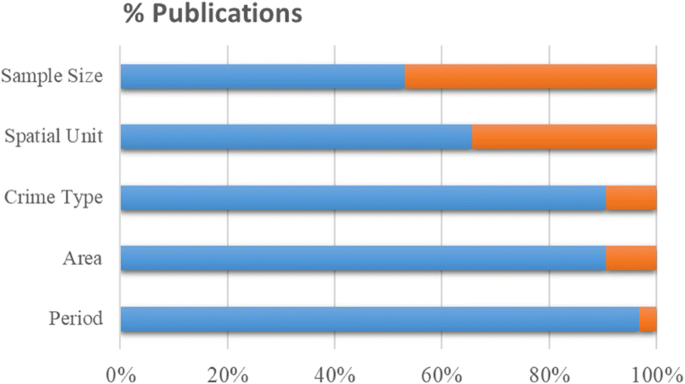
Percentages of all publications (n = 32) for describing basic information when reporting a spatial crime forecasting study. Blue: the item was properly defined; orange: the item was poorly defined or undefined
Most of the studies were conducted at the city level. In two studies, the forecasting area covered a county, which is the US administrative unit that usually expands across a city’s boundary. In one paper, predictions covered an entire country (US). New York City (US) was examined in four studies, Pittsburgh (US) was examined in three studies, and Portland (US), Natal (Brazil), and Chicago (US), were examined in two studies. All other publications were based on individual study areas.
The oldest crime data that were used in the 32 papers are from the year 1960 and the most recent crime data are from 2018. The sampling period ranges from 1 year up to 50 years. There is one paper with an undefined sampling period. However, from the article it can be inferred that the length of the sampling period is at least 1 year. Regarding the sample size of the crime data, it ranges from about 1400 incidents up to 6.6 million, which is relevant to the number of crime types as well as to the length of the sampling period. As for the number of crime types, there are four studies that aggregated and analysed all crime types together, twelve studies that focused on a particular crime type, fourteen studies that looked from two to up to 34 different crime types, and three studies with undefined information on the crime type analysed. Residential burglary was the crime type that was most often examined in studies that looked into only one crime type.
The last four data items in Table 3 describe details of the forecasted information, which we refer to as “inference”. The temporal unit is the time granularity of the inference and ranges from a fine granularity of 3 h up to 1 year. The most frequent temporal unit across all papers is 1 month (used in 12 papers). In addition, day and week have been used in eight studies and years in seven studies. Other less frequent temporal units are 3 h, daytime for 1 month, night-time for 1 month, 2 weeks, 2 months, 3 months, and 6 months. Similarly, the spatial unit is the space granularity of the inference and ranges from a small area of 75 m × 75 m grid cell size to a large area, such as police precincts or even countries. The spatial unit is difficult to analyse and to compare for two reasons. First, spatial units do not have a standard format like time and are two-dimensional. Thus, they can vary in size and shape. Second, for about one-third of the papers this information was poorly reported (Fig. 3 ). In the case of administrative units (e.g., census blocks or districts), the shape and size usually vary, but if someone is looking for further details or the units themselves, these can be in most cases retrieved by administrative authorities. However, spatial clusters may also vary in shape and size, but if details are not reported (e.g., the direction of ellipses, the range of clusters’ size, the average size of clusters) it is difficult to quantify and replicate such clusters. We also encountered cases where authors report dimensions of a grid cell size without mentioning the units of measurement. Nevertheless, the grid cell seems to be the preferable type of spatial unit and it is used in the majority of papers (n = 20).
The data items “inference” and “task” refer to the types of forecasted information and predictive task, respectively. Inference and task are defined according to the information that the authors evaluated and not to the output of a prediction algorithm. For example, an algorithm may derive crime intensity in space (i.e. the algorithm’s output), which the authors used to extract hotspots (i.e. processed output to be evaluated) from and then evaluate their results using a classification measure such as precision, accuracy, or others. Some predictive methods, such as random forest, can be used for both classification and regression tasks. It is unclear why some authors choose to apply a regression application of a method and then process, derive, and evaluate a classification output, although they could do this by directly applying a classification application of the same method. In addition, the inference “hotspots” in Table 3 includes the next four categories:
Concerning categories three and four, some authors refer to these areas as hotspots and others do not. We group all four categories together and define them as hotspots and non-hotspots, representing the output of a binary classification that separates space into areas for police patrolling that are alarming and non-alarming. We acknowledge that in the field of spatial crime analysis, hotspots are areas of high crime intensity. However, in our selected papers there does not seem to be a clear definition of the term “hotspot”.
The majority of the papers (n = 20) inferred hotspots as the outcome of a binary classification. Nine studies inferred the number of crimes or the crime rate in each spatial unit. However, three studies appear to be somehow different and unique from the rest. Huang et al. ( 2018 ) evaluated the forecasted category of crime as the output of a binary classification problem (e.g., is category A present in area X; yes or no). Ivaha et al. ( 2007 ) inferred the total number of crimes in a region, spatial clusters (or hotspots), and the share of crime within each cluster. Last, Rodríguez et al. ( 2017 ) evaluated the properties (i.e., location and size) of inferred clusters.
Spatial crime forecasting methods
The first three data items that were extracted to be analysed in this section are the proposed forecasting method, best proposed forecasting method , and the baseline forecasting method . The latter is the method used as a comparison measure of the proposed method. We analysed the frequency of the methods for each of the three forecasting types. The best proposed forecasting method is the one with the best performance throughout the conducted experiments. For example, if an experiment is evaluated separately on multiple types of crimes, we only count the method with the best performance for most cases. In case two methods perform similarly (as evidenced by statistical results and reported by the authors of the papers), both methods are considered. This was necessary because some papers proposed more than one method to be compared with a baseline method, but in the end, these papers propose the method with the best performance. In addition, this reduces biased frequency counts of proposed methods. On the other hand, we considered as a baseline the method, with which the authors wanted to compare the proposed methods. For instance, Zhuang et al. (2018) proposed three Deep Neural Networks and used an additional six machine learning algorithms as baseline methods to assess how much better the proposed methods were compared to the baseline methods. In this case, we counted the six machine learning algorithms as the baseline methods.
In Table 4 , we show “top” methods (i.e., frequently counted within the 32 selected papers) by each item. Random Forest (RF) is the most frequently used proposed method. Multilayer Perceptron (MLP) appears as a top method in all three items (i.e., proposed, best, baseline). Other best proposed methods are Kernel Density Estimation (KDE)-based and Risk Terrain Modelling (RTM). Interestingly, Support Vector Machines (SVM) have been proposed in five papers, but are not among the top four best-proposed methods. On the other hand, plenty well-known statistical models, are preferred as baseline methods, such as Autoregressive model (AR)-based, Logistic Regression, Autoregressive Integrated Moving Average model (ARIMA), and Linear Regression, as well as KDE-based and K Nearest Neighbours. In Additional file 1 C we added detailed tables that show for each paper the data items proposed method, best proposed method, and baseline method.
In the next sections, we categorize the proposed forecasting methods by type of algorithm (“ Algorithm type of proposed forecasting methods ” section) and by type of inputs they take (“ Proposed method input ” section). This task was challenging because there is no scientific consensus on a single taxonomy or categorization of analytical techniques and methods. Papamitsiou and Economides ( 2014 ) reviewed papers in educational analytics, categorizing data mining methods into classification, clustering, regression, text mining, association rule mining, social network analysis, “discovery with models”, visualization, and statistics. Other researchers would summarize all of these categories, for instance, as supervised learning, non-supervised learning, and exploratory data analysis. Vlahogianni et al. ( 2014 ) use different categorizations for reviewed papers in traffic forecasting, including aspects related to the model’s approach to treating inputs and other properties relevant to split the proposed methodologies. The right granularity of properties to define a useful categorization can be problematic and particular for each field.
Algorithm type of proposed forecasting methods
Another suitable characteristic to classify forecasting methods is the similarities between algorithms. We divide all algorithms used in the reported papers into (i) kernel-based (ii) point process, (iii) traditional machine learning, and (iv) deep learning, according to the following criteria. Kernel-based algorithms are particularly concerned with finding a curve of crime rate \(\lambda\) for each place \(g\) that fits a subset of data points within the boundaries of a given kernel (see Eq. 1 ). We observe that the main difference among kernel-based algorithms is the use of different kernel types. Hart and Zandbergen ( 2014 ) experimented with different kernel types, providing some useful conclusions. In our selected papers, six of them have used kernel-based algorithms with the most frequently used the simple two-dimensional Kernel Density Estimation (KDE) (n = 2). However, we observed that some methods are a variation from the simple KDE model, in the form of the Spatio-Temporal KDE (STKDE) used in the paper by Hu et al. ( 2018 ), the Network-Time KDE (NTKDE) proposed by Rosser et al. ( 2017 ), or the dynamic-covariance KDE (DCKDE) proposed by Malik et al. ( 2014 ). We also have considered the Exponential Smoothing method used in the paper of Gorr et al. ( 2003 ) as a kernel-based algorithm, since it works with a window function (kernel) on time series aggregation.
Point processes can be distinguished from kernel-based algorithms insofar as a background rate factor \(\mu\) that can be calculated stochastically, such as with a Poisson process, is considered. The background rate factor includes the modelling of covariates or features of the place \(g\) , such as demographic, economical, geographical, etc. variables (see Eq. 2 ). From the explanation made by Mohler ( 2014 ), we suppose that the introduction of the background rate makes the point process more suitable for multivariate modelling when compared to kernel-based algorithms. In the reviewed papers, algorithms can be distinguished among each other based on their mathematical formulations of \(\kappa\) and \(\mu\) , but also on their internal parameter selection, mostly based on likelihood maximization. Only three papers proposed such an algorithm, including the Marked Point Process from Mohler ( 2014 ), the maximum likelihood efficient importance sampling (ML-EIS) from Liesenfeld et al. ( 2017 ), and the Hawkes Process from Mohler et al. ( 2018 ).
In the case of machine learning algorithms, their formulation is often associated with finding a function \(f\) that maps feature vectors X to a given output Y. These algorithms are distinguished from each other in the way this function is estimated, some being more accurate and complex than others. We include in this category all algorithms that are explicitly associated with regression or classification. They differ from algorithms of previous categories, because \(f\) is constructed only after a training process. This training step aims to find a model that minimizes the estimation error between the predicted output and the original output. The majority of the reported papers (n = 20) was included in this class of algorithms. The most proposed traditional machine learning algorithms were RF and MLP (tied at n = 6), followed by SVM together with Logistic Regression (n = 4), and Negative Binomial Regression used in RTM studies together with (n = 3).
Although deep learning algorithms have the same formulation as traditional machine learning algorithms, they present a much more complex internal structure that affects their use. The deep layer structure makes the computational budget mainly needed during training. Additionally, the need for samples is also greater, than for the other approaches. Among the reported papers, the three that have used this type of algorithm argue that it has the best overall performance. This includes the Deep Neural Networks (DNN) fitted by Lin et al. ( 2018 ), the DeepCrime framework from Huang et al. ( 2018 ), and the Long Short-Term Memory (LSTM) architecture proposed by Zhuang et al. ( 2017 ). The paper by Huang et al. ( 2018 ) even presents a neural architecture dedicated to a feature-independent approach, with a recurrent layer dedicated to encoding the temporal dependencies directly from the criminal occurrences. Still, none of these papers has discussed computational time performance against other algorithms, nor sample sizes sufficient to obtain accurate models. At the time of this writing, we argue that there is no clear guidance on when one should conduct a deep neural networks approach, although in recent years evidence of its effectiveness has begun to emerge.
Proposed method input
Another split factor is the inputs of the forecasting methods, i.e. the independent variables. There are some forecasting methods that accept as input the latitude, longitude, and timestamp of criminal events (raw data), while others need to apply explicit aggregations or transformations before feeding their models. In this paper, we refer as feature engineering the process of crafting, scaling and selecting covariates or features to better explain a prediction variable which often requires considerable domain-specific knowledge. An example is the aggregation of criminal events into spatiotemporal series, which can be decomposed into autoregressive lags and used as features. This feature engineering can also be applied to ancillary data methodologies not directly related to crime. For instance, Lin et al. ( 2018 ) count landmarks on the grid by counting the number of items in each cell (spatial aggregation) and craft a new feature for each landmark type, while Huang et al. ( 2018 ) define a part of their algorithm being a region embedding layer for only processing the raw location of the city’s landmarks. We believe that the split factor by method inputs may be useful information for a potential researcher who wishes to perform spatial forecasting and consults this section of our paper. Data processing requires domain knowledge, and it is an expensive (timewise) task, especially when dealing with large spatiotemporal datasets. Thus, avoiding the feature-engineering process may be preferable by some researchers. On the other hand, one may prefer to use data to derive their variables with particular patterns.
We call methods that have an internal approach to aggregating crime events into spatiotemporal variables “feature-engineering independent” and “feature-engineering dependent”. In other words, these methods explicitly need aggregations to derive spatiotemporal variables from the raw data independently of the forecasting algorithm. The majority (n = 24) of reported papers have an explicit way to transform their crime events, as well as ancillary data, into features to feed their algorithms (i.e., feature-engineering dependent). Although we have found many different forms of data aggregation into features, both spatially and temporally, the procedure of assigning features is often insufficiently reported, making it difficult to reproduce the proposed methodology. Still, well-defined workflows or frameworks followed by feature-engineering dependent methods were detailed in Malik et al. ( 2014 ) and Araújo et al. ( 2018 ). They synthesized their forecasting methods in (1) aggregate raw data spatially, following a crime mapping methodology (e.g., counting events inside grid cells), (2) generate time series and their features, (3) fit a forecasting model using an algorithm, and (4) visualize the results. In feature-engineering dependent methods the aggregation and time series generation is done separately as processing steps before fitting a model, whereas this is not needed for the feature-engineering independent methods.
Considerations when analysing forecasting performance
In this section, we look at measures of forecasting performance (“ Overview of evaluation metrics ” section) and discuss which are used for each forecasting task, including for classification and regression (“ Metrics by forecasting task ” section). Then, we explore validation strategies by types of algorithms (“ Algorithms and validation strategies ” section). Finally, we summarize and discuss the main dependencies and limitations of the above subsections (“ Dependencies and limitations ” section).
Overview of evaluation metrics
As mentioned in “ Spatial crime forecasting methods ” section, selected papers include forecasting baseline models, novel models, or ensemble models proposed by respective authors. Evaluation metrics of such models are in general, well-known in criminology, GIScience, mathematics, or statistics. However, it is important to mention that few authors highlight the necessity of combining or using diverse evaluation metrics.
We cannot make a comparison of all evaluation results across the 32 papers due to various reasons, such as different spatial and temporal units, study areas, or forecasting methods applied. Yet, we can discuss certain similarities between them. Choosing an evaluation metric is highly dependent on the main prediction outcome, such as counts (e.g., for a Poisson distribution), normalized values or rates (e.g., for a continuous Gaussian distribution), or binary classification (crime is absent or present). The most frequent evaluation metrics used in the selected papers are the Prediction Accuracy (PA, n = 9), followed by the Prediction Accuracy Index (PAI, n = 7), the F1-Score (n = 7), Precision and Recall (n = 5), the Mean Squared Error (MSE, n = 4), the Root Mean Squared Error (RMSE, n = 3), R-squared (n = 3), the Recapture Rate Index (RRI, n = 3), the Hit Rate (n = 2), the Area Under the Curve (AUC, n = 2), and the Mean Absolute Forecast Error (MAFE, n = 2). Some additional metrics are used only once, namely the Spatio-Temporal Mean Root Square Estimate (STMRSE), the average RMSE (RMSE), the Regression Accuracy Score (RAS), the Regression Precision Score (RPS), the Ljung-Box test, the Mean Absolute Error (MAE), the Mean Absolute Percentage Error (MAPE), macro-F1, micro-F1, the Mean (Squared) Forecast Error (M(S)FE), the Pearson Correlation Coefficient, and the Nash coefficient. Generally, metrics derived from the confusion matrix, namely accuracy, precision, recall, and F1-Score, are used together to evaluate binary classifications.
We analysed the top three evaluation metrics (PA, PAI, F1-Score) in relation to their distribution among the data items of discipline, proposed forecasting algorithm type, forecasting inference, forecasting task, spatial unit, and temporal unit. Interestingly, we found that computer scientists exclusively use the PA, while criminologists prefer to apply the PAI. In addition, while the PA and the F1-Score have been preferably tested for short-term predictions (i.e., less or equal to 3 months), the PAI has been used for both short and long-term predictions. No other obvious pattern was detected among the other information elements regarding the usage and preference of these evaluation metrics.
Metrics by forecasting task
The most common forecasting task is binary classification (n = 21) for crime hotspots (n = 20) and the category of crime (n = 1). While the classification task is frequently discussed at the beginning of experiments, some articles consider in the performance evaluation a different item than in the output of the algorithm, thus transforming regression products into binary values. The most prominent examples include RTM models (Drawve et al. 2016 ; Dugato et al. 2018 ; Gimenez-Santana et al. 2018 ), where the output of the algorithm is a risk score. This score is later reclassified into a binary outcome (a positive or negative risk score) for the purpose of the evaluation. In addition, Rummens et al. ( 2017 ) propose a combined ensemble model consisting of LR and MLP that infers risk values, similar to RTM, where authors consider as crime hotspot, values with a risk higher than 20%.
The regression task (n = 11) is largely used for predicting the number of crimes (n = 8) and the performance is measured by various error measurements, such as MSE (n = 4) or RMSE (n = 3). Araujo et al. ( 2017 ) propose two new evaluation metrics, namely the Regression Accuracy Score (RAS), representing the percentage of success in predicting a sample, and the Regression Precision Score (RPS), which defines the RAS’s precision. The RPS measures the MSE of success samples normalized by the variance of the training sample (Araujo et al. ( 2017 )). Rodríguez et al. ( 2017 ) introduce the Nash–Sutcliffe Efficiency (NSE), which they derive from hydrological models forecasting, as a normalized statistic determining the relative magnitude of the residual variance compared to the measured data variance.
However, the number of crimes is not the only inference considered in regression models. For example, Ivaha et al. ( 2007 ) predict the percentage of crime in clusters, using spatial ellipses as spatial units, Rodríguez et al. ( 2017 ) investigate properties of clusters, while Shoesmith ( 2013 ) infers crime rates from historical crime data.
In addition to the above-mentioned evaluation metrics, three articles discuss surveillance plots for prediction evaluation. Mohler ( 2014 ) uses a surveillance plot metric showing the fraction of crime predicted over a time period versus the number of grid cells with real crimes for each day (Fig. 4 a). The same author mentions that this metric is similar to the receiver operating characteristic curve, or ROC curve, applied by Gorr ( 2009 ), but differs because it is not using an associated false positive rate on the x-axis. Similarly, Hu et al. ( 2018 ) apply the PAI curve, also a surveillance plot showing the area percentage on the x-axis, and the PAI or the hit rate value on the y-axis (Fig. 4 b, c). Similarly, Rosser et al. ( 2017 ) use hit rate surveillance plots, representing the mean hit rate against the coverage for the network and grid-based prediction approaches (Fig. 4 c). These plots are highly useful to visualize metrics’ values over the surveyed territory.
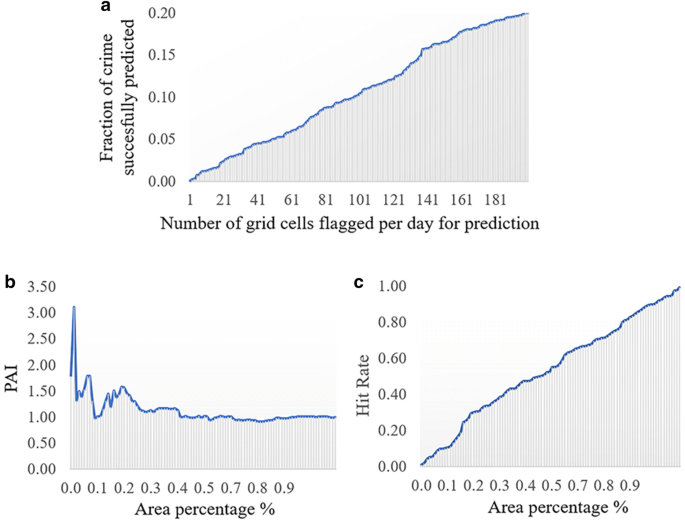
Comparable surveillance plots for evaluation metrics visualization in space (using dummy data). a ROC-like accuracy curve, b PAI curve, and c Hit rate curve
Algorithms and validation strategies
As mentioned in “ Spatial crime forecasting methods ” section, in many of the papers, the proposed forecasting method does not include a novel algorithm, but mostly applies new variables that have not previously been used before. When reminding us of the four types of algorithms, namely (i) kernel-based, (ii) point process, (iii) traditional machine learning, and (iv) deep learning, we note a diversity between the proposed forecasting and the baseline methods. In addition, validation strategies are diverse, as well. Half of the studies (n = 16) consider splitting the data into training and testing subsets. Most of these studies include 70% training (current) for 30% testing (future) sets. Johansson et al. ( 2015 ) use a combined approach, including rolling horizon, which is producing ten times the size of a sample for the KDE algorithm, containing 70% of the original crime dataset (keeping the 70/30 ratio). The final result is calculated as the mean of the ten measurements. Figure 5 gives a good overview of all algorithms and their validation strategies. This decision tree visualization includes five central data items, namely prediction task, proposed input forecasting method, proposed forecasting algorithm type, validation strategy, and evaluation metrics. Classification m refers to those evaluation metrics that are particularly used for classification tasks (e.g., PA, F1-score). Regression m is a composition of error metrics for regression analysis (e.g., MSE, RMSE, MAE), while Criminology m includes crime analysis metrics (e.g., PAI, RRI).
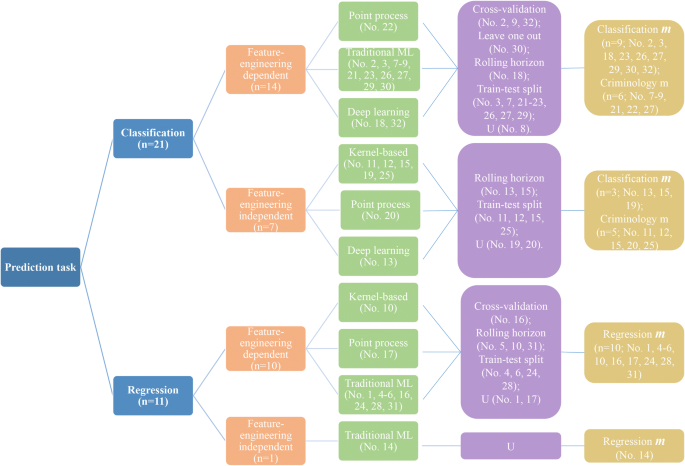
Overview of forecasting methods (see “ Spatial crime forecasting methods ” section) and their performance evaluation (see “ Considerations when analysing forecasting performance ” section) linked to the 32 selected papers. The papers’ references linked to their number are shown in Table 3 . The letter m denotes an evaluation metric. The letter “U” denotes an undefined item
Kernel-based algorithms are preferably used to predicting hotspots (n = 5) and the number of crimes (n = 1). Interestingly, Malik et al. ( 2014 ) bring into discussion the fact that regions with similar demographics tend to illustrate similar trends for certain crime types. This observation is included in their prediction model “Dynamic Covariance Kernel Density Estimation method (DSKDE)” and compared with the “Seasonal Trend decomposition based on Loess (STL)” baseline model. Hart and Zandbergen ( 2014 ) and Johansson et al. ( 2015 ) use a kernel-based KDE approach without comparing it with a baseline method, both considering the PAI as one of the evaluation metrics. Only two of the kernel-based studies consider ancillary data (Gorr et al. 2003 ; Rosser et al. 2017 ), yet both use different validation strategies (rolling-horizon and train-test split, respectively) and evaluation metrics (MAE, MSE, MAPE in the first publication and Hit Rate in the second publication). Thus, it is worth noting that, while using the same base algorithm, such as KDE, other components of the prediction process may be different.
Two out of three point process algorithms do not explain the validation strategy followed in the studies (Liesenfeld et al. 2017 ; Mohler 2014 ). Mohler ( 2014 ) shows an interesting point process approach using only historical crime data, capturing both short-term and long-term patterns of crime risk. This article includes the surveillance plot evaluation (see “ Metrics by forecasting task ” subsection), comparing chronic and dynamic hotspot components for homicides and all crime types.
The third category of forecasting algorithms, the traditional ML, is split up almost equally between classification and regression tasks. Only three articles discussing traditional ML algorithms do not mention information about the baseline comparison (Araújo et al. 2018 ; Rodríguez et al. 2017 ; Rummens et al. 2017 ). The majority of ML algorithms (n = 11) use the training–testing split validation strategy applied to the classification task. Interestingly, one of the articles (Yu et al. 2011 ) discusses a different validation approach, the “Leave-One-Month-Out” (LOMO), where instead of running the classification only once on the training and testing data sets, it is run on S − 1 sets (S = number of sets/months).
An increasing body of forecasting techniques are based on DL, however, for this review, we include only three articles, with all of them for short-term prediction and coming from the computer science discipline (Huang et al. 2018 ; Lin, Yen, and Yu 2018 ; Zhuang et al. 2017 ). Two of the three articles consider geographic ancillary variables and apply the rolling-horizon validation strategy, while the third article deals only with crime lags following a 10-fold cross-validation approach. All three articles consider a binary classification evaluated by metrics such as the PA and the F1-score. Zhuang et al. ( 2017 ) propose a spatio-temporal neural network (STNN) for forecasting crime occurrences, while embedding spatial information. They then compare the STNN with three state-of-the-art methods, including the Recurrent Neural Network (RNN), the Long Short-Term Memory (LSTM), and the Gated Recurrent Unit (GRU). Since the model is designed for all types of crime data, each crime type can lead to different performances of the STNN due to their variability in time and space. Presumably, challenges will appear for crime types with low data volumes, because neural networks require a sufficient amount of data for training.
Dependencies and limitations
Although most papers use standard evaluation metrics, such as PA for a binary outcome, they usually do not include complementary metrics, in order to ensure that every aspect of the prediction performance is covered. Often, the PA is used by itself to measure model performance (Araújo et al. 2018 ; Malik et al. 2014 ; Mu et al. 2011 ). Complementary metrics are needed, because whilst one method may have a higher evaluation score than others, they may provide additional information. For example, while showing a high PAI, the Prediction Efficiency Index (PEI) value (Hunt 2016 ) may be reduced. PEI is another evaluation metric that is calculated by the ratio of the PAI to the maximum possible PAI a model can achieve. The difference between the PAI and the PEI can be explained by both metrics having different dependencies on the cell size.
Complementary metrics also overcome limitations of some evaluation measures. For example, the PA is the sum of true positives and true negatives divided by the total number of instances, which represents the percentage that is classified correctly. However, this information may not be enough to judge the performance of a model, because it omits information about precision. The Hit rate and the PAI are obtained through a division, thus, when the denominator is small, both metrics are high. Consequently, when crime occurrences are low, results are heavily affected.
Furthermore, traditional metrics are global in nature, but in spatial prediction or forecasting, we are also interested in the spatial distribution of the prediction. There may be local areas of good and bad prediction performance, resulting in an average global value. A complementary metric for a regression outcome could be to calculate the Moran’s I of the prediction error and explore the variation of the predictive performance throughout the study area. Ideally, the prediction error should follow a random spatial distribution. Overall, we find a low to no interest in developing (local) spatial, temporal, or spatiotemporal evaluation metrics.
The relevance of evaluation metrics may be biased for various reasons. One example can be the class imbalance. A model can have high accuracy while predicting the locations without crime very well. In contrast, locations with crimes are not well forecasted. Some authors try to ameliorate the negative–positive ratio between crime and no crime cells, by adjusting the weight of hotspots and cold spots (Yu et al. 2011 ), or change the training set, while the test set keeps its original, real data (Rumi et al. 2018 ). Another dependency is the different kinds of aggregation that take place during modelling by time, space, or crime types attributes. For instance, while the majority of papers report to work with disaggregated crime types, some of them consider to aggregate crime types to, e.g., “violent crimes”, without specifying which types are included. In addition, the effects spatiotemporal aggregations have on the forecasting performance are typically not analysed, but could easily be conducted with a sensitivity analysis.
In this section, we perform a SWOT analysis of the most significant findings.
One of the strongest elements of current research efforts is the incorporation of spatial or spatiotemporal information into traditional prediction algorithms. The examples of this approach is STAR and STKDE (Shoesmith 2013 ; Rosser et al. 2017 ). Also, KDE, a traditional method in the field, has been adapted to consider sampling problems, such as sparse data (DCKDE) and grid format (NTKDE) (Malik et al. 2014 ; Rosser et al. 2017 ). Besides, the interest of the scientific community in the incorporation and effect of big data into prediction is evident from the related work section. This interest is also supported by the trend of introducing dynamic variables into the modelling process, such as calculating visitor entropy from Foursquare or ambient population from social networks and transportation. Regarding the performance evaluation, surveillance plots (Fig. 4 ) provide a more detailed picture of the accuracy of the forecasted information. Since they include the area coverage on the x-axis, they can be used by the police as a decision tool to identify the threshold that balances prediction accuracy with the size of patrolling areas.
Overall, significant details of study experiments are not always reported and commonly undefined items are the spatial unit of analysis and the sample size. Similarly, for feature-engineering dependent methods the crafting procedures are not sufficiently described. The above elements make a study difficult to reproduce or to compare its results with a possible future study. Furthermore, we did not find any open source tools that implement spatial crime forecasting using the best-proposed methods reported. Such a tool could enhance the possibility of reproducing results from an existing forecasting study. We suggest that all data items analysed in “ Overview of selected publications on spatial crime forecasting ” section (for an overview have a look at Table 3 ) should always be reported. However, a detailed “spatial forecasting protocol” could be developed similarly to protocols for other modelling approaches such as the ODD protocol (Grimm et al. 2010 ). Furthermore, the most common spatial unit is the grid cell, which may not necessarily align with places that policing resources are typically deployed to. So far, we did not encounter a study that sufficiently addresses this issue. Regarding the performance evaluation, most authors use standard metrics. A “global” standard metric, such as MAE, cannot describe the distribution of the prediction error across space, which can vary a lot. We thus propose to develop novel local spatial or spatiotemporal evaluation metrics. Finally, other modelling issues are hardly discussed, if at all, such as overfitting, multi-collinearity, sampling bias, and data sparsity.
Opportunities
There is a tremendous increase in spatial crime forecasting studies. From the pool of the 32 selected papers, 7 and 11 papers were published in 2017 and 2018, respectively, compared to about one paper per year between 2000 and 2016 (Fig. 2 ). This shows the growing interest of scholars from varying disciplines (compare Table 2 ) into this kind of research. The crime type that has been studied the most is residential burglary. It is unclear why this particular crime type and property crimes, in general, are more likely to be studied. A future opportunity could be to systematically test whether there is a pattern of property crimes to consistently outperforming other crime types and why. Furthermore, except for RTM and KDE, other spatial methods mentioned in the related work section (“ Related work ” section) were not used by the selected papers. The reason may be that authors have varying backgrounds, such as computer science and criminology, and may not be familiar with such methods. This opens a research opportunity to explore and compare less used spatial methods with traditional approaches, such as RTM or KDE. Another opportunity would be to compare the forecasting performance of the methods among each other. In this review, we presented methodological trends, but a fair comparison among spatial methods was not possible. First, some methods were not compared to a baseline method. Other authors compared the same method with a different set of features. Even if there were papers with a similar set of features a comparison among them would be biased due to variations of sample data, study areas, sampling periods, etc. Future empirical studies should focus on the comparison of algorithms, of which the number is constantly increasing. We merged the selected papers into four categories of forecasting algorithms, including the kernel-based, point processes, traditional machine learning, and deep learning. Traditional machine learning algorithms were present in most proposed methods, with MLP and RF being the most common ones, while AR models were the most used baselines methods. A suggestion is to compare new or recently developed algorithms to the most frequently proposed ones, instead of continuing to conduct further comparisons with traditional or simpler methods that have repeatedly shown to underperform.
We outlined that spatial crime forecasting studies lack coherent terminology, especially for terms such as “prediction”, “forecasting”, and “hotspots”. The predominant predictive task is the binary classification (n = 21) and the predominant forecasting inference is hotspots (n = 20). It is important to understand the rationale behind this trend. Is regression analysis less useful or more difficult to predict? Although we notice a constant increase in developing classification algorithms or features to be infused in the classification task, we acknowledge the importance of both prediction tasks. Also, for the display of an area’s crime picture, it is important to examine both hotspots and coldspots or a multiclass classification towards the hottest crime spots. However, none of these was the focus of the examined papers. We acknowledge that forecasting hotspots is important for police to allocate resources. Nevertheless, what about the information that can be derived by other types of spatial groupings such as coldspots, coldspot outliers, or hotspot outliers, commonly referred to as LL, LH, HL (low–low, low–high, high-low, respectively) and calculated by the local Moran statistic (Anselin 2005 )? Science needs to progress knowledge, which requires understanding and examining all aspects of a phenomenon. Finally, only a third of all papers performed long-term predictions. Although this trend is positive because law enforcement has an interest in almost real-time prediction, the long-term prediction should not be overlooked as playing an important role in the understanding of the crime risk and providing a broad picture for strategic planning.
In this paper, we focus on “Spatial Crime Forecasting”, which is an inference approach about crime both in time and in space. We conducted a systematic literature review that follows the reporting guidance “PRISMA” (Liberati et al. 2009 ) to understand and evaluate the state of the art concerning concepts and methods in empirical studies on crime with many applications and special attention to crime. We addressed several research questions that deal with the role of space in the forecasting procedure, the methods used, the predictive performance, and finally model validation strategies.
We identified five types of inference, namely (1) hotspots (the majority of the papers), (2) number of crime, (3) crime rate, (4) category of crime, (5) percent of crime in clusters, and (6), properties of clusters. With regards to forecasting methods, the authors proposed mostly traditional machine learning methods, but also kernel density estimation based approaches, and less frequently point process and deep learning approaches. When it comes to measuring performance, a plethora of metrics were used with the top three ones being the Prediction Accuracy, followed by the Prediction Accuracy Index, and the F1-Score. Finally, the most common validation approach was the train-test split while other approaches include the cross-validation, the leave one out, and the rolling horizon.
This study was driven by the increasing publication of spatial crime forecasting studies and (crime predictive analytics in general). More than half of the selected papers (n = 32) were published in the last 2 years. In specific, about one paper per year was published between 2000 and 2016, while 7 and 11 papers were published in 2017 and 2018, respectively. At the same time, there is a global growth of scientific publication outputs. Bornmann and Mutz ( 2015 ), fitted an exponential model to this growth and calculated an increasing rate of outputs of about 3% annually, while the volume is estimated to double in approximately 24 years. Yet the yearly patterns of the selected papers show a much greater increase that indicates the importance and future potential of studies related to spatial crime forecasting.
Furthermore, we would like to outline the main limitations that may prohibit reproducibility, and hence the advancement of this topic in the long term. First, the terminology being used is not consistent possibly due to the fact that scientists working on this topic have various backgrounds (e.g. criminology, computer science, geosciences, public policy, etc.). Second, significant details of study experiments are vaguely or not at all reported. With respect to the last point, we suggested reporting the following data items: study area, scale, sampling period, months, type, sample, inference, task, spatial unit, and temporal unit (in total 10 items). Additional items to be reported are proposed method, best - proposed method, baseline method, evaluation metrics, and validation strategy (in total 5 items).
Availability of data and materials
The list of manuscripts used for this research is mentioned in Table 3 . If needed, the authors can provide the list of 193 manuscripts that went through the eligibility phase.
JCR: https://clarivate.com/webofsciencegroup/solutions/journal-citation-reports/ .
Al Boni, M., & Gerber, M. S. (2016). Predicting crime with routine activity patterns inferred from social media. In IEEE International Conference on Systems, Man and Cybernetics (SMC) , (pp. 1233–1238). https://ieeexplore.ieee.org/stamp/stamp.jsp?arnumber=7844410 .
Anselin, L. (2005). Exploring spatial data with GeoDaTM: A workbook . Santa Barbara: Center for Spatially Integrated Social Science.
Google Scholar
Araújo, A., Cacho, N., Bezerra, L., Vieira, C., & Borges, J. (2018). Towards a crime hotspot detection framework for patrol planning. In 2018 IEEE 20th International Conference on High Performance Computing and Communications; IEEE 16th International Conference on Smart City; IEEE 4th International Conference on Data Science and Systems (HPCC/SmartCity/DSS) , (pp. 1256–1263). https://doi.org/10.1109/HPCC/SmartCity/DSS.2018.00211 .
Araujo, A. J., Cacho, N., Thome, A. C., Medeiros, A., & Borges, J. (2017). A predictive policing application to support patrol planning in smart cities. In International Smart Cities Conference (ISC2) . https://www.researchgate.net/profile/Adelson_Araujo2/publication/321236214_A_predictive_policing_application_to_support_patrol_planning_in_smart_cities/links/5c068339299bf169ae316a6f/A-predictive-policing-application-to-support-patrol-planning-in-smart-ci .
Bernasco, W., & Elffers, H. (2010). Statistical analysis of spatial crime data. In A. R. Piquero & D. Weisburd (Eds.), Handbook of quantitative criminology (pp. 699–724). New York: Springer. https://doi.org/10.1007/978-0-387-77650-7_33 .
Chapter Google Scholar
Bornmann, L., & Mutz, R. (2015). Growth Rates of Modern Science: A Bibliometric Analysis Based on the Number of Publications and Cited References. Journal of the Association for Information Science and Technology, 66 (11), 2215–2222.
Article Google Scholar
Bowen, D. A., Mercer Kollar, L. M., Wu, D. T., Fraser, D. A., Flood, C. E., Moore, J. C., Mays E. W. & Sumner, S. A. (2018). Ability of crime, demographic and business data to forecast areas of increased violence. International journal of injury control and safety promotion , 25 (4), 443–448. https://doi.org/10.1080/17457300.2018.1467461 .
Bramer, W. M., Rethlefsen, M. L., Kleijnen, J., & Franco, O. H. (2017). Optimal database combinations for literature searches in systematic reviews: A prospective exploratory study. Systematic Reviews, 6 (1), 245.
Brantingham, P. J., & Brantingham, P. L. (1984). Patterns in crime . New York: Macmillan.
Brayne, S. (2017). Big data surveillance: The case of policing. American Sociological Review, 82 (5), 977–1008.
Brown, D. E., & Oxford, R. B. (2001). Data mining time series with applications to crime analysis. In 2001 IEEE International Conference on Systems, Man and Cybernetics. e-Systems and e-Man for Cybernetics in Cyberspace (Cat. No. 01CH37236), Vol. 3 (pp. 1453–1458). IEEE. https://doi.org/10.1109/ICSMC.2001.973487 .
Bruinsma, G. J. N., & Johnson, S. D. (2018). The oxford handbook of environmental criminology . Oxford: Oxford University Press.
Book Google Scholar
Caplan, J. M., Kennedy, L. W., & Miller, J. (2011). Risk terrain modeling: brokering criminological theory and gis methods for crime forecasting. Justice Quarterly, 28 (2), 360–381. https://doi.org/10.1080/07418825.2010.486037 .
Chainey, S., Tompson, L., & Uhlig, S. (2008). The utility of hotspot mapping for predicting spatial patterns of crime. Security Journal., 21, 4–28.
Chauhan, C., & Sehgal, S. 2017. A review: crime analysis using data mining techniques and algorithms. In P. N. Astya, A. Swaroop, V. Sharma, M. Singh, & K Gupta, (Ed.), 2017 IEEE International Conference on Computing, Communication and Automation (ICCCA) , edited by , (pp. 21–25).
Chen, H. C., Chung, W. Y., Xu, J. J., Wang, G., Qin, Y., & Chau, M. (2004). Crime data mining: A general framework and some examples. Computer, 37 (4), 50–56. https://doi.org/10.1109/MC.2004.1297301 .
Cohen, J., Gorr, W. L., & Olligschlaeger, A. M. (2007). Leading indicators and spatial interactions: A crime‐forecasting model for proactive police deployment. Geographical Analysis , 39 (1), 105–127. https://doi.org/10.1111/j.1538-4632.2006.00697.x
Cressie, N. A. C. (1993). Statistics for spatial data ., New York: Wiley. https://doi.org/10.2307/2533238 .
Dash, S. K., Safro, I., & Srinivasamurthy, R. S. (2018). Spatio-temporal prediction of crimes using network analytic approach. In 2018 IEEE International Conference on Big Data (Big Data) (pp. 1912-1917). IEEE. https://doi.org/10.1109/BigData.2018.8622041 .
Drawve, G., Moak, S. C., & Berthelot, E. R. (2016). Predictability of gun crimes: A comparison of hot spot and risk terrain modelling techniques. Policing & Society, 26 (3), 312–331. https://doi.org/10.1080/10439463.2014.942851 .
Dugato, M., Favarin, S., & Bosisio, A. (2018). Isolating target and neighbourhood vulnerabilities in crime forecasting. European of Criminal Policy and Reserach., 24 (4 SI), 393–415. https://doi.org/10.1007/s10610-018-9385-2 .
Gerber, M. S. (2014). Predicting crime using twitter and kernel density estimation. Decision Support Systems., 61, 115–125. https://doi.org/10.1016/j.dss.2014.02.003 .
Gimenez-Santana, A., Caplan, J. M., & Drawve, G. (2018). Risk terrain modeling and socio-economic stratification: Identifying risky places for violent crime victimization in Bogota, Colombia. European of Criminal Policy and Reserach, 24 (4 SI), 417–431. https://doi.org/10.1007/s10610-018-9374-5 .
Gorr, W. L. (2009). Forecast accuracy measures for exception reporting using receiver operating characteristic curves. International Journal of Forecasting, 25 (1), 48–61.
Gorr, W., & Harries, R. (2003). Introduction to crime forecasting. International Journal of Forcasting 19. https://www.sciencedirect.com/science/article/pii/S016920700300089X .
Gorr, W., Olligschlaeger, A., & Thompson, Y. International Journal Of, and Undefined 2003. (2003). “Short-Term Forecasting of Crime.” International Journal of Forecasting . https://www.sciencedirect.com/science/article/pii/S016920700300092X .
Grimm, V., Berger, U., DeAngelis, D. L., Polhill, J. G., Giske, J., & Railsback, S. F. (2010). The ODD protocol: A review and first update. Ecological Modelling, 221 (23), 2760–2768.
Haddaway, N. R., Collins, A. M., Coughlin, D., & Kirk, S. (2015). The role of google scholar in evidence reviews and its applicability to grey literature searching. PloS ONE 10(9).
Hardyns, W., & Rummens, A. (2018). predictive policing as a new tool for law enforcement? Recent developments and challenges. European Journal on Criminal Policy and Research, 24 (3), 201–218. https://doi.org/10.1007/s10610-017-9361-2 .
Hart, T., & Zandbergen, P. (2014). Kernel density estimation and hotspot mapping examining the influence of interpolation method, grid cell size, and bandwidth on crime forecasting. Policing—An International Journal o FPolice Strategies & Management, 37 (2), 305–323. https://doi.org/10.1108/PIJPSM-04-2013-0039 .
Hassani, H., Huang, X., Silva, E. S., & Ghodsi, M. (2016). A review of data mining applications in crime. Statistical Analysis and Data Mining, 9 (3), 139–154. https://doi.org/10.1002/sam.11312 .
Holone, H. (2016). The filter bubble and its effect on online personal health information. Croatian Medical Journal, 57 (3), 298.
Hu, Y., Wang, F., Guin, C., Zhu, H. (2018). A spatio-temporal Kernel density estimation framework for predictive crime hotspot mapping and evaluation. Applied Geography 99:89–97. https://www.sciencedirect.com/science/article/pii/S0143622818300560 .
Huang, C., Zhang, J., Zheng, Y., & Chawla, N. V. (2018). DeepCrime: Attentive hierarchical recurrent networks for crime prediction. In Proceedings of the 27th ACM International Conference on Information and Knowledge Management , (pp. 1423–1432). CIKM’18. New York, NY, USA: ACM. https://doi.org/10.1145/3269206.3271793 .
Hunt, J. M. (2016). Do crime hot spots move? Exploring the effects of the modifiable areal unit problem and modifiable temporal unit problem on crime hot spot stability. American University.
Ivaha, C., Al-Madfai, H., Higgs, G., & Ware, J. A. (2007). The dynamic spatial disaggregation approach: A spatio-temporal modelling of crime. In World Congress on Engineering , (pp. 961–966). Lecture Notes in Engineering and Computer Science. http://www.iaeng.org/publication/WCE2007/WCE2007_pp961-966.pdf .
Johansson, E., Gåhlin, C., & Borg, A. (2015). Crime hotspots: An evaluation of the KDE spatial mapping technique. In 2015 European Intelligence and Security Informatics Conference , (pp. 69–74). https://doi.org/10.1109/EISIC.2015.22 .
Kadar, C., Brüngger, R. R., & Pletikosa, I. (2017). Measuring ambient population from location-based social networks to describe urban crime. In International Conference on Social Informatics , (pp. 521–35). Springer, New York.
Kadar, C., & Pletikosa, I. (2018). Mining large-scale human mobility data for long-term crime prediction. EPJ Data Science . https://doi.org/10.1140/epjds/s13688-018-0150-z .
Kennedy, L. W., & Caplan, J. M. (2012). A theory of risky places . Newark: Rutgers Center on Public Security.
Kennedy, L. W., & Dugato, M. (2018). “Forecasting crime and understanding its causes. Applying risk terrain modeling worldwide. European Journal on Criminal Policy and Research, 24 (4, SI), 345–350. https://doi.org/10.1007/s10610-018-9404-3 .
Kinney, J. B., Brantingham, P. L., Wuschke, K., Kirk, M. G., & Brantingham, P. J. (2008). Crime attractors, generators and detractors: Land use and urban crime opportunities. Built Environment, 34 (1), 62–74.
Liberati, A., Altman, D., Tetzlaff, J., Mulrow, C., Gøtzsche, P. C., Ioannidis, J. P. A., et al. (2009). The PRISMA statement for reporting systematic reviews and meta-analyses of studies that evaluate health care interventions: Explanation and elaboration. Journal of Clinical Epidemiology . https://doi.org/10.1016/j.jclinepi.2009.06.006 .
Liesenfeld, R., Richard, J. F., & Vogler, J. (2017). Likelihood-based inference and prediction in spatio-temporal panel count models for urban crimes. Journal of Applied Econometrics, 32 (3), 600–620. https://doi.org/10.1002/jae.2534 .
Lin, Y. L., Yen, M. F., & Yu, L. C. (2018). Grid-based crime prediction using geographical features. ISPRS International Journal of Geo-Information, 7 (8), 298.
Malik, A., Maciejewski, R., Towers, S., McCullough, S., & Ebert, D. S. (2014). Proactive spatiotemporal resource allocation and predictive visual analytics for community policing and law enforcement. IEEE Transactions on Visualization and Computer 20(12): 1863–72. https://www.computer.org/csdl/trans/tg/2014/12/06875970-abs.html .
Mohler, G. (2014). Marked point process hotspot maps for homicide and gun crime prediction in Chicago. International Journal of Forecasting, 30 (3), 491–497. https://doi.org/10.1016/j.ijforecast.2014.01.004 .
Mohler, G., & Porter, M. D. (2018). Rotational grid, PAI-maximizing crime forecasts. Statistical Analysis and Data Mining: The ASA Data Science Journal , 11 (5), 227-236. https://doi.org/10.1002/sam.11389 .
Mohler, G., Raje, R., Carter, J., Valasik, M., & Brantingham, J. (2018). A penalized likelihood method for balancing accuracy and fairness in predictive policing. In 2018 IEEE International Conference on Systems, Man, and Cybernetics (SMC) . https://ieeexplore.ieee.org/abstract/document/8616417/ .
Mohler, G. O., Short, M. B., Brantingham, P. J., Schoenberg, F. P., & Tita, G. E. (2011). Self-exciting point process modeling of crime. Journal of the American Statistical Association, 106 (493), 100–108.
Mohler, G. O., Short, M. B., Malinowski, S., Johnson, M., Tita, G. E., Bertozzi, A. L., et al. (2015). Randomized controlled field trials of predictive policing. Journal of the American Statistical Association, 110 (512), 1399–1411. https://doi.org/10.1080/01621459.2015.1077710 .
Mu, Y., Ding, W., Morabito, M., & Tao, D. (2011). Empirical discriminative tensor analysis for crime forecasting. In International Conference on Knowledge Science, Engineering and Management (pp. 293-304). Springer, Berlin, Heidelberg. https://doi.org/10.1007/978-3-642-25975-3_26 .
Ohyama, T., & Amemiya, M. (2018). applying crime prediction techniques to Japan: A comparison between risk terrain modeling and other methods. European Journal on Criminal Policy and Research, 24 (4), 469–487.
Ozkan, T. (2018). Criminology in the age of data explosion: new directions. The Social Science Journal . https://doi.org/10.1016/J.SOSCIJ.2018.10.010 .
Papamitsiou, Z., & Economides, A. A. (2014). Learning analytics and educational data mining in practice: A systematic literature review of empirical evidence. Journal of Educational Technology & Society, 17 (4), 49–64.
Perry, W. L. (2013). Predictive policing: The role of crime forecasting in law enforcement operations . Santa Monica: Rand Corporation.
Ratcliffe, J. (2015). What is the future… of predictive policing. Practice, 6 (2), 151–166.
Rodríguez, C. D., Gomez, D. M., & Rey, M. A. (2017). Forecasting time series from clustering by a memetic differential fuzzy approach: An application to crime prediction. In 2017 IEEE Symposium Series on Computational Intelligence (SSCI) , (pp. 3372–3379). https://ieeexplore.ieee.org/abstract/document/8285373 .
Rosser, G., Davies, T., Bowers, K. J., Johnson, D. S., & Cheng, T. (2017). Predictive crime mapping: Arbitrary grids or street networks? Journal of Quantitative Criminology, 33 (3), 569–594. https://doi.org/10.1007/s10940-016-9321-x .
Rumi, S. K., Deng, K., & Salim, F. D. EPJ Data Science, and Undefined 2018. (2018). Crime event prediction with dynamic features. EPJ Data Science . https://doi.org/10.1140/epjds/s13688-018-0171-7 .
Rummens, A., Hardyns, W., & Pauwels, L. (2017). The use of predictive analysis in spatiotemporal crime forecasting: Building and testing a model in an urban context. Applied Geography, 86, 255–261. https://doi.org/10.1016/j.apgeog.2017.06.011 .
Seele, P. (2017). Predictive sustainability control: A review assessing the potential to transfer big data driven ‘predictive policing’ to corporate sustainability management. Journal of Cleaner Production, 153, 73–86. https://doi.org/10.1016/j.jclepro.2016.10.175 .
Shamsuddin, N.H. M., Ali. N. A., & Alwee, R. (2017). An overview on crime prediction methods. In 6th ICT International Student Project Conference (ICT - ISPC), IEEE. https://ieeexplore.ieee.org/abstract/document/8075335/ .
Shoesmith, G. L. (2013). Space–time autoregressive models and forecasting national, regional and state crime rates. International Journal of Forecasting, 29 (1), 191–201. https://doi.org/10.1016/j.ijforecast.2012.08.002 .
Thongsatapornwatana, U. (2016). A survey of data mining techniques for analyzing crime patterns. In 2016 Second Asian Conference on Defence Technology (ACDT) , (pp. 123–28). https://ieeexplore.ieee.org/stamp/stamp.jsp?tp=&arnumber=7437655 .
Thongtae, P., & Srisuk, S. (2008). An analysis of data mining applications in crime domain. In X. He, Q. Wu, Q. V. Nguyen, & W. Ja (Ed.), 8th IEEE International Conference on Computer and Information Technology Workshops , (pp. 122–126). https://doi.org/10.1109/CIT.2008.Workshops.80 .
Vlahogianni, E. I., Karlaftis, M. G., & Golias, J. C. (2014). Short-term traffic forecasting: where we are and where we’re going. Transportation Research Part C: Emerging Technologies, 43, 3–19.
Wang, X., & Brown, D. E. (2011). The Spatio-Temporal Generalized Additive Model for Criminal Incidents. In Proceedings of 2011 IEEE International Conference on Intelligence and Security Informatics , (pp. 42–47). IEEE, New York.
Wang, X., Brown, D. E., Gerber, M.S. (2012). Spatio-temporal modeling of criminal incidents using geographic, demographic, and twitter-derived information. In 2012 IEEE International Conference on Intelligence and Security Informatics , (pp. 36–41). IEEE, New York.
Wang, H., Kifer, D., Graif, C., & Li, Z. (2016). Crime rate inference with big data. In Proceedings of the 22nd ACM SIGKDD International Conference on Knowledge Discovery and Data Mining , (pp. 635–644). KDD’16. New York, NY, USA: ACM. https://doi.org/10.1145/2939672.2939736 .
Williams, M. L., & Burnap, P. (2015). Cyberhate on social media in the aftermath of woolwich: A case study in computational criminology and big Data. British Journal of Criminology, 56 (2), 211–238.
Yang, D., Heaney, T., Tonon, A., Wang, L., & Cudré-Mauroux, P. (2018). CrimeTelescope: crime hotspot prediction based on urban and social media data fusion. World Wide Web , 21 (5), 1323–1347. https://doi.org/10.1007/s11280-017-0515-4 .
Yu, C. H., Ward, M. W., Morabito, M., & Ding, W. (2011). Crime forecasting using data mining techniques. In IEEE 11th International Conference on Data Mining Workshops , (pp. 779–786). IEEE, New York.
Zhao, X., & Tang, J. (2017). Modeling temporal-spatial correlations for crime prediction. In Proceedings of the 2017 ACM on Conference on Information and Knowledge Management , (pp. 497–506). CIKM’17. New York, NY, USA: ACM. https://doi.org/10.1145/3132847.3133024 .
Zhuang, Y., Almeida, M., Morabito, M., & Ding. W. (2017). Crime hot spot forecasting: A recurrent model with spatial and temporal information. In X. D, Wu, T. Ozsu, J. Hendler, R. Lu, (Ed.), IEEE International Conference on Big Knowledge (ICBK) , (pp. 143–150). https://doi.org/10.1109/ICBK.2017.3 .
Download references
This research was funded by the Austrian Science Fund (FWF) through the Doctoral College GIScience at the University of Salzburg (DK W 1237-N23).
Author information
Authors and affiliations.
Department of Geoinformation Processing, University of Twente, Enschede, The Netherlands
Ourania Kounadi
Doctoral College GIScience, Department of Geoinformatics-Z_GIS, University of Salzburg, Salzburg, Austria
Alina Ristea & Michael Leitner
Boston Area Research Initiative, School of Public Policy and Urban Affairs, Northeastern University, Boston, MA, USA
Alina Ristea
Department of Informatics and Applied Mathematics, Federal University of Rio Grande do Norte, Natal, RN, Brazil
Adelson Araujo Jr.
Department of Geography and Anthropology, Louisiana State University, Baton Rouge, LA, USA
Michael Leitner
You can also search for this author in PubMed Google Scholar
Contributions
OK designed the initial idea, conceived and designed all experiments, analyzed the data and wrote the paper. AR helped designing the paper and running experiments. She also helped writing parts of the paper, with a focus on forecasting performance. AAJr. gave technical support and helped with running experiments and conceptual framework. He also helped writing the manuscript, focusing on forecasting algorithms. ML supervised the work and edited the final manuscript. All authors discussed the results and implications and commented on the manuscript at all stages. All authors read and approved the final manuscript.
Corresponding author
Correspondence to Alina Ristea .
Ethics declarations
Competing interests.
The authors declare that they have no competing interests.
Additional information
Publisher's note.
Springer Nature remains neutral with regard to jurisdictional claims in published maps and institutional affiliations.
Supplementary information
Additional file 1..
Online survey on Risk of Bias across Studies.
Rights and permissions
Open Access This article is licensed under a Creative Commons Attribution 4.0 International License, which permits use, sharing, adaptation, distribution and reproduction in any medium or format, as long as you give appropriate credit to the original author(s) and the source, provide a link to the Creative Commons licence, and indicate if changes were made. The images or other third party material in this article are included in the article's Creative Commons licence, unless indicated otherwise in a credit line to the material. If material is not included in the article's Creative Commons licence and your intended use is not permitted by statutory regulation or exceeds the permitted use, you will need to obtain permission directly from the copyright holder. To view a copy of this licence, visit http://creativecommons.org/licenses/by/4.0/ . The Creative Commons Public Domain Dedication waiver ( http://creativecommons.org/publicdomain/zero/1.0/ ) applies to the data made available in this article, unless otherwise stated in a credit line to the data.
Reprints and permissions
About this article
Cite this article.
Kounadi, O., Ristea, A., Araujo, A. et al. A systematic review on spatial crime forecasting. Crime Sci 9 , 7 (2020). https://doi.org/10.1186/s40163-020-00116-7
Download citation
Received : 17 November 2019
Accepted : 11 May 2020
Published : 27 May 2020
DOI : https://doi.org/10.1186/s40163-020-00116-7
Share this article
Anyone you share the following link with will be able to read this content:
Sorry, a shareable link is not currently available for this article.
Provided by the Springer Nature SharedIt content-sharing initiative
- Forecasting
- Predictive policing
- Spatiotemporal
- Spatial analysis
Crime Science
ISSN: 2193-7680
- Submission enquiries: [email protected]
- General enquiries: [email protected]
Cybercrime: Victimization, Perpetration, and Techniques
- Published: 10 November 2021
- Volume 46 , pages 837–842, ( 2021 )
Cite this article
- James Hawdon ORCID: orcid.org/0000-0002-0273-2227 1
10k Accesses
3 Citations
5 Altmetric
Explore all metrics
Avoid common mistakes on your manuscript.
The creation of the World Wide Web revolutionized communication. At the turn of the twenty-first century, roughly 413 million people used the internet (Roser & Ortiz-Ospina, 2015 ). A mere 21 years later, nearly 4.7 billion people, or about 60% of the world’s population, actively use the internet (We Are Social, & DataReportal, & Hootsuite, 2021 ). The pace of innovation in information technology, from the introduction of email in the 1960s to the rise of multiple social media platforms in the early 2000s to the rise of the Internet of Things (Iot) and 5 g, has been astonishing. It is now almost inconceivable to imagine life without access to the internet. Yet the IT revolution, like all technological revolutions, has been a dual-edge sword. Indeed, the internet’s many benefits and drawbacks have been discussed in numerous forums, and these discussions will undoubtedly continue as long as we remain dependent on this technology. This special edition of the American Journal of Criminal Justice contributes to those discussions by considering one of the drawbacks: cybercime.
Cybercrime, or the use of computer technology or online networks to commit crimes, ranges from fraud and identity theft to threats and intimidation. Cybercrime and its many manifestations has clearly increased over the past 20 years. For example, cybercrime costs increased from approximately $3 trillion in 2015 to more than $6 trillion in 2021, and these are expected to increase to over $10.5 trillion by 2025 (Morgan, 2020 ). In the U.S. alone, approximately 23 percent of households experience some sort of cybercrime annually (Reinhart, 2018 ; Hawdon et al., 2020 ). Indeed, in the same way that larceny characterized the twentieth century, cybercrime is characterizing the twenty-first century (Albanese, 2005 ). And these facts just reflect the economic costs of cybercrime and do not account for the non-monetary harms caused by cyberviolence. Cyberstalking, online sexual exploitation, cyber-harassment and bullying, threats of violence, and online violent extremism are also commonly committed acts of cyberviolence (FBI, 2021 ).
In many ways, it is unsurprising that cybercrime has increased in recent years. As technology becomes more sophisticated, so do cybercriminals, and cybercriminals now target individuals, businesses, healthcare facilities, educational institutions, and governments. As more people engage in an ever-increasing variety of online activities and more businesses conduct their affairs online, it is predictable that there would be a rise in cybercrime. To use the familiar language of Routine Activity Theory (Cohen & Felson, 1979 ), we have a lot more suitable targets in insufficiently guarded space being victimized by an increasing number motivated offenders. It is also unsurprising that there is a growing body of literature dedicated to cybercrime as scholars scramble to understand the ever-evolving phenomena. Entire journals are now dedicated to its study, and new academic disciplines have been created to try to prevent it. While our understanding of cybercrime has accumulated quickly and impressively, there is so much about cybercrime that we still do not know. This special issue of the A merican Journal of Criminal Justice offers nine new articles to help fill that knowledge gap.
The articles included in this issue reflect three broad areas of cybercrime research: cybercrime victimization, cybercrime perpetration, and techniques and facilitators of cybercrime. While there is some overlap, the issue includes three papers focused on each of these three areas.
The first area covered in the special issue focuses on cybercrime victimization. This area has generated the most research to date. In part because victims of cybercrime are relatively easy to find, considerable research has been conducted on cybervictimization across a variety of cybercrimes. Three of the articles in this special issue focus on cybervictimization, and they add to the literature in interesting ways by providing cross-national perspectives, building on theoretical traditions, or providing systematic summaries of the state of field at this time.
The first article in this section by Michelle Wright and a team of colleagues investigates how adolescent from China, Cyprus, the Czech Republic, India, Japan, and the United States explain being a victim of cyberbully. The investigation compares if how adolescents explain victimization varies by setting (private vs. public), medium (offline vs cyber), and severity and if cultural differences alter these relationships. Their findings suggest the need for prevention and intervention efforts to consider the role of setting, medium, severity, and cultural values if they are to be successful.
The second paper focusing on victimization builds on the frequent finding that problematic social media use is associated with negative life experiences and provides empirical support for a theoretical link between problematic social media use and cybervictimization. The analysis, conducted by colleagues Eetu Marttila, Aki Koivula, and Pekka Räsänen, is framed in Routine Activity Theory/Lifestyle-Exposure Theory. The results indicate that not only is problematic social media use strongly correlated with cybervictimization in a between-subject analysis, but within-subject analyses also reveal that problematic social media use has a cumulative effect on victimization.
The third paper bridges research on cybercrime victimization and cybercrime perpetration and provides a glimpse at the state of knowledge about a specific form of cyberviolence. Catherine Marcum and George Higgins conduct a systematic review of literature investigating both offending and victimization of cyberstalking, cyberdating abuse, and interpersonal electronic surveillance. Using a number of electronic databases, the authors focus on 31 studies to identify correlates of involvement in these cybercrimes. Victims are disproportionately female. Other correlates of victimization include overall social media use, risky online behavior, and negative external factors such as being attached to abusive peers. Correlates of perpetration provide support for a number of leading criminological theories as perpetrators tend to have low levels of self-control, associate with delinquent peers, and have low levels of parental supervision. As more research is conducted, there is a great need for more systematic literature reviews so we can begin to better refine our understanding and identify the theoretical approaches that provide the most insight into the world of cybercrime.
There are another three articles included in this special issue that focus on cybercrime perpetration. All three articles test traditional criminological theories and find support for them. In the first, Adam Bossler uses Sykes and Matza’s ( 1957 ) techniques of neutralization to examine the effects of techniques of neutralization on college students’ willingness to commit cybercrime, specifically hacking websites to deface them or compromise foreign and domestic financial and government targets. An overall techniques of neutralization scale significantly predicts being willing to commit cyberattacks even after controlling for other relevant factors. In addition to the theoretical implications of finding strong support for Sykes and Matza’s framework, the findings also have implications for situational crime prevention efforts aimed at removing excuses for offenders.
In another article focusing on perpetration, Thomas Dearden and Katalin Parti use a national online sample of 1,109 participants and find strong support for social learning theory as measures of both online and offline social learning correlate with a measure of cyber-offending. However, the authors also argue that self-control will interact with social learning variables to further influence the likelihood of cyber-offending. Overall, they find that both social learning and self-control, individually and as an interaction, are good predictors of cyber-offending.
In the final article dedicated to investigating the perpetration of cybercrime, Ashley Reichelmann and Matthew Costello use a nationally representative sample to explore how various dimensions of American national identity relate to producing online hate materials. The analysis reveals that higher levels of salience and public self-regard are weakly related to producing online hate. However, the findings suggest that understanding the nuances of “what it means to be American” is important for fully understanding the phenomenon of cyberhate, especially in this polarizing time when what it means to “be American” is frequently questioned.
Another three articles deal with perpetrating cybercrimes or “pseudo-cybercrimes,” but their focus is on how these crimes are committed. That is, the investigations deal with using the Dark Web or the surface web to make illegal or pseudo-legal purchases of illegal or quasi-legal substances. In the first paper in the section, Eric Jardine provides a crime script for purchasing drugs on the Dark Web. The script involves four generic stages (i.e. Informational Accumulation; Account Formation; Market Exchange; Delivery/Receipt) and provides an opportunity to review known law enforcement interventions that have effectively targeted each stage of the script to reduce the use of these online markets. The paper highlights numerous steps that law enforcement could take to effectively reduce the illegal selling and purchasing of drugs on the Dark Web.
Next, Robert Perdue engages in green criminology and focuses on the illegal trade of endangered species. Noting that regulating this trade is a critical, and very difficult, challenge for conservationists and law enforcement agents, Perdue examines the role the Internet plays in critically endangered plant transactions, but instead of focusing on the Dark Web, he investigates eBay to understand the extent to which such trades occur in plain sight. He finds that nearly a third of the critically endangered plant species examined were for sale in some form on eBay. Yet, despite the evidence that there is a high degree of open trading in these species, the complexity of the international legal frameworks regulating these transactions makes it difficult to ascertain their legality. Nevertheless, at least a subset of these sales are probably unlawful.
Finally, J. Mitchell Miller and Holly Ventura Miller provide insight into the computer-facilitated gray market of pseudo-legal marijuana sales in Los Vegas, Nevada. The ethnographic study reveals how various cannabis products are illegally diverted from legal markets to the gray market, and how brokers use the Internet in clever ways to advertise their products and services to a public that is likely unaware that they are engaging in illegal activities by skirting the regulations and tight control of the legal market.
Taken together, these three papers highlight the tremendous difficulties with regulating e-commerce. While the Dark Web provides an environment to conduct illegal transactions with minimal risk, it turns out that the Dark Web may be unnecessary for many illegal cyber-purchases. Given the surface web is convenient, widely available, and scarcely policed, many cybercriminals simply commit their crimes in the open. Using the language of Routine Activity Theory again, the internet—Dark or Surface—is an environment largely devoid of capable guardians.
As a whole, I believe these nine papers speak to the current state and future promise of cybercriminology. Currently, we are building a large body of empirical studies that speak to patterns of victimization and perpetration. With respect to victimization, we have learned a lot about who is likely to be victimized and how the patterns of victimization vary by type of cybercrime. We also have a good understanding of the activities that increase the likelihood of victimization, the emotional and financial costs of being a victim, and how people view victims depending on the setting and type of victimization. The body of evidence supporting a slightly modified version of Routine Activity Theory/Lifestyle-Exposure Theory is increasingly impressive, and the papers by Marttila, Koivula, and Räsänen as well as the article by Marcum and Higgins offer additional support for aspects of this theoretical approach.
Similarly, our understanding of cybercrime perpetration has expanded exponentially in recent years. While finding samples of cybercriminals is always a challenge, the growing body of evidence suggests that the behavior of cybercriminals is largely explained by the same set of factors that can account for the behavior of more traditional criminals. That is, cybercriminals tend to have low levels of self and social control, are largely unsupervised, experience strains, and learn the how, when, and why of their crimes from their associates. The papers in this issue offer additional support for techniques of neutralization, social learning theory, and self-control theory. While there are nuanced differences in how some criminogenic factors play out in the virtual and offline worlds, our existing theories appear to be robust as many of our theories apply to both online and offline criminal behavior. A number of the differences that exist largely relate to the asynchronous nature of many online interactions. The fact that online interactions can occur synchronously as well as asynchronously expands our networks and provide additional opportunities for others beyond our immediate environment to influence us and for us to commit crimes. The full ramifications of these changes in social networks, criminogenic forces, and criminal opportunities are not understood; however, we understand these far better today than we did even just a few years ago.
We also have a far greater understanding of the techniques of committing cybercrimes. We know considerably more about the use of the Dark Web to find and purchase illegal goods and services, and we have learned that the Surface Web plays a significant role in computer-dependent crimes. Moreover, as the article by Miller and Miller highlights, information technology has helped blur the line between legal, pseudo-legal, and illegal behaviors. What work in this area really highlights is how difficult it is to monitor and police the internet. While there is certainly social control exercised on the internet, there are limits to the effectiveness of this control (see Hawdon et al., 2017 ). Yet, by understanding the patterns of victimization, the underlying causes of perpetration, and the techniques that facilitate cybercrime, we become better armed in designing strategies to prevent it, defend against it, mitigate its adverse effects, and prosecute those who commit it. All of the articles included in this issue further that understanding.
The Special Issue
The process of selecting the articles for this special issue was perhaps unusual but also rather intensive. The process began by me inviting a group of scholars to submit manuscripts for the special issue. I selected these scholars because I knew of their work and was confident they would submit quality papers that covered a wide range of topics in the area of cybercrime. After discussing their planned submissions with the authors to assure there would be good topic coverage, the authors submitted their paper. An anonymous scholar and I reviewed these initial submissions (the anonymous scholar served as a typical double-blind reviewer). Each contributing author also reviewed one or two of the included articles. Authors then revised their work based on the reviewers’ comments and resubmitted the papers. Each contributing author was then asked to read all nine revised papers. Then, the authors and I took advantage of the brief pause in the COVID-19 pandemic and gathered for a two-day workshop in Asheville, North Carolina as part of the Center for Peace Studies and Violence Prevention’s annual research workshop program. The lone exception to this was our Finnish colleagues who were unable to get a special visa to visit the U.S. at that time. These colleagues joined the workshop via Zoom. The authors/workshop participants then discussed and provided feedback on all of the articles. The authors then made final revisions to their papers based on these discussions. Thus, these papers have been through three rounds of revisions. As the editor of the special edition, I am proud of the finished product.
Albanese, J. S. (2005). Fraud: The characteristic crime of the 21st Century. Trends in Organized Crime, 8 , 5–16.
Article Google Scholar
Cohen, L. E., & Felson, M. (1979). Social change and crime rate trends: A routine activity approach. American Sociological Review, 44 (4), 588–608.
Federal Bureau of Investigation. (2021). 2020 Internet crime report . U.S. Government Printing Office.
Google Scholar
Hawdon, J., Costello, C., Ratliff, T., Hall, L., & Middleton, J. (2017). Conflict management styles and cybervictimization: An extension of routine activity theory. Sociological Spectrum, 37 (4), 250–266.
Hawdon, J., Parti, K., & Dearden, T. E. (2020). Cybercrime in America amid COVID-19: The initial results from a natural experiment. American Journal of Criminal Justice, 45 , 546–562.
Morgan, S. (2020). Cybercrime to cost the World $10.5 Trillion Annually by 2025. Cybercrime Magazine , November 13, 2020. https://cybersecurityventures.com/hackerpocalypse-cybercrime-report-2016/
Reinhart, R. J. (2018). One in four Americans have experienced cybercrime. Gallup Politics . https://news.gallup.com/poll/245336/one-four-americans-experienced-cybercrime.aspx
Roser, M. H. R. & Ortiz-Ospina, E. (2015). "Internet". Published online at OurWorldInData.org. Retrieved from: ' https://ourworldindata.org/internet ' [Online Resource]
Sykes, G. M., & Matza, D. (1957). Techniques of neutralization: A theory of delinquency. American Sociological Review, 22 (6), 664–670.
We Are Social, & DataReportal, & Hootsuite. (2021). Global digital population as of January 2021 (in billions) [Graph]. In Statista . Retrieved September 24, 2021, from https://www.statista.com/statistics/617136/digital-population-worldwide/
Download references
Author information
Authors and affiliations.
Virginia Tech, Blacksburg, VA, USA
James Hawdon
You can also search for this author in PubMed Google Scholar
Corresponding author
Correspondence to James Hawdon .
Additional information
Publisher's note.
Springer Nature remains neutral with regard to jurisdictional claims in published maps and institutional affiliations.
Rights and permissions
Reprints and permissions
About this article
Hawdon, J. Cybercrime: Victimization, Perpetration, and Techniques. Am J Crim Just 46 , 837–842 (2021). https://doi.org/10.1007/s12103-021-09652-7
Download citation
Received : 03 July 2021
Accepted : 03 August 2021
Published : 10 November 2021
Issue Date : December 2021
DOI : https://doi.org/10.1007/s12103-021-09652-7
Share this article
Anyone you share the following link with will be able to read this content:
Sorry, a shareable link is not currently available for this article.
Provided by the Springer Nature SharedIt content-sharing initiative
- Find a journal
- Publish with us
- Track your research

An official website of the United States government
The .gov means it’s official. Federal government websites often end in .gov or .mil. Before sharing sensitive information, make sure you’re on a federal government site.
The site is secure. The https:// ensures that you are connecting to the official website and that any information you provide is encrypted and transmitted securely.
- Publications
- Account settings
Preview improvements coming to the PMC website in October 2024. Learn More or Try it out now .
- Advanced Search
- Journal List
- Springer Nature - PMC COVID-19 Collection

Research trends in cybercrime victimization during 2010–2020: a bibliometric analysis
Huong thi ngoc ho.
1 School of Journalism and Communication, Huazhong University of Science and Technology, Wuhan, Hubei China
Hai Thanh Luong
2 School of Global, Urban and Social Studies, RMIT University, Melbourne, Australia
Associated Data
The datasets generated during and/or analyzed during the current study are available from the corresponding author on reasonable request.
Research on cybercrime victimization is relatively diversified; however, no bibliometric study has been found to introduce the panorama of this subject. The current study aims to address this research gap by performing a bibliometric analysis of 387 Social Science Citation Index articles relevant to cybercrime victimization from Web of Science database during the period of 2010–2020. The purpose of the article is to examine the research trend and distribution of publications by five main fields, including time, productive authors, prominent sources, active institutions, and leading countries/regions. Furthermore, this study aims to determine the global collaborations and current gaps in research of cybercrime victimization. Findings indicated the decidedly upward trend of publications in the given period. The USA and its authors and institutions were likely to connect widely and took a crucial position in research of cybercrime victimization. Cyberbullying was identified as the most concerned issue over the years and cyber interpersonal crimes had the large number of research comparing to cyber-dependent crimes. Future research is suggested to concern more about sample of the elder and collect data in different countries which are not only European countries or the USA. Cross-nation research in less popular continents in research map was recommended to be conducted more. This paper contributed an overview of scholarly status of cybercrime victimization through statistical evidence and visual findings; assisted researchers to optimize their own research direction; and supported authors and institutions to build strategies for research collaboration.
Introduction
To date, the debate of cybercrime definition has been controversial which is considered as one of the five areas of cyber criminology (Ngo and Jaishankar 2017 ; Drew 2020 ). 1 Several terms are used to illustrate ‘cybercrime’, such as ‘high-tech crime’ (Insa 2007 ), ‘computer crime’ (Choi 2008 ; Skinner and Fream 1997 ), ‘digital crime’ (Gogolin 2010 ), or ‘virtual crime’ (Brenner 2001 ). ‘Cybercrime’, however, has been the most popular in the public parlance (Wall 2004 ). A propensity considers crime directly against computer as cybercrime, while other tendency asserts that any crime committed via internet or related to a computer is cybercrime (Marsh and Melville 2008 ; Wall 2004 ). Hence, there is a distinction between ‘true cybercrime’ or ‘high-tech’ cybercrime and ‘low-tech’ cybercrime (Wagen and Pieters 2020 ). Council of Europe defines ‘any criminal offense committed against or with the help of a computer network’ as cybercrime (Abdullah and Jahan 2020 , p. 90). Despite different approaches, cybercrime generally includes not only new types of crimes which have just occurred after the invention of computer and internet (Holt and Bossler 2014 ; Drew 2020 ) but also traditional types of crimes which took the advantages of information communication technology (ICT) as vehicle for illegal behaviors (Luong 2021 ; Nguyen and Luong 2020 ; Luong et al. 2019 ). Two main cybercrime categories identified, respectively, are cyber-dependent crime (hacking, malware, denial of service attacks) and cyber-enable crime (phishing, identity theft, cyber romance scam, online shopping fraud). Nevertheless, there are several different classifications of cybercrime such as cybercrime against certain individuals, groups of individuals, computer networks, computer users, critical infrastructures, virtual entities (Wagen and Pieters 2020 ); cyber-trespass, cyber-deceptions, cyber-pornography, and cyber-violence (Wall 2001 ).
Due to the common prevalence of cybercrime, the increasing threats of cybercrime victimization are obviously serious. Cybercrime victimization has become a crucial research subfield in recent years (Wagen and Pieters 2020 ). It is difficult to differ “forms of online victimization” and “acts that actually constitute a crime”, then it is usual for researchers to focus less on perspective of criminal law and consider any negative experiences online as cybercrime (Näsi et al. 2015 , p. 2). It was likely to lead to practical gaps between theory and practice in terms of investigating the nexus of offender and victims on cyberspace. In the light of literature review, numerous specific aspects of cybercrime victimization were investigated by questionnaire surveys or interview survey such as the prevalence of cybercrime victimization (Näsi et al. 2015 ; Whitty and Buchanan 2012 ); causes and predictors of cybercrime victimization (Abdullah and Jahan 2020 ; Algarni et al. 2017 ; Ilievski 2016 ; Jahankhani 2013 ; Kirwan et al. 2018 ; Näsi et al. 2015 ; Reyns et al. 2019 ; Saad et al. 2018 ); and the relationship between social networking sites (SNS) and cybercrime victimization (Das and Sahoo 2011 ; Algarni et al. 2017 ; Benson et al. 2015 ; Seng et al. 2018 ). To some extent, therefore, the current study examines cybercrime victimization in the large scale, referring to any negative experiences on cyberspace or computer systems. Nevertheless, no bibliometric analysis was found to show the research trend and general landscape of this domain.
Bibliometric is a kind of statistical analysis which uses information in a database to provide the depth insight into the development of a specified area (Leung et al. 2017 ). The present study aims to address this research gap by providing a bibliometric review of the relevant SSCI articles in WoS database during the period of 2010–2020. The pattern of publications, the productivity of main elements (authors, journals, institutions, and countries/regions), statistic of citations, classification of key terms, research gaps, and other collaborations will be presented and discussed in section four and five after reviewing literatures and presenting our methods conducted. This article contributes an overview of research achievements pertaining to cybercrime victimization in the given period through statistical evidence and visual findings; assists researchers to perceive clearly about the key positions in research maps of this field, and obtain more suggestions to develop their own research direction.
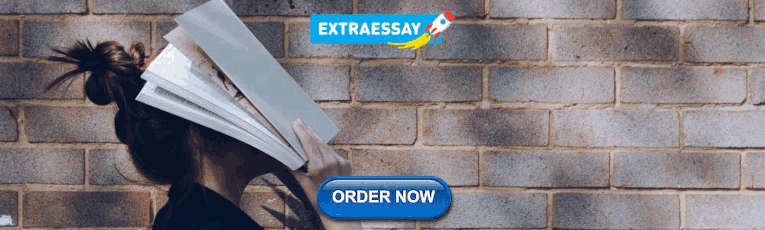
Literature review
Cybercrime victimization.
Cybercrime victimization may exist in two levels including institutional and individual level (Näsi et al. 2015 ). For the former, victim is governments, institutions, or corporations, whereas for the latter, victim is a specific individual (Näsi et al. 2015 ). A wide range of previous studies concerned about individual level of victim and applied Lifestyle Exposure Theory (LET), Routine Activity Theory (RAT) and General Theory of Crime to explain cybercrime victimization (Choi 2008 ; Holt and Bossler 2009 ; Ngo and Paternoster 2011 ). Basing on these theories, situational and individual factors were supposed to play an important role in understanding cybercrime victimization (Choi 2008 ; Van Wilsem 2013 ). However, there was another argument that situational and individual factors did not predict cybercrime victimization (Ngo and Paternoster 2011 ; Wagen and Pieters 2020 ). Overall, most of those studies just focused only one distinctive kind of cybercrime such as computer viruses, malware infection, phishing, cyberbullying, online harassment, online defamation, identity theft, cyberstalking, online sexual solicitation, cyber romance scams or online consumer fraud. Referring to results of the prior research, some supported for the applicability of mentioned theories but other did not share the same viewpoint (Leukfeldt and Yar 2016 ). It was hard to evaluate the effect of LET or RAT for explanation of cybercrime victimization because the nature of examined cybercrime were different (Leukfeldt and Holt 2020 ; Leukfeldt and Yar 2016 ).
Previous research determined that cybercrime victimization was more common in younger group compared to older group because the young is the most active online user (Näsi et al. 2015 ; Oksanen and Keipi 2013 ) and males tended to become victims of cybercrime more than females in general (Näsi et al. 2015 ). However, findings might be different in research which concerned specific types of cybercrime. Women were more likely to be victims of the online romance scam (Whitty and Buchanan 2012 ) and sexual harassment (Näsi et al. 2015 ), while men recorded higher rate of victimization of cyber-violence and defamation. Other demographic factors were also examined such as living areas (Näsi et al. 2015 ), education (Oksanen and Keipi 2013 ; Saad et al. 2018 ) and economic status (Oksanen and Keipi 2013 ; Saad et al. 2018 ). Furthermore, several prior studies focus on the association of psychological factors and cybercrime victimization, including awareness and perception (Ariola et al. 2018 ; Saridakis et al. 2016 ), personality (Kirwan et al. 2018 ; Orchard et al. 2014 ; Parrish et al. 2009 ), self-control (Ilievski 2016 ; Ngo and Paternoster 2011 ; Reyns et al. 2019 ), fear of cybercrime (Lee et al. 2019 ), online behaviors (Al-Nemrat and Benzaïd 2015 ; Saridakis et al. 2016 ). Psychological factors were assumed to have effects on cybercrime victimization at distinctive levels.
Another perspective which was much concerned by researchers was the relationship between cybercrime victimization and SNS. SNS has been a fertile land for cybercriminals due to the plenty of personal information shared, lack of guard, the availability of communication channels (Seng et al. 2018 ), and the networked nature of social media (Vishwanath 2015 ). When users disclosed their personal information, they turned themselves into prey for predators in cyberspace. Seng et al. ( 2018 ) did research to understand impact factors on user’s decision to react and click on suspicious posts or links on Facebook. The findings indicated that participants’ interactions with shared contents on SNS were affected by their relationship with author of those contents; they often ignored the location of shared posts; several warning signals of suspicious posts were not concerned. Additionally, Vishwanath ( 2015 ) indicated factors that led users to fall victims on the SNS; Algarni et al. ( 2017 ) investigated users’ susceptibility to social engineering victimization on Facebook; and Kirwan et al. ( 2018 ) determined risk factors resulting in falling victims of SNS scam.
Bibliometric of cybercrime victimization
“Bibliometric” is a term which was coined by Pritchard in 1969 and a useful method which structures, quantifies bibliometric information to indicate the factors constituting the scientific research within a specific field (Serafin et al. 2019 ). Bibliometric method relies on some basic types of analysis, namely co-authorship, co-occurrence, citation, co-citation, and bibliographic coupling. This method was employed to various research domains such as criminology (Alalehto and Persson 2013 ), criminal law (Jamshed et al. 2020 ), marketing communication (Kim et al. 2019 ), social media (Chen et al. 2019 ; Gan and Wang 2014 ; Leung et al. 2017 ; Li et al. 2017 ; You et al. 2014 ; Zyoud et al. 2018 ), communication (Feeley 2008 ), advertising (Pasadeos 1985 ), education (Martí-Parreño et al. 2016 ).
Also, there are more and more scholars preferring to use bibliometric analysis on cyberspace-related subject such as: cyber behaviors (Serafin et al. 2019 ), cybersecurity (Cojocaru and Cojocaru 2019 ), cyber parental control (Altarturi et al. 2020 ). Serafin et al. ( 2019 ) accessed the Scopus database to perform a bibliometric analysis of cyber behavior. All documents were published by four journals: Cyberpsychology, Behavior and Social Networking (ISSN: 21522723), Cyberpsychology and Behavior (ISSN: 10949313) , Computers in Human Behavior (ISSN: 07475632) and Human–Computer Interaction (ISSN: 07370024), in duration of 2000–2018. Findings indicated the use of Facebook and other social media was the most common in research during this period, while psychological matters were less concerned (Serafin et al. 2019 ). Cojocaru and Cojocaru ( 2019 ) examined the research status of cybersecurity in the Republic of Moldavo, then made a comparison with the Eastern Europe countries’ status. This study employed bibliometric analysis of publications from three data sources: National Bibliometric Instrument (database from Republic of Moldavo), Scopus Elsevier and WoS. The Republic of Moldavo had the moderate number of scientific publications on cybersecurity; Russian Federation, Poland, Romania, Czech Republic, and Ukraine were the leading countries in Eastern Europe area (Cojocaru and Cojocaru 2019 ). Altarturi et al. ( 2020 ) was interested in bibliometric analysis of cyber parental control, basing on publications between 2000 and 2019 in Scopus and WoS. This research identified some most used keywords including ‘cyberbullying’, ‘bullying’, ‘adolescents’ and ‘adolescence’, showing their crucial position in the domain of cyber parental control (Altarturi et al. 2020 ). ‘Cyber victimization’ and ‘victimization’ were also mentioned as the common keywords by Altarturi et al. ( 2020 ). Prior research much focus on how to protect children from cyberbullying. Besides, four online threats for children were determined: content, contact, conduct and commercial threats (Altarturi et al. 2020 ).
Generally, it has been recorded several published bibliometric analyses of cyber-related issues but remained a lack of bibliometric research targeting cybercrime victimization. Thus, the present study attempts to fill this gap, reviewing the achievements of existed publications as well as updating the research trend in this field.
In detail, our current study aims to address four research questions (RQs):
What is overall distribution of publication based on year, institutions and countries, sources, and authors in cybercrime victimization?
Which are the topmost cited publications in terms of cybercrime victimization?
Who are the top co-authorships among authors, institutions, and countries in research cybercrime victimization?
What are top keywords, co-occurrences and research gaps in the field of cybercrime victimization?
Data collection procedure
Currently, among specific approaches in cybercrime’s fileds, WoS is “one of the largest and comprehensive bibliographic data covering multidisciplinary areas” (Zyoud et al. 2018 , p. 2). This paper retrieved data from the SSCI by searching publications of cybercrime victimization on WoS database to examine the growth of publication; top keywords; popular topics; research gaps; and top influential authors, institutions, countries, and journals in the academic community.
This paper employed Preferred Reporting Items for Systematic Reviews and Meta-Analyses (PRISMA) for data collection procedure. For timeline, we preferred to search between 2010 and 2020 on the WoS system with two main reasons. First, when the official update of the 2009 PRISMA Statement had ready upgraded with the specific guidelines and stable techniques, we consider beginning since 2010 that is timely to test. Secondly, although there are several publications from the early of 2021 to collect by the WoS, its updated articles will be continued until the end of the year. Therefore, we only searched until the end of 2020 to ensure the full updates.
To identify publications on cybercrime victimization, the study accessed WoS and used two keywords for searching: ‘cybercrime victimization’ or ‘cyber victimization’ after testing and looking for some terminology-related topics. Accordingly, the paper applied a combination of many other searching terms besides two selected words such as “online victimization”, “victim of cybercrime”, “phishing victimization”, “online romance victimization”, “cyberstalking victim”, “interpersonal cybercrime victimization”, or “sexting victimization”, the results, however, were not really appropriate. A lot of papers did not contain search keywords in their titles, abstracts, keywords and were not relavant to study topic. After searching with many different terms and comparing the results, the current study selected the two search terms for the most appropriate articles. The query result consisted of 962 documents. Basing on the result from preliminary searching, retrieved publications were refined automatically on WoS by criteria of timespan, document types, language, research areas, and WoS Index as presented in Table Table1. 1 . Accordingly, the criteria for automatic filter process were basic information of an articles and classified clearly in WoS system so the results reached high accuracy. The refined results are 473 articles.
Criteria for automatic filter
After automatic filters, file of data was converted to Microsoft Excel 2016 for screening. The present study examined titles and abstracts of 473 articles to assess the eligibility of each publication according to the relevance with given topic. There are 387 articles are eligible,while 86 irrelevant publications were excluded.
Data analysis
Prior to data analysis, the raw data were cleaned in Microsoft Excel 2016. Different forms of the same author’s name were corrected for consistency, for example “Zhou, Zong-Kui” and “Zhou Zongkui”, “Van Cleemput, Katrien” and “Van Cleemput, K.”, “Williams, Matthew L.” and “Williams, Matthew”. Similarly, different keywords (single/plural or synonyms) used for the same concept were identified and standardized such as “victimization” and “victimisation”; “adolescent” and “adolescents”; “cyber bullying”, “cyber-bullying” and “cyberbullying”; “routine activity theory” and “routine activities theory”.
The data were processed by Microsoft Excel 2016 and VOS Viewer version 1.6.16; then it was analyzed according to three main aspects. First, descriptive statistic provided evidence for yearly distribution and growth trend of publications, frequency counts of citations, the influential authors, the predominant journals, the top institutions and countries/territories, most-cited publications. Second, co-authorship and co-occurrence analysis were constructed and visualized by VOS Viewer version 1.6.16 to explore the network collaborations. Finally, the current study also investigated research topics through content analysis of keywords. The authors’ keywords were classified into 15 themes, including: #1 cybercrime; #2 sample and demographic factors; #3 location; #4 theory; #5 methodology; #6 technology, platforms and related others; #7 psychology and mental health; #8 physical health; #9 family; #10 school; #11 society; #12 crimes and deviant behaviors; #13 victim; #14 prevention and intervention; and #15 others. Besides, the study also added other keywords from titles and abstracts basing on these themes, then indicated aspects examined in previous research.
In this section, all findings corresponding with four research questions identified at the ouset of this study would be illustrated (Fig. 1 ).

PRISMA diagram depicts data collection from WoS database
Distribution of publication
Distribution by year, institutions and countries.
Basing on retrieved data, it was witnessed an increasing trend of articles relevant to cybercrime victimization in SSCI list during the time of 2010–2020 but it had slight fluctuations in each year as shown in Fig. 2 . The total number of articles over this time was 387 items, which were broken into two sub-periods: 2010–2014 and 2015–2020. It is evident that the latter period demonstrated the superiority of the rate of articles (79.33%) compared to the previous period (20.67%). The yearly quantity of publications in this research subject was fewer than forty before 2015. Research of cybercrime victimization reached a noticeable development in 2016 with over fifty publications, remained the large number of publications in the following years and peaked at 60 items in 2018.

Annual distribution of publications
Distribution by institutions and countries
Table Table2 2 shows the top contributing institutions according to the quantity of publications related to cybercrime victimization. Of the top institutions, four universities were from the USA, two ones were from Spain, two institutions were from Australia and the rest ones were from Czech Republic, Belgium, Greece, and Austria. Specifically, Masaryk University (17 documents) became the most productive publishing institution, closely followed by Michigan State University (16 documents). The third and fourth places were University of Antwerp (13 documents) and Weber State University (10 documents). Accordingly, the institutions from The USA and Europe occupied the vast majority.
Top contributing institutions based on total publications
TP total publications, TC total citations for the publications reviewed, AC average citations per document
In Table Table2, 2 , University of Seville (total citations: 495, average citations: 70.71) ranked first and University of Cordoba (total citations: 484, average citations: 60.50) stayed at the second place in both total citations and average citations.
Referring to distribution of publications by countries, there were 45 countries in database contributing to the literature of cybercrime victimization. The USA recorded the highest quantity of papers, creating an overwhelming difference from other countries (159 documents) as illustrated in Fig. 3 . Of the top productive countries, eight European countries which achieved total of 173 publications were England (39 documents), Spain (34 documents), Germany (22 documents), Netherlands (18 documents), Italy (17 documents) and Czech Republic (17 documents), Belgium (14 documents), Greece (12 documents). Australia ranked the fourth point (32 documents), followed by Canada (30 documents). One Asian country which came out seventh place, at the same position with Netherlands was China (18 documents).

Top productive countries based on the number of publications
Distribution by sources
Table Table3 3 enumerates the top leading journals in the number of publications relevant to cybercrime victimization. The total publications of the first ranking journal— Computers in Human Behavior were 56, over twice as higher as the second raking journal— Cyberpsychology, Behavior and Social Networking (24 articles). Most of these journals have had long publishing history, starting their publications before 2000. Only three journals launched after 2000, consisting of Journal of School Violence (2002), Cyberpsychology: Journal of Psychosocial Research on Cyberspace (2007) and Frontiers in Psychology (2010). Besides, it is remarked that one third of the top journals focuses on youth related issues: Journal of Youth and Adolescence , Journal of Adolescence, School Psychology International and Journal of School Violence .
Top leading journals based on the quantity of publications
SPY Started Publication Year
In Table Table3, 3 , relating to total citations, Computers in Human Behavior remained the first position with 2055 citations. Journal of Youth and Adolescence had total 1285 citations, ranked second and followed by Aggressive Behavior with 661 citations. In terms of average citations per documents, an article of Journal of Youth and Adolescence was cited 67.63 times in average, much higher than average citations of one in Computers in Human Behavior (36.70 times). The other journals which achieved the high number of average citations per document were School Psychology International (59.00 times), Journal of Adolescence (44.83 times) and Aggressive Behavior (44.07 times).
Distribution by authors
Table Table4 4 displays ten productive authors based on article count; total citations of each author and their average citations per document are also included. Michelle F. Wright from Pennsylvania State University ranked first with twenty publications, twice as higher as the second positions, Thomas J. Holt (10 articles) from Michigan State University and Bradford W. Reyns (10 articles) from Weber State University. Rosario Ortega-Ruiz from University of Cordoba stayed at the third place in terms of total publications but the first place in aspect of total citations (483 citations) and the average citations (60.38 times).
Top productive authors based on article count
Of the most productive authors based on total publications, there were three authors from universities in the USA; one from the university in Canada (Brett Holfeld); the others were from institutions in Euro, including Spain (Rosario Ortega-Ruiz), Greece (Constantinos M. Kokkinos) and Belgium (Heidi Vandebosch), Netherlands (Rutger Leukfeldt) and Austria (Takuya Yanagida and Christiane Spiel).
Most-cited publications
The most-cited literature items are displayed in Table Table5. 5 . The article which recorded the highest number of citations was ‘Psychological, Physical, and Academic Correlates of Cyberbullying and Traditional Bullying’ (442 citations) by Robin M. Kowalski et al. published in Journal of Adolescent Health , 2013. Seven of ten most-cited articles were about cyberbullying; focused on youth population; made comparisons between cyberbullying and traditional bullying; analyzed the impact of several factors such as psychological, physical, academic factors or use of Internet; discussed on preventing strategies. The other publications studied victimization of cyberstalking and cyber dating abuse. All most-cited articles were from 2015 and earlier.
The most-cited publications in subject of cybercrime victimization during 2010–2020
Of the top productive authors, only Bradford W. Reyns had an article appeared in the group of most-cited publications. His article ‘Being Pursued Online: Applying Cyberlifestyle-Routine Activities Theory to Cyberstalking Victimization’ (2011) was cited 172 times.
Co-authorship analysis
“Scientific collaboration is a complex social phenomenon in research” (Glänzel and Schubert 2006 , p. 257) and becomes the increasing trend in individual, institutional and national levels. In bibliometric analysis, it is common to assess the productivity and international collaboration of research; identify key leading researchers, institutions, or countries (E Fonseca et al. 2016 ) as well as potential collaborators in a specific scientific area (Romero and Portillo-Salido 2019 ) by co-authorship analysis which constructs networks of authors and countries (Eck and Waltman 2020 ).
This section analyses international collaboration relevant to research of cybercrime victimization among authors, institutions, and countries during 2010–2020 through visualization of VOS Viewer software.
Collaboration between authors
Referring to the threshold of choose in this analysis, minimum number of documents of author is three and there were 80 authors for final results. Figure 4 illustrates the relationships between 80 scientists who study in subject of cybercrime victimization during 2010–2020. It shows several big groups of researchers (Wright’s group, Vandebosch’s group, or Holt’s group), while numerous authors had limited or no connections to others (Sheri Bauman, Michelle K. Demaray or Jennifer D. Shapka).

Collaboration among authors via network visualization (threshold three articles for an author, displayed 80 authors)
Figure 5 displayed a significant network containing 23 authors who were active in collaboration in detail. The displayed items in Fig. 5 are divided into five clusters coded with distinctive colors, including red, green, blue, yellow, and purple. Each author item was represented by their label and a circle; the size of label and circle are depended on the weight of the item, measured by the total publications (Eck and Waltman 2020 ). The thickness of lines depends on the strength of collaboration (Eck and Waltman 2020 ).

Collaboration among authors via network visualization (threshold three articles for an author, displayed 23 authors)
The most significant cluster was red one which is comprised of six researchers: Michelle F. Wright, Sebastian Wachs, Yan Li, Anke Gorzig, Manuel Gamez-Guadix and Esther Calvete. The remarked author for the red cluster was Michelle F. Wright whose value of total link strength is 24. She had the strongest links with Sebastian Wachs; closely link with Yan Li, Anke Gorzig, Manuel Gamez-Guadix and collaborated with authors of yellow cluster, including Shanmukh V. Kamble, Li Lei, Hana Machackova, Shruti Soudi as well as Takuya Yanagida of blue cluster. Michelle F. Wright who obtained the largest number of published articles based on criteria of this study made various connections with other scholars who were from many different institutions in the world. This is also an effective way to achieve more publications.
Takuya Yanagida was the biggest node for the blue cluster including Petra Gradinger, Daniel Graf, Christiane Spiel, Dagmar Strohmeier. Total link strength for Takuya Yanagida was 28; twelve connections. It is observed that Takuya Yanagida’ s research collaboration is definitely active. Besides, other research groups showed limited collaborations comparing with the red and blue ones.
Collaboration between institutions
The connections among 156 institutions which published at least two documents per one are shown in Fig. 6 . Interestingly, there is obvious connections among several distinctive clusters which were coded in color of light steel blue, orange, purple, steel blue, green, red, yellow, light red, dark turquoise, light blue, brown and light green. These clusters created a big chain of connected institutions and were in the center of the figure, while other smaller clusters or unlinked bubbles (gray color) were distributed in two sides. The biggest chain consisted of most of productive institutions such as Masaryk University, Michigan State University, University of Antwerp, Weber State University, University of Cordoba, Edith Cowan University, University of Cincinnati, University of Victoria, University of Vienna, and University of Seville.

Collaboration among institutions via network visualization (threshold two articles for an institution, 156 institutions were displayed)
Light steel blue and orange clusters presented connections among organizations from Australia. Light green included institutions from Netherland, while turquoise and light blue consisted of institutions from the USA. Yellow cluster was remarked by the various collaborations among institutions from China and Hong Kong Special Administrative Region (Renmin University of China and South China Normal University, University of Hong Kong, the Hong Kong Polytechnic University and the Chinese University of Hong Kong), the USA (University of Virginia), Cyprus (Eastern Mediterranean University), Japan (Shizuoka University), India (Karnataka University) and Austria (University Applied Sciences Upper Austria). Central China Normal University is another Chinese institution which appeared in Fig. 5 , linking with Ministry of Education of the People’s Republic of China, Suny Stony Brook and University of Memphis from the USA.
Masaryk University and Michigan State University demonstrated their productivity in both the quantity of publications and the collaboration network. They were active in research collaboration, reaching twelve and eleven links, respectively, with different institutions, but focused much on networking with institutions in the USA and Europe.
Collaboration between countries
The collaboration among 45 countries which published at least one SSCI documents of cybercrime victimization during the given period was examined in VOS Viewer but just 42 items were displayed via overlay visualization. Figure 7 depicts the international collaborations among significant countries. The USA is the biggest bubble due to its biggest number of documents and shows connections with 26 countries/regions in Euro, Asia, Australia, Middle East. Excepting European countries, England collaborate with the USA, Australia, South Korea, Japan, Thailand, Singapore, Sri Lanka, and Colombia. Spain and Germany almost focus on research network within Euro. China has the strongest tie with the USA, link with Australia, Germany, Czech Republic, Austria, Cyprus and Turkey, Japan, Indian, Vietnam.

Collaboration among countries via overlay visualization
Color bar in Fig. 7 is determined by the average publication year of each country and the color of circles based on it. It is unsurprised that the USA, Australia, England, or Spain shows much research experience in this field and maintain the large number of publications steadily. Interestingly, although the average publication year of South Korea or Cyprus was earlier than other countries (purple color), their quantities of documents were moderate. The new nodes (yellow circles) in the map included Vietnam, Norway, Pakistan, Ireland, Scotland, Switzerland.
Keywords and co-occurrence
The present paper examined the related themes and contents in research of cybercrime victimization during 2010–2020 through collecting author keywords, adding several keywords from tiles and abstracts. Besides, this study also conducted co-occurrence analysis of author keywords to show the relationships among these keywords.
The keywords were collected and categorized into 15 themes in Table Table6, 6 , including cybercrime; sample and demographic factors; location; theory; methodology; technology, platform, and related others; psychology and mental health; physical health; family; school; society; crimes and other deviant behaviors; victim; prevention and intervention; and others.
Statistic of keywords in themes
These keywords were most of author keyword, adding a few selected keywords from the titles and abstracts by the author of this current study
In the theme of cybercrime, there were numerous types of cybercrimes such as cyberbullying, cyber aggression, cyberstalking, cyber harassment, sextortion and other cyber dating crimes, cyber fraud, identity theft, phishing, hacking, malware, or ransomware. Generally, the frequency of interpersonal cybercrimes or cyber-enable crimes was much higher than cyber-dependent crimes. Cyberbullying was the most common cybercrime in research.
Relating to sample and demographic factors, there were sample of children, adolescent, adults, and the elder who were divided into more detail levels in each research; however, adolescent was the most significant sample. Besides, demographic factor of gender received a remarked concern from scholars.
It is usual that most of the research were carried out in one country, in popular it was the USA, Spain, Germany, England, Australia, Canada or Netherland but sometimes the new ones were published such as Chile, Vietnam, Thailand or Singapore. It was witnessed that some studies showed data collected from a group of countries such as two countries (Canada and the United State), three countries (Israel, Litva, Luxembourg), four countries (the USA, the UK, Germany, and Finland), or six Europe countries (Spain, Germany, Italy, Poland, the United Kingdom and Greece).
A wide range of theories were applied in this research focusing on criminological and psychological theories such as Routine Activities Theory, Lifestyle—Routine Activities Theory, General Strain Theory, the Theory of Reasoned Action or Self-control Theory.
Table Table6 6 indicated a lot of different research methods covering various perspective of cybercrime victimization: systematic review, questionnaire survey, interview, experiment, mix method, longitudinal study, or cross-national research; many kinds of analysis such as meta-analysis, social network analysis, latent class analysis, confirmatory factor analysis; and a wide range of measurement scales which were appropriate for each variable.
Topic of cybercrime victimization had connections with some main aspects of technology (information and communication technologies, internet, social media or technology related activities), psychology (self-esteem, fear, attitude, personality, psychological problems, empathy, perceptions or emotion), physical health, family (parents), school (peers, school climate), society (norms, culture, social bonds), victim, other crimes (violence, substance use), prevention and intervention.
Co-occurrence analysis was performed with keywords suggested by authors and the minimum number of occurrences per word is seven. The result showed 36 frequent keywords which clustered into five clusters as illustrated in Fig. 8 .

Co-occurrence between author keywords via network visualization (the minimum number of occurrences per word is seven, 36 keywords were displayed)
Figure 8 illustrates some main issues which were concerned in subject of cybercrime victimization, as well as the relationship among them. Fifteen most frequent keywords were presented by big bubbles, including: ‘cyberbullying’ (174 times), ‘cyber victimization’ (90 times), ‘adolescent’ (79 times), ‘bullying’ (66 times), ‘victimization’ (56 times), ‘cybercrime’ (40 times), ‘cyber aggression’ (37 times), ‘depression’ (23 times), ‘aggression’ (14 times), ‘routine activities theory’ (13 times), ‘cyberstalking’ (11 times), ‘gender’ (11 times), ‘longitudinal’ (10 times), ‘peer victimization’ (10 times) and ‘self-esteem’ (10 times).
‘Cyberbullying’ linked with many other keywords, demonstrating the various perspectives in research of this topic. The thick lines which linked ‘cyberbullying’ and ‘bullying’, ‘adolescent’, ‘cyber victimization’, ‘victimization’ showed the strong connections between them; there were close relationship between ‘cyber aggression’, ‘bystander”, ‘self-esteem’ or ‘moral disengagement’ and ‘cyberbullying’.
‘Cybercrime’ had strong links with ‘victimization’, ‘routine activities theory’. In Fig. 8 , the types of cybercrime which occurred at least seven times were: cyberbullying, cyber aggression, hacking, cyberstalking, and cyber dating abuse.
The increasing trend over the years reveals the increasing concern of scholarly community on this field, especially in the boom of information technology and other communication devices and the upward trend in research of cyberspace-related issues (Altarturi et al. 2020 ; Leung et al. 2017 ; Serafin et al. 2019 ). It predicts the growth of cybercrime victimization research in future.
Psychology was the more popular research areas in database, defeating criminology penology. As part of the ‘human factors of cybercrime’, human decision-making based on their psychological perspectives plays as a hot topic in cyber criminology (Leukfeldt and Holt 2020 ). Then, it is observed that journals in psychology field was more prevalent in top of productive sources. Besides, journal Computers in Human Behavior ranked first in total publications, but Journal of Youth and Adolescence ranked higher place in the average citations per document. Generally, top ten journals having highest number of publications on cybercrime victimization are highly qualified ones and at least 10 years in publishing industry.
The USA demonstrated its leading position in the studied domain in terms of total publications as well as the various collaborations with other countries. The publications of the USA occupied much higher than the second and third countries: England and Spain. It is not difficult to explain for this fact due to the impressive productivity of institutions and authors from the USA. A third of top twelve productive institutions were from the USA. Three leading positions of top ten productive authors based on document count were from institutions of the USA, number one was Michelle F. Wright; others were Thomas J. Holt and Bradford W. Reyns.
Furthermore, these authors also participated in significant research groups and become the important nodes in those clusters. The most noticeable authors in co-authors network were Michelle F. Wright. The US institutions also had strong links in research network. The USA was likely to be open in collaboration with numerous countries from different continents in the world. It was assessed to be a crucial partner for others in the international co-publication network (Glänzel and Schubert 2006 ).
As opposed to the USA, most of European countries prefer developing research network within Europe and had a limited collaboration with other areas. Australia, the USA, or Japan was in a small group of countries which had connections with European ones. Nevertheless, European countries still showed great contributions for research of cybercrime victimization and remained stable links in international collaboration. The prominent authors from Euro are Rosario Ortega-Ruiz, Constantinos M. Kokkinos or Rutger Leukfeldt.
It is obvious that the limited number of publications from Asia, Middle East, Africa, or other areas resulted in the uncomprehensive picture of studied subject. For example, in the Southeast Asia, Malaysia and Vietnam lacked the leading authors with their empirical studies to review and examine the nature of cybercrimes, though they are facing to practical challenges and potential threats in the cyberspace (Lusthaus 2020a , b ). The present study indicated that Vietnam, Ireland, or Norway was the new nodes and links in research network.
Several nations which had a small number of publications such as Vietnam, Thailand, Sri Lanka, or Chile started their journey of international publications. It is undeniable that globalization and the context of global village (McLuhan 1992 ) requires more understanding about the whole nations and areas. Conversely, each country or area also desires to engage in international publications. Therefore, new nodes and clusters are expected to increase and expand.
The findings indicated that cyberbullying was the most popular topic on research of cybercrime victimization over the given period. Over a half of most-cited publications was focus on cyberbullying. Additionally, ‘cyberbullying’ was the most frequent author keyword which co-occurred widely with distinctive keywords such as ‘victimization’, ‘adolescents’, ‘bullying’, ‘social media’, ‘internet’, ‘peer victimization’ or ‘anxiety’.
By reviewing keywords, several research gaps were indicated. Research samples were lack of population of the children and elders, while adolescent and youth were frequent samples of numerous studies. Although young people are most active in cyberspace, it is still necessary to understand other populations. Meanwhile, the elderly was assumed to use information and communication technologies to improve their quality of life (Tsai et al. 2015 ), their vulnerability to the risk of cybercrime victimization did not reduce. Those older women were most vulnerable to phishing attacks (Lin et al. 2019 ; Oliveira et al. 2017 ). Similarly, the population of children with distinctive attributes has become a suitable target for cybercriminals, particularly given the context of increasing online learning due to Covid-19 pandemic impacts. These practical gaps should be prioritized to focus on research for looking the suitable solutions in the future. Besides, a vast majority of research were conducted in the scope of one country; some studies collected cross-national data, but the number of these studies were moderate and focused much on developed countries. There are rooms for studies to cover several countries in Southeast Asia or South Africa.
Furthermore, although victims may be both individuals and organizations, most of research concentrated much more on individuals rather than organizations or companies. Wagen and Pieters ( 2020 ) indicated that victims include both human and non-human. They conducted research covering cases of ransomware victimization, Bonet victimization and high-tech virtual theft victimization and applying Actor-Network Theory to provide new aspect which did not aim to individual victims. The number of this kind of research, however, was very limited. Additionally, excepting cyberbullying and cyber aggression were occupied the outstanding quantity of research, other types of cybercrime, especially, e-whoring, or social media-related cybercrime should still be studied more in the future.
Another interesting topic is the impact of family on cybercrime victimization. By reviewing keyword, it is clear that the previous studies aimed to sample of adolescent, hence, there are many keywords linking with parents such as ‘parent-adolescent communication’, ‘parent-adolescent information sharing’, ‘parental mediation’, ‘parental monitoring of cyber behavior’, ‘parental style’. As mentioned above, it is necessary to research more on sample of the elder, then, it is also essential to find out how family members affect the elder’s cybercrime victimization.
It is a big challenge to deal with problems of cybercrime victimization because cybercrime forms become different daily (Näsi et al. 2015 ). Numerous researchers engage in understanding this phenomenon from various angles. The current bibliometric study assessed the scholarly status on cybercrime victimization during 2010–2020 by retrieving SSCI articles from WoS database. There is no study that applied bibliometric method to research on the examined subject. Hence, this paper firstly contributed statistical evidence and visualized findings to literature of cybercrime victimization.
Statistical description was applied to measure the productive authors, institutions, countries/regions, sources, and most-cited documents, mainly based on publication and citation count. The international collaborations among authors, institutions, and countries were assessed by co-authors, while the network of author keywords was created by co-occurrence analysis. The overall scholarly status of cybercrime victimization research was drawn clearly and objectively. The research trend, popular issues and current gaps were reviewed, providing numerous suggestions for policymakers, scholars, and practitioners about cyber-related victimization (Pickering and Byrne 2014 ). Accordingly, the paper indicated the most prevalent authors, most-cited papers but also made summary of contributions of previous research as well as identified research gaps. First, this article supports for PhD candidates or early-career researchers concerning about cybercrime victimization. Identifying the leading authors, remarked journals, or influencing articles, gaps related to a specific research topic is important and useful task for new researchers to start their academic journey. Although this information is relatively simple, it takes time and is not easy for newcomers to find out, especially for ones in poor or developing areas which have limited conditions and opportunities to access international academic sources. Thus, the findings in the current paper provided for them basic but necessary answers to conduct the first step in research. Secondly, by indicating research gaps in relevance to sample, narrow topics or scope of country, the paper suggests future study fulfilling them to complete the field of cybercrime victimization, especial calling for publications from countries which has had a modest position in global research map. Science requires the balance and diversity, not just focusing on a few developed countries or areas. Finally, the present study assists researchers and institutions to determined strategy and potential partners for their development of research collaborations. It not only improve productivity of publication but also create an open and dynamic environment for the development of academic field.
Despite mentioned contributions, this study still has unavoidable limitations. The present paper just focused on SSCI articles from WoS database during 2010–2020. It did not cover other sources of databases that are known such as Scopus, ScienceDirect, or Springer; other types of documents; the whole time; or articles in other languages excepting English. Hence it may not cover all data of examined subject in fact. Moreover, this bibliometric study just performed co-authorship and co-occurrence analysis. The rest of analysis such as citation, co-citation and bibliographic coupling have not been conducted. Research in the future is recommended to perform these kinds of assessment to fill this gap. To visualize the collaboration among authors, institutions, countries, or network of keywords, this study used VOS Viewer software and saved the screenshots as illustrations. Therefore, not all items were displayed in the screenshot figures.
Data availability
Declarations.
The authors declare that they have no competing interest.
1 In the ‘commemorating a decade in existence of the International Journal of Cyber Criminoogy’, Ngo and Jaishankar ( 2017 ) called for further research with focusing on five main areas in the Cyber Criminiology, including (1) defining and classifying cybercrime, (2) assessing the prevalence, nature, and trends of cybercrime, (3) advancing the field of cyber criminology, (4) documenting best practices in combating and preventing cybercrime, and (5) cybercrime and privacy issues.
Contributor Information
Huong Thi Ngoc Ho, Email: moc.liamg@252nhgnouH .
Hai Thanh Luong, Email: [email protected] .
- Abdullah ATM, Jahan I. Causes of cybercrime victimization: a systematic literature review. Int J Res Rev. 2020; 7 (5):89–98. [ Google Scholar ]
- Al-Nemrat A, Benzaïd C (2015) Cybercrime profiling: Decision-tree induction, examining perceptions of internet risk and cybercrime victimisation. In: Proceedings—14th IEEE International Conference on Trust, Security and Privacy in Computing and Communications, TrustCom 2015, vol 1, pp 1380–1385. 10.1109/Trustcom.2015.534
- Alalehto TI, Persson O. The Sutherland tradition in criminology: a bibliometric story. Crim Justice Stud. 2013; 26 (1):1–18. doi: 10.1080/1478601X.2012.706753. [ CrossRef ] [ Google Scholar ]
- Algarni A, Xu Y, Chan T. An empirical study on the susceptibility to social engineering in social networking sites: the case of Facebook. Eur J Inf Syst. 2017; 26 (6):661–687. doi: 10.1057/s41303-017-0057-y. [ CrossRef ] [ Google Scholar ]
- Altarturi HHM, Saadoon M, Anuar NB. Cyber parental control: a bibliometric study. Child Youth Serv Rev. 2020 doi: 10.1016/j.childyouth.2020.105134. [ CrossRef ] [ Google Scholar ]
- Ariola B, Laure ERF, Perol ML, Talines PJ. Cybercrime awareness and perception among Students of Saint Michael College of Caraga. SMCC Higher Educ Res J. 2018; 1 (1):1. doi: 10.18868/cje.01.060119.03. [ CrossRef ] [ Google Scholar ]
- Benson V, Saridakis G, Tennakoon H. Purpose of social networking use and victimisation: are there any differences between university students and those not in HE? Comput Hum Behav. 2015; 51 :867–872. doi: 10.1016/j.chb.2014.11.034. [ CrossRef ] [ Google Scholar ]
- Brenner SW. Is there such a thing as “rough”? Calif Crim Law Rev. 2001; 4 (1):348–349. doi: 10.3109/09637487909143344. [ CrossRef ] [ Google Scholar ]
- Chen X, Wang S, Tang Y, Hao T. A bibliometric analysis of event detection in social media. Online Inf Rev. 2019; 43 (1):29–52. doi: 10.1108/OIR-03-2018-0068. [ CrossRef ] [ Google Scholar ]
- Choi K. Computer Crime Victimization and Integrated Theory: an empirical assessment. Int J Cyber Criminol. 2008; 2 (1):308–333. [ Google Scholar ]
- Cojocaru I, Cojocaru I (2019) A bibliomentric analysis of cybersecurity research papers in Eastern Europe: case study from the Republic of Moldova. In: Central and Eastern European E|Dem and E|Gov Days, pp 151–161
- Das B, Sahoo JS. Social networking sites—a critical analysis of its impact on personal and social life. Int J Bus Soc Sci. 2011; 2 (14):222–228. [ Google Scholar ]
- Drew JM. A study of cybercrime victimisation and prevention: exploring the use of online crime prevention behaviours and strategies. J Criminol Res Policy Pract. 2020; 6 (1):17–33. doi: 10.1108/JCRPP-12-2019-0070. [ CrossRef ] [ Google Scholar ]
- E Fonseca B, Sampaio, de Araújo Fonseca MV, Zicker F (2016). Co-authorship network analysis in health research: method and potential use. Health Res Policy Syst 14(1):1–10. 10.1186/s12961-016-0104-5 [ PMC free article ] [ PubMed ]
- Feeley TH. A bibliometric analysis of communication journals from 2002 to 2005. Hum Commun Res. 2008; 34 :505–520. doi: 10.1111/j.1468-2958.2008.00330.x. [ CrossRef ] [ Google Scholar ]
- Gan C, Wang W. A bibliometric analysis of social media research from the perspective of library and information science. IFIP Adv Inf Commun Technol. 2014; 445 :23–32. doi: 10.1007/978-3-662-45526-5_3. [ CrossRef ] [ Google Scholar ]
- Glänzel W, Schubert A. Analysing scientific networks through co-authorship. Handb Quant Sci Technol Res. 2006 doi: 10.1007/1-4020-2755-9_12. [ CrossRef ] [ Google Scholar ]
- Gogolin G. The digital crime tsunami. Digit Investig. 2010; 7 (1–2):3–8. doi: 10.1016/j.diin.2010.07.001. [ CrossRef ] [ Google Scholar ]
- Holt TJ, Bossler AM. Examining the applicability of lifestyle-routine activities theory for cybercrime victimization. Deviant Behav. 2009; 30 (1):1–25. doi: 10.1080/01639620701876577. [ CrossRef ] [ Google Scholar ]
- Holt TJ, Bossler AM. An assessment of the current state of cybercrime scholarship. Deviant Behav. 2014; 35 (1):20–40. doi: 10.1080/01639625.2013.822209. [ CrossRef ] [ Google Scholar ]
- Ilievski A. An explanation of the cybercrime victimisation: self-control and lifestile/routine activity theory. Innov Issues Approaches Soc Sci. 2016; 9 (1):30–47. doi: 10.12959/issn.1855-0541.iiass-2016-no1-art02. [ CrossRef ] [ Google Scholar ]
- Insa F. The Admissibility of Electronic Evidence in Court (A.E.E.C.): Fighting against high-tech crime—results of a European study. J Digital Forensic Pract. 2007; 1 (4):285–289. doi: 10.1080/15567280701418049. [ CrossRef ] [ Google Scholar ]
- Jahankhani H. Developing a model to reduce and/or prevent cybercrime victimization among the user individuals. Strategic Intell Manag. 2013 doi: 10.1016/b978-0-12-407191-9.00021-1. [ CrossRef ] [ Google Scholar ]
- Jamshed J, Naeem S, Ahmad K (2020) Analysis of Criminal Law Literature: a bibliometric study from 2010–2019. Library Philos Pract
- Kim J, Kang S, Lee KH (2019) Evolution of digital marketing communication: Bibliometric analysis and networs visualization from key articles. J Bus Res
- Kirwan GH, Fullwood C, Rooney B. Risk factors for social networking site scam victimization among Malaysian students. Cyberpsychol Behav Soc Netw. 2018; 21 (2):123–128. doi: 10.1089/cyber.2016.0714. [ PubMed ] [ CrossRef ] [ Google Scholar ]
- Lee S-S, Choi KS, Choi S, Englander E. A test of structural model for fear of crime in social networking sites A test of structural model for fear of crime in social networking sites. Int J Cybersecur Intell Cybercrime. 2019; 2 (2):5–22. doi: 10.52306/02020219SVZL9707. [ CrossRef ] [ Google Scholar ]
- Leukfeldt R, Holt T, editors. The human factor of cybercrime. New York: Routledge; 2020. [ Google Scholar ]
- Leukfeldt ER, Yar M. Applying routine activity theory to cybercrime: a theoretical and empirical analysis. Deviant Behav. 2016; 37 (3):263–280. doi: 10.1080/01639625.2015.1012409. [ CrossRef ] [ Google Scholar ]
- Leung XY, Sun J, Bai B. Bibliometrics of social media research: a co-citation and co-word analysis. Int J Hosp Manag. 2017; 66 :35–45. doi: 10.1016/j.ijhm.2017.06.012. [ CrossRef ] [ Google Scholar ]
- Li Q, Wei W, Xiong N, Feng D, Ye X, Jiang Y. Social media research, human behavior, and sustainable society. Sustainability. 2017; 9 (3):384. doi: 10.3390/su9030384. [ CrossRef ] [ Google Scholar ]
- Lin T, Capecci DE, Ellis DM, Rocha HA, Dommaraju S, Oliveira DS, Ebner NC. Susceptibility to spear-phishing emails: effects of internet user demographics and email content. ACM Trans Comput-Hum Interact. 2019; 26 (5):1–28. doi: 10.1145/3336141. [ PMC free article ] [ PubMed ] [ CrossRef ] [ Google Scholar ]
- Luong TH. Prevent and combat sexual assault and exploitation of children on cyberspace in Vietnam: situations, challenges, and responses. In: Elshenraki H, editor. Combating the exploitation of children in cyberspace: emerging research and opportunities. Hershey: IGI Global; 2021. pp. 68–94. [ Google Scholar ]
- Luong HT, Phan HD, Van Chu D, Nguyen VQ, Le KT, Hoang LT. Understanding cybercrimes in Vietnam: from leading-point provisions to legislative system and law enforcement. Int J Cyber Criminol. 2019; 13 (2):290–308. doi: 10.5281/zenodo.3700724. [ CrossRef ] [ Google Scholar ]
- Lusthaus J (2020a) Cybercrime in Southeast Asia: Combating a Global Threat Locally. Retrieved from Canberra, Australia: https://s3-ap-southeast-2.amazonaws.com/ad-aspi/202005/Cybercrime%20in%20Southeast%20Asia.pdf?naTsKQp2jtSPYsWpSo4YmE1sVBNv_exJ
- Lusthaus J. Modelling cybercrime development: the case of Vietnam. In: Leukfeldt R, Holt T, editors. The human factor of cybercrime. New York: Routledge; 2020. pp. 240–257. [ Google Scholar ]
- Marsh I, Melville G. Crime justice and the media. Crime Justice Med. 2008 doi: 10.4324/9780203894781. [ CrossRef ] [ Google Scholar ]
- Martí-Parreño J, Méndez-Ibáñezt E, Alonso-Arroyo A (2016) The use of gamification in education: a bibliometric and text mining analysis. J Comput Assist Learn
- McLuhan M. The Global Village: Transformations in World Life and Media in the 21st Century (Communication and Society) Oxford: Oxford University Press; 1992. [ Google Scholar ]
- Näsi M, Oksanen A, Keipi T, Räsänen P. Cybercrime victimization among young people: a multi-nation study. J Scand Stud Criminol Crime Prev. 2015 doi: 10.1080/14043858.2015.1046640. [ CrossRef ] [ Google Scholar ]
- Ngo F, Jaishankar K. Commemorating a decade in existence of the international journal of cyber criminology: a research agenda to advance the scholarship on cyber crime. Int J Cyber Criminol. 2017; 11 (1):1–9. [ Google Scholar ]
- Ngo F, Paternoster R. Cybercrime victimization: an examination of individual and situational level factors. Int J Cyber Criminol. 2011; 5 (1):773. [ Google Scholar ]
- Nguyen VT, Luong TH. The structure of cybercrime networks: transnational computer fraud in Vietnam. J Crime Justice. 2020 doi: 10.1080/0735648X.2020.1818605. [ CrossRef ] [ Google Scholar ]
- Oksanen A, Keipi T. Young people as victims of crime on the internet: a population-based study in Finland. Vulnerable Child Youth Stud. 2013; 8 (4):298–309. doi: 10.1080/17450128.2012.752119. [ CrossRef ] [ Google Scholar ]
- Oliveira D, Rocha H, Yang H, Ellis D, Dommaraju S, Muradoglu M, Weir D, Soliman A, Lin T, Ebner N (2017) Dissecting spear phishing emails for older vs young adults: on the interplay of weapons of influence and life domains in predicting susceptibility to phishing. In: Conference on Human Factors in Computing Systems—Proceedings, 2017-May, 6412–6424. 10.1145/3025453.3025831
- Orchard LJ, Fullwood C, Galbraith N, Morris N. Individual differences as predictors of social networking. J Comput-Mediat Commun. 2014; 19 (3):388–402. doi: 10.1111/jcc4.12068. [ CrossRef ] [ Google Scholar ]
- Parrish JL, Jr, Bailey JL, Courtney JF. A personality based model for determining susceptibility to phishing attacks. Little Rock: University of Arkansas; 2009. pp. 285–296. [ Google Scholar ]
- Pasadeos Y. A bibliometric study of advertising citations. J Advert. 1985; 14 (4):52–59. doi: 10.1080/00913367.1985.10672971. [ CrossRef ] [ Google Scholar ]
- Pickering C, Byrne J. The benefits of publishing systematic quantitative literature reviews for PhD candidates and other early-career researchers. Higher Educ Res Devel ISSN. 2014; 33 (3):534–548. doi: 10.1080/07294360.2013.841651. [ CrossRef ] [ Google Scholar ]
- Reyns BW, Fisher BS, Bossler AM, Holt TJ. Opportunity and Self-control: do they predict multiple forms of online victimization? Am J Crim Justice. 2019; 44 (1):63–82. doi: 10.1007/s12103-018-9447-5. [ CrossRef ] [ Google Scholar ]
- Romero L, Portillo-Salido E. Trends in sigma-1 receptor research: a 25-year bibliometric analysis. Front Pharmacol. 2019 doi: 10.3389/fphar.2019.00564. [ PMC free article ] [ PubMed ] [ CrossRef ] [ Google Scholar ]
- Saad ME, Huda Sheikh Abdullah SN, Murah MZ. Cyber romance scam victimization analysis using Routine Activity Theory versus apriori algorithm. Int J Adv Comput Sci Appl. 2018; 9 (12):479–485. doi: 10.14569/IJACSA.2018.091267. [ CrossRef ] [ Google Scholar ]
- Saridakis G, Benson V, Ezingeard JN, Tennakoon H. Individual information security, user behaviour and cyber victimisation: an empirical study of social networking users. Technol Forecast Soc Change. 2016; 102 :320–330. doi: 10.1016/j.techfore.2015.08.012. [ CrossRef ] [ Google Scholar ]
- Seng S, Wright M, Al-Ameen MN (2018) Understanding users’ decision of clicking on posts in facebook with implications for phishing. Workshop on Technology and Consumer Protection (ConPro 18), May, 1–6
- Serafin MJ, Garcia-Vargas GR, García-Chivita MDP, Caicedo MI, Correra JC. Cyberbehavior: a bibliometric analysis. Annu Rev Cyber Ther Telemed. 2019; 17 :17–24. doi: 10.31234/osf.io/prfcw. [ CrossRef ] [ Google Scholar ]
- Skinner WF, Fream AM. A social learning theory analysis of computer crime among college students. J Res Crime Delinq. 1997; 34 (4):495–518. doi: 10.1177/0022427897034004005. [ CrossRef ] [ Google Scholar ]
- Tsai H, Yi S, Shillair R, Cotten SR, Winstead V, Yost E. Getting grandma online: are tablets the answer for increasing digital inclusion for older adults in the US? Educ Gerontol. 2015; 41 (10):695–709. doi: 10.1080/03601277.2015.1048165. [ PMC free article ] [ PubMed ] [ CrossRef ] [ Google Scholar ]
- van Eck NJ, Waltman L (2020) Manual for VOSviewer version 1.6.16
- Van Wilsem J. “Bought it, but never got it” assessing risk factors for online consumer fraud victimization. Eur Sociol Rev. 2013; 29 (2):168–178. doi: 10.1093/esr/jcr053. [ CrossRef ] [ Google Scholar ]
- van der Wagen W, Pieters W. The hybrid victim: re-conceptualizing high-tech cyber victimization through actor-network theory. Eur J Criminol. 2020; 17 (4):480–497. doi: 10.1177/1477370818812016. [ CrossRef ] [ Google Scholar ]
- Vishwanath A. Habitual Facebook use and its impact on getting deceived on social media. J Comput-Mediat Commun. 2015; 20 (1):83–98. doi: 10.1111/jcc4.12100. [ CrossRef ] [ Google Scholar ]
- Wall D. Crime and the Internet: Cybercrime and cyberfears. 1. London: Routledge; 2001. [ Google Scholar ]
- Wall D. What are cybercrimes? Crim Justice Matters. 2004; 58 (1):20–21. doi: 10.1080/09627250408553239. [ CrossRef ] [ Google Scholar ]
- Whitty MT, Buchanan T. The online romance scam: a serious cybercrime. Cyberpsychol Behav Soc Netw. 2012; 15 (3):181–183. doi: 10.1089/cyber.2011.0352. [ PubMed ] [ CrossRef ] [ Google Scholar ]
- You GR, Sun X, Sun M, Wang JM, Chen YW (2014) Bibliometric and social network analysis of the SoS field. In: Proceedings of the 9th International Conference on System of Systems Engineering: The Socio-Technical Perspective, SoSE 2014, 13–18. 10.1109/SYSOSE.2014.6892456
- Zyoud SH, Sweileh WM, Awang R, Al-Jabi SW. Global trends in research related to social media in psychology: Mapping and bibliometric analysis. Int J Ment Health Syst. 2018; 12 (1):1–8. doi: 10.1186/s13033-018-0182-6. [ PMC free article ] [ PubMed ] [ CrossRef ] [ Google Scholar ]
- Open access
- Published: 29 April 2021
Crime forecasting: a machine learning and computer vision approach to crime prediction and prevention
- Neil Shah 1 ,
- Nandish Bhagat 1 &
- Manan Shah ORCID: orcid.org/0000-0002-8665-5010 2
Visual Computing for Industry, Biomedicine, and Art volume 4 , Article number: 9 ( 2021 ) Cite this article
68k Accesses
46 Citations
4 Altmetric
Metrics details
A crime is a deliberate act that can cause physical or psychological harm, as well as property damage or loss, and can lead to punishment by a state or other authority according to the severity of the crime. The number and forms of criminal activities are increasing at an alarming rate, forcing agencies to develop efficient methods to take preventive measures. In the current scenario of rapidly increasing crime, traditional crime-solving techniques are unable to deliver results, being slow paced and less efficient. Thus, if we can come up with ways to predict crime, in detail, before it occurs, or come up with a “machine” that can assist police officers, it would lift the burden of police and help in preventing crimes. To achieve this, we suggest including machine learning (ML) and computer vision algorithms and techniques. In this paper, we describe the results of certain cases where such approaches were used, and which motivated us to pursue further research in this field. The main reason for the change in crime detection and prevention lies in the before and after statistical observations of the authorities using such techniques. The sole purpose of this study is to determine how a combination of ML and computer vision can be used by law agencies or authorities to detect, prevent, and solve crimes at a much more accurate and faster rate. In summary, ML and computer vision techniques can bring about an evolution in law agencies.
Introduction
Computer vision is a branch of artificial intelligence that trains the computer to understand and comprehend the visual world, and by doing so, creates a sense of understanding of a machine’s surroundings [ 1 , 2 ]. It mainly analyzes data of the surroundings from a camera, and thus its applications are significant. It can be used for face recognition, number plate recognition, augmented and mixed realities, location determination, and identifying objects [ 3 ]. Research is currently being conducted on the formation of mathematical techniques to recover and make it possible for computers to comprehend 3D images. Obtaining the 3D visuals of an object helps us with object detection, pedestrian detection, face recognition, Eigenfaces active appearance and 3D shape models, personal photo collections, instance recognition, geometric alignment, large databases, location recognition, category recognition, bag of words, part-based models, recognition with segmentation, intelligent photo editing, context and scene understanding, and large image collection and learning, image searches, recognition databases, and test sets. These are only basic applications, and each category mentioned above can be further explored. In ref. [ 4 ], VLFeat is introduced, which is a library of computer vision algorithms that can be used to conduct fast prototyping in computer vision research, thus enabling a tool to obtain computer vision results much faster than anticipated. Considering face detection/human recognition [ 5 ], human posture can also be recognized. Thus, computer vision is extremely attractive for visualizing the world around us.
Machine learning (ML) is an application that provides a system with the ability to learn and improve automatically from past experiences without being explicitly programmed [ 6 , 7 , 8 ]. After viewing the data, an exact pattern or information cannot always be determined [ 9 , 10 , 11 ]. In such cases, ML is applied to interpret the exact pattern and information [ 12 , 13 ]. ML pushes forward the idea that, by providing a machine with access to the right data, the machine can learn and solve both complex mathematical problems and some specific problems [ 14 , 15 , 16 , 17 ]. In general, ML is categorized into two parts: (1) supervised ML and (2) unsupervised ML [ 18 , 19 ]. In supervised learning, the machine is trained on the basis of a predefined set of training examples, which facilitates its capability to obtain precise and accurate conclusions when new data are given [ 20 , 21 ]. In unsupervised learning, the machine is given a set of data, and it must find some common patterns and relationships between the data its own [ 22 , 23 ]. Neural networks, which are important tools used in supervised learning, have been studied since the 1980s [ 24 , 25 ]. In ref. [ 26 ], the author suggested that different aspects are needed to obtain an exit from nondeterministic polynomial (NP)-completeness, and architectural constraints are insufficient. However, in ref. [ 27 ], it was proved that NP-completeness problems can be extended to neural networks using sigmoid functions. Although such research has attempted to demonstrate the various aspects of new ML approaches, how accurate are the results [ 28 , 29 , 30 ]?
Although various crimes and their underlying nature seem to be unpredictable, how unforeseeable are they? In ref. [ 31 ], the authors pointed out that as society and the economy results in new types of crimes, the need for a prediction system has grown. In ref. [ 32 ], crime trends and prediction technology called Mahanolobis and a dynamic time wrapping technique are given, delivering the possibility of predicting crime and apprehending the actual culprit. As described in ref. [ 33 ], in 1998, the United States National Institute of Justice granted five grants for crime forecasting as an extension to crime mapping. Applications of crime forecasting are currently being used by law enforcement in the United States, the United Kingdom, the Netherlands, Germany, and Switzerland [ 34 ]. Nowadays, criminal intellect with the help of advances in technology is improving with each passing year. Consequently, it has become necessary for us to provide the police department and the government with the means of a new and powerful machine (a set of programs) that can help them in their process of solving crimes. The main aim of crime forecasting is to predict crimes before they occur, and thus, the importance of using crime forecasting methods is extremely clear. Furthermore, the prediction of crimes can sometimes be crucial because it may potentially save the life of a victim, prevent lifelong trauma, and avoid damage to private property. It may even be used to predict possible terrorist crimes and activities. Finally, if we implement predictive policing with a considerable level of accuracy, governments can apply other primary resources such as police manpower, detectives, and funds in other fields of crime solving, thereby curbing the problem of crime with double the power.
In this paper, we aim to make an impact by using both ML algorithms and computer vision methods to predict both the nature of a crime and possibly pinpoint a culprit. Beforehand, we questioned whether the nature of the crime was predictable. Although it might seem impossible from the outside, categorizing every aspect of a crime is quite possible. We have all heard that every criminal has a motive. That is, if we use motive as a judgment for the nature of a crime, we may be able to achieve a list of ways in which crimes can be categorized. Herein, we discuss a theory where we combine ML algorithms to act as a database for all recorded crimes in terms of category, along with providing visual knowledge of the surroundings through computer vision techniques, and using the knowledge of such data, we may predict a crime before it occurs.
Present technological used in crime detection and prediction
Crime forecasting refers to the basic process of predicting crimes before they occur. Tools are needed to predict a crime before it occurs. Currently, there are tools used by police to assist in specific tasks such as listening in on a suspect’s phone call or using a body cam to record some unusual illegal activity. Below we list some such tools to better understand where they might stand with additional technological assistance.
One good way of tracking phones is through the use of a stingray [ 35 ], which is a new frontier in police surveillance and can be used to pinpoint a cellphone location by mimicking cellphone towers and broadcasting the signals to trick cellphones within the vicinity to transmit their location and other information. An argument against the usage of stingrays in the United States is that it violates the fourth amendment. This technology is used in 23 states and in the district of Columbia. In ref. [ 36 ], the authors provide insight on how this is more than just a surveillance system, raising concerns about privacy violations. In addition, the Federal Communicatons Commission became involved and ultimately urged the manufacturer to meet two conditions in exchange for a grant: (1) “The marketing and sale of these devices shall be limited to federal, state, local public safety and law enforcement officials only” and (2) “State and local law enforcement agencies must advance coordinate with the FBI the acquisition and use of the equipment authorized under this authorization.” Although its use is worthwhile, its implementation remains extremely controversial.
A very popular method that has been in practice since the inception of surveillance is “the stakeout”. A stakeout is the most frequently practiced surveillance technique among police officers and is used to gather information on all types of suspects. In ref. [ 37 ], the authors discuss the importance of a stakeout by stating that police officers witness an extensive range of events about which they are required to write a report. Such criminal acts are observed during stakeouts or patrols; observations of weapons, drugs, and other evidence during house searches; and descriptions of their own behavior and that of the suspect during arrest. Stakeouts are extremely useful, and are considered 100% reliable, with the police themselves observing the notable proceedings. However, are they actually 100% accurate? All officers are humans, and all humans are subject to fatigue. The major objective of a stakeout is to observe wrongful activities. Is there a tool that can substitute its use? We will discuss this point herein.
Another way to conduct surveillance is by using drones, which help in various fields such as mapping cities, chasing suspects, investigating crime scenes and accidents, traffic management and flow, and search and rescue after a disaster. In ref. [ 38 ], legal issues regarding the use of drones and airspace distribution problems are described. Legal issues include the privacy concerns raised by the public, with the police gaining increasing power and authority. Airspace distribution raises concerns about how high a drone is allowed to go.
Other surveillance methods include face recognition, license plate recognition, and body cams. In ref. [ 39 ], the authors indicated that facial recognition can be used to obtain the profile of suspects and analyze it from different databases to obtain more information. Similarly, a license plate reader can be used to access data about a car possibly involved in a crime. They may even use body cams to see more than what the human eye can see, meaning that the reader observes everything a police officer sees and records it. Normally, when we see an object, we cannot recollect the complete image of it. In ref. [ 40 ], the impact of body cams was studied in terms of officer misconduct and domestic violence when the police are making an arrest. Body cams are thus being worn by patrol officers. In ref. [ 41 ], the authors also mentioned how protection against wrongful police practices is provided. However, the use of body cams does not stop here, as other primary reasons for having a body camera on at all times is to record the happenings in front of the wearer in hopes of record useful events during daily activities or during important operations.
Although each of these methods is effective, one point they share in common is that they all work individually, and while the police can use any of these approaches individually or concurrently, having a machine that is able to incorporate the positive aspects of all of these technologies would be highly beneficial.
ML techniques used in crime prediction
In ref. [ 42 ], a comparative study was carried out between violent crime patterns from the Communities and Crime Unnormalized Dataset versus actual crime statistical data using the open source data mining software Waikato Environment for Knowledge Analysis (WEKA). Three algorithms, namely, linear regression, additive regression, and decision stump, were implemented using the same finite set of features on communities and actual crime datasets. Test samples were randomly selected. The linear regression algorithm could handle randomness to a certain extent in the test samples and thus proved to be the best among all three selected algorithms. The scope of the project was to prove the efficiency and accuracy of ML algorithms in predicting violent crime patterns and other applications, such as determining criminal hotspots, creating criminal profiles, and learning criminal trends.
When considering WEKA [ 43 ], the integration of a new graphical interface called Knowledge Flow is possible, which can be used as a substitute for Internet Explorer. IT provides a more concentrated view of data mining in association with the process orientation, in which individual learning components (represented by java beans) are used graphically to show a certain flow of information. The authors then describe another graphical interface called an experimenter, which as the name suggests, is designed to compare the performance of multiple learning schemes on multiple data sets.
In ref. [ 34 ], the potential of applying a predictive analysis of crime forecasting in an urban context is studied. Three types of crime, namely, home burglary, street robbery, and battery, were aggregated into grids of 200 m × 250 m and retrospectively analyzed. Based on the crime data of the previous 3 years, an ensemble model was applied to synthesize the results of logistic regression and neural network models in order to obtain fortnightly and monthly predictions for the year 2014. The predictions were evaluated based on the direct hit rate, precision, and prediction index. The results of the fortnightly predictions indicate that by applying a predictive analysis methodology to the data, it is possible to obtain accurate predictions. They concluded that the results can be improved remarkably by comparing the fortnightly predictions with the monthly predictions with a separation between day and night.
In ref. [ 44 ], crime predictions were investigated based on ML. Crime data of the last 15 years in Vancouver (Canada) were analyzed for prediction. This machine-learning-based crime analysis involves the collection of data, data classification, identification of patterns, prediction, and visualization. K-nearest neighbor (KNN) and boosted decision tree algorithms were also implemented to analyze the crime dataset. In their study, a total of 560,000 crime datasets between 2003 and 2018 were analyzed, and crime prediction with an accuracy of between 39% and 44% was obtained by predicting the crime using ML algorithms. The accuracy was low as a prediction model, but the authors concluded that the accuracy can be increased or improved by tuning both the algorithms and crime data for specific applications.
In ref. [ 45 ], a ML approach is presented for the prediction of crime-related statistics in Philadelphia, United States. The problem was divided into three parts: determining whether the crime occurs, occurrence of crime and most likely crime. Algorithms such as logistic regression, KNN, ordinal regression, and tree methods were used to train the datasets to obtain detailed quantitative crime predictions with greater significance. They also presented a map for crime prediction with different crime categories in different areas of Philadelphia for a particular time period with different colors indicating each type of crime. Different types of crimes ranging from assaults to cyber fraud were included to match the general pattern of crime in Philadelphia for a particular interval of time. Their algorithm was able to predict whether a crime will occur with an astonishing 69% accuracy, as well as the number of crimes ranging from 1 to 32 with 47% accuracy.
In ref. [ 46 ], the authors analyzed a dataset consisting of several crimes and predicted the type of crime that may occur in the near future depending on various conditions. ML and data science techniques were used for crime prediction in a crime dataset from Chicago, United States. The crime dataset consists of information such as the crime location description, type of crime, date, time, and precise location coordinates. Different combinations of models, such as KNN classification, logistic regression, decision trees, random forest, a support vector machine (SVM), and Bayesian methods were tested, and the most accurate model was used for training. The KNN classification proved to be the best with an accuracy of approximately 0.787. They also used different graphs that helped in understanding the various characteristics of the crime dataset of Chicago. The main purpose of this paper is to provide an idea of how ML can be used by law enforcement agencies to predict, detect, and solve crime at a much better rate, which results in a reduction in crime.
In ref. [ 47 ], a graphical user interface-based prediction of crime rates using a ML approach is presented. The main focus of this study was to investigate machine-learning-based techniques with the best accuracy in predicting crime rates and explore its applicability with particular importance to the dataset. Supervised ML techniques were used to analyze the dataset to carry out data validation, data cleaning, and data visualization on the given dataset. The results of the different supervised ML algorithms were compared to predict the results. The proposed system consists of data collection, data preprocessing, construction of a predictive model, dataset training, dataset testing, and a comparison of algorithms, as shown in Fig. 1 . The aim of this study is to prove the effectiveness and accuracy of a ML algorithm for predicting violent crimes.
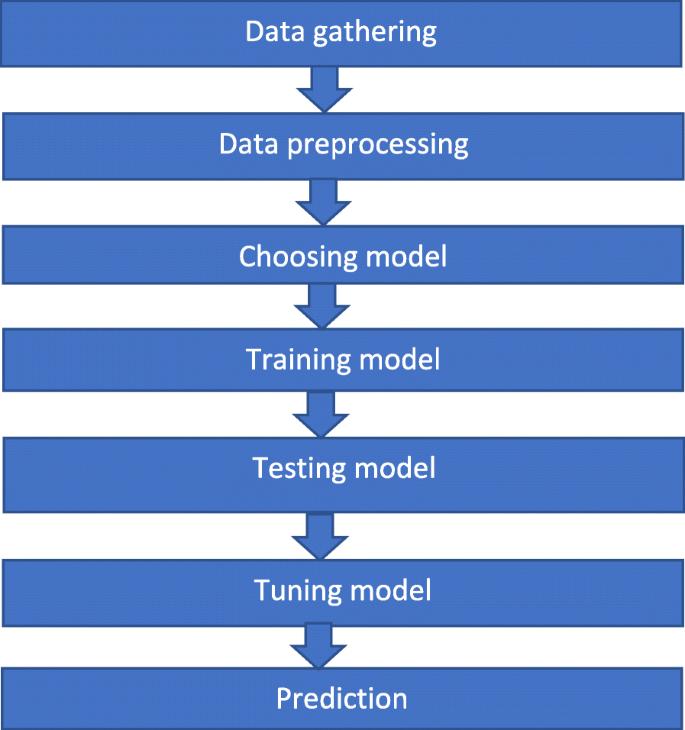
Dataflow diagram
In ref. [ 48 ], a feature-level data fusion method based on a deep neural network (DNN) is proposed to accurately predict crime occurrence by efficiently fusing multi-model data from several domains with environmental context information. The dataset consists of data from an online database of crime statistics from Chicago, demographic and meteorological data, and images. Crime prediction methods utilize several ML techniques, including a regression analysis, kernel density estimation (KDE), and SVM. Their approach mainly consisted of three phases: collection of data, analysis of the relationship between crime incidents and collected data using a statistical approach, and lastly, accurate prediction of crime occurrences. The DNN model consists of spatial features, temporal features, and environmental context. The SVM and KDE models had accuracies of 67.01% and 66.33%, respectively, whereas the proposed DNN model had an astonishing accuracy of 84.25%. The experimental results showed that the proposed DNN model was more accurate in predicting crime occurrences than the other prediction models.
In ref. [ 49 ], the authors mainly focused on the analysis and design of ML algorithms to reduce crime rates in India. ML techniques were applied to a large set of data to determine the pattern relations between them. The research was mainly based on providing a prediction of crime that might occur based on the occurrence of previous crime locations, as shown in Fig. 2 . Techniques such as Bayesian neural networks, the Levenberg Marquardt algorithm, and a scaled algorithm were used to analyze and interpret the data, among which the scaled algorithm gave the best result in comparison with the other two techniques. A statistical analysis based on the correlation, analysis of variance, and graphs proved that with the help of the scaled algorithm, the crime rate can be reduced by 78%, implying an accuracy of 0.78.

Functionality of proposed approach
In ref. [ 50 ], a system is proposed that predicts crime by analyzing a dataset containing records of previously committed crimes and their patterns. The proposed system works mainly on two ML algorithms: a decision tree and KNN. Techniques such as the random forest algorithm and Adaptive Boosting were used to increase the accuracy of the prediction model. To obtain better results for the model, the crimes were divided into frequent and rare classes. The frequent class consisted of the most frequent crimes, whereas the rare class consisted of the least frequent crimes. The proposed system was fed with criminal activity data for a 12-year period in San Francisco, United States. Using undersampling and oversampling methods along with the random forest algorithm, the accuracy was surprisingly increased to 99.16%.
In ref. [ 51 ], a detailed study on crime classification and prediction using ML and deep learning architectures is presented. Certain ML methodologies, such as random forest, naïve Bayes, and an SVM have been used in the literature to predict the number of crimes and hotspot prediction. Deep learning is a ML approach that can overcome the limitations of some machine-learning methodologies by extracting the features from the raw data. This paper presents three fundamental deep learning configurations for crime prediction: (1) spatial and temporal patterns, (2) temporal and spatial patterns, and (3) spatial and temporal patterns in parallel. Moreover, the proposed model was compared with 10 state-of-the-art algorithms on 5 different crime prediction datasets with more than 10 years of crime data.
In ref. [ 52 ], a big data and ML technique for behavior analysis and crime prediction is presented. This paper discusses the tracking of information using big data, different data collection approaches, and the last phase of crime prediction using ML techniques based on data collection and analysis. A predictive analysis was conducted through ML using RapidMiner by processing historical crime patterns. The research was mainly conducted in four phases: data collection, data preparation, data analysis, and data visualization. It was concluded that big data is a suitable framework for analyzing crime data because it can provide a high throughput and fault tolerance, analyze extremely large datasets, and generate reliable results, whereas the ML based naïve Bayes algorithm can achieve better predictions using the available datasets.
In ref. [ 53 ], various data mining and ML technologies used in criminal investigations are demonstrated. The contribution of this study is highlighting the methodologies used in crime data analytics. Various ML methods, such as a KNN, SVM, naïve Bayes, and clustering, were used for the classification, understanding, and analysis of datasets based on predefined conditions. By understanding and analyzing the data available in the crime record, the type of crime and the hotspot of future criminal activities can be determined. The proposed model was designed to perform various operations such as feature selection, clustering, analysis, prediction, and evaluation of the given datasets. This research proves the necessity of ML techniques for predicting and analyzing criminal activities.
In ref. [ 54 ], the authors incorporated the concept of a grid-based crime prediction model and established a range of spatial-temporal features based on 84 types of geographic locations for a city in Taiwan. The concept uses ML algorithms to learn the patterns and predict crime for the following month for each grid. Among the many ML methods applied, the best model was found to be a DNN. The main contribution of this study is the use of the most recent ML techniques, including the concept of feature learning. In addition, the testing of crime displacement also showed that the proposed model design outperformed the baseline.
In ref. [ 55 ], the authors considered the development of a crime prediction model using the decision tree (J48) algorithm. When applied in the context of law enforcement and intelligence analysis, J48 holds the promise of mollifying crime rates and is considered the most efficient ML algorithm for the prediction of crime data in the related literature. The J48 classifier was developed using the WEKA tool kit and later trained on a preprocessed crime dataset. The experimental results of the J48 algorithm predicted the unknown category of crime data with an accuracy of 94.25287%. With such high accuracy, it is fair to count on the system for future crime predictions.
Comparative study of different forecasting methods
First, in refs. [ 56 , 57 ], the authors predicted crime using the KNNs algorithm in the years 2014 and 2013, respectively. Sun et al. [ 56 ] proved that a higher crime prediction accuracy can be obtained by combining the grey correlation analysis based on new weighted KNN (GBWKNN) filling algorithm with the KNN classification algorithm. Using the proposed algorithm, we were able to obtain an accuracy of approximately 67%. By contrast, Shojaee et al. [ 57 ] divided crime data into two parts, namely, critical and non-critical, and applied a simple KNN algorithm. They achieved an astonishing accuracy of approximately 87%.
Second, in refs. [ 58 , 59 ], crime is predicted using a decision tree algorithm for the years 2015 and 2013, respectively. In their study, Obuandike et al. [ 58 ] used the ZeroR algorithm along with a decision tree but failed to achieve an accuracy of above 60%. In addition, Iqbal et al. [ 59 ] achieved a stunning accuracy of 84% using a decision tree algorithm. In both cases, however, a small change in the data could lead to a large change in the structure.
Third, in refs. [ 60 , 61 ], a novel crime detection technique called naïve Bayes was implemented for crime prediction and analysis. Jangra and Kalsi [ 60 ] achieved an astounding crime prediction accuracy of 87%, but could not apply their approach to datasets with a large number of features. By contrast, Wibowo and Oesman [ 61 ] achieved an accuracy of only 66% in predicting crimes and failed to consider the computational speed, robustness, and scalability.
Below, we summarize the above comparison and add other models to further illustrate this comparative study and the accuracy of some frequently used models (Table 1 ).
Computer vision models combined with machine and deep learning techniques
In ref. [ 66 ], the study focused on three main questions. First, the authors question whether computer vision algorithms actually work. They stated that the accuracy of the prediction is 90% over fewer complex datasets, but the accuracy drops to 60% over complex datasets. Another concern we need to focus on is reducing the storage and computational costs. Second, they question whether it is effective for policing. They determined that a distinct activity detection is difficult, and pinpointed a key component, the Public Safety Visual Analytics Workstation, which includes many capabilities ranging from detection and localization of objects in camera feeds to labeling actions and events associated with training data, and allowing query-based searches for specific events in videos. By doing so, they aim to view every event as a computer-vision trained, recognized, and labeled event. The third and final question they ask is whether computer vision impacts the criminal justice system. The answer to this from their end is quite optimistic to say the least, although they wish to implement computer vision alone, which we suspect is unsatisfactory.
In ref. [ 67 ], a framework for multi-camera video surveillance is presented. The framework is designed so efficiently that it performs all three major activities of a typical police “stake-out”, i.e., detection, representation, and recognition. The detection part mixes video streams from multiple cameras to efficiently and reliably extract motion trajectories from videos. The representation helps in concluding the raw trajectory data to construct hierarchical, invariant, and content-rich descriptions of motion events. Finally, the recognition part deals with event classification (such as robbery and possibly murder and molestation, among others) and identification of the data descriptors. For an effective recognition, they developed a sequence-alignment kernel function to perform sequence data learning to identify suspicious/possible crime events.
In ref. [ 68 ], a method is suggested for identifying people for surveillance with the help of a new feature called soft biometry, which includes a person’s height, built, skin tone, shirt and trouser color, motion pattern, and trajectory history to identify and track passengers, which further helps in predicting crime activities. They have gone further and discussed some absurd human error incidents that have resulted in the perpetrators getting away. They also conducted experiments, the results of which were quite astounding. In one case, the camera catches people giving piggyback rides in more than one frame of a single shot video. The second scenario shows the camera’s ability to distinguish between airport guards and passengers.
In ref. [ 69 ], the authors discussed automated visual surveillance in a realistic scenario and used Knight, which is a multiple camera surveillance and monitoring system. Their major targets were to analyze the detection, tracking, and classification performances. The detection, tracking, and classification accuracies were 97.4%, 96.7%, and 88%, respectively. The authors also pointed to the major difficulties of illumination changes, camouflage, uninteresting moving objects, and shadows. This research again proves the reliability of computer vision models.
It is well known that an ideal scenario for a camera to achieve a perfect resolution is not possible. In ref. [ 70 ], security surveillance systems often produce poor-quality video, which could be a hurdle in gathering forensic evidence. They examined the ability of subjects to identify targeted individuals captured by a commercially available video security device. In the first experiment, subjects personally familiar with the targets performed extremely well at identifying them, whereas subjects unfamiliar with the targets performed quite poorly. Although these results might not seem to be very conclusive and efficient, police officers with experience in forensic identification performed as poorly as other subjects unfamiliar with the targets. In the second experiment, they asked how familiar subjects could perform so well, and then used the same video device edited clips to obscure the head, body, or gait of the targets. Hiding the body or gait produced a small decrease in recognition performance. Hiding the target heads had a dramatic effect on the subject’s ability to recognize the targets. This indicates that even if the quality of the video is low, the head the target was seen and recognized.
In ref. [ 71 ], an automatic number plate recognition (ANPR) model is proposed. The authors described it as an “image processing innovation”. The ANPR system consists of the following steps: (1) vehicle image capture, (2) preprocessing, (3) number plate extraction, (4) character segmentation, and (5) character recognition. Before the main image processing, a pre-processing of the captured image is conducted, which includes converting the red, green and blue image into a gray image, clamor evacuation, and border enhancement for brightness. The plate is then separated by judging its size. In character segmentation, the letters and numbers are separated and viewed individually. In character recognition, optical character recognition is applied to a given database.
Although real-time crime forecasting is vital, it is extremely difficult to achieve in practice. No known physical models provide a reasonable approximation with dependable results for such a complex system. In ref. [ 72 ], the authors adapted a spatial temporal residual network to well-represented data to predict the distribution of crime in Los Angeles at an hourly scale in neighborhood-sized parcels. These experiments were compared with several existing approaches for prediction, demonstrating the superiority of the proposed model in terms of accuracy. They compared their deep learning approach to ARIMA, KNN, and the historical average. In addition, they presented a ternarization technique to address the concerns of resource consumption for deployment in the real world.
In ref. [ 73 ], the authors conducted a significant study on crime prediction and showed the importance of non-crime data. The major objective of this research was taking advantage of DNNs to achieve crime prediction in a fine-grain city partition. They made predictions using Chicago and Portland crime data, which were further augmented with additional datasets covering the weather, census data, and public transportation. In the paper they split each city into grid cells (beats for Chicago and square grid for Portland). The crime numbers are broken into 10 bins, and their model predicts the most likely bin for each spatial region at a daily level. They train these data using increasingly complex neural network structures, including variations that are suited to the spatial and temporal aspects of the crime prediction problem. Using their model, they were able to predict the correct bin for the overall number of crimes with an accuracy of 75.6% for Chicago and 65.3% for Portland. They showed that adding the value of additional non-crime data was an important factor. They found that days with higher amounts of precipitation and snow decreased the accuracy of the model slightly. Then, considering the impact of transportation, the bus routes and train routes were presented within their beats, and it was shown that the beat containing a train station is on average 1.2% higher than its neighboring beats. The accuracy of a beat that contained one or more train lines passing through it was 0.5% more accurate than its neighboring beats.
In ref. [ 74 ], the authors taught a system how to monitor traffic and identify vehicles at night. They used the bright spots of the headlights and tail lights to identify an object first as a vehicle, and the bright light is extracted with a segmentation process, and then processed by a spatial clustering and tracking procedure that locates and analyzes the spatial and temporal features of the vehicle light. They also conducted an experiment in which, for a span of 20 min, the detection scores for cars and bikes were 98.79% and 96.84%, respectively. In another part of the test, they conducted the same test under the same conditions for 50 min, and the detection scores for cars and bikes were 97.58% and 98.48%, respectively. It is good for machines to be built at such a beginning level. This technology can also be used to conduct surveillance at night.
In ref. [ 75 ], an important approach for human motion analysis is discussed. The author mentions that human motion analysis is difficult because appearances are extremely variable, and thus stresses that focusing on marker-less vision-based human motion analysis has the potential to provide a non-obtrusive solution for the evaluation of body poses. The author claims that this technology can have vast applications such as surveillance, human-computer interaction, and automatic annotation, and will thus benefit from a robust solution. In this paper, the characteristics of human motion analysis are discussed. We divide the analysis part into two aspects, modeling and an estimation phase. The modeling phase includes the construction of the likelihood function [including the camera model, image descriptors, human body model and matching function, and (physical) constraints], and the estimation phase is concerned with finding the most likely pose given the likelihood (function result) of the surface. We discuss the model-free approaches separately.
In ref. [ 76 ], the authors provided insight into how we can achieve crime mapping using satellites. The need for manual data collection for mapping is costly and time consuming. By contrast, satellite imagery is becoming a great alternative. In this paper, they attempted to investigate the use of deep learning to predict crime rates directly from raw satellite imagery. They trained a deep convolutional neural network (CNN) on satellite images obtained from over 1 million crime-incident reports (15 years of data) collected by the Chicago Police Department. The best performing model predicted crime rates from raw satellite imagery with an astounding accuracy of 79%. To make their research more thorough, they conducted a test for reusability, and used the tested and learned Chicago models for prediction in the cities of Denver and San Francisco. Compared to maps made from years of data collected by the corresponding police departments, their maps have an accuracy of 72% and 70%, respectively. They concluded the following: (1) Visual features contained in satellite imagery can be successfully used as a proxy indicator of crime rates; (2) ConvNets are capable of learning models for crime rate prediction from satellite imagery; (3) Once deep models are used and learned, they can be reused across different cities.
In ref. [ 77 ], the authors suggested an extremely intriguing research approach in which they claim to prove that looking beyond what is visible is to infer meaning to what is viewed from an image. They even conducted an interesting study on determining where a McDonalds could be located simply from photographs, and provided the possibility of predicting crime. They compared the human accuracy on this task, which was 59.6%, and the accuracy of using gradient-based features, which was 72.5%, with a chance performance (a chance performance is what you would obtain if you performed at random) of only 50%. This indicates the presence of some visual cues that are not easily spotted by an average human, but are able to be spotted by a machine, thus enables us to judge whether an area is safe. The authors indicated that numerous factors are often associated with our intuition, which we use to avoid certain areas because they may seem “shady” or “unsafe”.
In ref. [ 78 ], the authors describe in two parts how close we are to achieving a fully automated surveillance system. The first part views the possibility of surveillance in a real-world scenario where the installation of systems and maintenance of systems are in question. The second part considers the implementation of computer vision models and algorithms for behavior modeling and event detection. They concluded that the complete scenario is under discussion, and therefore many people are conducting research and obtaining results. However, as we look closely, we can see that reliable results are possible only in certain aspects, while other areas are still in the development process, such as obtaining information on cars and their owners as well as accurately understanding the behavior of a possible suspect.
Many times during criminal activities, convicts use hand gestures to signal messages to each other. In ref. [ 79 ], research on hand gesture recognition was conducted using computer vision models. Their application architecture is of extremely high quality and is easy to understand. They begin by capturing images, and then try detecting a hand in the background. They apply either computer aided manufacturing or different procedure in which they first convert a picture into gray scale, after which they set the image return on investment, and then find and extract the biggest contour. They then determine the convex hull of the contour to try and find an orientation around the bounded rectangle, and finally interpret the gesture and convert it into a meaningful command.
Crime hotspots or areas with high crime intensity are places where the future possibility of a crime exists along with the possibility of spotting a criminal. In ref. [ 80 ], the authors conducted research on forecasting crime hotspots. They used Google Tensor Flow to implement their model and evaluated three options for the recurrent neural network (RNN) architecture: accuracy, precision, and recall. The focus is on achieving a larger value to prove that the approach has a better performance. The gated recurrent unit (GRU) and long short-term memory (LSTM) versions obtained similar performance levels with an accuracy of 81.5%, precision of 86%–87%, recall of 75%, and F1-score of 0.8. Both perform much better than the traditional RNN version. Based on the area under the ROC curve (AUC) performance observations, the GRU version was 2% better than the RNN version. The LSTM version achieved the best AUC score, which was improved by 3% over the GRU version.
In ref. [ 81 ], a spatiotemporal crime network (STCN) is proposed that applies a CNN for predicting crime before it occurs. The authors evaluated the STCN using 311 felony datasets from New York from 2010 to 2015. The results were extremely impressive, with the STCN achieving an F1-score of 88% and an AUC of 92%, which confirmed that it exceeded the performance of the four baselines. Their proposed model achieved the best performance in terms of both F1 and AUC, which remained better than those of the other baselines even when the time window reached 100. This study provides evidence that the system can function well even in a metropolitan area.
Proposed idea
After finding and understanding various distinct methods used by the police for surveillance purposes, we determined the importance of each method. Each surveillance method can perform well on its own and produce satisfactory results, although for only one specific characteristic, that is, if we use a Sting Ray, it can help us only when the suspect is using a phone, which should be switched on. Thus, it is only useful when the information regarding the stake out location is correct. Based on this information, we can see how the ever-evolving technology has yet again produced a smart way to conduct surveillance. The introduction of deep learning, ML, and computer vision techniques has provided us with a new perspective on ways to conduct surveillance. This is an intelligent approach to surveillance because it tries to mimic a human approach, but it does so 24 h a day, 365 days a year, and once it has been taught how to do things it does them in the same manner repeatedly.
Although we have discussed the aspects that ML and computer vision can achieve, but what are these aspects essentially? This brings us to the main point of our paper discussion, i.e., our proposed idea, which is to combine the point aspects of Sting Ray, body cams, facial recognition, number plate recognition, and stakeouts. New features iclude core analytics, neural networks, heuristic engines, recursion processors, Bayesian networks, data acquisition, cryptographic algorithms, document processors, computational linguistics, voiceprint identification, natural language processing, gait analysis, biometric recognition, pattern mining, intel interpretation, threat detection, threat classification. The new features are completely computer dependent and hence require human interaction for development; however, once developed, it functions without human interaction and frees humans for other tasks. Let us understand the use of each function.
Core analytics: This includes having knowledge of a variety of statistical techniques, and by using this knowledge, predict future outcomes, which in our case are anything from behavioral instincts to looting a store in the near future.
Neural networks: This is a concept consisting of a large number of algorithms that help in finding the relation between data by acting similar to a human brain, mimicking biological nerve cells and hence trying to think on its own, thus understanding or even predicting a crime scene.
Heuristic engines: These are engines with data regarding antiviruses, and thus knowledge about viruses, increasing the safety of our system as it identifies the type of threat and eliminates it using known antiviruses.
Cryptographic algorithms: Such algorithms are used in two parts. First, they privately encode the known confidential criminal data. Second, they are used to keep the newly discovered potential crime data encrypted.
Recursion processors: These are used to apply the functions of our machine repeatedly to make sure they continuously work and never break the surveillance of the machine.
Bayesian networks: These are probabilistic acyclic graphical models that can be used for a variety of purposes such as prediction, anomaly detection, diagnostics, automated insight, reasoning, time series prediction, and decision making under uncertainty.
Data acquisition: This might be the most important part because our system has to possess the knowledge of previous crimes and learn from them to predict future possible criminal events.
Document processors: These are used after the data collection, primarily for going through, organizing, analyzing, and learning from the data.
Computer linguistics: Using algorithms and learning models, this method is attempting to give a computer the ability to understand human spoken language, which would be ground breaking, allowing a machine to not only identify a human but also understands what the human is saying.
Natural language processor: This is also used by computers to better understand human linguistics.
Voice print identification: This is an interesting application, which tries to distinguish one person’s voice from another, making it even more recognizable and identifiable. It identifies a target with the help of certain characteristics, such as the configuration of the speaker’s mouth and throat, which can be expressed as a mathematical formula.
Gait analysis: This will be used to study human motion, understanding posture while walking. It will be used to better understand the normal pace of a person and thus judge an abnormal pace.
Bio metric identification: This is used to identify individuals by their face, or if possible, identify them by their thumb print stored in few different databases.
Pattern mining: This is a subset of data mining and helps in observing patterns among routine activities. The use of this technology will help us identify if a person is seen an usual number of times behind a pharmacy window at particular time, allowing the machine to alert the authorities.
Intel interpretation: This is also used to make sense of the information gathered, and will include almost all features mentioned above, combining the results of each and making a final meaningful prediction.
Threat detection: A threat will be detected if during the intel processing a certain number of check boxes predefined when making the system are ticked.
Threat classification: As soon as a threat is detected, it is classified, and the threat can then be categorized into criminal case levels, including burglary, murder, or a possible terrorist attack; thus, based on the time line, near or distant future threats might be predictable.
Combining all of these features, we aim to produce software that has the capability of becoming a universal police officer, having eyes and ears everywhere. Obviously, we tend to use the CCTVs in urban areas during a preliminary round to see the functioning of such software in a real-world scenario. The idea is to train and make the software learn all previously recorded crimes whose footages are available (at least 5000 cases for optimum results), through supervised learning, unsupervised learning, semi-supervised learning, and reinforcement learning to help it to understand what a crime actually is. Thus, it will achieve a better understanding of criminality and can answer how crimes happen, as well as why and where. We do not propose simply making a world-class model to predict crimes, we also suggest making it understand previous crimes to better judge and therefore better predict them.
We aim to use this type of technology on two fronts: first and most importantly, for predicting crimes before they happen, followed by a thorough analysis of a crime scene allowing the system to possibly identify aspects that even a human eye can miss.
The most interesting cutting-edge and evolutionary idea that we believe should be incorporated is the use of scenario simulations. After analyzing the scene and using the 17 main characteristics mentioned above, the software should run at least 50 simulations of the present scenario presented in front of it, which will be assisted by previously learned crime recordings. The simulation will help the software in asserting the threat level and then accordingly recommend a course of action or alert police officials.
To visualize a possible scenario where we are able to invent such software, we prepared a flow chart (Fig. 3 ) to better understand the complete process.
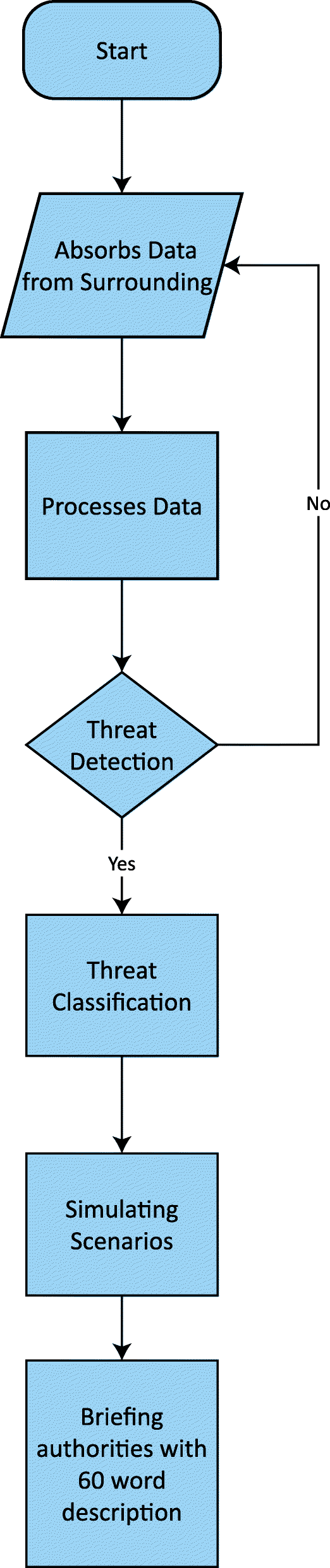
Flowchart of our proposed model. The data are absorbed from the surrounding with the help of cameras and microphones. If the system depicts an activity as suspicious, it gathers more intel allowing the facial algorithms to match against a big database such as a Social Security Number or Aadhaar card database. When it detects a threat, it also classifies it into categories such as the nature of the crime and time span within which it is possible to take place. With all the gathered intel and all the necessary details of the possible crime, it alerts the respective authority with a 60-word synopsis to give them a brief idea, allowing law enforcement agencies to take action accordingly
Although this paper has been implemented with high accuracy and detailed research, there are certain challenges that can pose a problem in the future. First, the correct and complete building of the whole system has to be done in the near future, allowing its implementation to take place immediately and properly. Furthermore, the implementation itself is a significant concern, as such technologies cannot be directly implemented in the open world. The system must first be tested in a small part of a metropolitan area, and only then with constant improvements (revisions of the first model) can its usage be scaled up. Hence, the challenges are more of a help in perfecting the model and thus gradually providing a perfect model that can be applied to the real world. Moreover, there are a few hurdles in the technological aspects of the model, as the size of the learning data will be enormous, and thus processing it will take days and maybe even weeks. Although these are challenges that need to be addressed, they are aspects that a collective team of experts can overcome after due diligence, and if so, the end product will be worth the hard work and persistence.
Future scope
This paper presented the techniques and methods that can be used to predict crime and help law agencies. The scope of using different methods for crime prediction and prevention can change the scenario of law enforcement agencies. Using a combination of ML and computer vision can substantially impact the overall functionality of law enforcement agencies. In the near future, by combining ML and computer vision, along with security equipment such as surveillance cameras and spotting scopes, a machine can learn the pattern of previous crimes, understand what crime actually is, and predict future crimes accurately without human intervention. A possible automation would be to create a system that can predict and anticipate the zones of crime hotspots in a city. Law enforcement agencies can be warned and prevent crime from occurring by implementing more surveillance within the prediction zone. This complete automation can overcome the drawbacks of the current system, and law enforcement agencies can depend more on these techniques in the near future. Designing a machine to anticipate and identify patterns of such crimes will be the starting point of our future study. Although the current systems have a large impact on crime prevention, this could be the next big approach and bring about a revolutionary change in the crime rate, prediction, detection, and prevention, i.e., a “universal police officer”.
Conclusions
Predicting crimes before they happen is simple to understand, but it takes a lot more than understanding the concept to make it a reality. This paper was written to assist researchers aiming to make crime prediction a reality and implement such advanced technology in real life. Although police do include the use of new technologies such as Sting Rays and facial recognition every few years, the implementation of such software can fundamentally change the way police work, in a much better way. This paper outlined a framework envisaging how the aspects of machine and deep learning, along with computer vision, can help create a system that is much more helpful to the police. Our proposed system has a collection of technologies that will perform everything from monitoring crime hotspots to recognizing people from their voice notes. The first difficulty faced will be to actually make this system, followed by problems such as its implementation and use, among others. However, all of these problems are solvable, and we can also benefit from a security system that monitors the entire city around-the-clock. In other words, to visualize a world where we incorporate such a system into a police force, tips or leads that much more reliable can be achieved and perhaps crime can be eradicated at a much faster rate.
Availability of data and materials
All relevant data and material are presented in the main paper.
Abbreviations
- Machine learning
Nondeterministic polynomial
Waikato Environment for Knowledge Analysis
K-nearest neighbor
Automatic number plate recognition
Deep neural network
Kernel density estimation
Support vector machine
Grey correlation analysis based on new weighted KNN
Autoregressive integrated moving average
Spatiotemporal crime network
Convolutional neural network
Area under the ROC curve
Recurrent neural network
Gated recurrent unit
Long short-term memory
Absolute percent error
Shah D, Dixit R, Shah A, Shah P, Shah M (2020) A comprehensive analysis regarding several breakthroughs based on computer intelligence targeting various syndromes. Augment Hum Res 5(1):14. https://doi.org/10.1007/s41133-020-00033-z
Patel H, Prajapati D, Mahida D, Shah M (2020) Transforming petroleum downstream sector through big data: a holistic review. J Pet Explor Prod Technol 10(6):2601–2611. https://doi.org/10.1007/s13202-020-00889-2
Szeliski R (2010) Computer vision: algorithms and applications. Springer-Verlag, Berlin, pp 1–979
MATH Google Scholar
Vedaldi A, Fulkerson B (2010) Vlfeat: an open and portable library of computer vision algorithms. Paper presented at the 18th ACM international conference on multimedia. ACM, Firenze. https://doi.org/10.1145/1873951.1874249
Le TL, Nguyen MQ, Nguyen TTM (2013) Human posture recognition using human skeleton provided by Kinect. In: Paper presented at the 2013 international conference on computing, management and telecommunications. IEEE, Ho Chi Minh City. https://doi.org/10.1109/ComManTel.2013.6482417
Ahir K, Govani K, Gajera R, Shah M (2020) Application on virtual reality for enhanced education learning, military training and sports. Augment Hum Res 5(1):7. ( https://doi.org/10.1007/s41133-019-0025-2 )
Talaviya T, Shah D, Patel N, Yagnik H, Shah M (2020) Implementation of artificial intelligence in agriculture for optimisation of irrigation and application of pesticides and herbicides. Artif Intell Agric 4:58–73. https://doi.org/10.1016/j.aiia.2020.04.002
Jha K, Doshi A, Patel P, Shah M (2019) A comprehensive review on automation in agriculture using artificial intelligence. Artif Intell Agric 2:1–12. https://doi.org/10.1016/j.aiia.2019.05.004
Kakkad V, Patel M, Shah M (2019) Biometric authentication and image encryption for image security in cloud framework. Multiscale Multidiscip Model Exp Des 2(4):233–248. https://doi.org/10.1007/s41939-019-00049-y
Pathan M, Patel N, Yagnik H, Shah M (2020) Artificial cognition for applications in smart agriculture: a comprehensive review. Artif Intell Agric 4:81–95. https://doi.org/10.1016/j.aiia.2020.06.001
Pandya R, Nadiadwala S, Shah R, Shah M (2020) Buildout of methodology for meticulous diagnosis of K-complex in EEG for aiding the detection of Alzheimer's by artificial intelligence. Augment Hum Res 5(1):3. https://doi.org/10.1007/s41133-019-0021-6
Dey A (2016) Machine learning algorithms: a review. Int J Comput Sci Inf Technol 7(3):1174–1179
Google Scholar
Sukhadia A, Upadhyay K, Gundeti M, Shah S, Shah M (2020) Optimization of smart traffic governance system using artificial intelligence. Augment Hum Res 5(1):13. https://doi.org/10.1007/s41133-020-00035-x
Musumeci F, Rottondi C, Nag A, Macaluso I, Zibar D, Ruffini M et al (2019) An overview on application of machine learning techniques in optical networks. IEEE Commun Surv Tutorials 21(2):1381–1408. https://doi.org/10.1109/COMST.2018.2880039
Patel D, Shah Y, Thakkar N, Shah K, Shah M (2020) Implementation of artificial intelligence techniques for cancer detection. Augment Hum Res 5(1):6. https://doi.org/10.1007/s41133-019-0024-3
Kundalia K, Patel Y, Shah M (2020) Multi-label movie genre detection from a movie poster using knowledge transfer learning. Augment Hum Res 5(1):11. https://doi.org/10.1007/s41133-019-0029-y
Article Google Scholar
Marsland S (2015) Machine learning: an algorithmic perspective. CRC Press, Boca Raton, pp 1–452. https://doi.org/10.1201/b17476-1
Jani K, Chaudhuri M, Patel H, Shah M (2020) Machine learning in films: an approach towards automation in film censoring. J Data Inf Manag 2(1):55–64. https://doi.org/10.1007/s42488-019-00016-9
Parekh V, Shah D, Shah M (2020) Fatigue detection using artificial intelligence framework. Augment Hum Res 5(1):5 https://doi.org/10.1007/s41133-019-0023-4
Gandhi M, Kamdar J, Shah M (2020) Preprocessing of non-symmetrical images for edge detection. Augment Hum Res 5(1):10 https://doi.org/10.1007/s41133-019-0030-5
Panchiwala S, Shah M (2020) A comprehensive study on critical security issues and challenges of the IoT world. J Data Inf Manag 2(7):257–278. https://doi.org/10.1007/s42488-020-00030-2
Simon A, Deo MS, Venkatesan S, Babu DR (2016) An overview of machine learning and its applications. Int J Electr Sci Eng 1(1):22–24.
Parekh P, Patel S, Patel N, Shah M (2020) Systematic review and meta-analysis of augmented reality in medicine, retail, and games. Vis Comput Ind Biomed Art 3(1):21. https://doi.org/10.1186/s42492-020-00057-7
Shah K, Patel H, Sanghvi D, Shah M (2020) A comparative analysis of logistic regression, random forest and KNN models for the text classification. Augment Hum Res 5(1):12. https://doi.org/10.1007/s41133-020-00032-0
Patel D, Shah D, Shah M (2020) The intertwine of brain and body: a quantitative analysis on how big data influences the system of sports. Ann Data Sci 7(1):1–16. https://doi.org/10.1007/s40745-019-00239-y
Judd S (1988) On the complexity of loading shallow neural networks. J Complex 4(3):177–192. https://doi.org/10.1016/0885-064X(88)90019-2
Article MathSciNet MATH Google Scholar
Blum AL, Rivest RL (1992) Training a 3-node neural network is NP-complete. Neural Netw 5(1):117–127. https://doi.org/10.1016/S0893-6080(05)80010-3
Gupta A, Dengre V, Kheruwala HA, Shah M (2020) Comprehensive review of text-mining applications in finance. Financ Innov 6(1):1–25. https://doi.org/10.1186/s40854-020-00205-1
Shah N, Engineer S, Bhagat N, Chauhan H, Shah M (2020) Research trends on the usage of machine learning and artificial intelligence in advertising. Augment Hum Res 5(1):19. https://doi.org/10.1007/s41133-020-00038-8
Naik B, Mehta A, Shah M (2020) Denouements of machine learning and multimodal diagnostic classification of Alzheimer's disease. Vis Comput Ind Biomed Art 3(1):26. https://doi.org/10.1186/s42492-020-00062-w
Chen P, Yuan HY, Shu XM (2008) Forecasting crime using the ARIMA model. In: Paper presented at the 5th international conference on fuzzy systems and knowledge discovery. IEEE, Ji'nan 18-20 October 2008. https://doi.org/10.1109/FSKD.2008.222
Rani A, Rajasree S (2014) Crime trend analysis and prediction using mahanolobis distance and dynamic time warping technique. Int J Comput Sci Inf Technol 5(3):4131–4135
Gorr W, Harries R (2003) Introduction to crime forecasting. Int J Forecast 19(4):551–555. https://doi.org/10.1016/S0169-2070(03)00089-X
Rummens A, Hardyns W, Pauwels L (2017) The use of predictive analysis in spatiotemporal crime forecasting: building and testing a model in an urban context. Appl Geogr 86:255–261. https://doi.org/10.1016/j.apgeog.2017.06.011
Bates A (2017) Stingray: a new frontier in police surveillance. Cato Institute Policy Analysis, No. 809
Joh EE (2017) The undue influence of surveillance technology companies on policing. N Y Univ Law Rev 92:101–130. https://doi.org/10.2139/ssrn.2924620
Vredeveldt A, Kesteloo L, Van Koppen PJ (2018) Writing alone or together: police officers' collaborative reports of an incident. Crim Justice Behav 45(7):1071–1092. https://doi.org/10.1177/0093854818771721
McNeal GS (2014) Drones and aerial surveillance: considerations for legislators. In: Brookings Institution: The Robots Are Coming: The Project On Civilian Robotics, November 2014, Pepperdine University Legal Studies Research Paper No. 2015/3
Fatih T, Bekir C (2015) Police use of technology to fight against crime. Eur Sci J 11(10):286–296
Katz CM, Choate DE, Ready JR, Nuňo L (2014) Evaluating the impact of officer worn body cameras in the Phoenix Police Department. Center for Violence Prevention & Community Safety, Arizona State University, Phoenix, pp 1–43
Stanley J (2015) Police body-mounted cameras: with right policies in place, a win for all. https://www.aclu.org/police-body-mounted-cameras-right-policies-place-win-all . Accessed 15 Aug 2015
McClendon L, Meghanathan N (2015) Using machine learning algorithms to analyze crime data. Mach Lear Appl Int J 2(1):1–12. https://doi.org/10.5121/mlaij.2015.2101
Frank E, Hall M, Trigg L, Holmes G, Witten IH (2004) Data mining in bioinformatics using Weka. Bioinformatics 20(15):2479–2481. https://doi.org/10.1093/bioinformatics/bth261
Kim S, Joshi P, Kalsi PS, Taheri P (2018) Crime analysis through machine learning. In: Paper presented at the IEEE 9th annual information technology, electronics and mobile communication conference. IEEE, Vancouver 1-3 November 2018. https://doi.org/10.1109/IEMCON.2018.8614828
Tabedzki C, Thirumalaiswamy A, van Vliet P (2018) Yo home to Bel-Air: predicting crime on the streets of Philadelphia. In: University of Pennsylvania, CIS 520: machine learning
Bharati A, Sarvanaguru RAK (2018) Crime prediction and analysis using machine learning. Int Res J Eng Technol 5(9):1037–1042
Prithi S, Aravindan S, Anusuya E, Kumar AM (2020) GUI based prediction of crime rate using machine learning approach. Int J Comput Sci Mob Comput 9(3):221–229
Kang HW, Kang HB (2017) Prediction of crime occurrence from multi-modal data using deep learning. PLoS One 12(4):e0176244. https://doi.org/10.1371/journal.pone.0176244
Bandekar SR, Vijayalakshmi C (2020) Design and analysis of machine learning algorithms for the reduction of crime rates in India. Procedia Comput Sci 172:122–127. https://doi.org/10.1016/j.procs.2020.05.018
Hossain S, Abtahee A, Kashem I, Hoque M, Sarker IH (2020) Crime prediction using spatio-temporal data. arXiv preprint arXiv:2003.09322. https://doi.org/10.1007/978-981-15-6648-6_22
Stalidis P, Semertzidis T, Daras P (2018) Examining deep learning architectures for crime classification and prediction. arXiv preprint arXiv: 1812.00602. p. 1–13
Jha P, Jha R, Sharma A (2019) Behavior analysis and crime prediction using big data and machine learning. Int J Recent Technol Eng 8(1):461–468
Tyagi D, Sharma S (2018) An approach to crime data analysis: a systematic review. Int J Eng Technol Manag Res 5(2):67–74. https://doi.org/10.29121/ijetmr.v5.i2.2018.615
Lin YL, Yen MF, Yu LC (2018) Grid-based crime prediction using geographical features. ISPRS Int J Geo-Inf 7(8):298. https://doi.org/10.3390/ijgi7080298
Ahishakiye E, Taremwa D, Omulo EO, Niyonzima I (2017) Crime prediction using decision tree (J48) classification algorithm. Int J Comput Inf Technol 6(3):188–195
Sun CC, Yao CL, Li X, Lee K (2014) Detecting crime types using classification algorithms. J Digit Inf Manag 12(8):321–327. https://doi.org/10.14400/JDC.2014.12.8.321
Shojaee S, Mustapha A, Sidi F, Jabar MA (2013) A study on classification learning algorithms to predict crime status. Int J Digital Content Technol Appl 7(9):361–369
Obuandike GN, Isah A, Alhasan J (2015) Analytical study of some selected classification algorithms in WEKA using real crime data. Int J Adv Res Artif Intell 4(12):44–48. https://doi.org/10.14569/IJARAI.2015.041207
Iqbal R, Murad MAA, Mustapha A, Panahy PHS, Khanahmadliravi N (2013) An experimental study of classification algorithms for crime prediction. Indian J Sci Technol 6(3):4219–4225. https://doi.org/10.17485/ijst/2013/v6i3.6
Jangra M, Kalsi S (2019) Crime analysis for multistate network using naive Bayes classifier. Int J Comput Sci Mob Comput 8(6):134–143
Wibowo AH, Oesman TI (2020) The comparative analysis on the accuracy of k-NN, naive Bayes, and decision tree algorithms in predicting crimes and criminal actions in Sleman regency. J Phys Conf Ser 1450:012076. https://doi.org/10.1088/1742-6596/1450/1/012076
Vanhoenshoven F, Nápoles G, Bielen S, Vanhoof K (2017) Fuzzy cognitive maps employing ARIMA components for time series forecasting. In: Czarnowski I, Howlett RJ, Jain LC (eds) Proceedings of the 9th KES international conference on intelligent decision technologies 2017, vol 72. Springer, Heidelberg, pp 255–264. https://doi.org/10.1007/978-3-319-59421-7_24
Chapter Google Scholar
Gorr W, Olligschlaeger AM, Thompson Y (2000) Assessment of crime forecasting accuracy for deployment of police. Int J Forecast 2000:743–754
Yu CH, Ward MW, Morabito M, Ding W (2011) Crime forecasting using data mining techniques. In: Paper presented at the 2011 IEEE 11th international conference on data mining workshops. IEEE, Vancouver 11-11 December 2011. https://doi.org/10.1109/ICDMW.2011.56
Alves LGA, Ribeiro HV, Rodrigues FA (2018) Crime prediction through urban metrics and statistical learning. Phys A Stat Mech Appl 505:435–443. https://doi.org/10.1016/j.physa.2018.03.084
Idrees H, Shah M, Surette R (2018) Enhancing camera surveillance using computer vision: a research note. Polic Int J 41(2):292–307. https://doi.org/10.1108/PIJPSM-11-2016-0158
Wu G, Wu Y, Jiao L, Wang YF, Chang EY (2003) Multi-camera spatio-temporal fusion and biased sequence-data learning for security surveillance. In: Paper presented at the 11th ACM international conference on multimedia. ACM, Berkeley 2-8 November 2003. https://doi.org/10.1145/957013.957126
Wang YF, Chang EY, Cheng KP (2005) A video analysis framework for soft biometry security surveillance. In: Paper presented at the 3rd ACM international workshop on video surveillance & sensor networks. ACM, Hilton 11 November 2005. https://doi.org/10.1145/1099396.1099412
Shah M, Javed O, Shafique K (2007) Automated visual surveillance in realistic scenarios. IEEE MultiMed 14(1):30–39. https://doi.org/10.1109/MMUL.2007.3
Burton AM, Wilson S, Cowan M, Bruce V (1999) Face recognition in poor-quality video: evidence from security surveillance. Psychol Sci 10(3):243–248. https://doi.org/10.1111/1467-9280.00144
Goyal A, Bhatia R (2016) Automated car number plate detection system to detect far number plates. IOSR J Comput Eng 18(4):34–40. https://doi.org/10.9790/0661-1804033440
Wang B, Yin PH, Bertozzi AL, Brantingham PJ, Osher SJ, Xin J (2019) Deep learning for real-time crime forecasting and its ternarization. Chin Ann Math Ser B 40(6):949–966. https://doi.org/10.1007/s11401-019-0168-y
Stec A, Klabjan D (2018) Forecasting crime with deep learning. arXiv preprint arXiv:1806.01486. p. 1–20
Chen YL, Wu BF, Huang HY, Fan CJ (2011) A real-time vision system for nighttime vehicle detection and traffic surveillance. IEEE Trans Ind Electron 58(5):2030–2044. https://doi.org/10.1109/TIE.2010.2055771
Poppe R (2007) Vision-based human motion analysis: an overview. Comput Vision Image Underst 108(1–2):4–18. https://doi.org/10.1016/j.cviu.2006.10.016
Najjar A, Kaneko S, Miyanaga Y (2018) Crime mapping from satellite imagery via deep learning. arXiv preprint arXiv:1812.06764. p. 1–8
Khosla A, An B, Lim JJ, Torralba A (2014) Looking beyond the visible scene. In: Paper presented at the of IEEE conference on computer vision and pattern recognition. IEEE, Columbus 23-28 June 2014. https://doi.org/10.1109/CVPR.2014.474
Dee HM, Velastin SA (2008) How close are we to solving the problem of automated visual surveillance? Mach Vis Appl 19(5–6):329–343. https://doi.org/10.1007/s00138-007-0077-z
Rautaray SS (2012) Real time hand gesture recognition system for dynamic applications. Int J Ubi Comp 3(1):21–31. https://doi.org/10.5121/iju.2012.3103
Zhuang Y, Almeida M, Morabito M, Ding W (2017) Crime hot spot forecasting: a recurrent model with spatial and temporal information. In: Paper presented at the IEEE international conference on big knowledge. IEEE, Hefei 9-10 August 2017. https://doi.org/10.1109/ICBK.2017.3
Duan L, Hu T, Cheng E, Zhu JF, Gao C (2017) Deep convolutional neural networks for spatiotemporal crime prediction. In: Paper presented at the 16th international conference information and knowledge engineering. CSREA Press, Las Vegas 17-20 July 2017
Download references
Acknowledgements
The authors are grateful to Department of Computer Engineering, SAL Institute of Technology and Engineering Research and Department of Chemical Engineering, School of Technology, Pandit Deendayal Energy University for the permission to publish this research.
Not applicable.
Author information
Authors and affiliations.
Department of Computer Engineering, Sal Institute of Technology and Engineering Research, Ahmedabad, Gujarat, 380060, India
Neil Shah & Nandish Bhagat
Department of Chemical Engineering, School of Technology, Pandit Deendayal Energy University, Gandhinagar, Gujarat, 382426, India
You can also search for this author in PubMed Google Scholar
Contributions
All the authors make substantial contribution in this manuscript; NS, NB and MS participated in drafting the manuscript; NS, NB and MS wrote the main manuscript; all the authors discussed the results and implication on the manuscript at all stages the author(s) read and approved the final manuscript.
Corresponding author
Correspondence to Manan Shah .
Ethics declarations
Competing interests.
The authors declare that they have no competing interests.
Additional information
Publisher’s note.
Springer Nature remains neutral with regard to jurisdictional claims in published maps and institutional affiliations.
Rights and permissions
Open Access This article is licensed under a Creative Commons Attribution 4.0 International License, which permits use, sharing, adaptation, distribution and reproduction in any medium or format, as long as you give appropriate credit to the original author(s) and the source, provide a link to the Creative Commons licence, and indicate if changes were made. The images or other third party material in this article are included in the article's Creative Commons licence, unless indicated otherwise in a credit line to the material. If material is not included in the article's Creative Commons licence and your intended use is not permitted by statutory regulation or exceeds the permitted use, you will need to obtain permission directly from the copyright holder. To view a copy of this licence, visit http://creativecommons.org/licenses/by/4.0/ .
Reprints and permissions
About this article
Cite this article.
Shah, N., Bhagat, N. & Shah, M. Crime forecasting: a machine learning and computer vision approach to crime prediction and prevention. Vis. Comput. Ind. Biomed. Art 4 , 9 (2021). https://doi.org/10.1186/s42492-021-00075-z
Download citation
Received : 18 July 2020
Accepted : 05 April 2021
Published : 29 April 2021
DOI : https://doi.org/10.1186/s42492-021-00075-z
Share this article
Anyone you share the following link with will be able to read this content:
Sorry, a shareable link is not currently available for this article.
Provided by the Springer Nature SharedIt content-sharing initiative
- Computer vision
- Crime forecasting
Crime Research Paper
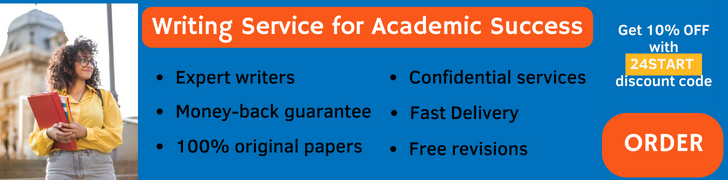
This sample crime research paper features: 3800 words (approx. 12 pages), an outline, and a bibliography with 12 sources. Browse other research paper examples for more inspiration. If you need a thorough research paper written according to all the academic standards, you can always turn to our experienced writers for help. This is how your paper can get an A! Feel free to contact our writing service for professional assistance. We offer high-quality assignments for reasonable rates.
Introduction
Civil and criminal divide, the positivistic approach to crime, legal moralism, social practice view, economic account, harm-based theory.
- Bibliography
A crime is a prohibited conduct that is punishable by law. It can also be an omission rather than an act, thus a failure to act when the law requires action. Historically, criminal activities have been limited to those that hurt the interests of others. Occasionally, though, a government will outlaw an act or omission because it is damaging to the perpetrator or because it is immoral. Such laws are referred to as “victimless” crimes. The notion of a crime without a victim highlights the fundamental distinction between criminal and civil law: a crime is an offense against the public good, whereas a civil wrong is an offense against private interests. The purpose of civil damages is to compensate the victim for the harm he has experienced at the hands of the offender, while the purpose of criminal punishment is to defend the state’s interest in the common good.
Academic Writing, Editing, Proofreading, And Problem Solving Services
Get 10% off with 24start discount code, more crime research papers:.
- Arson Research Paper
- Computer Crime Research Paper
- Domestic Violence Research Paper
- Drinking and Driving Research Paper
- Euthanasia Research Paper
- Gambling Research Paper
- Hate Crimes Research Paper
- Homicide Research Paper
- Juvenile Delinquency Research Paper
- Kidnapping Research Paper
- Organized Crime Research Paper
- Rape Research Paper
- Sexual Violence Research Paper
- Shoplifting Research Paper
- Stalking Research Paper
- Theft Research Paper
- Violence Research Paper
- White-Collar Crime Research Paper
After the Norman Conquest, the concept of the public wrong replaced what was effectively a system of private plea bargaining under the Anglo-Saxons. Prior to the conquest, a wrongdoer would give his victim a sum of money to purchase the victim’s right to retaliation. These payments, known as wer, wte, and bót, were not mandated by law, but instead were negotiated between the injurer and the victim. The fact that some injuries were bóteás, or beyond monetary compensation, and a man may be put to death for them reveals the concept of a public harm at this time. The jurisdictional idea of the “king’s peace” may have been a source of the offense against the state at a later time. Under this theory, the Crown reserved the ability to govern any violent acts that may have occurred along any route the king traveled. The introduction of a public police force made the concept of a public wrong institutionally viable, but this was an even later development.
In recent years, the line between civil and criminal wrongs has blurred substantially. On the civil side, for instance, there is the institution of “punitive damages,” whereby a person is penalized for the willful infliction of an injury or the malicious breach of a contract. Punitive damages are meant to penalize the wrongdoer, as opposed to compensatory damages, which cannot exceed the amount necessary to make the sufferer whole. On the criminal side, monetary sanctions in place of jail are becoming increasingly prevalent. Typically, such penalties are paid to the victim in the form of restitution. Also on the rise is the use of criminal sanctions against corporations. Given that a company can only be penalized with monetary punishments and that punitive damages are increasingly granted in civil trials, the distinction between civil and criminal in such situations is mostly academic. It appears to consist primarily of procedural differences, such as the various proof standards and rules of evidence. The concept that crime victims have a right to counsel in the prosecution of their perpetrators has spawned a current push to strengthen the victim’s involvement in criminal procedures. Most forcefully, the concept of victim’s rights suggests a departure from the notion of crime as a public offense. It implies that the offender’s punishment helps, at least in part, to satisfy the victim’s desire for revenge. This tendency toward the “privatization” of crime is also reflected in many institutional reform proposals, such as the proposal to privatize prisons.
The acceptability of these varied adjustments to the conventional concept of crime rests in part on our conception of what a crime is. Is a crime just a ban that appears under the name “criminal” in one of the state or federal penal codes? Or is the criminal category a more fundamental category, one that does not draw its meaning from any particular application of the concept of crime? The first is what we may term a “positivism” towards the concept of an offense. It sees crime as a purely legal concept. The second would be a normative posture towards the concept of a crime that identifies the criminal category through a theory of justified prohibition. Since there is no obligatory component to the concept of a crime, there can be no objection to punishing companies or enhancing the role of the victim from a positive perspective. On the other hand, from a normative perspective, there may be reasons to oppose to these adjustments to the conventional treatment of crime. For it may turn out that, according to our best theory of justifiable punishment, punishing an offender at the victim’s request, especially if accompanied with the payment of compensation, is not legitimate.
Positive thinking dominates the American legal system’s attitude to crime. Henry Hart once quipped, “a crime is anything that is called a crime, and a criminal penalty is simply the punishment imposed for committing anything that has been given that name.” (p. 404). In the last fifty or so years, the U.S. Supreme Court has articulated precisely this position by refusing to acknowledge constitutional limits on the concept of an offense. For instance, the Court has concluded that a legislator may penalize action without inserting a mental state element (mens rea) in the offense’s definition (U.S. v. Dotterweich; U.S. v. Balint). It has also determined that it is within the legislative discretion to include exonerating conditions such as insanity in the definition of the offense to which they apply or to regard them as so-called affirmative defenses. The former method would require the prosecution to prove, for instance, that the defendant was not insane at the time he committed the crime, whilst the latter would lay the burden on the defendant. In a case involving the defense of extreme emotional disturbance, the Supreme Court famously articulated its commitment to the positivistic approach to crime by upholding a New York statute that shifted the burden of proof to the defendant instead of requiring the prosecution to prove the absence of the defense beyond a reasonable doubt (Patterson v. New York). Given its premise, the reasoning of the Court was flawless: It contended that while a state has the ability to eliminate a defense entirely, it must also have the ability to transfer the burden of proof to the defendant, as “the greater power implies the lesser power” (p. 211). Even the most fundamental defense, self-defense, has been demonstrated to be susceptible to the identical reasoning. However, the Supreme Court has recently demonstrated a renewed willingness to limit state burden-shifting. The case involved a New Jersey hate crime statute that enabled significantly enhanced penalties for any defendant whose crime was motivated by racial animus. The Court ruled that the Act was unconstitutional on the grounds that it evaded the state’s responsibility to show mental state by treating racial bias as a sentencing factor rather than as an element of the offense. The implication of such a judgement is that legislatures lack unrestricted power to decide whether and how to criminalize, even outside the realm of fundamental rights. For if it is unconstitutional for a state to shift the burden onto a mental state element, it would seem that it does not have unrestricted discretion to include such mental state elements in its criminal definitions. The question thus is whether the Supreme Court’s recent decision in the area of burden of proof marks a fundamental movement away from the positivist approach to crime, or whether its influence will be limited to the area of burden of proof. Is the Court beginning on a new constitutional jurisprudence of substantive criminal law, or will it continue to avoid any genuine effort to limit the substantive criminal provisions that legislatures can enact?
While the positivistic approach to crime has triumphed, the Supreme Court has traditionally endeavored to narrow the concept of offense in a few discrete instances. As a result of the due process clauses of the Fifth and Fourteenth Amendments, the majority of these prohibitions have consisted of a series of formal constraints on how legislators may construct offenses. Despite the fact that these limits pretend to address only how action is criminalized and not what is criminalized, they frequently place substantial conditions on the definition of the offense. Consider, for instance, the four significant constraints listed below on the concept of a crime.
First, under the law of vagueness, criminal statutes must identify the forbidden conduct with sufficient precision to put potential defendants on notice that they may be subject to criminal prosecution. This approach has been most prominently applied to loitering statutes, many of which are seen to give police officers too much leeway to arrest persons based on their physical appearance or manner. In many instances, more specific phrasing would not eliminate the objection to such statutes. As the Supreme Court made plain in a recent decision involving a Chicago loitering ban, occasionally a statute intrudes too far and with insufficient explanation to be legally permissible (City of Chicago v. Morales). A second, related notion is that of overbreadth, which prohibits a legislator from crafting criminal statutes in such a way that prosecution and conviction for regular, noncriminal behavior is possible. The Court will primarily throw down criminal prohibitions on grounds of overbreadth when the restriction threatens encroaching upon freedom of speech and expression (R.A.V. v. City of St. Paul). A third notion, the doctrine of legality, is likewise expressed under the rubric of “due process.’ Ordinary persons must be afforded a fair opportunity to conform their conduct to the law, which necessitates that criminal statutes provide explicit notice of the possibility of criminal penalty. For instance, punishments cannot be retroactive and must be specific and certain. The Eighth Amendment prohibition on “cruel and unusual punishment” has been construed as incorporating a proportionality criterion that serves to limit the punishment selected for a given violation (Solem v. Helm; see Harmelin v. Michigan). In the past, this theory has served to guarantee that the discipline approved for a particular offense is roughly comparable to the sanction authorized for the same conduct in other jurisdictions and is acceptable in light of the sanction authorized for other offenses in the same jurisdiction.
Nonpositivist Approaches to Crime
The aforementioned constraints on the concept of an offense show that, while the positivistic approach to offense definition may be the prevailing one in our constitutional law, our adherence to it is questionable. We do not accept that any action that a legislature desires to criminalize is properly punished, and the limitations we set on the application of the criminal sanction cannot be fully explained by the first eight amendments to the Constitution. Some actions appear so unfit for criminal prohibition that we feel it is a stretch to apply the concept of crime to them. In severe situations, the point would be obvious: Statutes that made criminal punishment retroactive rather than prospective, that punished for thoughts without corresponding actions, that enacted a separate set of prohibitions for each separate member of the community, that established a separate count of theft for each thirty-second period that a thief withheld the stolen item from its owner, or that adopted an arbitrary class of subjects to whom the prohibition would apply, would be so out of step with the way we conduct ourselves as a society. In what way would these be considered crimes? The act for which a person is imprisoned and subjected to jail or other harsh treatment does not automatically render it unlawful. Even when the legislature has sanctioned the behavior in the form of a law, it does not do so. Although one would seek to limit the use of the criminal sanction in such instances by the types of ancillary constitutional constraints on legislative discretion outlined previously, these restrictions will prove insufficient to capture our current conception of crime. Therefore, it is possible that the concept of crime itself restricts what a government can ban and how it can assure compliance with such prohibitions.
Therefore, our understanding of crime is normative as well as descriptive, at least to some extent. In instance, there may be justifications that are integral to the concept of criminality. If this is true, then one of the things we mean when we refer to a criminal violation is that the statute-authorized restriction on liberty is justified by the necessity of promoting compliance with the criminal prohibition. This approach would imply not only that punishing a person for something he had no reason to know was prohibited is not, strictly speaking, punishment, but also that the behavior thus penalized could not be correctly labeled “criminal,” even if the legislature has labeled it a crime and attached the types of penalties typically associated with so-called criminal behavior. The normative approach to crime would therefore give a method for assessing legislative uses of the authority to criminalize by identifying criteria that are intrinsic to the concept of crime. Such criteria would make it possible to state unequivocally that the legislature erred in outlawing a given type of behavior and imposing severe penalties for its occurrence, on the grounds that the prohibited behavior is not a suitable target of criminal prohibition. And while governments may have considerable discretion in choosing the permissible objects of criminal prohibition, under a normative perspective on crime, their decision-making would be constrained by a number of broadly specified parameters.
In contrast to their judicial counterparts, criminal law researchers embrace normative approaches to the concept of an offense. However, there is no nonpositivist concept of crime that would garner their unanimity. The term “legal moralism” refers to a school of criminal philosophy. The legal moralist contends that a crime is an immoral act and that, thus, all immoral acts should be punished. Not only does the legal moralist believe that every crime is in some way an immoral act, or that it leads to generate an immoral act, but also that no immoral conduct should go unpunished. One category of crime, sometimes referred to as mala prohibita, appears to offer a difficulty for legal moralists. Mala prohibita crimes are deeds that are unlawful just because they are prohibited by law. Mala in se offences, on the other hand, prohibit bad conduct in and of themselves. The legal moralist has problems with this distinction since he appears to regard all crimes as mala in se, believing that it is the inherent immorality of an act that justifies criminal prohibition. Legal moralists attempt to answer the dilemma of mala prohibita crimes by asserting that the prohibited acts are instrumentally tied to a mala in se act or state of affairs. While it is not sinful to drive on the left side of the road, it is unethical to put others at great risk of injury. In this approach, the legal moralist justifies the law mandating left-hand driving in the United States and right-hand driving in Britain as a necessary prohibition to prevent the truly immoral act of crashing into oncoming vehicles.
A second nonpositivistic view of the concept of an offense views crimes as prohibited acts, with the explanation for these prohibitions being that they are prohibited by certain social practices or by those with the authority to make criminalization decisions in light of a social practice allocating the power to do so. For instance, H. L. A. Hart viewed criminal law as a series of “primary rules” designed to regulate behavior. He maintained that the primary rules are only law because they are created by officials whose authority is based on a social practice that determines when a rule becomes law. He claimed that the regulation requiring males to remove their hats in church indicates a social practice. However, not all social behaviors are legally binding. In contrast to customs and regular, quotidian norms, social rules that have the power of law are identified in a unique manner inside the practice as having legal force. Only rules developed by those authorized by “secondary rules” to create, interpret, and apply primary rules will be recognized as authoritative. The social practice view of crime may appear comparable to the positivistic approach since both consider crime as a collection of restrictions imposed by those with the authority to do so. Thus, it may be considered merely a variant of positivism. In contrast to the Supreme Court’s style of positivism on crime, Hart’s account would permit evaluative assessments of a legislature’s criminalization decisions based on their fidelity to an underlying concept of crime. A legislature that enacted draconian criminal prohibitions on the basis of a social practice perspective could be seen to have exceeded its jurisdiction as provided by the pertinent secondary norms.
The economic account of crime is a third significant nonpositivistic option. According to some theories, a criminal conduct is inefficient since it circumvents a free market. Criminal penalties are required to provide sufficient motivation for individuals to attain their desires through the market rather than by force. In this regard, criminal sanctions and civil penalties varied slightly. While the legal economist views civil and criminal liability rules as fulfilling the same objective, that of providing incentives for efficient action, the incentive structures required to promote efficiency for the two types of activities differ. According to the economic account of crime, criminal punishment should be applied to actions that are always inefficient. The criminal code must frighten potential defendants with severe enough punishments to deter them from committing crimes. In contrast, there are instances in which acts that contravene civil law are effective while being illegal. It is therefore occasionally efficient to permit individuals to breach a contract or endanger another person. In order to offer incentives for efficient behavior, unlike criminal sanctions, which must always force conformity, the penalty for civil wrongs need simply be equivalent to the damage caused. By requiring individuals who create harm or seek to breach a contract to “internalize” the cost of the damage they do, they will only inflict harm or breach if it is profitable to do so. Criminal sanctions are identical to civil sanctions, with the distinction that civil punishments must include a “kicker” in addition to the damage inflicted to ensure that it is never beneficial to breach the prohibitive norm. In fact, the narrowing gap between tort law and criminal law during the past decade may be evidence of the influence of law and economics on judicial and legislative procedure.
While the positivist perspective on crime enjoys a rhetorical edge in our system, the actual understanding of crime that our legal system entails appears to be a mixture of descriptive and normative truths. We rely on legislative proclamation to determine the content of the prohibitions we refer to as “crimes,” but we also make normative judgements about criminal statutes based on an implicit understanding of what constitutes a proper application of the concept of crime. Moreover, maybe because the conceptual boundaries of “crime” are pretty well-established in our public usage of the term, states do not strive to eradicate the defense of self-defense or, for the most part, make street corner conversation a crime.
Bentham is commonly regarded as the founder of legal positivism. But even Bentham acknowledged that the concept of crime must contain normative components. Bentham held the conventional positivist view that laws, and criminal laws in particular, are sovereign mandates. Everything that is ordered has the force of law. Bentham contended, however, that a command does not constitute law if it is not “complete.” For a law to be comprehensive, it must describe a specific damage or evil against which the legal restriction is directed. Thus, even according to Bentham, the concept of crime is founded on a pre-legislative concept, namely the concept of harm. Developing a theory of crime based on the concept of harm is the fourth nonpositivist approach. John Stuart Mill proposed the beginnings of such an account when he stated what has become known as the “harm principle.” In On Liberty, Mill stated, “The only purpose for which power may be legitimately exercised against the will of any member of a civilized community is to prevent harm to others.” (pp. 10–11). More recently, Joel Feinberg has elaborated on Mill’s fundamental strategy. However, he has maintained that injury may not be the only acceptable basis for imposing criminal sanctions. Even if Feinberg is correct that we do not adhere to the harm principle without exception, the harm principle may be central to American criminal law’s conception of a crime.
Bibliography:
- BENTHAM, JEREMY. Of Laws in General. New York: Oxford University Press, 1970.
- COLEMAN, JULES ‘‘Crimes, Kickers and Transaction Structures.’’ Repr. in Markets, Morals and the Law. New York: Cambridge University Press, 1988. Pages 153–166.
- FEINBERG, JOEL. The Moral Limits of the Criminal Law. 1–4. New York: Oxford University Press, 1984–1998.
- FINKELSTEIN, CLAIRE. ‘‘Positivism and the Notion of an Offense.’’ California Law Review 88: (2000): 335–394.
- FLETCHER, GEORGE With Justice For Some: Protecting Victims’ Rights in Criminal Trials. Reading, Mass.: Addison-Wesley Publishing Co., 1996.
- GOEBEL, JULIUS, JR. Felony and Misdemeanor: A Study in the History of English Criminal Procedure. University of Pennsylvania Press, 1937. Reprint, 1976.
- HART, H. L. A. Punishment and Responsibility. Oxford, U.K.: Clarendon Press, 1967.
- HART, HENRY ‘‘The Aims of the Criminal Law.’’ Law and Contemporary Problems 23 (1958): 401.
- MILL, JOHN STUART. On Liberty (1859). Reprint. New York: W. W. Norton, 1975.
- MOORE, MICHAEL. Placing Blame. New York: Oxford University Press, 1997.
- POLLOCK, SIR FREDERICK, AND MAITLAND, FREDERIC WILLIAM. The History of English Law before the Time of Edward I. Lawyer’s Literary Club Edition, 1959.
- POSNER, RICHARD. ‘‘An Economic Theory of the Criminal Law.’’ Columbia Law Review 85 (1985): 1193.
ORDER HIGH QUALITY CUSTOM PAPER

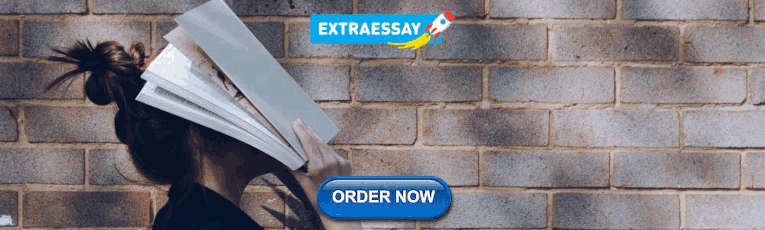
IMAGES
VIDEO
COMMENTS
The Journal of Research in Crime and Delinquency (JRCD), peer-reviewed and published bi-monthly, offers empirical articles and special issues to keep you up to date on contemporary issues and controversies in the study of crime and criminal-legal system responses.For more than sixty years, the journal has published work engaging a range of theoretical perspectives and methodological approaches ...
Table 4 presents the frequency of crime types studied in the collected research papers. As shown in the table, robbery is the most studied crime type, followed by murder/homicides and burglary with 51, 41, and 39 papers each, respectively. Most of the research papers studied more than one crime type.
The crucial social and social psychological aspects of crime, which include personal attitudes as well as the broader societal context. The investigation and management of crime. This increasingly includes careful consideration of the forms that crime is taking in contemporary society. The aftermath of crime, both for those who are convicted as ...
Examining What Is: Covid-19, Guns, and the Rise in Hate. Prior to the Covid-19 pandemic, crime rates were relatively low. As the graph in Figure 1 demonstrates, the rate of violent crime offenses declined from a peak in 1991 of 758.2 per year to 398.5 per year in 2020. 19 The rate of homicide over the same period also dropped significantly, from its highest level in 1991 compared with 2020. 20 ...
Criminology and Criminal Justice is a peer-reviewed journal that focuses on the broad field of criminology and criminal justice policy and practice. The journal publishes scholarly articles on all areas of criminology, crime and criminal justice. It includes theoretical pieces, as well as empirically-based analyses of policy and practice in areas that range from policing to sentencing ...
The COVID-19 pandemic of 2020 has impacted the world in ways not seen in generations. Initial evidence suggests one of the effects is crime rates, which appear to have fallen drastically in many communities around the world. We argue that the principal reason for the change is the government ordered stay-at-home orders, which impacted the routine activities of entire populations. Because these ...
The papers identified as review or related-work studies (a total of 13) date back to 2003 and are connected to the keyword strategy that we used (find further details in "Study selection" section). In addition, to review papers (a total of 9), we also include two editorials, one book chapter, and one research paper, because they contain an extensive literature review in the field of crime ...
Crime is a public wrong. It is an act of offense which violates. the law of the state and is strongly disapproved by the socie-. ty. Crime is defined as acts or omissions forbidden by law that ...
The field of machine learning is a subset of artificial intel-. ligence that uses statistical models and algorithms to analyze. and make predictions based on data. On the other hand, deep ...
The articles included in this issue reflect three broad areas of cybercrime research: cybercrime victimization, cybercrime perpetration, and techniques and facilitators of cybercrime. While there is some overlap, the issue includes three papers focused on each of these three areas. The first area covered in the special issue focuses on ...
Research investigating the link between mental health, crime and violence often rely on populations that are at a high-risk of violent and criminal behaviour, such as prison inmates and psychiatric patients. As a result of this selection bias, the relationship between mental health, criminal and violent behaviour is significantly over-estimated ...
Data are presented to examine the extent to which the policy and research discussions about organized crime match the organized crimes found in practice. In the article, the author stresses that what has been lacking thus far is a examination of actual cases of organized crime (i.e., investigated and prosecuted) to determine the extent to which ...
Crime Prediction and Analysis. February 2020. DOI: 10.1109/IDEA49133.2020.9170731. Conference: 2020 2nd International Conference on Data, Engineering and Applications (IDEA) Authors: Pratibha ...
Dr. Tamar Berenblum is the research director of the The Federmann Cyber Security Center - Cyber Law Program, Faculty of Law, the Hebrew University of Jerusalem, Israel, and the co-chair of the European Society of Criminology (ESC) Working Group on Cybercrime. Tamar is also a Post-Doc Research Fellow at the Netherlands Institute for the Study of Crime and Law Enforcement (NSCR), Netherlands ...
The current bibliometric study assessed the scholarly status on cybercrime victimization during 2010-2020 by retrieving SSCI articles from WoS database. There is no study that applied bibliometric method to research on the examined subject. Hence, this paper firstly contributed statistical evidence and visualized findings to literature of ...
Crime has been one of the main topics in news media for decades (e.g., Dominick, 1978; Graber, 1980; Johnson, 1998).In the United States, a study by Klite et al. (1997) revealed that more than 70% of the 100 news channels examined in the study opened with a crime-related news story. Similar trends are evident in number of other Western societies, for example, in the United Kingdom, the ...
A crime is a deliberate act that can cause physical or psychological harm, as well as property damage or loss, and can lead to punishment by a state or other authority according to the severity of the crime. The number and forms of criminal activities are increasing at an alarming rate, forcing agencies to develop efficient methods to take preventive measures. In the current scenario of ...
Note that, in general, a distinction can be made between cyber-crime, cyber-warfare, and cyber-attacks. Fig. 2 and Table 2 describes the distinction between cyber-crime, cyber-warfare, and cyber-attack that defines the conceptual distinction between them. Download : Download high-res image (324KB) Download : Download full-size image; Fig. 1.
There is a fast-growing body of critical literature on the proliferation of datafication in the form of the data-driven models now increasingly applied in justice systems across the world for predicting risk (Angwin and Larson, 2016), forecasting crime hotspots (Ensign et al., 2018; Lum and Isaac, 2016) and implementing the biometric identification of targeted individuals (Bennett Moses and ...
P.O. Box 5969, Safat 13060, Kuwait University, Kuwait. Abstract. The world has become more advanced in communication, espec ially after the invention of. the Internet. A key issue facing today's ...
This sample crime research paper features: 3800 words (approx. 12 pages), an outline, and a bibliography with 12 sources. Browse other research paper examples for more inspiration. If you need a thorough research paper written according to all the academic standards, you can always turn to our experienced writers for help.