2.1 Approaches to Sociological Research
Learning objectives.
By the end of this section, you should be able to:
- Define and describe the scientific method.
- Explain how the scientific method is used in sociological research.
- Describe the function and importance of an interpretive framework.
- Describe the differences in accuracy, reliability and validity in a research study.
When sociologists apply the sociological perspective and begin to ask questions, no topic is off limits. Every aspect of human behavior is a source of possible investigation. Sociologists question the world that humans have created and live in. They notice patterns of behavior as people move through that world. Using sociological methods and systematic research within the framework of the scientific method and a scholarly interpretive perspective, sociologists have discovered social patterns in the workplace that have transformed industries, in families that have enlightened family members, and in education that have aided structural changes in classrooms.
Sociologists often begin the research process by asking a question about how or why things happen in this world. It might be a unique question about a new trend or an old question about a common aspect of life. Once the question is formed, the sociologist proceeds through an in-depth process to answer it. In deciding how to design that process, the researcher may adopt a scientific approach or an interpretive framework. The following sections describe these approaches to knowledge.
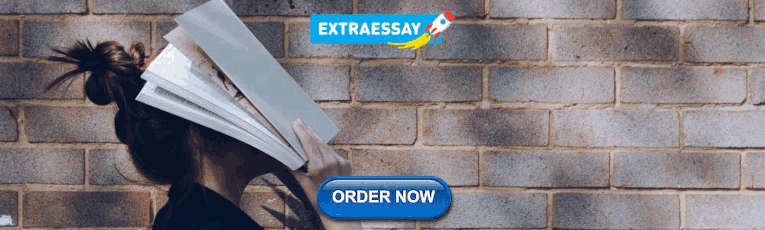
The Scientific Method
Sociologists make use of tried and true methods of research, such as experiments, surveys, and field research. But humans and their social interactions are so diverse that these interactions can seem impossible to chart or explain. It might seem that science is about discoveries and chemical reactions or about proving ideas right or wrong rather than about exploring the nuances of human behavior.
However, this is exactly why scientific models work for studying human behavior. A scientific process of research establishes parameters that help make sure results are objective and accurate. Scientific methods provide limitations and boundaries that focus a study and organize its results.
The scientific method involves developing and testing theories about the social world based on empirical evidence. It is defined by its commitment to systematic observation of the empirical world and strives to be objective, critical, skeptical, and logical. It involves a series of six prescribed steps that have been established over centuries of scientific scholarship.
Sociological research does not reduce knowledge to right or wrong facts. Results of studies tend to provide people with insights they did not have before—explanations of human behaviors and social practices and access to knowledge of other cultures, rituals and beliefs, or trends and attitudes.
In general, sociologists tackle questions about the role of social characteristics in outcomes or results. For example, how do different communities fare in terms of psychological well-being, community cohesiveness, range of vocation, wealth, crime rates, and so on? Are communities functioning smoothly? Sociologists often look between the cracks to discover obstacles to meeting basic human needs. They might also study environmental influences and patterns of behavior that lead to crime, substance abuse, divorce, poverty, unplanned pregnancies, or illness. And, because sociological studies are not all focused on negative behaviors or challenging situations, social researchers might study vacation trends, healthy eating habits, neighborhood organizations, higher education patterns, games, parks, and exercise habits.
Sociologists can use the scientific method not only to collect but also to interpret and analyze data. They deliberately apply scientific logic and objectivity. They are interested in—but not attached to—the results. They work outside of their own political or social agendas. This does not mean researchers do not have their own personalities, complete with preferences and opinions. But sociologists deliberately use the scientific method to maintain as much objectivity, focus, and consistency as possible in collecting and analyzing data in research studies.
With its systematic approach, the scientific method has proven useful in shaping sociological studies. The scientific method provides a systematic, organized series of steps that help ensure objectivity and consistency in exploring a social problem. They provide the means for accuracy, reliability, and validity. In the end, the scientific method provides a shared basis for discussion and analysis (Merton 1963). Typically, the scientific method has 6 steps which are described below.
Step 1: Ask a Question or Find a Research Topic
The first step of the scientific method is to ask a question, select a problem, and identify the specific area of interest. The topic should be narrow enough to study within a geographic location and time frame. “Are societies capable of sustained happiness?” would be too vague. The question should also be broad enough to have universal merit. “What do personal hygiene habits reveal about the values of students at XYZ High School?” would be too narrow. Sociologists strive to frame questions that examine well-defined patterns and relationships.
In a hygiene study, for instance, hygiene could be defined as “personal habits to maintain physical appearance (as opposed to health),” and a researcher might ask, “How do differing personal hygiene habits reflect the cultural value placed on appearance?”
Step 2: Review the Literature/Research Existing Sources
The next step researchers undertake is to conduct background research through a literature review , which is a review of any existing similar or related studies. A visit to the library, a thorough online search, and a survey of academic journals will uncover existing research about the topic of study. This step helps researchers gain a broad understanding of work previously conducted, identify gaps in understanding of the topic, and position their own research to build on prior knowledge. Researchers—including student researchers—are responsible for correctly citing existing sources they use in a study or that inform their work. While it is fine to borrow previously published material (as long as it enhances a unique viewpoint), it must be referenced properly and never plagiarized.
To study crime, a researcher might also sort through existing data from the court system, police database, prison information, interviews with criminals, guards, wardens, etc. It’s important to examine this information in addition to existing research to determine how these resources might be used to fill holes in existing knowledge. Reviewing existing sources educates researchers and helps refine and improve a research study design.
Step 3: Formulate a Hypothesis
A hypothesis is an explanation for a phenomenon based on a conjecture about the relationship between the phenomenon and one or more causal factors. In sociology, the hypothesis will often predict how one form of human behavior influences another. For example, a hypothesis might be in the form of an “if, then statement.” Let’s relate this to our topic of crime: If unemployment increases, then the crime rate will increase.
In scientific research, we formulate hypotheses to include an independent variables (IV) , which are the cause of the change, and a dependent variable (DV) , which is the effect , or thing that is changed. In the example above, unemployment is the independent variable and the crime rate is the dependent variable.
In a sociological study, the researcher would establish one form of human behavior as the independent variable and observe the influence it has on a dependent variable. How does gender (the independent variable) affect rate of income (the dependent variable)? How does one’s religion (the independent variable) affect family size (the dependent variable)? How is social class (the dependent variable) affected by level of education (the independent variable)?
Taking an example from Table 12.1, a researcher might hypothesize that teaching children proper hygiene (the independent variable) will boost their sense of self-esteem (the dependent variable). Note, however, this hypothesis can also work the other way around. A sociologist might predict that increasing a child’s sense of self-esteem (the independent variable) will increase or improve habits of hygiene (now the dependent variable). Identifying the independent and dependent variables is very important. As the hygiene example shows, simply identifying related two topics or variables is not enough. Their prospective relationship must be part of the hypothesis.
Step 4: Design and Conduct a Study
Researchers design studies to maximize reliability , which refers to how likely research results are to be replicated if the study is reproduced. Reliability increases the likelihood that what happens to one person will happen to all people in a group or what will happen in one situation will happen in another. Cooking is a science. When you follow a recipe and measure ingredients with a cooking tool, such as a measuring cup, the same results is obtained as long as the cook follows the same recipe and uses the same type of tool. The measuring cup introduces accuracy into the process. If a person uses a less accurate tool, such as their hand, to measure ingredients rather than a cup, the same result may not be replicated. Accurate tools and methods increase reliability.
Researchers also strive for validity , which refers to how well the study measures what it was designed to measure. To produce reliable and valid results, sociologists develop an operational definition , that is, they define each concept, or variable, in terms of the physical or concrete steps it takes to objectively measure it. The operational definition identifies an observable condition of the concept. By operationalizing the concept, all researchers can collect data in a systematic or replicable manner. Moreover, researchers can determine whether the experiment or method validly represent the phenomenon they intended to study.
A study asking how tutoring improves grades, for instance, might define “tutoring” as “one-on-one assistance by an expert in the field, hired by an educational institution.” However, one researcher might define a “good” grade as a C or better, while another uses a B+ as a starting point for “good.” For the results to be replicated and gain acceptance within the broader scientific community, researchers would have to use a standard operational definition. These definitions set limits and establish cut-off points that ensure consistency and replicability in a study.
We will explore research methods in greater detail in the next section of this chapter.
Step 5: Draw Conclusions
After constructing the research design, sociologists collect, tabulate or categorize, and analyze data to formulate conclusions. If the analysis supports the hypothesis, researchers can discuss the implications of the results for the theory or policy solution that they were addressing. If the analysis does not support the hypothesis, researchers may consider repeating the experiment or think of ways to improve their procedure.
However, even when results contradict a sociologist’s prediction of a study’s outcome, these results still contribute to sociological understanding. Sociologists analyze general patterns in response to a study, but they are equally interested in exceptions to patterns. In a study of education, a researcher might predict that high school dropouts have a hard time finding rewarding careers. While many assume that the higher the education, the higher the salary and degree of career happiness, there are certainly exceptions. People with little education have had stunning careers, and people with advanced degrees have had trouble finding work. A sociologist prepares a hypothesis knowing that results may substantiate or contradict it.
Sociologists carefully keep in mind how operational definitions and research designs impact the results as they draw conclusions. Consider the concept of “increase of crime,” which might be defined as the percent increase in crime from last week to this week, as in the study of Swedish crime discussed above. Yet the data used to evaluate “increase of crime” might be limited by many factors: who commits the crime, where the crimes are committed, or what type of crime is committed. If the data is gathered for “crimes committed in Houston, Texas in zip code 77021,” then it may not be generalizable to crimes committed in rural areas outside of major cities like Houston. If data is collected about vandalism, it may not be generalizable to assault.
Step 6: Report Results
Researchers report their results at conferences and in academic journals. These results are then subjected to the scrutiny of other sociologists in the field. Before the conclusions of a study become widely accepted, the studies are often repeated in the same or different environments. In this way, sociological theories and knowledge develops as the relationships between social phenomenon are established in broader contexts and different circumstances.
Interpretive Framework
While many sociologists rely on empirical data and the scientific method as a research approach, others operate from an interpretive framework . While systematic, this approach doesn’t follow the hypothesis-testing model that seeks to find generalizable results. Instead, an interpretive framework, sometimes referred to as an interpretive perspective , seeks to understand social worlds from the point of view of participants, which leads to in-depth knowledge or understanding about the human experience.
Interpretive research is generally more descriptive or narrative in its findings. Rather than formulating a hypothesis and method for testing it, an interpretive researcher will develop approaches to explore the topic at hand that may involve a significant amount of direct observation or interaction with subjects including storytelling. This type of researcher learns through the process and sometimes adjusts the research methods or processes midway to optimize findings as they evolve.
Critical Sociology
Critical sociology focuses on deconstruction of existing sociological research and theory. Informed by the work of Karl Marx, scholars known collectively as the Frankfurt School proposed that social science, as much as any academic pursuit, is embedded in the system of power constituted by the set of class, caste, race, gender, and other relationships that exist in the society. Consequently, it cannot be treated as purely objective. Critical sociologists view theories, methods, and the conclusions as serving one of two purposes: they can either legitimate and rationalize systems of social power and oppression or liberate humans from inequality and restriction on human freedom. Deconstruction can involve data collection, but the analysis of this data is not empirical or positivist.
As an Amazon Associate we earn from qualifying purchases.
This book may not be used in the training of large language models or otherwise be ingested into large language models or generative AI offerings without OpenStax's permission.
Want to cite, share, or modify this book? This book uses the Creative Commons Attribution License and you must attribute OpenStax.
Access for free at https://openstax.org/books/introduction-sociology-3e/pages/1-introduction
- Authors: Tonja R. Conerly, Kathleen Holmes, Asha Lal Tamang
- Publisher/website: OpenStax
- Book title: Introduction to Sociology 3e
- Publication date: Jun 3, 2021
- Location: Houston, Texas
- Book URL: https://openstax.org/books/introduction-sociology-3e/pages/1-introduction
- Section URL: https://openstax.org/books/introduction-sociology-3e/pages/2-1-approaches-to-sociological-research
© Jan 18, 2024 OpenStax. Textbook content produced by OpenStax is licensed under a Creative Commons Attribution License . The OpenStax name, OpenStax logo, OpenStax book covers, OpenStax CNX name, and OpenStax CNX logo are not subject to the Creative Commons license and may not be reproduced without the prior and express written consent of Rice University.
Have a language expert improve your writing
Run a free plagiarism check in 10 minutes, generate accurate citations for free.
- Knowledge Base
Methodology
- How to Write a Strong Hypothesis | Steps & Examples
How to Write a Strong Hypothesis | Steps & Examples
Published on May 6, 2022 by Shona McCombes . Revised on November 20, 2023.
A hypothesis is a statement that can be tested by scientific research. If you want to test a relationship between two or more variables, you need to write hypotheses before you start your experiment or data collection .
Example: Hypothesis
Daily apple consumption leads to fewer doctor’s visits.
Table of contents
What is a hypothesis, developing a hypothesis (with example), hypothesis examples, other interesting articles, frequently asked questions about writing hypotheses.
A hypothesis states your predictions about what your research will find. It is a tentative answer to your research question that has not yet been tested. For some research projects, you might have to write several hypotheses that address different aspects of your research question.
A hypothesis is not just a guess – it should be based on existing theories and knowledge. It also has to be testable, which means you can support or refute it through scientific research methods (such as experiments, observations and statistical analysis of data).
Variables in hypotheses
Hypotheses propose a relationship between two or more types of variables .
- An independent variable is something the researcher changes or controls.
- A dependent variable is something the researcher observes and measures.
If there are any control variables , extraneous variables , or confounding variables , be sure to jot those down as you go to minimize the chances that research bias will affect your results.
In this example, the independent variable is exposure to the sun – the assumed cause . The dependent variable is the level of happiness – the assumed effect .
Receive feedback on language, structure, and formatting
Professional editors proofread and edit your paper by focusing on:
- Academic style
- Vague sentences
- Style consistency
See an example
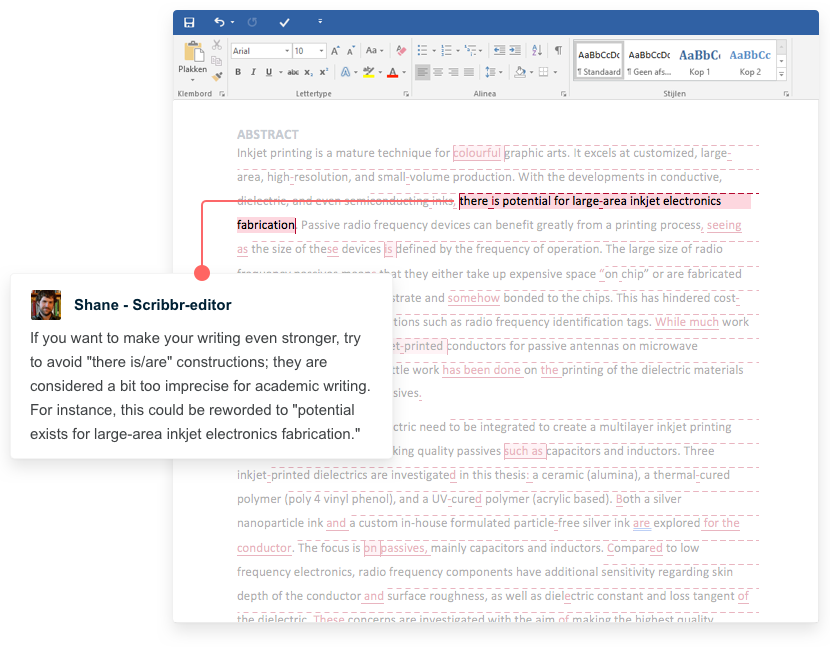
Step 1. Ask a question
Writing a hypothesis begins with a research question that you want to answer. The question should be focused, specific, and researchable within the constraints of your project.
Step 2. Do some preliminary research
Your initial answer to the question should be based on what is already known about the topic. Look for theories and previous studies to help you form educated assumptions about what your research will find.
At this stage, you might construct a conceptual framework to ensure that you’re embarking on a relevant topic . This can also help you identify which variables you will study and what you think the relationships are between them. Sometimes, you’ll have to operationalize more complex constructs.
Step 3. Formulate your hypothesis
Now you should have some idea of what you expect to find. Write your initial answer to the question in a clear, concise sentence.
4. Refine your hypothesis
You need to make sure your hypothesis is specific and testable. There are various ways of phrasing a hypothesis, but all the terms you use should have clear definitions, and the hypothesis should contain:
- The relevant variables
- The specific group being studied
- The predicted outcome of the experiment or analysis
5. Phrase your hypothesis in three ways
To identify the variables, you can write a simple prediction in if…then form. The first part of the sentence states the independent variable and the second part states the dependent variable.
In academic research, hypotheses are more commonly phrased in terms of correlations or effects, where you directly state the predicted relationship between variables.
If you are comparing two groups, the hypothesis can state what difference you expect to find between them.
6. Write a null hypothesis
If your research involves statistical hypothesis testing , you will also have to write a null hypothesis . The null hypothesis is the default position that there is no association between the variables. The null hypothesis is written as H 0 , while the alternative hypothesis is H 1 or H a .
- H 0 : The number of lectures attended by first-year students has no effect on their final exam scores.
- H 1 : The number of lectures attended by first-year students has a positive effect on their final exam scores.
If you want to know more about the research process , methodology , research bias , or statistics , make sure to check out some of our other articles with explanations and examples.
- Sampling methods
- Simple random sampling
- Stratified sampling
- Cluster sampling
- Likert scales
- Reproducibility
Statistics
- Null hypothesis
- Statistical power
- Probability distribution
- Effect size
- Poisson distribution
Research bias
- Optimism bias
- Cognitive bias
- Implicit bias
- Hawthorne effect
- Anchoring bias
- Explicit bias
A hypothesis is not just a guess — it should be based on existing theories and knowledge. It also has to be testable, which means you can support or refute it through scientific research methods (such as experiments, observations and statistical analysis of data).
Null and alternative hypotheses are used in statistical hypothesis testing . The null hypothesis of a test always predicts no effect or no relationship between variables, while the alternative hypothesis states your research prediction of an effect or relationship.
Hypothesis testing is a formal procedure for investigating our ideas about the world using statistics. It is used by scientists to test specific predictions, called hypotheses , by calculating how likely it is that a pattern or relationship between variables could have arisen by chance.
Cite this Scribbr article
If you want to cite this source, you can copy and paste the citation or click the “Cite this Scribbr article” button to automatically add the citation to our free Citation Generator.
McCombes, S. (2023, November 20). How to Write a Strong Hypothesis | Steps & Examples. Scribbr. Retrieved March 20, 2024, from https://www.scribbr.com/methodology/hypothesis/
Is this article helpful?
Shona McCombes
Other students also liked, construct validity | definition, types, & examples, what is a conceptual framework | tips & examples, operationalization | a guide with examples, pros & cons, unlimited academic ai-proofreading.
✔ Document error-free in 5minutes ✔ Unlimited document corrections ✔ Specialized in correcting academic texts
- Resources Home 🏠
- Try SciSpace Copilot
- Search research papers
- Add Copilot Extension
- Try AI Detector
- Try Paraphraser
- Try Citation Generator
- April Papers
- June Papers
- July Papers

The Craft of Writing a Strong Hypothesis

Table of Contents
Writing a hypothesis is one of the essential elements of a scientific research paper. It needs to be to the point, clearly communicating what your research is trying to accomplish. A blurry, drawn-out, or complexly-structured hypothesis can confuse your readers. Or worse, the editor and peer reviewers.
A captivating hypothesis is not too intricate. This blog will take you through the process so that, by the end of it, you have a better idea of how to convey your research paper's intent in just one sentence.
What is a Hypothesis?
The first step in your scientific endeavor, a hypothesis, is a strong, concise statement that forms the basis of your research. It is not the same as a thesis statement , which is a brief summary of your research paper .
The sole purpose of a hypothesis is to predict your paper's findings, data, and conclusion. It comes from a place of curiosity and intuition . When you write a hypothesis, you're essentially making an educated guess based on scientific prejudices and evidence, which is further proven or disproven through the scientific method.
The reason for undertaking research is to observe a specific phenomenon. A hypothesis, therefore, lays out what the said phenomenon is. And it does so through two variables, an independent and dependent variable.
The independent variable is the cause behind the observation, while the dependent variable is the effect of the cause. A good example of this is “mixing red and blue forms purple.” In this hypothesis, mixing red and blue is the independent variable as you're combining the two colors at your own will. The formation of purple is the dependent variable as, in this case, it is conditional to the independent variable.
Different Types of Hypotheses

Types of hypotheses
Some would stand by the notion that there are only two types of hypotheses: a Null hypothesis and an Alternative hypothesis. While that may have some truth to it, it would be better to fully distinguish the most common forms as these terms come up so often, which might leave you out of context.
Apart from Null and Alternative, there are Complex, Simple, Directional, Non-Directional, Statistical, and Associative and casual hypotheses. They don't necessarily have to be exclusive, as one hypothesis can tick many boxes, but knowing the distinctions between them will make it easier for you to construct your own.
1. Null hypothesis
A null hypothesis proposes no relationship between two variables. Denoted by H 0 , it is a negative statement like “Attending physiotherapy sessions does not affect athletes' on-field performance.” Here, the author claims physiotherapy sessions have no effect on on-field performances. Even if there is, it's only a coincidence.
2. Alternative hypothesis
Considered to be the opposite of a null hypothesis, an alternative hypothesis is donated as H1 or Ha. It explicitly states that the dependent variable affects the independent variable. A good alternative hypothesis example is “Attending physiotherapy sessions improves athletes' on-field performance.” or “Water evaporates at 100 °C. ” The alternative hypothesis further branches into directional and non-directional.
- Directional hypothesis: A hypothesis that states the result would be either positive or negative is called directional hypothesis. It accompanies H1 with either the ‘<' or ‘>' sign.
- Non-directional hypothesis: A non-directional hypothesis only claims an effect on the dependent variable. It does not clarify whether the result would be positive or negative. The sign for a non-directional hypothesis is ‘≠.'
3. Simple hypothesis
A simple hypothesis is a statement made to reflect the relation between exactly two variables. One independent and one dependent. Consider the example, “Smoking is a prominent cause of lung cancer." The dependent variable, lung cancer, is dependent on the independent variable, smoking.
4. Complex hypothesis
In contrast to a simple hypothesis, a complex hypothesis implies the relationship between multiple independent and dependent variables. For instance, “Individuals who eat more fruits tend to have higher immunity, lesser cholesterol, and high metabolism.” The independent variable is eating more fruits, while the dependent variables are higher immunity, lesser cholesterol, and high metabolism.
5. Associative and casual hypothesis
Associative and casual hypotheses don't exhibit how many variables there will be. They define the relationship between the variables. In an associative hypothesis, changing any one variable, dependent or independent, affects others. In a casual hypothesis, the independent variable directly affects the dependent.
6. Empirical hypothesis
Also referred to as the working hypothesis, an empirical hypothesis claims a theory's validation via experiments and observation. This way, the statement appears justifiable and different from a wild guess.
Say, the hypothesis is “Women who take iron tablets face a lesser risk of anemia than those who take vitamin B12.” This is an example of an empirical hypothesis where the researcher the statement after assessing a group of women who take iron tablets and charting the findings.
7. Statistical hypothesis
The point of a statistical hypothesis is to test an already existing hypothesis by studying a population sample. Hypothesis like “44% of the Indian population belong in the age group of 22-27.” leverage evidence to prove or disprove a particular statement.
Characteristics of a Good Hypothesis
Writing a hypothesis is essential as it can make or break your research for you. That includes your chances of getting published in a journal. So when you're designing one, keep an eye out for these pointers:
- A research hypothesis has to be simple yet clear to look justifiable enough.
- It has to be testable — your research would be rendered pointless if too far-fetched into reality or limited by technology.
- It has to be precise about the results —what you are trying to do and achieve through it should come out in your hypothesis.
- A research hypothesis should be self-explanatory, leaving no doubt in the reader's mind.
- If you are developing a relational hypothesis, you need to include the variables and establish an appropriate relationship among them.
- A hypothesis must keep and reflect the scope for further investigations and experiments.
Separating a Hypothesis from a Prediction
Outside of academia, hypothesis and prediction are often used interchangeably. In research writing, this is not only confusing but also incorrect. And although a hypothesis and prediction are guesses at their core, there are many differences between them.
A hypothesis is an educated guess or even a testable prediction validated through research. It aims to analyze the gathered evidence and facts to define a relationship between variables and put forth a logical explanation behind the nature of events.
Predictions are assumptions or expected outcomes made without any backing evidence. They are more fictionally inclined regardless of where they originate from.
For this reason, a hypothesis holds much more weight than a prediction. It sticks to the scientific method rather than pure guesswork. "Planets revolve around the Sun." is an example of a hypothesis as it is previous knowledge and observed trends. Additionally, we can test it through the scientific method.
Whereas "COVID-19 will be eradicated by 2030." is a prediction. Even though it results from past trends, we can't prove or disprove it. So, the only way this gets validated is to wait and watch if COVID-19 cases end by 2030.
Finally, How to Write a Hypothesis
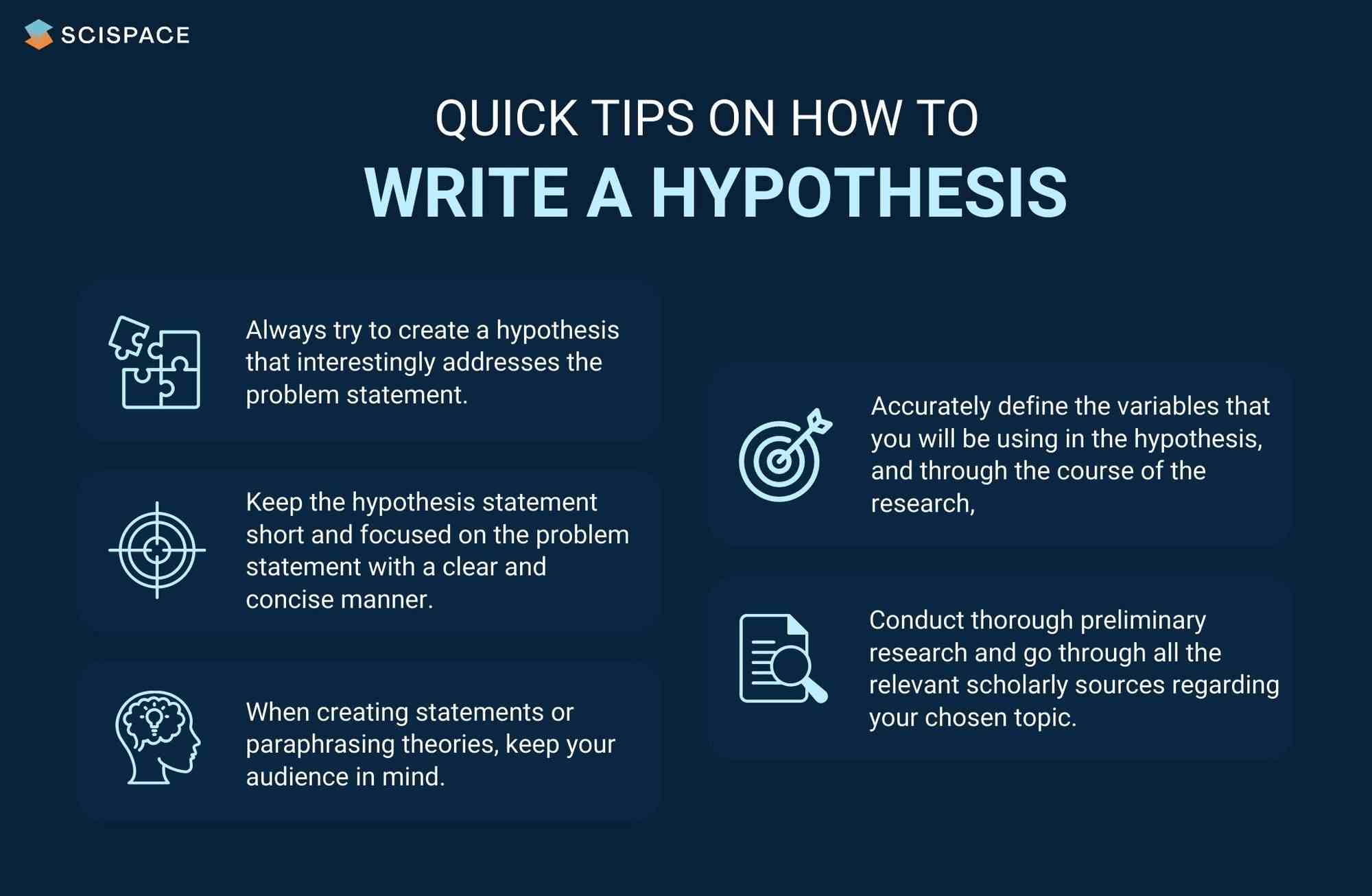
Quick tips on writing a hypothesis
1. Be clear about your research question
A hypothesis should instantly address the research question or the problem statement. To do so, you need to ask a question. Understand the constraints of your undertaken research topic and then formulate a simple and topic-centric problem. Only after that can you develop a hypothesis and further test for evidence.
2. Carry out a recce
Once you have your research's foundation laid out, it would be best to conduct preliminary research. Go through previous theories, academic papers, data, and experiments before you start curating your research hypothesis. It will give you an idea of your hypothesis's viability or originality.
Making use of references from relevant research papers helps draft a good research hypothesis. SciSpace Discover offers a repository of over 270 million research papers to browse through and gain a deeper understanding of related studies on a particular topic. Additionally, you can use SciSpace Copilot , your AI research assistant, for reading any lengthy research paper and getting a more summarized context of it. A hypothesis can be formed after evaluating many such summarized research papers. Copilot also offers explanations for theories and equations, explains paper in simplified version, allows you to highlight any text in the paper or clip math equations and tables and provides a deeper, clear understanding of what is being said. This can improve the hypothesis by helping you identify potential research gaps.
3. Create a 3-dimensional hypothesis
Variables are an essential part of any reasonable hypothesis. So, identify your independent and dependent variable(s) and form a correlation between them. The ideal way to do this is to write the hypothetical assumption in the ‘if-then' form. If you use this form, make sure that you state the predefined relationship between the variables.
In another way, you can choose to present your hypothesis as a comparison between two variables. Here, you must specify the difference you expect to observe in the results.
4. Write the first draft
Now that everything is in place, it's time to write your hypothesis. For starters, create the first draft. In this version, write what you expect to find from your research.
Clearly separate your independent and dependent variables and the link between them. Don't fixate on syntax at this stage. The goal is to ensure your hypothesis addresses the issue.
5. Proof your hypothesis
After preparing the first draft of your hypothesis, you need to inspect it thoroughly. It should tick all the boxes, like being concise, straightforward, relevant, and accurate. Your final hypothesis has to be well-structured as well.
Research projects are an exciting and crucial part of being a scholar. And once you have your research question, you need a great hypothesis to begin conducting research. Thus, knowing how to write a hypothesis is very important.
Now that you have a firmer grasp on what a good hypothesis constitutes, the different kinds there are, and what process to follow, you will find it much easier to write your hypothesis, which ultimately helps your research.
Now it's easier than ever to streamline your research workflow with SciSpace Discover . Its integrated, comprehensive end-to-end platform for research allows scholars to easily discover, write and publish their research and fosters collaboration.
It includes everything you need, including a repository of over 270 million research papers across disciplines, SEO-optimized summaries and public profiles to show your expertise and experience.
If you found these tips on writing a research hypothesis useful, head over to our blog on Statistical Hypothesis Testing to learn about the top researchers, papers, and institutions in this domain.
Frequently Asked Questions (FAQs)
1. what is the definition of hypothesis.
According to the Oxford dictionary, a hypothesis is defined as “An idea or explanation of something that is based on a few known facts, but that has not yet been proved to be true or correct”.
2. What is an example of hypothesis?
The hypothesis is a statement that proposes a relationship between two or more variables. An example: "If we increase the number of new users who join our platform by 25%, then we will see an increase in revenue."
3. What is an example of null hypothesis?
A null hypothesis is a statement that there is no relationship between two variables. The null hypothesis is written as H0. The null hypothesis states that there is no effect. For example, if you're studying whether or not a particular type of exercise increases strength, your null hypothesis will be "there is no difference in strength between people who exercise and people who don't."
4. What are the types of research?
• Fundamental research
• Applied research
• Qualitative research
• Quantitative research
• Mixed research
• Exploratory research
• Longitudinal research
• Cross-sectional research
• Field research
• Laboratory research
• Fixed research
• Flexible research
• Action research
• Policy research
• Classification research
• Comparative research
• Causal research
• Inductive research
• Deductive research
5. How to write a hypothesis?
• Your hypothesis should be able to predict the relationship and outcome.
• Avoid wordiness by keeping it simple and brief.
• Your hypothesis should contain observable and testable outcomes.
• Your hypothesis should be relevant to the research question.
6. What are the 2 types of hypothesis?
• Null hypotheses are used to test the claim that "there is no difference between two groups of data".
• Alternative hypotheses test the claim that "there is a difference between two data groups".
7. Difference between research question and research hypothesis?
A research question is a broad, open-ended question you will try to answer through your research. A hypothesis is a statement based on prior research or theory that you expect to be true due to your study. Example - Research question: What are the factors that influence the adoption of the new technology? Research hypothesis: There is a positive relationship between age, education and income level with the adoption of the new technology.
8. What is plural for hypothesis?
The plural of hypothesis is hypotheses. Here's an example of how it would be used in a statement, "Numerous well-considered hypotheses are presented in this part, and they are supported by tables and figures that are well-illustrated."
9. What is the red queen hypothesis?
The red queen hypothesis in evolutionary biology states that species must constantly evolve to avoid extinction because if they don't, they will be outcompeted by other species that are evolving. Leigh Van Valen first proposed it in 1973; since then, it has been tested and substantiated many times.
10. Who is known as the father of null hypothesis?
The father of the null hypothesis is Sir Ronald Fisher. He published a paper in 1925 that introduced the concept of null hypothesis testing, and he was also the first to use the term itself.
11. When to reject null hypothesis?
You need to find a significant difference between your two populations to reject the null hypothesis. You can determine that by running statistical tests such as an independent sample t-test or a dependent sample t-test. You should reject the null hypothesis if the p-value is less than 0.05.

You might also like

Consensus GPT vs. SciSpace GPT: Choose the Best GPT for Research
Literature Review and Theoretical Framework: Understanding the Differences

Types of Essays in Academic Writing
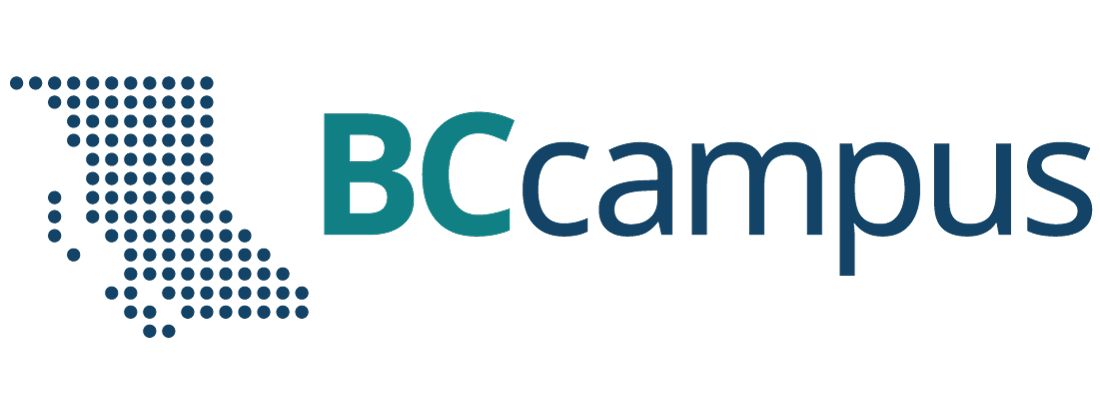
Want to create or adapt books like this? Learn more about how Pressbooks supports open publishing practices.
Developing a Research Question
18 Hypotheses
When researchers do not have predictions about what they will find, they conduct research to answer a question or questions, with an open-minded desire to know about a topic, or to help develop hypotheses for later testing. In other situations, the purpose of research is to test a specific hypothesis or hypotheses. A hypothesis is a statement, sometimes but not always causal, describing a researcher’s expectations regarding anticipated finding. Often hypotheses are written to describe the expected relationship between two variables (though this is not a requirement). To develop a hypothesis, one needs to understand the differences between independent and dependent variables and between units of observation and units of analysis. Hypotheses are typically drawn from theories and usually describe how an independent variable is expected to affect some dependent variable or variables. Researchers following a deductive approach to their research will hypothesize about what they expect to find based on the theory or theories that frame their study. If the theory accurately reflects the phenomenon it is designed to explain, then the researcher’s hypotheses about what would be observed in the real world should bear out.
Sometimes researchers will hypothesize that a relationship will take a specific direction. As a result, an increase or decrease in one area might be said to cause an increase or decrease in another. For example, you might choose to study the relationship between age and legalization of marijuana. Perhaps you have done some reading in your spare time, or in another course you have taken. Based on the theories you have read, you hypothesize that “age is negatively related to support for marijuana legalization.” What have you just hypothesized? You have hypothesized that as people get older, the likelihood of their support for marijuana legalization decreases. Thus, as age moves in one direction (up), support for marijuana legalization moves in another direction (down). If writing hypotheses feels tricky, it is sometimes helpful to draw them out. and depict each of the two hypotheses we have just discussed.
Note that you will almost never hear researchers say that they have proven their hypotheses. A statement that bold implies that a relationship has been shown to exist with absolute certainty and that there is no chance that there are conditions under which the hypothesis would not bear out. Instead, researchers tend to say that their hypotheses have been supported (or not) . This more cautious way of discussing findings allows for the possibility that new evidence or new ways of examining a relationship will be discovered. Researchers may also discuss a null hypothesis, one that predicts no relationship between the variables being studied. If a researcher rejects the null hypothesis, he or she is saying that the variables in question are somehow related to one another.
Quantitative and qualitative researchers tend to take different approaches when it comes to hypotheses. In quantitative research, the goal often is to empirically test hypotheses generated from theory. With a qualitative approach, on the other hand, a researcher may begin with some vague expectations about what he or she will find, but the aim is not to test one’s expectations against some empirical observations. Instead, theory development or construction is the goal. Qualitative researchers may develop theories from which hypotheses can be drawn and quantitative researchers may then test those hypotheses. Both types of research are crucial to understanding our social world, and both play an important role in the matter of hypothesis development and testing. In the following section, we will look at qualitative and quantitative approaches to research, as well as mixed methods.
Text Attributions
- This chapter has been adapted from Chapter 5.2 in Principles of Sociological Inquiry , which was adapted by the Saylor Academy without attribution to the original authors or publisher, as requested by the licensor. © Creative Commons Attribution-NonCommercial-ShareAlike 3.0 License .
An Introduction to Research Methods in Sociology Copyright © 2019 by Valerie A. Sheppard is licensed under a Creative Commons Attribution-NonCommercial-ShareAlike 4.0 International License , except where otherwise noted.
Share This Book
- Bipolar Disorder
- Therapy Center
- When To See a Therapist
- Types of Therapy
- Best Online Therapy
- Best Couples Therapy
- Best Family Therapy
- Managing Stress
- Sleep and Dreaming
- Understanding Emotions
- Self-Improvement
- Healthy Relationships
- Student Resources
- Personality Types
- Verywell Mind Insights
- 2023 Verywell Mind 25
- Mental Health in the Classroom
- Editorial Process
- Meet Our Review Board
- Crisis Support
How to Write a Great Hypothesis
Hypothesis Format, Examples, and Tips
Kendra Cherry, MS, is a psychosocial rehabilitation specialist, psychology educator, and author of the "Everything Psychology Book."
:max_bytes(150000):strip_icc():format(webp)/IMG_9791-89504ab694d54b66bbd72cb84ffb860e.jpg)
Amy Morin, LCSW, is a psychotherapist and international bestselling author. Her books, including "13 Things Mentally Strong People Don't Do," have been translated into more than 40 languages. Her TEDx talk, "The Secret of Becoming Mentally Strong," is one of the most viewed talks of all time.
:max_bytes(150000):strip_icc():format(webp)/VW-MIND-Amy-2b338105f1ee493f94d7e333e410fa76.jpg)
Verywell / Alex Dos Diaz
- The Scientific Method
Hypothesis Format
Falsifiability of a hypothesis, operational definitions, types of hypotheses, hypotheses examples.
- Collecting Data
Frequently Asked Questions
A hypothesis is a tentative statement about the relationship between two or more variables. It is a specific, testable prediction about what you expect to happen in a study.
One hypothesis example would be a study designed to look at the relationship between sleep deprivation and test performance might have a hypothesis that states: "This study is designed to assess the hypothesis that sleep-deprived people will perform worse on a test than individuals who are not sleep-deprived."
This article explores how a hypothesis is used in psychology research, how to write a good hypothesis, and the different types of hypotheses you might use.
The Hypothesis in the Scientific Method
In the scientific method , whether it involves research in psychology, biology, or some other area, a hypothesis represents what the researchers think will happen in an experiment. The scientific method involves the following steps:
- Forming a question
- Performing background research
- Creating a hypothesis
- Designing an experiment
- Collecting data
- Analyzing the results
- Drawing conclusions
- Communicating the results
The hypothesis is a prediction, but it involves more than a guess. Most of the time, the hypothesis begins with a question which is then explored through background research. It is only at this point that researchers begin to develop a testable hypothesis. Unless you are creating an exploratory study, your hypothesis should always explain what you expect to happen.
In a study exploring the effects of a particular drug, the hypothesis might be that researchers expect the drug to have some type of effect on the symptoms of a specific illness. In psychology, the hypothesis might focus on how a certain aspect of the environment might influence a particular behavior.
Remember, a hypothesis does not have to be correct. While the hypothesis predicts what the researchers expect to see, the goal of the research is to determine whether this guess is right or wrong. When conducting an experiment, researchers might explore a number of factors to determine which ones might contribute to the ultimate outcome.
In many cases, researchers may find that the results of an experiment do not support the original hypothesis. When writing up these results, the researchers might suggest other options that should be explored in future studies.
In many cases, researchers might draw a hypothesis from a specific theory or build on previous research. For example, prior research has shown that stress can impact the immune system. So a researcher might hypothesize: "People with high-stress levels will be more likely to contract a common cold after being exposed to the virus than people who have low-stress levels."
In other instances, researchers might look at commonly held beliefs or folk wisdom. "Birds of a feather flock together" is one example of folk wisdom that a psychologist might try to investigate. The researcher might pose a specific hypothesis that "People tend to select romantic partners who are similar to them in interests and educational level."
Elements of a Good Hypothesis
So how do you write a good hypothesis? When trying to come up with a hypothesis for your research or experiments, ask yourself the following questions:
- Is your hypothesis based on your research on a topic?
- Can your hypothesis be tested?
- Does your hypothesis include independent and dependent variables?
Before you come up with a specific hypothesis, spend some time doing background research. Once you have completed a literature review, start thinking about potential questions you still have. Pay attention to the discussion section in the journal articles you read . Many authors will suggest questions that still need to be explored.
To form a hypothesis, you should take these steps:
- Collect as many observations about a topic or problem as you can.
- Evaluate these observations and look for possible causes of the problem.
- Create a list of possible explanations that you might want to explore.
- After you have developed some possible hypotheses, think of ways that you could confirm or disprove each hypothesis through experimentation. This is known as falsifiability.
In the scientific method , falsifiability is an important part of any valid hypothesis. In order to test a claim scientifically, it must be possible that the claim could be proven false.
Students sometimes confuse the idea of falsifiability with the idea that it means that something is false, which is not the case. What falsifiability means is that if something was false, then it is possible to demonstrate that it is false.
One of the hallmarks of pseudoscience is that it makes claims that cannot be refuted or proven false.
A variable is a factor or element that can be changed and manipulated in ways that are observable and measurable. However, the researcher must also define how the variable will be manipulated and measured in the study.
For example, a researcher might operationally define the variable " test anxiety " as the results of a self-report measure of anxiety experienced during an exam. A "study habits" variable might be defined by the amount of studying that actually occurs as measured by time.
These precise descriptions are important because many things can be measured in a number of different ways. One of the basic principles of any type of scientific research is that the results must be replicable. By clearly detailing the specifics of how the variables were measured and manipulated, other researchers can better understand the results and repeat the study if needed.
Some variables are more difficult than others to define. How would you operationally define a variable such as aggression ? For obvious ethical reasons, researchers cannot create a situation in which a person behaves aggressively toward others.
In order to measure this variable, the researcher must devise a measurement that assesses aggressive behavior without harming other people. In this situation, the researcher might utilize a simulated task to measure aggressiveness.
Hypothesis Checklist
- Does your hypothesis focus on something that you can actually test?
- Does your hypothesis include both an independent and dependent variable?
- Can you manipulate the variables?
- Can your hypothesis be tested without violating ethical standards?
The hypothesis you use will depend on what you are investigating and hoping to find. Some of the main types of hypotheses that you might use include:
- Simple hypothesis : This type of hypothesis suggests that there is a relationship between one independent variable and one dependent variable.
- Complex hypothesis : This type of hypothesis suggests a relationship between three or more variables, such as two independent variables and a dependent variable.
- Null hypothesis : This hypothesis suggests no relationship exists between two or more variables.
- Alternative hypothesis : This hypothesis states the opposite of the null hypothesis.
- Statistical hypothesis : This hypothesis uses statistical analysis to evaluate a representative sample of the population and then generalizes the findings to the larger group.
- Logical hypothesis : This hypothesis assumes a relationship between variables without collecting data or evidence.
A hypothesis often follows a basic format of "If {this happens} then {this will happen}." One way to structure your hypothesis is to describe what will happen to the dependent variable if you change the independent variable .
The basic format might be: "If {these changes are made to a certain independent variable}, then we will observe {a change in a specific dependent variable}."
A few examples of simple hypotheses:
- "Students who eat breakfast will perform better on a math exam than students who do not eat breakfast."
- Complex hypothesis: "Students who experience test anxiety before an English exam will get lower scores than students who do not experience test anxiety."
- "Motorists who talk on the phone while driving will be more likely to make errors on a driving course than those who do not talk on the phone."
Examples of a complex hypothesis include:
- "People with high-sugar diets and sedentary activity levels are more likely to develop depression."
- "Younger people who are regularly exposed to green, outdoor areas have better subjective well-being than older adults who have limited exposure to green spaces."
Examples of a null hypothesis include:
- "Children who receive a new reading intervention will have scores different than students who do not receive the intervention."
- "There will be no difference in scores on a memory recall task between children and adults."
Examples of an alternative hypothesis:
- "Children who receive a new reading intervention will perform better than students who did not receive the intervention."
- "Adults will perform better on a memory task than children."
Collecting Data on Your Hypothesis
Once a researcher has formed a testable hypothesis, the next step is to select a research design and start collecting data. The research method depends largely on exactly what they are studying. There are two basic types of research methods: descriptive research and experimental research.
Descriptive Research Methods
Descriptive research such as case studies , naturalistic observations , and surveys are often used when it would be impossible or difficult to conduct an experiment . These methods are best used to describe different aspects of a behavior or psychological phenomenon.
Once a researcher has collected data using descriptive methods, a correlational study can then be used to look at how the variables are related. This type of research method might be used to investigate a hypothesis that is difficult to test experimentally.
Experimental Research Methods
Experimental methods are used to demonstrate causal relationships between variables. In an experiment, the researcher systematically manipulates a variable of interest (known as the independent variable) and measures the effect on another variable (known as the dependent variable).
Unlike correlational studies, which can only be used to determine if there is a relationship between two variables, experimental methods can be used to determine the actual nature of the relationship—whether changes in one variable actually cause another to change.
A Word From Verywell
The hypothesis is a critical part of any scientific exploration. It represents what researchers expect to find in a study or experiment. In situations where the hypothesis is unsupported by the research, the research still has value. Such research helps us better understand how different aspects of the natural world relate to one another. It also helps us develop new hypotheses that can then be tested in the future.
Some examples of how to write a hypothesis include:
- "Staying up late will lead to worse test performance the next day."
- "People who consume one apple each day will visit the doctor fewer times each year."
- "Breaking study sessions up into three 20-minute sessions will lead to better test results than a single 60-minute study session."
The four parts of a hypothesis are:
- The research question
- The independent variable (IV)
- The dependent variable (DV)
- The proposed relationship between the IV and DV
Castillo M. The scientific method: a need for something better? . AJNR Am J Neuroradiol. 2013;34(9):1669-71. doi:10.3174/ajnr.A3401
Nevid J. Psychology: Concepts and Applications. Wadworth, 2013.
By Kendra Cherry, MSEd Kendra Cherry, MS, is a psychosocial rehabilitation specialist, psychology educator, and author of the "Everything Psychology Book."
Research Hypothesis In Psychology: Types, & Examples
Saul Mcleod, PhD
Editor-in-Chief for Simply Psychology
BSc (Hons) Psychology, MRes, PhD, University of Manchester
Saul Mcleod, PhD., is a qualified psychology teacher with over 18 years of experience in further and higher education. He has been published in peer-reviewed journals, including the Journal of Clinical Psychology.
Learn about our Editorial Process
Olivia Guy-Evans, MSc
Associate Editor for Simply Psychology
BSc (Hons) Psychology, MSc Psychology of Education
Olivia Guy-Evans is a writer and associate editor for Simply Psychology. She has previously worked in healthcare and educational sectors.
On This Page:
A research hypothesis, in its plural form “hypotheses,” is a specific, testable prediction about the anticipated results of a study, established at its outset. It is a key component of the scientific method .
Hypotheses connect theory to data and guide the research process towards expanding scientific understanding
Some key points about hypotheses:
- A hypothesis expresses an expected pattern or relationship. It connects the variables under investigation.
- It is stated in clear, precise terms before any data collection or analysis occurs. This makes the hypothesis testable.
- A hypothesis must be falsifiable. It should be possible, even if unlikely in practice, to collect data that disconfirms rather than supports the hypothesis.
- Hypotheses guide research. Scientists design studies to explicitly evaluate hypotheses about how nature works.
- For a hypothesis to be valid, it must be testable against empirical evidence. The evidence can then confirm or disprove the testable predictions.
- Hypotheses are informed by background knowledge and observation, but go beyond what is already known to propose an explanation of how or why something occurs.
Predictions typically arise from a thorough knowledge of the research literature, curiosity about real-world problems or implications, and integrating this to advance theory. They build on existing literature while providing new insight.
Types of Research Hypotheses
Alternative hypothesis.
The research hypothesis is often called the alternative or experimental hypothesis in experimental research.
It typically suggests a potential relationship between two key variables: the independent variable, which the researcher manipulates, and the dependent variable, which is measured based on those changes.
The alternative hypothesis states a relationship exists between the two variables being studied (one variable affects the other).
A hypothesis is a testable statement or prediction about the relationship between two or more variables. It is a key component of the scientific method. Some key points about hypotheses:
- Important hypotheses lead to predictions that can be tested empirically. The evidence can then confirm or disprove the testable predictions.
In summary, a hypothesis is a precise, testable statement of what researchers expect to happen in a study and why. Hypotheses connect theory to data and guide the research process towards expanding scientific understanding.
An experimental hypothesis predicts what change(s) will occur in the dependent variable when the independent variable is manipulated.
It states that the results are not due to chance and are significant in supporting the theory being investigated.
The alternative hypothesis can be directional, indicating a specific direction of the effect, or non-directional, suggesting a difference without specifying its nature. It’s what researchers aim to support or demonstrate through their study.
Null Hypothesis
The null hypothesis states no relationship exists between the two variables being studied (one variable does not affect the other). There will be no changes in the dependent variable due to manipulating the independent variable.
It states results are due to chance and are not significant in supporting the idea being investigated.
The null hypothesis, positing no effect or relationship, is a foundational contrast to the research hypothesis in scientific inquiry. It establishes a baseline for statistical testing, promoting objectivity by initiating research from a neutral stance.
Many statistical methods are tailored to test the null hypothesis, determining the likelihood of observed results if no true effect exists.
This dual-hypothesis approach provides clarity, ensuring that research intentions are explicit, and fosters consistency across scientific studies, enhancing the standardization and interpretability of research outcomes.
Nondirectional Hypothesis
A non-directional hypothesis, also known as a two-tailed hypothesis, predicts that there is a difference or relationship between two variables but does not specify the direction of this relationship.
It merely indicates that a change or effect will occur without predicting which group will have higher or lower values.
For example, “There is a difference in performance between Group A and Group B” is a non-directional hypothesis.
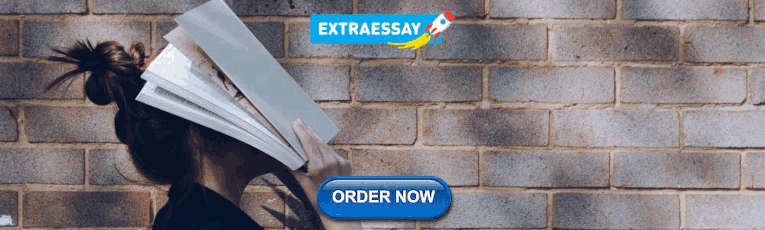
Directional Hypothesis
A directional (one-tailed) hypothesis predicts the nature of the effect of the independent variable on the dependent variable. It predicts in which direction the change will take place. (i.e., greater, smaller, less, more)
It specifies whether one variable is greater, lesser, or different from another, rather than just indicating that there’s a difference without specifying its nature.
For example, “Exercise increases weight loss” is a directional hypothesis.
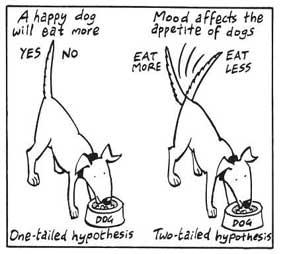
Falsifiability
The Falsification Principle, proposed by Karl Popper , is a way of demarcating science from non-science. It suggests that for a theory or hypothesis to be considered scientific, it must be testable and irrefutable.
Falsifiability emphasizes that scientific claims shouldn’t just be confirmable but should also have the potential to be proven wrong.
It means that there should exist some potential evidence or experiment that could prove the proposition false.
However many confirming instances exist for a theory, it only takes one counter observation to falsify it. For example, the hypothesis that “all swans are white,” can be falsified by observing a black swan.
For Popper, science should attempt to disprove a theory rather than attempt to continually provide evidence to support a research hypothesis.
Can a Hypothesis be Proven?
Hypotheses make probabilistic predictions. They state the expected outcome if a particular relationship exists. However, a study result supporting a hypothesis does not definitively prove it is true.
All studies have limitations. There may be unknown confounding factors or issues that limit the certainty of conclusions. Additional studies may yield different results.
In science, hypotheses can realistically only be supported with some degree of confidence, not proven. The process of science is to incrementally accumulate evidence for and against hypothesized relationships in an ongoing pursuit of better models and explanations that best fit the empirical data. But hypotheses remain open to revision and rejection if that is where the evidence leads.
- Disproving a hypothesis is definitive. Solid disconfirmatory evidence will falsify a hypothesis and require altering or discarding it based on the evidence.
- However, confirming evidence is always open to revision. Other explanations may account for the same results, and additional or contradictory evidence may emerge over time.
We can never 100% prove the alternative hypothesis. Instead, we see if we can disprove, or reject the null hypothesis.
If we reject the null hypothesis, this doesn’t mean that our alternative hypothesis is correct but does support the alternative/experimental hypothesis.
Upon analysis of the results, an alternative hypothesis can be rejected or supported, but it can never be proven to be correct. We must avoid any reference to results proving a theory as this implies 100% certainty, and there is always a chance that evidence may exist which could refute a theory.
How to Write a Hypothesis
- Identify variables . The researcher manipulates the independent variable and the dependent variable is the measured outcome.
- Operationalized the variables being investigated . Operationalization of a hypothesis refers to the process of making the variables physically measurable or testable, e.g. if you are about to study aggression, you might count the number of punches given by participants.
- Decide on a direction for your prediction . If there is evidence in the literature to support a specific effect of the independent variable on the dependent variable, write a directional (one-tailed) hypothesis. If there are limited or ambiguous findings in the literature regarding the effect of the independent variable on the dependent variable, write a non-directional (two-tailed) hypothesis.
- Make it Testable : Ensure your hypothesis can be tested through experimentation or observation. It should be possible to prove it false (principle of falsifiability).
- Clear & concise language . A strong hypothesis is concise (typically one to two sentences long), and formulated using clear and straightforward language, ensuring it’s easily understood and testable.
Consider a hypothesis many teachers might subscribe to: students work better on Monday morning than on Friday afternoon (IV=Day, DV= Standard of work).
Now, if we decide to study this by giving the same group of students a lesson on a Monday morning and a Friday afternoon and then measuring their immediate recall of the material covered in each session, we would end up with the following:
- The alternative hypothesis states that students will recall significantly more information on a Monday morning than on a Friday afternoon.
- The null hypothesis states that there will be no significant difference in the amount recalled on a Monday morning compared to a Friday afternoon. Any difference will be due to chance or confounding factors.
More Examples
- Memory : Participants exposed to classical music during study sessions will recall more items from a list than those who studied in silence.
- Social Psychology : Individuals who frequently engage in social media use will report higher levels of perceived social isolation compared to those who use it infrequently.
- Developmental Psychology : Children who engage in regular imaginative play have better problem-solving skills than those who don’t.
- Clinical Psychology : Cognitive-behavioral therapy will be more effective in reducing symptoms of anxiety over a 6-month period compared to traditional talk therapy.
- Cognitive Psychology : Individuals who multitask between various electronic devices will have shorter attention spans on focused tasks than those who single-task.
- Health Psychology : Patients who practice mindfulness meditation will experience lower levels of chronic pain compared to those who don’t meditate.
- Organizational Psychology : Employees in open-plan offices will report higher levels of stress than those in private offices.
- Behavioral Psychology : Rats rewarded with food after pressing a lever will press it more frequently than rats who receive no reward.
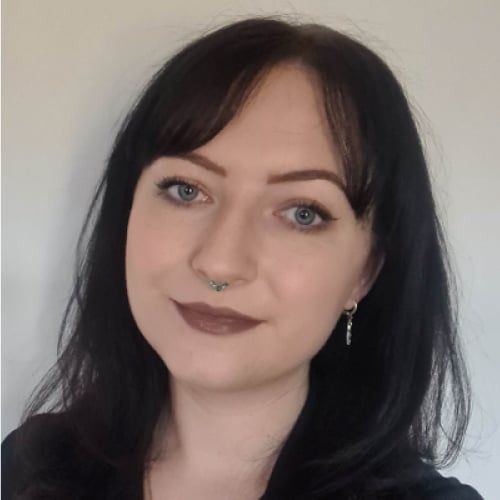

What Is A Research (Scientific) Hypothesis? A plain-language explainer + examples
By: Derek Jansen (MBA) | Reviewed By: Dr Eunice Rautenbach | June 2020
If you’re new to the world of research, or it’s your first time writing a dissertation or thesis, you’re probably noticing that the words “research hypothesis” and “scientific hypothesis” are used quite a bit, and you’re wondering what they mean in a research context .
“Hypothesis” is one of those words that people use loosely, thinking they understand what it means. However, it has a very specific meaning within academic research. So, it’s important to understand the exact meaning before you start hypothesizing.
Research Hypothesis 101
- What is a hypothesis ?
- What is a research hypothesis (scientific hypothesis)?
- Requirements for a research hypothesis
- Definition of a research hypothesis
- The null hypothesis
What is a hypothesis?
Let’s start with the general definition of a hypothesis (not a research hypothesis or scientific hypothesis), according to the Cambridge Dictionary:
Hypothesis: an idea or explanation for something that is based on known facts but has not yet been proved.
In other words, it’s a statement that provides an explanation for why or how something works, based on facts (or some reasonable assumptions), but that has not yet been specifically tested . For example, a hypothesis might look something like this:
Hypothesis: sleep impacts academic performance.
This statement predicts that academic performance will be influenced by the amount and/or quality of sleep a student engages in – sounds reasonable, right? It’s based on reasonable assumptions , underpinned by what we currently know about sleep and health (from the existing literature). So, loosely speaking, we could call it a hypothesis, at least by the dictionary definition.
But that’s not good enough…
Unfortunately, that’s not quite sophisticated enough to describe a research hypothesis (also sometimes called a scientific hypothesis), and it wouldn’t be acceptable in a dissertation, thesis or research paper . In the world of academic research, a statement needs a few more criteria to constitute a true research hypothesis .
What is a research hypothesis?
A research hypothesis (also called a scientific hypothesis) is a statement about the expected outcome of a study (for example, a dissertation or thesis). To constitute a quality hypothesis, the statement needs to have three attributes – specificity , clarity and testability .
Let’s take a look at these more closely.
Need a helping hand?
Hypothesis Essential #1: Specificity & Clarity
A good research hypothesis needs to be extremely clear and articulate about both what’ s being assessed (who or what variables are involved ) and the expected outcome (for example, a difference between groups, a relationship between variables, etc.).
Let’s stick with our sleepy students example and look at how this statement could be more specific and clear.
Hypothesis: Students who sleep at least 8 hours per night will, on average, achieve higher grades in standardised tests than students who sleep less than 8 hours a night.
As you can see, the statement is very specific as it identifies the variables involved (sleep hours and test grades), the parties involved (two groups of students), as well as the predicted relationship type (a positive relationship). There’s no ambiguity or uncertainty about who or what is involved in the statement, and the expected outcome is clear.
Contrast that to the original hypothesis we looked at – “Sleep impacts academic performance” – and you can see the difference. “Sleep” and “academic performance” are both comparatively vague , and there’s no indication of what the expected relationship direction is (more sleep or less sleep). As you can see, specificity and clarity are key.
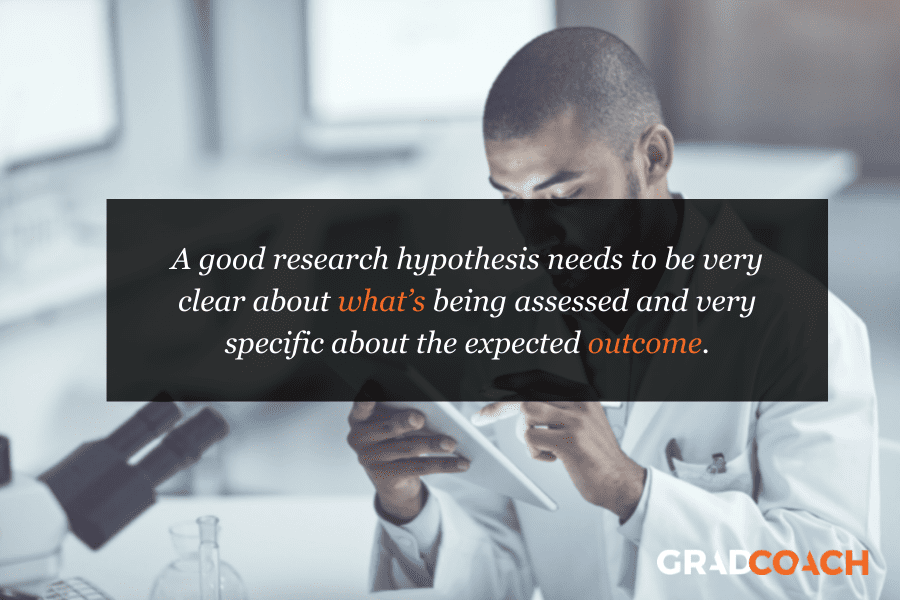
Hypothesis Essential #2: Testability (Provability)
A statement must be testable to qualify as a research hypothesis. In other words, there needs to be a way to prove (or disprove) the statement. If it’s not testable, it’s not a hypothesis – simple as that.
For example, consider the hypothesis we mentioned earlier:
Hypothesis: Students who sleep at least 8 hours per night will, on average, achieve higher grades in standardised tests than students who sleep less than 8 hours a night.
We could test this statement by undertaking a quantitative study involving two groups of students, one that gets 8 or more hours of sleep per night for a fixed period, and one that gets less. We could then compare the standardised test results for both groups to see if there’s a statistically significant difference.
Again, if you compare this to the original hypothesis we looked at – “Sleep impacts academic performance” – you can see that it would be quite difficult to test that statement, primarily because it isn’t specific enough. How much sleep? By who? What type of academic performance?
So, remember the mantra – if you can’t test it, it’s not a hypothesis 🙂
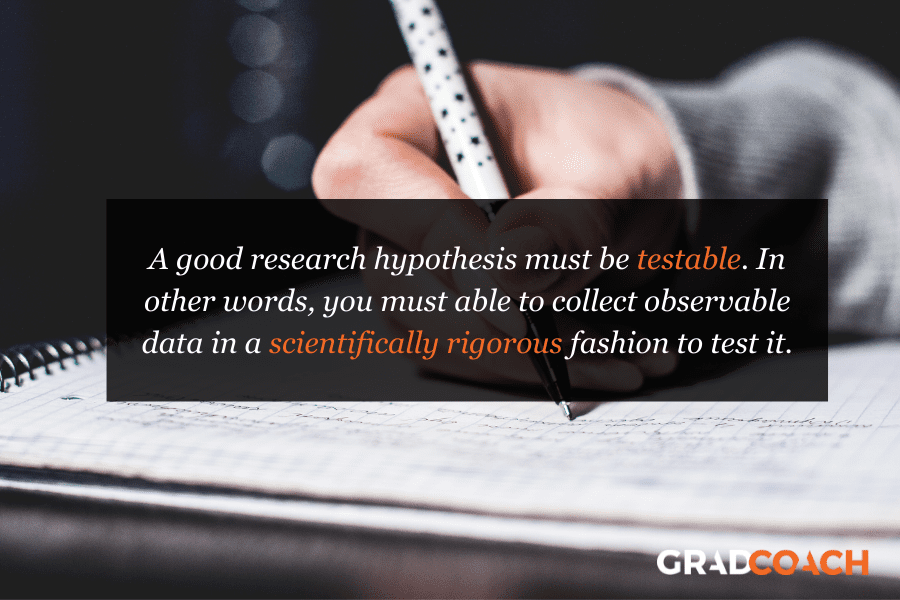
Defining A Research Hypothesis
You’re still with us? Great! Let’s recap and pin down a clear definition of a hypothesis.
A research hypothesis (or scientific hypothesis) is a statement about an expected relationship between variables, or explanation of an occurrence, that is clear, specific and testable.
So, when you write up hypotheses for your dissertation or thesis, make sure that they meet all these criteria. If you do, you’ll not only have rock-solid hypotheses but you’ll also ensure a clear focus for your entire research project.
What about the null hypothesis?
You may have also heard the terms null hypothesis , alternative hypothesis, or H-zero thrown around. At a simple level, the null hypothesis is the counter-proposal to the original hypothesis.
For example, if the hypothesis predicts that there is a relationship between two variables (for example, sleep and academic performance), the null hypothesis would predict that there is no relationship between those variables.
At a more technical level, the null hypothesis proposes that no statistical significance exists in a set of given observations and that any differences are due to chance alone.
And there you have it – hypotheses in a nutshell.
If you have any questions, be sure to leave a comment below and we’ll do our best to help you. If you need hands-on help developing and testing your hypotheses, consider our private coaching service , where we hold your hand through the research journey.
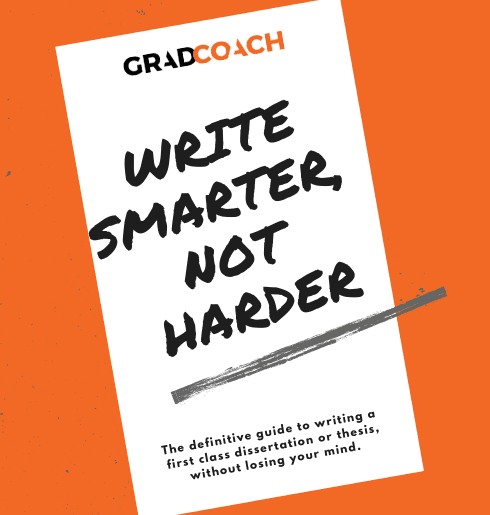
Psst… there’s more (for free)
This post is part of our dissertation mini-course, which covers everything you need to get started with your dissertation, thesis or research project.
You Might Also Like:
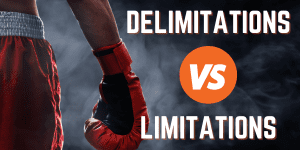
15 Comments
Very useful information. I benefit more from getting more information in this regard.
Very great insight,educative and informative. Please give meet deep critics on many research data of public international Law like human rights, environment, natural resources, law of the sea etc
In a book I read a distinction is made between null, research, and alternative hypothesis. As far as I understand, alternative and research hypotheses are the same. Can you please elaborate? Best Afshin
This is a self explanatory, easy going site. I will recommend this to my friends and colleagues.
Very good definition. How can I cite your definition in my thesis? Thank you. Is nul hypothesis compulsory in a research?
It’s a counter-proposal to be proven as a rejection
Please what is the difference between alternate hypothesis and research hypothesis?
It is a very good explanation. However, it limits hypotheses to statistically tasteable ideas. What about for qualitative researches or other researches that involve quantitative data that don’t need statistical tests?
In qualitative research, one typically uses propositions, not hypotheses.
could you please elaborate it more
I’ve benefited greatly from these notes, thank you.
This is very helpful
well articulated ideas are presented here, thank you for being reliable sources of information
Excellent. Thanks for being clear and sound about the research methodology and hypothesis (quantitative research)
I have only a simple question regarding the null hypothesis. – Is the null hypothesis (Ho) known as the reversible hypothesis of the alternative hypothesis (H1? – How to test it in academic research?
Trackbacks/Pingbacks
- What Is Research Methodology? Simple Definition (With Examples) - Grad Coach - […] Contrasted to this, a quantitative methodology is typically used when the research aims and objectives are confirmatory in nature. For example,…
Submit a Comment Cancel reply
Your email address will not be published. Required fields are marked *
Save my name, email, and website in this browser for the next time I comment.
- Print Friendly
Chapter 8: Inference for One Proportion
Hypothesis testing (2 of 5), learning objectives.
- Recognize the logic behind a hypothesis test and how it relates to the P-value.
In this section, our focus is hypothesis testing, which is part of inference. On the previous page, we practiced stating null and alternative hypotheses from a research question. Forming the hypotheses is the first step in a hypothesis test. Here are the general steps in the process of hypothesis testing. We will see that hypothesis testing is related to the thinking we did in Linking Probability to Statistical Inference .
Step 1: Determine the hypotheses.
The hypotheses come from the research question.
Step 2: Collect the data.
Ideally, we select a random sample from the population. The data comes from this sample. We calculate a statistic (a mean or a proportion) to summarize the data.
Step 3: Assess the evidence.
Assume that the null hypothesis is true. Could the data come from the population described by the null hypothesis? Use simulation or a mathematical model to examine the results from random samples selected from the population described by the null hypothesis. Figure out if results similar to the data are likely or unlikely. Note that the wording “likely or unlikely” implies that this step requires some kind of probability calculation.
Step 4: State a conclusion.
We use what we find in the previous step to make a decision. This step requires us to think in the following way. Remember that we assume that the null hypothesis is true. Then one of two outcomes can occur:
- One possibility is that results similar to the actual sample are extremely unlikely. This means that the data do not fit in with results from random samples selected from the population described by the null hypothesis. In this case, it is unlikely that the data came from this population, so we view this as strong evidence against the null hypothesis. We reject the null hypothesis in favor of the alternative hypothesis.
- The other possibility is that results similar to the actual sample are fairly likely (not unusual). This means that the data fit in with typical results from random samples selected from the population described by the null hypothesis. In this case, we do not have evidence against the null hypothesis, so we cannot reject it in favor of the alternative hypothesis.
Data Use on Smart Phones
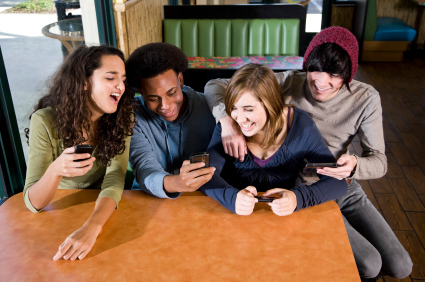
According to an article by Andrew Berg (“Report: Teens Texting More, Using More Data,” Wireless Week , October 15, 2010), Nielsen Company analyzed cell phone usage for different age groups using cell phone bills and surveys. Nielsen found significant growth in data usage, particularly among teens, stating that “94 percent of teen subscribers self-identify as advanced data users, turning to their cellphones for messaging, Internet, multimedia, gaming, and other activities like downloads.” The study found that the mean cell phone data usage was 62 MB among teens ages 13 to 17. A researcher is curious whether cell phone data usage has increased for this age group since the original study was conducted. She plans to conduct a hypothesis test.
The null hypothesis is often a statement of “no change,” so the null hypothesis will state that there is no change in the mean cell phone data usage for this age group since the original study. In this case, the alternative hypothesis is that the mean has increased from 62 MB.
- H 0 : The mean data usage for teens with smart phones is still 62 MB.
- H a : The mean data usage for teens with smart phones is greater than 62 MB.
The next step is to obtain a sample and collect data that will allow the researcher to test the hypotheses. The sample must be representative of the population and, ideally, should be a random sample. In this case, the researcher must randomly sample teens who use smart phones.
For the purposes of this example, imagine that the researcher randomly samples 50 teens who use smart phones. She finds that the mean data usage for these teens was 75 MB with a standard deviation of 45 MB. Since it is greater than 62 MB, this sample mean provides some evidence in favor of the alternative hypothesis. But the researcher anticipates that samples will vary when the null hypothesis is true. So how much of a difference will make her doubt the null hypothesis? Does she have evidence strong enough to reject the null hypothesis?
To assess the evidence, the researcher needs to know how much variability to expect in random samples when the null hypothesis is true. She begins with the assumption that H 0 is true – in this case, that the mean data usage for teens is still 62 MB. She then determines how unusual the results of the sample are: If the mean for all teens with smart phones actually is 62 MB, what is the chance that a random sample of 50 teens will have a sample mean of 75 MB or higher? Obviously, this probability depends on how much variability there is in random samples of this size from this population.
The probability of observing a sample mean at least this high if the population mean is 62 MB is approximately 0.023 (later topics explain how to calculate this probability). The probability is quite small. It tells the researcher that if the population mean is actually 62 MB, a sample mean of 75 MB or higher will occur only about 2.3% of the time. This probability is called the P-value .
Note: The P-value is a conditional probability, discussed in the module Relationships in Categorical Data with Intro to Probability . The condition is the assumption that the null hypothesis is true.
Step 4: Conclusion.
The small P-value indicates that it is unlikely for a sample mean to be 75 MB or higher if the population has a mean of 62 MB. It is therefore unlikely that the data from these 50 teens came from a population with a mean of 62 MB. The evidence is strong enough to make the researcher doubt the null hypothesis, so she rejects the null hypothesis in favor of the alternative hypothesis. The researcher concludes that the mean data usage for teens with smart phones has increased since the original study. It is now greater than 62 MB. ( P = 0.023)
Notice that the P-value is included in the preceding conclusion, which is a common practice. It allows the reader to see the strength of the evidence used to draw the conclusion.
How Small Does the P-Value Have to Be to Reject the Null Hypothesis?
A small P-value indicates that it is unlikely that the actual sample data came from the population described by the null hypothesis. More specifically, a small P-value says that there is only a small chance that we will randomly select a sample with results at least as extreme as the data if H 0 is true. The smaller the P-value, the stronger the evidence against H 0 .
But how small does the P-value have to be in order to reject H 0 ?
In practice, we often compare the P-value to 0.05. We reject the null hypothesis in favor of the alternative if the P-value is less than (or equal to) 0.05.
Note: This means that sampling variability will produce results at least as extreme as the data 5% of the time. In other words, in the long run, 1 in 20 random samples will have results that suggest we should reject H 0 even when H 0 is true. This variability is just due to chance, but it is unusual enough that we are willing to say that results this rare suggest that H 0 is not true.
Statistical Significance: Another Way to Describe Unlikely Results
When the P-value is less than (or equal to) 0.05, we also say that the difference between the actual sample statistic and the assumed parameter value is statistically significant . In the previous example, the P-value is less than 0.05, so we say the difference between the sample mean (75 MB) and the assumed mean from the null hypothesis (62 MB) is statistically significant. You will also see this described as a significant difference . A significant difference is an observed difference that is too large to attribute to chance. In other words, it is a difference that is unlikely when we consider sampling variability alone. If the difference is statistically significant, we reject H 0 .
Other Observations about Stating Conclusions in a Hypothesis Test
In the example, the sample mean was greater than 62 MB. This fact alone does not suggest that the data supports the alternative hypothesis. We have to determine that the data is not only larger than 62 MB but larger than we would expect to see in a random sampling if the population mean is 62 MB. We therefore need to determine the P-value. If the sample mean was less than or equal to 62 MB, it would not support the alternative hypothesis. We don’t need to find a P-value in this case. The conclusion is clear without it.
We have to be very careful in how we state the conclusion. There are only two possibilities.
- We have enough evidence to reject the null hypothesis and support the alternative hypothesis.
- We do not have enough evidence to reject the null hypothesis, so there is not enough evidence to support the alternative hypothesis.
If the P-value in the previous example was greater than 0.05, then we would not have enough evidence to reject H 0 and accept H a . In this case our conclusion would be that “there is not enough evidence to show that the mean amount of data used by teens with smart phones has increased.” Notice that this conclusion answers the original research question. It focuses on the alternative hypothesis. It does not say “the null hypothesis is true.” We never accept the null hypothesis or state that it is true. When there is not enough evidence to reject H 0 , the conclusion will say, in essence, that “there is not enough evidence to support H a .” But of course we will state the conclusion in the specific context of the situation we are investigating.
We compared the P-value to 0.05 in the previous example. The number 0.05 is called the significance level for the test, because a P-value less than or equal to 0.05 is statistically significant (unlikely to have occurred solely by chance). The symbol we use for the significance level is α (the lowercase Greek letter alpha). We sometimes refer to the significance level as the α-level. We call this value the significance level because if the P-value is less than the significance level, we say the results of the test showed a significance difference.
If the P-value ≤ α, we reject the null hypothesis in favor of the alternative hypothesis.
If the P-value > α, we fail to reject the null hypothesis.
In practice, it is common to see 0.05 for the significance level. Occasionally, researchers use other significance levels. In particular, if rejecting H 0 will be controversial or expensive, we may require stronger evidence. In this case, a smaller significance level, such as 0.01, is used. As with the hypotheses, we should choose the significance level before collecting data. It is treated as an agreed-upon benchmark prior to conducting the hypothesis test. In this way, we can avoid arguments about the strength of the data.
We look more at how to choose the significance level later. On this page we continue to use a significance level of 0.05.
First let’s look at some exercises that focus on the P-value and its meaning. Then we’ll try some that cover the conclusion.
Learn By Doing
For many years, working full-time has meant working 40 hours per week. Nowadays, it seems that corporate employers expect their employees to work more than this amount. A researcher decides to investigate this hypothesis.
- H 0 : The average time full-time corporate employees work per week is 40 hours.
- H a : The average time full-time corporate employees work per week is more than 40 hours.
To substantiate his claim, the researcher randomly selects 250 corporate employees and finds that they work an average of 47 hours per week with a standard deviation of 3.2 hours.
According to the Centers for Disease Control (CDC), roughly 21.5% of all high school seniors in the United States have used marijuana. (The data were collected in 2002. The figure represents those who smoked during the month prior to the survey, so the actual figure might be higher.) A sociologist suspects that the rate among African American high school seniors is lower. In this case, then,
- H 0 : The rate of African American high-school seniors who have used marijuana is 21.5% (same as the overall rate of seniors).
- H a : The rate of African American high-school seniors who have used marijuana is lower than 21.5%.
To check his claim, the sociologist chooses a random sample of 375 African American high school seniors,and finds that 16.5% of them have used marijuana.
- Concepts in Statistics. Provided by : Open Learning Initiative. Located at : http://oli.cmu.edu . License : CC BY: Attribution
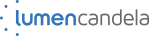
Privacy Policy
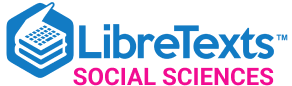
- school Campus Bookshelves
- menu_book Bookshelves
- perm_media Learning Objects
- login Login
- how_to_reg Request Instructor Account
- hub Instructor Commons
- Download Page (PDF)
- Download Full Book (PDF)
- Periodic Table
- Physics Constants
- Scientific Calculator
- Reference & Cite
- Tools expand_more
- Readability
selected template will load here
This action is not available.
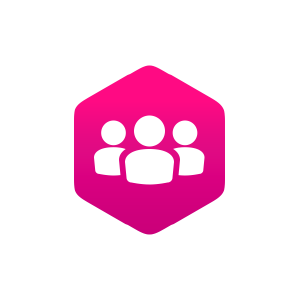
2: Sociological Research
- Last updated
- Save as PDF
- Page ID 564
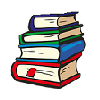
Social research is research conducted by social scientists following a systematic plan. Social research methodologies can be classified along a quantitative/qualitative dimension.
- 2.1: Introduction to Sociological Research People commonly try to understand the happenings in their world by finding or creating an explanation for an occurrence. Social scientists may develop a hypothesis for the same reason. A hypothesis is a testable educated guess about predicted outcomes between two or more variables; it’s a possible explanation for specific happenings in the social world and allows for testing to determine whether the explanation holds true in many instances, as well as among various groups or in different places.
- 2.2: Approaches to Sociological Research Sociologists often begin the research process by asking a question about how or why things happen in this world. It might be a unique question about a new trend or an old question about a common aspect of life. Once the sociologist forms the question, he or she proceeds through an in-depth process to answer it. In deciding how to design that process, the researcher may adopt a scientific approach or an interpretive framework. The following sections describe these approaches to knowledge.
- 2.3: Research Methods Sociologists use research methods to design a study—perhaps a detailed, systematic, scientific method for conducting research and obtaining data, or perhaps an ethnographic study utilizing an interpretive framework. Planning the research design is a key step in any sociological study. All studies shape the research design, while research design simultaneously shapes the study. Researchers choose methods that best suit their study topics and that fit with their overall approaches to research.
- 2.4: Ethical Concerns Many sociologists believe it is impossible to set aside personal values and retain complete objectivity. They caution readers, rather, to understand that sociological studies may, by necessity, contain a certain amount of value bias. It does not discredit the results but allows readers to view them as one form of truth rather than a singular fact. Some sociologists attempt to remain uncritical and as objective as possible when studying cultural institutions.
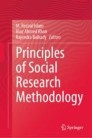
Principles of Social Research Methodology pp 43–55 Cite as
Theory in Social Research
- Mumtaz Ali 4 ,
- Maya Khemlani David 5 &
- Kuang Ching Hei 5
- First Online: 27 October 2022
1941 Accesses
In this chapter, the importance and link between social research and theory are discussed. Social research is taken as the sociological understanding of connections—connections between action, experience, and change—and it is the major vehicle for realizing these connections. The debate on using theory in any scope of social research is being deliberated by various scholars with many emphasizing the merits of using theory in social research. They argue that an appropriate theory clarifies the findings a researcher has uncovered in the study. Without a theory, the researcher could face difficulties in streamlining the study or the researcher may overlook particular phenomena or events from within the study. Thus, the researcher would be unable to relate the variables in the study. A theorist always tries to view things from his/her perspective. Upon reflection, the theorist may develop a refined framework which then becomes the intensive framework, hereby, called a theory. This phenomenon may not necessarily be agreed upon by every social scientist as can be illustrated by cases where, after using a theory, researchers have modified such a theory to suit their outcomes. The theory used in social research supports and facilitates the researcher to raise fundamental questions and facts which could serve as the common core or body of knowledge. From the basis of given facts and explanations, it can be claimed that the theory used in social research has a pivotal role to align the study. In social research, a researcher should make the matter of selecting a theory seriously as a good theory produces a better piece of research work. This chapter elaborates on the various aspects of using theory in social research. Several diagrams are used to make explanations clear.
- Social research
- Social process
- Social sciences
This is a preview of subscription content, log in via an institution .
Buying options
- Available as PDF
- Read on any device
- Instant download
- Own it forever
- Available as EPUB and PDF
- Compact, lightweight edition
- Dispatched in 3 to 5 business days
- Free shipping worldwide - see info
- Durable hardcover edition
Tax calculation will be finalised at checkout
Purchases are for personal use only
Bickman, L., & Rog, J. D. (1998). Handbook of applied social research methods . Sage Publications.
Google Scholar
Brown, K. W. P.C., Cozby, D., & Kee, W. (1999). Research methods in human development . California State University: Mayfield Publishing Company.
Bulmer, M., Gibbs, J., et al. (2010). Social measurement through social surveys: An applied approach. Ashgate Publishing Limited.
Burr, W. R. (1973). Theory construction and the sociology of the family . Wiley Interscience.
Fisher, L. (2008). Rock, paper, scissors: Game theory in everyday life . Basic Books. ISBN. 0786726938.
Gilbert, N. (2005). Researching social life . SAGE Publications Ltd.
Leedy, P. D., & Ormrod, J. E. (2005). Practical research planning and design (5th ed.). Pearson Merrill Prentice Hall.
Mladovsky, P., & Mossialos, E. (2008). A conceptual framework for community-based health insurance in low-income countries: Social capital and economic development. World Development, 36 (4), 590–607. https://doi.org/10.1016/j.worlddev.2007.04.018
Parsons, T. (1975). The present status of ‘structural-functional’ theory in sociology. In Social systems and the evolution of action theory, The free press.
Silver, P. (1983). Educational administration : Theoretical perspectives on practice and research. Harper & Row.
Tavallaei, M., & Abu Talib, M. (2010). A general perspective on role of theory in qualitative research. Uluslararası Sosyal Aratırmalar Dergisi, The Journal of International Social Research, 3 / 11 (Spring 2010), 570–578.
Download references
Author information
Authors and affiliations.
Department of Sociology, University of Sindh, Jamshoro, Sindh, Pakistan
University of Malaya, Kuala Lumpur, Malaysia
Maya Khemlani David & Kuang Ching Hei
You can also search for this author in PubMed Google Scholar
Corresponding author
Correspondence to Mumtaz Ali .
Editor information
Editors and affiliations.
Centre for Family and Child Studies, Research Institute of Humanities and Social Sciences, University of Sharjah, Sharjah, United Arab Emirates
M. Rezaul Islam
Department of Development Studies, University of Dhaka, Dhaka, Bangladesh
Niaz Ahmed Khan
Department of Social Work, School of Humanities, University of Johannesburg, Johannesburg, South Africa
Rajendra Baikady
Rights and permissions
Reprints and permissions
Copyright information
© 2022 The Author(s), under exclusive license to Springer Nature Singapore Pte Ltd.
About this chapter
Cite this chapter.
Ali, M., David, M.K., Hei, K.C. (2022). Theory in Social Research. In: Islam, M.R., Khan, N.A., Baikady, R. (eds) Principles of Social Research Methodology. Springer, Singapore. https://doi.org/10.1007/978-981-19-5441-2_4
Download citation
DOI : https://doi.org/10.1007/978-981-19-5441-2_4
Published : 27 October 2022
Publisher Name : Springer, Singapore
Print ISBN : 978-981-19-5219-7
Online ISBN : 978-981-19-5441-2
eBook Packages : Social Sciences
Share this chapter
Anyone you share the following link with will be able to read this content:
Sorry, a shareable link is not currently available for this article.
Provided by the Springer Nature SharedIt content-sharing initiative
- Publish with us
Policies and ethics
- Find a journal
- Track your research
- Search Menu
- Advance articles
- Author Interviews
- Research Curations
- Author Guidelines
- Open Access
- Submission Site
- Why Submit?
- About Journal of Consumer Research
- Editorial Board
- Advertising and Corporate Services
- Self-Archiving Policy
- Dispatch Dates
- Journals on Oxford Academic
- Books on Oxford Academic

Article Contents
- LITERATURE REVIEW
- HYPOTHESIS DEVELOPMENT
- OVERVIEW OF STUDIES
- STUDY 1: REAL EMPLOYEES’ BEHAVIORS
- STUDY 2: CAUSAL MANIPULATION OF HIERARCHY BASE
- STUDY 3: EVIDENCE FOR PROCESS VIA MEDIATION
- STUDY 4: BOUNDARY CONDITION
- STUDY 5: THE MODERATING EFFECT OF PRODUCT NORMATIVITY
- GENERAL DISCUSSION
- DATA COLLECTION INFORMATION
- Author notes
- < Previous
Dominance versus Prestige Hierarchies: How Social Hierarchy Base Shapes Conspicuous Consumption

- Article contents
- Figures & tables
- Supplementary Data
Perrine Desmichel, Derek D Rucker, Dominance versus Prestige Hierarchies: How Social Hierarchy Base Shapes Conspicuous Consumption, Journal of Consumer Research , Volume 50, Issue 5, February 2024, Pages 887–906, https://doi.org/10.1093/jcr/ucad024
- Permissions Icon Permissions
Consumers are known to seek out and display conspicuous goods—items that are exclusive and signal wealth and high social standing. Though many factors can drive such conspicuous consumption, the present work looks at an unexplored element: whether consumers find themselves in a dominance versus prestige-based hierarchy. Dominance-based hierarchies encourage consumers to use threatening, assertive, and manipulative behaviors to navigate the hierarchy. In contrast, prestige-based hierarchies encourage consumers to show their talent, skills, and motivation to operate within the hierarchy. The present research reveals how these two main bases of hierarchy shape consumers’ consumption of conspicuous goods. Specifically, dominance, relative to prestige hierarchies, is proposed to foster greater social anxiety, which leads people to seek conspicuous goods as a means of psychological security. Consequently, dominance-based hierarchies are more likely to encourage conspicuous consumption relative to prestige-based hierarchies. Multiple experiments, which utilize real employees’ behaviors, experimental manipulations of hierarchy, as well as incentivize-compatible decisions, reveal that dominance-based hierarchies, relative to prestige-based hierarchies, increase consumers’ preference for conspicuous goods. Moreover, evidence for a psychological mechanism in the form of social anxiety is demonstrated via both mediation and moderation.
Email alerts
Citing articles via.
- Recommend to your Library
Affiliations
- Online ISSN 1537-5277
- Print ISSN 0093-5301
- Copyright © 2024 Journal of Consumer Research Inc.
- About Oxford Academic
- Publish journals with us
- University press partners
- What we publish
- New features
- Open access
- Institutional account management
- Rights and permissions
- Get help with access
- Accessibility
- Advertising
- Media enquiries
- Oxford University Press
- Oxford Languages
- University of Oxford
Oxford University Press is a department of the University of Oxford. It furthers the University's objective of excellence in research, scholarship, and education by publishing worldwide
- Copyright © 2024 Oxford University Press
- Cookie settings
- Cookie policy
- Privacy policy
- Legal notice
This Feature Is Available To Subscribers Only
Sign In or Create an Account
This PDF is available to Subscribers Only
For full access to this pdf, sign in to an existing account, or purchase an annual subscription.
Read our research on: TikTok | Podcasts | Election 2024
Regions & Countries
How teens and parents approach screen time, most teens at least sometimes feel happy and peaceful when they don’t have their phone, but 44% say this makes them anxious. half of parents say they have looked through their teen’s phone.
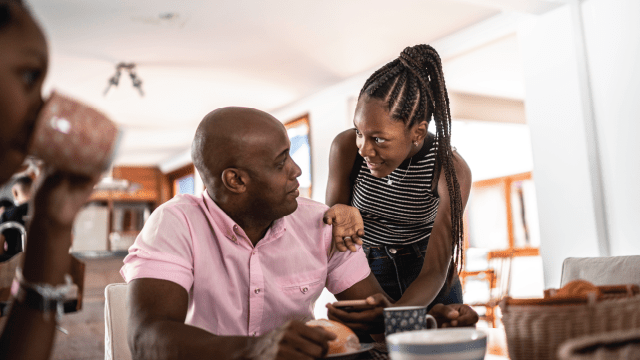
Pew Research Center conducted this study to better understand teens’ and parents’ experiences with screen time.
The Center conducted an online survey of 1,453 U.S. teens and parents from Sept. 26 to Oct. 23, 2023, through Ipsos. Ipsos invited one parent from each of a representative set of households with parents of teens in the desired age range from its KnowledgePanel . The KnowledgePanel is a probability-based web panel recruited primarily through national, random sampling of residential addresses. Parents were asked to think about one teen in their household (if there were multiple teens ages 13 to 17 in the household, one was randomly chosen). At the conclusion of the parent’s section, the parent was asked to have this chosen teen come to the computer and complete the survey in private.
The survey is weighted to be representative of two different populations: 1) parents with teens ages 13 to 17 and 2) teens ages 13 to 17 who live with parents. For each of these populations, they survey is weighted to be representative by age, gender, race and ethnicity, household income and other categories.
This research was reviewed and approved by an external institutional review board (IRB), Advarra, an independent committee of experts specializing in helping to protect the rights of research participants.
Here are the questions among parents and among teens used for this report, along with responses, and its methodology .
Today’s teenagers are more digitally connected than ever. Most have access to smartphones and use social media , and nearly half say they are online almost constantly. But how are young people navigating this “always on” environment?
To better understand their experiences, we surveyed both teens and parents on a range of screen time-related topics. Our questions explored the emotions teens tie to their devices, the impact of smartphones on youth, and the challenges parents face when raising children in the digital age.
Key findings from the survey:
- Phone-less: 72% of U.S. teens say they often or sometimes feel peaceful when they don’t have their smartphone; 44% say it makes them feel anxious.
- Good for hobbies, less so for socialization: 69% of teens say smartphones make it easier for youth to pursue hobbies and interests; fewer (30%) say it helps people their age learn good social skills.
- Parental snooping: Half of parents say they have looked through their teen’s phone.
- Smartphone standoffs: About four-in-ten parents and teens report regularly arguing with one another about time spent on their phone.
- Distracted parenting: Nearly half of teens (46%) say their parent is at least sometimes distracted by their phone when they’re trying to talk to them.
This Pew Research Center survey of 1,453 U.S. teens ages 13 to 17 and their parents was conducted Sept. 26-Oct. 23, 2023. 1
Jump to read about views among teens on: Screen time | Feelings when disconnected from phones | Thoughts on smartphones’ impact
Jump to read about views among parents on: Parenting in the smartphone age | Their own screen time struggles
Teens’ views on screen time and efforts to cut back
Fully 95% of teens have access to a smartphone, and about six-in-ten say they use TikTok, Snapchat or Instagram . But do teens think they spend too much time in front of screens?
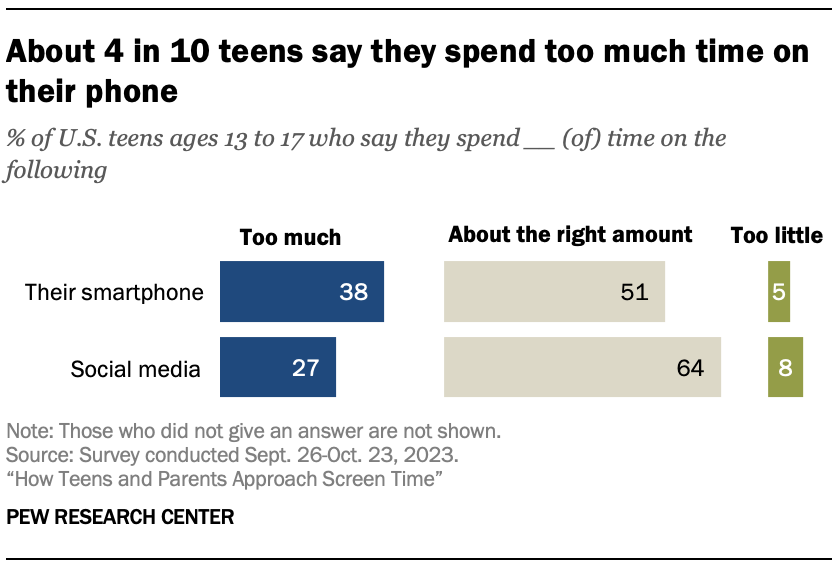
More teens say they spend too much time on their phone or social media than say they don’t spend enough time on them. We found that 38% of teens say they spend too much time on their smartphone. About a quarter say the same regarding their social media use. 2
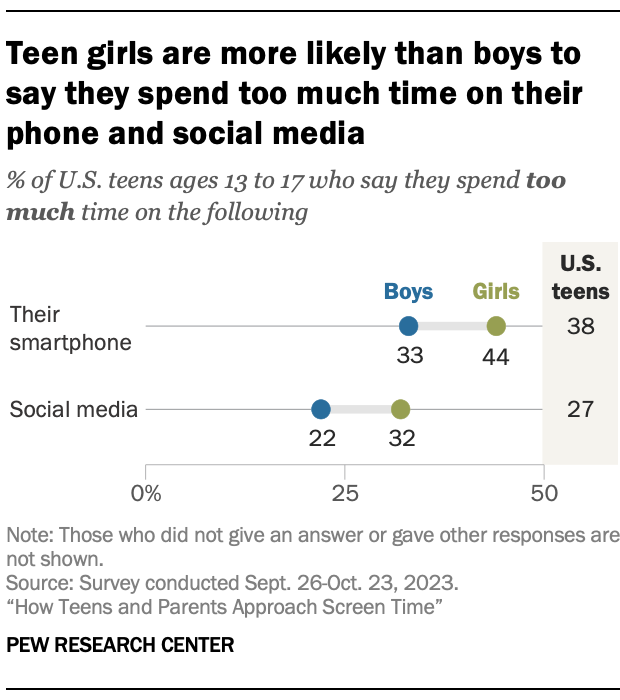
But the largest shares say the amount of time they spend on their phone (51%) or on social media (64%) is about right. Relatively few teens say they don’t spend enough time with these technologies.
Views on this differ by gender. Teen girls are more likely than boys to say they spend too much time on their smartphone (44% vs. 33%) or social media (32% vs. 22%).
Teens’ efforts to curb their screen time
A minority of teens have taken steps to reduce their screen time. Roughly four-in-ten teens (39%) say they have cut back on their time on social media. A similar share says the same about their phone (36%).
Still, most teens have not limited their smartphone (63%) or social media (60%) use.
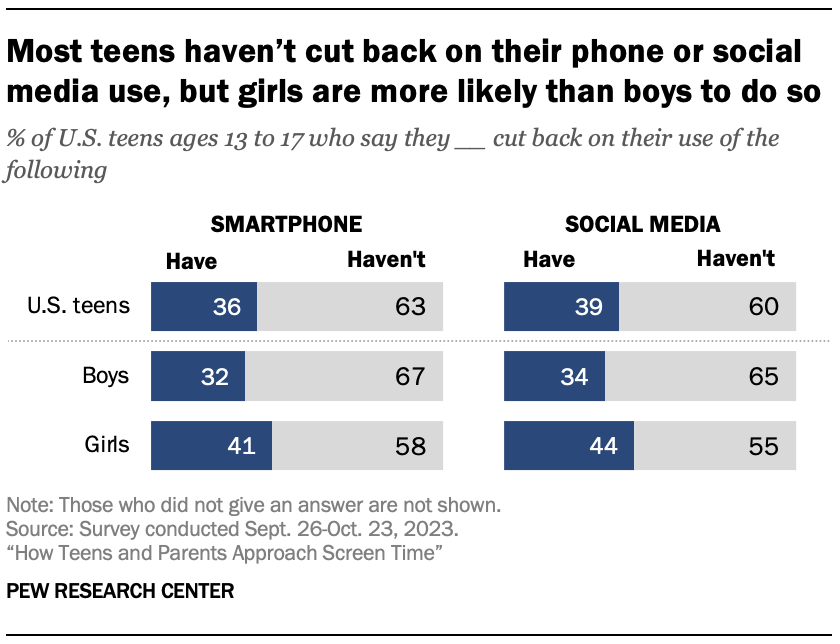
How teens’ behaviors vary by gender
About four-in-ten or more girls say they have cut back on their smartphone or social media use. For boys, those figures drop to roughly one-third.
How teens’ behaviors vary based on their screen time
Teens who report spending too much time on social media and smartphones are especially likely to report cutting back on each. For instance, roughly six-in-ten teens who say they are on social media too much say they have cut back (57%). This is far higher than the 32% among those who say they are on social media too little or the right amount.
How teens feel when they don’t have their phone
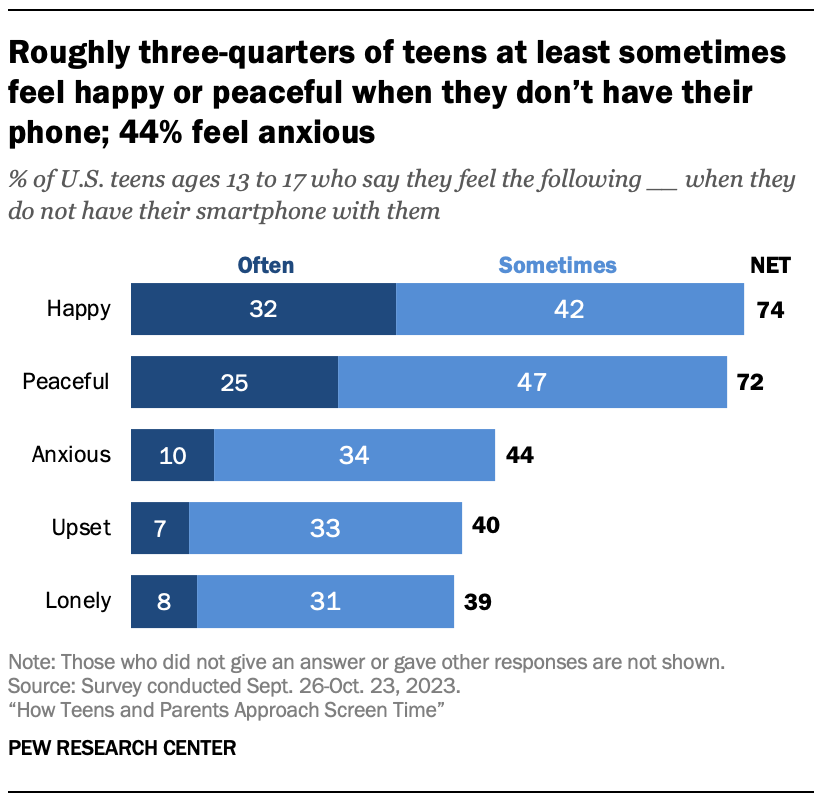
Teens encounter a range of emotions when they don’t have their phones, but we asked them about five specific ones. Roughly three-quarters of teens say it often or sometimes makes them feel happy (74%) or peaceful (72%) when they don’t have their smartphone.
Smaller but notable shares of teens equate not having their phone with more negative emotions. Teens say not having their phone at least sometimes makes them feel anxious (44%), upset (40%) and lonely (39%).
It is worth noting that only a minority of teens – ranging from 7% to 32% – say they often feel these emotions when they’re phone-less.
Teens’ feelings on this differ by some demographic factors:
- Age and gender: Older girls ages 15 to 17 (55%) are more likely than younger girls (41%) and teen boys who are younger (41%) and older (40%) to say they feel anxious at least sometimes when they don’t have their smartphone.
- Gender: 45% of teen girls say not having their phone makes them feel lonely regularly, compared with 34% of teen boys.
Do teens think smartphones are negatively impacting young people?
As smartphones have become a universal part of teen life, many have asked what impact, if any, phones are having on today’s youth.
Teens shared their perspectives on smartphones’ impact on people their age and whether these devices have made certain aspects of growing up more or less challenging.
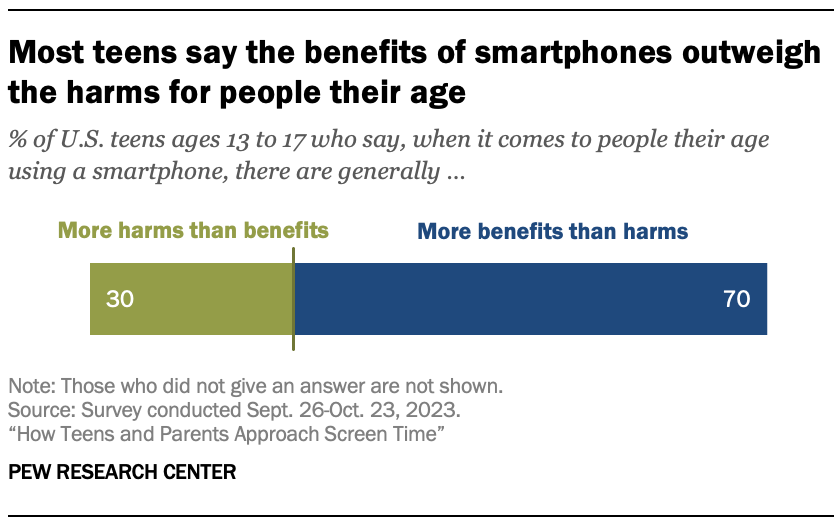
Most teens think the benefits of smartphones outweigh the harms for people their age. Seven-in-ten teens say smartphones provide more benefits than harms for people their age, while a smaller share (30%) take the opposing view, saying there are more harms than benefits.
Teens’ views, by gender and age
Younger girls ages 13 and 14 (39%) are more likely than older teen girls (29%) and teen boys who are younger (29%) and older (25%) to say that the harms of people their age using smartphones outweigh the benefits.
The survey also shows that teens see these devices’ impacts on specific aspects of life differently.
More teens believe smartphones make it easier, rather than harder, to be creative, pursue hobbies and do well in school. Majorities of teens say smartphones make it a little or a lot easier for people their age to pursue hobbies and interests (69%) and be creative (65%). Close to half (45%) say these devices have made it easier for youth to do well in school.
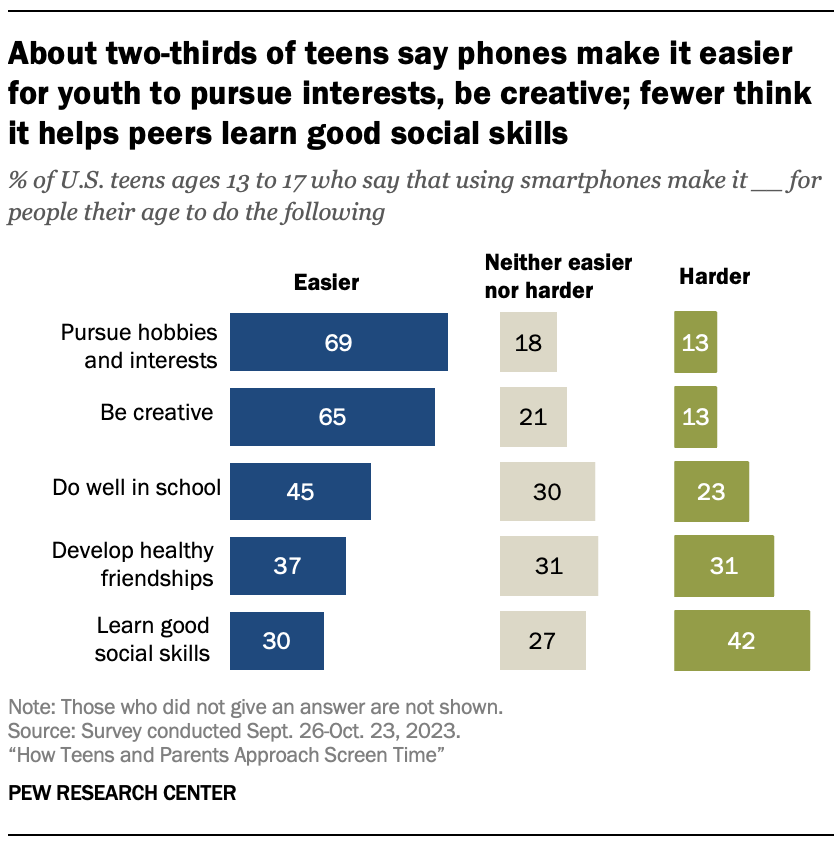
Views are more mixed when it comes to developing healthy friendships. Roughly four-in-ten teens say smartphones make it easier for teens to develop healthy friendships, while 31% each say they make it harder or neither easier nor harder.
But they think smartphones have a more negative than positive impact on teens’ social skills. A larger percentage of teens say smartphones make learning good social skills harder (42%) rather than easier (30%). About three-in-ten say it neither helps nor hurts.
How parents navigate raising teens in the smartphone age
With the rise of smartphones, today’s parents must tackle many questions that previous generations did not. How closely should you monitor their phone use? How much screen time is too much? And how often do phones lead to disagreements?
We developed a set of parallel questions to understand the perspectives of both parents and teens. Here’s what we found:
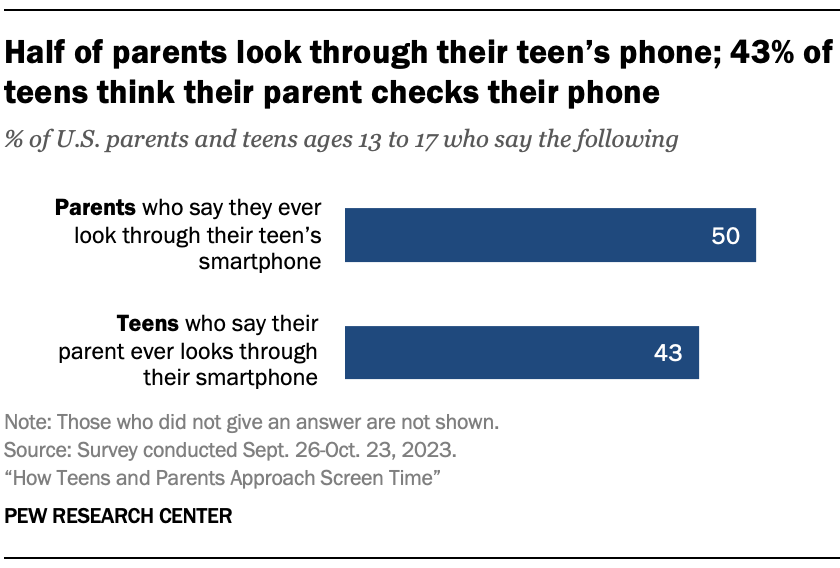
It’s common for parents to look through their teen’s phone – and many of their teens know it. Half of parents of teens say they look through their teen’s phone. When we asked teens if they thought their parents ever look through their phones, 43% believed this had happened.
Whether parents report looking through their child’s smartphone depends on their kid’s age. While 64% of parents of 13- to 14-year-olds say they look through their teen’s smartphone, this share drops to 41% among parents of 15- to 17-year-olds.
Teens’ accounts of this also vary depending on their age: 56% of 13- to 14-year-olds say their parent checks their smartphone, compared with 35% of teens ages 15 to 17.
How often do parents and teens argue about phone time?
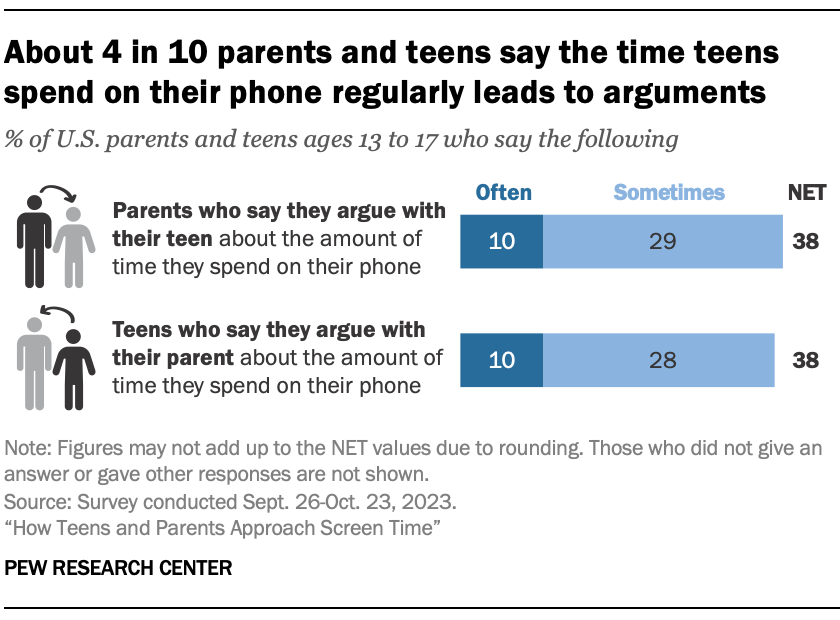
Parents and teens are equally likely to say they argue about phone use. Roughly four-in-ten parents and teens (38% each) say they at least sometimes argue with each other about how much time their teen spends on the phone. This includes 10% in each group who say this happens often .
Still, others say they never have these types of disagreements. One-quarter of parents say they never argue with their teen about this, while 31% of teens say the same.
Teens’ and parents’ views, by race and ethnicity
Hispanic Americans stand out for reporting having these disagreements often. While 16% of Hispanic teens say they often argue with their parent about how much time they’re spending on their phone, that share drops to 9% for White teens and 6% for Black teens. 3
A similar pattern is present among parents. Hispanic parents (19%) are more likely than White (6%) or Black (7%) parents to say they often argue with their teen about this.
Teens’ views, by frequency of internet use
The amount of time teens report being online is also a factor. About half (47%) of teens who report being online almost constantly say they at least sometimes argue with their parent about the amount of time they spend on their phone, compared with those who are online less often (30%).
How much do parents prioritize tracking their teen’s phone use?
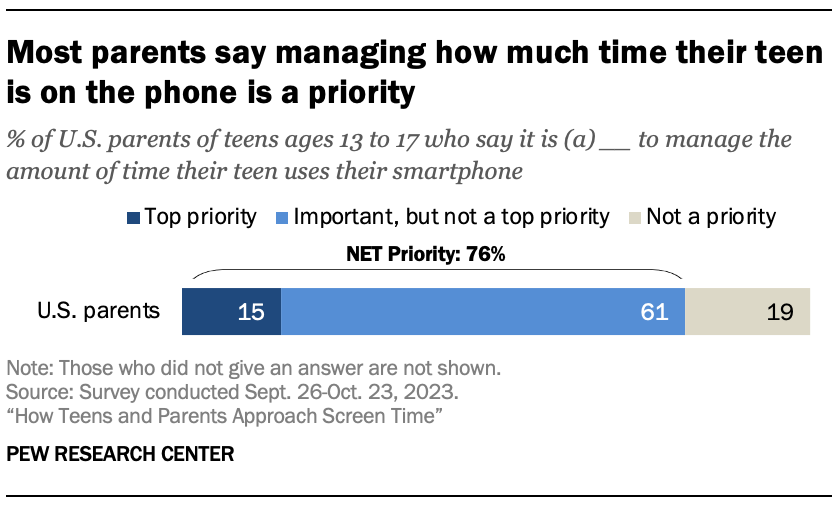
Most parents prioritize managing the amount of time their teen spends on the phone. Roughly three-quarters of parents (76%) say managing how much time their teen spends on the phone is an important or a top priority. Still, 19% of parents don’t consider this a priority.
Parents’ views, by race and ethnicity
Majorities of parents across racial and ethnic groups think of this as a priority. But some groups stand out for how much they prioritize this. For example, Hispanic (25%) or Black (24%) parents are more likely to say managing how much time their teen is on the phone is a top priority. That share drops to 10% among White parents.
Parents’ views, by household income
We also see differences between the lowest and highest income households: 22% of parents whose annual household income is less than $30,000 consider managing the amount of time their teen is on the phone a top priority, compared with 14% of those whose household income is $75,000 or more a year. Those whose household income is $30,000 to $74,999 a year do not meaningfully differ from either group.
Do parents set time limits on their teen’s phone use?
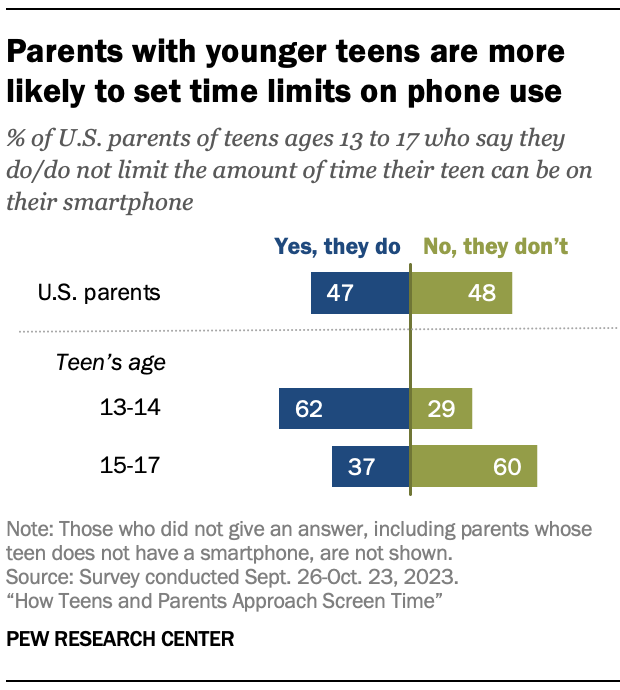
There’s a nearly even split between parents who restrict their teen’s time on their phone and those who don’t. About half of parents (47%) say they limit the amount of time their teen can be on their phone, while a similar share (48%) don’t do this.
Parents’ views, by teen’s age
Parents of younger teens are far more likely to regulate their child’s screen time. While 62% of parents of 13- to 14-year-olds say they limit how much time their teen can be on their phone, that share drops to 37% among those with a 15- to 17-year-old.
How difficult is it for parents to keep up with their teen’s phone use?
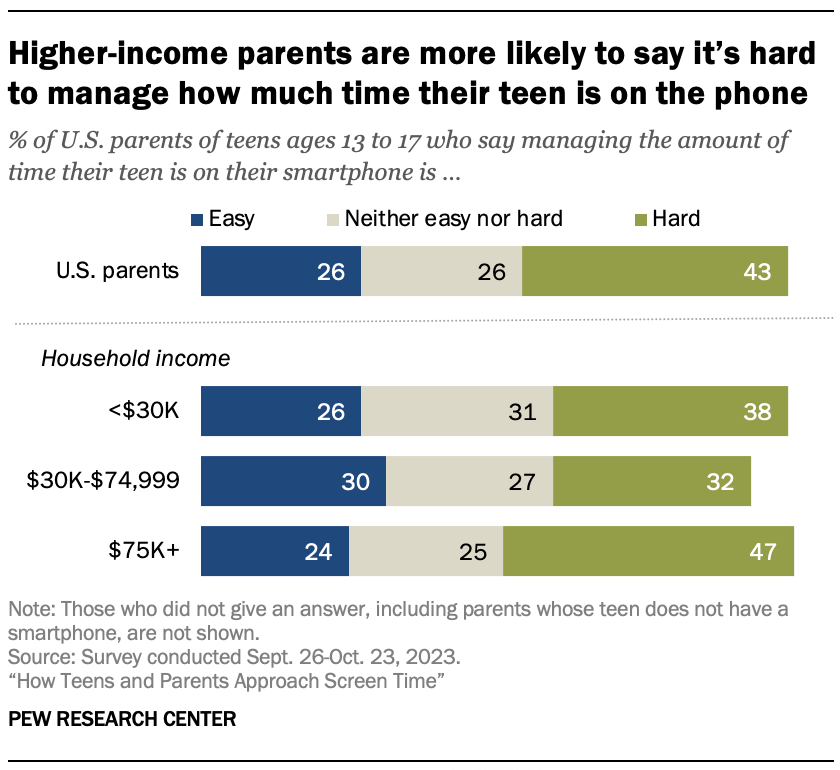
Managing screen time can feel like an uphill battle for some parents. About four-in-ten say it’s hard to manage how much time their teen spends on their phone. A smaller share (26%) says this is easy to do.
Another 26% of parents fall in the middle – saying it’s neither easy nor hard.
Higher-income parents are more likely to find it difficult to manage their teen’s phone time. Roughly half (47%) of parents living in households earning $75,000 or more a year say managing the amount of time their teen is on their phone is hard. These shares are smaller among parents whose annual household income falls below $30,000 (38%) or is between $30,000 and $74,999 (32%).
Parents’ own struggles with device distractions, screen time
Teens aren’t the only ones who can be glued to their phones. Parents, too, can find themselves in an endless cycle of checking emails , text messages and social media.
With that in mind, we asked parents to think about their own screen time – both the time they spend on their phone, and if it ever gets in the way of connecting with their teen.
Do parents think they spend too much time on their phone?
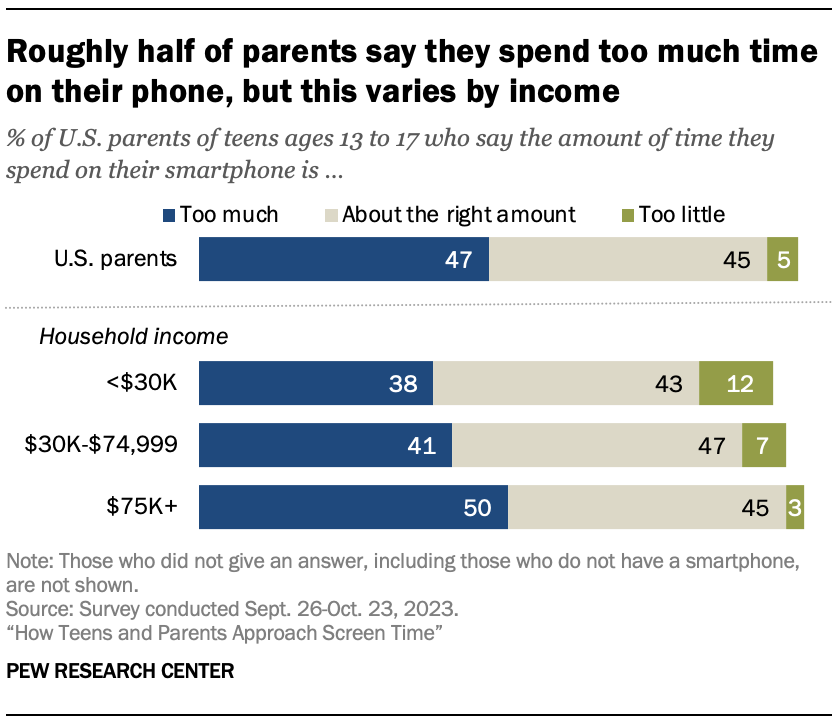
Like teens, parents are far more likely to say they spend too much rather than not enough time on their phone. About half of parents (47%) say they spend too much time on their smartphone. Just 5% think they spend too little time on it. And 45% believe they spend the right amount of time on their phone.
Parents’ views differ by:
- Household Income: 50% of parents with annual household incomes of $75,000 or more say they spend too much time on their phone. This share drops to 41% among those living in households earning $30,000 to $74,999 a year and 38% among those earning under $30,000.
- Race and ethnicity: 57% of White parents believe they spend too much time on their phone, compared with 38% of Black parents and 34% of Hispanic parents.
How often are parents distracted by their phone when talking with their teen?
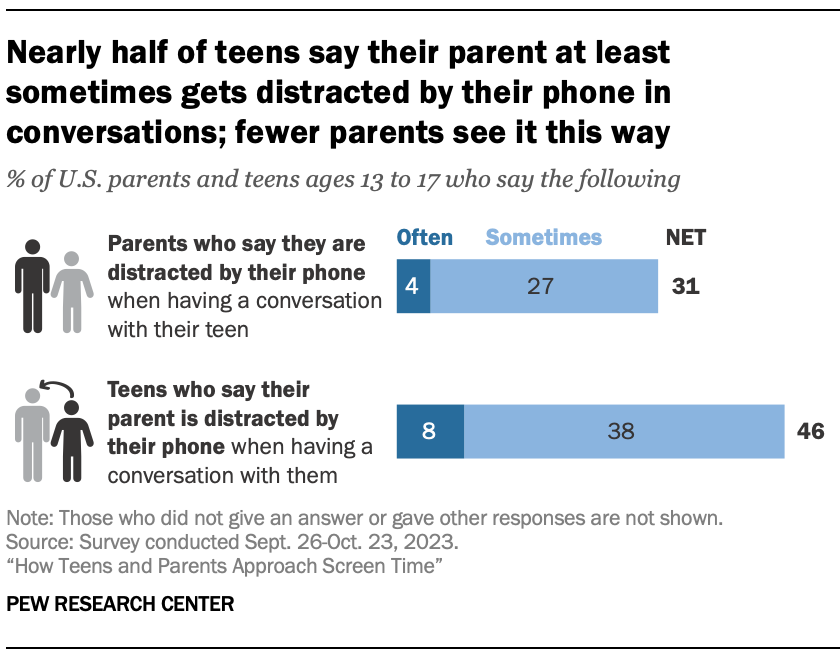
When it comes to distracted parenting, parents paint a rosier picture than teens. Nearly half of teens (46%) say their parent is at least sometimes distracted by their phone when they’re trying to talk to them, including 8% who say this happens often.
But when parents were asked to assess their own behavior, fewer – 31% – say this happens regularly.
- Throughout this report, “teens” refers to those ages 13 to 17 and “parents” refers to those with a child ages 13 to 17. ↩
- A 2018 Center survey also asked U.S teens some of the same questions about experiences and views related to smartphone and social media. Direct comparisons cannot be made across the two surveys due to mode, sampling and recruitment differences. Please read the Methodology section to learn more about how the current survey was conducted. ↩
- There were not enough Asian respondents in the sample to be broken out into a separate analysis. As always, their responses are incorporated into the general population figures throughout the report. ↩
Sign up for our Internet, Science and Tech newsletter
New findings, delivered monthly
Report Materials
Table of contents, teens and internet, device access fact sheet, teens and social media fact sheet, a declining share of adults, and few teens, support a u.s. tiktok ban, teens, social media and technology 2023, about 1 in 5 u.s. teens who’ve heard of chatgpt have used it for schoolwork, most popular.
About Pew Research Center Pew Research Center is a nonpartisan fact tank that informs the public about the issues, attitudes and trends shaping the world. It conducts public opinion polling, demographic research, media content analysis and other empirical social science research. Pew Research Center does not take policy positions. It is a subsidiary of The Pew Charitable Trusts .
The Federal Register
The daily journal of the united states government, request access.
Due to aggressive automated scraping of FederalRegister.gov and eCFR.gov, programmatic access to these sites is limited to access to our extensive developer APIs.
If you are human user receiving this message, we can add your IP address to a set of IPs that can access FederalRegister.gov & eCFR.gov; complete the CAPTCHA (bot test) below and click "Request Access". This process will be necessary for each IP address you wish to access the site from, requests are valid for approximately one quarter (three months) after which the process may need to be repeated.
An official website of the United States government.
If you want to request a wider IP range, first request access for your current IP, and then use the "Site Feedback" button found in the lower left-hand side to make the request.
- Reference Manager
- Simple TEXT file
People also looked at
Hypothesis and theory article, artificial cognition vs. artificial intelligence for next-generation autonomous robotic agents.
- Italian Institute of Technology, Cognitive Architecture for Collaborative Technologies (CONTACT) and Robotics, Brain and Cognitive Sciences (RBCS) Research Units, Genoa, Italy
The trend in industrial/service robotics is to develop robots that can cooperate with people, interacting with them in an autonomous, safe and purposive way. These are the fundamental elements characterizing the fourth and the fifth industrial revolutions (4IR, 5IR): the crucial innovation is the adoption of intelligent technologies that can allow the development of cyber-physical systems , similar if not superior to humans. The common wisdom is that intelligence might be provided by AI (Artificial Intelligence), a claim that is supported more by media coverage and commercial interests than by solid scientific evidence. AI is currently conceived in a quite broad sense, encompassing LLMs and a lot of other things, without any unifying principle, but self-motivating for the success in various areas. The current view of AI robotics mostly follows a purely disembodied approach that is consistent with the old-fashioned, Cartesian mind-body dualism, reflected in the software-hardware distinction inherent to the von Neumann computing architecture. The working hypothesis of this position paper is that the road to the next generation of autonomous robotic agents with cognitive capabilities requires a fully brain-inspired, embodied cognitive approach that avoids the trap of mind-body dualism and aims at the full integration of Bodyware and Cogniware. We name this approach Artificial Cognition (ACo) and ground it in Cognitive Neuroscience. It is specifically focused on proactive knowledge acquisition based on bidirectional human-robot interaction: the practical advantage is to enhance generalization and explainability. Moreover, we believe that a brain-inspired network of interactions is necessary for allowing humans to cooperate with artificial cognitive agents, building a growing level of personal trust and reciprocal accountability: this is clearly missing, although actively sought, in current AI. The ACo approach is a work in progress that can take advantage of a number of research threads, some of them antecedent the early attempts to define AI concepts and methods. In the rest of the paper we will consider some of the building blocks that need to be re-visited in a unitary framework: the principles of developmental robotics, the methods of action representation with prospection capabilities, and the crucial role of social interaction.
1 Introduction: artificial cognition vs. artificial intelligence
There is no doubt that AI (Artificial Intelligence) and ACo (Artificial Cognition) are frequently confused, in the engineering community as well as in the media, assuming that somehow are synonyms and functional for a common growing process of innovation in AI technologies. As a matter of fact, AI is a generic label that has been around since the 50’s of the last century, whereas the ACo label is quite recent, although the conceptual framework has been outlined at the same time of AI. Moreover, a similar confusion affects also the scientific community at large, including philosophy, psychology, and neuroscience: the issue is old and highly debated but still is far from allowing a shared definition of either Intelligence or Cognition, including the clear identification of similarities and differences. This also includes the use of quasi-oxymoronic expressions, as embodied intelligence, and is further clarified if we consider the etymology of intelligence and cognition.

Cognition comes from the Latin verb Cognoscere and it is defined as the faculty of knowing, in the sense of the ability of an agent to learn and evaluate the surrounding reality. It is a personal, not impersonal faculty. Knowledge is not in the “cloud” but is acquired through personal experience in a dynamical way, filtered through the moving/sensing body as well as from past personal experience.
Thus, the main differences between intelligence and cognition for cooperative robots of the next generation can be summarized as follows:
• Cognition is embodied – Intelligence is likely to be dis-embodied.
• Cognition rejects the mind-body dualism – Intelligence implies the mind-body dualism.
• The goal of intelligence is to reason about encyclopedic knowledge and bounded by data.
• The goal of cognition is to improve the chance of personal survival, in the Darwinian as well as in the Enactivist sense, within a strong social context and exploiting internal models.
In summary, we suggest that Intelligence and Cognition correspond to two very different approaches to the acquisition, representation, accumulation, and exploitation of knowledge: Intelligence aims at abstract, impersonal, encyclopedic knowledge, independent of the personal and/or social process of action and interaction between brain, body, and environment; in contrast, Cognition deals with the individual process of knowledge acquisition through personal experience, possibly mediated by social interaction, a process constrained and guided by dynamics (between brain, body, and environment), development (ontogenetic as well as phylogenetic) and a value system of some type that is intrinsically alien to Intelligence per se . For example, E = mc 2 is a piece of knowledge that captures the essence of a large variety of phenomena, independent of the humans that invented/discovered it and of the actual use by human society, e.g., understanding the nature of black holes vs. developing atomic bombs. This does not imply that Intelligence and Cognition are antithetic and mutually exclusive: on the contrary, they should be integrated carefully and “wisely,” in particular when we address the technological counterpart of such categories of knowledge, namely AI & ACo. On the other hand, it is not uncommon, in the neuroscience/cybernetic literature, to find expressions like “motor intelligence” that appear to contradict the distinction above and the crucial role of “embodiment.” In our opinion, this is just an example of the unavoidable ambiguity of natural language, including the language used in science: motor intelligence and related concepts are intrinsic embodied components of cognition and have little to share with disembodied, abstract intelligence. For AI a chair is a 3D shape while for ACo is a goal of the act of sitting.
At the same time, we should also consider a criticism of embodied cognition ( Goldinger et al., 2016 ), suggesting that cognitive science (the whole of mental life, articulated in terms of perception, attention, memory, reasoning, and language) has little to gain from the posited integration of body and mind typical of embodied cognition. The main point is that, according to the authors, different forms of disembodied cognition, somehow in a similar way with the disembodied outline of AI, are characterized by a substantial underestimation of the fundamental concept of action in relation with other cognitive concepts as perception, attention, memory and so on. The cognitive importance of this concept is highlighted by the need of prospection , namely the mental simulation of actions for evaluating their potential sensorimotor effects in the future, either positive or negative, and thus supporting an informed decision-making process that escapes and bypasses the trap of trial and error. For example, mentally simulating “sitting” as part of the process to find a chair.
Historically, the starting point of the debate between AI and ACo can be identified with the invention of Cybernetics ( Wiener, 1948 ), namely the discipline that deals with Communication and Control in the Animal and the Machine . Communication and Control are the basic ingredients when dealing with both Intelligence and Cognition and indeed Artificial Intelligence, including neural networks, is one of the many disciplines coming out from the cybernetic revolution. One of the hot issues that divided and still divides the cybernetic community at large is the degree of overlap between the application of cybernetic concepts to “Animals” or to “Machines”: in particular, to which extent engineers should focus on a bio or brain inspired approach for the design of intelligent/cognitive agents? Wiener himself was much in favor of a fundamental communality between natural and artificial prototypes/paradigms whereas the AI community was and is still oriented in the opposite direction, supporting the hypothesis that accelerating innovation methods of AI can approach a “different kind” of intelligence that, according to some “to be specified” metric, can even surpass human intelligence in many application areas, with a very thin degree of connection to biology and neuroscience.
The history of AI is marked by a number of booms and busts, driven by the dynamic interplay between science, technology, and economy. The last decade or so is clearly a booming period, marked by well publicized achievements in gaming (e.g., chess or go), computer vision and natural language technologies, driven by machine learning methodologies applied to deep neural networks. Artificial neural networks employed in AI are relatively simple and easy to use but are essentially “black boxes” whose connection weights can be refined through learning techniques, like back-propagation, without attempting any linkage with biology and neuroscience (another source of ambiguity of the term “neural networks”): the results are opaque for the designers and/or the user of the AI application, independent of the success of the app. In general, AI apps can make decisions or predictions based on parameters that the programmer has not defined and cannot deduce by looking at the output or the network code: the fundamental source of information behind the produced result is the intrinsic correlation hidden in the data used to learn. Two identical networks may behave in a quite different way depending on the random selection of the initial connection weights and, more importantly, on the choice of the big data set used for training the network as well as the order in which these data are acquired. This kind of opacity, due to the intrinsically passive nature of the learning mechanism, is clearly in contrast with standard engineering design methodologies related to safety and reliability and is likely to imply ethical and legal adoption problems as soon as the application domains include sensitive problems as medical diagnosis, autonomous driving, fraud detection, econometric analysis, and economic decision making. Moreover, the expansion of the application areas is motivating the growth of the number of layers and parameters of deep neural networks, supported by the continuous development of new software tools and the still increasing computational power: each layer of these networks represents the knowledge extracted from the training data at progressively more abstract levels, whose interpretation becomes more and more difficult to ascertain, highlighting fundamental limitations of the deep learning framework, such as catastrophic forgetting ( French, 1999 ) and never-ending learning ( Mitchell et al., 2018 ). Moreover, even in the specific application areas where AI technologies achieved unprecedented advancements, a strong impediment to the generally accepted adoption of such systems is “explainability,” namely the lack of transparency due to the black-box nature of these systems: they may provide powerful predictions that cannot be directly explained ( Adadi and Berrada, 2018 ; Rahwan et al., 2019 ). The causal relationship between the acquired training data is lost and does not emerge.
Such problems motivated the rise of a recent discipline on a side of AI: Explainable Artificial Intelligence (XAI) ( Barredo Arrieta et al., 2022 ). A major focus of XAI is not on the design and structural feature of such systems but on the audience for which explainability is sought and the different purposes of the explanations, e.g., explain to justify/control/improve performance/discover causal relationships. At the same time, the value of XAI is frequently called into question, claiming that the credibility of an AI system can be evaluated directly by monitoring the quality of the result over time and observing that modification of AI systems in such a way to explain every decision could decrease speed and efficiency. In any case, AI as well XAI research is far away from any brain-inspired formulation, somehow based on cognitive neuroscience.
Thus, it is somehow surprising that recent papers ( Taylor and Taylor, 2021 ; Iglesias, 2022 ) associated Artificial Intelligence with Artificial Cognition 1 proposing to “explain” the black boxes produced by AI technologies by means of the methods of analysis of the mind developed by Psychology and Cognitive Neuroscience, based on controlled stimuli and measurement of behaviour in various network architectures. The underlying rationale is that also the mind, investigated by such methods, can be treated as a black box and thus by importing cognitive methodology in the analysis of AI apps can allow to make causal inferences about the structure, architecture, and functioning of the black boxes generated by AI methods. This approach to XAI has been called Artificial Cognition and is based on the hope that experimental psychology can help generate explainable neural networks: the problem is that this is a post-hoc method that in no sense can be interpreted as a brain model.
In general, the definition of artificial cognition as a tool to measure decision making in artificial neural networks is clearly reductive, at best describing, not explaining, how the system works and does not consider a totally different approach for the development of cognitive architectures for autonomous agents that is fundamentally brain-inspired to start with and “proactive/exploratory” in nature. This approach can be traced back to the early times of AI although the name ACo was not specifically used and/or publicized but it is clearly behind the pioneering studies on Enactivism, Embodied Cognition and Self-Organization of living organisms ( Maturana and Varela, 1980 ; Varela et al., 1991 ), leading to a formulation of cognitive neuroscience arguing that cognition arises through a dynamic interaction between an acting organism and its environment, mediated by a sensing and acting body, namely a purposeful, proactive, exploratory interaction that emphasizes the importance of adaptability and compositionality in artificial cognition while mirroring a fundamental organizational principle of the brain expressed as neural reuse: “…the brain as a dynamical system where individual regions are functionally diverse and are used and reused in many different tasks across the cognitive domain” ( Anderson, 2010 ). In our opinion, this is the appropriate starting point for the development of reliable autonomous agents of the next generation, guided by the concept that acting is an aspect of decision making and perhaps the ultimate reason for a brain to exist.
In the following part of the paper, some crucial founding concepts and building blocks for conceiving and designing Artificial Cognitive agents will be outlined but we wish to emphasize that it is not explainability per se , as defined by XAI, the most urgent need for society, which demands to understand how and on what basis intelligent/cognitive agents support decisions that may affect everybody. One of the goals of AI is to achieve Artificial General Intelligence (AGI) that captures human cognitive abilities in general, aiming at a sort of “impersonal super-intelligence.” This would clearly overcome the understanding capability of any single individual, although there are reasons to doubt that this is an unrealistic and even impossible goal ( Fjelland, 2020 ). We believe that a decision-making process based essentially on correlation, having lost any causal relationship between training data cannot “explain” the “why” of its outcome ( Fjelland, 2020 ) but only “how” similar is the decision to past decisions. On the contrary, Cognitive Penetrability, in the sense defined by ( Pylyshyn, 1999 ) 2 , allows humans to communicate and interact on the basis of a shared value system and this is the basis for achieving mutual trust in a cooperating society: for this reason, cognitive penetrability rather than explainability should be the starting point for the development of Autonomous Robotic Agents of the next generation.
In summary, the ACo formulation of the development of autonomous agents is fundamentally brain-inspired but its biomimetic approach is not intended to imitate the fully developed brain of Nobel prize winners or other remarkable cultural leaders but the process of progressive knowledge and competence acquisition, leading to autonomous decision-making ability. Thus, a fundamental building block of ACo robots can be outlined by re-visiting the basic issues of evolutionary robotics, emphasizing the difference between the data-driven, passive nature of training deep and large neural networks (the AI/AGI approach) and the active exploratory-driven knowledge acquisition nature of sensory-motor-cognitive development of human cubs . As suggested by von Hofsten (2004) , “development is about systems with the urge to act and explore.” One crucial aspect of the developmental process is that it is made possible by internal and external constraints: the intrinsic plasticity of the brain and the persistent/sought interaction with the physical and social environment. The role and the computational mechanisms of the former type of interaction will be addressed in section 3 “Body model and Prospection capabilities for Artificial Cognition” and the latter type of interaction will be touched upon in section 4 “Social support of Developmental Artificial Cognition.” In particular, the issue of prospection will be linked to the memory system that accompanies and supports the accumulation of knowledge, at increasing levels of abstraction, on the basis of mental (covert) simulation of action and mental (covert) speech. Of course, this short list of items does not cover all the relevant components of ACo, such as the specific role of consciousness/awareness in artificial cognition and inner language in the development of cognition that are only briefly mentioned.
2 Principles of developmental robotics
In the context of artificial cognition, we suggest that a brain-inspired cognitive architecture for autonomous robotic agents of the next generation should consider general principles of Developmental Robotics. This is a multifaceted and interdisciplinary research area at the intersection of robotics, developmental psychology and developmental neuroscience. It is more than twenty years old ( Sandini et al., 1998 ; Asada et al., 2001 ; Lungarella et al., 2003 ; Schmidhuber, 2006 ; Cangelosi et al., 2015 ; Min et al., 2016 ; Huang et al., 2017 ) and it clearly represents a brain-inspired approach to the design of robots: in contrast with industrial robots that perform repetitive predefined tasks in a predefined environment: these robots are supposed to be dynamic models of how humans develop, explore, and quickly adapt in an open-ended manner to a changing environment through lifelong learning, in order to cope with unpredictable challenges. Thus, the biomimetic goal of developmental robotics that is pursued is not to imitate the brain per se , namely the performing brain of trained adults, but the process of progressive knowledge acquisition, leading to autonomous decision-making ability by interaction with the physical and social environment.
In particular, we suggest that developmental robotics is intrinsically based on two basic pillars: (1) personal embodiment and (2) personal/social (accumulation of) knowledge. In relation with the first pillar, analyzing the emergence of fetal and neonatal movements Asada et al. (2009) observed that predefined (“innate”) circuits exist in the nervous system: in particular, the stretch reflex in the spinal circuit and the oscillator neurons in the medulla that lead to central pattern generators involved in locomotion; in contrast, it appears that there is no predefined circuit specifying coordination of full-body actions. Thus, it is suggested that the observed synergies of adults emerge from an embodiment process, namely the interaction between the brain and the environment through the body, in the early development stage, and the body model, later on as a result of neural maturation and physical growth. Such internal representation of the body, for describing and predicting the evolution of actions, requires an abstraction from specific sensory modalities ( Squeri et al., 2012 ) and this is the result of a multisensory, multimodal integration-fusion process that is known to occur in late childhood ( Chen et al., 2004 ; Gori et al., 2008 , 2012a , 2012b ; Gori, 2015 ).
The general principles of developmental robotics are strongly interleaved with the central tenet of embodied cognition, namely that cognitive and behavioral processes emerge from the reciprocal, dynamic and evolving coupling between brain, body and environment throughout the entire life of an individual. This means, in particular, that cognition and intelligence cannot be captured by a complex computer software, such as a deep neural network, because the three-ways interaction mentioned above is intrinsically hybrid, with digital, analogic, and physical components.
As regards the personal/social nature of robot learning/training, we may observe that developmental robots are typically supposed to share the environment with humans, in the framework of a common goal or cooperative task. Therefore, it is beneficial for the robot to infer humans’ goals, imitate their behaviors and/or follow their suggestions in order to accelerate the autonomous acquisition of know-how, marked by the memorization of critical episodic occurrences. Curiosity, exploration and motivation are the essential ingredients to be combined in a synergic way, by linking intrinsic motivation to the attention for the human partner behavior; the role of social environment in this process will be expanded in section 4 “Social support of developmental artificial cognition.”
The crucial role of embodiment, including its ecological aspects, for developmental robotics is apparently challenged by “Vehicles” ( Braitenberg, 1986 ) and the notion of “intelligence without representation” ( Brooks, 1991 ): it is suggested that purposive behavior does not necessarily have to rely on accurate models of the environment, but rather might be the result of the close interaction of a simple control architecture with a complex world. In other words, according to such view there is no need to build enduring, full-scale internal models of the world, because the environment can be probed and re-probed as needed, thus closing the loop in real-time. More recently, Pfeifer and Scheier (1994) argued that a better global understanding of the perception–action cycle might be required. The authors propose an alternative view that breaking up perception, computation and action into different subsystems might be too strong a commitment. In other words, the minimal unit of processing should be a complete perception–action cycle that includes the environment in the loop ( Vernon et al., 2015b ).
We may also add that such computational kernel is likely to evolve during development, with multi-sensory fusion and progressive abstraction from the specific sensory modalities, maintaining and updating an internal eco-body model based on a unifying simulation/emulation theory of action with prospective capabilities ( Decety and Ingvar, 1990 ; Jeannerod, 2001 ; Grush, 2004 ; O’Shea and Moran, 2017 ; Ptak et al., 2017 ). Clearly, prospection cannot be obtained through the representation-less models of Brooks and Braitenberg that only operate in the present: prospection operates in the future to control the present in a purposive way. It is also worth considering that, despite his emphasis that in general quite complex “intelligent” behavior can be achieved without representation, Brooks accepts a role for representations as building blocks, emerging in a bottom-up fashion ( Brooks, 2002 ). This is a view that matches at the same time the fundamental role of embodied cognition and its ontogenetic evolution as a bottom-up process: internal representations could be introduced at different stages in service for action ( Clark, 1997 ; Steels, 2003 ). As examples of approaches following such a bottom-up approach we may also quote the DAC architecture ( Verschure and Althaus, 2003 ) or the walking agent model ( Schilling and Cruse, 2017 ; Schilling et al., 2021 ).
Sensory-Motor-Cognitive Development in humans is an incremental process, involving maturation, integration and adaptation, following the blueprints of a dynamic process moving through more and more skilled cognitive states ( Piaget, 1953 ) and mediated by social interaction ( Vygotsky, 1962 ). However, this process is somehow ragged and the genetically pre-programmed roadmap is steered and implemented by epigenetic phenomena affected by environment. This is related to the long-term discussion of the role of nature and nurture in the development of a personal agent. In other words, development is largely decentralized, event and environment driven, exhibiting the typical features of a self-organizing system. Moreover, there is ample evidence that such spontaneous self-organizing process is aided and amplified through social interactions with adults and peers ( Vygotsky, 1962 ). This includes various types of social support, as scaffolding, guidance, coaching and apprenticeship; moreover, mimetic processes such as mimicry, imitation and emulation are likely to play a central role in cognitive development. More specifically, the development of skilled actions in children can be investigated with three different but complementary approaches that focus, respectively, (1) on the intrinsic maturation of the nervous system ( Gesell, 1946 ), (2) on the information processing aspects, associated with the interaction of the developing nervous system with newly emerging cognitive processes, driven by the interaction with the environment ( Connolly, 1970 ), and (3) on the dynamics of such interaction, i.e., a dynamic systems approach. In summary, it is suggested that the acquisition of new motor skills is guided by the drive to explore (curiosity) and supported equally by the developing nervous system and its interactions with sensory-motor processes and the environment ( Bernstein, 1967 ; Gibson, 1979 ; Thelen and Smith, 1998 ; Oudeyer et al., 2016 ; Schillaci et al., 2016 ). Moreover, there is mounting evidence ( Alderson-Day and Fernyhough, 2015 ; Fernyhough and Borghi, 2023 ) that such process of cognitive development is accompanied by the evolution of inner or covert speech that associates a symbolic component to the maturation of subsymbolic sensorimotor capabilities. Such linguistic component has also been linked to the role of inner speech in working memory ( Miyake and Shah, 1999 ), namely the retention of information “online,” critical for a complex task.
If developmental robotics is to be taken seriously in the long run, it should be considered that human cognitive development (approached by means of the theoretical frameworks proposed either by Piaget or Vygotsky) is inexorably interleaved with educational issues and this should be the basis for training and educating autonomous robotic agents during development. The main motivation and attractive promise for pursuing this line of research is that it may allow to overcome the explainability issue, namely the generalized level of distrust affecting AGI and to give rise to mutual understanding. In other words, we may assume that humans may be readier to trust cognitive robotic agents if they are well educated (i.e., robotic agents and humans share a value system), according to clear and public training plans, including such things as a certified CV and reference list. Moreover, by relying on social education and training it will be possible to differentiate the type of skills achieved by the robotic agents in order to obtain some kind of social balance between human and robotic populations as well as to interact differently with human with different abilities (e.g., young or elderly persons).
On the other hand, in spite of the intense and wide research activities on developmental robotics in the last two decades ( Min et al., 2016 ; Huang et al., 2017 ; Wieser and Cheng, 2018 ; Naya-Varela et al., 2021 ; Rayyes et al., 2022 ), we are still far away from a level of understanding of the global implementation issues that may allow testing and evaluation in a sufficiently general manner. Certainly, there are still limitations regarding the “bodyware” capabilities of robots used to implement a developmental process inspired by humans, including the two most advanced child robots, namely iCub ( Sandini et al., 2004 ) and CB2 ( Minato et al., 2008 ). Their limited sensory and motor abilities is not the most relevant factor so far, to investigate the organization of Sensory-Motor-Cognitive Development in realistic robots, but the fact that most research has been focused on the biomimetic, time frozen implementations of specific aspects of human cognitive development, missing to address the large picture as well as its temporal continuity: in some sense, it is like implementing a specific ability of a three-year-old without figuring out where the system is coming from and how it may progress beyond. This strategy is in contrast with the theory of development, expressed by Vygotsky (1978) , describing the “zone of proximal development” as the distance between the actual developmental level and the level of potential development. The actual developmental level characterizes mental development retrospectively, while the Zone of Proximal Development characterizes mental development prospectively. For example, we may quote a representative list of studies in the two preceding decades that cover in isolation some of the specific issues of developmental robotics: Self-exploration and early imitation ( Kuniyoshi et al., 2003 ), Modeling joint attention ( Nagai, 2007 ), Scaffolding Robot Action Learning ( Nagai and Rohlfing, 2009 ), Affordance-based perception ( Min et al., 2016 ), Bootstrapping the semantics of tools ( Schoeler and Wörgötter, 2016 ), Perception of Localized Features ( Giagkos et al., 2017 ), Bootstrapping of Sensory-Motor Skills ( Wieser and Cheng, 2018 ), Developing Reaching Ability like human infants ( Luo et al., 2018 ), Sensorimotor Communication ( Donnarumma et al., 2012 , 2018 ; Pezzulo et al., 2019 ), Integration of Sensing, Cognition, Learning, and Control ( Li et al., 2019 ), Evaluation of Internal Models ( Smith and Herrmann, 2019 ), Emergence of symbolic representations ( Ugur et al., 2015 ; Taniguchi et al., 2019 ), Grounded affordances ( Saponaro et al., 2020 ), Bodily Expression of Emotion ( Tuyen et al., 2021 ), Morphological development ( Naya-Varela et al., 2021 ), Skill Learning Strategy with Dynamic Motion Primitives ( Li et al., 2021 ), Interest-driven exploration ( Rayyes et al., 2022 , 2023 ).
This “stroboscopic” view of development, even if advancing the field, completely misses the continuous dynamic process behind sensorimotor and cognitive development. To advance the field of developmental robotics we should focus on a wider view of cognition, making explicit the relationship between different cognitive skills and their shared functions, although we should not disregard bodyware technologies because, obviously, better bodies can be advantageous.
In spite of the intrinsic fragmentation of the growth/integration process that needs to be overcome to give developmental robotics its full potential, the alternative roadmap that aims at the design of fully developed “adult” cognitive architectures for autonomous robots is only apparently more direct and simpler: in contrast, we believe that it misses the crucial fact that it is precisely this being always “under construction” that characterizes human intelligence and its open cognitive development.
A recent review of forty years in Cognitive Architecture Research for autonomous robotics ( Kotseruba and Tsotsos, 2020 ) clearly shows that we are still far away from a solid platform. The problem is that it does not make sense to aim at a General-Adult Cognitive Architecture that (as the dream of AGI) can be easily adapted to any application area; at the same time, it is not very attractive to aim at a population of cognitive architectures, specifically designed for each application, without a well-defined common computational core. In contrast, the developmental roadmap, which is apparently more arduous, would take advantage of a close interaction with humans and the well-developed human tools for building, communicating and transmitting knowledge and culture ( Vernon, 2014 ).
One of the crucial problems that will challenge in the future the research in developmental robotics might be the identification of a minimal set of sensory-motor-cognitive kernels capable to bootstrap the growth, through self-organization, interaction, and training, of sensory-motor-cognitive abilities optimized for different application areas. With respect to this minimal set it has become clear that the apparent simplification offered by the analogy to human development, characterized by an incremental improvement of cognitive skills, on one side reduces the complexity of the learning processes by suggesting the sequence of the functions to be learned but on the other does not make explicit the complexity of the underlying computational/functional architecture of the system which is fully formed during the gestational period and expressed through highly structured brain connectivity. The baseline architecture represents the blueprint of evolution providing the scaffolding for innate behaviors as well as for continuous learning ( Zador, 2019 ). It is the opinion of the authors that without a baseline cognitive architecture with the potential to express all cognitive functions the advantage offered by a developmental approach to the design of ACo system will continue to produce temporally and functionally fragmented robots. It is worth mentioning that this baseline cognitive architecture is not the result of learning but is encoded in the genome and marginally modified epigenetically and, therefore, in ACo systems may require different computational tools than those used during the successive developmental and lifelong learning phases (among other differences its structure is only marginally affected by sensory and social interaction).
From the computational point of view, we may suggest that the cognitive architecture of cognitive agents will not be a closed piece of software running on a powerful but traditional von Neumann computer: in contrast, it can be conceived as a hybrid dynamical system that changes over time as an effect of learning and on-line interaction with humans or other cognitive agents. This growth process may take advantage of a number of possible tools, for example using neuromorphic computing technologies that may match better than traditional von Neumann design the need of self-organized growth ( Tang and Huang, 2018 ).
Moreover, we expect that the converging integration of technologies and methodologies will be facilitated by the development of the next generation of baby robots, following the first baby robot iCub ( Figure 1 ) with better sensory-motor-cognitive capabilities.
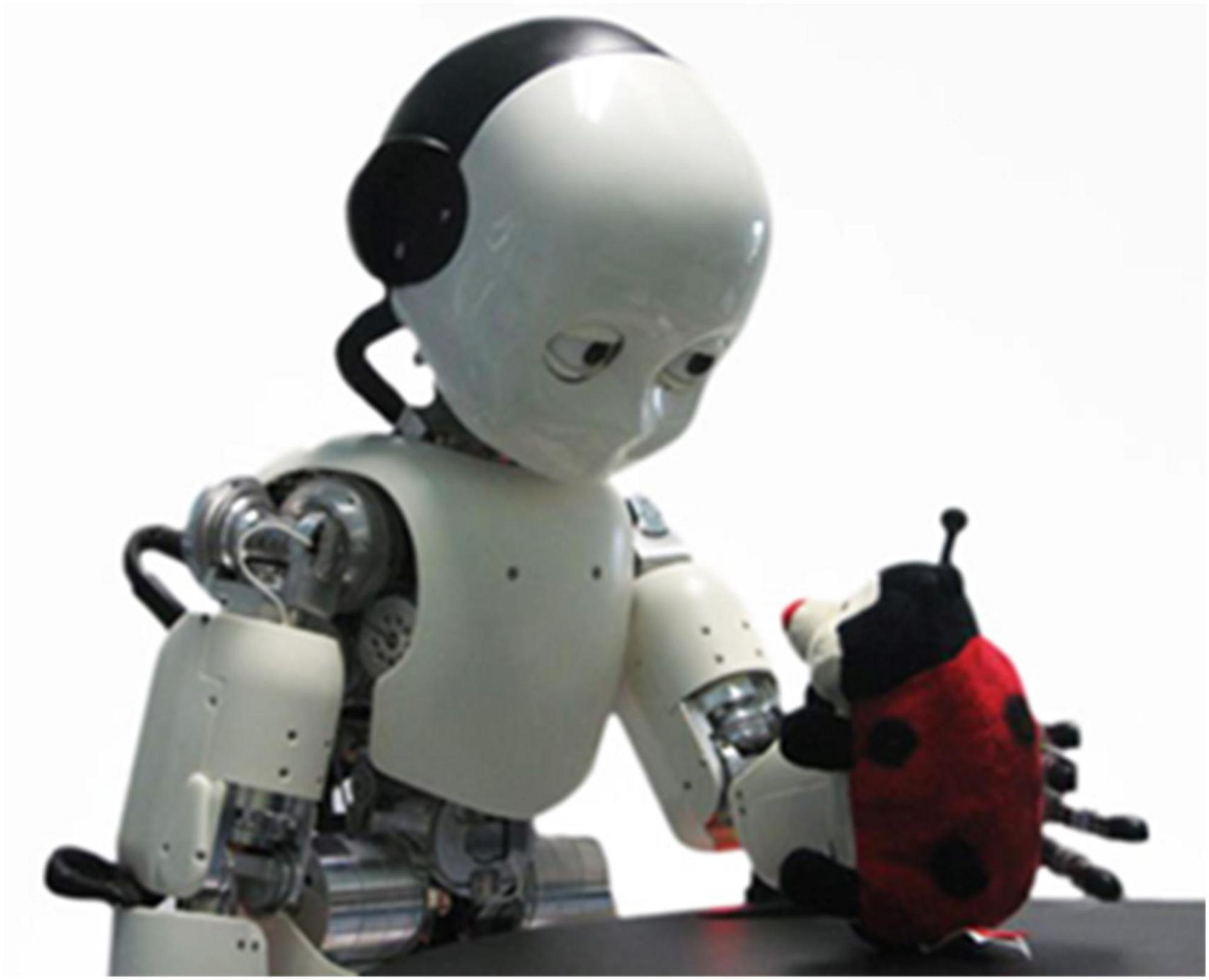
Figure 1. iCub: the first baby robot.
3 Body model and prospection capabilities for artificial cognition
Evolutionary pressure is behind the increase and expansion of motor redundancy in the human species, with emphasis on two functional areas: manipulation (through the availability of thumb opposition) and vocalization (through the development of a sophisticated vocal tract). Such bodily evolution has been accompanied by the emergence of specialized brain regions required to tame the abundance of DoFs, with a dual computational function: control of overt (real) actions and generation of covert (imagined) actions for allowing adult subjects to reason in a proactive way, in agreement with the fundamental cognitive capability of prospection ( Gilbert and Wilson, 2007 ; Seligman et al., 2013 ; Vernon et al., 2015a , b ). Prospection includes planning, prediction, imagination of hypothetical scenarios, and evaluation/assessment of possible future events. Prospective abilities – how much and how well a person is able to bring mental representations and evaluations of possible futures to bear on the selection of action – is a fundamental cognitive faculty akin to other basic faculties as language and reasoning.
The key achievement for cognitive mastering the physical interaction with the environment is prospection, which can also be described as “Mental Time Travel” ( Suddendorf and Corballis, 2007 ) in order to emphasize the extended role of memory in purposive actions: episodic memory (retrieving from the past events/situations, specifically relevant for the current state) as well as procedural memory (namely, the identification of the appropriate selection of tools and sub-actions as well as the prediction of the perceptual feedback); such memorized know-how can be combined for imagining future scenarios and evaluating alternative courses of action. Mental time travel allows cognitive agents to act skillfully by integrating and fusing in the performed action the past (through smart retrieval of goal-driven experiences), the present (through the activation of previously trained synergies), and the future (through the anticipated internal and external consequences of the action). Moreover, the propensity for prospection and mental time travel can be greatly enhanced by another intrinsically social mental ability, i.e., flexible communication and language, enhancing the potential predisposition of humans to cooperate by pooling information and resources ( Tomasello, 2009 ; Slocombe and Seed, 2019 ).
In relation with the unifying simulation/emulation theory of action ( Decety and Ingvar, 1990 ; Jeannerod, 2001 ; Grush, 2004 ; O’Shea and Moran, 2017 ; Ptak et al., 2017 ) we suggest that the basic computational module required for a cognitive agent to achieve prospection is an internal representation of the whole body or body schema. In neuroscience “body schema” is a label (with other similar labels, as body image etc.) that covers a scattered range of phenomena rather than a specific well-modeled neural mechanism ( Head and Holmes, 1911 ; Penfield and Boldrey, 1937 ; Gallagher, 1985 ; Graziano and Botvinick, 1999 ; Paillard, 1999 ; Holmes and Spence, 2004 ; Preester and Knockaert, 2005 ; Hoffmann et al., 2010 ; Morasso et al., 2015 ; Di Vita et al., 2016 ). However, there is an agreement on some computational features that are relevant for the development of cognitive robotic agents: the body schema (1) is spatially encoded (with multiple reference frames), (2) is intermodal/supramodal (including the dynamic integration of sensory and motor information ( Azañón et al., 2016 ), (3) is distributed and modular (in multiple cortical maps dynamically interconnected into networks such as the “default mode network”) ( Horn et al., 2013 ), (4) is characterized by a short-term plasticity and reorganization on the time scale of seconds, as shown by the quick integration of tools into the body schema for skilled performers ( Maravita and Iriki, 2004 ).
Moreover, there is ground to believe that the body schema is directly responsible for the kinematic-figural invariants that characterize the spatio-temporal features of biological motion ( Johansson, 1973 ). Such invariants are mainly related to the spatio-temporal features of the end-effectors rather than joint coordination, suggesting that the brain representation of action is more skill-oriented than purely movement/muscle-oriented. For example, Figure 2 shows that in reaching movements the trajectory of the end-effector is straight with a symmetrical bell-shape of the corresponding speed profile, independent of the starting position, direction and of the specific end-effector; in whole body gestures, as handwriting or drawing, the curvature and speed profiles are anti-correlated ( Morasso, 2022 ) and characterize what is known as “biological motion” (see below). Since these invariants are independent of the number of DoFs recruited in a given action, we may suggest that the computational machinery producing them is actually solving, at the same time and in an intrinsic manner, what Bernstein (1967) called the Degrees-of-Freedom-Problem.
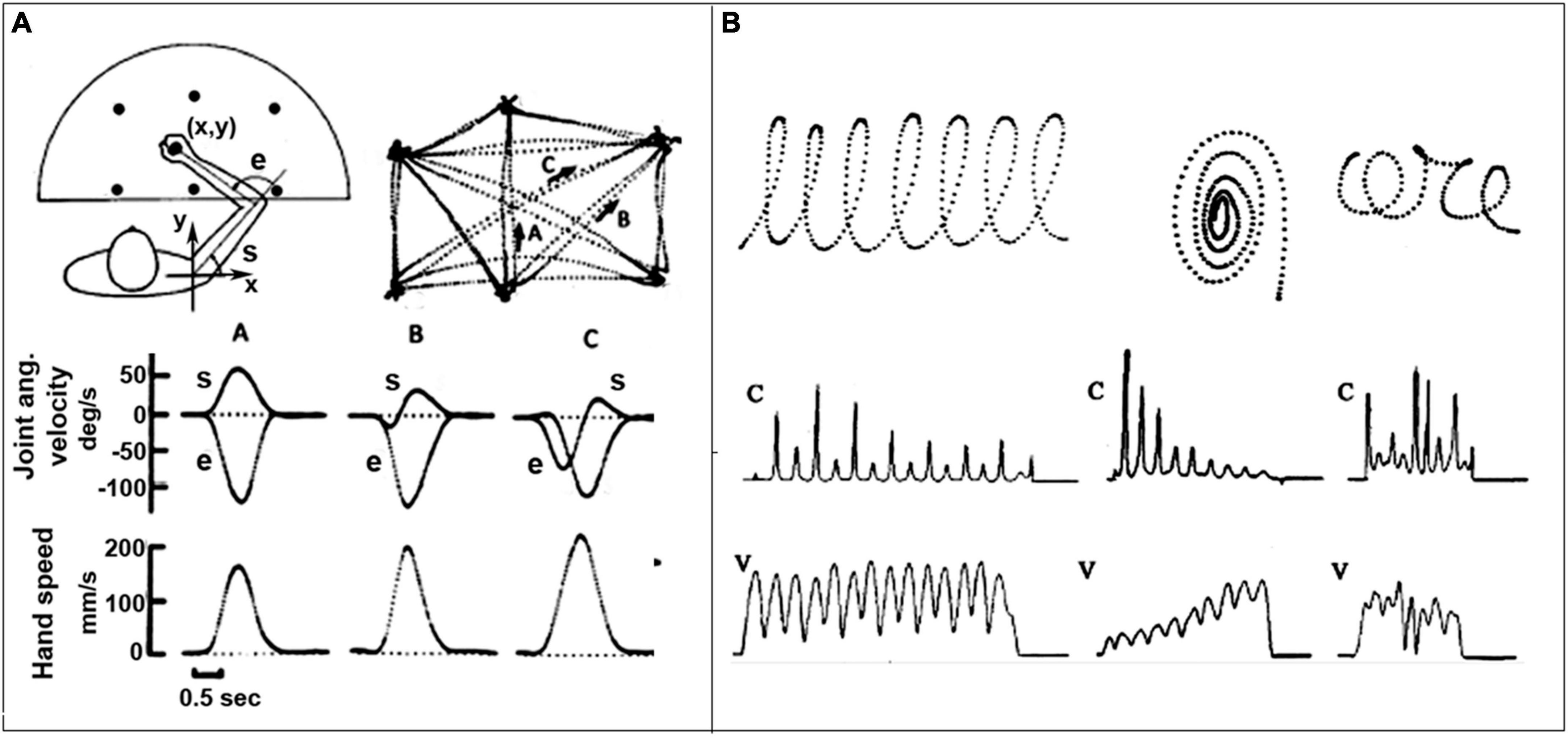
Figure 2. Spatio-temporal or figural-kinematic invariants in trajectory formation. (A) Planar reaching movements between six target points; note the invariant straight point-to-point trajectories and the invariant bell-shaped speed profiles. (B) Three examples of continuous hand gestures displayed as digitized trajectories, including the profiles of the velocity (V) and curvature (C); note the anti-correlation of the two profiles. From Morasso, 2022 .
It is also worth considering that the study of motor imagery provided evidence that kinematic invariants are also present in covert movements ( Decety and Jeannerod, 1995 ; Karklinsky and Flash, 2015 ). Such evidence, together with the finding that the same invariants characterize the actions of congenitally blind persons ( Sergio and Scott, 1998 ), suggests to focus the attention of the computational analysis of kinematic invariants from their phenomenological description [e.g., the minimization of jerk ( Flash and Hogan, 1985 ) or the 2/3 power law ( Lacquaniti et al., 1983 )] to the organization of internal models incorporating the intrinsic dynamics of the bodyware.
The Mental Simulation Theory ( Jeannerod, 2001 ) provides a powerful approach to the solution of this problem: it posits that the brain generates a mental flow of simulation-states in such a way as to simulate the activity produced during the same, executed action and this process “would put the action representation in a true motor format so that it would be regarded by the motor system as a real action.” A computational implementation of the mental simulation theory that can be easily integrated in the cognitive architecture of autonomous robots is the PMP model of trajectory formation (Passive Motion Paradigm: Mussa Ivaldi et al., 1988 , 1989 ; Mohan and Morasso, 2011 ; Mohan et al., 2019 ): its basic rationale is the same as the models of action representation and motor control based on a force-field approach, namely the idea that the motor coordination of multiple, redundant DoFs of the body is the consequence of (real or virtual) force fields applied to an internal representation of the body (the body model). The body model is viewed as a network of spring-like elements that individually store elastic potential energy, contributing to a global potential energy that recapitulates, in a smooth, analogic manner, the complex set of bodily interactions: the network is then characterized by an attractor neurodynamics that aims at equilibrium states of minimum potential energy, in a similar way to the dynamics of Hopfield networks ( Hopfield, 1982 ). The PMP model applies the concept of passive motion to active synergy formation by updating the control input of each element of the body model so as to cancel the “stress” induced by a simulated external perturbation, e.g., the attractive force field to a designated target.
There is also an interesting relationship between the Passive Motion Paradigm and Active Inference ( Friston et al., 2011 ): the anti-symmetry between active inference and passive motion speaks to the complementary but convergent view of how we use our forward models to generate predictions of sensed movements. This view is an example of Dennett’s “strange inversion” ( Dennett, 2009 ), in which motor commands no longer cause desired movements – but desired movements cause motor commands (in the form of the predicted consequences of movement). Thus, the PMP model can tame the abundance of degrees of freedom of the human body by using a small number of primitives (force fields, associated with specific end-effectors as a function of a given whole-body gesture): the diffusion of such fields throughout the internal body model distributes the activity to all the DoFs, with an attractor neurodynamics, driven by the instantiated force fields, that indirectly produces at the same time the kinematic invariants mentioned above for both the overt and covert actions.
At the end of the ontogenic development of adult subjects, we may assume that the redundant degrees of freedom of the human body are coordinated by the brain in such a way to exhibit the observed kinematic invariants by animating the body schema according to something similar to the PMP computational model. From what is known of the sensory-motor-cognitive development of humans we may exclude that the organization of the body model is somehow innate and genetically preprogrammed 3 . In contrast, we may expect that it is built and refined during development by exploiting plasticity, consolidating a physical self-awareness and conquering two crucial developmental targets; (1) multi-modal sensory fusion and calibration, for achieving perceptual abstraction ( Gori et al., 2008 , 2012a ) (2) generalized kinematic invariance, for general end-effectors and/or skilled tool-use.
As regards self-awareness/self-body-consciousness, it has been suggested ( Tononi, 2004 ) that it is the result of a general process of information integration carried out by the brain through the neural connectome ( Tononi, 2005 ) and, from a developmental point of view, is an emergent property of the interaction between brain, body and environment, with the crucial role of visuo-haptic information ( Morasso, 2007 ). Infants spontaneously touch their own body and reach to tactile targets on the skin already in the final part of pregnancy and with even more intensity in the post-natal period ( Figure 3 ), when touching and being touched occurs systematically, with the reinforcement effect of mother care ( Corbetta and Snapp-Childs, 2009 ; Di Mercurio et al., 2018 ): infants are active explorers of their own body as well as of their peripersonal space ( Cardinali et al., 2009 ) from the first days of life and such self-generation of multi-sensory information is likely to shape and adapt Tononi’s connectome. This primordial and self-generated sense of self-unity is suggested to be the crucial initial base from which learning and development may evolve ( Rochat, 2019 ). However, the self-exploration mentioned above in early childhood, although crucial for building a solid awareness for the emergence of a “body space,” with the surrounding “peri-personal space,” as well as a strong sense of unity is initially rather qualitative and sensory-modality dependent. As shown by Gori et al. (2008) , children before 8 years of age fail to integrate visual and haptic spatial information. More generally, Gori (2015) proposed a cross-sensory calibration theory, according to which before 8−10 years of age the more accurate sensory modality “teaches/calibrates” the more variable sensory source and only afterwards accurate and reliable multisensory information is made available by the CNS. In contrast, adults are able to optimally fuse multisensory information in an abstract form ( Ernst and Banks, 2002 ). An effective internal representation of the body, for describing and predicting the evolution of actions, requires an abstraction from specific sensory modalities as well the corresponding motor information flow ( Squeri et al., 2012 ).
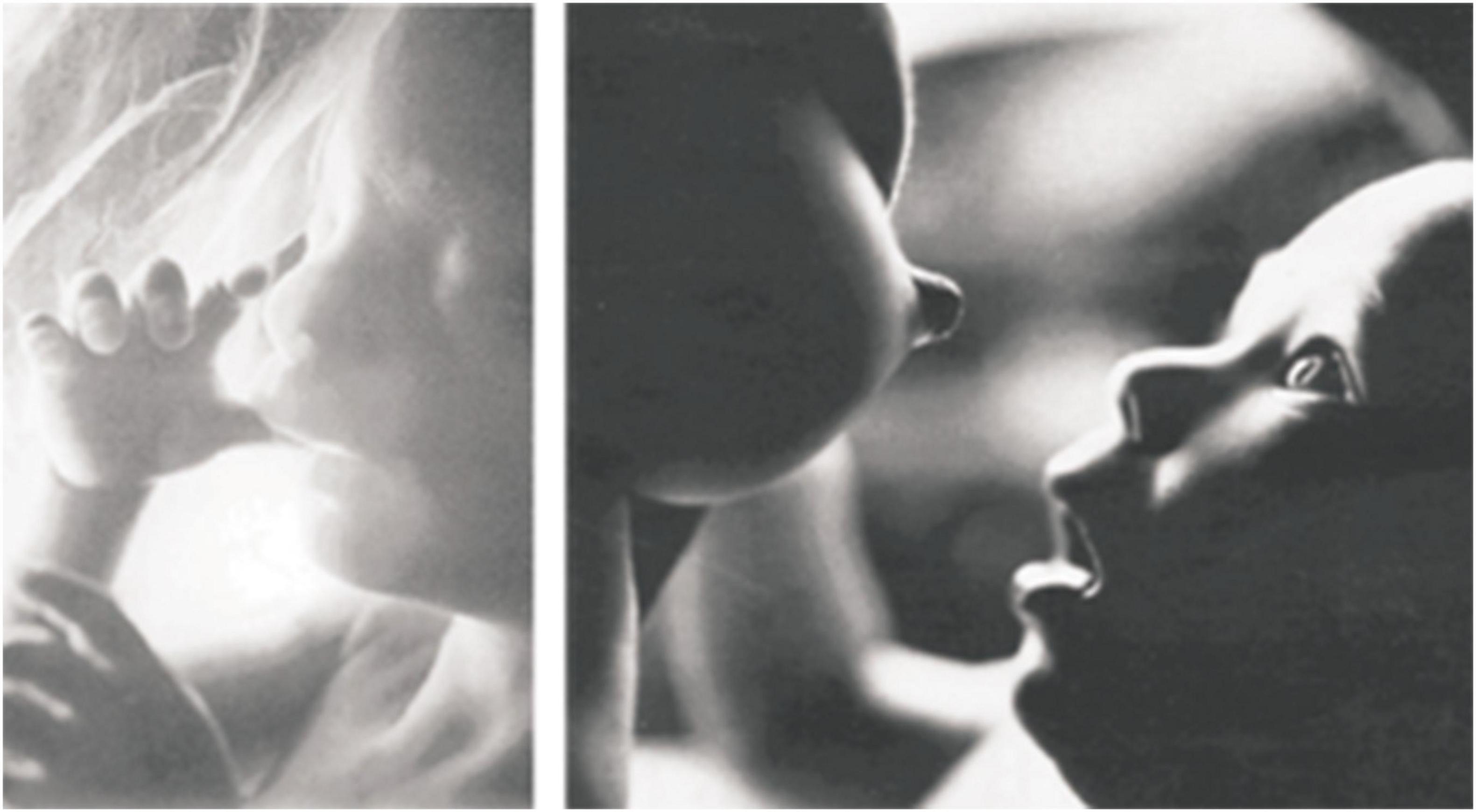
Figure 3. Pre-natal and post-natal visuo-haptic experience.
As regards the emergence of kinematic invariants, as indicators of the consolidation of the body schema, it is worth considering the maturation of the kinematic patterns of reaching movements. First of all, it has been observed that although infants reliably grasp for objects within their workspace 3−4 months after the onset of reaching, stereotypic kinematic motor patterns begin to emerge between the 2nd and 3rd year of life ( Konczak et al., 1995 ; Konczak and Dichgans, 1997 ; Gonçalves et al., 2013 ; Zhou and Smith, 2022 ): for example, only at that stage, the majority of trials exhibit a single peaked velocity profile of the hand. The observation of reaching movements beyond the 3rd year of life ( Schneiberg et al., 2002 ) reveals that development is characterized by a gradual decrease of the variability of Interjoint coordination and end-point trajectories and an increase of their smoothness: the highly stereotyped and stable patterns that characterize adults are reached only after 10−12 years of age and probably further consolidated in early adolescence ( Mitchell, 1998 ).
In general, the concept of body schema implies an internal awareness of the body ( Berlucchi and Aglioti, 1997 ) and the relationship of body parts to each other, encompassing cognitive aspects. In the framework of the Piaget theory of cognitive development that identifies four stages of maturation, the preliminary consolidation of the body schema as a working computational mechanism may be located at the end of the third stage ( concrete operational thinking , at the age of 11−12 years) that marks the beginning of abstract or operational thought: this means that the child can imagine things/situations internally in the mind, rather than physically try things out in the real world. However, such operational thought is only effective if the child is asked to reason about materials that are physically present. Only afterwards, at the later stage of formal operational thinking while entering adolescence, children may gain the ability to think in a more abstract and systematic manner, reasoning about what might be as well as what is, as an effect of their actions and the related reactions of the environment. As a matter of fact, such fourth stage of Piaget’s theory is open-ended and strongly dependent on the specific interaction with the physical/social environment as a life-long learning/training process ( Flesch et al., 2023 ).
In summary, we suggest that the Body Schema (animated through the mental simulation theory) and the Prospection capabilities, which are essential for the Artificial Cognition of the next generation of cognitive robots, may be achieved more naturally in a developmental, self-organized framework. The achievement of this goal will probably require the adoption of new computing technologies, beyond the traditional von Neumann approach, allowing the implementation of a kind of large connectome as the basic skeleton for the cognitive development of the robot. The connectome may support the emergence of a number of self-organizing maps, derived from the pioneering research of Kohonen ( Kohonen, 1982 ; Martinetz and Schulten, 1994 ): this self-adaptive neural substrate, evolving under the action of Hebbian learning mechanisms, should be driven by a built-in, spontaneous tendency to explore the body space and the peripersonal space: a generalized, spontaneous babbling strategy ( Kuperstein, 1991 ; Goldstein and Schwade, 2008 ). Babbling is a stage in child development (4−12 months of age) and a precursor to language acquisition: infants experiment with phonation by uttering sounds that not yet produce recognizable words but are essential to self-generation of training data for the phonatory system. A similar babbling strategy appears to be in operation in the same period, for experimenting manipulation and acquiring self-awareness of the body that initiates the construction of the body schema. Thus, we suggest that such generalized babbling mechanism, ranging from phonation to manipulation, is a crucial bootstrap mechanism for cognitive developmental robotics. At the same time, the maturation of prospection capabilities via the evolution of mechanisms for the mental simulation of covert actions can be integrated with the evolution of covert and overt speech ( Alderson-Day and Fernyhough, 2015 ) that associates a symbolic component to the maturation of subsymbolic sensorimotor capabilities. Moreover, reinforcement learning ( Neftci and Averbeck, 2019 ) can support the strategic organization of purposive actions for cooperative human-robot interaction.
4 Social support of developmental artificial cognition
For cognitive robots conceived in the framework of developmental robotics, cognitive capabilities are not preprogrammed but can be achieved and organized through interaction with the physical and social environment.
While the former sections have addressed the development of cognition mostly focusing on an individual agent, the current one underlines the importance of the social dimension and advocates the need of considering it since the beginning of the modeling process of artificial cognition. This claim draws parallels with human cognition, where the innate predisposition of humans for social interaction from early stages influences cognitive, emotional, and motor development. Then, a pathway to model core cognitive abilities in artificial agents based on a social perspective is proposed. It starts from the incorporation of social motives into artificial cognitive systems, moves on emphasizing the impact of bidirectional non-verbal linguistic interactions for effective cognitive development in robots and concludes by highlighting the role of social interaction in consolidating cooperation, teamwork, and the transmission of ethical norms and cultural values in cognitive agents.
Human beings show a marked predisposition to work together toward joint goals and in cooperation, to teach (and be taught by) others and to consider their perspectives and intentions ( Tomasello, 2009 , 2018 ; Slocombe and Seed, 2019 ). It has even been proposed that human beings have evolved special social-cognitive skills beyond those shared by primates in general for living and exchanging knowledge in cultural groups, by communicating, learning and “reading the mind” of others in complex ways ( Herrmann et al., 2007 ). In particular, the generalized capacity for teaching, which involves the use of shared attention and other forms of verbal and non-verbal information exchanges, is one of the features at the basis of the extreme capacity for cultural learning shown by humans ( Laland and Seed, 2021 ).
The predisposition to social interaction is apparent since the initial moments of human life. Already before birth, the direct interaction with the mother and, when present, with the twins ( Castiello et al., 2010 ) and with the social inputs coming from outside the womb (e.g., as sounds) play an important role in shaping the future interaction of the baby with the world and others ( Ciaunica et al., 2021 ).
Since birth, human neonates are attracted by other people and are endowed with skills that facilitate the establishment of an interaction, ranging from the preference for biological motion ( Simion et al., 2008 ) and for faces looking directly to them ( Farroni et al., 2002 ), with preference for human voices to other sounds ( Alegria and Noirot, 1978 ) and especially infant directed speech ( Cooper and Aslin, 1990 ). As soon as about half an hour after birth newborns can imitate facial expressions of happiness and surprise ( Meltzoff and Moore, 1977 , 1983 ; Field et al., 1983 ) and within the first following days, newborns look significantly longer at a happy facial expression than a fearful one, suggesting a sensitivity to the facial characteristics that maximize their chances of interacting with others ( Farroni et al., 2007 ).
It appears that particular high relevance is given since the earliest moments of development to elements that ensure the affiliation with conspecifics. This is not unexpected in a species where newborns are born with undeveloped skills and strongly depend on the support of their caregivers to survive and grow in the first portion of their life (i.e., an altricial species, in contrast to precocial ones ( Vernon, 2014 ).
Such social inclination at birth is not the result of supervised or unsupervised learning algorithms but an inherited predisposition to exploit social interaction for the development of cognitive abilities through the production and understanding of social signals, such as gestures, gaze direction and emotional displays. It is worth mentioning here that some of these innate “social signs” are in fact exapted functions ( Gould and Vrba, 1982 ) in the sense that the main motivation they appeared during evolution was not for their “social use.” For example, facial expression ( Murray et al., 2017 ) are supported by part of the system for regulating ingestion in relation to breathing and the need to control the direction of gaze is mainly motivated by the computational advantages of human space variant retina ( Sandini and Tagliasco, 1980 ). Starting from these innate skills the social competence of the newborn develops very rapidly. Around 3 months of age infants engage mutual gaze with adults, i.e., both agents attend to each other’s eyes simultaneously ( Kaplan and Hafner, 2006 ). At around 6 months of age infants can perceive the direction of attention of others, at least in term of discriminating whether the caregiver’s gaze is directed to the left or to the right ( Butterworth, 1991 ; Butterworth and Jarrett, 1991 ). In the sensitive period for social coordination, from 3 to 9 months of age, parents and infants establish social interaction by coordinating gaze, affect, vocalizations and touches. These reciprocal exchanges shape the child’s development, impacting not only the social domain, but also the emotional, cognitive and brain development ( Feldman, 2007 , 2012 ).
By the end of their first year of life infants start to understand pointing as an object-directed action ( Woodward and Guajardo, 2002 ) and to address it, their gazing and vocalizations more often to people than to inanimate objects, showing that they are aware of the person’s attentional state ( Legerstee and Barillas, 2003 ). Still during the first year of life infants demonstrate action understanding skills strongly correlated with the infants’ prior motor experience ( Sommerville and Woodward, 2005 ; Sommerville et al., 2005 ). For instance, the capability to execute and anticipate reaching ( Kanakogi and Itakura, 2011 ) or eating actions ( Kochukhova and Gredebäck, 2010 ) appears at 6 months-of-age, while at least 9−12 months of life are required in order to skillfully perform and anticipate the goal of a transport action ( Falck-Ytter et al., 2006 ).
Instrumental helping emerges already at 18 months of age, when toddlers altruistically try and help adults when they don’t manage to reach a certain goal (Warneken and Tomasello, 2009 ) and then increases its complexity and selectivity with age ( Slocombe and Seed, 2019 ).
The presence of innate skills and the rapid development of early abilities suggest a preparedness for social interaction and a primary importance of social relationships in the development of cognition. Such importance is confirmed also by studying the behavior of caregivers, as it has been proven that maternal postpartum behavior shapes children’s symbolic competence and cognitive skills ( Feldman et al., 2004 ; Feldman and Eidelman, 2009 ). Immediately after birth human mothers express specific newborn directed behaviors, such as gazing at the infant’s face and body, expressing positive affects, modulating their prosody by using high-pitched vocalizations (“motherese”), and affectionate touch. Importantly – and proper for the human species only – mothers show reciprocity, i.e., mothers adapt their behavioral intensity to the neonate’s moments of alertness ( Feldman and Eidelman, 2003 , 2007 ).
These findings suggest that, when attempting to model cognition, it is mandatory not only to address the individual agent and its relation with the environment, but also to consider the impact of being immersed in the social world and somehow predisposed to social interaction since the earliest moments of development. In this perspective, the necessity to thrive in a social context becomes a foundational element of how the agent’s cognition develops and of which are the basic abilities with which it needs to be endowed first.
A similar shift has recently informed the field of neuroscience with the call for a change in paradigms toward the so-called “second-person neuroscience” ( Schilbach et al., 2013 ), in which neural processes are examined within the context of a real-time reciprocal social interaction. Neuroimaging and psychophysiological studies have provided evidence that social cognition is fundamentally different when someone is involved in an interaction and emotionally engaged as compared to being just an observer ( Redcay and Schilbach, 2019 ).
Also studying artificial cognition could benefit from shifting from modeling of the individual agent and of its (passive) understanding of the social environment to a perspective where active social interaction is foundational and transformative, impacting the way the agent faces the complex problem of understanding and anticipating others and its cognition overall. Rather, approximating the development of cognition as sequential process where the agent first develops in isolation certain skills and then merely exploits them in its interaction with others might lead to overseeing some crucial components of what makes human-like cognition so advanced and capable of generalization.
Some authors have even proposed that social interaction might be the brain’s default mode ( Hari et al., 2015 ). Indeed, although social interaction represents one of the most complex functions that human cognition enables and one of the most difficult to implement on cognitive artificial agents, still it appears to us incredibly easy. A paradigmatic example is that of dialogue: turn taking during human-human conversation is naturally achieved effortlessly and with an extreme temporal accuracy, yet unmatched in human-agent verbal exchanges. It has been suggested ( Garrod and Pickering, 2004 ) that humans are “designed” for dialogs, endowed with unconscious interactive processing, seamlessly aligning the linguistic representations of the interlocutors. Furthermore, the evidence that children learn best during interaction than by observing others ( Moll and Meltzoff, 2011 ) has been proposed as another evidence in favor of the primacy of interaction ( Hari et al., 2015 ).
This is not to deny the principle that individual first-hand competence (e.g., action execution) plays a crucial role in shaping the understanding of the social world, which has received very strong support also from neuroscience. As an example, it has been proven that children have first to learn how to perform an action themselves before being able to automatically anticipate the outcome of the same action, when it is executed by another agent ( Falck-Ytter et al., 2006 ). This process maintains its validity also during adulthood ( Flanagan and Johansson, 2003 ). It’s only through extensive first-hand motor experience that athletes become extremely proficient at predicting the outcome of others’ actions in the same sport, e.g., guessing whether a basketball will enter the loop or whether a certain kick will lead to a goal. Sport journalist, though accumulating a similar amount of observational exposure, do not reach comparable anticipatory capabilities ( Aglioti et al., 2008 ).
However, the centrality of the reciprocity in infant-caregiver behaviors in the development of children cognition ( Feldman and Eidelman, 2009 ) and the evidence that a great component of human learning is inherently social and (almost uniquely in the human species) relies on teaching ( Csibra and Gergely, 2011 ; Laland and Seed, 2021 ), indicate that the traditional approach of “developing the individual” and then face it with the social environment could lead only to a partial comprehension of human-like cognition. Citing Hari et al. (2015) “If the goal is to achieve human-like behavior, it is not enough to build on the bottom-up stimulus-driven effects, but the centrality, eventually primacy, of social interaction should be incorporated to the models .”
Following this approach, it would be worth analyzing the core cognitive capabilities currently identified for Cognition (Perception, Attention, Action Selection, Memory, Learning, Reasoning, Metacognition and more recently Prospection: Vernon et al., 2007 ; Vernon et al., 2016 ; Kotseruba and Tsotsos, 2020 ) through a “social lens”: how they developed based on the necessity of being a social agent in a social world?
As a starting point, we may include social motives in the development of an artificial cognitive system. A motive defines the goal of the system and determines its decision making. The social motive guides the agent toward the research of comfort, security and satisfaction from the interaction with others, guiding to the exchanges of information and to the need of maintaining the interaction over long periods of time ( Vernon, 2014 ). This approach is being explored in some cognitive architecture. For instance, in the Clarion modularly structured cognitive architecture ( Sun, 2006 ) the motivational subsystem derives goals both from physiological needs (such as need for food, need for water, need to avoid danger, and need to avoid boredom) and so-called high-level drives, such as desire for social approval, desire for following social norms, desire for reciprocation, and desire for imitation of other individuals. More recent attempts in robotics have looked into social motivation where the need for social comfort drives the action selection and adaptivity ( Hiolle et al., 2014 ; Tanevska et al., 2020 ; Mongile et al., 2022 ). Including social elements, e.g., social norms, in the development of basic learning skills of an agent, is another step in this direction such as considering social constructs such as rivalry when learning to win a competitive game ( Barros et al., 2022 ) or ensuring legibility of the agent’s reinforcement learning process for the human teacher ( Matarese et al., 2021 ).
From the point of view of developmental cognition, we should then emphasize the critical role of pedagogy that is supposed to integrate subtle scaffolding, motivation, teaching, training and information transmission for children of primary school age ( Csibra, 2010 ; Csibra and Gergely, 2011 ). In particular, this also includes a combination of verbal and gestural/bodily linguistic interactions between the teacher and the pupil, aimed at grabbing and directing the attention of the pupils: for example, effective gestural language refers to pointing, with the fingers and/or the eye, and adopting facial expressions for expressing surprise, curiosity, interest, fear etc.
Developing systems that can understand and properly express such wide range of implicit cues ( Figure 4 ) becomes then crucial in facilitating the overall development of the skills of the cognitive system, not only of its social competences ( Sandini and Sciutti, 2018 ; Sciutti et al., 2018 ). Considering that in humans simpler forms of sensorimotor communication might have scaffolded more complex cognitive abilities, such as linguistic communication, endowing robots with sensorimotor communication abilities might aid in developing more advanced interaction capabilities Hence, the need of developing systems that are sensitive to the subtle variations of human movements of face, body and gaze ( Palinko et al., 2016 ; Vignolo et al., 2017 ; Cazzato et al., 2020 ; Barros and Sciutti, 2022 ; Barros et al., 2022 ) and that can embed in their own behavior the same subtle cues that make human action seamlessly understandable to the human partner ( Di Cesare et al., 2020 ; Lastrico et al., 2023 ).
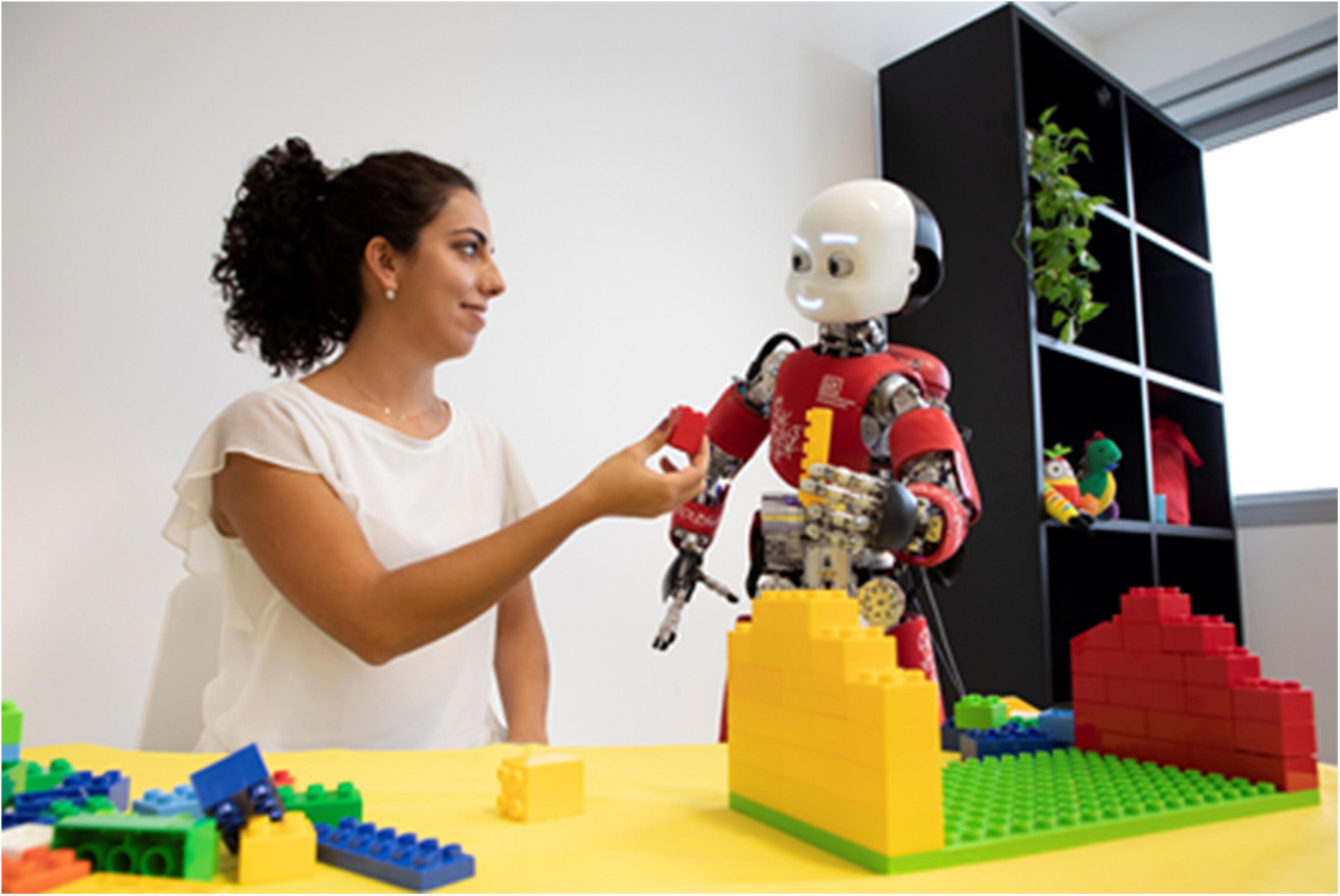
Figure 4. Mutual understanding, supported by the exchange of verbal and non-verbal signals, and a reciprocal interaction with the caregiver, are at the basis of children’s cognitive development. They might be as necessary for the development of Artificial Cognitive systems.
Moreover, such non-verbal linguistic interactions are strongly bidirectional: from the child to the adult and vice versa. Thus, if we aim at promoting the maturation of the cognitive abilities of developing robots we should refer more to pedagogists rather than to ICT experts.
At later stages of development, the role of social interaction should focus on consolidating the tendency of the cognitive agent to cooperate and integrate in a teamwork ( Tomasello, 2009 ), including the ability to represent the mental states (both of oneself or partners) and communicate/talk with the partners about knowledge, beliefs, desires, intentions, and so on. More generally, the reliability of ACo robots in the social context is determined by a shared value system that, to a great extent, can emerge from structured social interaction, capable to transmit ethical norms and cultural values ( Boyd and Richerson, 1985 ; Tomasello, 1999 ). Obviously, this is a highly sensitive issue that requires a great care and responsibility. Moreover, language is certainly a crucial tool in this process, both the inner speech for consolidating and retrieving sensory-motor-cognitive capabilities and the open speech for allowing cognitive agents to reason about cooperative tasks and openly explore different alternatives. Moreover, here is a possible link between ACo and AI in the sense of using LLMs as well-developed working tools.
5 More on ACo vs. AI in relation with cognitive neuroscience
After having explained in detail the main pillars of the ACo framework, it is worth explaining the differences between ACo and other attempts inspired by cognitive neuroscience and somehow linked to AI. In particular, comments are provided for clarifying why we believe that attempts to extend current AI in the sense of cognitive neuroscience miss crucial points. Such attempts are motivated by a preliminary, fully acceptable consideration, that current AI applications (shortly AIs) do not meet social expectations in many cases: for example, when a specific AI-based agent faces situations that their designers did not anticipate the result is failure or, even worse, irrational and unexplainable behaviors that evidentiate lack of understanding and ineffective interaction/cooperation with people or other agents. As regards the attempts to make AIs more compatible with neuroscience we already mentioned the issue of Explainable AI (XAI), namely the “explainability” problem of AI technologies, and the proposed solution ( Taylor and Taylor, 2021 ; Iglesias, 2022 ) to import assessment methods developed in Psychology and Cognitive Neuroscience: although this may be a useful method of analysis, it is of no help from the “generative” point of view, i.e., for the design of autonomous, prospective cognitive agents. Another stream of neuro-inspired AI is Embodied AIs ( Duan et al., 2022 ): please, note the plural, with the implicit assumption that Intelligence or Cognition is not the fundamental organizational principle from which all the specific competences are derived and integrated but is rather the result of the mere assembly of specific and substantially independent AIs, like perceiving, skilled gesturing, abstraction discovering, planning, modeling other agents, self and social awareness, interacting, and communicating. Embodied AI research diverges from conventional AI where learning is orchestrated on collecting big data and using them separately for different functions (perception, motor control, planning, etc.) with a third-person (impersonal) view of the acquired knowledge. In contrast, embodied AI is characterized by a first-person perspective that aims at mimicking human behavior during the interaction with the environment. However, this view of Embodied AI captures only a marginal part of Embodied Neuroscience: for example, in the radical formulation of the embodied brain ( Kiverstein and Miller, 2015 ) it is suggested that cognitive neuroscience should look to an ecological dynamical psychology where the functions associated to brain regions are dynamically changed over time and are based on the inseparability of cognitive and emotional processing; emotional states should be best understood in terms of action readiness (both overt and covert actions) in the context of the organism’s ongoing skillful and prospective engagement with the environment. States of action readiness involve the whole living body of the organism, including a dynamic body schema, and are elicited by possibilities for action in the environment (more generally affordances ) that matter to the organism. Thus, the egocentric characterization of Embodied AI captures only a small part to the fundamental properties of Embodied Cognition that allow a cognitive agent to operate in a successful and autonomous way in a changing and unpredictable environment: moreover, switching from a first-person to a third-person perspective and back is one of the crucial powerful cognitive features of prospection.
Let us consider another possible variation of neuro-inspired AI, namely Developmental AIs ( Stefik and Price, 2023 ) aimed at bootstrapping a process that may evolve from simple innate competences to intelligent, human-compatible AIs. The premise of both Embodied AIs and Developmental AIs is that a better approach for AIs to acquire what knowledge is needed for a given task is by observing and probing the environment, interacting with people and with socially developed information including online media. Moreover, bootstrapping developmental AIs is about creating socially aware human compatible AIs that learn from and teach others. It is suggested an approach that follows a bio-inspired trajectory for bootstrapping separately the different AIs: perceiving, understanding and manipulating objects; multi-step actions and abstraction discovery; curiosity and intrinsic motivation; imitation learning; imagination and play; communication and language. The bootstrapping approach tracks a competence trajectory where the competences are developed in small steps in parallel by embodied AIs. Although such items are certainly relevant in developmental robotics, developmental AI ignores/under evaluates a key issue, i.e., the fact that development is a self-organizing process where the different functions or AIs cannot be separated with the classical “divide and conquer” strategy. Both the proposed Embodied AIs and Developmental AIs implicitly assume a separation of Bodyware and Cogniware where Cogniware consists of a collection of software packages running on traditional von Neumann computational architectures. The Artificial Cognition approach supported in this work suggests a full integration of Bodyware and Cogniware, with a deep compatibility between artificial and human cognition that implicitly solves the explainability problem providing a common interaction language. Moreover, ACo is not the mere collection of Embodied AIs and Developmental AIs but is brain-inspired to the extent that Embodied and Developmental features are essential parts of its DNA. Thus, the cognitive architecture embedded and embodied in the Cogniware will provide a coherent baseline to facilitate convergent, cumulative progress in the development of an operational model of cognition and in parallel will facilitate experimental testing of core cognitive abilities and their dynamic and synergistic interplay as the robot interacts with its environment and other cognitive agents.
6 Conclusions
There is wide agreement that understanding the brain structure and function is one of the most substantial and challenging frontier scientific questions of the 21st century, as clearly expressed by several world-wide proposals of “brain projects” in the last two decades ( Jones and Mendell, 1999 ; Markram, 2006 ; Kandel et al., 2013 ; Okano et al., 2015 ; Poo et al., 2016 ; Ramos et al., 2019 ; Bjaalie et al., 2020 ; Yuan et al., 2022 ). In most cases, such projects have a double target: a medical target and a computational target. For example, in ( Yuan et al., 2022 ) it is stated that “the China Brain Project is structured as “one body and two wings”, with the goal of developing treatments for major brain disorders and promoting the development of a new generation of artificial intelligence.” While in the last two decades the research in the “first wing” has produced a number of interesting and useful results, the “second wing” is still far away from any preliminary conceptual framework for a bio-inspired and neuro-driven approach to the design of autonomous, cognitive, robotic agents: on one side, the current wave of AI research and development is totally dis-embodied and disconnected from any brain-like formulation and, on the other, the brain projects mentioned above do not really address the cognitive/computational issues in depth. For example, the EU-funded Human Brain Project has ended without fulfilling its promise to build an artificial simulation of the brain that could support the second “wing” of brain-related projects in a significant way.
As we discussed in this paper, we are convinced that the future generation of autonomous robotic agents supporting humans in everyday activities should be strongly neuro-inspired, not in the sense of exploiting the detailed simulation of the biophysics of the adult human brain but of exploiting the processing structure and functions supporting human ability to act prospectively and interact with others on the basis of mutual understanding. In relation with the general issues of the brain projects mentioned above it is worth considering briefly the relationship between the cognitive abilities of the human species and animals, with particular reference to monkeys and chimpanzees. Although the wide spread prejudice of human superiority, based on the contrast between human rationality and animal instinctuality, research into animal cognition ( Gallistel, 1989 ; Laland and Seed, 2021 ) has established striking similarities for all the typical cognitive abilities, such as memory, problem solving, tool construction and use, communication, and social interaction with proven superiority in short-term memory in chimpanzees ( Matsuzawa et al., 2006 ). The difference is more in the degree of sophistication and generalization, although there is still some debate on the ability to implement mental time travel by animal species ( Clayton et al., 2003 ; Suddendorf and Corballis, 2007 ). Maybe, we may consider cognitive robots as representative of an artificial humanoid species to be epigenetically designed, grown up, trained, and educated in such a way to be compatible with humans, human society, and human cultural values.
The detailed simulation of the biophysics of the adult human brain is certainly an interesting scientific endeavor, although it might be close to impossible, with the investigating tools we have today, but it is at the same time too much and too little for figuring out a feasible approach for the design of autonomous robotic agents that can be integrated usefully in the social and industrial environment of the 21st century: for convenience we may use the acronym COCOBOT, to refer to the dual requirements of CO-operation (with humans) and COgnition (which includes autonomous and prospection capabilities). Robots of this kind do not need to be world masters of chess or go and are not supposed to be fluent in any human language, although some rudimentary logical capacity (for autonomy) and some linguistic competence (for communication/interaction with human partners) is necessary. As a matter of fact, COBOTs or Collaborative robots are already in the market since more than a decade: they are designed to work in the vicinity of human workers, allowing close interaction with humans, without protective barriers, in a number of typical industrial applications, with the strict requirements of reactive-safety blocking the movements in case of unexpected collisions. Existing COBOTS can be programmed for specific tasks as pick&place, machine tool tending, packaging, assembly, surface finish, welding, dispensing of sealants, etc. However, they are designed to be efficient and not to be brain-inspired in any sense, lacking any degree of cognitive capability. On the other hand, the issue of safety is important and should be intrinsic also in the design of COCOBOTS; if applied to interaction with non-expert and/or fragile humans, safety can be achieved only in systems with cognitive abilities based on prospection. Safety is not limited to the Bodyware of COCOBOTS, involving a set of fast reactive mechanisms but is supposed to refer to proactive behaviors, real as well as mental, that identify the Cogniware of COCOBOTS.
In the previous sections of this paper we propose two pillars for the design of such Cogniware: (1) it should be embodied and (2) it should support adaptive behavior through dynamic lifelong processes starting from the analogy with a developmental process. This means, in particular, that Bodyware and Cogniware should be designed as a unitary organism, not two independent entities to be selected off the shelf and matched for any specific application. In particular, the developmental pillar suggests that a main challenge for the future research will be to identify a minimal embryonic structure, from which it is possible to bootstrap a developmental process of a prospection grounded system capable of action execution and simulation. Since we are still far away from any accepted and/or credible solution, it may be inspiring to listen to an imaginative novelist ( Calvino, 1988 ) when he defines an “imagination machine” as follows: “…a kind of electronic machine that keeps track of all possible combinations and selects those that suit a particular purpose, or simply those that are the most interesting or pleasing or amusing.” This simple definition emphasizes the role of causality, purposiveness and the existence of a value system. Calvino’s “electronic machine” might be in the form of a minimalistic cognitive architecture with the components needed for prospection to emerge and that, in spite of its simplicity, could trigger complex behaviors. However, starting from such initial implementation, the critical issue remains how to outline the evolution process in a self-organizing manner.
Moreover, it is worth stressing that the embodiment pillar refers to both the Bodyware and Cogniware of such embryonic agents stressing the importance of the relationship between structure and function in the design of cogniware and the potentials offered by neuromorphic hardware/wetware that, even if still does not exist, is an active research area. For example, organic computers which, unlike conventional digital computers using binary states, are based on neural tissue ( Vacher et al., 2008 ; Bray, 2009 ; Cai et al., 2023 ) or polymeric material ( Krauhausen et al., 2021 ; Sarkar et al., 2022 ) capable of instantiating thousands of states and communicating with each other in a self-organized way, constantly forming and reforming new connections. In principle, such technologies might provide substantially more energy-efficient computing and this feature is in sharp contrast with the notorious energy voracity of AI technologies. Moreover, for organic computing technologies the classical distinction between hardware and software of the von Neumann computing architecture is supposed to disappear because the nature of computation is not Boolean algebra on strings of bits but the dynamic modulation of connection and disconnection of “organic neural assemblies” as an effect of the interaction of the organic brain with the environment through an organic body. This should evolve as a self-organizing process driven by Hebbian learning, at the microscopic level, and reinforcement learning, at the behavioral level. It is also expected that such self-organizing process could lead to the formation of self-organized maps ( Kohonen, 1982 ) as well as topology representing networks ( Martinetz and Schulten, 1994 ) that might be the building blocks for the maturation of the body schema throughout development.
At the same time, the same organic technologies that are being investigated for organic computing are also being considered for the development of neuromorphic sensors ( Blaiszik et al., 2010 ; Hassan et al., 2015 ; Richardson and Cheneler, 2019 ; Krauhausen et al., 2021 ; Sarkar et al., 2022 ). Neuromorphic actuators or artificial muscles are also being investigated using a variety of technologies, such as semicrystalline polymer fibers, ionic-polymer/metal composites, twisted nanofibers, conducting polymers, etc., ( Mirvakili and Hunter, 2018 ) and probably the challenge is greater in this case than for neuromorphic sensors. In summary, we suggested that COCOBOTs might be the target for establishing Artificial Cognition as the crucial approach for developing the Next-Generation of Autonomous Robotic Agents rather than one of the many forms of Artificial Intelligence applications. Furthermore, we suggest that the evolution of COCOBOTs is a work in progress that requires a multidisciplinary converging approach, both in the scientific and technological sense, in order to establish Artificial Cognition as the crucial approach for developing the Next-Generation of Autonomous Robotic Agents that can fit the requirements of 5IR, namely “the harmonious human–machine collaborations, with a specific focus on the well-being of the multiple stakeholders” ( Noble et al., 2022 ).
Data availability statement
The original contributions presented in this study are included in this article/supplementary material, further inquiries can be directed to the corresponding author.
Author contributions
GS: Conceptualization, Formal Analysis, Methodology, Writing – review & editing. AS: Conceptualization, Formal Analysis, Methodology, Writing – review & editing. PM: Conceptualization, Formal Analysis, Methodology, Writing – original draft.
This work is related to the iCog Initiative of the Fondazione Istituto Italiano di Tecnologia, coordinated by the RBCS Research Unit. Financial support is provided by G.A. No 804388, wHiSPER (Starting Grant from the European Research Council under the European Union’s Horizon 2020 Research and Innovation Program) and by “Progetto “Future Artificial Intelligence Research (hereafter FAIR)”, codice PE00000013 Finanziato dall’Unione Europea – NextGenerationEU PNRR MUR - M4C2 – Investimento 1.3 - Avviso Creazione di “Partenariati estesi alle università, ai centri di ricerca, alle aziende per il finanziamento di progetti di ricerca di base” CUP J53C22003010006”.
Conflict of interest
The authors declare that the research was conducted in the absence of any commercial or financial relationships that could be construed as a potential conflict of interest.
The author(s) declared that they were an editorial board member of Frontiers, at the time of submission. This had no impact on the peer review process and the final decision.
Publisher’s note
All claims expressed in this article are solely those of the authors and do not necessarily represent those of their affiliated organizations, or those of the publisher, the editors and the reviewers. Any product that may be evaluated in this article, or claim that may be made by its manufacturer, is not guaranteed or endorsed by the publisher.
- ^ To our knowledge the two papers ( Taylor and Taylor, 2021 ; Iglesias, 2022 ) are the first ones to contain the term Artificial Cognition in the title.
- ^ Z.W. Pylyshyn: “if a system is cognitively penetrable then the function it computes is sensitive, in a semantically coherent way, to the organism’s goals and beliefs.”
- ^ This issue is related to the baseline architecture previously mentioned as the blueprint of evolution providing the scaffolding for innate behaviors ( Zador, 2019 ), such as the observed prenatal synergies possibly facilitated by the viscous amniotic liquid.
Adadi, A., and Berrada, M. (2018). Peeking inside the black-box: a survey on explainable Artificial Intelligence (XAI). IEEE Access 6, 52138–52160. doi: 10.1109/ACCESS.2018.2870052
Crossref Full Text | Google Scholar
Aglioti, S., Cesari, P., Romani, M., and Urgesi, C. (2008). Action anticipation and motor resonance in elite basketball players. Nat. Neurosci. 11, 1109–1116. doi: 10.1038/nn.2182
PubMed Abstract | Crossref Full Text | Google Scholar
Alderson-Day, B., and Fernyhough, C. (2015). inner speech: development, cognitive functions, phenomenology, and neurobiology. Psychol. Bull. 141, 931–965. doi: 10.1037/bul0000021
Alegria, J., and Noirot, E. (1978). Neonate orientation behaviour towards human voice. Int. J. Behav. Dev. 1, 291–312. doi: 10.1177/016502547800100401
Anderson, M. L. (2010). Neural reuse: a fundamental organizational principle of the brain. Behav. Brain Sci. 33, 245–266. doi: 10.1017/S0140525X10000853
Asada, M., Hosoda, K., Kuniyoshi, Y., Ishiguro, H., Inui, T., and Yoshikawa, Y. (2009). Cognitive developmental robotics: a survey. IEEE Trans Autonomous Mental Dev. 1, 12–34. doi: 10.1109/TAMD.2009.2021702
Asada, M., MacDorman, K. F., Ishiguro, H., and Kuniyoshi, Y. (2001). Cognitive developmental robotics as a new paradigm for the design of humanoid robots. Robot. Autonomous Syst. 37, 185–193. doi: 10.1016/S0921-8890(01)00157-9
Azañón, E., Tamè, L., Maravita, A., Linkenauger, S. A., Ferrè, E. R., Tajadura-Jiménez, A., et al. (2016). Multimodal contributions to body representation. Multisens. Res. 29, 635–661. doi: 10.1163/22134808-00002531
Barredo Arrieta, A., Díaz-Rodríguez, N., Del Ser, J., Bennetot, A., Tabik, S., Barbado, A., et al. (2022). Explainable Artificial Intelligence (XAI): concepts, taxonomies, opportunities and challenges toward responsible AI. Information Fusion 58, 82–115. doi: 10.1016/j.inffus.2019.12.012
Barros, P., and Sciutti, A. (2022). Across the universe: biasing facial representations toward non-universal emotions with the face-STN. IEEE Access 10, 103932–103947. doi: 10.1109/ACCESS.2022.3210183
Barros, P., Yalcin, O. N., Tanevska, A., and Sciutti, A. (2022). Incorporating rivalry in reinforcement learning for a competitive game. Neural Comput. Appl. 35, 16739–16752. doi: 10.1007/s00521-022-07746-9
Berlucchi, G., and Aglioti, S. (1997). The body in the brain: neural bases of corporeal awareness. Trends Neurosci. 20, 560–564.
Google Scholar
Bernstein, N. (1967). The Coordination and Regulation of Movements. Oxford: Pergamon Press.
Bjaalie, J. G., Okabe, S., and Richards, L. J. (2020). International brain initiative: an innovative framework for coordinated global brain research efforts. Neuron 105, 212–216. doi: 10.1016/j.neuron.2020.01.002
Blaiszik, B. J., Kramer, S. L. B., Olugebefola, S. C., Moore, J. S., Sottos, N. R., and White, S. R. A. (2010). Self-Healing polymers and composites. Rev. Mater. Res. 40, 179–211. doi: 10.1146/annurev-matsci-070909-104532
Boyd, R., and Richerson, P. J. (1985). Culture and the Evolutionary Process. Chicago: University Chicago Press.
Braitenberg, V. (1986). Vehicles Experiments in Synthetic Psychology. Cambridge, MA: MIT Press.
Bray, D. (2009). Wetware: a Computer in Every Living Cell. New Haven, CT: Yale University Press.
Brooks, R. (1991). Intelligence without representation. Artificial Intelligence 47, 139–159.
Brooks, R. A. (2002). Flesh and Machines: How Robots Will Change Us. New York: Pantheon.
Butterworth, G. (1991). “The ontogeny and phylogeny of joint visual attention,” in Natural Theories of Mind: Evolution, Development, and Simulation of Everyday Mind , ed. A. Whiten (Oxford: Blackwell).
Butterworth, G., and Jarrett, N. (1991). What minds have in common is space: spatial mechanisms serving joint visual attention in infancy. Br. J. Dev. Psychol. 9, 55–72. doi: 10.1111/j.2044-835X.1991.tb00862.x
Cai, H., Ao, Z., Tian, C., Wu, Z., Liu, H., Tchieu, J., et al. (2023). Brain organoid reservoir computing for artificial intelligence. Nat. Electronics 6, 1032–1039. doi: 10.1038/s41928-023-01069-w
Calvino, I. (1988). Six Memos for the Next Millennium. Chapter on Visibility. Cambridge, MA: Harvard Unervsity Press.
Cangelosi, A., Schlesinger, M., and Smith, L. B. (2015). Developmental Robotics: From Babies to Robots. Cambridge, MA: MIT Press.
Cardinali, L., Brozzoli, C., and Farne, A. (2009). Peripersonal space and body schema: two labels for the same concept? Brain Topogr. 21, 252–260. doi: 10.1007/s10548-009-0092-7
Castiello, U., Becchio, C., Zoia, S., Nelini, C., Sartori, L., Blason, L., et al. (2010). Wired to be social: the ontogeny of human interaction. PLoS One 5:e13199. doi: 10.1371/journal.pone.0013199
Cazzato, D., Leo, M., Distante, C., and Voos, H. (2020). When i look into your eyes: a survey on computer vision contributions for human gaze estimation and tracking. Sensors 20:3739. doi: 10.3390/s20133739
Chen, X., Striano, T., and Rakoczy, H. (2004). Auditory-oral matching behavior in newborns. Dev. Sci. 7, 42–47. doi: 10.1111/j.1467-7687.2004.00321.x
Ciaunica, A., Constant, A., Preissl, H., and Fotopoulou, K. (2021). The first prior: from co-embodiment to co-homeostasis in early life. Consciousness Cogn. 91:103117. doi: 10.1016/j.concog.2021.103117
Clark, A. (1997). Being There: Putting Brain, Body, and World Together Again. Cambridge Mass: MIT Press.
Clayton, N. S., Bussey, T. J., and Dickinson, A. (2003). Can animals recall the past and plan for the future? Nat. Rev. Neurosci. 4, 685–691. doi: 10.1038/nrn1180
Connolly, K. J. (1970). “Skill development: problems and plans,” in Mechanisms of Motor Skill Development , ed. K. Connolly (London: Academic Press).
Cooper, R. P., and Aslin, R. N. (1990). Preference for infant-directed speech in the first month after birth. Child Dev. 61, 1584–1595. doi: 10.1111/j.1467-7687.2007.00605.x
Corbetta, D., and Snapp-Childs, W. (2009). Seeing and touching: the role of sensory-motor experience on the development of infant reaching. Infant Behav. Dev. 32, 44–58. doi: 10.1016/j.infbeh.2008.10.004
Csibra, G. (2010). Recognizing communicative intentions in infancy. Mind Lang. 25, 141–168. doi: 10.1111/j.1468-0017.2009.01384.x
Csibra, G., and Gergely, G. (2011). Natural pedagogy as evolutionary adaptation. Philos. Trans. R. Soc. B 366, 1149–1157. doi: 10.1098/rstb.2010.0319
Decety, J., and Ingvar, D. H. (1990). Brain structures participating in mental simulation of motor behaviour: a neuropsychological interpretation. Acta Psychol. 73, 13–34. doi: 10.1016/0001-6918(90)90056-l
Decety, J., and Jeannerod, M. (1995). Mentally simulated movements in virtual reality: does Fitts’s law hold in motor imagery. Behav. Brain Res. 72, 127–134. doi: 10.1016/0166-4328(96)00141-6
Dennett, D. (2009). Darwin’s “strange inversion of reasoning”. Proc. Natl. Acad. Sci. U S A. 6, (Suppl. 1), 10061–10065. doi: 10.1073/pnas.0904433106
Di Cesare, G., Vannucci, F., Rea, F., Sciutti, A., and Sandini, G. (2020). How attitudes generated by humanoid robots shape human brain activity. Sci. Rep. 10:16928. doi: 10.1038/s41598-020-73728-3
Di Mercurio, A., Connell, J. P., Clark, M., and Corbetta, D. (2018). A naturalistic observation of spontaneous touches to the body and environment in the first 2 months of life. Front. Psychol. 9:2613. doi: 10.3389/fpsyg.2018.02613
Di Vita, A., Boccia, M., Palermo, L., and Guariglia, C. (2016). To move or not to move, that is the question! body schema and non-action oriented body representations: an fMRI meta-analytic study. Neurosci. Biobehav. Rev. 68, 37–46. doi: 10.1016/j.neubiorev.2016.05.005
Donnarumma, F., Dindo, H., and Pezzulo, G. (2018). Sensorimotor communication for humans and robots: improving interactive skills by sending coordination signals. IEEE Trans. Cognit. Develop. Syst. 10, 901–917. doi: 10.1109/TCDS.2017.2756107
Donnarumma, F., Prevete, R., and Trautteur, G. (2012). Programming in the brain: a neural network theoretical framework. Connection Sci. 24, 71–90.
Duan, J., Yu, S., Tan, L., and Zhu, H. (2022). A survey of embodied AI: from simulators to research tasks. IEEE Trans. Emerg. Top. Comput. Intelligence 6, 230–244. doi: 10.1109/TETCI.2022.3141105
Ernst, M. O., and Banks, M. S. (2002). Humans integrate visual and haptic information in a statistically optimal fashion. Nature 415, 429–433. doi: 10.1038/415429a
Falck-Ytter, T., Gredeback, G., and von Hofsten, C. (2006). Infants predict other people’s action goals. Nat. Neurosci. 9, 878–879. doi: 10.1038/nn1729
Farroni, T., Csibra, G., Simion, F., and Johnson, M. H. (2002). Eye contact detection in humans from birth. Proc. Natl. Acad. Sci. U S A. 99, 9602–9605. doi: 10.1073/pnas.152159999
Farroni, T., Menon, E., Rigato, S., and Johnson, M. H. (2007). The perception of facial expressions in newborns. Eur. J. Dev. Psychol. 4, 2–13. doi: 10.1080/17405620601046832
Feldman, R. (2007). Parent–infant synchrony: biological foundations and developmental outcomes. Curr. Dir. Psychol. Sci. 48, 329–354. doi: 10.1111/j.1467-8721.2007.00532.x
Feldman, R. (2012). Oxytocin and social affiliation in humans. Hormones Behav. 61, 380–391. doi: 10.1016/j.yhbeh.2012.01.008
Feldman, R., and Eidelman, A. I. (2003). Skin-to-skin contact (Kangaroo Care). accelerates autonomic and neurobehavioural maturation in preterm infants. Dev. Med. Child Neurol. 45, 274–281. doi: 10.1017/s0012162203000525
Feldman, R., and Eidelman, A. I. (2007). Maternal postpartum behavior and the emergence of infant–mother and infant–father synchrony in preterm and full-term infants: the role of neonatal vagal tone. Dev. Psychobiol. 49, 290–302. doi: 10.1002/dev.20220
Feldman, R., and Eidelman, A. I. (2009). Biological and environmental initial conditions shape the trajectories of cognitive and social-emotional development across the first years of life. Dev. Sci. 12, 194–200. doi: 10.1111/j.1467-7687.2008.00761.x
Feldman, R., Eidelman, A. I., and Rotenberg, N. (2004). Parenting stress, infant emotion regulation, maternal sensitivity, and the cognitive development of triplets: a model for parent and child influences in a unique ecology. Child Dev. 75, 1774–1791. doi: 10.1111/j.1467-8624.2004.00816.x
Fernyhough, C., and Borghi, A. M. (2023). Inner speech as language process and cognitive tool. Trends Cogn. Sci. 27, 1180–1193. doi: 10.1016/j.tics.2023.08.014
Field, T., Woodson, R., Cohen, D., Greenberg, R., Garcia, R., and Collins, K. (1983). Discrimination and imitation of facial expressions by term and preterm neonates. Infant Behav. Dev. 6, 485–490. doi: 10.1016/S0163-6383(83)90316-8
Fjelland, R. (2020). Why general artificial intelligence will not be realized. Hum. Soc. Sci. Commun. 7, 1–9. doi: 10.1057/s41599-020-0494-4
Flanagan, J. R., and Johansson, R. S. (2003). Action plans used in action observation. Nature 424, 769–771. doi: 10.1038/nature01861
Flash, T., and Hogan, N. (1985). The coordination of arm movements: an experimentally confirmed mathematical model. J. Neurosci. 5, 1688–1703. doi: 10.1523/JNEUROSCI.05-07-01688
Flesch, T., Saxe, A., and Summerfield, C. (2023). Continual task learning in natural and artificial agents. Trends Neurosci. 46, 199–210.
French, R. M. (1999). Catastrophic forgetting in connectionist networks. Trends Cogn. Sci. 3, 128–125. doi: 10.1016/S1364-6613(99)01294-2
Friston, K., Mattout, J., and Kilner, J. (2011). Action understanding and active inference. Biol. Cybern. 104, 137–160. doi: 10.1007/s00422-011-0424-z
Gallagher, S. (1985). Body image and body schema: a conceptual clarification. J. Mind Behav. 7, 541–554.
Gallistel, C. R. (1989). Animal cognition: the representation of space, time and number. Ann. Rev. Psychol. 40, 155–189. doi: 10.1146/annurev.ps.40.020189.001103
Garrod, S., and Pickering, M. J. (2004). Why is conversation so easy? Trends Cogn. Sci. 8, 8–11. doi: 10.1016/j.tics.2003.10.016
Gesell, A. (1946). “The ontogenesis of infant behavior,” in Manual of Child Psychology , ed. L. Carmichael (New York: Wiley).
Giagkos, A., Lewkowicz, D., Shaw, P., Kumar, S., Lee, M., and Shen, Q. (2017). Perception of localized features during robotic sensorimotor development. IEEE Trans. Cognit. Develop. Syst. 9, 127–140. doi: 10.1109/TCDS.2017.2652129
Gibson, J. J. (1979). The Ecological Approach to Visual Perception. Boston: Houghton-Mifflin.
Gilbert, D., and Wilson, T. (2007). Prospection: experiencing the future. Science 351, 1351–1354. doi: 10.1126/science.1144161
Goldinger, S. D., Papesh, M. H., Barnhart, A. S., Hansen, W. A., and Hout, M. C. (2016). The poverty of embodied cognition. Psychon. Bull. Rev. 23, 959–978. doi: 10.3758/s13423-015-0860-1
Goldstein, M. H., and Schwade, J. A. (2008). Social feedback to infants’ babbling facilitates rapid phonological learning. Psychol. Sci. 19, 515–523. doi: 10.1111/j.1467-9280.2008.02117.x
Gonçalves, R. V., Figueiredo, E. M., Mourão, C. B., Colosimo, E. A., Fonseca, S. T., and Mancini, M. C. (2013). Development of infant reaching behaviors: kinematic changes in touching and hitting. Infant. Behav. Dev. 36, 825–832. doi: 10.1016/j.infbeh.2013.09.009
Gori, M. (2015). Multisensory integration and calibration in children and adults with and without sensory and motor disabilities. Multisensory Res. 28, 71–99. doi: 10.1163/22134808-00002478
Gori, M., Del Viva, M., Sandini, G., and Burr, D. C. (2008). Young children do not integrate visual and haptic form information. Curr. Biol. 18, 694–698. doi: 10.1016/j.cub.2008.04.036
Gori, M., Giuliana, L., Sandini, D., and Burr, D. (2012a). Visual size perception and haptic calibration during development. Dev. Sci. 15, 854–862. doi: 10.1111/j.1467-7687.2012.01183.x

« RETURN TO NEWS
Doctoral Student Selected for 2023-24 Research Scholar Program
By Renee Douglas / 03/22/2024 College of Health and Human Sciences , Social Work , The Graduate College
- 4-H and Youth Development News
- Academic Affairs News
- Accounting and Finance News
- Administration and Instructional Services News
- Admissions News
- Agribusiness, Applied Economics and Agriscience Education News
- Agricultural and Natural Resources News
- Alumni News
- Animal Sciences News
- Applied Engineering Technology News
- Athletics News
- Biology News
- Built Environment News
- Business and Finance News
- Business Education News
- Chancellor's Speaker Series
- Chancellors Town Hall Series
- Chemical, Biological, and Bio Engineering News
- Chemistry News
- Civil, Architectural and Environmental Engineering News
- College News
- Community and Rural Development News
- Computational Science and Engineering News
- Computer Science News
- Computer Systems Technology News
- Cooperative Extension News
- Counseling News
- Criminal Justice News
- Deese College News
- Economics News
- Educator Preparation News
- Electrical and Computer Engineering News
- Employees News
- Energy and Environmental Systems News
- English Department News
- Family and Consumer Sciences News
- Graphic Design Technology News
- Hairston College News
- Headlines News
- History & Political Science News
- Honors College News
- Human Resources News
- Industrial and Systems Engineering News
- Information Technology Services News
- Innovation Station News
- Journalism & Mass Communication
- Kinesiology News
- Leadership Studies and Adult Education News
- Liberal Studies News
- Library News
- Magazine News
- Management News
- Marketing News
- Mathematics News
- Mechanical Engineering News
- Media Spotlight News
- Natural Resources and Environmental Design News
- News Categories
- Nursing News
- Psychology News
- Research and Economic Development News
- Social Work News
- Student Affairs News
- Students News
- The Graduate College News
- Transportation & Supply Chain
- University Advancement News
- Visual & Performing Arts News

EAST GREENSBORO, N.C. (March 22, 2024) – A student from North Carolina Agricultural and Technical State University has been chosen as a scholar for the 2023-24 All of Us Research Scholar Program.

This initiative, backed by the National Institutes of Health, pairs early career investigators with health research professionals to advance precision medicine research. The program is dedicated to high school, undergraduate, or graduate/professional students who aspire to pursue careers in the health field.
Dawson, a Ph.D. candidate in the Joint Program in Social Work between N.C. A&T and with the University of North Carolina Greensboro, is passionately committed to paving her path as a future health researcher. She holds an MSW from East Carolina University and a B.A. in psychology from the University of North Carolina at Chapel Hill. She successfully defended her dissertation in February and will graduate in May from A&T.
Prior to embarking on her doctoral degree, Dawson established herself as a licensed clinical social worker and clinical addiction specialist, with experience across various clinical, leadership and administrative roles. She is also a minority fellow of the Council of Social Work Education Program who is committed to furthering the mission of the Substance Abuse and Mental Health Services Administration (SAMHSA) to diminish the impact of substance abuse and mental illness in American communities.
Dawson will learn how to conduct scientific research using the All of Us Data Browser and Research Workbench, creating a specific project and poster, while sharpening the skills that they need to support the future of biomedical research.
Her research interests span health equity, digital health interventions, social determinants of health and disparities among marginalized groups with chronic illnesses and mental health disorders.
Media Contact Information: [email protected]
Latest News

03/22/2024 in College of Health and Human Sciences , Social Work , The Graduate College

Belk Launches Spring Collection Designed by A&T Fashion Alumna
03/21/2024 in Alumni , College of Agriculture and Environmental Sciences , Family and Consumer Sciences

A&T to Conduct Siren Test on Monday, April 1
03/20/2024 in Employees , Students
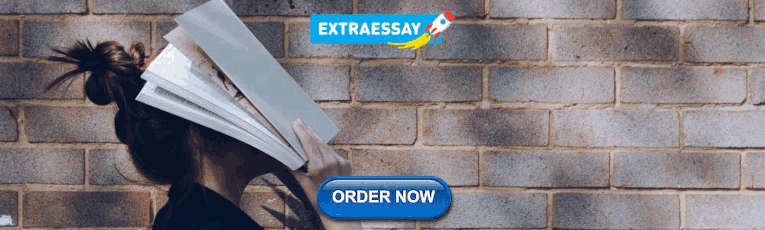
IMAGES
VIDEO
COMMENTS
3.4 Hypotheses. When researchers do not have predictions about what they will find, they conduct research to answer a question or questions with an open-minded desire to know about a topic, or to help develop hypotheses for later testing. In other situations, the purpose of research is to test a specific hypothesis or hypotheses.
Theories and Hypotheses. Before describing how to develop a hypothesis, it is important to distinguish between a theory and a hypothesis. A theory is a coherent explanation or interpretation of one or more phenomena. Although theories can take a variety of forms, one thing they have in common is that they go beyond the phenomena they explain by including variables, structures, processes ...
A hypothesis is an explanation for a phenomenon based on a conjecture about the relationship between the phenomenon and one or more causal factors. In sociology, the hypothesis will often predict how one form of human behavior influences another. For example, a hypothesis might be in the form of an "if, then statement."
2.3: Propositions and Hypotheses. Page ID. Anol Bhattacherjee. University of South Florida via Global Text Project. Figure 2.2 shows how theoretical constructs such as intelligence, effort, academic achievement, and earning potential are related to each other in a nomological network. Each of these relationships is called a proposition.
A hypothesis is an assumption or suggested explanation about how two or more variables are related. It is a crucial step in the scientific method and, therefore, a vital aspect of all scientific research. There are no definitive guidelines for the production of new hypotheses. The history of science is filled with stories of scientists claiming ...
6. Write a null hypothesis. If your research involves statistical hypothesis testing, you will also have to write a null hypothesis. The null hypothesis is the default position that there is no association between the variables. The null hypothesis is written as H 0, while the alternative hypothesis is H 1 or H a.
3. Simple hypothesis. A simple hypothesis is a statement made to reflect the relation between exactly two variables. One independent and one dependent. Consider the example, "Smoking is a prominent cause of lung cancer." The dependent variable, lung cancer, is dependent on the independent variable, smoking. 4.
Approaches to Sociological Research. Using the scientific method, a researcher conducts a study in five phases: asking a question, researching existing sources, formulating a hypothesis, conducting a study, and drawing conclusions. The scientific method is useful in that it provides a clear method of organizing a study.
18. Hypotheses. When researchers do not have predictions about what they will find, they conduct research to answer a question or questions, with an open-minded desire to know about a topic, or to help develop hypotheses for later testing. In other situations, the purpose of research is to test a specific hypothesis or hypotheses.
In social research, a hypothesis is defined as _____ a. an educated guess. b. a comprehensive explanation for why a particular social phenomenon occurs. c. the process of testing the relationship between an independent and dependent variable. d. a proposed relationship between two variables.
Simple hypothesis: This type of hypothesis suggests that there is a relationship between one independent variable and one dependent variable.; Complex hypothesis: This type of hypothesis suggests a relationship between three or more variables, such as two independent variables and a dependent variable.; Null hypothesis: This hypothesis suggests no relationship exists between two or more variables.
Abstract. Social research is often defined as a study of mankind that helps to identify the relations between social life and social systems. This kind of research usually creates new knowledge and theories or tests and verifies existing theories. However, social research is a broad spectrum that requires a discursive understanding of its ...
A research hypothesis, in its plural form "hypotheses," is a specific, testable prediction about the anticipated results of a study, established at its outset. It is a key component of the scientific method. Hypotheses connect theory to data and guide the research process towards expanding scientific understanding.
Social research is an organized, systematic, and scientific activity to critically investigate, explore, experiment, test, and analyse human society and the patterns and meanings of human behaviour (Henn et al., 2009). May (2011) discusses that most social research is conducted after identifying a problem that is regarded as a concern for society.
In social research, a hypothesis is best described as a(n) A. educated guess B. Description of why a particular social phenomenon occurs C. Proposed relationship between two or more variables D. Explanation for why two variables are coorelated. A. Validity.
A research hypothesis (also called a scientific hypothesis) is a statement about the expected outcome of a study (for example, a dissertation or thesis). To constitute a quality hypothesis, the statement needs to have three attributes - specificity, clarity and testability. Let's take a look at these more closely.
Step 1: Determine the hypotheses. The null hypothesis is often a statement of "no change," so the null hypothesis will state that there is no change in the mean cell phone data usage for this age group since the original study. In this case, the alternative hypothesis is that the mean has increased from 62 MB.
Study with Quizlet and memorize flashcards containing terms like In social research, a hypothesis is a(n): a. educated guess. b. proposed relationship between two or more variables. c. description for why a particular social phenomenon occurs. d. explanation for why two variables are correlated., When a researcher is successful at measuring what he or she intends to measure, this is called: a ...
2: Sociological Research is shared under a CC BY 4.0 license and was authored, remixed, and/or curated by OpenStax via source content that was edited to the style and standards of the LibreTexts platform; a detailed edit history is available upon request. Social research is research conducted by social scientists following a systematic plan.
Theory as a peg. In the context of social science, Gilbert ( 2005 ) defines research as a sociological understanding of connections—connections between action, experience, and change—and theory is the major vehicle for realizing these connections as is illustrated in Fig. 4.3. Theory- the major vehicle.
The Research Topic will serve as an outlet for social and personality psychology findings that might not otherwise see the light of day, including, but not limited to, results that contradict the initial hypothesis, a surprising moderator or pattern of moderation, and (provided statistical power is sufficient and methods are sound ...
Of note, some research suggests that being in a low social position can raise an individual's social anxiety compared to being in a high position (Aderka et al. 2009). Here, we propose that hierarchy base may impact social anxiety independent of an individual's social rank ( Bricker, Krimmel, and Ramcharan 2021 ; Wang et al. 2022 ).
Study with Quizlet and memorize flashcards containing terms like In social research, a hypothesis is best described as a(n):, Which of the following methods involves collecting data from written reports or other artifacts in order to discover patterns in behaviors or attitudes dating to an earlier time period?, A sociologist finds a strong relationship between education and income.
Key findings from the survey: Phone-less: 72% of U.S. teens say they often or sometimes feel peaceful when they don't have their smartphone; 44% say it makes them feel anxious. Good for hobbies, less so for socialization: 69% of teens say smartphones make it easier for youth to pursue hobbies and interests; fewer (30%) say it helps people their age learn good social skills.
If you are using public inspection listings for legal research, you should verify the contents of the documents against a final, official edition of the Federal Register. Only official editions of the Federal Register provide legal notice of publication to the public and judicial notice to the courts under 44 U.S.C. 1503 & 1507 .
In social research, a hypothesis is a(n): a. educated guess. b. proposed relationship between two or more variables. c. description for why a particular social phenomenon occurs. d. explanation for why two variables are correlated.
The social motive guides the agent toward the research of comfort, security and satisfaction from the interaction with others, guiding to the exchanges of information and to the need of maintaining the interaction over long periods of time (Vernon, 2014). This approach is being explored in some cognitive architecture.
Denise Dawson, a fifth-year doctoral student in social work from Hope Mills, North Carolina, will receive a stipend to participate in an eight-month program focused on professional development, hands-on health research experience, and access to opportunities. Additionally, she will have the privilege to attend the All of Us Research Convention.
A researcher interested in how many changes in the cells of the hippocampus (a structure in the brain related to learning and memory) are related to memory formation would be most likely to identify as a(n) _____ psychologist. A. Biological B. Health C. Clinical D. Social